
- Methodology
- Issues in Focus
- Country Case Studies
- Policy Recommendations
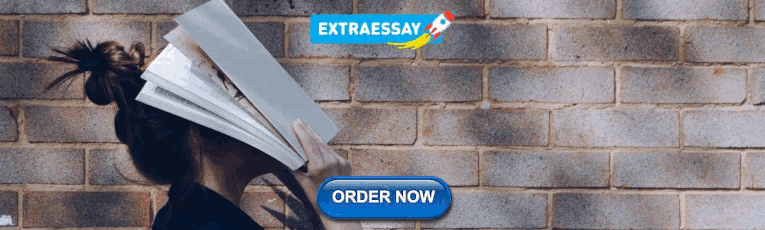
India: Making Food a Right for All
Making food a right for all, india has made considerable progress in tackling hunger and undernutrition in the past two decades, yet this pace of change has been uneven and many have been left behind. now is the time to assert the right to food for all and make zero hunger a reality for everyone..
When you have your godowns (warehouses) full and people are starving, what is the benefit? You cannot have two Indias. Dalveer Bhandari, Judge of India’s Supreme Court, 21 April 2011
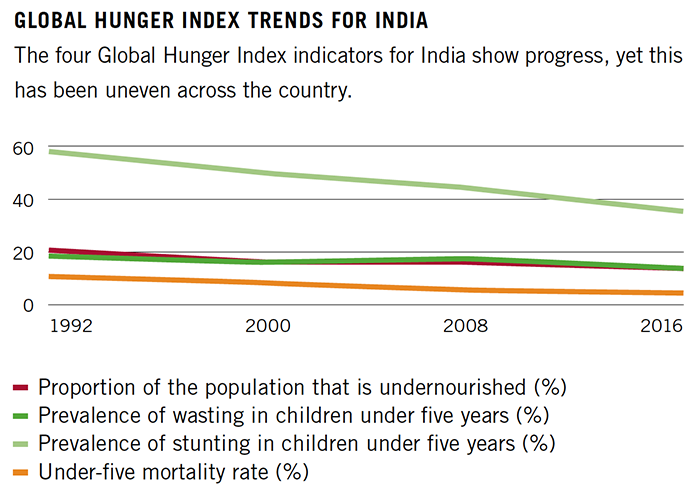
I ndia is a country of stark contrasts. In total, 22% of its population lives below the poverty line (Government of India 2013). At the same time, it is home to 84 of the world’s billionaires (Forbes 2016).
India’s top 1% own more than 50% of the country’s wealth. It is the world’s second largest food producer and yet is also home to the second-highest population of undernourished people in the world (FAO 2015).
One side of this story is clear from the score for India on the Global Hunger Index (GHI) – 28.5 (von Grebmer et al 2016). By contrast, Brazil, Russia, China and South Africa, all of whom share the BRICS high table with India, have a single-digit score. India’s neighbours, including Bangladesh, Nepal, Sri Lanka and Myanmar, have better GHI scores as well. Although the country has managed to reduce instances of stunting among children by nearly half in the past decade compared to the previous one (IFPRI 2015), India remains home to one third of the world's stunted children (UNICEF et al. 2016). It therefore falls into the ‘serious’ category in this year’s (2016) GHI.
Now, the 2030 Agenda for Sustainable Development is seeking to end hunger, achieve food security, improve nutrition and promote sustainable agriculture. The tangible outcomes will be to eradicate instances of stunting among children and guarantee every citizen with access to adequate food throughout the year through sustainable food systems, the doubling of smallholder productivity and income, and zero food loss or waste.
Although rainfed agriculture supports nearly 40% of India’s population (Government of India 2012), these farmers are highly sensitive to drought, which can cause crops to fail and lead to spiralling debt.
The key driver behind the goal to reach Zero Hunger and malnutrition is to ensure that no one is left behind in the pursuit of food and nutrition security. In the Indian context, this will also mean greatly improving the health of women and children.
The Government of India enacted the National Food Security Act (NFSA) in 2013, a law seeking to “provide for food and nutritional security […] by ensuring access to adequate quantity of quality food at affordable prices to people to live a life with dignity” (Ministry of Law and Justice 2013).
The 2013 NFSA created legal entitlements to existing governmental food and nutrition security programmes. Most significantly, it has changed the nature of discourse on food, making it a human right and putting the onus on the state to guarantee basic entitlements. However, the question is whether the quality of life has actually improved for everyone in the meantime. The food provided by the Government through its procurement and disbursement schemes serves the calorific requirement for some of the population. However, the system has also altered their food habits, made them dependent on rice and wheat and eliminated traditional diet diversity, thereby reducing the micronutrient content of the food on their plates.
Those Left Behind
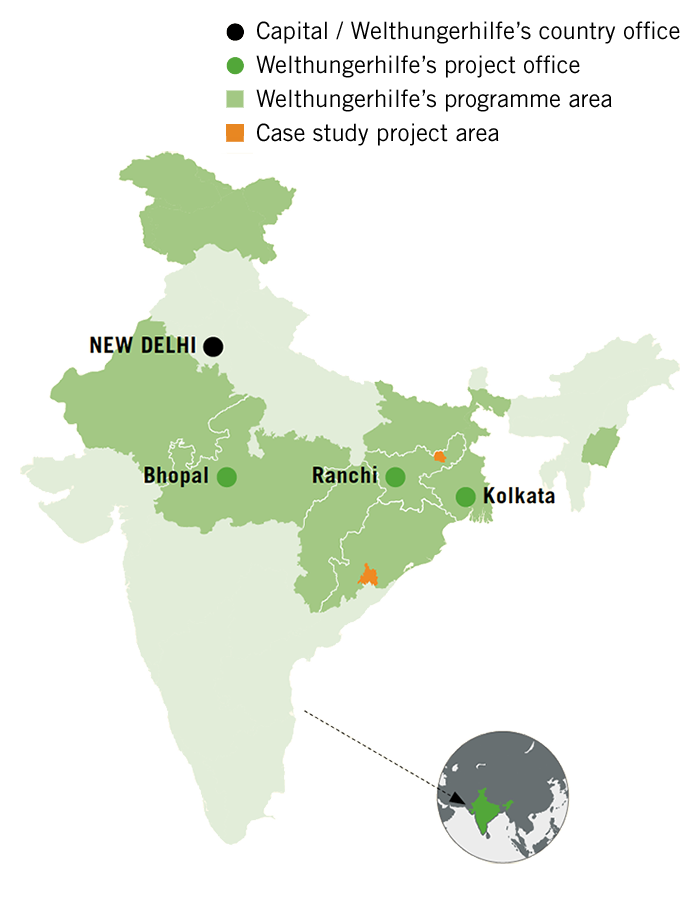
Among the poorest people in India are those who belong to Scheduled Castes and Scheduled Tribes – traditionally oppressed classes for whom the Indian constitution provides special affirmative provisions to promote and protect their social, educational and economic interests.
The Scheduled Castes include millions of Dalits, or ‘untouchables’, who continue to be subject to endemic discrimination. This is also the case for the Scheduled Tribes, which comprise indigenous people, also known as Adivasis, who are often disadvantaged, in part because of the forested geographies in which they live.
As a consequence, Dalits and Adivasis are over-proportionally affected by poverty. With 104 million people belonging to nearly 700 distinct ethnic groups, India has the second-largest tribal population in the world (Government of India 2011). 47% of the rural tribal population lives below the national poverty line, compared to the national average for rural areas of 28% (Rao 2012). The level of poverty and food and nutrition insecurity of the tribal people continues to be a major issue, despite the affirmative action put in place by the architects of India’s Constitution for their protection and welfare.
The Adivasis have borne witness to the appropriation of their lands, destruction of their environment and commoditisation of their traditional knowledge – a lopsided bargain which has come at the cost of their way of life and well-being, beginning with their health and the security of resources for future generations. Safeguards such as informed consent have been thrown to the winds in the rush to acquire and trade forest produce and land on a large scale.
The problems faced by the indigenous people of India are further iterated by a recent study conducted by the United Nations Children´s Fund (UNICEF). Covering 11 states, it shows that every second Adivasi child is stunted, 68% of Adivasi mothers are less than 20 years old, 48% are undernourished and 76% are anaemic. Furthermore, the study states that the risk of severe stunting is nearly twice as high among girls aged 6-23 months compared to boys (UNICEF 2014). This may be due to food distribution practices within households and gender discrimination, resulting in woman receiving less food or men being served the best portions.
The efforts of the Food Security Act and a range of other laws to tackle these issues have encountered many challenges. Adivasi hamlets are often remote and poorly connected, making logistics and monitoring difficult. Indeed, the plight of those who suffer from hunger is only addressed when deaths resulting from starvation momentarily lead to public outrage. These are the groups that need support most urgently.
Growing Rich Diversity with Limited Land
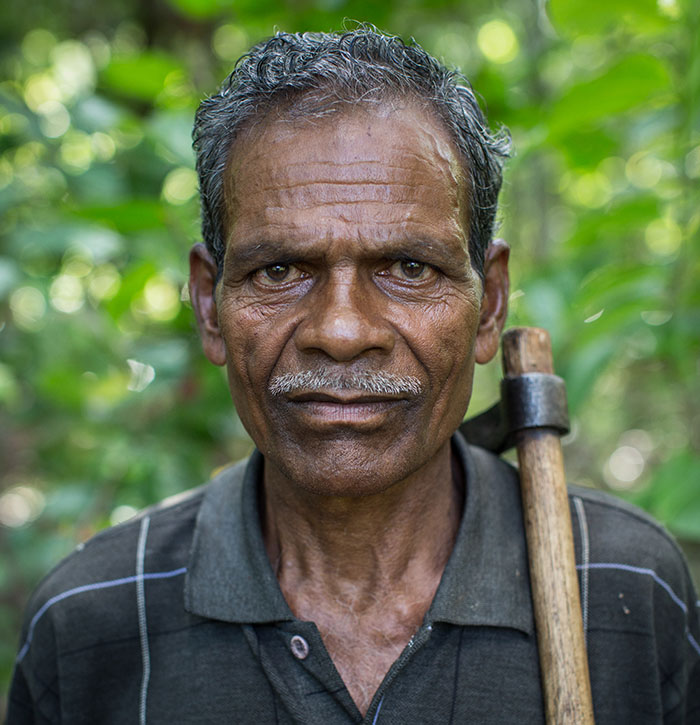
My friends were avoiding farming. I told them that we can’t afford to let the varieties of our village get lost. Last year, I grew 70 varieties. It sufficed us for the year. This year I have told fellow villagers to grow 80 varieties Adi Kumbruka, young farmer in Kanduguda village, Odisha
Welthungerhilfe has been working in India since 1965. As part of its current efforts, it is increasingly focusing on mobilising and raising awareness among the marginalised and poor rural communities in order to help them access their rights and entitlements in relation to hunger and poverty.
Welthungerhilfe’s approaches address the four pillars of food and nutrition security, namely ensuring the availability of food of sufficient quantity and quality, guaranteeing that people have physical and economic access to this food, providing health and sanitation conditions that enable them to truly benefit from this food, and ensuring that these factors are stable all year round.
Welthungerhilfe works with a number of civil society partners across the country implementing a rights-based approach that addresses these four aspects of food and nutrition security. Many of these projects are established in states and regions suffering from extremely high levels of malnutrition.
Living Farms , a partner NGO, works with landless, small and marginalised farmers in the dry, hilly region of the state of Odisha in Eastern India to help them assert their food sovereignty and improve their well-being by means of an ecological and sustainable approach to agriculture. To this end, Living Farms is working to re-establish the control of these farmers over food and farming systems through the conservation, renewal and rejuvenation of biodiversity. Availability of food is improved at household level by initiating a series of interventions to enhance productivity on the limited land they have.
In the Kerandiguda village of Rayagada, Living Farms is working with Loknath Nauri (pictured), a farmer in his sixties who draws inspiration from how tribal people practised agriculture decades ago. Loknath is a repository of wisdom. For example, he can tell the direction the yearly rains will come from based on how a local bird’s nest is positioned. He can also predict when it will rain purely using the beans in the pods of a local creeping plant. These are just two of the many lessons he shares with other farmers. Although Loknath owns just 2.5 acres (1ha) of land, his food stocks at home are plentiful. He grows 72 different varieties of crops on his farm:
Growing multiple varieties of crops reduces the risks from drought and other farm stress. I harvest from September until January and have vegetables throughout the year.
Living Farms has an extensive list of small land-holding farmers who grow over 50 different varieties on their farms. Thousands more cultivate over 20 crop varieties and no longer have to endure the type of crisis faced by farmers in other rain-fed agricultural regions around the country. This stability is important, as farmers can otherwise become lured into growing cash crops instead, such as cotton, cashew, palm oil, sugarcane and eucalyptus, which reduces food availability.
Debjeet Sarangi from Living Farms explains that the NGO is working with researchers to rediscover the virtues of traditional local crop varieties that can withstand erratic rainfall and soaring temperatures and still produce bountiful yields.
The community already has traditional rice varieties that are rich in micronutrients, zinc, iron, magnesium and calcium, while scientists are working on creating such seeds in laboratories around the world.
...Debjeet says, adding that the Adivasis avoid using chemicals on their farms and make their own compost instead.
In Jharkhand, Pravah, another Welthungerhilfe partner, encourages landless families to set up kitchen gardens in their homesteads and harness common fallows to grow food for the family. On the farmlands, diversity is returning through the ‘Sustainable Integrated Farming Systems’ approach, which functions according to the principle of farm planning and the use of all available resources, including time and space, as efficiently as possible. Hardy, drought-resilient millet crops are now being reintroduced to the cropping cycle. Waste from livestock, poultry and aquaculture is recycled through a bio-digester in order to provide rich farm manure, and agroforestry is practised to provide fodder for animals.
Space on the homestead as well as on the farm is used to grow different crops, sometimes in multiple tiers. Crops are planned in such a way that food is available throughout the year, thereby resulting in different food products for the market. In addition, this directly addresses micronutrient deficiency and leads to diet diversity among the population.
Pravah has worked closely with farmers like Nandlal Singh, who owns 2.5 acres of land. Nandlal’s story was similar to any other farmer’s in the region, namely one of debt, crop failure, migration and mortgages. His situation has now changed. Thanks to farm planning and integrated farming Nandlal has not only cleared his old debts, but has money in the bank. Pravah’s training on vermicomposting, organic farming and integrated pest and nutrient management techniques have proven successful and led to the production costs on Nandlal’s farm being reduced.
The family has a diverse diet which incorporates up to eight food groups, including cereals, lentils, fruits and vegetables. Nandlal grows these vegetables throughout the year while also rearing cattle, fish and ducks on what was once a patch of wasteland.
A striking feature of the work of both organisations is the low incidence of indebted households among the farmers involved in the programme. Small and marginal farmers like Nandlal Singh and Loknath are not short of the resources they need for this kind of farming. This reduces their reliance on loans and avoids the problems associated with debt. Debjeet Sarangi from Living Farms cites examples of how farmers who used to be impoverished now grow multiple crops per year and how, together with poultry and animal husbandry, they are able to safeguard their families against food and nutrition insecurity. But, most importantly, Debjeet says, they depend on forests.
Securing Forests to Secure Nutrition
of the Indian population is undernourished , meaning that they do not receive enough calories per day
of children under five are stunted (low height for their age), reflecting chronic undernutrition
of children under five are wasted (low weight for their height), reflecting acute undernutrition
of children die before the age of five
The diet of the Adivasi people used to be a highly diverse one. However, years of planning and the Government’s control over resources meant that these tribal farmers began to lose the ability to cultivate the seeds that kept their people fed for generations. One result of these developments is that the diversity of the crops grown and of the available forest resources has shrunk massively over the years.
...explains Debjeet. Debjeet alludes to evidence that vast swathes of forests are being acquired fraudulently:
Officials only see it as lost forest when in actual fact the loss of these forests is also affecting the eating habits and the nutrition of the tribal people. The range of forest fauna, flowers, fruits, vegetables and mushrooms previously consumed by tribal people has diminished over time due to governmental policies. Tribal people collect 25 varieties of roots and tubers, 35 kinds of fruits and various oil seeds from the forests. The forest also provides 40 different leaf vegetables, mushrooms and various birds, animals, edible insects and other food sources throughout the year. In addition, the bodies of water belonging to the forests are home to an abundance of snails, fish and crab.
This diversity offered by the forests is now under threat and entire species have disappeared, thus depriving families of a varied diet.
Women in Rayagada are now resisting the forest department’s attempts to plant commercial trees, demanding that multipurpose trees are planted instead. The move has led to the protection of forest cover and the revival of over 275 varieties of wild foods that provide food rich in micronutrients.
Rua Ulaka, a farmer from the village of Lanji, Rayagada, is part of this active citizenship movement, as evidenced by the level of care that she shows for her forests and its ecosystem. By working together, the Adivasis are able to ensure that this asset is not commercialised. Ultimately, the work carried out by Living Farms and Pravah has shown that families with very small landholdings and continued access to the forest are more than able to survive another year. With dignity.
Fighting the Day-to-Day Nutrition Crisis
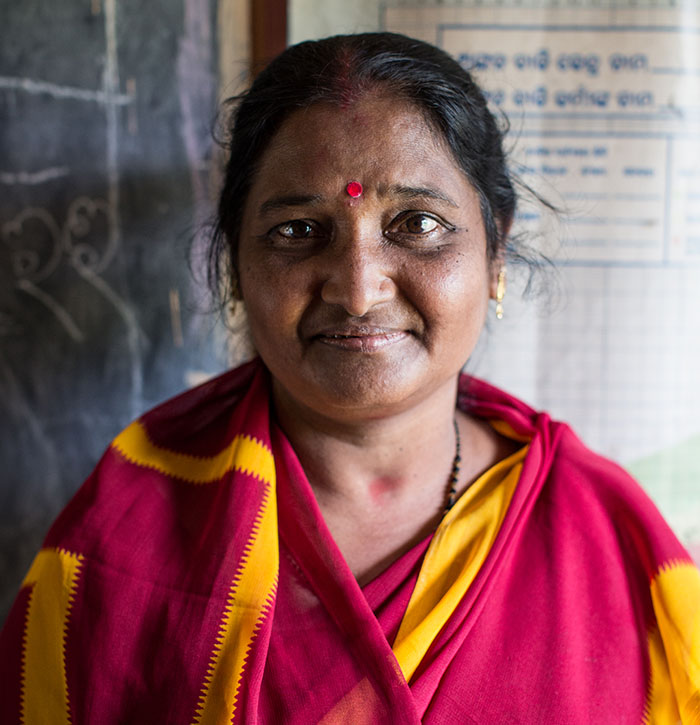
Previously, women would not have their babies weighed or breast-feed their newborns due to superstition. This has changed. Mothers now ask me how much their child weighs. Women wear slippers, families have toilets, girls are resisting early marriage and babies are being born in hospitals. Not a single child has died in this village over the past five years. Sharmishta Raj, Anganwadi worker at the center of Laxmipur village in Odisha
As well as working to ensure the availability of sufficient and nutritious food, both organisations promote awareness and changes in behaviour at community level in terms of health care and infant and young child feeding practices.
A promising approach introduced by Pravah in the villages of Jharkhand consists of ‘Positive Deviance Sessions’, whose aim is to improve the health of moderately malnourished children. With 15% of children below five years of age being classified as underweight for their height, India’s acute malnutrition rate is at the international threshold that indicates a nutrition emergency. Babita Sinha, Pravah’s Programme Manager, explains:
80% of the children in the nutrition camps have recorded weight gain and have shifted from the Moderate Acute Malnutrition (MAM) category to the category of healthy children. This is due in part to a 15-day hands-on camp to introduce young and pregnant mothers to new, nutritious recipes, gathering and using nutritious, uncultivated food, child-care practices and hand-washing.
These ‘Positive Deviance Camps’ have also implemented de-worming programmes and helped to change the behaviour of young parents in various villages. According to Babita, the mothers realised that their children were responding positively to these initiatives when they were weighed. She recalls:
Seeing a gain of 500 grams in the child’s weight gave the mothers tremendous joy.
The sensitisation process was revealing for the experts at Pravah as well. “We were able to understand why children in the red category (meaning those belonging to the lowest-weight-for-age section of the WHO Growth Monitoring Charts, signifying malnutrition) belonged to families from certain clusters of the villages,” states Sweta Banerjee, Nutrition Specialist with Welthungerhilfe in India. Sweta bore witness to how the process taught the village communities to link nutrition with good agriculture practices and proper management of natural resources.
As she states, “We realised it was not a coincidence that these children came from families that were either landless or owned land uphill that was not irrigated. As such, the nutrition programme had to be amended to benefit them.”
One key breakthrough was that women were able to grasp how the nutrition chain between generations could be broken by paying attention to the nutritional needs of different age and gender groups and by including adolescent girls, expectant mothers and women nursing children. The Pravah team noted that close to half of the households in the villages in which they were working have since improved their food and diet practices. There have also been visible changes in personal hygiene practices at household level. Combined, these actions will have a lasting impact on the health of the people living in these villages.
Putting the Right to Food into Action
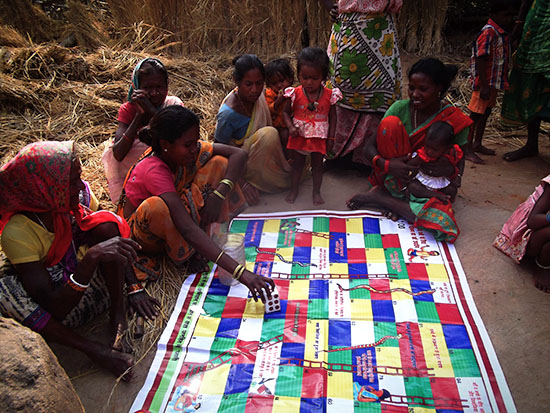
The Fight Hunger First Initiative implemented by Welthungerhilfe in cooperation with several Indian partner organisations, including Living Farms and Pravah, is based on the premise that it is only possible for people to break out of the cycle of inequality and discrimination permanently if adequate welfare systems are in place and basic rights are fulfilled.
This includes access to proper education, sufficient and adequate access to food and income, better health services and treatment as equal citizens by the state.
The right to food guaranteed by the Food Security Act is translated into a number of entitlements ensured through different programs. Examples include the Integrated Child Development Services (ICDS), which provides health and nutrition services to pregnant women and young children, and the Mid-Day Meals (MDM) scheme, which is aimed at providing free lunches and thereby improving the nutritional status and attendance of school children.
Meanwhile, the National Rural Employment Guarantee Act (NREGA) guarantees the provision of paid employment to rural families. In some cases, it has reduced reliance on the Public Distribution System (PDS), which distributes subsidised food rations to those who are most in need.
Enforcement of the National Food Security Act is a challenge, especially in far-flung villages. In addition, many families have little access to work for wages that could be used to buy food, educate children and cover other household expenses.
At national level, for example, households covered by the Rural Employment Act, on average, only received 41 days of work per year between 2011/12 and 2013/14 (Desai et al 2015). This equates to less than half the amount set out in the constitutional provisions. The situation is similar in Jharkhand. Rather ironically, the Government has increased the minimum number of days of work to which the households are entitled under the law to 150.
As part of the Fight Hunger First Initiative, community-based organisations are formed or strengthened and social accountability mechanisms such as community score cards are introduced as a means of empowering community members to access various forms of entitlements and holding service providers accountable. In the state of Jharkhand, Pravah successfully campaigned with 13 Non-Governmental Organisations (NGOs) for the inclusion of eggs in the Mid-Day-Meals at schools three times a week. Likewise, Living Farms has been able to persuade Government authorities to include millets in the ICDS programme, especially in the form of take-home rations for pregnant women. Persuading ICDS officials to appreciate community inputs has been a lesson in advocacy.
“Community members feel the services do not make sense. On the other hand, service providers also feel handicapped. The gap is evident and the community participation tools we have employed help to bridge this gap,” says Babita Sinha.
A federation of self-help groups promoted by Pravah offers numerous examples of how leadership has been nurtured among women, who are now able to confront agents working at public distribution system outlets or others charged with managing governmental service provision agencies. At the same time, workers at the Anganwadi centres are now on equal terms with the village women. Rua Ulaka is now aware of her rights and entitlements as a citizen, what she can expect from the village’s own self-governance institution, the Panchayat, and of her right to participate in the Gramsabha (village assembly).
Awareness of these aspects of governance ensures that Adivasi women can demand accountability from those governing them. As a result of the work carried out by Pravah and Living Farms, more households are now receiving work. Furthermore, community access to an array of welfare schemes run by the Government has vastly improved, thereby breaking the cycle of poverty and building the community’s resilience.
By engaging with the Village Health and Nutrition Days and working with institutions like the Village Health Sanitation & Nutrition Committees, the project also strengthens the government health service delivery mechanisms regarding its coverage and quality. Sharmishta Raj and her colleagues from the Anganwadi child care centre in Lakhimpur highlight the difference that working with Living Farms has made thanks to effective communication between the centre and the community.
“Not a single child has died in this village over the past five years,” she says, her face brimming with pride. This is a reflection of how much a small group of front-line government functionaries has achieved through a partnership with a civil society organisation in a remote corner of the country.
Ending a Nutrition Paradox
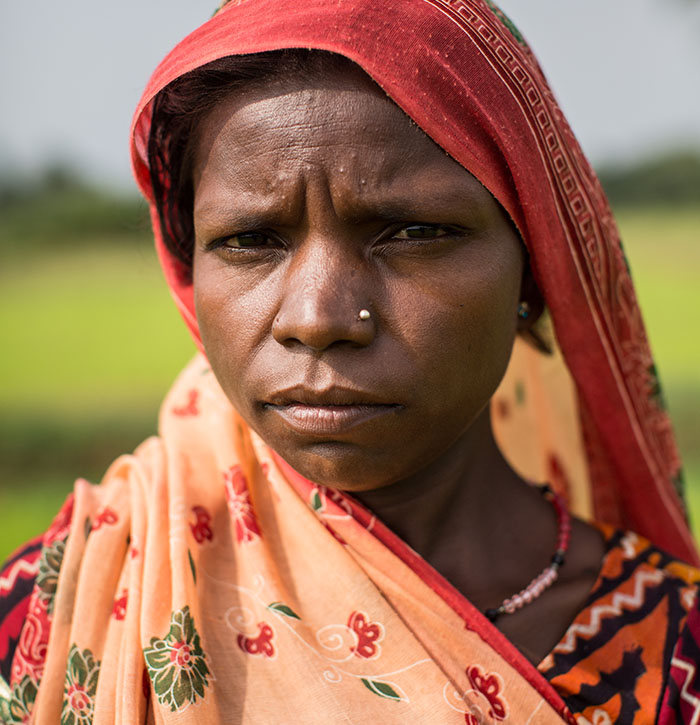
I grow enough and earn enough and can also take advantage of the government’s food schemes. We even have fish once a week and enough fruits and vegetables. Geeta Devya, Dhanway Naya village in Jharkhand
India’s agricultural growth rate increased phenomenally in the decades following the green revolution that turned the country from a “ship-to-mouth economy” into a land able to provide food security.
This growth was propelled by technological changes, major investment in infrastructure such as irrigation, markets and roads, the development of credit institutions, auxiliary services and the facilitation of pricing policies. However, the revolution has come with several significant limitations. As a result, a more ecologically and socially sustainable ‘evergreen revolution’ is needed.
India still faces a long road ahead in its quest to achieve Zero Hunger. Over 25 years since India ushered in its economic reforms, the country’s economy has undergone significant structural transformations, encouraging planners to turn their focus away from agriculture and instead towards the service and manufacturing sectors.
The priority now is to return attention to agriculture and its central role of providing food security, reducing poverty and generating employment. Turning one’s back on agriculture, particularly in a time when the climate is changing considerably, will put the food security of the 1.25 billion people living in India in jeopardy.
The Government has recently set an ambitious target to double the income of farmers by 2022 (The Economic Times 2016). This corresponds to targeted annual agricultural growth of more than 14% per year. More needs to be done to enhance the role that agriculture can play in improving nutrition outcomes, for example via the implementation of cross-sector policies and programmes at national and sub-national levels.
Efforts must also be made to ensure that small-scale, marginal and landless farmers are the true beneficiaries of these policies, as too many people are being left behind in India’s efforts to reach Zero Hunger. This goal can only be achieved when the people who are most excluded are placed at the centre of all action and thinking.
Indian civil society, including Welthungerhilfe’s partners, has been working with these communities to enable them to take control of their own lives and demand their right to food. It has also been working in close cooperation with the Government to implement a range of innovative ideas that address issues of food insecurity and malnutrition in remote corners of the country.
Above all, in this land of plenty it will only become possible to overcome the national nutrition paradox by challenging the social, economic and political structures that lead to the discrimination of the most vulnerable people in India.
About this Case Study
The United Nations Sustainable Development Goals are an inspiring and essential call to action. We are being called upon to end hunger by 2030 for everyone – forever. So how do we respond? How can we make this a reality?
This case study highlights the work of Welthungerhilfe as part of efforts towards achieving zero hunger. Drawing on many years of experience and well-founded evidence, we work with governments and partner organisations to scale up solutions which are both sustainable and sustained.
People are at the heart of these programmes. Their stories highlight the diversity of challenges faced by ordinary people every day: coping with conflict while building resilience, living with as well as tackling social inequality, and dealing with and mitigating the impacts of climate change. Although these challenges are enormous, so, too, is the potential to turn the ambition of the Sustainable Development Goals into a reality for everyone.
Taking an Ecosystems Approach: Samarthan’s Work on Rural Poverty in India
- Oct 07, 2016
September 2016 | by Brendan Halloran, IBP (adapted from a longer study by Padmaja Nair)
Despite years of strong economic growth in India, rural poverty still affects tens of millions of households. In 2005, the government created the Mahatma Gandhi National Rural Employment Guarantee Scheme (MGNREGS) to address rural poverty. The scheme, which commits the government to providing adult members of rural households with 100 days of paid work, should provide a revolutionary safety net for rural households. Yet corruption, insufficient administrative capacity, a lack of awareness among many rural inhabitants, and manipulation by influential political and economic actors have undermined it.
Samarthan, an organization dedicated to pursuing more inclusive development in the state of Madhya Pradesh, has worked to realize the scheme’s potentially transformative promise. It has pursued this aim through an evolving understanding of the challenges and opportunities inherent in the complex system of actors, processes, and tools. This case study summarizes Samarthan’s approach, the role of IBP in supporting the organization, and describes the impacts and lessons learned.
- Taking an Ecosystems Approach: Samarthan’s Work on Rural Poverty in India (September 2016)
- Taking an Ecosystems Approach: Support for Advocacy Training Initiatives’ (SATHI) Public Health Work in India (Case Study, September 2016)
- You Cannot Go it Alone: Learning from Cooperative Relationships in Civil Society Budget Campaigns (May 2016)
- Uganda: Winning Human Resources for Health (Case Study, December 2015)
ibp case study summary india samarthan rural poverty 2016.pdf
Brendan halloran.

Brendan Halloran is International Budget Partnership’s Head of Strategy and Learning. In this role, Brendan facilitates strategy and learning processes at IBP – both the internal production of learning insights and drawing on evidence and ideas from broader research and practice in the governance space. He’s particularly interested in complex change dynamics, and how to support organizations to both navigate and strengthen their accountability ecosystems.
Prior to joining IBP in 2016, Brendan lead the learning work of the Transparency and Accountability Initiative, where he played a role in shaping and interpreting evidence about what works, as well as supporting collective learning spaces, such as the TALEARN network. Before that, Brendan spent five years living, researching and working in Guatemala, most recently as a Governance Advisor for USAID. Brendan has a Ph.D. from Virginia Tech, and has published work in a variety of journals, think pieces, and blogs, including his own — Politics, Governance, and Development .
Advertisement
I. Introduction
Ii. the expenditure surveys, iii. nsso versus nas expenditure estimates, iv. the official poverty lines, v. controversies regarding poverty lines 3, vi. poverty at the national level, vii. poverty in the states: rural and urban, viii. poverty in the states by social group, ix. poverty in the states by religious group, x. inequality, xi. concluding remarks, a comprehensive analysis of poverty in india.
- Cite Icon Cite
- Open the PDF for in another window
- Permissions
- Article contents
- Figures & tables
- Supplementary Data
- Peer Review
- Search Site
Arvind Panagariya , Megha Mukim; A Comprehensive Analysis of Poverty in India. Asian Development Review 2014; 31 (1): 1–52. doi: https://doi.org/10.1162/ADEV_a_00021
Download citation file:
- Ris (Zotero)
- Reference Manager
This paper offers a comprehensive analysis of poverty in India. It shows that regardless of which of the two official poverty lines we use, we see a steady decline in poverty in all states and for all social and religious groups. Accelerated growth between fiscal years 2004–2005 and 2009–2010 also led to an accelerated decline in poverty rates. Moreover, the decline in poverty rates during these years has been sharper for the socially disadvantaged groups relative to upper caste groups so that we now observe a narrowing of the gap in the poverty rates between the two sets of social groups. The paper also provides a discussion of the recent controversies in India regarding the choice of poverty lines.
This paper provides comprehensive up-to-date estimates of poverty by social and religious groups in the rural and urban areas of the largest 17 states in India. The specific measure of poverty reported in the paper is the poverty rate or headcount ratio (HCR), which is the proportion of the population with expenditure or income below a pre-specified level referred to as the poverty line. In the context of most developing countries, the poverty line usually relates to a pre-specified basket of goods presumed to be necessary for above-subsistence existence.
In so far as prices vary across states and between rural and urban regions within the same state, the poverty line also varies in nominal rupees across states and between urban and rural regions within the same state. 1 Similarly, since prices rise over time due to inflation, the poverty line in nominal rupees in a given location is also adjusted upwards over time.
The original official poverty estimates in India, provided by the Planning Commission, were based on the Lakdawala poverty lines, so named after Professor D. T. Lakdawala who headed a 1993 expert group that recommended these lines. Recommendations of a 2009 expert committee headed by Professor Suresh Tendulkar led to an upward adjustment in the rural poverty line relative to its Lakdawala counterpart. Therefore, while the official estimates for earlier years were based on the lines and methodology recommended by the expert group headed by Lakdawala, those for more recent years were based on the line and methodology recommended by the Tendulkar Committee. Official estimates based on both methodologies exist for only two years, 1993–1994 and 2004–2005. These estimates are provided for the overall population, for rural and urban regions of each state, and for the country as a whole. The Planning Commission does not provide estimates by social or religious groups.
In this paper, we provide estimates using Lakdawala and Tendulkar lines for different social and religious groups in rural and urban areas in all major states and at the national level. Our estimates based on Lakdawala lines are computed for all years beginning in 1983 for which large or “thick” expenditure surveys have been conducted. Estimates based on the Tendulkar line and methodology are provided for the three latest large expenditure surveys, 1993–1994, 2004–2005, and 2009–2010.
Our objective in writing the paper is twofold. First, much confusion has arisen in the policy debates in India around certain issues regarding poverty in the country—for instance, whether or not growth has helped the poor (if yes, how much and over which time period) and whether growth is leaving certain social or religious groups behind. We hope that by providing poverty estimates for various time periods, social groups, religious groups, states, and urban and rural areas, this paper will help ensure that future policy debates are based on fact. Second, researchers interested in explaining how various policy measures impact poverty might find it useful to have the poverty lines and the associated poverty estimates for various social and religious groups and across India's largest states in rural and urban areas readily available in one place.
The literature on poverty in India is vast and many of the contributions or references to the contributions can be found in Srinivasan and Bardhan ( 1974 , 1988 ), Fields ( 1980 ), Tendulkar ( 1998 ), Deaton and Drèze ( 2002 ), Bhalla ( 2002 ), and Deaton and Kozel ( 2005 ). Panagariya ( 2008 ) provides a comprehensive treatment of the subject until the mid-2000s including the debates on whether or not poverty had declined in the post-reform era and whether or not reforms had been behind the acceleration in growth rates and the decline in poverty. Finally, several of the contributions in Bhagwati and Panagariya ( 2012a , 2012b ) analyze various aspects of poverty in India using the expenditures surveys up to 2004–2005. In particular, Cain, Hasan, and Mitra ( 2012 ) study the impact of openness on poverty; Mukim and Panagariya ( 2012 ) document the decline in poverty across social groups; Dehejia and Panagariya ( 2012 ) provide evidence on the growth in entrepreneurship in services sectors among the socially disadvantaged groups; and Hnatkovska and Lahiri ( 2012 ) provide evidence on and reasons for narrowing wage inequality between the socially disadvantaged groups and the upper castes.
To our knowledge, this is the first paper to systematically and comprehensively exploit the expenditure survey conducted in 2009–2010. This is important because growth was 2–3 percentage points higher between 2004–2005 and 2009–2010 surveys than between any other prior surveys. As such, we are able to study the differential impact accelerated growth has had on poverty alleviation both directly, through improved employment and wage prospects for the poor, and indirectly, through the large-scale redistribution program known as the National Rural Employment Guarantee Scheme, which enhanced revenues made possible. In addition, ours is also the first paper to comprehensively analyze poverty across religious groups. In studying the progress in combating poverty across social groups, the paper complements our previous work, Mukim and Panagariya ( 2012 ).
The paper is organized as follows. In Section II , we discuss the history and design of the expenditure surveys conducted by the National Sample Survey Office (NSSO), which form the backbone of all poverty analysis in India. In Section III , we discuss the rising discrepancy between average expenditures as reported by the NSSO surveys and by the National Accounts Statistics (NAS) of the Central Statistical Office (CSO). In Section IV , we describe in detail the evolution of official poverty lines in India, while in Section V we discuss some recent controversies regarding the level of the official poverty line. In Sections VI to Section IX , we present the poverty estimates. In Section X , we discuss inequality over time in rural and urban areas of the 17 states. In Section XI , we offer our conclusions.
The main source of data for estimating poverty in India is the expenditure survey conducted by the NSSO. India is perhaps the only developing country that began conducting such surveys on a regular basis as early as 1950–1951. The surveys have been conducted at least once a year since 1950–1951. However, the sample had been too small to permit reliable estimates of poverty at the level of the state until 1973–1974. A decision was made in the early 1970s to replace the smaller annual surveys by large-size expenditure (and employment–unemployment) surveys to be conducted every 5 years.
This decision led to the birth of “thick” quinquennial (5-yearly) surveys. Accordingly, the following 8 rounds of large-size surveys have been conducted: 27 (1973–1974), 32 (1978), 38 (1983), 43 (1987–1988), 50 (1993–1994), 55 (1999–2000), 61 (2004–2005), and 66 (2009–2010). Starting from the 42nd round in 1986–1987, a smaller expenditure survey was reintroduced. This was conducted annually except during the years in which the quinquennial survey was to take place. Therefore, with the exception of the 65th and 67th rounds in 2008–2009 and 2010–2011, respectively, an expenditure survey exists for each year beginning 1986–1987.
While the NSSO collects the data and produces reports providing information on monthly per-capita expenditures, it is the Planning Commission that computes the poverty lines and provides official estimates of poverty. The official estimates are strictly limited to quinquennial surveys. While they cover rural, urban, and total populations in different states and at the national level, estimates are not provided for specific social or religious groups. These can be calculated selectively for specific groups or specific years by researchers. With rare exceptions, discussions and debates on poverty have been framed around the quinquennial surveys even though the other survey samples are large enough to allow reliable estimates at the national level.
For each household interviewed, the survey collects data on the quantity of and expenditure on a large number of items purchased. For items such as education and health services, where quantity cannot be meaningfully defined, only expenditure data are collected. The list of items is elaborate. For example, the 66th round collected data on 142 items under the food category; 15 items under energy; 28 items under clothing, bedding, and footwear; 19 items under educational and medical expenses; 51 items under durable goods; and 89 in the other items category.
It turns out that household responses vary systematically according to the length of the reference period to which the expenditures are related. For example, a household could be asked about its expenditures on durable goods during the preceding 30 days or the preceding year. When the information provided in the first case is converted into annual expenditures, it is found to be systematically lower than when the survey directly asks households to report their annual spending. Therefore, estimates of poverty vary depending on the reference period chosen in the questionnaire.
Most quinquennial surveys have collected information on certain categories of relatively infrequently purchased items including clothing and consumer durables on the basis of both 30-day and 365-day reference periods. For other categories, including all food and fuel and consumer services, they have used a 30-day reference period. The data allow us to estimate two alternative measures of monthly per-capita expenditures that refer to the following: (i) a uniform reference period (URP) where all expenditure data used to estimate monthly per-capita expenditure are based on the 30-day reference period, and (ii) a mixed reference period (MRP) where expenditure data used to estimate the monthly per-capita expenditure are based on the 365-day reference period in the case of clothing and consumer durables and the 30-day reference period in the case of other items.
With rare exceptions, monthly per-capita expenditure associated with the MRP turns out to be higher than that associated with the URP. The Planning Commission's original estimate of poverty that employed the Lakdawala poverty lines had relied on the URP monthly per-capita expenditures. At some time prior to the Tendulkar Committee report, however, the Planning Commission decided to shift to the MRP estimates. Therefore, while recommending revisions that led to an upward adjustment in the rural poverty line, the Tendulkar Committee also shifted to the MRP monthly per-capita expenditures in its poverty calculations. Therefore, the revised poverty estimates available for 1993–1994, 2004–2005, and 2009–2010 are based on the Tendulkar lines and the MRP estimates of monthly per-capita expenditures.
We note an important feature of the NSSO expenditure surveys at the outset. The average monthly per-capita expenditure based on the surveys falls well short of the average private consumption expenditure separately available from the NAS of the CSO. Moreover, the proportionate shortfall has been progressively rising over successive surveys. These two observations hold regardless of whether we use the URP or MRP estimate of monthly per-capita expenditure available from the NSSO. Figure 1 graphically depicts this phenomenon in the case of URP monthly per-capita expenditure, which is more readily available for all quinquennial surveys since 1983.
NSSO Household Total URP Expenditure Estimate as % of NAS Total Private Consumption Expenditure
Precisely what explains the gap between the NSSO and NAS expenditures has important implications for poverty estimates. For example, if the gap in any given year is uniformly distributed across all expenditure classes as Bhalla ( 2002 ) assumes in his work, true expenditure in 2009–2010 is uniformly more than twice of what the survey finds. This would imply that many individuals currently classified as falling below the poverty line are actually above it. Moreover, a recognition that the proportionate gap between NSSO and NAS private expenditures has been rising over time implies that the poverty ratio is being overestimated by progressively larger margins over time. At the other extreme, if the gap between NSSO and NAS expenditures is explained entirely by underreporting of the expenditures by households classified as non-poor, poverty levels will not be biased upwards.
There are good reasons to believe, however, that the truth lies somewhere between these two extremes. The survey underrepresents wealthy consumers. For instance, it is unlikely that any of the billionaires, or most of the millionaires, are covered by the survey. Likewise, the total absence of error among households below the poverty line is highly unlikely. For example, recall that the expenditures on durables are systematically underreported for the 30-day reference period relative to that for 365-day reference period. Thus, in all probability, households classified as poor account for part of the gap so that there is some overestimation of the poverty ratio at any given poverty line. 2
The 1993 expert group headed by Lakdawala defined all-India rural and urban poverty lines in terms of per-capita total consumption expenditure at 1973–1974 market prices. The underlying consumption baskets were anchored to the per-capita calorie norms of 2,400 and 2,100 in rural and urban areas, respectively. The rural and urban poverty line baskets were based on different underlying baskets, which meant that the two poverty lines represented different levels of real expenditures.
State-level rural poverty lines were derived from the national rural poverty line by adjusting the latter for price differences between national and state-level consumer price indices for agricultural laborers. Likewise, state-level urban poverty lines were derived from the national urban poverty line by adjusting the latter for price differences between the national and state-level consumer price indices for industrial laborers. National and state-level rural poverty lines were adjusted over time by applying the national and state-level price indices for agricultural workers, respectively. Urban poverty lines were adjusted similarly over time.
Lakdawala lines served as the official poverty lines until 2004–2005. The Planning Commission applied them to URP-based expenditures in the quinquennial surveys to calculate official poverty ratios. Criticisms of these estimates on various grounds led the Planning Commission to appoint an expert group under the chairmanship of Suresh Tendulkar in December 2005 with the directive to recommend appropriate changes in methodology for computing poverty estimates. The group submitted its report in 2009.
In its report, the Tendulkar committee noted three deficiencies of the Lakdawala poverty lines (Government of India 2009 ). First, the poverty line baskets remained tied to consumption patterns observed in 1973–1974. But more than 3 decades later, these baskets had shifted, even for the poor. Second, the consumer price index for agricultural workers understated the true price increase. This meant that over time the upward adjustment in the rural poverty lines was less than necessary so that the estimated poverty ratios understated rural poverty. Finally, the assumption underlying Lakdawala lines that health and education would be largely provided by the government did not hold any longer. Private expenditures on these services had risen considerably, even for the poor. This change was not adequately reflected in the Lakdawala poverty lines.
To remedy these deficiencies, the Tendulkar committee began by noting that the NSSO had already decided to shift from URP-based expenditures to MRP-based expenditures to measure poverty. With this in view, the committee's first step was to situate the revised poverty lines in terms of MRP expenditures in some generally acceptable aspect of the existing practice. To this end, it observed that since the nationwide urban poverty ratio of 25.7%, calculated from URP-based expenditures in the 2004–2005 survey, was broadly accepted as a good approximation of prevailing urban poverty, the revised urban poverty line could be anchored to yield this same estimate using MRP-based per-capita consumption expenditure from the 2004–2005 survey. This decision led to MRP-based per-capita expenditure of the individual at the 25.7 percentile in the national distribution of per-capita MRP expenditures becoming the national urban poverty line.
The Tendulkar committee further argued that the consumption basket associated with the national urban poverty line also be accepted as the rural poverty line consumption basket. This implied the translation of the new urban poverty line using the appropriate price index to obtain the nationwide rural poverty line. Under this approach, rural and urban poverty lines became fully aligned. Applying MRP-based expenditures, the new rural poverty line yielded a rural poverty ratio of 41.8% in 2004–2005 compared with 28.3% under the old methodology.
It is important to note that even though the method of pegging the national urban poverty line in the manner done by the Tendulkar committee left the national urban poverty in 2004–2005 originally measured at the Lakdawala urban poverty line unchanged, it did impact state-level urban poverty estimates. The methodology required that the state-level rural and urban poverty lines be derived from the national urban poverty line by applying the appropriate price indices derived from the price information within the sample surveys. In some cases, the state-level shift was sufficiently large to significantly alter the estimate of urban poverty. For example, Lakdawala urban poverty line in Gujarat in 2004–2005 was Rs541.16 per-capita per month. The corresponding Tendulkar line turned out to be Rs659.18. This change led the urban poverty estimate in 2004–2005 to jump from 13.3% based on the Lakdawala line to 20.1% based on the Tendulkar line.
An important final point concerns the treatment of health and education spending by the Tendulkar Committee in recommending the revised poverty lines. On this issue, it is best to directly quote the Tendulkar Committee report (Government of India 2009 , p. 2):
Even while moving away from the calorie norms, the proposed poverty lines have been validated by checking the adequacy of actual private expenditure per capita near the poverty lines on food, education, and health by comparing them with normative expenditures consistent with nutritional, educational, and health outcomes. Actual private expenditures reported by households near the new poverty lines on these items were found to be adequate at the all-India level in both the rural and the urban areas and for most of the states. It may be noted that while the new poverty lines have been arrived at after assessing the adequacy of private household expenditure on education and health, the earlier calorie-anchored poverty lines did not explicitly account for these. The proposed poverty lines are in that sense broader in scope.
We address here the two rounds of controversies over the poverty line that broke out in the media in September 2011 and March 2012. The first round of controversy began with the Planning Commission filing an affidavit with the Supreme Court stating that the poverty line at the time had been on average Rs32 and Rs26 per person per day in urban and rural India, respectively. Being based on the Tendulkar methodology, these lines were actually higher than the Lakdawala lines on which the official poverty estimates had been based until 2004–2005. However, the media and civil society groups pounced on the Planning Commission for diluting the poverty lines so as to inflate poverty reduction numbers and to deprive many potential beneficiaries of entitlements. For its part, the Planning Commission did a poor job of explaining to the public precisely what it had done and why.
The controversy resurfaced in March 2012 when the Planning Commission released the poverty estimates based on the 2009–2010 expenditure survey. The Planning Commission reported that these estimates were based on average poverty lines of Rs28.26 and Rs22.2 per person per day in urban and rural areas, respectively. Comparing these lines to those previously reported to the Supreme Court, the media once again accused the Planning Commission of lowering the poverty lines. 4 The truth of the matter was that whereas the poverty lines reported to the Supreme Court were meant to reflect the price level prevailing in mid-2011, those underlying poverty estimates for 2009–2010 were based on the mid-point of 2009–2010. The latter poverty lines were lower because the price level at the mid-point of 2009–2010 was lower than that in mid-2011. In real terms, the two sets of poverty lines were identical.
While there was no basis to the accusations that the Planning Commission had lowered the poverty lines, the issue of whether the poverty lines remain excessively low despite having been raised does require further examination. In addressing this issue, it is important to be clear about the objectives behind the poverty line.
Potentially, there are two main objectives behind poverty lines: to track the progress made in combating poverty and to identify the poor towards whom redistribution programs can be directed. The level of the poverty line must be evaluated separately against each objective. In principle, we may want separate poverty lines for the two objectives.
With regard to the first objective, the poverty line should be set at a level that allows us to track the progress made in helping the truly destitute or those living in abject poverty, often referred to as extreme poverty. Much of the media debate during the two episodes focused on what could or could not be bought with the poverty-line expenditure. 5 There was no mention of the basket of goods that was used by the Tendulkar Committee to define the poverty line.
In Annex E of its report (Government of India 2009 ), the Tendulkar Committee gave a detailed itemized list of the expenditures of those “around poverty line class for urban areas in all India.” Unfortunately, it did not report the corresponding quantities purchased of various commodities. In this paper, we now compute these quantities from unit-level data where feasible and report them in Table 1 for a household consisting of five members. 6 Our implicit per-person expenditures on individual items are within Rs3 of their corresponding expenditures reported in Annex E of the report of the Tendulkar Committee.
Source: Authors’ calculations using unit-level data (supplied by Rahul).
We report quantities wherever the relevant data are available. In the survey, the quantities are not always reported in weights. For example, lemons and oranges are reported in numbers and not in kilograms. In these cases, we have converted the quantities into kilograms using the appropriate conversion factors. The main point to note is that while the quantities associated with the poverty line basket may not permit a comfortable existence, including a balanced diet, they allow above-subsistence existence. The consumption of cereals and pulses at 50.9 kilograms (kg) and 3.5 kg compared with 48 kg and 5.5 kg, respectively, for the mean consumption of the top 30% of the population. Likewise, the consumption of edible oils and vegetables at 2.7 kg and 23.9 kg for the poor compared with 4.5 kg and 35.5 kg, respectively, for the top 30% of the population. 7 This comparison shows that, at least in terms of the provision of two square meals a day, the poverty line consumption basket is compatible with above-subsistence level consumption.
We reiterate our point as follows. In 2009–2010, the urban poverty line in Delhi was Rs1,040.3 per person per month (Rs34.2 per day). For a family of five, this amount would translate to Rs5,201.5 per month. Assuming that each family member consumes 10 kg per month of cereal and 1 kg per month of pulses and the prices of the two grains are Rs15 and Rs80 per kilogram, respectively, the total expenditure on grain would be Rs1,150. 8 This would leave Rs4,051.5 for milk, edible oils, fuel, clothing, rent, education, health, and other expenditures. While this amount may not allow a fully balanced diet, comfortable living, and access to good education and health, it is consistent with an above-subsistence level of existence. Additionally, if we take into account access to public education and health, and subsidized grain and fuel from the public distribution system, the poverty line is scarcely out of line with the one that would allow exit from extreme poverty.
But what about the role of the poverty line in identifying the poor for purposes of redistribution? Ideally, this exercise should be carried out at the local level in light of resources available for redistribution, since the poor must ultimately be identified locally. Nevertheless, if the national poverty line is used to identify the poor, could we still defend the Tendulkar line as adequate? We argue in the affirmative.
Going by the urban and rural population weights of 0.298 and 0.702 implicit in the population projections for 1 January 2010, the average countrywide per-capita MRP expenditure during 2009–2010 amounts to Rs40.2 per person per day. Therefore, going by the expenditure survey data, equal distribution across the entire country would allow barely Rs40.2 per person per day in expenditures. Raising the poverty line significantly above the current level must confront this limit with regard to the scope for redistribution.
It could be argued that this discussion is based on data in the expenditure survey, which underestimates true expenditures. The scope for redistribution might be significantly greater if we go by expenditures as measured in the NAS. The response to this criticism is that the surveys underestimate not just the average national expenditure but also the expenditures of those identified as poor. Depending on the extent of this underestimation, the need for redistribution itself would be overestimated.
Even so, it is useful to test the limits of redistribution by considering the average expenditure according to the NAS. The total private final consumption expenditure at current prices in 2009–2010 was Rs37,959.01 billion. Applying the population figure of 1.174 billion as of 1 January 2010 in the NSSO 2009–2010 expenditure survey, this total annual expenditure translates to daily spending of Rs88.58 per person. This figure includes certain items such as imputed rent on owner-occupied housing and expenditures other than those by households such as the spending of civil society groups, which would not be available for redistribution. Thus, per-capita expenditures achievable through equal distribution, even when we consider the expenditures as per the NAS, is likely to be modest.
To appreciate further the folly of setting too high a poverty line for the purpose of identifying the poor, recall that the national average poverty line was Rs22.2 per person per day in rural areas and Rs28.26 in urban areas in 2009–2010. Going by the expenditure estimates for different spending classes in Government of India ( 2011a ), raising these lines to just Rs33.3 and Rs45.4, respectively, would place 70% of the rural population and 50% of the urban population in poverty in 2009–2010. If we went a little further and set the rural poverty line at Rs39 per day and the urban poverty line at Rs81 per day in 2009–2010, we would place 80% of the population in each region below the poverty line. Will the fate of the destitute not be compromised if the meager tax revenues available for redistribution were thinly spread on this much larger population?
Before we turn to reporting the poverty estimates, we should clarify that while we have defended the current poverty line in India for both purposes—tracking abject poverty and redistribution—in general, we believe a case exists for two separate poverty lines to satisfy the two objectives. The poverty line to track abject poverty must be drawn independently of the availability of revenues for redistribution purposes and should be uniform nationally. The poverty line for redistribution purposes would in general differ from this line and, indeed, vary in different jurisdictions of the same nation depending on the availability of revenues. This should be evident from the fact that redistribution remains an issue even in countries that have entirely eradicated abject poverty. 9
Official poverty estimates are available at the national and state levels for the entire population, but not by social or religious groups, for all years during which the NSSO conducted quinquennial surveys. These years include 1973–1974, 1977–1978, 1983, 1987–1988, 1993–1994, 2004–2005, and 2009–2010, but not 1999–2000, as that year's survey became noncomparable to other quinquennial surveys due to a change in sample design. The Planning Commission has published poverty ratios for the first six of these surveys based on the Lakdawala lines and for the last three based on the Tendulkar lines. These ratios were estimated for rural and urban areas at the national and state levels.
In this paper, we provide comparable poverty rates for all of the last five quinquennial surveys including 2009–2010 derived from Lakdawala lines. For this purpose, we update the 2004–2005 Lakdawala lines to 2009–2010 using the price indices implicit in the official Tendulkar lines for 2004–2005 and 2009–2010 at the national and state levels. We provide estimates categorized by social as well as religious groups for all quinquennial surveys beginning in 1983 based on the Lakdawala lines and for the years relating to the last three such surveys based on the Tendulkar lines at the national and state levels.
While we focus mainly on the evolution of poverty since 1983 in this paper, it is useful to begin with a brief look at the poverty profile in the early years. This is done in Figure 2 using the estimates in Datt ( 1998 ) for years 1951–1952 to 1973–1974. The key message of the graph is that the poverty ratio hovered between 50% and 60% with a mildly rising trend.
The Poverty Ratio in India, 1951–1952 to 1973–1974 (%)
This is not surprising, as India had been extremely poor at independence. Unlike economies such as Taipei, China; the Republic of Korea; Singapore; and Hong Kong, China, the country then grew very slowly. Growth in per-capita income during these years had been a mere 1.5% per year. Such low growth coupled with a very low starting per-capita income meant at best limited scope for achieving poverty reduction even through redistribution. As argued above, even today, after more than 2 decades of almost 5% growth in per-capita income, the scope for redistribution remains limited. 10
We are now in a position to provide the poverty rates for the major social groups based on the quinquennial expenditure surveys beginning 1983. The social groups identified in the surveys are scheduled castes (SC), scheduled tribes (ST), other backward castes (OBC), and the rest, which we refer to as forward castes (FC). In addition, we define the nonscheduled castes as consisting of the OBC, and FC. The NSSO began identifying the OBC beginning 1999–2000. Since we are excluding this particular survey due to its lack of comparability with other surveys, the OBC as a separate group begins appearing in our estimates from 2004–2005 only.
In Table 2 , we provide the poverty rates based on the Lakdawala lines in rural and urban areas and at the national level. Four features of this table are worthy of note. First, poverty rates have continuously declined for every single social group in both the rural and urban areas. Contrary to common claims, growth has been steadily helping the poor from every broad social group escape poverty rather than leaving the socially disadvantaged behind.
FC = forward castes, NS = non-scheduled, OBC = other backward castes, SC = scheduled castes, ST = scheduled tribes.
Source: Authors’ calculations.
Second, the rates in rural India have consistently been the highest for the ST followed by the SC, OBC, and FC in that order. This pattern also holds in urban areas but with some exceptions. In particular, in some years, poverty rates of scheduled tribes are lower than that of scheduled castes, but this is not of great significance since more than 90% of the scheduled tribe population live in rural areas.
Third, with growth accelerating to above 8% beginning 2003–2004, poverty reduction between 2004–2005 and 2009–2010 has also accelerated. The percentage point reduction during this period has been larger than during any other 5-year period. Most importantly, the acceleration has been the greatest for the ST and SC in that order so that at last, the gap in poverty rates between the scheduled and nonscheduled groups has declined significantly.
Finally, while the rural poverty rates were slightly higher than the urban poverty rates for all groups in 1983, the order switched for one or more groups in several of the subsequent years. Indeed, in 2009–2010, the urban rates turned out to be uniformly higher for every single group. This largely reflects progressive misalignment of the rural and urban poverty lines with the former becoming lower than the latter. It was this misalignment that led the Tendulkar Committee to revise the rural poverty line and realign it to the higher, urban line.
Table 3 reports the poverty estimates based on the Tendulkar lines. Recall that the Tendulkar line holds the urban poverty ratio at 25.7% in 2004–2005 when measuring poverty at MRP expenditures. Our urban poverty ratio in Table 3 reproduces this estimate within 0.1 of a percentage point.
The steady decline in poverty rates for the various social groups in rural as well as urban areas, which we noted based on the Lakdawala lines in Table 2 , remains valid at the Tendulkar lines. Moreover, rural poverty ratios turn out to be higher than their urban counterparts for each group in each year. As in Table 2 , the decline had been sharpest during the high-growth period between 2004–2005 and 2009–2010.
Finally and most importantly, the largest percentage-point decline between these years in rural and urban areas combined had been for the ST followed by the SC, OBC, and FC in that order. Given that scheduled tribes also had the highest poverty rates followed by scheduled castes and other backward castes in 2004–2005, the pattern implies that the socially disadvantaged groups have achieved significant catching up with the better-off groups. This is a major break with past trends.
Next, we report the national poverty rates by religious groups. In Table 4 , we show the poverty rates based on Lakdawala lines of rural and urban India and of the country taken as a whole. Three observations follow. First, at the aggregate level (rural plus urban), poverty rates show a steady decline for Hindus, Muslims, Christians, Jains, and Sikhs. Poverty among the Buddhists also consistently declined except for 1983 and 1987–1988. With one exception (Muslims in rural India between 1987–1988 and 1993–1994), the pattern of declining poverty rates between any two successive surveys also extends to the rural and urban poverty rates in the case of the two largest religious communities, Hindus and Muslims.
Second, going by the poverty rates in 2009–2010 in rural and urban areas combined, Jains have the lowest poverty rates followed by Sikhs, Christians, Hindus, Muslims, and Buddhists. Prosperity among Jains and Sikhs is well known, but not the lower level of poverty among Christians relative to Hindus. Also interesting is the relatively small gap of just 5.8 percentage points between poverty rates among Hindus and Muslims.
Finally, the impact of accelerated growth on poverty between 2004–2005 and 2009–2010 that we observed across social groups can also be seen across religious groups. Once again, we see a sharper decline in the poverty rate for the largest minority, the Muslims, relative to Hindus who form the majority of the population.
This broad pattern holds when we consider poverty rates by religious groups based on the Tendulkar line, as seen in Table 5 . Jains have the lowest poverty rates followed by Sikhs, Christians, Hindus, Muslims, and Buddhists. With one exception (Sikhs in rural India between 1993–1994 and 2004–2005), poverty had declined steadily for all religious groups in rural as well as urban India. The only difference is that the decline in poverty among Muslims in rural and urban areas combined between the periods 2004–2005 and 2009–2010 had not been as sharp as that estimated from the Lakdawala lines. As a result, we do not see a narrowing of the difference in poverty between Hindus and Muslims. We do see a narrowing of the difference in urban poverty but this gain is neutralized by the opposite movement in the rural areas due to a very sharp decline in poverty among Hindus, perhaps due to the rapid decline in poverty among scheduled castes and scheduled tribes.
Before we turn to poverty estimates by state, we should note that in this paper, we largely confine ourselves to reporting the extent of poverty measured based on the two poverty lines. Other than occasional references to the determinants of poverty such as growth and caste composition, we make no systematic effort to identify them. Evidently, many factors influence the decline in poverty. For instance, the acceleration in growth between 2004–2005 and 2009–2010 also led to increased revenue that made it possible for the government to introduce the National Rural Employment Guarantee Scheme under which one adult member of each rural household is guaranteed 100 days per year of employment at a pre-specified wage. The employment guarantee scheme may well have been a factor in the recent acceleration in poverty reduction.
In a similar vein, rural–urban migration may also impact the speed of decline of poverty. Once again, rapid growth, which inevitably concentrates disproportionately in urban areas, may lead to some acceleration in rural-to-urban migration. If, in addition, the rural poor migrate in proportionately larger numbers in search of jobs, poverty ratios could fall in both rural and urban areas. In the rural areas, the ratio could fall because proportionately more numerous poor than in the existing rural population migrate. In the urban areas, the decline may result from these individuals being gainfully employed at wages exceeding the urban poverty line. Migration may also reinforce the reduction in rural poverty by generating extra rural income through remittances. Evidence suggests that this effect may have been particularly important in the state of Kerala.
We now turn to the progress made in poverty alleviation in different states. Though our focus in this paper is on poverty by social and religious groups, we first consider poverty at the aggregate level in rural and urban areas. India has 28 states and 7 union territories. To keep the analysis manageable, we limit ourselves to the 17 largest states. 11 Together, these states account for 95% of the total population. We exclude all seven union territories including Delhi; the smallest six of the seven northeastern states (retaining only Assam); and the states of Sikkim, Goa, Himachal Pradesh, and Uttaranchal. Going by the expenditure survey of 2009–2010, each of the included states has a population exceeding 20 million while each of the excluded states has a population less than 10 million. Among the union territories, only Delhi has a population exceeding 10 million.
A. Rural and Urban Populations
We begin by presenting the total population in each of the 17 largest states and the distribution between rural and urban areas as revealed by the NSSO expenditure survey of 2009–2010 (Table 6 ). 12 The population totals in the expenditure survey are lower than the corresponding population projections by the registrar general and census commissioner of India (2006) as well as those implied by Census 2011. 13 Our choice is dictated by the principle that poverty estimates should be evaluated with reference to the population underlying the survey design instead of those suggested by external sources. For example, the urban poverty estimate in Kerala in 2009–2010 must be related to the urban population in the state covered by the expenditure survey in 2009–2010 instead of projections based on the censuses in 2001 and 2011. 14
As shown in Table 6 , 27% of the national population lived in urban areas, while the remaining 73% resided in rural areas in 2009–2010. This composition understates the true share of the urban population, revealed to be 31.2% in the 2011 census. The table shows 10 states having populations of more than 50 million (60 million according to the 2011 census). We will refer to these 10 states as the “large” states. They account for a little more than three-fourths of the total population of India. At the other extreme, eleven “small” states (excluded from our analysis and therefore not shown in Table 6 ) have populations of less than ten million (13 million according to the Census 2011) each. The remaining seven states, which we call “medium-size” states, have populations ranging from 36 million in Orissa to 22 million in Chhattisgarh (42 million in Orissa to 25.4 million in Chhattisgarh, according to the 2011 census).
Among the large states, Tamil Nadu, Maharashtra, Gujarat, and Karnataka, in that order, are the most urbanized with a rate of urbanization of 35% or higher. Bihar is the least urbanized among the large states, with an urbanization rate of just 10%. Among the medium-size states, only Punjab has an urban population of 35%. The rest have urbanization rates of 30% or less. Assam and Orissa, with an urban population of just 10% and 14%, respectively, are the least urbanized medium-size states.
B. Rural and Urban Poverty
We now turn to the estimates of rural and urban poverty in the 17 largest states. To conserve space, we confine ourselves to presenting the estimates based on the Tendulkar line. We report the estimates based on the Lakdawala lines in the Appendix. Recall that the estimates derived from the Tendulkar line are available for 3 years: 1993–1994, 2004–2005, and 2009–2010. Disregarding 1973–1974 and 1977–1978, which are outside the scope of our paper, estimates based on the Lakdawala lines are available for an additional 2 years: 1983 and 1987–1988.
Table 7 reports the poverty estimates with the states arranged in descending order of their populations. Several observations follow. First, taken as a whole, poverty fell in each of the 17 states between 1993–1994 and 2009–2010. When we disaggregate rural and urban areas within each state, we still find a decline in poverty in all states in each region over this period. Indeed, if we take the 10 largest states, which account for three-fourths of India's population, every state except Madhya Pradesh experienced a consistent decline in both rural and urban poverty. The reduction in poverty with rising incomes is a steady and nationwide phenomenon and not driven by the gains made in a few specific states or certain rural or urban areas of a given state.
Second, acceleration in poverty reduction in percentage points per year during the highest growth period (2004–2005 to 2009–2010) over that in 1993–1994 to 2004–2005 can be observed in 13 out of the total 17 states. The exceptions are Uttar Pradesh and Bihar among the large states and Assam and Haryana among medium-size states. Of these, Uttar Pradesh and Assam had experienced at best modest acceleration in gross state domestic product (GSDP) during the second period while Haryana had already achieved a relatively low level of poverty by 2004–2005. The most surprising had been the negligible decline in poverty in Bihar between 2004–2005 and 2009–2010, as GSDP in this state had grown at double-digit rates during this period.
Finally, among the large states, Tamil Nadu had the lowest poverty ratio followed by Andhra Pradesh and Gujarat. Tamil Nadu, Karnataka, and Andhra Pradesh—all of them from the south—made the largest percentage-point improvements in poverty reduction among the large states between 1993–1994 and 2009–2010. Among the medium-size states, Kerala and Haryana had the lowest poverty rates while Orissa and Jharkhand made the largest percentage-point gains during 1993–1994 to 2009–2010.
It is useful to relate poverty levels to per-capita spending. In Table 8 , we present per-capita expenditures in current rupees in the 17 states in the 3 years for which we have poverty ratios, with the states ranked in descending order of population. Ideally, we should have the MRP expenditures for all 3 years, but since they are available for only the last 2 years, we report the URP expenditures for 1993–1994. Several observations follow from a comparison of Tables 7 and 8 .
MRP = mixed reference period, URP = uniform reference period.
First, high per-capita expenditures are associated with low poverty ratios. Consider, for example, rural poverty in 2009–2010. Kerala, Punjab, and Haryana, in that order, have the highest rural per-capita expenditures. They also have the lowest poverty ratios, in the same order. At the other extreme, Chhattisgarh and Bihar have the lowest rural per-capita expenditures and also the highest rural poverty ratios. More broadly, the top nine states by rural per-capita expenditure are also the top nine states in terms of low poverty ratios. A similar pattern can also be found for urban per-capita expenditures and urban poverty. Once again, Kerala ranks at the top and Bihar at the bottom in terms of each indicator. Figure 3 offers a graphical representation of the relationship in rural and urban India in 2009–2010 using state level data.
Poverty and Per-capita MRP Expenditure in Rural and Urban Areas in Indian States, 2009–2010
One state that stands out in terms of low poverty ratios despite a relatively modest ranking in terms of per-capita expenditure is Tamil Nadu. It ranked eighth in terms of rural per-capita expenditure but fourth in terms of rural poverty in 2009–2010. In terms of urban poverty, it did even better, ranking a close second despite its ninth rank in urban per-capita expenditure. Gujarat also did very well in terms of urban poverty, ranking third in spite of the seventh rank in urban per-capita expenditure.
Finally, there is widespread belief that Kerala achieved the lowest rate of poverty despite its low per-capita income through more effective redistribution. Table 8 entirely repudiates this thesis. In 1993–1994, Kerala already had the lowest rural and urban poverty ratios and enjoyed the second highest rural per-capita expenditure and third highest urban per-capita expenditure among the 17 states. Moreover, in terms of percentage-point reduction in poverty, all other southern states dominate Kerala. For example, between 1993–1994 and 2004–2005, Tamil Nadu achieved a 27.4 percentage-point reduction in poverty compared to just 19.3 for Kerala. We may also add that Kerala experienced very high inequality of expenditures. In 2009–2010, the Gini coefficient associated with spending in the state was by far the highest among all states in rural as well as urban areas.
In this section we decompose population and poverty by social group. As previously mentioned, the expenditure surveys traditionally identified the social group of the households using a three-way classification: scheduled castes, scheduled tribes, and nonscheduled castes. However, beginning with the 1999–2000 survey, the last category had been further subdivided into other backward castes and the rest, the latter sometimes referred to as forward castes, a label that we use in this paper.
We begin by describing the shares of the four social groups in the total population of the 17 states.
A. Population Distribution by Social Group within the States
Table 9 reports the shares of various social groups in the 17 largest states according to the expenditure survey of 2009–2010. We continue to rank the states according to population from the largest to the smallest.
Source: Authors’ calculations from the NSSO expenditure survey conducted in 2009–2010.
Nationally, the Scheduled Tribes constitute 9% of the total population of India according to the expenditure survey of 2009–2010. In past surveys and the Census 2001, this proportion was 8%. The scheduled castes form 20% of the total population according to the NSSO expenditure surveys, though the Census 2001 placed this proportion at 16%. The OBC are not identified as a separate group in the censuses so that their proportion can be obtained from the NSSO surveys only. The figure has varied from 36% to 42% across the three quinquennial expenditure surveys since the OBC began to be recorded as a separate group.
The scheduled tribes are more unevenly divided across states than the remaining social groups. In so far as these groups had been very poor at independence and happened to be outside the mainstream of the economy, ceteris paribus, states with high proportions of ST population may be at a disadvantage in combating poverty. From this perspective, the four southern states enjoy a clear advantage: Kerala and Tamil Nadu have virtually no tribal populations while Andhra Pradesh and Karnataka have proportionately smaller tribal populations (5% and 9% of the total, respectively) than some of the northern states which had high concentrations.
Among the large states, Madhya Pradesh, Gujarat, and Rajasthan have proportionately the largest concentrations of ST populations. The ST constitute 20%, 17%, and 14% of their respective populations. Some of the medium-size states, of course, have proportionately even larger concentrations. These include Chhattisgarh, Jharkhand, and Orissa with the ST forming 30%, 29%, and 22% of their populations, respectively.
Since the traditional exclusion of the SC has meant they began with a very high incidence of abject poverty and low levels of literacy, states with high proportions of these groups also face an uphill task in combating poverty. Even so, since the SC populations are not physically isolated from the mainstream of the economy, there is greater potential for the benefits of growth reaching them than the ST. This is illustrated, for example, by the emergence of some rupee millionaires among the SC but not the ST during the recent high-growth phase (Dehejia and Panagariya 2012 ).
Once again, at 9%, Kerala has proportionately the smallest SC population among the 17 states listed in Table 9 . Among the largest 10 states, West Bengal, Uttar Pradesh, Bihar, Rajasthan, and Madhya Pradesh have the highest concentrations. Among the medium-size states, Punjab, Haryana, and Orissa in that order have proportionately the largest SC populations.
The SC and ST populations together account for as much as 40% and 35%, respectively, of the total state population in Madhya Pradesh and Rajasthan. At the other extreme, in Kerala, these groups together account for only 10% of the population. These differences mean that, ceteris paribus, Madhya Pradesh, and Rajasthan face a significantly more difficult battle in terms of combating poverty than Kerala.
The ST populations also differ from the SC in that they are far more heavily concentrated in rural areas than in urban areas. Table 10 illustrates this point. In 2009–2010, 89% of the ST population was classified as rural. The corresponding figure was 80% for the SC, 75% for the OBC, and 60% for FC.
An implication of the small ST population in the urban areas in all states and in both rural and urban areas in a large number of states is that the random selection of households results in a relatively small number of ST households being sampled. The problem is especially severe in many of the smallest states where the total sample size is small in the first place. A small sample translates into a large error in the associated estimate of the poverty ratio. We will present the poverty estimates in all states and regions as long as a positive group is sampled. Nevertheless, we caution the reader on the possibility of errors in Table 11 that may be associated with the number of ST households in the 2009–2010 survey.
B. Poverty by Social Group
We now turn to poverty estimates by social groups. We present statewide poverty ratios based on the Tendulkar line for the ST, SC, and nonscheduled castes in Table 12 . We present the ratios for the OBC and FC in Table 13 . As before, we arrange the states from the largest to the smallest according to population. Separate rural and urban poverty estimates derived from the Tendulkar lines and Lakdawala lines are relegated to the Appendix.
NS = non-scheduled, SC = scheduled castes, ST = scheduled tribes.
FC = forward castes, OBC = other backward castes.
With one exception, Chhattisgarh, the poverty ratio declines for each group in each state between 1993–1994 and 2009–1010. There is little doubt that rising incomes have helped all social groups nearly everywhere. In the vast majority of the states, we also observe acceleration in the decline in poverty between 2004–2005 and 2009–2010 compared to between 1993–94 and 2004–2005. Reassuringly, the decline in ST poverty among scheduled tribes and scheduled castes and SC poverty has sped up recently with the gap in poverty rates between these groups and the nonscheduled castes narrowing.
The negative relationship between poverty ratios and per-capita expenditures that we depicted in Figure 3 can also be observed for the social groups taken separately. Using rural poverty estimates by social group in the Appendix, we show this relationship between SC poverty and per capita rural expenditures in the left panel of Figure 4 and that between the ST poverty and per capita rural expenditures in the right panel. Figure 4 closely resembles Figure 3 . The fit in the right panel is poorer than that in the left panel as well as those in Figure 3 . This is partially because the ST are often outside the mainstream of the economy and therefore less responsive to rising per-capita incomes. This factor is presumably exacerbated by the fact that the number of observations in the case of the ST has been reduced to 11 due to the number of ST households in the sample dropping to below 100 in six of the 17 states.
Scheduled Caste and Scheduled Tribe Poverty Rates and Per-capita MRP Expenditures in Rural Areas, 2009–2010
For years 2004–2005 and 2009–2010, we disaggregate the nonscheduled castes into the OBC and FC. The resulting poverty estimates are provided in Table 13 . Taking the estimates in Tables 12 and 13 , one can see that on average poverty rates are at their highest for the ST followed by SC, OBC, and FC in that order. At the level of individual states, ranking of the poverty rates of scheduled castes and scheduled tribes is not clear-cut, but with rare exceptions, poverty rates of these two groups exceed systematically those of other backward castes, which in turn exceed rates of forward castes.
An interesting feature of the poverty rates of forward castes is their low level in all but a handful of the states. For example, in 2009–2010, the statistic computed to just 3.9% in Punjab, 5.9% in Kerala, 6.5% in Haryana, 6.9% in Tamil Nadu, and 10.5% even in Rajasthan. In 14 out of the largest 17 states, it fell below 25%. The states with low FC poverty rates generally also have low OBC poverty rates making the proportion of the SC and ST population the key determinant of the statewide rate.
This point is best illustrated by a comparison of poverty rates of Punjab and Kerala. Poverty rates for the nonscheduled caste population in 2009–2010 was 7.3% in Punjab and 10.4% in Kerala, while those for scheduled castes stood at 29.2% and 27.4%, respectively, in the two states. But since scheduled castes constitute 39% of the population in Punjab but only 9% in Kerala, statewide poverty rate turned out to be 15.8% in the former and 12% in the latter.
The caste composition also helps explain the differences in poverty rates between Maharashtra and Gujarat on the one hand and Kerala on the other. In 2009–2010, statewide poverty rates were 24.8% and 23.2%, respectively, in the former and 12% in the latter (Table 10 ). In part, the differences follow from the significantly higher per-capita expenditures in Kerala, as seen from Table 11 . 15 But Maharashtra and Gujarat also face a steeper uphill task in combating poverty on account of significantly higher proportions of the scheduled tribe and scheduled caste populations. These groups account for 17% and 11%, respectively, of the total population in Gujarat, and 10% and 15% in Maharashtra. In comparison, only 1% of the population comprises scheduled tribes in Kerala, while just 9% comprise scheduled castes (Table 9 ).
Finally, we turn to poverty estimates by religious group in the states. India is home to many different religious communities including Hindus, Muslims, Christians, Sikhs, Jains, and Zoroastrians. Additionally, tribes follow their own religious practices. Though tribal religions often have some affinity with Hinduism, many are independent in their own right.
Table 14 provides the composition of population by religious group as well as the rural–urban split of each religious group based on the expenditure survey of 2009–2010. Hindus comprise 82% of the population, Muslims 12.8%, Christians 2.3%, Sikhs 1.7%, Jains 0.3%, and Zoroastrians 0.016%. The remaining comprises just 0.3%.
Together, Hindus and Muslims account for almost 95% of India's total population. With 34% of the population in urban areas compared with 26% in the case of Hindus, Muslims are more urbanized than Hindus. Among the other communities, Jains and Zoroastrians are largely an urban phenomenon. Moreover, while Muslims can be found in virtually all parts of India, other smaller minority communities tend to be geographically concentrated. Sikhs cluster principally in Punjab, Christians in Kerala and adjoining southern states, Zoroastrians in Maharashtra and Gujarat, and Jains in Gujarat, Rajasthan, Karnataka, and Tamil Nadu.
Given their small shares in the total population and their geographical concentration, random sampling of households in the expenditure surveys yields less than 100 observations for minority religious communities other than Muslims in the vast majority of the states. Indeed, as Table 15 indicates, only 13 out of the 17 largest states had a sufficiently large number of households even for Muslims to allow poverty to be reliably estimated. Orissa, Haryana, Punjab, and Chhattisgarh each had fewer than 100 Muslim households in the survey. Thus, we attempt poverty estimates by religious groups in the states separately for Hindus and Muslims only. We do provide estimates for the catch-all “other” category but caution that, in many cases, these estimates are based on less than 100 observations and therefore subject to large statistical errors.
As before, we present the estimates for statewide poverty of the religious groups using the Tendulkar line, placing the more detailed estimates for rural and urban areas and estimates based on the Lakdawala lines in the Appendix. Table 15 reports the estimates for Hindus, Muslims, and other minority religion groups for the years 1993–1994, 2004–2005, and 2009–2010.
Religious groups replicate the broad pattern seen in the context of poverty by social group. Poverty has fallen in every single state between 1993–1994 and 2009–2010 for Hindus as well as for Muslims, though the change is not always monotonic. While the level of poverty in 2009–2010 is higher for Muslims than Hindus in the majority of the states, the reverse is true in Bihar, Tamil Nadu, Madhya Pradesh, and Karnataka. An anomaly is the marginal increase in the poverty rate between 2004–2005 and 2009–2010 in Bihar for Hindus and in Gujarat for Muslims. The observation is particularly surprising since we simultaneously observe a significant decline in poverty during the same period for Muslims in Bihar and for Hindus in Gujarat. Interestingly, as documented in the Appendix, poverty rates for both Hindus and Muslims decline in both states based on the Lakdawala lines between 2004–2005 and 2009–2010.
Although the focus of this paper is on poverty, we find it useful to briefly report the evolution of inequality at the state and national levels in rural and urban areas. At the outset, it is important to note that the issue of inequality is complex partly because it can be measured in numerous ways. 16 The potential list of measures is almost endless, and there is no guarantee that these different measures will move in the same direction. Therefore, it is quite easy to show simultaneously that inequality has risen as well as fallen depending on the choice of measure.
In this paper, we use one measure of overall inequality based on the same expenditure survey data we used to report poverty measures in the previous sections: specifically, the Gini coefficient of household expenditures in rural and urban areas in the 17 states and in India as a whole using URP expenditures in 1983, 1993–1994, 1999–2000, 2004–2005, and 2009–2010. Table 17 and Table 18 report the Gini coefficient in rural and urban areas, respectively. As before, we arrange the states in descending order of population size.
Source: Planning Commission website (accessed 4 February 2013).
An immediate observation from Tables 17 and 18 is that, with rare exceptions, rural inequality tends to be lower than urban inequality. At the national level in 2009–2010, the Gini coefficient was 0.291 in rural areas and 0.382 in urban areas. These values reflect a difference of 9 percentage points. This is not surprising. The vast majority of the villagers are small farmers or wage laborers. As a result, variation in their incomes and therefore expenditures are not large. In contrast, cities serve as home to much of the industry and formal sector services as well as to a large informal sector which attracts migrant workers. This results in greater variation in incomes and expenditures.
The tables show no clear trend in the Gini in rural areas but do show a tendency for it to rise in urban areas. At the national level, rural Gini fell between 1983 and 1999–2000, rose between 1999–2000 and 2004–2005, and fell again between 2004–2005 and 2009–2010, with a small net decline over the entire period. In contrast, the urban Gini has climbed steadily.
This is hardly surprising since rapid growth, which can produce increased inequality, is concentrated in urban areas. In the Indian case, a dualism of sorts exists within urban areas. Output growth has been concentrated in the formal sector, while employment has been disproportionately concentrated in the informal sector. Unlike the Republic of Korea and Taipei, China in the 1960s and 1970s and the People's Republic of China more recently, employment in the formal sector has not grown in India due to the poor performance of labor-intensive sectors. Growth in India has been concentrated in skilled labor and capital-intensive sectors.
The data do not support the hypothesis that high levels of poverty reflect high levels of inequality. At least in the Indian case, the two outcomes are at best unrelated and at worst negatively associated. For example, at the national level, rural inequality has remained more or less unchanged and urban inequality has risen, while both rural and urban poverty have steadily and significantly declined over time.
Looking at a cross section of the data, Kerala offers the most dramatic example. In 2009–2010, it had the lowest levels of rural and urban poverty and by far the highest rural and urban Gini coefficients. At the other extreme, Bihar had the second lowest rural Gini coefficient but the highest rural poverty ratio during the same period.
At a more aggregate level, the left panel in Figure 5 plots the rural Gini against the rural poverty ratio, while the right panel plots the urban Gini against the urban poverty ratio. The exponential trend line has a negative slope in each case, though the fit is poor. In other words, there is no evidence of a positive relationship between poverty and inequality, but there is some evidence of a negative relationship.
Gini Coefficients and Poverty Ratios in Rural and Urban Areas in Indian States, 2009–2010
In this paper, we have provided a comprehensive analysis of poverty in India along six different dimensions: across time, across states, between rural and urban areas, across social and religious groups, and based on two different poverty lines (Lakdawala and Tendulkar). To keep the exposition manageable, we have concentrated on estimates based on the Tendulkar line except when we discuss poverty at the national level. In the latter case, we report estimates in rural and urban India derived from both the Lakdawala and Tendulkar lines. Our detailed estimates by social and religious groups, by rural and urban areas, and by state based on both the Lakdawala and Tendulkar lines are provided in the Appendix.
The following are some of the key conclusions of the paper. First, poverty has declined between 1993–1994 and 2009–2010 along every dimension. Indeed, poverty has fallen for every social and religious group in every state and in rural and urban areas, separately as well as jointly. Estimates based on the Lakdawala line show that the decline can be observed steadily since 1983 for all social and religious groups in all 17 large states.
Second, acceleration in growth rates between 2004–2005 and 2009–2010 has been accompanied by acceleration in poverty reduction. Poverty rates have fallen rapidly for all major social and religious groups at the national level. This phenomenon also holds true for most states across various social and religious groups.
Third, for the first time, poverty reduction between 2004–2005 and 2009–2010 has been larger for the scheduled castes and scheduled tribes than the upper caste groups. Thus, the gap in poverty rates between the socially disadvantaged and upper caste groups has narrowed over time. This pattern provides clear evidence to refute the claim that reforms and growth have failed to help the socially disadvantaged or that they are leaving these groups behind. A continuation of this trend, bolstered by further reforms and higher growth rates, would help eliminate the difference in poverty rates between the historically disadvantaged and the privileged.
Fourth, interstate comparisons reveal that the states with large scheduled castes and scheduled tribe populations face a steeper climb in combating poverty. The point is most forcefully brought out by a comparison of Punjab and Kerala. When we compare poverty rates in 2009–2010 by social group, the two states have very similar poverty rates. But because the poverty rates for the scheduled castes are higher than those for the nonscheduled castes in both states and the scheduled castes account for a much larger proportion of the population, the aggregate poverty rate in Punjab turns out to be significantly higher.
Finally, we find that in the case of India, there is no robust relationship between inequality and poverty. Indeed, to the extent that such a relationship exists, this would suggest that more unequal states enjoy lower levels of poverty. Kerala offers the most dramatic example. It has had one of the highest Gini coefficients for rural as well as urban areas and also one of the lowest poverty ratios for both regions. In 2009–2010, its Gini coefficients were by far the highest among the large states in both rural and urban areas, while its poverty ratios were the smallest.
Given space limitations, we have deliberately limited ourselves to providing one specific indicator of poverty—the headcount ratio—in different states and for different social and religious groups based on the two official poverty lines. There are at least two broad complementary directions in which the work in this paper can be extended.
First, it may be desirable for certain purposes to estimate alternative indicators of poverty such as the poverty gap or its close cousin, the Foster-Greer-Thorbecke index. Such an index allows one to gauge the resources needed to bring all those below the poverty line to a level above it. In a similar vein, we have focused on progress in combating poverty among social and religious groups that are more vulnerable. Alternatively, we could focus on a different dimension of vulnerability such as male-headed versus female-headed households and evaluate the progress in combating poverty among female-headed households.
The second direction in which the work of this paper could be extended is towards explaining the determinants of poverty. Within this broad category, we have left many questions unanswered. For instance, it would be useful to separate the contributions of growth and redistribution policies in explaining the decline in poverty. Likewise, we may want to know what role, if any, rural-to-urban migration may have played—directly as well as through remittances. Similarly, we might ask what role the division of population among various social and religious groups plays in determining the progress in combating poverty. Finally, we might also wish to study the role that education plays in bringing down poverty. The recent work by Hnatkovska and Lahiri ( 2012 ) shows that education has indeed been pivotal in bridging the wage gap between scheduled castes and scheduled tribes on the one hand and nonscheduled castes on the other. This suggests an important role for education in eradicating poverty.
a Calculated by adjusting the 2004–2005 lines using the index implicit in the official Tendulkar lines for 2004–2005 and 2009–2010.
Source: Planning Commission, Government of India, Data Tables.
SC = scheduled castes, ST = scheduled tribes, URP = uniform reference period.
a Delhi is 95% urban. The SC and ST estimates in this case are based on too few households and therefore subject to substantial sampling errors.
FC = forward castes, NC = nonscheduled castes, OBC = other backward castes.
a Only 5% of Delhi by population is rural. SC and ST estimates in this case are based on too few households and therefore subject to substantial sampling errors.
FC = forward castes, NS = nonscheduled castes, OBC = other backward castes, URP = uniform reference period.
MRP = mixed reference period, SC = scheduled castes, ST = scheduled tribes.
Source: Authors’ calculations
FC = forward castes, MRP = mixed reference period, NS = nonscheduled castes, OBC = other backward castes.
URP = uniform reference period.
MRP = mixed reference period.
The views expressed in the paper are those of the authors and not of the World Bank. We thank an anonymous referee, P. V. Srinivasan, and participants of the first 2013 Asian Development Review conference held on 25–26 March 2013 at the Asian Development Bank headquarters in Manila, Philippines.
Prices could vary not just between urban and rural regions within a state but also across subregions within rural and subregions within urban regions of a state. Therefore, in principle, we could envision many different poverty lines within rural and within urban regions in each state. To keep the analysis manageable, we do not make such finer distinctions in the paper.
We do not go into the sources of underestimation of expenditures in NSSO surveys. These are analyzed in detail in Government of India ( 2008 ). According to the report (Government of India 2008 , p. 56), “The NSS estimates suffer from difference in coverage, underreporting, recall lapse in case of nonfood items or for the items which are less frequently consumed and increase in nonresponse particularly from affluent section of population. It is suspected that the household expenditure on durables is not fully captured in the NSS estimates, as the expensive durables are purchased more by the relatively affluent households, which do not respond accurately to the NSS surveys.” Two items, imputed rentals of owner-occupied dwellings and financial intermediation services indirectly measured, which are included in the NAS estimate, are incorporated into the NSSO expenditure surveys. But these account for only 7–9 percentage points of the discrepancy.
This section is partially based on Panagariya ( 2011 ).
See, for example, the report by the NDTV entitled “Planning Commission further lowers poverty line to Rs28 per day.” Available: http://www.ndtv.com/article/india/planning-commission-further-lowers-poverty-line-to-rs-28-per-day-187729
For instance, one commentator argued in a heated television debate that since bananas in Jor Bagh (an upmarket part of Delhi) cost Rs60 a dozen, an individual could barely afford two bananas per meal per day at poverty line expenditure of Rs32 per person per day.
We thank Rahul Ahluwalia for supplying us with Table 1 . The expenditures in the table represent the average of the urban decile class including the urban poverty line. Since the urban poverty line is at 25.7% of the population, the table takes the average over those between the 20th and 30th percentile of the urban population.
The consumption figures for the top 30% of the population are from Ganesh-Kumar et al. ( 2012 ).
These amounts of cereal and pulses equal or exceed their mean consumption levels according to the 2004–2005 NSSO expenditure survey.
Recently, Panagariya ( 2013 ) has suggested that if political pressures necessitate shifting up the poverty line, the government should opt for two poverty lines in India—the Tendulkar line, which allows it to track those in extreme poverty, and a higher one that is politically more acceptable in view of the rising aspirations of the people.
The issue is discussed at length in Bhagwati and Panagariya ( 2013 ).
Although Delhi has its own elected legislature and chief minister, it remains a union territory. For example, central home ministry has the effective control of the Delhi police through the lieutenant governor who is the de jure head of the Delhi government and appointed by the Government of India.
Our absolute totals for rural and urban areas of the states and India in Table 6 match those in Tables 1A-R and 1A-U, respectively, in Government of India ( 2011b ).
The Planning Commission derives the absolute number of poor from poverty ratios using census-based population projections. Therefore, the population figure underlying the absolute number of poor estimated by the Planning Commission are higher than those in Table 6 , which are based on the expenditure survey of 2009–2010.
This distinction is a substantive one in the case of states in which the censuses reveal the degree of urbanization to be very different from that underlying the design of the expenditure surveys. For example, the expenditure survey of 2009–2010 places the urban population in Kerala at 26% of the total in 2009–2010, but the census in 2011 finds the rate of urbanization in the state to be 47.7%.
This is true in spite of significantly higher per-capita GSDP in Maharashtra presumably due to large remittances flowing into Kerala. According to the Government of India ( 2011a ), one in every three households in both rural and urban Kerala reports at least one member of the household living abroad.
For instance, inequality could be measured as the ratio of the top 10% to bottom 10% of the population, the ratio of rural to urban per-capita incomes, the ratio of skilled to unskilled wages (or formal and informal sector wages), and through the Gini coefficient (nationally or across states).
Email alerts
Affiliations.
- Online ISSN 1996-7241
- Print ISSN 0116-1105
A product of The MIT Press
Mit press direct.
- About MIT Press Direct
Information
- Accessibility
- For Authors
- For Customers
- For Librarians
- Direct to Open
- Open Access
- Media Inquiries
- Rights and Permissions
- For Advertisers
- About the MIT Press
- The MIT Press Reader
- MIT Press Blog
- Seasonal Catalogs
- MIT Press Home
- Give to the MIT Press
- Direct Service Desk
- Terms of Use
- Privacy Statement
- Crossref Member
- COUNTER Member
- The MIT Press colophon is registered in the U.S. Patent and Trademark Office
This Feature Is Available To Subscribers Only
Sign In or Create an Account

An official website of the United States government
The .gov means it’s official. Federal government websites often end in .gov or .mil. Before sharing sensitive information, make sure you’re on a federal government site.
The site is secure. The https:// ensures that you are connecting to the official website and that any information you provide is encrypted and transmitted securely.
- Publications
- Account settings
Preview improvements coming to the PMC website in October 2024. Learn More or Try it out now .
- Advanced Search
- Journal List
- Wiley - PMC COVID-19 Collection

Poverty in India in the face of Covid‐19: Diagnosis and prospects *
Hai‐anh dang.
1 Development Data Group, the World Bank, Washington District of Columbia, USA
Peter Lanjouw
2 Department of Economics, Vrije Universiteit, Amsterdam the Netherlands
Elise Vrijburg
India has been hard‐hit by the Covid‐19 pandemic. The virus has exacted a heavy toll in terms of lives lost and deteriorating health outcomes. The economic consequences of the pandemic have been similarly grim. In this paper we attempt an initial, interim, assessment of the impacts of the crisis on poverty. We review the growing literature that considers emerging poverty impacts, noting that there remain significant knowledge gaps due to limited evidence on current welfare outcomes. We analyze pre‐Covid survey data to examine the incidence of chronic poverty and downward mobility during a period of rapid economic growth and declining poverty. A profile of poverty during such a period might offer a plausible, partial, window on population groups currently at risk. We suggest that, notwithstanding the severe initial impacts of the crisis on poverty, there are grounds for expecting further consequences going forward. As the virus has spread out of the relatively affluent cities, and as economic stagnation persists, rural areas, with historically higher rates of chronic poverty and vulnerability, may see particularly sharp increases in poverty. While recent vaccination developments offer some grounds for optimism, there remains an urgent need to identify, implement and amplify effective policy alleviation measures.
1. INTRODUCTION
The year 2020 marks a major break in the progress achieved throughout the world in reducing global poverty. Sustainable Development Goal 1, announced by the international development community in 2015, had proclaimed the ambitious objective of ending extreme global poverty by 2030. Success in meeting the Millennium Development Goal of halving global poverty during the 1990–2015 interval had undoubtedly emboldened the architects of the Sustainable Development Goals to pursue a poverty objective that, while clearly aspirational, did not appear to be entirely beyond reach. The onset of the Covid‐19 pandemic in early 2020 has dampened these ambitions. Nowhere is this more evident than in India, a country where poverty reduction during the first decades of the 2000s had been remarkable, but where the impact of the Covid pandemic has been staggering in terms of both public health and economic livelihoods.
Given that the Covid‐19 pandemic remains in full swing, it is impossible to arrive at a complete assessment of its full impact on poverty in India and how it may evolve going forward. The economic consequences of the crisis are still working their way through the Indian economy, and policy measures aimed at addressing both the public health and economic fallout from the crisis continue to be formulated and rolled out. Empirical evidence on the actual impact of the crisis also remains highly fragmented and incomplete.
Yet, to preserve as much as possible of the progress that India undeniably achieved in recent decades, policies that mitigate the most severe consequences of the crisis must be introduced. To this end, there is a need to anticipate the likely consequences of the crisis for poverty. Not only is it necessary to track how poverty is evolving in the face of the crisis, but it is important to also identify and understand the circumstances of those who face a heightened risk of falling into poverty as the crisis continues to unfold.
In this study we attempt to provide an assessment of how the fight to end extreme poverty in India has been disrupted by the arrival of the Covid‐19 crisis. In particular, we are interested in informing policy‐making by providing insights into possible population groups that are likely to have been most seriously affected by the crisis, or to be particularly exposed to its most pernicious consequences in the months and years to come.
We start in the next section with a brief review of progress in poverty reduction prior to the onset of the Covid‐19 crisis. We are severely handicapped in this regard in that nationally representative poverty data that underpin official estimates of poverty in India are not available beyond the year 2011/12, when the last publicly available National Sample Survey was published. Nonetheless, we document that, in the period between 2004/05 and 2011/12, poverty reduction in India was indeed significant. It seems likely that a trajectory of declining poverty persisted until the Covid‐19 crisis hit in 2020.
We then proceed in Section 3 to briefly describe the spread and dimensions of the Covid‐19 crisis in India and provide a timeline of the policy measures that were introduced in response to the spread of the virus. Section 4 summarizes findings from a desk review of reports on the poverty impacts of the crisis. Absent systematic and current statistical evidence, we rely on a variety of sources and often anecdotal evidence to arrive at an initial impression of the poverty consequences of the crisis and its economic manifestations.
We then turn in Section 5 to a statistical analysis of household survey data from the first decade of the 2000s to investigate patterns of poverty dynamics that occurred during that time. We employ methods to construct synthetic panels from cross‐sectional survey data to inquire into the characteristics of the chronically poor in India during this period of rapid economic growth and poverty reduction. It is likely that those who remained poor during that interval are among those who are also at greatest risk of destitution during the sharp economic downturn ushered in by the Covid‐19 crisis. We also scrutinize those who, during the period 2004/05–2011/12, faced a heightened risk of falling into poverty. Again, it seems reasonable to suppose that those population groups that are most vulnerable during such a period of rapid progress are likely to count among the most vulnerable during a period of tremendous economic stress. In Section 6 we offer some concluding remarks.
2. PRE‐COVID POVERTY TRENDS
The evolution of poverty in India has long been the subject of close attention. In particular, with the introduction of economic reforms in the early 1990s, there has been a strong interest in seeing how poverty outcomes fared as economic growth accelerated (Datt & Ravallion, 2011 ; Ravallion, 2011 ). Figure 1 illustrates that poverty fell sharply during this period, falling from nearly 50 per cent of the population in 1987/88 to just over 20 per cent in 2011/12 (Dang & Lanjouw, 2018 ). Although growth started to pick up in the mid‐1990s, the evidence suggests that poverty reduction only started to gather pace in the early 2000s, after the 2004/05 round of the National Sample Survey (NSS) and, in particular, between the 2009/10 and 2011/12 rounds. Interestingly, the dramatic falls in poverty between 2009/10 and 2011/12 occurred when per capita growth rates were in fact lower than during the 2004/05–2009/10 interval (Figure 1 ).

Trends in poverty and gross domestic product per capita for India, 1987/88–2011/12. Source: Dang and Lanjouw ( 2018 )
A question of considerable importance concerns how poverty evolved from 2011/12 up to the onset of the Covid‐19 crisis. Unfortunately, the data needed to answer such questions are not available. The subsequent “quinquennial” round of the NSS survey after 2011/12, fielded during the 2017/18 survey year, has not been released by the National Sample Survey Organization (NSSO). There is considerable debate as to the likely evolution of poverty, with many commentators suggesting that the pace of poverty reduction slowed post‐2011/12, alongside the lower per capita income growth rates for India as a whole. What seems unambiguous is that with the onset of the Covid‐19 crisis, the decline in poverty is likely to have halted altogether.
3. THE COVID‐19 PANDEMIC
The Covid‐19 pandemic, to which India became exposed at the beginning of 2020, has left a deep mark on the country. In absolute terms, the spread of the virus has been striking – particularly during the surge in the early months of 2021. With over 27.1 million reported cases (as of May 26, 2021), India lagged behind only the United States in terms of the absolute number of Covid‐19 infected persons (John Hopkins University Center for Systems Science & Engineering, 2021 ). Compared to its vast population, however, India's Covid‐19 outbreak seems less catastrophic. The country ranked 94th out of all 199 investigated countries on the number of cumulative confirmed Covid‐19 cases per million people as of May 25, 2021 (Our World in Data, 2021a ). However, the rapid spread of Covid‐19 during the spring of 2021 was particularly alarming (Figure 2 ). In late May, India topped the World Health Organization's (WHO) worldwide list of newly reported Covid‐19 cases in the last 24 hr with an astounding 222,315 cases on May 24 (WHO, 2021 ). Worryingly, these data may well have been underestimated. 1

Time‐map of daily confirmed Covid‐19 cases in India as of May 25, 2021. Source: Our World in Data ( 2021b )
Beyond the health crisis, India has also faced severe socioeconomic challenges. As noted earlier, the Indian economy was already slowing down before the recent pandemic. The two nationwide lockdowns introduced shortly after the onset of the pandemic, which have been rated as among the most stringent in the world, 2 dealt the Indian economy an additional blow. The first lockdown, starting on March 25, 2020, and lasting for 21 days, involved a total closure of almost every type of commercial entity (except for grocery outlets) (Ministry of Home Affairs, 2020a ; Singh et al., 2020 ). The second one comprised an extension of the first lockdown – initially for two weeks until May 3, followed by another 2 weeks, and finally extending until May 31 (Ministry of Home Affairs, 2020b , 2020c , 2020d ). During the second lockdown, districts were divided into three possible zones – red, yellow and green, depending on the spread of Covid‐19 in that area – that determined the severity of measures in those places (Ray & Subramanian, 2020 , p. 3). Within the red zones, the highest‐risk areas were furthermore classified as containment zones (Express News Service, 2020 ). Although states and Union territories carried the responsibility to demarcate containment zones and to declare appropriate measures, the measures were expected to involve at least a night curfew from 7 p.m. to 7 a.m. imposed by the national government (Home Secretary, 2020 ). Within the containment zones, the lockdown was further extended to June 30 and finally to July 31 (Ministry of Home Affairs, 2020e ).
While the lockdown was extended in the containment zones, the rest of India was slowly reopened in different unlock phases (Gangwar & Ray, 2021 , p. 435). More recently, many states reverted to lockdowns on their own as a response to the new Covid surge in early 2021.
The disruption to economic activity in India throughout the lockdown period has been investigated by Beyer et al. ( 2021 ) who scrutinize daily electricity consumption and nighttime light intensity for evidence of economic turmoil. These two indicators capture important inputs into a wide range of economic activities and data are available at short notice and at high resolution (Beyer et al., 2021 , pp. 4–5). Their model, which can explain 90 per cent of the variance in electricity consumption and light intensity in normal times, reveals that on the day the first lockdown was imposed (March 23, 2020), electricity levels were 21 per cent lower than their predicted normal value. It was not until the end of June, after the lockdown measures had been lifted, that deviations from the predicted normal electricity levels were no longer statistically significant (Beyer et al., 2021 , pp. 5–7). However, in an extended analysis for The Economist , Beyer and colleagues show that India's rapidly rising infections have reversed the economic recovery from June (Figure 3 ). Clearly, the second wave placed the Indian economy under further strain.

Deviations from predicted daily normal electricity consumption in India (%). Source: The Economist ( 2021 )
4. POVERTY IMPACTS: A DESK REVIEW
The socioeconomic consequences of the Covid‐19 crisis and the measures subsequently taken to address them are unlikely to be distributed uniformly among Indian citizens. It is thus useful to identify those population groups that are most exposed to the economic burden of the current crisis in India and to elaborate on the specific vulnerabilities they face. We begin by assembling insights gleaned from a review of research and media reports that have emerged since the onset of the pandemic.
One of the most widely observed vulnerable groups in India is informal workers. This segment of the labor force is vast. A report by the National Commission for Enterprises in the Unorganised Sector (NCEUS) shows that, based on sectoral statistics from 2004–2005, the informal sector contributed up to 50 per cent to India's national gross domestic product (NCEUS, 2009 , p. 23). What makes informal workers economically vulnerable is that they are excluded from social security benefits, thereby being left unprotected against sudden shocks such as illness or death (Unni & Rani, 2003 , p. 130). Moreover, informal employment often generates lower incomes than similar formal employment does (Al Dahdah et al., 2020 ). Based on data from India's national 2011–2012 Employment and Unemployment Survey studying over 450,000 individuals across India, Narayanan ( 2015 ) estimates the formal–informal wage gap at different quantiles of the wage distribution and finds that this gap is significant for both Indian males and females across each quantile. He suggests that informal workers are consistently punished for being informal workers, even when they have similar characteristics and skills to their formal worker counterparts. Lastly, informal workers often possess individual characteristics that draw them to this type of work and create other vulnerabilities simultaneously – such as being female or belonging to a lower caste (Unni & Rani, 2003 ). We touch further upon these cross‐dimensional characteristics below.
The Covid‐19 crisis has accentuated the vulnerability of informal workers in several ways. First and foremost, informal workers saw an immediate decline in their earnings as soon as the lockdown put their jobs on hold (Al Dahdah et al., 2020 ). Based on data from the 2017–2018 Periodic Labour Force Survey, Estupinan and Sharma ( 2020 , pp. 14–17) estimate that 89.5 per cent of those at risk of losing their job during the first national lockdown and 68 per cent of those at risk during the second national lockdown were informally employed. Moreover, they estimate that the average wage loss for informal employees throughout the entire lockdown period in 2020 was 22.62 per cent, compared to a relatively low 3.83 per cent for formal employees.
Although informal employment could technically be more easily restored after a lockdown than formal employment, the numbers disappoint. Using a combination of personal surveys, secondary reports from organizations engaged with informal employees and comprehensive fieldwork, a case study by Azim Premji University ( 2021a ) conducted in Bangalore showed that only 6 per cent of the informal employees interviewed had returned to their previous job with similar or higher wages, and 15 per cent had not returned to any sort of work by December 2020. Finally, one could expect a sudden loss of income to have a bigger impact on informal than on formal employees given their generally lower‐income status (Centre for Monitoring Indian Economy, 2020 ).
Beyond the loss of income, informal employees are vulnerable due to a lack of access to health care. With one of the largest private and unregulated health‐care insurance markets, only 37 per cent of the Indian population had any form of health insurance in the fiscal year 2017/18 (Chatterjee, 2016 ; Tikkanen et al., 2020 ). Although two‐thirds of the insured rely on public health insurance, informal employees might especially miss out on such chances (Tikkanen et al., 2020 ). The largest existing health insurance schemes in place – the Employees’ State Insurance Scheme and the Central Government Health Scheme – rely upon employment in the formal sector, and smaller initiatives meant to target informal employees have not quite lifted off yet due to their limited focus on surgical procedures and families below the poverty line (La Forgia & Nagpal, 2012 ). Informal employees thus risk facing high out‐of‐pocket payments when they fall ill with the coronavirus.
One particularly vulnerable subgroup of informal workers are migrant workers, who often reside in rural areas but travel intra‐ or inter‐state to urban areas for work (Rawal et al., 2020 ). In a paper by Srivastava ( 2020 , pp. 29–30), the total number of vulnerable migrant workers is estimated using data from the NSSO, the National Statistical Office and the Census of India. Defining vulnerable migrant workers as those workers who either migrate for a short duration (i.e. seasonal migrants) or longer‐term migrants from the four lowest consumption quantiles and five lowest occupational categories, 3 the paper estimates that there are about 128 million such migrant workers in India (Srivastava, 2020 , pp. 8–13).
It should be noted that even compared to other informal workers, migrant workers face a particularly high chance of losing employment. In the four‐hour interval following the announcement of the first lockdown in March 2020, most of these migrant workers were suddenly left without a job (Sengupta & Jha, 2020 , pp. 158–162). Systematic data on the effects of the two nationwide lockdowns on migrants and other vulnerable communities are scarce, but a purposive sampling study from 5,000 telephone interviews conducted in 12 Indian states by Kesar et al. ( 2020 ) reveals that 86.6 per cent of inter‐state migrant workers lost employment due to these lockdowns in 2020. Regression analysis further shows that migrant workers were significantly more likely to be laid off than similar non‐migrant workers – rural migrant workers were 73.9 per cent more likely and urban workers 50.7 per cent more likely to lose their job than their non‐migrant counterparts (Kesar et al., 2020 , pp. 19–22).
Presumably, these job losses were only partially recovered during the unlock phase. A survey of 372 migrant workers in the automobile, construction and garment sectors revealed that, in October 2020, 60 per cent of the migrant workers were still fully out of work (Seth, 2020 , p. 1). A survey conducted by Azim Premji University among 2,778 individuals across 13 states revealed that around 20 per cent of the informal workers interviewed who lost their job during the first two nationwide lockdowns were still out of work in October and November (Azim Premji University, 2021b , p. 17, n.d. ).
Alongside a heightened risk of losing their job, migrant workers are especially vulnerable to getting infected with Covid‐19. It is generally known that the housing conditions for migrant workers in their area of employment are often rudimentary and cramped, making it difficult for them to self‐isolate (Sengupta & Jha, 2020 , pp. 158–162). A cross‐sectional study by Babu et al. ( 2017 , pp. 336–338), using a sample of almost 50,000 internal migrant households from 13 different Indian cities, revealed that the majority of those (43.4 per cent) lived in non‐registered slums, followed by registered slums (32.7 per cent) and dwellings at the work or construction site (11.7 per cent). The majority of these workers (70.6 per cent) further resided in single‐room shelters, had no access to a private water tap (44.6 per cent) or their own private toilet (63.8 per cent). Under such conditions, the chances of getting infected with Covid‐19, paired with facing high out‐of‐pocket payments, are substantial.
Confronted with income shortfalls and the risk of getting infected with the coronavirus, national and local lockdowns compel migrant workers to go back to their rural homes, sometimes under hazardous conditions (Sengupta & Jha, 2020 , pp. 158–162). The first nationwide lockdowns (from March 25 to May 31) caused a flood of migrants attempting to return home. Estimates by the economist and demographer Amitabh Kundu and his colleagues put the number of internal migrants who returned home at around 12 million (Chishti, 2020 ). The issue was prominent enough for the national government to help individual states transport about 9.9 million migrants by means of designated buses and trains between May and June (Iyer, 2020 ). Unfortunately, the most vulnerable immigrant workers were unable to access these transportation opportunities as they were stranded in peripheral locations and on work sites, leaving them no alternative but to walk home (Srivastava, 2020 , p. 18). A database of media‐reported deaths in India up to July 3 showed that, out of the 961 deaths that resulted from the lockdowns (deaths resulting from Covid‐19 infection not included), 209 were caused by walking long distances or during migration (Thejesh GN, 2020 ). Despite a large number of these migrants returning to their work sites in the unlock phase, news reports fear that the new wave Covid‐19 and subsequent local lockdowns will cause another migrant push to rural India (Agarwal & Bellman, 2021 ; Gulati et al., 2021 , p. 24; Naik, 2021 ; The Indian Express, 2021 ).
When returning home, migrants may find themselves in an even more vulnerable position due to the absence of social protection measures and health facilities in rural areas. First of all, media reports show that in rural areas there is often a lack of awareness of appropriate measures against Covid‐19 or of what it means to quarantine. Furthermore, local efforts to inform rural residents about these matters are inadequate (Agrawal, 2020 ). Even when returning migrants and villagers are well informed, the quarantine arrangements in rural areas frequently fall short: they either involve being placed in poorly equipped quarantine centers or going into self‐quarantine, for which many migrants lack the means (Sengupta & Jha, 2020 ).
Second, numbers show that rural areas severely lack health‐care facilities compared to urban areas. Based on a nationwide survey conducted with 14,746 households from 12 different states, a report by the IMS Institute from 2013 revealed that inhabitants in rural areas all across India have to travel disproportionately further to access a health‐care facility: 63 per cent of the rural population have to travel over 5 kilometers to access a hospitalization unit, compared to 26 per cent of the urban population (IMS Institute for Healthcare Informatics, 2013 , p. 17). Moreover, the number of hospital beds in rural areas may be critically low. With data from the Directorate General of State Health Services, a study by Ghosh and Dinda ( 2017 , p. 110) reveals that the states whose rural areas have the fewest hospital beds per hundred thousand inhabitants are Bihar (5.685), Chhattisgarh (7.762), Uttar Pradesh (9.947), Haryana, (14.864), Madhya Pradesh (19.065) and Maharashtra (18.360). Three of these six states are among the top states home to inter‐state migrants. Professor Kundu from the Research and Information System estimated that, based on the 2011 Census of India, Uttar Pradesh would rank first (accounting for 25 per cent of the inter‐state migrants), Bihar second (accounting for 14 per cent) and Madhya Pradesh fourth (accounting for 5 per cent) (Singh & Magazine, 2020 ).
For rural areas, the threat of bad containment measures and inadequate health‐care provisions is severe. Data from the Backward Regions Grant Fund, dedicated to the development of backward (rural) districts, shows that the contribution of the 243 backward regions for which data were available to the number of infections has risen from 11.2 per cent during the first Covid‐19 wave to 16 per cent during the new second wave. Moreover, in absolute terms, the death toll from these regions has quadrupled compared to that of the first wave (Sinha, 2021 ; Ministry of Panchayati Raj, n.d. ). Though the new wave has initially spread most rapidly in urban areas, the consequences of the second wave may be hardest felt in the less well‐equipped and impoverished rural regions (Mehta & Jamkhandikar, 2021 ; Mitra et al., 2021 ).
Aside from serious health risks, another issue that awaits migrants when returning home is further income losses. In rural areas, poor households are typically unable to generate sufficient income from farming. With the nationwide lockdown falling exactly within the winter harvest season in 2020, rural families faced a shortage of labor and equipment and saw their crops being left unsold (Maggo, 2020 ). Based on key informant interviews among 1,515 farmers in two contrasting Indian states (Haryana and Odisha), Ceballos et al. ( 2020 , pp. 1–3) found that, in both states, the majority (61 per cent and 74 per cent, respectively) could not sell their crops immediately upon harvest. Thus, migrant workers and their rural‐based families have experienced a double‐dip in income from both a lack of wages and salaries and a lack of produce sales.
Such a double‐dip in income might lead to concerning levels of indebtedness. In a mixed‐methods‐based study including both data from the Networks, Employment, Debt, Mobility and Skills in India Survey and individual in‐depth surveys from 2016 to 2017 distributed among 2,692 individuals across 15 rural villages in Tamil Nadu, Guérin et al. ( 2020 , p. 11) shows that 99 per cent of the households were indebted, with a median of four outstanding loans per family and an average value of Rs. 58,000 (almost US$800) per loan. Informal interviews from the same study revealed that following the first‐wave lockdown, families were pressured by lenders to pay back their outstanding debts and faced difficulties finding new sources of credit (Guérin et al., 2020 , pp. 17–20).
4.1. Cross‐cutting dimensions
Certain dimensions, such as gender, religious status or caste, will affect one's vulnerability in the current crisis disproportionately. These cross‐cutting dimensions often coexist with one's (informal) employment situation to create an even more vulnerable profile (Ray & Subramanian, 2020 , pp. 46–47). We will touch upon three of these dimensions: gender, religion and caste.
First, women are likely to carry a higher burden during the current crisis than men. Despite only 20 per cent of all Indian women being employed, among those women who are employed, 90 per cent are involved in informal employment, thus facing the associated vulnerabilities (World Economic Forum, 2020 , p. 28; International Labour Organization, 2018 , p. 88). The current crisis is likely to disproportionately affect their employment status. Based on the Centre for Monitoring Indian Economy's Consumer Pyramids Household Survey, drawing on data from 174,405 households nationwide, Ashwini ( 2020 , pp. 3–4) found that women employed pre‐lockdown were approximately 20 per cent less likely to be employed post‐lockdown than pre‐lockdown employed men. Estimating the effect of being female in rural areas on the risk of employment loss, another study finds that being a rural‐based female increases one's chance of losing employment during the lockdown period by 75.7 per cent compared to being a rural‐based male (Kesar et al., 2020 , p. 22).
The vulnerability of women extends beyond a disproportionate loss of income. The literature suggests that, due to their lower social status, women in India are likely to suffer disproportionately from food shortages and price hikes, thus having higher chances than men of going hungry (Asadullah & Raghunathan, 2020 ; Global Hunger Index, 2010 , p. 14). Moreover, women often suffer from additional health risks (and accompanying high out‐of‐pocket payments). Research suggests that Indian households are more likely to prioritize, and devote more resources to, the health‐care needs of males than of females (Barcellos et al., 2014 ; Oster, 2006 ). Female‐headed households are also more likely to suffer economically from a health shock. A study by Dhanaraj ( 2014 , p. 15), which employs a logit model on a panel data set covering more than 3,000 households in the Indian state of Andhra Pradesh over 15 years, estimates that female‐headed households faced an 80 per cent higher risk of experiencing such a welfare loss relative to male‐headed households.
Muslims form another vulnerable group. First, they are more likely to be employed in vulnerable segments of the informal sector than other religious groups. According to the 2014 Post Sachar Evaluation Committee (PSEC) 4 report, a mere 23 per cent of the Muslim urban households earn their livelihoods through regular wage employment compared to 42 per cent of all urban households. Furthermore, their incidence of relying on non‐agricultural self‐employment is 25 per cent, compared to 14 per cent for Hindu households (PSEC, 2014 , p. 14). Moreover, Muslims often lack access to social resources available to other vulnerable communities and suffer from a lower distribution of public welfare services and benefits, making it more difficult to cope with a loss of earnings (Pandya, 2010 , pp. 16, 29). This could explain why, according to the Dhanaraj study ( 2014 , p. 15), Muslim households have an estimated 26.5 per cent higher chance of welfare loss due to serious illness or death than other religious groups. They are also more likely to sell assets or borrow money to cope with such a loss, which in the long run is believed to increase their economic vulnerability even further (Dhanaraj, 2014 , pp. 6, 17). Thus, the current Covid‐19 crisis might deteriorate their already low economic status.
Third, Scheduled Castes (SCs, more commonly known as Dalits) are a particularly vulnerable constituency. Households belonging to this group are often reliant on low‐paid informal jobs that require migrating or commuting to urban areas, thus exposing them to the vulnerabilities of informal employment (Ganguly, 2020 ). Estimates provided by the NSSO, based on surveys covering more than 2.5 million households in 2011/12, indicate that SCs account for the bulk of households dependent on income from casual labor (Ministry of Statistics & Programme Implementation, 2015 , pp. 21–25). In addition, SC members had limited access to public benefits even before the crisis, and are likely to be in even more desperate need of them now (Ganguly, 2020 ). The study by Kesar et al. ( 2020 , p. 34) revealed that 54 per cent of all SC members in the sample did not receive any type of cash transfer during the first nationwide lockdown, which is considerably higher than the percentage for the other socially disadvantaged castes, namely the Scheduled Tribes (46 per cent) and the Other Backward Classes (44 per cent). Unsurprisingly, then, SC members were more susceptible to going hungry than other groups. Using a telephone survey among 164 rural households across 13 states, Niyati and Vijayamba ( 2020 ) reveal that the proportion of households with less than usual food consumption in September 2020 was higher among SC members (56 per cent) than among households belonging to other castes (42 per cent).
5. POVERTY IMPACTS: INSIGHTS FROM HISTORICAL ANALYSIS OF POVERTY DYNAMICS
Documenting the poverty consequences of the Covid‐19 crisis with formal statistical analysis is difficult due to the non‐availability of the necessary household survey data. As noted above, some efforts have been made to produce interim results based on phone interviews, data on electricity consumption and via the recording of economic activity through night‐lights data (Beyer et al., 2020 ; Kesar et al., 2020 ). However, insights are still very limited and piecemeal.
One additional potential direction is to analyze pre‐Covid data to identify population groups in India that face a heightened likelihood of being chronically poor (i.e. long‐term poor) or that face a heightened risk of falling into poverty even if currently non‐poor. The working hypothesis here, is that vulnerability and chronic poverty observed during a period of rapidly rising living standards may point to population groups that are deprived in a fundamental sense, and are thus particularly likely to be hard hit when general economic conditions deteriorate.
Studying such poverty dynamics at the all‐India level is difficult, however, as the data underpinning official poverty estimates in India come from cross‐section rather than panel surveys. Dang and Lanjouw ( 2018 ) attempt to overcome this constraint by applying a “synthetic panel” method to NSSO data, and study welfare dynamics based on estimates derived from this approach. Their approach is outlined in the companion paper by Garcés‐Urzainqui et al. ( 2021 ) included in this symposium issue. We build on their analysis to assemble potential insights into the population groups particularly exposed to the impacts of the Covid‐19 crisis.
As described in Garcés‐Urzainqui et al. ( 2021 ), a key parameter needed to produce point estimates of poverty transitions on the basis of the synthetic panel approach is the correlation over time of the error terms from consumption models estimated with the two cross‐sectional rounds of data. The procedure for estimating this correlation from cross‐section data is not uncontroversial, with some observers casting doubt on the stability and reliability of the estimate that results from it (Elbers, 2021 ; Herault & Jenkins, 2019 ). Fortunately, in an earlier exercise employing these methods, Dang and Lanjouw ( 2018 ) were able to validate NSS‐based estimates of poverty dynamics for India employed in the present study by comparing them with those obtained from the India Human Development Survey (IHDS), an actual panel data set also covering the 2004/05–2011/12 period. They indicate that the error term correlation that they approximate using only cross‐sectional NSS data is not far from that observed in the IHDS panel data. While the IHDS could directly support analysis of poverty dynamics, the overall sample size is smaller than that of the NSS, and the latter also underpin the official poverty estimates in India. For this reason it was preferred, in the present study, to apply the synthetic panel method to the NSS data rather than to base the analysis on the IHDS survey. Dang and Lanjouw ( 2018 ) show that at the national‐level estimates of poverty mobility in India based on the IHDS and NSS line up very closely.
As indicated in Garcés‐Urzainqui et al. ( 2021 ), poverty dynamics can be explored by dividing the population into two groups: one poor and the other non‐poor. But the analysis can be expanded further by disaggregating the non‐poor group into two groups: the “vulnerable” (those that are non‐poor but still face a significant risk of falling into poverty) and the “secure” (or “middle class”). These two groups can be distinguished on the basis of a “vulnerability line” that lies above the poverty line and that separates the two non‐poor groups from one another. A common, but rather ad‐hoc, approach is to arbitrarily scale up the poverty line by a certain factor to obtain such a vulnerability line. For example, in India, vulnerability has in the past been proposed to occur within a fixed income range between 1.25 times and twice the national poverty line in India (NCEUS, 2007 ). This approach has the advantage of being simple and easily communicated, but it appears to be based on no underlying rationale.
A recent approach proposed in Dang and Lanjouw ( 2017 ) instead derives the vulnerability line from a specified probability of the non‐poor falling back into poverty. This approach estimates a vulnerability line that lies above the poverty line and below which the non‐poor population faces an average risk of falling back into poverty equal to some predetermined level (designated by Dang and Lanjouw as the vulnerability index). The vulnerability index itself has to be specified upfront and can be based on various criteria, including budgetary planning, social welfare objectives, or relative concepts of well‐being. In contrast to Pritchett et al. ( 2000 ) and Chaudhuri ( 2003 ), this approach to estimating vulnerability considers as “vulnerable” a segment of the population that is currently non‐poor, treating this population segment as distinct from the currently poor (even though the poor are certainly also likely to be vulnerable in a deeper sense).
Dang and Lanjouw ( 2018 ) analyze the dynamics of poverty and vulnerability in India based on the synthetic panels procedure outlined above. Their analysis covers multiple rounds of data between 1987 and 2012. We report here their findings for the period 2004/05–2011/12 (Table 1 ). Dang and Lanjouw ( 2018 ) estimate a vulnerability line that is based on a vulnerability index of 20 per cent. In other words, they derive a vulnerability line such that the risk, on average, of those who are located between the poverty and the vulnerability line is 20 per cent. This analysis yields a vulnerability line based on the 2004/05–2011/12 synthetic panel interval equal to Rs. 770 in 2004/05 prices and can be compared to the rural India poverty line in 2004/05 of Rs. 447 per person per month.
Welfare transition dynamics based on synthetic panel data, India, 2004/05–2011/12 (percent)
The vulnerability line is that which corresponds to a vulnerability index of 0.2 in 2004/05–2011/12 (i.e. Rs. 770). All numbers are in 2004 prices for all rural India. The rural India poverty line is Rs. 446.68 for 2004/05. All numbers are estimated with synthetic panel data and weighted with population weights, where the first survey round in each period is used as the base year. Bootstrap standard errors in parentheses are estimated with 1,000 bootstraps, adjusting for the complex survey design. Household head's age range is restricted to between 25 and 55 for the first survey and adjusted accordingly for the second survey in each period. Estimation sample sizes are 91,751 and 75,159 for the first and second periods, respectively.
Table 1 points to a fair amount of consumption mobility between 2004/05 and 2011/12. However, transitioning out of poverty and directly into the “secure” category is a very rare occurrence; most “escapes” from poverty landed the poor into the category of the vulnerable. Between 2004/05 and 2011/12, just under 18 per cent of the population was estimated to be chronically poor (in the sense of being poor in both periods). Of the 37 per cent of the population estimated to be poor in 2004/05, therefore, roughly half (48.8%) was unable to escape from poverty. At the same time, while just over 40 per cent of the population could be considered vulnerable in 2004/05, an estimated 6.4 per cent had dropped back into poverty by 2011/12 and another 19.4 per cent continued to face a heightened risk of falling back into poverty. Given that the economy of India was growing particularly strongly during this interval, and that overall poverty fell markedly (from an estimated 37 per cent to 25 per cent for this subset of the population, comprised of households with household heads aged between 25 and 55), it seems reasonable to suppose that the chronically poor and vulnerable possess characteristics and attributes that would make them particularly likely to experience reversals during a period of generalized contraction. It is also plausible that many of these characteristics and attributes would persist over time. If so, acquiring a better sense of the characteristics of the chronically poor and the vulnerable between 2004/05 and 2011/12 might point to the population groups also most at risk during the current Covid‐related economic downturn. Under that hypothesis we undertake such a profiling exercise below.
5.1. Who are the chronically poor and the downwardly mobile?
Our interest here is in studying chronic poverty and vulnerability during a time of rapid economic growth and deriving from that exercise some insights into how the current Covid‐19 pandemic might expose particular population groups to a heightened risk of poverty. In order to base our estimates on the actual experience of households during the 2004/05–2011/12 period, we present our findings in terms of the odds of belonging to the chronically poor and the odds of actually being downwardly mobile between 2004/05 and 2011/12. 5 In doing the latter we depart slightly from our concept of vulnerability, in that we look at the characteristics of those households who actually dropped into the status of poor in 2011/12 from the status of vulnerable or secure in 2004/05, combined with those households who fell into the status of vulnerable in 2011/12 from the status of secure in 2004/05. From Table 1 we can see that the average odds of downward mobility were thus 19.8 per cent (12.6/63.5 = 0.198). while the average odds of chronic poverty were 48.8 per cent (17.8/36.5 = 0.488).
We consider first in Table 2 the association between education and the odds of belonging to the category of either the chronically poor or the downwardly mobile. Table 2 indicates that the education level of the household head is closely associated with both chronic poverty and downward mobility. Households in which the household head is uneducated, or has less than primary school completion, are more likely than average to comprise the chronically poor or to be downwardly mobile. Chronic poverty becomes less likely once the household head has primary schooling or higher. And as education levels rise, the likelihood of chronic poverty diminishes further. The odds of downward mobility, on the other hand, only diminish appreciably (falling below 1) once the household head has completed secondary schooling or higher. Even a little education thus seems to help protect against chronic poverty, but more than a minimum is needed to guard against a heightened risk of downward mobility. From this, one might infer that protection measures during the Covid‐19 crisis should not fail to target those with moderate education levels as well. This is especially so since it is likely that overall education levels are likely to have increased since 2011/12.
Profile of the chronically poor or downwardly mobile, 2004/05–2011/12: Education
Estimates show the difference between the probability of falling into each category relative to the mean chronic poverty and vulnerability rates of 48.8% and 19.8%.
Table 3 examines patterns of employment of heads of households and the odds of being chronically poor or downwardly mobile. Considering first the chronically poor, we see that on the whole rural workers have a higher than average odds of belonging to the chronically poor, while urban workers are less exposed. Among rural workers, only those employed in the “others” (non‐labor) category have a lower than average odds of being chronically poor. Agricultural laborers in rural areas are particularly strongly linked to chronic poverty, while urban wage workers are least exposed. As noted above, the economic crisis induced by Covid‐19 was particularly strongly felt, at least initially, by informal sector workers in urban areas. To the extent that the patterns prevailing in 2004/05–2011/12 continued to hold, it would appear that the poorest of the poor at the all‐India level were not those who were initially hit the hardest by the crisis.
Profile of the chronically poor or downwardly mobile, 2004/05–2011/12: Employment
See explanatory notes to Table 2 .
Looking at patterns of downward mobility in Table 3 , we see that households headed by agricultural laborers were also particularly likely to experience downward mobility between 2004/05 and 2011/12, a time when rural incomes and employment were rising sharply. It is difficult to imagine that this vulnerability of agricultural laborers would not persist when the Indian economy experienced a sharp reversal as a result of Covid‐19. In urban areas, urban wage workers had the lowest odds of downward mobility, consistent with the booming, urban‐led, growth trajectory under way in India between 2004/05 and 2011/12.
Table 4 considers the likelihood of chronic poverty and downward mobility among different social groups between 2004/05 and 2011/12. Given that the Indian economy was growing strongly during this period, it is not surprising that Scheduled Tribes – the social group least likely to be full participants in the modernizing Indian economy – experienced the highest odds of chronic poverty. Relative to the average, the odds of chronic poverty were more than 25 per cent higher for this group. Compared to the Scheduled Tribes, Scheduled Castes face lower odds of chronic poverty, but are still significantly more likely than average to experience such poverty. This ranking of deprivation between Scheduled Tribes and Scheduled Castes also carries over to downward mobility. Scheduled Tribes were particularly likely to experience downward mobility between 2004/05 and 2011/12, followed by the Scheduled Castes. The other caste groups in the Indian population (Other Backward Classes and other castes) faced lower than average odds of chronic poverty and downward mobility.
Profile of the chronically poor or downwardly mobile, 2004/05–2011/12: Caste
Table 5 indicates that Muslim households were only slightly more highly represented than Hindu households among the chronically poor during the 2004/05–2011/12 period. However, the likelihood of downward mobility among Muslim households was considerably higher than among Hindu households. This finding resonates with the earlier discussion of the vulnerable position of Muslims in India during the Covid‐19 pandemic.
Profile of the chronically poor or downwardly mobile, 2004/05–2011/12: Religion
Table 6 examines the odds of chronic poverty and downward mobility by population groups defined in terms of dependency ratio. Households with a dependency ratio above 50 per cent are both slightly more likely than average to be chronically poor, and rather more likely than average to experience downward mobility.
Profile of the chronically poor or downwardly mobile, 2004/05–2011/12: Dependency ratio
We turn, finally, to the spatial distribution of chronic poverty and downward mobility across Indian states between 2004/05 and 2011/12. Table 7 indicates that of the major states, chronic poverty was relatively pronounced in Rajasthan, West Bengal, Jharkhand, Orissa, Chhattisgarh and Madhya Pradesh, and to a lesser extent Bihar. These are not states where Covid‐19 outbreaks were initially recorded. Indeed, the states of Maharashtra and Delhi are often described as having been confronted early on by the Covid‐19 crisis, but, with respective odds of chronic poverty between 2004/05 and 2011/12 of 0.932 and 0.715, would appear to have been relatively well protected in this regard. This lends some further support to the contention that the crisis affected a relatively better‐protected segment of the population – at least initially – than might have been feared. Again, however, this observation would need to be tempered by the fact that the large‐scale migration back to rural areas following the lockdown originated largely from such cities as Delhi and Mumbai, and would thus drive rising poverty rates in rural areas of such poor sending‐states such as Bihar, Jharkand, and Orissa.
Profile of the chronically poor or downwardly mobile, India, 2004/05–2011/12: Major states
When we examine the odds of downward mobility and consider those states with particularly large rural populations – regions within which there are fears that the Covid‐19 virus has more recently been spreading – these are also the states with the highest chronic poverty rates during prosperous times: Rajasthan, West Bengal, Jharkhand, Orissa, Chhattisgarh, Madhya Pradesh, joined now also by Bihar, Uttar Pradesh and Himachal Pradesh. In these states, the odds of downward mobility were all greater than average during the 2004/05–2011/12 period. To the extent that our conjecture is correct – that scrutinizing poverty dynamics during good times offers a perspective on looming trouble‐spots and particularly vulnerable groups during an economic downturn – we may take from this that poverty impacts in India will become more pronounced as the coronavirus spreads away from its initial points of entry into regions and among population groups that appeared initially to have been spared.
6. CONCLUDING REMARKS
India is suffering deeply from the Covid‐19 pandemic. Although the country had achieved major successes in its efforts to reduce poverty in the decades up to 2020, there are grounds for suspecting that the combined health and economic consequences of the spread of the coronavirus have brought that progress to a halt. It is reasonable to fear that progress in fighting poverty may have been significantly reversed.
Assessing the full implications of the crisis for poverty is hampered by the lack of solid evidence on poverty in the period immediately preceding the onslaught of the Covid‐19 crisis. Systematic statistical evidence on the evolution of poverty in the face of the crisis is also still pending. However, this paper shows that the rapidly growing literature documenting the spread of the virus, the corresponding impact on health outcomes, and the economic repercussions of the lockdown measures aimed at slowing the spread, provides ample grounds for suspecting a significant impact on poverty. This literature also documents population characteristics and identifies population subgroups that are facing sharply falling living standards.
The numerous studies assembled and reviewed in this paper point to the particular vulnerability of informal sector wage workers in urban areas. The studies document the phenomenon of mass migration by laid‐off temporary workers in urban centers – where the spread of the virus was first documented – back to their villages in rural areas. The literature further indicates that among those who have suffered a loss of employment and falling earnings, women, Scheduled Castes (Dalits) and religious minorities (notably Muslims) are disproportionately represented.
Because the greatest number of coronavirus cases have been documented in large urban centers such as Delhi and Mumbai, and because the phenomenon of mass migration has generally been of laid‐off urban workers, one may be inclined to view the poverty impact of the Covid‐19 crisis as largely confined to urban areas. There are grounds, however, for caution in drawing such a conclusion. First of all, the second Covid‐19 wave has spread far more rapidly and widely into rural regions than was initially experienced in the spring of 2020. Further, it is widely acknowledged that documentation of the spread of the virus is less comprehensive in rural areas than in urban centers, due to fewer resources, lower capacity, and a greater unwillingness of the rural population to submit to testing and the possibility of quarantine. As a result, the current incidence of Covid infections is likely to be both surging, and undercounted, in rural areas. Furthermore, the consequences of a rise in infections may be more difficult to carry for these areas due to their underdeveloped health‐care infrastructure.
Our analysis of poverty dynamics in India – based on pre‐Covid experience during an episode of rapid economic growth and poverty decline – indicates, moreover, that the risk of chronic (long‐term) poverty and the likelihood of falling into poverty are particularly high in rural areas, and in those states to which a majority of Covid‐driven migrants are moving. Our analysis is premised on the notion that poverty persistence and downward mobility during periods of generalized prosperity can help point to likely “hotspots” and particularly at‐risk population groups when economic downturns occur. We suggest, therefore, that while the initial impact of the Covid‐19 pandemic was on sectors and population groups that were relatively less poor, as the crisis spreads and the economic consequences of the crisis continue to reverberate, it is likely that the poverty impacts will become increasingly acute. Absent comprehensive and effective policy interventions to combat these poverty impacts, we suggest that they will be increasingly visible in rural areas.
The world, and India, remain in the midst of an unprecedentedly severe health and economic crisis. Recent progress in the development and application of a vaccine against Covid‐19 provides grounds for some optimism but is unlikely to dramatically alter circumstances on the ground for some time to come. The final consequences of the crisis on poverty are still unclear. There is a pressing need to continue to assemble and analyze emerging evidence to document the scale of the challenge and to identify those who are in greatest need of assistance. Policies must learn from emerging lessons and adapt in response to the evolving picture on the ground. Successful experience must be digested and documented, as it is not unlikely that new pandemics, calling for renewed intervention, will emerge in the future.
Dang, H.‐A. , Lanjouw, P. , & Vrijburg, E. (2021). Poverty in India in the face of Covid‐19: Diagnosis and prospects . Review of Development Economics , 25 , 1816–1837. 10.1111/rode.12833 [ PMC free article ] [ PubMed ] [ CrossRef ] [ Google Scholar ]
* This paper is part of the Oxford Policy Management DEEP project undertaken on behalf of the Foreign Commonwealth and Development Office of the UK. We are grateful to two reviewers for comments as well to Finn Tarp, guest editor of this symposium issue, for guidance. We are also grateful to Jehovannes Aikaeli, Ines Afonso Roque Ferreira, Chris Elbers, David Garcés‐Urzainqui, John Hoddinott, Kenneth Mdadila, Tseday Mekasha, Gerton Rongen, and Vincenzo Salvucci for helpful discussions. All errors are our own.
1 It should be noted that, in contrast to other countries, the number of PCR tests in India could not be isolated from the total number of tests performed, indicating that its test rate could also be based on other, less reliable tests (Our World in Data, 2021c ). According to the Hindustan Times , the more unreliable rapid antigen test made up about 49 per cent of the tests in November 2020 (Hindustan Times, 2020 ). Furthermore, other news reports indicate that massive testing seems to be largely done in low‐profile areas just to meet the high‐testing target (Menon, 2020 ; Express Web Desk, 2020 ).
2 See also the Oxford Covid‐19 Government Response Tracker (OxCGRT, n.d. ), which includes an index for the stringency of the government response to Covid‐19. India's government response received a score of 86.57 out of 100 ‘stringency points’ the day the first lockdown was announced. The following days this score rose quickly to 100.
3 Srivastava employs the 2004 National Classification of Occupational categories as defined by the National Career Service project, which is based upon the level of skill and education necessary to perform the occupation. The lowest five out of nine categories are: (5) service workers and shop and market sales workers; (6) skilled agricultural and fishery workers; (7) craft and related trades workers; (8) plant and machinery operators and assemblers; (9) elementary occupations (Srivastava, 2020 , p. 10; Ministry of Labour & Employment, 2015 , pp. 4–14).
4 The PSEC is a committee set up by the Prime Minister's Office on Socio‐Economic and Educational Status of the Muslim Community in India to evaluate the socioeconomic conditions of Muslim Indians (PSEC, 2014). The PSEC uses national data sets (such as from the NSSO) to infer its results.
5 The data that support the findings of this study are available from the corresponding author upon reasonable request.
The data that support the findings of this study are available from the corresponding author upon reasonable request
- Agarwal, V. , & Bellman, E. (2021). India’s migrants flee to their villages as Covid‐19 prompts new lockdown . Retrieved from https://www.wsj.com/articles/indias‐migrants‐flee‐to‐their‐villages‐as‐covid‐19‐prompts‐new‐lockdown‐11618392602 [ Google Scholar ]
- Agrawal, P. (2020). Bihar’s migrants return to face stigma, under‐prepared medical facilities . Retrieved from https://www.indiaspend.com/bihars‐migrants‐return‐to‐face‐stigma‐under‐prepared‐medical‐facilities/ [ Google Scholar ]
- Al Dahdah, M. , Ferry, M. , Guérin, I. , & Venkatasubramanian, G. (2020). The Covid‐19 crisis in India: A nascent humanitarian tragedy . Retrieved from https://booksandideas.net/The‐Covid‐19‐Crisis‐in‐India.html [ Google Scholar ]
- Asadullah, M. N. , & Raghunathan, K. (2020). Fighting gender inequality in India during the COVID‐19 pandemic . Retrieved from https://thediplomat.com/2020/06/fighting‐gender‐inequality‐in‐india‐during‐the‐covid‐19‐pandemic/ [ Google Scholar ]
- Ashwini, D. (2020). The Covid‐19 pandemic and lockdown: First order effects on gender gaps in employment and domestic time use in India . GLO Discussion Paper no. 607, Global Labor Organization (GLO), Essen. Retrieved from https://www.econstor.eu/handle/10419/222416 [ Google Scholar ]
- Azim Premji University (n.d.). COVID19: Analysis of impact and relief measures . Retrieved from https://cse.azimpremjiuniversity.edu.in/covid19‐analysis‐of‐impact‐and‐relief‐measures/#clips [ Google Scholar ]
- Azim Premji University (2021). Impact of COVID‐19 on livelihoods of informal sector workers and vulnerable groups in Bengaluru: A view from the ground . Retrieved from the Azim Premji University website: https://azimpremjiuniversity.edu.in/SitePages/pdf/COVID19‐Impact‐on‐Informal‐Sector‐Bengaluru.pdf [ Google Scholar ]
- Azim Premji University (2021). Reviving employment and livelihoods in India: COVID‐19 and after . Retrieved from the Azim Premji University website: http://publications.azimpremjifoundation.org/2531/1/CII%20Report%20‐%20Employment%20%20Livelihoods.pdf [ Google Scholar ]
- Babu, B. , Kusuma, Y. S. , Sivakami, M. , Kumar Lal, D. , Marimuth, P. , Babu Geddam, J. , Khanna, A. , Agarwal, M. , Sudhakar, G. , Sengupta, P. , Borhade, A. K. Z. , Kerketta, A. S. , & Akoijam, B. S. (2017). Living conditions of internal labour migrants: A nationwide study in 13 Indian cities . International Journal of Migration and Border Studies , 3 ( 4 ), 328–351. [ Google Scholar ]
- Barcellos, S. H. , Leandro, S. C. , & Lleras‐Muney, A. (2014). Child gender and parental investments In India: Are boys and girls treated differently? American Economic Journal: Applied Economics , 6 ( 1 ), 157–189. [ PMC free article ] [ PubMed ] [ Google Scholar ]
- Beyer, R. C. M. , Franco‐Bedoya, S. , & Galdo, V. (2021). Examining the economic impact of COVID‐19 in India through daily electricity consumption and nighttime light intensity . World Development , 140 ( 2021 ), 1–13. [ PMC free article ] [ PubMed ] [ Google Scholar ]
- Beyer, R. C. M. , Jain, T. , & Sinha, S. (2020). Lights out? Covid‐19 containment policies and economic activity . Policy Research Working Paper No. 9485. the World Bank. [ PMC free article ] [ PubMed ] [ Google Scholar ]
- Ceballos, F. , Kannan, S. , & Kramer, B. (2020). Impact of a national lockdown on smallholder farmers’ income and food security: Empirical evidence from two states in India . World Development , 136 ( 2020 ), 1–5. [ Google Scholar ]
- Centre for Monitoring Indian Economy (2020). An unhealthy recovery . Retrieved from the Centre for Monitoring Indian Economy website: https://www.cmie.com/kommon/bin/sr.php?kall=warticle&dt=2020‐08‐18%2011:02:19&msec=596 [ Google Scholar ]
- Chatterjee, M. (2016). Universal health coverage and occupational health and safety for informal workers: A view from India. In: R.M. Taylor, (Rapporteur), Forum on public‐private partnerships for global health and safety (pp. 30–39). Forum conducted at the meeting of the Board on Global Health; Institute of Medicine; National Academies of Sciences, Engineering, and Medicine. The NAtional Academies Press. [ PubMed ] [ Google Scholar ]
- Chaudhuri, S. (2003). ‘Assessing vulnerability to poverty: Concepts, empirical methods and illustrative examples’, mimeo . Department of Economics, Columbia University. Retrieved from https://www.researchgate.net/profile/Detlef_Brem/post/What_is_the_relationship_between_vulnerability_and_poverty_as_well_as_gender_and_poverty/attachment/59d6310fc49f478072ea0cff/AS:273621778403334@1442247923533/download/Assessing+Vulnerability+to+Poverty+theory+2003.pdf [ Google Scholar ]
- Chishti, S. (2020). Explained: How many migrant workers displaced? A range of estimates . Retrieved from https://indianexpress.com/article/explained/coronavirus‐how‐many‐migrant‐workers‐displaced‐a‐range‐of‐estimates‐6447840/ [ Google Scholar ]
- Dang, H. , & Lanjouw, P. (2017). Welfare dynamics measurement: Two definitions of a vulnerability line and the empirical application . Review of Income and Wealth , 63 ( 4 ), 633–660. [ Google Scholar ]
- Dang, H.‐A.H. , & Lanjouw, P. (2018). Welfare dynamics in India over a quarter‐century: Poverty, vulnerability, and mobility, 1987–2012 . WIDER Working Paper 2018/175. UNU‐WIDER. [ Google Scholar ]
- Datt, G. , & Ravallion, M. (2011). Has India’s economic growth become more pro‐poor in the wake of economic reforms? World Bank Economic Review , 25 ( 2 ), 157–189. [ Google Scholar ]
- Dhanaraj, S. (2014). Health shocks and coping strategies: State health insurance scheme of Andhra Pradesh, India (WIDER Working Paper 2014/003) . Retrieved from the UNU‐WIDER website: https://www.wider.unu.edu/publication/health‐shocks‐and‐coping‐strategies [ Google Scholar ]
- Elbers, C. (2021). A note on synthetic panels in poverty mapping, mimeo . School of Business and Economics, Vrije Universiteit. [ Google Scholar ]
- Estupinan, X. , & Sharma, M. (2020). Job and Wage Losses in Informal Sector due to the COVID‐19 Lockdown Measures in India . Retrieved from https://papers.ssrn.com/sol3/papers.cfm?abstract_id=3680379 [ Google Scholar ]
- Express News Service (2020). Explained: What are containment zones, how are they demarcated? Retrieved from https://indianexpress.com/article/explained/coronavirus‐cases‐india‐containment‐zones‐6487494/ [ Google Scholar ]
- Express Web Desk (2020). Coronavirus May 19 highlights: Record 1,08,233 samples tested in a day, says Health Ministry . Retrieved from https://indianexpress.com/article/india/coronavirus‐in‐india‐news‐live‐updates‐covid‐19‐state‐wise‐cases‐tracker‐latest‐news‐lockdown‐4‐guidelines‐6416396/ [ Google Scholar ]
- Ganguly, S. (2020). India’s coronavirus pandemic shines a light on the curse of caste . Retrieved from https://theconversation.com/indias‐coronavirus‐pandemic‐shines‐a‐light‐on‐the‐curse‐of‐caste‐139550 [ Google Scholar ]
- Gangwar, H. S. , & Ray, P. K. C. (2021). Geographic information system‐based analysis of COVID‐19 cases in India during pre‐lockdown, lockdown, and unlock phases . International Journal of Infectious Diseases , 105 ( 2021 ), 424–435. [ PMC free article ] [ PubMed ] [ Google Scholar ]
- Garcés‐Urzainqui, D. , Lanjouw, P. , & Rongen, G. (2021). Constructing synthetic panels for the purpose of studying poverty dynamics: A primer . Review of Development Economics . [ Google Scholar ]
- Ghosh, D. , & Dinda, S. (2017). Chapter 6: Health infrastructure and economic development in India. In Das R. C. (Ed.), Social, health, and environmental infrastructure for economic growth (pp. 99–119). IGI Global. [ Google Scholar ]
- Global Hunger Index (2010). The Challenge of Hunger: Focus on the Crisis of Child Undernutrition . Retrieved from the International Food Policy Research Institute site: https://www.ifpri.org/publication/2010‐global‐hunger‐index‐challenge‐hunger [ Google Scholar ]
- Guérin, I. , Michiels, S. , Natal, A. , Nordman, C. J. , & Venkatasubramanian, G. (2020). Surviving debt, survival debt in times of lockdown (CEB Working Paper, no. 20–009) . Retrieved from https://ideas.repec.org/p/sol/wpaper/2013‐309493.html [ Google Scholar ]
- Gulati, A. , Jose, S. , & Singh, B. B. (2021). COVID‐19: Emergence, spread and its impact on the India economy and migrant workers (ZEF Working Paper 207) . Retrieved from https://papers.ssrn.com/sol3/papers.cfm?abstract_id=3834328 [ Google Scholar ]
- Herault, N. , & Jenkins, S. (2019). How valid are synthetic panel estimates of poverty dynamics? Journal of Economic Inequality , 17 , 51–76. 10.1007/s10888-019-09408-8 [ CrossRef ] [ Google Scholar ]
- Hindustan Times . (2020). Rapid antigen tests account for close to 50% of Covid‐19 tests in India: Govt data . Retrieved from the Hindustan Times site: https://www.hindustantimes.com/health/rapid‐antigen‐tests‐account‐for‐close‐to‐50‐of‐covid‐19‐tests‐in‐india‐govt‐data/story‐WLh6mbgmXsZ92EBAA3zzBM.html [ Google Scholar ]
- Home Secretary . (2020). D.O. No. 40‐10/2020‐DM‐1(A) . Retrieved from https://prsindia.org/files/covid19/notifications/5976.IND_Implementation_of_Lockdown_May_21.pdf [ Google Scholar ]
- IMS Institute for Healthcare Informatics (2013). Understanding healthcare access in India: What is the current state? Retrieved from https://docshare04.docshare.tips/files/25555/255552955.pdf [ Google Scholar ]
- International Labour Organization (2018). Women and men in the informal economy: A statistical picture , 3rd ed. ILO. [ Google Scholar ]
- Iyer, M. (2020). Migration in India and the impact of the lockdown on migrants . Retrieved from https://www.prsindia.org/theprsblog/migration‐india‐and‐impact‐lockdown‐migrants [ Google Scholar ]
- John Hopkins University Center for Systems, Science and Engineering . (2021). COVID‐19 dashboard by the center for systems science and engineering (CSSE) at John Hopkins University . Retrieved from https://gisanddata.maps.arcgis.com/apps/opsdashboard/index.html#/bda7594740fd40299423467b48e9ecf6 [ Google Scholar ]
- Kesar, S. , Abraham, R. , Lahoti, R. , Nath, P. , & Basole, A. (2020). Pandemic, informality, and vulnerability: Impact of COVID‐19 on livelihoods in India . CSE Working Paper 2020‐01. Retrieved from the Centre for Sustainable Employment site: https://www.research‐collection.ethz.ch/handle/20.500.11850/428008 [ Google Scholar ]
- La Forgia, G. , & Nagpal, S. (2012). Government‐sponsored health insurance in India: Are you covered? . International Bank for Reconstruction and Development/The World Bank. [ Google Scholar ]
- Maggo, D. (2020). Impact of COVID‐19 on smallholder farmers – insights from India . Retrieved from the World Business Council of Sustainable Development site: https://www.wbcsd.org/Overview/News‐Insights/WBCSD‐insights/Impact‐of‐COVID‐19‐on‐smallholder‐farmers‐in‐India [ Google Scholar ]
- Mehta, T. , & Jamkhandikar, S. (2021). COVID‐19 spreads to rural India, villages ill‐equipped to fight it . Retrieved from https://www.reuters.com/world/india/india‐sees‐record‐daily‐rises‐covid‐19‐infections‐deaths‐2021‐05‐06/ [ Google Scholar ]
- Menon, S. (2020). Coronavirus: Is India’s test and tracing strategy working? Retrieved from the BBC news site: https://www.bbc.com/news/world‐asia‐india‐55083825 [ Google Scholar ]
- Ministry of Labour & Employment (2015). National classification of occupations‐2015 (Code Structure): Vol‐I . Retrieved from https://www.ncs.gov.in/Documents/National%20Classification%20of%20Occupations%20_Vol%20I‐%202015.pdf [ Google Scholar ]
- Ministry of Panchayati Raj (n.d.), Backward regions grant fund: Programme guidelines . Retrieved from https://mizorural.nic.in/file/BRGF/BRGF_GUIDELINES.pdf [ Google Scholar ]
- Ministry of Statistics & Programme Implementation (2015). Employment and unemployment situation among social groups in India (NSS Report No. 563(68/10/4) . Retrieved from http://mospi.nic.in/sites/default/files/publication_reports/nss_rep_563_13mar15.pdf [ Google Scholar ]
- Mitra, E. , Talreja, S. , & Yeung, J. (2021). India’s second wave is ravaging villages without hospitals or even doctors to fight it . Retrieved from https://edition.cnn.com/2021/05/11/india/india‐covid‐gujarat‐village‐intl‐hnk/index.html [ Google Scholar ]
- Naik, M. (2021). As the pandemic’s second wave rages, migrants trudge home again . Retrieved from https://indianexpress.com/article/opinion/columns/as‐the‐pandemics‐second‐wave‐rages‐migrants‐trudge‐home‐again‐7297303/ [ Google Scholar ]
- Narayanan, A. (2015). Informal employment in India: Voluntary choice or a result of labor market segmentation? Indian Journal of Labour Economics , 58 ( 1 ), 119–167. 10.1007/s41027-015-0009-9 [ CrossRef ] [ Google Scholar ]
- National Commission for Enterprises in the Unorganised Sector (2009). The challenge of employment in India: An informal economy perspective (Volume 1‐Main Report) . Retrieved from the Development Commissioner Ministry of Micro, Small and Medium Enterprises website: http://dcmsme.gov.in/The_Challenge_of_Employment_in_India.pdf [ Google Scholar ]
- Niyati, S. , & Vijayamba, R. (2020). Impact of the Covid‐19 pandemic on food security and indebtedness in rural India . Retrieved from https://www.researchgate.net/profile/Niyati‐Singaraju/publication/351082698_Impact_of_the_Covid‐19_Pandemic_on_Food_Security_and_Indebtedness_in_Rural_India/links/6083fce9907dcf667bbdff6c/Impact‐of‐the‐Covid‐19‐Pandemic‐on‐Food‐Security‐and‐Indebtedness‐in‐Rural‐India.pdf [ Google Scholar ]
- Ministry of Home Affairs (2020a). Order. No. 40‐3/2020‐DM‐I(A) . Retrieved from https://prsindia.org/files/covid19/notifications/144.IND_Citizens_Guidelines_Lockdown_Mar_24.pdf [ Google Scholar ]
- Ministry of Home Affairs (2020b). Order. No. 40‐3/2020‐DM‐I(A) . Retrieved from https://prsindia.org/files/covid19/notifications/2737.IND_Extension_Lockdown_Order_April_14.pdf [ Google Scholar ]
- Ministry of Home Affairs (2020c). Order. No. 40‐3/2020‐DM‐I(A) . Retrieved from https://prsindia.org/files/covid19/notifications/IND_Extension_Lockdown_May_1.pdf [ Google Scholar ]
- Ministry of Home Affairs (2020d). Order. No. 40‐3/2020‐DM‐I(A) . Retrieved from https://prsindia.org/files/covid19/notifications/IND_MHA_Lockdown_Extension_upto_May31_17052020.pdf [ Google Scholar ]
- Ministry of Home Affairs (2020e). Order. No. 40‐3/2020‐DM‐I(A) . Retrieved from https://prsindia.org/files/covid19/notifications/MHAOrder_29062020.pdf [ Google Scholar ]
- National Commision for Enterprises in the Unorganised Sector (NCEUS) (2007). Report on conditions of work and promotion of livelihoods in the unorganised sector . New Delhi, India. [ Google Scholar ]
- Oster, E. (2006). Does increased access increase equality? Gender and child health investments in India (NBER Working Paper No. 12743). Retrieved from the NBER site: http://www.nber.org/papers/w12743 [ Google Scholar ]
- Our World in Data (2021a). Cumulative confirmed COVID‐19 cases per million people, May 25, 2021 . Retrieved from https://ourworldindata.org/coronavirus‐data‐explorer?tab=map&zoomToSelection=true&country=GBR~USA~ESP~ITA~BRA~IND~KOR®ion=World&casesMetric=true&interval=total&hideControls=true&perCapita=true&smoothing=0&pickerMetric=location&pickerSort=asc [ Google Scholar ]
- Our World in Data (2021b). Daily new confirmed Covid‐19 cases . Retrieved from https://ourworldindata.org/explorers/coronavirus‐data‐explorer?zoomToSelection=true&hideControls=true&Metric=Confirmed+cases&Interval=New+per+day&Relative+to+Population=false&Align+outbreaks=false&country=~IND [ Google Scholar ]
- Our World in Data (2021c). Daily tests per thousand people (7‐day smoothed) . Retrieved from https://ourworldindata.org/coronavirus‐testing [ Google Scholar ]
- OxCGRT (n.d.). Relationship between number of COVID‐19 cases and government response . Retrieved from https://covidtracker.bsg.ox.ac.uk/stringency‐scatter [ Google Scholar ]
- Pandya, A. A. (2010). Muslim Indians: Struggle for Inclusion . Retrieved from the Stimson Regional Voices Website, https://www.stimson.org/wp‐content/files/file‐attachments/Muslim_Indians‐Complete_1.pdf [ Google Scholar ]
- Post Sachar Evaluation Committee (2014). Post Sachar evaluation committee report . Retrieved from https://www.sabrangindia.in/sites/default/files/audio_listing_images/kundu_commission_report_0.pdf?698 [ Google Scholar ]
- Pritchett, L. , Suryahadi, A. , & Sumarto, S. (2000). Quantifying vulnerability to poverty: A proposed measure, applied to Indonesia . World Bank Policy Research Working Paper No. 2437, The World Bank. [ Google Scholar ]
- Ravallion, M. (2011). A comparative perspective on poverty reduction in Brazil, China and India . World Bank Research Observer , 26 ( 1 ), 71–104. 10.1093/wbro/lkp031 [ CrossRef ] [ Google Scholar ]
- Rawal, V. , Kumar, M. , Verma, A. , & Pais, J. (2020). COVID‐19 Lockdown: Impact on agriculture and rural economy. SSER Monograph 20/3 . Retrieved from http://archive.indianstatistics.org/sserwp/sserwp2003.pdf [ Google Scholar ]
- Ray, D. , & Subramanian, S. (2020). India’s lockdown: An interim report . Retrieved from https://debrajray.com/wp‐content/uploads/2020/05/RaySubramanian.pdf [ PMC free article ] [ PubMed ] [ Google Scholar ]
- Sengupta, S. , & Jha, M. K. (2020). Social policy, COVID‐19 and impoverished migrants: Challenges and prospects in locked down India . The International Journal of Community and Social Development , 2 ( 2 ), 152–172. 10.1177/2516602620933715 [ CrossRef ] [ Google Scholar ]
- Seth, A. (2020). The post‐lockdown state of labour: Heading towards an institutionalization of exploitation and inequality . Retrieved from https://drive.google.com/file/d/1baXB35TNNlp‐ap6fMZtg4eIFg4Yxe8Mk/view [ Google Scholar ]
- Singh, K. D. , Goel, V. , Kumar, H. , & Gettleman, J. (2020). India, Day 1: World’s largest coronavirus lockdown begins . Retrieved from https://www.nytimes.com/2020/03/25/world/asia/india‐lockdown‐coronavirus.html [ Google Scholar ]
- Singh, S. , & Magazine, A. (2020). Explained: Indian migrants, across India . Retrieved from https://indianexpress.com/article/explained/coronavirus‐india‐lockdown‐migran‐workers‐mass‐exodus‐6348834/ [ Google Scholar ]
- Sinha, A. (2021). In second wave, Covid hits rural India: Cases and deaths quadruple . Retrieved from https://indianexpress.com/article/india/covid‐second‐wave‐rural‐india‐coronavirus‐cases‐7306422/ [ Google Scholar ]
- Srivastava, R. (2020). Understanding circular migration in India: Its nature and dimensions, the crisis under lockdown and the response of the state (Centre for Employment Studies WP 04/2020) . Retrieved from the Institute for Human Development website: http://www.ihdindia.org/working‐papers/2020/IHD‐CES_WP_04_2020.pdf [ Google Scholar ]
- The Economist . (2021). Tracking the economic impact of India’s second covid wave . Retrieved from The Economist website: https://www.economist.com/finance‐and‐economics/2021/05/01/tracking‐the‐economic‐impact‐of‐indias‐second‐covid‐wave [ Google Scholar ]
- The Indian Express (2021). Daily Briefing: Massive spread of Covid‐10 in rural India; nearly 100 bodies found floating in Ganga; and more . Retrieved from https://indianexpress.com/article/india/daily‐briefing‐covid‐19‐vaccination‐drive‐second‐wave‐may‐12‐7311703/ [ Google Scholar ]
- Thejesh, G. N. (2020). Non virus deaths . Retrieved from https://thejeshgn.com/projects/covid19‐india/non‐virus‐deaths/ [ Google Scholar ]
- Tikkanen, R. , Osborn, R. , Mossialos, E. , Djordjevic, A. , & Wharton, G. A. (2020). International health care system profiles: India . Retrieved from the Commonwealth Fund Website https://www.commonwealthfund.org/international‐health‐policy‐center/countries/india [ Google Scholar ]
- Unni, J. , & Rani, U. (2003). Social protection for informal workers in India: Insecurities, instruments and institutional mechanisms . Development and Change , 34 ( 1 ), 127–161. [ Google Scholar ]
- World Economic Forum (2020). Global gender gap report 2020 . Retrieved from the World Economic Forum Website: https://www.weforum.org/reports/gender‐gap‐2020‐report‐100‐years‐pay‐equality [ Google Scholar ]
- World Health Organization (2021). WHO coronavirus (COVID‐19) dashboard: Situation by Region, Country, Territory & Area . Retrieved from https://covid19.who.int/table [ Google Scholar ]
Click through the PLOS taxonomy to find articles in your field.
For more information about PLOS Subject Areas, click here .
Loading metrics
Open Access
Peer-reviewed
Research Article
Uneven burden of multidimensional poverty in India: A caste based analysis
Roles Conceptualization, Formal analysis, Methodology, Writing – original draft, Writing – review & editing
Affiliation Department of Humanities and Social Sciences, National Institute of Technology, Rourkela, India

Roles Data curation, Formal analysis, Methodology, Writing – review & editing
Roles Conceptualization, Supervision
* E-mail: [email protected]
- Itishree Pradhan,
- Binayak Kandapan,
- Jalandhar Pradhan
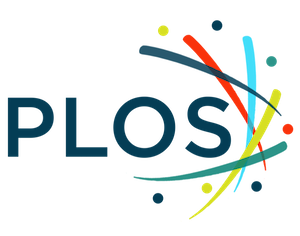
- Published: July 29, 2022
- https://doi.org/10.1371/journal.pone.0271806
- Reader Comments
Poverty is multifaceted. The global poverty profile shows 41% of multidimensionally poor people living in South Asian countries. Though castes and tribes are a more prevalent line of social stratification in India, and their socio-economic characteristics also vary remarkably, hardly any study has explored these dimensions while analysing multidimensional poverty in India. Hence, this study attempts to assess the multidimensional status of poverty among the social groups in India. National Family Health Survey, 2015–16 (NFHS-4) is a source of rich information on 579,698 households’ well-being for this analysis. Alkire- Foster technique was applied to decompose the Multidimensional Poverty Index (M 0 ) across its dimensions and indicators for all the social groups. Three broad dimensions of deprivation–Health, Education and Standard of Living–include 12 indicators, guided by the poverty literature, data availability and the country’s sustainable Development Goals (SDGs). There were three main findings in this study: (1) Scheduled Tribes (STs) are the most disadvantaged subgroup in India with remarkably high values of headcount (H = 0.444;), intensity (A = 0.486), and M 0 (0.216), followed by Scheduled Castes (SCs) (H = 0.292; A = 0.473; M 0 = 0.138), and Other Backward Classes (OBCs) (H = 0.245; A = 0.465; M 0 = 0.114); and Others category is the most privileged with very low values of H = 0.149, A = 0.463, and M 0 = 0.069; (2) STs contribute nearly twice their population share for both H and M 0 , and the SCs contribution is also noticeably higher than their population share; (3) States located in the central and eastern regions of India have the higher H, A and M 0 for all the social groups. This suggests that there is a need for a thorough assessment of poverty at specific levels to uncover the poverty situation in society, improve the effectiveness of evidence-based planning and effective policymaking.
Citation: Pradhan I, Kandapan B, Pradhan J (2022) Uneven burden of multidimensional poverty in India: A caste based analysis. PLoS ONE 17(7): e0271806. https://doi.org/10.1371/journal.pone.0271806
Editor: Deepak Dhamnetiya, Dr Baba Saheb Ambedkar Medical College and Hospital, INDIA
Received: April 4, 2022; Accepted: July 7, 2022; Published: July 29, 2022
Copyright: © 2022 Pradhan et al. This is an open access article distributed under the terms of the Creative Commons Attribution License , which permits unrestricted use, distribution, and reproduction in any medium, provided the original author and source are credited.
Data Availability: The data underlying the results presented in the study are available from the Demographic and Health Surveys (DHS) Program. It can be accessed from the public domain at https://dhsprogram.com/data/dataset/India_Standard-DHS_2015.cfm?flag=1 .
Funding: The author(s) received no specific funding for this work.
Competing interests: The authors have declared that no competing interests exist.
1. Introduction
Traditionally ‘Poverty’ is defined as scarcity of income by most of the countries worldwide [ 1 ] and it is understood by the financial status of a person or a household or a community [ 2 ]. Sen [ 3 ] described the income or unidimensional poverty measurement in his monumental work “Poverty: An Ordinal Approach to Measurement,”. However, he acknowledged that it is difficult to replicate this ordinal approach in day to day life. Since the mid-1970s, it has been widely accepted that poverty is nothing but the low level of income [ 4 ]. However, studies have shown that income cannot be used to determine a person’s well-being [ 2 ], because it does not account for nonmonetary deprivations such as lack of access to nutritious food, healthcare services, better education, safe drinking water, improved sanitation facilities, clean cooking fuel, electricity, adequate housing condition, financial security, basic information and so on, and thus fails to identify who is poor [ 3 , 5 – 8 ]. With time, various ways for identifying the poor gained prominence, including the ‘basic needs approach’, ‘social exclusion’, and ‘capability approach’. As a result, researchers have combined monetary and nonmonetary indices of deprivation to produce a more comprehensive picture of poverty [ 7 ]. In the word of Sen [ 9 ], “Human lives are battered and diminished in all kinds of different ways, and the first step is to accept that deprivations of very different kinds have to be accommodated within a general overarching framework”. Hence it is suggested that the multidimensional poverty measurement is more reliable than unidimensional poverty measurement [ 10 – 12 ]. Consequently, the poverty argument has shifted from a unidimensional (income) to a multidimensional prospective, resulting in a more comprehensive picture of poverty [ 1 ]. The Sustainable Development Goals (SDGs) of the United Nations (UN), often known as the 2030 Agenda for Sustainable Development, have reignited interest in multidimensional poverty reduction strategies [ 13 ]. The SDGs target 1.2 says “by 2030, reduce at least half the proportion of men, women and children of all ages living in poverty in all its dimensions according to national definitions” [ 14 ].
The global multidimensional poverty index in 2021 covers 5.9 billion people of 109 countries worldwide: 26 low-income, 80 middle-income and three high-income. The findings indicate one in every five persons are multidimensionally poor in these 109 countries combined, which represents approximately 1.3 billion people, and 41% (532 million) of them are living in the South Asian countries [ 15 ]. Despite experiencing the largest reduction in the number of multidimensionally poor people during the decade 2006–16 [ 16 ], India has more than 381 million of the multidimensionally poor, accounting for a whopping proportion of 72% multidimensionally poor in South Asia [ 15 ]. Though, India’s major economic growth over the past 30 years has continued to lift millions of people out of poverty, the unforeseen impact of COVID-19 may have potentially pushed more people into poverty [ 15 ]. Because, many people lost their jobs; they were unable to meet their essential needs; their consumption expenditure reduced; and they became impoverished. As a result, India may witness a significant increment in its poverty rate in the near future.
The household has been utilised as a unit of analysis in the majority of empirical studies on the multidimensional poverty measure [ 17 – 19 ]. Which considers all members of the household as multidimensionally poor if the household is multidimensionally poor [ 18 , 20 ]. The MPI creates a vivid image of household living in poverty within and across countries, regions and the world [ 21 ]. It calculates the severity of deprivation in poor populations using various deprivation indices [ 22 – 24 ]. In India, numerous studies have already been conducted using the multidimensional approach to define poverty and its trends and determinants. These studies are basically estimated poverty across the states and regions in India [ 25 – 32 ].
In India, the Scheduled Castes (SCs), and Scheduled Tribes (STs) minorities have historically been the most underprivileged [ 33 ]. Because, these groups have faced discrimination, and thus exclusion, in one way or another from the mainstream economic and social spheres. Despite the fact that the government initiated various projects and programmes for the uplift of these groups, which were backed by substantial financial support, their growth in terms of economic and social status has still stagnant [ 34 ]. According to global MPI estimates, five out of every six multidimensionally poor in India belongs to SC, ST or Other Backward Class (OBC) households: ST with more than 50% multidimensionally poor, followed by SC with 33.3% and OBC with 27.2% [ 15 ] Though castes and tribes are a more prevalent line of social stratification in India, and their socio-economic characteristics also varies remarkably, there is hardly any study that explored these dimensions while analyzing multidimensional poverty in India. Hence, this study attempts to assess the differential in multidimensional poverty amongst the social groups in India. Further, it also attempts to decompose the MPI across its dimensions and indicators for all the social groups.
2. Deprivation, dimensions and indicators
The UN’s proposal for a globally agreed upon definition of poverty justifies the selection of the dimensions and indicators for this study. According to UN’s proposal, all basic human rights and needs, such as access to nutritious food, safe drinking water, appropriate sanitation, proper health care facilities, shelter, and education, should be considered in the poverty analysis [ 14 ]. Further, the SDGs are also useful for determining which aspects and indicators should be utilised to measure poverty in a multidimensional prospective. Access to safe drinking water, appropriate sanitation, and primary education are all goals that must be met under SDGs. Based on these human’s need and necessities MPI was calculated using data from 12 indicators across three key dimensions: health, education and standard of living, all of which were given equal weightage. Selection of the 12 indicators for the three dimensions was adopted from National Institution for Transforming India (NITI) Aayog’s report [ 35 ]. The rationale behind selection of the 12 indicators is explained in details elsewhere [ 35 ]. Detailed information on the thresholds for poverty dimensions and indicator weights are shown in Table 1 .
- PPT PowerPoint slide
- PNG larger image
- TIFF original image
https://doi.org/10.1371/journal.pone.0271806.t001
2.1. Health
Health is considered as the central capability of overall well-being, and being healthy is not only a valuable achievement in itself, but also can help individuals to do many important things such as participating in social and sports activities [ 9 ]. Not being in good health often affects other capabilities such as hindering in the educational achievement and economic participation. Following the NITI Aayog report [ 35 ], the study includes nutrition, child & adolescent mortality and maternal health in the health domain. While, nutrition and child & adolescent mortality parameters are based on global MPI, the maternal health indicator is unique to India’s MPI. The maternal health indicator is a signatory to the Goal 3 of SDGs “Ensure healthy lives and promote well-being for all at all ages”, that intends to ensure rigorous adherence to the SDG targets of lowering maternal mortality and eliminating preventable new-born deaths [ 35 ]. The maternal health indicator is a union of two distinct components: Antenatal care (ANC) and assisted delivery, both of which are critical prerequisites for mothers and newborns to have a positive health outcome. With a significant percentage of maternal deaths occurring during the period of pregnancy, the four-visit antenatal care model outlined in the World Health Organization (WHO) clinical guidelines has been instrumental in the early identification of complications in pregnancy, monitoring of foetal growth and the management of complications through the referral of mothers to the appropriate facility for further treatment. The complications due to prematurity, intrapartum deaths, and neonatal infections accounts for nearly 80 percent of the new-born deaths, that can be identified and addressed for preventing death or life-long disability [ 36 ]. Since, ANC cannot be considered as prevention of intrapartum deaths, which requires quality care provided during childbirth, that is mostly characterized as the assistance of skilled health personnel during childbirth [ 35 ].
The indicators in the Health dimension are not evenly weighted. Malnutrition has significant consequences to early childhood development as well as to the health and overall wellbeing of adults, and nutritional status of an individual can be linked to almost all socio-economic development indicators. Hence, giving nutrition indicator a higher weight is justifiable. Nutrition carries half the dimension weight of 1/3 with a weight of 1/6. Similarly remaining dimension weight is divided evenly between Child-Adolescent Mortality and Maternal Health, with each indicator receiving a 1/12 weight.
2.2. Education
Like health, education is an important capability for improving people’s wellbeing. In most countries, especially low-income developing countries, people with more education earn more than those with less education [ 17 ]. At the very least, education has a private benefit, such as enabling people to take an active role in their social, economic, and political lives [ 37 ]. The two indicators selected under this dimension are in line with the goal 4 of SDGs “Ensure inclusive and equitable quality education and promote lifelong learning opportunities for all”, which is represented by school attendance of 6–14 years children and years of schooling of 10 years and above household members, with each indicator weighted at 1/6, representing half of the dimension weight (1/3). It is worth noting that, due to the nature of the indicator, an individual living in a ‘household with at least one member with six years of schooling’ is considered non-deprived, even if they haven’t attended school themselves. Because, even if one member of a family has more than six years of schooling, the positive effect of that education, be in terms of an increase in economic opportunities such as the ability to enter high-paying employment or improve social standing, is shared by all members of the household [ 35 ]. Similarly, an individual living in a ‘household where at least one child does not attend school’ is declared deprived in this indicator, even if they have completed their schooling. The reason for this is that a child who does not attend school is indicative of a larger set of deprivations that the family is experiencing, which acts as a barrier to the child’s education.
2.3. Standard of living
The ‘Standard of Living’ dimension includes several indicators that depict households’ living conditions. In this study, the ‘Standard of Living’ dimension includes seven metrics that describe a household’s access to essential services like electricity, clean cooking fuel, safe drinking water, improved sanitation, pucca housing (good flooring, roof and walls), bank account, and household assets. Except for the indicator for bank accounts, which is unique to India’s national MPI [ 35 ], all other indicators follow global definitions and cut-offs. A household’s access to a bank account is critical for availing the benefits of several flagship government programs aimed at reducing poverty, increasing access to education, and creating livelihoods–which often utilize direct benefit transfers.
It is well laid down that access to basic amenities such as safe drinking water, improved sanitation facilities, shelter, electricity, and improved cooking fuel are vital for the overall growth and development of any individual and eventually boost the economic growth of the respective country/ state [ 38 ]. The safe drinking water and improved sanitation facility are in line with the goal 6 of SDGs, that is, ensuring “availability and sustainable management of water and sanitation for all” [ 14 ]. Similarly, cooking fuel and electricity falls under the broad umbrella of goal 7 “Ensure access to affordable, reliable, sustainable and modern energy for all”, housing under the goal 11 “Make cities and human settlements inclusive, safe, resilient and sustainable”, assets and banking under the goal 1 “End poverty in all its forms everywhere” [ 14 ]. The dimension weight (1/3) is distributed evenly among the seven indicators, giving each a weight of 1/21.
3. Data and methodology
This study employs micro survey data from a nationally representative Indian Demographic Health Survey collected in 2015–16. The Indian DHS is the National Family Health Survey (NFHS), which is conducted by the International Institute of Population Sciences (IIPS) under the Ministry of Health and Family Welfare (MoHFW), Government of India [ 39 ]. The NFHS-4 survey covered a nationally representative sample of 2,869,043 individuals across 628,892 households. This study has collected precise data on health, nutritional status, mortality, sociodemographic characteristics, access to basic facilities, and household assets, all of which are required for MPI calculations. In this survey, the many caste groups are grouped into four major categories: SC, ST, OBC, and Others [ 39 ]. While SC includes Dalit communities, the category ‘Other’ include higher castes. The SC and ST communities have tended to be the most disadvantaged subgroups in India, followed by the OBC community. This study is based on a total of 2,703,773 individuals from 579,698 households. Detailed procedure of sample selection for this study is depicted in Fig 1 .
https://doi.org/10.1371/journal.pone.0271806.g001
3.1. Methodology
In 2010 the Oxford Poverty and Human Development Initiative (OPHI) and United Nations Development Program (UNDP) have launched the ‘global Multidimensional Poverty Index (MPI)’. It assesses the intricacies of poor people’s lives, both individually and collectively, each year and focuses on how multidimensional poverty has decreased. It represents a deprivation in the basic rights and needs of the people; it reveals a pattern of poverty other than income poverty [ 22 ] and its indicators are selected based on theSDGs.
While using the multidimensional poverty index technique, it is important to consider the magnitude of the dimensions and indicators of poverty. Because all three dimensions are equally vital, they are given the same weight. Education, standard of living, and health are the three key deprivation indicators analysed using the AF technique [ 21 ] These dimensions were also included in this study. After that, the indicators’ weights under each dimension are assigned. Since there are different numbers of indicators under each dimension, the indicators’ weight was unevenly assigned. As a result, the index for each individual ‘i’ is a number between 0 and 1.
In this study, we have employed Alkire-Foster counting methodology [ 23 ] to determine the multidimensional poverty among social groups across the nation. The AF technique’s proposed conceptual framework is based on Amartya Sen’s ‘capability approach,’ which reflects capability poverty [ 23 ]. Based on this methodology MPIs are constructed using two analytical steps: identification and aggregation. In a dual cut-off identification technique, deprivation and poverty cut-offs are both employed to identify poor individuals. A deprivation cut-off is employed for each indicator to determine whether a person is deprived in that particular indicator, and each person’s final deprivation score is obtained by summing up their weighted deprivation score of all the indicators. Next, a poverty cut-off is utilised to evaluate whether a person falls into the multidimensionally poor category. If a person’s deprivation score is more than or equal to that poverty cut-off, he or she is considered multidimensionally poor. Here the poverty cut-off is denoted as ‘k’ and following the global MPI cut-off of ‘1/3’, cut-off point for being multidimensionally poor is set at k = 0.333.
Where, ‘ C i ( k )’ is the deprivation score of the multidimensionally poor individual ‘ i ’; and ‘ q ’ ‘q’ is the number of people who were identified as multidimensionally poor.
Besides, M 0 satisfies the axioms of population subgroup decomposability, dimensional breakdown and ordinality. Subgroup decomposability relates subgroup to overall poverty levels; dimensional breakdown relates multidimensional poverty levels to dimensional components; and ordinality allows meaningful evaluations of poverty when variables are ordinal. In this study, M 0 was decomposed according to social groups (castes) and dimensions to facilitate comparisons of those castes and dimensions.
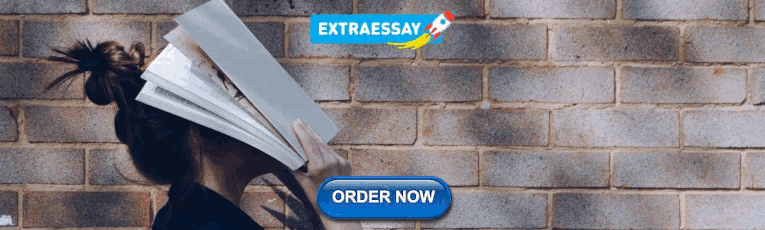
4.1. Deprivation by indicators across social groups
Out of the total sample of 28,69,043, this study utilised the information of 27,03,773 for MPI estimation at national, state and social group levels. Table 2 depicts the percentage of people deprived in each indicator across the social groups and not necessarily being multidimensionally poor. It is important because it helps to target sector-specific poverty. It is observed that overall, the rate of deprivation is highest in cooking fuel, followed by sanitation, housing, and nutrition, and a similar pattern was observed across all the social groups. However, there exists a significant social group differential in terms of magnitude of deprivation by indicators. STs have dramatically higher rate of deprivation in all the indicators compared to other social groups, followed by SCs, and SCs followed closely by the OBCs. It is noteworthy that, relatively the STs lags much behind in both the indicators of education dimension and safe drinking water, assets, housing and banking indicators of standard of living dimension. Though SCs and OBCs show a similar pattern of deprivation in most indicators, the relative differences in household assets, banking, and years of schooling are notably higher among the SCs. Interestingly, although the relative difference in social groups is noticeable in the dimension of health, it is not as wide as the relative differences in the indicators of other two dimensions.
https://doi.org/10.1371/journal.pone.0271806.t002
4.2. Multidimensional poverty by social groups
H, a, and mpi at national level..
The estimates for multidimensional poverty among the social groups of India are displayed in Table 3 . It shows the headcount ratio (H), the average deprivation score of the multidimensional poor or intensity (A), the adjusted headcount ratio or the MPI (M 0 ), weighted population share across the social groups in India. The headcount ratios reveal that nearly one-fourth (24.8%) of the Indian are multidimensionally poor; and among the social groups the headcount ratio estimated for the STs (44.4%) is stinkingly higher compared to the SCs (29.2%), OBCs (24.5%) and Others (14.9%). However, there exist merely any caste difference in the intensity of multidimensional poverty A, which ranges from 46.3% for Others to 48.6% for the STs. The STs exhibits the highest adjusted headcount ratio with M 0 = 0.216, followed by SCs (0.138) and OBCs (0.114). It can be concluded from the table that STs are the most disadvantaged subgroup in India with remarkably high values of H, A, and M 0 , and the other categories are the most privileged with very low values of H, A, and M 0 . Meanwhile, the multidimensional poverty estimates for SCs and OBCs show a similar picture but are slightly favoured for OBCs.
https://doi.org/10.1371/journal.pone.0271806.t003
H, A, and MPI across States and UTs.
Table 4 presents the H, A and MPI across the states and UTs of India by social groups. In overall, the result indicates MPI varies significantly from 0.002 in Kerala to 0.268 in Bihar. The highest MPI was estimated to be for the states of Bihar (0.268), followed by Jharkhand (0.201), Uttar Pradesh (0.18), Madhya Pradesh (0.172), Assam (0.158), Meghalaya (0.157), Rajasthan (0.139), Odisha (0.134), Chhattisgarh (0.125), Dadra & Nagar Haveli (0.121), and Nagaland (0.118). The extent of the MPI in the 11 states and UTs mentioned above was greater than at the national level. The lowest MPI was estimated to be in Kerala, followed by Puducherry, Lakshadweep, Sikkim, Goa, Delhi, and Tamil Nadu compared to other states and UTs. The states and UTs were divided into three categories according to their MPI and presented in Map 1 , the poor performing states were highlighted in red, and the better-performing ones are in blue. A similar breakdown of MPI for STs, SCs, OBCs and Others were presented in Map 2(A)–2(D) , respectively: orange indicates poor performers, pink indicates average performers, and blue indicates better performers.
Source: Authors’ compilation based on data from NFHS-4, 2015–16. The Map was developed by the authors using QGIS Version 3.24.0, and the map was cross verified with the India map and its States and Union Territories’ boundaries as shown in the official website of Survey of India: https://indiamaps.gov.in/soiapp/ .
https://doi.org/10.1371/journal.pone.0271806.g002
https://doi.org/10.1371/journal.pone.0271806.g003
https://doi.org/10.1371/journal.pone.0271806.t004
From Table 4 it can also be noticed that among the SCs, Bihar recorded the highest MPI, followed by Jharkhand, Uttar Pradesh, Madhya Pradesh, Rajasthan, Nagaland and Odisha. On the other hand, MPI is lowest in Kerala, Goa, Puducherry, Andaman and Nicobar Islands, Sikkim, Mizoram and Delhi. The highest MPI for the STs was recorded in Madhya Pradesh, Bihar and Uttar Pradesh; these states show almost similar MPI. Whereas lowest MPI was recorded in Puducherry followed by Lakshadweep, Sikkim, Andaman, Kerala and Goa. Data is not available for STs in Delhi and Punjab. Similarly, data on OBCs in Lakshadweep is not available. Among the OBCs across the states and UTs, the lowest MPI was recorded in Kerala followed by Goa and Puduchchery, meanwhile highest in Bihar followed by Uttar Pradesh and Jharkhand in OBC communities. The Others social group’s data were not available for Lakshadweep and Kerala. The states of Punjab, Puducherry and Tamil Nadu recorded the lowest MPI among the Others across the states and UTs, followed by Chandigarh, Goa and Sikkim, those have almost equal MPIs. The highest MPI for the Others social group is merely equal in Meghalaya, Bihar, Assam, Nagaland and Arunachal Pradesh, with Meghalaya slightly worse off than the above-mentioned states.
While comparing the ranks of 36 states and union territories in terms of Adjusted Headcount Ratio (M 0 ) and Headcount Ratio (H) for SCs, it is observed that Arunachal Pradesh, Kerala, Meghalaya, and Nagaland were ranked higher in MPI than their ranks in H. This indicates a greater intensity of poverty (A) among the SCs in these states compared to states with H values closer to the respective states. Similarly, among the STs in Haryana, OBCs in Mizoram and Punjab, and Others in Daman & Diu marked a higher intensity of poverty compared to the states and UTs with a nearby value to their H.
At the national level, STs is the most deprived social group, followed by SCs according to the MPI estimates according to the MPI estimates, and half of the States and UTs (18 out of 36) follow the same pattern. On the other hand, in six states and UTs, the SCs are the most deprived, followed by STs: Bihar, Himachal Pradesh, Sikkim, Damn and Diu, and Puducherry. Contrarily, in Arunachal Pradesh, Assam, Meghalaya, Mizoram, and Andaman and Nicobar Island the Others social group were estimated to have greater values for adjusted headcount ratio (M 0 ). In Uttarakhand and Delhi, both OBCs and SCs have the greater MPI values. States, where the STs and SCs outperform OBC and Others social groups in terms of MPI, are Arunachal Pradesh, Mizoram, and Andaman and Nicobar Island. It is also interesting to note that while STs of four of the eight north-eastern states (Arunachal Pradesh, Assam, Mizoram, and Nagaland) perform quite better compared to their other social group counterparts, in the rest four (Manipur, Meghalaya, Sikkim, and Tripura) STs’ MPI is noticeably greater than other social groups.
4.3. Decomposition of MPI by social groups, dimensions, and indicators
Social groups. The property of dimensional breakdown is relevant to multidimensional poverty which allows poverty to be broken down by deprivations in all subgroups, dimensions, and indicators among the poor [ 41 ]. Fig 2 demonstrates the social group-wise contribution to H and M 0 of India. It shows OBCs contribute more than two-fifths and SCs more than one-fifth to both H and M 0 , while the Others social group the least. However, when the population share of social groups is compared with their contribution, it is observed that STs contribute nearly twice their population share for both H and M 0 . And the SCs contribution is also noticeably higher than their population share. Whereas the contributions of OBCs and Others social groups is smaller than their population shares.
https://doi.org/10.1371/journal.pone.0271806.g004
Fig 3 illustrates the contribution of each dimension to MPI across the castes. At the national level both standard of living (38.4%) and health (39.2%) are the major contributor to multidimensional poverty with almost equal contribution. The dimensional contribution varied noticeably across the social groups. While for STs, standard of living is the greatest contributor, followed by health, for OBCs and Others social groups health dimension is the most significant contributor, followed by standard of living. On contrary, both standard of living and health contributes equally to the MPI for SCs.
https://doi.org/10.1371/journal.pone.0271806.g005
Table 5 depicts the contribution of each indicator to the MPI of each social group in India. Of the 12 indicators considered, the largest contribution to multidimensional poverty came from nutrition (28.4%), followed by years of schooling (14.9%), maternal health (9.7%), and cooking fuel (9.5%). And except for STs, all social groups show a similar order of contribution to MPI. For the STs, the maternal health’s contribution to the MPI is preceded by cooking fuel, sanitation and housing. It is also noticed, while drinking water contributes merely 2% to the MPIs of SCs, OBCs and Others, it contributes relatively higher proportion (4%) to the MPI for STs. While all of the 12 indicators show a similar pattern of contribution to MPIs of SCs, OBCs and Others, the contribution of nutrition and maternal health indicator to MPI of STs is noticeably lower, and the contribution of safe drinking water and household assets is remarkably higher compared to rest of the social groups.
https://doi.org/10.1371/journal.pone.0271806.t005
5. Discussion
The study’s objective is to assess India’s multidimensional poverty across social groups. This analysis adds to existing base of information in India about household-level multidimensional poverty measurement. This study contributed to a better understanding of poverty in India by offering a detailed analysis and data disaggregation in line with the SDGs. To the best of our knowledge this is the first ever study that comprehensively estimates multidimensional poverty among social groups across India and its states and UTs, and examines the contribution of indicators and dimensions to MPI in India as well as to MPI of the social groups. The salient findings of the study are discussed in this section.
The results reveal that nearly one-fourths of Indian population are living in households that considered as multidimensionally poor. The extent and nature of multidimensional poverty vary significantly across the four social groups in the population as well as across the States and UTs. The highest MPI was estimated for states located in the central region: Bihar, Jharkhand, Uttar Pradesh, Madhya Pradesh and Chhattisgarh; While Assam, Meghalaya, Rajasthan, Odisha and Nagaland are among others with higher MPI. Tripathi & Yenneti [ 42 ] have also observed these states have the greater MPIs but with different orders. and this finding is in line with Dehury & Mohanty [ 31 ]. These 10 states account for nearly three-fifths (57.1%) of the Indian population [ 43 ], hence have the potential to alter the multidimensional poverty at the national level. In addition, the states in the central region recorded the highest levels of multidimensional poverty among all social groups. For these reasons, this study suggests that there is a need for in-depth assessment of poverty in these states to uncover poverty conditions, improve the effectiveness of evidence-based planning and for effective policy making. In this way, interventions can be adapted to account for the heterogeneities of states and regions, as well as improve targeting of policy interventions. The lowest MPI were estimated to be in the state of Kerala, this finding is supported by Dehury & Mohanty [ 31 ] and Tripathi & Yenneti [ 42 ]. Other than Kerala, Puducherry, Lakshadweep, Sikkim, Goa, Delhi and Tamil Nadu have remarkably lower MPI compared to other states and UTs.
Regarding the social groups differential in MPI, this study found ST as the most deprived social group, with a dramatically higher number of multidimensionally poor people compared to their other social group counterparts, and Others social group are the most prosperous. This finding is consistent with the findings of the studies conducted by Bagli & Tewari [ 44 ] in Purulia district of West Bengal, Alkire et al. [ 33 ] in India, and in a recent study in urban India by Kaibarta et al. [ 45 ]. Our result that SCs and OBCs have nearly equal rates of multidimensional poverty is disproved by findings from another study [ 46 ], which show that OBCs have a far lower rate than SCs. When it comes to decomposing MPI across social groups, OBCs contribute the most, which can be linked to their population share being the highest of all social groups. However, it is worth noting that the STs contribute a significantly higher amount of the national MPI, around double their population share, whilst the SCs contribute around 1.25 times their population share. OBC and Other contributions, on the other hand, are lower than their population proportions.
MPI decomposition by dimensions and indicators yields some intriguing results. Both standard of living and health are key contributors to multidimensional poverty at the national level, with almost equal contributions. In their studies of 82 natural regions in India and urban India, Dehury & Mohanty [ 31 ] and Mohanty & Vasishtha [ 46 ], respectively, revealed that the health dimension provides the largest share to MPI, which is consistent with our findings. In contrast to our findings, Mohanty and Vasishtha [ 46 ] claim that standard of living has the smallest impact on MPI. The dimensional contribution varied noticeably between social groups. For OBCs and Others social groups health dimension contributes the highest, followed by standard of living. Whereas, for STs, standard of living is the greatest contributor, followed by health, which supported by the findings of Kaibarta et al. [ 45 ]. This finding backs up by Megbowon [ 46 ] and Espinoza-Delgado & Klasen [ 18 ], who argued that the standard of living dimension contributes the most to multidimensional poverty, particularly in poorer countries and rural areas. Because STs in India are usually located in hard-to-reach places and have historically been economically poorer than others, which restricts them from availing improved facilities in the standard of living dimension. On the contrary, for SCs, both standard of living and health play an equally role in MPI. Though SCs, like STs, are mostly located in rural areas and economically weaker, they have been settled closer to the society’s mainstream, allowing them to exercise their basic rights and adapt to better amenities to a larger extent than STs.
The decomposition of MPI with respect to the 12 indicators revealed that, the largest contribution to multidimensional poverty came from nutrition, followed by years of schooling, maternal health, and cooking fuel. Except for STs, all social groups, follow the similar sequence of contribution by 12 indicators to the MPI at the national level. While nutrition and years of schooling are greater concerns for STs’ MPI, unlike other three social groups the contribution of maternal health is preceded by cooking fuel, sanitation, and housing. In overall, the study recommends, the government should allocate more resources to improve the nutritional and health status of its citizens as well as their educational level and attainment. Because education is a critical area and its absence and inadequacy prevent individuals as well as the concerned households from realizing their basic rights [ 40 ]. Similarly, health has both intrinsic and instrumental value as well [ 22 ]; because it can affect several others capabilities, for instance, being not healthy can limit an individual’s capability to take part in social activities and prevent them to practice their profession [ 47 ].
6. Conclusion
In this era of globalization and the information technology revolution, poverty has no longer been considered as an absolute concept measured in money metrics. It has been realized that people may have better income, but may be lacking in health, nutrition or may not access proper day to day facilities or education or in any other dimension which prevents them to benefit from the mainstream economy. So, we call them multidimensional poor. This study explored the multidimensional poverty problem among social groups across India. The significant factors contributing to it are household used unimproved fuel for cooking purposes, unimproved sanitation facilities, lack of proper nutritional intake of children or men or women, improper maternal health and lack of education on the household level. The analysis across several categories provides essential information to policymakers since it indicates the particular areas of who the poor are, where they live, and how poor they are. Based on this finding, the policy should be formulated according to the deprivation pattern identified among the four social groups in each state with specifically designed plans and programs. Provision of providing access to clean cooking fuels, affordable and improved sanitation, providing safe and accessible drinking water, better free healthcare services, promote education for all, aid housing assets accumulation for the poor, electricity subsidies coverage etc. would facilitate in reducing the headcounts as well as the intensity of multidimensional poverty in these respective areas so that its economy may be placed on a high growth trajectory. Furthermore, disaggregating data analysis by these different segments allows for monitoring of the SDG’s commitment to halve the number of men, women, and children living in poverty in all of its aspects, as well as the ‘Leaving No One Behind’ commitment. From a multidimensional deprivation standpoint, the findings of this social group-based poverty study are not only an improvement over previous poverty measures that use money, but they also serve to address a knowledge gap. As emphasised by SDGs target 1.3, the Alkire-Foster methodology [ 23 ] for constructing the household-level multidimensional poverty measure assists policymakers in examining the poor’s joint deprivation, allowing them to implement nationally appropriate social protection systems and cover those left behind.
Acknowledgments
The authors are grateful to the Department of Humanities and Social Sciences, National Institute of Technology (NIT), Rourkela, for their support and encouragement, which helped improve this research paper. In particular, Miss. Itishree Pradhan would like to thank the Indian Council of Social Science Research (ICSSR), Govt. of India, for awarding her the Doctoral Fellowship for conducting her PhD research at NIT Rourkela.
- View Article
- Google Scholar
- PubMed/NCBI
- 7. Townsend P. Poverty in the United Kingdom. Harmondsworth: penguin Books; 1979.
- 8. Bourguignon F, Chakravarty SR. Multidimensional poverty orderings: Theory and applications. In: Basu K, Kanbur R, editors. Arguments for a better world: Essays in honor of Amartya Sen Ethics, welfare, and measurement. Oxford University Press; 2009. p. 337–362.
- 13. Messerli P, Murniningtyas E, Eloundou-Enyegue P, Foli EG, Furman E, Glassman A, et al. Global Sustainable Development Report 2019: The Future is Now–Science for Achieving Sustainable Development. New York: United Nations; 2019.
- 14. United Nations. Transforming our world: the 2030 Agenda for Sustainable Development. New York: United Nations; 2015.
- 17. Correa AF. An Individual-centred Approach to Multidimensional Poverty—The Case of Chile, Colombia, Ecuador and Peru. In: IARIW 33rd General Conference. Rotterdam, Netherlands: UNU-MERIT; 2014.
- 24. Alkire S, Seth S. Multidimensional Poverty Reduction in India between 1999 and 2006: Where and How? OPHI Working Paper 60. Oxford Poverty and Human Development Initiative, University of Oxford; 2013. Report No.: 60.
- 34. Baiju K, Sivaraman S. Multidimensional Poverty among Social Groups in Kerala: Incidence, Intensity and Disparity. Cambridge Scholars Publishing; 2018.
- 35. Aayog NITI. National Multidiemnsional Poverty Index Baseline Report: Based on the National Family Health Survey-4. India: NITI Aayog, Government of India; 2021.
- 36. WHO UNICEF. Every newborn: An action plan to end Preventable deaths: Executive summary Geneva: World Health Organization. [Internet]. Geneva; 2014. Available from: www.who.int/about/licensing/copyright_form/en/index.html .
- 37. Naveed A, Tanweer-ul-Islam . A New Methodological Framework for Measuring Poverty in Pakistan. Working paper. Islamabad, Pakistan: Sustainable Development Policy Institute; 2012. Report No.: 122.
- 39. IIPS ICF. National Family Health Survey (NFHS-4), 2015–16. Mumbai, India: International Institute for Population Sciences (IIPS); 2017.
- 41. Alkire S, Foster J, Seth S, Santos ME, Roche JM, Ballón P. The Alkire–Foster Counting Methodology [Internet]. Multidimensional Poverty Measurement and Analysis. Oxford University Press; 2015. p. 144–85. Available from: http://dx.doi.org/10.1093/acprof:oso/9780199689491.003.0005 .
- 43. Census of India. Census of India. Office of the Registrar General & Census Commissioner, Governement of India. 2011.
Advertisement
Multidimensional poverty in slums: an empirical study from urban India
- Published: 20 January 2022
- Volume 87 , pages 527–549, ( 2022 )
Cite this article
- Samyakami Kaibarta 1 ,
- Somnath Mandal ORCID: orcid.org/0000-0002-4353-3304 1 ,
- Pintu Mandal ORCID: orcid.org/0000-0002-7408-509X 1 ,
- Subhasis Bhattacharya ORCID: orcid.org/0000-0002-1607-6312 2 &
- Suman Paul ORCID: orcid.org/0000-0001-6666-0683 1
5 Citations
8 Altmetric
Explore all metrics
Poverty is the most important metric for determining the nature and sense of wellbeing in a given area. Most economists consider poverty to be an economic criterion for assessing many aspects of human development as well as overall social development; yet, society is multi-faceted in its many forms. To address this pressing societal issue, the current study used the Multidimensional poverty index (MPI). To analyse urban poverty among slum communities, the researchers used the Global MPI of the Oxford Poverty and Human Development Initiative and UNDP (following Alkire and Foster) techniques. Researchers attempted to create a Multidimensional poverty index (MPI) for impoverished households in Purulia's designated slums in this study. In the second phase, the multidimensional poverty of Purulia's urban poor households was assessed based on (a) location, (b) social groupings, and (c) length of stay. Finally, researchers have attempted to identify the factors that contribute to multidimensional poverty. Two indicators, the Head Count Ratio (H) and Intensity of Poverty, have been offered to better explain the nature of MPI (A). Based on slum population density and areal density, eight urban slum areas with 320 households has been taken from 8 selected slums based on Yamane’s methodology from Purulia Municipality's wards. A structured questionnaire, an oral history interview, and a focus group discussion were used as primary data sources, with secondary data acquired from several officially published sources. The study displays a decomposed multidimensional poverty picture in terms of overall condition, socioeconomic groups, and household age, with a quantitative methodology that is transparent. When the locations have been considered, a qualitative approach has been used to determine that the slums closest to the railway track are the most multidimensionally disadvantaged of the eight slums. Furthermore, the schedule caste population has been found to be more deprived across many socioeconomic groups, with Scheduled tribe (ST) households being the most deprived in terms of health on one hand (applied quantitative methodology) and multi-nominal regression (applied qualitative methodology) indicating a mix mode approach. This form of analysis, which combines quantitative and qualitative approaches, can aid stakeholders and policymakers in developing specific poverty-reduction policies at the regional level.
This is a preview of subscription content, log in via an institution to check access.
Access this article
Price includes VAT (Russian Federation)
Instant access to the full article PDF.
Rent this article via DeepDyve
Institutional subscriptions
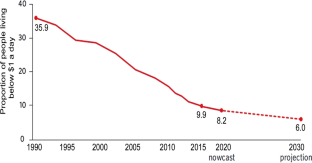
Source: Training material for producing nation human development report)
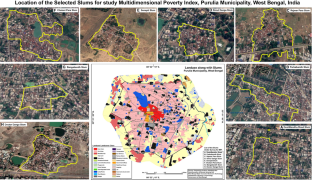
Similar content being viewed by others
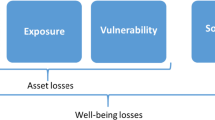
From Poverty to Disaster and Back: a Review of the Literature
Social Diversity, Gender, Equity and Public Policy
Health Inequity in the Philippines
Agarwal, S., & Taneja, S. (2005). All slums are not equal: Child health conditions among the urban poor. Indian Paediatrics, 42 (3), 233–244.
Google Scholar
Ahmed, I. (2016). Building resilience of urban slums in Dhaka, Bangladesh. Procedia - Social and Behavioral Sciences, 218 , 202–213.
Article Google Scholar
Alkire, S., Foster, J., 2007. Counting and Multidimensional Poverty Measurement. Working Paper No. VII, Oxford Poverty and Human Development Initiative (OPHI), New York.
Alkire, S., Santos, M.E., 2010. Acute Multidimensional Poverty: A New Index for Developing Countries. UNDP - Human Development Research Paper - 2010/11.
Batana, Y.M., 2008. Multidimensional Measurement of Poverty in Sub-Saharan Africa. Working Paper No. XIII, Oxford Poverty and Human Development Initiative (OPHI), New York
Bhan, G., & Jana, A. (2013). Of Slums or Poverty Notes of Caution from Census 2011. Economic and Political Weekly, 48 (13), 13–16.
CDE, 2014. Cities of hope: Young people and opportunity in South Africa’s cities. Johannesburg, South Africa: Centre for Development and Enterprise (CDE). (Round Table No. 24) ( http://www.cde.org.za/wpcontent/uploads/2014/03/CITIES_OF_ HOPE Young_people_and_ opportunity_in_South_Africas_cities.pdf〉 (Accessed 18 May 2020).
Chatterji, R. (2004). Plans, habitation and slum redevelopment: The production of community in Dharavi in Mumbai. Contribution to Indian Sociology, 39 , 201–217.
Coelho, K., & Maringanti, A. (2012). Urban Poverty in India: Tools, Treatment and Politics at the Neo-liberal Turn. Economic and Political Weekly, 47 (47/48), 39–43.
Datt. G., Ravallion, M., 1989. Regional Disparities, Targeting and Poverty in India. Working Paper No. CCCLXXV, Agriculture and Rural Development Department, World Bank, Washington.
Deka, N. (2018). Multidimensional poverty index for the poor in Guwahati city (Assam, India). Journal of Social and Economic Development, 20 (1), 1–32.
Desai, R., Mahadevia, D., Gogoi, T., Datey, A., Patel, T., & Mishra, A. (2012). Rental housing for the urban poor in Guwahati . Unpublished research report.
Foster, J.E., 2007. A Report on Mexican Multidimensional Poverty Measurement. Working Paper No. XXXX, Oxford Poverty and Human Development Initiative (OPHI), New York.
Government of India, 2002. Report of the Expert Group on Identification of Households Below Poverty Line (BPL Census 2002). Guidelines, Ministry of Rural Development, Government of India, New Delhi.
Haq, M. U. (1995). Reflections on human development . Oxford University Press.
Henderson, J. V., Storeygard, A., & Weil, D. N. (2012). Measuring economic growth from outer space. American Economic Review, 102 , 994–1028. https://doi.org/10.1257/aer.102.2.994
Jorgenson, A. K., & Rice, J. (2016). Slum prevalence and health in developing countries: Sustainable development challenges in the urban context. Sustainable Development, 24 , 53–63.
Khatun, D., & Roy, B. C. (2012). Rural livelihood diversification in West Bengal: determinants and constraints. Agricultural Economics Research Review, 25 , 115–124.
Kundu, A., & Sarangi, N. (2007). Migration, Employment Status and Poverty. Economic and Political Weekly, 42 (4), 299–306.
Marx, B., Stoker, T., & Suri, T. (2013). The economics of slums in the developing world. Journal of Economic Perspectives, 27 (4), 187–210.
Mathur, O.P., 2013. Urban poverty in Asia. Manila: Asian Development Bank. https://www.adb.org/sites/default/files/project-document/81002/urban-povertyasia.pdf Accessed 16 December 2021.
Mitlin, D., & Satterthwaite, D. (2013). Urban poverty in the global south: Scale and nature . Routledge.
Mitra, S. (2016). Synergies among monetary, multidimensional and subjective poverty: Evidence from Nepal. Social Indicators Research, 125 (1), 103–125.
Osrin, D., Das, S., Bapat, U., Alcock, G. A., Joshi, W., & More, N. S. (2011). A rapid assessment scorecard to identify informal settlements at higher maternal and child health risk in Mumbai. Journal of Urban Health, 88 (5), 919–932.
Panda, S., Chakraborty, M., & Misra, S. K. (2016). Assessment of social sustainable development in urban India by a composite index. International Journal of Sustainable Built Environment, 5 , 435–450.
Pimental, D., Cooperstein, S., Randell, H., Piliberto, D., Sorentimo, S., Kaye, B., Nicklin, C., Yagi, J., Brain, J., & Weinstein, C. (2007). Ecology of increasing diseases: Population growth and environmental degradation. Human Ecology, 35 , 653–668.
Primary Census Abstract, 2011. Census of India. Govt. of India, West Bengal Series.
Rashid, S. F. (2009). Strategies to reduce exclusion among populations living in urban slum settlements in Bangladesh. Journal of Health and Population Nutrition, 27 (4), 574–586.
Sathyanarayana, T., & N. (2015). Multidimensional Measurement of Poverty: A Study in Mysore. Indian Journal of Human Development, 9 (2), 235–259.
Sen, A. (1976). Poverty: An Ordinal Approach to Measurement. Econometrica, 44 (2), 219–231.
Sen, A. (1985). Commodities and Capabilities . North-Holland.
Sen, A. (1992). Inequality Re-examined . Harvard University Press.
Sen, A. (2000). A decade of Human Development. Journal of Human Development, 1 (1), 17–23.
Sen, A., 1995. The Political Economy of Targeting in Public Spending and the Poor: Theory and Evidence’, in D. van der Walle and K. Nead, Baltimore (eds): Johns Hopkins University Press, Maryland.
Sharma, G., 2009. Rapid growth of slums in Jammu city polluting the environment. Daily-Excelsior, 23rd June, p 6.
Smets, P., & Lindert, P. (2016). Sustainable housing and the urban poor. International Journal of Urban Sustainable Development, 8 (1), 1–9.
Subbaraman, R., O’Brien, T. J., Shitole, S., Sawant, K., Bloom, D. K., & Deshmukh, P. A. (2012). Off the map: The health and social implications of being a non-notified slum in India. Environment and Urbanization, 24 (2), 643–663.
The National Sample survey Organization (NSSO). [2012]. Government of India. no Report 56
Turok, I., Borel-Saladin, J., 2016. The theory and reality of urban slums: Pathways-out-of-poverty or cul-de-sacs? Urban Studies, 1–22.
Turok, I., Budlender, J., Visagie, J., 2017. The role of informal urban settlements in upward mobility. DPRU, University of Cape Town (Development Policy Research Unit Working Paper 201505).
Uddin, A. (2018). Assessing urban sustainability of slum settlements in Bangladesh: Evidence from Chittagong city. Journal of Urban Management, 7 , 32–42.
UNDP, 2019. The Sustainable Development Goals Report 2019. New York.
UN-Habitat, 2003a. The Challenge of Slums. London, UK: Earth scan
UN-Habitat, 2014b. Sustainable building design for tropical climates. Principles and applications for Eastern Africa. Nairobi: UN-Habitat.
UN-Habitat, 2015a. The role of ICT in the proposed urban sustainable development goal and the new urban agenda. ( https://unhabitat.org/the-role-of-ict-in-theproposed-urban-sustainable-development-goal-and-the-new-urban-agenda/ ) (Accessed 16 December 2021).
UN-Habitat, 2015b. Global urban indicators database 2015. UN-Habitat, Nairobi.
UN-Habitat, 2015c. The role of ICT in the proposed urban sustainable development goal and the new urban agenda. ( https://unhabitat.org/the-role-of-ict-in-theproposed-urban-sustainable-development-goal-and-the-new-urban-agenda/ ) (Accessed 20 May 2020).
United Nations Organization, 1995. Report of the World Summit for Social Development. New York.
Vakulabharanam, V., & Motiram, S. (2012). Understanding poverty and inequality in urban India since reforms bringing quantitative and qualitative approaches together. Economic and Political Weekly, 48 , 44–52.
Van, H.J., 2017. Urban sustainability and resilience. In P. DRAHOS (Ed.). Regulatory theory: foundations and applications (pp. 725–740). Acton ACT, Australia: ANU Press.
Yamane, T. (1973). Statistics: An introductory analysis . Harper and Row.
Download references
No funding is involved with this study.
Author information
Authors and affiliations.
Department of Geography, Sidho Kanho Birsha University, Purulia, West Bengal, India
Samyakami Kaibarta, Somnath Mandal, Pintu Mandal & Suman Paul
Department of Economics, Sidho Kanho Birsha University, Purulia, West Bengal, India
Subhasis Bhattacharya
You can also search for this author in PubMed Google Scholar
Corresponding author
Correspondence to Suman Paul .
Ethics declarations
Conflict of interest.
The authors declare that they have no known competing financial interests or personal relationships that could have appeared to influence the work reported in this paper.
Ethical approval
After going through the questionnaire one by one, Ethics Committee of the Sidho-Kanho-Birsha University, Purulia firmly considers the Ethical Clearance and conveys their best wishes also for fruitful results from it in near future.
Informed consent
Informed consent was obtained from all individual participants included in the study.
Additional information
Publisher's note.
Springer Nature remains neutral with regard to jurisdictional claims in published maps and institutional affiliations.
Rights and permissions
Reprints and permissions
About this article
Kaibarta, S., Mandal, S., Mandal, P. et al. Multidimensional poverty in slums: an empirical study from urban India. GeoJournal 87 (Suppl 4), 527–549 (2022). https://doi.org/10.1007/s10708-021-10571-7
Download citation
Accepted : 21 December 2021
Published : 20 January 2022
Issue Date : October 2022
DOI : https://doi.org/10.1007/s10708-021-10571-7
Share this article
Anyone you share the following link with will be able to read this content:
Sorry, a shareable link is not currently available for this article.
Provided by the Springer Nature SharedIt content-sharing initiative
- Urbanisation
- Alleviation
- Find a journal
- Publish with us
- Track your research
PEACE, JUSTICE & STRONG INSTITUTIONS
International diplomatic alliance.
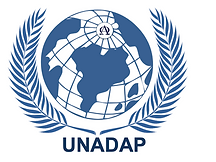
About | COP26 PRESIDENCY | PUBLISHING | Team | GWA | Clean energy | UN-SDG | Media | Contact | Internships | News
- Aug 17, 2018
India's stance on SDG 1: No Poverty
Updated: Aug 19, 2018

The Challenge
Around the world today, a staggering 800 million people still live in conditions of extreme poverty. With one in five people living on less than USD 1.25 a day, extreme poverty presents one of the most urgent crises of our times. While the number of people living in extreme poverty has declined by more than half since 1990, a great deal more needs to be done. Millions subsist on just over USD 1.25 a day, and many more remain at risk of slipping back into poverty. Young people are especially vulnerable. While 10.2% of all working adults live below the global poverty line of USD 1.9 a day as of 2015, this number rises to 16% when we consider the age group of 15-24 years. Children, too, are victims of global poverty, with 18,000 children dying every single day from poverty related causes.
Why is this important?
Poverty is more than just the lack of income or access to resources – it manifests itself in diminished opportunities for education, social discrimination and the inability to participate in decision-making processes. For instance, in developing countries, children in the poorest households are four times less likely to be in school than those of the richest. But extreme deprivation is not just about wellbeing and opportunity; it is a question of survival itself. In Latin America and East Asia, the poorest children are three times more likely to die by age 5 than the richest.
How can we address this?
Ending poverty in all its forms everywhere forms the first goal of the 2030 Sustainable Development agenda. It calls for ensuring social protection, enhancing access to basic services, and building resilience against the impacts of natural disasters which can cause severe damage to people’s resources and livelihoods. The international community agrees, through the Sustainable Development agenda for 2030, that economic growth must be inclusive, especially of the most poor and vulnerable, and aims to eradicate extreme poverty for all people everywhere in the next 15 years.
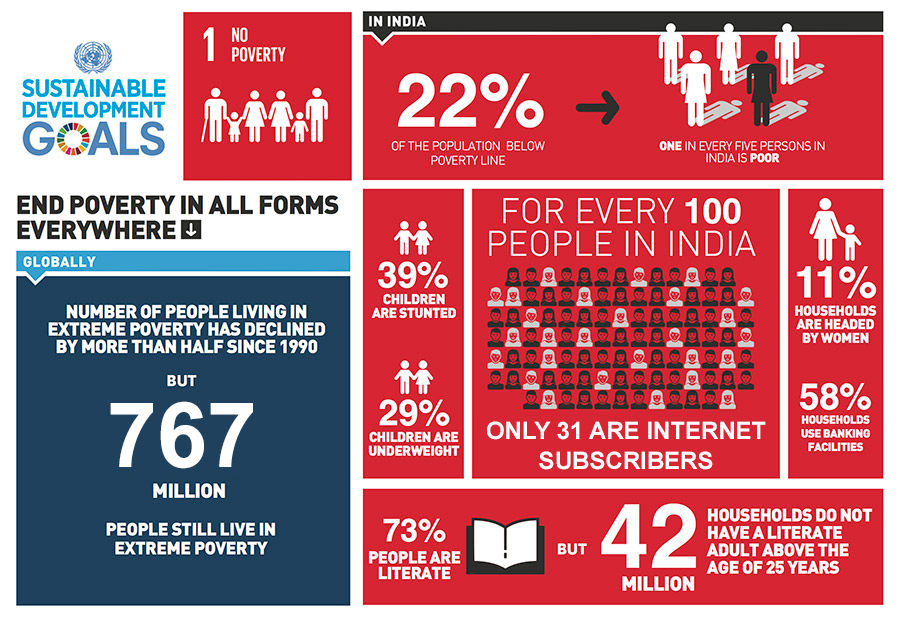
India and Goal 1
Between 2012-2013, global reduction in extreme poverty was driven mainly by Asia – notably China and India. Despite the fact that India made tremendous progress in halving its poverty head count ratio by 2011-2012, it still remains at 21% of the population. Nearly 80% of these poor live in rural areas and eradicating poverty is at the core of India’s national priorities. The Government of India has many progressive schemes, including the world’s largest employment guarantee scheme, the Mahatma Gandhi National Rural Employment Guarantee Scheme , and the National Social Assistance Programme .
By 2030, eradicate extreme poverty for all people everywhere, currently measured as people living on less than USD1.25 a day.
By 2030, reduce at least by half the proportion of men, women and children of all ages living in poverty in all its dimensions according to national definitions.
Implement nationally appropriate social protection systems and measures for all, including floors, and by 2030 achieve substantial coverage of the poor and the vulnerable.
By 2030, ensure that all men and women, in particular the poor and the vulnerable, have equal rights to economic resources, as well as access to basic services, ownership and control over land and other forms of property, inheritance, natural resources, appropriate new technology and financial services, including microfinance.
By 2030, build the resilience of the poor and those in vulnerable situations and reduce their exposure and vulnerability to climate- related extreme events and other economic, social and environmental shocks and disasters.
Ensure significant mobilization of resources from a variety of sources, including through enhanced development cooperation, in order to provide adequate and predictable means for developing countries, in particular least developed countries, to implement programmes and policies to end poverty in all its dimensions.
Create sound policy frameworks at the national, regional and international levels, based on pro-poor and gender-sensitive development strategies, to support accelerated investment in poverty eradication actions.
Copyright: UN India, SDG
TAGS: Dominic Dixon , United Nations , Dominic F Dixon , Dr. Dominic Dixon , UN SDG
- United Nations
Recent Posts
Human Rights Council election: 5 things you need to know about it
UNICEF and ADB join forces for children and young people
A super-charged typhoon took my family away. It can happen to you too.
Comentários

India’s poverty debate needs to move on: Let’s adopt new norms

- We ought to acknowledge India’s success in relieving poverty by norms framed back in the 1970s, but it’s also time to revise our poverty cut-off in accordance with what we consider a ‘decent standard of living’ today.
The National Sample Survey Office (NSSO) released summary results for the Household Consumer Expenditure Survey (HCES) earlier this year. This release re-ignited discussions on poverty, with commentators raising a range of issues. Using the Tendulkar Committee methodology, an SBI report estimated that poverty in India would be around 6.3% in 2022-23. Using the Rangarajan Committee methodology, C. Rangarajan and Mahendra Dev suggested that the all- India poverty ratio would be around 10%.
In either case, these estimates suggest that there has been a sharp reduction in poverty since 2011-12. Partly in response to these articles, other commentators have suggested revisiting the poverty line. They argue that changes in survey methodology in the recent HCES renders the application of earlier methodologies to HCES data inappropriate. These types of arguments were comprehensively rebutted by Surjit S. Bhalla in a column in this paper on 27 March 2024.
None of these discussions, however, examined the appropriateness of the existing methodologies for tracking poverty using consumption data.
Consumption-based poverty measurement in India traces its roots to a Working Group constituted by the Planning Commission in 1962, which was subsequently re-visited and revised by a task force in 1979. This task force provided a careful description of the reasoning it employed to arrive at a poverty line for India.
In brief, it defined the poverty line as a per-capita consumption expenditure level which could meet an average per capita daily calorie requirement of 2,400 Kcal in rural areas and 2,100 Kcal in urban areas, along with the associated quantum of expenditure on non-food items. This average calorie norm was based on an analysis of the demographic and activity-based composition of the population at that time.
The monetary value of this norm formed the basis of poverty lines in all subsequent revisions. However, the basic approach underlying this calculation was never seriously revisited until the Tendulkar and Rangarajan committees. Both of them noted that changes in the demographic and activity composition since the task force required changes in the calorie norm and expenditure level.
However, while they revisited the expenditure and nutritional dimensions of a poverty norm, they did not adequately address its non-food components. The essence of their argument was to assert that if spending in an expenditure class is adequate to meet nutritional norms, it will also be normatively adequate to meet the associated non-food components.
The problem with this assumption is that India has changed significantly since the task force on poverty was set up in the 1970s. Life expectancy at birth, which was 49.7 years in 1970 has risen to 69.4 in 2018. The population above 60 has risen from 6.1% to above 10.1% (as of 2021). India’s gross enrolment ratio (GER) at the primary level has risen from about 62% in 1971 to universal enrolment today. In higher education, our GER has risen from below 6% then to around 28% today.
These changes have implications for out-of-pocket expenditures on education and health. NSS surveys reveal this fact by showing the significant rise in expenditure on these accounts over the years. The reduction in mortality rates and rise in life expectancy have increased age heterogeneity in our population, with a rising share of the elderly. Changes in household composition (with the rise of unitary families) have also increased the proportion of the elderly who are living on their own. It is well documented that the medical requirements of the elderly are different from those of the young.
The implications of this changing age profile on the normative health expenditure requirements of this emerging group have thus far not been carefully addressed. Given that the proportion of the elderly is projected to rise even more sharply in the coming years, the adequacy of norms derived for a much younger population will become even more questionable.
The inclusion of a poll promise to extend the coverage of Ayushman Bharat to the elderly in the Bharatiya Janata Party manifesto is an indicator that the political class has understood this problem better than the academic community that specializes in studying poverty.
The success of policies that have resulted in a rise in enrolment ratios both at primary and higher education levels has meant that competition for aspirational jobs has become stiffer. This increased competition has led to increased out-of-pocket expenditure on supplementary tuition classes, as indicated by the mushrooming of coaching institutions across the country.
The burden of expenditure on private coaching is a major factor in creating what we may call ‘education poverty’ among families with young children. Addressing this problem is not merely about making financial resources available, but about changing the approach to education, as has at least been signalled in the National Education Policy, 2020.
The deeper problem is as follows. Consumption- based poverty measures are essentially capturing the average attributes of the population. While an average is a reasonable way to compare population attributes over time when the underlying population structure is stable, increased heterogeneity in the population makes the use of an average to describe attributes such as poverty seriously problematic.
For example, an elderly household may have enough income to meet the expenditure requirements of a nutrition norm, but not its healthcare requirements. Similarly, we could have a household with young children that can meet its food needs but not its aspirational requirements of private tuition.
Such examples reveal that households may exceed the thresholds of extreme poverty, but yet continue to lack resources for a ‘decent standard of living.’ In order to meet the Sustainable Development Goal of ending poverty in all its forms, we should acknowledge India’s success in relieving poverty by norms framed in the 1970s and move on, so that we can define fresh norms appropriate for Amrit Kaal.
MINT SPECIALS
Wait for it….
Log in to our website to save your bookmarks. It'll just take a moment.
You are just one step away from creating your watchlist!
Oops! Looks like you have exceeded the limit to bookmark the image. Remove some to bookmark this image.
Your session has expired, please login again.
Congratulations!
You are now subscribed to our newsletters. In case you can’t find any email from our side, please check the spam folder.

Subscribe to continue
This is a subscriber only feature Subscribe Now to get daily updates on WhatsApp

Open Demat Account and Get Best Offers
Start Investing in Stocks, Mutual Funds, IPOs, and more
- Please enter valid name
- Please enter valid mobile number
- Please enter valid email
- Select Location
I'm interested in opening a Trading and Demat Account and am comfortable with the online account opening process. I'm open to receiving promotional messages through various channels, including calls, emails & SMS.

The team will get in touch with you shortly
Main navigation
- Clinical Education
- O'Brien Fellowships
- Steinberg Fellowships
- CHRLP Article Lab
Gender Disparities In Organ Donation And Transplantation In India: A Call For Equality

- Add to calendar
- Tweet Widget
The 2021 statistics report of the National Organ and Tissue Transplant Organisation (NOTTO) of India has brought attention to a significant gender disparity: 80% of organ recipients were male, while, 75% of organ donors were female. This phenomenon has become entangled in the complex existing web of intersectional discrimination in India, which deviates from the principles of justice and equality enshrined in Articles 14 and 15 of the Indian Constitution. Moreover, the gender inequality in organ donation and transplantation in India reflects discriminatory practices and is a manifestation of patriarchal ideologies that marginalize women’s accessibility to healthcare facilities. This gender imbalance constitutes a violation of women’s right to health and medical care, which were recognized as essential aspects of the right to life under Article 21 of the Indian Constitution in the landmark case of Francies Coralie Mullin v Union Territory of Delhi.
Female organ donors are influenced by sociocultural factors and financial dependency. Financial independence may reduce their inclination to donate. Additionally, one of the psychological factors contributing to the gender gap in organ donation is women’s prior experiences, like childbirth, which enhance their trust in the medical system. Mothers are the primary organ donors among parents accounting for 73% of donations, while wives contribute 91% of donations in married couples. Regarding this gender inequality, Dr Pranjali Modi, the convenor of the State Organ and Tissue Transplant Organisation (SOTTO), has emphasized that patriarchal beliefs play a significant role in the general reluctance to accept organ donations from men, who are often considered to be the primary breadwinners in families. Moreover, when men donate organs, financial difficulties might arise during the evaluation, surgery, and recovery phases, which can result in the coercion of women to undergo organ donation surgery.
Relatedly, there is a subconscious prioritization of men over women for life-saving treatments, such as organ transplantation, based on the perceived economic importance of their roles. These biases are also apparent within medical institutions and professions, potentially affecting doctor-patient interactions and resulting in gender inequalities in organ transplant procedures. However, a notable scientific reason for the gender disparity in organ transplantation is that pregnancy sensitizes women’s immune systems, potentially diminishing the compatibility of their organs with those of their blood relatives, which are those individuals who are most inclined to donate organs. Further, women waiting to receive organ donations, due to their typically smaller stature, encounter a greater likelihood of being declined organ offers, leading to a higher risk of death or removal from the waitlist compared to small-stature men. This inherent bias in healthcare exacerbates gender inequalities in organ transplantation access for women. These factors, altogether, contribute to unequal access to organ transplantation, a critical healthcare intervention, as evidenced by a longitudinal study spanning 15 years which revealed that a mere 15% of female individuals were organ recipients in the nation.
This healthcare outcome contradicts the stipulations outlined in Article 12 of the International Covenant on Economic, Social and Cultural Rights (“ICESCR”), which acknowledges the entitlement of every individual to achieve “ the highest attainable standard of physical and mental health .” Additionally, the importance of guaranteeing equitable allocation of health facilities, goods and services among both men and women has been underscored in the remarkable case of Karukola Simhachalam vs Union of India and Ors (WP PIL No 164 of 2019). The case further emphasized the necessity of counteracting any hindrances to the full realisation of the right to health and eradicate discriminatory practices for all people. Nevertheless, any practice embedded in gender bias not only perpetuates disparities for women but also undermines the very bedrock of equality principles. This practice is vulnerable to legal scrutiny under the Universal Declaration of Human Rights , which accentuates the significance of legal egalitarianism, the right to health, and well-being.
It is imperative to confront gender imbalances to foster a society characterised by equality, inclusivity and the protection of women’s rights. Although the Transplantation of Human Organs Act, of 1994 ostensibly maintains a gender-neutral stance, the actual implementation unveils a fabric intricately woven with evident gender disparities and prejudices. The dissonance between legal intent and application of the legislation in practice underscores the need to erase gender stereotypes and inequalities within the organ transplantation and donation domain.
Addressing the issue of gender disparities in organ transplantation necessitates raising awareness as a foundational step. To mitigate disparities among disadvantaged groups, it is crucial to implement transplant centers and community initiatives targeting rural and marginalized areas and establish transplant centers in those places as well. The pre-transplant assessment should encompass psychosocial and economic dimensions to identify any potential negative motivations for donation, such as underlying abuse, coercion, threats and violence. Conducting research studies and collecting accurate statistics at the grassroots level is also imperative for developing policies that promote equality and fair access to organ transplantation. Additionally, overcoming biological barriers in transplantation procedures requires the implementation of science-backed policies.
Lastly, providing medical assistance to organ donors for the transplantation process and offering medical support to organ recipients from vulnerable communities are essential steps that Indian government should take. However, addressing gender imbalance requires more than just focusing on education and poverty alleviation. It necessitates a deeper examination of traditional gender roles and the roles of women within their families. Policies must be implemented to ensure that women receive equitable access to transplantation services, regardless of their societal status or financial standing within the family.

Department and University Information
Centre for human rights and legal pluralism.
- Faculty of Law
- Law Admissions - BCL/JD
- Law Admissions - graduate programs
- Law Student Affairs Office
- Law Career Development Office
- Nahum Gelber Law Library
- Focus online
- CHRLP Facebook page
- Business Law Platform
- Centre for Intellectual Property Policy
- Fortier Chair in Int'l Arbitration & Commercial Law
- Institute of Air & Space Law
- Jean Monnet Chair in International Economic Integration
- Labour Law and Development Research Laboratory
- Oppenheimer Chair in public international law
- Paul-André Crépeau Centre for Private & Comparative Law
- Peter MacKell Chair in Federalism
- Private Justice and the Rule of Law
- Research Group on Health & Law
- Rule of Law and Economic Development
- Stikeman Chair in Tax Law
- Wainwright Fund
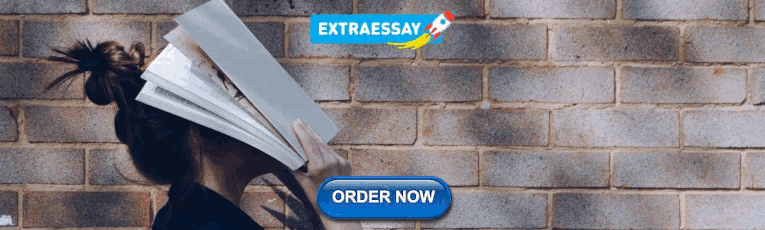
IMAGES
VIDEO
COMMENTS
A key argument for the introduction of the City Development Plans initiative (2007-2012) in India was to move away from national conceptions of and responses to poverty and to instead focus on engaging with local understandings of poverty. Through a case study of the City Development Plan initiative in Trivandrum, the capital city of the ...
The reason why poverty according to official and World Bank estimates appears to have declined in India, on the basis of which it is claimed that the link between poverty and hunger no longer holds, is because they use a "poverty line", a particular level of per capita money expenditure below which people are considered poor, which is ...
Abstract. This is a case study by Dr Manjit Sharma, about poverty in a village named Shergarh in the Bhatinda district of Punjab. Being a relatively better-performing state, all the 15 poorest households that are selected as samples for the research had self-owned houses, almost 3 meals a day etc.
The article surveys the reduction in poverty in India after Independence. It looks into the idea of development through inclusiveness of all sections of society. Important elements of inclusiveness and causes for low inclusive growth in India are explained. The methodologies adopted by Planning Commission of India to estimate poverty have been ...
India's top 1% own more than 50% of the country's wealth. It is the world's second largest food producer and yet is also home to the second-highest population of undernourished people in the world (FAO 2015). One side of this story is clear from the score for India on the Global Hunger Index (GHI) - 28.5 (von Grebmer et al 2016).
Panagariya (2012a, 2012b) analyze s of poverty using the in India various aspect expenditures surveys up to 200405. In particular, Cain, Hasan and Rana - study (2012) the impact of openness on poverty, Mukim and Panagariya (2012) document the decline in poverty across social groups, Dehejia and Panagariya (2012) provide evidence on the
The publication presents a case study of a program implemented in India to address rural poverty, including efforts to increase the effectiveness of social protection programs and improve public service delivery. ... ibp case study summary india samarthan rural poverty 2016.pdf. pdf, 0.26 MB. Download . Authors.
Abstract. This paper offers a comprehensive analysis of poverty in India. It shows that regardless of which of the two official poverty lines we use, we see a steady decline in poverty in all states and for all social and religious groups. Accelerated growth between fiscal years 2004-2005 and 2009-2010 also led to an accelerated decline in poverty rates. Moreover, the decline in poverty ...
We study the evolution of consumption-poverty measures for post-independence India, including 20 years since extensive economic reforms began in 1991. Progress against poverty was negligible until the mid-1970s, after which a downward trend in poverty measures emerged.
2. PRE‐COVID POVERTY TRENDS. The evolution of poverty in India has long been the subject of close attention. In particular, with the introduction of economic reforms in the early 1990s, there has been a strong interest in seeing how poverty outcomes fared as economic growth accelerated (Datt & Ravallion, 2011; Ravallion, 2011).Figure 1 illustrates that poverty fell sharply during this period ...
26. 31. 33. Paper presented at the IFPRI/World Bank Poverty Research Conference, October 1989. The paper draws on results of an ongoing World Bank research project, "Policy Analysis and Poverty: Appli;^able Methods and Case Studies" (RPO 675-04), based in the Agricultural Policies Division of the Agriculture and Rural Development Department ...
Accepted: 30 July 2021/Published online: 4 August 2021 The Author(s), under exclusive licence to Springer Nature B.V. 2021. Abstract The primary objective of this study is to investigate the regional disparities of multidimen-sional poverty (MPI) in the context of India. This study to our knowledge is the first of its kind which examined MPI ...
We focus in particular on the role of small and medium size conurbations in India, both as the urban sub-sector in which urban poverty is overwhelmingly concentrated, and as a sub-sector that could potentially stimulate rural-based poverty reduction. Second, in rural areas, we focus on the nature of intersectoral transformation out of ...
This analysis attempts to study the decline in poverty rates and number ... In such cases expenditure may surpass or fall short ... Poverty in rural India fell from 32.59% to 19.28% while in urban areas it declined from 8.65% to 5.27%. Thus, rural population witnessed a larger decline in poverty as compared ...
The primary objective of this study is to investigate the regional disparities of multidimensional poverty (MPI) in the context of India. This study to our knowledge is the first of its kind which examined MPI disparity at a regional level. The study has classified the geographic area of India into six regions: Northern, Eastern, North Eastern, Central, Western and Southern region. Further, we ...
This is a case study by Dr Manjit Sharma, about poverty in a village named Shergarh in the Bhatinda district of Punjab. Being a relatively better-performing state, all the 15 poorest households ...
Poverty is multifaceted. The global poverty profile shows 41% of multidimensionally poor people living in South Asian countries. Though castes and tribes are a more prevalent line of social stratification in India, and their socio-economic characteristics also vary remarkably, hardly any study has explored these dimensions while analysing multidimensional poverty in India. Hence, this study ...
The interface between their poverty and the real estate reality in India makes affordable housing a distant dream. The article, using the National Sample Survey Office 68th Round Consumer Expenditure Data and India's Consumer Economy (ICE 360) Data, tests Engel's law to determine the disposable income of the poor for housing.
An Assessment of Poverty Studies in India with Special Reference to Economic Reforms. BASANTA K. PRADHAN and M. R. SALUJA. 1. INTRODUCTION. As it is well-known, the study of poverty is extremely important on moral and philosophical and also, political grounds. Further, evidences are available to show that poverty affects growth adversely.
Based on the results of the survey, an official poverty line of rupees 356.30 person −1 month −1 in rural areas and rupees 538.60 person −1 month −1 in urban areas has been established for 2004/05. Urban areas need take 2,100 cal day −1 person −1, whereas rural areas should consume 2,400 cal day −1 person −1.
to severe hunger and malnutrition, 10.6 million died in 2003 before they reached the age of 5 (or roughly. 29,000 children per day). Almost half the world - over three billion people live on ...
Case Study A person is a victim of poverty when they are forced or left out with no other choice or alternatives but to live or spend their life without basic shelter on the streets, under ... India@ 75, CII; Maitri,NGO. My area of Interest lies on issues related to Children. I am going to work with organizations that are working on the issues ...
Millions subsist on just over USD 1.25 a day, and many more remain at risk of slipping back into poverty. Young people are especially vulnerable. While 10.2% of all working adults live below the global poverty line of USD 1.9 a day as of 2015, this number rises to 16% when we consider the age group of 15-24 years.
Using the Rangarajan Committee methodology, C. Rangarajan and Mahendra Dev suggested that the all- India poverty ratio would be around 10%. In either case, these estimates suggest that there has ...
The 2021 statistics report of the National Organ and Tissue Transplant Organisation (NOTTO) of India has brought attention to a significant gender disparity: 80% of organ recipients were male, while, 75% of organ donors were female. This phenomenon has become entangled in the complex existing web of intersectional discrimination in India, which deviates from the principles of justice and ...