Custom Essay, Term Paper & Research paper writing services
- testimonials
Toll Free: +1 (888) 354-4744
Email: [email protected]
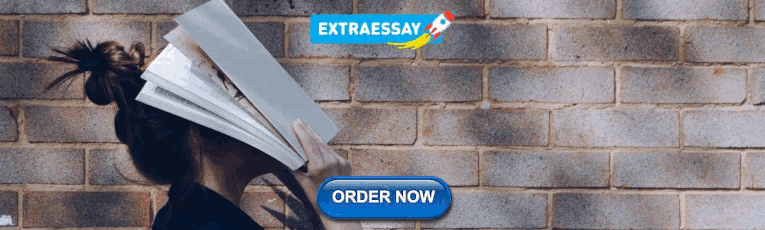
Writing custom essays & research papers since 2008
154 hot astronomy research topics for a-grade papers.
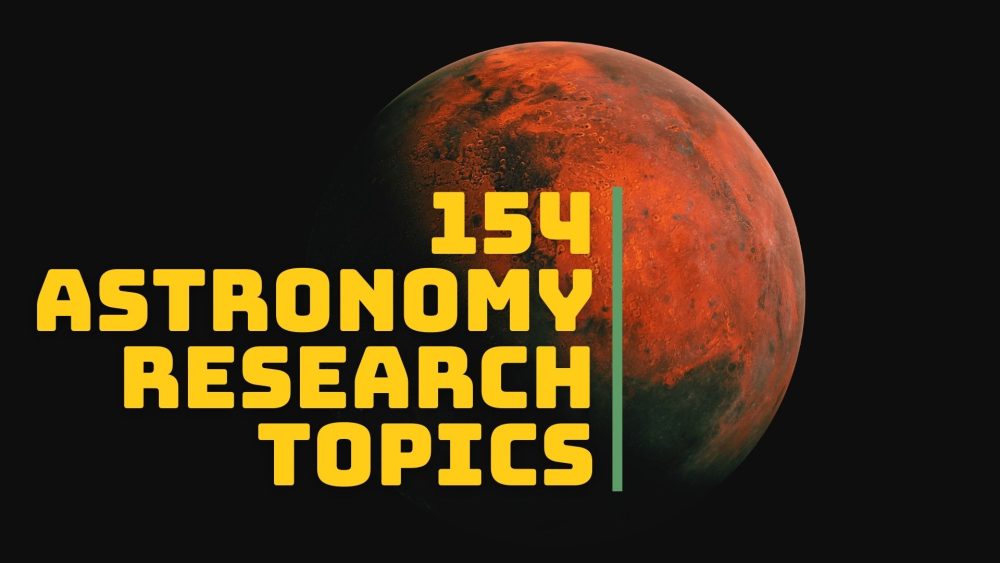
Do you have a college astronomy paper or essay and have been wondering how to get the best topic? You might also be stuck with the paper, wondering how to go about it.
The truth about astronomy is that getting interesting space topics is never easy, but how do you address the challenge? There is no need to worry anymore because we are here to help.
In this post, we list 154 astronomy paper topics and further highlight the traits of a great research paper. Why get content with standard or low-quality paper when you can get the best in your class by checking out the guide and topics, as well as getting lab report help ?
What Is Astronomy?
Before looking at the leading space science topics for your university assignment, let’s start with the definition. Astronomy is the study concerned with researching and understanding everything that takes place beyond the earth’s atmosphere. Although the advances in technology, especially on telescopes, satellites, and manned space vehicles, have helped people to peep deeper into space, this is just a scratch on the surface. There is a lot more waiting to be explored, including the controversial question, “Is there life on other planets?”
Characteristics Of A Good Astronomy Research Paper
From the definition of astronomy, it is clear that you can have a long list of astronomy project topics or ideas. Once you have picked the preferred option from our research topics in cosmology, the next step is preparing your paper. Here are the main characteristics of a good school research paper in astronomy:
- Systematic: This means that your research paper should be structured per clearly defined rules. So, students should start by reviewing requirements by their professors or teachers and think creatively of how to make their papers professional.
- Logical: This implies that the student carefully reasons all the points to ensure they support the selected topic. Although there is so much one can write on a specific topic, you must narrow it down to those points that are current and that support your topic.
- Comprehensive: Your paper also needs to be as comprehensive as possible. So, you must exhaustively identify the core points in a selected topic. It should also fit well in the current literature on the same topic, helping to advance the discipline.
- Plagiarism-Free: All universities out there have very strict rules on plagiarism. Therefore, your work must be 100% original.
- Clearly flowing points and free from errors: Finally, your paper should be arranged well to ensure that all the points flow logically from the start to the end. Again, it should be proofread to ensure it is free from errors.
Best Astronomy Essay Topics
Now that we have looked at the main characteristics of a high quality astronomy paper or essay, it is time to dig deeper into the main topics. Check out our list of the leading astronomy research topics for top grades.
Top Astronomy Research Topics
- What is the future of space exploration?
- A closer review of the big bang theory.
- Compare two theories that explain the origin of the universe.
- Stephen Hawking theories.
- Space Challenger disaster: What are the sociological impacts?
- A review of the recent space exploration breakthroughs.
- The moon landing.
- The Mars landing of space rovers.
- A deeper look at the history of astronomy.
- Reviewing the heliocentric model of the galaxy.
- Analyzing the lifecycle of a star.
- What impact does the moon have on the earth?
- Space debris and its impact on the solar system.
- What impact do humans have on the solar system?
- The rise of space tourism: What impact will it have on space exploration?
- Is space tourism a good thing?
- What could go wrong with space tourism?
- Space manufacturing: Is it a good thing?
- The mythologies associated with heavenly bodies.
- What impact do the stars have on earth?
Unique Astronomy Research Paper Topics
- A review of the Hubble telescope.
- A closer look at the Haley’s comet.
- Through the mind of early astronomers: Galileo Aristotle, and Ptolemy.
- What are the advantages of exploring space?
- The race to explore space and the cold war.
- Reviewing the first astronauts to visit the moon.
- What lessons did NASA learn from its first mission to the moon?
- Can life exist on the moon?
- What is the biggest difference between earth and moon?
- Explaining the earth’s outlook as viewed from space.
- The design of space vehicles: Are the modern models riskier compared to those used in the 20th century?
- What impact will private companies like SpaceX and Blue Origin have in astronomy?
- If we have a space station where scientists travel often, is the idea of space hotels far-fetched? A closer look at Blue Origin’s idea of a space hotel.
- Looking beyond the Milky Way.
- A review of Pluto: How does it compare to other planets?
- How does earth compare to Jupiter?
- Explain the sun’s source of heat and light for millions of years.
- Analyzing the rings of Saturn.
- A review of astronauts physical and health preparedness before setting off for space exploration.
- What effects does long stay in space have on the human body?
- What can astronauts do to reduce the danger of muscle atrophy?
- Zero gravity in space.
Awesome Astronomy Topics To Write About
- What are wormholes?
- A review of the evolution of space exploration changes in history.
- Speed of light travel: what are the implications?
- A closer look at time travel: Theory versus fiction.
- Zero gravity: What impact does it have on astronauts’ health over time?
- The interdisciplinary perspectives of space.
- Astrophysics: A review of the main controversies.
- Explore the possibility of having life on other planets.
- What implications would life on other planets have on planet earth?
- Think of yourself as an astronaut: What would be your reaction upon encounter with aliens?
- Stars and how people use them for navigation.
- Comparing different theories that explain the origin of life on planet earth.
- Space weather.
- How does space weather compare to the earth’s weather?
- Global warming: An astronaut’s view.
- The sun and its relationship with the earth.
- Comparing the sun’s relationship with Saturn and Pluto.
- Robotic space exploration: Is it a good idea?
- Constellations: A review of human interpretations.
- A review of emerging business opportunities in space.
- Space travel for non-astronauts: Is it a good idea?
- Comparing space travelling scientists to tourists: What is the difference?
Engaging Space Research Paper Topics
- What is the difference between planets and asteroids?
- How did the “northern lights” come about?
- Capture hypothesis: A review.
- What caused the Challenger to explode after take-off?
- The challenger shuttle disaster: A review of the preparations.
- What lessons did we learn from the challenger disaster?
- Was the Challenger the greatest failure in NASA’s history?
- Analyzing President Ronald Reagan’s speech after the Challenger disaster.
- Space Challenger disaster analysis: Why we are on the blink of another bigger and deadlier disaster.
- Are the current space policies ample to guide the new era of space travel?
- Dennis Tito: Looking at the experience of the first space tourists.
- Space politics: Is competition a good thing when it comes to space exploration?
- Reimagining the space: What would happen if we suddenly discovered that it was possible to inhabit the moon?
- Space will form the next generation combat zone for superpowers in competition for new resources.
- Factoring the distance and other logistics: Would mining in space be viable?
Great Space Exploration Topics
- A review of three biggest planets that orbit outside the solar system.
- Comparing the characteristics of gas planets to terrestrial planets.
- Fission Hypothesis by George Darwin.
- A review of the Giant Impact Theory.
- Exploring the theories that explain the origin of the moon.
- How long does it take for a new planet to form?
- Imagining a Marxist society living on Mars.
- Exploring the process of formation of the biggest stars in the cosmos.
- Is it possible for light to escape from the black hole?
- Determining the moon’s diameter: How accurate is the method used for calculation?
- Is the Big Bang Theory the best explanation of the origin of the universe?
- Reviewing the fate of the universe.
- Gravitational waves: Why their discovery is so important.
- Monitoring the State of the Environment using Ecologically Clean devices.
- Reviewing the doctrine of Noosphere.
- The legends of Starry Sky.
- The importance of mathematics in space explorations.
Astrophysics Research Topics For Debate
- Relativity theory and gravity.
- What is a variable star?
- Gravity and eclipse.
- Venus: Reviewing its formation.
- The mass of matter and nebulae.
- The Big Bang Theory.
- Brown Dwarf.
- Space manufacturing: What materials and products are manufactured in space?
- What happens during a solar eclipse?
- Celestial mechanics.
- Manned space shuttles.
Discussion Topics In Astronomy
- A thematic review of the heliocentric theory.
- The conflicting theories on the origin of the earth: science versus religion.
- Expecting the worst: What could go wrong with space missions?
- Is the cost and effort for space exploration worth it?
- Beyond the visible universe: What should we expect?
- How does the lunar cycle function?
- The dimensions of light in space: How does it differ after entering the earth’s atmosphere?
- What is astrophysics?
- Nonlinear, slow mode, and fast mode effects.
- Grand unification theories.
- What impact does the moon have on the oceans?
- The longitudes and latitudes of the earth.
- What are the different types of stars?
- The formation and destruction of clouds in the Galaxies.
- Meridian and transit circles: What are they?
- The galaxy cluster growth.
- A review of the molecular cloud.
Investigative Astrophysics Research Topics
- How long does it take to travel to space?
- Which is the most prominent of all planets? Is it earth?
- Survival on other planets? How to make it possible.
- Pluto should not be considered another planet: Discuss.
- Journey to Mars: Should we open it to all?
- Comparing the journeys to the International Space Station (ISS) and the Moon.
- Space keeps expanding: Explain.
- The best defense against killer asteroids.
- How to relate interplanetary matter with space activities.
- The 2012 transit of Venus: A detailed review.
- What do astronauts eat when travelling to space?
Controversial Astronomy Topics For Research Paper
- Militarization of space: Is it avoidable?
- Asteroid mining: Is it a good idea?
- A review of space exploration issues and connection to women.
- State exploration is very important: Approve or disapprove this statement.
- Colonizing other planets: Is it ethical?
- Terraforming on Mars.
- The security challenges of space explorations.
- Space exploration: Does it have any impacts on planet earth.
- Using VR and AR should replace humans for space explorations.
Other Cool Astronomy Topics
- The impact of the sun on water bodies.
- Interstellar extinction: What is the cause?
- What is the deep impact mission?
- Essential requirements for space travel.
- Preparing for space travel.
- Is there an earth-like planet that is habitable?
- Solar systems with two stars: How do they operate?
- Comparing the preparation for space tourists and astronauts.
- Where do asteroids come from?
- What is antimatter?
Seek Help From The Best Paper Writing Service
Now that we have listed the characteristics of a great research paper, are you ready to write the assignment? If you find it challenging, know that you are not alone. A lot of students find it tough because the topics are very broad and require a lot of research. “I need a paper written for me” is a thought of many, not just you. Well, no matter the reason why preparing the paper is challenging, you should seek help from online expert writers.
Our service works with professionals, and you can count on them to the best grade in your paper. They are native English writers with a lot of experience in writing custom astronomy papers. The service is also secure and trustworthy, implying that no one other than you can know that the paper was bought. The writers are also excellent in editing, cheap and fast. They will handle even your papers with very tight deadlines. All that you need is to visit our site and tell us to “ write my paper .”
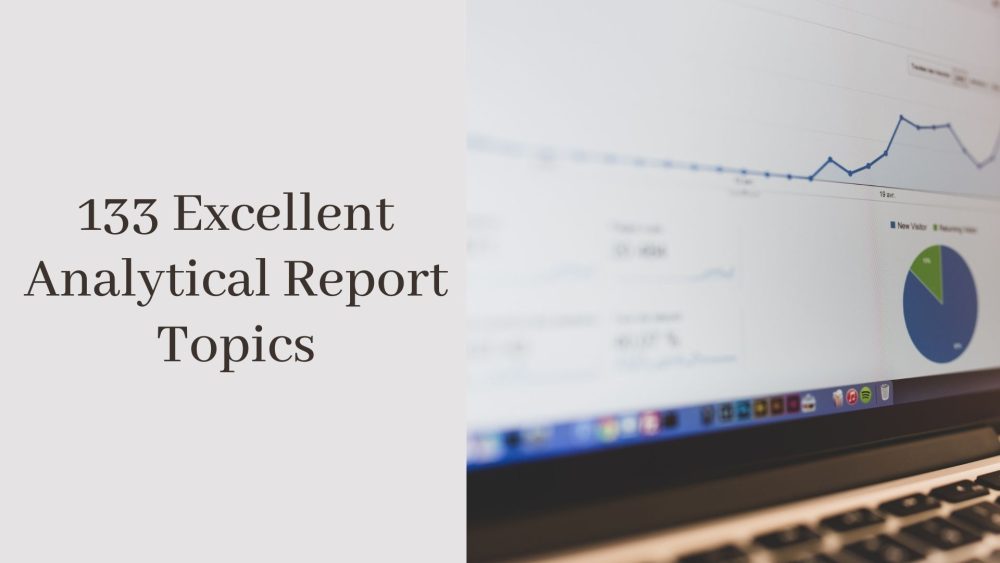
Thesis Helpers

Find the best tips and advice to improve your writing. Or, have a top expert write your paper.
Top 50 Astronomy Topics for Students
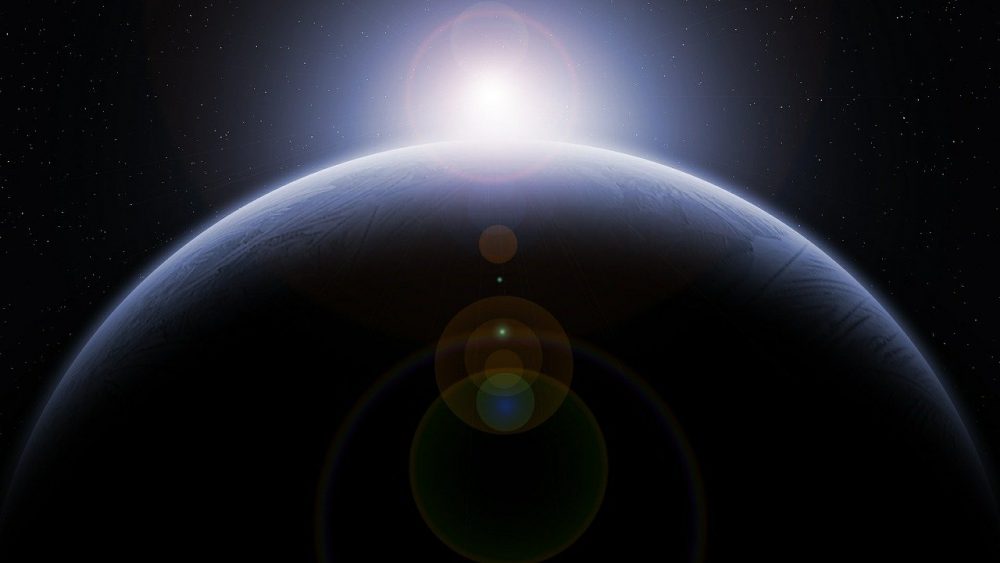
Let’s face it: astronomy topics are not easy to come by these days. And even when you find some, there is a very big chance they have been already taken by some of your classmates. Even finding some different simple topics to talk about in astronomy can prove to be a challenge. You definitely don’t want to write an essay on a topic that has been beaten to death already.
You want your topic to be of interest. You want it to be relevant and stand out from all the others. This is precisely why you need our list of excellent astronomy topics. We have 50 right here, and you can use them for free. Periodically, our thesis writers are updating the list to introduce new topics as frequently as possible.
Why Pick Only the Best Astronomy Research Topics?
OK, but why would you need to find the best astronomy research topics? The truth is that finding some excellent astronomy paper topics is one of the easiest ways to get a top grade. Here are some of the benefits of picking an idea from our astronomy topics list:
Your astronomy paper will be unique because nobody will probably think about the topic you choose. This means that your paper will stand out from the rest instantly. Professors love interesting astronomy ideas. In other words, they will read your paper from start to finish. Pique the interest of your professor and you will surely keep him reading. You can get bonus points on your astronomy research paper if the topic is original and highly interesting. Professors award students who demonstrate originality. Picking one of our astronomy topics to write about saves you a lot of time and effort. Why spend hours doing research when you can pick a topic and start writing right now?
One more thing you need to keep in mind though is that even the best of astronomy research topics can’t save you from a low grade if you don’t write the paper the right way. The topic can earn you some bonus points, but your professor will look for many other things in your essay. Make sure you know how to write a proper essay or, if you don’t, get some quick help from our degree-holding ENL writers.
Where to Search for Topics in Astronomy
There are several places where you can find astronomy essay topics, of course. One can try the library and read astronomy journals and papers until he or she finds a good idea. Another interesting choice would be to ask around on forums and social media. Maybe somebody will help you. Of course, you can also search for astronomy project topics online, but your classmates have probably beaten you to the best ones out there.
Our list of topics of astronomy is updated periodically, so there is a very big chance your peers have missed at least some of the topics. Our academic writers have extensive experience writing astronomy essays, so our supply of topics is virtually endless.
Most Interesting Astronomy Topics
If you want to write an excellent essay, you need only the most interesting astronomy topics. Check out these great topics:
- Methods used to detect exoplanets today.
- What lies beyond our Milky Way?
- What happens during a Sun eclipse?
- The full history of manned space flight.
Astronomy Topics for Research Projects
Are you looking for astronomy topics for research projects? We have the best solution for you. Pick one of our topics below:
- Determining the angular diameter of the Moon (experiment).
- How does the Sun affect our water bodies?
- Latest space tech and breakthroughs.
- Why are Musk’s Startlink satellites important?
Fresh Astronomy Paper Topics
Even though all our astronomy topics are updated for 2020, here are some new, fresh astronomy paper topics for you:
- Listening to the echoes of a solar storm.
- Legends that involve the start in the sky.
- How did our planet came to be?
- What causes a star to go supernova?
- Latest developments in NASA’s Shuttle Program.
Cool Astronomy Topics
Do you want your astronomy essay to stand out from the rest? Pick one of our cool astronomy topics:
- Here is how the universe is constantly expanding.
- Can we discover a way to travel in time?
- How do the Mars rovers work?
- Problems with satellite communications.
Astrophysics Essay Topics
Astrophysics is a very difficult field, we know. You need the best topics possible for a top grade. Here are some astrophysics essay topics for you:
- What is dark matter and where can it be found?
- Why are moons orbiting planets?
- The light spectrum of stars in distant galaxies.
- Can physics find what we call the multiverse?
Complex Astronomy Project Ideas
If you want to write a complex paper that will earn you a very good grade, you need to see our amazing list of astronomy topics for projects:
- The ins and outs of robotic space exploration.
- The future of space exploration.
- Making business in space.
- Define and explain star clusters.
- What does the Mars colonization mean for the human race?
Easy Astronomy Science Project Ideas
Maybe you don’t want to spend a few days writing the astronomy essay. Here are some easy astronomy science project ideas that will help you write it faster:
- How is a star born?
- Proof that supports the Big Bang theory.
- Searching for life on other planets.
- The effects of the Sun on our planet.
- Satellites and 5G wireless data transfer.
Best Astronomy Topics for Presentation
Our writers have selected the absolute best astronomy topics for presentation. Pick any of them and use it for free:
- This is how the Universe was born.
- Top 3 reasons we need to explore our solar system.
- Why is Pluto not a planet?
- Kepler’s contribution to astronomy.
Astronomy Topics for College Students
Our astronomy topics for college students are a bit more difficult, but are still relatively easy to research and write. Pick one now:
- The process of planet melting.
- Calculating the mass and density of our solar system.
- Why time-travel is impossible to discover.
- How did ancient humans see the most important constellations?
- What is an exoplanet?
Excellent Astronomy Research Paper Topics
If you need to write a research paper on astronomy, you definitely need to take a look at our list of excellent astronomy research paper topics:
- The slow process of black hole birth.
- What does it take for humans to survive on Mars?
- Did we really land on the Moon?
- The life of an unremarkable star.
- Is light-speed travel possible in the near future?
Astronomy Topics for High Schools Students
If you are in high school, we have some easier topics for you. Pick one of our astronomy topics for high schools students and start writing:
- What causes light pollution?
- Why are some of the galaxies “active”?
- Copernicus’s heliocentric model.
- What is dark energy?
If you didn’t find the topics in astronomy you were looking for on this page, explore more academic topics . You can also return to the blog post later because we update the topics frequently. However, your best option would be to get in touch with us directly. Our academic writers can make a list of 100% original topics for you in no time. And the best part is that these people know exactly what your professor wants. Order a fresh list of interesting astronomy topics and get a top grade on your next essay!
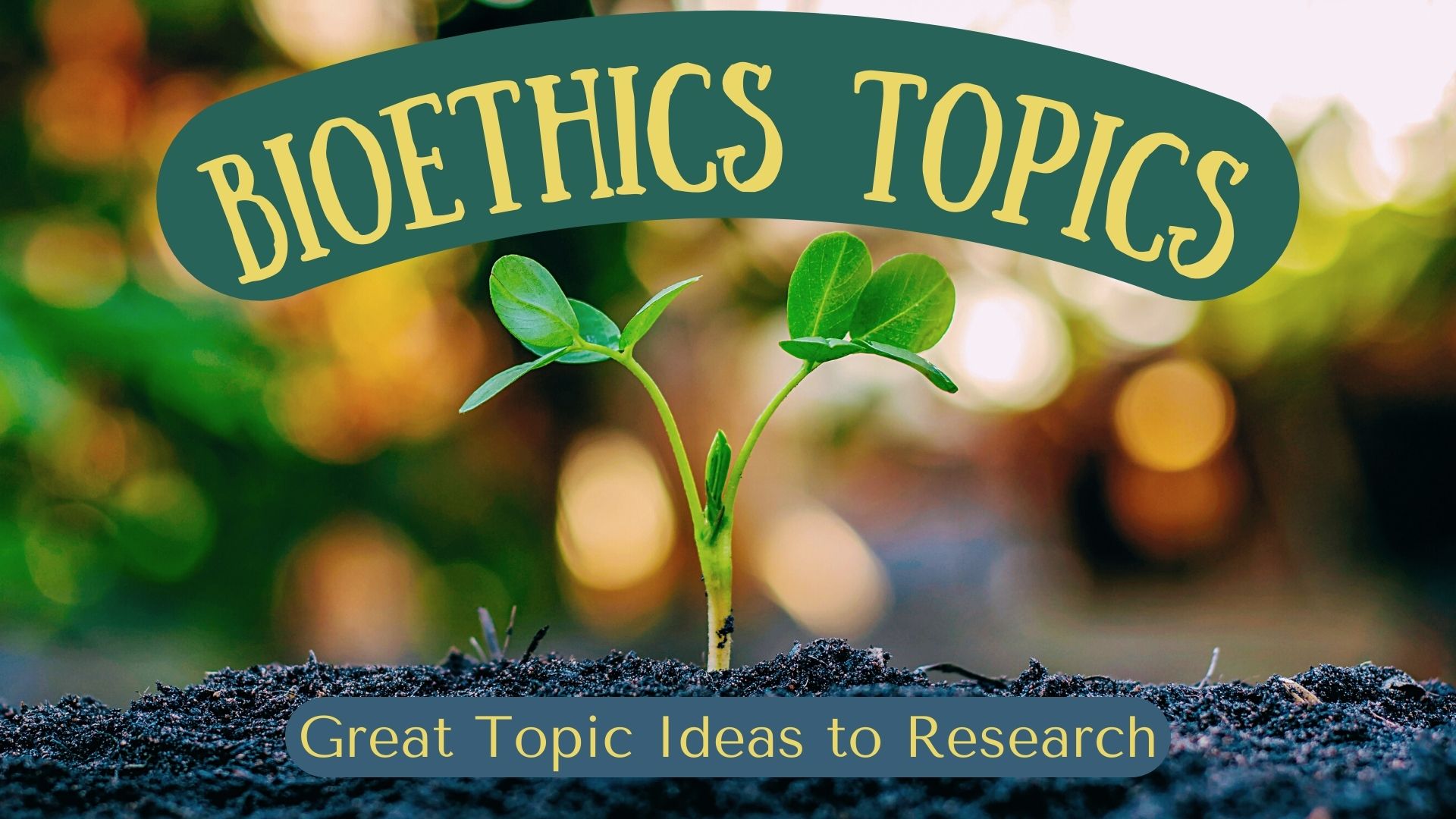
Make PhD experience your own
Leave a Reply Cancel reply
Your email address will not be published. Required fields are marked *
- How It Works
- PhD thesis writing
- Master thesis writing
- Bachelor thesis writing
- Dissertation writing service
- Dissertation abstract writing
- Thesis proposal writing
- Thesis editing service
- Thesis proofreading service
- Thesis formatting service
- Coursework writing service
- Research paper writing service
- Architecture thesis writing
- Computer science thesis writing
- Engineering thesis writing
- History thesis writing
- MBA thesis writing
- Nursing dissertation writing
- Psychology dissertation writing
- Sociology thesis writing
- Statistics dissertation writing
- Buy dissertation online
- Write my dissertation
- Cheap thesis
- Cheap dissertation
- Custom dissertation
- Dissertation help
- Pay for thesis
- Pay for dissertation
- Senior thesis
- Write my thesis
170 Fantastic Astronomy Topics For High Scoring Thesis
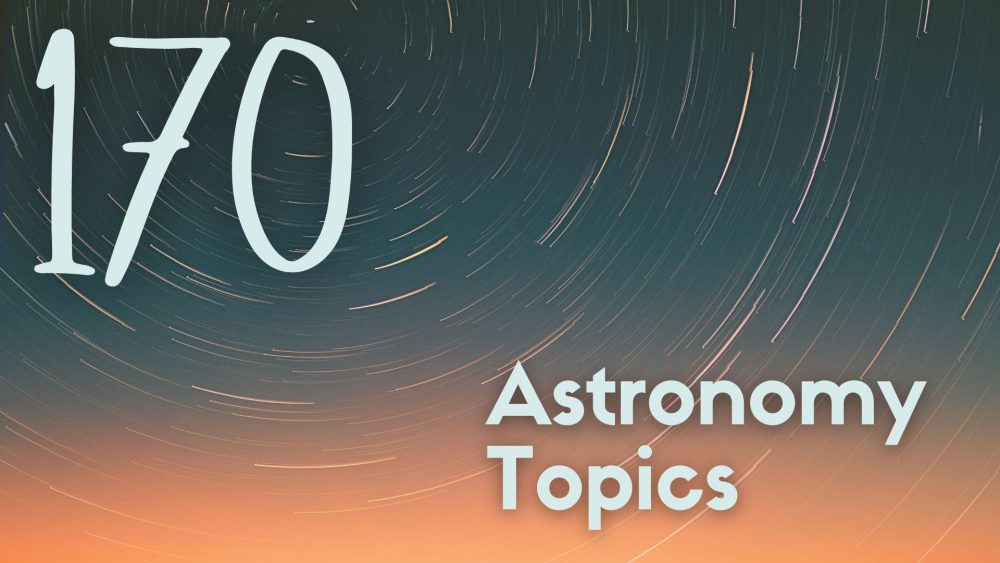
Astronomy, which is the study of celestial objects and phenomena, has indeed captured the imaginations of humanity since time immemorial. From unraveling the mysteries of distant galaxies to investigating the nature of dark matter, astronomy, interestingly, indeed encompasses a wide range of exciting research topics.
But before we look at a wide range of excellent astronomy-related research topics that college students, professors, teachers, and other professionals will find useful, let us take a small detour to what astronomy really is.
What Is Astronomy?
Astronomy is the scientific discipline that essentially focuses on the study of celestial objects such as stars, planets, galaxies, and other phenomena in the universe. In other words, astronomy is the observation, theoretical modeling, and interpretation of astronomical data for the purposes of deepening our understanding of the cosmos.
Characteristics Of A Good Astronomy Research Paper
Here are some characteristics of a good astronomy paper:
Originality : A good astronomy research paper should provide unique insights and be able to address unanswered questions.
Clarity : A good astronomy research paper should be able to simplify and communicate complex concepts effectively.
Scientific-rigor : A good astronomy research paper should be able to present a well-defined methodology and provide reliable data.
Relevance : A good astronomy research paper should be relevant. It should be able to explore emerging areas of study or address current astronomical issues.
Contribution : A good astronomy research paper should be able to advance existing knowledge and provide avenues for further research.
Astronomy Research Topics
Astronomy research topics, in a nutshell, encompass various research areas within astronomy. These research areas include but are not limited to: the study of gravitational waves, extraterrestrial intelligence, magnetic fields in celestial objects, stellar evolution, and high-energy astrophysics. Here are great astronomy research topics to consider for your school or university paper:
- Gravitational waves and their impact on Astronomy
- Stellar evolution and life cycle of stars
- The role of magnetic fields in shaping celestial objects
- The role of magnetic fields in star formation
- Origin and evolution of supermassive black holes
- Impact of Space weather on Earth’s atmosphere
- Investigating the nature of fast radio transients
- Evolution of galaxies in different environments
- Probing the intergalactic medium using quasar absorption lines
- Cosmic rays and their effects on astronaut health
- Detection and characterization of fast radio bursts
- Study of Exomoons: Moons orbiting exoplanets
- High energy astrophysics and cosmic rays
Astronomy Topics
These topics cover a wide range of astronomical subjects such as galaxies and their evolution, planetary atmospheres, the study of asteroids and comets, phenomena like gamma-ray bursts, and the formation of the solar system to mention a few. Students who need dissertation help will find the following topic suggestions interesting:
- The formation of the solar system
- Exploring the mysteries of gamma-ray bursts
- Planetary atmospheres and climate change
- The study of asteroids and comets
- Types, interactions, and evolutions of galaxies
- Stellar populations in different galactic environments
- Dynamics and formation of globular clusters
- The role of black holes in galaxy evolution
- Exploring the cosmic microwave background radiation
- Probing the origins of ultra-high energy cosmic rays
- The formation and evolution of planetary nebulae
- Investigating the origins of First Radio Bursts (FRBs)
- The interstellar medium and star formation
Space Research Paper Topics
These focus on topics related to space exploration and observations. Some high-quality interesting topics that native writers, students, and class teachers will find useful include:
- Space telescopes and their contribution to astronomy
- The study of space weather and its effects on earth
- Investigating the mysteries of black holes using space-based observatories
- Space missions to explore Mars and other planets
- The search for exoplanets and habitable environments
- Space debris: Assessment, mitigation, and future challenges
- Space tourism: potential market growth and safety regulations
- Space weather and its impacts on Earth’s technological systems
- Asteroid mining: Opportunities, challenges, and ethical considerations
- Interplanetary dust and its significance for planetary science
- Space colonization: Challenges and prospects for human settlement on other planets
- Lunar exploration and resource utilization for sustainable space missions
- Space telescopes: advancements in observational astronomy
Interesting Astronomy Topics
These topics delve into the intriguing aspects of astronomy. Here are interesting astronomy topics to consider:
- The concept of time in astronomy and relativity
- Exploring the origins of cosmic rays
- The possibility of life on other planets
- Pulsars: Nature’s most precise cosmic clocks
- Astrobiology: The study of life in the universe
- Studying the relationship between supermassive black holes and galaxy evolution
- Probing the origins and composition of interstellar dust
- Investigating the mystery of dark energy in the universe
- Unveiling the secrets of neutron stars and pulsars
- Investigating the role of magnetic fields in star formation and stellar evolution
- Examining the dynamics of composition of planetary atmospheres in our solar system and beyond
- Understanding the processes and consequences of stellar explosions (supernovae)
- Investigating the potential habitability of exoplanets and the search for life beyond earth
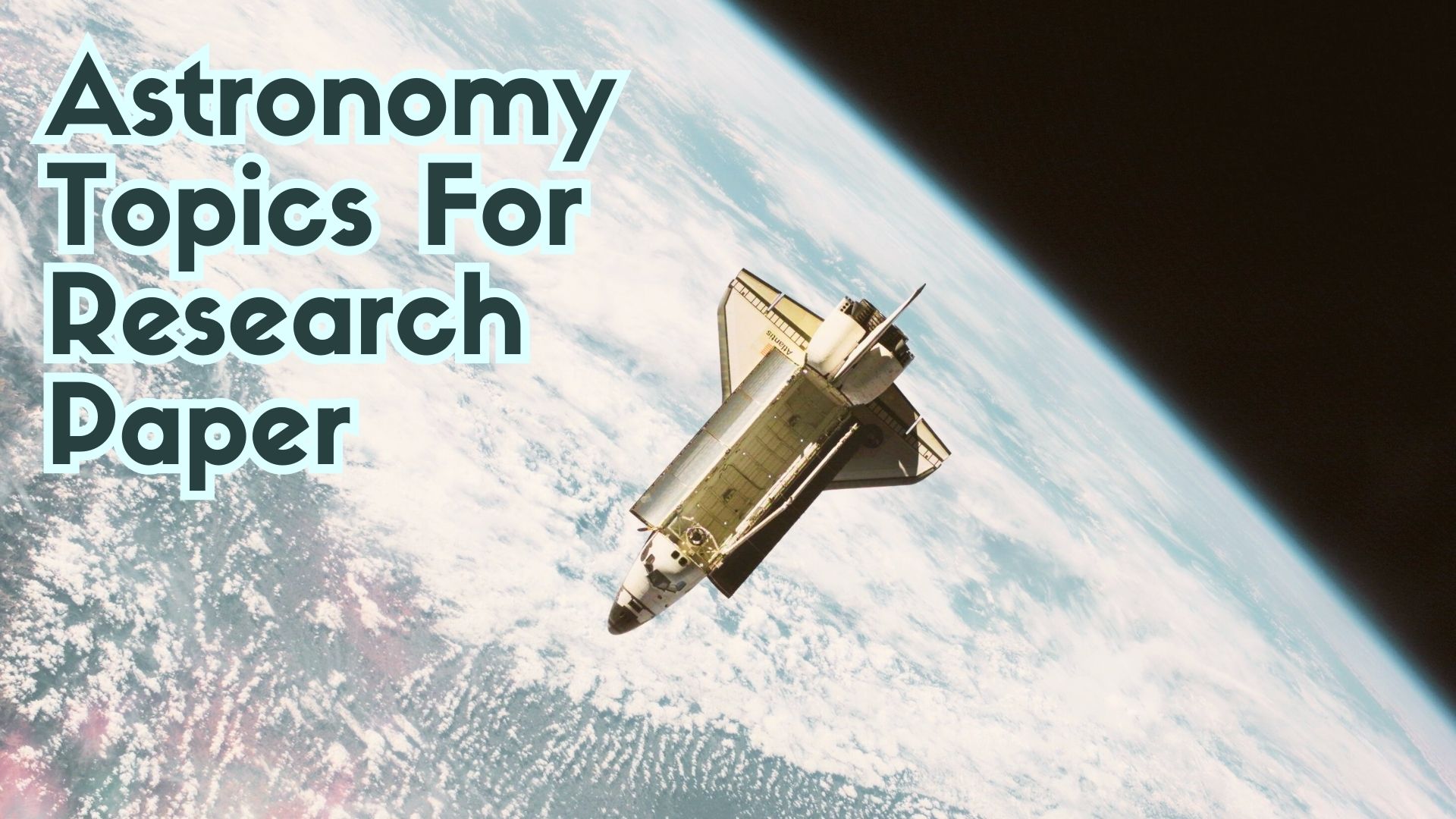
Astronomy Topics For Research Paper
This particular category suggests topics that are suitable for research papers in astronomy. Professionals, online writers, class teachers, college and university students, as well as professors will find the following topics excellent:
- The role of dark matter in galaxy formation
- Investigating the nature of dark energy
- Probing the mysteries of neutron
- Supernovae and their importance in the universe
- The discovery and characterization of exoplanets
- Stellar evolution and supernovae: Investigating the life cycles of stars, including their birth, evolution, and explosive deaths as supernovae
- The search for primordial black holes
- Stellar abundances: Tracing chemical evolution
- Interstellar medium: Composition, dynamics, and evolution
- Gravitational lensing: Probing the mass distribution of galaxy clusters
- Protoplanetary disks and planet formation
- Dwarf galaxies: formation and dark matter content
- Supernovae Remnants: probing stellar explosions
Space Science Topics
These topics encompass a broad range of scientific investigations related to space. Interesting topics include:
- Studying the dynamics of planetary atmospheres and weather patterns
- The exploration of moons in our solar system
- Exploring the nature of gravitational interactions in multiple star systems
- Investigating the nature of black hole mergers
- Investigating the origins of cosmic magnetic fields
- The role of space weather in satellite communications
- The study of planetary geology and surface features
- Studying the atmospheres of exoplanets using spectroscopy
- Probing the mysteries of white dwarfs and their evolutionary paths
- Exploring the possibilities of asteroid mining
- Understanding the formation of planetary rings
- The study of space debris and its impact on space exploration
- Investigating the physics of solar flares and coronal mass ejections
Astrophysics Research Topics
This particular topic focuses on research topics within astrophysics which entail the study of celestial objects and phenomena. The best topics to consider are:
- Understanding the physics of gamma-ray astronomy and its implications
- Probing the physics of stellar explosions: Supernovae and gamma-ray bursts
- Exploring the properties of interplanetary magnetic fields and solar wind
- The study of high-energy astrophysical phenomena: Ray binaries and pulsars
- Investigating the physics of stellar oscillations and asteroseismology
- Understanding the physics of accretion disks around black holes
- The study of interstellar turbulence and its impact on star formation
- Investigating the nature of dark energy through cosmic microwave background
- Understanding the dynamics of galactic collisions and mergers
- Exploring the mechanisms behind stellar magnetic fields and activity
- Probing the physics of gravitational waves and their sources
- The study of relativistic jets from active galactic nuclei
- Investigating the role of magnetic fields in star formation processes
Space Exploration Topics
These are topics that revolve around the exploration of space and entail:
- The future of human colonization of Mars and other celestial bodies
- Investigating the formation and evolution of planetary systems beyond our solar system
- Investigating the potential for life on moons of gas giants
- Exploring the potential for resource utilization on the moon and asteroids
- The study of space tourism and its challenges
- The study of space-based gravitational wave detectors
- Exploring the possibilities of interstellar travel and propulsion systems
- Understanding the challenges and prospects of manned deep space missions
- The role of robotic missions in planetary exploration
- Investigating the feasibility of asteroid deflection strategies
- Investigating the potential for terraforming other planets
- Exploring the mysteries of the outer heliosphere and heliopause
- The study of space-based telescopes and observatories
Research Topics In Cosmology
This particular category explores research topics within cosmology which is essentially the study of the origin of evolution and the structure of the universe. Some good topics to consider for scientific thesis writing include:
- Understanding the nature and properties of cosmic neutrinos
- The study of the cosmic microwave background radiation and its implications
- Exploring the physics of dark energy and its implications
- Understanding the large-scale structure of the universe
- Investigating the Role of baryonic acoustic oscillations in Cosmology
- Investigating the nature of primordial gravitational waves
- The study of the early universe and the era of recombination
- Exploring the possibilities of a multiverse
- Probing the nature of cosmic strings and other topological defects
- The study of cosmic voids and their impact on galaxy formation
- Investigating the physics of inflationary models
- Understanding the formation and evolution of cosmic filaments
- Probing the nature and properties of dark matter candidates
Astronomy Topics To Write About
If you are a student looking for the best astronomy topics to write about, here are excellent suggestions:
- The formation and evolution of galaxies
- The study of cosmic rays and high-energy particles
- The life cycle of stars: From birth to death
- Investigating the origins and evolution of the solar system
- The search for extraterrestrial intelligence (SETI)
- The Impact of Asteroids collisions on Earth’s History
- The Role of dark matter in the Universe
- Exploring the magnetic fields of celestial objects
- Exoplanet discoveries and characterization
- The structure and dynamics of spiral galaxies
- The nature of black holes: Mysteries and discoveries
- The detection and study of gravitational waves
- The Origins of the Universe: big bang cosmology
Cool Astronomy Topics
- Time dilation: The effects of relativity on observations
- Stellar Archeology: Unraveling the Secrets of old stars
- Stellar Nurseries: birthplaces of new stars
- The evolution of galaxies in galaxy clusters
- Exoplanet atmosphere: Clues for habitable worlds
- Strange Planets: Exotic discoveries beyond our solar system
- The Origins of Fast Radio Bursts (FRBs)
- The potential existence of a parallel universe
- The Kuiper Belt: Exploring the Icy Worlds Beyond Neptune
- Exploring supermassive black holes at the centers of galaxies
- Quasars: cosmic powerhouses and lighthouses
- The surprising behaviors of pulsars and magnetars
- The great attractor: Unveiling the Mysteries of cosmic flows
Interesting Space Topics
- Space debris: challenges and solutions for orbital cleanup
- Space mining: opportunities and ethical considerations
- Space-based telescopes: Expanding our view of the universe
- Space tourism: commercial ventures beyond Earth’s atmosphere
- Space weather: impacts on satellite communications and Earth’s environment
- The James Webb space telescope: A new era of observing the cosmos
- The search for water on Mars and its implication for life
- Mars colonization: challenges and benefits of establishing a human presence
- The lunar gateway: NASA’s Gateway to deep space exploration
- Astrobiology: Investigating the potential for life in extreme environments
- Space elevators: novel concepts for space transportation
- The Fermi Paradox: why haven’t we found extraterrestrial life yet?
- Planetary protection: safeguarding terrestrial bodies from contamination
Topics In Astronomy
- Stellar evolution: from protostars to supernovae
- The role of spiral density waves in shaping galaxies’ structures
- Galactic dynamics: The structure and evolution of galaxies
- The study of globular clusters: ancient stellar systems
- Observational techniques in astronomy: from telescopes to space missions
- White dwarfs and planetary nebulae: The final stages of stellar evolution
- Cosmic microwave background radiation: clues to the early universe
- Gamma-ray bursts: The most energetic explosions in the universe
- The Hertzsprung-Russell diagram: understanding stellar properties
- The Drake equation: estimating the number of technologically advanced civilizations
- The Doppler effect: Measuring the motion of celestial objects
- The role of interstellar dust in the absorption and scattering of light
- Variable stars: exploring the changing brightness of astronomical objects
- Astrochemistry: Understanding the chemistry of space
In conclusion, astronomy offers a vast realm of research possibilities ranging from the mysteries of dark matter and dark holes to the explorations of exoplanets. We understand that the field of astronomy can be a complex area to write a research paper on as a student. If you experience difficulties and look for someone to write a thesis for you , we do custom astronomy research papers, editing, and deliver them within no time. Whether you want a research paper or need thesis help, we have professional writers at your beck and call. We are cheap, fast, and trustworthy, and deliver high-quality and well-written research papers at a moment’s notice.
Make sure to check our posts with other topics before you leave:
- 163 Unique Artificial Intelligence Topics For Your Dissertation
- 121 Original Neuroscience Research Topics
- 211 Of The Most Interesting Earth Science Topics
- 214 Best Big Data Research Topics For Your Thesis
- 211 Interesting Engineering Research Paper Topics
- Experiment vs Observational Study: A Deeper Look
- 101 Best Computer Science Topics
What is the Big Bang Theory?
The big bang theory suggests that the universe originated from an extremely hot and dense state around 13.8 billion years ago. It goes further to explain the expansion and evolution of the cosmos including the formation of the stars, the galaxies, and the planets.
What is a black hole?
A black hole refers to a region in space with an intense gravitational pull that prevents anything, including light, from escaping. A black hole is formed when massive stars collapse under their own gravity forming a singularity surrounded by an event horizon.
What are exoplanets?
Exoplanets refer to planets that exist outside our solar system, orbiting stars other than the sun. The discovery of exoplanets has totally changed our understanding of the planetary system and the potential for habitable worlds beyond the Earth.
What is a dark matter?
Dark matter refers to a hypothetical form of matter that doesn’t interact with light or other electromagnetic radiation. It is generally inferred from its gravitational effects on visible matter and plays an instrumental role in explaining the dynamics of galaxies and the large-scale structure of the universe.
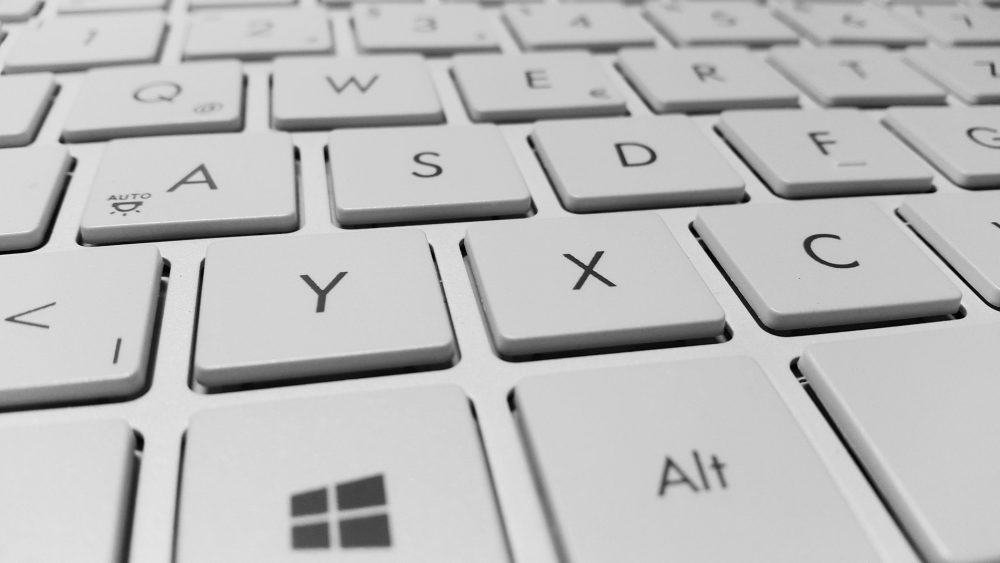
Leave a Reply Cancel reply
Your email address will not be published. Required fields are marked *
Comment * Error message
Name * Error message
Email * Error message
Save my name, email, and website in this browser for the next time I comment.
As Putin continues killing civilians, bombing kindergartens, and threatening WWIII, Ukraine fights for the world's peaceful future.
Ukraine Live Updates
185 Astronomy Research Topics & Interesting Ideas
18 January 2024
last updated
Astronomy research topics encompass a wide range of fascinating themes. These topics include cosmic microwave background study to explore the universe’s origins and exoplanetary research to identify potentially habitable worlds. People can also investigate dark matter and energy investigations, which attempt to explain the unobservable 95% of the universe. They can study possible extraterrestrial life forms or examine star lifecycles and behaviors. Moreover, scientists can provide new insights into celestial collisions or learn about celestial bodies through radio frequency analysis. In turn, astronomy research topics continue to shape the human understanding of the cosmos, challenging perceptions and reshaping the existing knowledge of the universe.
Best Astronomy Research Paper Topics
- Evidence Supporting the Multiverse Theory
- Black Holes: Exploring their Role in Galaxy Formation
- Life Beyond Earth: A Scientific Search for Extraterrestrial Existence
- Analyzing Cosmic Microwave Background Radiation: Insights Into the Big Bang
- Terraforming Mars: Theoretical Approaches and Practical Limitations
- Stellar Evolution: Unraveling the Life Cycles of Stars
- Space-Time Warps: Understanding the Theory of General Relativity
- Venus’ Atmosphere: A Comprehensive Study on Its Composition and Climate
- Jupiter’s Moons: Potential Habitability and Life-Sustaining Conditions
- Dark Matter: Its Inferred Presence and Theoretical Implications
- Impacts of Solar Flares on Earth’s Electromagnetic Field
- Kepler Mission Findings: A Closer Look at Exoplanets
- Pulsars: Nature’s Precise Cosmic Clocks
- Probing Into the Enigma of Quasars
- Dark Energy: Its Role in the Accelerated Expansion of the Universe
- Astrobiology: Defining the Parameters of Life in the Universe
- Significance of the Hubble Constant in Cosmology
- Cosmochemistry: Understanding the Elemental Abundance in the Universe
- Gravitational Lensing: Exploring the Mass of the Universe
- Asteroids: Evaluating the Risk and Potential Resources
- Interstellar Medium: Understanding Its Role in Star Formation
Easy Astronomy Research Paper Topics
- Dwarf Galaxies: A Key to Understanding Galaxy Formation
- Unraveling the Mystery of Fast Radio Bursts
- Theories and Evidence of Cosmic Inflation
- Habitability of Titan: A Potential Cradle for Life
- Tidal Forces: Impact on Planetary Bodies and Their Moons
- White Dwarfs: Windows Into Stellar Death
- Magnetars: Examining the Strongest Magnetic Fields in the Universe
- Comets: Probes Into the Early Solar System
- Impacts of Space Weather on Satellite Operations
- Neutrinos: Ghost Particles of the Universe
- Exploring the Dynamics of Binary Star Systems
- Red Giants: Understanding the Late Stages of Stellar Evolution
- Cosmic Rays: Origin and Implications for Earth’s Atmosphere
- Gamma-Ray Bursts: The Universe’s Most Luminous Explosions
- Active Galactic Nuclei: Powerhouses of the Universe
- Cepheid Variables: Standard Candles in Distance Measurement
- Exoplanet Atmospheres: Hints of Alien Life
- Neutron Stars: Compact Objects with Extreme Conditions
- Oort Cloud: The Outermost Boundary of the Solar System
- Supernovae: Cataclysmic Endings of Stellar Lives
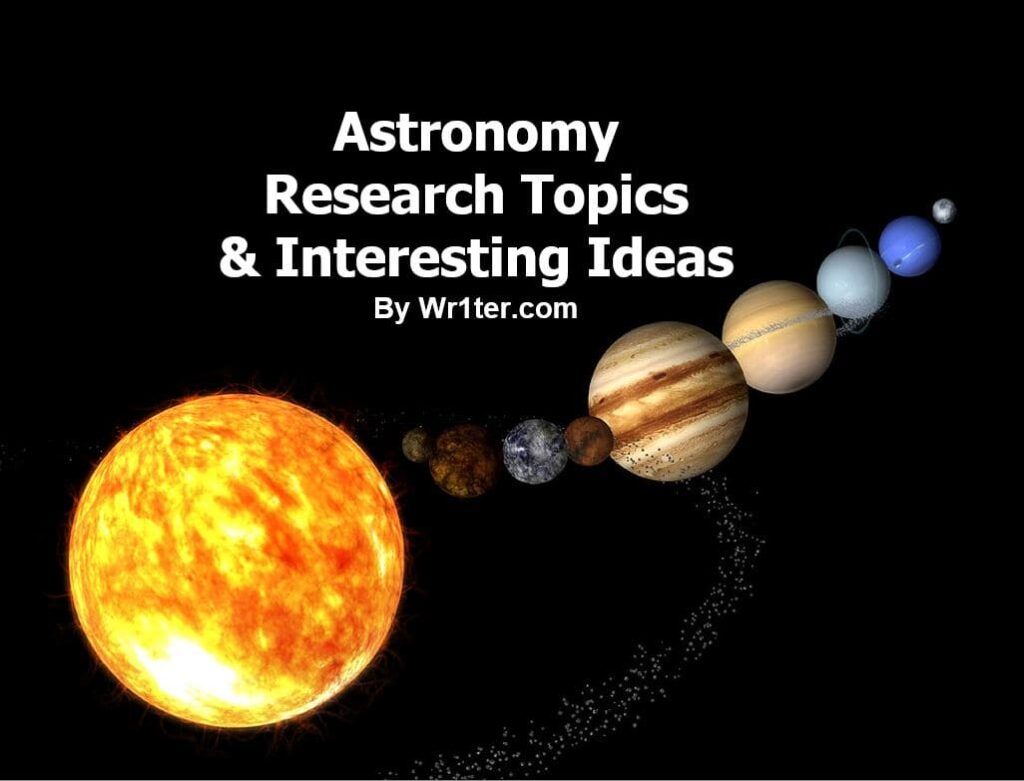
Interesting Astronomy Research Paper Topics
- Mercury’s Magnetic Field: Puzzling Aspects and Their Causes
- Radio Astronomy: Unveiling the Invisible Universe
- Spectroscopy: Decoding the Chemical Composition of Distant Stars
- Theories on the End of the Universe: Heat Death, Big Rip, or Something Else?
- Evolution of Galactic Structures in the Universe
- Roles of Dark Matter in Cosmic Structure Formation
- Enceladus: Investigating Potential Subsurface Life
- Interplay Between Cosmic Rays and Supernovae
- The James Webb Space Telescope: Anticipated Discoveries
- Theories on the Origin of the Moon
- Astrophysical Jets: High Energy Phenomena in the Universe
- Circumstellar Disks: Birthplaces of Planetary Systems
- Large Scale Structures in the Universe: Clusters and Superclusters
- Astronomical Impact on Earth’s Climate: A Long-Term View
- The Mystery of Ultra-High-Energy Cosmic Particles
- Supermassive Black Holes: Formation and Influence on Galaxy Evolution
- Quantum Gravity: Bridging the Gap Between Quantum Mechanics and General Relativity
- Investigation of Potential Life on Europa, Jupiter’s Ice Moon
- Kuiper Belt Objects: Probing the Edges of Our Solar System
- Merging Neutron Stars: Producers of Heavy Elements
- The Interplay of Gravitational Waves and Binary Black Holes
Astronomy Topics for High School
- Significance of Mars Colonization: A Comprehensive Study
- Investigating the Potential for Life on Jupiter’s Moon, Europa
- Pulsars: Lighthouses of the Universe
- Venus: The Hottest Planet’s Unique Atmosphere and Climate
- The Formation and Lifecycle of Stars
- Supermassive Black Holes: Engines of Galactic Centers
- Astrobiology: The Search for Extraterrestrial Life
- Comet Composition: Clues to the Early Solar System
- Harnessing Solar Energy: The Sun’s Role in Future Power
- Astrophotography: Capturing the Universe’s Stunning Phenomena
- Saturn’s Rings: Origin, Composition, and Evolution
- Dwarf Planets in the Solar System: An In-Depth Analysis
- Galactic Evolution: The Life and Death of Galaxies
- The Role of Telescopes in Shaping Modern Astronomy
- Supernova Explosions: Catastrophes that Shape the Universe
- The Impact of Space Debris on Earth’s Orbit
- Aurora Borealis: Unveiling the Northern Lights’ Secrets
- Exoplanets: The Hunt for Alien Worlds
- The Mystery of Fast Radio Bursts: A Cosmic Enigma
Astronomy Research Topics for College Students
- Origins of Galactic Structure: Unraveling the Formation of Spiral Galaxies
- Investigating Exoplanetary Systems: Analyzing Planetary Dynamics and Habitability
- Exploring Black Holes: Probing the Mysteries of Gravitational Singularities
- Unveiling the Secrets of Dark Matter: A Comprehensive Study on its Nature and Distribution
- Stellar Evolution: Tracing the Life Cycle of Stars From Birth to Death
- The Search for Extraterrestrial Intelligence: Examining Strategies and Technologies
- Analyzing Cosmic Microwave Background Radiation: Insights Into the Early Universe
- Solar Flares and Space Weather: Understanding the Impact on Earth and Astronauts
- The Great Attractor: Investigating the Mysterious Force Shaping the Local Universe
- Mapping the Large-Scale Structure of the Universe: Cosmological Surveys and Analysis
- Unraveling the Mystery of Fast Radio Bursts: Origins and Characteristics
- Interstellar Medium: Studying the Cosmic Material Between Stars and Its Influence
- Unveiling the Dark Energy: Constraints on its Nature and Implications for the Universe
- Stellar Archaeology: Examining Ancient Stars to Understand Galactic History
- Investigating the Kuiper Belt and Oort Cloud: Origins and Composition of Trans-Neptunian Objects
- Understanding Gamma-Ray Bursts: Probing the Most Energetic Explosions in the Cosmos
- Asteroid Mining: Assessing Feasibility and Implications for Future Space Exploration
- Proxima Centauri b: Analyzing the Exoplanet’s Potential for Habitability
- Galactic Collisions: Studying the Interactions and Mergers of Galaxies
- Quantum Cosmology: Examining the Role of Quantum Mechanics in the Early Universe
Astronomy Research Topics for University
- The Formation of Planetary Systems: Insights from Protoplanetary Disks
- Supernovae: Investigating the Explosive Deaths of Massive Stars
- Stellar Populations: Understanding the Diversity of Stars in Different Environments
- Space Telescopes: Advancements in Observation Techniques and Instrumentation
- The Transient Universe: Tracking and Analyzing Variable Celestial Objects
- Pulsars: Unraveling the Physics of Neutron Stars and Their Emissions
- Astrochemistry: Investigating the Chemical Processes in Space
- Cosmic Rays: Origins, Composition, and Effects on Astronomical Objects
- Exploring the Dynamics of Galactic Nuclei: Central Supermassive Black Holes
- The Role of Tidal Forces in Shaping Celestial Bodies: Tidal Interactions in the Solar System
- Galactic Archaeology: Tracing the Formation and Evolution of the Milky Way
- The Dark Side of the Moon: Unraveling Lunar Mysteries and Exploration Potential
- Supernova Remnants: Probing the Aftermath of Stellar Explosions
- Exoplanet Atmospheres: Characterizing Composition and Detecting Biosignatures
- Gravitational Waves: Detecting and Analyzing Ripples in Space-Time
- The Role of Magnetic Fields in Astrophysical Jets: Origin and Propagation
- Cosmic Inflation: Examining Early Universe Expansion and its Observational Consequences
- The Galactic Habitable Zone: Assessing Conditions for Life in the Milky Way
- Interplanetary Dust: Investigating Its Origin and Effects on Planetary Environments
- Solar System Dynamics: Orbital Evolution and Stability of Celestial Bodies
Astrophysics Essay Topics & Ideas
- Unraveling the Mystery of Dark Matter in the Universe
- Black Holes: Engines of Destruction or Creation?
- Implications of Einstein’s Theory of Relativity in Modern Astrophysics
- Understanding the Phenomenon of Quantum Entanglement on the Cosmic Scale
- Exoplanets: Searching for Earth’s Twin in the Universe
- The Role of Supernovae in Galaxy Formation
- Are We Alone? The Search for Extraterrestrial Intelligence
- The Enigma of Fast Radio Bursts: Theories and Observations
- Exploring the Potential of Interstellar Travel
- Gravitational Waves: Echoes of Cosmic Events
- The Life and Death of Stars: Stellar Evolution
- Time Dilation in Space: A Closer Look at Special Relativity
- Examining the Impact of Solar Flares on Earth’s Magnetosphere
- Cosmological Inflation: Exploring the First Seconds of the Universe
- The Expansion of the Universe: Beyond the Big Bang Theory
- Multiverse Theory: Are We Just One of Many Universes?
- Mysteries of Dark Energy and the Accelerating Universe
- Roles of Cosmic Microwave Background in Understanding the Universe’s Origins
- Space-Time Curvature: Unveiling the Secrets of Gravity
Astronomy Research Topics About Modern Problems in the Study
- Unraveling Dark Matter: Investigations and Implications
- Cosmic Microwave Background Radiation: What Does It Tell Us?
- Gravitational Waves: Detecting Cosmic Collisions
- Terraforming Mars: Scientific Feasibility and Challenges
- Mitigating Space Debris: Solutions for the Crowded Skies
- Enceladus’ Potential for Life: Recent Discoveries and Future Exploration
- Identifying Rogue Planets: Implications for Cosmic Formation Theories
- Climate of Venus: A Cautionary Tale for Earth’s Future
- Asteroid Mining: Environmental and Ethical Considerations
- Quantum Entanglement: Its Role in Understanding the Universe
- Probing the Edge of the Universe: The Limits of Observational Technology
- Galaxies Far, Far Away: Observing the Early Universe
- Neutrino Astronomy: Challenges and Opportunities
- Exoplanets’ Atmospheres: Hunting for Signs of Life
- Space Tourism: Ethical Dilemmas and Sustainability Issues
- Cosmic Rays: Unveiling Their Mysterious Origins
- Evolution of Stars: Paradoxes and Puzzles
- String Theory and Multiverses: Scientific Speculation or Reality?
- Black Holes: Exploring Their Mysteries and Misconceptions
- Interstellar Travel: Evaluating the Technological Hurdles
- Harnessing Solar Power from Space: Opportunities and Challenges
- Magnetars and Pulsars: Decoding Their Bizarre Behaviors
Astronomy Research Paper Topics for Space Exploration
- Tracing the Evolution of Stars: From Protostars to Supernovas
- The Enigma of Dark Matter: Unraveling Universal Mysteries
- Mars Colonization: Feasibility, Challenges, and Potential Benefits
- Roles of Astronomy in Predicting Climate Change on Earth
- The Influence of Solar Flares on Earth’s Satellite Technology
- Significance of Exoplanets: Possibilities for Alien Life and Habitable Conditions
- Comets, Asteroids, and Meteoroids: Cosmic Debris and Their Impact on Earth
- Interstellar Travel: Concept, Technologies, and Prospects for the Future
- Unveiling the Secrets of Black Holes: Their Formation and Role in the Universe
- Cosmic Microwave Background: Evidence for the Big Bang Theory
- Investigating the Kuiper Belt: Pluto and Beyond
- Extraterrestrial Atmospheres: Comparative Planetology of the Solar System
- Pulsars and Quasars: Extreme Conditions in the Universe
- Gravitational Waves: Detecting Ripples in Space-Time
- Comet Chasing: Understanding the Composition and Life Cycle of Comets
- Hazards of Space Debris: Mitigating the Risks for Space Travel
- Harnessing Solar Power: The Future of Energy in Space
- Astronomical Spectroscopy: Decoding Light From Distant Stars
- Interplanetary Dust: Studying the Smallest Particles in Our Solar System
- Neutrinos From Space: Detecting Subatomic Particles From Beyond our Galaxy
- Exoplanet Hunting: Techniques and Discoveries
To Learn More, Read Relevant Articles
115 british literature research paper topics & ideas, 218 anatomy & physiology topics to research.
- Privacy Policy
Buy Me a Coffee

Home » 500+ Astronomy Research Topics
500+ Astronomy Research Topics

Astronomy is a fascinating field of study that has captivated human beings for centuries. From the movement of the stars in the sky to the mysteries of black holes and dark matter, there is always something new and exciting to discover in the vast universe that surrounds us. As technology advances, so too does our ability to explore and understand the cosmos, leading to new discoveries and insights into the nature of our existence. If you’re interested in exploring the frontiers of astronomy research, there are a wide range of topics to choose from, including planetary science, astrophysics, cosmology, and more. Whether you’re an amateur stargazer or a seasoned astronomer, the world of astronomy research is full of wonder and excitement, waiting to be explored. In this post we will cover some interesting Astronomy Research Topics.
Astronomy Research Topics
Astronomy Research Topics are as follows:
- The formation and evolution of stars
- The structure and dynamics of galaxies
- The nature of dark matter and dark energy
- The search for exoplanets and habitable zones
- The properties of black holes and their effects on their surroundings
- The origin and distribution of cosmic rays
- The study of the cosmic microwave background radiation
- The detection and characterization of gravitational waves
- The study of high-energy astrophysical phenomena, such as gamma-ray bursts and supernovae
- The origin and evolution of the universe
- The structure and dynamics of the Milky Way galaxy
- The properties of interstellar gas and dust
- The study of planetary atmospheres and weather patterns
- The search for extraterrestrial life
- The study of the early universe, including the era of cosmic inflation
- The study of the intergalactic medium
- The formation and evolution of galaxies in the early universe
- The study of star formation and protoplanetary disks
- The study of the interstellar medium in nearby galaxies
- The study of the structure and evolution of galaxy clusters
- The study of the structure and dynamics of planetary systems
- The search for Earth-like planets in the habitable zone
- The study of the atmospheres of exoplanets
- The study of the formation and evolution of planetary systems
- The study of planetary geology and surface features
- The study of the magnetic fields of planets and stars
- The study of the chemical evolution of the universe
- The study of the role of massive stars in the evolution of galaxies
- The study of the impact of cosmic rays on planetary atmospheres
- The study of the effect of magnetic fields on star formation and evolution
- The study of the interstellar medium in starburst galaxies
- The study of the effects of mergers and interactions on galaxies
- The study of the properties of intergalactic gas and the intergalactic medium
- The study of the chemical composition of stars and galaxies
- The study of the formation and evolution of clusters of galaxies
- The study of the distribution of dark matter in galaxies
- The study of the formation of supermassive black holes
- The study of the evolution of the interstellar medium in the Milky Way galaxy
- The study of the structure and evolution of the Local Group of galaxies
- The study of the properties of high-redshift galaxies
- The study of the properties of globular clusters and their stellar populations
- The study of the structure and dynamics of the solar wind
- The study of the structure and dynamics of the heliosphere
- The study of the properties of the solar corona
- The study of the solar cycle and its effects on the Earth’s climate
- The study of the properties of near-Earth asteroids and comets
- The study of the origin and evolution of the Kuiper Belt
- The study of the formation and evolution of the Oort Cloud
- The study of the effects of meteorite impacts on planetary surfaces
- The study of the formation and evolution of the Martian atmosphere
- The study of the properties of the Martian polar ice caps
- The study of the origin and evolution of the Jovian moons
- The study of the properties of the Jovian magnetosphere
- The study of the formation and evolution of Saturn’s rings
- The study of the properties of Titan’s atmosphere
- The study of the properties of the Galilean moons
- Dark matter and dark energy
- The physics of magnetic reconnection in AGN jets
- The study of the interstellar medium in radio galaxies
- The study of magnetic fields in accretion disks
- The study of cosmic ray acceleration in galaxy clusters
- The study of magnetic fields in protostellar disks
- The study of the interstellar medium in high-redshift galaxies
- The study of cosmic rays in the heliosphere
- The study of magnetic fields in protostellar outflows
- The study of the interstellar medium in galaxy groups
- The study of magnetic fields in the intergalactic medium
- The study of cosmic ray propagation in the heliosphere
- The study of magnetic fields in the solar corona
- The study of the interstellar medium in nearby galaxy clusters
- The study of cosmic ray transport in the heliosphere
- The study of magnetic fields in starburst galaxies
- The study of cosmic ray acceleration in protostellar outflows
- The study of the interstellar medium in active galaxies
- The study of cosmic ray acceleration in AGN jets
- The study of magnetic fields in galaxy mergers
- The study of the interstellar medium in galaxy clusters
- The study of magnetic fields in galaxy clusters using radio observations
- The study of cosmic ray transport in the interstellar medium
- The study of magnetic fields in the Milky Way using polarimetry
- The study of cosmic ray acceleration in gamma-ray bursts
- The study of the interstellar medium in low surface brightness galaxies
- The study of magnetic fields in the interstellar medium using synchrotron emission
- The study of cosmic ray acceleration in the vicinity of black holes
- The study of magnetic fields in the intergalactic medium using Faraday rotation
- The study of the interstellar medium in the Magellanic Clouds
- The study of cosmic ray acceleration in supernova shock waves
- The study of magnetic fields in the interstellar medium using Zeeman splitting
- The study of the interstellar medium in the outskirts of galaxies
- The study of cosmic ray acceleration in astrophysical jets.
- The nature and behavior of black holes
- Formation and evolution of galaxies
- Exoplanet discovery and characterization
- The origin and nature of the cosmic microwave background radiation
- The search for life beyond Earth
- The structure and composition of the Sun
- The formation and evolution of the solar system
- The properties of comets and asteroids
- The role of magnetic fields in astrophysical phenomena
- The study of supernovae and their progenitors
- The dynamics of star clusters and their evolution
- The nature and behavior of pulsars and neutron stars
- The origin and properties of gamma-ray bursts
- The properties of high-energy cosmic rays and their sources
- The formation and evolution of planetary systems
- The physics of star formation
- The nature and properties of interstellar dust and gas
- The study of planetary atmospheres
- The properties and behavior of active galactic nuclei
- The study of cosmic magnetism
- The search for dark matter particles
- The study of gravitational waves and their sources
- The dynamics of binary and multiple star systems
- The formation and evolution of massive stars
- The properties and behavior of cosmic rays in the interstellar medium
- The study of protostars and protoplanetary disks
- The properties and behavior of supermassive black holes
- The study of the early universe
- The properties of the interstellar medium in external galaxies
- The physics of star clusters and their evolution
- The properties and behavior of quasars
- The study of the cosmic web
- The dynamics of galaxy clusters and their evolution
- The study of stellar atmospheres
- The formation and evolution of dwarf galaxies
- The study of cosmic ray acceleration mechanisms
- The physics of magnetic reconnection in astrophysical plasmas
- The study of active solar phenomena
- The properties and behavior of coronal mass ejections
- The study of cosmic ray propagation
- The study of the interstellar medium in the Milky Way
- The study of the heliosphere and its interaction with the interstellar medium
- The study of the Sun’s magnetic field
- The study of the interstellar medium in the Local Group of galaxies
- The physics of turbulence in astrophysical fluids
- The study of the Sun’s atmosphere
- The properties and behavior of solar flares
- The study of the interstellar medium in the Virgo cluster of galaxies
- The study of magnetic fields in galaxy clusters
- The physics of the solar wind
- The properties and behavior of solar prominences
- The study of coronal loops and their dynamics
- The study of cosmic rays in the Galaxy and their sources
- The study of the interstellar medium in distant galaxies
- The study of sunspots and their dynamics
- The study of cosmic ray acceleration in supernova remnants
- The study of magnetic fields in star-forming regions
- The study of the interstellar medium in merging galaxies
- The physics of magnetic reconnection in solar flares
- The study of cosmic ray transport in the Galaxy
- The study of magnetic fields in the interstellar medium
- The study of magnetic fields in AGN jets
- The study of magnetic fields in the circumgalactic medium
- The study of cosmic ray propagation in galaxy clusters
- The study of the interstellar medium in quasar host galaxies
- The study of magnetic fields in pulsars
- The study of the interstellar medium in galaxy mergers
- The study of magnetic fields in galaxy clusters using weak gravitational lensing
- The study of cosmic ray acceleration in the vicinity of hot spots in radio galaxies
- The study of cosmic ray transport in galactic winds
- The study of the interstellar medium in the outer regions of galaxy clusters using Sunyaev-Zeldovich effect
- The study of magnetic fields in the circumgalactic medium using Lyman-alpha forest observations
- The study of cosmic ray acceleration in the vicinity of supermassive black hole binaries
- The study of the interstellar medium in galactic warps
- The study of magnetic fields in the interstellar medium using Faraday depolarization
- The study of cosmic ray transport in galaxy clusters using Sunyaev-Zeldovich effect
- The study of the interstellar medium in the outskirts of galaxy groups
- The study of magnetic fields in the circumnuclear region of low-luminosity AGN
- The study of cosmic ray acceleration in the vicinity of galaxy mergers
- The study of the interstellar medium in galaxy clusters using metal absorption lines
- The study of magnetic fields in the interstellar medium using the Hanle effect
- The study of cosmic ray transport in the Local Group
- The study of the interstellar medium in the circumnuclear region of quasars
- The study of magnetic fields in the interstellar medium using Zeeman broadening
- The study of cosmic ray acceleration in the vicinity of star-forming galaxies
- The study of the interstellar medium in galaxy clusters using gravitational lensing
- The study of magnetic fields in the circumgalactic medium using galaxy-galaxy weak lensing
- The study of cosmic ray transport in the Galactic halo using gamma-ray observations
- The study of the interstellar medium in the outskirts of galaxy clusters using gravitational lensing
- The study of magnetic fields in the interstellar medium using anomalous microwave emission
- The study of cosmic ray acceleration in the vicinity of the Galactic center
- The study of the interstellar medium in galaxy groups using metal absorption lines
- The study of magnetic fields in the circumnuclear region of Seyfert galaxies
- The study of cosmic ray transport in the interstellar medium using gamma-ray observations
- The study of the interstellar medium in the circumnuclear region of low-metallicity galaxies
- The study of magnetic fields in the interstellar medium using the Cotton-Mouton effect
- The study of cosmic ray acceleration in the vicinity of massive black hole binaries
- The study of the interstellar medium in galaxy clusters using radio halos
- The study of magnetic fields in the circumgalactic medium using galaxy cluster weak lensing
- The study of cosmic ray transport in the intergalactic medium using gamma-ray observations
- The study of the interstellar medium in the circumnuclear region of radio galaxies
- The study of magnetic fields in the interstellar medium using the Hanle depolarization
- The study of cosmic ray acceleration in the vicinity of quasar host galaxies
- The study of the interstellar medium in the circumnuclear region of LINERs
- The study of cosmic ray transport in galaxy clusters using cosmic microwave background
- The study of cosmic ray acceleration in the vicinity of pulsars
- The study of the interstellar medium in low-metallicity galaxies
- The study of magnetic fields in the circumnuclear region of AGN
- The study of the interstellar medium in the Galactic Center
- The study of magnetic fields in the interstellar medium using dust polarization
- The study of cosmic ray transport in the Galactic halo
- The study of the interstellar medium in star-forming galaxies
- The study of magnetic fields in galactic outflows
- The study of cosmic ray acceleration in gamma-ray bursts using neutrino observations
- The study of the interstellar medium in dwarf galaxies
- The study of magnetic fields in the Milky Way using pulsar dispersion measures
- The study of cosmic ray transport in the Galactic magnetic field
- The study of the interstellar medium in interacting galaxies
- The study of magnetic fields in the interstellar medium using molecular line observations
- The study of cosmic ray acceleration in AGN using gamma-ray observations
- The study of the interstellar medium in radio galaxies using Faraday rotation
- The study of magnetic fields in galaxy clusters using X-ray observations
- The study of cosmic ray acceleration in the vicinity of black holes using gravitational wave observations
- The study of the interstellar medium in galactic bulges
- The study of magnetic fields in high-redshift galaxies using Lyman-alpha forest observations
- The study of cosmic ray transport in galaxy clusters using X-ray observations
- The study of the interstellar medium in the outer regions of galaxies
- The study of magnetic fields in galactic halos
- The study of cosmic ray acceleration in galaxy clusters using radio observations
- The study of the interstellar medium in the Local Group
- The study of magnetic fields in the interstellar medium using multi-wavelength observations
- The study of cosmic ray acceleration in the vicinity of magnetars
- The study of the interstellar medium in circumstellar disks
- The study of magnetic fields in AGN jets using VLBI observations
- The study of cosmic ray transport in the Galactic Center
- The study of the interstellar medium in the outskirts of galaxy clusters
- The study of magnetic fields in the interstellar medium using molecular clouds
- The study of cosmic ray acceleration in the vicinity of young stellar objects
- The study of the interstellar medium in the circumgalactic medium using absorption lines
- The study of magnetic fields in the circumnuclear region of starburst galaxies
- The study of cosmic ray acceleration in the vicinity of massive stars
- The study of the interstellar medium in high-velocity clouds
- The study of magnetic fields in the interstellar medium using polarization of starlight
- The study of cosmic ray transport in the intergalactic medium
- The study of the interstellar medium in galaxy groups using X-ray observations
- The study of magnetic fields in the interstellar medium using interstellar scintillation
- The study of cosmic ray acceleration in the vicinity of protostars
- The study of the interstellar medium in the vicinity of supermassive black holes
- The study of magnetic fields in the interstellar medium using dust extinction
- The study of magnetic fields in the circumgalactic medium using quasar-galaxy pairs
- The study of cosmic ray transport in the intergalactic medium using the Sunyaev-Zeldovich effect
- The study of the interstellar medium in the circumnuclear region of high-ionization nuclear emission-line regions (HINERs)
- The study of magnetic fields in the interstellar medium using Faraday rotation measurements
- The study of cosmic ray acceleration in the vicinity of black hole binaries
- The study of magnetic fields in the circumnuclear region of compact elliptical galaxies
- The study of the interstellar medium in the circumnuclear region of AGN with double-peaked emission lines
- The study of cosmic ray acceleration in the vicinity of microquasars
- The study of the interstellar medium in the circumnuclear region of green pea galaxies
- The study of the interstellar medium in the outskirts of galaxy clusters using radio observations
- The study of magnetic fields in the circumnuclear region of X-ray bright AGN
- The study of cosmic ray acceleration in the vicinity of massive star clusters
- The study of magnetic fields in the circumgalactic medium using galaxy-galaxy strong lensing
- The study of cosmic ray transport in the intergalactic medium using X-ray observations of galaxy clusters
- The study of the interstellar medium in the circumnuclear region of AGN with complex emission lines
- The study of magnetic fields in the interstellar medium using Zeeman effect measurements
- The study of cosmic ray acceleration in the vicinity of gamma-ray bursts with extended emission
- The study of the interstellar medium in the outskirts of galaxy clusters using deep optical surveys
- The study of magnetic fields in the circumnuclear region of broad-line radio galaxies
- The study of cosmic ray transport in the Galactic disk using supernova remnants
- The study of the interstellar medium in the circumnuclear region of post-starburst galaxies
- The study of magnetic fields in the circumgalactic medium using weak lensing of the cosmic microwave background radiation
- The study of cosmic ray acceleration in the vicinity of shock waves in galaxy clusters
- The study of the interstellar medium in the circumnuclear region of Seyfert 2 galaxies
- The study of magnetic fields in the interstellar medium using the rotation measure synthesis technique
- The study of cosmic ray transport in the interstellar medium using HII region surveys
- The study of the interstellar medium in the outskirts of galaxy clusters using submillimeter observations
- The study of magnetic fields in the circumnuclear region of radio galaxies with diffuse emission
- The study of cosmic ray acceleration in the vicinity of gamma-ray emitting blazars
- The study of the interstellar medium in the circumnuclear region of galaxies with ionization cones
- The study of magnetic fields in the circumgalactic medium using simulations of galaxy formation
- The study of cosmic ray transport in the Galactic halo using diffuse gamma-ray emission
- The study of cosmic ray acceleration in the vicinity of gamma-ray bursts
- The study of the interstellar medium in the circumnuclear region of high-redshift galaxies
- The study of magnetic fields in the interstellar medium using polarimetry
- The study of cosmic ray transport in the Galactic disk using pulsar wind nebulae
- The study of the interstellar medium in the outskirts of galaxy clusters using X-ray observations
- The study of magnetic fields in the circumgalactic medium using quasar absorption line systems
- The study of cosmic ray acceleration in the vicinity of gamma-ray emitting supernovae
- The study of the interstellar medium in the circumnuclear region of ultra-luminous infrared galaxies
- The study of magnetic fields in the interstellar medium using the Chandrasekhar-Fermi method
- The study of cosmic ray transport in the intergalactic medium using the Lyman-alpha forest
- The study of the interstellar medium in the circumnuclear region of dusty quasars
- The study of magnetic fields in the circumgalactic medium using Lyman-alpha absorption
- The study of the interstellar medium in the outskirts of galaxy groups using X-ray observations
- The study of magnetic fields in the circumnuclear region of radio-loud quasars
- The study of cosmic ray transport in the Galactic halo using pulsar timing
- The study of the interstellar medium in the circumnuclear region of low-ionization nuclear emission-line regions (LINERs)
- The study of magnetic fields in the interstellar medium using the polarization of cosmic microwave background radiation
- The study of cosmic ray acceleration in the vicinity of tidal disruption events
- The study of the interstellar medium in the circumnuclear region of submillimeter galaxies
- The study of magnetic fields in the circumgalactic medium using galaxy-galaxy lensing
- The study of the interstellar medium in the circumnuclear region of infrared luminous galaxies
- The study of cosmic ray acceleration in the vicinity of supernova remnants
- The study of the interstellar medium in the outskirts of galaxy clusters using X-ray and Sunyaev-Zeldovich effect observations
- The study of magnetic fields in the circumnuclear region of obscured AGN
- The study of cosmic ray transport in the interstellar medium using dust emission
- The study of the interstellar medium in the circumnuclear region of broad absorption line quasars
- The study of magnetic fields in the circumgalactic medium using high-resolution spectroscopy
- The study of cosmic ray acceleration in the vicinity of fast radio bursts
- The study of the interstellar medium in the circumnuclear region of high-metallicity galaxies
- The study of magnetic fields in the interstellar medium using pulsar polarization measurements
- The study of cosmic ray transport in the Galactic disk using HII regions
- The study of the interstellar medium in the outskirts of galaxy clusters using optical and infrared observations
- The study of magnetic fields in the circumnuclear region of compact steep spectrum radio sources
- The study of cosmic ray acceleration in the vicinity of pulsar wind nebulae
- The study of the interstellar medium in the circumnuclear region of radio-quiet quas
About the author
Muhammad Hassan
Researcher, Academic Writer, Web developer
You may also like

200+ Funny Research Topics

500+ Sports Research Topics

300+ American History Research Paper Topics

500+ Cyber Security Research Topics

500+ Environmental Research Topics

500+ Economics Research Topics
201 Astronomy Research Topics To Take You To The Stars

Are you looking for the best astronomy research topics for 2023? It would be best if you looked no more. This article has 200 of them. After offering years of paper writing services , we have the best research paper topics for you.
Table of Contents
Astronomy Research Topics: Solar System, Dark Matter & More
No matter your theme for astronomy research topics, we are here with aid if you’re writing a research paper .
Astronomy Research Topics About Solar System
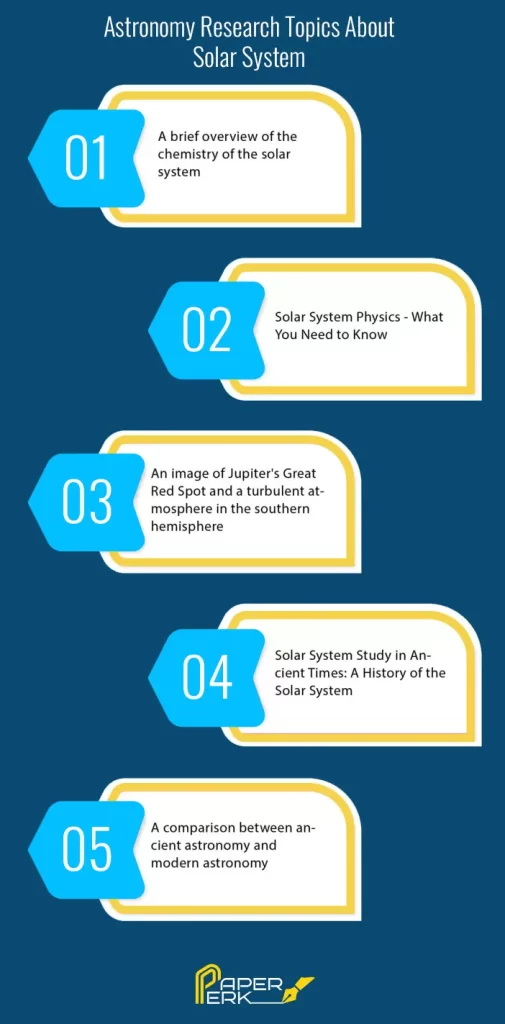
Here are some excellent astronomy research paper topics about the solar system.
- A brief overview of the chemistry of the solar system
- Solar System Physics – What You Need to Know
- An image of Jupiter’s Great Red Spot and a turbulent atmosphere in the southern hemisphere
- Solar System Study in Ancient Times: A History of the Solar System
- A comparison between ancient astronomy and modern astronomy
- Why Roman Astronomy differs from the Astronomy of the Middle Ages in several ways
- The Life of an Astronomer in the Middle Ages
- A brief description of the motivations and purposes behind the study of the solar system
- Models based on heliocentric versus models based on geocentricity
- The contribution of Kepler to the understanding of the solar system
- A contribution made by Galileo to the understanding of the solar system
- What makes the solar system different from other planetary systems?
- Mercury’s temperature and the possible implications of it
- What was the reason for Pluto’s extermination from the solar system?
- Does Mars have the potential to be habitable? Are there any reasons why this is or is not the case?
- What are the feasibility and realistic outlook for Elon Musk’s Mars terraforming project?
- NASA’s role in exploring the solar system and discovering new things about it
- The contributions of SpaceX to the discovery and exploration of the solar system
- A study of gas giants or the planets of the Jovian system
- Helium and hydrogen form Jupiter’s atmosphere
- Studying the atmosphere and planetary conditions of Jupiter.
- Great Red Spot on Jupiter, research, and study
- Instruments used by space probes and telescopes
- The role of computerized imagery in accelerating discoveries in astronomy
- Role of artificial intelligence in astronomical development
- Planets and the gravitational force on the objects orbiting them
- Dwarf planets in the Solar System
- Discoveries made with the help of the Galileo space probe
Astronomy Research Topics: Dark Matter
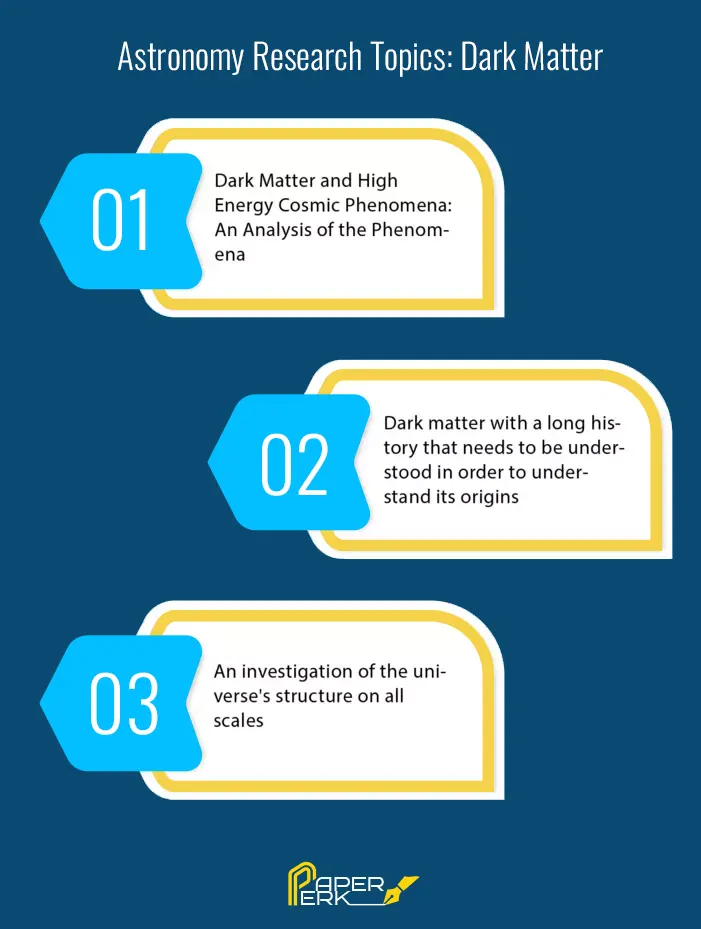
Dark matter is one of the most remarkable scientific discoveries made to that date. Here are some excellent astronomy research paper topics for you about dark matter.
- Dark Matter and High Energy Cosmic Phenomena: An Analysis of the Phenomena
- Dark matter with a long history that needs to be understood to understand its origins
- An investigation of the Universe’s structure on all scales
- Creating plantlets out of dust from interstellar space
- The structure and properties of dark matter and antimatter: A comparison
- A study of the early formation of galaxies and clusters of galaxies
- The laboratory studies star formation and interstellar phenomena such as dark energy and matter.
- Dark Matter: A study of its creation and study
- Discovery of Dark Matter
- The effects of dark matter on the Universe as a whole
- Dark energy, dark matter, antimatter: The four horsemen of the universal structure
- Facts and myths about dark matter
- The portrayal of dark matter in movies, between accuracy and fallacy
- Black holes, relativistic jets, energy, and interactions
- Can black holes be the source of dark matter?
- Dark matter vs. Dark energy: A comparative study
- What are the chances of there being dark matter on Earth? Is it possible or not?
- An examination of the dark matter hypothesis, its authenticity, and the truth behind it
- How do dark matter and antimatter differ from one another? What is the difference between them?
- Observing dark matter in deep space as a means of studying it
- The role of dark energy in the spread of the Universe
Astronomy Research Topics About Planets
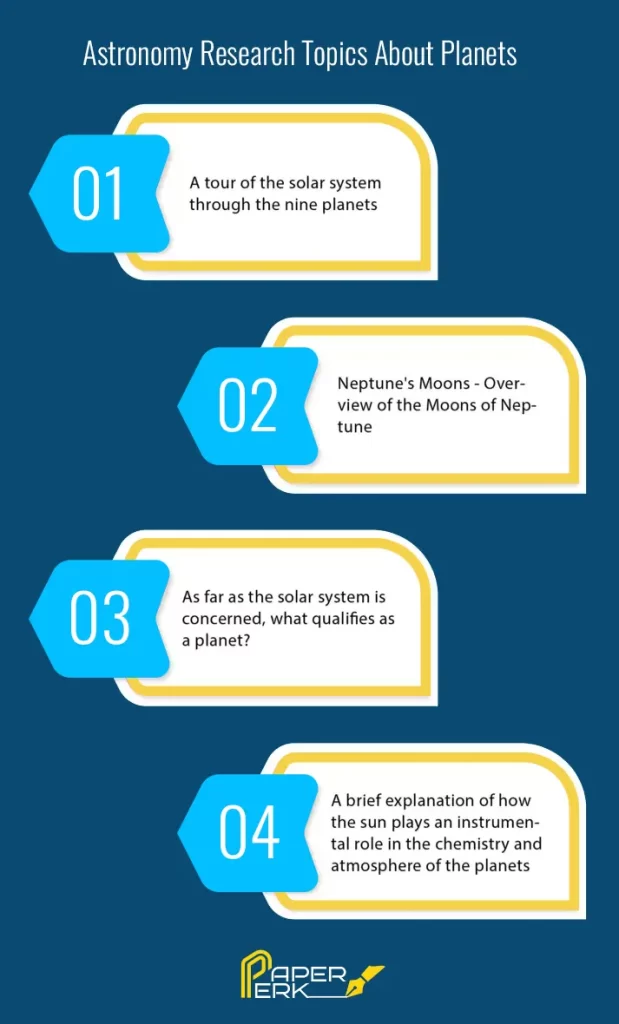
Some great astronomy research paper topics about planets can make your day.
- A tour of the solar system through the nine planets
- Neptune’s Moons – Overview of the Moons of Neptune
- As far as the solar system is concerned, what qualifies as a planet?
- A brief explanation of how the Sun plays an instrumental role in the chemistry and atmosphere of the planets
- All the planets in our solar system
- The rocky planets of the solar system
- Solar heat transfer between the poles and the equator
- The discovered planets that could be habitable
- Discovered planets outside the solar system
- An overview of the atmosphere of Saturn
- Importance of Earth In Our Solar System
- The gaseous planets of the solar system
- Astrophysics of Jupiter and the physical chemistry of the moon
- Physical chemistry of Mars: A research study
- A research analysis of Neptune’s atmospheric chemistry
- An overview of the planetary sciences
- The distribution of solar energy on Earth
- The old classic relationship between astronomy and quantum physics
- Structure of celestial bodies: planets, stars, and other cosmological objects
- Our moon and the moons of other planets in the solar system, a comparison.
- Atmosphere and density of planet Earth compared to other planets of the solar system
- The solar system is a planetary system
- What are the main characteristics of our planet?
- The Universe and the origin of the Solar System
- What is the solar system made of?
Astronomy Research Topics For Students
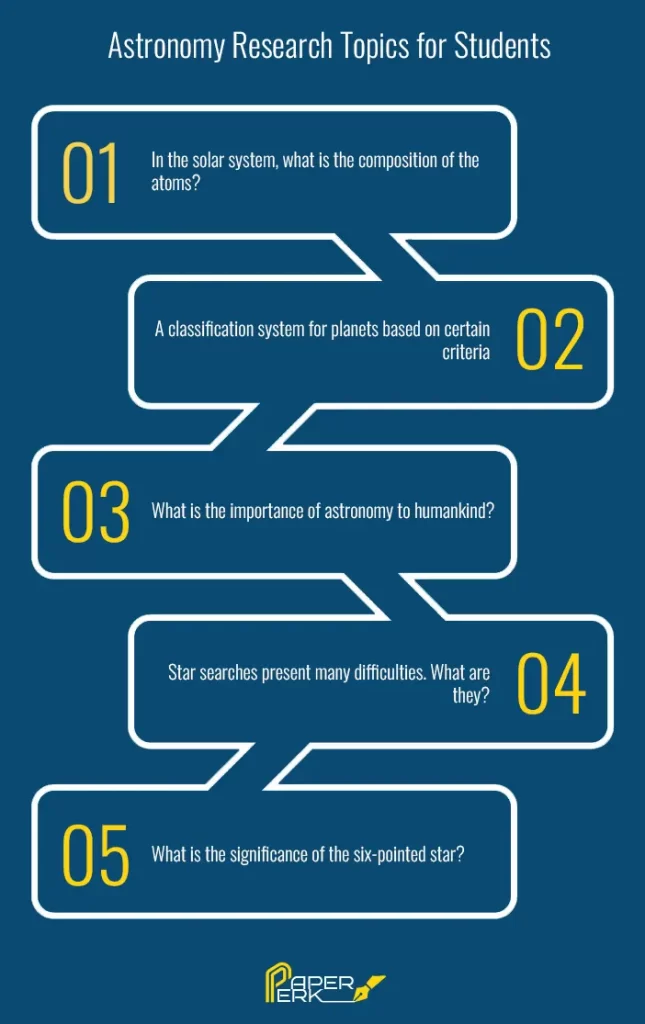
Living on the high school team and suddenly needing astronomy research paper topics to inspire the best paper? Or are you looking for some astronomy essay topics?? We got your back. Here are some great topics for school and college students for the next astronomy breakthrough.
- In the solar system, what is the composition of the atoms?
- A classification system for planets based on specific criteria
- What is the importance of astronomy to humankind?
- Star searches present many difficulties. What are they?
- Importance and need for space exploration
- What is the significance of the six-pointed star?
- What is the importance of rockets in space research?
- We see the Sun and the Moon as nearly the same size, yet their sizes differ enormously.
- Why Pluto is no longer a planet in the solar system
- What are the subjects and issues that astronomy seeks to address?
- An explanation is given of the history of the planets in the solar system
- Do we still have a planet named Pluto in our solar system? Is there a reason or not?
- Exploration of space and the history of astronomy
- Taking the first steps on the moon by man
- In what sense is the North Star defined? The concept in literature viewed from a scientific perspective
- Expectations from astronomy in 2023
- The development of new instruments for the discovery of astronomical phenomena
- How to properly observe the stars in the sky?
- What are astronomy’s significant discoveries, and how do they help us improve lives?
- Why should developing countries invest more in exploration? astronomy
- Importance and significance of Dark energy in the Universe
- Supermassive black holes discovered by American scientists
- A brief history of young planets discovered in the 2000s
- How have we determined the age of the Universe? A thorough analysis
- What is the speed difference of the planets around the Sun?
Find more science research topics: 146 Best Science Research Topics .
Astrophysics Research Topics
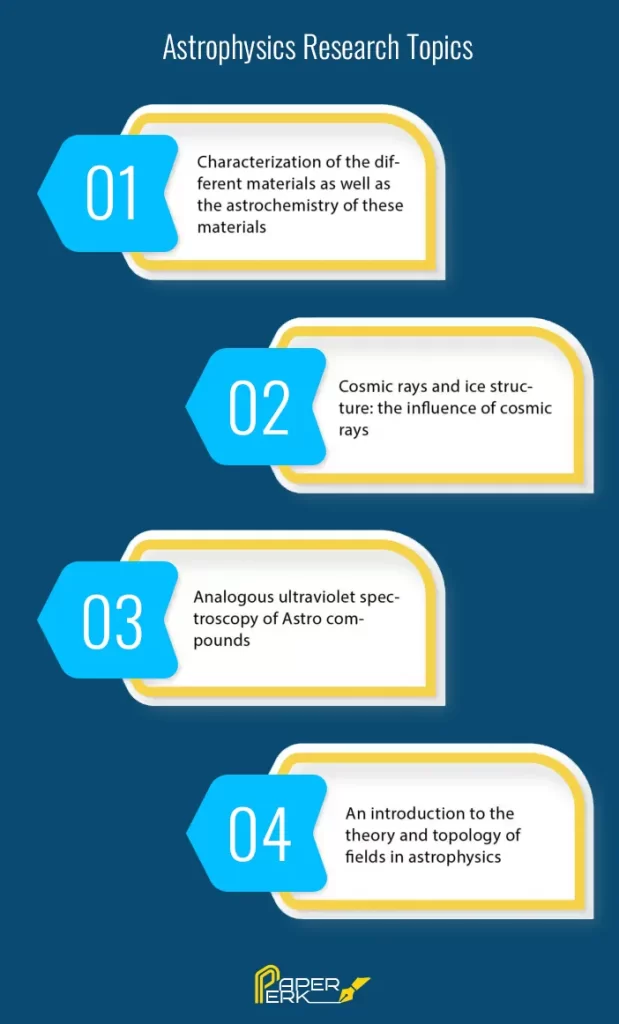
Here are some exciting astronomy topics for you if you are an astrophysics student.
- Characterization of the different materials, as well as the astrochemistry of these materials
- Cosmic rays and ice structure: the influence of cosmic rays
- Analogous ultraviolet spectroscopy of Astro compounds
- Breakthroughs in astrophysics made with robotic space exploration and experimentations on space debris
- Teaching students regarding the idea to explore space and other planets
- An introduction to the theory and topology of fields in astrophysics
- Detailed studies of exact solutions and black holes are carried out
- Space near blackholes is filled with energetic processes that are taking place
- An analysis of geometric quantization
- Solar system origins and the evolution of the planets around it
- The detection of gravitational waves in the laboratory
- An introduction to string theories and instantons
- Galactic sources produce cosmic rays
- Role of astrophysicists in the space exploration
- DarkMirage Energy: A mysterious energy that exists in space
- Developments in the physics of modern astrophysics
- The creation of the Universe was in part due to the contribution of hydrogen
- A detailed physical analysis of the exoplanets discovered to date
- An analysis of the solar atmosphere’s temperature profile
- Analysis of soluble macromolecular components in astrophysics
- Solar planets and the interaction between their silicate surfaces and their gaseous atmospheres
- The link between reflection and extraterrestrial matter in the solar system
- The formulation of the problem in a model based on astrophysics
- Working with General Relativity in the field of astrophysics
- An application of field theory in the field of space sciences
- Advances in astrophysics in the last few years
- An analysis of how astrophysics differs from astrochemistry, with a detailed breakdown
Astronomy Research Topics For University
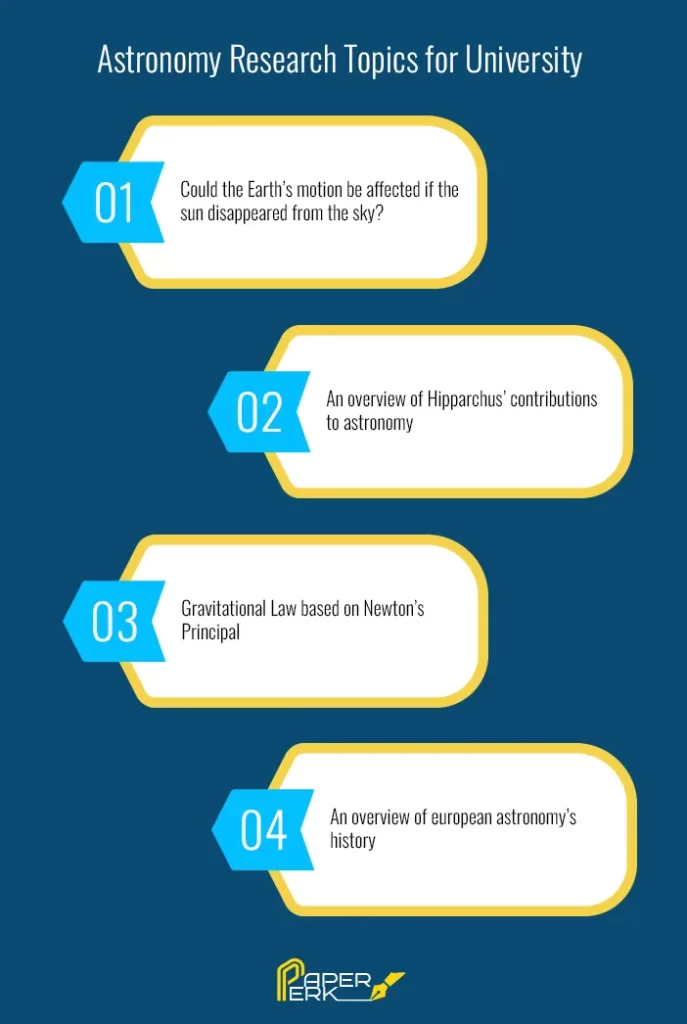
Being a university student and writing a research paper could be challenging when studying astronomy. It gets easier when you have exciting astronomy topics like these:
- Could the Earth’s motion be affected if the Sun disappeared from the sky? Would the Earth’s motion be altered as a result?
- An overview of Hipparchus’ contributions to astronomy
- Gravitational Law based on Newton’s Principle
- An overview of European astronomy’s history
- Astronomy essay on dark energy
- Scientists to explain star clusters and heavenly bodies
- Learning to survive zero gravity and space weather for the upcoming space race
- Using astronomical concepts in modern science is essential.
- Observing a lunar eclipse by observing its phases
- A brief history of the heliocentric theory
- Exploring the Sun’s effect with the scientific method
- The primary idea of Kepler’s Laws
- Astronomy essay on space travel
- In what ways did ancient civilizations contribute to the study of astronomy?
- New habitats are found through extraterrestrial activities.
- Research paper on space shuttle challenger disaster
- An overview of Galileo’s contributions to astronomy
- Outer space: Human interpretation vs. human mythology
- Early astronomers among human beings to discover dark matter
- Space exploration for all! Is Elon Musk’s idea going to work?
- Discoveries and contributions of Einstein to astronomy
- Writing science fiction about terrestrial planets inhabited by ancient humans.
- The gravitational interaction between the Earth and the Sun
- Planetary atmosphere differences
- The difference between a space probe and a spacecraft
- Egyptian astronomy in the ancient world
- Indian astronomy in the ancient world
- An ancient Greek view of astronomy
- Discovery of big bang theory
- How Kepler contributed to modern astronomy’s greatness
- A scientific approach to astronomy that applies to everyday life
- The discovery of gravitational waves
- An experimental study of thermal and soft matter physics in astronomy
Looking for research topics in general: 402 Best Research Paper Topics
High School Astronomy Research Topics
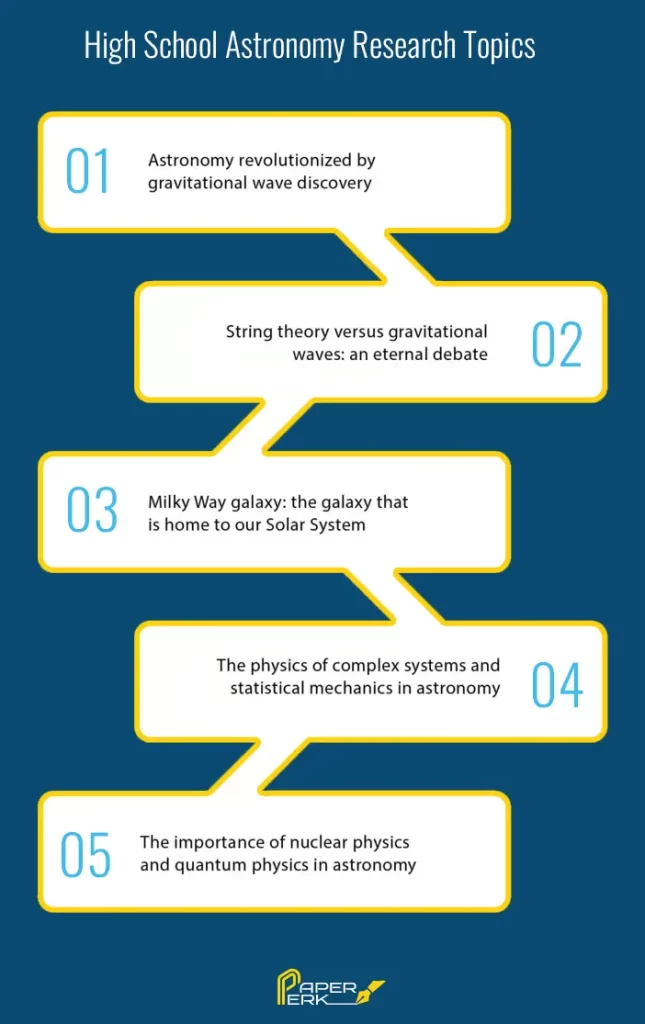
If you are a high school student looking for exciting astronomy topics, Santa just came early this year!
- Astronomy revolutionized by gravitational wave discovery
- String theory versus gravitational waves: an eternal debate
- Milky Way galaxy: the galaxy that is home to our Solar System
- The physics of complex systems and statistical mechanics in astronomy
- The importance of nuclear physics and quantum physics in astronomy
- Mathematical and theoretical physics are used in astronomy
- An overview of Big Bang Theory history
- Stars with helium as their main component
- Exciting supernova stars in distant galaxies
- A sub-millimeter study of interstellar turbulence and magnetism
- Astrophysics achievements in recent years
- Milestones achieved related to space exploration in recent days
- The relationship between plasma physics and astrophysics
- Space around the celestial sphere
- Writing a paper with your work in research related to black holes in the Milky Way galaxy
- Astronomy experiments with other methods
- Science of astronomy: theoretical developments
- Research on the dark matter based on collected data
- An experimental protocol for astronomy
- A review of recent advancements in astrochemistry
- The formulation of a hypothesis for space probes
- An analysis of the importance of link modeling in astronomy
- Brown dwarf spectra are affected by Earth’s atmosphere
- Motion catalog for the statistical study of white dwarf stars
Astronomy Research Topics For Thesis
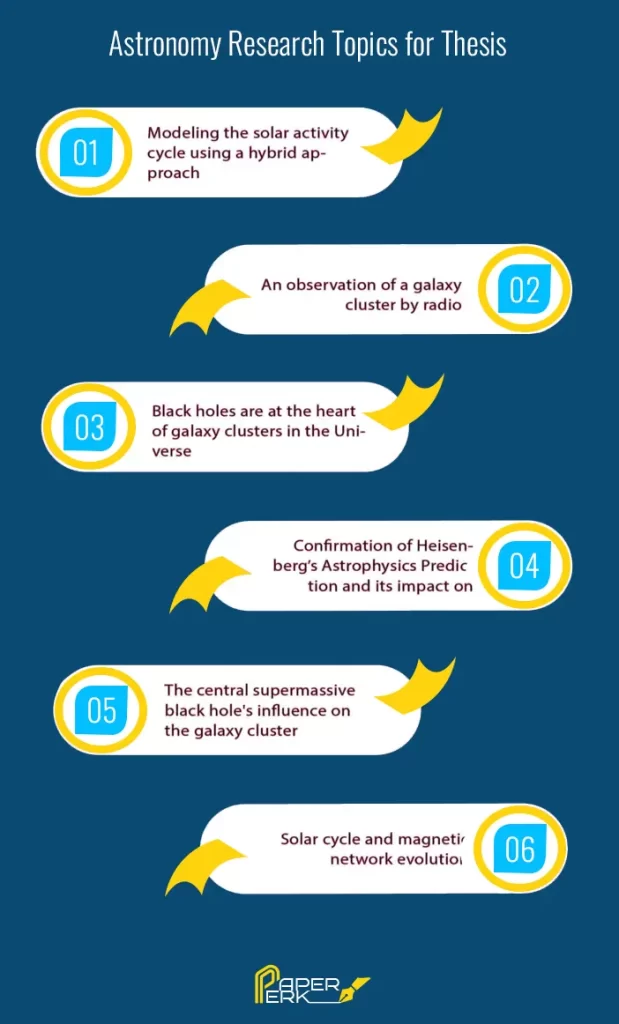
Writing a thesis could be the biggest challenge of your life. We know the feeling. Some interesting astronomy topics can make this challenge easier for you.
- Modeling the solar activity cycle using a hybrid approach
- An observation of a galaxy cluster by radio
- Black holes are at the heart of galaxy clusters in the Universe
- Confirmation of Heisenberg’s Astrophysics Prediction and its impact on modern astronomy
- The central supermassive black hole’s influence on the galaxy cluster
- Solar cycle and magnetic network evolution
- Spectral solar irradiance and its applications to terrestrial stratospheric chemistry
- A study of galaxies and clusters of galaxies using observations and machine learning
- The magnetic flux in the photospheric environment and the internal dynamo
- Cycles of stellar activity modeled using a dynamo
- White dwarf stars: harnessing their seismic potential
- Cold white dwarfs with high-density photospheres
- Stars with heavy elements that are magnetic white dwarfs
- Imaging exoplanets and their characterization in high contrast
- An analysis of white dwarfs with hydrogen-rich surfaces
- The Gaia sample and the white dwarf
- How does the mars rover work differently from space vehicles?
- The reflection of light from exoplanets
- A model of anelastic convection and a method of assimilation of data
- The motion of the plasma in an active region of the Sun
- Identifying and analyzing young low-mass stars around the Sun
- Stars with a low mass and significant separation of their exoplanets
Astronomy Research Topics For Research Paper
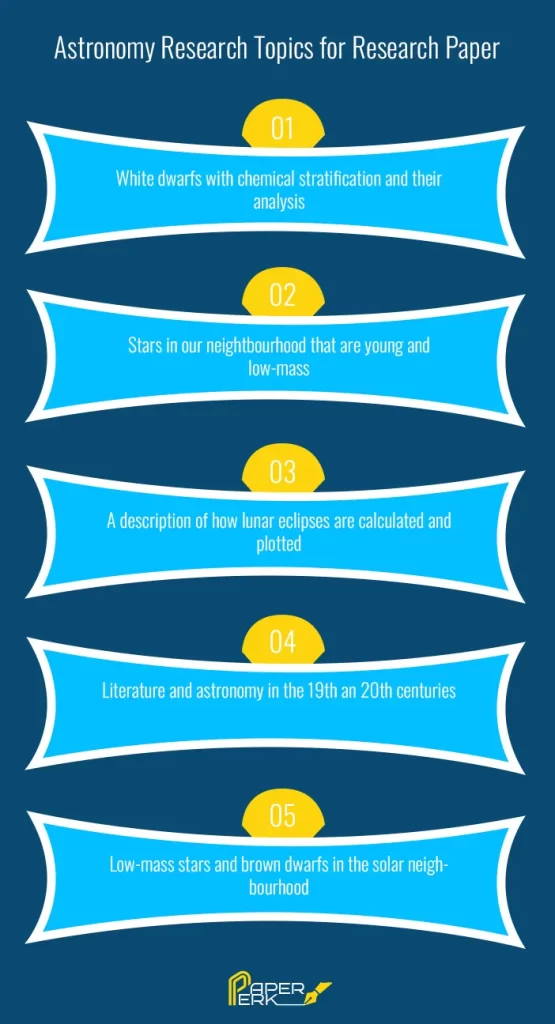
If you are writing a research paper or doing an astronomy project, these astronomy project topics could play a significant role in igniting a spark of inspiration.
- White dwarfs with chemical stratification and their analysis
- Stars in our neighborhood that are young and low-mass
- A description of how lunar eclipses are calculated and plotted
- Literature and astronomy in the 19th and 20th centuries
- Low-mass stars and brown dwarfs in the solar neighborhood
- Observatory and telescopes for optical observations
- Variable stars: How to observe them
- Gravitational waves across the milky way: What it means for the human race?
- Observation of space objects using artificial intelligence
- What can secondary schools do to address astronomy’s pedagogical challenges?
- An introduction to astronomy for the general public and scientists
- An astronomy project material kit for preparing the practical work
- Astrophysics projects involve analyzing objects and tasks
- An observational astronomy project at a university
- The telescopes available to universities and the ideal targets for them
- The construction of a laboratory for the study of astronomy at a secondary school
- Study of celestial objects throughout history
- In the world of digital art and astronomy
- Developing new technologies to aid in astronomy
- Astronomy to be revolutionized by artificial intelligence
- Constellation-related astronomical research
We are confident that you have enough ideas to ace your astronomy research paper by now. If you still face challenges and need academic writing help, contact us at Paper Perk, and we’ll help you.
Order Original Papers & Essays
Your First Custom Paper Sample is on Us!

Timely Deliveries

No Plagiarism & AI

100% Refund
Try Our Free Paper Writing Service
Related blogs.
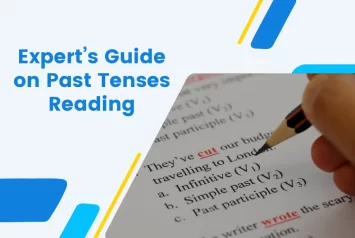
Connections with Writers and support
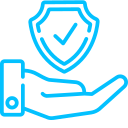
Privacy and Confidentiality Guarantee
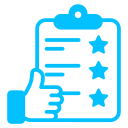
Average Quality Score
Research Topics
Scientists and engineers at the Center for Astrophysics | Harvard & Smithsonian collaborate across a broad variety of scientific disciplines, from astronomy and astrophysics to related areas of physics and geophysics, in advancing humanity’s understanding of the universe. Learn more about the full spectrum of research covered at the CfA.

- From the Director
- Values and Code of Conduct
- Equity and Inclusion
- Annual Reports
- Scientific Staff
- Postdoctoral Fellows
- Graduate Students
- Research Professionals
- Institute Staff
- Cosmic Structure
- Extreme Astrophysics
- Physics of the Universe
- Stellar, Interstellar and Planetary Astrophysics
- Research Interests of Senior Members
- Publications
- Events Calendar
- Upcoming Events
- Astrophysics Colloquium
- Cosmology Seminar
- Public Lectures
- Opportunities
- Postdoctoral opportunities
- Prospective Graduate Students
- Post-baccalaureate Fellowship
- Undergraduate Research
- Request a Tea Talk
- Getting Here
- Lecture Archive
- Latest from KIPAC
- Research Highlights
- In the News
- Newsletters
- New to the KIPAC Community?
- COVID Protocols
Research topics
Astrophysical magnetism and the interstellar medium.

What fills the space between the stars? In addition to stars, planets, and dark matter, galaxies are home to vast reservoirs of gas and dust, high-energy particles, and magnetic fields. This is the interstellar medium (ISM): the stuff between the stars. The interstellar medium is the material from which new stars are born.
Black Holes

Cosmic Microwave Background

Dark Energy

One of the most important and surprising scientific discoveries of the twentieth century is that the expansion of space is not slowing down, but speeding up—contrary to what we expect the gravitational pull of all the matter in the Universe to do. The driver of this accelerating expansion has been labeled "dark energy," but there is much about the phenomenon that researchers don’t understand.
Dark Matter

First Stars and Galaxies

Roughly 400,000 years after the Big Bang, the Universe—bathing in the afterglow of radiation that we see today as the cosmic microwave background—began to enter the cosmic “dark ages,” so named because the luminous stars and galaxies we see today had yet to form.
Galaxy Clusters

Galaxy Formation

Gravitational Lensing

Neutron Stars and Pulsars

Optical Surveys

In the traditional model of astronomical observation, individual or small teams of astronomers study a select class of objects in a small region of sky. However, some of the most exciting cosmological and astrophysical results in recent years have required the study of millions of galaxies over thousands of square degrees of sky.
Particle Acceleration

Scientific Visualization and Data Analysis

KIPAC's visualization and data analysis facilities provide hardware and software solutions that help users at KIPAC and SLAC to analyze their large-scale scientific data sets.
Solar Physics

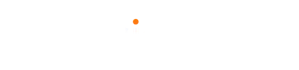
160 Outstanding Astronomy Research Topics to Consider
Table of Contents
Would you have to submit an astronomy research paper? Are you searching for the top astronomy research topics for your assignment? If yes, then this blog post is for you. Here, for your convenience, we have listed 150 interesting astronomy research ideas on various themes involved in the subject. Explore and pick any topic that matches your needs.

What is Astronomy?
Astronomy is a science that studies everything about celestial objects, space, and other things present in the universe beyond Earth’s atmosphere. It is one of the interesting subjects that are closely associated with physics, mathematics, chemistry, and molecular biology.
Astronomy Research Paper Topic Selection
If you are a student who is pursuing a degree course in astronomy, astrophysics, meteorology, or atmospheric science, then for your research project, you can consider any topic related to astronomy. However, choosing one ideal topic from numerous astronomy research ideas available is not at all easy. So, to make your astronomy research paper topic selection phase easier, here we have shared some key tips. Follow them all, whenever you are asked to choose an astronomy research paper topic of your interest.
- Always pick an astronomy topic in which you have strong knowledge.
- Never go with a topic that is too vast or too narrow.
- Pick a topic only if it has a wide scope of discussion and several credible sources of reference.
- In case, the topic you have chosen is broad, make sure to divide it down into smaller subtopics and concentrate on it.
- Select only the topic that allows you to complete the entire discussion before the deadline.
- Choose a topic that is not only interesting but also informative to your audience.
- Finalize the topic only if it matches the research paper writing guidelines shared by your university.
- In addition to that, consult with your supervisor and get approval for the topic you have chosen so that you can improve the quality of your paper as per their suggestions.
List of the Best Astronomy Research Topics
For writing your astronomy research paper, you can very well choose a topic from areas such as space science, astrophysics, planetary science, or any other astronomy-related sub-discipline. If you run short of ideas, then take a look at the list presented below. In the list, you will find 150+ fascinating astronomy research paper topic ideas deserving of an A+ grade.
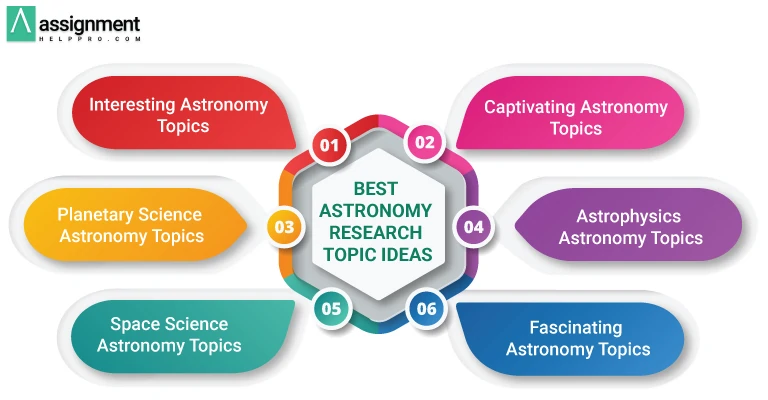
Interesting Astronomy Research Topics
- Why are Musk’s Starlink satellites important?
- How does the Sun affect our water bodies?
- What causes a star to go supernova?
- Determining the angular diameter of the Moon
- Latest developments in NASA’s Shuttle Program.
- Listening to the echoes of a solar storm.
- Problems with satellite communications.
- Can we discover a way to travel in time?
- How is a star born?
- Beyond the Milky Way
- What are called Dwarf galaxies and how many of them currently exist in the galaxy?
- Difference between the Hubble telescope and Kepler telescope along with their use in astronomy
- Who is called the father of modern astronomy?
- Discuss about the formation of galaxies
- What is the difference between astronomy and cosmology?
- What is the future of space exploration?
- A closer review of the Big Bang theory.
- Compare two theories that explain the origin of the universe.
- Stephen Hawking theories.
- Discuss about Quasars and their importance
- Compare and contrast Clashing branes and Cosmic ghost
- Briefly discuss the importance of relativity in astronomy
- Why Asteroid Extinction theory is considered one of the most terrifying theories in astronomy?
- What are called Exploding Stars?
Captivating Astronomy Topics for Research
- The Big Bang theory.
- How did ancient humans see the most important constellations?
- Is light-speed travel possible in the near future?
- The slow process of the black hole birth.
- The Rings of Saturn: An analysis
- Nebulae and masses of matter
- Quantum physics and astronomy
- Cosmic Microwave Background
- Star Gazing
- What are called Black Holes and how many of them are there in the space?
- Discuss the AGB (Asymptotic giant branch) phase of a star
- What is called a Supernova in astronomy?
- What do asteroids mean in astrology and how do they affect astronomy?
- What is known as dark energy in astronomy?
- What impact do humans have on the solar system?
- The rise of space tourism: What impact will it have on space exploration?
- Is space tourism a good thing?
- What could go wrong with space tourism?
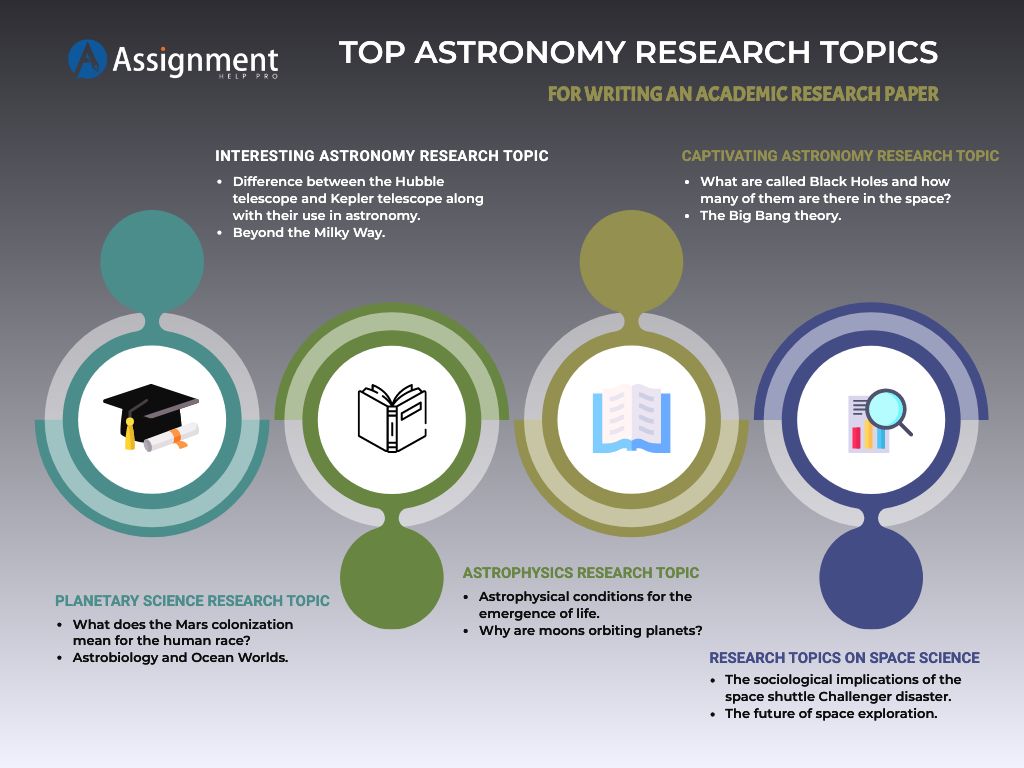
Planetary Science Research Topics
- Astrobiology and Ocean Worlds
- Planetary Interiors and Geophysics
- Geosciences
- Planetary and Exoplanetary Atmospheres
- Small Bodies of the Solar System
- The basis for the classification of planets
- The Coronado Expedition
- Martian Cratering Studies
- Fireballs that produce Meteorites
- The Origin of the Moon
- Iridescent clouds
- Multi-ring impact basins on the Moon
- Methods used to detect exoplanets
- Searching for life on other planets
- Planetary Remote Sensing and Spectroscopy.
- Geochemistry, Petrology and Mineralogy.
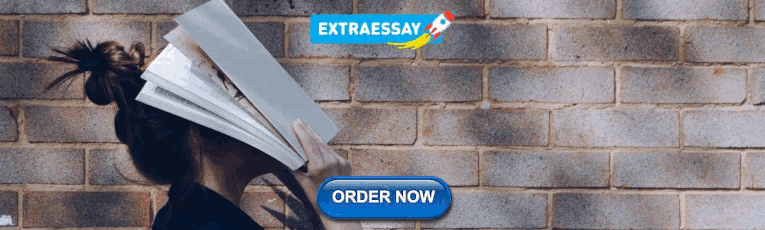
Additional Research Paper Topics on Planetary Science
- Colonization of other planets
- Implications of life on planets aside from Earth
- Similarities between Earth And Mars
- Similarities between Venus and Earth
- The implications of the moon on Earth
- The sun and its relationship to the Earth
- The origins of life on planet Earth
- How does the Mars rover work?
- What does the Mars colonization mean for the human race?
- The First Mars Landing
- The possibility of finding life on Mars
- What does it take for humans to survive on Mars?
- Why is Pluto not a planet?
- Hot black ice
- The process of the planet melting
- Discuss the secrets behind Quasars
- Describe the recently discovered planets by NASA
- What are called G-type stars?
- Discuss the second Earth-Size World in the Planetary System discovered by NASA
- What is called Planetary Remote Sensing?
Read more: Interesting Science Research Paper Topics To Deal With
Astrophysics Research Topics
- Why are moons orbiting planets?
- Can physics find what we call the multiverse?
- Controversies in astrophysics
- What is dark matter and where can it be found?
- The light spectrum of stars in distant galaxies.
- High energy processes and space plasmas
- Astrophysics : Interdisciplinary views of space
- Astrophysical conditions for the emergence of life
- Stellar evolution and nuclear astrophysics
- Spectroscopy in hot bodies
Research Topics on Space Science
- The latest space tech and breakthroughs.
- The future of space exploration.
- The full history of manned space flight.
- Making business in space.
- The ins and outs of robotic space exploration.
- Space debris and human impact on the solar system
- The future of NASA and space exploration
- The Space Race and the Cold War
- Understanding space-time
- Why explore space?
- Active Experiments in Space: Past, Present, and Future
- The space weather
- Natural processes that take place in the outer space
- The human exploration of space
- Higher Plants, Algae, and Cyanobacteria in Space Environments
- Wound Management and Healing in Space
- Space Traffic Management
- Astronauts and Space
- Biology in Space: Challenges and Opportunities
Fascinating Astronomy Research Topics
- Rocket launches
- The effects of zero gravity on humans
- Life cycles of stars
- Military Space Technology
- The Hubble telescope
- The great crunch
- Heavenly bodies and human mythology
- The similarities between asteroids and comets
- Cosmology: Cosmic ages and distance scales
- Galaxy formation
- Are extraterrestrials real?
- The Galactic Evolution
- The cause of the Northern Lights
- Remote sensing
- Cosmic Time And Distance
- Astrobiology and Plant Formation
- Unidentified Flying Objects and Extraterrestrials
Simple Astronomy Research Topics
- What happens during a solar eclipse?
- Proof that supports the Big Bang theory.
- Discuss the unsolved problems in astronomy.
- Copernicus’s heliocentric model.
- Discuss the effects of the sun on our planet.
- How do the stars shine?
- Theories about the origin of fast radio bursts.
- What is the most possible end of the universe?
- Compare the Big Bang theory and Steady State theory.
- Explain the impact of solar activity on the biosphere of the earth.
Read more: Excellent Forensic Science Research Topics For Students To Consider
Excellent Astronomy Research Ideas
- Describe the ancient peoples’ views on the most important constellations.
- Kepler model and the Eratosthenes model are compared and contrasted.
- Talk about how astronomy uses the geocentric model and the earth orbit model.
- Talk about the societal effects of the Space Shuttle Challenger disaster.
- space trash and the solar system as influenced by humans.
- calculating the density and mass of the solar system.
- the reasons why time travel is not conceivable.
- How did early humans perceive the most important constellations?
- Examine the first contact to understand the effects of life on other planets.
- Data transmission over 5G wireless and satellites
Top-rated Astronomy Research Topics
- Which issues are astrophysicists now working to resolve as the most crucial ones?
- What are the most significant astrophysical puzzles yet unanswered?
- What are the biggest problems that astrophysics is now facing?
- Stars in far-off galaxies have a wide range of light.
- Is the multiverse something that physics can detect?
- What current developments in astrophysics are the most significant?
- What are the most crucial astrophysical future directions?
- The enigmas surrounding dark matter and dark energy
Amazing Astronomy Research Topics
- Discuss the major influence of 5G wireless data transfer.
- Explain the sun’s source of heat and light for millions of years.
- Compare space weather and Earth’s weather.
- Evaluate the existence of an unremarkable star.
- Explain the earth’s outlook as viewed from space.
- What caused the Challenger to explode after take-off?
- Review the doctrine of Noosphere.
Final Words
Out of the numerous ideas suggested above, choose any topic of your choice and draft a high-quality astronomy research paper. If you want any other impressive astronomy research topics or if you are confused about how to write an engaging astronomy research paper, then reach out to us for assignment writing assistance. We have a team of professional writers who are experts in the field of astronomy to help you write a brilliant research paper on the latest astronomy research topics.
Just place your order by writing your requirements to us and get a plagiarism-free research paper matching your expectations at an affordable rate.
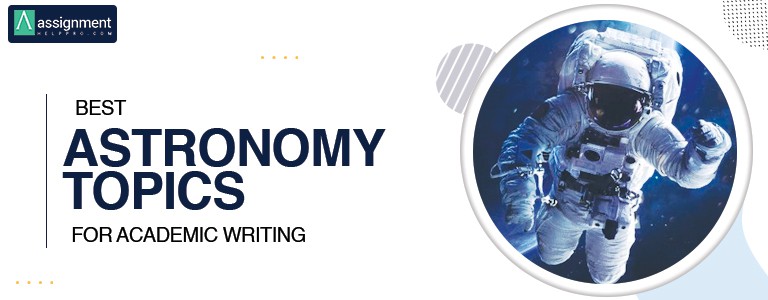
Related Post

220 Amazing Religious Research Paper Topics and Ideas
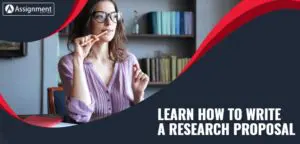
Read and Understand How to Write a Research Proposal
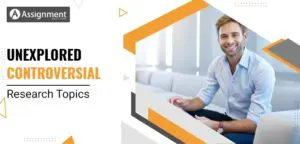
100+ Controversial Research Topics and Ideas to Focus On
About author.
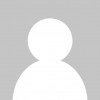
Jacob Smith
I am an Academic Writer and have affection to share my knowledge through posts’. I do not feel tiredness while research and analyzing the things. Sometime, I write down hundred of research topics as per the students requirements. I want to share solution oriented content to the students.
Leave a Reply Cancel reply
You must be logged in to post a comment.
- Featured Posts
140 Unique Geology Research Topics to Focus On
200+ outstanding world history topics and ideas 2023, 190 excellent ap research topics and ideas, 150+ trending group discussion topics and ideas, 170 funny speech topics to blow the minds of audience, who invented exams learn the history of examination, how to focus on reading 15 effective tips for better concentration, what is a rhetorical analysis essay and how to write it, primary school teacher in australia- eligibility, job role, career options, and salary, 4 steps to build a flawless business letter format, get help instantly.
Raise Your Grades with Assignment Help Pro
Frontiers | Science News
- Science News
Research Topics
Reach for the stars: research topics on space exploration.
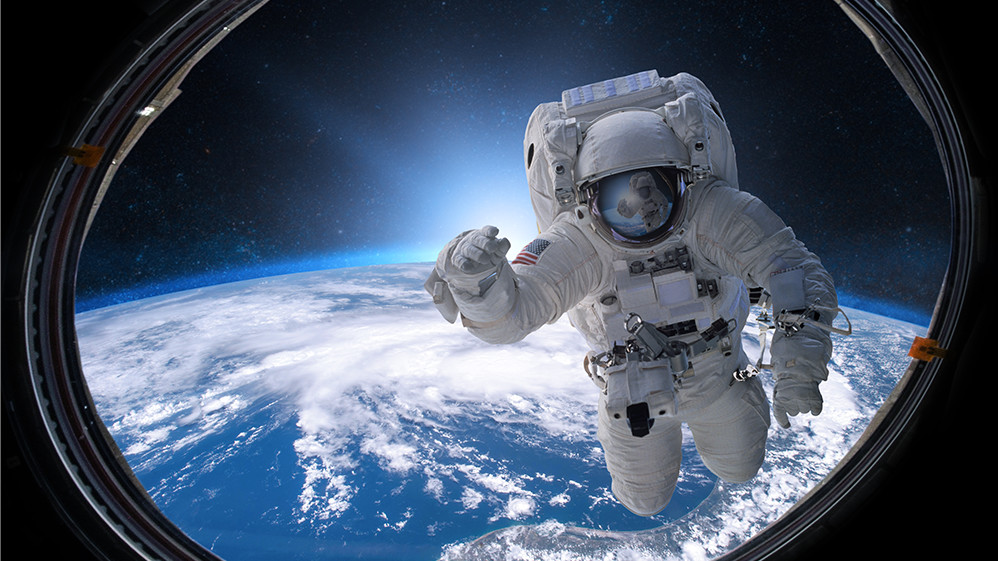
With recent advances in commercial space exploration, we have curated a list of our best Research Topics on outer space. Explore collections edited by experts from NASA, The Goddard Space Flight Center, Space Science Institute, German Aerospace Center, Canadian Space Agency, National Space Science Center, European Space Agency, International Space University, and many more.
Research Topics:
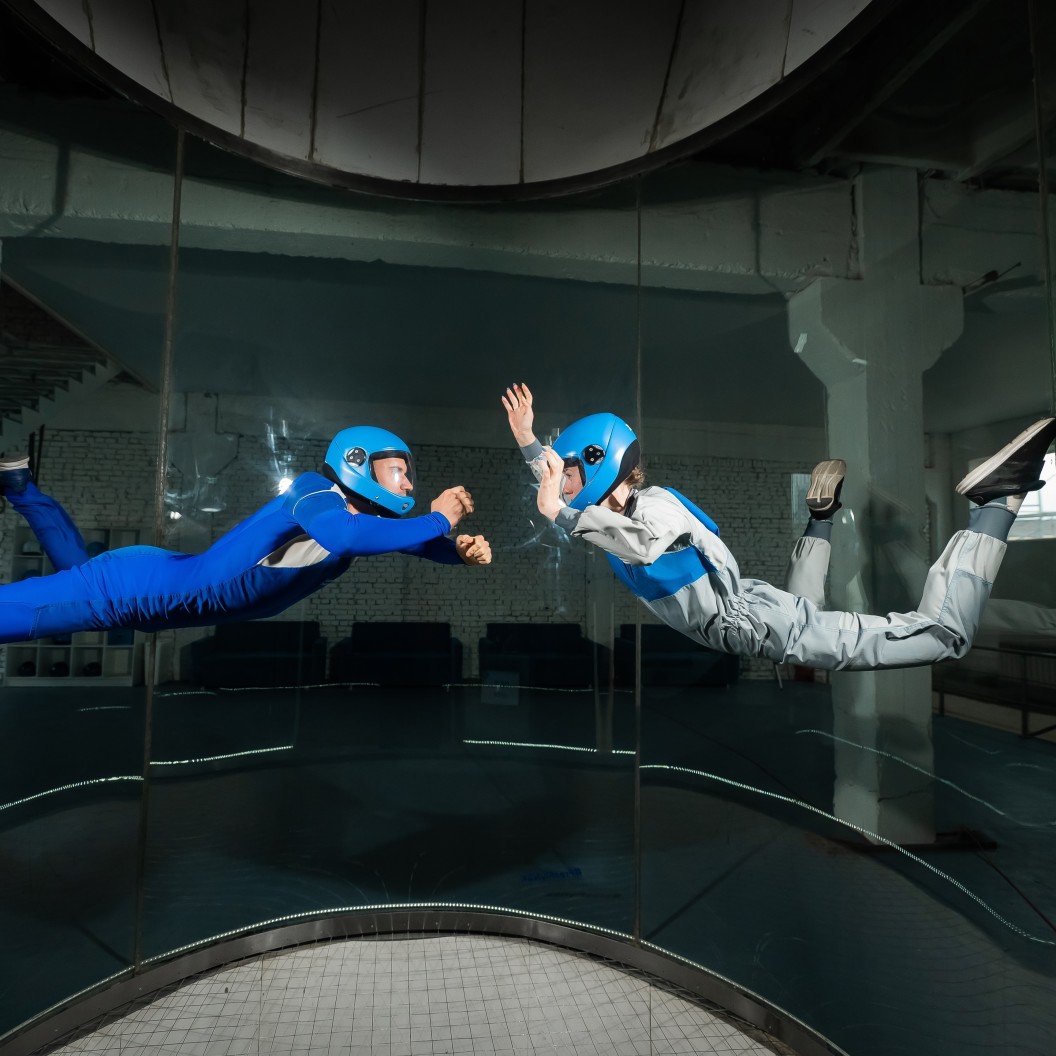
Optimization of Exercise Countermeasures for Human Space Flight – Lessons from Terrestrial Physiology and Operational Implementation
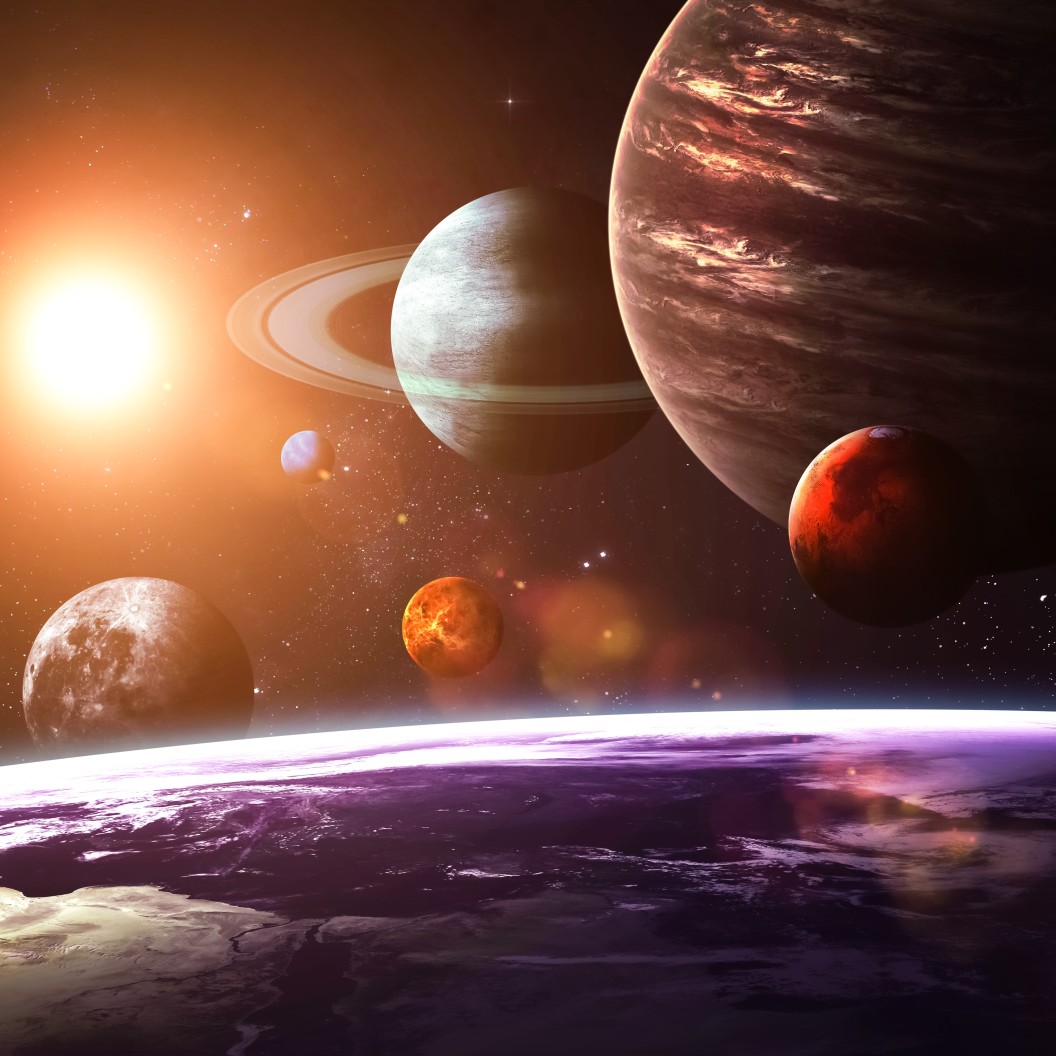
Biology in Space: Challenges and Opportunities
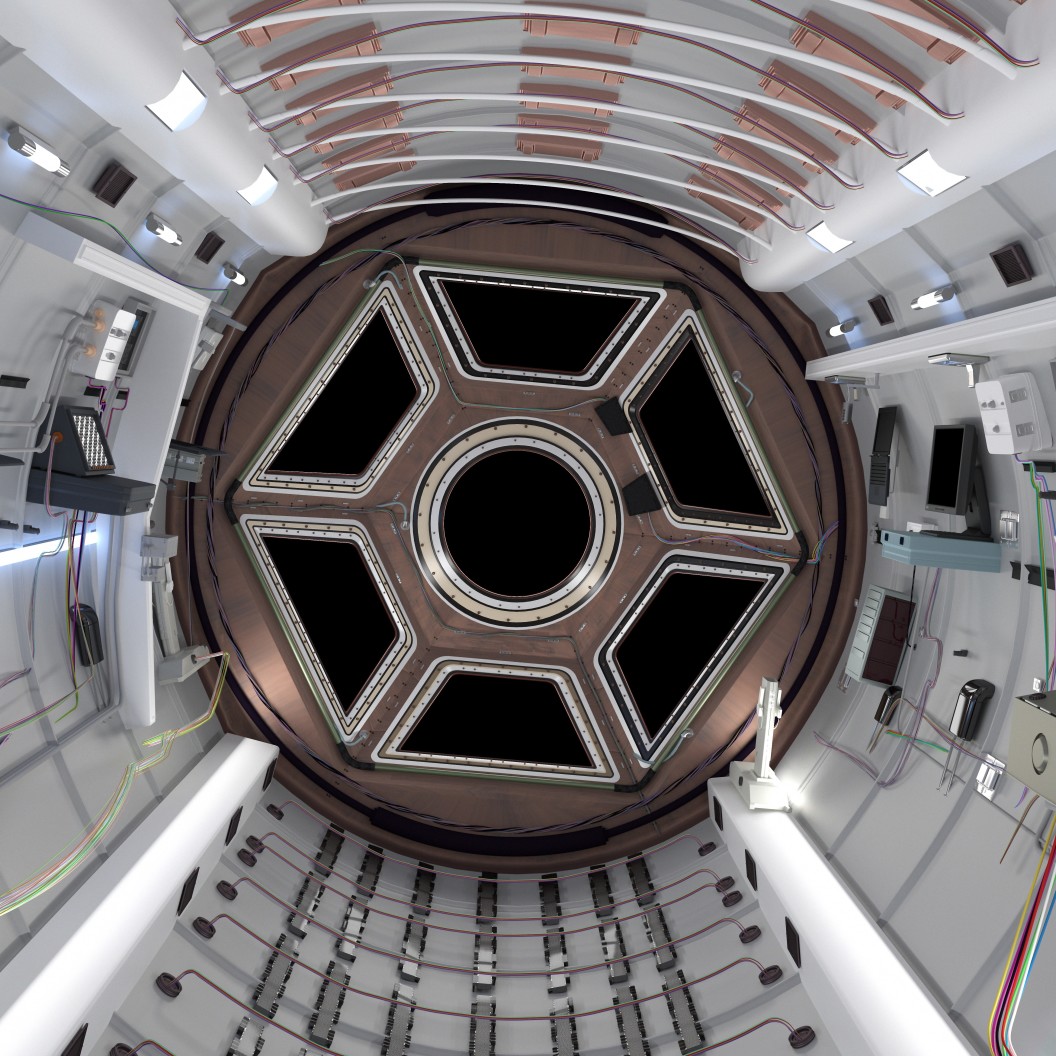
Microbiology of Extreme and Human-Made Confined Environments (Spacecraft, Space Stations, Cleanrooms, and Analogous Sites)
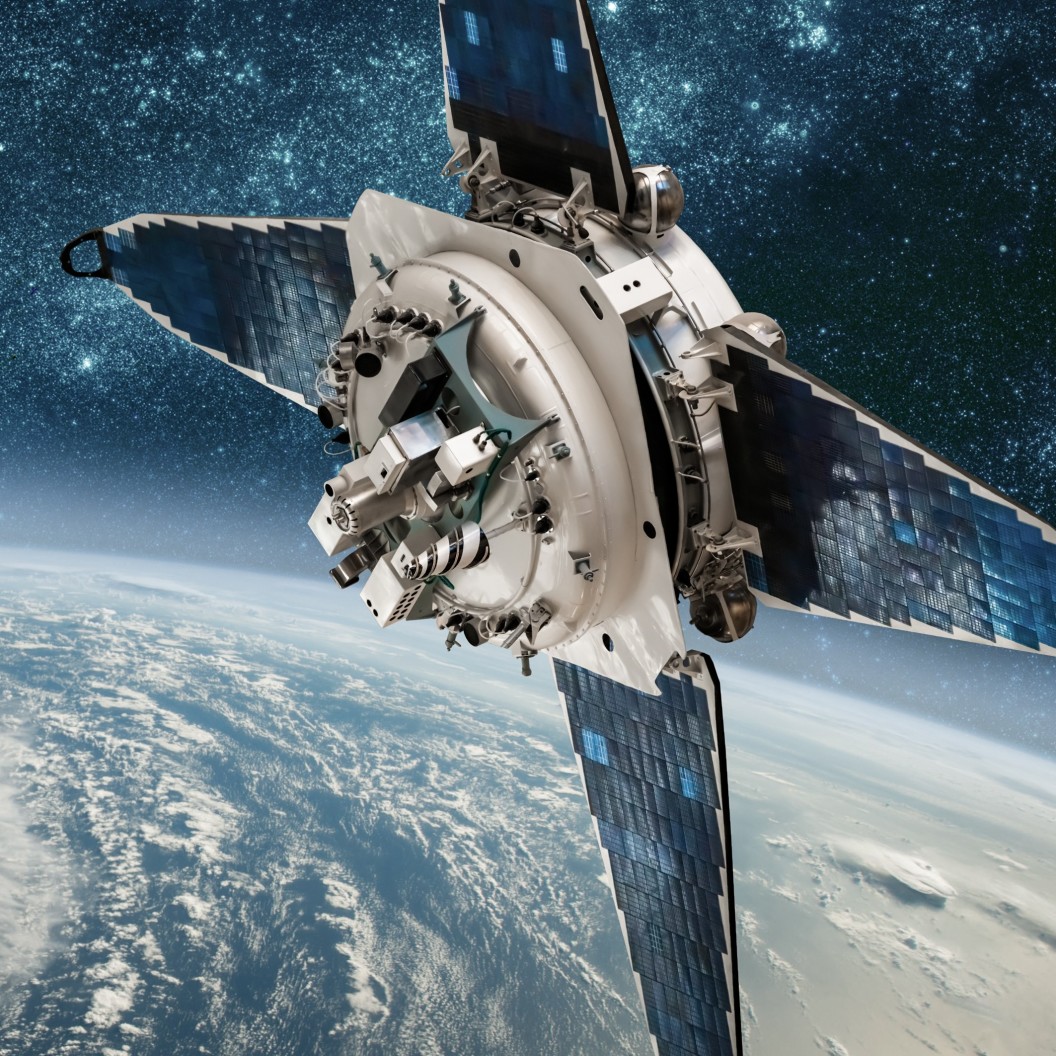
Geospace Observation of Natural Hazards
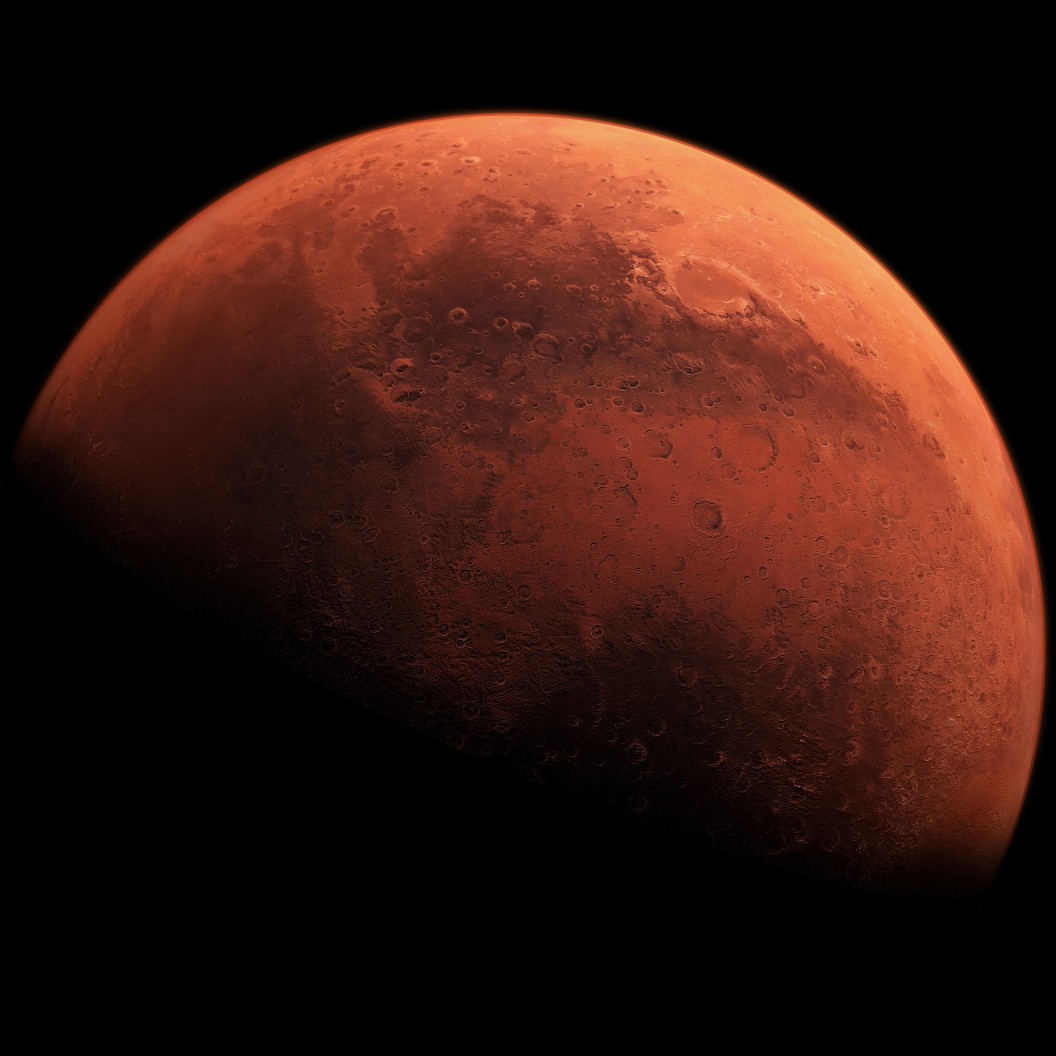
Astrobiology of Mars, Europa, Titan and Enceladus - Most Likely Places for Alien Life
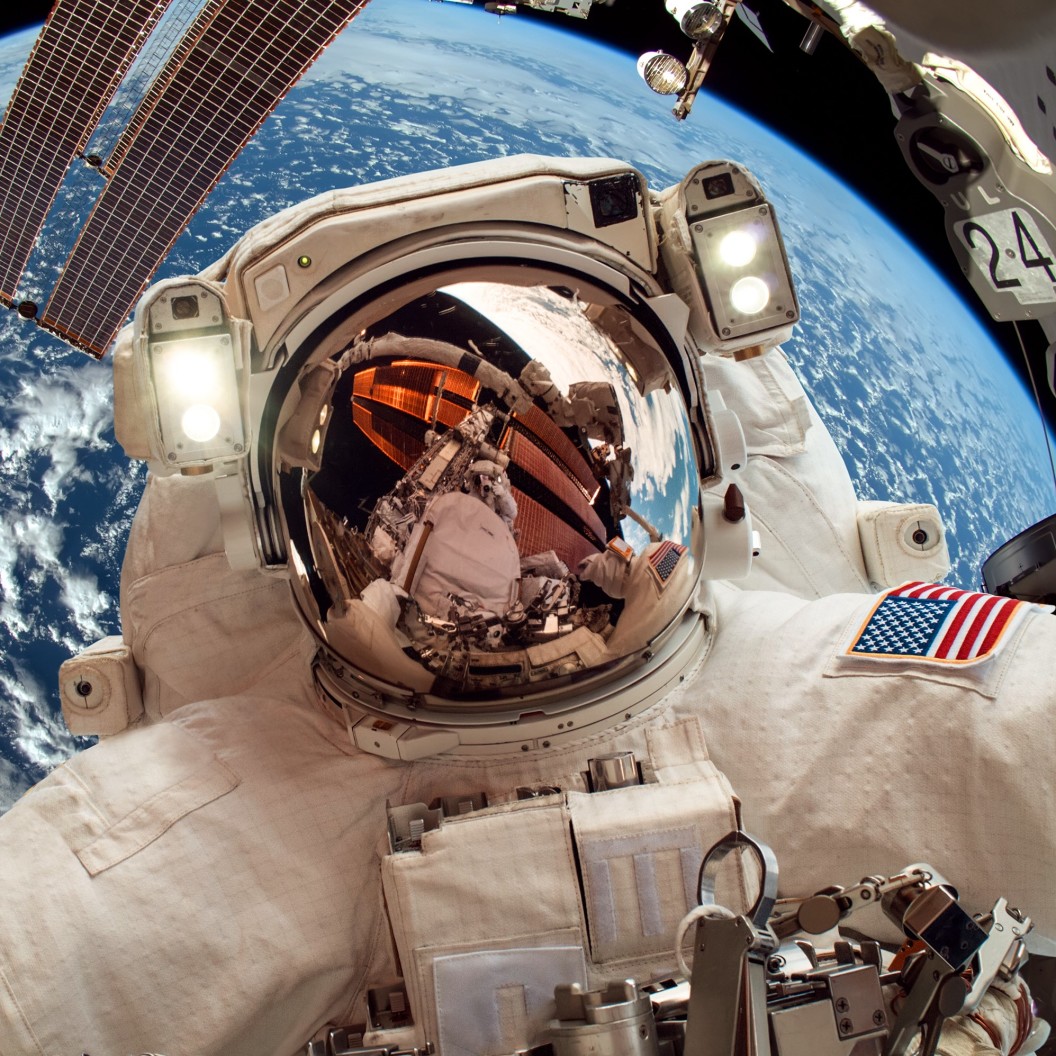
Imagining the Future of Astronomy and Space Science
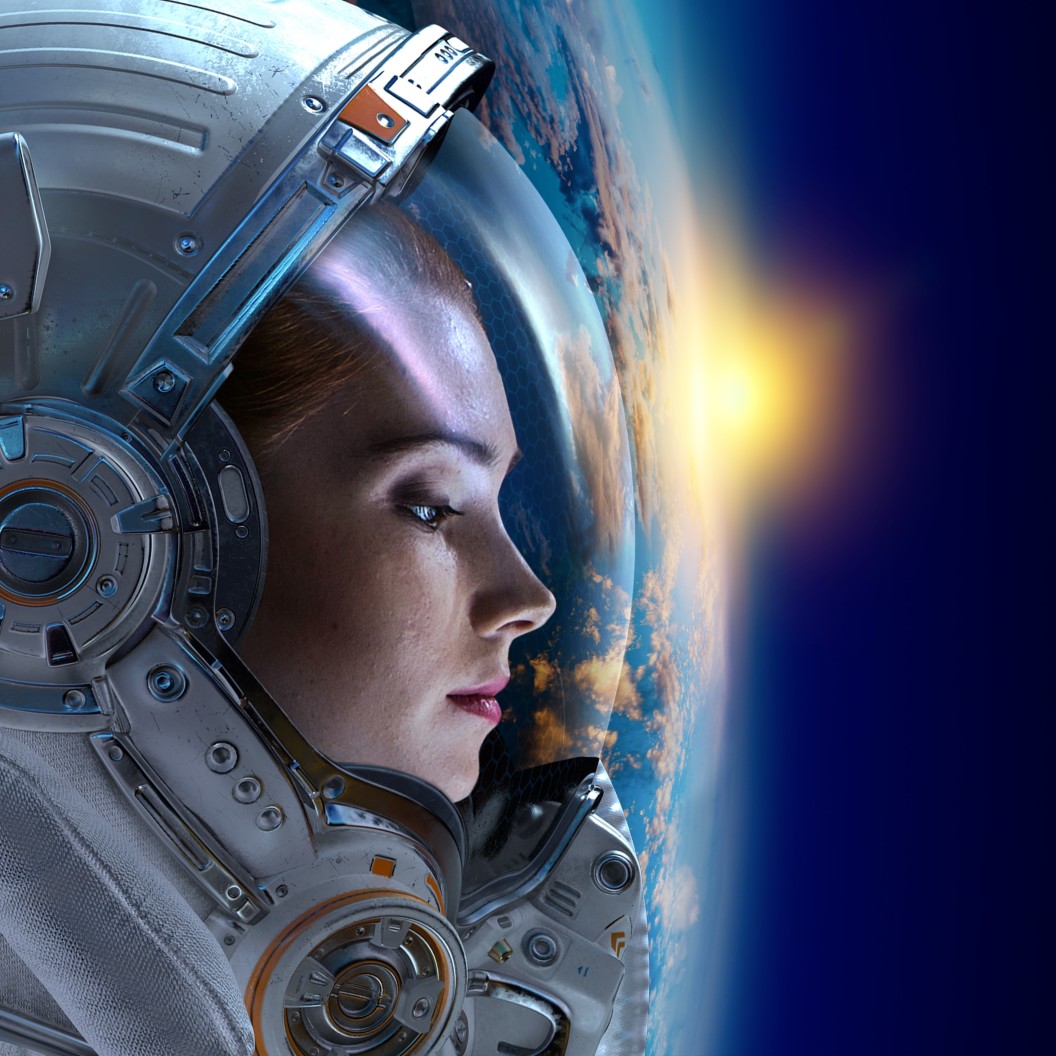
Brains in Space: Effects of Spaceflight on the Human Brain and Behavior
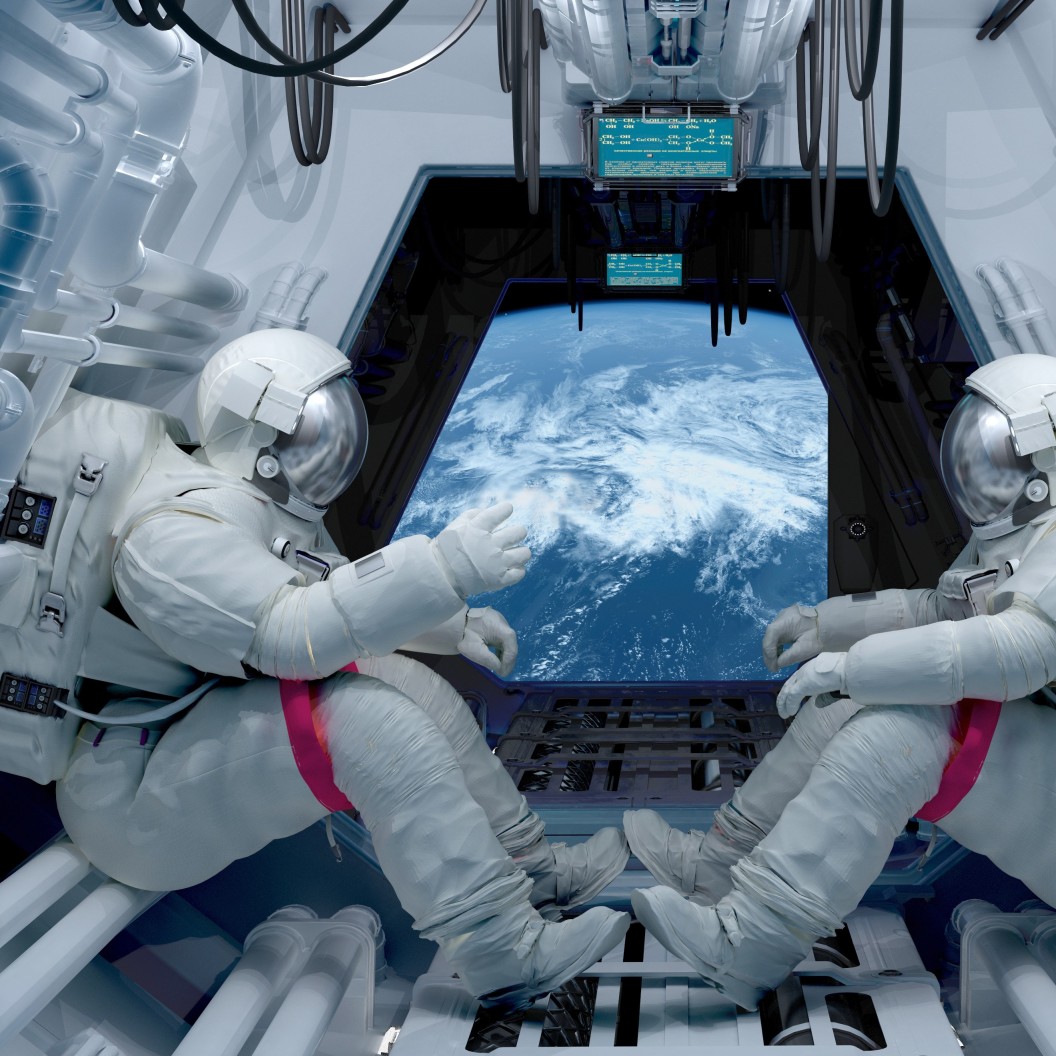
Creative Performance in Extreme Human Environments: Astronauts and Space
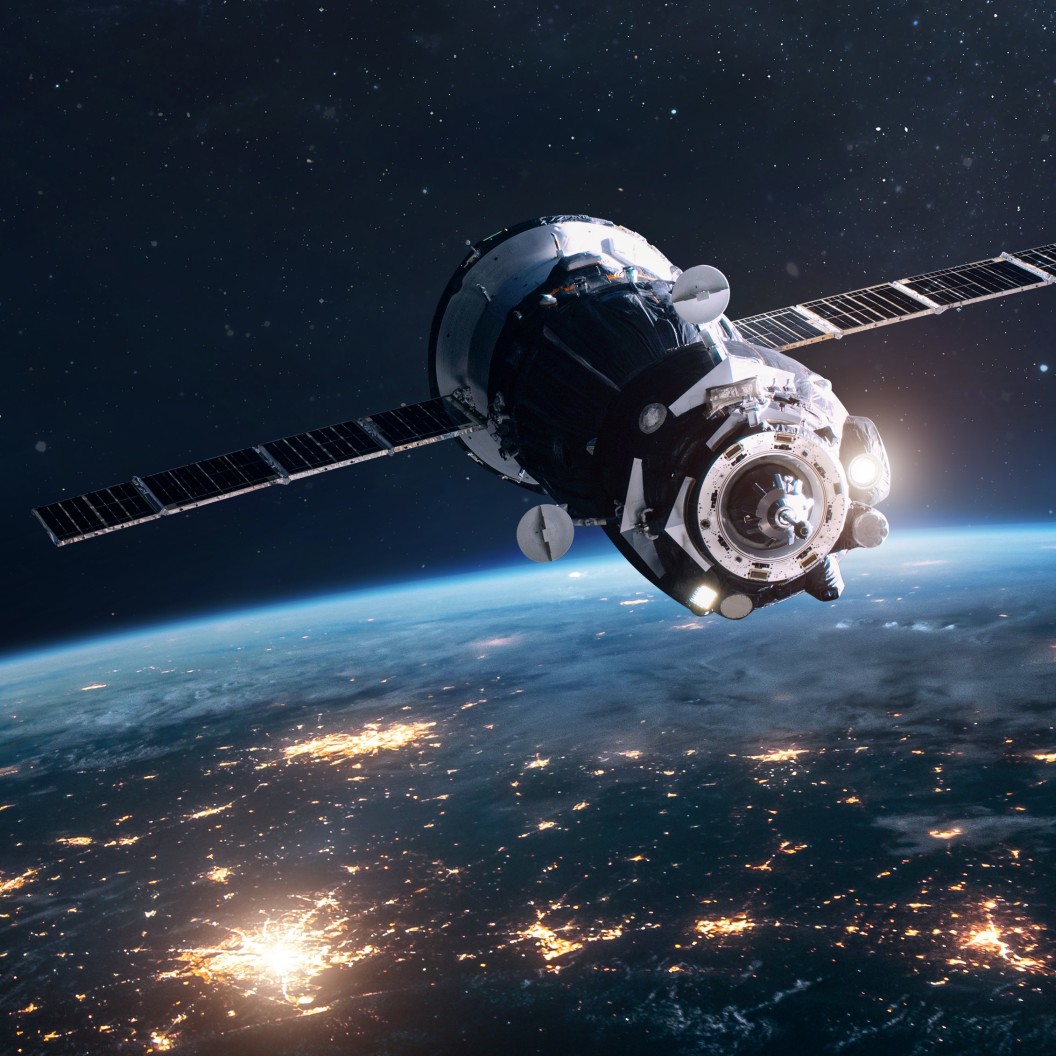
Space Traffic Management: a new era in Earth orbit
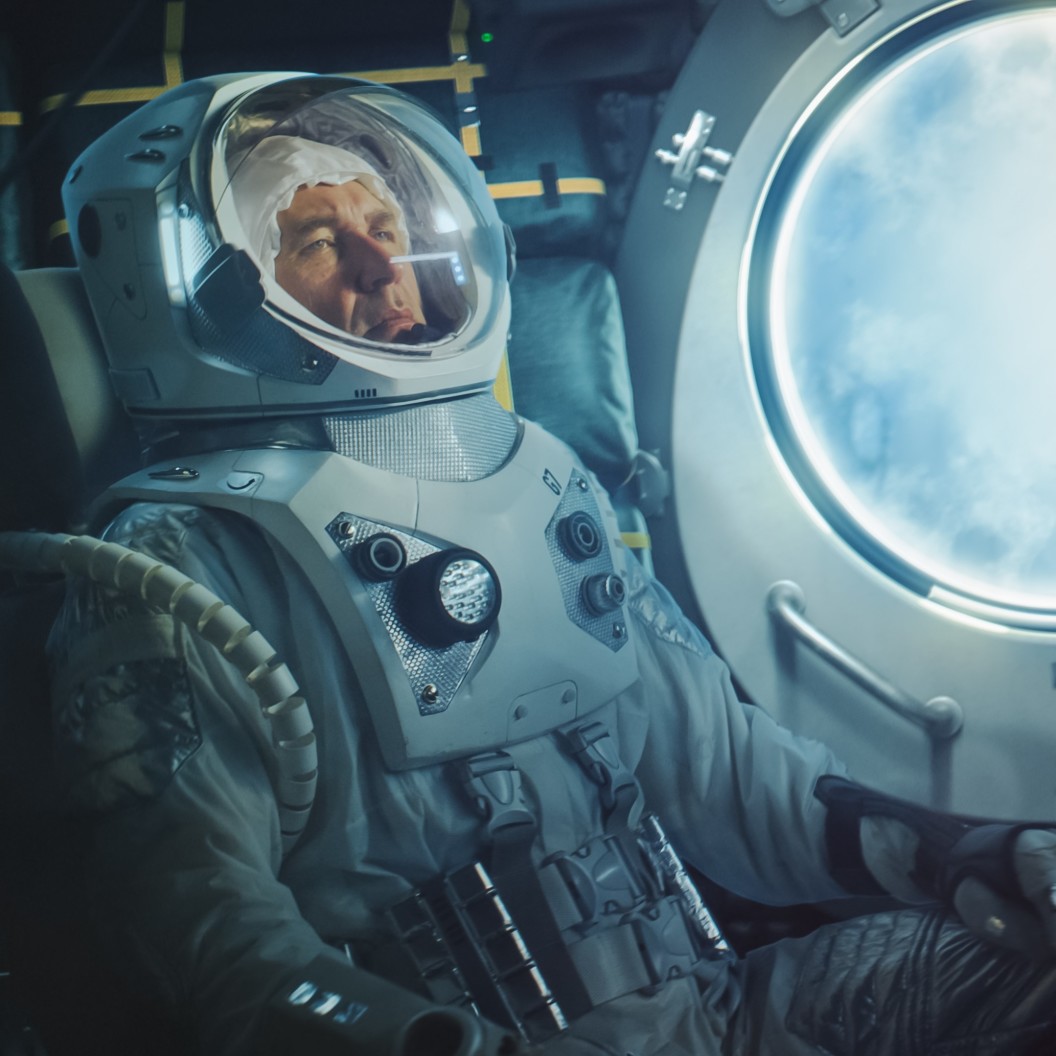
Wound Management and Healing in Space
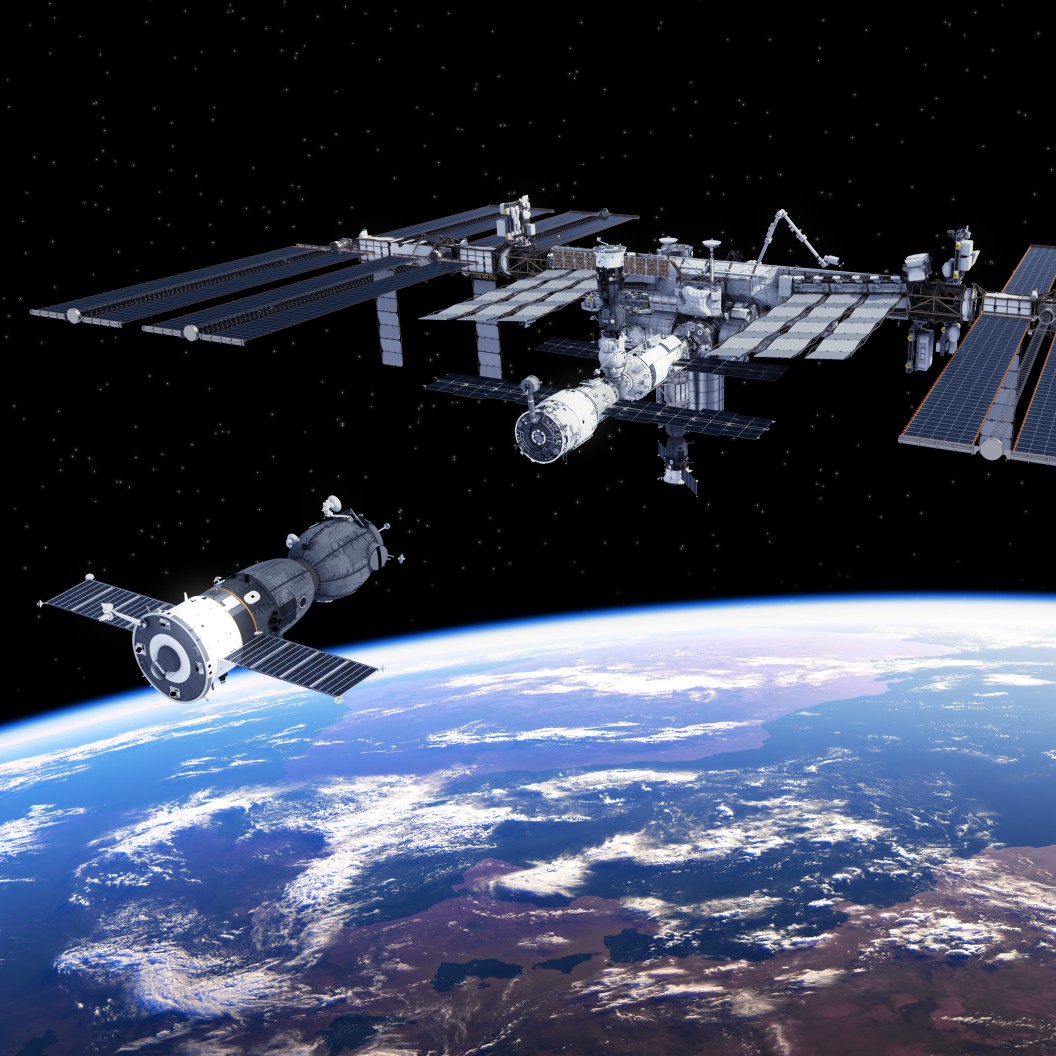
Robotic Manipulation and Capture in Space
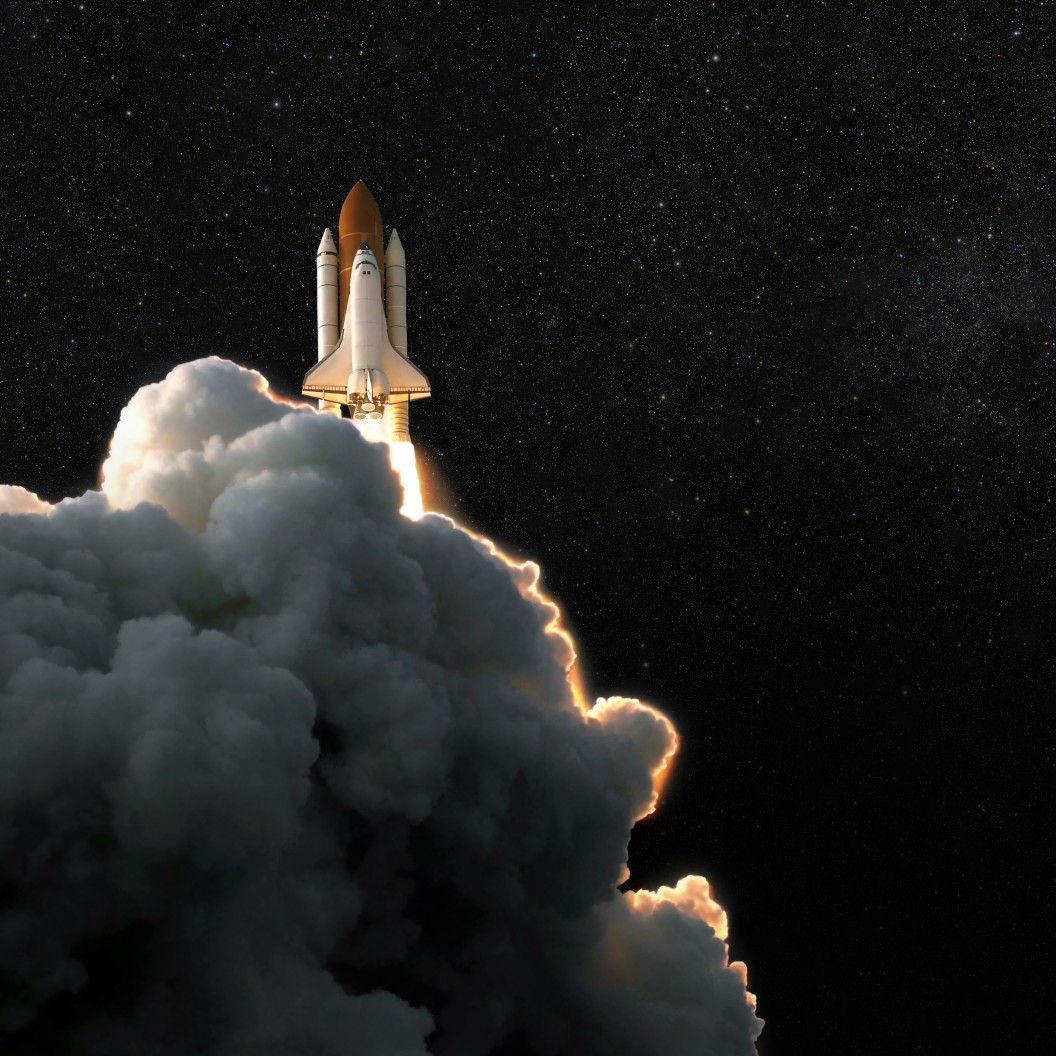
A Multidisciplinary Approach to designing Sensorimotor Adaptation countermeasures for space exploration missions
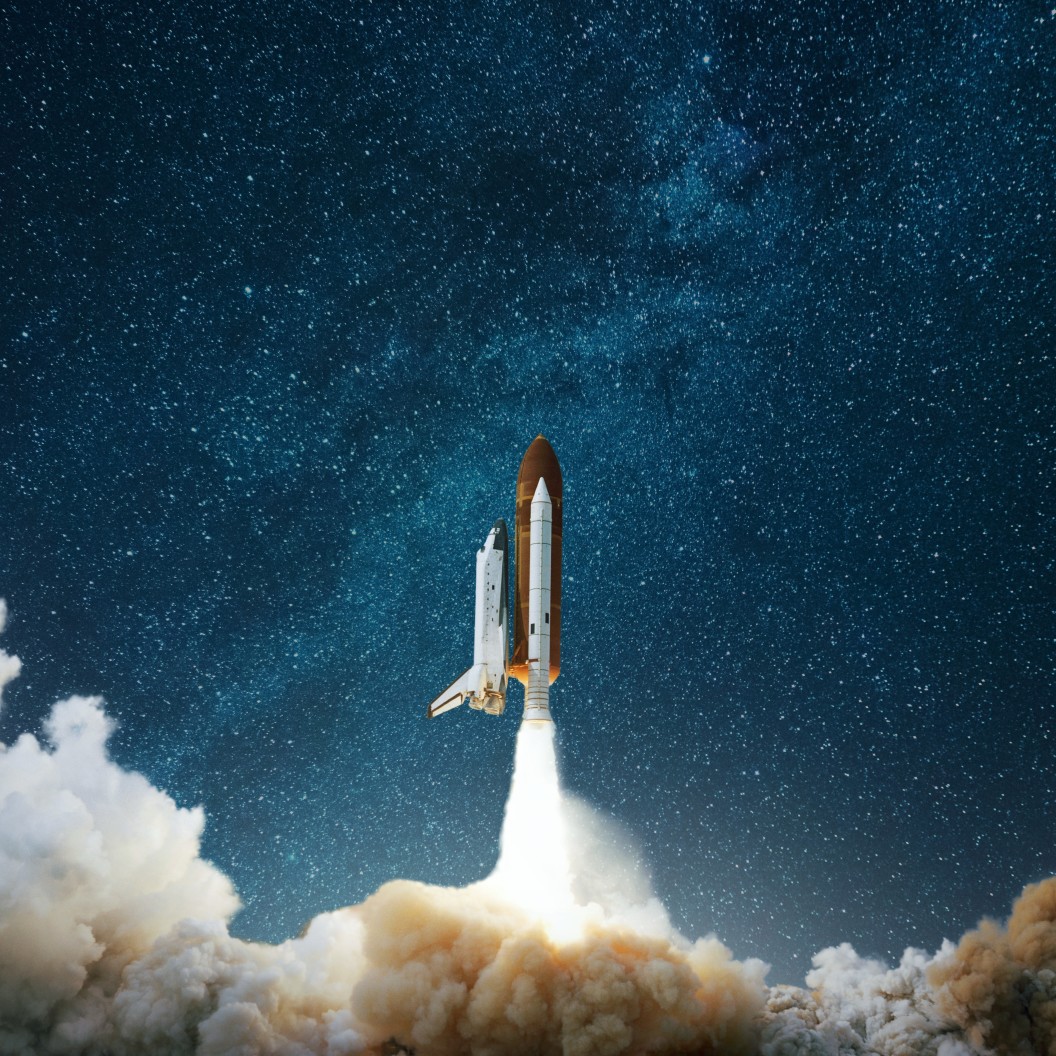
Active Experiments in Space: Past, Present, and Future
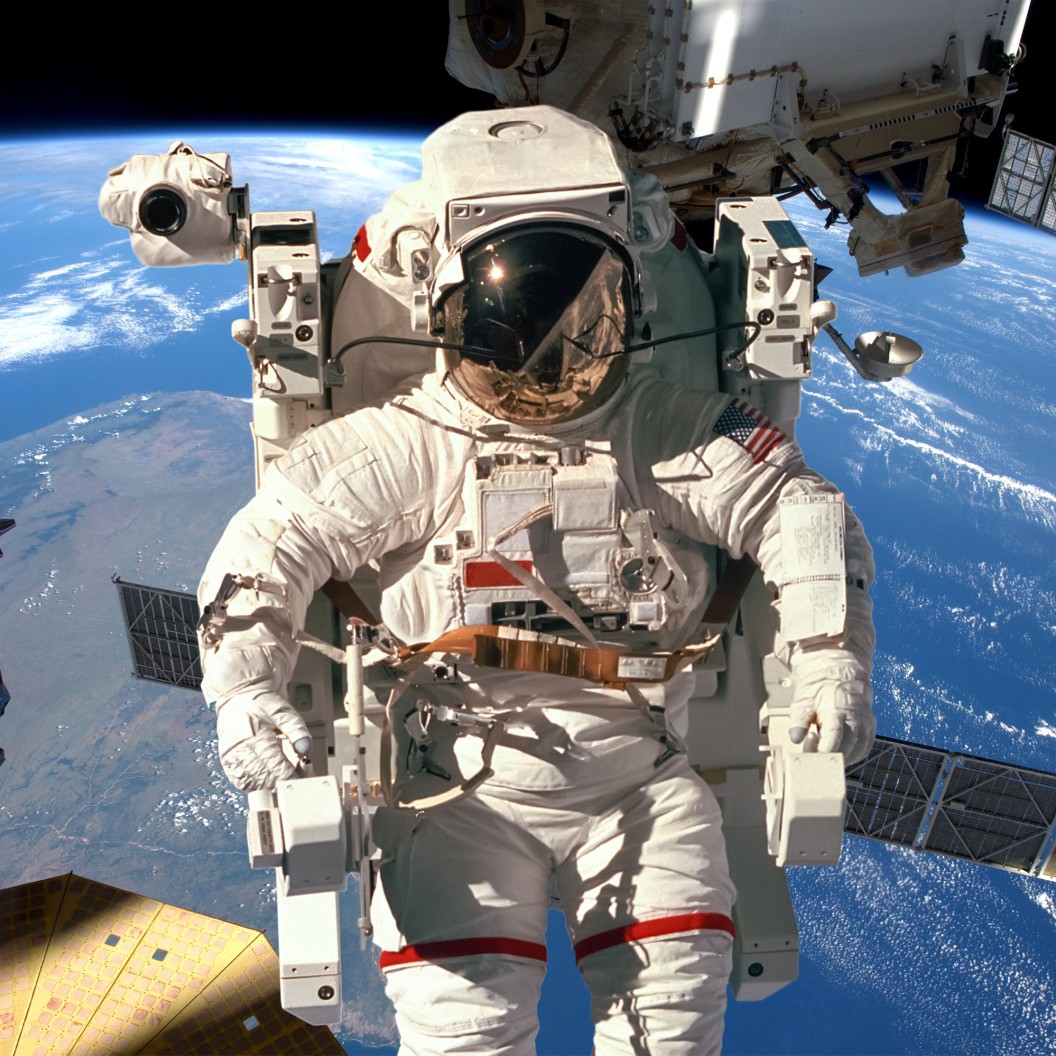
On-orbit Manufacturing and Assembly Technologies for Future Space Activities
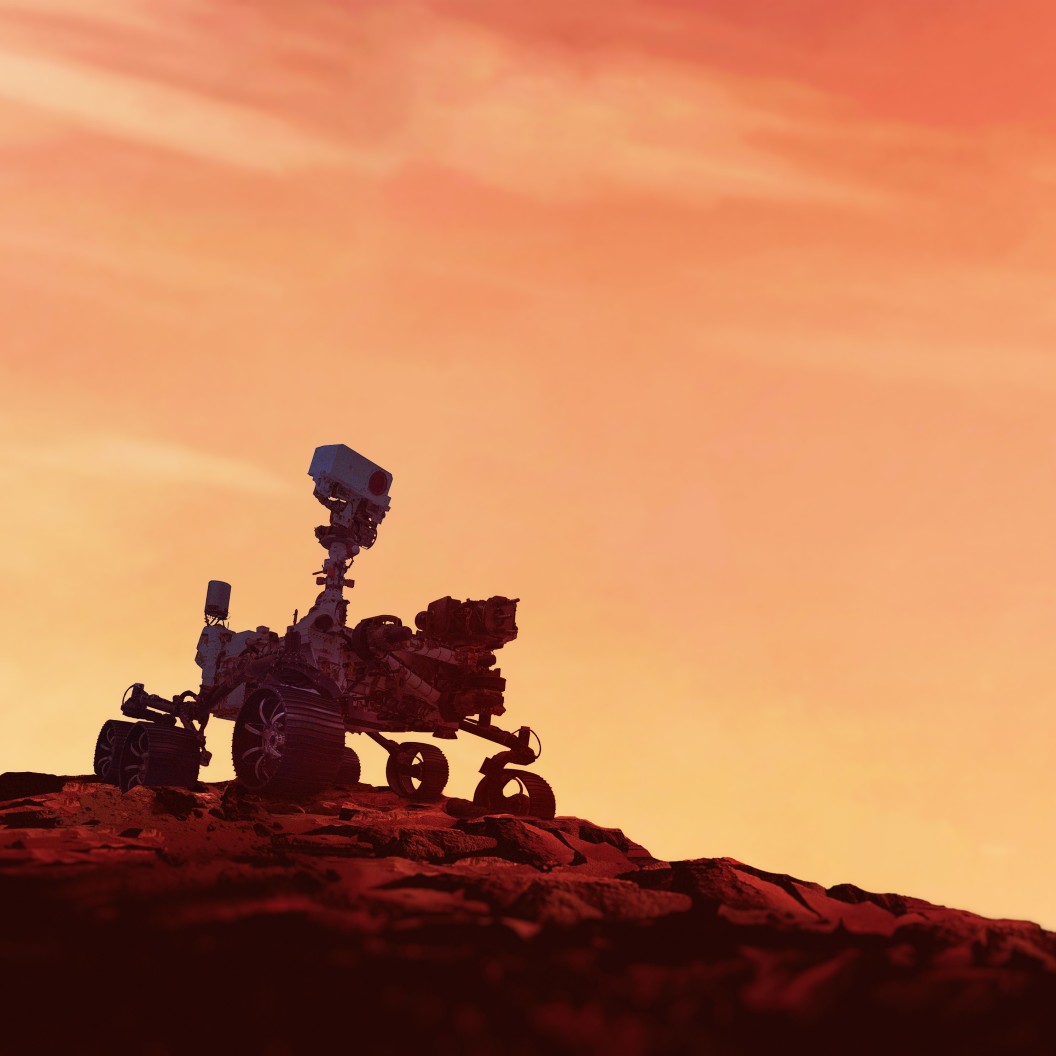
Current and Future Instrumentation for the Detection and Identification of Signatures of Life on Mars and Beyond
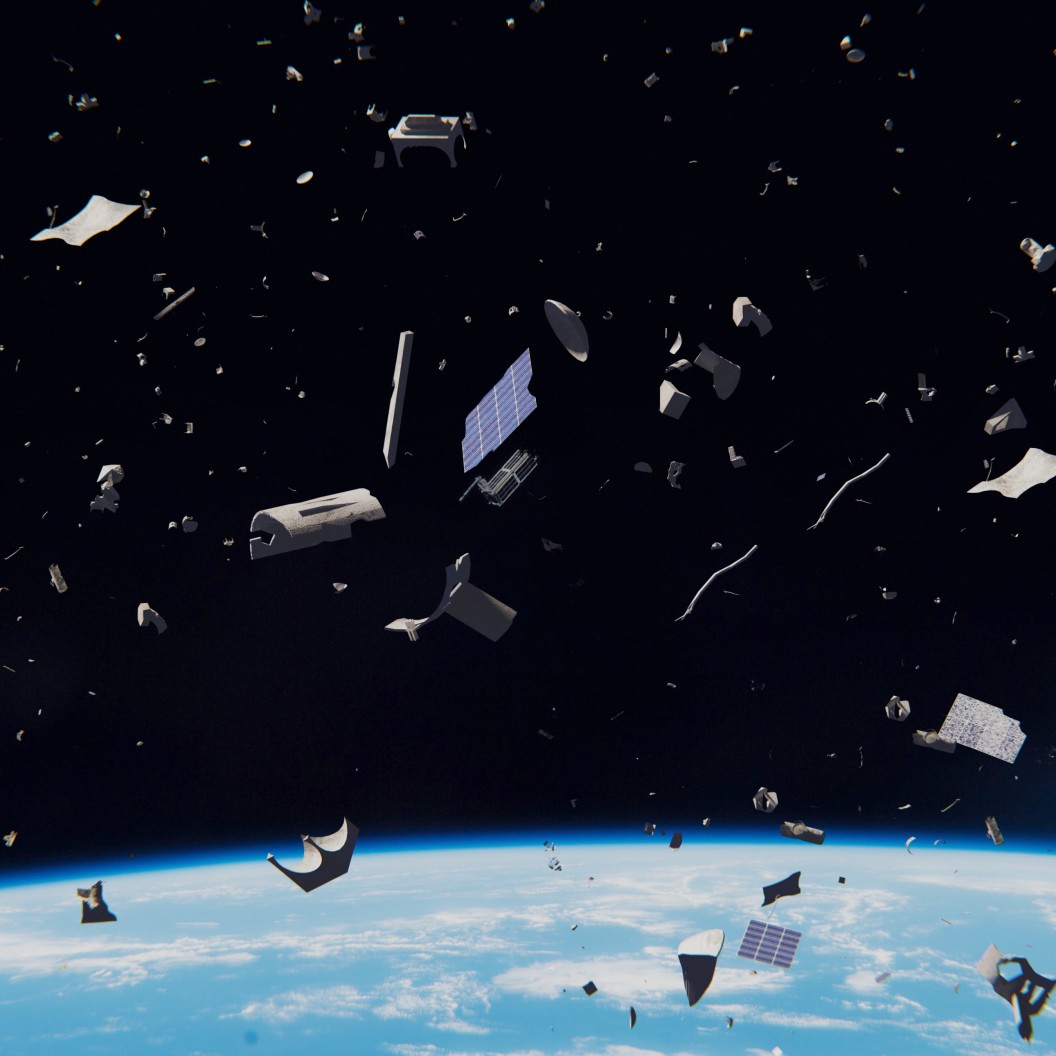
On-Orbit Servicing and Active Debris Removal: Enabling a Paradigm Shift in Spaceflight
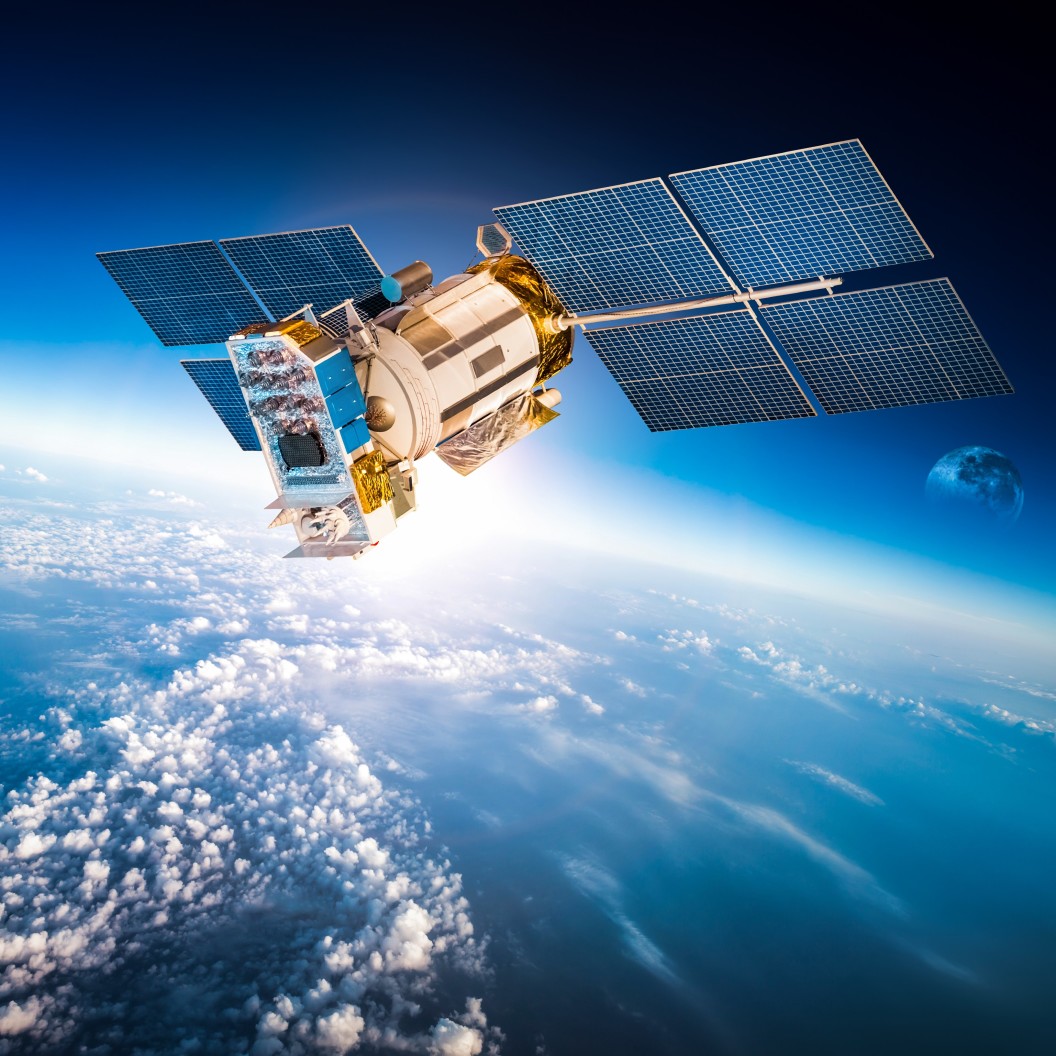
Space Weather with Small Satellites
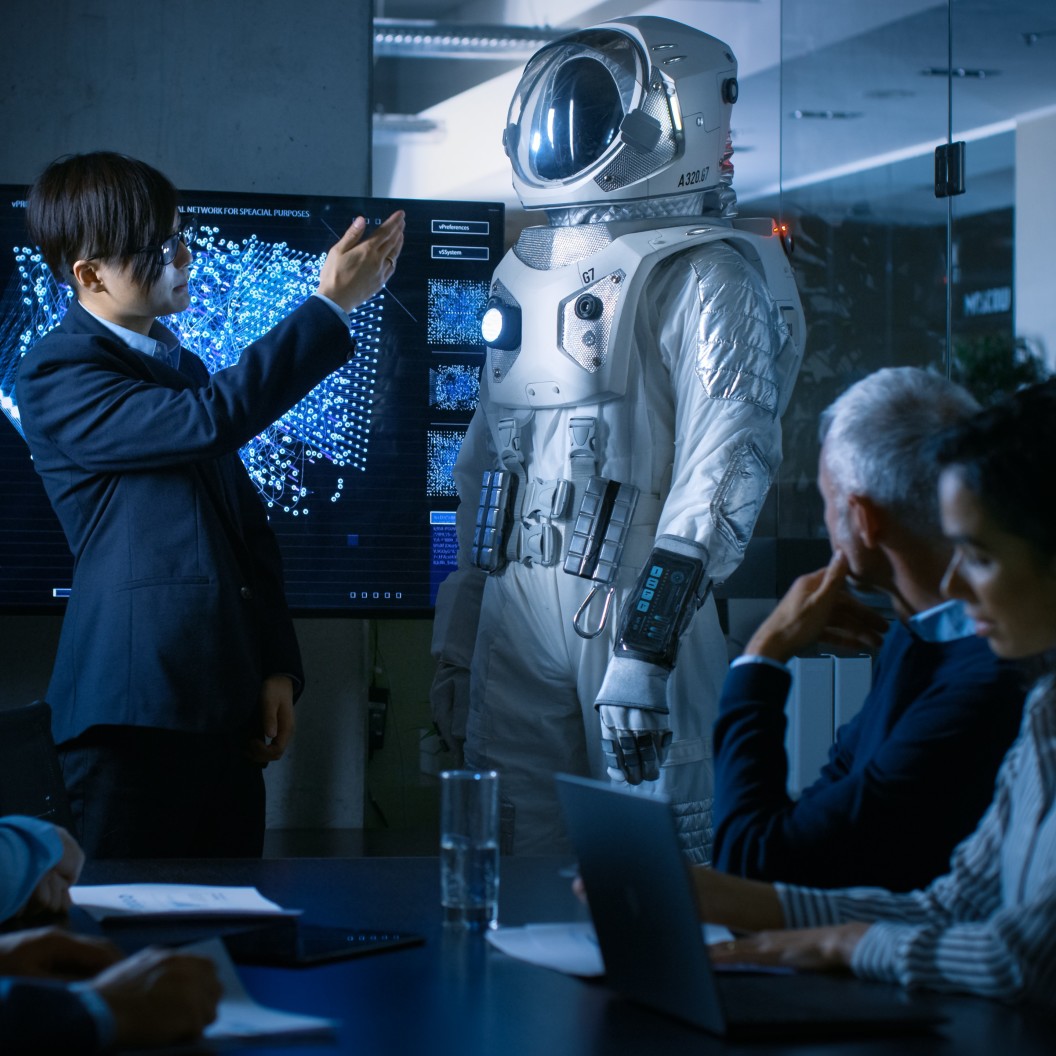
AI in the Space Sciences
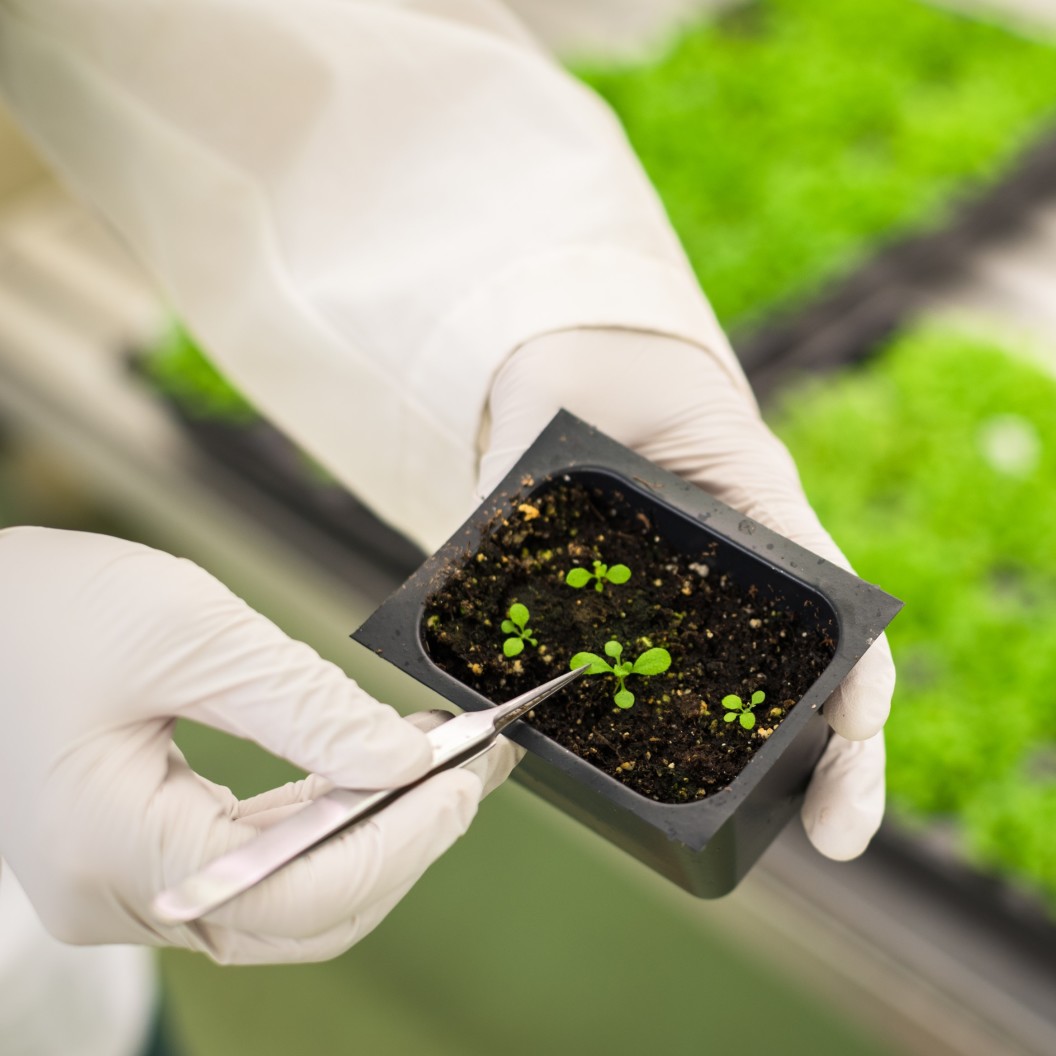
Higher Plants, Algae and Cyanobacteria in Space Environments
Post related info
October 11, 2021
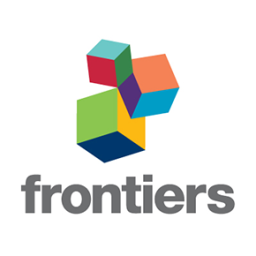
Frontiers Communications
Post categories, space sciences and astronomy, related subjects, research topics, related content.
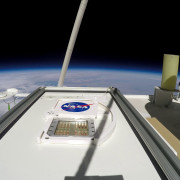
Life from Earth could temporarily survive on Mars
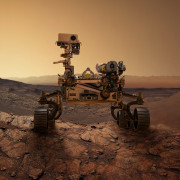
Meet a team of scientists working to prevent interplanetary pollution that could pose a threat to life on Earth and other planets
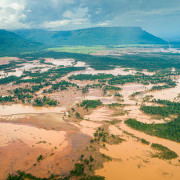
NASA space data can cut disaster response times, costs
Latest posts.
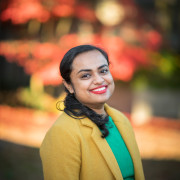
Kaveri Mayra - Unmasking the hidden violence around pregnancy and birth in obstetric settings
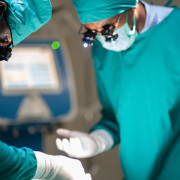
Pig hearts kept alive outside the body for more than 24 hours offers hope for many humans needing a transplant
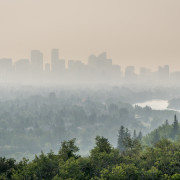
Scientists call for urgent action to prevent immune-mediated illnesses caused by climate change and biodiversity loss
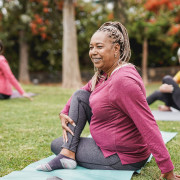
Frontiers ebook releases: March 2024
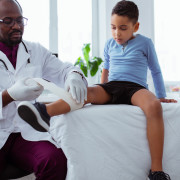
Opening health for all: 7 Research Topics shaping a healthier world

100+ Space Research Topics [Updated]
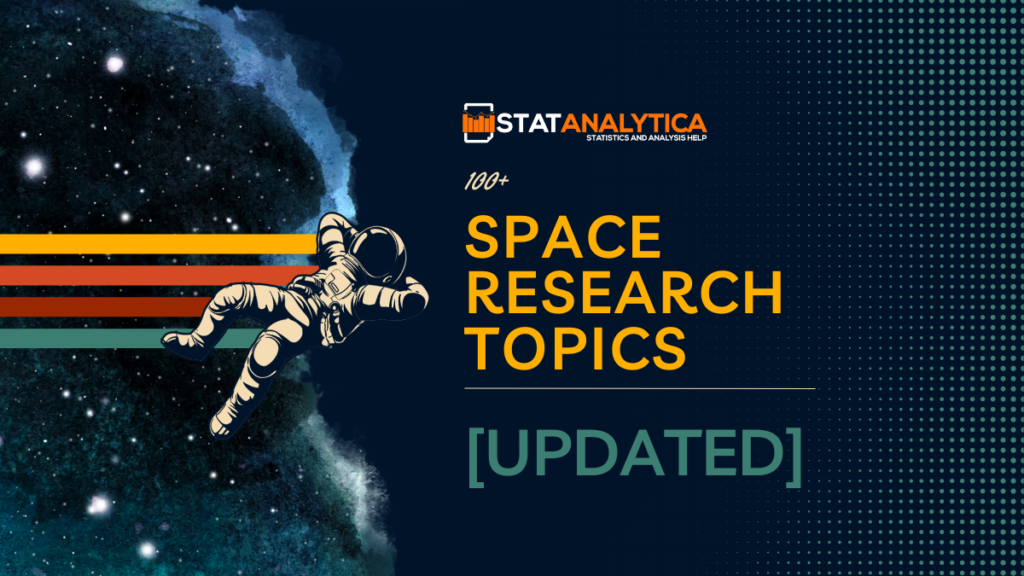
Space has always attracted humanity’s imagination. The vastness of the cosmos, with its twinkling stars, mysterious planets, and enigmatic black holes, beckons us to explore its depths. But why do we study space? What are the research topics that drive scientists to reach for the stars? In this blog, we’ll delve into the fascinating world of space research topics, exploring key topics that continue to inspire and challenge researchers around the globe.
Why Do We Study Space?
Table of Contents
Here are some key points explaining why we study space:
- Understanding our Origins: Space research helps us uncover the origins of the universe, including how galaxies, stars, and planets formed.
- Advancing Scientific Knowledge: Studying space leads to breakthroughs in physics, astronomy, and other scientific fields, expanding our understanding of the cosmos.
- Technological Innovation: Space exploration drives the development of new technologies, such as satellite communication and medical imaging, benefiting society as a whole.
- Exploration and Discovery: Humans are inherently curious, and space offers a vast frontier for exploration, fueling our desire to discover new worlds and phenomena.
- Earth Observation: Space-based observations provide valuable data on Earth’s climate, weather patterns, and environmental changes, aiding in disaster management and conservation efforts.
- Search for Life: Investigating other planets and celestial bodies helps us understand the conditions necessary for life, potentially leading to the discovery of extraterrestrial life forms.
- Inspiration and Education: Space exploration inspires future generations of scientists, engineers, and explorers, fostering innovation and curiosity about the universe.
100+ Space Research Topics: Category Wise
Astronomy and astrophysics.
- Exoplanet detection methods and recent discoveries
- The life cycle of stars: from birth to death
- Supermassive black holes and their role in galaxy formation
- Gravitational waves: detection and implications
- Dark matter and dark energy: understanding the mysteries of the universe
- Supernovae explosions: studying the aftermath of stellar deaths
- Galactic dynamics: exploring the structure and evolution of galaxies
- Cosmic microwave background radiation: insights into the early universe
- Gamma-ray bursts: uncovering the most energetic explosions in the cosmos
- The search for extrasolar planets with potential habitable conditions
Planetary Science
- Martian geology and the search for signs of past life
- Jupiter’s Great Red Spot: dynamics and longevity
- Saturn’s rings: composition, structure, and origin
- Lunar exploration: past missions and future prospects
- Venusian atmosphere: understanding the greenhouse effect and extreme conditions
- Io, Europa, Ganymede, and Callisto: Jupiter’s diverse moons
- Titan: Saturn’s moon with an Earth-like atmosphere and hydrocarbon lakes
- The Kuiper Belt and Oort Cloud: reservoirs of comets and icy bodies
- Dwarf planets: Pluto, Eris, Haumea, Makemake, and Ceres
- Planetary volcanism: processes and consequences on various celestial bodies
Space Technology and Engineering
- Satellite constellations for global internet coverage
- CubeSats: miniaturized satellites for scientific research and technology demonstration
- Space debris mitigation strategies and technologies
- Ion propulsion systems: efficient propulsion for deep space missions
- Space telescopes: next-generation observatories for astronomy and astrophysics
- Space-based solar power: harvesting solar energy in orbit
- Asteroid mining: extracting resources from near-Earth objects
- In-situ resource utilization on other planets and moons
- Additive manufacturing (3D printing) in space exploration
- Autonomous spacecraft navigation and control for long-duration missions
Astrobiology and the Search for Life
- Extremophiles: organisms thriving in extreme environments on Earth and their implications for extraterrestrial life
- Biosignatures: markers of past or present life on other planets
- Methanogenesis on Mars: potential evidence for subsurface microbial life
- Europa’s subsurface ocean: exploring the possibility of life beneath the ice
- Enceladus: hydrothermal vents and the search for life in its subsurface ocean
- The habitability of exoplanets: assessing conditions for life beyond the solar system
- Panspermia: the transfer of life between celestial bodies
- Astrobiology field research in extreme environments on Earth
- SETI: the search for extraterrestrial intelligence and communication
- The implications of discovering microbial life on Mars or other celestial bodies
Space Policy and Ethics
- International collaboration in space exploration and research
- The Outer Space Treaty: principles governing the use of outer space
- Space tourism regulations and safety considerations
- Space law and jurisdiction: legal frameworks for activities in space
- Military applications of space technology and potential arms race in space
- Space resource utilization and ownership rights
- Space environmentalism: advocating for the protection of celestial bodies and their environments
- Space colonization ethics and implications for human societies
- Space governance beyond national boundaries
- Cultural heritage preservation on the Moon and other celestial bodies
Challenges and Future Directions
- Funding challenges and opportunities in space research and exploration
- Space radiation hazards and mitigation strategies for astronauts
- Interplanetary communication and navigation for deep space missions
- Long-duration spaceflight: physiological and psychological effects on astronauts
- Terraforming Mars: engineering a habitable environment on the Red Planet
- Space elevator concept: a revolutionary approach to space access
- Next-generation space launch vehicles and propulsion technologies
- Nuclear propulsion for crewed missions to Mars and beyond
- Space settlement design and infrastructure requirements
- Advancing artificial intelligence and robotics for autonomous space exploration
Space Weather and Space Environment
- Solar flares and coronal mass ejections: impacts on Earth’s magnetosphere and technology
- Space weather forecasting and its applications in satellite operations
- Magnetospheres of Earth and other planets: comparative studies and dynamics
- Solar wind interactions with planetary atmospheres and magnetospheres
- Aurora phenomena on Earth and other planets
- Radiation belts: understanding and mitigating hazards for spacecraft and astronauts
- Cosmic rays: sources, composition, and effects on space missions
- Space climate change: long-term variations in solar activity and their consequences
- Space weather effects on satellite communications, navigation, and power systems
- Space weather monitoring and prediction networks
Space Exploration and Missions
- Mars Sample Return mission: challenges and scientific objectives
- Artemis program: NASA’s plans for returning astronauts to the Moon
- Asteroid impact mitigation strategies and planetary defense initiatives
- The James Webb Space Telescope: capabilities and scientific goals
- Europa Clipper mission: exploring Jupiter’s icy moon for signs of habitability
- China’s Chang’e lunar exploration program: past achievements and future missions
- Commercial crew and cargo transportation to the International Space Station
- Voyager and Pioneer missions: the farthest human-made objects in space
- Space missions to study near-Earth objects and potential asteroid mining targets
- International Mars exploration collaborations and missions
Space Communication and Navigation
- Deep space communication networks and relay satellites
- Laser communication technology for high-speed data transmission in space
- Satellite-based navigation systems: GPS, Galileo, and GLONASS
- Interplanetary Internet: protocols and architectures for space communications
- Radio astronomy and interferometry: probing the universe with radio waves
- Quantum communication in space: secure and ultra-fast communication channels
- Delay-tolerant networking for deep space missions
- Autonomous navigation systems for spacecraft and rovers
- Optical communications for small satellites and CubeSats
- Space-to-ground communication systems for remote sensing and Earth observation satellites
Space Medicine and Human Spaceflight
- Microgravity effects on human physiology and health
- Countermeasures for mitigating bone and muscle loss in space
- Psychological challenges of long-duration space missions
- Space food technology: nutrition and sustainability in space
- Medical emergencies in space: protocols and procedures for astronaut health care
- Radiation shielding and protection for crewed missions beyond Earth orbit
- Sleep and circadian rhythms in space: optimizing astronaut performance
- Artificial gravity concepts for maintaining crew health on long-duration missions
- Telemedicine applications for space exploration missions
- Bioastronautics research: advancing human spaceflight capabilities and safety
Space Industry and Commercialization
- NewSpace companies: the rise of private space exploration ventures
- Satellite constellation deployments for global internet coverage
- Space tourism: opportunities, challenges, and market trends
- Commercial spaceports and launch facilities around the world
- Space manufacturing and in-space assembly techniques
Tips To Write Space Research Papers
Crafting space research papers can be a thrilling and fulfilling pursuit, yet it demands meticulous planning and implementation to guarantee that your efforts effectively convey your discoveries and make meaningful contributions to the discipline. Here are some tips to help you write space research papers:
- Choose a Narrow Topic: Space is a vast field with numerous sub-disciplines. Narrow down your topic to something specific and manageable, ensuring that it aligns with your interests and expertise.
- Conduct Thorough Research: Before you start writing, immerse yourself in the existing literature on your chosen topic. Familiarize yourself with key concepts, theories, and recent discoveries to provide context for your research.
- Develop a Clear Thesis Statement: Define the central argument or hypothesis of your paper in a concise and focused thesis statement. This statement should guide your writing and serve as the foundation for your research.
- Outline Your Paper: Create a detailed outline outlining the structure of your paper, including the introduction, literature review, results, and conclusion sections. This will help you organize your thoughts and ensure that your paper flows logically.
- Write a Compelling Introduction: Begin your paper with a captivating introduction that offers context about your subject, underscores its importance, and delineates the paper’s framework . Grab the reader’s interest and inspire them to delve further into your work.
- Provide a Comprehensive Literature Review: Synthesize the existing research on your topic in a literature review section. Examine pertinent research, theories, methodologies, and results, pinpointing any disparities or deficiencies in the existing literature that your study seeks to rectify.
- Detail Your Methodology: Describe the methods you used to conduct your research, including data collection, analysis, and interpretation techniques. Provide enough detail for readers to understand how your study was conducted and to evaluate its validity and reliability.
- Present Your Results Clearly: Present your research findings in a clear, concise manner, using tables, figures, and charts to illustrate key data points. Interpret your results objectively and discuss their implications in relation to your research question or hypothesis.
- Engage in Critical Analysis: Analyze your findings in the context of existing literature, discussing their significance, strengths, limitations, and potential implications for future research. Be critical and objective in your evaluation, acknowledging any potential biases or limitations in your study.
- Craft a Strong Conclusion: Summarize the main findings of your research and reiterate their significance in the conclusion section. Discuss any implications for theory, practice, or policy and suggest avenues for future research.
- Proofread and Revise: Before submitting your paper, carefully proofread it for spelling, grammar, and punctuation errors. Edit your writing to ensure clarity, coherence, and consistency, guaranteeing that your points are adequately backed and logically organized.
- Follow Formatting Guidelines: Follow the formatting instructions provided by the journal or conference to which you intend to submit your paper. Pay attention to details such as font size, margins, citation style, and reference formatting to ensure that your paper meets the publication requirements.
Space research offers a window into the vastness of the cosmos, revealing the beauty and complexity of the universe we inhabit. From the depths of space to the surfaces of distant planets, scientists are uncovering new wonders and answering age-old questions about our place in the universe. As we look to the stars, let us be inspired by the spirit of exploration and discovery that drives humanity ever onward, towards new horizons and unknown worlds. I hope you find the best space research topics from the above list.
Related Posts
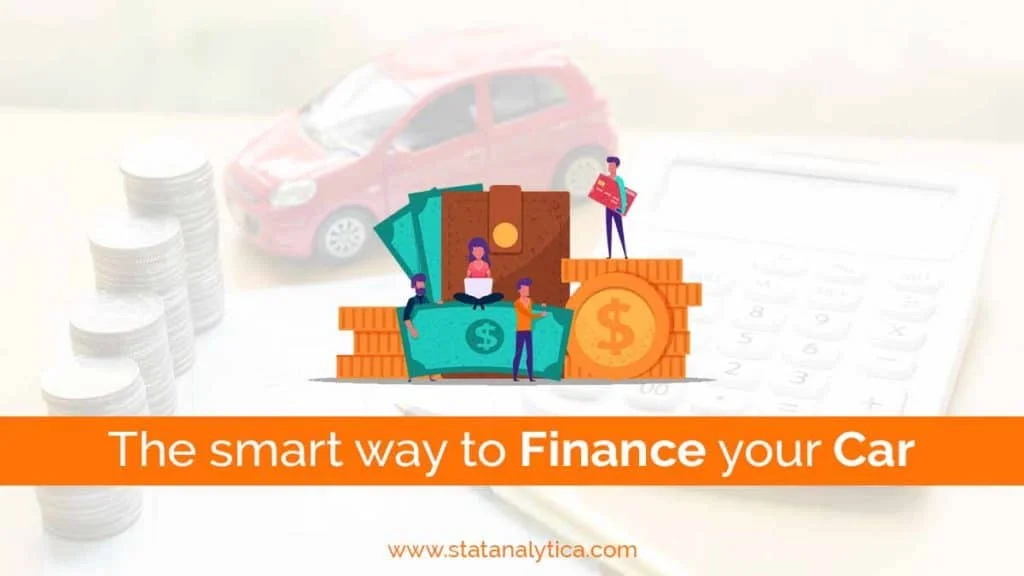
Step by Step Guide on The Best Way to Finance Car
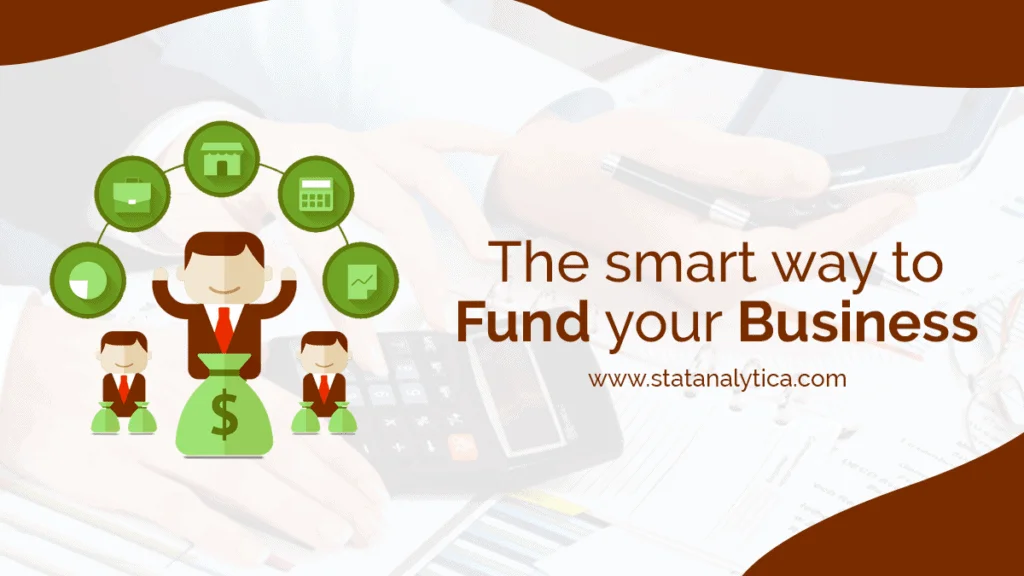
The Best Way on How to Get Fund For Business to Grow it Efficiently
Leave a comment cancel reply.
Your email address will not be published. Required fields are marked *
Writing papers doesn't always come easy, ordering essays from us does.
My Paper Writer
154 Astronomy Research Topics: Rocket To Your Marvelous Grades

Every student knows that the key to writing an excellent research assignment starts with choosing an interesting topic that captures the reader’s attention and explores new areas within the discipline. Some research topics come to students naturally, but often coming up with a good topic to research and write about can be difficult. This article provides 154 of the best astronomy topics for college and university students. They cover a variety of areas and are sure to impress even the toughest professors.
Table of Contents
What is astronomy, characteristics of a good research paper, astronomy research topics for all levels, easy astronomy research paper topics, a set of interesting astronomy topics, great astrophysics research topics, great research topics in cosmology, excellent space exploration topics.
Astronomy is a branch of natural science that deals with celestial objects, space, and the physical universe as a whole. Students use a combination of mathematics, physics, and chemistry to explain the origins and evolution of planets, stars, nebulae, galaxies, and comets. Ancient civilizations made observations and measurements of the night sky, making astronomy one of the oldest natural sciences.
A great research paper in any subject should meet common criteria. The work that goes into conducting research should be systematic, logical, tangible, replicable, and comprehensive. All of this requires an in-depth literature review and background data. The five characteristics a teacher or professor in any class would expect:
- Specific – A research paper should focus on a central question or issue in which a problem is clearly defined.
- Measurable – The information used to form an opinion related to the central question should be open to analysis and evaluation.
- Attainable – The information should also be available to other researchers for verification and review.
- Realistic – The opinion formed based on the information cannot be manipulated or modified to fit a hypothesis or theory.
- Formatting – A research paper should follow a specific format (e.g., APA or MLA) and be fully edited and proofread.
Whether or not you have a five-page paper or a twenty-page paper due, our expert writers and editors have scoured the net to find great topics for your consideration. These are completely free to use and modify in any way to suit your specific assignment needs. Teachers will be impressed with any of these cool astronomy topics which will improve your chances of earning a high score on your next assignment. However if you don’t want to go through the writing process yourself, you can always hire experts to write research papers for money .
These astronomy topics to write about are great when you don’t have the time or resources to conduct in-depth research. You can generally find a lot of information on the web and can find trustworthy sources at the library without having to request items through interlibrary loan:
- Will we find evidence of water on Mars?
- Are any planets in our Solar System habitable?
- What are the biggest differences between the inner and outer planets?
- Are any of the giant moons habitable?
- What are dwarf galaxies and what role do they play?
- What have we learned about Haley’s comet and its composition?
- How does the moon affect the Earth’s oceans?
- What does the Hubble telescope show us about the distant universe?
- What do we know about how planets formed in our galaxy?
- Why is the study of comets so important?
- How does astronomy help us measure time?
- How do constellations help determine the age of the Universe?
- What are the fundamentals of astronomy?
- How was our solar system formed?
- What impact does space debris have on the Solar System?
- How does the moon affect the Earth’s gravity?
- How do neighboring galaxies affect the Milky Way?
- What impact does the Sun have on climate change?
- What can we learn from clusters of galaxies?
- What are black holes and why should we worry?
- Why did the Catholic Church feel challenged by Newton?
- How did ancient civilizations utilize astronomy?
- What do we know about the life cycles of stars?
- How does the moon affect the Earth’s weather?
- What impact did Isaac Newton have on astronomy?
- How much influence did the early astronomers have on our understanding of space?
- How have humans affected the solar system?
- What is the Great Crunch in astronomy?
- What lies beyond the Milky Way?
- Why is Pluto a controversial planet?
- How have science fiction writers influenced astronomy?
If you are looking toward going into the field of astronomy, you will have to have a strong background in all things related to the discipline. These interesting space topics cover a broad range of questions you can explore. All the space science topics listed here will challenge you to conduct ample research:
- How accessible is Stephen Hawking’s research work?
- Can a black hole destroy the Milky Way?
- How influential is Hawking’s “A Brief History in Time”?
- Which method for detecting exoplanets is the most effective?
- How does the Sun impact the way major bodies of water move on Earth?
- What causes solar and lunar eclipses?
- How did heavenly bodies influence early mythologies?
- What purpose do black holes serve?
- What are the differences between the Copernicus and Heliocentric models?
- What do the rings of Saturn tell us about the planet’s composition?
- What are the origins of our solar system?
- How does the Moon’s orbit around Earth affect climate?
- How can we better understand the relationship between space and time?
- How fast are we moving when we stand still?
- How do zero-gravity conditions affect humans?
- What are the differences between dark energy and dark matter?
- What lies between the Milky Way and Andromeda?
- Are there any future business opportunities in space?
- Why does the Moon orbit around the Earth?
- What are the different views of interstellar space?
- Can life exist outside of the Solar System?
- What would first contact mean for the human race?
- How do sunspots affect the weather on earth?
- Why is it important to learn astronomy in college?
- How did the Sun form and how old is it?
- How do humans interpret constellations?
- How many galaxies are known to humans?
- What happens when galaxies collide?
- What is the most effective way to determine angular diameter in space objects?
- What do we understand about the universe’s age?
- What is the likelihood that the Earth could be hit by an asteroid?
The astronomy project topics listed here require you to employ the methods and principles of physics and chemistry to explain the birth of celestial objects and the universe. You will need to conduct a lot of in-depth research using scientific resources to tackle these astrophysics research topics:
- What are the differences between dark matter and dark energy?
- What role does philosophy play in astrophysics?
- How do celestial objects affect seasonal change on Earth?
- How did astrophysics change the way we look at the relationship between celestial objects?
- Where do twin stars originate from?
- What are the differences between black holes and quasars?
- What are the most important questions astrophysics answers?
- What can be understood about the death of a star?
- What are some of the major controversies in astrophysics?
- What are the differences between black-body radiation and thermal equilibrium?
- What are the basic properties of giant stars?
- What has astrophysics taught us about the lifespan of stars?
- How is an accelerating universe affected by dark matter?
- What contributions did the Kepler mission make to astronomy?
- How is stellar evolution measured using the HR diagram?
- Why do black holes remain dormant for thousands of years?
- Are there dangers with the Hadron Collider experiments?
- Can we view dead stars before they turn into black holes?
- How is radiative transport affected by gas emissions?
- What are the best theories supporting time travel?
- What do we know about the origins of the Milky Way?
- What are the astrophysical implications of black holes?
- How can we measure the size of a black hole?
- What are wormholes and what do they tell us about space travel?
- When do black holes begin to consume matter?
- What are the origins of astrophysics?
- How does astrophysics help us understand time travel?
- How has quantum physics helped us understand the universe?
- How can we better understand nebulae and masses of matter?
- What are the implications of the speed of light travel?
- How will the Sun’s evolution affect Earth?
Unlike the other astronomy paper topics we have listed, cosmology deals with the beginnings of the universe. The dominating theory is the Big Bang, but there are other schools of thought that can be considered controversial but certainly pique readers’ interests. Here are some great astronomy topics for research paper to think about:
- What is the Big Bang Theory?
- What is the current research landscape of string theory?
- What role does dark matter play in measuring the universe’s expansion?
- In what ways has mythological cosmology changed over the years?
- What is the difference between dark energy and dark matter?
- What is dark matter and what does it tell us about the universe’s origins?
- What causes eternal inflation in the string theory landscape?
- What has the COBE revealed about the universe’s origins?
- What are the gravitational waves and signatures of the early universe?
- What are the main characteristics of string theory?
- What do we know definitively about the origins of the Universe?
- What does the Hubble eXtreme Deep Field tell us about the furthest galaxies?
- How does the cosmic microwave background help us understand origins?
- Why is dark matter important to our understanding of the universe?
- What do scientists believe lies beyond the Milky Way?
- How does the size of the Universe tell us about its beginnings?
- How does dark energy interact with interplanetary objects?
This is one of the most important areas of astronomy because it deals with technologies used to carry out physical exploration done by both manned and unmanned spacecraft. Some of the space research paper topics listed here deal with the history of exploration, while the rest deal with current and future missions:
- What did we learn about the Challenger disaster?
- Why were the moon missions so important for space exploration?
- Is space exploration a philosophical question that needs answers?
- How did the moon explorations help us understand how the Earth was formed?
- Why are Musk’s Starlink technologies important for space travel?
- Why were the Apollo missions important for NASA’s survival?
- What are the major advancements in space exploration over the last 10 years?
- Is space exploration necessary and what are its ramifications?
- Should governments continue to use funds to pay for space exploration?
- What elements exist beyond the known universe?
- How did the space race change international politics?
- How have manned space flights changed over the decades?
- What are some of the challenges the Apollo 11 mission faced?
- Can space exploration lead to medical advancements?
- What are the benefits of space exploration?
- Will we ever colonize other planets in our Solar System?
- What are the challenges of space exploration for third-world countries?
- Should the U.S. government invest in a Mars manned mission?
- Why impact did the space race have on the Cold War?
- What does the future hold for NASA and space exploration?
- How can we optimize exercise for extended human space flight?
- What are the possibilities of colonizing the moon?
- Should funding space missions be a government priority?
- How does spaceflight affect bone density in astronauts?
- How likely is a manned mission to Mars in the next decade?
- How does spaceflight affect brain function in astronauts?
- What are the challenges international agencies face with space exploration?
- What are the challenges of colonizing other planets?
- What are the challenges and opportunities of biology in space?
- What impact has space debris had on space exploration?
- Why is space exploration so important?
- What role do robots play in space exploration?
- What are some of the current technologies for the detection of life?
- What role did the Apollo missions have on space exploration?
- How can deep space satellites be used to study exoplanets?
- What role does the international space station have on deep space exploration?
- What are the possibilities of finding life on other planets?
- What impact do space environments have on algae and cyanobacteria?
- What is the possibility of finding cures for diseases in space?
- How important is AI in space exploration?
- How does spaceflight affect creative performance?
- What impact did the Mercury missions have on the space race?
- What are the most important space breakthroughs in history?
- What impact will Elon Musk’s contribution to space travel have?
Need Someone to Write Your Paper?
As we mentioned above, a good research paper requires a lot of preparation and several days (sometimes weeks) of hard work. For a variety of reasons, many students are not able to complete a research project on their own and look toward outside assistance to get their work done.
We are a paper writing service that provides end-to-end help writing college papers for students at all levels. Our professionals are all native English speakers and writers that have earned advanced degrees in a variety of disciplines and have undergone extensive testing to ensure they deliver custom high-quality work. Unlike many other online services, we work fast and deliver assignments that earn students the highest scores for cheap prices. Our secure payment system Contact our trustworthy team by chat, email, or phone 24 hours a day year-round and let us show you how we can help you succeed.
189 Social Media Research Paper Topics To Top Your Paper

Social media has been around since the late 1990s and refers to the means of interaction and communication between groups of people from all over the world. It allows them to create, share, and exchange information, ideas, and conversations in virtual communities over the internet. Society embraced social media and it was made popular by individuals that wanted to connect with others but quickly became a tool used by businesses and organizations to promote products and services and is now a vital component in building relationships, broadcasting, and marketing at small- and large-scale levels.
- Different Types Of Social Media
- What Goes Into The Structure Of Great Social Media Paper
- Excellent Social Media Research Topics
- Easy Research Topics About Social Media
- Research Papers On Social Media For College
- Social Networks Topics For Graduate Students
- Popular List Of Social Networks Topics For 2023
I requested the editor as I wanted my essay to be proofread and revised following the teacher’s comments. Edits were made very quickly. I am satisfied with the writer’s work and would recommend her services. I requested the editor as I wanted my essay to be proofread and revised following the teacher’s comments. Edits were made very quickly. I am satisfied with the writer’s work and would recommend her services.
Leave a Reply Cancel reply
Secure the top grades, with vetted experts at your fingertips.
Questions? Call us:
Email:
- How it works
- Testimonials
Essay Writing
- Essay service
- Essay writers
- College essay service
- Write my essay
- Pay for essay
- Essay topics
Term Paper Writing
- Term paper service
- Buy term papers
- Term paper help
- Term paper writers
- College term papers
- Write my term paper
- Pay for term paper
- Term paper topic
Research Paper Writing
- Research paper service
- Buy research paper
- Research paper help
- Research paper writers
- College research papers
- Write my research paper
- Pay for research paper
- Research paper topics
Dissertation Writing
- Dissertation service
- Buy dissertation
- Dissertation help
- Dissertation writers
- College thesis
- Write my dissertation
- Pay for dissertation
- Dissertation topics
Other Services
- Custom writing services
- Speech writing service
- Movie review writing
- Editing service
- Assignment writing
- Article writing service
- Book report writing
- Book review writing
Popular request:
165 fascinating astronomy topics to research and learn about.
October 29, 2021
Astronomy is a branch of science dealing with celestial objects, space, and the physical universe. Despite this field being fascinating, some titles are confusing and complex.
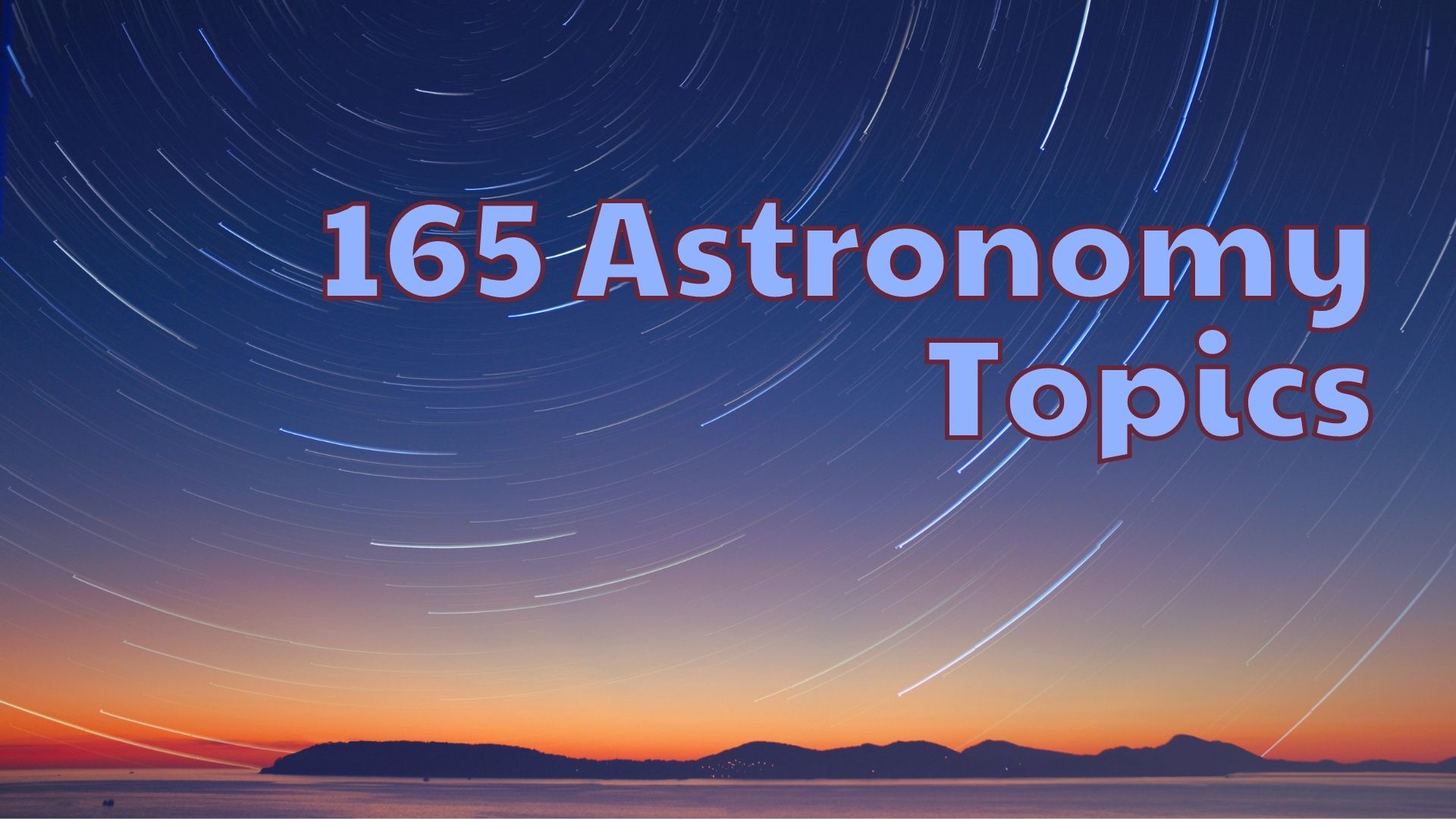
Essentially, astronomy topics revolve around material science exceeding beyond the earth’s atmosphere. Consequently, these topics revolve around things beyond the context of what most humans are familiar with, making writing about them difficult. With technological advancements in satellites, space travel, and telescopes, humans can peer past the earth.
How to Write a Good Astronomy Research Paper
Writing a good astronomy paper entails comprehensive research, careful planning, and information organization using a complete structure. Here’s how you can write do a good astronomy project to impress the teacher and earn the top grade in your class.
- Select a topic. Students have many astronomy project topics to consider in this subject. Choosing an ideal title is an essential aspect of writing a fantastic paper. Therefore, pick a topic that is direct to the point and straightforward. Also, pick a topic for which you can find relevant information to support your argument.
- Research the topic. Take adequate time to research the topic you select and gather all the necessary information to support your argument. A practical investigation will enable you to father critical ideas and gaps between the past studies.
- Outline your paper. An outline should steer the direction of your research while providing sense in tackling your main argument. Creating an outline enables you to determine the primary points and claims to include in the paper. What’s more, creating a structure makes the writing process enjoyable and smooth. It also enables you to incorporate coherent and explicit content in the essay.
Once you have a topic, an outline, and information from your research, you can start writing the paper.
- Start with an introduction: Here, introduce your topic and state your main argument. Begin your paper with a catchy phrase to raise the reader’s interest and encourage them to read further. Also, include background information and a thesis statement.
- Write the body: The body of an astronomy paper incorporates information supporting your statements. It also provides evidence to your claim. The total number of paragraphs to include in the body depends on your teacher’s instructions. However, this section enables you to argue different points. In this section, present information logically and orderly. Ensure seamless flow of information to ensure proper evaluation, clear understanding, and proper thesis defense. Use the outline to guide you in presenting information in the body section.
- Conclude your paper: In the conclusion, sum up all the points and claims you made in the body. The conclusion paragraph should bring all arguments together in a single section. It should also restate your thesis statement while proving it’s logical and valid.
Once you’ve written your astronomy paper, proofread and edit it. Also, include a reference to all information sources that you use in the essay. But the essential aspect of writing this paper is selecting an amazing topic. That’s why this article lists ideas that you can consider once your teacher or college professor assigns you this task.
Best Astronomy Paper Topics
Perhaps, you’re looking for a brilliant topic to research and write a high-quality paper about to ensure your academic excellence. In that case, consider these topic ideas for your astronomy paper.
- The sun’s nature and how it relates to earth
- Why Wien’s Law is essential to astronomers
- Kepler’s Planetary Motion Laws and how they relate to objects moving in space
- Using examples, describe the seasons’ phenomenon that varies in different latitudes
- How zero gravity affects humans
- What are the northern lights?- Explain what causes this natural display
- Astrophysics controversies about their effect on the humans’ universe understanding
- What are the causes and impacts of light pollution on astronomy?
- Big bang theory’s latest version and its implications on the universe’s future
- What are the variations and similarities between asteroids and comets?
- Between asteroids and comets, which one is a more significant threat to our planet?
- Why can’t planet Mars support life?
- Why there is no liquid water on planet Mars
- How humans have interpreted the important constellations over the years
- Theories negating and supporting time-travel
- The significant assumptions that humans make about star clusters- How do astronomers apply them?
- Various seasons- Why Australians’ Christmas is in summer
- Why Galileo, Kepler, and Newtown’s contribution to astronomy matters
- The four motion types the earth endures and their impacts on different seasons on earth
- The main categories of active galaxies- Why astronomers call them active
- What do active galaxies hint about galactic evolution?
- The apparent sun motion as seen from the earth in a day and the annual course
- The sun’s change and how it affects the planet
- Why do astronomers think planet earth keeps expanding
- Star’s death and the residual
- Planet melting and events that took place in the early solar system’s history
- Diameter, density, mass, and surface gravity of planets in the solar system
- The stars’ motion in various parts of the sky as viewed from varying latitudes on the planet
- The discovery and comparison of Exoplanets to the planets in the solar system
- Stars formation from gas clouds
- Why low-mass stars formation is slower than that of massive stars
- Different planet classes and their classification
- Differences and similarities between Earth and Venus
- Factors contributing to differences between Earth and Venus
- The space exploration history leading to the first man’s landing on Mars
These are good topics in astronomy to consider for your research paper. Nevertheless, take adequate time to research your preferred topic to write an informative and interesting piece.
Interesting Astronomy Essay Topics
Maybe the educator has asked you to write an astronomy essay. If so, you’re looking for an exciting topic to explore in your writing. Here are some of the topic ideas to consider for your assignment.
- Explain the rate at which the universe is expanding
- Can the sun turn into a black hole upon its death?
- Is Master time travel possible?
- Describe the stars’ average lifespan
- Describe the universe and its origin
- Describe planet Mars
- What is galactic evolution?
- How accurate are extra-terrestrials?
- Can humans colonize other planets?
- Dark energy and dark matter
- Astrophysics- Is it about the interdisciplinary opinions of space?
- Past the milky way
- Distance and cosmic time
- Astronomy history
- Why Hubble’s Law matter in astronomical study
- The Apollo missions history
- Big Bang theory cosmology
- Astronomy and quantum physics
- Heliocentric galaxy models and Copernicus
- Early astronomers- Galileo, Aristotle, Ptolemy, and Copernicus
- What are the black holes?
- The background of Cosmic Microwave
- Implications of potential life on other planets apart from earth
- Future opportunities for business beyond the earth
- Relativity theory and how it governs gravity
- What careers can people pursue in astronomy?
- Cosmology: Distance scales and cosmic ages
- Fundamentals: Constellations relativity, eclipses, and gravity
- Planet formation and astrobiology
- The stars’ life cycles
- Navigation and stars
- Why do humans explore space?
- Hale’s comet
- Human mythology and heavenly bodies
- Archaeo-astronomy and astronomy history
- Speed of light’s implications
- Natural processes occurring in the outer space
- Exoplanets and their properties
- How humans detect exoplanets
- Human opinions about the universe
- NASA and space exploration’s future
- The importance of human-crewed space flight
- Instrumentation: Telescopes astronomy, gamma-ray astronomy, ultraviolet astronomy, and radio astronomy
- Describe the Hubble telescope
- The rings of Saturn analysis
- The significance of the moon to earth
- Life origin on earth
- How the sun relates to earth
- What is the great crunch?
- How life began on earth
- Time travel- Theory versus fiction
- Understanding the space race
- Galaxies- The Galactic structure, galaxy formation, active galaxies, and clusters of galaxies
- Matter masses and nebulae
- How long will the sun last?
- Using robots in space exploration
- The size of black holes
- Space travel representations in fiction movies
- Understanding the solar systems- The moon, Space debris, and the eight planets
- How humans affect the solar system
- Kepler’s Planetary Motion laws and its impact on earth motions
- What is the basis of planets’ classification
- How celestial objects affect the planet
- Astronomical milestones that humans have made in history
- How the universe relates to planet earth
These are interesting astronomy topics to consider for your essay. However, spend adequate time conducting in-depth research to develop a paper that will impress the teacher to award you an excellent grade.
Space Research Paper Topics
Maybe you find topics about space exploration interesting. In that case, this category comprises the best research topics about space that you should consider for your paper.
- The discovery of the space black hole
- What humans might not know about life in the outer space
- How learning about space can be more enjoyable
- Telescope upgrading to view space objects
- How space technology can help with hazard prevention
- Necessary improvements to green aviation
- How technology can help in discovering unknown galaxies
- How humans have changed the way they travel to space
- Investigating the relationship between earth and moon
- What are the components of a comet?
- How climate change affects space technology advancement
- The black holes’ formation
- Can anything survive on Mars?
- The influence of solar activity on earth
- Can other planets in the solar system support life?
- The Bing Ban theory and the universe origin
- Why humans should continue to explore the space
- Satellites usage in wireless communication
- Galaxies overview and their definition
- Should humans reinstate Pluto’s planet status?
- Describe the stars formation process
- Space exploration history from the first time humans watched the stars until the first landing on mars
- History and promises of space exploration for the future
These are exciting space science topics to consider for your paper or essay. Nevertheless, select your subject carefully to write a winning paper.
Astrophysics Research Topics
Astrophysics is a science branch that uses physics’ principles and methods in studying astronomical phenomena and objects. Consider these topics if this is one of the astronomy subjects you would love to explore.
- Investigating stellar dynamics and star clusters
- Can physics help in finding the multiverse?
- The stars light spectrum in distant galaxies
- Why the moons orbit planets
- Where to find the dark matter
- Define astrophysics- Why is it important?
Astrophysics is a complex study field. Therefore, take adequate time to identify the best topic for your paper to enjoy the research and writing process.
Cool Astronomy Topics
Perhaps, you’re looking for astronomy topics to write about, and your paper stands out. In that case, consider the following topic ideas for your essay.
- Understanding how the Mars rovers function
- Satellite communication problems
- Can humans travel in time?
- How the universe continues to expand
- Understanding what happens during the solar eclipse
- Factors that cause the solar eclipse
- What lies past the visible universe
- The dimension of light in space
- Understanding the planets, the sun, and the solar system
- Define the transit circle and the meridian
- Clouds evaluation, formation, and destruction in galaxies
- Understanding galaxy cluster growth and cosmic acceleration
- What astronauts eat during space exploration
- Why earth is the most prominent planet
These are excellent astronomy topics for research. However, they require some research, like the ideas in the other categories.
More Topics for the Solar System Research Projects
Perhaps, you’ve not found a brilliant and fun topic to research and write about in this list. If so, here are more ideas that you might consider for your paper.
- Define the cooler index
- The impact of the sun on the water bodies
- Describe the origin of asteroids
- How a solar system that has two stars only operate
- Does the nearest solar system has an Earth-like planet
- Describe the interstellar extinction
- Did cosmic impact cause the death of dinosaurs?
- Does the galaxy have intelligent life?
- Which planet is the most promising to have a life?
- Describe the formation or origin of the moon
- What are the star clusters?
- Mars colonization- What is its implication for humans?
- Why the solar system’s exploration matters
- How ancient humans saw essential constellations
- Calculating the density and mass of the solar system
- The unremarkable star’s life
- Can humans excel in light-speed travel?
- Describe the heliocentric model by Copernicus
- Explain why humans misinterpret astronomy in most cases
- Discuss the history of astronomy and Neptune
This astronomy topics list is not exhaustive. However, you can find a good idea to research and write a high-quality paper. Nevertheless, take adequate time to research your topic and write the essay.
Use a Professional Writing Service
Maybe you can’t find time to research your topic and write your astronomy paper. In that case, hire professionals to write a custom paper for you online. Our staff comprises the most qualified experts to write your essay. We offer fast and cheap writing assistance to learners across academic levels. If stuck with astronomy paper, don’t hesitate to seek our assistance. We guarantee you a top-notch service once you enlist our help with your astronomy paper.
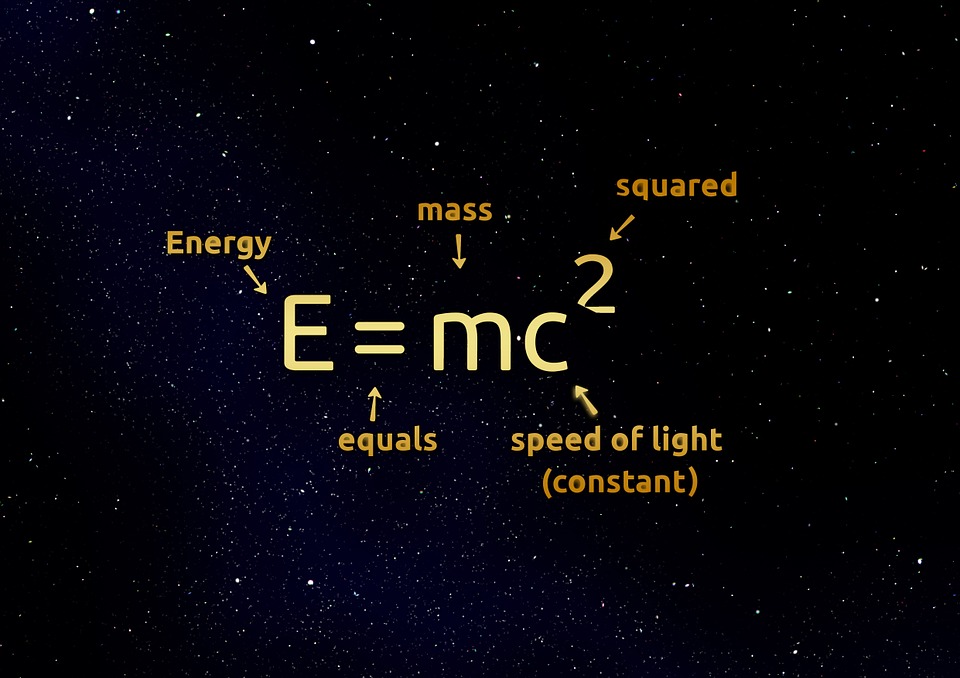
Take a break from writing.
Top academic experts are here for you.
- How To Write An Autobiography Guideline And Useful Advice
- 182 Best Classification Essay Topics To Learn And Write About
- How To Manage Stress In College: Top Practical Tips
- How To Write A Narrative Essay: Definition, Tips, And A Step-by-Step Guide
- How To Write Article Review Like Professional
- Great Problem Solution Essay Topics
- Creating Best Stanford Roommate Essay
- Costco Essay – Best Writing Guide
- How To Quote A Dialogue
- Wonderful Expository Essay Topics
- Research Paper Topics For 2020
- Interesting Persuasive Essay Topics
Research Topics
Scientists and engineers at the Center for Astrophysics | Harvard & Smithsonian collaborate across a broad variety of scientific disciplines, from astronomy and astrophysics to related areas of physics and geophysics, in advancing humanity’s understanding of the universe. Learn more about the full spectrum of research covered at the CfA.
Help | Advanced Search
Astrophysics > Instrumentation and Methods for Astrophysics
Title: how to write and develop your astronomy research paper.
Abstract: Writing is a vital component of a modern career in scientific research. But how to write correctly and effectively is often not included in the training that young astronomers receive from their supervisors and departments. We offer a step-by-step guide to tackle this deficiency, published as a set of two papers. In the first, we addressed how to plan and outline your paper and decide where to publish. In the current second paper, we describe the various sections that constitute a typical research paper in astronomy, sharing best practice for the most efficient use of each of them. We also discuss a selection of issues that often cause trouble to writers, from sentence to paragraph structure, the `writing mechanics' used to develop a manuscript. Our two-part guide is aimed primarily at master's and PhD level students who are presented with the daunting task of writing their first scientific paper, but more senior researchers or writing instructors may well find the ideas presented here useful.
Submission history
Access paper:.
- Other Formats
References & Citations
- Google Scholar
- Semantic Scholar
1 blog link
Bibtex formatted citation.

Bibliographic and Citation Tools
Code, data and media associated with this article, recommenders and search tools.
- Institution
arXivLabs: experimental projects with community collaborators
arXivLabs is a framework that allows collaborators to develop and share new arXiv features directly on our website.
Both individuals and organizations that work with arXivLabs have embraced and accepted our values of openness, community, excellence, and user data privacy. arXiv is committed to these values and only works with partners that adhere to them.
Have an idea for a project that will add value for arXiv's community? Learn more about arXivLabs .
Thank you for visiting nature.com. You are using a browser version with limited support for CSS. To obtain the best experience, we recommend you use a more up to date browser (or turn off compatibility mode in Internet Explorer). In the meantime, to ensure continued support, we are displaying the site without styles and JavaScript.
- View all journals
Astronomy and astrophysics articles from across Nature Portfolio
Astronomy and astrophysics are the study of objects and phenomena that are found beyond our solar system. This combines theoretical simulations and observation with both terrestrial and space-craft-borne instruments of the electromagnetic radiation and high-energy particles emitted by celestial bodies.
Related Subjects
- Astronomical instrumentation
- Astrophysical disks
- Astrophysical dust
- Astrophysical magnetic fields
- Compact astrophysical objects
- Computational astrophysics
- Dark energy and dark matter
- Early universe
- Galaxies and clusters
- General relativity and gravity
- High-energy astrophysics
- Interstellar medium
- Laboratory astrophysics
- Particle astrophysics
- Stellar evolution
- Time-domain astronomy
- Transient astrophysical phenomena
Latest Research and Reviews
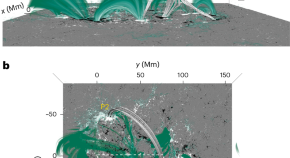
A model for heating the super-hot corona in solar active regions
A state-of-the-art simulation reveals that the long-lasting 10 MK plasma in solar active regions can be heated by magnetic reconnections driven by continuous flux emergence that repeatedly deposit impulsive heating into the coronal plasma.
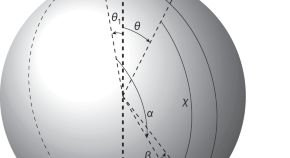
A freely precessing magnetar following an X-ray outburst
A radio-loud magnetar, XTE J1810–197, has been observed to precess shortly after an X-ray outburst. The precession decayed over the subsequent few months, which probably rules out freely precessing magnetars as the source of the fast radio bursts.
- Gregory Desvignes
- Patrick Weltevrede
- Jérôme Pétri
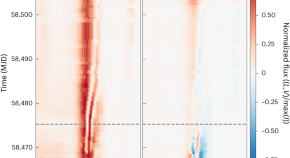
Linear to circular conversion in the polarized radio emission of a magnetar
Radio pulses from a rare, radio-loud magnetar, XTE J1810−197, are seen to have undergone a conversion in their polarization state. This change can be linked to the magnetar’s magnetic field geometry, and has commonalities with an effect also seen in fast radio bursts.
- Marcus E. Lower
- Simon Johnston
- Benjamin W. Stappers
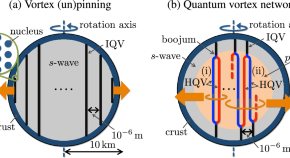
Pulsar glitches from quantum vortex networks
- Giacomo Marmorini
- Shigehiro Yasui
- Muneto Nitta
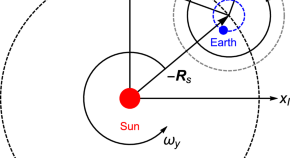
Tangential velocity constraint for orbital maneuvers with Theory of Functional Connections
- A. K. de Almeida Jr.
- T. Vaillant
- A. C. M. Correia
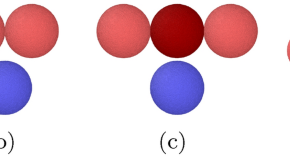
An atomistic study of sticking, bouncing, and aggregate destruction in collisions of grains with small aggregates
- Maureen L. Nietiadi
- Herbert M. Urbassek
- Yudi Rosandi
News and Comment
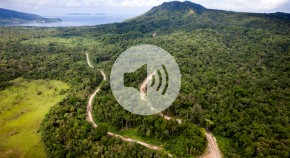
The ‘ghost roads’ driving tropical deforestation
Researchers find that a huge number of roads that don’t appear on official maps, and the protein that could determine whether someone is left-handed.
- Nick Petrić Howe
- Benjamin Thompson
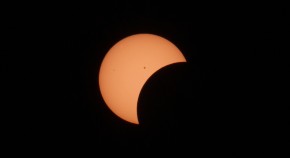
Total solar eclipse 2024: what dazzled scientists
Amateur and professional astronomers share with Nature what they observed and what data they collected when the Moon blocked the Sun.
- Sumeet Kulkarni
- Lauren Wolf
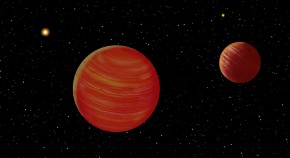
Smallest known starquakes are detected with a subtle shift of colour
An unusual technique picks up the slow vibration of a faint star.
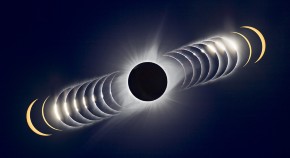
Total solar eclipse 2024: how it will help scientists to study the Sun
The Sun’s mysterious outer atmosphere, the corona, will become easier to view from Earth on 8 April.
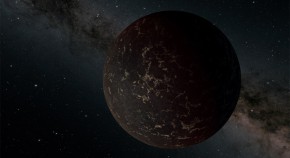
This super-Earth is the first planet confirmed to have a permanent dark side
Convincing evidence of 1:1 tidal locking had been absent until a new analysis of the exoplanet LHS 3844b.
- Joseph Howlett
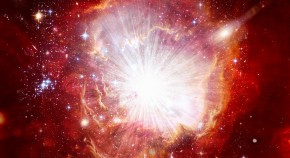
How did the Big Bang get its name? Here’s the real story
Astronomer Fred Hoyle supposedly coined the catchy term to ridicule the theory of the Universe’s origins — 75 years on, it’s time to set the record straight.
- Helge Kragh
Quick links
- Explore articles by subject
- Guide to authors
- Editorial policies

Astronomy Topics: Get Original Ideas Here
07 December, 2021
13 minutes read
Author: Kate Smith
Astronomy is the study of the universe and the physical entities surrounding it. These celestial objects include the moon, sun, stars, planets, comets, and meteorites. It incorporates several subject disciplines that allow students to understand the concept of the course. That is why it provides an extensive foundation for research papers in colleges and universities. Astronomy is an exciting subject with broad, complex topics. Since the subject concentrates on the universe and space, most students find the themes unfamiliar and confusing.
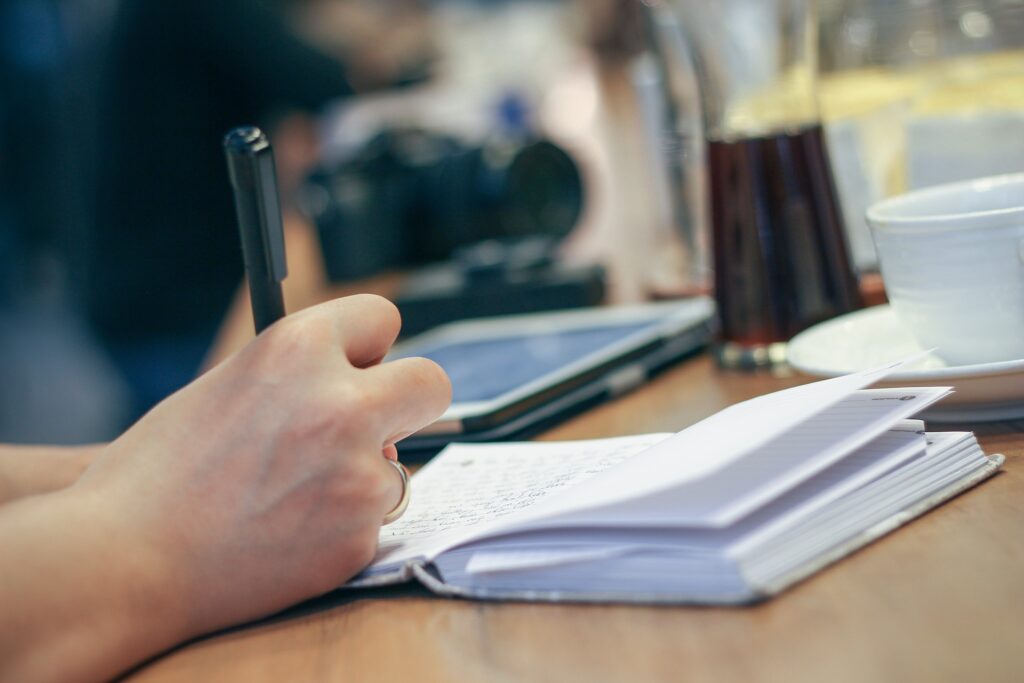
Professors usually assign numerous research papers to students to evaluate their understanding of the subjects. However, many learners get stuck when it comes to essay compositions. Choosing relevant astronomy research topics is crucial since it directs the entire research. With various concepts to grasp and topics to choose from, research writing becomes a hectic process.
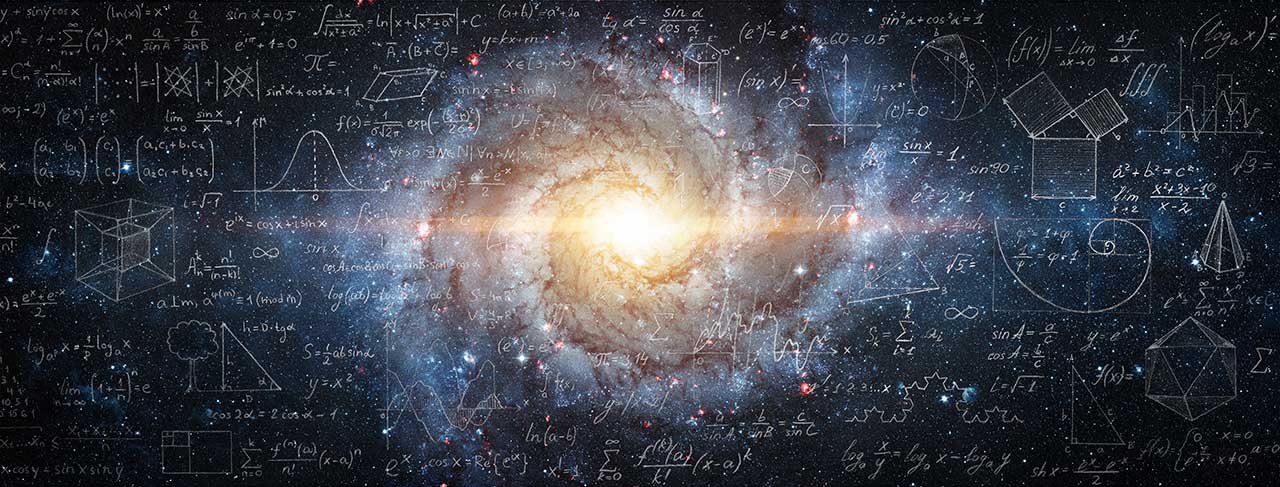
That is why we have compiled lists of interesting astronomy topics to inspire students and broaden their selection. You will enjoy writing and score A+ grades without pressure.
Astronomy Research Paper Definition & Purpose
Astronomy is quite a broad subject that covers the planetary objects and occurrences in the universe. In the past, the primary purpose of astronomy was to predict and note the rotating positions of the planets, moon, and sun for calendrical calculations . But over time, scientists used it for navigational purposes and to advance research studies.
Today, Astronomy entails broader concepts like the solar system, galaxies, and the calculations of distance between entities in the universe. Thus, you can define it as a scientific study of the universe extending beyond the Earth’s atmosphere.
Therefore, it is essential to pick topics in astronomy within the realms of what human scientists have explored and discovered. Having a relevant theme with adequate, verifiable research content will help you craft an exceptional paper.
Astronomy Is a Complex Subject – Main Branches & Research Fields
The main reason why it is a complex subject is that it has several branches and numerous research fields. It is majorly divided into two branches – observational and theoretical. Scientists often use telescopes and cameras for data collection, while theoretical analysis uses the collected data to explain how the celestial bodies function in space.
When it comes to the subject fields, Astronomy covers diverse disciplines. For instance, math, chemistry, physics, and even biology. These disciplines are interrelated to form both practical and theoretical investigations.
Thus, tutors often ask students to develop logical arguments from controversial themes. While space exploration still has human limitations, learners can use many astronomy topics for research to craft outstanding essays.
What Astronomy Topics Will Suit Your Paper The Best
When it comes to topic selection, you need to be keen and conduct an in-depth brainstorming session. Picking a suitable topic is a challenging task for many learners who don’t know where to start. To attain top grades, choosing excellent astronomy essay topics that reflect your research question is essential.
First, ensure you write down several ideas on paper before settling on one theme. Create a mind map that will help in narrowing down the scope to only relevant ideas. It will also save time when you search for content to write in your paper during the research phase.
Avoid going for too broad or too narrow subjects; you might get too much information or too little that will not be suitable for your paper. During the prewriting phase, remember to take enough time to capture important astronomy topics for research paper and the critical aspects you will include during the main composition.
Furthermore, make sure your research paper meets the required writing standards. Create an outline to guide you during the composition phase. Not forgetting to use proper grammar with no typos, including correct citations and the specified formatting style.
Here are some advantages of using our astronomy research paper topics:
- You will get unique and captivating topics that translate to original research papers. No other student will come up with a similar topic.
- Tutors often prefer reading exciting research papers. Thus, select one of our topic ideas and impress your astronomy tutor.
- You can be sure of getting top scores and bonus points on your paper from our original topic ideas.
- Selecting our topics will save you time and effort. Avoid spending hours of research looking for a suitable topic when you can just pick one from our list.
Ultimately, picking the best astronomy presentation topics will not protect you from bad grades if you have poor writing skills. You can get the topic right, but your tutor grades your paper by looking at various aspects such as language, style, logic, verifiable facts, etc.
Therefore, make sure you understand the entire research writing process and what it takes to craft an outstanding paper.
However, if you are stuck and have no idea where to start, get professional help from our site. We have expert MA and Ph.D. writers who are willing to help you with your astronomy paper. Do not let the academic pressure weigh you down. Let our experts help you compose remarkable essays, from topic selection to the bibliography section.
Reach out to us and enjoy top-of-the-class grades with no stress.
25 Interesting Astronomy Topics in Astrophysics
Astrophysics is a branch of astronomical studies providing students with a rich ground for research. Learners find it challenging to get the best topic to develop their analysis.
Below are interesting research astronomy project topics to help you jumpstart your astronomy paper:
- Evaluate the origin of dark matter.
- Why the moon rotates the planets.
- Discuss the galaxies and the formation of stars.
- The relationship between physics and the multiverse.
- Astrophysics: An analysis of space.
- What is the Milky Way?
- Discuss the Earth’s distance and the cosmic space.
- The historical view of astronomy.
- Explain the significance of Hubble’s law in astrophysics.
- Explore the Apollo missions relating to astrophysics.
- Discuss the big bang theory.
- An analysis of controversial concepts in astrophysics.
- The relationship between quantum physics and astrophysics.
- Evaluate the different galaxy models.
- An analysis of famous astronomers in history.
- What are black holes in astrophysics?
- Pros and cons of cosmic microwaves.
- Is there life on other planets apart from Earth?
- Discuss the future of space exploration.
- Discuss the theory of relativity.
- Why astronomy is a lucrative career.
- Analyze different astronomical careers.
- What is cosmology?
- Explore the fundamentals of eclipse formation.
- The relationship between constellations’ relativity and gravity.
25 Brilliant Astronomy Topics in Astrometry
Astrometry deals with specific measurements of celestial bodies and their movements. The primary purpose is to track the objects that rotate close to Earth, and scientists also use it to discover other extrasolar planets.
Let us explore some of the brilliant astronomy topics for presentation you can use to compose a great research paper:
- The navigation of stars in space.
- Discuss the significance of space exploration.
- The impact of celestial bodies and human traditions.
- Importance of stars and their lifecycles.
- What is Haley’s comet?
- An analysis of Astrometry and its impact.
- Discuss Stephen Hawking’s contribution to astronomy.
- Analyze human exploration of the moon.
- The role of NASA in advancing space research.
- Analyze the disaster in the space shuttle challenger.
- Discuss the galaxy models: Heliocentric and Copernicus.
- Importance of the Hubble telescope.
- The distance between the moon and the Earth.
- Effects of human activities on the solar system.
- How does space debris impact the sun
- An analysis of the great crunch.
- Discuss the investigations of Galileo and Aristotle in Astronomy.
- The significance of space investigation.
- Evaluate Saturn and its rings.
- An in-depth analysis of Pluto.
- Discuss the controversies in Astrometry.
- Does modern science support time travel?
- An evaluation of wormholes.
- Discuss the relationship between space and time.
- Significance of gravity on human activities.
25 Good Astronomy Topics in Astrogeology
Astrogeology is an astronomical branch dealing with the geology of objects in the universe, and it covers different celestial bodies and their functions. The study helps learners to know more about the Earth and its relationship with other universal objects.
Here are good astronomy topics to write about for your paper:
- Discuss the dynamics of planetary evolution.
- The impact of nitrogen cycles on the Earth’s surface.
- An analysis of how caldera forms in Mars.
- Discuss the law of newton and its impact on astronomy.
- Classify astrology since the early days.
- An analysis of astrogeology and celestial objects.
- Discuss the religious concept behind astrogeology.
- Influence of philosophy on astrogeology.
- Impact of excessive heat on the Earth’s atmosphere.
- An analysis of the Manhattan project.
- Explore Bohr’s radius and its importance in astrogeology.
- The impact of advanced astrological systems.
- Astrogeology: Origin and evolution.
- Influence of astrogeology on human activities.
- The study of astrogeology with supporting examples.
- Analyze the features of black holes.
- Compare and contrast the moon and the sun.
- An analysis of Kepler’s laws in astrogeology.
- Description of how astronomers conduct space exploration.
- Compare and contrast between Earth and Mars.
- An analysis of the solar system and its impact on humans.
- Analyze the origin of exoplanets.
- Evaluate the relationship between asteroids and comets.
- Explore the different celestial bodies.
- Analyze the significant inventions in astronomy.
25 Compelling Astronomy Topics in Astrobiology
Astrobiology is another field in astronomy that advances research about life on other planets and human detection methods. It is a discipline that requires an extensive comprehension of the universe and biological phenomena.
Here are some compelling astronomy topics for projects to inspire your research composition and help you develop compelling arguments:
- What is astrobiology, and what does it entail?
- Impact of the sun on the Earth’s biosphere.
- Discuss the importance of astrobiology.
- Evaluate the theories in cosmology.
- Discuss the existence of giant planets.
- Analyze the navigation of the moon.
- An analysis of planet discoveries.
- Discuss the galaxy formation.
- How can scientists predict the age of the universe?
- Explore the significant astrobiology experiments.
- Analyze Giordano Bruno’s theory.
- Can human beings survive on Mars?
- Importance of stars in navigation.
- Discuss the major purpose of space exploration.
- The future of space travel and its possibilities.
- Analyze the connection between the Earth and the sun.
- Define space-time in astrobiology.
- The role of robots in planet discoveries.
- The significance of the sun on Earth’s existence.
- Can the Earth survive if the sun explodes?
- Will the moon hit the Earth, and why?
- Will humans survive if the Earth’s core cools down?
- Impact of black holes on the galaxy.
- Discuss the influence of Mars colonization by humans.
- What if the biggest star caused an explosion?
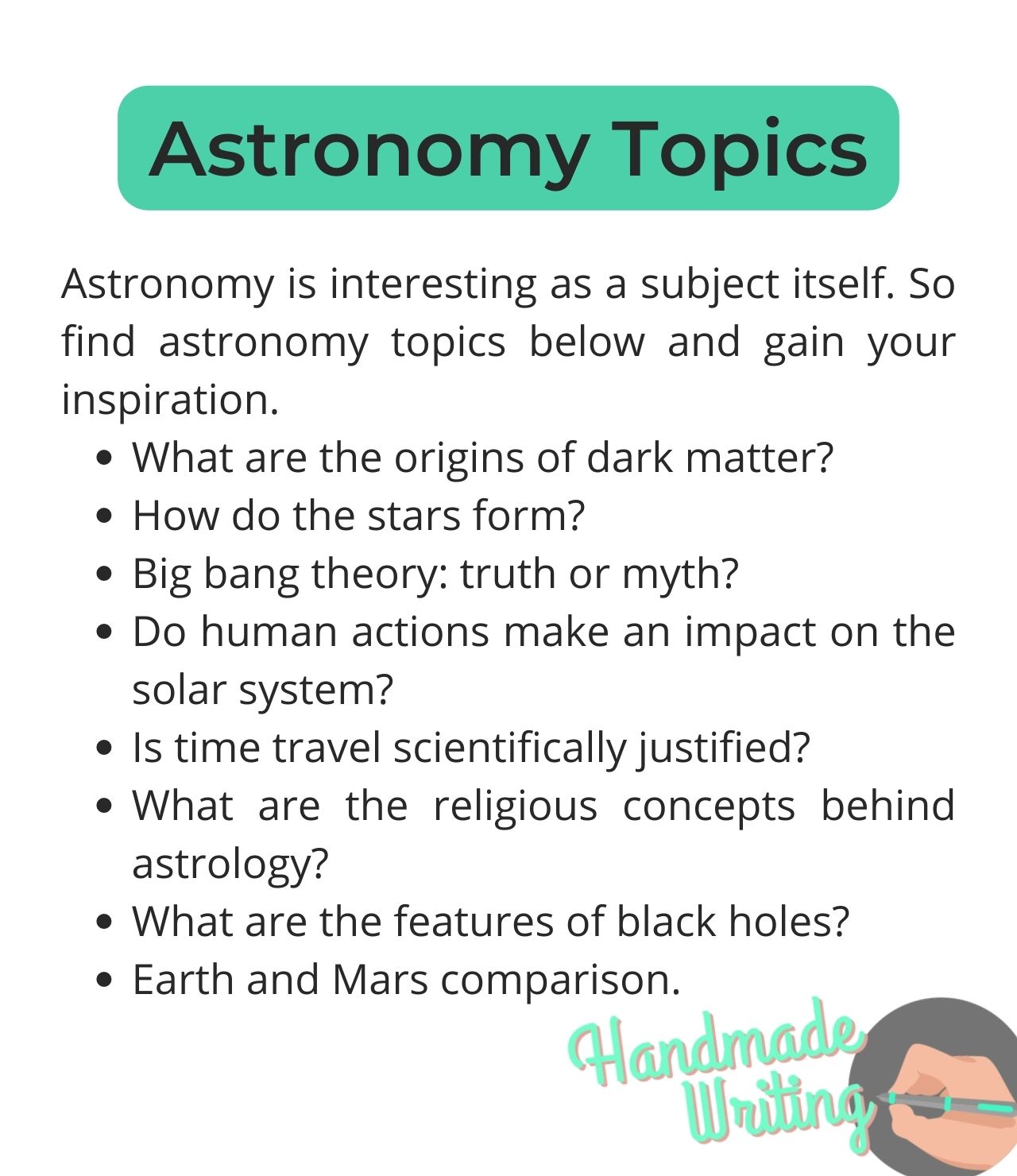
Our Expert Writers Are Available 24/7!
Need help with your astronomy paper? Don’t stress yourself anymore. Our qualified professional essay writers are on standby, ready to provide exceptional assistance to students seeking writing help. We provide an all-inclusive, tailored service that ensures you get your paper as per the specifications. With top ratings and many positive reviews from customers, we guarantee 100% client satisfaction.
Moreover, our subject-oriented writers are highly qualified with impressive research and writing skills. You can never go wrong if you trust us with your academics. Top-notch results, punctual deliveries, and pocket-friendly rates all in one service. Besides, our customer support agents are always online and offer speedy responses to inquiries and issues.
What more are you looking for in a writing service? We have all it takes to ensure you boost your overall performance with original papers and stellar grades. Stop wasting time and contact us now for cool astronomy topics and premium services.
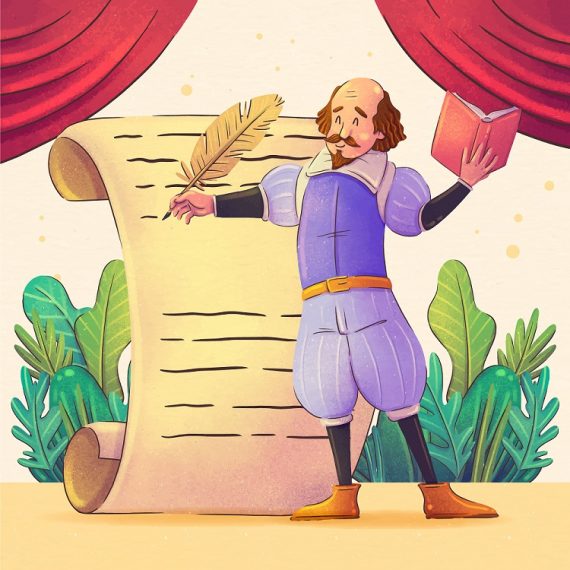
A life lesson in Romeo and Juliet taught by death
Due to human nature, we draw conclusions only when life gives us a lesson since the experience of others is not so effective and powerful. Therefore, when analyzing and sorting out common problems we face, we may trace a parallel with well-known book characters or real historical figures. Moreover, we often compare our situations with […]
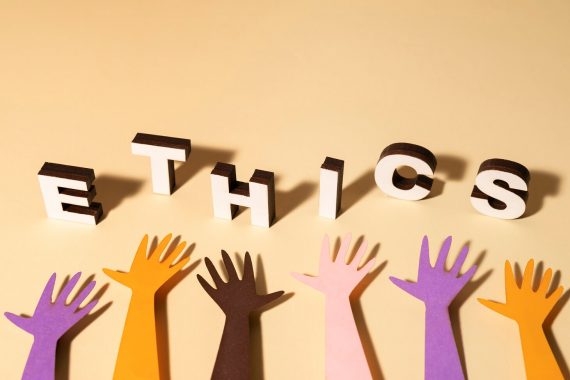
Ethical Research Paper Topics
Writing a research paper on ethics is not an easy task, especially if you do not possess excellent writing skills and do not like to contemplate controversial questions. But an ethics course is obligatory in all higher education institutions, and students have to look for a way out and be creative. When you find an […]
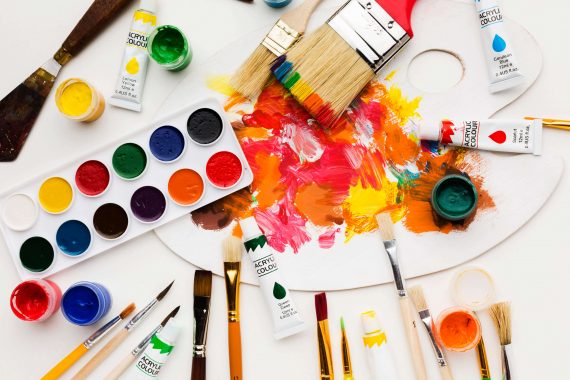
Art Research Paper Topics
Students obtaining degrees in fine art and art & design programs most commonly need to write a paper on art topics. However, this subject is becoming more popular in educational institutions for expanding students’ horizons. Thus, both groups of receivers of education: those who are into arts and those who only get acquainted with art […]
Interesting Astronomy Research Paper Topics and Writing Tips to Get You Started
Note: Only 'PhD' academic level option is available for Dissertation
29 Jan 2020
Astronomy has always attracted attention and high interest from many people across the globe. This scientific discipline, which deals with the study of stars, galaxies, and other celestial objects in the universe, is a never-ending source of mysteries and discoveries. If you are an Astronomy student or study any of the related fields, sooner or later, you will be assigned a research paper about the secrets of the cosmos.
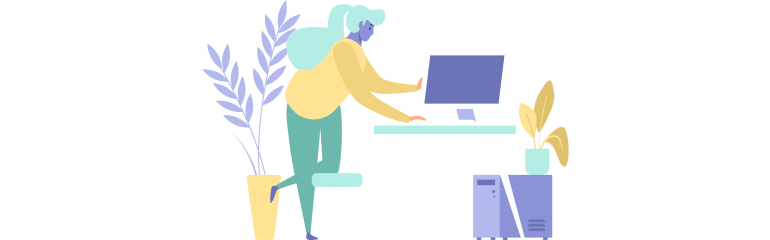
Any astronomy research has all chances of becoming a fascinating space journey for both freshmen and senior students who have the true passion for lifting the veil and exploring something unknown. Take the process of writing seriously: even if the results of your study aren’t a scientific leap forward, they still might be of some use in a broad range of other disciplines. However, students who decide to craft a paper on astronomy should stay confident as a spaceship captain and not be afraid of challenging tasks. Be ready to devote several hours to looking for appropriate materials, producing a strong outline, and filling your astronomy research paper with interesting, yet informative results.
Alternatively, you can it all done by a professional writer. Our experts can craft an entire piece from title to conclusions in a matter of days or – if needed – hours!
You can use it on one order over $

How to Choose Winning Astronomy Topics for Research Paper
Selecting a practical idea for the astronomy research paper can be a quite intimidating and enduring process, especially to students outside of this academic field. For non-majors, picking up the astronomy topics can include looking at the discipline from a different angle. For instance, students with a history major can start with a historical analysis of astronomy themes like ‘Contribution of early astronomers: Aristotle, Ptolemy, Copernicus, and Galileo.’ In a similar manner, literature students can focus on astronomy-related aspects like ‘Portrayal of space travel and exploration in science fiction: Frederik Pohl and Dan Simmons.’ These examples involve studying astronomy through the lenses of the interdisciplinary approach. Admittedly, for students of astrophysics or other pure researchers of astronomy, scrutinizing specific phenomena such as wormholes or dark matter would be a recommended course of action.
When choosing a topic for research, students should begin with determining the lens of inquiry connected to their academic discipline or area of interest, as described above, and then deciding on the degree to which this particular subject can be presented. To put it simply, the amount of information covered in a 100-page thesis should be much more broad and extensive than that of a short research paper. Below is the list of questions that fall within the scope of the astronomy field. It’s by no means exhaustive; rather, it's a small sampling of directions in which you can go deeper for your exploration of astronomy subjects.
Easy Astronomy Research Paper Topics for College Students
One of the toughest decisions to be made during the writing process is making a final choice among the variety of possible topics for your astronomy research paper or dissertation. There is quite a wide range of aspects to explore that can be the following: The Big Bang Theory, the Universe, the Moon, Aurora Borealis, Exploration of the Solar System, Supernova and many, many more. Let’s examine the most exciting and at the same time simple ideas a bit closer.
- How does solar activity influence the Earth?
- Can there be life on other planets of our solar system?
- The origin of the Universe and the Big Bang theory.
- What are the reasons why people should explore space?
- The use of satellites in wireless communication.
- A comprehensive overview of galaxies and what they are.
- Should Pluto’s planet status be reinstated?
- How are stars formed?
- The relevance of the contribution to the astronomy field by Newton, Kepler, and Galileo.
- History of space exploration from watching the stars up to the first Mars landing.
Fresh Ideas for Astronomy Research Papers
If the range of astronomy-related aspects you would like to delve into in your academic writing is as vast as space itself, we suggest checking the list in which our professional writers have gathered some of the most topical and discussable astronomy issues.
- What are the future business opportunities in space?
- What do astronomers believe that the universe is continuously expanding and how they came to this conclusion?
- Will time travel be ever possible?
- What are the star clusters and how they can be applied in astronomy and other fields?
- Is mars colonization a dream or a necessity?
- Robotic space exploration.
- What is the identity of dark matter?
- Can physicists ever prove the multiverse is real?
- Gaps between theory and application of satellite communications.
- Space exploration: history and promises for the future.
Now you know how the essential points to choose an excellent topic for your piece of writing and have a list of ideas to get started – you are fully prepared for your space odyssey. So, fasten your belts and be ready to get the highest score. Nevertheless, if you still find yourself thinking, ‘I need someone to write my research paper ’ – feel free to get in touch with us any time. As one of the best research paper writing websites on the market, WePapers.com does know how to produce a fabulous content you will be proud of!
Related posts:
- Independent Literature Reviews
- Defeat Literature Reviews
- Conservation Literature Reviews
- Opposite Literature Reviews
- Sales Literature Reviews
- Bachelor Literature Reviews
- Sin Literature Reviews
- Body Language Literature Reviews
- Usability Literature Reviews
- Increase Case Studies
- Instance Case Studies
- Allocation Case Studies
- Incentive Case Studies
- Input Case Studies
- Target Case Studies
- Housing Case Studies
- Immune Case Studies
- Infection Case Studies
- System Case Studies
- Broadcast Case Studies
- Social Issues Case Studies
- Information Case Studies
- Discrimination Case Studies
- Money Case Studies
- Interview Case Studies
- Human Case Studies
- Attitude Case Studies
- Discipline Case Studies
- Immigration Case Studies
- Gap Case Studies
- Police Case Studies
- Driving Case Studies
- House Case Studies
- Competition Case Studies
- Website Case Studies
- Variety Case Studies
- Software Case Studies
- Infrastructure Case Studies
- Enterprise Case Studies
- Identification Case Studies
- Protocol Case Studies
- Disaster Case Studies
- Music Case Studies
- Host Case Studies
- Competition Reports
- Activities Of Daily Living Essays
- Agricultural Practices Essays
- Angel Essays
- Attentiveness Essays
- Ballinger Essays
- Bar Chart Essays
- Belief Essays
- Call The Police Essays
- Champaign Essays
- Domestic Violence Essays
- Double Standards Essays
- Egyptian Civilization Essays
- Integrated Marketing Communication Essays
- Just Noticeable Difference Essays
- Literature Review Essays
- Manufacture Essays
- Miscue Essays
- Morality Essays
- Nipa Essays
- Production Essays
- Reductio Essays
- Temperate Climate Essays
- Theater Essays
- Wireless Essays
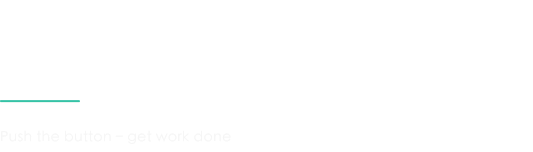
We use cookies to improve your experience with our site. Please accept before continuing or read our cookie policy here .
Wait, have you seen our prices?

TODAY'S HOURS:
Research Topic Ideas
- Picking a Topic
- Area & Interdisciplinary Studies
- Behavioral & Social Sciences
- Business, Economics, & Management
- Current Events and Controversial Issues
- Education & Social Work
- Health Sciences
Astronomy Topics
Biology topics, chemistry and biochemistry topics, computer science and information technology & informatics topics, engineering topics, geography, planning, & environment, mathematics topics, physics topics, related guides.
- Research Process by Liz Svoboda Last Updated Mar 1, 2024 6323 views this year
- Big Bang theory
- Black holes
- Curiosity Rover
- Dwarf galaxies
- Earth-size planets
- Gravitational lensing
- Hubble telescope
- Kepler telescope
- Philae probe
- Remote sensing
- Sagan effect
- Spectroscopy
- Stellar spectra
- Superclusters
Related subject guide and suggested database
- Physics and Astronomy: A Guide to Library Research by Reference Librarians Last Updated Nov 3, 2023 61 views this year

Online books and journals, primarily in the life and physical sciences, technology, and medicine, as well as business and the social sciences
Includes over 2,000 journals and 35,000 books published by Elsevier Science and its subsidiary publishers, including Academic Press, Cell Press, Pergamon, Mosby, and Saunders journals. Coverage is particularly strong for the life and physical sciences, medicine, and technical fields, but also includes some social sciences and humanities. Includes chemistry, chemical engineering, clinical medicine, computer science, earth & planetary sciences, economics, engineering, energy & technology, environmental science & technology, life sciences, materials science, mathematics, physics, astronomy.
- Algae blooms
- Biological warfare
- Bioluminescence
- Butterfly migration
- Circadian rhythm
- Coral reefs
- De-extinction
- Dismal Swamp
- Endangered species
- Fertilizers
- Genetically modified organisms (GMOs)
- Human genome map
- Invasive species
- Mass extinction
- Mutagenesis
- Neural systems
- Neurobiology of sleep
- Oil spill effects
- Organic farming
- Primate language
- Rainforest conservation
- Teratogenesis
- Wetland restoration
- Biology: A Guide to Library Research by Reference Librarians Last Updated Mar 12, 2024 78 views this year
Collection of five major collections in biology, environmental sciences, ecology, and agriculture.
Includes these collections:
- Agricola: Millions of records from the U.S. Department of Agriculture's National Agricultural Library dating back to 1970. The citations include journal articles, monographs, theses, patents, software, audiovisual materials, and technical reports related to agriculture.
- Biological & Agricultural Index Plus: 1983-present. Agriculture, animal husbandry, botany, cytology, ecology, entomology, environmental science, fishery sciences, food science, forestry, genetics, horticulture, microbiology, plant pathology, soil science, veterinary medicine, zoology.
- Environment Complete: Coverage in applicable areas of agriculture, ecosystem ecology, energy, renewable energy sources, natural resources, marine & freshwater science, geography, pollution & waste management, environmental technology, environmental law, public policy, social impacts, urban planning, and more.
- GreenFILE: Articles, books and government documents on global warming, environmental protection and renewable energy.
- Wildlife & Ecology Studies Worldwide: Covers 1935 & earlier to the present, and is the world's largest index to literature on wild mammals, birds, reptiles, and amphibians. Most of the records in this database are from Wildlife Review Abstracts, which offers a global perspective and is the most comprehensive resource on wildlife information. Major topic areas include studies of individual species, habitat types, hunting, economics, wildlife behavior, management techniques, diseases, ecotourism, zoology, taxonomy and much more.
- Alkali metals
- Bioenergetics
- Buffer solutions
- Carbon cature
- Carbon dating
- Chemical carcinogens
- Chemical warfare
- Desalinization
- Electromagnetic radiation
- Oxidation numbers
- Periodic table
- Pyrotechnics
- Radioactive waste
- Silver iodide
- Synthetic DNA
- Water fluoridation
- Chemistry: A Guide to Library Research by Reference Librarians Last Updated Dec 13, 2023 101 views this year
- Artificial intelligence
- Biometric signature
- Computer animation
- Computer composed music
- Computer forensics
- Computer modeling
- Gamification
- General Data Protection Regulation
- Intelligent databases
- Internet of things
- Machine language
- Mobile computing
- Social media literacy
- Ubiquitous design
- Voice recognition
- Computer Science: A Guide to Library Research by Reference Librarians Last Updated Mar 5, 2024 51 views this year
Full-text access to IEEE transactions, journals, magazines, conference proceedings, standards, and online courses, plus related e-books. Covers engineering, computer science, technology, and physics.
IEEE Courses uses the best educational courses from IEEE conferences and workshops around the world and delivers them in interactive video format. You can earn Continuing Education Unit (CEU) credits and Professional Development Hours (PDH). To set up remote access to IEEE Xplore on a mobile device, please follow these steps:
- On the mobile device, use a browser to visit the IEEE Xplore website through the U-M Library's access.
- Click on the blue tab "My Settings" in the middle of the screen, then choose "Remote Access."
- Follow the instructions to pair your device. Users will be asked to sign in with an IEEE personal account before pairing.
- This device can now be used off-site for 90 days to access IEEE Xplore. Users must go to IEEE Xplore on the paired device and log in with their IEEE personal account to access the roaming subscription.
Please note that after 90 days, users will be prompted to again pair their device and can follow the same steps as the initial pairing.
- 3D printing
- Additive design
- Aeroacoustics
- Aerothermodynamics
- Air turbulence
- Analytical mechanics
- Boundary layer
- Carbon capture
- Composite materials
- Computer-aided design (CAD)
- Computer-aided engineering (CAE)
- Computer-aided manufacturing (CAM)
- Design optimization
- Electric cars
- Fiber optics
- Finite element analysis (FEA)
- Fluid dynamics
- Flutter phenomena
- Information theory
- Geoengineering
- High horsepower in cars
- Hybrid vehicles
- Hydroforming
- Laser applications
- Liquid crystals
- Nanomaterials
- Nanotechnology
- Naturalistic decision making
- Nuclear power
- Stereo imaging
- Engineering: A Guide to Library Research by Reference Librarians Last Updated Mar 12, 2024 54 views this year
- Air pollution
- Algae bloom
- Alternative fuels
- Beach erosion
- Carbon footprint
- Climate forcing
- Compostable plastic
- Dymaxion House
- Earth-sheltered structures
- Green cities
- Green infrastructure
- Natual resource conservation
- Nitrogen farming
- Ozone depletion
- Pollution reduction
- Rip currents
- Soil contamination
- Sustainable design
- Three Gorges Dam
- Tiny houses
- Toxic dumping in oceans
- Water conservation
- Water pollution
- Geography, Planning, and Environment: A Guide to Library Research by Reference Librarians Last Updated Feb 23, 2024 48 views this year
- Applied statistics
- Bioinformatics
- Convex geometry
- Game theory
- Millennium Prize problems
- Probability theory
- Random walks
- Representation theory
- Riemannian geometry
- Theory of chaos
- Turing, Alan
- Turing patterns
- Mathematics: A Guide to Library Research by Reference Librarians Last Updated Mar 6, 2024 30 views this year
Online access to Mathematical Reviews , the journal of record which indexes, reviews, and abstracts the published mathematical research literature. 1940-present, with some earlier content.
- Antiparticles
- Bohr's radius
- Bose–Einstein condensate
- Dark matter
- Gravitational waves
- Heat death of the universe
- Higgs boson
- Manhattan Project
- Nanofabrication
- Quantum entanglement
- Space elevator
- Superstring theory
- Thermodynamics
- Wave-particle duality
World-wide literature (mainly journal articles and conference proceedings papers) in astronomy, physics, electronics and electrical engineering, computers and control, and information technology.
Hosted on Engineering Village platform. Covers physics, electrical & electronic engineering, communications, computer science, control engineering, information technology, manufacturing & mechanical engineering, operations research, material science, oceanography, engineering mathematics, nuclear engineering, environmental science, geophysics, nanotechnology, biomedical technology, and biophysics.
- << Previous: Humanities
- Last Updated: Mar 1, 2024 1:06 PM
- URL: https://libguides.umflint.edu/topics
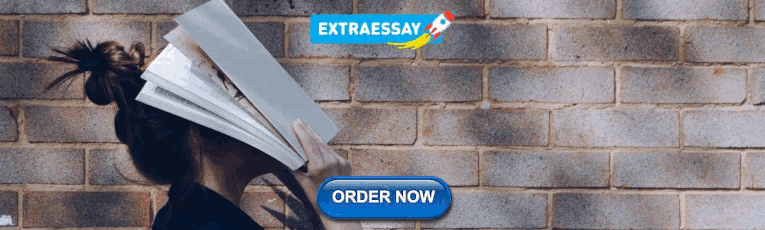
IMAGES
VIDEO
COMMENTS
From the definition of astronomy, it is clear that you can have a long list of astronomy project topics or ideas. Once you have picked the preferred option from our research topics in cosmology, the next step is preparing your paper. Here are the main characteristics of a good school research paper in astronomy:
Complex Astronomy Project Ideas. If you want to write a complex paper that will earn you a very good grade, you need to see our amazing list of astronomy topics for projects: The ins and outs of robotic space exploration. The future of space exploration. Making business in space.
Here are great astronomy research topics to consider for your school or university paper: Gravitational waves and their impact on Astronomy. Stellar evolution and life cycle of stars. The role of magnetic fields in shaping celestial objects. The role of magnetic fields in star formation. Origin and evolution of supermassive black holes.
185 Astronomy Research Topics & Interesting Ideas. Astronomy research topics encompass a wide range of fascinating themes. These topics include cosmic microwave background study to explore the universe's origins and exoplanetary research to identify potentially habitable worlds. People can also investigate dark matter and energy ...
Astronomy Research Topics. Astronomy Research Topics are as follows: The formation and evolution of stars. The structure and dynamics of galaxies. The nature of dark matter and dark energy. The search for exoplanets and habitable zones. The properties of black holes and their effects on their surroundings.
Here are some excellent astronomy research paper topics about the solar system. A brief overview of the chemistry of the solar system. Solar System Physics - What You Need to Know. An image of Jupiter's Great Red Spot and a turbulent atmosphere in the southern hemisphere. Solar System Study in Ancient Times: A History of the Solar System.
Research Topics. Scientists and engineers at the Center for Astrophysics | Harvard & Smithsonian collaborate across a broad variety of scientific disciplines, from astronomy and astrophysics to related areas of physics and geophysics, in advancing humanity's understanding of the universe. Learn more about the full spectrum of research covered ...
The accretion of a solar mass per day by a 17-billion solar mass black hole. A black hole at the centre of a quasar at a redshift of z = 4 is accreting the mass of the Sun every day. The quasar ...
6. Fill in the paragraphs and (sub)sections with a rough first draft of the text. You may want to start with the easiest sections (usually sample, data, methods) and leave the hardest for later ...
Frontiers in Astronomy and Space Sciences: A Decade of Discovery and Advancement - 10th anniversary conference. Julio Navarro. Alberto Fairén. Joseph E Borovsky. CRISTINA PUZZARINI.
Ideally, it should capture the main message of the paper, the 'narrative', or story, in around 15 words. The title needs to be accurate and needs to match the abstract and the rest of the ...
Research at KIPAC is revealing the lifecycle of galaxies: how galaxies were born in the darkness of the early universe, how their different components interact as they live and grow, and how they die. KIPAC scientists are studying early galaxies with computer simulations to understand the formation of the first galaxies and stars, and use a ...
For writing your astronomy research paper, you can very well choose a topic from areas such as space science, astrophysics, planetary science, or any other astronomy-related sub-discipline. If you run short of ideas, then take a look at the list presented below. In the list, you will find 150+ fascinating astronomy research paper topic ideas ...
Type at least 3 characters. Reach for the stars: Research Topics on space exploration. With recent advances in commercial space exploration, we have curated a list of our best Research Topics on outer space. Explore collections edited by experts from NASA, The Goddard Space Flight Center, Space Science Institute, German Aerospace Center ...
Here are some key points explaining why we study space: Understanding our Origins: Space research helps us uncover the origins of the universe, including how galaxies, stars, and planets formed. Advancing Scientific Knowledge: Studying space leads to breakthroughs in physics, astronomy, and other scientific fields, expanding our understanding of the cosmos.
154 Astronomy Research Topics: Rocket To Your Marvelous Grades. Every student knows that the key to writing an excellent research assignment starts with choosing an interesting topic that captures the reader's attention and explores new areas within the discipline. Some research topics come to students naturally, but often coming up with a ...
165 Fascinating Astronomy Topics To Research And Learn About. October 29, 2021. Astronomy is a branch of science dealing with celestial objects, space, and the physical universe. Despite this field being fascinating, some titles are confusing and complex. Essentially, astronomy topics revolve around material science exceeding beyond the earth ...
Research Topics. Scientists and engineers at the Center for Astrophysics | Harvard & Smithsonian collaborate across a broad variety of scientific disciplines, from astronomy and astrophysics to related areas of physics and geophysics, in advancing humanity's understanding of the universe. Learn more about the full spectrum of research covered ...
Submitted to Nature Astronomy as Paper 2 in a two-part mini-series of 'Perspectives' articles. Paper 1 in the series is 'How to plan your astronomy research paper in ten steps' by Chamba, Knapen & Black. Kindly cite and/or acknowledge this work and Paper 1 ( arXiv:2207.12959) if you use them to plan and write your paper.
Astronomy and astrophysics are the study of objects and phenomena that are found beyond our solar system. This combines theoretical simulations and observation with both terrestrial and space ...
Here are some advantages of using our astronomy research paper topics: You will get unique and captivating topics that translate to original research papers. No other student will come up with a similar topic. Tutors often prefer reading exciting research papers. Thus, select one of our topic ideas and impress your astronomy tutor.
Deadline. Approx. price. $6. Proceed to order. 29 Jan 2020. Astronomy has always attracted attention and high interest from many people across the globe. This scientific discipline, which deals with the study of stars, galaxies, and other celestial objects in the universe, is a never-ending source of mysteries and discoveries.
Includes over 2,000 journals and 35,000 books published by Elsevier Science and its subsidiary publishers, including Academic Press, Cell Press, Pergamon, Mosby, and Saunders journals. Coverage is particularly strong for the life and physical sciences, medicine, and technical fields, but also includes some social sciences and humanities.