- Skip to main content
- Skip to primary sidebar
- Skip to footer
- QuestionPro

- Solutions Industries Gaming Automotive Sports and events Education Government Travel & Hospitality Financial Services Healthcare Cannabis Technology Use Case NPS+ Communities Audience Contactless surveys Mobile LivePolls Member Experience GDPR Positive People Science 360 Feedback Surveys
- Resources Blog eBooks Survey Templates Case Studies Training Help center

Home Market Research
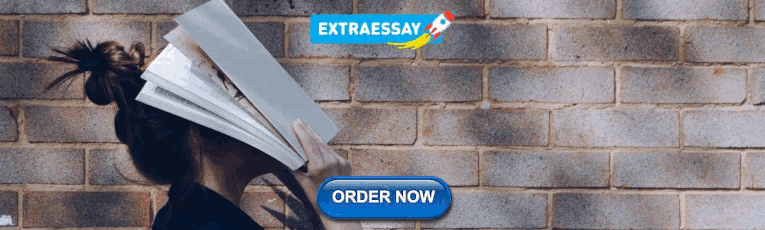
Data Analysis in Research: Types & Methods
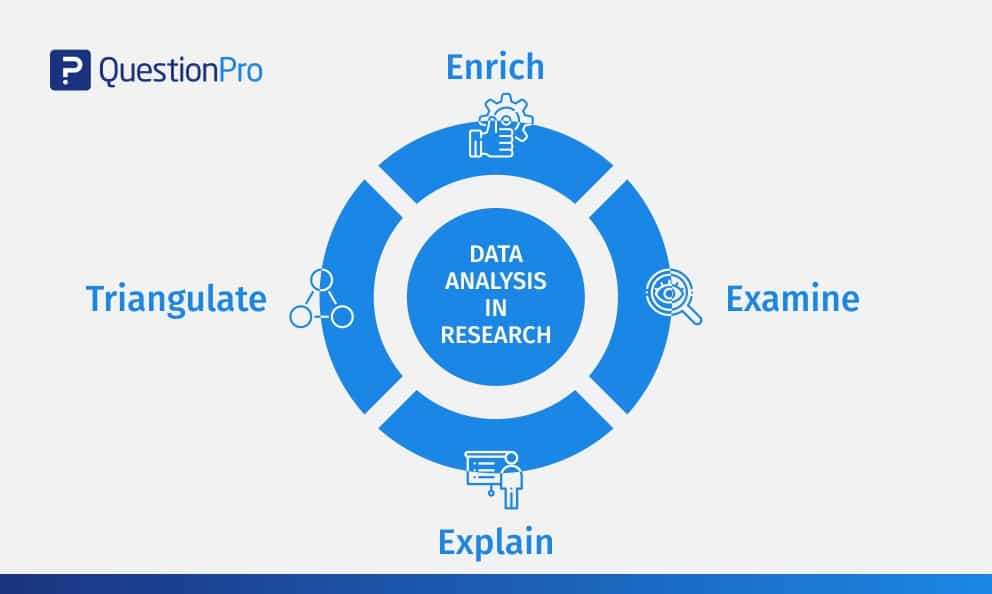
Content Index
Why analyze data in research?
Types of data in research, finding patterns in the qualitative data, methods used for data analysis in qualitative research, preparing data for analysis, methods used for data analysis in quantitative research, considerations in research data analysis, what is data analysis in research.
Definition of research in data analysis: According to LeCompte and Schensul, research data analysis is a process used by researchers to reduce data to a story and interpret it to derive insights. The data analysis process helps reduce a large chunk of data into smaller fragments, which makes sense.
Three essential things occur during the data analysis process — the first is data organization . Summarization and categorization together contribute to becoming the second known method used for data reduction. It helps find patterns and themes in the data for easy identification and linking. The third and last way is data analysis – researchers do it in both top-down and bottom-up fashion.
LEARN ABOUT: Research Process Steps
On the other hand, Marshall and Rossman describe data analysis as a messy, ambiguous, and time-consuming but creative and fascinating process through which a mass of collected data is brought to order, structure and meaning.
We can say that “the data analysis and data interpretation is a process representing the application of deductive and inductive logic to the research and data analysis.”
Researchers rely heavily on data as they have a story to tell or research problems to solve. It starts with a question, and data is nothing but an answer to that question. But, what if there is no question to ask? Well! It is possible to explore data even without a problem – we call it ‘Data Mining’, which often reveals some interesting patterns within the data that are worth exploring.
Irrelevant to the type of data researchers explore, their mission and audiences’ vision guide them to find the patterns to shape the story they want to tell. One of the essential things expected from researchers while analyzing data is to stay open and remain unbiased toward unexpected patterns, expressions, and results. Remember, sometimes, data analysis tells the most unforeseen yet exciting stories that were not expected when initiating data analysis. Therefore, rely on the data you have at hand and enjoy the journey of exploratory research.
Create a Free Account
Every kind of data has a rare quality of describing things after assigning a specific value to it. For analysis, you need to organize these values, processed and presented in a given context, to make it useful. Data can be in different forms; here are the primary data types.
- Qualitative data: When the data presented has words and descriptions, then we call it qualitative data . Although you can observe this data, it is subjective and harder to analyze data in research, especially for comparison. Example: Quality data represents everything describing taste, experience, texture, or an opinion that is considered quality data. This type of data is usually collected through focus groups, personal qualitative interviews , qualitative observation or using open-ended questions in surveys.
- Quantitative data: Any data expressed in numbers of numerical figures are called quantitative data . This type of data can be distinguished into categories, grouped, measured, calculated, or ranked. Example: questions such as age, rank, cost, length, weight, scores, etc. everything comes under this type of data. You can present such data in graphical format, charts, or apply statistical analysis methods to this data. The (Outcomes Measurement Systems) OMS questionnaires in surveys are a significant source of collecting numeric data.
- Categorical data: It is data presented in groups. However, an item included in the categorical data cannot belong to more than one group. Example: A person responding to a survey by telling his living style, marital status, smoking habit, or drinking habit comes under the categorical data. A chi-square test is a standard method used to analyze this data.
Learn More : Examples of Qualitative Data in Education
Data analysis in qualitative research
Data analysis and qualitative data research work a little differently from the numerical data as the quality data is made up of words, descriptions, images, objects, and sometimes symbols. Getting insight from such complicated information is a complicated process. Hence it is typically used for exploratory research and data analysis .
Although there are several ways to find patterns in the textual information, a word-based method is the most relied and widely used global technique for research and data analysis. Notably, the data analysis process in qualitative research is manual. Here the researchers usually read the available data and find repetitive or commonly used words.
For example, while studying data collected from African countries to understand the most pressing issues people face, researchers might find “food” and “hunger” are the most commonly used words and will highlight them for further analysis.
LEARN ABOUT: Level of Analysis
The keyword context is another widely used word-based technique. In this method, the researcher tries to understand the concept by analyzing the context in which the participants use a particular keyword.
For example , researchers conducting research and data analysis for studying the concept of ‘diabetes’ amongst respondents might analyze the context of when and how the respondent has used or referred to the word ‘diabetes.’
The scrutiny-based technique is also one of the highly recommended text analysis methods used to identify a quality data pattern. Compare and contrast is the widely used method under this technique to differentiate how a specific text is similar or different from each other.
For example: To find out the “importance of resident doctor in a company,” the collected data is divided into people who think it is necessary to hire a resident doctor and those who think it is unnecessary. Compare and contrast is the best method that can be used to analyze the polls having single-answer questions types .
Metaphors can be used to reduce the data pile and find patterns in it so that it becomes easier to connect data with theory.
Variable Partitioning is another technique used to split variables so that researchers can find more coherent descriptions and explanations from the enormous data.
LEARN ABOUT: Qualitative Research Questions and Questionnaires
There are several techniques to analyze the data in qualitative research, but here are some commonly used methods,
- Content Analysis: It is widely accepted and the most frequently employed technique for data analysis in research methodology. It can be used to analyze the documented information from text, images, and sometimes from the physical items. It depends on the research questions to predict when and where to use this method.
- Narrative Analysis: This method is used to analyze content gathered from various sources such as personal interviews, field observation, and surveys . The majority of times, stories, or opinions shared by people are focused on finding answers to the research questions.
- Discourse Analysis: Similar to narrative analysis, discourse analysis is used to analyze the interactions with people. Nevertheless, this particular method considers the social context under which or within which the communication between the researcher and respondent takes place. In addition to that, discourse analysis also focuses on the lifestyle and day-to-day environment while deriving any conclusion.
- Grounded Theory: When you want to explain why a particular phenomenon happened, then using grounded theory for analyzing quality data is the best resort. Grounded theory is applied to study data about the host of similar cases occurring in different settings. When researchers are using this method, they might alter explanations or produce new ones until they arrive at some conclusion.
LEARN ABOUT: 12 Best Tools for Researchers
Data analysis in quantitative research
The first stage in research and data analysis is to make it for the analysis so that the nominal data can be converted into something meaningful. Data preparation consists of the below phases.
Phase I: Data Validation
Data validation is done to understand if the collected data sample is per the pre-set standards, or it is a biased data sample again divided into four different stages
- Fraud: To ensure an actual human being records each response to the survey or the questionnaire
- Screening: To make sure each participant or respondent is selected or chosen in compliance with the research criteria
- Procedure: To ensure ethical standards were maintained while collecting the data sample
- Completeness: To ensure that the respondent has answered all the questions in an online survey. Else, the interviewer had asked all the questions devised in the questionnaire.
Phase II: Data Editing
More often, an extensive research data sample comes loaded with errors. Respondents sometimes fill in some fields incorrectly or sometimes skip them accidentally. Data editing is a process wherein the researchers have to confirm that the provided data is free of such errors. They need to conduct necessary checks and outlier checks to edit the raw edit and make it ready for analysis.
Phase III: Data Coding
Out of all three, this is the most critical phase of data preparation associated with grouping and assigning values to the survey responses . If a survey is completed with a 1000 sample size, the researcher will create an age bracket to distinguish the respondents based on their age. Thus, it becomes easier to analyze small data buckets rather than deal with the massive data pile.
LEARN ABOUT: Steps in Qualitative Research
After the data is prepared for analysis, researchers are open to using different research and data analysis methods to derive meaningful insights. For sure, statistical analysis plans are the most favored to analyze numerical data. In statistical analysis, distinguishing between categorical data and numerical data is essential, as categorical data involves distinct categories or labels, while numerical data consists of measurable quantities. The method is again classified into two groups. First, ‘Descriptive Statistics’ used to describe data. Second, ‘Inferential statistics’ that helps in comparing the data .
Descriptive statistics
This method is used to describe the basic features of versatile types of data in research. It presents the data in such a meaningful way that pattern in the data starts making sense. Nevertheless, the descriptive analysis does not go beyond making conclusions. The conclusions are again based on the hypothesis researchers have formulated so far. Here are a few major types of descriptive analysis methods.
Measures of Frequency
- Count, Percent, Frequency
- It is used to denote home often a particular event occurs.
- Researchers use it when they want to showcase how often a response is given.
Measures of Central Tendency
- Mean, Median, Mode
- The method is widely used to demonstrate distribution by various points.
- Researchers use this method when they want to showcase the most commonly or averagely indicated response.
Measures of Dispersion or Variation
- Range, Variance, Standard deviation
- Here the field equals high/low points.
- Variance standard deviation = difference between the observed score and mean
- It is used to identify the spread of scores by stating intervals.
- Researchers use this method to showcase data spread out. It helps them identify the depth until which the data is spread out that it directly affects the mean.
Measures of Position
- Percentile ranks, Quartile ranks
- It relies on standardized scores helping researchers to identify the relationship between different scores.
- It is often used when researchers want to compare scores with the average count.
For quantitative research use of descriptive analysis often give absolute numbers, but the in-depth analysis is never sufficient to demonstrate the rationale behind those numbers. Nevertheless, it is necessary to think of the best method for research and data analysis suiting your survey questionnaire and what story researchers want to tell. For example, the mean is the best way to demonstrate the students’ average scores in schools. It is better to rely on the descriptive statistics when the researchers intend to keep the research or outcome limited to the provided sample without generalizing it. For example, when you want to compare average voting done in two different cities, differential statistics are enough.
Descriptive analysis is also called a ‘univariate analysis’ since it is commonly used to analyze a single variable.
Inferential statistics
Inferential statistics are used to make predictions about a larger population after research and data analysis of the representing population’s collected sample. For example, you can ask some odd 100 audiences at a movie theater if they like the movie they are watching. Researchers then use inferential statistics on the collected sample to reason that about 80-90% of people like the movie.
Here are two significant areas of inferential statistics.
- Estimating parameters: It takes statistics from the sample research data and demonstrates something about the population parameter.
- Hypothesis test: I t’s about sampling research data to answer the survey research questions. For example, researchers might be interested to understand if the new shade of lipstick recently launched is good or not, or if the multivitamin capsules help children to perform better at games.
These are sophisticated analysis methods used to showcase the relationship between different variables instead of describing a single variable. It is often used when researchers want something beyond absolute numbers to understand the relationship between variables.
Here are some of the commonly used methods for data analysis in research.
- Correlation: When researchers are not conducting experimental research or quasi-experimental research wherein the researchers are interested to understand the relationship between two or more variables, they opt for correlational research methods.
- Cross-tabulation: Also called contingency tables, cross-tabulation is used to analyze the relationship between multiple variables. Suppose provided data has age and gender categories presented in rows and columns. A two-dimensional cross-tabulation helps for seamless data analysis and research by showing the number of males and females in each age category.
- Regression analysis: For understanding the strong relationship between two variables, researchers do not look beyond the primary and commonly used regression analysis method, which is also a type of predictive analysis used. In this method, you have an essential factor called the dependent variable. You also have multiple independent variables in regression analysis. You undertake efforts to find out the impact of independent variables on the dependent variable. The values of both independent and dependent variables are assumed as being ascertained in an error-free random manner.
- Frequency tables: The statistical procedure is used for testing the degree to which two or more vary or differ in an experiment. A considerable degree of variation means research findings were significant. In many contexts, ANOVA testing and variance analysis are similar.
- Analysis of variance: The statistical procedure is used for testing the degree to which two or more vary or differ in an experiment. A considerable degree of variation means research findings were significant. In many contexts, ANOVA testing and variance analysis are similar.
- Researchers must have the necessary research skills to analyze and manipulation the data , Getting trained to demonstrate a high standard of research practice. Ideally, researchers must possess more than a basic understanding of the rationale of selecting one statistical method over the other to obtain better data insights.
- Usually, research and data analytics projects differ by scientific discipline; therefore, getting statistical advice at the beginning of analysis helps design a survey questionnaire, select data collection methods, and choose samples.
LEARN ABOUT: Best Data Collection Tools
- The primary aim of data research and analysis is to derive ultimate insights that are unbiased. Any mistake in or keeping a biased mind to collect data, selecting an analysis method, or choosing audience sample il to draw a biased inference.
- Irrelevant to the sophistication used in research data and analysis is enough to rectify the poorly defined objective outcome measurements. It does not matter if the design is at fault or intentions are not clear, but lack of clarity might mislead readers, so avoid the practice.
- The motive behind data analysis in research is to present accurate and reliable data. As far as possible, avoid statistical errors, and find a way to deal with everyday challenges like outliers, missing data, data altering, data mining , or developing graphical representation.
LEARN MORE: Descriptive Research vs Correlational Research The sheer amount of data generated daily is frightening. Especially when data analysis has taken center stage. in 2018. In last year, the total data supply amounted to 2.8 trillion gigabytes. Hence, it is clear that the enterprises willing to survive in the hypercompetitive world must possess an excellent capability to analyze complex research data, derive actionable insights, and adapt to the new market needs.
LEARN ABOUT: Average Order Value
QuestionPro is an online survey platform that empowers organizations in data analysis and research and provides them a medium to collect data by creating appealing surveys.
MORE LIKE THIS
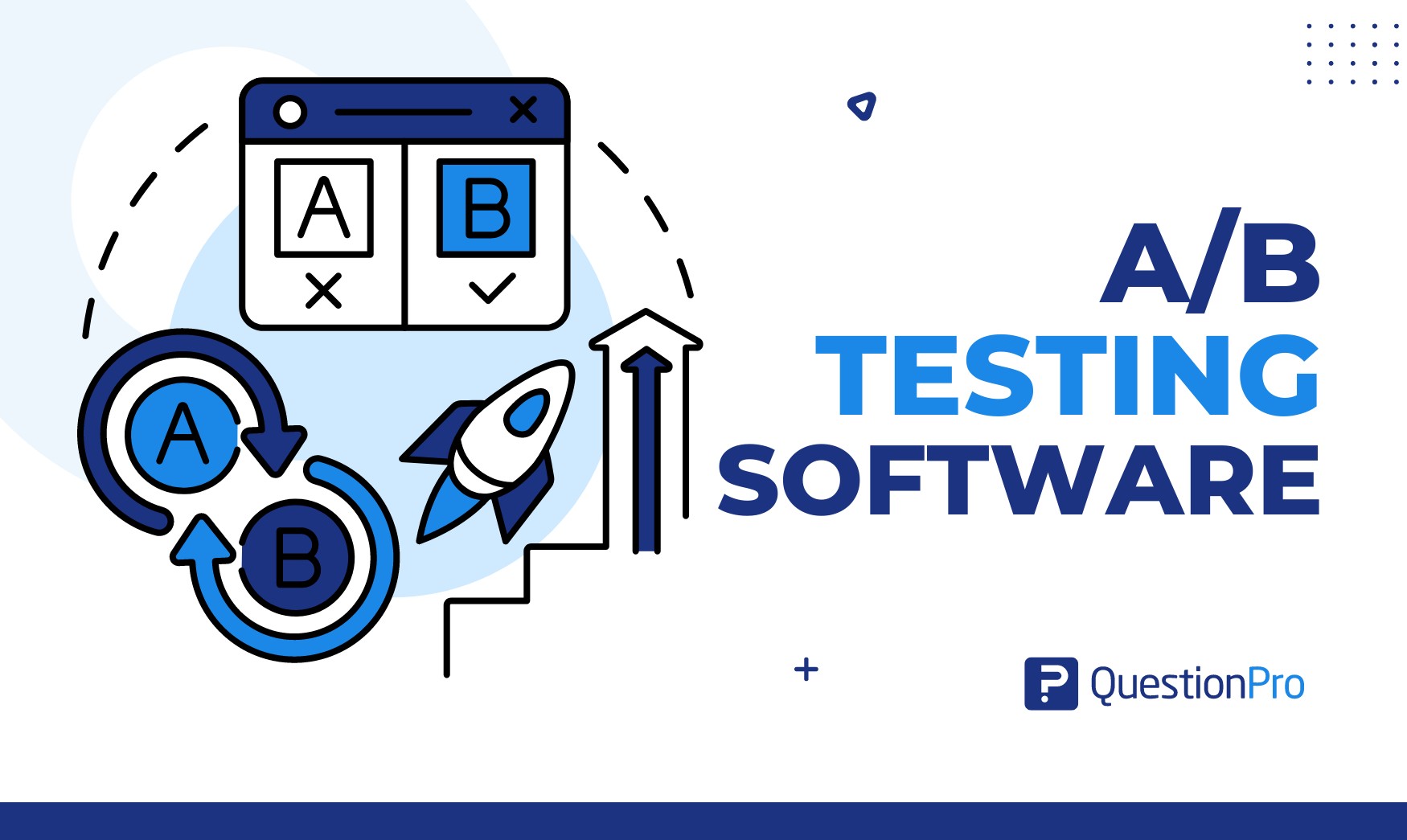
Top 13 A/B Testing Software for Optimizing Your Website
Apr 12, 2024
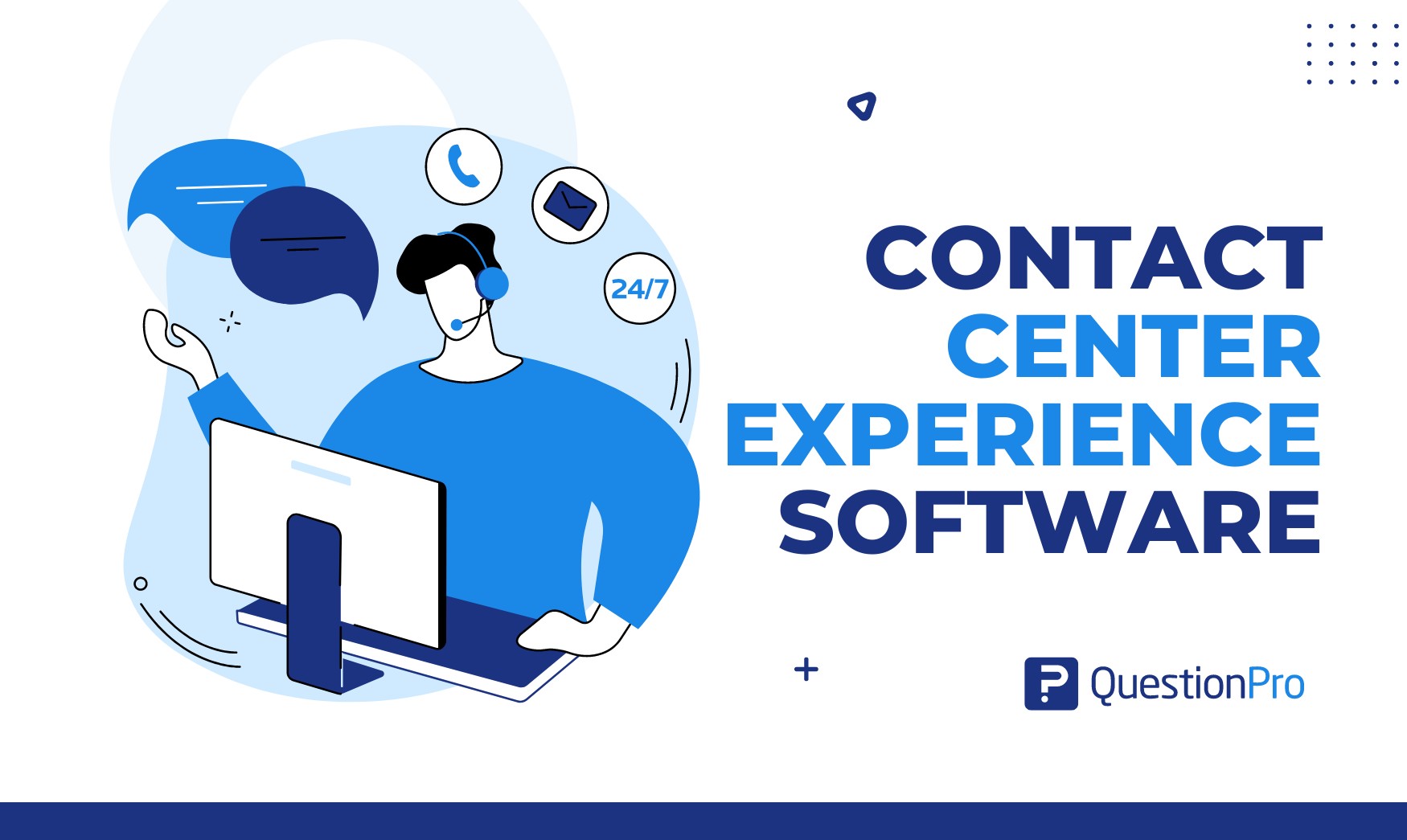
21 Best Contact Center Experience Software in 2024
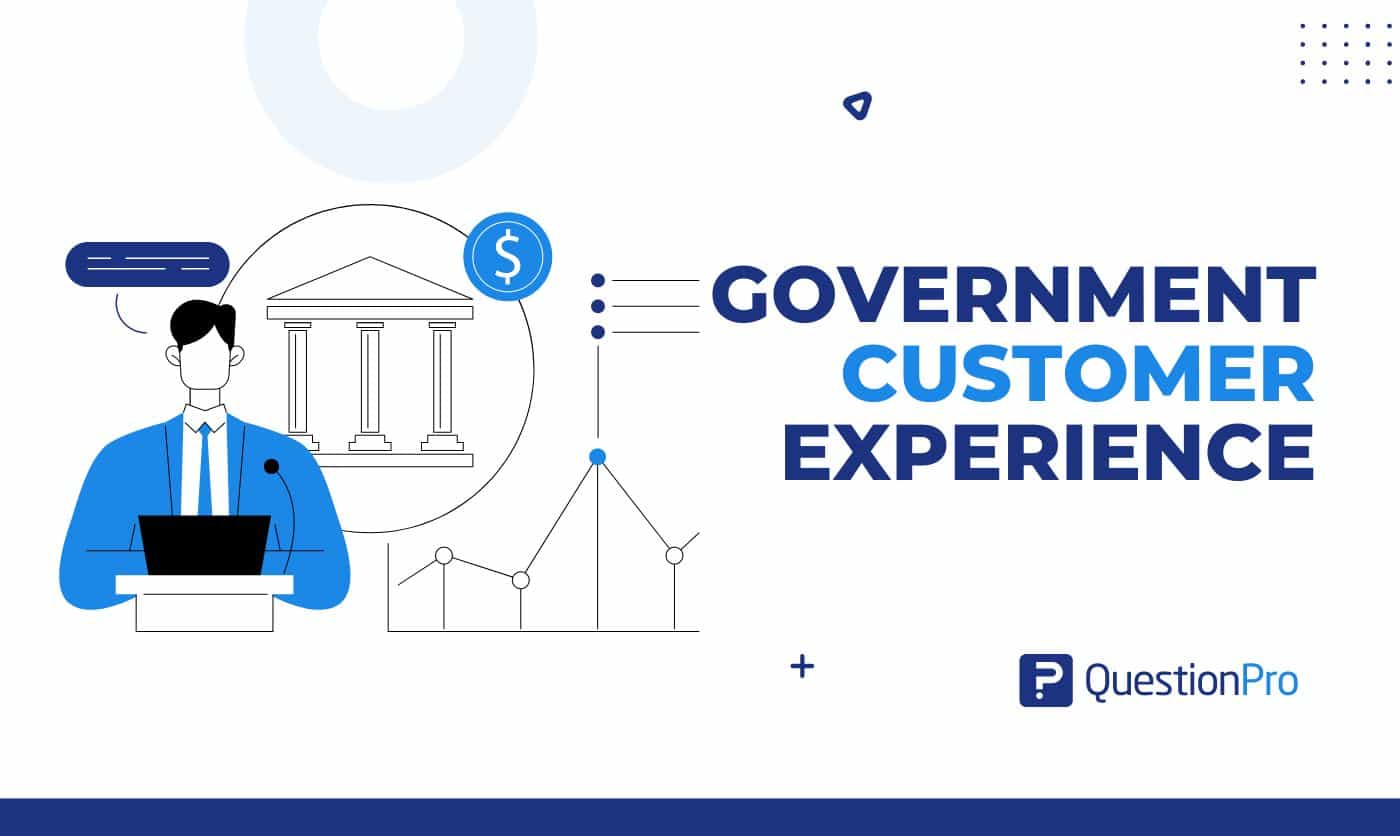
Government Customer Experience: Impact on Government Service
Apr 11, 2024
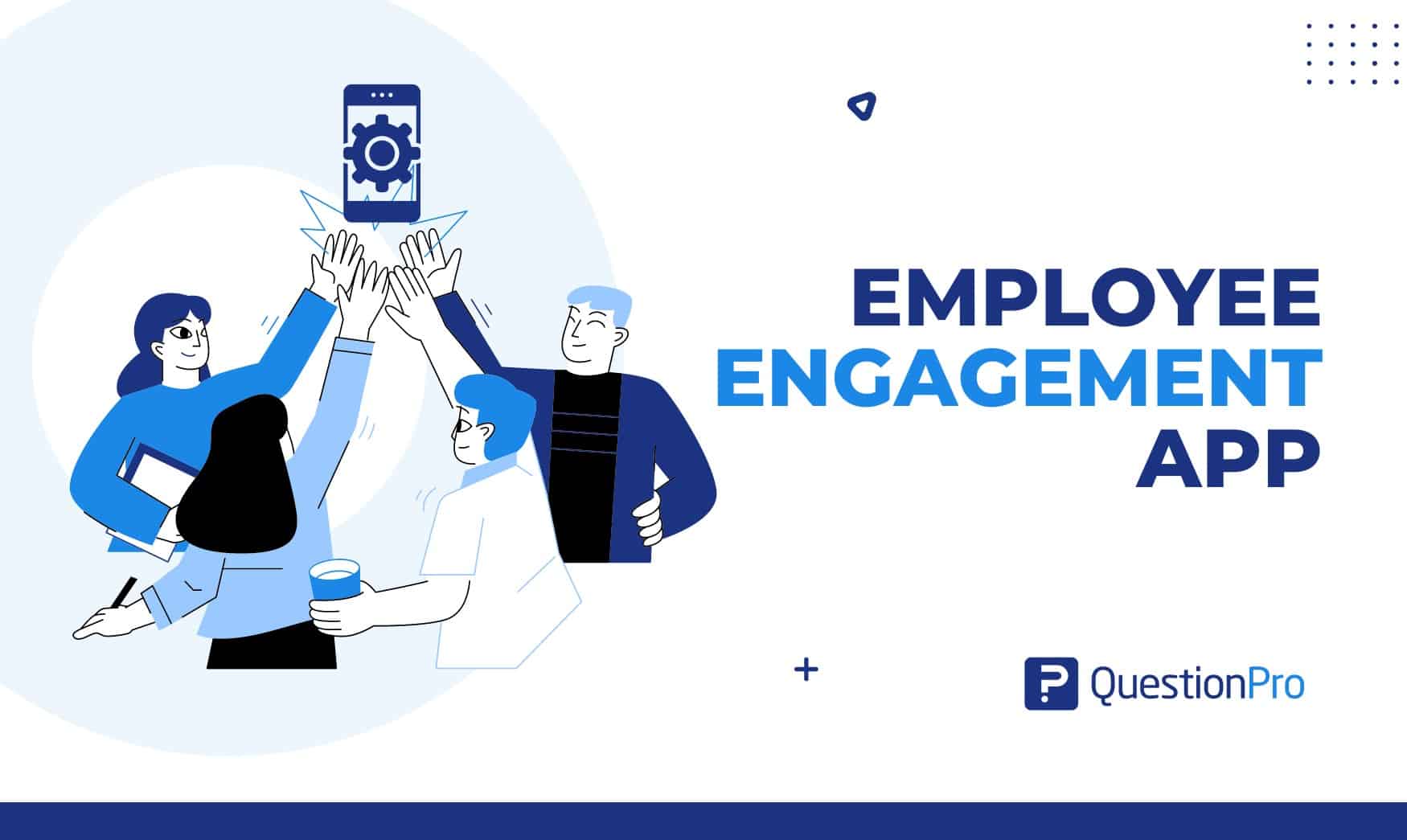
Employee Engagement App: Top 11 For Workforce Improvement
Apr 10, 2024
Other categories
- Academic Research
- Artificial Intelligence
- Assessments
- Brand Awareness
- Case Studies
- Communities
- Consumer Insights
- Customer effort score
- Customer Engagement
- Customer Experience
- Customer Loyalty
- Customer Research
- Customer Satisfaction
- Employee Benefits
- Employee Engagement
- Employee Retention
- Friday Five
- General Data Protection Regulation
- Insights Hub
- Life@QuestionPro
- Market Research
- Mobile diaries
- Mobile Surveys
- New Features
- Online Communities
- Question Types
- Questionnaire
- QuestionPro Products
- Release Notes
- Research Tools and Apps
- Revenue at Risk
- Survey Templates
- Training Tips
- Uncategorized
- Video Learning Series
- What’s Coming Up
- Workforce Intelligence
Your Modern Business Guide To Data Analysis Methods And Techniques

Table of Contents
1) What Is Data Analysis?
2) Why Is Data Analysis Important?
3) What Is The Data Analysis Process?
4) Types Of Data Analysis Methods
5) Top Data Analysis Techniques To Apply
6) Quality Criteria For Data Analysis
7) Data Analysis Limitations & Barriers
8) Data Analysis Skills
9) Data Analysis In The Big Data Environment
In our data-rich age, understanding how to analyze and extract true meaning from our business’s digital insights is one of the primary drivers of success.
Despite the colossal volume of data we create every day, a mere 0.5% is actually analyzed and used for data discovery , improvement, and intelligence. While that may not seem like much, considering the amount of digital information we have at our fingertips, half a percent still accounts for a vast amount of data.
With so much data and so little time, knowing how to collect, curate, organize, and make sense of all of this potentially business-boosting information can be a minefield – but online data analysis is the solution.
In science, data analysis uses a more complex approach with advanced techniques to explore and experiment with data. On the other hand, in a business context, data is used to make data-driven decisions that will enable the company to improve its overall performance. In this post, we will cover the analysis of data from an organizational point of view while still going through the scientific and statistical foundations that are fundamental to understanding the basics of data analysis.
To put all of that into perspective, we will answer a host of important analytical questions, explore analytical methods and techniques, while demonstrating how to perform analysis in the real world with a 17-step blueprint for success.
What Is Data Analysis?
Data analysis is the process of collecting, modeling, and analyzing data using various statistical and logical methods and techniques. Businesses rely on analytics processes and tools to extract insights that support strategic and operational decision-making.
All these various methods are largely based on two core areas: quantitative and qualitative research.
To explain the key differences between qualitative and quantitative research, here’s a video for your viewing pleasure:
Gaining a better understanding of different techniques and methods in quantitative research as well as qualitative insights will give your analyzing efforts a more clearly defined direction, so it’s worth taking the time to allow this particular knowledge to sink in. Additionally, you will be able to create a comprehensive analytical report that will skyrocket your analysis.
Apart from qualitative and quantitative categories, there are also other types of data that you should be aware of before dividing into complex data analysis processes. These categories include:
- Big data: Refers to massive data sets that need to be analyzed using advanced software to reveal patterns and trends. It is considered to be one of the best analytical assets as it provides larger volumes of data at a faster rate.
- Metadata: Putting it simply, metadata is data that provides insights about other data. It summarizes key information about specific data that makes it easier to find and reuse for later purposes.
- Real time data: As its name suggests, real time data is presented as soon as it is acquired. From an organizational perspective, this is the most valuable data as it can help you make important decisions based on the latest developments. Our guide on real time analytics will tell you more about the topic.
- Machine data: This is more complex data that is generated solely by a machine such as phones, computers, or even websites and embedded systems, without previous human interaction.
Why Is Data Analysis Important?
Before we go into detail about the categories of analysis along with its methods and techniques, you must understand the potential that analyzing data can bring to your organization.
- Informed decision-making : From a management perspective, you can benefit from analyzing your data as it helps you make decisions based on facts and not simple intuition. For instance, you can understand where to invest your capital, detect growth opportunities, predict your income, or tackle uncommon situations before they become problems. Through this, you can extract relevant insights from all areas in your organization, and with the help of dashboard software , present the data in a professional and interactive way to different stakeholders.
- Reduce costs : Another great benefit is to reduce costs. With the help of advanced technologies such as predictive analytics, businesses can spot improvement opportunities, trends, and patterns in their data and plan their strategies accordingly. In time, this will help you save money and resources on implementing the wrong strategies. And not just that, by predicting different scenarios such as sales and demand you can also anticipate production and supply.
- Target customers better : Customers are arguably the most crucial element in any business. By using analytics to get a 360° vision of all aspects related to your customers, you can understand which channels they use to communicate with you, their demographics, interests, habits, purchasing behaviors, and more. In the long run, it will drive success to your marketing strategies, allow you to identify new potential customers, and avoid wasting resources on targeting the wrong people or sending the wrong message. You can also track customer satisfaction by analyzing your client’s reviews or your customer service department’s performance.
What Is The Data Analysis Process?

When we talk about analyzing data there is an order to follow in order to extract the needed conclusions. The analysis process consists of 5 key stages. We will cover each of them more in detail later in the post, but to start providing the needed context to understand what is coming next, here is a rundown of the 5 essential steps of data analysis.
- Identify: Before you get your hands dirty with data, you first need to identify why you need it in the first place. The identification is the stage in which you establish the questions you will need to answer. For example, what is the customer's perception of our brand? Or what type of packaging is more engaging to our potential customers? Once the questions are outlined you are ready for the next step.
- Collect: As its name suggests, this is the stage where you start collecting the needed data. Here, you define which sources of data you will use and how you will use them. The collection of data can come in different forms such as internal or external sources, surveys, interviews, questionnaires, and focus groups, among others. An important note here is that the way you collect the data will be different in a quantitative and qualitative scenario.
- Clean: Once you have the necessary data it is time to clean it and leave it ready for analysis. Not all the data you collect will be useful, when collecting big amounts of data in different formats it is very likely that you will find yourself with duplicate or badly formatted data. To avoid this, before you start working with your data you need to make sure to erase any white spaces, duplicate records, or formatting errors. This way you avoid hurting your analysis with bad-quality data.
- Analyze : With the help of various techniques such as statistical analysis, regressions, neural networks, text analysis, and more, you can start analyzing and manipulating your data to extract relevant conclusions. At this stage, you find trends, correlations, variations, and patterns that can help you answer the questions you first thought of in the identify stage. Various technologies in the market assist researchers and average users with the management of their data. Some of them include business intelligence and visualization software, predictive analytics, and data mining, among others.
- Interpret: Last but not least you have one of the most important steps: it is time to interpret your results. This stage is where the researcher comes up with courses of action based on the findings. For example, here you would understand if your clients prefer packaging that is red or green, plastic or paper, etc. Additionally, at this stage, you can also find some limitations and work on them.
Now that you have a basic understanding of the key data analysis steps, let’s look at the top 17 essential methods.
17 Essential Types Of Data Analysis Methods
Before diving into the 17 essential types of methods, it is important that we go over really fast through the main analysis categories. Starting with the category of descriptive up to prescriptive analysis, the complexity and effort of data evaluation increases, but also the added value for the company.
a) Descriptive analysis - What happened.
The descriptive analysis method is the starting point for any analytic reflection, and it aims to answer the question of what happened? It does this by ordering, manipulating, and interpreting raw data from various sources to turn it into valuable insights for your organization.
Performing descriptive analysis is essential, as it enables us to present our insights in a meaningful way. Although it is relevant to mention that this analysis on its own will not allow you to predict future outcomes or tell you the answer to questions like why something happened, it will leave your data organized and ready to conduct further investigations.
b) Exploratory analysis - How to explore data relationships.
As its name suggests, the main aim of the exploratory analysis is to explore. Prior to it, there is still no notion of the relationship between the data and the variables. Once the data is investigated, exploratory analysis helps you to find connections and generate hypotheses and solutions for specific problems. A typical area of application for it is data mining.
c) Diagnostic analysis - Why it happened.
Diagnostic data analytics empowers analysts and executives by helping them gain a firm contextual understanding of why something happened. If you know why something happened as well as how it happened, you will be able to pinpoint the exact ways of tackling the issue or challenge.
Designed to provide direct and actionable answers to specific questions, this is one of the world’s most important methods in research, among its other key organizational functions such as retail analytics , e.g.
c) Predictive analysis - What will happen.
The predictive method allows you to look into the future to answer the question: what will happen? In order to do this, it uses the results of the previously mentioned descriptive, exploratory, and diagnostic analysis, in addition to machine learning (ML) and artificial intelligence (AI). Through this, you can uncover future trends, potential problems or inefficiencies, connections, and casualties in your data.
With predictive analysis, you can unfold and develop initiatives that will not only enhance your various operational processes but also help you gain an all-important edge over the competition. If you understand why a trend, pattern, or event happened through data, you will be able to develop an informed projection of how things may unfold in particular areas of the business.
e) Prescriptive analysis - How will it happen.
Another of the most effective types of analysis methods in research. Prescriptive data techniques cross over from predictive analysis in the way that it revolves around using patterns or trends to develop responsive, practical business strategies.
By drilling down into prescriptive analysis, you will play an active role in the data consumption process by taking well-arranged sets of visual data and using it as a powerful fix to emerging issues in a number of key areas, including marketing, sales, customer experience, HR, fulfillment, finance, logistics analytics , and others.

As mentioned at the beginning of the post, data analysis methods can be divided into two big categories: quantitative and qualitative. Each of these categories holds a powerful analytical value that changes depending on the scenario and type of data you are working with. Below, we will discuss 17 methods that are divided into qualitative and quantitative approaches.
Without further ado, here are the 17 essential types of data analysis methods with some use cases in the business world:
A. Quantitative Methods
To put it simply, quantitative analysis refers to all methods that use numerical data or data that can be turned into numbers (e.g. category variables like gender, age, etc.) to extract valuable insights. It is used to extract valuable conclusions about relationships, differences, and test hypotheses. Below we discuss some of the key quantitative methods.
1. Cluster analysis
The action of grouping a set of data elements in a way that said elements are more similar (in a particular sense) to each other than to those in other groups – hence the term ‘cluster.’ Since there is no target variable when clustering, the method is often used to find hidden patterns in the data. The approach is also used to provide additional context to a trend or dataset.
Let's look at it from an organizational perspective. In a perfect world, marketers would be able to analyze each customer separately and give them the best-personalized service, but let's face it, with a large customer base, it is timely impossible to do that. That's where clustering comes in. By grouping customers into clusters based on demographics, purchasing behaviors, monetary value, or any other factor that might be relevant for your company, you will be able to immediately optimize your efforts and give your customers the best experience based on their needs.
2. Cohort analysis
This type of data analysis approach uses historical data to examine and compare a determined segment of users' behavior, which can then be grouped with others with similar characteristics. By using this methodology, it's possible to gain a wealth of insight into consumer needs or a firm understanding of a broader target group.
Cohort analysis can be really useful for performing analysis in marketing as it will allow you to understand the impact of your campaigns on specific groups of customers. To exemplify, imagine you send an email campaign encouraging customers to sign up for your site. For this, you create two versions of the campaign with different designs, CTAs, and ad content. Later on, you can use cohort analysis to track the performance of the campaign for a longer period of time and understand which type of content is driving your customers to sign up, repurchase, or engage in other ways.
A useful tool to start performing cohort analysis method is Google Analytics. You can learn more about the benefits and limitations of using cohorts in GA in this useful guide . In the bottom image, you see an example of how you visualize a cohort in this tool. The segments (devices traffic) are divided into date cohorts (usage of devices) and then analyzed week by week to extract insights into performance.
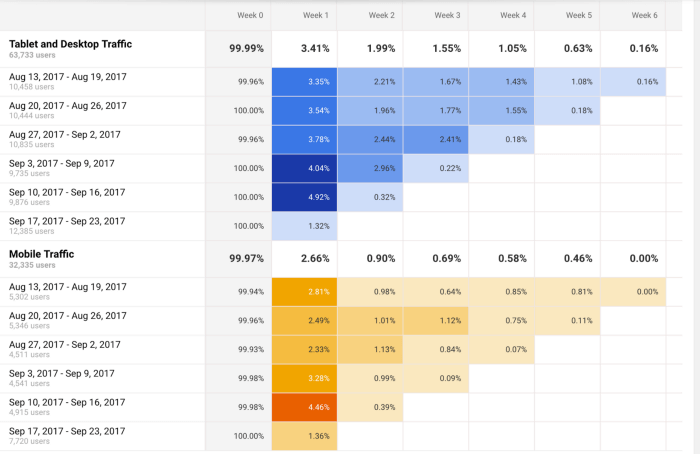
3. Regression analysis
Regression uses historical data to understand how a dependent variable's value is affected when one (linear regression) or more independent variables (multiple regression) change or stay the same. By understanding each variable's relationship and how it developed in the past, you can anticipate possible outcomes and make better decisions in the future.
Let's bring it down with an example. Imagine you did a regression analysis of your sales in 2019 and discovered that variables like product quality, store design, customer service, marketing campaigns, and sales channels affected the overall result. Now you want to use regression to analyze which of these variables changed or if any new ones appeared during 2020. For example, you couldn’t sell as much in your physical store due to COVID lockdowns. Therefore, your sales could’ve either dropped in general or increased in your online channels. Through this, you can understand which independent variables affected the overall performance of your dependent variable, annual sales.
If you want to go deeper into this type of analysis, check out this article and learn more about how you can benefit from regression.
4. Neural networks
The neural network forms the basis for the intelligent algorithms of machine learning. It is a form of analytics that attempts, with minimal intervention, to understand how the human brain would generate insights and predict values. Neural networks learn from each and every data transaction, meaning that they evolve and advance over time.
A typical area of application for neural networks is predictive analytics. There are BI reporting tools that have this feature implemented within them, such as the Predictive Analytics Tool from datapine. This tool enables users to quickly and easily generate all kinds of predictions. All you have to do is select the data to be processed based on your KPIs, and the software automatically calculates forecasts based on historical and current data. Thanks to its user-friendly interface, anyone in your organization can manage it; there’s no need to be an advanced scientist.
Here is an example of how you can use the predictive analysis tool from datapine:

**click to enlarge**
5. Factor analysis
The factor analysis also called “dimension reduction” is a type of data analysis used to describe variability among observed, correlated variables in terms of a potentially lower number of unobserved variables called factors. The aim here is to uncover independent latent variables, an ideal method for streamlining specific segments.
A good way to understand this data analysis method is a customer evaluation of a product. The initial assessment is based on different variables like color, shape, wearability, current trends, materials, comfort, the place where they bought the product, and frequency of usage. Like this, the list can be endless, depending on what you want to track. In this case, factor analysis comes into the picture by summarizing all of these variables into homogenous groups, for example, by grouping the variables color, materials, quality, and trends into a brother latent variable of design.
If you want to start analyzing data using factor analysis we recommend you take a look at this practical guide from UCLA.
6. Data mining
A method of data analysis that is the umbrella term for engineering metrics and insights for additional value, direction, and context. By using exploratory statistical evaluation, data mining aims to identify dependencies, relations, patterns, and trends to generate advanced knowledge. When considering how to analyze data, adopting a data mining mindset is essential to success - as such, it’s an area that is worth exploring in greater detail.
An excellent use case of data mining is datapine intelligent data alerts . With the help of artificial intelligence and machine learning, they provide automated signals based on particular commands or occurrences within a dataset. For example, if you’re monitoring supply chain KPIs , you could set an intelligent alarm to trigger when invalid or low-quality data appears. By doing so, you will be able to drill down deep into the issue and fix it swiftly and effectively.
In the following picture, you can see how the intelligent alarms from datapine work. By setting up ranges on daily orders, sessions, and revenues, the alarms will notify you if the goal was not completed or if it exceeded expectations.
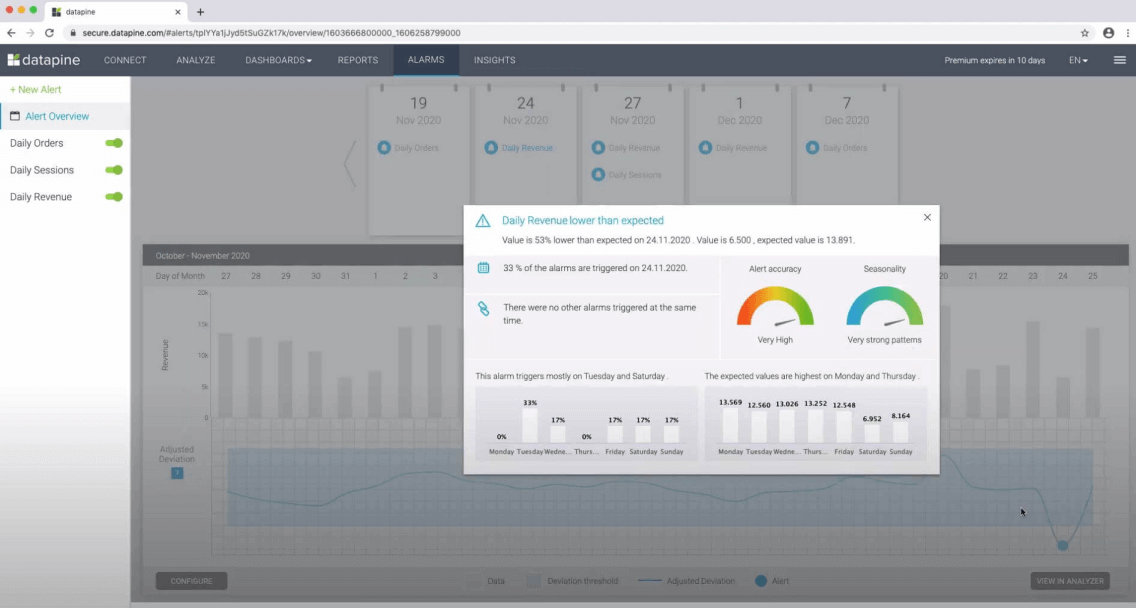
7. Time series analysis
As its name suggests, time series analysis is used to analyze a set of data points collected over a specified period of time. Although analysts use this method to monitor the data points in a specific interval of time rather than just monitoring them intermittently, the time series analysis is not uniquely used for the purpose of collecting data over time. Instead, it allows researchers to understand if variables changed during the duration of the study, how the different variables are dependent, and how did it reach the end result.
In a business context, this method is used to understand the causes of different trends and patterns to extract valuable insights. Another way of using this method is with the help of time series forecasting. Powered by predictive technologies, businesses can analyze various data sets over a period of time and forecast different future events.
A great use case to put time series analysis into perspective is seasonality effects on sales. By using time series forecasting to analyze sales data of a specific product over time, you can understand if sales rise over a specific period of time (e.g. swimwear during summertime, or candy during Halloween). These insights allow you to predict demand and prepare production accordingly.
8. Decision Trees
The decision tree analysis aims to act as a support tool to make smart and strategic decisions. By visually displaying potential outcomes, consequences, and costs in a tree-like model, researchers and company users can easily evaluate all factors involved and choose the best course of action. Decision trees are helpful to analyze quantitative data and they allow for an improved decision-making process by helping you spot improvement opportunities, reduce costs, and enhance operational efficiency and production.
But how does a decision tree actually works? This method works like a flowchart that starts with the main decision that you need to make and branches out based on the different outcomes and consequences of each decision. Each outcome will outline its own consequences, costs, and gains and, at the end of the analysis, you can compare each of them and make the smartest decision.
Businesses can use them to understand which project is more cost-effective and will bring more earnings in the long run. For example, imagine you need to decide if you want to update your software app or build a new app entirely. Here you would compare the total costs, the time needed to be invested, potential revenue, and any other factor that might affect your decision. In the end, you would be able to see which of these two options is more realistic and attainable for your company or research.
9. Conjoint analysis
Last but not least, we have the conjoint analysis. This approach is usually used in surveys to understand how individuals value different attributes of a product or service and it is one of the most effective methods to extract consumer preferences. When it comes to purchasing, some clients might be more price-focused, others more features-focused, and others might have a sustainable focus. Whatever your customer's preferences are, you can find them with conjoint analysis. Through this, companies can define pricing strategies, packaging options, subscription packages, and more.
A great example of conjoint analysis is in marketing and sales. For instance, a cupcake brand might use conjoint analysis and find that its clients prefer gluten-free options and cupcakes with healthier toppings over super sugary ones. Thus, the cupcake brand can turn these insights into advertisements and promotions to increase sales of this particular type of product. And not just that, conjoint analysis can also help businesses segment their customers based on their interests. This allows them to send different messaging that will bring value to each of the segments.
10. Correspondence Analysis
Also known as reciprocal averaging, correspondence analysis is a method used to analyze the relationship between categorical variables presented within a contingency table. A contingency table is a table that displays two (simple correspondence analysis) or more (multiple correspondence analysis) categorical variables across rows and columns that show the distribution of the data, which is usually answers to a survey or questionnaire on a specific topic.
This method starts by calculating an “expected value” which is done by multiplying row and column averages and dividing it by the overall original value of the specific table cell. The “expected value” is then subtracted from the original value resulting in a “residual number” which is what allows you to extract conclusions about relationships and distribution. The results of this analysis are later displayed using a map that represents the relationship between the different values. The closest two values are in the map, the bigger the relationship. Let’s put it into perspective with an example.
Imagine you are carrying out a market research analysis about outdoor clothing brands and how they are perceived by the public. For this analysis, you ask a group of people to match each brand with a certain attribute which can be durability, innovation, quality materials, etc. When calculating the residual numbers, you can see that brand A has a positive residual for innovation but a negative one for durability. This means that brand A is not positioned as a durable brand in the market, something that competitors could take advantage of.
11. Multidimensional Scaling (MDS)
MDS is a method used to observe the similarities or disparities between objects which can be colors, brands, people, geographical coordinates, and more. The objects are plotted using an “MDS map” that positions similar objects together and disparate ones far apart. The (dis) similarities between objects are represented using one or more dimensions that can be observed using a numerical scale. For example, if you want to know how people feel about the COVID-19 vaccine, you can use 1 for “don’t believe in the vaccine at all” and 10 for “firmly believe in the vaccine” and a scale of 2 to 9 for in between responses. When analyzing an MDS map the only thing that matters is the distance between the objects, the orientation of the dimensions is arbitrary and has no meaning at all.
Multidimensional scaling is a valuable technique for market research, especially when it comes to evaluating product or brand positioning. For instance, if a cupcake brand wants to know how they are positioned compared to competitors, it can define 2-3 dimensions such as taste, ingredients, shopping experience, or more, and do a multidimensional scaling analysis to find improvement opportunities as well as areas in which competitors are currently leading.
Another business example is in procurement when deciding on different suppliers. Decision makers can generate an MDS map to see how the different prices, delivery times, technical services, and more of the different suppliers differ and pick the one that suits their needs the best.
A final example proposed by a research paper on "An Improved Study of Multilevel Semantic Network Visualization for Analyzing Sentiment Word of Movie Review Data". Researchers picked a two-dimensional MDS map to display the distances and relationships between different sentiments in movie reviews. They used 36 sentiment words and distributed them based on their emotional distance as we can see in the image below where the words "outraged" and "sweet" are on opposite sides of the map, marking the distance between the two emotions very clearly.

Aside from being a valuable technique to analyze dissimilarities, MDS also serves as a dimension-reduction technique for large dimensional data.
B. Qualitative Methods
Qualitative data analysis methods are defined as the observation of non-numerical data that is gathered and produced using methods of observation such as interviews, focus groups, questionnaires, and more. As opposed to quantitative methods, qualitative data is more subjective and highly valuable in analyzing customer retention and product development.
12. Text analysis
Text analysis, also known in the industry as text mining, works by taking large sets of textual data and arranging them in a way that makes it easier to manage. By working through this cleansing process in stringent detail, you will be able to extract the data that is truly relevant to your organization and use it to develop actionable insights that will propel you forward.
Modern software accelerate the application of text analytics. Thanks to the combination of machine learning and intelligent algorithms, you can perform advanced analytical processes such as sentiment analysis. This technique allows you to understand the intentions and emotions of a text, for example, if it's positive, negative, or neutral, and then give it a score depending on certain factors and categories that are relevant to your brand. Sentiment analysis is often used to monitor brand and product reputation and to understand how successful your customer experience is. To learn more about the topic check out this insightful article .
By analyzing data from various word-based sources, including product reviews, articles, social media communications, and survey responses, you will gain invaluable insights into your audience, as well as their needs, preferences, and pain points. This will allow you to create campaigns, services, and communications that meet your prospects’ needs on a personal level, growing your audience while boosting customer retention. There are various other “sub-methods” that are an extension of text analysis. Each of them serves a more specific purpose and we will look at them in detail next.
13. Content Analysis
This is a straightforward and very popular method that examines the presence and frequency of certain words, concepts, and subjects in different content formats such as text, image, audio, or video. For example, the number of times the name of a celebrity is mentioned on social media or online tabloids. It does this by coding text data that is later categorized and tabulated in a way that can provide valuable insights, making it the perfect mix of quantitative and qualitative analysis.
There are two types of content analysis. The first one is the conceptual analysis which focuses on explicit data, for instance, the number of times a concept or word is mentioned in a piece of content. The second one is relational analysis, which focuses on the relationship between different concepts or words and how they are connected within a specific context.
Content analysis is often used by marketers to measure brand reputation and customer behavior. For example, by analyzing customer reviews. It can also be used to analyze customer interviews and find directions for new product development. It is also important to note, that in order to extract the maximum potential out of this analysis method, it is necessary to have a clearly defined research question.
14. Thematic Analysis
Very similar to content analysis, thematic analysis also helps in identifying and interpreting patterns in qualitative data with the main difference being that the first one can also be applied to quantitative analysis. The thematic method analyzes large pieces of text data such as focus group transcripts or interviews and groups them into themes or categories that come up frequently within the text. It is a great method when trying to figure out peoples view’s and opinions about a certain topic. For example, if you are a brand that cares about sustainability, you can do a survey of your customers to analyze their views and opinions about sustainability and how they apply it to their lives. You can also analyze customer service calls transcripts to find common issues and improve your service.
Thematic analysis is a very subjective technique that relies on the researcher’s judgment. Therefore, to avoid biases, it has 6 steps that include familiarization, coding, generating themes, reviewing themes, defining and naming themes, and writing up. It is also important to note that, because it is a flexible approach, the data can be interpreted in multiple ways and it can be hard to select what data is more important to emphasize.
15. Narrative Analysis
A bit more complex in nature than the two previous ones, narrative analysis is used to explore the meaning behind the stories that people tell and most importantly, how they tell them. By looking into the words that people use to describe a situation you can extract valuable conclusions about their perspective on a specific topic. Common sources for narrative data include autobiographies, family stories, opinion pieces, and testimonials, among others.
From a business perspective, narrative analysis can be useful to analyze customer behaviors and feelings towards a specific product, service, feature, or others. It provides unique and deep insights that can be extremely valuable. However, it has some drawbacks.
The biggest weakness of this method is that the sample sizes are usually very small due to the complexity and time-consuming nature of the collection of narrative data. Plus, the way a subject tells a story will be significantly influenced by his or her specific experiences, making it very hard to replicate in a subsequent study.
16. Discourse Analysis
Discourse analysis is used to understand the meaning behind any type of written, verbal, or symbolic discourse based on its political, social, or cultural context. It mixes the analysis of languages and situations together. This means that the way the content is constructed and the meaning behind it is significantly influenced by the culture and society it takes place in. For example, if you are analyzing political speeches you need to consider different context elements such as the politician's background, the current political context of the country, the audience to which the speech is directed, and so on.
From a business point of view, discourse analysis is a great market research tool. It allows marketers to understand how the norms and ideas of the specific market work and how their customers relate to those ideas. It can be very useful to build a brand mission or develop a unique tone of voice.
17. Grounded Theory Analysis
Traditionally, researchers decide on a method and hypothesis and start to collect the data to prove that hypothesis. The grounded theory is the only method that doesn’t require an initial research question or hypothesis as its value lies in the generation of new theories. With the grounded theory method, you can go into the analysis process with an open mind and explore the data to generate new theories through tests and revisions. In fact, it is not necessary to collect the data and then start to analyze it. Researchers usually start to find valuable insights as they are gathering the data.
All of these elements make grounded theory a very valuable method as theories are fully backed by data instead of initial assumptions. It is a great technique to analyze poorly researched topics or find the causes behind specific company outcomes. For example, product managers and marketers might use the grounded theory to find the causes of high levels of customer churn and look into customer surveys and reviews to develop new theories about the causes.
How To Analyze Data? Top 17 Data Analysis Techniques To Apply

Now that we’ve answered the questions “what is data analysis’”, why is it important, and covered the different data analysis types, it’s time to dig deeper into how to perform your analysis by working through these 17 essential techniques.
1. Collaborate your needs
Before you begin analyzing or drilling down into any techniques, it’s crucial to sit down collaboratively with all key stakeholders within your organization, decide on your primary campaign or strategic goals, and gain a fundamental understanding of the types of insights that will best benefit your progress or provide you with the level of vision you need to evolve your organization.
2. Establish your questions
Once you’ve outlined your core objectives, you should consider which questions will need answering to help you achieve your mission. This is one of the most important techniques as it will shape the very foundations of your success.
To help you ask the right things and ensure your data works for you, you have to ask the right data analysis questions .
3. Data democratization
After giving your data analytics methodology some real direction, and knowing which questions need answering to extract optimum value from the information available to your organization, you should continue with democratization.
Data democratization is an action that aims to connect data from various sources efficiently and quickly so that anyone in your organization can access it at any given moment. You can extract data in text, images, videos, numbers, or any other format. And then perform cross-database analysis to achieve more advanced insights to share with the rest of the company interactively.
Once you have decided on your most valuable sources, you need to take all of this into a structured format to start collecting your insights. For this purpose, datapine offers an easy all-in-one data connectors feature to integrate all your internal and external sources and manage them at your will. Additionally, datapine’s end-to-end solution automatically updates your data, allowing you to save time and focus on performing the right analysis to grow your company.
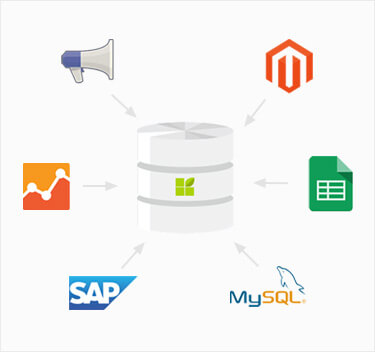
4. Think of governance
When collecting data in a business or research context you always need to think about security and privacy. With data breaches becoming a topic of concern for businesses, the need to protect your client's or subject’s sensitive information becomes critical.
To ensure that all this is taken care of, you need to think of a data governance strategy. According to Gartner , this concept refers to “ the specification of decision rights and an accountability framework to ensure the appropriate behavior in the valuation, creation, consumption, and control of data and analytics .” In simpler words, data governance is a collection of processes, roles, and policies, that ensure the efficient use of data while still achieving the main company goals. It ensures that clear roles are in place for who can access the information and how they can access it. In time, this not only ensures that sensitive information is protected but also allows for an efficient analysis as a whole.
5. Clean your data
After harvesting from so many sources you will be left with a vast amount of information that can be overwhelming to deal with. At the same time, you can be faced with incorrect data that can be misleading to your analysis. The smartest thing you can do to avoid dealing with this in the future is to clean the data. This is fundamental before visualizing it, as it will ensure that the insights you extract from it are correct.
There are many things that you need to look for in the cleaning process. The most important one is to eliminate any duplicate observations; this usually appears when using multiple internal and external sources of information. You can also add any missing codes, fix empty fields, and eliminate incorrectly formatted data.
Another usual form of cleaning is done with text data. As we mentioned earlier, most companies today analyze customer reviews, social media comments, questionnaires, and several other text inputs. In order for algorithms to detect patterns, text data needs to be revised to avoid invalid characters or any syntax or spelling errors.
Most importantly, the aim of cleaning is to prevent you from arriving at false conclusions that can damage your company in the long run. By using clean data, you will also help BI solutions to interact better with your information and create better reports for your organization.
6. Set your KPIs
Once you’ve set your sources, cleaned your data, and established clear-cut questions you want your insights to answer, you need to set a host of key performance indicators (KPIs) that will help you track, measure, and shape your progress in a number of key areas.
KPIs are critical to both qualitative and quantitative analysis research. This is one of the primary methods of data analysis you certainly shouldn’t overlook.
To help you set the best possible KPIs for your initiatives and activities, here is an example of a relevant logistics KPI : transportation-related costs. If you want to see more go explore our collection of key performance indicator examples .

7. Omit useless data
Having bestowed your data analysis tools and techniques with true purpose and defined your mission, you should explore the raw data you’ve collected from all sources and use your KPIs as a reference for chopping out any information you deem to be useless.
Trimming the informational fat is one of the most crucial methods of analysis as it will allow you to focus your analytical efforts and squeeze every drop of value from the remaining ‘lean’ information.
Any stats, facts, figures, or metrics that don’t align with your business goals or fit with your KPI management strategies should be eliminated from the equation.
8. Build a data management roadmap
While, at this point, this particular step is optional (you will have already gained a wealth of insight and formed a fairly sound strategy by now), creating a data governance roadmap will help your data analysis methods and techniques become successful on a more sustainable basis. These roadmaps, if developed properly, are also built so they can be tweaked and scaled over time.
Invest ample time in developing a roadmap that will help you store, manage, and handle your data internally, and you will make your analysis techniques all the more fluid and functional – one of the most powerful types of data analysis methods available today.
9. Integrate technology
There are many ways to analyze data, but one of the most vital aspects of analytical success in a business context is integrating the right decision support software and technology.
Robust analysis platforms will not only allow you to pull critical data from your most valuable sources while working with dynamic KPIs that will offer you actionable insights; it will also present them in a digestible, visual, interactive format from one central, live dashboard . A data methodology you can count on.
By integrating the right technology within your data analysis methodology, you’ll avoid fragmenting your insights, saving you time and effort while allowing you to enjoy the maximum value from your business’s most valuable insights.
For a look at the power of software for the purpose of analysis and to enhance your methods of analyzing, glance over our selection of dashboard examples .
10. Answer your questions
By considering each of the above efforts, working with the right technology, and fostering a cohesive internal culture where everyone buys into the different ways to analyze data as well as the power of digital intelligence, you will swiftly start to answer your most burning business questions. Arguably, the best way to make your data concepts accessible across the organization is through data visualization.
11. Visualize your data
Online data visualization is a powerful tool as it lets you tell a story with your metrics, allowing users across the organization to extract meaningful insights that aid business evolution – and it covers all the different ways to analyze data.
The purpose of analyzing is to make your entire organization more informed and intelligent, and with the right platform or dashboard, this is simpler than you think, as demonstrated by our marketing dashboard .

This visual, dynamic, and interactive online dashboard is a data analysis example designed to give Chief Marketing Officers (CMO) an overview of relevant metrics to help them understand if they achieved their monthly goals.
In detail, this example generated with a modern dashboard creator displays interactive charts for monthly revenues, costs, net income, and net income per customer; all of them are compared with the previous month so that you can understand how the data fluctuated. In addition, it shows a detailed summary of the number of users, customers, SQLs, and MQLs per month to visualize the whole picture and extract relevant insights or trends for your marketing reports .
The CMO dashboard is perfect for c-level management as it can help them monitor the strategic outcome of their marketing efforts and make data-driven decisions that can benefit the company exponentially.
12. Be careful with the interpretation
We already dedicated an entire post to data interpretation as it is a fundamental part of the process of data analysis. It gives meaning to the analytical information and aims to drive a concise conclusion from the analysis results. Since most of the time companies are dealing with data from many different sources, the interpretation stage needs to be done carefully and properly in order to avoid misinterpretations.
To help you through the process, here we list three common practices that you need to avoid at all costs when looking at your data:
- Correlation vs. causation: The human brain is formatted to find patterns. This behavior leads to one of the most common mistakes when performing interpretation: confusing correlation with causation. Although these two aspects can exist simultaneously, it is not correct to assume that because two things happened together, one provoked the other. A piece of advice to avoid falling into this mistake is never to trust just intuition, trust the data. If there is no objective evidence of causation, then always stick to correlation.
- Confirmation bias: This phenomenon describes the tendency to select and interpret only the data necessary to prove one hypothesis, often ignoring the elements that might disprove it. Even if it's not done on purpose, confirmation bias can represent a real problem, as excluding relevant information can lead to false conclusions and, therefore, bad business decisions. To avoid it, always try to disprove your hypothesis instead of proving it, share your analysis with other team members, and avoid drawing any conclusions before the entire analytical project is finalized.
- Statistical significance: To put it in short words, statistical significance helps analysts understand if a result is actually accurate or if it happened because of a sampling error or pure chance. The level of statistical significance needed might depend on the sample size and the industry being analyzed. In any case, ignoring the significance of a result when it might influence decision-making can be a huge mistake.
13. Build a narrative
Now, we’re going to look at how you can bring all of these elements together in a way that will benefit your business - starting with a little something called data storytelling.
The human brain responds incredibly well to strong stories or narratives. Once you’ve cleansed, shaped, and visualized your most invaluable data using various BI dashboard tools , you should strive to tell a story - one with a clear-cut beginning, middle, and end.
By doing so, you will make your analytical efforts more accessible, digestible, and universal, empowering more people within your organization to use your discoveries to their actionable advantage.
14. Consider autonomous technology
Autonomous technologies, such as artificial intelligence (AI) and machine learning (ML), play a significant role in the advancement of understanding how to analyze data more effectively.
Gartner predicts that by the end of this year, 80% of emerging technologies will be developed with AI foundations. This is a testament to the ever-growing power and value of autonomous technologies.
At the moment, these technologies are revolutionizing the analysis industry. Some examples that we mentioned earlier are neural networks, intelligent alarms, and sentiment analysis.
15. Share the load
If you work with the right tools and dashboards, you will be able to present your metrics in a digestible, value-driven format, allowing almost everyone in the organization to connect with and use relevant data to their advantage.
Modern dashboards consolidate data from various sources, providing access to a wealth of insights in one centralized location, no matter if you need to monitor recruitment metrics or generate reports that need to be sent across numerous departments. Moreover, these cutting-edge tools offer access to dashboards from a multitude of devices, meaning that everyone within the business can connect with practical insights remotely - and share the load.
Once everyone is able to work with a data-driven mindset, you will catalyze the success of your business in ways you never thought possible. And when it comes to knowing how to analyze data, this kind of collaborative approach is essential.
16. Data analysis tools
In order to perform high-quality analysis of data, it is fundamental to use tools and software that will ensure the best results. Here we leave you a small summary of four fundamental categories of data analysis tools for your organization.
- Business Intelligence: BI tools allow you to process significant amounts of data from several sources in any format. Through this, you can not only analyze and monitor your data to extract relevant insights but also create interactive reports and dashboards to visualize your KPIs and use them for your company's good. datapine is an amazing online BI software that is focused on delivering powerful online analysis features that are accessible to beginner and advanced users. Like this, it offers a full-service solution that includes cutting-edge analysis of data, KPIs visualization, live dashboards, reporting, and artificial intelligence technologies to predict trends and minimize risk.
- Statistical analysis: These tools are usually designed for scientists, statisticians, market researchers, and mathematicians, as they allow them to perform complex statistical analyses with methods like regression analysis, predictive analysis, and statistical modeling. A good tool to perform this type of analysis is R-Studio as it offers a powerful data modeling and hypothesis testing feature that can cover both academic and general data analysis. This tool is one of the favorite ones in the industry, due to its capability for data cleaning, data reduction, and performing advanced analysis with several statistical methods. Another relevant tool to mention is SPSS from IBM. The software offers advanced statistical analysis for users of all skill levels. Thanks to a vast library of machine learning algorithms, text analysis, and a hypothesis testing approach it can help your company find relevant insights to drive better decisions. SPSS also works as a cloud service that enables you to run it anywhere.
- SQL Consoles: SQL is a programming language often used to handle structured data in relational databases. Tools like these are popular among data scientists as they are extremely effective in unlocking these databases' value. Undoubtedly, one of the most used SQL software in the market is MySQL Workbench . This tool offers several features such as a visual tool for database modeling and monitoring, complete SQL optimization, administration tools, and visual performance dashboards to keep track of KPIs.
- Data Visualization: These tools are used to represent your data through charts, graphs, and maps that allow you to find patterns and trends in the data. datapine's already mentioned BI platform also offers a wealth of powerful online data visualization tools with several benefits. Some of them include: delivering compelling data-driven presentations to share with your entire company, the ability to see your data online with any device wherever you are, an interactive dashboard design feature that enables you to showcase your results in an interactive and understandable way, and to perform online self-service reports that can be used simultaneously with several other people to enhance team productivity.
17. Refine your process constantly
Last is a step that might seem obvious to some people, but it can be easily ignored if you think you are done. Once you have extracted the needed results, you should always take a retrospective look at your project and think about what you can improve. As you saw throughout this long list of techniques, data analysis is a complex process that requires constant refinement. For this reason, you should always go one step further and keep improving.
Quality Criteria For Data Analysis
So far we’ve covered a list of methods and techniques that should help you perform efficient data analysis. But how do you measure the quality and validity of your results? This is done with the help of some science quality criteria. Here we will go into a more theoretical area that is critical to understanding the fundamentals of statistical analysis in science. However, you should also be aware of these steps in a business context, as they will allow you to assess the quality of your results in the correct way. Let’s dig in.
- Internal validity: The results of a survey are internally valid if they measure what they are supposed to measure and thus provide credible results. In other words , internal validity measures the trustworthiness of the results and how they can be affected by factors such as the research design, operational definitions, how the variables are measured, and more. For instance, imagine you are doing an interview to ask people if they brush their teeth two times a day. While most of them will answer yes, you can still notice that their answers correspond to what is socially acceptable, which is to brush your teeth at least twice a day. In this case, you can’t be 100% sure if respondents actually brush their teeth twice a day or if they just say that they do, therefore, the internal validity of this interview is very low.
- External validity: Essentially, external validity refers to the extent to which the results of your research can be applied to a broader context. It basically aims to prove that the findings of a study can be applied in the real world. If the research can be applied to other settings, individuals, and times, then the external validity is high.
- Reliability : If your research is reliable, it means that it can be reproduced. If your measurement were repeated under the same conditions, it would produce similar results. This means that your measuring instrument consistently produces reliable results. For example, imagine a doctor building a symptoms questionnaire to detect a specific disease in a patient. Then, various other doctors use this questionnaire but end up diagnosing the same patient with a different condition. This means the questionnaire is not reliable in detecting the initial disease. Another important note here is that in order for your research to be reliable, it also needs to be objective. If the results of a study are the same, independent of who assesses them or interprets them, the study can be considered reliable. Let’s see the objectivity criteria in more detail now.
- Objectivity: In data science, objectivity means that the researcher needs to stay fully objective when it comes to its analysis. The results of a study need to be affected by objective criteria and not by the beliefs, personality, or values of the researcher. Objectivity needs to be ensured when you are gathering the data, for example, when interviewing individuals, the questions need to be asked in a way that doesn't influence the results. Paired with this, objectivity also needs to be thought of when interpreting the data. If different researchers reach the same conclusions, then the study is objective. For this last point, you can set predefined criteria to interpret the results to ensure all researchers follow the same steps.
The discussed quality criteria cover mostly potential influences in a quantitative context. Analysis in qualitative research has by default additional subjective influences that must be controlled in a different way. Therefore, there are other quality criteria for this kind of research such as credibility, transferability, dependability, and confirmability. You can see each of them more in detail on this resource .
Data Analysis Limitations & Barriers
Analyzing data is not an easy task. As you’ve seen throughout this post, there are many steps and techniques that you need to apply in order to extract useful information from your research. While a well-performed analysis can bring various benefits to your organization it doesn't come without limitations. In this section, we will discuss some of the main barriers you might encounter when conducting an analysis. Let’s see them more in detail.
- Lack of clear goals: No matter how good your data or analysis might be if you don’t have clear goals or a hypothesis the process might be worthless. While we mentioned some methods that don’t require a predefined hypothesis, it is always better to enter the analytical process with some clear guidelines of what you are expecting to get out of it, especially in a business context in which data is utilized to support important strategic decisions.
- Objectivity: Arguably one of the biggest barriers when it comes to data analysis in research is to stay objective. When trying to prove a hypothesis, researchers might find themselves, intentionally or unintentionally, directing the results toward an outcome that they want. To avoid this, always question your assumptions and avoid confusing facts with opinions. You can also show your findings to a research partner or external person to confirm that your results are objective.
- Data representation: A fundamental part of the analytical procedure is the way you represent your data. You can use various graphs and charts to represent your findings, but not all of them will work for all purposes. Choosing the wrong visual can not only damage your analysis but can mislead your audience, therefore, it is important to understand when to use each type of data depending on your analytical goals. Our complete guide on the types of graphs and charts lists 20 different visuals with examples of when to use them.
- Flawed correlation : Misleading statistics can significantly damage your research. We’ve already pointed out a few interpretation issues previously in the post, but it is an important barrier that we can't avoid addressing here as well. Flawed correlations occur when two variables appear related to each other but they are not. Confusing correlations with causation can lead to a wrong interpretation of results which can lead to building wrong strategies and loss of resources, therefore, it is very important to identify the different interpretation mistakes and avoid them.
- Sample size: A very common barrier to a reliable and efficient analysis process is the sample size. In order for the results to be trustworthy, the sample size should be representative of what you are analyzing. For example, imagine you have a company of 1000 employees and you ask the question “do you like working here?” to 50 employees of which 49 say yes, which means 95%. Now, imagine you ask the same question to the 1000 employees and 950 say yes, which also means 95%. Saying that 95% of employees like working in the company when the sample size was only 50 is not a representative or trustworthy conclusion. The significance of the results is way more accurate when surveying a bigger sample size.
- Privacy concerns: In some cases, data collection can be subjected to privacy regulations. Businesses gather all kinds of information from their customers from purchasing behaviors to addresses and phone numbers. If this falls into the wrong hands due to a breach, it can affect the security and confidentiality of your clients. To avoid this issue, you need to collect only the data that is needed for your research and, if you are using sensitive facts, make it anonymous so customers are protected. The misuse of customer data can severely damage a business's reputation, so it is important to keep an eye on privacy.
- Lack of communication between teams : When it comes to performing data analysis on a business level, it is very likely that each department and team will have different goals and strategies. However, they are all working for the same common goal of helping the business run smoothly and keep growing. When teams are not connected and communicating with each other, it can directly affect the way general strategies are built. To avoid these issues, tools such as data dashboards enable teams to stay connected through data in a visually appealing way.
- Innumeracy : Businesses are working with data more and more every day. While there are many BI tools available to perform effective analysis, data literacy is still a constant barrier. Not all employees know how to apply analysis techniques or extract insights from them. To prevent this from happening, you can implement different training opportunities that will prepare every relevant user to deal with data.
Key Data Analysis Skills
As you've learned throughout this lengthy guide, analyzing data is a complex task that requires a lot of knowledge and skills. That said, thanks to the rise of self-service tools the process is way more accessible and agile than it once was. Regardless, there are still some key skills that are valuable to have when working with data, we list the most important ones below.
- Critical and statistical thinking: To successfully analyze data you need to be creative and think out of the box. Yes, that might sound like a weird statement considering that data is often tight to facts. However, a great level of critical thinking is required to uncover connections, come up with a valuable hypothesis, and extract conclusions that go a step further from the surface. This, of course, needs to be complemented by statistical thinking and an understanding of numbers.
- Data cleaning: Anyone who has ever worked with data before will tell you that the cleaning and preparation process accounts for 80% of a data analyst's work, therefore, the skill is fundamental. But not just that, not cleaning the data adequately can also significantly damage the analysis which can lead to poor decision-making in a business scenario. While there are multiple tools that automate the cleaning process and eliminate the possibility of human error, it is still a valuable skill to dominate.
- Data visualization: Visuals make the information easier to understand and analyze, not only for professional users but especially for non-technical ones. Having the necessary skills to not only choose the right chart type but know when to apply it correctly is key. This also means being able to design visually compelling charts that make the data exploration process more efficient.
- SQL: The Structured Query Language or SQL is a programming language used to communicate with databases. It is fundamental knowledge as it enables you to update, manipulate, and organize data from relational databases which are the most common databases used by companies. It is fairly easy to learn and one of the most valuable skills when it comes to data analysis.
- Communication skills: This is a skill that is especially valuable in a business environment. Being able to clearly communicate analytical outcomes to colleagues is incredibly important, especially when the information you are trying to convey is complex for non-technical people. This applies to in-person communication as well as written format, for example, when generating a dashboard or report. While this might be considered a “soft” skill compared to the other ones we mentioned, it should not be ignored as you most likely will need to share analytical findings with others no matter the context.
Data Analysis In The Big Data Environment
Big data is invaluable to today’s businesses, and by using different methods for data analysis, it’s possible to view your data in a way that can help you turn insight into positive action.
To inspire your efforts and put the importance of big data into context, here are some insights that you should know:
- By 2026 the industry of big data is expected to be worth approximately $273.4 billion.
- 94% of enterprises say that analyzing data is important for their growth and digital transformation.
- Companies that exploit the full potential of their data can increase their operating margins by 60% .
- We already told you the benefits of Artificial Intelligence through this article. This industry's financial impact is expected to grow up to $40 billion by 2025.
Data analysis concepts may come in many forms, but fundamentally, any solid methodology will help to make your business more streamlined, cohesive, insightful, and successful than ever before.
Key Takeaways From Data Analysis
As we reach the end of our data analysis journey, we leave a small summary of the main methods and techniques to perform excellent analysis and grow your business.
17 Essential Types of Data Analysis Methods:
- Cluster analysis
- Cohort analysis
- Regression analysis
- Factor analysis
- Neural Networks
- Data Mining
- Text analysis
- Time series analysis
- Decision trees
- Conjoint analysis
- Correspondence Analysis
- Multidimensional Scaling
- Content analysis
- Thematic analysis
- Narrative analysis
- Grounded theory analysis
- Discourse analysis
Top 17 Data Analysis Techniques:
- Collaborate your needs
- Establish your questions
- Data democratization
- Think of data governance
- Clean your data
- Set your KPIs
- Omit useless data
- Build a data management roadmap
- Integrate technology
- Answer your questions
- Visualize your data
- Interpretation of data
- Consider autonomous technology
- Build a narrative
- Share the load
- Data Analysis tools
- Refine your process constantly
We’ve pondered the data analysis definition and drilled down into the practical applications of data-centric analytics, and one thing is clear: by taking measures to arrange your data and making your metrics work for you, it’s possible to transform raw information into action - the kind of that will push your business to the next level.
Yes, good data analytics techniques result in enhanced business intelligence (BI). To help you understand this notion in more detail, read our exploration of business intelligence reporting .
And, if you’re ready to perform your own analysis, drill down into your facts and figures while interacting with your data on astonishing visuals, you can try our software for a free, 14-day trial .
Have a language expert improve your writing
Run a free plagiarism check in 10 minutes, automatically generate references for free.
- Knowledge Base
- Methodology
Research Design | Step-by-Step Guide with Examples
Published on 5 May 2022 by Shona McCombes . Revised on 20 March 2023.
A research design is a strategy for answering your research question using empirical data. Creating a research design means making decisions about:
- Your overall aims and approach
- The type of research design you’ll use
- Your sampling methods or criteria for selecting subjects
- Your data collection methods
- The procedures you’ll follow to collect data
- Your data analysis methods
A well-planned research design helps ensure that your methods match your research aims and that you use the right kind of analysis for your data.
Table of contents
Step 1: consider your aims and approach, step 2: choose a type of research design, step 3: identify your population and sampling method, step 4: choose your data collection methods, step 5: plan your data collection procedures, step 6: decide on your data analysis strategies, frequently asked questions.
- Introduction
Before you can start designing your research, you should already have a clear idea of the research question you want to investigate.
There are many different ways you could go about answering this question. Your research design choices should be driven by your aims and priorities – start by thinking carefully about what you want to achieve.
The first choice you need to make is whether you’ll take a qualitative or quantitative approach.
Qualitative research designs tend to be more flexible and inductive , allowing you to adjust your approach based on what you find throughout the research process.
Quantitative research designs tend to be more fixed and deductive , with variables and hypotheses clearly defined in advance of data collection.
It’s also possible to use a mixed methods design that integrates aspects of both approaches. By combining qualitative and quantitative insights, you can gain a more complete picture of the problem you’re studying and strengthen the credibility of your conclusions.
Practical and ethical considerations when designing research
As well as scientific considerations, you need to think practically when designing your research. If your research involves people or animals, you also need to consider research ethics .
- How much time do you have to collect data and write up the research?
- Will you be able to gain access to the data you need (e.g., by travelling to a specific location or contacting specific people)?
- Do you have the necessary research skills (e.g., statistical analysis or interview techniques)?
- Will you need ethical approval ?
At each stage of the research design process, make sure that your choices are practically feasible.
Prevent plagiarism, run a free check.
Within both qualitative and quantitative approaches, there are several types of research design to choose from. Each type provides a framework for the overall shape of your research.
Types of quantitative research designs
Quantitative designs can be split into four main types. Experimental and quasi-experimental designs allow you to test cause-and-effect relationships, while descriptive and correlational designs allow you to measure variables and describe relationships between them.
With descriptive and correlational designs, you can get a clear picture of characteristics, trends, and relationships as they exist in the real world. However, you can’t draw conclusions about cause and effect (because correlation doesn’t imply causation ).
Experiments are the strongest way to test cause-and-effect relationships without the risk of other variables influencing the results. However, their controlled conditions may not always reflect how things work in the real world. They’re often also more difficult and expensive to implement.
Types of qualitative research designs
Qualitative designs are less strictly defined. This approach is about gaining a rich, detailed understanding of a specific context or phenomenon, and you can often be more creative and flexible in designing your research.
The table below shows some common types of qualitative design. They often have similar approaches in terms of data collection, but focus on different aspects when analysing the data.
Your research design should clearly define who or what your research will focus on, and how you’ll go about choosing your participants or subjects.
In research, a population is the entire group that you want to draw conclusions about, while a sample is the smaller group of individuals you’ll actually collect data from.
Defining the population
A population can be made up of anything you want to study – plants, animals, organisations, texts, countries, etc. In the social sciences, it most often refers to a group of people.
For example, will you focus on people from a specific demographic, region, or background? Are you interested in people with a certain job or medical condition, or users of a particular product?
The more precisely you define your population, the easier it will be to gather a representative sample.
Sampling methods
Even with a narrowly defined population, it’s rarely possible to collect data from every individual. Instead, you’ll collect data from a sample.
To select a sample, there are two main approaches: probability sampling and non-probability sampling . The sampling method you use affects how confidently you can generalise your results to the population as a whole.
Probability sampling is the most statistically valid option, but it’s often difficult to achieve unless you’re dealing with a very small and accessible population.
For practical reasons, many studies use non-probability sampling, but it’s important to be aware of the limitations and carefully consider potential biases. You should always make an effort to gather a sample that’s as representative as possible of the population.
Case selection in qualitative research
In some types of qualitative designs, sampling may not be relevant.
For example, in an ethnography or a case study, your aim is to deeply understand a specific context, not to generalise to a population. Instead of sampling, you may simply aim to collect as much data as possible about the context you are studying.
In these types of design, you still have to carefully consider your choice of case or community. You should have a clear rationale for why this particular case is suitable for answering your research question.
For example, you might choose a case study that reveals an unusual or neglected aspect of your research problem, or you might choose several very similar or very different cases in order to compare them.
Data collection methods are ways of directly measuring variables and gathering information. They allow you to gain first-hand knowledge and original insights into your research problem.
You can choose just one data collection method, or use several methods in the same study.
Survey methods
Surveys allow you to collect data about opinions, behaviours, experiences, and characteristics by asking people directly. There are two main survey methods to choose from: questionnaires and interviews.
Observation methods
Observations allow you to collect data unobtrusively, observing characteristics, behaviours, or social interactions without relying on self-reporting.
Observations may be conducted in real time, taking notes as you observe, or you might make audiovisual recordings for later analysis. They can be qualitative or quantitative.
Other methods of data collection
There are many other ways you might collect data depending on your field and topic.
If you’re not sure which methods will work best for your research design, try reading some papers in your field to see what data collection methods they used.
Secondary data
If you don’t have the time or resources to collect data from the population you’re interested in, you can also choose to use secondary data that other researchers already collected – for example, datasets from government surveys or previous studies on your topic.
With this raw data, you can do your own analysis to answer new research questions that weren’t addressed by the original study.
Using secondary data can expand the scope of your research, as you may be able to access much larger and more varied samples than you could collect yourself.
However, it also means you don’t have any control over which variables to measure or how to measure them, so the conclusions you can draw may be limited.
As well as deciding on your methods, you need to plan exactly how you’ll use these methods to collect data that’s consistent, accurate, and unbiased.
Planning systematic procedures is especially important in quantitative research, where you need to precisely define your variables and ensure your measurements are reliable and valid.
Operationalisation
Some variables, like height or age, are easily measured. But often you’ll be dealing with more abstract concepts, like satisfaction, anxiety, or competence. Operationalisation means turning these fuzzy ideas into measurable indicators.
If you’re using observations , which events or actions will you count?
If you’re using surveys , which questions will you ask and what range of responses will be offered?
You may also choose to use or adapt existing materials designed to measure the concept you’re interested in – for example, questionnaires or inventories whose reliability and validity has already been established.
Reliability and validity
Reliability means your results can be consistently reproduced , while validity means that you’re actually measuring the concept you’re interested in.
For valid and reliable results, your measurement materials should be thoroughly researched and carefully designed. Plan your procedures to make sure you carry out the same steps in the same way for each participant.
If you’re developing a new questionnaire or other instrument to measure a specific concept, running a pilot study allows you to check its validity and reliability in advance.
Sampling procedures
As well as choosing an appropriate sampling method, you need a concrete plan for how you’ll actually contact and recruit your selected sample.
That means making decisions about things like:
- How many participants do you need for an adequate sample size?
- What inclusion and exclusion criteria will you use to identify eligible participants?
- How will you contact your sample – by mail, online, by phone, or in person?
If you’re using a probability sampling method, it’s important that everyone who is randomly selected actually participates in the study. How will you ensure a high response rate?
If you’re using a non-probability method, how will you avoid bias and ensure a representative sample?
Data management
It’s also important to create a data management plan for organising and storing your data.
Will you need to transcribe interviews or perform data entry for observations? You should anonymise and safeguard any sensitive data, and make sure it’s backed up regularly.
Keeping your data well organised will save time when it comes to analysing them. It can also help other researchers validate and add to your findings.
On their own, raw data can’t answer your research question. The last step of designing your research is planning how you’ll analyse the data.
Quantitative data analysis
In quantitative research, you’ll most likely use some form of statistical analysis . With statistics, you can summarise your sample data, make estimates, and test hypotheses.
Using descriptive statistics , you can summarise your sample data in terms of:
- The distribution of the data (e.g., the frequency of each score on a test)
- The central tendency of the data (e.g., the mean to describe the average score)
- The variability of the data (e.g., the standard deviation to describe how spread out the scores are)
The specific calculations you can do depend on the level of measurement of your variables.
Using inferential statistics , you can:
- Make estimates about the population based on your sample data.
- Test hypotheses about a relationship between variables.
Regression and correlation tests look for associations between two or more variables, while comparison tests (such as t tests and ANOVAs ) look for differences in the outcomes of different groups.
Your choice of statistical test depends on various aspects of your research design, including the types of variables you’re dealing with and the distribution of your data.
Qualitative data analysis
In qualitative research, your data will usually be very dense with information and ideas. Instead of summing it up in numbers, you’ll need to comb through the data in detail, interpret its meanings, identify patterns, and extract the parts that are most relevant to your research question.
Two of the most common approaches to doing this are thematic analysis and discourse analysis .
There are many other ways of analysing qualitative data depending on the aims of your research. To get a sense of potential approaches, try reading some qualitative research papers in your field.
A sample is a subset of individuals from a larger population. Sampling means selecting the group that you will actually collect data from in your research.
For example, if you are researching the opinions of students in your university, you could survey a sample of 100 students.
Statistical sampling allows you to test a hypothesis about the characteristics of a population. There are various sampling methods you can use to ensure that your sample is representative of the population as a whole.
Operationalisation means turning abstract conceptual ideas into measurable observations.
For example, the concept of social anxiety isn’t directly observable, but it can be operationally defined in terms of self-rating scores, behavioural avoidance of crowded places, or physical anxiety symptoms in social situations.
Before collecting data , it’s important to consider how you will operationalise the variables that you want to measure.
The research methods you use depend on the type of data you need to answer your research question .
- If you want to measure something or test a hypothesis , use quantitative methods . If you want to explore ideas, thoughts, and meanings, use qualitative methods .
- If you want to analyse a large amount of readily available data, use secondary data. If you want data specific to your purposes with control over how they are generated, collect primary data.
- If you want to establish cause-and-effect relationships between variables , use experimental methods. If you want to understand the characteristics of a research subject, use descriptive methods.
Cite this Scribbr article
If you want to cite this source, you can copy and paste the citation or click the ‘Cite this Scribbr article’ button to automatically add the citation to our free Reference Generator.
McCombes, S. (2023, March 20). Research Design | Step-by-Step Guide with Examples. Scribbr. Retrieved 9 April 2024, from https://www.scribbr.co.uk/research-methods/research-design/
Is this article helpful?
Shona McCombes

Quantitative Data Analysis 101
The lingo, methods and techniques, explained simply.
By: Derek Jansen (MBA) and Kerryn Warren (PhD) | December 2020
Quantitative data analysis is one of those things that often strikes fear in students. It’s totally understandable – quantitative analysis is a complex topic, full of daunting lingo , like medians, modes, correlation and regression. Suddenly we’re all wishing we’d paid a little more attention in math class…
The good news is that while quantitative data analysis is a mammoth topic, gaining a working understanding of the basics isn’t that hard , even for those of us who avoid numbers and math . In this post, we’ll break quantitative analysis down into simple , bite-sized chunks so you can approach your research with confidence.
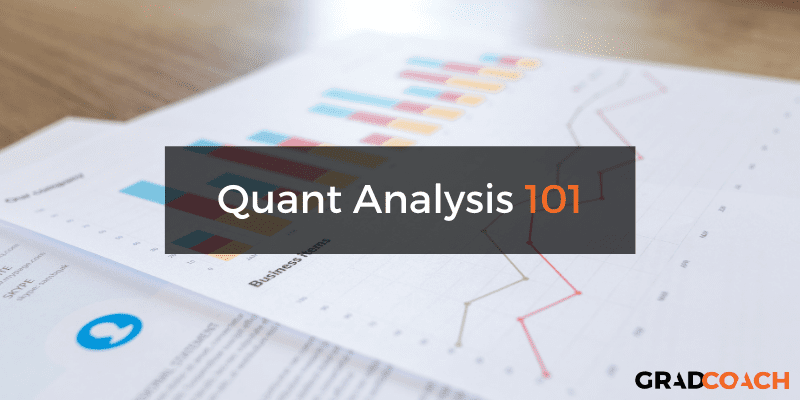
Overview: Quantitative Data Analysis 101
- What (exactly) is quantitative data analysis?
- When to use quantitative analysis
- How quantitative analysis works
The two “branches” of quantitative analysis
- Descriptive statistics 101
- Inferential statistics 101
- How to choose the right quantitative methods
- Recap & summary
What is quantitative data analysis?
Despite being a mouthful, quantitative data analysis simply means analysing data that is numbers-based – or data that can be easily “converted” into numbers without losing any meaning.
For example, category-based variables like gender, ethnicity, or native language could all be “converted” into numbers without losing meaning – for example, English could equal 1, French 2, etc.
This contrasts against qualitative data analysis, where the focus is on words, phrases and expressions that can’t be reduced to numbers. If you’re interested in learning about qualitative analysis, check out our post and video here .
What is quantitative analysis used for?
Quantitative analysis is generally used for three purposes.
- Firstly, it’s used to measure differences between groups . For example, the popularity of different clothing colours or brands.
- Secondly, it’s used to assess relationships between variables . For example, the relationship between weather temperature and voter turnout.
- And third, it’s used to test hypotheses in a scientifically rigorous way. For example, a hypothesis about the impact of a certain vaccine.
Again, this contrasts with qualitative analysis , which can be used to analyse people’s perceptions and feelings about an event or situation. In other words, things that can’t be reduced to numbers.
How does quantitative analysis work?
Well, since quantitative data analysis is all about analysing numbers , it’s no surprise that it involves statistics . Statistical analysis methods form the engine that powers quantitative analysis, and these methods can vary from pretty basic calculations (for example, averages and medians) to more sophisticated analyses (for example, correlations and regressions).
Sounds like gibberish? Don’t worry. We’ll explain all of that in this post. Importantly, you don’t need to be a statistician or math wiz to pull off a good quantitative analysis. We’ll break down all the technical mumbo jumbo in this post.
Need a helping hand?
As I mentioned, quantitative analysis is powered by statistical analysis methods . There are two main “branches” of statistical methods that are used – descriptive statistics and inferential statistics . In your research, you might only use descriptive statistics, or you might use a mix of both , depending on what you’re trying to figure out. In other words, depending on your research questions, aims and objectives . I’ll explain how to choose your methods later.
So, what are descriptive and inferential statistics?
Well, before I can explain that, we need to take a quick detour to explain some lingo. To understand the difference between these two branches of statistics, you need to understand two important words. These words are population and sample .
First up, population . In statistics, the population is the entire group of people (or animals or organisations or whatever) that you’re interested in researching. For example, if you were interested in researching Tesla owners in the US, then the population would be all Tesla owners in the US.
However, it’s extremely unlikely that you’re going to be able to interview or survey every single Tesla owner in the US. Realistically, you’ll likely only get access to a few hundred, or maybe a few thousand owners using an online survey. This smaller group of accessible people whose data you actually collect is called your sample .
So, to recap – the population is the entire group of people you’re interested in, and the sample is the subset of the population that you can actually get access to. In other words, the population is the full chocolate cake , whereas the sample is a slice of that cake.
So, why is this sample-population thing important?
Well, descriptive statistics focus on describing the sample , while inferential statistics aim to make predictions about the population, based on the findings within the sample. In other words, we use one group of statistical methods – descriptive statistics – to investigate the slice of cake, and another group of methods – inferential statistics – to draw conclusions about the entire cake. There I go with the cake analogy again…
With that out the way, let’s take a closer look at each of these branches in more detail.
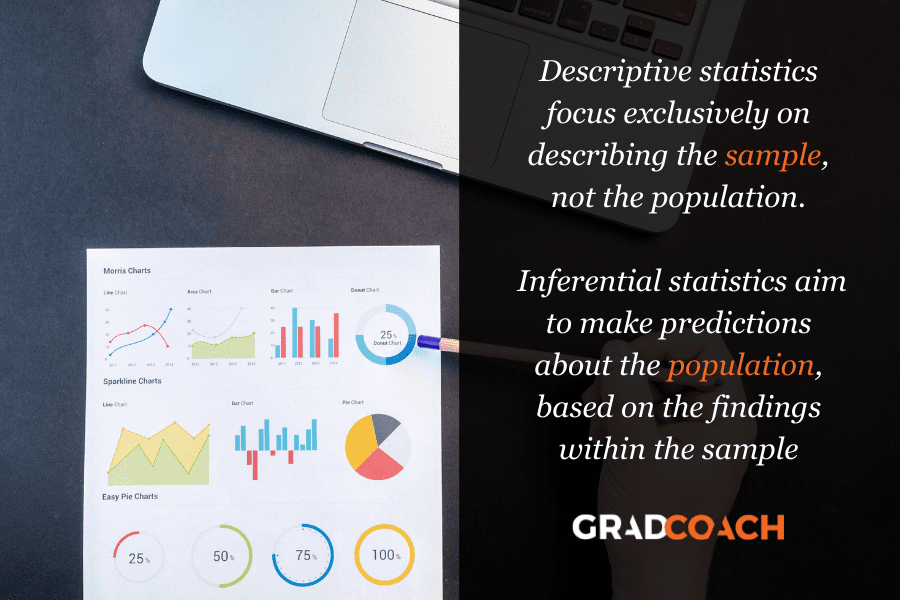
Branch 1: Descriptive Statistics
Descriptive statistics serve a simple but critically important role in your research – to describe your data set – hence the name. In other words, they help you understand the details of your sample . Unlike inferential statistics (which we’ll get to soon), descriptive statistics don’t aim to make inferences or predictions about the entire population – they’re purely interested in the details of your specific sample .
When you’re writing up your analysis, descriptive statistics are the first set of stats you’ll cover, before moving on to inferential statistics. But, that said, depending on your research objectives and research questions , they may be the only type of statistics you use. We’ll explore that a little later.
So, what kind of statistics are usually covered in this section?
Some common statistical tests used in this branch include the following:
- Mean – this is simply the mathematical average of a range of numbers.
- Median – this is the midpoint in a range of numbers when the numbers are arranged in numerical order. If the data set makes up an odd number, then the median is the number right in the middle of the set. If the data set makes up an even number, then the median is the midpoint between the two middle numbers.
- Mode – this is simply the most commonly occurring number in the data set.
- In cases where most of the numbers are quite close to the average, the standard deviation will be relatively low.
- Conversely, in cases where the numbers are scattered all over the place, the standard deviation will be relatively high.
- Skewness . As the name suggests, skewness indicates how symmetrical a range of numbers is. In other words, do they tend to cluster into a smooth bell curve shape in the middle of the graph, or do they skew to the left or right?
Feeling a bit confused? Let’s look at a practical example using a small data set.
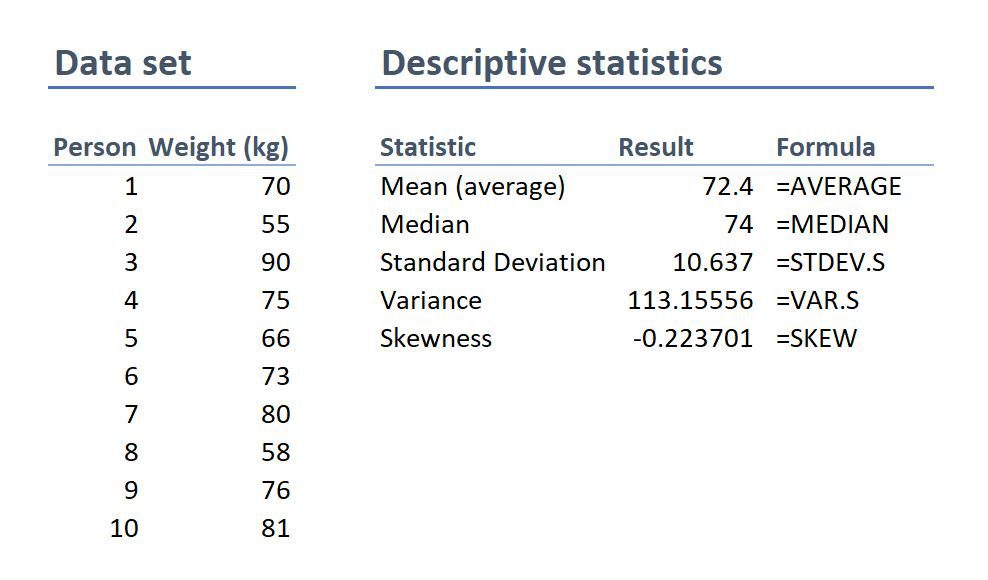
On the left-hand side is the data set. This details the bodyweight of a sample of 10 people. On the right-hand side, we have the descriptive statistics. Let’s take a look at each of them.
First, we can see that the mean weight is 72.4 kilograms. In other words, the average weight across the sample is 72.4 kilograms. Straightforward.
Next, we can see that the median is very similar to the mean (the average). This suggests that this data set has a reasonably symmetrical distribution (in other words, a relatively smooth, centred distribution of weights, clustered towards the centre).
In terms of the mode , there is no mode in this data set. This is because each number is present only once and so there cannot be a “most common number”. If there were two people who were both 65 kilograms, for example, then the mode would be 65.
Next up is the standard deviation . 10.6 indicates that there’s quite a wide spread of numbers. We can see this quite easily by looking at the numbers themselves, which range from 55 to 90, which is quite a stretch from the mean of 72.4.
And lastly, the skewness of -0.2 tells us that the data is very slightly negatively skewed. This makes sense since the mean and the median are slightly different.
As you can see, these descriptive statistics give us some useful insight into the data set. Of course, this is a very small data set (only 10 records), so we can’t read into these statistics too much. Also, keep in mind that this is not a list of all possible descriptive statistics – just the most common ones.
But why do all of these numbers matter?
While these descriptive statistics are all fairly basic, they’re important for a few reasons:
- Firstly, they help you get both a macro and micro-level view of your data. In other words, they help you understand both the big picture and the finer details.
- Secondly, they help you spot potential errors in the data – for example, if an average is way higher than you’d expect, or responses to a question are highly varied, this can act as a warning sign that you need to double-check the data.
- And lastly, these descriptive statistics help inform which inferential statistical techniques you can use, as those techniques depend on the skewness (in other words, the symmetry and normality) of the data.
Simply put, descriptive statistics are really important , even though the statistical techniques used are fairly basic. All too often at Grad Coach, we see students skimming over the descriptives in their eagerness to get to the more exciting inferential methods, and then landing up with some very flawed results.
Don’t be a sucker – give your descriptive statistics the love and attention they deserve!
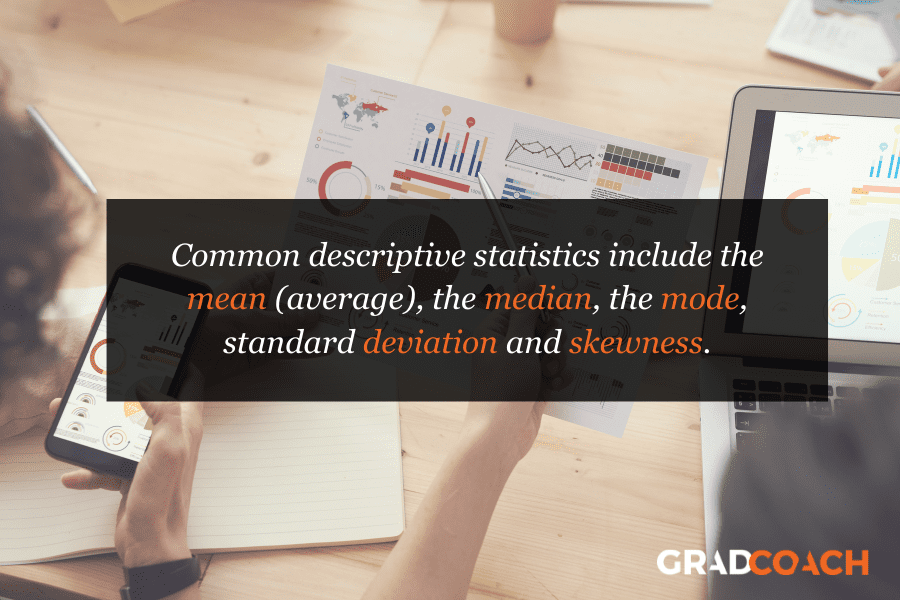
Branch 2: Inferential Statistics
As I mentioned, while descriptive statistics are all about the details of your specific data set – your sample – inferential statistics aim to make inferences about the population . In other words, you’ll use inferential statistics to make predictions about what you’d expect to find in the full population.
What kind of predictions, you ask? Well, there are two common types of predictions that researchers try to make using inferential stats:
- Firstly, predictions about differences between groups – for example, height differences between children grouped by their favourite meal or gender.
- And secondly, relationships between variables – for example, the relationship between body weight and the number of hours a week a person does yoga.
In other words, inferential statistics (when done correctly), allow you to connect the dots and make predictions about what you expect to see in the real world population, based on what you observe in your sample data. For this reason, inferential statistics are used for hypothesis testing – in other words, to test hypotheses that predict changes or differences.
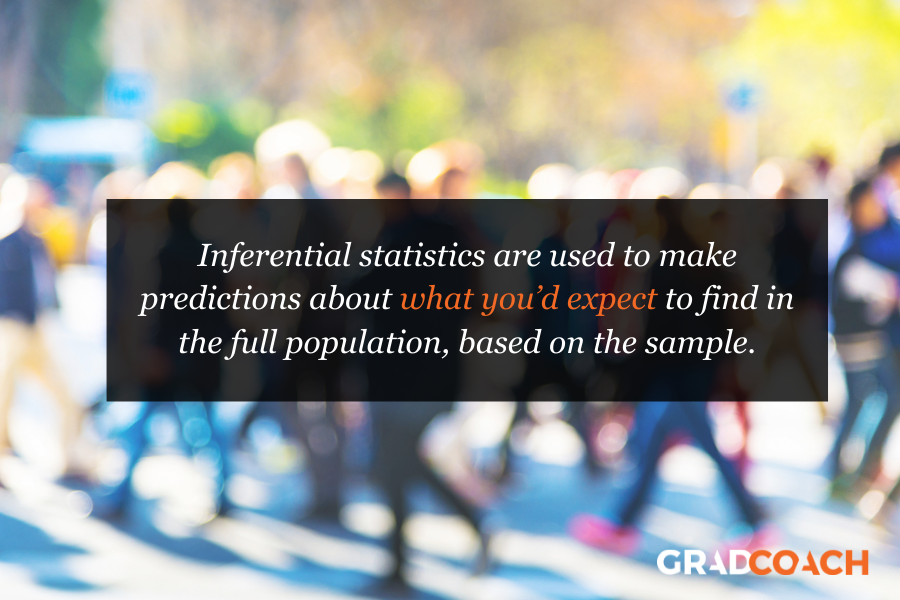
Of course, when you’re working with inferential statistics, the composition of your sample is really important. In other words, if your sample doesn’t accurately represent the population you’re researching, then your findings won’t necessarily be very useful.
For example, if your population of interest is a mix of 50% male and 50% female , but your sample is 80% male , you can’t make inferences about the population based on your sample, since it’s not representative. This area of statistics is called sampling, but we won’t go down that rabbit hole here (it’s a deep one!) – we’ll save that for another post .
What statistics are usually used in this branch?
There are many, many different statistical analysis methods within the inferential branch and it’d be impossible for us to discuss them all here. So we’ll just take a look at some of the most common inferential statistical methods so that you have a solid starting point.
First up are T-Tests . T-tests compare the means (the averages) of two groups of data to assess whether they’re statistically significantly different. In other words, do they have significantly different means, standard deviations and skewness.
This type of testing is very useful for understanding just how similar or different two groups of data are. For example, you might want to compare the mean blood pressure between two groups of people – one that has taken a new medication and one that hasn’t – to assess whether they are significantly different.
Kicking things up a level, we have ANOVA, which stands for “analysis of variance”. This test is similar to a T-test in that it compares the means of various groups, but ANOVA allows you to analyse multiple groups , not just two groups So it’s basically a t-test on steroids…
Next, we have correlation analysis . This type of analysis assesses the relationship between two variables. In other words, if one variable increases, does the other variable also increase, decrease or stay the same. For example, if the average temperature goes up, do average ice creams sales increase too? We’d expect some sort of relationship between these two variables intuitively , but correlation analysis allows us to measure that relationship scientifically .
Lastly, we have regression analysis – this is quite similar to correlation in that it assesses the relationship between variables, but it goes a step further to understand cause and effect between variables, not just whether they move together. In other words, does the one variable actually cause the other one to move, or do they just happen to move together naturally thanks to another force? Just because two variables correlate doesn’t necessarily mean that one causes the other.
Stats overload…
I hear you. To make this all a little more tangible, let’s take a look at an example of a correlation in action.
Here’s a scatter plot demonstrating the correlation (relationship) between weight and height. Intuitively, we’d expect there to be some relationship between these two variables, which is what we see in this scatter plot. In other words, the results tend to cluster together in a diagonal line from bottom left to top right.
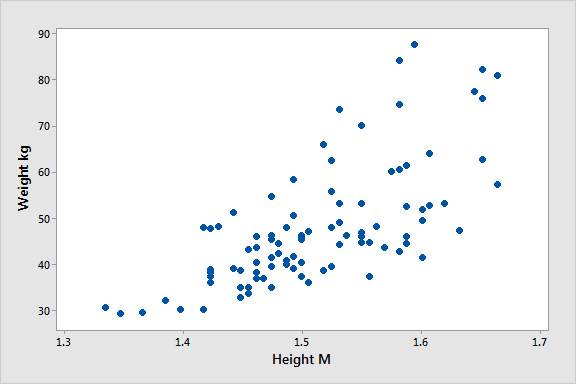
As I mentioned, these are are just a handful of inferential techniques – there are many, many more. Importantly, each statistical method has its own assumptions and limitations.
For example, some methods only work with normally distributed (parametric) data, while other methods are designed specifically for non-parametric data. And that’s exactly why descriptive statistics are so important – they’re the first step to knowing which inferential techniques you can and can’t use.
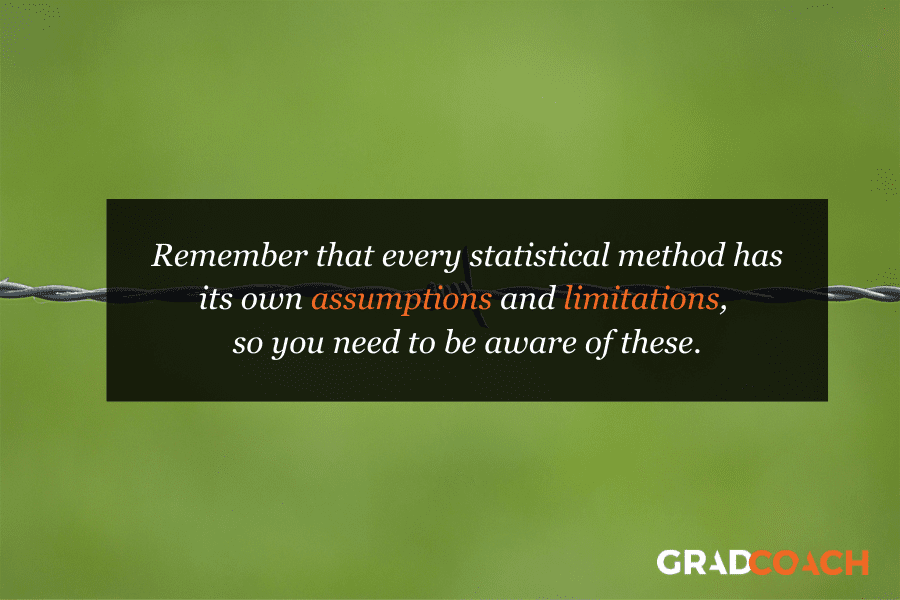
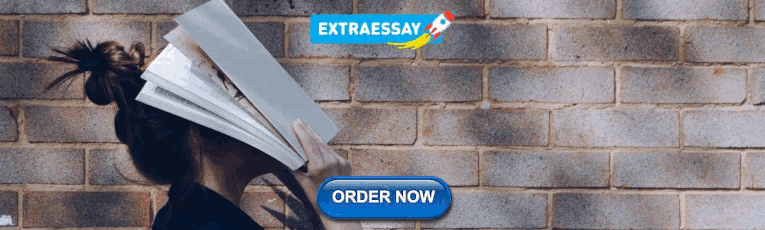
How to choose the right analysis method
To choose the right statistical methods, you need to think about two important factors :
- The type of quantitative data you have (specifically, level of measurement and the shape of the data). And,
- Your research questions and hypotheses
Let’s take a closer look at each of these.
Factor 1 – Data type
The first thing you need to consider is the type of data you’ve collected (or the type of data you will collect). By data types, I’m referring to the four levels of measurement – namely, nominal, ordinal, interval and ratio. If you’re not familiar with this lingo, check out the video below.
Why does this matter?
Well, because different statistical methods and techniques require different types of data. This is one of the “assumptions” I mentioned earlier – every method has its assumptions regarding the type of data.
For example, some techniques work with categorical data (for example, yes/no type questions, or gender or ethnicity), while others work with continuous numerical data (for example, age, weight or income) – and, of course, some work with multiple data types.
If you try to use a statistical method that doesn’t support the data type you have, your results will be largely meaningless . So, make sure that you have a clear understanding of what types of data you’ve collected (or will collect). Once you have this, you can then check which statistical methods would support your data types here .
If you haven’t collected your data yet, you can work in reverse and look at which statistical method would give you the most useful insights, and then design your data collection strategy to collect the correct data types.
Another important factor to consider is the shape of your data . Specifically, does it have a normal distribution (in other words, is it a bell-shaped curve, centred in the middle) or is it very skewed to the left or the right? Again, different statistical techniques work for different shapes of data – some are designed for symmetrical data while others are designed for skewed data.
This is another reminder of why descriptive statistics are so important – they tell you all about the shape of your data.
Factor 2: Your research questions
The next thing you need to consider is your specific research questions, as well as your hypotheses (if you have some). The nature of your research questions and research hypotheses will heavily influence which statistical methods and techniques you should use.
If you’re just interested in understanding the attributes of your sample (as opposed to the entire population), then descriptive statistics are probably all you need. For example, if you just want to assess the means (averages) and medians (centre points) of variables in a group of people.
On the other hand, if you aim to understand differences between groups or relationships between variables and to infer or predict outcomes in the population, then you’ll likely need both descriptive statistics and inferential statistics.
So, it’s really important to get very clear about your research aims and research questions, as well your hypotheses – before you start looking at which statistical techniques to use.
Never shoehorn a specific statistical technique into your research just because you like it or have some experience with it. Your choice of methods must align with all the factors we’ve covered here.
Time to recap…
You’re still with me? That’s impressive. We’ve covered a lot of ground here, so let’s recap on the key points:
- Quantitative data analysis is all about analysing number-based data (which includes categorical and numerical data) using various statistical techniques.
- The two main branches of statistics are descriptive statistics and inferential statistics . Descriptives describe your sample, whereas inferentials make predictions about what you’ll find in the population.
- Common descriptive statistical methods include mean (average), median , standard deviation and skewness .
- Common inferential statistical methods include t-tests , ANOVA , correlation and regression analysis.
- To choose the right statistical methods and techniques, you need to consider the type of data you’re working with , as well as your research questions and hypotheses.
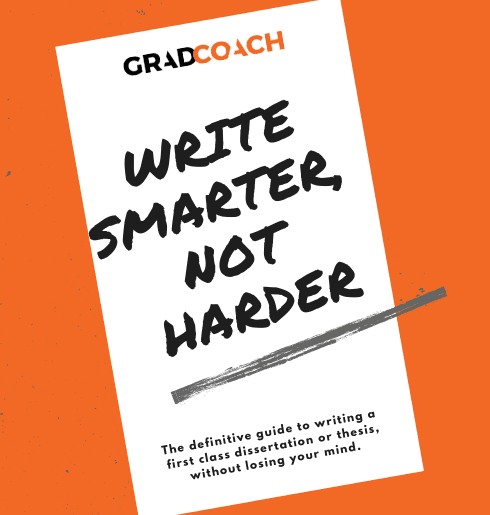
Psst… there’s more (for free)
This post is part of our dissertation mini-course, which covers everything you need to get started with your dissertation, thesis or research project.
You Might Also Like:
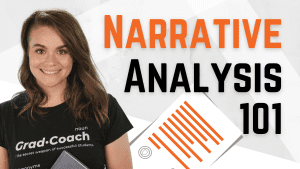
74 Comments
Hi, I have read your article. Such a brilliant post you have created.
Thank you for the feedback. Good luck with your quantitative analysis.
Thank you so much.
Thank you so much. I learnt much well. I love your summaries of the concepts. I had love you to explain how to input data using SPSS
Amazing and simple way of breaking down quantitative methods.
This is beautiful….especially for non-statisticians. I have skimmed through but I wish to read again. and please include me in other articles of the same nature when you do post. I am interested. I am sure, I could easily learn from you and get off the fear that I have had in the past. Thank you sincerely.
Send me every new information you might have.
i need every new information
Thank you for the blog. It is quite informative. Dr Peter Nemaenzhe PhD
It is wonderful. l’ve understood some of the concepts in a more compréhensive manner
Your article is so good! However, I am still a bit lost. I am doing a secondary research on Gun control in the US and increase in crime rates and I am not sure which analysis method I should use?
Based on the given learning points, this is inferential analysis, thus, use ‘t-tests, ANOVA, correlation and regression analysis’
Well explained notes. Am an MPH student and currently working on my thesis proposal, this has really helped me understand some of the things I didn’t know.
I like your page..helpful
wonderful i got my concept crystal clear. thankyou!!
This is really helpful , thank you
Thank you so much this helped
Wonderfully explained
thank u so much, it was so informative
THANKYOU, this was very informative and very helpful
This is great GRADACOACH I am not a statistician but I require more of this in my thesis
Include me in your posts.
This is so great and fully useful. I would like to thank you again and again.
Glad to read this article. I’ve read lot of articles but this article is clear on all concepts. Thanks for sharing.
Thank you so much. This is a very good foundation and intro into quantitative data analysis. Appreciate!
You have a very impressive, simple but concise explanation of data analysis for Quantitative Research here. This is a God-send link for me to appreciate research more. Thank you so much!
Avery good presentation followed by the write up. yes you simplified statistics to make sense even to a layman like me. Thank so much keep it up. The presenter did ell too. i would like more of this for Qualitative and exhaust more of the test example like the Anova.
This is a very helpful article, couldn’t have been clearer. Thank you.
Awesome and phenomenal information.Well done
The video with the accompanying article is super helpful to demystify this topic. Very well done. Thank you so much.
thank you so much, your presentation helped me a lot
I don’t know how should I express that ur article is saviour for me 🥺😍
It is well defined information and thanks for sharing. It helps me a lot in understanding the statistical data.
I gain a lot and thanks for sharing brilliant ideas, so wish to be linked on your email update.
Very helpful and clear .Thank you Gradcoach.
Thank for sharing this article, well organized and information presented are very clear.
VERY INTERESTING AND SUPPORTIVE TO NEW RESEARCHERS LIKE ME. AT LEAST SOME BASICS ABOUT QUANTITATIVE.
An outstanding, well explained and helpful article. This will help me so much with my data analysis for my research project. Thank you!
wow this has just simplified everything i was scared of how i am gonna analyse my data but thanks to you i will be able to do so
simple and constant direction to research. thanks
This is helpful
Great writing!! Comprehensive and very helpful.
Do you provide any assistance for other steps of research methodology like making research problem testing hypothesis report and thesis writing?
Thank you so much for such useful article!
Amazing article. So nicely explained. Wow
Very insightfull. Thanks
I am doing a quality improvement project to determine if the implementation of a protocol will change prescribing habits. Would this be a t-test?
The is a very helpful blog, however, I’m still not sure how to analyze my data collected. I’m doing a research on “Free Education at the University of Guyana”
tnx. fruitful blog!
So I am writing exams and would like to know how do establish which method of data analysis to use from the below research questions: I am a bit lost as to how I determine the data analysis method from the research questions.
Do female employees report higher job satisfaction than male employees with similar job descriptions across the South African telecommunications sector? – I though that maybe Chi Square could be used here. – Is there a gender difference in talented employees’ actual turnover decisions across the South African telecommunications sector? T-tests or Correlation in this one. – Is there a gender difference in the cost of actual turnover decisions across the South African telecommunications sector? T-tests or Correlation in this one. – What practical recommendations can be made to the management of South African telecommunications companies on leveraging gender to mitigate employee turnover decisions?
Your assistance will be appreciated if I could get a response as early as possible tomorrow
This was quite helpful. Thank you so much.
wow I got a lot from this article, thank you very much, keep it up
Thanks for yhe guidance. Can you send me this guidance on my email? To enable offline reading?
Thank you very much, this service is very helpful.
Every novice researcher needs to read this article as it puts things so clear and easy to follow. Its been very helpful.
Wonderful!!!! you explained everything in a way that anyone can learn. Thank you!!
I really enjoyed reading though this. Very easy to follow. Thank you
Many thanks for your useful lecture, I would be really appreciated if you could possibly share with me the PPT of presentation related to Data type?
Thank you very much for sharing, I got much from this article
This is a very informative write-up. Kindly include me in your latest posts.
Very interesting mostly for social scientists
Thank you so much, very helpfull
You’re welcome 🙂
woow, its great, its very informative and well understood because of your way of writing like teaching in front of me in simple languages.
I have been struggling to understand a lot of these concepts. Thank you for the informative piece which is written with outstanding clarity.
very informative article. Easy to understand
Beautiful read, much needed.
Always greet intro and summary. I learn so much from GradCoach
Quite informative. Simple and clear summary.
I thoroughly enjoyed reading your informative and inspiring piece. Your profound insights into this topic truly provide a better understanding of its complexity. I agree with the points you raised, especially when you delved into the specifics of the article. In my opinion, that aspect is often overlooked and deserves further attention.
Absolutely!!! Thank you
Thank you very much for this post. It made me to understand how to do my data analysis.
Submit a Comment Cancel reply
Your email address will not be published. Required fields are marked *
Save my name, email, and website in this browser for the next time I comment.
- Print Friendly
- Search Menu
- Browse content in Arts and Humanities
- Browse content in Archaeology
- Anglo-Saxon and Medieval Archaeology
- Archaeological Methodology and Techniques
- Archaeology by Region
- Archaeology of Religion
- Archaeology of Trade and Exchange
- Biblical Archaeology
- Contemporary and Public Archaeology
- Environmental Archaeology
- Historical Archaeology
- History and Theory of Archaeology
- Industrial Archaeology
- Landscape Archaeology
- Mortuary Archaeology
- Prehistoric Archaeology
- Underwater Archaeology
- Urban Archaeology
- Zooarchaeology
- Browse content in Architecture
- Architectural Structure and Design
- History of Architecture
- Residential and Domestic Buildings
- Theory of Architecture
- Browse content in Art
- Art Subjects and Themes
- History of Art
- Industrial and Commercial Art
- Theory of Art
- Biographical Studies
- Byzantine Studies
- Browse content in Classical Studies
- Classical History
- Classical Philosophy
- Classical Mythology
- Classical Literature
- Classical Reception
- Classical Art and Architecture
- Classical Oratory and Rhetoric
- Greek and Roman Epigraphy
- Greek and Roman Law
- Greek and Roman Papyrology
- Greek and Roman Archaeology
- Late Antiquity
- Religion in the Ancient World
- Digital Humanities
- Browse content in History
- Colonialism and Imperialism
- Diplomatic History
- Environmental History
- Genealogy, Heraldry, Names, and Honours
- Genocide and Ethnic Cleansing
- Historical Geography
- History by Period
- History of Emotions
- History of Agriculture
- History of Education
- History of Gender and Sexuality
- Industrial History
- Intellectual History
- International History
- Labour History
- Legal and Constitutional History
- Local and Family History
- Maritime History
- Military History
- National Liberation and Post-Colonialism
- Oral History
- Political History
- Public History
- Regional and National History
- Revolutions and Rebellions
- Slavery and Abolition of Slavery
- Social and Cultural History
- Theory, Methods, and Historiography
- Urban History
- World History
- Browse content in Language Teaching and Learning
- Language Learning (Specific Skills)
- Language Teaching Theory and Methods
- Browse content in Linguistics
- Applied Linguistics
- Cognitive Linguistics
- Computational Linguistics
- Forensic Linguistics
- Grammar, Syntax and Morphology
- Historical and Diachronic Linguistics
- History of English
- Language Acquisition
- Language Evolution
- Language Reference
- Language Variation
- Language Families
- Lexicography
- Linguistic Anthropology
- Linguistic Theories
- Linguistic Typology
- Phonetics and Phonology
- Psycholinguistics
- Sociolinguistics
- Translation and Interpretation
- Writing Systems
- Browse content in Literature
- Bibliography
- Children's Literature Studies
- Literary Studies (Asian)
- Literary Studies (European)
- Literary Studies (Eco-criticism)
- Literary Studies (Romanticism)
- Literary Studies (American)
- Literary Studies (Modernism)
- Literary Studies - World
- Literary Studies (1500 to 1800)
- Literary Studies (19th Century)
- Literary Studies (20th Century onwards)
- Literary Studies (African American Literature)
- Literary Studies (British and Irish)
- Literary Studies (Early and Medieval)
- Literary Studies (Fiction, Novelists, and Prose Writers)
- Literary Studies (Gender Studies)
- Literary Studies (Graphic Novels)
- Literary Studies (History of the Book)
- Literary Studies (Plays and Playwrights)
- Literary Studies (Poetry and Poets)
- Literary Studies (Postcolonial Literature)
- Literary Studies (Queer Studies)
- Literary Studies (Science Fiction)
- Literary Studies (Travel Literature)
- Literary Studies (War Literature)
- Literary Studies (Women's Writing)
- Literary Theory and Cultural Studies
- Mythology and Folklore
- Shakespeare Studies and Criticism
- Browse content in Media Studies
- Browse content in Music
- Applied Music
- Dance and Music
- Ethics in Music
- Ethnomusicology
- Gender and Sexuality in Music
- Medicine and Music
- Music Cultures
- Music and Religion
- Music and Media
- Music and Culture
- Music Education and Pedagogy
- Music Theory and Analysis
- Musical Scores, Lyrics, and Libretti
- Musical Structures, Styles, and Techniques
- Musicology and Music History
- Performance Practice and Studies
- Race and Ethnicity in Music
- Sound Studies
- Browse content in Performing Arts
- Browse content in Philosophy
- Aesthetics and Philosophy of Art
- Epistemology
- Feminist Philosophy
- History of Western Philosophy
- Metaphysics
- Moral Philosophy
- Non-Western Philosophy
- Philosophy of Science
- Philosophy of Language
- Philosophy of Mind
- Philosophy of Perception
- Philosophy of Action
- Philosophy of Law
- Philosophy of Religion
- Philosophy of Mathematics and Logic
- Practical Ethics
- Social and Political Philosophy
- Browse content in Religion
- Biblical Studies
- Christianity
- East Asian Religions
- History of Religion
- Judaism and Jewish Studies
- Qumran Studies
- Religion and Education
- Religion and Health
- Religion and Politics
- Religion and Science
- Religion and Law
- Religion and Art, Literature, and Music
- Religious Studies
- Browse content in Society and Culture
- Cookery, Food, and Drink
- Cultural Studies
- Customs and Traditions
- Ethical Issues and Debates
- Hobbies, Games, Arts and Crafts
- Lifestyle, Home, and Garden
- Natural world, Country Life, and Pets
- Popular Beliefs and Controversial Knowledge
- Sports and Outdoor Recreation
- Technology and Society
- Travel and Holiday
- Visual Culture
- Browse content in Law
- Arbitration
- Browse content in Company and Commercial Law
- Commercial Law
- Company Law
- Browse content in Comparative Law
- Systems of Law
- Competition Law
- Browse content in Constitutional and Administrative Law
- Government Powers
- Judicial Review
- Local Government Law
- Military and Defence Law
- Parliamentary and Legislative Practice
- Construction Law
- Contract Law
- Browse content in Criminal Law
- Criminal Procedure
- Criminal Evidence Law
- Sentencing and Punishment
- Employment and Labour Law
- Environment and Energy Law
- Browse content in Financial Law
- Banking Law
- Insolvency Law
- History of Law
- Human Rights and Immigration
- Intellectual Property Law
- Browse content in International Law
- Private International Law and Conflict of Laws
- Public International Law
- IT and Communications Law
- Jurisprudence and Philosophy of Law
- Law and Politics
- Law and Society
- Browse content in Legal System and Practice
- Courts and Procedure
- Legal Skills and Practice
- Primary Sources of Law
- Regulation of Legal Profession
- Medical and Healthcare Law
- Browse content in Policing
- Criminal Investigation and Detection
- Police and Security Services
- Police Procedure and Law
- Police Regional Planning
- Browse content in Property Law
- Personal Property Law
- Study and Revision
- Terrorism and National Security Law
- Browse content in Trusts Law
- Wills and Probate or Succession
- Browse content in Medicine and Health
- Browse content in Allied Health Professions
- Arts Therapies
- Clinical Science
- Dietetics and Nutrition
- Occupational Therapy
- Operating Department Practice
- Physiotherapy
- Radiography
- Speech and Language Therapy
- Browse content in Anaesthetics
- General Anaesthesia
- Neuroanaesthesia
- Browse content in Clinical Medicine
- Acute Medicine
- Cardiovascular Medicine
- Clinical Genetics
- Clinical Pharmacology and Therapeutics
- Dermatology
- Endocrinology and Diabetes
- Gastroenterology
- Genito-urinary Medicine
- Geriatric Medicine
- Infectious Diseases
- Medical Toxicology
- Medical Oncology
- Pain Medicine
- Palliative Medicine
- Rehabilitation Medicine
- Respiratory Medicine and Pulmonology
- Rheumatology
- Sleep Medicine
- Sports and Exercise Medicine
- Clinical Neuroscience
- Community Medical Services
- Critical Care
- Emergency Medicine
- Forensic Medicine
- Haematology
- History of Medicine
- Browse content in Medical Dentistry
- Oral and Maxillofacial Surgery
- Paediatric Dentistry
- Restorative Dentistry and Orthodontics
- Surgical Dentistry
- Browse content in Medical Skills
- Clinical Skills
- Communication Skills
- Nursing Skills
- Surgical Skills
- Medical Ethics
- Medical Statistics and Methodology
- Browse content in Neurology
- Clinical Neurophysiology
- Neuropathology
- Nursing Studies
- Browse content in Obstetrics and Gynaecology
- Gynaecology
- Occupational Medicine
- Ophthalmology
- Otolaryngology (ENT)
- Browse content in Paediatrics
- Neonatology
- Browse content in Pathology
- Chemical Pathology
- Clinical Cytogenetics and Molecular Genetics
- Histopathology
- Medical Microbiology and Virology
- Patient Education and Information
- Browse content in Pharmacology
- Psychopharmacology
- Browse content in Popular Health
- Caring for Others
- Complementary and Alternative Medicine
- Self-help and Personal Development
- Browse content in Preclinical Medicine
- Cell Biology
- Molecular Biology and Genetics
- Reproduction, Growth and Development
- Primary Care
- Professional Development in Medicine
- Browse content in Psychiatry
- Addiction Medicine
- Child and Adolescent Psychiatry
- Forensic Psychiatry
- Learning Disabilities
- Old Age Psychiatry
- Psychotherapy
- Browse content in Public Health and Epidemiology
- Epidemiology
- Public Health
- Browse content in Radiology
- Clinical Radiology
- Interventional Radiology
- Nuclear Medicine
- Radiation Oncology
- Reproductive Medicine
- Browse content in Surgery
- Cardiothoracic Surgery
- Gastro-intestinal and Colorectal Surgery
- General Surgery
- Neurosurgery
- Paediatric Surgery
- Peri-operative Care
- Plastic and Reconstructive Surgery
- Surgical Oncology
- Transplant Surgery
- Trauma and Orthopaedic Surgery
- Vascular Surgery
- Browse content in Science and Mathematics
- Browse content in Biological Sciences
- Aquatic Biology
- Biochemistry
- Bioinformatics and Computational Biology
- Developmental Biology
- Ecology and Conservation
- Evolutionary Biology
- Genetics and Genomics
- Microbiology
- Molecular and Cell Biology
- Natural History
- Plant Sciences and Forestry
- Research Methods in Life Sciences
- Structural Biology
- Systems Biology
- Zoology and Animal Sciences
- Browse content in Chemistry
- Analytical Chemistry
- Computational Chemistry
- Crystallography
- Environmental Chemistry
- Industrial Chemistry
- Inorganic Chemistry
- Materials Chemistry
- Medicinal Chemistry
- Mineralogy and Gems
- Organic Chemistry
- Physical Chemistry
- Polymer Chemistry
- Study and Communication Skills in Chemistry
- Theoretical Chemistry
- Browse content in Computer Science
- Artificial Intelligence
- Computer Architecture and Logic Design
- Game Studies
- Human-Computer Interaction
- Mathematical Theory of Computation
- Programming Languages
- Software Engineering
- Systems Analysis and Design
- Virtual Reality
- Browse content in Computing
- Business Applications
- Computer Security
- Computer Games
- Computer Networking and Communications
- Digital Lifestyle
- Graphical and Digital Media Applications
- Operating Systems
- Browse content in Earth Sciences and Geography
- Atmospheric Sciences
- Environmental Geography
- Geology and the Lithosphere
- Maps and Map-making
- Meteorology and Climatology
- Oceanography and Hydrology
- Palaeontology
- Physical Geography and Topography
- Regional Geography
- Soil Science
- Urban Geography
- Browse content in Engineering and Technology
- Agriculture and Farming
- Biological Engineering
- Civil Engineering, Surveying, and Building
- Electronics and Communications Engineering
- Energy Technology
- Engineering (General)
- Environmental Science, Engineering, and Technology
- History of Engineering and Technology
- Mechanical Engineering and Materials
- Technology of Industrial Chemistry
- Transport Technology and Trades
- Browse content in Environmental Science
- Applied Ecology (Environmental Science)
- Conservation of the Environment (Environmental Science)
- Environmental Sustainability
- Environmentalist Thought and Ideology (Environmental Science)
- Management of Land and Natural Resources (Environmental Science)
- Natural Disasters (Environmental Science)
- Nuclear Issues (Environmental Science)
- Pollution and Threats to the Environment (Environmental Science)
- Social Impact of Environmental Issues (Environmental Science)
- History of Science and Technology
- Browse content in Materials Science
- Ceramics and Glasses
- Composite Materials
- Metals, Alloying, and Corrosion
- Nanotechnology
- Browse content in Mathematics
- Applied Mathematics
- Biomathematics and Statistics
- History of Mathematics
- Mathematical Education
- Mathematical Finance
- Mathematical Analysis
- Numerical and Computational Mathematics
- Probability and Statistics
- Pure Mathematics
- Browse content in Neuroscience
- Cognition and Behavioural Neuroscience
- Development of the Nervous System
- Disorders of the Nervous System
- History of Neuroscience
- Invertebrate Neurobiology
- Molecular and Cellular Systems
- Neuroendocrinology and Autonomic Nervous System
- Neuroscientific Techniques
- Sensory and Motor Systems
- Browse content in Physics
- Astronomy and Astrophysics
- Atomic, Molecular, and Optical Physics
- Biological and Medical Physics
- Classical Mechanics
- Computational Physics
- Condensed Matter Physics
- Electromagnetism, Optics, and Acoustics
- History of Physics
- Mathematical and Statistical Physics
- Measurement Science
- Nuclear Physics
- Particles and Fields
- Plasma Physics
- Quantum Physics
- Relativity and Gravitation
- Semiconductor and Mesoscopic Physics
- Browse content in Psychology
- Affective Sciences
- Clinical Psychology
- Cognitive Psychology
- Cognitive Neuroscience
- Criminal and Forensic Psychology
- Developmental Psychology
- Educational Psychology
- Evolutionary Psychology
- Health Psychology
- History and Systems in Psychology
- Music Psychology
- Neuropsychology
- Organizational Psychology
- Psychological Assessment and Testing
- Psychology of Human-Technology Interaction
- Psychology Professional Development and Training
- Research Methods in Psychology
- Social Psychology
- Browse content in Social Sciences
- Browse content in Anthropology
- Anthropology of Religion
- Human Evolution
- Medical Anthropology
- Physical Anthropology
- Regional Anthropology
- Social and Cultural Anthropology
- Theory and Practice of Anthropology
- Browse content in Business and Management
- Business Strategy
- Business Ethics
- Business History
- Business and Government
- Business and Technology
- Business and the Environment
- Comparative Management
- Corporate Governance
- Corporate Social Responsibility
- Entrepreneurship
- Health Management
- Human Resource Management
- Industrial and Employment Relations
- Industry Studies
- Information and Communication Technologies
- International Business
- Knowledge Management
- Management and Management Techniques
- Operations Management
- Organizational Theory and Behaviour
- Pensions and Pension Management
- Public and Nonprofit Management
- Strategic Management
- Supply Chain Management
- Browse content in Criminology and Criminal Justice
- Criminal Justice
- Criminology
- Forms of Crime
- International and Comparative Criminology
- Youth Violence and Juvenile Justice
- Development Studies
- Browse content in Economics
- Agricultural, Environmental, and Natural Resource Economics
- Asian Economics
- Behavioural Finance
- Behavioural Economics and Neuroeconomics
- Econometrics and Mathematical Economics
- Economic Systems
- Economic History
- Economic Methodology
- Economic Development and Growth
- Financial Markets
- Financial Institutions and Services
- General Economics and Teaching
- Health, Education, and Welfare
- History of Economic Thought
- International Economics
- Labour and Demographic Economics
- Law and Economics
- Macroeconomics and Monetary Economics
- Microeconomics
- Public Economics
- Urban, Rural, and Regional Economics
- Welfare Economics
- Browse content in Education
- Adult Education and Continuous Learning
- Care and Counselling of Students
- Early Childhood and Elementary Education
- Educational Equipment and Technology
- Educational Strategies and Policy
- Higher and Further Education
- Organization and Management of Education
- Philosophy and Theory of Education
- Schools Studies
- Secondary Education
- Teaching of a Specific Subject
- Teaching of Specific Groups and Special Educational Needs
- Teaching Skills and Techniques
- Browse content in Environment
- Applied Ecology (Social Science)
- Climate Change
- Conservation of the Environment (Social Science)
- Environmentalist Thought and Ideology (Social Science)
- Natural Disasters (Environment)
- Social Impact of Environmental Issues (Social Science)
- Browse content in Human Geography
- Cultural Geography
- Economic Geography
- Political Geography
- Browse content in Interdisciplinary Studies
- Communication Studies
- Museums, Libraries, and Information Sciences
- Browse content in Politics
- African Politics
- Asian Politics
- Chinese Politics
- Comparative Politics
- Conflict Politics
- Elections and Electoral Studies
- Environmental Politics
- European Union
- Foreign Policy
- Gender and Politics
- Human Rights and Politics
- Indian Politics
- International Relations
- International Organization (Politics)
- International Political Economy
- Irish Politics
- Latin American Politics
- Middle Eastern Politics
- Political Methodology
- Political Communication
- Political Philosophy
- Political Sociology
- Political Behaviour
- Political Economy
- Political Institutions
- Political Theory
- Politics and Law
- Public Administration
- Public Policy
- Quantitative Political Methodology
- Regional Political Studies
- Russian Politics
- Security Studies
- State and Local Government
- UK Politics
- US Politics
- Browse content in Regional and Area Studies
- African Studies
- Asian Studies
- East Asian Studies
- Japanese Studies
- Latin American Studies
- Middle Eastern Studies
- Native American Studies
- Scottish Studies
- Browse content in Research and Information
- Research Methods
- Browse content in Social Work
- Addictions and Substance Misuse
- Adoption and Fostering
- Care of the Elderly
- Child and Adolescent Social Work
- Couple and Family Social Work
- Developmental and Physical Disabilities Social Work
- Direct Practice and Clinical Social Work
- Emergency Services
- Human Behaviour and the Social Environment
- International and Global Issues in Social Work
- Mental and Behavioural Health
- Social Justice and Human Rights
- Social Policy and Advocacy
- Social Work and Crime and Justice
- Social Work Macro Practice
- Social Work Practice Settings
- Social Work Research and Evidence-based Practice
- Welfare and Benefit Systems
- Browse content in Sociology
- Childhood Studies
- Community Development
- Comparative and Historical Sociology
- Economic Sociology
- Gender and Sexuality
- Gerontology and Ageing
- Health, Illness, and Medicine
- Marriage and the Family
- Migration Studies
- Occupations, Professions, and Work
- Organizations
- Population and Demography
- Race and Ethnicity
- Social Theory
- Social Movements and Social Change
- Social Research and Statistics
- Social Stratification, Inequality, and Mobility
- Sociology of Religion
- Sociology of Education
- Sport and Leisure
- Urban and Rural Studies
- Browse content in Warfare and Defence
- Defence Strategy, Planning, and Research
- Land Forces and Warfare
- Military Administration
- Military Life and Institutions
- Naval Forces and Warfare
- Other Warfare and Defence Issues
- Peace Studies and Conflict Resolution
- Weapons and Equipment

- < Previous chapter
- Next chapter >
15 Data Analysis I: Overview of Data Analysis Strategies
Julia Brannen is Professor of Sociology of the Family, Thomas Coram Research Unit, Institute of Education, University of London. Her substantive research has focused on the family lives of parents, children and young people in Britain and Europe, working families and food, and intergenerational relations. She is an academician of the Academy of Social Science in the UK. She has a particular interest in methodology including mixed methods, biographical and narrative approaches and comparative research. Co-founder of The International Journal of Social Research Methodology, she coedited the journal for 17 years with Rosalind Edwards and she is an Associate editor of The Journal of Mixed Methods. An early exponent of MMR, in 1992 she edited Mixing Methods: Qualitative and Quantitative Research (London: Gower). She has written many books journal articles and contributions to methodological texts. Recent books include: The Handbook of Social Research Methods (Sage 2010) Work, Family and Organizations in Transition: A European Perspective (Policy 2009), Transitions to Parenthood in Europe: A comparative life course perspective (Policy 2012).
Rebecca O’Connell is a Senior Research Officer at the Thomas Coram Research Unit (TCRU), Institute of Education, University of London, UK. She is a social anthropologist whose research interests focus on the intersection of care and work, particularly foodwork and childcare. She is currently Principal Investigator on two studies: ‘Families, Food and Work: taking a long view’, a multi-methods longitudinal study funded by the Department of Health and the Economic and Social Research Council (ESRC); and ‘Families and Food in Hard Times, a subproject of the ESRC's National Centre for Research Methods ‘Novella’ node, which is led by Professor Ann Phoenix. Professor Julia Brannen is co-investigator on both studies. From May 2014 Rebecca leads a study of ‘Families and food poverty in three European countries in an age of austerity’, a five-year project funded by the European Research Council. Rebecca is also co-convenor of the British Sociological Association Food Study Group.
- Published: 19 January 2016
- Cite Icon Cite
- Permissions Icon Permissions
This chapter enables the reader to consider issues that are likely to affect the analysis of mixed method research (MMMR). It identifies the ways in which data from multimethod and mixed methods research can be integrated in principle and gives detailed examples of different strategies in practice. Furthermore, it examines a particular type of MMMR and discusses an exemplar study in which national survey data are analyzed alongside a longitudinal qualitative study whose sample is drawn from the same national survey. By working through three analytic issues, it shows the complexities and challenges involved in integrating qualitative and quantitative data: issues about linking data sets, similarity (or not) of units of analysis, and concepts and meaning. It draws some conclusions and sets out some future directions for MMMR.
Introduction
This chapter begins with a consideration of the conditions under which integration is possible (or not). A number of factors that need to be considered before a researcher can decide that integration is possible are briefly discussed. This discussion is followed by a consideration of Caracelli and Greene’s (1993) analysis strategies. Examples of mixed method studies that involve these strategies are described, including the ways they attempt to integrate different data, in particular data transformation, examine typologies and outlier cases, and merge data sets. It is shown that these strategies are not always standalone but can merge into each other. The chapter concludes with a discussion of an extended example of the ways in which a study we carried out called Families, Food and Work (2009–2014) sought to employ analysis of relevant questions from different large-scale data sets with data from a qualitative study of how working parents and children negotiate food and eating ( O’Connell & Brannen, 2015 ).
Issues to Consider Before Conducting Mixed Method Research and Analysis
The researcher should consider a number of issues that need to be revisited during the analysis of the data before embarking on multimethod and mixed methods research (MMMR).
The first concerns the ontological and epistemological assumptions underpinning the choice of methods used to generate the data. Working from the principle that the choice of method is not made in a philosophical void, the data should be thought about in relation to epistemological assumptions underpinning the aspect of the research problem/question being addressed (see, e.g., Barbour, 1999 ). Thus in terms of best practice, researchers may be well advised to consider what kind of knowledge they seek to generate. Most multimethod and mixed methods researchers, while not necessarily thinking of themselves as pragmatists in a philosophical sense, adopt a pragmatic approach ( Bryman, 2008 ). Pragmatism dominates in MMMR ( Omwuegbuzie & Leech, 2005 ), especially among those from more applied fields of the social sciences (in which MMMR has been most widespread). However, pragmatism in this context connotes its common-sense meaning, sidelining philosophical issues so that MMMR strategies are employed as a matter of pragmatics ( Bryman, 2008 ). Some might argue that if different questions are addressed in a study that require different types of knowledge, then the data cannot be integrated unproblematically in the analysis phase. However, it depends on what one means by “integration,” as we later discuss.
The second issue concerns the level of reality under study. Some research questions are about understanding social phenomena at the micro level while others are concerned with social phenomena at the macro level. Thus researchers in the former group emphasize the agency of those they study through an emphasis on studying individuals’ subjective interpretations and perspectives and have allegiances to interpretivist and postmodernist epistemologies. Those working at the macro level are concerned with identifying larger scale patterns and trends and seek to hypothesize or create structural explanations, which may call on realist epistemologies. However, all researchers aim to focus to some extent on the relation between individuals and society. If one is to transcend conceptually the micro and the macro levels, then methods must be developed to reflect this transcendence ( Kelle, 2001 ). For example, in qualitative research that focuses on individuals’ perspectives, it is important to set those perspectives in their social structural and historical contexts. Whether those who apply a paradigm of rationality will apply both qualitative and quantitative methods will depend on the extent to which they seek to produce different levels and types of explanation. This will mean interrogating the linkages between the data analyzes made at these levels.
The third issue relates to the kinds of human experience and social action that the study’s research questions are designed to address. For example, if one is interested in life experiences over long periods of time, researchers will employ life story or other narrative methods. In this case, they need to take into account the way stories are framed, and in particular how temporal perspectives, the purposes of the narrator, and the way stories are told influence the stories. The data the researchers will collect are therefore narrative data. Hence how these stories fit, for example, with quantitative data collected as part of a MMMR approach will require close interrogation in the analysis of the two data sets, taking into account both interpretive and realist historical approaches.
The fourth issue to consider is whether the data are primary or secondary and, in the latter case, whether they are subjected to secondary analysis. Secondary data are by definition collected by other people, although access to them may not be straightforward. If the data have already been coded and the original data are not available, the types of secondary analysis possible will be limited. Moreover, the preexistence of these data may influence the timetabling of the MMMR project and may also shape the questions that are framed in any subsequent qualitative phase and in the data analysis. Depending on the nature and characteristics of the data, one data set may prove intrinsically more interesting; thus more time and attention may be given to its analysis. A related issue therefore concerns the possibilities for operationalizing the concepts employed in relation to the different parts of the MMMR inquiry. Preexisting data, especially those of a quantitative type, may make it difficult to reconceptualize the problem. At a practical level, the questions asked in a survey may poorly relate to those that fit the MMMR inquiry, as we later illustrate. Since one does not know what one does not know, it may be only at later stages that researchers working across disciplines and methodologies may come to realize which questions cannot be addressed and which data are missing.
The fifth issue relates to the environments in which researchers are located. For example, are the research and the researcher operating within the same research setting, for example the same discipline, the same theoretical and methodological tradition, or the same policy social context? MMMR fits with the political currency accorded to “practical inquiry” that speaks to policy and policymakers and that informs practice, as distinct from scientific research ( Hammersley, 2000 ). However, with respect to policy, this has to be set in the context of the continued policy importance afforded to large-scale data but also the increased scale of these data sets and the growth in the availability of official administrative data. In turn, these trends have been matched by the increased capacity of computing power to manage and analyze these data ( Brannen & Moss, 2013 ) and the increased pressure on social scientists to specialize in high-level quantitative data analysis. As more such data accrue, the apparent demand for quantitative analysis increases ( Brannen & Moss, 2013 ). However, MMMR strategies are also often employed alongside such quantitative analysis, especially in policy-driven research. For example, in cross-national research, governmental organizations require comparative data to assess how countries are doing in a number of different fields, a process that has become an integral part of performance monitoring. But, equally, there is a requirement for policy analysis and inquiries into how policies work in particular local conditions. Such micro-level analysis will require methods like documentary analysis, discourse analysis, case study designs, and intensive research approaches. Furthermore, qualitative data are thought useful to “bring alive” research for policy and practitioner audiences ( O’Cathain, 2009 ).
Another aspect of environment relates to the sixth issue concerning the constitution of the research team and the extent to which it is inter or transdisciplinary . Research teams can be understood as “communities of practice” ( Denscombe, 2008 ). While paradigms are pervasive ways of dividing social science research, as Morgan (2007) argues, we need to think in terms of shared beliefs within communities of researchers. This requires an ethic of “precarity” to prevail ( Ettlinger, 2007 , p. 319), through which researchers are open to others’ ideas and can relinquish entrenched positions. However, the success of communities of practice will depend on the political context, their composition, and whether they are democratic ( Hammersley, 2005 ). Thus in the analysis of MMMR, it is important to be cognizant of the power relations with such communities of practice since they will influence the researcher’s room for maneuvering in determining directions and outputs of the data analysis. At the same time, these political issues affect analysis and dissemination in research teams in which members share disciplinary approaches.
Finally, there are the methodological preferences, skills, and specialisms of the researcher, all of which have implications for the quality of the data and the data analysis. MMMR offers the opportunity to learn about a range of methods and thus to be open to new ways of addressing research questions. Broadening one’s methodological repertoire mitigates against “trained incapacities” as Reiss (1968) termed the issue—the entrenchment of researchers in particular types of research paradigms, as well as questions, research methods, and types of analysis.
The Context of Inquiry: Research Questions and Research Design
The rationale for MMMR must be clear both in the phase of the project’s research design (the context of the inquiry) and in the analysis phase (the context of justification). At the research design phase, researchers wrestle with such fundamental methodological questions as to what kinds of knowledge they seek to generate, such as whether to describe and understand a social phenomenon or seek to explain it. Do we wish to do both, that is, to understand and explain? In the latter case, the research strategy will typically translate itself into employing a mix of qualitative and quantitative methods, which some argue is the defining characteristic of mixed method research (MMR) ( Tashakorri & Creswell, 2007 ).
If a MMR strategy is employed, this generally implies that a number of research questions will address a substantive issue. MMMR is also justified in terms of its capacity to address different aspects of a research question. This is turns leads researchers to consider how to frame their research questions and how these determine the methods chosen. Typically research questions are formulated in the research proposal. However, they should also be amenable to adaptation ( Harrits, 2011 , citing Dewey, 1991 ); adaptations may be necessary as researchers respond to the actual conditions of the inquiry. According to Law (2004) , research is an “assemblage,” that is, something not fixed in shape but incorporating tacit knowledge, research skills, resources, and political agenda that are “constructed” as they are woven together (p. 42). Methodology should be rebuilt during the research process in a way that responds to research needs and the conditions encountered—what Seltzer-Kelly, Westwood, and Pena-Guzman (2012) term “a constructivist stance at the methodological level” (p. 270). This can also happen at the phase when data are analyzed.
Developing a coherent methodology with a close link between the research question and the research strategy holds out the best hope for answering a project’s objectives and questions ( Woolley, 2009 , p. 8). Thus Yin (2006) would say that to carry out an MMMR analysis it is essential to have an integrated set of research questions. However, it is not easy to determine what constitutes coherence. For example, the research question concerning the link between the quality of children’s diet in the general population and whether mothers are in paid employment may be considered a very different and not necessarily complementary question to the research question about the conditions under which the children of working mothers are fed. Thus we have to consider here how tightly or loosely the research questions interconnect.
The framing of the research question influences the method chosen that, in turn, influences the choice of analytic method. Thus in our study of children’s food that examined the link between children’s diet and maternal employment, we examined a number of large-scale data sets and carried out statistical analyzes on these, while in studying the conditions under which children in working families get fed, we carried out qualitative case analysis on a subset of households selected from one of the large-scale data sets.
The Context of Justification: The Analysis Phase
In the analysis phase of MMMR, the framing of the research questions becomes critical, affecting when, to what extent, and in what ways data from different methods are integrated. So, for example, we have to consider the temporal ordering of methods. For example, quantitative data on a research topic may be available and the results already analyzed. This analysis may influence the questions to be posed in the qualitative phase of inquiry.
Thus it is also necessary to consider the compatibility between the units of analysis in the quantitative phase and the qualitative phase of the study, for example, between variables studied in a survey and the analytic units studied in a qualitative study. Are we seeking analytic units that are equivalent (but not similar), or are we seeking to analyze a different aspect of a social phenomenon? If the latter, how do the two analyses relate? This may become more critical if the same population is covered in both the qualitative and quantitative phases. What happens when a nested or integrated sampling strategy is employed, as in the case of a large-scale survey analysis and a qualitative analysis based on a subsample of the survey?
A number of frameworks have been suggested for integrating data produced by quantitative and qualitative methods ( Brannen, 1992 ; Caracelli & Greene, 1993 ; Greene, Caracelli, & Graham, 1989 ). While these may provide a guide to the variety of ways to integrate data, they should not be used as fixed templates. Indeed, they may provide a basis for reflection after the analysis has been completed.
Corroboration —in which one set of results based on one method are confirmed by those gained through the application of another method.
Elaboration or expansion —in which qualitative data analysis may exemplify how patterns based on quantitative data analysis apply in particular cases. Here the use of one type of data analysis adds to the understanding gained by another.
Initiation —in which the use of a first method sparks new hypotheses or research questions that can be pursued using a different method.
Complementarity —in which qualitative and quantitative results are regarded as different beasts but are meshed together so that each data analysis enhances the other ( Mason, 2006 ). The data analyses from the two methods are juxtaposed and generate complementary insights that together create a bigger picture.
Contradiction —in which qualitative data and quantitative findings conflict. Exploring contradictions between different types of data assumed to reflect the same phenomenon may lead to an interrogation of the methods and to discounting one method in favor of another (in terms of assessments of validity or reliability). Alternatively, the researcher may simply juxtapose the contradictions for others to explore in further research. More commonly, one type of data may be presented and assumed to be “better,” rather than seeking to explain the contradictions in relation to some ontological reality ( Denzin & Lincoln, 2005 ; Greene et al., 1989 ).
As Hammersley (2005) points out, all these ways of combining different data analyses to some extent make assumptions that there is some reality out there to be captured, despite the caveats expressed about how each method constructs the data differently. Thus, just as seeking to corroborate data may not lead us down the path of “validation,” so too the complementarity rationale for mixing methods may not complete the picture either. There may be no meeting point between epistemological positions. As Hammersley (2008) suggests, there is a need for a dialogue between them in the recognition that absolute certainty is never justified and that “we must treat knowledge claims as equally doubtful or that we should judge them on grounds other than their likely truth” (p. 51).
Multimethod and Mixed Methods Research Analysis Strategies: Examples of Studies
Caracelli and Greene (1993) suggest analysis strategies for integrating qualitative and quantitative data. In practice these strategies are not always standalone but blur into each other. Moreover, as Bryman (2008) has observed, it is relatively rare for mixed method researchers to give full rationales for MMMR designs. They can involve data transformation in which, for example, qualitative data are treated quantitatively. They may involve typology development in which cases are categorized in patterns and outlier cases are scrutinized. They may involve data merging in which both data sets are treated in similar ways, for instance, by creating similar variables or equivalent units of analysis across data sets. In this section, drawing on the categorization of Caracelli and Greene, we give some examples of studies in which qualitative and quantitative data are integrated in these different ways (Table 15.1 ). These are not intended to be exhaustive, nor are the studies pure examples of these strategies.
Qualitative Data Are Transformed into Quantitative Data or Vice Versa
In survey research, in order to test how respondents understand questions it is commonplace to transform qualitative data into quantitative data. This is termed cognitive testing . The aim here is to find a fit between responses given in both the survey and the qualitative testing. For example, most personality scales are based on prior clinical research. An example of data transformation on a larger scale is taken from a program of research on the wider benefits of adult learning ( Hammond, 2005 ). The rationale for the study was that the research area was underresearched and the research questions relatively unformulated (p. 241). Qualitative research was carried out to identify variables to test on an existing national longitudinal data set. The qualitative phase involved biographical interviews with adult learners. The quantitative data consisted of data from an existing UK cohort study (the 1958 National Child Development Study). A main justification for using these latter data concerned the further exploitation of data that are expensive to collect. The qualitative component was conceived as a “mapping” exercise carried out to inform the research design and the implementation of the quantitative phase, that is, the identification of variables for quantitative analysis ( Hammond, 2005 , p. 243). This approach has parallels with qualitative pilot work carried out as a prologue to a survey, although the qualitative material was also analyzed in its own right. However, while the qualitative data were used with the aim of finding common measures that fit with the quantitative inquiry, Hammond also insisted that the qualitative data not be used to explain quantitatively derived outcomes but to interrogate them further ( Hammond, 2005 , p. 244). Inevitably, contradictions between the respective findings arose. For example, Hammond reported that the effect of adult learning on life satisfaction (the transformed measure) found in the National Child Development Study cohort analysis was greater for men than for women, while women reported themselves in the biographical interview responses to be positive about the courses they had taken. On this issue, the biographical interviews were regarded as being “more sensitive” than the quantitative measure. Hammond also suggested that the interview data showed that an improved sense of well-being (another transformed measure) experienced by the respondents in the present was not necessarily incompatible with having a negative view of the future. The quantitative data conflated satisfaction with “life so far” and with “life in the future.” Contradictions were also explained in terms of the lack of representativeness of the qualitative study (the samples did not overlap). In addition, it is possible that priority was given by the researcher to the biographical interviews and may have placed more trust in this approach. Another possibly relevant factor was that the researcher had no stake in creating or shaping the quantitative data set. In any event, the biographical interviews were conducted before the quantitative analyses and were used to influence the decisions about which analyses to focus on in the quantitative analysis. Hence the qualitative data threw up hypotheses that the quantitative data were used to reject or support. What is interesting about using qualitative data to test on quantitative evidence is the opportunity it offers to pose or initiate new lines of questioning ( Greene et al., 1989 )—a result not necessarily anticipated at the outset of this research.
Typologies, Deviant, Negative, or Outlier Cases Are Subjected to Further Scrutiny Later or in Another Data Set
A longitudinal or multilayered design provides researchers with opportunities to examine the strength of the conclusions that can be drawn about the cases and the phenomena under study ( Nilsen & Brannen, 2010 ). For an example of this strategy, we turn to the classic study carried by Glueck and Glueck (1950 , 1968 ). The study Five Hundred Criminal Careers was based on longitudinal research of delinquents and nondelinquents (1949–1965). The Gluecks studied the two groups at three age points; 14, 25, and 32. The study had a remarkably high (92%) response rate when adjusted for mortality at the third wave. The Gluecks collected very rich data on a variety of dimensions and embedded open-ended questions within a survey framework. Interviews with respondents and their families, as well as key informants (social workers, school teachers, employers, and neighbors), were carried out, together with home observations and the study of official records and criminal histories. Some decades later, Laub and Sampson (1993 , 1998) reanalyzed these data longitudinally (the Gluecks’ original analyzes were cross-sectional).
Laub and Sampson (1998) note that the Gluecks’ material “represents the comparison, reconciliation and integration of these multiple sources of data” (p. 217) although the Gluecks did not treat the qualitative data in their own right. The original study was firmly grounded in a quantitative logic where the purpose was to arrive at causal explanations and the ability to predict criminal behavior . However, the Gluecks were carrying out their research in a pre-computer age, a fact that facilitated reanalysis of the material. When Laub and Sampson came to recode the raw data many years later, they rebuilt the Gluecks’ original data set and used their coding schemes to validate their original analyzes. Laub and Sampson then constructed the criminal histories of the sample, drawing on and integrating the different kinds of data available. This involved merging data.
Next they purposively selected a subset of cases for intensive qualitative analysis in order to explore consistencies and inconsistencies between the original findings and the original study’s predictions for the delinquents’ future criminal careers—what happened to them some decades later. They examined “off diagonal” and “negative cases” that did not fit the quantitative results and predictions. In particular, they selected individuals who, on the basis of their earlier careers, were expected to follow a life of crime but did not and those expected to cease criminality but did not.
Citing Jick (1979) , Laub and Sampson (1998) suggest how divergence can become an opportunity for enriching explanations (p. 223). By examining deviant cases on the basis of one data analysis and interrogating these in a second data analysis, they demonstrated complex processes of individual pathways into and out of crime, including identified pathways, that take place over long time periods ( Laub & Sampson, 1998 , p. 222). They argued that “without qualitative data, discussions of continuity often mask complex and rich qualitative processes” (Sampson & Laub, 1997, quoted in Laub & Sampson 1998 , p. 229).
In addition they supported a biographical approach that enables the researcher to interpret data in historical context, in this case to understand criminality in relation to the type and level of crime prevalent at the time. Laub and Sampson (1998) selected a further subsample of the original sample of delinquents, having managed to trace them after 50 years ( Laub & Sampson, 1993 ) and asked them to review their past lives. The researchers were particularly interested in identifying turning points to understand what had shaped the often unexpected discontinuities and continuities in the careers of these one-time delinquents.
This is an exemplar study of the analytic strategy of subjecting typologies to deeper scrutiny. It also afforded an opportunity to theorize about the conditions concerning cases that deviated from predicted trajectories.
Data Merging: The Same Set of Variables Is Created Across Quantitative and Qualitative Data Sets
Here assumptions are made that the phenomena under study are similar in both the qualitative and quantitative parts of an inquiry, a strategy exemplified in the following two studies. The treatment of the data in both parts of the study was seamless, so that one type of data is transformed into the other. In a longitudinal study, Blatchford (2005) examined the relationship between classroom size and pupils’ educational achievement. Blatchford justifies using a mixed method strategy in terms of the power of mixed methods to reconciling inconsistencies found in previous research. The rationale given for using qualitative methods was the need to assess the relationships between the same variables but in particular case studies. Blatchford notes that “priorities had to be set and some areas of investigation received more attention than others” (p. 204). The dominance of the quantitative analysis occurred despite the collection of “fine grained data on classroom processes” that could have lent themselves to other kinds of analysis, such as understanding how students learn in different classroom environments. The qualitative data were in fact put to limited use and were merged with the quantitative data.
Sammons et al. (2005) similarly employed a longitudinal quantitative design to explore the effects of preschool education on children’s attainment and development at entry to school. Using a purposive rationale, they selected a smaller number of early education centers from their original sample on the basis of their contrasting profiles. Sammons et al. coded the qualitative data in such a way that the “reduced data” (p. 219) were used to provide statistical explanations for the outcomes produced in the quantitative longitudinal study. Thus, again, the insights derived from the qualitative data analysis were merged with the quantitative variables, which were correlated with outcome variables on children’s attainment. The researchers in question could have drawn on both the qualitative and quantitative data for different insights, as is required in case study research ( Yin, 2006 ) and as suggested in their purposive choice of preschool centers.
Using Quantitative and Qualitative Data: A Longitudinal Study of Working Families and Food
In this final part of the chapter we take an example from our own work in which we faced a number of methodological issues in integrating and meshing different types of data. In this section we discuss some of the challenges involved in the collection and analysis of such data.
The study we carried out is an example of designing quantitative and qualitative constituent parts to address differently framed questions. Its questions were, and remain, currently highly topical in the Western world and concern the influences of health policy on healthy eating, including in childhood, and its implications for obesity. 1 Much of the health evidence is unable to explain why it is that families appear to ignore advice and continue to eat in unhealthy ways. The project arose in the context of some existing research that suggests an association between parental (maternal) employment and children’s (poor) diet ( Hawkins, Cole, & Law, 2009 ). We pursued these issues by framing the research phenomenon in different ways and through the analysis of different data sets.
The project was initiated in a policy context in which we tendered successfully for a project that enabled us to exploit a data set commissioned by government to examine the nation’s diet. Somewhat of a landmark study in the UK, the project is directly linked to the National Diet and Nutrition Survey (NDNS) funded by the UK’s Food Standards Agency and Department of Health, a study largely designed by those from public health and nutritionist perspectives. These data, from the first wave of the new rolling survey, were unavailable to others at that time. We were also facilitated in selecting a subsample of households with children from the NDNS that we subjected to a range of qualitative methods. The research team worked closely with the UK government to gain access to the data collected and managed by an independent research agency in the identification of a subsample to meet the research criteria and in seeking the consent of the survey subsample participants.
Applying anthropological and sociological lenses, the ethnographically trained researchers in the team sought to explore inductively parents’ experiences of negotiating the demands of “work” and “home” and domestic food provisioning in families. We therefore sought to understand the contextual and embodied meanings of food practices and their situatedness in different social contexts (inside and outside the home). We also assumed that children are agents in their own lives, and therefore we included children in the study and examined the ways in which children reported food practices and attributed meaning to food. The main research questions (RQ) for the study were:
What is the relationship between parental employment and the diets of children (aged 1.5 to 10 years)?
How does food fit into working family life and how do parents experience the demands of “work” and “home” in managing food provisioning?
How do parents and children negotiate food practices?
What foods do children of working parents eat in different contexts—home, childcare, and school—and how do children negotiate food practices?
The study not only employed a MMMR strategy but was also longitudinal, a design that is rarely discussed in the MMMR literature. We conducted a follow-up study (Wave 2) approximately two years later, which repeated some questions and additionally asked about social change, the division of food work, and the social practice of family meals. The first research question was to be addressed through the survey data while RQ 2, 3, and 4 were addressed through the qualitative study. In the qualitative study, a variety of ethnographic methods were to be deployed with both parents and children ages 2 to 10 years. The ethnographic methods included a range of interactive research tools, which were used flexibly with the children since their age span is wide: interviews, drawing methods, and, with some children, photo elicitation interviews in which children photographed foods and meals consumed within and outside the home and discussed these with the researcher at a later visit. Semistructured interviews were carried out with parents who defined themselves as the main food providers and sometimes with an additional parent or care-provider who was involved in food work and also wished to participate.
In the context of existing research that suggests an association between parental (maternal) employment and household income with children’s (poor) diet ( Hawkins et al., 2009 ) carried out on a different UK data set and also supported by some US research (e.g. Crepinsek & Burstein, 2004 ; McIntosh et al., 2008 ), it was important to investigate whether this association was born out elsewhere. In addition and in parallel, we therefore carried out secondary analysis on the NDNS Year 1 (2008/2009) data and on two other large-scale national surveys, the Health Survey for England (surveys, 2007, 2008) and the Avon Longitudinal Study of Parents and Children (otherwise known as “Children of the Nineties”) (data sweeps 1995/1996, 1996/1997, and 1997/1999) to examine the first research question. This part of the work was not straightforward. First we found that, contrary to a previous NDNS (1997) survey that had classified mothers’ working hours as full or part-time, neither mothers’ hours of work nor full/part-time status had been collected in the new rolling NDNS survey. Rather, this information was limited in most cases to whether a mother was or was not in paid employment. Thus it was not possible to disentangle the effects of mothers working full-time from those doing part-time hours on children’s diets. This was unfortunate since the NDNS provided very detailed data on children’s nutrition based on food diaries, unlike the Millennium Cohort Study, which collected only mothers’ reports of children’s snacking between meals at home ( Hawkins et al., 2009 ). While the Millennium Cohort Study analysis found a relationship between long hours of maternal employment and children’s dietary intake, no association between mothers’ employment and children’s dietary intake was found in the NDNS ( O’Connell, Brannen, Mooney, Knight, & Simon, 2011 ; Simon et al., forthcoming ). However, it is possible that a relationship might have been found if we had been able to disaggregate women’s employment by hours.
In the following we describe three instances of data analysis in this longitudinal MMMR study in relation to some of the key analytic issues set out in the research questions described previously (see Table 15.2 ).
Studying children’s diets in a MMMR design
Examining the division of household food work in a MMMR design
Making sense of family meals in a MMMR design
Linking Data in a Longitudinal Multimethod and Mixed Methods Research Design: Studying Children’s Diets
The research problem.
Together with drawing a sample for qualitative study from the national survey, we aimed to carry out secondary analysis on the NDNS data in order to generate patterns of “what” is eaten by children and parents and to explore associations with a range of independent variables, notably mothers’ employment. The NDNS diet data were based on four-day unweighed food diaries that recorded detailed information about quantities of foods and drinks consumed, as well as where, when, and with whom foods were eaten ( Bates, Lennox, Bates, & Swan, 2011 ). On behalf of the NDNS survey, the diaries were subjected by researchers at Human Nutrition Research, Cambridge University, to an analysis of nutrient intakes using specialist dietary recording and analysis software ( Bates et al., 2011 ; Data In Nutrients Out [DINO]).
Note: NDNS = National Diet and Nutrition Survey; HSE = Health Survey for England; ALSPAC = Avon Longitudinal Study of Pregnancy and Childhood.
Methodological challenges
These nutritional data proved challenging for us as social scientists to use, and they involved discussion with nutrition experts from within and outside Human Nutrition Research who created different dietary measures for the use of the team in the secondary analysis, thereby involving some interesting cross-disciplinary discussion. Working with nutritionists, we developed a unique diet quality index that compared intakes for children in different age ranges to national guidelines, giving an overall diet “score”—a composite measure that could be used to sample children from the survey and also as an outcome measure in the regression analysis described earlier, which set out to answer the first research question on the relationship between maternal employment and children’s dietary intakes ( Simon, O’Connell, & Stephen, 2012 ). While the usefulness of the diet data was constrained by the fact that no data had been collected about mothers’ working hours (nor indeed maternal education, an important cofounder), an important impact of our study has been to have these added to the annual survey from 2015 to increase the study’s usefulness to social science and social policy.
As noted, another aim of using the NDNS was to help us draw a purposive subsample of children ( N = 48) in which cases of children with healthier and less healthy diets were equally represented (as well as to select the sample on demographic variables). However, we encountered a challenge because of the small number of children in the age range in which we were interested; we thus had to include a wider age range of children (1.5–10 years) than would have been ideal.
We also sought to link the quantitative diary data from NDNS with the ethnographic and interview data from parents and children concerning their reported food practices. However, while the NDNS dietary data and the diary method used are considered the “gold standard” in dietary surveys ( Stephen, 2007 ), they were less useful for us at the level of the qualitative sample, and in practice this proved not to be feasible. First, the scores were based on dietary data collected over a single brief period of time (four days; Bates et al., 2011 ). Also, the whole survey was conducted over an extended time period (one year), with a mixture of weekdays and weekend days surveyed, which was unproblematic at the aggregate level. However, at the individual level, it was clear that these four days were not generalizable to dietary intakes over a longer time period. One parent in the qualitative study, for example, said the diary had been collected over a weekend break in Scotland where the family had indulged in holiday eating, including plenty of chips. In addition, since the data we had was about nutrient intakes—we did not have the resources (time or expertise) to examine the raw diary data, which could potentially have been provided—we had no idea what children were actually eating. Furthermore, there was a time lag between when the diet data were collected and when we did the fieldwork for the qualitative study (around six months later). We could have waited for all the NDNS data to be cleaned and analyzed, which would have given us additional information about children’s food intakes (e.g., their consumption of fruit and vegetables), but this would have caused a far greater time delay in starting the qualitative study. Given the rapidity with which children in the younger age range change their preferences and habits, the diet data would then have been “out of date” by the time we conducted qualitative interviews. Our decision to construct a diet quality index was therefore a pragmatic one, largely determined in practice by the data available to us within a reasonable time from the diary data collection—those provided as feedback to the study participants after the NDNS visits. 2
As we were also interested in the foods children were eating, we asked parents and children in the qualitative interviews to describe what they ate on the last weekday and on the last weekend day and about typicality, rather than repeating the diet diaries, which would have been highly resource intensive. Mothers were also asked to assess their children’s diets. We could not compare mothers’ descriptions and assessments of their child’s diet with diaries since we did not have access to the latter. However, in comparing these assessments with the child’s NDNS diet score there appeared in some cases to be corroboration, while others appeared to bear no relation. Indeed, some of the apparently “worst” cases, according to mothers’ assessments, did not necessarily have scores suggesting poor diets. Although hours of employment were asked in the qualitative study, no patterns were found in these data between hours of employment or other characteristics such as social class. 3 In analyzing other research questions about patterns of family meals and child versus adult control, for example, diet scores did not generally appear to be related to patterns found in the qualitative data. This may have been explained by the small sample or by lack of validity of the diet data at the individual level or by changes in children’s diet between the survey and qualitative study. In a follow-up qualitative study we are conducting with families two years later we will be able to compare analyses over two time points using the same questions put to parents and children about children’s food and eating.
In terms of linking dietary data in a MMMR design, the NDNS survey data suffered from a number of limitations. Even though we set aside our particular epistemological assumptions, theoretical interests, and research objectives, which were different from those of the survey, these affected the integration of the data. The usefulness of the NDNS data for addressing the research questions at the aggregate and individual level was questionable, notably the lack of data on the working hours of mothers and the difficulties of accessing detailed diary data collected at one time point as an individual measure of nutrition. The NDNS had rather small numbers of the groups in which we were interested, which compounded the selection of the qualitative subsample. There were also issues concerning the employment of different methods at different time points; this was especially challenging methodologically given the focus on younger children’s food tastes and diets, which can change dramatically within a short period of time. A further issue concerned conceptualization across different data sets, in particular relating to issues around food practices such as healthy eating. As noted, in the case of the survey data composite, measures of children’s nutrient intake at a particular moment in time were created using food diary data, and the measurements were then compared to national guidelines. In contrast, in the qualitative study latitude was given to parents to make their own judgments about their child’s diet from their perspectives while we were able to compare what parents reported at two time points and so take a longer term view. Therefore in integrating and interpreting both sets of data, we wrestled with the epistemological and ontological assumptions that underpinned the study’s main research questions concerning the meaning and significance of food and our own expectations about the kinds of “essential” sociodemographic data that we would expect any survey to collect.
Nonetheless, we had to overcome these tensions and demonstrate the societal impact of research that focused on an emotive and politically sensitive topic—maternal employment and diets of young children. In practice, the study’s outputs remained divided by approach, with papers drawing on mainly qualitative ( Brannen, O’Connell, & Mooney, 2013 ; Knight, O’Connell, & Brannen, 2014 ; O’Connell & Brannen, 2014 ) or quantitative ( Simon et al., forthcoming ) findings or describing methodological approaches (e.g., Brannen & Moss, 2013 ; O’Connell, 2013 ; Simon et al., 2012 ).
Similar Units of Analysis in a Multimethod and Mixed Methods Research Longitudinal Design: Examining the Division of Household Food Work
Mothers’ employment is only one small part of the picture of how food and work play out in households in which there are children. UK evidence suggests that men are more likely to cook regularly and share responsibility for feeding the family when women are absent, usually because of paid employment. However, this research was conducted some time ago (e.g., Warde & Hetherington, 1994 ).
The analysis
The more recent evidence that we analyzed is from the NDNS and the 40,000 UK Household Panel study called Understanding Society. The NDNS (Year 1: 2008/2009) survey findings suggest that mothers are the “main food providers” in 93% of families with a child 18 months to 10 years, with no significant differences according to work status or social class. Data from Understanding Society (Wave 2: 2010/2011) provide data on parental hours of work (10,236 couples with a child age zero to 14 years). Our secondary analysis of these data suggests that mothers working part-time are significantly less likely to share cooking with their partners, compared with mothers working full-time (but not those working 48 or more hours per week). 4 Complementing this, the secondary analysis also found that, in general, the longer the hours worked by a father, the less likely he was to share cooking with his spouse or partner.
In the qualitative study we asked questions (mainly to mothers) about who took charge of the food work, including cooking and food shopping. At the follow-up study (Wave 2) we asked about whether this was the same/had changed, whether children were encouraged to do more as they got older, and how the participants felt about how food work was shared. In their responses, the participants, usually mothers, mentioned other aspects of food work such as planning for meals, washing up, and loading the dishwasher. In classifying the cases, we drew on DeVault’s (1991) concept of “domestic food provisioning” and did not limit food work to cooking but also included shopping, cleaning up, and less visible aspects such as meal planning and feeling “responsible” for children’s diets. (At Wave 2 we asked a question about which parent worried more about the target child’s diet, thus eliciting responses about “responsibility.”)
The methodological challenges
The treatment of the households as cases was intrinsic to the way we approached the qualitative analysis. We plotted the households according to the level of fathers’ involvement in food provisioning on a continuum. This resulted in a more refined analysis compared with the quantitative data analysis (in the UK panel study Understanding Society). It enabled us to identify features of family life, not only mothers’ and fathers’ working hours—albeit these were mentioned most often—which were important in explaining the domestic division of food work ( Metcalfe, Dryden, Johnson, Owen, & Shipton, 2009 , pp. 109–111). Moreover, because we investigated the division of food work over time (two years), we were also able to explore continuities and discontinuities at the household level. Parents accounted for changes in the division of food work accordingly: a mother becoming ill, moving house, the birth of an additional child, loss of energy, children being older and easier to cook for, the loss of other help, and health concerns. We found, therefore, that patterns within households do change, with some fathers doing more food work and some doing less in response to circumstances in their lives (within and beyond the household), albeit only a minority do equal amounts or more. The conceptual approach that was adopted included a focus on routine practices and on accounting for practices to help shift the gaze away from a narrow behavioral “working hours perspective” toward understanding how family (food) practices are influenced by the interpenetration of public and private spheres (home and workplace) and how people make sense of (and thus reproduce or redefine) patterns of paid and unpaid work. Food practices, like other family practices, are shaped by gendered cultural expectations about motherhood and fatherhood—what it means to be “a good mother” and “a good father”—as well as by material constraints of working hours.
In addition to providing a more refined analysis than the quantitative data, the qualitative data also provided a way of examining outliers or cases that did not fit the general pattern shown in the survey results (according to Caracelli & Greene’s [1993] categories described earlier). Although the general trend was for a man to share cooking more when his spouse worked longer hours and to do less sharing when he worked longer hours, the qualitative data provided examples of where this did not fit (as well as where it did). For example, in one case, a father worked fewer hours than his wife but did almost no food work as he was said by his wife to lack competence, while another father who worked longer hours took most responsibility for food work as this fitted with his and his wife’s shift patterns.
In addressing the research question of how parental employment influences the division of food work, the use of both the survey data and the qualitative material together proved relatively successful since the unit of analysis in both referred to behavior. To some extent the MMMR approach provided corroborating evidence while the qualitative material refined and elaborated on the quantitative analysis. Broadly, the results were comparable and complementary, albeit the research questions relating to each method were somewhat different; notably in the qualitative study, there was a concern to understand the respondents’ accounts for the division of food work and to examine food work in the context of the families more holistically and the meaning of mothering and fathering more generally. By contrast, in the survey, food work was conceptualized behaviorally and broken down into constituent “tasks” such as cooking (cf. DeVault, 1991 ).
Concepts and Meaning in a Multimethod and Mixed Methods Research Longitudinal Design: The Study of Family Meals
Studies have identified an association between frequency of “family meals” and children’s and adolescents’ body mass index, nutritional status, social well-being, and physical and mental health. These studies suggest that children who eat fewer family meals have poorer health, nutrition, and behavioral outcomes than those who eat more meals with their families (e.g., Neumark-Sztainer, Hannon, Story, Croll, & Perry, 2003 ). Some longitudinal research implies causality rather than mere association and that family meals are “protective” against a range of less optimal nutritional and psychosocial outcomes, especially for girls (e.g., Neumark-Sztainer et al., 2003 ). There is widespread agreement about the reduced frequency of eating dinner as a family as children age (e.g., Gilman et al., 2000). Some studies also find an association with socioeconomic status and mothers’ paid employment (e.g., Neumark-Stzainer et al., 2003 ). In Wave 1 of the study we wanted to examine via the qualitative data set the relationship between children’s participation in family meals and their parents’ employment to establish whether maternal employment seemed important in explaining the social dimension of children’s eating practices (in this case meals). We asked about eating patterns on the previous work and nonwork day and their typicality. We also asked about the presence of different family members on different days of the week.
These questions enabled us to develop a typology of eating patterns in the working week: eating together most days, the modified family meal in which children ate with one parent, and a third situation in which eating together never occurred. In addition we asked what participants understood by the term family meal and whether family meals were important to them. Most people suggested that family meals were important, but fewer managed to eat them on most working days. We drew on the concept of synchronicity to shed light on how meals and mealtimes were coordinated in family life and the facilitators and constraints on coordination ( Brannen et al., 2013 ). We found that whether families ate together during the week, on the weekend only, or more rarely was not only influenced by parents’ work-time schedules but also by children’s timetables relating to their age and bodily tempos, their childcare regimes, their extracurricular activities, and the problem of coordinating different food preferences and tastes. While we did not report it, as the numbers were small, there was very little difference between the average diet score of children in each group (meals, no meals, and modified meals), which, as explained previously, is perhaps to be expected given that the differences within each group meant there were many factors involved.
At Wave 2 we aimed to extend this analysis by examining quantitatively the relationship between children eating family meals and sociodemographic variables (e.g., child age, maternal employment, social class) and nutritional intake at the aggregate level. To do so we aimed to explore a unique aspect of the archived NDNS data set, which has currently only been analyzed in one other study ( Mak et al., 2012 ). These data are “contextual” in relation to the food and nutrition data in that participants were asked as part of the food diaries to record, in relation to each eating occasion, not only what was eaten but also the time of eating, where, with whom, and whether the television was on ( Bates et al., 2011 ).
The main advantage of using these data was that, in contrast to dietary surveys that include a measure of “family meal frequency” and take the meaning of family meal for granted, these data were not collected by retrospective self-reports. Given the normative status of “the family meal,” as set out in the sociological literature (e.g., Jackson, Olive, & Smith, 2009 ; Murcott, 1997 , 2010 ) and our own qualitative study (Wave 1), we thought that these data were advantageous. 5 In addition, since the NDNS contains information about overall dietary intake, we could link family meal frequency to diet quality using our score. However, there were also disadvantages, namely that the sociodemographic data (especially maternal education and hours of employment) had not been collected. There were also other methodological challenges for the team, specifically in designing an operationalizable definition of a family meal. In short, we had to define “what is a meal?” and “what is a family?” in relation to the data available. This was limited by the following factors, among others. First, in relation to the variable “who eaten with,” we found little within-group variation in that most children were not reported as eating alone. While we thought it feasible to create a dichotomous variable—family/not family—decisions about how to do this were tricky given the data had been coded into categories that were not mutually exclusive (“alone,” “family,” “friend’s,” “parent(s)/care-provider,” “siblings,” “parent(s)/care-provider & siblings,” “care-provider & other children,” “other”). Second, the number of possible eating occasions throughout the day was considerable, involving consideration of which “time slot” to examine for a possible “family meal.” We opted to look at the family evening meal, as this is implied if not explicitly spelled out in popular understanding and in research about family meals, and, furthermore, we had established that 5 pm to 8 pm was the most common time slot for children’s eating in the NDNS data. However, we were aware that this might exclude some younger children who might eat earlier.
Other problems not limited to this data set were that we could not know from these data whether those present were actually eating with the child or whether they were eating the same foods (both of which are thought to be potentially important in explaining any association between family meal frequency and children’s and adolescents’ overall dietary intakes; (e.g., Centre for Research on Families and Relationships, 2012 ; Skafida, 2013 ).
In operationalizing family meals in the NDNS data set, we therefore recognized that we had moved away somewhat from the idea of a family meal as held by most people. While we were avoiding the problem of asking participants to define this themselves, we were creating new problems in that any conclusions would not relate to family meals as they are popularly understood but would rather relate to very particular practices—a child eating between 5 pm and 8 pm with an adult member of his or her family present (or not).
Thus in examining the topic of family meals via a MMMR design, we sought through the qualitative data to explore similar issues to those examined in the quantitative data, namely to determine the frequency of parental presence and child presence at family mealtimes. However, we were also able to tease out whether children and parents were both eating and whether they were eating the same foods and the conditions under which they did and did not do so ( Brannen et al., 2013 ). The qualitative study also provided insight into the symbolic and moral aspects surrounding the concept of family meals as well as practices of eating together, while in the analysis of the quantitative data set the onus was on us to define what constituted eating together.
Given the risk inherent in the experimental nature of our analysis of NDNS data and the political appetite for statistical findings, we sought also to analyze participation in family meal frequency and sociodemographic factors in two other UK large-scale surveys that have asked about children and adults eating together: the Millennium Cohort Survey and Understanding Society. Since these were not dietary surveys, we could not examine associations of self-reported family meal frequency with diet outcomes, but we could examine the relationship with factors such as hours of maternal employment. Albeit the data were limited by their reliance on self-report based on the assumption of a shared understanding of the concept of family meal as outlined earlier, in combining results with findings from our complementary analyses of the qualitative data and the NDNS “contextual data” we hope to foreground the importance of methodology and highlight the complexities of measuring children’s participation in family meals and any association with sociodemographic factors or health and behavioral outcomes.
In disrupting common sense and taken for granted assumptions about the association between family meals and other factors such as mothers’ work and children’s overall diets, our findings based on applying a MMMR approach—while unsettling in the sense of raising methodological uncertainties—speak directly to political and policy concerns in that they caution against the idea that family meals are some sort of “magic bullet,” although they are a convenient way for politicians to (dis)place responsibility for children’s food intake onto parents ( O’Connell & Simon, 2013 ; Owen, 2013 ).
We hope we have demonstrated some of the benefits as well as the methodological issues in multimethod research. In particular it is important to take into account that quantitative and qualitative methods each suffer from their own biases and limitations. Survey diary data, while advantageous in measuring behaviors (e.g., what children ate), have the disadvantage that they do not address issues of meaning (in the previous example concerning the study of family meals). Qualitative methods, while providing contextual and symbolic meanings (about food and meals, for example), may not provide detailed information about what is eaten and how much.
However, the combination of these methods may not provide a total solution either, as we have demonstrated with particular reference to our own study of food and families. Qualitative and quantitative methods may not together succeed in generating the knowledge that the researcher is seeking. In researching everyday, taken-for-granted practices, both methods can suffer from similar disadvantages. In surveys, practices may not easily be open to accurate recall or reflection. Likewise, qualitative methods, even when a narrative approach is adopted, may not produce recall but instead provoke normative accounts or justifications. While survey data can provide a “captive” sample for a qualitative study and the opportunity to analyze extensive contextual data about that sample, the two data samples may not be sufficiently comparable. Survey data conducted at one moment in time may not connect with qualitative data when they are collected at another time point (this is critical, for example, in studying children’s diets). Thus it may be necessary to build resources into the qualitative phase of the inquiry for reassessing children’s diet using a method based on that adopted in the survey. This may prove costly and require bringing in the help of nutritionists.
Many social practices are clothed in moral discourses and are thereby difficult to study by whatever method. Surveys are renowned for generating socially acceptable answers, but in interviews respondents may not want to admit that they do not measure up to normative ideals. In discussing methodological issues in MMMR, it is all too easy to segment qualitative and quantitative approaches artificially ( Schwandt, 2005 ). As already stressed, MMMR can provide an articulation between different theoretical levels as in macro, meso, and micro contexts. However, these theoretical levels typically draw on different logics of interpretation and explanation, making it necessary to show how different logics can be integrated ( Kelle, 2001 ). Moreover, we should not adopt a relativist approach but continue to subject our findings to scrutiny in order to draw fewer false conclusions.
Translating research questions across different methods of data collection may involve moving between different epistemologies and logics of inquiry and is likely to affect the data and create problems of interpretation, as has been discussed in the example of families and food. Quantitative and qualitative analyzes do not necessarily map on to each other readily; they may be based on different forms of explanation. While sometimes they may complement one another, in other instances analyzes are dissonant. However, we should also expect that findings generated by different methods (or conducted at different points in time) do not match up. It is, for example, one thing to respond to an open-ended question in a face-to-face interview context and quite another to tick an item from a limited set of alternatives in a self-completion questionnaire.
Given the complexities of linking quantitative and qualitative data sets, we suggest a narrative approach be adopted in reporting the data analyses. By this we mean that when writing up their results, researchers should give attention to the ways in which the data have been integrated, the issues that arise in interpreting the different data both separately and in combination, and how the use of different methods have benefited or complicated the process. This is particularly important where MMMR is carried out in a policy context so that policymakers and other stakeholder groups may be enlightened about the caveats associated with different types of data and, in particular, the advantages and issues of employing more than one type of research methodology (typically quantitative data are preferred by policymakers; Brannen & Moss, 2013 ).
In addition, the researcher should be attentive to the ways in which the processes of “translation” involved in interpreting data are likely, often unwittingly, to reflect rather than reveal the contexts in which the research is carried out. Data have to be understood and interpreted in relation to the contexts in which the research is funded (by whom and for whom), the research questions posed, the theoretical frameworks that are fashionable at the time of study, and the methods by which the data are produced ( Brannen, 2005a , 2005b ).
Just as when we use or reuse archived data, it is important in primary research to take into account the broad historical and social contexts of the data and the research inquiry. All data analyses require contextualization, and whether this is part of a mixed method or multimethod research strategy, it is necessary to have recourse to diverse data sources and data collected in different ways. MMMR is not only a matter of strategy. It is not a tool-kit or a technical fix, nor is it a belt-and-braces approach. MMMR requires as much if not more reflexivity than other types of research. This means that researchers need to examine their own presumptions and preferences about different methods and the results that derive from each method and be open to shifting away from entrenched positions to which they cling—theoretical, epistemological, and methodological. At a practical level, the multimethod researcher should be prepared to learn new skills and engage with new communities of practice.
Future Directions
In the future, social science research is likely to become increasingly expensive. Primary research may also prove more difficult to do for other reasons, for example the restrictions imposed by ethics committees. Many researchers will have recourse to secondary analysis of existing contemporary data or turn to older archived data. MMMR research will therefore increasingly involve the analysis of different types of data rather than the application of different methods. For example, in our own work we have become increasingly engaged not only in examining data from different surveys but also in interrogating the assumptions that underlie the variables created in those surveys and reconciling (or not) these variables with new qualitative data we have collected.
Another possible trend that is likely to promote the use of MMMR is the growth in demand for interdisciplinary research that embraces disciplines beyond the social sciences. This trend will require even more emphasis on researchers working outside their traditional comfort zones and intellectual and methodological silos. For example, in our own research on families and children’s food practices we have been required to engage with nutritionists.
A third trend concerns the growing external pressure on social scientists to justify the importance of their work to society at large, while from within social sciences there is pressure on them to reassert the role of social science as publically and politically engaged. In the UK, we are increasingly required by funding bodies—research councils and government—and by universities to demonstrate the societal “impact” of our research. Part and parcel of this mission is the need to demonstrate the credibility of our research findings and to educate the wider world about the rigor and robustness of our methods. In order to understand the conditions of society in a globalized world, it will indeed be necessary to deepen, develop, and extend our methodological repertoire. MMMR is likely to continue to develop an increasingly high profile in this endeavor.
Discussion Questions
Discuss the benefits and challenges of linking a qualitative sample to a survey.
Identify within a MMMR study a research question that can be addressed both by qualitative and quantitative methods and a research question that can be addressed via one method only; discuss some different ways of integrating the data from these methods.
Discuss two or three methodological strategies for integrating dissonant research results based on quantitative and qualitative data.
Create a research design for a longitudinal research project that employs MMMR as part of that design.
Suggested Websites
http://eprints.ncrm.ac.uk
UK’s National Centre of Research Methods website, where its “e-print” series of working and commissioned papers are found.
http://www.qualitative-research.net/fqs
Website of a European online qualitative journal, FQS.
The study titled Food Practices and Employed Families with Younger Children was funded as part of a special initiative funded by the UK’s Economic and Social Research Council and the UK’s Food Standards Agency (RES-190–25-0010) and subsequently with the Department of Health (ES/J012556/1). The current research team includes Charlie Owen and Antonia Simon (statisticians and secondary data analysts), the principal investigator Dr. Rebecca O’Connell (anthropologist), and Professor Julia Brannen (sociologist). Katie Hollinghurst and Ann Mooney were formerly part of the team (psychologists).
A “Dietary Feedback” report was provided to each individual participant about his or her own intake within 3 months of the diet diary being completed. This provided information about the individual intakes of fat, saturated fat, non-milk extrinsic sugars, dietary fiber (as non-starch polysaccharide), Vitamin C, folate, calcium, iron and energy (Table 15.1 ) relative to average intakes for each of these items for children in the UK, these being based on the results for children of this age from the NDNS conducted in the 1990s ( Gregory & Hinds, 1995 ; Gregory et al., 2000 ).
However at the aggregate level, secondary analysis of the NDNS for 2008–2010 ( National Centre for Social Research, Medical Research Council Resource Centre for Human Nutrition Research, & University College London Medical School, 2012 ), a combined data set of respondents from Year 1 (2008–2009) and Year 2 (2009–2010) found that social class and household income were related to children’s fruit and vegetable consumption and overall diet quality (as measured by our nutritional score; Simon et al., forthcoming ).
A feasible explanation for this finding is that mothers working these long hours are more likely to use paid or unpaid childcare in addition to sharing with a partner or spouse.
They avoid two key problems associated with self-report of family meals in the extant literature in which “family meals” are not usually defined by interviewers but by interviewees themselves (cf. Hammons & Fiese, 2011 ): that people will answer the same response but mean different things and, additionally, also because of the normativity surrounding family meals, some participants may overreport their participation in them. In short, such data seemed advantageous compared to poorly designed survey questions that are associated with known problems related to reliability, validity, and bias (cf. Hammons & Fiese, 2011 ).
Bates, B. , Lennox, A. , Bates, C. , & Swan, G. (Eds.). ( 2011 ). National Diet & Nutrition Survey: Headline results from Years 1 & 2 (combined) of the rolling programme 2008/9–2009/10. Appendix A. Dietary data collection and editing. London, England: Food Standards Agency and Department of Health.
Google Scholar
Google Preview
Blatchford, P. ( 2005 ). A multi-method approach to the study of school class size differences. International Journal of Social Research Methodology , 8 (3), 195–205.
Brannen, J. ( 1992 ). Mixing methods: Qualitative and quantitative research . Aldershot, UK: Ashgate.
Brannen, J. ( 2005 a). Mixed methods research: A discussion paper. NCRM Methods Review Papers NCRM/005. Southampton, UK: National Center for Research Methods. Retrieved from http://eprints.ncrm.ac.uk/89/
Brannen, J. ( 2005 b). Mixing methods: The entry of qualitative and quantitative approaches into the research process. International Journal of Social Research Methodology [Special Issue], 8 (3), 173–185.
Brannen, J. , & Moss, G. ( 2013 ). Critical issues in designing mixed methods policy research. American Behavioral Scientist , 7 , 152–172.
Brannen, J. , O’Connell, R. , & Mooney, A. ( 2013 ). Families, meals and synchronicity: Eating together in British dual earner families. Community, Work and Family , 16 (4), 417–434. Retrieved from http://www.tandfonline.com/doi/abs/10.1080/13668803.2013.776514 doi:10.1080/13668803.2013.776514
Bryman, A. ( 2008 ). Why do researchers integrate/combine/mesh/blend/mix/merge/fuse quantitative and qualitative research? In M. Bergman (Ed.), Advances in Mixed methods research: Theories and applications (pp. 87–100). London, England: Sage.
Caracelli, V.J. , & Greene, J. ( 1993 ). Data analysis strategies for mixed-method evaluation designs. Educational Evaluation and Policy Analysis , 15 (2), 195–207.
Centre for Research on Families and Relationships. ( 2012 ). Is there something special about family meals? Exploring how family meal habits relate to young children’s diets. Briefing 62. Edinburgh, UK: Author. Retrieved from http://www.era.lib.ed.ac.uk/bitstream/1842/6554/1/briefing%2062.pdf
Crepinsek, M. , & Burstein, N. ( 2004 ). Maternal employment and children’s nutrition: Vol. 2. Other nutrition-related outcomes . Washington, DC: Economic Research Service, US Department of Agriculture.
Denscombe, M. ( 2008 ). Communities of practice: A research paradigm for the mixed method approach. Journal of Mixed Methods Research , 2 , 270–284.
Denzin, N. , & Lincoln, Y. (Eds.). ( 2005 ). The SAGE handbook of qualitative research . Thousand Oaks, CA: Sage.
DeVault, D. L. ( 1991 ). Feeding the family: The social organization of caring as gendered work . Chicago, IL: University of Chicago Press.
Dewey, J. ( 1991 ). The pattern of inquiry. In J. Dewey (Ed.), The later works (pp. 105–123). Carbondale: Southern University Illinois Press.
Ettlinger, N. ( 2007 ). Precarity unbound. Alternatives , 32 , 319–340.
Gillman, M. W. , S. L. Rifas-Shiman , A. Lindsay Frazier , H. R. H. Rockett , C. A. Camargo Jr ., A. E. Field , . . . G. A. Colditz . ( 2000 ). Family dinner and diet quality among older children and adolescents. Archives of Family Medicine , 9 , 235–240.
Glueck, S. , & Glueck, E. ( 1950 ). Unravelling juvenile delinquency . New York, NY: Commonwealth Fund.
Glueck, S. , & Glueck, E. ( 1968 ). Delinquents and nondelinquents in perspective . Cambridge, MA: Harvard University Press.
Greene, J. , Caracelli, V. J. , & Graham, W. F. ( 1989 ). Towards a conceptual framework for mixed-method evaluation designs. Education , Evaluation and Policy Analysis , 11 (3), 255–274.
Gregory J. R. , & Hinds K. ( 1995 ). National Diet and Nutrition Survey: Children aged 1 1⁄2 to 4 1⁄2 Years . London, England: Stationery Office.
Gregory, J. R. , Lowe S. , Bates, C. J. , Prentice, A. , Jackson, L. V. , Smithers, G. , . . . Farron, M. ( 2000 ). National Diet and Nutrition Survey: Young people aged 4 to 18 years: Vol. 1. Report of the diet and nutrition survey . London, England: Stationery Office.
Hammersley, M. ( 2000 ). Varieties of social research: A typology. International Journal of Social Research Methodology , 3 (3), 221–231.
Hammersley, M. ( 2005 ). The myth of research-based practice: The critical case of educational inquiry. International Journal for Multiple Research Approaches , 8 (4), 317–331.
Hammersley, M. ( 2008 ). Troubles with triangulation. In M. Bergman (Ed.), Advances in mixed methods research: Theories and applications (pp. 22–37). London, England: Sage.
Hammond, C. ( 2005 ). The wider benefits of adult learning: An illustration of the advantages of multi-method research. International Journal of Social Research Methodology , 8 (3), 239–257.
Hammons, A. , & Fiese, B. ( 2011 ). Is frequency of shared family meals related to the nutritional health of children and adolescents? Pediatrics , 127 (6), e1565–e1574.
Harrits, G. ( 2011 ). More than method? A discussion of paradigm differences within mixed methods research. Journal of Mixed Methods Research , 5 (2), 150–167.
Hawkins, S. , Cole, T. , & Law, C. ( 2009 ). Examining the relationship between maternal employment and health behaviours in 5-year-old British children. Journal of Epidemiology and Community Health , 63 (12), 999–1004.
Jackson, P. Olive, S. , & Smith, G. ( 2009 ). Myths of the family meal: Re-reading Edwardian life histories. In P. Jackson (Ed.), Changing families, changing food , (pp. 131–145). Basingstoke, UK: Palgrave Macmillan.
Jick, T. ( 1979 ). Mixing qualitative and quantitative methods: Triangulation in action. Administrative Science Quarterly , 24 , 602–611.
Kelle, U. ( 2001 ). Sociological explanations between micro and macro and the integration of qualitative and quantitative methods. FQS , 2 (1). Retrieved from http://www.qualitative-research.net/fqs-eng.htm
Knight, A. , O’Connell, R. , & Brannen, J. ( 2014 ). The temporality of food practices: Intergenerational relations, childhood memories and mothers’ food practices in working families with young children. Families , Relationships and Societies , 3 (2), 303–318.
Laub, J. , & Sampson, R. ( 1993 ). Turning points in the life course: Why change matters to the study of crime, Criminology , 31 , 301–325.
Laub, J. , & Sampson, R. ( 1998 ). Integrating qualitative and quantitative data. In J. Giele & G. Elder (Eds.), Methods of life course research: Qualitative and quantitative approaches (pp. 213–231). London, England: Sage.
Law, J. ( 2004 ). After method: Mess in social science research . New York, NY: Routledge.
Mak, T. , Prynne, C. , Cole, D. , Fitt, E. , Roberts, C. , Bates, B. , & Stephen, A. ( 2012 ). Assessing eating context and fruit and vegetable consumption in children: New methods using food diaries in the UK National Diet and Nutrition Survey Rolling Programme. International Journal of Behavioral Nutrition and Physical Activity , 9 , 126.
Mason, J. ( 2006 ). Mixing methods in a qualitatively driven way,” Qualitative Research , 6 (1), 9–26.
Metcalfe, A. , Dryden, C. , Johnson, M. , Owen, J. , & Shipton, G. ( 2009 ). Fathers, food and family life. In P. Jackson (Ed.), Changing families, changing food . Basingstoke, UK: Palgrave Macmillan.
McIntosh, A. , Davis, G. , Nayga, R. , Anding, J. , Torres, C. , Kubena, K. , . . . You, W. ( 2008 ). Parental time, role strain, and children’s fat intake and obesity-related outcomes . Washington, DC: US Department of Agriculture, Economic Research Service.
Morgan, D. ( 2007 ). Paradigms lost and pragmatism regained; Methodological implications of combining qualitative and quantitative methods. Journal of Mixed Methods Research , 1 (1), 48–76.
Murcott, A. ( 1997 ). Family meals—A thing of the past? In P. Caplan (Ed.), Food, identity and health (pp. 32–49). London, England: Routledge.
Murcott, A. ( 2010 , March 9). Family meals: Myth, reality and the reality of myth . Myths and Realities: A New Series of Public Debates. London, England: British Library.
National Centre for Social Research, Medical Research Council Resource Centre for Human Nutrition Research, & University College London Medical School. ( 2012 ). National Diet and Nutrition Survey, 2008–2010 [Computer file]. 3rd ed. Essex, UK: UK Data Archive [distributor]. Retrieved from http://dx.doi.org/10.5255/UKDA-SN-6533-1
Neumark-Sztainer, D. , Hannon, P. J. , Story, M. , Croll, J. , & Perry, C. , ( 2003 ). Family meal patterns: Associations with socio-demographic characteristics and improved dietary intake among adolescents. Journal of the American Dietetic Association , 103 , 317–322.
Nilsen, A. , & Brannen, J. ( 2010 ). The use of mixed methods in biographical research. In A. Tashakorri & C. Teddlie (Eds.), SAGE handbook of mixed methods research in social & behavioral research (2nd ed., pp. 677–696). London, England: Sage.
O’Cathain, A. ( 2009 ). Reporting results. In S. Andrew & E. Halcomb (Eds.), Mixed methods research for nursing and the health sciences (pp. 135–158). Oxford, UK: Blackwell.
O’Connell, R. ( 2013 ). The use of visual methods with children in a mixed methods study of family food practices. International Journal of Social Research Methodology , 16 (1), 31–46.
O’Connell, R. , & Brannen, J. ( 2014 ). Children’s food, power and control: Negotiations in families with younger children in England. Childhood , 21 (1), 87–102.
O’Connell, R. , & Brannen, J. ( 2015 ). Food, Families and work . London, England: Bloomsbury.
O’Connell, R. , Brannen, J. , Mooney, A. , Knight, A. , & Simon, A. (2011). Food and families who work: A summary. Available at http://www.esrc.ac.uk/my-esrc/grants/RES-190-25-0010/outputs/Read/e7d99b9f-eafd-4650-9fd2-0efcf72b4555
O’Connell, R. , & Simon, A. (2013, April). A mixed methods approach to meals in working families: Addressing lazy assumptions and methodologies difficulties . Paper presented at the British Sociological Association Annual Conference: Engaging Sociology. London, England.
Omwuegbuzie, A. , & Leech, N. ( 2005 ). On becoming a pragmatic researcher: The importance of combining quantitative and qualitative research methodologies. International Journal of Social Research Methodology , 8 (5), 375–389.
Owen, C. (2013, July). Do the children of employed mothers eat fewer “family meals”? Paper presented at the Understanding Society Conference, University of Essex.
Reiss, A. L. ( 1968 ). Stuff and nonsense about social surveys and participant observation. In H. L. Becker , B. Geer , D. Riesman , & R. S. Weiss (Eds.), Institutions and the person: Papers in memory of Everett C. Hughes (pp. 351–367). Chicago, IL: Aldine.
Sammons, P. , Siraj-Blatchford, I. , Sylva, K. , Melhuish, E. , Taggard, B. , & Elliot, K. ( 2005 ). Investigating the effects of pre-school provision: Using mixed methods in the EPPE research. International Journal of Social Research Methodology , 8 (3), 207–224.
Schwandt, T. ( 2005 ). A diagnostic reading of scientifically based research for education. Educational Theory , 55 , 285–305.
Seltzer-Kelly, D. , Westwood, D. , & Pena-Guzman, M. ( 2012 ). A methodological self-study of quantitizing: Negotiated meaning and revealing multiplicity. Journal of Mixed Methods Research , 6 (4), 258–275.
Simon, A. , O’Connell, R. , & Stephen, A. M. ( 2012 ). Designing a nutritional scoring system for assessing diet quality for children aged 10 years an under in the UK. Methodological Innovations Online , 7 (2), 27–47.
Simon, A. , Owen, C. , O’Connell, R. , & Stephen, A. ( forthcoming ). Exploring associations between children’s diets and maternal employment through an analysis of the UK National Diet and Nutrition Survey.
Skafida, V. ( 2013 ). The family meal panacea: Exploring how different aspects of family meal occurrence, meal habits and meal enjoyment relate to young children’s diets. Sociology of Health and Illness , 25 (6), 906–923. doi:10.1111/1467-9566.12007
Stephen, A. ( 2007 ). The case for diet diaries in longitudinal studies. International Journal of Social Research Methodology , 10 (5), 365–377.
Tashakorri, A. , & Creswell, J. ( 2007 ). Exploring the nature of research questions in mixed methods research. Journal of Mixed Methods Research , 1 (3), 207–211.
Woolley, C. ( 2009 ). Meeting the methods challenge of integration in a sociological study of structure and agency. Journal of Mixed Methods Research , 3 (1), 7–26.
Yin, R. ( 2006 ). Mixed methods research: Are the methods genuinely integrated or merely parallel? Research in the Schools , 13 (1), 41–47.
Warde, A. , & Hetherington, K. ( 1994 ). English households and routine food practices: A research note. Sociological Review , 42 (4), 758–778.
- About Oxford Academic
- Publish journals with us
- University press partners
- What we publish
- New features
- Open access
- Institutional account management
- Rights and permissions
- Get help with access
- Accessibility
- Advertising
- Media enquiries
- Oxford University Press
- Oxford Languages
- University of Oxford
Oxford University Press is a department of the University of Oxford. It furthers the University's objective of excellence in research, scholarship, and education by publishing worldwide
- Copyright © 2024 Oxford University Press
- Cookie settings
- Cookie policy
- Privacy policy
- Legal notice
This Feature Is Available To Subscribers Only
Sign In or Create an Account
This PDF is available to Subscribers Only
For full access to this pdf, sign in to an existing account, or purchase an annual subscription.
Have a language expert improve your writing
Run a free plagiarism check in 10 minutes, generate accurate citations for free.
- Knowledge Base
Methodology
Research Methods | Definitions, Types, Examples
Research methods are specific procedures for collecting and analyzing data. Developing your research methods is an integral part of your research design . When planning your methods, there are two key decisions you will make.
First, decide how you will collect data . Your methods depend on what type of data you need to answer your research question :
- Qualitative vs. quantitative : Will your data take the form of words or numbers?
- Primary vs. secondary : Will you collect original data yourself, or will you use data that has already been collected by someone else?
- Descriptive vs. experimental : Will you take measurements of something as it is, or will you perform an experiment?
Second, decide how you will analyze the data .
- For quantitative data, you can use statistical analysis methods to test relationships between variables.
- For qualitative data, you can use methods such as thematic analysis to interpret patterns and meanings in the data.
Table of contents
Methods for collecting data, examples of data collection methods, methods for analyzing data, examples of data analysis methods, other interesting articles, frequently asked questions about research methods.
Data is the information that you collect for the purposes of answering your research question . The type of data you need depends on the aims of your research.
Qualitative vs. quantitative data
Your choice of qualitative or quantitative data collection depends on the type of knowledge you want to develop.
For questions about ideas, experiences and meanings, or to study something that can’t be described numerically, collect qualitative data .
If you want to develop a more mechanistic understanding of a topic, or your research involves hypothesis testing , collect quantitative data .
You can also take a mixed methods approach , where you use both qualitative and quantitative research methods.
Primary vs. secondary research
Primary research is any original data that you collect yourself for the purposes of answering your research question (e.g. through surveys , observations and experiments ). Secondary research is data that has already been collected by other researchers (e.g. in a government census or previous scientific studies).
If you are exploring a novel research question, you’ll probably need to collect primary data . But if you want to synthesize existing knowledge, analyze historical trends, or identify patterns on a large scale, secondary data might be a better choice.
Descriptive vs. experimental data
In descriptive research , you collect data about your study subject without intervening. The validity of your research will depend on your sampling method .
In experimental research , you systematically intervene in a process and measure the outcome. The validity of your research will depend on your experimental design .
To conduct an experiment, you need to be able to vary your independent variable , precisely measure your dependent variable, and control for confounding variables . If it’s practically and ethically possible, this method is the best choice for answering questions about cause and effect.
Prevent plagiarism. Run a free check.
Your data analysis methods will depend on the type of data you collect and how you prepare it for analysis.
Data can often be analyzed both quantitatively and qualitatively. For example, survey responses could be analyzed qualitatively by studying the meanings of responses or quantitatively by studying the frequencies of responses.
Qualitative analysis methods
Qualitative analysis is used to understand words, ideas, and experiences. You can use it to interpret data that was collected:
- From open-ended surveys and interviews , literature reviews , case studies , ethnographies , and other sources that use text rather than numbers.
- Using non-probability sampling methods .
Qualitative analysis tends to be quite flexible and relies on the researcher’s judgement, so you have to reflect carefully on your choices and assumptions and be careful to avoid research bias .
Quantitative analysis methods
Quantitative analysis uses numbers and statistics to understand frequencies, averages and correlations (in descriptive studies) or cause-and-effect relationships (in experiments).
You can use quantitative analysis to interpret data that was collected either:
- During an experiment .
- Using probability sampling methods .
Because the data is collected and analyzed in a statistically valid way, the results of quantitative analysis can be easily standardized and shared among researchers.
Receive feedback on language, structure, and formatting
Professional editors proofread and edit your paper by focusing on:
- Academic style
- Vague sentences
- Style consistency
See an example
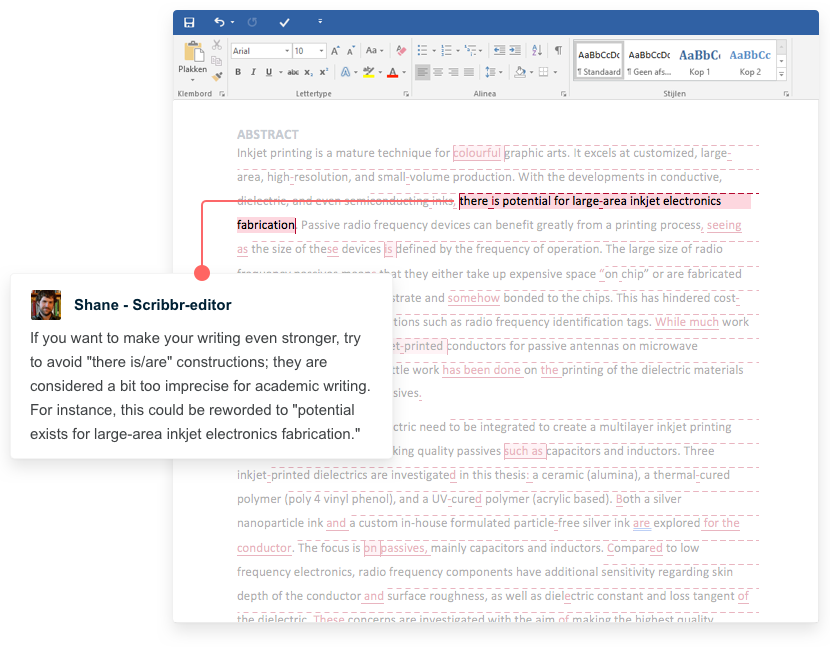
If you want to know more about statistics , methodology , or research bias , make sure to check out some of our other articles with explanations and examples.
- Chi square test of independence
- Statistical power
- Descriptive statistics
- Degrees of freedom
- Pearson correlation
- Null hypothesis
- Double-blind study
- Case-control study
- Research ethics
- Data collection
- Hypothesis testing
- Structured interviews
Research bias
- Hawthorne effect
- Unconscious bias
- Recall bias
- Halo effect
- Self-serving bias
- Information bias
Quantitative research deals with numbers and statistics, while qualitative research deals with words and meanings.
Quantitative methods allow you to systematically measure variables and test hypotheses . Qualitative methods allow you to explore concepts and experiences in more detail.
In mixed methods research , you use both qualitative and quantitative data collection and analysis methods to answer your research question .
A sample is a subset of individuals from a larger population . Sampling means selecting the group that you will actually collect data from in your research. For example, if you are researching the opinions of students in your university, you could survey a sample of 100 students.
In statistics, sampling allows you to test a hypothesis about the characteristics of a population.
The research methods you use depend on the type of data you need to answer your research question .
- If you want to measure something or test a hypothesis , use quantitative methods . If you want to explore ideas, thoughts and meanings, use qualitative methods .
- If you want to analyze a large amount of readily-available data, use secondary data. If you want data specific to your purposes with control over how it is generated, collect primary data.
- If you want to establish cause-and-effect relationships between variables , use experimental methods. If you want to understand the characteristics of a research subject, use descriptive methods.
Methodology refers to the overarching strategy and rationale of your research project . It involves studying the methods used in your field and the theories or principles behind them, in order to develop an approach that matches your objectives.
Methods are the specific tools and procedures you use to collect and analyze data (for example, experiments, surveys , and statistical tests ).
In shorter scientific papers, where the aim is to report the findings of a specific study, you might simply describe what you did in a methods section .
In a longer or more complex research project, such as a thesis or dissertation , you will probably include a methodology section , where you explain your approach to answering the research questions and cite relevant sources to support your choice of methods.
Is this article helpful?
Other students also liked, writing strong research questions | criteria & examples.
- What Is a Research Design | Types, Guide & Examples
- Data Collection | Definition, Methods & Examples
More interesting articles
- Between-Subjects Design | Examples, Pros, & Cons
- Cluster Sampling | A Simple Step-by-Step Guide with Examples
- Confounding Variables | Definition, Examples & Controls
- Construct Validity | Definition, Types, & Examples
- Content Analysis | Guide, Methods & Examples
- Control Groups and Treatment Groups | Uses & Examples
- Control Variables | What Are They & Why Do They Matter?
- Correlation vs. Causation | Difference, Designs & Examples
- Correlational Research | When & How to Use
- Critical Discourse Analysis | Definition, Guide & Examples
- Cross-Sectional Study | Definition, Uses & Examples
- Descriptive Research | Definition, Types, Methods & Examples
- Ethical Considerations in Research | Types & Examples
- Explanatory and Response Variables | Definitions & Examples
- Explanatory Research | Definition, Guide, & Examples
- Exploratory Research | Definition, Guide, & Examples
- External Validity | Definition, Types, Threats & Examples
- Extraneous Variables | Examples, Types & Controls
- Guide to Experimental Design | Overview, Steps, & Examples
- How Do You Incorporate an Interview into a Dissertation? | Tips
- How to Do Thematic Analysis | Step-by-Step Guide & Examples
- How to Write a Literature Review | Guide, Examples, & Templates
- How to Write a Strong Hypothesis | Steps & Examples
- Inclusion and Exclusion Criteria | Examples & Definition
- Independent vs. Dependent Variables | Definition & Examples
- Inductive Reasoning | Types, Examples, Explanation
- Inductive vs. Deductive Research Approach | Steps & Examples
- Internal Validity in Research | Definition, Threats, & Examples
- Internal vs. External Validity | Understanding Differences & Threats
- Longitudinal Study | Definition, Approaches & Examples
- Mediator vs. Moderator Variables | Differences & Examples
- Mixed Methods Research | Definition, Guide & Examples
- Multistage Sampling | Introductory Guide & Examples
- Naturalistic Observation | Definition, Guide & Examples
- Operationalization | A Guide with Examples, Pros & Cons
- Population vs. Sample | Definitions, Differences & Examples
- Primary Research | Definition, Types, & Examples
- Qualitative vs. Quantitative Research | Differences, Examples & Methods
- Quasi-Experimental Design | Definition, Types & Examples
- Questionnaire Design | Methods, Question Types & Examples
- Random Assignment in Experiments | Introduction & Examples
- Random vs. Systematic Error | Definition & Examples
- Reliability vs. Validity in Research | Difference, Types and Examples
- Reproducibility vs Replicability | Difference & Examples
- Reproducibility vs. Replicability | Difference & Examples
- Sampling Methods | Types, Techniques & Examples
- Semi-Structured Interview | Definition, Guide & Examples
- Simple Random Sampling | Definition, Steps & Examples
- Single, Double, & Triple Blind Study | Definition & Examples
- Stratified Sampling | Definition, Guide & Examples
- Structured Interview | Definition, Guide & Examples
- Survey Research | Definition, Examples & Methods
- Systematic Review | Definition, Example, & Guide
- Systematic Sampling | A Step-by-Step Guide with Examples
- Textual Analysis | Guide, 3 Approaches & Examples
- The 4 Types of Reliability in Research | Definitions & Examples
- The 4 Types of Validity in Research | Definitions & Examples
- Transcribing an Interview | 5 Steps & Transcription Software
- Triangulation in Research | Guide, Types, Examples
- Types of Interviews in Research | Guide & Examples
- Types of Research Designs Compared | Guide & Examples
- Types of Variables in Research & Statistics | Examples
- Unstructured Interview | Definition, Guide & Examples
- What Is a Case Study? | Definition, Examples & Methods
- What Is a Case-Control Study? | Definition & Examples
- What Is a Cohort Study? | Definition & Examples
- What Is a Conceptual Framework? | Tips & Examples
- What Is a Controlled Experiment? | Definitions & Examples
- What Is a Double-Barreled Question?
- What Is a Focus Group? | Step-by-Step Guide & Examples
- What Is a Likert Scale? | Guide & Examples
- What Is a Prospective Cohort Study? | Definition & Examples
- What Is a Retrospective Cohort Study? | Definition & Examples
- What Is Action Research? | Definition & Examples
- What Is an Observational Study? | Guide & Examples
- What Is Concurrent Validity? | Definition & Examples
- What Is Content Validity? | Definition & Examples
- What Is Convenience Sampling? | Definition & Examples
- What Is Convergent Validity? | Definition & Examples
- What Is Criterion Validity? | Definition & Examples
- What Is Data Cleansing? | Definition, Guide & Examples
- What Is Deductive Reasoning? | Explanation & Examples
- What Is Discriminant Validity? | Definition & Example
- What Is Ecological Validity? | Definition & Examples
- What Is Ethnography? | Definition, Guide & Examples
- What Is Face Validity? | Guide, Definition & Examples
- What Is Non-Probability Sampling? | Types & Examples
- What Is Participant Observation? | Definition & Examples
- What Is Peer Review? | Types & Examples
- What Is Predictive Validity? | Examples & Definition
- What Is Probability Sampling? | Types & Examples
- What Is Purposive Sampling? | Definition & Examples
- What Is Qualitative Observation? | Definition & Examples
- What Is Qualitative Research? | Methods & Examples
- What Is Quantitative Observation? | Definition & Examples
- What Is Quantitative Research? | Definition, Uses & Methods
Unlimited Academic AI-Proofreading
✔ Document error-free in 5minutes ✔ Unlimited document corrections ✔ Specialized in correcting academic texts
University Library, University of Illinois at Urbana-Champaign

Qualitative Data Analysis: Qualitative Data Analysis Strategies
- Atlas.ti web
- R for text analysis
- Microsoft Excel & spreadsheets
- Other options
- Planning Qual Data Analysis
- Free Tools for QDA
- QDA with NVivo
- QDA with Atlas.ti
- QDA with MAXQDA
- PKM for QDA
- QDA with Quirkos
- Working Collaboratively
- Qualitative Methods Texts
- Transcription
- Data organization
- Example Publications
Defining Strategies for Qualitative Data Analysis
Analysis is a process of deconstructing and reconstructing evidence that involves purposeful interrogation and critical thinking about data in order to produce a meaningful interpretation and relevant understanding in answer to the questions asked or that arise in the process of investigation (Bazeley, 2021, p. 3)
When we analyze qualitative data, we need systematic, rigorous, and transparent ways of manipulating our data in order to begin developing answers to our research questions. We also need to keep careful track of the steps we've taken to conduct our analysis in order to communicate this process to readers and reviewers.
Beyond coding, it is not always clear of what steps you should take to analyze your data. In this series of pages, I offer some basic information about different strategies you might use to analyze your qualitative data, as well as information on how you can use these strategies in different QDA software programs.
Useful Resources on QDA Strategies
QDA strategies in this guide
- Data Organization
Cited on this page
Bazeley, P. (2021). Qualitative data analysis: Practical strategies (2nd ed.). Sage.
- << Previous: Qualitative Methods Texts
- Next: Transcription >>
- Last Updated: Apr 5, 2024 2:23 PM
- URL: https://guides.library.illinois.edu/qualitative

An official website of the United States government
The .gov means it’s official. Federal government websites often end in .gov or .mil. Before sharing sensitive information, make sure you’re on a federal government site.
The site is secure. The https:// ensures that you are connecting to the official website and that any information you provide is encrypted and transmitted securely.
- Publications
- Account settings
Preview improvements coming to the PMC website in October 2024. Learn More or Try it out now .
- Advanced Search
- Journal List
- Indian J Anaesth
- v.60(9); 2016 Sep
Basic statistical tools in research and data analysis
Zulfiqar ali.
Department of Anaesthesiology, Division of Neuroanaesthesiology, Sheri Kashmir Institute of Medical Sciences, Soura, Srinagar, Jammu and Kashmir, India
S Bala Bhaskar
1 Department of Anaesthesiology and Critical Care, Vijayanagar Institute of Medical Sciences, Bellary, Karnataka, India
Statistical methods involved in carrying out a study include planning, designing, collecting data, analysing, drawing meaningful interpretation and reporting of the research findings. The statistical analysis gives meaning to the meaningless numbers, thereby breathing life into a lifeless data. The results and inferences are precise only if proper statistical tests are used. This article will try to acquaint the reader with the basic research tools that are utilised while conducting various studies. The article covers a brief outline of the variables, an understanding of quantitative and qualitative variables and the measures of central tendency. An idea of the sample size estimation, power analysis and the statistical errors is given. Finally, there is a summary of parametric and non-parametric tests used for data analysis.
INTRODUCTION
Statistics is a branch of science that deals with the collection, organisation, analysis of data and drawing of inferences from the samples to the whole population.[ 1 ] This requires a proper design of the study, an appropriate selection of the study sample and choice of a suitable statistical test. An adequate knowledge of statistics is necessary for proper designing of an epidemiological study or a clinical trial. Improper statistical methods may result in erroneous conclusions which may lead to unethical practice.[ 2 ]
Variable is a characteristic that varies from one individual member of population to another individual.[ 3 ] Variables such as height and weight are measured by some type of scale, convey quantitative information and are called as quantitative variables. Sex and eye colour give qualitative information and are called as qualitative variables[ 3 ] [ Figure 1 ].

Classification of variables
Quantitative variables
Quantitative or numerical data are subdivided into discrete and continuous measurements. Discrete numerical data are recorded as a whole number such as 0, 1, 2, 3,… (integer), whereas continuous data can assume any value. Observations that can be counted constitute the discrete data and observations that can be measured constitute the continuous data. Examples of discrete data are number of episodes of respiratory arrests or the number of re-intubations in an intensive care unit. Similarly, examples of continuous data are the serial serum glucose levels, partial pressure of oxygen in arterial blood and the oesophageal temperature.
A hierarchical scale of increasing precision can be used for observing and recording the data which is based on categorical, ordinal, interval and ratio scales [ Figure 1 ].
Categorical or nominal variables are unordered. The data are merely classified into categories and cannot be arranged in any particular order. If only two categories exist (as in gender male and female), it is called as a dichotomous (or binary) data. The various causes of re-intubation in an intensive care unit due to upper airway obstruction, impaired clearance of secretions, hypoxemia, hypercapnia, pulmonary oedema and neurological impairment are examples of categorical variables.
Ordinal variables have a clear ordering between the variables. However, the ordered data may not have equal intervals. Examples are the American Society of Anesthesiologists status or Richmond agitation-sedation scale.
Interval variables are similar to an ordinal variable, except that the intervals between the values of the interval variable are equally spaced. A good example of an interval scale is the Fahrenheit degree scale used to measure temperature. With the Fahrenheit scale, the difference between 70° and 75° is equal to the difference between 80° and 85°: The units of measurement are equal throughout the full range of the scale.
Ratio scales are similar to interval scales, in that equal differences between scale values have equal quantitative meaning. However, ratio scales also have a true zero point, which gives them an additional property. For example, the system of centimetres is an example of a ratio scale. There is a true zero point and the value of 0 cm means a complete absence of length. The thyromental distance of 6 cm in an adult may be twice that of a child in whom it may be 3 cm.
STATISTICS: DESCRIPTIVE AND INFERENTIAL STATISTICS
Descriptive statistics[ 4 ] try to describe the relationship between variables in a sample or population. Descriptive statistics provide a summary of data in the form of mean, median and mode. Inferential statistics[ 4 ] use a random sample of data taken from a population to describe and make inferences about the whole population. It is valuable when it is not possible to examine each member of an entire population. The examples if descriptive and inferential statistics are illustrated in Table 1 .
Example of descriptive and inferential statistics

Descriptive statistics
The extent to which the observations cluster around a central location is described by the central tendency and the spread towards the extremes is described by the degree of dispersion.
Measures of central tendency
The measures of central tendency are mean, median and mode.[ 6 ] Mean (or the arithmetic average) is the sum of all the scores divided by the number of scores. Mean may be influenced profoundly by the extreme variables. For example, the average stay of organophosphorus poisoning patients in ICU may be influenced by a single patient who stays in ICU for around 5 months because of septicaemia. The extreme values are called outliers. The formula for the mean is

where x = each observation and n = number of observations. Median[ 6 ] is defined as the middle of a distribution in a ranked data (with half of the variables in the sample above and half below the median value) while mode is the most frequently occurring variable in a distribution. Range defines the spread, or variability, of a sample.[ 7 ] It is described by the minimum and maximum values of the variables. If we rank the data and after ranking, group the observations into percentiles, we can get better information of the pattern of spread of the variables. In percentiles, we rank the observations into 100 equal parts. We can then describe 25%, 50%, 75% or any other percentile amount. The median is the 50 th percentile. The interquartile range will be the observations in the middle 50% of the observations about the median (25 th -75 th percentile). Variance[ 7 ] is a measure of how spread out is the distribution. It gives an indication of how close an individual observation clusters about the mean value. The variance of a population is defined by the following formula:

where σ 2 is the population variance, X is the population mean, X i is the i th element from the population and N is the number of elements in the population. The variance of a sample is defined by slightly different formula:

where s 2 is the sample variance, x is the sample mean, x i is the i th element from the sample and n is the number of elements in the sample. The formula for the variance of a population has the value ‘ n ’ as the denominator. The expression ‘ n −1’ is known as the degrees of freedom and is one less than the number of parameters. Each observation is free to vary, except the last one which must be a defined value. The variance is measured in squared units. To make the interpretation of the data simple and to retain the basic unit of observation, the square root of variance is used. The square root of the variance is the standard deviation (SD).[ 8 ] The SD of a population is defined by the following formula:

where σ is the population SD, X is the population mean, X i is the i th element from the population and N is the number of elements in the population. The SD of a sample is defined by slightly different formula:

where s is the sample SD, x is the sample mean, x i is the i th element from the sample and n is the number of elements in the sample. An example for calculation of variation and SD is illustrated in Table 2 .
Example of mean, variance, standard deviation

Normal distribution or Gaussian distribution
Most of the biological variables usually cluster around a central value, with symmetrical positive and negative deviations about this point.[ 1 ] The standard normal distribution curve is a symmetrical bell-shaped. In a normal distribution curve, about 68% of the scores are within 1 SD of the mean. Around 95% of the scores are within 2 SDs of the mean and 99% within 3 SDs of the mean [ Figure 2 ].

Normal distribution curve
Skewed distribution
It is a distribution with an asymmetry of the variables about its mean. In a negatively skewed distribution [ Figure 3 ], the mass of the distribution is concentrated on the right of Figure 1 . In a positively skewed distribution [ Figure 3 ], the mass of the distribution is concentrated on the left of the figure leading to a longer right tail.

Curves showing negatively skewed and positively skewed distribution
Inferential statistics
In inferential statistics, data are analysed from a sample to make inferences in the larger collection of the population. The purpose is to answer or test the hypotheses. A hypothesis (plural hypotheses) is a proposed explanation for a phenomenon. Hypothesis tests are thus procedures for making rational decisions about the reality of observed effects.
Probability is the measure of the likelihood that an event will occur. Probability is quantified as a number between 0 and 1 (where 0 indicates impossibility and 1 indicates certainty).
In inferential statistics, the term ‘null hypothesis’ ( H 0 ‘ H-naught ,’ ‘ H-null ’) denotes that there is no relationship (difference) between the population variables in question.[ 9 ]
Alternative hypothesis ( H 1 and H a ) denotes that a statement between the variables is expected to be true.[ 9 ]
The P value (or the calculated probability) is the probability of the event occurring by chance if the null hypothesis is true. The P value is a numerical between 0 and 1 and is interpreted by researchers in deciding whether to reject or retain the null hypothesis [ Table 3 ].
P values with interpretation

If P value is less than the arbitrarily chosen value (known as α or the significance level), the null hypothesis (H0) is rejected [ Table 4 ]. However, if null hypotheses (H0) is incorrectly rejected, this is known as a Type I error.[ 11 ] Further details regarding alpha error, beta error and sample size calculation and factors influencing them are dealt with in another section of this issue by Das S et al .[ 12 ]
Illustration for null hypothesis

PARAMETRIC AND NON-PARAMETRIC TESTS
Numerical data (quantitative variables) that are normally distributed are analysed with parametric tests.[ 13 ]
Two most basic prerequisites for parametric statistical analysis are:
- The assumption of normality which specifies that the means of the sample group are normally distributed
- The assumption of equal variance which specifies that the variances of the samples and of their corresponding population are equal.
However, if the distribution of the sample is skewed towards one side or the distribution is unknown due to the small sample size, non-parametric[ 14 ] statistical techniques are used. Non-parametric tests are used to analyse ordinal and categorical data.
Parametric tests
The parametric tests assume that the data are on a quantitative (numerical) scale, with a normal distribution of the underlying population. The samples have the same variance (homogeneity of variances). The samples are randomly drawn from the population, and the observations within a group are independent of each other. The commonly used parametric tests are the Student's t -test, analysis of variance (ANOVA) and repeated measures ANOVA.
Student's t -test
Student's t -test is used to test the null hypothesis that there is no difference between the means of the two groups. It is used in three circumstances:

where X = sample mean, u = population mean and SE = standard error of mean

where X 1 − X 2 is the difference between the means of the two groups and SE denotes the standard error of the difference.
- To test if the population means estimated by two dependent samples differ significantly (the paired t -test). A usual setting for paired t -test is when measurements are made on the same subjects before and after a treatment.
The formula for paired t -test is:

where d is the mean difference and SE denotes the standard error of this difference.
The group variances can be compared using the F -test. The F -test is the ratio of variances (var l/var 2). If F differs significantly from 1.0, then it is concluded that the group variances differ significantly.
Analysis of variance
The Student's t -test cannot be used for comparison of three or more groups. The purpose of ANOVA is to test if there is any significant difference between the means of two or more groups.
In ANOVA, we study two variances – (a) between-group variability and (b) within-group variability. The within-group variability (error variance) is the variation that cannot be accounted for in the study design. It is based on random differences present in our samples.
However, the between-group (or effect variance) is the result of our treatment. These two estimates of variances are compared using the F-test.
A simplified formula for the F statistic is:

where MS b is the mean squares between the groups and MS w is the mean squares within groups.
Repeated measures analysis of variance
As with ANOVA, repeated measures ANOVA analyses the equality of means of three or more groups. However, a repeated measure ANOVA is used when all variables of a sample are measured under different conditions or at different points in time.
As the variables are measured from a sample at different points of time, the measurement of the dependent variable is repeated. Using a standard ANOVA in this case is not appropriate because it fails to model the correlation between the repeated measures: The data violate the ANOVA assumption of independence. Hence, in the measurement of repeated dependent variables, repeated measures ANOVA should be used.
Non-parametric tests
When the assumptions of normality are not met, and the sample means are not normally, distributed parametric tests can lead to erroneous results. Non-parametric tests (distribution-free test) are used in such situation as they do not require the normality assumption.[ 15 ] Non-parametric tests may fail to detect a significant difference when compared with a parametric test. That is, they usually have less power.
As is done for the parametric tests, the test statistic is compared with known values for the sampling distribution of that statistic and the null hypothesis is accepted or rejected. The types of non-parametric analysis techniques and the corresponding parametric analysis techniques are delineated in Table 5 .
Analogue of parametric and non-parametric tests

Median test for one sample: The sign test and Wilcoxon's signed rank test
The sign test and Wilcoxon's signed rank test are used for median tests of one sample. These tests examine whether one instance of sample data is greater or smaller than the median reference value.
This test examines the hypothesis about the median θ0 of a population. It tests the null hypothesis H0 = θ0. When the observed value (Xi) is greater than the reference value (θ0), it is marked as+. If the observed value is smaller than the reference value, it is marked as − sign. If the observed value is equal to the reference value (θ0), it is eliminated from the sample.
If the null hypothesis is true, there will be an equal number of + signs and − signs.
The sign test ignores the actual values of the data and only uses + or − signs. Therefore, it is useful when it is difficult to measure the values.
Wilcoxon's signed rank test
There is a major limitation of sign test as we lose the quantitative information of the given data and merely use the + or – signs. Wilcoxon's signed rank test not only examines the observed values in comparison with θ0 but also takes into consideration the relative sizes, adding more statistical power to the test. As in the sign test, if there is an observed value that is equal to the reference value θ0, this observed value is eliminated from the sample.
Wilcoxon's rank sum test ranks all data points in order, calculates the rank sum of each sample and compares the difference in the rank sums.
Mann-Whitney test
It is used to test the null hypothesis that two samples have the same median or, alternatively, whether observations in one sample tend to be larger than observations in the other.
Mann–Whitney test compares all data (xi) belonging to the X group and all data (yi) belonging to the Y group and calculates the probability of xi being greater than yi: P (xi > yi). The null hypothesis states that P (xi > yi) = P (xi < yi) =1/2 while the alternative hypothesis states that P (xi > yi) ≠1/2.
Kolmogorov-Smirnov test
The two-sample Kolmogorov-Smirnov (KS) test was designed as a generic method to test whether two random samples are drawn from the same distribution. The null hypothesis of the KS test is that both distributions are identical. The statistic of the KS test is a distance between the two empirical distributions, computed as the maximum absolute difference between their cumulative curves.
Kruskal-Wallis test
The Kruskal–Wallis test is a non-parametric test to analyse the variance.[ 14 ] It analyses if there is any difference in the median values of three or more independent samples. The data values are ranked in an increasing order, and the rank sums calculated followed by calculation of the test statistic.
Jonckheere test
In contrast to Kruskal–Wallis test, in Jonckheere test, there is an a priori ordering that gives it a more statistical power than the Kruskal–Wallis test.[ 14 ]
Friedman test
The Friedman test is a non-parametric test for testing the difference between several related samples. The Friedman test is an alternative for repeated measures ANOVAs which is used when the same parameter has been measured under different conditions on the same subjects.[ 13 ]
Tests to analyse the categorical data
Chi-square test, Fischer's exact test and McNemar's test are used to analyse the categorical or nominal variables. The Chi-square test compares the frequencies and tests whether the observed data differ significantly from that of the expected data if there were no differences between groups (i.e., the null hypothesis). It is calculated by the sum of the squared difference between observed ( O ) and the expected ( E ) data (or the deviation, d ) divided by the expected data by the following formula:

A Yates correction factor is used when the sample size is small. Fischer's exact test is used to determine if there are non-random associations between two categorical variables. It does not assume random sampling, and instead of referring a calculated statistic to a sampling distribution, it calculates an exact probability. McNemar's test is used for paired nominal data. It is applied to 2 × 2 table with paired-dependent samples. It is used to determine whether the row and column frequencies are equal (that is, whether there is ‘marginal homogeneity’). The null hypothesis is that the paired proportions are equal. The Mantel-Haenszel Chi-square test is a multivariate test as it analyses multiple grouping variables. It stratifies according to the nominated confounding variables and identifies any that affects the primary outcome variable. If the outcome variable is dichotomous, then logistic regression is used.
SOFTWARES AVAILABLE FOR STATISTICS, SAMPLE SIZE CALCULATION AND POWER ANALYSIS
Numerous statistical software systems are available currently. The commonly used software systems are Statistical Package for the Social Sciences (SPSS – manufactured by IBM corporation), Statistical Analysis System ((SAS – developed by SAS Institute North Carolina, United States of America), R (designed by Ross Ihaka and Robert Gentleman from R core team), Minitab (developed by Minitab Inc), Stata (developed by StataCorp) and the MS Excel (developed by Microsoft).
There are a number of web resources which are related to statistical power analyses. A few are:
- StatPages.net – provides links to a number of online power calculators
- G-Power – provides a downloadable power analysis program that runs under DOS
- Power analysis for ANOVA designs an interactive site that calculates power or sample size needed to attain a given power for one effect in a factorial ANOVA design
- SPSS makes a program called SamplePower. It gives an output of a complete report on the computer screen which can be cut and paste into another document.
It is important that a researcher knows the concepts of the basic statistical methods used for conduct of a research study. This will help to conduct an appropriately well-designed study leading to valid and reliable results. Inappropriate use of statistical techniques may lead to faulty conclusions, inducing errors and undermining the significance of the article. Bad statistics may lead to bad research, and bad research may lead to unethical practice. Hence, an adequate knowledge of statistics and the appropriate use of statistical tests are important. An appropriate knowledge about the basic statistical methods will go a long way in improving the research designs and producing quality medical research which can be utilised for formulating the evidence-based guidelines.
Financial support and sponsorship
Conflicts of interest.
There are no conflicts of interest.
- Open access
- Published: 17 April 2023
Adoptive neoantigen-reactive T cell therapy: improvement strategies and current clinical researches
- Ruichen Huang 1 na1 ,
- Bi Zhao 1 na1 ,
- Qian Zhang 3 ,
- Xiaoping Su 4 &
- Wei Zhang 1
Biomarker Research volume 11 , Article number: 41 ( 2023 ) Cite this article
2808 Accesses
2 Citations
2 Altmetric
Metrics details
Neoantigens generated by non-synonymous mutations of tumor genes can induce activation of neoantigen-reactive T (NRT) cells which have the ability to resist the growth of tumors expressing specific neoantigens. Immunotherapy based on NRT cells has made preeminent achievements in melanoma and other solid tumors. The process of manufacturing NRT cells includes identification of neoantigens, preparation of neoantigen expression vectors or peptides, induction and activation of NRT cells, and analysis of functions and phenotypes. Numerous improvement strategies have been proposed to enhance the potency of NRT cells by engineering TCR, promoting infiltration of T cells and overcoming immunosuppressive factors in the tumor microenvironment. In this review, we outline the improvement of the preparation and the function assessment of NRT cells, and discuss the current status of clinical trials related to NRT cell immunotherapy.
Recently, groundbreaking immunotherapies have revolutionized the schemes of cancer treatment. Conventional immunotherapies include immune checkpoint inhibitors (ICIs), adoptive cell therapy (ACT) and cancer vaccines, all of which improve the capability of immune system of recognizing and attacking cancer cells [ 1 , 2 ]. However, due to the heterogeneity of tumors, Immunotherapy targeting single antigen may also result in the generation of target-irrelevant tumor cell clones and tumor immune escape, which has been reviewed in reference [ 3 ]. Therefore, it is urgent to develop multi-targeted immunotherapy. The term “neoantigen” means a new epitope of autoantigens generated by somatic non-synonymous mutations [ 4 ]. And cancer neoantigens will be generated by this kind of DNA mutations accumulated in tumor cells [ 5 ]. These antigens have tumor specificity and are absolutely absent in normal cells; they also possess the ability to stimulate autoimmune response and are not subject to central immune tolerance [ 6 ]. Targeting multiple neoantigens can be a significant measure to deal with the challenge of tumor immune escape. Since adoptive therapy with tumor infiltrating lymphocytes (TILs) emerged in the 1980s, neoantigens have been found as the major targets of TILs to exert specific antitumor function. Researches showed that these neoantigens can induce neoantigen-specific T cells, also called “neoantigen-reactive T cells” or “NRT” cells. Researches on NRT-based immunotherapies, including neoantigen vaccine and NRT cell adoptive therapy, have made remarkable achievements in melanoma and other solid tumors [ 7 , 8 ]. The common point of these therapies is to recognize and kill neoplastic cells with autologous or heterologous NRT cells. However, Zhuting Hu et al. noted that neoantigen vaccine cannot induce adaptive immunity if not combined with appropriate adjuvants in the review of [ 6 ]. Even after activation, this vaccine still upregulates the immunosuppressive signaling of cancer, leading to the formation of suppressive tumor microenvironment (TME) [ 9 ]. What is more, weak immune induction is the most obvious defect of neoantigen vaccine in the treatment of advanced solid tumors. By contrast, a recent review has revealed that NRT cells can directly infiltrate into tumors after cultivation, and overcome the inhibition from TME by genetic modification of signal molecules [ 10 ]. For these reasons, developing NRT cell adoptive therapy can be a more effective method in treating solid tumors. This review focuses on the development history, preparation process, and preclinical as well as clinical researches of NRT cell therapy. It also explores the methods to enhance the anti-tumor effect of NRT cells.
Development history of NRT cell therapy
In the 1980s, De Plaen E. et al. first explored a neoantigen deriving from a single nucleotide variant which could be recognized by cytolytic T cells [ 11 ]. Subsequently, numerous cancer-related mutations that can be recognized by T cells were identified, including tumor associated antigens (TAAs), tumor specific antigens (TSAs), and cancer or testis antigens [ 12 , 13 , 14 , 15 , 16 ]. Among them, TSAs, especially neoantigens, are considered as the optimal tumor targets because they are never expressed in normal tissues and have a low probability of inducing tolerance. In a recent review, this group of antigens were divided into three types: Guarding neoantigens can induce antitumor immune response independently; restrained neoantigens have immune checkpoint-dependent immunogenicity; ignored neoantigens lack spontaneous immunogenicity [ 17 ]. The majority of neoantigens belong to the “ignored neoantigen” type, regarded as “the reserve of neoantigens”, which can be prepared as vaccine to induce autologous NRT cells [ 17 ]. In 2004, Rosenberg and his colleagues completed the first case of adoptive cell therapy, which showed that the tumor in metastatic lesions of patients with malignant melanoma regressed completely after adoptive transfer of TILs [ 18 ]. In another study, they also demonstrated that this therapy with two identified neoantigens can promote tumor infiltration of NRT cells and prolong their persistence [ 19 ]. The emergence of the next-generation sequencing technology has brought a new dawn for screening tumor neoantigens. This technique, combined with major histocompatibility complex (MHC) binding prediction approach based on silico algorithms, facilitates the selection of optimal missense genes and has become the mainstream method in neoantigen-identification [ 20 , 21 ]. Patrick A. Ott et al. observed that neoantigen vaccine, another neoantigen-based immunotherapy, induces significant anti-tumor immune response in melanoma patients [ 22 ]. This therapy provides another reasonable, safe and durable anti-tumor method in a more individualized mode, but it has failed to achieve clinical benefits in a wider spectrum of cancer patients, which is addressed in the review of [ 23 ]. However, the combined treatment of neoantigen vaccines and immune checkpoint inhibitors at least partly provides a reference scheme for enhancing the clinical response of NRT cell treatment [ 24 ]. Currently, The focus of NRT cell therapy has shifted from melanoma to other solid tumors [ 25 , 26 , 27 , 28 , 29 ]. However, the efficacy of this therapy in solid tumors is limited, which is related to tumor immune escape, immune cell exhaustion or dysfunction, and immunosuppressive state of the tumor microenvironment (TME). Current NRT therapy mainly stems from improvement on TILs adoptive therapy. Neoantigen vaccines, adoptive transfer of NRT cells, TCR-engineered T cells and chimeric receptor T cell therapy have gradually emerged in clinical individualized antitumor treatment. Encouraging results from clinical studies highlight the importance of NRT cells in antitumor immunity. However, because few researches have studied adopting NRT cell therapy, very limited information of how to increase the efficacy of NRT cells adoptive therapy can be obtained from completed clinical trials hitherto. More endeavors are therefore needed to dissect the relationship between tumor immunity, neoantigens, and immune cells.
Introduction of neoantigen detecting platforms
Unlike overexpressed or abnormally expressed tumor-associated antigens, neoantigens are absent in the normal human genome [ 30 ]. The high-throughput sequencing technology and algorithmic prediction platforms render neoantigen identification more rapid and accurate (Fig. 1 B, C) [ 31 ]. As for high-throughput sequencing, whole exome sequencing (WES) becomes the keystone of neoantigen identification [ 32 ]. Besides, mass spectrometry technology provides a large number of peptide data for training of MHC prediction platforms [ 33 ] (Fig. 1 B). As for algorithmic prediction platforms, machine learning and artificial intelligence platforms (Fig. 2 A) help to precisely predict potential MHC binding epitopes and MHC-peptide binding affinity based on sequencing outcomes. Some common prediction software, algorithms, and databases are listed in Table 1 . Mutation screening is the first step in neoantigen identification. Mutation calling tools include Burrows-Wheeler Alignment tool (BWA), ANNOVAR, MuTect, SomaticSniper, VarScan, and FusionCatcher [ 34 , 35 , 36 , 37 , 38 ]. Differences between mutant sequence and wild sequences can be identified using the differential agretopic index (DAI) [ 39 ]. It is necessary to predict MHC binding ligands and binding affinity to determine whether mutations can form neoantigens. Published software represented by NetMHCpan, MHCflurry, HLAthena, MHCnuggets and ProGeo-Neo shows favorable outcomes in neoantigen prediction [ 40 , 41 , 42 , 43 , 44 ]. Immune Epitope Database (IEDB) is a primary epitope database and nealy all the prediction tools use the data from it [ 45 ]. The company of this database recently developed TCRMatch, which can identify T cell epitopes with unknown specificity based on optimized T cell epitope data of IEDB [ 46 ]. However, the data of IEDB are mostly from virus resources, which lead to the deviation of cancer neoantigen prediction. Novel database-Tumor Neoantigen Selection Alliance (TESLA)-based on tumor sequencing data will improve the precision of tumor neoantigen prediction [ 47 ]. The platforms widely applied in predicting MHC ligands are trained on neoepitope prediction with the data from literature or online databases. Each platform has the limitations of prediction objects and methods, while collaboration of multiple platforms will improve the specificity and accuracy. It is reasonable to prospect that these techniques will help to solve the difficulty in choosing the optimal neoantigen to activate antitumor NRT cells, which may be conducive to the efficacy improvement of immunotherapy.
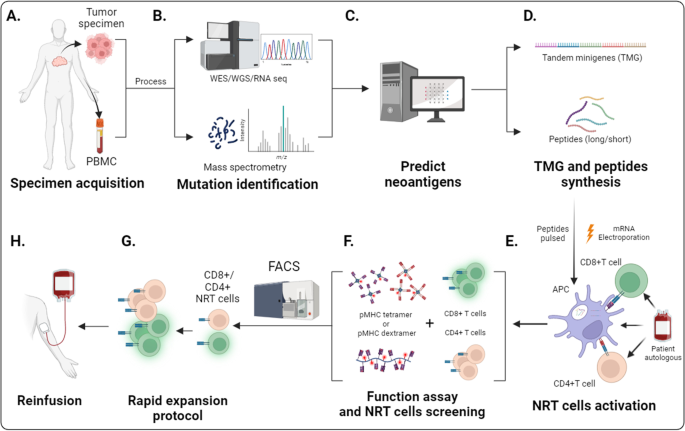
Process of NRT cell manufacturing and adoptive therapy. NRT cells are manufactured via the following steps: A acquisition and cultivation of tumor specimens and peripheral blood mononuclear cells; B mutation identification with WES/WGS/RNA sequencing (seq), potential antigen detection with mass spectrometry; C neoantigen prediction; D design and synthesis of neoantigen-encoding mRNA in tandem minigene configuration or neoantigen peptides; E pulsing DCs directly with peptides, or transfection of neoantigen-encoding mRNA into DCs by electroporation, followed by the co-incubation of neoantigen-loaded DCs and PBMC-derived T cells, F flow cytometry-based neoantigen-specific T cell sorting; G rapid expansion protocol (REP) of NRT cells, H reinfusion of NRT cells into patients or mouse model
Process of NRT cell induction
The major purpose of predicting neoantigens precisely is to induce the immune response of NRT cells, which is the critical element for antitumor immunotherapy. To induce NRT cells, the wide accepted protocols mainly include: specimen acquisition and isolation (Fig. 1 A), identification of non-synonymous mutation through WES/WGS/RNA sequencing (seq), and detection of potential antigens with mass spectrometry (Fig. 1 B), neoantigen prediction utilizing bioinformation technology (Fig. 1 C), design and synthesis of neoantigen-encoding mRNA in tandem minigene configuration or neoantigen peptides (Fig. 1 D), pulsing DCs directly with peptides, or transfection of neoantigen-encoding mRNA into DCs by electroporation, followed by the co-incubation of neoantigen-loaded DCs and PBMC-derived T cells (Fig. 1 E), NRT cell functional assay and sorting through flow cytometry (Fig. 1 F), rapid expansion protocol (REP) of NRT cells (Fig. 1 G) before reinfusion into patients or mouse model (Fig. 1 H) [ 55 , 56 ]. Then, efficacy assessments of NRT cell adoptive therapy will be performed. In this part, we will introduce the improvement strategies of NRT cell induction specifically.
Ameliorated technologies, such as microinjection, micro-electroporation and nano-delivery, should be taken into account to elevate transfection efficiency (Fig. 2 B a, b, c) [ 57 , 58 ]. Because of the limited capacity of antigen presentation and long duration of the induction process when using autologous DCs, modified strategies of allogeneic APCs have been put forward (Fig. 2 B d). Synthetic APCs, including magnetic and polymer compound beads covered with anti-CD3/CD28 antibody and HLA-Ig, also can be used to activate NRT cells [ 59 , 60 ]. Nanoparticle-based artificial antigen presenting cell can mimic DCs to effectively prime and expand T cells. The following are some ways whereby this APC can be engineered: adding co-receptors, synthesizing nanoparticles coated with molecule-labeled DC membrane and T cell targeted antigens, modifying the shape of nanoparticles and endowing anti-phagocytosis ability [ 61 , 62 , 63 , 64 ]. Introduction of IL-2, and low-level IL-7 and IL-15 can be a time-saving method for NRT cell priming in expanding process, and these two cytokines promote the formation of the effector phenotype and the central memory phenotype while IL-15 additionally induces the stem cell memory phenotype of T cells (Fig. 2 C a) [ 65 , 66 ]. Cultivating TILs with agonistic CD137 (4-1BB) monoclonal antibodies increases frequency of CD8 + TILs, as well as amplification rate and quantity of T cell subclone types (Fig. 2 C b) [ 67 ]. The “feeder cell”, including immortalized B cells and K562 cells, can be modified to express signals for T cell proliferation, which wins the favor of researchers in the REP process(Fig. 2 C c) [ 68 , 69 ]. In addition, inhibiting AICD signaling cascade and preventing the aging of T cells (“young” T cultivation method) in the process of REP will enhance the activity and prolong the persistence of adoptively transferred T cells (Fig. 2 C d, e) [ 70 , 71 ]. Another strategy is to cultivate NRT cells with tumor organoids in a personalized manner (Fig. 2 C f) [ 72 ]. This patient-specific cell culture method develops a platform for better exploring the interaction between T cells, tumor cells and other immune cells from native environment. The study of NRT cells based on multi-omics analysis has confirmed its feasibility [ 73 ]. Generally, a more efficient inducing process of NRT cells has important implications for NRT cell therapy. The above-mentioned strategies seek to improve the efficiency of vector transduction, and promote the amplification and activation of T cells.
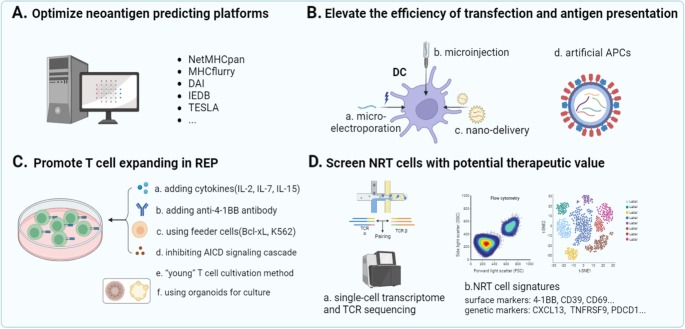
Feasible improvement for the manufacture of NRT cells. A Optimize neoantigen predicting platforms to promote the efficiency and accuracy of prediction. B Micro-electroporation (a), microinjection (b) and nano-delivery c can be applied to elevate transfection efficiency. Artificial APCs can be used to increase the efficiency of antigen presentation. C Promote NRT cell expansion in rapid expanding protocol (REP) through adding cytokines (IL-2, IL-7 and IL-15) (a) or anti-4-1BB antibody (b), using feeder cells (Bcl-xL, K562) (c), inhibiting AICD signaling cascade (d), or adopting the culture method of “young” T cells (e) or organoids (f). D Screen NRT cells with surface or genetic markers via single-cell transcriptome and TCR sequencing
Detection of neoantigen-reactive T cell populations
Florian Kast et al. reviewed that using pMHC tetramer and dextramer binding assay based on flow cytometry can strengthen the binding forces between single pMHC and TCR, and elevate the efficiency of NRT cells screening (Fig. 1 F) [ 4 ]. However, due to its low sensitivity, this technique cannot detect rare T cell clones containing NRT cells. Single-cell transcriptome and TCR sequencing play essential roles in the discovery of novel tumor-reactive T cell subclones, and further aid in the analysis and filteration of potential NRT cells within these subclones (Fig. 2 E). Currently, the most common single-cell sequencing method of T cells mainly adopts microwell or microfluidic technology [ 74 , 75 ]. Then, reverse transcription and PCR amplification are performed before transcriptomic and TCR sequencing. The sequencing data can be integrated and analyzed to identify T cell subclones and reconstruct TCR chains for pairing TCR with specific T cell clones [ 75 , 76 ]. With the support of bioinformatic analysis, it is more convenient to find potential therapeutic targets and novel biomarkers with prognostic value, which facilitates efficacy evaluation of immunotherapies tailored to individuals. The feasibility of this novel detecting method has been demonstrated in several studies, and NRT cell populations have been successfully identified and isolated [ 77 , 78 ]. The latest research also revealed that NeoTCR signatures can be used to identify specific antitumor NRT cells via single-cell transcriptome and single-cell TCR sequencing [ 79 ].
The value of screening signatures in NRT cell identification
Currently accepted surface markers of NRT cell include CTLA-4, PD-1, LAG-3, TIM-3 and TIGIT. 4-1BB/CD137 is transiently expressed on T cells, which is regarded as specific activating signature of NRT cells and extensively used in NRT cell population screening [ 80 , 81 ]. High frequency of CD39 + tumor reactive T cells is relevant to better prognosis in cancer patients [ 82 ]. NRT cells of stem-like double negative (CD39- CD69-) phenotype show stronger antitumor activity and longer persistence despite the rarity of these cells [ 83 ]. In addition, other novel NRT cell surface markers have been identified, including CXCL13 and CD200 [ 84 , 85 , 86 ]. Particularly, the expression of CXCL13 is significantly different between NRT cells and bystander T cells. Common gene signatures include PDCD1, ENTPD1, LAG3, TIGHT, TNFRSF9, HAVCR2, BATF, GZMA/B/K, IFNA/B/G and CXCL13 gene [ 79 , 84 ]. In order to elevate sensitivity and specificity of NRT cell screening, a combination of surface markers and transcriptome markers is recommended for identifying NRT cells. This approach not only facilitates the cell screening process, but also circumvents the influence of functional assays on the viability of the cells.
Feasible engineering strategies for NRT cells
The process of TCR recognizing and binding to MHC molecules is of great importance for T cells to perform antitumor function. The challenges are how to make T cells recognize tumor cells more effectively, how to enhance TCR function without increasing toxicity, and how to counteract the problems of T cell exhaustion as well as dysfunction. Therefore, we summarize some feasible engineering strategies for NRT cells to address these issues (Fig. 3 A). Currently, the three most common engineering objects are TCR signals, co-stimulated signals and cytokines of T cells. Using transgenic TCR, co-expressing CD8 αβ with TCR and upregulating adhesion molecules can enhance MHC-TCR binding avidity and elevate signal-transducing efficiency [ 87 , 88 , 89 ]. Besides, engineering co-stimulatory signals is proposed to prolong T cell persistence and enhance anti-tumor activity, which can be achieved by coupling T cell activating signals (CD3ζ) with co-stimulating signals (CD28, OX40, 4-1BB), or using chimeric switch receptors which link exodomain of CTLA-4, PD-1 or TIGHT to intradomain of CD28 [ 90 , 91 , 92 , 93 , 94 , 95 ]. In addition, engineering cytokine receptors represented by orthogonal IL-2 has been found to enhance T cell antitumor function while attenuating the side effects caused by cytokine pleiotropy [ 96 , 97 , 98 ]. And T cells engineered to secrete additional cytokines (IL-7/12/15/18/23 and Flt3L) or chemokines (CXCL9/10/11 and CCL19/21) also show enhancement of activity and function [ 99 , 100 , 101 , 102 , 103 , 104 , 105 , 106 , 107 , 108 , 109 ]. These strategies improve the function of autologous tumor-reactive T cells, promote their phenotype switching, and, meanwhile, recruit other immune cells (such as NK cells and DCs) to exert antitumor effects. The abovementioned engineering strategies are used in CAR-T cells. CAR-T cells can also be designed to release enzymes, express multiple immunomodulators, deliver endogenous RNA, maximize the diversity of functions and minimize “off-target” toxicity, which are summarized as comprehensive strategies of armored CAR-T (Fig. 3 B). These strategies have been reviewed in [ 110 , 111 , 112 , 113 ]. Engineering strategies of TCR T cells can also draw on this idea to extend persistence, promote homing and penetration into tumors, and enable T cells to target tumor cells and activate multiple immune cells simultaneously. Overall, the above researches highlight the necessity of T cell signaling in priming, proliferation and exerting function. Engineering strategies for receptors and cytokines expressed by T cells help to improve the persistence and antitumor function of T cells. We propose that these T cell modification methodologies can be also used to improve the antitumor activity as well as persistence of NRT cells, and promote infiltration of immune cells into solid tumors to limit their growth more effectively.
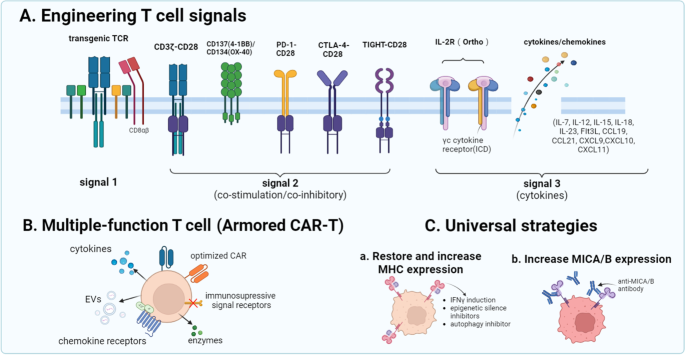
Feasible strategies for the improvement of T cell antitumor function. A Engineer T cell signals. Signal 1: edit TCR genes or make TCR and CD8 αβ co-expression. Signal 2: join CD28 to CD3ζ combined with 4-1BB or OX40 to enhance activation signals, construct the chimeric switch receptor (e. g., CD28 linked to PD-1,CTLA-4 and TIGHT) to reverse inhibitory signals. Signal 3: modify cytokine receptors (e. g., IL-2 orthogonal receptor) and increase the expression of autologous or heterologous cytokines or chemokines. B Produce multiple-function T cells: optimize CARs, secrete cytokines and enzymes, release extracellular vesicles containing RNAs, express multiple chemokine receptors, and modify immunosuppressive signal receptors. C Universal strategies to restore and increase the expression of MHC (inducing IFNγ production, using epigenetic silence or autophagy inhibitors (a)) and MICA/B (anti-MICA/B antibody (b))
Universal strategies for NRT cell therapeutic enhancement
MHC-TCR recognition pattern is the primary mechanism of NRT cell therapy. Under certain circumstances, however, tumor escape will occur when classical MHC molecules are downregulated or lose expression, which may be caused by gene reconstruction or mutation and deletion of functional components, loss of transcription factor, epigenetic silence, and pre-or post-transcriptional inhibitory regulation [ 114 , 115 ]. Deficient expression of MHC also leads to dysfunction in the neoantigen presentation process [ 116 ]. This phenomenon will eventually affect the ability of T cells to recognize tumor cells. Since traditional TCR engineered T cells have MHC -restriction, modification of autologous T cells can be cumbersome and expensive. It is a tendency to use universal methods to enhance the antitumor function of T cells (Fig. 3 C). These strategies can be used as auxiliary means to improve the efficacy of NRT cell therapy. The first strategy is to restore and increase expression of MHC molecules (Fig. 3 C a). Previous researches have shown that IFN-γ can increase MHC expression [ 117 ]. Adopting epigenetic silence inhibitors, such as DNA methyltransferase inhibitors and histone deacetylase inhibitors, also has a pronounced effect on restoring or increasing MHC molecular expression [ 118 , 119 , 120 , 121 ]. Besides, reduction of MHC expression caused by autophagy can be another common tumor escape mechanism in a solid tumor. Autophagy inhibition recovers the MHC level of the tumor cell surface and promotes T cell activation [ 122 ]. NK cells acquire disinhibition when tumor cells decrease MHC molecular expression, and become activated when detecting ligands of activating receptors. Thus, exploiting the NK-involved antitumor mechanism can be a feasible and reasonable strategy to counteract tumor escape. A vaccine designed to induce antibodies that anchor MICA/B has been demonstrated to prevent tumor escape and enhance the function of tumor-reactive T cells and APCs (Fig. 3 C b) [ 123 ]. Notably, this vaccine targeting MHC-I expressing tumors can be applied in clinical ACT as an inexpensive and effective “off-the-shelf” drug.
Influence of T cell infiltration and tumor microenvironment on the efficacy of immunotherapy
Although adoptive therapy of engineered T cells shows remarkable efficacy in clinical therapy, the interaction among malignant cells, immune cells, and other stromal components also requires deep exploration, which will offer feasible approaches to improving the infiltration and potency of T cells, preferably NRT. In some recent reviews, turning “cold tumors (immune-excluded and deserted tumors)” into “hot tumors (immune-inflamed tumors)” has become a research hot spot to strengthen immunotherapy efficacy [ 124 , 125 ]. Compelling evidence proves that infiltration of antigen-specific T cells within tumors promotes favorable clinical outcomes. However, tumor-infiltrating T lymphocytes expressing high-level inhibitory receptors trigger downregulation of antitumor response, and low-level expression of chemokine receptors leads to poor T cell infiltration [ 126 ]. Strategies to improve T cell infiltration have been proposed to overcome these challenges (Fig. 4 A). With radiotherapy and chemotherapy, tumor cells undergo immunogenic cell death (ICD), and release multiple cytokines and chemokines [ 127 , 128 ]. Radiotherapy and thermal ablation can directly kill tumor cells or induce their apoptosis, as well as increase expression of MHC on the surface of the antigen presenting cells. [ 129 , 130 ]. Compared with monotherapy, combination of NRT cell adoptive therapy or neoantigen vaccine with ICIs (Fig. 5 ) has been proven to achieve impressive outcomes [ 22 , 24 , 26 ]. Suppressive tumor microenvironment (TME) is composed of fibroblasts, immunosuppressive cells, abnormal proliferating vasculature and extracellular matrix, which may negatively impact host immune cell infiltration. Eliminating the physical barrier effect of extracellular matrix (ECM) by using ECM targeting agents can promote T cell infiltration into tumors (Fig. 4 B), which has been addressed in the reviews of [ 131 , 132 ]. The most common strategy for depleting the stroma is to use albumin-bound paclitaxel to facilitate T cell infiltration [ 133 , 134 ]. Research showed that combinational therapy with nab-paclitaxel and gemcitabine or nab-paclitaxel and atezolizumab significantly improves tumor control and patient survival [ 135 ]. Aberrant growth of tumor vasculature will result in the formation of hypoxia and immunosuppressive TME [ 136 ]. Thus, normalizing the originally abnormal tumor vasculature via antiangiogenic agents (such as VEGFR inhibitor) and ICIs treatment will reduce hypoxia and remodel TME for a more favorable treating condition and potentiate antitumor immune cell activation (Fig. 4 C) [ 136 , 137 ]. Immune suppressive cells, such as Tregs, myeloid-derived suppressor cells (MDSCs) and tumor-associated macrophages (TAMs), are important study subjects in researches on overcoming resistance from TME. Depletion is the most common strategy for decreasing the quantity of both Tregs and MDSCs (Fig. 4 D). Using anti-CCR4-antibody can deplete suppressive Treg cells in TILs and enhance antitumor-specific function of T cells [ 138 ]. For MDSCs, gemtuzumab can deplete intratumoral MDSCs, and the CXCR2 antagonist can block migration of MDSCs into tumors [ 139 , 140 ]. Besides, targeting signaling pathway can also inhibit the proliferation and function of Tregs or MDSCs. Tregs can be inhibited by deleting transcription factor Blimp1 [ 141 ], while MDSCs can be inhibited by upregulating LXR expression [ 142 ], downregulating PEKR expression [ 143 ] or blocking CaMKK2 signal pathway [ 144 ]. In addition, another research hot spot is re-polarization of tumor-associated macrophages (TAM) from an anti-inflammatory (M2) phenotype to a pro-inflammatory (M1) one (Fig. 4 E). This can be realized by using CpG-ODN [ 145 ] or non-coding RNAs as the immune regulator [ 146 ], or inhibiting the metabolism of lipid [ 147 ]. Tertiary lymphoid structures (TLSs) have a significant association with immune cell infiltration and cancer prognosis (Fig. 4 F). In the two reviews of TLSs, the authors believed that solid tumors with more TLSs present a large quantity of effector memory T cells and cytotoxic T cells [ 148 , 149 ]. Activated B cells in TLSs, except Bregs, can present antigens, stimulate activating signals, and secret cytokines to activate T cells and augment their antitumor function. Researches have demonstrated that the synergy work of B cells and T cells, together with the cooperation of humoral and cellular immunity, impacts the immune response and survival of patients [ 150 , 151 ]. The number of TLSs is positively related to the efficacy of immunotherapy. And the induction of TLSs formation can be realized by applying ICIs [ 149 , 152 ], vaccines [ 9 ], lymphoid chemokines or stromal cells [ 153 , 154 ]. The abovementioned strategies strive to facilitate T cell infiltration, reinvigorate and augment the function of effector T cells, induce memory T cell generation, and eventually remodel adverse TME. These strategies can be performed by inhibiting immunosuppressive signals, increasing chemokine expression, removing barriers from TME, depleting or remodeling immunosuppressive cells, re-directing TAMs toward antitumor phenotype, and inducing formation of TLSs within the tumor. These measures dedicated to overcoming extracellular resistance can also improve the efficacy of adoptive NRT cell therapy in solid tumor treatment.
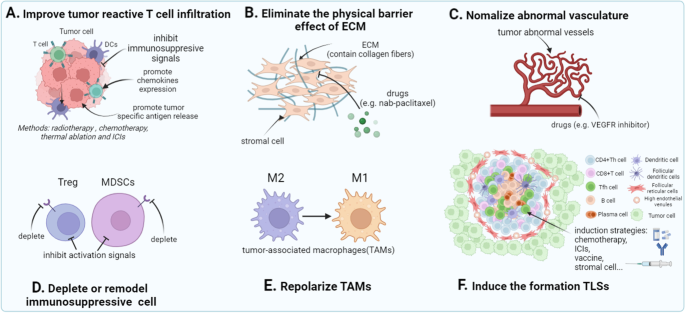
Strategies for promoting NRT cell tumor infiltration and modifying suppressive TME. A Improve tumor reactive T cell infiltration through inhibiting immunosuppressive signals, and promoting the expression of chemokines and the release of tumor specific antigens. B Eliminate the physical barrier effect of extracellular matrix(ECM) using drugs such as nab-paclitaxel. C Normalize abnormal vasculature by using VEGFR inhibitor. D Deplete immunosuppressive cells(Treg and MDSCs) and inhibit their activation signals. E Repolarize tumor-associated macrophages(TAM) from M2 towards M1. F Induce the formation of tertiary lymphoid structures(TLSs)(chemotherapy, ICIs, vaccine and stromal cell)
Clinical Trials for NRT cell therapy
We have summarized the clinical trials of NRT cell adoptive therapy and other NRT cell-related immunotherapies. Twenty-six eligible researches are incorporated, among which six are on NRT cell adoptive therapy, one on NRT cell adoptive therapy plus neoantigen vaccine (Neovax), one on neoantigen dendritic cell vaccine (Neo-DCVac) plus NRT cell adoptive therapy, seven on NeoVax, two on Neovax plus ICIs, three on tumor infiltrating lymphocytes (TILs), one on TIL plus ICIs, two on Neo-DCVac, one on Neo-DCVac plus ICIs and two on ICIs monotherapy. Almost all these researches are in phase I or II, and the majority are applied in melanoma due to its high mutant frequency. We have found that patients receiving NRT cell adoptive therapy or neoantigen vaccine therapy combined with ICIs outperform those who only receive monotherapy. In the following paragraphs we will mainly introduce clinical trials of NRT cell therapy. Other researches of NRT cell-related immunotherapy will be listed in Table 2 . Schemes of feasible NRT cell combinational therapy are shown in Fig. 5 .
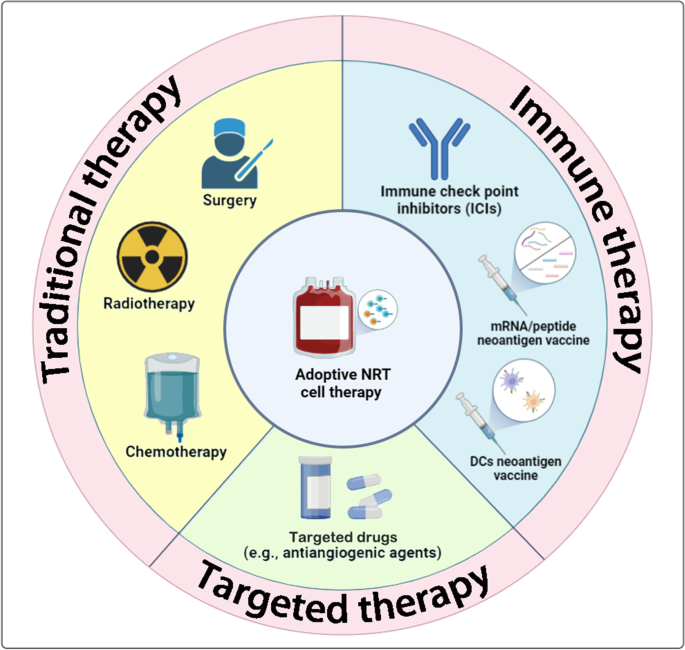
Feasible combinational therapy strategies of adoptive NRT cell therapy. Feasible combinational therapy strategies are shown in this figure. Adoptive NRT cell therapy can be combined with such strategies: immune therapy (immune check point inhibitors (ICIs), mRNA/peptide neoantigen vaccine, DC neoantigen vaccine), targeted drugs (e.g., antiangiogenic agents) and traditional therapy (surgery, radiotherapy and chemotherapy)
The majority of traditional engineered TCR T cells are designed to target tumor-associated antigens (TAAs) while relatively few teams have conducted researches on neoantigens [ 89 , 176 ]. It is difficult for these T cells to eliminate tumor cells thoroughly due to their heterogeneity, and patients also show limited clinical responses or even suffer autoimmune diseases caused by the “off-target” effect [ 177 , 178 , 179 , 180 ]. Rosenberg’s team is devoted to exploring NRT cell populations targeting shared antigens and developing engineered neoantigen-targeted TCR T cells as “off-the-shelf” products. Their first NRT cell therapy case was a metastatic cholangiocarcinoma patient who received ERBB2IP-targeted CD4 + T cell therapy and achieved disease stability for more than one year after twice reinfusions [ 155 ]. KRAS-G12D-targeted NRT cells in gastrointestinal cancers have also been screened [ 181 , 182 ]. Recently, a case report showed that a pancreatic cancer patient who received KRAS-G12D NRT cell therapy obtained a 6-month partial objective response accompanied by long-term existence of effector T cells [ 156 ]. Besides, twelve patients in two clinical trials who harbored TP53 mutation received NRT cell therapy. Among them, two patients exhibited a partial response, and another patient with chemo-refractory breast cancer realized tumor regression that lasted for at least six months [ 157 ]. Another research team conducted two pioneering clinical trials, showing the value of transferring NRT cells in treating advanced and refractory solid tumors. In the first study, researchers compared the therapeutic effects of two sources of neoantigens-de novo and shared library. In three patients treated with NRT cells manufactured by the de novo pattern, the overall response rate of T cells to neoantigens was lower than 34%. However, using a shared neoantigen library could significantly increase the efficiency and accuracy of hot spot mutation identification. Six patients using NRT cells made by this pattern achieved one CR, one PR, and four SD [ 27 ]. In the second study, a patient with advanced hepatocellular carcinoma (HCC) received NRT cell therapy combined with radiotherapy and ICI therapy, and realized partial response and complete regression in the new lesion [ 25 ]. This study completed the first comprehensive NRT cell therapy in an advanced HCC patient who benefited from prolonged survival without severe side effects. In addition, other studies have also shown favorable clinical results of NRT cell therapy. Nikolaos Zacharakis et al. presented a case of a breast cancer patient with complete regression after reinfusion of NRT cells targeting four individual somatic mutations combined with ICIs [ 26 ]. Our team also reported a case of a collecting duct carcinoma (CDC) patient who obtained SD with decreased tumor loads and regression of metastatic lesions after administration of NRT immunotherapy [ 28 ]. More than 92% of the neoantigens in this research could fully stimulate reactive T cells in PBMC. The activation proportion of NRT elevated from 1.92% to 7.92%. The latest phase II clinical trial used NRT cell therapy combined with DC neoantigen vaccine, chemotherapy, radiofrequency ablation, and ICIs to treat hepatocellular carcinoma [ 158 ]. Fifty percent of the patients obtained disease stability without relapse for two years. Other patients failed to respond due to depletion of tumor neoantigen and generation of new neoantigen epitope. The overall safety of adoptive NRT cell therapy is good and no prominently serious adverse events have been observed. Only two among the seven studies of NRT cell therapy reported minor adverse reactions of grade 1–2 [ 27 , 157 ]. These results demonstrate that this therapy is feasible and safe for the activation of autologous NRT cells to eliminate tumor cells.
Furthermore, some recruiting studies on NRT cell therapy and engineering TCR neoantigen T cells are listed in Table 3 . All these researches aim at solid tumors, including three in phase I, six in phase I/II, and two in phase II clinical trials. Four studies use shared NRT cells, while two use de novo NRT cells. Six studies apply NRT cells combined with ICIs, one of which also adds CDX-1140, a monoclonal antibody targeting CD40. The feasibility and safety of these researches need to be confirmed by the publication of the latest results.
The studies above show that NRT cell therapy realizes favorable tumor regression and long-term antitumor effect, especially for patients of end-stage melanoma or refractory solid tumors, in a more individualized or “off-the-shelf” way. However, due to the inaccuracy of prediction algorithms or suppression of TME, the overall response to NRT cell therapy is limited. In some cases, shared antigens are not included in the top alternative neoantigens, which means driver gene peptides are not the optimal targets in some cancer patients. The efficacy of adoptive NRT cell therapy will be improved by both traditional therapy and other immunotherapies, which can broaden the repertoire and augment the function of autologous NRT cells.
Limitations of NRT cell therapy
Although adoptive NRT cell therapy has superiority in effectiveness and safety in advanced tumor treatment, it still has some limitations. Under the pressure of immune editing, the consequence of the tumor cell evolution is that the quantity of cancer neoantigens originating from driver mutation will decrease while the number of those deriving from passenger mutation will increase. Thus, NRT cells designed to target single driver gene mutations (e.g., KRAS, TP53) fail to achieve complete regression of primary tumors. Moreover, unlike driver mutation-derived neoantigens, passenger mutation-derived neoantigens are different in each patient, suggesting that the cell products for each patient need to be tailored. Besides, the deficiency of predicting platforms leads to the deviation of neoantigen prediction and dissatisfactory treatment efficacy. Compared with the neoantigen vaccine, adoptive NRT cell therapy targets fewer neoantigens and induces a narrower breadth of the immune response [ 5 , 183 ], and the process of NRT cell manufacturing is complicated, time-consuming and costly. Existent evidence has shown that ex vivo cultivation will increase the proportion of the terminal differential phenotype of T cells and reduce activity and proliferation of NRT cells [ 184 , 185 , 186 ], whereas neoantigen vaccine will not result in these problems due to it induces NRT cells activation directly in vivo. Furthermore, potential cytokine release syndrome requires additional attention in NRT cell-based therapy, and IL-1 and IL-6 receptor antagonists or blockades are needed to cope with this problem [ 187 , 188 ].
The discovery of neoantigens boosts the development of individually-tailored immunotherapy, including adoptive therapy with T cells. With more efficient and precise therapeutic potency, T cells stimulated by neoantigens exhibit powerful antitumor capability. Based on bio-information technology, T cell screening and engineering techniques, modified NRT cells can be implemented more economically and conveniently. Although the feasibility and safety of NRT-related immunotherapy have been verified, the majority of researches are still in the initial stage, and the overall treatment results are unsatisfactory. Improved methods have been proposed to meet the urgent demand for improvement of therapy effectiveness and development of novel platforms as well as of multiple-drug combinatorial therapy. Current challenges to adoptive NRT cell therapy are the high cost and difficulty in realizing individualization, which renders industrial mass production unlikely and needs to be solved by future technological innovation. However, generally speaking, we are convinced that NRT cell-based immunotherapy has the effectiveness and safety to realize enduring tumor elimination and significantly prolonged survival that benefit patients with advanced solid tumors.
Availability of data and materials
Not applicable.
Abbreviations
- Adoptive cell therapy
Activation-induced T cell death
Antigen presenting cell
Chimeric antigen receptor-T cell
Collecting duct carcinoma
Complete response
Dendritic cell
Extracellular matrix
Hepatocellular carcinoma
Immunogenic cell death
Immune checkpoint inhibitor
Myeloid-derived suppressor cell
Major histocompatibility complex
MHC class I polypeptide–related sequence A/B
Neoantigen dendritic cell vaccine
Neoantigen vaccine
Neoantigen-reactive T cells
Peripheral blood mononuclear cell
Partial response
Rapid expansion protocol
Stable disease
Tumor associated antigen
Tumor-associated macrophages
T cell receptor
Tumor infiltrating lymphocyte
Tertiary lymphoid structure
Tumor microenvironment
Tumor specific antigen
Vascular endothelial growth factor receptor
Whole exome sequencing
Kennedy LB, Salama AKS. A review of cancer immunotherapy toxicity. CA Cancer J Clin. 2020;70(2):86–104.
Article PubMed Google Scholar
Rosenberg SA, Restifo NP. Adoptive cell transfer as personalized immunotherapy for human cancer.pdf. Science. 2015;348(6230):62–8.
Article CAS PubMed PubMed Central Google Scholar
McGranahan N, Swanton C. Clonal heterogeneity and tumor evolution: past, present, and the future. Cell. 2017;168(4):613–28.
Article CAS PubMed Google Scholar
Kast FKC, Umaña P, Gros A, Gasser S. Advances in identification and selection of personalized neoantigen/T-cell pairs for autologous adoptive T cell therapies. Oncoimmunology. 2021;10(1):1869389.
Article PubMed PubMed Central Google Scholar
Schumacher TN, Scheper W, Kvistborg P. Cancer neoantigens. Annu Rev Immunol. 2019;37:173–200.
Hu Z, Ott PA, Wu CJ. Towards personalized, tumour-specific, therapeutic vaccines for cancer. Nat Rev Immunol. 2018;18(3):168–82.
Leko V, Rosenberg SA. Identifying and targeting human tumor antigens for T cell-based immunotherapy of solid tumors. Cancer Cell. 2020;38(4):454–72.
Davis L, Tarduno A, Lu YC. Neoantigen-reactive T cells: the driving force behind successful melanoma immunotherapy. Cancers (Basel). 2021;13(23):6061.
Lutz ER, Wu AA, Bigelow E, Sharma R, Mo G, Soares K, Solt S, Dorman A, Wamwea A, Yager A, et al. Immunotherapy converts nonimmunogenic pancreatic tumors into immunogenic foci of immune regulation. Cancer Immunol Res. 2014;2(7):616–31.
Restifo NP, Dudley ME, Rosenberg SA. Adoptive immunotherapy for cancer: harnessing the T cell response. Nat Rev Immunol. 2012;12(4):269–81.
De Plaen E, Lurquin C, Van Pel A, Mariamé B, Szikora JP, Wölfel T, Sibille C, Chomez P, Boon T. Immunogenic (tum-) variants of mouse tumor P815: cloning of the gene of tum-antigen P91A and identification of the tum- mutation. Proc Natl Acad Sci U S A. 1988;85(7):2274–8.
Brichard V, Van Pel A, Wölfel T, Wölfel C, De Plaen E, Lethé B, Coulie P, Boon T. The tyrosinase gene codes for an antigen recognized by autologous cytolytic T lymphocytes on HLA-A2 melanomas. J Exp Med. 1993;178(2):489–95.
Gaugler B, Van den Eynde B, van der Bruggen P, Romero P, Gaforio JJ, De Plaen E, Lethé B, Brasseur F, Boon T. Human gene MAGE-3 codes for an antigen recognized on a melanoma by autologous cytolytic T lymphocytes. J Exp Med. 1994;179(3):921–30.
Boël P, Wildmann C, Sensi ML, Brasseur R, Renauld JC, Coulie P, Boon T, van der Bruggen P. BAGE: a new gene encoding an antigen recognized on human melanomas by cytolytic T lymphocytes. Immunity. 1995;2(2):167–75.
Wang RF, Robbins PF, Kawakami Y, Kang XQ, Rosenberg SA. Identification of a gene encoding a melanoma tumor antigen recognized by HLA-A31-restricted tumor-infiltrating lymphocytes. J Exp Med. 1995;181(3):1261.
CAS Google Scholar
Kawakami Y, Wang X, Shofuda T, Sumimoto H, Tupesis J, Fitzgerald E, Rosenberg S. Isolation of a new melanoma antigen, MART-2, containing a mutated epitope recognized by autologous tumor-infiltrating T lymphocytes. J Immunol. 2001;166(4):2871–7.
Lang F, Schrors B, Lower M, Tureci O, Sahin U. Identification of neoantigens for individualized therapeutic cancer vaccines. Nat Rev Drug Discov. 2022;21(4):261–82.
Huang J, El-Gamil M, Dudley ME, Li YF, Rosenberg SA, Robbins PF. T cells associated with tumor regression recognize frameshifted products of the CDKN2A tumor suppressor gene locus and a mutated HLA class I gene product. J Immunol. 2004;172(10):6057–64.
Zhou J, Dudley ME, Rosenberg SA, Robbins PF. Persistence of multiple tumor-specific T-cell clones is associated with complete tumor regression in a melanoma patient receiving adoptive cell transfer therapy. J Immunother. 2005;28(1):53–62.
Hoof I, Peters B, Sidney J, Pedersen LE, Sette A, Lund O, Buus S, Nielsen M. NetMHCpan, a method for MHC class I binding prediction beyond humans. Immunogenetics. 2009;61(1):1–13.
Stranzl T, Larsen MV, Lundegaard C, Nielsen M. NetCTLpan: pan-specific MHC class I pathway epitope predictions. Immunogenetics. 2010;62(6):357–68.
Ott PA, Hu Z, Keskin DB, Shukla SA, Sun J, Bozym DJ, Zhang W, Luoma A, Giobbie-Hurder A, Peter L, et al. An immunogenic personal neoantigen vaccine for patients with melanoma. Nature. 2017;547(7662):217–21.
Yang JC, Rosenberg SA. Adoptive T-cell therapy for cancer. Adv Immunol. 2016;130:279–94.
Ott PA, Hu-Lieskovan S, Chmielowski B, Govindan R, Naing A, Bhardwaj N, Margolin K, Awad MM, Hellmann MD, Lin JJ, et al. A phase Ib trial of personalized neoantigen therapy plus anti-PD-1 in patients with advanced melanoma, non-small cell lung cancer, or bladder cancer. Cell. 2020;183(2):347-362.e24.
Liu C, Shao J, Dong Y, Xu Q, Zou Z, Chen F, Yan J, Liu J, Li S, Liu B, et al. Advanced HCC patient benefit from neoantigen reactive T cells based immunotherapy: a case report. Front Immunol. 2021;12: 685126.
Zacharakis N, Chinnasamy H, Black M, Xu H, Lu YC, Zheng Z, Pasetto A, Langhan M, Shelton T, Prickett T, et al. Immune recognition of somatic mutations leading to complete durable regression in metastatic breast cancer. Nat Med. 2018;24(6):724–30.
Chen F, Zou Z, Du J, Su S, Shao J, Meng F, Yang J, Xu Q, Ding N, Yang Y, et al. Neoantigen identification strategies enable personalized immunotherapy in refractory solid tumors. J Clin Invest. 2019;129(5):2056–70.
Zeng Y, Zhang W, Li Z, Zheng Y, Wang Y, Chen G, Qiu L, Ke K, Su X, Cai Z, et al. Personalized neoantigen-based immunotherapy for advanced collecting duct carcinoma: case report. J Immunother Cancer. 2020;8(1): e000217.
Parkhurst MR, Robbins PF, Tran E, Prickett TD, Gartner JJ, Jia L, Ivey G, Li YF, El-Gamil M, Lalani A, et al. Unique neoantigens arise from somatic mutations in patients with gastrointestinal cancers. Cancer Discov. 2019;9(8):1022–35.
Schumacher TN, Schreiber RD. Neoantigens in cancer immunotherapy. Science. 2015;348(6230):69–74.
Blaha DT, Anderson SD, Yoakum DM, Hager MV, Zha Y, Gajewski TF, Kranz DM. High-throughput stability screening of neoantigen/HLA complexes improves immunogenicity predictions. Cancer Immunol Res. 2019;7(1):50–61.
Ng SB, Turner EH, Robertson PD, Flygare SD, Bigham AW, Lee C, Shaffer T, Wong M, Bhattacharjee A, Eichler EE, et al. Targeted capture and massively parallel sequencing of 12 human exomes. Nature. 2009;461(7261):272–6.
Purcell AW, Ramarathinam SH, Ternette N. Mass spectrometry-based identification of MHC-bound peptides for immunopeptidomics. Nat Protoc. 2019;14(6):1687–707.
Li H, Durbin R. Fast and accurate short read alignment with burrows-wheeler transform. Bioinformatics. 2009;25(14):1754–60.
Wang K, Li M, Hakonarson H. ANNOVAR: functional annotation of genetic variants from high-throughput sequencing data. Nucleic Acids Res. 2010;38(16): e164.
Cibulskis K, Lawrence MS, Carter SL, Sivachenko A, Jaffe D, Sougnez C, Gabriel S, Meyerson M, Lander ES, Getz G. Sensitive detection of somatic point mutations in impure and heterogeneous cancer samples. Nat Biotechnol. 2013;31(3):213–9.
Larson DE, Harris CC, Chen K, Koboldt DC, Abbott TE, Dooling DJ, Ley TJ, Mardis ER, Wilson RK, Ding L. SomaticSniper: identification of somatic point mutations in whole genome sequencing data. Bioinformatics. 2012;28(3):311–7.
Koboldt DC, Zhang Q, Larson DE, Shen D, McLellan MD, Lin L, Miller CA, Mardis ER, Ding L, Wilson RK. VarScan 2: somatic mutation and copy number alteration discovery in cancer by exome sequencing. Genome Res. 2012;22(3):568–76.
Duan F, Duitama J, Al Seesi S, Ayres CM, Corcelli SA, Pawashe AP, Blanchard T, McMahon D, Sidney J, Sette A, et al. Genomic and bioinformatic profiling of mutational neoepitopes reveals new rules to predict anticancer immunogenicity. J Exp Med. 2014;211(11):2231–48.
Zhang H, Lundegaard C, Nielsen M. Pan-specific MHC class I predictors: a benchmark of HLA class I pan-specific prediction methods. Bioinformatics. 2009;25(1):83–9.
O’Donnell TJ, Rubinsteyn A, Bonsack M, Riemer AB, Laserson U, Hammerbacher J. MHCflurry: open-source class I MHC binding affinity prediction. Cell Syst. 2018;7(1):129-132.e4.
Sarkizova S, Klaeger S, Le PM, Li LW, Oliveira G, Keshishian H, Hartigan CR, Zhang W, Braun DA, Ligon KL, et al. A large peptidome dataset improves HLA class I epitope prediction across most of the human population. Nat Biotechnol. 2020;38(2):199–209.
Shao XM, Bhattacharya R, Huang J, Sivakumar IKA, Tokheim C, Zheng L, Hirsch D, Kaminow B, Omdahl A, Bonsack M, et al. High-throughput prediction of MHC class I and II neoantigens with MHCnuggets. Cancer Immunol Res. 2020;8(3):396–408.
Liu C, Zhang Y, Jian X, Tan X, Lu M, Ouyang J, Liu Z, Li Y, Xu L, Chen L, et al. ProGeo-neo v2.0: a one-stop software for neoantigen prediction and filtering based on the proteogenomics strategy. Genes (Basel). 2022;13(5):783.
Vita R, Mahajan S, Overton JA, Dhanda SK, Martini S, Cantrell JR, Wheeler DK, Sette A, Peters B. The Immune epitope database (IEDB): 2018 update. Nucleic Acids Res. 2019;47(D1):D339–43.
Chronister WD, Crinklaw A, Mahajan S, Vita R, Kosaloglu-Yalcin Z, Yan Z, et al. TCRMatch: predicting T-cell receptor specificity based on sequence similarity to previously characterized receptors. Front Immunol. 2021;12:640725.
Wells DK, van Buuren MM, Dang KK, et al. Key parameters of tumor epitope immunogenicity revealed through a consortium approach improve neoantigen prediction. Cell. 2020;183(3):818–34.
Jurtz VPS, Andreatta M, Marcatili P, Peters B, Nielsen M. NetMHCpan 4.0 Improved peptide-MHC class I interaction predictions integrating eluted ligand and peptide binding affinity data1. J Immunol. 2017;199(9):3360–8.
Jensen KK, Andreatta M, Marcatili P, Buus S, Greenbaum JA, Yan Z, Sette A, Peters B, Nielsen M. Improved methods for predicting peptide binding affinity to MHC class II molecules. Immunology. 2018;154(3):394–406.
O’Donnell TJ, Rubinsteyn A, Laserson U. MHCflurry 2.0: improved pan-allele prediction of MHC class I-presented peptides by incorporating antigen processing. Cell Syst. 2020;11(1):42–8.
Bassani-Sternberg M, Chong C, Guillaume P, Solleder M, Pak H, Gannon PO, Kandalaft LE, Coukos G, Gfeller D. Deciphering HLA-I motifs across HLA peptidomes improves neo-antigen predictions and identifies allostery regulating HLA specificity. PLoS Comput Biol. 2017;13(8): e1005725.
Larsen MV, Lundegaard C, Lamberth K, Buus S, Lund O, Nielsen M. Large-scale validation of methods for cytotoxic T-lymphocyte epitope prediction. BMC Bioinformatics. 2007;8:424.
Roth A, Khattra J, Yap D, Wan A, Laks E, Biele J, Ha G, Aparicio S, Bouchard-Cote A, Shah SP. PyClone: statistical inference of clonal population structure in cancer. Nat Methods. 2014;11(4):396–8.
Bjerregaard AM, Nielsen M, Hadrup SR, Szallasi Z, Eklund AC. MuPeXI: prediction of neo-epitopes from tumor sequencing data. Cancer Immunol Immunother. 2017;66(9):1123–30.
Ali M, Foldvari Z, Giannakopoulou E, et al. Induction of neoantigen-reactive T cells from healthy donors. Nat Protoc. 2019;14(6):1926–43.
Dudley ME, Wunderlich JR, Shelton TE, Even J, Rosenberg SA. Generation of tumor-infiltrating lymphocyte cultures for use in adoptive transfer therapy for melanoma patients. J Immunother. 2003;26(4):332–42.
Chow YT, Chen S, Wang R, Liu C, Kong CW, Li RA, Cheng SH, Sun D. Single cell transfection through precise microinjection with quantitatively controlled injection volumes. Sci Rep. 2016;6:24127.
Kumar ARK, Shou Y, Chan B, Krishaa L, Tay A. Materials for Improving immune cell transfection. Adv Mater. 2021;33(21): e2007421.
Zappasodi R, Di Nicola M, Carlo-Stella C, Mortarini R, Molla A, Vegetti C, Albani S, Anichini A, Gianni AM. The effect of artificial antigen-presenting cells with preclustered anti-CD28/-CD3/-LFA-1 monoclonal antibodies on the induction of ex vivo expansion of functional human antitumor T cells. Haematologica. 2008;93(10):1523–34.
Turtle CJRS. Artificial antigen-presenting cells for use in adoptive immunotherapy. Cancer J. 2010;16(4):374–81.
Ichikawa J, Yoshida T, Isser A, Laino AS, Vassallo M, Woods D, Kim S, Oelke M, Jones K, Schneck JP, et al. Rapid expansion of highly functional antigen-specific T cells from patients with melanoma by nanoscale artificial antigen-presenting cells. Clin Cancer Res. 2020;26(13):3384–96.
Xiao P, Wang J, Zhao Z, Liu X, Sun X, Wang D, Li Y. Engineering nanoscale artificial antigen-presenting cells by metabolic dendritic cell labeling to potentiate cancer immunotherapy. Nano Lett. 2021;21(5):2094–103.
Song S, Jin X, Zhang L, Zhao C, Ding Y, Ang Q, Khaidav O, Shen C. PEGylated and CD47-conjugated nanoellipsoidal artificial antigen-presenting cells minimize phagocytosis and augment anti-tumor T-cell responses. Int J Nanomedicine. 2019;14:2465–83.
Meyer RA, Sunshine JC, Perica K, Kosmides AK, Aje K, Schneck JP, Green JJ. Biodegradable nanoellipsoidal artificial antigen presenting cells for antigen specific T-cell activation. Small. 2015;11(13):1519–25.
Kato T, Matsuda T, Ikeda Y, Park JH, Leisegang M, Yoshimura S, Hikichi T, Harada M, Zewde M, Sato S, Hasegawa K, Kiyotani K, Nakamura Y. Effective screening of T cells recognizing neoantigens and construction of T-cell receptor-engineered T cells. Oncotarget. 2018;9(13):11009–19.
Chan JD, Lai J, Slaney CY, Kallies A, Beavis PA, Darcy PK. Cellular networks controlling T cell persistence in adoptive cell therapy. Nat Rev Immunol. 2021;21(12):769–84.
Sakellariou-Thompson D, Forget MA, Creasy C, Bernard V, Zhao L, Kim YU, Hurd MW, Uraoka N, Parra ER, Kang Y, et al. 4–1BB agonist focuses CD8(+) tumor-infiltrating T-cell growth into a distinct repertoire capable of tumor recognition in pancreatic cancer. Clin Cancer Res. 2017;23(23):7263–75.
Kwakkenbos MJ, Bakker AQ, van Helden PM, Wagner K, Yasuda E, Spits H, Beaumont T. Genetic manipulation of B cells for the isolation of rare therapeutic antibodies from the human repertoire. Methods. 2014;65(1):38–43.
Forget MA, Malu S, Liu H, Toth C, Maiti S, Kale C, Haymaker C, Bernatchez C, Huls H, Wang E, Marincola FM, Hwu P, Cooper LJ, Radvanyi LG. Activation and propagation of tumor-infiltrating lymphocytes on clinical-grade designer artificial antigen-presenting cells for adoptive immunotherapy of melanoma. J Immunother. 2014;37(9):448–69.
Scheffel MJ, Scurti G, Simms P, Garrett-Mayer E, Mehrotra S, Nishimura MI, Voelkel-Johnson C. Efficacy of adoptive T-cell therapy is improved by treatment with the antioxidant n-acetyl cysteine, which limits activation-induced T-cell death. Cancer Res. 2016;76(20):6006–16.
Tran KQ, Zhou J, Durflinger KH, Langhan MM, Shelton TE, Wunderlich JR, Robbins PF, Rosenberg SA, Dudley ME. Minimally cultured tumor-infiltrating lymphocytes display optimal characteristics for adoptive cell therapy. J Immunother. 2008;31(8):742–51.
Dijkstra KK, Cattaneo CM, Weeber F, Chalabi M, van de Haar J, Fanchi LF, Slagter M, van der Velden DL, Kaing S, Kelderman S, et al. Generation of tumor-reactive T cells by co-culture of peripheral blood lymphocytes and tumor organoids. Cell. 2018;174(6):1586-1598.e12.
Wang W, Yuan T, Ma L, Zhu Y, Bao J, Zhao X, Zhao Y, Zong Y, Zhang Y, Yang S, et al. Hepatobiliary tumor organoids reveal HLA class I neoantigen landscape and antitumoral activity of neoantigen peptide enhanced with immune checkpoint inhibitors. Adv Sci (Weinh). 2022;9:e2105810.
Prakadan SM, Shalek AK, Weitz DA. Scaling by shrinking: empowering single-cell “omics” with microfluidic devices. Nat Rev Genet. 2017;18(6):345–61.
Papalexi E, Satija R. Single-cell RNA sequencing to explore immune cell heterogeneity. Nat Rev Immunol. 2018;18(1):35–45.
Pai JA, Satpathy AT. High-throughput and single-cell T cell receptor sequencing technologies. Nat Methods. 2021;18(8):881–92.
Pasetto A, Gros A, Robbins PF, Deniger DC, Prickett TD, Matus-Nicodemos R, Douek DC, Howie B, Robins H, Parkhurst MR, et al. Tumor- and neoantigen-reactive T-cell receptors can be identified based on their frequency in fresh tumor. Cancer Immunol Res. 2016;4(9):734–43.
Bobisse S, Genolet R, Roberti A, Tanyi JL, Racle J, Stevenson BJ, Iseli C, Michel A, Le Bitoux MA, Guillaume P, et al. Sensitive and frequent identification of high avidity neo-epitope specific CD8 (+) T cells in immunotherapy-naive ovarian cancer. Nat Commun. 2018;9(1):1092.
Lowery FJ, Krishna S, Yossef R, et al. Molecular signatures of antitumor neoantigen-reactive T cells from metastatic human cancers. Science. 2022;375(6583):877–84.
Chester C, Sanmamed MF, Wang J, Melero I. Immunotherapy targeting 4–1BB: mechanistic rationale, clinical results, and future strategies. Blood. 2018;131(1):49–57.
Parkhurst M, Gros A, Pasetto A, Prickett T, Crystal JS, Robbins P, Rosenberg SA. Isolation of T-cell receptors specifically reactive with mutated tumor-associated antigens from tumor-infiltrating lymphocytes based on CD137 expression. Clin Cancer Res. 2017;23(10):2491–505.
Duhen T, Duhen R, Montler R, Moses J, Moudgil T, de Miranda NF, Goodall CP, Blair TC, Fox BA, McDermott JE, et al. Co-expression of CD39 and CD103 identifies tumor-reactive CD8 T cells in human solid tumors. Nat Commun. 2018;9(1):2724.
Krishna S, Lowery FJ, Copeland AR, Bahadiroglu E, Mukherjee R, Jia L, Anibal JT, Sachs A, Adebola SO, Gurusamy D, Yu Z, Hill V, Gartner JJ, Li YF, Parkhurst M, Paria B, Kvistborg P, Kelly MC, Goff SL, Altan-Bonnet G, Robbins PF, Rosenberg SA. Stem-like CD8 T cells mediate response of adoptive cell immunotherapy against human cancer. Science. 2020;370(6522):1328–34.
Hanada KI, Zhao C, Gil-Hoyos R, Gartner JJ, Chow-Parmer C, Lowery FJ, Krishna S, Prickett TD, Kivitz S, Parkhurst MR, et al. A phenotypic signature that identifies neoantigen-reactive T cells in fresh human lung cancers. Cancer Cell. 2022;40(5):479-493.e6.
He J, Xiong X, Yang H, Li D, Liu X, Li S, Liao S, Chen S, Wen X, Yu K, Fu L, Dong X, Zhu K, Xia X, Kang T, Bian C, Li X, Liu H, Ding P, Zhang X, Liu Z, Li W, Zuo Z, Zhou P. Defined tumor antigen-specific T cells potentiate personalized TCR-T cell therapy and prediction of immunotherapy response. Cell Res. 2022;32(6):530–42.
Veatch JR, Lee SM, Shasha C, Singhi N, Szeto JL, Moshiri AS, Kim TS, Smythe K, Kong P, Fitzgibbon M, et al. Neoantigen-specific CD4+ T cells in human melanoma have diverse differentiation states and correlate with CD8+ T cell, macrophage, and B cell function. Cancer Cell. 2022;40(4):393-409.e9.
Bajwa G, Lanz I, Cardenas M, Brenner MK, Arber C. Transgenic CD8alphabeta co-receptor rescues endogenous TCR function in TCR-transgenic virus-specific T cells. J Immunother Cancer. 2020;8(2):e001487.
Shenderov E, Kandasamy M, Gileadi U, Chen J, Shepherd D, Gibbs J, Prota G, Silk JD, Yewdell JW, Cerundolo V. Generation and characterization of HLA-A2 transgenic mice expressing the human TCR 1G4 specific for the HLA-A2 restricted NY-ESO-1157-165 tumor-specific peptide. J Immunother Cancer. 2021;9(6): e002544.
Rath JA, Arber C. Engineering strategies to enhance TCR-based adoptive T cell therapy. Cell. 2020;9(6):1485.
Article CAS Google Scholar
Halim L, Das KK, Larcombe-Young D, Ajina A, Candelli A, Benjamin R, Dillon R, Davies DM, Maher J. Engineering of an avidity-optimized CD19-specific parallel chimeric antigen receptor that delivers dual CD28 and 4–1BB Co-stimulation. Front Immunol. 2022;13: 836549.
Roselli E, Boucher JC, Li G, Kotani H, Spitler K, Reid K, et al. 4-1BB and optimized CD28 co-stimulation enhances function of human mono-specific and bi-specific third-generation CAR T cells. J Immunother Cancer. 2021;9(10):e003354.
Guercio M, Orlando D, Di Cecca S, Sinibaldi M, Boffa I, Caruso S, Abbaszadeh Z, Camera A, Cembrola B, Bovetti K, et al. CD28. OX40 co-stimulatory combination is associated with long in vivo persistence and high activity of CAR CD30 T-cells. Haematologica. 2021;106(4):987–99.
Fedorov VD, Themeli M, Sadelain M. PD-1- and CTLA-4-based inhibitory chimeric antigen receptors (iCARs) divert off-target immunotherapy responses. Sci Transl Med. 2013;5(215):215ra172.
Liu H, Lei W, Zhang C, Yang C, Wei J, Guo Q, Guo X, Chen Z, Lu Y, Young KH, et al. CD19-specific CAR T cells that express a PD-1/CD28 chimeric switch-receptor are effective in patients with PD-L1-positive B-cell lymphoma. Clin Cancer Res. 2021;27(2):473–84.
Hoogi S, Eisenberg V, Mayer S, Shamul A, Barliya T, Cohen CJ. A TIGIT-based chimeric co-stimulatory switch receptor improves T-cell anti-tumor function. J Immunother Cancer. 2019;7(1):243.
Zhang Q, Hresko ME, Picton LK, Su L, Hollander MJ, Nunez-Cruz S, Zhang Z, Assenmacher CA, Sockolosky JT, Garcia KC, et al. A human orthogonal IL-2 and IL-2Rbeta system enhances CAR T cell expansion and antitumor activity in a murine model of leukemia. Sci Transl Med. 2021;13(625):eabg6986.
Kalbasi A, Siurala M, Su LL, Tariveranmoshabad M, Picton LK, Ravikumar P, Li P, Lin JX, Escuin-Ordinas H, Da T, et al. Potentiating adoptive cell therapy using synthetic IL-9 receptors. Nature. 2022;612(7938):E10.
Kagoya Y, Tanaka S, Guo T, Anczurowski M, Wang C-H, Saso K, Butler MO, Minden MD, Hirano N. A novel chimeric antigen receptor containing a JAK–STAT signaling domain mediates superior antitumor effects. Nat Med. 2018;24(3):352–9.
Luo H, Su J, Sun R, Sun Y, Wang Y, Dong Y, Shi B, Jiang H, Li Z. Coexpression of IL7 and CCL21 increases efficacy of CAR-T cells in solid tumors without requiring preconditioned lymphodepletion. Clin Cancer Res. 2020;26(20):5494–505.
Kerkar SP, Muranski P, Kaiser A, Boni A, Sanchez-Perez L, Yu Z, Palmer DC, Reger RN, Borman ZA, Zhang L, et al. Tumor-specific CD8+ T cells expressing interleukin-12 eradicate established cancers in lymphodepleted hosts. Cancer Res. 2010;70(17):6725–34.
Zhang L, Morgan RA, Beane JD, Zheng Z, Dudley ME, Kassim SH, Nahvi AV, Ngo LT, Sherry RM, Phan GQ, et al. Tumor-infiltrating lymphocytes genetically engineered with an inducible gene encoding interleukin-12 for the immunotherapy of metastatic melanoma. Clin Cancer Res. 2015;21(10):2278–88.
Krenciute G, Prinzing BL, Yi Z, Wu MF, Liu H, Dotti G, Balyasnikova IV, Gottschalk S. Transgenic expression of IL15 improves antiglioma activity of IL13Ralpha2-CAR T cells but results in antigen loss variants. Cancer Immunol Res. 2017;5(7):571–81.
Drakes DJ, Rafiq S, Purdon TJ, Lopez AV, Chandran SS, Klebanoff CA, Brentjens RJ. Optimization of T-cell receptor-modified T cells for cancer therapy. Cancer Immunol Res. 2020;8(6):743–55.
Ma X, Shou P, Smith C, Chen Y, Du H, Sun C, Porterfield Kren N, Michaud D, Ahn S, Vincent B, et al. Interleukin-23 engineering improves CAR T cell function in solid tumors. Nat Biotechnol. 2020;38(4):448–59.
Adachi K, Kano Y, Nagai T, Okuyama N, Sakoda Y, Tamada K. IL-7 and CCL19 expression in CAR-T cells improves immune cell infiltration and CAR-T cell survival in the tumor. Nat Biotechnol. 2018;36(4):346–51.
Lai J, Mardiana S, House IG, Sek K, Henderson MA, Giuffrida L, Chen AXY, Todd KL, Petley EV, Chan JD, et al. Adoptive cellular therapy with T cells expressing the dendritic cell growth factor Flt3L drives epitope spreading and antitumor immunity. Nat Immunol. 2020;21(8):914–26.
Wang G, Zhang Z, Zhong K, Wang Z, Yang N, Tang X, Li H, Lu Q, Wu Z, Yuan B, et al. CXCL11-armed oncolytic adenoviruses enhance CAR-T cell therapeutic efficacy and reprogram tumor microenvironment in glioblastoma. Mol Ther. 2023;31(1):134–53.
Li X, Lu M, Yuan M, Ye J, Zhang W, Xu L, Wu X, Hui B, Yang Y, Wei B, et al. CXCL10-armed oncolytic adenovirus promotes tumor-infiltrating T-cell chemotaxis to enhance anti-PD-1 therapy. Oncoimmunology. 2022;11(1):2118210.
Tian Y, Wen C, Zhang Z, Liu Y, Li F, Zhao Q, Yao C, Ni K, Yang S, Zhang Y. CXCL9-modified CAR T cells improve immune cell infiltration and antitumor efficacy. Cancer Immunol Immunother. 2022;71(11):2663–75.
Depil S, Duchateau P, Grupp SA, Mufti G, Poirot L. ‘Off-the-shelf’ allogeneic CAR T cells: development and challenges. Nat Rev Drug Discovery. 2020;19(3):185–99.
Johnson LR, Lee DY, Eacret JS, Ye D, June CH, Minn AJ. The immunostimulatory RNA RN7SL1 enables CAR-T cells to enhance autonomous and endogenous immune function. Cell. 2021;184(19):4981-4995.e14.
Rafiq S, Hackett CS, Brentjens RJ. Engineering strategies to overcome the current roadblocks in CAR T cell therapy. Nat Rev Clin Oncol. 2020;17(3):147–67.
Tokarew N, Ogonek J, Endres S, von Bergwelt-Baildon M, Kobold S. Teaching an old dog new tricks: next-generation CAR T cells. Br J Cancer. 2019;120(1):26–37.
Dhatchinamoorthy K, Colbert JD, Rock KL. Cancer immune evasion through loss of MHC class I antigen presentation. Front Immunol. 2021;12:636568.
Shklovskaya E, Rizos H. MHC class I deficiency in solid tumors and therapeutic strategies to overcome it. Int J Mol Sci. 2021;22(13):6741.
Rosenthal R, Cadieux EL, Salgado R, Bakir MA, Moore DA, Hiley CT, Lund T, Tanic M, Reading JL, Joshi K, et al. Neoantigen-directed immune escape in lung cancer evolution. Nature. 2019;567(7749):479–85.
Zhou F. Molecular mechanisms of IFN-gamma to up-regulate MHC class I antigen processing and presentation. Int Rev Immunol. 2009;28(3–4):239–60.
Ugurel S, Spassova I, Wohlfarth J, Drusio C, Cherouny A, Melior A, Sucker A, Zimmer L, Ritter C, Schadendorf D, et al. MHC class-I downregulation in PD-1/PD-L1 inhibitor refractory Merkel cell carcinoma and its potential reversal by histone deacetylase inhibition: a case series. Cancer Immunol Immunother. 2019;68(6):983–90.
Luo N, Nixon MJ, Gonzalez-Ericsson PI, Sanchez V, Opalenik SR, Li H, Zahnow CA, Nickels ML, Liu F, Tantawy MN, et al. DNA methyltransferase inhibition upregulates MHC-I to potentiate cytotoxic T lymphocyte responses in breast cancer. Nat Commun. 2018;9(1):248.
Ennishi D, Takata K, Beguelin W, Duns G, Mottok A, Farinha P, Bashashati A, Saberi S, Boyle M, Meissner B, et al. Molecular and genetic characterization of MHC deficiency identifies EZH2 as therapeutic target for enhancing immune recognition. Cancer Discov. 2019;9(4):546–63.
Briere D, Sudhakar N, Woods DM, Hallin J, Engstrom LD, Aranda R, Chiang H, Sodre AL, Olson P, Weber JS, et al. The class I/IV HDAC inhibitor mocetinostat increases tumor antigen presentation, decreases immune suppressive cell types and augments checkpoint inhibitor therapy. Cancer Immunol Immunother. 2018;67(3):381–92.
Yamamoto K, Venida A, Yano J, Biancur DE, Kakiuchi M, Gupta S, Sohn ASW, Mukhopadhyay S, Lin EY, Parker SJ, et al. Autophagy promotes immune evasion of pancreatic cancer by degrading MHC-I. Nature. 2020;581(7806):100–5.
Badrinath S, Dellacherie MO, Li A, Zheng S, Zhang X, Sobral M, Pyrdol JW, Smith KL, Lu Y, Haag S, et al. A vaccine targeting resistant tumours by dual T cell plus NK cell attack. Nature. 2022;606(7916):992–8.
Hegde PS, Chen DS. Top 10 challenges in cancer immunotherapy. Immunity. 2020;52(1):17–35.
Liu YT, Sun ZJ. Turning cold tumors into hot tumors by improving T-cell infiltration. Theranostics. 2021;11(11):5365–86.
Ahmadzadeh M, Johnson LA, Heemskerk B, Wunderlich JR, Dudley ME, White DE, Rosenberg SA. Tumor antigen-specific CD8 T cells infiltrating the tumor express high levels of PD-1 and are functionally impaired. Blood. 2009;114(8):1537–44.
Grassberger C, Ellsworth SG, Wilks MQ, Keane FK, Loeffler JS. Assessing the interactions between radiotherapy and antitumour immunity. Nat Rev Clin Oncol. 2019;16(12):729–45.
Galluzzi L, Humeau J, Buque A, Zitvogel L, Kroemer G. Immunostimulation with chemotherapy in the era of immune checkpoint inhibitors. Nat Rev Clin Oncol. 2020;17(12):725–41.
McLaughlin M, Patin EC, Pedersen M, Wilkins A, Dillon MT, Melcher AA, Harrington KJ. Inflammatory microenvironment remodelling by tumour cells after radiotherapy. Nat Rev Cancer. 2020;20(4):203–17.
Chu KF, Dupuy DE. Thermal ablation of tumours: biological mechanisms and advances in therapy. Nat Rev Cancer. 2014;14(3):199–208.
Roma-Rodrigues C, Mendes R, Baptista PV, Fernandes AR. Targeting tumor microenvironment for cancer therapy. Int J Mol Sci. 2019;20(4):840.
Zhu Y, Qian Y, Li Z, Li Y, Li B. Neoantigen-reactive T cell: An emerging role in adoptive cellular immunotherapy. MedComm (2020). 2021;2(2):207–20.
PubMed Google Scholar
Frese KK, Neesse A, Cook N, Bapiro TE, Lolkema MP, Jodrell DI, Tuveson DA. nab-Paclitaxel potentiates gemcitabine activity by reducing cytidine deaminase levels in a mouse model of pancreatic cancer. Cancer Discov. 2012;2(3):260–9.
Yardley DA. nab-Paclitaxel mechanisms of action and delivery. J Control Release. 2013;170(3):365–72.
Von Hoff DD, Ervin T, Arena FP, Chiorean EG, Infante J, Moore M, Seay T, Tjulandin SA, Ma WW, Saleh MN, et al. Increased survival in pancreatic cancer with nab-paclitaxel plus gemcitabine. N Engl J Med. 2013;369(18):1691–703.
Article Google Scholar
Huang Y, Kim BYS, Chan CK, Hahn SM, Weissman IL, Jiang W. Improving immune-vascular crosstalk for cancer immunotherapy. Nat Rev Immunol. 2018;18(3):195–203.
Tian L, Goldstein A, Wang H, Ching Lo H, Sun Kim I, Welte T, Sheng K, Dobrolecki LE, Zhang X, Putluri N, et al. Mutual regulation of tumour vessel normalization and immunostimulatory reprogramming. Nature. 2017;544(7649):250–4.
Sugiyama D, Nishikawa H, Maeda Y, Nishioka M, Tanemura A, Katayama I, Ezoe S, Kanakura Y, Sato E, Fukumori Y, et al. Anti-CCR4 mAb selectively depletes effector-type FoxP3+CD4+ regulatory T cells, evoking antitumor immune responses in humans. Proc Natl Acad Sci U S A. 2013;110(44):17945–50.
Fultang L, Panetti S, Ng M, Collins P, Graef S, Rizkalla N, Booth S, Lenton R, Noyvert B, Shannon-Lowe C, et al. MDSC targeting with Gemtuzumab ozogamicin restores T cell immunity and immunotherapy against cancers. EBioMedicine. 2019;47:235–46.
Highfill SL, Cui Y, Giles AJ, Smith JP, Zhang H, Morse E, Kaplan RN, Mackall CL. Disruption of CXCR2-mediated MDSC tumor trafficking enhances anti-PD1 efficacy. Sci Transl Med. 2014;6(237):237ra267.
Dixon ML, Luo L, Ghosh S, Grimes JM, Leavenworth JD, Leavenworth JW. Remodeling of the tumor microenvironment via disrupting Blimp1(+) effector Treg activity augments response to anti-PD-1 blockade. Mol Cancer. 2021;20(1):150.
Tavazoie MF, Pollack I, Tanqueco R, Ostendorf BN, Reis BS, Gonsalves FC, Kurth I, Andreu-Agullo C, Derbyshire ML, Posada J, et al. LXR/ApoE activation restricts innate immune suppression in cancer. Cell. 2018;172(4):825-840.e18.
Mohamed E, Sierra RA, Trillo-Tinoco J, Cao Y, Innamarato P, Payne KK, de Mingo PA, Mandula J, Zhang S, Thevenot P, Biswas S, Abdalla SK, Costich TL, Hänggi K, Anadon CM, Flores ER, Haura EB, Mehrotra S, Pilon-Thomas S, Ruffell B, Munn DH, Cubillos-Ruiz JR, Conejo-Garcia JR, Rodriguez PC. The unfolded protein response mediator PERK governs myeloid cell-driven immunosuppression in tumors through inhibition of STING signaling. Immunity. 2020;52(4):668-682.e7.
Huang W, Liu Y, Luz A, Berrong M, Meyer JN, Zou Y, et al. Calcium/Calmodulin dependent protein Kinase Kinase 2 regulates the expansion of tumor-induced Myeloid-derived suppressor cells. Front Immunol. 2021;12:754083.
Han S, Wang W, Wang S, Yang T, Zhang G, Wang D, Ju R, Lu Y, Wang H, Wang L. Tumor microenvironment remodeling and tumor therapy based on M2-like tumor associated macrophage-targeting nano-complexes. Theranostics. 2021;11(6):2892–916.
Mohapatra S, Pioppini C, Ozpolat B, Calin GA. Non-coding RNAs regulation of macrophage polarization in cancer. Mol Cancer. 2021;20(1):24.
Liu C, Chikina M, Deshpande R, Menk AV, Wang T, Tabib T, Brunazzi EA, Vignali KM, Sun M, Stolz DB, et al. Treg cells promote the SREBP1-dependent metabolic fitness of tumor-promoting macrophages via repression of CD8+ T cell-derived interferon-γ. Immunity. 2019;51(2):381-397.e6.
Sautes-Fridman C, Petitprez F, Calderaro J, Fridman WH. Tertiary lymphoid structures in the era of cancer immunotherapy. Nat Rev Cancer. 2019;19(6):307–25.
Cabrita R, Lauss M, Sanna A, Donia M, Skaarup Larsen M, Mitra S, Johansson I, Phung B, Harbst K, Vallon-Christersson J, et al. Tertiary lymphoid structures improve immunotherapy and survival in melanoma. Nature. 2020;577(7791):561–5.
Wouters MCA, Nelson BH. Prognostic significance of tumor-infiltrating B cells and plasma cells in human cancer. Clin Cancer Res. 2018;24(24):6125–35.
Kroeger DR, Milne K, Nelson BH. Tumor-infiltrating plasma cells are associated with tertiary lymphoid structures, cytolytic t-cell responses, and superior prognosis in ovarian cancer. Clin Cancer Res. 2016;22(12):3005–15.
Meylan M, Petitprez F, Becht E, Bougouin A, Pupier G, Calvez A, Giglioli I, Verkarre V, Lacroix G, Verneau J, et al. Tertiary lymphoid structures generate and propagate anti-tumor antibody-producing plasma cells in renal cell cancer. Immunity. 2022;55(3):527-541.e5.
Delvecchio FR, Fincham REA, Spear S, Clear A, Roy-Luzarraga M, Balkwill FR, Gribben JG, Bombardieri M, Hodivala-Dilke K, Capasso M, et al. Pancreatic cancer chemotherapy is potentiated by induction of tertiary lymphoid structures in mice. Cell Mol Gastroenterol Hepatol. 2021;12(5):1543–65.
Zhu G, Nemoto S, Mailloux AW, Perez-Villarroel P, Nakagawa R, Falahat R, Berglund AE, Mule JJ. Induction of tertiary lymphoid structures with antitumor function by a lymph node-derived stromal cell line. Front Immunol. 2018;9:1609.
Tran E, Turcotte S, Gros A, Robbins PF, Lu YC, Dudley ME, Wunderlich JR, Somerville RP, Hogan K, Hinrichs CS, Parkhurst MR, Yang JC, Rosenberg SA. Cancer immunotherapy based on mutation-specific CD4+ T cells in a patient with epithelial cancer. Science. 2014;344(6184):641–5.
Leidner R, Sanjuan Silva N, Huang H, Sprott D, Zheng C, Shih YP, Leung A, Payne R, Sutcliffe K, Cramer J, et al. Neoantigen T-cell receptor gene therapy in pancreatic cancer. N Engl J Med. 2022;386(22):2112–9.
Kim SP, Vale NR, Zacharakis N, Krishna S, Yu Z, Gasmi B, Gartner JJ, Sindiri S, Malekzadeh P, Deniger DC, et al. Adoptive cellular therapy with autologous tumor-infiltrating lymphocytes and T-cell receptor-engineered t cells targeting common p53 neoantigens in human solid tumors. Cancer Immunol Res. 2022;10(8):932–46.
Peng S, Chen S, Hu W, Mei J, Zeng X, Su T, Wang W, Chen Z, Xiao H, Zhou Q, et al. Combination neoantigen-based dendritic cell vaccination and adoptive T-cell transfer induces antitumor responses against recurrence of hepatocellular carcinoma. Cancer Immunol Res. 2022;10(6):728–44.
Sahin U, Derhovanessian E, Miller M, Kloke BP, Simon P, Lower M, Bukur V, Tadmor AD, Luxemburger U, Schrors B, et al. Personalized RNA mutanome vaccines mobilize poly-specific therapeutic immunity against cancer. Nature. 2017;547(7662):222–6.
Keskin DB, Anandappa AJ, Sun J, Tirosh I, Mathewson ND, Li S, Oliveira G, Giobbie-Hurder A, Felt K, Gjini E, et al. Neoantigen vaccine generates intratumoral T cell responses in phase Ib glioblastoma trial. Nature. 2019;565(7738):234–9.
Cafri G, Gartner JJ, Zaks T, Hopson K, Levin N, Paria BC, Parkhurst MR, Yossef R, Lowery FJ, Jafferji MS, et al. mRNA vaccine-induced neoantigen-specific T cell immunity in patients with gastrointestinal cancer. J Clin Invest. 2020;130(11):5976–88.
Fang Y, Mo F, Shou J, Wang H, Luo K, Zhang S, Han N, Li H, Ye S, Zhou Z, et al. A pan-cancer clinical study of personalized neoantigen vaccine monotherapy in treating patients with various types of advanced solid tumors. Clin Cancer Res. 2020;26(17):4511–20.
Kloor M, Reuschenbach M, Pauligk C, Karbach J, Rafiyan MR, Al-Batran SE, Tariverdian M, Jager E, von Knebel DM. A frameshift peptide neoantigen-based vaccine for mismatch repair-deficient cancers: a phase I/IIa clinical trial. Clin Cancer Res. 2020;26(17):4503–10.
Mueller S, Taitt JM, Villanueva-Meyer JE, Bonner ER, Nejo T, Lulla RR, Goldman S, Banerjee A, Chi SN, Whipple NS, et al. Mass cytometry detects H3 .3K27M-specific vaccine responses in diffuse midline glioma. J Clin Invest. 2020;130(12):6325–37.
Cai Z, Su X, Qiu L, Li Z, Li X, Dong X, Wei F, Zhou Y, Luo L, Chen G, et al. Personalized neoantigen vaccine prevents postoperative recurrence in hepatocellular carcinoma patients with vascular invasion. Mol Cancer. 2021;20(1):164.
Veatch JR, Lee SM, Fitzgibbon M, Chow IT, Jesernig B, Schmitt T, Kong YY, Kargl J, Houghton AM, Thompson JA, et al. Tumor-infiltrating BRAFV600E-specific CD4+ T cells correlated with complete clinical response in melanoma. J Clin Invest. 2018;128(4):1563–8.
van den Berg JH, Heemskerk B, van Rooij N, Gomez-Eerland R, Michels S, van Zon M, et al. Tumor Infiltrating Lymphocytes (TIL) therapy in metastatic melanoma: boosting of neoantigen-specific T cell reactivity and long-term follow-up. J Immunother Cancer. 2020;8(2):e000848.
Kristensen NP, Heeke C, Tvingsholm SA, Borch A, Draghi A, Crowther MD, Carri I, Munk KK, Holm JS, Bjerregaard AM, et al. Neoantigen-reactive CD8+ T cells affect clinical outcome of adoptive cell therapy with tumor-infiltrating lymphocytes in melanoma. J Clin Invest. 2022;132(2): e150535.
Creelan BC, Wang C, Teer JK, Toloza EM, Yao J, Kim S, Landin AM, Mullinax JE, Saller JJ, Saltos AN, et al. Tumor-infiltrating lymphocyte treatment for anti-PD-1-resistant metastatic lung cancer: a phase 1 trial. Nat Med. 2021;27(8):1410–8.
Carreno BMMV, Becker-Hapak M, Kaabinejadian S, Hundal J, Petti AA, Ly A, Lie WR, Hildebrand WH, Mardis ER, Linette GP. Cancer immunotherapy: A dendritic cell vaccine increases the breadth and diversity of melanoma neoantigen-specific T cells. Science. 2015;348(6236):803–8.
Carreno BM, Becker-Hapak M, Huang A, Chan M, Alyasiry A, Lie WR, Aft RL, Cornelius LA, Trinkaus KM, Linette GP. IL-12p70-producing patient DC vaccine elicits Tc1-polarized immunity. J Clin Invest. 2013;123(8):3383–94.
Ding Z, Li Q, Zhang R, Xie L, Shu Y, Gao S, Wang P, Su X, Qin Y, Wang Y, et al. Personalized neoantigen pulsed dendritic cell vaccine for advanced lung cancer. Signal Transduct Target Ther. 2021;6(1):26.
Tanyi JLBS, Ophir E, Tuyaerts S, Roberti A, Genolet R, Baumgartner P, Stevenson BJ, Iseli C, Dangaj D, Czerniecki B, Semilietof A, Racle J, Michel A, Xenarios I, Chiang C, Monos DS, Torigian DA, Nisenbaum HL, Michielin O, June CH, Levine BL, Powell DJ Jr, Gfeller D, Mick R, Dafni U, Zoete V, Harari A, Coukos G, Kandalaft LE. Personalized cancer vaccine effectively mobilizes antitumor T cell immunity in ovarian cancer. Sci Transl Med. 2018;10(436):eaao5931.
Holm JS, Funt SA, Borch A, Munk KK, Bjerregaard AM, Reading JL, Maher C, Regazzi A, Wong P, Al-Ahmadie H, et al. Neoantigen-specific CD8 T cell responses in the peripheral blood following PD-L1 blockade might predict therapy outcome in metastatic urothelial carcinoma. Nat Commun. 2022;13(1):1935.
Fehlings M, Jhunjhunwala S, Kowanetz M, O’Gorman WE, Hegde PS, Sumatoh H, Lee BH, Nardin A, Becht E, Flynn S, et al. Late-differentiated effector neoantigen-specific CD8+ T cells are enriched in peripheral blood of non-small cell lung carcinoma patients responding to atezolizumab treatment. J Immunother Cancer. 2019;7(1):249.
Zhang J, Wang L. The emerging world of TCR-T cell trials against cancer: a systematic review. Technol Cancer Res Treat. 2019;18:1533033819831068.
Jamal-Hanjani M, Quezada SA, Larkin J, Swanton C. Translational implications of tumor heterogeneity. Clin Cancer Res. 2015;21(6):1258–66.
Ping Y, Liu C, Zhang Y. T-cell receptor-engineered T cells for cancer treatment: current status and future directions. Protein Cell. 2018;9(3):254–66.
Huang J, Khong HT, Dudley ME, El-Gamil M, Li YF, Rosenberg SA, Robbins PF. Survival, persistence, and progressive differentiation of adoptively transferred tumor-reactive T cells associated with tumor regression. J Immunother. 2005;28(3):258–67.
Chandran SS, Paria BC, Srivastava AK, Rothermel LD, Stephens DJ, Dudley ME, Somerville R, Wunderlich JR, Sherry RM, Yang JC, et al. Persistence of CTL clones targeting melanocyte differentiation antigens was insufficient to mediate significant melanoma regression in humans. Clin Cancer Res. 2015;21(3):534–43.
Tran E, Ahmadzadeh M, Lu YC, Gros A, Turcotte S, Robbins PF, Gartner JJ, Zheng Z, Li YF, Ray S, Wunderlich JR, Somerville RP, Rosenberg SA. Immunogenicity of somatic mutations in human gastrointestinal cancers. Science. 2015;350(6266):1387–90.
Tran E, Robbins PF, Lu YC, Prickett TD, Gartner JJ, Jia L, Pasetto A, Zheng Z, Ray S, Groh EM, et al. T-cell transfer therapy targeting mutant KRAS in cancer. N Engl J Med. 2016;375(23):2255–62.
Zhao X, Pan X, Wang Y, Zhang Y. Targeting neoantigens for cancer immunotherapy. Biomark Res. 2021;9(1):61.
Gattinoni L, Klebanoff CA, Palmer DC, Wrzesinski C, Kerstann K, Yu Z, Finkelstein SE, Theoret MR, Rosenberg SA, Restifo NP. Acquisition of full effector function in vitro paradoxically impairs the in vivo antitumor efficacy of adoptively transferred CD8+ T cells. J Clin Invest. 2005;115(6):1616–26.
Hinrichs CS, Borman ZA, Gattinoni L, Yu Z, Burns WR, Huang J, Klebanoff CA, Johnson LA, Kerkar SP, Yang S, Muranski P, Palmer DC, Scott CD, Morgan RA, Robbins PF, Rosenberg SA, Restifo NP. Human effector CD8+ T cells derived from naive rather than memory subsets possess superior traits for adoptive immunotherapy. Blood. 2011;117(3):808–14.
Crompton JG, Sukumar M, Restifo NP. Uncoupling T-cell expansion from effector differentiation in cell-based immunotherapy. Immunol Rev. 2014;257(1):264–76.
Giavridis T, van der Stegen SJC, Eyquem J, Hamieh M, Piersigilli A, Sadelain M. CAR T cell-induced cytokine release syndrome is mediated by macrophages and abated by IL-1 blockade. Nat Med. 2018;24(6):731–8.
Norelli M, Camisa B, Barbiera G, Falcone L, Purevdorj A, Genua M, Sanvito F, Ponzoni M, Doglioni C, Cristofori P, et al. Monocyte-derived IL-1 and IL-6 are differentially required for cytokine-release syndrome and neurotoxicity due to CAR T cells. Nat Med. 2018;24(6):739–48.
Download references
Acknowledgements
The Basic Medical Research Project of the First Affiliated Hospital of Second Military Medical University (Grant No. 2021JCMS16), The San Hang Program of the Second Military Medical University, The Project of Disciplines of Excellence, Shanghai Municipal Health Commission (Grant No. 20224Z0037).
Author information
Ruichen Huang and Bi Zhao contributed equally to this work.
Authors and Affiliations
Department of Respiratory and Critical Care Medicine, the First Affiliated Hospital of Second Military Medical University, Shanghai, 200433, People’s Republic of China
Ruichen Huang, Bi Zhao & Wei Zhang
Department of Biophysics, College of Basic Medical Sciences, Second Military Medical University, 800 Xiangyin Road, Shanghai, 200433, People’s Republic of China
National Key Laboratory of Medical Immunology, Institute of Immunology, Second Military Medical University, 800 Xiangyin Road, Shanghai, 200433, People’s Republic of China
School of Basic Medicine, Wenzhou Medical University, Wenzhou, 325000, People’s Republic of China
Xiaoping Su
You can also search for this author in PubMed Google Scholar
Contributions
RH and BZ contributed equally to this review. RH, BZ, XS and WZ conceived the framework and content of this manuscript. RH and BZ collected the published literature and researches data and drafted the manuscript. SH, QZ, XS and WZ provided critical comments and edited the manuscript. All the authors read and approved the final manuscript.
Corresponding authors
Correspondence to Xiaoping Su or Wei Zhang .
Ethics declarations
Ethics approval and consent to participate, consent for publication, competing interests.
The authors declare no competing interests.
Additional information
Publisher’s note.
Springer Nature remains neutral with regard to jurisdictional claims in published maps and institutional affiliations.
Rights and permissions
Open Access This article is licensed under a Creative Commons Attribution 4.0 International License, which permits use, sharing, adaptation, distribution and reproduction in any medium or format, as long as you give appropriate credit to the original author(s) and the source, provide a link to the Creative Commons licence, and indicate if changes were made. The images or other third party material in this article are included in the article's Creative Commons licence, unless indicated otherwise in a credit line to the material. If material is not included in the article's Creative Commons licence and your intended use is not permitted by statutory regulation or exceeds the permitted use, you will need to obtain permission directly from the copyright holder. To view a copy of this licence, visit http://creativecommons.org/licenses/by/4.0/ . The Creative Commons Public Domain Dedication waiver ( http://creativecommons.org/publicdomain/zero/1.0/ ) applies to the data made available in this article, unless otherwise stated in a credit line to the data.
Reprints and permissions
About this article
Cite this article.
Huang, R., Zhao, B., Hu, S. et al. Adoptive neoantigen-reactive T cell therapy: improvement strategies and current clinical researches. Biomark Res 11 , 41 (2023). https://doi.org/10.1186/s40364-023-00478-5
Download citation
Received : 21 December 2022
Accepted : 21 March 2023
Published : 17 April 2023
DOI : https://doi.org/10.1186/s40364-023-00478-5
Share this article
Anyone you share the following link with will be able to read this content:
Sorry, a shareable link is not currently available for this article.
Provided by the Springer Nature SharedIt content-sharing initiative
- Neoantigen-reactive T cell
- Immunotherapy
Biomarker Research
ISSN: 2050-7771
- General enquiries: [email protected]
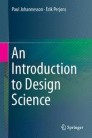
An Introduction to Design Science pp 39–73 Cite as
Research Strategies and Methods
- Paul Johannesson 3 &
- Erik Perjons 3
- First Online: 01 January 2014
23k Accesses
1 Citations
1 Altmetric
Researchers have since centuries used research methods for supporting the creation of reliable knowledge based on empirical evidence and logical arguments. This chapter offers an overview of established research strategies and methods with a focus on empirical research in the social sciences. The chapter discusses research strategies, such as experiment, survey, case study, ethnography, grounded theory, action research, and phenomenology. Research methods for data collection are also described, including questionnaires, interviews, focus groups, observations, and documents. Qualitative and quantitative methods for data analysis are discussed. Finally, the use of research strategies and methods in design science is investigated.
This is a preview of subscription content, log in via an institution .
Buying options
- Available as PDF
- Read on any device
- Instant download
- Own it forever
- Available as EPUB and PDF
- Compact, lightweight edition
- Dispatched in 3 to 5 business days
- Free shipping worldwide - see info
Tax calculation will be finalised at checkout
Purchases are for personal use only
Bhattacherjee A (2012) Social science research: principles, methods, and practices, 2nd edn. CreateSpace Independent Publishing Platform, Tampa, FL
Google Scholar
Blake W (2012) Delphi complete works of William Blake, 2nd edn. Classics, Delphi
Bradburn NM, Sudman S, Wansink B (2004) Asking questions: the definitive guide to questionnaire design—for market research, political polls, and social and health questionnaires, Revised edition. Jossey-Bass
Bryman A (2012) Social research methods (4 edn). Oxford University Press, Oxford
Charmaz K (2014) Constructing grounded theory, 2nd edn. Sage, Thousand Oaks, CA
Coghlan D, Brannick T (2014) Doing action research in your own organization (4th edn). Sage, London
Creswell JW (2013) Research design: qualitative, quantitative, and mixed methods approaches, 4th edn. Sage, Thousand Oaks
Denscombe M (2014) The good research guide: for small scale research projects, 5th revised edn. Open University Press
Dey I (2003) Qualitative data analysis: a user friendly guide for social scientists. Routledge, London
Fairclough N (2013) Critical discourse analysis: the critical study of language, 2nd edn. Routledge, London
Field A, Hole DGJ (2003) How to design and report experiments, 1st edn. Sage, London
Fowler FJ (2013) Survey research methods, 5th edn. Sage, Thousand Oaks, CA
Glaser BG, Strauss AL (2009) The discovery of grounded theory: strategies for qualitative research. Aldine Transaction
Kemmis S, McTaggart R, Nixon R (2013) The action research planner: doing critical participatory action research, 2014 edn. Springer
Krippendorff KH (2012) Content analysis: an introduction to its methodology, 3rd edn. Sage, Los Angeles
LeCompte MD, Schensul JJ (2010) Designing and conducting ethnographic research: an introduction, 2nd edn. AltaMira Press, Lanham, MD
McNiff J (2013) Action research: principles and practice, 3rd edn. Routledge, Abingdon
Oates BJ (2006) Researching information systems and computing. Sage, London
Peterson RA (2000) Constructing effective questionnaires. Sage, Thousand Oaks, CA
Prior L (2008) Repositioning documents in social research. Sociology 42:821–836. doi: 10.1177/0038038508094564
Article Google Scholar
Seidman I (2013) Interviewing as qualitative research: a guide for researchers in education and the social sciences, 4th edn. Teachers College Press, New York
Silverman D (2013) Doing qualitative research, 4th edn. Sage
Stephens L (2004) Advanced statistics demystified, 1 edn. McGraw-Hill Professional
Urdan TC (2010) Statistics in plain English, 3rd edn. Routledge, New York
MATH Google Scholar
Yin RK (2013) Case study research: design and methods, 5th edn. Sage, Los Angeles
Download references
Author information
Authors and affiliations.
Stockholm University, Kista, Sweden
Paul Johannesson & Erik Perjons
You can also search for this author in PubMed Google Scholar
Rights and permissions
Reprints and permissions
Copyright information
© 2014 Springer International Publishing Switzerland
About this chapter
Cite this chapter.
Johannesson, P., Perjons, E. (2014). Research Strategies and Methods. In: An Introduction to Design Science. Springer, Cham. https://doi.org/10.1007/978-3-319-10632-8_3
Download citation
DOI : https://doi.org/10.1007/978-3-319-10632-8_3
Published : 12 September 2014
Publisher Name : Springer, Cham
Print ISBN : 978-3-319-10631-1
Online ISBN : 978-3-319-10632-8
eBook Packages : Computer Science Computer Science (R0)
Share this chapter
Anyone you share the following link with will be able to read this content:
Sorry, a shareable link is not currently available for this article.
Provided by the Springer Nature SharedIt content-sharing initiative
- Publish with us
Policies and ethics
- Find a journal
- Track your research
ORIGINAL RESEARCH article
Rhetorical strategies in chinese and english talk show humour: a comparative analysis.
- 1 Zunyi Medical University, Zunyi, China
- 2 Department of Foreign Languages, Faculty of Modern Language and Communication, Putra Malaysia University, Serdang, Malaysia
The final, formatted version of the article will be published soon.
Select one of your emails
You have multiple emails registered with Frontiers:
Notify me on publication
Please enter your email address:
If you already have an account, please login
You don't have a Frontiers account ? You can register here
Humour is a kind of cognitive psychology activity, and it is diverse among individuals.One of the main characteristics of talk shows is to produce humorous discourse to make the audience laugh; however, rare studies have made a deeper comparative investigation on the rhetorical strategies in different language humorous utterances. Therefore, the current study adopted a mixed method of sequential explanatory design to identify the types of rhetorical strategies in the monologue verbal humour of Chinese and English talk shows, examine their similarities and differences. 200 monologue samples from 2016 to 2022, which consisted of 100 monologues of Chinese talk shows (CTS) and 100 monologues of English talk shows (ETS), were downloaded from the internet as language corpus. Berger's theory was adopted to identify the types of rhetorical strategies. Based on the obtained findings, this study found that both language talk show hosts use a variety type of rhetorical strategies to produce humorous discourse. The comparison of similarities and differences revealed that the most frequently used rhetorical strategies in both talk shows were almost similar (e.g., satire, exaggeration, facetiousness, and ridicule), but the percentage of usage of these various rhetorical strategies in both talk shows was slightly different. Interestingly, misunderstanding occurred twenty times in CTS but was not found in ETS. Meanwhile, simile and personification were used more often in ETS. Conclusively, this study contributes valuable insights on the use of different types of rhetorical strategies to create verbal humour in different language contexts.
Keywords: rhetorical strategies, Talk show, Humour, comparative analysis, Chinese and English
Received: 02 Feb 2024; Accepted: 12 Apr 2024.
Copyright: © 2024 ZHOU and Chen. This is an open-access article distributed under the terms of the Creative Commons Attribution License (CC BY) . The use, distribution or reproduction in other forums is permitted, provided the original author(s) or licensor are credited and that the original publication in this journal is cited, in accordance with accepted academic practice. No use, distribution or reproduction is permitted which does not comply with these terms.
* Correspondence: Shiyue Chen, Department of Foreign Languages, Faculty of Modern Language and Communication, Putra Malaysia University, Serdang, 43400, Malaysia
Disclaimer: All claims expressed in this article are solely those of the authors and do not necessarily represent those of their affiliated organizations, or those of the publisher, the editors and the reviewers. Any product that may be evaluated in this article or claim that may be made by its manufacturer is not guaranteed or endorsed by the publisher.
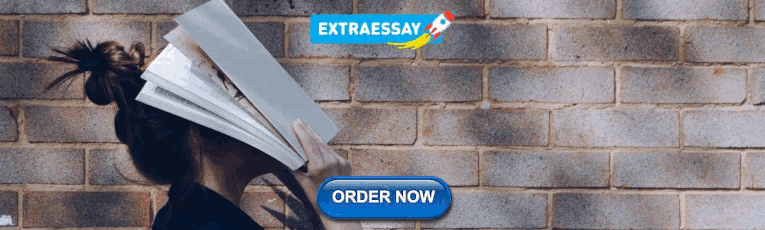
IMAGES
VIDEO
COMMENTS
Definition of research in data analysis: According to LeCompte and Schensul, research data analysis is a process used by researchers to reduce data to a story and interpret it to derive insights. The data analysis process helps reduce a large chunk of data into smaller fragments, which makes sense. Three essential things occur during the data ...
For many researchers unfamiliar with qualitative research, determining how to conduct qualitative analyses is often quite challenging. Part of this challenge is due to the seemingly limitless approaches that a qualitative researcher might leverage, as well as simply learning to think like a qualitative researcher when analyzing data. From framework analysis (Ritchie & Spencer, 1994) to content ...
This article is a practical guide to conducting data analysis in general literature reviews. The general literature review is a synthesis and analysis of published research on a relevant clinical issue, and is a common format for academic theses at the bachelor's and master's levels in nursing, physiotherapy, occupational therapy, public health and other related fields.
e) Prescriptive analysis - How will it happen. Another of the most effective types of analysis methods in research. Prescriptive data techniques cross over from predictive analysis in the way that it revolves around using patterns or trends to develop responsive, practical business strategies.
Step 6: Decide on your data analysis strategies. On their own, raw data can't answer your research question. The last step of designing your research is planning how you'll analyse the data. Quantitative data analysis. In quantitative research, you'll most likely use some form of statistical analysis. With statistics, you can summarise ...
This chapter provides an overview of selected qualitative data analysis strategies with a particular focus on codes and coding. Preparatory strategies for a qualitative research study and data management are first outlined. Six coding methods are then profiled using comparable interview data: process coding, in vivo coding, descriptive coding ...
Quantitative data analysis is one of those things that often strikes fear in students. It's totally understandable - quantitative analysis is a complex topic, full of daunting lingo, like medians, modes, correlation and regression.Suddenly we're all wishing we'd paid a little more attention in math class…. The good news is that while quantitative data analysis is a mammoth topic ...
Abstract. This chapter provides an overview of selected qualitative data analytic strategies with a particular focus on codes and coding. Preparatory strategies for a qualitative research study and data management are first outlined. Six coding methods are then profiled using comparable interview data: process coding, in vivo coding ...
However, MMMR strategies are also often employed alongside such quantitative analysis, especially in policy-driven research. For example, in cross-national research, governmental organizations require comparative data to assess how countries are doing in a number of different fields, a process that has become an integral part of performance ...
What Is Analysis in Qualitative Research? A classic definition of analysis in qualitative research is that the "analyst seeks to provide an explicit rendering of the structure, order and patterns found among a group of participants" (Lofland, 1971, p. 7). Usually when we think about analysis in research, we think about it as a stage in the ...
Statistical analysis means investigating trends, patterns, and relationships using quantitative data. It is an important research tool used by scientists, governments, businesses, and other organizations. ... A research design is your overall strategy for data collection and analysis. It determines the statistical tests you can use to test your ...
2.1 Step 1: defining the research question. The first step in conducting a meta-analysis, as with any other empirical study, is the definition of the research question. Most importantly, the research question determines the realm of constructs to be considered or the type of interventions whose effects shall be analyzed.
A research design is a strategy for answering your research question using empirical data. Creating a research design means making decisions about: Your overall research objectives and approach. Whether you'll rely on primary research or secondary research. Your sampling methods or criteria for selecting subjects. Your data collection methods.
A research strategy is an overall plan for conducting a research study. A research strategy guides a researcher in planning, executing, and monitoring the study. While the research strategy provides useful support at a high level, it needs to be complemented with research methods that can guide the research work at a more detailed level.
The first step in a data analysis plan is to describe the data collected in the study. This can be done using figures to give a visual presentation of the data and statistics to generate numeric descriptions of the data. Selection of an appropriate figure to represent a particular set of data depends on the measurement level of the variable.
Research methods are specific procedures for collecting and analyzing data. Developing your research methods is an integral part of your research design. When planning your methods, there are two key decisions you will make. First, decide how you will collect data. Your methods depend on what type of data you need to answer your research question:
Thematic analysis is a research method used to identify and interpret patterns or themes in a data set; it often leads to new insights and understanding (Boyatzis, 1998; Elliott, 2018; Thomas, 2006).However, it is critical that researchers avoid letting their own preconceptions interfere with the identification of key themes (Morse & Mitcham, 2002; Patton, 2015).
Resources on conducting qualitative data analysis. Analysis is a process of deconstructing and reconstructing evidence that involves purposeful interrogation and critical thinking about data in order to produce a meaningful interpretation and relevant understanding in answer to the questions asked or that arise in the process of investigation (Bazeley, 2021, p.
Qualitative data analysis seeks to make general statements on how categories or themes of data are related and their meaning. It consists of identifying, coding, and categorizing patterns found in ...
Abstract. Statistical methods involved in carrying out a study include planning, designing, collecting data, analysing, drawing meaningful interpretation and reporting of the research findings. The statistical analysis gives meaning to the meaningless numbers, thereby breathing life into a lifeless data. The results and inferences are precise ...
What is Strategic Analysis? Strategic analysis refers to the process of conducting research on a company and its operating environment to formulate a strategy. The definition of strategic analysis may differ from an academic or business perspective, but the process involves several common factors:
This article addresses the complex challenges that public organizations face in designing, implementing, and evaluating their strategies, where public interest and regulatory compliance often intertwine with strategic objectives. This research investigates the application of ontologies in the field of public sector strategy management to ...
Reproducibility is a "proteomic dream" yet to be fully realized. A typical data analysis workflow utilizing extracted ion chromatograms (XICs) often treats the information path from identification to quantification as a one-way street. Here, we propose an XIC-centric approach in which the data flow is bidirectional: identifications are used to derive XICs whose information is in turn ...
This strategy builds on those strengths to respond urgently to the new era of strategic competition and a renewed focus to align with the Department of Defense (DoD) S&T strategy, to better team with our allies, Congress, partner nations, and DoD research community. We recognize the need for improvements to
Strategic Analysis Latest Articles. Submit an article Journal homepage. 0 Views 0 CrossRef citations to date 0. Altmetric Book Review. Changing the Narrative: Information Campaigns, Strategy and Crisis Escalation in the Digital Age ... Related Research . People also read lists articles that other readers of this article have read.
A case study is one of the most commonly used methodologies of social research. This article attempts to look into the various dimensions of a case study research strategy, the different epistemological strands which determine the particular case study type and approach adopted in the field, discusses the factors which can enhance the effectiveness of a case study research, and the debate ...
The most common strategy for depleting the stroma is to use albumin-bound paclitaxel to facilitate T cell infiltration [133, 134]. Research showed that combinational therapy with nab-paclitaxel and gemcitabine or nab-paclitaxel and atezolizumab significantly improves tumor control and patient survival .
Green development represents a critical pathway to achieving high-quality growth. From a regional perspective, examining the impact of regional integration on urban green economic development holds significant importance. This study leverages a quasi-natural experiment of regional integration strategy implementation, utilizing unbalanced panel data from China's ten major urban agglomerations ...
A research strategy is an overall plan for conducting a research study. A research strategy guides a researcher in planning, executing, and monitoring the study. While the research strategy provides useful support on a high level, it needs to be complemented with research methods that can guide the research work on a more detailed level.
Therefore, the current study adopted a mixed method of sequential explanatory design to identify the types of rhetorical strategies in the monologue verbal humour of Chinese and English talk shows, examine their similarities and differences. 200 monologue samples from 2016 to 2022, which consisted of 100 monologues of Chinese talk shows (CTS ...