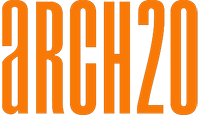
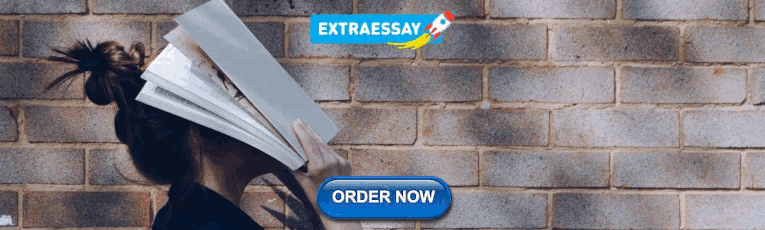
Triodos Bank | RAU
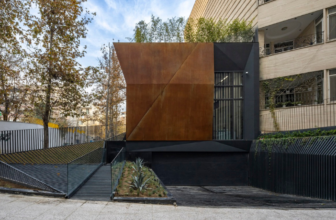
Karafarin Bank l NextOffice–Alireza Taghaboni
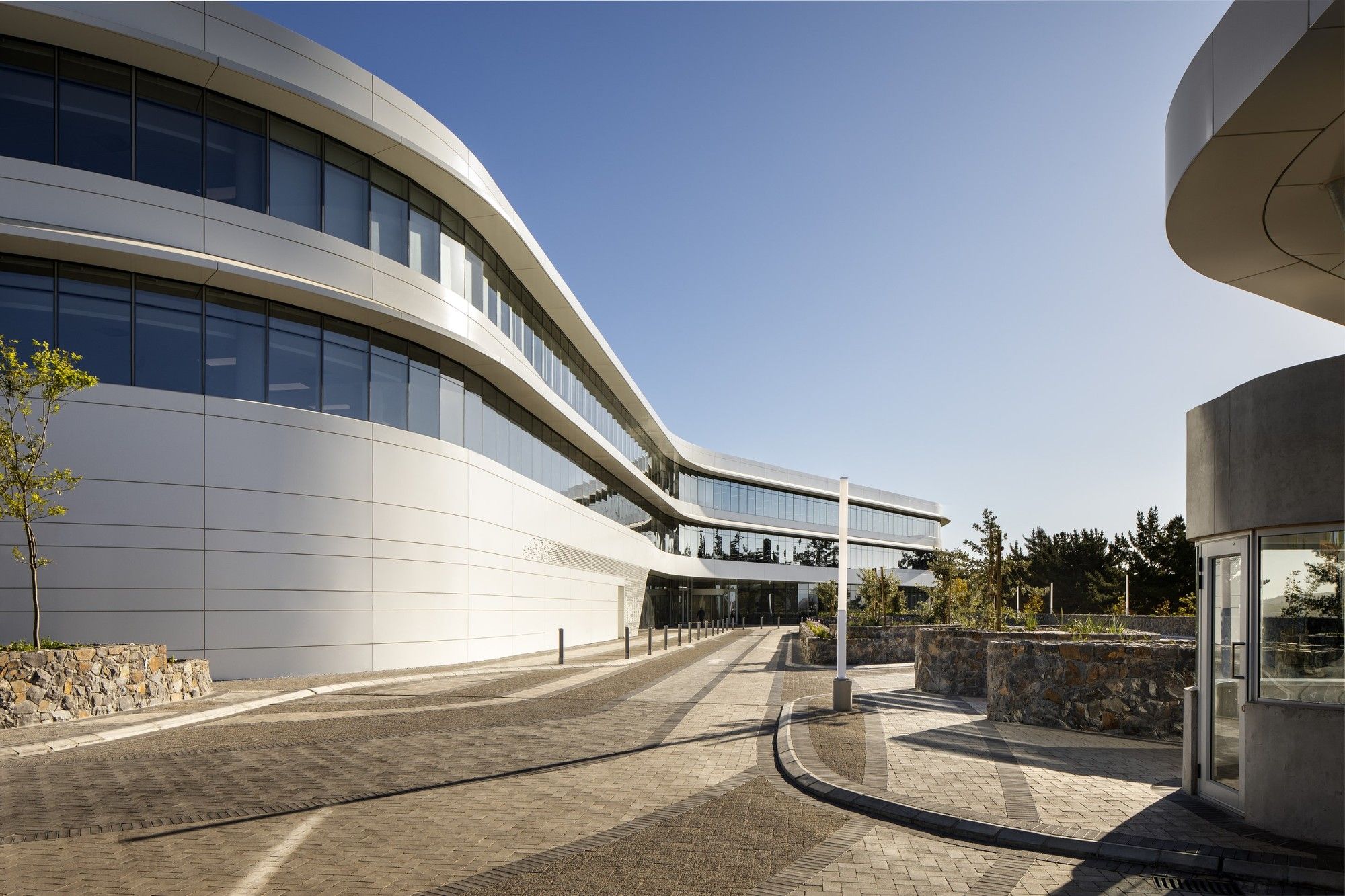
Capitec Bank Headquarters | dhk Architects
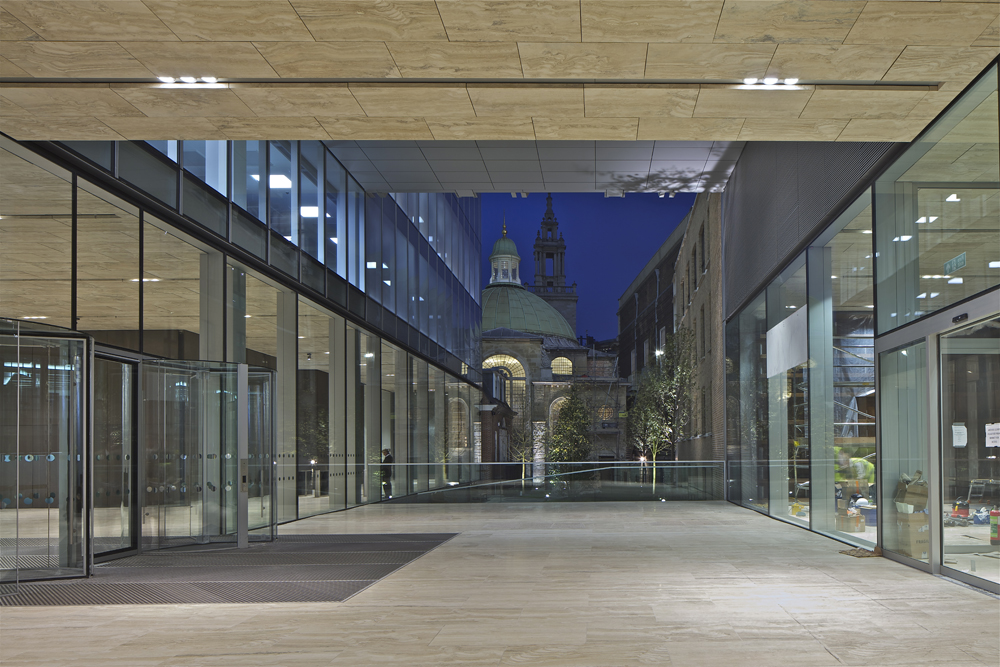
Rothschild Bank Headquarters | OMA
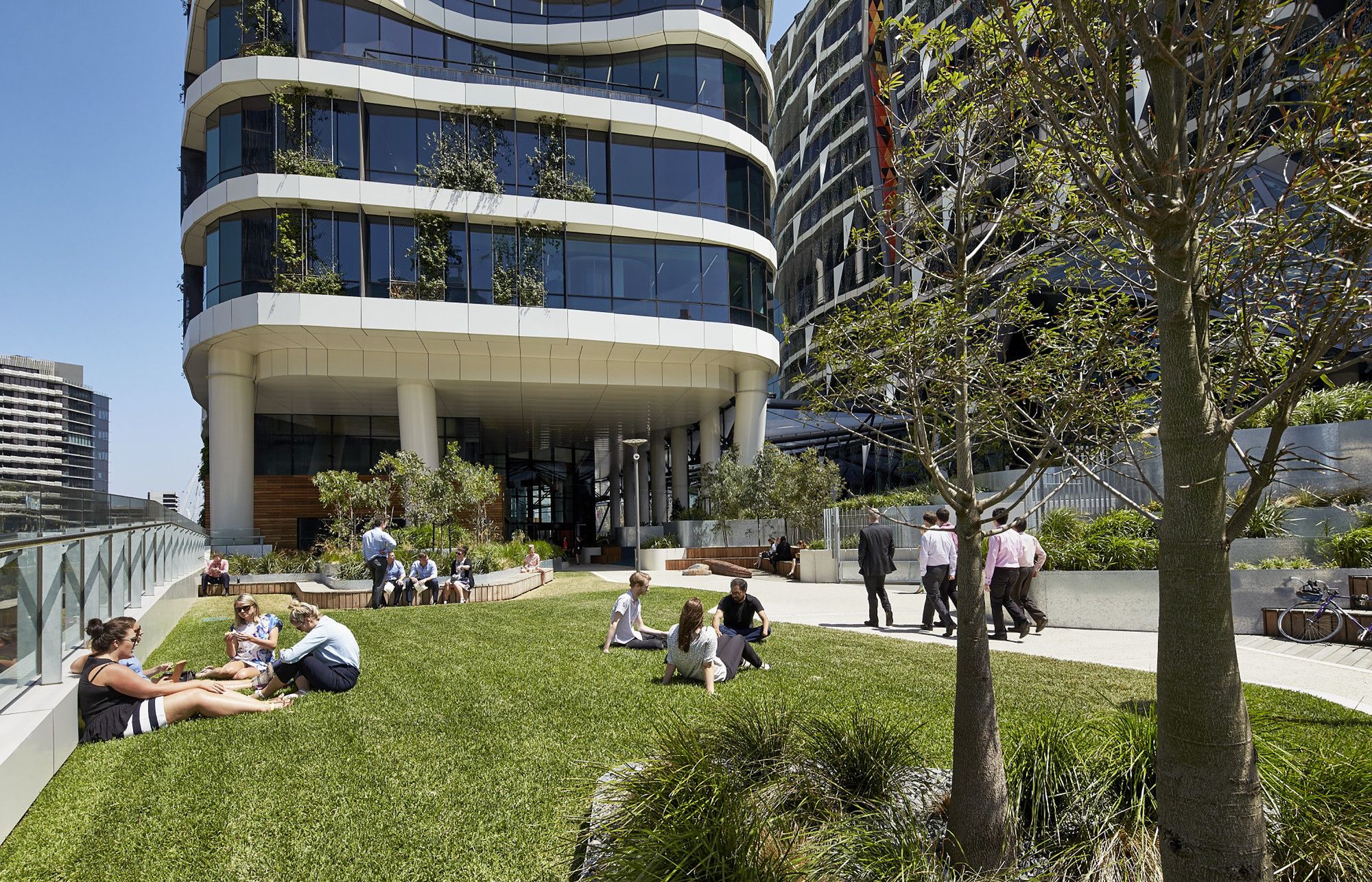
Medibank | Hassell
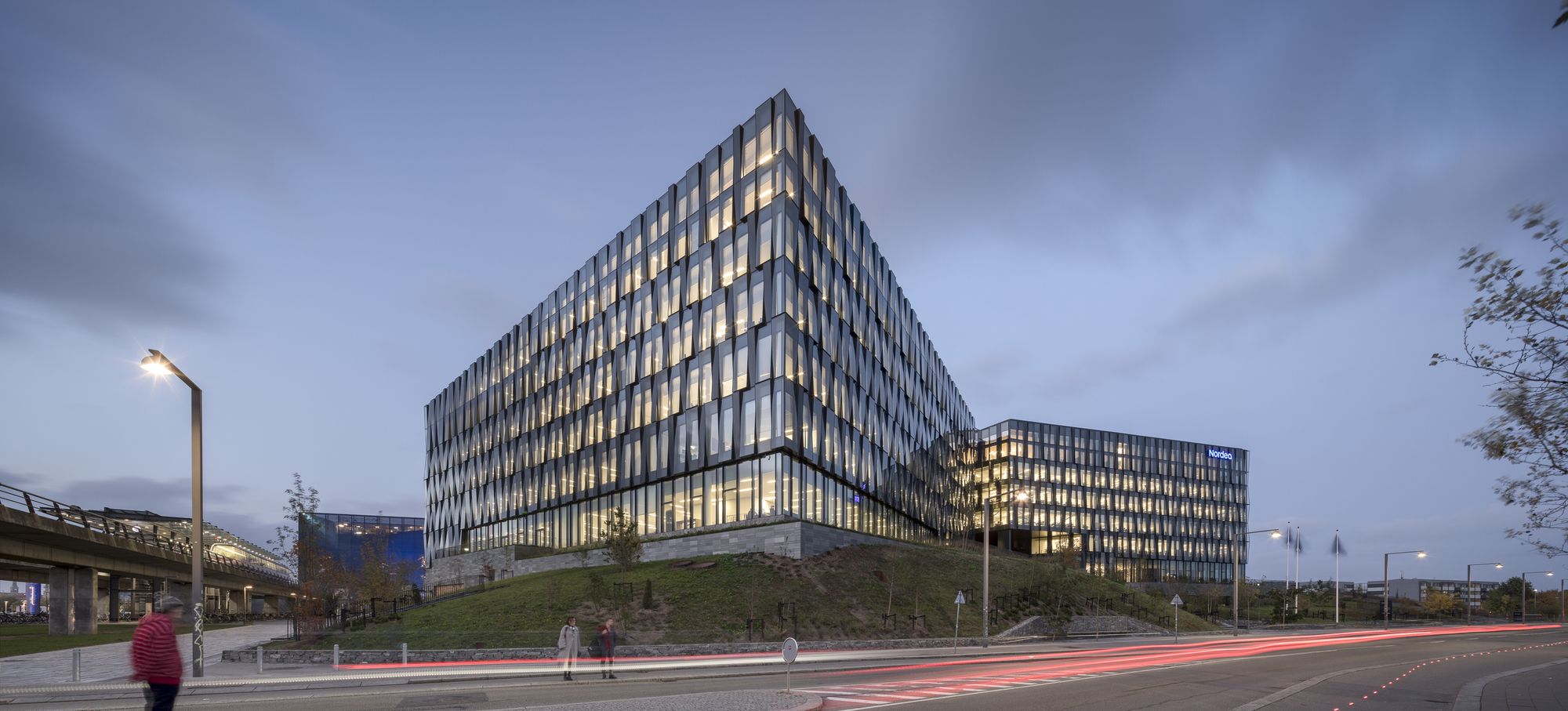
Nordea Bank | Henning Larsen
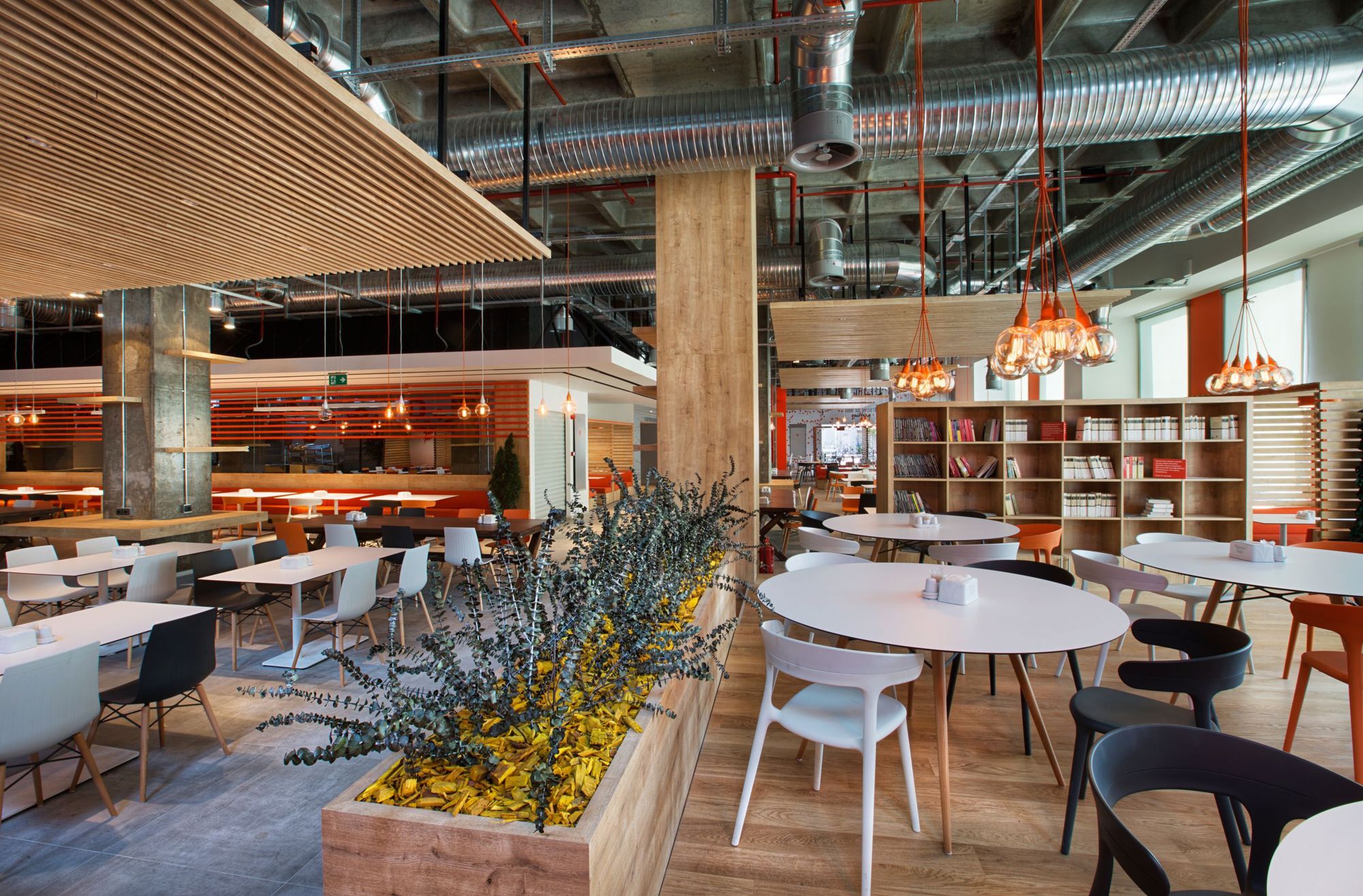
ING Bank Turkey HQ | Bakirkure Architects
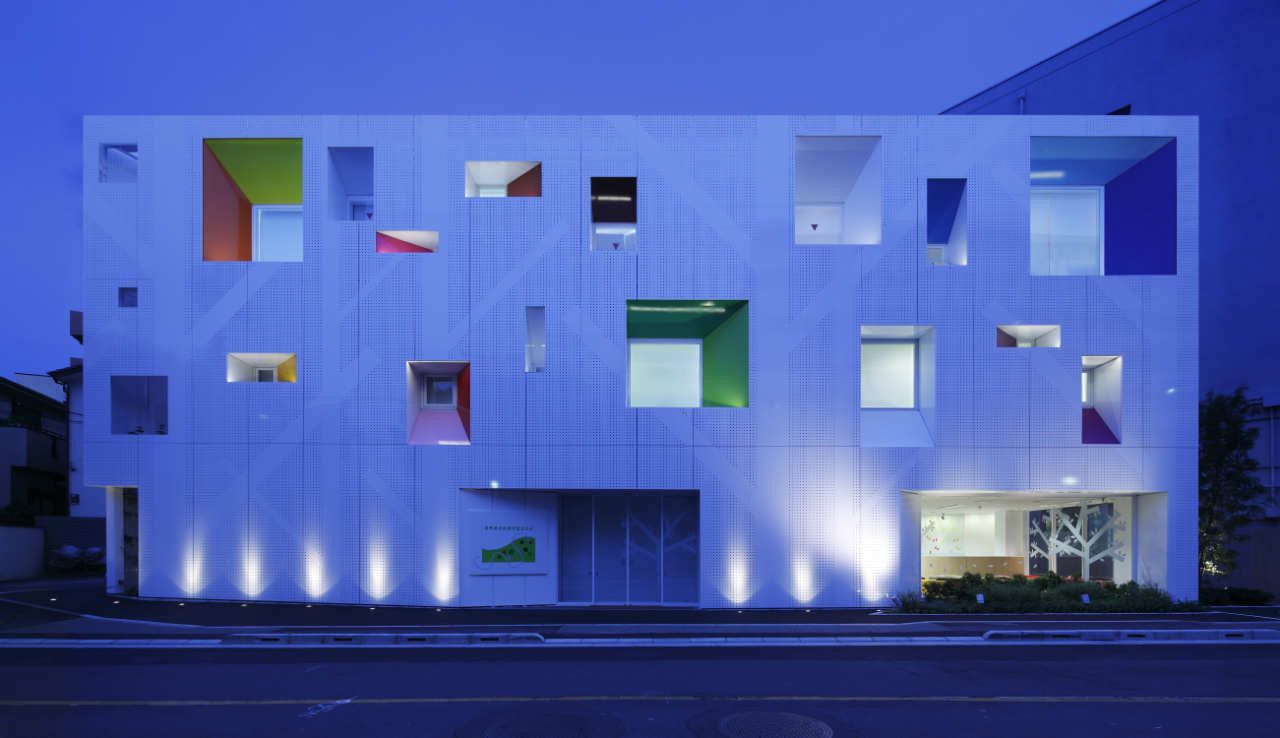
Sugamo Shinkin Bank, Tokiwadai Branch | Emmanuelle Moureaux Architecture + Design
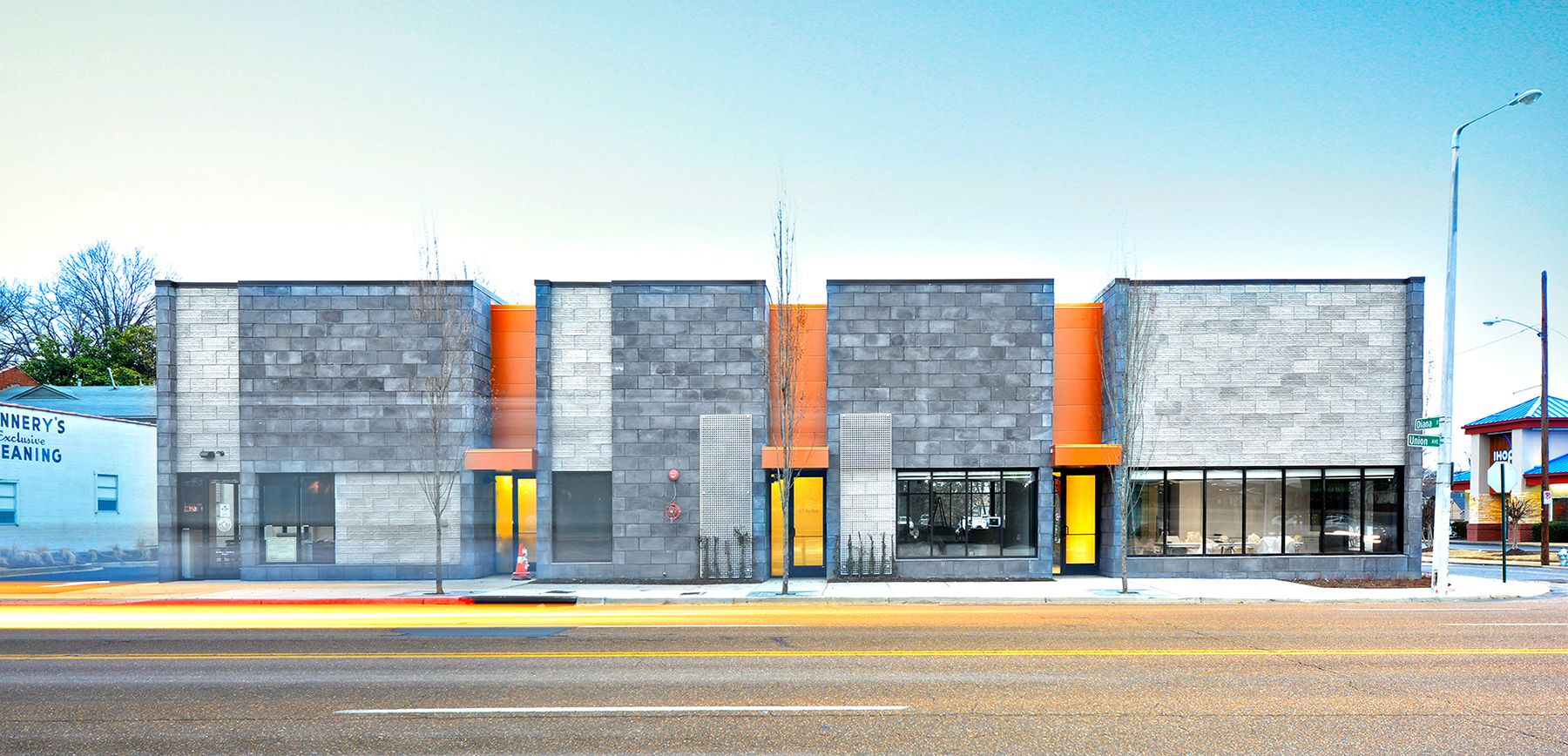
Renasant Bank – Shell + Interiors | brg3s Architects
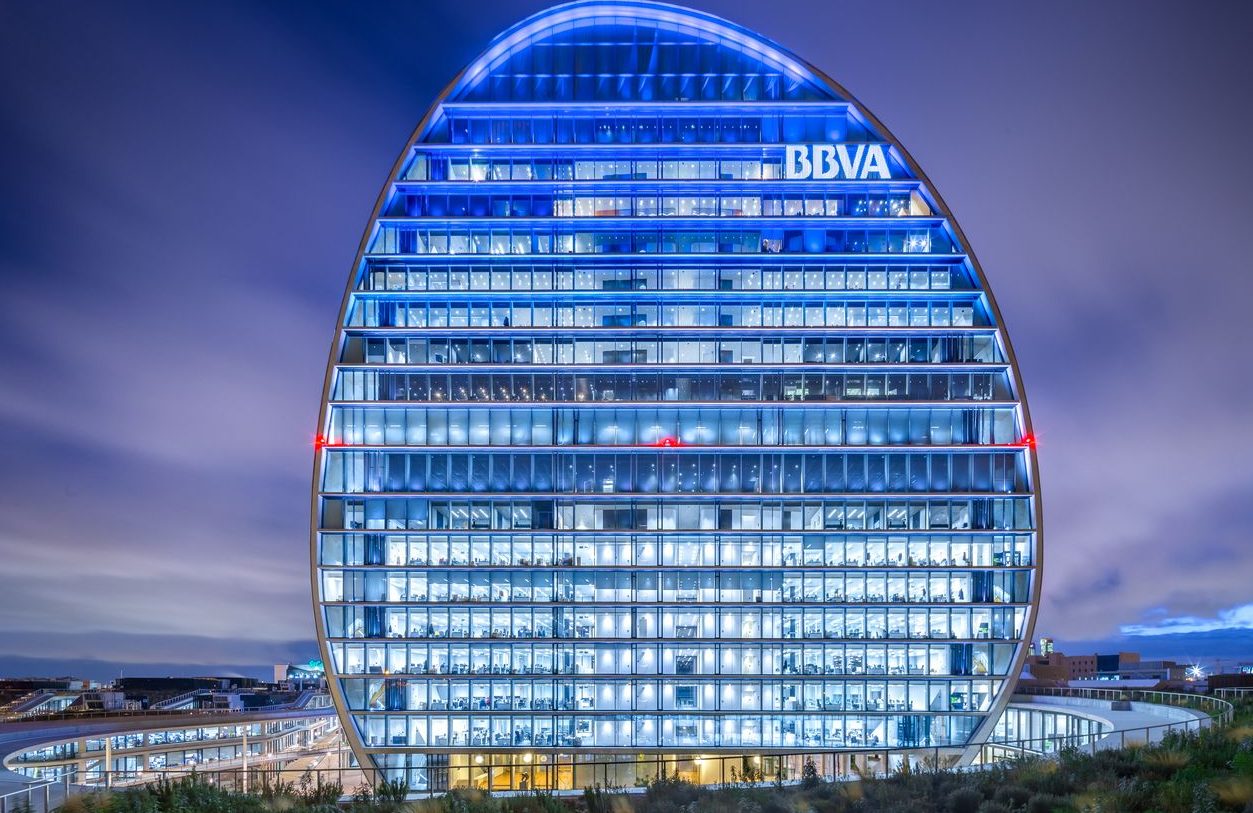
BBVA Headquarters | Herzog & de Meuron
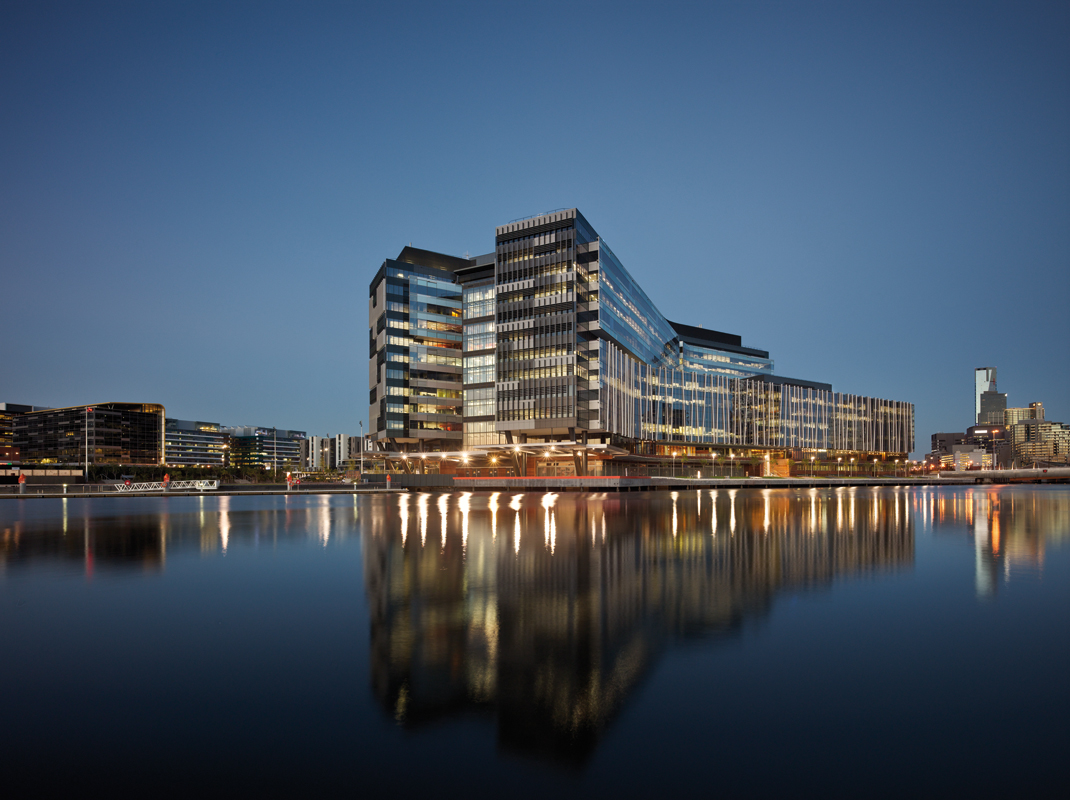
ANZ Centre | Hassell
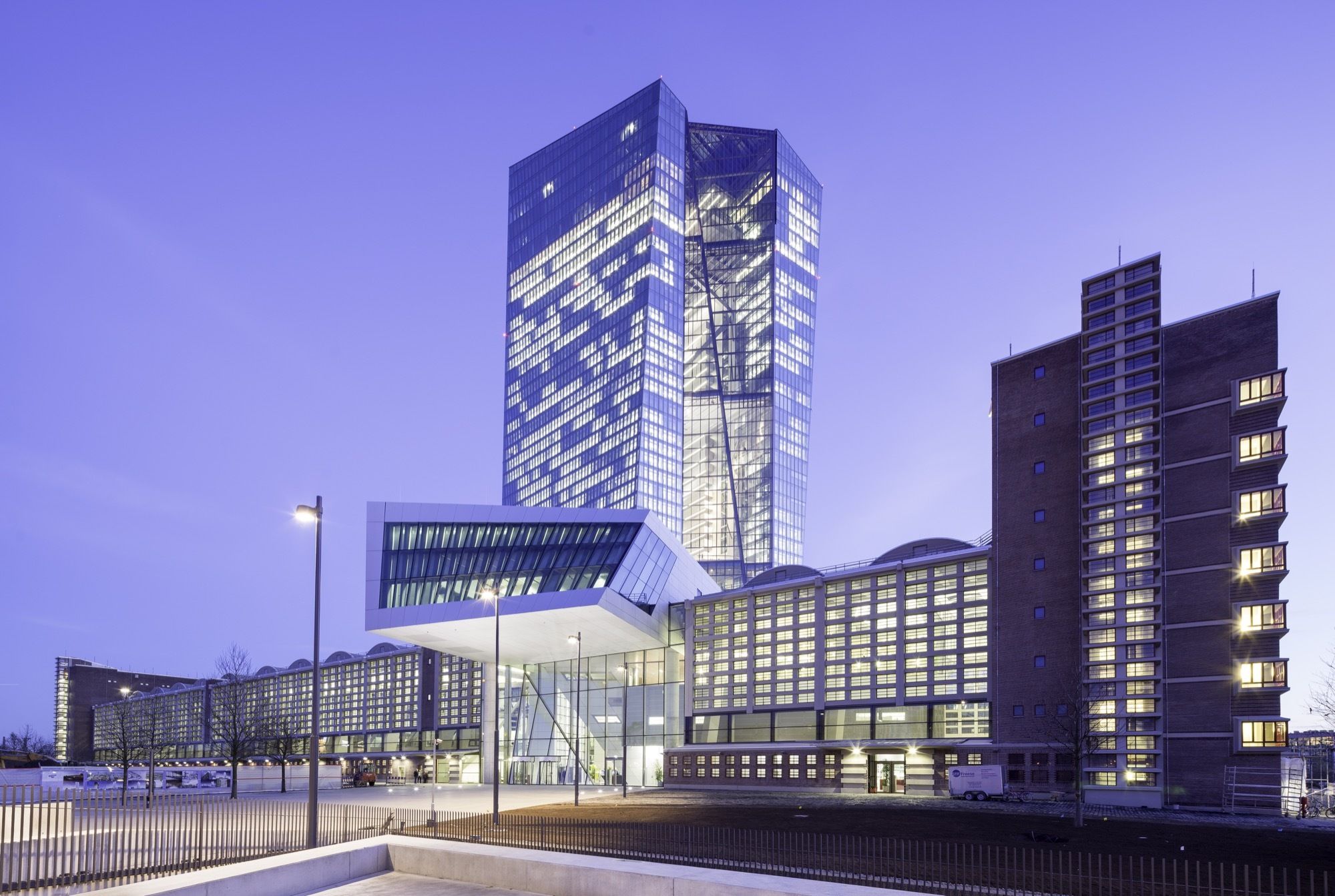
European Central Bank | Coop Himmelb(l)au
- Next Page »
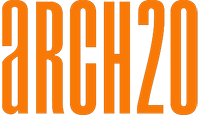
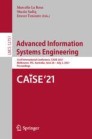
International Conference on Advanced Information Systems Engineering
CAiSE 2021: Advanced Information Systems Engineering pp 536–550 Cite as
A Multi Case Study on Legacy System Migration in the Banking Industry
- Hasan Emre Hayretci 11 , 12 &
- Fatma Başak Aydemir 12
- Conference paper
- First Online: 24 June 2021
2916 Accesses
4 Citations
Part of the book series: Lecture Notes in Computer Science ((LNISA,volume 12751))
Advances in technology, changing customer requirements, and pressure from business goals are the main drivers for innovation in the banking industry. Legacy architectures with monolithic structures prevent banks from implementing new generation banking models. To stay competitive, banks migrate to modular and scalable architectures. This migration has a significant impact on banks’ technical and organizational infrastructures, so it is crucial to devise an end-to-end migration strategy and plan the transformation. This paper reports our observations on the legacy system migration of three large retail banks between 2014 and 2020, focusing on the evaluation and prioritization criteria for their application portfolio to be migrated. We compare and contrast the motivations, migration strategies, and migration prioritization methods and discuss key takeaways from these high scale migration projects.
- Legacy migration
- Next-generation architectures
- Migration prioritization
This is a preview of subscription content, log in via an institution .
Buying options
- Available as PDF
- Read on any device
- Instant download
- Own it forever
- Available as EPUB and PDF
- Compact, lightweight edition
- Dispatched in 3 to 5 business days
- Free shipping worldwide - see info
Tax calculation will be finalised at checkout
Purchases are for personal use only
Banking industry architecture network. http://bian.org
Migrating applications to the cloud: Rehost, refactor, revise, rebuild, or replace? https://www.gartner.com/en/documents/1485116/migrating-applications-to-the-cloud-rehost-refactor-revi
Agievich, V., Skripkin, K.: Enterprise architecture migration planning using the matrix of change. In: ITQM, pp. 231–235. Elsevier (2014)
Google Scholar
Aitken, I.: Value-driven IT management. Routledge, Milton (2012)
Book Google Scholar
Balalaie, A., Heydarnoori, A., Jamshidi, P.: Microservices architecture enables DevOps: migration to a cloud-native architecture. IEEE Softw. 33 (3), 42–52 (2016)
Article Google Scholar
Bucchiarone, A., Dragoni, N., Dustdar, S., Larsen, S.T., Mazzara, M.: From monolithic to microservices: an experience report from the banking domain. IEEE Softw. 35 (3), 50–55 (2018)
Chilipirea, C., Laurentiu, G., Popescu, M., Radoveneanu, S., Cernov, V., Dobre, C.: A comparison of private cloud systems. In: 2016 30th International Conference on Advanced Information Networking and Applications Workshops (WAINA), pp. 139–143. IEEE (2016)
Di Francesco, P., Lago, P., Malavolta, I.: Migrating towards microservice architectures: an industrial survey. In: 2018 IEEE International Conference on Software Architecture (ICSA), pp. 29–2909. IEEE (2018)
Dragoni, N., Dustdar, S., Larsen, S.T., Mazzara, M.: Microservices: Migration of a mission critical system. arXiv preprint arXiv:1704.04173 (2017)
Erl, T.: Service-Oriented Architecture: Concepts, Technology, and Design. Pearson Education India, Bengaluru (1900)
Fan, C.Y., Ma, S.P.: Migrating monolithic mobile application to microservice architecture: an experiment report. In: 2017 IEEE International Conference on AI & Mobile Services (AIMS), pp. 109–112. IEEE (2017)
Furda, A., Fidge, C., Zimmermann, O., Kelly, W., Barros, A.: Migrating enterprise legacy source code to microservices: on multitenancy, statefulness, and data consistency. IEEE Softw. 35 (3), 63–72 (2017)
Gholami, M.F., Daneshgar, F., Beydoun, G., Rabhi, F.: Challenges in migrating legacy software systems to the cloud – an empirical study. Inf. Syst. 67 , 100–113 (2017). https://doi.org/10.1016/j.is.2017.03.008 , https://www.sciencedirect.com/science/article/pii/S0306437917301564
Gouigoux, J.P., Tamzalit, D.: From monolith to microservices: lessons learned on an industrial migration to a web oriented architecture. In: 2017 IEEE International Conference on Software Architecture Workshops (ICSAW), pp. 62–65. IEEE (2017)
Hariharan, N., Reeshma, K.: Challenges of core banking systems. Mediterr. J. Soci. Sci. 6 (5), 24 (2015)
Hu, S.J.: Method and system for integrating core banking business processes, 24 Jan 2006, uS Patent 6,990,466
Jamshidi, P., Pahl, C., Mendonça, N.C.: Pattern-based multi-cloud architecture migration. Softw. Pract. Experience 47 (9), 1159–1184 (2017)
Kerzner, H., Saladis, F.P., et al.: Value-Driven Project Management, vol. 1. John Wiley & Sons, Hoboken (2011)
Liu, Y., Hu, E., Chen, X.: Architecture of information system combining SOA and BPM. In: 2008 International Conference on Information Management, Innovation Management and Industrial Engineering, vol. 1, pp. 42–45. IEEE (2008)
Orban, S.: 6 strategies for migrating to the cloud. https://aws.amazon.com/blogs/enterprise-strategy/6-strategies-for-migrating-applications-to-the-cloud/ (2016)
Pérez-Castillo, R., Mas, B., Pizka, M.: Understanding legacy architecture patterns. In: 2015 International Conference on Evaluation of Novel Approaches to Software Engineering (ENASE), pp. 282–288. IEEE (2015)
Runeson, P., Höst, M.: Guidelines for conducting and reporting case study research in software engineering. Empir. Softw. Eng. 14 (2), 131 (2009)
Steffens, A., Lichter, H., Döring, J.S.: Designing a next-generation continuous software delivery system: concepts and architecture. In: 2018 IEEE/ACM 4th International Workshop on Rapid Continuous Software Engineering (RCoSE), pp. 1–7. IEEE (2018)
Taibi, D., Lenarduzzi, V., Pahl, C.: Processes, motivations, and issues for migrating to microservices architectures: an empirical investigation. IEEE Cloud Comput. 4 (5), 22–32 (2017)
Thönes, J.: Microservices. IEEE Softw. 32 (1), 116 (2015)
Verner, J.M., Sampson, J., Tosic, V., Bakar, N.A., Kitchenham, B.A.: Guidelines for industrially-based multiple case studies in software engineering. In: 2009 Third International Conference on Research Challenges in Information Science, pp. 313–324. IEEE (2009)
Zimmermann, A., Schmidt, R., Sandkuhl, K., Jugel, D., Bogner, J., Möhring, M.: Evolution of enterprise architecture for digital transformation. In: 2018 IEEE 22nd International Enterprise Distributed Object Computing Workshop (EDOCW), pp. 87–96. IEEE (2018)
Download references
Acknowledgments
The second author has been partially supported by the Scientific and Technological Research Council of Turkey through BIDEB 2232 grant 118C255, Requirements Engineering for Digital Transformation.
Author information
Authors and affiliations.
DefineX Consulting Technology Labs, Istanbul, Turkey
Hasan Emre Hayretci
Boğaziçi University, Istanbul, Turkey
Hasan Emre Hayretci & Fatma Başak Aydemir
You can also search for this author in PubMed Google Scholar
Corresponding author
Correspondence to Fatma Başak Aydemir .
Editor information
Editors and affiliations.
The University of Melbourne, Melbourne, VIC, Australia
Marcello La Rosa
The University of Queensland, St Lucia, QLD, Australia
Shazia Sadiq
Universitat Politècnica de Catalunya, Barcelona, Spain
Ernest Teniente
Rights and permissions
Reprints and permissions
Copyright information
© 2021 Springer Nature Switzerland AG
About this paper
Cite this paper.
Hayretci, H.E., Aydemir, F.B. (2021). A Multi Case Study on Legacy System Migration in the Banking Industry. In: La Rosa, M., Sadiq, S., Teniente, E. (eds) Advanced Information Systems Engineering. CAiSE 2021. Lecture Notes in Computer Science(), vol 12751. Springer, Cham. https://doi.org/10.1007/978-3-030-79382-1_32
Download citation
DOI : https://doi.org/10.1007/978-3-030-79382-1_32
Published : 24 June 2021
Publisher Name : Springer, Cham
Print ISBN : 978-3-030-79381-4
Online ISBN : 978-3-030-79382-1
eBook Packages : Computer Science Computer Science (R0)
Share this paper
Anyone you share the following link with will be able to read this content:
Sorry, a shareable link is not currently available for this article.
Provided by the Springer Nature SharedIt content-sharing initiative
- Publish with us
Policies and ethics
- Find a journal
- Track your research

- Subscribers
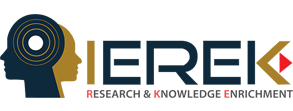
Sustainable Architecture: The Bank of America (Case Study)
Sustainable architecture focuses on how energy generates from the building itself and to it. That is through making an effective structure and ideal insulation to give air, water, and other facilities.
Resulting in, the building won’t need external air conditioners or other facilities. Sustainable Architecture provides the environment with green buildings.
So let’s know more about the features of Green buildings:
- Relying on solar energy or other renewable sources of energy.
- It’s a recycled building that decreases the wastes.
- Lowering the cement usage that reflects on the earth.
- Focusing on energy conservation.
Besides, Green buildings not only enhance the earth by the eco-friendly alternative to conventional construction techniques but also added energy efficiency, innovative designs and superior style which led to many sustainable buildings becoming known for their impressive looks.
There’s no doubt that sustainable architecture is the way of the future and will become more common as the concern for the environment vegetates because of it:
- Is providing air, water, and other resources
- Saves human health and enhance productivity
- Cuts pollution and wasted materials
- Saves money on operational costs
Wherefore, The Bank of America is the greenest project of New York in 2008, so we can say that an environmentally friendly building. It’s at One Bryant Park in the Midtown area of Manhattan in New York City.
As the greenest high-rise building, it is expounding the case of the art in:
- Energy efficiency
- Internal air quality
- Sustainable materials
- Environmentally conscious construction, operations, and maintenance rules.
- It’s made of recycled and recyclable materials
- The building has 55 stories high and has 2,100,000 square feet (195,096 m2) of office space.
- The tower’s architectural spire is 255.5 ft. (77.9 m) tall and place
As a result of these, The Bank of America Tower is the first skyscraper designed to attain a Platinum LEED Certification. https://www.youtube.com/watch?v=40xPO7bYc7U
Moreover, here is a brief about technologies which were used in the Bank of America construction process:
- Reduces thermal loss
- Lowering energy consumption
- Increasing transparency
- Carbon dioxide sensors signal increased fresh air ventilation when higher levels of carbon dioxide are detected in the building.
- Are estimated to save 8,000,000 US gal (30,000,000 l) of water per year.
- Reduce CO2 emissions by 144,000 lb. (65,000 kg) per year (as calculated with the Pacific Institute water-to-air model).
- It’s constructed using a concrete manufactured with slag, a byproduct of blast furnaces. The mixture used in the building concrete is 55% cement and 45% slag.
- Conditioned air for the residents provided by many air column units located in the lessee space that delivers 50 °F air into a raised access floor plenum.
This underfloor air system provides users with the ability to control their space temperature as well as improving ventilation effectiveness.
When building churn occurs, workstation moves can be performed more easily with lower cost and less product waste. The cooling system produces and stores ice during off-peak hours, and allows the ice to melt to help cool the building during peak load.
So we can conclude the uniqueness of Bank of America in many bullet points which present the building features:
- The tower provides part of the base-load energy need by a 4.6-megawatt cogeneration plant.
- Waterless urinals save 10.3 million gallons.
- The gray water system will capture, store, and re-use 100% of rainwater and recycle wastewater and planted roofs.
- Reducing the urban heat island effect.
- Reducing energy consumption by 50%. potable water consumption by 50%, and create net-zero carbon dioxide emissions.
Green Urbanism (GU) – International Conference
The 4th international conference on “Green Urbanism (GU)” is taking place in Rome, Italy , from 24 to 26 November 2020 .
GU-2020 aims to bring together, scientists, urban planners, architects and other stakeholders from across the globe to discuss the latest research findings in all aspects of sustainability .
Moreover, GU international conference avails the opportunity for interested researchers , scholars , scientists , architects , and engineers from around the globe to submit their research papers and get them published in highly-ranked international journals by the esteemed publishers Springer and IEREK Press . Noting that submitted researches must be in the scope of at least one of the official conference topics and abiding the submission instructions .
Many critical topics will be discussed during the GU conference, such as:
- The Evolution of Green Urbanism
- Formulating the Principles of Green Urbanism
- Urban Design for Nature, Conservation of Natural Resources
- Passive Designs: Green Buildings for Sustainable Use
- Green Districts: Bike, Cycles, and Pedestrians’ Corridors
- Urban Growth of Developing Cities
- City Urban Form and Natural Resources
- Landscape, Gardens, Streetscapes, and Urban Biodiversity
- Urbanization of Rural Area: Energy, Water, and Food Security
- Urban Governance, Leadership, Best Practices, Research, and Awareness
- Green Infrastructure and Sustainable Environment
- Regeneration of the City Center and Urban Transit Hubs
- Waste Management, Pollution, and Health Risks
- Energy Efficiency in the Built Environment
- Effects of Sustainable Environments on Human Health
- Advancing Solutions towards Zero Waste Cities
- Transformation to Green Environment
For more information & registration, visit the conference’s official page: https://www.ierek.com/events/GU4th
Coastal Landscape Identity
Dr. Nabil Khelifi’s workshop | Malaysia
The Vital Role of a Research Director in Fostering Academic Excellence
Italy’s Architectural Marvels: Crafting Cultural Identity Through Time
Unraveling the Dynamics of Macroeconomics, International Trade
The Evolution of Modern Architecture in Dubai
You must be logged in to post a comment.
How To Design The IT Architecture of a Digital Bank
The evolution of banking has gone digital, so banks need to design core banking systems architecture for the online and mobile environments that support their customers' needs. Learn how to design a digital banking IT architecture that works for the bank and its customers.
Necessary IT architecture is vital in the process of making a bank digital. According to Forbes , digital transformation remained an important trend in banking in 2021. Digital technology continues to reshape banking services at every level.
Therefore, banks should embrace digital transformation to provide customer services. It will translate into greater customer experience besides increasing operational efficiency. Also, the IT infrastructure of a digital bank should be responsive to client needs.
More importantly, banks should endeavor to sustain digital transformation to reap the benefits. For instance, employees can be relieved of some pressure by automating certain banking tasks. In return, their productivity and efficiency increase.
In this article, you will learn how to design your secure, scalable and flexible IT architecture for digital banking. You will also learn about the viable solutions for IT architecture design in the current market, considering the right mix of cloud or on-premise software solutions.
Let's start by looking at the basics of IT architecture for digital banking.
What Is IT Architecture For Digital Banking?
IT architecture for digital banking refers to the use of a set of rules or frameworks that enable a list of banking services. These services can be for the banks' or customers' internal use.
IT architecture involves all those frameworks enabling digital banks to run their operations smoothly.
Digital banking is revolutionizing banks' operations, and their technology solutions must keep up. Banks get challenged to build their IT architecture for digital banking in ways that allow them to gain a competitive edge.
Cloud solutions are emerging to provide the agility, flexibility, and scalability banks need to grow profitably. However, as the industry continues to mature, there is also a need for greater security and compliance. Banks' IT is required to strike the right balance of these competing needs based on the requirements of their end users.
Blockchain technology offers banks new opportunities to increase revenue and profitability through new business models. Blockchain-based initiatives provide improved liquidity, cost savings, and operational efficiencies while reducing costs and risks associated with legacy banking solutions.
Many banks are looking to blockchain as the solution for streamlining existing processes and enabling new business models. Banks' IT must be designed with the ability to adapt its architecture to meet the needs of its customers.
Here is how to design the Information Technology architecture of an online bank.
Digital Banking IT Infrastructure Layers
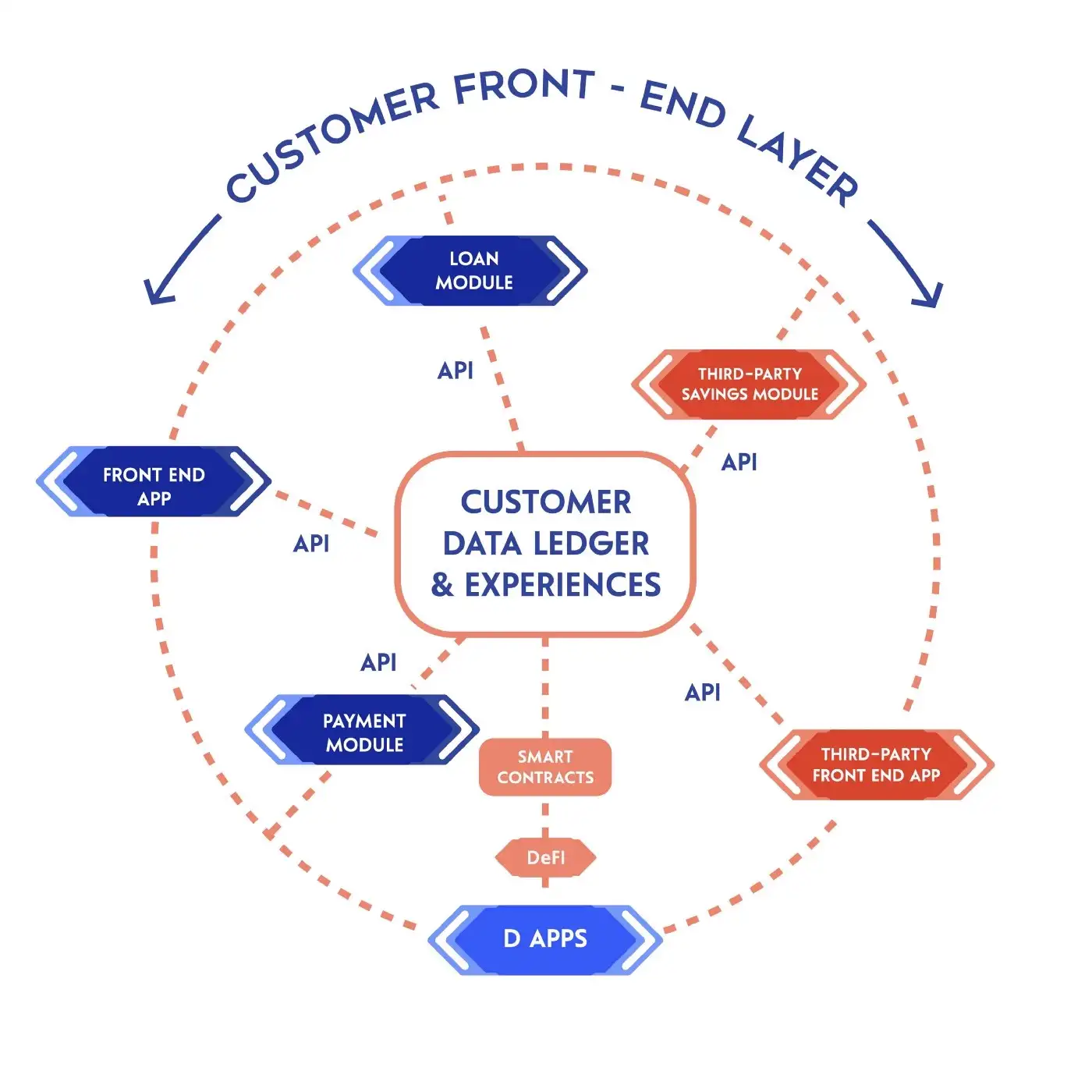
There are four digital banking IT infrastructure layers banks can adopt. Here are details about each IT architecture layer.
Back End Layer
The core banking systems architecture involving back-office processes stores and manipulates customer data. Therefore, all information about client transactions, accounting systems, and loan issuing are part of this core IT layer. It ensures that the basic operations of a digital bank run smoothly.
Banks may use an in-house online banking architecture or source it from service providers. But modern service providers and Fintech services are more innovative. They provide flexible and cost-effective core banking systems customized for digital banking.
In addition, the providers are keen on constantly developing their software and core banking technology. Thus, it provides an effective and tailored customer experience.
Many customers still need to be convinced about digital banking. So, banks should assure clients of digital banking safety. Then they can develop the architecture of a digital bank and roll it out.
Middleware Layer
The software layer links the front end with the back end, plus the rest of the banking applications. It is responsible for interpreting, managing, and transmitting information between the front and back end.
Several technology providers can offer necessary middleware software. That includes business applications for card issuing, instant notifications, and more. It connects various levels, eliminating the need for a central banking system.
Front-end Layer
The layer provides the platform through which clients can interact with the bank. It includes online, web, or mobile banking app architecture networks for digital banking. Digital banks should ensure the interface is user-friendly for exceptional customer experience.
Digital banks may develop their in-house front-end or in-source them from third-party software providers. An in-sourcing interface may be preferable due to its flexibility, lower costs, and ease of customization.
Some of the front-end platforms include:
- Third-party payment gateways
- Mobile banking channels
Bank API Layer
It is a layer that forms a linkage between internal and external applications. Hence, this layer seamlessly integrates the services of third-party application providers.
Incorporating third-party applications is an essential part of the Information Technology architecture of an online bank. Digital banks need proper investment in the API layer before they become fully integrated. Through API access, digital banks can reap the maximum benefits of collaborations.
Microservices Architecture in Banking
Microservices architecture is a fundamental style for distributed systems. It is a method of building applications that decouples the components of an application and allows them to self-configure, self-heal and scale independently as the system scales.
Microservices architecture provides the agility and flexibility necessary to meet the needs of banking customers while addressing regulatory compliance issues, improving performance and lowering costs. By building applications on microservices architecture, banks can provide end users with a better user experience. Also, the ability to interact with their bank more straightforwardly. Furthermore, banks can integrate new business models into their current systems. It presents a new opportunity for banks to increase revenue.
Technology Stack for Digital Banking
Architecture of a digital bank should guarantee security and privacy requirements while meeting the demands of today's examples of digital banking customers. The technology stack for digital banking depends on how well IT is structured to respond to current and emerging requirements. Banks need a technology stack that can support their strategic goals and allow them to adapt to changes like mobile banking app architecture in the market environment. Here is the technology stack for digital banking.
1. Java and Spring Framework
Java and Spring Framework are widely used in banking applications. Java is a general-purpose programming language for developing applications and Server-side components such as servlets, JSPs, JSF, and Struts. The Java IDE covers a wide range of functionality. Developers widely use it to build applications in Java. Spring Framework supports building modular Java and XML solutions that can run in the cloud or on-premise.
2. Hibernate and Spring JDBC
Hibernate is a Java open-source persistence framework used to persist data in the relational database. Spring JDBC provides the functionality necessary to send and receive data from the database. Most banks adopt a hybrid approach to their application architecture with a mixture of on-premises and cloud-based offerings.
Zimbra is an open-source email, calendaring and collaboration suite that offers secure e-mail, fax, contacts management, scheduling, file sharing and collaboration capabilities for departments or organizations of all sizes. The suite is written in Java and uses a REST API to interact with the various components of the suite.
MongoDB is a document-oriented database popular for online transaction and analytical processing. MongoDB is compatible with JSON and the JSON specification makes it easy to use any programming language without modifications.
Java Persistent Objects is a Java API for persistence. JPA is a standard for using an object model in Java to represent persistent data. In many cases, such as web development, JPA provides the easiest way to access and modify data. This API gets successfully used in a variety of applications. Some popular frameworks that use JPA are Java EE, Spring Framework, and Spring Boot.
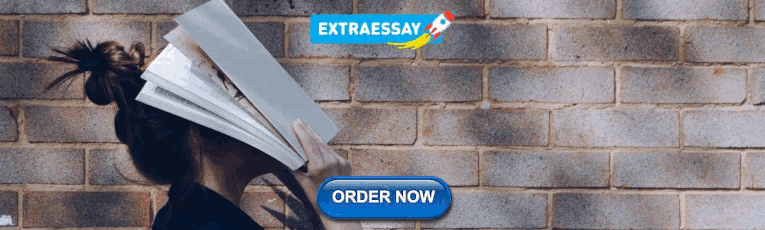
How to Design Your IT Architecture for Digital Banking
Digital Banking Architecture platforms must adhere to best practices for security and comply with regulatory guidelines. The IT architecture of a digital bank must be flexible, scalable, secure and manageable. The major challenges that digital banking faces include increasing regulatory compliance, adapting to changing customer needs, and creating a competitive edge against your competitors.
1. Security
Banks are required to maintain compliance with security regulations. Security of the IT infrastructure is essential to protect sensitive customer data, comply with regulatory requirements and ensure that the bank has a secure IT environment. Banks must ensure that their cloud providers have adequate cyber-security controls and practices. Cloud solutions can help banks improve their security posture by distributing workloads across multiple data centers.
Digital banking platforms must support compliance with regulations such as UDAAP or GDPR. An organization may use one or more tiers of security controls, depending on its risk profile and business needs. Security includes encryption at rest and in transit, digital identity and privacy management, user authentication and authorization, website security, application security, and security monitoring capabilities. In particular, banks should have a secure digital banking platform with appropriate isolation of consumer data. A bank's digital banking platform must also consider any compliance standards or guidelines to which the bank's activities are subject.
Data encryption technologies, API, and AI can help digital banks enhance their security. These tools can keep customer information secure as they move across multiple channels, devices, or apps and also manage data internally.
2. Standards Compliance
Compliance refers to an organization's ability to comply with regulations required by its operating environment, such as laws and internal policies. An IT architecture for digital banking should conform to the technology standards, especially in customer-facing applications. Many banks are required to use the PCI DSS for their core systems.
Ensuring compliance is easiest with tech tools like automation and AI. They can take care of manual tasks while also tracking compliance procedures in one location.
Agility refers to responding quickly and effectively to change. One example of a change that requires agility is the need for an increase or decrease in a system's capacity or ability to handle new types of data or workloads. It can be an issue for traditional banking systems, as they involve time-consuming manual work.
Cloud technologies, robotic processing automation (RPA), API, AI, and machine learning are some of the best tech for operational agility. They provide a robust management solution and predictive insights, allowing banks to streamline operations to maximize efficiency.
4. Flexibility
Flexibility refers to the ability to adapt to environmental changes. Flexibility is important for a bank because it must be capable of adapting to new regulations or customer needs and must respond quickly.
Microservice architecture is one popular example visible in many digital business sectors. It is used in other industries to make IT architecture systems more flexible by using independent services that are lightweight and require low overhead.
5. Scalability
Scalability is the ability of a system to process an increasing number of transactions without growing in size too slowly. Banking applications must be capable of handling both increasing and decreasing workloads. Banking applications must have the ability to handle workload peaks, such as the need for more transactions during peak months, and be able to reduce capacity during periods of low usage.
Cloud services are an ideal solution for increasing scaling capabilities. The best part is that the cloud doesn’t require as much management as a large-scale storage infrastructure would.
6. Risk Management
Risk management refers to managing the risk associated with a set of activities. A bank must develop and implement processes for managing risk. These processes should help ensure that the bank can respond effectively when risks materialize, such as a vulnerability in a software component or an unexpected increase in workload.
Your best options for managing and minimizing business risks are AI, machine learning, and cloud computing technologies.
7. Maintainability
Maintainability refers to the ability of a system to be modified to meet the future needs of an organization. Digital banking platforms must allow rapid deployment of new applications and capabilities. Cloud-native technologies for digital banking architectures are one solution that ensures continuous development and software development and creates new environments using code.
8. Usability
A system's usability is defined as the ease of using a system or the extent to which users can effectively use it. Usability refers to the ease with which users can access, use and understand a system.
Technology tools such as APIs allow banks to participate in digital ecosystems. They enable banks to integrate their products and services with third-party applications. This lets the bank provide a seamless service to customers across various devices, channels, apps, and services.
9. Reliability
Reliability means that a system will perform its intended functions without failure. While security and manageability are important, reliability is the key to a successful IT architecture for digital banking.
The basics of building modern digital banks are largely similar. However, each bank can adopt a different tech stack. While some banks will opt for in-house developed capabilities, others outsource their IT architecture.
A digital bank should design an IT infrastructure that seamlessly shifts between various layers. So, employees and users can effortlessly interact with the IT architecture.
Developing a bank's IT architecture should consider precise business objectives. In that way, the bank can achieve the intended outcome from its shrewd investments.
Also, the bank should consider the worthy proposals appropriate to the target audience. A thorough assessment of the bank's business history, operations, strategy, and capabilities is necessary.
Consequently, the bank architecture lays the foundations for future data and technology architecture. Technology architecture will aid practical and physical applications, data components, and the vision of bank architecture.
The IT architecture is usually centered on the following core IT architecture principles:
- Infrastructure scalability – Ensures that existing bank business operations are adaptable and agile to shifts in market dynamics.
- The solution's flexibility – To enable incorporation with other platforms and evolving technologies. All this with minimal changes and interruptions to processes while supporting new business ideas.
- System resilience and robustness – Has brief but clear IT governance to address various technological and cybersecurity threats.
- A bank will realize a future-proof technology stack if it implements these principles. Such technology will also be flexible to internal and external change and interruption.
Banks can adopt modern digital innovations like artificial intelligence (AI), natural language processing, and data analytics as part of their IT strategy. The innovations will enhance customer experience besides cutting operational costs.
To ensure the system is reliable, consider solutions like blockchain and RPA. Blockchain can eliminate the need for intermediaries while still keeping the system secure. And RPA can deal with tedious processes with better precision and accuracy.
Digitized Banking Architecture Technology Roadmap
A bank needs to prepare its IT architecture and operations based on well-defined business purposes and worthy propositions appropriate to its target audience to accomplish the desired outcome and make practical financial investments.
Beyond the fundamentals of organization and innovation design, you should think about integrating extra kinds of digital innovation into your IT strategy, with the core goal of enhancing customer experience, decreasing functional prices, or boosting risk management. AI, natural language processing, robotics, and real-time data analytics are among the latest growth areas.
Suppose you don't range your digital financial business from the very beginning but have already inserted some technology in place. In that case, it's essential to think of any type of space in today's IT landscape and how to fix them.
You also need to consider the challenges and limitations that your digital banking architecture may encounter in the future. These can include:
- Managing cybersecurity risks
- Processing transactions and other updates in real-time
- Upgrading legacy systems
- Minimizing transaction processing risks
- Understanding potentially disruptive impacts of processes or technology
- Integrating legacy systems with new technologies
- Understanding different cost drivers
- Ensuring seamless user experience across devices and platforms
- Complying with internal controls, compliance, and regulatory agenda
Lastly, when it concerns an online bank's tech stack, there is no one-size-fits-all service, specifically when changing typical old frameworks. You'll typically require a complete makeover strategy and roadmap to develop your bank properly.
Digital banks need a vision of the future and a strategic plan that gets them there. Of course, moving from vision to action may be easier said than done. Hence, only a roadmap can outline and prioritize a bank's digitization initiatives.
Every strategic plan in an organization requires practical and flexible planning to succeed. That is what a roadmap is all about. A roadmap for digital banking architecture will utilize precise technology solutions to bring together short-term and long-term goals.
A digital banking technology roadmap provides a common understanding for effective alignment and scheduling of changes. It also provides corrections for unforeseen changes in technology and market dynamics.
Want some real-world examples to understand the IT architecture for digital banking better?
Check these examples.
- PayPal (Third-party platform and system integrations)
- Monzo (Microservice core banking system)
- BBVA, Banco Bilbao Vizcaya Argentaria (Third-party platform and system integrations)
- Nubank (Fintech-friendly regulatory agenda and customer data usage)
- TymeBank (Linking core banking platforms and interfaces and combining online and offline customer interaction)
- Liv. (Third-party partnership integration)
- TMRW (Technology partnerships and data analytics)
Concluding Remarks
The banks deal with some routine, easy-to-digitize operations. Automation of such tasks will save time and improve service delivery. Overall, digital banks provide a more remarkable customer experience.
Digital banking platforms must be flexible and resilient. It is important to consider the use cases of digital banking platforms and the applications you will run on them. The architecture for digital banking must be designed with security and compliance in mind.
This architecture should allow your bank to be agile, flexible, and secure. It must also make it easy for the IT team to manage the platform and deploy new applications rapidly.
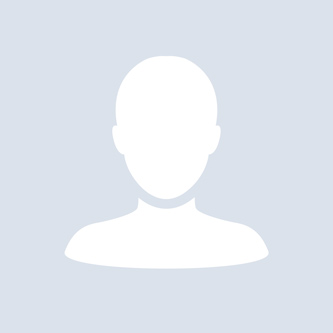
Get New Posts to Your Inbox
You might also like, 11 best ai tools for developers to boost coding efficiency, 14 best ai website builders and generators in 2024, the future of web development concerning artificial intelligence, 7 practical uses of ai in web development (with examples), how to build a fitness website in 9 steps, how to start using ai in software development.
Virtusa | Fast-track and future-proof core banking transformation leveraging BIAN
Zafin integral to the success of bian’s second coreless banking proof of concept, redhat | build a modern core banking platform, tcs | bian: powering purpose-driven, future-ready banks.
In the race to stay ahead, provide best-in-class customer service, and meet ever increasing market demands, banks have bolted on digital capabilities in an ad hoc manner introducing considerable complexity into the underlying IT architecture. Today, most traditional banks operate with a complex, unmanageable IT architecture with duplicate systems and data impeding speed-to-market for new products and services. Increasingly inflexible legacy systems have resulted in business silos and monolithic applications that hinder agility and adversely impact the pace of key transformation initiatives.
To stay relevant, incumbent banks must foray into areas beyond traditional banking spaces by stepping into their customers’ lives at the right time with the right product. This will require banks to embrace purpose-driven business models through new partnerships with larger ecosystem partners, which in turn will require architectural readiness and plug-and-play integrations to enable ecosystem play. While banking channels are increasingly adopting digitalization to deliver beyond banking services, pain points around legacy architecture remain. In our view, to address these pain points, transition to purpose-driven ecosystem models and become future-ready, banks must adopt the Banking Industry Architecture Network (BIAN) standard. This article focuses on BIAN adoption trends and journeys.
Video: TCS | BIAN Business IT Alignment Adoption TCS | BIAN APIs and Microservices Adoption
Cognizant | Core Banking Transformation at a top North American bank leveraging BIAN
Bian member case studies.
Download an overall summary of Adoption examples
Case Studies
ArchiMate’ Modeling Notation for the Financial Industry Reference Model: Banking Industry Architecture Network (BIAN)
This document provides guidance on how the ArchiMate Specification, a standard of The Open Group, can be used to exploit the value of the Banking Industry Architecture Network (BIAN) Financial Industry Reference Model.
It is designed to provide a guide to anyone involved or interested in how to manage the transition to a digital financial institution. It guides an Enterprise Architecture organization to develop an agile, lean, and stable banking architecture using the ArchiMate language and BIAN.
Patrick Derde, EnVizion, BIAN & Martine Alaerts, EnVizion
Archi Banking Group: Combining the BIAN Reference Model, ArchiMate’ Modeling Notation, and the TOGAF’ Framework
This Case Study is a fictitious example developed to illustrate the combined use of the Banking Industry Architecture Network (BIAN) Reference Model with the ArchiMate’ modeling notation and the TOGAF’ framework (both standards of The Open Group). The ArchiMate and TOGAF concepts used in this Case Study can be applied to different situations. The use of the BIAN Reference Model supports addressing typical financial industry concerns.
PNC Financial Services Group
The BIAN model fits perfectly in line with how we view enterprise architecture (EA) at PNC. One of the first steps we took as an organisation was to bring a business perspective to enterprise architecture. To us, technology is not just a collection of servers and software, but rather a set of technical solutions that are aligned to specific business capabilities and functions. Steven Van Wyk, Executive Vice President, Head of Technology and Operations, PNC Financial Services Group Read case study
Cognizant Technology Solutions, led by Sanghosh Bhalla, Niloy Sengupta and Akshaya Bhargava from the firm’s Banking and Financial Services Consulting practice, recently helped a top three North American bank, adopt BIAN and optimize their enterprise portfolio of applications that support business functions across all of its business units. Sanghosh Bhalla, Niloy Sengupta and Akshaya Bhargava, Cognizant Read Case Study
As a vendor that grew through acquisitions, we inherited a rich collection of applications that have their application specific interfaces. Applications that had similar scope ended up having their specific interfaces for essentially the same responsibilities. One of our strategic goals was to cut integration time and cost and over time achieve plug-and-play interoperability between different applications in our portfolio. Aleksandar Milosevic, Chief Software Architect at banking software provider Asseco SEE Read case study
A generative AI reset: Rewiring to turn potential into value in 2024
It’s time for a generative AI (gen AI) reset. The initial enthusiasm and flurry of activity in 2023 is giving way to second thoughts and recalibrations as companies realize that capturing gen AI’s enormous potential value is harder than expected .
With 2024 shaping up to be the year for gen AI to prove its value, companies should keep in mind the hard lessons learned with digital and AI transformations: competitive advantage comes from building organizational and technological capabilities to broadly innovate, deploy, and improve solutions at scale—in effect, rewiring the business for distributed digital and AI innovation.
About QuantumBlack, AI by McKinsey
QuantumBlack, McKinsey’s AI arm, helps companies transform using the power of technology, technical expertise, and industry experts. With thousands of practitioners at QuantumBlack (data engineers, data scientists, product managers, designers, and software engineers) and McKinsey (industry and domain experts), we are working to solve the world’s most important AI challenges. QuantumBlack Labs is our center of technology development and client innovation, which has been driving cutting-edge advancements and developments in AI through locations across the globe.
Companies looking to score early wins with gen AI should move quickly. But those hoping that gen AI offers a shortcut past the tough—and necessary—organizational surgery are likely to meet with disappointing results. Launching pilots is (relatively) easy; getting pilots to scale and create meaningful value is hard because they require a broad set of changes to the way work actually gets done.
Let’s briefly look at what this has meant for one Pacific region telecommunications company. The company hired a chief data and AI officer with a mandate to “enable the organization to create value with data and AI.” The chief data and AI officer worked with the business to develop the strategic vision and implement the road map for the use cases. After a scan of domains (that is, customer journeys or functions) and use case opportunities across the enterprise, leadership prioritized the home-servicing/maintenance domain to pilot and then scale as part of a larger sequencing of initiatives. They targeted, in particular, the development of a gen AI tool to help dispatchers and service operators better predict the types of calls and parts needed when servicing homes.
Leadership put in place cross-functional product teams with shared objectives and incentives to build the gen AI tool. As part of an effort to upskill the entire enterprise to better work with data and gen AI tools, they also set up a data and AI academy, which the dispatchers and service operators enrolled in as part of their training. To provide the technology and data underpinnings for gen AI, the chief data and AI officer also selected a large language model (LLM) and cloud provider that could meet the needs of the domain as well as serve other parts of the enterprise. The chief data and AI officer also oversaw the implementation of a data architecture so that the clean and reliable data (including service histories and inventory databases) needed to build the gen AI tool could be delivered quickly and responsibly.

Creating value beyond the hype
Let’s deliver on the promise of technology from strategy to scale.
Our book Rewired: The McKinsey Guide to Outcompeting in the Age of Digital and AI (Wiley, June 2023) provides a detailed manual on the six capabilities needed to deliver the kind of broad change that harnesses digital and AI technology. In this article, we will explore how to extend each of those capabilities to implement a successful gen AI program at scale. While recognizing that these are still early days and that there is much more to learn, our experience has shown that breaking open the gen AI opportunity requires companies to rewire how they work in the following ways.
Figure out where gen AI copilots can give you a real competitive advantage
The broad excitement around gen AI and its relative ease of use has led to a burst of experimentation across organizations. Most of these initiatives, however, won’t generate a competitive advantage. One bank, for example, bought tens of thousands of GitHub Copilot licenses, but since it didn’t have a clear sense of how to work with the technology, progress was slow. Another unfocused effort we often see is when companies move to incorporate gen AI into their customer service capabilities. Customer service is a commodity capability, not part of the core business, for most companies. While gen AI might help with productivity in such cases, it won’t create a competitive advantage.
To create competitive advantage, companies should first understand the difference between being a “taker” (a user of available tools, often via APIs and subscription services), a “shaper” (an integrator of available models with proprietary data), and a “maker” (a builder of LLMs). For now, the maker approach is too expensive for most companies, so the sweet spot for businesses is implementing a taker model for productivity improvements while building shaper applications for competitive advantage.
Much of gen AI’s near-term value is closely tied to its ability to help people do their current jobs better. In this way, gen AI tools act as copilots that work side by side with an employee, creating an initial block of code that a developer can adapt, for example, or drafting a requisition order for a new part that a maintenance worker in the field can review and submit (see sidebar “Copilot examples across three generative AI archetypes”). This means companies should be focusing on where copilot technology can have the biggest impact on their priority programs.
Copilot examples across three generative AI archetypes
- “Taker” copilots help real estate customers sift through property options and find the most promising one, write code for a developer, and summarize investor transcripts.
- “Shaper” copilots provide recommendations to sales reps for upselling customers by connecting generative AI tools to customer relationship management systems, financial systems, and customer behavior histories; create virtual assistants to personalize treatments for patients; and recommend solutions for maintenance workers based on historical data.
- “Maker” copilots are foundation models that lab scientists at pharmaceutical companies can use to find and test new and better drugs more quickly.
Some industrial companies, for example, have identified maintenance as a critical domain for their business. Reviewing maintenance reports and spending time with workers on the front lines can help determine where a gen AI copilot could make a big difference, such as in identifying issues with equipment failures quickly and early on. A gen AI copilot can also help identify root causes of truck breakdowns and recommend resolutions much more quickly than usual, as well as act as an ongoing source for best practices or standard operating procedures.
The challenge with copilots is figuring out how to generate revenue from increased productivity. In the case of customer service centers, for example, companies can stop recruiting new agents and use attrition to potentially achieve real financial gains. Defining the plans for how to generate revenue from the increased productivity up front, therefore, is crucial to capturing the value.
Upskill the talent you have but be clear about the gen-AI-specific skills you need
By now, most companies have a decent understanding of the technical gen AI skills they need, such as model fine-tuning, vector database administration, prompt engineering, and context engineering. In many cases, these are skills that you can train your existing workforce to develop. Those with existing AI and machine learning (ML) capabilities have a strong head start. Data engineers, for example, can learn multimodal processing and vector database management, MLOps (ML operations) engineers can extend their skills to LLMOps (LLM operations), and data scientists can develop prompt engineering, bias detection, and fine-tuning skills.
A sample of new generative AI skills needed
The following are examples of new skills needed for the successful deployment of generative AI tools:
- data scientist:
- prompt engineering
- in-context learning
- bias detection
- pattern identification
- reinforcement learning from human feedback
- hyperparameter/large language model fine-tuning; transfer learning
- data engineer:
- data wrangling and data warehousing
- data pipeline construction
- multimodal processing
- vector database management
The learning process can take two to three months to get to a decent level of competence because of the complexities in learning what various LLMs can and can’t do and how best to use them. The coders need to gain experience building software, testing, and validating answers, for example. It took one financial-services company three months to train its best data scientists to a high level of competence. While courses and documentation are available—many LLM providers have boot camps for developers—we have found that the most effective way to build capabilities at scale is through apprenticeship, training people to then train others, and building communities of practitioners. Rotating experts through teams to train others, scheduling regular sessions for people to share learnings, and hosting biweekly documentation review sessions are practices that have proven successful in building communities of practitioners (see sidebar “A sample of new generative AI skills needed”).
It’s important to bear in mind that successful gen AI skills are about more than coding proficiency. Our experience in developing our own gen AI platform, Lilli , showed us that the best gen AI technical talent has design skills to uncover where to focus solutions, contextual understanding to ensure the most relevant and high-quality answers are generated, collaboration skills to work well with knowledge experts (to test and validate answers and develop an appropriate curation approach), strong forensic skills to figure out causes of breakdowns (is the issue the data, the interpretation of the user’s intent, the quality of metadata on embeddings, or something else?), and anticipation skills to conceive of and plan for possible outcomes and to put the right kind of tracking into their code. A pure coder who doesn’t intrinsically have these skills may not be as useful a team member.
While current upskilling is largely based on a “learn on the job” approach, we see a rapid market emerging for people who have learned these skills over the past year. That skill growth is moving quickly. GitHub reported that developers were working on gen AI projects “in big numbers,” and that 65,000 public gen AI projects were created on its platform in 2023—a jump of almost 250 percent over the previous year. If your company is just starting its gen AI journey, you could consider hiring two or three senior engineers who have built a gen AI shaper product for their companies. This could greatly accelerate your efforts.
Form a centralized team to establish standards that enable responsible scaling
To ensure that all parts of the business can scale gen AI capabilities, centralizing competencies is a natural first move. The critical focus for this central team will be to develop and put in place protocols and standards to support scale, ensuring that teams can access models while also minimizing risk and containing costs. The team’s work could include, for example, procuring models and prescribing ways to access them, developing standards for data readiness, setting up approved prompt libraries, and allocating resources.
While developing Lilli, our team had its mind on scale when it created an open plug-in architecture and setting standards for how APIs should function and be built. They developed standardized tooling and infrastructure where teams could securely experiment and access a GPT LLM , a gateway with preapproved APIs that teams could access, and a self-serve developer portal. Our goal is that this approach, over time, can help shift “Lilli as a product” (that a handful of teams use to build specific solutions) to “Lilli as a platform” (that teams across the enterprise can access to build other products).
For teams developing gen AI solutions, squad composition will be similar to AI teams but with data engineers and data scientists with gen AI experience and more contributors from risk management, compliance, and legal functions. The general idea of staffing squads with resources that are federated from the different expertise areas will not change, but the skill composition of a gen-AI-intensive squad will.
Set up the technology architecture to scale
Building a gen AI model is often relatively straightforward, but making it fully operational at scale is a different matter entirely. We’ve seen engineers build a basic chatbot in a week, but releasing a stable, accurate, and compliant version that scales can take four months. That’s why, our experience shows, the actual model costs may be less than 10 to 15 percent of the total costs of the solution.
Building for scale doesn’t mean building a new technology architecture. But it does mean focusing on a few core decisions that simplify and speed up processes without breaking the bank. Three such decisions stand out:
- Focus on reusing your technology. Reusing code can increase the development speed of gen AI use cases by 30 to 50 percent. One good approach is simply creating a source for approved tools, code, and components. A financial-services company, for example, created a library of production-grade tools, which had been approved by both the security and legal teams, and made them available in a library for teams to use. More important is taking the time to identify and build those capabilities that are common across the most priority use cases. The same financial-services company, for example, identified three components that could be reused for more than 100 identified use cases. By building those first, they were able to generate a significant portion of the code base for all the identified use cases—essentially giving every application a big head start.
- Focus the architecture on enabling efficient connections between gen AI models and internal systems. For gen AI models to work effectively in the shaper archetype, they need access to a business’s data and applications. Advances in integration and orchestration frameworks have significantly reduced the effort required to make those connections. But laying out what those integrations are and how to enable them is critical to ensure these models work efficiently and to avoid the complexity that creates technical debt (the “tax” a company pays in terms of time and resources needed to redress existing technology issues). Chief information officers and chief technology officers can define reference architectures and integration standards for their organizations. Key elements should include a model hub, which contains trained and approved models that can be provisioned on demand; standard APIs that act as bridges connecting gen AI models to applications or data; and context management and caching, which speed up processing by providing models with relevant information from enterprise data sources.
- Build up your testing and quality assurance capabilities. Our own experience building Lilli taught us to prioritize testing over development. Our team invested in not only developing testing protocols for each stage of development but also aligning the entire team so that, for example, it was clear who specifically needed to sign off on each stage of the process. This slowed down initial development but sped up the overall delivery pace and quality by cutting back on errors and the time needed to fix mistakes.
Ensure data quality and focus on unstructured data to fuel your models
The ability of a business to generate and scale value from gen AI models will depend on how well it takes advantage of its own data. As with technology, targeted upgrades to existing data architecture are needed to maximize the future strategic benefits of gen AI:
- Be targeted in ramping up your data quality and data augmentation efforts. While data quality has always been an important issue, the scale and scope of data that gen AI models can use—especially unstructured data—has made this issue much more consequential. For this reason, it’s critical to get the data foundations right, from clarifying decision rights to defining clear data processes to establishing taxonomies so models can access the data they need. The companies that do this well tie their data quality and augmentation efforts to the specific AI/gen AI application and use case—you don’t need this data foundation to extend to every corner of the enterprise. This could mean, for example, developing a new data repository for all equipment specifications and reported issues to better support maintenance copilot applications.
- Understand what value is locked into your unstructured data. Most organizations have traditionally focused their data efforts on structured data (values that can be organized in tables, such as prices and features). But the real value from LLMs comes from their ability to work with unstructured data (for example, PowerPoint slides, videos, and text). Companies can map out which unstructured data sources are most valuable and establish metadata tagging standards so models can process the data and teams can find what they need (tagging is particularly important to help companies remove data from models as well, if necessary). Be creative in thinking about data opportunities. Some companies, for example, are interviewing senior employees as they retire and feeding that captured institutional knowledge into an LLM to help improve their copilot performance.
- Optimize to lower costs at scale. There is often as much as a tenfold difference between what companies pay for data and what they could be paying if they optimized their data infrastructure and underlying costs. This issue often stems from companies scaling their proofs of concept without optimizing their data approach. Two costs generally stand out. One is storage costs arising from companies uploading terabytes of data into the cloud and wanting that data available 24/7. In practice, companies rarely need more than 10 percent of their data to have that level of availability, and accessing the rest over a 24- or 48-hour period is a much cheaper option. The other costs relate to computation with models that require on-call access to thousands of processors to run. This is especially the case when companies are building their own models (the maker archetype) but also when they are using pretrained models and running them with their own data and use cases (the shaper archetype). Companies could take a close look at how they can optimize computation costs on cloud platforms—for instance, putting some models in a queue to run when processors aren’t being used (such as when Americans go to bed and consumption of computing services like Netflix decreases) is a much cheaper option.
Build trust and reusability to drive adoption and scale
Because many people have concerns about gen AI, the bar on explaining how these tools work is much higher than for most solutions. People who use the tools want to know how they work, not just what they do. So it’s important to invest extra time and money to build trust by ensuring model accuracy and making it easy to check answers.
One insurance company, for example, created a gen AI tool to help manage claims. As part of the tool, it listed all the guardrails that had been put in place, and for each answer provided a link to the sentence or page of the relevant policy documents. The company also used an LLM to generate many variations of the same question to ensure answer consistency. These steps, among others, were critical to helping end users build trust in the tool.
Part of the training for maintenance teams using a gen AI tool should be to help them understand the limitations of models and how best to get the right answers. That includes teaching workers strategies to get to the best answer as fast as possible by starting with broad questions then narrowing them down. This provides the model with more context, and it also helps remove any bias of the people who might think they know the answer already. Having model interfaces that look and feel the same as existing tools also helps users feel less pressured to learn something new each time a new application is introduced.
Getting to scale means that businesses will need to stop building one-off solutions that are hard to use for other similar use cases. One global energy and materials company, for example, has established ease of reuse as a key requirement for all gen AI models, and has found in early iterations that 50 to 60 percent of its components can be reused. This means setting standards for developing gen AI assets (for example, prompts and context) that can be easily reused for other cases.
While many of the risk issues relating to gen AI are evolutions of discussions that were already brewing—for instance, data privacy, security, bias risk, job displacement, and intellectual property protection—gen AI has greatly expanded that risk landscape. Just 21 percent of companies reporting AI adoption say they have established policies governing employees’ use of gen AI technologies.
Similarly, a set of tests for AI/gen AI solutions should be established to demonstrate that data privacy, debiasing, and intellectual property protection are respected. Some organizations, in fact, are proposing to release models accompanied with documentation that details their performance characteristics. Documenting your decisions and rationales can be particularly helpful in conversations with regulators.
In some ways, this article is premature—so much is changing that we’ll likely have a profoundly different understanding of gen AI and its capabilities in a year’s time. But the core truths of finding value and driving change will still apply. How well companies have learned those lessons may largely determine how successful they’ll be in capturing that value.

The authors wish to thank Michael Chui, Juan Couto, Ben Ellencweig, Josh Gartner, Bryce Hall, Holger Harreis, Phil Hudelson, Suzana Iacob, Sid Kamath, Neerav Kingsland, Kitti Lakner, Robert Levin, Matej Macak, Lapo Mori, Alex Peluffo, Aldo Rosales, Erik Roth, Abdul Wahab Shaikh, and Stephen Xu for their contributions to this article.
This article was edited by Barr Seitz, an editorial director in the New York office.
Explore a career with us
Related articles.

The economic potential of generative AI: The next productivity frontier
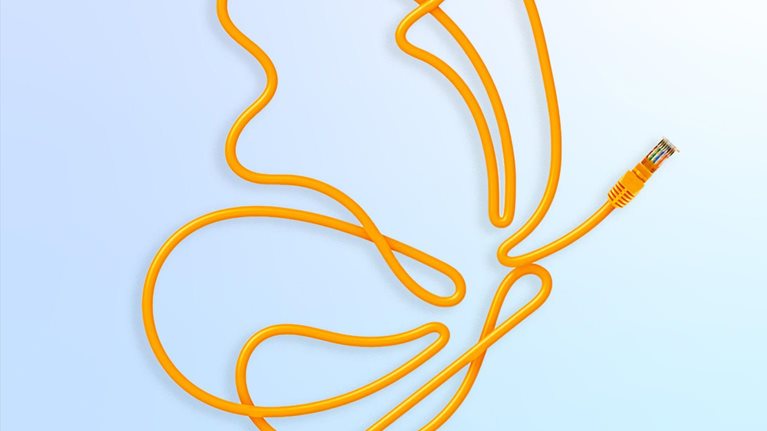
Rewired to outcompete

Meet Lilli, our generative AI tool that’s a researcher, a time saver, and an inspiration
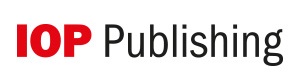
We apologize for the inconvenience...
To ensure we keep this website safe, please can you confirm you are a human by ticking the box below.
If you are unable to complete the above request please contact us using the below link, providing a screenshot of your experience.
https://ioppublishing.org/contacts/
- Hispanoamérica
- Work at ArchDaily
- Terms of Use
- Privacy Policy
- Cookie Policy
Capital Bank of Jordan / Paradigm DH
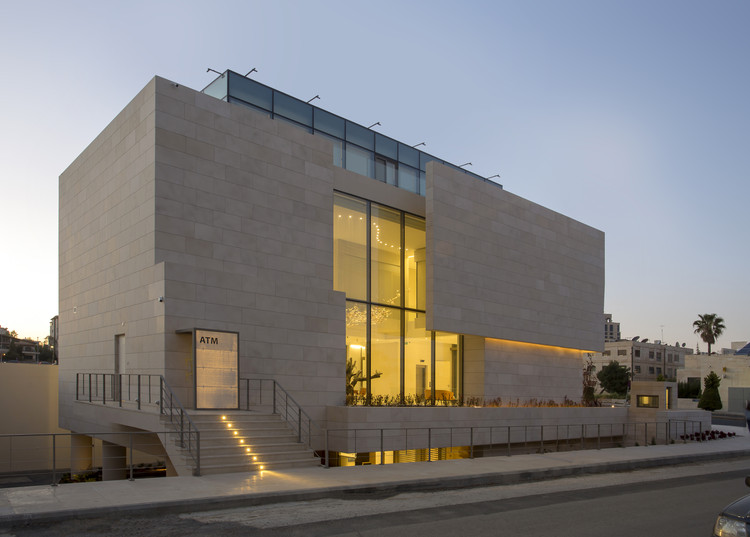
- Curated by Fernanda Castro
- Architects: Paradigm DH
- Area Area of this architecture project Area: 1100 m²
- Year Completion year of this architecture project Year: 2018
- Photographs Photographs: Nabil Qutteneh , Sireen Khateeb
- Manufacturers Brands with products used in this architecture project Manufacturers: Fantoni , Linea Light Group , Lucem Lichtbeton , Poltrona Frau , interstull , preciosa
- Lead Architects: Principal Architect Saja Nashashibi
- Contractor : Al wajih Contracting Company
- Electromechanical Consultant : EMCC
- Structure Consultant : Engineering Solutions and Consulting – Esc
- Lighting Consultant & Supplier : ICC – Ideal Concepts Co.
- Client: Capital Bank of Jordan
- City: Amman
- Country: Jordan
- Did you collaborate on this project?
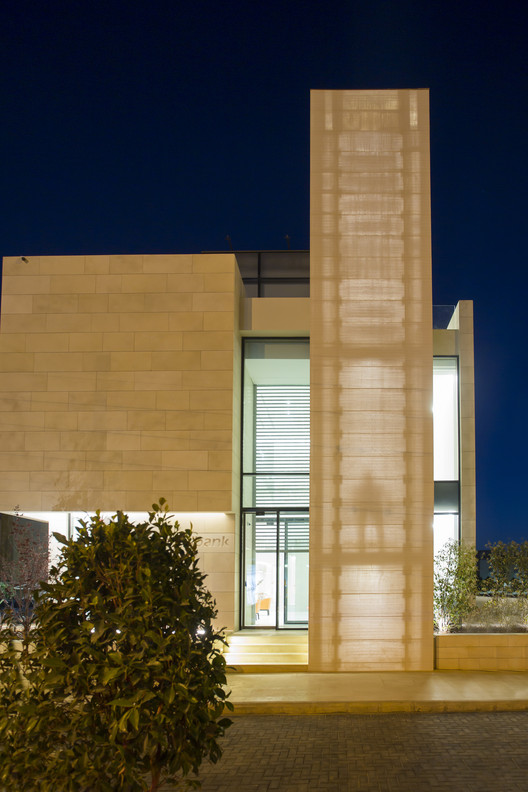
Text description provided by the architects. Capital Select is located in a residential area in one of the most exclusive neighborhoods of Jordan’s capital Amman in Abdoun. Creating a "boutique bank" experience triggered the design concept of this branch introducing a sense of belonging and a welcoming design influenced by nature.
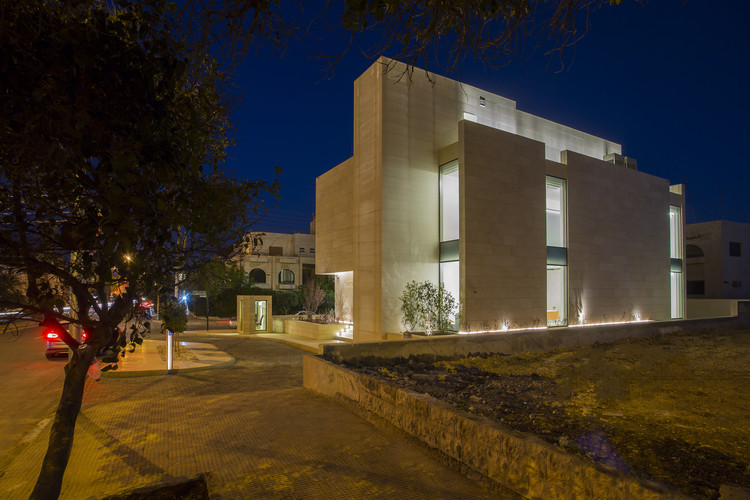
To create a “boutique” experience, the traditional bank experience had to be replaced with an approach that is private, luxurious and catered to the customer’s needs. The teller counters and waiting areas with traditional queuing approach, were replaced with individual office "boxes", lobby and reception areas which were designed to accommodate for all the customer's needs. Nature theme was incorporated in the design by first placing separate masses for the offices within the main mass of the building around a double volume void that contained an inner court which was influenced by Japanese Zen gardens. It was emphasized by a crystal chandelier flowing above a sculptural olive tree that acts as a focal point for the entire space, in addition to the artwork layer. The office masses were then individually shifted from each other in order for the void to become more fluid and thusly create unique interior views and spaces as the user walks through the circulation corridor.
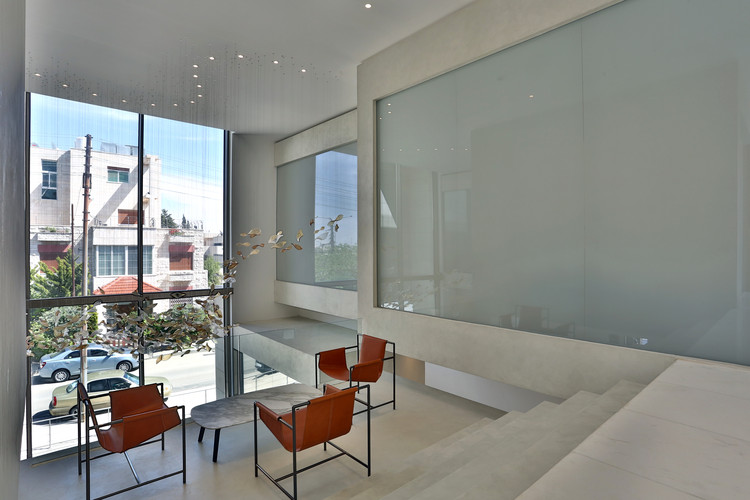
The previously mentioned shifting masses of the offices translated into shifted planes and masses of the exterior main front and side façades. The remaining side & back facades had minimal treatment with minimal openings and were cladded with Taffouh Stone, this was to respect the adjacent neighbors’ privacy as well as the branch users’ privacy maintaining the alignment with the local vernacular stone architecture. However, the main side elevation’s transparent glass curtain wall opens to the inner court, further enhancing the continuation of space from the exterior to the interior and vice versa.
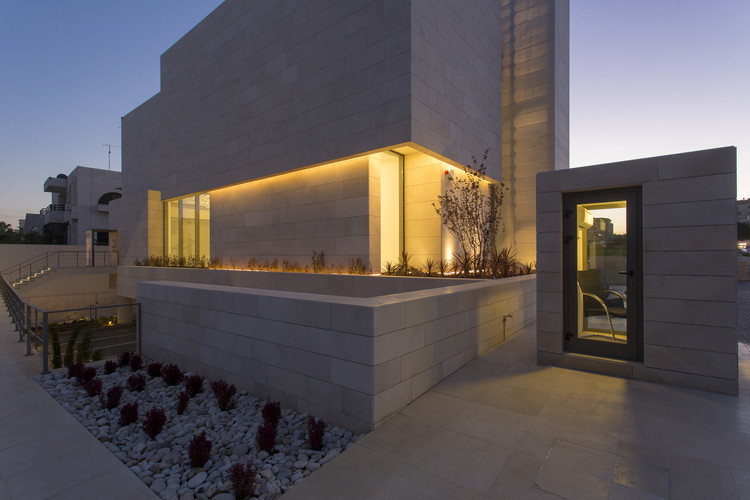
As for the main elevation Paradigm DH Team has decided to use LUCEM translucent concrete panels, as self-Supporting panels, one of a kind walls on the external façade, these concrete panels are made with fiber optics where light can pass through. This material was used for the first time using this technique in Capital Select, free of any boundaries and as opaque as it could be. In addition to the use of translucent concrete panels, PDH decided to use Plain concrete panels without fiber-optics to cover non-translucent parts of the façade using the same cement / sand mixture while maintaining similar surface finish matching the color of the stone used on the facades to maintain its harmony with the residential surroundings.
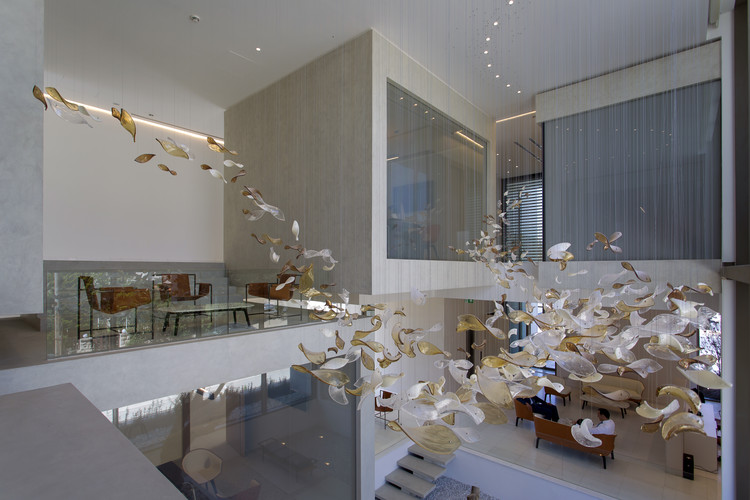
The result was achieved by cladding a 14-meter-high stairwell with translucent concrete panels that are mechanically fixed, keeping the supporting structure exposed from the inside with the lights that illuminate the translucent concrete, PDH opted for vertical lights that span the full height of the stairwell while having this 30mm thick LUCEM panels mounted on steel/wood columns and incorporated into the design of the handrail. The reception counters and ATM areas were also made with a backlit translucent concrete Panels.
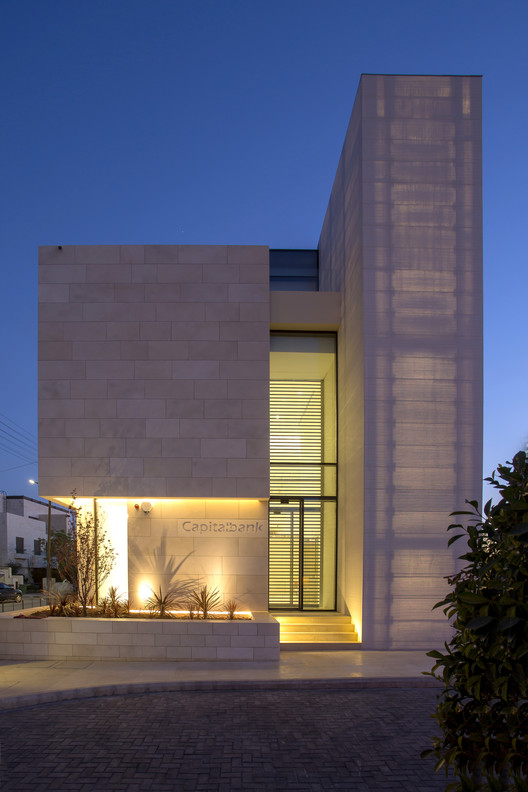
The idea of nature that flows through the staircase in the form of light and shadow plays was the main idea of the design. With the use of translucent concrete, the architects and lighting engineers are setting a striking example of how external walls can solve the contradiction between massiveness and lightness through translucency.
Project gallery
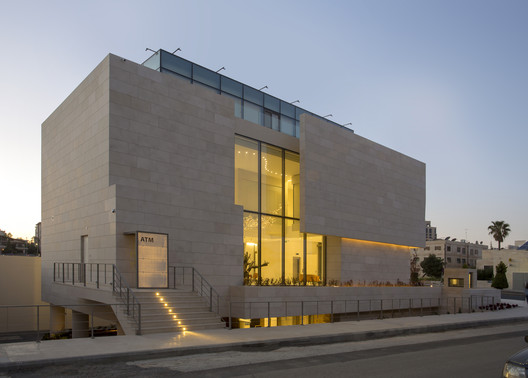
Project location
Address: capital bank, queen rania al abdullah st 317, amman, jordan.
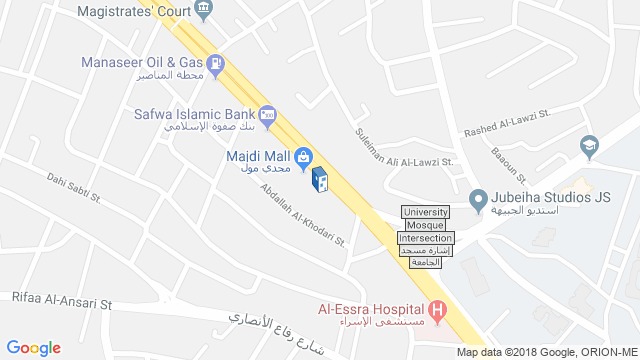
Materials and Tags
- Sustainability
世界上最受欢迎的建筑网站现已推出你的母语版本!
想浏览archdaily中国吗, you've started following your first account, did you know.
You'll now receive updates based on what you follow! Personalize your stream and start following your favorite authors, offices and users.
Check the latest Mobile Workstations
Check the latest Bureaus
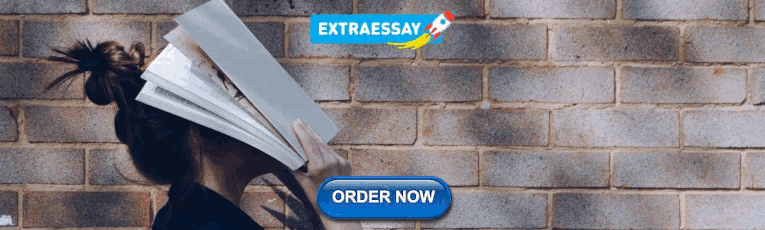
IMAGES
VIDEO
COMMENTS
SOM unveils "breathing" Shenzhen bank tower enclosed in diagrid. Architecture firm SOM has completed its headquarters for Shenzhen 's Rural Commercial Bank, a naturally ventilated steel-wrapped ...
6 Case Study 18 References 19 About the Authors 19 Table of Contents ... Drivers for Bank Architecture Simplification Customer-focused concerns are among the most important external drivers for architecture transformation such as regulations, competitive differentiation, and new
Top architecture projects recently published on ArchDaily. The most inspiring residential architecture, interior design, landscaping, urbanism, and more from the world's best architects. Find ...
Published on June 27, 2012. Share. The Bank of America Tower at One Bryant Park in midtown New York, designed by Cook + Fox Architects, is the first commercial high-rise to achieve LEED Platinum ...
European Central Bank | Coop Himmelb (l)au. Architecture. Stories about the design and architecture of Bank projects from around the world. In the list below, you'll find the most recent Bank news, and ideas that arouse the most interest in our audience.
In this paper, a system architecture for an MSA-based core banking system was proposed by particularly taking performance efficiency into account. The justification of this architecture was made through a real-world case study in a commercial bank having a large-scale legacy core banking monolith.
In our case studies, the main migration problem is the one from the monolithic legacy system to modular architecture. Migration to cloud is a part of the problem, but not the main focus. Balalaie et al. [ 5 ] report on the migration to a cloud-native microservices architecture of a single company.
Chapter 4: Location. The Importance of Bank Locations. Too many banks have been—and are still being—built in the wrong locations. Unlike a lot of the other concepts in this guide, which are made up of many smaller decisions, choosing a bank building location is a single decision that carries a lot of weight.
Wherefore, The Bank of America is the greenest project of New York in 2008, so we can say that an environmentally friendly building. It's at One Bryant Park in the Midtown area of Manhattan in New York City. As the greenest high-rise building, it is expounding the case of the art in: Energy efficiency; Internal air quality; Sustainable materials
Bank. Karafarin Bank / NextOffice Architecture Classics: Colpatria TO / Obregón y Valenzuela & C... Exo Towers Ruifeng Digital Finance Center / UUA (United Units ...
The IT architecture of a digital bank must be flexible, scalable, secure and manageable. The major challenges that digital banking faces include increasing regulatory compliance, adapting to changing customer needs, and creating a competitive edge against your competitors. 1. Security.
Address: The Mall, Boulevard "Tsarigradsko shose" 115и, 1784 м. Къро, Sofia, Bulgaria. Location to be used only as a reference. It could indicate city/country but not exact address. Share ...
Case Study. PNC Financial Services Group. The BIAN model fits perfectly in line with how we view enterprise architecture (EA) at PNC. One of the first steps we took as an organisation was to bring a business perspective to enterprise architecture. To us, technology is not just a collection of servers and software, but rather a set of technical ...
This intense, thorough work session laid the foundation for Granite Bank's metamorphosis. The InVision process helped unveil the Bank's desire to form a new work culture and laid out the possibilities and solutions for developing it. The process also helped chart a strategy to establish a new community relationship at the Bank's new location.
The main purpose of the study is design the cloud computing architecture for Dashen bank, which will reduce the labor need for managing IT infrastructure and system and enhance the use of technology with the required security verification. The study focused on designing aspects of cloud computing. The study used interview, observation and document
St.George Bank is Australia's 5 largest retail bank with a market capitilisation of th. AU$15B1. In 2007 St.George undertook a project to document its IT infrastructure using Enterprise Architect, a CASE tool developed by Sparx Systems. St.George wasn't looking for an "encyclopedic" style of documentation. They wanted something intranet ...
Building for scale doesn't mean building a new technology architecture. But it does mean focusing on a few core decisions that simplify and speed up processes without breaking the bank. Three such decisions stand out: Focus on reusing your technology. Reusing code can increase the development speed of gen AI use cases by 30 to 50 percent.
The solution assimilates architecture and engineering simultaneously, involving an asymmetrical tower that informs both skyline and street. The Bank of China Tower stands 70 stories tall, reaching ...
The study shows that residential areas - built-up l ands - grow much faster than the population size. From 2010 to 2017 only, the area of Belgorod metropolis expanded by 4%, built-up areas grew by
Urban sprawl in the regional settlement system: a case study of Belgorod and Stary Oskol-Gubkin metropolitan areas; Analysis of natural prerequisites for the development of ecological tourism in the Belgorod region; Historical and architectural monuments of wooden architecture in the territory of historical settlements in Belgorod region
Producers of iron ore. The company is engaged in manufacturing and processing raw materials for metallurgical enterprises. Its main facility, the Stoilensky Mining and Beneficiation Plant. The main products of the plant - iron ore and iron sintering ore.
Address: Capital Bank, Queen Rania Al Abdullah St 317, Amman, Jordan. Location to be used only as a reference. It could indicate city/country but not exact address. Share. About this office ...
IOP Conference Series: Earth and Environmental Science PAPER OPEN ACCESS 8UEDQVSUDZOLQWKHUHJLRQDOVHWWOHPHQWV\VWHP D FDVHVWXG\RI%HOJRURGDQG6WDU\2VNRO *XENLQ