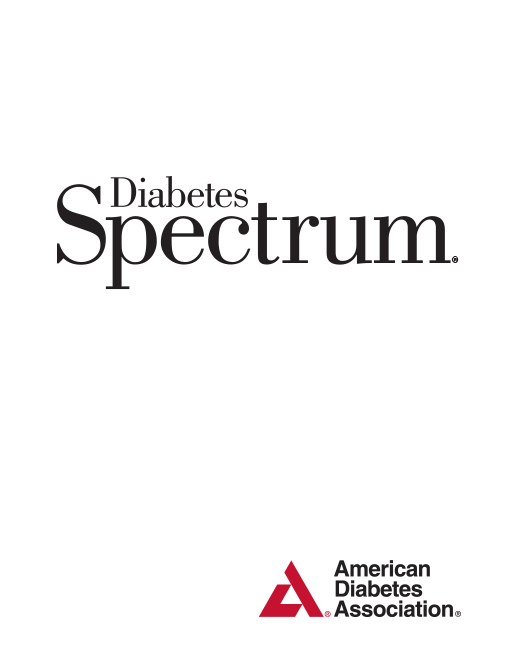
- Previous Article
- Next Article
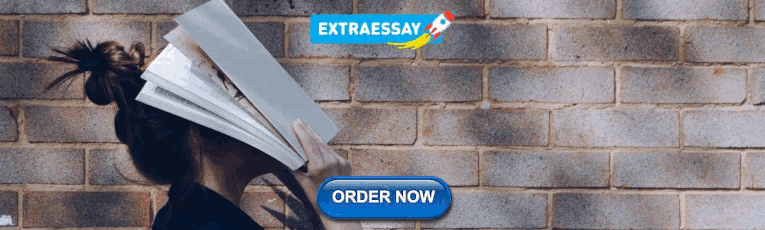
Case Presentation
Case study: a patient with uncontrolled type 2 diabetes and complex comorbidities whose diabetes care is managed by an advanced practice nurse.
- Split-Screen
- Article contents
- Figures & tables
- Supplementary Data
- Peer Review
- Open the PDF for in another window
- Cite Icon Cite
- Get Permissions
Geralyn Spollett; Case Study: A Patient With Uncontrolled Type 2 Diabetes and Complex Comorbidities Whose Diabetes Care Is Managed by an Advanced Practice Nurse. Diabetes Spectr 1 January 2003; 16 (1): 32–36. https://doi.org/10.2337/diaspect.16.1.32
Download citation file:
- Ris (Zotero)
- Reference Manager
The specialized role of nursing in the care and education of people with diabetes has been in existence for more than 30 years. Diabetes education carried out by nurses has moved beyond the hospital bedside into a variety of health care settings. Among the disciplines involved in diabetes education, nursing has played a pivotal role in the diabetes team management concept. This was well illustrated in the Diabetes Control and Complications Trial (DCCT) by the effectiveness of nurse managers in coordinating and delivering diabetes self-management education. These nurse managers not only performed administrative tasks crucial to the outcomes of the DCCT, but also participated directly in patient care. 1
The emergence and subsequent growth of advanced practice in nursing during the past 20 years has expanded the direct care component, incorporating aspects of both nursing and medical care while maintaining the teaching and counseling roles. Both the clinical nurse specialist (CNS) and nurse practitioner (NP) models, when applied to chronic disease management, create enhanced patient-provider relationships in which self-care education and counseling is provided within the context of disease state management. Clement 2 commented in a review of diabetes self-management education issues that unless ongoing management is part of an education program, knowledge may increase but most clinical outcomes only minimally improve. Advanced practice nurses by the very nature of their scope of practice effectively combine both education and management into their delivery of care.
Operating beyond the role of educator, advanced practice nurses holistically assess patients’ needs with the understanding of patients’ primary role in the improvement and maintenance of their own health and wellness. In conducting assessments, advanced practice nurses carefully explore patients’ medical history and perform focused physical exams. At the completion of assessments, advanced practice nurses, in conjunction with patients, identify management goals and determine appropriate plans of care. A review of patients’ self-care management skills and application/adaptation to lifestyle is incorporated in initial histories, physical exams, and plans of care.
Many advanced practice nurses (NPs, CNSs, nurse midwives, and nurse anesthetists) may prescribe and adjust medication through prescriptive authority granted to them by their state nursing regulatory body. Currently, all 50 states have some form of prescriptive authority for advanced practice nurses. 3 The ability to prescribe and adjust medication is a valuable asset in caring for individuals with diabetes. It is a crucial component in the care of people with type 1 diabetes, and it becomes increasingly important in the care of patients with type 2 diabetes who have a constellation of comorbidities, all of which must be managed for successful disease outcomes.
Many studies have documented the effectiveness of advanced practice nurses in managing common primary care issues. 4 NP care has been associated with a high level of satisfaction among health services consumers. In diabetes, the role of advanced practice nurses has significantly contributed to improved outcomes in the management of type 2 diabetes, 5 in specialized diabetes foot care programs, 6 in the management of diabetes in pregnancy, 7 and in the care of pediatric type 1 diabetic patients and their parents. 8 , 9 Furthermore, NPs have also been effective providers of diabetes care among disadvantaged urban African-American patients. 10 Primary management of these patients by NPs led to improved metabolic control regardless of whether weight loss was achieved.
The following case study illustrates the clinical role of advanced practice nurses in the management of a patient with type 2 diabetes.
A.B. is a retired 69-year-old man with a 5-year history of type 2 diabetes. Although he was diagnosed in 1997, he had symptoms indicating hyperglycemia for 2 years before diagnosis. He had fasting blood glucose records indicating values of 118–127 mg/dl, which were described to him as indicative of “borderline diabetes.” He also remembered past episodes of nocturia associated with large pasta meals and Italian pastries. At the time of initial diagnosis, he was advised to lose weight (“at least 10 lb.”), but no further action was taken.
Referred by his family physician to the diabetes specialty clinic, A.B. presents with recent weight gain, suboptimal diabetes control, and foot pain. He has been trying to lose weight and increase his exercise for the past 6 months without success. He had been started on glyburide (Diabeta), 2.5 mg every morning, but had stopped taking it because of dizziness, often accompanied by sweating and a feeling of mild agitation, in the late afternoon.
A.B. also takes atorvastatin (Lipitor), 10 mg daily, for hypercholesterolemia (elevated LDL cholesterol, low HDL cholesterol, and elevated triglycerides). He has tolerated this medication and adheres to the daily schedule. During the past 6 months, he has also taken chromium picolinate, gymnema sylvestre, and a “pancreas elixir” in an attempt to improve his diabetes control. He stopped these supplements when he did not see any positive results.
He does not test his blood glucose levels at home and expresses doubt that this procedure would help him improve his diabetes control. “What would knowing the numbers do for me?,” he asks. “The doctor already knows the sugars are high.”
A.B. states that he has “never been sick a day in my life.” He recently sold his business and has become very active in a variety of volunteer organizations. He lives with his wife of 48 years and has two married children. Although both his mother and father had type 2 diabetes, A.B. has limited knowledge regarding diabetes self-care management and states that he does not understand why he has diabetes since he never eats sugar. In the past, his wife has encouraged him to treat his diabetes with herbal remedies and weight-loss supplements, and she frequently scans the Internet for the latest diabetes remedies.
During the past year, A.B. has gained 22 lb. Since retiring, he has been more physically active, playing golf once a week and gardening, but he has been unable to lose more than 2–3 lb. He has never seen a dietitian and has not been instructed in self-monitoring of blood glucose (SMBG).
A.B.’s diet history reveals excessive carbohydrate intake in the form of bread and pasta. His normal dinners consist of 2 cups of cooked pasta with homemade sauce and three to four slices of Italian bread. During the day, he often has “a slice or two” of bread with butter or olive oil. He also eats eight to ten pieces of fresh fruit per day at meals and as snacks. He prefers chicken and fish, but it is usually served with a tomato or cream sauce accompanied by pasta. His wife has offered to make him plain grilled meats, but he finds them “tasteless.” He drinks 8 oz. of red wine with dinner each evening. He stopped smoking more than 10 years ago, he reports, “when the cost of cigarettes topped a buck-fifty.”
The medical documents that A.B. brings to this appointment indicate that his hemoglobin A 1c (A1C) has never been <8%. His blood pressure has been measured at 150/70, 148/92, and 166/88 mmHg on separate occasions during the past year at the local senior center screening clinic. Although he was told that his blood pressure was “up a little,” he was not aware of the need to keep his blood pressure ≤130/80 mmHg for both cardiovascular and renal health. 11
A.B. has never had a foot exam as part of his primary care exams, nor has he been instructed in preventive foot care. However, his medical records also indicate that he has had no surgeries or hospitalizations, his immunizations are up to date, and, in general, he has been remarkably healthy for many years.
Physical Exam
A physical examination reveals the following:
Weight: 178 lb; height: 5′2″; body mass index (BMI): 32.6 kg/m 2
Fasting capillary glucose: 166 mg/dl
Blood pressure: lying, right arm 154/96 mmHg; sitting, right arm 140/90 mmHg
Pulse: 88 bpm; respirations 20 per minute
Eyes: corrective lenses, pupils equal and reactive to light and accommodation, Fundi-clear, no arteriolovenous nicking, no retinopathy
Thyroid: nonpalpable
Lungs: clear to auscultation
Heart: Rate and rhythm regular, no murmurs or gallops
Vascular assessment: no carotid bruits; femoral, popliteal, and dorsalis pedis pulses 2+ bilaterally
Neurological assessment: diminished vibratory sense to the forefoot, absent ankle reflexes, monofilament (5.07 Semmes-Weinstein) felt only above the ankle
Lab Results
Results of laboratory tests (drawn 5 days before the office visit) are as follows:
Glucose (fasting): 178 mg/dl (normal range: 65–109 mg/dl)
Creatinine: 1.0 mg/dl (normal range: 0.5–1.4 mg/dl)
Blood urea nitrogen: 18 mg/dl (normal range: 7–30 mg/dl)
Sodium: 141 mg/dl (normal range: 135–146 mg/dl)
Potassium: 4.3 mg/dl (normal range: 3.5–5.3 mg/dl)
Lipid panel
• Total cholesterol: 162 mg/dl (normal: <200 mg/dl)
• HDL cholesterol: 43 mg/dl (normal: ≥40 mg/dl)
• LDL cholesterol (calculated): 84 mg/dl (normal: <100 mg/dl)
• Triglycerides: 177 mg/dl (normal: <150 mg/dl)
• Cholesterol-to-HDL ratio: 3.8 (normal: <5.0)
AST: 14 IU/l (normal: 0–40 IU/l)
ALT: 19 IU/l (normal: 5–40 IU/l)
Alkaline phosphotase: 56 IU/l (normal: 35–125 IU/l)
A1C: 8.1% (normal: 4–6%)
Urine microalbumin: 45 mg (normal: <30 mg)
Based on A.B.’s medical history, records, physical exam, and lab results, he is assessed as follows:
Uncontrolled type 2 diabetes (A1C >7%)
Obesity (BMI 32.4 kg/m 2 )
Hyperlipidemia (controlled with atorvastatin)
Peripheral neuropathy (distal and symmetrical by exam)
Hypertension (by previous chart data and exam)
Elevated urine microalbumin level
Self-care management/lifestyle deficits
• Limited exercise
• High carbohydrate intake
• No SMBG program
Poor understanding of diabetes
A.B. presented with uncontrolled type 2 diabetes and a complex set of comorbidities, all of which needed treatment. The first task of the NP who provided his care was to select the most pressing health care issues and prioritize his medical care to address them. Although A.B. stated that his need to lose weight was his chief reason for seeking diabetes specialty care, his elevated glucose levels and his hypertension also needed to be addressed at the initial visit.
The patient and his wife agreed that a referral to a dietitian was their first priority. A.B. acknowledged that he had little dietary information to help him achieve weight loss and that his current weight was unhealthy and “embarrassing.” He recognized that his glucose control was affected by large portions of bread and pasta and agreed to start improving dietary control by reducing his portion size by one-third during the week before his dietary consultation. Weight loss would also be an important first step in reducing his blood pressure.
The NP contacted the registered dietitian (RD) by telephone and referred the patient for a medical nutrition therapy assessment with a focus on weight loss and improved diabetes control. A.B.’s appointment was scheduled for the following week. The RD requested that during the intervening week, the patient keep a food journal recording his food intake at meals and snacks. She asked that the patient also try to estimate portion sizes.
Although his physical activity had increased since his retirement, it was fairly sporadic and weather-dependent. After further discussion, he realized that a week or more would often pass without any significant form of exercise and that most of his exercise was seasonal. Whatever weight he had lost during the summer was regained in the winter, when he was again quite sedentary.
A.B.’s wife suggested that the two of them could walk each morning after breakfast. She also felt that a treadmill at home would be the best solution for getting sufficient exercise in inclement weather. After a short discussion about the positive effect exercise can have on glucose control, the patient and his wife agreed to walk 15–20 minutes each day between 9:00 and 10:00 a.m.
A first-line medication for this patient had to be targeted to improving glucose control without contributing to weight gain. Thiazolidinediones (i.e., rosiglitizone [Avandia] or pioglitizone [Actos]) effectively address insulin resistance but have been associated with weight gain. 12 A sulfonylurea or meglitinide (i.e., repaglinide [Prandin]) can reduce postprandial elevations caused by increased carbohydrate intake, but they are also associated with some weight gain. 12 When glyburide was previously prescribed, the patient exhibited signs and symptoms of hypoglycemia (unconfirmed by SMBG). α-Glucosidase inhibitors (i.e., acarbose [Precose]) can help with postprandial hyperglycemia rise by blunting the effect of the entry of carbohydrate-related glucose into the system. However, acarbose requires slow titration, has multiple gastrointestinal (GI) side effects, and reduces A1C by only 0.5–0.9%. 13 Acarbose may be considered as a second-line therapy for A.B. but would not fully address his elevated A1C results. Metformin (Glucophage), which reduces hepatic glucose production and improves insulin resistance, is not associated with hypoglycemia and can lower A1C results by 1%. Although GI side effects can occur, they are usually self-limiting and can be further reduced by slow titration to dose efficacy. 14
After reviewing these options and discussing the need for improved glycemic control, the NP prescribed metformin, 500 mg twice a day. Possible GI side effects and the need to avoid alcohol were of concern to A.B., but he agreed that medication was necessary and that metformin was his best option. The NP advised him to take the medication with food to reduce GI side effects.
The NP also discussed with the patient a titration schedule that increased the dosage to 1,000 mg twice a day over a 4-week period. She wrote out this plan, including a date and time for telephone contact and medication evaluation, and gave it to the patient.
During the visit, A.B. and his wife learned to use a glucose meter that features a simple two-step procedure. The patient agreed to use the meter twice a day, at breakfast and dinner, while the metformin dose was being titrated. He understood the need for glucose readings to guide the choice of medication and to evaluate the effects of his dietary changes, but he felt that it would not be “a forever thing.”
The NP reviewed glycemic goals with the patient and his wife and assisted them in deciding on initial short-term goals for weight loss, exercise, and medication. Glucose monitoring would serve as a guide and assist the patient in modifying his lifestyle.
A.B. drew the line at starting an antihypertensive medication—the angiotensin-converting enzyme (ACE) inhibitor enalapril (Vasotec), 5 mg daily. He stated that one new medication at a time was enough and that “too many medications would make a sick man out of me.” His perception of the state of his health as being represented by the number of medications prescribed for him gave the advanced practice nurse an important insight into the patient’s health belief system. The patient’s wife also believed that a “natural solution” was better than medication for treating blood pressure.
Although the use of an ACE inhibitor was indicated both by the level of hypertension and by the presence of microalbuminuria, the decision to wait until the next office visit to further evaluate the need for antihypertensive medication afforded the patient and his wife time to consider the importance of adding this pharmacotherapy. They were quite willing to read any materials that addressed the prevention of diabetes complications. However, both the patient and his wife voiced a strong desire to focus their energies on changes in food and physical activity. The NP expressed support for their decision. Because A.B. was obese, weight loss would be beneficial for many of his health issues.
Because he has a sedentary lifestyle, is >35 years old, has hypertension and peripheral neuropathy, and is being treated for hypercholestrolemia, the NP performed an electrocardiogram in the office and referred the patient for an exercise tolerance test. 11 In doing this, the NP acknowledged and respected the mutually set goals, but also provided appropriate pre-exercise screening for the patient’s protection and safety.
In her role as diabetes educator, the NP taught A.B. and his wife the importance of foot care, demonstrating to the patient his inability to feel the light touch of the monofilament. She explained that the loss of protective sensation from peripheral neuropathy means that he will need to be more vigilant in checking his feet for any skin lesions caused by poorly fitting footwear worn during exercise.
At the conclusion of the visit, the NP assured A.B. that she would share the plan of care they had developed with his primary care physician, collaborating with him and discussing the findings of any diagnostic tests and procedures. She would also work in partnership with the RD to reinforce medical nutrition therapies and improve his glucose control. In this way, the NP would facilitate the continuity of care and keep vital pathways of communication open.
Advanced practice nurses are ideally suited to play an integral role in the education and medical management of people with diabetes. 15 The combination of clinical skills and expertise in teaching and counseling enhances the delivery of care in a manner that is both cost-reducing and effective. Inherent in the role of advanced practice nurses is the understanding of shared responsibility for health care outcomes. This partnering of nurse with patient not only improves care but strengthens the patient’s role as self-manager.
Geralyn Spollett, MSN, C-ANP, CDE, is associate director and an adult nurse practitioner at the Yale Diabetes Center, Department of Endocrinology and Metabolism, at Yale University in New Haven, Conn. She is an associate editor of Diabetes Spectrum.
Note of disclosure: Ms. Spollett has received honoraria for speaking engagements from Novo Nordisk Pharmaceuticals, Inc., and Aventis and has been a paid consultant for Aventis. Both companies produce products and devices for the treatment of diabetes.
Email alerts
- Advanced Practice Care: Advanced Practice Care in Diabetes: Epilogue
- Advanced Practice Care: Advanced Practice Care in Diabetes: Preface
- Online ISSN 1944-7353
- Print ISSN 1040-9165
- Diabetes Care
- Clinical Diabetes
- Diabetes Spectrum
- Standards of Medical Care in Diabetes
- Scientific Sessions Abstracts
- BMJ Open Diabetes Research & Care
- ShopDiabetes.org
- ADA Professional Books
Clinical Compendia
- Clinical Compendia Home
- Latest News
- DiabetesPro SmartBrief
- Special Collections
- DiabetesPro®
- Diabetes Food Hub™
- Insulin Affordability
- Know Diabetes By Heart™
- About the ADA
- Journal Policies
- For Reviewers
- Advertising in ADA Journals
- Reprints and Permission for Reuse
- Copyright Notice/Public Access Policy
- ADA Professional Membership
- ADA Member Directory
- Diabetes.org
- X (Twitter)
- Cookie Policy
- Accessibility
- Terms & Conditions
- Get Adobe Acrobat Reader
- © Copyright American Diabetes Association
This Feature Is Available To Subscribers Only
Sign In or Create an Account
Learn how UpToDate can help you.
Select the option that best describes you
- Medical Professional
- Resident, Fellow, or Student
- Hospital or Institution
- Group Practice
- Patient or Caregiver
- Find in topic
RELATED TOPICS
The patient has been treated for hypertension for 10 years, currently with amlodipine 10 mg by mouth daily. She was once told that her cholesterol value was "borderline high" but does not know the value.
She denies symptoms of diabetes, chest pain, shortness of breath, heart disease, stroke, or circulatory problems of the lower extremities.
She estimates her current weight at 165 lbs (75 kg). She thinks she weighed 120 lbs (54 kg) at age 21 years but gained weight with each of her three pregnancies and did not return to her nonpregnant weight after each delivery. She weighed 155 lbs one year ago but gained weight following retirement from her job as an elementary school teacher. No family medical history is available because she was adopted. She does not eat breakfast, has a modest lunch, and consumes most of her calories at supper and in the evening.
On examination, blood pressure is 140/85 mmHg supine and 140/90 mmHg upright with a regular heart rate of 76 beats/minute. She weighs 169 lbs, with a body mass index (BMI) of 30.9 kg/m 2 . Fundoscopic examination reveals no evidence of retinopathy. Vibratory sensation is absent at the great toes, reduced at the medial malleoli, and normal at the tibial tubercles. Light touch sensation is reduced in the feet but intact more proximally. Knee jerks are 2+ bilaterally, but the ankle jerks are absent. The examination is otherwise within normal limits.
- Open access
- Published: 17 January 2024
Nutrient patterns and risk of diabetes mellitus type 2: a case-control study
- Morteza haramshahi 1 ,
- Thoraya Mohamed Elhassan A-Elgadir 2 ,
- Hamid Mahmood Abdullah Daabo 3 ,
- Yahya Altinkaynak 4 ,
- Ahmed Hjazi 5 ,
- Archana Saxena 6 ,
- Mazin A.A. Najm 7 ,
- Abbas F. Almulla 8 ,
- Ali Alsaalamy 9 &
- Mohammad Amin Kashani 10
BMC Endocrine Disorders volume 24 , Article number: 10 ( 2024 ) Cite this article
1653 Accesses
1 Altmetric
Metrics details
Backgrounds
Although the significance of diet in preventing or managing diabetes complications is highlighted in current literature, there is insufficient evidence regarding the correlation between nutrient patterns and these complications. The objective of this case-control study is to investigate this relationship by analyzing the dietary intake of nutrients in participants with and without type 2 diabetes (T2D).
A case-control study was conducted at the Tabriz Center of Metabolism and Endocrinology to investigate the relationship between nutrient patterns and type 2 diabetes (T2D). The study enrolled 225 newly diagnosed cases of T2D and 225 controls. The dietary intake of nutrients was assessed using a validated semi-quantitative food frequency questionnaire (FFQ). Principal component analysis using Varimax rotation was used to obtain nutrient patterns. Logistic regression analysis was performed to estimate the risk of T2D.
The participants’ mean (SD) age and BMI were 39.8 (8.8) years and 27.8 (3.6) kg/m2, respectively. The results identified three major nutrient patterns. The first nutrient pattern was characterized by high consumption of sucrose, animal protein, vitamin E, vitamin B1, vitamin B12, calcium, phosphorus, zinc, and potassium. The second nutrient pattern included fiber, plant protein, vitamin D, Riboflavin, Vitamin B5, copper, and Magnesium. The third nutrient pattern was characterized by fiber, plant protein, vitamin A, riboflavin, vitamin C, calcium, and potassium. Individuals in the highest tertile of nutrient pattern 3 (NP3) had a lower risk of T2D compared to those in the lowest tertile after adjusting for confounders. The odds ratio was 0.52 with a 95% confidence interval of 0.30–0.89 and a P_trend of 0.039.
This study found that conforming to a nutrient pattern consisting of plant protein, vitamin C, vitamin A, vitamin B2, potassium, and calcium is linked to a lower likelihood of developing T2D.The initial results suggest that following a nutrient pattern that includes these nutrients may reduce the risk of T2D. However, further research is required to confirm the relationship between nutrient patterns and T2D.
Peer Review reports
Type 2 diabetes is a significant concern for public health in developed nations. It leads to high rates of illness and death and places a significant financial burden on healthcare systems [ 1 , 2 ]. In the past few decades, there has been a sharp increase in the occurrence of diabetes, and is expected to continue increasing, with an estimated 693 million people living with the disease by 2045 [ 1 ]. Complications associated with type 2 diabetes can also contribute to premature death. A concerning aspect of the disease is that a significant proportion of cases (40%) go undetected [ 3 ], and there is also an increasing prevalence of prediabetes, which raises the risk of developing type 2 diabetes and other chronic diseases [ 1 ].
The connection between diet and type 2 diabetes has been extensively studied, including the examination of dietary patterns and individual foods or nutrient patterns [ 4 , 5 , 6 , 7 ]. Various sources have suggested that chronic diseases may be influenced by a combination of nutrients [ 8 ]. In the field of nutritional epidemiology, the examination of dietary patterns has emerged as a viable approach to investigate the correlation between diet and disease. This method involves using statistical techniques to combine multiple foods or nutrients into dietary or nutrient patterns, which are believed to provide a more detailed understanding of the connection between diet and disease. It has been suggested that the impact of individual nutrients or foods on chronic disease may be too subtle to detect, but their collective effect within a pattern may be more indicative [ 9 ].
There have been some recent studies examining the effect of nutrient patterns on chronic disease such as, non-alcoholic fatty liver, breast and gastric cancer, Polycystic Ovary Syndrome (PCOs) and metabolic syndrome [ 10 , 11 , 12 , 13 , 14 ]. For example, it was found that a nutrient pattern consisting mainly of protein, carbohydrates, and various sugars was linked to a higher risk of Metabolic Syndrome (MetS) in both men and women, whereas a pattern characterized by copper, selenium, and several vitamins was linked to greater odds of MetS [ 14 ]. A prospective study conducted among participants of the Tehran Lipid and Glucose Study indicates that a nutrient pattern rich in vitamin A, vitamin C, vitamin B6, potassium, and fructose is associated with a reduced risk of insulin-related disorders [ 15 ]. Although there have been limited investigations on the connection between nutrient patterns and the likelihood of developing diabetes, the present study seeks to explore this relationship by analyzing the adherence to different nutrient patterns and its effect on the risk of type 2 diabetes.
Study population
This study utilized a case-control design and involved participants between the ages of 18 and 60 who had been diagnosed with type 2 diabetes within the previous six months based on specific glucose level criteria (FBS levels of ≥ 126 mg/dl and 2 h-PG levels of ≥ 200 mg/dl [ 17 ]). Healthy individuals within the same age range were also included, with specific glucose level criteria (FBS levels of < 100 mg/dl and 2 h-PG levels of < 200 mg/dl [ 17 ]). The study excluded individuals with certain chronic diseases, Type 1 Diabetes, gestational diabetes, those following specific dietary patterns or taking certain medications, pregnant and breastfeeding women, those with a family history of diabetes or hypertension, and those who did not complete the food frequency questionnaire (more than 35 items) or whose reported energy intake was outside of a specific range (range of 800–4200 kcal [ 18 ]).
This study enrolled 450 adult participants, with 225 individuals in the case group and 225 in the control group. The case group was selected using a simple sampling method from patients diagnosed with diabetes at the Tabriz Center of Metabolism and Endocrinology as a referral center affiliated to tabriz University of Medical Sciences from January 2021 to March 2022, as well as through a two-stage cluster sampling method among patients referred to private endocrinologists to enhance the sample’s external validity. Participants in the control group were also selected through a two-stage cluster sampling method from individuals who had undergone blood glucose checkups at the Tabriz Center of Metabolism and Endocrinology, a referral center affiliated with Tabriz University of Medical Sciences, within the past six months. All participants provided informed consent at the beginning of the study. The study was financially supported by Tabriz University of Medical Sciences and is related to project NO. 1400/63,145.
Dietary assessment
To collect dietary intake information, personal interviews and a semi-quantitative food frequency questionnaire (FFQ) consisting of 168 food items were used [ 16 ]. The FFQ asked about the frequency of consumption for each item over the course of one year, with the year before diagnosis for the case group and the year before the interview for the control group. Participants were also asked about the frequency of consumption (per day, week, month, or year) for each type of food. to ensure consistency in measurements, a nutritionist provided instructions on converting the size of reported food items from household measures to grams using four scales. The quantity of food consumed by each individual was calculated based on their intake in grams and reported on a daily basis. The nutrient composition of all foods was derived by using modified nutritionist IV software.
Nutrient pattern assessment
We conducted factor analyses using a comprehensive set of 34 nutrients, encompassing various macronutrients, micronutrients, and other dietary components. These included sucrose, lactose, fructose, fiber, animal protein, plant protein, saturated fatty acids, monounsaturated fatty acids, polyunsaturated fatty acids, cholesterol, as well as an array of vitamins and minerals such as A, D, E, K, C, thiamine (B1), riboflavin (B2), niacin (B3), pantothenic acid (B5), pyridoxine (B6), folate (B9), B12, calcium, phosphorus, iron, zinc, copper, magnesium, manganese, chromium, selenium, sodium, potassium, and caffeine. The dietary intake of these 34 nutrients per 1,000 Kcal of energy intake was computed and utilized as input variables. Subsequently, nutrient patterns (NPs) were derived through principal component analysis (PCA) with varimax rotation, based on the correlation matrix. Factor scores for each participant were then calculated by aggregating the frequency of consumption and multiplying it by the factor loadings across all 34 nutrients. To assess the statistical correlation between variables and evaluate the adequacy of the sample size, we employed the Bartlett test of sphericity ( P < 0.001) and the Kaiser-Mayer-Olkin test (0.71), respectively.
Assessment of other variables
To obtain the participants’ anthropometric measurements, weight and height were measured using a seca scale, and the participants’ BMI was determined by dividing their weight in kilograms by the square of their height in meters. Waist circumference was measured using a metal anthropometric tape, and the participants’ hip circumference was measured using a metal anthropometric tape while standing [ 17 ]. Daily physical activity was measured using a physical activity questionnaire [ 18 ], and personal questioning was employed to gather information on population and socioeconomic characteristics, including marital status, academic degree, and smoking.
Statistical analysis
Statistical analysis was performed using the Statistical Package Software for Social Science, version 21. The normality of the data was assessed using Kolmogorov-Smirnov’s test and histogram chart. The characteristics and dietary intakes of the case and control groups were presented as mean ± SD or median and frequency (percentages). Independent sample t-tests and chi-square tests were used to compare continuous and categorical variables, respectively, between the case and control groups.
The participants’ mean (SD) age and BMI were 39.8 (8.8) years and 27.8 (3.6) kg/m2, respectively. The mean (SD) BMI in the case group was 30.5 ± 4.1, and in the control group, it was 25.2 ± 3.2 kg/m2. The mean (SD) physical activity in the case group was 1121 ± 611 MET/min/week, and in the control group, it was 1598 ± 940 MET/min/week. There were significant differences in BMI and physical activity between the two groups. The mean (SD) waist circumference in the case group was 109.32 ± 10.28 cm, and in the control group, it was 87.25 ± 9.35 cm. The mean (SD) hip circumference in the case group was 107.25 ± 8.61 cm, and in the control group, it was 91.44 ± 6.17 cm. The study identified three primary nutrient patterns (NPs) with eigenvalues greater than 2. Table 1 displays the factor loadings for nutrient patterns, which accounted for 56.11% of the total nutrient variation. The high intake of sucrose, animal protein, phosphorus, zinc, potassium, calcium, vitamin E, vitamin B1 and vitamin B12 were the distinguishing features of the first pattern. The second nutrient pattern was positively associated with copper, magnesium, fiber, vitamin D, B2, B5 and plant protein but had a negative correlation with lactose and saturated fatty acids. On the other hand, the high intake of fiber, vitamin A, B2, vitamin C, plant protein and potassium were the distinguishing features of the third pattern.
The following are the characteristics of T2D patients compared to the control group, as shown in Table 2 : Higher BMI, More likely to be smokers, Lower physical activity levels, higher FBS, HbA1C, Insulin ( p < 0.05). Other variables did not differ significantly between the two groups ( p > 0.05). Additionally, T2D patients had a greater intake of energy and vitamin B3 but consumed less plant protein, vitamin A, vitamin E, vitamin B2, and zinc ( p < 0.05).
Table 3 summarizes the partial correlation coefficient between NPs and food sources, with NP1 showing a strong positive correlation with low-fat dairy, NP2 with refined grains, and NP3 with fruits and vegetables.
Table 4 demonstrates the relationships between NPs and T2D. After adjusting for age and sex, there was no significant link between each nutrient pattern (NP) and T2D. However, when adjusting for other factors such as BMI, physical activity, smoking, and energy intake, individuals in the highest tertile of NP1 and NP2 did not show a significant association with T2D compared to those in the lowest tertile. On the other hand, those in the highest tertile of NP3 had a lower probability of developing T2D than those in the lowest tertile (OR: 0.52, 95%CI: 0.30–0.89, P_trend = 0.039).
In this study, three major NPs were identified. After adjusting for potential confounders, we observed a significant inverse association between the Third NP and the odds of T2D. The high intake of fiber, vitamin A, B2, vitamin C, plant protein and potassium were the distinguishing features of the third pattern.
Dietary patterns, such as healthy, Mediterranean, traditional, and Western dietary patterns, have recently received significant attention in studying the connection between diet and health. When looking at the relationship between nutrients and disease incidence, it is more challenging to evaluate when considering individual foods and the metabolism of all nutrients together [ 19 ]. It is therefore more effective to take a broader view and consider diet as a whole. Dietary and nutrient patterns can have a greater impact on health than specific nutrients or nutritional groups. There is supporting evidence that links high calorie or high glycemic index foods with an increased risk of T2D. The quality of one’s diet is also associated with the risk, progression, and side effects of T2D [ 20 ]. Establishing a desirable food pattern has become a priority in public health efforts to prevent T2D. By studying dietary and nutrient patterns, we can gain a comprehensive understanding of an individual’s overall diet beyond just the consumption of specific nutrients and food groups. Moreover, it is easier for people to understand health recommendations when presented as dietary patterns rather than focusing solely on individual nutrients [ 19 ].
A previous cross-sectional study investigated the relationship between NPs and fasting glucose and glycated hemoglobin levels among apparently healthy black South Africans. The study stratified 2,010 participants by gender and urban/rural status and identified three nutrient patterns per stratum. In rural women, a nutrient pattern driven by starch, dietary fiber, and B vitamins was significantly associated with lower fasting glucose and glycated hemoglobin levels. A nutrient pattern that included vitamin B1, zinc, and plant protein was linked to notable decreases in glycated hemoglobin and fasting glucose levels in rural men. These findings suggest that nutrient patterns that are plant-based are linked to lower levels of fasting glucose and glycated hemoglobin [ 21 ].
Iwasaki et al. found that specific nutrient patterns were associated with lower risks of MetS. One nutrient pattern high in potassium, fiber, and vitamins, while another pattern high in vitamin B2, saturated fatty acids and calcium [ 22 ]. A recent study found that a nutrient pattern characterized by high intake of calcium, potassium, fats, cholesterol, vitamins B2, B12, A, D, K and C was positively linked to MetS [ 23 ]. Salehi-Sahlabadi et al. found that adhering to a nutrient pattern rich in potassium, vitamin A, fructose, vitamin C and vitamin B6 was negatively associated with the likelihood of NAFLD [ 11 ]. A nutrient pattern high in potassium, vitamin A, vitamin B6, vitamin C and fructose was associated with a reduced risk of hyperinsulinemia, IR, and dyslipidemia among participants in Tehran, according to a prospective study [ 11 , 24 , 25 ].
Due to several variations among studies exploring NPs linked to chronic diseases, including differences in the number of nutrients, populations, study designs and outcomes there has been a considerable diversity in the identified NPs, with only a few NPs being replicated across studies. Our study is the first of its kind to explore the correlation between nutrient patterns and T2D in this context.
In our study, there was no association between NPs 1 and 2 and T2D. This lack of correlation may be attributed to the absence of harmful nutrients or food categories linked to diabetes in these NPs. NP3 in this study, unlike other NPs, is positively associated with beneficial food groups such as nuts, fruits, plant oil and vegetables, and negatively associated with unhealthy food groups like red-processed meat, snacks, high-fat dairy and refined grains. A recent systematic review and meta-analysis found that individuals who consumed higher amounts of fruits and vegetables had a lower risk of developing type 2 diabetes [ 26 ]. Moreover, the consumption of vegetables was found to have an inverse relationship with ALT, TC and LDL levels among adults, while fruit consumption was associated with a positive reduction in visceral fat [ 27 , 28 ]. Another study suggested that an increased intake of vegetables and fruits could potentially lower the risk of MetS [ 29 ]. According to a study, greater nut consumption was significantly linked to a reduced prevalence of T2D [ 30 ]. Consuming fruits and vegetables is a crucial component of a healthful dietary pattern that can lower the risk of type 2 diabetes [ 31 ]. On the other hand, Consuming a Western dietary pattern, which primarily consists of fast foods, high-fat dairy, refined grains, soft drinks and processed meat has been found to be correlated with an increased risk of type 2 diabetes [ 31 ].
Several mechanisms have been identified that explain the positive associations between the components of NP 3 and T2D or its risk factors. Vitamin intake has been shown to play a role in the development of T2D through various pathways. Consuming vitamin C has been found to have beneficial effects in reducing the risk of type 2 diabetes mellitus. These effects can be attributed to the following actions of vitamin C: vasodilator, cytoprotective, platelet anti-aggregator and anti-mutagenic. To achieve this, the body increases the production of several substances including prostaglandin E1, PGI2, endothelial nitric oxide, and lipoxin A4. Additionally, the body restores the Arachidonic Acid content to normal levels [ 32 ]. Vitamin A has a multifaceted role in cell regulation beyond its antioxidant function. It contributes to gene regulation, epithelial cell integrity, and resistance to infection. Research suggests that vitamin A also enhances antioxidant enzyme function in the body. Research has indicated a link between vitamin A deficiency and type 2 diabetes mellitus (T2DM), which suggests that vitamin A may have a role in the biology of T2DM [ 33 ]. Moreover, a meta-analysis has found that replacing animal protein with plant protein can lead to minor improvements in glycemic control for individuals with diabetes [ 34 ]. According to a recent meta-analysis, increasing the consumption of fruits, especially berries, yellow vegetables, cruciferous vegetables, green leafy vegetables is associated with a lower risk of developing type 2 diabetes. These results support the recommendation to incorporate more fruits and vegetables into the diet as a way to prevent various chronic diseases, including type 2 diabetes [ 35 ]. A study showed that maintaining adequate potassium intake could regulate insulin secretion and carbohydrate metabolism, leading to the prevention of obesity and metabolic syndrome (MetS) [ 36 ].
A number of research studies conducted in the Western societies have shown that Western dietary pattern including higher intake of red meat, processed meat, and refined grains is significantly associated with increased risk of T2D [ 37 , 38 ]. For example, in the 12-years cohort prospective study, van Dam et al. investigated dietary pattern of 42,504 American white men at the age range of 40–75 years old using the FFQ. After controlling the confounders, the risk of T2D increased 60% in people adherent to the western-like dietary pattern [ 38 ]. The rapid process of change in lifestyle, diets, and physical activity that have been occurred as a result of extended urbanization, improved economic status, change of work pattern toward jobs, and change in the processes of producing and distributing nutrients during the recent years in developing countries have led people to more consumption of fast food and processed foods [ 20 ].
Significant research has been conducted on the impact of nutrient type and sequence on glucose tolerance. Multiple studies have shown that manipulating the sequence of food intake can enhance glycemic control in individuals with type 2 diabetes in real-life situations. The glucose-lowering effect of preload-based nutritional strategies has been found to be more pronounced in type 2 diabetes patients compared to healthy individuals. Moreover, consuming carbohydrates last, as part of meal patterns, has been proven to improve glucose tolerance and reduce the risk of weight gain [ 39 ]. Recent findings on meal sequence further emphasize the potential of this dietary approach in preventing and managing type 2 diabetes [ 40 ].
Several studies have shown that food from a short supply chain has a significant impact on metabolic syndrome. The length of the food supply chain is important in determining the risk of metabolic syndrome in a population [ 41 ]. Research indicates that people who consume food from short supply chains have a lower prevalence of metabolic syndrome compared to those who consume food from long supply chains. Specifically, food from short supply chains is associated with lower levels of triglycerides and glucose, which leads to a reduced occurrence of metabolic syndrome [ 42 ]. Adhering to the Mediterranean diet with a short supply chain is also found to significantly reduce the prevalence of metabolic syndrome. Therefore, these studies provide evidence that food from short supply chains positively affects metabolic parameters and the occurrence of metabolic syndrome [ 41 ].
The study we conducted presented several advantages. It was the first case-control research to investigate the correlation between nutrient patterns and the likelihood of developing type 2 diabetes (T2D). While numerous studies have explored the relationship between dietary patterns and diabetes, there is a scarcity of research specifically focusing on nutrient patterns in individuals with type 2 diabetes. Furthermore, the collection of dietary intake data was carried out through face-to-face interviews conducted by trained dieticians to minimize measurement errors. However, this study also had some limitations. Case-control studies are susceptible to selection and recall biases. Additionally, the use of factor analysis to identify patterns, and the potential influence of research decisions on the number of factors and nutrient factor loadings in each pattern, should be considered. Lastly, despite the use of a validated semi-quantitative FFQ (food frequency questionnaire), there remains a possibility of measurement error due to dietary recall. The study’s findings and limitations contribute to the ongoing discourse on the role of nutrient patterns in the development of T2D and the importance of considering these factors in future research and preventive strategies.
Conclusions
The results of this study indicate that conforming to a nutrient pattern consisting of plant protein, vitamin C, vitamin A, vitamin B2, potassium, and calcium is linked to a lower likelihood of developing T2D. Our investigation did not reveal any significant correlation between other nutrient patterns and T2D risk. However, additional research is necessary to authenticate these initial findings and establish the correlation between nutrient patterns and T2D.
Data availability
Upon reasonable request, the corresponding author can provide the datasets that were produced and analyzed during the current study.
Ogurtsova K, Guariguata L, Barengo NC, Ruiz PL-D, Sacre JW, Karuranga S, et al. IDF Diabetes Atlas: global estimates of undiagnosed diabetes in adults for 2021. Diabetes Res Clin Pract. 2022;183:109118.
Article PubMed Google Scholar
Teo ZL, Tham Y-C, Yu M, Chee ML, Rim TH, Cheung N, et al. Global prevalence of diabetic retinopathy and projection of burden through 2045: systematic review and meta-analysis. Ophthalmology. 2021;128(11):1580–91.
Sugiyama T, Yanagisawa-Sugita A, Tanaka H, Ihana-Sugiyama N, Imai K, Ohsugi M, et al. Different incidences of diabetic retinopathy requiring treatment since diagnosis according to the course of diabetes diagnosis: a retrospective cohort study. Sci Rep. 2023;13(1):10527.
Article CAS PubMed PubMed Central Google Scholar
Hodge AM, English DR, O’Dea K, Giles GG. Dietary patterns and diabetes incidence in the Melbourne Collaborative Cohort Study. Am J Epidemiol. 2007;165(6):603–10.
Jannasch F, Kröger J, Schulze MB. Dietary patterns and type 2 diabetes: a systematic literature review and meta-analysis of prospective studies. J Nutr. 2017;147(6):1174–82.
Article CAS PubMed Google Scholar
Sami W, Ansari T, Butt NS, Ab Hamid MR. Effect of diet on type 2 diabetes mellitus: a review. Int J Health Sci. 2017;11(2):65.
Google Scholar
Miller V, Micha R, Choi E, Karageorgou D, Webb P, Mozaffarian D. Evaluation of the quality of evidence of the association of foods and nutrients with cardiovascular disease and diabetes: a systematic review. JAMA Netw open. 2022;5(2):e2146705–e.
Article PubMed PubMed Central Google Scholar
Salehi-Sahlabadi A, Teymoori F, Jabbari M, Momeni A, Mokari-Yamchi A, Sohouli M, et al. Dietary polyphenols and the odds of non-alcoholic fatty liver disease: a case-control study. Clin Nutr ESPEN. 2021;41:429–35.
Salehi-Abargouei A, Esmaillzadeh A, Azadbakht L, Keshteli AH, Feizi A, Feinle-Bisset C, et al. Nutrient patterns and their relation to general and abdominal obesity in Iranian adults: findings from the SEPAHAN study. Eur J Nutr. 2016;55:505–18.
Panjeshahin A, Salehi-Abargouei A, Ghadiri-Anari A, Rasouli A, Hosseinzadeh M. The Association between nutrient patterns and polycystic ovary syndrome: a case-control study. J Nutr Food Secur. 2022.
Salehi-Sahlabadi A, Teymoori F, Ahmadirad H, Mokhtari E, Azadi M, Seraj SS, et al. Nutrient patterns and non-alcoholic fatty liver disease in Iranian Adul: a case-control study. Front Nutr. 2022;9:977403.
Fereidani SS, Eini-Zinab H, Heidari Z, Jalali S, Sedaghat F, Rashidkhani B. Nutrient patterns and risk of breast cancer among Iranian women: a case-control study. Asian Pac J cancer Prevention: APJCP. 2018;19(9):2619.
CAS Google Scholar
Narmcheshm S, Sasanfar B, Hadji M, Zendehdel K, Toorang F, Azadbakht L. Patterns of nutrient intake in relation to gastric cancer: a case control study. Nutr Cancer. 2022;74(3):830–9.
Khayyatzadeh SS, Moohebati M, Mazidi M, Avan A, Tayefi M, Parizadeh SMR, et al. Nutrient patterns and their relationship to metabolic syndrome in Iranian adults. Eur J Clin Invest. 2016;46(10):840–52.
Teymoori F, Mokhtari E, Salehi P, Hosseini-Esfahani F, Mirmiran P, Azizi F. A nutrient pattern characterized by vitamin A, C, B6, potassium, and fructose is associated with reduced risk of insulin-related disorders: a prospective study among participants of Tehran lipid and glucose study. Diabetol Metab Syndr. 2021;13(1):12.
Esmaillzadeh A, Azadbakht L. Major dietary patterns in relation to general obesity and central adiposity among Iranian women. J Nutr. 2008;138(2):358–63.
Wang J, Thornton JC, Bari S, Williamson B, Gallagher D, Heymsfield SB, et al. Comparisons of waist circumferences measured at 4 sites. Am J Clin Nutr. 2003;77(2):379–84.
Committee IR. Guidelines for data processing and analysis of the International Physical Activity Questionnaire (IPAQ)-short and long forms. http://www.ipaq.ki.se/scoring.pdf ; 2005.
Mandalazi E, Drake I, Wirfält E, Orho-Melander M, Sonestedt E. A high diet quality based on dietary recommendations is not associated with lower incidence of type 2 diabetes in the Malmö Diet and Cancer Cohort. Int J Mol Sci. 2016;17(6):901.
Beigrezaei S, Ghiasvand R, Feizi A, Iraj B. Relationship between dietary patterns and incidence of type 2 diabetes. Int J Prev Med. 2019;10:122.
Chikowore T, Pisa PT, Van Zyl T, Feskens EJ, Wentzel-Viljoen E, Conradie KR. Nutrient patterns associated with fasting glucose and glycated haemoglobin levels in a black South African population. Nutrients. 2017;9(1):9.
Iwasaki Y, Arisawa K, Katsuura-Kamano S, Uemura H, Tsukamoto M, Kadomatsu Y, et al. Associations of nutrient patterns with the prevalence of metabolic syndrome: results from the baseline data of the Japan multi-institutional collaborative cohort study. Nutrients. 2019;11(5):990.
Sadeghi O, Sadeghi A, Mozaffari-Khosravi H, Shokri A. The association between nutrient patterns and metabolic syndrome among Iranian adults: cross-sectional analysis of Shahedieh cohort study. Public Health Nutr. 2021;24(11):3379–88.
Mottaghian M, Salehi P, Teymoori F, Mirmiran P, Hosseini-Esfahani F, Azizi F. Nutrient patterns and cardiometabolic risk factors among Iranian adults: Tehran lipid and glucose study. BMC Public Health. 2020;20:1–12.
Article Google Scholar
Teymoori F, Mokhtari E, Salehi P, Hosseini-Esfahani F, Mirmiran P, Azizi F. A nutrient pattern characterized by vitamin A, C, B6, potassium, and fructose is associated with reduced risk of insulin-related disorders: a prospective study among participants of Tehran lipid and glucose study. Diabetol Metab Syndr. 2021;13(1):1–13.
Halvorsen RE, Elvestad M, Molin M, Aune D. Fruit and vegetable consumption and the risk of type 2 diabetes: a systematic review and dose–response meta-analysis of prospective studies. BMJ Nutr Prev Health. 2021;4(2):519.
Mollahosseini M, Daneshzad E, Rahimi MH, Yekaninejad MS, Maghbooli Z, Mirzaei K. The association between fruit and vegetable intake and liver enzymes (aspartate and alanine transaminases) in Tehran, Iran. Ethiop J Health Sci. 2017;27(4):401–10.
Plaz Torres MC, Bodini G, Furnari M, Marabotto E, Zentilin P, Giannini EG. Nuts and non-alcoholic fatty liver disease: are nuts safe for patients with fatty liver disease? Nutrients. 2020;12(11):3363.
Lee M, Lim M, Kim J. Fruit and vegetable consumption and the metabolic syndrome: a systematic review and dose–response meta-analysis. Br J Nutr. 2019;122(7):723–33.
Muley A, Fernandez R, Ellwood L, Muley P, Shah M. Effect of tree nuts on glycemic outcomes in adults with type 2 diabetes mellitus: a systematic review. JBI Evid Synthesis. 2021;19(5):966–1002.
Beigrezaei S, Ghiasvand R, Feizi A, Iraj B. Relationship between dietary patterns and incidence of type 2 diabetes. Int J Prev Med. 2019;10.
Das UN. Vitamin C for type 2 diabetes mellitus and hypertension. Arch Med Res. 2019;50(2):11–4.
Iqbal S, Naseem I. Role of vitamin A in type 2 diabetes mellitus biology: effects of intervention therapy in a deficient state. Nutrition. 2015;31(7–8):901–7.
Viguiliouk E, Stewart SE, Jayalath VH, Ng AP, Mirrahimi A, De Souza RJ, et al. Effect of replacing animal protein with plant protein on glycemic control in diabetes: a systematic review and meta-analysis of randomized controlled trials. Nutrients. 2015;7(12):9804–24.
Wang PY, Fang JC, Gao ZH, Zhang C, Xie SY. Higher intake of fruits, vegetables or their fiber reduces the risk of type 2 diabetes: a meta-analysis. J Diabetes Invest. 2016;7(1):56–69.
Cai X, Li X, Fan W, Yu W, Wang S, Li Z, et al. Potassium and obesity/metabolic syndrome: a systematic review and meta-analysis of the epidemiological evidence. Nutrients. 2016;8(4):183.
van Dam RM, Rimm EB, Willett WC, Stampfer MJ, Hu FB. Dietary patterns and risk for type 2 diabetes mellitus in US men. Ann Intern Med. 2002;136(3):201–9.
Fung TT, Schulze M, Manson JE, Willett WC, Hu FB. Dietary patterns, meat intake, and the risk of type 2 diabetes in women. Arch Intern Med. 2004;164(20):2235–40.
Wheeler ML, Dunbar SA, Jaacks LM, Karmally W, Mayer-Davis EJ, Wylie-Rosett J, et al. Macronutrients, Food groups, and eating patterns in the management of diabetes: a systematic review of the literature, 2010. Diabetes Care. 2012;35(2):434–45.
Dwibedi C, Mellergård E, Gyllensten AC, Nilsson K, Axelsson AS, Bäckman M, et al. Effect of self-managed lifestyle treatment on glycemic control in patients with type 2 diabetes. Npj Digit Med. 2022;5(1):60.
Santulli G, Pascale V, Finelli R, Visco V, Giannotti R, Massari A, et al. We are what we eat: impact of food from short supply chain on metabolic syndrome. J Clin Med. 2019;8(12):2061.
De Rosa M, Giannotti R, Pascale A, Finelli R, Ilario M, Ciccarelli M, et al. P6280 food of short supply chain impacts metabolism and cardiovascular risk. A survey in Southern Italy. Eur Heart J. 2018;39(suppl1):ehy566. P6280.
Download references
Acknowledgements
The researchers express their gratitude towards all the individuals who volunteered to take part in the study.
This research received no external funding.
Author information
Authors and affiliations.
Faculty of medicine, Tabriz University of medical sciences, Tabriz, Iran
Morteza haramshahi
Department of clinical biochemistry, College of medicine, King Khalid University, Abha, Saudi Arabia
Thoraya Mohamed Elhassan A-Elgadir
Fharmacy Department, Duhok polytechnic, University Duhok, Kurdistan, Iraq
Hamid Mahmood Abdullah Daabo
Department of Medical Services and Techniques, Ardahan University, Ardahan, Turkey
Yahya Altinkaynak
Department of Medical Laboratory Sciences, College of Applied Medical Sciences, Prince Sattam bin Abdulaziz University, Jeddah, Saudi Arabia
Ahmed Hjazi
Department of Management, Uttaranchal Institute of Management, Uttaranchal University, Dehradun, Uttarakhand, India
Archana Saxena
Pharmaceutical Chemistry Department, College of Pharmacy, Al-Ayen University, Thi-Qar, Iraq
Mazin A.A. Najm
College of technical engineering, The Islamic University, Najaf, Iraq
Abbas F. Almulla
College of technical engineering, Imam Ja’afar Al-Sadiq University, Al‐Muthanna, 66002, Iraq
Ali Alsaalamy
Department of Medicinal Chemistry, Faculty of Pharmacy, Tehran Medical Sciences, Islamic Azad University, Tehran, Iran
Mohammad Amin Kashani
You can also search for this author in PubMed Google Scholar
Contributions
The study’s protocol was designed by M.K., M.H., and T.E., while H.A., Y.A., and A.H. carried out the research. A.S. analyzed the data and prepared the initial draft of the manuscript. M.N., A.FA., and A.A. interpreted the data and provided critical feedback on the manuscript. All authors reviewed and approved the final version of the manuscript.
Corresponding author
Correspondence to Mohammad Amin Kashani .
Ethics declarations
Ethics approval and consent to participate.
This study was performed in line with the principles of the Declaration of Helsinki. Informed consent was obtained from all participants or their legal guardians. Approval was granted by the Research Ethics Committee of Islamic Azad University of Medical Sciences (Approval number: IR.AUI.MEDICINE. REC.1401.147).
Consent for publication
Not applicable.
Competing interests
The authors declared no conflicts of interest.
Additional information
Publisher’s note.
Springer Nature remains neutral with regard to jurisdictional claims in published maps and institutional affiliations.
Rights and permissions
Open Access This article is licensed under a Creative Commons Attribution 4.0 International License, which permits use, sharing, adaptation, distribution and reproduction in any medium or format, as long as you give appropriate credit to the original author(s) and the source, provide a link to the Creative Commons licence, and indicate if changes were made. The images or other third party material in this article are included in the article’s Creative Commons licence, unless indicated otherwise in a credit line to the material. If material is not included in the article’s Creative Commons licence and your intended use is not permitted by statutory regulation or exceeds the permitted use, you will need to obtain permission directly from the copyright holder. To view a copy of this licence, visit http://creativecommons.org/licenses/by/4.0/ . The Creative Commons Public Domain Dedication waiver ( http://creativecommons.org/publicdomain/zero/1.0/ ) applies to the data made available in this article, unless otherwise stated in a credit line to the data.
Reprints and permissions
About this article
Cite this article.
haramshahi, M., A-Elgadir, T.M.E., Daabo, H.M.A. et al. Nutrient patterns and risk of diabetes mellitus type 2: a case-control study. BMC Endocr Disord 24 , 10 (2024). https://doi.org/10.1186/s12902-024-01540-5
Download citation
Received : 04 November 2023
Accepted : 09 January 2024
Published : 17 January 2024
DOI : https://doi.org/10.1186/s12902-024-01540-5
Share this article
Anyone you share the following link with will be able to read this content:
Sorry, a shareable link is not currently available for this article.
Provided by the Springer Nature SharedIt content-sharing initiative
- Nutrient pattern
BMC Endocrine Disorders
ISSN: 1472-6823
- General enquiries: [email protected]
- Diabetes & Primary Care
- Vol:23 | No:02
Interactive case study: Making a diagnosis of type 2 diabetes
- 12 Apr 2021
Share this article + Add to reading list – Remove from reading list ↓ Download pdf
Diabetes & Primary Care ’s series of interactive case studies is aimed at GPs, practice nurses and other professionals in primary and community care who would like to broaden their understanding of type 2 diabetes.
The three mini-case studies presented with this issue of the journal take you through what to consider in making an accurate diagnosis of type 2 diabetes.
The format uses typical clinical scenarios as tools for learning. Information is provided in short sections, with most ending in a question to answer before moving on to the next section.
Working through the case studies will improve your knowledge and problem-solving skills in type 2 diabetes by encouraging you to make evidence-based decisions in the context of individual cases.
Crucially, you are invited to respond to the questions by typing in your answers. In this way, you are actively involved in the learning process, which is a much more effective way to learn.
By actively engaging with these case histories, I hope you will feel more confident and empowered to manage such presentations effectively in the future.
Colin is a 51-year-old construction worker. A recent blood test shows an HbA 1c of 67 mmol/mol. Is this result enough to make a diagnosis of diabetes?
Rao, a 42-year-old accountant of Asian origin, is currently asymptomatic but has a strong family history of type 2 diabetes. Tests have revealed a fasting plasma glucose level of 6.7 mmol/L and an HbA 1c of 52 mmol/mol. How would you interpret these results?
43-year-old Rachael has complained of fatigue. She has a BMI of 28.4 kg/m 2 and her mother has type 2 diabetes. Rachael’s HbA 1c is 46 mmol/mol. How would you interpret her HbA 1c measurement?
By working through these interactive cases, you will consider the following issues and more:
- The criteria for the correct diagnosis of diabetes and non-diabetic hyperglycaemia.
- Which tests to use in different circumstances to determine a diagnosis.
- How to avoid making errors in classification of the type of diabetes being diagnosed.
- The appropriate steps to take following diagnosis.
How to diagnose and treat hypertension in adults with type 2 diabetes
Diabetes distilled: statin heart benefits outweigh diabetes risks, interactive case study: non-diabetic hyperglycaemia – prediabetes, diabetes distilled: smoking cessation cuts excess mortality rates after as little as 3 years, impact of freestyle libre 2 on diabetes distress and glycaemic control in people on twice-daily pre-mixed insulin, updated guidance from the pcds and abcd: managing the national glp-1 ra shortage, diabetes distilled: fib-4 – a diagnostic and prognostic marker for liver and cardiovascular events and mortality.
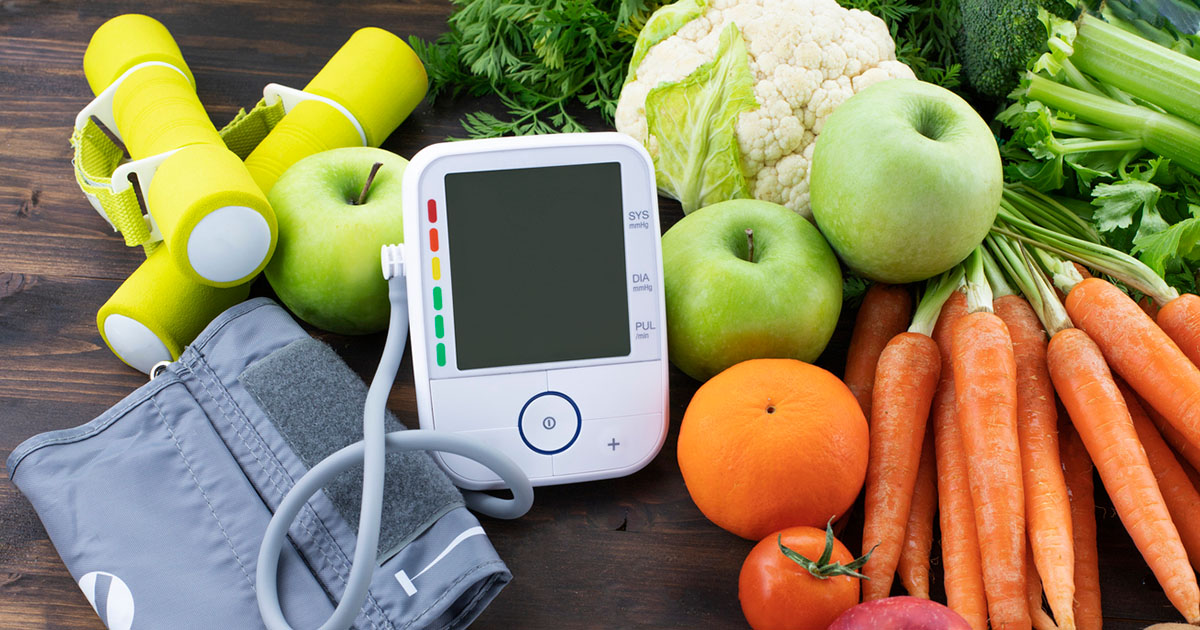
Diagnosing and treating hypertension in accordance with updated NICE guidelines.
24 Apr 2024
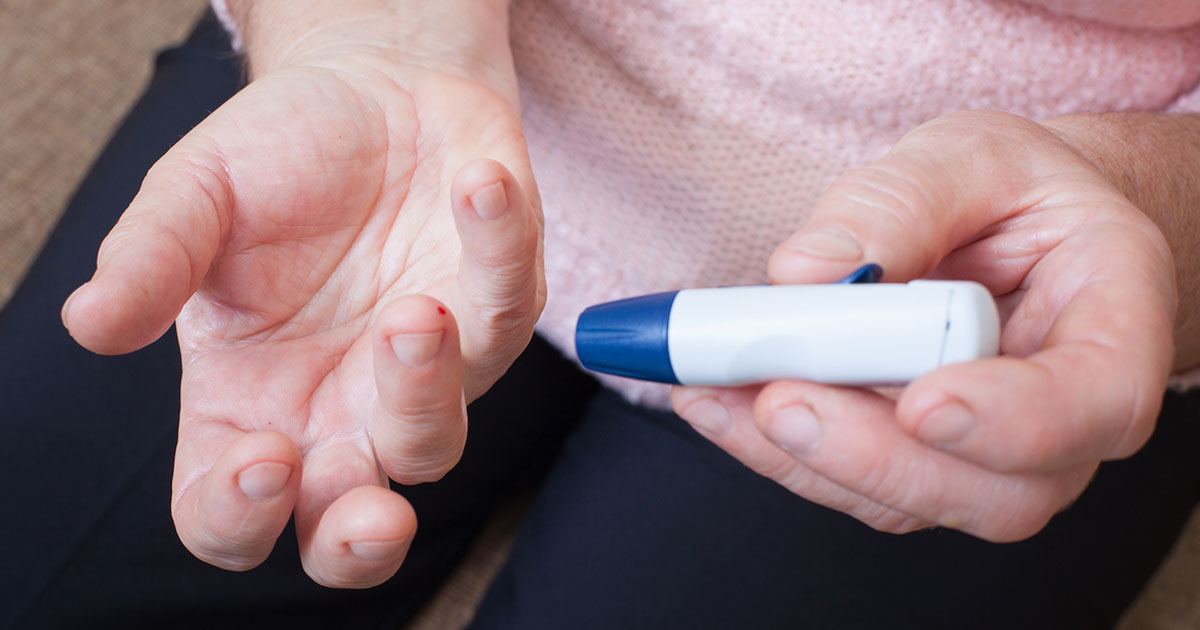
Quantifying the risk of worsening glycaemia, and how should healthcare professionals respond?
22 Apr 2024
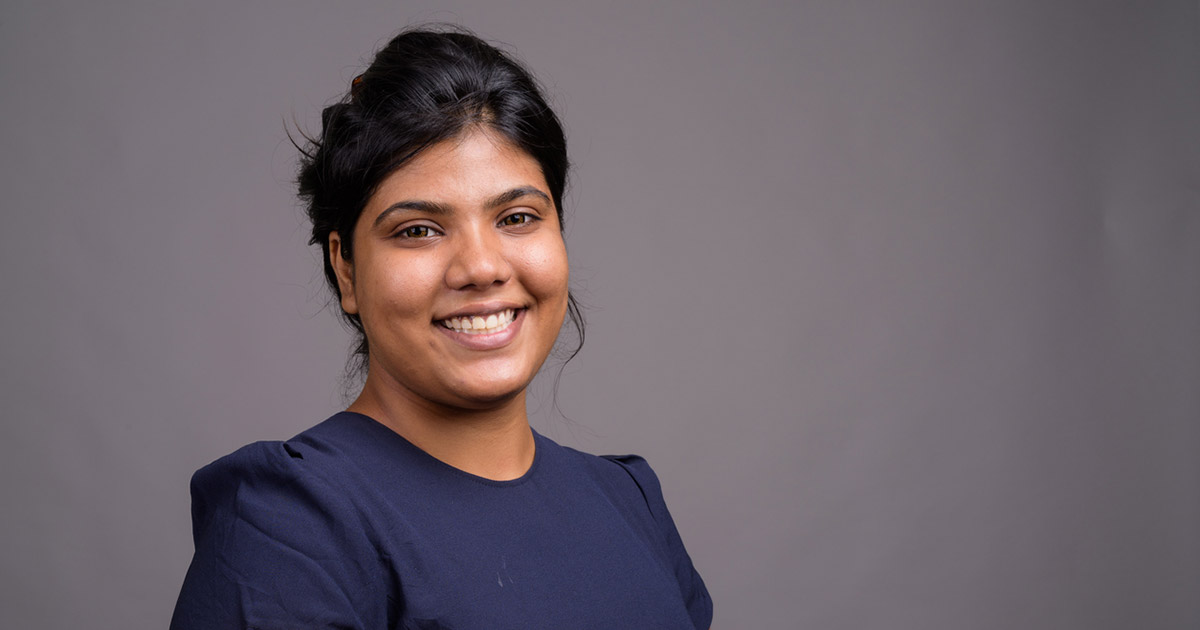
Diagnosing and managing non-diabetic hyperglycaemia.
17 Apr 2024
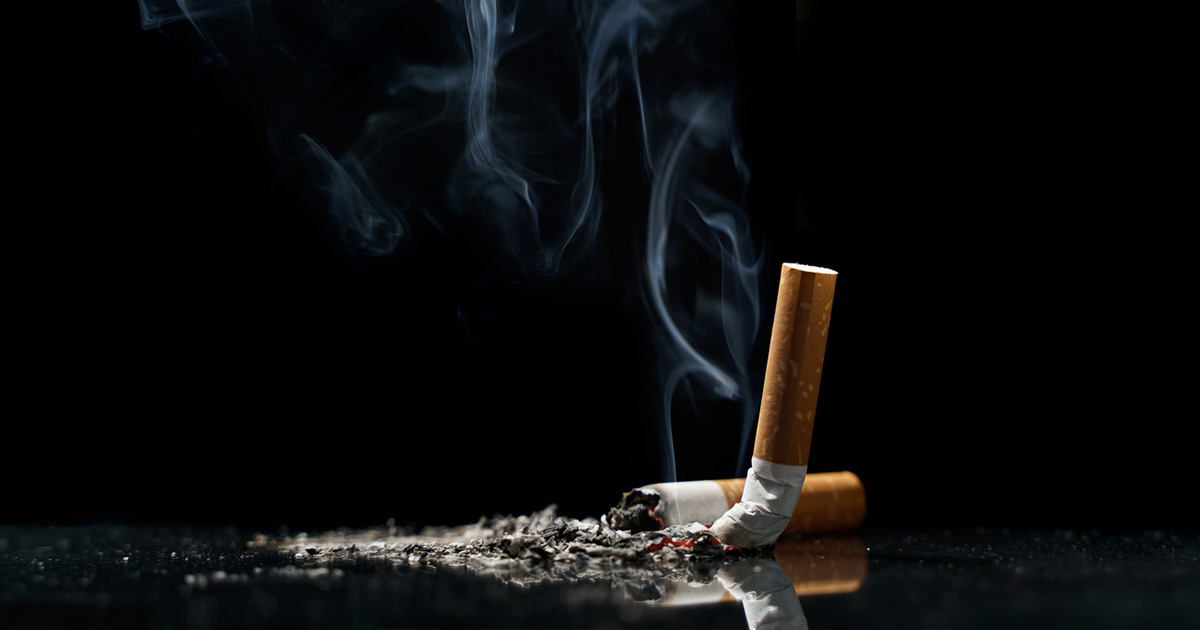
The mortality benefits of smoking cessation may be greater and accrue more rapidly than previously understood.
Sign up to all DiabetesontheNet journals
- CPD Learning
- Journal of Diabetes Nursing
- Diabetes Care for Children & Young People
- The Diabetic Foot Journal
- Diabetes Digest
Useful information
- Terms and conditions
- Privacy policy
- Editorial policies and ethics
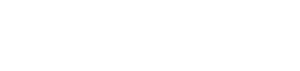
By clicking ‘Subscribe’, you are agreeing that DiabetesontheNet.com are able to email you periodic newsletters. You may unsubscribe from these at any time. Your info is safe with us and we will never sell or trade your details. For information please review our Privacy Policy .
Are you a healthcare professional? This website is for healthcare professionals only. To continue, please confirm that you are a healthcare professional below.
We use cookies responsibly to ensure that we give you the best experience on our website. If you continue without changing your browser settings, we’ll assume that you are happy to receive all cookies on this website. Read about how we use cookies .
Type 2 diabetes: a case study
Affiliation.
- 1 Queen's University Belfast, Belfast, Northern Ireland.
- PMID: 25270482
- DOI: 10.7748/ns.29.5.37.e9142
Increased prevalence of diabetes in the community has been accompanied by an increase in diabetes in hospitalised patients. About a quarter of these patients experience a hypoglycaemic episode during their admission, which is associated with increased risk of mortality and length of stay. This article examines the aetiology, pathophysiology, diagnosis and treatment of type 2 diabetes using a case study approach. The psychosocial implications for the patient are also discussed. The case study is based on a patient with diabetes who was admitted to hospital following a hypoglycaemic episode and cared for during a practice placement. The importance of early diagnosis of diabetes and the adverse effects of delayed diagnosis are discussed.
Keywords: Blood glucose; case study; diabetes; glucose testing; hyperglycaemia; hypoglycaemia; insulin resistance; sulfonylureas; type 2 diabetes.
Publication types
- Case Reports
- Anxiety / complications
- Depression / complications
- Diabetes Mellitus, Type 2 / diagnosis*
- Diabetes Mellitus, Type 2 / physiopathology
- Diabetes Mellitus, Type 2 / therapy
- Hypoglycemic Agents / therapeutic use
- Middle Aged
- Hypoglycemic Agents
Advertisement
Sarcopenia in patients with type 2 diabetes mellitus: a case–control study in Maracaibo city, Venezuela
- Original Article
- Published: 27 August 2021
- Volume 42 , pages 203–210, ( 2022 )
Cite this article
- Onofre Pineda 1 ,
- Victoria Stepenka 1 ,
- Alejandra Rivas-Motenegro 2 ,
- Nelson Villasmil-Hernandez 3 ,
- Roberto Añez 2 &
- Juan Salazar ORCID: orcid.org/0000-0003-4211-528X 1 , 3
277 Accesses
Explore all metrics
Background/purpose of the study
Several studies implicate skeletal muscle as a physio-pathological target among the metabolic disturbances of diabetes, or with the mechanisms shared by sarcopenia and type 2 diabetes mellitus (T2DM). The present study aimed to determine the relationship between sarcopenia and T2DM in outpatients of the Zulian Diabetes Institute, Maracaibo, Venezuela.
A case–control study was performed on patients diagnosed with T2DM that went to the Zulian Diabetes Institute between January and June 2019. After obtaining a final sample of 131 patients with T2DM, control subjects were selected in a 1:1 ratio according to sex. The European Working Group on Sarcopenia in Older People (EWGSOP) criteria were followed to diagnose sarcopenia, so muscle mass, muscle strength, and muscle performance were determined.
A total of 261 patients were evaluated (131 with T2DM and 131 controls), with no differences in mean age (with T2DM 63.6 ± 9.3 vs. controls 63.6 ± 9.4 years old; p = 0.99). The prevalence of sarcopenia in control subjects was 9.9% and in subjects with diabetes 25.2%; p < 0.01; decreased muscle strength was the component with the most significant difference within the two groups (controls 16.8% vs. T2DM 49.6%; p < 0.01). In the multivariate analysis, subjects with worse metabolic control had a greater risk of sarcopenia (OR, 3.31; 95%CI, 1.10–9.97; p = 0.03).
The prevalence of sarcopenia was higher in subjects with T2DM, especially in those with worse metabolic control and normal weight status.
This is a preview of subscription content, log in via an institution to check access.
Access this article
Price includes VAT (Russian Federation)
Instant access to the full article PDF.
Rent this article via DeepDyve
Institutional subscriptions
Similar content being viewed by others
Sarcopenia: a chronic complication of type 2 diabetes mellitus.
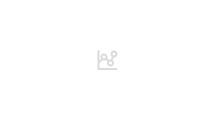
Type 2 diabetes mellitus is associated with increased risks of sarcopenia and pre-sarcopenia in Chinese elderly
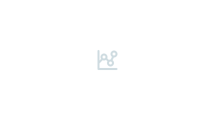
Sarcopenia in elderly patients with type 2 diabetes mellitus: prevalence and related clinical factors
La vejez HA. ¿Un paradigma de enfermedad?”. Revista Hospital Clínico de la Universidad de Chile. Rev Hosp Clín Univ Chile. 2000;11(1):5–8.
Rush EC, Freitas I, Plank LD. Body size, body composition and fat distribution: comparative analysis of European, Maori, Pacific Island and Asian Indian adults. Br J Nutr. 2009;102:632–41.
Article CAS Google Scholar
Campos AC, Ferreira EF, Vargas AM, Gonçalves LH. Healthy aging profile in octogenarians in Brazil. Rev Latino-Am Enfermagem. 2016;24:e2724.
Article Google Scholar
Kyle UG, Genton L, Hans D, et al. Age-related differences in fat-free mass, skeletal muscle, body cell mass and fat mass between 18 and 94 years. Eur J Clin Nutr. 2001;55:663–72.
Guo SS, Zeller C, Chumlea WC, Siervogel RM. Aging, body composition, and lifestyle: the Fels Longitudinal Study. Am J Clin Nutr. 1999;70:405–11.
Delmonico MJ, Harris TB, Lee JS, et al. Alternative definitions of sarcopenia, lower extremity performance, and functional impairment with aging in older men and women. J Am Geriatr Soc. 2007;55:769–74.
Shock NW. Physiologic aspects of aging. J Am Diet Assoc. 1970;56:491–496.
Rosenberg IH. Sarcopenia: origins and clinical relevance. J Nutr. 1997;127:990S–991S.
Khamseh ME, Malek M, Aghili R, Emami Z. Sarcopenia and diabetes: pathogenesis and consequences. Br J Diabetes Vasc Dis. 2011;11:230–4.
Vellas B, Villars H, Abellan G, Soto ME, Rolland Y, Guigoz Y, Morley JE, Chumlea W, Salva A, Rubenstein LZ, Garry P. Overview of the MNA–Its history and challenges. J Nutr Health Aging. 2006;10(6):456–63.
CAS PubMed Google Scholar
Cruz-Jentoft AJ, Baeyens JP, Bauer JM, et al. Sarcopenia: European consensus on definition and diagnosis: report of the European Working Group on Sarcopenia in Older People. Age Ageing. 2010;39(4):412–23.
Masanés F, Culla A, Navarro-González M, Navarro-López M, Sacanella E, Torres B, et al. Prevalence of sarcopenia in healthy community-dwelling elderly in an urban area of Barcelona (Spain). J Nutr Health Aging. 2012;16:184–7.
American Diabetes Association. Standards of Medical Care in Diabetes 2018. Diabetes Care. 2018;41(Suppl. 1):S55–64.
Landi F, Onder G, Bernabei R. Sarcopenia and diabetes: two sides of the same coin. J Am Med Dir Assoc. 2013;14:540–1.
Gao L, Jiang J, Yang M, Hao Q, Luo L, Dong B. Prevalence of sarcopenia and associated factors in Chinese community-dwelling elderly: comparison between rural and urban areas. J Am Med Dir Assoc. 2015;16(1003):e1001-1006.
Google Scholar
Trierweiler H, Kisielewicz G, Hoffman T, Rasmussen R, Aguiar C, Cochenski V. Sarcopenia: a chronic complication of type 2 diabetes mellitus. Diabetol Metab Syndr. 2018;10:25.
Wang T, Feng X, Zhou J, Gong H, Xia S, Wei Q, et al. Type 2 diabetes mellitus is associated with increased risks of sarcopenia and pre-sarcopenia in Chinese elderly. Sci Rep. 2016;6:38937.
Kim TN, Park MS, Yang SJ, Yoo H, Kang H, Song W, et al. Prevalence and determinant factors of sarcopenia in patients with type 2 diabetes: the Korean Sarcopenic Obesity Study (KSOS). Diabetes Care. 2010;33:1497–9.
Vergara J, Muñoz M, Alcalá I, Sanz M. Sarcopenia y dinapenia en pacientes con diabetes mellitus tipo 2 en un área rural de Castilla-La Mancha. Rev Clín Med Fam. 2017;10(2):86–95.
Dejo C, Palacios M. Asociación entre Sarcopenia y Diabetes Mellitus tipo 2 en adultos mayores. Tesis para optar al título de Licenciada en nutrición y dietética. Lima, 2015.
Park SW, Goodpaster BH, Lee JS, Kuller LH, Boudreau R, Rekeneire N. Excessive loss of skeletal mass in older adults with type 2 diabetes mellitus. Diabetes Care. 2009;32:1993–7.
Kalyani RR, Metter EJ, Egan J, Golden S, Ferrucci L. Hyperglycemia predicts persistently lower muscle strength with aging. Diabetes Care. 2015;38:82–90.
Akpinar TS, Tayfur M, Tufan F, et al. Uncomplicated diabetes does not accelerate age-related Sarcopenia. Aging Male. 2014;17(4):205–10.
He Q, Wang X, Yang C, et al. Metabolic and nutritional characteristics in middle-aged and elderly sarcopenia patients with type 2 diabetes. J Diabetes Res. 2020;2020:6973469.
Lin Y, Zhang Y, Shen X, Huang L, Yan S. Influence of glucose, insulin fluctuation, and glycosylated hemoglobin on the outcome of sarcopenia in patients with type 2 diabetes mellitus. J Diabetes Complications. 2021;35(6):107926.
Izzo A, Massimino E, Riccardi G, Della PG. A narrative review on sarcopenia in type 2 diabetes mellitus: prevalence and associated factors. Nutrients. 2021;13(1):183.
Velázquez-Alva MC, Irigoyen-Camacho ME, Zepeda-Zepeda MA, Lazarevich I, Arrieta-Cruz I, D’Hyver C. Sarcopenia, nutritional status and type 2 diabetes mellitus: a cross-sectional study in a group of Mexican women residing in a nursing home. Nutr Diet. 2020;77(5):515–22.
Download references
Author information
Authors and affiliations.
Instituto Zuliano de Diabetes, Programa de Especialización en Medicina Interna, Hospital General del Sur “Dr. Pedro Iturbe”, Maracaibo, Venezuela
Onofre Pineda, Victoria Stepenka & Juan Salazar
Department of Endocrinology and Nutrition, Gregorio Marañón General University Hospital, Madrid, Spain
Alejandra Rivas-Motenegro & Roberto Añez
Endocrine and Metabolic Diseases Research Center, School of Medicine, University of Zulia, Maracaibo, 4004, Venezuela
Nelson Villasmil-Hernandez & Juan Salazar
You can also search for this author in PubMed Google Scholar
Corresponding author
Correspondence to Juan Salazar .
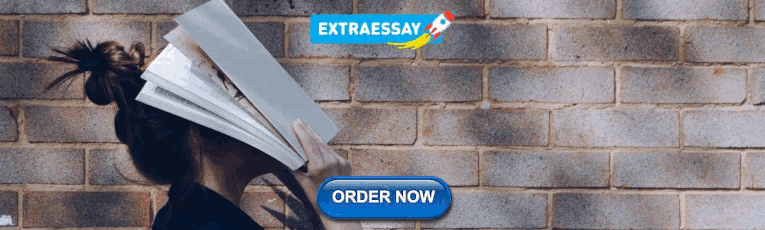
Ethics declarations
Informed consent.
Each patient selected to be included in the study received a comprehensive explanation of the study, and their authorization was requested via a written informed consent before they participated in the study.
Additional information
Publisher’s note.
Springer Nature remains neutral with regard to jurisdictional claims in published maps and institutional affiliations.
Rights and permissions
Reprints and permissions
About this article
Pineda, O., Stepenka, V., Rivas-Motenegro, A. et al. Sarcopenia in patients with type 2 diabetes mellitus: a case–control study in Maracaibo city, Venezuela. Int J Diabetes Dev Ctries 42 , 203–210 (2022). https://doi.org/10.1007/s13410-021-00989-7
Download citation
Received : 15 February 2021
Accepted : 22 July 2021
Published : 27 August 2021
Issue Date : April 2022
DOI : https://doi.org/10.1007/s13410-021-00989-7
Share this article
Anyone you share the following link with will be able to read this content:
Sorry, a shareable link is not currently available for this article.
Provided by the Springer Nature SharedIt content-sharing initiative
- Type 2 diabetes mellitus
- Muscle function
- Glycemic control
- Find a journal
- Publish with us
- Track your research
Ohio State nav bar
The Ohio State University
- BuckeyeLink
- Find People
- Search Ohio State
Diabetes Type 2
- Adrienne Franklin
- Sarah Garee
- Kristina Hughes
- Mitchell McManis
Our rationale for choosing this condition
Type II Diabetes affects multiple body systems and has increased in prevalence over the past decade.
- Open access
- Published: 23 April 2024
Diabetes and osteoporosis: a two-sample mendelian randomization study
- Yu-Dun Qu 1 ,
- Zhao-Hua Zhu 2 ,
- Jia-Xuan Li 3 ,
- Wei Zhang 4 ,
- Qi Chen 4 ,
- Chang-Liang Xia 4 ,
- Jun-Nan Ma 4 ,
- Shuan-Ji Ou 4 ,
- Yang Yang 4 ,
- Yong Qi 4 &
- Chang-Peng Xu 3
BMC Musculoskeletal Disorders volume 25 , Article number: 317 ( 2024 ) Cite this article
171 Accesses
Metrics details
The effects on bone mineral density (BMD)/fracture between type 1 (T1D) and type 2 (T2D) diabetes are unknown. Therefore, we aimed to investigate the causal relationship between the two types of diabetes and BMD/fracture using a Mendelian randomization (MR) design.
A two-sample MR study was conducted to examine the causal relationship between diabetes and BMD/fracture, with three phenotypes (T1D, T2D, and glycosylated hemoglobin [HbA1c]) of diabetes as exposures and five phenotypes (femoral neck BMD [FN-BMD], lumbar spine BMD [LS-BMD], heel-BMD, total body BMD [TB-BMD], and fracture) as outcomes, combining MR-Egger, weighted median, simple mode, and inverse variance weighted (IVW) sensitivity assessments. Additionally, horizontal pleiotropy was evaluated and corrected using the residual sum and outlier approaches.
The IVW method showed that genetically predicted T1D was negatively associated with TB-BMD ( β = -0.018, 95% CI: -0.030, -0.006), while T2D was positively associated with FN-BMD ( β = 0.033, 95% CI: 0.003, 0.062), heel-BMD ( β = 0.018, 95% CI: 0.006, 0.031), and TB-BMD ( β = 0.050, 95% CI: 0.022, 0.079). Further, HbA1c was not associated with the five outcomes ( β ranged from − 0.012 to 0.075).
Conclusions
Our results showed that T1D and T2D have different effects on BMD at the genetic level. BMD decreased in patients with T1D and increased in those with T2D. These findings highlight the complex interplay between diabetes and bone health, suggesting potential age-specific effects and genetic influences. To better understand the mechanisms of bone metabolism in patients with diabetes, further longitudinal studies are required to explain BMD changes in different types of diabetes.
Peer Review reports
Diabetes mellitus, a prevalent noncommunicable chronic disease [International Diabetes Federation (IDF), Available at: http://www.diabetesatlas.org ], poses a global public health challenge and is associated with severe disability and mortality [ 1 ]. In 2019, its worldwide prevalence was approximately 9.3%, rising to 9.6% in the Western Pacific, and is projected to affect 693 million individuals by 2045, a 50% increase from 2017 [ 2 ]. The two main forms of diabetes are type 1 (T1D) and type 2 (T2D). T1D is caused by the immune system’s elimination of pancreatic beta cells [ 3 ], which results in a dramatic decrease in blood insulin levels. Contrarily, T2D is more prevalent among adults and older adults and constitutes roughly 90% of all cases of diabetes [ 4 ]. Morbidity and mortality in diabetes mainly stem from complications of the macrovascular (cardiovascular disease) and microvascular (diabetic kidney disease), retinopathy, and neuropathy systems [ 5 ].
Osteoporosis (OP) is characterized by low bone mass and altered bone architecture, leading to compromised bone strength and an increased risk of fracture [ 6 ]. Often termed a “silent disease,” OP frequently manifests no signs until the occurrence of the first fracture [ 7 ]. Clinically, OP is diagnosed by measuring bone mineral density (BMD), with a T score of -2.5 as the cutoff [ 8 ], which remains the strongest predictor of fracture risk. Globally, an osteoporotic fracture occurs every 3 s, resulting in over 8.9 million fractures annually [ 9 ]. The disease inflicts considerable emotional, physical, and financial burden on patients, often leading to disability, diminished quality of life, and mortality [ 10 ]. Furthermore, the prevalence of both diabetes and OP is on the rise due to population aging and increased life expectancy among patients with diabetes [ 11 ].
Over 60 years ago, Albright and Reifenstein proposed a potential link between diabetes mellitus and OP, suggesting that diabetes might contribute to bone mass loss leading to OP [ 12 ]. This topic has since garnered significant attention and investigation [ 13 ]. A 2019 observational study involving 9238 adults with diabetes and 99,980 individuals without diabetes found a significant association between diabetes and OP (1.2 [1.1–1.4], P = 0.010) [ 14 ]. Specifically, diabetes was linked to a decreased BMD, elevating the risk of bone fracture [ 15 ]. While most studies suggest a modest reduction in BMD associated with diabetes [ 16 ], some do not confirm this finding [ 17 ]. Recent cohort studies have indicated a 4–12-fold increase in the risk of hip fracture among individuals with diabetes [ 18 , 19 ], contrasting earlier case-control investigations that found no elevated risk [ 20 , 21 ].
To evaluate potential causal links, Mendelian randomization (MR) offers a valuable alternative method [ 22 ]. It aims to reduce confounding effects and prevent reverse causation bias since genotypes are independent of postnatal lifestyle and environmental variables and precede the onset of disease [ 23 ]. To investigate the quantitative impact of diabetes (both T1D and T2D) and associated glycemic characteristics (glycosylated hemoglobin [HbA1c]) on various aspects of bone health, including femoral neck BMD (FN-BMD), lumbar spine BMD (LS-BMD), total body BMD (TB-BMD), and fracture, we conducted an analysis using MR and genome-wide association study (GWAS) data analysis.
Study design and data sources
In 2021, the American Diabetes Association released the following new diagnostic criteria for diabetes [ 24 ]: (1) fasting plasma glucose level ≥ 126 mg/dL (7.0 mmol/L), (2) 2-h plasma glucose level ≥ 200 mg/dL (11.1 mmol/L) during Diabetes Control and Complications Trial (OGTT), (3) HbA1c level ≥ 6.5% (48 mmol/mol), and (4) random plasma glucose level ≥ 200 mg/dL (11.1 mmol/L). Compared to T2D, T1D typically presents at a younger age at diagnosis (< 35 years) with a lower body mass index (BMI, < 25 kg/m 2 ) and is characterized by positivity for insulin-related antibodies [ 25 ]. This study employs a two-sample MR design adhering to STROBE-MR guidelines [ 26 ] (Figs. 1 and 2 ). Utilizing publicly available summary statistics from GWAS consortia, MR leverages genetic variants associated with the exposures of interest to examine their associations with disease outcomes. As genetic predisposition to a trait is not affected by potential confounders, this approach is considered to be less prone to confounding compared to traditional observational analyses.
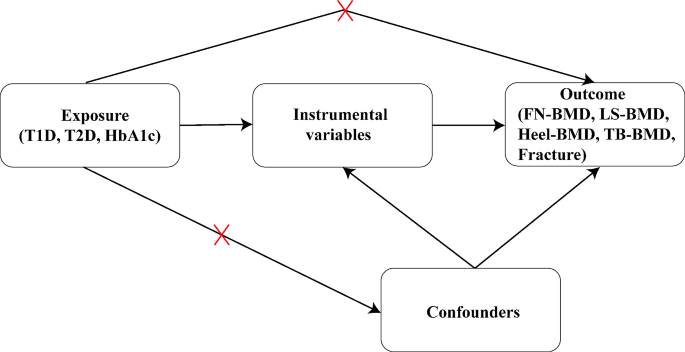
Illustration of the two-sample Mendelian randomization analysis. T1D: type 1 diabetes; T2D: type 2 diabetes; HbA1c: glycosylated hemoglobin; FN: femoral neck; LS: lumbar spine; TB: total body
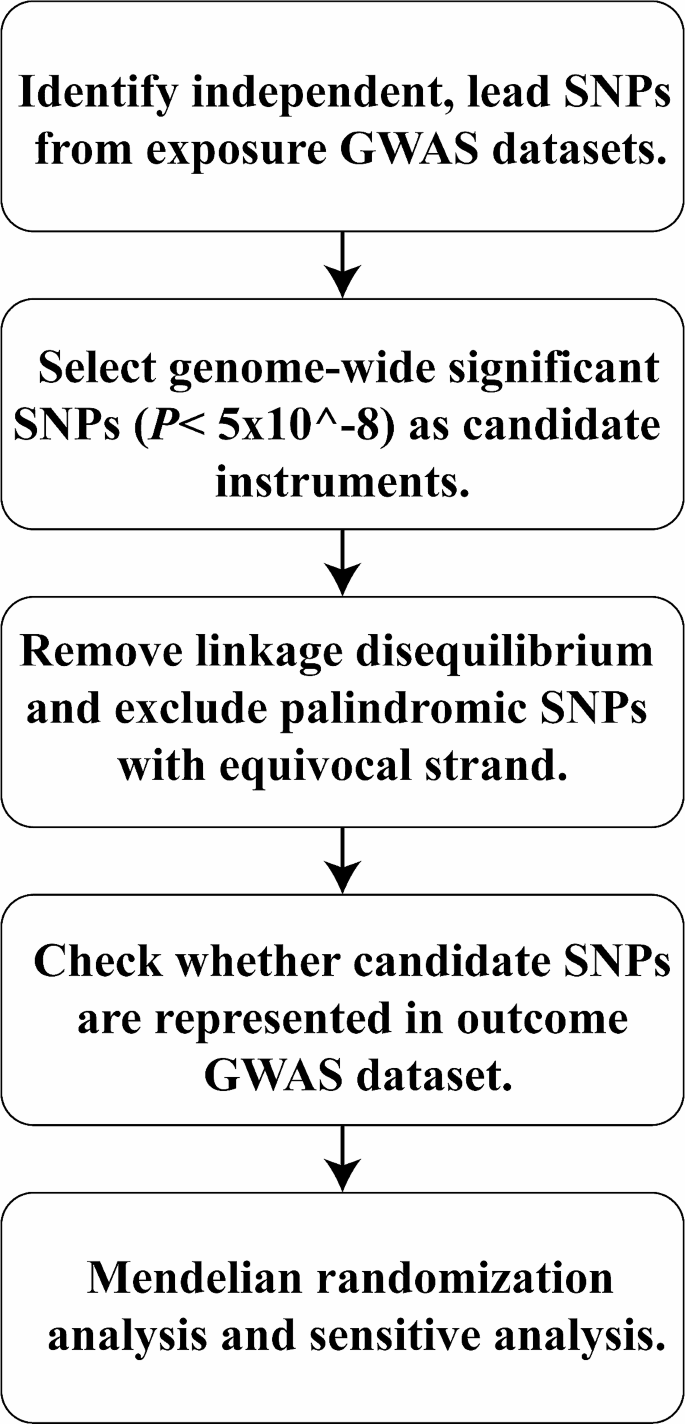
Flowchart of a Mendelian randomization research. GWAS: Genome wide association study; SNPs: Single-nucleotide polymorphisms
Selection of instrumental variables
Using this strategy, we examined GWAS data from the largest investigations of the genetic causes of T1D that are currently available ( N = 15,573 cases and N = 158,408 controls) [ 27 ], T2D ( N = 265,678 cases) [ 28 ], and HbA1c ( N = 46,368 cases) [ 29 ]. Three types of genetic susceptibility to diabetes were adjusted for age, sex, weight, and height. Five phenotypes (FN-BMD, LS-BMD, heel-BMD, TB-BMD, and fracture) were used as outcomes. Table 1 presents additional details of the exposures and outcomes.
For MR estimates, instrumental variables (IVs) were derived from summary-level GWAS data. Genetic variations linked to diabetes were identified as instrumental single nucleotide polymorphisms (SNPs). From our analyses (Table 1 ), we selected a set of independent genome-wide significant genetic variants for T1D, T2D, and HbA1c as IVs ( P < 5 × 10 − 8 ). We used linkage disequilibrium (LD) [ 33 ] clustering with a threshold of r 2 > 0.001 and excluded variations within a 1 Mb distance from other SNPs with stronger connections to ensure independence among instrumental SNPs for each exposure. Additionally, we standardized the effects of these instrumental SNPs whenever feasible and excluded those not present in the GWAS of the outcomes to ensure alignment of all associated risk factors and resulting alleles on the same strand. Ultimately, we selected SNPs associated with each exposure (T1D = 47, T2D = 57 and HbA1c = 10).
Associations with outcomes
Clinically, OP is defined by measuring BMD with a T score below − 2.5, which remains the single best predictor of fractures [ 34 ]. In this research, BMD and fracture data were used to characterize OP phenotypes at various anatomical sites, including FN ( n = 32,735), LS ( n = 28,498), systemic TB ( n = 66,628), heel ( n = 426,824), and fracture ( n = 416,795). Data from the Genetic Factors for Osteoporosis meta-analysis of FN-BMD and LS-BMD in the European population (32,735 and 28,498 individuals, respectively, in 2012) were included [ 35 ]. Additionally, summary statistics from a GWAS meta-analysis involving 66,628 European participants were included for TB-BMD [ 31 ]. The UK Biobank conducted a comprehensive study on the genetic influences on human heel-BMD and fracture risk, encompassing 426,824 and 416,795 individuals for the GWAS dataset for heel-BMD and fracture [ 32 ], respectively. Further, BMD phenotypes (per standard deviation) were adjusted for age, sex, weight, and height in the previous GWAS studies.
MR analysis
The causal relationship between each exposure and outcome was assessed using the inverse variance weighted (IVW) approach with a fixed-effect model. We excluded IVs that were substantially linked with outcome. The IVW approach is often considered the most reliable indicator in MR analysis when evidence of directional pleiotropy is absent ( P for MR-Egger intercept > 0.05). When each genetic variation meets the IV hypothesis, the IVW method can yield a consistent estimate of the exposure’s causal effect on the outcome. Cochran’s Q statistics were employed to evaluate the IV heterogeneity.
To further validate MR estimates, we used the MR-Pleiotropy Residual Sum and Outlier (MR-PRESSO) techniques, which identify and remove potential pleiotropic IVs, providing outlier-adjusted estimates for IVW analysis ( P < 0.05). Additionally, we employed complementary analysis methods including weighted median, MR-Egger, simple mode, and weighted mode, using random-effect model estimation to test the robustness of the IVW method. The weighted median estimate, utilizing aggregate data, offers protection against ineffective instruments and provides reliable estimates of causation if at least 50% of the weight originates from IVs [ 36 ].
Sensitivity analysis
In addition to employing the MR-PRESSO technique to identify and eliminate potential pleiotropic IVs and offer outlier-adjusted estimates to the IVs for IVW analysis ( P < 0.05), we used the Single Nucleotide Polymorphisms Annotator tool ( https://snipa.helmholtz-muenchen.de/snipa3/ ) to analyze the pleiotropy of potential confounders [ 37 ]. Cochran’s Q statistics were utilized to evaluate the IV heterogeneity, and loci displaying considerable heterogeneity were eliminated to further confirm the reliability of our MR estimations. Furthermore, we employed weighted median, weighted mode, simple mode, and MR-Egger as complementary analysis methods to assess the robustness of the IVW method using random-effect model estimation.
Subgroup analysis of age
Considering the potential impact of using summary data from GWAS analyses across all age groups on the accuracy of MR analyses, we performed a more detailed analysis by age groups for TB-BMD. Further, TB-BMD data were stratified into five age stages: 0–15 years ( N = 11,807), 15–30 years ( N = 4180), 30–45 years ( N = 10,062), 45–60 years ( N = 18,805), and over 60 years ( N = 22,504). The MR analysis method was employed to estimate the correlation between diabetes and TB-BMD at each respective age stage.
MR analysis of the effects of diabetes on BMD/fracture
In our initial investigation, we assessed the causal associations between diabetes and BMD/fracture utilizing a two-sample MR approach. We identified 47 IVs for T1D, 57 for T2D, and 10 for HbA1c, all of which reached genome-wide significance ( P < 5 × 10 − 8 ) in GWAS analyses after removing some missing data. These IVs were selected based on their independence from LD effects (r 2 < 0.001). Heterogeneity tests revealed no significant heterogeneity among the selected IVs (Q- P > 0.05, Supplementary Tables S1 - S3 in Additional file 1 ), demonstrating that neither horizontal pleiotropy nor heterogeneity influenced our MR results. Additionally, supplementary Tables S6 - S8 in Additional file 1 provide details on the power of selected IVs.
The IVW method showed that genetically predicted T1D was negatively associated with TB-BMD ( β = -0.018, 95% CI: -0.030, -0.006) (Fig. 3 (a)). Consistent findings were observed with causal estimates from MR-Egger, weighted median, and weighted mode for T1D’s effect on TB-BMD. Although T1D displayed heterogeneity for TB-BMD (Cochran’s Q- P < 0.05), the heterogeneity was reduced after processing using the random effect model (IVW, P > 0.05). The MR-PRESSO test showed no horizontal pleiotropic effect ( P > 0.05). Interestingly, genetically predicted T1D was not associated with FN-BMD ( β = 0.007, 95% CI: -0.008, 0.021), LS-BMD ( β = 0.010, 95% CI: -0.006, 0.026), heel-BMD ( β = 0.002, 95% CI: -0.004, 0.007), or fracture ( β = -0.001, 95% CI: -0.012, 0.010) (Supplementary Table S1 in Additional file 1 ).
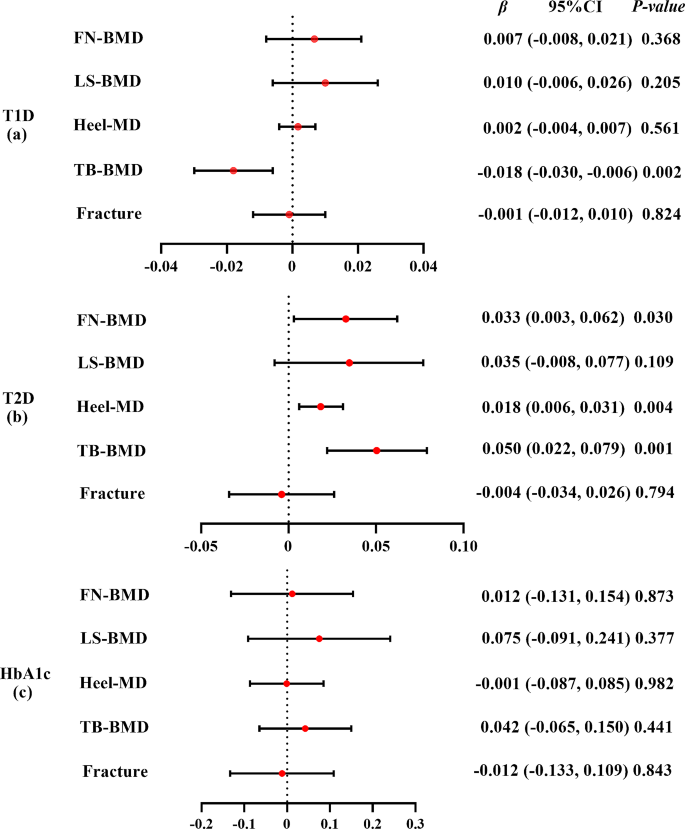
Mendelian randomization estimates the effect of T1D/T2D/HbA1c risk on bone mineral density and fracture. T1D: type 1 diabetes; T2D: type 2 diabetes; HbA1c: glycosylated hemoglobin; FN: femoral neck; LS: lumbar spine; TB: total body; BMD: bone mineral density. Detailed data are provided in Additional file 1 (Supplementary Tables S1 – S3 )
The IVW method showed that genetically predicted T2D was positively associated with FN-BMD ( β = 0.033, 95% CI: 0.003, 0.062), heel-BMD ( β = 0.018, 95% CI: 0.006, 0.031), and TB-BMD ( β = 0.050, 95% CI: 0.022, 0.079) but not with LS-BMD ( β = 0.035, 95% CI: -0.008, 0.077) or fracture ( β = -0.004, 95% CI: -0.034, 0.026) (Fig. 3 (b)). The causative effect of T2D on heel-BMD was disclosed by causal estimates from MR-Egger and weighted median, and the causal effect of T2D on TB-BMD was also indicated by causal estimates from weighted median and weighted mode. The heterogeneity (Cochran’s Q- P < 0.05) of T2D on heel-BMD and TB-BMD existed and was persistent after processing using the random effect model (IVW P < 0.05). The MR-PRESSO test showed no horizontal pleiotropic effect ( P > 0.05). Weighted mode showed potential causal association between T2D and FN-BMD ( β = 0.053, 95% CI: 0.005, 0.100). However, no causal relationship between T2D and FN-BMD, LS-BMD, TB-BMD, or fracture was observed by MR-Egger (Supplementary Table S2 in Additional file 1 ).
The IVW method showed that genetically predicted HbA1c was not associated with the five outcomes ( β ranged from − 0.012 to 0.075) (Fig. 3 (c)). The causal estimates from MR-Egger, weighted median, simple mode, and weighted mode were highly similar ( β ranged from − 0.183 to 0.075) (Supplementary Table S3 in Additional file 1 ). Although HbA1c displayed heterogeneity for heel-BMD (Cochran’s Q- P < 0.05), the heterogeneity reduced after processing using the random effect model (IVW, P > 0.05). The MR-PRESSO test showed no horizontal pleiotropic effect ( P > 0.05).
Subgroup analysis by age
Results of the subgroup analyses of the association between T1D and TB-BMD according to age are shown in Fig. 4 (a). The weighted median indicated a causal association between T1D and TB-BMD ( β = -0.027, 95% CI: -0.052, -0.002) between 45 and 60 years of age. However, causal estimates from MR-Egger, weighted median, simple mode, and weighted mode did not reveal a significant association between them. The estimates from IVW method, MR-Egger, simple median, and weighted median showed that genetically predicted T1D was not associated with any of the five outcomes ( β ranged from − 0.006 to 0.181) (Supplementary Table S4 in Additional file 1 ).
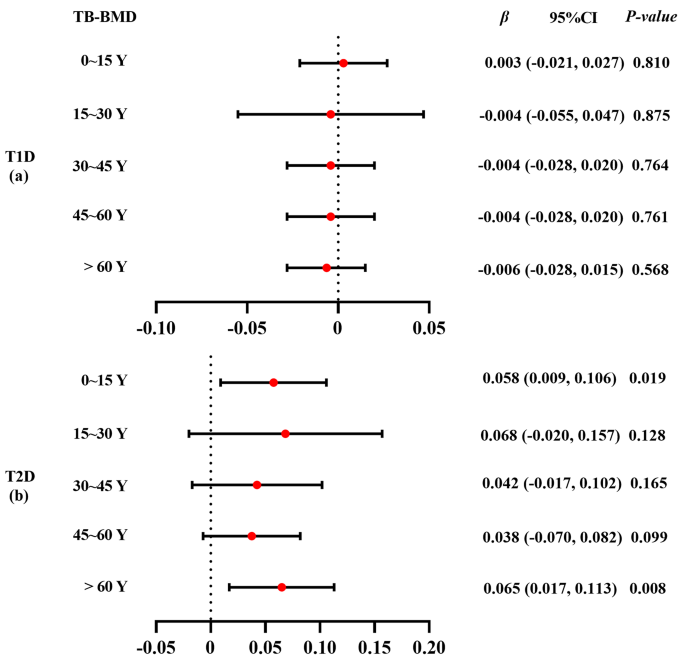
Subgroup analysis using Mendelian randomization estimates for T1D/T2D on total-body bone mineral density by age. TB: total body; BMD: bone mineral density. Detailed data are provided in Additional file 1 (Supplementary Tables S4 – S5 )
Results of the subgroup analyses of the association between T2D and TB-BMD according to age are presented in Fig. 4 (b). The IVW method showed that genetically predicted T2D was positively associated with TB-BMD under 15 years ( β = 0.058, 95% CI: 0.009, 0.106). The causal estimates from IVW, weighted median, and weighted mode revealed a causal association between T2D and TB-BMD in individuals over 60 years. However, no causal effect of T2D and TB-BMD was found in individuals aged 15–60-years (Supplementary Table S5 in Additional file 1 ).
Using a two-sample MR method, we revealed a causal association of T1D and T2D risk with TB-BMD. Additionally, we found that T2D risk was associated with FN-BMD and heel-BMD. However, no clear evidence of a causal relationship between HbA1c and OP/fracture was found. The subgroup analyses by age revealed that T2D has a stronger causal effect on TB-BMD than does T1D, especially in individuals aged > 60 years. Notably, this MR study yielded consistent results even after adjusting for diabetes-related characteristics.
Our study indicated that genetically increased T1D risk was significantly associated with decreased TB-BMD but not with other outcomes (FN-BMD, LS-BMD, and heel-BMD)/fracture. Although T1D commonly occurs between the ages of 9 and 14 years [ 38 ], a critical period for optimal bone development in children and adolescents [ 39 ], subgroup analysis by age revealed that the causal effect of T1D on TB-BMD was only evident in individuals aged 45–60 years.
A case-control study involving 32 children with T1D found that TB-BMD was significantly reduced in children with T1D, but not in the lumbar spine, suggesting a negative association between T1D and TB-BMD, albeit with different ages [ 40 ]. Similarly, a 2021 cross-sectional study of BMD using dual X-ray densitometry at the femoral neck, entire hip, lumbar spine, and whole body reported that men with T1D had compromised bone material strength and microarchitecture, and individuals with T1D showed a modest decline in BMD and low bone turnover [ 41 ]. Other studies have also found reduced BMD in patients with T1D [ 42 ]. However, a newly published MR study did not find an association between T1D and fracture/OP [ 43 ]. Although previous studies have revealed that the deficiency in bone mass in T1D may be apparent at the time of diagnosis [ 44 ], whether the duration of the disease or any other clinical aspects of diabetes or its management are linked to unfavorable skeletal morbidity remains unknown. Increasingly, experts recognize that a thorough evaluation of bone health necessitates examining bone microarchitecture alongside bone density. This recognition stems from the fact that the greatly increased fracture risk in individuals with T1D is disproportionate to the barely detectable decline in BMD [ 45 ].
While T2D demonstrated no significant association with LS-BMD or fracture risk, a notable association was observed between genetically predicted T2D and FN-BMD, heel-BMD, and TB-BMD. Recently, an MR study also found that T2D can reduce the incidence of OP, that is, increase BMD ( P = 0.0056) [ 46 ]. The 2017 MR study on T2D and BMD revealed a weak positive correlation between the two, whereas no link between T2D and LS-BMD was reported, and these findings were independent to BMI [ 47 ]. Secondary analysis of a cross-sectional data from youth aged 10–23 years (55% African American, 70% female) with T2D ( N = 90), obesity (BMI > 95th; N = 128), or normal weight (BMI 85th; N = 197) revealed that the obesity and T2D groups had significantly higher BMD Z-scores than those of the normal weight group [ 48 ]. Given that T2D may have an asymptomatic phase prior to diagnosis, we observed an increase in BMD around the time of T2D diagnosis [ 49 ]. However, we did not find evidence of increased heel-BMD in the T2D population, suggesting the need for further investigation to confirm this observation. Notably, subgroup analysis by age showed that the causal effect of T2D on TB-BMD was stronger in individuals over 60 years than in those under 15 years. This finding is supported by a prospective observational study [ 50 ] in which all patients with T2D newly diagnosed through normal health care procedures were identified between May 1, 1996 and June 30, 1998. The mean age at T2D diagnosis was 62.9 years for men and higher for women [ 50 ]. The considerable regional variation in bone microstructure across the skeleton and the known distinct effects of T2D on the cortical and trabecular bones [ 42 ] may be associated with a suggested mechanism for site-specific effects of T2D on BMD [ 51 ]. Several hypotheses exist regarding the correlation between T2D and BMD and bone quality. One hypothesis suggests that low bone mass results from insulin insufficiency in type-1 diabetes, but increasing insulin levels in individuals with T2D may increase BMD because insulin signals the bone’s osteoblasts to become more active [ 52 ]. However, patients with T2D may develop insulin resistance, which may compromise the physiological effects of insulin on bones. Additionally, it has been hypothesized that hyperglycemia, which characterizes T2D [ 53 ], impacts bone integrity, presumably by elevating the quantities of advanced glycation end products and collagen cross-linking, which have been associated with an increased risk of fractures [ 54 ]. . A 2019 prospective cohort study offers a relatively plausible explanation for the Diabetes Bone Paradox [ 55 ] (high risk of fracture but normal or increased BMD) in type 2 diabetes [ 56 ]. In a previous study, frailty status was measured by the Camos-based algorithm for Frailty Index (FI) of deficit accumulation [ 57 ]. Guowei Li et al. found that the increased fracture risk in patients with T2D may be related to the frailty of the patients themselves, and T2D exacerbates this process.
In the present MR analysis, HbA1c did not show a causal effect on FN-BMD, LS-BMD, heel-BMD, TB-BMD, and fracture. This observation implies that the influence of metabolic management on BMD may not be directly proportional over extended durations. This is because the detrimental effects of diabetes on BMD may have a more substantial impact than what can be solely attributed to levels of HbA1C [ 58 ]. Although long-term glycemic management is not linked to BMD, this does not rule out the possibility that patients with T1D may experience short-term, reversible alterations in BMD that can be treated to reverse them [ 58 ].
Notably, this study revealed that T1D had a weak negative correlation with BMD, whereas T2D had a positive correlation with BMD. Recent research, including two meta-analyses, has demonstrated a considerably higher risk of fracture (six studies, 35,925 individuals with T1D), reduced BMD (16 studies, 966 adults with T2D), and OP in individuals with T1D [ 59 ]. The status of BMD in patients with T2D is debatable, and some meta-analyses have found that the risk of fractures is high in patients with T2D [ 60 ]. The risk of hip fracture has been shown to increase by 1.3–2.1 times [ 61 ], and the risk of other fractures reportedly increases by 1.2 times [ 60 ], while the risk of vertebral fractures does not increase [ 60 ]. Further, BMD is enhanced in patients with T2D (lumbar spine Z-score + 0.41, total hip Z-score + 0.27), even though the fracture risk is high [ 62 ]. Considering that nearly all patients with T2D are obese, the same processes likely contribute to the greater BMD these patients than in individuals with obesity but without diabetes. Further, BMD is positively associated with BMI [ 63 ], and skeletal mass adapts to the current mechanical demands, reflecting a physiological process. In addition, diabetes treatment may also modulate BMD [ 64 ]. Sulfonylureas and metformin reportedly have neutral or marginally protective relationships with fracture risk [ 64 ]. T1D is mediated by T lymphocytes [ 65 ], leading to autoimmune insulitis and characterized by selective islet beta cell damage. In contrast, T2D results from the interaction between genetic and environmental factors, giving rise to the development of a complex polygenic hereditary disease characterized by insulin resistance and defective islet beta cell function (insulin hyposecretion) [ 66 ].
This study has certain limitations. First, the relationship between diabetes and BMD/fracture may be confounded by the pleiotropic effect of diabetes-associated SNPs [ 67 ]. Therefore, our results may represent a shared genetic basis between diabetes and BMD/fracture rather than a causal relationship. Second, the GWAS summary data mostly included individuals of European ancestry, raising caution in generalizing our findings to populations with different racial and ethnic backgrounds. Third, due to insufficient sex-stratified GWAS summary data on BMD, we were unable to conduct separate analyses for men and women. Lastly, our MR analysis results were solely based on bioinformatics analyses, limiting our ability to elucidate the underlying mechanisms. Further confirmation of our findings would necessitate in vitro and in vivo investigations in future studies.
Our study revealed that T1D and T2D have different effects on BMD at the genetic level. Additionally, BMD decreased in patients with T1D and increased in patients with T2D. These findings underscore the complex relationship between diabetes and bone health, highlighting the need for tailored interventions. Future research is warranted to elucidate underlying mechanisms and validate these associations across diverse populations, potentially informing targeted therapies and preventive strategies for individuals with diabetes.
Data availability
The datasets analyzed during the current study are available in the GWAS catalog (GWAS Catalog (ebi.ac.uk)). All data generated during this study are included in supplementary files.
Abbreviations
- Bone mineral density
- Osteoporosis
Type 1 diabetes
Type 2 diabetes
Glycosylated hemoglobin
- Mendelian randomization
Femoral neck
Lumbar spine
Body mass index
Inverse variance weighted
MR-Pleiotropy Residual Sum and Outlier
Instrumental variables
Linkage disequilibrium
Genome wide association study
Single nucleotide polymorphisms
Global regional. National disability-adjusted life-years (DALYs) for 359 diseases and injuries and healthy life expectancy (HALE) for 195 countries and territories, 1990–2017: a systematic analysis for the global burden of Disease Study 2017. Lancet (London England). 2018;392(10159):1859–922.
Article Google Scholar
Cho NH, Shaw JE, Karuranga S, Huang Y, da Rocha Fernandes JD, Ohlrogge AW, Malanda B. IDF Diabetes Atlas: global estimates of diabetes prevalence for 2017 and projections for 2045. Diabetes Res Clin Pract. 2018;138:271–81.
Article CAS PubMed Google Scholar
van Belle TL, Coppieters KT, von Herrath MG. Type 1 diabetes: etiology, immunology, and therapeutic strategies. Physiol Rev. 2011;91(1):79–118.
Article PubMed Google Scholar
Khosla S, Samakkarnthai P, Monroe DG, Farr JN. Update on the pathogenesis and treatment of skeletal fragility in type 2 diabetes mellitus. Nat Reviews Endocrinol. 2021;17(11):685–97.
Article CAS Google Scholar
Morrish NJ, Wang SL, Stevens LK, Fuller JH, Keen H. Mortality and causes of death in the WHO multinational study of Vascular Disease in Diabetes. Diabetologia. 2001;44(Suppl 2):S14–21.
Ogurtsova K, da Rocha Fernandes JD, Huang Y, Linnenkamp U, Guariguata L, Cho NH, Cavan D, Shaw JE, Makaroff LE. IDF Diabetes Atlas: global estimates for the prevalence of diabetes for 2015 and 2040. Diabetes Res Clin Pract. 2017;128:40–50.
Johnston CB, Dagar M. Osteoporosis in older adults. Med Clin N Am. 2020;104(5):873–84.
Kanis JA, Oden A, Johnell O, Johansson H, De Laet C, Brown J, Burckhardt P, Cooper C, Christiansen C, Cummings S, et al. The use of clinical risk factors enhances the performance of BMD in the prediction of hip and osteoporotic fractures in men and women. Osteoporos International: J Established as Result Cooperation between Eur Foundation Osteoporos Natl Osteoporos Foundation USA. 2007;18(8):1033–46.
Johnell O, Kanis JA. An estimate of the worldwide prevalence and disability associated with osteoporotic fractures. Osteoporos International: J Established as Result Cooperation between Eur Foundation Osteoporos Natl Osteoporos Foundation USA. 2006;17(12):1726–33.
Lane NE. Epidemiology, etiology, and diagnosis of osteoporosis. Am J Obstet Gynecol. 2006;194(2 Suppl):S3–11.
Salari N, Ghasemi H, Mohammadi L, Behzadi MH, Rabieenia E, Shohaimi S, Mohammadi M. The global prevalence of osteoporosis in the world: a comprehensive systematic review and meta-analysis. J Orthop Surg Res. 2021;16(1):609.
Article PubMed PubMed Central Google Scholar
Abdulameer SA, Sulaiman SA, Hassali MA, Subramaniam K, Sahib MN. Osteoporosis and type 2 diabetes mellitus: what do we know, and what we can do? Patient Prefer Adherence. 2012;6:435–48.
Gunczler P, Lanes R, Paoli M, Martinis R, Villaroel O, Weisinger JR. Decreased bone mineral density and bone formation markers shortly after diagnosis of clinical type 1 diabetes mellitus. J Pediatr Endocrinol Metabolism: JPEM. 2001;14(5):525–8.
Rehling T, Bjørkman AD, Andersen MB, Ekholm O, Molsted S. Diabetes Is Associated with Musculoskeletal Pain, Osteoarthritis, Osteoporosis, and Rheumatoid Arthritis. Journal of diabetes research 2019, 2019:6324348.
Rakic V, Davis WA, Chubb SA, Islam FM, Prince RL, Davis TM. Bone mineral density and its determinants in diabetes: the Fremantle Diabetes Study. Diabetologia. 2006;49(5):863–71.
Botushanov NP, Orbetzova MM. Bone mineral density and fracture risk in patients with type 1 and type 2 diabetes mellitus. Folia Medica. 2009;51(4):12–7.
PubMed Google Scholar
Olmos JM, Pérez-Castrillón JL, García MT, Garrido JC, Amado JA, González-Macías J. Bone densitometry and biochemical bone remodeling markers in type 1 diabetes mellitus. Bone Miner. 1994;26(1):1–8.
Nicodemus KK, Folsom AR. Type 1 and type 2 diabetes and incident hip fractures in postmenopausal women. Diabetes Care. 2001;24(7):1192–7.
Forsén L, Meyer HE, Midthjell K, Edna TH. Diabetes mellitus and the incidence of hip fracture: results from the Nord-Trøndelag Health Survey. Diabetologia. 1999;42(8):920–5.
Heath H 3rd, Melton LJ 3rd, Chu CP. Diabetes mellitus and risk of skeletal fracture. N Engl J Med. 1980;303(10):567–70.
Melchior TM, Sørensen H, Torp-Pedersen C. Hip and distal arm fracture rates in peri- and postmenopausal insulin-treated diabetic females. J Intern Med. 1994;236(2):203–8.
Davey Smith G, Hemani G. Mendelian randomization: genetic anchors for causal inference in epidemiological studies. Hum Mol Genet. 2014;23(R1):R89–98.
Article CAS PubMed PubMed Central Google Scholar
Fan J, Zhu J, Sun L, Li Y, Wang T, Li Y. Causal association of adipokines with osteoarthritis: a mendelian randomization study. Rheumatology (Oxford). 2021;60(6):2808–15.
ElSayed NA, Aleppo G, Aroda VR, Bannuru RR, Brown FM, Bruemmer D, Collins BS, Hilliard ME, Isaacs D, Johnson EL, et al. 2. Classification and diagnosis of diabetes: standards of Care in Diabetes-2023. Diabetes Care. 2023;46(Suppl 1):S19–40.
Davies MJ, Aroda VR, Collins BS, Gabbay RA, Green J, Maruthur NM, Rosas SE, Del Prato S, Mathieu C, Mingrone G, et al. Management of hyperglycemia in type 2 diabetes, 2022. A Consensus Report by the American Diabetes Association (ADA) and the European Association for the Study of Diabetes (EASD). Diabetes Care. 2022;45(11):2753–86.
Skrivankova VW, Richmond RC, Woolf BAR, Yarmolinsky J, Davies NM, Swanson SA, VanderWeele TJ, Higgins JPT, Timpson NJ, Dimou N, et al. Strengthening the reporting of Observational studies in Epidemiology using mendelian randomization: the STROBE-MR Statement. JAMA. 2021;326(16):1614–21.
Censin JC, Nowak C, Cooper N, Bergsten P, Todd JA, Fall T. Childhood adiposity and risk of type 1 diabetes: a mendelian randomization study. PLoS Med. 2017;14(8):e1002362.
Zhao W, Rasheed A, Tikkanen E, Lee JJ, Butterworth AS, Howson JMM, Assimes TL, Chowdhury R, Orho-Melander M, Damrauer S, et al. Identification of new susceptibility loci for type 2 diabetes and shared etiological pathways with coronary heart disease. Nat Genet. 2017;49(10):1450–7.
Soranzo N, Sanna S, Wheeler E, Gieger C, Radke D, Dupuis J, Bouatia-Naji N, Langenberg C, Prokopenko I, Stolerman E, et al. Common variants at 10 genomic loci influence hemoglobin A 1 (C) levels via glycemic and nonglycemic pathways. Diabetes. 2010;59(12):3229–39.
Estrada K, Styrkarsdottir U, Evangelou E, Hsu YH, Duncan EL, Ntzani EE, Oei L, Albagha OM, Amin N, Kemp JP, et al. Genome-wide meta-analysis identifies 56 bone mineral density loci and reveals 14 loci associated with risk of fracture. Nat Genet. 2012;44(5):491–501.
Medina-Gomez C, Kemp JP, Trajanoska K, Luan J, Chesi A, Ahluwalia TS, Mook-Kanamori DO, Ham A, Hartwig FP, Evans DS, et al. Life-Course Genome-Wide Association Study Meta-Analysis of Total Body BMD and Assessment of Age-Specific effects. Am J Hum Genet. 2018;102(1):88–102.
Morris JA, Kemp JP, Youlten SE, Laurent L, Logan JG, Chai RC, Vulpescu NA, Forgetta V, Kleinman A, Mohanty ST, et al. An atlas of genetic influences on osteoporosis in humans and mice. Nat Genet. 2019;51(2):258–66.
Wang Y, Li T, Fu L, Yang S, Hu YQ. A Novel Method for mendelian randomization analyses with pleiotropy and linkage disequilibrium in genetic variants from Individual Data. Front Genet. 2021;12:634394.
Johnell O, Kanis JA, Oden A, Johansson H, De Laet C, Delmas P, Eisman JA, Fujiwara S, Kroger H, Mellstrom D, et al. Predictive value of BMD for hip and other fractures. J bone Mineral Research: Official J Am Soc Bone Mineral Res. 2005;20(7):1185–94.
Zheng HF, Forgetta V, Hsu YH, Estrada K, Rosello-Diez A, Leo PJ, Dahia CL, Park-Min KH, Tobias JH, Kooperberg C, et al. Whole-genome sequencing identifies EN1 as a determinant of bone density and fracture. Nature. 2015;526(7571):112–7.
Bowden J, Davey Smith G, Haycock PC, Burgess S. Consistent estimation in mendelian randomization with some Invalid instruments using a weighted median estimator. Genet Epidemiol. 2016;40(4):304–14.
Arnold M, Raffler J, Pfeufer A, Suhre K, Kastenmüller G. SNiPA: an interactive, genetic variant-centered annotation browser. Bioinf (Oxford England). 2015;31(8):1334–6.
Google Scholar
Lanzinger S, Zimmermann A, Ranjan AG, Gani O, Pons Perez S, Akesson K, Majidi S, Witsch M, Hofer S, Johnson S et al. A collaborative comparison of international pediatric diabetes registries. Pediatric diabetes 2022.
Baxter-Jones AD, Faulkner RA, Forwood MR, Mirwald RL, Bailey DA. Bone mineral accrual from 8 to 30 years of age: an estimation of peak bone mass. J bone Mineral Research: Official J Am Soc Bone Mineral Res. 2011;26(8):1729–39.
Chen SC, Shepherd S, McMillan M, McNeilly J, Foster J, Wong SC, Robertson KJ, Ahmed SF. Skeletal fragility and its clinical determinants in children with type 1 diabetes. J Clin Endocrinol Metab. 2019;104(8):3585–94.
Syversen U, Mosti MP, Mynarek IM, Vedal TSJ, Aasarød K, Basso T, Reseland JE, Thorsby PM, Asvold BO, Eriksen EF, et al. Evidence of impaired bone quality in men with type 1 diabetes: a cross-sectional study. Endocr Connections. 2021;10(8):955–64.
Napoli N, Chandran M, Pierroz DD, Abrahamsen B, Schwartz AV, Ferrari SL. Mechanisms of diabetes mellitus-induced bone fragility. Nat Reviews Endocrinol. 2017;13(4):208–19.
Tang Y, Zhang L, Ye D, Zhao A, Liu Y, Zhang M. Causal relationship between type 1 diabetes and osteoporosis and fracture occurrence: a two-sample mendelian randomization analysis. Osteoporos International: J Established as Result Cooperation between Eur Foundation Osteoporos Natl Osteoporos Foundation USA. 2023;34(6):1111–7.
Weber DR, Schwartz G. Epidemiology of Skeletal Health in type 1 diabetes. Curr Osteoporos Rep. 2016;14(6):327–36.
McComb C, Harpur A, Yacoubian C, Leddy C, Anderson G, Shepherd S, Perry C, Shaikh MG, Foster J, Ahmed SF. MRI-based abnormalities in young adults at risk of adverse bone health due to childhood-onset metabolic & endocrine conditions. Clin Endocrinol. 2014;80(6):811–7.
Cheng L, Wang S, Tang H. Type 2 diabetes mellitus plays a protective role against osteoporosis --mendelian randomization analysis. BMC Musculoskelet Disord. 2023;24(1):444.
Ahmad OS, Leong A, Miller JA, Morris JA, Forgetta V, Mujammami M, Richards JB. A mendelian randomization study of the effect of Type-2 diabetes and glycemic traits on bone Mineral Density. J bone Mineral Research: Official J Am Soc Bone Mineral Res. 2017;32(5):1072–81.
Kindler JM, Gallo S, Khoury PR, Urbina EM, Zemel BS. Diet Quality and Bone Density in Youth with Healthy Weight, obesity, and type 2 diabetes. Nutrients 2021, 13(9).
de Klift LII, de Laet M, van Daele CE, Hofman PL, Pols A. Bone mineral density and fracture risk in type-2 diabetes mellitus: the Rotterdam Study. Osteoporos International: J Established as Result Cooperation between Eur Foundation Osteoporos Natl Osteoporos Foundation USA. 2005;16(12):1713–20.
Gatling W, Guzder RN, Turnbull JC, Budd S, Mullee MA. The Poole Diabetes Study: how many cases of type 2 diabetes are diagnosed each year during normal health care in a defined community? Diabetes Res Clin Pract. 2001;53(2):107–12.
Chen H, Zhou X, Fujita H, Onozuka M, Kubo KY. Age-related changes in trabecular and cortical bone microstructure. Int J Endocrinol. 2013;2013:213234.
Thrailkill KM, Lumpkin CK Jr., Bunn RC, Kemp SF, Fowlkes JL. Is insulin an anabolic agent in bone? Dissecting the diabetic bone for clues. Am J Physiol Endocrinol Metabolism. 2005;289(5):E735–745.
Hamann C, Kirschner S, Günther KP, Hofbauer LC. Bone, sweet bone–osteoporotic fractures in diabetes mellitus. Nat Reviews Endocrinol. 2012;8(5):297–305.
Patsch JM, Burghardt AJ, Yap SP, Baum T, Schwartz AV, Joseph GB, Link TM. Increased cortical porosity in type 2 diabetic postmenopausal women with fragility fractures. J bone Mineral Research: Official J Am Soc Bone Mineral Res. 2013;28(2):313–24.
Botella Martínez S, Varo Cenarruzabeitia N, Escalada San Martin J, Calleja Canelas A. The diabetic paradox: bone mineral density and fracture in type 2 diabetes. Endocrinologia Y Nutricion: organo de la Sociedad Esp De Endocrinologia Y Nutricion. 2016;63(9):495–501.
Li G, Prior JC, Leslie WD, Thabane L, Papaioannou A, Josse RG, Kaiser SM, Kovacs CS, Anastassiades T, Towheed T, et al. Frailty and Risk of fractures in patients with type 2 diabetes. Diabetes Care. 2019;42(4):507–13.
Kennedy CC, Ioannidis G, Rockwood K, Thabane L, Adachi JD, Kirkland S, Pickard LE, Papaioannou A. A Frailty Index predicts 10-year fracture risk in adults age 25 years and older: results from the Canadian Multicentre osteoporosis study (CaMos). Osteoporos International: J Established as Result Cooperation between Eur Foundation Osteoporos Natl Osteoporos Foundation USA. 2014;25(12):2825–32.
Joad S, Ballato E, Deepika F, Gregori G, Fleires-Gutierrez AL, Colleluori G, Aguirre L, Chen R, Russo V, Fuenmayor Lopez VC, et al. Hemoglobin A1c threshold for reduction in bone turnover in men with type 2 diabetes Mellitus. Front Endocrinol. 2021;12:788107.
Thong EP, Herath M, Weber DR, Ranasinha S, Ebeling PR, Milat F, Teede H. Fracture risk in young and middle-aged adults with type 1 diabetes mellitus: a systematic review and meta-analysis. Clin Endocrinol. 2018;89(3):314–23.
Dytfeld J, Michalak M. Type 2 diabetes and risk of low-energy fractures in postmenopausal women: meta-analysis of observational studies. Aging Clin Exp Res. 2017;29(2):301–9.
Fan Y, Wei F, Lang Y, Liu Y. Diabetes mellitus and risk of hip fractures: a meta-analysis. Osteoporos International: J Established as Result Cooperation between Eur Foundation Osteoporos Natl Osteoporos Foundation USA. 2016;27(1):219–28.
Vestergaard P. Discrepancies in bone mineral density and fracture risk in patients with type 1 and type 2 diabetes–a meta-analysis. Osteoporos International: J Established as Result Cooperation between Eur Foundation Osteoporos Natl Osteoporos Foundation USA. 2007;18(4):427–44.
De Laet C, Kanis JA, Odén A, Johanson H, Johnell O, Delmas P, Eisman JA, Kroger H, Fujiwara S, Garnero P, et al. Body mass index as a predictor of fracture risk: a meta-analysis. Osteoporos International: J Established as Result Cooperation between Eur Foundation Osteoporos Natl Osteoporos Foundation USA. 2005;16(11):1330–8.
Palermo A, D’Onofrio L, Eastell R, Schwartz AV, Pozzilli P, Napoli N. Oral anti-diabetic drugs and fracture risk, cut to the bone: safe or dangerous? A narrative review. Osteoporos International: J Established as Result Cooperation between Eur Foundation Osteoporos Natl Osteoporos Foundation USA. 2015;26(8):2073–89.
de Jong VM, van der Slik AR, Laban S, van ‘t Slot R, Koeleman BP, Zaldumbide A, Roep BO. Survival of autoreactive T lymphocytes by microRNA-mediated regulation of apoptosis through TRAIL and Fas in type 1 diabetes. Genes Immun. 2016;17(6):342–8.
Prasad RB, Groop L. Genetics of type 2 diabetes-pitfalls and possibilities. Genes. 2015;6(1):87–123.
Prudente S, Trischitta V. Editorial: the pleiotropic effect of the ENPP1 (PC-1) gene on insulin resistance, obesity, and type 2 diabetes. J Clin Endocrinol Metab. 2006;91(12):4767–8.
Download references
Acknowledgements
The authors thank all the participants and staff who made this study possible, especially participants who have contributed data to the UK Biobank.
This work was supported by the National Natural Science Foundation of China (No. 81972083), Science and Technology Planning Project of Guangzhou (No. 202201020303, 202102080052, 202102010057, 201804010226), and Foundation of Guangdong Second Provincial General Hospital (No. 3D-A2020004, 3D-A2020002, YQ2019-009, C2020019).
Author information
Authors and affiliations.
The Second School of Clinical Medicine, Guangdong Second Provincial General Hospital, Southern Medical University, Guangzhou, Guangdong, P.R. China
Clinical Research Centre, Zhujiang Hospital, Southern Medical University, Guangzhou, Guangdong, China
Zhao-Hua Zhu
Department of Orthopaedics, Guangdong Second Provincial General Hospital, The Second School of Clinical Medicine, Southern Medical University, Guangzhou, Guangdong, P.R. China
Jia-Xuan Li & Chang-Peng Xu
Department of Orthopaedics, Guangdong Second Provincial General Hospital, No. 466 Xingang Road, Haizhu District, Guangzhou, 510317, Guangdong, People’s Republic of China
Wei Zhang, Qi Chen, Chang-Liang Xia, Jun-Nan Ma, Shuan-Ji Ou, Yang Yang & Yong Qi
You can also search for this author in PubMed Google Scholar
Contributions
YQ, ZZ, and XC designed the study. YQ, XC, and ZZ managed and analyzed the data. YQ drafted the initial study protocol. YQ wrote the first draft of the article. JL, WZ, QC, CX, JM, SO, YY, and YQ contributed to data interpretation and preparation of the report. All the writers contributed ideas to the paper and gave their consent for submission. The corresponding author attests that all authors mentioned in the text meet the criteria for authorship, and that no authors who meet the criteria have been left out.
Corresponding authors
Correspondence to Yong Qi or Chang-Peng Xu .
Ethics declarations
Ethics approval and consent to participate.
Not applicable.
Consent for publication
Competing interests.
All other authors declare no competing interests.
Transparency
The manuscript is an authentic, accurate, and transparent account of the study being reported, according to the lead authors (YQ/CX/ZZ). We also affirm that no significant aspects of the study have been left out and that any discrepancies from the study’s original design have been adequately explained.
Additional information
Publisher’s note.
Springer Nature remains neutral with regard to jurisdictional claims in published maps and institutional affiliations.
Electronic supplementary material
Below is the link to the electronic supplementary material.
Supplementary Material 1
Rights and permissions.
Open Access This article is licensed under a Creative Commons Attribution 4.0 International License, which permits use, sharing, adaptation, distribution and reproduction in any medium or format, as long as you give appropriate credit to the original author(s) and the source, provide a link to the Creative Commons licence, and indicate if changes were made. The images or other third party material in this article are included in the article’s Creative Commons licence, unless indicated otherwise in a credit line to the material. If material is not included in the article’s Creative Commons licence and your intended use is not permitted by statutory regulation or exceeds the permitted use, you will need to obtain permission directly from the copyright holder. To view a copy of this licence, visit http://creativecommons.org/licenses/by/4.0/ . The Creative Commons Public Domain Dedication waiver ( http://creativecommons.org/publicdomain/zero/1.0/ ) applies to the data made available in this article, unless otherwise stated in a credit line to the data.
Reprints and permissions
About this article
Cite this article.
Qu, YD., Zhu, ZH., Li, JX. et al. Diabetes and osteoporosis: a two-sample mendelian randomization study. BMC Musculoskelet Disord 25 , 317 (2024). https://doi.org/10.1186/s12891-024-07430-0
Download citation
Received : 16 September 2023
Accepted : 09 April 2024
Published : 23 April 2024
DOI : https://doi.org/10.1186/s12891-024-07430-0
Share this article
Anyone you share the following link with will be able to read this content:
Sorry, a shareable link is not currently available for this article.
Provided by the Springer Nature SharedIt content-sharing initiative
BMC Musculoskeletal Disorders
ISSN: 1471-2474
- Submission enquiries: [email protected]
- General enquiries: [email protected]

An official website of the United States government
The .gov means it’s official. Federal government websites often end in .gov or .mil. Before sharing sensitive information, make sure you’re on a federal government site.
The site is secure. The https:// ensures that you are connecting to the official website and that any information you provide is encrypted and transmitted securely.
- Publications
- Account settings
Preview improvements coming to the PMC website in October 2024. Learn More or Try it out now .
- Advanced Search
- Journal List
- J Epidemiol Glob Health
- v.10(1); 2020 Mar

Epidemiology of Type 2 Diabetes – Global Burden of Disease and Forecasted Trends
Moien abdul basith khan.
1 Department of Family Medicine, College of Medicine and Health Sciences, United Arab Emirates University, Al-Ain, United Arab Emirates
Muhammad Jawad Hashim
Jeffrey kwan king, romona devi govender, halla mustafa, juma al kaabi.
2 Department of Internal Medicine, College of Medicine and Health Sciences, United Arab Emirates University, Al-Ain, United Arab Emirates
The rising burden of type 2 diabetes is a major concern in healthcare worldwide. This research aimed to analyze the global epidemiology of type 2 diabetes. We analyzed the incidence, prevalence, and burden of suffering of diabetes mellitus based on epidemiological data from the Global Burden of Disease (GBD) current dataset from the Institute of Health Metrics, Seattle. Global and regional trends from 1990 to 2017 of type 2 diabetes for all ages were compiled. Forecast estimates were obtained using the SPSS Time Series Modeler. In 2017, approximately 462 million individuals were affected by type 2 diabetes corresponding to 6.28% of the world’s population (4.4% of those aged 15–49 years, 15% of those aged 50–69, and 22% of those aged 70+), or a prevalence rate of 6059 cases per 100,000. Over 1 million deaths per year can be attributed to diabetes alone, making it the ninth leading cause of mortality. The burden of diabetes mellitus is rising globally, and at a much faster rate in developed regions, such as Western Europe. The gender distribution is equal, and the incidence peaks at around 55 years of age. Global prevalence of type 2 diabetes is projected to increase to 7079 individuals per 100,000 by 2030, reflecting a continued rise across all regions of the world. There are concerning trends of rising prevalence in lower-income countries. Urgent public health and clinical preventive measures are warranted.
1. INTRODUCTION
Type 2 diabetes is recognized as a serious public health concern with a considerable impact on human life and health expenditures. Rapid economic development and urbanization have led to a rising burden of diabetes in many parts of the world [ 1 ]. Diabetes affects individuals’ functional capacities and quality of life, leading to significant morbidity and premature mortality [ 2 ]. Recently, concerns have been raised that more than one-third of the diabetes-related deaths occur in people under the age of 60 [ 3 ]. Increased consumption of unhealthy diets and sedentary lifestyles, resulting in elevated Body Mass Index (BMI) and fasting plasma glucose, have been blamed for these trends [ 4 ]. In particular, persons with higher BMI are more likely to have type 2 diabetes [ 5 ]. The aging of the human population is another contributor, as diabetes tends to affect older individuals [ 6 ]. The cost of diabetes care is at least 3.2 times greater than the average per capita healthcare expenditure, rising to 9.4 times in presence of complications [ 7 ]. Control of blood glucose, blood pressure, and other targets remains suboptimal for many patients [ 8 ]. This has been partly attributed to the lack of awareness and health promotion needed for diabetes control [ 9 ].
Unfortunately, the global epidemiology of diabetes has not been re-evaluated since the availability of recent high-quality data [ 10 ]. We found no studies providing global forecasts for the intermediate future, which would be a critical piece of information for health policymakers.
This research project examines the latest dataset of the Global Burden of Disease (GBD) to assess the burden of type 2 diabetes worldwide. The aim is to study the current global epidemiology of diabetes and highlight the current distribution of disease and emerging epidemiologic trends.
2. MATERIALS AND METHODS
We analyzed descriptive epidemiological data from the GBD dataset managed by the Institute of Health Metrics and Evaluation at the University of Washington, Seattle [ 11 ]. The GBD dataset is actively maintained and updated based on research data, epidemiology studies, and governmental publications from more than 100,000 sources. As a systematic public health project, it carefully builds models and statistical estimates for health loss due to illness, injury, and risk factors based on empirical data. GBD produces annual estimates of disease measures, such as prevalence, incidence, deaths, and Disability-Adjusted Life Years (DALYs). DALYs combine years of life lost due to premature death and years lived with disability, and are a more accurate reflection of human suffering resulting from a disease than prevalence or mortality alone.
We used the latest data refresh from GBD (the 2017 update). This dataset includes annual figures from 1990 to 2017 for type 2 diabetes in all countries and regions. We selected four world regions (Asia, Europe, America, and Africa) instead of other classification schemes based on economic development. All data were directly retrieved from GBD without any adjustments. Estimates were not age adjusted for differences in underlying population age distributions. Thus, the rates for different countries represent the actual burden on their respective health systems.
2.1. Statistical Data Analysis
Forecasting was conducted using IBM SPSS version 25 (IBM Corp., Armonk, NY, USA). The Time Series Modeler was used to develop a forecast model using the Expert Modeler option without any events. None of the observed values were marked as outliers.
Globally, an estimated 462 million individuals are affected by type 2 diabetes, corresponding to 6.28% of the world’s population ( Table 1 ). More than 1 million deaths were attributed to this condition in 2017 alone, ranking it as the ninth leading cause of mortality. This is an alarming rise when compared with 1990, when type 2 diabetes was ranked as the eighteenth leading cause of deaths. In terms of human suffering (DALYs), diabetes ranks as the seventh leading disease.
Disease burden of type 2 diabetes, 2017
The prevalence of type 2 diabetes shows a distribution pattern that matches socio-economic development ( Figure 1 ). Developed regions, such as Western Europe, show considerably higher prevalence rates that continue to rise despite public health measures ( Figure 2 ). The rate of increase does not appear to be slowing down.

Global distribution of diabetes mellitus type 2 prevalence. Note: Colors indicate prevalence rates per 100,000 population in 2017.

Trends in the prevalence of type 2 diabetes. Note: Forecast estimates using SPSS Time Series Modeler (Ljung Box Q, p = 0.16). Dotted lines indicate upper and lower confidence limits.
Remarkably, certain regions, such as Pacific Ocean island nations, are sustaining the highest prevalence of disease. These countries include Fiji (20,277 per 100,000), Mauritius (18,545), American Samoa (18,312), and Kiribati (17,432). Southeast Asian countries, such as Indonesia, Malaysia, Thailand, and Vietnam, have moved up the ranks in the last two decades. Owing to their large population sizes, China (88.5 million individuals with type 2 diabetes), India (65.9 million), and the US (28.9 million) retain the top spots as the countries with the greatest total number of individuals with this condition.
Males show a slightly higher prevalence than females (6219 compared with 5898 cases per 100,000), although this difference is within the margin of uncertainty. The age of onset of new diagnosis is also somewhat earlier among males and shows expected patterns of rising prevalence with increasing age, whereas the incidence peaks at 55–59 years ( Figure 3 ). There appears to be no major shift in the age distribution from 1990 to 2017.

Age distribution of diabetes mellitus type 2, worldwide. (A) Incidence vs. prevalence (both 2017). (B) Incidence in 1990 vs. 2017. p < 0.0001, chi-square test.
Even though it afflicts individuals later in life, type 2 diabetes ranks seventh among the leading causes of disability and years of life lost (DALYs). It has jumped ranks from nineteenth position in 1990, indicating a global transition in disease patterns toward noncommunicable diseases.
Statistical forecasting using a model based on the 1990–2017 data showed that global diabetes prevalence could increase to 7079 per 100,000 by 2030 and 7862 by 2040. This estimate for 2040 is flanked by an upper confidence limit of 9904 and a lower limit of 5821 per 100,000.
4. DISCUSSION
This study reports on the current trends in the global burden of diabetes with emphasis on the burden of human suffering. The high prevalence of type 2 diabetes worldwide continues to rise, and there are no signs of it stabilizing. A concerning finding is the rapidly rising burden in lower-income countries. These findings have implications for health policy planners, physicians, healthcare professionals, and the public.
The burden of suffering due to diabetes, as measured by DALYs, is increasing despite significant investment in clinical care and pharmaceutical research. This increase is in excess of population growth and aging. Notably, Western Europe has a rate of increase greater than that of global and Asian averages. Even with the high levels of clinical and public health expenditure, this region is losing the battle against diabetes. One explanation might be non-modifiable risk factors, such as age and family history [ 12 ]. However, factors like a highly processed, calorie-dense western diet and a sedentary lifestyle may also be contributing. Developed countries like Italy and the US endure the highest burdens of human suffering (DALYs) due to diabetes. Advanced economies in Asia, such as South Korea and Taiwan, are joining the ranks of these countries, based on GBD data. Thus, our findings support the correlation between diabetes and economic development [ 13 ]. We speculate that our current approach to diabetes management, which focuses on expensive oral medications and insulin, is not working. Lowering blood glucose levels is perhaps not sufficient by itself nor effective in reducing all-cause mortality among these patients.
Prevention of new cases of diabetes appears to be not working as well based on our findings from global data. Although research is ongoing to reduce the progression from metabolic syndrome and prediabetes to diabetes, most interventions being tried seem to be unsuccessful in affecting the incidence. According to our data, there is no evidence of a decrease in incidence. Alarmingly high incidence rates recorded in island nations in the Pacific region are an indication of the interaction between genetic predisposition and the effect of rapid nutritional change on these indigenous populations. Meanwhile, the sheer number of individuals with diabetes is testing health systems in China, India, and the US to the limit. Rapid urbanization and its effects on diet and lifestyle has been implicated [ 14 ]. These findings have direct implications for health systems planning and resource allocation. Clearly, hospital-based management and subspecialist care are not sustainable strategies. Resource allocation in healthcare budgets for prevention of diabetes needs to be comparable to expenditures on treatment. Strengthening of primary care and community restructuring for active lifestyles and healthy nutrition are perhaps more likely to be cost effective [ 15 ]. Sadly, the rising tide of type 2 diabetes is out pacing preventive efforts by a wide margin [ 16 ].
The rising incidence of type 2 diabetes at earlier ages warrants closer attention. Previous clinic-based studies have reported a high number of young adults being diagnosed with type 2 diabetes, most of whom are obese [ 17 ]. There appears to be an age gradient with early-onset type 2 diabetes patients (those younger than 45) showing more obesity, dyslipidemia, smoking, sedentary lifestyles, and low-grade inflammation [ 18 ]. In our study, although the incidence of diabetes in young adults has increased over the past decades, the rise is across all ages. Thus, there appears to be no clear indication that the age of onset of type 2 diabetes has shifted to younger age groups. In any case, rising life expectancy in many countries will lead to a substantially greater burden of diabetes in the elderly.
The main limitations of our study include reliance on secondary data, which in turn is affected by the accuracy of measurement, changes in case definition, and heterogeneity in study designs. Yet as GBD evolves and matures, its estimation techniques have become more accurate and reliable. These statistical estimates provide a more complete and continuous picture of disease epidemiology than relying on raw data from isolated studies [ 11 ]. Ultimately, the goal is to guide decision making in clinical care and public health policy.
5. CONCLUSION
Type 2 diabetes continues to increase in prevalence, incidence, and as a leading cause of human suffering and deaths. Despite significant investments in clinical care, research, and public health interventions, there appears to be no sign of reduction in the rate of increase. Certain regions of the world, such as Western Europe and island states in the Pacific, are experiencing a disproportionately high burden. This epidemic will require an urgent and unwavering commitment to aggressive solutions at national levels with public policies, public health funding, and economic incentives for local communities to start diabetes prevention programs. Healthy eating options need to be subsidized, and unhealthy foods need to be taxed or otherwise disincentivized. Healthcare organizations and individual healthcare providers from multiple disciplines (doctors, nurses, pharmacists, dieticians, and diabetes educators) must be given time and resources to collaborate as they educate and care for individual and groups of patients. Unless urgent measures are instituted to reduce unhealthy eating, sedentary lifestyles, rapid urbanization, and other factors related to economic development, the burden of diabetes is expected to continue rising.
ACKNOWLEDGMENT
We would like to thank the Institute of Health Metrics, Seattle for compiling global epidemiological statistics and allowing access to data.
Data availability statement: The data that support the findings of this study are openly available in Global Health Data Exchange by the Institute of Health Metrics at http://ghdx.healthdata.org/gbd-results-tool .
CONFLICTS OF INTEREST
The authors declare they have no conflicts of interest.
AUTHORS’ CONTRIBUTION
MK contributed to writing the manuscript including the literature review. MJH designed the study/basic concept, wrote sections of the manuscript, analyzed the data, and provided overall supervision of the study. JK wrote parts of the manuscripts, proofread, and provided insights into the interpretation. RDG revised the manuscript and provided additional interpretation of results. HM compiled data and wrote the table. JAK revised and proofread the manuscript and provided additional interpretation of results.
This study did not receive any external grants from government, private or commercial sources.
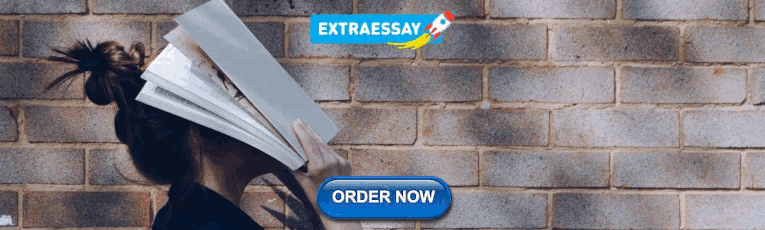
IMAGES
VIDEO
COMMENTS
In diabetes, the role of advanced practice nurses has significantly contributed to improved outcomes in the management of type 2 diabetes,5 in specialized diabetes foot care programs,6 in the management of diabetes in pregnancy,7 and in the care of pediatric type 1 diabetic patients and their parents.8,9 Furthermore, NPs have also been ...
PRESENTATION OF CASE. Dr. Max C. Petersen (Medicine): A 34-year-old woman was evaluated in the diabetes clinic of this hospital for hyperglycemia. Eleven years before this presentation, the blood glucose level was 126 mg per deciliter (7.0 mmol per liter) on routine laboratory evaluation, which was performed as part of an annual well visit.
One year before the current presentation, the weight was 48 kg, and the BMI was 19.4. Medications for diabetes included subcutaneous insulin glargine (34 units daily), subcutaneous insulin lispro ...
CASE. A 47-year-old woman was found to have hyperglycemia at a health fair when a random blood glucose level was 227 mg/dL (12.6 mmol/L). Several days later, a fasting blood glucose value was 147 mg/dL (8.2 mmol/L). She has no previous history of diabetes, is alarmed by the possibility of having this disorder, and seeks your advice.
A case-control study was conducted at the Tabriz Center of Metabolism and Endocrinology to investigate the relationship between nutrient patterns and type 2 diabetes (T2D). The study enrolled 225 newly diagnosed cases of T2D and 225 controls. The dietary intake of nutrients was assessed using a validated semi-quantitative food frequency ...
Results. Results of the lunch study for a type 2 diabetic subject indicate that the recovery time of the post-prandial blood glucose level can be adjusted to 4 hours, which is comparable to the typical time interval for non-diabetics: 3 to 4 hours. A moderate lifestyle adjustment of light supper coupled with morning swimming of 20 laps in a 25 ...
Study population. This study utilized a case-control design and involved participants between the ages of 18 and 60 who had been diagnosed with type 2 diabetes within the previous six months based on specific glucose level criteria (FBS levels of ≥ 126 mg/dl and 2 h-PG levels of ≥ 200 mg/dl []).Healthy individuals within the same age range were also included, with specific glucose level ...
The three mini-case studies presented with this issue of the journal take you through what to consider in making an accurate diagnosis of type 2 diabetes. The format uses typical clinical scenarios as tools for learning. Information is provided in short sections, with most ending in a question to answer before moving on to the next section.
Diabetes mellitus currently affects 6.4% or 285 million adults worldwide, and that number is expected to increase to 7.7% or 439 million by 2030. 1 In the United States, the prevalence of diabetes in adults increased from 11.3% in 2010 to 12.3% in 2012. 2 The current type 2 diabetes mellitus (T2DM) epidemic is closely associated with a parallel obesity epidemic, with more than 85% of patients ...
This article examines the aetiology, pathophysiology, diagnosis and treatment of type 2 diabetes using a case study approach. The psychosocial implications for the patient are also discussed. The case study is based on a patient with diabetes who was admitted to hospital following a hypoglycaemic episode and cared for during a practice placement.
Presentation of Case. Dr. Max C. Petersen (Medicine): A 34-year-old woman was evaluated in the diabetes clinic of this hospital for hyperglycemia. Eleven years before this presentation, the blood ...
Treatment. Treatment of type 2 diabetes mellitus (T2DM) focuses on decreasing blood glucose, increasing insulin secretion, or countering insulin resistance. Treatment of symptoms, such as diabetic retinopathy, nephropathy, or neuropathy requires additional and involved patient education, medications, and therapies.
Background/purpose of the study Several studies implicate skeletal muscle as a physio-pathological target among the metabolic disturbances of diabetes, or with the mechanisms shared by sarcopenia and type 2 diabetes mellitus (T2DM). The present study aimed to determine the relationship between sarcopenia and T2DM in outpatients of the Zulian Diabetes Institute, Maracaibo, Venezuela. Methods A ...
Diabetes mellitus (DM) is a chronic metabolic disorder characterized by persistent hyperglycemia. It may be due to impaired insulin secretion, resistance to peripheral actions of insulin, or both. According to the International Diabetes Federation (IDF), approximately 415 million adults between the ages of 20 to 79 years had diabetes mellitus in 2015.[1] DM is proving to be a global public ...
The specialized role of nursing in the care and education of people with diabetes has been in existence for more than 30 years and the emergence and subsequent growth of advanced practice in nursing during the past 20 years has expanded the direct care component, incorporating aspects of both nursing and medical care while maintaining the teaching and counseling roles. The specialized role of ...
type 2 diabetes mellitus (T2DM) has been well described. According to a survey performed in. five European countries, nearly one-third of people with T2DM were overweight (where one's. body mass ...
Diabetes Mellitus Tipo 2, also known as type 2 diabetes, is a chronic metabolic disorder characterized by high blood sugar levels. It is a prevalent condition affecting millions of people worldwide. In this comprehensive case study, we will delve into the causes, symptoms, and effective management strategies for Diabetes Mellitus Tipo 2.
Diabetes Mellitus Type 2. Pathophysiology Case Study. Home; History and Assessment; Differential Diagnoses; Pathophysiology; Evidence Based Management; Reference; Posts Diabetes Type 2. September 23, 2014 at 1:36am November 12, 2014 by Ning Sun. Creators: Wendy Born;
Methods. PubMed ®, Cochrane and Web of Science data bases were searched for randomized controlled clinical trials.Studies evaluating pharmaceutical care-based interventions in type 2 diabetes mellitus published between 2012 and 2017 were included. Glycated hemoglobin was defined as the primary endpoint; blood pressure, triglycerides and cholesterol as secondary endpoints.
Based on key terms such as diabetes mellitus, cost of disease, cost control, cost analysis, healthcare cost, and type 2 diabetes, the study results were divided into themes. The study's major goals were to calculate type 2 diabetes mellitus (T2DM) direct expenses, estimate per capita costs, and assess T2DM's effects on employment, income ...
Background The effects on bone mineral density (BMD)/fracture between type 1 (T1D) and type 2 (T2D) diabetes are unknown. Therefore, we aimed to investigate the causal relationship between the two types of diabetes and BMD/fracture using a Mendelian randomization (MR) design. Methods A two-sample MR study was conducted to examine the causal relationship between diabetes and BMD/fracture, with ...
Global and regional trends from 1990 to 2017 of type 2 diabetes for all ages were compiled. Forecast estimates were obtained using the SPSS Time Series Modeler. In 2017, approximately 462 million individuals were affected by type 2 diabetes corresponding to 6.28% of the world's population (4.4% of those aged 15-49 years, 15% of those aged ...