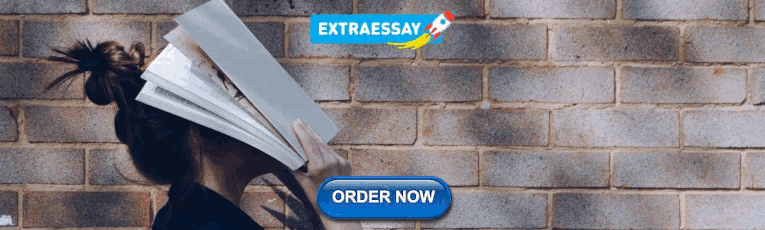
Why education matters for economic development
Harry a. patrinos.
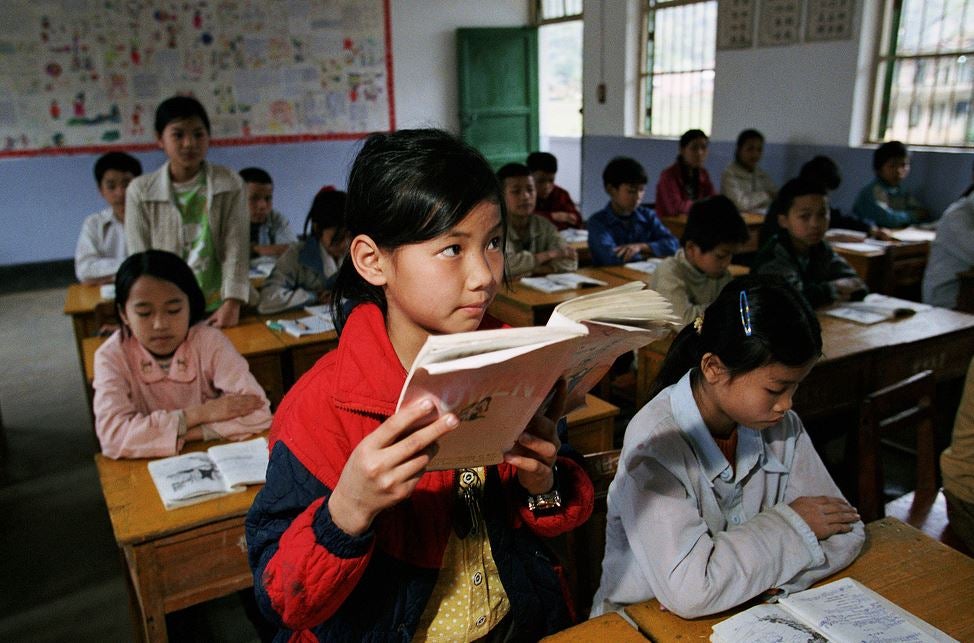
Senior Adviser, Education
Join the Conversation
- Share on mail
- comments added
Why education is the key to development
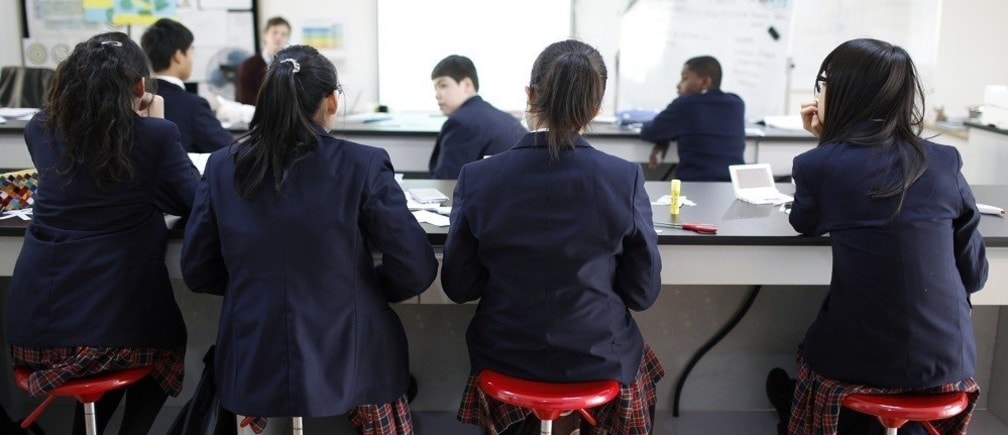
.chakra .wef-1c7l3mo{-webkit-transition:all 0.15s ease-out;transition:all 0.15s ease-out;cursor:pointer;-webkit-text-decoration:none;text-decoration:none;outline:none;color:inherit;}.chakra .wef-1c7l3mo:hover,.chakra .wef-1c7l3mo[data-hover]{-webkit-text-decoration:underline;text-decoration:underline;}.chakra .wef-1c7l3mo:focus,.chakra .wef-1c7l3mo[data-focus]{box-shadow:0 0 0 3px rgba(168,203,251,0.5);} Børge Brende
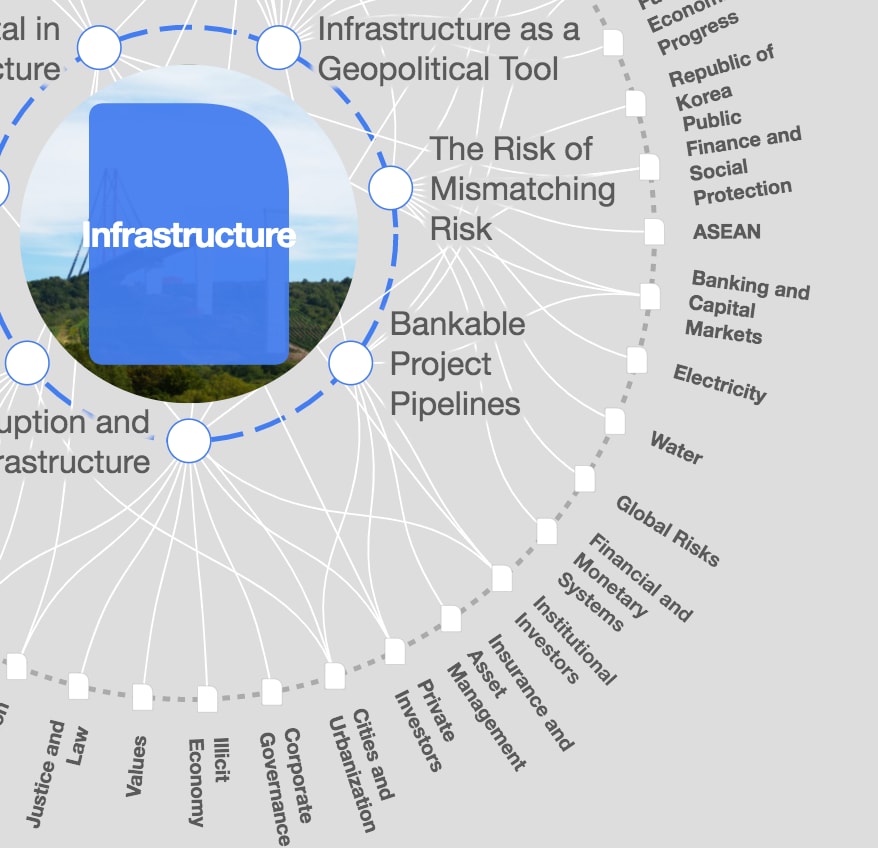
.chakra .wef-9dduvl{margin-top:16px;margin-bottom:16px;line-height:1.388;font-size:1.25rem;}@media screen and (min-width:56.5rem){.chakra .wef-9dduvl{font-size:1.125rem;}} Explore and monitor how .chakra .wef-15eoq1r{margin-top:16px;margin-bottom:16px;line-height:1.388;font-size:1.25rem;color:#F7DB5E;}@media screen and (min-width:56.5rem){.chakra .wef-15eoq1r{font-size:1.125rem;}} Infrastructure is affecting economies, industries and global issues
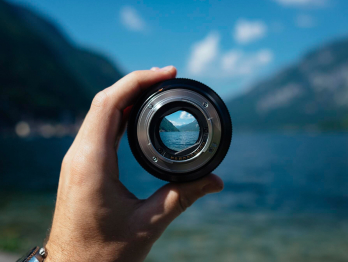
.chakra .wef-1nk5u5d{margin-top:16px;margin-bottom:16px;line-height:1.388;color:#2846F8;font-size:1.25rem;}@media screen and (min-width:56.5rem){.chakra .wef-1nk5u5d{font-size:1.125rem;}} Get involved with our crowdsourced digital platform to deliver impact at scale
Stay up to date:, infrastructure.
Education is a human right. And, like other human rights, it cannot be taken for granted. Across the world, 59 million children and 65 million adolescents are out of school . More than 120 million children do not complete primary education.
Behind these figures there are children and youth being denied not only a right, but opportunities: a fair chance to get a decent job, to escape poverty, to support their families, and to develop their communities. This year, decision-makers will set the priorities for global development for the next 15 years. They should make sure to place education high on the list.
The deadline for the Millennium Development Goals is fast approaching. We have a responsibility to make sure we fulfill the promise we made at the beginning of the millennium: to ensure that boys and girls everywhere complete a full course of primary schooling.
The challenge is daunting. Many of those who remain out of school are the hardest to reach, as they live in countries that are held back by conflict, disaster, and epidemics. And the last push is unlikely to be accompanied by the double-digit economic growth in some developing economies that makes it easier to expand opportunities.
Nevertheless, we can succeed. Over the last 15 years, governments and their partners have shown that political will and concerted efforts can deliver tremendous results – including halving the number of children and adolescents who are out of school. Moreover, most countries are closing in on gender parity at the primary level. Now is the time to redouble our efforts to finish what we started.
But we must not stop with primary education. In today’s knowledge-driven economies, access to quality education and the chances for development are two sides of the same coin. That is why we must also set targets for secondary education, while improving quality and learning outcomes at all levels. That is what the Sustainable Development Goal on education, which world leaders will adopt this year, aims to do.
Addressing the fact that an estimated 250 million children worldwide are not learning the basic skills they need to enter the labor market is more than a moral obligation. It amounts to an investment in sustainable growth and prosperity. For both countries and individuals, there is a direct and indisputable link between access to quality education and economic and social development.
Likewise, ensuring that girls are not kept at home when they reach puberty, but are allowed to complete education on the same footing as their male counterparts, is not just altruism; it is sound economics. Communities and countries that succeed in achieving gender parity in education will reap substantial benefits relating to health, equality, and job creation.
All countries, regardless of their national wealth, stand to gain from more and better education. According to a recent OECD report , providing every child with access to education and the skills needed to participate fully in society would boost GDP by an average 28% per year in lower-income countries and 16% per year in high-income countries for the next 80 years.
Today’s students need “twenty-first-century skills,” like critical thinking, problem solving, creativity, and digital literacy. Learners of all ages need to become familiar with new technologies and cope with rapidly changing workplaces.
According to the International Labour Organization, an additional 280 million jobs will be needed by 2019. It is vital for policymakers to ensure that the right frameworks and incentives are established so that those jobs can be created and filled. Robust education systems – underpinned by qualified, professionally trained, motivated, and well-supported teachers – will be the cornerstone of this effort.
Governments should work with parent and teacher associations, as well as the private sector and civil-society organizations, to find the best and most constructive ways to improve the quality of education. Innovation has to be harnessed, and new partnerships must be forged.
Of course, this will cost money. According to UNESCO, in order to meet our basic education targets by 2030, we must close an external annual financing gap of about $22 billion. But we have the resources necessary to deliver. What is lacking is the political will to make the needed investments.
This is the challenge that inspired Norway to invite world leaders to Oslo for a Summit on Education for Development , where we can develop strategies for mobilizing political support for increasing financing for education. For the first time in history, we are in the unique position to provide education opportunities for all, if only we pull together. We cannot miss this critical opportunity.
To be sure, the responsibility for providing citizens with a quality education rests, first and foremost, with national governments. Aid cannot replace domestic-resource mobilization. But donor countries also have an important role to play, especially in supporting least-developed countries. We must reverse the recent downward trend in development assistance for education, and leverage our assistance to attract investments from various other sources. For our part, we are in the process of doubling Norway’s financial contribution to education for development in the period 2013-2017.
Together, we need to intensify efforts to bring the poorest and hardest to reach children into the education system. Education is a right for everyone. It is a right for girls, just as it is for boys. It is a right for disabled children, just as it is for everyone else. It is a right for the 37 million out-of-school children and youth in countries affected by crises and conflicts. Education is a right regardless of where you are born and where you grow up. It is time to ensure that the right is upheld.
This article is published in collaboration with Project Syndicate . Publication does not imply endorsement of views by the World Economic Forum.
To keep up with the Agenda subscribe to our weekly newsletter .
Author: Erna Solberg is Prime Minister of Norway. Børge Brende is Norway’s Minister of Foreign Affairs.
Image: Students attend a class at the Oxford International College in Changzhou. REUTERS/Aly Song.
Share this:
- Share on Facebook (Opens in new window)
- Click to share on Twitter (Opens in new window)
- Click to share on LinkedIn (Opens in new window)
- Click to share on WhatsApp (Opens in new window)
Don't miss any update on this topic
Create a free account and access your personalized content collection with our latest publications and analyses.
License and Republishing
World Economic Forum articles may be republished in accordance with the Creative Commons Attribution-NonCommercial-NoDerivatives 4.0 International Public License, and in accordance with our Terms of Use.
The views expressed in this article are those of the author alone and not the World Economic Forum.
Related topics:
The agenda .chakra .wef-n7bacu{margin-top:16px;margin-bottom:16px;line-height:1.388;font-weight:400;} weekly.
A weekly update of the most important issues driving the global agenda
.chakra .wef-1dtnjt5{display:-webkit-box;display:-webkit-flex;display:-ms-flexbox;display:flex;-webkit-align-items:center;-webkit-box-align:center;-ms-flex-align:center;align-items:center;-webkit-flex-wrap:wrap;-ms-flex-wrap:wrap;flex-wrap:wrap;} More on Economic Growth .chakra .wef-17xejub{-webkit-flex:1;-ms-flex:1;flex:1;justify-self:stretch;-webkit-align-self:stretch;-ms-flex-item-align:stretch;align-self:stretch;} .chakra .wef-nr1rr4{display:-webkit-inline-box;display:-webkit-inline-flex;display:-ms-inline-flexbox;display:inline-flex;white-space:normal;vertical-align:middle;text-transform:uppercase;font-size:0.75rem;border-radius:0.25rem;font-weight:700;-webkit-align-items:center;-webkit-box-align:center;-ms-flex-align:center;align-items:center;line-height:1.2;-webkit-letter-spacing:1.25px;-moz-letter-spacing:1.25px;-ms-letter-spacing:1.25px;letter-spacing:1.25px;background:none;padding:0px;color:#B3B3B3;-webkit-box-decoration-break:clone;box-decoration-break:clone;-webkit-box-decoration-break:clone;}@media screen and (min-width:37.5rem){.chakra .wef-nr1rr4{font-size:0.875rem;}}@media screen and (min-width:56.5rem){.chakra .wef-nr1rr4{font-size:1rem;}} See all
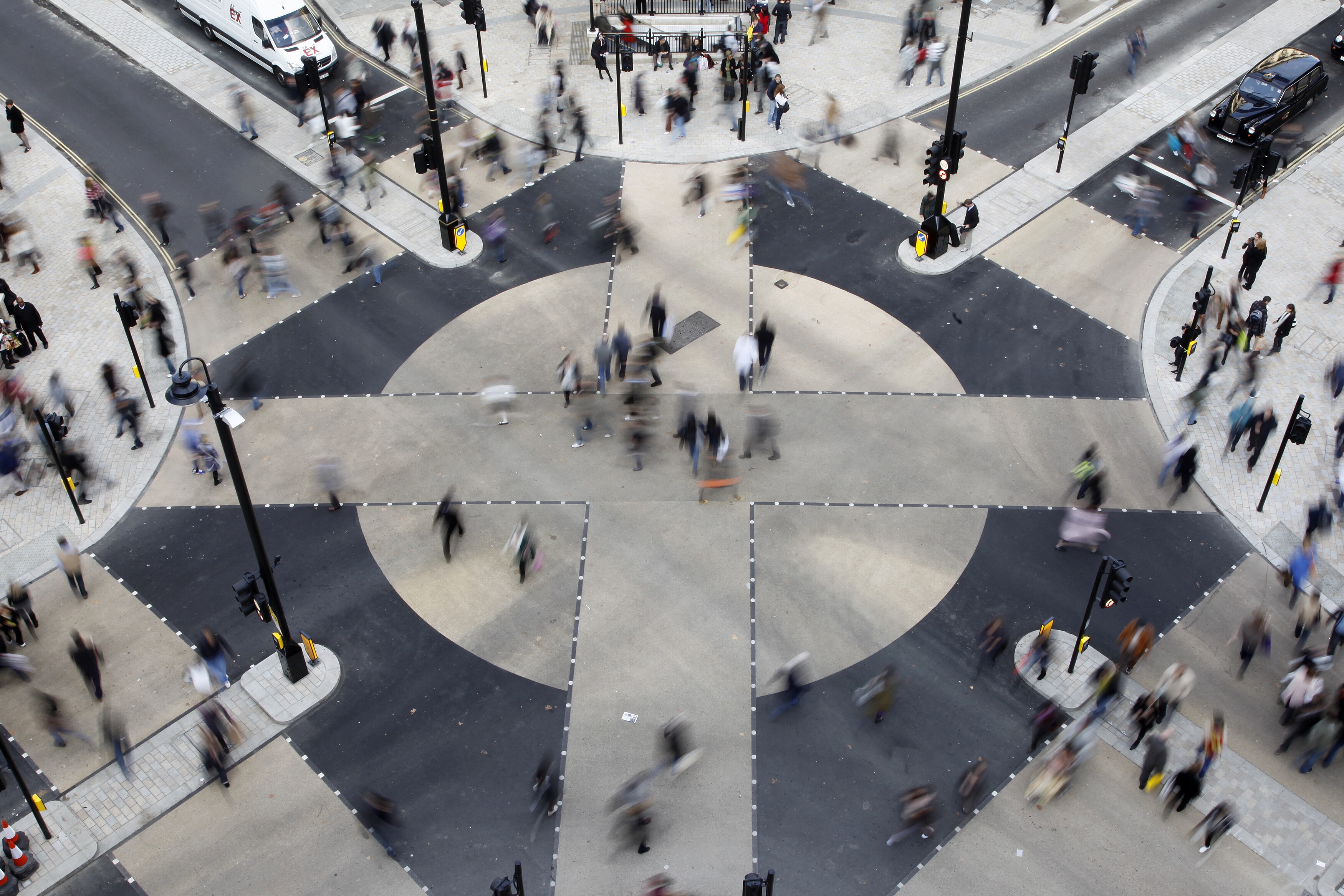
Why shifting from prediction to foresight can help us plan for future disruption
Roger Spitz
May 3, 2024
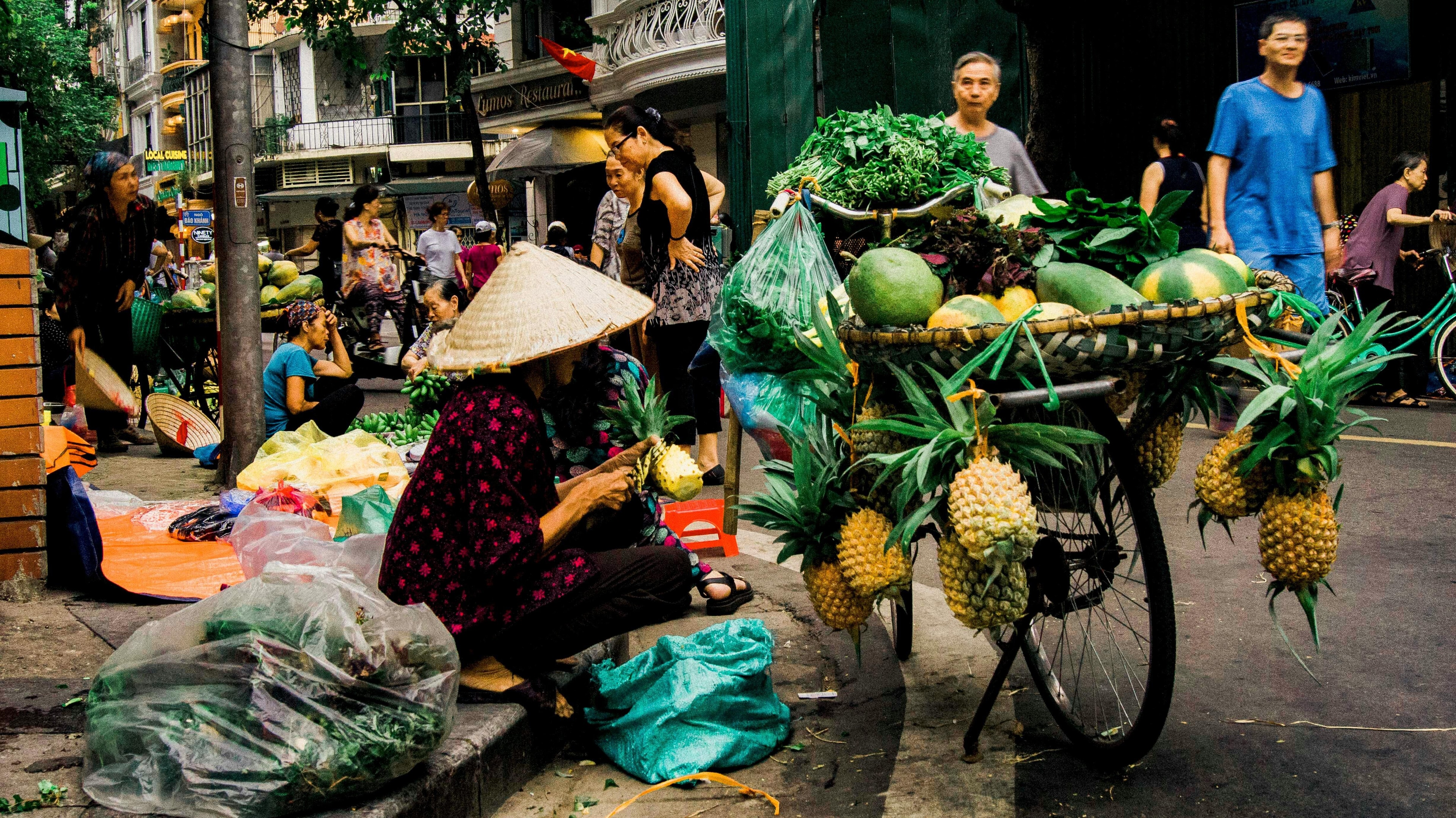
5 ways businesses can help to alleviate poverty
Sreevas Sahasranamam and Vivek Soundararajan
The state of world prosperity: 4 key data points you need to understand
Wolfgang Fengler and Homi Kharas

Money matters: Your guide to financial literacy
Meagan Andrews and Haleh Nazeri
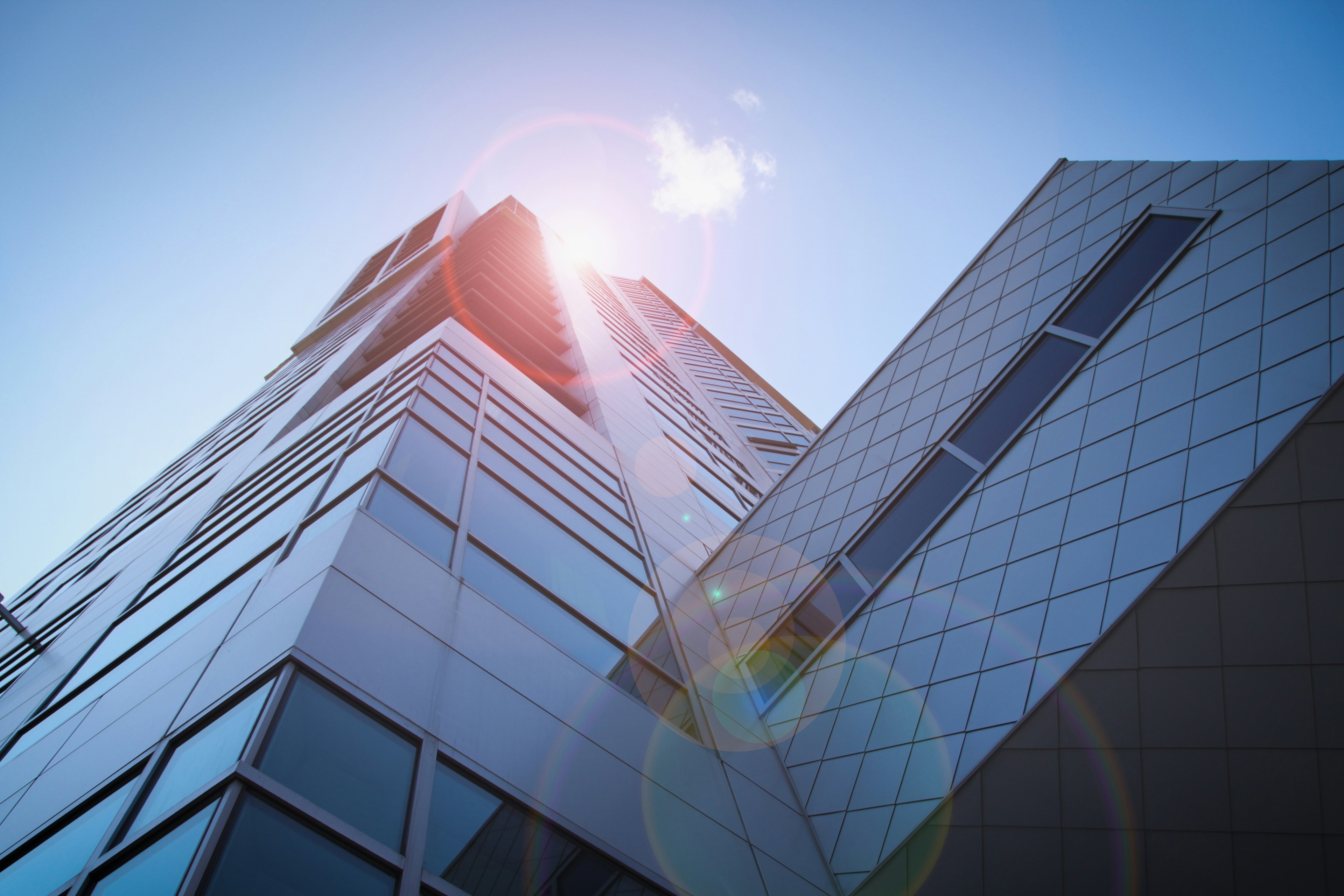
OECD upgrades global economic outlook, and other economics stories to read
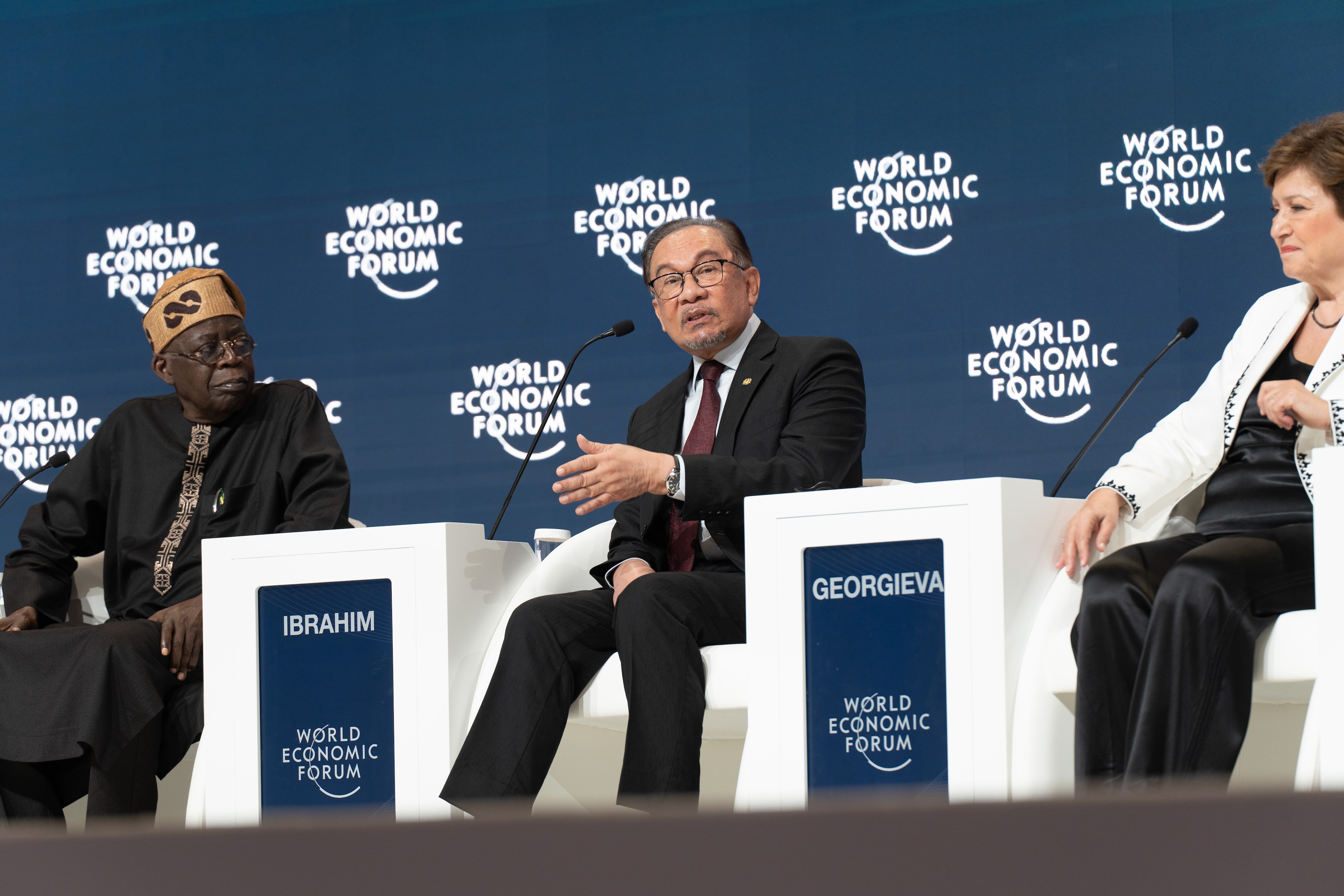
Why investing in people is essential to revive growth: Key quotes from leaders at the #SpecialMeeting24
Gayle Markovitz and Kate Whiting
May 2, 2024
2021 Theses Doctoral
Essays in the Economics of Education
Nguyen, Dieu Hoa Thi
Education is at the center of upskilling human capital in developing countries, thereby positively influencing economic growth and development. For decades, many education policies targeted at developing countries have been narrowly focused on improving access to basic education (Barrett et al., 2015). However, access to education does not always translate into educational attainment. Thus, beyond the initial goal of expanding access to education in developing countries, there has been a growing focus on delivering quality education on the development agenda for developing countries in recent years. One popular policy instrument in enhancing education quality has been school choice. Analysis of school choice and the subsequent academic performance outcomes can provide new insight on the economics of education to policymakers, schools, parents and students alike. This dissertation consists of three essays, which focus on understanding the demand for public schools and the returns to school quality in a merit-based competitive school assignment system. In particular, these papers investigate how positive recognition of ability through awards can affect the students’ decision-making process; what the students might gain from attending a more selective school; and how students balance between their preferences for school characteristics and maximizing their chances of admission in a competitive school choice market. Altogether, this dissertation highlights the role of information as well as educational background in explaining differences in school choice decisions and achievement outcomes. In chapter 1, I examine the role of positive recognition on students’ school choice decisions and achievement outcomes in the context of academic competitions. Academic competitions are an essential aspect of education. Given the prevalence and the amount of resources spent organizing them, a natural question that arises is the extent of the impact on winners’ education outcomes when their talent is recognized. I exploit the award structure in Vietnam’s annual regional academic competitions to answer this question. By leveraging the pre-determined share of awards, I apply a regression discontinuity design to assess the effects of receiving a Prize and receiving an Honorable Mention. I find that both types of awards lead to improvements in educational outcomes, and the results are persistent after three years. I also find some evidence of specialization associated with receiving a Prize Award. I hypothesize that long-term effects can be partially explained by school choice: winners are significantly more likely to apply to and consequently enroll in higher-quality schools. There are also prominent differences in educational choices and outcomes along gender lines: female students are more sensitive to award receipts than male students. These findings underscore the positive motivational effects of awards, even among the top performers in a highly competitive schooling market. In chapter 2, I explore the impacts of attending a selective school on students’ educational outcomes. Students in Vietnam are assigned to public high schools based on their performance in a placement exam as well as their ranked choice of schools. Public schools are often oversubscribed, which contributes to exogeneous admission score cutoffs below which students are not considered for admission. By applying a regression discontinuity research design to these admission score cutoffs, I find that students who are marginally admitted to their top-choice public schools are exposed to significantly higher-achieving peers while finding themselves at the bottom of the ability distribution. They experience some improvements in standardized test scores at the end of their high school, but fare worse in school-based achievements and graduation outcomes. These findings highlight the importance of the potential trade-offs between attending more selective schools with better peer quality while receiving a lower ordinal rank in the ability distribution in the assigned school. In addition, the impacts of selective schools on students vary along the lines of the students’ own attitude towards studying as well as their middle school educational background. This substantial heterogeneity collectively highlights the importance of considering the students’ past educational background in interpreting how selective schools might impact students’ outcomes. In chapter 3, I investigate students’ preferences, strategic behaviors and welfare outcomes under a competitive school choice market by conducting a survey on school choice participants in two school districts in Vietnam. The original survey data on school choice participants, coupled with administrative data, afford me the opportunity to understand true preferences and strategies without involving strong assumptions on the students’ beliefs. In order to balance out their own preferences and chance of admission in such a competitive setting, the majority of students exhibit strategic behaviors. However, students from less advanced educational backgrounds tend to have large belief errors and are more likely to make strategic mistakes. Consequently, these students are at a disadvantage, as they find themselves among lower-achieving peers in their new schools. With preference data from the survey, I estimate the students’ preferences for school characteristics and find evidence of heterogeneity in students’ preferences for school characteristics: students from more advanced educational backgrounds value school selectivity and teacher qualification more than their peers. Using these estimates to evaluate students’ welfare under the current assignment mechanism as well as a counterfactual strategy-proof deferred acceptance algorithm, I find that switching to deferred acceptance algorithm can be welfare-improving, particularly for high-performing students. Overall, this paper provides a starting point to directly study the drawbacks of manipulable assignment mechanisms by using survey data and highlight the potential disparity in preferences and application strategies that can further widen the gap in educational mobility.
Geographic Areas
- Education--Economic aspects
- Public schools
- School choice--Econometric models
- School contests
This item is currently under embargo. It will be available starting 2026-05-26.
More About This Work
- DOI Copy DOI to clipboard
The Role of Education in Development
- First Online: 30 August 2019
Cite this chapter
- Tristan McCowan 6
Part of the book series: Palgrave Studies in Global Higher Education ((PSGHE))
1719 Accesses
1 Citations
Understanding the role of education in development is highly complex, on account of the slippery nature of both concepts, and the multifaceted relationship between them. This chapter provides a conceptual exploration of these relationships, laying the groundwork for the rest of the book. First, it assesses the role of education as a driver of development, including aspects of economic growth, basic needs and political participation. Second, it looks at the constitutive perspective, involving education as national status, human right and human development. Finally, it assesses the ‘other face’ of education and its negative impacts, as well as the specificities of higher education in relation to other levels.
This is a preview of subscription content, log in via an institution to check access.
Access this chapter
- Available as PDF
- Read on any device
- Instant download
- Own it forever
- Available as EPUB and PDF
- Compact, lightweight edition
- Dispatched in 3 to 5 business days
- Free shipping worldwide - see info
- Durable hardcover edition
Tax calculation will be finalised at checkout
Purchases are for personal use only
Institutional subscriptions
Almond, G., & Verba, S. (1963). The civic culture: Political attitudes and democracy five nations . London: Sage.
Book Google Scholar
Becker, G. S. (1962, October). Investment in human capital: A theoretical analysis. Journal of Political Economy, 70 (Suppl.), 9–49.
Article Google Scholar
Beckett, K. (2011). R. S. Peters and the concept of education. Educational Theory, 61 (3), 239–255.
Bengtsson, S., Barakat, B., & Muttarak, R. (2018). The role of education in enabling the sustainable development agenda . London: Routledge.
Boni, A., Lopez-Fogues, A., & Walker, M. (2016). Higher education and the post 2015 agenda: A contribution from the human development approach. Journal of Global Ethics, 12 (1), 17–28.
Google Scholar
Boni, A., & Walker, M. (2013). Human development and capabilities: Re-imagining the university of the twenty-first century . London: Routledge.
Boni, A., & Walker, M. (2016). Universities and global human development: Theoretical and empirical insights for social change . London: Routledge.
Bowles, S., & Gintis, H. (1976). Schooling in capitalist America: Educational reform and the contradictions of economic life . New York: Basic Books.
Bush, K., & Saltarelli, D. (2000). The two faces of education in ethnic conflict . Paris: UNICEF Innocenti Research Centre.
Brundtland Commission. (1987). Our common future (Report of the World Commission on Environment and Development). Oxford: Oxford University Press.
Bynner, J., Dolton, P., Feinstein, L., Makepiece, G., Malmberg, L., & Woods, L. (2003). Revisiting the benefits of higher education: A report by the Bedford Group for Lifecourse and Statistical Studies, Institute of Education . Bristol: Higher Education Funding Council for England.
Castells, M. (1994). The university system: Engine of development in the new world economy. In J. Salmi & A. Verspoor (Eds.), Revitalizing higher education (pp. 14–40). Oxford: Pergamon.
Coleman, J. S., Campbell, E. Q., Hobson, C. F., McPartland, J., Mood, A. M., Weinfeld, F. D., et al. (1966). Equality of educational opportunity . Washington, DC: U. S. Office of Education.
Dewey, J. (1966 [1916]). Democracy and education . New York: MacMillan.
Diamond, J. M. (1997). Guns germs and steel: The fate of human societies . New York: W. W. Norton.
Fraser, N. (1998). From redistribution to recognition? Dilemmas of justice in a ‘post-socialist’ age. In A. Philipps (Ed.), Feminism and politics . New York: Oxford University Press.
Freire, P. (1970). Pedagogy of the oppressed . London: Penguin Books.
Garrod, N., & Macfarlane, B. (2009). Challenging boundaries: Managing the integration of post-secondary education . Abingdon: Routledge.
Giroux, H., & McLaren, P. (1986). Teacher education and the politics of engagement: The case for democratic schooling. Harvard Educational Review, 56 (3), 213–240.
Green, A. (1990). Education and state formation: the rise of education systems in England, France and the USA . London: Macmillan.
Hanushek, E. (2013). Economic growth in developing countries: The role of human capital. Economics of Education Review, 73, 204–212.
Hanushek, E., & Woessmann, L. (2008). The role of cognitive skills in economic development. Journal of Economic Literature, 46 (3), 607–668.
hooks, b. (1994). Teaching to transgress: Education as the practice of freedom . London: Routledge.
Huntington, S., & Nelson, J. (1976). No easy choice: Political participation in developing countries . Cambridge, MA: Harvard University Press.
Klees, S. J. (2016). Human capital and rates of return: Brilliant ideas or ideological dead ends? Comparative Education Review, 60 (4), 644–672.
Lucas, R. E. (1988). On the mechanics of development. Journal of Monetary Economics, 22 (1), 3–42.
Luescher-Mamashela, T. M., Kiiru, S., Mattes, R., Mwollo-ntallima, A., Ng’ethe, N., & Romo, M. (2011). The university in Africa and democratic citizenship: Hothouse or training ground? Wynberg: Centre for Higher Education Transformation.
Mamdani, M. (2018). The African university. London Review of Books, 40 (14), 29–32.
Marginson, S. (2016). The worldwide trend to high participation higher education: Dynamics of social stratification in inclusive systems. Higher Education, 72, 413–434.
Marginson, S. (2017). Limitations of human capital theory. Studies in Higher Education, 44 (2), 287–301.
Martin, C. (2018). Political authority, personal autonomy and higher education. Philosophical Inquiry in Education, 25 (2), 154–170.
Mazrui, A. A. (1975). The African university as a multinational corporation: Problems of penetration and dependency. Harvard Educational Review, 45, 198.
McCowan, T. (2004). The growth of private higher education in Brazil: Implications for equity and quality. Journal of Education Policy, 19 (4), 453–472.
McCowan, T. (2009). Rethinking citizenship education: A curriculum for participatory democracy . London: Continuum.
McCowan, T. (2013). Education as a human right: Principles for a universal entitlement to learning . London: Bloomsbury.
McCowan, T. (2015). Theories of development. In T. McCowan & E. Unterhalter (Eds.), Education and international development: An introduction . London: Bloomsbury.
McCowan, T., Ananga, E., Oanda, I., Sifuna, D., Ongwenyi, Z., Adedeji, S., et al. (2015). Students in the driving seat: Young people’s views on higher education in Africa (Research Report). Manchester: British Council.
McGrath, S. (2010). The role of education in development: An educationalist’s response to some recent work in development economics. Comparative Education, 46, 237–253.
McGrath, S. (2014). The post-2015 debate and the place of education in development thinking. International Journal of Educational Development, 39, 4–11.
McMahon, W. W. (2009). Higher learning, greater good . Baltimore: John Hopkins Press.
Milbrath, L., & Goel, M. (1977). Political participation: How and why do people get involved in politics? (2nd ed.). Chicago: Rand McNally College.
Milton, S. (2019). Syrian higher education during conflict: Survival, protection, and regime security. International Journal of Educational Development, 64, 38–47.
Milton, S., & Barakat, S. (2016). Higher education as the catalyst of recovery in conflict-affected societies. Globalisation, Societies and Education, 14 (3), 403–421.
Mincer, J. (1981). Human capital and economic growth (Working Paper 80). Cambridge, MA: National Bureau of Economic Research. http://www.nber.org/papers/w0803.pdf .
Newman, J. H. (1947 [1852]). The idea of the university: Defined and illustrated . London: Longmans, Green.
Novelli, M., & Lopez Cardozo, M. (2008). Conflict, education and the global south: New critical directions. International Journal of Educational Development, 28 (4), 473–488.
Nussbaum, M. (2000). Women and human development: The capabilities approach . Cambridge, MA: Cambridge University Press.
Oketch, M. O., McCowan, T., & Schendel, R. (2014). The impact of tertiary education on development: A rigorous literature review . London: Department for International Development.
Parsons, S., & Bynner, J. (2002). Basic skills and political and community participation: Findings from a study of adults born in 1958 and 1970 . London: Basic Skills Agency.
Perkin, H. (2007). History of universities. In J. J. F. Forest & P. G. Altbach (Eds.), International handbook of higher education (pp. 159–206). Dordrecht: Springer.
Peters, R. S. (1966). Ethics and education . London: George Allen and Unwin.
Pherali, T., & Lewis, A. (2019). Developing global partnerships in higher education for peacebuilding: A strategy for pathways to impact. Higher Education. https://doi.org/10.1007/s10734-019-00367-7 .
Prakash, M. S., & Esteva, G. (2008). Escaping education: Living as learning in grassroots cultures (2nd ed.). New York: Peter Lang.
Rist, G. (2002). The history of development: From western origins to global faith . London: Zed Books.
Robertson, S. (2016). Piketty, capital and education: A solution to, or problem in, rising social inequalities? British Journal of Sociology of Education, 37 (6), 823–835.
Romer, P. M. (1986). Increasing returns and long-run growth. The Journal of Political Economy, 94 (5), 1002–1037.
Sachs, J. D. (2008). Common wealth: Economics . New York: Penguin Press.
Santos, B. de S. (2015). Epistemologies of the south: Justice against epistemicide . New York: Routledge.
Schultz, T. W. (1961). Investment in human capital. American Economic Review, 51, 1–17.
Sen, A. (1992). Inequality re-examined . Oxford: Clarendon Press.
Sen, A. (1999a). Development as freedom . New York: Oxford University Press.
Sen, A. (1999b). Democracy as a universal value. Journal of Democracy, 10 (3), 3–17.
Smith, A., & Vaux, T. (2003). Education, conflict and international development . London: DFID.
Stewart, F. (1985). Planning to meet basic needs . London: Macmillan.
Stiglitz, J. E. (2007). Making globalization work . London: Allen Lane.
Streeten, P. (1977). The basic features of a basic needs approach to development. International Development Review, 3, 8–16.
Takayama, K., Sriprakash, A., & Connell, R. (2015). Rethinking Knowledge production and circulation in comparative and international education: Southern theory, postcolonial perspectives, and alternative epistemologies. Comparative Education Review , 59 (1), v–viii.
Tilak, J. B. G. (2003). Higher education and development in Asia. Journal of Educational Planning and Administration, 17 (2), 151–173.
Tomaševski, K. (2001). Human rights obligations: Making education available, accessible, acceptable and adaptable. Right to Education Primers No. 3. Gothenburg: Novum Grafiska.
Unterhalter, E. (2003). The capabilities approach and gendered education: An examination of South African complexities. Theory and Research in Education, 1 (1), 7–22.
Willis, P. (1978). Learning to Labour: How working class kids get working class jobs . Aldershot Gower: Saxon House/Teakfield.
Winch, C. (2006). Graduate attributes and changing conceptions of learning. In P. Hager & S. Holland (Eds.), Graduate attributes, learning and employability (pp. 67–89). Dordrecht: Springer.
Wolff, J., & de-Shalit, A. (2013). On fertile functionings: A response to Martha Nussbaum. Journal of Human Development and Capabilities, 14(1), 161–165.
World Bank. (1999). Knowledge for development. World Development Report. New York: Oxford University Press.
Download references
Author information
Authors and affiliations.
UCL Institute of Education, London, UK
Tristan McCowan
You can also search for this author in PubMed Google Scholar
Corresponding author
Correspondence to Tristan McCowan .
Rights and permissions
Reprints and permissions
Copyright information
© 2019 The Author(s)
About this chapter
McCowan, T. (2019). The Role of Education in Development. In: Higher Education for and beyond the Sustainable Development Goals. Palgrave Studies in Global Higher Education. Palgrave Macmillan, Cham. https://doi.org/10.1007/978-3-030-19597-7_2
Download citation
DOI : https://doi.org/10.1007/978-3-030-19597-7_2
Published : 30 August 2019
Publisher Name : Palgrave Macmillan, Cham
Print ISBN : 978-3-030-19596-0
Online ISBN : 978-3-030-19597-7
eBook Packages : Education Education (R0)
Share this chapter
Anyone you share the following link with will be able to read this content:
Sorry, a shareable link is not currently available for this article.
Provided by the Springer Nature SharedIt content-sharing initiative
- Publish with us
Policies and ethics
- Find a journal
- Track your research
Thank you for visiting nature.com. You are using a browser version with limited support for CSS. To obtain the best experience, we recommend you use a more up to date browser (or turn off compatibility mode in Internet Explorer). In the meantime, to ensure continued support, we are displaying the site without styles and JavaScript.
- View all journals
- My Account Login
- Explore content
- About the journal
- Publish with us
- Sign up for alerts
- Open access
- Published: 17 April 2024
How popularising higher education affects economic growth and poverty alleviation: empirical evidence from 38 countries
- Jian Li ORCID: orcid.org/0000-0002-3228-8163 1 na1 ,
- Eryong Xue ORCID: orcid.org/0000-0002-7079-5027 2 na1 ,
- Yukai Wei ORCID: orcid.org/0000-0002-5202-7307 2 &
- Yunshu He ORCID: orcid.org/0000-0003-4814-9835 2
Humanities and Social Sciences Communications volume 11 , Article number: 520 ( 2024 ) Cite this article
1016 Accesses
3 Altmetric
Metrics details
The popularisation of higher education supports UNESCO’s aim of developing inclusive and equitable quality education to achieve the fourth Sustainable Development Goal. However, the effect of popularising higher education on economic growth and poverty alleviation remains unexplored. Therefore, this study investigated the effects of higher education and adult education within populations (popularisation of higher education) on economic growth (gross domestic product; GDP) and the poverty line using panel data from 38 countries. OLS and quantile regression were performed using data for the period 1995–2021 extracted from the OECD and World Bank databases. The results showed that the population segments with higher education had a significantly positive impact on GDP growth. Moreover, an increased proportion of the population with higher education, of working age, was found to be a contributing factor to GDP growth. Popularising higher education also played a positive role during the initial stage of social and economic development. This study also highlighted that popularising higher education play a key role to influence a country’s educational development and scientific and technological innovation drives the deepening of a country’s economy. It suggested that both national and local governments worldwide should pay much attention to the popularisation degree of higher education to greatly improve the innovative ability of talents and scientific and technological innovation in higher education for both the economic growth and poverty alleviation.
Similar content being viewed by others
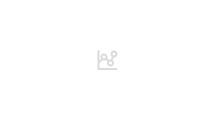
The impact of artificial intelligence on employment: the role of virtual agglomeration
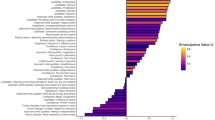
Worldwide divergence of values
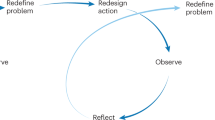
Participatory action research
Introduction.
The popularisation of higher education critically contributes to UNESCO’s efforts to realise the fourth Sustainable Development Goal of inclusive and equitable quality education (Ainscow, 2020 ; Bamberger and Kim, 2022 ).Popularisation of higher education expands the scale of higher education and its high growth rate introduces considerable challenges to the management structure of higher education, triggering a series of theoretical and practical concerns relating to the nature and function of higher education (Balestra and Ruiz, 2015 ; Brand, 2021 ). Given that education and social and economic development are mutually reinforcing, the expansion of higher education leads to an ascending spiral of development for individuals and/or economies. By contrast, a lack of education or early withdrawal from education leads to a downward spiral for them (Camilla, 2023 ). This relationship between education and development undergirds the model of poverty alleviation based on the return on education (Decancq, 2017 ). The previous studies emphasise the importance of the return on education as a multidimensional anti-poverty mechanism and thus a key factor in poverty alleviation (Fang et al., 2022 ; Chelli et al., 2022 ; Garritzmann, 2016 ). For example, return on education is the key factor enabling a transition from poverty alleviation through education to poverty alleviation through education (Gillian et al., 2021 ; Gong and Hong, 2021 ). Poverty alleviation is realised through an interlinking of these two processes and the promotion of the latter (Granata, 2022 ; Habibi and Zabardast, 2020 ). The educational resources can meet the needs of the poor mainly through the return on education at the levels of survival and life quality. In addition, the previous studies highlighted that, with a continuous expansion in the scale of higher education, its economic effect gradually appears to become marginal (Hoeller et al., 2014 ). The density of colleges and universities worldwide has increased considerably in recent years, but it is still inadequate to meet increasing demands resulting from the ongoing popularisation of higher education (Jericho, 2016 ). The increase in the number of colleges and universities has a positive effect in promoting economic development but with marginal benefits. (Julian, 2018 ).
Through reviewed the current relevant studies, it is found that there have limited studies that have simultaneously explored the effects of popularising higher education on economic growth and poverty alleviation. The previous research revealed that most studies have focused on the relations between popularisation of higher education and economic growth. However, a few empirical investigations have examined the effect of population segments with higher education and adult education (popularisation of higher education) on economic growth (GDP) and poverty reduction. Considering the scope and limitations of previous studies, it aimed to address the above research gap by investigating the effect of a population segment with high levels of higher education and adult education (popularisation of higher education) on economic growth (GDP) and the poverty line at a wide scale using panel data from 38 countries. The main research questions addressed in this study are as follows.
Q1: What is the effect of a population segment with higher education on GDP growth?
Q2: What is the effect of adult education on GDP growth?
Q3: What impact does a population segment with higher education have on reducing the proportion of those experiencing poverty?
Q4: What is the relation between an increased level of adult education and the proportion of the population experiencing poverty?
All these questions are relevant to an exploration of the effect of the population segment with higher education and adult education (popularisation of higher education) on economic growth (GDP) and the poverty line. This study is divided into several sections: the first section concentrates on examining the effect of popularising higher education on economic growth and the poverty line, the relationship between popularisation of higher education and poverty alleviation, and the relationship between popularisation of higher education and poverty alleviation. In the second section of method, to address this research gap, this study performed OLS and quantile regressions using data extracted from the OECD and World Bank databases for the period 1995–2021. An OLS regression model and a panel quantile model were used to analyse the effect of a population segment with higher education and adult education (popularisation of higher education) on economic growth (GDP) and the poverty line within 38 OECD countries. The impact of the proportion of people aged 24–64 years and 25–34 years who had completed higher education in relation to their peers on GDP and the proportion of people living in poverty in 38 OECD countries have been measured and analysed. The results and discussion have been provided at the last.
Literature review
The effect of popularising higher education on economic growth.
The population segment with higher education is regarded as an important contributor to economic growth, generating scientific knowledge and providing labour, which in turn increases human capital and productivity (Jungblut, 2017 ; Kalamova, 2020 ; Liu, 2017 ). As the scale of higher education expands, the emergence of knowledge power as a large-scale global phenomenon reflects the important role of an expanded educated labour force in the advancement of science and technology and the economy. For example, the relationship between higher education and economic development in European Union countries between 1997 and 2016 was analysed. Their findings revealed a statistically significant correlation between expanding higher education and economic growth in the selected countries. The one-way cause-and-effect relationship between education and economic development in these countries suggests that an increase in the proportion of the population enroled in higher education boosts economic performance. In addition, using a survey sample of 35 households, a retrospective study in Brazil, examined the role of educational expansion in reducing income inequality and poverty. Its findings suggest that it would take decades to reduce inequality and poverty in this country and that this outcome could only be achieved through a major expansion of the higher education sector. The growth needed to achieve this outcome would be considerable (Lamichhane et al., 2021 ). This reduction in inequality and poverty could only be achieved if optimistic assumptions about growth, matching job skills and the return on education do not fall short. In brief, education is not a panacea for reducing poverty and inequality. How three major stages of education contributed to the growth in labour productivity in 125 countries during the period 1999–2014 was also explored. They found that human capital is consistent with the educational returns of an average number of years of formal education at the levels of primary, secondary, and higher education. Their analysis showed that higher education had the greatest impact on labour productivity in the economies under study (Ledger et al., 2019 ). In addition, popularising higher education plays an important role in promoting economic growth, as the scale of higher education can guarantee the scale of human resources development by improving the quality of human resources and cultivating and distributing innovative scientific and technological talents. The scale of higher education guarantees the spread of science and technology and the popularisation of scientific and technological achievements (Mathias, 2023 ; Megyesiova and Lieskovska, 2018 ). The expanded scale of higher education worldwide has a spatial spillover effect on economic growth, which is strengthened through international cooperation in the fields of science and technology.
Popularising higher education also plays a direct role in cultivating and transporting scientific and technological talents to promote international scientific and technological cooperation (Mitic, 2018 ; Özdoğan Özbal, 2021 ; OECD, 2022 ; Pinheiro and Pillay, 2016 ). The scale of postgraduate education inhibited the total number of scientific and technological innovation achievements, indicating that there may be a trade-off between ‘quantity expansion’ and ‘quality upgrading’ of scientific and technological innovation achievements. Nevertheless, the positive effect on the number of high-tech innovation outcomes is significant, indicating that the supporting effect of graduate education on scientific and technological innovation is mainly concentrated in the high-tech fields (Pinheiro and Pillay, 2016 ; Rowe, 2019 ; Sahnoun and Abdennadher, 2022 ). The ‘talent increment’ of regional expansion and the ‘resource stock’ of graduate education have a combined promoting effect on high-tech innovation. There are differences in the effect of graduate education supporting high-tech innovation among provinces with different characteristics relating to the development of graduate education. The incremental expansion of high-quality talent is essential for enhancing the efficiency of material capital and stabilising the advantage of resource stocks. Using education statistics from OECD countries, Russia, and several other countries that participate in OECD education research, comparative and correlational analysis methods were applied to analyse how the scale of growth in young people’s participation in higher education is reflected in changes in their employment and economic activity. The results of their analysis showed that the growth in economic activity involving young graduates with a master’s degree exceeded that of college graduates after the 2009 financial crisis, and graduates fared better in the 2020 crisis, which was triggered by the COVID-19 pandemic.
The effect of popularisation of higher education on poverty alleviation
Popularisation of higher education is regarded as an essential factor contributing to poverty alleviation (Samo, 2022 ; Adams, 2013 ; Zapp, 2022 ). The higher education’s role in promoting economic growth can only be fully realised through the cultivation of talents suitable for the actual development situation of the country. Countries with food shortages, for example in Africa, also need to procure and train the right agricultural talent. Key drivers of sustainable agricultural production include access to improved technologies, sustainable growth of human, biological and natural resource capital, improvements in institutional performance and a favourable economic policy environment. Higher education graduates with the necessary ‘soft skills and business skills constitute an important pillar. Chakeredza ( 2008 ), who explored the effect of popularising higher education on poverty alleviation, suggested that the number of hungry people in Africa will continue to increase. Higher education in agriculture must be transformed, and efforts must focus on retaining faculty and on reviewing and redesigning institutional management systems, curriculum content and education delivery.
There are many reasons for poverty, with a lack of education being an important one. Insufficient quality education leads to educational poverty. Using PISA data, Agasisti et al. ( 2021 ) investigated the extent of educational poverty in European countries, considering its incidence, breadth, depth and severity. For this study, they adopted an additive multidimensional poverty measure proposed by Alkirew and Foster. Their findings indicated that between 2006 and 2015, the depth and severity of poverty decreased in most of the countries under study. Moreover, the incidence of educational poverty in many European countries was related mainly to student characteristics and school factors. The expansion of higher education has a positive effect on economic development and poverty reduction by improving work skills within the labour force. Increased enrolment in higher education encourages individuals born in families with low education levels to avail of higher education opportunities. Evidently, the expanded scale of higher education in the process of promoting economic growth has enhanced the equity effect of intergenerational social mobility. The expansion of higher education improves total factor productivity, thus promoting economic transformation and advancement globally (Samo, 2022 ; Adams, 2013 ; Zapp, 2022 ). Furthermore, the previous studies have shown that the structure of higher education talent training has a significant impact on economic development. Therefore, government departments need to make constant efforts to improve relevant systems and promote the optimisation and upgrading of the structure of higher education talent training to meet the needs of future economic development.
Theoretical underpinnings
The relationship between education and economic growth is a classic issue in the study of educational economics. For example, in Solow’s view, the growth of per capita output comes from per capita capital stock and technological progress, but capital investment has the problem of diminishing marginal returns, and the long-term sustainable development of the economy depends on technological progress (Solow, 1957 ). The emphasis on technological progress is a very important point in Solow’s growth theory. It was Schultz who systematically analyzed the contribution of education to economic growth. Influenced by the progress of economic growth theory and national accounting methods, Schulz proposed human capital theory in the process of explaining Solow residuals (Schultz, 1961 ). believes that once human capital is included in economic growth, it will solve the paradoxes and puzzles faced in economic growth research. Starting with the difference in income of different types of workers in the labour market, he found that education and health factors are the main reasons for the income difference, and further clarified that the reason for the income difference is the difference in labor productivity (Schultz, 1961 ). Schultz ( 1961 ) believes that human resources include the quantity and quality of labor, and he mainly focuses on the skills and knowledge of people who can improve labor productivity. As for how to measure human capital investment, Schulz believes that the cost of human capital can be measured in the same way as physical capital. Lucas ( 1988 ) focuses on the mechanism of human capital accumulation and why human capital does not show diminishing marginal returns like physical capital. Lucas divides the effect of human capital into internal effect and external effect. Romer ( 1990 ) internalised technological progress, revealed the relationship between human capital and technological progress, and proposed that the stock of human capital determines the economic growth rate, and it is human capital rather than population that determines economic growth. Romer starts with three hypotheses: first, technological progress is central to long-term economic growth; Second, technological progress is formed by people’s response to market incentives, and market incentives determine technological progress. Third, technology is a special kind of product, and once the cost of the initial input is produced, the technology can be reproduced indefinitely at no cost or very low cost.
In other words, higher education is more about improving students’ ability and productivity, thereby increasing students’ income, and promoting economic growth. Higher education mainly affects economic growth through two aspects: one is the same as Schulz’s improvement of individual ability, and the internal effect of human capital, which directly affects the production process (Schultz, 1961 ). Second, Lucas emphasised the external effect of human capital, and the comprehensive effect of human capital on the whole society, which has the characteristics of increasing marginal benefit (Lucas, 1988 ). It emphasises that the human capital invested in technological innovation and the existing knowledge and technology stock of the whole society jointly determine technological innovation.
Research hypotheses and analytical model
In this study, an OLS regression model and a panel quantile model were used to analyse the effect of a population segment with higher education and adult education (popularisation of higher education) on economic growth (GDP) and the poverty line within 38 OECD countries. The study’s hypotheses were as follows:
Hypothesis 1: The effect of a population segment with higher education has a positive impact on GDP growth.
Hypothesis 2: Some level of adult education has a positive impact on GDP growth.
Hypothesis 3: A population segment with higher education has a positive impact by reducing the proportion of the population experiencing poverty.
Hypothesis 4: An increase in the level of adult education has a positive impact by reducing the proportion of the population experiencing poverty.
The widely used Mankiw-Romer-Weil model was applied in this study. The overall level of development of higher education and the popularisation of higher education were considered core elements that independently promote economic development and alleviate poverty. The following model was constructed by incorporating the variable of quality higher education into the Solow model:
where Y it refers to the output of i country in t year. The independent variables Qit and P it respectively represent the scale of development and the degree of popularisation of higher education in i country in t year. The following specific model was constructed:
The independent variables were the proportion of people aged 25–64 years with higher education (A) and the proportion of people aged 25–34 years with higher education within the same age group (B). The first variable reflects the population segment that has completed higher education and can work in the corresponding age group. The second reflects the degree of popularisation of higher education. The proportion of those who have completed higher education in relation to their peers is in the normal state, which can reflect the enrolment rate for the previous process of higher education, thus indicating the degree of popularisation of higher education.
The dependent variables were GDP and the poverty line (D). GDP is a measure the overall level of a country’s economic and social development. The poverty line refers to the proportion of people living on less than US$1.25 a day as a percentage of the country’s total population or the proportion of people living in poverty. Thus, it reflects the level of equity in social development. The figure of US$2.15 is used in the World Bank’s index and is based on the purchasing power parity in 2017 (see Table 1 ).
Data sources and selection of variables
This study measured the impact of the proportion of people aged 24–64 years and 25–34 years who had completed higher education in relation to their peers on GDP and the proportion of people living in poverty in 28 OECD countries. Specifically, this study assessed the impact of the overall level of development of higher education and the degree of its popularisation (the breadth of development of higher education) on GDP (the height of development of economic and social development) and the poverty line (the breadth of development of economic and social development). Data were sourced from the OECD database and the World Bank website covering the period 1995–2021. This study selected 38 OECD countries for this study: the United States, UK, France, Germany, Italy, Canada, Ireland, the Netherlands, Belgium, Luxembourg, Austria, Switzerland, Norway, Iceland, Denmark, Sweden, Spain, Portugal, Greece, Turkey, Japan, Finland, Australia, New Zealand, Mexico, the Czech Republic, Hungary, Poland, South Korea, Slovakia, Chile, Slovenia, Estonia, Israel, Latvia, Lithuania Colombia and Costa Rica. Figure 1 shows the distribution of the 38 OECD countries. Of these countries, 20 were founding members of the OECD when it was established in 1961, while the remaining 18 subsequently became members. After 1994, OECD membership expanded rapidly. Five new members were added within three years. OECD then entered a period of accelerated development, and its operations and advancement reached an optimal stage. Therefore, this study selected data from the OECD database and the World Bank website covering the period 1995–2021 to explore the relationship between higher education and economic and social development in OECD member countries.
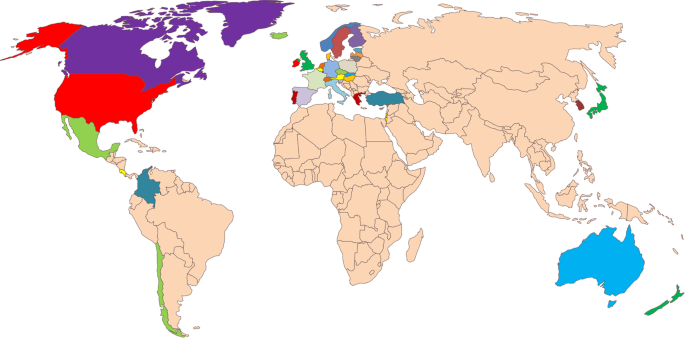
It expresses the geographical relations of the Atlantic region and simplifies the latitude and longitude lines and country symbols, highlighting the geographical distribution by highlighting OECD countries in color and other countries in apricot color.
The impact of the population segment with higher education on GDP growth
This study explored the impact of the population segment with higher education on GDP, taking the proportion of people aged 25–34 years who had completed higher education (B) and the proportion of people aged 25–64 years who had completed higher education (A) as the independent variables for the OLS regression. The square value of model R was 0.097, indicating that the two independent variables could explain 9.73% of the change in GDP. The model passed an F test ( F = 46.137, p = 0.000 < 0.05), indicating that at least one of the two independent variables impacted the GDP regression coefficient (C). The following formula was used:
The final analysis revealed that the regression coefficient value of A was 1.553 and the significance level was 0.01 ( t = 7.141, p = 0.000 < 0.01). Therefore, A had a significantly positive influence on C. Accordingly, the proportion of the population aged 25–64 years who had completed higher education, that is, the overall level of development of higher education was found to have a positive impact on GDP. The influence coefficient value was 1.533, indicating that an increase in the proportion of the population with completed higher education led to an increase in GDP.
The regression coefficient value of B was −0.813 at a 0.01 level of significance ( t = −4.300, p = 0.000 < 0.01), indicating that B had a significantly negative influence on C. The proportion of the population aged 25–34 years who had completed higher education, that is, the degree of popularisation of higher education had a negative effect on GDP, and the influence coefficient value was −0.813.
The negative impact on economic and social development caused by an increase in the popularity of higher education and the proportion of young people’s higher education experience may be attributed to the excess capacity of higher education. The development of higher education should be adapted to the national context. An excess of higher education and a lack of investment lead to a rise in the social cost of education and a decline in social outputs, which hinder social and economic development. At the same time, young people aged between 25 and 34 years occupy the position of’ export’ in the education process. With the increasing popularity of higher education, the supply of talents in the labour market generated through the recruitment of former higher education exceeds the demand for graduates with higher education within recruiting organisations. Consequently, issues such as wasted educational resources and knowledge, unemployment, excessive education, excess talents, an imbalance in the structure of higher education, excessive expansion and decreasing compatibility undermine economic operations and hinder GDP growth.
In this study, the variance decomposition and Pearson coefficient based on covariance calculation were analyzed. The variable of the number of 25–34-year-old who have completed higher education as a percentage of their peers explains 50.74% of the change in GDP. The variable of the proportion of 25–64-year-old who have completed higher education explains 49.26% of the change in GDP. The variable of 25- to 34-year-olds who completed higher education as a percentage of their peers explained 45.88% of the change in poverty line. The variable of the proportion of people aged 25–64 who have completed higher education explains 54.12% of the change in GDP (See Table 2 ).
The proportion of people aged 25–34 who have completed higher education in their peers and the proportion of people aged 25–64 who have completed higher education in their peers, GDP and poverty line showed significant correlation coefficients. The correlation between the proportion of people who have completed higher education at the age of 25–34 and the proportion of people who have completed higher education at the age of 25–64 is 0.931, and shows a significance of 0.01, which indicates that there is a significant positive correlation between the proportion of people who have completed higher education at the age of 25–34 and the proportion of people who have completed higher education at the age of 25–64. The correlation between the proportion of the number of people who have completed higher education at the age of 25–34 and the GDP is 0.209, and the significance is 0.01, which indicates that there is a significant positive correlation between the number of people who have completed higher education at the age of 25–34 and the GDP. The correlation between the number of people who have completed higher education and the poverty line at the age of 25–34 is −0.365, with a significance of 0.01, indicating a significant negative correlation between the number of people who have completed higher education and the poverty line at the age of 25–34 (See Table 2 ).
White test and BP test were used in this study. The test null hypothesis is that the model has no heteroscedasticity. The table above shows that both tests reject the null hypothesis ( p < 0.05), indicating that the model does have heteroscedasticity. When there is a heteroscedasticity problem, Robust and robust standard false regression is used (See Table 3 ).
The impact of a population segment with higher education on the poverty line
This study also explored the impact of a population segment with higher education on the poverty line. Specifically, this study performed an OLS regression in which the proportion of people aged 25–34 years who had completed higher education (B) and the proportion of those aged 25–64 years who had completed higher education (A) were the independent variables. As Table 2 shows, the R squared value was 0.134. This means that variables A and B could explain 13.37% of the change in the poverty line (D). The model passed the F test ( F = 48.771, p = 0.000 < 0.05), which means that at least one variable (A or B) had an impact on the poverty line. The formula for the change in the poverty line was expressed as follows:
The final analysis revealed that the regression coefficient value of the proportion of people aged 25–64 years who had completed higher education (A) was 0.005 but with no significance ( t = 0.428, p = 0.669 > 0.05), indicating that the population segment with higher education did not have an impact on the poverty line.
The regression coefficient value of the proportion of people aged 25–34 years who had completed higher education (B) was −0.048 at a significance level of 0.01 ( t = −4.305, p = 0.000 < 0.01), which means that in relation to their peers, the proportion of people aged 25–34 years who had completed higher education had a significantly negative impact on the proportion of poor people. A higher proportion of people aged 25–34-years who had completed higher education corresponded to a higher penetration rate of higher education and a lower proportion of those living in poverty. This phenomenon can be attributed to OECD’s support for the development of higher education in various countries. When the development of higher education reaches a certain level, the reduction of the proportion of the population segment experiencing poverty will no longer be affected by a simple expansion of the scale of extended higher education and the superposition of the total number of highly educated human resources. It will be influenced more by the reasonable distribution of educational resources and educational equity within higher education and its popularisation, that is, the increase in the proportion of the school-aged population aged 25–34 years based on the increase of the previous enrolment rate (see Table 4 ).
The effect of adult education on GDP growth
For quantile regression analysis, a total of nine models (with decimal points ranging from 0.10 to 0.90 and at intervals of 0.10) were estimated in this analysis, which aimed to explore the impact of the independent variables A and B on the dependent variable, GDP (C). When the quantile value was between 0.1 and 0.3, the proportion of the population aged 25–64 years who had completed higher education (A) had no significant positive impact on GDP growth, indicating that the development of higher education did not significantly affect economic and social development in poorer OECD countries. When the quantile value was between 0.4 and 0.6, the level of development of higher education had a significantly negative impact on economic and social development. Thus, for a country that had developed over a period, the advancement of higher education required multiple inputs, such as capital, material, and human resources.
During the early stage of the development of higher education, such inputs may, however, have a negative and weakening impact on social and economic development. The added cost of education and the lag between the output of educational achievements and the input of talents puts increased pressure on economic and social development during a certain period. When the quantile value was 0.7 or higher, the improvement of the overall level of higher education had a significantly positive impact on GDP growth, indicating the realisation of the talent training outcomes of higher education. Teaching and research outcomes were thus transformed into socially productive resources and power, with talents with higher education contributing to economic and social development.
When the quantile value was 0.1, the proportion of people aged 25–34 years who had completed higher education in relation to their peers (variable B), indicating the popularisation of higher education, had no significant impact on GDP growth. Thus, in extremely backward countries, the popularisation of higher education had little effect on economic and social development. When the quantile value ranged between 0.2 and 0.6, the popularisation of higher education had a significantly positive effect on GDP growth, indicating its contribution to economic growth.
When the quantile value was 0.7, the influence of variable B on variable C was no longer significant, indicating that social development would soon face the problem of overcapacity in higher education. When it exceeded 0.7, the ratio of eligible people aged 25–34 years who had completed higher education in relation to their peers had a significantly negative impact on GDP growth, revealing that with the development of the economy, society and education, higher education had become overexpanded. Thus, the cost of investing in education exceeded the social benefits, leading to overcapacity whereby the supply of higher education talents exceeded the demand. This situation led to wasted educational resources and excessive competition of talents, hindering economic growth (See Table 5 ).
The increased level of adult education and the proportion of the population experiencing poverty
Using the same model, this study explored the influence of the independent variables, A and B, on the poverty line (dependent variable D). The proportion of the population aged 25–64 years who had completed higher education (independent variable A) had no significant influence on the proportion of the population living in poverty, indicating that popularisation of education and economic and social development have been achieved to a certain extent in OECD countries, and improvements targeting the population experiencing poverty could no longer be achieved simply by increasing the volume and quantity of higher education. When the quantile value was 0.1, the proportion of people aged 25–34 years who had completed higher education in relation to their peers (independent variable B) had no significant effect on the proportion of the population experiencing poverty (dependent variable D). Therefore, the strategy of increasing higher education enrolment and the ratio of the eligible population through the fair allocation of educational resources, and thus the popularisation of education, would not be effective for a small population segment experiencing poverty. In other words, the population segment experiencing poverty in highly developed countries is less receptive to the popularisation of higher education. When the quantile value was 0.2, the independent variable, B, had a significantly positive impact on the dependent variable D, that is, an increase in the popularity of higher education led to an increase in the population segment experiencing poverty. This phenomenon can be interpreted as reflecting the inherent disadvantages of the welfare state in the field of education. A rise in the number of eligible young people aged 25–34 years who have completed higher education reflects the development trend of higher education towards fairness and popularisation following the redistribution of higher education resources.
The fair distribution of higher education resources leads to a lack of competition in the areas of teaching and career development. To a certain extent, reducing students’ willingness and enthusiasm to work may lead to poverty caused by the failure to achieve teaching results. When the quantile value was between 0.3 and 0.4, the independent variable, B, had no significant influence on the dependent variable D. In relatively poor countries, the popularisation of higher education contributes little to reducing the degree of poverty, so it may be necessary to explore ways of alleviating poverty from the perspective of improving the overall level and expanding the scale of basic higher education. When the quantile value was 0.5 or above, the independent variable B had a significantly negative impact on the dependent variable D, indicating that for countries with a relatively high proportion of their population experiencing poverty, the following strategy would be more effective.
Considering the quantile data, this study deemed that the degree of sensitivity of countries at different stages of economic development to the level of development and popularisation of higher education could be more intuitively evaluated using a radar map (see Fig. 2 ). Countries with sub-points 0.1–0.9 were defined along a spectrum as extremely backward, backward, moderately backward, slightly backward, moderate, preliminarily developed, moderately developed, developed, and highly developed. From the perspective of economic development, increasing the proportion of young people who complete higher education and popularising higher education had an obvious positive effect in backward and medium-developed countries, whereas the effect in highly developed countries was not obvious. Overall, the sensitivity of OECD countries to the high level of education penetration was found to be higher than the level of development of higher education. From the perspective of equitable economic development, the overall level of development of higher education had no significant impact on the poverty link in OECD countries, whereas OECD countries with differing economic development backgrounds and at varying stages of development evidenced relatively significant and stable sensitivity to the proportion of young and middle-aged people who completed higher education and the popularisation of higher education.
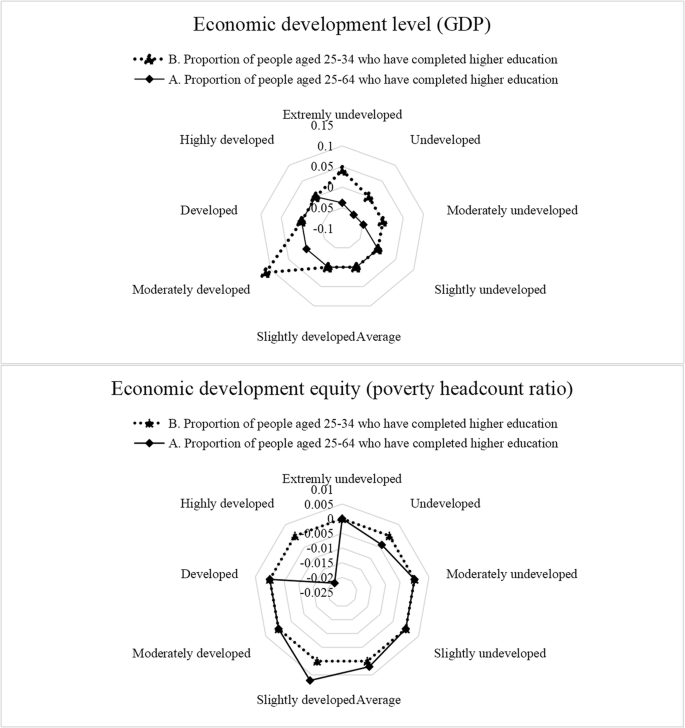
The dashed line represents the proportion of people aged 25–34 years who have completed higher education. The solid line represents the proportion of people aged 25–64 years who have completed higher education, the impact of the overall level of higher education.
Our findings indicated that population segments with higher education had a significantly positive impact on GDP growth in 38 OECD countries. An increase in the proportion of the population segment of working age who completed higher education was found to contribute to GDP growth. Moreover, an improvement in the popularity of higher education played a positive role during the initial stage of economic and social development.
At the same time, oversupply and overcapacity may result from a continuous improvement of higher education. A very large number of young people who have completed higher education can lead to excessive competition and wasted academic qualifications (Mathias, 2023 ; Megyesiova and Lieskovska, 2018 ). In turn, higher education knowledge unemployment, overinvestment, a structural imbalance, disorderly expansion and wasted resources can occur, which have detrimental impacts on economic operations.
Some studies have shown that strengthening the quality of higher education helps to improve cognitive abilities within the labour force, thereby enhancing the growth of the knowledge economy (Ainscow, 2020 ; Bamberger and Kim, 2022 ). Other studies have reported regional heterogeneity relating to the marginal effect of improving the quality of higher education on economic growth. Some scholars have analysed the influence of the quality of higher education on economic development from the perspective of human capital investment theory. Their findings indicate that the quality of higher education determines the composition and growth trend of social human capital. Because of differences in the degrees of development of different economies, the quality of higher education has a phased influence on economic growth (Balestra and Ruiz, 2015 ; Brand, 2021 ). Case studies of African developing countries by international scholars have revealed that quality factors are key to realising the economic development function of higher education. From the perspectives of both efficient financial investments by states in education poverty alleviation and the effects of economic, time and emotional investments of poor families and individuals in education poverty alleviation, it is necessary to take the return on education into consideration. Moreover, it is important to respond to reasonable concerns regarding the return on education for poor people and to strengthen their cognitive capacities to rationalise as well as their expectations regarding returns on education (Li et al., 2023 ). In this way, the intention to participate and behaviour of anti-poverty education will be generated, and the strategic upgrading of poverty alleviation combined with the promotion of aspirations and cognitive capacities will be emphasised.
Implications
Our use of panel data from 38 countries to deepen understanding of the effect of popularising higher education on economic growth and poverty reduction also has practical implications. The economic, social, and higher education undertakings in OECD countries evidence a certain level of development. The population segment with higher education has no significant impact on reducing the proportion of the population segment experiencing poverty. Simply increasing the proportion of people who complete higher education and expanding the scale of higher education will not effectively reduce poverty (Li and Xue, 2021 ). Providing more educational opportunities to poor people through the slanting of educational resources can help to reduce the proportion of poor people (Ainscow, 2020 ; Bamberger and Kim, 2022 ). For example, popularising higher education plays a key role to influence a country’s development level and scientific and technological innovation drives the deepening of a country’s economy (Bamberger and Kim, 2022 ). Technological progress is the core of economic growth, scientific and technological innovation brings technological change and development in all aspects, human capital promotes economic growth, and higher education trains talents and improves the capital attribute of human (Camilla, 2023 ). For endogenous economic growth theory, the economy does not rely on external forces to achieve sustained growth, and endogenous technological progress determines sustained economic growth. Popularising higher education worldwide brings the accumulation of human capital, improves the quality of workers, and scientific and technological innovation makes technological progress and high-quality economic development, practically. Human capital accumulation is also the process of continuous input of labour force, which covers the accumulation of human capital by labour force factors in formal education, training, and other learning processes. From the perspective of human capital, popularising higher education is the most direct and efficient way to promote the accumulation of human capital and improve the quality of labour force (Balestra and Ruiz, 2015 ; Brand, 2021 ). The popularisation degree of higher education is one of the important indicators to measure the development level of a country’s economic, and it is also the common trend of the development of higher education in all countries after World War II. In this transitional era, how to continue the achievements of higher education in the popular era and solve the existing problems as soon as possible is the heavy responsibility of our times. Therefore, at the initial stage of popularisation of higher education, it is necessary to re-examine the process of higher education popularisation globally and explore the internal logics between the popularisation of higher education and Sustainable Development Goal of inclusive and equitable quality education (Ainscow, 2020 ; Bamberger and Kim, 2022 ).
For policy suggestions, this study suggests that both national and local governments worldwide should pay much attention to the popularisation degree of higher education to greatly improve the innovative ability of talents and scientific and technological innovation in higher education. For example, they could promote scientific and technological innovation in an organised manner to serve national and regional economic and social development. Faced with the current situation in which global higher education has entered a stage of popularisation and new challenges and problems in serving regional economic and social development, national governments should continue to optimise the distribution and structure of higher education resources to support different regions, focusing on the major strategy of enhancing national competitiveness, serving economic and social development, and promoting common prosperity.
Contributions
This study novelty contributes on examining how popularising higher education affects economic growth and poverty alleviation, conceptually, methodologically, and practically. For instance, this study focuses on epitomising the conceptual and analytical model to explore the effects of higher education and adult education within populations (popularisation of higher education) on economic growth (gross domestic product; GDP) and the poverty line. In addition, this study novelty combines both Mankiw-Romer-Weil model Solow model to investigate the effects of higher education and adult education within populations on economic growth and the poverty through OLS regression model and quantile model. For the practical aspect, this study practically uncovers the implicit significance of the popularisation of higher education for advocating UNESCO’s aim of developing inclusive and equitable quality education to achieve the fourth Sustainable Development Goal.
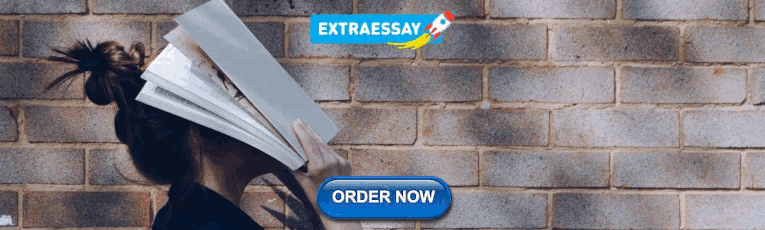
Limitations
This study had some limitations. Data could have been collected from a larger sample of OECD countries to explore the effect of population segments with higher education and adult education (popularisation of higher education) on economic growth (GDP) and the poverty line. In addition, a qualitative component could be included in future studies to uncover the cultural and historical contexts of the effect of popularising higher education on economic growth and poverty reduction at the local level. Future studies should also investigate the causal relationship between the popularisation of higher education and economic growth. Additional empirical data and advanced research methods can be used to enable a shift from correlation to causality.
In conclusion, this study examined the effect of the population segment with higher education and adult education (popularisation of higher education) on economic growth (GDP) and the poverty line using panel data from 38 countries. The population segment with higher education was found to have a significant positive impact on promoting GDP growth. An increase in the proportion of the working-age population segment that had completed higher education was evidently conducive to GDP growth. Popularisation of higher education was also found to play a positive role in the initial stage of economic and social development.
Data availability
The data of OECD country GDP is retrieved from https://data.worldbank.org/indicator/NY.GDP.MKTP.CD?locations=1W , The data of OECD country poverty line is retrieved from https://data.worldbank.org/indicator/SI.POV.DDAY?locations=1W&start=1984&view=chart , The data of OECD country Population with tertiary education 25–34-year-old is retrieved from https://data.oecd.org/eduatt/population-with-tertiary-education.htm#indicator-chart , The data of OECD country Percentage of 25–64-year old’s who have completed higher education (%) is retrieved from https://data.oecd.org/eduatt/adult-education-level.htm#indicator-chart , The datasets generated during and/or analysed during the current study are available in Harvard Dataverse https://doi.org/10.7910/DVN/TP43QS .
Adams, R (2013). Education: poverty and inequality blamed as UK sinks in adult literacy league: OECD survey flags up big problems for England: Post-16 education blamed for failure to address issue. The Guardian . (2013, October 9). London, England
Agasisti T, Longobardi S, Prete V, Russo F (2021) The relevance of educational poverty in europe: determinants and remedies. J Policy Model 43(3):692–709. https://doi.org/10.1016/j.jpolmod.2020.03.015
Article Google Scholar
Ainscow M (2020) Promoting inclusion and equity in education: lessons from international experiences. Nord J Stud Educ Policy 6(1):7–16
Balestra C, Ruiz N (2015) Scale-invariant measurement of inequality and welfare in ordinal achievements: an application to subjective well-being and education in OECD Countries. Soc Indic Res 123(2):479–500. https://doi.org/10.1007/s11205-014-0751-2
Bamberger, A, & Kim, MJ (2022) The OECD’s influence on national higher education policies: internationalisation in Israel and South Korea. Comp Educ 18. https://doi.org/10.1080/03050068.2022.2147635
Brand M (2021) The OECD poverty rate: lessons from the russian case. Glob Soc Policy 21(1):144–147
Camilla S. (2023) The design of upper secondary education across OECD countries: Managing choice, coherence and specialisation. OECD Education Working Papers. https://doi.org/10.1787/158101f0-en
Chakeredza S, Temu A, Saka J, Munthali D, Muir-Leresche K, Akinnifesi F, Ajayi O, Sileshi G (2008) Tailoring Tertiary Agricultural Education for Sustainable Development in Sub-Saharan Africa: Opportunities and Challenges. J Sci Res Essay 3(8):326–332
Google Scholar
Chelli F, Ciommi M, Mariani F, Polinesi G, Recchioni MC, Lamonica GR, Salvati L (2022) A story of strengths and weaknesses in tertiary education: evaluating “Mobility” and “Opportunities” in OECD countries with composite indicators. Sustainability 14(24):16463. https://doi.org/10.3390/su142416463
Decancq, K (2017) Measuring multidimensional inequality in the OECD member countries with a distribution-sensitive better life index. Soc Indic Res 131(3):1057–1086. https://doi.org/10.1007/s11205-016-1289-2
Fang W, Farooq U, Liu Z, Lan J, Iram R (2022) Measuring energy efficiency financing: a way forward for reducing energy poverty through financial inclusion in OECD. Environ Sci Pollut Res 29(47):71923. https://doi.org/10.1007/s11356-022-20139-8
Garritzmann, JL (2016) The political economy of higher education finance: the politics of tuition fees and subsidies in OECD Countries, 1945–2015. Garritzmann. Germany, Berlin: Springer International Publishing
Gillian G, Lisa T, & Thomas W (2021) How are higher education systems in OECD countries resourced?: Evidence from an OECD Policy Survey.OCDE. https://doi.org/10.1787/0ac1fbad-en
Gong HJ, Hong JE (2021) Does postsecondary education attainment matter in community service engagement? Evidence from Across 18 OECD Countries. Educ Sci 11(3):1–17. https://doi.org/10.3390/educsci11030096
Article MathSciNet Google Scholar
Granata, M (2022) The OECD and technical education in post-war Mediterranean Europe. Labor History. https://doi.org/10.1080/0023656X.2022.2057459
Habibi, F & Zabardast, MA (2020) Digitalization, education and economic growth: a comparative analysis of Middle East and OECD countries. Technol Soc 63. https://doi.org/10.1016/j.techsoc.2020.101370
Hoeller P, Joumard I, Koske I (2014) Reducing income inequality while boosting economic growth: can it be done? Evidence from OECD Countries. Singap Econ Rev 59(1):1–22
Jericho, G (2016) Australia didn’t have a ‘great recession’? Tell that to young people; an OECD report has found a rise in youth not in employment, education or training (Neets) since 2008. While historically the rate isn’t high, benefits are so low that youth are now more likely to be living in poverty. Guardian. London, England. https://link.gale.com/apps/doc/A463552813/AONE?u=unimelb&sid=bookmark-AONE&xid=701996a9
Julian LG (2018) The political economy of higher education finance. the politics of tuition fees and subsidies in OECD Countries, 1945–2015. Czech Sociol Rev 54(3):479–482
Jungblut J (2017) The political economy of higher education finance. The politics of tuition fees and subsidies in OECD countries, 1945–2015. Eur J High Educ 7(1):96–99. https://doi.org/10.1080/21568235.2017.1265789
Kalamova, M (2020) Resourcing higher education: challenges, choices and consequences. Higher Education Policy Team. Paris: OECD Publishing
Lamichhane, S, Egilmez, G, Gedik, R, Bhutta, MKS, & Erenay, B (2021) Benchmarking OECD countries’ sustainable development performance: a goal-specific principal component analysis approach. J Clea Prod 287. https://doi.org/10.1016/j.jclepro.2020.125040
Ledger, S, Thier, M, Bailey, L, & Pitts, C (2019) OECD’s approach to measuring global competency: powerful voices shaping education. Teachers College Record, 121(8):1–40
Li, J & Xue. E (2021) Returnee faculty responses to internationalizing “Academic Ecology” for Creating World-class Universities in China’ Elite Universities. Higher Education. 81(5), 1063–1078
Li, J, & Xue. E, Liu, C, Li, X (2023) Integrated macro and micro analyses of student burden reduction policies in China: call for a collaborative “family–school–society” model. Humanities & Social Sciences Communications. 10, 184 (2023). https://doi.org/10.1057/s41599-023-01695-x
Liu, L (2017) Exploring the relationship between education economy and individual civic attitudes: a cross-national analysis in England, Spain, Sweden, Poland and Chinese Taipei
Lucas R (1988) On the mechanics of economic development. J. Monetary Econ 22(1):342
Mathias B (2023). The assessment of students’ creative and critical thinking skills in higher education across OECD countries: a review of policies and related practices. OECD Education Working Papers. https://doi.org/10.1787/35dbd439-en
Megyesiova, S, & Lieskovska, V (2018) Analysis of the sustainable development indicators in the OECD Countries. Sustainability 10(12). https://doi.org/10.3390/su10124554
Mitic RR (2018) The political economy of higher education finance: the politics of tuition fees and subsidies in OECD Countries, 1945-2015. Rev High Educ 41(3):484–487
OECD (2022) Education at a Glance 2022: OECD Indicators, OECD Publishing, Paris, https://doi.org/10.1787/3197152b-en
Özdoğan Özbal E (2021) Dynamic effects of higher education expenditures on human capital and economic growth: an evaluation of OECD countries. Policy Rev High Educ 5(2):174–196. https://doi.org/10.1080/23322969.2021.1893125
Pinheiro R, Pillay P (2016) Higher education and economic development in the OECD: policy lessons for other countries and regions. J High Educ Policy Manag 38(2):150
Romer M (1990) Endogenous technological change. J Political Econ 98(5):S71–S102
Rowe E (2019) Capitalism without capital: the intangible economy of education reform. Discourse 40(2):271
Sahnoun M, Abdennadher C (2022) Returns to investment in education in the OECD Countries: does governance quality matter? J Knowldege Econ 13(3):1819–1842. https://doi.org/10.1007/s13132-021-00783-0
Samo V. (2022). A snapshot of equity and inclusion in OECD education systems: findings from the Strength through Diversity Policy Survey. OECD Eduction Working Papers. https://doi.org/10.1787/801dd29b-en
Schultz (1961) Investment in human capital. Am Econ Rev 51(1):1–17
Solow RM (1957) Technical change and the aggregate production function. Rev Econ Stat 39(3):312–320
Zapp M (2022) Revisiting the global knowledge economy: the worldwide expansion of research and development personnel, 1980–2015. Minerva 60(2):181–208. https://doi.org/10.1007/s11024-021-09455-4
Article PubMed PubMed Central Google Scholar
Download references
Acknowledgements
This study is funded by 2021 National Social Science Foundation of Higher Education Ideological and Political Course research (Key project) Ideological and Political Education System Construction System Mechanism Research in New Era (No.: 21VSZ004).
Author information
These authors contributed equally: Jian Li, Eryong Xue.
Authors and Affiliations
Institute of International and Comparative Education, Beijing Normal University, Beijing, 100875, China
China Institute of Education Policy, Faculty of Education, Beijing Normal University, Beijing, 100875, China
Eryong Xue, Yukai Wei & Yunshu He
You can also search for this author in PubMed Google Scholar
Substantial contributions to the conception or design of the work: Jian Li and Eryong Xue; the acquisition, analysis, or interpretation of data for the work: Jian Li and Eryong Xue; drafting the work or revising it critically for important intellectual content: Eryong Xue, Jian Li, Yukai Wei, and Yunshu He; final approval of the version to be published: Eryong Xue and Jian Li.
Corresponding authors
Correspondence to Jian Li or Eryong Xue .
Ethics declarations
Competing interests.
The authors declare no competing interests.
Ethical approval
Ethical approval was not required as the study did not involve human participants. It was not required as this article used published data.
Informed consent
This article does not contain any studies with human participants performed by any of the authors.
Additional information
Publisher’s note Springer Nature remains neutral with regard to jurisdictional claims in published maps and institutional affiliations.
Rights and permissions
Open Access This article is licensed under a Creative Commons Attribution 4.0 International License, which permits use, sharing, adaptation, distribution and reproduction in any medium or format, as long as you give appropriate credit to the original author(s) and the source, provide a link to the Creative Commons licence, and indicate if changes were made. The images or other third party material in this article are included in the article’s Creative Commons licence, unless indicated otherwise in a credit line to the material. If material is not included in the article’s Creative Commons licence and your intended use is not permitted by statutory regulation or exceeds the permitted use, you will need to obtain permission directly from the copyright holder. To view a copy of this licence, visit http://creativecommons.org/licenses/by/4.0/ .
Reprints and permissions
About this article
Cite this article.
Li, J., Xue, E., Wei, Y. et al. How popularising higher education affects economic growth and poverty alleviation: empirical evidence from 38 countries. Humanit Soc Sci Commun 11 , 520 (2024). https://doi.org/10.1057/s41599-024-03013-5
Download citation
Received : 29 June 2023
Accepted : 02 April 2024
Published : 17 April 2024
DOI : https://doi.org/10.1057/s41599-024-03013-5
Share this article
Anyone you share the following link with will be able to read this content:
Sorry, a shareable link is not currently available for this article.
Provided by the Springer Nature SharedIt content-sharing initiative
Quick links
- Explore articles by subject
- Guide to authors
- Editorial policies

Education and economic growth: an empirical analysis of nonlinearities
Applied Economic Analysis
ISSN : 2632-7627
Article publication date: 23 August 2019
Issue publication date: 23 October 2019
Might a country’s economic growth performance differ depending on the evolution of its human capital? This paper aims to consider education as a channel for human capital improvement and then for economic growth. The authors hypothesize the existence of a threshold for education, after which point the characteristics of economic growth change.
Design/methodology/approach
To address this question, the authors turn from a linear framework to a nonlinear one by applying smooth transition specifications.
This empirical analysis for Spain points to the existence of nonlinearities in the relationship between education and economic growth at country level, for both secondary and tertiary education. Next, as different patterns emerge in different regions, the authors provide a regional analysis for a number of representative Spanish regions. The results show that both secondary and tertiary education matter for economic growth and that nonlinearities in this relationship should be taken into account.
Practical implications
What is learnt from using Smooth Transition Regression models for the education-economic growth link is that the educational level of the population can be understood as a source of nonlinearities in the economic activity of a country (and of a region). Thus, depending on national and regional educational levels, economic growth behaves differently.
Originality/value
Although the importance of nonlinearities has been identified, linearity is usually assumed in this field of the literature. This paper calls into question the linearity assumption by using time series techniques for 1971-2013 in Spain, an OECD country, and testing whether the results at country level hold for different regions within Spain as a robustness check.
- Economic growth
- Nonlinearities
Marquez-Ramos, L. and Mourelle, E. (2019), "Education and economic growth: an empirical analysis of nonlinearities", Applied Economic Analysis , Vol. 27 No. 79, pp. 21-45. https://doi.org/10.1108/AEA-06-2019-0005
Emerald Publishing Limited
Copyright © 2019, Laura Marquez-Ramos and Estefanía Mourelle.
Published in Applied Economic Analysis . Published by Emerald Publishing Limited. This article is published under the Creative Commons Attribution (CC BY 4.0) licence. Anyone may reproduce, distribute, translate and create derivative works of this article (for both commercial and non-commercial purposes), subject to full attribution to the original publication and authors. The full terms of this licence may be seen at http://creativecommons.org/licences/by/4.0/legalcode
1. Introduction
This paper analyses the relationship between education and economic growth in Spain. It makes a contribution to the literature by analysing the existence of nonlinearities in this relationship. The direction of the causality that we account for is from education to economic growth. In some cases, an increase in education might have a positive (or large) effect on GDP growth and, in other cases, the effect might be negative (or small). Because the effect of education might differ in different stages, nonlinearities gain importance in the analysis of the relationship between education and economic growth. Specifically, we hypothesise that a country’s economic growth performance differs depending on the evolution of its human capital and hence, on its educational achievement.
Education is a key determinant of economic well-being and increases the human capital inherent in the labour force of a country ( Hanushek and Wößmann, 2010 ). In this paper, we start from the assumption that a country with a population that is capable of exploiting new knowledge will perform better; as such, education is considered a channel for economic growth since it constitutes an intrinsic mechanism for knowledge absorption. We focus on both secondary and tertiary education, and use time series techniques to analyse the existence of nonlinearities.
Cohen and Levinthal (1990) introduced the concept of absorptive capacity, which is the ability to recognise the value of new, external information, to assimilate it and to apply it. Education improves this ability and so absorptive capacity can explain why a nonlinear relationship between education and growth might be expected, i.e. why a country’s economic growth performance might differ depending on its educational achievement[ 1 ].
Our research has a bearing on the literature on education and economic growth. Previous studies have analysed the causal effect of education on countries’ economic performance using a variety of measures and quantitative tools for cross-country, time series and panel data ( Hanushek and Wößmann, 2010 ). From the literatures on growth, education and development, we know that the difference between having a primary education and being illiterate has a relatively higher impact on individual wages than having a secondary or tertiary education (i.e. there are decreasing returns to education over certain thresholds). Thus, nonlinearities exist at the micro level, and the macro literature has also explored diverse effects. For example, previous studies have revealed country heterogeneity, showing that estimated returns to education are, in general, higher in developing countries than in developed countries ( Duflo, 2001 ).
Moreover, there is a very large macro (cross-country and panel) literature analysing the relevance of education and economic growth, which has focused on different types of education. Within this research line, Sunde and Vischer (2015) find that there is a weak empirical effect of human capital on economic growth in existing cross-country studies, which is partly due to inappropriate specifications. Previously, Kalaitzidakis et al. (2001) found a nonlinear effect of human capital on economic growth. Kalaitzidakis et al. (2001) point out that their evidence “is consistent with the theoretical suggestion that there exist threshold levels of human capital and the growth experience of a country may well differ according to which side of the threshold it finds itself in” (p. 251).
The importance of nonlinearities is illustrated by Krueger and Lindahl (2001) . These authors divide their sample of countries into three subsamples based on their initial human capital endowment, finding a positive association only in the subsample of countries with the lowest educational level. For the group of countries in the middle of the education distribution, they either find no effect of education on growth or a negative relationship. The relationship is also negative in countries with a higher educational level. In other words, the authors find that “the relationship is inverted-U shaped, with a peak at 7.5 years of education” (p. 1130). Given that the average years of education in OECD countries was 8.4 in the year of the study, these authors point out that “the average OECD country is on the downward-sloping segment of the education-growth profile” (p. 1130).
Although the importance of nonlinearities has been identified, linearity is usually assumed in this field of the literature ( Self and Grabowski, 2004 ; Cohen and Soto, 2007 ; Afzal et al. , 2011 ; Armellini, 2012 ; Jalil and Idrees, 2013 ). This paper calls into question the linearity assumption by using time series techniques for 1971-2013 in Spain, an OECD country, and testing whether the results at country level hold for different regions within Spain as a robustness check[ 2 ].
When analysing the relationship between education and economic growth, it must be acknowledged that Spain is one country where over -education might be a significant issue. Given the high unemployment rate in Spain (resulting in underemployment), the impact of education on economic growth might be expected to be close to zero. That is, increasing educational levels might not necessarily be associated with higher economic growth in Spain. Regarding to the specific hypothesis on the relationship between education and economic growth in Spain, it is worth noting that the answer to this research question is ambiguous, i.e. this relationship can be positive, negative or non-significant.
In our empirical analysis, we incorporate a number of explanatory variables that are closely related to both education and economic growth, as they also affect this relationship; based on the related literature, we account for the potential effects that physical capital, labour force and public expenditure on education may have on the economic activity. We are able to provide evidence of a positive correlation between education and economic growth, as well of the existence of a nonlinear relationship at both country and regional level. This analysis helps us to shed light on the question posed by the existing literature about the positive, although not yet universally accepted, impact of education on economic growth.
The rest of the paper is organised as follows. Section 1 presents the methodology employed. Section 2 details the empirical results obtained at country level, while Section 3 presents the results at regional level. Finally, Section 4 concludes.
2. Methodology
One common assumption when analysing the relationship between economic growth and education is that of linearity. However, this assumption would mean that the parameters in this relationship do not change over time[ 3 ]. Thus, we go beyond the conventional use of linear models by exploring nonlinear specifications that might more accurately explain the relationship between education and economic growth.
Threshold regressions are one of the most common specifications used for reflecting regime changes. As these models consider abrupt changes between regimes, we adopt smooth transition (ST) specifications and, in particular, the more generalised smooth transition regression (STR). In this case, the variable is assumed to vary between two extreme regimes and the smoothness of the transition is estimated from the data. This framework provides us with three main advantages. First, it offers more flexibility in studying the dynamics of the relationship between economic growth and education, not only enabling a wide range of nonlinear behaviours to be described, but also allowing for a continuum of intermediate regimes. Second, it allows us to analyse the potential existence of a threshold that determines the behaviour of economic growth according to education. Finally, it permits the incorporation of exogenous variables in addition to the endogenous structure[ 4 ].
Let y t be a stationary ergodic process and, without loss of generality, only one exogenous variable x t . The model is given by: (1) y t = w t ' π + w t ' θ F s t ; γ , c + u t , where w t = 1 , y t - 1 , … , y t - p 1 ; x t , x t - 1 , … , x t - p 2 ' is a vector of regressors; π = ( π 0 , π 1 ,…, π p ' and θ = ( θ 0 , θ 1 , …, θ p ' are parameter vectors (p = p 1 + p 2 + 1); and u t is an error process, u t ∼ Niid (0, σ 2 ). Likewise, F(.) is a transition function customarily bounded between 0 and 1, implying that the STR coefficients vary between π j and π j + θ j (j = 0, …, p), respectively. The regime at each t is determined by the transition variable, s t , and the associated value F(s t ); the transition variable can be a lagged endogenous variable, an exogenous variable or just another variable.
In this study, we select the logistic transition, as it appears to be the most suitable for describing the relationship between education and economic growth, as there is no reason to assume that a positive and a negative variation in a country’s educational level will have a similar effect on its economic activity. The slope parameter γ defines the smoothness of the transition from one regime to the other, such that the higher the value, the more rapid the change; and the location parameter c indicates the threshold between the two regimes. The logistic case involves that F(-∞) = 0 and F(∞) = 1; this means that the extreme regimes (related to F = 0 and F = 1) are associated with s t values far above or below c, where dynamics may be different.
Regarding to the modelling procedure, we follow a well-established strand of the literature that focuses on an extensive search of STR models. The validation of the estimated models is the core part of the procedure. Accordingly, we apply the three specific tests designed by Eitrheim and Teräsvirta (1996) for STs as misspecification tests. In terms of diagnostic statistics, we employ the adjusted coefficient of determination and we pay particular attention to the variance ratio of the residuals from the nonlinear model and the best linear regression estimated by ordinary least squares (OLS), as it provides relevant information on the explanatory power of both specifications. Finally, these evaluation tests are completed with an analysis of the estimated residuals to describe the behaviour of the STR models more in depth. Appendix 1 provides a detailed explanation of the model, as well as of the modelling procedure.
It is worth mentioning that, beyond the better econometric properties shown by STR models compared to linear ones, the use of nonlinear models allows us for characterising the “economic growth-education” dynamics in a more complete manner than linear models. It is well-known that the linear model is very restrictive; were the relationship between economic growth and education linear, a change in education would be associated to a particular change in economic growth, independently of the level of education reached. Under the linear framework, only one state is possible. However, scenarios with multiple states seem much more realistic.
In our case, and following the related literature, it makes sense to think that the economy might behave differently depending on the educational level of the population. This asymmetric behaviour cannot be captured by a linear model, so the search for nonlinearities becomes a crucial question. In this sense, ST models allow us for defining different regimes regarding the educational level so that we can determine whether they affect the economic activity in a different manner. Thus, these nonlinear models give us more information on the dynamics of the relationship education-economic growth than linear models. For instance, we are able to know how rapidly economic growth reacts to changes in education.
3. Empirical analysis
3.1 data and descriptive analysis.
We use data for Spain and carry out both an aggregate study at country level and a disaggregated analysis for a group of regions to test the robustness of the main results. Table AI in Appendix 2 displays the variables used in the empirical analysis, their definitions and sources.
GDP is traditionally used as a measure of economic activity. We proxy education by using enrolment ratios for both secondary and tertiary education. We define one proxy for labour force for each educational level; specifically, we calculate the proportion of the active population with secondary (and with tertiary) education over the total active population in Spain. Physical capital might exert a relevant direct effect on economic growth[ 5 ]. Thus, as a proxy for physical capital, we use real Gross Fixed Capital Formation. Finally, we use the government expenditure on education (as a percentage of GDP)[ 6 ]. The sample spans the period from 1971 to 2013 and the data are annual.
To provide an overview of the selected variables, we first provide brief descriptive statistics in Table I , then display their evolution in Figure 1 .
Looking at Table I and Figure 1 , we can highlight several features. The education variables show a positive trend over time. This is especially remarkable for tertiary education, which increases at a higher rate than secondary education; the latter even registers negative variations in a number of periods. Higher education shows a steady evolution, with important increases in the mid-80s, mid-90s and late 2000s. In general, all variables display an upward trend in recent decades, in particular after the end of the Franco dictatorship and the economic crisis of the 1970s. Thus, the mid-1970s is a crucial period for public government expenditure on education, with the proportion of the labour force with tertiary education starting to rise, and an almost continuous upward trend in the labour force with secondary education. In the 1980s, physical capital registers a substantial increase, which continues until the breakout of the last economic crisis. The upward trend in GDP is accompanied by a similar trend in the remaining variables. It is therefore challenging to isolate the effect of a causal relationship between economic growth and education using a time series approach.
Before focusing on the relationship between economic growth and education, a statistical processing of the information is required, to carry out the suitable transformations of the variables. First, we use a logarithmic transformation of the original data; namely, a particular type of Box–Cox transformation customarily used to solve problems of nonstationarity in variance. Second, one assumption of ST models is that all the variables involved in the study must be stationary.
In this paper, we have applied the Ng and Perron (2001) unit root tests to analyse the order of integration of our variables. These authors propose several unit root tests (MZα, MZt, MSB and MPt) with the aim of improving the performance of existing ones, in particular regarding size and power features. In this paper, the Ng–Perron tests include intercept and linear trend as deterministic components, and the lag length has been selected by means of the modified Akaike Information Criterion proposed by the authors. The null hypothesis is the existence of unit roots. The test statistics are displayed in Table AIII (Appendix 3) . Considering the asymptotic critical values defined in Ng and Perron (2001) , all the variables analysed in the study are unit root processes. These results point to the need to apply regular differences in all (the logarithms of) our variables in the regression analysis.
3.2 Main results
Four explanatory variables are initially included in the regression analysis: education, physical capital, labour force, and public government expenditure on education. We consider up to two lags of the variables, so as to account for the effect of their most recent history on economic growth. In our paper, linearity tests involve working with a great number of cross products and our sample size is not very large, so the potential results should be taken with caution ( Teräsvirta, 1998 ; and Skalin and Teräsvirta, 2002). As the results would not be conclusive for the two educational levels, the modelling strategy will be based on the abovementioned extensive search of STR models and the core part of the modelling process will be at the validation stage[ 7 ].
The starting point of the modelling strategy consists of finding out the linear specification that would best describe the behaviour of the series under study. OLS estimation is carried out; all parameters are introduced initially, but those that are not significant at the 0.05 level are successively excluded. The next stage focuses on the estimation of the nonlinear models. We achieve valid STR specifications for secondary and tertiary education.
The traditional modelling cycle determines the transition variable by carrying out tests on all explanatory variables; however, following the literature, it can also be selected on the basis of the rationale for the analysis or the economic theory ( Cheikh, 2012 ). As our aim is to study the effect of education on economic growth considering nonlinearities, the enrolment ratio for secondary and tertiary education is chosen as the transition variable. This decision is thus made on the basis of testing a theoretical hypothesis. In any case, any inadequacy arising in the first stages is likely to be later revealed in the modelling cycle ( Teräsvirta, 1998 ). Thus, this variable plays a dual role in the explanation of the economic growth dynamics. On the one hand, it represents the source of nonlinearities in the evolution of economic growth; on the other hand, it is also a determining factor of the dynamics of GDP growth. The estimated linear and STR models are presented in Table II , along with several diagnostic statistics and misspecification tests.
The estimated models for secondary and tertiary education are reflected in columns one to four, taking into account the explanatory variables listed in column (0). For each educational level, the first column (Columns 1 and 3) presents the linear model and the second column is for the STR specification (Columns 2 and 4). With respect to the nonlinear models, the upper part of the table presents the regression coefficients (joint with their standard errors) corresponding to the “lower regime” (F = 0); the regression coefficients for the “upper regime” (F = 1) are the result of summing up the values in the upper and the lower parts of the table (i.e. interactions and no-interactions with F), as commented in Section 1. The smoothness of the transition between regimes is given by the slope parameter γ and c represents the estimated threshold.
Regarding the results of the linear specification, we might expect to find a negative or a positive association between higher education and economic growth in Spain. It is worth mentioning that reverse causality might be present. On the one hand, when GDP grows steadily at the same time as rising youth employment (as in the boom stage from 1996 to 2008), the opportunity cost of investing in education increases and the demand for post-compulsory studies, such as college, declines. On the other hand, when the crisis hits and the probability of a young person having a job falls abruptly, the opportunity cost of investing in tertiary education is reduced and the demand for higher education increases. This establishes not only an association between GDP growth (effect) and enrolment education (cause), but also an association between GDP growth (cause) and enrolment education (effect). The direction of the causality that we account for is from education to economic growth, but in some stages, an increase in education might have a positive (or large) effect on GDP growth and, in other stages, the effect might be negative (or small)[ 8 ]. Because the effect of education on growth might differ in different stages, nonlinearities gain importance in the analysis of the relationship between education and economic growth.
In the linear model, both secondary and tertiary education present a positive and significant association with economic growth. According to the obtained results, a 10 per cent increase in secondary education leads to a 1.5 per cent increase in economic growth, while an increase of 10 per cent in tertiary education increases economic growth by 0.9 per cent, ceteris paribus .
Regarding the model including secondary education, the nonlinear model shows that economic growth depends on its own recent history (GDP), as well as on physical capital (PHY), labour force (see LABS/T in column 2) and public government expenditure on education (EXP). However, secondary education does not seem to be a significant factor determining economic growth, according to the final explanatory variables included in the model (see ENRS/T in column 2). Turning to tertiary education, results indicate that the estimated STR parameters are significant: recent history of economic growth has a significant effect on its current state (GDP), as do tertiary education (see ENRS/T in column 4), physical capital, labour force (see LABS/T in column 4) and government expenditure on education (the latter is not relevant for explaining economic growth in the linear specification). In contrast to secondary education, tertiary education is a significant determinant of economic growth dynamics in Spain (see ENRS/T in column 4).
Nonlinear models point to asymmetric effects of the different variables on economic growth. As shown in Table II , most variables exhibit a different sign when the variation in the enrolment ratio exceeds the location parameter (c equals 1.5 per cent for secondary and 5.2 per cent for tertiary education) and when it is below this threshold. This is proven by comparing the coefficients for the lower regime (F = 0) and those for the upper regime (F = 1); the latter are the results of summing up the coefficients for the lower regime and those for the interactions with the transition function.
Thus, for relatively large levels of secondary education enrolment (upper regime), we expect an overall negative effect of investment and labour on economic growth, but overall positive when the enrolment ratios are relatively low. When focusing on tertiary education, we observe how labour has always a positive effect (regardless of the regime) on economic growth. Physical capital affects in a positive manner especially when tertiary education enrolment is relatively high (upper regime). Interestingly, if the country shows large tertiary education enrolment ratios, their increase strengthens the role of education, as the economic activity improves (see upper regime); in case tertiary education enrolment were relatively low, its increase does not help economic growth in an overall sense (note the opposite signs for ENRS/T in moments t and t-1 in the last column). However, it should be noted that the interpretation of ST models merely based on the estimated coefficients can be misleading, as they do not completely account for the dynamics ( Mejía-Reyes et al. , 2010 ).
As obtained for the linear model, results of the estimated coefficients obtained with the nonlinear specification indicate that higher education is a significant determinant of economic growth in Spain; however, in this specification, secondary education is not statistically significant[ 9 ]. Interestingly, both educational levels play a role in generating asymmetric effects on economic growth. In other words, while tertiary education plays the double role of being a transition and an explanatory variable, secondary education acts as a transition variable only. In any case, this result does not diminishes the relevance of secondary education on economic growth, as the behaviour of economic activity is determined by the regime education is.
Figure A1 in Appendix 3 depicts the estimated transition functions[ 10 ]. Regarding the secondary education case, the transition between regimes is logistic and smooth, according to the value of γ, and the delay is one year. Regarding the value for c (the estimated threshold), the Spanish economic activity will exhibit different dynamics when the enrolment ratio rises above 1.5 per cent (upper extreme regime) than when it goes at slower pace, that is, below that threshold (lower extreme regime). This function shows wide variation, allowing more flexibility in the dynamics of economic growth. The estimated value for the threshold is very close to the enrolment ratio mean (2 per cent), so that the left side of the logistic function contains fewer observations (66 per cent of the total).
With respect to tertiary education, we can define a lower extreme regime, ranging from negative growth to 5.2 per cent variation in the enrolment ratio (the estimated threshold, c), and an upper extreme regime, for variations greater than 5.2 per cent. This value is remarkably close to the mean of the transition variable (5.7 per cent), so there is a near equal distribution between the left and right sides of the logistic function. In this case, regime changes are extremely rapid (due to the large value of γ, which equals 66.65), a fact that suggests a need for threshold specifications and strengthens the importance of using STR models[ 11 ].
According to the estimated values of the slope parameter (γ) in Table II , economic growth appears to evolve more rapidly from one extreme regime to the other when considering tertiary education than secondary education, that is, economic growth shows a more immediate reaction to shocks in tertiary education than to shocks in secondary education. As stated in Section 1, the larger the value for γ, the more rapid the transition between regimes (γ equals 2.17 in secondary education and 66.65 in tertiary education). A possible cause may be the importance traditionally attributed in Spain to the pursuit of a university degree.
Following the evaluation tests, there are no indications of misspecification in the STR models[ 12 ], so we conclude that the proposed STR is adequate. Finally, a comparison analysis of the estimated residuals is carried out, comparing the residuals of the linear and nonlinear specifications for secondary and tertiary education. Figure A2 in Appendix 3 depicts the differences, in absolute values, between the residuals from the linear model and the STR specification over time. In both cases, the residuals of the STR model are lower (in absolute values) than those of the linear regression; in particular, the nonlinear model reduces overall the highest residuals of the linear specification in a reasonable way. Moreover, differences between residuals are mainly positive, thus supporting the use of the STR specification. These signs are a further indication of the superior performance of the nonlinear model relative to the linear model.
4. Results at regional level
To test whether results are robust to the degree of territorial aggregation chosen, in a first step, we construct a classification matrix to choose a representative sample of Spanish regions for the analysis. We classify regions by regional income per capita and regional government expenditure per capita. The information in Table AII in Appendix 2 is used to determine whether the different Spanish regions are above or below average in terms of both income and government expenditure on education. For example, when income per capita in a specific region is above the average over the analysed period, then this region is considered to be in a different cell of the classification matrix than a region that is below the average. A representative region is selected from each of the four groups of regions constructed (see Table AII , Appendix 2 ). We select four representative Autonomous Communities: Castilla y León, Cataluña, Comunidad Valenciana and País Vasco. In three of these regions (Castilla y León, Cataluña and País Vasco), the average public expenditure on fundamental public services per capita for 2009-2015 was above the average of the communities with a common system (i.e. all Autonomous Communities, excluding País Vasco and Navarra, which have a foral system[ 13 ]). Conversely, spending on basic public services per capita in Comunidad Valenciana is below the average of the communities with a common system [ Instituto Valenciano de Investigaciones Económicas (IVIE) (2017) ].
Two methodological issues regarding regional-level data should be mentioned. First, as gross enrolment ratios are not available for Spanish regions, we use a proxy variable proposed by de la Fuente and Doménech (2015) , which is constructed as the proportion of the population for which the maximum level of education achieved is either secondary or tertiary[ 14 ]. Second, with respect to government expenditure on education, we derive the value for each region by applying a ratio (the weight of each region’s GDP over the total Spanish GDP) to the total expenditure for the country[ 15 ]. In addition, the sample ranges from 1971 to 2013 except for education, which goes from 1971 to 2011, and for labour force, which starts in 1977 and goes until 2013. Data are annual in all cases. All variables are used in their logarithmic transformation.
As in the country-level analysis, in a first step we carry out the Ng–Perron unit root tests for the different variables at regional level. As for the aggregate, all variables are used in their first differences[ 16 ].
Regarding nonlinearities, we observe how the estimated model including secondary education reflects the dependence of the economic growth on its own past, educational level of the population, physical capital, labour force and (regional) government expenditure on education. Education plays the dual role of being a determining factor of economic growth as well as the force driving its nonlinear behaviour. We also find evidence of asymmetric effects of the variables on economic growth in the regional case; there is a notable difference regarding the sign when comparing the coefficients for the lower and the upper regimes (especially in Castilla y Léon and Cataluña).
Two issues arise in the regional analysis for secondary education. First, this variable is found to be a significant factor for the economic activity at regional level, though it was not statistically significant at country level. Second, overall, the labour force variable does not seem to exert the same influence on economic growth at regional level as it does at country level. The observable relevance of other factors such as physical capital or public expenditure on education might be diminishing the effect of labour force.
Turning to the nonlinear specification for tertiary education, all the variables considered are relevant factors explaining economic growth in the regional analysis. Once more, the educational level plays a key role acting as the transition variable as well as a relevant factor explaining the economic growth. Asymmetries are again found (in particular, for Castilla y León and Cataluña).
One aspect to mention about nonlinear models in the regional analysis is the remarkable influence of physical capital, which is often present in all models, while labour force appears as an explanatory variable to a greater extent in the aggregate analysis than in the disaggregated analysis. Conversely, public spending on education more often appears as an explanatory variable at regional level than at country level. One explanation for this might be that the analysis using the aggregated data could be masking the importance of public spending on education for economic growth in Spain.
Regarding transition functions, one remarkable difference from the aggregate analysis for secondary education is the estimated value for the slope parameter. At regional level, the transition between the lower and the upper regime takes place at a higher speed than in the country-level analysis. The location parameters that determine the thresholds between the extreme regimes are remarkably close to the mean of the transition variable (5-6 per cent), except for Cataluña. Finally, the estimated functions generally display a wide variation range (except for Cataluña).
Regarding tertiary education, the transition between the extreme regimes is smoother at regional than at country level. Nevertheless, the changes between regimes occur at a notable speed. The estimated thresholds between the extreme regimes range from 3 to 6 per cent; these values are very close to the respective enrolment ratio means (mainly around 4 per cent). Thus, the left and the right sides of the logistic function are fairly well balanced in terms of the number of observations. As observed in the secondary education case, there is wide variation in the estimated transition functions. It is worth mentioning the relatively rapid transition between the extreme regimes we observe in the regional analysis. As noted in the country-level analysis, this fact points to the need for threshold specifications, thus reinforcing the relevance of STR models.
Focusing on the evaluation stage, the estimated models for the four regions present no evidence of misspecification following the diagnostic statistics. Furthermore, as in the country analysis, the explanatory power of the nonlinear models substantially outweighs that of the linear specifications.
Finally, just as we did for the aggregate analysis, we examine the residuals of both linear and nonlinear models for the four regions under study. According to the results obtained, the pattern of behaviour at regional level resembles that observed in the country-level analysis, although in some regions (Comunidad Valenciana and Castilla y León) there is even stronger evidence in favour of the nonlinear model.
5. Conclusions and discussion
In this paper, the assumption of linearity in the relationship between education and economic growth is called into question. We opt for a time series framework (analysing the period from 1971 to 2013) and, more specifically, we estimate STR models for Spain. The estimated models reflect how the variation in education (contemporary or one or two periods before) generates nonlinear effects on current economic growth.
We proceed in two stages. First, we focus on the role of secondary and tertiary education in economic growth in Spain (aggregated or country-level analysis). Second, as different solutions emerge in different regions, we perform our nonlinear analysis for a number of representative Spanish regions (disaggregated or regional analysis). We can then test for the existence of regional heterogeneity. Using these two steps, we test whether our initial findings hold up or are sensitive to the level of territorial aggregation used.
According to the obtained results, we find that both the aggregated and disaggregated analyses lead to consistent and coherent results. The linear analysis has shown evidence in favour of a positive correlation between education and economic growth: the higher the educational level (both secondary and tertiary), the higher the economic growth in Spain. When analysing nonlinearities, we observe a number of differences regarding the importance of secondary education as an explanatory variable, as well as the role of other variables. Also, there are some variables that might seem more relevant than they really are as a consequence of statistical aggregation (e.g. labour force, once education is controlled for), while others might seem to be less relevant (e.g. public spending on education).
Both secondary and tertiary education are relevant for economic growth[ 17 ]. The obtained results point to the need for a nonlinear model, as there are abrupt shifts between regimes; importantly, we evidence how education plays the dual role of being a determining factor of economic growth as well as the force driving its nonlinear behaviour. However, our aim here is not to provide an answer as to which level of education is more important to boost economic growth in Spain, as we have not focused on comparing the magnitudes of estimated coefficients for different educational levels, and we have not included primary education in our analysis, though this might also be relevant for economic growth in Spain. We leave this important issue for further research.
What we learn from using STR models for the education-economic growth link is that the educational level of the population can be understood as a source of nonlinearities in the economic activity of a country (and of a region). Thus, depending on national and regional educational levels, economic growth behaves differently. By means of these models, we have been able to detect how rapidly economic growth reacts to the variable determining the transition, that is, education.
Evolution of the selected variables over time (in levels)
Estimated transition functions
Differences between the absolute values of the estimated residuals (linear and STR model)
Descriptive statistics for the selected variables (in levels)
All variables are first differences of the logarithmic transformation. Values after regression coefficients are SEs of the estimates; R ¯ 2 is the adjusted coefficient of determination; s 2 /s 2 L is the variance ratio of the residuals from the STR model and the linear regression; AUTO is the test for residual autocorrelation of order 1; NL is the test for no remaining nonlinearity; PC is the general parameter constancy test. Numbers in parentheses after misspecification tests statistics are p -values. This table reports the estimated models for secondary and tertiary education using the explanatory variables listed in the first column. For each educational level, the first column presents the linear model and the second column is for the STR specification. Regarding the nonlinear model, the upper part of the table corresponds to the “lower” regime (F = 0), and the next part introduces the interactions with the transition function [ equation (1) ]. The smoothness of the transition between regimes is given by the slope parameter γ and c represents the estimated threshold
Source: Own elaboration
Our paper is linked to the literature on absorptive capacity as it could be argued that human capital is a key element in a country’s absorptive capacity ( Cohen and Levinthal, 1990 ; Zahra and George, 2002 , and Márquez-Ramos and Martínez-Zarzoso, 2010 ). See, for example, the illustration of the role of education in the aerospace sector for establishing sufficient levels of absorptive capacity in Asia ( van der Heiden et al. , 2015 ).
Spain is an interesting country to explore at regional level because of its high degree of decentralization, through which different solutions emerge in different regions.
Under certain circumstances, economic theory or/and data might suggest that a given variable is generated by a nonlinear process. In this sense, it is quite usual for a variable to behave differently according to the values of another variable; for example, it has been shown that the dynamic properties of GDP growth are different in expansion and recession periods. Thus, there would be a change of regimes and the data generating process to be modelled would be a linear process that switches between regimes according to a rule.
STR has been mainly used for evidencing asymmetries in economic variables; some leading empirical applications deal with interest rates, unemployment or exchange rates, among other variables. However, the application of STs is not restricted to the economic context. For example, Hall et al. (2001 ) demonstrate how a Smooth Transition Auto Regression (STAR) model fits better than an Autoregressive (AR) specification when describing climate turbulence periods, and Abril-Salcedo et al. (2016 ) estimate the impact of weather conditions on food inflation growth by means of STR models, attempting to account for the fact that the effects of weather anomalies vary over time due to climate change.
Note that, for example, in Lucas’ model, aggregate output depends on physical capital and human capital ( Krueger and Lindahl, 2001 ).
Kalaitzidakis et al. (2001 ) refer to the proportion of government expenditures on education as a measure employed by several researchers for capturing those resources used as inputs into the educational process.
Likewise, linearity tests have been carried out and further information on the results is provided in Table AIV (Appendix 3) .
We are grateful to two anonymous reviewers that helped us to develop this argument.
Note that secondary education is found to be significant in the linear model. We anticipate that secondary education is a significant determinant of economic growth in different regions within Spain (see Section 3).
Note that each dot in Figure C.1 represents one observation in the sample.
The comparatively large value for the standard deviation reflects the numerical difficulties in obtaining an accurate value for γ when it is large, as in this case F functions mimic step functions, and many observations close to the location parameter are needed [see, for example, van Dijk et al. (2002 )].
The specific validation tests do not detect serial dependence in the estimated residuals, there is no need for a second transition function and constancy in the parameters is demonstrated.
In a foral system, the autonomous communities are granted the power to maintain, establish and regulate their own tax regimes (as opposed to the common system, where the State is responsible).
Note that the results obtained at the national level are not strictly comparable with those obtained in the regional analysis because in the former we use the gross enrolment ratios, whereas in the latter, we use the population for which the maximum level achieved is either secondary or tertiary.
Note that we considered a more accurate proxy for regional public spending in education by considering the series provided by the Ministry of Education (“Recursos económicos. Gasto Público”, available at www.mecd.gob.es/servicios-al-ciudadano-mecd/estadisticas/educacion/recursos-economicos/gasto-publico.html ). However, due to the limitation of the available time series, we rely on the constructed alternative proxy.
Full results for the regional analysis are not reported to save space. In Appendix 4, we report the estimated STR models for secondary and tertiary education. The rest of tests and results are available from the authors upon request.
The results show that the main difference regarding secondary education in the aggregate and the regional analyses is that this educational level seems to play a more relevant role at a regional scale, where it is both a transition and an explanatory variable.
Smooth Transition (ST) models belong to the family of state-dependent models where the data-generating process is linear but switches between a certain number of regimes according to a rule (see, for example, Teräsvirta , 1994, 1998 , and Potter, 1999 ). This parameterisation allows for capturing different types of behaviour that a linear model cannot appropriately characterise; once the state is given, the model is locally linear, involving an easy interpretation of the local dynamics. In contrast to other regime-switching models (such as Markov–Switching or threshold models), STs consider that the change between regimes is smooth over time, rather than abrupt, which is normally a more realistic situation; in any case, STs nest some threshold models as particular cases.
In recent decades, STs have been a popular choice for economic time series, performing well in capturing cyclical behaviour in macroeconomic variables ( Teräsvirta and Anderson, 1992 ; Mejía-Reyes et al. , 2010 ; Cuestas and Mourelle, 2011 , among others). For further details on these models, see Teräsvirta (1994 , 1998 ) and van Dijk et al. (2002) .
In its basic version, the regime-switching STR specification considers two distinct regimes corresponding to F = 0 and F = 1, and the transition from one regime to the other is smooth over time, meaning that parameters in (1) gradually change with the transition variable. Two formulations are generally used for F: the logistic and the exponential function. In the logistic model, the extreme regimes are associated with s t values far above or below c, where the dynamics may be different; conversely, in the exponential case, the extreme regimes are related to low and high absolute values of s t , with rather similar dynamics, which may differ in the transition period.
With regards the modelling procedure, the STR modelling cycle has traditionally relied on the iterative methodology proposed by Teräsvirta (1994) for the univariate case: specification, estimation and evaluation of the model. The usual starting point is identifying the linear model that best characterises the behaviour of the series under study; once this specification has been determined, its suitability regarding the relationship being analysed is tested. If the null hypothesis of a linear process against the alternative of an STR one is rejected, a preliminary specification of the nonlinear model is defined. Then, the parameters of the STR specification are estimated by nonlinear least squares.
Nevertheless, an important branch of the literature on this specification does not follow the above procedure so strictly, as, among other issues, linearity tests suffer from size and power problems under certain circumstances ( van Dijk et al. , 2002 ). In this sense, it is argued that it is possible to develop nonlinear formulations that improve the fit of the linear ones without having to do the previous tests, i.e. the data themselves would reveal the potential existence of nonlinearities; the validation process will determine whether or not the model has correctly captured the nonlinear behaviour ( Granger and Teräsvirta, 1993 ).
This alternative procedure is done through an extensive search of STR models by defining a grid for (γ, c). This is the strategy proposed by several authors, such as Öcal and Osborn (2000) , or Sensier et al. (2002) , among others, and the one we adopt in our study; we try different values of γ and use the sample mean of the transition variable for c. Where parameter convergence is reached, models are subjected to further refinement; cross-parameter restrictions are evaluated to increase efficiency and non-significant coefficients are dropped to conserve degrees of freedom.
As mentioned above, less emphasis is given to the initial stages of the modelling process and more attention is paid to the validation of the estimated model, as it will reveal any possible specification inadequacy ( van Dijk et al. , 2002 ). Most of the tests commonly applied to dynamic models are also valid in the STR framework. Besides, STR estimation is based on the assumption of no residual autocorrelation and parameter constancy, making it necessary to test these hypotheses. Eitrheim and Teräsvirta (1996) develop several evaluation tests especially derived for ST models, such as the test of residual serial independence against processes of different orders and the test of parameter constancy against changing parameters under the alternative, which refer to the two previous assumptions. In addition, these authors define the test of no remaining nonlinearity in the residuals.
Abril-Salcedo , D.S. , Melo-Velandia , L.F. and Parra-Amado , D. ( 2016 ), “ Impactos de los fenómenos climáticos sobre el precio de los alimentos en Colombia ”, Ensayos Sobre Política Económica , Vol. 34 No. 80 , pp. 146 - 158 .
Afzal , M. , Rehman , H.U. , Farooq , M.S. and Sarwar , K. ( 2011 ), “ Education and economic growth in Pakistan: a cointegration and causality analysis ”, International Journal of Educational Research , Vol. 50 Nos 5/6 , pp. 321 - 335 .
Armellini , M. ( 2012 ), “ The democratic factor in the education‐growth relationship ”, Kyklos , Vol. 65 No. 3 , pp. 285 - 312 .
Cheikh , N.B. ( 2012 ), “ Non-linearities in exchange rate pass-through: evidence from smooth transition models ”, MPRA Paper no. 39258 .
Cohen , D. and Soto , M. ( 2007 ), “ Growth and human capital: good data, good results ”, Journal of Economic Growth , Vol. 12 No. 1 , pp. 51 - 76 .
Cohen , W.M. and Levinthal , D.A. ( 1990 ), “ Absorptive capacity: a new perspective on learning and innovation ”, Administrative Science Quarterly , Vol. 35 No. 1 , pp. 128 - 152 .
Cuestas , J. and Mourelle , E. ( 2011 ), “ Nonlinearities in real exchange rate determination: do African exchange rates follow a random walk? ”, Applied Economics , Vol. 43 No. 2 , pp. 243 - 258 .
De la Fuente , A. ( 2017 ), “ Series enlazadas de algunos agregados económicos regionales, 1955-2014. Parte I: metodología ”, VAB, PIB y puestos de trabajo. RegDat Versión 5.0-I. FEDEA, Estudios sobre la Economía Española no. 2017-08 .
De la Fuente , A. and Doménech , R. ( 2015 ), “ El nivel educativo de la población en españa y sus regiones (1960-2011) ”, [ RegDatEdu_v40_1960_2011]. FEDEA and BBVA Research, Estudios sobre la Economía Española no. 2015/05 .
Duflo , E. ( 2001 ), “ Schooling and labor market consequences of school construction in Indonesia: evidence from an unusual policy experiment ”, American Economic Review , Vol. 91 No. 4 , pp. 795 - 813 .
Eitrheim , Ø. and Teräsvirta , T. ( 1996 ), “ Testing the adequacy of smooth transition autoregressive models ”, Journal of Econometrics , Vol. 74 No. 1 , pp. 59 - 75 .
Granger , C.W.J. and Teräsvirta , T. ( 1993 ), Modelling Nonlinear Economic Relationships , Oxford University Press , Oxford .
Hall , A.D. , Skalin , J. and Teräsvirta , T. ( 2001 ), “ A nonlinear time series model of El niño ”, Environmental Modelling and Software , Vol. 16 No. 2 , pp. 139 - 146 .
Hanushek , E.A. and Wößmann , L. ( 2010 ), “ Education and economic growth ”, in Peterson , P. , Baker , E. and McGaw , B. (Eds), International Encyclopedia of Education , Vol. 2 , Elsevier , Oxford , pp. 245 - 252 .
Instituto Valenciano de Investigaciones Económicas (IVIE) ( 2017 ), “ Insuficiencia de recursos Para servicios públicos fundamentales ”, Papeles de Financiación Autonómica no. 2, November 2017 .
Jalil , A. and Idrees , M. ( 2013 ), “ Modeling the impact of education on the economic growth: evidence from aggregated and disaggregated time series data of Pakistan ”, Economic Modelling , Vol. 31 , pp. 383 - 388 .
Kalaitzidakis , P. , Mamuneas , T.P. , Savvides , A. and Stengos , T. ( 2001 ), “ Measures of human capital and nonlinearities in economic growth ”, Journal of Economic Growth , Vol. 6 No. 3 , pp. 229 - 254 .
Krueger , A.B. and Lindahl , M. ( 2001 ), “ Education for growth: why and for whom? ”, Journal of Economic Literature , Vol. 39 No. 4 , pp. 1101 - 1136 .
Márquez-Ramos , L. and Martínez-Zarzoso , I. ( 2010 ), “ The effect of technological innovation on international trade ”, Economics: The Open-Access, Open-Assessment E-Journal , Vol. 4 Nos 2010/11 , pp. 1 - 37 .
Mejía-Reyes , P. , Osborn , D.R. and Sensier , M. ( 2010 ), “ Modelling real exchange rate effects on output performance in Latin America ”, Applied Economics , Vol. 42 No. 19 , pp. 2491 - 2503 .
Ng , S. and Perron , P. ( 2001 ), “ Lag length selection and the construction of unit root tests with good size and power ”, Econometrica , Vol. 69 No. 6 , pp. 1519 - 1554 .
Öcal , N. and Osborn , D.R. ( 2000 ), “ Business cycle non-linearities in UK consumption and production ”, Journal of Applied Econometrics , Vol. 15 No. 1 , pp. 27 - 43 .
Potter , S.M. ( 1999 ), “ Nonlinear time series modelling: an introduction ”, Journal of Economic Surveys , Vol. 13 No. 5 , pp. 505 - 528 .
Self , S. and Grabowski , R. ( 2004 ), “ Does education at all levels cause growth? India, a case study ”, Economics of Education Review , Vol. 23 No. 1 , pp. 47 - 55 .
Sensier , M. , Öcal , N. and Osborn , D.R. ( 2002 ), “ Asymmetric interest rate effects for the UK real economy ”, Oxford Bulletin of Economics and Statistics , Vol. 64 No. 4 , pp. 315 - 339 .
Sunde , U. and Vischer , T. ( 2015 ), “ Human Capital and growth: specification matters ”, Economica , Vol. 82 No. 326 , pp. 368 - 390 .
Teräsvirta , T. ( 1994 ), “ Specification, estimation, and evaluation of smooth transition autoregressive models ”, Journal of the American Statistical Association , Vol. 89 No. 425 , pp. 208 - 218 .
Teräsvirta , T. ( 1998 ), “ Modeling economic relationships with smooth transition regressions ”, in Ullah , A. and Giles , D.E.A. (Eds), Handbook of Applied Economic Statistics , Marcel Dekker , New York, NY , pp. 507 - 552 .
Teräsvirta , T. and Anderson , H.M. ( 1992 ), “ Characterizing nonlinearities in business cycles using smooth transition autoregressive models ”, Journal of Applied Econometrics , Vol. 7 , pp. S119 - 136 .
van der Heiden , P. , Pohl , C. , Mansor , S.B. and van Genderen , J. ( 2015 ), “ The role of education and training in absorptive capacity of international technology transfer in the aerospace sector ”, Progress in Aerospace Sciences , Vol. 76 , pp. 42 - 54 .
van Dijk , D. , Franses , P.H. and Lucas , A. ( 1999 ), “ Testing for smooth transition nonlinearity in the presence of outliers ”, Journal of Business and Economic Statistics , Vol. 17 No. 2 , pp. 217 - 235 .
van Dijk , D. , Teräsvirta , T. and Franses , P.H. ( 2002 ), “ Smooth transition autoregressive models-a survey of recent developments ”, Econometric Reviews , Vol. 21 No. 1 , pp. 1 - 47 .
Zahra , S.A. and George , G. ( 2002 ), “ Absorptive capacity: a review, reconceptualization, and extension ”, Academy of Management Review , Vol. 27 No. 2 , pp. 185 - 203 .
Corresponding author
Related articles, we’re listening — tell us what you think, something didn’t work….
Report bugs here
All feedback is valuable
Please share your general feedback
Join us on our journey
Platform update page.
Visit emeraldpublishing.com/platformupdate to discover the latest news and updates
Questions & More Information
Answers to the most commonly asked questions here
- School guide
- Social Science Notes
- History Notes
- Geography Notes
- Political Science Notes
- Economics Notes
- Science and Technology Notes
- Social Science Solutions
- English Grammar
- Science Solution
- Biology Solutions
- Mathematics Solutions
- R.D.Sharma Solutions
Role of Education in Economic Development
- Introduction to Development Economics
- National Development
- Role of Agriculture in Indian Economy
- Role of Banks in Economy of India
- Advantages and Problems of Education System in India
- Top 10 Benefits of Co-Education
- Economic Effects of Public Debt
- Role of Human Capital on Economic Growth
- Evaluation (Achievements and Failures) of Economic Planning till 1991
- What is the basic goal of Human Development?
- Difference Between Traditional and Online Education
- How does transport play an important role in the Economic development of a country?
- Education System in America
- Rural Development: Meaning, Significance, Process and Evaluation
- Skill Development and Employment Opportunity in India
- Entrepreneurship Development Process
- The Gandhian Model of Development -Five Year Plan
- Indian Economic Development
- CBSE Class 12 Indian Economic Development Notes
- Difference between Training, Development and Education
- Contribution of Family in Value Education
- Dichotomy of Development
- How Public Sector Contributes to the Economic Development of a Nation?
- Factors Caused For Rapid Growth in Economic Development in China
- Ethics In Economic Life
- What do you mean by Training and Development?
- Economic Policies of the British in India
- The United Nations - Sustainable Development Goals
- Role of Rivers in Indian Economy
Until recently, economists considered physical capital to be the most significant component affecting economic growth, proposing that the rate of physical capital formation in developing nations be raised to expedite the process of economic growth and boost people’s living standards. However, economic research over the last three decades has demonstrated the relevance of education as a critical determinant in economic development. The development of human abilities and knowledge of the population or labor force is referred to as education.
The key to economic progress is not only the numeric increase of educational possibilities but also the qualitative enhancement of the sort of education provided to the labor force. Education has been referred to as human capital due to its substantial contribution to economic growth, and public expenditure on education has been referred to as an investment in man or human capital.
Education and Economic Growth
Several empirical studies conducted in developed countries, on the sources of growth, or, in other words, the contributions made by various factors such as physical capital, man-hours (i.e., physical labor), education, and so on, have revealed that education or the development of human capital is a significant source of economic growth.
Rate of Return Technique
The rate of return approach has also been used to assess the contribution of education to economic growth. The rate of return in this technique is determined by individual education spending and the flow of a person’s future earnings predicted to emerge from schooling.
It should be noted that estimations of the rate of return on education investment are based on private rates of return to persons obtaining an education. However, because disparities in incomes in a market economy reflect inequalities in productivity, the rate of return on education investment is assumed to be the influence of education on the country’s production.
Expenditure on Education and Income
Another method for calculating education’s contribution is to examine the link between education spending and income. Using this method, the link between education spending and consumer income, as well as the relationship between education spending and physical capital production He discovered that “the resources dedicated to education climbed nearly three and a half times (a) relative to consumer income (b) compared to the gross production of physical capital
Education’s Consumption Advantages
We have already discussed the investment benefits of education, as well as its influence on productivity and national production. However, investment rewards are not the only ones that come from education. Education also has consumption advantages for the individual since it allows him to “enjoy” more education and get more enjoyment from his current and future personal life.
If the welfare of society is dependent on the welfare of its individual members, then the welfare of society as a whole benefit from the higher consumption advantages of people who receive more education. Economic theory can also help us estimate the consumption advantages of education.
External Benefits of Education
We have already discussed the investment and consumption benefits of more education for both individuals and society. The benefit analysis was founded on the assumption that individuals’ private interests are congruent with the societal good.
Education, Inequality, and Poverty
An assessment of the function of education in economic development must consider not just its influence on production growth, but also its impact on the structure and pattern of economic development, as well as on income distribution and poverty alleviation.
The fact that poor children and boys are unable to complete their secondary education, combined with large income or wage disparities between different people of different levels of education, explains why education in developing economies tends to increase income inequalities and perpetuate poverty rather than help to reduce it.
What function does education play in economic development?
As follows, education has an important influence on economic development:
- Education enhances people’s access to current and scientific concepts.
- It improves people’s efficiency and capacity to absorb new technologies.
- It raises knowledge of potential possibilities and labor mobility.
- Education helps people obtain information, skills, and attitudes that will allow them to grasp societal changes and scientific advances.
- Education investment is one of the primary sources of human capital that enables inventions and discoveries.
- A country’s ability to adopt sophisticated technologies is aided by a well-educated labor population.
Sample Questions
Question 1: What role does education play in economic development?
Answer :
Consistent income for the working class offers financial stability for them, their families, and their communities. Education is regarded as human capital due of its importance to economic growth. Any sort of educational investment creates chances for national economic development.
Question 2: Why is education considered human capital?
Answer:
Education is regarded as human capital due of its importance to economic growth. Any sort of educational investment creates chances for national economic development.
Question 3: What is the value of education to a country?
Education is the sole key to a nation’s success in today’s globe. It causes significant changes in a society’s social, political, and economic realms of existence. It is the only means by which mankind may develop as a whole and achieve world peace.
Please Login to comment...
Similar reads.
- School Economics
- School Learning

Improve your Coding Skills with Practice
What kind of Experience do you want to share?
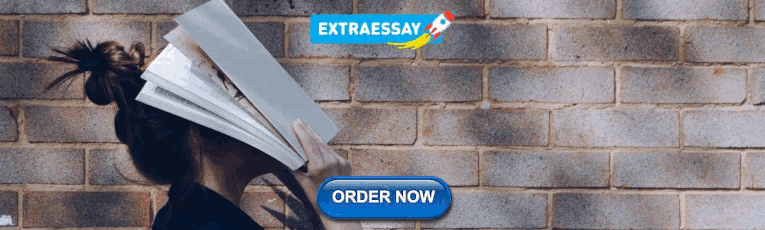
COMMENTS
Here are five things you should know about the pivotal role of education in economic development: Education is an investment. The importance of knowledge and learning has been recognized since the beginning of time. Plato wrote: "If a man neglects education, he walks lame to the end of his life.".
1.1 Introduction. The main purpose of this paper is to show the role of education in economic development and the effect of education on labour productivity, poverty, trade, technology, health, income distribution and family structure. Education provides a foundation for development, the groundwork on which much of our economic and social well ...
Conclusions. Economic growth determines the future economic well-being of a country, making understanding the determinants of growth a high-priority area of economic research. The analysis of how education affects growth makes a strong case that the skills of the population are by far the most important factor.
Education raises people's productivity and creativity and promotes entrepreneurship and technological advances. In addition it plays a very crucial role in securing economic and social progress and improving income distribution. Keywords: Human Development, Economic Growth, Poverty, Labour Productivity, Education, Technology, Trade, Health ...
The World Economic Forum is an independent international organization committed to improving the state of the world by engaging business, political, academic and other leaders of society to shape global, regional and industry agendas. Incorporated as a not-for-profit foundation in 1971, and headquartered in Geneva, Switzerland, the Forum is tied to no political, partisan or national interests.
Professor Asim Khwaja Professor Michael Kremer. Author: Stephanie Majerowicz. Essays in Education and Development Economics. Abstract. Despite large improvements in access to schooling across developing countries, there are large remaining gaps in educational quality: many students are in school but not learning.
Essays in the Economics of Education. Education is at the center of upskilling human capital in developing countries, thereby positively influencing economic growth and development. For decades, many education policies targeted at developing countries have been narrowly focused on improving access to basic education (Barrett et al., 2015).
First, it assesses the role of education as a driver of development, including aspects of economic growth, basic needs and political participation. Second, it looks at the constitutive perspective, involving education as national status, human right and human development.
Many economists believe education is the key to economic development [1]. Ozturk [2] ... This essay focuses on the relationship between education and the economy, the level of education needed in ...
The effect of popularising higher education on economic growth. The population segment with higher education is regarded as an important contributor to economic growth, generating scientific ...
Education in every sense is one of the fundamental factors of development. No country can achieve sustainable economic development without substantial investment in human capital. Education enriches people's understanding of themselves and world. It improves the quality of their lives and leads to broad social benefits to individuals and society. Education raises people's productivity and ...
Findings. This empirical analysis for Spain points to the existence of nonlinearities in the relationship between education and economic growth at country level, for both secondary and tertiary education. Next, as different patterns emerge in different regions, the authors provide a regional analysis for a number of representative Spanish regions.
History provides a useful example of the adaptation needed in skills development due to changes in technology and the labor market. Goldin and Katz reviewed the U.S.'s 20 th century response to ...
Education is the only means to enhance man from ignorance and superstition and to help him master his environment.2 Bolino states that economists now see man as ""the essential prerequisite for economic advancement. It is he who shapes the environment to serve the unlimited demands. of the human imagination."3.
Education and economic growth Anna Valero ISSN 2042-2695. Abstract . ... Overall, there is little doubt over the centrality of human capital for growth and development. However, ... several papers (for example, Klenow and Rodríguez-Clare, 1997; Hall and Jones, 1999) express the growth accounting equation in per worker or ...
Hanushek and Woessman n (2008) explain that education can have a. positive influence on growth by enhancing human capital which in turn enhances the productivity of labor while in endogenous ...
Development education, education for sustainable development and global citizenship education. More than a century ago, Durkheim (Citation 1885, 445) declared that the 'aim of public education is not 'a matter of training workers for the factory or accountants for the warehouse but citizens for society'.From a US perspective, Feinberg (Citation 2006, xi) draws attention to the 'shared ...
economic development, the process whereby simple, low-income national economies are transformed into modern industrial economies.Although the term is sometimes used as a synonym for economic growth, generally it is employed to describe a change in a country's economy involving qualitative as well as quantitative improvements.The theory of economic development—how primitive and poor ...
The essay discusses various pathways through which economic development might lead to women's empowerment (variously equated with increased work opportunities, reduced time burdens, improvements in health and education, and women's rights).
Personal Finance for the Middle School Classroom is a five-day professional development course for K-12 educators taught by Federal Reserve economic education specialists and staff from the state centers for economic education. The course covers how to teach students about money, banking, and the Federal Reserve System.
ISSN:1473-236X. Education and Economic Development in India. Monojit Chatterji. University of Dundee. February 2008. ABSTRACT JEL I2, O53. This brief survey examines the returns to education in ...
As follows, education has an important influence on economic development: Education enhances people's access to current and scientific concepts. It improves people's efficiency and capacity to absorb new technologies. It raises knowledge of potential possibilities and labor mobility. Education helps people obtain information, skills, and ...
This note provides general guidance on the operationalization of the strategy for IMF engagement on social spending. Social spending plays a critical role as a key lever for promoting inclusive growth, addressing inequality, protecting vulnerable groups during structural change and adjustment, smoothing consumption over the lifecycle, and stabilizing demand during economic shocks. Social ...