- Architecture and Design
- Asian and Pacific Studies
- Business and Economics
- Classical and Ancient Near Eastern Studies
- Computer Sciences
- Cultural Studies
- Engineering
- General Interest
- Geosciences
- Industrial Chemistry
- Islamic and Middle Eastern Studies
- Jewish Studies
- Library and Information Science, Book Studies
- Life Sciences
- Linguistics and Semiotics
- Literary Studies
- Materials Sciences
- Mathematics
- Social Sciences
- Sports and Recreation
- Theology and Religion
- Publish your article
- The role of authors
- Promoting your article
- Abstracting & indexing
- Publishing Ethics
- Why publish with De Gruyter
- How to publish with De Gruyter
- Our book series
- Our subject areas
- Your digital product at De Gruyter
- Contribute to our reference works
- Product information
- Tools & resources
- Product Information
- Promotional Materials
- Orders and Inquiries
- FAQ for Library Suppliers and Book Sellers
- Repository Policy
- Free access policy
- Open Access agreements
- Database portals
- For Authors
- Customer service
- People + Culture
- Journal Management
- How to join us
- Working at De Gruyter
- Mission & Vision
- De Gruyter Foundation
- De Gruyter Ebound
- Our Responsibility
- Partner publishers

Your purchase has been completed. Your documents are now available to view.
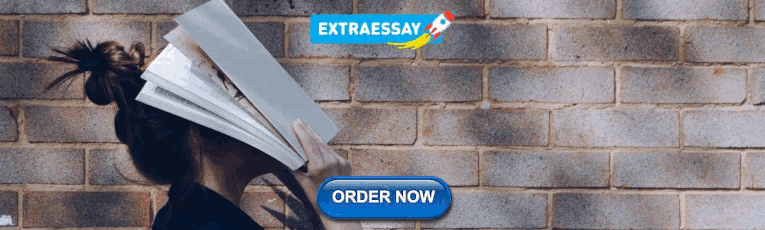
A Systematic Overview of Blockchain Research
Blockchain has been receiving growing attention from both academia and practices. This paper aims to investigate the research status of blockchain-related studies and to analyze the development and evolution of this latest hot area via bibliometric analysis. We selected and explored 2451 papers published between 2013 and 2019 from the Web of Science Core Collection database. The analysis considers different dimensions, including annual publications and citation trends, author distribution, popular research themes, collaboration of countries (regions) and institutions, top papers, major publication journals (conferences), supportive funding agencies, and emerging research trends. The results show that the number of blockchain literature is still increasing, and the research priorities in blockchain-related research shift during the observation period from bitcoin, cryptocurrency, blockchain, smart contract, internet of thing, to the distributed ledger, and challenge and the inefficiency of blockchain. The findings of this research deliver a holistic picture of blockchain research, which illuminates the future direction of research, and provides implications for both academic research and enterprise practice.
1 Introduction
With the era of bitcoin, digital cash denoted as BTC makes it possible to store and transmit value through the bitcoin network [ 1 ] . And therewith, blockchain, the technology underlying bitcoin, which adopts a peer-to-peer network to authenticate transactions, has been gaining growing attention from practices, especially Libra, a global currency and financial infrastructure launched by Facebook, and digital currency electronic payment. Currently, blockchain is also an increasingly important topic in the academic field. Blockchain research has considerably progressed, attracting attention from researchers, practitioners, and policy-makers [ 2 , 3 , 4 , 5 , 6 , 7 , 8 , 9 ] .
Considering the huge potential benefits that blockchain would bring in various aspects of industries, for instance, finance and economy [ 10 , 11 , 12 ] , internet of things [ 13 , 14 , 15 ] , energy [ 16 , 17 ] , supply chain [ 18 , 19 ] , and other areas. It is often compared with the Internet and is even referred to as a new form of the Internet. As a result, the number of publications in the blockchain is growing rapidly. According to an initial search on the Web of Science Core Collection, over 2000 scientific papers published are related to blockchain.
Under the circumstances where the number of research publications in the blockchain is quickly increasing, although studies have tried to provide some insights into the blockchain research via literature reviews [ 20 , 21 , 22 , 23 , 24 ] . Comprehensive scientometric analysis of academic articles published in influential journals are beneficial to the further development of blockchain research. This research conducts a bibliometric visualization review and attempts to deliver an overview of the research in this fast-growing field.
The objectives of this research are as follows. First, we intend to build an overview of the distribution of blockchain-related research by time, authors, journals, institutions, countries (regions), and areas in the blockchain academic community. Second, we probe the key research topics of blockchain study, for which purpose, we conduct keyword co-occurrence analysis. Third, we picture the intellectual structure of blockchain study based on co-citation analysis of articles and author co-citation analysis. Finally, we identify the direction for the evolution of blockchain study. We adopt Citespace to detect and visualize emerging trends in blockchain study. To achieve these targets, we posed the following research questions:
Q1: What is the distribution pattern of blockchain publications and citations over recent years? Q2: Which are the main international contributing countries (regions) and institutions in blockchain research, and the collaboration network among them? Q3: What are the characteristics of the authorship distribution pattern? Q4: What are the key blockchain subjects based on the number of publications? Q5: Which are the major journals or conferences for blockchain-related research? Q6: Which are the most influential papers in blockchain research based on the number of citations? Q7: Who are the most influential authors in blockchain research according to the author co-citation network? Q8: What are the research trends in blockchain? Q9: What are the most supportive funding agencies for blockchain research?
Our intended contributions in this research are twofold. First, it is an attempt of adopting co-citation analysis to provide comprehensive and up-to-date developing trends in the lasted hot area, blockchain. Second, this study depicts a state-of-the-art blockchain research development and gives enlightenment on the evolution of blockchain. The findings of this research will be illuminating for both academic researchers, entrepreneurs, as well as policymakers.
The rest of the article is organized as follows. The literature review mainly summarizes related work. The “Data and methodology” section describes the data source and methodological process. The “Results” section presents the main results based on the bibliometric analysis as well as statistical analysis. “Conclusions and implications” conclude this research provides answers to the aforementioned research questions and poses directions for further work.
2 Literature Review
Scientometric analysis, also known as bibliometric network visualization analysis has been widely adopted in numerous areas to identify and visualize the trends in certain fields. For instance, Bonilla, et al. analyzed the development of academic research in economics in Latin America based on a scientometric analysis [ 25 ] . Li, et al. conducted research on emerging trends in the business model study using co-citation analysis [ 26 ] . Gaviriamarin, et al. applied bibliometric analysis to analyze the publications on the Journal of Knowledge Management [ 27 ] .
Since the birth of bitcoin, as the foundation of which, blockchain has gained an increasing amount of attention in academic research and among practices. The research papers focus on the blockchain are quite abundant and are continuing to emerge. Among a host of papers, a few studies investigate the research trend of blockchain-based on a bibliometric analysis [ 22 , 23 , 28 , 29 , 30 ] .
Table 1 presents a summary of these bibliometric studies that summarized some findings on blockchain research, yet very few investigated the co-citation network and the evolution of popular topics in a timeline view. The number of papers these articles analyzed is relatively small, which may be because they used simple retrieval formula in searching blockchain-related articles, and it could pose a threat to bibliometric analysis. Therefore, this research aims to conduct a comprehensive analysis of the status of blockchain research, which is beneficial to future research and practices.
An overview of existing bibliometric studies on blockchain research
Note: NP = number of publications; WOS = Web of Science Core Collection; CNKI = China National Knowledge Infrastructure Databases; EI = EI Compendex, an engineering bibliographic database published by Elsevier; Scopus = Elsevier’s abstract and citation database.
3 Data and Methodology
This section elaborates steps to conduct a comprehensive bibliometric-based analysis: 1) data collection, 2) methodological process. The overall approach and methodology are shown in Figure 1 , the details could be seen as follows.

Research methodology
3.1 Data and Collection
As the leading database for science and literature, the Web of Science Core Collection has been widely used in bibliometrics analysis. It gives access to multidisciplinary information from over 18,000 high impact journals and over 180,000 conference proceedings, which allows for in-depth exploration of the complete network of citations in any field.
For the sake of acquiring enough articles that are relative to the blockchain, we select keywords from Wikipedia and industry information of blockchain, and some existing research literature [ 1 , 20 , 23 , 30 ] . Moreover, in consideration of that, there are a host of blockchain research papers in various fields, in fact, although some papers use keywords in abstract or the main body, blockchain is not the emphasis of the researches. Therefore, in order to get more accurate research results, we choose to conduct a title search instead of a topic search. Table 2 presents the retrieval results with different keywords in the titles, we find that among publications that are relative to the blockchain, the number of Proceeding Papers is the biggest, which is closely followed by articles, and a few reviews. Based on the comparison of five search results in Table 2 . In addition, for accuracy and comprehensiveness, we manually go through the abstract of all the papers form conducting a title search, and choose papers that are related to blockchain. Finally, a dataset with 2451 articles is used in the subsequent analysis.
The dataset we choose has good representativeness, although it may not completely cover all papers on the blockchain, it contains core papers, and in bibliometric analysis, core papers are enough to provide a holistic view for a comprehensive overview of blockchain research.
Blockchain research article characteristics by year from 2013 to 2019
Note: Document type include: Article(A), Proceedings Paper(P), Review(R); Timespan = 2013 ∼ 2019, download in May 31, 2019; Indexes = SCI-EXPANDED, SSCI, A&HCI, CPCI-S, CPCI-SSH, ESCI, CCR-EXPANDED, IC.
3.2 Methodological Process
The bibliometric approach has received increasing attention in many research domains. In this study, the methodological process mainly includes three methods: 1) descriptive statistical analysis, 2) article co-citation, author co-citation, and cluster analysis on co-cited articles; 3) time-zone analysis on co-cited keywords.
Descriptive statistical analysis displays an overall status of the research development in the target field, which mainly presents an overview by publication years, document types, the research area of published journals, number of citations, and in terms of most cited paper, influential author, institutions and countries. Co-citation analysis helps to identify the frequency of co-cited papers and authors and provides crucial insights into the intellectual structure of certain research fields [ 31 ] . Time-zone analysis helps to understand the flow of information and research trends in the target area [ 32 ] .
Various visualization tools have been designed and developed as computer software such as Citespace and VOSviewer. In this study, we use Citespace for co-citation analysis and timezone analysis, VOSviewer is adopted for social network analysis and visualization, we also apply other tools such as Excel and Tableau for basic statistical analysis and the visualization of the bibliometric results. Notably, in Citespace, core nodes are displayed as “citation tree-rings”, which contain abundant information of an article, for instance, the color of a citation ring denotes the year of corresponding citations, and the rule of colors in Citespace is the oldest in dark blue and newest in light orange with a spectrum of colors in between, the thickness of a ring is proportional to the number of citations in a time slice [ 33 ] . Figure 2 illustrates the details of the citation tree-rings. In addition, Citespace adopts a time-slicing mechanism to produce a synthesized network visualization [ 34 ] .
![research and analysis of blockchain data Figure 2 Citation tree-rings[33]](https://www.degruyter.com/document/doi/10.21078/JSSI-2021-205-34/asset/graphic/j_JSSI-2021-205-34_fig_002.jpg)
Citation tree-rings [ 33 ]
4.1 Distribution by Publication Year
Table 3 illustrates several characteristics of blockchain-related publications sorted by the year of publication. The annual number of articles and countries has been growing continuously since the proposing of Nakamoto’s paper in 2008 [ 1 ] , and the first blockchain research paper was published in 2013. By examining the published papers over time, there were only eight articles published in 2013. Afterward, with a continuous increase, a peak of 1,148 articles was published in 2018, and the number of publications is likely to grow ever since. Meanwhile, the annual number of countries taking part in blockchain research has also rapidly increased from 6 to 93 between 2013 and 2017, whereas the average number of Times Cited for single articles declined from 34.00 to 1.73 between 2013 and 2018. Over the observation period, 97 countries took part in the research on the blockchain with a sample of 44 in the H-index of our paper.
Statistical description of Blockchain research article from 2013 to 2019
Note: NP = number of publications; No.CO = number of countries; AV.TC = average number of Times Cited.
Figure 3 presents the cumulative numbers of published articles and citations from 2013 to 2019. There was a drastic increase in the number of papers published annually after 2016. As for the cumulative number of citations, there was no citation of blockchain literature before 2013, and 272 citations in 2013. By 2018, this number has grown over 10,000, which implies a widespread influence and attention of blockchain study in recent years.

Cumulative growth in blockchain publications and citations, 2013–2019
The exponential growth is a typical characteristic of the development of research fields [ 35 ] . The model can be expressed as:
where C is the cumulative number of articles or citations, Y is the publication or citation year, α , and β are parameters. In this study period, the cumulative articles and citations in the filed grow exponentially by R articles 2 = 0.9463 and R citations 2 = 0.8691 respectively. This shows that the research quantity curve of the blockchain is like an exponential function, which means the attention of academic circles on the blockchain has been increasing in recent years.
4.2 Distribution and International Collaboration Among Countries/Regions
A total of 97 countries/areas have participated in blockchain research during the observation period. Table 4 shows the number of articles for each country (region) contributing to publications. Remarkably, an article may be written by several authors from different countries/areas, therefore, the sum of articles published by each country is large than the total number of articles. As can be seen from Table 4 , the USA and China play leading roles amongst all countries/areas observed, with publications of 532 (20.94%) and 489 (19.24%) articles respectively, followed by the UK, which published 214 (8.42%) articles.
Blockchain research country (region) ranked by number of articles (top 25)
Note: NP = number of publications; No.TC = number of total Times Cited; AV.TC = average number of Times Cited; No.CA = number of Citing Articles.
From the perspective of citations, according to country/area distribution in Table 4 , we also find that USA-authored papers were cited by 1,810 papers with 3,709 (36.57%) citations, accounting for 36.57% of total citations. Meanwhile, articles from the USA also have a very high average number of citations per paper with a frequency of 6.97, which ranks third among the top 25 countries/ areas. Interestingly, the articles from Austria and Singapore appeared with the highest average number of citations per paper, with a frequency of 7.44 and 7.16 respectively, whereas the number of publications from these two countries was relatively low compared with the USA. The second was China, following the USA, papers were cited by 753 articles with 1,357 (13.38%) citations. Although the number of articles from China is close to the USA, the average number of citations per paper is lower with a frequency of 2.78. The subsequent countries include the UK, Germany, and Italy. The results indicate that the USA is the most influential country in blockchain.
International collaboration in science research is both a reality and a necessity [ 36 ] . A network consisting of nodes with the collaborating countries (regions) during the observation period is shown in Figure 4 . The network is created with the VOS viewer in which the thickness of the linking lines between two countries (regions) is directly proportional to their collaboration frequency. We can see from Figure 4 that the USA has the closest collaborative relationships with China, the UK, Australia, Germany, and Canada. China has the closest collaborative relationships with the USA, Australia, Singapore, UK, and South Korea. UK has the closest collaborative relationships with the USA, China, France, and Switzerland. Overall, based on the collaboration network, collaboration mainly emerges in highly productive countries (regions).

International collaboration network of the top 25 countries (territories), 2013–2019
4.3 Institution Distribution and Collaboration
A total of 2,190 institutions participated in blockchain-related research, and based on the number of publications, the top 25 of the most productive institutions are shown in Table 5 . Chinese Academy of Sciences had the highest number of publications with 43 papers, followed by the University of London with 42 papers, and Beijing University of Posts Telecommunications ranked third with 36 papers. The subsequent institutions included the University of California System and the Commonwealth Scientific Industrial Research Organization (CSIRO). In terms of the number of total Times Cited, Cornell University is cited most with 499 citations, and the average number of Times Cited is 20.79. Massachusetts Institute of Technology followed closely with 407 citations and with an average number of Times Cited of 22.61. The University of California System ranks third with 258 citations and an average number of Times Cited of 8.06. ETH Zurich ranked fourth with 257 citations and an average number of Times Cited of 10.28. It is notable that the National University of Singapore also had a high average number of Times Cited of 12.56. These results indicate that most of the influential institutions are mainly in the USA and Europe and Singapore. The number of publications from institutions in China is large, whereas few of the papers are highly recorded in average Times Cited. Papers from the National University of Defense Technology China took the highest of average Times Cited of 7.79.
Blockchain research country (territory) ranked by number of articles (top 25)
To further explore data, the top 186 institutions with at least 5 articles each are chosen for collaboration network analysis. The collaboration network map is shown in Figure 5 , the thickness of linking lines between two institutions is directly proportional to their collaboration frequency. As seen from the cooperation network in the Chinese Academy of Sciences, Cornell University, Commonwealth Scientific Industrial Research Organization (CSIRO), University of Sydney, and ETH Zurich cooperated widely with other institutions. This shows that collaboration between institutions may boost the research of blockchain which echoes with extant research that proposes with-institution collaboration and international collaboration may all contribute to article quality [ 37 ] .

Collaboration network for institutions, 2013–2019
4.4 Authorship Distribution
The total number of authors who contribute to the publications of blockchain is 5,862. Remarkably, an article may be written by several authors from different countries (regions) or institutions. Therefore, the total number of authors is bigger than the total number of articles. In fact, during the observation period, the average number of authors per paper is 2.4 articles. Reveals the distribution of the number of authors with different numbers of papers. As seen from the results, most of the authors had a tiny number of papers, i.e., among 5,862 authors, 4,808 authors have only one paper, 662 authors have two papers, and 213 authors have three papers.
According to the participation number of articles, the most productive author in the blockchain is Choo, Kim-Kwang Raymond from Univ Texas San Antonio, who took part in 14 articles in blockchain, followed by Marchesi, Michele from Univ of Cagliari, who took part in 13 articles related to blockchain. The third most productive author is Bouri, Elie from the Holy Spirit University of Kaslik, and David Roubaud from Montpellier Business School. Miller, Andrew, Shetty, Sachin, and Xu, Xiwei ranked fourth, who took part in 10 articles related to blockchain.
The distribution of number of author with different numbers of articles
Note: No.AU = number of author; No.AR = number of articles.
Figure 6 displays the collaboration network for authors. The thickness of the linking lines between the two authors is directly proportional to their collaboration frequency. As we can see from Figure 6 , it indicates the most productive authors cooperate widely with others.

Collaboration network for authors, 2013–2019
4.5 Distribution of Subject Categories
Table 7 presents the top 25 blockchain categories ranked in terms of the number of articles published. As can be seen from Table 7 , among the top 10 categories, six are related to the Computer Science field, which indicates that blockchain-related researches are more abundant in the field of Computer Science compared with other research fields. Besides, there are also publications in the category of Business & Economics with 385 records.
The top 25 blockchain categories ranked by the number of publications
Figure 7 illustrates the betweenness centrality network of papers of the above categories by using Citespace after being simplified with Minimum Spanning Tree network scaling, which remains the most prominent connections. We can see from Figure 7 , the centrality of Computer Science, Engineering Electrical Electronic, Telecommunications, Engineering, and Business & Economics are notable.

Categories involved in blockchain, 2013–2019
4.6 Journal Distribution
The research of blockchain is published in 1,206 journals (conferences), the top 25 journals (conferences) are displayed in Table 8 . Blockchain research papers are concentrated in these top journals (conferences) and with a concentration ratio of nearly 20%. The major blockchain research journals include Lecture Notes in Computer Science, IEEE Access, Economics Letters, Future Generation Computer Systems, and Finance Research Letters, with more than 20 articles in each one. Meanwhile, the major blockchain research conferences include IEEE International Conference on Hot Information-Centric Networking, International Conference on Parallel and Distributed Systems Proceedings, International Conference on New Technologies Mobility, and Security, and Financial Cryptography and Data Security, with at least 14 articles published in each of these.
The top 25 blockchain publication journals (conferences)
Note: NP = number of papers; No.TC = number of total Times Cited; Italic represents conference.
4.7 Intellectual Structure of Blockchain
Since the notion of co-citation was introduced, there are a host of researchers have adopted the visualization of co-citation relationships. The work is followed by White and Griffith [ 38 ] , who identified the intellectual structure of science, researches then broaden the unit of analysis from articles to authors [ 39 , 40 ] . There are two major types of co-citation analysis, namely, article cocitation analysis and author co-citation analysis, which are commonly adopted to visualize the intellectual structure of the research field. In this study, we explore the intellectual structure of blockchain by using both article co-citation analysis and author co-citation analysis. We apply Citespace to analyze and visualize the intellectual structure [ 41 ] .
In this study, mining spanning trees was adopted to present the patterns in the author cocitation network, a visualization of the network of author co-citation is demonstrated in Figure 8 . In the visualization of the co-citation network, pivot points are highlighted with a purple ring, and landmark nodes are identified with a large radius. From Figure 8 , there are six pivot nodes and landmark nodes: Nakamoto S, Buterin V, Eyal I, Wood G, Swan M, Christidis K. These authors truly played crucial roles during the development of blockchain research. Table 9 shows the ranking of author citation counts, as well as their prominent publications.

Network of author co-citation, 2013–2019
The top 15 co-cited author ranked by citation counts
Nakamoto S, as the creator of bitcoin, authored the bitcoin white paper, created and deployed bitcoin’s original reference implementation, is not surprised at the top of the co-citation count ranking, and has 1,202 citations in our dataset. Buterin V, a Russian-Canadian programmer, and writer primarily are known as a co-founder of ethereum and as a co-founder of Bitcoin Magazine, follows Nakamoto S, receives 257 citations. Eyal I, an assistant professor in technion, is a third of the ranking, with a representative article is “majority is not enough: Bitcoin mining is vulnerable”. Wood G, the ethereum founder, and free-trust technologist ranks fourth with 244 citations. The other core author with high citations includes Swan M, Christidis K, Bonneau J, Szabo N, Zyskind G, Castro M, and Meiklejohn S, with more than 150 citations of each person, and the typical publications of there are present in Table 9 .
To further investigate the features of the intellectual structure of blockchain research, we conducted an article co-citation analysis, using cluster mapping of co-citation articles networks to complete a visualization analysis of the evolution in the research field of blockchain. According to the article co-citation network, we adopted Citespace to divide the co-citation network into several clusters of co-cited articles. The visualization of clusters of co-cited articles is displayed in Figure 9 .

Clusters of co-cited articles, 2013–2019
As we mentioned earlier in the “Data and Methodology” section, the colors of citation rings and links are corresponding to the different time slices. Therefore, the deeper purple cluster (Cluster #1) is relatively old, and the prominent clusters (Cluster #0 and #2) are more recent. Cluster #0 is the youngest and Cluster #1 is the oldest. Cluster labels are identified based on burst terms extracted from titles, abstracts, keywords of bibliographic records [ 26 , 41 ] . Table 10 demonstrates six predominant clusters by the number of members in each cluster.
Results show that the research priorities of the clusters keep changing during the observation period. From the earlier time (Cluster # 1), bitcoin and bitcoin network are the major priorities of researchers, then some researchers changed the focuses onto cryptocurrency in blockchain research. Notably, more researchers are most interested in blockchain technology and public ledger recently.
According to the characteristics of pivot nodes and landmark nodes in the co-citation article network. The landmark and pivot nodes in co-citation articles are shown in Figure 10 , Five pivot nodes are Nakamoto S [ 1 ] , Wood G [ 44 ] , Kosba A [ 51 ] , Eyal I [ 12 ] and Maurer B [ 55 ] . The main landmark nodes are Christidis K [ 45 ] . Swan M [ 2 ] , Zyskind G [ 48 ] Nakamoto S [ 1 ] , Kosba A [ 51 ] , Notably, some nodes can be landmark and pivot at the same time.

Landmark and pivot nodes, 2013–2019
Summary of the largest 6 blockchain clusters
Details of the largest cluster (Cluster #0, top10)
Details of the largest cluster (Cluster #1, top10)
Details of the largest cluster (Cluster #2, top10)
As seen from Table 10 , Cluster #0 is the largest cluster, containing 36 nodes, for the sake of obtaining more information about these clusters, we explored the details of the largest clusters. Table 11 illustrates the details of the Cluster 0#.
We also explored Cluster #1 and #2 in more detail. Table 12 and Table 13 present the details of Cluster #1 and Cluster #2 respectively, it is notable that the most active citation in Cluster #1 is “bitcoin: A peer-to-peer electronic cash system”, and the most active citation in Cluster #2 is “bitcoin: Economics, technology, and governance”. The core members of Cluster #1 and Cluster #2 deliver milestones of blockchain research related to the bitcoin system and cryptocurrency.
Table 14 lists the first 10 most cited blockchain research articles indexed by the Web of Science. These articles are ranked according to the total number of citations during the observation period. Among these articles, the publication of “blockchains and smart contracts for the internet of things” by Christidis is identified as the most cited paper of 266 citations. The paper also has the highest average number of citations per year.
The top 10 cited blockchain articles
4.8 Keywords Co-Citation Analysis
According to Callon, et al. [ 77 ] co-word analysis is a useful way of examining the evolution of science. In our study, among 2,451 articles related to blockchain, we obtained 4,834 keywords, 594 keywords appeared 3 times, 315 keywords appeared 5 times, and 130 keywords appeared 10 times. Table 15 presents the most important keywords according to frequency. As seen, ‘blockchain’ ranks first with an occurrence frequency of 1,105, followed by ‘bitcoin’ of 606. The other high occurrence frequency keywords include: ‘cryptocurrency’, ‘smart contract’, and ‘iot’ (internet of thing).
The top 25 keywords ranked by frequency
For the sake of further exploration of the relation amongst the major keywords in blockchain research papers, we adopted the top 315 keywords with a frequency no less than 5 times for co-occurrence network analysis. The keywords co-occurrence network is illustrated in Figure 11 . In a co-occurrence network, the size of the node represents the frequency of the keywords co-occurrence with other keywords. The higher the co-occurrence frequency of the two keywords, the closer the relationship between them.

The keywords co-occurrence network, 2013–2019
We can see from Figure 11 , the size of blockchain and bitcoin are the largest among all keywords. This means, in general, blockchain and bitcoin have more chances to co-occurrence with other keywords. Besides, blockchain is closer with a smart contract, iot, Ethereum, security, internet, and privacy, whereas bitcoin is closer with digital currency and cryptocurrency.
Figure 12 displays the time-zone view of co-cited keywords, which puts nodes in order from left to right according to their years being published. The left-sided nodes were published in the last five years, and on the right-hand side, they were published in recent two years. Correspondingly, some pivot nodes of keywords are listed in the boxes. We hope to show the evolution of blockchain in general and the changes of focuses in blockchain study.

The time-zone view of co-cited keywords, 2013–2019
The results suggest that, in 2013, when blockchain research begins to surface, bitcoin dominated the blockchain research field. Reasonably, the bitcoin is the first cryptocurrency based on blockchain technology, and the influential essays include quantitative analysis of the full bitcoin transaction graph [ 54 ] ; a fistful of bitcoins: Characterizing payments among men with no
names [ 50 ] ; and bitcoin meets google trends and Wikipedia: Quantifying the relationship between phenomena of the internet era [ 69 ] . Afterward, as various altcoins appeared, cryptocurrency and digital currency are widely discussed in blockchain-related research. The high-citation article is Zerocash: Decentralized anonymous payments from bitcoin [ 74 ] and privacy, which is the prominent characteristic of cryptocurrency. In 2015, blockchain and smart contract become a hotspot, the core publications include blockchain: A blueprint for a new economy [ 2 ] ; decentralizing privacy: Using blockchain to protect personal data [ 48 ] ; at the same time, some researchers also focus on the volatility and mining of cryptocurrency. In 2016, a growing number of researchers focus on the internet of things. The most popular article is blockchains and smart contracts for the internet of things [ 45 ] . In 2017, distributed ledger and blockchain technology become a research focus point. From 2018 onward, research focus on the challenge, and the inefficiency of blockchain appear.
4.9 Funding Agencies of Blockchain-Related Research
Based on all 2451 funding sources we analyzed in this study, the National Natural Science Foundation of China (NSFC) has supported the biggest number of publications with 231 papers, followed by the National Key Research and Development Program of China, which supported the publication of 88 papers. Comparatively, the National Science Foundation of the USA has only supported 46 papers. It is remarkable that the “Ministry of Science and Technology Taiwan” supported 22 papers, which is more than the European Union. Table 16 illustrates the top 20 funding agencies for blockchain research ranked by the number of supported papers. The results indicate that China is one of the major investing countries in Blockchain research with the biggest number of supporting articles.
The top 20 funding agencies of blockchain-related research
5 Conclusions and Implications
5.1 conclusions.
This research comprehensively investigates blockchain-related publications based on the Web of Science Core Collection and provides a quick overview of blockchain research. In this study, a coherent comprehensive bibliometric evaluation framework is adopted to investigate the hot and promising blockchain domain. We outline the core development landscape of blockchain, including the distribution of publications over time, by authors, journals, categories, institutions, countries (territories), intellectual structure, and research trends in the blockchain academic community. Combining the results of statistical analysis and co-cited articles, authors, and keywords, we formulate the answers to the following research questions:
RQ1 What is the distribution pattern of blockchain publications and citations over recent years?
The published blockchain papers significantly increased since 2013, when the first blockchain paper was published. An increasing number of articles were published since. In 2018, 1,148 articles were published at the peak, and the number of publications is likely to continuously grow. As for the cumulative number of citations, there were only 272 citations in 2013. By 2018 this number has grown to more than 10,000, which implies a widespread influence and attention attracted by blockchain study in recent years.
RQ2 Which are the main international contributing countries (regions) and institutions in blockchain research, as well as collaboration networks among them?
A total of 97 countries (regions) participated in blockchain research during the observation period. USA and China play the leading roles among all countries (regions), with publications of 532 (20.94%) and 489 (19.24%) articles respectively, followed by the UK, Germany, Italy, and Australia. From the aspect of citations, USA-authored papers were cited by 1,810 papers with 3,709 (36.57%) citations, accounting for 36.57% of total citations. Articles from the USA also have a very high average number of citations per paper with a frequency of 6.97. Although the number of articles from China is close to the USA, the average number of citations per paper is lower with a frequency of 2.78. The results indicate that the USA is the most influential country in the field of blockchain.
A total of 2,190 institutions participated in blockchain-related research. Among them, the Chinese Academy of Sciences has the highest number of publications with 43 papers, followed by the University of London, Beijing University of Posts Telecommunications, University of California System, Commonwealth Scientific Industrial Research Organization (CSIRO), Beihang University, University of Texas System, ETH Zurich. In respect of the number of total Times Cited and the average number of Times Cited, Cornell University is cited the most with 499 citations, and the average number of Times Cited is 20.79. followed by the Massachusetts Institute of Technology, University of California System, and ETH Zurich. The number of publications forms institutions in China is large, whereas few papers own high average Times Cited.
In terms of collaboration networks among different institutions, we found that the Chinese Academy of Sciences, Cornell University, Commonwealth Scientific Industrial Research Organization (CSIRO), University of Sydney, and ETH Zurich cooperated widely with other institutions.
RQ3 What are the characteristics of the authorship distribution?
The total number of authors who contribute to the publications of blockchain is 5,862. the average number of authors per paper is 2.4. Among 5,862 authors, 4,808 authors have only one paper, 662 authors have two papers, and 213 authors have three papers. Based on the number of participated papers, the most productive author in the field of blockchain is Choo, Kim-Kwang Raymond from Univ Texas San Antonio, who participated in 14 articles in the field of blockchain, followed by Marchesi M, Bouri E, David R, Miller A, Shetty S and Xu X.
RQ4 What are the core blockchain subjects and journals based on the number of publications?
Blockchain-related researches are more abundant in the field of Computer Science compared with other categories. Other major fields include Engineering, Business & Economics, Telecommunications, and Business & Economics.
RQ5 What are the major journals or conferences for blockchain-related research?
The research of blockchain is published in 1,206 journals (conferences), the major blockchain research journals include Lecture Notes In Computer Science, IEEE Access, Economics Letters, Future Generation Computer Systems, and Finance Research Letters. Meanwhile, the major blockchain research conferences include IEEE International Conference on Hot Information-Centric Networking, International Conference on Parallel and Distributed Systems Proceedings, International Conference on New Technologies Mobility and Security, and Financial Cryptography and Data Security.
RQ6 What are the most influential papers in blockchain research based on the number of citations?
Ranked by the total number of citations during the observation period, the publication: “blockchains and smart contracts for the internet of things” by Christidis and Devetsikiotis [ 45 ] is identified as the most cited paper with 266 citations, which also has a highest average number of citation per year, followed by decentralizing privacy: Using blockchain to protect personal data [ 48 ] with 169 citations and 33.80 average number of citations per year.
According to the number of times co-cited, the top five influential publications are as follows: Bitcoin: A peer-to-peer electronic cash system [ 1 ] , A next-generation smart contract and decentralized application platform [ 42 ] , Majority is not enough: Bitcoin mining is vulnerable [ 12 ] , Ethereum: A secure decentralised generalised transaction ledger [ 44 ] , Blockchain: Blueprint for a new economy [ 2 ] .
RQ7 Who are the most influential authors in blockchain research according to the author co-citation network?
Some authors played a crucial role during the development of blockchain research, Nakamoto S, as the creator of Bitcoin, and the author of the bitcoin white paper, created and deployed bitcoin’s original reference, therefore is not surprised at the top of the co-citation count ranking and got 1,202 citations in our dataset. Buterin V, a Russian-Canadian, programmer, and writer, primarily known as a co-founder of Ethereum and as a co-founder of Bitcoin Magazine who follows Nakamoto S and receives 257 citations. Other core authors with high citations include Eyal I, Wood G, Swan M, Christidis K, Bonneau J, Szabo N, Zyskind G, Castro M, and Meiklejohn S.
According to co-cited articles clusters, the research priorities in blockchain-related research keep changing during the observation period. Bitcoin and bitcoin network are the main priorities of researchers, then some researchers changed to focus on cryptocurrency in blockchain research.
RQ8 What are the research trends of blockchain?
The research priorities in blockchain-related research evolve during the observation period. As early as 2013, when the research on blockchain first appears, bitcoin dominated the blockchain research field. Then only one year later, as various altcoins begin to appear, cryptocurrency and digital currency are widely discussed in blockchain-related research. In 2015, blockchain and smart contracts become a hotspot till 2016 when a growing body of researches begin to focus on the internet of things. In 2017, distributed ledger and blockchain technology become the research focal point. From 2018 onward, research focus on the challenge and inefficiency of blockchain.
RQ9 What are the most supportive funding agencies of blockchain research?
The most supportive funding agency of blockchain research is the National Natural Science Foundation of China (NSFC) which has supported the publication of 231 papers. The results indicate that China is one of the major investing countries in Blockchain research with the biggest number of supporting articles.
Given the potential power of blockchain, it is noticeable that governments, enterprises, and researchers all pay increasing attention to this field. The application of blockchain in various industries, the supervision of cryptocurrencies, the newly rising central bank digital currency and Libra, are becoming the central issues of the whole society.
In our research, we conducted a comprehensive exploration of blockchain-related research via a bibliometrics analysis, our results provide guidance and implications for academic research and practices. First, the findings present a holistic view of research in the blockchain domain which benefits researchers and practitioners wanting to quickly obtain a visualized overview of blockchain research. Second, according to our findings of the evolution and trends in blockchain research, researchers could better understand the development and status of blockchain, which is helpful in choosing valuable research topics, the distributed ledger, the discussions on the inefficiency and challenges of blockchain technology, the supervision of cryptocurrencies, the central bank digital currency are emerging research topics, which deserve more attention from the academic community.
5.2 Limitations and Future Work
As with any research, the design employed incorporates limitations that open avenues for future research. First, this study is based on 2,451 articles retrieved from the Web of Science of Core Collection, although the Web of Science of Core Collection is truly a powerful database for bibliometric analysis, we can’t ignore the limitation brought by a unique data source. Future research can deal with this limitation by merging the publications from other sources, for instance, Scopus, CNKI, as well as patent database and investment data of blockchain, and it could help to validate the conclusion. Second, we mainly adopt the frequency indicator to outline the state-of-the art of blockchain research, although the frequency is most commonly used in the bibliometric analysis, and we also used H-index, citation to improve our analysis, some other valuable indicators are ignored, such as sigma and between centrality, therefore, it’s beneficial to combine those indicators in future research. Besides, it should be noted that, in co-citation analysis, a paper should be published for a certain period before it is cited by enough authors [ 26 ] , the newest published papers may not include in co-citation analysis, it’s also an intrinsic drawback of bibliometric methods.
Supported by the National Natural Science Foundation of China (71872171), and the Open Project of Key
Laboratory of Big Data Mining and Knowledge Management, Chinese Academy of Sciences
[1] Nakamoto S. Bitcoin: A peer-to-peer electronic cash system. https://bitcoin.org/bitcoin.eps 2008. Search in Google Scholar
[2] Swan M. Blockchain: Blueprint for a new economy. O’Reilly, 2015. Search in Google Scholar
[3] Weber I, Xu X, Riveret R, et al. Untrusted business process monitoring and execution using blockchain. Business Process Management, 2016: 329–347. 10.1007/978-3-319-45348-4_19 Search in Google Scholar
[4] Mettler M. Blockchain technology in healthcare: The revolution starts here. International Conference on E-health Networking, Applications and Services, 2016: 1–3. 10.1109/HealthCom.2016.7749510 Search in Google Scholar
[5] Paech P. The governance of blockchain financial networks. Modern Law Review, 2016, 80(6): 1073–1110. 10.1111/1468-2230.12303 Search in Google Scholar
[6] Yue X, Wang H, Jin D, et al. Healthcare data gateways: Found healthcare intelligence on blockchain with novel privacy risk control. Journal of Medical Systems, 2016, 40(10): 1–8. 10.1007/s10916-016-0574-6 Search in Google Scholar PubMed
[7] Maupin J. Mapping the global legal landscape of blockchain and other distributed ledger technologies. https://ssrn.com/abstract=2930077 2017. 10.2139/ssrn.2930077 Search in Google Scholar
[8] Sachin K, Angappa G, Himanshu A. Understanding the blockchain technology adoption in supply chains. International Journal of Production Research, 2018: 1–25. Search in Google Scholar
[9] Tasca P, Tessone C J. A taxonomy of blockchain technologies: Principles of identification and classification. Ledger, 2019, 4: 140. 10.5195/ledger.2019.140 Search in Google Scholar
[10] Catalini C, Gans J S. Some simple economics of the blockchain. Communications of the ACM, 2016, 63(7): 80–90. 10.3386/w22952 Search in Google Scholar
[11] Csoka P, Herings P J. Decentralized clearing in financial networks. Management Science, 2017, 64(5): 4681–4699. 10.1287/mnsc.2017.2847 Search in Google Scholar
[12] Eyal I. Blockchain technology: Transforming libertarian cryptocurrency dreams to finance and banking realities. Computer, 2017, 50(9): 38–49. 10.1109/MC.2017.3571042 Search in Google Scholar
[13] Huckle S, Bhattacharya R, White M, et al. Internet of things, blockchain and shared economy applications. Procedia Computer Science, 2016: 461–466. 10.1016/j.procs.2016.09.074 Search in Google Scholar
[14] Bahga A, Madisetti V K. Blockchain platform for industrial internet of things. Journal of Software Engineering and Applications, 2016, 9(10): 533–546. 10.4236/jsea.2016.910036 Search in Google Scholar
[15] Dorri A, Kanhere S S, Jurdak R. Towards an optimized blockchain for IoT. IEEE/ACM Second International Conference on Internet-of-Things Design and Implementation (IoTDI), 2017: 173–178. 10.1145/3054977.3055003 Search in Google Scholar
[16] Aitzhan N Z, Svetinovic D. Security and privacy in decentralized energy trading through multi-signatures, blockchain and anonymous messaging streams. IEEE Transactions on Dependable and Secure Computing, 2018, 15(5): 840–852. 10.1109/TDSC.2016.2616861 Search in Google Scholar
[17] Mengelkamp E, Notheisen B, Beer C, et al. A blockchain-based smart grid: Towards sustainable local energy markets. Computer Science — Research and Development, 2018: 207–214. 10.1007/s00450-017-0360-9 Search in Google Scholar
[18] Korpela K, Hallikas J, Dahlberg T. Digital supply chain transformation toward blockchain integration. Hawaii International Conference on System Sciences, 2017: 1–10. 10.24251/HICSS.2017.506 Search in Google Scholar
[19] Toyoda K, Mathiopoulos P T, Sasase I, et al. A novel blockchain-based product ownership management system (POMS) for anti-counterfeits in the post supply chain. IEEE Access, 2017: 17465–17477. 10.1109/ACCESS.2017.2720760 Search in Google Scholar
[20] Yuan Y, Wang F Y. Blockchain: The state of the art and future trends. Acta Automatica Sinica, 2016, 42(4): 481–494. Search in Google Scholar
[21] Yli-Huumo J, Ko D, Choi S, et al. Where is current research on blockchain technology? — A systematic review. PLoS One, 2016, 11(10): e0163477. 10.1371/journal.pone.0163477 Search in Google Scholar PubMed PubMed Central
[22] Zeng S, Ni X. A bibliometric analysis of blockchain research. IEEE Intelligent Vehicles Symposium, 2018: 102–107. 10.1109/IVS.2018.8500606 Search in Google Scholar
[23] Miau S, Yang J. Bibliometrics-based evaluation of the blockchain research trend: 2008–2017. Technology Analysis & Strategic Management, 2018, 30(9): 1029–1045. 10.1080/09537325.2018.1434138 Search in Google Scholar
[24] Hawlitschek F, Notheisen B, Teubner T. The limits of trust-free systems: A literature review on blockchain technology and trust in the sharing economy. Electronic Commerce Research and Applications, 2018: 50–63. 10.1016/j.elerap.2018.03.005 Search in Google Scholar
[25] Bonilla C A, Merigo J M, Torres-Abad C. Economics in Latin America: A bibliometric analysis. Scientometrics, 2015, 105(2): 1239–1252. 10.1007/s11192-015-1747-7 Search in Google Scholar
[26] Li X, Qiao H, Wang S. Exploring evolution and emerging trends in business model study: A co-citation analysis. Scientometrics, 2017, 111(2): 869–887. 10.1007/s11192-017-2266-5 Search in Google Scholar
[27] GaviriamarinM,Merigo JM, Popa S. Twenty years of the journal of knowledge management: A bibliometric analysis. Journal of Knowledge Management, 2018, 22(8): 1655–1687. 10.1108/JKM-10-2017-0497 Search in Google Scholar
[28] Faming W, Meijuan Z. Bibliometric analysis on the research hotspots of blockchain in China. Journal of Intelligence, 2017, 36(12): 69–74. Search in Google Scholar
[29] Mu-Nan L. Analyzing intellectual structure of related topics to blockchain and bitcoin: From co-citation clustering and bibliographic coupling perspectives. Acta Automatica Sinica, 2017, 43(9): 1501–1519. Search in Google Scholar
[30] Dabbagh M, Sookhak M, Safa N S. The evolution of blockchain: A bibliometric study. IEEE Access, 2019: 19212–19221. 10.1109/ACCESS.2019.2895646 Search in Google Scholar
[31] Chen C, Ibekwesanjuan F, Hou J. The structure and dynamics of co citation clusters: A multiple perspective co-citation analysis. Journal of the Association for Information Science and Technology, 2010, 61(7): 1386–1409. Search in Google Scholar
[32] Chen C. Visualizing scientific paradigms: An introduction. Journal of the Association for Information Science and Technology, 2003, 54(5): 392–393. 10.1002/asi.10224 Search in Google Scholar
[33] Chen C. CiteSpace II: Detecting and visualizing emerging trends and transient patterns in scientific literature. Journal of the Association for Information Science and Technology, 2006, 57(3): 359–377. 10.1002/asi.20317 Search in Google Scholar
[34] Chen C, Morris S A. Visualizing evolving networks: Minimum spanning trees versus pathfinder networks. IEEE Symposium on Information Visualization, 2003: 67–74. Search in Google Scholar
[35] Guan J, Ma N. China’s emerging presence in nanoscience and nanotechnology: A comparative bibliometric study of several nanoscience giants. Research Policy, 2007, 36(6): 880–886. 10.1016/j.respol.2007.02.004 Search in Google Scholar
[36] Wagner C S, Leydesdorff L. Network structure, self-organization, and the growth of international collaboration in science. Research Policy, 2005, 34(10): 1608–1618. 10.1016/j.respol.2005.08.002 Search in Google Scholar
[37] He Z, Geng X, Campbellhunt C. Research collaboration and research output: A longitudinal study of 65 biomedical scientists in a New Zealand University. Research Policy, 2009, 38(2): 306–317. 10.1016/j.respol.2008.11.011 Search in Google Scholar
[38] White H D, Griffith B C. Author cocitation: A literature measure of intellectual structure. Journal of the American Society for Information Science, 1981, 32(3): 163–171. 10.1002/asi.4630320302 Search in Google Scholar
[39] Chen C. Searching for intellectual turning points: Progressive knowledge domain visualization. Proceedings of the National Academy of Sciences of the United States of America, 2004: 5303–5310. 10.1073/pnas.0307513100 Search in Google Scholar PubMed PubMed Central
[40] Borner K, Chen C, Boyack K W. Visualizing knowledge domains. The Artist and Journal of Home Culture, 2005, 37(1): 179–255. 10.1002/aris.1440370106 Search in Google Scholar
[41] Chen C. CiteSpace II: Detecting and visualizing emerging trends and transient patterns in scientific literature. Journal of the Association for Information Science and Technology, 2006, 57(3): 359–377. 10.1002/asi.20317 Search in Google Scholar
[42] Buterin V. A next-generation smart contract and decentralized application platform. https://ethereum.org/en/whitepaper/ 2014. Search in Google Scholar
[43] Eyal I, Sirer E G. Majority is not enough: Bitcoin mining is vulnerable. International Conference on Financial Cryptography and Data Security, 2014: 436–454. 10.1145/3212998 Search in Google Scholar
[44] Wood G. Ethereum: A secure decentralised generalised transaction ledger. Ethereum Project Yellow Paper, 2014, 151: 1–32. Search in Google Scholar
[45] Christidis K, Devetsikiotis M. Blockchains and smart contracts for the internet of things. IEEE Access, 2016: 2292–2303. 10.1109/ACCESS.2016.2566339 Search in Google Scholar
[46] Bonneau J, Miller A, Clark J, et al. Sok: Research perspectives and challenges for bitcoin and cryptocurrencies. IEEE Symposium on Security and Privacy, 2015: 104–121. 10.1109/SP.2015.14 Search in Google Scholar
[47] Szabo N. Formalizing and securing relationships on public networks. First Monday, 1997. 10.5210/fm.v2i9.548 Search in Google Scholar
[48] Zyskind G, Nathan O, Pentland A. Decentralizing privacy: Using blockchain to protect personal data. IEEE Security and Privacy Workshops, 2015: 180–184. 10.1109/SPW.2015.27 Search in Google Scholar
[49] Castro M, Liskov B. Practical byzantine fault tolerance and proactive recovery. ACM Transactions on Computer Systems (TOCS), 2002, 20(4): 398–461. 10.1145/571637.571640 Search in Google Scholar
[50] Meiklejohn S, Pomarole M, Jordan G, et al. A fistful of bitcoins: Characterizing payments among men with no names. Internet Measurement Conference, 2013: 127–140. 10.1145/2504730.2504747 Search in Google Scholar
[51] Kosba A, Miller A, Shi E, et al. Hawk: The blockchain model of cryptography and privacy-preserving smart contracts. IEEE Symposium on Security and Privacy, 2016: 839–858. 10.1109/SP.2016.55 Search in Google Scholar
[52] Reid F, Harrigan M. An analysis of anonymity in the bitcoin system. Security and Privacy in Social Networks, 2013: 197–223. 10.1109/PASSAT/SocialCom.2011.79 Search in Google Scholar
[53] Luu L, Narayanan V, Zheng C, et al. A secure sharding protocol for open blockchains. ACM SIGSAC Conference on Computer and Communications Security, 2016: 17–30. 10.1145/2976749.2978389 Search in Google Scholar
[54] Ron D, Shamir A. Quantitative analysis of the full bitcoin transaction graph. International Conference on Financial Cryptography and Data Security, 2013: 6–24. 10.1007/978-3-642-39884-1_2 Search in Google Scholar
[55] Maurer B, Nelms T C, Swartz L. When perhaps the real problem is money itself? The practical materiality of bitcoin. Social Semiotics, 2013, 23(2): 261–277. 10.1080/10350330.2013.777594 Search in Google Scholar
[56] Tschorsch F, Scheuermann B. Bitcoin and beyond: A technical survey on decentralized digital currencies. IEEE Communications Surveys & Tutorials, 2016, 18(3): 2084–2123. 10.1109/COMST.2016.2535718 Search in Google Scholar
[57] Radziwill N. Blockchain revolution: How the technology behind bitcoin is changing money, business, and the world. The Quality Management Journal, 2018, 25(1): 64–65. 10.1080/10686967.2018.1404373 Search in Google Scholar
[58] Azaria A, Ekblaw A, Vieira T, et al. Medrec: Using blockchain for medical data access and permission management. International Conference on Open and Big Data (OBD), 2016: 25–30. 10.1109/OBD.2016.11 Search in Google Scholar
[59] Narayanan A, Bonneau J, Felten E, et al. Bitcoin and cryptocurrency technologies: A comprehensive introduction. Princeton University Press, 2016. Search in Google Scholar
[60] Miers I, Garman C, Green M, et al. Zerocoin: Anonymous distributed e-cash from bitcoin. IEEE Symposium on Security and Privacy, 2013: 397–411. 10.1109/SP.2013.34 Search in Google Scholar
[61] Ober M, Katzenbeisser S, Hamacher K. Structure and anonymity of the bitcoin transaction graph. Future Internet, 2013, 5(2): 237–250. 10.3390/fi5020237 Search in Google Scholar
[62] Moore T, Christin N. Beware the middleman: Empirical analysis of bitcoin-exchange risk. International Conference on Financial Cryptography and Data Security, 2013: 25–33. 10.1007/978-3-642-39884-1_3 Search in Google Scholar
[63] Androulaki E, Karame G O, Roeschlin M, et al. Evaluating user privacy in bitcoin. International Conference on Financial Cryptography and Data Security, 2013: 34–51. 10.1007/978-3-642-39884-1_4 Search in Google Scholar
[64] Barber S, Boyen X, Shi E, et al. Bitter to better — How to make bitcoin a better currency. International International Conference on Financial Cryptography and Data Security, 2012: 399–414. 10.1007/978-3-642-32946-3_29 Search in Google Scholar
[65] Böhme R, Christin N, Edelman B, et al. Bitcoin: Economics, technology, and governance. Journal of Economic Perspectives, 2015, 29(2): 213–238. 10.1257/jep.29.2.213 Search in Google Scholar
[66] Cheah E T, Fry J. Speculative bubbles in bitcoin markets? An empirical investigation into the fundamental value of bitcoin. Economics Letters, 2015, 130: 32–36. 10.1016/j.econlet.2015.02.029 Search in Google Scholar
[67] Urquhart A. The inefficiency of bitcoin. Economics Letters, 2016, 148: 80–82. 10.1016/j.econlet.2016.09.019 Search in Google Scholar
[68] Dyhrberg A H. Bitcoin, gold and the dollar — A GARCH volatility analysis. Finance Research Letters, 2016, 16: 85–92. 10.1016/j.frl.2015.10.008 Search in Google Scholar
[69] Ciaian P, Rajcaniova M, Kancs D A. The economics of bitcoin price formation. Applied Economics, 2016, 48(19): 1799–1815. 10.1080/00036846.2015.1109038 Search in Google Scholar
[70] Kristoufek L. BitCoin Meets Google Trends and Wikipedia: Quantifying the relationship between phenomena of the internet era. Scientific Reports, 2013, 3(1): 3415–3415. 10.1038/srep03415 Search in Google Scholar PubMed PubMed Central
[71] Dwyer G P. The economics of bitcoin and similar private digital currencies. Journal of Financial Stability, 2015, 17: 81–91. 10.1016/j.jfs.2014.11.006 Search in Google Scholar
[72] Nadarajah S, Chu J. On the inefficiency of bitcoin. Economics Letters, 2017, 150: 6–9. 10.1016/j.econlet.2016.10.033 Search in Google Scholar
[73] Katsiampa P. Volatility estimation for bitcoin: A comparison of GARCH models. Economics Letters, 2017, 158: 3–6. 10.1016/j.econlet.2017.06.023 Search in Google Scholar
[74] Bouri E, Gupta R, Tiwari A K, et al. Does bitcoin hedge global uncertainty? Evidence from wavelet-based quantile-in-quantile regressions. Finance Research Letters, 2017, 23: 87–95. 10.1016/j.frl.2017.02.009 Search in Google Scholar
[75] Sasson E B, Chiesa A, Garman C, et al. Zerocash: Decentralized anonymous payments from bitcoin. IEEE Symposium on Security and Privacy, 2014: 459–474. 10.1109/SP.2014.36 Search in Google Scholar
[76] Garay J, Kiayias A, Leonardos N. The bitcoin backbone protocol: Analysis and applications. International Conference on the Theory and Applications of Cryptographic Techniques, 2015: 281–310. 10.1007/978-3-662-46803-6_10 Search in Google Scholar
[77] Callon M, Courtial J P, Laville F. Co-word analysis as a tool for describing the network of interactions between basic and technological research: The case of polymer chemistry. Scientometrics, 1991, 22(1): 155–205. 10.1007/BF02019280 Search in Google Scholar
© 2021 Walter de Gruyter GmbH, Berlin/Boston
This work is licensed under the Creative Commons Attribution 4.0 International License.
- X / Twitter
Supplementary Materials
Please login or register with De Gruyter to order this product.
Journal and Issue
Articles in the same issue.
Thank you for visiting nature.com. You are using a browser version with limited support for CSS. To obtain the best experience, we recommend you use a more up to date browser (or turn off compatibility mode in Internet Explorer). In the meantime, to ensure continued support, we are displaying the site without styles and JavaScript.
- View all journals
- My Account Login
- Explore content
- About the journal
- Publish with us
- Sign up for alerts
- Data Descriptor
- Open access
- Published: 07 April 2022
Deciphering Bitcoin Blockchain Data by Cohort Analysis
- Yulin Liu 1 , 4 na1 ,
- Luyao Zhang ORCID: orcid.org/0000-0002-1183-2254 1 , 2 na1 &
- Yinhong Zhao 1 , 3 na1
Scientific Data volume 9 , Article number: 136 ( 2022 ) Cite this article
7893 Accesses
9 Citations
18 Altmetric
Metrics details
- Interdisciplinary studies
- Research data
Bitcoin is a peer-to-peer electronic payment system that has rapidly grown in popularity in recent years. Usually, the complete history of Bitcoin blockchain data must be queried to acquire variables with economic meaning. This task has recently become increasingly difficult, as there are over 1.6 billion historical transactions on the Bitcoin blockchain. It is thus important to query Bitcoin transaction data in a way that is more efficient and provides economic insights. We apply cohort analysis that interprets Bitcoin blockchain data using methods developed for population data in the social sciences. Specifically, we query and process the Bitcoin transaction input and output data within each daily cohort. This enables us to create datasets and visualizations for some key Bitcoin transaction indicators, including the daily lifespan distributions of spent transaction output (STXO) and the daily age distributions of the cumulative unspent transaction output (UTXO). We provide a computationally feasible approach for characterizing Bitcoin transactions that paves the way for future economic studies of Bitcoin.
Similar content being viewed by others
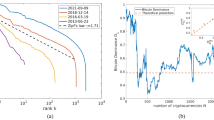
Modeling innovation in the cryptocurrency ecosystem
Giordano De Marzo, Francesco Pandolfelli & Vito D. P. Servedio
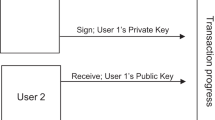
Blockchain vehicles for efficient Medical Record management
Anuraag A. Vazirani, Odhran O’Donoghue, … Edward Meinert
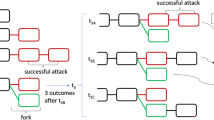
Statistical detection of selfish mining in proof-of-work blockchain systems
Sheng-Nan Li, Carlo Campajola & Claudio J. Tessone
Background & Summary
Bitcoin is a peer-to-peer electronic payment system that has rapidly grown in popularity in recent years 1 , 2 , 3 , 4 . As a distributed ledger technology (DLT), Bitcoin records newly generated transactions in a decentralized way, eliminating the need for intermediaries like banks and reducing transaction costs 5 , 6 , 7 .
Bitcoin relies on recording the unspent transaction outputs (UTXO) to efficiently verify newly generated transactions 8 , 9 , 10 , 11 . An illustrative example of UTXO is shown in Fig. 1 . A UTXO can be generated either as block rewards or outputs of transactions. Block rewards are newly minted bitcoins (BTC) distributed to miners for their work to maintain the network, such as routing transactions and validating blocks. In fact, all UTXOs can be dated back to block rewards. The timestamp is recorded when a UTXO is generated. A UTXO is spent and converted into a spent transaction output (STXO) when it is used as the input of a transaction. A timestamp is again recorded when the UTXO is spent, and each UTXO can be spent only once. Such a unique feature allows us to calculate the age of each UTXO and the lifespan of each STXO as we do in population data. Take Fig. 1 as an example. As of July 1, 2020, UTXOs 1–3 are 8.5-years, 1-year, and 1-day old, respectively. Immediately after Alice’s payment to Bob on January 1, 2021, UTXOs 1–3 are converted to STXOs with ages of 9 years, 1.5 years, and 0.5 years and 1-day old, respectively.
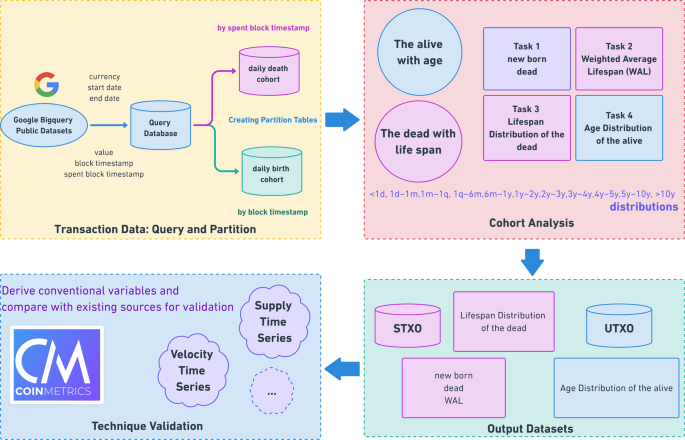
An example of UTXO birth and death. UTXOs 1, 2, and 3 were spent in a transaction taking place between Alice and Bob and were transformed to UTXOs 4 and 5. UTXOs 1, 2, and 3 became STXOs after the transaction.
Noticing the unique structure of the Bitcoin blockchain data, we apply cohort analysis 12 , 13 , 14 , 15 , 16 , originally developed for population data, to analyze it. To continue the analogy with the population data, we say a UTXO is born when it is generated as block rewards or the output of a transaction, and we say a UTXO is dead when it is spent as the input of another transaction. In this way, all UTXOs generated on the same day form a daily birth cohort, and all UTXOs spent on the same day form a daily death cohort. We define the age of a UTXO as the difference between “now” (the date on which we are working) and the time when it was born. We define the lifespan of an STXO as the difference between the time when the STXO was dead and the time when it was born. Thus, all UTXOs within an age range form an age cohort, and all STXOs within a lifespan range form a lifespan cohort. With this framework, we naturally replicate in Bitcoin blockchain data a trinity of birth, death, and age cohorts using population cohort analysis.
Usually, we need to query the complete history of Bitcoin blockchain data to acquire variables with economic meaning. With over 1.6 billion historical transactions on the Bitcoin blockchain, it has become increasingly difficult and computationally intensive now to download the complete Bitcoin blockchain records. It is thus important to query Bitcoin transaction data in a way that is more efficient and provides economic insights 17 . Cohort analysis provides a new perspective from which we can analyze data within each cohort separately before integrating them into a time series.
Our workflow is displayed in Fig. 2 . We query and process Bitcoin transaction input and output data within each daily cohort. By doing so, we successfully create datasets and visualizations for some key Bitcoin transactions indicators, including the daily lifespan distributions of STXOs as percentages (Fig. 3 ) and the cumulative daily age distributions of UTXOs (Fig. 4 ). These visualizations can be used to study the functions of bitcoin (BTC) as a currency. The three functions of a currency include acting as a store of value, unit of account, and medium of exchange. For example, Fig. 4 shows the number of BTCs in UTXOs (i.e., BTCs that have not been spent) by age distribution. By the end of 2020, approximately 2 million BTCs had not been transacted for more than 10 years. In the past 5–10 years, 2–5 years, and 1–2 years, approximately 2 million, 4.5 million, and 3 million BTCs, respectively, remained inactive. This equals approximately 11.5 million BTCs not having been transacted for more than 1 year. These BTCs serve as a time deposit and act as a store of value. Moreover, approximately 5 million BTCs are alive for 1 month to 1 year. These BTCs are similar to a demand deposit. Frequently transacted BTCs are those with ages between 1 day and 1 month (2 million) and less than 1 day (0.2 million). These BTCs act as a medium of exchange.
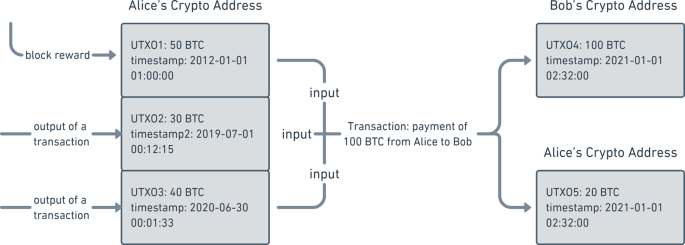
Workflow of cohort analysis on BTC UTXO data.
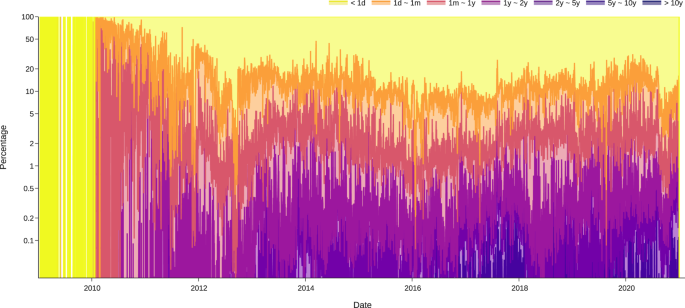
Lifespan distribution of BTC STXOs. The figure shows the log percentage of spent transaction outputs with different lifespans in each day until Feb. 2021. For example, by Feb. 2021, the STXOs with lifespans of less than one day accounted for 80% of all STXOs, while those with lifespans between 1 day and 1 month accounted for another 15%.
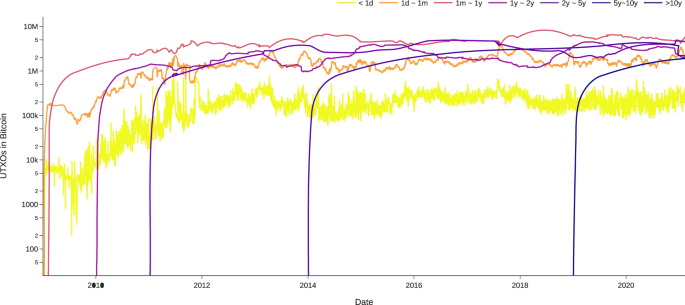
Number of BTC UTXOs by age. The figure shows the total cumulative unspent transaction outputs by age. For example, by Feb. 2021, there were approximately 200k UTXOs less than 1 day old used as the medium of exchange and approximately 2 million UTXOs more than 10 years old lost or used as store of value.
Our final datasets include one dataset that characterizes STXOs and one that characterizes UTXOs, which are both smaller than 1 MB. Moreover, cohort analysis keeps data querying and processing to a minimum for future updates and enables automated updates. We thus provide a computationally feasible approach for characterizing BTC transactions, which paves the way for future economic studies of Bitcoin. Our methods can be generally applied to other cryptocurrencies that adopt UTXO protocols, including Litecoin, Dash, Zcash, Dogecoin, and Bitcoin Cash.
While the Bitcoin transaction output data are publicly available on its blockchain, we find the size of the raw data (approximately 1.3 TB) overwhelming to process, even with cloud computing platforms. To improve the efficiency of computation, we first retrieve the data relevant to the study to create a more manageable data table of only 45 GB. By partitioning this data table into daily birth and death cohorts, we can analyze the STXOs and UTXOs in each cohort separately to summarize the daily characteristics of transaction outputs and create visualizations based on the cohort summary. Our method can be adapted to the creation of future blocks — we only need to process the transaction output data from the latest cohort and append the summary to the current version.
Creating partitioned tables
Our primary workplace is Google Colaboratory (Colab), a Jupyter Notebook hosted environment from Google, and BigQuery, a data warehouse from Google Cloud Platform. We first query the columns of interest from the public dataset crypto-bitcoin on BigQuery 18 , which includes the input and output data of Bitcoin. We then join the data queried from input and output data to create a data table that includes the value of UTXO ( value ), the timestamp when the UTXO was created ( block_timestamp ), and the timestamp when the UTXO was spent as an input of another transaction ( spent_block_timestamp ) (this column is left null if the transaction output is unspent). As the UTXO in a transaction is counted in satoshi (1 satoshi = 10 −8 BTCs), the actual number of BTCs in a UTXO can be computed by # UTXO = value *10 −8 , where the value represents the number of BTCs in satoshi. We rely on this derived data table (1.6 billion rows, 45 GB) to conduct further analysis.
To save the cost of the query, we create two partitioned tables based on the derived data table, one by the date in block_timestamp and one by the date in spent_block_timestamp . This means that the data entries are partitioned either by the date when the UTXOs were created or by the date when the UTXOs were spent. In this way, the program queries only the entries with timestamps in a specific range, which saves a notable amount of computational power. This step can significantly improve query performance and reduce query cost 19 .
Querying and processing cohort data
The data structure of partitioned tables coincides with our need to process cohort data. The table partitioned by date in block_timestamp naturally divides the derived data into birth cohorts that include the segment of transaction outputs created on the same date, and the table partitioned by date in spent_block_timestamp divides the derived data into death cohorts that include the segment of transaction outputs spent on the same date.
We query and process each birth cohort and each death cohort with a loop program following the procedure described in Fig. 2 . For each specific date after 2009-01-03, when the first block of Bitcoin was created, the birth cohort data and the death cohort data of that date are queried and imported to Colab from BigQuery. As in Task 1, we compute the total number of BTCs in UTXOs created and spent on that date by summing the number of BTCs in UTXOs in the birth cohort data and the death cohort data respectively. Task 2 focuses on the weighted average lifespan (WAL) on the date, defined as the average lifespan (the difference between the time when the output was spent and the time when the output was created) weighted by the number of BTCs contained in the transaction outputs. WAL can be computed from the death cohort data by the formula:
where Lifespan = spent_block_timestamp-block_timestamp .
As in Task 3, we compute the distribution of lifespan with death cohort data on that date by first categorizing UTXOs based on lifespan and then summing the number of BTCs in UTXOs in each category. In Task 4, we apply a more complicated partitioning method to compute the age distribution for each specific date. The age of a UTXO is defined as age = working_date_block_timestamp , where working date means the date of interest for the data cohort being studied. Each UTXO that remains alive on a specific date must satisfy both conditions: a) its block_timestamp must be smaller than the end of the working date, which means that the UTXO was created sometime before or on the date, and b) its spent_block_timestamp must either be null, which means the UTXO was not spent before 2021-02-10, or be larger than the end of the working date, which means that the UTXO was spent sometime after the working date but before 2021-02-10. Thus, we cannot simply interpret this information as either birth or death cohort data. Instead, we must first query the data needed to compute the age distribution for a twelve-month or six-month period depending on the size of the data in each year and then split the queried data into daily cohorts in the Python program. We compute the age distribution of each daily cohort by categorizing the age of each UTXO and summing up the number of BTCs in UTXOs in each category.
Visualizing the time series
The result of our analysis is condensed into time-series data that include the number of BTCs in UTXOs created and spent, the weighted average lifespan, the lifespan distribution, and the age distribution on each date from 2009-01-03 to 2021-02-10. Many visualizations can potentially be generated from this informative time series. For example, BTC token velocity, which we define below as the number of BTCs spent in the last 30 days divided by the circulating supply of BTCs, can be computed by
Our method can be adapted to the creation of future blocks. The time-series data for the past dates are not subject to changes as new blocks are created. As time goes on, we need only query and process the latest data cohorts to extend the time series. We will update the visualizations according to the latest development of Bitcoin, and researchers may easily repeat our work in part or in whole based on their needs.
Data Records
The final data records are stored and published on the Harvard Dataverse 20 . The records consist of the UTXO and the STXO datasets in csv format. Data ranges from 2009-01-03 to 2021-02-10, and the data frequency is daily ( n = 4421). The timezone used in the data is UTC + 0. In addition to examining Bitcoin, we apply the same cohort analysis to five other cryptocurrencies and generate twelve datasets in total. Detailed information on these data files is presented in Appendix. We also provide supplementary figures in Appendix.
Technical Validation
To further verify the validity of our methods, we use our data to calculate other variables, including block reward and circulating supply of BTCs, and check whether the results are consistent with descriptions in the Bitcoin white paper 1 and external data sources. We compute the circulating supply of BTC by computing the cumulative net new UTXOs with the formula
Figure 5 visualizes the block rewards and the circulating supply. Block rewards are the BTC awarded to the miner who wins the right to record a block of transactions by proof-of-work. Supply of the BTCs originates from the block rewards, so the cumulative sum of block rewards is the total number of BTCs in UTXOs, i.e., the circulating supply of BTC. The Bitcoin block reward was initially set at 50 BTCs per block in 2009, which means approximately 7,200 newly minted BTCs every 24 hours. The block reward halves every 210,000 blocks, roughly every four years, until the total BTC supply reaches 21 million 1 . As of the time of writing, the daily block reward amounts to approximately 900, and the circulating BTC supply is 18.9 million.
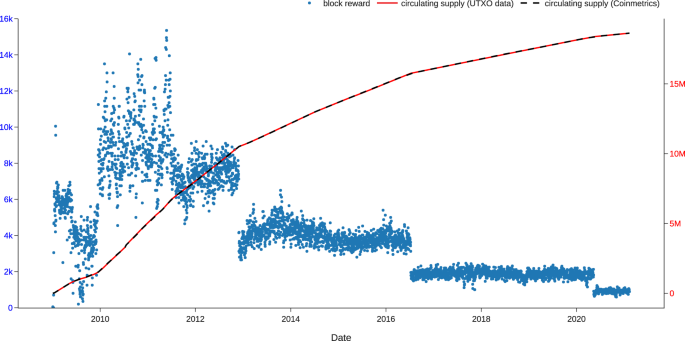
Block reward and circulating BTC supply. The figure shows that the block reward (net BTC supply) roughly corresponds to the halving pattern written in the Bitcoin white paper. It also shows that the circulating supply we compute with our UTXO data coincides exactly with the circulating supply from Coinmetrics.
In addition, we calculate the circulating supply of BTCs by summing all UTXOs in different age cohorts because existing BTC are essentially just UTXOs of different ages. We then compare the circulating supply we compute with the circulating supply data obtained from CoinMetrics ( https://github.com/coinmetrics/data ), a widely used blockchain database. As shown in Fig. 5 , the two measures of circulating supply match exactly with each other. Hence, the validity of our data is verified.
Usage Notes
Applicability.
Our data can inspire research in finance, computer science, and macroeconomics. Our data can produce new technical indicators for financial studies to predict cryptocurrency bubbles 21 , 22 , measure cryptocurrency volatility and systematic risk 23 , 24 , design investment strategies 25 , 26 , 27 and implement portfolio managements 28 , 29 . For instance, Liu and Zhang 17 used our data to design automated trading strategies for BTC investment that outperform conventional approaches. In computer science, we can apply the UTXO and STXO data to evaluate blockchain security and scalability 30 , 31 , 32 . Wang et al . 33 cite our data to demonstrate the scalability issues of the BTC blockchain and propose an efficient storage scheme. Our data can also contribute to event studies that evaluate the effect of macro policies on BTC transactions 34 .
Limitations and future research
In this section, we identify the limitations of our current results and directions for future research. First, although the frequency of our data is on a daily level, our cohort analysis can produce data with higher frequencies. Table 1 shows several other cryptocurrencies to which our methodology can be easily applied. The granularity of the data can reach different levels (75 seconds to 10 minutes) depending on the block time of each cryptocurrency.
Second, the age distribution of UTXOs is a limited measure for BTC as a store of value. UTXOs might accumulate ages for at least two reasons other than being a store of value: First, the owner of the UTXOs has lost the private key, or second, the amount of UTXOs in the owner’s account is less than the transaction fee. Owners do not transact these dust UTXOs for cost-benefit reasons. In neither case is age accumulation a sign that BTC acts as a store of value. However, scientific methods to identify the two types of UTXOs have yet to be found.
Third, the cohort analysis we designed and implemented was for UTXO-based blockchains. However, account-based blockchains, such as Ethereum, Polkadot, and Dfinity, adopt a different accounting method. In the UTXO model, crypto tokens are akin to banknotes issued by central banks; in the account model, crypto tokens are akin to balances in commercial bank accounts. Future research could extend the cohort analysis for account-based blockchains. Moreover, Ethereum, a Turing-complete blockchain, has two types of accounts: externally owned accounts (EOA) and contract accounts, which can be analogized to private and corporate accounts in commercial banks. A comparative study of the two accounts by cohort analysis could be an exciting direction for future research.
Code availability
The code used for the cohort analysis is available on GitHub ( https://github.com/SciEcon/UTXO ). The GitHub repository is also archived by Zenodo 35 , with the code available in Python and written in Google Colab Notebook with Markdown. first release created on Github: 22 Apr 2021; license: GPL-3.0 License
Nakamoto, S. Bitcoin: A peer-to-peer electronic cash system. Decentralized Bus. Rev . 21260, https://www.debr.io/article/21260-bitcoin-a-peer-to-peer-electronic-cash-system (2008).
Böhme, R., Christin, N., Edelman, B. & Moore, T. Bitcoin: Economics, technology, and governance. J. Econ. Perspectives 29 , 213–238, https://doi.org/10.1257/jep.29.2.213 (2015).
Article Google Scholar
Halaburda, H. Blockchain revolution without the blockchain? Commun. ACM 61 , 27–29, https://doi.org/10.1145/3225619 (2018).
Subacchi, P. From gold to bitcoin and beyond. Nat . 597 , 626–627, https://doi.org/10.1038/d41586-021-02615-2 (2021).
Ornes, S. Core concept: Blockchain offers applications well beyond bitcoin but faces its own limitations. Proc. Natl. Acad. Sci . 116 , 20800–20803, https://doi.org/10.1073/pnas.1914849116 (2019).
Townsend, R. M. Distributed Ledgers: Design and Regulation of Financial Infrastructure and Payment Systems (The MIT Press, 2020).
Harvey, C. R., Ramachandran, A. & Santoro, J. Defi And The Future Of Finance . (John Wiley, 2021).
Delgado-Segura, S., Pérez-Solà, C., Navarro-Arribas, G. & Herrera-Joancomartí, J. Analysis of the bitcoin utxo set. Financial Cryptogr. Data Secur . 78–91, https://doi.org/10.1007/978-3-662-58820-86 (2019).
Urquhart, A. The inefficiency of bitcoin. Econ. Lett . 148 , 80–82, https://doi.org/10.1016/j.econlet.2016.09.019 (2016).
Chakravarty, M. M. T. et al . The extended utxo model. Financial Cryptogr. Data Secur . 525–539, https://doi.org/10.1007/978-3-030-54455-337 (2020).
Pérez-Solà, C., Delgado-Segura, S., Navarro-Arribas, G. & Herrera-Joancomartí, J. Another coin bites the dust: an analysis of dust in utxo-based cryptocurrencies. Royal Soc. Open Sci . 6, 180817, https://doi.org/10.1098/rsos.180817 (2019).
Glenn, N. D. Cohort analysis (Sage Publications, 2005).
Mason, K. O., Mason, W. M., Winsborough, H. H. & Poole, W. K. Some methodological issues in cohort analysis of archival data. Am. Sociol. Rev . 38, 242, https://doi.org/10.2307/2094398 (1973).
Breslow, N. E., Lubin, J. H., Marek, P. & Langholz, B. Multiplicative models and cohort analysis. J. Am. Stat. Assoc . 78, 1–12, https://www.jstor.org/stable/2287093 . https://doi.org/10.2307/2287093 (1983).
Jiang, D. et al . Cohort query processing. Proc. VLDB Endow . 10, 1–12, https://doi.org/10.14778/3015270.3015271 (2016).
Omidvar-Tehrani, B., Amer-Yahia, S. & Lakshmanan, L. V. Cohort representation and exploration. IEEE 5th Int. Conf. on Data Sci. Adv. Anal. (DSAA) , https://doi.org/10.1109/dsaa.2018.00027 (2018).
Liu, Y. & Zhang, L. Cryptocurrency valuation: An explainable ai approach. SSRN Electron. J. https://doi.org/10.2139/ssrn.3657986 (2021).
Day, A., Medvedev, E., AK, N. & Price, W. Introducing six new cryptocurrencies in bigquery public datasets—and how to analyze them, https://cloud.google.com/blog/products/data-analytics/introducing-six-new-cryptocurrencies-in-bigquery-public-datasets-and-how-to-analyze-them (2019).
Introduction to partitioned tables | bigquery | google cloud, https://cloud.google.com/bigquery/docs/partitioned-tables (2021).
Liu, Y., Zhang, L. & Zhao, Y. Replication data for: “deciphering bitcoin blockchain data by cohort analysis”. Harv. Dataverse. https://doi.org/10.7910/DVN/XSZQWP (2021).
Shu, M. & Zhu, W. Real-time prediction of bitcoin bubble crashes. Phys. A: Stat. Mech. its Appl . 548, 124477, https://doi.org/10.1016/j.physa.2020.124477 (2020).
Li, T. R., Chamrajnagar, A. S., Fong, X. R., Rizik, N. R. & Fu, F. Sentiment-based prediction of alternative cryptocurrency price fluctuations using gradient boosting tree model. Front. Phys . 7, https://doi.org/10.3389/fphy.2019.00098 (2019).
Giudici, P. & Pagnottoni, P. Vector error correction models to measure connectedness of bitcoin exchange markets. Appl. Stoch. Model. Bus. Ind . 36 , 95–109, https://doi.org/10.1002/asmb.2478 (2019).
Giudici, P., Leach, T. & Pagnottoni, P. Libra or librae? basket based stablecoins to mitigate foreign exchange volatility spillovers. Finance Res. Lett . 102054, https://doi.org/10.1016/j.frl.2021.102054 (2021).
Pagnottoni, P. Neural network models for bitcoin option pricing. Front. Artif. Intell . 2 , https://doi.org/10.3389/frai.2019.00005 (2019).
Karalevicius, V., Degrande, N. & De Weerdt, J. Using sentiment analysis to predict interday bitcoin price movements. The J. Risk Finance 19, 56–75, https://doi.org/10.1108/jrf-06-2017-0092 (2018).
Resta, M., Pagnottoni, P. & De Giuli, M. E. Technical analysis on the bitcoin market: Trading opportunities or investors’ pitfall? Risks 8, 44, https://doi.org/10.3390/risks8020044 (2020).
Daniel, K., Mota, L., Rottke, S. & Santos, T. The cross-section of risk and returns. The Rev. Financial Stud . 33, 1927–1979, https://doi.org/10.1093/rfs/hhaa021 (2020).
Griffin, J. M. & Shams, A. Is bitcoin really untethered? The J. Finance 75, 1913–1964, https://doi.org/10.1111/jofi.12903 (2020).
Croman, K. et al . On scaling decentralized blockchains. Financial Cryptogr. Data Secur . 106–125, https://doi.org/10.1007/978-3-662-53357-48 (2016).
Gervais, A. et al . On the security and performance of proof of work blockchains. Proc. 2016 ACM SIGSAC Conf. on Comput. Commun. Secur. - CCS’16 , https://doi.org/10.1145/2976749.2978341 (2016).
Pagnotta, E. S. Decentralizing money: Bitcoin prices and blockchain security. The Rev. Financial Stud , https://doi.org/10.1093/rfs/hhaa149 (2021).
Wang, X., Wang, C., Zhou, K. & Cheng, H. Ess: An efficient storage scheme for improving the scalability of bitcoin network. IEEE Transactions on Netw. Serv. Manag. https://doi.org/10.1109/tnsm.2021.3127187 (2021).
Karau, S. Monetary policy and cryptocurrencies. SSRN Electron. J , https://doi.org/10.2139/ssrn.3949549 (2021).
Zhang, L. & Zhao, Y. Sciecon/utxo: Preprint: Deciphering bitcoin blockchain data by cohort analysis. Zenodo , https://doi.org/10.5281/zenodo.4708453 (2021).
Download references
Acknowledgements
We have benefited from the comments by discussions at the SciEcon Research Accelerator Seminar. We thank two anonymous referees at Scientific Data for their insightful comments that helped us revise the manuscript. We thank Duke Kunshan University for its continued support in interdisciplinary research and in cultivating undergraduate research. Duke Kunshan University is the corresponding institution for this article. Duke Kunshan University provides the funding support for the article processing charge (APC) to facilitate the great cause of open access publication. Yinhong Zhao was a visiting undergraduate student hosted by Duke Kunshan University, during which he started to partake in the co-authorship as the research assistant of Professor Luyao Zhang during the Covid-19 global pandemic.
Author information
These authors contributed equally: Yulin Liu, Luyao Zhang, Yinhong Zhao.
Authors and Affiliations
SciEcon CIC, London, WC2H 9JQ, United Kingdom
Yulin Liu, Luyao Zhang & Yinhong Zhao
Data Science Research Center and Social Science Division, Duke Kunshan University, Kunshan, Jiangsu, 215316, China
Luyao Zhang
Duke University, Durham, NC, 27708, United States
Yinhong Zhao
Bochsler Finance, Zug, 6300, Switzerland
You can also search for this author in PubMed Google Scholar
Contributions
Each author contributed equally to this research. Yulin Liu led the discussion on the blockchain mechanism and the economic meaning of UTXO data. Luyao Zhang designed the cohort analysis methods applying to UTXO-based blockchains. Yinhong Zhao queried and processed the data. Each author contributed significantly to the draft and revisions of the manuscript.
Corresponding author
Correspondence to Luyao Zhang .
Ethics declarations
Competing interests.
The authors declare no competing interests.
Additional information
Publisher’s note Springer Nature remains neutral with regard to jurisdictional claims in published maps and institutional affiliations.
Supplementary information
Rights and permissions.
Open Access This article is licensed under a Creative Commons Attribution 4.0 International License, which permits use, sharing, adaptation, distribution and reproduction in any medium or format, as long as you give appropriate credit to the original author(s) and the source, provide a link to the Creative Commons license, and indicate if changes were made. The images or other third party material in this article are included in the article’s Creative Commons license, unless indicated otherwise in a credit line to the material. If material is not included in the article’s Creative Commons license and your intended use is not permitted by statutory regulation or exceeds the permitted use, you will need to obtain permission directly from the copyright holder. To view a copy of this license, visit http://creativecommons.org/licenses/by/4.0/ .
Reprints and permissions
About this article
Cite this article.
Liu, Y., Zhang, L. & Zhao, Y. Deciphering Bitcoin Blockchain Data by Cohort Analysis. Sci Data 9 , 136 (2022). https://doi.org/10.1038/s41597-022-01254-0
Download citation
Received : 30 April 2021
Accepted : 11 March 2022
Published : 07 April 2022
DOI : https://doi.org/10.1038/s41597-022-01254-0
Share this article
Anyone you share the following link with will be able to read this content:
Sorry, a shareable link is not currently available for this article.
Provided by the Springer Nature SharedIt content-sharing initiative
Quick links
- Explore articles by subject
- Guide to authors
- Editorial policies
Sign up for the Nature Briefing newsletter — what matters in science, free to your inbox daily.

Visual Analysis of Research on Blockchain
Ieee account.
- Change Username/Password
- Update Address
Purchase Details
- Payment Options
- Order History
- View Purchased Documents
Profile Information
- Communications Preferences
- Profession and Education
- Technical Interests
- US & Canada: +1 800 678 4333
- Worldwide: +1 732 981 0060
- Contact & Support
- About IEEE Xplore
- Accessibility
- Terms of Use
- Nondiscrimination Policy
- Privacy & Opting Out of Cookies
A not-for-profit organization, IEEE is the world's largest technical professional organization dedicated to advancing technology for the benefit of humanity. © Copyright 2024 IEEE - All rights reserved. Use of this web site signifies your agreement to the terms and conditions.
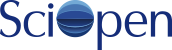
Logging off

Blockchain Data Analysis from the Perspective of Complex Networks: Overview

Cryptocurrency based on blockchain technology has gradually become a choice for people to invest in, and several users have participated in the accumulation of massive transaction data. Complete transaction records in blockchains and the openness of data provide researchers with opportunities to mine and analyze data in blockchains. Network modeling and analysis of cryptocurrency transaction records are common methods in blockchain data analysis. The analysis of attribute graphs can provide insights into various economic indicators, illegal activities, and general Internet security, among others. Accordingly, this article aims to summarize and analyze the literature on cryptocurrency transaction data from the perspective of complex networks. To provide systematic guidance for researchers, we put forward a blockchain data analysis framework based on the introduction of the relevant background and reviewed the work from five aspects: blockchain data model, data acquisition on blockchains, existing analysis tools, available insights, and common analysis methods. For each aspect, we introduce the research problems, summarize the methods, and discuss the results and findings. Finally, we present future research points and several open questions in the study of cryptocurrency transaction networks.
FullText for HTML: https://doi.org/.....
References( 108 )
Publication history, acknowledgements.
This work was partially funded by the Key Research and Development Program of Shandong Province (Nos. 2017GGX10142, 2019GNC106027, and 2019JZZY010134); and the Natural Science Foundation of Shandong Province (Nos. ZR2020MF058 and ZR2020MF029).
Rights and permissions
The articles published in this open access journal are distributed under the terms of the Creative Commons Attribution 4.0 International License (http://creativecommons.org/licenses/by/4.0/).

京ICP备 10035462号-42


An official website of the United States government
The .gov means it’s official. Federal government websites often end in .gov or .mil. Before sharing sensitive information, make sure you’re on a federal government site.
The site is secure. The https:// ensures that you are connecting to the official website and that any information you provide is encrypted and transmitted securely.
- Publications
- Account settings
Preview improvements coming to the PMC website in October 2024. Learn More or Try it out now .
- Advanced Search
- Journal List
- Springer Nature - PMC COVID-19 Collection

A critical analysis of the integration of blockchain and artificial intelligence for supply chain
Vincent charles.
1 CENTRUM Católica Graduate Business School, Lima, Peru
2 Pontifical Catholic University of Peru, Lima, Peru
Ali Emrouznejad
3 Surrey Business School, University of Surrey, Guildford, Surrey UK
Tatiana Gherman
4 Faculty of Business and Law, University of Northampton, Northampton, UK
The integration between blockchain and artificial intelligence (AI) has gained a lot of attention in recent years, especially since such integration can improve security, efficiency, and productivity of applications in business environments characterised by volatility, uncertainty, complexity, and ambiguity. In particular, supply chain is one of the areas that have been shown to benefit tremendously from blockchain and AI, by enhancing information and process resilience, enabling faster and more cost-efficient delivery of products, and augmenting products’ traceability, among others. This paper performs a state-of-the-art review of blockchain and AI in the field of supply chains. More specifically, we sought to answer the following three principal questions: Q1—What are the current studies on the integration of blockchain and AI in supply chain?, Q2—What are the current blockchain and AI use cases in supply chain?, and Q3—What are the potential research directions for future studies involving the integration of blockchain and AI? The analysis performed in this paper has identified relevant research studies that have contributed both conceptually and empirically to the expansion and accumulation of intellectual wealth in the supply chain discipline through the integration of blockchain and AI.
Introduction
Traditionally dispersed geographically, supply chains have always been difficult to manage. Supply chain complexity is caused by a variety of factors and its long-term viability necessitates effective maintenance, repair, and operations management, among others. In supply chain networks, everything from link maintenance and regulatory policies to cultural norms and human behaviour makes evaluating information and managing risk a difficult task (Ivanov et al., 2019 ). Trust can be easily undermined by inefficient transactions, fraud, theft, and weak supply chains, which highlights the need for better information sharing and verifiability (Saberi et al., 2019 ).
In today’s business environment, traceability is becoming a necessity and a competitive advantage in many supply chain industries. Without transparency in the supply chain, stakeholders cannot properly assess and validate the true value of items. The cost of dealing with intermediaries, as well as their dependability and transparency, make managing supply chain traceability even more difficult, leading to strategic and reputational competitive issues (Saberi et al., 2019 ).
There are a number of issues with today's supply chains because they rely so heavily on central, sometimes disparate, and stand-alone systems of information management, such as enterprise resource planning systems (Saberi et al., 2019 ). The single point failure of centralised information systems is a drawback of such systems, which in turn makes the entire system vulnerable to error, hacking, corruption, or attack (Dong et al., 2017 ). Without doubt, there must be a high level of trust present for supply chain entities to entrust their sensitive and valuable data to a single organisation or broker (Abeyratne & Monfared, 2016 ).
In addition, there are continuous pressures on supply chain practice to recognise and certify the sustainability of supply chains. Environmental, social, and business aspects must all be considered in order to achieve sustainability, as part of the triple-bottom-line concept (Seuring et al., 2008 ). As a strategic and competitive issue, supply chain sustainability requires confirming and verifying that supply chain processes, products, and activities meet certain sustainability criteria and certifications (Grimm et al., 2016 ).
Existing supply chain information systems must be examined to determine if they can provide the secure, transparent, and reliable data needed to track the timely origin of goods and services. The key to resolving these difficult matters is to improve supply chain security, transparency, long-term viability, and process integrity. Blockchain technology could be the solution to this problem. New technological breakthroughs and applications based on the blockchain concept have made these objectives more attainable from an organisational, technological, and financial standpoint (Abeyratne & Monfared, 2016 ). With its decentralised ‘trustless’ database characteristics, blockchain technology can facilitate global-scale transaction and process disintermediation and decentralisation among a variety of different stakeholders (Crosby et al., 2016 ; Saberi et al., 2019 ).
As Saberi et al. ( 2019 ) noted, although the number of blockchain use cases has grown over time, blockchain, like any potentially disruptive system or technology, faces a number of challenges and barriers in terms of adoption and implementation by supply chain networks. Blockchain is still in its early stages of development, posing a number of challenges in terms of behavioural, organisational, technological, and policy-related issues.
Artificial intelligence (AI) promises to solve some of the above-mentioned problems. As a matter of fact, the integration of blockchain and AI is estimated to bring a number of significant various advantages, such as more robust deliverables (Odekanle et al., 2022 ). With such integration, parties can share massive amounts of data for the purposes of analysis, learning, and decision-making without the need for a central authority or third-party intermediaries.
By automating the entire workflow, the use of AI technology in the blockchain system has the potential to redefine the supply chain. Using a combined AI and blockchain approach, useful information can be extracted from historical purchase data and other sources, allowing for the identification of data characteristics and the performance of predictive analysis tasks such as future demand and sales forecasting (Zhang et al., 2021a , b ).
In spite of its importance and relevance, to the best of our knowledge, there is, at the time of conducting this research, no systematic literature review of studies on the integration of blockchain and AI for supply chain. Our research contributes to the operations management field by addressing this gap through a critical evaluation of such studies. The three principal questions we aim to answer are:
As such, the aim is to not only investigate the current state of studies focusing on the integration of both technologies, but also to highlight how such integration can revolutionise “business-as-usual” practices in supply chain management. The analysis performed in this paper has identified relevant research studies that have contributed both conceptually and empirically to the expansion and accumulation of intellectual wealth in the supply chain discipline through the integration of blockchain and AI. As we will show, the majority of the literature is conceptual rather than empirical in nature, indicating that blockchain and AI integration is still in its early stages. Nonetheless, by highlighting current trends and interests in the field, it is expected that this study will assist academics, practitioners, and policymakers in understanding the current state-of-the-art on the topic and aid their decision-making to engage in empirical studies focusing on the actual deployment of AI-driven blockchain technology for supply chain and its implications for long-term performance. As a result, the current work will serve as a foundation for future research studies.
The remainder of the paper is organised as follows. In Sect. 2 , we lay out the theoretical framework by defining our key terms, namely blockchain, artificial intelligence, and supply chain. In Sect. 3 , we first detail the steps followed in identifying relevant studies (Sect. 3.1 ). The results of the bibliometric analysis are presented in Sect. 3.2 in order to obtain an overall view of the publications available in the literature on the topic. In Sect. 3.3 , we then narrow down the pool of studies identified by means of defining inclusion and exclusion criteria. In Sect. 4 , we discuss the identified thematic clusters, which are indicative of the current research trends on blockchain and AI integration in supply chain. Section 5 concludes the paper with final thoughts, limitations, and future research directions.
Theoretical framework: blockchain and artificial intelligence for supply chain
From the procurement stage to the product stage, the supply chain encompasses all activities involving the production of goods and the delivery of finished goods. Or, in the words of Pimenidis et al. ( 2021 ), “in the realm of manufacturing, a supply chain is the process of the flow of goods from the upper echelons of value creation to the end customer consumption. It is a form of symbiotic connection in which customers and suppliers work together to achieve the best interests of each other, buying, converting, distributing and selling goods and services to create specific final products and to add value to their organisations” (p. 369). In this sense then, regardless of industry, the supply chain has a complex architecture, on which a significant portion of business productivity and profits is based.
Sharing information securely, effectively, and efficiently is critical to running supply chains smoothly. A good supply chain requires efficiency and transparency at every level of the supply chain, as well as trust among stakeholders. Moreover, supply chains must become more adaptable and responsive, while also increasing their resilience and traceability, in order to be sustainable. Yet again, innovation and technology will be central to supply chain success (Baucherel, 2018 ). It is in this context that blockchain has emerged as a critical technology that has the potential to improve supply chain operations’ flexibility and agility (Cole et al., 2019 ). Through the use of blockchain technology, all stakeholders in the ecosystem can actively engage, share, and verify all types of information and data (Gohil & Thakker, 2021 ).
Satoshi Nakamoto is credited with the invention of the concept of “blockchain”. In 2008, Nakamoto ( 2008 ) published a paper wherein he presented the concept of the first peer-to-peer electronic cash system aided by digital currency, dubbed as “bit-coin”. Transactions are recorded as interlinked blocks that are linked to one another. In this context, the term “blockchain” refers to a chain of interconnected transactions. Blockchain enables parties who do not know each other to deal securely without the need for a centrally trusted middleman, lowering legal and transaction expenses (Pilkington, 2016 ). The name “distributed ledger” comes from the fact that records can be shared with different parties and maintained in multiple locations.
In other words, blockchain technology is a decentralised, distributed database of records or shared public/private digital ledgers that exists across a network and that is used to record transactions that have been executed and shared among participating agents (Crosby et al., 2016 ). Four key characteristics of blockchain technology distinguish it from most existing information system designs: non-localisation (decentralisation), security, auditability, and smart execution (Saberi et al., 2019 ; Steiner & Baker, 2015 ).
The way that the blockchain works is that a blockchain agent creates a new transaction to be added to the blockchain. This new transaction is transmitted to the network for auditing, and once it has been approved by a majority of nodes, it is added to the chain as a new block. It is further saved in several distributed nodes for security reasons. The smart contract, a key feature of blockchain technology, allows for trustworthy transactions to be executed without third-party involvement. Real-time visibility of transactions throughout the supply chain and a reduced risk of data manipulation and fraud are thus among the many advantages of blockchain technology (Cottrill, 2018 ; Partida, 2018 ).
The key properties of this technology that make it a valuable proposition to handle transactions, according to Rodríguez-Espíndola et al. ( 2020 ), are:
- Immutable: All transactions will be recorded and stored by blockchain, and each transaction will be protected against deletion, tampering, and revision. The software code in the blocks automatically records any changes as new transactions that are linked to the previous transaction.
- Distributed: Each of the blockchain network’s participants has an identical copy of the ledger on their computer (frequently called nodes). Cash management and the parties involved in the transaction are more easily spotted as a result of the increased visibility provided by this.
- Decentralised: Transactions between the blockchain network entities will be easier with this property, as it eliminates the need for a central intermediary and speeds up processing time.
- Automated: The code running in the blockchain automatically records and cryptographically verifies each transaction, ensuring transaction authenticity. This renders the entire process incorruptible and error-free. Transaction times are reduced as a result of the automation.
- A single unified ledger: If a permissionless blockchain network is used and all transactions are recorded in a single immutable public ledger, grouping them together will be easier.
- Self-reviewing: When a transaction occurs, the blockchain automatically updates and records the information, ensuring that every node (network participant) has an up-to-date ledger copy of the information available.
AI is a way of getting a machine or programme to perform tasks that would normally be performed by human intelligence; hence, it is used to develop intelligent computers and machines that behave like humans. There are two types of AI, namely General AI and Narrow AI. Narrow AI, or AI programmes capable of solving a single problem or task, is still the prevalent type of AI today. General AI, on the other hand, which can be described as an AI programme that can solve any problem it is given, is still a work in progress.
When integrated with AI, blockchain technology can assist the supply chain in various ways. A supply chain powered by blockchain and AI could be the answer to securing the operations of a local or regional supply chain and providing the intelligence required to enhance operational efficacy (Pimenidis et al., 2021 ). It can increase data security, data efficiency, and contribute to making smart decisions (Banerjee et al., 2018 ). Blockchain securely stores a large amount of data, while AI helps analyse and produce insights from data and generates new scenarios and patterns based on data behaviour (Ahmed et al., 2022 ). In order to make supply chains more adaptable, responsive, and efficient, while also maintaining transaction transparency for the benefit of all members, blockchain and AI algorithms can help (Pimenidis et al., 2021 ). All in all, the integration between blockchain technology and AI can strengthen applications in terms of reliability, security, transparency, and trust. To the best of our knowledge, papers have looked into the use of blockchain and AI in supply chains, but they have generally treated the two technologies separately, as if they were on opposite ends of a spectrum. The current paper, on the other hand, examines the integration of the two concepts.
Methods and materials
Extracting relevant literature.
To address our research questions, a systematic literature review was carried out. To this aim, the Scopus search database, which is the abstract and citation database of Elsevier, was used as the main scientific database. Scopus is well-known and widely used for literature mapping (e.g., Fahimnia et al., 2019 ). Despite the fact that there are other databases available, Scopus was chosen as one of the best options because of its consistent citation metrics and precision in locating authors and institutions (Charles et al., 2022a , b ). Scopus also provides a more comprehensive coverage of articles in Business, Economics, Management, and Social Sciences in general (Martín-Martín et al., 2018 ). Hence, it was deemed that the works identified in the Scopus database can provide a reasonably good overview of what has been published on the topic of blockchain and artificial intelligence integration for supply chain that are relevant to this study.
Figure 1 depicts the flowchart of the approach followed in this paper. In the first phase, to conduct the search for relevant literature, we devised a set of keywords that would cover all relevant publications in the Scopus database to the greatest extent possible. The Scopus search algorithm was TITLE-ABS-KEY (“blockchain” OR “block chain”) AND (“artificial intelligence” OR “machine learning” OR “neural network” OR “deep learning”) AND “supply chain”). These keywords were looked up in each publication’s title, abstract, and keyword list. The search returned 280 document results, all published between 2017 and 2022. Data were downloaded on 27 March 2022. These documents were analysed using bibliometric analysis.

Flowchart of the systematic literature review with bibliometric analysis and thematic analysis
The application of the above criteria for identifying relevant material was left intentionally broad, so that we could get a sense of what researchers in the field are interested in right now and in the future. Moreover, only 280 results were returned, which is a relatively low number. On the other hand, we considered excluding conference papers from our analysis because they are frequently written to present preliminary findings and thus constitute works in progress rather than complete papers (Mubin et al., 2018 ). A similar consideration was made with regards to book chapters, conference reviews, and reviews, as they serve a different purpose and do different work than journal articles. However, a decision was made not to exclude such work. The reasoning was that, because the field is still young, it is understandable that more research is ongoing rather than finished; also, that more work is conceptual or theoretical in nature rather than empirical. As a result, it would be ideal at this point to provide an overview of the entire pool of documents that we came across in order to better capture all ongoing research endeavours. The 280 records were subjected to a co-occurrence analysis using the VOSviewer software for bibliometric analysis (Sect. 3.2 ).
In the subsequent phase (Sect. 3.3 ), the 280 records were screened, and only journal articles (as complete, published, and peer-reviewed research work) were selected for further analysis; this yielded 75 research articles. Then, a set of inclusion and exclusion criteria was developed, and a manual review of the 75 research articles was conducted to determine whether or not they complied with the set criteria and clearly addressed the integration of blockchain and AI for supply chain. This step resulted in a final pool of 42 research articles, which was subjected to a thematic analysis.
Bibliometric analysis of the results
Bibliometric analysis is a useful research technique for identifying global research trends and predicting future research directions (Liu et al., 2011 ). Through bibliometric analysis, this paper reveals the characteristics and trends in studies integrating blockchain and AI in the field of supply chain. The results are analysed using summary statistics.
The number of publications on blockchain and AI for supply chain has increased over time, as shown in Fig. 2 . It is worth noting that all 280 documents identified were published after 2017, implying that there were no publications combining the three keywords before this year, at least according to the Scopus database. Second, in recent years, there has been an increase in interest in the topic, with a peak in the year 2021 (124 records).

Annual scientific production.
(Source: Scopus 2022)
Figure 3 depicts the annual document volume broken down by information source. It can be observed that top five preferred outlets for publications on blockchain and artificial intelligence for supply chain are IFIP Advances in Information and Communication Technology (17 documents), Advances in Intelligent Systems and Computing (11 documents), Communications in Computer and Information Science (10 documents), Lecture Notes in Computer Science including Subseries Lecture Notes in Artificial Intelligence and Lecture Notes in Bioinformatics (10 documents), and Sustainability Switzerland (9 documents).

Documents per year per source.
Figure 4 shows the documents by affiliation, with the first 12 affiliations compared to the total number of documents. Affiliation-wise, the University of the West of England (Great Britain) leads the ranking with 5 publications and is closely followed by Hong Kong Polytechnic University (Hong Kong) and Peter the Great St. Petersburg Polytechnic University (Russia), each with 4 publications. The subsequent 9 institutions shown in the figure account for 3 publications each. It is interesting to note how these institutions reflect a mix of countries from around the world.

Documents by affiliation
Figure 5 compares the document counts for 13 countries/territories to show which have the most publications. The countries of origin were determined by the corresponding author’s country. It can be easily observed that India dominates the ranking, with 51 publications, followed by China and the United States, with 38 and 34 publications, respectively. A notable observation is that these first three countries together account for almost half (i.e., 44%) of the total number of publications. The fourth position is held by the United Kingdom, with 22 publications and the fifth place belongs to Australia, with 12 publications. France and Germany occupy the sixth and seventh place, respectively, both with 10 publications, followed by Hong Kong and South Korea (each with 7 publications), Italy and Taiwan (each with 6 publications) and Finland, Greece, and Iran (each with 5 publications).

Documents by country or territory.
Table Table1 1 shows the number of documents by publication type. In this regard, we can observe that conference papers predominate in the literature (86 documents, which constitute approx. 30% of the publications). The high number of conference papers in the literature could be attributed to the novelty of the research topic as well as the fact that the field is still in its early stages. Then there are articles (75 documents, representing approx. 27% of the publications). Conference reviews comprise 68 documents, or approximately 24% of the publications. Book chapters and reviews each have 22 documents, while books and editorials have the fewest (4 and 3 documents, respectively).
Documents by publication type
Finally, an analysis of published documents by subject area (Table (Table2) 2 ) reveals that the subject area of “computer science” concentrates most of the research on the topic, with a whopping 171 documents or 29% of the publications. This is followed by the area of “engineering”, with 114 documents or approx. 19% of the publications, and the area of “decision sciences”, with 67 documents or approx. 11% of the publications. The areas of “business, management, and accounting” and “social sciences” occupies the fourth position, with 47 documents, or 8% the publications.
Documents by subject area
A publication can be classified under more than one subject area
The VOSviewer software was used to create maps of co-authorship and keyword co-occurrence based on bibliographic data. The co-authorship analysis required at least five documents per country (Fig. 6 ); 14 of the 63 countries identified met this threshold. In this regard, Fig. 6 depicts the network map of international collaboration among major countries (those with the highest total link strength) engaged in blockchain and AI for supply chain research. The colours denote the clusters to which countries are assigned according to the strength of their relationships, while the surface area of the circles indicates the number of publications secured by each country. We can see that there are a total of six clusters.

Co-authorship network of countries
Using index keywords as the unit of analysis, a minimum of six occurrences per keyword and full counting as the counting method, a keyword co-occurrence analysis (Fig. 7 ) was performed. Of the 1210 keywords, 35 met the threshold. The total strength of the co-occurrence links with other keywords was calculated for each of the 35 keywords. We chose the keywords with the most total link strength. Keywords are denoted by coloured frames, the size of which is proportional to the number of times the keyword appears in the document.

Network map showing the relations between various topics in the literature on blockchain and AI for supply chain (280 documents)
Moreover, these keywords are grouped into four clusters that seem thus to assume a prominent role vis-à-vis “food and agriculture” (green cluster), “risk, resilience, and sustainability” (blue cluster), “security and ethical governance” (red cluster), and “emerging technologies and their benefits” (yellow cluster). This is indicative of the thematic areas that have captured the interest of researchers in the area of blockchain and AI for supply chains.
Pool of research articles on the integration of blockchain and AI
After obtaining an overall view of “what is out there” in terms of existing literature on the topic, that is, the 280 records previously discussed in Sect. 3.2 , we added, as previously mentioned, a second stage in which we narrowed our search results in order to bring additional accuracy and identify only those studies that specifically dealt with the integration of blockchain and AI for supply chain. On this occasion, it was decided to look only at research articles in order to clearly identify complete and published research work that had also been peer-reviewed. This consideration resulted in the selection of 75 research articles for further analysis (see previous Table 1 ). Following that, the authors manually reviewed these 75 research articles to determine whether or not they dealt with the integration of blockchain and AI for supply chain.
Studies were considered for further analysis if they met the following criteria:
- The articles treated the topics of “blockchain”, “AI” (or any of its proxies, “machine learning”, “neural network”, or “deep learning”), and “supply chain” as a core question.
- Regardless of whether the studies were literature reviews, conceptual works, empirical, case studies, or experimental, the studies clearly focused on the integration of blockchain and AI for supply chain.
- The studies included research that was published in English, regardless of the year of publication.
Articles were excluded if:
- The topics of “blockchain” and “AI” (or any of its proxies, “machine learning”, “neural network”, and “deep learning”) were treated separately, with no emphasis on their integration.
- Because the full text was not available, we were unable to verify the content.
- The journal publication was discontinued, which also meant that the full text was no longer available (hence, we were unable to verify the content).
- Despite being labelled as research articles, the records were discovered to be other types of publications, such as editorials.
- The articles used the terms “blockchain” and/or “AI” (or any of its proxies, “machine learning”, “neural network”, or “deep learning”) to analyse empirical data in the context of a supply chain, which was outside the scope of our focus.
The use of the aforementioned criteria resulted in a final pool of 42 research articles. Table Table3 3 summarises the characteristics of the studies examined. Surprisingly, a manual content analysis of these 42 studies revealed that the previously identified thematic clusters can still be used to categorise this pool of studies. In this regard, it was discovered that 18 studies could be classified as belonging to the “Emerging Technologies and Their Benefits” cluster, 13 studies as belonging to the “Food and Agriculture” cluster, 8 studies as belonging to the “Risk, Resilience, and Sustainability” cluster, and 3 as belonging to the “Security and Ethical Governance” cluster.
Critical taxonomy of articles dealing with the integration of blockchain and AI for supply chain
It is also worth noting that the vast majority of these studies (30 articles) are conceptual in nature, followed by experimental (15 articles), literature reviews (8 articles), case studies (6 articles), and, finally, empirical studies (5 articles). Of course, with the caveat that some articles combine conceptual and literature review, conceptual and empirical, and so on (see Table Table3). 3 ). Such findings emphasise the still-emerging nature of studies focusing on the integration of blockchain and AI for supply chain, indicating that more empirically completed studies are still required to fully understand what such a field has to offer from a very practical standpoint. In the next section, we proceed to interpret the identified thematic clusters. To be noted that additional searches for materials relevant to our discussion were further conducted using the referenced works of the main pool of 42 articles (snowball effect) and included herein. Section 4 thus complements the information presented in Table Table3 3 .
Interpreting clusters
Cluster 1: food and agriculture.
AI and machine learning (ML), big data analytics, cloud computing, IoT, robotics, and blockchain are examples of disruptive information and communication technologies that can help to solve problems like increasing productivity and yield, conserving water, ensuring soil and plant health, and improving environmental stewardship. According to Lezoche et al. ( 2020 ), these technologies “will let the agriculture to evolve in a data-driven, intelligent, agile and autonomous connected system of systems”.
Apart from the sustainability benefits, AI-ML applications in particular can help to improve supply chain transparency, visibility, and product traceability (Sharma et al., 2020a , b ). However, all these are ongoing efforts and practitioners will need to investigate the possibilities of combining various sources of AI-ML data with blockchain technology and other technologies to a greater extent (Kamble et al., 2018 ; Sharma et al., 2018 ). Studies have started to emerge. Using RFID (Radio-Frequency IDentification) and Blockchain technology, Tian ( 2016 ), for example, gathered and transferred trusted information to reduce agri-food risk in order to build a supply chain traceability system. It is worth noting that our review revealed that building product traceability systems is one of the most common research endeavours that researchers are currently engaged in.
Smart contracts and cybersecurity are two critical applications of blockchain in the domain of agriculture. The Internet of Things enables precise sensing throughout the supply chain. With smart contracts, all transactions are documented in a distributed manner. Immutable transaction histories from raw material suppliers to consumers would aid in food quality control, increase traceability, and, ultimately, resolve food safety concerns. Blockchain-based smart contract technologies are expected to enable the agri-food supply chain's digital transformation, resulting in a “traceable, transparent, trustful, and intelligent ecosystem” (Liu et al., 2021a , b , p. 4330).
Tripoli and Schmidhuber ( 2018 ) highlighted how data generated by the IoT can be used to enhance the details of blockchain transactions. Then, the precise data provided by blockchain technology can be used to power AI applications (Rabah et al., 2018 ). Moreover, AI can enhance IoT by developing applications that use machine learning algorithms to analyse data captured by sensors in real time (Reshma & Pillai, 2018 ).
Sharma et al., ( 2020a , b ) performed a systematic literature review of machine learning applications in agricultural supply chains and found that all three ML algorithms, that is, supervised, unsupervised, and reinforcement learning, are used to develop sustainable agricultural supply chains. However, the study does not discuss the integration between ML algorithms and blockchain. On the other hand, Putri et al. ( 2020 ) noted that in the future, hyperledger blockchains can be developed and implemented using AI, allowing for the prediction, classification, and clustering of existing data and transactions based on their time period.
Although most of materials identified represent a theoretical treatment of the subject, there are a few studies that demonstrate successful integration of blockchain and AI techniques for supply chain traceability improvement, such as the study by Chen ( 2018 ). In this sense, Chen ( 2018 ) proposed a novel approach called Takagi–Sugeno Fuzzy cognitive maps artificial neural network as a traceability chain algorithm.
Cluster 2: Risk, resilience, and sustainability
Supply chain network complexity, combined with external factors, has resulted in supply chain disruptions over the last few years (Fan & Stevenson, 2018 ). Most recently, the pandemic has focused renewed attention on sustainability. As a matter of fact, no recent event has highlighted the vulnerability of supply chains as much as the COVID-19 outbreak in early 2020 (Pournader et al., 2020 ; Spieske & Birkel, 2021 ).
The medical supply chain, especially for pharmaceutical drugs, has been particularly affected. COVID-19’s sudden emergence and uncontrolled global spread has exposed the inadequacies of the current healthcare systems across the world in responding to public health emergencies in a timely manner. Breakthrough technologies like blockchain and AI have emerged as viable and sustainable solutions for combating the pandemic in such circumstances (Baz et al., , 2021 ) and this has evolved into a common research endeavour that has recently piqued the interest of researchers. In this context, research has emerged looking at medical supply chain and drugs and pharmaceutical supply chain management.
The COVID-19 outbreak has the potential to strain existing healthcare systems. At the moment, there is a dearth of a reliable data surveillance system capable of providing relevant healthcare organisations with real-time information about potential outbreaks. Indeed, the majority of existing Covid-19 data originates from a wide range of sources, including the general public, hospitals, and clinical laboratories, and contains a substantial amount of incorrect information that has not been thoroughly monitored (Nguyen et al., 2021 ).
Blockchain technology can assist in combating the COVID-19 pandemic by facilitating early detection of outbreaks, ensuring a secure drug supply chain and expediting drug delivery, and establishing consensus on the ordering of Covid-19 data records (Baz et al . , 2021 ). Additionally, AI-based supervised and unsupervised ML techniques enable the development of intelligent solutions for real-time epidemic outbreak monitoring, pandemic trend forecasting, coronavirus symptom identification for treatment, and drug manufacturing support.
Nguyen et al. ( 2021 ) conducted a comprehensive survey on the use of blockchain and AI in the fight against the recent pandemic. The authors introduced a novel conceptual architecture that integrates blockchain and AI to combat Covid-19 and reviewed recent studies on the use of blockchain and AI to combat Covid-19 in a multitude of scenarios, including the medical supply chain.
Blockchain-based smart contracts are computer programmes that carry out the terms of a contract when certain objectives are satisfied (Griggs et al., 2018 ). Automating auditing processes, medical supply chain management, outbreak tracking, and remote patient monitoring could all be done using smart contracts based on blockchain technology (Griggs et al., 2018 ; Roosan et al., 2022 ). Using blockchain-based smart contracts, pharmaceutical supply chains can be improved, and their quality and regulatory compliance can be verified, all while automating auditing processes (Angeles, 2018 ).
These combined technologies were further examined by Jabarulla and Lee ( 2021 ) to see if they could be used to help with the conventional public health strategies for combating Covid-19, such as contact tracing, outbreak estimation, coronavirus detection and analysis, as well as the management of clinical data and the supply chain, among others.
The patient-centred approach to healthcare could be reshaped by integrating blockchain and AI technology (Chen et al., 2019 ; Jabarulla & Lee, 2021 ; Ploug & Holm, 2020 ). In turn, it is possible that a patient-centred approach to dealing with the coronavirus pandemic could be useful in distributing treatment and managing pandemics (Jabarulla & Lee, 2021 ).
The combination of blockchain and AI technologies enables the development of an all-encompassing predictive system that could help to keep the threat of a pandemic at bay within a country’s borders (Fusco et al., 2020 ). Moreover, the public surveillance system can be made more effective and robust by combining blockchain technology with AI and geographic information systems (Sharma et al., 2020a , b ).
Another research strand that has been gaining momentum is the use of blockchain-AI integration for circular economy and sustainability. Studies like Ebinger and Omondi’s ( 2020 ) report on the current use of digital technologies (such as blockchain, cloud computing, and AI) in sustainable supply chain management provide further evidence of the progress being made in this area. A blockchain-enabled and Intelligent Agent-supported supply chain community that is intelligent and responsive, as well as secure and sustainable has been proposed by Pimenidis et al. ( 2021 ). Chidepatil et al. ( 2020 ) discussed how blockchain and multi-sensor-driven AI can transform the circular economy of plastic waste. When it comes to the protection and preservation of the global environment, including life on land, life underground, and climate change, Sivarethinamohan and Sujatha ( 2021 ) looked at how AI-driven blockchain technology could be put to use for this purpose.
Cluster 3: Security and ethical governance
Recent surges in security breaches and digital surveillance have highlighted the need for enhanced privacy and security, particularly with regard to users’ personal data (Heister & Yuthas, 2021 ). Blockchain technologies enable novel methods of protecting user data through decentralised identity and other privacy mechanisms. These systems can empower users by providing tools that enable them to own and handle their data. On the other hand, AI opens up new avenues for improving system and user security.
As Heister and Yuthas ( 2021 ) highlighted, “ blockchain provides new mechanisms, such as decentralized identities and zero-knowledge proofs, that enable data to be shared in ways that maintain the privacy of the individual and allow users to maintain control over their own data. These advances can provide both increased cybersecurity and more ethical use of personal data. Blockchain participants can realize these outcomes through careful development of governance frameworks and mechanisms ”. As a result, advancements in these technologies open up new avenues for the ethical use of data.
Furthermore, by improving the quality, transparency, traceability, and security of data, blockchain can improve the capabilities of other technologies and techniques such as AI and IoT in-process monitoring, trend prediction and decision-making, among others (Sun & Zhang, 2020 ). The combination of blockchain, AI, and supply chains may result in an improvement in information management for interconnected devices. A recent study by Unal et al. ( 2021 ) presented a practical approach for integrating blockchain with federated learning in order to provide private and secure big data analytics services.
One of the driving forces behind blockchain and AI in supply chains is information management. Supply chains can use blockchain technology to organise a reliable and secure digital transition in which all operations can potentially be digitised. Implementing blockchain and AI on information management tasks can result in administrative benefits such as time savings, improved information quality, and increased security. All of these developments are currently the subject of ongoing research efforts.
Cluster 4: Emerging technologies and their benefits
Blockchain, AI, cloud computing, IoT, cyber-physical systems, and robotics are examples of recent technological developments (components of Industry 4.0) that enable the integration of disparate supply chain advancements into intelligent and connected Systems of Systems. These technologies have the capability for strengthening the supply chain management and will assist the relevant sectors in becoming data-driven, agile, intelligent, and automated. Table Table3 3 showcases recent studies, with applications ranging from healthcare, business, and financial services to the automobile industry and humanitarian logistics.
Rodríguez-Espíndola et al. ( 2020 ), for example, argued that integrating disparate technologies is critical for the humanitarian supply chain to reap real gains. To accomplish this, the authors proposed a framework for enhancing the flow of information, products, and financial resources in humanitarian supply chains through the integration of three emerging disruptive technologies: blockchain, AI, and 3D printing. Their analysis demonstrates that the framework has the potential to alleviate supply chain congestion, improve simultaneous collaboration among various stakeholders, reduce lead times, improve accountability, traceability, and transparency of material and financial resources, and empower victims to contribute to their own needs fulfilment.
Applications can also combine blockchain with machine learning (Ramezani & Camarinha-Matos, 2020 ). This combination of technologies would enable systems to improve continuously, respond more quickly and effectively to disruptions, and predict potential failures.
Given recent advancements in these fields, the adoption of smart products based on IoT and other technologies may necessitate systems with high data integrity and user privacy, which can only be furnished by blockchain technology (Chanson et al., 2019 ). Moreover, by integrating AI and blockchain with IoT sensors and edge devices, supplies chains can work toward becoming “smart supply chains”.
These technologies also make intelligent transportation systems possible. Self-driving cars can use IoT sensors to constantly monitor and, in some cases, predict developments using AI, while also incorporating blockchain wallets that allow passengers to pay for rides, rentals, tolls, and other services without revealing personal information (Heister & Yuthas, 2021 ).
Conclusions and future research directions
Blockchain and AI technologies are rapidly evolving, opening up new avenues for working with data that were previously unimaginable. They are setting the pace of innovation and introducing a radical shift in almost every industry. On their own, blockchain and AI are cutting-edge technologies, but when combined, they can be truly revolutionary, of course, as long as such integration is underpinned by a problem-centric thinking approach (Charles et al., 2022a , b ). Each of them has the potential to improve the other’s capabilities, allowing for better oversight and accountability.
Although blockchain and AI have been explored in the extant literature on operations management, no state-of-the-art review of blockchain and AI integration in the field of supply chain was identified. This article is, therefore, the first to provide an overview and assessment of such studies. More specifically, we sought to answer the following three principal research questions: Q1—What are the studies on the integration of blockchain and AI in supply chain?, Q2—What are the blockchain and AI use cases in supply chain?, and Q3—What are the potential research directions for future studies involving the integration of blockchain and AI?
To answer the first two questions, we queried the SCOPUS database. Our search turned up 280 results, all of which were published between 2017 and 27 March 2022. Early on, it was noted that the majority of the material published was conceptual in nature rather than empirical. This indicated that the field of blockchain and AI convergence is still in its infancy. Given the field’s nascent status, as evidenced also by the large number of publications other than completed research articles, we decided not to remove any of the materials we came across. This was done so that we could get a sense of what researchers in the field are currently interested in. A bibliometric analysis of the materials revealed four main themes that have piqued researchers’ interest. These themes are “Emerging Technologies and Their Benefits”, “Food and Agriculture”, “Risk, Resilience, and Sustainability”, and “Security and Ethical Governance”, which are also indicative of the most significant use cases. Based on the findings of this study, a number of future research directions have been outlined below, which helps us to answer the third research question.
First, as previously mentioned, most of the works reviewed are conceptual rather than empirical in nature. In other words, empirical studies aimed at implementing blockchain-AI applications in real life are still lacking. This, in turn, limits the full validity of conversations on the topic. Blockchain is still in its infancy, and so is the integration of blockchain with other emerging technologies such as AI. Therefore, many flaws must be addressed before any benefits and long-term effects can be observed in practice. Empirical studies focusing on the actual deployment of AI-driven blockchain technology for supply chain and its implications on long-term performance make thus an interesting and promising future research direction.
Second, the integration between blockchain and AI can prove to be highly advantageous especially for the healthcare sector, while at the same time tackling existing ethical issues. The Covid-19 pandemic has compelled medical researchers and technologists alike to look into methods of rapidly collecting information about virus exposure and transmission while maintaining user privacy. Testing patients who may have been exposed to the virus quickly with point-of-care diagnostics has proven effective in tracing its spread and mitigating its effects. Blockchain and AI can help by collecting the data on blockchain infrastructure and then quickly analysing it using AI. Moreover, blockchain and AI can be combined with other technologies, such as cloud computing, to create more comprehensive and efficient systems. High computational power and resourceful storage are two of the most important aspects of the cloud that can aid in AI analytics. The integration of these promising technologies can be further explored in the near future to create highly advanced medical systems to combat future coronavirus-like pandemics.
Third, our analysis reveals that more studies are needed to explore the integration of blockchain and AI from an environmental sustainability, social, and economic points of view (triple bottom line perspective). In the end, blockchain and AI are merely tools, and their long-term effects are largely determined by the underlying vision and strategies that companies choose to govern their daily operations. As a result, more research is needed to optimise the range of business initiatives and strategies as well as the technology choices in order to achieve the United Nation’s Sustainable Development Goals. The needs of multiple stakeholders and the complexity of multi-layered supply chains should be taken into account in future analyses.
Finally, because the integration of blockchain and AI for supply chain is still in its early stages, more cross- and inter-disciplinary empirically grounded research, particularly research approaches to study practice in truly insightful and impactful ways, is required (Charles & Gherman, 2018 ). As the concept of distributed ledger technologies evolves over time, new AI-based emerging technologies surface, and new sustainability debates emerge, such endeavours will necessitate the participation of a wide range of stakeholders, from researchers to practitioners and policymakers (Charles et al., 2019 ).
The present paper is not without limitations. Our analysis and findings are limited to the Scopus scientific database, which was chosen for its consistent citation metrics and precision in locating authors and institutions. Despite this, the Scopus database is the largest database of peer-reviewed literature and further offers a comprehensive coverage of articles in Business, Economics, Management, and Social Sciences in general. As a result, we are confident that the works identified and included in this paper provide a reasonably good overview of what has been published on the topic of blockchain and artificial intelligence integration for supply chain, as well as current and future interests on the topic. Future studies can, of course, complement the current study by including other databases. Additionally, future studies can expand the scope of the present study by detailing the challenges and limitations of existing researches. We hope, however, that the current work serves as a foundation for future research studies.
Acknowledgements
The authors are thankful to the Editor-in-Chief and the anonymous reviewers for their insightful comments and suggestions on an earlier version of this manuscript.
Publisher's Note
Springer Nature remains neutral with regard to jurisdictional claims in published maps and institutional affiliations.
Contributor Information
Vincent Charles, Email: ep.pcup@selrahcv .
Ali Emrouznejad, Email: [email protected] .
Tatiana Gherman, Email: [email protected] .
- Abbas K, Afaq M, Khan TA, Song W-C. A blockchain and machine learning-based drug supply chain management and recommendation system for smart pharmaceutical industry. Electronics (switzerland) 2020; 9 (5):852. [ Google Scholar ]
- Abeyratne SA, Monfared RP. Blockchain ready manufacturing supply chain using distributed ledger. International Journal of Renewable Energy Technology. 2016; 5 (9):1–10. [ Google Scholar ]
- Ahamed NN, Vignesh R. Smart agriculture and food industry with blockchain and artificial intelligence. Journal of Computer Science. 2022; 181 (1):1–17. doi: 10.3844/jcssp.2022.1.17. [ CrossRef ] [ Google Scholar ]
- Ahmed I, Zhang Y, Jeon G, Lin W, Khosravi MR, Qi L. A blockchain-and artificial intelligence-enabled smart IoT framework for sustainable city. International Journal of Intelligent Systems. 2022; 37 (9):5473–6605. doi: 10.1002/int.22852. [ CrossRef ] [ Google Scholar ]
- Angeles R. Blockchain-based healthcare: Three successful proof-of-concept pilots worth considering. Journal of Information Technology Management. 2018; 27 (3):47–83. [ Google Scholar ]
- Bamakan SMH, Faregh N, Zareravasan A. Di-ANFIS: An integrated blockchain-IoT-big data-enabled framework for evaluating service supply chain performance. Journal of Computational Design and Engineering. 2021; 8 (2):676–690. doi: 10.1093/jcde/qwab007. [ CrossRef ] [ Google Scholar ]
- Banerjee M, Lee J, Choo KKR. A blockchain future for internet of things security: A position paper. Digital Communications and Networks. 2018; 4 (3):149–160. doi: 10.1016/j.dcan.2017.10.006. [ CrossRef ] [ Google Scholar ]
- Baucherel K. Blockchain from hype to help. ITNOW. 2018; 60 (4):4–7. doi: 10.1093/itnow/bwy087. [ CrossRef ] [ Google Scholar ]
- Baz M, Khatri S, Baz A, Alhakami H, Agrawal A, Khan RA. Blockchain and artificial intelligence applications to defeat COVID-19 pandemic. Computer Systems Science and Engineering. 2021; 40 (2):691–702. doi: 10.32604/csse.2022.019079. [ CrossRef ] [ Google Scholar ]
- Bechtsis D, Tsolakis N, Iakovou E, Vlachos D. Data-driven secure, resilient and sustainable supply chains: Gaps, opportunities, and a new generalised data sharing and data monetisation framework. International Journal of Production Research. 2022; 60 (14):4397–4417. doi: 10.1080/00207543.2021.1957506. [ CrossRef ] [ Google Scholar ]
- Bragadeesh SA, Umamakeswari A. Secured vehicle life cycle tracking using blockchain and smart contract. Computer Systems Science and Engineering. 2022; 41 (1):1–18. doi: 10.32604/csse.2022.019842. [ CrossRef ] [ Google Scholar ]
- Chanson M, Bogner A, Bilgeri D, Fleisch E, Wortmann F. Blockchain for the IoT: Privacy-preserving protection of sensor data. Journal of the Association for Information Systems. 2019; 20 (9):1272–1307. doi: 10.17705/1jais.00567. [ CrossRef ] [ Google Scholar ]
- Charles V, Gherman T. Big data and ethnography: Together for the greater good. In: Emrouznejad A, Charles V, editors. Big data for the greater good. Springer; 2018. pp. 19–34. [ Google Scholar ]
- Charles V, Gherman T, Paliza JC. Stakeholder involvement for public sector productivity enhancement: Strategic considerations. ICPE Public Enterprise Half-Yearly Journal. 2019; 24 (1):77–86. doi: 10.21571/pehyj.2019.2401.05. [ CrossRef ] [ Google Scholar ]
- Charles V, Emrouznejad A, Gherman T, Cochran J. Why data analytics is an art. Significance. 2022; 19 (6):42–45. doi: 10.1111/1740-9713.01707. [ CrossRef ] [ Google Scholar ]
- Charles V, Gherman T, Emrouznejad A. Characteristics and trends in big data for service operations management research: A blend of descriptive and bibliometric analysis. In: Emrouznejad A, Charles V, editors. Big data and blockchain for service operations management. Studies in big data. Springer; 2022. pp. 1–18. [ Google Scholar ]
- Chen RY. A traceability chain algorithm for artificial neural networks using T-S fuzzy cognitive maps in blockchain. Future Generation Computer Systems. 2018; 80 :198–210. doi: 10.1016/j.future.2017.09.077. [ CrossRef ] [ Google Scholar ]
- Chen H, Chen Z, Lin F, Zhuang P. Effective management for blockchain-based agri-food supply chains using deep reinforcement learning. IEEE Access. 2021; 9 (9363883):36008–36018. doi: 10.1109/ACCESS.2021.3062410. [ CrossRef ] [ Google Scholar ]
- Chen HS, Jarrell JT, Carpenter KA, Cohen DS, Huang X, Hospital MG. Blockchain in healthcare: A patient-centered model. Biomedical Journal of Scientific & Technical Research. 2019; 20 :15017–15022. [ PMC free article ] [ PubMed ] [ Google Scholar ]
- Chidepatil A, Bindra P, Kulkarni D, Qazi M, Kshirsagar M, Sankaran K. From trash to cash: How blockchain and multi-sensor-driven artificial intelligence can transform circular economy of plastic waste? Administrative Sciences. 2020; 10 (2):23. doi: 10.3390/admsci10020023. [ CrossRef ] [ Google Scholar ]
- Cole R, Stevenson M, Aitken J. Blockchain technology: Implications for operations and supply chain management. Supply Chain Management: International Journal. 2019; 24 (4):469–483. doi: 10.1108/SCM-09-2018-0309. [ CrossRef ] [ Google Scholar ]
- Cottrill K. The benefits of blockchain: Fact or wishful thinking? Supply Chain Management Review. 2018; 22 (1):20–25. [ Google Scholar ]
- Crosby M, Pattanayak P, Verma S, Kalyanaraman V. Blockchain technology: Beyond bitcoin. Applied Innovation. 2016; 2 :6–9. [ Google Scholar ]
- Dadi V, Nikhil SR, Mor RS, Agarwal T, Arora S. Agri-food 4.0 and innovations: Revamping the supply chain operations. Production Engineering Archives. 2021; 27 (2):75–89. doi: 10.30657/pea.2021.27.10. [ CrossRef ] [ Google Scholar ]
- Dey S, Saha S, Singh AK, McDonald-Maier K. SmartNoshWaste: Using blockchain, machine learning, cloud computing and QR Code to reduce food waste in decentralized web 3.0. Enabled Smart Cities Smart Cities. 2022; 5 (1):162–176. doi: 10.3390/smartcities5010011. [ CrossRef ] [ Google Scholar ]
- Dillenberger DN, Novotny P, Zhang Q, Jayachandran P, Gupta H, Hans S, Verma D, Chakraborty S, Thomas JJ, Walli MM, Vaculin R, Sarpatwar K. Blockchain analytics and artificial intelligence. IBM Journal of Research and Development. 2019; 63 (2):8645631. [ Google Scholar ]
- Dong F, Zhou P, Liu Z, Shen D, Xu Z, Luo J. Towards a fast and secure design for enterprise-oriented cloud storage systems. Concurrency and Computation: Practice and Experience. 2017; 29 (19):e4177. doi: 10.1002/cpe.4177. [ CrossRef ] [ Google Scholar ]
- Dwivedi SK, Roy P, Karda C, Agrawal S, Amin R. Blockchain-based internet of things and industrial IoT: A comprehensive survey. Security and Communication Networks. 2021; 2021 :7142048. doi: 10.1155/2021/7142048. [ CrossRef ] [ Google Scholar ]
- Ebinger F, Omondi B. Leveraging digital approaches for transparency in sustainable supply chains: A conceptual paper. Sustainability. 2020; 12 :6129. doi: 10.3390/su12156129. [ CrossRef ] [ Google Scholar ]
- Eluubek I, Song H, Vajdi A, Wang Y, Zhou J. Blockchain for consortium: A practical paradigm in agricultural supply chain system. Expert Systems with Applications. 2021; 184 :115425. doi: 10.1016/j.eswa.2021.115425. [ CrossRef ] [ Google Scholar ]
- Fahimnia B, Pournader M, Siemsen E, Bendoly E, Wang C. Behavioral operations and supply chain management—A review and literature mapping. Decision Sciences. 2019; 50 (6):1127–1183. doi: 10.1111/deci.12369. [ CrossRef ] [ Google Scholar ]
- Fan Y, Stevenson M. A review of supply chain risk management: Definition, theory, and research agenda. International Journal of Physical Distribution & Logistics Management. 2018; 48 (3):205–230. doi: 10.1108/IJPDLM-01-2017-0043. [ CrossRef ] [ Google Scholar ]
- Fusco A, Dicuonzo G, Dell'Atti V, Tatullo M. Blockchain in healthcare: Insights on COVID-19. International Journal of Environmental Research and Public Health. 2020; 17 (19):7167. doi: 10.3390/ijerph17197167. [ PMC free article ] [ PubMed ] [ CrossRef ] [ Google Scholar ]
- Gohil D, Thakker SV. Blockchain-integrated technologies for solving supply chain challenges. Modern Supply Chain Research and Applications. 2021; 3 (2):78–97. doi: 10.1108/MSCRA-10-2020-0028. [ CrossRef ] [ Google Scholar ]
- Griggs KN, Ossipova O, Kohlios CP, Baccarini AN, Howson EA, Hayajneh T. Healthcare blockchain system using smart contracts for secure automated remote patient monitoring. Journal of Medical Systems. 2018; 42 :1–7. doi: 10.1007/s10916-018-0982-x. [ PubMed ] [ CrossRef ] [ Google Scholar ]
- Grimm JH, Hofstetter JS, Sarkis J. Exploring sub-suppliers’ compliance with corporate sustainability standards. Journal of Cleaner Production. 2016; 112 :1971–1984. doi: 10.1016/j.jclepro.2014.11.036. [ CrossRef ] [ Google Scholar ]
- Heister S, Yuthas K. How blockchain and AI enable personal data privacy and support cybersecurity. In: Fernández-Caramés TM, Fraga-Lamas P, editors. Advances in the convergence of blockchain and artificial intelligence. IntechOpen; 2021. [ Google Scholar ]
- Hopkins JL. An investigation into emerging industry 4.0 technologies as drivers of supply chain innovation in Australia. Computers in Industry. 2021; 125 :103323. doi: 10.1016/j.compind.2020.103323. [ CrossRef ] [ Google Scholar ]
- Ivanov D, Dolgui A, Sokolov B. The impact of digital technology and industry 4.0 on the ripple effect and supply chain risk analytics. International Journal of Production Research. 2019; 57 (3):829–846. doi: 10.1080/00207543.2018.1488086. [ CrossRef ] [ Google Scholar ]
- Jabarulla MY, Lee H-N. A blockchain and artificial intelligence-based, patient centric healthcare system for combating the COVID-19 pandemic: Opportunities and applications. Healthcare. 2021; 9 :1019. doi: 10.3390/healthcare9081019. [ PMC free article ] [ PubMed ] [ CrossRef ] [ Google Scholar ]
- Kamble SS, Gunasekaran A, Gawankar SA. Sustainable industry 4.0 frame- work: A systematic literature review identifying the current trends and future perspectives. Process Safety and Environmental Protection. 2018; 117 :408–425. doi: 10.1016/j.psep.2018.05.009. [ CrossRef ] [ Google Scholar ]
- Kamble SS, Gunasekaran A, Parekh H, Mani V, Belhadi A, Sharma R. Digital twin for sustainable manufacturing supply chains: Current trends, future perspectives, and an implementation framework. Technological Forecasting and Social Change. 2022; 176 :121448. doi: 10.1016/j.techfore.2021.121448. [ CrossRef ] [ Google Scholar ]
- Khadke S, Gupta P, Rachakunta S, Mahata C, Dawn S, Sharma M, Verma D, Pradhan A, Krishna AMS, Ramakrishna S, Chakrabortty S, Saianand G, Sonar P, Biring S, Dash JK, Dalapati GK. Efficient plastic recycling and remolding circular economy using the technology of trust–blockchain. Sustainability (switzerland) 2021; 13 (16):9142. doi: 10.3390/su13169142. [ CrossRef ] [ Google Scholar ]
- Khan PW, Byun Y-C, Park N. IoT-blockchain enabled optimized provenance system for food industry 4.0 using advanced deep learning. Sensors (switzerland) 2020; 20 (10):2990. doi: 10.3390/s20102990. [ PMC free article ] [ PubMed ] [ CrossRef ] [ Google Scholar ]
- Lezoche M, Hernandez JE, Diaz MDMEA, Panetto H, Kacprzyk J. Agri-food 4.0: a survey of the supply chains and technologies for the future agriculture. Computers in Industry. 2020; 117 :103187. doi: 10.1016/j.compind.2020.103187. [ CrossRef ] [ Google Scholar ]
- Lee C-H, Yang H-C, Wei Y-C, Hsu W-K. Enabling blockchain based scm systems with a real time event monitoring function for preemptive risk management. Applied Sciences (switzerland) 2021; 11 (11):4811. doi: 10.3390/app11114811. [ CrossRef ] [ Google Scholar ]
- Li Z, Guo H, Barenji AV, Wang WM, Guan Y, Huang GQ. A sustainable production capability evaluation mechanism based on blockchain, LSTM, analytic hierarchy process for supply chain network. International Journal of Production Research. 2020; 58 (24):7399–7419. doi: 10.1080/00207543.2020.1740342. [ CrossRef ] [ Google Scholar ]
- Liu Y, Ma X, Shu L, Hancke GP, Abu-Mahfouz AM. From industry 4.0 to agriculture 4.0: Current status, enabling technologies, and research challenges. IEEE Transactions on Industrial Informatics. 2021; 17 (6):4322–4334. doi: 10.1109/TII.2020.3003910. [ CrossRef ] [ Google Scholar ]
- Liu Y, Zhang S, Chen M, Wu Y, Chen Z. The sustainable development of financial topic detection and trend prediction by data mining. Sustainability (switzerland) 2021; 13 (14):7585. doi: 10.3390/su13147585. [ CrossRef ] [ Google Scholar ]
- Liu XJ, Zhang LA, Hong S. Global biodiversity research during 1900–2009: A bibliometric analysis. Biodiversity and Conservation. 2011; 20 (4):807–826. doi: 10.1007/s10531-010-9981-z. [ CrossRef ] [ Google Scholar ]
- Luo S, Choi T-M. Operational research for technology-driven supply chains in the industry 4.0 Era: Recent development and future studies. Asia-Pacific Journal of Operational Research. 2022; 39 (1):2040021. doi: 10.1142/S0217595920400217. [ CrossRef ] [ Google Scholar ]
- Mao D, Wang F, Hao Z, Li H. Credit evaluation system based on blockchain for multiple stakeholders in the food supply chain. International Journal of Environmental Research and Public Health. 2018; 15 (8):1627. doi: 10.3390/ijerph15081627. [ PMC free article ] [ PubMed ] [ CrossRef ] [ Google Scholar ]
- Martín-Martín A, Orduna-Malea E, Thelwall M, Delgado López-Cózar E. Google scholar, web of science, and Scopus: A systematic comparison of citations in 252 subject categories. Journal of Informetrics. 2018; 12 (4):1160–1177. doi: 10.1016/j.joi.2018.09.002. [ CrossRef ] [ Google Scholar ]
- Martínez J, Durán JM. Software supply chain attacks, a threat to global cybersecurity: SolarWinds’ case study. International Journal of Safety and Security Engineering. 2021; 11 (5):537–545. doi: 10.18280/ijsse.110505. [ CrossRef ] [ Google Scholar ]
- Monteiro ES, Righi RDR, Barbosa JLV, Alberti AM. APTM: A model for pervasive traceability of agrochemicals. Applied Sciences (switzerland) 2021; 11 (17):8149. doi: 10.3390/app11178149. [ CrossRef ] [ Google Scholar ]
- Mubin O, Arsalan M, Al Mahmud A. Tracking the follow-up of work in progress papers. Scientometrics. 2018; 114 :1159–1174. doi: 10.1007/s11192-017-2631-4. [ PMC free article ] [ PubMed ] [ CrossRef ] [ Google Scholar ]
- Nakamoto, S. (2008). Bitcoin: A peer-to-peer electronic cash system. Retrieved from https://bitcoin.org/en/bitcoin-paper .
- Nandi, S., Hervani, A. A., Helms, & M. M., Sarkis, J. (2021). Conceptualising Circular economy performance with non-traditional valuation methods: Lessons for a post-Pandemic recovery. International Journal of Logistics Research and Applications . (in Press).
- Nguyen DC, Ding M, Pathirana PN, Seneviratne A. Blockchain and AI-based solutions to combat coronavirus (COVID-19)-like epidemics: A survey. IEEE Access. 2021; 9 :95730–95753. doi: 10.1109/ACCESS.2021.3093633. [ PMC free article ] [ PubMed ] [ CrossRef ] [ Google Scholar ]
- Odekanle EL, Fakinle BS, Falowo OA, Odejobi OJ. Challenges and benefits of combining AI with blockchain for sustainable environment. In: Kaushik K, Tayal A, Dahiya S, Salau AO, editors. Sustainable and advanced applications of blockchain in smart computational technologies. Chapman and Hall/CRC; 2022. pp. 43–62. [ Google Scholar ]
- Partida B. Blockchain's great potential: Blockchain’s potential is immense, but most organizations have not yet made the investment. Supply Chain Management Review. 2018; 22 (1):51–53. [ Google Scholar ]
- Pilkington M. Blockchain technology: Principles and applications. In: Olleros FX, Zhegu M, editors. Research handbook on digital transformations. Edward Elgar; 2016. pp. 225–253. [ Google Scholar ]
- Pimenidis E, Patsavellas J, Tonkin M. Blockchain and artificial intelligence managing a secure and sustainable supply chain. In: Jahankhani H, Jamal A, Lawson S, editors. Cybersecurity, privacy and freedom protection in the connected world advanced sciences and technologies for security applications. Springer; 2021. [ Google Scholar ]
- Ploug T, Holm S. The four dimensions of contestable AI diagnostics—A patient-centric approach to explainable AI. Artificial Intelligence in Medicine. 2020; 107 :101901. doi: 10.1016/j.artmed.2020.101901. [ PubMed ] [ CrossRef ] [ Google Scholar ]
- Pournader M, Kach A, Talluri S. A review of the existing and emerging topics in the supply chain risk management literature. Decision Sciences. 2020; 51 (4):867–919. doi: 10.1111/deci.12470. [ PMC free article ] [ PubMed ] [ CrossRef ] [ Google Scholar ]
- Putri AN, Hariadi M, Wibawa AD. Smart agriculture using supply chain management based on hyperledger blockchain. Conference Series: Earth and Environmental Science. 2020; 466 :1–9. [ Google Scholar ]
- Rabah K, Research M, Nairobi K. Convergence of AI, IoT, big data and blockchain: A review. The Lake Institute Journal. 2018; 1 (1):1–18. [ Google Scholar ]
- Ramezani J, Camarinha-Matos LM. Approaches for resilience and antifragility in collaborative business ecosystems. Technological Forecasting and Social Change. 2020; 151 :119846. doi: 10.1016/j.techfore.2019.119846. [ CrossRef ] [ Google Scholar ]
- Reshma, S. R. J., & Pillai, A. S. (2018). Proceedings of the Eighth International Conferenceon Soft Computing and Pattern Recognition (SoCPaR 2016) , 614, no. SoCPaR 2016.
- Reyes PM, Visich JK, Jaska P. Managing the dynamics of new technologies in the global supply chain. IEEE Engineering Management Review. 2020; 48 (1):156–162. doi: 10.1109/EMR.2020.2968889. [ CrossRef ] [ Google Scholar ]
- Rodríguez-Espíndola O, Chowdhury S, Beltagui A, Albores P. The potential of emergent disruptive technologies for humanitarian supply chains: The integration of blockchain, artificial intelligence and 3D printing. International Journal of Production Research. 2020; 58 (15):4610–4630. doi: 10.1080/00207543.2020.1761565. [ CrossRef ] [ Google Scholar ]
- Roosan D, Wu Y, Tatla V, Li Y, Kugler A, Chok J, Roosan MR. Framework to enable pharmacist access to health care data using Blockchain technology and artificial intelligence. Journal of the American Pharmacists Association. 2022; 62 (4):1124–1132. doi: 10.1016/j.japh.2022.02.018. [ PubMed ] [ CrossRef ] [ Google Scholar ]
- Runzel MAS, Hassler EE, Rogers REL, Formato G, Cazier JA. Designing a smart honey supply chain for sustainable development. IEEE Consumer Electronics Magazine. 2021; 10 (4):69–78. doi: 10.1109/MCE.2021.3059955. [ CrossRef ] [ Google Scholar ]
- Saberi S, Kouhizadeh M, Sarkis J, Shen L. Blockchain technology and its relationships to sustainable supply chain management. International Journal of Production Research. 2019; 57 (7):2117–2135. doi: 10.1080/00207543.2018.1533261. [ CrossRef ] [ Google Scholar ]
- Saurabh S, Dey K. Blockchain technology adoption, architecture, and sustainable agri-food supply chains. Journal of Cleaner Production. 2021; 284 :124731. doi: 10.1016/j.jclepro.2020.124731. [ CrossRef ] [ Google Scholar ]
- Seuring S, Sarkis J, Müller M, Rao P. Sustainability and supply chain management—an introduction to the special issue. Journal of Cleaner Production. 2008; 16 (15):1545–1551. doi: 10.1016/j.jclepro.2008.02.002. [ CrossRef ] [ Google Scholar ]
- Sgantzos K, Grigg I. Artificial intelligence implementations on the blockchain. Use cases and future applications. Future Internet. 2019; 11 (8):170. doi: 10.3390/fi11080170. [ CrossRef ] [ Google Scholar ]
- Shahbazi Z, Byun Y-C. A procedure for tracing supply chains for perishable food based on blockchain, machine learning and fuzzy logic. Electronics (switzerland) 2021; 10 (14):1–21. [ Google Scholar ]
- Sharma A, Bahl S, Bagha AK, Javaid M, Shukla DK, Haleem A. Blockchain technology and its applications to combat COVID-19 pandemic. Research on Biomedical Engineering. 2020; 38 (1):173–180. doi: 10.1007/s42600-020-00106-3. [ CrossRef ] [ Google Scholar ]
- Sharma R, Kamble SS, Gunasekaran A. Big GIS analytics framework for agriculture supply chains: A literature review identifying the current trends and future perspectives. Computers and Electronics in Agriculture. 2018; 155 :103–120. doi: 10.1016/j.compag.2018.10.001. [ CrossRef ] [ Google Scholar ]
- Sharma R, Kamble SS, Gunasekaran A, Kumar V, Kumar A. A systematic literature review on machine learning applications for sustainable agriculture supply chain performance. Computers and Operations Research. 2020; 119 :104926. doi: 10.1016/j.cor.2020.104926. [ CrossRef ] [ Google Scholar ]
- Singh, P. D., Kaur, R., Dhiman, G., Bojja, G. R. (2021). BOSS: A new QoS aware blockchain assisted framework for secure and smart healthcare as a service. Expert Systems, e12838.
- Sivarethinamohan R, Sujatha S. Unraveling the potential of artificial intelligence-driven blockchain technology in environment management. In: Manik G, Kalia S, Sahoo SK, Sharma TK, Verma OP, editors. Advances in mechanical engineering. Lecture notes in mechanical engineering. Springer; 2021. [ Google Scholar ]
- Sobb T, Turnbull B, Moustafa N. Supply chain 4.0: A survey of cyber security challenges, solutions and future directions. Electronics (switzerland) 2020; 9 (11):1–31. [ Google Scholar ]
- Spieske A, Birkel H. Improving supply chain resilience through industry 4.0: A systematic literature review under the impressions of the COVID-19 pandemic. Computers & Industrial Engineering. 2021; 158 :107452. doi: 10.1016/j.cie.2021.107452. [ PMC free article ] [ PubMed ] [ CrossRef ] [ Google Scholar ]
- Steiner, J., & Baker, J. (2015). Blockchain: The solution for transparency in product supply chains. https://www.provenance.org/whitepaper .
- Sun M, Zhang J. Research on the application of block chain big data platform in the construction of new smart city for low carbon emission and green environment. Computer Communications. 2020; 149 :332–342. doi: 10.1016/j.comcom.2019.10.031. [ CrossRef ] [ Google Scholar ]
- Suroso AI, Rifai B, Hasanah N. Traceability system in hydroponic vegetables supply chain using blockchain technology. International Journal of Information and Management Sciences. 2021; 32 (4):347–361. [ Google Scholar ]
- Tian, F. (2016). An agri-food supply chain traceability system for china based on RFID & blockchain technology . In 13th International Conference on Service Systems and Service Management (ICSSSM).
- Tripoli, M., & Schmidhuber, J. (2018). Emerging opportunities for the application of blockchain in the agri-food industry agriculture. Food and Agriculture Organization of the United Nations (August).
- Unal D, Hammoudeh M, Khan MA, Abuarqoub A, Epiphaniou G, Hamila R. Integration of federated machine learning and blockchain for the provision of secure big data analytics for Internet of Things. Computers and Security. 2021; 109 :102393. doi: 10.1016/j.cose.2021.102393. [ CrossRef ] [ Google Scholar ]
- Wang Y. Research on supply chain financial risk assessment based on blockchain and fuzzy neural networks. Wireless Communications and Mobile Computing. 2021; 2021 :5565980. [ Google Scholar ]
- Wong S, Yeung JKW, Lau Y-Y, So J. Technical sustainability of cloud-based blockchain integrated with machine learning for supply chain management. Sustainability (switzerland) 2021; 13 (15):8270. doi: 10.3390/su13158270. [ CrossRef ] [ Google Scholar ]
- Yong B, Shen J, Liu X, Li F, Chen H, Zhou Q. An intelligent blockchain-based system for safe vaccine supply and supervision. International Journal of Information Management. 2020; 52 :102024. doi: 10.1016/j.ijinfomgt.2019.10.009. [ CrossRef ] [ Google Scholar ]
- Zhang P, Liu X, Li W, Yu X. Pharmaceutical cold chain management based on blockchain and deep learning. Journal of Internet Technology. 2021; 22 (7):1531–1542. doi: 10.53106/160792642021122207007. [ CrossRef ] [ Google Scholar ]
- Zhang Z, Song X, Liu L, Yin J, Wang Y, Lan D. Recent Advances in blockchain and artificial intelligence integration: Feasibility analysis, research issues, applications, challenges, and future work. Security and Communication Networks. 2021; 2021 :9991535. [ Google Scholar ]
Click through the PLOS taxonomy to find articles in your field.
For more information about PLOS Subject Areas, click here .
Loading metrics
Open Access
Peer-reviewed
Research Article
Towards secure and efficient integration of blockchain and 6G networks
Roles Funding acquisition
* E-mail: [email protected]
Affiliation School of Information Science and Engineering, Henan University of Technology, Zhengzhou, Henan, China

Roles Conceptualization, Data curation, Methodology, Software, Writing – original draft, Writing – review & editing
Roles Formal analysis, Investigation
Roles Project administration, Resources, Supervision
Roles Supervision, Validation, Visualization
- Yang Liu,
- Song Peng,
- Miaomiao Zhang,
- Shidong Shi,
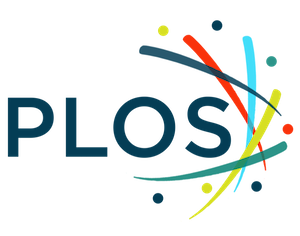
- Published: April 11, 2024
- https://doi.org/10.1371/journal.pone.0302052
- Reader Comments
The future of communication systems is undergoing a transformative shift towards intelligence, efficiency, and flexibility. Presently, the amalgamation of blockchain technology and the sixth-generation mobile communication network (6G) has garnered significant attention, as their fusion is poised to profoundly impact the digital economy and society at large. However, the convergence of blockchain and 6G networks poses challenges pertaining to security and performance. In this article, we propose an approach based on the design of secure mechanisms and performance optimization to delve into the key issues surrounding the integration of blockchain and 6G networks from both security and performance perspectives. Specifically, we first introduce the application scenarios of 6G networks and blockchain’s empowerment of them to highlight the necessity of combining blockchain technology with 6G. Subsequently, in order to ensure the security of communication and data transmission between blockchain and 6G networks, we have investigated the design requirements for security mechanisms. Furthermore, we discuss the efficient realization of the amalgamation between blockchain and 6G networks by proposing a solution based on Directed Acyclic Graph (DAG) for blockchain’s asynchronous consensus protocol, alongside optimization strategies for storage and communication to meet the desired characteristics and requirements of 6G networks. Lastly, we provide valuable research directions that serve as references and guidance for the future development of the integration between blockchain and 6G networks.
Citation: Liu Y, Peng S, Zhang M, Shi S, Fu J (2024) Towards secure and efficient integration of blockchain and 6G networks. PLoS ONE 19(4): e0302052. https://doi.org/10.1371/journal.pone.0302052
Editor: Mohammed Shuaib, Universiti Teknologi Malaysia, MALAYSIA
Received: October 25, 2023; Accepted: March 27, 2024; Published: April 11, 2024
Copyright: © 2024 Liu et al. This is an open access article distributed under the terms of the Creative Commons Attribution License , which permits unrestricted use, distribution, and reproduction in any medium, provided the original author and source are credited.
Data Availability: All relevant data are within the manuscript and its Supporting information files.
Funding: This work has received funding from significant scientific projects in Henan Province (No. 201300210100, No. 201300210200), key research projects in universities in Henan Province (No. 23ZX017), key collaborative innovation projects in Zhengzhou City (No. 21ZZXTCX07), and key scientific research projects in Henan Province (No. 232102211082). The funders had no role in study design, data collection and analysis, decision to publish, or preparation of the manuscript.
Competing interests: The authors have declared that no competing interests exist.
Introduction
The 6G network is the next generation of wireless communication technology, built upon the foundation of the 5G network [ 1 – 3 ]. Compared to 5G, 6G offers higher spectrum efficiency, lower latency, faster data rates, broader coverage, and the ability to connect a larger number of devices [ 4 – 6 ]. The development of 6G networks aims to establish a seamless global connectivity infrastructure, enabling efficient interconnection of all devices and systems [ 7 ]. Its technical architecture will rely on smarter technologies such as Software-Defined Networking (SDN), Network Function Virtualization (NFV), Mobile Edge Computing (MEC), big data, artificial intelligence (AI), and quantum communication to deliver more efficient, secure, and reliable communication services [ 8 ].
The 6G network is dedicated to a user-centric approach, emphasizing user experience, and excelling in network performance, service quality, and application scenarios [ 9 ]. Furthermore, the 6G network possesses robust heterogeneous support capabilities, enabling multimodal connections that cover different spectrums, network types, and devices [ 10 ]. From a technological perspective, the 6G network will be a highly heterogeneous network comprising numerous small intelligent devices and sensors. It will find applications in various scenarios such as the Internet of Things (IoT), smart cities, intelligent manufacturing, healthcare, and autonomous driving. The overarching goal is to achieve ubiquitous connectivity, comprehensive coverage, extensive perception, and complete intelligence. Additionally, the development of the 6G network will drive technological innovation and industrial upgrading in several domains, including novel antenna technologies, terahertz communications, and artificial intelligence [ 11 ].
However, in the evolving trends of the digital information era, 6G networks are moving towards a higher degree of decentralization. Yet, in this process, they face a series of intricate issues such as trust establishment, resource sharing, secure data exchange, access control, privacy protection, and effective governance [ 8 ]. Traditional network security techniques often rely on centralized institutions and encryption algorithms to address data security, transparency, and trust issues. However, in the large-scale distributed systems of the 6G environment, these methods may not meet the requirements of real-time operation, scalability, and decentralization. At this juncture, the unique advantages of blockchain technology come to the fore: its decentralization, immutability, and traceability offer potential solutions to address these challenges, promising to significantly enhance the security and reliability of 6G networks [ 12 ].
The integration of blockchain with 6G networks not only effectively addresses the information security demands of the new era but also has the potential to catalyze entirely new digital economic and social models, leading to profound societal impacts [ 13 – 15 ]. However, this convergence also faces a series of technical challenges and issues, such as security and privacy protection, performance and scalability of consensus mechanisms, and efficient and low-latency data and communication [ 16 ]. The motivation of this paper is precisely based on this backdrop, aiming to design a solution that combines security mechanisms with performance optimization. This solution seeks to ensure the secure and efficient integration of blockchain technology within the 6G network environment. The main contributions of this article are as follows:
- We are committed to exploring how to securely and efficiently integrate blockchain technology in 6G networks. By proposing methods based on secure mechanism design and performance optimization, we have conducted in-depth analyses of the application of blockchain technology in 6G networks, focusing on both security and performance optimization aspects, and put forward feasible solutions.
- We have conducted an in-depth study of the design requirements for security mechanisms. By thoroughly analyzing the security threats and attack types faced by the integration of blockchain and 6G networks, we have researched a range of design requirements for security mechanisms to ensure the security and reliability of communication and data transmission between blockchain and 6G networks.
- Our focus lies on the efficient implementation of the integration between blockchain and 6G networks. We have proposed a solution based on DAG for blockchain asynchronous consensus protocol. This solution aims to meet the desired characteristics of low latency, high throughput, and high reliability in the 6G network. Additionally, we have provided optimization strategies in various aspects such as storage and communication to accommodate the substantial data exchange and processing requirements in the 6G network.
The applications and challenges of 6G networks
As an integral component of the next generation of communication networks, 6G possesses not only application potential in traditional communication domains but also significant roles to play in various fields such as healthcare, military, entertainment, and industry. Below, we will delve into the specific application scenarios of 6G networks in these domains and the transformative impact they bring, while also highlighting the key security and performance challenges accompanying their rapid development.
6G networks have facilitated the digitization of the healthcare industry, significantly enhancing the efficiency, reliability, and security of medical services. Through remote diagnosis and surgical techniques, physicians can improve their workflow efficiency and reduce the need for in-person patient visits. Leveraging the high-speed data transmission capabilities of 6G networks, real-time analysis of patient health data allows doctors to gain a more accurate understanding of patient conditions, thereby providing more precise treatment plans. Additionally, smart wearable devices (such as smartwatches and smart clothing) utilize 6G networks for real-time monitoring of vital signs, offering unprecedented accuracy in personal health management. Nevertheless, as medical information flows rapidly across 6G networks, ensuring data security, protecting privacy, and addressing potential security threats emerge as critical challenges.
With its capabilities for massive-scale, high-speed, and real-time data processing, 6G networks have greatly enhanced the efficiency and combat capabilities of military operations. Battlefield intelligence collection and analysis can be conducted rapidly and efficiently, enabling commanders to gain a deeper understanding of the battlefield situation and make more accurate and timely decisions based on this information. However, while enhancing military effectiveness, ensuring the high security and stability of military intelligence transmission under 6G networks is crucial. Preventing potential network attacks and information leaks has become one of the core challenges in technological development.
Entertainment culture
The entertainment and cultural industries are benefiting from 6G networks, undergoing a profound transformation from traditional models to digitization and intelligence. Particularly in the virtual reality and augmented reality industries, the high-definition and low-latency characteristics of 6G networks have elevated user experiences to new heights. However, for applications with high bandwidth demands, maintaining stable high-performance services and effectively preventing issues such as illegal access and data tampering are technical challenges that 6G networks must address.
Industrial automation and IoT
The development of industrial automation and IoT also relies heavily on the support of 6G networks. Its high-speed and low-latency characteristics greatly drive innovation in various fields, including industrial robotics, smart manufacturing, intelligent transportation systems, smart logistics, and smart homes. However, with the massive influx of devices and data exchange, 6G networks need to continuously enhance processing capabilities and optimize resource allocation while ensuring stability and security in complex environments [ 17 , 18 ]. This is a crucial challenge that needs to be overcome urgently in the practical application process.
Furthermore, with the development of 6G networks, they will provide enhanced services for fields such as remote education, smart agriculture, intelligent buildings, and tourism. For instance, 6G networks can enable real-time online interactions and virtual laboratories for remote education applications. In smart agriculture, 6G networks facilitate data collection and transmission for real-time weather monitoring, farmland data analysis, and crop growth tracking. Intelligent buildings can benefit from the connectivity of various sensors and devices through 6G networks, enabling automated control and data analysis. Moreover, 6G networks offer smoother network connections and enrich the tourism industry with enhanced online experiences, injecting new vitality into the sector. However, ensuring that 6G networks can meet the specific needs of various industries while still addressing universal security and performance optimization remains a central topic for future research and development efforts.
Blockchain empowering 6G networks
With the rapid development of the digital economy and digital society, the application of blockchain technology in fields such as IoT, and healthcare has garnered extensive attention and exploration [ 19 – 23 ]. As a distributed ledger technology, blockchain achieves secure data interchange and value transfer through a decentralized approach, significantly enhancing the efficiency and transparency of information exchange. In the context of 6G networks, blockchain technology can bring higher security, transparency, and reliability to the network while enabling various new application scenarios and business models [ 24 ].
The fundamental components of blockchain encompass distributed ledger structure, cryptographic algorithms, consensus mechanisms, and smart contracts. These theoretical underpinnings collectively bolster blockchain technology to provide a higher level of security assurance for 6G networks. Specifically, by dispersing transaction records among numerous network nodes and employing encryption algorithms to ensure data integrity and immutability, blockchain significantly mitigates the risks of data fraud and tampering. Furthermore, based on consensus mechanisms, blockchain can achieve network state consistency and trustworthiness, further enhancing the network’s resilience against attacks [ 25 ].
In the context of 6G network environments, the decentralized nature of blockchain technology is paramount for enhancing network security. By incorporating network transaction records and identity verification processes into blockchain, not only does it improve transaction processing speed, but it also establishes a trust framework with highly anti-tampering characteristics. The functionality of smart contracts in blockchain further applies automatically executed security rules to the network’s operational layer, effectively preventing malicious activities and rapidly responding to various network threats [ 26 ].
Addressing the key features of 6G networks, such as ultra-low latency, extremely high-speed transmission, massive connectivity, and network intelligence, blockchain technology offers unique theoretical support and solutions. For instance, integrating blockchain’s distributed ledger enables real-time, fair, and traceable resource allocation, optimizing network resource management [ 27 ]. Through the automation of decision-making and execution mechanisms in smart contracts, network service response speed and management efficiency can be enhanced while ensuring strict control over data access and exchange authorization, thereby safeguarding user privacy and data security.
The security mechanisms of blockchain-6G network
This section will focus on how to ensure the security requirements for the combination of blockchain and 6G networks.
Analysis of security threats and attack types
The essence of blockchain technology lies in achieving distributed consensus through decentralization, thereby ensuring the security and reliability of transactions. However, this decentralized nature also makes blockchain networks vulnerable to malicious attacks. Take Bitcoin as an example [ 28 ], where an attacker with control over more than 51% of the network’s computational power can modify transaction records on the blockchain, effectively taking control of the entire network. Additionally, since participants in a blockchain network can be anonymous, malicious actors can exploit this anonymity to carry out attacks. For instance, they may propagate malicious or forged transactions, thereby posing threats to the reliability and security of transactions.
Furthermore, smart contracts are one of the core features of blockchain technology, enabling automated execution without the need for centralized intervention. However, smart contracts are susceptible to various vulnerabilities, such as reentrancy attacks, race conditions, integer overflow, and more. Malicious actors can exploit these vulnerabilities to carry out malicious operations, including double-spending and denial-of-service attacks, posing threats to blockchain networks. Additionally, protocol-level vulnerabilities are also a common avenue for attacking blockchain networks. Attackers can attack blockchain networks by maliciously constructing messages, tampering with messages, faking identities, etc. to gain improper benefits.
In the future 6G networks, their high speed and low latency characteristics make them potential targets for attackers. Attackers can exploit the high-speed transmission feature of 6G networks to launch various forms of network attacks. For instance, distributed denial-of-service (DDoS) attacks can leverage the high bandwidth of 6G networks to flood the network with a large volume of traffic, depleting network resources and causing network paralysis. Additionally, network phishing attacks may take advantage of the high-speed nature of 6G networks to masquerade as trustworthy entities and deceive users into submitting sensitive information. Moreover, the low latency feature of 6G networks can be exploited by attackers for real-time attacks such as intrusion attacks and port scanning.
Security mechanism design requirements
To ensure the security of the integration between blockchain and 6G network, it is imperative to develop a comprehensive set of security mechanisms. As depicted in Fig 1 , these mechanisms encompass not only identity authentication and authorization but also encrypted communication and data privacy protection, among other aspects.
- PPT PowerPoint slide
- PNG larger image
- TIFF original image
https://doi.org/10.1371/journal.pone.0302052.g001
Identity authentication and authorization serve as fundamental prerequisites for the integration of blockchain and 6G networks. Within the realm of 6G networks, users can acquire network access privileges through identity authentication mechanisms, while smart contracts within the blockchain ensure compliance with established rules. In the context of applications combining blockchain and 6G networks, identity authentication mechanisms further guarantee that only authorized users can access shared data.
To ensure the data security within applications combining blockchain and 6G networks, the implementation of encryption communication mechanisms is of paramount importance. In the context of 6G networks, where wireless channels are susceptible to attacks, employing encryption communication can effectively enhance the confidentiality and integrity of transmitted data. Various encryption algorithms, such as hybrid encryption and lightweight encryption, along with key management mechanisms, can be utilized to further strengthen data security. Simultaneously, measures need to be taken to safeguard user privacy and prevent data theft or misuse. Zero-knowledge proof techniques can be employed to verify that users’ data adheres to certain rules without revealing the actual data information.
Furthermore, to prevent malicious nodes from disrupting the blockchain network, employing trust-based security mechanisms is highly necessary. Reputation assessment and trust management mechanisms are common security measures in this regard. By evaluating the reputation and trustworthiness of each node, these mechanisms can better prevent attacks from malicious nodes. Additionally, smart contracts are a vital component of blockchain networks, and thus, it is crucial to implement security measures to ensure their correctness and safety. One common approach is to utilize formal verification techniques to check for vulnerabilities and errors in smart contracts, thereby avoiding potential attacks. Furthermore, multi-signature technology can enhance the security of smart contracts and prevent the execution of malicious contracts. Lastly, conducting regular security audits and vulnerability scans is essential. This helps in identifying and promptly resolving potential vulnerabilities, thereby mitigating the risk of attackers exploiting these vulnerabilities for their malicious activities.
Efficient implementation of blockchain-6G network
In this section, we will delve into the detailed discussion on achieving an efficient integration of blockchain and 6G networks.
The adaptation of blockchain consensus protocols to 6G networks
In wireless networks, especially in the context of 6G communication environments, blockchain technology has demonstrated significant security advantages. However, it faces a prominent challenge of high energy consumption in its application, particularly for nodes with limited battery capacity. The complex consensus process accelerates energy consumption, which may lead to premature battery depletion and offline nodes, thereby affecting network scalability and reliability. Addressing this core issue, innovative solutions like the Green Sharding (GS) [ 29 ] scheme employ geolocation-based node sharding strategies to restrict wireless nodes’ participation in global communication, effectively reducing unnecessary energy consumption. This approach has shown promising energy efficiency and performance in scenarios involving the terahertz (THz) and the millimeter wave (mmWave) signals.
However, even with optimization solutions like GS to alleviate energy consumption issues, when integrating blockchain with 6G networks, the adaptation between blockchain consensus protocols and 6G networks is essential, as it directly affects the overall system’s performance and efficiency. Previous research endeavors aimed at integrating blockchain into wireless communication, such as employing Proof of Work (PoW) [ 28 ], Proof of Stake (PoS) [ 30 ], or Practical Byzantine Fault Tolerance (PBFT) [ 31 ] consensus protocols. However, these protocols face challenges in handling high concurrency transactions and low-latency demands [ 25 , 29 , 32 ]. As evident from Table 1 , traditional consensus protocols typically prioritize ensuring strong consistency at the expense of lower throughput, higher latency, and poorer scalability. Particularly in wireless network environments, the synchronous or semi-synchronous communication models and high-energy consumption characteristics required by these protocols make them difficult to adapt to the large-scale connectivity, low latency, and high energy-efficiency demands of 6G networks. For instance, PoW and PoS often rely on chain-like structures for transaction recording, limiting their processing capabilities in high-concurrency environments. Moreover, as the number of nodes increases in large-scale networks, energy consumption and latency issues become more pronounced. Therefore, in order to better apply blockchain technology in the 6G network environment, the development of new consensus protocols is necessary to cater to the requirements of high-speed network environments.
https://doi.org/10.1371/journal.pone.0302052.t001
In contrast, DAG-based consensus protocol offers numerous advantages, including high concurrency, low latency, and high throughput, which align well with the requirements of 6G networks. The asynchronous communication model and non-linear transaction confirmation method employed by DAG consensus significantly enhance system throughput and reduce latency, while also providing better scalability and energy efficiency(EE) [ 33 ]. DAG consensus allows for parallel processing of multiple transactions, overcoming the bottlenecks associated with traditional chain-like structures. Furthermore, DAG adopts a finality consistency model, ensuring data correctness while processing transactions more rapidly. However, this optimization may entail sacrificing some immediate determinism in extreme cases. Nevertheless, in many real-time scenarios where high immediacy is required and a certain degree of time lag in consistency is acceptable, DAG consensus protocols demonstrate their unique advantages. Therefore, the DAG-based consensus protocol holds promise as a favorable choice for 6G networks, particularly in supporting high-concurrency applications.
Based on the aforementioned considerations and to better adapt to real-world asynchronous network environments with network communication failures and unpredictable network conditions, we propose a solution for a DAG-based asynchronous consensus protocol in blockchain. Additionally, we employ sharding techniques to further enhance transaction throughput and reduce the burden on participating nodes. The blockchain network is divided into multiple shards that concurrently process different sets of transactions. Within each shard, nodes maintain a consistent DAG structure based on rounds (constants) locally. In each round, two types of messages are broadcasted: blocks containing specific transactions and events containing block hashes. These events serve to prove the legitimacy of blocks and facilitate inter-shard communication. When a node receives a sufficient number of events for the current round, it proceeds to the next round and creates a new block, which is then broadcasted to neighboring nodes within the same shard. Nodes receiving the block validate it, and once the block is validated by a majority of nodes, the node that created the block generates a corresponding event to prove its legitimacy. The DAG structure is illustrated in Fig 2 .
https://doi.org/10.1371/journal.pone.0302052.g002
Specifically, within each shard, nodes employ a structured DAG to facilitate parallel broadcasting and transaction processing. Nodes only broadcast and store transaction blocks of their local shard, thereby enhancing transaction processing efficiency and reducing storage costs. To ensure intra-shard consistency, we introduce a randomized approach to order the blocks within the shard. In more detail, we utilize an active threshold signature scheme [ 34 ] to obtain shard-consistent random coins, which are used to determine leader blocks. By sorting the ancestor blocks of the leader block, we establish a consistent block order within the shard. Furthermore, to ensure global consistency, inter-shards consensus is required, nodes send the batch of events corresponding to the blocks that have been confirmed in the intra-shard consensus to the nodes within other shards through inter-shards communication, instead of the blocks themselves. This significantly reduces inter-shard communication overhead. Additionally, batch merging reduces network bandwidth consumption and accelerates confirmation speed. Through this approach, nodes in different shards can maintain a consistent global block event state, effectively addressing the security downgrade caused by sharding. The architecture of the consensus mechanism is illustrated in Fig 3 .
https://doi.org/10.1371/journal.pone.0302052.g003
We have set up a local experimental environment for evaluating the performance of the consensus protocol. This experimental environment consists of four high-performance servers, each equipped with an Intel(R) Xeon(R) 5218R CPU running at 2.10GHz, 128GB of memory, and 40 CPU cores, running the Linux Ubuntu Server 20.04 operating system. To evaluate its performance in scenarios involving a large number of transactions and high workloads, we conducted tests on the protocol’s performance under different numbers of shards and input rates. The experimental results, shown in Fig 4 , depict the protocol’s throughput and latency for configurations with 2, 4, 8, and 16 shards, as well as input rates of 50,000 tx/s, 100,000 tx/s, 150,000 tx/s, and 200,000 tx/s. Here, the input rate represents the rate at which clients submit transactions to the system. The experimental results demonstrate a significant improvement in throughput and effective control of latency as the number of shards and input rate increase. Particularly, in the configuration with 16 shards and an input rate of 200,000 tx/s, the protocol achieves a throughput of over 190,000 tx/s with a latency of less than 2 seconds. The findings indicate that the protocol exhibits excellent adaptability and performance in future 6G environments characterized by large-scale and high workload scenarios.
(Data presented in S1 and S2 Tables in S1 File ).
https://doi.org/10.1371/journal.pone.0302052.g004
Optimization strategies for blockchain storage and communication based on 6G networks
Given the high data rates and large-scale data processing requirements of 6G networks, effective optimization of storage and communication of blockchain systems is essential. Taking into account the characteristics of 6G networks, we have devised a set of strategies to optimize storage and communication. These strategies are aimed at ensuring that the blockchain system performs well and remains stable in high-load and large-scale transaction scenarios.
Storage optimization.
To support the vast storage demands of blockchain, data compression technology emerges as a crucial means. In scenarios where blockchain storage entails a massive amount of data, data compression techniques can significantly reduce the occupancy of storage space. Moreover, with the advancement of bandwidth and transmission speeds in 6G networks, compression algorithms can better facilitate the application of blockchain technology. For instance, by utilizing compression algorithms, large-scale blockchain data files can be compressed into smaller ones, thereby conserving storage space. Furthermore, compression techniques can also be employed to compress transaction data, reducing the volume of stored and transmitted data, thereby further enhancing system efficiency and performance.
Furthermore, in blockchain technology, sharding is a common method of data storage optimization. By dividing the blockchain data into multiple shards, the amount of data that each node needs to store can be reduced. To ensure load balancing in storage, the size and quantity of shards can be dynamically adjusted based on the node’s workload. When a node needs to access data from other shards, it can request the data from other nodes and perform merging operations. However, to maintain data consistency, certain synchronization operations may be required.
Communication optimization.
To optimize P2P communication, attention should be given to both node selection and message routing. Regarding node selection, a comprehensive evaluation of node quality can be conducted, taking into account factors such as network topology, bandwidth, and response time. Prioritizing high-quality nodes in this process can effectively reduce message transmission latency and enhance the overall system efficiency. For instance, algorithms like Distributed Hash Tables (DHT) can be utilized to identify the most optimal nodes. In terms of message routing, more efficient routing can be achieved by employing distance-based routing algorithms such as Kademlia [ 35 ] and other related technologies, enabling rapid identification of the nearest nodes and improving message transmission efficiency.
Future research directions
Here, we propose several research directions aimed at promoting the deep integration of blockchain and 6G networks, and further enhancing network efficiency, security, and scalability.
The combination of intelligent blockchain and 6G network
The future 6G network will no longer be merely an infrastructure for device connectivity but a more intelligent and adaptive network. This implies that the integration of blockchain and 6G networks will require even more intelligent approaches. Therefore, future research directions should explore the combination of blockchain technology and AI to achieve a more intelligent and adaptive integration of blockchain and 6G networks. One research direction is to leverage machine learning techniques to enhance the selection and data processing of blockchain nodes, enabling more efficient communication and data storage. Furthermore, the application of blockchain technology and AI can be explored to achieve more intelligent network management and security mechanisms, thereby enhancing the security and reliability of the network.
Flexible and efficient blockchain consensus protocol
In the realm of 6G networks, the consensus protocol of blockchain necessitates meeting heightened performance requirements to facilitate expedited transaction processing and large-scale data transmission. Concurrently, given the multidimensional architecture of 6G networks encompassing space, aerial, terrestrial, underground, and underwater domains, security and privacy concerns become more intricate and pivotal. Hence, future research endeavors can converge on devising consensus protocols that are more secure, efficient, and privacy-preserving, aligning with the demands of 6G networks. Pertinent research areas encompass, but are not limited to, leveraging cryptographic techniques to enhance the resilience and interference resistance of consensus protocols, crafting innovative consensus protocols tailored to the 6G network architecture, and exploring avenues to expedite blockchain transactions and data processing while upholding stringent security prerequisites.
Post-quantum era security safeguards
With the advancements in quantum computing technology, traditional public key encryption algorithms have become vulnerable to attacks, posing significant security challenges to the practical application of blockchain technology and 6G networks. Therefore, exploring the integration of blockchain and 6G networks with quantum security technology to enhance network security is an important research direction. Specifically, the use of encryption methods based on quantum key distribution can be explored to protect the communication and storage processes of blockchain, thereby improving its security. Additionally, researchers can investigate leveraging quantum technology to enhance the randomness and security of blockchain, such as using quantum random number generators to generate high-quality random numbers, thereby enhancing the strength and security of cryptography.
Furthermore, addressing the challenges posed by the continually evolving complex network environments and dynamic load conditions, deepening the research on the adaptability and stability of consensus algorithms is crucial. This encompasses, but is not limited to, developing more intelligent dynamic sharding strategies to balance system loads, designing flexible and efficient adaptive parameter adjustment mechanisms, and exploring the integration of Trusted Execution Environments (TEEs) and other emerging hardware technologies into consensus algorithms to enhance the security and efficiency of the consensus process. Additionally, within the broader context of the blockchain ecosystem increasingly emphasizing interoperability, innovation in consensus algorithms for cross-chain communication scenarios emerges as one of the core trends for future development. By studying consensus mechanisms applicable to interactions between different blockchains, it becomes possible to effectively promote value exchange, information dissemination, and resource coordination, thereby driving the interconnectedness of the entire blockchain ecosystem. Furthermore, to expand the scope of blockchain technology applications and the range of service recipients, in-depth exploration of the design and implementation methods of lightweight clients is particularly crucial. This lightweight participation model enables devices with limited resources to easily access blockchain networks, not only lowering the user threshold but also providing strong support for the widespread adoption and deep integration of blockchain technology in various fields such as healthcare, IoT, and smart cities, thus truly achieving its broad dissemination and deep integration in the 6G network environment.
The present manuscript presents a method based on the design of security mechanisms and performance optimization to achieve a secure and efficient integration of blockchain and 6G networks. Initially, we delve into the application scenarios of 6G and the empowerment of blockchain in the 6G context, conducting a detailed analysis of the advantages of 6G networks and the synergistic effects of blockchain and 6G. Subsequently, we have explored a security mechanism suitable for the integration of blockchain and 6G networks. Through a detailed analysis of security threats and attack types, we have conducted an in-depth study of the design requirements for security mechanisms in this combined scenario. Furthermore, we introduce a DAG-based blockchain asynchronous consensus solution, aiming to fulfill the desired characteristics of 6G networks. Additionally, we provide optimization strategies pertaining to storage, communication to better cater to the extensive data exchange and processing requirements in 6G networks. Lastly, we offer valuable research directions to guide the future development of the integration between blockchain and 6G networks.
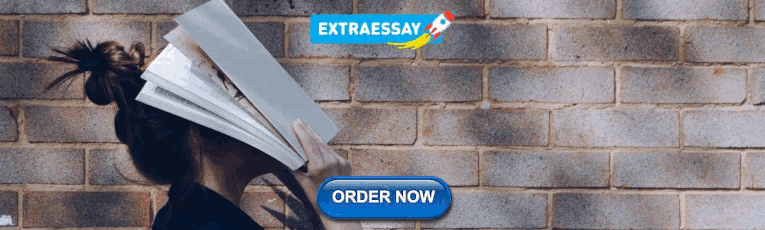
Supporting information
S1 file. towards secure and efficient integration of blockchain and 6g networks..
https://doi.org/10.1371/journal.pone.0302052.s001
Acknowledgments
We would like to thank members of the distributed computing and blockchain research team, for their helpful feedback in drafting previous versions of this manuscript.
- View Article
- Google Scholar
- PubMed/NCBI
- 31. Castro M, Liskov B, et al. Practical byzantine fault tolerance. In: OsDI. vol. 99; 1999. p. 173–186.
- 34. Boldyreva A. Threshold signatures, multisignatures and blind signatures based on the gap-Diffie-Hellman-group signature scheme. In: International Workshop on Public Key Cryptography. Springer; 2002. p. 31–46.
- 35. Maymounkov P, Mazieres D. Kademlia: A peer-to-peer information system based on the xor metric. In: Peer-to-Peer Systems: First InternationalWorkshop, IPTPS 2002 Cambridge, MA, USA, March 7–8, 2002 Revised Papers. Springer; 2002. p. 53–65.
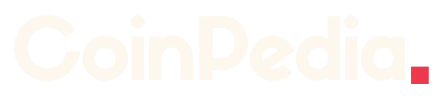
$70,621.97 (1.04%)
$3,519.05 (0.12%), $606.50 (0.08%).

Track your Portfolio
Weekly web3, blockchain, and crypto analysis, author: qadir ak qadir ak.
Qadir Ak is the founder of Coinpedia. He has over a decade of experience writing about technology and has been covering the blockchain and cryptocurrency space since 2010. He has also interviewed a few prominent experts within the cryptocurrency space.

This analysis report, backed by quality data, covers the major developments the Web3, Blockchain and Crypto sector has experienced this week.
1. Breaking News This Week
- Paypal Expands International Transfers with PYUSD to USD Conversions
PayPal’s recent announcement allows users in the United States to engage in international money transfers using the PYUSD stablecoin. This expansion broadens the spectrum of global payment options available to US users, facilitating smoother transactions with friends and family overseas through currency conversion.
- Pyth Network Introduces New Price Feeds for W and USDB
Pyth Network, a notable price feed oracle and market data provider, has introduced fresh price feeds for two blockchain tokens: W and USDB. These newly integrated feeds are now accessible across approximately 50 blockchains that have integrated Pyth, enhancing the availability and accuracy of market data for users.
- Paradigm in Talks to Rise $750-$850 Million for New Crypto Fund
Paradigm, a prominent crypto venture capital firm co-founded by Fred Ehrsam and Matt Huang, is reportedly in discussion with investors to rise between $750 million and $850 million for a new crypto fund. This initiative aims to capitalise on increasing institutional interest in the crypto space following a lull in VC investing.
- Binance to Shut Down Bitcoin NFT Marketplace
Binance , a leading cryptocurrency exchange, has announced its decision to close its Bitcoin NFT marketplace, ceasing support for traders and deposits starting April 18. Users are required to withdraw their NFTs by May 18, marking the end of Binance’s brief venture into the realm of Bitcoin collectibles.
- Ripple Plans to Launch 1:1 USD-Pegged Stablecoin
Ripple , a prominent provider for enterprise blockchain and crypto solutions, has unveiled plans to introduce a stablecoin pegged 1:1 to the US dollar (USD). Backed by a reserve of US dollar deposits, short-term US government treasuries, and other cash equivalents, this stablecoin aims to provide stability and reliability in transactions.
- Coinbase Partners with Lightspark for Lightning Network Implementation
Coinbase , the largest crypto exchange in the United States, has selected Lightspark as its partner to spearhead the implementation of Bitcoin’s lightning network. Led by PayPal co-founder David Marcus, Lightspark’s expertise will aid in enhancing transaction scalability and efficiency within Coinbase’s platform.
- CrowdSwap Launches First Decentralised Crypto ETF
CrowdSwap has introduced the Bull Run dETF, marking the debut of the first decentralised crypto exchange-trading fund ever. Comprising ten digital assets, including Bitcoin and Ether, this innovative fund offers investors a diversified portfolio while maintaining decentralised and secure processes amidst a growing market for such products.
- US Authorities Possibly Selling More Bitcoin
Reports suggest that US authorities may be considering selling additional portions of the nation’s significant Bitcoin stash, potentially impacting the crypto market. With approximately 2000 BTC recently transferred from the government’s holdings to Coinbase, concerns arise regarding the implications of increased sell pressure on market dynamics.
- Argentina Requires Local Crypto Firms to Register with Regulatory Body
The Argentine government has mandated all local crypto firms to register with the newly established Registry of Virtual Asset Service Providers. Failure to comply could result in cessation of operations, aligning with recommendations from the Financial Action Task Force as Argentina aims to regulate its crypto sector more comprehensively.
- OFAC Tightens Grip on Russian Individuals and Entities in Virtual Assets
The Office of Foreign Assets Control has intensified enforcement efforts targeting individuals and entities operating within Russia’s financial services and technology sectors, particularly those involved in virtual assets. This crackdown aims to curb activities facilitating evasion of US sanctions, tightening regulatory oversight in this domain.
- Grayscale Unveils Grayscale Dynamic Income Fund
Grayscale, a leading institutional crypto asset management firm, has introduced its first actively managed investment product, the Grayscale Dynamic Income Fund (GDIF). This new fund focuses on optimising income through staking rewards associated with proof-of-stake crypto assets, offering investors exposure to innovative yield-generating strategies.
- Prisma Finance Hacker Claims “Whitehat Rescue” of Stolen Funds
The hacker responsible for the $11.6 million theft from Prisma Finance has asserted that the exploit was a ‘Whitehat Rescue’ attempt. Seeking guidance on how to return the funds, the hacker characterises their actions as an endeavour to identify and rectify security vulnerabilities in the DeFi protocol, emphasising ethical intent.
2. Blockchain Performance
In this section, we will analyse two factors primarily: the top-performing blockchains based solely on their 7-day change and the top performers among the top five blockchains with the highest TVL.
2.1. Top Blockchain Performers by 7-Day Change
This week’s top blockchain performers, based on their 7-day change, are Core, Mantle, Chiliz, Linea and Base.
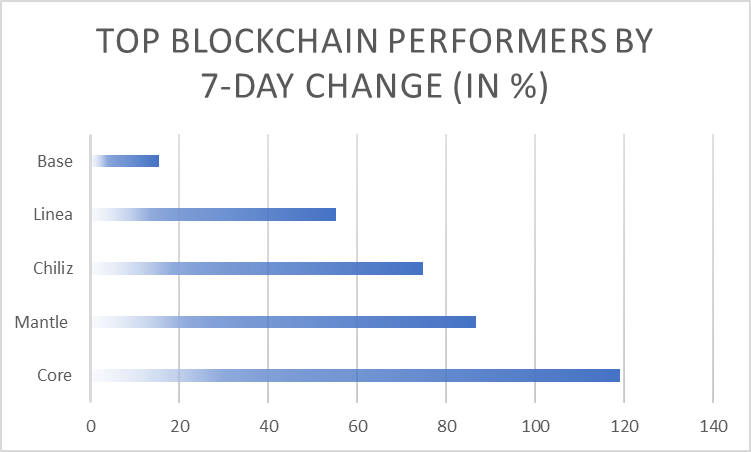
Among blockchains, this week, Core led with a remarkable 119.1% increase, followed closely by Mantle at 86.7% and Chiliz at 74.7%. Linea showed a substantial 55.1% rise, while Base recorded a modest 15.3% growth.
2.2. Top Performers: 7-Day Change in Top 5 Blockchains with Highest TVL
Ethereum, TRON, BNB Smart Chain, Solana, and Arbitrum One are the top five blockchains in the market on the basis of TVL and market dominance. Let’s see how the top five blockchains have performed this week, using 7-day TVL change.
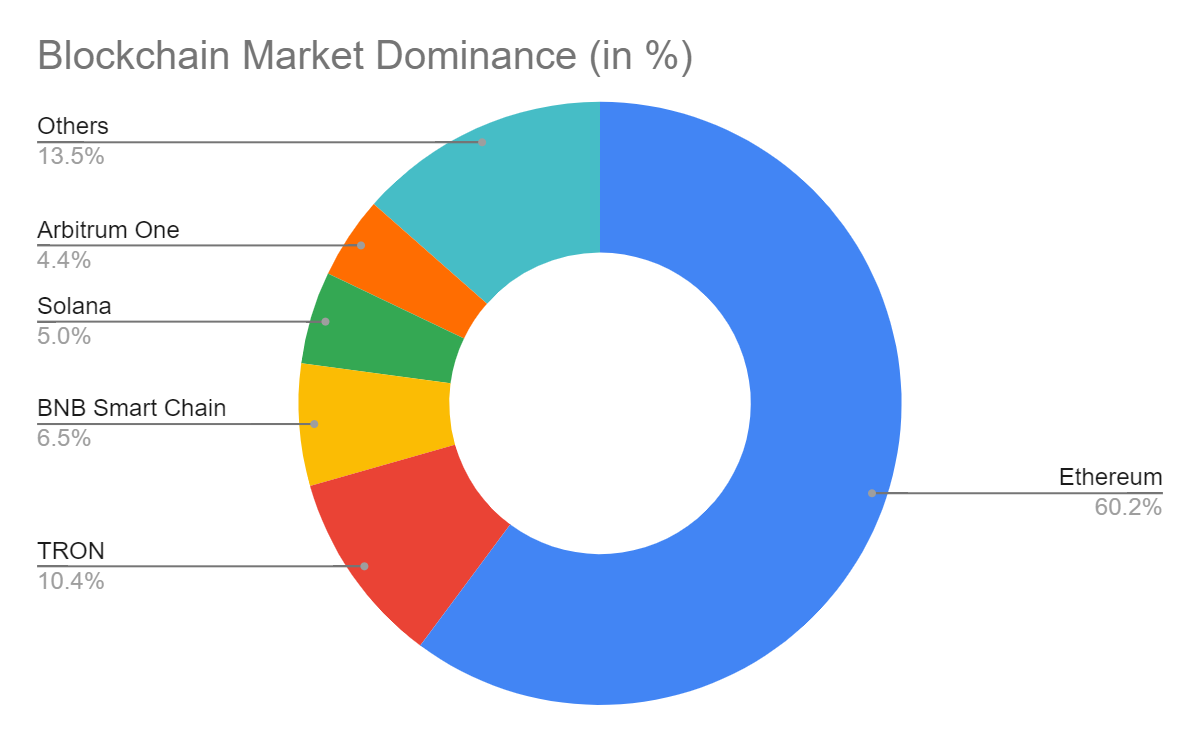
This week, BNB Smart Chain experienced the most significant decline at -8.6%, followed by Solana at -6.8%, Tron at -3.7%, Arbitrum One at -3.5%, and Ethereum at -1.0%. All the top five blockchains saw negative trends, suggesting potential market volatility or shifts in sentiment.
3. Crypto Market Analysis
The crypto 7-day price change and dominance analysis and top gains and losers analysis are the two prime areas of the crypto market analysis.
3.1. Crypto 7-D Price Change and Dominance Analysis
Bitcoin, Ethereum, Tether, BNB, and Solana are the top cryptocurrencies as per the market cap and dominance indices. Let’s analyse their seven-day price change.
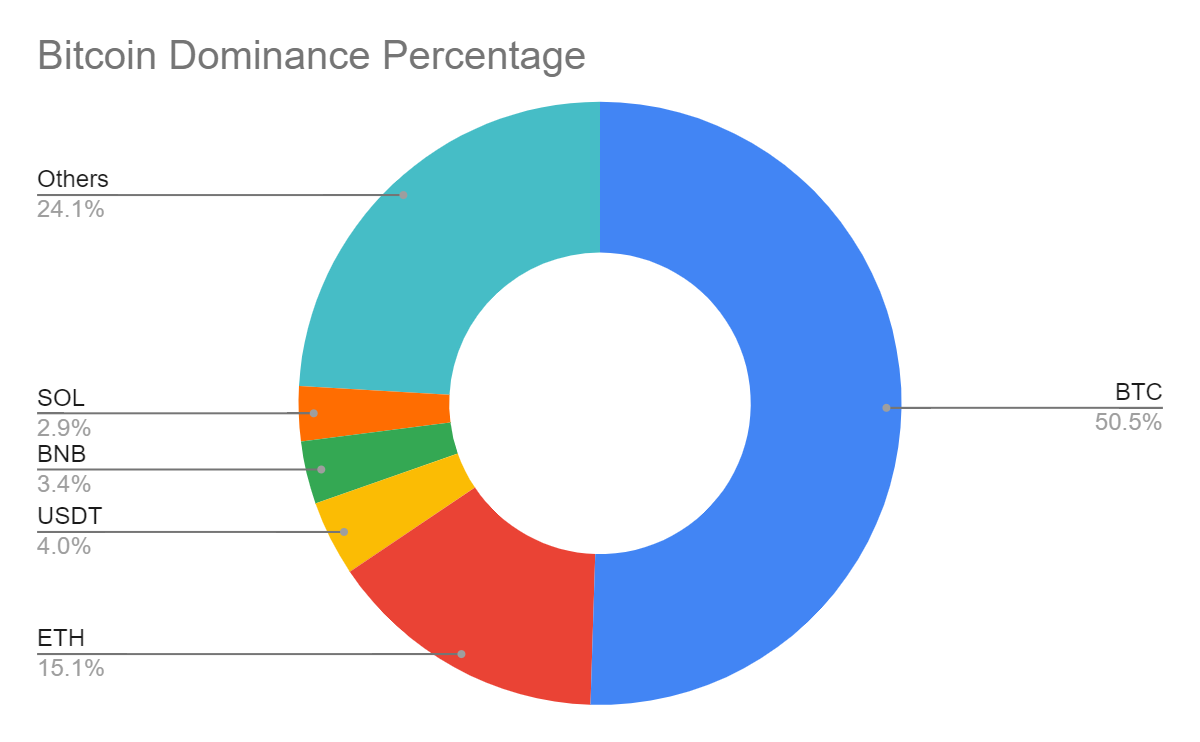
Among the top cryptocurrencies, Solana exhibited the most significant decline with a -10.7% decrease, followed by Ethereum at -4.7%, and BNB at -4.5%. Bitcoin experienced a comparatively milder decline at -2.8%, while Tether remained stable with a +0.0% change.
3.2. Top Gainers & Losers of the Week in Crypto Market
Here is the list of top gainers and top losers of the week in the cryptocurrency market. The analysis is made using the 7-day Gain and 7-day Lose indices.
3.2.1. Top Gainers of the Week in Crypto
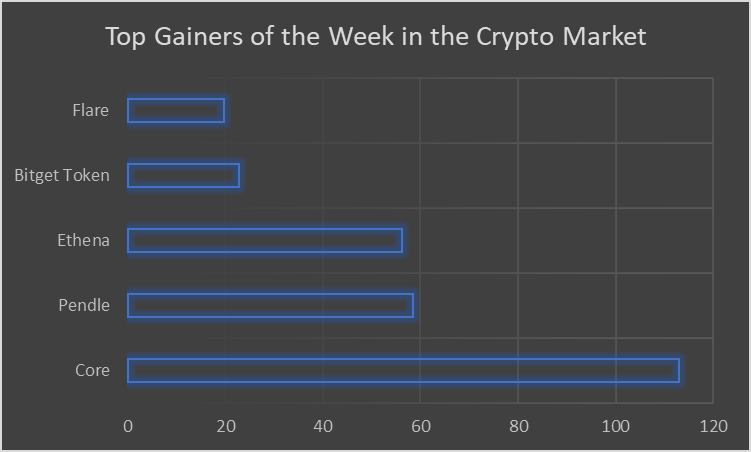
This week’s top cryptocurrency gainers include Core with a remarkable +112.91% increase, followed by Pendle at +58.87% and Ethena at +56.24%. Bitget Token and Flare also saw gains of +22.78% and +19.77% respectively.
Also Read : Crypto Hack Report Q1 2024: Trends, Losses, and Recovery Efforts
3.2.2. Top Losers of the Week in Crypto
This week’s top cryptocurrency losers include Wormhole with a significant loss of -28.11%, followed by dogwifhat at -26.27% and Conflux at -24.83%. Axelar and Aptos also experienced notable losses at -24.51% and -22.16% respectively.
3.3. Stablecoin Weekly Analysis
Tether, USDC, DAI, First Digital USD, and Ethena USDe are the top stablecoins in the market in terms of market capitalization. Let’s analyse their weekly performance using seven-day market capitalisation, market dominance and trading volume indices.
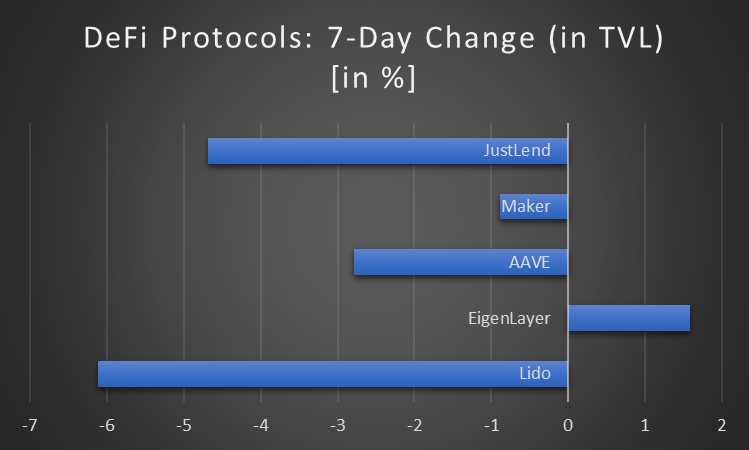
Tether dominates the stablecoin market with 70.36% market dominance, followed by USDC at 21.74%. Dai holds 3.33%, while First Digital USD and Ethena USDe have 2.33% and 1.33% respectively. Tether’s significant lead reflects its widespread use and stability, while USDC’s strong position indicates growing adoption.
3.3.1. Stablecoin Weekly Price Analysis
Let’s analyse the weekly performance of the top stablecoins further using the seven-day price change index.
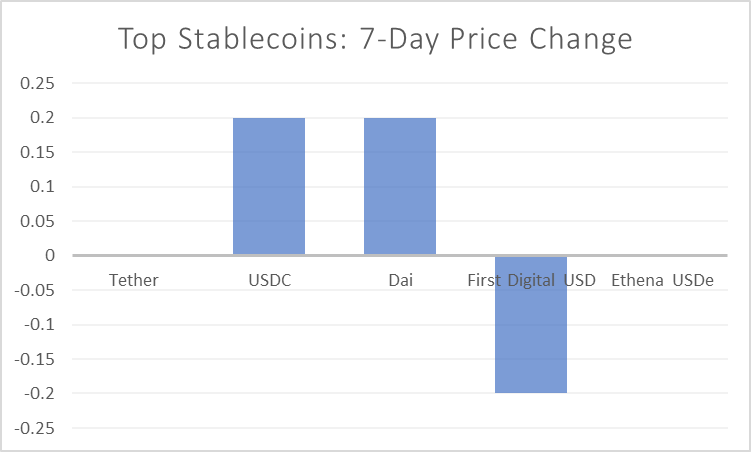
Among the top stablecoins, Tether and Ethena USDe remained stable with no change, while USDC and Dai experienced a slight increase of +0.2%. Conversely, First Digital USD saw a minor decrease of -0.2%.
4. Bitcoin ETF Weekly Analysis
Bitcoin Futures ETFs and Bitcoin Spot ETFs should be analysed separately, in order to get the right picture of the Bitcoin ETF market, as they represent two different segments. Let’s start!
4.1. Bitcoin Futures ETF Weekly Analysis
ProShares (BITO), VanEck (XBTF), Valkyrie (BTF), Global X (BITS), and Ark/21 Shares (ARKA) are the top Bitcoin Future ETFs, as per the Asset Under Management index. Let’s use the price change percentage index to analyse these ETFs.
ProShares (BITO) experienced the largest decline among top Bitcoin Futures ETFs at -1.73%, followed by Ark/21 Shares at -1.64%. Valkyrie (BTF) and Global X (BITS), both, saw a -1.54% decrease. Conversely, VanEck (XBTF) showed a modest gain of +0.33%.
4.2. Bitcoin Spot ETF Weekly Analysis
Grayscale (GBTC), Blackrock (IBIT), Fidelity (FBTC), Ark/21 Shares (ARKB), and Bitwise (BITB) are the top Bitcoin Spot ETFs, as per the Asset Under Management index. Let’s analyse them using the price change index.
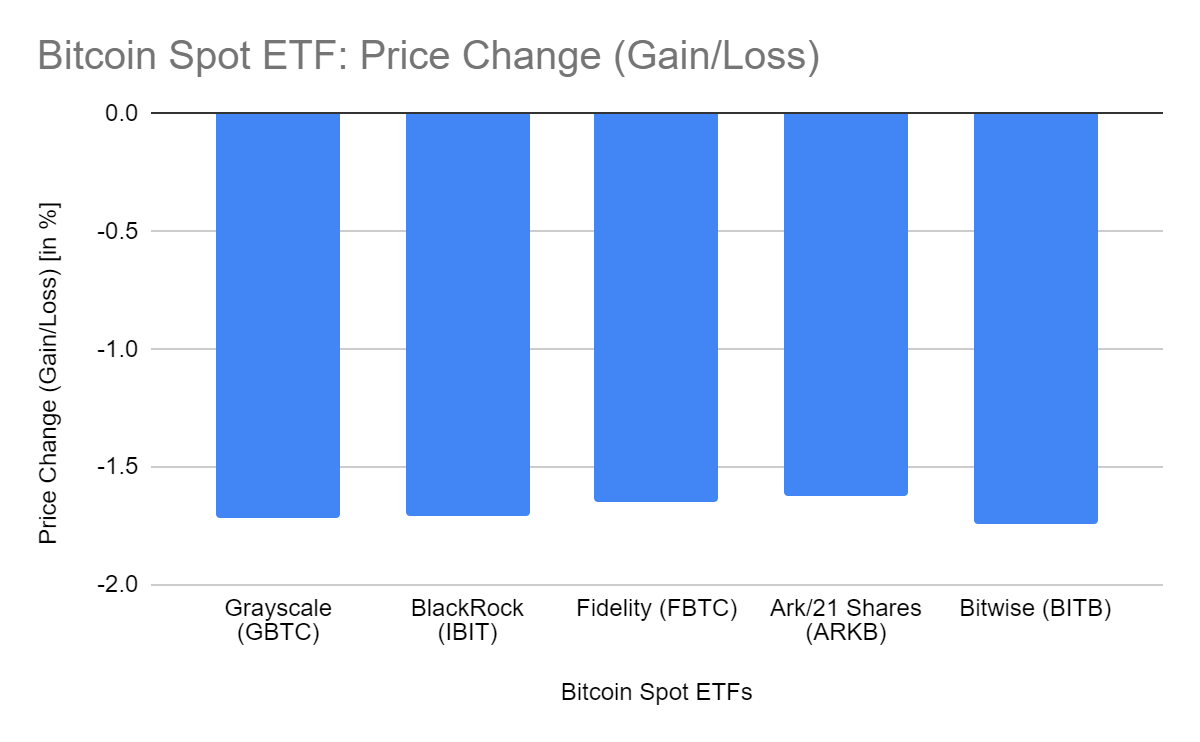
Among the top Bitcoin Spot ETFs, Bitwise (BITB) faced the largest decrease at -1.74%, followed closely by Grayscale (GBTC) and BlackRock (IBIT) at -1.72% and -1.71% respectively. Fidelity (FBTC) and Ark/21 Shares (ARKB) also experienced declines at -1.65% and -1.62%.
5. DeFi Market Weekly Status Analysis
Lido, EigenLayer, AAVE, Maker, JustLend are the five top DeFi protocols on the basis of TVL. Let’s analyse its weekly performance using the 7d Change index.
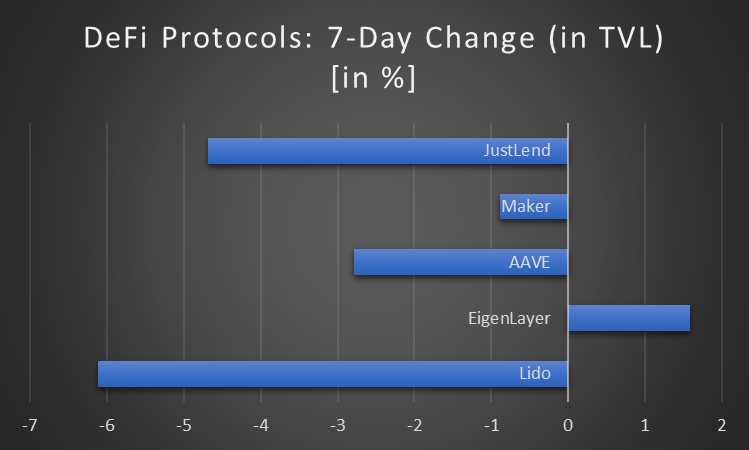
Among the top DeFi protocols, only EigenLayer exhibited an increase. It experienced an increase of +1.58%. Meanwhile, Lido saw a notable decline of -6.11%. JustLend and AAVE also experienced losses at -4.69% and -2.79% respectively. Notably, Maker also showed a decrease, but only a marginal loss of -0.88%.
6. NFT Marketplace: A Basic Weekly Analysis
Blur, Blur Aggregator, Opensea, Cryptopunks, and X2Y2 are the top NFT marketplaces on the basis of market share. Let’s analyse them using the Volume Change (change of last 7d volume over the previous 7d volume) index.
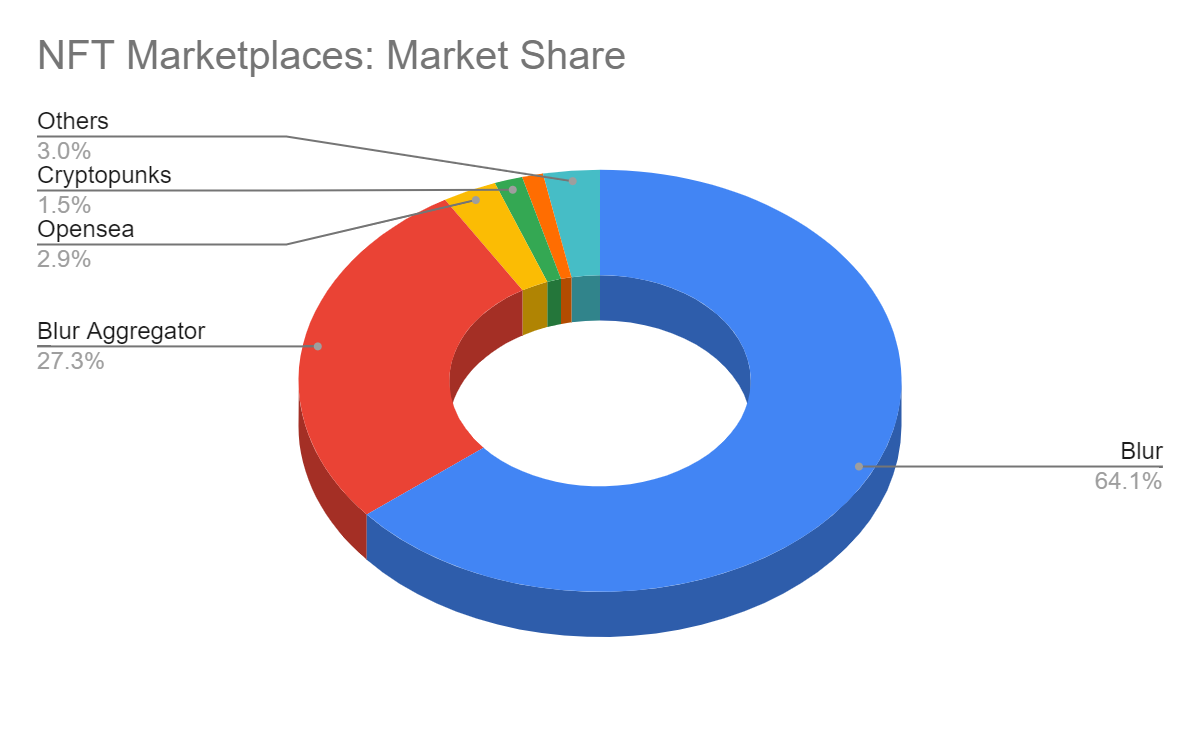
Among the top NFT marketplaces, Cryptopunks and X2Y2 saw volume increase of +11.89% and +9.96% respectively, indicating heightened trading activity. Blur Aggregator showed a modest increase at +1.44%. However, Opensea experienced a significant decline of -78.22%, while Blur saw a comparatively modest decrease of -15.48%.
6.1. Top NFT Collectible Sales this Week
Bored Ape Yacht Club #591, Azuki #6525, Azuki #1836, CryptoPunks #5448, and Bitcoin Shrooms #a0e8054 are the top NFT collectable sales reported this week in the NFT market landscape.
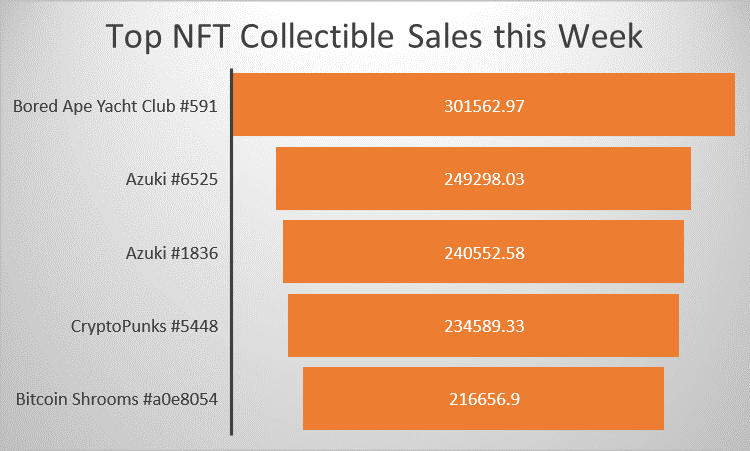
This week’s top NFT collectibles sales showcase Bored Ape Yacht Club #591 leading at $301,562.97, followed by Azuki #6525 at $249,298.03 and Azuki #1836 at $240,552.58. CryptoPunks #5448 sold for $234,589.33, while Bitcoin Shrooms #a0e8054 fetched $216,656.90.
7. Web3 Weekly Funding Analysis
7.1. ico landscape: a weekly overview .
Versus-X, Sensay, Ethena, BonusBlock, SatoshiSync, and Wormhole are the major ICOs that ended this week. Let’s analyse how much amount they have received.
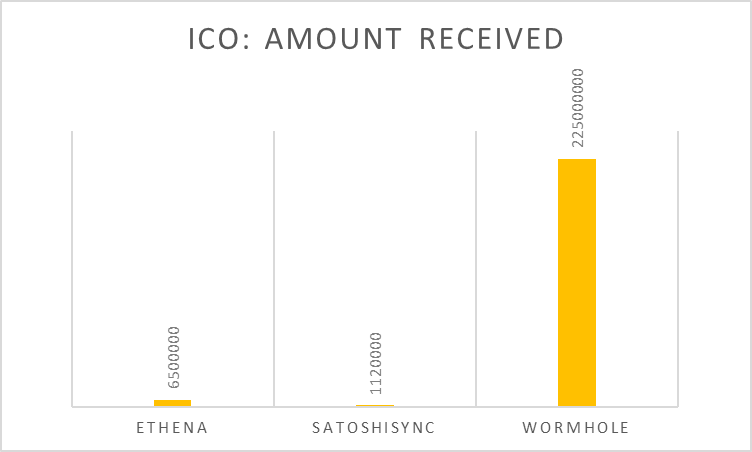
Wormhole received the highest amount at $225,000,000, indicating significant investor interest and project potential. Ethena secured $6,500,000, reflecting a medium-scale project, while SatoshiSync received $1,120,000, suggesting a smaller-scale project.
Also Check Out The : Bitcoin ETF March Monthly Report: Insights into Performance Trends
8. Weekly Blockchain Hack Analysis
As of April 6, 2024, hackers have stolen a significant $7.77 billion. The majority, approximately $5.85 billion, targeted decentralised finance platforms. Another portion, roughly $2.83 billion, was taken from bridges connecting different blockchain networks.
Fortunately, no major hacks have been reported this month. However, late last month, the Web 3, Blockchain and Crypto ecosystem experienced three significant breaches. On March 29, 2024, Lava lost approximately $0.34 million to hackers. On the preceding day, March 26, 2024, Prisma Finance fell victim to hackers, losing $11.6 million. Also on March 26, 2024, Munchables was hacked using a storage slot exploit, resulting in a staggering $62.5 million loss, the largest reported this year.
Fortunately, the hackers returned the stolen Ether from the Munchables exploit. Additionally, the hackers behind the Prisma Finance theft claimed it was a ‘Whitehat Rescue’ effort. And, they sought guidance on returning the funds, describing their actions as aimed at identifying and fixing DeFi protocol security flaws, emphasising ethical intent.
Endnote
In this week’s comprehensive analysis of Web3, Blockchain and Crypto, we have brought out powerful insights, which can be used to stay-to-data about the market developments. As the landscape evolves, the strategic integration of technology and data-driven decision-making becomes paramount for stakeholders navigating the dynamic realms of decentralised technologies.
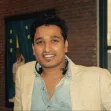
Was this writing helpful?
Tell us why.
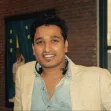
Related Articles
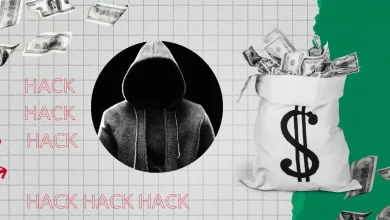
Crypto Hack Report Q1 2024: Trends, Losses, and Recovery Efforts
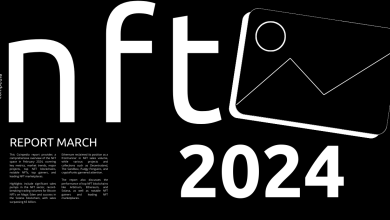
March 2024 NFT Report: Trends, Market Dynamics, and Future Projections
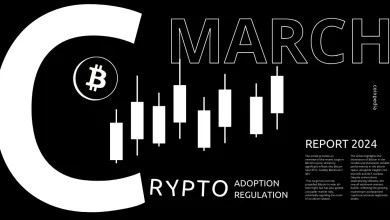
March 2024 Crypto Adoption Report: Global Trends, Top Nations, and Regulatory Insights
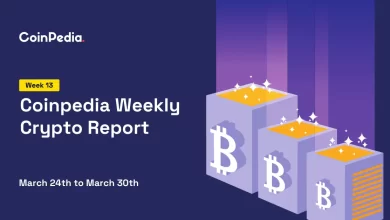
Top Crypto News Weekly: ETFs, Blockchain Developments, Altcoin Surges, and Bitcoin Trends
Login to coinpedia., or continue with, you do not have permission to login. please contact administrator for more details. (email: [email protected] ), create coinpedia account.
A Platform to over 1 million + Crypto and Blockchain enthusiasts in the world.
Full Name *
Connect wallet.
Click here to connect Wallet
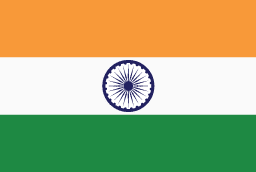
Referral ID
By creating account with us, you clarify you have read and accepted the Privacy policy and Terms & Conditions
Select a Country

- No related data found for - " ".
Verify your email
A code has been sent to . Enter it below to verify your email. The code is valid for Resend OTP

Registration Successful !
Hello ,Welcome to Coinpedia! Now, you can join contests, ace quizzes, read exclusive crypto insights, and unlock your potential in the cryptocurrency world with us.
AI Index Report
The AI Index Report tracks, collates, distills, and visualizes data related to artificial intelligence. Our mission is to provide unbiased, rigorously vetted, broadly sourced data in order for policymakers, researchers, executives, journalists, and the general public to develop a more thorough and nuanced understanding of the complex field of AI. The report aims to be the world’s most credible and authoritative source for data and insights about AI.
Subscribe to receive the 2024 report in your inbox!
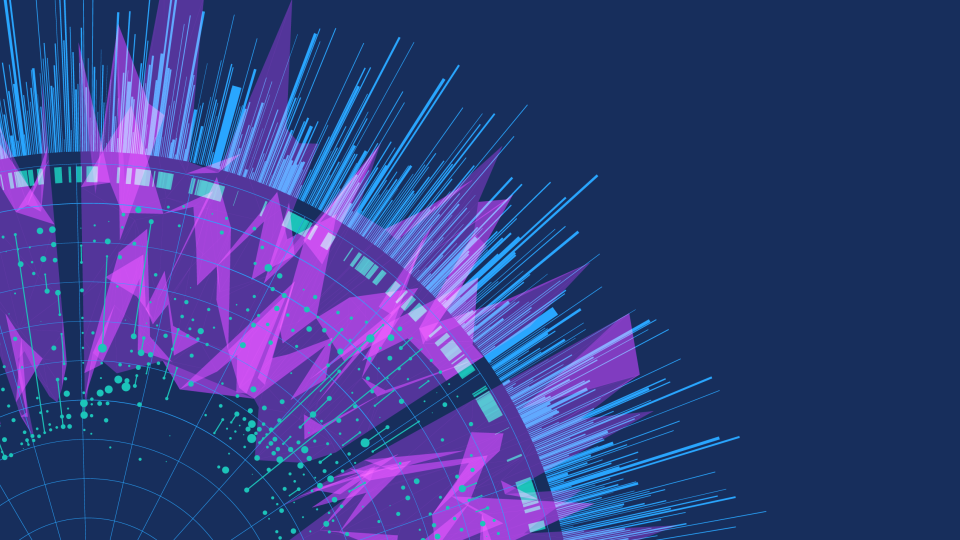
Coming Soon: 2024 AI Index Report!
The 2024 AI Index Report will be out April 15! Sign up for our mailing list to receive it in your inbox.
Steering Committee Co-Directors
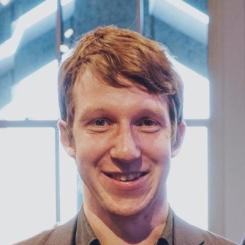
Ray Perrault
Steering committee members.
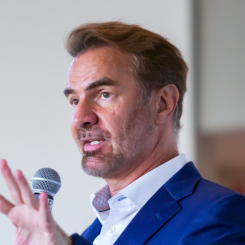
Erik Brynjolfsson
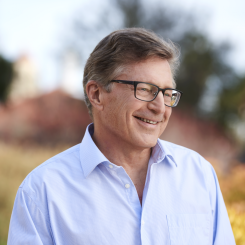
John Etchemendy
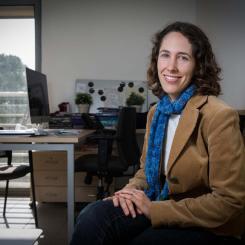
Katrina Ligett
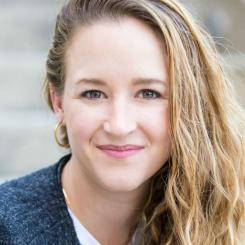
Terah Lyons
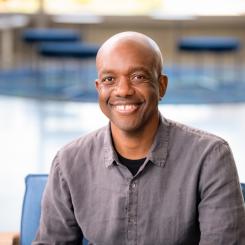
James Manyika
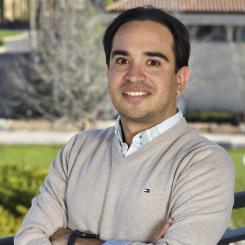
Juan Carlos Niebles
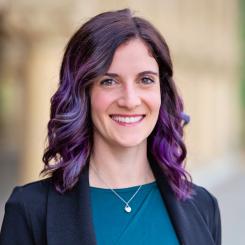
Vanessa Parli
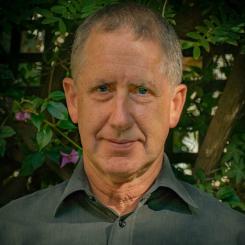
Yoav Shoham
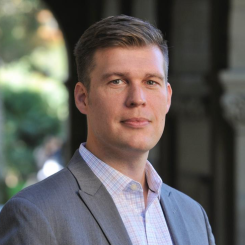
Russell Wald
Staff members.
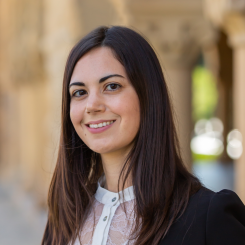
Loredana Fattorini
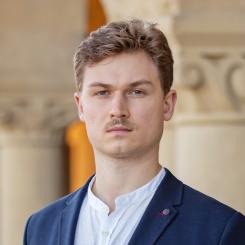
Nestor Maslej
Letter from the co-directors.
AI has moved into its era of deployment; throughout 2022 and the beginning of 2023, new large-scale AI models have been released every month. These models, such as ChatGPT, Stable Diffusion, Whisper, and DALL-E 2, are capable of an increasingly broad range of tasks, from text manipulation and analysis, to image generation, to unprecedentedly good speech recognition. These systems demonstrate capabilities in question answering, and the generation of text, image, and code unimagined a decade ago, and they outperform the state of the art on many benchmarks, old and new. However, they are prone to hallucination, routinely biased, and can be tricked into serving nefarious aims, highlighting the complicated ethical challenges associated with their deployment.
Although 2022 was the first year in a decade where private AI investment decreased, AI is still a topic of great interest to policymakers, industry leaders, researchers, and the public. Policymakers are talking about AI more than ever before. Industry leaders that have integrated AI into their businesses are seeing tangible cost and revenue benefits. The number of AI publications and collaborations continues to increase. And the public is forming sharper opinions about AI and which elements they like or dislike.
AI will continue to improve and, as such, become a greater part of all our lives. Given the increased presence of this technology and its potential for massive disruption, we should all begin thinking more critically about how exactly we want AI to be developed and deployed. We should also ask questions about who is deploying it—as our analysis shows, AI is increasingly defined by the actions of a small set of private sector actors, rather than a broader range of societal actors. This year’s AI Index paints a picture of where we are so far with AI, in order to highlight what might await us in the future.
- Jack Clark and Ray Perrault
Our Supporting Partners
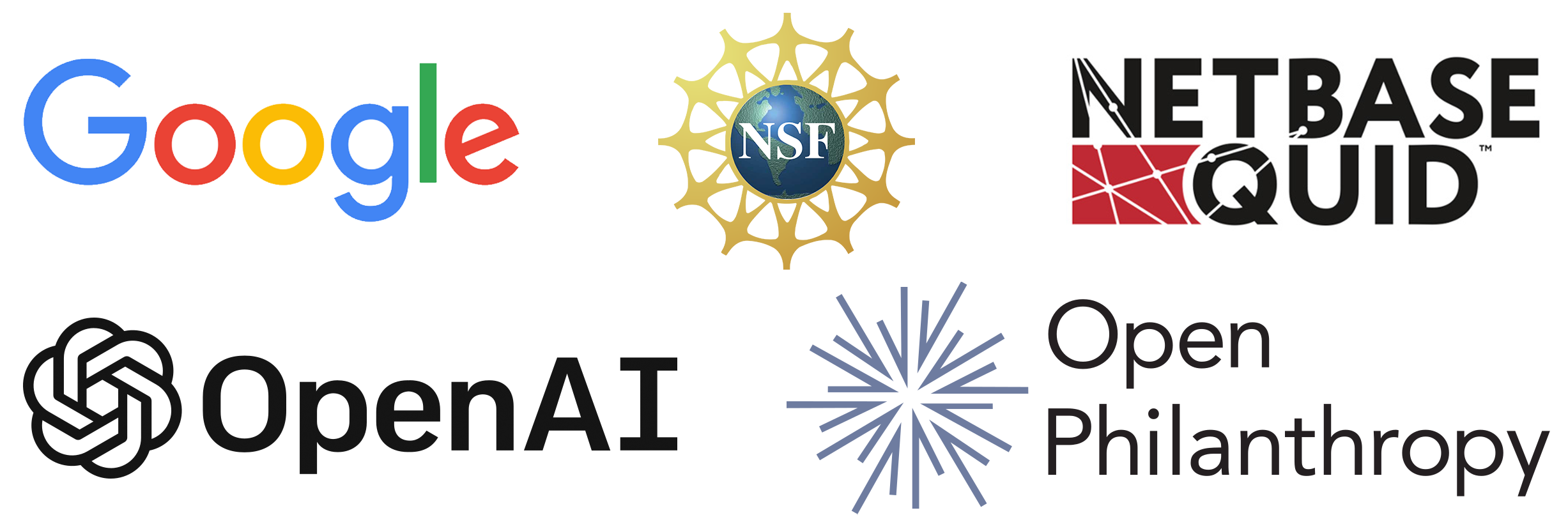
Analytics & Research Partners

Stay up to date on the AI Index by subscribing to the Stanford HAI newsletter.
An Analysis of Blockchain and GDPR under the Data Lifecycle Perspective
- Published: 29 August 2020
- Volume 26 , pages 266–276, ( 2021 )
Cite this article
- Gislaine Parra Freund 1 ,
- Priscila Basto Fagundes ORCID: orcid.org/0000-0002-9461-311X 1 &
- Douglas Dyllon Jeronimo de Macedo 1
1399 Accesses
16 Citations
1 Altmetric
Explore all metrics
The purpose of this article is to present an analysis of the privacy principles of personal data prescribed in the General Data Protection Regulation and the treatment of data in Blockchain technology in its primary version, guided by the phases of the Data LifeCycle. The Data Life Cycle presents the stages in which the data act in a given process and are related to each other, forming a chain of dependence between them. The General Data Protection Regulation, on the other hand, presents privacy principles that contemplate the necessary treatment for data at all stages of its life cycle. This analysis made it possible to identify the influence that the phases of the Data Lifecycle have on the adequacy of the principles of the General Data Protection Regulation and the treatment of the data performed on the Blockchain technology associated with the phases, presenting an approach to lead the process of adapting the technology to compliance with the legislation. In this context, some data treatment options were presented for the phases that presented gaps, making it possible to conclude that the result of this analysis can be used as a support tool to systematize the process of adapting to the law by organizations that use or intend to adopt Blockchain technology.
This is a preview of subscription content, log in via an institution to check access.
Access this article
Price includes VAT (Russian Federation)
Instant access to the full article PDF.
Rent this article via DeepDyve
Institutional subscriptions
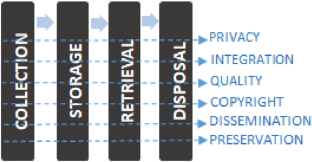
Similar content being viewed by others
Blockchain and Data Protection: An Evaluation of the Challenges and Solutions Mentioned by German Stakeholders
Blockchain and the GDPR: New Challenges for Privacy and Security
An Introduction to Blockchain Technology and Their Applications in the Actuality with a View of Its Security Aspects
GDPR (General Data Protection Regulation) (2016) Regulation (EU) 2016/679 of the European Parliament and of the Council. https://eur-lex.europa.eu/eli/reg/2016/679/oj. Accessed 24 April 2020
Collins R (2016) Blockchain: A New Architecture for Digital Content, EContent. http://www.econtentmag.com/Articles/Editorial/Commentary/Blockchain-A-New-Architecture-for-Digital-Content-114161.htm. Accessed 20 April 2020
Hernon P (1994) Information lifecycle: its place in the management of US government information resources. Gov Inf Q 11(2):143–170. https://doi.org/10.1016/0740-624X(94)90002-7
Article Google Scholar
Kreutz D, Malichevskyy O, Feitosa E, Cunha H, da Rosa RR, de Macedo DDJ (2016) A cyber-resilient architecture for critical security services. J Netw Comput Appl 63:173–189. https://doi.org/10.1016/j.jnca.2015.09.014
Wallauer J, von Wangenheim A, Andrade R, de Macedo DDJ (2008). A telemedicine network using secure techniques and intelligent user access control. 21st IEEE international symposium on computer-based medical systems, pp. 105-107. https://doi.org/10.1109/CBMS.2008.124
de Macedo DDJ, von Wangenheim A, Dantas MA (2015) A data storage approach for large-scale distributed medical systems. Ninth international conference on complex, intelligent, and software intensive systems. Pp. 486-490. https://doi.org/10.1109/CISIS.2015.88
Gomes EH, Dantas MA, de Macedo DDJ, Rolt CRD, Dias J, Foschini L (2018) An infrastructure model for smart cities based on big data. International Journal of Grid and Utility Computing 9(4):322–332. https://doi.org/10.1504/IJGUC.2018.095435
de Souza IA, Andrade R, von Wangenheim A, and Macedo DDJ (2014). Designing an information retrieval system for the STT/SC. 16th international conference on e-health networking, applications and services (Healthcom), pp. 500–505. https://doi.org/10.1109/HealthCom.2014.7001893
Sant’Ana RCG (2016) Data life cycle: A perspective from the Information Science. Informação & Informação 21(2):116–142. https://doi.org/10.5433/1981-8920.2016v21n2p116
Freund GP, Fagundes PB, Macedo DDJ (2020) identification of the relationships between the stages of the data lifecycle andthe principles of the Brazilian general data protection act. Lecture notes of the Institute for Computer Sciences, social informatics and telecommunications engineering. (Ed.): DIONE 2020, LNICST 319, pp. 1–10, 2020. https://doi.org/10.1007/978-3-030-50072-6_7 (in press)
Voigt P, Von dem Bussche A (2017) The EU general data protection regulation (GDPR): a practical guide, 1st edn. Springer International Publishing, Cham
Book Google Scholar
Hoofnagle CJ, Van der Sloot B, Borgesius FZ (2019) The European Union general data protection regulation: what it is and what it means. Information & Communications Technology Law 28(1):65–98. https://doi.org/10.1080/13600834.2019.1573501
Mercer S (2020) The limitations of European data protection as a model for global privacy regulation. AJIL Unbound 114:20–25. https://doi.org/10.1017/aju.2019.83
Ruparelia NB (2010) Software development lifecycle models. SIGSOFT Softw. Eng Notes 35(3):8–13. https://doi.org/10.1145/1764810.1764814
Nayak A, Dutta K (2017) Blockchain: the perfect data protection tool. International conference on intelligent computing and control (I2C2) pp. 1-3. https://doi.org/10.1109/I2C2.2017.8321932
Macrinici D, Cartofeanu C, Gao S (2018) Smart contract applications within Blockchain technology: a systematic mapping study. Telematics Inform 35(8):2337–2354. https://doi.org/10.1016/j.tele.2018.10.004
Lacity M (2018) Addressing key challenges to making Enterprise Blockchain applications a reality. MIS Q Exec 17(3):201–222 https://aisel.aisnet.org/misqe/vol17/iss3/3/ .
Google Scholar
Gupta V. A brief history of Blockchain. Harv Bus Rev https://hbr.org/2017/02/a-brief-history-of-blockchain . Accessed 20 April 2020
Bernabe JB, Canovas JL, Hernandez-Ramos JL, Moreno RT, Skarmeta A (2019) Privacy-preserving solutions for Blockchain: review and challenges. IEEE Access 7:164908–164940. https://doi.org/10.1109/ACCESS.2019.2950872
Suzuki S, Murai J (2017) Blockchain as an audit-able Communication Channel. Conference: 2017 IEEE 41st annual computer software and applications conference (COMPSAC), pp. 516-522. https://doi.org/10.1109/COMPSAC.2017.72
Christidis K, Devetsikiotis M (2016) Blockchains and smart contracts for the internet of things. IEEE Access 4:2292–2303. https://doi.org/10.1109/ACCESS.2016.2566339
Zheng Z, Xie S, Daí H, Chen X, Wang H (2017) An overview of Blockchain technology: architecture, consensus and future trends. 2017 IEEE international congress on big data, (BigData congress), pp. 557–564 https://ieeexplore.ieee.org/stamp/stamp.jsp?tp=&arnumber=8029379 . Accessed 16 April 2020
Bayle A, Koscina M, Manset D, Perez-Kempner O (2018) When Blockchain meets the right to be forgotten: technology versus law in the healthcare industry. 2018 IEEE/WIC/ACM International Conference on Web Intelligence (WI) pp 788–792 https://doi.org/10.1109/WI.2018.00133
Zemler F, Westner M (2019) Blockchain and GDPR: Application Scenarios and Compliance Requirements. Portland International Conference on Management of Engineering and Technology (PICMET), pp. 1–8. https://doi.org/10.23919/PICMET.2019.8893923
Rieger A, Guggenmos F, Locki J, Fridgen G, Urbach N (2019) Building a blockchain application that complies with the eu general data protection regulation. MIS Quarterly Executive 18(4):263–279. https://doi.org/10.17705/2msqe.00020
Millard C (2018) Blockchain and law: incompatible codes? Computer Law & Security Review 34(4):843–846. https://doi.org/10.1016/j.clsr.2018.06.006
Article MathSciNet Google Scholar
Moerel L (2018) Blockchain & data protection…and why they are not on a collision course. European review of private law 26(6 ): 825-851. http://www.kluwerlawonline.com/abstract.php?area=Journals&id=ERPL2018057
Powell RR, Connaway LS (2004) Basic research methods for librarians (4th ed.) Westport, CT: libraries unlimited
Download references
Acknowledgements
This research was partially supported by Coordenação de Aperfeiçoamento de Pessoal de Nivel Superior (CAPES) and the Santa Catarina Research Foundation (FAPESC) by grant Public Note FAPESC N° 03/2017.
Author information
Authors and affiliations.
Department of Information Science (CIN), Federal University of Santa Catarina (UFSC), Florianópolis, Santa Catarina, Brazil
Gislaine Parra Freund, Priscila Basto Fagundes & Douglas Dyllon Jeronimo de Macedo
You can also search for this author in PubMed Google Scholar
Corresponding author
Correspondence to Gislaine Parra Freund .
Additional information
Publisher’s note.
Springer Nature remains neutral with regard to jurisdictional claims in published maps and institutional affiliations.
Rights and permissions
Reprints and permissions
About this article
Freund, G.P., Fagundes, P.B. & de Macedo, D.D.J. An Analysis of Blockchain and GDPR under the Data Lifecycle Perspective. Mobile Netw Appl 26 , 266–276 (2021). https://doi.org/10.1007/s11036-020-01646-9
Download citation
Published : 29 August 2020
Issue Date : February 2021
DOI : https://doi.org/10.1007/s11036-020-01646-9
Share this article
Anyone you share the following link with will be able to read this content:
Sorry, a shareable link is not currently available for this article.
Provided by the Springer Nature SharedIt content-sharing initiative
- Data lifecycle
- General data protection regulation
- Data privacy
- Find a journal
- Publish with us
- Track your research
2024 Identity Fraud Study: Resolving the Shattered Identity Crisis
- Date: April 10, 2024
- Suzanne Sando
- Report Details: 48 pages, 27 graphics
- Research Topic(s):
- Fraud & Security
- Fraud Management
- PAID CONTENT
The financial landscape experienced meaningful innovation and expansion over the past few decades. The most notable acceleration in advancement has happened in just the past few years. Digital banking, especially in the form of mobile banking apps, is a must for financial institutions to stay relevant with on-the-go consumers. Brick-and-mortar stores have moved into the e-commerce space (with many businesses moving away from physical stores to online-only offerings). Online loan origination is transforming the auto and mortgage industries. The list is endless.
Whether it was the growing adoption of cryptocurrency and digital assets, real-time payments innovation, or frugal savers taking advantage of interest rate increases to employ strategic saving in 2023, consumers seemed to be taking advantage of forward economic momentum.
And criminals surely took advantage of this forward momentum. Fraud-related resolution hours skyrocketed in 2023. The average amount of time consumers spent in 2022 resolving issues stemming from identity fraud clocked in at six hours, but in 2023, fraud resolution hours rose steeply, jumping to a nearly 10-hour average, a major disruption for consumers and financial institutions alike.
Skyrocketing Resolution Hours Create Headaches for Consumers
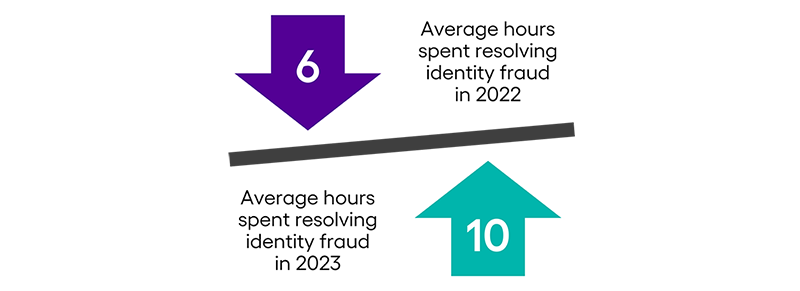
Traditional identity fraud losses amounted to nearly $23 billion in 2023, resulting in a 13% increase in overall losses for U.S. adult victims of identity fraud. And since Javelin began tracking financial losses attributable to scams in 2021, there has been a steady yet nearly imperceptible drop in financial loss. Scams orchestrated by criminals resulted in just over $20 billion in fraud losses to victims.
Javelin makes a distinction between traditional identity fraud and identity fraud scam losses to add perspective to the landscape of identity fraud and provide accurate historical information and relevant recommendations to financial institutions, fintechs, third-party fraud solutions providers, and even consumers. But it’s vital to remember that, to identity fraud victims, it doesn’t matter how the losses are analyzed and categorized.
What matters is how their fraud and scam encounter is managed by the organizations they trust and with which they choose to do business, how they are treated throughout the resolution process, and how they feel after suffering a financial loss and a breach of trust. This must always be top of mind for organizations as they work to improve their efforts to detect and prevent further damage from identity fraud.
2024 Identity Fraud Study Sponsors
The Javelin Strategy & Research 2024 Identity Fraud Study provides a comprehensive analysis of fraud trends in the context of a changing technological and payments landscape. Its goal is to inform consumers, financial institutions, and businesses about the most effective means of controlling identity fraud. The study began in 2003 and serves as the nation’s longest-running analysis of identity fraud, with more than 105,000 consumers surveyed. This study is independently produced by Javelin and made possible with support from the following sponsors:
Methodology
Survey data collection.
This ID fraud survey was conducted online among 5,000 U.S. adults over the age of 18; this sample is representative of the U.S. census demographics distribution. Data collection took place Oct. 23-Nov. 28, 2023. Data is weighted using 18-plus U.S. population benchmarks on age, gender, race/ethnicity, education, census region, and metropolitan status from the most current CPS targets. Due to rounding errors, the percentages on graphs may add up to 100% plus or minus 1%. To preserve the independence and objectivity of this annual report, the sponsors of this project were not involved in the tabulation, analysis, or reporting of final results.
Comparing Research Findings Across Organizations: Please Anticipate Natural Variances in Key Findings Javelin cautions readers to understand the context behind increases and decreases in key findings as they apply to the annual Identity Fraud Report, especially when comparing your own organization’s experiences or comparing research outcomes from other companies or agencies. It is impossible to compare identity fraud key findings across multiple sources and expect to see universal alignment. Key findings never line up across organizations due to how data is collected. Sample sizes (the number of consumers) also vary a great deal.
Learn More About This Report & Javelin
Related content, pig butchering scams: how banks can stop the slaughter.
Pig butchering is a devastating, multilayered, drawn-out investment scam that leaves victims with nothing. By implementing robust customer education, employing effective fraud dete...
Customer Contact Centers: Heroes in Cybercrime Remediation, Fraud Prevention
Criminals increasingly use cyberattacks and scams to target consumers, and FI call centers are often relied upon for victim assistance. The key will be FI customer-oriented contact...
2024 Authentication and Identity-Proofing Vendor Solutions Scorecard
Identity-proofing, a fundamental need of financial services companies, is a space in considerable flux. Fintech companies that cut their teeth as e-commerce payments platforms are ...
Make informed decisions in a digital financial world

Maternal and Infant Research Electronic Data Analysis (MIREDA): A protocol for creating a common data model for federated analysis of UK birth cohorts and the life course.
- Find this author on Google Scholar
- Find this author on PubMed
- Search for this author on this site
- ORCID record for Michael Seaborne
- For correspondence: [email protected]
- ORCID record for Hope Jones
- ORCID record for Neil Cockburn
- ORCID record for Stevo Durbaba
- ORCID record for Tom Giles
- ORCID record for Arturo Gonzalez-Izquierdo
- ORCID record for Amy Hough
- ORCID record for Dan Mason
- ORCID record for Armando Mendez-Villalon
- ORCID record for Carlos Sanchez-Soriano
- ORCID record for Chris Orton
- ORCID record for David Ford
- ORCID record for Phillip Quinlan
- ORCID record for Krish Nirantharakumar
- ORCID record for Lucilla Poston
- ORCID record for Rebecca Reynolds
- ORCID record for Gillian Santorelli
- ORCID record for Sinead Brophy
- Info/History
- Preview PDF
Introduction: Birth cohorts are valuable resources for studying early life, the determinants of health, disease, and development. They are essential for studying life course. Electronic cohorts are live, dynamic longitudinal cohorts using anonymised, routinely collected data. There is no selection bias through direct recruitment, but they are limited to health and administrative system data and may lack contextual information. The MIREDA (Maternal and Infant Research Electronic Data Analysis) partnership creates a UK-wide birth cohort by aligning existing electronic birth cohorts to have the same structure, content, and vocabularies, enabling UK-wide federated analyses. Objectives: 1) Create a core dynamic, live UK-wide electronic birth cohort with approximately 100,000 new births per year using a common data model (CDM). 2) Provide data linkage and automation for long-term follow up of births from MuM-PreDiCT and the Born-in initiatives of Bradford, Wales, Scotland, and South London for comparable analyses. Methods: We will establish core data content and collate linkable data. Use a suite of extraction, transformation, and load (ETL) tools will be used to transform the data for each birth cohort into the CDM. Transformed datasets will remain within each cohorts trusted research environment (TRE). Metadata will be uploaded for the public to the Health Data Research (HDRUK) Innovation Gateway. We will develop a single online data access request for researchers. A cohort profile will be developed for researchers to reference the resource. Ethics: Each cohort has approval from their TRE through compliance with their project application processes and information governance. Dissemination: We will engage with researchers in the field to promote our resource through partnership networking, publication, research collaborations, conferences, social media, and marketing communications strategies.
Competing Interest Statement
The authors have declared no competing interest.
Funding Statement
This work was supported by an MRC Partnership Grant [MR/X02055X/1], MatCHNet pump-priming [U20005/302873] and an MRC Programme Grant [MR/X009742/1].
Author Declarations
I confirm all relevant ethical guidelines have been followed, and any necessary IRB and/or ethics committee approvals have been obtained.
The details of the IRB/oversight body that provided approval or exemption for the research described are given below:
Access to data is granted according to the information governance requirements of each TRE. The Data Protection Act 2018 is not applicable to anonymised data and the OMOP CDM will be anonymised and provide aggregated data and statistics only. Each TRE has ethical approval for its operation and use, thus no additional ethical approval was required beyond the standard project approval by official channels.
I confirm that all necessary patient/participant consent has been obtained and the appropriate institutional forms have been archived, and that any patient/participant/sample identifiers included were not known to anyone (e.g., hospital staff, patients or participants themselves) outside the research group so cannot be used to identify individuals.
I understand that all clinical trials and any other prospective interventional studies must be registered with an ICMJE-approved registry, such as ClinicalTrials.gov. I confirm that any such study reported in the manuscript has been registered and the trial registration ID is provided (note: if posting a prospective study registered retrospectively, please provide a statement in the trial ID field explaining why the study was not registered in advance).
I have followed all appropriate research reporting guidelines, such as any relevant EQUATOR Network research reporting checklist(s) and other pertinent material, if applicable.
The formatting of the abstract on the website removed spaces between headings and text so I have amended to add punctuation to make it clearer. The paper itself is unchanged.
Data Availability
Data will be available upon reasonable request through the Health Data Research (HDRUK) Innovation Gateway.
View the discussion thread.
Thank you for your interest in spreading the word about medRxiv.
NOTE: Your email address is requested solely to identify you as the sender of this article.

Citation Manager Formats
- EndNote (tagged)
- EndNote 8 (xml)
- RefWorks Tagged
- Ref Manager
- Tweet Widget
- Facebook Like
- Google Plus One
Subject Area
- Public and Global Health
- Addiction Medicine (316)
- Allergy and Immunology (620)
- Anesthesia (160)
- Cardiovascular Medicine (2284)
- Dentistry and Oral Medicine (280)
- Dermatology (201)
- Emergency Medicine (370)
- Endocrinology (including Diabetes Mellitus and Metabolic Disease) (805)
- Epidemiology (11593)
- Forensic Medicine (10)
- Gastroenterology (681)
- Genetic and Genomic Medicine (3600)
- Geriatric Medicine (337)
- Health Economics (618)
- Health Informatics (2311)
- Health Policy (916)
- Health Systems and Quality Improvement (865)
- Hematology (335)
- HIV/AIDS (753)
- Infectious Diseases (except HIV/AIDS) (13170)
- Intensive Care and Critical Care Medicine (758)
- Medical Education (360)
- Medical Ethics (100)
- Nephrology (391)
- Neurology (3368)
- Nursing (191)
- Nutrition (508)
- Obstetrics and Gynecology (652)
- Occupational and Environmental Health (647)
- Oncology (1764)
- Ophthalmology (526)
- Orthopedics (210)
- Otolaryngology (284)
- Pain Medicine (223)
- Palliative Medicine (66)
- Pathology (441)
- Pediatrics (1008)
- Pharmacology and Therapeutics (422)
- Primary Care Research (407)
- Psychiatry and Clinical Psychology (3076)
- Public and Global Health (6004)
- Radiology and Imaging (1227)
- Rehabilitation Medicine and Physical Therapy (715)
- Respiratory Medicine (811)
- Rheumatology (367)
- Sexual and Reproductive Health (356)
- Sports Medicine (318)
- Surgery (390)
- Toxicology (50)
- Transplantation (171)
- Urology (142)
Office of the Vice President for Research
Four clas faculty researchers secure prestigious early career awards.
Continuing an upward trend of University of Iowa faculty securing prestigious early-career grants, four investigators from the Departments of Physics and Astronomy and Computer Science have been awarded notable grant awards to advance their careers.
DeRoo, Hoadley advance space instrumentation with Nancy Grace Roman Technology Fellowships in Astrophysics for Early Career Researchers
Casey DeRoo and Keri Hoadley , both assistant professors in the Department of Physics and Astronomy, each received a Nancy Grace Roman Technology Fellowship in Astrophysics for Early Career Researchers. The NASA fellowship provides each researcher with $500,000 over two years to support their research in space-based instrumentation.
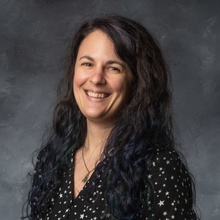
Hoadley’s research is two-pronged. She will design and ultimately prototype a mirror-based vacuum ultraviolet polarizer, which will allow researchers to access polarized light from space below 120-nanometer wavelength. Polarizing light at such a low wavelength is crucial to building optics for NASA’s future Habitable World Observatory (HWO), the agency’s next flagship astrophysics mission after the Nancy Grace Roman Space Telescope.
“Our vacuum ultraviolet polarizer project is meant to help set up our lab to propose to NASA for one or more follow-up technology programs, including adapting this polarizer for use in vacuum systems, duplicating it and measuring its efficiency to measure additional flavors of polarized UV light, quantifying the polarization effects introduced by UV optical components that may be used on HWO, and building an astronomical instrument to measure the polarization of UV from around massive stars and throughout star-forming regions,” said Hoadley.
In addition, Hoadley and her team will build a facility to align, calibrate, and integrate small space telescopes before flight, using a vacuum chamber and wavelengths of light typically only accessible in space, which could help the university win future small satellite and suborbital missions from NASA.
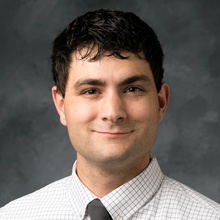
DeRoo will work to advance diffraction gratings made with electron beams that pattern structures on a nanometer scale. Like a prism, diffraction gratings spread out and direct light coming from stars and galaxies, allowing researchers to deduce things like the temperature, density, or composition of an astronomical object.
The fellowship will allow DeRoo to upgrade the university’s Raith
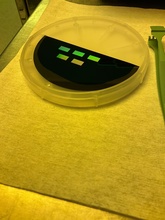
Voyager tool, a specialized fabrication tool hosted by OVPR’s Materials Analysis, Testing and Fabrication (MATFab) facility.
“These upgrades will let us perform algorithmic patterning, which uses computer code to quickly generate the patterns to be manufactured,” DeRoo said. “This is a major innovation that should enable us to make more complex grating shapes as well as make gratings more quickly.” DeRoo added that the enhancements mean his team may be able to make diffraction gratings that allow space instrument designs that are distinctly different from those launched to date.
“For faculty who develop space-based instruments, the Nancy Grace Roman Technology Fellowship is on par with the prestige of an NSF CAREER or Department of Energy Early Career award,” said Mary Hall Reno, professor and department chair. “Our track record with the program elevates our status as a destination university for astrophysics and space physics missions.”
Uppu pursues building blocks quantum computing with NSF CAREER Award
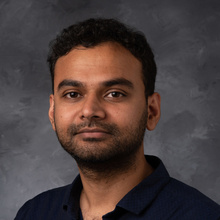
Ravitej Uppu, assistant professor in the Department of Physics and Astronomy, received a 5-year NSF CAREER award of $550,000 to conduct research aimed at amplifying the power of quantum computing and making its application more practical.
Uppu and his team will explore the properties of light-matter interactions at the level of a single photon interacting with a single molecule, enabling them to generate efficient and high-quality multiphoton entangled states of light. Multiphoton entangled states, in which photons become inextricably linked, are necessary for photons to serve as practical quantum interconnects, transmitting information between quantum computing units, akin to classical cluster computers.
“ In our pursuit of secure communication, exploiting quantum properties of light is the final frontier,” said Uppu. “However, unavoidable losses that occur in optical fiber links between users can easily nullify the secure link. Our research on multiphoton entangled states is a key building block for implementing ‘quantum repeaters’ that can overcome this challenge.”
Jiang tackles real-world data issues with NSF CAREER Award
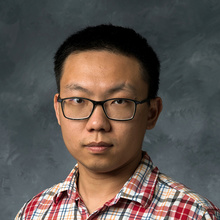
Peng Jiang, assistant professor in the Department of Computer Science, received an NSF CAREER Award that will provide $548,944 over five years to develop tools to support the use of sampling-based algorithms.
Sampling-based algorithms reduce computing costs by processing only a random selection of a dataset, which has made them increasingly popular, but the method still faces limited efficiency. Jiang will develop a suite of tools that simplify the implementation of sampling-based algorithms and improve their efficacy across wide range of computing and big data applications.
“ A simple example of a real-world application is subgraph matching,” Jiang said. “For example, one might be interested in finding a group of people with certain connections in a social network. The use of sampling-based algorithms can significantly accelerate this process.”
In addition to providing undergraduate students the opportunity to engage with this research, Jiang also plans for the project to enhance projects in computer science courses.
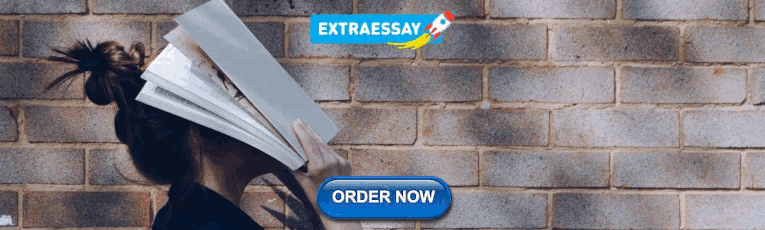
IMAGES
VIDEO
COMMENTS
Abstract. Blockchain technology is characterized by anti-counterfeiting, non-tampering and easy to implement smart contracts, and is known as a new technology that will lead to social change. Therefore, the study of data in blockchain has important theoretical and practical significance. The author proposes a three-layer model from the ...
of blockchain data is also in the exploratory stage, and there is a lack of research on blockchain data. Organizational structure of this paper: Section 1 briefly intro duced the basic concepts of ...
Data analytics is the process of analyzing massive data through an algorithmic or mechanical process to understand the correlations between patterns, trends & associations and draw conclusions ...
Additionally, the research topic of blockchain analysis Footnote 6 [1, 31], shows us that (old) new problems, such as fast data querying and management, arise from the volume of data generated by blockchain networks, where data is often churned in order to extract extra information from it. Some examples of applications are: (i) Detecting ...
Blockchain has been receiving growing attention from both academia and practices. This paper aims to investigate the research status of blockchain-related studies and to analyze the development and evolution of this latest hot area via bibliometric analysis. We selected and explored 2451 papers published between 2013 and 2019 from the Web of Science Core Collection database.
The architecture of the framework is shown in Fig. 1. The Data collector first gathers raw data on concerning blockchain networks and stores it in a cloud database. The raw data, including blocks, transactions, and events, is the core of the networks and can be reused in multiple analysis projects.
In this work, we systematically reviewed 76 blockchain data visualization. sources—14 visualization articles, 17 data analysis articles, and 45 online web-based tools. W e classified those ...
Data ranges from 2009-01-03 to 2021-02-10, and the data frequency is daily ( n = 4421). The timezone used in the data is UTC + 0. In addition to examining Bitcoin, we apply the same cohort ...
Blockchain technology has a great potential to radically change our socio-economical systems. Even if several applications are already leveraging its disruptive potentials, further research and development is required for several challenging aspects like scalability, efficiency, privacy and support of complex queries, both from a theoretical and applicative point of view.
Moreover, they listed current challenges and future development trends of blockchain technology. Based on data gathered from the Web of Science (WoS) database, Guo conducted a visual analysis on blockchain research including its research status and trends by using Citespace software. However, blockchain technology is developing with each new ...
Cryptocurrency based on blockchain technology has gradually become a choice for people to invest in, and several users have participated in the accumulation of massive transaction data. Complete transaction records in blockchains and the openness of data provide researchers with opportunities to mine and analyze data in blockchains. Network modeling and analysis of cryptocurrency transaction ...
data is an emerging area of research. While many graph analysis works on the publicly available Ethereum blockchain data have emerged, to the best of our knowledge, ours is the first in-depth survey of the literature that conducted graph analysis with the Ethereum blockchain data. We first introduce Ethereum's heterogeneous ecosystem
With the development of big data, people focus on data security and privacy, blockchain has been paid more and more attention. Compared to pre-2013, the number of blockchain literature publications has increased. Scholars from different research fields have deeply explored blockchain technology. In this paper, we collected blockchain literature data from 2013 to 2020, and then we conducted a ...
As an emerging technology enabling economic, social, and environmental sustainability, blockchain has drawn considerable attention from the academic community in management and economics. It is essential to clarify this field's research status, hotspots, and evolution trends. This study took 642 pieces of literature on blockchain in management and economics from the Web of Science (WoS) as ...
A critical analysis of current research and implementations will reveal remaining gaps and opportunities to realise this promising convergence. 3.4 Blockchain Technology: Key Principles and Characteristics. Blockchain is a distributed ledger technology (DLT) that allows for synchronised sharing of data across multiple nodes .
Cryptocurrency based on blockchain technology has gradually become a choice for people to invest in, and several users have participated in the accumulation of massive transaction data. Complete transaction records in blockchains and the openness of data provide researchers with opportunities to mine and analyze data in blockchains. Network modeling and analysis of cryptocurrency transaction ...
blockchain data. Therefore, the study of blockchain data has important theoretical and practical significance. At the same time, since the blockchain technology is still in the initial stage, the analysis of blockchain data is also in the exploratory stage, and there is a lack of research on blockchain data.
The First Institute of Resources and Environment Investigation of Henan Province, Zhengzhou 450000, China. * Correspondence: [email protected]. Abstract: Blockchain has the potential to ...
They identify the evolution of blockchain research and propose emerging topics for future research. Yu, ... (2012-2016), cryptocurrency and market efficiency (2017-2018), and technical analysis, big data, data privacy, and the connection between Bitcoin and financial markets (2019-2022).
This article takes the secure IoT-blockchain data of Industry 4.0 in the food sector as a research object. It proposes a hybrid model based on recurrent neural networks using ADL techniques. ... Recent Advances in blockchain and artificial intelligence integration: Feasibility analysis, research issues, applications, challenges, and future work ...
One research direction is to leverage machine learning techniques to enhance the selection and data processing of blockchain nodes, enabling more efficient communication and data storage. Furthermore, the application of blockchain technology and AI can be explored to achieve more intelligent network management and security mechanisms, thereby ...
In this week's comprehensive analysis of Web3, Blockchain and Crypto, we have brought out powerful insights, which can be used to stay-to-data about the market developments. As the landscape evolves, the strategic integration of technology and data-driven decision-making becomes paramount for stakeholders navigating the dynamic realms of ...
The research results showed that the efficient aspect of "how much the burden of admission management is reduced" should be considered as the most important factor in accepting Blockchain technology into the university admissions system. In this study, we explored the acceptance factors of Blockchain technology, a fourth industrial innovation technology, for efficient management of basic ...
AI Index Report. The AI Index Report tracks, collates, distills, and visualizes data related to artificial intelligence. Our mission is to provide unbiased, rigorously vetted, broadly sourced data in order for policymakers, researchers, executives, journalists, and the general public to develop a more thorough and nuanced understanding of the ...
The purpose of this article is to present an analysis of the privacy principles of personal data prescribed in the General Data Protection Regulation and the treatment of data in Blockchain technology in its primary version, guided by the phases of the Data LifeCycle. The Data Life Cycle presents the stages in which the data act in a given process and are related to each other, forming a chain ...
2024 Identity Fraud Study Sponsors. The Javelin Strategy & Research 2024 Identity Fraud Study provides a comprehensive analysis of fraud trends in the context of a changing technological and payments landscape. Its goal is to inform consumers, financial institutions, and businesses about the most effective means of controlling identity fraud.
Data analytics in the Blockchain is a new field, with only a few studies looking into the difficulties involving Blockchain data analytics. In this article, a Systematic analysis presents how data ...
The MIREDA (Maternal and Infant Research Electronic Data Analysis) partnership creates a UK-wide birth cohort by aligning existing electronic birth cohorts to have the same structure, content, and vocabularies, enabling UK-wide federated analyses. Objectives: 1) Create a core dynamic, live UK-wide electronic birth cohort with approximately ...
A test array of gratings printed with Raith Voyager tool. Photo courtesy of Casey DeRoo. Voyager tool, a specialized fabrication tool hosted by OVPR's Materials Analysis, Testing and Fabrication (MATFab) facility. "These upgrades will let us perform algorithmic patterning, which uses computer code to quickly generate the patterns to be manufactured," DeRoo said.
The global data center transformer market is expected to grow at a CAGR of 6.5% during the forecast period of 2024 to 2032. The data center transformer market is poised for continued expansion ...