- Skip to global NPS navigation
- Skip to this park navigation
- Skip to the main content
- Skip to this park information section
- Skip to the footer section
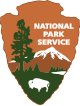
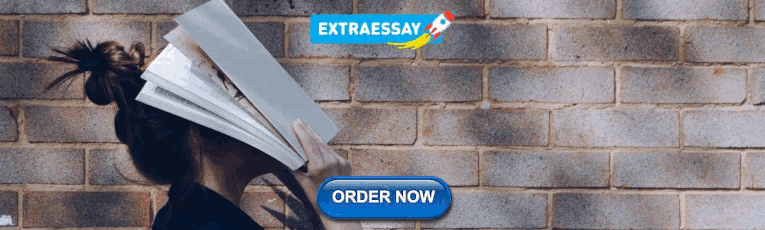
Exiting nps.gov
Alerts in effect, conducting research in yellowstone.
Last updated: April 5, 2024
Park footer
Contact info, mailing address:.
PO Box 168 Yellowstone National Park, WY 82190-0168
307-344-7381
Stay Connected
- Search Menu
- Advance articles
- Editor's Choice
- In Remembrance
- High-Impact Collection
- ASM History
- Author Guidelines
- Submission Site
- Open Access
- Why Publish with Us?
- About Journal of Mammalogy
- About the American Society of Mammalogists
- Editorial Board
- Advertising and Corporate Services
- Journals Career Network
- Self-Archiving Policy
- Dispatch Dates
- Journals on Oxford Academic
- Books on Oxford Academic

Article Contents
Brief history of wolves in yellowstone, materials and methods, acknowledgments, literature cited, wolves for yellowstone: dynamics in time and space.

- Article contents
- Figures & tables
- Supplementary Data
Mark S Boyce, Wolves for Yellowstone: dynamics in time and space, Journal of Mammalogy , Volume 99, Issue 5, 10 October 2018, Pages 1021–1031, https://doi.org/10.1093/jmammal/gyy115
- Permissions Icon Permissions
The reintroduction of gray wolves ( Canis lupus ) to Yellowstone National Park is the most celebrated ecological experiment in history. As predicted by population models, the rapid recovery of a wolf population caused both temporal and spatial variability in wolf–ungulate interactions that likewise generated temporal and spatial variation in the expression of trophic cascades. This has amplified spatial variation in vegetation in Yellowstone, particularly with willow ( Salix spp.) and cottonwood ( Populus spp.) in riparian areas, with associated changes in food webs. Increasing influences of grizzly bears ( Ursus arctos ), cougars ( Puma concolor ), and bison ( Bison bison ) are making what initially was predominantly an elk–wolf interaction into an increasingly complex system. Outside Yellowstone, however, humans have a dominant influence in western North America that overwhelms trophic cascades resulting in what appear to be bottom-up influences on community structure and function. Complex and unexpected ecosystem responses to wolf recovery in Yellowstone reinforce the value of national parks and other protected areas as ecological baseline reserves.
The reintroduction of gray wolves ( Canis lupus ) into Yellowstone National Park is a well-known ecological experiment, albeit with a lack of replication, randomization, and controls ( Kauffman et al. 2013 ; Ford and Goheen 2015 ). The trophic cascade that resulted in vegetation being released from herbivory caused by wolf predation on elk ( Cervus elaphus ) is purported to be among the most significant advances in conservation biology of this century ( Estes et al. 2011 ; Ripple and Beschta 2012 ). I began research on large mammals in the Greater Yellowstone Ecosystem in 1977, thus my involvement in the ecology of the area spans more than 40 years. I will draw from this long-term perspective to reflect on the ecology of wolf recovery in Yellowstone, framed largely on my research and that of my students and colleagues.
Although Yellowstone was designated as a national park in 1871, early park management did not provide the protection for wildlife that it does today ( Schullery 2003 ). In fact, the park deliberately eliminated wolves and cougars ( Puma concolor ) by 1926. Within a few years, numbers of elk in Yellowstone had increased substantially, and by the 1930s, park managers recognized that herbivory was altering vegetation, leading park managers to implement culls to contain the elk population ( Houston 1982 ; Barmore 2003 ). Public pressure prompted Senator Gale McGee from Wyoming to insist in 1967 that the National Park Service terminate the elk culls. Terminating the elk cull inside the park initiated a period when elk numbers were allowed to reach levels where their abundance would undergo “natural regulation” imposed by the interaction between herbivory and vegetation, i.e., extensive starvation of elk during severe winters ( Houston 1982 ; Merrill and Boyce 1991 ). Consistent with a review of management of wildlife in national parks ( Leopold et al. 1963 ), the park management committed to restoring wolves to limit elk abundance ( Weaver 1978 ; Despain et al. 1986 ). Eventually, in 1995, 14 wolves from Alberta were released in Yellowstone, supplemented by another 17 Canadian wolves in 1996 ( Smith and Ferguson 2012 ).
Modeling wolf recovery
During the 1980s, the National Park Service began accumulating research and public consultations required to reintroduce wolves. Because of previous research that we had done on population dynamics of elk in the Greater Yellowstone Ecosystem ( Sauer and Boyce 1983 ; Boyce 1989 ; Merrill and Boyce 1991 ), in 1988, John Varley (Director, Yellowstone Center for Resources) persuaded me to develop a model to anticipate probable consequences of wolf recovery to populations of wild ungulates in the park. I proceeded to build a computer simulation model of a predator–prey system focusing primarily on elk–wolf interactions but including alternative prey of bison ( Bison bison ), moose ( Alces alces ), and mule deer ( Odocoileus hemionus ) that were sufficiently abundant to potentially influence wolf recovery. Predation on multiple prey was modeled using a multispecies Holling Type III functional response ( Abrams and Allison 1982 ) to accommodate learning by wolves and density dependence ( Maynard Smith 1974 ).
Parameterization of the elk components of the model relied extensively on data compiled by Houston (1982) for elk populations in the park, and studies of the interaction between wolves and elk in Riding Mountain National Park ( Carbyn 1980 ). Data on predation by wolves on deer ( Odocoileus spp.) and moose were based on studies in Michigan, Minnesota, Canada, and Alaska ( Pimlott 1967 ; Mech 1970 ; Garton et al. 1990 ), whereas the only data for wolf predation on bison were from Wood Buffalo National Park ( Carbyn and Trottier 1987 ). John Varley later asked me to expand my original model focused on the Northern Range ( Boyce 1990 ) to include the Jackson elk herd and the population along the North Fork of the Shoshone River east of the park ( Boyce and Gaillard 1992 ).
Stabilizing features of the model included density dependence for ungulates presumed to be limited by their interaction with vegetation ( Houston 1982 ; Sauer and Boyce 1983 ; Merrill and Boyce 1991 ), the logistic functional response affording density-dependent predation at low densities, and density dependence for wolves because of territorial behavior ( Cubaynes et al. 2014 ). In addition, Montana Department of Fish, Wildlife, and Parks had published guidelines for hunter quotas that were density dependent with more licenses being issued when elk numbers on the Northern Range were high (table 2 in Varley and Boyce 2006 ).
Based on studies of elk ecology on Yellowstone’s Northern Range, key to the simulation models was stochastic variation in winter severity ( Houston 1982 ) and summer forage production ( Merrill and Boyce 1991 ). These climate-based perturbations to carrying capacity ensured realistic levels of variation among model predictions; indeed, it was nearly impossible to obtain the same simulation result twice. I programmed a user-friendly interface and we distributed the model on 5 ¼-inch floppy diskettes to high schools, universities, and government agency personnel, encouraging users to explore alternative decisions on how wolf recovery might be managed.
Monitoring dynamics
For the decade following the reintroduction of wolves, we participated in monitoring elk movements and abundance ( Fortin et al. 2005a , 2005b ; Mao et al. 2005 ). Elk were captured in winters of 2000–2002 using net-gunning and collared initially with VHF transmitters, and later with GPS telemetry then monitored by Julie Mao ( Mao et al. 2005 ) and Shaney Evans ( Evans et al. 2006 ). Elk on the Northern Range were monitored by the Northern Yellowstone Cooperative Wildlife Working Group in most years using aerial surveys for minimum estimates of abundance not corrected for variation in sightability. Wolves were monitored by park biologists led by Douglas W. Smith following the releases in 1995 and 1996, and beginning in 2004 wolves also were monitored using GPS telemetry.
We used resource selection functions ( Mao et al. 2005 ), step-selection functions ( Fortin et al. 2005a ), and state-based models ( Forester et al. 2007 ) to document patterns of habitat selection in the context of the distribution of wolves. In addition, we used resource selection functions to study the distribution of sites where wolves killed elk across the Northern Range and performed that analysis each year 1996–2005 to study vulnerability of elk to the spatial distribution of wolves and vegetation ( Kauffman et al. 2007 ). More recently, Kohl et al. (2018) used our telemetry data for elk and data for wolves collected and maintained by park biologists in an analysis of the daily variation in activity by elk and wolves to reveal that they interact in diel patterns of movement and habitat selection.
We revisited the simulation model 10 years after wolf reintroduction in the context of adaptive management ( Walters 1986 ), i.e., to evaluate how well we predicted system dynamics and to adjust the model based on new data ( Varley and Boyce 2006 ).
Vegetation responses
In the context of trophic cascades, I focus attention on woody plants, in particular aspen ( Populus tremuloides ), willow ( Salix spp.), and cottonwood ( P . trichocarpa and P. angustifolia and hybrids) that are heavily browsed by both elk and bison.
To study responses of willows to herbivory, 18 stands of willows across Yellowstone’s Northern Range were identified, and stems were sampled in 2001. Thin cross-sections of stems were used to measure growth by Salix boothii and S. geyeriana each year from 1989 to 2001. Variation in growth of willows was modeled relative to browsing, snow depth, elevation, and watershed area, and information-theoretic methods were used to select the best statistical models ( Beyer et al. 2007 ). During the winter of 2003–2004, 7 stands of S. geyeriana were monitored 7 times from December through March to document the seasonal pattern of browsing by elk ( Varley 2007 ).
Hydrology is an important factor for riparian vegetation. Marshall et al. (2013) performed 4 replicates of an experimental manipulation of browsing with fenced exclosures and dams to simulate the effect of beavers during 2001–2010. Height and biomass of individually marked willow stems were recorded annually for replicated controls, fenced exclosures, dammed sites, and dammed sites with exclosures. All research was approved by university animal care and conformed to ASM guidelines ( Sikes et al. 2011 ).
The wolf–ungulate model was presented to the United States Congress in support of proposed wolf recovery ( Boyce 1990 ; Boyce and Gaillard 1992 ). Model predictions depended on the management alternatives selected by the user, but always resulted in a reduction in the number of elk ( Boyce 1995 ). Yet, density-dependent mechanisms for ungulates and wolves ensured that the model predicted long-term persistence of wolves and all ungulates in the park. Indeed, in over 100,000 iterations of the model, all 4 ungulates and wolves persisted for 100 years ( Boyce 1995 ). Including additional subpopulations in the model also contributed to the stability of the system because fluctuations were not entirely synchronous; therefore, fluctuations in one subpopulation helped to offset those in others ( Boyce and Gaillard 1992 ; Boyce 1995 ). Consistently, wolf numbers throughout the entire park have been remarkably constant for the past decade at about 100 animals ( Fig. 1 ).

Census of wolves ( Canis lupus ) in Yellowstone National Park, 1995–2017, including a breakdown for the Northern Range and the interior portions of the park. Courtesy of Yellowstone National Park.
Simulations correctly predicted elk and wolf numbers observed during the first decade after wolf reintroduction ( Fig. 2 ). Early years after wolf reintroduction were dominated by a simple wolf–elk interaction, so the model was most likely to work during the initial period. However, despite these good predictions, we learned that several assumptions and components of the original model ( Boyce 1990 ; Boyce and Gaillard 1992 ) were incorrect. First, we underestimated the extent to which wolves specialized on elk as prey ( White and Garrott 2005 ), which required revision to the multispecies functional response. Another shortcoming was failure to recognize the strong age selectivity by wolves and hunters ( Fig. 3 ). Hunters preferentially kill bulls but when they kill cow elk they primarily kill prime-age females of high reproductive value ( Wright et al. 2006 ); cows learn to avoid hunters to the extent that individuals older than about 9–10 are essentially “bullet proof” ( Thurfjell et al. 2017 ). In contrast, wolves killed primarily young and old and as a consequence the per capita influence of hunters on elk populations was much greater than for those killed by wolves ( Vucetich et al. 2005 ; Wright et al. 2006 ). Progressively more elk also began wintering outside the park on winter ranges secured in part by the Rocky Mountain Elk Foundation, expanding the winter range for elk by about 40% ( Taper and Gogan 2002 ). These deficiencies in the original model structure were accommodated in a revised model that included elk population structure ( Varley and Boyce 2006 ).

Time series projections from the WOLF5 model ( Boyce and Gaillard 1992 ; Boyce 1995 ) compared with survey data for the Northern Range elk ( Cervus elaphus ) population, 1995–2004 (from Varley and Boyce 2006 ).

Age distributions of female elk ( Cervus elaphus ) killed by hunters versus wolves ( Canis lupus ), 1995–2001. Elk calves killed by wolves is 49% of the total. The line is the reproductive value for females of each age class (adapted from Wright et al. 2006 ).
Even after making these adjustments, however, during the second decade after wolf reintroduction, the number of elk averaged lower than predicted by the simulation model ( MacNulty et al. 2016 ). I believe that this is because bear ( Ursus arctos and U. americanus ) and cougar ( P. concolor ) predation was higher than we had anticipated. In particular, grizzly bears have been shown to be highly effective predators on elk calves ( Singer et al. 1997 ; Barber-Meyer et al. 2008 ), reducing recruitment by about 7 calves/100 cows ( Lukacs et al. 2018 ). Numbers of bears have not increased appreciably within the park subsequent to wolf reintroduction ( Boyce et al. 2001a , 2001b ; van Manen et al. 2016 ), but predation by bears on elk calves has increased ( Barber-Meyer et al. 2008 ; Griffin et al. 2011 ). Displacement of wolves by bears at kill sites also does not appear to be compensated by increased kill rate by wolves ( Tallian et al. 2017 ). Cougars are highly capable predators on elk and compete with wolves for prey, although they appear to be a subordinate predator ( Elbroch et al. 2015 ).
Another major change in the large mammal community in the past decade has been that the number of bison using the Northern Range has increased to over 4,000 animals, possibly due to reduced competition with elk. Wolves certainly kill bison ( Carbyn and Trottier 1987 ), but bison are more formidable and dangerous prey; thus, when elk are available they are preferred over bison. Consequently, change in the distribution of large herbivores in the park has been one of the most significant responses to wolf predation and this was not anticipated in our predator–prey models.
We found that that the interaction between elk and wolves was highly seasonal. During spring and summer, wolf activity was greatest near dens and rendezvous sites, and resource selection functions for elk showed that they avoided wolves by selecting higher elevations, less-open habitats, more-burned forest, and steeper slopes after wolf reintroduction. During winter, however, wolves appeared to track the distribution of elk so that elk were unable to select habitats where they might avoid wolves; consequently winter habitat selection by elk did not change much before and after wolf reintroduction ( Mao et al. 2005 ), although in the presence of wolves elk tended to move out of aspen stands toward open grasslands or conifer forests ( Fortin et al. 2005a ).
Consistent with these elk-based patterns of habitat selection, wolves showed highly seasonal patterns of habitat selection, but also a substantial year effect, meaning that habitat selection by wolves varied over time ( Uboni et al. 2015 ). Kill sites varied as the wolf population became established and began to saturate the Northern Range. During the first 5 years after wolf reintroduction, the distribution of elk kills could be attributed in part to the distribution of wolf packs, with remaining variation attributable to landscape and vegetation variables. However, as the Northern Range became saturated with wolf pack territories by year 2000, only landscape and vegetation variables contributed to the distribution of kill sites ( Kauffman et al. 2007 ), again amplifying how the predator–prey system changed over time.
Using our data on elk, Kohl et al. (2018) emphasized that not only was the interaction between wolves and elk highly seasonal, but it also varied within the day, as had been shown by Forester et al. (2007) . Wolf and elk activity are highly crepuscular ( Boyce et al. 2010 ), and the “landscape of fear” is very different during the day and night than during these dawn and dusk activity periods ( Kohl et al. 2018 ). This does not diminish the landscape of fear patterns as reflected by the influence of topography and vegetation on the distribution of wolf kills ( Kauffman et al. 2007 ), but informs a dynamic landscape of fear helping to interpret behavioral observations where elk appeared to ignore wolves during the day.
Foraging by herbivores, especially elk and bison, has substantial consequences to vegetation in Yellowstone National Park ( Houston 1982 ; National Research Council [NRC] 2002 ; Barmore 2003 ). Indeed, concern over excessive herbivory precipitated elk culling programs during 1930–1967 and ultimately contributed to wolf reintroduction. Willows, aspen, and cottonwoods were most severely browsed by elk and bison, and reduced browsing resulting from wolf recovery is the expression of a trophic cascade ( Ripple and Beschta 2012 ). Herbaceous grassland plants are important forage for elk and bison as well ( Merrill and Boyce 1991 ; Merrill et al. 1993 ), but these plants appear to be resilient to grazing ( Frank et al. 2016 ). Yet, grazing amplifies sensitivity of plants to site variation in moisture and nutrients ( Frank et al. 2017 ).
Browsing by elk prior to wolf reintroduction had suppressed growth of willows across Yellowstone’s Northern Range ( Beyer et al. 2007 ; Varley 2007 ). Cross-sectioned willow stem annuli that reflect plant growth for S. boothii and S. geyeriana show an overall increase in growth at 17 sites across the Northern Range during years following wolf reintroduction, presumably due to reduced browsing by elk ( Fig. 4 ). The best statistical models of willow growth included covariates for elevation, climatic conditions, and the presence of wolves ( Beyer et al. 2007 ). Most important, however, is the enormous variation among sites. This reflects substantial site variation relative to soil type, soil moisture, topography, and vegetation ( Tercek et al. 2010 ). Some sites were released from herbivory, whereas other sites continued to experience substantial herbivory, largely by elk ( Varley 2007 ). Note that these results in Fig. 4 from the analysis by Beyer et al. (2007) are through 2001, before the elk population had begun to decline as a numerical response to wolves, contrary to the interpretation by Creel and Christianson (2009) .

Growth by 2 species of willow, Salix boothii and S. geyeriana , before and after wolf ( Canis lupus ) reintroduction in 1995 measured by dendrochronology ( Beyer et al. 2007 ). Each line is a separate sampling site illustrating substantial variation in the response of willows to wolf recovery. Courtesy of H. Beyer.
Experimental manipulations of the water table and herbivory revealed clear results ( Fig. 5 ). Exclosures to eliminate herbivory enhanced willow growth above that in the control sites, and even stronger response was observed when dams were constructed to simulate beaver dams. The most exaggerated growth by willows was observed at plots behind dams inside exclosures ( Marshall et al. 2013 ).

Height response of willows ( Salix spp.) to 4 replicated treatments of herbivory using exclusion fences to eliminate grazing and dams to simulate beaver ( Castor canadensis ) dams. White lines are mean response with shading of 95% prediction intervals for controls in gray, unbrowsed in red, dammed in blue, and both grazing exclosure and dammed in purple. Dashed line at 200 cm is height required to escape herbivory (from Marshall et al. 2013 ).
I visited the Northern Range of Yellowstone in October 2012 at the nadir of elk abundance following wolf reintroduction. Site variation in willow recovery was highly evident. Figure 6 illustrates that willow growth on the Blacktail Plateau is vigorous at a site where willows were suppressed prior to wolf reintroduction. However, south of Mammoth, elk and bison continued to suppress willows, revealed by dramatic growth of willows inside an exclosure built in 1958, but no willows persisting outside the exclosure ( Fig. 7 ). I visited this site in 1985 with Don Despain (plant ecologist, Yellowstone Center for Resources), who pointed out that although willow were browsed to the ground every year, the density of stems outside the exclosure was the same as inside. By 2012, however, no willow sprouts could be found outside the exclosure although willows remained robust within the exclosure. Persistent heavy browsing appeared to have killed the plants.

Willow growth evident in the Blacktail Plateau of Yellowstone National Park, October 2012. A bedded bison ( Bison bison ) is in the middle of the photograph for scale. Photo by M. S. Boyce.

An exclosure 1 km south of Mammoth showing tall willow inside the fenced exclosure, constructed in 1958. I could find no evidence of willow stems outside the exclosure when this photo was taken in October 2012. Photo by M. S. Boyce.
Browsing had largely eliminated cottonwoods from Yellowstone with only a few old trees remaining ( NRC 2002 ; Beschta 2005 ). However, several flooding events from 1995–2008 resulted in widespread establishment and recruitment of over 1.3 million cottonwoods and an expectation that some of these will survive to form mature stands ( Rose and Cooper 2017 ). Occasional flooding is crucial to the establishment of riparian cottonwoods and release from herbivory is needed for recruitment. Thus, for cottonwoods as well as for willows, we have observed a trophic cascade, albeit associated with flooding conditions required for cottonwood establishment.
Most controversial, however, is the nature of a trophic cascade for aspen. Like cottonwoods, aspen require exceptional conditions to permit recruitment of young trees, especially from seed. Based on sampling of aspen conducted in August and September 1999, Ripple et al. (2001) suggested that due to elk avoiding wolves, aspen might escape herbivory. This could be facilitated by post-fire suckering of aspen ( Romme et al. 1995 ). Aspen were browsed so heavily after the fires of 1988, however, that few saplings were able to escape herbivory ( Forester et al. 2007 ; Romme et al. 2011 ), and high-predation-risk sites were browsed as well ( Kauffman et al. 2010 ).
Consequences of herbivory can be very different among seasons, e.g., elk are typically grazers but browsing is most likely to occur in winter ( Fig. 8 ). Thus, sampling aspen in late summer is not likely to show much site variation in browsing. The ability for a trophic cascade to be expressed also is easily overwhelmed by site and seasonal influences ( Marshall et al. 2013 ). Topography, soils, hydrology, and vegetation all interact to create variation among sites that influences both prey and predator ( Bilyeu et al. 2008 ). Recently, with reduced elk browsing, aspen appear to be recovering in a few stands, especially in eastern portions of the Northern Range ( Painter et al. 2015 ), but not on a broad scale across the Northern Range ( Kimble et al. 2011 ; Kauffman et al. 2013 ). Again, the outcome is high spatial variability caused by site characteristics and elk herbivory.

Accumulated browsing by elk ( Cervus elaphus ) on willows at 7 stands on the Northern Range, 2003–2004 (from Varley 2007 ). Patterns of browse removal are indicated by symbols as: early pattern (▲), abrupt pattern (◊), and gradual pattern (● ).
Several topics regarding wolves in Yellowstone have attracted debate and differences of interpretation, mostly surrounding trophic cascades ( Terborgh and Estes 2010 ). During the first few years after wolf reintroduction, wolves were few and elk still were abundant, leading Beyer et al. (2007) to conclude that behavioral responses released selected willow stands from herbivory. Creel and Christianson (2009) failed to recognize that no decline in elk abundance had occurred during the period studied by Beyer et al. (2007) and incorrectly suggested that the willow response could have been a numerical response. Likewise, during the first 5 years after wolf reintroduction, the distribution of wolf home ranges contributed to the distribution of elk kills on the Northern Range ( Kauffman et al. 2007 ). But after that initial period, the landscape became saturated with wolf home ranges and we found that the distribution of wolf-killed elk was related to topography and vegetation ( Kauffman et al. 2007 ), e.g., elk are easier prey in riparian areas ( Bergman et al. 2006 ). The upshot is that both behavioral and numerical responses have been documented in Yellowstone and these have varied in space and time. I believe that the consensus will be that numerical declines in elk have contributed more to trophic cascades than has behavioral avoidance of wolves, yet behavioral responses clearly exist ( Kohl et al. 2018 ). As vegetation has recovered at some sites, we are now witnessing complex interactions between predation risk and attraction to forage ( Gallagher et al. 2017 ). Clearly, habitat selection by wolves in Yellowstone has varied over time ( Kauffman et al. 2007 ; Uboni et al. 2015 ; Kohl et al. 2018 ). Likewise, habitat selection by elk varies seasonally ( Mao et al. 2005 ) and differs among scales ( Boyce et al. 2003 ). Indeed, this heterogeneity in space and time is a hallmark of the expression of a trophic cascade.
My simulation models worked well during the first decade after wolf reintroduction, when the system was dominated by the simple predator–prey interaction between wolves and elk. Density dependence for wolves was debated, and my earlier models were questioned for assuming density dependence in wolves based on their well-known territorial behavior. Yet, this assumption clearly was supported after wolf populations had stabilized and intraspecific interactions were shown to be the most common cause of mortality among wolves ( Cubaynes et al. 2014 ). My assumption of a Type III (logistic) functional response will always be challenging to verify statistically because at low densities we seldom have the sample sizes to show the convex portions of the logistic curve ( Marshal and Boutin 1999 ). The logistic functional response is an important assumption because it contributes to the stability of the system and is highly likely to occur in mammalian carnivores where learning is a mechanism behind the form of the functional response ( Maynard Smith 1974 ; Boyce 2005 ).
Responses of vegetation to herbivory show remarkable spatial variation. The willow story appears reasonably clear: some drainages began to support good willow growth and populations of willows and songbirds recovered ( Beschta and Ripple 2016 ). However, other sites continue to receive heavy browsing even at reduced elk densities, and might require many decades to recover from 70 years of heavy browsing by elk and loss of beavers ( Hobbs and Cooper 2013 ). Likewise, cottonwoods are recovering not only due to release from elk but largely in response to perturbations created by occasional flooding events ( Rose and Cooper 2017 ); again, this is creating a highly heterogeneous riparian landscape in the park.
The dynamics of aspen and elk continue to perplex us. Beetle (1979) and Gruell (1979) noted that declining aspen could be attributed to heavy browsing by elk as well as an absence of fire. Small fires were ineffective because resprouting aspen attracted heavy herbivory, preventing recovery ( Bartos and Mueggler 1979 ). So, we presumed that what was needed were large fires so that aspen had opportunity to escape elk herbivory. The extensive fires of 1988 gave us the presumed remedy, but elk numbers were so high that aspen could not escape herbivory even though there was extensive resprouting and aspen seed establishment ( Romme et al. 2011 ). Subsequent to wolf recovery, we observed that in the presence of wolves, elk tended to move away from aspen stands toward either open grasslands or coniferous forests ( Fortin et al. 2005a ). Yet, Kauffman et al. (2010) found that aspen stands were still suffering heavy elk browsing even in high-predation-risk sites. I believe that there are 3 probable explanations: 1) our radiocollars were set to a 5-h fix schedule and with such a long period between fixes, we could not obtain good resolution in a step-selection function ( Fortin et al. 2005a ); 2) interactions between wolves and elk are highly dynamic in space and time; and 3) we treated all aspen stands as equal in the analysis, whereas in reality there is substantial variation in the perceived threat of wolf approach among stands because of variation in cover and topography. Nevertheless, Painter et al. (2015) present evidence that some stands of aspen in Yellowstone are now recovering, but there is not convincing evidence that this is driven by a behaviorally mediated response by elk to wolves ( Kauffman et al. 2013 ). Clearly, resolution the dynamics of herbivory and trophic cascades in aspen warrants continuing study.
Although the demonstration that wolves in Yellowstone National Park have shaped a trophic cascade is fascinating ecology, it is not clear that trophic cascades will occur in other areas. We also cannot be confident that these observations have conservation implications for wolf management and ecosystem restoration elsewhere ( Estes et al. 2011 ; Ripple and Beschta 2012 ; Wolf and Ripple 2018 ). We need to be cautious about overextending the ecological results from wolf reintroduction in Yellowstone to applications outside parks, where livestock management ( Morehouse and Boyce 2011 ) and hunting have a major influence on ecosystem structure and function ( Mech 2012 ; Muhly et al. 2013 ).
A principle emerging from wolf recovery in Yellowstone is that trophic cascades have caused increased spatial and temporal heterogeneity. This is evident in the large spatial and temporal variation in growth of willows ( Beyer et al. 2007 ; Varley 2007 ; Marshall et al. 2013 ), aspen ( Kimble et al. 2011 ; Brodie et al. 2012 ; Painter et al. 2015 ), and cottonwoods ( Rose and Cooper 2017 ). Spatial and temporal variation in predation risk means that the landscape of fear creates an additional source of variation ( Abrams 2000 ). Movement ecology demonstrates how both predators and prey interact in a landscape driven by spatial variation in both energy resources and risk ( Fortin et al. 2005a , 2005b ; Harvey and Fortin 2013 ; Gallagher et al. 2017 ). For example, vegetation resources attract elk to riparian habitats and aspen stands, but these can be risky places because wolves are able to approach and kill prey in these areas ( Fortin et al. 2005a ; Bergman et al. 2006 ; Beyer 2006 ; Ripple and Beschta 2012 ). In addition, site variation in topography and hydrology create variation in soil moisture that has a large influence on growth of vegetation and thereby the attractiveness of sites to herbivores ( Fortin et al. 2005b ; Tercek et al. 2010 ; Brodie et al. 2012 ; Marshall et al. 2013 ; Frank et al. 2017 ; Raynor et al. 2017 ).
The interplay between predator–prey interactions and spatial heterogeneity includes important connections to scale ( Boyce et al. 2003 , 2017 ). Classic studies of predator–prey interactions showed how spatial heterogeneity can stabilize population dynamics and enhance persistence ( Huffaker 1958 ), as we observed when modeling multiple wolf–ungulate populations in the Greater Yellowstone ( Boyce and Gaillard 1992 ). Yet, at finer scales in Yellowstone, we have seen trophic-level interactions amplifying spatial heterogeneity through a trophic cascade. Movement among foraging patches might constitute a “shell game” by bison ( Harvey and Fortin 2013 ) and elk ( Boyce et al. 2003 ; Seidel and Boyce 2016 ) to avoid wolves, another mechanism amplifying spatial and temporal variation in herbivory. The Huffaker effect applies to wolf–ungulate systems at large scales, where some subpopulations might wink in and out (e.g., Isle Royale and Michipicoten Island in Lake Superior), whereas at finer scales, trophic cascades amplify spatial variation in predation risk and consequent herbivory. Indeed, spatial heterogeneity arising from trophic cascades at small scales could be what stabilizes the system at the larger population scale.
National parks and protected areas serve a crucial function as ecological baseline reserves from which we can evaluate how humans are affecting nature ( Sinclair 1983 ; Boyce 1991 , 1992 , 1998 ). The National Park Service has adopted ecological process management, minimizing interference when possible, allowing predation, herbivory, fire, and flooding to shape the ecosystem ( White et al. 2013 ), and this policy allowed wolf recovery with the fascinating dynamics that have emerged. The most immediate threat to this park policy is the increasing bison population that has precipitated political pressure to limit their abundance for fear that heavy grazing and browsing by bison might “damage” vegetation. We do not know how bison will affect Yellowstone, but surely we will learn a great deal more if we allow the bison population to take its course rather than intervening in a fashion that will be arbitrary to the underlying ecological system. Whatever influence we can have as scientists, we must insist that the National Park Service maintain its policy of ecological process management for their Crown Jewell that is Yellowstone National Park.
I am honored to have been selected by the American Society of Mammalogists for the C. Hart Merriam Award. I thank my students for field study and data analysis including H. Beyer, D. Fortin, J. M. Gaillard, C. J. Johnson, J. S. Mao, A. T. Morehouse, J. Sauer, and N. Varley. I am indebted to colleagues for insights and collaboration: J. Fryxell, D. Houston, M. Haroldson, N. T. Hobbs, M. J. Kauffman, D. R. McNulty, L. D. Mech, E. H. Merrill, B. Patterson, J. Peek, G. Plumb, P. Turchin, M. G. Turner, R. O. Peterson, A. R. E. Sinclair, D. W. Smith, J. Varley, and P. J. White. Thanks to J. Mao, D. Fortin, N. Varley, and E. Heske for helpful review comments on the manuscript. Funding has been provided by the Campfire Conservation Fund, National Park Service, National Science Foundation (grant #0078130), Alberta Conservation Association, Natural Sciences and Engineering Research Council of Canada (grant #364323-07), Safari Club International, University of Wyoming, and University of Alberta.
Abrams , P. A . 2000 . The impact of habitat selection on the spatial heterogeneity of resources in varying environments . Ecology 81 : 2902 – 2913 .
Google Scholar
Abrams , P. A. , and T. B. Allison . 1982 . Complexity, stability, and functional response . American Naturalist 119 : 240 – 249 .
Barber-Meyer , S. M. , L. D. Mech , and P. J. White . 2008 . Elk calf survival and mortality following wolf restoration to Yellowstone National Park . Wildlife Monographs 169 : 1 – 30 .
Barmore , W. J . 2003 . Ecology of ungulates and their winter range in northern Yellowstone National Park: research and synthesis, 1962–1970 . Yellowstone Center for Resources , Mammoth, Wyoming .
Google Preview
Bartos , D. L. , and W. F. Mueggler . 1979 . Influence of fire on vegetation production in the aspen ecosystem in western Wyoming . Pp. 75 – 79 in North American elk: ecology, behavior and management (M. S. Boyce and L. D. Hayden-Wing , eds.). University of Wyoming , Laramie .
Beetle , A. A . 1979 . Jackson Hole elk herd: a summary after 25 years of study . Pp. 259 – 262 in North American elk: ecology, behavior and management (M. S. Boyce and L. D. Hayden-Wing , eds.). University of Wyoming , Laramie .
Bergman , E. J. , R. A. Garrott , S. Creel , J. J. Borkowski , R. Jaffe , and F. G. R. Watson . 2006 . Assessment of prey vulnerability through analysis of wolf movements and kill sites . Ecological Applications 16 : 273 – 284 .
Beschta , R. L . 2005 . Reduced cottonwood recruitment following extirpation of wolves in Yellowstone’s Northern Range . Ecology 86 : 391 – 403 .
Beschta , R. L. , and W. J. Ripple . 2016 . Riparian vegetation recovery in Yellowstone: the first two decades after wolf reintroduction . Biological Conservation 198 : 93 – 103 .
Beyer , H. L . 2006 . Wolves, elk and willow on Yellowstone’s National Park’s Northern Range . M.Sc. thesis, University of Alberta , Edmonton, Alberta, Canada .
Beyer , H. L. , E. H. Merrill , N. Varley , and M. S. Boyce . 2007 . Willow on Yellowstone’s Northern Range: evidence for a trophic cascade ? Ecological Applications 17 : 1563 – 1571 .
Bilyeu , D. M. , D. J. Cooper , and N. T. Hobbs . 2008 . Water tables constrain height recovery of willow on Yellowstone’s Northern Range . Ecological Applications 18 : 80 – 92 .
Brodie , J. , E. Post , F. Watson , and J. Berger . 2012 . Climate change intensification of herbivore impacts on tree recruitment . Proceedings of the Royal Society B 279 : 1366 – 1370 .
Boyce , M. S . 1989 . The Jackson elk herd: intensive wildlife management in North America . Cambridge University Press , Cambridge, United Kingdom .
Boyce , M. S . 1990 . Wolf recovery for Yellowstone National Park: a simulation model . Pp. 3–5 to 3 – 58 in Wolves for Yellowstone? A report to the United States Congress, vol. 2. Research and analysis (J. D. Varley and S. H. Fritts, eds.) . National Park Service, Yellowstone National Park , Mammoth, Wyoming .
Boyce , M. S . 1991 . Natural regulation or the control of nature . Pp. 183 – 208 in The greater Yellowstone ecosystem: redefining America’s wilderness heritage (R. B. Keiter and M. S. Boyce , eds.). Yale University Press , New Haven, Connecticut .
Boyce , M. S . 1992 . Intervention versus natural regulation philosophies for managing wildlife in national parks . Oecologia Montana 1 : 49 – 50 .
Boyce , M. S . 1995 . Anticipating consequences of wolves in Yellowstone: model validation . Pp. 199 – 209 in Ecology and conservation of wolves in a changing world (L. Carbyn , S. Fritts , and D. Seip , eds.). Canadian Circumpolar Institute , Edmonton, Alberta, Canada .
Boyce , M. S . 1998 . Ecological-process management and ungulates: Yellowstone’s conservation paradigm . Wildlife Society Bulletin 26 : 391 – 398 .
Boyce , M. S . 2005 . Wolves are consummate predators . Quarterly Review of Biology 80 : 87 – 92 .
Boyce , M. S. , B. M. Blanchard , R. R. Knight , and C. Servheen . 2001a . Population viability for grizzly bears: a critical review . International Association for Bear Research and Management, Monograph Series No. 4 .
Boyce , M. S. , and J.-M. Gaillard . 1992 . Wolves in Yellowstone, Jackson Hole, and the North Fork of the Shoshone River: simulating ungulate consequences of wolf recovery . Pp. 4/71 – 4/111 in Wolves for Yellowstone? A report to the United States Congress, vol. 4. Research and analysis (J. D. Varley and W. G. Brewster , eds.). National Park Service, Yellowstone National Park , Mammoth, Wyoming .
Boyce , M. S. , D. I. MacKenzie , B. F. J. Manly , M. Haroldson , and D. Moody . 2001b . Negative binomial models for abundance estimation of multiple closed populations . Journal of Wildlife Management 65 : 498 – 509 .
Boyce , M. S. , C. Mallory , A. Morehouse , C. Prokopenko , M. Scrafford , and C. Warbington . 2017 . Defining landscapes and scales to model landscape–organism interactions . Current Landscape Ecology Reports 2 : 89 – 95 .
Boyce , M. S. , et al. 2003 . Scale and heterogeneity in habitat selection by elk in Yellowstone National Park . Écoscience 10 : 421 – 431 .
Boyce , M. S. , et al. 2010 . Temporal autocorrelation functions for movement rates from global positioning system radiotelemetry data . Philosophical Transactions of the Royal Society B 365 : 2213 – 2219 .
Carbyn , L. N . 1980 . Ecology and management of wolves in Riding Mountain National Park, Manitoba . Canadian Wildlife Service , Edmonton, Alberta, Canada .
Carbyn , L. N. , and T. Trottier . 1987 . Responses of bison on their calving grounds to predation by wolves in Wood Buffalo National Park . Canadian Journal of Zoology 65 : 2072 – 2078 .
Creel , S. , and D. Christianson . 2009 . Wolf presence and increased willow consumption by Yellowstone elk: implications for trophic cascades . Ecology 90 : 2454 – 2466 .
Cubaynes , S. , D. R. MacNulty , D. R. Stahler , K. A. Quimby , D. W. Smith , and T. Coulson . 2014 . Density-dependent intraspecific aggression regulates survival in northern Yellowstone wolves ( Canis lupus ) . Journal of Animal Ecology 83 : 1344 – 1356 .
Despain , D. , D. Houston , M. Meagher , and P. Schullery . 1986 . Wildlife in transition: man and nature on Yellowstone’s Northern Range . Roberts Rinehart , Boulder, Colorado .
Elbroch , L. M. , P. E. Lendrum , J. Newby , H. Quigley , and D. J. Thompson . 2015 . Recolonizing wolves influence the realized niche of resident cougars . Zoology Studies 54 : 41 .
Estes , J. A. , et al. 2011 . Trophic downgrading of planet earth . Science 333 : 301 – 306 .
Evans , S. , L. D. Mech , P. J. White , and G. A. Sergeant . 2006 . Survival of adult female elk in Yellowstone following wolf restoration . Journal of Wildlife Management 70 : 1372 – 1378 .
Ford , A. T. , and J. R. Goheen . 2015 . Trophic cascades by large carnivores: a case for strong inference and mechanism . Trends in Ecology and Evolution 30 : 725 – 735 .
Forester , J. D. , et al. 2007 . State-space models link elk movement to landscape characteristics in Yellowstone National Park . Ecological Monographs 77 : 285 – 299 .
Fortin , D. , H. Beyer , M. S. Boyce , D. W. Smith , and J. S. Mao . 2005a . Wolves influence elk movements: behavior shapes a trophic cascade in Yellowstone National Park . Ecology 86 : 1320 – 1330 .
Fortin , D. , J. M. Morales , and M. S. Boyce . 2005b . Elk winter foraging at fine scales in Yellowstone National Park . Oecologia 145 : 335 – 343 .
Frank , D. A. , R. L. Wallen , E. W. Hamilton III , P. J. White , and J. D. Fridley . 2017 . Manipulating the system: how large herbivores control bottom-up regulation of grasslands . Journal of Ecology 106 : 434 – 443 .
Frank , D. A. , R. L. Wallen , and P. J. White . 2016 . Ungulate control of grassland production: grazing intensity and ungulate species composition in Yellowstone Park . Ecosphere 7 : e01603 .
Gallagher , A. J. , S. Creel , R. P. Wilson , and S. J. Cooke . 2017 . Energy landscapes and the landscape of fear . Trends in Ecology & Evolution 32 : 88 – 96 .
Garton , E. O. , R. L. Crabtree , B. B. Ackerman , and G. Wright . 1990 . The potential impact of a reintroduced wolf population on the northern Yellowstone elk herd . Pp. 3 – 59 to 3 – 91 in Wolves for Yellowstone? A report to the United States Congress, vol. 2. Research and analysis (J. D. Varley and S. H. Fritts , eds.). National Park Service, Yellowstone National Park , Mammoth, Wyoming .
Griffin , K. A. , et al. 2011 . Neonatal mortality of elk driven by climate, predator phenology and predator community composition . Journal of Animal Ecology 80 : 1246 – 1257 .
Gruell , G. E . 1979 . Wildlife habitat investigations and management implications on the Bridger-Teton National Forest . Pp. 63 – 74 in North American elk: ecology, behavior and management (M. S. Boyce and L. D. Hayden-Wing , eds.). University of Wyoming , Laramie .
Harvey , L. , and D. Fortin . 2013 . Spatial heterogeneity in the strength of plant-herbivore interactions under predation risk: the tale of bison foraging in wolf country . PLoS ONE 8 : e73324 .
Hobbs , N. T. , and D. J. Cooper . 2013 . Have wolves restored riparian willows in northern Yellowstone ? Pp. 179 – 194 in Yellowstone’s wildlife in transition (P. J. White , R. A. Garrott , and G. E. Plumb , eds.). Harvard University Press , Cambridge, Massachusetts .
Houston , D. B . 1982 . The northern Yellowstone elk: ecology and management . Macmillan , New York .
Huffaker , C. B . 1958 . Experimental studies on predation: dispersion factors and predator-prey oscillations . Hilgardia 27 : 795 – 835 .
Kauffman , M. J. , J. F. Brodie , and E. S. Jules . 2010 . Are wolves saving Yellowstone’s aspen? A landscape-level test of a behaviorally mediated trophic cascade . Ecology 91 : 2742 – 2755 .
Kauffman , M. J. , J. F. Brodie , and E. S. Jules . 2013 . Are wolves saving Yellowstone’s aspen? A landscape-level test of a behaviorally mediated trophic cascade: reply . Ecology 94 : 1425 – 1431 .
Kauffman , M. J. , N. Varley , D. W. Smith , D. R. Stahler , D. R. MacNulty , and M. S. Boyce . 2007 . Landscape heterogeneity shapes predation in a newly restored predator–prey system . Ecology Letters 10 : 690 – 700 .
Kimble , D. S. , D. B. Tyers , J. Robison-Cox , and B. F. Sowell . 2011 . Aspen recovery since wolf reintroduction on the northern Yellowstone winter range . Rangeland Ecology and Management 64 : 119 – 130 .
Kohl , M. T. , et al. 2018 . Diel predator activity drives a dynamic landscape of fear . Ecological Monographs . doi: 10.1002/ecm.1313
Leopold , A. S. , S. A. Cain , C. M. Cottam , I. N. Gabrielson , and T. L. Kimball . 1963 . Wildlife management in the national parks . Transactions of the North American Wildlife and Natural Resources Conference 28 : 28 – 45 .
Lukacs , P. M. , et al. 2018 . Factors influencing elk recruitment across ecotypes in the western United States . Journal of Wildlife Management 82 : 698 – 710 .
MacNulty , D. R. , D. R. Stahler , C. T. Wyman , J. Ruprecht , and D. W. Smith . 2016 . The challenge of understanding northern Yellowstone elk dynamics after wolf reintroduction . Yellowstone Science 24 : 25 – 33 .
Mao , J. S. , et al. 2005 . Habitat selection by elk before and after wolf reintroduction in Yellowstone National Park . Journal of Wildlife Management 69 : 1691 – 1707 .
Marshal , J. P. , and S. Boutin . 1999 . Power analysis of wolf-moose functional responses . Journal of Wildlife Management 63 : 396 – 402 .
Marshall , K. N. , N. T. Hobbs , and D. J. Cooper . 2013 . Stream hydrology limits recovery of riparian ecosystems after wolf reintroduction . Proceedings Royal Society B 280 : 20122977 .
Maynard Smith , J . 1974 . Models in ecology . Cambridge University Press , Cambridge, United Kingdom .
Mech , L. D . 1970 . The wolf: the ecology and behavior of an endangered species . Natural History Press , Garden City, New York .
Mech , L. D . 2012 . Is science in danger of sanctifying the wolf ? Biological Conservation 150 : 143 – 149 .
Merrill , E. H. , and M. S. Boyce . 1991 . Summer range and elk population dynamics in Yellowstone National Park . Pp. 263 – 273 in The greater Yellowstone ecosystem: redefining America’s wilderness heritage (R. B. Keiter and M. S. Boyce , eds.). Yale University Press , New Haven, Connecticut .
Merrill , E. H. , M. K. Bramble-Brodahl , R. W. Marrs , and M. S. Boyce . 1993 . Estimation of green herbaceous phytomass from Landsat MSS data in Yellowstone National Park . Journal of Range Management 46 : 151 – 156 .
Morehouse , A. T. , and M. S. Boyce . 2011 . From venison to beef: seasonal changes in wolf diet composition in a livestock grazing landscape . Frontiers in Ecology and the Environment 9 : 440 – 445 .
Muhly , T. , M. Hebblewhite , M. S. Boyce , D. Paton , J. A. Pitt , and M. Musiani . 2013 . Humans strengthen bottom-up effects and weaken trophic cascades in a terrestrial food web . PLoS ONE 8 : e64311 .
National Research Council (NRC) . 2002 . Ecological dynamics on Yellowstone’s Northern Range . National Academy Press , Washington, D.C .
Painter , L. E. , R. L. Beschta , E. J. Larsen , and W. J. Ripple . 2015 . Recovering aspen follow changing elk dynamics in Yellowstone: evidence of a trophic cascade ? Ecology 96 : 252 – 263 .
Pimlott , D. H . 1967 . Wolf predation and ungulate populations . American Zoologist 7 : 267 – 278 .
Raynor , E. J. , H. L. Beyer , J. M. Briggs , and A. Joern . 2017 . Complex variation in habitat selection strategies among individuals driven by extrinsic factors . Ecology and Evolution 7 : 1802 – 1822 .
Ripple , W. J. , and R. L. Beschta . 2012 . Trophic cascades in Yellowstone: the first 15 years after wolf reintroduction . Biological Conservation 145 : 205 – 213 .
Ripple , W. J. , E. J. Larsen , R. A. Renkin , and D. W. Smith . 2001 . Trophic cascades among wolves, elk, and aspen on Yellowstone National Park’s Northern Range . Biological Conservation 102 : 227 – 234 .
Romme , W. H. , M. G. Turner , L. L. Wallace , and J. S. Walker . 1995 . Aspen, elk and fire on the Northern Range of Yellowstone National Park . Ecology 76 : 2097 – 2106 .
Romme , W. H. , et al. 2011 . Twenty years after the 1988 Yellowstone fires: lessons about disturbance and ecosystems . Ecosystems 14 : 1196 – 1215 .
Rose , J. R. , and D. J. Cooper . 2017 . The influence of floods and herbivory on cottonwood establishment and growth in Yellowstone National Park . Ecohydrology 10 : e1768 .
Sauer , J. R. , and M. S. Boyce . 1983 . Density-dependence and survival of elk in Northwestern Wyoming . Journal of Wildlife Management 47 : 31 – 37 .
Schullery , P . 2003 . The Yellowstone wolf: a guide and sourcebook . University of Oklahoma Press , Norman .
Seidel , D. , and M. S. Boyce . 2016 . Varied tastes: home range implications of foraging patch selection . Oikos 125 : 39 – 49 .
Sikes, R. S., and the Animal Care and Use Committee of the American Society of Mammalogists. 2016. 2016 Guidelines of the American Society of Mammalogists for the use of wild mammals in research and education. Journal of Mammalogy 97:663–688.
Sinclair , A. R. E . 1983 . Management of conservation areas as ecological baseline controls . Pp. 13 – 22 in Management of large mammals in African conservation areas (R. N. Owen-Smith , ed.). Haum , Pretoria, South Africa .
Singer , F. J. , A. Harting , K. K. Symonds , and M. B. Coughenour . 1997 . Density dependence, compensation and environmental effects on elk calf mortality in Yellowstone National Park . Journal of Wildlife Management 61 : 12 – 25 .
Smith , D. W. , and G. Ferguson . 2012 . Decade of the wolf, revised and updated: returning the wild to Yellowstone . Globe Pequot Press/Lyon Press , Guilford, Connecticut .
Tallian , A. , et al. 2017 . Competition between apex predators? Brown bears decrease wolf kill rate on two continents . Proceedings Royal Society B 284 : 20162368 .
Taper , M. L. , and P. J. P. Gogan . 2002 . The northern Yellowstone elk: density dependence and climatic conditions . Journal of Wildlife Management 66 : 106 – 122 .
Terborgh , J. , and J. A. Estes . 2010 . Trophic cascades: predators, prey, and the changing dynamics of Nature . Island Press , Washington, D.C .
Tercek , M. T. , R. Stottlemyer , and R. Renkin . 2010 . Bottom-up factors influencing riparian willow recovery in Yellowstone National Park . Western North American Naturalist 70 : 387 – 399 .
Thurfjell , H. , S. Ciuti , and M. S. Boyce . 2017 . Learning from the mistakes of others: how female elk ( Cervus elaphus ) adjust behaviour with age to avoid hunters . PLoS ONE 12 : e0178082 .
Uboni , A. , D. W. Smith , J. S. Mao , D. R. Stahler , and J. A. Vucetich . 2015 . Long- and short-term temporal variability in habitat selection of a top predator . Ecosphere 6 : 51 .
Van Manen , F. T. , et al. 2016 . Density dependence, whitebark pine, and vital rates of grizzly bears . Journal of Wildlife Management 80 : 300 – 313 .
Varley , N . 2007 . Gray wolf (Canis lupus) effects on ecological communities . Ph.D. dissertation , University of Alberta , Edmonton, Alberta, Canada .
Varley , N. , and M. S. Boyce . 2006 . Adaptive management for reintroductions: updating a wolf recovery model for Yellowstone National Park . Ecological Modelling 193 : 315 – 339 .
Vucetich , J. A. , D. W. Smith , and D. R. Stahler . 2005 . Influence of harvest, climate, and wolf predation on Yellowstone elk, 1961–2004 . Oikos 111 : 259 – 270 .
Walters, C. J. 1986. Adaptive management of renewable resources. Macmillan, New York.
Weaver , J . 1978 . The wolves of Yellowstone. Natural resources report no. 14 . National Park Service, United States Government Printing Office , Washington, D.C .
White , P. J. , and R. A. Garrott . 2005 . Yellowstone’s ungulates after wolves—expectations, realizations, and predictions . Biological Conservation 125 : 141 – 152 .
White , P. J. , R. A. Garrott , and G. E. Plumb . 2013 . Ecological process management . Pp. 3 – 9 in Yellowstone’s wildlife in transition (P. J. White , R. A. Garrott , and G. E. Plumb , eds.). Harvard University Press , Cambridge, Massachusetts .
Wolf , C. , and W. J. Ripple . 2018 . Rewilding the world’s large carnivores . Royal Society Open Science 5 : 172235 .
Wright , G. J. , R. O. Peterson , D. W. Smith , and T. O. Lemke . 2006 . Selection of northern Yellowstone elk by gray wolves and hunters . Journal of Wildlife Management 70 : 1070 – 1078 .
Email alerts
Citing articles via.
- Recommend to your Library
Affiliations
- Online ISSN 1545-1542
- Print ISSN 0022-2372
- Copyright © 2024 American Society of Mammalogists
- About Oxford Academic
- Publish journals with us
- University press partners
- What we publish
- New features
- Open access
- Institutional account management
- Rights and permissions
- Get help with access
- Accessibility
- Advertising
- Media enquiries
- Oxford University Press
- Oxford Languages
- University of Oxford
Oxford University Press is a department of the University of Oxford. It furthers the University's objective of excellence in research, scholarship, and education by publishing worldwide
- Copyright © 2024 Oxford University Press
- Cookie settings
- Cookie policy
- Privacy policy
- Legal notice
This Feature Is Available To Subscribers Only
Sign In or Create an Account
This PDF is available to Subscribers Only
For full access to this pdf, sign in to an existing account, or purchase an annual subscription.
Thank you for visiting nature.com. You are using a browser version with limited support for CSS. To obtain the best experience, we recommend you use a more up to date browser (or turn off compatibility mode in Internet Explorer). In the meantime, to ensure continued support, we are displaying the site without styles and JavaScript.
- View all journals
- My Account Login
- Explore content
- About the journal
- Publish with us
- Sign up for alerts
- Open access
- Published: 09 April 2024
Hot springs viruses at Yellowstone National Park have ancient origins and are adapted to thermophilic hosts
- L. Felipe Benites 1 ,
- Timothy G. Stephens 1 ,
- Julia Van Etten ORCID: orcid.org/0000-0002-4012-7271 1 , 2 ,
- Timeeka James 1 ,
- William C. Christian ORCID: orcid.org/0000-0003-1682-8083 3 ,
- Kerrie Barry ORCID: orcid.org/0000-0002-8999-6785 4 ,
- Igor V. Grigoriev ORCID: orcid.org/0000-0002-3136-8903 4 , 5 ,
- Timothy R. McDermott 6 &
- Debashish Bhattacharya ORCID: orcid.org/0000-0003-0611-1273 1
Communications Biology volume 7 , Article number: 312 ( 2024 ) Cite this article
1366 Accesses
26 Altmetric
Metrics details
- Classification and taxonomy
- Evolutionary genetics
Geothermal springs house unicellular red algae in the class Cyanidiophyceae that dominate the microbial biomass at these sites. Little is known about host-virus interactions in these environments. We analyzed the virus community associated with red algal mats in three neighboring habitats (creek, endolithic, soil) at Lemonade Creek, Yellowstone National Park (YNP), USA. We find that despite proximity, each habitat houses a unique collection of viruses, with the giant viruses, Megaviricetes, dominant in all three. The early branching phylogenetic position of genes encoded on metagenome assembled virus genomes (vMAGs) suggests that the YNP lineages are of ancient origin and not due to multiple invasions from mesophilic habitats. The existence of genomic footprints of adaptation to thermophily in the vMAGs is consistent with this idea. The Cyanidiophyceae at geothermal sites originated ca. 1.5 Bya and are therefore relevant to understanding biotic interactions on the early Earth.
Similar content being viewed by others
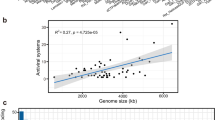
Viruses in deep-sea cold seep sediments harbor diverse survival mechanisms and remain genetically conserved within species
Yongyi Peng, Zijian Lu, … Xiyang Dong
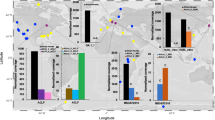
Lytic archaeal viruses infect abundant primary producers in Earth’s crust
Janina Rahlff, Victoria Turzynski, … Alexander J. Probst
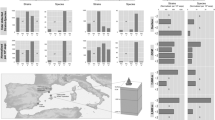
Unexpected myriad of co-occurring viral strains and species in one of the most abundant and microdiverse viruses on Earth
Francisco Martinez-Hernandez, Awa Diop, … Manuel Martinez-Garcia
Introduction
Extreme environments such as acidic geothermal springs (hot springs) are thought to have been “cradles of microbial life” on the early Earth 1 . In contrast, on the modern Earth, these sites represent biology at the extremes, comprising isolated “islands”, usually surrounded by mesophilic life 2 . A key question is whether species that currently inhabit hot springs have anciently derived adaptations that can provide insights into primordial life. Specialized archaeal and bacterial taxa thrive and dominate hot springs, whereas eukaryotes are often absent 3 , 4 . However, in more moderate downstream habitats, polyextremophilic unicellular Rhodophyta (red algae) that split from their mesophilic sister taxa ca. 1.5 billion years ago 5 form dense, conspicuous mats overshadowing prokaryotic life. And, where there is life, there are viruses. Although in lower densities than in other environments 5 , 6 , viruses appear to play a pivotal role in microbial host mortality in other hot springs, often being the only infectious agents in these sites 7 , 8 . Bacterial and archaeal viruses are abundant 8 , 9 , 10 , however less is known about eukaryotic viruses, with some exceptions 11 , 12 , 13 , 14 . And whether eukaryotic viruses modulate adaptation to these extreme environments by reprogramming host metabolism and/or through interdomain gene transfer is currently an open question 15 .
Thermoacidophilic red algae include the genera Cyanidioschyzon and Galdieria (class Cyanidiophyceae), which, in hot springs 16 such as Yellowstone National Park (YNP), detoxify arsenic and other heavy metals 17 . The major driver of polyextremophilic adaptation in these algae is horizontal gene transfer (HGT) from prokaryotic donors 5 , 18 , 19 , 20 , although there exists some evidence for viral HGTs 21 , 22 . Thus far, no viruses infecting these algae have been identified. Microbial mats generally comprise lineages that are phylogenetically worlds apart, whereby related viral groups may interact with unrelated hosts 23 . Furthermore, microbial mat viruses may act as drivers of mat formation by triggering regeneration due to nutrient fluxes from host mortality 24 , 25 .
The “hot start” hypothesis posits that early life flourished in a hot environment and later adapted to the cooling Earth 26 , which is supported by the ancient splits of thermophilic organisms in gene trees 27 , 28 , 29 . Therefore, modern hot springs are ideal systems for investigating adaptations that could have been present in extreme environments in the early Earth. Specifically, longer-term infections within the mats may have led to thermal signatures of ancient associations in viral genomes (Hypothesis [H] 1). Alternatively, these environments may have been invaded more recently by viruses from mesophilic habitats (H2) and lack signatures of thermophily. To discriminate between these two hypotheses, we investigated viruses infecting red algal mats in a hot spring environment with the over-arching goals of characterizing viral community composition, elucidating local adaptation and potential role in cellular communities, and understanding virus evolutionary history. We analyzed metagenomic data from three adjacent environments at Lemonade Creek, YNP (Fig. 1a ): a creek fed by an acidic hot spring, neighboring endoliths, and acidic soils (Fig. 1b ). Their taxonomic diversity, phylogenetic position, and landmark genomic signatures suggest ancient and persistent adaptations by YNP viruses to geothermal habitats.
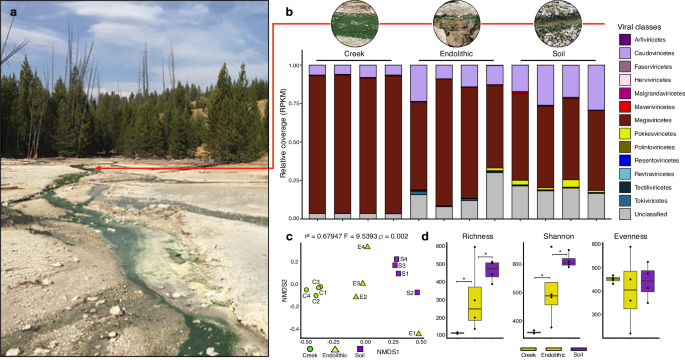
a Lemonade Creek, Yellowstone National Park (YNP) and the virus community at the sampled sites (see red arrow). b Abundance of virus classes (legend shown at right) reflecting relative coverage (reads per kilobase million, RPKM) at the creek, endolithic, and soil sites with four replicates/site. c Beta diversity was calculated using a non-metric multidimensional scaling (NMDS) ordination of virus classes in the creek (green circles), endolithic (yellow triangles), and soil (purple squares) communities, based on Bray–Curtis dissimilarity. d Alpha diversity calculated using the richness (number of virus classes), Shannon diversity, and evenness of virus communities in the three environments. The asterisks show significant statistical differences between groups.
Overview of virus community composition at Lemonade Creek, YNP
From 12 metagenomic samples spanning the three environments, we assembled 7,886,883 scaffolds of which 6,390 were predicted to be viral. After scaffold dereplication and clustering (see methods), following standard procedures for the identification of viral operational taxonomic units (vOTUs) 30 , 31 , we found 3679 vOTUs that encode 17,102 proteins. To reconstruct larger and more complete genomes, we binned these vOTU scaffolds and recovered 25 viral metagenome-assembled genomes (vMAGs), ranging from high ( n = 5), medium ( n = 14), to low completeness ( n = 6) according to CheckV 32 , with lengths ranging from 1.63 kb to 2.2 Mb (Supplementary Data 1 , Supplementary Data 2 ).
Virus taxonomy, distribution, and abundance at Lemonade Creek
Of the 3679 vOTUs, the majority were from the class Megaviricetes ( n = 890), eukaryotic DNA viruses that infect algae, other protists, and some metazoans 33 . Other frequent classes were Caudoviricetes ( n = 711), containing bacterial and archaeal viruses, Pokkesviricetes ( n = 70) that infect Metazoa and dinoflagellate algae 33 , Maveriviricetes ( n = 61) that are “virophages”, parasites from giant eukaryotic viruses 34 , and Tectiliviricetes ( n = 27), associated with Bacteria, Archaea, and metazoan hosts 35 . However, 51.05% of the vOTUs ( n = 1878) were unclassified, suggesting the presence of many diverged viruses (Supplementary Data 1 ).
To compare vOTU abundance profiles across samples, we calculated RPKM (Reads Per Kilobase Million) values for viral sequences at the class level (Fig. 1b ). This analysis revealed that Megaviricetes (69.9%) and Caudoviricetes (14.15%) are most abundant, followed by Unclassified viruses (12.85%) that are present in all the studied environments (although the latter group are more abundant in soil samples), whereas all others represent ∼ 1% or less, and are specific to environments. Maveriviricetes, although only comprising 0.5% of the total vOTU count, are present in all environments, which is expected given the high abundance of their known viral hosts (Megaviricetes). It is noteworthy that the most abundant viral classes observed at these sites are known to infect eukaryotic hosts.
To study viral diversity and abundance and their relationship to the environment, we performed alpha and beta diversity analyses of vOTUs at the class level. Soil has the highest diversity, whereas the creek has the lowest diversity, overall richness, and Shannon diversity among the environments (Fig. 1d ). Non-metric multidimensional scaling (NMDS) (p(perm) = 0.002) analysis of beta diversity from each metagenome sample shows that samples grouped according to environment (Fig. 1c ). However, the endolithic site shows higher variation of the viral community, whereby samples are positioned at different extremes of the NMDS2 axis. Endolithic samples also contain most viral classes, whereas, in the creek, samples are more tightly clustered, driven by the dominance of the Megaviricetes. This suggests that despite being adjacent, the studied environments contain unique distributions of viral classes, with the endolithic environment harboring a high and non-homogeneous diversity of viruses. This may be explained by endoliths containing unique internal microhabitats, which are better protected from low pH, high temperature, and the damaging UV irradiation associated with the other two environments in YNP (i.e., the park has an average elevation of ca. 8000 ft) [ https://www.nps.gov ].
Functional profile of the Lemonade Creek virus community
Of the 17,102 proteins encoded by the 3679 vOTUs, 15.7% have assigned functions based on GO (gene ontology), KEGG (Kyoto Encyclopedia of Genes and Genomes), and PFAM (protein families) analysis (Fig. 2a–c , respectively) (Supplementary Data 3 ). We analyzed the top 10 annotations for each gene to investigate their putative functions. Proteins encoding cellular metabolic processes, viral genome replication, nitrogen metabolism, DNA and protein binding, and transferase activity are most frequent, although the soil contained a larger number of proteins encoding host cellular and intracellular components, as well as symbiotic and interspecies interactions. Pathways involved in RNA polymerase, DNA repair, purine and pyrimidine metabolism, drug metabolism, and homologous recombination, and, curiously, cellular structural development are the most frequent. Regarding PFAM domains, the most frequent domains are associated with viral replication, ATPases, glycosyltransferases, HNH (histidine and asparagine domain) endonucleases and protein kinases, and those involved in viral structures such as major capsid proteins (MCPs) and genome maintenance via helicases.
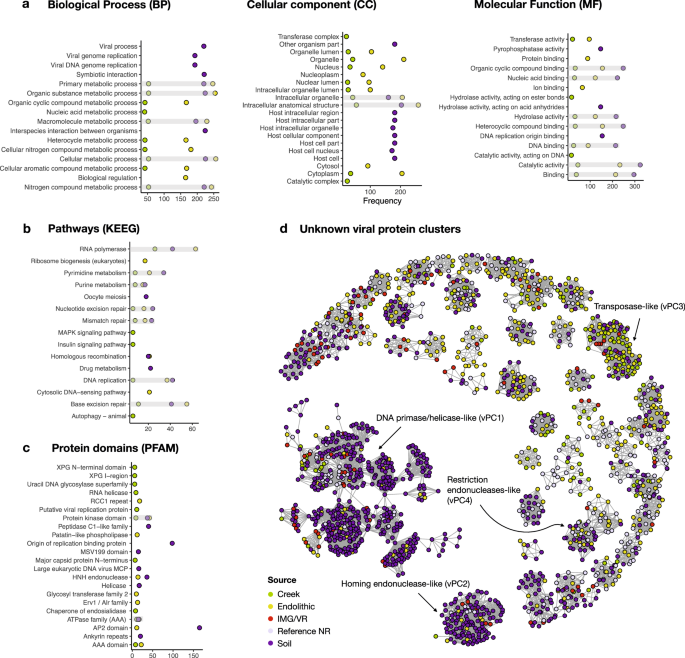
a Gene ontologies (GO terms) describing the categories for biological process (BP), cellular component (CC), and molecular function (MF); b KEGG pathways (Kyoto Encyclopedia of Genes and Genomes); c protein families (PFAM) according to InterPro database. d Sequence similarity networks containing 86 unknown viral protein clusters (vPCs). The color key shows the sampled site: creek (green), endolithic (yellow), and soil (purple), IMG/VR (Integrated Microbial Genomes and uncultivated viruses) (red), Reference/NR (lavender) denotes GenBank viral RefSeq and non-redundant databases.
To explore the proteins with unknown functions ( n = 14,415) (Supplementary Data 4 ), we retrieved protein matches to build a sequence similarity network (SSN) containing viral protein clusters (vPCs). To reduce the total protein space, we first clustered all unknown sequences with their matches in viral databases at the threshold of 60% identity and 80% coverage, which resulted in 13,543 proteins. These vPCs allowed us to transfer putative functions to a fraction of unknown proteins and evaluate their distribution and composition from the sampled environments. After removing singletons and poorly connected clusters (degrees < 8), 86 vPCs remained (Fig. 2d ), with just four of the largest vPCs being functionally classifiable. The largest (vPC1) is dominated by proteins encoding DNA primase/helicase, or the origin of replication binding. vPC2 comprises homologs of homing endonucleases. In vPC3, we find a single sequence encoding a transposase with the remainder having unknown functions, whereas vPC4 contains homologs encoding restriction endonucleases. The remaining 82 vPCs lack annotated functions. These results suggest that hot springs harbor many uncharacterized proteins that may play specific ecological roles in each environment.
HGT in the Lemonade Creek virus community
We investigated the impact of HGT on generating novelty (i.e., to the best of our knowledge) in Lemonade Creek viruses and explored their potential role as gene transfer agents between cellular taxa via viral transduction. HGTs can also provide clues of past and present virus-host associations 36 . For this aim, we evaluated the proportion of protein sequences with cellular homologs for each vOTU and calculated their alien and HGT indices (AI and hU) (Fig. 3 a, b, respectively). We identified 921 unique HGT candidates (534 using AI and 855 using hU) from 5734 sequences (representing 16% of this total) with hits to genes in cellular taxa (Fig. 3c ). Bacteria was the major HGT source ( n = 658), followed by Eukarya ( n = 196), and Archaea ( n = 67). We found evidence of virus-host associations. In Revtraviricetes, a class that infects Fungi 37 , all HGTs ( n = 2) are from fungal donors, in Tokiviricetes, which infect Archaeal hosts 38 , all HGTs acquisitions are from Archaea, and an intriguing result in the unclassified viruses, 3 HGTs (one hypothetical protein and two GDP-D-mannose-3’,5’-epimerase) are from Galdieria (Cyanidiophyceae) (Supplementary Data 5 ). Most HGT-derived cellular proteins are hypothetical/uncharacterized/unnamed ( n = 512), followed by those in the TIGR03118 family ( n = 13), which has a C-terminal putative exosortase interaction domain (InterPro id: TIGR03118) with unknown function, and DUF2341 domain-containing proteins ( n = 11), which are found in bacterial proteins such as proton channels and transport proteins (InterPro id: IPR018765). Other proteins contain LysM domains ( n = 8) (InterPro id: IPR018392), used by plants for pathogen defense and symbiosis and by some bacteriophages to degrade bacterial cell walls 39 . Other proteins, such as DNA methyltransferases ( n = 7), phage portal proteins ( n = 7), which may be remnants of lysogenic viruses, and timeless family proteins ( n = 7) that regulate eukaryotic circadian rhythms, stress responses, and may be responsible for maintenance of viral latency 40 , 41 , are less frequent. Although these results provide a lower bound for HGTs, given the conservative measures we used, the finding of bacterial genes in eukaryotic vMAGs, and vice versa, suggests that viruses act as HGT vectors in hot spring mats, implicating a role in interdomain gene acquisition, and potentially host adaptations.
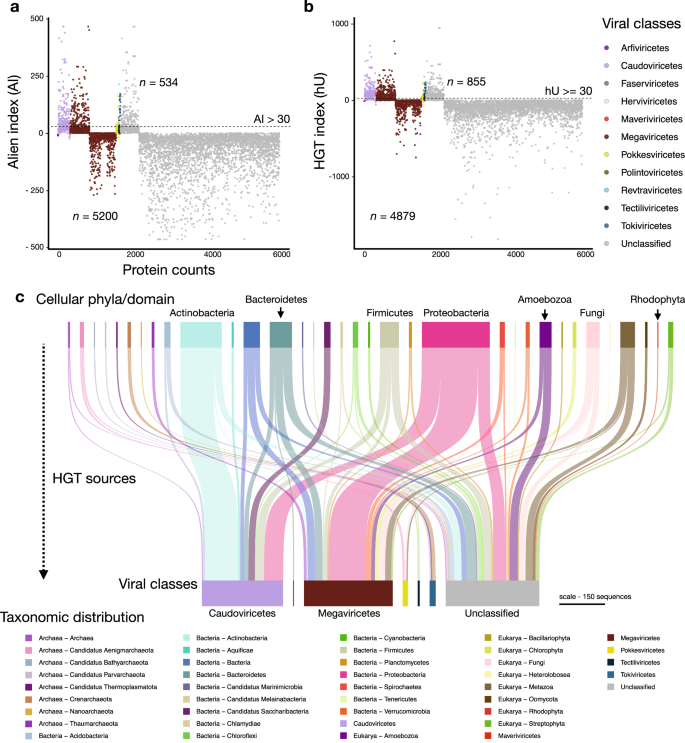
a HGT measured using the alien index (AI). b , HGT measured using the HGT index (hU). Proteins with indices greater than the threshold (dashed lines at AI > 30 and hU ≥ 30) are vHGT candidates. The key at right shows virus classes. c Sankey diagram showing the cellular sources of HGTs (top) for each virus class (bottom). The key on the bottom shows archaeal, bacterial, eukaryotic, and viral taxonomic groups and is ordered alphabetically in the same direction as the Sankey diagram. The scale bar represents the amount of putative HGT-derived genes.
Viral metagenome-assembled genomes (vMAGs)
The 25 vMAGs produced from binning of the vOTUs were grouped into five viral families: Circoviridae ( n = 2), Mimiviridae ( n = 2), Pandoraviridae ( n = 1), Pithoviridae ( n = 7), and Tectiviridae ( n = 1) (Supplementary Data 2 ). However, 12 bins were initially unclassified. The vMAGs have varied completeness, with five having 100% completeness, 14 medium, and 6 low. The largest vMAG (bin787; Pithoviridae-like) is of size 2.2 Mb, followed by bin800 (Pandoravirus-like) of size 1.36 Mb (Fig. 4b ), and the smallest ranged from 7.8 kb (bin1029) to 1.6 kb (bin901). We searched for viral markers and found 11 vMAGs that contain putative capsid and replication-associated proteins (rep), characteristic of CRESS DNA viruses (Eukaryotic Circular Rep-Encoding Single-Stranded virus). A vMAG (bin250) assigned to Tectiviridae encoded a major capsid protein and a terminase large subunit, among other proteins, confirming its initial classification. We were unable to confidently classify one vMAG (bin871), that contained hits with both unclassified viruses (Pacmanvirus, Faustovirus), Nucleocytoviricota and Caudoviricetes viruses. Although there were no major hallmark genes from the database “giant virus orthologous groups” (GVOGs), we found highly duplicated “Nucleo-Cytoplasmic Virus Orthologous Groups” (NCVOGs database) genes, as “Superfamily II helicase related to herpesvirus replicative helicase” ( n = 96) and HNH endonuclease ( n = 87) (Supplementary Data 6 ). CheckV did not identify contamination in this vMAG, therefore we could not determine if it is a novel (i.e., to the best of our knowledge) chimeric virus, like Mirusviruses 42 (despite no significant hits with these viruses) or a database primary misannotation.
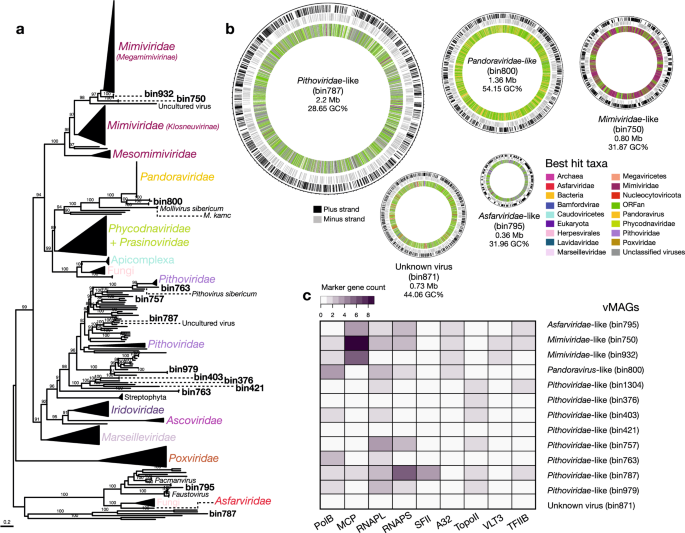
a Maximum likelihood phylogenetic tree of the marker protein, B DNA Polymerase (PolB), from the vMAGs and reference viral genomes, with bootstrap support values ≥ 90% shown. b Circular vMAG representation with approximate sizes of selected viruses shown in the first and second outer rings (black and gray lines, respectively), the third (inner) ring is the taxonomic affiliation of the best BLAST hit. c Marker gene presence/absence in the vMAGs.
Putative giant Nucleocytoviricota virus homologs were found in the majority of vMAGs ( n = 12) (Fig. 4c ). To delineate families within this group, we searched for markers using GVOGs) 33 and NCVOGs 43 databases (Supplementary Data 6 ), together with homologs in databases, to build maximum likelihood (ML) phylogenies. Individual phylogenies for nine marker genes (Fig. 4a , Supplementary Fig. 1 ) confirmed the initial taxonomic identification for some vMAGs as Nucleocytoviricota and placed two unclassified vMAGs (bin787 and bin795) into the Pithoviridae and Asfarviridae families, respectively. We resolved their phylogenetic positions within these families and found that vMAGs affiliated with Mimiviridae-like families clustered with Tupanviruses isolated from the deep-sea 44 , Pithoviridae-like vMAGs clustered with Pithoviruses also retrieved from deep-sea, in the Loki’s Castle hydrothermal vent system 45 , Pandoravirus-like clustered at the base of Pandoraviruses isolated from Siberian permafrost and from aquatic environments 46 , 47 , and one Asfarviridae-like vMAG clustered at the base of the main group, near Faustoviruses 48 and Pacmanviruses 49 that are distantly related to Asfarviridae. Despite their inclusion in established giant virus families, vMAG average amino acid identities (AAI) indicate high divergence when compared to viral reference genomes (Supplementary Data 9 ). Given their relative phylogenetic positions, it appears that hot springs giant vMAGs split early on from most of their relatives. These findings, together with the vast geographic distribution of viruses within these viral families from deep-sea locations in South America, the North Sea, and the Siberian peninsula, are consistent with the ancient splits of vMAGs.
Footprints of genome-wide adaptation to extremophily at Lemonade Creek
The genomes and proteomes of thermophilic bacterial and archaeal species differ from those in mesophilic species due to local adaptation 50 , 51 , 52 . We investigated whether the same holds for hot springs vMAGs ( n = 25) by comparing their genomes to those of phylogenetically closely related viruses, not from Lemonade Creek ( n = 46) (Supplementary Data 7 ). To achieve this aim, we compared amino acid frequencies, including the thermophilic amino acid signature (IVYWREL—Ile, Val, Tyr, Trp, Arg, Glu, and Leu) 50 , relative synonymous codon usage (RSCU), protein isoelectric point (pI) and length, purine-loading index (PLI), guanine-cytosine percentage composition (GC%), and predicted optimal growth temperatures (pOGT).
We find that hot springs vMAGs have a marginally higher frequency of the amino acids Cys, Gln, Glu, His, Leu, Phe, Pro, Ser, The, Trp, and Tyr, and lower Ala, Asp, Gly, Ile, Lys, Met, and Val content, with similar frequencies of Arg when compared to viral genomes from other environments. However, none of these values are statistically significant (Fig. 5a ). Comparison of the amino acids IVYWREL also showed no statistical difference ( p = 0.3888), although there was a higher mean frequency in hot spring vMAGs (5.62%) than in non-hot springs virus genomes (5.42%). For RSCU (Fig. 5b ), we found significant differences between groups, with AGG and ATA as preferred codons in hot spring vMAGs, whereas CGC, CTC, GGC, TAC, TCG, TGC, and TTT are preferred in non-hot spring viral genomes ( p < 0.0005). We also found that hot spring vMAGs have a higher protein pI ( p < 0.0005) (Fig. 5c left), higher PLI ( p < 2.538e −11 ) (Fig. 5c right), lower GC% (mean 38.09%; non-hot spring viruses 41.87%) ( p < 2.2e −16 ), and shorter protein lengths ( p < 2.2e −16 ) (Fig. 5d ). Lastly, we predicted optimal growth temperatures (pOGT) for the longest vMAGs ( n = 5) and non-hot springs viruses ( n = 12) (Fig. 5e ) and found that mean pOGT for the former (55.3 °C) was significantly higher than for the latter (46.6 °C) (W = 52, p = 0.01939; Supplementary Data 7 ). These findings indicate that Lemonade Creek vMAGs have genomic signatures of thermophilic adaptation, suggesting a long-term relationship with hot spring environments.
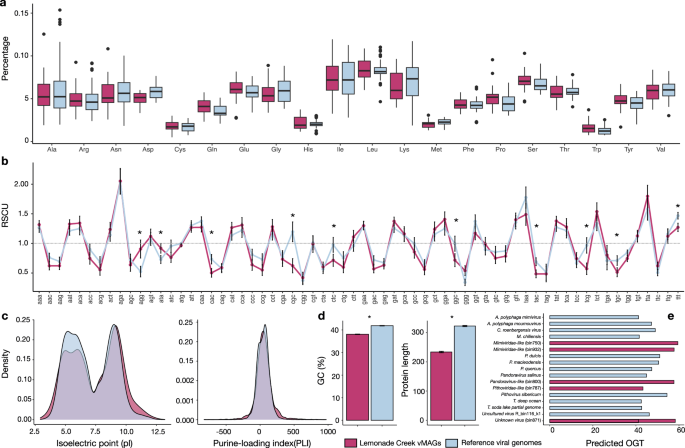
a Analysis of differences in amino acid frequencies. b Analysis of relative synonymous codon usage (RSCU) of 64 codons as a measure of codon usage bias between groups, where the dashed line represents the threshold of values if >1.0, the codon is used more often than expected, and when <1.0, the codon is used less often than expected. c Density plots of protein isoelectric point (pL) (left) and purine-loading index (PLI) (right) distributions between groups. d Guanine–cytosine percentage (GC%) (left) of protein-coding sequences (CDSs) and protein lengths (right). e Predicted optimal growth temperature of five vMAGs (bins) and twelve reference genomes, where the x -axis corresponds to temperature (in Celsius). In each panel, asterisks represent significant statistical differences between the two virus groups (i.e., vMAGs versus reference viral genomes).
A major finding of our study is that even though different habitats at hot springs may be adjacent (within centimeters of each other) and visually dominated by Cyanidiophyceae, multiple, divergent virus classes exist at these sites that are often habitat specific (Fig. 1c ). Megaviricetes is the dominant class at Lemonade Creek, suggesting they may infect and influence red algal population dynamics. This idea is supported by the finding of vHGTs within this group 21 , 22 , 53 . Most virus proteins in our dataset are unclassified, indicating remarkable novelty (i.e., based on the current data) at geothermal sites, with some having roles in genome mobility (e.g., endonucleases, transposases).
When comparing virus genomes from Lemonade Creek to those of related lineages isolated from mesophilic sites, our results demonstrate the existence of genome and protein signatures associated with a thermophilic lifestyle. The early branching phylogenetic position of the most abundant Nucleocytoviricota vMAGs strongly supports Hypothesis 1 (H1) that we posed, whereby hot springs viruses are ancient lineages that have adapted to, and likely remained in geothermal environments, at least 1.5 Bya, since the time of Cyanidiophyceae origin 5 . These splits likely occurred when their hosts initially colonized extreme environments, emphasizing the ancient and divergent nature of hot springs vMAGs when compared to their virus relatives. Our work also expands to terrestrial thermal springs, the distribution of giant viruses that were previously identified in different extreme environments, such as the deep sea.
It has been proposed that the ancestral state of prokaryotes, and life itself, was thermophilic 54 , 55 , 56 because cells originated in the late Hadean or early Archean eon on the early Earth 57 , 58 , potentially in a hot springs environment 59 . Recently, a major deep-diverging thermophilic bacterial clade from hydrothermal vents was reported 60 . However, a non-thermophilic origin was also suggested by placing the root of these lineages within Chloroflexota or Candidate Phyla Radiation (CPR). In another study, the root was placed between Gracilicutes and Terrabacteria, both of which contain thermophiles, but the majority are mesophilic 61 , 62 , 63 . Although we do not know if the ancestors of Lemonade Creek viruses were thermophilic, our results indicate that for at least 3 different viral groups (Nucleocytoviricota, CRESS DNA viruses, and Tectiliviruses), the shift to thermophilic environments was an early event in their evolution.
Thermophilic organisms evolved several features to enhance genome and protein thermostability, such that proteomes and single protein ortholog pairs can be differentiated from homologs in mesophilic species based on sequence and composition properties 64 . Specifically, the summed frequency of the Ile, Val, Tyr, Trp, Arg, Glu, and Leu amino acids are correlated with the optimal growth temperature (OGT) and thermal adaptation in Bacteria, Archaea, and Eukarya 50 , 65 . The average length of proteins is much shorter 66 , 67 , 68 , and a higher protein isoelectric point (pI) of thermophilic Bacteria and Archaea reflects not only high temperature but also adaptation to acidic environments 69 , 70 . Codon bias in thermophiles is related to protein thermostability by enhancing the number of ionic interactions and salt bridges 71 , reflected by the preference for AGR codons (AGG, AGA), that encode arginine (Arg), contrasting to CGN codons preferred by mesophiles 72 . A high purine-loading index (PLI - the difference between purines and pyrimidines) prevents the formation of double-stranded RNAs generated by RNA-RNA interactions that occur with increasing temperatures 73 . In prokaryotes, the OGT is related to the average PLI of open reading frames and is strongly correlated with codon usage bias 50 , as in plastid genomes from the extremophilic red alga Cyanidium caldarium 74 .
Our findings are consistent with previous studies on extremophilic cellular organisms and reveal a fundamental principle of biology at the extremes: hot spring viruses are not simply different with respect to minor features from relatives inhabiting mesophilic environments, rather, they have evolved distinct molecular signatures characteristic of their hosts, i.e., thermophilic Bacteria, Archaea, and possibly Eukarya. This suggests that adaptation at the extremes follows the same underlying rules, regardless of the domain, specifically, ancient origins, compact proteins, stable molecular bonds, optimal growth at higher temperatures, and potentially, communal interactions through infection or syntrophy. Our study provides the first assessment of viruses associated with polyextremophilic red algae that form extensive microbial mats in YNP and are common worldwide at geothermal sites 2 , 5 , 75 . Our study identified only four vOTUs that map to RNA viruses (Riboviria), likely due to a bias favoring DNA viral genome amplification rather than the absence of these viruses in hot springs 76 , 77 , 78 . Despite the challenges posed by extreme conditions in YNP, such as high heat and acidity that may compromise RNA stability, further exploration of RNA viruses in these mats is necessary to understand their ecology and potential genome adaptations under extreme conditions.
Finally, our metagenomic data demonstrate that viruses from red algal mats at Lemonade Creek are phylogenetically diverse, abundant, and appear to have persisted in extreme environments at least for as long as the Cyanidiophyceae have existed 79 . Although more recent than the earliest forms of prokaryotic life on our planet, these eukaryotic algal mats span a long period of the Earth’s history, surviving and thriving in some of the most challenging ecosystems for both cellular life and viruses.
Site description and sampling
The geothermal study site in Yellowstone National Park (YNP) is referred to as Lemonade Creek (Lat.: 44° 48’05” N, Long.: 110° 43’44” W). During the spring months, it drains run-off from snow melt (March–May), and in the following months of the year, it is completely derived from four geothermal tributary features (Fig. 1a ). Samples were taken downstream of the four tributaries to reflect the inputs from all sources. Three environments were sampled at this site: (1) the lush green biofilms formed mainly by Cyanidioschyzon in the creek channel (Fig. 1 b), (2) the soils adjacent to the creek channel, and (3) the endolithic materials also adjacent to the creek channel. On the day of sampling, the creek water pH and temperature were 2.55 and 44 °C, respectively. The adjacent soil pH was 2.1, and the temperature was ~32 °C. Replicate ( n = 4) samples were taken from each environment on the same day. For the creek biofilm samples, small stream bed rocks were loaded into 50 ml sterile Falcon tubes along with ~15 ml of creek water and then shaken vigorously (~10 s) to generate a thick suspension of biomass. Rocks and grit were allowed to settle (~10 s), and then the suspension was decanted into a 15 ml Falcon tube and immediately centrifuged (2000× g for 3 min). The resulting pellet was saved, and the supernatant was discarded. Soil samples were taken by scraping the upper ~0.5 cm of surface soil, being careful to avoid soil below the discrete algal layer (Fig. 1b ). Endolithic material was acquired by breaking off chips of stream-side rock. Immediately after the acquisition, the samples were flash-frozen in an ethanol-dry ice bath and kept on dry ice during transport back to the lab, where they were kept at −80 °C until nucleic acid extraction. The fieldwork and sample collection were conducted by Timothy McDermott under NPS research permit YELL-2022-SCI-5364.
Nucleic acid extraction
Total DNA was extracted from the samples with the Qiagen DNeasy PowerSoil Pro kit (Hilden, Germany). Samples were checked for quality, purity, and concentration using the Nanodrop 3000C and Qubit 2.0. They were stored at −80 °C and shipped on dry ice to the DOE Joint Genome Institute (JGI) for sequencing. Due to the challenges associated with extracting biological material from the endolithic site, sample E4 underwent PCR amplification during library preparation to increase DNA concentration to a usable level.
Sample sequencing and metagenome assembly
DNA samples were sequenced by the DOE Joint Genome Institute (JGI). For each sample, an Illumina library was constructed and sequenced 2 × 151 using the Illumina NovaSeq S4 platform following SOP 1064 (the number of reads and bases sequenced for each sample are listed in Supplementary Data 1 ). BBDuk (v38.94) 80 was used to remove contaminants, trim reads that contained adapter sequence, trim G homopolymers of size 5 or more at the ends of the reads, and “right quality” trim reads where quality drops to 0. BBDuk was used to remove reads that contained 4 or more ‘N’ bases, had an average quality score across the read less than 3, or had a minimum length ≤51 bp or 33% of the full read length. Reads mapped with BBMap 80 to masked human, cat, dog, and mouse references at 93% identity were removed from downstream analysis, as were reads that aligned to common microbial contaminants (following SOP 1077). The reads retained after filtering were corrected using bbcms (v38.90; “-Xmx100g mincount=2 highcountfraction=0.6”) and assembled independently (i.e., the corrected reads from each library were assembled separately) using spades v3.15.2 (“-m 2000 --only-assembler -k 33,55,77,99,127 --meta”) 81 .
Identification of viral scaffolds
Viral scaffolds were identified in each of the 12 assembled metagenomes by taking scaffolds longer than 1.5 kb and applying VirSorter2 (“--min-score 0.9 --min-length 1500 --hallmark-required-on-short”) 82 , geNomad v1.3.0 (“end-to-end --min-score 0.7 --cleanup --splits 8”; https://github.com/apcamargo/genomad ) 83 , and a custom pipeline using DIAMOND v2.0.15 BLASTx ( e -value 1e-5, “--ultra-sensitive”) 84 against the viral protein reference sequence database (v211) from GenBank and the IMG/VR v4 database 85 . The latter approach was used to retrieve shorter viral scaffolds with no hallmark genes that would normally be excluded from downstream analysis by VirSorter2 and geNomad. We combined the results of these tools and extracted scaffolds resulting from these searches; Prodigal v2.9.0 (“-p meta”) 86 was used to predict proteins in the resulting scaffolds. To remove false positives, we aligned the predicted proteins with DIAMOND BLASTp against the non-redundant GenBank database (NR), with taxonomic information for each protein included in the output – specifically the highest taxonomic level classification (i.e., the “sskingdoms” field) for each hit. We combined this blast search with results from the previous blast analysis against the IMG/VR v4 database, keeping only the scaffolds where most protein top hits (≥60%) per scaffold were with viruses. Finally, we used CheckV v1.1 87 to flag contaminant scaffolds (“checkv end_to_end”, default parameters) which were subsequently removed. The resulting scaffolds that passed CheckV filtering were designated as viral scaffolds; this final set of scaffolds had an average scaffold length of 3.97 kb, with 703 of the scaffolds derived from the creek samples, 2239 from the endolithic samples, and 3348 from the acidic soil samples.
Taxonomic classification of virus scaffolds
Taxonomic classification of viral scaffolds was done using the parsed geNomad output and a majority rule approach using DIAMOND BLASTp top hits from the predicted proteins against the non-redundant Genbank (NR) and IMG/VR v4 databases, processed using a custom Python script ( https://github.com/LFelipe-B/YNP_Lemonade_Creek_viruses ).
Clustering of virus scaffolds into virus operational taxonomic units (vOTUs)
Predicted viral scaffolds that shared >95% average nucleotide identity and >85% alignment fraction were clustered and dereplicated into vOTUs using BLASTn (“-outfmt ‘6 std qlen slen’ -max_target_seqs 10000”) and the anicalc and aniclust python scripts (“--min_ani 95 --min_tcov 85 --min_qcov 0”) available with CheckV ( https://bitbucket.org/berkeleylab/checkv/src/master/scripts/ ). Clustering thresholds were chosen according to community standards 88 .
vOTU abundance across samples
The abundance of the identified vOTUs was computed by CoverM v0.6.1 (“--min-read-percent-identity 98 --min-covered-fraction 0”; https://github.com/wwood/CoverM ) using corrected metagenome reads aligned with BBMap v38.87 (“ambiguous=random”) 80 against the vOTU sequences as well as contigs from other cellular organisms identified in the samples. The Reads Per Kilobase per Million mapped reads (RPKM) value was extracted for each metagenome sample and used for downstream analysis. We chose to perform downstream abundance analysis at the “class” taxonomic rank as this is the most complete rank across all viral lineages. That is, some viral groups, such as Caudoviricetes, lack an updated lower taxonomic classification, making its analysis and comparison with other lineages with, for example, family-level classifications, challenging. Taxonomic annotations where no “class” level information is described (i.e., environmental samples, unclassified archaeal viruses, unclassified viruses, or where only the realm was present) are considered “Unclassified” in our analysis.
vOTU alpha and beta diversity community analysis
Alpha diversity was estimated by calculating richness (Chao1 index), diversity (Shannon), and evenness with vegans at the class taxonomic level. To test the effect of sample type on the virus composition, we run a Permanova analysis (999 permutations) on a Bray–Curtis dissimilarity matrix constructed from the viral classes using the adonis2 function from the vegan 89 R package. To assess the sample clustering profiles, we then use the same dissimilarity matrix in a non-metric multidimensional scaling (NMDS) analysis using Vegan, with the dimensions plotted using the ggplot2 R package. We used the Shapiro test to assess the normality of the data. When the data were not normally distributed, we ran the non-parametric Kruskal-Wallis test, followed by the post-hoc Tukey–Kramer–Nemenyi test with p -values adjusted using Bonferroni correction.
Functional prediction of vOTU proteins
We used eggNOG-mapper v2.1.6 (“--pfam_realign denovo --report_orthologs”) 90 , 91 to functionally annotate the proteins predicted in the vOTUs. Gene ontology terms (GO terms; http://geneontology.org/docs/download-ontology/ ) were used to evaluate the functional landscape of the vOTUs, discarding the “obsolete” and generic terms before analysis: biological process (GO:0008150), biosynthetic process (GO:0009058), cellular process (GO:0009987), cellular component (GO:0005575), molecular function (GO:0003674), metabolic process (GO:0008152). We also use the KEGG (Kyoto Encyclopedia of Genes and Genomes — https://www.genome.jp/kegg/kegg1.html and PFAM (Protein families; https://www.ebi.ac.uk/interpro/entry/pfam/ ) databases to evaluate biological pathways and protein domains for vOTU predicted proteins (Supplementary Data 4 ).
Sequence similarity network construction with unclassified vOTU proteins
Viral protein clusters (vPCs) were derived from sequence similarity networks constructed using unclassified vOTU proteins combined with their top hits against GenBank NR and IMG/VR v4 databases. Before clustering, CD-HIT v4.8.1 92 , run using a 60% identity and 80% coverage cutoff (-c 0.60 -s 0.80 -n 4), was used to cluster similar proteins. The representative sequence from the CD-HIT analysis was then compared together using an all-against-all DIAMOND BLASTp ( e -value 1e-5, “--ultra-sensitive”) search, with best reciprocal blast hits removed using a custom python script (available at https://github.com/Lfelipe-B/YNP_Lemonade_Creek_viruses ). The results were constructed into a network by the igraph v4.2.1R package 93 , with singleton proteins and less connected components with degrees < 8, removed from the final network before visualization (Supplementary Data 4 ).
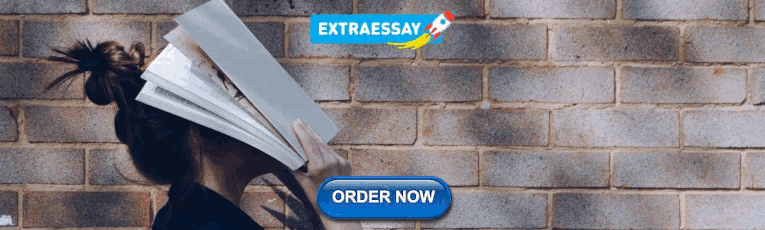
vOTU horizontal gene transfer analysis
To search for horizontal gene transfer (HGT) candidates between cellular life and viruses, we calculated the Alien index (AI) 94 and the HGT index (hU) 95 for each protein in the vOTUs, grouping the results by viral class. We parse the full taxonomic annotations of the subject accession numbers from the DIAMOND BLASTp outputs against the non-redundant GenBank database (NR) using custom Python scripts (available at https://github.com/LFelipe-B/YNP_Lemonade_Creek_viruses ). Hits were categorized as “non-alien” if the subject sequence was from a virus protein and as “alien” if the subject sequence was from a eukaryote, bacteria, or archaea; hits to the same viral phylogenetic group of the vOTU sequences were omitted from the subsequent calculations to avoid self-hits with sequences from the same or similar taxa already submitted to GenBank. vOTU proteins that had AI and hU indices ≥30, indicating that they have better scoring hits to non-viral proteins than to viral proteins, were considered putative candidates for HGT between viruses and cellular taxa. The putative sources of HGTs in each viral class was evaluated using the domain and/or phylum taxonomic rank of the best cellular hits from each putative HGT (Supplementary Data 5 ). Available scripts for this analysis at https://github.com/LFelipe-B/YNP_Lemonade_Creek_viruses .
Virus scaffold binning into virus metagenome-assembled genomes (vMAGs)
The corrected reads from each of the metagenome samples were aligned against each of the metagenome assemblies using BBMap v38.87 80 (“ambiguous=random rgid=filename”), with the resulting mapped reads sorted using samtools sort 96 v1.11. For each metagenome assembly, the “jgi_summarize_bam_contig_depths” tool from MetaBAT2 97 v2.15 was used to calculate the scaffold read mapping depth in each of the corresponding bam files produced from the mapping of the corrected metagenomic reads. The depth information for each of the vOTUs was extracted from their respective metagenome assemblies and used by Metabat2 to produce viral bins, which were assessed for quality and completeness using CheckV. Bins that were considered with completeness as low (30–50%), medium (50–90%), high (>90%), or complete (100%) according to CheckV quality score were retained for downstream analysis and subsequently referred to as viral metagenome-assembled genomes (vMAGs). There were, in total, 1082 vOTUs recruited into the vMAGs. Taxonomic annotation as performed by a majority consensus rule using top hits from all the predicted ORFs in each vMAG, with the lowest taxonomic rank that still satisfies the majority threshold (60%) assigned as a vMAG’s final classification.
vMAG viral marker genes and phylogenetic profile
We screened vMAGs for the presence of viral marker genes to delineate lower taxonomic ranks (below class level) and solve their specific phylogenetic relationships. For this we checked the predicted annotations, DIAMOND BLASTp output files from previous initial searches, and for the presence of giant virus hallmark genes from the Nucleo-Cytoplasmic Virus Orthologous Groups (NCVOGs) 43 using DIAMOND BLASTp, and for Giant Virus Orthologous Groups (GVOGs) using the tool “ncldv_markersearch” ( https://github.com/faylward/ncldv_markersearch ) 98 . Final taxonomic annotations, environmental and host sources of viral hits were retrieved from Giant Virus Database ( https://faylward.github.io/GVDB/ ), IMG/V ( https://img.jgi.doe.gov ) and Virus Host database ( https://www.genome.jp/virushostdb ). To confirm the presence of NCVOGs and GVOGs in specific vMAGs we subjected candidate marker proteins from vMAGs to a BLASTp search using the online suite ( https://blast.ncbi.nlm.nih.gov/Blast.cgi ), removing false positives (i.e., wrong marker gene or non-viral sequence). We keep only nine markers that overlapped in both NCVOGs and GVOGs databases since these are the most well-conserved genes for reconstructing evolutionary relationships within this viral group 99 : B DNA Polymerase (PolB), A32-like packaging ATPase (A32), virus late transcription factor 3 (VLTF3), superfamily II helicase (SFII), major capsid protein (MCP), the large and small RNA polymerase subunits (RNAPL and RNAPS, respectively), TFIIB transcriptional factor (TFIIB), and Topoisomerase family II (TopoII) (Supplementary Data 6 , Supplementary Data 8 ). Next, we combined proteins from GenBank and IMGV/V4 with vMAG candidates and performed a multiple sequence alignment (MSA) using MAFFT v7.305b (L-INS-i algorithm: “--localpair --maxiterate 1000”) 100 , with the resulting alignments trimmed using TrimAI v1.2 in automated mode (-automated1) 101 . Individual maximum likelihood phylogenies were built using IQ-TREE v2.0.3 102 with the ModelFinder option (-m TEST) 103 and 1000 bootstrap replicates (UFBoot) 104 . Phylogenies were midpoint rooted, visualized, and edited using the R packages ape v5.6-2 105 , phangorn v2.10.0 106 , ggtree v3.4.4 107 , phytools v1.2-0 108 (Revell 2012), and ggnewscale v0.4.9 ( https://github.com/eliocamp/ggnewscale ) in the R environment (version 4.2.1). Average amino acid identity (AAI) when compared to reference viral genomes was calculated using the tool “AAI calculator from http://enve-omics.ce.gatech.edu/aai/ (Supplementary Data 9 ).
Virus genomic signature analysis
We downloaded complete viral reference genomes from GenBank ( https://www.ncbi.nlm.nih.gov/nuccore ) for species that showed proximity to our viral proteins in individual phylogenetic marker gene trees or that had top hits against our vMAGs (full list and accession numbers in Supplementary Data 7 ). Amino acid frequency and protein isoelectric point (pI) were calculated for each protein per genome using the functions “AAstat” and “computePI” from the R v4.2.1 package seqinr v4.2-30 109 . To calculate relative synonymous codon usage (RSCU) tables from predicted coding sequences (CDS) we use the uco function (‘index = “rscu”’) from the seqinr package. The purine loading index (PLI) per CDS was calculated using the formula from Cristillo et al. 108 and Lambros et al. 73 , where we first counted the proportion of purines (A + G) and pyrimidines (C + T), we then calculated Δ W = ( A − T )/ N * 1000 and Δ S = ( G − C )/ N * 1000, where N is the total number of bases in the CDS and 1000 is to convert it to per kilobase, and finally the PLI = Δ W + Δ S . To calculate cytosine and guanine percentage (GC%) from each CDSs we used a custom Python script available at https://github.com/LFelipe-B/YNP_Lemonade_Creek_viruses . For the prediction of optimal growth temperatures for vMAGs and viral reference genomes, we use the tool “CnnPOGTP” 109 available at www.orgene.net/CnnPOGTP , which is based on deep learning of k -mers distribution from genomic sequence.
Statistics and reproducibility
To test for differences in amino acid representation and codon usage between vMAGs and viral reference genomes, we used ANOVA, followed by Tukey’s post hoc test. To compare genomic signatures among vMAGs and reference viral genomes, we used t -test or ANOVA, Wilcoxon rank test, or Kruskal–Wallis rank test in the case data was not normally distributed according to the Shapiro–Wilk normality test, with all p -values adjusted for multiple testing. Statistical analysis was conducted in R v4.2.1. The reproducibility of experiments is not relevant here. However, details about sample sizes and number of replicates and how replicates were defined are described above in the Methods section.
Reporting summary
Further information on research design is available in the Nature Portfolio Reporting Summary linked to this article.
Data availability
Files containing vOTUs and vMAGs fasta sequences, and vMAG newick phylogenetic trees are available at https://doi.org/10.5281/zenodo.10465700 .
Code availability
Scripts to run parts of this analysis are available at https://github.com/LFelipe-B/YNP_Lemonade_Creek_viruses .
Allioux, M. et al. A metagenomic insight into the microbiomes of geothermal springs in the Subantarctic Kerguelen Islands. Sci. Rep. 12 , 22243 (2022).
Article ADS PubMed PubMed Central Google Scholar
Castenholz, R. W. & McDermott, T. R. The Cyanidiales: Ecology, Biodiversity, and Biogeography. in Red Algae in the Genomic Age (eds. Seckbach, J. & Chapman, D. J.) 357–371 (Springer Netherlands, Dordrecht, 2010).
Inskeep, W. P. et al. Metagenomes from high-temperature chemotrophic systems reveal geochemical controls on microbial community structure and function. PLoS ONE 5 , e9773 (2010).
Bolduc, B., Wirth, J. F., Mazurie, A. & Young, M. J. Viral assemblage composition in Yellowstone acidic hot springs assessed by network analysis. ISME J. 9 , 2162–2177 (2015).
Article CAS PubMed PubMed Central Google Scholar
Cho, C. H. et al. Genome-wide signatures of adaptation to extreme environments in red algae. Nat. Commun. 14 , 10 (2023).
Article ADS CAS PubMed PubMed Central Google Scholar
López-López, O., Cerdán, M. E. & González-Siso, M. I. Hot spring metagenomics. Life Open Access J. 3 , 308–320 (2013).
Google Scholar
Breitbart, M., Wegley, L., Leeds, S., Schoenfeld, T. & Rohwer, F. Phage community dynamics in hot springs. Appl. Environ. Microbiol. 70 , 1633–1640 (2004).
Schoenfeld, T. et al. Assembly of viral metagenomes from Yellowstone Hot Springs. Appl. Environ. Microbiol. 74 , 4164–4174 (2008).
Dávila-Ramos, S. et al. A review on viral metagenomics in extreme environments. Front. Microbiol . 10 , 2403 (2019).
Munson-McGee, J. H., Rooney, C. & Young, M. J. An uncultivated virus infecting a nanoarchaeal parasite in the hot springs of Yellowstone National Park. J. Virol. 94 , e01213–e01219 (2020).
Márquez, L. M., Redman, R. S., Rodriguez, R. J. & Roossinck, M. J. A virus in a fungus in a plant: three-way symbiosis required for thermal tolerance. Science 315 , 513–515 (2007).
Article ADS PubMed Google Scholar
Zhou, J. et al. Three novel virophage genomes discovered from Yellowstone Lake Metagenomes. J. Virol. 89 , 1278–1285 (2014).
Article PubMed PubMed Central Google Scholar
Zhang, W. et al. Four novel algal virus genomes discovered from Yellowstone Lake metagenomes. Sci. Rep. 5 , 15131 (2015).
Yoshikawa, G. et al. Medusavirus, a Novel large DNA virus discovered from hot spring water. J. Virol . https://doi.org/10.1128/jvi.02130-18 (2019).
Breitbart, M., Bonnain, C., Malki, K. & Sawaya, N. A. Phage puppet masters of the marine microbial realm. Nat. Microbiol. 3 , 754–766 (2018).
Article CAS PubMed Google Scholar
Reysenbach, A.-L. & Cady, S. L. Microbiology of ancient and modern hydrothermal systems. Trends Microbiol. 9 , 79–86 (2001).
Qin, J. et al. Biotransformation of arsenic by a Yellowstone thermoacidophilic eukaryotic alga. Proc. Natl Acad. Sci. USA 106 , 5213–5217 (2009).
Schönknecht, G. et al. Gene transfer from bacteria and archaea facilitated evolution of an extremophilic eukaryote. Science 339 , 1207–1210 (2013).
Qiu, H. et al. Adaptation through horizontal gene transfer in the cryptoendolithic red alga Galdieria phlegrea. Curr. Biol. 23 , R865–R866 (2013).
Rossoni, A. W. et al. The genomes of polyextremophilic cyanidiales contain 1% horizontally transferred genes with diverse adaptive functions. eLife 8 , e45017 (2019).
Wang, L. et al. Endogenous viral elements in algal genomes. Acta Oceanol. Sin. 33 , 102–107 (2014).
CAS Google Scholar
Nelson, D. R. et al. Large-scale genome sequencing reveals the driving forces of viruses in microalgal evolution. Cell Host Microbe 29 , 250–266.e8 (2021).
Hwang, Y., Roux, S., Coclet, C., Krause, S. J. E. & Girguis, P. R. Viruses interact with hosts that span distantly related microbial domains in dense hydrothermal mats. Nat. Microbiol. 8 , 946–957 (2023).
Carreira, C. et al. Microscale spatial distributions of microbes and viruses in intertidal photosynthetic microbial mats. SpringerPlus 4 , 239 (2015).
Fernández, L., Rodríguez, A. & García, P. Phage or foe: an insight into the impact of viral predation on microbial communities. ISME J. 12 , 1171–1179 (2018).
Nguyen, V. et al. Evolutionary drivers of thermoadaptation in enzyme catalysis. Science 355 , 289–294 (2017).
Article ADS CAS PubMed Google Scholar
Ciccarelli, F. D. et al. Toward automatic reconstruction of a highly resolved tree of life. Science 311 , 1283–1287 (2006).
Gaucher, E. A., Govindarajan, S. & Ganesh, O. K. Palaeotemperature trend for Precambrian life inferred from resurrected proteins. Nature 451 , 704–707 (2008).
Akanuma, S. et al. Experimental evidence for the thermophilicity of ancestral life. Proc. Natl Acad. Sci. USA 110 , 11067–11072 (2013).
Roux, S., Emerson, J. B., Eloe-Fadrosh, E. A. & Sullivan, M. B. Benchmarking viromics: an in silico evaluation of metagenome-enabled estimates of viral community composition and diversity. PeerJ 5 , e3817 (2017).
Roux, S. et al. Minimum Information about an Uncultivated Virus Genome (MIUViG). Nat. Biotechnol. 37 , 29–37 (2019).
Nayfach, S. et al. CheckV assesses the quality and completeness of metagenome-assembled viral genomes. Nat. Biotechnol. 39 , 578–585 (2021).
Aylward, F. O., Moniruzzaman, M., Ha, A. D. & Koonin, E. V. A phylogenomic framework for charting the diversity and evolution of giant viruses. PLoS Biol. 19 , e3001430 (2021).
Roux, S. et al. Updated virophage taxonomy and distinction from Polinton-like viruses. Biomolecules 13 , 204 (2023).
Woo, A. C., Gaia, M., Guglielmini, J., Da Cunha, V. & Forterre, P. Phylogeny of the Varidnaviria morphogenesis module: congruence and incongruence with the tree of life and viral taxonomy. Front. Microbiol. 12 , 704052 (2021).
Patel, M. R., Emerman, M. & Malik, H. S. Paleovirology—ghosts and gifts of viruses past. Curr. Opin. Virol. 1 , 304–309 (2011).
Kondo, H., Botella, L. & Suzuki, N. Mycovirus diversity and evolution revealed/inferred from recent studies. Annu. Rev. Phytopathol. 60 , 307–336 (2022).
Wang, F. et al. Structure of a filamentous virus uncovers familial ties within the archaeal virosphere. Virus Evol. 6 , veaa023 (2020).
Oguiza, J. A. LysM proteins in mammalian fungal pathogens. Fungal Biol. Rev. 40 , 114–122 (2022).
Article CAS Google Scholar
Dheekollu, J. & Lieberman, P. M. The replisome pausing factor Timeless is required for episomal maintenance of latent Epstein-Barr virus. J. Virol. 85 , 5853–5863 (2011).
Vipat, S. & Moiseeva, T. N. The TIMELESS roles in genome stability and beyond. J. Mol. Biol. 436 , 168206 (2024).
Gaïa, M. et al. Mirusviruses link herpesviruses to giant viruses. Nature 616 , 783–789 (2023).
Yutin, N., Wolf, Y. I., Raoult, D. & Koonin, E. V. Eukaryotic large nucleo-cytoplasmic DNA viruses: clusters of orthologous genes and reconstruction of viral genome evolution. Virol. J. 6 , 223 (2009).
Abrahão, J. et al. Tailed giant Tupanvirus possesses the most complete translational apparatus of the known virosphere. Nat. Commun. 9 , 749 (2018).
Bäckström, D. et al. Virus genomes from deep sea sediments expand the ocean megavirome and support independent origins of viral gigantism. mBio 10 , e02497–18 (2019).
Legendre, M. et al. Diversity and evolution of the emerging Pandoraviridae family. Nat. Commun. 9 , 2285 (2018).
Rigou, S., Santini, S., Abergel, C., Claverie, J.-M. & Legendre, M. Past and present giant viruses diversity explored through permafrost metagenomics. Nat. Commun. 13 , 5853 (2022).
Benamar, S. et al. Faustoviruses: comparative genomics of new Megavirales family members. Front. Microbiol. 7 , 3 (2016).
Andreani, J. et al. Pacmanvirus, a New giant icosahedral virus at the crossroads between Asfarviridae and faustoviruses. J. Virol. 91 , e00212-17 (2017).
Zeldovich, K. B., Berezovsky, I. N. & Shakhnovich, E. I. Protein and DNA sequence determinants of thermophilic adaptation. PLoS Comput. Biol. 3 , e5 (2007).
Sabath, N., Ferrada, E., Barve, A. & Wagner, A. Growth temperature and genome size in bacteria are negatively correlated, suggesting genomic streamlining during thermal adaptation. Genome Biol. Evol. 5 , 966–977 (2013).
Alcorta, J., Alarcón-Schumacher, T., Salgado, O. & Díez, B. Taxonomic novelty and distinctive genomic features of hot spring cyanobacteria. Front. Genet. 11 , 568223 (2020).
Benites, L. F. & Alves-Lima, C. Viruses of Rhodophyta: lack of cultures and genomic resources pose a threat to the growing red algal aquaculture industry. Appl. Phycol. 3 , 285–299 (2022).
Article Google Scholar
Di Giulio, M. The ancestor of the Bacteria domain was a hyperthermophile. J. Theor. Biol. 224 , 277–283 (2003).
Article ADS MathSciNet PubMed Google Scholar
Pollo, S. M. J., Zhaxybayeva, O. & Nesbø, C. L. Insights into thermoadaptation and the evolution of mesophily from the bacterial phylum Thermotogae. Can. J. Microbiol. 61 , 655–670 (2015).
Weiss, M. C. et al. The physiology and habitat of the last universal common ancestor. Nat. Microbiol. 1 , 16116 (2016).
Abramov, O. & Mojzsis, S. J. Microbial habitability of the Hadean Earth during the late heavy bombardment. Nature 459 , 419–422 (2009).
Sleep, N. H. The Hadean-Archaean environment. Cold Spring Harb. Perspect. Biol. 2 , a002527 (2010).
Damer, B. & Deamer, D. The Hot Spring Hypothesis for an origin of life. Astrobiology 20 , 429–452 (2020).
Leng, H., Wang, Y., Zhao, W., Sievert, S. M. & Xiao, X. Identification of a deep-branching thermophilic clade sheds light on early bacterial evolution. Nat. Commun. 14 , 4354 (2023).
Castelle, C. J. & Banfield, J. F. Major new microbial groups expand diversity and alter our understanding of the tree of life. Cell 172 , 1181–1197 (2018).
Zhu, Q. et al. Phylogenomics of 10,575 genomes reveals evolutionary proximity between domains Bacteria and Archaea. Nat. Commun. 10 , 5477 (2019).
Coleman, G. A. et al. A rooted phylogeny resolves early bacterial evolution. Science 372 , eabe0511 (2021).
Taylor, T. J. & Vaisman, I. I. Discrimination of thermophilic and mesophilic proteins. BMC Struct. Biol. 10 , S5 (2010).
van Noort, V. et al. Consistent mutational paths predict eukaryotic thermostability. BMC Evol. Biol. 13 , 7 (2013).
Thompson, M. J. & Eisenberg, D. Transproteomic evidence of a loop-deletion mechanism for enhancing protein thermostability. J. Mol. Biol. 290 , 595–604 (1999).
Lobry, J. R. & Necşulea, A. Synonymous codon usage and its potential link with optimal growth temperature in prokaryotes. Gene 385 , 128–136 (2006).
Gao, J. & Wang, W. Analysis of structural requirements for thermo-adaptation from orthologs in microbial genomes. Ann. Microbiol. 62 , 1635–1641 (2012).
Kawashima, T. et al. Archaeal adaptation to higher temperatures revealed by genomic sequence of Thermoplasma volcanium . Proc. Natl Acad. Sci. USA 97 , 14257–14262 (2000).
Seshadri, R. et al. Complete genome sequence of the Q-fever pathogen Coxiella burnetii . Proc. Natl Acad. Sci. USA 100 , 5455–5460 (2003).
Khan, M. F. & Patra, S. Deciphering the rationale behind specific codon usage pattern in extremophiles. Sci. Rep. 8 , 15548 (2018).
De Farias, S. T. & Bonato, M. C. M. Preferred codons and amino acid couples in hyperthermophiles. Genome Biol. 3 , PREPRINT0006 (2002).
Article PubMed Google Scholar
Lambros, R. J., Mortimer, J. R. & Forsdyke, D. R. Optimum growth temperature and the base composition of open reading frames in prokaryotes. Extrem. Life Extrem. Cond. 7 , 443–450 (2003).
Lao, P. J. & Forsdyke, D. R. Thermophilic bacteria strictly obey Szybalski’s transcription direction rule and politely purine-load RNAs with both adenine and guanine. Genome Res. 10 , 228–236 (2000).
Van Etten, J., Cho, C. H., Yoon, H. S. & Bhattacharya, D. Extremophilic red algae as models for understanding adaptation to hostile environments and the evolution of eukaryotic life on the early earth. Semin. Cell Dev. Biol. 134 , 4–13 (2023).
Bolduc, B. et al. Identification of novel positive-strand RNA viruses by metagenomic analysis of archaea-dominated Yellowstone hot springs. J. Virol. 86 , 5562–5573 (2012).
Stedman, K. M., Kosmicki, N. R. & Diemer, G. S. Codon usage frequency of RNA virus genomes from high-temperature acidic-environment metagenomes. J. Virol. 87 , 1919 (2013).
Le Lay, C., Stott, M. B., Shi, M., Sadiq, S. & Holmes, E. C. A metatranscriptomic analysis of geothermal hot springs reveals diverse RNA viruses including the phylum Lenarviricota. Virology 587 , 109873 (2023).
Yoon, H. S., Hackett, J. D., Ciniglia, C., Pinto, G. & Bhattacharya, D. A molecular timeline for the origin of photosynthetic eukaryotes. Mol. Biol. Evol. 21 , 809–818 (2004).
Bushnell, B. BBMap. https://sourceforge.net/projects/bbmap (2022).
Nurk, S., Meleshko, D., Korobeynikov, A. & Pevzner, P. A. metaSPAdes: a new versatile metagenomic assembler. Genome Res. 27 , 824–834 (2017).
Guo, J. et al. VirSorter2: a multi-classifier, expert-guided approach to detect diverse DNA and RNA viruses. Microbiome 9 , 37 (2021).
Camargo, A. P. et al. Identification of mobile genetic elements with geNomad. Nat. Biotechnol . https://doi.org/10.1038/s41587-023-01953-y (2023).
Buchfink, B., Xie, C. & Huson, D. H. Fast and sensitive protein alignment using DIAMOND. Nat. Methods 12 , 59–60 (2015).
Camargo, A. P. et al. IMG/VR v4: an expanded database of uncultivated virus genomes within a framework of extensive functional, taxonomic, and ecological metadata. Nucleic Acids Res. 51 , D733–D743 (2023).
Hyatt, D. et al. Prodigal: prokaryotic gene recognition and translation initiation site identification. BMC Bioinforma. 11 , 119 (2010).
Oksanen, F. J. et al. Vegan: Community Ecology Package. R package Version 2.4-3. https://CRAN.R-project.org/package=vegan (2017).
Huerta-Cepas, J. et al. eggNOG 5.0: a hierarchical, functionally and phylogenetically annotated orthology resource based on 5090 organisms and 2502 viruses. Nucleic Acids Res. 47 , D309–D314 (2019).
Cantalapiedra, C. P., Hernández-Plaza, A., Letunic, I., Bork, P. & Huerta-Cepas, J. eggNOG-mapper v2: functional annotation, orthology assignments, and domain prediction at the metagenomic scale. Mol. Biol. Evol. 38 , 5825–5829 (2021).
Fu, L., Niu, B., Zhu, Z., Wu, S. & Li, W. CD-HIT: accelerated for clustering the next-generation sequencing data. Bioinforma. Oxf. Engl. 28 , 3150–3152 (2012).
Csardi, G., Nepusz, T. The igraph software package for complex network research. InterJ. Complex Syst. https://igraph.org (2006).
Gladyshev, E. A., Meselson, M. & Arkhipova, I. R. Massive horizontal gene transfer in bdelloid rotifers. Science 320 , 1210–1213 (2008).
Boschetti, C. et al. Biochemical diversification through foreign gene expression in bdelloid rotifers. PLoS Genet. 8 , e1003035 (2012).
Danecek, P. et al. Twelve years of SAMtools and BCFtools. GigaScience 10 , giab008 (2021).
Kang, D. D. et al. MetaBAT 2: an adaptive binning algorithm for robust and efficient genome reconstruction from metagenome assemblies. PeerJ 7 , e7359 (2019).
Moniruzzaman, M., Martinez-Gutierrez, C. A., Weinheimer, A. R. & Aylward, F. O. Dynamic genome evolution and complex virocell metabolism of globally-distributed giant viruses. Nat. Commun. 11 , 1710 (2020).
Koonin, E. V. & Yutin, N. Multiple evolutionary origins of giant viruses. F1000Research 7 , F1000 (2018). Faculty Rev-1840.
Katoh, K. & Standley, D. M. MAFFT multiple sequence alignment software version 7: improvements in performance and usability. Mol. Biol. Evol. 30 , 772–780 (2013).
Capella-Gutiérrez, S., Silla-Martínez, J. M. & Gabaldón, T. trimAl: a tool for automated alignment trimming in large-scale phylogenetic analyses. Bioinformatics 25 , 1972–1973 (2009).
Nguyen, L.-T., Schmidt, H. A., von Haeseler, A. & Minh, B. Q. IQ-TREE: a fast and effective stochastic algorithm for estimating maximum-likelihood phylogenies. Mol. Biol. Evol. 32 , 268–274 (2015).
Kalyaanamoorthy, S., Minh, B. Q., Wong, T. K. F., von Haeseler, A. & Jermiin, L. S. ModelFinder: fast model selection for accurate phylogenetic estimates. Nat. Methods 14 , 587–589 (2017).
Minh, B. Q., Nguyen, M. A. T. & von Haeseler, A. Ultrafast approximation for phylogenetic bootstrap. Mol. Biol. Evol. 30 , 1188–1195 (2013).
Paradis, E. & Schliep, K. ape 5.0: an environment for modern phylogenetics and evolutionary analyses in R. Bioinforma. Oxf. Engl. 35 , 526–528 (2019).
Schliep, K. P. phangorn: phylogenetic analysis in R. Bioinformatics 27 , 592–593 (2011).
Yu, G., Smith, D. K., Zhu, H., Guan, Y. & Lam, T. T.-Y. ggtree: an r package for visualization and annotation of phylogenetic trees with their covariates and other associated data. Methods Ecol. Evol. 8 , 28–36 (2017).
Revell, L. J. phytools: an R package for phylogenetic comparative biology (and other things). Methods Ecol. Evol. 3 , 217–223 (2012).
Charif, D. & Lobry, J. R. SeqinR 1.0-2: A contributed package to the R project for statistical computing devoted to biological sequences retrieval and analysis. in Structural Approaches to Sequence Evolution: Molecules, Networks, Populations (eds. Bastolla, U., Porto, M., Roman, H. E. & Vendruscolo, M.) 207–232 (Springer, Berlin, Heidelberg, 2007).
Cristillo, A. D., Mortimer, J. R., Barrette, I. H., Lillicrap, T. P. & Forsdyke, D. R. Double-stranded RNA as a not-self alarm signal: to evade, most viruses purine-load their RNAs, but some (HTLV-1, Epstein-Barr.) pyrimidine-load. J. Theor. Biol. 208 , 475–491 (2001).
Wang, S. et al. CnnPOGTP: a novel CNN-based predictor for identifying the optimal growth temperatures of prokaryotes using only genomic k-mers distribution. Bioinformatics 38 , 3106–3108 (2022).
Article MathSciNet CAS PubMed Google Scholar
Download references
Acknowledgements
L.F.B. was supported by the NASA Postdoctoral Program at Rutgers University, New Brunswick, administered by Oak Ridge Associated Universities under contract with NASA. J.V.E. was supported by National Aeronautics and Space Administration Future Investigators in NASA Earth and Space Science and Technology (FINESST grant 80NSSC19K1542). T.G.S. and D.B. were supported by a grant from NASA (80NSSC19K0462) awarded to D.B. D.B. was also supported by a NIFA-USDA Hatch grant (NJ01180). T.R.M. was supported by a grant from NASA (80NSSC21K0487). We would also like to acknowledge our collaboration with the JGI. The work (proposal: 10.46936/10.25585/60000481) conducted by the U.S. Department of Energy Joint Genome Institute ( https://ror.org/04xm1d337 ), a DOE Office of Science User Facility, is supported by the Office of Science of the U.S. Department of Energy operated under Contract No. DE-AC02-05CH11231. Sampling at Lemonade Creek, YNP was conducted under Research Permit YELL-2020-SCI-5364 issued to T.R.M.
Author information
Authors and affiliations.
Department of Biochemistry and Microbiology, Rutgers, The State University of New Jersey, New Brunswick, NJ, 08901, USA
L. Felipe Benites, Timothy G. Stephens, Julia Van Etten, Timeeka James & Debashish Bhattacharya
Graduate Program in Ecology and Evolution, Rutgers, The State University of New Jersey, New Brunswick, NJ, 08901, USA
Julia Van Etten
Department of Land Resources and Environmental Sciences, Montana State University, Bozeman, Montana, USA
William C. Christian
U.S. Department of Energy Joint Genome Institute, Lawrence Berkeley National Laboratory, Berkeley, CA, 94720, USA
Kerrie Barry & Igor V. Grigoriev
Department of Plant and Microbial Biology, University of California Berkeley, Berkeley, CA, 94720, USA
Igor V. Grigoriev
Department of Chemistry and Biochemistry, Montana State University, Bozeman, Montana, USA
Timothy R. McDermott
You can also search for this author in PubMed Google Scholar
Contributions
D.B., L.F.B., and T.R.M. conceived and designed the project, sampling, and scope. K.B. and I.V.G supervised data collection at the Joint Genome Institute. T.R.M. and W.C.C. performed sampling at Yellowstone National Park. L.F.B, T.G.S, J.V.E., and T.J. analyzed data. L.F.B, T.G.S., J.V.E., T.R.M., and D.B. wrote the paper, and all authors reviewed and approved the final version of this paper.
Corresponding author
Correspondence to Debashish Bhattacharya .
Ethics declarations
Competing interests.
The authors declare no competing interests.
Peer review
Peer review information.
Communications Biology thanks Frank Aylward, Marike Palmer, and Emily Chase for their contribution to the peer review of this work. Primary Handling Editor: Luke R. Grinham. A peer review file is available.
Additional information
Publisher’s note Springer Nature remains neutral with regard to jurisdictional claims in published maps and institutional affiliations.
Supplementary information
Peer review file, supplementary fig. 1, supplementary data 1, supplementary data 2, supplementary data 3, supplementary data 4, supplementary data 5, supplementary data 6, supplementary data 7, supplementary data 8, supplementary data 9, reporting summary, rights and permissions.
Open Access This article is licensed under a Creative Commons Attribution 4.0 International License, which permits use, sharing, adaptation, distribution and reproduction in any medium or format, as long as you give appropriate credit to the original author(s) and the source, provide a link to the Creative Commons licence, and indicate if changes were made. The images or other third party material in this article are included in the article’s Creative Commons licence, unless indicated otherwise in a credit line to the material. If material is not included in the article’s Creative Commons licence and your intended use is not permitted by statutory regulation or exceeds the permitted use, you will need to obtain permission directly from the copyright holder. To view a copy of this licence, visit http://creativecommons.org/licenses/by/4.0/ .
Reprints and permissions
About this article
Cite this article.
Felipe Benites, L., Stephens, T.G., Van Etten, J. et al. Hot springs viruses at Yellowstone National Park have ancient origins and are adapted to thermophilic hosts. Commun Biol 7 , 312 (2024). https://doi.org/10.1038/s42003-024-05931-1
Download citation
Received : 15 November 2023
Accepted : 16 February 2024
Published : 09 April 2024
DOI : https://doi.org/10.1038/s42003-024-05931-1
Share this article
Anyone you share the following link with will be able to read this content:
Sorry, a shareable link is not currently available for this article.
Provided by the Springer Nature SharedIt content-sharing initiative
By submitting a comment you agree to abide by our Terms and Community Guidelines . If you find something abusive or that does not comply with our terms or guidelines please flag it as inappropriate.
Quick links
- Explore articles by subject
- Guide to authors
- Editorial policies
Sign up for the Nature Briefing newsletter — what matters in science, free to your inbox daily.

Loading metrics
Open Access
Perspective
The Perspective section provides experts with a forum to comment on topical or controversial issues of broad interest.
See all article types »
Yellowstone Wolves and the Forces That Structure Natural Systems
* E-mail: [email protected]
Affiliations Department of Ecology and Evolutionary Biology, Princeton University, Princeton, New Jersey, United States of America, Santa Fe Institute, Santa Fe, New Mexico, United States of America
- Andy P. Dobson
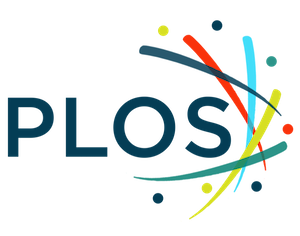
Published: December 23, 2014
- https://doi.org/10.1371/journal.pbio.1002025
- Reader Comments
Since their introduction in 1995 and 1996, wolves have had effects on Yellowstone that ripple across the entire structure of the food web that defines biodiversity in the Northern Rockies ecosystem. Ecological interpretations of the wolves have generated a significant amount of debate about the relative strength of top-down versus bottom-up forces in determining herbivore and vegetation abundance in Yellowstone. Debates such as this are central to the resolution of broader debates about the role of natural enemies and climate as forces that structure food webs and modify ecosystem function. Ecologists need to significantly raise the profile of these discussions; understanding the forces that structure food webs and determine species abundance and the supply of ecosystem services is one of the central scientific questions for this century; its complexity will require new minds, new mathematics, and significant, consistent funding.
Citation: Dobson AP (2014) Yellowstone Wolves and the Forces That Structure Natural Systems. PLoS Biol 12(12): e1002025. https://doi.org/10.1371/journal.pbio.1002025
Copyright: © 2014 Andy P. Dobson. This is an open-access article distributed under the terms of the Creative Commons Attribution License , which permits unrestricted use, distribution, and reproduction in any medium, provided the original author and source are credited.
Funding: A grant from the James McDonnell Foundation supports my work on food webs. The funders had no role in study design, data collection and analysis, decision to publish, or preparation of the manuscript.
Competing interests: The author has declared that no competing interests exist.
In the half moonlight at dawn on a sharply cold January morning, they looked like small ponies galloping beside the old railroad at the northern entrance of Yellowstone National Park. They weren't ponies. This was the “Eight Mile” wolf pack, each member huge, healthy, and vigorous, romping through the light snow on a morning quest for elk, bison, or anyone too slow to get out of their way. It was an incredible moment, one that evoked feelings shared by the hundreds of wolf watchers who come to Yellowstone every month of the year hoping to experience even a glimpse of the wolves.
The enthusiasm of the wolf watchers is almost totally reversed by the many local ranchers who live outside the park and regard wolves as varmints, best used for target practice. The pack that I heard howling outside the cabin every night was quickly dispatched by the local rancher soon after I left; their pelts could be found for sale in one of the souvenir stores at the entrance to Yellowstone.
Scientists initially appear as polarized in their opinions of the role of wolves and large predators in ecosystems as the wolf watchers and ranchers are about their value to the local economy. Wolves were introduced back into Yellowstone following the development of a huge environmental impact assessment (EIA) that attempted to predict the outcome of their reintroduction. The EIA, a 4-ft-deep pile of documents, provided solid testimony to the need for a deeper empirical and theoretical understanding of how ecological food webs respond to species additions and losses. At the time, even the suggestion of introducing wolves created huge discord in the ranching community surrounding Yellowstone; most ranchers (and some ecologists) were convinced wolves would feed exclusively on cattle and sheep; the ranching industry was dead set against reintroduction. A curious event then occurred: photographers started getting photographs of wolves that had naturally colonized the park. As any natural colonization would provide the wolves with full legal protection under the United States Endangered Species Act, the Ranchers Association hastily made a U-turn and supported introduction on the grounds that experimentally introduced wolves were nonnative and could be shot if they left the park. I know of no better environmental example of nonlinear political expediency.
Once introduced in 1995 and 1996, the wolf population grew rapidly. At the time, the elk population was declining from an all-time high and provided a large supply of prey to fuel wolf reproduction; the population increased at close to the maximum rate ever recorded [1] . As the wolf numbers increased, the elk numbers decreased, but at a rate that was more parsimoniously explained by a prolonged drought and levels of human harvest, the decline in abundance far exceeding that which could be accounted for purely in terms of elk consumed by wolves [2] , [3] . Significant evidence does suggest that the elk had changed their feeding habits in the presence of wolves, avoiding areas where they could readily be ambushed [3] – [8] . This allowed vegetation in riparian areas to recover; photographs taken at a variety of locations showed considerable recovery of aspen in areas where it had become overgrazed in the years when elk were abundant [1] , [9] . Although these riparian areas cover only a small area of the ecosystem (<2%), the park was witnessing the first significant growth of aspen for over half a century. More recent data suggest that similar recoveries are being seen in cottonwoods and willows [1] ; this in turn has led to an increase in the abundance and diversity of riparian bird species [10] . All of this evidence suggests that wolves have a strong top-down effect on trophic structure of the ecosystem ( Fig. 1 ).
- PPT PowerPoint slide
- PNG larger image
- TIFF original image
Photo credit: Daniel Stahler/National Park Service (NPS) photo from http://www.yellowstonewolf.org/ , a site at which information and pictures of Yellowstone wolves can be found.
https://doi.org/10.1371/journal.pbio.1002025.g001
Alternatively, climate has been argued to be the principal driver of ecosystem change, not wolves; changes in vegetation may have been driven by bottom-up changes in water availability due to changes in snow melt patterns [11] . Wolf population expansion occurred at a time when the Yellowstone region was entering a prolonged drought that also reduced forage available to elk; this combined with human harvest contributed significantly to the declines in ungulate abundance. Furthermore, climate change has lengthened the growing season for willows and aspen by around 27 days in the last couple of decades [12] , while the vegetation in many areas of the park is dominated by conifer forest that has simultaneously been recovering from the fires of 1989. Thus, it is not straightforward to differentiate between postfire recovery and the indirect effects of carnivores on vegetation regeneration.
Concomitant to wolf introduction, the grizzly bear population was increasing, creating the potential for indirect competition between bears and wolves as the latter selectively prey on old or injured elk in the winter. This predation reduces the number of elk that would otherwise die and become available for grizzlies emerging from hibernation in the spring. This absence of “frozen meals” caused grizzlies to switch to feeding on elk calves as an alternative spring food source when recovering from their long winter fast [13] . As elk numbers declined following the triple assault of drought, wolves, and bears, both grizzlies and some wolf packs switched their attention to bison [14] , which require larger packs to make an effective kill but ultimately provide a larger meal. All of the extra carcasses have provided a new bounty of food for ravens and golden eagles, both of which have increased in abundance [2] .
Less well understood is the impact of wolves on coyotes, the numbers of which may have declined since wolves were reintroduced [2] ; carnivores are aggressive to other carnivores of similar but slightly smaller body size. As coyotes were the primary predators of sheep, you would think that the sheep ranchers would applaud wolves for the reduced loss of stock to predators; they have been noticeably silent on this front. More subtly, the presence of wolves may help reduce the threat posed by chronic wasting disease (CWD), an emerging prion pathogen that is spreading from elk and deer to cattle and is arguably the biggest biological threat to ranching in the region [15] . Unfortunately, the ranching community does not recognize that the wolves may be doing them a huge favor by removing sick elk and mule deer infected with CWD (and elk and bison infected with brucellosis) from the wild reservoir of infection. If CWD or Brucella enters cattle herds in the states bordering Yellowstone, then federal mandates will hugely restrict movement of cattle in and out of these states.
We may have to wait at least another ten years before the impact of wolves on the Yellowstone ecosystem is fully quantified. Although many strong patterns are observed, several of these may be correlation without causation (for example, the increase in beaver abundance is more likely to be a consequence of beaver introductions to the north of Yellowstone National Park [NP] [12] ). Furthermore, although there is considerable pressure from the conservation community to sanctify the wolves as bringing only benefits to the ecosystem [12] , there is still a need for stronger data to support some of the beneficial claims made for wolf reintroduction. Some of this will come from Yellowstone, but this needs to be combined with studies of wolf reintroduction, or natural reestablishment, in other ecosystems. If the patterns observed in Yellowstone are repeated, as preliminary evidence suggests, then hard-core wolf haters are going to need to reconsider the labelling of wolves as varmints.
The research and debates surrounding the role of wolves in modifying the behavior and abundance of species on multiple trophic levels in Yellowstone illustrates the complex interactions between the forces that structure patterns of abundance in natural ecosystems. The debate gets to the heart of one of the central scientific challenge of ecology: how can we understand the structure of food webs? Central to any discussion of food-web dynamics and ecosystem management is the relative importance of top-down roles played by large predators and pathogens and bottom-up forces driven by the climatological processes that determine plant growth. All of the work from Yellowstone cries out for the development of next-generation, population-based ecosystem models that focus on interactions between climate, vegetation, and the dominant herbivore and carnivore species in the park. In particular, food-web ecologists need to more aggressively move beyond descriptions of the network geometry of food webs and grasp the thistle of food-web dynamics. More generally, we cannot afford for this debate to become polarized; that simply suggests to funding agencies and the general public that ecologists do not know how ecological systems function. Instead, we need to frame the discussion as a major scientific challenge that requires significant international and national funding.
There are curious and unexplored parallels between work on food webs and trophic interactions and that of physicists who are trying to understand the forces that determine the way the universe is structured at either the atomic or astronomical level. At both scales, a series of nested forces hold increasingly large particles together using a mixture of centripetal and gravitational forces, which operate essentially as bottom-up forces (although this is almost a metaphysical debating point!). Seen from this perspective, the current controversy about ecosystem-level effects of wolf reintroduction to Yellowstone NP is every bit as scientifically exciting as the recent discovery of the Higgs boson. Determining the strength of the forces produced by the loss or addition of particles or species to these very different systems are key scientific questions for the 21st century. Although each discipline uses very different types of equipment, budgets, and collaborations to undertake experiments that generate data for subsequent analysis, they each seek the answer to the same questions: “What are the fundamental forces that structure the universe in which we live, how do they operate, and how can we measure them?”
The hunt for the Higgs boson—an infinitely tiny particle whose energy is required to hold the interior particles of atoms in orbit—was an international research collaboration with a budget that exceeded all funding for ecology over the last ten years, perhaps even over the last century! In contrast, funding for work on natural ecological systems is usually cobbled together from a mixture of government and individual research funds; it is rarely clear from year to year when, or if, funds will appear for the next year's salaries. One benefit of working in national parks is that management occasionally allows experimental introductions, or removals, of species that permit investigation of the impact of these changes at ecosystem-level scales. However, the results of management experiments are rarely clear-cut and often ambiguous. It is all too easy to be critical about lack of controls and absence of replication (which is nontrivially a function of trivial budgets!), but understanding how food webs in national parks react to the addition and loss of species is as scientifically challenging as searching for tiny particles using very expensive particle accelerators. The central problem is ecological budgets are tiny compared to those for “big science,” so we need to use all sources of information that are available, including management exercises, to interpret findings at the appropriate ecosystem-level scale.
If we agree that physicists and ecologists are both trying to understand the forces that determine the structure of the universe, what are the major scientific differences between their approaches? Ecologists are focusing on understanding these forces at the spatial and temporal scales intermediate to that of physicists—less heroic, perhaps, but the scale that is directly relevant to humans. Less heroic or not, from the perspective of systems with interacting components, ecosystems and their constituent species will always be as complicated as those exhibited by atoms and bosons or galaxies and planets, perhaps more so; food webs have many different types of “particles” (species) that interact, evolve, and behave nonlinearly in a huge variety of time, and spatial, scales. Ultimately, we need to arrive at a realization that the mathematics of food webs and ecosystems is as complicated as that found in any of the problems of atomic and galactic structure studied in physics. Increasingly, we are realizing that the quality of human life on the planet depends on a deep functional understanding of the forces that structure the dynamics of food webs and the ecosystem services they provide to the human economy. We may even need new mathematics to deal with these levels and layers of complexity.
The current controversy about the role that wolves play in modifying the behavior and dynamics of other species in Yellowstone is a classic case study in this broader class of problems: it is about understanding how to measure forces and processes that act between operators at a variety of different spatial and temporal rates within a natural ecosystem that contains a diversity of natural heterogeneities (that initially appear to confound the search for broad patterns). If we re-pose ecology as the science that examines the forces that structure the central part of the universe in which we live, then more funding might be available to address these complexities; we would also simultaneously attract more bright minds willing to grapple with complexity.
From a much broader perspective, we need many more ecosystem-level studies of how species interactions between predators, parasites, and prey change the patterns of spatial heterogeneity in vegetation that ultimately drive levels of biodiversity at higher trophic levels. This is an exercise that requires a new generation of spatial, multispecies, multitrophic models and many more debates such as the current one about the role of wolves in Yellowstone. Resolving these discussions will allow ecologists to present a much stronger case to funding agencies and the general public for ecology to be recognized as the central scientific discipline of the 21st century. Ecology's mathematical problems are as complex as anything in physics, and their solutions are required with increasing urgency, particularly if we want to test these assumptions and predictions against viable natural ecosystems.
Acknowledgments
Time spent at the Santa Fe Institute constantly stimulates me to think about the need to understand problems of “complexity.” The ideas described here were developed from many hours spent in Yellowstone in conversation with Peter Hudson, Emily Almberg, Tim Coulson, Paul Cross, Mary Meagher, Doug Smith, Dan McNulty, Dan Stahler, Bridgett van Holdt, and Robert Wayne. Anieke van Leeuwen, Annarie Lyles, and Mercedes Pascual commented extensively on an earlier draft. The opinions expressed here are purely my own.
- View Article
- Google Scholar
- 2. Smith DW, Ferguson G (2012) Decade of the Wolf, revised and updated edition: Returning the Wild to Yellowstone. Guilford (Connecticut): Globe Pequot Press.

An official website of the United States government
Here’s how you know
Official websites use .gov A .gov website belongs to an official government organization in the United States.
Secure .gov websites use HTTPS A lock ( Lock A locked padlock ) or https:// means you’ve safely connected to the .gov website. Share sensitive information only on official, secure websites.
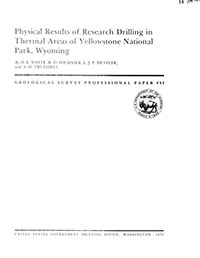
Physical results of research drilling in thermal areas of Yellowstone National Park, Wyoming
- Document: Report
- Download citation as: RIS | Dublin Core

- Apply to UW
- Programs & Majors
- Cost & Financial Aid
- Current Students
- UW Libraries
- Online Degrees
- Catalogs & Courses
- Degree Plans
- Advising & Career Services
- UW College of Law
- Honors College
- Academic Affairs
- Geological Museum
- All Colleges
- Campus Recreation
- Campus Maps
- Housing & Dining
- Transit & Parking
- University Store
- Student Organizations
- Campus Activities
- Campus Safety
- Diversity, Equity & Inclusion
- Research & Economic Dev.
- Wyoming INBRE
- Neuroscience Center
- Technology Business Center
- National Parks Service
- Research Production Center
- Supercomputing
- Water Research
- WY EPSCoR/IDeA
- American Heritage Center
- Where We Shine
- About Laramie
- Student Stories
- Campus Fact Book
- UWYO Magazine
- Marketing & Brand Center
- Administrative Resources
- Strategic Plan
- +Application Login
- UW Homepage
UW-Led Research: Yellowstone Lake Ice Cover Unchanged Despite Warming Climate
- News Releases
- Back to 2023 Archive
Institutional Communications Bureau of Mines Building, Room 137 Laramie, WY 82071 Phone: (307) 766-2929 Email: [email protected]

Published April 09, 2024
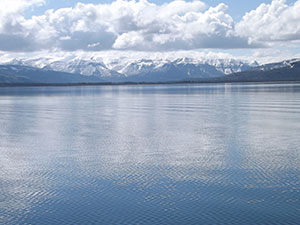
The length of time that Yellowstone Lake is covered by ice each year has not changed in the past century, despite warming temperatures in the region, according to new research led by University of Wyoming scientists.
That is an unexpected finding, as most lakes around the world are experiencing shorter durations of ice cover, the scientists note in a new paper published in the journal Environmental Research Letters .
“We show that contrary to expectation, the ice phenology of Yellowstone Lake has been uniquely resistant to climate change,” wrote the scientists, led by Lusha Tronstad, lead invertebrate zoologist with UW’s Wyoming Natural Diversity Database and Department of Zoology and Physiology, and Isabella Oleksy, a former UW postdoctoral researcher now on the University of Colorado-Boulder faculty. “The unchanging ice phenology of Yellowstone Lake stands in stark contrast to similar lakes in the Northern Hemisphere.”
Other researchers involved in the study are from Utah State University, Colorado State University and Colorado Mesa University.
Situated at 7,733 feet above sea level in the heart of Yellowstone National Park, Yellowstone Lake is North America’s largest high-elevation lake, roughly 20 miles long and 14 miles wide with a surface area of 132 square miles. It freezes over completely in late December or early January and usually thaws in late May or early June.
Records for the lake’s ice-off date have been recorded each year by Lake Village Ranger Station staff since 1927, and the ice-on date has been recorded since 1931. In addition to studying those records, the scientists analyzed climate data for the same period, 1927-2022, including air temperatures and precipitation. They also compared Yellowstone Lake’s data with seven similar lakes in northern Europe.
The lack of long-term change in the duration of Yellowstone Lake’s ice cover was unexpected because the Yellowstone region has seen a warming climate, the researchers say. Since 1950, annual temperatures have increased by 1.8 degrees Fahrenheit throughout the greater Yellowstone ecosystem. The changes are particularly pronounced at the high elevation of Yellowstone Lake, where air temperatures increased by about 2.5 degrees Fahrenheit between 1980-2018.
“Using local weather data, we found some evidence for increased summer, fall and spring temperatures, primarily in the last three decades,” the scientists wrote about air temperatures at Yellowstone Lake. “Given the key role of air temperatures in driving ice formation and break-up, it is noteworthy that we did not find evidence for corresponding shifts in ice phenology.”
Why the apparent discrepancy?
While it is possible that fall minimum temperatures -- which are important in predicting ice formation -- are not rising as quickly as overall temperature trends in the region, a more likely explanation is that increased snowfall at Yellowstone Lake has served as a buffer against warmer weather, the scientists say.
Snow cover, particularly in spring, can delay ice break-up. Cumulative spring snow, which was strongly correlated with delayed ice-off dates, has nearly doubled over the last century at Yellowstone Lake, the research showed. In general, precipitation has increased in spring and fall there.
That differs from the Upper Green River Basin to the south, where snowfall has declined or been relatively stable at high elevations.
“Shifts in local precipitation, especially increases in fall and spring snow, appear to be buffering (Yellowstone Lake) ice phenology against warming temperatures,” the researchers wrote.
But, the scientists are not sure how long this phenomenon will last, noting projections of continued warming and shifting precipitation regimes in the high Rocky Mountains.
“Our results, paired with recent analyses of climate projections, suggest a ‘tipping point’ may be coming when ice phenology abruptly changes for Yellowstone Lake,” they wrote. “This tipping point will largely stem from the ongoing shift from snow- to rain-dominated precipitation regimes in the fall and spring.
“… Increased spring rainfall has not yet caused a detectable long-term trend toward earlier ice break-up, potentially because of the counteracting effects of increased spring snow. As temperatures warm further, and fall and spring snowfall decreases, ice phenology may rapidly change on Yellowstone Lake.”
If that happens, “there may be wide-ranging consequences for nutrient cycling, lake productivity, fisheries and recreation,” the scientists concluded.

An official website of the United States government
Here's how you know
Official websites use .gov A .gov website belongs to an official government organization in the United States.
Secure .gov websites use HTTPS A lock ( ) or https:// means you’ve safely connected to the .gov website. Share sensitive information only on official, secure websites.
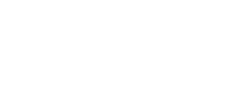
- Digg
Latest Earthquakes | Chat Share Social Media
Historic Water Chemistry Data for Thermal Features, Streams, and Rivers in the Yellowstone National Park Area, 1883-2021
Yellowstone National Park (YNP; Wyoming, Montana, and Idaho, USA) contains more than 10,000 hydrothermal features, several lakes, and four major watersheds. For more than 140 years, researchers at the U.S. Geological Survey and other scientific institutions have investigated the chemical compositions of hot springs, geysers, fumaroles, mud pots, streams, rivers, and lakes in YNP and surrounding areas. Water chemistry studies have revealed a range of compositions including waters with pH values ranging from about 1 to 10, surface temperatures from ambient to superheated values of 95°C, and elevated concentrations of silica, lithium, boron, fluoride, mercury, and arsenic. Hydrogeochemical data from YNP research have led to insights on subsurface conditions of temperature and chemistry, water-rock-gas interactions and processes of high-temperature mineral alteration with dissolution and precipitation, redox processes, thermophilic microbial metabolism under extreme conditions and effects of thermal water chemistry on river systems. In this Data Release, water chemistry data for 4,918 water samples are reported for numerous thermal features, rivers, streams, lakes, drillholes, and precipitation in and around YNP. The data for these samples were originally located in 38 reports published between 1888 and 2022 and in multiple unpublished documents. Spanning more than 600 unique sampling sites throughout the YNP region, this dataset includes samples collected as early as 1883 (Gooch & Whitfield, 1888) and as recently as 2021 (McCleskey, et al, 2022). The thermal features sampled most frequently include Cistern Spring (180 samples) and Echinus Geyser (73 samples) in Norris Geyser Basin and Ojo Caliente Spring (143 samples) in the Lower Geyser Basin, while more than 500 sites have 5 samples or fewer. Water chemistry data from thermal features, rivers, and streams are most represented, comprising 75% (thermal) and 17% (rivers/streams) of the dataset. Across all major areas of the park, Norris Geyser Basin has been sampled more than any other basin, with more than 1,100 samples reported in this dataset.
Citation Information
Related content, r. blaine mccleskey, research chemist, shaul hurwitz, research hydrologist, d. kirk nordstrom, hydrogeochemist emeritus.

Where to Find Yellowstone’s Captivating Wildlife, Indigenous Heritage, and Uncrowded Places
Here’s how to explore yellowstone, according to the experts..
- Copy Link copied

Indigenous people referred to Yellowstone National Park’s 10,000 thermal features as the “land of the burning ground.”
Courtesy of Morgan Newnham/Unsplash
The Greater Yellowstone Ecosystem offers plenty to see, including volcanic plateaus, alpine valleys, and mountain ranges—and thanks to the volcanic caldera hidden beneath the ground, geothermal features you won’t see anywhere else on Earth.
The 3,472-square-mile park in Northwestern Wyoming (and small sections of Montana and Idaho) is also home to the largest concentration of mammals in the lower 48, including the famous reintroduced wolves. In short, there are many reasons Yellowstone National Park pulls in more than 3 million visitors per year. Here are some insider tips on what you should know and where to go on your trip.

Be on the lookout for bighorn sheep and wildflowers on your way up Mount Washburn.
Photo by Jurekz/Shutterstock
Explore Yellowstone’s geological features
The first volcanic eruption in what scientists call the “ sleeping giant ” miles below Yellowstone took place 2.1 million years ago, when up to a foot of ash covered the entire western section of the United States. (Don’t worry, the last eruption was 70,000 years ago.)
At the National Historic Landmark of Obsidian Cliff , the molten lava that oozed beneath the park cooled into spiky black obsidian and was even used for toolmaking more than 11,000 years ago. Take in all the translucent dark cliffs of obsidian here along the roughly 20-mile-section of Grand Loop Road between Mammoth Hot Springs and Norris Geyser Basin .
Next up? Hike for about four hours past wildflowers and bighorn sheep to the 10,219-foot summit of nearby Mount Washburn , named after former U.S. Representative Henry Washburn. For dramatic bright blues and greens, head a couple of hours southwest to the waters of the Grand Prismatic Spring at Midway Geyser Basin.
Your trip to the world’s greatest concentration of geysers, hot springs, mud pots, and steam vents wouldn’t be complete without a stroll on the boardwalks of the hottest and oldest, Norris Geyser Basin , located in the north of the park next to the remains of a 600,000-year-old volcanic explosion, known as Mammoth Hot Springs .
“It’s always worth seeing Old Faithful and the other geysers in that area, but I would also recommend also visiting Fountain Paint Pots and Artists Paint Pots mud pots [hot springs filled with boiling mud] to get a good sense of the variety of hydrothermal features at Yellowstone,” says U.S. Geological Survey and Volcano Science Center geologist Mark Stelten, who is researching the magma stored under Yellowstone and triggers for potential future eruption.
And while many visitors know about Old Faithful, one of the best places to see the geyser is from the porch of the Yellowstone Tribal Heritage Center , which opened in 2022 to honor the region’s tribal communities through stories and artwork. Here, you can listen to presenters with ancestral ties to the tribes associated with the park, according to Alyssa McGeeley, a member of the Muscogee Nation with Yuchi heritage who is developing a strategic plan for tribal engagement in the park. McGeeley also recommends other spots with Indigenous history: “Sheepeater Cliff [in the northern part of the park] was once home to traditional wickiup lodges made of wooden poles, and Nez Perce Creek [in the park’s northeast] is a tranquil spot that holds echoes of the 1877 Flight of the Nez Perce .”

Although the bison population increased in the 20th century, some bison still face livestock disease.
Photo by Andrea Izzotti/Shutterstock
Spot a diverse range of wildlife
Wildlife conservation is further along than it’s ever been in Yellowstone’s history, despite habitat disruption from rising temperatures and unpredictable precipitation from man-made climate change . In 2003, it was removed from UNESCO’s list of World Heritage sites in danger, a sign of progress.
One prime spot for wildlife is Hayden Valley , named after Ferdinand Hayden, who completed the first federally funded geological survey in 1871, convincing the United States to sign a new law making Yellowstone the first national park. Pull over at one of the scenic road stops in this centrally located 50-square-mile section of the park to catch a glimpse of coyotes, grizzly bears, wolves, and bison.
In the Lamar Valley , near the park’s northern entrance, you’re likely to see elk, moose, grizzly bears, badgers, bald eagles, pronghorn, osprey, deer, coyotes, and the park’s largest herd of wild bison. (And if you’re lucky, maybe you’ll stumble on the burly beasts in the Tower-Roosevelt area in the northern part of the park too.) Considered to be the last wild herd of American plains bison, this species has continuously lived in Yellowstone since prehistoric times. The National Park Service saved the herd from extinction, raising 21 bison in Lamar Valley to recover the dwindling bison population in the 20th century.
Another success story in the Lamar Valley belongs to the gray wolves. They were completely killed off in 1926 by park rangers, but in 1995 these intelligent and loyal animals were reintroduced from western Canada, and they were eventually taken off the endangered list in 2017. (For a blissfully quiet experience, consider seeing this valley in the winter .)

Inside the landmark historic Old Faithful Inn next to the famous geyser in Yellowstone National Park
Photo by EQRoy/Shutterstock
Sleep in and around the park
- Book now: Old Faithful Inn ; Canyon Lodge and Cabins; Mammoth Hot Springs Hotel & Cabins ; Under Canvas West Yellowstone; Mountain Sky Guest Ranch
The 1903-opened Old Faithful Inn is one of the country’s few remaining log hotels and the largest log structure in the world, an architectural marvel that influenced the exposed log-and-wood frame architecture we see throughout U.S. national parks today. For sprawling eco-friendly charm, check into Canyon Lodge and Cabins , and visit Mammoth Hot Springs Hotel & Cabins , one of Yellowstone’s year-round properties.
Feel like venturing outside the park? Glamp at Under Canvas West Yellowstone , a 10-minute drive from the West Entrance, or roam around a dude ranch 30 miles away at Mountain Sky Guest Ranch in Paradise Valley, Montana.
A Fascinating Journey through Yellowstone National Park
Y ellowstone National Park, located primarily in the U.S. state of Wyoming, is a captivating destination that offers a wide range of adventures for nature enthusiasts and outdoor lovers. With its diverse landscapes, geothermal wonders, and abundant wildlife, a visit to Yellowstone is an unforgettable experience.
One of the most popular activities in Yellowstone National Park is wildlife watching. The park is home to a variety of animals, including grizzly bears, wolves, elk, bison, and moose. Visitors can spot these magnificent creatures in their natural habitat, either by taking a guided tour or exploring on their own. It is essential to remember that these are wild animals, and maintaining a safe distance is crucial for both their well-being and your own safety.
Exploring the geothermal features is another must-do adventure in Yellowstone. The park boasts more than 10,000 geothermal features, including the famous Old Faithful geyser. Witnessing an eruption of boiling water shooting up into the air is a sight like no other. The park also features colorful hot springs, mud pots, and fumaroles, each with its unique characteristics.
For those seeking a unique experience, backcountry camping and hiking are highly recommended. Yellowstone offers over 900 miles of hiking trails, ranging from easy nature walks to challenging multi-day treks. Backpacking through the wilderness allows visitors to truly immerse themselves in the pristine beauty of the park and discover hidden gems off the beaten path.
Hiking trails in Yellowstone
Yellowstone National Park is a haven for hiking enthusiasts, with an extensive network of trails that cater to all skill levels. Whether you are a beginner or an experienced hiker, you will find trails that suit your preferences and abilities.
One of the most iconic hikes in Yellowstone is the Grand Canyon of the Yellowstone trail. This trail offers breathtaking views of the Yellowstone River as it cascades through the vibrant-colored canyon. The trail covers approximately 6 miles and is known for its stunning waterfalls and dramatic cliffs.
If you are looking for a more challenging hike, the Mount Washburn trail is an excellent option. This trail takes you to the summit of Mount Washburn, offering panoramic views of the surrounding landscapes. The trail is approximately 6 miles round trip and is known for its wildflower meadows and opportunities to spot wildlife such as bighorn sheep and mountain goats.
For those seeking a shorter and easier hike, the Fairy Falls trail is a great choice. This 5-mile round trip trail takes you to a beautiful waterfall surrounded by lush greenery. It is the perfect spot for a picnic or a peaceful moment amidst nature.
When hiking in Yellowstone, it is essential to come prepared with proper gear, including sturdy hiking boots, a map, plenty of water, and bear spray. Additionally, always check the weather conditions and trail closures before embarking on your hike.
Best attractions in Yellowstone
Yellowstone National Park is renowned for its incredible natural wonders and attractions. Here are some of the must-visit places within the park:
1. Old Faithful: The most famous geyser in Yellowstone, Old Faithful, attracts visitors from around the world. It erupts approximately every 90 minutes, shooting a column of hot water high into the air. Witnessing this natural phenomenon is an absolute highlight of any visit to the park.
2. Grand Prismatic Spring: Known for its vibrant colors, the Grand Prismatic Spring is the largest hot spring in the United States and one of the most photographed features in the park. The vivid hues, ranging from deep blues to bright oranges, create a mesmerizing spectacle that is a must-see for any visitor.
3. Mammoth Hot Springs: These terraces of travertine are formed by the precipitation of hot springs water saturated with calcium carbonate. The result is a stunning display of intricate and ever-changing mineral formations. Visitors can explore the boardwalks that wind their way through the terraces, offering unique perspectives of this geological wonder.
4. Lamar Valley: Known as the “Serengeti of North America,” Lamar Valley is a haven for wildlife enthusiasts. It is often referred to as the best place in Yellowstone to spot wolves, as well as other animals such as bison, elk, and pronghorn. The vast open landscapes and diverse wildlife make for an unforgettable experience.
5. Norris Geyser Basin: This geothermal area is one of the most active in Yellowstone, with a large number of geysers, hot springs, and steam vents. The boardwalks allow visitors to explore the otherworldly landscapes and witness the power and beauty of Yellowstone's geothermal features up close.
These are just a few of the many attractions that Yellowstone National Park has to offer. Exploring the park at your own pace and immersing yourself in its natural wonders is the key to an unforgettable journey.
In conclusion, a journey through Yellowstone National Park is a truly fascinating experience. From the diverse adventures and hiking trails to the breathtaking attractions, there is something for everyone to enjoy. Whether you are a nature lover, a wildlife enthusiast, or simply seeking awe-inspiring landscapes, Yellowstone will leave you in awe. Plan your visit, venture into the wilderness, and embark on a journey you will cherish forever.
The post A Fascinating Journey through Yellowstone National Park appeared first on Things That Make People Go Aww .
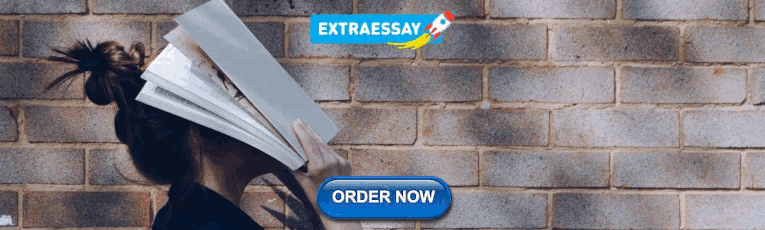
IMAGES
COMMENTS
Number in Yellowstone. 2019: 145 permits were issued. All scientists in Yellowstone work under research permits and are closely supervised by National Park Service staff. Types of Research. In 2019, permitted research included. Physical Sciences: 27%. Biology (wildlife, vegetation): 31%. Microbiology: 23%. Ecology: 15%.
The reintroduction of gray wolves (Canis lupus) into Yellowstone National Park is a well-known ecological experiment, albeit with a lack of replication, randomization, and controls (Kauffman et al. 2013; Ford and Goheen 2015).The trophic cascade that resulted in vegetation being released from herbivory caused by wolf predation on elk (Cervus elaphus) is purported to be among the most ...
Understanding Demographics and Experience of Visitors in Yellowstone National Park through Social Media 1 Introduction . National parks have been hailed as "America's best idea" (Wallace Stegner, 1983). In 2016, U.S. national parks attracted 331 million national and international visitors, a sharp increase from
a Lemonade Creek, Yellowstone National Park (YNP) and the virus community at the sampled sites (see red arrow).b Abundance of virus classes (legend shown at right) reflecting relative coverage ...
Here, we provide evidence of cascading interactions across the aquatic-terrestrial food web of the Yellowstone Lake watershed, a highly protected landscape within Yellowstone National Park and the Bridger-Teton wilderness of Wyoming (>3200 km 2; fig. S1).The cascade was driven by the invasion of a previously nonexistent apex predator, lake trout (Salvelinus namaycush), which exhibited direct ...
Yellowstone National Park (YNP) is world famous and sets precedents across the globe. The broader region, the Greater Yellowstone Ecosystem (GYE), also embodies an "idea" and an "ideal": a formula of the wild, and of the human-wildlife nexus, where people might live sustainably within the ecosystem.
Abstract. Comparison of precise leveling measurements made in 1923 with those made in 1975, 1976, and 1977 reveals that the 600,000-year-old Yellowstone caldera is being uplifted relative to its surroundings. Maximum relative uplift since 1923 is in excess of 700 millimeters—about 14 millimeters vertically per year.
Yellowstone National Park is the first protected area to have ... There is already a fairly substantial body of work pertaining to research on national parks, as well as park management. For example ... , Kartawijaya, Yulianto, Prasetia, & Clifton, 2013; Yahnke, 1998), they are quantitative and/or focused on a single park. In this paper, we ...
The research and debates surrounding the role of wolves in modifying the behavior and abundance of species on multiple trophic levels in Yellowstone illustrates the complex interactions between the forces that structure patterns of abundance in natural ecosystems. ... Clark W, Jaffe R (2008) Wolf-bison interactions in Yellowstone National Park ...
(b) Isle Royale National Park wolf‐moose population fluctuations 1900-2020. (c) Wolf-elk population fluctuations in Yellowstone National Park since wolf reintroduction 1995-2019.
In Bob Rye and Alfred Truesdell's paper, the question of recharge to the deep thermal reservoir underlying the geysers and hot springs of Yellowstone National Park is discussed. Irving Friedman and Dan Norton report on the chloride flux emissions from Yellowstone in their paper questioning whether Yellowstone is losing its steam.
Gas and isotope chemistry of thermal features in Yellowstone National Park, Wyoming. U.S. Geological Survey Scientific Investigations Report , 2011-5012, 26 p. Morgan, L. (Ed.) (2007).
Sapphire Pool, in Biscuit Basin, steams on a stormy morning. National Park Service photo by Jacob W. Frank, July 20, 2020. Scientists have studied water chemistry in Yellowstone for more than 140 years. At hydrothermal features, rivers, streams, lakes, drillholes, and precipitation gages, as well as in laboratories across the country, researchers measure the chemical constituents of ...
Physical results of research drilling in thermal areas of Yellowstone National Park, Wyoming. Professional Paper 892. By: Donald Edward White, R.O. Fournier, L. J . P. Muffler, and A.H ... Physical results of research drilling in thermal areas of Yellowstone National Park, Wyoming: Series title: Professional Paper: Series number: 892: DOI: 10. ...
Abstract. The Yellowstone plateau volcanic field is less than 2 million years old, lies in a region of intense tectonic and hydrothermal activity, and probably has the potential for further volcanic activity. The youngest of three volcanic cycles in the field climaxed 600,000 years ago with a voluminous ashflow eruption and the collapse of two ...
Mullis was awarded the 1993 Nobel Prize in Chemistry for his groundbreaking work on PCR. Continuing this legacy, about 25% of the Yellowstone research permits issued annually investigate the unique microbial communities thriving in hydrothermal features. Thermophiles from Doublet Pool in the Upper Geyser Basin, Yellowstone National Park.
Situated at 7,733 feet above sea level in the heart of Yellowstone National Park, Yellowstone Lake is North America's largest high-elevation lake, roughly 20 miles long and 14 miles wide with a surface area of 132 square miles.
The theory was exciting and quickly grabbed headlines. Fifteen years after wolves were reintroduced to Yellowstone National Park in 1995, some scientists pointed to the resurgence of aspen growth ...
Yellowstone National Park (YNP; Wyoming, Montana, and Idaho, USA) contains more than 10,000 hydrothermal features, several lakes, and four major watersheds. For more than 140 years, researchers at the U.S. Geological Survey and other scientific institutions have investigated the chemical compositions of hot springs, geysers, fumaroles, mud pots, streams, rivers, and lakes in YNP and surrounding ar
The 3,472-square-mile park in Northwestern Wyoming (and small sections of Montana and Idaho) is also home to the largest concentration of mammals in the lower 48, including the famous reintroduced wolves. In short, there are many reasons Yellowstone National Park pulls in more than 3 million visitors per year. Here are some insider tips on what ...
Yellowstone National Park, located primarily in the U.S. state of Wyoming, is a captivating destination that offers a wide range of adventures for nature enthusiasts and outdoor lovers. With its ...