- Search Menu
- Advance Articles
- Perspectives
- Knowledgebase and Database Resources
- Nobel Laureates Collection
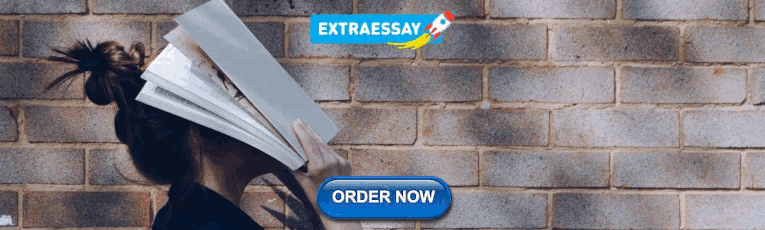
China Virtual Outreach Webinar
Neurogenetics, fungal genetics and genomics.
- Multiparental Populations
- Genomic Prediction
- Plant Genetics and Genomics
Genetic Models of Rare Diseases
- Genomic Data Analyses In Biobanks
- Why Publish
- Author Guidelines
- Submission Site
- Open Access Options
- Full Data Policy
- Self-Archiving Policy
- About Genetics
- About Genetics Society of America
- Editorial Board
- Early Career Reviewers
- Guidelines for Reviewers
- Advertising & Corporate Services
- Journals on Oxford Academic
- Books on Oxford Academic

Editor-in-Chief
Howard Lipshitz
Executive Editor
Tracey DePellegrin
Managing Editor
Ruth Isaacson
Scientific Editor and Program Manager
Opportunities and challenges for genomic data analyses in biobanks: a call for papers.
The GSA Journals are calling for submissions of papers on biobank-scale genomic data analyses. The closing date for submissions is May 31 2024.
Why publish with GENETICS?
Why publish in genetics.
Learn more about why GENETICS is the perfect home for your research, and submit today to join our celebrated author community.
Why publish?
Series and Collections accepting papers
Submit your work to one of GSA’s ongoing series and collections.
Currently accepting submissions
Meet the Editorial Board
See who handles papers for GENETICS by topic.
Editorial board
Re-watch the recent China Virtual Outreach Webinar where you will learn more about publishing your work in the journal.
Watch the webinar
Latest articles
Series & collections.

Genes and variants of interest in rare diseases often benefit from modelling in cellular assays or genetic models to aid in understanding molecular and cellular mechanisms of disfunction. Model organisms are useful for the discovery of new genetic diseases and key to understanding variant effects, and modelling a disease gene in a genetic model means that researchers can perform an in-depth exploration of gene or variant function. The GSA Journals are pleased to publish a series highlighting ongoing advances in rare disease discovery and mechanisms by presenting key research findings and new discoveries.
Plant Genetics and Genomics
Plant science has generated many discoveries and advances in genetics and genomics research. These contributions reflect the ingenuity and rigor of the plant science community, as well as the rich diversity of plants and their biology. To showcase this critical work, GENETICS and G3: Genes|Genomes|Genetics has launched the Plant Genetics and Genomics series with a collection of fourteen research articles and an accompanying editorial.

Neurogenetics lies at the intersection of Neuroscience and Genetics, where genetic approaches are applied to the study of nervous system development, function, and plasticity. Overseen by Series Editors Oliver Hobert, Cecilia Moens, and Kate O’Connor Giles, this new series aims to make the GSA Journals a home for cutting-edge, robust research in neurogenetics.

The fungal kingdom is remarkable in its breadth and depth of impact on global health, agriculture, biodiversity, ecology, manufacturing, and biomedical research. Overseen by editors Leah Cowen and Joseph Heitman, this series aims to report and thereby further stimulate advances in genetics and genomics across a diversity of fungal species.

FlyBook from GENETICS is a comprehensive compendium of review articles presenting the current state of knowledge in Drosophila research.
Browse FlyBook

WormBook from GENETICS features a comprehensive compendium of review articles presenting the current state of knowledge in C. elegans research. WormBook articles will span the breadth of the biology, genetics, genomics, and evolutionary biology of C. elegans .
Browse WormBook

The YeastBook series from GENETICS features a comprehensive compendium of reviews that presents the current state of knowledge of the molecular biology, cellular biology, and genetics of the yeast Saccharomyces cerevisiae .
Browse YeastBook
More from GSA

G3: Genes|Genomes|Genetics
G3, a Genetics Society of America journal, provides a forum for the publication of high-quality foundational research-particularly research that generates useful genetic and genomic information, as well as genome reports, mutant screens, and advances in methods and technology.
Find out more

GSA members of all career stages receive member benefits including access to professional development programs, discounted meeting registration, and eligibility for travel awards. Members also receive a personal subscription to GENETICS, as well as discounted publication fees in both GSA journals.

Conferences
GSA conferences have long served as community hubs for researchers focused on particular organisms or topics. GSA also hosts The Allied Genetics Conference (TAGC) , a unique meeting that brings together multiple research communities for collaboration and synthesis.
Attend a conference

Career Development
GSA professional development programs provide rich opportunities for scientists to gain skills, experience, mentors, and networks. Our initiatives and resources range from peer review training to inclusive public engagement, newsletters, webinars, a job board, leadership programs, and much more.
Browse Opportunities

Email alerts
Register to receive email alerts as soon as new content from GENETICS is published online.

Recommend to your library
Fill out our simple online form to recommend GENETICS to your library. Recommend now

Author resources
Learn about how to submit your article, our publishing process, and tips on how to promote your article.
Related Titles
- Recommend to Your Librarian
- Advertising and Corporate Services
- Journals Career Network
Affiliations
- Online ISSN 1943-2631
- Copyright © 2024 Genetics Society of America
- About Oxford Academic
- Publish journals with us
- University press partners
- What we publish
- New features
- Open access
- Institutional account management
- Rights and permissions
- Get help with access
- Accessibility
- Advertising
- Media enquiries
- Oxford University Press
- Oxford Languages
- University of Oxford
Oxford University Press is a department of the University of Oxford. It furthers the University's objective of excellence in research, scholarship, and education by publishing worldwide
- Copyright © 2024 Oxford University Press
- Cookie settings
- Cookie policy
- Privacy policy
- Legal notice
This Feature Is Available To Subscribers Only
Sign In or Create an Account
This PDF is available to Subscribers Only
For full access to this pdf, sign in to an existing account, or purchase an annual subscription.
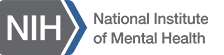
Transforming the understanding and treatment of mental illnesses.
Información en español
Celebrating 75 Years! Learn More >>
- Science News
- Meetings and Events
- Social Media
- Press Resources
- Email Updates
- Innovation Speaker Series
Genomic Data From More Than 41,000 People Shed New Light on Bipolar Disorder
September 29, 2021 • Research Highlight
In the largest genome-wide association study of bipolar disorder to date, researchers found about twice as many genetic locations associated with bipolar disorder as reported in previous studies. These and other genome-wide findings help improve our understanding of the biological origins of bipolar disorder and suggest some promising genes for further research.
The study, led by the Psychiatric Genomics Consortium bipolar disorder working group, is published in Nature Genetics . The Psychiatric Genomics Consortium is a global collaborative effort consisting of more than 800 investigators, including researchers in the National Institute of Mental Health (NIMH) Intramural Research Program and extramural scientists conducting NIMH-supported research.
Bipolar disorder is a mental illness characterized by episodes of mania and depression that can seriously impair day-to-day functioning. Affecting up to 50 million people worldwide, bipolar disorder is a major public health concern. Although evidence suggests that genes play an important role in the development of bipolar disorder, researchers still do not have a clear understanding of the disorder’s specific biological causes. Examining common genetic variations in the genomes (or complete set of DNA) of people with bipolar disorder is a way that scientists can home in on the genetic factors that are likely to play a causal role in the disorder.
For this study, the researchers analyzed genomic data from 57 groups of participants across Europe, North America, and Australia. These cohorts included individuals receiving clinical care for bipolar disorder and individuals classified as having bipolar disorder based on data from health registries, electronic health records, or repositories. The total combined sample included 41,917 individuals with bipolar disorder and 371,549 individuals without bipolar disorder.
The researchers used an approach known as a genome-wide association study (GWAS) , which allowed them to identify common genetic variations that are more likely to occur in people with bipolar disorder. Identifying these variations can provide important clues about the biological pathways and processes that are likely to be involved in the disorder.
According to the GWAS results, a total of 64 genomic locations, or risk loci , were associated with bipolar disorder even after accounting for all the variations studied across the genome. These 64 risk loci included 33 that had not been reported in previous bipolar disorder studies. Among the novel loci, the researchers found that bipolar disorder was associated with the major histocompatibility complex, which is a large group of genes involved in immune function. They also found a correlation between bipolar disorder and loci linked to other psychiatric disorders, including schizophrenia, major depression, and childhood-onset disorders such as attention-deficit/hyperactivity disorder (ADHD).
The study findings also revealed genome-wide genetic overlaps, or correlations, between bipolar disorder and certain traits. For example, the results showed a genetic correlation between bipolar disorder and both alcohol use and smoking, as well as genetic correlations with some aspects of sleep (daytime sleepiness, insomnia, and sleep duration).
The researchers also compared genetic overlap between the two types of bipolar disorder: bipolar I disorder (which includes manic episodes and, typically, depressive episodes) and bipolar II disorder (which includes depressive episodes and hypomanic episodes). As expected, the results indicated a substantial but incomplete genetic overlap between the two types. Comparing the two types and their associations with other psychiatric disorders, the researchers found that bipolar I disorder showed a stronger genetic correlation with schizophrenia, whereas bipolar II disorder was more closely correlated with major depression. Additional studies with detailed trait data for large cohorts will be essential for further understanding the genetic components of these bipolar disorder types.
Drawing from the GWAS results, the researchers found that the 64 risk loci contained at least 161 individual genes. Some of these genes play a role in how neurons signal to each other in the brain. Some of these genes are also known to be targets for certain types of drugs currently used to treat bipolar disorder, such as antipsychotics, mood stabilizers, and antiepileptics. And some genes are known to be targets for other drug types, including calcium channel blockers (typically used to treat high blood pressure) and certain anesthetics.
The researchers then used an analytic technique called “fine-mapping” to connect risk loci with specific genes that are most likely to play a causal role in bipolar disorder. This technique identified 15 genes with the strongest evidence, which suggests they are promising candidates for further study.
Overall, the study findings confirmed many of the risk loci and genetic correlations reported in previous studies. But the study also represents an advance for the field, as a 1.5-fold increase in the number of participants effectively doubled the number of loci identified as associated with bipolar disorder. According to the researchers, this marks an “inflection point” in discovery because it indicates that the number of loci identified will continue to increase rapidly with the addition of new cohorts.
Taken together, these findings establish a more detailed picture of the genetic factors that underlie bipolar disorder and suggest possible biological targets for new treatments.
Mullins, N., Forstner, A. J., O’Connell, K. S., Coombes, B., Coleman, J. R., Qiao, Z., Als, T. D., Bigdeli, T. B., Børte, S., Bryois, J., Charney, A. W., Drange, O. K., Gandal, M. J., Hagenaars, S. P., Ikeda, M., Kamitaki, N., Kim, M., Krebs, K., Panagiotaropoulou, G.,…Andreassen, O.A. (2021). Genome-wide association study of more than 40,000 bipolar disorder cases provides new insights into the underlying biology. Nature Genetics, 53, 817–829. https://doi.org/10.1038/s41588-021-00857-4
MH109528 , MH094421 , MH085520
Clinical Trials
Genetic testing.
Displaying 49 studies
The purpose of this study is to provide descriptive information on patients with hereditary hemochromatosis, including their predominant symptoms, time to diagnosis, lifestyle habits, physician counseling, and use of direct-to-consumer (DTC) genetic testing.
The purpose of this study is to establish a patient and clinical information database, and a genetic material biobank to better characterize the symptoms and unknown genetic causes of Angelman syndrome
The purpose of this study is to establish the prevalence and severity of specific morbid indicators of disease severity such as specific organ system involvement, degree of cognitive disability, and case-fatality associated with various congenital disorders of glycosylation (CDG), and establish a dynamic platform to effectively disperse clinically relevant findings to families, non-expert clinicians and researchers, as well as provide a verified method to link these individuals to experts in CDG.
The goal of this study is to evaluate the integration of cancer genetic testing into a community cancer clinical practice aimed toward patients of various ethnic populations in north Florida including, but not limited to, African Americans, Hispanic/Latino, Native American/Alaskan and Asian, by self-report.
The purpose of this project will examine the feasibility of offering whole genome sequencing (WGS), and developing a Rapid WGS protocol, for a fetus or neonate with multiple anomalies.
The purpose of this study is to identify novel genetic variants that predispose to Type 1 Diabetes.
The purpose of this study is to measure the clinical impact of re-testing (quantifying the percentage of those who subsequently test positive, which genes are identified, and quantifying the percentage of those who have not received recommended clinical management as well as those for whom new clinical management options exist).
The purpose of this research is to find out how genetic variations in GLP1R, alters insulin secretion, in the fasting state and when blood sugars levels are elevated. Results from this study may help us identify therapies to prevent or reverse type 2 diabetes mellitus.
The purpose of this research is to develop a collection of known mutation carriers to analyze cancer risk and long-term clinical outcomes.
The purpose of this research study is to understand the views and experiences of African American women with a diagnosis or who support a family member with breast or ovarian cancer. We also want to know participant thoughts on genetic testing for cancer risk.
This is a qualitative interview study. Study participation involves talking on the phone or videoconference (e.g., Zoom) for about 1 hour with a researcher.
The electronic Medical Records and GEnomics (eMERGE) Network Genomic Risk Assessment Study is a multisite study funded by the National Human Genome Research Institute (NHGRI) to investigate the clinical utility of polygenic risk scores for common conditions in adults and children. eMERGE researchers aim to learn about how people’s family history and genetic makeup affect their risk of developing common conditions such as heart disease, diabetes, and cancer, and about how to use this information to help them reduce their risk. The study will recruit 25,000 participants from around the country, including groups who are underrepresented in biomedical ...
The purpose of this study is to investigate the experience and understanding of patients with ovarian cancer in relation to genetic testing by means of one-on-one qualitative interviews and survey.
This is an interview study to understand the views of people with the lived experience of 10 different genetic conditions on gene modification therapies, and specifically on prenatal gene editing. Prenatal gene editing is not happening now, but it is possible that prenatal gene editing will be available in the next few years, at least in a research setting, and we want to know your thoughts about the direction this technology is going. We to hope speak with many different stakeholders (patients and their families, clinicians, scientists) with diverse perspectives to understand values and priorities for prenatal gene ...
The objectives of this study are to perform molecular and/or biochemical testing in individuals with suspected hereditary disorders, and/or their family members, to further elucidate possible mechanisms of disease and/or inheritance, and/or to help yield a final laboratory diagnosis at the judgement of the original ordering healthcare provider.
The purpose of this study is to look at function of ACLP in cultured fibroblasts and to gain better understanding on the role of AEBP1 in human disease.
The overall hypothesis is that the early detection of genetic variants leads to early interventions in cancer development. This will lead to lower rates of cancer development and will eventually result in lower death rate from cancer. In the short term, healthcare utilization and costs will increase due to the early interventions and treatments. In the long term, benefit will start to emerge due to the lower disease development, lower death rate, and retaining of the quality of life and life expectancy.
The purpose of this study is to assess the ability of the hyperphagia questionnaires to identify children and young adults with early-onset obesity for genetic testing to identify variants in 36 genes of interest associated with MC4R pathway genetic obesity
Can the genetic makeup of an individual who does not yet have cancer provide an indication of the risk of future cancer?
The study will be performed in two phases: Phase I will be performed for biologic validation of marker candidates from a discovery cohort and phase II will be performed to evaluate the discrimination (sensitivity/specificity) of best candidate markers when assayed from blood of cases with CAP and controls without history of cancer.
The long term research goal and objective of this application is to improve the clinical outcomes of patients with biliary tract cancers and germ cell tumors. There is an imminent need to identify novel targets suitable for drug development in patients with these cancers given the need for novel therapeutics for patients diagnosed with BTCs and GCTs.
The purpose of this study is to collect natural history and treatment-related data of patients diagnosed with congenital disorders of glycosylation.
The purpose of this study is to form processes to get findings from targeted gene sequencing that can be acted on using two common actionable genetic disorders–familial hyperlipidemia and familial colorectal cancer.
The purpose of this study is to validate the effectiveness and clinical use of array and next generation sequencing tests in hopes of adopting these tests as standard of care and advancing glioma patient care and clinical practice at Mayo Clinic and beyond.
The purpose of this study is to better understand the impact of predictive genomic testing in the healthy adult population by assessing decisional satisfaction/regret, understanding of disease risk, lifestyle modifications, and satisfaction with the testing experience.
The purpose of this study is to identify genetic causes for epilepsy (sometimes referred to as seizure disorders) and associated co-morbidities such as developmental delay (DD), intellectual disability (ID), and other neurological or neurodevelopmental disorders. We are collecting genetic and clinical information from individuals who have undergone exome/genome sequencing as part of their regular medical care or as part of a research study.
Aims, purpose, or objectives:
1) Develop hypotheses related to how the human genome and genetic variants affect hearing loss in children and adults.
2) Understand phenotypes of hearing loss on a deeper level by combining and analyzing information from audiometric, genetic, and radiologic data.
3) Explore genetic variants with an unknown significance to develop an understanding of their potential significance and explore other genes may influence an individual's hearing ability.
The purpose of this study is to examine the genomic testing practices by Hematology/Oncology physicians at Mayo Clinic in Florida in an effort to 1) catalogue the number and type of panel-based tests used as well as 2) determine how often the test findings informed the clinical decision making for the physician and their patient.
The purpose of this study is to analyze genome-wide methylation patterns in DNA from patients with suspected hereditary disorders but for whom all previous genetic testing has been negative and/or equivocal.
The purpose of this study is to evaluate the proposed risks and benefits of predictive genomic sequencing, including short-and long-term impacts on:
(i) health-related outcomes; (ii) healthcare utilization; (iii) psychosocial impact, and (iv) physician acceptance.
The purpose of this study is to explore the experiences of consumers in receiving results on genetic risk of malignant hyperthermia and pseudocholinesterase deficiency via an online direct-to-consumer portal that includes robust consumer education and guidance on physician consultation, and to explore the experiences of consumers of MCGG in purchasing and using the product.
The goal of this study is to assess the diagnostic yield of concomitant germline and somatic tumor profile testing in a population of cancer patients unselected for family cancer history. In this study, we will retrospectively perform TMP on all cancer patients enrolled into the IRB 18-00326 who underwent germline genetic testing and have tumor tissue available within the Mayo Clinic Tissue Archives. TMP will be performed using a 435 gene platform, along with assessment of tumor mutational burden and microsatellite instability status, among other analyses. Paraffin-embedded tissues will be obtained from the Mayo Tissue Registry and will ...
The purpose of this study is to perform RNA (genetic) sequencing on biopsies taken from the esophagus of adult patients who have active eosinophilic esophagitis, and look for potential therapeutic targets.
The purpose of the study is to determine whether somatic mutations in T cells contribute to the pathogenesis of rheumatoid arthritis (RA).
The purpose of this study is to evaluate the immune regulation at the point where maternal genes meet fetal genes in the tissues of the placenta. Specimens from the placentas of healthy pregnancies will be collected, and genetic arrays from both areas, maternal and fetal, will be prepared and compared.
This study will use a 22 gene pharmacogenomics panel on 30 children with persistent Gastroesophageal Reflux Disease (GERD) who have not responded to therapy.
The purpose of this study is for The Center for Individualized Medicine (CIM) at Mayo Clinic to discover and translate genomic and molecular science into personalized medical applications
The researchers are trying to identify molecular mechanisms that control spine deformity and degenerative changes that can be used for therapeutic strategies.
The purpose of this study is to evauate how well genetic testing works in guiding treatment for patients with solid tumors that have spread to the brain. Several genes have been found to be altered or mutated in brain metastases such as NTRK, ROS1, CDK or PI3K. Medications that target these genes such as abemaciclib, GDC-0084, and entrectinib may stop the growth of tumor cells by blocking some of the enzymes needed for cell growth. Genetic testing may help doctors tailor treatment for each mutation.
The goal of the study is to create a database of clinical information and a repository of biological specimens for genetic, molecular and microbiological research to better understand hereditary cancer and help develop new therapies and preventive strategies.
The purpose of this study is to determine the prevalence of genetic mutations in cancer patients from various ethnic populations seeking care at Mayo Clinic cancer clinics.
The study aims are to develop a consent-based IRB proposal that will enroll newborns who have imperforate anus. The proposal will include permission for chart review and database information storage, to develop a database that will include MRN, imperforate anus phenotype, information on other congenital malformations, syndromic diagnosis if available, demographic information, and to develop a biobank of DNA and white blood cell samples from infants with imperforate anus. If patients are undergoing a skin biopsy for a medically indicated reason, cells will be requested.
Imperforate Anus, also known as anal atresia, is a rare birth defect. ...
This research trial studies genetic testing in screening patients with stage IB-IIIA non-small cell lung cancer that has been or will be removed by surgery. Studying the genes in a patient's tumor cells may help doctors select the best treatment for patients that have certain genetic changes.
This laboratory study is looking at genetic mutations and environmental exposure in young patients with retinoblastoma and in their parents and young healthy unrelated volunteers. Gathering information about gene mutations and environmental exposure may help doctors learn more about the causes of retinoblastoma in young patients.
The goal of this study is to examine the current and (potential) future therapeutic relevance of pharmacogenomics (PGx) testing for a group of patients in order to improve patient clinical care at Mayo Clinic with more effective and efficient prescribing of medications.
Biomarkers from circulating cell-free tumor DNA in peripheral blood will identify patients with metastatic prostate cancer diagnosed with C11 choline PET/CT who will benefit from metastasis-directed radiation, ablative therapies, and/or surgery. Tissue and blood will be collected before treatment. If patients receive androgen deprivation, then blood will be collected after neoadjuvant androgen deprivation but before radiation, ablative therapies, or surgery. Subsequent samples will be obtained at 3 months and 6 months following treatment, after which no further patient contact will occur.
The purpose of this study is to determine the prevalence of genetic mutations of cancer in kidney transplant patients receiving care at Mayo Clinic Arizona.
The primary aim of this study is to determine if the addition of an individual polygenic risk score (PRS) in addition to the Breast Cancer Risk Assessment Tool (BCRAT) or Tyrer-Cuzick (IBIS) score will aid women at risk of breast cancer in making a decision to take (or not take) medications to prevent breast cancer.
The purpose of this study is to improve the interpretation of mutations in breast cancer predisposition genes. This will be accomplished by recruiting members of families found to carry deleterious (mainly protein truncating) mutations and evaluating co-segregation of the mutations with cancer within families.
The purpose of this study is to to evaluate the integration of cancer genetic testing into a mammography practice aimed toward women at intermediate- to-high lifetime risk of breast cancer. If successful, this will provide an opportunity for cancer risk stratification and individualized screening.
Mayo Clinic Footer
- Request Appointment
- About Mayo Clinic
- About This Site
Legal Conditions and Terms
- Terms and Conditions
- Privacy Policy
- Notice of Privacy Practices
- Notice of Nondiscrimination
- Manage Cookies
Advertising
Mayo Clinic is a nonprofit organization and proceeds from Web advertising help support our mission. Mayo Clinic does not endorse any of the third party products and services advertised.
- Advertising and sponsorship policy
- Advertising and sponsorship opportunities
Reprint Permissions
A single copy of these materials may be reprinted for noncommercial personal use only. "Mayo," "Mayo Clinic," "MayoClinic.org," "Mayo Clinic Healthy Living," and the triple-shield Mayo Clinic logo are trademarks of Mayo Foundation for Medical Education and Research.
- Alzheimer's disease & dementia
- Arthritis & Rheumatism
- Attention deficit disorders
- Autism spectrum disorders
- Biomedical technology
- Diseases, Conditions, Syndromes
- Endocrinology & Metabolism
- Gastroenterology
- Gerontology & Geriatrics
- Health informatics
- Inflammatory disorders
- Medical economics
- Medical research
- Medications
- Neuroscience
- Obstetrics & gynaecology
- Oncology & Cancer
- Ophthalmology
- Overweight & Obesity
- Parkinson's & Movement disorders
- Psychology & Psychiatry
- Radiology & Imaging
- Sleep disorders
- Sports medicine & Kinesiology
- Vaccination
- Breast cancer
- Cardiovascular disease
- Chronic obstructive pulmonary disease
- Colon cancer
- Coronary artery disease
- Heart attack
- Heart disease
- High blood pressure
- Kidney disease
- Lung cancer
- Multiple sclerosis
- Myocardial infarction
- Ovarian cancer
- Post traumatic stress disorder
- Rheumatoid arthritis
- Schizophrenia
- Skin cancer
- Type 2 diabetes
- Full List »
share this!
April 5, 2024
This article has been reviewed according to Science X's editorial process and policies . Editors have highlighted the following attributes while ensuring the content's credibility:
fact-checked
trusted source
Study reveals genetic drivers of metabolic dysfunction-associated steatotic liver disease
by Becky Ham, Translational Genomics Research Institute
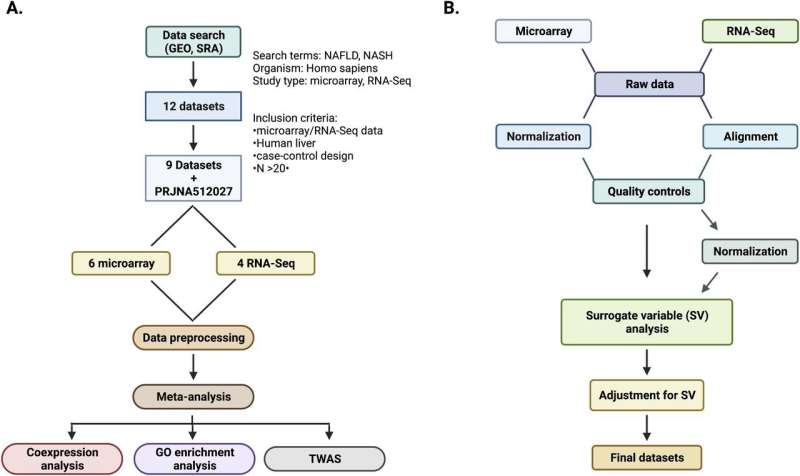
In the largest study of its kind, researchers from the Translational Genomics Research Institute (TGen), part of City of Hope, have identified genes that appear to drive the progression of metabolic dysfunction-associated steatotic liver disease (MASLD) and metabolic dysfunction-associated steatohepatitis (MASH).
Formerly known as non-alcoholic fatty liver disease , MASLD affects about 30% of the global population , a number that is expected to nearly double by 2040. People with MASH, the more severe form of the disease, have a greater risk of deadly and disabling conditions such as fibrosis, cirrhosis, and hepatocellular carcinoma.
The absence of pharmacological interventions for MASH until the recent FDA approval in March 2024 underscores the urgent need addressed by this research.
In their report published April 2 in Life Science Alliance TGen researchers Johanna K. DiStefano, Ph.D., and Ignazio S. Piras, Ph.D., analyzed liver gene expression datasets derived from ten diverse cohorts comprising 1,058 patients and controls to find out more about the molecular drivers of MASLD. Their meta-analysis is the largest study of gene expression from liver biopsies to date, comparing more than 12,000 shared genes across the datasets.
Combining data from different datasets allowed the researchers to obtain a more robust perspective of gene expression patterns from the individual studies alone, which are more prone to false positive and negative results, explained Piras.
"Given the complex nature of MASLD, which is influenced by diverse genetic, lifestyle, demographic, and environmental factors , we would expect many genes across a number of different pathways to be involved," said DiStefano, Professor and Head of the TGen Diabetes and Fibrotic Disease Unit. "However, our investigation is the first to look at drivers of this disease progression from this unique perspective."
Among their findings, the scientists identified two different networks of genes co-expressed in the liver disease—one comprised of genes that were already known to be involved in MASLD, but also a second network of genes that had never been linked to the disease.
"Based on our rigorous statistical analysis, these are genes that are great candidates for driving disease progression," said DiStefano, "and they highlight promising candidates for targeted interventions, opening the door for the development of new treatments to prevent MASH," she added.
The researchers also found different gene expression patterns in MASLD and MASH. The differences between the two conditions were even larger than those between MASLD or MASH and healthy liver tissue.
"Some of those genes may exhibit differential expression due to the compromised function of the liver in MASH compared to MASLD," DiStefano explained. "Additionally, these genes could be specifically activated, contributing directly to the development of MASH."
Not everyone with MASLD progresses to MASH, although predictors include conditions such as excess adiposity, type 2 diabetes and metabolic syndrome.
Learning more about the genetic mechanisms underlying disease progression could help clinicians better predict which patients might be more likely to develop MASH, Piras noted.
Explore further
Feedback to editors

AI model has potential to detect risk of childbirth-related PTSD
2 hours ago

Case study of 4-year-old with Down syndrome and sleep apnea suggests procedure can be effective at young ages
7 hours ago

Study finds esketamine injection just after childbirth reduces depression in new mothers
12 hours ago

A new screening protocol can detect aggressive prostate cancers more selectively
14 hours ago

How a new drug prototype regenerates lung tissue

Why some people with rheumatoid arthritis have pain without inflammation
15 hours ago

Researchers show chemical found naturally in cannabis may reduce anxiety-inducing effects of THC
16 hours ago

'Virtual biopsy' lets clinicians analyze skin noninvasively

Research team discovers new way to generate human cartilage

Filling in genomic blanks for disease studies works better for some groups than others
Related stories.

Association between metabolic dysfunction-associated steatohepatitis and mortality in organ transplant recipients
Jan 16, 2024

Researchers discover novel drug candidate to combat fatty liver disease
Apr 3, 2024

Experts help bring first-of-its-kind drug for metabolic liver disease to the clinic
Mar 15, 2024

Aspirin cuts liver fat in clinical trial
Mar 25, 2024

Liver cancer and severe liver disease found to be more common if a close relative has fatty liver disease
Sep 8, 2023

Involvement of brain peptide dynamics in the pathology of fatty liver disease
Nov 3, 2023
Recommended for you

How the body switches out of 'fight' mode: Study shows hormones reprogram metabolism of immune cells
18 hours ago

Newly found genetic variant defends against Alzheimer's disease
20 hours ago

Researchers explore role of androgens in shaping sex differences

New insight into combating drug-resistant prostate cancer
21 hours ago
Let us know if there is a problem with our content
Use this form if you have come across a typo, inaccuracy or would like to send an edit request for the content on this page. For general inquiries, please use our contact form . For general feedback, use the public comments section below (please adhere to guidelines ).
Please select the most appropriate category to facilitate processing of your request
Thank you for taking time to provide your feedback to the editors.
Your feedback is important to us. However, we do not guarantee individual replies due to the high volume of messages.
E-mail the story
Your email address is used only to let the recipient know who sent the email. Neither your address nor the recipient's address will be used for any other purpose. The information you enter will appear in your e-mail message and is not retained by Medical Xpress in any form.
Newsletter sign up
Get weekly and/or daily updates delivered to your inbox. You can unsubscribe at any time and we'll never share your details to third parties.
More information Privacy policy
Donate and enjoy an ad-free experience
We keep our content available to everyone. Consider supporting Science X's mission by getting a premium account.
E-mail newsletter
How pregnancy may speed up the aging process

The fatigue and pangs of pregnancy have made many women feel older than their years. Now there’s new research that suggests pregnancy may, in fact, accelerate the aging process.
Two new studies of genetic markers in the blood cells of pregnant women suggest that their cells seem to age at an exaggerated clip, adding extra months or even years to a woman’s so-called biological age as her pregnancy progresses.
But one of the studies also suggests this process may reverse itself once a woman gives birth, rewinding time so that some mothers’ cells seemingly end up biologically younger afterward than they’d been during gestation, especially if a mother breastfeeds her baby.
Together, the studies underscore how physically demanding pregnancy is. But they also raise important questions about aging itself and whether it really can be sped up, slowed or reversed by pregnancy.
Aging in pregnancy
The newest of the studies , published today in Proceedings of the National Academy of Sciences, found pregnancy “has a big impact on a woman’s body” and biological age, said Calen P. Ryan, an associate research scientist at the Columbia Aging Center at Columbia University in New York, who led the new research.
In it, scientists used several different biological-age “clocks” and other measures to analyze DNA markers in blood samples. These clocks aren’t timepieces but, instead, algorithms, developed using artificial intelligence programs, that examine the patterns of specialized chemical markers found on the outside of some genes. These markers accumulate and change in response to our age, health and lifestyles, a process known as epigenetics.
The algorithms can use these epigenetic markers to estimate the relative age of cells. This measure, often referred to as biological age, can differ from someone’s chronological age, which just means how long he or she’s been alive.
In the new study, the researchers checked blood samples from 825 young women in the Philippines, all born in the same year. Some had been or were pregnant, and others hadn’t conceived. Analyzing these samples, the epigenetic clocks broadly agreed that the biological age of the young women who’d been or were pregnant tended to be higher than that of the others, by at least several months, even after the researchers controlled for economic disparities and other social and health factors.
Pregnancy as a stress test
Similarly, the other new study, published in March in Cell Metabolism , used several different epigenetic clocks to estimate the changing internal age of pregnant women at several points during their pregnancies.
“We were very interested in looking at the impacts of pregnancy as a naturally occurring stress test,” said Kieran J. O’Donnell, an assistant professor at the Yale Child Study Center and Yale School of Medicine, who oversaw one of the new studies.
Get Well+Being tips straight to your inbox

With blood samples from 119 pregnant American women and five different clocks, the researchers tracked the epigenetic changes related to the women’s biological age, starting early in gestation and ending three months after they’d given birth.
The clocks again agreed that pregnancy seemed to be aging the incipient moms as they approached full term, making their blood cells’ DNA appear to be as much as two years older than it had been earlier in the pregnancy.
More encouraging, though, O’Donnell said, is that this aging seemed to reverse for most of the women within three months after birth. In general, their patterns of DNA markers soon reverted to an earlier, more-youthful state, and for some new moms who’d breastfed exclusively in the first three months postpartum, overshot the mark, leaving them apparently “younger” biologically than before, by as much as eight years, the study’s authors wrote, “indicating a pronounced reversal of biological aging.”
Disagreement about aging
But some researchers who study aging, longevity and epigenetics are skeptical of the studies’ findings and conclusions. “It seems unlikely to me that pregnancy induces a whole-body acceleration of biological aging which is then reversed soon after pregnancy,” said Matt Kaeberlein, a longtime longevity researcher who serves as CEO of Optispan, a company that promotes longevity and produces the “Optispan” podcast.
Charles Brenner, who studies metabolism, cancer and aging at the Beckman Research Institute of the City of Hope National Medical Center in California was blunter in an email. “100%, it’s a misuse of aging biomarkers,” he wrote.
Both scientists, as well as others who’ve discussed the studies online, speculate that the epigenetic shifts seen during pregnancy probably reflect the profound physiological demands of carrying a child. They’re “a transient response to the stress of pregnancy, particularly in the immune system,” Kaeberlein said.
What they aren’t is evidence that pregnant women suddenly get older and then younger, these researchers say, or experience lasting effects that could directly shorten or lengthen their life spans.
But the cellular changes being picked up and analyzed by the epigenetic clocks might someday be useful health indicators. If a pregnant woman’s epigenetic markers don’t soon bounce back once she’s no longer pregnant, she and her doctor might want to closely monitor her blood pressure, blood sugar and other standard measures of health, not because she suddenly seems older after becoming pregnant, but because, Ryan said, “pregnancy is such a big deal, physically.”
Do you have a fitness question? Email [email protected] and we may answer your question in a future column.
Read more from Well+Being
Well+Being shares news and advice for living well every day. Sign up for our newsletter to get tips directly in your inbox.
Are you taking your meds wrong ? Many patients make these common mistakes.
Centenarians give their advice about everything.
The wall sit is a simple exercise that can lower your blood pressure.
Tart cherries — more specifically, tart cherry juice — may help with inflammation and pain.
Do you self-sabotage ? Here’s how to stop.

Premium Content
Just one pregnancy can add months to your biological age
A landmark new study confirms that growing a human being in nine months takes a toll—and multiple pregnancies can have a cumulative effect.
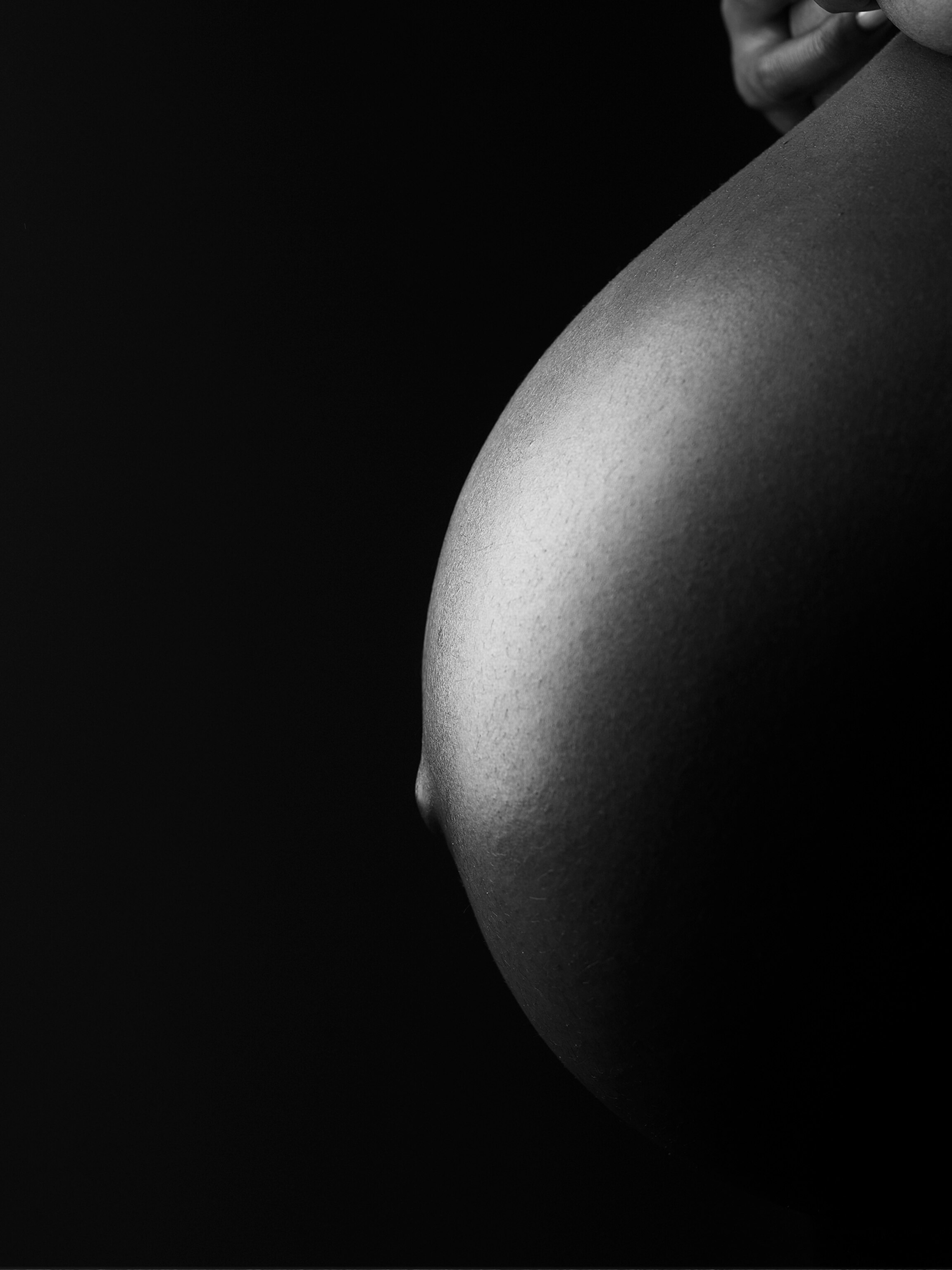
Surprising no one who has ever been pregnant, scientists have found that growing a human being from scratch makes your body “older."
New research suggests that a single pregnancy can add between two to 14 months to your biological age.
“Pregnancy has a cost that appears to be detectable even" as early as your 20s, says study leader Calen Ryan , a human biologist at Columbia University’s school of public health in New York City.
It’s a “landmark study” that reaffirms what women already know—pregnancy takes a tremendous toll on the body, says Yousin Suh , a Columbia University professor who researches how pregnancy affects aging and wasn’t involved in the study, published April 8 in Proceedings of the National Academy of Sciences .
Your chronological age—or the number of trips you’ve made around the sun—may be different than your biological age, which is how old your cells and organs seem based on their biochemistry.
Ryan studies the reasons why our bodies may age faster or slower than we expect them too, and a lot of that comes down to epigenetics, or how and when our bodies decide to turn genes on and off. (Read how scientists are finally studying women's bodies—and what they're learning.)
Certain life events—including major illnesses, trauma, or periods of intense stress —seem to cause “jumps” in epigenetic age as the body redirects energy and resources toward coping with these challenges.
And since there are few biological functions more arduous than growing an entire person in just nine months, the recent study confirms the scientists’ suspicion that pregnancy—particularly multiple pregnancies—come at a cost to biological age.
Your epigenetic clock
If our genome is an instruction manual, the epigenome is a complex system of bookmarks, highlights, and underlines that tells our cells which genes to read and when. This often happens through methylation, a process by which tiny chemical tags called methyl groups attach to a section of DNA .
Which genes need to be active changes constantly in response to our environment and experiences, so those methyl groups need frequent moving and replacing. Yet as we age, this maintenance machinery appears to start making errors, causing methylations to accumulate in some places and disappear in others. (Read how influencing your genes could help you live longer.)
By taking a blood sample and tallying methyl bookmarks in key locations along the genetic code, scientists can calculate a person’s epigenetic age via a suite of algorithms called “clocks.” These clocks predict your risk of death and health complications, but less known is how fertility impacts your biological age .
To learn more, Ryan and his colleagues turned to a long-running study on intergenerational health in the Philippines . In 2005, they analyzed blood samples from 825 women participants between the ages of 20 and 22. (Learn about simple innovations that could help millions of pregnant women.)
The scientists identified a striking difference—the number of epigenetic changes in their DNA revealed that women who had been pregnant were between four and 14 months were biologically older than their peers who hadn’t, even after controlling for factors such as income level and smoking habits.
A cumulative effect
Despite being close in age, the women in the study were already on very different fertility trajectories—some had never been pregnant, some reported one or more previous pregnancies, and some were pregnant at the time the samples were collected.
You May Also Like
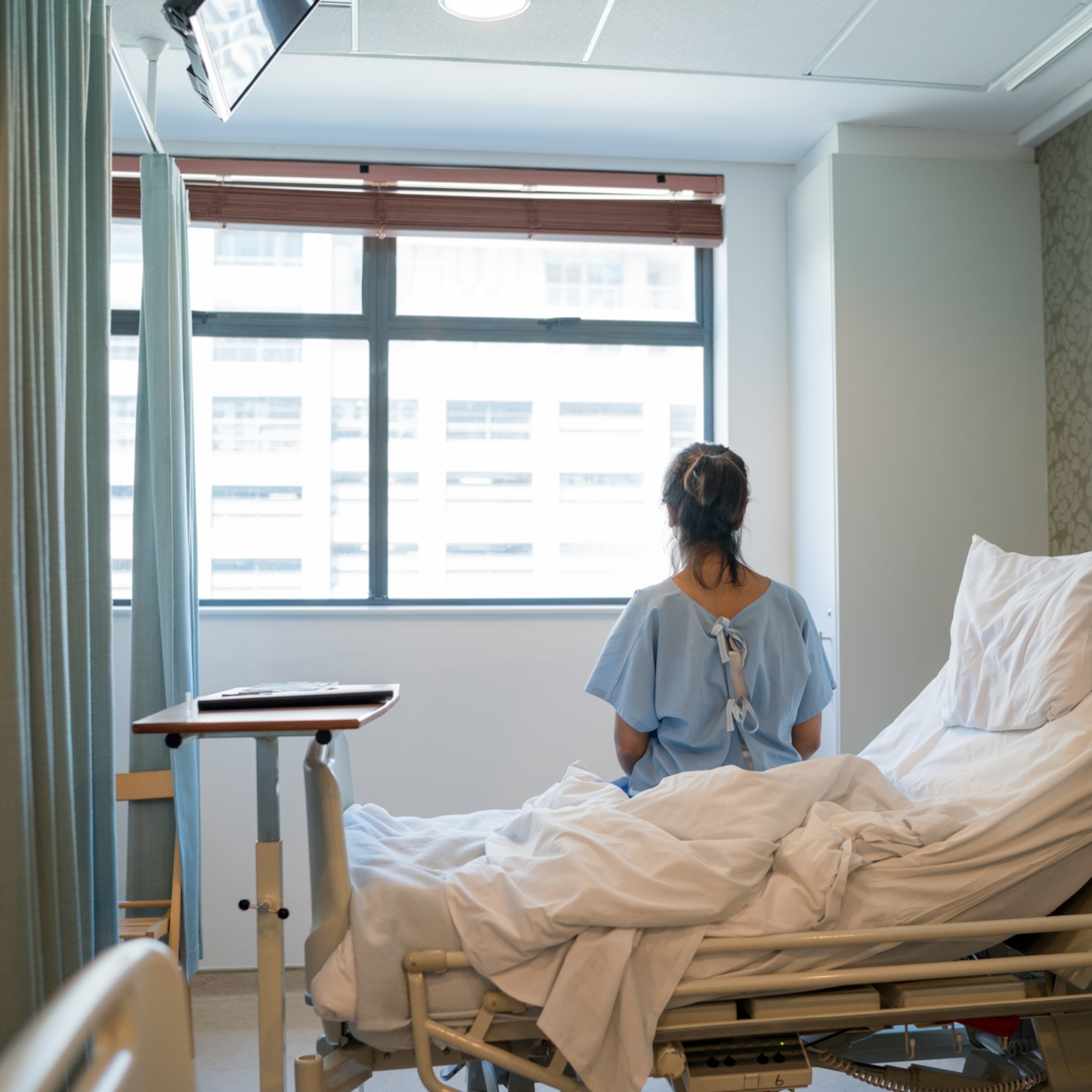
Scientists are finally studying women’s bodies. This is what we’re learning.
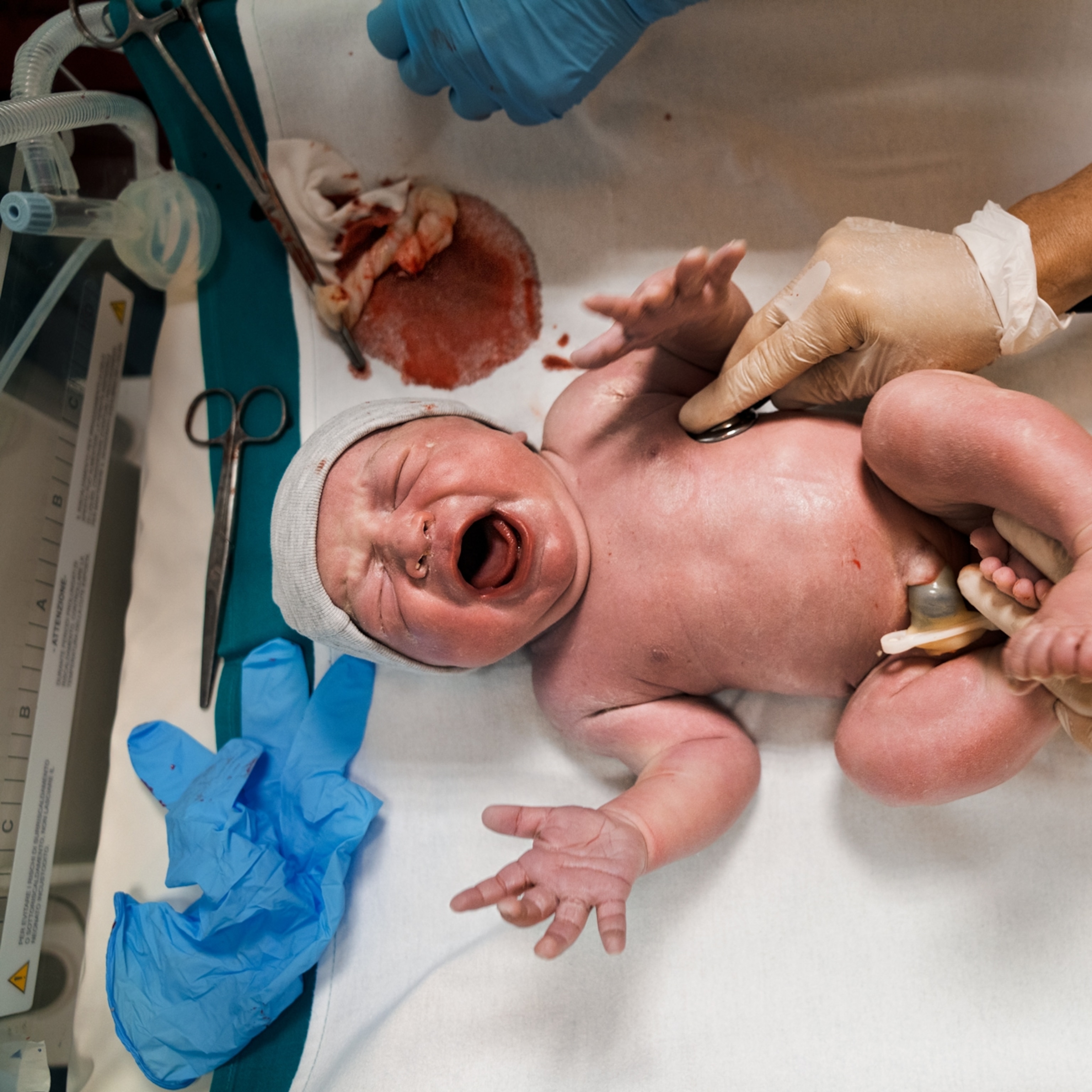
Can aging be cured? Scientists are giving it a try
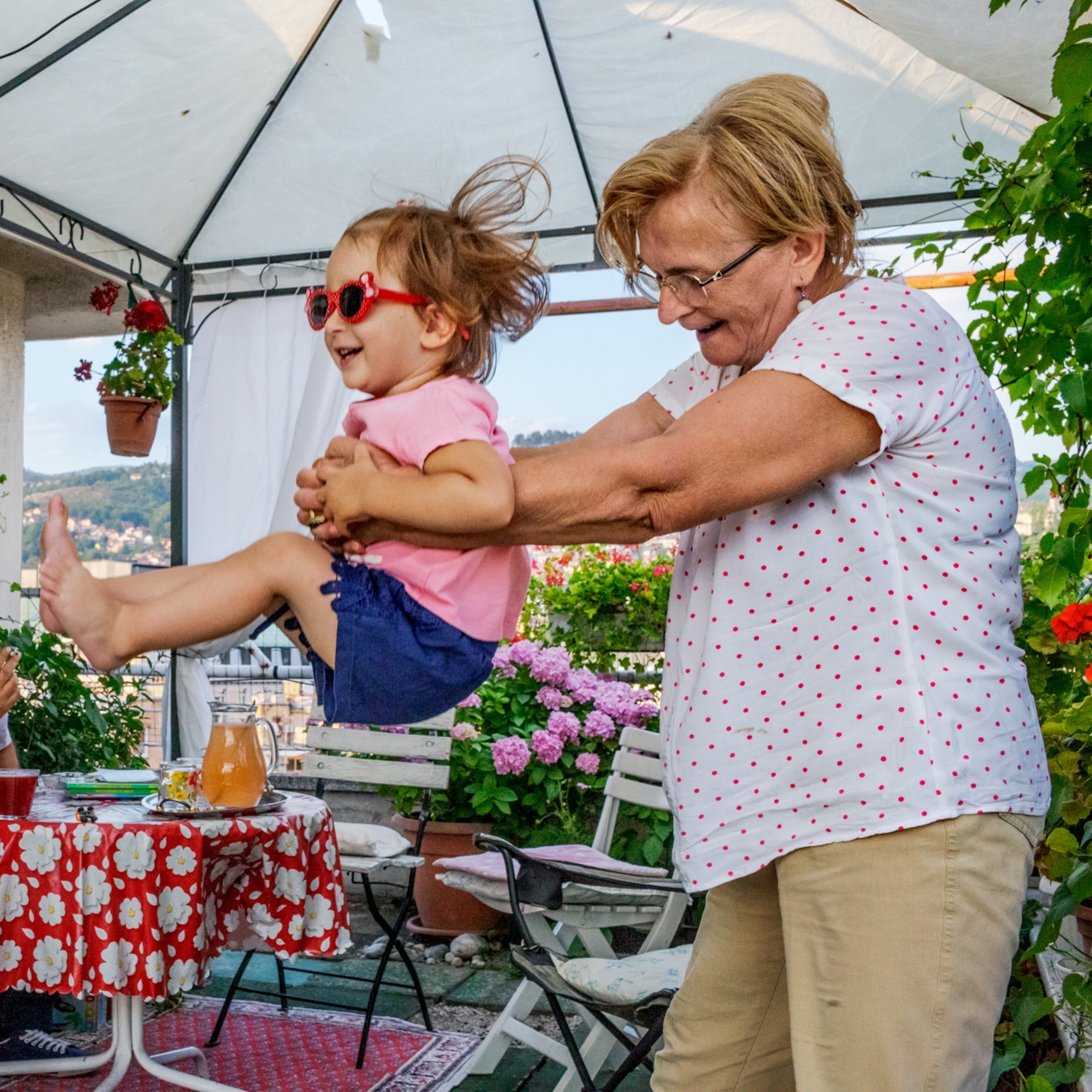
It's not your life span you need to worry about. It's your health span.
That raised a crucial question: Did multiple pregnancies create a cumulative effect of aging, with each additional pregnancy further raising the mother’s epigenetic age?
Using the first blood samples as a baseline, the researchers collected new samples from 331 of the same women while they were pregnant between four and nine years later. (Learn how babies develop in the womb.)
By comparing the two snapshots of each woman’s epigenetic age, Ryan and his team calculated the impact of each additional pregnancy during the intervening years.
“Women who had more pregnancies during that time had more change in epigenetic aging,” Ryan says, with each pregnancy tacking on two to three months to the parent’s biological age.
Suh, who studies the cost of reproduction on the human body, says Ryan’s findings represent an important advancement in our understanding of how multiple pregnancies affect biological age, as the bulk of existing research has examined just one pregnancy.
The new research, she says, squares with what we know about high birthrates—that experiencing many pregnancies can lead to a shorter life span and higher risk of cardiovascular disease.
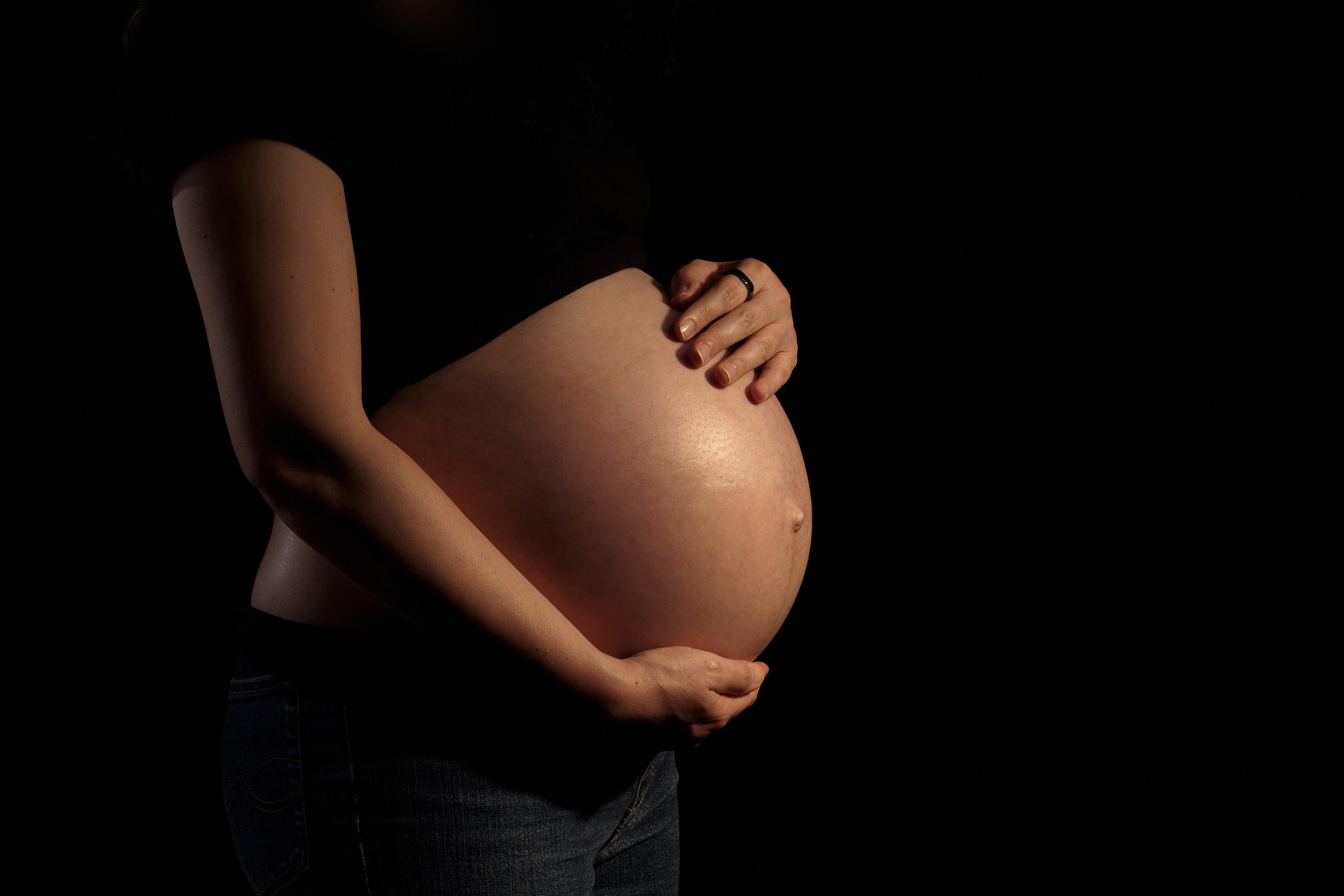
Reason for optimism
But would-be parents shouldn’t despair, Suh and Ryan agree—it’s not certain that a slightly higher epigenetic age during your childbearing years will lead to complications decades down the road.
In fact, some research suggests there may be a “sweet spot” for fertility, Suh says. For instance, one or two pregnancies may be better than none in some cases, as pregnancy is linked to lower risks of certain cancers and having at least one child is associated with a slightly longer life expectancy .
As scientists learn more about aging and fertility, “we can work towards identifying people who might be at higher risk,” Ryan adds, and come up with strategies to lessen the negative impacts of pregnancy.
Recent studies indicate the epigenetic cost of pregnancy may differ by country and culture, suggesting that parental support and access to healthcare may play a significant role—improving these could soften pregnancy’s blow to epigenetic age.
Suh adds more research will be needed to untangle the impact of child- rearing from childbirth on epigenetic age, as well as investigate whether the burden of pregnancy is greater when parents are older than those in the study.
While it may feel like common knowledge that pregnancy ages you, it’s a relatively new concept in the scientific literature—and Suh says that research like Ryan’s is long overdue.
“I’m so encouraged that this kind of study is now being done,” she adds.
Related Topics
- FAMILY LIFE
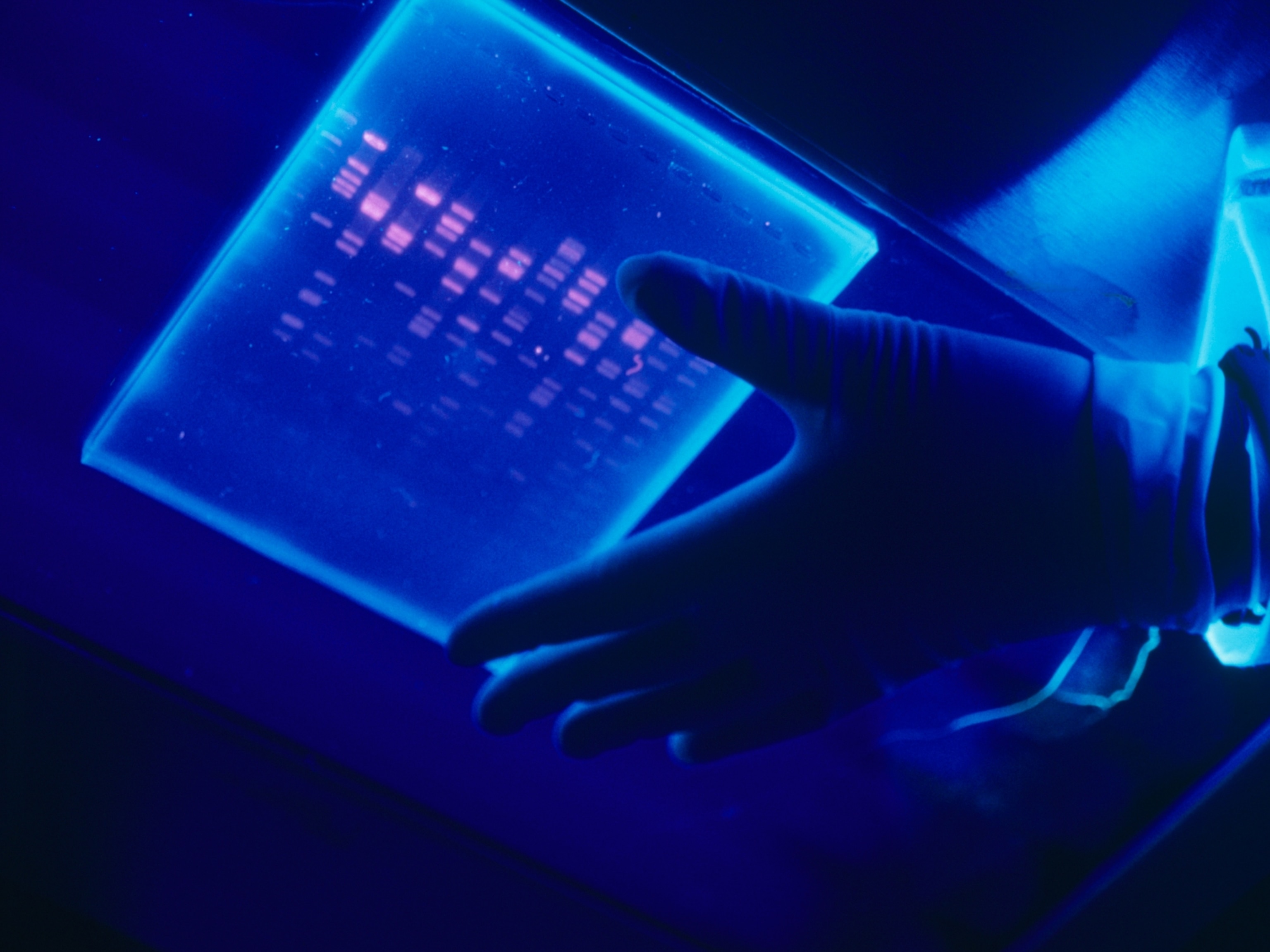
What can your DNA say about your risk of opioid addiction?
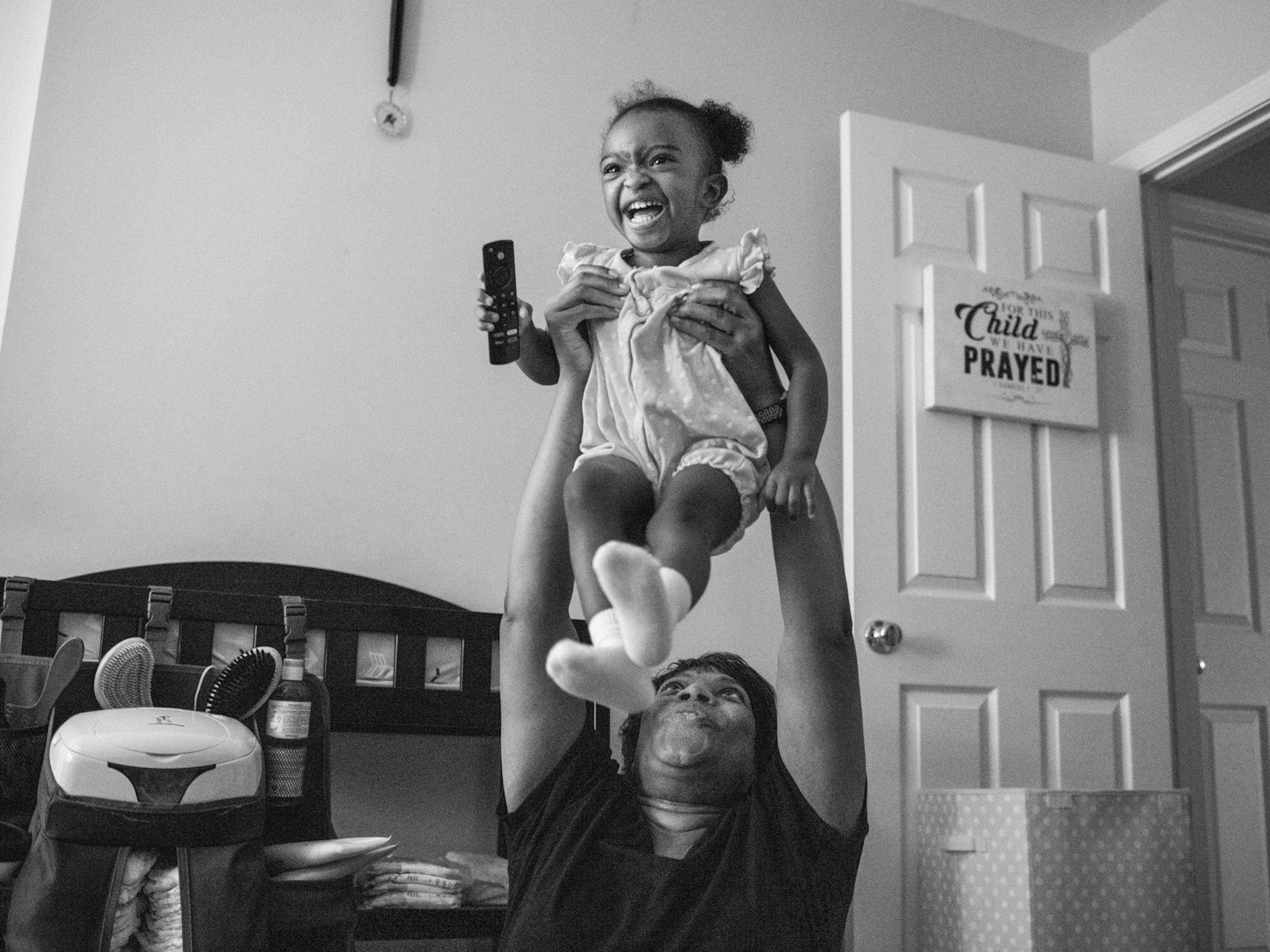
IVF revolutionized fertility. Will these new methods do the same?
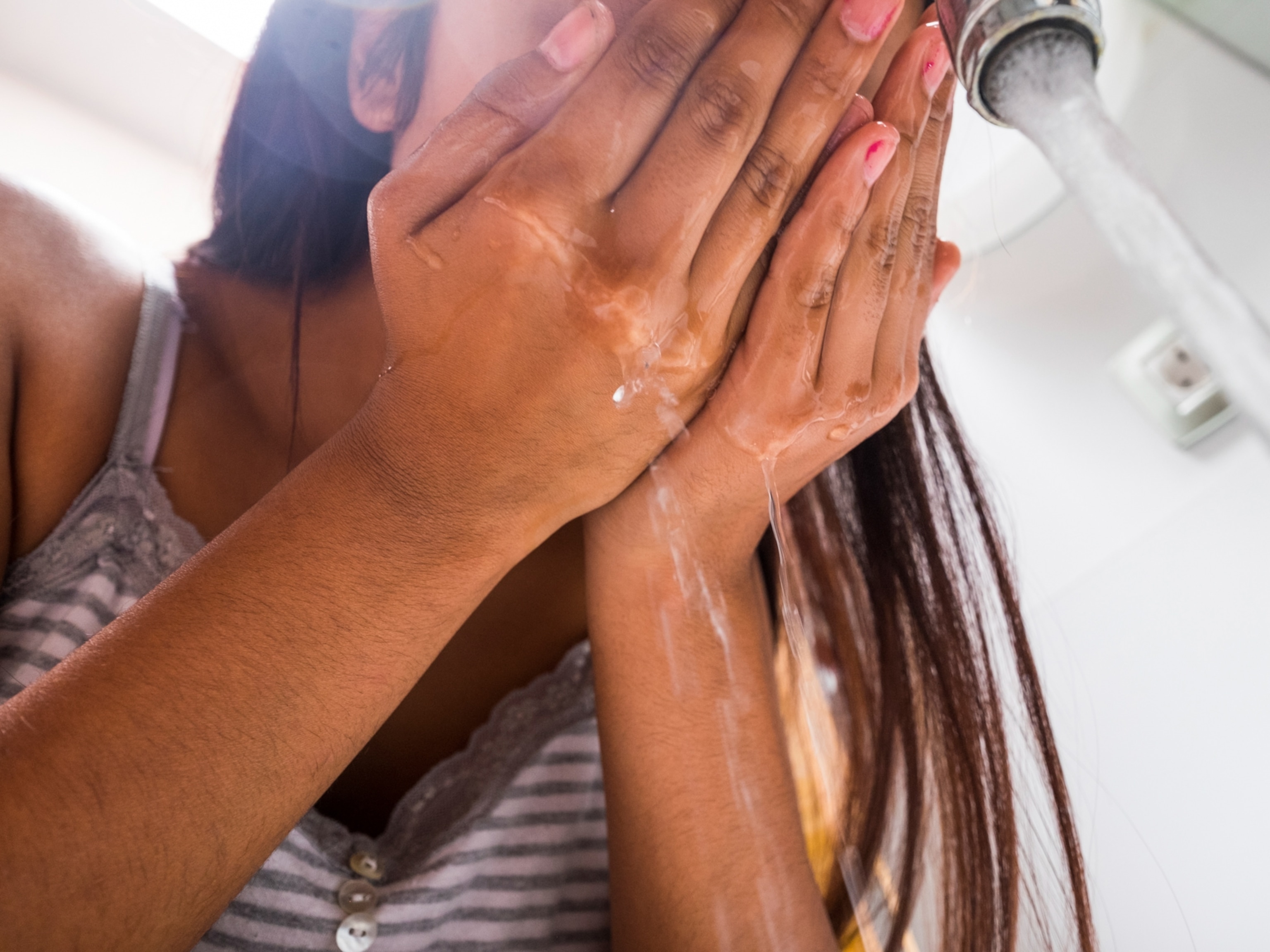
What is our fear of aging doing to our kids’ mental health?
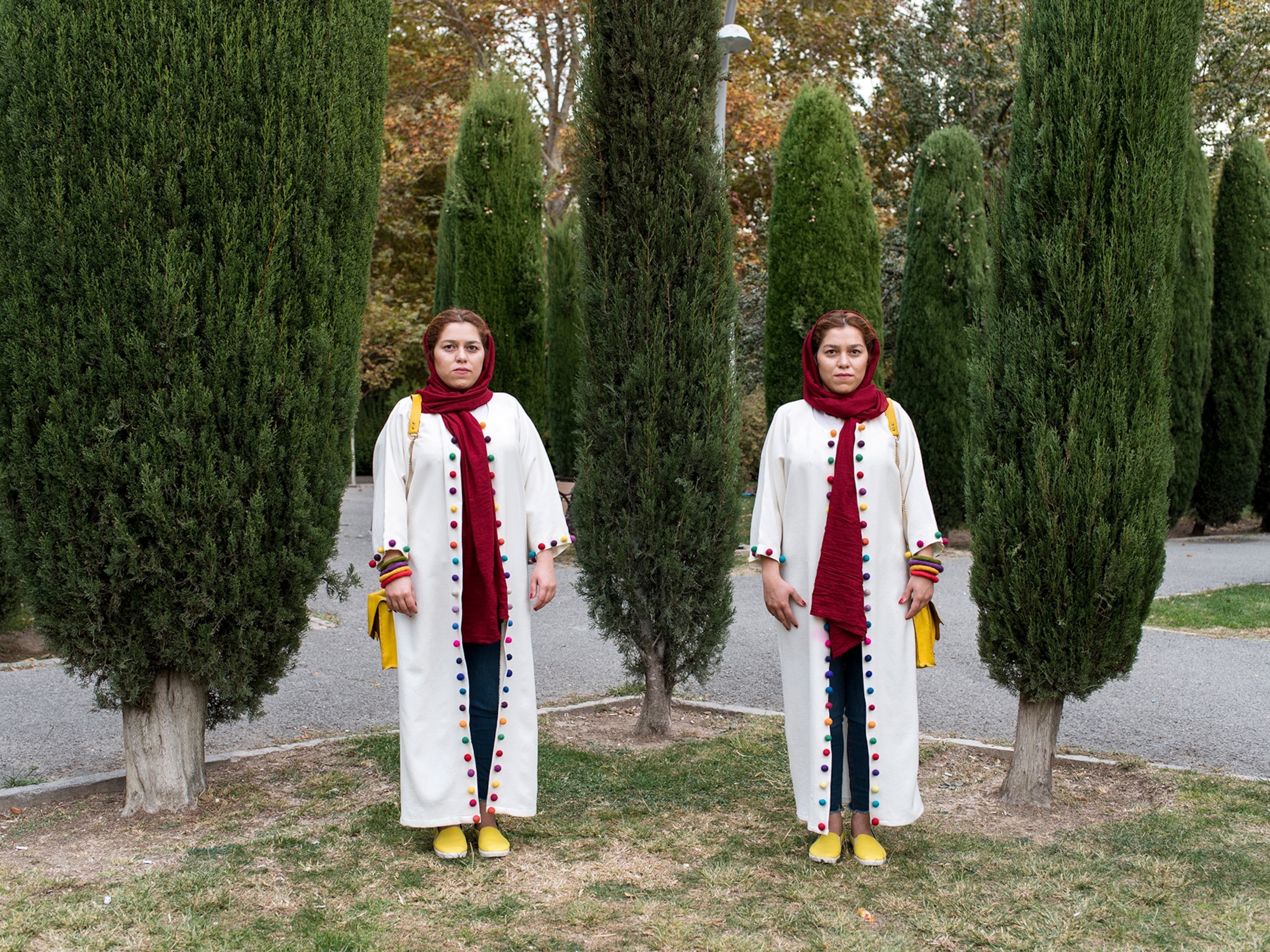
Twins can become ‘unidentical’—and more fascinating twin facts
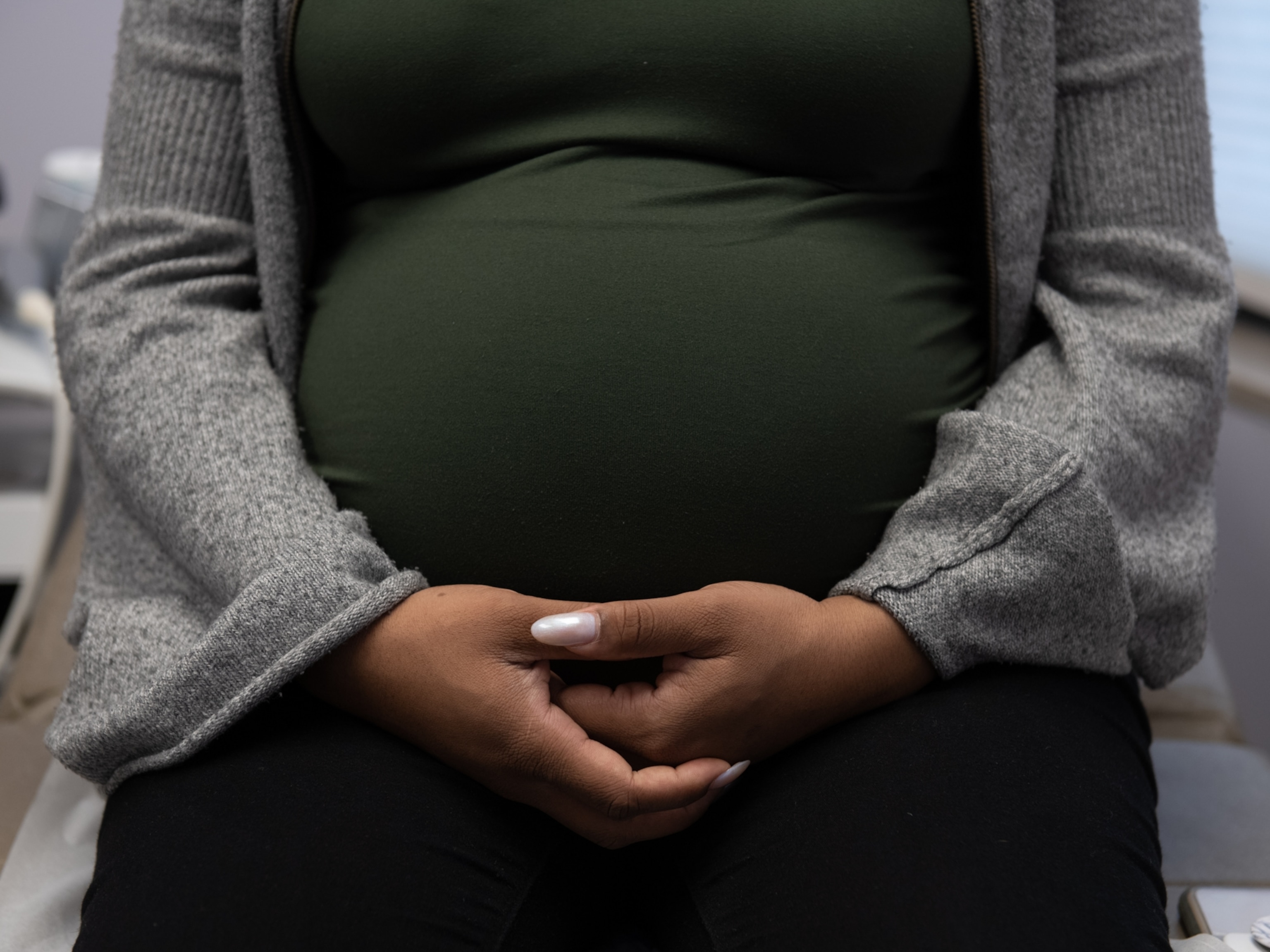
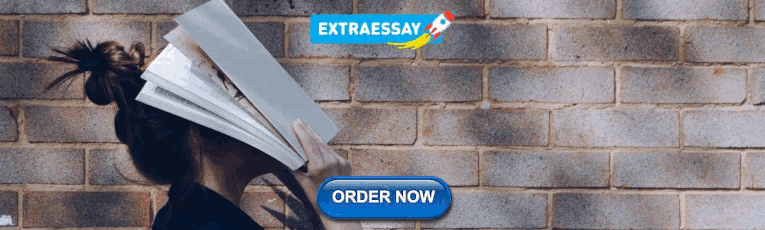
This is one of the leading causes of maternal mortality. A new test could change that.
- History & Culture
- Environment
- Paid Content
History & Culture
- History Magazine
- Mind, Body, Wonder
- Terms of Use
- Privacy Policy
- Your US State Privacy Rights
- Children's Online Privacy Policy
- Interest-Based Ads
- About Nielsen Measurement
- Do Not Sell or Share My Personal Information
- Nat Geo Home
- Attend a Live Event
- Book a Trip
- Inspire Your Kids
- Shop Nat Geo
- Visit the D.C. Museum
- Learn About Our Impact
- Support Our Mission
- Advertise With Us
- Customer Service
- Renew Subscription
- Manage Your Subscription
- Work at Nat Geo
- Sign Up for Our Newsletters
- Contribute to Protect the Planet
Copyright © 1996-2015 National Geographic Society Copyright © 2015-2024 National Geographic Partners, LLC. All rights reserved
- Introduction to Genomics
- Educational Resources
- Policy Issues in Genomics
- The Human Genome Project
- Funding Opportunities
- Funded Programs & Projects
- Division and Program Directors
- Scientific Program Analysts
- Contact by Research Area
- News & Events
- Research Areas
- Research investigators
- Research Projects
- Clinical Research
- Data Tools & Resources
- Genomics & Medicine
- Family Health History
- For Patients & Families
- For Health Professionals
- Jobs at NHGRI
- Training at NHGRI
- Funding for Research Training
- Professional Development Programs
- NHGRI Culture
- Social Media
- Broadcast Media
- Image Gallery
- Press Resources
- Organization
- NHGRI Director
- Mission & Vision
- Policies & Guidance
- Institute Advisors
- Strategic Vision
- Leadership Initiatives
- Diversity, Equity, and Inclusion
- Partner with NHGRI
- Staff Search
ADHD Genetic Research Study
The Attention Deficit/Hyperactivity Disorder (ADHD) Genetic Research Study is exploring the genetic factors that contribute to ADHD, with the hope of improving treatment for this condition in the future.
ADHD often appears to run in families, and research studies have suggested that there may be a genetic component to this disorder. Individuals diagnosed with ADHD may have close blood relatives with the disorder. Scientists believe that ADHD is a complex disorder that probably involves at least two genes. Non-genetic causes such as abnormal brain development, brain injury or environmental factors are also believed to play a role in the disorder.
One of the long-range goals of this study is to facilitate the diagnosis of ADHD and the development of improved treatments, possibly including individually tailored treatments. Discovering the genes that are related to ADHD can only be done with the assistance of children that have ADHD and their families. By volunteering for this type of study, we believe that you and your family will help to bring about the benefits of research: improved diagnoses and treatment for future generations and, eventually, prevention.
The study is not a diagnostic service for ADHD, a treatment or advice service, or a "second opinion" service.
Eligibility
Because this is a genetic study, the children must be related by blood (not adopted or fostered) to the parents and to each other. Eligible families must have more than one child. At least one of the children between the ages of 7 and 18 must have a diagnosis of ADD or ADHD. Other children in the family may be either affected (have ADHD) or unaffected (not have ADHD), but must be at least 7 years of age at the time of enrollment. We would like to enroll entire families, both biological parents included, whenever possible.
No. There is no cost to you, the participant. NIH will cover screening and blood draw costs. In addition, we will provide a "thank you" gift ($10) to each participant for his or her involvement once we have received blood samples.
If you are interested in learning more about the study and/or participating in the study, please fill out the downloadable questionnaire and send it to us using the contact information below, or call the toll free number (1-888-226-6249). We will contact you as soon as possible. Participation is completely voluntary. Indicating interest in the study does not obligate you to participate. Even if at first you decide to participate but later change your mind, you can withdraw at any time.
What's Involved?
If you are interested, one of our team members will call you to take screening information and to explain details of the study and to answer your questions. If we determine that your family might qualify for the study, you will be sent informed consent forms and questionnaires in the mail for each potential enrollee in the study. The questionnaires will ask about past medical and behavioral histories. These questionnaires take approximately 10 to 20 minutes each to complete.
An additional telephone questionnaire with a staff member may also take place, which may take about 45 minutes for each child enrolled.
Once all information is obtained, it will be reviewed by the ADHD team members. If your family is eligible for the next level of our study, a blood collection kit will be sent to you to take to your health care provider to have your blood drawn and sent to us. All the instructions for obtaining the blood and mailing it back to us will be in the kit.
Personal information that is given to us by study participants is kept confidential. Blood samples are given a code that does not include your name. Information about you is kept in locked cabinets or password protected computer files. Research results from blood sample analysis will not be made available to participating families.
As with all of our research studies, participation is completely voluntary. You may decide to participate in the study but later change your mind. You may withdraw your consent to participate at any time.
Contact Information
By Phone (Toll Free): 1-800-411-1222
By Fax: Fax: (301) 480-7825 Attention: Research Coordinator/ADHD Genetic Research Study Download and complete the contact form.
By Mail: Research Coordinator/ADHD Genetic Research Study NHGRI / NIH Building 10, Room 10C103 10 Center Drive Bethesda, MD 20892
Last updated: March 17, 2014

Researchers discover how we perceive bitter taste
Humans can sense five different tastes: sour, sweet, umami, bitter, and salty, using specialized sensors on our tongues called taste receptors. Other than allowing us to enjoy delicious foods, the sensation of taste allows us to determine the chemical makeup of food and prevents us from consuming toxic substances.
Researchers at the UNC School of Medicine, including Bryan Roth, MD, PhD, the Michael Hooker Distinguished Professor of Pharmacology, and Yoojoong Kim, PhD, a postdoctoral researcher in the Roth Lab, recently set out to address one very basic question: "How exactly do we perceive bitter taste?"
A new study, published in Nature , reveals the detailed protein structure of the TAS2R14 bitter taste receptor. In addition to solving the structure of this taste receptor, the researchers were also able to determine where bitter-tasting substances bind to TAS2R14 and how they activate them, allowing us to taste bitter substances.
"Scientists know very little about the structural make up of sweet, bitter, and umami taste receptors," said Kim. "Using a combination of biochemical and computational methods, we now know the structure of the bitter taste receptor TAS2R14 and the mechanisms that initializes the sensation of bitter taste in our tongues."
This detailed information is important for discovering and designing drug candidates that can directly regulate taste receptors, with the potential to treat metabolic diseases such as obesity and diabetes.
From Chemicals to Electricity to Sensation
TAS2R14s are members of the G protein-coupled receptor (GPCR) family of bitter taste receptors. The receptors are attached to a protein known as a G protein. TAS2R14 stands out from the others in its family because it can identify more than 100 distinct substances known as bitter tastants.
Researchers found that when bitter tastants come into contact with TAS2R14 receptors, the chemicals wedge themselves into to a specific spot on the receptor called an allosteric site, this causes the protein to change its shape, activating the attached G protein.
This triggers a series of biochemical reactions within the taste receptor cell, leading to activation of the receptor, which can then send signals to tiny nerve fibers -- through the cranial nerves in the face -- to an area of the brain called the gustatory cortex. It is here where the brain processes and perceives the signals as bitterness. And of course, this complex signaling system occurs almost instantaneously.
Cholesterol's Role in Bitter Taste Reception
While working to define its structure, researchers found another unique feature of TAS2R14 -- that cholesterol is giving it a helping hand in its activation.
"Cholesterol was residing in another binding site called the orthosteric pocket in TAS2R14, while the bitter tastant binds to the allosteric site," said Kim. "Through molecular dynamics simulations, we also found that the cholesterol puts the receptor in a semi-active state, so it can be easily activated by the bitter tastant."
Bile acids, which are created in the liver, have similar chemical structures with cholesterol. Previous studies have suggested that bile acids can bind and activate TAS2R14, but little is known about how and where they bind in the receptor.
Using their newfound structure, researchers found that bile acids might be binding to the same orthosteric pocket as cholesterol. While the exact role of bile acid or cholesterol in TAS2R14 remains unknown, it may play a role in the metabolism of these substances or in relation to metabolic disorders such as obesity or diabetes.
How This Can Help Drug Development
The discovery of this novel allosteric binding site for bitter tasting substances is unique.
The allosteric binding region is located between TAS2R14 and its coupled G protein is called G-protein alpha. This region is critical to form a signaling complex, which helps to transfer the signal from the taste receptor to the G-protein to the taste receptor cells.
"In the future, this structure will be key to discovering and designing drug candidates that can directly regulate G proteins through the allosteric sites," said Kim. "We also have the ability to affect specific G-protein subtypes, like G-protein alpha or G-protein beta, rather than other G-protein pathways that we don't want to cause any other side effects."
Roth and Kim have made a number of new discoveries, but some leave more questions than answers. While running a genomics study, they found that the TAS2R14 protein in complex with the GI is expressed outside the tongue, especially in the cerebellum in the brain, the thyroid, and the pancreas. Researchers are planning future studies to elucidate the function these proteins may have outside of the mouth.
This work was supported by the NIH Illuminating the Druggable Genome Initiative .
- Cholesterol
- Nervous System
- Diseases and Conditions
- Molecular Biology
- Cell Biology
- Biotechnology
- Birch bracket
- Sensory system
- Radiography
- Excitotoxicity and cell damage
- Sugar substitute
Story Source:
Materials provided by University of North Carolina Health Care . Note: Content may be edited for style and length.
Journal Reference :
- Yoojoong Kim, Ryan H. Gumpper, Yongfeng Liu, D. Dewran Kocak, Yan Xiong, Can Cao, Zhijie Deng, Brian E. Krumm, Manish K. Jain, Shicheng Zhang, Jian Jin, Bryan L. Roth. Bitter taste receptor activation by cholesterol and an intracellular tastant . Nature , 2024; DOI: 10.1038/s41586-024-07253-y
Cite This Page :
Explore More
- 3D Mouth of an Ancient Jawless Fish
- Connecting Lab-Grown Brain Cells
- Device: Self-Healing Materials, Drug Delivery
- How We Perceive Bitter Taste
- Next-Generation Digital Displays
- Feeling Insulted? How to Rid Yourself of Anger
- Pregnancy Accelerates Biological Aging
- Tiny Plastic Particles Are Found Everywhere
- What's Quieter Than a Fish? A School of Them
- Do Odd Bones Belong to Gigantic Ichthyosaurs?
Trending Topics
Strange & offbeat.
- Search the site GO Please fill out this field.
- Newsletters
Study: People With Higher Genetic Risk for Obesity May Need More Daily Steps to Manage Weight
:max_bytes(150000):strip_icc():format(webp)/DavidJMartinPhotographyMastroiannipic-BrianMastroianni-c80cebc86ab94699949737ab91911ede.jpg)
- For people looking to avoid obesity, the number of daily steps they should take depends on each person’s body mass index and genetic risk of obesity, new research found.
- Data suggested people with a high obesity risk may need to take more than 11,000 daily steps to lower that added risk.
- People can increase their daily step count by creating new habits and gradually increasing the distance walked each day, experts said.
How many steps should you take each day to manage your weight? The answer may depend on your risk for obesity , according to new research.
A study published on March 27 in JAMA Network Open found that those with a higher genetic risk of obesity had to log more daily steps than individuals who had a lower genetic propensity for obesity.
“It seems intuitive that the risk of obesity would be related to how much activity one engages in and their genetic background. So I wouldn’t say I was surprised that our findings were consistent with those ideas,” said lead study author Evan Brittain, MD, associate professor of medicine in the Division of Cardiovascular Medicine at Vanderbilt University Medical Center.
“What’s new and exciting is that we were able to estimate the actual amount of activity needed to prevent obesity in a way that accounts for both genetic risk of obesity and one’s current body mass index (BMI) ,” Brittain told Health .
Here’s what experts had to say about the new research, how a person’s genetic risk for obesity is determined, and how movement can help you manage your weight .
Trinette Reed / Stocksy
A Closer Look at the Study’s Findings
The research team behind the study looked at National Institute of Health data from 3,124 people of European ancestry. Participants were, on average, 53 years old, owned a Fitbit fitness tracker, and didn’t have obesity up to 6 months into tracking their activity.
On average, these individuals recorded their physical activity for 5.4 years and walked about 8,326 steps each day.
In addition to this tracker data, researchers calculated participants’ polygenic risk score (PRS)—a measure of genetic risk for a disease, in this case, obesity. Participants were then put in different percentile groups based on that genetic risk.
Over the course of the study, 13% of people in the lowest genetic risk group developed obesity, as compared to 43% of people in the highest risk group.
Brittain and his colleagues found that for each group to have a comparable risk of developing obesity, those with higher polygenic risk scores would need to walk more steps each day. People with a PRS in the 75th percentile would need an extra 2,280 steps per day—for a total of 11,020 steps—to have a risk of obesity similar to those with a PRS in the 50th percentile. Meanwhile, those with a PRS in the 25th percentile could get away with walking just 5,080 steps a day.
Step count recommendations also varied based on a person’s BMI. Those in the 75th PRS percentile who had baseline BMIs of 22, 24, 26, and 28 needed to walk an additional 3,460, 4,430, 5,380, and 6,350 more steps each day, respectively, in order to have a similar obesity risk to their study peers who fell in that 25th percentile.
Though the study was able to provide specific step count recommendations for participants, there are some important caveats when it comes to applying the findings more broadly.
First, 95% of the participants were white and 73% were women. Also, individuals had fitness trackers to monitor their behavior, something that not everyone has access to.
“The population we studied was self-selected in that they chose to purchase and wear a Fitbit device,” Brittain explained. “This population is, on the whole, healthier than the general population. So one might speculate that the step counts we derived for reducing obesity risk were higher than what might be needed for a less healthy population.”
Can You Determine Your Genetic Risk for Obesity?
Researchers can calculate PRS for obesity by looking at previous research that tested the influence of genetics on BMI, Brittain explained.
“Each gene gets a ‘weight’ and those weights are averaged for each individual into a ‘genetic risk score,’ or ‘polygenic risk score,’” he said. “In that way, we used the entire genome rather than just genes known to be associated with weight.”
This is important, as there isn’t just one specific gene that can cause obesity, said José Ordovás, PhD, senior scientist and leader of the Nutrition and Genomics Team at the Jean Mayer USDA Human Nutrition Research Center on Aging at Tufts University.
Looking at all of these genes in sum helps paint a picture of someone’s predisposition to gaining weight, he explained.
But Brittain acknowledges that this kind of genetic assessment isn’t necessarily accessible to everyone.
“One’s PRS is not commonly available. But such data are increasingly being returned to patients from commercial sources—like 23andMe,” Brittain added. “So we anticipate a future in which such data may be accessible to both the public and healthcare providers.”
The Healthiest Amount of Daily Steps Depend on the Individual
Though everyone may not know their PRS for obesity, the study results hit on a larger point about how the disease is managed in medicine and in society at large.
While public health guidelines encourage people to embrace physical activity to prevent obesity, those blanket recommendations may not be effective for everyone, Ordovás said.
“These findings could lead to more personalized recommendations, where physical activity guidelines take into account a person's genetic susceptibility to obesity,” he told Health.
Essentially, treating and preventing obesity isn’t a monolithic problem—it varies greatly from person to person.
In the future, as genetic testing likely becomes more widely available, Brittain said he sees a future where “doctors will be able to take advantage of this information to provide more personalized ‘prescriptions’ for activity to prevent obesity and other chronic diseases associated with sedentary behavior.”
Increasing Step Count and Other Ways to Prevent Obesity
When looking at these findings, the thought of incorporating 8,000 to 11,000 steps each day might be intimidating for many people, especially for those who aren’t regularly physically active.
That increase would have to be intentional, Brittain said, but could likely be achieved through habitual changes such as taking a walk during phone calls or parking further away from store entrances. But changing your physical activity routine can be difficult and takes time, Ordovás added.
“For someone who isn’t used to walking much during the day, gradually increasing the number of steps is usually more sustainable than making a sudden jump to 10,000 steps,” Ordovás said. “They might start by adding a short walk to their routine and then increasing the distance over time.”
If fitting walks into your schedule isn’t possible, look for other ways to get movement—this could include taking short breaks during work to stretch or walk, or doing high-intensity interval training (HIIT) for a short but effective workout, Ordovás recommended.
Since managing weight is multi-faceted, oftentimes exercise alone isn't enough. Diet coupled with activity can play an important role in managing obesity, especially for those with greater genetic risk, Ordovás said.
“Studies on diet and obesity PRS often find that people with a higher genetic risk for obesity might need to follow stricter dietary guidelines to manage their weight effectively,” he said.
This could mean eating a balanced diet, rich in whole food and low in processed foods, Ordovás added.
Ultimately, Brittain said that this research shows that one’s genetics don’t necessarily mean that personal behavioral changes can’t alter one’s overall health.
“Our data suggest that genetics are not destiny," he said. "The influence of genetics on obesity risk may be mitigated by increasing activity."
Brittain EL, Han L, Annis J, et al. Physical activity and incident obesity across the spectrum of genetic risk for obesity . JAMA Netw Open . 2024;7(3):e243821. doi:10.1001/jamanetworkopen.2024.3821
Related Articles
Thank you for visiting nature.com. You are using a browser version with limited support for CSS. To obtain the best experience, we recommend you use a more up to date browser (or turn off compatibility mode in Internet Explorer). In the meantime, to ensure continued support, we are displaying the site without styles and JavaScript.
- View all journals
- My Account Login
- Explore content
- About the journal
- Publish with us
- Sign up for alerts
- Expert Review
- Open access
- Published: 02 February 2021
Genetic variation, brain, and intelligence differences
- Ian J. Deary ORCID: orcid.org/0000-0002-1733-263X 1 na1 ,
- Simon R. Cox ORCID: orcid.org/0000-0003-4036-3642 1 na1 &
- W. David Hill 1 na1
Molecular Psychiatry volume 27 , pages 335–353 ( 2022 ) Cite this article
58k Accesses
38 Citations
479 Altmetric
Metrics details
- Neuroscience
Individual differences in human intelligence, as assessed using cognitive test scores, have a well-replicated, hierarchical phenotypic covariance structure. They are substantially stable across the life course, and are predictive of educational, social, and health outcomes. From this solid phenotypic foundation and importance for life, comes an interest in the environmental, social, and genetic aetiologies of intelligence, and in the foundations of intelligence differences in brain structure and functioning. Here, we summarise and critique the last 10 years or so of molecular genetic (DNA-based) research on intelligence, including the discovery of genetic loci associated with intelligence, DNA-based heritability, and intelligence’s genetic correlations with other traits. We summarise new brain imaging-intelligence findings, including whole-brain associations and grey and white matter associations. We summarise regional brain imaging associations with intelligence and interpret these with respect to theoretical accounts. We address research that combines genetics and brain imaging in studying intelligence differences. There are new, though modest, associations in all these areas, and mechanistic accounts are lacking. We attempt to identify growing points that might contribute toward a more integrated ‘systems biology’ account of some of the between-individual differences in intelligence.
Similar content being viewed by others
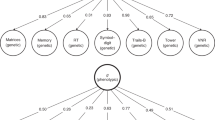
A general dimension of genetic sharing across diverse cognitive traits inferred from molecular data
Javier de la Fuente, Gail Davies, … Ian J. Deary
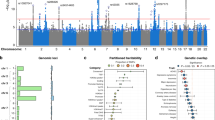
Genome-wide meta-analysis of brain volume identifies genomic loci and genes shared with intelligence
Philip R. Jansen, Mats Nagel, … Danielle Posthuma
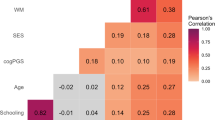
Schooling substantially improves intelligence, but neither lessens nor widens the impacts of socioeconomics and genetics
Nicholas Judd, Bruno Sauce & Torkel Klingberg
Individual differences in human intelligence
This article is about some new contributions toward understanding the aetiology of individual differences in human intelligence. The focus is on genetic variation and brain imaging-derived differences, including where those two sources overlap. For more than a century, the field of research that studies intelligence differences has had some controversies (Box 1 ). Notwithstanding these, research findings on intelligence have much consensus, based on robust findings. The first part of this article summarises some of the findings from which reductionist approaches—including brain imaging and genetics—to intelligence differences proceed.
Box 1 Some controversies and some consensuses in intelligence
Here is an abbreviated litany of controversies about human intelligence differences. Galton [ 130 ], who suggested that cognitive capabilities might be general, normally distributed, and somewhat heritable (modern data show some support for these suggestions), was also, notoriously, an originator of eugenics, and invented that word (see www.ucl.ac.uk/provost/inquiry-history-eugenics-ucl ). Spearman [ 4 ] discovered the positive matrix of correlations among cognitive performance assessments, and developed a two-factor theory of intelligence, which had ‘general intelligence’, which he called g , and specific abilities, which he called ‘ s ’. Researchers such as Thurstone [ 131 ] and Gardner [ 132 ] disagreed, and thought there were several separate intelligences. Thomson [ 133 ] produced an ingenious theory about how the positive matrix of cognitive test correlations might occur without there being a g factor. Whether g is found (it is a replicable statistical finding [ 5 ]) and what it means (which is not known) have been discussed since then. Henry Herbert Goddard imported Binet’s intelligence test (the first one to be invented) to the USA and is documented to have over- and mis-applied it (Zenderland) [ 134 ]. Gould [ 135 ] strongly criticised the g factor in intelligence—stating that it was a necessary outcome of the statistical analytic methods applied (which is incorrect)—and the association between intelligence and brain size in his famous book, The Mismeasure of Man . The book has been criticised for having got both of these wrong (Carroll) [ 136 ]. Flynn [ 137 ] found that intelligence test scores increased across the years and generations of the middle two quarters of the 20th century. However, Flynn also made it clear that this does not alter the within-cohort reliability, validity, and heritability of intelligence test scores. Nevertheless, the cause(s) of the ‘Flynn’ effect on intelligence test scores still remains mysterious. Herrnstein and Murray wrote a book called The Bell Curve [ 138 ]. They analysed data from the USA’s National Longitudinal Survey of Youth 1979 and found that higher intelligence in late adolescence/early adulthood was related to better life outcomes by the 30 s. The book was strongly and widely criticised, especially for its dealing with ethnic group differences, and for not having published its analyses via peer review.
Calming, consensual oil was poured on intelligence’s stormy waters in 1996, by the American Psychological Association (APA). As a result of the controversy caused by The Bell Curve , the APA put together a Task Force, chaired by cognitive psychology doyen Neisser [ 139 ], to tell non-experts what was (solidly) known and (as-yet) unknown about intelligence test score differences. The 11 persons in the Task Force—who became co-authors of an agreed article—surprised many. They were experts who were diverse in their viewpoints—for example, there were some individuals who were more environmentally inclined and some who were more genetically inclined, some who were associated with the hierarchical model of intelligence differences including g and some with different models of intelligence, etc. Yet, they wrote a still-useful article on some of the solid ground in intelligence research. Among many topics addressed, they recognised the prominence of the psychometric testing approach to intelligence differences, and the hierarchy with the g factor at the apex; they summarised the stability of intelligence test scores, their predictive validity for education, work and other life outcomes, their having environmental and genetic origins, the Flynn effect, and various types of group differences. The APA Task Force Report is still a must-read for obtaining a mostly disinterested and consensual summary about intelligence. Their list of intelligence’s unknowns are still mostly in that state; one of them was genetics, which we address here and which has moved on considerably. We recommend reading other, more recent summaries about knowns and unknowns in intelligence, though we wish to orient the reader that some come from more socially/environmentally inclined groups of authors (Nisbett et al.) [ 129 ], some from more genetically inclined (Gottfredson) [ 2 ], and two from one of the present authors (Deary) [ 27 , 140 ]. Progress in finding social and environmental causes of intelligence has arguably been less successful than the biological research summarised herein, though the Nisbett et al. review discusses many growth points. Moreover, the Neisser et al., and Nisbett et al., summaries also deal with brain imaging and genetics, providing useful background to the present overview, as does Haier’s book, The Neuroscience of Intelligence [ 141 ]. Lest the reader makes the error of over-extending intelligence’s demesne and importance, the APA Task Force ended by emphasising—as do we—that there are many cognitive and non-cognitive aspects of human differences that are not captured by intelligence tests and general intelligence. g might be important, but it is far from being all that matters.
Describing the phenotype of intelligence
We should make it clear to the reader that ‘intelligence’ is just one of the terms that are used to describe humans’ differences in thinking skills; others, sometimes used as near-synonyms, include cognitive ability, cognitive performance, cognitive functioning, and mental ability. Sometimes IQ (intelligence quotient) is used, although that has a specific meaning within the field of psychometrics. Intelligence (or the other terms listed in the previous sentence), as a human phenotype, is measured using cognitive tests, of which there are thousands. This hands the cynic a weapon that, to the ignorant, can glibly dismiss the field of research because, as Boring [ 1 ] famously wrote in 1923, “…intelligence as a measurable capacity must at the start be defined as the capacity to do well in an intelligence test. Intelligence is what the tests test.” That much-quoted last short sentence was not Boring’s opinion; rather, it was his saying that that is what one would think if one did not know the research findings. His next sentence starts, “This is a narrow definition, but it is the only point of departure for a rigorous discussion of the tests”. We shall have that rigorous discussion here. Before that, we offer another, much-cited definition: “Intelligence is a very general mental capability that, among other things, involves the ability to reason, plan, solve problems, think abstractly, comprehend complex ideas, learn quickly, and learn from experience. It is not merely book learning, a narrow academic skill, or test-taking smarts. Rather, it reflects a broader and deeper capability for comprehending our surroundings—‘catching on’, ‘making sense’ of things, or ‘figuring out’ what to do’ [ 2 ]. More succinctly, intelligence has been described as, “rapid and accurate problem solving” [ 3 ].
Cognitive ability differences form a hierarchy of variances. This grew from the finding that all cognitive tests are positively correlated; people who score well on one cognitive test tend to score well on all the others, no matter how different the cognitive skills being assessed appear to be. This finding has been replicated consistently since Charles Spearman discovered it in 1904 [ 4 ]. For example, John Carroll re-analysed correlation matrices of diverse cognitive tests from 400+ studies conducted in the 20th century [ 5 ]. These included studies by many of the most prominent researchers over that time, including those who had claimed not have found a general intelligence factor. Carroll found that, in all studies, the cognitive tests’ scores correlated positively, and that each study contained a general component that accounted for around 40%, sometimes more, of people’s differences in performance [ 5 ]. There was also variance at the level of cognitive domains, such as memory, reasoning, and speed; i.e., some cognitive tests correlate more highly with some tests—that have contents similar to theirs—than with others. And there was variance at the level of the individual tests. In summary, as shown in Fig. 1 , the reasons that people do well on any cognitive test are that: they are generally intelligent; they are good at that type of test; that they are good at the specific skills in that test; and we should not forget the error in the measurement, and just having a good day for whatever reason.
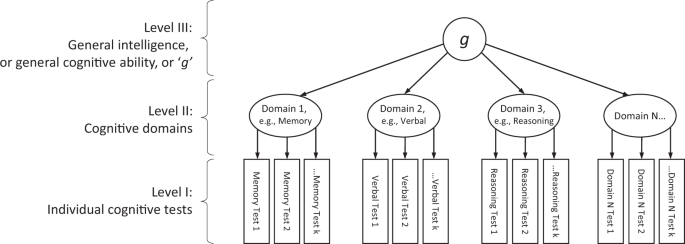
At the bottom, on level 1, individuals differ with regard to their performance on specific cognitive tests. Here we have shown that multiple tests (up to a number k ) are used to assess each domain of cognitive capability. Scores on all tests of cognitive ability correlate positively. However, there are especially strong correlations among tests that tax the same cognitive domain (level 2). Level 2 illustrates three example cognitive domains: memory, processing speed, and verbal. There could be more (up to a number ‘ N ’), depending on the types of specific tests included in the battery. The names applied to domains are common-sense labels based on the apparently shared contents of the specific tests that contribute to them. It is possible to have tests that contribute to more than one domain (a possibility not shown in the Figure). Individual differences are observable at the domain level. However, people’s scores on any one cognitive domain correlate with their scores in other domains. This means that there is a third level describing the variance common across all the domains and, thereby, across all the individual cognitive tests. This is denoted, at Level 3, by general cognitive ability, general intelligence, or just ‘ g ’. It is important to note that this three-level structure emerges from the data and is not imposed on it. g tends to account for about 40% of the total test score variance when a battery of diverse cognitive tests is administered to a sample of people with a wide range of cognitive capabilities.
General intelligence ( g ), as a statistical phenomenon, is a universal finding from different batteries of cognitive tests. However, there is still mystery about what causes the covariation. If a large sample of people take two different, diverse sets of cognitive tests, the two g factors from them correlate highly [ 6 ]; thus, g is not idiosyncratic, and its ranking of individuals does not differ substantially depending on the test battery. Among the sources of between-individual variance that are extractable from a set of cognitive test scores, it tends to be the g factor that leads the way in being associated with life outcomes, and with some possible origins, such as brain imaging indices and genetic variants. When researchers measure general intelligence they tend to use one of the following indices: ideally, the first unrotated principal component or factor from a battery of diverse cognitive tests; a total score from a test that has heterogeneous items covering diverse cognitive skills; or a test with a more homogeneous set of items that loads highly on the first unrotated principal component or general factor of several cognitive tests.
Stability of intelligence differences and mean scores
Measures of general intelligence have high test-retest reliability. Taking an extreme example, the stability co-efficient of intelligence test scores is between 0.6 and 0.7 from age 11 years to about 80, even before correcting for measurement error [ 7 ].
The other type of stability—stability of age-related means—shows a well-reproduced pattern. Tests that involve the recall of learned information (called crystallised intelligence)—such as vocabulary, general knowledge, and some number skills—are relatively stable in mean levels from young adulthood to older age [ 8 , 9 ]. Tests that involve active mental work (aspects of fluid intelligence) decline in mean levels from young or middle adulthood to older age [ 8 , 9 ]. These include cognitive domains such as processing speed (e.g. in tests of coding numbers into symbols at speed), memory (e.g. delayed recall of a story or a list of words, or a working memory test, such as backward digit span), visuo-spatial ability (such as the replication of a 2-dimensional pattern using blocks in the Wechsler Block Design test), and abstract reasoning (such as the inductive reasoning from abstract patterns that is required in Raven’s Progressive Matrices). These more age-sensitive cognitive domains tend to age in concert, with half or more of the individual differences in their age-related declines accounted for by the ageing of general fluid intelligence [ 10 ]. There are individual differences in the ageing of intelligence, which has growing importance as populations have more older people who live longer [ 9 ]; that is, it is important to understand the determinants (especially the modifiable ones) of successful cognitive ageing.
Predictive validity of intelligence: ‘healthy, wealthy, and wise’
Intelligence test scores at the end of primary schooling—at about 11 years of age—are highly correlated with educational outcomes several years later, whether that is measured as scores on standardised national examinations at 16 (where correlations up to 0.8 have been reported), years of education undertaken, or the highest qualification obtained [ 11 , 12 ]. Probably, intelligence is causal to experiencing longer and more complex education, and there appears to be a small effect in the opposite direction too [ 13 ]. Thus, intelligence and education probably have a dynamic bi-directional, and possibly causal, association.
Intelligence is one of the best (and cheapest) predictors of performing well on a job, and of learning well on a job, with moderate correlations [ 14 ]. This applies to all levels of job complexity, though the correlations are somewhat higher with more complex occupations. Higher childhood intelligence is moderately related to moving upward in occupational status from one’s parents (usually father) [ 15 ]. Intelligence is one among many other variables that are associated with socioeconomic status differences in the UK [ 15 , 16 ]. More affluent parental socioeconomic status and more education are among other variables that independently contribute; the former effect is relatively small, and it is not certain to what extent education acts as a proxy for prior intelligence.
There is a robust and consistently sized association between higher intelligence measured in childhood or youth and longer life and better health [ 17 ]. Studies on this topic include unusually impressive samples, including a country’s almost-whole year-of-birth population [ 17 ] and samples that contain up to millions of subjects [ 18 , 19 ]. People with higher intelligence in early life are, up to several decades later, less likely to suffer from poor health and then die from all causes, and, specifically, from heart disease, stroke, respiratory disease, smoking-related cancers, digestive diseases, dementia, accidents, and suicide, among other causes. A typical effect size for this field—cognitive epidemiology—is that a standard deviation (15 IQ-type points) advantage in intelligence in youth is associated with 20–25% lower risk of these illness and mortality outcomes up to several decades later. Expressed as a correlation, the association between childhood cognitive test scores and all-cause mortality is typically between about 0.15 and 0.2.
Therefore, intelligence, as operationalised by cognitive test scores, has a robustly characterised phenotype, high test-retest stability, and some predictive validity for education, work, and health; all are contributions to broader construct validity. However, there is a lack when it comes to understanding why some people are more intelligent than others. We have written about these matters previously. A book-length treatment in 2000 [ 20 ] examined possible origins of intelligence differences in so called ‘elementary’ cognitive components, brain parameters, and genetic variation. There are robust associations between intelligence test scores and apparently simpler processing speed measures such as reaction times [ 21 ] and the psychophysical procedure called inspection time [ 22 ]. We do not focus on these here, because we judge that they afford less-tractable possible causes of some of the between-individual differences in intelligence than genetic variation and brain structure and functioning. We previously summarised genetics and brain imaging associations with intelligence test scores in 2010 [ 23 ]. However, at that time there were no genome-wide association studies (GWAS) of intelligence and, since then, brain imaging studies have larger samples, new brain parameters, and have been linked with molecular genetic studies.
Intelligence differences and genetic variation
Heritability and genetic architecture of intelligence differences.
Twin and family studies report that genetic differences are associated with individual differences in intelligence test scores (Box 2 ). If studies from all ages are taken together, genetic differences account for about 50% (standard error [SE] about 2%) of the variation in intelligence [ 24 ]. Higher heritability (see Glossary) estimates are found in samples of adults (where it can be 70% or slightly more) than in children (where estimates as low as 20–30% have been reported) [ 24 , 25 , 26 , 27 ]. The finding that intelligence is heritable has been replicated across multiple data sets sourced from different countries and times [ 28 ]. Our emphasis herein is on results from the newer, DNA-based studies rather than on traditional twin and family studies.
DNA-based studies have shown that a pattern of hierarchical variance is evident at the genetic as well as the phenotypic level. Using genomic structural equation modelling [ 29 ] it was found that a genetic general factor explained, on average, 58.4% (SE = 4.8%, ranging from 9 to 95% for individual tests) of the genetic variance across seven cognitive tests in people with European ancestry. This provides some support for the idea that the phenotypic structure of intelligence is in part due to genetic effects that act on a general factor of intelligence and also at more specific cognitive levels.
Since 2011, the heritability of intelligence has been investigated by direct testing of DNA in large numbers of unrelated individuals [ 30 ]. This is mostly based on the testing of genetic variants called single nucleotide polymorphisms (SNPs) (see Glossary). The statistical-genetic method used to estimate heritability is called genome-based restricted maximum likelihood single component (GREML-SC) (Box 2 ). This tests how closely people’s similarity in cognitive test scores associates with their genetic similarity, the latter being based on hundreds of thousands of SNPs. In such studies, heritability estimates are about 20–30% (SEs < 1% in recent studies) [ 31 , 32 ]. The lower estimates of heritability found using GREML-SC are probably due to the technique’s being better at capturing variance from genetic variants in linkage disequilibrium (LD) with common SNPs rather than those that are less common or in lower LD [ 33 ]. This difference in estimated heritability of intelligence between twin-based studies and DNA-based studies using SNPs has been recovered using DNA testing and the GREML-KIN analysis method in a large cohort of individuals that included families (Box 2 ) [ 32 ].
Non-additive genetic variation, including dominance and epistasis, has been postulated as a partial explanation for the gap in heritability estimates derived using twin and family methods compared with those derived using DNA-SNPs. However, one study found dominance effects were linked to less than 4% of phenotypic variance in complex traits [ 34 ]. Furthermore, quantitative genetics theory predicts that epistasis is unlikely to be associated with a substantial amount of phenotypic variance [ 35 ]. Moreover, the results of GREML-KIN have been replicated in unrelated individuals by deriving heritability estimates using high-quality imputation panels [ 32 ], indicating that non-additive genetic effects, if present for intelligence, are not a major contributing factor to intelligence differences.
Although GREML-KIN might recover some of the heritability that is attributable to genetic variants that are in poor LD with common genotyped SNPs, it cannot determine the proportion of the heritability estimate that is due to dynastic effects [ 36 ]. Dynastic effects include instances where the genotype of a parent is associated with the phenotype of the offspring, including via alleles that are not passed from parent to offspring; this has been termed genetic nurture [ 37 ]. Whereas the presence of dynastic effects does not indicate a bias in methods that also capture indirect genetic effects, it does however hamper efforts to understand how genetic differences can give rise to phenotypic differences. This is because the resulting heritability estimate is, potentially, a combination of direct genetic effects (genetic variation in an organism that is associated with phenotypic variation in the same organism) and indirect genetic effects (where genetic endowment of one organism is associated with the phenotype of another organism).
The presence of dynastic effects has been indicated for education. In one study, its SNP-based heritability estimate was 29.2% (SE = 4.4%) before indirect genetic effects were removed, and 17% (SE = 9.4%) afterwards [ 36 ]. Moreover, environmental influence on the heritability of education was suggested by finding that a polygenic score (see below) predicting education in non-adoptees accounted for twice the phenotypic variance of a polygenic risk score applied to adoptees [ 38 ]. The variance accounted for by a polygenic score captures both direct genetic effects and indirect, environmentally mediated, genetic effects. When predicting education with polygenic scores in adopted individuals, the link between the rearing environment provided by the genetically related parent and the phenotype of the offspring is broken. Because intelligence is highly genetically and phenotypically associated with education (see below), it appears likely that indirect genetic effects influence intelligence too.
Box 2 Heritability of intelligence: why different methods give different results
Heritability describes the proportion (often expressed as a percentage) of phenotypic variation in a tested sample of people that can be accounted for by genetic variation [ 142 ]. Note that heritability estimates apply to a sample at a given time; the estimate might be different in other groups, and in the same group at other times. Often, in human studies, only additive genetic factors are considered. Different methods are used to estimate heritability of intelligence (and other phenotypes). They give different estimates. These are not contradictory; rather, they are a reflection of the sources of genetic variation to which the methods afford access, as we explain below.
Twin and family methods
Twin- and family-based estimates of the heritability use the expected proportion of alleles shared between the participants as the estimate of genetic variance within the sample. They can include comparisons between monozygotic twins and dizygotic twins, as well as studies that include families consisting of parents, siblings, and other relationships. In each instance, a genetic effect on a trait is inferred if individuals who are more genetically similar are also more similar in terms of their intelligence test score. For intelligence, substantial (50% or more, unless the study is of young children) heritability estimates are found in twin studies [ 24 ] and family studies [ 143 ]. We note that twin studies assume that dizygotic twins have just as similar shared environments as monozygotic twins, a potential limitation that does not affect DNA-based studies.
Genome-based restricted maximum likelihood single component (GREML-SC)
GREML-SC, sometimes referred to as ‘the GCTA method’, was the first DNA-based genetic method used to derive an estimate of heritability for intelligence [ 30 ]. As with twin and family-based methods, genetic similarity is compared with phenotypic similarity. However, genetic similarity is measured, rather than inferred, using a genomic relationship matrix constructed from genotyped common SNPs. Importantly, closely related individuals are excluded from the analysis (typically those who are more than 0.025 similar, i.e. closer than a second cousin). This is an attempt to ensure that the similarity in environment between family members is not captured by genetic the genomic relationship matrix, which can result in an inflation of the heritability estimate. Heritability estimates of intelligence that use GREML-SC have typically been between 20 and 30% [ 30 , 31 , 143 ]. One of the major assumptions of GREML-SC is that genetic similarity is uncorrelated with environmental similarity. Whereas this assumption has been found not to hold in some situations, the inflation of the resulting heritability estimate is thought to be negligible [ 144 ]. Furthermore, GREML-SC assumes an infinitesimal, or polygenic, model whereby the trait examined is associated with a very large number of variants each making an infinitesimal contribution to phenotypic variance. GREML-SC assumes that SNP effects are normally distributed as well as independent of LD (see Glossary), and inversely proportionate to minor allele frequency [ 33 ].
Genome-based restricted maximum likelihood kinship (GREML-KIN)
GREML-KIN was introduced to capture the effects of rarer and less common genetic variants that are not captured using GREML-SC. Importantly, whereas it uses the same genetic data as GREML-SC, GREML-KIN uses samples with a dense and known pedigree to derive additional matrices to capture additional sources of variance from genetic variants that are in poor LD with common genotyped SNPs and to control for the effect of environmental influences. When GREML-KIN was applied to the study of intelligence, 54% of intelligence test variation was accounted for [ 32 ]; therefore, the DNA-based heritability of intelligence was about the same as those derived using twins. GREML-KIN has the same assumptions as GREML-SC. In addition, GREML-KIN uses closely related individuals, and the data it is applied to must contain a sufficiently dense pedigree in order to prevent an inflation of the heritability estimates due to shared environmental influences between those closely related individuals.
Genome-wide association studies (GWAS) of intelligence: finding loci
Heritability analyses suggest the presence of genetic influence on a trait. They do not indicate which genetic variants are associated with trait variation. Initially, candidate gene designs (see Glossary) were used to test for associations between genetic variants and intelligence test scores. However, these designs were underpowered and produced no replicable results [ 39 ]. The qualified exception is that possession of the e4 allele of the gene for Apolipoprotein E ( APOE ) is reliably associated with slightly lower cognitive function at older ages, accounting for around 1% of the variance [ 40 , 41 ]. No other SNP-based genetic variant comes close to this effect size in accounting for intelligence differences. The association might occur because APOE is involved in neuronal repair, and there is more repairing to do—and probably more individual differences (variance) in neurodegeneration—at older ages. The field changed to the conducting of GWAS (see Glossary) that are agnostic regarding which, if any, loci are associated with the trait of interest. This was driven by the availability of affordable arrays of hundreds of thousands of SNPs covering the genome, alongside the collection of large samples sizes and the formation of multi-sample consortia.
The first GWAS of intelligence with N > 3000, from 2011, detected no significantly associated loci [ 30 ]. However, it included the first DNA-based (GREML-SC-derived) heritability estimate of intelligence and showed that genotyped SNPs do account—collectively—for some of its variation [ 30 ]. For the next 6 years, GWASs conducted on intelligence test scores were largely unsuccessful in identifying associated genetic loci [ 42 , 43 , 44 , 45 ]. In 2018, three studies, using substantially overlapping samples, attained sample sizes of over 200,000 participants and found hundreds of genetic loci significantly associated with intelligence [ 31 , 46 , 47 ].
The first of the three studies had a sample size of 248,428; it found 187 (172 novel when it appeared online) independent regions of the genome that were associated with intelligence [ 46 ]. A major contribution to this study was from the large UK Biobank sample’s short Verbal and Numerical Reasoning test (VNR; called the ‘fluid’ test by UK Biobank, which is a misnomer). This study used a meta-analytic method (MTAG) to combine data sets using different indices of cognitive ability, and including educational attainment to increase statistical power. However, whereas a proxy-phenotype approach was used previously to identify SNPs that showed a joint association with education and intelligence [ 48 ], the meta-analytic method used in MTAG is different because it was designed to detect genetic associations with the target trait of intelligence and not those specific to educational attainment [ 46 , 49 ].
The second of the three studies to appear found 148 loci (53 novel when it appeared online) associated with intelligence, with a sample size of 300,046 participants [ 31 ]. This study also used the UK Biobank VNR test and several other samples that formed a general intelligence component from three-or-more, mostly fluid intelligence-type tests. The third study to appear identified 205 loci (84 novel when it appeared online) using 269,867 participants [ 47 ]. This study was conditioned on socioeconomic status, and combined tests of cognitive ability and scores on scholastic aptitude tests.
It has become clear, therefore, that the genetic contribution to intelligence differences is highly polygenic, i.e. there are large numbers of independent genetic variants, each of which accounts for a tiny proportion of intelligence variation.
The three studies above used polygenic scores to provide out-of-sample predictions of intelligence based solely on DNA-SNP data [ 31 , 46 , 47 ]. A polygenic score is an individual-level predictor derived from the sum of effect alleles at a SNP, weighted by the regression co-efficient describing each SNP’s level of association with the trait, in this case intelligence. The polygenic scores predicted 4–7% of intelligence variance in independent samples; another study predicted 10.6% [ 50 ]. Thus, a blood sample at birth in these samples predicts intelligence with about the same effect size as parental socioeconomic status, i.e. they do not predict well; neither is of practical use for predicting the intelligence of an individual. The proportion of variance explained by polygenic scores rises with sample size, so the predictive power is likely to rise as sample sizes increase [ 51 ]. This raises ethical issues—outlined more elsewhere [ 52 ]—which should be addressed by well-informed professionals and lay people from appropriate interest groups and areas of expertise. We emphasise that the results above apply to the samples tested, all of which were of European ancestry, and relatively few of which, probably, were from very deprived situations. Therefore, results reported here may not be assumed to apply to other populations, or to the same populations at other times.
After GWAS of intelligence: clues to mechanisms?
Finding genetic loci whose variants are associated with intelligence differences only helps to understand these differences if we understand the mechanistic consequences of the genetic variation. GWAS data sets’ results on intelligence have found associations between SNP variation and tissue-specific gene expression across many of the brain’s cortical regions (Fig. 2 ) [ 31 , 46 , 47 ]. SNP variation associated with intelligence has been linked to tissue-specific gene expression in specific classes of neuron, including pyramidal neurons of the somatosensory cortex, the CA1 region of the hippocampus, midbrain embryonic GABAergic neurons, [ 53 ] and medium spiny neurons [ 47 ]. These associations indicate that, rather than any one specific area, the association between genetic and intelligence variation is probably mediated in part by individual differences in gene expression across the cortex.
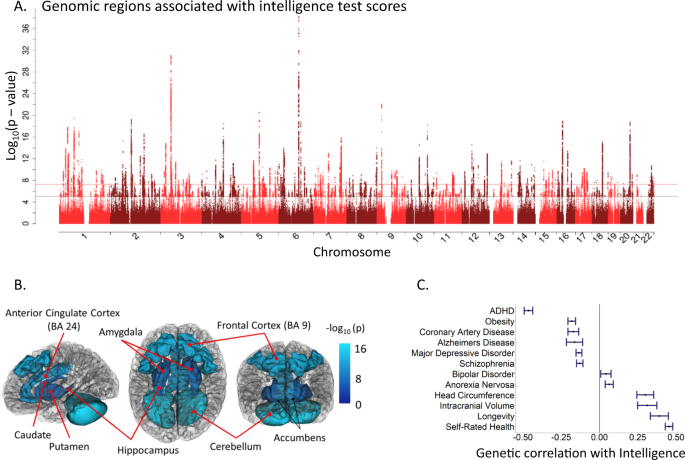
A Manhattan plot displaying 187 regions of the genome associated with intelligence test scores. The chromosomes are on the x axis and the −log 10 P value is on the y axis. Each dot represents a single nucleotide polymorphism. The horizontal red line indicates the genome-wide significant threshold of P = 5 × 10 −8 and the horizontal black line represents genome-wide suggestive variants at P = 1 × 10 −5 . B The relationship between specific gene expression profiles in the cortex and intelligence-gene associations. Illustrated here is the finding that tissue-specific expression in and across the brain is associated with intelligence; the full list of associations is in the original report [ 46 ]. C Some of the genetic correlations between intelligence test scores and phenotypes linked to health, mental health, and measures of brain size [ 46 ]. A positive genetic correlation indicates that the genetic variants associated with higher intelligence test scores are associated with a greater value of the trait or a higher likelihood of developing the disorder. Longevity, intracranial volume, self-rated health, and head circumference all show positive genetic correlations with intelligence. A negative genetic correlation describes instances where the genetic variants associated with higher intelligence are also those that are associated with a lower value of the trait or a lower likelihood of developing the disorder. Traits such as ADHD, obesity, coronary artery disease, major depressive disorder, and Alzheimer’s disease show negative genetic correlations with intelligence.
Genetic variants associated with intelligence test scores tend to cluster in groups of genes linked with neurogenesis, the synapse, neuron differentiation, and oligodendrocyte differentiation [ 46 ]. These results are consistent with previous studies that found an association between intelligence and brain-expressed genes [ 54 ], as well as the genes expressed in the postsynaptic density and its associated components of the NMDA-receptor signalling complex [ 55 ] and Arc complex [ 56 ] more specifically. Together, these studies highlight the role of the synapse, and possibly the postsynaptic density and/or its associated components, as being biological systems that, when perturbed by common genetic variation, are associated with some of phenotypic differences in intelligence. However, the variance accounted for by the intelligence-associated SNPs found in these biologically plausible tissues is probably very small. Nevertheless, efforts to understand DNA versus intelligence phenotype associations at levels such as neuron types and gene systems are ways to tame the huge number of cognition-related SNPs, each of which has a miniscule effect size.
Pleiotropic associations and intelligence
Pleiotropy (see Glossary) describes instances where variation at a region of the genome is associated with multiple phenotypes. Widespread pleiotropy between two phenotypes can be detected by deriving a genetic correlation (see Glossary) between the two phenotypes. A genetic correlation describes the average genetic effect shared between two traits as well as whether it is positive or negative; i.e. a positive genetic correlation occurs when genetic effects associated with an increase in one trait are also associated with an increase in a second trait, and a negative genetic correlation occurs when genetic effects associated with an increase in one trait are also associated with a decrease in a second trait. A genetic correlation co-efficient is derived using all SNPs from a GWAS regardless of the SNPs’ levels of association with a trait. Genetic correlations can be derived using two independent samples; this has the advantage that any genetic correlations found between intelligence and disease, for example, will not be due to individuals’ having the disease’s symptoms (i.e. if the intelligence GWAS has been conducted in healthy individuals). Polygenic scores can also make predictions across traits by deriving a polygenic score for intelligence and using it to predict health, or brain imaging traits, for example. However, these shared genetic associations—detected by genetic correlations or polygenic scores—can arise due to vertical pleiotropy, horizontal pleiotropy, or spurious pleiotropy, which we explain below [ 57 ].
Genetic correlations derived using GWAS data sets have demonstrated that genetic variants associated with higher intelligence test scores are, on average, also associated with, for example, longevity [ 46 ], better physical health [ 58 ], and more advantaged socioeconomic position [ 16 , 50 , 59 ]. Genetic variants associated with higher intelligence are more likely to be associated with lower levels of traits associated with mental health problems (Fig. 2 ) [ 60 ]. On the other hand, genetic variants associated with higher intelligence test scores are, typically, slightly positively associated with autism spectrum disorder and anorexia nervosa [ 46 ].
Mendelian randomisation (MR; see Glossary) studies move beyond associations between intelligence and health variables to seeking evidence that one phenotype might be causally related to another. MR results have indicated that intelligence and education probably have a bi-directional causal relationship [ 61 ], and that intelligence might have some causal association with, for example, Alzheimer’s disease that is independent of any protective effects of education [ 61 ]. Such results from MR should be interpreted cautiously as they can be biased by dynastic effects [ 62 ] known to influence education [ 36 ], which is highly genetically correlated with intelligence. The presence of dynastic effects violates the independence assumption of MR, as they induce a correlation between the environment in which a child is raised and their genetic inheritance.
Assortative mating, the tendency to select a partner based on heritable traits similar to one’s own, can bias the results of MR [ 63 ]. Education and intelligence are traits with evidence of assortative mating; there are reports of cross-spouse correlations of r = 0.40 for intelligence and r = 0.60 for education [ 64 ]. These contrast with measures of personality where correlations of r = 0.10 are found [ 65 ]. Biases from assortative mating can be induced by cross-trait assortative mating whereby, for example, more highly educated women might select partners who are taller, resulting in an apparent ‘finding’ that height is causally associated with education [ 63 ]. Bias due to dynastic effects and assortative mating can be controlled for by performing MR within families [ 66 ].
GWASs of educational attainment show high genetic correlations with intelligence (rg = 0.70–0.80 [ 46 ]) and have identified 1271 independent genome-wide significant SNPs [ 50 ]. Bioinformatic analyses of these data have identified associations with genes expressed in the brain and other cortical tissues as well as genes whose level of expression is elevated both pre- and postnatally. Furthermore, many of the genes identified encode proteins that are involved in synaptic functions such as synaptic plasticity, and neurotransmitter secretion, consistent with what has been identified for intelligence [ 46 , 55 , 67 ]. However, the use of education as a proxy phenotype for intelligence in genetic studies should be interpreted with caution. For example, whereas genetic correlations with schizophrenia indicate that the genetic variation that is associated with higher intelligence test scores is also associated with lower risk of schizophrenia, the genetic variants associated with attaining a longer and higher-level education are associated with higher risk of schizophrenia [ 46 ]. In a study investigating this phenomenon, SNPs associated with lower intelligence test scores, less education, and an increased risk for schizophrenia were also associated with early developmental processes [ 68 ]. This contrasted with the SNPs associated with lower education, and a lower risk of schizophrenia which were associated with biological processes of mature synapses.
A complementary explanation of some of the difference in genetic correlations between intelligence and education with schizophrenia focussed on “non-cognitive skills” [ 69 ]. The study used genomic structural equation modelling [ 70 ] to perform a GWAS by subtraction, resulting in associations specific to education once variance attributable to differences in intelligence was removed. The resulting GWAS of so-called “non-cognitive skills” found a heritability of 6.6% (SE = 0.2%), and a positive genetic correlation with schizophrenia of rg = 0.26 (SE = 0.02). However, whereas the cognitive traits captured by a GWAS of education were negatively genetically correlated with schizophrenia, the “non-cognitive traits” were genetically associated with a greater schizophrenia risk. A single cognitive performance test was used (UK Biobank’s VNR). This short test is unlikely to have captured all variance associated with intelligence and, so, this uncaptured cognitive ability variance would also be included in the “non-cognitive skills” (which would therefore be a misnomer) as evidenced by a genetic correlation of rg = 0.31 (SE = 0.06) with other measures of intelligence.
Intelligence and the brain
Intelligence and brain volume.
There is a well-replicated, modest positive association between brain size and intelligence test scores. Brain size is usually measured as total volume, assessed in magnetic resonance imaging (MRI) scans. A meta-analysis of data from over 148 studies across more than 8000 individuals [ 71 ] estimated the association at r = 0.24. A re-analysis of those data including only healthy adults estimated the association at r = 0.31; this rose to r = 0.39 when it included only the studies judged to have used better-quality intelligence testing [ 72 ]. In a single sample of 18,426 middle- and older-aged participants of the UK Biobank (age range 44–81 years), the association between intelligence and total brain volume was estimated at r = 0.276 (95% CI = 0.252, 0.300) [ 73 ]. This is about halfway between the two previous estimates, and has the benefit of eliminating cross-cohort heterogeneity that can influence meta-analytic results.
There are many other ways to interrogate brain differences, beyond overall brain size, which, on its own, is not informative about the brain’s complexity. For example, the fact that there are substantial sex differences in brain size [ 74 ] but very small or no sex differences in mean intelligence [ 75 , 76 ] is likely to be because multiple aspects of the brain’s structure, function, and connectivity are compensatory for any apparent brain size difference. Notably, there do not appear to be sex differences in the size of the brain-intelligence correlation [ 73 , 74 ]. There is evidence that the magnitude of associations with intelligence vary as a function of brain tissue type and locus. A study that included brain cortical characteristics (volume, area, and thickness), total volume of subcortical structures, and measures of white matter macro- and micro-structure found that, together, they accounted for up to 18% of the variance in general intelligence in 73-year-olds [ 77 ]. In the wider age range of UK Biobank, multiple structural measures accounted for more of the variance in intelligence in older-age (13.6%) compared to middle-age participants (5.4%), only outperforming the single variable of total brain volume in the former group [ 73 ].
The Parieto-Frontal Integration Theory attempted to summarise the intelligence-related brain regions implicated by structural, functional, and diffusion imaging studies of the brain [ 78 ] (Box 3 ). It identified that variations in the structure and function of lateral and medial frontal, parietal, lateral temporal, and lateral occipital cortex, and underlying white matter connectivity (such as the arcuate fasciculus) were associated with individual differences in intelligence. The available neuroimaging research at the time (37 studies) was hampered by small sample sizes, variable methods of measuring intelligence, and the-then limited number of diffusion MRI (dMRI) papers. This probably contributed to the relatively weak convergence of findings; even the most strongly implicated regions were supported by ≤60% of the papers surveyed. P-FIT theory receives support from some newer empirical findings (Fig. 3 ). We shall consider more recent studies in the light of it.
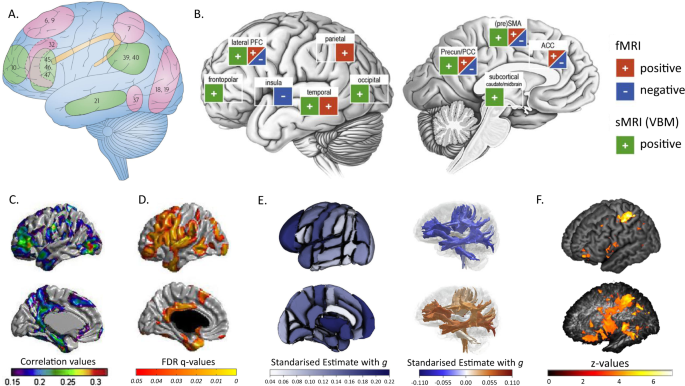
A The Parieto-Frontal Integration Theory (P-FIT) of intelligence differences, proposed by Jung and Haier [ 78 ]; reproduced with permission from [ 23 ]. B A meta-analysis of functional and structural (voxel-based morphometry only) studies of intelligence; reproduced with permission from [ 85 ]. C Associations between cortical thickness and intelligence in children (age range 6–18 years; N = 216); reproduced with permission from [ 83 ]. D Associations between cortical thickness and intelligence in older adults (age 73 years; N = 588); reproduced with permission from [ 79 ]. E Associations between intelligence test scores and regional cortical volume (left), and white matter tract fractional anisotropy (upper right, blue) and mean diffusivity (lower right, orange) in middle-aged and older adults (age range 44–81 years; N range = 7201–18,426); reproduced with permission from [ 73 ]. F Associations between lesion locus and intelligence in cortical (top) and subcortical (bottom) loci (age M = 49, SD = 16 years; N = 241); reproduced with permission from [ 80 ].
Box 3 Routes from the brain to theories of intelligence
P-FIT and beyond
We mention the parieto-frontal integration theory (P-FIT) [ 78 ] in the main text as a trellis upon which to hang diverse research results relating brain measures to intelligence test scores. Here we describe other theories that are not necessarily aimed specifically at where intelligence differences reside in the brain (as was the P-FIT), but they are nonetheless informative of the neuroscience of intelligence differences. For example, a narrative synthesis of several divergent traditions within the functional brain imaging literature identified a number of regions consistently showing convergent activations across multiple executive function tasks [ 145 ]. There are variations in the nomenclature (e.g. Multiple Demand Network [MDN], fronto-parietal control network, the superordinate cognitive control network, and the extrinsic mode network [ 145 ]), yet these schemas commonly comprise dorso-medial and lateral prefrontal cortex, insula, and parietal cortex. The more recent extended MDN [ 145 ] also includes the putamen, thalamus, and more dorsolateral aspects of prefrontal cortex. Though these results are based on convergent functional activations across multiple tasks (rather than patterns of activations from a general factor of intelligence indicated by multiple tasks), the regions implicated from these functional studies bear a striking resemblance to the P-FIT. Integration of structural and functional data (along with other modalities) will be central to testing broader theories about the nature of intelligence. It will provide important biological constraints to questions about the degree to which intelligence is a single construct in the brain, or arises from a number of overlapping brain networks that support the variety of psychological processes that are required by the multiple tests from which intelligence is derived (e.g. [ 146 ]). It will also allow insights into the hypothesis that cortical connectivity facilitated by white matter is a biological substrate for individual differences in processing speed, which is hierarchically subordinate to fluid intelligence [ 92 , 147 ].
Contributions of longitudinal and lesion studies
If intelligence is supported by a large distributed network of brain regions and their connections, there may well be many potential cerebral routes to intelligence differences, and to intellectual changes. This is a matter on which neuroimaging can offer important insights. Data from lesion studies and the ageing process can be seen as helpful in triangulating whether certain brain regions might be necessary for higher intelligence, rather than being related due to confounding factors (such as pre-existing, lifetime differences in brain volumes, in the example of ageing). Nonetheless, both methods are still limited.
Longitudinal studies offer a more stringent test of causal hypotheses than do cross-sectional studies. For example, if white matter health is important for higher intelligence, we would expect declines in both to be correlated [ 94 ]. However, even finding correlated changes between intelligence test scores and changes in a given brain region or pathway is still potentially confounded. A region that is not central to intellectual function could still exhibit correlated changes because its structural decline is simply correlated with regions that do support processes central to intelligence. Improved characterisation of longitudinal brain ageing will elucidate the degree to which this (the magnitude of correlated regional brain change) is an issue for localisation of intelligence.
Lesion studies offer valuable data on individuals in whom there has been a sudden and specific focal insult, which can be linked to differences in intelligence [ 80 , 81 ]. Those brain loci more strongly linked to lower intelligence can allow mapping of the relative importance of specific regions. However, lesion studies may be limited by the numbers required to eliminate statistical power variability across the brain; i.e., the regional coverage of lesion loci in the sample might be heterogeneous. The fact that lesions often involve both grey and white matter is a further issue. Parsing their relative importance for a behavioural outcome (and identifying the underlying connective pathways affected in each case) at a particular locus requires many participants with selective (grey or white) tissue damage.
Brain grey matter and intelligence
Associations between higher intelligence test scores and greater brain cortical volume and thickness in adults [ 73 , 79 ], as well as data from lesion studies [ 80 , 81 ] show stronger magnitudes across areas cited by the P-FIT (Box 3 ), though with consistently small effect sizes. The intelligence-cortical thickness relationship changes over the life course, with negative associations reported in 10-year-olds [ 82 , 83 , 84 ]. In the largest of these recent adult studies ( N = 18,426, ages 44–81 [ 73 ]), some of the strongest associations were also found between intelligence and the volumes of the insula, posterior cingulate/precuneus ( r < 0.20). These regions were not implicated in the initial P-FIT, but were identified in a meta-analytic update [ 78 ] using both functional and structural MRI data. However, considering only structural voxel-based morphometry analyses in easily-meta-analysable common space meant other types of structural study were excluded [ 85 ]. The large UK Biobank-based study [ 73 ] found that the intelligence associations with the volume of the thalamus ( r = 0.25) were 1.5 times larger than for any other subcortical structure ( r 0.06–0.17). The caudate, which had also previously been implicated in intelligence differences, albeit in smaller samples [ 86 , 87 ], was also correlated with intelligence in the UK Biobank analysis ( r ≈ 0.13), but only hippocampal ( r = 0.05) and thalamic volumes ( r = 0.19) showed unique associations with intelligence in a multivariate model including all subcortical structures. These findings are in line with the extensive cortical connectivity profiles of both insula and thalamus [ 88 , 89 ].
Brain white matter and intelligence
The last 20 years have witnessed an explosion of white matter brain imaging studies, due to the advent of dMRI. By exploiting the influence of various white matter properties (axonal myelination and diameter, among others [ 90 ]) on the motion of water molecular diffusion, dMRI enables inferences about white matter microstructure. Measures such as fractional anisotropy (FA; an index of the directional coherence of diffusion) and mean diffusivity (MD; the average magnitude of diffusion) are commonly used metrics. Both show general and regional ageing effects: on average, FA goes down with age, and MD goes up [ 91 ].
When assessed as summary measures across multiple parts of white matter, more directionally coherent water molecular diffusion (higher FA) and lower overall magnitude of diffusion (lower MD) are associated with higher intelligence test scores in studies together covering ages 8–81 years [ 73 , 92 , 93 ], typically with small effect sizes. FA and fluid cognitive ability show significantly coupled declines even over a 3-year period in older age ( r = 0.31; [ 94 ]). Whereas we acknowledge inconsistencies in white matter tract nomenclature and identification, there is emerging evidence that long range cortico-cortical (association pathways), the genu (more so than splenium) of the corpus callosum, and subcortico-cortical (mainly thalamic) pathways show numerically larger associations with intelligence than projection fibres (though all r < 0.11; [ 73 ]). The identification of these pathways as associated with intelligence accords with the grey matter findings above, as these are broadly the connections that facilitate frontal, parietal, lateral temporal (and subcortical) interconnectivity. Age-related accrual of white matter damage (measured by white matter hyperintensities [WMHs]) is associated with lower ( r = −0.106) and longitudinally coupled declines ( r ≤ −0.334) in cognitive function [ 95 , 96 ]. WMHs might interfere with selective pathways, because they tend to accumulate predominantly superior to the lateral ventricles [ 97 ].
Larger effect sizes for brain-intelligence associations can be found with multiple measures of white matter microstructure. One study derived a general factor of white matter integrity, across 12 white matter tracts, using three diffusion-based metrics: FA, longitudinal relaxation time, and magnetisation transfer ratio [ 92 ]. These three general factors showed only small correlations with each other, indicating that they might capture non-overlapping aspects of white matter microstructure. Together, they accounted for about 10% of intelligence differences in 73-year-olds. This link between white matter integrity and intelligence was fully mediated by the cognitive domain of processing speed.
It appears that the brain regions and their underlying connections that are associated with individual differences in our most complex cognitive abilities are also those which might: (i) show greater areal expansion as a function of increasing brain size [ 98 ]; (ii) be those that are latest to develop [ 99 ]; and (iii) be those most susceptible to brain ageing [ 91 ] and potential determinants thereof, such as vascular risk [ 100 ]. It is important to note that, even when brain regional measures are used, effect sizes remain relatively low, at an upper limit of ~ r = 0.30 among well-powered studies; however, these estimates appear robust and replicable in large samples [ 73 ]. These findings illustrate the small but significant associations of multiple facets of brain structure with intelligence differences. This appears especially at older ages when a larger proportion of the variance in these measures is probably driven by differences in age-related neurodegenerative processes. Modest associations should not be surprising given the macro-scale of the brain variables, and that measures discussed above are only few of the potentially large number of brain properties that might be measured.
Newer approaches to brain-intelligence associations
Emerging approaches have begun to model multivariate cross-tissue contributions to intelligence across selective grey matter regions and white matter pathways. For example, FA in the forceps minor and fronto-polar volume mediated 18.2% of the age association with fluid intelligence [ 101 ]. Such approaches have the potential to more directly test the specificity of brain-based network theories of intelligence, such as the P-FIT. Exploiting the brain’s structural connectome offers the chance to assess such network-based analyses with greater fidelity than via the measurement of fewer, larger pathways (though this brings a different set of limitations, e.g. [ 102 ]). Global measures such as connectomic efficiency [ 103 ], or variation in the ‘degree’ of nodes in morphometric similarity networks, have shown potential to predict intelligence differences (up to a remarkable 40% in one study with an N of 296 young adults [ 104 ]). Resting-state fMRI connectivity matrices predicted 20% of the variance in intelligence among young adults ( N = 884; [ 105 ]). These results need replicating. It is therefore of interest to continue the development of clear and interpretable integration of structural and function brain connectomes [ 106 ] to inform our understanding of intelligence differences and the brain.
The next period of research should try to explain the associations between brain indices (which are sometimes rather crude) and intelligence, in addition to seeking and testing new brain variables. Having larger volumes of brain (and tissues therein) appears to be relatively strongly related to having greater numbers of neurons [ 107 ]. Cortical thickness differences are related to neuronal density, columnar arrangement, alongside dendritic arbour and glial properties [ 108 ]. Another promising metric may be the diffusion characteristics of grey matter, assessed using neurite orientation dispersion and density imaging, which are putative markers of dendritic density and arborisation and exhibit some P-FIT-like regional associations with intelligence (| r | <0.25) [ 109 ]. Yet, much work is required before we understand whether and how such specific microscopic features (some of which are estimated with neuroimaging) might specifically give rise to intelligence differences. Whereas new methods, larger sample sizes, and out-of-sample prediction designs have the potential to add further insights and complementary contributions to intelligence variation, we judge it will be important to: (i) test the incremental validity of newer measures beyond more conventional metrics (e.g. [ 110 ]); (ii) be critical regarding whether the newer measures offer more biologically tractable variables for understanding brain differences; and, if they do, (iii) integrate these new indices with other levels of explanation, bridging the spectrum of macrostructure to cellular.
Toward a better integration of genes, brains, and intelligence
We structured this review into sections on phenotypic issues about intelligence, the genetic associations with intelligence test scores, and their brain correlates. This partly reflects the division of labour among the authors, but also reflects the current prevailing separation of these lines of enquiry. We should have liked there to have been more amalgamated brain-cognition-genetics studies, and we should have liked more integrated sections, examples and ideas to review. However, we consider that to be a lesson for us, and for the field, about the sorts of studies that could be done. These divisions are also in part because research on the statistical phenotypic nature of intelligence has been going on for more than a century. Genetics and brain imaging are relative youngsters, and methodologically fast-moving. New methods have blossomed in these fields, and debates and enquiry into the merits of these will continue apace. To mention a few examples, there are debates about both the diffusion and fMRI work in terms of cross-lab stability for neuroimaging [ 111 , 112 ], and there are discussions about the causal nature, if any, of the observed genetic correlations between intelligence and other phenotypes, as well as the role of non-transmitted genetic effects. The phenotypic nature of intelligence, too, has areas of contention: some areas of the neuroscience and cognitive literature focus on experimental cognitive tasks that assess aspects of so-called executive functioning, in contrast to the psychometric tests upon which intelligence scores are based. Consequently, reviews that focus solely on, say, executive function(s) or working memory might not fully bring out their strong phenotypic [ 8 , 113 , 114 , 115 ], genotypic [ 116 , 117 ] and neurostructural (Box 3 ) overlaps with psychometric intelligence. It would be of interest to develop a theoretical superstructure to unite these cognitive disciplines; it is unfortunate that cognitive neuropsychologists and psychometricians do not bring their tests and constructs together more when, empirically, they are strongly related [ 114 ]. In this section we summarise some recent studies that have integrated genes and brains to try to understand intelligence differences, before discussing their implications and potential future directions for a more integrative and nuanced account of some biological underpinnings of intelligence differences.
Looking at all three of genes, brains, and intelligence
Genetic correlations find that the genetic variants associated with intelligence are shared in part with those associated with volumetric measures of brain structure, such as intracranial volume (rg = 0.27), total brain volume (rg = 0.23), grey matter volume (rg = 0.08), white matter volume (rg = 0.08) [ 31 ], and volume of the left posterior cingulate cortex (rg = 0.23) [ 118 ]. Positive genetic correlations have been identified between healthier brain white matter microstructure and higher intelligence [ 119 ]. Reaction time—in which higher values are worse—had genetic correlations of −0.18 on average with brain white matter health/integrity, based on FA, as well as widespread positive genetic correlations of 0.17, on average, with axial diffusivity, MD, mode of anisotropy, and radial diffusivity [ 119 ].
A study which found that a polygenic score for intelligence predicted 3–5% of intelligence differences in a new sample also reported that this association was partially mediated to a small extent by brain cortical thickness and surface area of the anterior cingulate cortex, the prefrontal cortex, the insula, and the medial temporal cortex [ 120 ]. The results are consistent with the notion that the genetic variants’ associations with intelligence test scores might be accounted for partly through their associations with variation in the structure of the brain in many of the areas already linked in phenotypically with intelligence differences.
The high level of polygenicity of both intelligence [ 46 ] and structural brain measures [ 118 , 119 ] may indicate that a wealth of biological systems are associated with individual differences in both. This may prove an obstacle for uncovering a mechanistic account of how genetic variation is associated with brain and intelligence differences, as any biological system associated with both is likely to explain only a fraction of phenotypic variance in both brain and intelligence measures. However, despite the small effect sizes likely to be associated with each mechanism separately, future work aiming to produce a more mechanistic account of intelligence differences should examine the relative importance of any biological system identified, as well as examining its association with brain imaging measures.
The pleiotropy identified between intelligence and cortical measures also requires further examination. Specifically, the relative importance of vertical pleiotropy and horizontal pleiotropy in the generation of genetic correlations between cognitive ability and brain variables is currently unknown. By understanding the forms of pleiotropy responsible for such genetic correlations, more mechanistic accounts of intelligence differences can be formulated. For example, should vertical pleiotropy drive the genetic correlation between intelligence and brain structure then it could indicate that brain structure is causal in intelligence differences; however the opposite scenario is possible, i.e. where intelligence partly drives brain differences. Should these genetic correlations be the result of horizontal pleiotropy this would indicate that the same genetic loci are associated with both brain structure and intelligence with no causal relationship between the two.
A related difficulty in the interpretation of genetic correlations between intelligence and brain morphology arises from non-transmitted genetic effects [ 36 , 37 ]. Here, genetic correlations between intelligence and cortical measures may, in part, be the result of the genotype of the parent being linked to rearing practices that support both healthy brain development, and intellectual growth. Within-family GWAS will provide future opportunities to identify these effects [ 66 ] and gauge the magnitude of the residual genetic relationship between intelligence and brain structure, in the absence of the effect that parental genotype may have on both these variables.
The finding of a genetic g factor [ 29 ] also has implications for the functional annotation of the loci identified as being associated with intelligence. Specifically, loci found to be associated with cognitive abilities might be associated with the variance from a general factor or they might be associations specific to the domain examined, or even to the specific test within the given domain. This can be seen most clearly when examining genetic loci previously associated with the Trails B cognitive test; it was shown [ 29 ] that Trails B’s scores’ associations with genetic loci were shared with other tests of cognitive ability, and so were more general than initially assumed. This is a potential issue for examining more mechanistic accounts of intelligence differences, as variance that is both common across cognitive tests, and the variance that is specific to any particular testing domain or single test might be included together. By using techniques such as genomic SEM to distil these associations into those that are general across cognitive domains, and those that are specific to each domain or test could help in identifying plausible biological mechanisms linked with each.
Beyond just gen -‘omics’
There are, as yet, few studies that directly integrate genetic variation, brain imaging indices, and intelligence test scores. We barely have even a rudimentary understanding of how variation in the huge number of genetic variants identified as being associated with intelligence test scores and brain indices might directly relate to mechanisms (e.g. protein expression) and how these go on to facilitate the neurobiological machinery whose properties can be estimated and tested for any associations with differences in general cognitive ability. The explanatory gap between genetic loci and cognitive test scores is massive, and liable to induce a range of responses from the pleasure and terror of the Burkean sublime (a job to be done, though daunting), to less helpful routes of either premature and simplistic reductionism, or hopelessness.
There are fields that might help to bridge the gap, and contribute toward understanding intelligence differences, such as epigenetics [ 121 , 122 ], transcriptomics, proteomics, virtual histology, and others. Compared with, say GWAS methods, these are newer still, and will take time to even partly fill in the spaces between the triumvirate of genes, brains, and intelligence. However, genome-wide methylation studies examining intelligence and brain structure have begun to show some converging results. An association was identified between cg12507869 on chromosome 10 in the INPP5A gene and cognitive ability, as measured using the Mini-Mental State Examination, and with phonemic verbal fluency [ 121 ]. Methylation probes in the INPP5A gene have also been shown to be associated with hippocampal volume, cg25594319, although this did not withstand correction for multiple testing across the brain regions examined [ 122 ]. Nevertheless, we judge that poly-epigenetic approaches (e.g. EWAS and resultant epigenetic scores created out-of-sample) and larger sample sizes, particularly in epigenetic-neuroimaging studies [ 123 ] will yield further progress. For example, epigenetic signatures of smoking, mortality, and inflammation exhibit cognitive associations and regional cortical correlates in older age that overlap with P-FIT areas [ 124 , 125 , 126 ].
‘Virtual histological’ methods—which exploit histological data on regional gene expression [ 127 ]—also have potential for understanding how genes and brain measures relate to each other in the context of cognitive differences. Unifying information on (i) gene expression patterns and (ii) brain structural information at the same specific spatial level (e.g. cortical parcels) allows researchers to ask whether brain regional differences in brain structure-expression associations are relevant for other correlates of brain structure, using a correlation-of-correlations approach. For example, those brain cortical regions that showed a stronger relationship between greater triacylglycerol expression and greater cortical thickness were also the regions where thickness was more strongly associated with general cognitive ability [ 128 ]. Multivariate and systems-specific approaches (which consider multiple proteins together) will further illuminate the unique contributions of specific proteins and the underlying mechanisms via which they might relate to the underlying neurobiological bases of cognitive differences.
Finally, we want to be clear about ‘prediction’ of intelligence using genetic or brain imaging variables. We advocate testing replication across samples; that is, if a set of genetic variants or one or more brain imaging variables is associated with psychometric intelligence in a discovery sample then it is prudent to test whether the association holds in replication samples. This is a means to validating and understanding the generalisability of the genetic and cerebral correlates of intelligence from a starting sample. Thus, replication via out of sample prediction into another sample is a tool via which we can enhance our understanding of reasons why people differ in their cognitive abilities. On the other hand, prediction of the intelligence of an individual from genetic or neuroimaging variables is not a practical or, in our view, desirable aim.
Brain imaging and genetic associations with intelligence test score differences made progress in the last 10 years, with a raft of results based on new methods and large samples. Imaging and genetic variables account for a minority of intelligence variation. In both fields we conclude that: additional sources of variation should be sought; there is still a large explanatory gap separating us from even a partial mechanistic account of why people differ in intelligence; and the associations should not be taken to mean that there are immutable contributions to intelligence. When, or maybe if, we understand these and future associations, there might be hints as to what tends to make optimal cognitive development and healthy cognitive ageing. We recognise and encourage research on other substantial sources of variation in intelligence, social as well as biological [ 129 ].
Boring EG. Intelligence as the tests test it. N. Repub. 1923;35:35–7.
Google Scholar
Gottfredson LS. Mainstream science on intelligence: an editorial with 52 signatories, history, and bibliography. Intelligence. 1997;24:13–23.
Article Google Scholar
Jung RE, Chohan MO. Three individual difference constructs, one converging concept: adaptive problem solving in the human brain. Curr Opin. Behav Sci. 2019;27:163–8.
Spearman C. “General Intelligence,” objectively determined and measured. Am J Psychol. 1904;15:201–92.
Carroll JB. Human cognitive abilities: a survey of factor-analytic studies. Cambridge, UK: Cambridge University Press; 1993.
Johnson W, Bouchard TJ, Krueger RF, McGue M, Gottesman RI. Just one g: consistent results from three test batteries. Intelligence. 2004;32:95–107.
Deary IJ. The stability of intelligence from childhood to old age. Curr Dir Psychol Sci. 2004;23:239–45.
Salthouse TA. Selective review of cognitive aging. J Int Neuropsychol Soc. 2010;16:754–60.
Article PubMed PubMed Central Google Scholar
Tucker-Drob EM. Cognitive aging and dementia: a life-span perspective. Annu Rev Dev Psychol. 2019;1:177–96.
Tucker-Drob EM, Brandmaier AM, Lindenberger U. Coupled cognitive changes in adulthood: a meta-analysis. Psychol Bull. 2019;145:273–301.
Deary IJ, Strand S, Smith P, Fernandes C. Intelligence and educational achievement. Intelligence. 2007;35:13–21.
Strenze T. Intelligence and socioeconomic success: a meta-analytic review of longitudinal research. Intelligence. 2007;35:401–26.
Ritchie SJ, Tucker-Drob EM. How much does education improve intelligence? A meta-analysis. Psychol Sci. 2018;29:1358–69.
Schmidt FL, Hunter J. General mental ability in the world of work: occupational attainment and job performance. J Pers Soc Psychol. 2004;86:162–73.
Article PubMed Google Scholar
Deary IJ, Taylor MD, Hart CL, Wilson V, Davey Smith G, Blane D, et al. Intergenerational social mobility and mid-life status attainment: Influences of childhood intelligence, childhood social factors, and education. Intelligence. 2005;33:455–72.
Hill WD, Davies NM, Ritchie SJ, Skene NG, Bryois J, Bell S, et al. Genome-wide analysis identifies molecular systems and 149 genetic loci associated with income. Nat Commun. 2019;10:5741.
Article CAS PubMed PubMed Central Google Scholar
Calvin CM, Batty GD, Der G, Brett CE, Taylor A, Pattie A, et al. Childhood intelligence in relation to major causes of death in 68 year follow-up: prospective population study. BMJ. 2017;357:j2708.
Twig G, Tirosh A, Derazne E, Haklai Z, Goldberger N, Afek A, et al. Cognitive function in adolescence and the risk for premature diabetes and cardiovascular mortality in adulthood. Cardiovasc Diabetol. 2018;17:154.
Christensen GT, Mortensen EL, Christensen K, Osler M. Intelligence in young adulthood and cause-specific mortality in the Danish Conscription Database—A cohort study of 728,160 men. Intelligence. 2016;59:64–71.
Deary IJ. Looking down on human intelligence: from psychometrics to the brain. Oxford, UK: Oxford University Press; 2000.
Der G, Deary IJ. The relationship between intelligence and reaction time varies with age: Results from three representative narrow-age age cohorts at 30, 50 and 69 years. Intelligence. 2017;64:89–97.
Deary IJ, Johnson W, Starr JM. Are processing speed tasks biomarkers of cognitive aging? Psychol Aging. 2010;25:219–28.
Deary IJ, Penke L, Johnson W. The neuroscience of human intelligence differences. Nat Rev Neurosci. 2010;11:201–11.
Article CAS PubMed Google Scholar
Haworth CMA, Wright MJ, Luciano M, Martin NG, de Geus EJC, van Beijsterveldt CEM, et al. The heritability of general cognitive ability increases linearly from childhood to young adulthood. Mol Psychiatry. 2009;15:1112–20.
Lee T, Henry JD, Trollor JN, Sachdev PS. Genetic influences on cognitive functions in the elderly: a selective review of twin studies. Brain Res. 2010;64:1–13.
Article CAS Google Scholar
Plomin R, Deary IJ. Genetics and intelligence differences: five special findings. Mol Psychiatry. 2014;20:98–108.
Deary IJ. Intelligence. Annu Rev Psychol. 2012;63:453–82.
Polderman TJC, Benyamin B, de Leeuw CA, Sullivan PF, van Bochoven A, Visscher PM, et al. Meta-analysis of the heritability of human traits based on fifty years of twin studies. Nat Genet. 2015;47:702–9.
de la Fuente J, Davies G, Grotzinger AD, Tucker-Drob EM, Deary IJ. A general dimension of genetic sharing across diverse cognitive traits inferred from molecular data. Nat Commun. 2020. https://doi.org/10.1038/s41562-020-00936-2 .
Davies G, Tenesa A, Payton A, Yang J, Harris SE, Liewald D, et al. Genome-wide association studies establish that human intelligence is highly heritable and polygenic. Mol Psychiatry. 2011;16:996–1005.
Davies G, Lam M, Harris SE, Trampush JW, Luciano M, Hill WD, et al. Study of 300,486 individuals identifies 148 independent genetic loci influencing general cognitive function. Nat Commun. 2018;9:2098.
Hill WD, Arslan RC, Xia C, Luciano M, Amador C, Navarro P, et al. Genomic analysis of family data reveals additional genetic effects on intelligence and personality. Mol Psychiatry. 2018;23:2347–62.
Evans LM, Tahmasbi R, Vrieze SI, Abecasis GR, Das S, Gazal S, et al. Comparison of methods that use whole genome data to estimate the heritability and genetic architecture of complex traits. Nat Genet. 2018;50:737–45.
Zhu Z, Bakshi A, Vinkhuyzen AAE, Hemani G, Lee SH, Nolte IM, et al. Dominance genetic variation contributes little to the missing heritability for human complex traits. Am J Hum Genet. 2015;96:377–85.
Mäki-Tanila A, Hill WG. Influence of gene interaction on complex trait variation with multilocus models. Genetics. 2014;198:355–67.
Young AI, Frigge ML, Gudbjartsson DF, Thorleifsson G, Bjornsdottir G, Sulem P, et al. Relatedness disequilibrium regression estimates heritability without environmental bias. Nat Genet. 2018;50:1304–10.
Kong A, Thorleifsson G, Frigge ML, Vilhjalmsson BJ, Young AI, Thorgeirsson TE, et al. The nature of nurture: Effects of parental genotypes. Science. 2018;359:424–8.
Cheesman R, Hunjan A, Coleman JRI, Ahmadzadeh Y, Plomin R, McAdams TA, et al. Comparison of adopted and nonadopted individuals reveals gene–environment interplay for education in the UK Biobank. Psychol Sci. 2020. https://doi.org/10.1177/0956797620904450 .
Chabris CF, Hebert BM, Benjamin DJ, Beauchamp J, Cesarini D, van der Loos M, et al. Most reported genetic associations with general intelligence are probably false positives. Psychol Sci. 2012;23:1314–23.
Davies G, Harris SE, Reynolds CA, Payton A, Knight HM, Liewald DC, et al. A genome-wide association study implicates the APOE locus in nonpathological cognitive ageing. Mol Psychiatry. 2014;19:76–87.
Schiepers OJG, Harris SE, Gow AJ, Pattie A, Brett CE, Starr JM, et al. APOE E4 status predicts age-related cognitive decline in the ninth decade: longitudinal follow-up of the Lothian Birth Cohort 1921. Mol Psychiatry. 2012;17:315–24.
Davies G, Armstrong N, Bis JC, Bressler J, Chouraki V, Giddaluru S, et al. Genetic contributions to variation in general cognitive function: a meta-analysis of genome-wide association studies in the CHARGE consortium (N=53 949). Mol Psychiatry. 2015;20:183–92.
Davies G, Marioni RE, Liewald DC, Hill WD, Hagenaars SP, Harris SE, et al. Genome-wide association study of cognitive functions and educational attainment in UK Biobank (N=112 151). Mol Psychiatry. 2016;21:758–67.
Trampush JW, Yang MLZ, Yu J, Knowles E, Davies G, Liewald DC, et al. GWAS meta-analysis reveals novel loci and genetic correlates for general cognitive function: a report from the COGENT consortium. Mol Psychiatry. 2017;22:336–45.
Sniekers S, Stringer S, Watanabe K, Jansen PR, Coleman JRI, Krapohl E, et al. Genome-wide association meta-analysis of 78,308 individuals identifies new loci and genes influencing human intelligence. Nat Genet. 2017;49:1107–12.
Hill W, Marioni RE, Maghzian O, Ritchie SJ, Hagenaars SP, McIntosh AM, et al. A combined analysis of genetically correlated traits identifies 187 loci and a role for neurogenesis and myelination in intelligence. Mol Psychiatry. 2019;2:169–81.
Savage JE, Jansen PR, Stringer S, Watanabe K, Bryois J, de Leeuw CA, et al. Genome-wide association meta-analysis in 269,867 individuals identifies new genetic and functional links to intelligence. Nat Genet. 2018;50:912–9.
Rietveld CA, Esko T, Davies G, Pers TH, Turley P, Benyamin B, et al. Common genetic variants associated with cognitive performance identified using the proxy-phenotype method. Proc Natl Acad Sci USA. 2014;111:13790–4.
Turley P, Walters R, Maghzian O, Okbay A, Lee JJ, Fontana MA, et al. Multi-trait analysis of genome-wide association summary statistics using MTAG. Nat Genet. 2018;50:229–37.
Lee JJ, Wedow R, Okbay A, Kong E, Maghzian O, Zacher M, et al. Gene discovery and polygenic prediction from a genome-wide association study of educational attainment in 1.1 million individuals. Nat Genet. 2018;50:1112–21.
Dudbridge F. Power and predictive accuracy of polygenic risk scores. PLOS Genet. 2013;9:e1003348.
Plomin R, von Stumm S. The new genetics of intelligence. Nat Rev Genet. 2018;19:148–59.
Coleman JRI, Bryois J, Gaspar HA, Jansen PR, Savage J, Skene N, et al. Biological annotation of genetic loci associated with intelligence in a meta-analysis of 87,740 individuals. Mol Psychiatry. 2019;24:182–97.
Johnson MR, Shkura K, Langley SR, Delahaye-Duriez A, Srivastave P, Hill WD, et al. Systems genetics identifies a convergent gene network for cognition and neurodevelopmental disease. Nat Neurosci. 2015;19:223–32.
Hill WD, Davies G, van de Lagemaat LN, Christoforou A, Marioni RE, Fernandes CPD, et al. Human cognitive ability is influenced by genetic variation in components of postsynaptic signalling complexes assembled by NMDA receptors and MAGUK proteins. Transl Psychiatry. 2014;4:e341.
Fernández E, Collins MO, Frank RAW, Zhu F, Kopanitsa MV, Nithianantharajah J, et al. Arc requires PSD95 for assembly into postsynaptic complexes involved with neural dysfunction and intelligence. Cell Rep. 2017;21:679–91.
van Rheenen W, Peyrot WJ, Schork AJ, Lee SH, Wray NR. Genetic correlations of polygenic disease traits: from theory to practice. Nat Rev Genet. 2019;20:567–81.
Deary IJ, Harris SE, Hill WD. What genome-wide association studies reveal about the association between intelligence and physical health, illness, and mortality. Curr Opin Psychol. 2019;27:6–12.
Hill WD, Hagenaars SP, Marioni RE, Harris SE, Liewald DC, Davies G, et al. Molecular genetic contributions to social deprivation and household income in UK Biobank. Curr Biol. 2016;26:3083–9.
Hill WD, Harris SE, Deary IJ. What genome-wide association studies reveal about the association between intelligence and mental health. Curr Opin Psychol. 2019;27:25–30.
Anderson EL, Howe LD, Wade KH, Ben-Shlomo Y, Hill WD, Deary IJ, et al. Education, intelligence and Alzheimer’s disease: Evidence from a multivariable two-sample Mendelian randomization study. Int J Epidemiol. 2020;49:1163–72.
Koellinger PD, de Vlaming R. Mendelian randomization: the challenge of unobserved environmental confounds. Int J Epidemiol. 2019;48:665–71.
Hartwig FP, Davies NM, Davey Smoth G. Bias in Mendelian randomization due to assortative mating. Genet Epidemiol. 2018;42:608–20.
Jensen AR. The g factor: The science of mental ability. Westport, CT, USA: Praeger; 1998.
Vandenberg SG. Assortative mating, or who marries whom? Behav Genet. 1972;2:127–57.
Brumpton B, Sanderson E, Heibron K, Hartwig FP, Harrison S, Vie GA, et al. Avoiding dynastic, assortative mating, and population stratification biases in Mendelian randomization through within-family analyses. Nat Commun. 2020;11:3519.
Malanchini M, Rimfeld K, Allegrini AG, Ritchie SJ, Plomin R. Cognitive ability and education: how behavioural genetic research has advanced our knowledge and understanding of their association. Neurosci Biobehav Rev. 2020;111:229–45.
Lam M, Hill WD, Trampush JW, Yu J, Knowles E, Davies G, et al. Pleiotropic meta-Analysis of cognition, education, and schizophrenia differentiates roles of early neurodevelopmental and adult synaptic pathways. Am J Hum Genet. 2019;105:334–50.
Demange PA, Malanchini M, Mallard TT, Biroli P, Cox SR, Grotzinger AD, et al. Investigating the genetic architecture of non-cognitive skills using GWAS-by-subtraction. bioRxiv. 2020. https://doi.org/10.1101/2020.01.14.905794v1 .
Grotzinger AD, Rhemtulla M, de Vlaming R, Ritchie SJ, Mallard TT, Hill WD, et al. Genomic structural equation modelling provides insights into the multivariate genetic architecture of complex traits. Nat Hum Behav. 2019;3:513–25.
Pietschnig J, Penke L, Wicherts JM, Zeiler M, Voracek M. Meta-analysis of associations between human brain volume and intelligence differences: How strong are they and what do they mean? Neurosci Biobehav Rev. 2015;57:411–32.
Gignac GE, Bates TC. Brain volume and intelligence: the moderating role of intelligence measurement quality. Intelligence. 2017;64:18–29.
Cox SR, Ritchie SJ, Fawns-Ritchie C, Tucker-Drob EM, Deary IJ. Structural brain imaging correlates of general intelligence in UK Biobank. Intelligence. 2019;10:101376.
Ritchie SJ, Cox SR, Shen X, Lombardo MV, Reus LM, Alloza C, et al. Sex differences in the adult human brain: Evidence from 5216 UK Biobank participants. Cereb Cortex. 2018;28:2959–75.
Deary IJ, Irwing P, Der G, Bates TC. Brother–sister differences in the g factor in intelligence: analysis of full, opposite-sex siblings from the NLSY1979. Intelligence. 2007;35:451–6.
Johnson W, Carothers A, Deary IJ. Sex differences in variability in general intelligence: a new look at the old question. Perspect Psychol Sci. 2008;3:518–31.
Ritchie SJ, Booth T, Hernández MCV, Corley J, Maniega SM, Gow AJ, et al. Beyond a bigger brain: Multivariable structural brain imaging and intelligence. Intelligence. 2015;51:47–56.
Jung RE, Haier RJ. The Parieto-Frontal Integration Theory (P-FIT) of intelligence: converging neuroimaging evidence. Behav Brain Sci. 2007;30:135–54.
Karama S, Bastin ME, Murray C, Royle NA, Penke L, Maniega SM, et al. Childhood cognitive ability accounts for associations between cognitive ability and brain cortical thickness in old age. Mol Psychiatry. 2014;19:555–9.
Gläscher J, Rudrauf D, Colom R, Paul LK, Tranel D, Damasio H, et al. Distributed neural system for general intelligence revealed by lesion mapping. Proc Natl Acad Sci USA. 2010;107:4705–9.
Barbey AK, Colom R, Solomon J, Krueger F, Forbes C, Grafman J. An integrative architecture for general intelligence and executive function revealed by lesion mapping. Brain. 2012;135:1154–64.
Burgaleta M, Johnson W, Waber D, Colom R, Karama S. Cognitive ability changes and dynamics of cortical thickness development in healthy children and adolescents. NeuroImage. 2014;84:810–9.
Karama S, Ad-Dab’bagh Y, Haier RJ, Deary IJ, Lyttelton OC, Lepage C, et al. Positive association between cognitive ability and cortical thickness in a representative US sample of healthy 6 to 18 year-olds. Intelligence. 2009;37:145–55.
Schnack HG, van Haren NEM, Brouwer RM, Evans A, Durston S, Boomsma DI, et al. Changes in thickness and surface area of the human cortex and their relationship with intelligence. Cereb Cortex. 2014;25:1608–17.
Basten U, Hilger K, Fiebach CJ. Where smart brains are different: a quantitative meta-analysis of functional and structural brain imaging studies on intelligence. Intelligence. 2015;51:10–27.
Rhein C, Mühle C, Richter-Schmidinger T, Alexopoulos P, Doerfler A, Kornhuber J. Neuroanatomical correlates of intelligence in healthy young adults: the role of basal ganglia volume. PLOS One. 2014;9:e93623.
Grazioplene RG, Ryman SG, Gray JR, Rustichini A, Jung RE, DeYoung CG. Subcortical intelligence: Caudate volume predicts IQ in healthy adults. Hum Brain Mapp. 2015;36:1407–16.
Nomi JS, Schettini E, Broce I, Dick AS, Uddin LQ. Structural connections of functionally defined human insular subdivisions. Cereb Cortex. 2018;28:3445–56.
Aggleton JP, O’Mara SM, Vann SD, Wright NF, Tsanov M, Erichsen JT. Hippocampal–anterior thalamic pathways for memory: uncovering a network of direct and indirect actions. Eur J Neurosci. 2010;31:2292–307.
Jones DK, Knösche TR, Turner R. White matter integrity, fiber count, and other fallacies: the do’s and don’ts of diffusion MRI. NeuroImage. 2013;73:239–54.
Cox SR, Ritchie SJ, Tucker-Drob EM, Liewald DC, Hagenaars SP, Davies G, et al. Ageing and brain white matter structure in 3,513 UK Biobank participants. Nat Commun. 2016;7:13629.
Penke L, Maniega SM, Bastin ME, Hernández MCV, Murray C, Royle NA, et al. Brain white matter tract integrity as a neural foundation for general intelligence. Mol Psychiatry. 2012;17:1026–30.
Tamnes CK, Østby Y, Walhovd KB, Westlye LT, Due-Tønnessen, Fjell AM. Intellectual abilities and white matter microstructure in development: a diffusion tensor imaging study. Hum Brain Mapp. 2010;31:1609–25.
Ritchie SJ, Bastin ME, Tucker-Drob EM, Maniega SM, Engelhardt LE, Cox SR, et al. Coupled changes in brain white matter microstructure and fluid intelligence in later life. J Neurosci. 2015;35:8672–82.
Puzo C, Labriola C, Sugarman MA, Tripodis Y, Martin B, Palmisano JN, et al. Independent effects of white matter hyperintensities on cognitive, neuropsychiatric, and functional decline: a longitudinal investigation using the National Alzheimer’s Coordinating Center Uniform Data Set. Alzheimer’s Res Ther. 2019;11:64.
Ritchie SJ, Dickie DA, Cox SR, Hernández MCV, Corley J, Royle NA, et al. Brain volumetric changes and cognitive ageing during the eighth decade of life. Hum Brain Mapp. 2015;36:4910–25.
Muñoz Maniega S, Meijboom R, Chappell FM, Hernández MCV, Starr JM, Bastin ME, et al. Spatial gradient of microstructural changes in normal-appearing white matter in tracts affected by white matter hyperintensities in older age. Front Neurol. 2019;10:784.
Reardon PK, Seidlitz J, Vandekar S, Liu S, Patel R, Park MTM, et al. Normative brain size variation and brain shape diversity in humans. Science. 2018;360:1222–7.
Fjell AM, McEvoy L, Holland D, Dale AM, Walhovd KB. Alzheimer’s disease neuroimaging initiative. what is normal in normal aging? effects of aging, amyloid and Alzheimer’s disease on the cerebral cortex and the hippocampus. Prog Neurobiol. 2014;117:20–40.
Cox SR, Lyall DM, Ritchie SJ, Bastin ME, Harris MA, Buchanan CR, et al. Associations between vascular risk factors and brain MRI indices in UK Biobank. Eur Heart J. 2019;40:2290–300.
Kievit RA, Davis SW, Mitchell DJ, Taylor JR, Duncan J. Cam-CAN Research team, et al. Distinct aspects of frontal lobe structure mediate age-related differences in fluid intelligence and multitasking. Nat Commun. 2014;5:5658.
Buchanan CR, Bastin ME, Ritchie SJ, Liewald DC, Madole JW, Tucker-Drob EM, et al. The effect of network thresholding and weighting on structural brain networks in the UK Biobank. NeuroImage. 2020;211:116443.
Bathelt J, Scerif G, Nobre AC, Astle DE. Whole-brain white matter organization, intelligence, and educational attainment. Trends Neurosci Educ. 2019;15:38–47.
Seidlitz J, Váša F, Shinn M, Romero-Garcia R, Whitaker J, Vértes PE, et al. Morphometric similarity networks detect microscale cortical organization and predict inter-individual cognitive variation. Neuron. 2018;97:231–47.
Dubois J, Galdi P, Paul LK, Adolphs R. A distributed brain network predicts general intelligence from resting-state human neuroimaging data. Philos Trans R Soc Lond B Biol Sci. 2018;373:20170284.
Zimmermann J, Griffiths JD, McIntosh AR. Unique mapping of structural and functional connectivity on cognition. J Neurosci. 2018;38:9658–67.
Pakkenberg B, Gundersen HJG. Neocortical neuron number in humans: Effect of sex and age. J Comp Neurol. 1997;384:312–20.
la Fougère C, Grant S, Kostikov A, Schirrmacher R, Gravel P, Schipper HM, et al. Where in-vivo imaging meets cytoarchitectonics: the relationship between cortical thickness and neuronal density measured with high-resolution [18F]flumazenil-PET. Neuroimage. 2011;56:951–60.
Genç E, Fraenz C, Schlüter C, Friedrich P, Hossiep R, Voelkle MC, et al. Diffusion markers of dendritic density and arborization in gray matter predict differences in intelligence. Nat Commun. 2018;9:1905.
Deary IJ, Ritchie SJ, Maniega SM, Cox SR, Hernández MCV, Luciano M, et al. Brain peak width of skeletonized mean diffusivity (PSMD) and cognitive function in later life. Front Psychiatry. 2019;10:524.
Elliott ML, Knodt AR, Ireland D, Morris ML, Poulton R, Ramrakha S, et al. What is the test-retest reliability of common task-functional MRI measures? New empirical evidence and a meta-analysis. Psychol Sci. 2020;31:792–806.
Schilling KG, Petit L, Rheault F, Remedios S, Pierpaoli C, Anderson AW, et al. Brain connections derived from diffusion MRI tractography can be highly anatomically accurate—if we know where white matter pathways start, where they end, and where they do not go. Brain Struct Funct. 2020;225:2387–402.
Diamond A. Executive functions. Annu Rev Psychol. 2013;64:135–68.
Engle R. Working memory and executive functions: a revisit. Perspect Psychol Sci. 2018;13:190–3.
Duncan J, Emslie H, Williams P, Johnson R, Freer C. Intelligence and the frontal lobe: the organisation of goal-directed behavior. Cogn Psychol. 1996;30:257–303.
Engelhardt LE, Mann FD, Briley DA, Church JA, Harden KP, Tucker-Drob EM. Strong genetic of overlap between executive functions and intelligence. J Exp Psychol Gen. 2016;145:1141–59.
Friedman NP, Miyake A. Unity and diversity of executive functions: Individuals differences as a window on cognitive function. Cortex. 2017;86:186–204.
Zhao B, Luo T, Li T, Li Y, Zhang J, Shan Y, et al. Genome-wide association analysis of 19,629 individuals identifies variants influencing regional brain volumes and refines their genetic co-architecture with cognitive and mental health traits. Nat Genet. 2019;51:1637–44.
Zhao B, Zhang J, Ibrahim JG, Luo T, Santelli RC, Li Y, et al. Large-scale GWAS reveals genetic architecture of brain white matter microstructure and genetic overlap with cognitive and mental health traits (n = 17,706). Mol Psychiatry. 2019. https://doi.org/10.1038/s41380-019-0569-z .
Lett TA, Vogel BO, Ripke S, Wackerhagen C, Erk S, Awasthi S, et al. Cortical surfaces mediate the relationship between polygenic scores for intelligence and general intelligence. Cereb Cortex. 2019;30:2708–19.
Article PubMed Central Google Scholar
Marioni RE, McCrae AF, Bressler J, Colicino E, Hannon E, Li S, et al. Meta-analysis of epigenome-wide association studies of cognitive abilities. Mol Psychiatry. 2018;23:2133–44.
Jia T, Chu C, Liu Y, van Dongen J, Papastergios E, Armstrong NJ, et al. Epigenome-wide meta-analysis of blood DNA methylation and its association with subcortical volumes: findings from the ENIGMA epigenetics working group. Mol Psychiatry. 2019. https://doi.org/10.1038/s41380-019-0605-z .
Wheater ENW, Stoye DQ, Cox SR, Wardlaw JM, Drake AJ, Bastin ME, et al. DNA methylation and brain structure and function across the life course: a systematic review. Neurosci Biobehav Rev. 2020;113:113–56.
Corley J, Cox SR, Harris SE, Hernandez MCV, Maniega SM, Bastin ME, et al. Epigenetic signatures of smoking associate with cognitive function, brain structure, and mental and physical health outcomes in the Lothian Birth Cohort 1936. Transl Psychiatry. 2019;9:248.
Hillary RF, Stevenson AJ, Cox SR, McCartney DL, Harris SE, Seeboth A, et al. An epigenetic predictor of death captures multi-model measures of brain health. Mol Psychiatry. 2019. https://doi.org/10.1038/s41380-019-0616-9 .
Conole ELS, Stevenson AJ, Green C, Harris SE, Maniega SM, Hernández MCV, et al. An epigenetic proxy of chronic inflammation outperforms serum levels as a biomarker of brain ageing. medRxiv. 2020. https://doi.org/10.1101/2020.10.08.20205245 .
Shin J, French L, Xu T, Leonard G, Perron M, Pike GB, et al. Cell-specific gene-expression profiles and cortical thickness in the human brain. Cereb Cortex. 2018;28:3267–77.
Sliz E, Shin J, Syme C, Black S, Seshadri S, Paus T, et al. Thickness of the cerebral cortex shows positive association with blood levels of tricylglycerols carrying 18-carbon fatty acids. Commun Biol. 2020;3:456.
Nisbett RE, Aronson J, Blair C, Dickens W, Flynn J, Halpern, et al. Intelligence: new findings and theoretical developments. Am Psychol. 2012;67:130–59.
Galton F. Hereditary genius: An inquiry into its laws and consequences. London, UK: Macmillan; 1869.
Thurstone L. Primary mental abilities. Psychometric Monographs No. 1. Chicago, IL, USA: The University of Chicago Press; 1938
Gardner H Frames of mind: The theory of multiple intelligences. New York, NY, USA: Basic Books; 1993.
Thomson GH. A hierarchy without a general factor. Br J Psychol. 1916;8:271–81.
Zenderland L. Measuring minds: Henry Herbert Goddard and the origins of American intelligence testing. Cambridge, UK: Cambridge University Press; 1998.
Gould S. The mismeasure of man. New York, NY, USA: WW Norton & Company; 1981, 2nd edition 1996.
Carroll JB. Reflections on Stephen Jay Gould’s the mismeasure of man (1981): a retrospective review. Intelligence. 1995;21:121–34.
Flynn JR. Massive IQ gains in 14 nations: What IQ tests really measure. Psychol Bull. 1987;101:171–91.
Herrnstein RJ, Murray C. The bell curve: Intelligence and class structure in American life. New York, NY, USA: Free Press; 1994.
Neisser U, Boodoo G, Bouchard TJ, Boykin AW, Brody N, Ceci SJ, et al. Intelligence: knowns and unknowns. Am Psychol. 1996;51:77–101.
Deary IJ. Intelligence: A very short introduction (2nd edition). Oxford, UK: Oxford University Press; 2020.
Haier RJ. The neuroscience of intelligence. Cambridge, UK: Cambridge University Press; 2016.
Visscher PM, Hill WG, Wray NR. Heritability in the genomics era—concepts and misconceptions. Nat Rev Genet. 2008;9:255–66.
Marioni RE, Davies G, Hayward C, Liewald D, Kerr SM, Campbell A, et al. Molecular genetic contributions to socioeconomic status and intelligence. Intelligence. 2014;44:26–32.
Conley D, Siegal ML, Domingue, Harris KM, McQueen MB, Boardman JD. Testing the key assumption of heritability estimates based on genome-wide genetic relatedness. J Hum Genet. 2014;59:342–5.
Camilleri JA, Muller VI, Fox P, Laird AR, Hoffstaedter F, Kalenscher T, et al. Definition and characterization of an extended multiple-demand network. NeuroImage. 2018;165:138–47.
Kovacs K, Conway ARA. Process overlap theory: a unified account of the general factor of intelligence. Psychol Inq. 2016;27:151–77.
Kievit RA, Davis SW, Griffiths J, Correia MM, Cam-CAN, Henson RN. A watershed model of individual differences in fluid intelligence. Neuropsychologia. 2016;91:186–98.
Download references
Acknowledgements
IJD and SRC were supported by the UKRI Medical Research Council (MR/R024065/1) and the United States Department of Human Sciences National Institutes of Health (1R01AG054628-01A1). IJD and WDH were supported by Age UK (Disconnected Mind grant).
Author information
These authors contributed equally: Ian J. Deary, Simon R. Cox, W. David Hill
Authors and Affiliations
Lothian Birth Cohorts group, Department of Psychology, University of Edinburgh, 7 George Square, Edinburgh, EH8 9JZ, UK
Ian J. Deary, Simon R. Cox & W. David Hill
You can also search for this author in PubMed Google Scholar
Corresponding author
Correspondence to Ian J. Deary .
Ethics declarations
Conflict of interest.
The authors declare that they have no conflict of interest.
Additional information
Publisher’s note Springer Nature remains neutral with regard to jurisdictional claims in published maps and institutional affiliations.
This design focusses on variants in specific genes that are thought to be linked to the trait of interest due to the biological functions of the genes and genetic variants that are selected.
This describes the standardised average genetic effect shared between two phenotypes. It is a correlation between the tested genetically heritable elements of each trait.
This study design is used to identify loci throughout the genome associated with a trait or disease state. GWAS can include millions of mostly common single nucleotide polymorphisms from across the genome. These are each tested for association with the phenotype of interest. The problem of type 1 statistical errors in such a large number of tests is controlled for by adopting a stringent p value cut off, typically of 5 × 10 −8 .
This is the proportion of phenotypic variance that is attributable to the variance found in genetic factors. In the context of GWAS it is typically only the additive genetic factors that are considered from variants that are in linkage disequilibrium (see below) with the common SNPs used, and so the heritability estimate is the narrow-sense heritability.
This is the non-random association of alleles located in at least two distinct genetic loci.
MR is a statistical technique that uses genetic variants, which are unchanged from conception, to test for possible causal relationships between an exposure and an outcome. MR typically uses SNPs that have attained genome wide significance for an exposure trait, such as smoking, as proxy variables for the exposure trait, i.e. forming an instrument for it. Such instrumental variables are defined by three key assumptions. First, that they are associated with the exposure. Second, they are associated with the outcome only through the exposure. Third, that they are not associated with confounders with respect to the outcome.
This describes the finding that multiple phenotypes can be associated with genetic variation at the same locus. First, this may be due to the locus’s having an independent causal effect on each phenotype, which is termed horizontal or biological pleiotropy. Second, it can describe instances whereby one phenotype is causally associated with a second phenotype, meaning that any genetic associations found with the first phenotype will be shared with the second; this is termed vertical or mediated pleiotropy.
SNP is pronounced ‘snip’. This is the most common type of genetic variation between people. Each SNP is a substitution of a single nucleotide at a specific position in the genome. In a GWAS, a SNP is treated as the statistical unit of association.
Rights and permissions
Open Access This article is licensed under a Creative Commons Attribution 4.0 International License, which permits use, sharing, adaptation, distribution and reproduction in any medium or format, as long as you give appropriate credit to the original author(s) and the source, provide a link to the Creative Commons license, and indicate if changes were made. The images or other third party material in this article are included in the article’s Creative Commons license, unless indicated otherwise in a credit line to the material. If material is not included in the article’s Creative Commons license and your intended use is not permitted by statutory regulation or exceeds the permitted use, you will need to obtain permission directly from the copyright holder. To view a copy of this license, visit http://creativecommons.org/licenses/by/4.0/ .
Reprints and permissions
About this article
Cite this article.
Deary, I.J., Cox, S.R. & Hill, W.D. Genetic variation, brain, and intelligence differences. Mol Psychiatry 27 , 335–353 (2022). https://doi.org/10.1038/s41380-021-01027-y
Download citation
Received : 23 July 2020
Revised : 28 December 2020
Accepted : 11 January 2021
Published : 02 February 2021
Issue Date : January 2022
DOI : https://doi.org/10.1038/s41380-021-01027-y
Share this article
Anyone you share the following link with will be able to read this content:
Sorry, a shareable link is not currently available for this article.
Provided by the Springer Nature SharedIt content-sharing initiative
This article is cited by
Biomarkers of preschool children with autism spectrum disorder: quantitative analysis of whole-brain tissue component volumes, intelligence scores, ados-css, and ages of first-word production and walking onset.
- Wu-Sheng Lin
World Journal of Pediatrics (2024)
A General Cognitive Ability Factor for the UK Biobank
- Camille Michèle Williams
- Ghislaine Labouret
- Franck Ramus
Behavior Genetics (2023)
Multivariate genetic analysis of personality and cognitive traits reveals abundant pleiotropy
- Guy Hindley
- Alexey A. Shadrin
- Ole A. Andreassen
Nature Human Behaviour (2023)
The impact of digital media on children’s intelligence while controlling for genetic differences in cognition and socioeconomic background
- Bruno Sauce
- Magnus Liebherr
- Torkel Klingberg
Scientific Reports (2022)
- Nicholas Judd
npj Science of Learning (2022)
Quick links
- Explore articles by subject
- Guide to authors
- Editorial policies


An official website of the United States government
The .gov means it’s official. Federal government websites often end in .gov or .mil. Before sharing sensitive information, make sure you’re on a federal government site.
The site is secure. The https:// ensures that you are connecting to the official website and that any information you provide is encrypted and transmitted securely.
- Publications
- Account settings
Preview improvements coming to the PMC website in October 2024. Learn More or Try it out now .
- Advanced Search
- Journal List
- Evol Med Public Health
- v.9(1); 2021
Race and genetics versus ‘race’ in genetics
Theresa m duello.
Department of Obstetrics and Gynecology, School of Medicine and Public Health, University of Wisconsin – Madison, Madison, WI 53706, USA
Shawna Rivedal
Colton wickland, annika weller, associated data, lay summary.
A review of the medical literature since the completion of the Human Genome Project in 2003 revealed that race, a social construct created to justify slavery, continues to be used as a genetic category, due to a lack of understanding of the continuous nature of human genetic variation.
Social scientists have long understood race to be a social category invented to justify slavery and evolutionary biologists know the socially constructed racial categories do not align with our biological understanding of genetic variation. The completion of the Human Genome Project in 2003 confirmed humans are 99.9% identical at the DNA level and there is no genetic basis for race. A systematic review of the PubMed medical literature published since 2003 was conducted to assess the use of African ancestry to denote study populations in genetic studies categorized as clinical trials, to examine the stated rationale for its use and to assess the use of evolutionary principles to explain human genetic diversity. We searched for papers that included the terms ‘African’, ‘African American’ or ‘Black’ in studies of behavior (20 papers), physiological responses, the pharmacokinetics of drugs and/or disease associations (62 papers), and as a genetic category in studies, including the examination of genotypes associated with life stress, pain, stuttering and drug clearance (126 papers). Of these, we identified 74 studies in which self-reported race alone or in combination with admixture mapping was used to define the study population. However, none of these studies provided a genetic explanation for the use of the self-identified race as a genetic category and only seven proffered evolutionary explanations of their data. The concept of continuous genetic variation was not clearly articulated in any of these papers, presumably due to the paucity of evolutionary science in the college and medical school curricula.
INTRODUCTION
Social scientists have long understood race to be a social construct used in its most benign form to categorize groups of people according to a small group of phenotypes and cultural differences and in its most insidious form to assign value to a social hierarchy. The concept and significance of race varies around the world with the USA first creating and assigning value to racial categories to justify slavery. Biologists and geneticists have historically been divided as to whether ‘race’ also defines distinct biological and genetic categories. Herein, race without notations is used to denote its use as a social construct, while ‘race’ denotes its use as a biological or genetic entity. Definitions of these latter terms vary widely. Genetic ‘race’ has been viewed as a result of human migration with genetic isolation leading to the development of distinct populations that share DNA as the result of common descent. While the terms genetic ‘race’ and biological ‘race’ have been used interchangeably, Templeton [ 1 ] defines biological race as (i) geographically defined populations within a species that have sharp boundaries that separate them from other species or as (ii) distinct evolutionary lineages within a species characterized by a continuous line of descent. He emphasizes that genetic differentiation alone is insufficient to define a subspecies or race under either of these definitions as both require that genetic differentiation exists across sharp boundaries and not as gradual changes.
As a taxonomic term, race defines an informal subdivision of subspecies which are physically and genetically different. However, the species Homo sapiens cannot be further subdivided into subspecies which are physically and genetically different. Thus, for H.sapiens , the species and subspecies are the same— H.sapiens sapiens ( Box 1 ). In 2003, Phase 1 of the Human Genome Project (HGP) demonstrated that humans populating the earth today are on average 99.9% identical at the DNA level, there is no genetic basis for race, and there is more genetic variation within a race than between them [ 2 ]. In addition, genetic isolation, sharp boundaries and distinct evolutionary lineages of ‘races’ do not exist. Thus, the idea of ‘race’ as a genetic category was presumably put to rest. The continued acceptance of ‘race’ as an appropriate biological category would have to be predicated on data indicating there are genes distinct to one ‘race’ that are transcribed in one ‘race’, but not another and human genetic variation is not continuous. This is distinct from small differences in allele frequencies due to mutations in a given family’s genetic lineage as will be discussed later in this manuscript.
Box 1 . Taxonomy of Homo sapiens sapiens
Kingdom— Animalia
Phylum— Chordata
Class— Mammalia
Subclass— Eutheria
Order— Primates
Family— Hominidae
Genus— Homo
Species— Sapiens
Subspecies— Sapiens
Humans— Homo sapiens sapiens
Evolutionary biologist Joseph L. Graves Jr sought to resolve the misunderstandings and misconceptions with the publication of The Emperor’s New Clothes: Biological Theories of Race at the Millennium 2 years prior to the sequencing of the human genome. It discussed the origins of the race concept, scientific racism, the misapplications of Darwinism, eugenics and the fallacies of the association of race with IQ and disease [ 3 ]. This was followed by publication of The Race Myth: Why We Pretend Race Exists in America in 2005, which discussed how the socially constructed racial categories do not align with our biological understanding of genetic variation [ 4 ].
At this same time that the human genome was sequenced and the concept of biological ‘race’ and racism were under renewed scrutiny, the FDA and the US Patent Office approved a drug named BiDil ® to treat heart failure in ‘self-identified African Americans’ [ 5 , 6 ]. In brief, the US Patent Office had issued a method patent in 1989 to a company as they had combined two generic anti-hypertensive drugs that had each been in use for 20 years into one medication. Eight years later the company submitted a New Drug Application to the FDA as they had demonstrated the combined drugs were as biopotent as the co-administered drugs. The application was denied as there were too many variables as primary endpoints to interpret two decades of clinical trials data with any certainty. However, in 2000, the company submitted a new patent application for the use of the drug by African Americans. In 2002, the US Patent Office granted the patent for ‘methods for treating and preventing mortality associated with heart failure in an African-American patient’ and in 2005, the FDA approved BiDil ® for ‘self-identified African Americans’.
Examination of the rationale for the approval of this drug provides insight into problems of experimental design, but provided no insight into the rationale to view African Americans as physiologically distinct ( Table 1 ). The report of a 2:1 black/white mortality rate due to heart failure at age 35–74 was an erroneous calculation as it does not take into account that 71% of Caucasian American mortality due to heart failure occurred after age 74. When corrected, the ratio was ∼1.1:1. The report of the lack of effectiveness of angiotensin-converting enzyme (ACE) inhibitors in African Americans based on blood pressure measurements was also disputed as other publications reported ACE inhibitors to be equally effective in African Americans compared to Caucasian Americans [ 7 , 8 ]. The single study of nitric oxide turnover examined umbilical endothelial cells in culture collected from only 13 black women and 12 white women without acknowledging that the endothelial cells were fetal in origin, not maternal [ 9 ]. In the two studies of ACE inhibitors and the kinetic study of nitric oxide turnover the rationale for the study of black individuals was epidemiological, rather than biological, that is, the authors cited the greater incidence of hypertension and heart disease in the black population compared to the white population as the rationale for their concept of biological ‘race’.
Rationale for approval of BiDil and assessment of rationale
A portion of the biomedical community heralded BiDil ® as a great discovery, while others voiced strong objections. The immediate response was the publication of articles, editorials, letters to the editor and commentaries in medical journals expounding on issues of ethics, law, commerce, racial categorization and racial profiling raised by the approval of this drug for ‘self-identified African Americans’. Of particular interest was the range of perspectives from the scientific community on the use of ‘race’ as a biological category ( Table 2 ). While the extremes were that ‘race’ was or was not a legitimate biological category, some considered it to be a legitimate proxy for a biological category, while others believed its use, though not ideal, would inevitably continue as it was so entrenched in the biomedical literature. Yet others believed its use would be obviated by the advent of ‘individualized medicine’ at some point in the future, though they did not clarify how population data by race would be applied to individuals. The long-term response has been a growing body of literature addressing the problems associated with the biologization of race and the racialization of medicine [ 10–21 ].
Perspectives on use of ‘race’ as a biological category
A decade following this controversy the issue of ‘race’ as a genetic category or as a proxy for a genetic category persists. In 2016, the NIH ‘Workshop on the Use of Race and Ethnicity in Genomics and Biomedical Research’ was sponsored by the National Human Genome Research Institute (NHGRI) and the National Institute on Minority Health and Health Disparities (NIMHD) [ 22 ]. It was convened to discuss the use of race and ethnicity data in genomics, biomedical and clinical research and their application to minority health and health disparities. The meeting summary emphasized the importance of the discussion to facilitate rigorous scientific study design in order to influence how scientists and the public conceptualize, discuss and react to human differences. It warns that misuse of population descriptors in biomedical research has the potential to perpetuate misinformation, stigmatize certain groups and simplify the complex relationships between individual identity, genetics and health. This warning was never more urgent than it is now during the COVID-19 pandemic, which is underlining the centuries of health disparities burdening African Americans.
EXAMINATION OF THE USE OF AFRICAN ANCESTRY IN GENETIC STUDIES: SYSTEMATIC REVIEW METHODS
We undertook a systematic review of the medical literature to better understand the use of race in biomedical research. To do this, we searched the literature published since 2003 to assess the use of African ancestry and categorization of subjects into African/African American/black populations. We focused on genetic studies categorized as clinical trials since completion of Phase I of the HGP in 2003.
At the outset a National Library of Medicine PubMed database search of the biomedical literature was used to identify papers of interest where Africa, African Americans or blacks were participants in genetics clinical trials. Study of PubMed MeSH terms in consultation with a University of Wisconsin Health Sciences librarian revealed ‘African Continental Ancestry’ to be the MeSH term which encompassed African ancestry, black, African and African American. The terms ‘genetics’ and ‘clinical trials’ were intentionally selected for their breadth to not restrict the breadth of the papers cataloged under ‘African Continental Ancestry’.
A PubMed search of the biomedical literature published from January 2003 to December 2019 was then conducted using the following specific search terms: African continental ancestry, genetics and clinical trials. In each publication the (i) specific use of African continental ancestry, African, African American or black was examined and (ii) the rationale for the use of ‘race’ as a genetic category was examined. (iii) The use of evolutionary explanations of human genetic variation was also assessed to determine if the respective authors framed the population as a biological ‘race’ ( Box 2 ).
Box 2 . Evolutionary processes responsible for human genetic variation
Genetic drift: Variation in the relative frequency of different alleles due to the chance disappearance of particular genes as individuals die or do not reproduce.
Gene flow : Transfer of genetic material from one population to another.
Natural selection Survival of the fittest.
Founder effect: The loss of genetic variation that results when a new population is established by a small number of individuals separate from a larger population.
Mutations: The change in the structure of a gene, resulting in a variant form that may be transmitted to subsequent generations, caused by the alteration of single base units in DNA, or the deletion, insertion or rearrangement of larger sections of genes or chromosomes.
A total of 208 full-length, peer-reviewed papers published during this 17-year period were identified [ 23–61 ] ( Supplementary Material ). Each publication was reviewed to identify studies that used African ancestry, African, African American or black (i) as a social construct, (ii) to correlate race with responses or outcomes or (iii) as a genetic category ( Table 3 ). The publications were evaluated by two authors to determine the stated rationale for study of individuals of African ancestry and to assess evolutionary explanations of genetic differences. In addition, a Portable Document Format of the publications was searched using Preview software for the following keywords related to evolutionary processes, family lineage, genetic lineage and admixture: race, African, African ancestry, African American, black, self-identified, self-reported, ethnic, admixture, ancestry informative marker, ancestry proportion, association, Hardy-Weinberg, family, parent, grandfather, grandmother, grandparent, genetic variation, GWAS, linkage, evolution, genetic drift, gene flow, natural selection, founder effect and mutation. Data were entered into a Filemaker database to facilitate analysis.
Uses of African ancestry
The terms African, African ancestry, African American or black were used as a social construct/category in 9.3% (20) of the 208 papers reviewed [ 23–61 ] ( Supplementary Material ). An additional 28.4% (61) of the studies used these terms to describe studies of physiological responses, the pharmacokinetics of drugs and/or disease associations. In the remaining 58.6% (126 papers) of the studies, African ancestry, African, African American or black were used to define a population in studies including the examination of genotypes associated with life stress, pain, stuttering and drug clearance. This latter group is the subject of further analysis in this review as we wish to determine the author’s conceptions of ‘race’. In these studies, the terms race and ethnicity were often used interchangeably to denote a population of African origin and/or a black study population, though they are not equivalent terms, ethnicity encompassing common ancestry, shared beliefs, cultural traditions, religion and language as well as race. The number of genotyping studies since 2003 does not reflect a specific pattern, the same research group being responsible for multiple papers ( Fig. 1 ). However, it does indicate that there was not a precipitous decline upon completion of Phase I of the HGP.

Number of African ancestry genetic studies by year since completion of Phase I of the Human Genome Project in 2003
CHARACTERIZATION OF AFRICAN ANCESTRY IN STUDY PARTICIPANTS: SUMMARY FINDINGS
The systematic review assessed (i) the use of African ancestry to denote study populations in genetic studies categorized as clinical trials, (ii) examined the stated rationale for its use and (iii) assessed the use of evolutionary principles to explain human genetic diversity.
African ancestry to denote study populations
Self-reported race.
Self-reported race, surrogate-reported race or self-reported skin color were used in 58.7% of the studies to identify and include individuals of African ancestry, while a single study used self-identified race to exclude participants of African ancestry [ 23 ] ( Table 3 ). A portion of the studies included individuals who self-identified as African American or black only if they also reported that three or four grandparents self-identified as African American or black [ 24–26 ]. Self-identification was also used in combination with admixture mapping using ancestry informative markers [ 27–37 ]. Two studies excluded individuals when there was a mismatch between self-identification and genetically inferred ethnicity [ 38 , 39 ].
Comparison of black and white populations
Black populations only were studied in 38.9%, including large cohort studies, such as the African-American Heart Failure Trial, African-American Study of Kids and Strong African-American Families [ 50–56 ]. Black populations were compared to white reference populations in 61.6% (77) of the studies.
Identification of related study participants
To determine whether study participants were related and therefore genetically similar, the studies were also reviewed to determine whether two or more members of the same family were studied. Table 3 shows that different approaches were taken ranging from parents and one to three children or sibling pairs. While it was stated that African-American families were studied, it was not always clear whether two or more members of the same family were studied and whether those two members were direct ancestors, that is, a child, parents and grandparents, but not aunts, uncles and cousins. An example is the study of Musani et al. [ 57 ], who indicates the population included 22% family members; however, it is not possible to determine which portion of the data are based on direct ancestors or whether all data were pooled across seven families.
The size of study populations
A subset of 117 of the studies focused on African Americans/American blacks was further examined to assess the size of the study populations. Thirty-one studies had 100 or fewer participants, 87 fewer than 500 participants and 30 over 500 participants (range 502–5047). There was no discussion as to whether the data from these studies could be generalized to any other African American/black population.
Rationale for use of African ancestry as a genetic category
The studies that undertook genotyping of an African/African American/black population did not state their rationales for using ‘race’ as a genetic category with the exception of the study by Horowitz et al. [ 40 ], where the authors acknowledged that, while race is a social construct, ancestry has important biological implications; thus, they used ‘race’ as a genetic group. However, biological and genetic are not equivalent terms as biological impact does not necessarily occur through a genetic mechanism. In fact, social determinants of poor health—primarily income, education, occupation—are largely responsible for the majority of biological outcomes that impact black and brown people as the result of poor air, water, food, soil and housing quality. Thus, it is not clear whether the biological implications to which Horowitz et al. referred specifically indicated the need for a genetic approach.
The introductions of the remainder of the publications often cited previous literature which correlated the incidence of a disease with people of African ancestry, suggestive of a genetic basis. Thus, this was an epidemiological, not a causal, justification for a genetic study. Alternatively, papers cited previous genetic studies of people of African ancestry that also did not justify the use of African ancestry as a genetic group.
Assessment of the use of evolutionary principles to explain human genetic diversity
To better understand how the authors viewed ‘race’, we reviewed each genetic study to determine if evolutionary explanations for human genetic variation were offered to determine or infer the author’s position on biological/genetic ‘race’.
In 71 of the 126 genetic studies of African or African-American population, Hardy-Weinberg equilibrium (HWE) was assessed to determine whether or not the gene was evolving. When a population is in HWE for a gene, the population is by definition not evolving. This assumes there is random mating, no mutation, no gene flow, an infinite population size and no selection. If the Hardy-Weinberg value is >0.01, it is concluded that a gene is not evolving. Conversely, if the gene is not in equilibrium (<0.01), the differences are due to mutation, non-random mating, gene flow, finite population size (genetic drift) and/or natural selection. In the 71 studies, polymorphisms were determined to be in equilibrium or excluded if they were not. A portion indicated HWE was assessed, but did not indicate in the results whether the polymorphisms were in equilibrium.
In five studies, evolutionary principles were offered to explain the results or to indicate that evolutionary explanations could not be ruled out. Elhassan et al. [ 41 ] described an episode of genetic drift to define the migration of a large east African population out of Africa based on mitochondrial cytochrome C oxidase subunit II (MT-CO2) sequence analysis and genome-wide microsatellite data. East Africans were shown to possess more ancestral lineages in comparison to various other continental populations, concluding that east Africa as the likely spot from which migration toward Asia took place, placing this population at the root of the human evolutionary tree.
Thompson et al. [ 42 ] analyzed the contribution of cysteinyl leukotriene 2 receptor gene variation to the development of asthma in the inhabitants of the south Atlantic island of Tristan da Cunha, a population characterized by both a founder effect and a 47% prevalence of atopy, the tendency to develop allergic diseases.
In a study by Sinues et al. [ 43 ] of 317 Mestizo Ecuadoreans, Ecuadoreans of combined Spanish and South American Indian descent, revealed CYP3A5*3 allele frequency to be significantly lower in Ecuadorians than in Spaniards and other white populations and higher than in Central Americans, Asians and blacks CYP3A4*1B was more common in Ecuadorians than in the Caucasian or Asian reference populations, but less present compared to a black reference population. The authors presume that the presence of the negative selection factor has been less present in Central and South America. In addition to differential selection, a founder effect and genetic drift could not be excluded.
Ryckman et al. [ 44 ] examined genetic polymorphisms in interleukin-1α, -1β, -6 and -8, and tumor necrosis factor-α and their receptors for association with cervical cytokine concentrations in a population of African Americans and European Americans. The goal was to determine if these variants ‘interact’ with polymorphisms in toll-like receptor 4, which was previously shown to associate with pro-inflammatory cervical cytokine concentrations, and to determine if findings are affected by bacterial vaginosis. Several SNPs in IL-1RAP and IL-1R2 were associated with IL-1α or IL-1 β concentrations in African Americans, while only the IL-1RAP SNP was associated with cervical cytokine concentrations in European Americans. They speculate the observed differences in allele frequencies between African Americans and those of European descent may represent convergent evolution and partially explain population disparity in pregnancy-related phenotypes that are cytokine concentration-dependent.
van Zyl et al. [ 45 ] identified twenty-five single nucleotide polymorphisms in the low-density lipoprotein receptor (LDLR) gene in a black South African population. One rare variant of the gene (rs17249141) was significantly associated with lower low-density lipoprotein cholesterol levels, while four variants (rs2738447, rs14158, rs2738465 and rs3180023) were significantly associated with elevated low-density lipoprotein cholesterol levels. All of the polymorphisms were in HWE, except the rs6413503 variant of the LDLR gene. However, this was thought to be due to an excess of homozygote mutants as a result of the genotyping assay and the fact that a large number of heterozygotes were not included in the analysis. It was therefore not suspected to be due to genetic drift, non-random mating, selection, or population structure.
INTERPRETATIONS AND RECOMMENDATIONS: THE WAY FORWARD
This systematic review indicated that the completion of Phase I of the Human Genome Project did not uniformly trigger a re-evaluation of the use of ‘race’ in genetic studies. Instead, the papers reviewed cited epidemiological data as the justification for a genetic approach or publication of a prior genetic study that either preceded the HGP or failed to take its findings into consideration. While the burden of poor health or a specific disease to those of African ancestry is of great concern, it is not in and of itself a justification for a genetic approach, given the multitude of social factors that impact biological outcomes.
In the absence of a stated rationale or proffered evolutionary explanations in the papers reviewed, we infer the authors of these studies believe African ancestry denotes a biological ‘race’ of people of common descent who share DNA unique from the rest of mankind. Presumably they do not accept that all people who populate the earth today had common ancestors who migrated out of Africa 30 000–50 000 years ago or that common ancestors did migrate, but subsequently isolated to form distinct evolutionary lineages with distinct gene sequences that differentiate ‘white’ from ‘black’ disease.
We conclude that an understanding of evolutionary biology, specifically the continuous nature of human genetic variation, is missing [ 62–72 ] compounded by a misreading of federal directives to include minorities in medical research. Fortunately, both of these misunderstandings can be remedied.
The required understanding of the continuous nature of human genetic variation
To understand the continuous nature of human variation, it is useful for the initiate to consider the concept of a cline, a term proposed by Huxley in 1938 [ 73 ]. A cline is a measurable gradient in a single characteristic of a species across its geographical range. Cline is not a term used frequently in biology, but greatly facilitates a basic understanding of the gradient of genetic variation in humans as we migrated out of Africa. In brief, a portion of the African population migrated out of Africa ∼30 000–50 000 years ago, settled at some distance from the parent population, and became a reproductive group. A portion of this second group migrated, settled at some distance and again reproduced. Migration, settling, reproduction and further migration resulted in a gradient of alleles across a geographical range defining clines, hence, the term clinal genetic variation. A cline in what is present day Turkey had a different allele frequency from a cline in present day China or India, even if all of the occupants are considered Asian. Similarly, a cline in what is present day Morocco had a different allele frequency different from what is represented today in Atlanta, Georgia. Thus, it is impossible to specify a specific allele or an allele frequency that would typify ‘African ancestry’ for 1.3 billion Africans on the African continent or 45 million African Americans in the USA.
Because allele frequencies vary across geographical space, it follows that people of ‘African ancestry’ do not represent a homogeneous group. Only those who share direct ancestors—parents, grandparents and all generations of great grandparents—are genetically related. Figure 2 demonstrates biological lineage coalescence as described by Jackson [ 74 ]. The number of direct ancestors is plotted as a function 20-year generations. As the number of ancestors increases exponentially going back in time, the number of actual humans on the planet decreases. Therefore, it is apparent that biological lineages must converge around shared ancestors, thus increasing the potential for genetic similarity among all modern humans. The genetic contribution of the gametes of all direct ancestors coalesce to contribute to the genome of the individual. Any individual’s genome is the result of reproduction of direct ancestors, direct ancestors who are 99.9% identical at the DNA level. The issue is genetic lineage. Not genealogy. Not skin color. Thus, if authors use African ancestry as a genetic category or as a proxy for a genetic category, it implies that a mutation in a direct ancestor occurred at a specific point in time and this allele was inherited by everyone who shares anywhere from 1% to 99% African ancestry and ‘self-identifies’ as having African heritage. This is the genetic version of the one-drop rule [ 75 ].

Biological lineage coalescence. Note that the number of ancestors increases exponentially going back in time but that the number of actual humans on the planet decreases. Therefore, it is obvious that biological lineages must converge around shared ancestors, thus, increasing the potential for genetic similarity among all modern humans. This figure was reproduced with the permission of Oxford University Press from the work of Dr Fatimah Jackson
Given this heterogeneity in allele frequencies, the only way any specific African ancestry study can be reproduced is if the exact same cohort of ‘self-identified’ Africans or African Americans are studied again. Any different combination of people of African ancestry would result in different findings. Thus, it is not at all surprising that a portion of the studies reported here showed no or very small statistical differences. Even those genetic studies that showed statistically significant differences may not if the study were conducted on any other random cohort of ‘self-identified’ people of African ancestry. Thus, the scientific gold standard of reproducibility is not met when ‘race’ is used as a genetic category in these studies. Even if ‘race’ were used as a proxy, it is not clear how the findings of genetic studies of fewer than 500 participants of ‘self-identified’ African ancestry could be generalized to everyone of African descent. Nor can statistical significance be equated with clinical significance given the host of societal and environmental factors which impact expression of a symptom or disease. None of the papers reviewed address when this hypothetical mutation in direct ancestors took place, such that all black people are genetically similar with the same propensities to certain diseases unique from other ‘races’. There is not a plausible explanation.
As stated above, the majority of the health disparities experienced by African Americans are due to social determinants of health, thus, while genetic discoveries and new technologies offer great promise, the public should not be led to believe that genetic solutions to health disparities are imminent given that 34 million Americans have not completed high school, 38.1 million live below the poverty level, 13.55 million are unemployed and 44 million are without health insurance [ 75 ]. Extreme caution must also be exercised in positing that race-based genetics is the path to individualized medicine. In individualized medicine, will the genome scan of an African-American individual only be assessed for alleles believed to reflect African ancestry? Will this be done only if the individual ‘self-identifies’ as such? Will the same mutation not be sought in a ‘white’ person with the same disease? Misconceptions of ‘race’ and errors could result in missed diagnoses, stigmatization of entire populations or remedies proffered to one population over another, errors that would only further divide our nation.
Re-education on guidelines for inclusion of minorities in medical research
There has been insufficient education of scientist, physicians, physician-scientists and the public regarding the rationale for inclusion of minorities in medical research, particularly Office of Management and Budget (OMB) Directive 15 [ 76 ], the Guidelines for the Inclusion of Women and Minorities in Medical Research [ 77 , 78 ] and the Belmont Report to expand human subjects protection [ 79 ].
While slavery officially ended with the Emancipation Proclamation in 1862, the Civil Rights Act was not passed for another 104 years. Thereafter, the federal government deemed it necessary to monitor discrimination of minorities in housing, banking and education by collecting demographic data by race and ethnicity. In 1977, the US OMB Directive 15 clarified that these federal classifications were for record keeping, collection and presentation of data on race and ethnicity in Federal program administrative reports and statistical analyses [ 76 ]. The Directive specifically states that these classifications should not be interpreted as being scientific or anthropological in nature, though they have been. Then in 1986 the NIH issued guidelines encouraging inclusion of women in clinical research as historically women were underrepresented in studies and clearly differed biologically from white men on whom most medical research had been conducted [ 77 ]. In 1989, NIH expanded the guidelines calling for the inclusion of both women and minorities in clinical research, absent an explanation that minorities did not differ biologically from white men [ 78 ]. Thus, an assumption among scientists and physicians was perpetuated that people of color, like women, are biologically different from white men.
Federal funding agencies in the USA also require scientists to report the race and ethnicity of their study populations. In social science studies where race is studied as a social construct, racial and ethnic minorities would logically be included in a study at a level to achieve statistical significance. In biological and genetic studies, some scientists and physicians have followed suit, despite race being a social category. Thus, in genetics black people and white people have been included to achieve a level of statistical significance and viewed as different ‘races’. However, all investigators who conduct studies on human beings are required to have taken a human subjects tutorial. These tutorials explain that human subject guidelines initially focused on ‘Do no harm’, but were expanded in 1979 to include respect for persons, beneficence and social justice [ 79 ]. Thus, minorities are not to be included because they are innately biologically or genetically different, but because social justice dictates all Americans share the risks and benefits of medical research.
SUMMATION AND REMEDIES
In 2004, the Director of the NHGRI wrote ‘“Race” and “ethnicity” are poorly defined terms that serve as flawed surrogates for multiple environmental and genetic factors in disease causation. Research must move beyond these weak and imperfect proxy relationships to define the more proximate factors that influence health’ [ 80 ]. Many of these more proximate factors are not genetic. In 2016, NHGRI and NIMHD co-sponsored the ‘Workshop on the Use of Race and Ethnicity in Genomics and Biomedical Research’ to explore how genomics and biomedical research can describe research participant’s diverse backgrounds and experiences in ways that are scientifically and socially meaningful [ 22 ]. Participants included genomic, clinical, epidemiologic and social science researchers in addition to NIH and government stakeholders. The workshop did not aim to create unanimous recommendations or formal consensus, but did produce a summary upon which it was reported there was broad agreement. However, it fell far short of addressing and resolving misconceptions of ‘race’, focusing instead on instructions on collecting and reporting race and ethnicity as to not limit the ways in which a population can be reported. The summary also discouraged the use of race and ethnicity as a proxy for ‘expanded data categories’. Here we would encourage extreme caution against the selection of expanded data categories where the causes, effects and answers are not in the primary DNA sequence.
It is unfortunate it did not clarify that one can self-identify one’s cultural identity, religious identity and sexual identity, but one does not self-identify one’s DNA. We also regret that alternative models were not summarized that are able to simultaneously consider race/ethnicity, culture, genetics, disease incidence and geography as distinct, but very important, categories as demonstrated by the Ethnogenetic Layering Approach of Jackson in 2004 [ 74 ]. Her model acknowledges the genetic makeup present at birth as well as environmental factors that impact expressed genotype while taking into consideration the many cultural factors. The model acknowledges the rich contribution of each factor without conflating ‘race’ and self-identification with genetics.
Table 4 lists issues to be remedied by individual investigators as well as issues to be remedied at the systemic level. The first step for individuals in either group is to re-examine and question their own individual beliefs and assumptions as to what race is and is not, the same exercise the entire nation is facing. The next step is to embrace the findings of the HGP and replace misconceptions with an understanding of evolutionary biology and a corrected view of federal directives. Though it is difficult to give up strongly held beliefs, the transition will be greatly facilitated by layering new learning to supplant the incorrect information that ‘race’ is genetic. Table 5 lists the highlights of the new understanding achieved. ‘Race’ is not genetic. ‘Race’ nor ethnicity is suitable proxies for a genetic category. The scientific community and institutions need to discontinue its use in genetics immediately and embrace the findings of the HGP. No substitute term is required to divide us into genetic categories as we are all H.sapiens sapiens . One can self-identify one’s cultural, religious and sexual identity, but not one’s DNA. We are not better people if we attempt to infuse genetics with cultural humility or political correctness. The biomedical community must get its own house in order before it can hope to educate the public and restore the much-needed public trust of science. Only then can the promises of individualized medicine be realized in the future in an equitable and ethical manner.
Remedies to Correct Misconceptions of ‘Race’
Corrected conceptions of race
Supplementary data
Supplementary data is available at EMPH online.
Supplementary Material
Eoab018_supplementary_data, acknowledgements.
The authors wish to thank Drs Fatimah Jackson, Joseph L. Graves Jr and John Pool for helpful scientific discussions and James Barney and Emanuel Scarbrough for many helpful discussions on lay understandings of race.
Conflict of interest: None declared.
Screening & Prevention
Guides & resources, salt lake city, utah county, community clinics, our cancer center, faculty & students, volunteering.

The Surprising Connection Between Male Infertility and Family Cancer Risk
Read Time: 3 minutes

In a recent study , researchers at Huntsman Cancer Institute at the University of Utah (the U) found a surprising trend in families with male infertility: an increased risk of certain cancers. This discovery could lead to a more personalized approach to cancer risk assessments, making cancer prevention more effective.
According to the National Institutes of Health, around 9% of men at reproductive age have experienced fertility problems .
“We know that men who experience infertility tend to have more health issues like cardiovascular disease, autoimmune conditions, earlier mortality, chronic health conditions, and cancer,” says Joemy Ramsay, PhD , the study's lead investigator, researcher at Huntsman Cancer Institute, and assistant professor in the Division of Urology at the U. “We wanted to look at whether the family members of these men were at higher risk for these conditions.”
Ramsay has a background in public health, specializing in occupational and environmental exposure. This study represents the first step in determining family members’ correlated risk levels to diseases, such as cancer. Ramsay explains that since family members share similar genetic factors, environments, and lifestyles, it would be easier to identify other things impacting their cancer risk. Once general risk has been assessed, etiological factors can be more accurately evaluated in determining the part they play in a diagnosis.
Using the Utah Population Database , one of the world’s richest sources of genetic and public health information, Ramsay and her team, which included Heidi Hanson, MS, PhD , Nicola Camp, PhD , and Myke Madsen , looked at parents, siblings, children, and even aunts, uncles, and cousins, of men who have been diagnosed with infertility.
“It is important to have these conversations with our families and bring your concerns to your medical team.”
By observing several types of cancer at once, the team was able to develop an algorithm that clusters similar things together. This algorithm made it possible to identify roughly 13 characteristic patterns. The patterns were based on families possessing similar multi-cancer risks, instead of looking at only one cancer type at a time.
“Both cancer and subfertility are complex diseases and processes,” says Ramsay. “This method helps create similar family groups, making it easier to uncover the reason behind a family being at high risk for certain diseases over others.”
For families with male infertility, these findings may prompt additional conversations with their doctors.
“While the link is still not fully understood, it is important to have these conversations with our families and bring your concerns to your medical team,” says Ramsay.
Further research is needed to continue to establish a link between male infertility and cancer risk. Understanding the reason behind a risk may ultimately lead to more personalized courses of treatment, screening, and prevention.
Huntsman Cancer Institute leads the way in educating patients on how to prevent and treat cancer. For more information on genetic testing, visit our Family Cancer Assessment Clinic .
This study was supported by the National Institutes of Health/National Cancer Institute including P30 CA042014 and Huntsman Cancer Foundation . The chatbots were developed in a recently completed trial funded by the Inherited Cancer Syndrome Collaborative of the Cancer Moonshot initiative.
Media Contact
Heather Simonsen Public Relations Huntsman Cancer Institute Email Us 801 581-3194
About Huntsman Cancer Institute at the University of Utah
Huntsman Cancer Institute at the University of Utah (the U) is the National Cancer Institute-designated Comprehensive Cancer Center for Utah, Idaho, Montana, Nevada, and Wyoming. With a legacy of innovative cancer research, groundbreaking discoveries, and world-class patient care, we are transforming the way cancer is understood, prevented, diagnosed, treated, and survived. Huntsman Cancer Institute focuses on delivering a cancer-free frontier to all communities in the area we serve . We have more than 300 open clinical trials and 250 research teams studying cancer at any given time. More genes for inherited cancers have been discovered at Huntsman Cancer Institute than at any other cancer center. Our scientists are world-renowned for understanding how cancer begins and using that knowledge to develop innovative approaches to treat each patient’s unique disease. Huntsman Cancer Institute was founded by Jon M. and Karen Huntsman.
Resources for Media
Cancer touches all of us..
- Cancer Research
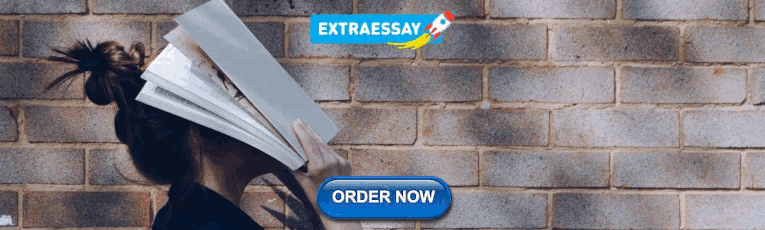
IMAGES
VIDEO
COMMENTS
Genetics research is the scientific discipline concerned with the study of the role of genes in traits such as the development of disease. It has a key role in identifying potential targets for ...
Participants in clinical studies help current and future generations. Through these studies, researchers develop new diagnostic tests, more effective treatments, and better ways of managing diseases with genetic components. Participants in studies are actively involved in understanding their disorder and current research.
The future of genomics and genetics: What to expect. The study of genomics and genetics is rapidly advancing, meaning it will likely play an increasingly important role in human disease research and prevention. Some of the emerging trends in genomics and genetics include: Direct-to-consumer genetic testing
Genomic research has evolved from seeking to understand the fundamentals of the human genetic code to examining the ... Much work remains to be done to enhance the study of human genetic variation
Each DNA strand is made of four chemical units, called nucleotide bases, which comprise the genetic "alphabet." The bases are adenine (A), thymine (T), guanine (G), and cytosine (C). Bases on opposite strands pair specifically: an A always pairs with a T; a C always pairs with a G. The order of the As, Ts, Cs and Gs determines the meaning of ...
About the National Human Genome Research Institute. At NHGRI, we are focused on advances in genomics research. Building on our leadership role in the initial sequencing of the human genome, we collaborate with the world's scientific and medical communities to enhance genomic technologies that accelerate breakthroughs and improve lives. By ...
E.R. SmithN Engl J Med 2024;390:1022-1028. Cerebral cavernous malformations occur in 0.5% of the population; 85% are sporadic, and 15% are familial or radiation-induced. Several genetic variants ...
Neurogenetics lies at the intersection of Neuroscience and Genetics, where genetic approaches are applied to the study of nervous system development, function, and plasticity. Overseen by Series Editors Oliver Hobert, Cecilia Moens, and Kate O'Connor Giles, this new series aims to make the GSA Journals a home for cutting-edge, robust research ...
Genetics, study of heredity in general and of genes in particular. Genetics forms one of the central pillars of biology and overlaps with many other areas, such as agriculture, medicine, and biotechnology. ... All present research in genetics can be traced back to Mendel's discovery of the laws governing the inheritance of traits. The word ...
The study findings also revealed genome-wide genetic overlaps, or correlations, between bipolar disorder and certain traits. For example, the results showed a genetic correlation between bipolar disorder and both alcohol use and smoking, as well as genetic correlations with some aspects of sleep (daytime sleepiness, insomnia, and sleep duration).
A recent study in a neonatal intensive care unit in Texas studied outcomes for 278 infants who were referred for clinical exome sequencing, and found that 36.7% received a genetic diagnosis, and medical management was affected for 52% of infants with diagnoses [ ].
However, a few genes and genetic loci have been identified for this trait in the recent studies [10,11,12]. Gene coding for apolipoprotein (APOE) has been reported by multiple genome-wide association studies (GWAS) [10,11,12]. Telomeres are the protective caps at the termini of human and other eukaryotic chromosomes.
Genetics Research is a fully open access journal providing a key forum for original research on all aspects of human and animal genetics, reporting key findings on genomes, genes, mutations, developmental, evolutionary, and population genetics as well as ethical, legal and social aspects. ... This study included 66 patients with CLL, diagnosed ...
A Study of Symptoms, Lifestyle Habits, Physician Counseling, and Direct-to Consumer Genetic Testing in Patients with Hereditary Hemochromatosis Jacksonville, FL . The purpose of this study is to provide descriptive information on patients with hereditary hemochromatosis, including their predominant symptoms, time to diagnosis, lifestyle habits, physician counseling, and use of direct-to ...
DOI: 10.26508/lsa.202302517. In the largest study of its kind, researchers from the Translational Genomics Research Institute (TGen), part of City of Hope, have identified genes that appear to ...
Clarifying the nature of shared genetic influences between psychiatric disorders and with other traits and diseases has become an important research area in psychiatric genetics. This research could inform ongoing processes aiming to reconceptualize psychiatric nosology173, 174, increase the understanding of the pervasive comorbidity and shared ...
Two new studies of genetic markers in the blood cells of pregnant women suggest that their cells seem to age at an exaggerated clip, adding extra months or even years to a woman's so-called ...
In celebration of the 20th anniversary of Nature Reviews Genetics, we asked 12 leading researchers to reflect on the key challenges and opportunities faced by the field of genetics and genomics.
New research suggests that a single pregnancy can add between two to 14 months to your biological age. "Pregnancy has a cost that appears to be detectable even" as early as your 20s, says study ...
Background . Investigative genetic genealogy (IGG) is a technique that involves uploading genotypes developed from perpetrator DNA left at a crime scene, or DNA from unidentified remains, to public genetic genealogy databases to identify genetic relatives and, through the creation of a family tree, the individual who was the source of the DNA.
ADHD often appears to run in families, and research studies have suggested that there may be a genetic component to this disorder. Individuals diagnosed with ADHD may have close blood relatives with the disorder. Scientists believe that ADHD is a complex disorder that probably involves at least two genes. Non-genetic causes such as abnormal ...
Genetic counseling research, which strives to improve the quality and availability of genetic counseling services, benefits from these clinical data. Original articles contributed to a large proportion of the publication outputs and increased significantly over the last decade. ... Lastly, the research themes in this study were derived from ...
A new study reveals the detailed protein structure of the TAS2R14, a bitter taste receptor that allows us to perceive bitter taste. In addition to solving the structure of this taste receptor, the ...
A study published on March 27 in JAMA Network Open found that those with a higher genetic risk of obesity had to log more daily steps than individuals who had a lower genetic propensity for obesity.
Twin and family studies report that genetic differences are associated with individual differences in intelligence test scores (Box 2 ). If studies from all ages are taken together, genetic ...
Even those genetic studies that showed statistically significant differences may not if the study were conducted on any other random cohort of 'self-identified' people of African ancestry. Thus, the scientific gold standard of reproducibility is not met when 'race' is used as a genetic category in these studies. ... Research must move ...
In a recent study, researchers at Huntsman Cancer Institute at the University of Utah (the U) found a surprising trend in families with male infertility: an increased risk of certain cancers. This discovery could lead to a more personalized approach to cancer risk assessments, making cancer prevention more effective. According to the National Institutes of Health, around 9% of men at ...