Have a language expert improve your writing
Run a free plagiarism check in 10 minutes, generate accurate citations for free.
- Knowledge Base
Methodology
- Stratified Sampling | Definition, Guide & Examples
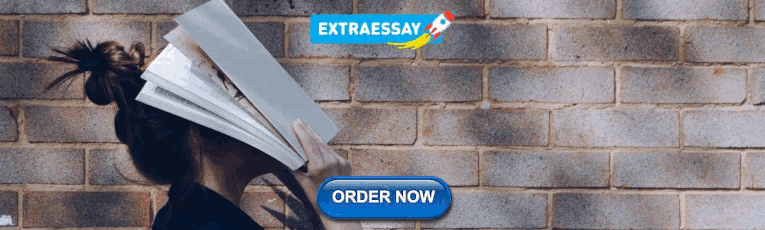
Stratified Sampling | Definition, Guide & Examples
Published on September 18, 2020 by Lauren Thomas . Revised on June 22, 2023.
In a stratified sample , researchers divide a population into homogeneous subpopulations called strata (the plural of stratum) based on specific characteristics (e.g., race, gender identity, location, etc.). Every member of the population studied should be in exactly one stratum.
Each stratum is then sampled using another probability sampling method, such as cluster sampling or simple random sampling , allowing researchers to estimate statistical measures for each sub-population.
Researchers rely on stratified sampling when a population’s characteristics are diverse and they want to ensure that every characteristic is properly represented in the sample. This helps with the generalizability and validity of the study, as well as avoiding research biases like undercoverage bias .
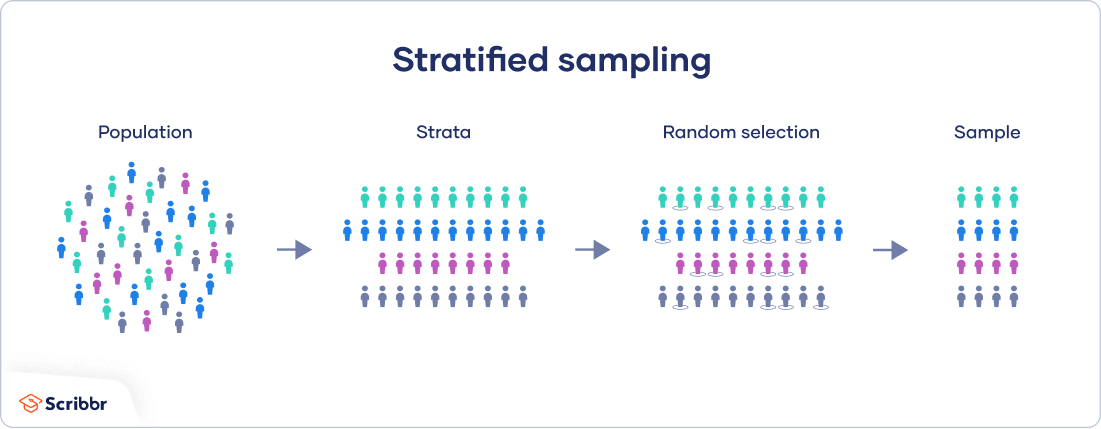
Table of contents
When to use stratified sampling, step 1: define your population and subgroups, step 2: separate the population into strata, step 3: decide on the sample size for each stratum, step 4: randomly sample from each stratum, other interesting articles, frequently asked questions about stratified sampling.
To use stratified sampling, you need to be able to divide your population into mutually exclusive and exhaustive subgroups. That means every member of the population can be clearly classified into exactly one subgroup.
Stratified sampling is the best choice among the probability sampling methods when you believe that subgroups will have different mean values for the variable(s) you’re studying. It has several potential advantages:
Ensuring the diversity of your sample
A stratified sample includes subjects from every subgroup, ensuring that it reflects the diversity of your population. It is theoretically possible (albeit unlikely) that this would not happen when using other sampling methods such as simple random sampling .
Ensuring similar variance
If you want the data collected from each subgroup to have a similar level of variance , you need a similar sample size for each subgroup.
With other methods of sampling, you might end up with a low sample size for certain subgroups because they’re less common in the overall population.
Lowering the overall variance in the population
Although your overall population can be quite heterogeneous, it may be more homogenous within certain subgroups.
For example, if you are studying how a new schooling program affects the test scores of children, both their original scores and any change in scores will most likely be highly correlated with family income. The scores are likely to be grouped by family income category.
In this case, stratified sampling allows for more precise measures of the variables you wish to study, with lower variance within each subgroup and therefore for the population as a whole.
Allowing for a variety of data collection methods
Sometimes you may need to use different methods to collect data from different subgroups.
For example, in order to lower the cost and difficulty of your study, you may want to sample urban subjects by going door-to-door, but rural subjects using mail.
Because only a small proportion of this university’s graduates have obtained a doctoral degree, using a simple random sample would likely give you a sample size too small to properly compare the differences between men, women, and those who do not identify as men or women with a doctoral degree versus those without one.
Prevent plagiarism. Run a free check.
Like other methods of probability sampling , you should begin by clearly defining the population from which your sample will be taken.
Choosing characteristics for stratification
You must also choose the characteristic that you will use to divide your groups. This choice is very important: since each member of the population can only be placed in only one subgroup, the classification of each subject to each subgroup should be clear and obvious.
Stratifying by multiple characteristics
You can choose to stratify by multiple different characteristics at once, so long as you can clearly match every subject to exactly one subgroup. In this case, to get the total number of subgroups, you multiply the numbers of strata for each characteristic.
For instance, if you were stratifying by both race and gender identity, using four groups for the former and three for the latter, you would have 4 x 3 = 12 groups in total.
Next, collect a list of every member of the population, and assign each member to a stratum.
You must ensure that each stratum is mutually exclusive (there is no overlap between them), but that together, they contain the entire population.
Combining these characteristics, you have nine groups in total. Each graduate must be assigned to exactly one group.
First, you need to decide whether you want your sample to be proportionate or disproportionate.
Proportionate versus disproportionate sampling
In proportionate sampling, the sample size of each stratum is equal to the subgroup’s proportion in the population as a whole.
Subgroups that are less represented in the greater population (for example, rural populations, which make up a lower portion of the population in most countries) will also be less represented in the sample.
In disproportionate sampling, the sample sizes of each strata are disproportionate to their representation in the population as a whole.
You might choose this method if you wish to study a particularly underrepresented subgroup whose sample size would otherwise be too low to allow you to draw any statistical conclusions.
Sample size
Next, you can decide on your total sample size. This should be large enough to ensure you can draw statistical conclusions about each subgroup.
If you know your desired margin of error and confidence level as well as estimated size and standard deviation of the population you are working with, you can use a sample size calculator to estimate the necessary numbers.
Here's why students love Scribbr's proofreading services
Discover proofreading & editing
Finally, you should use another probability sampling method , such as simple random or systematic sampling , to sample from within each stratum.
If properly done, the randomization inherent in such methods will allow you to obtain a sample that is representative of that particular subgroup.
If you want to know more about statistics , methodology , or research bias , make sure to check out some of our other articles with explanations and examples.
- Student’s t -distribution
- Normal distribution
- Null and Alternative Hypotheses
- Chi square tests
- Confidence interval
- Quartiles & Quantiles
- Cluster sampling
- Data cleansing
- Reproducibility vs Replicability
- Peer review
- Prospective cohort study
Research bias
- Implicit bias
- Cognitive bias
- Placebo effect
- Hawthorne effect
- Hindsight bias
- Affect heuristic
- Social desirability bias
Probability sampling means that every member of the target population has a known chance of being included in the sample.
Probability sampling methods include simple random sampling , systematic sampling , stratified sampling , and cluster sampling .
In stratified sampling , researchers divide subjects into subgroups called strata based on characteristics that they share (e.g., race, gender, educational attainment).
Once divided, each subgroup is randomly sampled using another probability sampling method.
You should use stratified sampling when your sample can be divided into mutually exclusive and exhaustive subgroups that you believe will take on different mean values for the variable that you’re studying.
Using stratified sampling will allow you to obtain more precise (with lower variance ) statistical estimates of whatever you are trying to measure.
For example, say you want to investigate how income differs based on educational attainment, but you know that this relationship can vary based on race. Using stratified sampling, you can ensure you obtain a large enough sample from each racial group, allowing you to draw more precise conclusions.
Yes, you can create a stratified sample using multiple characteristics, but you must ensure that every participant in your study belongs to one and only one subgroup. In this case, you multiply the numbers of subgroups for each characteristic to get the total number of groups.
For example, if you were stratifying by location with three subgroups (urban, rural, or suburban) and marital status with five subgroups (single, divorced, widowed, married, or partnered), you would have 3 x 5 = 15 subgroups.
Cite this Scribbr article
If you want to cite this source, you can copy and paste the citation or click the “Cite this Scribbr article” button to automatically add the citation to our free Citation Generator.
Thomas, L. (2023, June 22). Stratified Sampling | Definition, Guide & Examples. Scribbr. Retrieved April 9, 2024, from https://www.scribbr.com/methodology/stratified-sampling/
Is this article helpful?
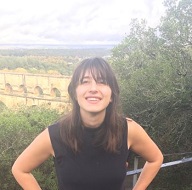
Lauren Thomas
Other students also liked, sampling methods | types, techniques & examples, simple random sampling | definition, steps & examples, cluster sampling | a simple step-by-step guide with examples, "i thought ai proofreading was useless but..".
I've been using Scribbr for years now and I know it's a service that won't disappoint. It does a good job spotting mistakes”
Have a language expert improve your writing
Run a free plagiarism check in 10 minutes, automatically generate references for free.
- Knowledge Base
- Methodology
Stratified Sampling | A Step-by-Step Guide with Examples
Published on 3 May 2022 by Lauren Thomas .
In a stratified sample , researchers divide a population into homogeneous subpopulations called strata (the plural of stratum) based on specific characteristics (e.g., race, gender identity, location). Every member of the population studied should be in exactly one stratum.
Each stratum is then sampled using another probability sampling method, such as cluster or simple random sampling, allowing researchers to estimate statistical measures for each subpopulation.
Researchers rely on stratified sampling when a population’s characteristics are diverse and they want to ensure that every characteristic is properly represented in the sample.
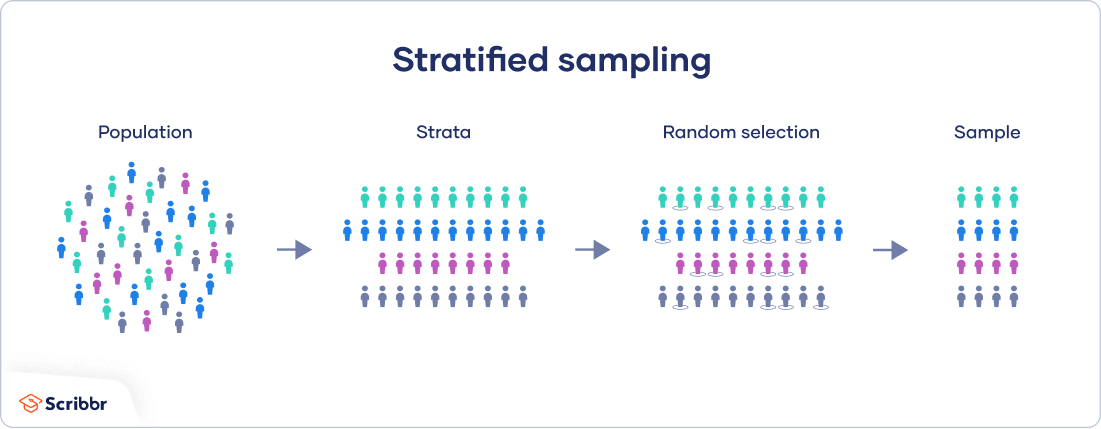
Table of contents
When to use stratified sampling, step 1: define your population and subgroups, step 2: separate the population into strata, step 3: decide on the sample size for each stratum, step 4: randomly sample from each stratum, frequently asked questions about stratified sampling.
To use stratified sampling, you need to be able to divide your population into mutually exclusive and exhaustive subgroups. That means every member of the population can be clearly classified into exactly one subgroup.
Stratified sampling is the best choice among the probability sampling methods when you believe that subgroups will have different mean values for the variable(s) you’re studying. It has several potential advantages:
Ensuring the diversity of your sample
A stratified sample includes subjects from every subgroup, ensuring that it reflects the diversity of your population. It is theoretically possible (albeit unlikely) that this would not happen when using other sampling methods such as simple random sampling .
Ensuring similar variance
If you want the data collected from each subgroup to have a similar level of variance , you need a similar sample size for each subgroup.
With other methods of sampling, you might end up with a low sample size for certain subgroups because they’re less common in the overall population.
Lowering the overall variance in the population
Although your overall population can be quite heterogeneous, it may be more homogenous within certain subgroups.
For example, if you are studying how a new schooling program affects the test scores of children, both their original scores and any change in scores will most likely be highly correlated with family income. The scores are likely to be grouped by family income category.
In this case, stratified sampling allows for more precise measures of the variables you wish to study, with lower variance within each subgroup and therefore for the population as a whole.
Allowing for a variety of data collection methods
Sometimes you may need to use different methods to collect data from different subgroups.
For example, in order to lower the cost and difficulty of your study, you may want to sample urban subjects by going door to door, but rural subjects by post.
Because only a small proportion of this university’s graduates have obtained a doctoral degree, using a simple random sample would likely give you a sample size too small to properly compare the differences between men, women, and those who do not identify as men or women with a doctoral degree vs those without one.
Prevent plagiarism, run a free check.
As with other methods of probability sampling , you should begin by clearly defining the population from which your sample will be taken.
Choosing characteristics for stratification
You must also choose the characteristic that you will use to divide your groups. This choice is very important: since each member of the population can only be placed in only one subgroup, the classification of each subject to each subgroup should be clear and obvious.
Stratifying by multiple characteristics
You can choose to stratify by multiple different characteristics at once, so long as you can clearly match every subject to exactly one subgroup. In this case, to get the total number of subgroups, you multiply the numbers of strata for each characteristic.
For instance, if you were stratifying by both race and gender identity, using four groups for the former and three for the latter, you would have 4 × 3 = 12 groups in total.
Next, collect a list of every member of the population, and assign each member to a stratum.
You must ensure that each stratum is mutually exclusive (there is no overlap between them), but that together, they contain the entire population.
Combining these characteristics, you have nine groups in total. Each graduate must be assigned to exactly one group.
First, you need to decide whether you want your sample to be proportionate or disproportionate.
Proportionate vs disproportionate sampling
In proportionate sampling, the sample size of each stratum is equal to the subgroup’s proportion in the population as a whole.
Subgroups that are less represented in the greater population (for example, rural populations, which make up a lower portion of the population in most countries) will also be less represented in the sample.
In disproportionate sampling, the sample sizes of each strata are disproportionate to their representation in the population as a whole.
You might choose this method if you wish to study a particularly underrepresented subgroup whose sample size would otherwise be too low to allow you to draw any statistical conclusions.
Sample size
Next, you can decide on your total sample size. This should be large enough to ensure you can draw statistical conclusions about each subgroup.
If you know your desired margin of error and confidence level as well as estimated size and standard deviation of the population you are working with, you can use a sample size calculator to estimate the necessary numbers.
Finally, you should use another probability sampling method , such as simple random or systematic sampling , to sample from within each stratum.
If properly done, the randomisation inherent in such methods will allow you to obtain a sample that is representative of that particular subgroup.
In stratified sampling , researchers divide subjects into subgroups called strata based on characteristics that they share (e.g., race, gender, educational attainment).
Once divided, each subgroup is randomly sampled using another probability sampling method .
You should use stratified sampling when your sample can be divided into mutually exclusive and exhaustive subgroups that you believe will take on different mean values for the variable that you’re studying.
Using stratified sampling will allow you to obtain more precise (with lower variance ) statistical estimates of whatever you are trying to measure.
For example, say you want to investigate how income differs based on educational attainment, but you know that this relationship can vary based on race. Using stratified sampling, you can ensure you obtain a large enough sample from each racial group, allowing you to draw more precise conclusions.
Yes, you can create a stratified sample using multiple characteristics, but you must ensure that every participant in your study belongs to one and only one subgroup. In this case, you multiply the numbers of subgroups for each characteristic to get the total number of groups.
For example, if you were stratifying by location with three subgroups (urban, rural, or suburban) and marital status with five subgroups (single, divorced, widowed, married, or partnered), you would have 3 × 5 = 15 subgroups.
Probability sampling means that every member of the target population has a known chance of being included in the sample.
Probability sampling methods include simple random sampling , systematic sampling , stratified sampling , and cluster sampling .
Cite this Scribbr article
If you want to cite this source, you can copy and paste the citation or click the ‘Cite this Scribbr article’ button to automatically add the citation to our free Reference Generator.
Thomas, L. (2022, May 03). Stratified Sampling | A Step-by-Step Guide with Examples. Scribbr. Retrieved 9 April 2024, from https://www.scribbr.co.uk/research-methods/stratified-sampling-method/
Is this article helpful?
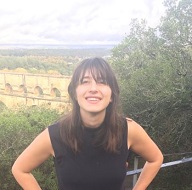
Lauren Thomas
Other students also liked, sampling methods | types, techniques, & examples, cluster sampling | a simple step-by-step guide with examples, simple random sampling | definition, steps & examples.
Stratified Random Sampling: Definition, Method & Examples
Julia Simkus
Editor at Simply Psychology
BA (Hons) Psychology, Princeton University
Julia Simkus is a graduate of Princeton University with a Bachelor of Arts in Psychology. She is currently studying for a Master's Degree in Counseling for Mental Health and Wellness in September 2023. Julia's research has been published in peer reviewed journals.
Learn about our Editorial Process
Saul Mcleod, PhD
Editor-in-Chief for Simply Psychology
BSc (Hons) Psychology, MRes, PhD, University of Manchester
Saul Mcleod, PhD., is a qualified psychology teacher with over 18 years of experience in further and higher education. He has been published in peer-reviewed journals, including the Journal of Clinical Psychology.
Olivia Guy-Evans, MSc
Associate Editor for Simply Psychology
BSc (Hons) Psychology, MSc Psychology of Education
Olivia Guy-Evans is a writer and associate editor for Simply Psychology. She has previously worked in healthcare and educational sectors.
On This Page:
Stratified random sampling is a method of selecting a sample in which researchers first divide a population into smaller subgroups, or strata, based on shared characteristics of the members and then randomly select among each stratum to form the final sample.
These shared characteristics can include gender, age, sex, race, education level, or income.
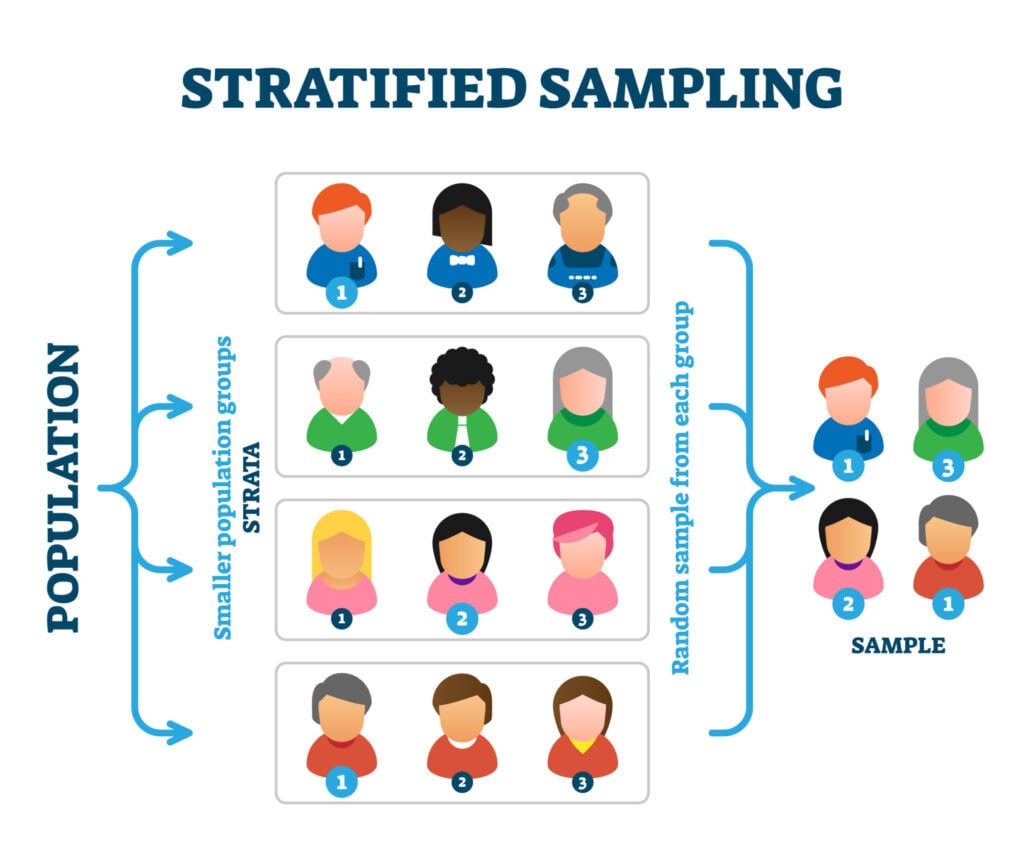
The process of classifying the population into groups before sampling is called stratification. The strata must be mutually exclusive, and all members of the population can only be in one stratum.
When stratifying, researchers tend to use proportionate sampling, where they maintain the correct proportions to represent the population as a whole.
For example, if the larger population contains 40% history majors and 60% English majors, the final sample should reflect these percentages.
Disproportionate sampling is typically only used when studying an underrepresented group.
Applications
- When studying polling of elections, population demographics, or life expectancy.
- When studying the income of varying populations or the income for different jobs across a nation.
- When time is limited, or budgeting is tight as stratified sampling is quicker and cheaper than many other sampling methods.
- When the samples of a population vary drastically as forming strata helps organize a group of people.
- When researchers do not have access to an entire population.
- Define your population of interest and choose the characteristic(s) that you will use to divide your groups.
- Divide your sample into strata depending on the relevant characteristic(s). Each stratum must be mutually exclusive, but together, they must represent the entire population.
- Define the sample size for each stratum and decide whether your sample will be proportionate or disproportionate. The sample size in each stratum should ideally be in proportion to the members of that group within the target population or sampling frame.
- Draw a random sample from each stratum and combine them to form your final sample.
Example Situations
- Public Health Studies: To understand the incidence of disease across different age groups, the population could be stratified into different age brackets (e.g., 0-18, 19-35, 36-50, 51+).
- Investigating the relationship between average travel frequency, trip mode structure, and the characteristics of residential areas (Shi, 2015).
- Examining the prevalence and psychological sequelae of childhood sexual and physical abuse in adults from the general population (Briere & Elliott, 2003).
- Evaluating the usefulness of personality traits in explaining and predicting entrepreneurship (Llewellyn & Wilson, 2003).
- Examining women’s involvement in multiple roles in relation to 3 stress indices: role overload, role conflict, and anxiety (Barnett & Baruch, 1985).
- Studying perceptions of drinking water quality at four locations in Western Australia (Syme & Williams, 1993).
Efficient and manageable
By organizing a population into groups with similar characteristics, researchers save data collection time and can better manage a sample that would otherwise be too large to analyze.
The research costs for this sampling method are minimized as researchers save money by dividing a large population into smaller groups containing similar members rather than sampling every individual of a larger population.
Stratified sampling can produce more precise estimates than simple random sampling when members of the subpopulations are homogeneous relative to the entire population. This gives a study more statistical power.
Limitations
Too many differences within the population.
A population can’t be organized into subgroups if there are too many differences within the population or if there is not enough information about the population at hand.
Researchers must ensure that every member of the population fits into only one stratum and that all the strata collectively contain every member of the greater population. This involves extra planning and information gathering that simple random sampling does not require.
Sampling errors
Sampling errors can occur when the sample does not accurately represent the population as a whole. If this occurs, the researcher would need to restart the sampling process.
Cluster Sampling vs. Stratified Sampling
Stratified sampling and cluster sampling both involve dividing a large population into smaller groups and then selecting randomly among the subgroups to form a sample.
However, the main difference is that researchers in stratified sampling divide the population into groups based on age, religion, ethnicity, or income level and randomly choose from these strata to form a sample.
Alternatively, researchers in cluster sampling will use naturally divided groups to separate the population (i.e., city blocks or school districts) and then randomly select elements from these clusters to be a part of the sample.
Stratified Sampling vs. Quota Sampling
Quota sampling and stratified sampling both involve dividing a population into mutually exclusive subgroups and sampling a predetermined number of individuals from each.
However, the most significant difference between these two techniques is that quota sampling is a non-probability sampling method, while stratified sampling is a probability sampling method.
In a stratified sample, individuals within each stratum are selected randomly, while in a quota sample, researchers choose the sample instead of randomly selecting it.
Stratified sampling is also known as quota random sampling.
- A sample is the participants you select from a target population (the group you are interested in) to make generalizations about. As an entire population tends to be too large to work with, a smaller group of participants must act as a representative sample.
- Representative means the extent to which a sample mirrors a researcher’s target population and reflects its characteristics (e.g., gender, ethnicity, socioeconomic level). In an attempt to select a representative sample and avoid sampling bias (the over-representation of one category of participant in the sample), psychologists utilize a variety of sampling methods.
- Generalisability means the extent to which their findings can be applied to the larger population of which their sample was a part.
Barnett, R. C., & Baruch, G. K. (1985). Women’s involvement in multiple roles and psychological distress. Journal of Personality and Social Psychology, 49(1), 135–145.
Briere, J., & Elliott, D. M. (2003). Prevalence and psychological sequelae of self-reported childhood physical and sexual abuse in a general population sample of men and women. Child abuse & neglect, 27(10), 1205-1222.
How to use stratified random sampling to your advantage. Qualtrics. (n.d.). Retrieved from https://www.qualtrics.com/experience-management/research/stratified-random-sampling/
Llewellyn, D. J., & Wilson, K. M. (2003). The controversial role of personality traits in entrepreneurial psychology. Education+ Training.
Nickolas, S. (2021, May 19). How stratified random sampling works. Investopedia. Retrieved January 27, 2022, from https://www.investopedia.com/ask/answers/032615/what-are-some-examples-stratified-random-sampling.asp
Shi, F. (2015). Study on a stratified sampling investigation method for resident travel and the sampling rate. Discrete Dynamics in Nature and Society, 2015.
Syme, G. J., & Williams, K. D. (1993). The psychology of drinking water quality: an exploratory study. Water Resources Research, 29(12), 4003-4010.

Stratified Sampling: An Introduction With Examples

If you’ve ever been to the beach, especially the beaches of the coastal U.S. southeast, you might be familiar with the terrifying feeling of seeing something globular and undulating in a fast-approaching wave during your dip in the ocean. Jellyfish pervade the local waters, and doubly so during the months when ocean swimming is best. For a creature without a brain , this certainly seems well-coordinated for maximal inconvenience. Besides being little more than hunks of floating ooze, some species of jellyfish also boast stingers, and a subset of these species can produce stings that are potentially life-threatening.
Most stings, though, however unpleasant, are not life-threatening. For this reason, they are not recorded as medical statistics. So, if researchers wanted to collect information on how much of the U.S. population has been stung by jellyfish, the best option would be to ask a sample of people and extrapolate the findings population-wide.
In this case, if researchers surveyed people nationwide and simply aggregated their results, however, those results would be misleading. Most of the U.S. is landlocked. Surveying individuals in states with no coastline inherently involves a different probability of being stung by a jellyfish than individuals who live near a beach. A nationwide, random survey of Americans would tend to overrepresent individuals with higher likelihoods of never being stung.
What Is Stratified Sampling?
More From Edward Hearn Ordinal Data Versus Nominal Data: What’s the Difference?
In situations like this, stratification produces sharper, more precise estimates than other methods. To stratify means to subdivide a population into a collection of non-overlapping groups along some metric. Individuals within these subgroups — or “strata” — can then be randomly surveyed. Lastly, researchers aggregate survey results pertaining to each subgroup with statistical weights built from the information researchers used to subdivide the individual strata.
Take, for example, the hapless researchers who forgot to tailor their jellyfish-sting survey to the specific parts of the U.S. most likely to contain jellyfish (outside of an aquarium setting). A potential option these researchers could use to stratify their nationwide population is by state, and then weight their aggregated statewide proportion of stings by how many miles of coastline each state has. Doing so would upweight individuals’ answers to the question “Have you ever been stung by a jellyfish?” if they live in states near which jellyfish are also more likely to reside.
This example assumes, of course, that individuals who have been stung by a jellyfish and reside in Nebraska, say, got stung in Nebraska. Such an outcome is unlikely, however, unless the person in question were wildly unlucky or given to swimming through aquarium tanks. Clearly, stratification is not immune to bad assumptions!
Stratified Sampling: An Example
Another illustration of stratification that potentially speaks to a wider swath of the U.S. population concerns another type of pest, this one closer to home. The U.S. Census Bureau reports that nearly 15 million American households reported seeing rodents in their dwellings last year. Rats and mice, while arguably more adorable than stinging sea jellies, are Americans’ most familiar uninvited house guests. But how many rodents are there in a given house? It’s tough to say, given that rodents typically avoid making their presence known to homeowners. Stratified sampling can help solve this dilemma.
As of 2020, the median size of U.S. houses is 2,261 square feet . Assume a just-above-median, 2,400-square-foot house has five rooms, each with a different square-foot area. Rats can be in any of these rooms. The homeowners want to know how many total rats there are in the house. So, they purchase traps to set in each of the five rooms to determine the likely total size of their rat infestation. Since larger rooms provide both more room for rats to live in but also more area for rats to evade traps, the homeowners decide to stratify the total area of their house by room size.
The table below contains room size information, along with the results of the “survey” of traps (it should be pointed out that these owners are sampling rodents without replacing them, due to the lethality of trapping).
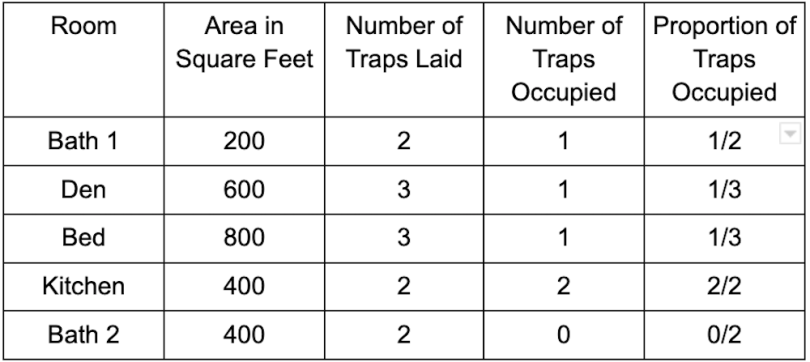
The homeowners, in their haste to estimate the scale of their rodent infestation, quickly take an average of the five proportions: (1/5)(1/2) + (1/5)(1/3) + (1/5)(1/3) + (1/5)(2/2) + (1/5)(0/2) = 13/30 or about 43 percent of traps occupied. Multiplying this proportion by the total traps set gives an estimated total size of the rat population across all rooms: (.43)(12) = 5.2 rats.
“But wait,” says one of the homeowners, “didn’t we say that bigger rooms are less likely to have occupied traps? Shouldn’t we account for this by weighting our estimate by room size?” The other homeowners agree. They re-compute their estimate, this time weighting by the proportions of room sizes to total house size: (200/2,400)(1/2) + (600/2,400)(1/3) + (800/2,400)(1/3) + (400/2,400)(2/2) + (400/2,400)(0/2) = 40 percent of traps occupied. This results in a rat population estimate of (.40)(12) = 4.8 rats.
These estimated totals seem close, but are not quite as proximate as they initially appear. A half-rat discrepancy might not make a lot of difference for a 2,400 square-foot home, but imagine estimating total rats across several warehouses or a fleet of cruise ships or a large hospital system. In these cases, the estimated total could be dozens or hundreds of rats off simply because researchers failed to account for relevant information at subpopulation levels.
Understand Our Data-Driven World What Is Data Warehousing? Understand the Importance of Data Structures and Architecture.
When Should You Use Stratified Sampling?
So, when should researchers employ stratification in their sampling process? In the second edition of her textbook, Sampling: Design and Analysis , the statistician Sharon Lohr lists four primary reasons for stratification.
4 Reasons for Stratified Sampling
- To prevent taking a bad sample.
- Knowledge of subgroups improves estimate precision.
- Stratification makes research easier and cheaper.
- Stratification reduces noise in a sample.
First, researchers should stratify when they want to shield themselves from taking a horrible sample. As Lohr puts it, when taking a simple random sample (i.e. taking a sample of everyone in a population without accounting for subgroups) of size 100 from a population of 2,000 males and females divided evenly, it is possible that the simple random sample includes no or few members of one sex. Knowledge of the proportionate breakdown between sexes in this population results in researchers randomly sampling 50 males and 50 females. Stratification by sex precludes a non-representative sample that is made up of no or few members of one of the sexes (the keen eye will note that the homeowners set their traps this way — larger rooms got more traps than smaller rooms).
Knowledge about subgroups can improve estimate precision, which is the second reason Lohr gives in favor of stratification. In their 1992 work on gender and engineering careers, Judith Samsom McIlwee and J. Gregg Robinson sought to measure workplace outcomes for male and female mechanical and electrical engineering graduates. At the time the authors sampled engineering graduates, however, there were many more men than women in these fields. McIlwee and Robinson used this information to oversample (sampling the under-represented group more than the over-represented group) women to ensure that the precisions of estimated outcomes were the same across genders. Without stratifying and oversampling, estimated outcomes for female engineers would have been more uncertain than estimates for their male counterparts.
A third reason to employ stratification techniques is that they might make surveying easier or cheaper. Researchers can tailor survey types by subgroups to drive down administrative costs. For instance, internet-based surveys are more affordable for large corporations than traditional phone screening or site visits. Researchers could then focus more effort on contacting smaller businesses via telephone or visitation to collect information. Both of these techniques are more expensive than internet-based surveying but can better capture under-represented strata. By targeting specific under-represented subgroups, stratification can result in higher precision for the same cost as larger, simple random surveys or the same precision at lower costs.
This leads directly to the last reason researchers might consider stratification: It frequently yields less noisy estimates of population means and totals. It’s almost always the case that members of the same subgroup of a population are more similar than a random sample of members of all subgroups. Accounting for these within-stratum similarities and then pooling information across strata typically yields estimates with lower standard errors than randomly surveying an identical number of individuals population-wide.
Knowledge of how to construct strata can inject more information about individual responses into aggregated measures than simple random surveying. Thus, correctly identifying appropriate strata is a low-cost method by which to improve statistical estimates of populations. Assuming researchers choose strata that contain even small amounts of relevant information pertaining to all members of population subgroups, stratification proves a crucial tool to sharpen empirical estimation and, thereby, produce more accurate inferences; it’s a lot more honey for a little more sting.
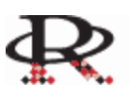
Resolution Economics
Built In’s expert contributor network publishes thoughtful, solutions-oriented stories written by innovative tech professionals. It is the tech industry’s definitive destination for sharing compelling, first-person accounts of problem-solving on the road to innovation.
Great Companies Need Great People. That's Where We Come In.
- Research article
- Open access
- Published: 10 November 2020
Area based stratified random sampling using geospatial technology in a community-based survey
- Carrie R. Howell ORCID: orcid.org/0000-0002-6554-6237 1 ,
- Ariann F. Nassel 2 ,
- April A. Agne 1 &
- Andrea L. Cherrington 1
BMC Public Health volume 20 , Article number: 1678 ( 2020 ) Cite this article
26k Accesses
18 Citations
Metrics details
Most studies among Hispanics have focused on individual risk factors of obesity, with less attention on interpersonal, community and environmental determinants. Conducting community based surveys to study these determinants must ensure representativeness of disparate populations. We describe the use of a novel Geographic Information System (GIS)-based population based sampling to minimize selection bias in a rural community based study.
We conducted a community based survey to collect and examine social determinants of health and their association with obesity prevalence among a sample of Hispanics and non-Hispanic whites living in a rural community in the Southeastern United States. To ensure a balanced sample of both ethnic groups, we designed an area stratified random sampling procedure involving three stages: (1) division of the sampling area into non-overlapping strata based on Hispanic household proportion using GIS software; (2) random selection of the designated number of Census blocks from each stratum; and (3) random selection of the designated number of housing units (i.e., survey participants) from each Census block.
The proposed sample included 109 Hispanic and 107 non-Hispanic participants to be recruited from 44 Census blocks. The final sample included 106 Hispanic and 111 non-Hispanic participants. The proportion of Hispanic surveys completed per strata matched our proposed distribution: 7% for strata 1, 30% for strata 2, 58% for strata 3 and 83% for strata 4.
Utilizing a standardized area based randomized sampling approach allowed us to successfully recruit an ethnically balanced sample while conducting door to door surveys in a rural, community based study. The integration of area based randomized sampling using tools such as GIS in future community-based research should be considered, particularly when trying to reach disparate populations.
Peer Review reports
Obesity is a leading risk factor for the development of diabetes, cardiovascular illness, cancer and other chronic conditions that cause significant morbidity and mortality as well as increased health care costs [ 1 ]. Hispanics are the largest and fastest growing racial/ethnic minority group in the United States, comprising 17.3% of the population in 2014 [ 2 ], with disproportionately high obesity rates. Among adults living in the United States in 2015, the prevalence of obesity was 47% among Hispanics compared to 38% among non-Hispanic whites [ 3 ], highlighting the need to examine factors that contribute to this increased risk. To date, most studies among Hispanics have focused on individual risk factors of obesity, with less attention on interpersonal, community and environmental determinants. In order to conduct community level surveys to collect this type of data, it is crucial to ensure representativeness of both Hispanic and non-Hispanic populations in the study sample. Here we describe the use of a novel GIS-based population based sampling approach to minimize selection bias in a community based study.
Sampling for cross-sectional survey studies can be probability based or non-probability based. Probability based (e.g. random sampling) requires a defined population, where each possible unit has a known possibility of being selected [ 4 ]. Non-probability sampling methods (e.g. convenience sampling) have no known inclusion probabilities [ 5 ], producing bias and unbalanced sample representation [ 6 , 7 , 8 , 9 , 10 , 11 , 12 , 13 , 14 ]. Simple random sampling can also pose a problem for studies conducting research in minority populations. This method targets the whole population of interest and often results in minority under-representation. Stratified random sampling increases sample representativeness by dividing the study population into strata based on characteristics that are of interest to the researcher [ 15 ]. Random samples are then drawn from each strata to ensure adequate sampling of all groups. This approach reduces sampling bias; allows researchers to estimate within and between strata outcomes; and improves accuracy of results [ 15 , 16 ].
Sampling design is important in large population studies with several national surveys utilizing stratified approaches to minimize bias. The US Census Bureau conducts the American Community Survey (ACS) to produce annually updated census data estimates based on geographic units (e.g. census tract and block group). The complex sampling design consists of first stratifying the US population by census block, then calculating population based sampling rates. Appropriate weights are applied in the analytical phase so that estimates represent the full population [ 17 ]. Similarly, the National Health and Nutrition Examination Survey (NHANES) employs a stratified, multistage cluster design that oversamples specific subgroups to increase precision in health outcome estimates [ 18 ]. Smaller scale community based population studies should draw upon and incorporate aspects of these rigorous sampling designs to reduce sampling error and increase precision in estimates.
In recent years, technologies such as Geographic Information System (GIS) have been used to facilitate the sampling process in community-based research. Typically, GIS software have been used for data analysis and visualization [ 19 ]; however, health researchers have begun to realize its potential in facilitating the sampling and recruitment process, particularly in rural, developing countries [ 20 , 21 , 22 ]. To aid in sampling, GIS has been used to define populations in areas without formal census data [ 21 , 22 ]; create clusters [ 22 ]; and stratify populations [ 20 ]. Area stratified random sampling methods use area units as the strata, such as census blocks, and produce samples comparable to random digit dialing recruitment approaches [ 20 , 23 , 24 ]. This method provides an innovative way to conduct community-based health survey research, particularly when the study area is small in population. Blending aspects of complex sampling design, such as those used in national surveys, with GIS methods has the potential to strengthen community based research. Here, we describe how geospatial data and Geographic Information Systems (GIS) were used to develop an area stratified random sampling protocol that ensured demographic balance in conducting a community-based, interviewer administered survey. The study’s main aim was to examine social determinants of health and their association with obesity prevalence among a sample of Hispanics and non-Hispanic whites living in a rural community in the Southeastern United States.
Participants and setting
The population of interest resided in Albertville, Alabama where researchers had previously conducted a cervical screening study aimed at Hispanic women [ 25 ]. Located in Marshall County in the northeastern side of the state, Albertville has a population of 21,160 with 64.7% non-Hispanic white and 30.2% Hispanic as of the 2010 Census [ 26 ]. The city has two zip codes and is 26 square miles with a population density of 817 per square mile. The nearest metropolitan city with a population of over 150,000 is located 38 miles away. The median yearly income of Albertville is lower than Alabama as a whole ($35,878 vs. 40,489). The Hispanic population is concentrated to approximately 17% of the households in the city (Table 1 ).
Data was collected from participants interviewed by trained research interviewers in door-to-door canvas between June and December 2013. To be included, participants had to be at least 19 years of age, not pregnant, speak English or Spanish fluently, and self-identify as non-Hispanic white or as Hispanic/Latino. Participants were compensated with a gift card for their time. All study procedures were reviewed and approved by the University of Alabama at Birmingham’s Institutional Review Board.
Area stratified random sampling for recruitment
The goal to recruit an equal number of Hispanic and non-Hispanic participants would have been difficult to achieve by employing a completely random sampling procedure across the entire city. Therefore, a stratified random sampling procedure was created based on the Center for Disease Control and Prevention’s (CDC) Community Assessment for Public Health Emergency Responses (CASPER) sampling methodology [ 27 ]. The CASPER approach was developed using cross-sectional epidemiological principles and is a form of a community needs assessment that provides a systematic approach to collecting household information on community public health status. The cluster sampling design involves two stages: selecting clusters based on household proportions and then interviewing a set random number of households in each cluster. The CDC recommends using GIS software in the selection of the sampling frame to allow users to select portions (clusters) of geographically defined areas, such as counties or cities. In addition, GIS software provides the ability to easily develop maps for community interviewers based on the selected clusters. For this reason, CASPER provides a toolbox for use in ArcGIS software to facilitate this methodology. Using this approach in our study involved three stages: (1) division of the sampling area into non-overlapping strata based on Hispanic household proportion; (2) random selection of the designated number of Census blocks from each stratum; and (3) random selection of the designated number of housing units (i.e., survey participants) from each Census block.
Stage 1: Divide the sampling area into non-overlapping strata based on Hispanic household proportion
To ensure that the interviewers would be able to reach sufficient Hispanic households, all Census blocks within Albertville were divided into four strata based on percentage of Hispanic households using GIS software. Since Albertville city boundaries and Census block boundaries do not perfectly align with each other, a centroid criterion was used to determine whether or not a Census block belonged to Albertville city. As a result, 647 Census blocks were assigned to Albertville city. Of those, only 455 blocks contained households and the other 192 blocks were non-residential. Since the Hispanic population was concentrated in a relatively small geographic area, the 455 blocks were further divided into four unbalanced strata identified by Hispanic household proportion: < 10% Hispanic households, 10–30% Hispanic, 30–50% Hispanic, and ≥ 50% Hispanic. Roughly 60% of the blocks were assigned to the ≤10% of Hispanic households stratum, with 7% ( N = 32) of the blocks assigned to the > 50% of Hispanic households stratum (see Table 2 and Fig. 1 ).
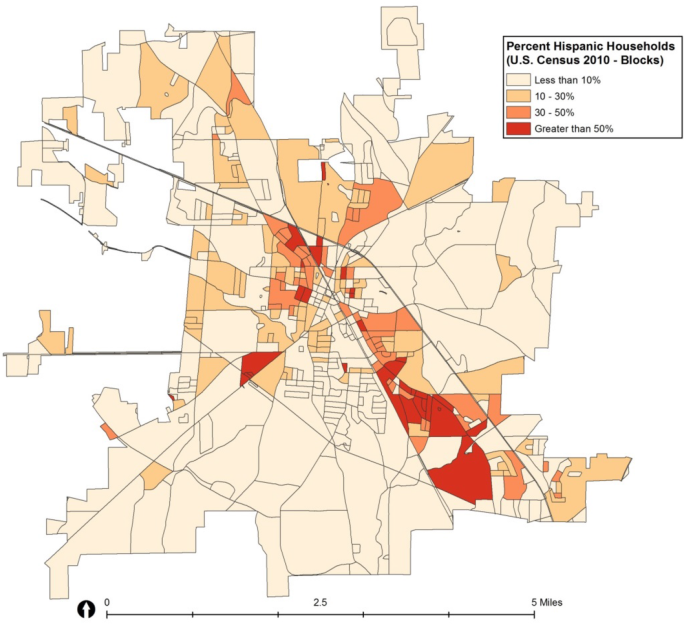
Census blocks in Albertville, AL by Hispanic household proportion. Map of census block groups in Albertville, AL. Darker shading indicates higher Hispanic household proportions. Map developed using licensed ArcGIS software
Stage 2: Randomly select the designated number of Census blocks from each stratum
Our goal was to recruit a total of 200 participants, with a distribution of 50% Hispanic and 50% non-Hispanic white (1:1 ratio). Maps denoted that the Hispanic population was largely concentrated in small area blocks (Fig. 1 ). Although smaller blocks suggest higher population density, they also contain fewer individuals and households compared with larger blocks. Since Hispanics comprised a smaller proportion of total households (17%), we needed to oversample blocks with higher concentrations of Hispanic households in order to reach an equal number of Hispanic and Non-Hispanic surveys. For these reasons we took the following approach to determine the number of Census blocks to select from each group, and the number of housing units to select from each Census block.
Considering the varying population size across blocks, it was determined to be more feasible to plan fewer surveys per block in more Hispanic population concentrated areas (i.e., strata 3 & 4 in Table 2 ), and more surveys per block in more non-Hispanic population concentrated areas (i.e., strata 1 & 2 in Table 2 ). As a result, we selected 10 blocks with 6 surveys per block from strata 1 and 2 and 12 blocks with 4 surveys per block from strata 3 and 4. These numbers were somewhat arbitrary, balancing the concern that selecting too many blocks which would increase cost, while taking care to not plan for an unrealistic quota of surveys per block when not feasible (e.g. the smallest block in the study area contained only 8 households).
For strata 1 and 2, distribution of Hispanic versus non-Hispanic surveys within each block roughly reflected the proportions of Hispanic and non-Hispanic households in the corresponding group. Since oversampling of the Hispanic population was needed to achieve the recruitment goal, proportions of Hispanic surveys in strata 3 and 4 were set higher than the actual proportions of Hispanic households. Table 2 shows the proposed number of blocks to select from each group and numbers of Hispanic versus non-Hispanic surveys projected within each block. In total, we proposed 109 Hispanic surveys and 107 non-Hispanic surveys from 44 blocks.
Once the number of blocks from each group were determined, the CASPER toolkit developed by the CDC was utilized to generate random samples [ 27 ]. We used an add-on program developed for ArcGIS by the CDC to generate random samples using a polygon layer that represents the sampling area and non-overlapping clusters within the sampling area. In our study, the four strata were our sampling areas with Census blocks the non-overlapping clusters, accounting for the number of housing units within each cluster. The random sampling procedure was repeated four times, once for each stratum. Figure 2 shows the 44 random blocks selected from the entire study area using this approach.
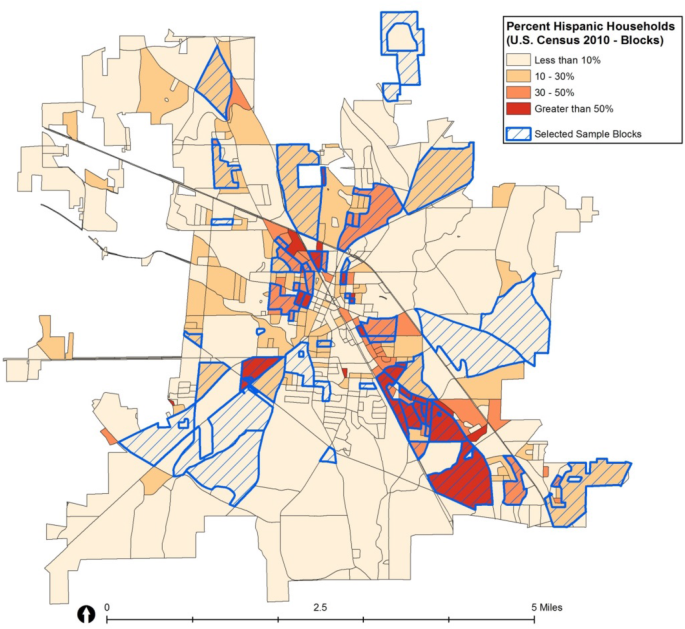
Census blocks selected for recruitment. Map of the 44 census block groups randomly selected in Albertville, AL using an area stratified random sampling approach. Blue outline indicates block group selected. Map developed using licensed ArcGIS software
Stage 3: Randomly select the designated number of housing units from each Census block
Interviewers were provided with satellite maps (Fig. 3 ) for each block randomized with detailed instructions regarding how to randomly select the designated number of housing units within each block. The systematic random sampling method described in the CASPER toolkit [ 27 ] was adapted and modified to develop the study’s survey protocol:
A starting point (address) for each sampling block was provided. This was the first house for the interviewers to survey.
After completing the first survey, interviewers would walk or drive in either direction to the next N th house. This would be the next household for the interviewers to survey.
If no one answers the door, continue to the next N th house.
Continue traveling through the sampling block, selecting every N th house until they have completed the designated number of surveys for that sampling block.
If the interviewers circled back to the starting point and had not completed the designated number of surveys, they would then proceed through the block again and select every (N + 1) th house. For example, if Block A had an N of 8, in the next pass the interviewer would approach every 9th house.
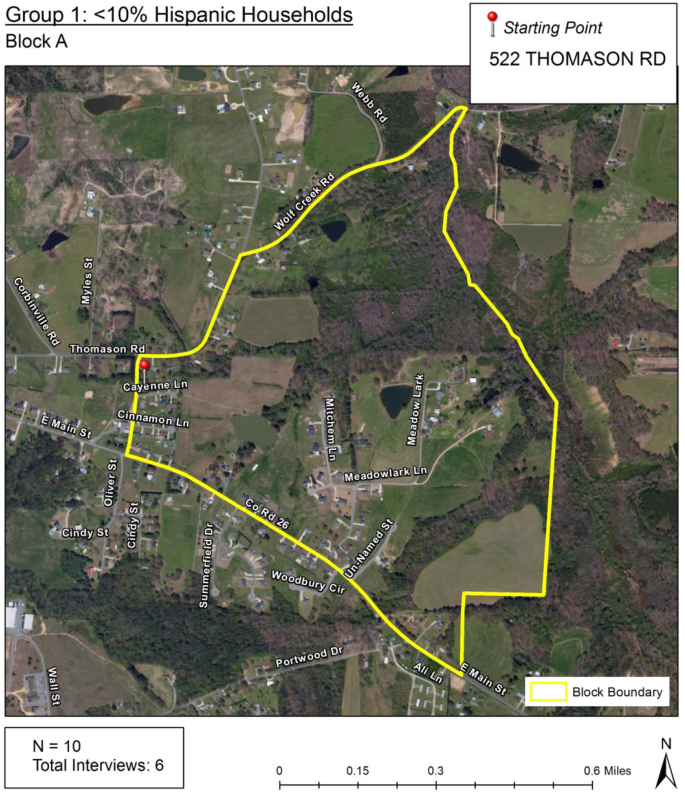
Field interviewer block map. An example of the satellite image map provided to interviewers to conduct field surveys. Map data image provided by© 2013 Google; Imagery© 2013 MaxarTechnologies
The N used in the protocol was determined by dividing the total number of housing units by the designated number of surveys to complete in each block, and thus could vary from block to block. For example, if a block contained 50 housing units and the designated number of surveys was 6 for that block, the N would be 8. Values of N for each individual block were provided in the instructions to the interviewers. Additional instructions with regards to abandoned homes, businesses, duplexes and apartment complexes, multiple family homes, and trailer parks were also provided.
The proposed sample included 109 Hispanic and 107 non-Hispanic participants to be recruited from 44 Census blocks. After exhausting all 44 blocks, interviewers were unable to meet recruitment goals for the proposed number of surveys in each block. Twenty additional blocks were selected using the same random sampling procedure described above, including two from strata 1 (≤10% of Hispanic households), two from strata 2 (10–30% of Hispanic households), six from strata 3 (30–50% of Hispanic households), and ten from strata 4 (> 50% of Hispanic households). More blocks with higher Hispanic population density were selected because field interviewers found that recruitment of Hispanic participants was particularly challenging. The final sample included 106 Hispanic and 111 non-Hispanic participants. The number of surveys completed from each block ranged from 0 to 11, with an average of 3.4 surveys per block (Table 3 and Fig. 4 ).
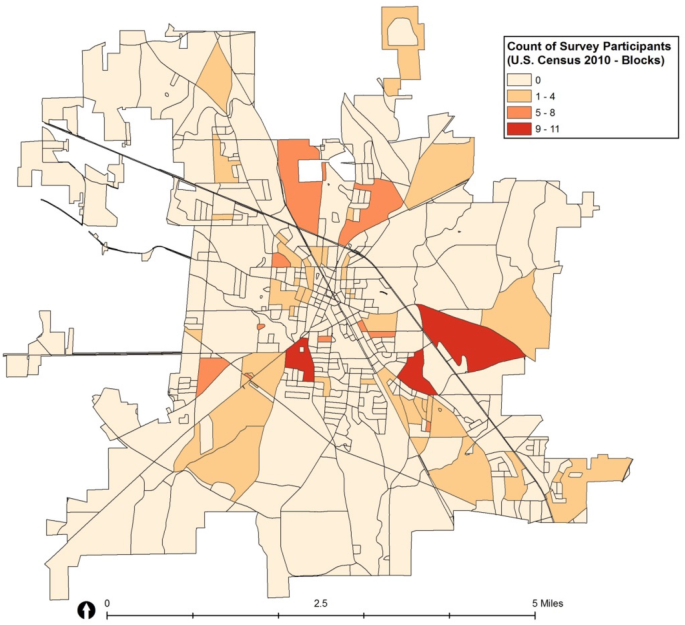
Number of participants by Census block. Map of census block groups in Albertville, AL with the number of participants who completed a survey. Darker shading indicates more participants. Map developed using licensed ArcGIS software
Post-hoc chi-square and Fishers exact tests were used to test the proposed distribution of surveys by ethnicity status to the proportions of surveys completed. P -values > 0.05 indicate that actual proportions did not differ from proposed population based proportions. The proportion of Hispanic surveys completed per strata were similar to our proposed distribution for strata 1–3: 7% for strata 1 ( p = 1.0), 30% for strata 2 ( p = 0.71), and 58% for strata 3 ( p = 0.07). Although Strata 4 (83% Hispanic surveys, p = 0.002) had statistically different proportions, this was expected due to the need to oversample Hispanic surveys from this strata.
Here we demonstrate the successful use of a novel area stratified random sampling technique utilizing GIS that ensured ethnic balance in the recruitment of our community canvased study sample. Field recruitment in community studies presents challenges in minimizing selection bias and ensuring demographic representation. Here, integrating GIS based technology with census data provided a standardized and objective approach to recruitment to address these issues. Specifically, we utilized GIS to create and visualize non-overlapping strata to determine individual stratums and to randomly select Census blocks within those strata. Our approach ensured the 1:1 ratio of Hispanics to non-Hispanics in our study, minimized selection bias, and provided an approach that was easy for the ‘boots on the ground’ interviewers to implement. Moreover, the distribution of completed Hispanic surveys by stratum closely matched our original proposed proportions (defined based on percentage of Hispanic households in block), giving our sample geographic representation by Albertville block.
Utilizing GIS to facilitate community-based research, such as targeting areas for program planning or ensuring random sampling of survey respondents [ 28 ], has been implemented in recent population based studies. This method has been particularly useful in rural, developing countries [ 20 , 21 , 22 , 29 ]. Defar et al. used GIS methods to conduct a cross-sectional survey in Ethiopia on maternal and child health care utilization in a similar two-stage process as the current study [ 29 ] while Wampler et al. used GIS to facilitate the random selection of households in specific areas in Haiti for water quality research [ 22 ]. Akin to the results here, a study that compared simple random sampling to stratified sampling by zip code and census tract found that area based stratified sampling ensured a higher representativeness of Hispanic residents in audits of tobacco retailers in an urban area [ 30 ]. In the public health realm, Lafontaine et al. developed a spatial random sampling method to conduct neighborhood built environment audits and concluded that this approach was more cost and time effective [ 31 ]. Likewise, using the approach herein resulted in recruiting our Hispanic sample in a more efficient manner.
It is important to note that we selected the number of blocks for randomization and recruitment based on feasibility but nonetheless in an arbitrary fashion. While this resulted in a balanced sample for our study, this will likely not translate into other scenarios. Since stratification by design results in subgroups that are over or under represented compared to the overall population [ 15 ], taking the actual population weights of each census tract into account when selecting blocks would have been more appropriate. Since the ultimate goal in sampling is to select a study sample that is representative of the population, applying population sampling weights and using model-based approaches such as raking prior to analysis are essential. Raking adjusts the sampling weights by forcing the survey totals to match proportions in the known population [ 32 ].
Our approach was not without challenges or limitations. When conducting the door to door surveys, interviewers were provided with detailed protocol and satellite maps. However, multiple issues arose. First, there was a significant number of houses that provided “no answer” and we had to implement the N + 1 sampling multiple times to reach recruitment targets. Time constraints also impacted interviewers. Some blocks sampled had a count number that was large ( N > 14), which decreased sampling efficiency as driving from one house to the next could exceed 10 min. Another limitation of the study is that we used the population and household counts from the 2010 Decennial Census data, which may have underestimated the number of Hispanics in Albertville at the time of data collection (2013). Further, the criterion used to divide the study area was Census block group and 2010 Census estimates were likely different than the true distribution of Hispanic households by block in 2013. Lastly, it is important to note that CASPER was designed for use in the United States and associated territories and uses data collected from the census bureau to create population based sampling areas and clusters. However, since CASPER was developed based on an epidemiological two-stage cluster sampling approach, it is possible to conduct this type of sampling in other countries where census type data are available using the CASPER protocol as a guide.
Overall, we developed a standardized area based randomized sampling protocol that allowed us to successful recruit an ethnically balanced sample while conducting door to door community surveys. Minimizing selection bias in community-based surveys can be difficult; however, advancement in technological tools such as GIS provides novel approaches to address these biases. Based on our results here, we advocate the integration of area based randomized sampling in future community-based research, particularly when trying to reach disparate populations.
Availability of data and materials
Data sharing is not applicable to this article as no datasets were generated or analyzed during the current study.
Abbreviations
- Geographic information systems
Community Assessment for Public Health Emergency Response
Centers for Disease Control and Prevention
American Community Survey
National Health and Nutrition Examination Study
World Health Organization. Obesity and overweight fact sheet no. 311. 2013. Available from: http://www.who.int/mediacentre/factsheets/fs311/en/index.html .
Stepler R, Brown A. 2014, Hispanics in the United States statistical portrait. 2016. Available from: https://www.pewresearch.org/hispanic/2016/04/19/2014-statistical-information-on-hispanics-in-united-states/ .
Google Scholar
Hales CM, Carroll MD, Fryar CD, Ogden CL. Prevalence of obesity among adults and youth: United States, 2015-2016. NCHS Data Brief. 2017;(288):1–8.
Tashakkori A, Teddlie C. Handbook on mixed methods in the behavioral and social sciences. Thousand Oaks: Sage; 2003.
Doherty M. Probability versus non-probability sampling in sample surveys. N Z Stat Rev. 1994;4:21–8.
Fanzana B, Srunv E. A venue-based method for sampling hard-to-reach populations. Public Health Rep. 2001:216–22.
Klein JD, Thomas RK, Sutter EJ. Self-reported smoking in online surveys: prevalence estimate validity and item format effects. Med Care. 2007;45(7):691–5.
Article Google Scholar
Roster CA, Rogers RD, Albaum G, Klein D. A comparison of response characteristics from web and telephone surveys. Int J Mark Res. 2004;46(3):359–73.
Schillewaert N, Meulemeester P. Comparing response distributions of offline and online. Int J Mark Res. 2005;47(2):163–78.
Schonlau M, Zapert K, Simon LP, Sanstad KH, Marcus SM, Adams J, et al. A comparison between responses from a propensity-weighted web survey and an identical RDD survey. Soc Sci Comput Rev. 2004;22(1):128–38.
Spijkerman R, Knibbe R, Knoops K, Van De Mheen D, Van Den Eijnden R. The utility of online panel surveys versus computer assisted interviews in obtaining substance use prevalence estimates in the Netherlands. Addiction. 2009;104(10):1641–5.
Bethell C, Fiorillo J, Lansky D, Hendryx M, Knickman J. Online consumer surveys as a methodology for assessing the quality of the United States health care system. J Med Internet Res. 2004;6(1):e2.
Chang L, Krosnick JA. National surveys via RDD telephone interviewing versus the internet: comparing sample representativeness and response quality. Public Opin Q. 2009;73(4):641–78.
Malhotra N, Krosnick JA. The effect of survey mode and sampling on inferences about political attitudes and behavior: comparing the 2000 and 2004 ANES to internet surveys with nonprobability samples. Polit Anal. 2007;15(3):286–323.
Teddlie C, Yu F. Mixed methods sampling: a typology with examples. J Mixed Methods Res. 2007;1(1):77–100.
Elfil M, Negida A. Sampling methods in clinical research; an educational review. Emerg (Tehran). 2017;5(1):e52.
American Community Survey Design and Methodology. Chapter 4: Sample design and selection. 2014. https://www2.census.gov/programs-surveys/acs/methodology/design_and_methodology/acs_design_methodology_ch04_2014.pdf?# . Accessed 20 July 2020.
National Health and Nutrition Examination Survey, 2015−2018: Sample Design and Estimation Procedures. https://www.cdc.gov/nchs/data/series/sr_02/sr02-184-508.pdf . Accessed 20 July 2020.
Cromley EK, McLafferty SL. GIS and public health: Guilford Press; 2011.
Kondo MC, Bream KD, Barg FK, Branas CC. A random spatial sampling method in a rural developing nation. BMC Public Health. 2014;14:338.
Lin Y, Kuwayama DP. Using satellite imagery and GPS technology to create random sampling frames in high risk environments. Int J Surg. 2016;32:123–8.
Wampler PJ, Rediske RR, Molla AR. Using ArcMap, Google Earth, and Global Positioning Systems to select and locate random households in rural Haiti. Int J Health Geogr. 2013;12:3.
Aquilino WS, Wright DL. Substance use estimates from RDD and area probability samples: impact of differential screening methods and unit nonresponse. Public Opin Q. 1996;60(4):563–73.
Lete C, Holly EA, Roseman DS, Thomas DB. Comparison of control subjects recruited by random digit dialing and area survey. Am J Epidemiol. 1994;140(7):643–8.
Scarinci IC, Garces-Palacio IC, Morales-Aleman MM, McGuire A. Sowing the seeds of health: training of community health advisors to promote breast and cervical cancer screening among Latina immigrants in Alabama. J Health Care Poor Underserved. 2016;27(4):1779–93.
US Census Bureau. QuickFacts: Albertville, AL. https://www.census.gov/quickfacts/fact/table/albertvillecityalabama/PST045218 .
Centers for Disease Control and Prevention. Community Assessment for Public Health Emergency Response (CASPER), sampling methodology. https://www.cdc.gov/nceh/casper/sampling-methodology.htm .
Quon Huber MS, Van Egeren LA, Pierce SJ, Foster-Fishman PG. GIS applications for community-based research and action: mapping change in a community-building initiative. J Prev Interv Community. 2009;37(1):5–20.
Defar A, Okwaraji YB, Tigabu Z, Persson LA, Alemu K. Geographic differences in maternal and child health care utilization in four Ethiopian regions; a cross-sectional study. Int J Equity Health. 2019;18(1):173.
Lee JGL, Shook-Sa BE, Bowling JM, Ribisl KM. Comparison of sampling strategies for tobacco retailer inspections to maximize coverage in vulnerable areas and minimize cost. Nicotine Tob Res. 2018;20(11):1353–8.
Lafontaine SJ, Sawada M, Kristjansson E. A direct observation method for auditing large urban centers using stratified sampling, mobile GIS technology and virtual environments. Int J Health Geogr. 2017;16(1):6.
Battaglia MP, Izrael D, Hoaglin DC, Frankel MR. Practical considerations in raking survey data. Surv Pract. 2009;2(5):1–10.
Download references
Acknowledgements
We especially thank Matthew Carle, Morgan Griesemer Lepard, Ynhi Thai, Meghan Meehan, Amancia Carrera, Sylvia Alavarez Mancinas, Susan Henry Barber, and Chris Caudill for their tireless efforts to canvas neighborhoods and interviews participants. We would also like to thank all our participants, the office of the Mayor of Albertville, the Albertville Police Department, support staff, and others who helped make this study possible.
This work was supported by grants from the University of Alabama with funding from the National Institute of Minority Health and Health Disparities (U54MD008176) and support from the National Institutes of Health, National Institute of Diabetes and Digestive and Kidney Diseases, UAB Diabetes Research Center [1P60DK079626–01]. The content is solely the responsibility of the authors and does not necessarily represent the official views of the National Institute of Minority Health and Health or National Institute of Diabetes and Digestive and Kidney Diseases or the National Institutes of Health or others supporting this work. All sources of funding had no role in study design; collection, analysis, and interpretation of data; writing the report; or the decision to submit the report for publication.
Author information
Authors and affiliations.
Department of Medicine, Division of Preventive Medicine, University of Alabama at Birmingham, Medical Towers 62, 1717 11th Avenue South, Birmingham, AL, 35205, USA
Carrie R. Howell, April A. Agne & Andrea L. Cherrington
School of Public Health, University of Alabama at Birmingham, 1665 University Blvd, Birmingham, AL, 35233, USA
Wei Su & Ariann F. Nassel
You can also search for this author in PubMed Google Scholar
Contributions
WS, AC made substantial contributions to the design of the work. CH, WS, AC made substantial contributions to the analysis, interpretation of data and drafted the work. AN and AA made substantial contributions to the acquisition and interpretation of data. All authors read and approved the final manuscript and are accountable for the accuracy and integrity of the work presented.
Corresponding author
Correspondence to Carrie R. Howell .
Ethics declarations
Ethics approval and consent to participate.
This study was approved by the University of Alabama at Birmingham Institutional Review board and documented written informed consent was obtained from all participants prior to participation.
Consent for publication
Not applicable.
Competing interests
The authors declare they have no competing interests or financial relationships relevant to this article to disclose.
Additional information
Publisher’s note.
Springer Nature remains neutral with regard to jurisdictional claims in published maps and institutional affiliations.
Rights and permissions
Open Access This article is licensed under a Creative Commons Attribution 4.0 International License, which permits use, sharing, adaptation, distribution and reproduction in any medium or format, as long as you give appropriate credit to the original author(s) and the source, provide a link to the Creative Commons licence, and indicate if changes were made. The images or other third party material in this article are included in the article's Creative Commons licence, unless indicated otherwise in a credit line to the material. If material is not included in the article's Creative Commons licence and your intended use is not permitted by statutory regulation or exceeds the permitted use, you will need to obtain permission directly from the copyright holder. To view a copy of this licence, visit http://creativecommons.org/licenses/by/4.0/ . The Creative Commons Public Domain Dedication waiver ( http://creativecommons.org/publicdomain/zero/1.0/ ) applies to the data made available in this article, unless otherwise stated in a credit line to the data.
Reprints and permissions
About this article
Cite this article.
Howell, C.R., Su, W., Nassel, A.F. et al. Area based stratified random sampling using geospatial technology in a community-based survey. BMC Public Health 20 , 1678 (2020). https://doi.org/10.1186/s12889-020-09793-0
Download citation
Received : 22 May 2020
Accepted : 29 October 2020
Published : 10 November 2020
DOI : https://doi.org/10.1186/s12889-020-09793-0
Share this article
Anyone you share the following link with will be able to read this content:
Sorry, a shareable link is not currently available for this article.
Provided by the Springer Nature SharedIt content-sharing initiative
- Stratified random sampling
- Hispanic population
- Rural population
- Community based methods
BMC Public Health
ISSN: 1471-2458
- Submission enquiries: [email protected]
- General enquiries: [email protected]
Click through the PLOS taxonomy to find articles in your field.
For more information about PLOS Subject Areas, click here .
Loading metrics
Open Access
Peer-reviewed
Research Article
Stratified Sampling of Neighborhood Sections for Population Estimation: A Case Study of Bo City, Sierra Leone
Independent consultant, Fairfax, Virginia, United States of America
Affiliation Information Technology Division, Naval Research Laboratory, Washington, District of Columbia, United States of America
Affiliation Department of Global and Community Health, George Mason University, Fairfax, Virginia, United States of America
Affiliations Njala University, Bo, Sierra Leone, Mercy Hospital Research Laboratory, Bo, Sierra Leone
Affiliation Mercy Hospital Research Laboratory, Bo, Sierra Leone
* E-mail: [email protected]
Affiliation Center for Bio/Molecular Science and Engineering, Naval Research Laboratory, Washington, District of Columbia, United States of America
- Roger Hillson,
- Joel D. Alejandre,
- Kathryn H. Jacobsen,
- Rashid Ansumana,
- Alfred S. Bockarie,
- Umaru Bangura,
- Joseph M. Lamin,
- David A. Stenger
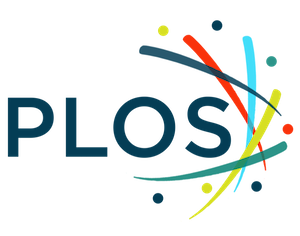
- Published: July 15, 2015
- https://doi.org/10.1371/journal.pone.0132850
- Reader Comments
There is a need for better estimators of population size in places that have undergone rapid growth and where collection of census data is difficult. We explored simulated estimates of urban population based on survey data from Bo, Sierra Leone, using two approaches: (1) stratified sampling from across 20 neighborhoods and (2) stratified single-stage cluster sampling of only four randomly-sampled neighborhoods. The stratification variables evaluated were (a) occupants per individual residence, (b) occupants per neighborhood, and (c) residential structures per neighborhood. For method (1), stratification variable (a) yielded the most accurate re-estimate of the current total population. Stratification variable (c), which can be estimated from aerial photography and zoning type verification, and variable (b), which could be ascertained by surveying a limited number of households, increased the accuracy of method (2). Small household-level surveys with appropriate sampling methods can yield reasonably accurate estimations of urban populations.
Citation: Hillson R, Alejandre JD, Jacobsen KH, Ansumana R, Bockarie AS, Bangura U, et al. (2015) Stratified Sampling of Neighborhood Sections for Population Estimation: A Case Study of Bo City, Sierra Leone. PLoS ONE 10(7): e0132850. https://doi.org/10.1371/journal.pone.0132850
Editor: Maciej F. Boni, University of Oxford, VIET NAM
Received: February 2, 2015; Accepted: June 19, 2015; Published: July 15, 2015
This is an open access article, free of all copyright, and may be freely reproduced, distributed, transmitted, modified, built upon, or otherwise used by anyone for any lawful purpose. The work is made available under the Creative Commons CC0 public domain dedication
Data Availability: All relevant tabular data are within the paper and its Supporting Information files. GIS data are available on OpenStreetMap ( http://osm.org/go/am_ZKeeU- ).
Funding: This work was funded by the Defense Threat Reduction Agency, Joint Science and Technology Office ( http://www.dvidshub.net/unit/DTRA-CB#.UoUqZ9wo5zk ) via contract to myself at the Naval Research Laboratory. A subcontract from NRL with George Mason University was used to provide support for contractors (Rashid Ansumana, Alfred Bockarie, Umaru Bangura and Joseph Lamin) working at Mercy Hospital Research Laboratory in Bo, Sierra Leone. There is no past, present or future Intellectual Property associated with the work described in the paper, and none of the authors have any financial interests or conflicts in the outcome of the study. The funders had no role in study design, data collection and analysis, decision to publish, or preparation of the manuscript.
Competing interests: The authors have declared that no competing interests exist.
Introduction
The population of a region of interest must be estimated if one’s goal is to convert incidence counts into rates. This conversion is not always necessary, because some epidemiological parameters can now be estimated from incidence counts alone, including the interval between successive cases, and the reproductive number R 0 , which is the average number of secondary cases attributable to a primary cause [ 1 , 2 ]. If these parameters are insufficient to evaluate the models, it may be necessary to calculate the total population N . The 5 brief examples that follow illustrate both the necessity of doing so, and some of the difficulties that may be encountered.
In resource-limited environments, it may be possible to use both aerial imagery and limited residential survey data to estimate the population of a region of interest, as shown in the first two examples. Using ground truth data for the measured population of 20 sections in Bo City, Sierra Leone, we compared the uncertainty of estimating the population using survey data for either (1) occupants per residence or (2) rooftop area per resident. The latter variable was computed by manually digitizing the rooftop areas of residential structures in 5 sections of Bo, and calculating the ratio of rooftop area per occupant for each residence [ 3 ]. The ability to rapidly estimate the population of both temporary and unplanned settlements is critical for planning resource allocation for refugee and internally displaced populations as well as for places undergoing rapid unplanned urbanization, since in these settings there is usually not a stable residential population. Checchi et al. [ 4 ] have developed a two-step method for estimating a refugee population that requires (1) estimating the number of temporary residential structures from satellite imagery and (2) estimating the mean occupancy per structure. The product of the estimate (1) “number of structures” and (2) “mean number of persons per structure” yields an estimate of the total refugee population.
As shown in the next 2 examples, if salient population data are available either directly or by interpolation; derived rates of infection, immunity, or morbidity may be calculated. The standard SEIR (Susceptible, Exposed, Infectious, Recovered) compartmental epidemiological model [ 5 , 6 ] requires N as a parameter. Glasser et al. [ 6 ] simulated the implementation of two different influenza vaccination policies, in order to predict their effect on both the incidence of infection and the rate of morbidity. They applied a SEIR model parameterized by demographic parameters for the United States (2005), including the total population stratified by age. The age-specific death rates attributable to pneumonia and influenza were estimated, as were the death rates from all other remaining causes. Gomez-Elipe et al. [ 7 ] have developed a model for forecasting the incidence of Malaria in Karuzi, Burundi (1997–2003). To convert the reported instances of malaria to a rate, the investigators divided the rate by the 2006 population census, after rescaling (decrementing) by the population growth factors for the intervals from 1995–2000 (growth factor = 1.32) and 2000–2005 (growth factor = 3.29).
In demographically-diverse environments, different methods may be required to estimate the population at different locations, as shown in our final example. The GRUMPv1 (Global Rural-Urban Mapping Project, Version One) , separates the urban population density estimates from the population of the surrounding areas. In addition to enumerated city population data, city footprints can be established by analyzing nighttime satellite images, but this approach may fail to capture small informal settlements in Africa and rural Asia [ 8 ] (page 9). Accordingly, several corrections are applied for poorly illuminated settlements [ 8 ] (page 9), and point estimates are provided for settlement populations exceeding 1,000. Many models utilize GRUMP for epidemiological modeling, including [ 8 , 9 ].
Proposed analysis
In a previous study [ 3 ], a Finite Population Bootstrap (FPB) [ 10 ] (page 92) was used to compare the relative uncertainty of two population estimators: an occupancy-based estimator and a rooftop area-based estimator. For the region of interest, the former was estimated as the product of (1) the average number of persons per residential structure multiplied by (2) the total number of residential structures; and the latter was calculated as (1) the average number of persons per rooftop area (i.e., persons per m 2 ) multiplied by (2) the total estimated rooftop area in m 2 . Both estimators were effective, but the uncertainty was about 20% less for the occupancy-based estimator [ 3 ] (page 10). Both the occupancy-based and rooftop area-based population estimators were evaluated by simulating simple random sampling without replacement (SRSWOR).
The analysis in this current paper will evaluate the use of stratified sampling for population estimation, and will demonstrate the reduction in the uncertainty of the population estimate achievable relative to SRSWOR. Two different stratification designs will be explored: (1) optimal stratification by “persons per structure” and (2) stratified single-stage cluster sampling. The relative advantages and restrictions of both methods will be discussed. The city of Bo itself is approximately 30.1 km 2 in area, and is divided into 68 uniquely-shaped neighborhoods or sections [ 11 ](see Fig 1 in [ 3 ] and Table 1 ). These sections vary in size from 0.02 km 2 (Toubu) to 2.33 km 2 (Bo Government Reservation). For 20 of the 68 sections, residential survey data are also available [ 3 ] (see Table 1 ). The ground truth survey data for these 20 sections will provide the basis for simulated sampling using different stratification protocols, and for quantifying the reduction in the uncertainty of the population estimate achievable.
- PPT PowerPoint slide
- PNG larger image
- TIFF original image
https://doi.org/10.1371/journal.pone.0132850.t001
The first approach, optimal stratification by persons per structure, requires that the number of persons per structure be already known for all residential structures; possibly from a prior survey or census data. The objective is to exploit this prior data to design an improved stratification protocol for re-estimating the population, and to demonstrate a significant reduction in the uncertainty of the population estimate relative to random sampling. Single-stage cluster sampling is useful if the number of sections that can actually be sampled is restricted, perhaps because of cost or schedule limitations. In our examples, the simulated cluster sampling will be restricted to 4 of the 20 available sections. We will investigate the reduction in uncertainty that can be achieved by using a stratified cluster sampling protocol, rather than random selection, to select the 4 sections on each simulation trial. Each section will be completely sampled.
Note that choice of population estimators is independent of the stratified sampling protocol selected for simulated data collection. A stratified Horvitz-Thompson [ 12 ] population estimator will be evaluated for all examples. We have also extended our original FPB model to support stratified sampling [ 10 ], and partial results from the latter will be contrasted with estimates obtained using the stratified Horvitz-Thompson estimator. Neither the stratified FPB nor the Horvitz-Thompson estimator were used in the prior study.
- What reduction in the uncertainty of the population estimate can be achieved by stratified sampling—relative to simple random sampling of all sections—if the residential survey records are first partitioned into mutually-exclusive strata with non-overlapping ranges of “persons per residential structure?”
- Can any reduction in uncertainty be achieved—again relative to simple random sampling of all sections—if the sections are partitioned into mutually-exclusive and exhaustive strata, rather than partitioning the individual records (PSUs) into strata?
- For single-stage cluster sampling, if the sections (clusters) are partitioned into mutually-exclusive strata by “total residential structures per section,” what is the relative reduction in uncertainty that can achieved using stratified cluster sampling, rather than unclassified cluster sampling?
- Does stratification by the “total persons per section”—if known—further reduce the uncertainty of the single-stage cluster population estimates?
We will use a single dataset developed previously in [ 3 ] (see Table 1 ). This dataset contains individual records for each of 1,979 residential structures surveyed. Each record includes the number of persons in the structure, a variable that we will utilize in this paper. The survey methodology and data collection methods used to construct the dataset analyzed in this manuscript were all developed previously. The original articles [ 3 , 11 ] should be consulted for a complete discussion. The current article complements and extends these prior studies, but does not supplant them.
The utility of these methods for the 5 initial examples, which were presented to establish the importance of estimating the population of a region of interest, will depend upon the availability of partial survey data for occupancy, the existence of adequate estimates of the total number of residential structures, and the presence of stable patterns of residential occupation. Neither method is likely to be useful for improved estimation or re-estimation of the population of a highly transient population living in temporary shelters as described by Checchi et al. [ 4 ].
Model development
The simulations described in this investigation were written in the programming language R [ 13 ]. Supporting functions from multiple R libraries were used, including [ 14 – 16 ]. Additional custom code was written and tested by the first author. The R package stratification [ 16 , 17 ] provides algorithms for finding the optimal boundaries for a variable Y , based on criteria proposed by Lavallée and Hidiroglou [ 18 ]. This package supports several different heuristics, including Kozak’s algorithm [ 19 , 20 ] which can also find the optimized boundaries for a specified sample size n .
In all of the examples presented here, the true optimal boundaries were found through exhaustive search. Given the relatively small size of the dataset (1,979 records), all possible combination of strata boundaries were tested to determine which set minimized the uncertainty of the population estimate as a function of sample size [ 17 ] (page 33).
Survey methodology and dataset development
Ethics statement..
All data collection involving human subjects was approved by a total of three independent Human Subjects Research Institutional Review Boards: Njala University, George Mason University, and the U.S. Naval Research Laboratory. Written informed consent was obtained from each household representative who participated in the survey. Survey data were obtained as part of a broader study to determine not only population demographics but health metrics and health care utilization trends.
Terminology.
Structures in Bo City were divided into two categories. “Nonresidential” structures included governmental, commercial, and nonprofit organizational structures such as places of worship. “Residential” structures included all structures used as sleeping quarters. Fig 1 in [ 3 ] shows the 20 sections in which the surveys were conducted. Some surveyors were staff of Mercy Hospital Research Laboratory (MHRL); most were Master of Public Health students at Njala University. The surveyors received several days of training, including instruction on geographic data collection using hand-held GPS units, interviewing techniques, and research ethics—including an emphasis on confidentiality. During the interviews, one representative—an adult of either sex—served as a representative of each household. Each residential record lists the number of persons reported living within the same residential structure, and the number of separate households. No attempt was made to differentiate between persons based on gender, age, or household affiliation.
Protection of human subjects.
This field work was a joint task of Njala University, George Mason University, and the U.S. Naval Research Laboratory. Institutional review boards (IRB) at all three institutions approved the data collection methodology.
Bo City dataset.
Our sampling frame is a list of 1,979 residential structures encompassing 20 of the 68 sections in Bo City. For each residential structure, there is a unique single record listing the number of persons and households; because these records can be randomly selected, this database will provide the basis for simulated sampling of residential structures. By definition, each residential structure is also a Primary Sampling Unit (PSU). A cluster is defined as a logical collection of PSUs [ 21 ](page 24); in this study, a cluster and a Bo City section will be treated as synonymous in the context of single-stage cluster sampling.
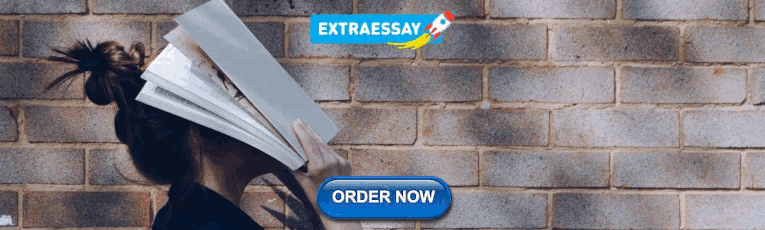
Overview of stratified sampling
The flowchart in Fig 1 summarizes the algorithms and simulations that will be developed in the text. The objective of this study is to investigate alternative approaches for stratified sampling of the residential structures in a resource-limited environment, and to determine the relative reduction in the uncertainty of the estimate of the total population—if any—that results. In all cases, it is assumed that at least the number of residential structures in each section are known. This flowchart may be referenced as the two major protocols are developed and simulated in detail.
This figure summarizes all of the optimization and control protocols for stratified sampling developed in this study. See text for a summary of each major protocol and its corresponding steps through the flow chart. The light brown parallelogram is the starting point for all protocols, the yellow diamonds are decision boxes, and the light green squares denote the process end states.
https://doi.org/10.1371/journal.pone.0132850.g001
Optimal stratification by persons per residence.
As with any stratified sampling scheme, the PSUs (Primary Sampling Units) —the 1,979 individual residential structures (see Table 1 )—must first be divided into mutually-exclusive and exhaustive strata [ 21 ] (page 121). After the stratification boundaries have been determined, simulated sampling can be executed. Based on pilot studies, we determined that 4 levels of stratification would be sufficient for proof of concept. The stratification and estimation algorithms will be summarized later. The survey variable X and the stratification variable Y are the same—specifically, the number of persons per residential structure. For this reason, it was not necessary to model the relationship between Y , the measured survey variable (persons per residential structure), and X , the stratification variable [ 17 ].
- reduce the uncertainty of the estimated population as a function of sample size relative to random sampling without replacement: (1) → (2) → (3) → (4 a ) → (5).
- and/or find the minimum sample size needed to minimize the Coefficient of Variation (CV) below some specified threshold: (1) → (2) → (3) → (4 b ) → (5)
Stratified single-stage cluster sampling.
When schedule or resources restrict the survey to a subset of sections within the region of interest, single-stage cluster sampling can be applied. (If there is no restriction on the number of sections to be sampled, all sections can be sampled without replacement for a given sample size.) Assume that the number of residential structures per section is known, but not the number of persons per section. The 20 sections will first be partitioned into the desired number of mutually-exclusive strata, using the section sizes (i.e., total residential structures per section) as the stratification variable; see Table 1 for these values. Each residence in a section will be assigned to the same stratum. For each trial of the stratified single-stage clustering protocol, one section will be selected from each stratum, and all of the residences in the selected sections will be completely sampled. For the control case, the same number of sections will be selected, but the stratification boundaries will be ignored. In effect, in the control case, all sections will be assigned to a single stratum.
- (1) → (2 a ) → (4′ a ) → (5′ a )
- (1) → (2) → (4′ b ) → (5′ b )
- No auxiliary data is required other than a count of residential structures in each of the 20 sections under consideration. If the total population of each section is available, an even more efficient design can be realized.
- A cluster design permits a trade-off between the size of the survey, the number of sections sampled, and the uncertainty of the population estimate.
Optimal stratification.
Let L strata be defined on the stratification variable X , the number of persons per residential structure. Number the strata h = 1, 2…, L . Define the boundaries of the strata as b h = 1 , b h = 2 , …, b h = L . Stratum h will include all values of X in the interval [ b h −1 , b h ) such that b h −1 < X ≤ b h .
- N L is the size of stratum h
- W h = N h / N is the proportion of the total units (records) in N assigned to stratum h
- S h is the standard deviation of the stratification variable Y in stratum h
- c is the CV (coefficient of variation) of the survey variable Y
- N is the total number of records or units being partitioned into strata
Allocation selection.
When the strata boundaries are optimized for a given sample size n , the coefficient of variation of Y is minimized [ 17 ]. Note that the constraint for optimization is dependent not only on the distribution of the stratification variable Y , but also upon the allocation rule used. The allocation rule chosen will determine the weights W h . The allocation rule used in the R package stratification [ 16 ] is developed in [ 22 ].
Setting q 1, q 2 and q 3 to (0.5, 0.0, 0.5) parameterizes Neyman’s allocation for each stratum, while (0.5, 0.0, 0.0) corresponds to proportional allocation. When Neyman’s allocation is used, a sample size n h may be equal to or greater than the number of available PSU’s N h . The stratum may then be categorized as a “take-all” stratum [ 17 ], and every record (i.e. PSU) in the stratum will be selected, rather than a subset of the stratum records. If necessary, the sample sizes of one or more of the remaining strata are transparently incremented to realize the desired total sample size n .
The Horvitz-Thompson estimator.
This expression could be simplified, but the double summation makes clear that the total population estimate is the sum of the weighted estimates for the individual strata.
Optimal stratification for resampling.
In our first set of demonstrations, we evaluated a design for resampling a known population for which complete survey data exists [ 19 ]. Using the optimization approach described earlier, the 1,979 units were divided into 4 strata, using the number of persons per residential structure as the sampling variate Y . The choice of L = 4 as a reasonable number of strata was based on the findings from preliminary simulation studies. Five different random sample sizes were selected: 330, 660, 990, 1,320, and 1,650 records, out of the total 1,979 records available. Simulations were run using both proportional and Neyman allocation.
For each sample size, 1,000 random trials were run. In each trial, a stratified sample was selected, and the Horvitz-Thompson population estimate calculated. The inclusion probability π h for each record in the sample was calculated as shown in Table 2 .
https://doi.org/10.1371/journal.pone.0132850.t002
Stratified finite population bootstrap.
The samples drawn for each stratum were also concatenated and resampled [ 10 , page 97], [ 14 , 24 ] creating a bootstrap sample of size n h for each strata. The n h samples from each strata were then combined to create a single sample of size n (330, 660, 990, …), and the total population was estimated using the FPB. For the control group and the proportional allocation case, the estimated population obtained using the FPB was compared with the results from the Horvitz-Thompson estimations. (Neyman allocation could not be compared, since the individual bootstrap estimates for each stratum required proportional allocation.)
The FPB model mirrored the decrease in uncertainty observed with the H-T estimator using optimal proportional allocation, but the variance of the FPB is greater. The average ratio of the 0.95 confidence intervals between the H-T estimator and FPB estimator was approximately 0.70 for the control group, and 0.58 when comparing the estimators for optimal proportional allocation. A paired t-test was used to compare the intervals, and P < 0.001 in both cases. For the control case, 67% of the H-T estimators fell within the 0.50 confidence interval for the FPB, quantifying the greater uncertainty of the FPB estimator. Likewise, comparing the proportionally-allocated 4 strata case, 76% of the H-T estimators fell within the 0.50 confidence interval for the FPB. The FPB used is one of a family of finite population bootstrap algorithms. A recent study [ 24 ] compared the variance characteristics of different implementations of the FPB, and proposed a new FPB algorithm may present reduced uncertainty relative to the implementation used here.
Relative uncertainty of the population estimates.
Fig 2 illustrates the [0.25, 0.75] quantile boxplots as a function of sample size for the H-T estimator for the single-stratum control case (A), and using proportional (B) and Neyman (C) allocation, respectively. The mean ratios of the 0.95 confidence intervals were 0.58 and 0.19, respectively ( P < 0.001 and P < .005). In summary, the uncertainty using optimal stratification with Neyman allocation was roughly 20% of the uncertainty observed for the single stratum control group, averaged over 1,000 simulations.
Quantile boxplots (0.25, 0.75) showing the distribution of the stratified Horvitz-Thompson population estimates as a function of sample size and stratification protocol. The bar in each box is the median value of the estimate, while outliers deviating by one or more quantiles from the median are denoted as discrete points. (A) control—all 20 sections are placed in a single stratum (B) 4 strata, with proportional allocation for sample selection (C) 4 strata, with Neyman allocation for sample selection. Persons per residence was used as the stratification variable, and there were 1,000 simulations for each boxplot.
https://doi.org/10.1371/journal.pone.0132850.g002
Coefficient of Variation optimization.
- stratum 1: 9.5
- stratum 2: 17.5
- stratum 3: 31.5
- stratum 4: 86.0
Single-stage cluster sampling.
Table 3 shows the results of applying the Neyman stratification algorithm. For a sample of some specified number of clusters (sections), the recommended number of sections to select are given for each stratum. The variable bh [ h ] specifies the upper boundary in “residential structures per section” for each stratum h . The stratification algorithm actually returns the first three boundaries, since the upper boundary of the 4th stratum is the maximum possible value of the stratification variable, which is 208—the number of residential structures in the New London section. The variable nh [ h ] indicates the allocated number of clusters that should be selected from each stratum for a balanced sample of a given size in clusters (sections). Given 4 stratification levels, the minimum number of clusters that can be selected is 4, and the recommended sample allocation is (1, 1, 1, 1). A comparable table was generated for proportional allocation, and for an allocation of (1, 1, 1, 1) sections per stratum, the stratification partition was identical. Table 4 shows the stratification by section for the 4-section allocation (1, 1, 1, 1), which was used in the simulations, and the 10-section allocation (2, 1, 6, 1) provided for comparison.
https://doi.org/10.1371/journal.pone.0132850.t003
https://doi.org/10.1371/journal.pone.0132850.t004
- y i = the total number of persons for i th cluster (section)
- π i = the probability of the i th cluster being sampled during this trial
- v = the total number of clusters sampled (i.e., 20)
This estimator provides an unbiased estimate of the total population.
Optimal stratified sampling
https://doi.org/10.1371/journal.pone.0132850.t005
The Levene test [ 25 , 26 ]was used to compare the variances of the stratified protocols with the variance of the unstratified control group. The paired comparisons were blocked by sample size. The null hypothesis for the Levene test is that the ratio of 2 specified variances is equal to 1.0. For all tests, σ x | N = n 2 was the variance for 1,000 simulated trials for sample size of n (e.g., 330, 660, 990 …) using 4-level Neyman or proportional allocation, and σ c | N = n 2 the variance 1,000 simulated trials for the comparable unstratified control case. The differences between the variances were statistically significant, with p < 0.001 for all comparisons, and the hypothesis that the ratio σ x | N = n 2 /σ c | N = n 2 = 1.0 was rejected for all tests.
Single-stage cluster sampling
Fig 3 shows the box histograms for the single-stage cluster sampling simulations. The uncertainty of the population estimation using stratified cluster selection is about 48% of the uncertainty of the estimation based on random cluster selection, as measured by comparing the [0.25, 0.75] quantile intervals. This difference is significant at P < 0.001 (paired t-test).
Quantile boxplots for 1,000 stratified 4-level simulated single-stage cluster sampling trials using H-T estimation. The bar in each box is the median value of the estimate, while outliers deviating by one or more quantiles from the median are denoted as discrete points. Four selected sections are completely sampled on each simulation trial. (1) “Survey” is the measured value of the population of the 20 sections (25,954 persons). (2) 4 L /4 C (pers.)—4 cluster sample, sections stratified by “persons per section.” (3) 4 L /4 C (strs.)—4 cluster sample, sections stratified by “residential structures per section.” (4) 1 L /4 C —4 clusters selected at random from the 20 available sections.
https://doi.org/10.1371/journal.pone.0132850.g003
In single-stage sampling, if a section is selected from one of the four strata, all residences in the section are then included in the sample. Each stratum contains a mutually-exclusive subset of the 20 sections, with non-overlapping ranges of buildings per section between the strata. Because a single section is selected from each stratum for each one-stage survey sample, the sample allocation is balanced with respect to the stratification variable “residential structures per section.” See Table 4 , column 4.
Table 6 compares of the variance and standard error of the mean (SEM) of the Horvitz-Thompson estimator for 1,000 simulated sampling trials, selecting 4 sections on each trial. The average number of residences selected per trial are shown in the table. For the unstratified control case, all sections were assigned to a single stratum, in contrast to 4-level optimal stratification using either proportional or Neyman allocation. The variance ratios were again compared between all three protocols using the Levene test. The differences between the variances were statistically significant, with p < 0.001 for all comparisons.
https://doi.org/10.1371/journal.pone.0132850.t006
The above calculation is consistent with the simulation results, in which 119 balanced 4-strata samples were drawn in 1,000 random trials. A comparable argument applies to the simulations using the number of persons per section as the stratification variable.
Stratification by section for non-cluster sampling
If “persons per structure” are known, optimal stratification boundaries and allocations can be found [ 18 ]. Each stratum will contain residences from one or more sections. In single-stage cluster analysis, the sections are partitioned into strata by either “(a) residential structures per section” or “(b) total persons per section,” and one or more sections are selected on each trial from each stratum for complete sampling. As a third possibility, if “persons per structure” are unknown, we may ask whether either of the stratification variables (a) or (b) could be used to efficiently partition the 20 sections into mutually-exclusive strata for non -cluster sampling. All of the residences in a given section would be assigned to the same stratum, and a given stratum would contain all of the residential records from the subset of sections assigned to it. A sample of residential records would be drawn from each stratum on a given trial, usually without completely sampling any one section. This protocol could prove advantageous if the proposed partitioning is more efficient than simple random sampling without replacement, even if it is less efficient than optimal stratification by “persons per structure.”
There are two difficulties with attempting to stratify the data at the section level, rather than at the level of the individual record. For any stratification plan to be viable, the units within a stratum must be relatively coherent with respect to the stratification variable selected. If the stratification variable is “persons per section,” this goal will be difficult to achieve. Fig 4 shows the quantile boxplots for the number of buildings per section, arranged from left to right in order of decreasing number of persons per section. The upper and lower “hinges” correspond to the first and third quartiles (the 25th and 75th percentiles), and the band inside the box is the 2nd quartile (i.e., the median) value of the number of persons per residential structure. The width of each box is proportional to the square root of the number of residential structures (i.e., records) in the section [ 27 ]. Roma appears to be anomalous because, although there are only 4 residential structures in this section, there are a total of 139 persons, because these structures are apartment complexes, rather than individual homes. As can be seen, there will be significant overlap between the the ranges of persons per structure for virtually any partitioning of the 20 sections used.
For each section, a quantile boxplot (0.25, 0.75) shows the distribution of the number of persons per residence, arranged in descending order of total section population. The bar in each box is the median value, while outliers deviating by one or more quantiles from the median are denoted as discrete points. The width of each box is proportional to the square root of the number of residential structures (i.e., records) in the section. Roma is an anomaly with 4 residential structures, and 139 total persons.
https://doi.org/10.1371/journal.pone.0132850.g004
To clarify the above discussion, two experimental simulations were run. The same 4-level partition used for the single-stage cluster sampling was used to define a non-clustered random sampling protocol. Every record in a section was then assigned to the same designated stratum. For example, all records for Kulanda Town, Nduvuibu, and New London were assigned to stratum 4—see Table 4 . Residences were then randomly selected from all 4 strata, and the number of residences selected from each stratum was proportional to the total number of residences the stratum contains. 1,000 simulated sampling trials were run, using the same sequence of 5 sample sizes used for the optimal stratification analysis (see Table 2 ). Because each stratum contained records from multiple sections, each sample typically contained records from multiple sections. Conversely, none of the sections were completely sampled on a given trial, in contrast to the protocol for the single-stage cluster model. For a second simulation, the stratification variable “persons per section” was used, rather than “residential structures per section.” See columns 4 and 5 in Table 4 . The results are summarized in the next paragraph, but are not presented in a table or figure.
Relative to simple random sampling without replacement of all strata, which was also simulated as a control, the reduction in uncertainty for section-based non-cluster stratification was minimal and statistically insignificant. Levene’s test was again used to compare the ratio of the σ 2 s. The ratio of σ x 2 /σ c 2 , where x denotes the stratification variable, and c denotes the unstratified control case, was 0.95 for stratification by “total persons per section,” and 0.98 for stratification by “residential structures per section,” averaged over the 5 sample sizes. For either stratification method, the hypothesis that the σ 2 were the same for the 1,000 trial comparisons of the stratified and unstratified population estimates could not be rejected for p < 0.05 for any of the 5 sample sizes.
- By design, all residences with a stratum were subsampled, rather than selecting a single section from each stratum to achieve balanced sampling across strata, as was done using a single-stage cluster sampling protocol.
- There will be considerable overlap in the variable “persons per residential structure” for any possible partition (see Fig 4 ), although the range of section sizes (i.e., number of residences per section) for each stratum was distinct in the constructed example.
In this context, it is also instructive to compare Figs 5 and 6 . Fig 5 shows the distribution of the unit records (i.e., persons per residence) as a function of the stratification boundaries for a Neyman allocation for a sample of size 990. See Table 2 . All 1,979 records are shown in the box histograms. In each stratum, the records can be selected from any of the 20 eligible sections. Note that there is complete separation between the 4 stratum-specific distributions of the stratification variable “persons per residence.” In contrast, Fig 6 shows the comparable distributions of the unit records as a function of the 4-level stratification by residential structures per section (A) and persons per section (B) to support single-stage cluster sampling. In both cases, the records within a section are assigned to a single stratum, which results in considerable overlap between the number of persons per residence within the same stratum. Although there is an apparent grouping, the coherence within the strata is relatively weak, and the strata are not well separated, as in Fig 5 . Stratification by “persons per section” is relatively efficient for single-stage cluster sampling because a single section will be completely sampled from each stratum, and the ranges of residential structures per section are non-overlapping between strata.
The 4-level stratification variable is “persons per residence” ( Table 2-d ). The quantile boxplots [0.25, 0.75] show the partitioning of the records by stratum for all 1,979 records. The bar in each box is the median value of persons per residence, while outliers deviating by one or more quantiles from the median are denoted as discrete points. The samples in a given stratum may be assigned from any of the 20 eligible sections. The optimized Neyman allocation has completely separated the 4 strata with respect to overlapping values of the stratification variable.
https://doi.org/10.1371/journal.pone.0132850.g005
(A) For the single-stage cluster sampling, the 20 sections were partitioned into 4 proportionally-allocated stratification levels. Within each stratum, the sections are arranged in descending order of total persons. The stratification variable is the total number of residential buildings per section (see Table 4 ). The quantile boxplots show the partitioning by stratum of the 1,979 records in the database, although only a subset of 4 sections will be drawn on a single simulation trial. The bar in each box is the median value of “persons per residence,” while outliers deviating by one or more quantiles from the median are denoted as discrete points. (B) Quantile boxplots showing stratification by total persons per section. This stratification approach requires that the population of each section be known, in contrast to stratification by residential structures per section.
https://doi.org/10.1371/journal.pone.0132850.g006
The second difficulty is operational, and not specific to this dataset. The stratification boundaries were determined as a function of the number of residential structures per section. But all sections contain both residential and non-residential structures, as shown in Table 1 . If a survey of all sections is first required to enumerate the number of residential and non-residential structures, the apparent simplicity of the single-stage cluster sampling design is reduced. In our previous paper, [ 3 ] we discuss this issue is more detail.
Summary and Conclusions
We have developed and modeled two different but complementary approaches for stratified sampling in resource-limited environments. Their relative efficiencies have been discussed, and illustrated graphically and numerically. It does not seem likely that significant additional improvements can be achieved with respect to the stratification of the variable “persons per residential structure” demonstrated herein. Conversely, the single-stage cluster sampling method could well be the subject of additional research and application.
The stratification approach used for the latter was based on the partitioning of sections (clusters) into strata as a function of the number of residential structures per section. Alternative stratification variables could also be explored. As a hypothetical example, the section data available in this study encompasses 20 randomly-selected sections of the 68 sections comprising Bo City. Given data for all 68 sections, it would be possible to divide Bo City into a complete 68 section grid. Sections could then be assigned to strata as a function of the radial distance from the center of the city, or some other rule relating to geographical location or proximity.
Answers to Key Questions
The objective of the current study was to examine methods for either re-estimating the population following a complete survey, or for estimating the population in a new environment under conditions which—for reasons of schedule or funding—preclude undertaking similar surveys. The ground truth data used for the simulations came from a larger field survey that collected data for the 20 municipal sections described in this paper [ 28 – 30 ]. The first method used proportional and Neyman-allocated optimal stratification, and the latter achieved a reduction in uncertainty of the population estimation of about 80% in 1,000 simulated sampling trials. For proportional allocation only, the simulations were also validated by comparing the estimates obtained using a stratified finite population bootstrap with comparable estimates using an unbiased Thompson-Horovitz estimator. The second method explored the use of single-stage cluster sampling. The uncertainty of the population estimates for the latter protocol was significantly improved by first stratifying the 20 sections into 4 strata as a function of section size (i.e., number of residential structures per section). If the total number of persons per section was used as the stratification variable, a further reduction in uncertainty was observed, but this variable may not be known prior to conducting a survey.
- If the 1,979 residential survey records are first partitioned into mutually-exclusive strata using “persons per residential structure” as the stratification variable, there is a reduction in uncertainty of about 80% relative to the estimate obtained using random sampling. The strata are cleanly separated by non-overlapping ranges of “persons per structure,” as shown in Fig 5 . Because the variable “persons per residential structure’ must be known in advance, presumably from prior survey data, this protocol is potentially useful for re-estimating a population.
- If the strata are created by partitioning the 20 sections into mutually-exclusive groups, using either residential structures or individual persons per section as the stratification variable, no statistically significant reduction in uncertainty is observed. The distributions of “persons per residential structure” overlap significantly between strata, and the strata are no longer well separated. Compare Fig 4 with Fig 5 .
- For 4-section single-stage cluster sampling, if the 20 sections are partitioned into mutually-exclusive strata by “total residential structures per section,” the uncertainty (H-T variance) of the population estimate is about 50% of the uncertainty for unstratified sampling. See Table 6 and Fig 6A .
- If the sections are instead stratified by “total persons per section” the uncertainty of the population estimate is reduced to about 6% of the uncertainty of the unstratified case for single-stage cluster sampling. See Table 6 and Fig 6B .
Future applications and research
For the single-stage cluster sampling, the sections were stratified by either total number of buildings per section, or by total persons per section. As an alternative, Bo could divided up into equal squares using a grid. There is a reasonably well-defined center of Bo, just are there are reasonably well-defined high-population-density centers that could be visually identified from aerial photographs of most cities. It is clear that if a grid was overlaid on a map of Bo, the cells farther from dense population areas would have fewer residential structures and a lower population density. If a Neyman stratification algorithm were to be applied, we would hypothesize that cells would be assigned to strata as a rough function of their distance from the center of the city. It would be interesting to compare the efficiency of this protocol for stratification with our existing results for single-stage cluster sampling, looking for possible improvement. At this time, we do not have sufficient data to test this hypothesis.
In summary, the ability to quickly estimate the total population size with reasonable precision in resource-limited environments can be of high value for demography, epidemiology, and health and social services research. The two approaches analyzed here are both of potential value in achieving these goals. Although the optimal stratification by residential occupancy is highly efficient, a single-stage cluster sampling protocol requires minimal data in advance, while minimizing the number of sections that must be surveyed.
Supporting Information
S1 file. related manuscript [ 3 ]..
https://doi.org/10.1371/journal.pone.0132850.s001
Acknowledgments
The views expressed herein are those of the individual authors and do not reflect views of the Department of the Navy or the Department of Defense.
Author Contributions
Conceived and designed the experiments: RH. Performed the experiments: RH. Analyzed the data: RH JDA. Contributed reagents/materials/analysis tools: KHJ RA ASB UB JML DAS. Wrote the paper: RH KHJ JDA RA DAS.
- View Article
- PubMed/NCBI
- Google Scholar
- 10. Davison AC, Hinkley DV. Bootstrap Methods and Their Application. Cambridge University Press; 1997.
- 13. Dalgaard P. Introductory Statistics with R. New York, NY 2013.: Springer; 2008.
- 14. Ripley B, Canty A. Package boot: Bootstrap R (S-Plus) Functions—R Package; 2013. Available from: http://cran.r-project.org/web/packages/boot/index.html .
- 15. Tillé Y, Matei A. Package sampling R (S-Plus) Functions—R package;. Available from: http://cran.r-project.org/web/packages/sampling/index.html .
- 16. Baillargeon S, Rivest LP. Package stratification R (S-Plus) Functions—R package; 2012. Available from: http://cran.r-project.org/web/packages/stratification/index.html .
- 21. Levey PS, Lemeshow S. Sampling of Population—Methods and Applications. 4th ed. Hoboken, New Jersey.: John Wiley & Sons, Inc.; 2008.
- 23. Tillé Y, Matei A. Teaching Survey Sampling With the R Package Sampling. In: ICOTS, The 8th International Conference on Teaching Statistics, Ljubljana.; 2010..
- 25. Croarkin C, Guthrie W. NIST/SEMATECH e-Handbook of Statistical Methods. 2015. Available from: http://www.itl.nist.gov/div898/handbook/ .
- 26. Fox J, Weisberg S. Package CAR: companion to applied regression R (S-Plus) Functions—R Package; 2014. Available from: http://cran.r-project.org/web/packages/car/car.pdf .
If you're seeing this message, it means we're having trouble loading external resources on our website.
If you're behind a web filter, please make sure that the domains *.kastatic.org and *.kasandbox.org are unblocked.
To log in and use all the features of Khan Academy, please enable JavaScript in your browser.
Statistics and probability
Course: statistics and probability > unit 6.
A Science and Risk-Based Pragmatic Methodology for Blend and Content Uniformity Assessment
- Brief/Technical Note
- Published: 06 December 2017
- Volume 19 , pages 1483–1492, ( 2018 )
Cite this article
- Naheed Sayeed-Desta 1 ,
- Ajay Babu Pazhayattil 1 ,
- Jordan Collins 1 &
- Chetan Doshi 1
786 Accesses
5 Citations
2 Altmetric
Explore all metrics
This paper describes a pragmatic approach that can be applied in assessing powder blend and unit dosage uniformity of solid dose products at Process Design, Process Performance Qualification, and Continued/Ongoing Process Verification stages of the Process Validation lifecycle. The statistically based sampling, testing, and assessment plan was developed due to the withdrawal of the FDA draft guidance for industry “Powder Blends and Finished Dosage Units—Stratified In-Process Dosage Unit Sampling and Assessment.” This paper compares the proposed Grouped Area Variance Estimate (GAVE) method with an alternate approach outlining the practicality and statistical rationalization using traditional sampling and analytical methods. The approach is designed to fit solid dose processes assuring high statistical confidence in both powder blend uniformity and dosage unit uniformity during all three stages of the lifecycle complying with ASTM standards as recommended by the US FDA.
This is a preview of subscription content, log in via an institution to check access.
Access this article
Price includes VAT (Russian Federation)
Instant access to the full article PDF.
Rent this article via DeepDyve
Institutional subscriptions
Guidance for Industry, “Powder Blends and Finished Dosage Units—Stratified In-Process Dosage Unit Sampling and Assessment”. U.S. Department of Health and Human Services, Food and Drug Administration, Center for Drug Evaluation and Research (CDER), October 2003, Pharmaceutical CGMPs.
Question and Answers on Current Good Manufacturing Practices, good guidance practices, level 2 guidance—Production and Process Controls, CDER/OC Office of Manufacturing and Product Quality: CGMP Subject Matter Contacts, 6 August 2013. https://www.fda.gov/Drugs/GuidanceComplianceRegulatoryInformation/Guidances/ucm124782.htm#18 .
Garcia T, Bergum J, Prescott J, Tejwani R, Parks T, Clark J, et al . Recommendations for the assessment of blend and content uniformity: modifications to withdrawn FDA draft stratified sampling guidance. J Pharm Innov. 2015;10(1):76–83. https://doi.org/10.1007/s12247-014-9207-0 .
Article Google Scholar
Brittain HG. Particle size distribution II: the problem of sampling powdered solids. Pharm Technol. 2002;26(7):67–73.
Alexander A, Sudah O, Arratia P, Duong N-H., Reynolds S, Muzzio F. Characterization of the performance of bin blenders part 3 of 3: cohesive powders. Pharm Technol. 2004;28(9):54–74.
Alexander A, Arratia P, Goodridge C, Sudah O, Brone D, Muzzio F. Characterization of the performance of bin blenders part 1 of 3: methodology. Pharm Technol. 2004;13(5):70–86.
Google Scholar
Alexander A, Sudah O, Arratia P, Goodridge C, Alani L, Muzzio F. Characterization of the performance of bin blenders part 2 of 3: free flowing mixtures. Pharm Technol. 2004;28(7):56–7.
Sayeed-Desta N, Pazhayattil A, Chowdari S. “Determining minimum batch size,” APIs, excipients, and manufacturing supplement to. Pharm Technol. 2016;40:s16–9.
Collins J, Sayeed-Desta N, Pazhayattil AB, Doshi C. A novel metric for continuous improvement during stage three. BioPharm Int. 2017;30(6):32–5.
Bergum J, Parks T, Prescott J, Tejwani R, Clark J, Brown W, et al . Assessment of blend and content uniformity. Technical discussion of sampling plans and application of ASTM E2709/E2810. J Pharm Innov. 2015;10(1):84–97. https://doi.org/10.1007/s12247-014-9208-z .
Sayeed-Desta N, Pazhayattil AB, Collins J, Chen S, Ingram M, Spes J. Assessment methodology for process validation lifecycle stage 3A. AAPS PharmSciTech. 2017;18(5):1881–6. https://doi.org/10.1208/s12249-016-0641-9 .
Article PubMed Google Scholar
Lewis RA, Fan A. Improved acceptance limits for ASTM standard E2810. Stat Biopharm Res. 2016;8(1):40–8. https://doi.org/10.1080/19466315.2015.1093959 .
Nunnally B. Variance component analysis to determine sources of variation for vaccine drug product assays. J Validation Technol. 2009;15(3):78–88.
Gelman A. Prior distributions for variance parameters in hierarchical models. Bayesian Anal. 2006;1(3):515–33. https://doi.org/10.1214/06-BA117A .
Madsen C. Statistical Methods for Assessment of Blend Homogeneity. PhD Thesis for the department of Informatics and Mathematical Modelling, Technical University of Denmark;2002.
Snijders TAB. Power and sample size in multilevel linear models. In: Everitt BS, Howell DC, editors. Encyclopedia of statistics in behavioral science, vol. 3. Chicester: Wiley; 2005. p. 1570–3. https://doi.org/10.1002/0470013192.bsa492 .
Maas CJM, Hox JJ. Sufficient sample sizes for multilevel modeling. Methodology. 2005;1(3):86–92.
Bell BA, Ferron JM, Kromrey J.‘Cluster size in multilevel models: the impact of sparse data structures on point and interval estimates in two-level models’ Joint Statistical Meetings—Section on Survey Research Methods;2008 .
Download references
Author information
Authors and affiliations.
Apotex Inc., 150 Signet Drive, Toronto, Ontario, M9L 1T9, Canada
Naheed Sayeed-Desta, Ajay Babu Pazhayattil, Jordan Collins & Chetan Doshi
You can also search for this author in PubMed Google Scholar
Corresponding author
Correspondence to Ajay Babu Pazhayattil .
Ethics declarations
Conflict of interest.
The authors declare that they have no competing interests.
Rights and permissions
Reprints and permissions
About this article
Sayeed-Desta, N., Pazhayattil, A.B., Collins, J. et al. A Science and Risk-Based Pragmatic Methodology for Blend and Content Uniformity Assessment. AAPS PharmSciTech 19 , 1483–1492 (2018). https://doi.org/10.1208/s12249-017-0925-8
Download citation
Received : 14 October 2017
Accepted : 21 November 2017
Published : 06 December 2017
Issue Date : April 2018
DOI : https://doi.org/10.1208/s12249-017-0925-8
Share this article
Anyone you share the following link with will be able to read this content:
Sorry, a shareable link is not currently available for this article.
Provided by the Springer Nature SharedIt content-sharing initiative
- blend uniformity
- content uniformity
- dosage uniformity
- process validation
- lifecycle stages
- Find a journal
- Publish with us
- Track your research

Sampling Methods & Strategies 101
Everything you need to know (including examples)
By: Derek Jansen (MBA) | Expert Reviewed By: Kerryn Warren (PhD) | January 2023
If you’re new to research, sooner or later you’re bound to wander into the intimidating world of sampling methods and strategies. If you find yourself on this page, chances are you’re feeling a little overwhelmed or confused. Fear not – in this post we’ll unpack sampling in straightforward language , along with loads of examples .
Overview: Sampling Methods & Strategies
- What is sampling in a research context?
- The two overarching approaches
Simple random sampling
Stratified random sampling, cluster sampling, systematic sampling, purposive sampling, convenience sampling, snowball sampling.
- How to choose the right sampling method
What (exactly) is sampling?
At the simplest level, sampling (within a research context) is the process of selecting a subset of participants from a larger group . For example, if your research involved assessing US consumers’ perceptions about a particular brand of laundry detergent, you wouldn’t be able to collect data from every single person that uses laundry detergent (good luck with that!) – but you could potentially collect data from a smaller subset of this group.
In technical terms, the larger group is referred to as the population , and the subset (the group you’ll actually engage with in your research) is called the sample . Put another way, you can look at the population as a full cake and the sample as a single slice of that cake. In an ideal world, you’d want your sample to be perfectly representative of the population, as that would allow you to generalise your findings to the entire population. In other words, you’d want to cut a perfect cross-sectional slice of cake, such that the slice reflects every layer of the cake in perfect proportion.
Achieving a truly representative sample is, unfortunately, a little trickier than slicing a cake, as there are many practical challenges and obstacles to achieving this in a real-world setting. Thankfully though, you don’t always need to have a perfectly representative sample – it all depends on the specific research aims of each study – so don’t stress yourself out about that just yet!
With the concept of sampling broadly defined, let’s look at the different approaches to sampling to get a better understanding of what it all looks like in practice.
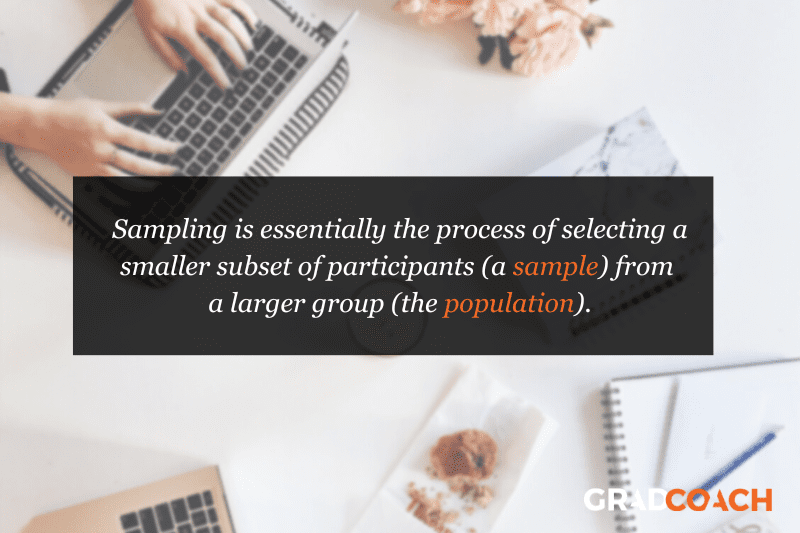
The two overarching sampling approaches
At the highest level, there are two approaches to sampling: probability sampling and non-probability sampling . Within each of these, there are a variety of sampling methods , which we’ll explore a little later.
Probability sampling involves selecting participants (or any unit of interest) on a statistically random basis , which is why it’s also called “random sampling”. In other words, the selection of each individual participant is based on a pre-determined process (not the discretion of the researcher). As a result, this approach achieves a random sample.
Probability-based sampling methods are most commonly used in quantitative research , especially when it’s important to achieve a representative sample that allows the researcher to generalise their findings.
Non-probability sampling , on the other hand, refers to sampling methods in which the selection of participants is not statistically random . In other words, the selection of individual participants is based on the discretion and judgment of the researcher, rather than on a pre-determined process.
Non-probability sampling methods are commonly used in qualitative research , where the richness and depth of the data are more important than the generalisability of the findings.
If that all sounds a little too conceptual and fluffy, don’t worry. Let’s take a look at some actual sampling methods to make it more tangible.
Need a helping hand?
Probability-based sampling methods
First, we’ll look at four common probability-based (random) sampling methods:
Importantly, this is not a comprehensive list of all the probability sampling methods – these are just four of the most common ones. So, if you’re interested in adopting a probability-based sampling approach, be sure to explore all the options.
Simple random sampling involves selecting participants in a completely random fashion , where each participant has an equal chance of being selected. Basically, this sampling method is the equivalent of pulling names out of a hat , except that you can do it digitally. For example, if you had a list of 500 people, you could use a random number generator to draw a list of 50 numbers (each number, reflecting a participant) and then use that dataset as your sample.
Thanks to its simplicity, simple random sampling is easy to implement , and as a consequence, is typically quite cheap and efficient . Given that the selection process is completely random, the results can be generalised fairly reliably. However, this also means it can hide the impact of large subgroups within the data, which can result in minority subgroups having little representation in the results – if any at all. To address this, one needs to take a slightly different approach, which we’ll look at next.
Stratified random sampling is similar to simple random sampling, but it kicks things up a notch. As the name suggests, stratified sampling involves selecting participants randomly , but from within certain pre-defined subgroups (i.e., strata) that share a common trait . For example, you might divide the population into strata based on gender, ethnicity, age range or level of education, and then select randomly from each group.
The benefit of this sampling method is that it gives you more control over the impact of large subgroups (strata) within the population. For example, if a population comprises 80% males and 20% females, you may want to “balance” this skew out by selecting a random sample from an equal number of males and females. This would, of course, reduce the representativeness of the sample, but it would allow you to identify differences between subgroups. So, depending on your research aims, the stratified approach could work well.
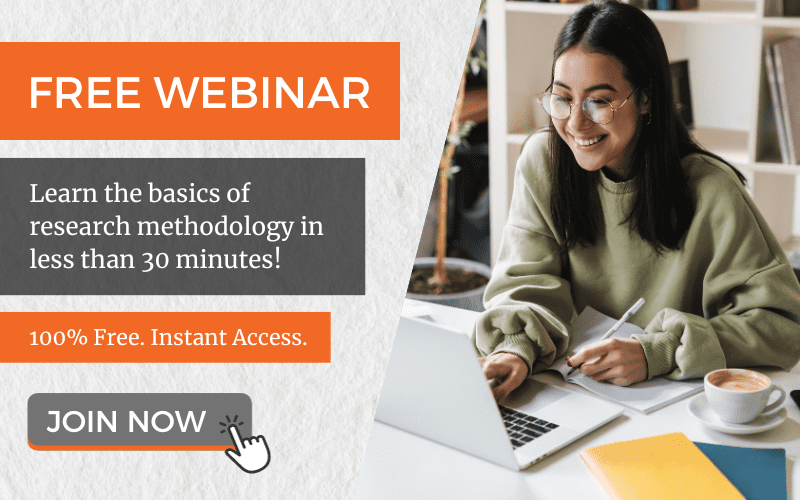
Next on the list is cluster sampling. As the name suggests, this sampling method involves sampling from naturally occurring, mutually exclusive clusters within a population – for example, area codes within a city or cities within a country. Once the clusters are defined, a set of clusters are randomly selected and then a set of participants are randomly selected from each cluster.
Now, you’re probably wondering, “how is cluster sampling different from stratified random sampling?”. Well, let’s look at the previous example where each cluster reflects an area code in a given city.
With cluster sampling, you would collect data from clusters of participants in a handful of area codes (let’s say 5 neighbourhoods). Conversely, with stratified random sampling, you would need to collect data from all over the city (i.e., many more neighbourhoods). You’d still achieve the same sample size either way (let’s say 200 people, for example), but with stratified sampling, you’d need to do a lot more running around, as participants would be scattered across a vast geographic area. As a result, cluster sampling is often the more practical and economical option.
If that all sounds a little mind-bending, you can use the following general rule of thumb. If a population is relatively homogeneous , cluster sampling will often be adequate. Conversely, if a population is quite heterogeneous (i.e., diverse), stratified sampling will generally be more appropriate.
The last probability sampling method we’ll look at is systematic sampling. This method simply involves selecting participants at a set interval , starting from a random point .
For example, if you have a list of students that reflects the population of a university, you could systematically sample that population by selecting participants at an interval of 8 . In other words, you would randomly select a starting point – let’s say student number 40 – followed by student 48, 56, 64, etc.
What’s important with systematic sampling is that the population list you select from needs to be randomly ordered . If there are underlying patterns in the list (for example, if the list is ordered by gender, IQ, age, etc.), this will result in a non-random sample, which would defeat the purpose of adopting this sampling method. Of course, you could safeguard against this by “shuffling” your population list using a random number generator or similar tool.
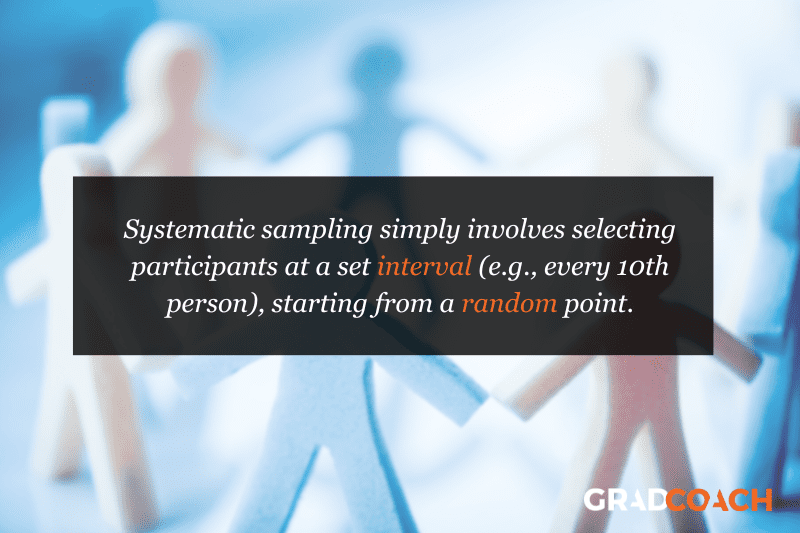
Non-probability-based sampling methods
Right, now that we’ve looked at a few probability-based sampling methods, let’s look at three non-probability methods :
Again, this is not an exhaustive list of all possible sampling methods, so be sure to explore further if you’re interested in adopting a non-probability sampling approach.
First up, we’ve got purposive sampling – also known as judgment , selective or subjective sampling. Again, the name provides some clues, as this method involves the researcher selecting participants using his or her own judgement , based on the purpose of the study (i.e., the research aims).
For example, suppose your research aims were to understand the perceptions of hyper-loyal customers of a particular retail store. In that case, you could use your judgement to engage with frequent shoppers, as well as rare or occasional shoppers, to understand what judgements drive the two behavioural extremes .
Purposive sampling is often used in studies where the aim is to gather information from a small population (especially rare or hard-to-find populations), as it allows the researcher to target specific individuals who have unique knowledge or experience . Naturally, this sampling method is quite prone to researcher bias and judgement error, and it’s unlikely to produce generalisable results, so it’s best suited to studies where the aim is to go deep rather than broad .
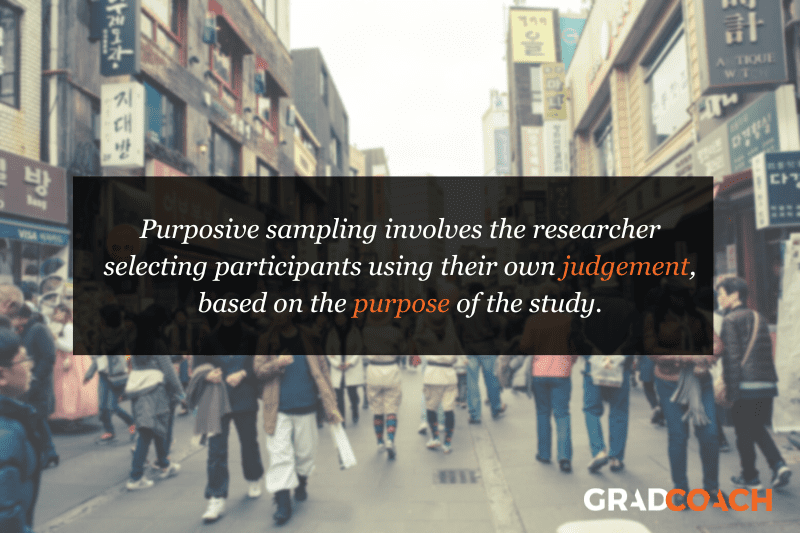
Next up, we have convenience sampling. As the name suggests, with this method, participants are selected based on their availability or accessibility . In other words, the sample is selected based on how convenient it is for the researcher to access it, as opposed to using a defined and objective process.
Naturally, convenience sampling provides a quick and easy way to gather data, as the sample is selected based on the individuals who are readily available or willing to participate. This makes it an attractive option if you’re particularly tight on resources and/or time. However, as you’d expect, this sampling method is unlikely to produce a representative sample and will of course be vulnerable to researcher bias , so it’s important to approach it with caution.
Last but not least, we have the snowball sampling method. This method relies on referrals from initial participants to recruit additional participants. In other words, the initial subjects form the first (small) snowball and each additional subject recruited through referral is added to the snowball, making it larger as it rolls along .
Snowball sampling is often used in research contexts where it’s difficult to identify and access a particular population. For example, people with a rare medical condition or members of an exclusive group. It can also be useful in cases where the research topic is sensitive or taboo and people are unlikely to open up unless they’re referred by someone they trust.
Simply put, snowball sampling is ideal for research that involves reaching hard-to-access populations . But, keep in mind that, once again, it’s a sampling method that’s highly prone to researcher bias and is unlikely to produce a representative sample. So, make sure that it aligns with your research aims and questions before adopting this method.
How to choose a sampling method
Now that we’ve looked at a few popular sampling methods (both probability and non-probability based), the obvious question is, “ how do I choose the right sampling method for my study?”. When selecting a sampling method for your research project, you’ll need to consider two important factors: your research aims and your resources .
As with all research design and methodology choices, your sampling approach needs to be guided by and aligned with your research aims, objectives and research questions – in other words, your golden thread. Specifically, you need to consider whether your research aims are primarily concerned with producing generalisable findings (in which case, you’ll likely opt for a probability-based sampling method) or with achieving rich , deep insights (in which case, a non-probability-based approach could be more practical). Typically, quantitative studies lean toward the former, while qualitative studies aim for the latter, so be sure to consider your broader methodology as well.
The second factor you need to consider is your resources and, more generally, the practical constraints at play. If, for example, you have easy, free access to a large sample at your workplace or university and a healthy budget to help you attract participants, that will open up multiple options in terms of sampling methods. Conversely, if you’re cash-strapped, short on time and don’t have unfettered access to your population of interest, you may be restricted to convenience or referral-based methods.
In short, be ready for trade-offs – you won’t always be able to utilise the “perfect” sampling method for your study, and that’s okay. Much like all the other methodological choices you’ll make as part of your study, you’ll often need to compromise and accept practical trade-offs when it comes to sampling. Don’t let this get you down though – as long as your sampling choice is well explained and justified, and the limitations of your approach are clearly articulated, you’ll be on the right track.
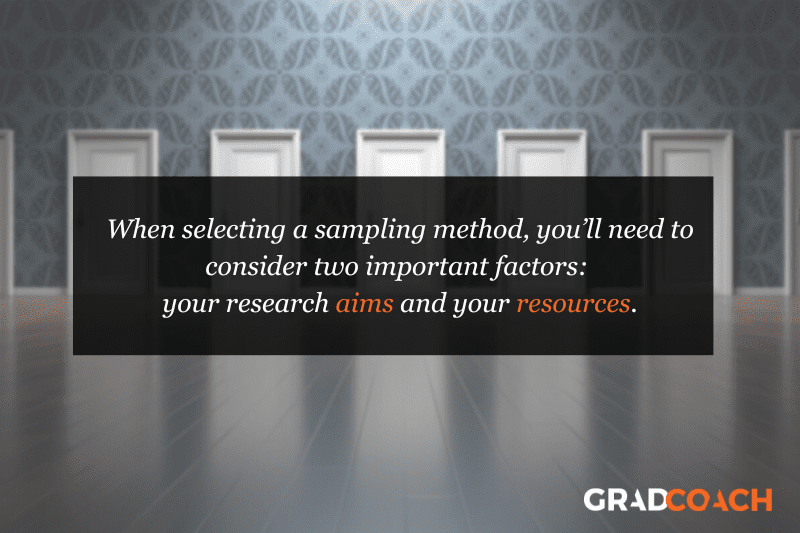
Let’s recap…
In this post, we’ve covered the basics of sampling within the context of a typical research project.
- Sampling refers to the process of defining a subgroup (sample) from the larger group of interest (population).
- The two overarching approaches to sampling are probability sampling (random) and non-probability sampling .
- Common probability-based sampling methods include simple random sampling, stratified random sampling, cluster sampling and systematic sampling.
- Common non-probability-based sampling methods include purposive sampling, convenience sampling and snowball sampling.
- When choosing a sampling method, you need to consider your research aims , objectives and questions, as well as your resources and other practical constraints .
If you’d like to see an example of a sampling strategy in action, be sure to check out our research methodology chapter sample .
Last but not least, if you need hands-on help with your sampling (or any other aspect of your research), take a look at our 1-on-1 coaching service , where we guide you through each step of the research process, at your own pace.
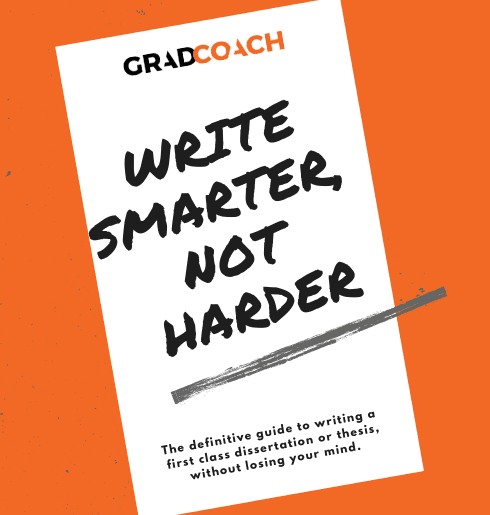
Psst… there’s more (for free)
This post is part of our dissertation mini-course, which covers everything you need to get started with your dissertation, thesis or research project.
You Might Also Like:
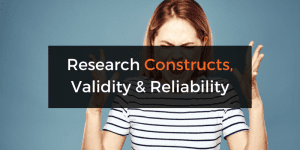
Excellent and helpful. Best site to get a full understanding of Research methodology. I’m nolonger as “clueless “..😉
Excellent and helpful for junior researcher!
Grad Coach tutorials are excellent – I recommend them to everyone doing research. I will be working with a sample of imprisoned women and now have a much clearer idea concerning sampling. Thank you to all at Grad Coach for generously sharing your expertise with students.
Submit a Comment Cancel reply
Your email address will not be published. Required fields are marked *
Save my name, email, and website in this browser for the next time I comment.
- Print Friendly

An official website of the United States government
The .gov means it’s official. Federal government websites often end in .gov or .mil. Before sharing sensitive information, make sure you’re on a federal government site.
The site is secure. The https:// ensures that you are connecting to the official website and that any information you provide is encrypted and transmitted securely.
- Publications
- Account settings
Preview improvements coming to the PMC website in October 2024. Learn More or Try it out now .
- Advanced Search
- Journal List

Stratified Sampling of Neighborhood Sections for Population Estimation: A Case Study of Bo City, Sierra Leone
Roger hillson.
1 Information Technology Division, Naval Research Laboratory, Washington, District of Columbia, United States of America
Joel D. Alejandre
Kathryn h. jacobsen.
2 Department of Global and Community Health, George Mason University, Fairfax, Virginia, United States of America
Rashid Ansumana
3 Njala University, Bo, Sierra Leone
4 Mercy Hospital Research Laboratory, Bo, Sierra Leone
Alfred S. Bockarie
Umaru bangura, joseph m. lamin, david a. stenger.
5 Center for Bio/Molecular Science and Engineering, Naval Research Laboratory, Washington, District of Columbia, United States of America
Conceived and designed the experiments: RH. Performed the experiments: RH. Analyzed the data: RH JDA. Contributed reagents/materials/analysis tools: KHJ RA ASB UB JML DAS. Wrote the paper: RH KHJ JDA RA DAS.
Associated Data
All relevant tabular data are within the paper and its Supporting Information files. GIS data are available on OpenStreetMap ( http://osm.org/go/am_ZKeeU- ).
There is a need for better estimators of population size in places that have undergone rapid growth and where collection of census data is difficult. We explored simulated estimates of urban population based on survey data from Bo, Sierra Leone, using two approaches: (1) stratified sampling from across 20 neighborhoods and (2) stratified single-stage cluster sampling of only four randomly-sampled neighborhoods. The stratification variables evaluated were (a) occupants per individual residence, (b) occupants per neighborhood, and (c) residential structures per neighborhood. For method (1), stratification variable (a) yielded the most accurate re-estimate of the current total population. Stratification variable (c), which can be estimated from aerial photography and zoning type verification, and variable (b), which could be ascertained by surveying a limited number of households, increased the accuracy of method (2). Small household-level surveys with appropriate sampling methods can yield reasonably accurate estimations of urban populations.
Introduction
The population of a region of interest must be estimated if one’s goal is to convert incidence counts into rates. This conversion is not always necessary, because some epidemiological parameters can now be estimated from incidence counts alone, including the interval between successive cases, and the reproductive number R 0 , which is the average number of secondary cases attributable to a primary cause [ 1 , 2 ]. If these parameters are insufficient to evaluate the models, it may be necessary to calculate the total population N . The 5 brief examples that follow illustrate both the necessity of doing so, and some of the difficulties that may be encountered.
In resource-limited environments, it may be possible to use both aerial imagery and limited residential survey data to estimate the population of a region of interest, as shown in the first two examples. Using ground truth data for the measured population of 20 sections in Bo City, Sierra Leone, we compared the uncertainty of estimating the population using survey data for either (1) occupants per residence or (2) rooftop area per resident. The latter variable was computed by manually digitizing the rooftop areas of residential structures in 5 sections of Bo, and calculating the ratio of rooftop area per occupant for each residence [ 3 ]. The ability to rapidly estimate the population of both temporary and unplanned settlements is critical for planning resource allocation for refugee and internally displaced populations as well as for places undergoing rapid unplanned urbanization, since in these settings there is usually not a stable residential population. Checchi et al. [ 4 ] have developed a two-step method for estimating a refugee population that requires (1) estimating the number of temporary residential structures from satellite imagery and (2) estimating the mean occupancy per structure. The product of the estimate (1) “number of structures” and (2) “mean number of persons per structure” yields an estimate of the total refugee population.
As shown in the next 2 examples, if salient population data are available either directly or by interpolation; derived rates of infection, immunity, or morbidity may be calculated. The standard SEIR (Susceptible, Exposed, Infectious, Recovered) compartmental epidemiological model [ 5 , 6 ] requires N as a parameter. Glasser et al. [ 6 ] simulated the implementation of two different influenza vaccination policies, in order to predict their effect on both the incidence of infection and the rate of morbidity. They applied a SEIR model parameterized by demographic parameters for the United States (2005), including the total population stratified by age. The age-specific death rates attributable to pneumonia and influenza were estimated, as were the death rates from all other remaining causes. Gomez-Elipe et al. [ 7 ] have developed a model for forecasting the incidence of Malaria in Karuzi, Burundi (1997–2003). To convert the reported instances of malaria to a rate, the investigators divided the rate by the 2006 population census, after rescaling (decrementing) by the population growth factors for the intervals from 1995–2000 (growth factor = 1.32) and 2000–2005 (growth factor = 3.29).
In demographically-diverse environments, different methods may be required to estimate the population at different locations, as shown in our final example. The GRUMPv1 (Global Rural-Urban Mapping Project, Version One) , separates the urban population density estimates from the population of the surrounding areas. In addition to enumerated city population data, city footprints can be established by analyzing nighttime satellite images, but this approach may fail to capture small informal settlements in Africa and rural Asia [ 8 ] (page 9). Accordingly, several corrections are applied for poorly illuminated settlements [ 8 ] (page 9), and point estimates are provided for settlement populations exceeding 1,000. Many models utilize GRUMP for epidemiological modeling, including [ 8 , 9 ].
Proposed analysis
In a previous study [ 3 ], a Finite Population Bootstrap (FPB) [ 10 ] (page 92) was used to compare the relative uncertainty of two population estimators: an occupancy-based estimator and a rooftop area-based estimator. For the region of interest, the former was estimated as the product of (1) the average number of persons per residential structure multiplied by (2) the total number of residential structures; and the latter was calculated as (1) the average number of persons per rooftop area (i.e., persons per m 2 ) multiplied by (2) the total estimated rooftop area in m 2 . Both estimators were effective, but the uncertainty was about 20% less for the occupancy-based estimator [ 3 ] (page 10). Both the occupancy-based and rooftop area-based population estimators were evaluated by simulating simple random sampling without replacement (SRSWOR).
The analysis in this current paper will evaluate the use of stratified sampling for population estimation, and will demonstrate the reduction in the uncertainty of the population estimate achievable relative to SRSWOR. Two different stratification designs will be explored: (1) optimal stratification by “persons per structure” and (2) stratified single-stage cluster sampling. The relative advantages and restrictions of both methods will be discussed. The city of Bo itself is approximately 30.1 km 2 in area, and is divided into 68 uniquely-shaped neighborhoods or sections [ 11 ](see Fig 1 in [ 3 ] and Table 1 ). These sections vary in size from 0.02 km 2 (Toubu) to 2.33 km 2 (Bo Government Reservation). For 20 of the 68 sections, residential survey data are also available [ 3 ] (see Table 1 ). The ground truth survey data for these 20 sections will provide the basis for simulated sampling using different stratification protocols, and for quantifying the reduction in the uncertainty of the population estimate achievable.
A summary of the residential and household survey data for 20 municipal sections of Bo (1), showing the area of each section (2); the total number of residential structures, combined residential and non-residential structures, households, and persons per section (3–6); and the mean occupancy per residential structure (7) [ 3 , 11 ].
The first approach, optimal stratification by persons per structure, requires that the number of persons per structure be already known for all residential structures; possibly from a prior survey or census data. The objective is to exploit this prior data to design an improved stratification protocol for re-estimating the population, and to demonstrate a significant reduction in the uncertainty of the population estimate relative to random sampling. Single-stage cluster sampling is useful if the number of sections that can actually be sampled is restricted, perhaps because of cost or schedule limitations. In our examples, the simulated cluster sampling will be restricted to 4 of the 20 available sections. We will investigate the reduction in uncertainty that can be achieved by using a stratified cluster sampling protocol, rather than random selection, to select the 4 sections on each simulation trial. Each section will be completely sampled.
Note that choice of population estimators is independent of the stratified sampling protocol selected for simulated data collection. A stratified Horvitz-Thompson [ 12 ] population estimator will be evaluated for all examples. We have also extended our original FPB model to support stratified sampling [ 10 ], and partial results from the latter will be contrasted with estimates obtained using the stratified Horvitz-Thompson estimator. Neither the stratified FPB nor the Horvitz-Thompson estimator were used in the prior study.
More specifically, we will address the following 4 questions:
- What reduction in the uncertainty of the population estimate can be achieved by stratified sampling—relative to simple random sampling of all sections—if the residential survey records are first partitioned into mutually-exclusive strata with non-overlapping ranges of “persons per residential structure?”
- Can any reduction in uncertainty be achieved—again relative to simple random sampling of all sections—if the sections are partitioned into mutually-exclusive and exhaustive strata, rather than partitioning the individual records (PSUs) into strata?
- For single-stage cluster sampling, if the sections (clusters) are partitioned into mutually-exclusive strata by “total residential structures per section,” what is the relative reduction in uncertainty that can achieved using stratified cluster sampling, rather than unclassified cluster sampling?
- Does stratification by the “total persons per section”—if known—further reduce the uncertainty of the single-stage cluster population estimates?
We will use a single dataset developed previously in [ 3 ] (see Table 1 ). This dataset contains individual records for each of 1,979 residential structures surveyed. Each record includes the number of persons in the structure, a variable that we will utilize in this paper. The survey methodology and data collection methods used to construct the dataset analyzed in this manuscript were all developed previously. The original articles [ 3 , 11 ] should be consulted for a complete discussion. The current article complements and extends these prior studies, but does not supplant them.
The utility of these methods for the 5 initial examples, which were presented to establish the importance of estimating the population of a region of interest, will depend upon the availability of partial survey data for occupancy, the existence of adequate estimates of the total number of residential structures, and the presence of stable patterns of residential occupation. Neither method is likely to be useful for improved estimation or re-estimation of the population of a highly transient population living in temporary shelters as described by Checchi et al. [ 4 ].
Model development
The simulations described in this investigation were written in the programming language R [ 13 ]. Supporting functions from multiple R libraries were used, including [ 14 – 16 ]. Additional custom code was written and tested by the first author. The R package stratification [ 16 , 17 ] provides algorithms for finding the optimal boundaries for a variable Y , based on criteria proposed by Lavallée and Hidiroglou [ 18 ]. This package supports several different heuristics, including Kozak’s algorithm [ 19 , 20 ] which can also find the optimized boundaries for a specified sample size n .
In all of the examples presented here, the true optimal boundaries were found through exhaustive search. Given the relatively small size of the dataset (1,979 records), all possible combination of strata boundaries were tested to determine which set minimized the uncertainty of the population estimate as a function of sample size [ 17 ] (page 33).
Survey methodology and dataset development
Ethics statement.
All data collection involving human subjects was approved by a total of three independent Human Subjects Research Institutional Review Boards: Njala University, George Mason University, and the U.S. Naval Research Laboratory. Written informed consent was obtained from each household representative who participated in the survey. Survey data were obtained as part of a broader study to determine not only population demographics but health metrics and health care utilization trends.
Terminology
Structures in Bo City were divided into two categories. “Nonresidential” structures included governmental, commercial, and nonprofit organizational structures such as places of worship. “Residential” structures included all structures used as sleeping quarters. Fig 1 in [ 3 ] shows the 20 sections in which the surveys were conducted. Some surveyors were staff of Mercy Hospital Research Laboratory (MHRL); most were Master of Public Health students at Njala University. The surveyors received several days of training, including instruction on geographic data collection using hand-held GPS units, interviewing techniques, and research ethics—including an emphasis on confidentiality. During the interviews, one representative—an adult of either sex—served as a representative of each household. Each residential record lists the number of persons reported living within the same residential structure, and the number of separate households. No attempt was made to differentiate between persons based on gender, age, or household affiliation.
Protection of human subjects
This field work was a joint task of Njala University, George Mason University, and the U.S. Naval Research Laboratory. Institutional review boards (IRB) at all three institutions approved the data collection methodology.
Bo City dataset
Our sampling frame is a list of 1,979 residential structures encompassing 20 of the 68 sections in Bo City. For each residential structure, there is a unique single record listing the number of persons and households; because these records can be randomly selected, this database will provide the basis for simulated sampling of residential structures. By definition, each residential structure is also a Primary Sampling Unit (PSU). A cluster is defined as a logical collection of PSUs [ 21 ](page 24); in this study, a cluster and a Bo City section will be treated as synonymous in the context of single-stage cluster sampling.
Overview of stratified sampling
The flowchart in Fig 1 summarizes the algorithms and simulations that will be developed in the text. The objective of this study is to investigate alternative approaches for stratified sampling of the residential structures in a resource-limited environment, and to determine the relative reduction in the uncertainty of the estimate of the total population—if any—that results. In all cases, it is assumed that at least the number of residential structures in each section are known. This flowchart may be referenced as the two major protocols are developed and simulated in detail.

This figure summarizes all of the optimization and control protocols for stratified sampling developed in this study. See text for a summary of each major protocol and its corresponding steps through the flow chart. The light brown parallelogram is the starting point for all protocols, the yellow diamonds are decision boxes, and the light green squares denote the process end states.
Optimal stratification by persons per residence
As with any stratified sampling scheme, the PSUs (Primary Sampling Units) —the 1,979 individual residential structures (see Table 1 )—must first be divided into mutually-exclusive and exhaustive strata [ 21 ] (page 121). After the stratification boundaries have been determined, simulated sampling can be executed. Based on pilot studies, we determined that 4 levels of stratification would be sufficient for proof of concept. The stratification and estimation algorithms will be summarized later. The survey variable X and the stratification variable Y are the same—specifically, the number of persons per residential structure. For this reason, it was not necessary to model the relationship between Y , the measured survey variable (persons per residential structure), and X , the stratification variable [ 17 ].
On each simulation trial, a subset of the PSUs were randomly selected from each stratum as a function of (1) the total sample size and (2) the allocation algorithm selected. This step created a stratified sample of the PSUs. A stratified Horvitz-Thompson estimator was then used to re-estimate the total population of the 20 pooled sections [ 12 , 17 , 21 ]. Referring to Fig 1 , the objective was to use the previously collected survey data to design a survey protocol that would:
(1) → (2) → (3) → (4 a ) → (5).
(1) → (2) → (3) → (4 b ) → (5)
Stratified single-stage cluster sampling
When schedule or resources restrict the survey to a subset of sections within the region of interest, single-stage cluster sampling can be applied. (If there is no restriction on the number of sections to be sampled, all sections can be sampled without replacement for a given sample size.) Assume that the number of residential structures per section is known, but not the number of persons per section. The 20 sections will first be partitioned into the desired number of mutually-exclusive strata, using the section sizes (i.e., total residential structures per section) as the stratification variable; see Table 1 for these values. Each residence in a section will be assigned to the same stratum. For each trial of the stratified single-stage clustering protocol, one section will be selected from each stratum, and all of the residences in the selected sections will be completely sampled. For the control case, the same number of sections will be selected, but the stratification boundaries will be ignored. In effect, in the control case, all sections will be assigned to a single stratum.
- (1) → (2 a ) → (4′ a ) → (5′ a )
If the total population of each cluster is known, “total persons per section” can be used as the stratification variable, rather than “total residential structures per section.” The relative uncertainty of the population estimate for single-stage cluster sampling will be further reduced.
- (1) → (2) → (4′ b ) → (5′ b )
Single-stage cluster sampling may also be executed without stratification, but in the simulations that follow, the uncertainty of the population estimate will be roughly doubled for the unstratified case. The cluster sampling protocol is appropriate when financial or schedule constraints impose limits on the number of sections to be sampled. The advantages of stratified cluster sampling are:
- No auxiliary data is required other than a count of residential structures in each of the 20 sections under consideration. If the total population of each section is available, an even more efficient design can be realized.
- A cluster design permits a trade-off between the size of the survey, the number of sections sampled, and the uncertainty of the population estimate.
Optimal stratification
Let L strata be defined on the stratification variable X , the number of persons per residential structure. Number the strata h = 1, 2…, L . Define the boundaries of the strata as b h = 1 , b h = 2 , …, b h = L . Stratum h will include all values of X in the interval [ b h −1 , b h ) such that b h −1 < X ≤ b h .
Assume that there is a total of N units or records that are being stratified. An optimal solution of the values b 1 ≤ b 2 ≤ b L −1 for a sample of size n minimizes the following objective function [ 19 ] ( Eq 3 ):
- N L is the size of stratum h
- W h = N h / N is the proportion of the total units (records) in N assigned to stratum h
- S h is the standard deviation of the stratification variable Y in stratum h
- Y ‾ is the population mean of the survey variable Y
- c is the CV (coefficient of variation) of the survey variable Y
- N is the total number of records or units being partitioned into strata
Allocation selection
When the strata boundaries are optimized for a given sample size n , the coefficient of variation of Y is minimized [ 17 ]. Note that the constraint for optimization is dependent not only on the distribution of the stratification variable Y , but also upon the allocation rule used. The allocation rule chosen will determine the weights W h . The allocation rule used in the R package stratification [ 16 ] is developed in [ 22 ].
Let a h be the proportion of samples assigned to the h th stratum. Then:
Given a total sample size n , the sample sizes for each “take-some” stratum will be:
Setting q 1, q 2 and q 3 to (0.5, 0.0, 0.5) parameterizes Neyman’s allocation for each stratum, while (0.5, 0.0, 0.0) corresponds to proportional allocation. When Neyman’s allocation is used, a sample size n h may be equal to or greater than the number of available PSU’s N h . The stratum may then be categorized as a “take-all” stratum [ 17 ], and every record (i.e. PSU) in the stratum will be selected, rather than a subset of the stratum records. If necessary, the sample sizes of one or more of the remaining strata are transparently incremented to realize the desired total sample size n .
Neyman allocation will minimize the variance (i.e. uncertainty) of the stratified population estimator. The Neyman allocation for a sample of size n is equivalent to the expression below [ 21 ] (page 158):
The Horvitz-Thompson estimator
The Horvitz-Thompson (H-T) estimator provides an unbiased estimate of the total population from either a stratified or unstratified sample, provided the inclusion probabilities are greater than zero for each unit sampled [ 23 ]. Let the sample size be n , the value of the i th individual record or unit be y i , and define π i = n h / N h as the inclusion probability for the i th record in strata h . (For the important special case where all units are assigned to a single stratum, π i = n / N , and all units are assigned the same probability of inclusion. In the text, this is referred to as the control case.) For simple random sampling without replacement, the Horvitz-Thompson estimator is then:
This expression could be simplified, but the double summation makes clear that the total population estimate is the sum of the weighted estimates for the individual strata.
Optimal stratification for resampling
In our first set of demonstrations, we evaluated a design for resampling a known population for which complete survey data exists [ 19 ]. Using the optimization approach described earlier, the 1,979 units were divided into 4 strata, using the number of persons per residential structure as the sampling variate Y . The choice of L = 4 as a reasonable number of strata was based on the findings from preliminary simulation studies. Five different random sample sizes were selected: 330, 660, 990, 1,320, and 1,650 records, out of the total 1,979 records available. Simulations were run using both proportional and Neyman allocation.
For each sample size, 1,000 random trials were run. In each trial, a stratified sample was selected, and the Horvitz-Thompson population estimate calculated. The inclusion probability π h for each record in the sample was calculated as shown in Table 2 .
Table 2a: Optimal samples per stratum as a function of sample size. Table 2b: Optimal allocation of residential structures per stratum as a function of sample size. Table 2c: The inclusion probability π h = h [ h ]/ Nh [ h ] as a function of sample size. Table 2d: The upper strata boundaries as a function of sample size.
Table 2a lists the number of residential structures to be sampled in each stratum for optimal stratification of the variable “persons per residential structure.” Table 2b is the total number of residential structures per stratum, while Table 2c specifies the ratios of samples per stratum divided by the total number of residential structures per stratum. These ratios are not constant for each sample size because the optimization was constrained by Neyman allocation, rather than proportional allocation. Table 2d lists the upper boundary limits as a function of sample size.
Stratified finite population bootstrap
The samples drawn for each stratum were also concatenated and resampled [ 10 , page 97], [ 14 , 24 ] creating a bootstrap sample of size n h for each strata. The n h samples from each strata were then combined to create a single sample of size n (330, 660, 990, …), and the total population was estimated using the FPB. For the control group and the proportional allocation case, the estimated population obtained using the FPB was compared with the results from the Horvitz-Thompson estimations. (Neyman allocation could not be compared, since the individual bootstrap estimates for each stratum required proportional allocation.)
The FPB model mirrored the decrease in uncertainty observed with the H-T estimator using optimal proportional allocation, but the variance of the FPB is greater. The average ratio of the 0.95 confidence intervals between the H-T estimator and FPB estimator was approximately 0.70 for the control group, and 0.58 when comparing the estimators for optimal proportional allocation. A paired t-test was used to compare the intervals, and P < 0.001 in both cases. For the control case, 67% of the H-T estimators fell within the 0.50 confidence interval for the FPB, quantifying the greater uncertainty of the FPB estimator. Likewise, comparing the proportionally-allocated 4 strata case, 76% of the H-T estimators fell within the 0.50 confidence interval for the FPB. The FPB used is one of a family of finite population bootstrap algorithms. A recent study [ 24 ] compared the variance characteristics of different implementations of the FPB, and proposed a new FPB algorithm may present reduced uncertainty relative to the implementation used here.
Relative uncertainty of the population estimates
Fig 2 illustrates the [0.25, 0.75] quantile boxplots as a function of sample size for the H-T estimator for the single-stratum control case (A), and using proportional (B) and Neyman (C) allocation, respectively. The mean ratios of the 0.95 confidence intervals were 0.58 and 0.19, respectively ( P < 0.001 and P < .005). In summary, the uncertainty using optimal stratification with Neyman allocation was roughly 20% of the uncertainty observed for the single stratum control group, averaged over 1,000 simulations.

Quantile boxplots (0.25, 0.75) showing the distribution of the stratified Horvitz-Thompson population estimates as a function of sample size and stratification protocol. The bar in each box is the median value of the estimate, while outliers deviating by one or more quantiles from the median are denoted as discrete points. (A) control—all 20 sections are placed in a single stratum (B) 4 strata, with proportional allocation for sample selection (C) 4 strata, with Neyman allocation for sample selection. Persons per residence was used as the stratification variable, and there were 1,000 simulations for each boxplot.
Coefficient of Variation optimization
A single example will be provided for optimizing the Coefficient of Variation, as illustrated schematically in Fig 1 . The CV is equal to the RRMSE , the Relative Root Mean Squared Error. The target value of the CV was set to be ≤ 0.01. When 4 levels of stratification were requested, Neyman allocation was enabled, and “persons per residential structure” was selected as the stratification variable. The critical minimal sample size returned by the stratification algorithm was 456. The upper stratification boundaries (persons per residential structure) were:
- stratum 1: 9.5
- stratum 2: 17.5
- stratum 3: 31.5
- stratum 4: 86.0
Single-stage cluster sampling
Table 3 shows the results of applying the Neyman stratification algorithm. For a sample of some specified number of clusters (sections), the recommended number of sections to select are given for each stratum. The variable bh [ h ] specifies the upper boundary in “residential structures per section” for each stratum h . The stratification algorithm actually returns the first three boundaries, since the upper boundary of the 4th stratum is the maximum possible value of the stratification variable, which is 208—the number of residential structures in the New London section. The variable nh [ h ] indicates the allocated number of clusters that should be selected from each stratum for a balanced sample of a given size in clusters (sections). Given 4 stratification levels, the minimum number of clusters that can be selected is 4, and the recommended sample allocation is (1, 1, 1, 1). A comparable table was generated for proportional allocation, and for an allocation of (1, 1, 1, 1) sections per stratum, the stratification partition was identical. Table 4 shows the stratification by section for the 4-section allocation (1, 1, 1, 1), which was used in the simulations, and the 10-section allocation (2, 1, 6, 1) provided for comparison.
Stratification variables for selecting clusters (sections) for one-stage stratified cluster sampling with 4 levels of stratification ( L = 4). The entries in each column are (1) the number of clusters to be selected (2) the minimum number of PSU’s (i.e. residential structures) spanned by the selected clusters if the allocation nh [ h ] is drawn (3) the number of clusters (sections) nh [ h ] to be drawn from each stratum (4) the total number of clusters Nh [ h ] in each stratum and (5) the upper boundary bh [ h ] in units of “residential structures per cluster” for each of the four strata. A comparable table was constructed for stratification by population per section, but is not shown for the sake of brevity.
4-level Neyman stratification boundaries for the cluster (section) list stratified by the number of residential structures per section (cols. 4–5), and the number of persons per section (cols. 6–7). In practice, the latter may be unknown. An allocation of (1, 1, 1, 1) sections per stratum is optimal for a sample size of 4 sections, which was used in our examples. The (2, 1, 6, 1) allocation, shown for comparison, is optimal for a 10-section sample. See Table 3 .
In our implementation of simulated single-stage cluster sampling, the allocation used on each trial was (1, 1, 1, 1), because the objective was to estimate the population while minimizing the number of sections sampled. A single section was selected from each stratum, as discussed previously. In the control protocol, 4 sections were selected randomly without replacement from the unconstrained population of 20 sections. The form of the Horvitz-Thompson for single-stage cluster sampling is [ 21 ] (page 336):
- y i = the total number of persons for i th cluster (section)
- π i = the probability of the i th cluster being sampled during this trial
- v = the total number of clusters sampled (i.e., 20)
This estimator provides an unbiased estimate of the total population.
Optimal stratified sampling
The relative efficiency of optimal stratification by persons per residential structure has already been discussed. As shown in Fig 2 , the uncertainty of the population estimation with Neyman allocation was roughly 20% of the uncertainty observed for the unstratified control group, averaged over 1,000 simulations. Table 5 compares the variance and standard error of the mean (SEM) of the Horvitz-Thompson estimators for 1,000 simulated single-stage cluster sampling trials, selecting a fixed sample size of 990 records.
A comparison of the variance σ 2 and the SEM ( Standard Error of the Mean ) of the Horvitz-Thompson (H-T) estimator for 1,000 simulated sampling trials, and a fixed sample size of 990. For the unstratified control case (A), all sections were assigned to a single stratum, in contrast to 4-level optimal stratification using either proportional (B) or Neyman allocation (C). The stratification variable is “persons per residential structure” and Table 2 , subtable 2a, specifies the samples per stratum.
The Levene test [ 25 , 26 ]was used to compare the variances of the stratified protocols with the variance of the unstratified control group. The paired comparisons were blocked by sample size. The null hypothesis for the Levene test is that the ratio of 2 specified variances is equal to 1.0. For all tests, σ x | N = n 2 was the variance for 1,000 simulated trials for sample size of n (e.g., 330, 660, 990 …) using 4-level Neyman or proportional allocation, and σ c | N = n 2 the variance 1,000 simulated trials for the comparable unstratified control case. The differences between the variances were statistically significant, with p < 0.001 for all comparisons, and the hypothesis that the ratio σ x | N = n 2 /σ c | N = n 2 = 1.0 was rejected for all tests.
Fig 3 shows the box histograms for the single-stage cluster sampling simulations. The uncertainty of the population estimation using stratified cluster selection is about 48% of the uncertainty of the estimation based on random cluster selection, as measured by comparing the [0.25, 0.75] quantile intervals. This difference is significant at P < 0.001 (paired t-test).

Quantile boxplots for 1,000 stratified 4-level simulated single-stage cluster sampling trials using H-T estimation. The bar in each box is the median value of the estimate, while outliers deviating by one or more quantiles from the median are denoted as discrete points. Four selected sections are completely sampled on each simulation trial. (1) “Survey” is the measured value of the population of the 20 sections (25,954 persons). (2) 4 L /4 C (pers.)—4 cluster sample, sections stratified by “persons per section.” (3) 4 L /4 C (strs.)—4 cluster sample, sections stratified by “residential structures per section.” (4) 1 L /4 C —4 clusters selected at random from the 20 available sections.
In single-stage sampling, if a section is selected from one of the four strata, all residences in the section are then included in the sample. Each stratum contains a mutually-exclusive subset of the 20 sections, with non-overlapping ranges of buildings per section between the strata. Because a single section is selected from each stratum for each one-stage survey sample, the sample allocation is balanced with respect to the stratification variable “residential structures per section.” See Table 4 , column 4.
Table 6 compares of the variance and standard error of the mean (SEM) of the Horvitz-Thompson estimator for 1,000 simulated sampling trials, selecting 4 sections on each trial. The average number of residences selected per trial are shown in the table. For the unstratified control case, all sections were assigned to a single stratum, in contrast to 4-level optimal stratification using either proportional or Neyman allocation. The variance ratios were again compared between all three protocols using the Levene test. The differences between the variances were statistically significant, with p < 0.001 for all comparisons.
Comparison of the standard deviation σ , the variance σ 2 , and the SEM ( Standard Error of the Mean ) for the single-stage sampling protocol. The uncertainty of the Horvitz-Thompson population estimate decreases as a function of the protocol used to partition the 20 sections of Bo City into 4 strata: (A) unstratified single-stage sampling (B) stratification by number of buildings per section (C) stratification by the total number of persons per section. There are 1,979 residential structures in the 20 sections, and a measured population of 25,954 persons.
If the 4 sections for the single-stage protocol are chosen at random, rather than in accordance with the stratification partition, the uncertainty of the population estimate for 1,000 simulation trials is roughly doubled ( Fig 3 ). This occurs because the sampling protocol is no longer balanced with respect to the number of buildings per section. The probability of selecting a single section from each of the 4 strata is now 11%, rather than 100% ( Eq 13 ). Conversely, almost 90% of the samples drawn will consist of sections drawn from 3 strata or fewer. The theoretical probability that a section will be selected from each of the 4 sections on a given trial is:
The above calculation is consistent with the simulation results, in which 119 balanced 4-strata samples were drawn in 1,000 random trials. A comparable argument applies to the simulations using the number of persons per section as the stratification variable.
Stratification by section for non-cluster sampling
If “persons per structure” are known, optimal stratification boundaries and allocations can be found [ 18 ]. Each stratum will contain residences from one or more sections. In single-stage cluster analysis, the sections are partitioned into strata by either “(a) residential structures per section” or “(b) total persons per section,” and one or more sections are selected on each trial from each stratum for complete sampling. As a third possibility, if “persons per structure” are unknown, we may ask whether either of the stratification variables (a) or (b) could be used to efficiently partition the 20 sections into mutually-exclusive strata for non -cluster sampling. All of the residences in a given section would be assigned to the same stratum, and a given stratum would contain all of the residential records from the subset of sections assigned to it. A sample of residential records would be drawn from each stratum on a given trial, usually without completely sampling any one section. This protocol could prove advantageous if the proposed partitioning is more efficient than simple random sampling without replacement, even if it is less efficient than optimal stratification by “persons per structure.”
There are two difficulties with attempting to stratify the data at the section level, rather than at the level of the individual record. For any stratification plan to be viable, the units within a stratum must be relatively coherent with respect to the stratification variable selected. If the stratification variable is “persons per section,” this goal will be difficult to achieve. Fig 4 shows the quantile boxplots for the number of buildings per section, arranged from left to right in order of decreasing number of persons per section. The upper and lower “hinges” correspond to the first and third quartiles (the 25th and 75th percentiles), and the band inside the box is the 2nd quartile (i.e., the median) value of the number of persons per residential structure. The width of each box is proportional to the square root of the number of residential structures (i.e., records) in the section [ 27 ]. Roma appears to be anomalous because, although there are only 4 residential structures in this section, there are a total of 139 persons, because these structures are apartment complexes, rather than individual homes. As can be seen, there will be significant overlap between the the ranges of persons per structure for virtually any partitioning of the 20 sections used.

For each section, a quantile boxplot (0.25, 0.75) shows the distribution of the number of persons per residence, arranged in descending order of total section population. The bar in each box is the median value, while outliers deviating by one or more quantiles from the median are denoted as discrete points. The width of each box is proportional to the square root of the number of residential structures (i.e., records) in the section. Roma is an anomaly with 4 residential structures, and 139 total persons.
To clarify the above discussion, two experimental simulations were run. The same 4-level partition used for the single-stage cluster sampling was used to define a non-clustered random sampling protocol. Every record in a section was then assigned to the same designated stratum. For example, all records for Kulanda Town, Nduvuibu, and New London were assigned to stratum 4—see Table 4 . Residences were then randomly selected from all 4 strata, and the number of residences selected from each stratum was proportional to the total number of residences the stratum contains. 1,000 simulated sampling trials were run, using the same sequence of 5 sample sizes used for the optimal stratification analysis (see Table 2 ). Because each stratum contained records from multiple sections, each sample typically contained records from multiple sections. Conversely, none of the sections were completely sampled on a given trial, in contrast to the protocol for the single-stage cluster model. For a second simulation, the stratification variable “persons per section” was used, rather than “residential structures per section.” See columns 4 and 5 in Table 4 . The results are summarized in the next paragraph, but are not presented in a table or figure.
Relative to simple random sampling without replacement of all strata, which was also simulated as a control, the reduction in uncertainty for section-based non-cluster stratification was minimal and statistically insignificant. Levene’s test was again used to compare the ratio of the σ 2 s. The ratio of σ x 2 /σ c 2 , where x denotes the stratification variable, and c denotes the unstratified control case, was 0.95 for stratification by “total persons per section,” and 0.98 for stratification by “residential structures per section,” averaged over the 5 sample sizes. For either stratification method, the hypothesis that the σ 2 were the same for the 1,000 trial comparisons of the stratified and unstratified population estimates could not be rejected for p < 0.05 for any of the 5 sample sizes.
This approach failed to reduce the uncertainty of the estimate because:
- By design, all residences with a stratum were subsampled, rather than selecting a single section from each stratum to achieve balanced sampling across strata, as was done using a single-stage cluster sampling protocol.
- There will be considerable overlap in the variable “persons per residential structure” for any possible partition (see Fig 4 ), although the range of section sizes (i.e., number of residences per section) for each stratum was distinct in the constructed example.
In this context, it is also instructive to compare Figs Figs5 5 and and6. 6 . Fig 5 shows the distribution of the unit records (i.e., persons per residence) as a function of the stratification boundaries for a Neyman allocation for a sample of size 990. See Table 2 . All 1,979 records are shown in the box histograms. In each stratum, the records can be selected from any of the 20 eligible sections. Note that there is complete separation between the 4 stratum-specific distributions of the stratification variable “persons per residence.” In contrast, Fig 6 shows the comparable distributions of the unit records as a function of the 4-level stratification by residential structures per section (A) and persons per section (B) to support single-stage cluster sampling. In both cases, the records within a section are assigned to a single stratum, which results in considerable overlap between the number of persons per residence within the same stratum. Although there is an apparent grouping, the coherence within the strata is relatively weak, and the strata are not well separated, as in Fig 5 . Stratification by “persons per section” is relatively efficient for single-stage cluster sampling because a single section will be completely sampled from each stratum, and the ranges of residential structures per section are non-overlapping between strata.

The 4-level stratification variable is “persons per residence” ( Table 2-d ). The quantile boxplots [0.25, 0.75] show the partitioning of the records by stratum for all 1,979 records. The bar in each box is the median value of persons per residence, while outliers deviating by one or more quantiles from the median are denoted as discrete points. The samples in a given stratum may be assigned from any of the 20 eligible sections. The optimized Neyman allocation has completely separated the 4 strata with respect to overlapping values of the stratification variable.

(A) For the single-stage cluster sampling, the 20 sections were partitioned into 4 proportionally-allocated stratification levels. Within each stratum, the sections are arranged in descending order of total persons. The stratification variable is the total number of residential buildings per section (see Table 4 ). The quantile boxplots show the partitioning by stratum of the 1,979 records in the database, although only a subset of 4 sections will be drawn on a single simulation trial. The bar in each box is the median value of “persons per residence,” while outliers deviating by one or more quantiles from the median are denoted as discrete points. (B) Quantile boxplots showing stratification by total persons per section. This stratification approach requires that the population of each section be known, in contrast to stratification by residential structures per section.
The second difficulty is operational, and not specific to this dataset. The stratification boundaries were determined as a function of the number of residential structures per section. But all sections contain both residential and non-residential structures, as shown in Table 1 . If a survey of all sections is first required to enumerate the number of residential and non-residential structures, the apparent simplicity of the single-stage cluster sampling design is reduced. In our previous paper, [ 3 ] we discuss this issue is more detail.
Summary and Conclusions
We have developed and modeled two different but complementary approaches for stratified sampling in resource-limited environments. Their relative efficiencies have been discussed, and illustrated graphically and numerically. It does not seem likely that significant additional improvements can be achieved with respect to the stratification of the variable “persons per residential structure” demonstrated herein. Conversely, the single-stage cluster sampling method could well be the subject of additional research and application.
The stratification approach used for the latter was based on the partitioning of sections (clusters) into strata as a function of the number of residential structures per section. Alternative stratification variables could also be explored. As a hypothetical example, the section data available in this study encompasses 20 randomly-selected sections of the 68 sections comprising Bo City. Given data for all 68 sections, it would be possible to divide Bo City into a complete 68 section grid. Sections could then be assigned to strata as a function of the radial distance from the center of the city, or some other rule relating to geographical location or proximity.
Answers to Key Questions
The objective of the current study was to examine methods for either re-estimating the population following a complete survey, or for estimating the population in a new environment under conditions which—for reasons of schedule or funding—preclude undertaking similar surveys. The ground truth data used for the simulations came from a larger field survey that collected data for the 20 municipal sections described in this paper [ 28 – 30 ]. The first method used proportional and Neyman-allocated optimal stratification, and the latter achieved a reduction in uncertainty of the population estimation of about 80% in 1,000 simulated sampling trials. For proportional allocation only, the simulations were also validated by comparing the estimates obtained using a stratified finite population bootstrap with comparable estimates using an unbiased Thompson-Horovitz estimator. The second method explored the use of single-stage cluster sampling. The uncertainty of the population estimates for the latter protocol was significantly improved by first stratifying the 20 sections into 4 strata as a function of section size (i.e., number of residential structures per section). If the total number of persons per section was used as the stratification variable, a further reduction in uncertainty was observed, but this variable may not be known prior to conducting a survey.
We can now briefly answer the 4 questions raised in the section “Proposed Analysis.”
- If the 1,979 residential survey records are first partitioned into mutually-exclusive strata using “persons per residential structure” as the stratification variable, there is a reduction in uncertainty of about 80% relative to the estimate obtained using random sampling. The strata are cleanly separated by non-overlapping ranges of “persons per structure,” as shown in Fig 5 . Because the variable “persons per residential structure’ must be known in advance, presumably from prior survey data, this protocol is potentially useful for re-estimating a population.
- If the strata are created by partitioning the 20 sections into mutually-exclusive groups, using either residential structures or individual persons per section as the stratification variable, no statistically significant reduction in uncertainty is observed. The distributions of “persons per residential structure” overlap significantly between strata, and the strata are no longer well separated. Compare Fig 4 with Fig 5 .
- For 4-section single-stage cluster sampling, if the 20 sections are partitioned into mutually-exclusive strata by “total residential structures per section,” the uncertainty (H-T variance) of the population estimate is about 50% of the uncertainty for unstratified sampling. See Table 6 and Fig 6A .
- If the sections are instead stratified by “total persons per section” the uncertainty of the population estimate is reduced to about 6% of the uncertainty of the unstratified case for single-stage cluster sampling. See Table 6 and Fig 6B .
Future applications and research
For the single-stage cluster sampling, the sections were stratified by either total number of buildings per section, or by total persons per section. As an alternative, Bo could divided up into equal squares using a grid. There is a reasonably well-defined center of Bo, just are there are reasonably well-defined high-population-density centers that could be visually identified from aerial photographs of most cities. It is clear that if a grid was overlaid on a map of Bo, the cells farther from dense population areas would have fewer residential structures and a lower population density. If a Neyman stratification algorithm were to be applied, we would hypothesize that cells would be assigned to strata as a rough function of their distance from the center of the city. It would be interesting to compare the efficiency of this protocol for stratification with our existing results for single-stage cluster sampling, looking for possible improvement. At this time, we do not have sufficient data to test this hypothesis.
In summary, the ability to quickly estimate the total population size with reasonable precision in resource-limited environments can be of high value for demography, epidemiology, and health and social services research. The two approaches analyzed here are both of potential value in achieving these goals. Although the optimal stratification by residential occupancy is highly efficient, a single-stage cluster sampling protocol requires minimal data in advance, while minimizing the number of sections that must be surveyed.
Supporting Information
The views expressed herein are those of the individual authors and do not reflect views of the Department of the Navy or the Department of Defense.
Funding Statement
This work was funded by the Defense Threat Reduction Agency, Joint Science and Technology Office ( http://www.dvidshub.net/unit/DTRA-CB#.UoUqZ9wo5zk ) via contract to myself at the Naval Research Laboratory. A subcontract from NRL with George Mason University was used to provide support for contractors (Rashid Ansumana, Alfred Bockarie, Umaru Bangura and Joseph Lamin) working at Mercy Hospital Research Laboratory in Bo, Sierra Leone. There is no past, present or future Intellectual Property associated with the work described in the paper, and none of the authors have any financial interests or conflicts in the outcome of the study. The funders had no role in study design, data collection and analysis, decision to publish, or preparation of the manuscript.
Data Availability
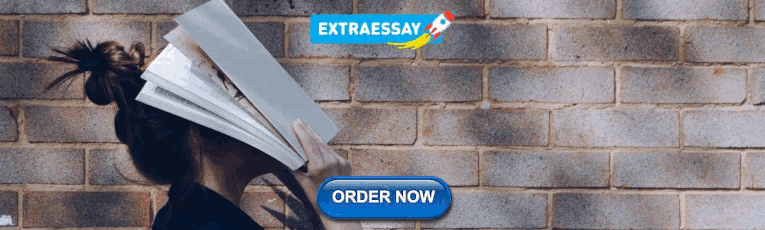
IMAGES
VIDEO
COMMENTS
This helps with the generalizability and validity of the study, as well as avoiding research biases like undercoverage bias. Table of contents. When to use stratified sampling; Step 1: Define your population and subgroups ... In this case, stratified sampling allows for more precise measures of the variables you wish to study, with lower ...
In this case, stratified sampling allows for more precise measures of the variables you wish to study, with lower variance within each subgroup and therefore for the population as a whole. Allowing for a variety of data collection methods; Sometimes you may need to use different methods to collect data from different subgroups.
When members of the subpopulations are relatively homogeneous relative to the entire population, stratified sampling can produce more precise estimates of those subgroups than simple random sampling. In this case, the strata have lower standard deviations than the entire population. The strata are the subpopulations in the study.
Purposive sampling has a long developmental history and there are as many views that it is simple and straightforward as there are about its complexity. The reason for purposive sampling is the better matching of the sample to the aims and objectives of the research, thus improving the rigour of the study and trustworthiness of the data and ...
Stratified sampling method in statistics. Research on sample collecting data in scientific survey techniques. Example Situations. Public Health Studies: To understand the incidence of disease across different age groups, the population could be stratified into different age brackets (e.g., 0-18, 19-35, 36-50, 51+).
Stratified sampling can help solve this dilemma. As of 2020, the median size of U.S. houses is 2,261 square feet. Assume a just-above-median, 2,400-square-foot house has five rooms, each with a different square-foot area. Rats can be in any of these rooms. The homeowners want to know how many total rats there are in the house.
Most studies among Hispanics have focused on individual risk factors of obesity, with less attention on interpersonal, community and environmental determinants. Conducting community based surveys to study these determinants must ensure representativeness of disparate populations. We describe the use of a novel Geographic Information System (GIS)-based population based sampling to minimize ...
For this study, we consider 2010 Census data for Brazil obtained in Sample questionnaire composed of 20635472 people interviewed. For this data set applying complex proportional stratified ...
Stratified Sampling. Stratification refers to dividing a population into groups, called strata, such that pairs of population units within the same stratum are deemed more similar ( homogeneous) than pairs from different strata. The strata are mutually exclusive (non-overlapping) and exhaustive of the population.
Stratified sampling, also sometimes called quota sampling, is akin to systematic sampling in that a predetermined number of samples are taken from each of M subregions, but the method of selection N m is quite different. As with systematic sampling, one seeks. (5.45) 〈 z 〉 = ∫ V z ( x) f ( x) d x = ∑ m = 1 M ∫ V m z ( x) f ( x) d x.
The case studies explained the key factors to consider when choosing a purposive sample and these included many of the principles of sampling as outlined by Kemper et al. (2003). Of these principles, the cases did not seem to address principle 4 explicitly: the sampling strategy must be ethical. For healthcare research, this would be an ...
The single case study designs are called N-of-1 type clinical trials [4,5]. The N-of-1 study design is being increasingly applied in healthcare-related research. ... Probability sampling can be separated into four types that include simple random sampling, systematic sampling, stratified sampling, and cluster sampling, as shown in Figure ...
There is a need for better estimators of population size in places that have undergone rapid growth and where collection of census data is difficult. We explored simulated estimates of urban population based on survey data from Bo, Sierra Leone, using two approaches: (1) stratified sampling from across 20 neighborhoods and (2) stratified single-stage cluster sampling of only four randomly ...
Download Citation | Stratified Sampling | Stratified sampling is a probability sampling method that is implemented in sample surveys. ... including datasets of case-control studies or real-world ...
So one can divide all the students into different subpopulations or strata such as Students of class 1, 2 and 3: Stratum 1 Students of class 4, 5 and 6: Stratum 2 Students of class 7, 8 and 9: Stratum 3 Students of class 10, 11 and 12: Stratum 4 Now draw the samples by SRS from each of the strata 1, 2, 3 and 4.
Cluster sampling- she puts 50 into random groups of 5 so we get 10 groups then randomly selects 5 of them and interviews everyone in those groups --> 25 people are asked. 2. Stratified sampling- she puts 50 into categories: high achieving smart kids, decently achieving kids, mediumly achieving kids, lower poorer achieving kids and clueless ...
Study on a Stratified Sampling Investigation Method for. Resident Travel and the Sampling Rate. Fei Shi. Depart ment of Urban Planning and Desig n, Nanjing University, Nanjing 210093, Chin a ...
Sampling types. There are two major categories of sampling methods ( figure 1 ): 1; probability sampling methods where all subjects in the target population have equal chances to be selected in the sample [ 1, 2] and 2; non-probability sampling methods where the sample population is selected in a non-systematic process that does not guarantee ...
Since the withdrawal of the US FDA draft guidance document for industry, "Powder Blends and Finished Dosage Units—Stratified In-Process Dosage Unit Sampling and Assessment" on August, 2013 (), the pharmaceutical industry required a science and risk-based approach, justified sampling, and testing plans to ensure drug product uniformity.. Although a draft, the guidance was extensively used ...
In this article, you will learn how to use stratified sampling and see some case studies that illustrate its benefits and challenges. Top experts in this article Selected by the community from 2 ...
Stratified random sampling. Stratified random sampling is similar to simple random sampling, but it kicks things up a notch. As the name suggests, stratified sampling involves selecting participants randomly, but from within certain pre-defined subgroups (i.e., strata) that share a common trait.For example, you might divide the population into strata based on gender, ethnicity, age range or ...
Similarly, in the classic case study of Union Democracy by Lipset, Coleman and Trow (1956), which studies the internal democracy within a single trade union, the authors make use of stratified random sampling 5 (Yin, 2014, p. 118).
The package implements the stratified proportional hazards regression model with piecewise constant hazard within each stratum. The package allows the historical data to inform the treatment effect parameter, parameter effects for other covariates in the regression model, as well as the baseline hazard parameters.
Proposed analysis. In a previous study [], a Finite Population Bootstrap (FPB) [] (page 92) was used to compare the relative uncertainty of two population estimators: an occupancy-based estimator and a rooftop area-based estimator.For the region of interest, the former was estimated as the product of (1) the average number of persons per residential structure multiplied by (2) the total number ...