
An official website of the United States government
The .gov means it’s official. Federal government websites often end in .gov or .mil. Before sharing sensitive information, make sure you’re on a federal government site.
The site is secure. The https:// ensures that you are connecting to the official website and that any information you provide is encrypted and transmitted securely.
- Publications
- Account settings
Preview improvements coming to the PMC website in October 2024. Learn More or Try it out now .
- Advanced Search
- Journal List
- BMJ Open Access

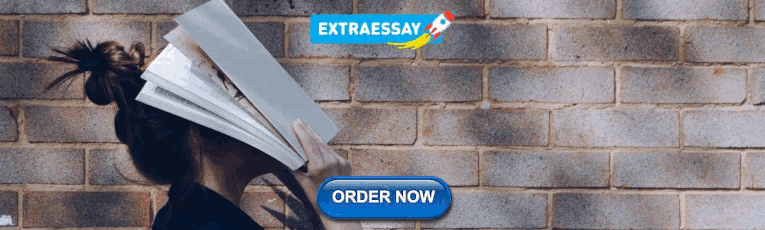
Analytical studies: a framework for quality improvement design and analysis
Conducting studies for learning is fundamental to improvement. Deming emphasised that the reason for conducting a study is to provide a basis for action on the system of interest. He classified studies into two types depending on the intended target for action. An enumerative study is one in which action will be taken on the universe that was studied. An analytical study is one in which action will be taken on a cause system to improve the future performance of the system of interest. The aim of an enumerative study is estimation, while an analytical study focuses on prediction. Because of the temporal nature of improvement, the theory and methods for analytical studies are a critical component of the science of improvement.
Introduction: enumerative and analytical studies
Designing studies that make it possible to learn from experience and take action to improve future performance is an essential element of quality improvement. These studies use the now traditional theory established through the work of Fisher, 1 Cox, 2 Campbell and Stanley, 3 and others that is widely used in biomedicine research. These designs are used to discover new phenomena that lead to hypothesis generation, and to explore causal mechanisms, 4 as well as to evaluate efficacy and effectiveness. They include observational, retrospective, prospective, pre-experimental, quasiexperimental, blocking, factorial and time-series designs.
In addition to these classifications of studies, Deming 5 defined a distinction between analytical and enumerative studies which has proven to be fundamental to the science of improvement. Deming based his insight on the distinction between these two approaches that Walter Shewhart had made in 1939 as he helped develop measurement strategies for the then-emerging science of ‘quality control.’ 6 The difference between the two concepts lies in the extrapolation of the results that is intended, and in the target for action based on the inferences that are drawn.
A useful way to appreciate that difference is to contrast the inferences that can be made about the water sampled from two different natural sources ( figure 1 ). The enumerative approach is like the study of water from a pond. Because conditions in the bounded universe of the pond are essentially static over time, analyses of random samples taken from the pond at a given time can be used to estimate the makeup of the entire pond. Statistical methods, such as hypothesis testing and CIs, can be used to make decisions and define the precision of the estimates.

Environment in enumerative and analytical study. Internal validity diagram from Fletcher et al . 7
The analytical approach, in contrast, is like the study of water from a river. The river is constantly moving, and its physical properties are changing (eg, due to snow melt, changes in rainfall, dumping of pollutants). The properties of water in a sample from the river at any given time may not describe the river after the samples are taken and analysed. In fact, without repeated sampling over time, it is difficult to make predictions about water quality, since the river will not be the same river in the future as it was at the time of the sampling.
Deming first discussed these concepts in a 1942 paper, 8 as well as in his 1950 textbook, 9 and in a 1975 paper used the enumerative/analytical terminology to characterise specific study designs. 5 While most books on experimental design describe methods for the design and analysis of enumerative studies, Moen et al 10 describe methods for designing and learning from analytical studies. These methods are graphical and focus on prediction of future performance. The concept of analytical studies became a key element in Deming's ‘system of profound knowledge’ that serves as the intellectual foundation for improvement science. 11 The knowledge framework for the science of improvement, which combines elements of psychology, the Shewhart view of variation, the concept of systems, and the theory of knowledge, informs a number of key principles for the design and analysis of improvement studies:
- Knowledge about improvement begins and ends in experimental data but does not end in the data in which it begins.
- Observations, by themselves, do not constitute knowledge.
- Prediction requires theory regarding mechanisms of change and understanding of context.
- Random sampling from a population or universe (assumed by most statistical methods) is not possible when the population of interest is in the future.
- The conditions during studies for improvement will be different from the conditions under which the results will be used. The major source of uncertainty concerning their use is the difficulty of extrapolating study results to different contexts and under different conditions in the future.
- The wider the range of conditions included in an improvement study, the greater the degree of belief in the validity and generalisation of the conclusions.
The classification of studies into enumerative and analytical categories depends on the intended target for action as the result of the study:
- Enumerative studies assume that when actions are taken as the result of a study, they will be taken on the material in the study population or ‘frame’ that was sampled.
More specifically, the study universe in an enumerative study is the bounded group of items (eg, patients, clinics, providers, etc) possessing certain properties of interest. The universe is defined by a frame, a list of identifiable, tangible units that may be sampled and studied. Random selection methods are assumed in the statistical methods used for estimation, decision-making and drawing inferences in enumerative studies. Their aim is estimation about some aspect of the frame (such as a description, comparison or the existence of a cause–effect relationship) and the resulting actions taken on this particular frame. One feature of an enumerative study is that a 100% sample of the frame provides the complete answer to the questions posed by the study (given the methods of investigation and measurement). Statistical methods such as hypothesis tests, CIs and probability statements are appropriate to analyse and report data from enumerative studies. Estimating the infection rate in an intensive care unit for the last month is an example of a simple enumerative study.
- Analytical studies assume that the actions taken as a result of the study will be on the process or causal system that produced the frame studied, rather than the initial frame itself. The aim is to improve future performance.
In contrast to enumerative studies, an analytical study accepts as a given that when actions are taken on a system based on the results of a study, the conditions in that system will inevitably have changed. The aim of an analytical study is to enable prediction about how a change in a system will affect that system's future performance, or prediction as to which plans or strategies for future action on the system will be superior. For example, the task may be to choose among several different treatments for future patients, methods of collecting information or procedures for cleaning an operating room. Because the population of interest is open and continually shifts over time, random samples from that population cannot be obtained in analytical studies, and traditional statistical methods are therefore not useful. Rather, graphical methods of analysis and summary of the repeated samples reveal the trajectory of system behaviour over time, making it possible to predict future behaviour. Use of a Shewhart control chart to monitor and create learning to reduce infection rates in an intensive care unit is an example of a simple analytical study.
The following scenarios give examples to clarify the nature of these two types of studies.
Scenario 1: enumerative study—observation
To estimate how many days it takes new patients to see all primary care physicians contracted with a health plan, a researcher selected a random sample of 150 such physicians from the current active list and called each of their offices to schedule an appointment. The time to the next available appointment ranged from 0 to 180 days, with a mean of 38 days (95% CI 35.6 to 39.6).
This is an enumerative study, since results are intended to be used to estimate the waiting time for appointments with the plan's current population of primary care physicians.
Scenario 2: enumerative study—hypothesis generation
The researcher in scenario 1 noted that on occasion, she was offered an earlier visit with a nurse practitioner (NP) who worked with the physician being called. Additional information revealed that 20 of the 150 physicians in the study worked with one or more NPs. The next available appointment for the 130 physicians without an NP averaged 41 days (95% CI 39 to 43 days) and was 18 days (95% CI 18 to 26 days) for the 20 practices with NPs, a difference of 23 days (a 56% shorter mean waiting time).
This subgroup analysis suggested that the involvement of NPs helps to shorten waiting times, although it does not establish a cause–effect relationship, that is, it was a ‘hypothesis-generating’ study. In any event, this was clearly an enumerative study, since its results were to understand the impact of NPs on waiting times in the particular population of practices. Its results suggested that NPs might influence waiting times, but only for practices in this health plan during the time of the study. The study treated the conditions in the health plan as static, like those in a pond.
Scenario 3: enumerative study—comparison
To find out if administrative changes in a health plan had increased member satisfaction in access to care, the customer service manager replicated a phone survey he had conducted a year previously, using a random sample of 300 members. The percentage of patients who were satisfied with access had increased from 48.7% to 60.7% (Fisher exact test, p<0.004).
This enumerative comparison study was used to estimate the impact of the improvement work during the last year on the members in the plan. Attributing the increase in satisfaction to the improvement work assumes that other conditions in the study frame were static.
Scenario 4: analytical study—learning with a Shewhart chart
Each primary care clinic in a health plan reported its ‘time until the third available appointment’ twice a month, which allowed the quality manager to plot the mean waiting time for all of the clinics on Shewhart charts. Waiting times had been stable for a 12-month period through August, but the manager then noted a special cause (increase in waiting time) in September. On stratifying the data by region, she found that the special cause resulted from increases in waiting time in the Northeast region. Discussion with the regional manager revealed a shortage of primary care physicians in this region, which was predicted to become worse in the next quarter. Making some temporary assignments and increasing physician recruiting efforts resulted in stabilisation of this measure.
Documenting common and special cause variation in measures of interest through the use of Shewhart charts and run charts based on judgement samples is probably the simplest and commonest type of analytical study in healthcare. Such charts, when stable, provide a rational basis for predicting future performance.
Scenario 5: analytical study—establishing a cause–effect relationship
The researcher mentioned in scenarios 1 and 2 planned a study to test the existence of a cause–effect relationship between the inclusion of NPs in primary care offices and waiting time for new patient appointments. The variation in patient characteristics in this health plan appeared to be great enough to make the study results useful to other organisations. For the study, she recruited about 100 of the plan's practices that currently did not use NPs, and obtained funding to facilitate hiring NPs in up to 50 of those practices.
The researcher first explored the theories on mechanisms by which the incorporation of NPs into primary care clinics could reduce waiting times. Using important contextual variables relevant to these mechanisms (practice size, complexity, use of information technology and urban vs rural location), she then developed a randomised block, time-series study design. The study had the power to detect an effect of a mean waiting time of 5 days or more overall, and 10 days for the major subgroups defined by levels of the contextual variables. Since the baseline waiting time for appointments varied substantially across practices, she used the baseline as a covariate.
After completing the study, she analysed data from baseline and postintervention periods using stratified run charts and Shewhart charts, including the raw measures and measures adjusted for important covariates and effects of contextual variables. Overall waiting times decreased 12 days more in practices that included NPs than they did in control practices. Importantly, the subgroup analyses according to contextual variables revealed conditions under which the use of NPs would not be predicted to lead to reductions in waiting times. For example, practices with short baseline waiting times showed little or no improvement by employing NPs. She published the results in a leading health research journal.
This was an analytical study because the intent was to apply the learning from the study to future staffing plans in the health plan. She also published the study, so its results would be useful to primary care practices outside the health plan.
Scenario 6: analytical study—implementing improvement
The quality-improvement manager in another health plan wanted to expand the use of NPs in the plan's primary care practices, because published research had shown a reduction in waiting times for practices with NPs. Two practices in his plan already employed NPs. In one of these practices, Shewhart charts of waiting time by month showed a stable process averaging 10 days during the last 2 years. Waiting time averaged less than 7 days in the second practice, but a period when one of the physicians left the practice was associated with special causes.
The quality manager created a collaborative among the plan's primary care practices to learn how to optimise the use of NPs. Physicians in the two sites that employed NPs served as subject matter experts for the collaborative. In addition to making NPs part of their care teams, participating practices monitored appointment supply and demand, and tested other changes designed to optimise response to patient needs. Thirty sites in the plan voluntarily joined the collaborative and hired NPs. After 6 months, Shewhart charts indicated that waiting times in 25 of the 30 sites had been reduced to less than 7 days. Because waiting times in these practices had been stable over a considerable period of time, the manager predicted that future patients would continue to experience reduced times for appointments. The quality manger began to focus on a follow-up collaborative among the backlog of 70 practices that wanted to join.
This project was clearly an analytical study, since its aim was specifically to improve future waiting-time performance for participating sites and other primary care offices in the plan. Moreover, it focused on learning about the mechanisms through which contextual factors affect the impact of NPs on primary care office functions, under practice conditions that (like those in a river) will inevitably change over time.
Statistical theory in enumerative studies is used to describe the precision of estimates and the validity of hypotheses for the population studied. But since these statistical methods provide no support for extrapolation of the results outside the population that was studied, the subject experts must rely on their understanding of the mechanisms in place to extend results outside the population.
In analytical studies, the standard error of a statistic does not address the most important source of uncertainty, namely, the change in study conditions in the future. Although analytical studies need to take into account the uncertainty due to sampling, as in enumerative studies, the attributes of the study design and analysis of the data primarily deal with the uncertainty resulting from extrapolation to the future (generalisation to the conditions in future time periods). The methods used in analytical studies encourage the exploration of mechanisms through multifactor designs, contextual variables introduced through blocking and replication over time.
Prior stability of a system (as observed in graphic displays of repeated sampling over time, according to Shewhart's methods) increases belief in the results of an analytical study, but stable processes in the past do not guarantee constant system behaviour in the future. The next data point from the future is the most important on a graph of performance. Extrapolation of system behaviour to future times therefore still depends on input from subject experts who are familiar with mechanisms of the system of interest, as well as the important contextual issues. Generalisation is inherently difficult in all studies because ‘whereas the problems of internal validity are solvable within the limits of the logic of probability statistics, the problems of external validity are not logically solvable in any neat, conclusive way’ 3 (p. 17).
The diverse activities commonly referred to as healthcare improvement 12 are all designed to change the behaviour of systems over time, as reflected in the principle that ‘not all change is improvement, but all improvement is change.’ The conditions in the unbounded systems into which improvement interventions are introduced will therefore be different in the future from those in effect at the time the intervention is studied. Since the results of improvement studies are used to predict future system behaviour, such studies clearly belong to the Deming category of analytical studies. Quality improvement studies therefore need to incorporate repeated measurements over time, as well as testing under a wide range of conditions (2, 3 and 10). The ‘gold standard’ of analytical studies is satisfactory prediction over time.
Conclusions and recommendations
In light of these considerations, some important principles for drawing inferences from improvement studies include 10 :
- The analysis of data, interpretation of that analysis and actions taken as a result of the study should be closely tied to the current knowledge of experts about mechanisms of change in the relevant area. They can often use the study to discover, understand and evaluate the underlying mechanisms.
- The conditions of the study will be different from the future conditions under which the results will be used. Assessment by experts of the magnitude of this difference and its potential impact on future events should be an integral part of the interpretation of the results of the intervention.
- Show all the data before aggregation or summary.
- Plot the outcome data in the order in which the tests of change were conducted and annotate with information on the interventions.
- Use graphical displays to assess how much of the variation in the data can be explained by factors that were deliberately changed.
- Rearrange and subgroup the data to study other sources of variation (background and contextual variables).
- Summarise the results of the study with appropriate graphical displays.
Because these principles reflect the fundamental nature of improvement—taking action to change performance, over time, and under changing conditions—their application helps to bring clarity and rigour to improvement science.
Acknowledgments
The author is grateful to F Davidoff and P Batalden for their input to earlier versions of this paper.
Competing interests: None.
Provenance and peer review: Not commissioned; externally peer reviewed.
North America
Analytical and Measuring Instruments
- About Shimadzu
- Sales Rep Locator
- Global Links
JavaScript Disabled
Our website uses JavaScript. Please confirm that JavaScript is enabled in your browser.
AR&D / Method Development
- X (Twitter)
Add Bookmark
- Contact a Sales Rep
- Request More Info
- Tech Support
- Request a Virtual Demo
Featured Solutions & Applications
Related products.
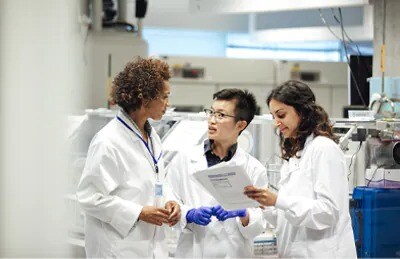
The process of analytical method development begins early in drug discovery and evolves in terms of needs and goals as a candidate approaches final release. Initial method development done to determine racemic content or quickly separate major impurities is honed as compounds become fewer in number and the focus shifts to making robust and repeatable analyses. As a candidate moves from early stage development toward formulation and stability concerns, the need for continuing method development persists and the challenges of separating related or interfering compounds increases. The power of sophisticated chromatography and detection techniques, such as comprehensive multi-dimensional liquid chromatography (LC) and time-of-flight mass spectrometry (TOF-MS), can be employed to the challenges of advanced method development
To meet these needs, Shimadzu offers a variety of solutions including chromatographic method development platforms, TOF and quadrupole mass spectrometry, a full suite of molecular spectroscopy equipment, and powerful software tools based on LabSolutions. With workflows to support photo diode array peak deconvolution, impurities discovery, and LC method transfer, we give you insight to catch coeluting impurities even without separation and move methods from system to system. Add in compliance-enabled integration across multiple instrument types including balances, physical testing, LC, GC, LC-MS, GC-MS, ICP-OES, ICP-MS, FTIR, UV, Fluorometry, and TOC, to see how Shimadzu and LabSolutions can help you create efficient and robust analytical methods that move from development to QA/QC with ease.
Request More Information
Return to Pharma & Biopharma Industry Page
Method Scouting Solution for UHPLC/HPLC/SFC
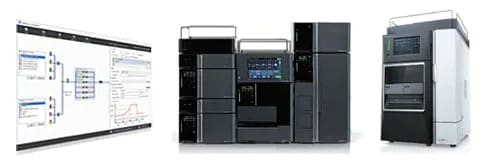
Method Scouting System is a method development system based on Shimadzu’s UHPLC technology. The combination with Method Scouting Solution dedicated control software achieves a fast and accurate method scouting workflow, offering excellent support for method development.
Structural analysis of impurities in pharmaceutical ingredients using trap-free 2D-LC high-resolution accurate mass spectrometry
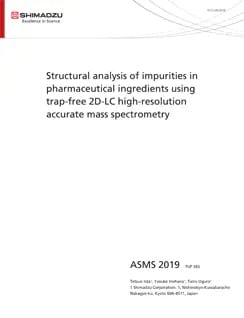
High performance liquid chromatography (HPLC) is the primary means for impurity testing in pharmacopeia. Solutions containing non-volatile salts are frequently used as a mobile phase because of the irreplaceable advantage to achieve better chromatographic performances. However, non-volatile mobile phases are not compatible with mass spectrometry which is commonly used for structural analysis of impurities. Trap-free two-dimensional HPLC (trap-free 2D-LC) can fill this gap by exchanging a non-volatile mobile phase with a volatile one compatible for mass spectrometry. In this study, we demonstrate how structural analysis of impurities in pharmaceutical ingredients is feasible by using trap-free 2D-LC coupled to quadrupole time-of-flight mass spectrometry (Q-TOF MS) without modifying the original method mobile phases.
Simplified approach for structural elucidation and quantitation for pharmaceutical API and related impurities
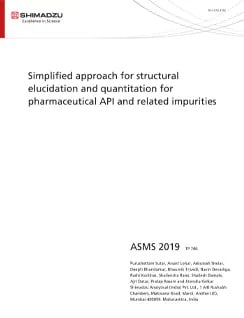
Various guidelines require the impurities of drug substance and drug product be structurally identified and quantitated based on dosage. Source of these impurities could be innumerable based on raw material, process, product, packaging etc. In this study, a workflow is demonstrated (Figure 1) for deducing probable structure of Paracetamol related impurities using Shimadzu LCMS-9030 and Insight Explore software.
Improved Analytical Workflow for Phospholipids by Nexera-e and Co-Sense for Impurities
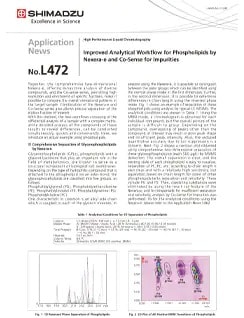
Smart Metabolites Database registers MRM information of 475 metabolites mainly contained in biological samples such as blood, urine and cells. It enables simultaneous measurement of 475 metabolites using MRM mode. This application data sheet presents an analysis of metabolites in standard human plasma using the scan and MRM methods included in the Smart Metabolites Database, as well as a comparison of the results.
Isolation and identification of Atorvastatin degradation impurities by UFPLC
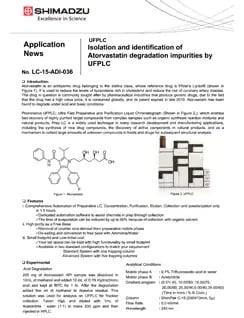
Atorvastatin is an antilipemic drug belonging to the statins class, whose reference drug is Pfizer’s Lipitor. It is used to reduce the levels of lipoproteins rich in cholesterol and reduce the risk of coronary artery disease. The drug in question is commonly sought after by pharmaceutical industries that produce generic drugs, due to the fact that the drug has a high value price, it is consumed globally, and its patent expired in late 2010. Atorvastatin has been found to degrade under acid and basic conditions. Prominence UFPLC, Ultra Fast Preparative and Purification Liquid Chromatograph, which enables fast recovery of highly purified target compounds from complex samples such as organic synthesis reaction mixtures and natural products. Prep LC is a widely used technique in many research development and manufacturing applications, including the synthesis of new drug compounds, the discovery of active components in natural products, and as a mechanism to collect large amounts of unknown compounds in foods and drugs for subsequent structural analysis.
Isolation and identification of Fluticasone degradation impurities by UFPLC
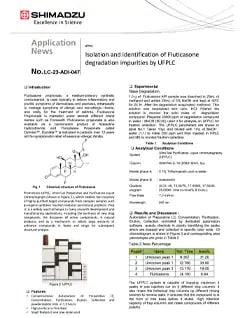
The Prominence UFPLC seamlessly integrates traditional Prep LC with novel fraction trapping for upto five compounds of interest. The instrument is controlled by a dedicated walk-up software designed to empower nonexpert users to easily set conditions for chromatographic separation and isolation of target compounds, trapping, purifying, eluting and collecting highly purified compounds in as little as 90 minutes. For applications involving the isolation of low concentration targets, replicate injection and collection to the same trapping column to increase the amount of compound trapped on column prior to elution is easily accomplished.
Method Transfer and High-Speed Analysis of Impurities of Drug by Prominence-i
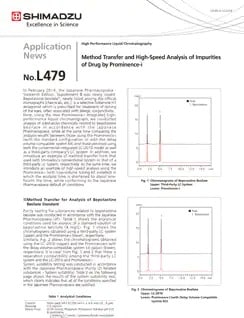
Here, using the new Prominence-i integrated high-performance liquid chromatograph, we conducted analysis of substances chemically related to bepotastine besilate in accordance with the Japanese Pharmacopeia, while at the same time comparing the analysis results between those using the Prominence-i and those obtained using both the conventional integrated lC-2010 model as well as a third-party company's LC system. In addition, we introduce an example of method transfer from that used with Shimadzu's conventional system or that of a third-party LC system, respectively. At the same time, we introduce an example of high-speed analysis using the Prominence-i in which the analysis time is shortened to about one-fourth the time, while conforming to the Japanese Pharmacopoeia default of conditions.
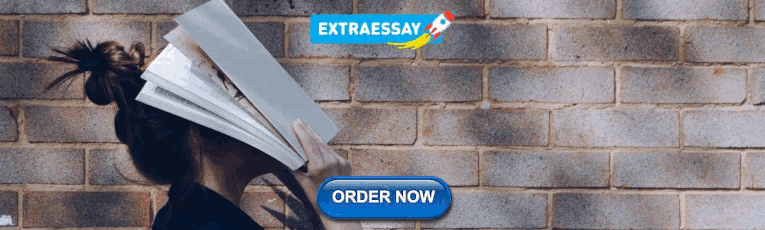
Improvement of Quantitative Performance for Ibuprofen Using UV Cut-Off Filter on SPD-M40
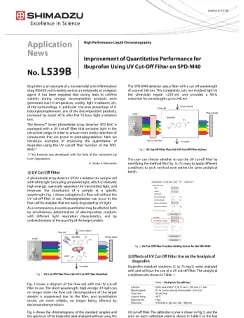
Ibuprofen is an example of a nonsteroidal anti-inflammatory drug (NSAID) and is widely used as an antipyretic or analgesic agent. It has been reported that during tests to confirm stability during storage, decomposition products were generated due to temperature, acidity, light irradiation, etc. of the surroundings. In particular, the area percentage of 4-isobutylacetophenone, one of the decomposition products, increased by about 40% after the 72-hour light irradiation test. The Nexera Series photodiode array detector SPD-M40 is equipped with a UV cut-off filter that exlcudes light in the ultraviolet range, in order to ensure more stable detection of compounds that are prone to photodegradation. We are introducing examples of improving the quantitation of ibuprofen using the UV cut-off filter function of the SPD-M40.
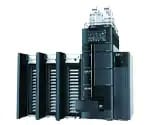
High Performance Liquid Chromatography (HPLC & UHPLC)
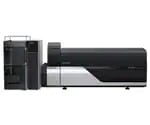
Triple Quadrupole LC-MS/MS Instruments
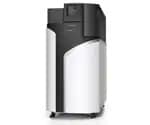
LCMS-9030 Q-TOF LC/MS System
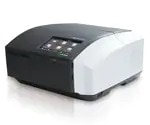
Molecular Spectroscopy
News / Events
AAPS Pharm Sci 360 2024
October 21-23 Salt Palace Convention Center Salt Lake City, Utah Booth #2107
October 21, 2024
ASMS (American Society for Mass Spectrometry) 2024
June 2-6 Anaheim Convention Center Anaheim, California
June 2, 2024
- Search Search Please fill out this field.
- What Is R&D?
- Understanding R&D
- Types of R&D
- Pros and Cons
- Considerations
- R&D vs. Applied Research
- Who Spends the Most?
The Bottom Line
- Business Essentials
Research and Development (R&D) Definition, Types, and Importance
:max_bytes(150000):strip_icc():format(webp)/wk_headshot_aug_2018_02__william_kenton-5bfc261446e0fb005118afc9.jpg)
Investopedia / Ellen Lindner
What Is Research and Development (R&D)?
The term research and development (R&D) is used to describe a series of activities that companies undertake to innovate and introduce new products and services. R&D is often the first stage in the development process. Companies require knowledge, talent, and investment in order to further their R&D needs and goals. The purpose of research and development is generally to take new products and services to market and add to the company's bottom line .
Key Takeaways
- Research and development represents the activities companies undertake to innovate and introduce new products and services or to improve their existing offerings.
- R&D allows a company to stay ahead of its competition by catering to new wants or needs in the market.
- Companies in different sectors and industries conduct R&D—pharmaceuticals, semiconductors, and technology companies generally spend the most.
- R&D is often a broad approach to exploratory advancement, while applied research is more geared towards researching a more narrow scope.
- The accounting for treatment for R&D costs can materially impact a company's income statement and balance sheet.
Understanding Research and Development (R&D)
The concept of research and development is widely linked to innovation both in the corporate and government sectors. R&D allows a company to stay ahead of its competition. Without an R&D program, a company may not survive on its own and may have to rely on other ways to innovate such as engaging in mergers and acquisitions (M&A) or partnerships. Through R&D, companies can design new products and improve their existing offerings.
R&D is distinct from most operational activities performed by a corporation. The research and/or development is typically not performed with the expectation of immediate profit. Instead, it is expected to contribute to the long-term profitability of a company. R&D may often allow companies to secure intellectual property, including patents , copyrights, and trademarks as discoveries are made and products created.
Companies that set up and employ departments dedicated entirely to R&D commit substantial capital to the effort. They must estimate the risk-adjusted return on their R&D expenditures, which inevitably involves risk of capital. That's because there is no immediate payoff, and the return on investment (ROI) is uncertain. As more money is invested in R&D, the level of capital risk increases. Other companies may choose to outsource their R&D for a variety of reasons including size and cost.
Companies across all sectors and industries undergo R&D activities. Corporations experience growth through these improvements and the development of new goods and services. Pharmaceuticals, semiconductors , and software/technology companies tend to spend the most on R&D. In Europe, R&D is known as research and technical or technological development.
Many small and mid-sized businesses may choose to outsource their R&D efforts because they don't have the right staff in-house to meet their needs.
Types of R&D
There are several different types of R&D that exist in the corporate world and within government. The type used depends entirely on the entity undertaking it and the results can differ.
Basic Research
There are business incubators and accelerators, where corporations invest in startups and provide funding assistance and guidance to entrepreneurs in the hope that innovations will result that they can use to their benefit.
M&As and partnerships are also forms of R&D as companies join forces to take advantage of other companies' institutional knowledge and talent.
Applied Research
One R&D model is a department staffed primarily by engineers who develop new products —a task that typically involves extensive research. There is no specific goal or application in mind with this model. Instead, the research is done for the sake of research.
Development Research
This model involves a department composed of industrial scientists or researchers, all of who are tasked with applied research in technical, scientific, or industrial fields. This model facilitates the development of future products or the improvement of current products and/or operating procedures.
$42.7 billion of research and development costs later, Amazon was granted 2,244 new patents in 2020. Their patents included advancements in artificial intelligence, machine learning, and cloud computing.
Advantages and Disadvantages of R&D
There are several key benefits to research and development. It facilitates innovation, allowing companies to improve existing products and services or by letting them develop new ones to bring to the market.
Because R&D also is a key component of innovation, it requires a greater degree of skill from employees who take part. This allows companies to expand their talent pool, which often comes with special skill sets.
The advantages go beyond corporations. Consumers stand to benefit from R&D because it gives them better, high-quality products and services as well as a wider range of options. Corporations can, therefore, rely on consumers to remain loyal to their brands. It also helps drive productivity and economic growth.
Disadvantages
One of the major drawbacks to R&D is the cost. First, there is the financial expense as it requires a significant investment of cash upfront. This can include setting up a separate R&D department, hiring talent, and product and service testing, among others.
Innovation doesn't happen overnight so there is also a time factor to consider. This means that it takes a lot of time to bring products and services to market from conception to production to delivery.
Because it does take time to go from concept to product, companies stand the risk of being at the mercy of changing market trends . So what they thought may be a great seller at one time may reach the market too late and not fly off the shelves once it's ready.
Facilitates innovation
Improved or new products and services
Expands knowledge and talent pool
Increased consumer choice and brand loyalty
Economic driver
Financial investment
Shifting market trends
R&D Accounting
R&D may be beneficial to a company's bottom line, but it is considered an expense . After all, companies spend substantial amounts on research and trying to develop new products and services. As such, these expenses are often reported for accounting purposes on the income statement and do not carry long-term value.
There are certain situations where R&D costs are capitalized and reported on the balance sheet. Some examples include but are not limited to:
- Materials, fixed assets, or other assets have alternative future uses with an estimable value and useful life.
- Software that can be converted or applied elsewhere in the company to have a useful life beyond a specific single R&D project.
- Indirect costs or overhead expenses allocated between projects.
- R&D purchased from a third party that is accompanied by intangible value. That intangible asset may be recorded as a separate balance sheet asset.
R&D Considerations
Before taking on the task of research and development, it's important for companies and governments to consider some of the key factors associated with it. Some of the most notable considerations are:
- Objectives and Outcome: One of the most important factors to consider is the intended goals of the R&D project. Is it to innovate and fill a need for certain products that aren't being sold? Or is it to make improvements on existing ones? Whatever the reason, it's always important to note that there should be some flexibility as things can change over time.
- Timing: R&D requires a lot of time. This involves reviewing the market to see where there may be a lack of certain products and services or finding ways to improve on those that are already on the shelves.
- Cost: R&D costs a great deal of money, especially when it comes to the upfront costs. And there may be higher costs associated with the conception and production of new products rather than updating existing ones.
- Risks: As with any venture, R&D does come with risks. R&D doesn't come with any guarantees, no matter the time and money that goes into it. This means that companies and governments may sacrifice their ROI if the end product isn't successful.
Research and Development vs. Applied Research
Basic research is aimed at a fuller, more complete understanding of the fundamental aspects of a concept or phenomenon. This understanding is generally the first step in R&D. These activities provide a basis of information without directed applications toward products, policies, or operational processes .
Applied research entails the activities used to gain knowledge with a specific goal in mind. The activities may be to determine and develop new products, policies, or operational processes. While basic research is time-consuming, applied research is painstaking and more costly because of its detailed and complex nature.
Who Spends the Most on R&D?
Companies spend billions of dollars on R&D to produce the newest, most sought-after products. According to public company filings, these companies incurred the highest research and development spending in 2020:
- Amazon: $42.7 billion
- Alphabet.: $27.6 billion
- Huawei: $22.0 billion
- Microsoft: $19.3 billion
- Apple: $18.8 billion
- Samsung: $18.8 billion
- Facebook: $18.5 billion
What Types of Activities Can Be Found in Research and Development?
Research and development activities focus on the innovation of new products or services in a company. Among the primary purposes of R&D activities is for a company to remain competitive as it produces products that advance and elevate its current product line. Since R&D typically operates on a longer-term horizon, its activities are not anticipated to generate immediate returns. However, in time, R&D projects may lead to patents, trademarks, or breakthrough discoveries with lasting benefits to the company.
What Is an Example of Research and Development?
Alphabet allocated over $16 billion annually to R&D in 2018. Under its R&D arm X, the moonshot factory, it has developed Waymo self-driving cars. Meanwhile, Amazon has spent even more on R&D projects, with key developments in cloud computing and its cashier-less store Amazon Go. At the same time, R&D can take the approach of a merger & acquisition, where a company will leverage the talent and intel of another company to create a competitive edge. The same can be said with company investment in accelerators and incubators, whose developments it could later leverage.
Why Is Research and Development Important?
Given the rapid rate of technological advancement, R&D is important for companies to stay competitive. Specifically, R&D allows companies to create products that are difficult for their competitors to replicate. Meanwhile, R&D efforts can lead to improved productivity that helps increase margins, further creating an edge in outpacing competitors. From a broader perspective, R&D can allow a company to stay ahead of the curve, anticipating customer demands or trends.
There are many things companies can do in order to advance in their industries and the overall market. Research and development is just one way they can set themselves apart from their competition. It opens up the potential for innovation and increasing sales. But it does come with some drawbacks—the most obvious being the financial cost and the time it takes to innovate.
NASDAQ. " Which Companies Spend the Most in Research and Development (R&D)? "
Strategy+Business. " WHAT THE TOP INNOVATORS GET RIGHT ."
:max_bytes(150000):strip_icc():format(webp)/research_pharma-5bfc322b46e0fb0051bf11a0.jpg)
- Terms of Service
- Editorial Policy
- Privacy Policy
- Your Privacy Choices
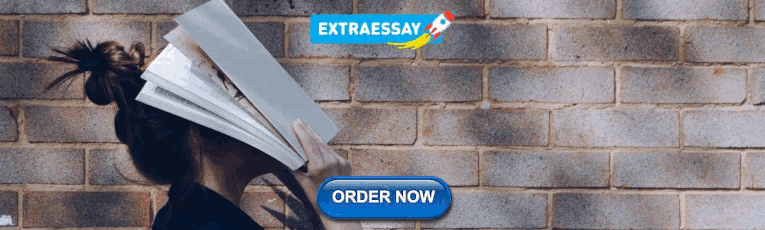
IMAGES
VIDEO
COMMENTS
An analytical study is one in which action will be taken on a cause system to improve the future performance of the system of interest. The aim of an enumerative study is estimation, while an analytical study focuses on prediction. Because of the temporal nature of improvement, the theory and methods for analytical studies are a critical ...
AR&D / Method Development. The process of analytical method development begins early in drug discovery and evolves in terms of needs and goals as a candidate approaches final release. Initial method development done to determine racemic content or quickly separate major impurities is honed as compounds become fewer in number and the focus ...
Research And Development - R&D: Research and development (R&D) refers to the investigative activities a business conducts to improve existing products and procedures or to lead to the development ...