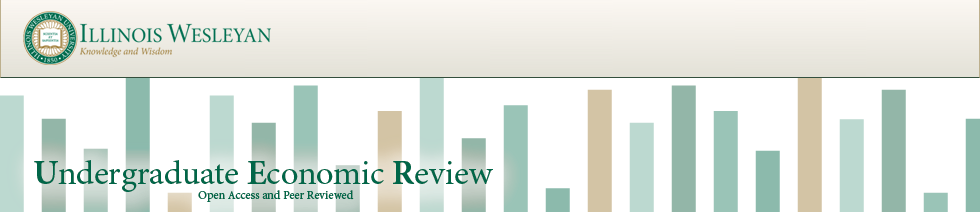
Home > ECONOMICS > UER
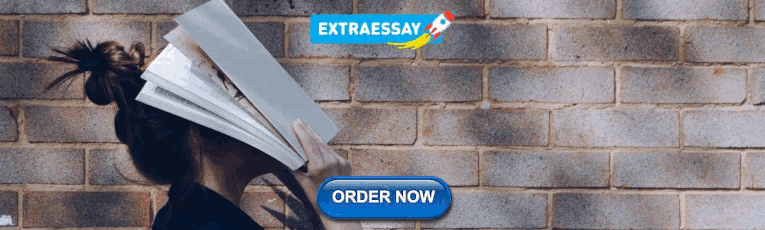
Most Popular Papers *
The Role of Entrepreneurship in Economic Growth Daniel Smith
The Strength of Religious Beliefs is Important for Subjective Well-Being Enrique Colón-Bacó
Impact of Exchange Rate Regimes on Economic Growth Brigitta Jakob
The Impact of Sustainability Reporting on Firm Profitability Lancee L. Whetman
Do Mandatory Minimums Increase Racial Disparities in Federal Criminal Sentencing? Caroline Gillette
A Data Analysis of the World Happiness Index and its Relation to the North-South Divide Charles Alba
Impact of Privatization on Economic Growth Adnan Filipovic
Does the Economy Determine the President? A Regression Model For Predicting US Presidential Elections Roy K. Roth
Determinants of Bank Profitability in Ukraine Antonina Davydenko
Crisis: Capitalism, Economics and the Environment Raj Navanit Patel Mr
* Based on the average number of full-text downloads per day since the paper was posted. » Updated as of 11/29/23.
- Journal Home
- About This Journal
- Journal FAQ
- Editorial Board
- Most Popular Papers
- Receive Email Notices or RSS
Advanced Search
- Log into SelectedWorks
- Economics Department
Home | About | FAQ | My Account | Accessibility Statement
Privacy Copyright
- Search Menu
- Advance articles
- Featured articles
- Virtual Issues
- Prize-Winning Articles
- Browse content in A - General Economics and Teaching
- Browse content in A1 - General Economics
- A11 - Role of Economics; Role of Economists; Market for Economists
- A12 - Relation of Economics to Other Disciplines
- Browse content in B - History of Economic Thought, Methodology, and Heterodox Approaches
- Browse content in B4 - Economic Methodology
- B41 - Economic Methodology
- Browse content in C - Mathematical and Quantitative Methods
- Browse content in C1 - Econometric and Statistical Methods and Methodology: General
- C10 - General
- C11 - Bayesian Analysis: General
- C12 - Hypothesis Testing: General
- C13 - Estimation: General
- C14 - Semiparametric and Nonparametric Methods: General
- C18 - Methodological Issues: General
- Browse content in C2 - Single Equation Models; Single Variables
- C22 - Time-Series Models; Dynamic Quantile Regressions; Dynamic Treatment Effect Models; Diffusion Processes
- C23 - Panel Data Models; Spatio-temporal Models
- C26 - Instrumental Variables (IV) Estimation
- Browse content in C3 - Multiple or Simultaneous Equation Models; Multiple Variables
- C31 - Cross-Sectional Models; Spatial Models; Treatment Effect Models; Quantile Regressions; Social Interaction Models
- C32 - Time-Series Models; Dynamic Quantile Regressions; Dynamic Treatment Effect Models; Diffusion Processes; State Space Models
- C33 - Panel Data Models; Spatio-temporal Models
- C36 - Instrumental Variables (IV) Estimation
- Browse content in C4 - Econometric and Statistical Methods: Special Topics
- C40 - General
- C44 - Operations Research; Statistical Decision Theory
- C45 - Neural Networks and Related Topics
- Browse content in C5 - Econometric Modeling
- C52 - Model Evaluation, Validation, and Selection
- C53 - Forecasting and Prediction Methods; Simulation Methods
- C55 - Large Data Sets: Modeling and Analysis
- Browse content in C6 - Mathematical Methods; Programming Models; Mathematical and Simulation Modeling
- C61 - Optimization Techniques; Programming Models; Dynamic Analysis
- C62 - Existence and Stability Conditions of Equilibrium
- C63 - Computational Techniques; Simulation Modeling
- Browse content in C7 - Game Theory and Bargaining Theory
- C70 - General
- C71 - Cooperative Games
- C72 - Noncooperative Games
- C73 - Stochastic and Dynamic Games; Evolutionary Games; Repeated Games
- C78 - Bargaining Theory; Matching Theory
- Browse content in C8 - Data Collection and Data Estimation Methodology; Computer Programs
- C81 - Methodology for Collecting, Estimating, and Organizing Microeconomic Data; Data Access
- Browse content in C9 - Design of Experiments
- C90 - General
- C91 - Laboratory, Individual Behavior
- C92 - Laboratory, Group Behavior
- C93 - Field Experiments
- C99 - Other
- Browse content in D - Microeconomics
- Browse content in D0 - General
- D00 - General
- D01 - Microeconomic Behavior: Underlying Principles
- D02 - Institutions: Design, Formation, Operations, and Impact
- D03 - Behavioral Microeconomics: Underlying Principles
- Browse content in D1 - Household Behavior and Family Economics
- D11 - Consumer Economics: Theory
- D12 - Consumer Economics: Empirical Analysis
- D13 - Household Production and Intrahousehold Allocation
- D14 - Household Saving; Personal Finance
- D15 - Intertemporal Household Choice: Life Cycle Models and Saving
- D18 - Consumer Protection
- Browse content in D2 - Production and Organizations
- D21 - Firm Behavior: Theory
- D22 - Firm Behavior: Empirical Analysis
- D23 - Organizational Behavior; Transaction Costs; Property Rights
- D24 - Production; Cost; Capital; Capital, Total Factor, and Multifactor Productivity; Capacity
- Browse content in D3 - Distribution
- D30 - General
- D31 - Personal Income, Wealth, and Their Distributions
- D33 - Factor Income Distribution
- Browse content in D4 - Market Structure, Pricing, and Design
- D40 - General
- D42 - Monopoly
- D43 - Oligopoly and Other Forms of Market Imperfection
- D44 - Auctions
- D47 - Market Design
- Browse content in D5 - General Equilibrium and Disequilibrium
- D50 - General
- D52 - Incomplete Markets
- Browse content in D6 - Welfare Economics
- D60 - General
- D61 - Allocative Efficiency; Cost-Benefit Analysis
- D62 - Externalities
- D63 - Equity, Justice, Inequality, and Other Normative Criteria and Measurement
- D64 - Altruism; Philanthropy
- Browse content in D7 - Analysis of Collective Decision-Making
- D70 - General
- D71 - Social Choice; Clubs; Committees; Associations
- D72 - Political Processes: Rent-seeking, Lobbying, Elections, Legislatures, and Voting Behavior
- D73 - Bureaucracy; Administrative Processes in Public Organizations; Corruption
- D74 - Conflict; Conflict Resolution; Alliances; Revolutions
- D78 - Positive Analysis of Policy Formulation and Implementation
- Browse content in D8 - Information, Knowledge, and Uncertainty
- D80 - General
- D81 - Criteria for Decision-Making under Risk and Uncertainty
- D82 - Asymmetric and Private Information; Mechanism Design
- D83 - Search; Learning; Information and Knowledge; Communication; Belief; Unawareness
- D84 - Expectations; Speculations
- D85 - Network Formation and Analysis: Theory
- D86 - Economics of Contract: Theory
- D87 - Neuroeconomics
- D89 - Other
- Browse content in D9 - Micro-Based Behavioral Economics
- D90 - General
- D91 - Role and Effects of Psychological, Emotional, Social, and Cognitive Factors on Decision Making
- D92 - Intertemporal Firm Choice, Investment, Capacity, and Financing
- Browse content in E - Macroeconomics and Monetary Economics
- Browse content in E0 - General
- E01 - Measurement and Data on National Income and Product Accounts and Wealth; Environmental Accounts
- E02 - Institutions and the Macroeconomy
- E1 - General Aggregative Models
- Browse content in E2 - Consumption, Saving, Production, Investment, Labor Markets, and Informal Economy
- E20 - General
- E21 - Consumption; Saving; Wealth
- E22 - Investment; Capital; Intangible Capital; Capacity
- E23 - Production
- E24 - Employment; Unemployment; Wages; Intergenerational Income Distribution; Aggregate Human Capital; Aggregate Labor Productivity
- E25 - Aggregate Factor Income Distribution
- E26 - Informal Economy; Underground Economy
- E27 - Forecasting and Simulation: Models and Applications
- Browse content in E3 - Prices, Business Fluctuations, and Cycles
- E30 - General
- E31 - Price Level; Inflation; Deflation
- E32 - Business Fluctuations; Cycles
- E37 - Forecasting and Simulation: Models and Applications
- Browse content in E4 - Money and Interest Rates
- E43 - Interest Rates: Determination, Term Structure, and Effects
- E44 - Financial Markets and the Macroeconomy
- Browse content in E5 - Monetary Policy, Central Banking, and the Supply of Money and Credit
- E51 - Money Supply; Credit; Money Multipliers
- E52 - Monetary Policy
- E58 - Central Banks and Their Policies
- Browse content in E6 - Macroeconomic Policy, Macroeconomic Aspects of Public Finance, and General Outlook
- E61 - Policy Objectives; Policy Designs and Consistency; Policy Coordination
- E62 - Fiscal Policy
- E63 - Comparative or Joint Analysis of Fiscal and Monetary Policy; Stabilization; Treasury Policy
- E65 - Studies of Particular Policy Episodes
- Browse content in F - International Economics
- Browse content in F0 - General
- F01 - Global Outlook
- F02 - International Economic Order and Integration
- Browse content in F1 - Trade
- F10 - General
- F11 - Neoclassical Models of Trade
- F12 - Models of Trade with Imperfect Competition and Scale Economies; Fragmentation
- F13 - Trade Policy; International Trade Organizations
- F14 - Empirical Studies of Trade
- F15 - Economic Integration
- F16 - Trade and Labor Market Interactions
- F17 - Trade Forecasting and Simulation
- Browse content in F2 - International Factor Movements and International Business
- F20 - General
- F21 - International Investment; Long-Term Capital Movements
- F22 - International Migration
- F23 - Multinational Firms; International Business
- Browse content in F3 - International Finance
- F31 - Foreign Exchange
- F32 - Current Account Adjustment; Short-Term Capital Movements
- F33 - International Monetary Arrangements and Institutions
- F34 - International Lending and Debt Problems
- F35 - Foreign Aid
- Browse content in F4 - Macroeconomic Aspects of International Trade and Finance
- F41 - Open Economy Macroeconomics
- F42 - International Policy Coordination and Transmission
- F43 - Economic Growth of Open Economies
- F45 - Macroeconomic Issues of Monetary Unions
- Browse content in F5 - International Relations, National Security, and International Political Economy
- F50 - General
- F51 - International Conflicts; Negotiations; Sanctions
- F52 - National Security; Economic Nationalism
- F55 - International Institutional Arrangements
- Browse content in F6 - Economic Impacts of Globalization
- F60 - General
- F61 - Microeconomic Impacts
- F66 - Labor
- F68 - Policy
- Browse content in G - Financial Economics
- Browse content in G0 - General
- G01 - Financial Crises
- Browse content in G1 - General Financial Markets
- G10 - General
- G11 - Portfolio Choice; Investment Decisions
- G12 - Asset Pricing; Trading volume; Bond Interest Rates
- G15 - International Financial Markets
- Browse content in G2 - Financial Institutions and Services
- G20 - General
- G21 - Banks; Depository Institutions; Micro Finance Institutions; Mortgages
- G24 - Investment Banking; Venture Capital; Brokerage; Ratings and Ratings Agencies
- G28 - Government Policy and Regulation
- Browse content in G3 - Corporate Finance and Governance
- G30 - General
- G31 - Capital Budgeting; Fixed Investment and Inventory Studies; Capacity
- G32 - Financing Policy; Financial Risk and Risk Management; Capital and Ownership Structure; Value of Firms; Goodwill
- G33 - Bankruptcy; Liquidation
- G34 - Mergers; Acquisitions; Restructuring; Corporate Governance
- Browse content in G4 - Behavioral Finance
- G41 - Role and Effects of Psychological, Emotional, Social, and Cognitive Factors on Decision Making in Financial Markets
- Browse content in G5 - Household Finance
- G51 - Household Saving, Borrowing, Debt, and Wealth
- G53 - Financial Literacy
- Browse content in H - Public Economics
- Browse content in H0 - General
- H00 - General
- Browse content in H1 - Structure and Scope of Government
- H11 - Structure, Scope, and Performance of Government
- H12 - Crisis Management
- Browse content in H2 - Taxation, Subsidies, and Revenue
- H20 - General
- H21 - Efficiency; Optimal Taxation
- H22 - Incidence
- H23 - Externalities; Redistributive Effects; Environmental Taxes and Subsidies
- H24 - Personal Income and Other Nonbusiness Taxes and Subsidies; includes inheritance and gift taxes
- H25 - Business Taxes and Subsidies
- H26 - Tax Evasion and Avoidance
- Browse content in H3 - Fiscal Policies and Behavior of Economic Agents
- H30 - General
- H31 - Household
- Browse content in H4 - Publicly Provided Goods
- H41 - Public Goods
- H42 - Publicly Provided Private Goods
- H44 - Publicly Provided Goods: Mixed Markets
- Browse content in H5 - National Government Expenditures and Related Policies
- H50 - General
- H51 - Government Expenditures and Health
- H52 - Government Expenditures and Education
- H53 - Government Expenditures and Welfare Programs
- H54 - Infrastructures; Other Public Investment and Capital Stock
- H55 - Social Security and Public Pensions
- H56 - National Security and War
- H57 - Procurement
- Browse content in H6 - National Budget, Deficit, and Debt
- H62 - Deficit; Surplus
- H63 - Debt; Debt Management; Sovereign Debt
- H68 - Forecasts of Budgets, Deficits, and Debt
- Browse content in H7 - State and Local Government; Intergovernmental Relations
- H71 - State and Local Taxation, Subsidies, and Revenue
- H72 - State and Local Budget and Expenditures
- H75 - State and Local Government: Health; Education; Welfare; Public Pensions
- H76 - State and Local Government: Other Expenditure Categories
- H77 - Intergovernmental Relations; Federalism; Secession
- Browse content in H8 - Miscellaneous Issues
- H87 - International Fiscal Issues; International Public Goods
- Browse content in I - Health, Education, and Welfare
- Browse content in I1 - Health
- I10 - General
- I11 - Analysis of Health Care Markets
- I12 - Health Behavior
- I14 - Health and Inequality
- I15 - Health and Economic Development
- I18 - Government Policy; Regulation; Public Health
- I19 - Other
- Browse content in I2 - Education and Research Institutions
- I20 - General
- I21 - Analysis of Education
- I22 - Educational Finance; Financial Aid
- I23 - Higher Education; Research Institutions
- I24 - Education and Inequality
- I25 - Education and Economic Development
- I26 - Returns to Education
- I28 - Government Policy
- Browse content in I3 - Welfare, Well-Being, and Poverty
- I30 - General
- I31 - General Welfare
- I32 - Measurement and Analysis of Poverty
- I38 - Government Policy; Provision and Effects of Welfare Programs
- Browse content in J - Labor and Demographic Economics
- Browse content in J0 - General
- J00 - General
- J01 - Labor Economics: General
- J08 - Labor Economics Policies
- Browse content in J1 - Demographic Economics
- J10 - General
- J11 - Demographic Trends, Macroeconomic Effects, and Forecasts
- J12 - Marriage; Marital Dissolution; Family Structure; Domestic Abuse
- J13 - Fertility; Family Planning; Child Care; Children; Youth
- J14 - Economics of the Elderly; Economics of the Handicapped; Non-Labor Market Discrimination
- J15 - Economics of Minorities, Races, Indigenous Peoples, and Immigrants; Non-labor Discrimination
- J16 - Economics of Gender; Non-labor Discrimination
- J17 - Value of Life; Forgone Income
- J18 - Public Policy
- Browse content in J2 - Demand and Supply of Labor
- J20 - General
- J21 - Labor Force and Employment, Size, and Structure
- J22 - Time Allocation and Labor Supply
- J23 - Labor Demand
- J24 - Human Capital; Skills; Occupational Choice; Labor Productivity
- J26 - Retirement; Retirement Policies
- J28 - Safety; Job Satisfaction; Related Public Policy
- Browse content in J3 - Wages, Compensation, and Labor Costs
- J31 - Wage Level and Structure; Wage Differentials
- J32 - Nonwage Labor Costs and Benefits; Retirement Plans; Private Pensions
- J33 - Compensation Packages; Payment Methods
- Browse content in J4 - Particular Labor Markets
- J42 - Monopsony; Segmented Labor Markets
- J43 - Agricultural Labor Markets
- J45 - Public Sector Labor Markets
- J46 - Informal Labor Markets
- J47 - Coercive Labor Markets
- Browse content in J5 - Labor-Management Relations, Trade Unions, and Collective Bargaining
- J50 - General
- J51 - Trade Unions: Objectives, Structure, and Effects
- J53 - Labor-Management Relations; Industrial Jurisprudence
- Browse content in J6 - Mobility, Unemployment, Vacancies, and Immigrant Workers
- J61 - Geographic Labor Mobility; Immigrant Workers
- J62 - Job, Occupational, and Intergenerational Mobility
- J63 - Turnover; Vacancies; Layoffs
- J64 - Unemployment: Models, Duration, Incidence, and Job Search
- J65 - Unemployment Insurance; Severance Pay; Plant Closings
- J68 - Public Policy
- Browse content in J7 - Labor Discrimination
- J71 - Discrimination
- Browse content in K - Law and Economics
- Browse content in K1 - Basic Areas of Law
- K10 - General
- K12 - Contract Law
- K14 - Criminal Law
- Browse content in K3 - Other Substantive Areas of Law
- K36 - Family and Personal Law
- Browse content in K4 - Legal Procedure, the Legal System, and Illegal Behavior
- K40 - General
- K41 - Litigation Process
- K42 - Illegal Behavior and the Enforcement of Law
- Browse content in L - Industrial Organization
- Browse content in L0 - General
- L00 - General
- Browse content in L1 - Market Structure, Firm Strategy, and Market Performance
- L11 - Production, Pricing, and Market Structure; Size Distribution of Firms
- L12 - Monopoly; Monopolization Strategies
- L13 - Oligopoly and Other Imperfect Markets
- L14 - Transactional Relationships; Contracts and Reputation; Networks
- L15 - Information and Product Quality; Standardization and Compatibility
- L16 - Industrial Organization and Macroeconomics: Industrial Structure and Structural Change; Industrial Price Indices
- Browse content in L2 - Firm Objectives, Organization, and Behavior
- L22 - Firm Organization and Market Structure
- L25 - Firm Performance: Size, Diversification, and Scope
- L26 - Entrepreneurship
- Browse content in L3 - Nonprofit Organizations and Public Enterprise
- L31 - Nonprofit Institutions; NGOs; Social Entrepreneurship
- L32 - Public Enterprises; Public-Private Enterprises
- L33 - Comparison of Public and Private Enterprises and Nonprofit Institutions; Privatization; Contracting Out
- Browse content in L4 - Antitrust Issues and Policies
- L41 - Monopolization; Horizontal Anticompetitive Practices
- L42 - Vertical Restraints; Resale Price Maintenance; Quantity Discounts
- L44 - Antitrust Policy and Public Enterprises, Nonprofit Institutions, and Professional Organizations
- Browse content in L5 - Regulation and Industrial Policy
- L51 - Economics of Regulation
- L52 - Industrial Policy; Sectoral Planning Methods
- Browse content in L6 - Industry Studies: Manufacturing
- L60 - General
- L67 - Other Consumer Nondurables: Clothing, Textiles, Shoes, and Leather Goods; Household Goods; Sports Equipment
- Browse content in L8 - Industry Studies: Services
- L81 - Retail and Wholesale Trade; e-Commerce
- L82 - Entertainment; Media
- L83 - Sports; Gambling; Recreation; Tourism
- L86 - Information and Internet Services; Computer Software
- Browse content in L9 - Industry Studies: Transportation and Utilities
- L91 - Transportation: General
- L94 - Electric Utilities
- L96 - Telecommunications
- L98 - Government Policy
- Browse content in M - Business Administration and Business Economics; Marketing; Accounting; Personnel Economics
- Browse content in M1 - Business Administration
- M10 - General
- M12 - Personnel Management; Executives; Executive Compensation
- M13 - New Firms; Startups
- Browse content in M2 - Business Economics
- M21 - Business Economics
- Browse content in M3 - Marketing and Advertising
- M30 - General
- M31 - Marketing
- Browse content in M5 - Personnel Economics
- M50 - General
- M51 - Firm Employment Decisions; Promotions
- M52 - Compensation and Compensation Methods and Their Effects
- M55 - Labor Contracting Devices
- Browse content in N - Economic History
- Browse content in N1 - Macroeconomics and Monetary Economics; Industrial Structure; Growth; Fluctuations
- N10 - General, International, or Comparative
- N12 - U.S.; Canada: 1913-
- N13 - Europe: Pre-1913
- N14 - Europe: 1913-
- N15 - Asia including Middle East
- Browse content in N2 - Financial Markets and Institutions
- N20 - General, International, or Comparative
- N23 - Europe: Pre-1913
- N26 - Latin America; Caribbean
- Browse content in N3 - Labor and Consumers, Demography, Education, Health, Welfare, Income, Wealth, Religion, and Philanthropy
- N30 - General, International, or Comparative
- N32 - U.S.; Canada: 1913-
- N33 - Europe: Pre-1913
- N34 - Europe: 1913-
- N35 - Asia including Middle East
- Browse content in N4 - Government, War, Law, International Relations, and Regulation
- N40 - General, International, or Comparative
- N41 - U.S.; Canada: Pre-1913
- N42 - U.S.; Canada: 1913-
- N43 - Europe: Pre-1913
- N44 - Europe: 1913-
- N45 - Asia including Middle East
- Browse content in N5 - Agriculture, Natural Resources, Environment, and Extractive Industries
- N50 - General, International, or Comparative
- N51 - U.S.; Canada: Pre-1913
- N53 - Europe: Pre-1913
- N55 - Asia including Middle East
- N57 - Africa; Oceania
- Browse content in N6 - Manufacturing and Construction
- N63 - Europe: Pre-1913
- Browse content in N7 - Transport, Trade, Energy, Technology, and Other Services
- N70 - General, International, or Comparative
- N71 - U.S.; Canada: Pre-1913
- N72 - U.S.; Canada: 1913-
- N73 - Europe: Pre-1913
- N75 - Asia including Middle East
- Browse content in N9 - Regional and Urban History
- N90 - General, International, or Comparative
- N92 - U.S.; Canada: 1913-
- N94 - Europe: 1913-
- N95 - Asia including Middle East
- Browse content in O - Economic Development, Innovation, Technological Change, and Growth
- Browse content in O1 - Economic Development
- O10 - General
- O11 - Macroeconomic Analyses of Economic Development
- O12 - Microeconomic Analyses of Economic Development
- O13 - Agriculture; Natural Resources; Energy; Environment; Other Primary Products
- O14 - Industrialization; Manufacturing and Service Industries; Choice of Technology
- O15 - Human Resources; Human Development; Income Distribution; Migration
- O16 - Financial Markets; Saving and Capital Investment; Corporate Finance and Governance
- O17 - Formal and Informal Sectors; Shadow Economy; Institutional Arrangements
- O18 - Urban, Rural, Regional, and Transportation Analysis; Housing; Infrastructure
- O19 - International Linkages to Development; Role of International Organizations
- Browse content in O2 - Development Planning and Policy
- O22 - Project Analysis
- O24 - Trade Policy; Factor Movement Policy; Foreign Exchange Policy
- O25 - Industrial Policy
- Browse content in O3 - Innovation; Research and Development; Technological Change; Intellectual Property Rights
- O30 - General
- O31 - Innovation and Invention: Processes and Incentives
- O32 - Management of Technological Innovation and R&D
- O33 - Technological Change: Choices and Consequences; Diffusion Processes
- O34 - Intellectual Property and Intellectual Capital
- O38 - Government Policy
- O39 - Other
- Browse content in O4 - Economic Growth and Aggregate Productivity
- O40 - General
- O41 - One, Two, and Multisector Growth Models
- O43 - Institutions and Growth
- O44 - Environment and Growth
- O47 - Empirical Studies of Economic Growth; Aggregate Productivity; Cross-Country Output Convergence
- Browse content in O5 - Economywide Country Studies
- O50 - General
- O52 - Europe
- O53 - Asia including Middle East
- O55 - Africa
- Browse content in P - Economic Systems
- Browse content in P0 - General
- P00 - General
- Browse content in P1 - Capitalist Systems
- P14 - Property Rights
- P16 - Political Economy
- Browse content in P2 - Socialist Systems and Transitional Economies
- P26 - Political Economy; Property Rights
- Browse content in P3 - Socialist Institutions and Their Transitions
- P39 - Other
- Browse content in P4 - Other Economic Systems
- P48 - Political Economy; Legal Institutions; Property Rights; Natural Resources; Energy; Environment; Regional Studies
- Browse content in P5 - Comparative Economic Systems
- P50 - General
- P51 - Comparative Analysis of Economic Systems
- Browse content in Q - Agricultural and Natural Resource Economics; Environmental and Ecological Economics
- Browse content in Q1 - Agriculture
- Q12 - Micro Analysis of Farm Firms, Farm Households, and Farm Input Markets
- Q14 - Agricultural Finance
- Q15 - Land Ownership and Tenure; Land Reform; Land Use; Irrigation; Agriculture and Environment
- Q16 - R&D; Agricultural Technology; Biofuels; Agricultural Extension Services
- Q17 - Agriculture in International Trade
- Q18 - Agricultural Policy; Food Policy
- Browse content in Q2 - Renewable Resources and Conservation
- Q23 - Forestry
- Q28 - Government Policy
- Browse content in Q3 - Nonrenewable Resources and Conservation
- Q32 - Exhaustible Resources and Economic Development
- Q33 - Resource Booms
- Browse content in Q4 - Energy
- Q41 - Demand and Supply; Prices
- Q48 - Government Policy
- Browse content in Q5 - Environmental Economics
- Q51 - Valuation of Environmental Effects
- Q52 - Pollution Control Adoption Costs; Distributional Effects; Employment Effects
- Q53 - Air Pollution; Water Pollution; Noise; Hazardous Waste; Solid Waste; Recycling
- Q54 - Climate; Natural Disasters; Global Warming
- Q55 - Technological Innovation
- Q56 - Environment and Development; Environment and Trade; Sustainability; Environmental Accounts and Accounting; Environmental Equity; Population Growth
- Q58 - Government Policy
- Browse content in R - Urban, Rural, Regional, Real Estate, and Transportation Economics
- Browse content in R1 - General Regional Economics
- R10 - General
- R11 - Regional Economic Activity: Growth, Development, Environmental Issues, and Changes
- R12 - Size and Spatial Distributions of Regional Economic Activity
- R13 - General Equilibrium and Welfare Economic Analysis of Regional Economies
- R15 - Econometric and Input-Output Models; Other Models
- Browse content in R2 - Household Analysis
- R21 - Housing Demand
- R23 - Regional Migration; Regional Labor Markets; Population; Neighborhood Characteristics
- Browse content in R3 - Real Estate Markets, Spatial Production Analysis, and Firm Location
- R30 - General
- R31 - Housing Supply and Markets
- Browse content in R4 - Transportation Economics
- R41 - Transportation: Demand, Supply, and Congestion; Travel Time; Safety and Accidents; Transportation Noise
- Browse content in R5 - Regional Government Analysis
- R52 - Land Use and Other Regulations
- Browse content in Z - Other Special Topics
- Browse content in Z1 - Cultural Economics; Economic Sociology; Economic Anthropology
- Z10 - General
- Z12 - Religion
- Z13 - Economic Sociology; Economic Anthropology; Social and Economic Stratification
- Z19 - Other
- Browse content in Z2 - Sports Economics
- Z20 - General
- Author Guidelines
- Submission Site
- Open Access
- Self-Archiving Policy
- About The Economic Journal
- About the Royal Economic Society
- Editorial Board
- Advertising and Corporate Services
- Journals on Oxford Academic
- Books on Oxford Academic

Editorial board
The Economic Journal is one of the founding journals of modern economics first published in 1891. The journal remains one of the top journals in the profession and provides a platform for high quality, innovative, and imaginative economic research, publishing papers in all fields of economics for a broad international readership.

Latest Impact Factor
The Economic Journal remains a highly read and cited publication by researchers, as demonstrated by our latest Impact Factor. To celebrate this continued success, we have curated a free collection of the most cited papers published in recent years. Use this collection to support your current work, or get up to date with important discussions in the field of economics.
Read the papers
Prize-winning articles
The RES prize is the prize given for the best paper published in the year.
The Austin Robinson prize is awarded to the best paper published in The Economic Journal in a given year by an author (or multiple authors) who is (or are) within 5 years of receiving his or her (or their) PhD at the time of acceptance.
Read the prize-winning articles
The Economics of Conflict

Economic History Supplements: 1926 - 1940
A fascinating window into the past has been opened with the release of newly-digitalised Economic History supplements of The Economic Journal . The archives contain articles published between 1926 - 1940, covering studies in economic history and the history of economic thought.
Explore the first issue
Latest articles
Most cited (past 5 years), latest posts on x, on the oupblog.
Fading signs of son preference
Son preference is a phenomenon that has strong historical roots in many western and non-western cultures. In some domains of life, is such preference already a thing of the past?
Read the blog post | Read the article
How paternity leave can help couples stay together
The birth of a child is accompanied by many changes in a couple’s life. The first few weeks and months are a time of acquiring new skills and creating new habits which allow parents to carry on with their other responsibilities while also caring for the new family member...
Read the blog post | Read the article
Natural disasters make people more religious
Philosophers once predicted that religion would die out as societies modernize. This has not happened. Today, more than four out of every five people on Earth believe in God. Religion seems to be serving a purpose that modernization does not replace...

How helping disabled people find employment affects the job market
Policy makers have long been concerned with helping people on disability benefits find some employment as this group has grown dramatically in recent decades. In the UK, as in several other countries, there are now many more people on disability benefits than on unemployment benefits...
Royal Economic Society Lectures
RES 2021 Past President's Address: Lord Nicholas Stern
RES 2021 EJ Lecture: Rachel Griffith
At the 2021 Royal Economic Society Annual Conference, former RES President Rachel Griffith delivered a lecture on her research paper 'Obesity, Poverty and Public Policy', published in The Economic Journal . View her address to delegates above, or read the full research paper.
Read the full research article
RES 2019 EJ Lecture: Eliana La Ferrara
Eliana La Ferrara gave the 2019 Economic Journal Lecture at the Royal Economic Society Annual Conference, in which she discussed her research into enforcing conditional welfare programs. Watch her address above, or read the full research article 'Learning Spillovers in Conditional Welfare Programmes: Evidence from Brazil' at the link below.
RES 2019 Past President's Address: Peter Neary
At the 2019 Royal Economic Society Annual Conference, former RES President Peter Neary delivered a lecture on his research paper 'Gravity without Apologies: The Science of Elasticities, Distance, and Trade', published in The Economic Journal. View his address to delegates above, or read the full research paper.

Trending articles
Discover the top articles from The Economic Journal sorted by Altmetric Score, mentioned online in the past three months.
Explore trending articles

Submissions
The Editors of The Economic Journal welcome high-quality papers in economics from any field. Submissions should be technically well-crafted, make a substantial contribution to the subject, and be of broad interest to economists at large.
Find out more and submit

Royal Economic Society
The Royal Economic Society is one of the oldest and most prestigious economic associations in the world. It is a learned society, founded in 1890 to promote the study of economic science. The Society publishes two leading Economic titles: The Economic Journal and The Econometrics Journal .
Related Titles
- Recommend to your Librarian
Affiliations
- Online ISSN 1468-0297
- Print ISSN 0013-0133
- Copyright © 2024 Royal Economic Society
- About Oxford Academic
- Publish journals with us
- University press partners
- What we publish
- New features
- Open access
- Institutional account management
- Rights and permissions
- Get help with access
- Accessibility
- Advertising
- Media enquiries
- Oxford University Press
- Oxford Languages
- University of Oxford
Oxford University Press is a department of the University of Oxford. It furthers the University's objective of excellence in research, scholarship, and education by publishing worldwide
- Copyright © 2024 Oxford University Press
- Cookie settings
- Cookie policy
- Privacy policy
- Legal notice
This Feature Is Available To Subscribers Only
Sign In or Create an Account
This PDF is available to Subscribers Only
For full access to this pdf, sign in to an existing account, or purchase an annual subscription.
Advertisement
- Next Article
I. Introduction
Ii. historical context, iii. data and empirical strategy, iv. main results, v. what drives our results exploring mechanisms, vi. who drives our results heterogeneous effects by workers’ characteristics, vii. conclusion, migration and knowledge diffusion: the effect of returning refugees on export performance in the former yugoslavia.
This paper benefited from helpful comments at various stages by Ran Abramitzky, Simone Bertoli, Clément De Chaisemartin, Michael Clemens, Wolfgang Dauth, David Yanagizawa-Drott, Esther Duflo, Peter Egger, Albrecht Glitz, Simon Goerlach, Bill Kerr, Florian Lehmer, Britta Matthes, Anna Maria Mayda, Thierry Mayer, Nathan Nunn, Ariell Reshef, and participants in many different seminars and conferences who provided valuable comments. We are grateful to Valentin Todorov from UNIDO for data guidance. Rotem Weinberg provided excellent research assistance. H.R, and C.Ö. acknowledge the support of EUR grant ANR-17-EURE-0001. All errors are our own.
A supplemental appendix is available online at https://doi.org/10.1162/rest_a_01165 .
- Cite Icon Cite
- Open the PDF for in another window
- Permissions
- Article contents
- Figures & tables
- Supplementary Data
- Peer Review
- Search Site
Dany Bahar , Andreas Hauptmann , Cem Özgüzel , Hillel Rapoport; Migration and Knowledge Diffusion: The Effect of Returning Refugees on Export Performance in the Former Yugoslavia. The Review of Economics and Statistics 2024; 106 (2): 287–304. doi: https://doi.org/10.1162/rest_a_01165
Download citation file:
- Ris (Zotero)
- Reference Manager
During the early 1990s, Germany offered temporary protection to 700,000 Yugoslavian refugees fleeing war. By 2000, many had been repatriated. We exploit this natural experiment to investigate the role of returning migrants in boosting export performance upon their return. Using confidential German administrative data, we find that industries with 10% more returning refugees exhibit larger exports between the pre- and postwar periods by 1% to 1.6%. We use exogenous allocation rules for asylum seekers within Germany as an instrument to deal with endogeneity concerns. We show evidence pointing to productivity shifts as the main mechanism behind our results. Consistently, we find our results are driven by refugees in occupations more likely to transfer knowledge, technologies, and best practices.
AS of today, there are over 26 million refugees hosted in a foreign country. This figure is likely to keep increasing as sources of displacement such as ongoing conflict and climate change pose important challenges to stability around the globe. For the most part, refugees in host countries face hurdles to integrate due to negative perceptions of their effect on local labor markets, crime levels, or social cohesion. Despite the negative public sentiment, refugees, and migrants alike, represent important economic opportunities for both their host communities and home countries, as evidenced by a small but growing literature. 1 Yet to the best of our knowledge, there is no rigorous study documenting the crucial role refugees can play in shaping large-scale economic outcomes (such as a country’s industrial or export structure) in their home countries upon return. This paper fills this gap by documenting the long-run effect of returning migrants on industry-level exports driven by productivity shifts, using the case of Yugoslavian refugees during the 1990s.
We exploit a natural experiment and document a systematic relationship between refugees spending time and gaining work experience in a foreign country and the subsequent export performance at home of the same sectors in which they had worked while abroad upon their return. The context is the early 1990s, when about 700,000 citizens of what was then Yugoslavia fled to Germany escaping war. Most Yugoslavian migrants in the first half of the 1990s were given a Duldung status (German for “toleration”), in effect a temporary protection status, or more specifically, a “suspended deportation” permit. After the Dayton peace agreement was signed in 1995, the protection status of temporary migrants was revoked, forcing them to leave the country. By 2000, the majority of these migrants had been repatriated back to their home country or to other territories of the dissolved Yugoslavia.
Using confidential administrative data from the Institute for Employment Research (IAB), we identify returning migrants as Yugoslavian workers who entered the German labor force (by industry) between 1991 and 1995 and had left it by the year 2000. In this sense, our “treatment” reflects the extent to which Yugoslavian refugees were exposed to German productive knowledge. We link this information to standard industry-level international trade data and employ a difference-in-differences methodology to estimate changes in export values from the former Yugoslavia to the rest of the world. The different data sources we rely on limit us from linking both exports and migrants to the different nations that emerged after the dissolution of Yugoslavia. We overcome this limitation, however, by aggregating data for all former Yugoslavian nations in order to construct an industry-level panel data. We find that the industries that perform better in terms of exports in the years following the repatriation are the very same ones in which refugees worked while in Germany.
In order to address concerns of endogeneity due to, for example, self-selection of workers into certain industries, we instrument for the actual number of returning workers per industry using a spatial dispersal policy that exogenously allocated asylum seekers across the different administrative regions of Germany upon arrival.
Our main finding is that industries with a 1% increase in return migration (as defined by our treatment) experienced an increase in exports to the rest of the world of 0.1% to 0.16% between the pre- and postwar periods. Based on all the returning refugees in our sample, this represents up to 6% of all the export growth of former Yugoslavian nations between 1990 and 2005, most of it occurring after 2000.
Our results are robust to an estimation in the form of an event-study, which shows that there are no existing previous trends for industries treated at different levels of intensity and that the treatment effect becomes larger with time. In addition, we complement our main difference-in-difference results by applying the synthetic control method (Abadie & Gardeazabal, 2003 ; Abadie et al., 2010 , 2015 ) to create a counterfactual for every Yugoslavian export industry in our sample. We find that real Yugoslavian industries outperform their synthetic counterparts, on average, with this pattern being particularly strong for industries associated with more intense treatment.
When exploring mechanisms driving our main findings, we find robust evidence that points to industry-specific productivity shifts brought on by returning refugees as the main driving force of export performance. In particular, our findings are robust to substituting actual exports with a constructed measure of revealed comparative advantage based on Costinot et al. ( 2012 ), which arguably reflects productivity dynamics, and we replicate our analysis using more aggregated data to study changes in labor productivity (as measured by output per worker, added value per worker, and wages) using data from the UN Industrial Development Organization (UNIDO), as well as using sectoral data on exports per worker and size (firms and workers) for the specific case of Bosnia. We find that all these complementary results are consistent with our main findings.
Through numerous empirical tests, we also rule out a number of alternative explanations, which include our results being driven by purely scale effects or convergence patterns in structural transformation. We also rule out channels that the literature links to migration flows and could also explain export performance, such as capital formation and reduction of international trade transaction costs due to migrant networks. While exploring these channels provides us with additional insights, we find no evidence in them to invalidate the results that point to productivity shifts as a mechanism driving our results.
Consistently with productivity shifts, we then document that our results are particularly driven by certain types of workers and occupations, which would be more likely to transfer knowledge, technologies, and best practices across borders, and thus able to induce productivity improvements. For instance, our results are driven by workers with high educational attainment, in occupations intensive in analytical tasks (as opposed to manual ones), occupations that can be classified as professional and/or skill intensive, and occupations that have managerial characteristics. We also find that our results are stronger when looking at workers who, while abroad, experienced faster wage growth and were employed by the top-paying firms within each industry.
Our findings contribute to the economic literature at large by documenting substantial industry-specific productivity increases, resulting in changes in the export industrial composition of a country as a whole, as a result of returning migrants who were exposed to foreign knowledge, technologies, and best practices. To the best of our knowledge, this is the first paper documenting such large-scale and country-level industrial productivity shifts induced by return migration in the context of a natural experiment. 2
Our paper contributes to a number of strands of the literature. First, we contribute to the literature on migrants as drivers of international knowledge diffusion back to their home countries (Kerr, 2008 ; Agrawal et al., 2006 , 2011 ; Breschi et al., 2017 ; Bahar & Rapoport, 2018 ; Miguélez, 2018 ; Miguelez & Noumedem Temgoua, 2020 ; Bahar et al., 2019 ) and, relatedly, to a burgeoning literature that looks at migrants as drivers of productivity shifts (Scoville, 1952a , 1952b ; Markusen & Trofimenko, 2009 ; Poole, 2013 ; Choudhury, 2016 ; Hornung, 2014 ; Malchow-Møller et al., 2017 ; Bryan & Morten, 2019 ). Second, our results speak to the important link between migration and economic development, given the substantial role of migrants affecting structural transformation, an important driver of economic growth (Imbs & Wacziarg, 2003 ; Hausmann et al., 2006 ; Hidalgo et al., 2007 ; Koren & Tenreyro, 2007 ; Cadot et al., 2011 ). Third, we contribute to the growing literature emphasizing the role of managerial skills as a crucial determinant of productivity (Bloom & Van Reenen, 2007 ; Bloom et al., 2013 ; Giorcelli, 2019 ) and of exports (Bloom et al., 2021 ). Finally, we contribute to a growing number of studies that focus on consequences of forced displacement for the refugees’ home countries (Waldinger, 2010 , 2012 ; Acemoglu et al., 2011 ; Grosfeld et al., 2013 ; Akbulut-Yuksel & Yuksel, 2015 ; Bharadwaj et al., 2015 ; Pascali, 2016 ; Huber et al., 2021 ; Testa, 2020 ; Mayda et al., 2022 ), as well as to the literature of the economic consequences of return migration (McCormick & Wahba, 2001 ; Dustmann & Kirchkamp, 2002 ; Mesnard, 2004 ; Wahba & Zenou, 2012 ; Batista et al., 2017 ). 3
The rest of the paper is organized as follows. Section II provides a description of the historical context of the Yugoslavian refugee crisis. Section III details the data sources and the empirical strategy. Section IV presents the main results and performs a series of robustness tests. Section V explores possible mechanisms driving our results, and section VI exploits heterogeneity effects based on the characteristics and occupations of the refugees. Section VII concludes. The paper is accompanied by an online appendix, which we refer to throughout the text.
A. The Refugee Crisis and Integration in Germany
In June 1991, the Socialist Federal Republic of Yugoslavia started to disintegrate following several armed conflicts and ethnic civil wars. Fighting began with the “Ten-Day War” in summer 1991 after Slovenia declared its independence. Soon after, the conflict spread to Croatia and later, in 1992, to Bosnia and Herzegovina. It was only in December 1995, with the signing of the Dayton Peace Accord involving President Clinton, that the armed conflict officially ended.
During the armed conflict, around 3.7 million people (roughly 16% of the Yugoslavian population) were displaced and fled from their homes, making this episode the largest migration flow in Europe since the end of World War II (Radovic, 2005 ). While many affected by the war became internally displaced, about 800,000 people resettled outside the boundaries of the former Yugoslavia, hoping to find refuge in other countries (Lederer, 1997 ). 4 Among these countries, Germany was one of the best suited to receive these refugees because of the already significant Yugoslavian community residing there and Germany’s ability and willingness to provide protection to those fleeing the war. 5 The flow of refugees into Germany responded to the dynamics of the conflict: in the early stages of the war, involving mostly Croatians, about 100,000 of them arrived in Germany; later, when the war spread to Bosnia, acts of systematic violence triggered massive outflows from those areas, and Germany hosted some 350,000 Bosnian refugees. Simultaneously, Germany also received another 250,000 Yugoslavians mainly from Serbia and Kosovo. Thus, overall during the first half of the 1990s, Germany received roughly 700,000 migrants from Yugoslavia, making it by far the largest recipient foreign country (see Lederer, 1997 , for a detailed account of these flows).
Most of Yugoslavian refugees who arrived in Germany were given a temporary protection status, known as Duldung , which can be translated to English as “toleration.” 6 The temporary character of the Duldung status constituted a “suspended deportation” status. In other words, Duldung holders were allowed to remain in Germany until the permit expired, after which they were obliged to leave the country immediately. While the Duldung duration upon issuance was set to six months, the authorities had the option to renew it. De facto, the Duldung status was renewed for all holders as long as the war continued.
A less popular option for Yugoslavians fleeing the war and arriving in Germany was to apply for asylum. According to Article 16(a) of the German Basic Law (Grundgesetz), an individual is eligible to seek asylum if he or she faces individual persecution and is able to prove so. If granted asylum, the individual enters a path toward permanent residency (Hailbronner, 2003 ). Asylum recognition rates, however, were very low for citizens from the former Yugoslavia (Lederer, 1997 , documents that between 1992 and 1995, only 1% of Bosnian applicants were granted asylum). This is because most of them could not prove to the German authorities they were facing individual persecution at home following the standards set by the German authorities at the time (Dimova, 2006 ). Importantly enough, however, asylum seekers whose request was denied also were able to receive Duldung status, since the German authorities were not deporting these refugees back into active war zones.
A large number of Yugoslavian refugees integrated into the German labor force after their arrival. For instance, in 1992 alone, the number of workers from (former) Yugoslavia rose by 15.3% to 375,000 (Deutscher-Bundestag, 1994 ). Overall, both Duldung holders and asylum seekers had relatively good access to the labor market. 7 But there was an important difference between the two statuses concerned with respect to their mobility. Duldung holders faced no geographical limitation. But asylum seekers were subjected to mandatory residency ( Residenzpflicht ) within the region in which their application was initiated while it was processed. 8 The decision on which region would process the application was made by the authorities based on preestablished quotas. This limitation on geographic mobility for asylum seekers is an important part of our identification strategy, which we detail in section IIID .
Generally refugees found employment across diverse sectors and relied on different channels to secure their jobs. Some were able to use their network of friends and family relatives, some relied on local employment agencies, and some found work by themselves (Walker, 2010 ; Ruben et al., 2009 ).
B. End of the War and Deportation
The signature of the Dayton Peace Accord in December 1995 officially marked the end of the war that started in 1991. After that date, the German authorities had no reason to renew the Duldung status of refugees and indeed enacted the imminent deportation of refugees back to the former Yugoslavia. 9 In fact, only one day after the signing of the Dayton Accord, Germany formally announced a repatriation plan through which Duldung refugees were gradually forced to leave the country (Dimova, 2006 ), often simultaneously rolling out assisted repatriation programs (Bosswick, 2000 ). 10
Repatriation was planned in two main phases. The first phase targeted single adults and childless couples, as well as people with family back in their home country. The second phase targeted the rest of the refugees. By summer 1996, letters requesting deportation were sent out, and by the end of 1996, deportations had begun. Repatriation and deportations continued until 2000, though most of them had happened by 1998. Figures by international organizations and independent academic research suggest that about 75% of Yugoslavian civil war refugees returned to their home country or to another former Yugoslavian nation, with an additional 15% settling in third countries and only about 10% remaining in Germany (UNHCR, 2005 ; Ruhl & Lederer, 2001 ; Lederer, 1997 ). 11 With respect to Croatian refugees, Lederer ( 1997 , 310) explains: “During the Croatian-Serbian War (1991 to 1993) numerous Croatians were also admitted to the Federal Republic of Germany. According to information from the Federal Ministry of the Interior of 9 October 1996, most of the original 100,000 Croatian refugees should have returned to their homeland within the framework of the repatriation process that began in 1994. However, the Federal Ministry of the Interior notes that there is no precise information on this from the federal states.”
Figure 1a plots the evolution of the population size of Yugoslavia (as a whole) over time during the period of our study. It shows, consistent with the historic accounts, overall population drops significantly after 1991, and following the end of the war (1995) it grows again. In 2005 the population of the former Yugoslavian countries combined was comparable to the population of Yugoslavia before the war, in 1990.
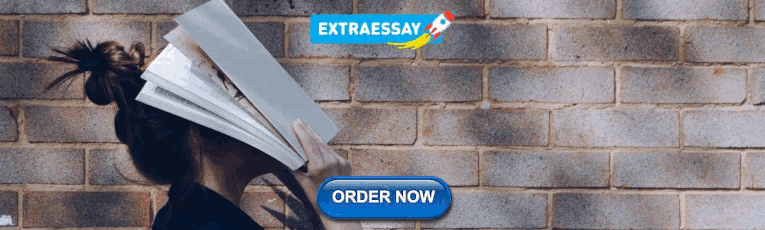
Population in Yugoslavia and Migration to Germany

Panel a plots the population of the (former) Yugoslavia over time, sourced from the Maddison Project Database 2018. Panel b shows the net inflow, stock, and asylum requests of migrants from the (former) Yugoslavia into Germany from 1980 until 2010. The number of migrant stocks by nationality is based on the Central Register of Foreigners (Ausländerzentralregister). The data have been downloaded from the online database of the German Federal Statistical Office ( Statistisches Bundesamt ). Data on migration flows and asylum applications by nationality are from the German Federal Statistical Office and the Federal Office for Migration and Refugees (Bundesamt für Migration und Flüchtlinge), respectively, and sent to us upon request.
Figure 1b plots the “mirror image”: the inflow of around 700,000 refugees from the former Yugoslavia who arrived in Germany in the first half of the 1990s. In 1980, there were already about 600,000 Yugoslavians residing in Germany. This stock remained steady until the late 1980s when the net inflow of Yugoslavian migrants started to grow at a rate of 25,000 per year, including until the year 1990. This rate skyrocketed to 168,000, 250,000, and 165,000 during 1991, 1992, and 1993, respectively. The sharp increase in the net inflow of migrants was fueled by refugees escaping war. We also see a sharp increase in asylum requests from Yugoslavian citizens during the same years.
Consistent with the accounts, the number of Yugoslavians in Germany sharply declines starting in 1996, after the Dayton treaty was signed. By 2000, close to 350,000 Yugoslavians had left the country. While some of them left to a third country, it has been estimated that the majority of them returned to countries of the (by then) former Yugoslavia (UNHCR, 2005 ; Ruhl & Lederer, 2001 ; Lederer, 1997 ), consistent with figure 1a .
In the German labor force, we see patterns consistent with the historical narrative described so far: both the inflow of Yugoslavian workers into the tradable sector labor force between the years 1991 and 1995 and their outflow in the years that followed are highly unusual as compared to foreign workers from other nationalities, as figure 2 shows. The figure plots the yearly share of Yugoslavian workers entering and exiting the labor force of Germany’s tradable sector. The share is always computed using the total number of all foreign workers entering and exiting the labor force in those same years at the denominator. It becomes clear from figure 2 that Yugoslavians entered the labor force in much higher proportions during the first half of the 1990s compared to the same proportion in years before 1990 and after 2000. We also see that Yugoslavian workers exited the German labor force in higher proportion during the latter half of the 1990s, consistent with the historical events.
Yugoslavian Workers’ Yearly Entry to and Exit from

The graph shows the yearly share (out of all foreign workers) of Yugoslavians entering and exiting the labor force of Germany’s tradable sector.
A. Data Sources
We link a number of data sets together for our study. First, we use data on exports for the period 1984 to 2014, which comes from bilateral trade data compiled by Feenstra et al. ( 2005 ), with extensions and corrections suggested by Hausmann et al. ( 2014 ; the original source of the trade data is UN Comtrade). In most cases our dependent variable is exports by industry from Yugoslavia to the rest of the world, excluding to Germany. We exclude Germany as a first attempt to reduce the possibility that our results are driven by lower transaction costs caused by migrant networks between the former Yugoslavian countries and Germany (Rauch & Trindade, 2002 ; Parsons & Vézina, 2018 ). 12
Industries are defined using the four-digit Standard Industry Trade Classification (SITC) revision 2 and list 786 different varieties. This industry classification provides a disaggregation level that enables a meaningful discussion about export diversification patterns. Some examples of industries in this level of disaggregation are, for example, “Knitted/Crocheted Fabrics Elastic or Rubberized” (SITC 6553) and “Electrical Measuring, Checking, Analyzing Instruments” (SITC 8748). Following Hausmann et al. ( 2014 ), we exclude countries below 1 million citizens and total trade below USD $1 billion in 2010. Other variables created using trade data are explained as they are introduced into the analysis.
The data on migrant workers in Germany are based on records from the German social security system and comprise all persons employed subject to social security contributions, with the exception of self-employed and civil servants. 13 The records indicate the industry where the workers are employed. Our sample is restricted to 40% random draws of foreign nationals observed on June 30 of each year from 1975 to 2014, augmented by the employment history of each individual for our sampling period. This amounts to about 2.4 million workers per year on average, a large enough number for the random draws to form a representative sample. 14 Moreover, since we can observe the full employment history, we can determine whether an individual was employed before or after any given year in Germany, which we exploit to construct our treatment. Beyond individual information such as age, nationality, and educational attainment, the data include detailed occupational codes categorized in more than 300 different occupations (see more details on this data set in online appendix section B).
In order to construct an instrument to deal with endogeneity issues, we use inflow quotas mandated by the government that define the regional distribution of asylum seekers ( Königsteiner Schlüssel ). These quotas are determined yearly by the Joint Science Conference (Gemeinsame Wissenschaftskonferenz, GWK). The GWK sent to us, in response to our request, yearly data between 1990 and 2016.
With these data sets we are able to match the exports of Yugoslavia to the rest of the world with the number of Yugoslavian workers in Germany working in the same industry category. 15
B. Main Outcome and Treatment Variables
Our main unit of analysis, which we use as our dependent variable in our baseline specification, is the combined exports by industry of Yugoslavia to the rest of the world, excluding to Germany (to avoid results being driven by lower transactions costs, as explained above). To construct this variable we combine per industry exports of Yugoslavia as a nation until 1991, with exports by industry of all countries combined that formed Yugoslavia after 1992: Bosnia and Herzegovina, Croatia, FYR of Macedonia, Montenegro, Serbia, and Slovenia (excluding trade within these countries). 16 We focus on all former Yugoslavian countries as one following the disintegration because the German data used to construct our treatment do not allow us to distinguish which is the region of origin of the refugees within Yugoslavia (e.g., we only see that they entered the labor force with a Yugoslavian passport).
The main independent variable, the treatment, is the number of Yugoslavian refugees by (tradable) sector who (i) joined the German labor force between 1991 and 1995, 17 (ii) had not been recorded in our data in 1990 or before, and (iii) had not been recorded in our data in 2000 or after. 18 Applying this filter allows us to proxy for returning refugees with a high degree of certainty, consistent with the historical accounts described above. 19
As already explained, a limitation of our data is that we cannot distinguish with full certainty whether these workers with Yugoslavian passports who left the labor force indeed returned to the former Yugoslavia. Thus, in our calculation of return migration, we are including workers who, for instance, stayed in Germany working in the informal sector or went to a third country. Yet all of these possibilities work against us in our estimation, implying that our estimates are to some extent understating the true effect of return migration. However, plenty of historic evidence suggests that indeed those who were repatriated went back and worked in related industries. For instance, Ruben et al. ( 2009 ) note that those who worked during their time in Germany were more likely to be employed when they returned to the former Yugoslavia, with many finding jobs in industries in which they had worked before the war. There is anecdotal evidence, too, suggesting that after returning to their home countries, refugees subsequently worked (or founded companies) in the same sector they had worked at in Germany (or other countries such as Sweden or Austria). In online appendix section A, we summarize some of the evidence we’ve collected on this. As these stories show, refugees benefited from their experiences in Germany in many ways, such as applying the acquired knowledge and skills about different production methods.
Figure 3 describes the treatment variable. It plots the number of Yugoslavian workers who entered the German workforce between 1991 and 1995 (horizontal axis) and the number of those workers who remain in the labor force beyond year 2000 (vertical axis), by four-digit SITC code. All observations are below the 45 degree line, simply because the number of migrants who stay in each industry beyond the year 2000 is a subset of all those who arrived between 1991 and 1995. Thus, the treatment is the difference between each one of the observations and the 45 degree line. As can be seen in the graph, there is variation in the treatment across industries. Some of the codes that stand out as having a large number of worker returnees are 8,219 (furniture parts), 6,911 (iron and steel structures), 5,989 (chemical products), and 2,482 (worked wood of coniferous). Based on our sample (which is limited to wage earners in the tradable sector), it is noticeable that “rate of return” as measured through our filter is substantial (about 30%), but not as high as what the historical acccounts suggest as documented in official reports from international organizations (e.g., UNHCR, 2005 ). 20 The fact that some Yugoslavians stayed beyond 2000 for whatever reason is not a threat to our identification strategy, as long as these cases were not more or less frequent in some industries than in others. We expand on this in section IIID .
Yugoslavians in the German Workforce, by Industry

The figure shows the number of Yugoslavian workers in the German workforce who arrived between 1991 and 1995 against those who remain in year 2000 and beyond. Marker labels indicate four-digit industries.
With the treatment defined but before we turn to the econometrics, we report whether industries associated with a larger treatment experienced better export performance in the former Yugoslavian countries upon their return. Using only raw data, figure 4 visualizes the total value of exports of industries linked to different levels of treatment (i.e., quartiles), year after year. The figure shows until 1995 (the year where our “treatment” begins), industries in the four different quartiles had somewhat parallel trends. However, after 1995, the third and fourth quartiles in terms of treatment intensity diverge quite significantly from the first two quartiles. This visualization not only provides some descriptive evidence of the results holding with raw data, but also shows that the parallel trends assumption required for the difference-in-differences methodology is a reasonable one. In any event, we address pretrend issues more thoroughly in the next section.
Exports for Industries with Different Levels of Treatment

The figure plots the cumulative value of exports of the former Yugoslavia to the rest of the world (vertical axis) across years. Treatment is defined as the number of return migrants from Germany by 2000 as defined in the text.
C. Summary Statistics
Table 1 presents the summary statistics. In our sample are 786 industries, and since we use two points in time for the differences-in-differences estimation, the initial empirical analysis will use up to 1,572 observations. The table presents summary statistics for the main variables in the regression. The first two lines of the table present data for the average export value from former Yugoslavian countries to the rest of the world in the years 1990 and 2005, all in million U.S. dollars (note that we do not adjust these values for inflation, which is accounted for by our year fixed effect). These two points in time are the ones used in the main specification, which correspond to years before and after the war. However, we also present results for a multiperiod analysis as well that uses export data for all the years in between.
Summary Statistics
This table presents the sample summary statistics for the variables used to estimate specification ( 1 ).
Table 1 also summarizes the treatment. The third row in the table presents statistics for the number of sampled workers with Yugoslavian passport that joined the German labor force at some point between 1991 and 1995. The average industry had 74 Yugoslavian workers who, arguably, arrived at Germany because of the war and joined the labor force. The next row is a subset of that group and corresponds to our main treatment variable: the number of workers with Yugoslavian passport who had joined the German labor force sometime between 1991 and 1995 and had dropped from it by the year 2000. The value for this variable, averaged across all industries, is 21.6. Our treatment exploits variation across industries, which we see in the table varies from 0 to 778. 21 All in all, our treatment is based on roughly 17,000 Yugoslavian workers across all industries, representative of the actual distribution. 22
Note that our sample of Yugoslavians employed in the tradable industry shows that the rate of return was roughly 30%, substantially lower than the anecdotical 75% figure, which applies to the Yugoslavian refugee population as a whole (UNHCR, 2005 ; Ruhl & Lederer, 2001 ; Lederer, 1997 ). This discrepancy, however, poses no problem for our identification strategy as long as the rate of return is not biased toward certain industries, which we discuss in section IIID . Also note that despite presenting the summary statistics in nominal values, unless otherwise stated, all right-hand-side variables are rescaled using the inverse hyperbolic sine for estimation purposes.
D. Empirical Strategy
The variable of interest, t r e a t p , is the treatment as explained above, which corresponds to the number of likely returning refugees for industry p , according to the definition detailed earlier. Given the fat-tailed distribution of this variable, we rescale it using the inverse hyperbolic sine, which behaves similar to a log transformation but has the benefit of being defined at 0. The interpretation of regression estimators in the form of the inverse hyperbolic sine is similar to the interpretation of a log-transformed variable (see MacKinnon & Magee, 1990 ).
We estimate this regression using two periods: “before,” which corresponds to 1990, just before the war started, and “after,” which corresponds to 2005, five years after most Yugoslavian refugees had returned. 23 Thus, a f t e r t is a dummy variable that equals 1 for the observations corresponding to the second period.
As for the other terms, η p represents industry fixed effects while α t represents year fixed effects. The two fixed effects are perfectly multicollinear with the terms t r e a t p and a f t e r t , and so there is no need to add the interacted terms separately. ɛ p , t represents the error term. Our estimations cluster standard errors at the industry level, the level at which the treatment varies (Besley & Burgess, 2004 ; Bertrand et al., 2004 ).
Given the fact that the left-hand side is calculated in U.S. dollars, we are required to use a monotonic transformation to deal with the fat-tailed distribution. All of our results are presented using three different transformations: log ( e x p o r t s p , t ) , log ( e x p o r t s p , t + 1 ) , and asinh ( e x p o r t s p , t ) . The first one is undefined for values where e x p o r t s p , t = 0 , and therefore, when using log ( e x p o r t s p , t ) as the dependent variable, the sample size is reduced. The two other transformations deal with the occasions where e x p o r t s p , t = 0 by either adding US$1 before the transformation or by computing instead the inverse hyperbolic sine ( a s i n h ), respectively. 24 Given these monotonic log-type transformations, β D I D represents the elasticity of exports to returnee workers. That is, industries with a 1% larger pool of returnee workers have larger export value by β D I D percent larger between the before and after periods compared to industries with no returnee workers. Bear in mind that since this is a difference-in-difference setting, our results reflect relative differences in levels across industries based on their exposure to the treatment.
Our identification relies on the exogeneity of arrival and exit of refugees into and out of the German labor force with regard to industry-level export trends back in Yugoslavia. There are two main endogeneity concerns: the possibility that upon arrival, refugees self-selected into particular tradable sectors after anticipating their future postwar growth potential in Yugoslavia and the possibility that the exit of refugees from the German labor force by year 2000—even if it was enforced by across-the-board repatriation efforts by the German authorities—happened more or less frequently in particular industries in a way that is correlated with export dynamics in Yugoslavia. We address each of these possible endogeneity concerns below.
Self-selection into industries upon arrival.
In order to deal with the possibility that migrants self-selected into particular industries in a way that correlates with future Yugoslavian exports, we construct an instrumental variable that estimates the share of asylum seekers working in each industry by exploiting a spatial dispersal policy. While asylum requests were being processed, asylum seekers were sent to different parts of the country following the Königstein State Convention (Königsteiner Staatsabkommen) which was signed in 1949 by all German federal states and defined cost-sharing rules between states in jointly financed projects. The dispersal of asylum seekers is regulated at the federal level by the Asylum Procedure Act (Asylverfahrensgesetz), where each state is allocated a certain number of asylum seekers according to its “Königstein” quota (Königsteiner Schlüssel). The quota is based on the weighted sum of population (one-third) and tax revenues (two-thirds), and is recalculated annually. For example, Nordrhein-Westfalen is the state that should have received the largest numbers of asylum seekers in 1995, followed by Bayern and Baden-Württemberg, while states such as Bremen and Saarland received a very small share.
Our identification strategy is based on the premise that this allocation was exogenous to the Yugoslavian asylum seekers entering the country and, furthermore, to the future dynamics of Yugoslavian exports. In practice, an asylum seeker into the German territory is absorbed by a reception center in the state of arrival if there is any remaining capacity to receive more people, according to the quota described above; alternatively, the person is allocated to the reception center in a different state with the most vacancies according to the quota. The residence obligation ends as soon as asylum is granted. The average duration of the application procedure was between six months and two years. 25
Difference-in-Difference Estimation
This table shows results of the estimation for specification ( 1 ) using different monotonic transformations for e x p o r t s p , t in each column. The estimation uses average exports for years 1988 to 1990 in the before period and average exports for years 2005 to 2007 in the after period. The first three columns report results from an OLS estimation, while the last three columns report results from a 2SLS estimation. The lower panel presents the results of the first-stage estimation when appropriate. All columns include industry fixed effects and year fixed effects. Standard errors clustered at the industry level presented in parentheses. * p < 0 . 10 , * * p < 0 . 05 , and * * * p < 0 . 01 .
There are two main reasons for which this strong correlation between the instrument and the endogenous variable is not surprising. First, the geographic allocation of asylum seekers is relevant for all refugees who request asylum, even if the asylum request turns out not to be approved. That is, all refugees who eventually got a Duldung status but who originally requested asylum had to comply with this exogenous geographic allocation while their asylum status was being reviewed by the authorities. Second, the exogenous allocation of the share of Yugoslavians who actually requested asylum might as well be explanatory of the location choice of those who received Duldung even if they did not request asylum to begin with due to pull factors induced by migrant networks.
Our main assumption regarding the second condition—the exclusion restriction—is that both the quota of asylum seekers per state and year (defined by the German federal authorities), as well as the relative size of industries in Germany in 1990, are not correlated with future industry-specific export trends of former Yugoslavian countries to the rest of the world other than through the migrants themselves. We have no reason to think that this assumption could be violated. 28
Self-selection out of industries upon exit.
The other remaining concern is that the exit of Yugoslavian Duldung holders from the German labor force was endogenous. For example, if workers left the labor force more massively in some industries than in others, in a way that correlates with their future export potential in Yugoslavia, then this could invalidate our identification strategy.
This was not the case, as shown in figure 5 . The figure compares the proportion of Yugoslavians who arrived between 1991 and 1995 working in each four-digit SITC industry on the vertical axis, against the proportion of Yugoslavian who had returned by 2000 (based on the definition of the treatment) for each industry on the horizontal axis. The dashed line represents the 45 degree line. If exit from the labor force by Yugoslavians was completely random, we would see a perfect alignment of those dots along the 45 degree line: the share of workers entering each industry must be the same as the share of workers leaving it. Barring some exceptions, the graph does approximate this scenario. In fact, the correlation between both shares is close to 0.9.
Share of Arrival versus Exit from the German Labor Force by Industry

The figure plots the share of Yugoslavian workers in each industry out of the total that joined the labor force between 1991 and 1995 on the vertical axis against the share of Yugoslavian workers in each industry out of the total who dropped out of the labor force by 2000 (according to the way we define the treatment).
Given that few observations seem to be outliers, we also compute the distance between each value in the plot and the 45 degree line and correlate it with the log-growth rate of Yugoslavian exports by industry between 1990 and 2005. We find small, negative, and statistically insignificant correlation coefficients across the board ( - 0.0295 when computing the growth using logs, and - 0.0133 when computing growth using the inverse hyperbolic sine).
Finally, as a way to relieve possible concerns due to endogeneity with respect to exit, it is important to note that our results are robust to using the number of Yugoslavian refugees workers per industry in 1995, without the exit component, which directly addresses this concern (see online appendix table C1).
A. Baseline Results
Results for specification ( 1 ) are presented in table 2 . The first three columns report results using an OLS estimation, while the last three columns report results using a 2SLS estimation, making use of the instrumental variable described in section IIID . The table reports results using log ( e x p o r t s p , t ) , log ( e x p o r t s p , t + 1 ) and asinh ( e x p o r t s p , t ) as dependent variables. Since the regressor t r e a t p is rescaled using the inverse hyperbolic sine transformation, which behaves similar to a log transformation, we interpret β D I D as an elasticity. 29
In the first three columns, we find all estimates to be positive and statistically different from 0 for all different monotonic transformations of the dependent variable. The standard errors are clustered at the industry level, which is the level of disaggregation of the treatment.
Column 1 of table 2 presents the estimates when using the natural logarithmic transformation for the dependent variable. The point estimate in the first column is about two-thirds the size of those in the other two columns. This is not surprising as the first column excludes zeros and therefore excludes instances in which industries are more likely to grow faster if they have a non-zero value in the second period. Yet this difference says something more: the fact that the point estimates in columns 2 and 3 are positive and significant, which include instances where an industry was inexistent in the export basket of Yugoslavia by 1995, and are larger than the point estimate in column 1, implies that the effect of return migration on comparative advantage is valid at the extensive margin (e.g., opening a new line of exports) as well as at the intensive margin (e.g., growth of already existing export lines), along the lines of Bahar and Rapoport ( 2018 ). In either case, the results show that the elasticity of exports to return workers ranges from 0.09 to 0.14, depending on the transformation of the left-hand-side variable used (and, thus, on whether zeros are included).
Columns 4, 5, and 6 present the analogous 2SLS estimates (and the lower panel presents the corresponding first-stage estimations). For those columns, we also report the F -statistics, which measure the strength of the first stage; they are all above 14, so we can reject the possibility of weak instrumentation. The elasticities estimated through 2SLS are positive, statistically significant, and quite similar to the OLS results with point estimates that are only slightly larger. Given the standard errors, however, we cannot reject the hypothesis that the OLS and the 2SLS estimates are equal. Given the setting of the natural experiment and the use of an instrumental variable, we interpret these results as causal. Thus, based on the 2SLS results, we find that Yugoslavian industries that received 10% more return migrants from Germany (who worked in those same industries), exhibited higher exports by 1% to 1.6% during the period of the study. We show in online appendix section C.2.1 that our results are not sensitive to any particular industry.
Back-of-the-envelope calculations based on our sample size imply that returning refugees explain up to 6% of all Yugoslavian export growth between 1990 and 2005. In fact, since most of the export growth between 1990 and 2005 happens after the year 2000, most of this effect is concentrated between 2000 to 2005. 30
In the presence of self-selection of workers we would expect results to decrease in magnitude once we instrument. What may then explain why the 2SLS estimates are in fact slightly larger than the OLS ones? One possible explanation is that the 2SLS estimation uses variation in the treatment that is disproportionately coming from refugees allocated to areas in Western Germany (and specifically western and southern German states) where the most productive firms (and workers) are located.
B. Event Study Estimation
Can our results be explained by a previous trend in exports? Given the availability of exports data across several years, we turn to estimate the effect of return migration in an event study setting. To account for the typical volatility of exports on a yearly basis, we perform the estimation using five-year averages for the dependent variable and estimate β D I D for six different periods, from 1985–1989 to 2010–2014. To do this, we simply reestimate specification ( 1 ), this time substituting the dummy a f t e r t for several dummies, each one signaling a time period, along the lines of Autor et al. ( 2003 ). We define 1990 to 1994 as the base period, and therefore the other point estimates are relative to it.
The estimation (using 2SLS) is summarized in figure 6 , which shows in the upper panel the evolution of the expected value of exports (across our three different measures) by periods for two groups of industries: those for which the value of t r e a t p equals 0, and those for which t r e a t p equals 1 standard deviation of the treatment (denoted by σ ). The figures in the lower panel show the difference between the two groups, and it can be seen how the effect becomes positive and statistically significant starting in the period where the refugees start returning, 1995 to 1999. Note that based on the standard errors (as measured by the whiskers representing 95% confidence intervals), we cannot reject the hypothesis that the trends for both groups in periods before 1995 are statistically the same.
Event Study (2SLS), Five-Year Periods

The top panel plots exports over time for two groups: industries for which t r e a t p equals 0 and for which t r e a t p equals 1 standard deviation of the treatment. The dependent variable is the five-year average of exports (each column uses a different linear transformation and the period 1990 to 1994 is used as the base year). The bottom figure plots estimates for β D I D for each five-year period, which corresponds to the difference between the two groups of industries plotted in the top panel. The results are estimated using 2SLS and include the convergence control. Ninety-five percent confidence intervals for the estimation are represented by the whiskers.
These findings suggest an important result: the marginal effect of return migration on the emergence of new exports becomes stronger over time. The figure also reveals that the average industry experiences a drop in export performance during the war as compared to the base period. Treated industries recover and reach back their 1990–1994 level in the 2000 to 2004 period (or even earlier, when using the log transformation), while nontreated ones recover later. In that sense, part of what our effect is capturing in the first few postconflict years is that treated industries, on average, shrunk less than nontreated ones. 31
C. Falsification Test Using the Synthetic Control Method
Our main strategy compares different industry lines based on the intensity of the treatment. We complement those results with implementing the synthetic control method (Abadie & Gardeazabal, 2003 ; Abadie et al., 2010 , 2015 ) in the context of our empirical strategy. In other words, we create a synthetic industry for every Yugoslavian industry that mimics export dynamics until 1995 (the year our treatment starts kicking in), based on over one hundred other countries. We use five main predictors to construct each synthetic industry, using data for the period 1986 to 1995: export value of the industry itself (only for years 1990, 1993, and 1995), its export share, and the export share of its corresponding one-digit SITC, as well as GDP per capita, and population size. We then apply the synth algorithm that computes a synthetic control for each industry based on a weighted combination of countries other than Yugoslavia (see Abadie et al., 2011 ).
Figure 7 visualizes the main results of this exercise, plotting the difference between the export value of the real and synthetic industries. The top panel shows the evolution of each one of these differences: until 1995, there is almost no difference between the real and synthetic industries, but after 1995, we see all types of dynamics. The bottom panel groups industries in two, averaging the difference between their real and synthetic export values: low treatment and high treatment, which corresponds to the first and fourth quartiles of the distribution of t r e a t p as defined above (analogous to figure 4 ). The plot shows how, on average, real Yugoslavian industries outperform the synthetic ones, particularly among the high-treatment industries. Results using regression analysis are consistent and presented in online appendix C.3.
Difference in Export Value, Real versus Synthetic Industries

The top panel presents the evolution of the difference in export value between real and synthetic for all industries in the data set. The bottom panel groups the differences in high versus low treatment (4th versus 1st quartile of the treatment) across years. Results are robust to the inclusion of a small number of outlier industries that were removed from this plot for visualization purposes.
There are a number of possible candidate explanations for our results, which we explore in this section.
A. Industry-Level Productivity Shifts
Our analysis that follows consistently suggests that the main driver of our results are productivity shifts caused by the inflows of returning workers, who were exposed to better practices and technologies while in Germany. This is supported by a number of tests, which we expand on below, as well as those in section VI , where we show that the workers driving our results are those with characteristics or occupations more likely to transfer knowledge, technology, best practices, and more that have the potential to shift productivity.
Industry-level Ricardian comparative advantage.
First, we note that our results are consistent when using an industry-level measure of comparative advantage, which in theory responds to productivity parameters. In practice, we reestimate our specification using as the dependent variable a Ricardian comparative advantage parameter for Yugoslavia ( Φ p , t ), estimated following Costinot et al. ( 2012 ) and Leromain and Orefice ( 2014 ). 32 Analogous to our baseline results, we use the same two different monotonic transformations for Φ p , t (we skip the log ( Φ p , t + 1 ) transformation since there are no zeros). Results are presented in table 3 .
DID, Costinot et al. ( 2012 ) Measures in LHS
This table shows the results of the estimation for specification ( 1 ) using different monotonic transformations for Φ p , t in each column. Φ p , t is a measure that quantifies Ricardian comparative advantage estimated following Costinot et al. ( 2012 ). The first two columns report results from an OLS estimation, and the last two columns report results from a 2SLS estimation. All columns include industry fixed effects and year fixed effects. Standard errors clustered at the industry level are presented in parentheses. * p < 0 . 10 , * * p < 0 . 05 , and * * * p < 0 . 01 .
Labor productivity, wages, and industry size.
We replicate our main specification using production data from UNIDO for sectors in Yugoslavian countries (consistent with the main results, we aggregate all countries into a single one). We focus on outcomes such as output (production) per worker, value added per worker, and average wage, all in U.S. dollars. As opposed to the exports data used above, having the number of employees per sector allows us to compute outputs relative to the size of the sector. The data have complete information for only eighteen two-digit ISIC sectors, and as such we are limited to a low number of observations, which we acknowledge is a limitation of this test. We merge these data to the treatment variable, which was a trivial task given the one-to-one correspondence between ISIC/NACE and W93 sector classifications.
Results of OLS estimations based on the main specification are presented in table 4 , panel A. Columns 1 and 2 use as dependent variable measures of labor productivity, output (production) per worker and value added per worker, while column 3 uses as dependent variable the average wage of the sector, which also proxies for labor productivity of the sector as a whole. The results are consistent with the idea that productivity shifts are a central mechanism behind our main findings. Sectors with 10% more returnees tend to be 0.9% to 1.1% more productive depending on the measure used.
DID, Outcomes from UNIDO
Panel A shows results of the estimation for specification ( 1 ), focusing only on outcomes of production and wages using data from UNIDO for former Yugoslavian republics combined, using variation of two-digit sectors. Column 1 uses output or production per worker as the dependent variable, column 2 uses value added per worker, and column 3 uses average wages, all in U.S. dollars and transformed using the inverse hyperbolic sine. Panel B shows results of the estimation for specification ( 1 ), focusing only on outcomes for the Bosnian economy, using variation of two-digit sectors. Column 1 uses exports per worker in U.S. dollars as the dependent variable, column 2 uses number of firms, and column 3 uses number of workers, all transformed using the inverse hyperbolic sine. All columns include sector fixed effects and year fixed effects. Standard errors clustered at the industry level presented in parentheses. * p < 0 . 10 , * * p < 0 . 05 , and * * * p < 0 . 01 .
We were able to collect and digitize a number of sector-level indicators for the Republic of Bosnia and Herzegovina (the country with the largest number of refugees hosted by Germany) before and after the war. In particular, we gather sectoral data for export values, number of firms, and number of workers for the years 1990 and 2010 (the latter being the first postwar year for which such data are available). The sources of our collection are the Statistical Almanac ( Statisticki Godisnjak ) and the Structural Business Statistics reports ( Strukturne Poslovne Statistike ) published by the Statistical Agency of Bosnia and Herzegovina (Agencija za Statistiku BiH).
Given that these data are particularly for Bosnia and Herzegovina, we use our main data source to construct variations of the treatment meant to capture more precisely the number of Bosnian returning refugees (as opposed to Yugoslavians in general). In particular, we count workers who had been registered as having a Bosnian passport at some point during their stay in Germany and who had arrived sometime between 1991 and 1995 and had left by 2000. Note that while some of these workers entered the German labor force as Bosnian nationals, others obtained Bosnian citizenship during their stay in Germany and reported it. 33 We link this treatment to the outcomes described by sector relying on the equivalence between the German classification WZ 93 (the format for the treatment data) and the two-digit NACE sectoral classification (the format for the Bosnian data we collected). We end up for with a sample of 22 sectors and 44 observations (using data for each sector in 1990 and 2005). Similar to the previous results, we acknowledge that the small number of observations poses a limitation for these particular results.
Consistent with previous estimations, we transform all the variables using the inverse hyperbolic sine and based our estimations on specification ( 1 ). Table 4 , panel B, presents the OLS results using the treatment variable described above. Column 1 presents estimates when using Bosnian exports per worker as the dependent variable (in U.S. dollars), while columns 2 and 3 estimate the effect of the treatment on the number of firms and the number of workers per sector, respectively. The point estimates are positive and significant, implying that a higher number of returnees translates into higher labor productivity, as proxied by exports per worker, as well as sectors that are larger in terms of both firms and workers. We note that the point estimates are particularly high compared to our baseline results, but since these results are for Bosnia only, and not for Yugoslavia as a whole, a comparison is possible to only a limited extent. Moreover, a simple look at the coefficients suggest that on average, the treated sectors end up having firms that are smaller in terms of workers, which is typically the case for new firms. Consistently, there is rich anecdotal evidence on the creation of new firms by returnees, some of which we compile in online appendix section A.
B. Alternative Explanations
We go through a number of alternative explanations of our results, other than productivity shifts, that we are able to rule out, to the best of our ability. It is important to mention, however, that for any variable to be an alternative explanation in our empirical setting, it must comply with one important condition: that it can systematically explain cross-industry variation in export dynamics in ways that also correlate with the treatment variable (or in 2SLS specifications with our instrument). To the extent that alternative explanations do not comply with this condition, accounting for them won’t result in results that are very different from the main ones.
Scale effects: Workers returning simply are able to produce—and thus export—more quantity. For more details on how we address these concerns, see online appendix section C.4.1.
Convergence in structural transformation: Our effects are being driven by pure “convergence” in terms of structural transformation such that Yugoslavia’s productive structure in the late 2000s reflects that of Germany in the early 1990s. This concern is addressed in online appendix section C.4.2. Note that the results using the synthetic controls presented in section IVC address this concern too.
Capital and investment: Returning refugees are going into industries that are experiencing capital inflows, simultaneously, and our results are indeed explained by the latter. We address these concerns in online appendix section C.4.3.
Bilateral transaction trade costs: Our results are being driven by possible reductions of transaction costs of exporting to Germany caused by migrant networks. For more details on how we address this concern, see online appendix section C.4.4.
Skilled versus unskilled workers based on their education levels. As unskilled, we define workers without postsecondary education and skilled as workers with education beyond high school, including vocational training, college degree or more. Since education does not devalue, we simply use the highest educational information attached to each worker at any point during the period of observation.
We distinguish migrants with occupations intensive in manual tasks versus occupations intensive in analytical and cognitive tasks, using the classification provided by Dengler et al. ( 2014 ), which formalizes German occupations into five task categories, similar to Autor et al. ( 2003 ).
We classify occupations as low skilled and high skilled based on Blossfeld’s ( 1987 ) classification of professions. For example, high-skilled occupations include managerial ones as well as professionals (e.g., engineers, lawyers, technicians, accountants, lab technicians), and low-skilled occupations as, for example, drivers, carpenters, and textile processing operatives.
We distinguish workers by the supervisory intensity of their occupation based on the German Qualifications and Career Survey (BIBB/BAuA) of 1999. In particular we use the workers’ responses regarding their supervisory status (based on the answer to the question: “Do you have coworkers for whom you are the direct supervisor?”) and assign to each occupation both the share of workers who self-report acting as supervisors and the share of those who report the opposite. 34
We distinguish workers based on whether they worked in the top 25% paying firms in terms of average wages or in the bottom 75% paying firms. Typically, top-paying firms are the most productive ones by being able to attract the best workers and innovating or adopting innovations that help workers be more productive.
We distinguish workers based on the average growth in their wage during their stay in Germany as proxy for productivity improvements. We separate workers within each industry into two groups: workers with wage growth (based on the compound average growth rate, CAGR) below median and those with wage growth above the median, based on all returnees in our treatment.
The summary of our results is presented in table 5 . 35 Each column presents results using a different monotonic transformation of the dependent variable, consistent with all previous results in the paper. Columns show the estimated value of β i D I D for each of the constructed treatments belonging to each of the categories described above (we only present results using OLS, as we don’t have instruments for more than one endogenous variable at a time).
DID, Workers’ Education and Occupations
This table shows the results of the OLS estimation for specification ( 1 ) using treatments constructed by aggregating workers by groups based on their skills and/or occupations. The table presents OLS estimations. Each group of results uses different monotonic transformations for e x p o r t s p , t in different columns. All columns include the convergence control, as well as industry fixed effects and year fixed effects. Standard errors clustered at the industry level are presented in parentheses. * p < 0 . 10 , * * p < 0 . 05 , and * * * p < 0 . 01 .
The first row replicates the main results using the total number of returnees per industry, for comparison purposes. Overall, based on the size of point estimates, results reveal that our findings are particularly driven by workers with higher educational attainment (skilled), workers in occupations that are intensive in analytical tasks (as opposed to manual ones), workers in skilled occupations (as opposed to unskilled ones), and workers in supervisory occupations. We also find that the results are particularly driven by workers who worked in the top-paying firms during their stay in Germany and find that workers for whom wages grew faster during their stay in Germany correlate with a higher export performance. Note that while some of these results are statistically significant (namely, skilled workers, occupations intensive in analytical and cognitive tasks, and workers in high-paying firms), while the other point estimates are less precise.
Note, however, that the point estimates are to be interpreted in terms of elasticity and thus ultimately, the marginal effect of one worker belonging to each of the categories driving the results is much larger than what the point estimate suggests. This is because the types of workers driving the results are a smaller share of all workers when looking at the within-industry composition of workers in the sample. The idea that a small number of workers can have such an important effect on exports of a whole industry in such little time might seem implausible at first, but some anecdotal evidence documented by others seems to strongly support that idea. For instance, Rhee and Belot ( 1990 ) and Easterly ( 2001 ) document the story of the success of the garment sector in Bangladesh. Between 1980 and 1986, the share of garments in Bangladesh’s total exports rose from 0.5% to 28.3%. The unprecedented take-off of the garment export sector is often attributed to 130 Bangladeshi workers—only four of them in management positions—who spent eight months in 1979 working and being trained in Korea as part of an agreement between their company, Desh of Bangladesh, and the Korean firm Daewoo. The know-how acquired by these workers seems to have been crucial in making Desh a highly successful exporter firm. Perhaps more important, such know-how eventually spilled over as workers moved to other firms or created new ones, contributing to the massive success of garment exports as one of Bangladesh’s most significant export sectors.
In this context, we believe our findings pointing to productive knowledge and managerial know-how as the main mechanisms driving the export dynamics, as well as the magnitudes of the coefficients we report, are aligned with other studies in the literature.
The Balkan wars of the early 1990s created massive forced displacement from and within the former Yugoslavia. Most internationally displaced refugees ended up in Germany, where they could work under temporary protection status. A majority of them eventually returned home after the Dayton peace agreement of December 1995 and the repatriations that followed. We exploit this natural experiment that resulted in exposure to German industrial know-how, technology, and best practices, to investigate the role of returning refugees in explaining the export performance of their home countries. Using confidential German social security data, we find that Yugoslavian exports performed significantly better during the postwar period in industries that returnees had worked in while in Germany. Furthermore, we find that productivity is the underlying mechanism driving export performance in our setting. This is backed by the fact that our results are particularly driven by returnees with characteristics and in occupations more likely to generate productivity improvements.
Our results contribute to a burgeoning literature that emphasizes that migrants can serve as drivers of the diffusion of knowledge, technologies, and best practices resulting in productivity shifts. To the best of our knowledge, we are first to find such evidence using a natural experiment, especially in a context of returning forced migration.
In terms of policy implications, our results speak to the importance of allowing refugees full labor integration in their receiving countries. This is not only for the obvious reasons of the benefits to local hosting communities, but also because of how such policy can be a crucial determinant of the reconstruction of their home countries upon their eventual return.
More generally, the ability of a worker to become more productive has to do with his or her accumulated experience and his or her ability to learn from others while on the job. Migration therefore is an important vehicle in the process of knowledge transfers across locations. 36
Better understanding this process and identifying channels through which these dynamics occur, such as the policy and institutional environments that facilitate the diffusion of knowledge through migration, are important missing pieces in the literature and an active part of our future research agenda.
See Becker and Ferrara ( 2019 ) for a comprehensive literature review on the economic consequences of forced displacement.
Previous studies have focused on the role that return migrants play (mostly through capital accumulation while abroad) in explaining small-scale entrepreneurship. Rapoport and Docquier ( 2006 ) provide a review of this literature. Yet our paper differs from these other studies as we document large-scale effects on industry-level outcomes driven by productivity, as opposed to small-scale effects driven by capital accumulation.
Our results also relate to the determinants of postconflict reconstruction literature in general, using the case of former Yugoslavian countries (Black, 2001 ; Black & Gent, 2006 ).
See Angrist and Kugler ( 2003 ) for a summary of the migration of Yugoslavian nationals to different European destinations (in the context of a study on the impact on local labor markets).
It is important to note, however, that while we refer to Yugoslavians fleeing the civil conflict as “refugees” throughout this paper, we are using the term loosely, as most of them did not receive formal refugee status by Germany (as we explain below), which is the outcome of a legal process that is considered on a case-by-case basis according to the definitions agreed on and stated in the Geneva Convention on Refugees of 1951 and the Protocol Relating to the Status of Refugees of 1967 (among other country- or region-specific definitions). Despite the exact legal definitions, throughout the paper, we often use the words refugees and migrants interchangeably to refer to the same people.
In the early 1990s, as a response to the legal difficulties faced by the hundreds of thousands of refugees seeking protection, of whom almost none were eligible for asylum, the German government applied this status on a large scale. In principle, from 1993 onward there existed a legal status for civil war refugees, which granted temporary protection without a case-by-case assessment, but this status was not applied until 1999 because federal and state governments could not agree on distributing the costs. The Duldung status was also granted relatively quickly, making it possible for Germany to process large numbers of arrivals. Compared to other European countries, this was a considerable humanitarian gesture on the part of the German government (Dimova, 2006 ; Lederer, 1997 ).
Labor market access conditions for asylum seekers and Duldung holders changed a few times. Until 1991, immediate access to the labor market was possible. Between 1991 and 1997, they could apply for a general work permit and with an additional waiting period of three months for asylum seekers. Modifications in the law in 1997 further restricted access to the labor market. This changed again in 2001 when one-year waiting time was introduced. For details, see Liedtke ( 2002 ).
The rules on mobility while the application was being processed were defined by local governments. Some states restricted movement of the asylum seeker to a district, while others allowed free mobility within the state.
For the Croatians, however, the deportations started following the signature of the cease-fire agreement known as the Vance Plan in January 1992. By the end of 1994, almost all of the Croatian refugees had returned (Lederer, 1997 ).
Voluntary returns were mainly realized as a part of the program of German government through REAG (Program for Reintegration and Emigration for Claimants of Asylum in Germany) and GARP (Government Program of Assistance to Repatriation), which was implemented in cooperation with the International Organization for Migration (IOM) whose target was to support voluntary return. Both programs were completed in 2001 (Nenadic et al., 2005 ).
These numbers are confirmed when looking at the return rates with respect to the different nationalities and ethnicities involved in the conflict. For example, when it comes to Bosnian refugees, Rühl and Lederer ( 2001 , 50) write that “the number of Bosnian war refugees fell from 345,000 to approximately 28,000 by December 2000, more than 260,000 of which went voluntarily to Bosnia-Herzegovina. About 51,000 have migrated on to other countries (to the USA, Canada and Australia). The proportion of forced repatriations is well below 2% (approximately 5,500 cases).”
Social networks have also be shown to affect aggregate economic outcomes (Burchardi & Hassan, 2013 ).
These records have been assembled by the Institute of Employment Research (IAB) into the Employment History (BeH) data file (IAB, 2015 ). The data or variants of it have been widely used to study a variety of labor market aspects (Card et al., 2013 ; Dustmann & Glitz, 2015 ; Dustmann et al., 2017 ).
For privacy issues, the sample used in this paper is an anonymized version of the original database. In order to comply with data privacy rules, sensitive values—industry-period observations with fewer than 20 workers—have been replaced with different moments of the distribution of the number of migrant workers in the same industry and year. The number of cells affected depends of course on the level of disaggregation of worker characteristics such as education, occupation, and skill. More details on this procedure can be provided on request. The results presented here, however, are robust to using the nonanonymized version instead.
Using our employment sample, we compute the number of workers in Germany by nationality and year for all SITC four-digit industry categories. We use the nationality of the worker based on the passport recorded at his or her first appearance in the database. To compute the number of workers by industry we rely on the concordance tables produced by the United Nations Statistical Office and the work by Dauth et al. ( 2014 ) that matches German three-digit WZ industry codes to four-digit SITC (rev. 2) industries. When match is one-to-many, we create our own concordance following the same procedure as Cuñat and Melitz ( 2012 ) described in their footnote 24. In particular, we use the distribution of German exports in 1995 as a proxy for the distribution of employment across different four-digit SITC industries that correspond to a single three-digit WZ German industry. Further details on the employment sample, variable descriptions, and auxiliary data are provided in online appendix section B.
Very few people left Slovenia while almost none left the FYR of Macedonia as both countries obtained their independence with limited or no armed conflict. While Slovenia was the republic with highest GDP per capita and a much more diversified export basket than the rest of the countries to begin with, Macedonia was one of the poorest republics of the former Yugoslavia with few exports. Our results are robust to excluding both Slovenia and Macedonia from the exports data (see online appendix section C.2.4).
About 10% of the Yugoslavian workers we see entering the labor force between 1991 and 1995 were 18 years or younger on the year of entry. In contrast, 75% were 20 or older and 50% were 25 or older. This rules out the possibility that the entry of Yugoslavian into the labor force is mostly driven by locals with Yugoslavian passports joining the labor force at a young age rather than by refugees arriving to Germany.
Finding no entry for a person in our data implies that this person was not employed in any job, industry, or occupation subject to social security contributions on June 30 of any given year.
Our data also show that about half of these Yugoslavian workers who arrived between 1991 and 1995 are still active in the German labor force by 2014. Presumably, these “stayers” were not Duldung holders (and therefore, were not subject to deportation). Some of them could have been holders but were allowed to stay for humanitarian reasons.
However, when focusing on those migrants we can identify in our data as Bosnians, even though they are a smaller share, the dropout rate of the labor force is more than 50%, closer to the global official figures.
Noninteger number of workers in an industry is a result of the use of weights based on industry code concordances during the data construction stage. For more information see online appendix section B.
Between 1991 and 1995, 210,000 Yugoslavian workers appear the first time in our data. If the total flow was of 700,000 people, it is reasonable that about half of them were of working age. Our sample is, of course, smaller than the total population; thus the 210,000 figure seems reasonable. Of the 210,000 in our sample, 35% (or 75,000 workers) had exited the sample before the year 2000. Of those, roughly 75,000, only 22% (around 17,000 workers) had a job in tradable industries during the 1990s.
More precisely, we use average exports per industry between 1988 and 1990 for the before period, and the average between 2005 and 2007 for the after period, given the high volatility of yearly export data. Our results, however, are robust to using only data for the actual years for which the before and after periods are defined: 1990 and 2005.
Since exports are aggregated across all destinations, the number of zeroes in the data is not as large as when using bilateral trade data. We explore this in detail in online appendix section C.1.
Concerns might remain given that the geographic allocation of refugees is exogenous conditional on the reception center at the port of arrival being at full capacity. However, in online appendix section C.2.3, we present results using an instrument that excludes the most common ports of arrivals in the calculation and find them to be robust to our main results.
In fact, since we don’t have data pre-1995 for states of East Germany, we set those at 0 for 1991 to 1994. However, it turns out that this lack of variation is not critical. According to the employment data, in 1995 there were over 367,000 Yugoslavians employed in West German states across all industries, as compared to only 1,400 in East Germany, or just 0.38%. Thus, that lack of variation should not affect the relevance of our instrument.
We are aware of the critique by Jaeger et al. ( 2018 ) regarding using past spatial distributions of migrants to instrument for current distribution, though in our paper, it lacks relevance given that our dependent variable does not reflect economic activity in the same location of the migrants’ destination but rather in their country of origin.
One possible violation for this exclusion restriction is convergence in terms of structural transformation: Yugoslavia in the 2000s moved toward industries that were large in Germany in 1990. In robustness tests detailed below (and expanded on in online appendix section C.4.2) we rule out this possibility by including the relevant controls in the baseline specification.
The continuous character of our treatment implies, arguably, that our estimator can be characterized as a fuzzy differences-in-differences one (see De Chaisemartin & D’Haultfoeuille, 2018 ). In our setting, the “control” group is stable over time (e.g., there are no control group “switchers”), which implies our estimation only relies on the common trends assumption. In other words, our setting allows us not to require the “stable treatment over time” or the “homogeneous treatment effect between groups” assumptions (assumptions 5 and 6 in De Chaisemartin & D’Haultfoeuille, 2018 ). While relaxing assumption 5 in our setting is straightforward, doing the same with assumption 6 might not be. Thus, as a robustness test, we compute the Wald DID estimator following De Chaisemartin and D’Haultfoeuille ( 2018 ), defining the treated units as those above the 25th percentile in terms of treatment intensity. We find our results reassuring: the Wald DID point estimates are between 0.15 and 0.28, depending on the monotonic transformation used, and are all statistically significant at the 10% level. The point estimates are slightly larger than the OLS ones reported in table 2 , but they all fall within the statistical margin of error of the estimators. We thank Clement De Chaisemartin for his guidance on this exercise.
According to our estimates, 10% of returning refugees for the average industry can explain larger exports by 1.6%. For the average industry, these numbers are two people and roughly US$200,000 (based on 1990’s exports), respectively. Given that all of our sample corresponds to 17,000 returning refugees, that would represent an increase in exports of nearly US$2 billion. This is about 6% of the difference in total exports between 1990 and 2005, which corresponds to US$34 billion. In our sample, we note that the difference in exports between the years 2000 and 2005 is nearly the same (i.e., exports for the average industry are of similar orders of magnitude both in 1990 and in 2000).
Online appendix section C.2.5 exploits the timing of returns of refugees in an event study setting and finds that industries start outperforming earlier when we identify that our treatment kicks in earlier.
According to Costinot et al. ( 2012 ), Φ p , t = e ( φ p , t / 6 . 53 ) , where figure 6 .53 is their estimation of the elasticity of (adjusted) bilateral exports with respect to observed productivity and φ p , t is estimated as the country-industry specific productivity parameters for Yugoslavia using the following specification and using the complete matrix of bilateral trade (where Yugoslavia is one of the c countries in the data set): asinh ( exp c , c ' , p , t ) = φ c , p , t + Ω c ' , p , t + Ψ c , c ' , p + ɛ c , c ' , p , t . In the specification exp c , c ' , p , t is the export value from country c to country c ' of industry p in year t , φ c , p , t is an exporter-industry-year fixed effect, Ω c ' , p , t is an importer-industry-year fixed effect, Ψ c , c ' , p is an exporter-importer-industry fixed effect, and ɛ c , c ' , p , t is the error term.
As noted above, a large share of people in our data are recorded as Yugoslavian nationals. Bosnia and Herzegovina is the most important successor state of the former Yugoslavia in our setting and also the only one for which we have a sufficient number of cases that we can recalculate the treatment variable specifically for Bosnians (as opposed to other nationalities).
Online appendix section B.2 summarizes the values of these characteristics for each one of the occupations in our data set, along with the number of workers in our sample in each occupation.
See online appendix section C.5 for tables with all the estimations by group, including both univariate and multivariate regressions. While there is multicollinearity, the relative size of the point estimates remains consistent in univariate and multivariate regressions.
In online appendix section C.6, we present results expanding the exercise to all countries as a way to explore external validity, and find consistent results.
Author notes
Supplementary data, email alerts, related articles, related book chapters, affiliations.
- Online ISSN 1530-9142
- Print ISSN 0034-6535
A product of The MIT Press
Mit press direct.
- About MIT Press Direct
Information
- Accessibility
- For Authors
- For Customers
- For Librarians
- Direct to Open
- Open Access
- Media Inquiries
- Rights and Permissions
- For Advertisers
- About the MIT Press
- The MIT Press Reader
- MIT Press Blog
- Seasonal Catalogs
- MIT Press Home
- Give to the MIT Press
- Direct Service Desk
- Terms of Use
- Privacy Statement
- Crossref Member
- COUNTER Member
- The MIT Press colophon is registered in the U.S. Patent and Trademark Office
This Feature Is Available To Subscribers Only
Sign In or Create an Account
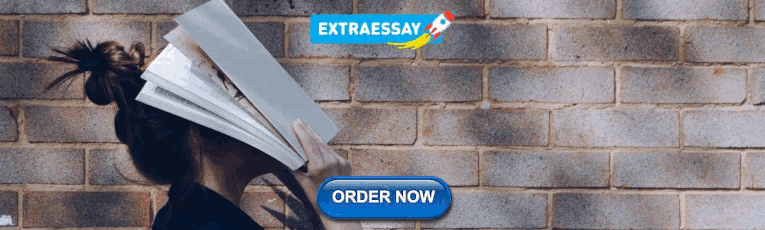
IMAGES
VIDEO
COMMENTS
Welcome to EconPapers! EconPapers provides access to RePEc, the world's largest collection of on-line Economics working papers, journal articles and software. We have: 1,196,327 Working Papers (1,010,566 downloadable) in 5,589 series 3,181,957 Journal Articles (3,111,707 downloadable) in 4,121 journals 5,293 Software Items (5,280 downloadable) in 36 series
Sharpening your economics writing skills is crucial in communicating top-notch research effectively. Remember, your paper's impact may suffer if your writing is: • grammatically flawed, • unclear, or • excessively journalistic. Writing an economics paper without proper grammar is like balancing an economic model on a
What Is An Economics Research Paper? How Does One Write An Economics Research Paper? Summary Reminders for Next Week Theoretical Research Papers Example 1: Intermediate implications: When the price of AA guns goes up, the demand curve for AA bullets will shift leftward. When the demand curve for AA bullets shifts leftward, the
NATIONAL BUREAU OF ECONOMIC RESEARCH 1050 Massachusetts Avenue Cambridge, MA 02138 June 2020 I have no disclosures to report. The research did not receive funding from external sources. ... National Bureau of Economic Research. NBER working papers are circulated for discussion and comment purposes. They have not been peer-
PDF. Crisis: Capitalism, Economics and the Environment Raj Navanit Patel Mr * Based on the average number of full-text downloads per day since the paper was posted. » Updated as of 11/29/23. Journal Home About This Journal Journal FAQ Editorial Board Undergraduate Research Commons. Most Popular Papers Receive Email Notices or RSS ...
II, "Researching Economic Topics," tries to explain the scholarly and analytical approach behind economics papers. The third part, "Genres of Economics Writing," briefly surveys some of the kinds of papers and essays economists write. It is in the fourth part, "Writing Economics," that the manual homes in on discipline-specific writing.
and doing research in economics. Chapters One through Four take you through the various aspects of writing a research paper, and Chapter Five guides you on putting all your work together into creating a coherent, interesting, thorough research paper. In addition, three appendices provide useful information for your research process. Appendix A ...
to succeed as a writer of economics and offers an overview of the writing process from beginning to end. Chapter 2 describes the basic methods economists use to analyze data and communicate their ideas. Chapter 3 offers suggestions for finding and focusing your topic, including standard economic sources and techniques for doing economic research.
Symposium on Inequality. Browse research from the latest Oxford Economic Papers Symposium on Inequality.Articles discuss selective schooling systems, increased income inequality in advanced economies since the 1980s, and the impact of wealth on children's exposure to environmental pollutants.
Citation of such a paper should account for its provisional character. A revised version may be available directly from the author. ISSN: 2365-9793. Schaumburg-Lippe-Straße 5-9 53113 Bonn, Germany. IZA - Institute of Labor Economics. Phone: +49-228-3894- Email: [email protected] www.iza.org.
General principles RePEc (Research Papers in Economics) is an initiative that seeks to enhance the dissemination of research in Economics and related areas.We want to make research more accessible both for the authors and the readers. RePEc is a crowd-sourced effort: a) thousands of people and organizations contribute the underlying data, b) a core team of contributors manage the system, and c ...
The Economic Journal is one of the founding journals of modern economics first published in 1891. The journal remains one of the top journals in the profession and provides a platform for high quality, innovative, and imaginative economic research, publishing papers in all fields of economics for a broad international readership. Find out more.
magnitude of health and economic shocks are not homogeneous across the student population. The average of the principal component for the economic and health shocks is about 0.3-0.4 standard deviations higher for students from lower-income families. Importantly, we nd that the disparate economic and health impacts
ago, but as of 2016-18 nearly a third of DSGE papers incorporate heterogeneous agents. Fourth, research using models characterized by frictionless, competitive markets has become increasingly rare. The vast majority of papers published in recent years incorporate some form of
Applied research can also vary according to the nature of method. used in analysis. There are mainly four categories of applied research: 1) statistical and econometric. analysis 2) calibration ...
This paper surveys the existing literature on the relationship between inflation and economic growth in developed and developing countries, highlighting the theoretical and empirical indications ...
1 See especially the UNDP's Human Development Reports, starting in 1990. 2 See e.g. Sen, 1984; Streeten et al., 1981; Fei, Ranis and Stewart, 1985. 3 The first UNDP Human Development Report stated that: 'The basic objective of development is to create an enabling environment for people to enjoy long, healthy and creative lives' (UNDP, 1990, p. 9), and defined human development as 'a process of ...
On average, a one per cent increase in per capita income reduced poverty by 1.7 per cent (see Figure 1).2. Among these 14 countries, the reduction in poverty was particularly spectacular in Vietnam, where poverty fell by 7.8 per cent a year between 1993 and 2002, halving the poverty rate from 58 per cent to 29 per cent.
This paper examines the impact of financial development, economic growth and income inequality on poverty in India from 1970 to 2015 by employing the autoregressive distributed lag (ARDL) bounds ...
Economic Papers features a balance of quality research in applied economics and economic policy analysis. The intended audience is the broad range of economists working in business, government and academic communities within Australia and internationally who are interested in economic and social issues related to Australia and the Asia-Pacific region.
Center for generous research support. The data and Stata programs used in this paper are available for download from the authors' homepages. The views expressed herein are those of the author(s) and do not necessarily reflect the views of the National Bureau of Economic Research. NBER working papers are circulated for discussion and comment ...
Five-page review of literature relevant to research paper topic due at the beginning of class. •March 20. Five-page description of model or data to be used in research paper due at the beginning of class. •April 10. Five-page summary of research paper results due at the beginning of class. •May 1. Completed research paper (drawing on but
Economic Research Working Paper No. 23 The Use of Intellectual Property in Brazil 2014 WIPO Economics & Statistics Series December Vivian Barcelos Marina Filgueiras Jorge Bruno Le Feuvre Felipe Lopes Sergio Medeiros Paulino de Carvalho Vera Pinheiro Julio Raffo Leonardo Ribeiro. 1
Abstract. During the early 1990s, Germany offered temporary protection to 700,000 Yugoslavian refugees fleeing war. By 2000, many had been repatriated. We exploit this natural experiment to investigate the role of returning migrants in boosting export performance upon their return. Using confidential German administrative data, we find that industries with 10% more returning refugees exhibit ...