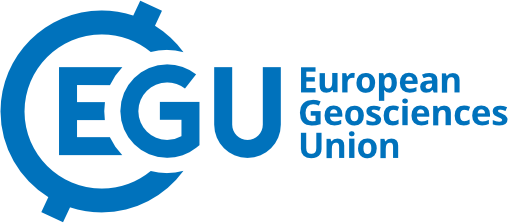
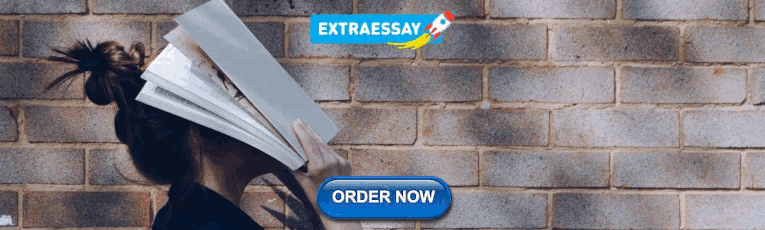
Article
- Volume 21, issue 5
- NHESS, 21, 1513–1530, 2021
- Peer review
- Related articles
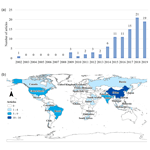
Review article: A systematic review and future prospects of flood vulnerability indices
Luana lavagnoli moreira, mariana madruga de brito, masato kobiyama.
Despite the increasing body of research on flood vulnerability, a review of the methods used in the construction of vulnerability indices is still missing. Here, we address this gap by providing a state-of-art account on flood vulnerability indices, highlighting worldwide trends and future research directions. A total of 95 peer-reviewed articles published between 2002–2019 were systematically analyzed. An exponential rise in research effort is demonstrated, with 80 % of the articles being published since 2015. The majority of these studies (62.1 % ) focused on the neighborhood followed by the city scale (14.7 % ). Min–max normalization (30.5 % ), equal weighting (24.2 % ), and linear aggregation (80.0 % ) were the most common methods. With regard to the indicators used, a focus was given to socioeconomic aspects (e.g., population density, illiteracy rate, and gender), whilst components associated with the citizen's coping and adaptive capacity were slightly covered. Gaps in current research include a lack of sensitivity and uncertainty analyses (present in only 9.5 % and 3.2 % of papers, respectively), inadequate or inexistent validation of the results (present in 13.7 % of the studies), lack of transparency regarding the rationale for weighting and indicator selection, and use of static approaches, disregarding temporal dynamics. We discuss the challenges associated with these findings for the assessment of flood vulnerability and provide a research agenda for attending to these gaps. Overall, we argue that future research should be more theoretically grounded while, at the same time, considering validation and the dynamic aspects of vulnerability.
- Article (PDF, 1061 KB)
- Supplement (220 KB)
- Included in Encyclopedia of Geosciences
- Article (1061 KB)

Moreira, L. L., de Brito, M. M., and Kobiyama, M.: Review article: A systematic review and future prospects of flood vulnerability indices, Nat. Hazards Earth Syst. Sci., 21, 1513–1530, https://doi.org/10.5194/nhess-21-1513-2021, 2021.
Floods affect billions of people worldwide (Zarekarizi et al., 2020). Indeed, according to the Emergency Events Database (CRED, 2019), around 50 000 people died and approximately 10 % of the world population was affected by floods between 2009 and 2019. Due to population growth and climate change, more frequent and widespread floods are anticipated (Hirsch and Archfield, 2015; Leung et al., 2019). Therefore, flood risk management is required for mitigating potential damages.
Nowadays there is a consensus that risk (i.e., the potential for adverse impacts) is not driven solely by natural hazards (e.g., floods, droughts) but depends on the interactions between hazards, exposure, and vulnerability (IPCC, 2012, 2014). In this regard, vulnerability plays an important role in flood risk assessment. It encompasses multiple social, economic, physical, cultural, environmental, and institutional characteristics which influence the susceptibility of the exposed elements to the impact of hazards (Birkmann et al., 2013; UNDRR, 2017). Due to its importance, the need to understand and assess flood vulnerability has been highlighted by international initiatives such as the Sendai Framework for Disaster Risk Reduction 2015–2030 (UNISDR, 2015).
In response to this, numerous studies have been undertaken to better understand flood vulnerability. Nevertheless, both the terminology and methodology used in these assessments are still a subject of discussion (Aroca-Jiménez et al., 2020; Kelman, 2018). In fact, some consider vulnerability as being a function of exposure and susceptibility (Balica et al., 2009; IPCC, 2001; Turner et al., 2003; UNDP, 2014), while others separate these concepts (Dilley et al., 2005; Fedeski and Gwilliam, 2007) as it is possible to be exposed to a hazard and not be vulnerable. For instance, a person may live in an area prone to natural hazards but have sufficient alternatives to modify the structure of their house to prevent potential losses (Cardona et al., 2012).
A wide range of approaches have been proposed for assessing flood vulnerability. The most commonly used methods are stage damage functions (Papathoma-Köhle et al., 2012, 2017; Tarbotton et al., 2015), damage matrices (Bründl et al., 2009; Papathoma-Köhle et al., 2017), and vulnerability indices (Birkmann, 2006; de Brito et al., 2017; Kappes et al., 2012; Moreira et al., 2021). The first two methods assess only the physical vulnerability, neglecting the social vulnerability of their inhabitants (Koks et al., 2015). However, the capacity of households to cope, adapt, and respond to hazards is equally important for assessing the potential impacts of floods (de Brito et al., 2018). Therefore, given the importance of holistic studies on vulnerability to ensure a better representation of reality, the use of vulnerability indices is recommended (Balica et al., 2013; Birkmann et al., 2013; Fuchs et al., 2011; Nasiri et al., 2016). Indices serve as a summary of complex and multidimensional issues to assist decision-makers, to facilitate the interpretation of a phenomenon, and to increase public interest through a summary of the results. Flood vulnerability indices are, therefore, a tool for measuring the vulnerability degree throughout the aggregation of several indicators or variables. Despite their advantages, indices can present misleading messages if they are poorly constructed or misinterpreted. Hence, a clear understanding of the normalization, weighting, and aggregation methods used to build an index is required (Moreira et al., 2021).
Over the past few years, several review articles about flood vulnerability have been published. For instance, Rufat et al. (2015) reviewed 67 articles to identify the leading drivers of social vulnerability to floods. Rehman et al. (2019) and Fatemi et al. (2017) reviewed different methodologies used for assessing flood vulnerability. Jurgilevich et al. (2017) systematically reviewed 42 climate risk and vulnerability assessments. More recently, Diaz-Sarachaga and Jato-Espino (2020) evaluated 72 articles related to the appraisal of vulnerability in different types of hazards in urban areas. Some studies also analyzed different methods and indexed construction designs to understand which decisions have the greatest influence on the vulnerability outcomes. For instance, Nasiri et al. (2016) compared damage curves, computer modeling, and indicators to evaluate flood vulnerability. Similarly, Schmidtlein et al. (2008) and Tate (2012, 2013) examined the sensitivity of the results to changes in the construction of the vulnerability index.
Notwithstanding these advances, to the best of our knowledge, no study has conducted a systematic review of flood vulnerability indices with a focus on the different stages involved in the construction of flood vulnerability indices. The investigation of the methods used for normalizing, weighting, aggregation, and validation and the implications for each choice for vulnerability assessment have received little attention so far. In addition, even though there have been recent advancements in the field (e.g., Cutter and Derakhshan, 2020), the temporal dynamics of flood vulnerability have not been tackled by the existing reviews. This is particularly important given that certain adaptation policies and strategies may reduce short-term risk probability but increase long-term vulnerability and exposure (Cardona et al., 2012). Therefore, a better understanding of the methods used in each step of the index construction, the vulnerability temporal dynamics (e.g., pre- and post-flood), and the uncertainty involved is needed for advancing research on flood vulnerability assessments.
Considering the aforementioned gaps, and given the proliferation of methods for building vulnerability indices, it is pertinent to review the development of this field. Hence, here, we carried out a systematic literature review of indices used to assess flood vulnerability. The focus is given to urban and riverine floods. The following questions guided the analysis: (1) which spatial scale was considered? (2) Which indicators were most commonly used to measure flood vulnerability? (3) How were the temporal dynamics of vulnerability addressed (e.g., pre- or post-flood event)? (4) Which methods were most commonly applied in each stage of the index building process (i.e., normalization, weighting, or aggregation)? (5) To which extent did these studies conduct validation and apply uncertainty and sensitivity analysis? In addition to highlighting existing challenges, we also point out directions for further research.
In general, indicators consist of various pieces of data capable of synthesizing the characteristics of a system. When these indicators are aggregated, they are called index or composite indicators (Saisana and Tarantola, 2002). Overall, the construction of an index comprehends seven steps (Fig. 1). First, the phenomenon to be measured is defined, so that the index results can provide a clear understanding of this phenomenon (Nardo et al., 2008). Then, the indicators used to measure the phenomenon are selected. This should be done carefully as the results reflect the quality of the selected indicators.
Table 1 Characteristics of the main normalization methods used for building indices.
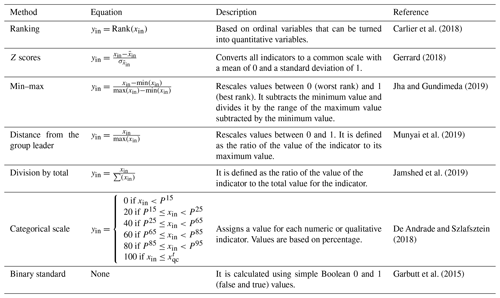
Note: y is the transformed variable of x for indicator i for unit n . P i is the i th percentile of the distribution of the indicator x in , and p is an arbitrary threshold around the mean.
Download Print Version | Download XLSX

Figure 1 Overview of the different steps involved in constructing an index.
In the third step, the relationships between the selected indicators are identified. Indicators with similar characteristics can be grouped, aiming to reduce the number of variables. To this end, statistical analysis (e.g., principal component analysis – PCA) or expert knowledge can be used to decide whether the indicators are sufficient or appropriate for describing the phenomenon (Nardo et al., 2008). After selecting the indicators, they need to be normalized to a common scale before being aggregated into an index as they usually have different units of measurement (see Table 1 for the main normalization methods). By doing so, problems with outliers can also be reduced (Jacobs et al., 2004).
The fifth step comprises the weighting and aggregation of the indicators. Weights can be assigned to indicators to demonstrate their importance in relation to the studied phenomenon (see Table 2 for the main weighting methods). Given that it may be difficult to find an acceptable weighting scheme, equal weights are often used, which implies that all criteria are worth the same (de Brito et al., 2018). Alternatively, an equal weighting scheme could be the result of a lack of knowledge about the indicators' importance. After the indicators are weighted, they are aggregated. The most common aggregation methods are linear and geometric. The linear method consists of the weighted and normalized sum of indicators, whereas the geometric aggregation represents the output of the indicators for which the exponent is their assigned weight (Nardo et al., 2008).
The sixth step consists of sensitivity and uncertainty analyses (see Table 3 for the main uncertainty and sensitivity methods). The first evaluates the contribution of the uncertainty source of each indicator to the variance of the results, while the latter focuses on how the uncertainty of each indicator propagates through the index structure and affects the outputs (Saisana et al., 2005; Saisana and Tarantola, 2002).
Table 2 Characteristics of the main weighting methods used for building indices.
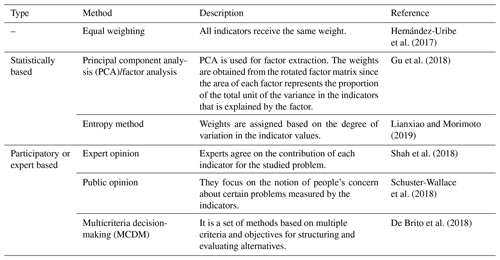
Table 3 Characteristics of the main methods for uncertainty and sensitivity analysis used for building indices.
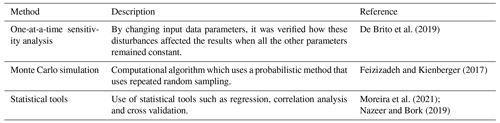
The final step comprises the validation of the index results. This is crucial for verifying if they are consistent with the real system and have a satisfactory precision range. Validation can be achieved by using independent secondary data that refer to observable outcomes. Since vulnerability is not a directly observable phenomenon, its validation requires the use of proxies such as mortality and built environment damage (Schneiderbauer and Ehrlich, 2006), post-event surveys (Fekete, 2009), number of disasters (Debortoli et al., 2017), and emergency service requests (Kontokosta and Malik, 2018).
A bibliographic search was performed by focusing on studies that constructed flood vulnerability indexes. The Web of Science (WoS) database was used to identify peer-reviewed articles published since 1945, using the following Boolean keywords: (“flood” OR “flooding”) AND (“index” OR “composite indicator”) AND (“vulnerability” NOT “coast*”). Only the abstract, title, and keywords were searched. This narrowed the search space substantially.
These queries elicited over 348 articles published between January 2002 and December 2019. At first, the title, abstract, and keywords were screened manually to exclude articles that were not useful for the purpose of the present study. After this preselection, the full text of 84 selected papers was revised in detail. An additional 11 key articles were included. They were not found in our original search even though they built vulnerability indices. This occurred because the keywords “index” or “composite indicator” were not mentioned in the article's abstract, title, or keywords. Hence, this limitation should be acknowledged, as relevant articles may have been disregarded.
Following their selection, the articles were classified according to (1) publication year, (2) study area country, (3) spatial scale (e.g., neighborhood, household, or city), (4) region classification (e.g., urban, rural, 1 or both), (5) number of indicators, (6) whether or not there was a reduction in the indicators (e.g., PCA and expert knowledge), (7) temporal dynamics (pre- or post-flood), (8) normalization, aggregation, and weighting methods used, and (9) if there uncertainty and validation analysis were performed. A complete list of the reviewed papers is presented in the Supplement.
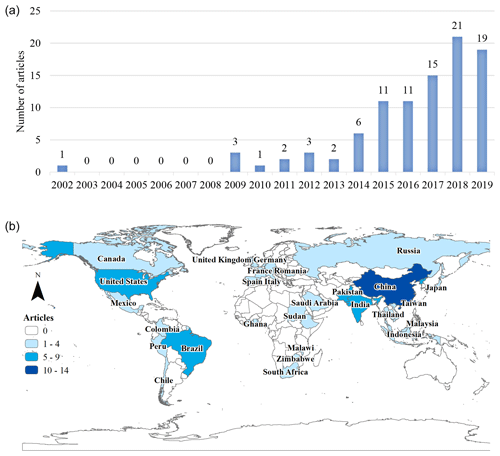
Figure 2 Flood vulnerability index studies. (a) Temporal distribution from 2002 to 2019. For the standardized number of articles according to the total number of publications in the WoS database, see Appendix A (Fig. A1). (b) Geographical distribution.
4.1 Flood vulnerability indices at a glance
An increasing number of studies that built flood vulnerability indices can be observed in recent years, with about 80 % ( n =76 ) of the articles being published since 2015 (Fig. 2a), which is also the year that the Sendai Framework for Disaster Risk Reduction (UNISDR, 2016) was agreed among several member states. Therefore, the growing number of publications may result from the increasing awareness of flood disaster prevention and reduction policies. The increasing number of vulnerability indices studies could also be attributed to the ease of using indices to address complex and multidimensional issues such as flood vulnerability in contrast to methods that demand more data (e.g., damage curves). Alternatively, this increase may just match a general rise in published papers. To investigate this, we calculated the increase in flood vulnerability studies in relative terms, based on a normalization according to the number of all flood publications in the WoS database. Results show that the increase in research on flood vulnerability indices is significantly greater than the increase in published flood articles (Appendix A; Fig. A1).
Overall, most of the assessments were conducted in Asia (45.3 % ), followed by the Americas (24.2 % ), and encompassing 38 countries (Fig. 2b). This was expected as, according to the Emergency Events Database (EM-DAT) statistics, between 2002 and 2019 Asia showed the highest number of deaths caused by floods (1027 deaths; CRED, 2019). As such, the studies are highly concentrated in a few countries, namely China ( n =14 ), Brazil ( n =8 ), India ( n =6 ), Pakistan ( n =6 ), and the United States ( n =6 ). Meanwhile, there were fewer studies in East and West Africa, despite the frequent occurrence of floods and the high mortality they cause across these regions.
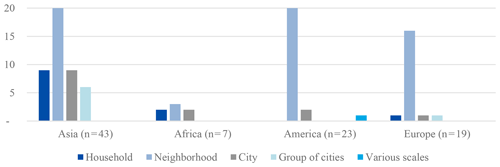
Figure 3 Classification of papers of flood vulnerability in terms of scale by continents.
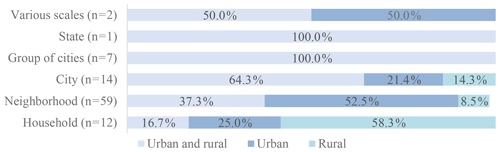
Figure 4 Classification of studies in terms of rural and urban areas and spatial scale.
In terms of spatial scale, most of the studies were conducted at the neighborhood scale (62.1 % ), followed by city (14.7 % ), household (12.6 % ), group of cities (7.4 % ), various scales (2.1 % ), and state scale (1.1 % ). Similar outcomes were obtained by Diaz-Sarachaga and Jato-Espino (2020), who found out that vulnerability studies at national and regional scales are infrequent. The neighborhood scale was the dominant scale in all continents (Fig. 3) as it is the smallest unit for which data are available for large areas, generally through census data. Only eight studies (8.4 % ) were conducted at the basin level (i.e., group of cities), and a few articles ( n =2 ) conducted assessments across various scales. For instance, Balica et al. (2009) evaluated the vulnerability at the basin, subbasin, and city scales. Similarly, Remo et al. (2016) compared three scales (i.e., census blocks, communities, and counties) and found out that the results generally mirrored each other. None of the considered articles draw conclusions at the national or global level.
Around 40.0 % of the studies were applied to urban areas, 15.8 % to rural areas, and 44.2 % to both. The high prevalence of studies that consider both urban and rural areas is related to the data availability, as the census tracks usually encompass the entire perimeter of a municipality. At the neighborhood scale, most studies considered only urban areas (53.4 % ; Fig. 4). Conversely, studies at the household scale were developed mainly in rural areas (58.3 % ). This can be explained by a lower availability of detailed geospatial data in rural areas worldwide (Zhang and Zhu, 2018; Zielstra and Zipf, 2010). Therefore, in these cases, it is necessary to collect data via household surveys.
4.2 Indicators used to characterize flood vulnerability
Table 4 shows the most frequent indicators grouped into social, economic, physical, and coping capacity dimensions. In summary, social and economic indicators such as population density (37.9 % ), illiteracy rate (32.6 % ), unemployment rate (29.5 % ), female rate (28.4 % ), per capita income (25.3 % ), and elderly rate (22.1 % ) were the most commonly used vulnerability indicators (Table 4). This is similar to the results obtained by Rufat et al. (2015), who found out that the most used indicators are poverty and deprivation, per capita income, unemployment rate, the elderly, and children. Nevertheless, widely used indicators found by the authors were not identified or were rarely used in our sample. These include, for example, stress and mental health, hygiene and sanitation, social networks, and experience with floods (Schneiderbauer and Ehrlich, 2006).
Table 4 Most commonly used flood vulnerability indicators. Only indicators used in at least five articles are shown here. This cut-off point was defined for clarity purposes as more than 600 different indicators were mentioned in the 95 reviewed articles.
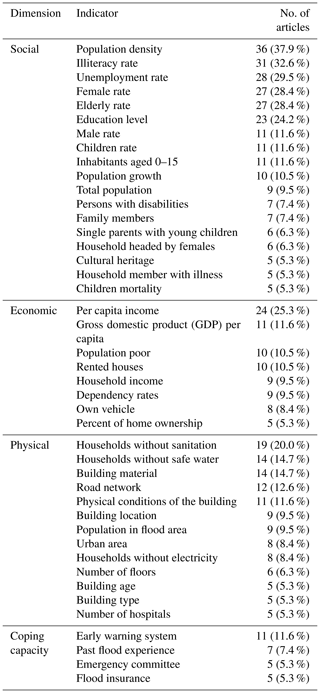
The studies used a median of 16 indicators. Although 32.6 % ( n =31 ) of the studies used more than 20 indicators (e.g., Sam et al., 2017), most of them (58.0 % ) did not apply any method for reducing the number of variables. Among the studies which conducted reduction, the most used technique was the PCA, which was applied to 35.5 % ( n =11 ) of the indices that used more than 20 indicators (e.g., Aroca-Jimenez et al., 2017; Grosso et al., 2015; Török, 2018). In addition to PCA, some studies used other approaches, for example, based on expert opinion (e.g., de Brito et al., 2018) or based on indicators with a high Pearson correlation (e.g., Kotzee and Reyers, 2016).
4.3 Temporal dynamics
In order to identify if the articles included the temporal dynamics of vulnerability, the indices were classified into pre-event (before), event (during) and post-event (after; Kobiyama et al., 2006). Most of the studies focused on assessing past vulnerability trends (88.4 % ) and only 12.6 % explored post-event flood vulnerability (e.g., Carlier et al., 2018; Miguez and Veról, 2017). None focused on the vulnerability during the event or elaborated on projections for future vulnerabilities.
Table 5 Indicators used for flood vulnerability assessment through post-event approach.
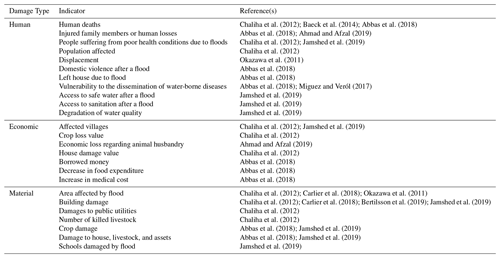
The indicators used are different according to the temporal scale considered. Post-event indices encompassed human, economic, and material damages to quantify the flood vulnerability (Table 5). Variables such as mitigation, damages, and coping behavior after experiencing a flood were often considered (Abbas et al., 2018). For instance, Rogelis et al. (2016) compared the results of the most vulnerable areas by ranking the basins according to the observed impacts from highest to lowest damage in terms of number of fatalities, injured people, evacuated people, and number of affected houses.
4.4 Indicator normalization, weighting, and aggregation
Concerning the indicators normalization, the most used approach was min–max (30.5 % ), followed by Z score (12.6 % ) and distance from the group leader (12.6 % ; Table 6a). A total of five studies applied other methods. For example, Aroca-Jimenez et al. (2017, 2018) expressed the indicators' values in percentage or per capita value, and de Brito et al. (2018) used fuzzy functions to normalize the indicators. It is important to note that most papers did not specify the normalization method used (11.6 % ), which limits the reproducibility of the study results.
Among the weighing approach types, statistical methods were the most applied (30.5 % ), especially the PCA method (21.1 % ). The high use of PCA can be attributed to the pioneering work by Cutter et al. (2003), who recommended the use of a factor analytic approach. Other less common statistical methods include dividing the indicator values by the total or maximum value (Okazawa et al., 2011), hot spot analysis (Kubal et al., 2009), and the unequal weighting method (Kablan et al., 2017).
Many authors recommend the use of participatory methods for weighing the indicators, such as the use of multicriteria decision-making (MCDM) tools (Evers et al., 2018). It is assumed that, if practitioners and experts are involved in creating an index that they find useful, it is more likely that they will trust its results (Oulahen et al., 2015). Furthermore, participation is believed to be a key component in fostering effective disaster risk reduction (Fekete et al., 2021). In the present study, the analytical hierarchy process (AHP) was the most common MCDM technique, which corroborates the results by de Brito and Evers (2016). These authors attributed this preference to the fact that AHP is a straightforward and flexible method. This method was applied separately in 10 papers and together with other methods in five papers, totaling 16.0 % of the reviewed articles. Among the less common MCDM methods, Promethee (Preference Ranking Organization METHod for Enrichment of Evaluations; Daksiya et al., 2017) and the analytical network process (de Brito et al., 2018) techniques are worth mentioning.
A total of seven articles used other weighting methods, including the entropy method (Lianxiao and Morimoto, 2019), Delphi technique (Yang et al., 2018b), and expert scoring (Wu et al., 2015). Furthermore, about one-quarter (24.2 % ) of the papers attributed equal weighting, and 6.3 % did not specify the weighting method used (Table 6b). Some authors preferred not to weight indicators because they assumed that these indicators are equally important for the vulnerability calculation (Yoon, 2012), whereas others pointed out that there is insufficient evidence to attribute importance to one factor over another (Fekete, 2011).
In terms of aggregation, the majority of the articles (80.0 % ) used linear aggregation, followed by geometric aggregation (10.5 % ) and mixed methods (4.2 % ). The linear method is useful when all indicators have the same unit or after they have been normalized. The geometric aggregation is preferred when the interest is assessing the degree of non-compensation between the indicators. In linear aggregation, compensation is constant, while in geometric aggregation the compensation is lower for indices with low values (Nardo et al., 2008). Nevertheless, the geometric method has a limitation when indicators with very low scores are compensated by indicators with high scores (Gan et al., 2017).
Table 6 Normalization and weighting methods.
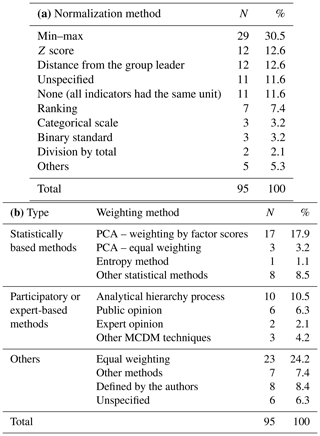
It is important to mention other aggregation methods used (5.3 % ). For instance, Abebe et al. (2018) used the Bayesian belief network, which is formed by a graphical network representing the cause–effect relationships between the different indicators (Pearl, 1988). Yang et al. (2018b, a) applied the Shannon entropy method. More recently, Amadio et al. (2019) used a non-compensatory aggregation method to compensate for the low performance of one indicator with some higher performance of another indicator. Finally, Chiu et al. (2014) used the fuzzy comprehensive evaluation method to aggregate the indicators.
4.5 Uncertainty, sensitivity, and validation
Each step in the construction of flood vulnerability indices carries uncertainty (Saisana et al., 2005), which is added to the ones associated with the randomness of flood events (Merz et al., 2008). Therefore, to ensure a better index quality and verify the results' robustness, uncertainty analysis should be conducted. Despite its importance, only three (3.2 % ) of the reviewed papers performed uncertainty analysis. Nazeer and Bork (2019) observed variations in the final results that changed input variables, and Feizizadeh and Kienberger (2017) and Guo et al. (2014) applied a Monte Carlo simulation and set pair analysis, respectively.
With respect to the sensitivity analysis (SA), only nine papers (9.5 % ) performed it. Most articles applied local SA by comparing the results obtained by changing input methods, such as choosing different weights (Müller et al., 2011; Nazeer and Bork, 2019; Rogelis et al., 2016), aggregation methods (Fernandez et al., 2016; Nazeer and Bork, 2019), or indicators (Rogelis et al., 2016; Zhang and You, 2014). In addition, Abebe et al. (2018) quantified sensitivity through variance reduction and mutual information. Spatial SA was conducted by de Brito et al. (2019) by computing the vulnerability class switches when the indicator weights were changed. Only Feizizadeh and Kienberger (2017) performed the global sensitivity analysis.
Although the number of flood vulnerability studies has increased, few studies attempted to validate the obtained outcomes (Fekete, 2009). Of the reviewed articles, only 11 (11.6 % ) validated the results, mostly using maps with flooded areas ( n =7 ), flood damage ( n =3 ), and expert opinion ( n =1 ).
Despite the increasing amount of research on flood vulnerability indices since 2015, a series of persistent knowledge gaps of methodological nature were found in our systematic review (Table 7). Here, we summarize these gaps and provide a research agenda with needs that should be addressed in the future.
The first challenge refers to the spatial scale, as vulnerability is not only site specific but also scale dependent (Ciurean et al., 2013). The choice of the spatial scale in the reviewed articles was mostly driven by data availability, and hence, most of them were applied at the neighborhood level using census tracks. Despite the availability of census data at the country level, there were no studies at the national level, and only eight papers (8.4 % ) constructed vulnerability indices using data at the basin scale. Nevertheless, these scales are often used for flood risk management and are necessary to enable the comparability of regions and to define hot spot areas where intervention is needed (Balica et al., 2009; Fekete et al., 2010). Conversely, studies at the household level were rare in our sample ( n =12 ). Yet, aspects related to the citizens' coping capacities can only be captured at this spatial scale.
An additional issue is the problem of down- or upscaling that implies different levels of generalization. Hence, multilevel and cross-scale studies are needed. They allow for a better understanding of scale implications, including their benefits and drawbacks. A better understanding of urban–rural linkages is also required, instead of studying them in isolation. To this end, the framework proposed by Jamshed et al. (2020) could be used. This framework considers, either qualitatively or quantitatively, how rural–urban linkages can influence the occurrence of floods and how these shape the vulnerability of rural households. It considers rural areas not as secluded units but rather as being interlinked with cities.
A further gap is that indicators related to the populations' coping and adaptive capacity were rarely used. The focus was given to social indicators that increase people's vulnerability. Similar to the scale choice, the preference for these indicators is driven due to data availability, as social indicators (e.g., age and gender) are easily accessible. Nevertheless, the capacity of people to anticipate, cope with, resist, and recover from disasters is equally important for understanding the risk. In fact, even poor and vulnerable people have the capacity to cope (Wisner et al., 2012). Therefore, when dealing with flood vulnerability, other relevant indicators, such as risk perception (Carlier et al., 2018) and past flood experience (Beringer and Kaewsuk, 2018), are important. However, data on these are often not readily available, thus requiring local research, which demands time, financial resources, and a multidisciplinary team. Indeed, information on citizens' adaptive behavior and perception requires longitudinal or quasi-experimental studies that allow the capturing of behavioral dynamics over time (Kuhlicke et al., 2020). For instance, recent advancements have been made by applying geostatistical methods to psychosocial survey data (Guardiola-Albert et al., 2020). As an alternative, people's risk perception could be derived from widely available data sources, including, for instance, Google trends (e.g., Kam et al. 2019) and Twitter statistics (Dyer and Kolic, 2020). Nevertheless, it should be noted that such approaches can be considered only where the use of social media and search engines are prevalent across the society. In countries where the use of digital technologies is not widespread, there is the risk that the marginalized population is left out of the analysis.
Table 7 Summary of the identified knowledge gaps and need for building flood vulnerability indicators.
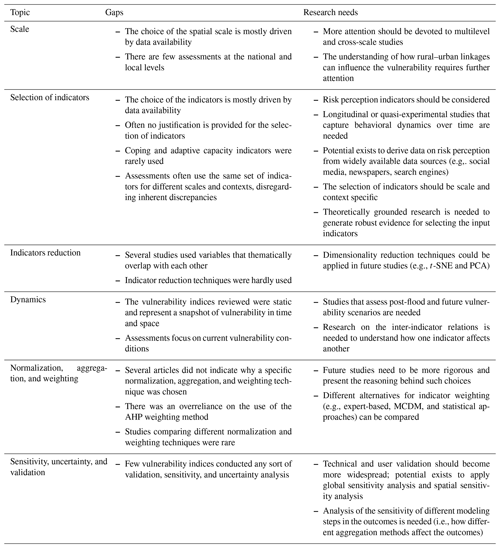
Still with regard to the indicators used, many studies used variables that thematically overlap with each other. In this context, some indices used more than 20 indicators to measure flood vulnerability and did not apply any technique (e.g., PCA or expert based) to reduce this number. This can decrease the explanatory power of the index. In this context, besides PCA, the potential exists to apply dimensionality reduction techniques such as the t -distributed stochastic neighbor embedding ( t -SNE; Anowar et al., 2021). A further issue is that the reason for variable selection was often not given, or it was justified based on previous studies, without taking into consideration the study area specificities or conceptual frameworks. Due to the difficulty and time involved in developing indicators, low-quality databases are normally used (Freudenberg, 2003). For adequate indicator selection, the analytical soundness, measurability, relevance to the phenomenon being measured, and the relationships to each other (e.g., interrelationships and feedback loops) should be taken into account. Furthermore, more theoretically grounded research is needed to generate robust evidence for selecting the input indicators.
All of the vulnerability indices reviewed here are static and represent a snapshot of vulnerability. Hence, they do not capture the complexities and temporal dynamics of vulnerability. Few studies focused on measuring flood vulnerability post-event. Nevertheless, the drivers of vulnerability can vary considerably over time. Results by Kuhlicke et al. (2011) and Reiter et al. (2018) show that the exposed citizens (e.g., the elderly and children) may be less vulnerable during the preparatory phase of a flood but highly vulnerable during the recovery phase. Hence, incorporating the phase of the flood disaster is key for improving the validity of vulnerability indices (Rufat et al., 2015). To account for temporal context, the indicators can be differentiated according to the different phases of a disaster, i.e., preparedness, response, and recovery phases. Such a phase-oriented approach could inform variable selection and weighting. In addition to this, there is a need for research looking into future vulnerabilities, and a forward-looking perspective is needed for preventive flood risk reduction (Birkmann et al., 2013; Garschagen and Kraas, 2010). These could make use of, for instance, population growth projections or employ tools such as qualitative futuring techniques (Hoffman et al., 2021). Nevertheless, it is important to notice that this can further increase the uncertainty of the vulnerability modeling outcomes. Still, exercises like this can provide a glimpse of plausible futures.
Similar to the selection of the indicators, several articles did not indicate why a specific normalization and weighting technique was chosen. Additionally, some did not explicitly specify any normalization (11.6 % ) or weighting (6.3 % ) method. Nevertheless, the use of arbitrary techniques without testing different methods and their assumptions increases the subjective judgment error. Hence, it is imperative for future studies to be more rigorous and present the reasoning behind such choices. Furthermore, there was an overreliance on the use of the AHP weighting method, and studies comparing different normalization and weighting techniques were rare (7.4 % ). Future research should tackle this by exploring different alternatives for evaluating indicator weights (e.g., expert-based, MCDM, or statistical approaches) and compare the findings by means of validation and sensitivity analyses.
A final persisting gap is that few vulnerability indices conducted any sort of validation, sensitivity, or uncertainty analysis. Fewer than 14 % of the studies validated the obtained results. To this end, impact data were often used (e.g., Rezende et al., 2019). Only 9.5 % conducted sensitivity or uncertainty analysis. This can lead to vulnerability outputs that are incoherent with the local reality by either over- or underestimating the vulnerability. This, in turn, has direct implications for flood risk management. In this regard, Fekete (2009) points out the difficulty in finding empirical evidence about vulnerability because vulnerability is multidimensional and not directly observable. Thus, further research is needed on the validation of vulnerability outcomes (including technical and user validation) and analysis of the sensitivity of the contribution of individual indicators to the obtained results. The potential exists to apply a global sensitivity analysis, which is already widely applied for building composite indicators for other fields of study (Luan et al., 2017; Saisana and Saltelli, 2008).
Besides the aforementioned methodological gaps, it is important to emphasize that the theoretical framework adopted influences the methodological choices that are made when constructing vulnerability indices. Even though we have not analyzed the theoretical constructs used by each study, when reading the articles it became clear that several of them do not specify how they conceptualize vulnerability. Furthermore, there are ambiguities in how vulnerability is understood (Kelman, 2018). For instance, some authors consider coping and adaptive capacity as components of flood vulnerability (e.g., de Brito et al., 2018; Feizizadeh and Kienberger, 2017). Others include flood hazard characteristics or exposure (e.g., Carlier et al., 2018; Chaliha et al., 2012) as part of vulnerability. Hence, we argue that a stronger theoretical underpinning of research is needed for producing scientifically rigorous and comparable research. Within this context, future work could investigate how different terminologies and theoretical constructs are defined and applied across different flood vulnerability case studies. Future reviews could also look into the methodology used to collect information on vulnerability indicators (e.g., surveys and public databases) as this influences the choices that can be made at each stage of the index construction.
The present study reviewed 95 articles from 38 countries that constructed flood vulnerability indices. In summary, despite the increasing number of studies and advances made, the review has revealed and reconfirmed a number of persistent knowledge gaps. Temporal dynamic aspects of vulnerability were often disregarded. Only 11.6 % of the studies focused on indicators that address post-event conditions related to flood damage and consequences, and none of them investigated future vulnerabilities. Coping and adaptive capacity indicators were frequently ignored, as obtaining these data demands time and effort. Most did not apply sensitivity (90.5 % ) and uncertainty analyses (96.8 % ) nor did they perform results validation (86.3 % ). This demonstrates a limitation in the reliability of these indices. It is clear from the literature that the challenge for further research is to foster the development of dynamic vulnerability assessments that consider the coping capacity of citizens and take the uncertainty involved in all steps of the index building process into account, including the selection of indicators, normalization, weighting, and aggregation. This is required in order to advance our understanding of flood vulnerability and support pathways towards flood risk reduction.
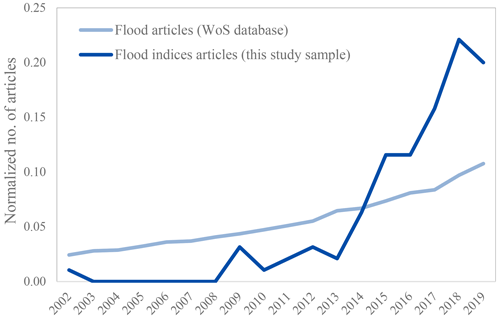
Figure A1 Normalized number of flood vulnerability indices and flood articles according to the Web of Science database. For the flood articles search, the keyword “flood*” was used.
The underlying research data are available in the Supplement.
The supplement related to this article is available online at: https://doi.org/10.5194/nhess-21-1513-2021-supplement .
LLM contributed to the conceptualization, data curation, investigation, methodology and writing (original draft preparation and review and editing). MMB contributed to the methodology, supervision, validation and writing (review and editing). MK contributed to project administration, methodology, supervision and writing (review and editing).
The authors declare that they have no conflict of interest.
The authors thank CNPq for the research scholarship.
This research has been supported by the Conselho Nacional de Desenvolvimento Científico e Tecnológico (grant no. 141387/2019-0). The article processing charges for this open-access publication were covered by the Helmholtz Centre for Environmental Research – UFZ.
This paper was edited by Sven Fuchs and reviewed by three anonymous referees.
Abbas, A., Amjath-Babu, T. S., Kächele, H., Usman, M., Amjed Iqbal, M., Arshad, M., Adnan Shahid, M., and Müller, K.: Sustainable survival under climatic extremes: linking flood risk mitigation and coping with flood damages in rural Pakistan, Environ. Sci. Pollut. R., 25, 32491–32505, https://doi.org/10.1007/s11356-018-3203-8 , 2018.
Abebe, Y., Kabir, G., and Tesfamariam, S.: Assessing urban areas vulnerability to pluvial flooding using GIS applications and Bayesian Belief Network model, J. Clean. Prod., 174, 1629–1641, https://doi.org/10.1016/j.jclepro.2017.11.066 , 2018.
Ahmad, D. and Afzal, M.: Household vulnerability and resilience in flood hazards from disaster-prone areas of Punjab, Pakistan, Nat. Hazards, 99, 337–354, https://doi.org/10.1007/s11069-019-03743-9 , 2019.
Amadio, M., Mysiak, J., and Marzi, S.: Mapping Socioeconomic Exposure for Flood Risk Assessment in Italy, Risk Anal., 39, 829–845, https://doi.org/10.1111/risa.13212 , 2019.
Anowar, F., Sadaoui, S., and Selim, B.: Conceptual and empirical comparison of dimensionality reduction algorithms (PCA, KPCA, LDA, MDS, SVD, LLE, ISOMAP, LE, ICA, t -SNE), Comput. Sci. Rev., 40, 100378, https://doi.org/10.1016/j.cosrev.2021.100378 , 2021.
Aroca-Jimenez, E., Bodoque, J. M., Garcia, J. A., and Diez-Herrero, A.: Construction of an integrated social vulnerability index in urban areas prone to flash flooding, Nat. Hazards Earth Syst. Sci., 17, 1541–1557, https://doi.org/10.5194/nhess-17-1541-2017 , 2017.
Aroca-Jiménez, E., Bodoque, J. M., García, J. A., and Díez-Herrero, A.: A quantitative methodology for the assessment of the regional economic vulnerability to flash floods, J. Hydrol., 565, 386–399, https://doi.org/10.1016/j.jhydrol.2018.08.029 , 2018.
Aroca-Jiménez, E., Bodoque, J. M., and García, J. A.: How to construct and validate an Integrated Socio-Economic Vulnerability Index: Implementation at regional scale in urban areas prone to flash flooding, Sci. Total Environ., 746, 140905, https://doi.org/10.1016/j.scitotenv.2020.140905 , 2020.
Baeck, S. H., Choi, S. J., Choi, G. W., and Lee, D. R.: A study of evaluating and forecasting watersheds using the flood vulnerability assessment index in Korea, Geomatics, Nat. Hazards Risk, 5, 208–231, https://doi.org/10.1080/19475705.2013.803268 , 2014.
Balica, S. F., Douben, N., and Wright, N. G.: Flood vulnerability indices at varying spatial scales, Water Sci. Technol., 60, 2571–2580, https://doi.org/10.2166/wst.2009.183 , 2009.
Balica, S. F., Popescu, I., Beevers, L., and Wright, N. G.: Parametric and physically based modelling techniques for flood risk and vulnerability assessment: A comparison, Environ. Model. Softw., 41, 84–92, https://doi.org/10.1016/j.envsoft.2012.11.002 , 2013.
Beringer, A. L. and Kaewsuk, J.: Emerging livelihood vulnerabilities in an urbanizing and climate uncertain environment for the case of a secondary city in Thailand, Sustainability, 10, 1452, https://doi.org/10.3390/su10051452 , 2018.
Bertilsson, L., Wiklund, K., de Moura Tebaldi, I., Rezende, O. M., Veról, A. P., and Miguez, M. G.: Urban flood resilience – A multi-criteria index to integrate flood resilience into urban planning, J. Hydrol., 573, 970–982, https://doi.org/10.1016/j.jhydrol.2018.06.052 , 2019.
Birkmann, J.: Indicators and criteria for measuring vulnerability: Theoretical bases and requirements, in: Measuring Vulnerability to Natural Hazards: Towards Disaster Resilient Societies, vol. 02, United Nations University Press, Tokyo, New York, Paris, 55–77, 2006.
Birkmann, J., Cardona, O. D., Carreño, M. L., Barbat, A. H., Pelling, M., Schneiderbauer, S., Kienberger, S., Keiler, M., Alexander, D., Zeil, P., and Welle, T.: Framing vulnerability, risk and societal responses: the MOVE framework, Nat. Hazards, 67, 193–211, https://doi.org/10.1007/s11069-013-0558-5 , 2013.
Bründl, M., Romang, H. E., Bischof, N., and Rheinberger, C. M.: The risk concept and its application in natural hazard risk management in Switzerland, Nat. Hazards Earth Syst. Sci., 9, 801–813, https://doi.org/10.5194/nhess-9-801-2009 , 2009.
Cardona, O.-D., Aalst, M. K. van, Birkmann, J., Fordham, M., McGregor, G., Perez, R., Pulwarty, R. S., Schipper, E. L. F., and Sinh, B. T.: Determinants of risk: Exposure and vulnerability, in: Managing the Risks of Extreme Events and Disasters to Advance Climate Change Adaptation, vol. 34, Cambridge University Press, Cambridge, UK, and New York, NY, USA, 65–108, 2012.
Carlier, B., Puissant, A., Dujarric, C., and Arnaud-Fassetta, G.: Upgrading of an index-oriented methodology for consequence analysis of natural hazards: application to the Upper Guil catchment (southern French Alps), Nat. Hazards Earth Syst. Sci., 18, 2221–2239, https://doi.org/10.5194/nhess-18-2221-2018 , 2018.
Chaliha, S., Sengupta, A., Sharma, N., and Ravindranath, N. H.: Climate variability and farmer's vulnerability in a flood-prone district of Assam, Int. J. Clim. Chang. Str., 4, 179–200, https://doi.org/10.1108/17568691211223150 , 2012.
Chiu, R., Lin, L., and Ting, S.: Evaluation of green port factors and performance: a fuzzy AHP analysis, Math. Probl. Eng., 2014, 1–12, 2014.
Ciurean, R. L., Schröter, D., and Glade, T.: Conceptual Frameworks of Vulnerability Assessments for Natural Disasters Reduction, in Approaches to Disaster Management – Examining the Implications of Hazards, Emergencies and Disasters completely, Intech., London, 31 pp., 2013.
CRED: EM-DAT: The international disasters database, available at: https://www.emdat.be/database , last access: 8 December 2019.
Cutter, S. L. and Derakhshan, S.: Temporal and spatial change in disaster resilience in US counties, 2010–2015, Environ. Hazards, 19, 10–29, https://doi.org/10.1080/17477891.2018.1511405 , 2020.
Cutter, S. L., Boruff, B. J., and Shirley, W. L.: Social vulnerability to environmental hazards, Soc. Sci. Quart., 84, 242–261, https://doi.org/10.1111/1540-6237.8402002 , 2003.
Daksiya, V., Su, H. T., Chang, Y. H., and Lo, E. Y. M.: Incorporating socio-economic effects and uncertain rainfall in flood mitigation decision using MCDA, Nat. Hazards, 87, 515–531, https://doi.org/10.1007/s11069-017-2774-x , 2017.
de Andrade, M. M. N. and Szlafsztein, C. F.: Vulnerability assessment including tangible and intangible components in the index composition: An Amazon case study of flooding and flash flooding, Sci. Total Environ., 630, 903–912, https://doi.org/10.1016/j.scitotenv.2018.02.271 , 2018.
Debortoli, N. S., Camarinha, P. I. M., Marengo, J. A., and Rodrigues, R. R.: An index of Brazil's vulnerability to expected increases in natural flash flooding and landslide disasters in the context of climate change, Nat. Hazards, 86, 557–582, https://doi.org/10.1007/s11069-016-2705-2 , 2017.
de Brito, M. M. and Evers, M.: Multi-criteria decision-making for flood risk management: a survey of the current state of the art, Nat. Hazards Earth Syst. Sci., 16, 1019–1033, https://doi.org/10.5194/nhess-16-1019-2016 , 2016.
de Brito, M. M., Evers, M., and Höllermann, B.: Prioritization of flood vulnerability, coping capacity and exposure indicators through the Delphi technique: A case study in Taquari-Antas basin, Brazil, Int. J. Disast. Risk Re., 24, 119–128, https://doi.org/10.1016/j.ijdrr.2017.05.027 , 2017.
de Brito, M. M., Evers, M., and Almoradie, A. D. S.: Participatory flood vulnerability assessment: a multi-criteria approach, Hydrol. Earth Syst. Sci., 22, 373–390, https://doi.org/10.5194/hess-22-373-2018 , 2018.
de Brito, M. M., Almoradie, A., and Evers, M.: Spatially-explicit sensitivity and uncertainty analysis in a MCDA-based flood vulnerability model, Int. J. Geogr. Inf. Sci., 33, 1788–1806, https://doi.org/10.1080/13658816.2019.1599125 , 2019.
Diaz-Sarachaga, J. M. and Jato-Espino, D.: Analysis of vulnerability assessment frameworks and methodologies in urban areas, Nat. Hazards, 100, 437–457, https://doi.org/10.1007/s11069-019-03805-y , 2020.
Dilley, M., Chen, R. S., Deichmann, U., Lerner-Lam, A., Arnold, M., Agwe, J., Buys, P., Kjekstad, O., Lyon, B., and Yetman, G.: Natural disaster hotspots: A global risk analysis, World Bank and Columbia University, Washington, D.C., 2005.
Dyer, J. and Kolic, B.: Public risk perception and emotion on Twitter during the Covid-19 pandemic, Appl. Netw. Sci., 5, 99, https://doi.org/10.1007/s41109-020-00334-7 , 2020.
Evers, M., Almoradie, A., and de Brito, M. M.: Enhancing Flood Resilience Through Collaborative Modelling and Multi-criteria Decision Analysis (MCDA), in: The Urban Book Series, Springer International Publishing, New York, 221–236, 2018.
Fatemi, F., Ardalan, A., Aguirre, B., Mansouri, N., and Mohammadfam, I.: Social vulnerability indicators in disasters: Findings from a systematic review, Int. J. Disast. Risk Re., 22, 219–227, https://doi.org/10.1016/j.ijdrr.2016.09.006 , 2017.
Fedeski, M. and Gwilliam, J.: Urban sustainability in the presence of flood and geological hazards: The development of a GIS-based vulnerability and risk assessment methodology, Landscape Urban Plan., 83, 50–61, https://doi.org/10.1016/j.landurbplan.2007.05.012 , 2007.
Feizizadeh, B. and Kienberger, S.: Spatially explicit sensitivity and uncertainty analysis for multicriteria-based vulnerability assessment, J. Environ. Plann. Man., 60, 2013–2035, https://doi.org/10.1080/09640568.2016.1269643 , 2017.
Fekete, A.: Validation of a social vulnerability index in context to river-floods in Germany, Journal of the British Academy, 9, 393–403, https://doi.org/10.5871/jba/001.151 , 2009.
Fekete, A.: Spatial disaster vulnerability and risk assessments: Challenges in their quality and acceptance, Nat. Hazards, 61, 1161–1178, https://doi.org/10.1007/s11069-011-9973-7 , 2011.
Fekete, A., Damm, M. and Birkmann, J.: Scales as a challenge for vulnerability assessment, Nat. Hazards, 55, 729–747, https://doi.org/10.1007/s11069-009-9445-5 , 2010.
Fekete, A., Aslam, A. B., de Brito, M. M., Dominguez, I., Fernando, N., Illing, C. J., KC, A. K., Mahdavian, F., Norf, C., Platt, S., Santi, P. A., and Tempels, B.: Increasing flood risk awareness and warning readiness by participation – But who understands what under `participation'?, Int. J. Disast. Risk Re., 57, 102157, https://doi.org/10.1016/j.ijdrr.2021.102157 , 2021.
Fernandez, P., Mourato, S., Moreira, M., and Pereira, L.: A new approach for computing a flood vulnerability index using cluster analysis, Phys. Chem. Earth, 94, 47–55, https://doi.org/10.1016/j.pce.2016.04.003 , 2016.
Freudenberg, M.: Composite Indicators of Country Performance: A Critical Assessment, Organisation for Economic Co-operation and Development, Paris, 2003.
Fuchs, S., Kuhlicke, C. and Meyer, V.: Editorial for the special issue: Vulnerability to natural hazards-the challenge of integration, Nat. Hazards, 58, 609–619, https://doi.org/10.1007/s11069-011-9825-5 , 2011.
Gan, X., Fernandez, I. C., Guo, J., Wilson, M., Zhao, Y., Zhou, B., and Wu, J.: When to use what: Methods for weighting and aggregating sustainability indicators, Ecol. Indic., 81, 491–502, https://doi.org/10.1016/j.ecolind.2017.05.068 , 2017.
Garbutt, K., Ellul, C., and Fujiyama, T.: Mapping social vulnerability to flood hazard in Norfolk, England, Environ. Hazards, 14, 156–186, https://doi.org/10.1080/17477891.2015.1028018 , 2015.
Garschagen, M. and Kraas, F.: Assessing Future Resilience to Natural Hazards – The Challenge of Capturing Dynamic Changes under Conditions of Transformation and Climate Change, in: International Disaster and Risk Conference, IDRC, Davos, 2010.
Gerrard, R. E. C.: Developing an index of community competence in flood response for flood-affected rural parishes on the Somerset Levels and Moors using composite and spatial datasets, Area, 50, 344–352, https://doi.org/10.1111/area.12416 , 2018.
Grosso, N., Dias, L., Costa, H. P., Santos, F. D., and Garrett, P.: Continental Portuguese Territory Flood Social Susceptibility Index, Nat. Hazards Earth Syst. Sci., 15, 1921–1931, https://doi.org/10.5194/nhess-15-1921-2015 , 2015.
Gu, H., Du, S., Liao, B., Wen, J., Wang, C., Chen, R., and Chen, B.: A hierarchical pattern of urban social vulnerability in Shanghai, China and its implications for risk management, Sustain. Cities Soc., 41, 170–179, https://doi.org/10.1016/j.scs.2018.05.047 , 2018.
Guardiola-Albert, C., Díez-Herrero, A., Amerigo Cuervo-Arango, M., Bodoque, J. M., García, J. A., Naranjo-Fernández, N., and Aroca-Jiménez, E.: Analysing flash flood risk perception through a geostatistical approach in the village of Navaluenga, Central Spain, J. Flood Risk Manag., 13, 1–16, https://doi.org/10.1111/jfr3.12590 , 2020.
Guo, E., Zhang, J., Ren, X., Zhang, Q., and Sun, Z.: Integrated risk assessment of flood disaster based on improved set pair analysis and the variable fuzzy set theory in central Liaoning Province, China, Nat. Hazards, 74, 947–965, https://doi.org/10.1007/s11069-014-1238-9 , 2014.
Hernández-Uribe, R. E., Barrios-Piña, H., and Ramírez, A. I.: Análisis de riesgo por inundación: Metodología y aplicación a la cuenca Atemajac, Tecnol. Cienc. Agua, 8, 5–25, 2017.
Hirsch, R. M. and Archfield, S. A.: Not higher but more often, Nat. Clim. Change, 5, 198–199, https://doi.org/10.1038/nclimate2551 , 2015.
Hoffman, J., Pelzer, P., Albert, L., Béneker, T., Hajer, M., and Mangnus, A.: A futuring approach to teaching wicked problems, J. Geogr. High. Educ., https://doi.org/10.1080/03098265.2020.1869923 , in press, 2021.
IPCC: Climate Change 2001: Impacts, Adaptation, and Vulnerability, 1st edn., edited by: McCarthy, J. J., Canziani, O. F., Leary, N. A., Dokken, D. J., and White, K. S., Cambridge University Press, Cambridge, 2001.
IPCC: Managing the Risks of Extreme Events and Disasters to Advance Climate Change Adaptation. A Special Report of Working Groups I and II of the Intergovernmental Panel on Climate Change, edited by: Field, C. B., Barros, V., Stocker, T. F., Qin, D., Dokken, D. J., Ebi, K. L., Mastrandrea, M. D., Mach, K. J., Plattner, G., Allen, S. K., Tignor, M., Midgley, P. M., Cambridge University Press, New York, 2012.
IPCC: Climate change 2014: Impacts, Adaptation, and Vulnerability, Cambridge University Press, Cambridge, UK and New York, USA, 2014.
Jacobs, R., Smith, P., and Goddard, M.: Measuring performance: An examination of composite performance indicators, Centre for Health Economics University of York, York, 2004.
Jamshed, A., Rana, I. A., Mirza, U. M., and Birkmann, J.: Assessing relationship between vulnerability and capacity: An empirical study on rural flooding in Pakistan, Int. J. Disast. Risk Re., 36, 101109, https://doi.org/10.1016/j.ijdrr.2019.101109 , 2019.
Jamshed, A., Birkmann, J., Feldmeyer, D., and Rana, I. A.: A Conceptual Framework to Understand the Dynamics of Rural–Urban Linkages for Rural Flood Vulnerability, Sustainability, 12, 2894, https://doi.org/10.3390/su12072894 , 2020.
Jha, R. K. and Gundimeda, H.: An integrated assessment of vulnerability to floods using composite index – A district level analysis for Bihar, India, Int. J. Disast. Risk Re., 35, 101074, https://doi.org/10.1016/j.ijdrr.2019.101074 , 2019.
Jurgilevich, A., Räsänen, A., Groundstroem, F., and Juhola, S.: A systematic review of dynamics in climate risk and vulnerability assessments, Environ. Res. Lett., 12, 013002, https://doi.org/10.1088/1748-9326/aa5508 , 2017.
Kablan, M. K. A., Dongo, K., and Coulibaly, M.: Assessment of social vulnerability to flood in urban Côte d'Ivoire using the MOVE framework, Water, 9, 292, https://doi.org/10.3390/w9040292 , 2017.
Kam, J., Stowers, K., and Kim, S.: Monitoring of Drought Awareness from Google Trends: A Case Study of the 2011–17 California Drought, Weather. Clim. Soc., 11, 419–429, https://doi.org/10.1175/WCAS-D-18-0085.1 , 2019.
Kappes, M. S., Papathoma-Köhle, M., and Keiler, M.: Assessing physical vulnerability for multi-hazards using an indicator-based methodology, Appl. Geogr., 32, 577–590, https://doi.org/10.1016/j.apgeog.2011.07.002 , 2012.
Kelman, I.: Lost for Words Amongst Disaster Risk Science Vocabulary?, Int. J. Disast. Risk Sc., 9, 281–291, https://doi.org/10.1007/s13753-018-0188-3 , 2018.
Kobiyama, M., Mendonça, M., Moreno, D. A., Marcelino, I. P. V. de O., Marcelino, E. V., Gonçalves, E. F., Brazetti, L. L. P., Goerl, R. F., Molleri, G. S. F., and Rudorff, F. de M.: Prevenção de desastres naturais: conceitos básicos, 1st edn., Organic Trading, Florianópolis, 2006.
Koks, E. E., Jongman, B., Husby, T. G., and Botzen, W. J. W.: Combining hazard, exposure and social vulnerability to provide lessons for flood risk management, Environ. Sci. Policy, 47, 42–52, https://doi.org/10.1016/j.envsci.2014.10.013 , 2015.
Kontokosta, C. E. and Malik, A.: The Resilience to Emergencies and Disasters Index: Applying big data to benchmark and validate neighborhood resilience capacity, Sustain. Cities Soc., 36, 272–285, https://doi.org/10.1016/j.scs.2017.10.025 , 2018.
Kotzee, I. and Reyers, B.: Piloting a social-ecological index for measuring flood resilience: A composite index approach, Ecol. Indic., 60, 45–53, https://doi.org/10.1016/j.ecolind.2015.06.018 , 2016.
Kubal, C., Haase, D., Meyer, V., and Scheuer, S.: Integrated urban flood risk assessment – adapting a multicriteria approach to a city, Nat. Hazards Earth Syst. Sci., 9, 1881–1895, https://doi.org/10.5194/nhess-9-1881-2009 , 2009.
Kuhlicke, C., Scolobig, A., Tapsell, S., Steinführer, A., and De Marchi, B.: Contextualizing social vulnerability: findings from case studies across Europe, Nat. Hazards, 58, 789–810, https://doi.org/10.1007/s11069-011-9751-6 , 2011.
Kuhlicke, C., Seebauer, S., Hudson, P., Begg, C., Bubeck, P., Dittmer, C., Grothmann, T., Heidenreich, A., Kreibich, H., Lorenz, D. F., Masson, T., Reiter, J., Thaler, T., Thieken, A. H ., and Bamberg, S.: The behavioral turn in flood risk management, its assumptions and potential implications, WIREs Water, 7, 1–22, https://doi.org/10.1002/wat2.1418 , 2020.
Leung, J. Y. S., Russell, B. D., and Connell, S. D.: Summary for Policymakers, available at: https://www.ipcc.ch/site/assets/uploads/sites/2/2019/05/SR15_SPM_version_report_LR.pdf (last access: 10 May 2021), 2019.
Lianxiao and Morimoto, T.: Spatial analysis of social vulnerability to floods based on the MOVE framework and information entropy method: Case study of Katsushika Ward, Tokyo, Sustainability, 11, 529, https://doi.org/10.3390/su11020529 , 2019.
Luan, W., Lu, L., Li, X., and Ma, C.: Weight Determination of Sustainable Development Indicators Using a Global Sensitivity Analysis Method, Sustainability, 9, 303, https://doi.org/10.3390/su9020303 , 2017.
Merz, V. B., Kreibich, H., and Apel, H.: Flood risk analysis: Uncertainties and validation, Österreichische Wasser- und Abfallwirtschaft, 60, 89–94, https://doi.org/10.1007/s00506-008-0001-4 , 2008.
Miguez, M. G. and Veról, A. P.: A catchment scale Integrated Flood Resilience Index to support decision making in urban flood control design, Environ. Plann. B, 44, 925–946, https://doi.org/10.1177/0265813516655799 , 2017.
Moreira, L. L., de Brito, M. M., and Kobiyama, M.: Effects of Different Normalization, Aggregation, and Classification Methods on the Construction of Flood Vulnerability Indexes, Water, 13, 98, https://doi.org/10.3390/w13010098 , 2021.
Müller, A., Reiter, J., and Weiland, U.: Assessment of urban vulnerability towards floods using an indicator-based approach – a case study for Santiago de Chile, Nat. Hazards Earth Syst. Sci., 11, 2107–2123, https://doi.org/10.5194/nhess-11-2107-2011 , 2011.
Munyai, R. B., Musyoki, A., and Nethengwe, N. S.: An assessment of flood vulnerability and adaptation: A case study of Hamutsha-Muungamunwe village, Makhado municipality, Jamba J. Disaster Risk Stud., 11, 1–8, https://doi.org/10.4102/jamba.v11i2.692 , 2019.
Nardo, M., Saisana, M., Saltelli, A., and Tarantola, S.: Handbook of Contructing Compsoite Indicators: Methodology and user guide, Secretary-General of the OECD, Paris, 2008.
Nasiri, H., Mohd Yusof, M. J., and Mohammad Ali, T. A.: An overview to flood vulnerability assessment methods, Sustain. Water Resour. Manag., 2, 331–336, https://doi.org/10.1007/s40899-016-0051-x , 2016.
Nazeer, M. and Bork, H. R.: Flood vulnerability assessment through different methodological approaches in the context of North-West Khyber Pakhtunkhwa, Pakistan, Sustainability, 11, 6695, https://doi.org/10.3390/su11236695 , 2019.
Okazawa, Y., Yeh, P. J.-F., Kanae, S., and Oki, T.: Development of a global flood risk index based on natural and socio-economic factors, Hydrolog. Sci. J., 56, 789–804, https://doi.org/10.1080/02626667.2011.583249 , 2011.
Oulahen, G., Mortsch, L., Tang, K., and Harford, D.: Unequal Vulnerability to Flood Hazards: “Ground Truthing” a Social Vulnerability Index of Five Municipalities in Metro Vancouver, Canada, Ann. Assoc. Am. Geogr., 105, 473–495, https://doi.org/10.1080/00045608.2015.1012634 , 2015.
Papathoma-Köhle, M., Keiler, M., Totschnig, R., and Glade, T.: Improvement of vulnerability curves using data from extreme events: Debris flow event in South Tyrol, Nat. Hazards, 64, 2083–2105, https://doi.org/10.1007/s11069-012-0105-9 , 2012.
Papathoma-Köhle, M., Gems, B., Sturm, M., and Fuchs, S.: Matrices, curves and indicators: A review of approaches to assess physical vulnerability to debris flows, Earth-Sci. Rev., 171, 272–288, https://doi.org/10.1016/j.earscirev.2017.06.007 , 2017.
Pearl, J.: Probabilistic Reasoning in Intelligent Systems: Networks of Plausible Inference, Morgan Kaufmann Publishers, United States, 1988.
Rehman, S., Sahana, M., Hong, H., Sajjad, H., and Ahmed, B. Bin: A systematic review on approaches and methods used for flood vulnerability assessment: framework for future research, Nat. Hazards, 96, 975–998, https://doi.org/10.1007/s11069-018-03567-z , 2019.
Reiter, J., Wenzel, B., Dittmer, C., Lorenz, D. F., and Voss, M.: The 2013 flood in the community of Elbe-Havel-Land in the eyes of the population, Research report of the quantitative survey, KFS Working Paper, KFS, Berlin, 2018.
Remo, J. W. F., Pinter, N., and Mahgoub, M.: Assessing Illinois's flood vulnerability using Hazus-MH, Nat. Hazards, 81, 265–287, https://doi.org/10.1007/s11069-015-2077-z , 2016.
Rezende, O. M., de Franco, A. B. R. da C., de Oliveira, A. K. B., Jacob, A. C. P., and Miguez, M. G.: A framework to introduce urban flood resilience into the design of flood control alternatives, J. Hydrol., 576, 478–493, https://doi.org/10.1016/j.jhydrol.2019.06.063 , 2019.
Rogelis, M. C., Werner, M., Obregón, N., and Wright, N.: Regional prioritisation of flood risk in mountainous areas, Nat. Hazards Earth Syst. Sci., 16, 833–853, https://doi.org/10.5194/nhess-16-833-2016 , 2016.
Rufat, S., Tate, E., Burton, C. G., and Maroof, A. S.: Social vulnerability to floods: Review of case studies and implications for measurement, Int. J. Disast. Risk Re., 14, 470–486, https://doi.org/10.1016/j.ijdrr.2015.09.013 , 2015.
Saisana, M. and Saltelli, A.: Expert Panel Opinion and Global Sensitivity Analysis for Composite Indicators, in: Computational Methods in Transport: Verification and Validation, Lecture Notes in Computational Science and Engineering, vol. 62, edited by: Graziani, F., Springer, Berlin, Heidelberg, https://doi.org/10.1007/978-3-540-77362-7_11 , 2008.
Saisana, M. and Tarantola, S.: State-of-the-art Report on Current Methodologies and Practices for Composite Indicator Development, European Commission Joint Research Centre, Ispra, 1–72, 2002.
Saisana, M., Tarantola, S., and Saltelli, A.: Uncertainty and sensitivity techniques as tools for the analysis and validation of composite indicators, J. R. Stat. Soc., 168, 307–323, 2005.
Sam, A. S., Kumar, R., Kächele, H., and Müller, K.: Vulnerabilities to flood hazards among rural households in India, Nat. Hazards, 88, 1133–1153, https://doi.org/10.1007/s11069-017-2911-6 , 2017.
Schmidtlein, M. C., Deutsch, R. C., Piegorsch, W. W., and Cutter, S. L.: A Sensitivity Analysis of the Social Vulnerability Index, Risk Anal., 28, 1099–1114, https://doi.org/10.1111/j.1539-6924.2008.01072.x , 2008.
Schneiderbauer, S. and Ehrlich, D.: Social levels and hazard (in)dependence in determining vulnerability, in: Measuring Vulnerability to Natural Hazards: Towards Disaster Resilie nt Societies, United Nations University Press, Tokyo, New York, Paris, 78–102, 2006.
Schuster-Wallace, C. J., Murray, S. J., and McBean, E. A.: Integrating Social Dimensions into Flood Cost Forecasting, Water Resour. Manag., 32, 3175–3187, https://doi.org/10.1007/s11269-018-1983-8 , 2018.
Shah, A. A., Ye, J., Abid, M., Khan, J., and Amir, S. M.: Flood hazards: household vulnerability and resilience in disaster-prone districts of Khyber Pakhtunkhwa province, Pakistan, Nat. Hazards, 93, 147–165, https://doi.org/10.1007/s11069-018-3293-0 , 2018.
Tarbotton, C., Osso, F. D., Dominey-howes, D., and Goff, J.: The use of empirical vulnerability functions to assess the response of buildings to tsunami impact: Comparative review and summary of best practice, Earth-Sci. Rev., 142, 120–134, https://doi.org/10.1016/j.earscirev.2015.01.002 , 2015.
Tate, E.: Social vulnerability indices: a comparative assessment using uncertainty and sensitivity analysis, Nat. Hazards, 63, 325–347, https://doi.org/10.1007/s11069-012-0152-2 , 2012.
Tate, E.: Uncertainty Analysis for a Social Vulnerability Index, Ann. Assoc. Am. Geogr., 103, 526–543, https://doi.org/10.1080/00045608.2012.700616 , 2013.
Török, I.: Qualitative assessment of social vulnerability to flood hazards in Romania, Sustainability, 10, 3780, https://doi.org/10.3390/su10103780 , 2018.
Turner, B. L., Kasperson, R. E., Matson, P. A., Mccarthy, J. J., and Corell, R. W.: A framework for vulnerability analysis in sustainability science, P. Natl. Acad. Sci. USA, 100, 8074–8079, https://doi.org/10.1073/pnas.1231335100 , 2003.
UNDP: Disaster resilience measurements: Stocktaking of ongoing efforts in developing systems for measuring resilience, available at: https://www.preventionweb.net/files/37916_disasterresiliencemeasurementsundpt.pdf (last access: 10 May 2021), 2014.
UNDRR: Terminology on Disaster Risk Reduction, UNISDR, Geneva, 2017.
UNISDR: Sendai Framework for Disaster Risk Reduction 2015–2030, Sendai, 2015.
UNISDR: Report of the open-ended intergovernmental expert working group on indicators and terminology relating to disaster risk reduction, available at: https://www.preventionweb.net/files/50683_oiewgreportenglish.pdf (last access: 10 May 2021), 2016.
Wisner, B., Gaillard, J. C., and Kelman, I.: Framing disaster: Theories and stories seeking to understand hazards, vulnerability and risk, in: Handbook of Hazards and Disaster Risk Reduction, Routledge, Abingdon, 18–34, 2012.
Wu, Y., Zhong, P. an, Zhang, Y., Xu, B., Ma, B., and Yan, K.: Integrated flood risk assessment and zonation method: A case study in Huaihe River basin, China, Nat. Hazards, 78, 635–651, https://doi.org/10.1007/s11069-015-1737-3 , 2015.
Yang, W., Xu, K., Lian, J., Ma, C., and Bin, L.: Integrated flood vulnerability assessment approach based on TOPSIS and Shannon entropy methods, Ecol. Indic., 89, 269–280, https://doi.org/10.1016/j.ecolind.2018.02.015 , 2018a.
Yang, W., Xu, K., Lian, J., Bin, L., and Ma, C.: Multiple flood vulnerability assessment approach based on fuzzy comprehensive evaluation method and coordinated development degree model, J. Environ. Manage., 213, 440–450, https://doi.org/10.1016/j.jenvman.2018.02.085 , 2018b.
Yoon, D. K.: Assessment of social vulnerability to natural disasters: A comparative study, Nat. Hazards, 63, 823–843, https://doi.org/10.1007/s11069-012-0189-2 , 2012.
Zarekarizi, M., Srikrishnan, V., and Keller, K.: Neglecting uncertainties biases house-elevation decisions to manage riverine flood risks, Nat. Commun., 11, 1–11, https://doi.org/10.1038/s41467-020-19188-9 , 2020.
Zhang, G. and Zhu, A.-X.: The representativeness and spatial bias of volunteered geographic information: a review, Annales of GIS, 24, 151–162, https://doi.org/10.1080/19475683.2018.1501607 , 2018.
Zhang, Y. L. and You, W. J.: Social vulnerability to floods: A case study of Huaihe River Basin, Nat. Hazards, 71, 2113–2125, https://doi.org/10.1007/s11069-013-0996-0 , 2014.
Zielstra, D. and Zipf, A.: A comparative study of proprietary geodata and volunteered geographic information for Germany, in: 13th AGILE International Conference on Geographic Information Science, vol. 2010, Guimarães, 1–15, available at: http://agile2010.dsi.uminho.pt/pen/shortpapers_pdf/142_doc.pdf (last access: 3 January 2021), 2010.
Here, rural areas are defined as sparsely populated areas, whereas urban areas are considered densely populated regions.
- Introduction
- Overview of indicators and indices
- Results and discussion
- Persisting gaps and future research
- Conclusions
- Data availability
- Author contributions
- Competing interests
- Acknowledgements
- Financial support
- Review statement
- Full-text XML

An official website of the United States government
The .gov means it’s official. Federal government websites often end in .gov or .mil. Before sharing sensitive information, make sure you’re on a federal government site.
The site is secure. The https:// ensures that you are connecting to the official website and that any information you provide is encrypted and transmitted securely.
- Publications
- Account settings
Preview improvements coming to the PMC website in October 2024. Learn More or Try it out now .
- Advanced Search
- Journal List
- v.11(2); 2019

An assessment of flood vulnerability and adaptation: A case study of Hamutsha-Muungamunwe village, Makhado municipality
Rendani b. munyai.
1 Department of Geography and Geo-Information Sciences, University of Venda, Thohoyandou, South Africa
Agnes Musyoki
Nthaduleni s. nethengwe, associated data.
Data sharing is not applicable to this article as no new data were created or analysed in this study.
This study assesses flood vulnerability, levels of vulnerability, determinants of flood vulnerability and coping strategies for flood hazards. The vulnerability and resilience of the local communities are key concepts in this study. Most households are vulnerable to flood hazards. It is therefore important to measure their levels of vulnerability and assess their responses for current and future planning. A flood vulnerability index was used to measure the extent of flood vulnerability. Key informant interviews, field surveys and household questionnaires were used to collect the data. The results show that vulnerability to flood in this community is determined by the nature of soil, dwelling type, employment, education and amount of rainfall in a season. Social and economic components scored higher than the physical environment, while social factors are higher than the economic factors. Contextual coping strategies in this community were temporary relocation, evacuation to a safe area and waiting for government and neighbours to help. The study recommends that public awareness campaigns, early warning systems and improved disaster management strategies must take into consideration differentiated levels of vulnerability and community coping mechanisms and preferences.
Introduction
Floods are among the most devastating natural hazards and cost many lives every year (Dilley et al. 2005 :43). To reduce the damage of floods, both structural measures, such as the building of dams and dikes, and non-structural measures, such as forecasting and education, are often employed (Jelmer 2013 :1). Drogue et al. ( 2004 :355) postulated that the frequency of floods has been rising every year. An increase in the frequency of floods resulted in loss of people’s lives, damage to property and infrastructure, as well as the destruction of the natural environment.
The number of people at risk has been growing each year and the majority are in developing countries with high poverty levels, making them more vulnerable to disasters (UN/ISDR 2004 :95). However, communities and societies have specific ways of responding to floods, which has resulted in various ways of coping with the flood phenomenon. Cardona ( 2003 :7) noted that individuals and communities are differently exposed and are vulnerable to floods because of the socio-economic factors, such as wealth, education, race, ethnicity, religion, gender, age, class, disability and health status. This is because flood vulnerability and adaptations are firmly related to the context of the natural environment and socio-economic factors of a specific area. The assessment of both vulnerability and adaptation are of great importance globally.
In South Africa, the annual risk of flooding is 83.3% and the level of vulnerability is high because of economic factors and geographical location (Zuma et al. 2012 :127). According to Prevention Web (PRW 2011 ), ‘[i]n Eastern Cape, KwaZulu-Natal, the North-West and Limpopo provinces of South Africa; 77 flood disaster events were recorded in between 1980 and 2010’. This means that of all natural hazards, floods are the most frequently experienced disaster. Losses have been experienced by various communities around the world because of floods. Flood hazard is not only a local or regional issue but also a global issue, which should be planned and prepared for at international, national, provincial and local levels. Every year the World Health Organization (WHO) records various events of flood disasters in various regions of the world.
Floods can be caused by the natural environment through heavy rainfall, storms and cyclones, yet human activities such as development and settlement planning are key attributes. Nevertheless, climate change has become one of the important causes of floods, as it is believed that the rise in global temperatures will result in severe floods in several regions of the world. These changes are associated with the variability of weather and climate, such as the El-Nino Southern Oscillation (ENSO).
The aim of this study was to assess flood vulnerability and adaptation strategies of the Hamutsha-Muungamunwe rural community. To achieve the objective, the study set out to answer the following research questions: ‘what are the contextual determinants of flood vulnerability?’, ‘what is the extent of flood vulnerability?’ and ‘how do communities cope with floods in Hamutsha-Muungamunwe village?’
This study adopted the conceptual framework of Turner et al. ( 2003 :3), which illustrates that vulnerability is a function of exposure, sensitivity and adaptive capacity (see Figure 1 ).

The conceptual framework of Turner et al.
Exposure, sensitivity and resilience are the key factors of vulnerability. Exposure refers to the alteration of the operational system, operating out of its normality operation. Judy et al. ( 2011 :6) stated that it is the state and change in external stresses that a system is exposed to. The system is now predisposed to harm; these are also the present natural conditions and societal aspects.
Susceptibility is the potential or the likelihood of a hazard to have impacts in the system. According to Samuels et al. ( 2009 :1), susceptibility is the probability of negative consequences of floods to the environment and society. Both socio-economic and the natural environments might be susceptible to a hazard. Resilience is the capacity of a community to adapt to changes in a hazardous area by modifying itself to achieve an acceptable structural and functional level (Galderisi et al. 2005 ). This means that the system must bounce back after disturbances, that is, the ability to retain the operation and function of the system is resilient.
According to the Intergovernmental Panel on Climate Change (IPCC 2007 :881), ‘[S]ensitivity refers to the degree to which a system is affected, adversely or beneficially, by a given exposure’. Socio-economic and environmental systems have different sensitivities. Sensitiveness is mostly concerned with impacts of floods. A system can be sensitive to direct (physical) impacts (e.g. a given change in rainfall which affects the water supply of a city) and indirect (socio-economic) impacts (e.g. age structure of a population which influences the degree to which mortality increases during a heatwave) (Judy et al. 2011 :6).
Vulnerability is therefore the degree to which a system is susceptible and unable to cope with adverse effects of climate change (IPCC 2007 :21). Adaptation is the adjustment in natural or human systems in response to actual or expected climatic stimuli or their effects, which moderates harm or exploits beneficial opportunities (IPCC 2007 :867). Literally, the definition of flood vulnerability is firmly rooted in how people or societies are likely to be affected by flood phenomena – that is, the sensitivity of the community or people to flooding considering the socio-economic, environmental and physical components. These components can be understood through an assessment of flood vulnerability components and factors.
Flood vulnerability is influenced by personal or group characteristics in terms of their capacity to anticipate and cope with the impacts of flood (Scoones 1998 :8). Vulnerability quantifies the associated risks within the context of environmental and socio-economic capacity to adapt to flood events. Different social groups or classes within a society are differentially at risk, both in terms of probability of occurrence of an extreme flood event and helping different classes to recover (Cardona 2003 ; Nethengwe 2007 :2). Ngie ( 2012 :52) postulates that for vulnerability to exist, the capacity of the population to absorb, respond and recover from the impacts must be taken into consideration. This study therefore assessed the vulnerability of a community in a rural setting – Hamutsha-Muungamunwe community in the Vhembe district of the Limpopo province.
Methods and materials
Qualitative and quantitative research designs were implemented in this study. Qualitative methods seek to better understand respondents’ own perceptions of vulnerability and capacities to cope with and adapt to possible threatening climatic events, as opposed to quantitative modes of inquiry (Jean-Baptiste et al. 2010 :48). In quantitative research, the design is more deterministic in methodological approaches with fixed basics, determining what strategy or design the research should implement.
The target population included community leaders and members of the Vhembe District Disaster Risk Management Centre who were purposively selected. The total number of households was 810, and 60 were sampled through systematic random sampling and questionnaires were administered. Qualitative key informant interviews, questionnaires and field observations were used to collect the data. The key informant interviews were held with two community leaders and one member of the Disaster Management Centre. Babbie and Mouton ( 2001 :476) postulated that the basic objective of a questionnaire is to obtain facts and opinions about a phenomenon from people who are informed on a specific issue. The questionnaire covered flood vulnerability determinants; indicators of flood vulnerability; impacts of flood on socio-economic status such as education, agriculture, health, infrastructure, housing and properties and water. Census (2011) provided useful information on flood vulnerability indicators such as population density, total population, types of sanitation and dwelling types. Meanwhile, the South African Weather Service (SAWS) provided rainfall data.
Data were entered into an Statistical Package for the Social Sciences (SPSS) spreadsheet wherein cross-tabulation was performed to interlink various variables in order to deduce any relationships between them. Descriptive statistics were applied (mostly frequencies) to enable the comparison of results either in percentages or in frequencies. The data were then grouped and presented in the form of tables, charts (bar or pie) and bar graphs.
A flood vulnerability index (FVI) was applied to measure the extent of flood vulnerability. The FVI method uses three factors of flood vulnerability, namely, exposure (E), susceptibility (S) and resilience (R). Exposure and susceptibility positively influence vulnerability, whereas resilience negatively influences vulnerability. Because of exposure, susceptibility and resilience have an influence on flood vulnerability. Indicators belonging to exposure and susceptibility increase the FVI; therefore, they are placed in the numerator. Indicators belonging to resilience decrease the FVI; therefore, they are placed in the denominator (Quang et al. 2012 :103).
There are four major components of flood vulnerability, namely, social, economic, environmental and physical components. The three factors of vulnerability index are aligned with the components to reveal indicators. The general formula for FVI is calculated by classifying the components into three groups of indicators, namely, exposure, susceptibility and resilience (Balica et al. 2012 :68). The formula for FVI is as follows:
where E is exposure, S is susceptibility and R is resilience.
Pilot surveys, questionnaires, key informant interviews, Census 2011 and field observations were useful sources of indicators. The first aspect in the selection of an indicator was the provision of predetermined indicators, such as the level of income, type of house and questionnaires. Predetermined indicators were adopted from the study of Balica ( 2012 :53). These indicators were then reviewed and merged to suit the study area. Table 1 presents the analysis and interpretation of the FVI. The index gives a number from 0 to 1, signifying low or high flood vulnerability.
Interpretation of flood vulnerability index.
Source: Balica et al. ( 2012 ).
Factors that determine flood vulnerability
Five factors that determine flood vulnerability are identified in Hamutsha-Muungamunwe village. These include the nature of soil, dwelling type, employment status, education and rainfall. All these factors were ranked according to their importance by using a five-point ranking scale, from most important to second, third, fourth and least important. The nature of the soil was ranked as the most important factor, followed by dwelling types. Respondents’ rankings were influenced by the collapsed and cracking of their houses that occurred during the flood event. The majority of respondents have experienced house collapse (see Figure 2 ). Employment status was ranked third, while education was ranked fourth and rainfall was ranked as the least important factor in determining flood vulnerability.

(b & c) Collapsed houses and (a & d) cracks in Hamutsha-Muungamunwe village.
Source: (a - b) Photographs taken by Rendani B. Munyai, at Hamutsha-Muungamunwe village, unkown date, published with permission from Rendani B. Munyai
The extent of flood vulnerability
Flood vulnerability index was applied to the Hamutsha-Muungamunwe community to measure the extent of their vulnerability to floods. Table 2 shows all indicators selected, components, factors of flood vulnerability, function and relationship with flood vulnerability. Fourteen indicators were identified from household level; the researcher used a deductive approach adopted from Balica ( 2012 :58) and Jelmer ( 2013 :8).
Vulnerability indicators, components, factors and relationship with vulnerability.
CH, cultural heritage; WS, warning system; FI, flood insurance.
where A/P: awareness/preparedness; ED: education level/literacy; PD: population density; CH: cultural heritage; EM: emergency service; and WS: warning system.
where UM: unemployment rate; QDI: quality of dwellings or infrastructure; LU: land use; FI: flood insurance; DSC: dam and storage capacity.
where FO: frequency of flood occurrence; T: topography; HR: heavy rainfall; and DSC: dam and storage capacity.
The FVI results of Hamutsha-Muungamunwe village are presented in Table 3 . Social and economic components scored higher vulnerability to floods than the physical environment, whereas social factors are specifically higher than economic factors in terms of vulnerability. This means that socially Hamutsha-Muungamunwe community has a very high vulnerability to floods. This is because of the high population density, lack of early warning systems for flood and poor or slow emergency services. Economically, Hamutsha-Muungamunwe community has a ‘high to very high’ vulnerability to floods. The physical environment contributes the least, with ‘small vulnerability to floods’ because of fewer days of heavy precipitation and plain landscape.
Hamutsha-Muungamunwe flood vulnerability index results.
FVI, flood vulnerability index.
Coping strategies
Considering all impacts of floods, all coping strategies were assessed. Figure 3 presents the coping strategies implemented against floods. The most preferred coping strategy was temporarily relocating, as confirmed by 44% of respondents. The second coping strategy was relying on neighbours and government help to withstand flood impacts, as indicated by 32% of respondents. The third strategy was evacuation, which was stated by 20% of the respondents. The remaining 4% of respondents stated other strategies, including making a small furrow to redirect flood water and planting lawn grass in and around the constructed houses.

Coping strategies adopted by the community in Hamutsha-Muungamunwe.
In this section, we discuss the flood vulnerability determinants, namely, nature of the soil, dwelling type, employment status, education and amount of rainfall. These determinants do not function independently but are interconnected with each other. Pelling ( 1997 :202) found that income level, dwelling type, health and resource accessibility are factors that determined flood vulnerability in Georgetown, Guyana. However, the result of this study is different because instead of income level, health and resources accessibility as the determinants of flood vulnerability, the nature of soil, employment, education and rainfall were apparent. The only similarity is the presence of dwelling type; however, it was ranked as the second important factor determining flood vulnerability in this community.
The nature of soil is identified as the most important factor determining flood vulnerability in Hamutsha-Muungamunwe village. This is because of the dominance of clayey soils in this area. The topsoil is composed of sand, while the bottom part is clay and can hold water preventing any permeability process to take place. The exposure to flood is also directly related with flood flow (Karmaka et al. 2010 :129). Clayey soil influences the probability of flood occurrence and vulnerability of people to floods. More permeable soil has more infiltration capacity and therefore reduces surface run-off, whereas less permeable soil has less infiltration capacity and is more prone to water logging (Grosshans et al. 2005 :40). The findings of this study revealed similarities with the study conducted by Karmaka et al. ( 2010 :129), where the nature of the soil was found as one of the major factors determining vulnerability to floods. A small downpour of rain can cause floods because of the nature of soil in this area.
Studies conducted by Balica ( 2012 ), Jelmer ( 2013 ) and Samuels et al. ( 2009 ) showed that rainfall and education level rankings are among the most important factors that determine flood vulnerability; however, in this study, they are found the least. This proves that vulnerability is rooted within the context of the socio-economic characteristics and the physical environment. The study of flood vulnerability should not be generalised because findings of a certain area might not be relevant to another area. The Hamutsha-Muungamunwe community should be aware of the nature of soils in their area and this should always be considered always in any construction and settlement processes. It also applies to the type of dwelling that should be built in this area because a poor type of dwelling increases the vulnerability to hazards.
Social factors influenced the vulnerability to floods more than economic and physical environments. The total vulnerability of the study community is within the parameter of ‘high vulnerability to floods’. Even though social factors increase vulnerability than both economic and physical environments, there is sufficient evidence to recognise the contribution of economic factors in their vulnerability because the value of FVI social is 0.801, while the value of FVI economy is 0.752. This means that socio-economically, this area is highly vulnerable to floods.
However, the incorporation of flood vulnerability designations is probably the most difficult of all variables to include in the vulnerability index (Balica et al. 2012 :45). A very high vulnerability to floods is associated with high extreme potential for loss in both the socio-economic and physical environments. This kind of vulnerability can also result in catastrophic phenomenon. High vulnerability indicates that there is high potential for damage to properties and loss of life, and this is normally based on the indicator of the lack of flood warning system. High vulnerability is assigned to a case where there is a high chance for loss of life, while medium vulnerability is allocated in a case where medium potential of harm to people’s lives and properties is apparent. A small vulnerability is assigned if there is only small potential of harm and damage to the socio-economy of a place, while very small vulnerability is concerned with a very small potential damage and harm upon various systems within a particular place. These losses and damages occur in both the socio-economy and the physical environment. The existence of quantifiable data is important for flood vulnerability measurement.
The high vulnerability of Hamutsha-Muungamunwe village is closely associated with the community’s capacity to cope with floods; however, poverty also plays a significant role.
From the above discussion, it is clear that vulnerability is not only a physical condition but also that people are significantly exposed to hazards socially. The most critical part about these socio-economic characteristics is that they are very contextual (Balica 2012 :68). Vulnerability of a certain place cannot be generalised to other settings; otherwise, the results will be misleading.
Balica et al. ( 2012 :14) found that social and economic components are more significant than the physical environmental component, which is similar to the findings of our study. This reveals that levels of social and economic vulnerability are important because of the ability of these factors in assisting people to resist and return to their normal state of operations. Stronger socio-economic characteristics of a specific area influence better resilience to floods. However, the similarities between the findings of these two studies do not mean that every FVI would result in higher social and economic indices than the physical environmental factor. This is because flood vulnerability is rooted within the parameter of scale and time, and it is dynamic to change in space, time and place (Balica et al. 2012 :74).
The main problem associated with these indicators as listed in the literature is the availability of the data. Groundwater level was not included in this study, although significant, because of their unavailability. Any future studies would have to take them into consideration. This study found that exposure, susceptibility and resilience influence flood vulnerability in the study area.
Various coping strategies were assessed based on the respondents’ capability of living and resisting floods. Socio-economic characteristics of the respondents play a key role in determining their ability and capacity to respond during flood events. Various studies have identified different coping strategies; for example, Ngie ( 2012 :62) found that relocating to a safer area and evacuation were the most practised coping strategies in the study that was conducted in Diepsloot. The present study has similarities with Ngie’s finding because it revealed that evacuation and relocation are part of the coping strategies in Hamutsha-Muungamunwe community. In addition to similar findings, ‘waiting for government and neighbours to help’ was also part of the coping strategies practised in this community.
High rate of unemployment and low income among the respondents have contributed to the lack of resilience of Hamutsha-Muungamunwe community. This is because resource availability influences the level of resilience and recovery. Adequate resources lead to lower vulnerability, whilst a lack of them makes people more exposed and vulnerable to floods because it would take a long period for people to recover from damage. Temporary relocation was the most adopted coping strategy because of good communication and interconnection between neighbours and relatives. This coping strategy is rooted within the parameter of relationships between people, meaning that where there is a lack of communication and interconnection, this coping strategy is not possible. A person cannot temporarily relocate to a neighbour’s or relative’s house without any good relations with them.
However, some respondents prefer to wait for the government and neighbours to help. This is unadvisable because the municipality has insufficient resources for recovery and responses during flood events. Sometimes they are unable to access relief funds because of a lack of capacity for assessing flood impacts to lodge a declaration of disaster with the National Centre for Disaster Management. The majority of the respondents therefore did not receive any help from the municipality. With the very high vulnerability to floods by the community, there is a need to develop a strong resilience system to meet these high levels of vulnerability to flood.
Tsi-Hamutsha, a community organisation, helps in recovery and response. The critical point is that few respondents have interacted with this organisation. Most of the members rely on neighbours and relatives for relocation when their houses collapsed and inform the community leader. Even though the organisation is not made specifically for flood support, it is quite helpful because most of the respondents are not aware of other disaster risk management options available to them.
Conclusion and recommendation
The main objective of this article was to assess flood vulnerability and adaptation strategies of Hamutsha-Muungamunwe village. The findings articulated the following factors that determine flood vulnerability, in order of importance: soil nature, dwelling type, employment status, education and amount of rainfall. The present socio-economic characteristics of Hamutsha-Muungamunwe village have influenced the vulnerability of this area. The findings also revealed that this area is socially, economically and environmentally vulnerable to floods at different levels. Meanwhile, the physical and environmental components have minor contribution in the vulnerability of this area to floods. The overall finding of Hamutsha-Muungamunwe village’s vulnerability to flood indicated that the area has high vulnerability to floods. The main reason of this high vulnerability to floods is the lack of resilience.
There are many coping strategies that the community is using and intends to use against floods. Of all the identified strategies, temporary relocation to safer places such as houses of relatives and neighbours, doing nothing and waiting for neighbours and government to help and evacuating to a safer area were among the most practised coping strategies.
To build resilience against floods, the following initiatives are recommended:
- There is a need for public awareness campaigns, particularly the development of efficient early warning systems. The availability of an early flood warning system will assist households in the expansion of their knowledge. Indigenous knowledge systems should be taken into consideration when developing these systems.
- The community needs to develop strong collaboration with traditional leaders, municipal officials, Makhado municipality, Vhembe district and households.
- The municipality should commit more funds for disaster reduction activities.
- It is important to build dwellings with durable materials, which requires channelling of extra funding to the community for housing.
- Community leaders should not allocate plots in the floodplain area. Hence, there is a need for floodplain mapping.
- The community leaders should develop response and recovery mechanisms by setting aside money for flood response and recovery.
Acknowledgements
This paper was presented at the first National Conference on Disaster Risk Science and Management in ‘South Africa’s Response in a Changing Global Environment’, 02–04 March 2015, at the Ranch Resort, Polokwane, South Africa. The conference was jointly hosted by the School of Environmental Sciences, University of Venda, South Africa and the National Disaster Management Centre (representing the Department of Cooperative Governance), South Africa. Mr Tendayi Gondo, Prof. Agnes Musyoki and Mr Edmore Kori were the faculty collaborators.
Competing interests
The authors declare that they have no financial or personal relationships that may have inappropriately influenced them in writing this article.
Authors’ contributions
Most of the data collection, analysis and write-up was done by R.B.M., and A.M. provided guidance throughout the study whilst N.S.N. reviewed a draft version of the paper and provided useful comments.
Funding information
Funding for this study was provided by the National Research Foundation of South Africa.
Data availability statement
The views and opinions expressed in this article are those of the authors and do not necessarily reflect the official policy or position of any affiliated agency of the authors.
Ethical consideration
The ethical clearance was obtained from the community leaders in the study area.
How to cite this article: Munyai, R.B., Musyoki, A. & Nethengwe, N.S., 2019, ‘An assessment of flood vulnerability and adaptation: A case study of Hamutsha-Muungamunwe village, Makhado municipality’, Jàmbá: Journal of Disaster Risk Studies 11(2), a692. https://doi.org/10.4102/jamba.v11i2.692
- Babbie E. & Mouton J, 2001, The practice of social research , Oxford University Press, Cape Town. [ Google Scholar ]
- Balica S.T, 2012, Applying the flood vulnerability index as a knowledge base for food risk assessment , UNESCO-IHE, Institute for Water Education, Delft, Netherlands. [ Google Scholar ]
- Balica S.F., Popescu I., Beevers L. & Wright N.G, 2012, Parametric and physically based modelling techniques for flood risk and vulnerability assessment: A comparison , UNESCO-IHE, Institute for Water Education, Delft, Netherlands. [ Google Scholar ]
- Balica S.F., Wright N.G. & Van Der Meulen F, 2012, ‘ A flood vulnerability index for coastal cities and its use in assessing climate change impacts ’, Natural Hazards 64 ( 1 ), 73–105. 10.1007/s11069-012-0234-1 [ CrossRef ] [ Google Scholar ]
- Cardona O, 2003, A need for rethinking the concept of vulnerability and risk from a holistic perspective: A necessary review and criticism for effective risk management , viewed 08 November 2014, from http://www.desenredando.org/public/articulos/2003/nrcvrfhp/nrcvrfhp_ago-042003.pdf .
- Dilley M., Chen R.S., Deichmann U., Lerner-Lam A.L., Arnold M., Agwe J. et al., 2005, Natural disasters hotspots: A global risk analysis , Synthesis report, The World Bank, Hazard Management Unit, Washington, DC. [ Google Scholar ]
- Drogue G., Pfister L., Leviandier T., El Idrissi A., Iffly J.F., Matgen P. et al., 2004, ‘ Simulating the spatio-temporal variability of streamflow response to climate change scenarios in a meso scale basin ’, Journal of Hydrology 293 , 255–269. 10.1016/j.jhydrol.2004.02.009 [ CrossRef ] [ Google Scholar ]
- Galderisi A., Ceudech A. & Pistucci M, 2005, ‘ Integrated vulnerability assessment: The relevance “to” and “of” urban planning ’, in Proceedings (CD format) of the 1st ARMONIA Project Conference ‘Multi-hazards: Challenges for risk assessment, mapping and management’, Barcelona, Spain, 05–06 December, 2005, pp. 5–6. [ Google Scholar ]
- Grosshans R., Venema H. & Barg S, 2005, Geographical analysis of cumulative threats to prairie water resources: Mapping water availability, water Quality, and water use stresses , p. 40, International Institute for Sustainable Development, Winnipeg. [ Google Scholar ]
- Intergovernmental Panel on Climate Change (IPCC) , 2007, Climate change: Impacts, adaptation and vulnerability , Cambridge University Press, Cambridge. [ Google Scholar ]
- Jean-Baptiste N., Kuhlicke C., Kunath A. & Kabisch S, 2011, ‘Review and evaluation of existing vulnerability indicators in order to obtain an appropriate set of indicators for assessing climate related vulnerability’, CLUVA Deliverable D, 2 , p. 11, Helmholtz Centre for Environmental Research – UFZ, Munich. [ Google Scholar ]
- Jelmer V, 2013, Flood vulnerability assessment on a commune level in Vietnam , University of Twente, Enschede. [ Google Scholar ]
- Judy W.L., Tegg S., Reisinger A. & Quade D, 2011, Vulnerability and adaptation to increased flood risk with climate change , Hutt Valley report 02, The New Zealand Climate Change Research Institute, Victoria University, Wellington. [ Google Scholar ]
- Karmakar S., Simonovic S.P., Peck A. & Black J, 2010, ‘ An information system for risk-vulnerability assessment to flood ’, Journal of Geographic Information Systems 2 ( 3 ), 129. [ Google Scholar ]
- Nethengwe N.S, 2007, ‘ Integrating participatory GIS and political ecology to study flood vulnerability in the Limpopo Province of South Africa ’, PhD thesis, West Virginia University, Department of Geology and Geography, Morgantown. [ Google Scholar ]
- Ngie A, 2012, A GIS approach for flood vulnerability and adaptation analysis in Diepsloot , University of Johannesburg, Johannesburg. [ Google Scholar ]
- Pelling M, 1997, ‘ What determines vulnerability to floods: A case study in Georgetown, Guyana ’, Environment and Urbanization 9 , 203–226. 10.1630/095624797101287354 [ CrossRef ] [ Google Scholar ]
- Prevention Web (PRW) 2011. South Africa – Disaster Statistics . Available at: http://www.preventionweb.net/english/countries/statistics/index_region.php?rid=1 . (Accessed 15th November 2015).
- Quang D., Balica S., Popescu I. & Jonoski A, 2012, ‘ Climate change impact on flood hazard, vulnerability and risk of the Long Xuyen Quadrangle in the Mekong Delta ’, International Journal of River Basin Management 10 ( 1 ), 103–120. 10.1080/15715124.2012.663383 [ CrossRef ] [ Google Scholar ]
- Republic of South Africa , 2011, Census 2011, Statistical Release P0301.4 , viewed n.d., from www.statssa.gov.za/publications/P03014/P030142011.pdf .
- Samuels P., Gouldby B., Klijn F., Messner F., van Os A., Sayers P. et al., 2009, Language of risk – Project definitions , Floodsite project report T32-04-01, 2nd edn, viewed n.d., from www.foodsite.net/html/partner_area/project_docs/T32_04_01_FLOODsite_Language_of_Risk_D32_2_v5_2_P1.pdf .
- Scoones I, 1998, Sustainable rural livelihoods: A framework for analysis , IDS Working Paper 72, Institute of Development Studies, Brighton. [ Google Scholar ]
- Turner B.L., Kasperson R.E., Matson P.A., McCarthy J.J., Corell R.W., Christensen L. et al., 2003, ‘ A framework for vulnerability analysis in sustainability science ’, Proceedings of the National Academy of Sciences of the United States of America 100 ( 14 ), 8074–8079. 10.1073/pnas.1231335100 [ PMC free article ] [ PubMed ] [ CrossRef ] [ Google Scholar ]
- UN/ISDR , 2004, Living with risk: A global review of disaster reduction initiatives , United Nations, Geneva. [ Google Scholar ]
- Zuma B.D., Luyt C.D., Chirenda T. & Tandlich R, 2012, Flood disaster management in South Africa: Legislative framework and current challenges’ , International conference on Applied Life Sciences (ICALS2012), Konya, Turkey, September 10–12, 2012, n.p. [ Google Scholar ]
Thank you for visiting nature.com. You are using a browser version with limited support for CSS. To obtain the best experience, we recommend you use a more up to date browser (or turn off compatibility mode in Internet Explorer). In the meantime, to ensure continued support, we are displaying the site without styles and JavaScript.
- View all journals
- My Account Login
- Explore content
- About the journal
- Publish with us
- Sign up for alerts
- Open access
- Published: 16 January 2020
A Place-based Assessment of Flash Flood Hazard and Vulnerability in the Contiguous United States
- Sepideh Khajehei 1 ,
- Ali Ahmadalipour 1 ,
- Wanyun Shao ORCID: orcid.org/0000-0002-1609-7383 2 &
- Hamid Moradkhani ORCID: orcid.org/0000-0002-2889-999X 1
Scientific Reports volume 10 , Article number: 448 ( 2020 ) Cite this article
10k Accesses
73 Citations
16 Altmetric
Metrics details
- Natural hazards
Flash flood is among the most catastrophic natural hazards which causes disruption in the environment and societies. Flash flood is mainly initiated by intense rainfall, and due to its rapid onset (within six hours of rainfall), taking action for effective response is challenging. Building resilience to flash floods require understanding of the socio-economic characteristics of the societies and their vulnerability to these extreme events. This study provides a comprehensive assessment of socio-economic vulnerability to flash floods and investigates the main characteristics of flash flood hazard, i.e. frequency, duration, severity, and magnitude. A socio-economic vulnerability index is developed at the county level across the Contiguous United States (CONUS). For this purpose, an ensemble of social and economic variables from the US Census and the Bureau of Economic Analysis were analyzed. Then, the coincidence of socio-economic vulnerability and flash flood hazard were investigated to identify the critical and non-critical regions. Results show that the southwest U.S. experienced severe flash flooding with high magnitude, whereas the Northern Great Plains experience lower severity and frequency. Critical counties (high-vulnerable-hotspot) are mostly located in the southern and southwestern parts of the U.S. The majority of counties in the Northern Great Plains indicate a non-critical status.
Similar content being viewed by others
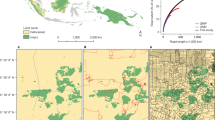
Ghost roads and the destruction of Asia-Pacific tropical forests
Jayden E. Engert, Mason J. Campbell, … William F. Laurance
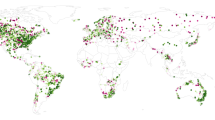
Global prediction of extreme floods in ungauged watersheds
Grey Nearing, Deborah Cohen, … Yossi Matias
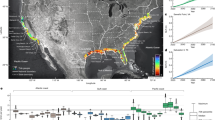
Disappearing cities on US coasts
Leonard O. Ohenhen, Manoochehr Shirzaei, … Robert J. Nicholls
Introduction
Flash floods impose extensive damage and disruption to societies, and they are among the deadliest natural hazards worldwide. Several studies have assessed the impacts of flash flood events around the world with regards to substantial financial losses, destruction of infrastructures, displacement, and fatalities 1 , 2 , 3 . Climate change is expected to increase extreme rainfalls and heavy river discharge, which will in turn exacerbate the likelihood of frequent flash floods with amplified severity 4 , 5 , 6 . There is an extensive body of research on the effects of development and construction in riverine floodplains, whereas less attention has been given to flash flooding and its impacts 7 , 8 .
The compound effects of population growth, inappropriate land-use planning and management, and environmental degradation altogether with the impacts of climate change are the main drivers of increasing losses triggered by the incident of natural hazards 9 , 10 , 11 . Flash flood is identified as a natural hazard with the highest capacity to generate damages to the human society on a global scale 12 , 13 . The high risk associated with the flash flood is due to its main characteristic: rapid onset and happening in a relatively short time, which significantly limits the warning and response time of the affected population and concerned agencies 14 , 15 .
Compound effect of the sensitivity of a population and its capability to respond to and recover from the consequences of a natural hazard construct the socio economic vulnerability 16 , 17 . Socioeconomic vulnerability is an integral variable that is influenced by a variety of factors, and thus it is essential to understand the societies’ characteristics and investigate their susceptibility to the hazards’ impact 18 , 19 , 20 .
Despite the significant advances in defining and formulating vulnerability 21 , 22 , 23 , 24 , there still remains disparities in interpretation of vulnerability between the risk/hazard research and human-environmental research communities. However, both communities acknowledge that vulnerability is composed of exposure, sensitivity, and response or resilience to the compound effects of both human and environmental systems 25 , 26 , 27 , 28 .
Vulnerability analyses are mainly classified into two groups that either (1) investigate the natural hazard characteristics and the physical attributes that aggravate the loses, which can be summarized as biophysical vulnerability, or (2) address the compound effect of socio-economic status and exposure, which directly affect the impacts 29 . Multitude of studies have focused on the elements of exposure due to less complexity compared to the socio-economic vulnerability. However, socio-economic vulnerability can address the root causes of damages to societies by investigating the social status, economic growth, population limitations, and several other characteristics 16 . Several other authors have discussed, in much greater details, many of the important aspects of social vulnerability and its measures 23 , 27 , 30 , 31 .
A region with advanced socio-economic status, and thus more efficient coping mechanisms in place, is generally less vulnerable to disasters 32 . Indicators of social and economic status often include percentage of vulnerable population such as the youth and the elderly, per capita income, extent of access to public amenities, and other socio-economic variables 33 , 34 . To this end, an index-based vulnerability assessment is a practical tool that helps comparing and ranking areas in terms of their vulnerability 16 , 35 , 36 .
Špitalar et al . 37 evaluated the fatalities from 2006 to 2012 in the United States caused by flash floods and realized most of the fatalities accrued in rural areas. However, when a flash flood occurs in an urban area, human impacts (i.e., injuries and fatalities per event) would be higher. Notably, the frequency and number of flood fatalities are likely to increase over the U.S. in the coming decades due to two primary reasons 15 . Urbanization has increasing trend in the U.S. 38 and urbanized basins are more prone to impacts from intense rainfall with reduced infiltration and the absence of natural defenses such as vegetation. Second, climate change studies have projected an intensifying hydrologic cycle under future emission scenarios, resulting in more intense rainfall events and exacerbated potential for flash floods 39 , 40 , 41 , 42 .
Very few studies have particularly evaluated the vulnerability to flash flood events. Milansei et al . 43 investigated the flash flood vulnerability for risk mitigation of large buildings, whereas Karahiorgos et al . 44 implemented a statistical model to countify vulnerability to flash floods. However, it is essential to investigate the socio-economic status and its interaction. with flash flood characteristics. Such a study can help advance flash flood risk mitigation planning as well as socio-hydrologic management to reduce the adverse impact of flash floods in the United States and beyond. As a result, it is critical to develop a comprehensive indicator of the disaster risk and vulnerability to flash floods. The vulnerability to flash floods depends on both biophysical and socio-economic factors. An indicator of flash flood vulnerability should be able to help the decision makers assess the potential impacts of flash floods and identify the most vulnerable social group and areas 45 .
The current study provides a comprehensive and multi-dimensional assessment of socio-economic vulnerability and its coincidence with flash flood characteristics over the contiguous United States (CONUS). Built upon previous analyses 16 , this study improves the measure of socio-economic vulnerability by utilizing Probabilistic Principal Components Analysis (PPCA) that handles the issue of missing values in the data. We then integrate the socio-economic vulnerability and flash flood characteristics at the county level to analyze the spatial distribution of flash flooding across the CONUS. Therefore, two main components of risk (i.e. vulnerability and hazard) are assessed here for flash floods. The results will indicate the resemblance and heterogeneity of flash flood spatial clustering and vulnerability of the regions over the CONUS. Identifying these spatial patterns will assist policy makers reach informed and effective decisions for planning and allocating resources.
Socio-economic data
The categories that develop the basis of Socio-Economic Vulnerability Index (SEVI) in this study are identified as: (1) demographic socioeconomic status, (2) race and ethnicity, (3) age, (4) employment and gender, (5) housing and transportation, and (6) industrial economy. The data from the first five categories are named social variables and are acquired from the 2015 American Community Survey (ACS) 5-year Estimates ( https://factfinder.census.gov/faces/nav/jsf/pages/index.xhtml ). The industrial economy variables are collected from the Bureau of Economic Analysis ( https://www.bea.gov/iTable/index_regional.cfm ). Descriptions of the chosen variables are summarized in Table 1 .
Flash flood data
The Unified Flash Flood Database was assembled by the Hydrometeorology and Remote Sensing (HyDROS) group at the University of Oklahoma. The Unified Flash Flood Database is composed of data from a variety of sources including the gauge measurements of streamflow by the United States Geological Survey (USGS), flash flooding reports in the National Weather Service (NWS) Storm Events Database, and public survey responses on flash flood impacts collected during the Severe Hazards Analysis and Verification Experiment (SHAVE) 46 , 47 .
This study has used the USGS automated streamflow measurements (which is a primary data source in the FLASH database developed by HyDROS) for investigating flash flood hazard characteristics. The flash flood characteristics were extracted from the USGS streamflow measurements collected by the HyDROS for the period of 1950 to 2017 over 3,490 stations. Based on the USGS database, flood events are defined when streamflow exceeds the defined action stage for a particular gauge. These action stages are derived based on the historical streamflow record at the location of interest as well as the physical characteristics of the area and the potential flood protection structures (e.g. levees). The USGS database that is used in this study provides the following information for each gauge: the USGS gauge identifier (ID), latitude (decimal degrees), longitude (decimal degrees), start time (UTC) at which the flow first exceeded the action stage threshold, end time (UTC) when the flow receded below the threshold, peak flow magnitude (cms), peak time (UTC) at which peak flow occurred, and the flood rise time (hr) defined as the difference between the start time and peak time.
This study focuses on four characteristics of flash flood at each station including the frequency, magnitude, duration, and severity. Frequency is extracted from the USGS database based on the number of times that flash flood is reported for a specific station. The peak streamflow is treated as the magnitude, whereas the flood rise time is considered for duration. Severity is defined as the flash flood magnitude divided by the duration.
Methodology
Socio-economic vulnerability index (sevi).
Cutter et al . 16 introduced the Social Vulnerability Index (SoVI) to examine the spatial patterns of social vulnerability to natural hazards at the county level in the United States. The SoVI was developed by collecting the social characteristics consistently identified within the research literature as contributors to vulnerability. These variables should explain the socioeconomic status of the given region as well as its capacity for recovery from the impacts of natural hazards. Selection of the specific variables which represent the socio-economic vulnerability is specific to the study objective. However, the most common characteristics include demographic socioeconomic status, the quality of human settlements, and the built environment 16 . Due to the high number of variables describing the social vulnerability, statistical procedures are commonly used to reduce data dimensions. The Principal Component Analysis (PCA) 48 has been widely utilized for decreasing the dimension of data in order to create a single and consolidated index of social variables 16 , 21 , 49 , 50 , 51 , 52 . Based on PCA, the components which explain the majority of the variance in the data will be chosen to calculate the SEVI. The most important step in constructing the index is scaling the chosen components. Each component represents a different element of vulnerability, therefore positive values raise the vulnerability index and negative values reduce it 53 . One shortcoming of using PCA-based index is that this procedure falls short in dealing with missing values. To overcome this problem, a few studies have suggested replacing the missing values with a value of zero 16 , 21 , 52 . However, a zero value cannot accurately represent the true vulnerability based on the particular variable of interest, and it would underestimate the level of vulnerability for the affected regions.
This study introduces a new algorithm for building the socio-economic vulnerability, which is based on Probabilistic Principal Components Analysis (PPCA) introduced by Tipping and Bishop 54 , which overcomes the shortcomings of PCA. The PPCA is a probabilistic formulation of PCA based on a Gaussian latent variable model, which has the capability to estimate the missing values, and therefore, there is no need for replacing the missing values with zero. PPCA retains the characteristics of PCA such as the principle scores and loadings. The expectation maximization (EM) algorithm is used to estimate the parameters of PPCA model, which will consequently allow the framework to deal with the missing values 55 , 56 .
In more detail, PPCA represents a constrained form of Gaussian distribution in which the number of free parameters can be restricted while still allowing the mode to capture the dominant correlation in a dataset. It is expressed as the maximum likelihood solution of a probabilistic latent variable model.
Given a PPCA model M, one can use it to transform observations into latent variables, as:
Where x refers to observation matrix, W is the factor loadings or weight matrix, and I reparenting the identity matrix. The model parameters W, σ, and μ can be estimated by maximizing the likelihood function. For further information on PPCA readers are referred to 54 .
To generate the SEVI, the social and economic variables were chosen based on the previous studies 16 , 57 , and they are presented in the Data section. These variables include demographic socioeconomic status, race and ethnicity, age, employment and gender, housing and transportation, and industrial economy 20 . The demographic socioeconomic status demonstrates the ability of a society to absorb losses and enhance resilience to hazards 58 . In addition, wealth enables communities to absorb and recover from losses more quickly due to insurance and social safety nets 59 . Race and ethnicity impose language and cultural barriers that may affect access to post-disaster funding and residential locations in hazardous areas 60 . Extreme age spectrum affects mobility. For example, parents spend time and money caring for children when daycare facilities are affected. The potential loss of employment following a disaster exacerbates the number of unemployed workers in a community, contributing to a slower recovery from the disaster. Loss of sewers, bridges, water, communications, and transportation infrastructure compounds potential disaster losses. The loss of infrastructure may place an insurmountable financial burden on smaller communities that lack the financial resources to rebuild 16 . The income from the industries provides an indicator of the state of economic health of the community and longer term issues with recovery after an event 61 .
All the input variables should be normalized at first, therefore the z-scores were calculated for all the data with zero mean and unit standard deviation. The PPCA was performed with the normalized input variables. PPCA returns a set of orthogonal components that are the linear combinations of the original variables. In this step, it is necessary to choose the number of components representing the chosen variables. In this study, the Kaiser criterion 62 was used to select the number of components, which retains the components with eigenvalues greater than 1. Then, the varimax rotation was applied to the retaining components in order to reduce the number of high-loading variables on each component. Before formulating the SEVI, the resulting components should be scaled based on their influence on the socio-economic vulnerability the influence of the dominating socio-economic factor, as if it has positive or negative sign. The influence of the chosen variables is presented in Table 1 . For this step, an output of the loadings of each variable on each factor was used to determine if high levels of a given factor tend to increase or decrease social vulnerability. If a factor tends to show high levels for low social vulnerability (e.g., higher wealth improves the socio-economic status), the corresponding factor scores are multiplied by –1. In some cases, both high and low levels may increase social vulnerability (e.g., a mature population decreases the socio-economic status and abundance of children increases the status) and in this case the absolute value of the corresponding factor score was used for calculating SEVI. Following the suggestion of Schmidtlein et al . 53 , the component scores were combined by weighted sum using explainable variance. Finally, the resulting score was normalized between 0 and 1 to calculate the final SEVI.
In summary, the SEVI is calculated using the following steps:
1. Standardize all input variables to z-scores, each with zero mean and unit standard deviation.
2. Perform the PPCA with the standardized input variables.
3. Select the number of components to be further used based on the unrotated solution.
4. Rotate the initial PPCA solution.
5. Interpret the resulting components on how they may influence the social vulnerability and assign signs to the components accordingly.
6. Combine the selected component scores into a univariate score using weighting scheme on the explained variance.
7. Standardize the resulting scores to mean 0 and standard deviation 1.
Figure 1 presents the schematic flowchart for SEVI development procedure in this study.
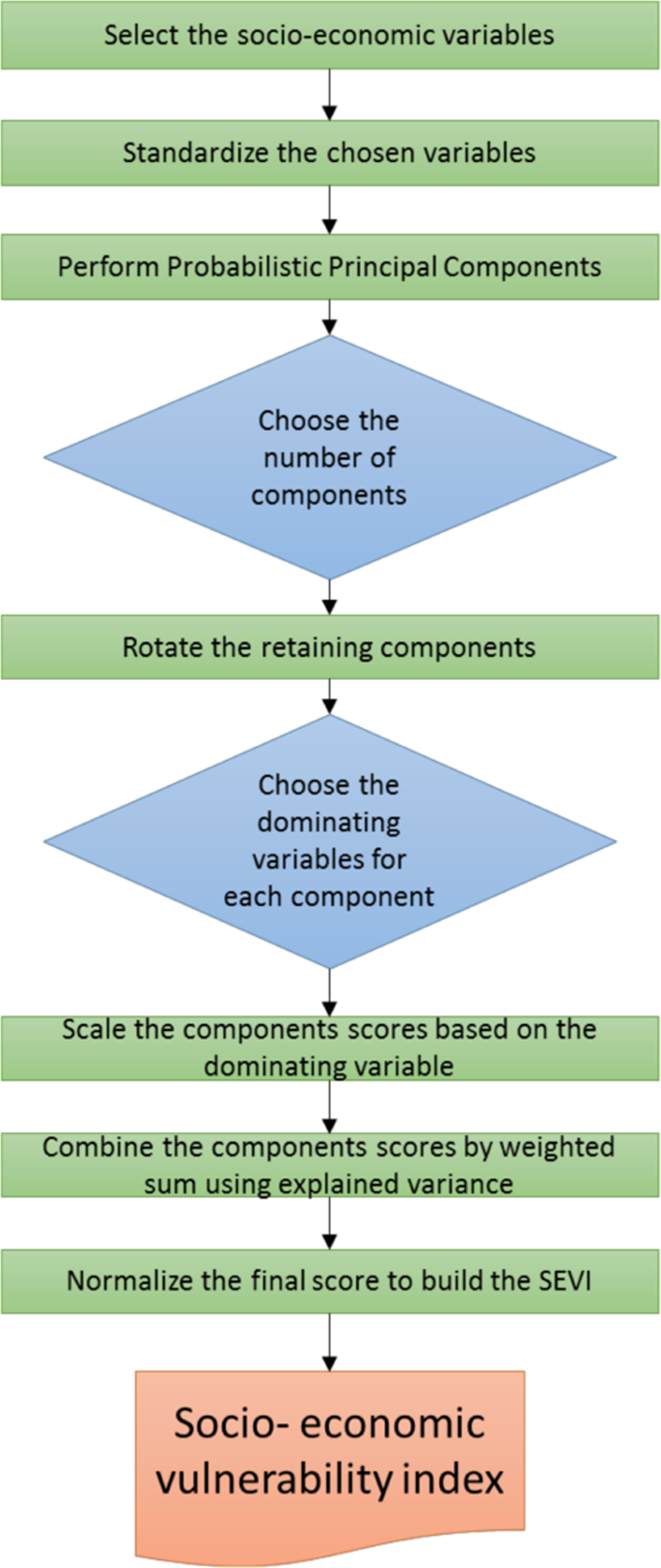
The methodology employed to calculate the Socio-Economic Vulnerability Index (SEVI).
The methodology was applied to the socio-economic variables at county level, and for clear presentation, the regional units (i.e. counties) were divided into three categories; low vulnerable (SEVI <= 10 th Percentile), medium vulnerable (10 th Percentile < SEVI < 90 th Percentile) and high vulnerable (SEVI => 90 th Percentile). The calculated socio-vulnerability is a unitless spatial measure, and it is designed to be employed as a comparative value across geographic locations.
Flash flood clustering
The United States Geological Survey (USGS) stations were spatially clustered based on each flash flood characteristic (i.e., magnitude, duration, frequency, and severity). This study has employed the Getis-Ord hotspot analysis for this purpose 63 . The Getis-Ord hotspot analysis is a parametric technique which can capture the local hotspots. The hotspot analysis uses the flash flood characteristics to identify the locations of statistically significant hot spots and cold spots in the USGS data.
A high Z-score and small P value for a region indicates a significant hot spot (i.e. significant clusters of high values). A low negative Z-score with a high absolute value and small P value indicates a significant cold spot (i.e. significant clusters of low values). In simple words, hotspots are the regions that the variable of interest (e.g. flash flood magnitude or frequency) is high and the surrounding regions also indicate large values. Therefore, they are associated with higher hazard. The higher the Z-score (absolute value), the denser is the clustering. A Z-score near zero means no spatial clustering.
Give the station i, in total number of n stations, the Getis-Ord local statistic is given as:
Where x j is the attribute value for feature j, w i,j is the spatial weight (i.e, the distance between the stations) between stations i and j, and n is the total number of stations and:
Results and Discussion
The results of socio-economic vulnerability analysis and its spatial relation with flash flood characteristic are divided into two sections. The first section investigates the characteristics of SEVI over the U.S. counties. Then, the resemblance of the flash flood characteristics and the SEVI is evaluated over the CONUS.
Socio-Economic Vulnerability over the CONUS
The SEVI is calculated by feeding the PPCA with the 32 social and economic variables described in section 2.1. The PPCA analysis of socioeconomic vulnerability resulted in retaining nine components, which explain 78% of the total variance among the U.S. counties. Each of the components explains between 3 to 17 percent of the total variance. The dominant categories for each retaining component (i.e., the variables with the highest value of loading) are presented in Table 2 .
Results of Table 2 indicate that the demographic socioeconomic status dominates the SEVI with more than 30% of the variance explained. This finding is in agreement with the results from Cutter and Finch 21 , in which the socioeconomic status explained approximately 20% of the variance in five decades at a county level. The calculated SEVI of each county is classified into three classes of high vulnerable, medium vulnerable, and low vulnerable. The low and high vulnerable counties are the ones placed in the 10 th and 90 th percentile of the calculated index. Figure 2 shows the SEVI at the county level.
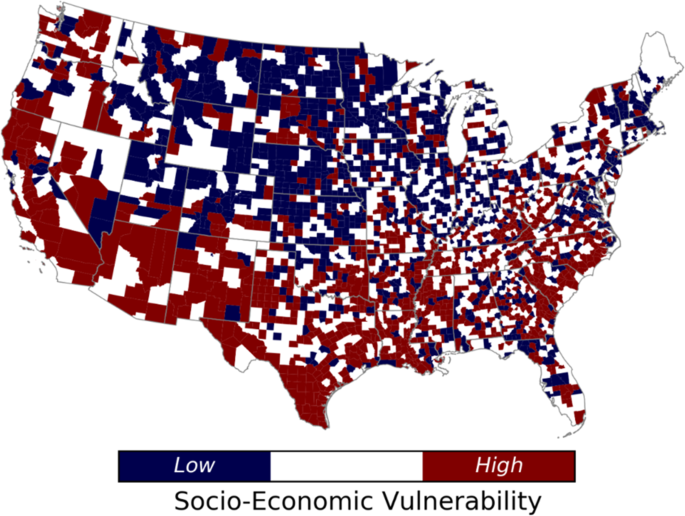
The spatial distribution of Socio-Economic Vulnerability Index (SEVI) at county level across the CONUS.
As shown in Fig. 2 , the most vulnerable counties are concentrated in the southwest and the southern Plains (i.e, Texas and Louisiana) as well as along the U.S.–Mexico border regions of Texas. The least vulnerable counties are located in New England and the upper Great Lakes. Results from the previous assessments 16 , 21 , 52 indicate similar spatial geographical patterns. Cutter and Finch 21 showed that the vulnerability is increasing in the southwest parts toward the U.S.–Mexico border regions of Texas in the last four decades, which can be due to the clustering of high vulnerable counties in that region, based on the SEVI calculated in this study.
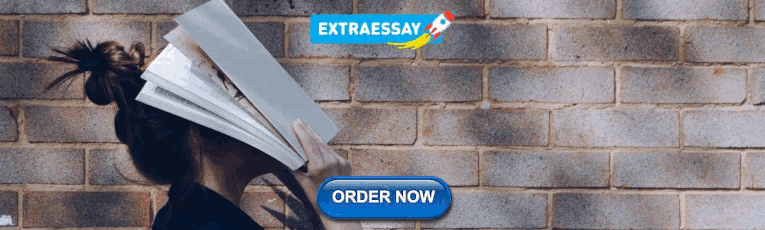
Spatial distribution of flash flood characteristics
This study targets four characteristics of flash flood including frequency, magnitude, duration, and severity. Results from the hotspot analysis are summarized in Fig. 3 through Fig. 4 . Hotspots represent the spatial clustering of high values for a given characteristic, and the spatial clustering of low values are indicated by the cold spots.
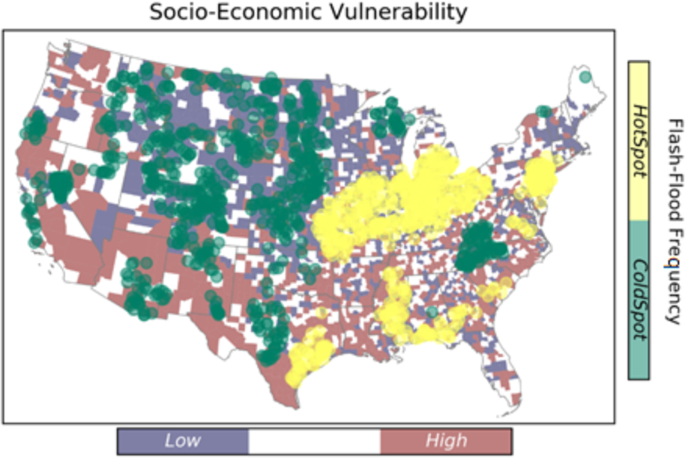
Spatial clustering of flash flood frequency over the CONUS. The underlying map shows the distribution of social vulnerability at county level.
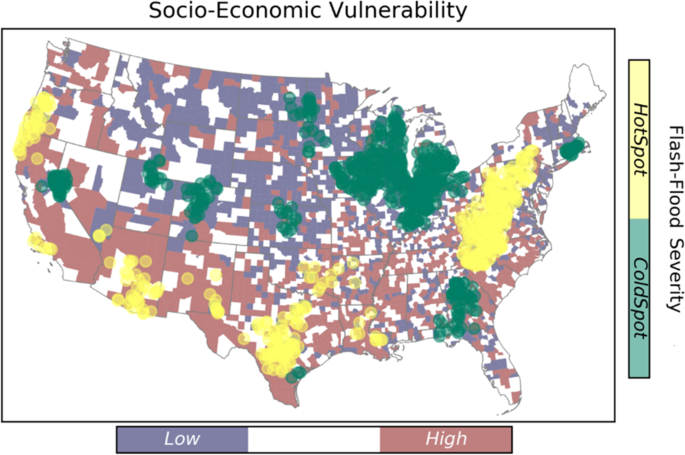
Spatial clustering of flash flood severity over the CONUS.
As shown in Fig. 3 , the Midwest experiences the largest values of flash flood frequency, whereas the cold spots are located in the Great Plains region. Eastern and southern parts of the U.S. show smaller hotspot clusters, and scattered coldspots are visible in the southwest and pacific regions.
The flash flood magnitude has the smallest clusters comparing to the rest of characteristics (Fig. 5 ). The hotspot clusters of flash flood magnitude are located in the Missouri Valley, East coast, and Oregon. The largest coldspot of flash flood magnitude is located in the Upper North region. This indicates that the magnitude of flash flood varies substantially throughout the CONUS.
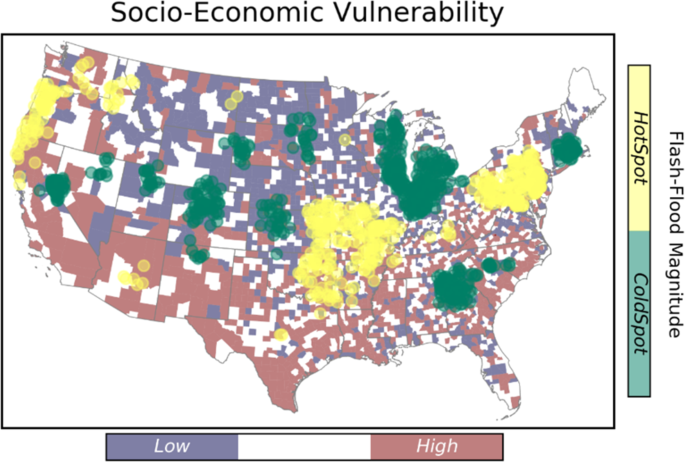
Spatial clustering of flash flood magnitude over the CONUS.
Figure 6 represents the results for flash flood duration. A considerably large coldspot cluster is located in the Northeastern and part of Southeastern regions. The hotspot clusters are spread out in the lower part of Southeast, Midwest, and Great Plains. Disperse clusters of short flash flood duration are located in the Central U.S., Southwest, and pacific regions.
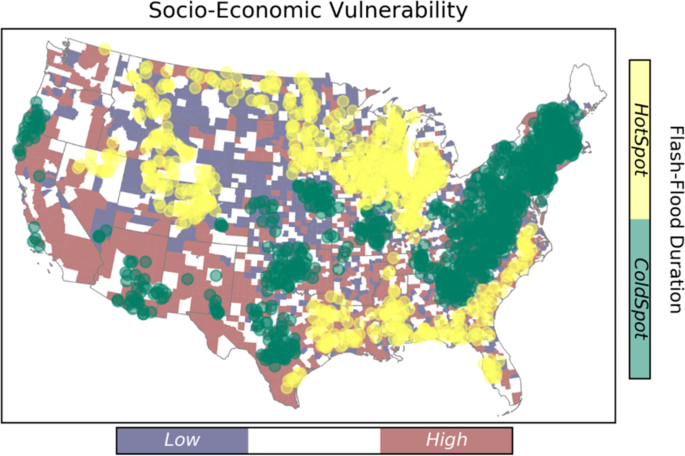
Spatial clustering of flash flood duration over the CONUS.
Similar to the flash flood magnitude, flash flood severity has small number of clusters, as it depends on the magnitude (Fig. 4 ). The hotspot clusters of flash flood severity are located in the Appalachians, Texas, Arizona, and West coast. On the other hand, the Missouri Valley is where the coldspot of flash flood severity is located. This spatial pattern is observed in a recent study by Saharia et al . 64 , and they investigated the flash flood severity over the United States by introducing a new index called the flashiness. Flashiness was defined as the difference between the peak discharge and action stage discharge normalized by the flooding rise time and basin area.
One of the porpuses of this study is to invesigate the coincide of flash flood chararacteristics and socio-economic vulnerability over the CONUS. Therefore, the flash flood characteristis (i.e., frequency, duration, magnitude, and severity) should be converted to county-scale from the station data, which was carried out by employing the overlay tools in ArcMap. Figure 7 represents the flash flood hazard characteristics on the county level. The orange counties are the ones where the stations show hotspots for that specific flash flood characteristic and the blue color indicates the coldspots.
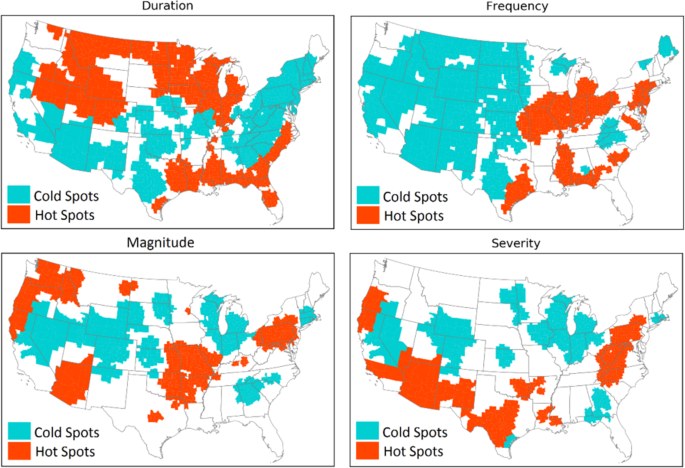
Flash flood hazard characteristics converted from gauge station to the county-scale.
The counties that are located in the Pacific Northwest region indicate hotspots for the flash flood magnitude and severity, whereas it is not the case for the duration and frequency. The majority of counties in the Northern Great Plains are located in the hotspots for flash flood duration and cold spot for frequency. The western and central couties experience less frequent flash flood compared to the Southeast and Northern U.S. The Southwest and Southern Great Plains experienced more severe flash floods with shorter duration.
Analogy between socio-economic vulnerability and flash flood hazard
A two-way cross tabulation is used to map the coincidence of SEVI and flash flood hazard characteristics, and the results are presented in Fig. 8 . Comparing the results with respect to flash flood duration and frequency, the high-vulnerable-hotspot counties for frequency are located in the lower Southeast. Whereas, the high-vulnerable-hotspot counties for duration are located in the Southwest and Pacific Northwest.
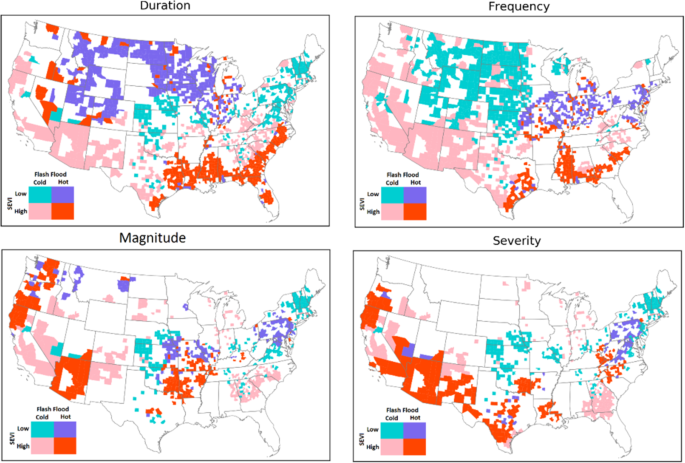
Maps of the counties where flashflood extremes coincide with socio-economic vulnerability extremes.
From Fig. 8 , the majority of high-vulnerable-coldspot counties (i.e., the regions associated with high vulnerability and low hazard) are concentrated in the Southwest for flash flood duration, frequency and magnitude, however; they are scattered in regard to severity. The low-vulnerable-coldspot counties are densely located at the Northern Great Plains and Midwest, comparing the frequency with other flash flood characteristics. Focusing on flash flood duration, the low-vulnerable-hotspots are located in the Northern Great Plains. In case of a large flash flood event with vast spatial scale, the hotspot-high vulnerable regions such at Southwest (shown in orange) will probably be affected the most, and since they indicate cluster of areas that will probably be highly impacted, they are unlikely to receive immediate aid from adjacent counties either.
Overall, the majority of areas located in hot spots of rapid floods exhibit low SEVI. However, hot spots and high SEVI do intersect and affect a considerable number of counties in the United States. For instance, counties that are located in the southwest regions are prone to severe floods and they have poor socio-economic status, especially in Nevada. Whereas, the southeastern counties with high SEVI are facing more frequent flooding. The high risk counties in the south-east region indicate an overlapping situation of the hot-spots of flooding duration and frequency. In summary, the counties that are located in these regions can benefit from additional flood management assistance, which are mostly located in the southern United States.
Concluding Remarks
This study presented a thorough assessment of socio-economic vulnerability and its interaction with four main flash flood characteristics over the CONUS. The evaluation of socio-economic vulnerability usually consists of the construction of indices representing the inherent characteristics or qualities of social systems that are susceptible to a potential impact 12 , 21 , 22 . For instance, by understanding the vulnerability of a region to flash flooding, it is possible to assign various evacuation policies for different regions accordingly. A region (i.e. county) with high levels of vulnerability should be asked to evacuate at lower flash flood hazards, compared to the regions that have low vulnerability. In addition, special programs can be initiated to assist the regions that are associated with high level of hazard (frequent and intense flash floods) as well as high vulnerability.
In this study, vulnerability was assessed at the county level by employing 36 social and economic variables. Probabilistic Principal Component Analysis (PPCA) was utilized to quantify socio-economic vulnerability index (SEVI). The flash flood characteristics including frequency, magnitude, duration, and severity were considered for evaluating flash flood hazard. The spatial distribution of flash floods was assessed by using hotspot analysis, and the intersection of SEVI and flash flood characteristics were mapped using the cross-tabulation. The southwest region shows severe flash flooding with high magnitude, whereas the Northern Great Plains indicate lower duration and frequency. Critical counties (high-vulnerable-hotspots) are mostly located in the southern parts of the U.S., whereas the majority of counties in the Northern Great Plains are in the non-critical status.
This study aimed to generate a comprehensive SEVI; however, it still has some limitations. Although social vulnerability indices can efficiently describe broad-scale vulnerability, they fall short on integrating localized information related to exposure, sensitivity, and adaptive capacity that is often better collected using qualitative methods such as empirical methods and surveying 52 , 65 . Moreover, the calculated SEVI is based on only one point in time (the recent past), and the spatial patterns of socioeconomic vulnerability that were identified in this study represent the recent past. In addition, it would be beneficial to look at the SEVI as the simple weighted sum of the physical variables and compare the outcome with the current SEVI. The socio-economic status and flash flood potential inevitably change over time, due to climate change, migration, and social development. Future studies may consider updating these maps or extending them to longer time frames, especially if/when new extreme events occur. It can also be beneficial to validate the calculated socio-economic vulnerability by comparing the post-hazard outcomes such as economic damages and fatalities with the pre-hazard vulnerability in a spatial context.
Lastly, it is essential to quantify the uncertainty associated with the SEVI, in the future studies. The uncertainty in SEVI can raise from multiple sources including the data used, the chosen variables, period of interest, the methodology, and the weighting technique to integrate the variables and calculate the index. An accurate and reliable cascade of uncertainty for SEVI requires that many permutations of each source of uncertainty be investigated in order to reliably capture the range of uncertainty, and then the fraction of total variance caused by each factor can be determined.
Kotzee, I. & Reyers, B. Piloting a social-ecological index for measuring flood resilience: A composite index approach. Ecol. Indic. 60 , 45–53 (2016).
Article Google Scholar
Ashley, S. T. & Ashley, W. S. Flood fatalities in the United States. J. Appl. Meteorol. Climatol. 47 , 805–818 (2008).
Article ADS Google Scholar
Armah, F. A., Yawson, D. O., Yengoh, G. T., Odoi, J. O. & Afrifa, E. K. A. Impact of floods on livelihoods and vulnerability of natural resource dependent communities in Northern Ghana. Water 2 , 120–139 (2010).
Borga, M., Anagnostou, E. N., Blöschl, G. & Creutin, J.-D. Flash flood forecasting, warning and risk management: the HYDRATE project. Environ. Sci. Policy 14 , 834–844 (2011).
Kleinen, T. & Petschel-Held, G. Integrated assessment of changes in flooding probabilities due to climate change. Clim. Change 81 , 283–312 (2007).
Habibi, H. et al . High Resolution Flash Flood Forecasting for the Dallas-Fort Worth Metroplex. J. Water Manag. Model (2016).
Cutter, S. L., Emrich, C. T., Gall, M. & Reeves, R. Flash Flood Risk and the Paradox of Urban Development. Nat. Hazards Rev. 19 , 5017005 (2017).
Hapuarachchi, H. A. P., Wang, Q. J. & Pagano, T. C. A review of advances in flash flood forecasting. Hydrol. Process. 25 , 2771–2784 (2011).
Aroca-Jiménez, E., Bodoque, J. M., García, J. A. & Díez-Herrero, A. A quantitative methodology for the assessment of the regional economic vulnerability to flash floods. J. Hydrol. 565 , 386–399 (2018).
Yin, J., Ye, M., Yin, Z. & Xu, S. A review of advances in urban flood risk analysis over China. Stoch. Environ. Res. risk Assess. 29 , 1063–1070 (2015).
Li, G.-F., Xiang, X.-Y., Tong, Y.-Y. & Wang, H.-M. Impact assessment of urbanization on flood risk in the Yangtze River Delta. Stoch. Environ. Res. risk Assess. 27 , 1683–1693 (2013).
Terti, G., Ruin, I., Anquetin, S. & Gourley, J. J. Dynamic vulnerability factors for impact-based flash flood prediction. Nat. Hazards 79 , 1481–1497 (2015).
Yin, J., Yu, D., Yin, Z., Liu, M. & He, Q. Evaluating the impact and risk of pluvial flash flood on intra-urban road network: A case study in the city center of Shanghai, China. J. Hydrol. 537 , 138–145 (2016).
Javelle, P. et al . Improving flash flood forecasting and warning capabilities (2016).
Ahmadalipour, A. & Moradkhani, H. A data-driven analysis of flash flood hazard, fatalities, and damages over the CONUS during 1996-2017. J. Hydrol . 124106 (2019).
Cutter, S. L., Boruff, B. J. & Shirley, W. L. Social vulnerability to environmental hazards. Soc. Sci. Q. 84 , 242–261 (2003).
Ge, Y. et al . Assessment of social vulnerability to natural hazards in the Yangtze River Delta, China. Stoch. Environ. Res. Risk Assess. 27 , 1899–1908 (2013).
Council, N. R. Facing hazards and disasters: Understanding human dimensions . (National Academies Press, 2006).
Center, H. Human links to coastal disasters. H. John Heinz III Cent (2002).
Peduzzi, P., Dao, H., Herold, C. & Mouton, F. Assessing global exposure and vulnerability towards natural hazards: the Disaster Risk Index. Nat. Hazards Earth Syst. Sci. 9 , 1149–1159 (2009).
Cutter, S. L. & Finch, C. Temporal and spatial changes in social vulnerability to natural hazards. Proc. Natl. Acad. Sci. 105 , 2301–2306 (2008).
Article ADS CAS PubMed PubMed Central Google Scholar
Cutter, S. L. Hazards vulnerability and environmental justice . (Routledge, 2012).
Adger, W. N. Vulnerability. Glob. Environ. Chang. 16 , 268–281 (2006).
Folke, C. Resilience: The emergence of a perspective for social–ecological systems analyses. Glob. Environ. Chang. 16 , 253–267 (2006).
Kasperson, R. E. Ecosystems and Human Well-Being. Current State and Trends, eds. Hassan RM, Scholes R, Ash N. (2005).
Blaikie, P., Cannon, T., Davis, I. & Wisner, B. At risk: natural hazards, people’s vulnerability and disasters . (Routledge, 1994).
Turner, B. L. et al . A framework for vulnerability analysis in sustainability science. Proc. Natl. Acad. Sci. 100 , 8074–8079 (2003).
Ming, X. et al . Quantitative multi-hazard risk assessment with vulnerability surface and hazard joint return period. Stoch. Environ. Res. risk Assess. 29 , 35–44 (2015).
Cutter, S. L. Vulnerability to environmental hazards. Prog. Hum. Geogr. 20 , 529–539 (1996).
Blaikie, P., Cannon, T., Davis, I. & Wisner, B. At risk: natural hazards, people’s vulnerability and disasters . (Routledge, 2005).
Eakin, H. & Luers, A. L. Assessing the vulnerability of social-environmental systems. Annu. Rev. Environ. Resour. 31 , 365–394 (2006).
Klein, R. J. T., Nicholls, R. J. & Thomalla, F. Resilience to natural hazards: How useful is this concept? Glob. Environ. Chang. Part B Environ. Hazards 5 , 35–45 (2003).
Hahn, M. B., Riederer, A. M. & Foster, S. O. The Livelihood Vulnerability Index: A pragmatic approach to assessing risks from climate variability and change—A case study in Mozambique. Glob. Environ. Chang. 19 , 74–88 (2009).
Malakar, K. & Mishra, T. Assessing socio-economic vulnerability to climate change: a city-level index-based approach. Clim. Dev. 9 , 348–363 (2017).
Kelkar, U., Balachandra, P. & Gurtoo, A. Assessing Indian cities for vulnerability to climate change. In Proceedings of the 2nd international conference on environmental science and development IPCBEE 4, (2011).
Ahmadalipour, A. & Moradkhani, H. Multi-dimensional assessment of drought vulnerability in Africa: 1960–2100. Sci. Total Environ. 644 , 520–535 (2018).
Article ADS CAS PubMed Google Scholar
Špitalar, M. et al . Analysis of flash flood parameters and human impacts in the US from 2006 to 2012. J. Hydrol. 519 , 863–870 (2014).
Economic, U. N. D. of. World population prospects : The 2006 revision . 261, (United Nations Publications, 2007).
Schelf K.E., H. Moradkhani, and U. Lall, Atmospheric Circulation Patterns Associated with extreme United States Floods Identified via Machine Learning, Scientific Reports https://doi.org/10.1038/s41598-019-43496-w (2019).
Wu, Y., Zhong, P., Xu, B., Zhu, F. & Ma, B. Changing of flood risk due to climate and development in Huaihe River basin, China. Stoch. Environ. Res. risk Assess. 31 , 935–948 (2017).
Trenberth, K. E., Dai, A., Rasmussen, R. M. & Parsons, D. B. The changing character of precipitation. Bull. Am. Meteorol. Soc. 84 , 1205–1217 (2003).
Prein, A. F. et al . The future intensification of hourly precipitation extremes. Nat. Clim. Chang. 7 , 48 (2017).
Milanesi, L., Pilotti, M., Belleri, A., Marini, A. & Fuchs, S. Vulnerability to flash floods: a simplified structural model for masonry buildings. Water Resour. Res. 54 , 7177–7197 (2018).
Karagiorgos, K., Heiser, M., Thaler, T., Hübl, J. & Fuchs, S. Micro-sized enterprises: vulnerability to flash floods. Nat. Hazards 84 , 1091–1107 (2016).
Birkmann, J. et al . Framing vulnerability, risk and societal responses: the MOVE framework. Nat. hazards 67 , 193–211 (2013).
Gourley, J. J., Erlingis, J. M., Smith, T. M., Ortega, K. L. & Hong, Y. Remote collection and analysis of witness reports on flash floods. J. Hydrol. 394 , 53–62 (2010).
Gourley, J. J. et al . The FLASH Project: improving the tools for flash flood monitoring and prediction across the united states. Bull. Am. Meteorol. Soc. 98 , 361–372 (2017).
Manly, B. F. J. & Alberto, J. A. N. Multivariate statistical methods: a primer . (Chapman and Hall/CRC, 2016).
Filmer, D. & Pritchett, L. Estimating wealth effects without expenditure data—or tears. In Policy Research Working Paper 1980, The World (Citeseer, 1998).
McKenzie, D. J. Measuring inequality with asset indicators. J. Popul. Econ. 18 , 229–260 (2005).
Vyas, S. & Kumaranayake, L. Constructing socio-economic status indices: how to use principal components analysis. Health Policy Plan. 21 , 459–468 (2006).
Article PubMed Google Scholar
Wigtil, G. et al . Places where wildfire potential and social vulnerability coincide in the coterminous United States. Int. J. Wildl. fire 25 , 896–908 (2016).
Schmidtlein, M. C., Deutsch, R. C., Piegorsch, W. W. & Cutter, S. L. A sensitivity analysis of the social vulnerability index. Risk Anal. An Int. J. 28 , 1099–1114 (2008).
Tipping, M. E. & Bishop, C. M. Probabilistic principal component analysis. J. R. Stat. Soc. Ser. B (Statistical Methodol. 61, , 611–622 (1999).
Article MathSciNet MATH Google Scholar
Nasrabadi, N. M. Pattern recognition and machine learning. J. Electron. Imaging 16 , 49901 (2007).
Nyamundanda, G., Brennan, L. & Gormley, I. C. Probabilistic principal component analysis for metabolomic data. BMC Bioinformatics 11 , 571 (2010).
Article PubMed PubMed Central Google Scholar
Cutter, S. L. The vulnerability of science and the science of vulnerability. Ann. Assoc. Am. Geogr. 93 , 1–12 (2003).
Cutter, S. L., Mitchell, J. T. & Scott, M. S. Revealing the vulnerability of people and places: a case study of Georgetown County, South Carolina. Ann. Assoc. Am. Geogr. 90 , 713–737 (2000).
White, G. F., Kates, R. W. & Burton, I. Knowing better and losing even more: the use of knowledge in hazards management. Glob. Environ. Chang. Part B Environ. Hazards 3 , 81–92 (2001).
Pulido, L. Geographies of race and ethnicity 1: White supremacy vs white privilege in environmental racism research. Prog. Hum. Geogr. 39 , 809–817 (2015).
Webb, G. R., Tierney, K. J. & Dahlhamer, J. M. Businesses and disasters: Empirical patterns and unanswered questions. Nat. Hazards Rev. 1 , 83–90 (2000).
Humphreys, L. G. & Ilgen, D. R. Note on a criterion for the number of common factors. Educ. Psychol. Meas. 29 , 571–578 (1969).
Ord, J. K. & Getis, A. Local spatial autocorrelation statistics: distributional issues and an application. Geogr. Anal. 27 , 286–306 (1995).
Saharia, M. et al . Mapping flash flood severity in the United States. J. Hydrometeorol. 18 , 397–411 (2017).
Fischer, A. P., Paveglio, T., Carroll, M., Murphy, D. & Brenkert-Smith, H. Assessing Social Vulnerability to Climate Change in Human Communities near Public Forests and Grasslands: A Framework for Resource Managers and Planners. J. For. 111 , 357–365 (2013).
Google Scholar
Download references
Author information
Authors and affiliations.
Center for Complex Hydrosystems Research, Department of Civil, Construction and Environmental Engineering, University of Alabama, Tuscaloosa, AL, USA
Sepideh Khajehei, Ali Ahmadalipour & Hamid Moradkhani
Center for Complex Hydrosystems Research, Department of Geography, University of Alabama, Tuscaloosa, AL, USA
Wanyun Shao
You can also search for this author in PubMed Google Scholar
Contributions
S.K. carried out the assessment and prepared the initial draft of the article. H.M. initiated the study and supervised it throughout. S.K., A.A., W.S. and H.M. contributed in writing the paper and discussing the results.
Corresponding author
Correspondence to Hamid Moradkhani .
Ethics declarations
Competing interests.
The authors declare no competing interests.
Additional information
Publisher’s note Springer Nature remains neutral with regard to jurisdictional claims in published maps and institutional affiliations.
Rights and permissions
Open Access This article is licensed under a Creative Commons Attribution 4.0 International License, which permits use, sharing, adaptation, distribution and reproduction in any medium or format, as long as you give appropriate credit to the original author(s) and the source, provide a link to the Creative Commons license, and indicate if changes were made. The images or other third party material in this article are included in the article’s Creative Commons license, unless indicated otherwise in a credit line to the material. If material is not included in the article’s Creative Commons license and your intended use is not permitted by statutory regulation or exceeds the permitted use, you will need to obtain permission directly from the copyright holder. To view a copy of this license, visit http://creativecommons.org/licenses/by/4.0/ .
Reprints and permissions
About this article
Cite this article.
Khajehei, S., Ahmadalipour, A., Shao, W. et al. A Place-based Assessment of Flash Flood Hazard and Vulnerability in the Contiguous United States. Sci Rep 10 , 448 (2020). https://doi.org/10.1038/s41598-019-57349-z
Download citation
Received : 11 June 2019
Accepted : 23 December 2019
Published : 16 January 2020
DOI : https://doi.org/10.1038/s41598-019-57349-z
Share this article
Anyone you share the following link with will be able to read this content:
Sorry, a shareable link is not currently available for this article.
Provided by the Springer Nature SharedIt content-sharing initiative
This article is cited by
Linkages between riverine flooding risk and economic damage over the continental united states.
- Cisneros-Pineda Alfredo
- Hertel Thomas
Natural Hazards (2024)
Block-level vulnerability assessment reveals disproportionate impacts of natural hazards across the conterminous United States
- Farnaz Yarveysi
- Atieh Alipour
- Hamid Moradkhani
Nature Communications (2023)
Indicator-based approach for fluvial flood risk assessment at municipal level in Slovakia
- Matej Vojtek
Scientific Reports (2023)
Evaluating flash flood simulation capability with respect to rainfall temporal variability in a small mountainous catchment
- Xuemei Wang
- Xiaoyan Zhai
Journal of Geographical Sciences (2023)
Study on spatial divergence of rural resilience and optimal governance paths in oasis: the case of Yongchang County in the Hexi Corridor of China
- Tianmin Tao
- Wenbo Zhang
Environment, Development and Sustainability (2023)
By submitting a comment you agree to abide by our Terms and Community Guidelines . If you find something abusive or that does not comply with our terms or guidelines please flag it as inappropriate.
Quick links
- Explore articles by subject
- Guide to authors
- Editorial policies
Sign up for the Nature Briefing newsletter — what matters in science, free to your inbox daily.

You are using an outdated browser. Please upgrade your browser to improve your experience.
Assessing Drainage Capacity and Managing Flood Vulnerability: Case Studies from Guyana's East Berbice-Corentyne Area
- Michael L. Hackett
Description
This study examines the recurrent issue of flooding in various regions of Guyana during the biannual rainy season and proposes strategies to enhance water resilience and mitigate the impact of flooding. The affected areas experience floods of varying depths during heavy rainfall events. These floods disrupt human activities, resulting in substantial economic losses across residential, commercial, agricultural, and industrial sectors. This study delves into the historical context of drainage systems established by European colonizers and plantation owners, originally designed for optimal drainage under specific rainfall conditions. The study underscores the vulnerability of these systems to “short duration high-intensity rainfall” which leads to pluvial flooding. Two case studies, focusing on Black Bush Polder and John’s-Port Mourant, provide detailed insight into flooding scenarios and their causes. Inadequate drainage capacities, siltation, and canal networks are identified as factors contributing to flooding. The study emphasizes the need for maintaining efficient and clean drainage infrastructure to prevent flood exacerbation. In response to the challenges of flooding, the study recommends a multi-pronged approach to building water resilience. Strategies include educating the population about flood risks, implementing warning systems, maintaining emergency supplies, enforcing building codes for flood resilience, designing permeable urban areas, and modernizing drainage systems. The study concludes that while flooding cannot be eliminated, a combination of resilient infrastructure, community awareness, and innovative technologies can significantly mitigate its adverse effects. By implementing these measures, Guyana can achieve enhanced water resilience and be better prepared for flood challenges.
Keywords:- Cusecs (Cubic Feet Per Second), Discharge Capacity, Drainage And Irrigation Area, Pluvial Flooding, Pooling, Drainage Coefficient, Short Duration High- Intensity Rainfall.
IJISRT24FEB1010.pdf
Files (942.3 kb), additional details.
This site uses cookies. Find out more on how we use cookies
Social vulnerability to floods: a case study of Huaihe River Basin
- Original Paper
- Published: 19 December 2013
- Volume 71 , pages 2113–2125, ( 2014 )
Cite this article
- Yong-Ling Zhang 1 , 2 &
- Wen-Jiao You 1 , 2
1395 Accesses
42 Citations
8 Altmetric
Explore all metrics
Since ancient times, floods occurred frequently in Huaihe River with significant casualties and economic losses. In developing measures for disaster prevention or emergency response for disaster relief, the study of social vulnerability to floods in Huaihe River Basin should be strengthened. Based on the latest socioeconomic data, the index system of social vulnerability to floods was constructed from three dimensions: population, economy, and flood prevention. Sensitive indexes were identified from the original indexes by principal component analysis, and the social vulnerability index for floods was calculated for Huaihe River Basin. The results described the characteristics of the spatial distribution. It also demonstrated that vulnerability manifests itself as a regional phenomenon, with significant changes from city to city across the Huaihe River Basin. Understanding the impacts of changes in vulnerability was crucial in developing measures to prevent floods.
This is a preview of subscription content, log in via an institution to check access.
Access this article
Price includes VAT (Russian Federation)
Instant access to the full article PDF.
Rent this article via DeepDyve
Institutional subscriptions
Similar content being viewed by others
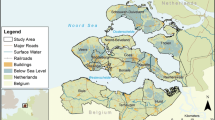
Assessing Social Vulnerability to Flood Hazards in the Dutch Province of Zeeland
Ryan H. Kirby, Margaret A. Reams, … D. Q. P. Fundter
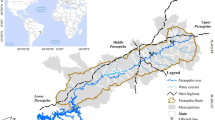
Social vulnerability to environmental disasters in the Paraopeba River Basin, Minas Gerais, Brazil
Mariane Paulina Batalha Roque, José Ambrósio Ferreira Neto, … Arthur Telles Calegario
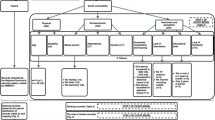
Flood hazards, social vulnerability and societal risks in Russia
Viacheslav Lipatov, Nadira Mavlyanova & John Tiefenbacher
Barry EF, Edward WG, Elaine JH et al (2011) A social vulnerability index for disaster management. J Homel Secur Emerg Manag 8(1):1–22
Google Scholar
Candice AM, Tim S, Joachim S (2008) Social vulnerability and migration in the wake of disaster: the case of hurricanes Katrina and Rita. Popul Environ 29:271–291
Article Google Scholar
Clark GE, Moser SC, Ratick SJ et al (1998) Assessing the vulnerability of coastal communities to extreme storms: the case of revere, MA, USA. Mitig Adapt Strateg Glob Chang 3:59–82
Cutter SL, Boruff BJ, Shirley WL (2003) Social vulnerability to environmental hazards. Soc Sci Q 84(2):242–261
Ge Y, Shi PJ, Liu J et al (2005) Improvement and application of vulnerability assessment methodology for flood hazards in China: using Changsha prefecture as a case study. J Nat Disasters 14(6):54–58
Huaihe River Commission, Ministry of Water Resources (2007) History of Huaihe River. Science Press, Beijing
Ivar S, Lujala, Jan KR (2011) Social vulnerability assessment for Norway: a quantitative approach. Nor Geogr Tidsskr 65:1–17
Mathew CS, Roland CD, Walter WP et al (2008) A sensitivity analysis of the social vulnerability index. Risk Anal 28(4):1099–1114
Shi Y, Xu SY, Shi C et al (2011) Progress in research on vulnerability of natural disasters. J Nat Disasters 20(2):131–137
Yook DK (2012) Assessment of social vulnerability to natural disasters: a comparative study. Nat Hazards 63:823–843
Download references
Acknowledgments
This study is financially supported by the MOE (Ministry of Education in China) Project of Humanities and Social Sciences (Project No. 10YJAZH091). We also would like to thank Xiaolin Yang for their assistance in the data analysis.
Author information
Authors and affiliations.
Safety and Emergency Management Research Center, Henan Polytechnic University, Jiaozuo, 454000, Henan, China
Yong-Ling Zhang & Wen-Jiao You
Emergency Management School, Henan Polytechnic University, Jiaozuo, 454000, Henan, China
You can also search for this author in PubMed Google Scholar
Corresponding author
Correspondence to Yong-Ling Zhang .
Rights and permissions
Reprints and permissions
About this article
Zhang, YL., You, WJ. Social vulnerability to floods: a case study of Huaihe River Basin. Nat Hazards 71 , 2113–2125 (2014). https://doi.org/10.1007/s11069-013-0996-0
Download citation
Received : 01 October 2013
Accepted : 07 December 2013
Published : 19 December 2013
Issue Date : April 2014
DOI : https://doi.org/10.1007/s11069-013-0996-0
Share this article
Anyone you share the following link with will be able to read this content:
Sorry, a shareable link is not currently available for this article.
Provided by the Springer Nature SharedIt content-sharing initiative
- Huaihe River
- Social vulnerability
- Find a journal
- Publish with us
- Track your research
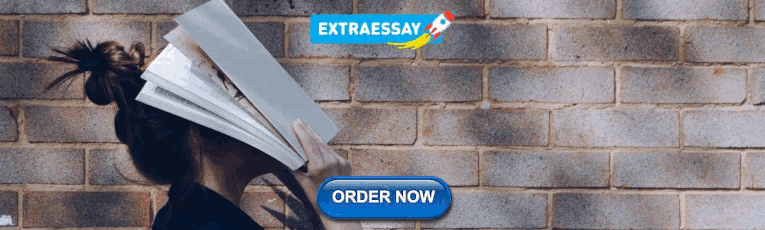
IMAGES
VIDEO
COMMENTS
Many researchers have also made substantial attempts to explain the damage to floods (Chang and Baiamonte, 2002) (Cannon, 2004) (Chen et al., 2015) (Chakraborty and Joshi, 2016) (Canevari-Luzardo et al., 2017); (Vazire, 2018) evaluated the flood vulnerability from the perspective of case studies and observed that flood vulnerability is an ...
Across the case studies, risk perception was most frequently identified as a social vulnerability driver during the mitigation and response phases of flood disasters, and in more developed national settings (Table 2). Flood awareness and prior experience were the primary perceptual aspects explored in the articles, and to a lesser extent, trust ...
Case study research on flood disasters has significantly contributed to understanding of the relationship between floods and social vulnerability, but translation of findings to the development of flood-specific social indicators is not well established (Rufat et al. 2015).
On the social vulnerability side, through a meta-analysis of 67 flood disaster case studies from 1997 to 2013, Rufat et al. summarized demographic, socioeconomic, and health metrics as the main indicators of social vulnerability to flood events. Among the existing works on social vulnerability, most tend to apply an indexing approach.
The study introduces a method of integrating gridded estimates of social vulnerability into high-resolution global flood risk maps demonstrating new insights into the geography of flood risk ...
The rest of the paper is devoted to the flood loss and damage analysis methodology (section 2), including the case study, data preparation, and damage functions. Research results along with discussion are presented in section 3. The conclusion and further analysis follow these sections. 2. Methodology2.1. Case study
Numerous studies have focused on quantifying future flood losses at scales from local to global; all of which involve a translation from the physical phenomenon of flooding (e.g., extent and depth ...
The present study reviewed 95 articles from 38 countries that constructed flood vulnerability indices. In summary, despite the increasing number of studies and advances made, the review has revealed and reconfirmed a number of persistent knowledge gaps. Temporal dynamic aspects of vulnerability were often disregarded.
Ngie A, 2012, A GIS approach for flood vulnerability and adaptation analysis in Diepsloot, University of Johannesburg, Johannesburg. [Google Scholar] Pelling M, 1997, ' What determines vulnerability to floods: A case study in Georgetown, Guyana ', Environment and Urbanization 9, 203-226. 10.1630/095624797101287354 [Google Scholar]
& He, Q. Evaluating the impact and risk of pluvial flash flood on intra-urban road network: A case study in the city center of Shanghai, China. J. Hydrol. 537 , 138-145 (2016).
This method is widely used in flood vulnerability studies and preferred by policy makers for its clarified vulnerability image over space, a depiction which aims to priorities measures and plan for the risk response in specified region. ... Khan S (2012) Vulnerability assessments and their planning implications: a case study of the Hutt Valley ...
Floods are one of the most destructive natural hazards to which Australia is exposed. The frequency of extreme rainfall events and consequential floods are projected to increase into the future as a result of anthropogenic climate change. This highlights the need for more holistic risk assessments of flood affected regions. Flood risk assessments (FRAs) are used to inform decision makers and ...
For presenting a brief review on various works on flood vulnerability, several studies were selected from different journals at global level (Table 1).A time period (1990-2018) was considered for reviewing earlier published works (Fig. 1).Science citation index journals were used for selecting the studies and identifying diverse ways to address vulnerability to flood (Fig. 2).
Many previous flood vulnerability studies have focused on respective SETS domains, yet recognize a need for integrated vulnerability assessments across these domains. ... Social vulnerability to floods: Review of case studies and implications for measurement. International Journal of Disaster Risk Reduction, 14 (2015), pp. 470-486, 10.1016/j ...
AMA Style. Diaz ND, Lee Y, Kothuis BLM, Pagán-Trinidad I, Jonkman SN, Brody SD. Mapping the Flood Vulnerability of Residential Structures: Cases from The Netherlands, Puerto Rico, and the United States.
Extreme sea levels and coastal flooding are projected to be among the most uncertain and severe consequences of climate change. In response, a wide development of coastal vulnerability assessment methodologies has been observed in research to support societal resilience to future coastal flood risks. This work aims to explore the scope of application of index-based methodologies for coastal ...
flood vulnerability nor the total population and property loss of flooding to date, except for a few studies that examined the effectiveness of green infrastructure or only wetlands in flood regulation. The proposed research sought to understand if the existing pattern and composition of NbS can mitigate flood vulnerability and loss of flooding ...
Malaysia is frequently affected by the annual flooding event caused by the seasonal monsoon which accounts for significant losses. Flood risk, exposure and damage potential are increasing, causing the level of poverty and vulnerability to rise. The annual occurrence of the flood hazard has forced residents to prepare beforehand to help them spring back to their daily life faster. This study ...
Two U.S.-based multi-scale flood vulnerability studies revealed that finer spatial scales had identified more vulnerable communities overlooked at larger spatial ... This research seeks to address the knowledge gap in understanding and applying indices of social vulnerability and flood risk. Utilizing the case study area of coastal Virginia, we ...
The study underscores the vulnerability of these systems to "short duration high-intensity rainfall" which leads to pluvial flooding. Two case studies, focusing on Black Bush Polder and John's-Port Mourant, provide detailed insight into flooding scenarios and their causes.
This study aims at providing expertise for preparing public-based flood mapping and estimating flood risks in growing urban areas. To model and predict the magnitude of flood risk areas, an integrated Analytical Hierarchy Process (AHP) and Geographic Information System (GIS) analysis techniques are used for the case of Eldoret Municipality in Kenya. The flood risk vulnerability mapping follows ...
Through a case study of Peshawar, a district of KP, this study aims to assess the household vulnerability of communities to floods in Town II (northern part of the district). In order to identify the factors that drive people's vulnerability and to have better understanding of the situation, various proxy indicators were identified and ...
Moreover, flood vulnerability models have limited applicability for other cities. Therefore, this case study of Wangchengpo, Changsha, presents a flood risk assessment model targeting mesoscale urban land use to identify the key areas where flood hazards and economic vulnerability intersect.
Social vulnerability is a combination of the three subgroups, reflecting a city's comprehensive ability when facing floods. In the case of Jining, for example, the low ranking on social vulnerability is a function of extremely low values on all three indexes. For the other cities, such as Yancheng, the social vulnerability is more of a ...
An approach for measuring social vulnerability in context: the case of flood hazards in Muzarabani district, Zimbabwe. Geoforum, 86 (2017), pp. 103-117. ... Social vulnerability to floods: review of case studies and implications for measurement. International journal of disaster risk reduction, 14 (2015), pp. 470-486.