- Search Menu
- Advance Articles
- Author Guidelines
- Submission Site
- Open Access Options
- Self-Archiving Policy
- Why Submit?
- About Protein & Cell
- Editorial Board
- Advertising & Corporate Services
- Journals on Oxford Academic
- Books on Oxford Academic
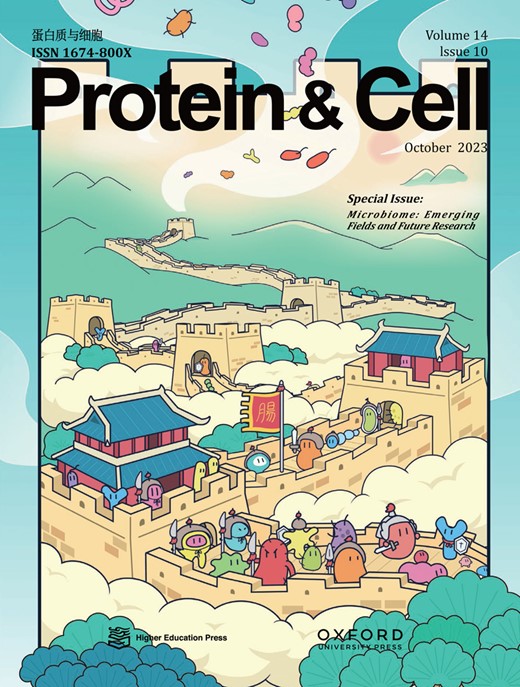
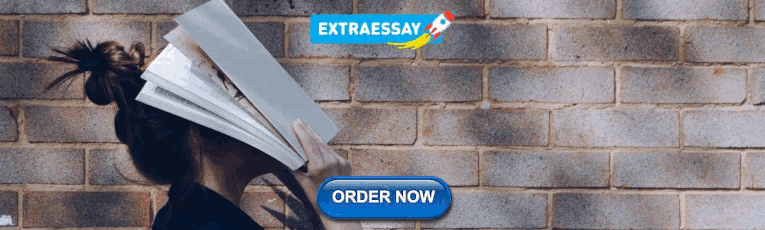
Article Contents
Global trends and advancements in microbiome research: from composition to functionality, unraveling the mysteries of the microbiome: impact on human health and disease, exploring the frontiers of microbiome research: unlocking the advanced tools to revolutionize human health, acknowledgements, conflict of interest, consent for publication, author contributions, code availability, data availability, microbiome research outlook: past, present, and future.

- Article contents
- Figures & tables
- Supplementary Data
Yunyun Gao, Danyi Li, Yong-Xin Liu, Microbiome research outlook: past, present, and future, Protein & Cell , Volume 14, Issue 10, October 2023, Pages 709–712, https://doi.org/10.1093/procel/pwad031
- Permissions Icon Permissions
With its critical role in human health and disease, the microbiome has transformed modern biology. Over the past few years, microbiome research has evolved rapidly, with microbiologists gradually shifting their focus from cataloging microorganisms in the human microbiome to understanding their functional roles and how they interact with the host. Here, we present the global trends in microbiome research and summarize the past and current work on microbiome published in Protein & Cell . In closing, we highlight some of the major advancements in microbiome research, including technical, practical, and conceptual advancements, that aim to enhance disease diagnosis, medicine development, and personalized interventions.
Microbiome research has experienced major technical, practical, and conceptual progress in recent years ( Lynch et al., 2019 ; Liu et al., 2022 ). During the early days, the initial focus was to describe the composition and diversity of microbiomes and to identify correlations between microbes and host phenotypes. However, current research has shifted toward refined studies dedicated to gaining functional insights into the microbiome, deciphering its mechanisms of action, and studying its co-evolution with the host ( Li et al., 2022 ). These ongoing efforts will advance our understanding of how the microbiome influences various aspects of the host ( Fig. 1A ).

The trends and growth of microbiome research over the past years. Data were extracted from Web of Science Core Collection database on March 29th, 2023. (A) Keywords cluster map illustrating the hot spots in microbiome research, with 638 terms occurred at least 100 times since 1985. (B) Tree map indicating the geographical distribution of publications related to microbiome since 1985. (C) Bar graph displaying the year-over-year growth rate of the five countries with the most microbiome-related publications over the past 5 years. Year-over-year growth rate is calculated as follows: (the amount of the year’s publications—the amount of the last-year’s publications)/the amount of the last-year’s publications × 100%; (D) Bubble plot showing the citation indexes of journals citing publications from the first two special issues of Protein & Cell . Citation index is calculated as follows: the number of citations that a journal has made to the publications × the journal impact factor (IF) in 2021. (A) is drawn by VOSviewer.
According to a search of the Web of Science Core Collection (data extracted on March 29th, 2023), most global research publications on the microbiome originated from North America, Asia, and Europe ( Fig. 1B ), and over two-thirds of the publications were released in the past 5 years. Notably, scientists from China have made an enormous contribution to the field since 2018, with a sustained increase in the year-over-year growth rate of publications ( Figs. 1C and S1 ).
Protein & Cell offers a platform for publishing high-quality research and reviews on the microbiome. In the journal’s first two microbiome-themed special issues, several papers have received widespread acclaim worldwide ( Fig. S2A ), top of which are two reviews on the roles of the gut and oral microbiomes in disease diagnostics and therapy ( Gao et al., 2018 ; Wang and Zhao, 2018 ), as well as a pipeline for acquiring microbiome data ( Liu et al., 2020 ). These special issues have garnered more than 1,500 citations since 2018, with an average citation rate over three times that of other publications in this journal of the same period ( Fig. S2B ), and have featured contributions to publications in esteemed journals such as Science , Cell , and Nature Medicine ( Figs. 1D and S2A ).
In the current special issue of Protein & Cell , scientists are seeking to unravel the mysteries of microbiome on human health and disease ( Fig. 2 ). Prof. Hongwei Liu characterizes the chemical structures of gut bacterial cell wall-derived molecules and proposes the role of microbiome-derived components in the training of the human immune system ( Yin et al., 2023 ). Prof. Shu Zhu summarizes how components of gut microbiome and microbiota-derived metabolites are sensed by the host immune system and their involvement in the maintenance of human gut homeostasis ( Wan et al., 2023 ). Gut microbiota plays an important role in gut–brain interactions, and two reviews discuss the underlying mechanisms, with Prof. Xingyin Liu focusing on the current research in neurodevelopmental disorders ( Wang et al., 2023 ) and Prof. Liping Duan emphasizing on the study of irritable bowel syndrome ( Zheng et al., 2023 ). Prof. Gong Cheng reviews the mechanisms of both the mosquito and host microbiomes against mosquito-borne diseases ( Shi et al., 2023 ). Prof. Jinfeng Wang reconceptualizes the concept of maternal and infant health from a microbiome perspective ( Gao and Wang, 2023 ). Prof. Jusheng Zheng provides extensive insights into the interplay between nutrition and microbiome for human health ( Gou et al., 2023 ).
Meanwhile, to effectively manage the expanding volume of microbiome data and complex statistical software, a systematic approach is crucial for sorting and interpreting the results. Prof. Yong-Xin Liu conducts a comparative analysis and organization of commonly used R packages for analyzing microbiome data, culminating in a selection of tools that are both capable and efficient, also readily accessible to microbiome researchers ( Wen et al., 2023 ).
Soon, we believe that there will be major advancements in microbiome research, owing to practical, conceptual, and technical improvements. The practical improvements in high-molecular-weight DNA extraction ( Maghini et al., 2021 ) and high-throughput culturomics techniques ( Zhang et al., 2021 ) will revolutionize the workflow of microbiome research, facilitating extensive study of the functions and mechanisms of the microbiome. With the ever-increasing number of studies, a focus on understanding the microbiome patterns in health and disease will continue to be a crucial area of investigation ( Wilmanski et al., 2021 ). The pan-microbiome concept will be a promising strategy for identifying the core microbiome and microbiome patterns, which will be helpful to explain and understand the observed heterogeneity among individual hosts. And greater attention should be put on studying the “dark matter” of the microbiota such as fungi, viruses, archaea, and protozoa in the coming years, as these microorganisms have been largely overlooked in the past but may have as critical roles as that of bacteria. Furthermore, the introduction of cutting-edge sequencing technologies, such as long-read sequencing, single-cell sequencing, and metatranscriptomic sequencing, will allow higher resolution at the level of species or strain ( Fig. 3A ). And the development of cloud platforms ( Chen et al., 2021 , 2022 ) and R packages ( Wen et al., 2022 ) will greatly facilitate data exploration in microbiome research in terms of analysis.
![microbiome research reports impact factor The framework of the current microbiome special issue. This framework covers seven important aspects of microbiome research, including best practices for analyzing microbiomes [inner circle, Wen et al. (2023)], the regulation of gut microbiomes on the human immune system [upper outer circle, Yin et al. (2023)], how gut microbiomes affect human immune sensing (lower outer circle, Wan et al. (2023)), the role of microbiomes in gut–brain interaction [upper left, Wang et al. (2023), Zheng et al. (2023)], how microbiomes can interfere with the spread of mosquito-borne diseases in both mosquitoes and humans (upper right, Shi et al. (2023)), the application of microbiomes in maternal and newborn health [lower left, Gao and Wang (2023)], and the study of nutri-microbiome epidemiology [lower right, Gou et al. (2023)]. The gut and brain of the figure are drawn with Figdraw.](https://oup.silverchair-cdn.com/oup/backfile/Content_public/Journal/proteincell/14/10/10.1093_procel_pwad031/2/m_pwad031_fig2.jpeg?Expires=1716313494&Signature=AfiweGxC83XcTVN725rMRSY2XB4DB9Xhppt2lVEDmWw6IveFnzA88v08DHrBSqqaSzB5fP6OsdkRKpI5WV02hESsyjLw8uwYUUVovLFCXn8W-fbKJ22PA8~EXR1ZckrV6eaWpcu-Eiegvht2kZgLP7iOYOyV2GqY02WVXQOLQIVKdNlNxmj-NFDzttbnAA7ACSn~zbwMGL~g-3At5sRJPaSyWICEmKHuiK6wXuzt2cbraJiimR57aYfgFSVKd4-1KJixxKJh677iAq-ST8yL7RuxFat4oyMpFGbyxFjfAqfVdqnO8ogrO-fmaFgXiY0HobOdXhA69vcoawsEZ2hXKA__&Key-Pair-Id=APKAIE5G5CRDK6RD3PGA)
The framework of the current microbiome special issue. This framework covers seven important aspects of microbiome research, including best practices for analyzing microbiomes [inner circle, Wen et al. (2023) ], the regulation of gut microbiomes on the human immune system [upper outer circle, Yin et al. (2023) ], how gut microbiomes affect human immune sensing (lower outer circle, Wan et al. (2023) ), the role of microbiomes in gut–brain interaction [upper left, Wang et al. (2023) , Zheng et al. (2023) ], how microbiomes can interfere with the spread of mosquito-borne diseases in both mosquitoes and humans (upper right, Shi et al. (2023) ), the application of microbiomes in maternal and newborn health [lower left, Gao and Wang (2023) ], and the study of nutri-microbiome epidemiology [lower right, Gou et al. (2023) ]. The gut and brain of the figure are drawn with Figdraw.

Future direction of microbiome research. (A) The flow chart presents an overview of the practical, conceptual, and technical advances in microbiome for studying its function and mechanisms. (B) The research data of microbiome is essential for hypothesis generation, evaluation, and validation.
Based on these developments, it is conceivable that more hypotheses regarding the relationships between microbiome and host will be generated, and in vitro and in vivo experiments should be conducted before validating them in humans ( Fig. 3B ). Ultimately, we may use the microbiome as a versatile tool for disease diagnosis, medicine development, and personalized interventions. Upon unlocking this toolkit, we are on the cusp of a revolution in microbiome research that will transform the field and improve human health in unprecedented ways.
We thank Dr. Wenqi Yin for revised the manuscript, and Mr. Kai Peng for plotting world map.
This study was financially supported by the Agricultural Science and Technology Innovation Program (CAAS-ZDRW202308), and the Natural Science Foundation of China (U21A20182).
The authors declare no competing interests related to the content of this paper.
All authors agree to publish.
Y.L. and D.L. conceived the paper. Y.G. wrote the paper. Y.L. and D.L. revised the paper.
All data and draw code are available in GitHub github.com/YunyunGao374/ProteinCell2023MicrobiomeReview.
No new sequencing data generated by this project.
Chen T , Zhang H , Liu Y et al. . EVenn: easy to create repeatable and editable Venn diagrams and Venn networks online . J Genet Genom 2021 ; 48 : 863 – 866 .
Google Scholar
Chen T , Liu Y-X , Huang L . ImageGP: an easy-to-use data visualization web server for scientific researchers . iMeta 2022 ; 1 : e5 .
Gao S , Wang J. Maternal and infant microbiome: next-generation indicators and targets for Perinatal Health Care . Protein Cell 2023 ; doi:10.1093/procel/pwad031.
Gao L , Xu T , Huang G et al. . Oral microbiomes: more and more importance in oral cavity and whole body . Protein Cell 2018 ; 9 : 488 – 500 .
Gou W , Miao Z , Deng K et al. . Nutri-microbiome epidemiology, an emerging field to disentangle the interplay between nutrition and microbiome for human health . Protein Cell 2023 ; doi:10.1093/procel/pwad023.
Li W , Wang L , Li X et al. . Sequence-based functional metagenomics reveals novel natural diversity of functioning CopA in environmental microbiomes . Genom Proteom Bioinform 2022 ; doi:10.1101/2022.02.12.480192.
Liu Y-X , Qin Y , Chen T et al. . A practical guide to amplicon and metagenomic analysis of microbiome data . Protein Cell 2020 ; 12 : 315 – 330 .
Liu Y-X , Chen T , Li D et al. . iMeta: integrated meta-omics for biology and environments . iMeta 2022 ; 1 : e15 .
Lynch SV , Ng SC , Shanahan F et al. . Translating the gut microbiome: ready for the clinic ? Nat Rev Gastroenterol Hepatol 2019 ; 16 : 656 – 661 .
Maghini DG , Moss EL , Vance SE et al. . Improved high-molecular-weight DNA extraction, nanopore sequencing and metagenomic assembly from the human gut microbiome . Nat Protoc 2021 ; 16 : 458 – 471 .
Shi H , Yu X , Cheng G. Impact of the microbiome on mosquito-borne diseases . Protein Cell 2023 ; doi:10.1093/procel/pwad021.
Wan T , Wang Y , He K et al. . Microbial sensing in the intestine . Protein Cell 2023 : doi:10.1093/procel/pwad028.
Wang Z , Zhao Y. Gut microbiota derived metabolites in cardiovascular health and disease . Protein Cell 2018 ; 9 : 416 – 431 .
Wang Q , Yang Q , Liu X. The microbiota–gut–brain axis and neurodevelopmental disorders . Protein Cell 2023 ; doi:10.1093/procel/pwad026.
Wen T , Xie P , Yang S et al. . ggClusterNet: an R package for microbiome network analysis and modularity-based multiple network layouts . iMeta 2022 ; 1 : e32 .
Wen T , Niu G , Chen T et al. . The best practice for microbiome analysis using R . Protein Cell 2023 ; doi:10.1093/procel/pwad024.
Wilmanski T , Diener C , Rappaport N et al. . Gut microbiome pattern reflects healthy ageing and predicts survival in humans . Nat Metab 2021 ; 3 : 274 – 286 .
Yin R , Wang T , Dai H et al. . Immunogenic molecules associated with gut bacterial cell walls: chemical structures, immune-modulating functions, and mechanisms . Protein Cell 2023 ; doi:10.1093/procel/pwad016.
Zhang J , Liu Y-X , Guo X et al. . High-throughput cultivation and identification of bacteria from the plant root microbiota . Nat Protoc 2021 ; 16 : 988 – 1012 .
Zheng H , Zhang C , Zhang J et al. . “Sentinel or accomplice”—gut microbiota and microglia cross-talk in disorders of gut-brain interaction . Protein Cell 2023 ; doi:10.1093/procel/pwad020.
Supplementary data
Email alerts, citing articles via.
- About Protein & Cell
- Advertising and Corporate Services
Affiliations
- Online ISSN 1674-8018
- Print ISSN 1674-800X
- Copyright © 2024 Higher Education Press, Biophysical Society of China
- About Oxford Academic
- Publish journals with us
- University press partners
- What we publish
- New features
- Open access
- Institutional account management
- Rights and permissions
- Get help with access
- Accessibility
- Advertising
- Media enquiries
- Oxford University Press
- Oxford Languages
- University of Oxford
Oxford University Press is a department of the University of Oxford. It furthers the University's objective of excellence in research, scholarship, and education by publishing worldwide
- Copyright © 2024 Oxford University Press
- Cookie settings
- Cookie policy
- Privacy policy
- Legal notice
This Feature Is Available To Subscribers Only
Sign In or Create an Account
This PDF is available to Subscribers Only
For full access to this pdf, sign in to an existing account, or purchase an annual subscription.
You are using an outdated browser. Please upgrade your browser or activate Google Chrome Frame to improve your experience.
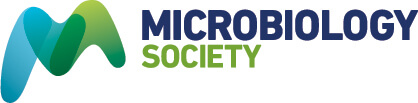
Journal metrics update 2021
21 September 2021
The Microbiology Society is pleased to provide an update on our journal and article metrics. Our latest updates include a suite of metrics at both the journal level, and the article level, and demonstrate the continued excellence of the content published in the journals.
We would like to thank our authors and Society members for continuing to spread the word about the journals and the value of publishing for the community. Publishing in our journals directly supports all of the other activities undertaken by the Microbiology Society, including our events, policy work and grants and prizes.
We saw general increases in the vast majority of the metrics we track regularly, and as signatories of the San Francisco Declaration on Research Assessment, we will always be providing them together with no particular emphasis given to any one metric.
Below you will find metrics highlighting journal-level performance. Definitions of each metric are provided at the end.
In addition to the journal-level statistics, we also collate a number of stats based on the performance of the articles published therein. While these give a snapshot of the general level of interest in each article, we will always recommend individual articles be evaluated on their own merits - on our article pages we provide widgets highlighting the citations and Altmetric attention generated, and readers can see how many others have read the article by clicking on the Info tab at the top.
- 2-year Journal Impact Factor : The Journal Impact Factor is defined as all citations to the journal in the current JCR year to items published in the previous two years, divided by the total number of scholarly items (these comprise articles, reviews, and proceedings papers) published in the journal in the previous two years.
- 5-year Impact Factor : The 5-year journal Impact Factor is the average number of times articles from the journal published in the past five years have been cited in the JCR year. It is calculated by dividing the number of citations in the JCR year by the total number of articles published in the five previous years.
- Immediacy Index : The Immediacy Index is the average number of times an article is cited in the year it is published.
- Cited half-life : The median age of the articles that were cited in the JCR year. Half of a journal's cited articles were published more recently than the cited half-life.
- CiteScore : CiteScore measures average citations received per document published in the serial.
- SNIP (Source Normalized Impact per Paper) : The SNIP measures actual citations received relative to citations expected for the serial’s subject field.
- Altmetrics : Altmetrics are non-traditional metrics proposed as an alternative to citation impact metrics. A paper’s Altmetric score is a weighted measure of its mentions in the press and on social media, among others, and is displayed on each article page.
Supporting authors with fee-free Open Access Publishing
The Society is expanding its Publish and Read programme to help more researchers benefit from Open Access (OA) publishing. Publish and Read is designed to offer a frictionless OA experience for authors, maximum value for institutions, with minimum administration.
Image: Dennis Kunkel .

An official website of the United States government
The .gov means it’s official. Federal government websites often end in .gov or .mil. Before sharing sensitive information, make sure you’re on a federal government site.
The site is secure. The https:// ensures that you are connecting to the official website and that any information you provide is encrypted and transmitted securely.
- Publications
- Account settings
Preview improvements coming to the PMC website in October 2024. Learn More or Try it out now .
- Advanced Search
- Journal List
- ACS AuthorChoice

Microbiome and Human Health: Current Understanding, Engineering, and Enabling Technologies
Nikhil aggarwal.
† NUS Synthetic Biology for Clinical and Technological Innovation (SynCTI), National University of Singapore, Singapore 117456, Singapore
‡ Synthetic Biology Translational Research Programme, Yong Loo Lin School of Medicine, National University of Singapore, Singapore 117456, Singapore
Shohei Kitano
Ginette ru ying puah.
§ Wilmar-NUS (WIL@NUS) Corporate Laboratory, National University of Singapore, Singapore 117599, Singapore
∥ Wilmar International Limited, Singapore 138568, Singapore
Sandra Kittelmann
In young hwang.
⊥ Department of Biochemistry, Yong Loo Lin School of Medicine, National University of Singapore, Singapore 117596, Singapore
# Singapore Institute of Technology, Singapore 138683, Singapore
Matthew Wook Chang

The human microbiome is composed of a collection of dynamic microbial communities that inhabit various anatomical locations in the body. Accordingly, the coevolution of the microbiome with the host has resulted in these communities playing a profound role in promoting human health. Consequently, perturbations in the human microbiome can cause or exacerbate several diseases. In this Review, we present our current understanding of the relationship between human health and disease development, focusing on the microbiomes found across the digestive, respiratory, urinary, and reproductive systems as well as the skin. We further discuss various strategies by which the composition and function of the human microbiome can be modulated to exert a therapeutic effect on the host. Finally, we examine technologies such as multiomics approaches and cellular reprogramming of microbes that can enable significant advancements in microbiome research and engineering.
1. Introduction
With advances that have enabled the sequencing of the whole genomes of organisms, we have since acquired an exponential amount of genome sequencing information from microbes. Over 130 000 complete or near-complete bacterial genomes have been sequenced. Meanwhile, there are more than 20 000 metagenomic projects publicly available, and many terabytes of sequencing data have been produced. This spectacular expansion of information regarding the genomic architecture of microbes has laid the foundation for truly revolutionary advances in our knowledge of microbial systems. We are now able to understand the interacting networks of biological molecules—including genes and proteins—at the systems level, and on the basis of this understanding, we can effectively engineer complex biological systems that perform desired functions. This technological advancement, along with the development of other key enabling techniques like gene synthesis, has contributed to the birth of the new interdisciplinary research field named synthetic biology.
However, microbes in the natural world are rarely found on their own; they invariably form a microbial community with each occupying a given niche. In addition, their habitats cover a wide range of abiotic and biotic environments. Through a long evolutionary cohabitation with the human body, this community of microbes, termed the microbiome, has established a profound role in its host’s physiological functions such as metabolism, immune development, and behavioral responses ( section 2 ). Due to the intricate relationship between microbial communities and the living host, unsurprisingly a disruption in one often results in the disruption of the other. That is, a disturbed microbiome—known as dysbiosis—can be observed in an array of the host’s disease states, ranging from metabolic to immune and mood disorders. There has been a dramatic increase in human microbiome research and its association with different diseases in recent years. As the importance of the relationship between human-associated microbial communities and disease development becomes evident, there is a growing interest in engineering microbiomes to reshape and reprogram the composition and function of the gut microbiome as a novel therapeutic modality.
In general, modulating the function of the microbiome, or performing “microbiome engineering”, can be achieved by altering the gut microbial composition or its metabolomic function ( section 3 ). Such alterations are reported to be largely mediated by providing a specific microbe (or consortia of microorganisms), prebiotics, or bioactive metabolites to elicit a change in the composition and functions of the microbiome to correct the disrupted metabolic function. In addition, engineered probiotics or synthetic consortia of microbes can be used to provide a more rational and precise therapeutic intervention. Since the early days of engineering probiotics for such interventions, various genetic tools have been identified and developed for the more precise and complex execution of therapeutic activities ( section 4 ).
The goal of this Review is to provide a comprehensive understanding of advances in the microbiome–host relationship for human health. Additionally, this Review aims to provide a nonexhaustive list of studies covering the manipulation of the human microbiome to prevent or treat human disease, with a special focus on multiomics approaches and the cellular reprogramming of microbes to enable in-depth microbiome research and robust microbiome engineering.
2. Human Microbiome
Microbiome research has advanced rapidly over the past few decades and has now become a topic of great scientific and public interest. Historically, the field of microbiome research emerged from environmental microbiome research and later evolved into viewing eukaryotes as inseparable from the microbial community with which they share space. After all, the human body is an ecosystem where trillions of tiny organisms coexist with the host. The scientific term “microbiome” therefore refers to the set of genes of all microorganisms that inhabit almost all human body parts. The microbiome is thus considered as a second genome that has a symbiotic relationship with the host. This relationship may be positive or beneficial, negative or pathogenic, or neutral; hence, microbiome interactions play a key role in human health. The complex and diversified microbiome operates as a functional expansion of host genomes with an estimate of 50- to 100-fold more genes. 1 These extra genes contribute to the regulation of host physiology by possessing various types of enzymatic proteins, influencing the produced metabolites and thus affecting host metabolism. 1
Over the years, instead of looking into the relationship between one specific microorganism with its host, a holistic approach based on the holobiont theory has been applied. 2 , 3 The beneficial interplay of the host and its microbiome is responsible for maintaining the host’s health, whereas disease development is often correlated with microbial dysbiosis, or a shift in the microbiota. As such, pathogens therefore represent only a tiny fraction of microorganisms, whereby the altered composition of the microbiome promotes the emergence and outbreak of pathogens. 2 , 3 The vast majority of microbes are crucial for ecosystem functioning as well as beneficial interactions with other microbes, contributing to population dynamics and functional activities. Thus, opportunistic pathogens show that host–microbe interactions depend not only on the host but also on the entire microbiome.
The microbiota comprises all living members that form the microbiome, which encompasses bacteria, archaea, fungi, algae, and small protists. The members of microbiome also extend to viruses, phages, and mobile genetic elements—one of the most controversial inclusions in the definition of a microbiome. 4 However, the microbiome has since been further defined to pertain to not only the community of microorganisms but also the whole spectrum of molecules produced by microorganisms, including their structural elements, metabolites, and molecules produced by the coexisting host.
Generally, microbial composition varies among different anatomical parts, and it is highly personalized as the microbiome’s composition also varies among individuals. The exact definition of a healthy microbiota has yet to be defined, but studies have shown that the use of probiotics, prebiotics, and synbiotics are beneficial by maintaining healthy body flora or by altering the microbiome toward a healthy microbial ecosystem.
Therefore, defining the core microbiota is crucial as it facilitates the discrimination of an intermittent or temporal microbiome that is affected by specific environmental conditions. 4 The core microbiota is the microbial community that is constantly associated with a given host genotype or a specific environment, whereas transient microbiota changes over time. By identifying these differences, an appropriate experimental, methodological, and statistical design can be applied to refine the approach taken in microbiome studies for therapeutic applications.
2.1. Factors Influencing the Human Microbiome
Microorganisms reside in their preferred environment depending on their optimal growth conditions. They can be found on the human body’s external and internal parts as well as entrance sites. The external sites that house microorganisms include the skin, eyes, and even the exposed sites under the nails. The portals of entry for microorganisms are the respiratory tract (mouth and nose), gastrointestinal tract (oral cavity), urogenital tract, and breaks in the skin surface. Meanwhile, the internal parts of the body that are occupied by microbes include the lungs, gut, bladder, kidneys, and vagina.
Microbes tend to thrive in an environment that is suitable for them. Hence, these microorganisms are predicted to have mechanisms for adapting to conditions in the human microbiome that resemble their preferred natural environment. Environmental factors such as temperature, pH, oxygen concentration, pressure, osmolarity, and nutrient source contribute to the diversity and abundance of microorganisms at different sites of the body. For instance, our body temperature is optimal for housing many different types of microbes. Other factors, such as the presence of nutrient sources like sebum, change the skin’s pH and also act as a carbon source, facilitating the growth of certain groups of microbes. 5 Interestingly, the dense layer of mucus that covers the intestinal epithelium not only serves as a carbon source for microbes but also provides attachment sites for bacterial adhesion. 6
The abundance and diversity of the human microbiota is dependent on intrinsic and extrinsic factors. Intrinsic factors include the nature of body environments, as previously described, as the physiology of habitat sites facilitates the growth of some microbes. Other intrinsic factors that contribute to the microbiome’s composition include genetics, ethnicity, gender, and age. The human microbiome is generally stable and resistant once the microorganism has adapted to the environment. On top of intrinsic factors that may cause a shift in the microbiome over time, extrinsic factors such as diet, lifestyle, medication, geographic location, climate, and seasonality may cause changes to the microbial community. Moreover, the mode of delivery during birth has been shown to influence the microbiome. For example, newborns delivered via the vaginal versus Caesarean delivery possess different groups of dominating gut microbiome. However, at the age of 3, the gut microbiome changes to resemble that of the adult’s gut microbiome. 7 As people reach beyond the age of 70, the ability to digest food and absorb nutrients in the gut changes, affecting the composition of the gut microbiome. With decreasing immune activity in older adults, this also contributes to changes in the overall microbiome as they are more susceptible to pathogens—thereby influencing the core microbiome. As Bifidobacterium spp. stimulates the immune system and metabolic processes, a decrease in Bifidobacteria may result in malnutrition and low systemic inflammatory status in older adults. 8 Altogether, the human microbiome thrives in optimal growth conditions, depending on the natural environment of the body. When the natural environment of the body is altered, this results in microbial composition and diversity shifting to adapt to the changing environment, potentially resulting in disease.
2.2. Microbiota in Different Body Parts and Its Relationship with Health/Disease
2.2.1. digestive system, 2.2.1.1. oral.
The human oral cavity harbors one of the most versatile microbiomes, including bacteria, fungi, viruses, and protozoa, among others. There are two regions in the oral cavity colonized by microorganisms—dentures, or the hard surfaces of the teeth, and the soft tissue of the oral mucosa. The main bacterial genera of oral cavities include Streptococcus , Granulicatella , Gemella , Actinomyces , Corynebacterium , Rothia , Veillonella , Fusobacterium , Prevotella , Porphyromonas , Capnocytophaga , Neisseria , Haemophilus , Treponema , Eikenella , Leptotrichia , Lactobacillus , Peptostreptococcus , Staphylococcus , Eubacterium , and Propionibacterium . 9 Meanwhile, predominant fungal genera include Candida , Cladosporium , Saccharomyces , Fusarium , Aspergillus , and Cryptococcus . 10 Disease-related viruses such as mumps, rabies, and human papillomaviruses 11 are also found in the mouth, as well as protozoa such as Trichomonas tenax and Entamoeba gingivalis . 12
The oral cavity is the principal entry point to the human body, and thus, microbes residing in this area can potentially spread to different body sites and cause disease. The composition of the oral microbiome therefore plays a vital role in providing immunity for human health. For instance, nitrate metabolism by the microbiome reduces nitrate to nitrite. Nitrite is then converted to nitric oxide, which has an antimicrobial effect and is crucial for vascular health. 10 Some oral microorganisms such as Streptococcus salivarius strain K12 contribute to host defense by creating an unfavorable environment that prevents the colonization of pathogenic bacteria. It produces a bacteriocin that restrains the growth of Gram-negative species associated with periodontitis disease. 13
The most prevalent oral disease is dental caries, commonly known as tooth decay. The bacteria involved in dental caries are Streptococcus mutans , Streptococcus sobrinus , and Lactobacillus acidophilus . Other species such as Veillonella , Bifidobacterium , Propionibacterium , Actinomyces , Atopobium , and Scardovia have also been found to be associated with dental caries. 14 , 15 Dental caries manifest when acid-producing bacteria residing in the oral cavity interact with the fermentable carbohydrate found in food. When the supragingival biofilm matures, it creates a low pH environment, demineralizing the tooth and eventually leading to cavitation. 16 − 18 Without adequate oral hygiene, certain microorganisms produce pathogenic characteristics, causing gingivitis. When this condition persists through chronic bacterial infections, the subgingival plaque accumulation rearranges the microflora from a healthy to diseased state, affecting the gingiva and causing damage to the supporting connective tissue and the bone that fixes the teeth to the jaws. 17 , 19 , 20
The oral microbiome has been recognized as a vital player in systemic health, with the disruption of the oral microbiome potentially contributing to several chronic diseases such as endocarditis, osteoporosis, and rheumatoid arthritis. 21 − 23 Oral health has also been found to play a role in the development and progression of noncommunicable diseases (NCDs) such as obesity, diabetes, cancers, 24 − 26 and neuropsychiatric disorders (NPDs). 27 − 30 Thus, it has been proposed that the oral microbiome could potentially be used to assess the risk for certain diseases. Similar to the widely studied gut microbiome, oral microbiome research is shifting to a holistic, systems-level understanding of its functions and interactions with the human body. 31 − 33 Future studies will likely shed light on how the oral microbiome can be restored to a healthy state.
2.2.1.2. Gastric
The stomach was previously believed to be a sterile organ due to its inhospitality to bacteria. Such factors include its acidic environment, reflux of bile acids, thickness of the mucus layer, and conversion of food to nitrite by Lactobacilli present in the oral cavity, which then transforms into the antimicrobial nitric oxide. However, the lack of simple and reliable diagnostic tests has hampered the study of the gastric microbiome. 34 , 35 With the discovery of Helicobacter pylori by Barry Marshall and Robin Warren in 1982, this notion has since been refuted. The most highly represented phyla in the gastric mucosa under normal conditions are Proteobacteria, Firmicutes (recently renamed to Bacillota 36 ), Bacteroidetes (recently renamed to Bacteroidota 36 ), Actinobacteria, and Fusobacteria. 37 − 39 The gastric juice has a diverse microbial community that differs from the gastric mucosa. The dominating phyla in gastric juice are Firmicutes, Actinobacteria, and Bacteroidetes, whereas Proteobacteria and Firmicutes are dominant in the gastric mucosa. 37 , 40 , 41 Furthermore, bacteria found in the oral cavity and duodenum such as Veillonella , Lactobacillus , and Clostridium can transiently colonize the stomach. 40 , 42
Unsurprisingly, H. pylori is the predominant bacterium in the stomach of H. pylori -infected patients, 43 and most H. pylori strains can modulate the gastric environment, thus altering the habitat of resident microorganisms. 44 Furthermore, alterations in the gastric microbiome community can increase the risk for developing gastric cancer. 39 It was also reported that eradicating H. pylori increased microbial diversity in the stomach. 45 Even though interactions between H. pylori and commensal bacteria in the stomach are not fully understood, the discovery of its direct effect on the healthy gastric microbiome may shed some light on ways to modulate the gastric microbiome to prevent progression to severe disease.
2.2.1.3. Intestines
The gut is the most densely and diversely colonized organ, with a bacterial-to-host cell ratio of 1:1. A vast majority of commensal bacteria reside in the colon, whereas a lower bacterial population is found in the stomach and small intestine. The main bacterial phyla present in the gut are Firmicutes and Bacteroides, which make up 90% of the gut microbiota. 46 Other phyla that exist in the gut environment are Actinobacteria, Proteobacteria, Fusobacteria, and Verrucomicrobia. 46 Notably, there are 200 different genera found under the Firmicutes phylum, with some examples including Bacillus , Lactobacillus , Enterococcus , Clostridium , and Ruminococcus . Although lactobacilli are beneficial to health, some Firmicutes species such as Staphylococcus aureus and Clostridium perfringens are harmful to the body when overgrown. Meanwhile, the predominant genera in Bacteroidota are Bacteroides and Prevotella . The less abundant Actinobacteria phylum is largely represented by Bifidobacterium , and this genus is known to have a positive impact on health. Under the Proteobacteria phylum, some well-known pathogens include Enterobacter , Helicobacter , Shigella , Salmonella , and Escherichia coli .
The composition of the gut microbiota changes at three stages in life: from birth to weaning; from weaning to obtaining a normal diet; and finally, during old age. Facultative anaerobes are the first to colonize the gut at birth, and these bacteria create anaerobic conditions that promote the growth of obligate anaerobes, starting with Bifidobacterium and Bacteroides spp., within 2 weeks. 47 Infants born naturally are inoculated by the mother’s vaginal and fecal microbiota during birth, whereas those born by Caesarean section are initially exposed to the skin microbiota as well as the microbiome found in the environment. 48 At 3 days, infants who were naturally delivered possessed a greater abundance and variety of Bifidobacterium spp. than the Caesarean-born babies. 49 , 50 Moreover, babies who were solely breastfed until weaning were observed to generally have a more stable and less diverse bacterial community, with higher proportions of bifidobacteria than babies fed by formula milk. 51 − 54 After being introduced to solid food, their gut microbiome diversified and the abundance of Firmicutes increased. 54 − 56 The microbiomes of breastfed and formula-fed babies become indistinguishable by around 18 months of age. By the age of 3, their microbiomes resemble that of an adult. 7 , 54 At old age, there is reportedly a decline in microbiota diversity, with reduced numbers of Bifidobacteria and an increase in Enterobacteriaceae. 57 , 58 Likewise, the abundance of Bacteroidetes increases, whereas the Firmicutes becomes less abundant in elderly adults (>65 years). 59
Apart from age, gut microbiome composition is also greatly influenced by the environment in different anatomical locations. The large intestine has slow flow rates, and the pH level ranges from mildly acidic to neutral. Thus far, it comprises the largest microbial community dominated by obligate anaerobes. The large intestine comprises several microenvironments wherein microorganisms reside. The epithelial surface and inner mucin layer harbors minimal colonization during the healthy state, whereas the diffuse mucin layer has specialist colonizers such as Akkermansia muciniphila . The liquid phase of the gut lumen comprises a diversity of microorganisms and specialized primary colonizers like Ruminococcus spp. depending upon the dietary fibers found in the gut lumen. 60 Given that the small intestine has a fairly short transit time of ∼3–5 h in digestion, the presence of high bile concentrations that possess antimicrobial activity 61 makes the small intestine a challenging environment for microbial colonizers. 61 Molecular analysis has revealed that the jejunal and ileal components comprise mainly facultative anaerobes, including the bacterial phyla Proteobacteria and Bacteroides and the Streptococci , Lactobacilli , and Enterococci species. 62 , 63
Gut bacteria are crucial for regulating digestion along the gastrointestinal tract. The commensal bacteria play a key role in processing nutrients and metabolites such as short-chain fatty acids (SCFAs), bile acids, amino acids, etc. 64 By doing so, some of these bacteria facilitate host energy harvesting and metabolic efficiency. 65 Some of these members also play an important immune function against pathogenic bacteria and prevent bacterial invasion by maintaining intestinal epithelium integrity. 66 Although the composition of the microbiome species performs a key role in metabolism, the community’s metabolic output is also dependent on the availability of substrates to the microbiota 67 , 68 or when extrinsic factors such as diet influence the gut microbiome. Microbe-synthesized metabolites potentially mediate crosstalk between the metabolic, immune, and neuroendocrine systems, thus governing host wellness. 69
In addition to regulating digestion, dominant, nonpathogenic gut microorganisms occupy a specific niche, suppressing pathogenic colonization and growth. However, when the balance of the gut microbiome is perturbed, gut permeability increases. This change in permeability allows opportunistic pathogens to invade and colonize empty niches, changing the gut environment. This may lead to the production of dysregulated metabolites that are potentially harmful to the host, causing a range of diseases. Increased gut permeability also permits the entrance of microbe-derived products such as metabolites, virulence factors, and other luminal components, disrupting the gut microbiome’s normal function and contributing to aberrant immune-inflammatory responses such as inflammation, allergy, and autoimmune disorders mediated by molecular mimicry and a dysregulated T cell response. 70
Sometimes the source of the opportunistic pathogens comes from the resident site of the microbiome, and this occurs when the healthy nondisease state of the gut microbiome is disturbed, causing the failure of colonization resistance against the pathogenic member. An example is Clostridium difficile , which exists in the normal gut microbiota but becomes pathogenic when the healthy nondisease microbiome state is disrupted. C. difficile may damage the cytoskeleton and colonic epithelial barrier integrity, inducing aberrant inflammatory response and cell death. 71 C. difficile infection (CDI)-associated symptoms include diarrhea, pseudomembranous colitis, sepsis, and death. 71 It is proposed that the dominant gut microbiota in the healthy nondisease state confers protection to the host by preventing the overgrowth of C. difficile as it is often related to antibiotic-associated diarrhea compared to other pathogens such as Salmonella species. 72 , 73
Another commonly studied gut microbiome-associated disease is inflammatory bowel disease (IBD). It is a group idiopathic, chronic, and relapsing gastrointestinal inflammation with two common forms: ulcerative colitis (UC) and Crohn’s disease (CD). 74 Inflammation occurs at any location along the entire GI tract in CD. Meanwhile in UC, inflammation is restricted to the large intestine. Both conditions are associated with recurring fever, diarrhea, and abdominal pain. It was suggested that dysbiosis in the gut potentially contributes to IBD pathogenesis. 75 An example is a reduction in the abundance of Firmicutes such as Faecalibacterium prausnitzii and Roseburia spp. 76 − 78 These are butyrate-producing bacteria, with butyrate being the primary energy substrate for colonocytes. Thus, a decrease in Firmicutes could heighten local inflammation by decreasing anti-inflammatory cytokines. 78 , 79 As such, F. prausnitzii has been explored as a probiotic for therapeutic use. 76
Aside from IBD, other intestinal disorders associated with the dysbiosis of the gut microbiota include irritable bowel syndrome (IBS), celiac disease, and colorectal cancer (CRC). A study of fecal samples from IBS patients exhibited a significant reduction in the concentration of Lactobacillus species as compared to healthy controls. 80 Other studies have revealed that there is an increase in the ratio of Firmicutes to Bacteroidetes in IBS patients as compared to healthy individuals. 81 , 82 There was also a decrease in some Firmicutes families such as Lactobacilli and Faecalibacterium , as well as Bifidobacteria and Collinsella under the Actinobacteria population. In IBS patients, there was an increase in abundance in some Firmicutes families ( Veillonella , Streptococci , and Ruminococcus spp.) and in Proteobacteria ( Enterobacteriaceae spp.). These findings reveal that there is a loss of microbes associated with epithelial barrier function in IBS patients. 81 , 82 Although many diseases are hypothesized to have an association or correlation with the microbiome, some studies have also suggested the causation factors of the disease based on microbial activities. With this information, therapeutics advances can be developed.
Other microorganisms that live in the gut are viruses and bacteriophages that make up the vast majority of gut microbiota’s viral components. Dominant archaeal species such as Methanosphaera stadtmanae and Methanobrevibacter smithii are also found in the gut microbiome. 83 Longitudinal studies of the gut have shown that specific species of an individual’s microbiota are very stable and persist for a year or more. 84 , 85 The specific communities of human gut microbiomes are influenced by interindividual and intraindividual variation throughout the life cycle. Examples of some factors that affect variations in the microbiome include the intestine’s anatomical regions, mode of delivery, method of milk feeding, weaning period, age, diet, and antibiotic treatments. The gut environment varies between different anatomical regions in terms of physiology, digesta flow rates, substrate availability, host secretions, pH, and oxygen tension.
2.2.2. Respiratory System (Nasal, Airway, and Lungs)
2.2.2.1. nasal.
The nasal cavity is an essential interface to the external environment. During inhalation, the airways are exposed to the environment, which comprises microorganisms, pollutants, aeroallergens, and more. A wide variety of potential pathogenic and harmless bacteria reside in the nose, and this diversity may be attributed to localized factors such as temperature and humidity. The position in the respiratory tract may also contribute to the diversity of the nasal microbiome. For instance, the anterior nares have decreased levels of microbiome biodiversity in comparison to the middle meatus and sphenoethmoidal recesses. The anterior nares are lined with keratinized squamous epithelium and sebaceous glands that produce sebum and may impact bacterial diversity. 86 However, a recent study did not detect any significant differences in bacterial diversity among the middle meatus, inferior turbinate, and anterior nares from healthy individuals, 87 and thus, further studies may be required to obtain comparable information.
The microbiome of the anterior nares in healthy adults has been observed to be dominated by three phyla: Actinobacteria, Firmicutes, and Proteobacteria. 88 The anterior nares are further classified into four distinct genus profiles comprising Staphylococcus , Propionibacterium , Corynebacterium , or Moraxella . 89 The middle meatus possesses a high abundance of Staphylococcus aureus , Staphylococcus epidermidis , and Propionibacterium acnes . 90 The nasal microbiome in the unhealthy disease state has not been well-characterized, making further research necessary. Thus far, Staphylococcus aureus has been identified as one bacterial species that potentially functions in the development of the nasal disease chronic rhinosinusitis (CRS). Colonization of the nasal cavity and sinus with S. aureus may be associated with the presence of nasal polyps or disease severity in CRS. 91 An increased abundance of S. aureus has been observed in CRS participants with nasal polyps, compared to participants without the polyps. 90 With this preliminary information in hand, further studies on the clinical relevance of the nasal microbiome in CRS and the functional role of S. aureus in CRS development should be explored in future research.
2.2.2.2. Pharynx, Larynx, and Trachea
The respiratory tract has long been thought to be sterile, largely due to the difficulty of culturing bacteria from the tract. However, microbes from the environment may first enter the upper tract (pharynx and larynx) followed by the lower tract (trachea) through the oral or nasal routes. As such, the upper respiratory tract has a greater abundance of bacteria compared to the lower region. 92 , 93 Given the current ease of sample collection, future respiratory tract microbiome studies may be explored further to obtain a consistent microbiome among healthy individuals. Nevertheless, studies have shown that healthy individuals have a lower abundance of Proteobacteria as compared to patients with mild asthma. 94 It was also reported that asymptomatic neonates whose throats are colonized with Streptococcus pneumoniae , Haemophilus influenzae , or Moraxella catarrhalis are at an increased risk for recurrent wheezing and asthma early in life. 95 These bacteria have consistently been associated with exacerbations of both asthma 96 and chronic obstructive pulmonary disease (COPD). 97 So far, there are still limited studies on the respiratory tract microbiome, and further research is required.
2.2.2.3. Lungs
In many textbooks, it is commonly held that the lungs are normally sterile. However, during respiration, the lungs are continuously exposed to a wide range of environmental microbes. In the past, incompatible culture conditions have led to the absence of bacteria in respiratory specimens, supporting the misinterpretation that healthy lungs are free of bacteria. 98 The invasive procedures involved in obtaining clinical samples also contributed to the delay in the systemic investigation of the lung microbiome. 98 While the most commonly used approach to study bacterial communities is via high-throughput sequencing of amplicons of the 16S rRNA gene, this technique presents technical challenges when bacteria with a low biomass are unable to mask any potential contaminants. 98 Healthy lungs contain a highly diverse interkingdom community of bacteria including Prevotella , Veillonella , Streptococcus , Haemophilus , Neisseria , and Corynebacteria . 99 − 101 In addition to these, many viruses such as Adenovirus , Rhinovirus , influenza, Epstein - Barr, and measles, among others, as well as fungi species ( Aspergillus spp., Candida albicans , Candida immitis , Candida neoformans , etc.) are also associated with the respiratory tract. 98
In every lung disease, the composition of the lung microbiome is altered compared to healthy controls. It is unknown if an altered lung microbiome drives the progression of lung disease or if it is a secondary consequence of the altered growth environment of the lungs. In some disease states, an increased airway wall permeability and mucus production introduces nutrient supply to the normally sparse lung environment. The mucus introduces pockets of increased temperature and decreased oxygen tension, selectively favoring the growth of disease-associated microbes. 102 , 103 In the event of enhanced immunogenicity, the airways and alveoli are exposed to pathogen-associated molecular patterns and microbial metabolites that provoke further inflammation, which in turn further alters airway conditions. 104 The generation of intraalveolar catecholamines and inflammatory cytokines promotes the growth of select bacterial species such as P. aeruginosa , S. pneumoniae , Staphylococcus aureus , and Burkholderia cepacia complex, whereas the recruitment and activation of inflammatory cells kills and clears bacteria with variable, species-specific effectiveness. 105 − 108
It has been proposed that respiratory exacerbations are acute events of respiratory dysbiosis—that is, the disorder and dysregulation of the respiratory ecosystem—accompanied by a dysregulated host immune response, eliciting negative effects on the host. 98 This is supported by a study that found that bacterial communities in the patients’ airways shift away from Bacteroidetes—the most abundant phylum in healthy subjects—toward Proteobacteria and other disease-associated bacteria at the time of exacerbation. 109 Exacerbations are activated by an inflammatory state that initiates a cascade of inflammatory responses that escalates the dysbiosis–inflammation cycle, and homeostasis is only restored after the disconnection of the positive feedback loop. 98
2.2.3. Skin
The skin is the largest and most exposed organ in the human body. Despite having plenty of transient interactions with the environment, the composition of the skin microbiota remains surprisingly stable. The diversity and relative abundance of the skin’s microbiome varies among individuals and the physiology of the skin sites. Generally, the microbial community has been categorized into three broad groups: oily, moist, and dry. 110 In some cases, “feet” is separately categorized from the three broad groups because it has a distinct microbial signature and is in regular contact with the ground, constituting unstable microflora. 111
These characteristics create many possibilities for the skin to house numerous commensal bacteria, fungi, viruses, archaea, and mites. 110 They exist in different compositions and densities at various skin sites, and altogether these microorganisms are defined as the skin microbiome. The composition and abundance of microorganisms are dependent on the physiology of the skin site. For healthy adults, sebum-rich sites were dominated by lipophilic Cutibacterium (formerly Propionibacterium ) species, whereas bacteria such as Staphylococcus and Corynebacterium species thrive in humid and moist areas such as the armpit, bends of the elbow, and feet. 112 − 115 In contrast to bacteria, the fungal community was not affected by the physiology of the skin. As such, the predominant fungi at the core body and arm sites are the genus Malassezia , while the feet’s skin is colonized by a diverse community of Malassezia spp., Aspergillus spp., Cryptococcus spp., Rhodotorula spp., Epicoccum spp., and others. 115 , 116 Across skin sites, bacteria were more abundant compared to fungi; however, as there are less fungal reference genomes compared to bacteria, this may partly contribute to the difference in the abundance. 110 Unlike bacteria and fungi, the colonization of eukaryotic viruses is not dependent on the anatomical site. 117 Currently, studies on the interaction of the skin virome with the host and bacteriophages is limited and will benefit from future research. For instance, a study has revealed that a eukaryotic virus may cause a rare but aggressive form of skin cancer. 118 In contrast, bacterial and fungal communities found at sebum-rich areas were found to be the most stable, whereas those at the foot sites were the least. 117 , 119 This instability may be due to the transient presence of fungi in the environment. 110 Eukaryotic DNA viruses, on the other hand, varied the most over time. 117 , 119
The skin has comparatively less nutrients compared to the nutrient-rich environment of the intestines, with its available resources comprising sweat, sebum, and the stratum corneum. 120 As such, this promotes Propionibacterium acnes to thrive in the anoxic sebaceous gland. 121 This facultative anaerobe also utilizes proteases to obtain amino acids from skin proteins, 121 as well as lipases to degrade triglycerides that retrieve free fatty acids, facilitating bacterial adherence. 122 − 125 In facial samples, the abundance of Propionibacterium spp. positively correlates with the cheek’s sebum levels. 126 Interestingly, auxotrophic species such as Malassezia and Corynebacterium employ the lipids found in sebum and from the stratum corneum as they are unable to produce their own lipids for certain functional roles. 120 Thus, this may be one reason for the dominance of the Malassezia species in the adult skin mycobiome. 110 Likewise, Staphylococcus spp. harbors strategies for surviving on the skin, including halotolerance and utilizing urea found in sweat as a nitrogen source. 120 Staphylococcus spp. also produces proteases that retrieve nutrients from the stratum corneum and adherens that facilitate skin adhesion. 120
Similar to the association of age with the gut microbiome, the skin microbiome is also significantly affected by age. During puberty, the increased level of hormones stimulates the sebaceous glands to produce additional sebum. This results in the skin of postpubescent individuals favoring the growth of lipophilic microorganisms such as Propionibacterium spp., Corynebacterium spp., 127 and fungal Malassezia spp. 128 , 129 On the other hand, prepubescent children have a higher abundance of Firmicutes ( Streptococcaceae spp.), Bacteroidetes, and Proteobacteria (betaproteobacteria and gammaproteobacteria) as well as a more diverse fungal community. 127 , 128 This reflects the association between one’s age and the skin microbiome and, hence, relates to the tendency to develop certain diseases at different ages. For instance, in prepubescent children, cases of atopic dermatitis related to Staphylococcus dropped, whereas Malassezia -related tinea versicolor is more prominent in adults as compared to children. 130 − 132
To prevent colonization by pathogens, the skin’s resident microbial members interact with each other. However, in some conditions, bacteria that were originally beneficial may exhibit pathogenicity associated with changes in the microbiota, otherwise known as dysbiosis. For example, the bacterium P. acnes , the most abundant microorganism present in the skin of healthy adults, is associated with the acne vulgaris commonly seen among teenagers. 133 , 134 Even though P. acnes is present in almost all adults, only a minority have acne issues, indicating that the gene expression profile varies at the functional level and that skin physiology—such as the level of sebum production and its secretion rate—correlates with the severity of clinical symptoms. 135 , 136 In addition, it was reported that the presence of P. acnes in the follicles and its formation of biofilms are associated with acne development. 137
S. aureus is commonly cultured from the skin of individuals with atopic dermatitis (AD), 138 also known as eczema. There are factors supporting the hypothesis that the skin microbiome has an influential role in disease pathogenesis. In the event of AD flares, it was demonstrated that there is a decline in microbiome diversity and a dramatic increase in the abundance of S. aureus compared to the healthy or postflare state. 139 − 141 Additionally, the relative abundance of staphylococci advanced closely with the severity of the AD flare. Even though the correlation of S. aureus with AD during active disease exacerbation is known, the functional role of staphylococci in driving disease states is still poorly understood. Furthermore, it is also unknown if S. aureus contributes to disease initiation due to dysbiosis or if the changes in the microbial community are a consequence of the disease state.
2.2.4. Urinary System
The urinary bladder was traditionally considered sterile as any bacteria found in the bladder was assumed to be pathogenic. However, with the discovery of the existence of nonpathogenic microbes in the human body, this notion has been abolished. 142 Due to advances in sampling and DNA sequencing techniques, commensal microbes have been identified in the urinary tract. 143 However, research on the urinary microbiome, or the urobiome, remains limited and understudied. In general, the abundance and diversity of the microbiome in the urine is lower compared to the gut by ∼10 6 –10 7 times. 144 The detection of the urobiome remains limited by the sampling method used. For example, some bladder mucosa-associated bacteria are undetectable in urine samples, and invasive methods are necessary for detection. The urobiome is similar for both genders, and the majority of the bacteria found belong to the phylum Firmicutes. Other phyla found in the urobiome are Actinobacteria, Bacteroidetes, and Proteobacteria. 145 Common genera for both genders are Escherichia , Enterococcus , Prevotella , Streptococcus , and Citrobacter . 146 Pseudomonas was detected only in males, whereas Corynebacterium and Streptococcus were more abundant in males compared to females. 146 , 147 On the other hand, the abundance of Lactobacillus was found to be higher in females compared to males. 146 , 147 Even though Lactobacillus is generally known as a probiotic, some species are associated with certain pathologies. For instance, Lactobacillus gasseri is associated with urgency urinary incontinence (UUI). 144 Moreover, a decrease in the abundance of Lactobacillus facilitates the colonization of disease-causing uropathogens. 147 Gardnerella is second to Lactobacillus in terms of abundance among the urobiome in females. The most abundant species is Gardnerella vaginalis , with some pathogenic strains, causing urinary tract infections (UTIs) in women, which is comparatively less frequent in men. 144 , 148 In general, the dominant genera found in the female urinary microbiome are Atopobium , Citrobacter , Enterococcus , Escherichia , Gardnerella , Lactobacillus , Prevotella , Shigella , Sneathia , and Streptococcus , with the dominating species exclusive to healthy women being Lactobacillus crispatus , Gardnerella vaginalis , and Atopobium vaginae . 149 However, reports on the male urobiome are significantly fewer compared to the female urobiome, and the small sample size may hinder the identification of differences in the urobiome of both populations. 150 Finally, in healthy males, it is known that Staphylococcus haemolyticus is an abundant species. 151
The anatomical proximity and physiology of body sites influences the microbial community and their abundance. Unlike males, the proximities between the opening of the reproductive organ and the urinary tract are closer to each other in females. Thus, the vagina might be the main source of the microbial community in the urinary tract. In two studies, the existence of a common urogenital microbiota in both vaginal and urine samples was reported. 152 , 153 However, some differences were also observed. For instance, the genera Tepidimonas and Flavobacterium were found to be present in the urobiome, even though they are absent in the vaginal microbiome. 153 Other urobiomes such as the urinary fungal community have not been well-characterized, though the presence of Candida spp. has been reported in healthy individuals. 144 To date, only one species of archaea ( Methanobrevibacter smithii ) has been reported to be associated with urinary infection. 154 A urinary virome has also been detected, including lytic bacteriophages such as a Pseudomonas aeruginosa -infecting phage isolated from kidney stones 155 or Escherichia coli -infecting phages isolated from the bladder of females suffering from UUI. 156
UTIs are one of the most common bacterial infections found in humans, especially among women due to the design of the female anatomy. UTIs have been commonly associated with Escherichia coli , but other commensal members are found in the gut microbiota, such as Enterococcus and Staphylococcus . 157 Interestingly, there seems to be a correlation between an increase in the intestinal abundance of these genera and a higher prevalence of UTI. 158 , 159 E. coli is also part of the commensal urobiome, and hence, it has been detected in healthy individuals. However, there are some differences in the motility genes between the isolates found in UTI patients and those in healthy individuals. 160 Moreover, E. coli has greater pathogenicity when it is isolated together with Enterococcus ; however, the mechanisms underlying this coinfection are not yet well-understood. 161 , 162
The vaginal microbiota may also impact the host’s susceptibility to UTI. For instance, women with recurrent UTI become resistant if their vaginal microbiome is altered by the administration of probiotics, especially Lactobacillus crispatus . 163 Furthermore, women with bacterial vaginosis caused by the overgrowth of anaerobic species such as Gardnerella vaginalis suffer more UTIs than women with microbiomes composed mainly of Lactobacillus . 164 Studies have shown that temporary exposure to some strains of Gardnerella vaginalis triggers the activation of E. coli from dormant intracellular reservoirs in the bladder, enhancing the chance of developing recurrent UTI through the induction of apoptosis and interleukin 1-receptor-mediated injury in bladder epithelial cells. 165 These results extend the classic concept of UTI pathogenesis, suggesting that the disease may be driven by occasional exposures of the urinary tract to gut or vagina-associated bacteria that are not traditionally considered as uropathogenic.
2.2.5. Reproductive System
2.2.5.1. vaginal.
The human vaginal microbiome differs from other body sites as it is dominated by a single genus, Lactobacillus . 166 − 168 Because Lactobacillus spp. lower vaginal pH, they inhibit the growth of many pathogens and beneficially impact the host epithelium, modulating the immune system. 169 − 172 It was reported that ∼25% of women in North America possess vaginal microbiomes that are not dominated by Lactobacillus . 173 Instead, their microbiomes are composed of an even population of obligate and facultative anaerobes–namely, species in the genera Gardnerella , Prevotella , Atopobium , Sneathia , Megasphaera , and Peptoniphilus . 166 , 168 , 173 − 175 Interestingly, having such a vaginal microbiome correlates with the higher tendency of being diagnosed with bacterial vaginosis (BV), 176 , 177 a bacterial infection resulting from the imbalance of beneficial and harmful bacteria. Thus, epidemiological studies have associated microbiomes that are not dominated by Lactobacillus with an increased risk of acquiring sexually transmitted infections (STIs) 178 − 181 and preterm birth. 182 − 186 This also suggests that having a non- Lactobacillus -dominated community may be less protective toward developing adverse health outcomes. 187
The vaginal epithelium is coated in a cervical mucus layer that is regulated by hormones. 188 The mucus is composed of protein, lipids, water, and glycoproteins, also referred to as mucins. 189 Mucins have been hypothesized to possess a protective role in the vaginal epithelium and may also serve as a nutrient source for the vaginal microbiome. 190 − 193 Mucin levels change throughout the menstrual cycle and similarly, the level of glycogen fluctuates throughout the cycle too. 194 − 196 Glycogen is produced by the vaginal epithelium, and epithelial cells consist of a high level of glycogen compared to other epithelial tissues. 197 Similar to mucin, glycogen is also thought to be a nutrient source for the vaginal microbiome. 198 , 199 The characteristics of vaginal physiology are influenced by hormonal changes. Therefore, during menopause, the levels of cervical mucus and glycogen decline, and the usual acidic environment of the vagina changes, contributing to the modified microenvironment for the vaginal microbiome. 200
Vaginal microbiota with a lower abundance of Lactobacillus and a higher proportion of facultative and obligate anaerobes such as Gardnerella , Prevotella , Atopobium , and Sneathia are associated with acquiring diseases like STIs and human immunodeficiency virus. 178 , 201 This vaginal microbiome profile has also been linked to both the incidence and prevalence of human papillomavirus. 202 , 203 Despite continuous research seeking to establish the association between vaginal microbiota and health, there is still insufficient information for connecting casual mechanisms and pathways. Nevertheless, an exploratory study that used vaginal microbiota transplants (VMTs) has demonstrated long-term remission of women with recurrent bacterial vaginosis, 204 and such an approach may be employed in the future to gain insights into the modulation of the vaginal microbiome for therapeutic purposes.
2.3. Summary
In this section, we described how the large and diverse groups of microorganisms that reside in various parts of the human body ( Table 1 ) have a highly coevolved relationship with human health. Microbiome research has highlighted the importance of human-microbiota ecosystems in the promotion of health and various disease-causing processes. This also suggests that the microbiome is a potential target for disease management. In the following section, we will present various strategies by which the composition and function of the microbiome can be modulated for therapeutic outcomes. With more studies revealing the mechanistic insights of the microbiome in relation to health, therapeutics applications can be refined.
3. Strategies To Engineer the Microbiome for Therapeutic Applications
As described in section 2 , the human microbiome plays a crucial role in health maintenance as it can influence the development of various diseases. This knowledge has led to the emergence of new therapeutic approaches that target both acute and chronic diseases by modulating the host microbiome. The rapid increase in the availability of robust, broad-spectrum, and easy-to-use synthetic biology tools (as discussed in section 4 ) has further contributed to unlocking the potential of engineering the microbiome to prevent and treat diseases.
In this section, we present different methods by which the human microbiome can be rationally engineered. We also discuss examples that demonstrate that microbiome engineering is a viable way to target diseases and enhance human health.
3.1. Changing the Population Dynamics of the Microbiome
The composition of the human microbiome is unique to every individual and is constantly fluctuating due to factors such as age, diet, host genetics, and medication. Nevertheless, distinct microbiome profiles have been associated with specific diseases by comparing the differences between patients and healthy controls. These differences can occur at any level of the taxonomic rank, with previous reports showing phylum-level to species-level associations. 205 Several methods have been applied to correct microbiome differences with varying specificity and magnitude, as discussed later.
3.1.1. Increasing the Abundance of Specific Members of the Microbiome
A low microbial diversity of the human microbiome is significantly associated with several diseases. 206 However, the changes observed in the microbiome composition might be dissimilar in different populations. For example, Dutch and Belgian cohorts showed a negative correlation between the Bacteroidetes enrichment and diversity, 207 whereas a positive correlation was observed between Bacteroidetes and diversity in African individuals, 208 underscoring the need for population-specific comparisons of the microbiome between healthy individuals and patients. The ratio of the two most dominant phyla in the microbiome, namely, Firmicutes/Bacteroidetes, is one of the most important parameters representing microbiome diversity, at least in the gut. Previous reports have shown a high ratio of Firmicutes/Bacteroidetes in obese Ukrainian adults compared to their lean counterparts. 209 A similar observation was also made in Dutch 210 and Japanese 211 individuals with a systematic review of 32 studies across varied populations, confirming the positive correlation between the Firmicutes/Bacteroidetes ratio and obesity. 212 In contrast, a decreased Firmicutes/Bacteroidetes ratio has been observed in patients with inflammatory bowel disease (IBD). Manichanh et al. reported a significant reduction in the proportion of Firmicutes in the microbiome of patients with Crohn’s disease (CD) compared to the healthy microbiome. 213 The alteration in the gut microbiome was also associated with disease activity and severity, with lower Firmicutes observed in patients with active ulcerative colitis (UC) compared to the inactive disease and in aggressive CD compared to the nonaggressive disease. 214 Firmicutes in the gut, particularly the genus Faecalibacterium , were also reduced in patients with major depressive disorder and bipolar disorder, 215 as well as chronic fatigue syndrome. 216 Apart from Firmicutes and Bacteroidetes, a lower abundance of other phyla in the gut, such as Actinobacteria, have also been associated with several diseases. Bifidobacterium is one of the most important genera belonging to the Actinobacteria, with lower counts of bifidobacteria found in celiac disease, 217 irritable bowel syndrome, 218 and Alzheimer’s disease. 219
The correlation between the decrease in microbiome diversity and disease development is not only limited to the gut; it has been observed in other anatomical locations as well. Kong et al. reported reduced skin microbiome diversity in patients with atopic dermatitis, with an enrichment of Staphylococcus sequences and depletion of Actinobacteria. 220 In the lung, reduced microbial diversity and an abundance of Firmicutes was found to be significantly associated with the progression of idiopathic pulmonary fibrosis. 221 The depletion of Lactobacillus spp., predominant members of the healthy urine microbiome in females, was observed in patients with UUI, 144 a predisposition to UTI, 222 and overactive bladder. 223
3.1.1.1. Probiotic Supplementation
The studies mentioned earlier demonstrate that decreased levels of specific phyla or genera of microbes in the microbiome are significantly associated with disease development and progression. Therefore, engineering the microbiome to correct this imbalance is imperative to alleviate associated disorders and promote health. This can potentially be achieved by exogenously supplementing beneficial bacteria, such as probiotics, which can rebalance the microbiome. Such strategies have been evaluated previously and were found to be successful in some cases.
Joung et al. studied the effect of oral administration of L. plantarum K50 or L. rhamnosus GG to obese mice on a high-fat diet for 12 weeks. 224 At the end of the intervention, they showed that treated mice had reduced body weight and serum triglyceride levels as well as increased high-density lipoprotein cholesterol levels. A high Firmicutes/Bacteroidetes ratio was seen in nontreated obese mice, as seen in obese human individuals, which significantly reduced after treatment with the probiotic strains. 224 In another study, the administration of L. rhamnosus GG to mice on a high-fat diet led to the reversal of resistance to leptin (an appetite-regulating hormone), an increase in fecal microbiome diversity, and a reduction in the Proteobacteria phylum. 225 A meta-analysis of 15 clinical trials comprising a total of 957 participants concluded that probiotic intervention resulted in a significant reduction of body weight, fat percentage, and body mass index but not fat mass when compared to the placebo. 226 Although most clinical trials conducted did not perform microbiome analyses, changes in the composition of the fecal microbiome post- L. salivarius Ls-33 administration in obese adolescents were reported by Larsen et al., with a significant decrease in the Firmicutes/Bacteroidetes ratio. 227 However, the study did not find any changes in anthropometric and inflammatory parameters, 228 making the correlation between changes in the gut microbiome and obesity ambiguous in humans.
Apart from the commonly used Lactobacillus and Bifidobacterium strains, Akkermansia muciniphila has found wide applications in treating cardiometabolic diseases, including obesity and diabetes. A. muciniphila is one of the most abundant species of the human gut microbiome; its depletion has been reported in obese and diabetic mice and, more importantly, in humans with pathologies such as obesity, type 2 diabetes, hypertension, and IBD. 229 Due to this clear negative correlation between A. muciniphila and cardiometabolic diseases, the safety and efficacy of A. muciniphila supplementation in counteracting obesity and diabetes was evaluated in a randomized, double-blind, placebo-controlled study with 40 overweight or obese individuals. 230 It was observed that, compared to the placebo, both live and pasteurized A. muciniphila (10 10 CFU per day) were safe and tolerable for the study duration (3 months) with no reported adverse events. After 3 months, the group receiving pasteurized A. muciniphila showed improved insulin sensitivity as well as reduced cholesterol and body weight compared to the placebo. A reduction in the levels of markers for liver dysfunction was also seen in pasteurized A. muciniphila but not in the live microbe group. No change in the gut microbiome composition was seen in either of the groups. Strikingly, the pasteurization of A. muciniphila exacerbated its beneficial effects, raising interesting questions about the bacteria’s mechanism of action. Although an outer membrane protein called Amuc_1100 was found to partly recapitulate A. muciniphila ’s beneficial effects, 231 further elucidation of the mechanism is warranted.
As for obesity, the administration of probiotics was evaluated for IBD management. Wang et al. showed that administering a mixture of L. plantarum ZDY2013 from acid beans and B. bifidum WBIN03 from infant feces to dextran sodium sulfate (DSS)-induced UC in mice led to the downregulation of the pro-inflammatory cytokines and upregulation of antioxidant factors. 232 Subsequently, the microbiome analysis of fecal samples from the mice revealed an increase in the abundance of unidentified Firmicutes and a decrease in Bacteroidetes. L. fermentum strains isolated from healthy individuals also demonstrated similar effects on the innate immune system in mice with DSS-induced colitis. 233 The administration of L. fermentum KBL374 and KBL375 resulted in decreased levels of inflammatory cytokines and increased levels of the anti-inflammatory interleukin (IL)-10. L. fermentum administration also reshaped the gut microbiome of mice by increasing the abundance of Lactobacillus and Akkermansia spp. and decreasing Bacteroides numbers. 233 Despite these reports of probiotics ameliorating IBD in mice, multiple clinical trial results have been discouraging. In a randomized, double-blind, placebo-controlled clinical trial comprising 142 patients with asymptomatic UC or CD, treatment with a multistrain probiotic cocktail showed no improvement in quality of life and other laboratory parameters, with a reduction in the fecal calprotectin levels of UC patients being the only significant finding. 234 Another clinical trial comprising 56 UC patients showed that administering B. longum 536 resulted in a significant decrease in the disease activity score after 8 weeks, but it was not statistically different from the placebo group at the end of the treatment. 235 Improvements in rectal bleeding and endoscopic score were observed in the probiotic group, but not in the placebo group. Moreover, the meta-analyses of clinical trials with UC and CD patients given probiotics concluded that probiotics are somewhat beneficial in UC, particularly in maintaining remission, but not in CD patients. 236 , 237 This might be due to the insufficient period of treatment or delays in intervention. 237
The bacterium F. prausnitzii , which belongs to the phylum Firmicutes, is a prominent member of the gut microbiome, accounting for 5% of total fecal bacteria. 238 A negative association between the abundance of F. prausnitzii and IBD has been confirmed by a meta-analysis of 16 studies encompassing 1700 CD or UC patients. 239 It was found that both CD and UC patients had a lower abundance of F. prausnitzii compared to healthy controls. Furthermore, patients with active CD and UC had reduced F. prausnitzii when compared to patients with CD and UC in remission, respectively. The importance of F. prausnitzii was further confirmed by in vivo studies in which the administration of bacteria to mice models of colitis led to reduced disease severity. 240 , 241 Surprisingly, no clinical trial involving the supplementation of this bacteria to IBD patients has been conducted yet, likely due to F. prausnitzii ’s extreme oxygen sensitivity, making it difficult to cultivate even in an anaerobic environment. 242
Few studies have evaluated changes in the fungal diversity and virome of patients with IBD. Sokol et al. reported a decrease in the levels of Saccharomyces cerevisiae and an increase in Candida albicans in IBD patients compared to healthy subjects. 243 Malassezia restricta , a common skin fungus, was also found in abundance in the gut of CD patients. 244 This fungus is known to elicit the release of inflammatory cytokines from innate immune cells, thus contributing to IBD development. 244 Similarly, the gut microbiome of UC and CD patients had a significant expansion of Caudovirales bacteriophages, which may contribute to intestinal inflammation. 245 Previous clinical trials have shown that S. boulardii , a closely related yeast to S. cerevisiae , can be used as an adjuvant therapy to induce remission or prevent the relapse of IBD in remission. 246 However, no clinical trial has evaluated S. boulardii as a standalone IBD treatment.
Atopic dermatitis (AD) is a common skin allergic condition associated with a dysbiotic skin microbiome with a higher abundance of S. aureus . Studies on culturable Gram-negative bacteria from the skin of AD patients and healthy individuals revealed that Roseomonas mucosa from healthy volunteers, and not AD patients, was associated with the amelioration of AD in a mice model. 247 On the basis of these results, a clinical trial comprising children below 7 years of age with AD (the most common group suffering from the disease) treated with R. mucosa isolated from healthy individuals was conducted. 248 Treatment with the commensal bacteria led to an improvement in the skin epithelial barrier function, lowered S. aureus burden, increased the skin’s microbial diversity, and reduced the requirement of topical steroids for treatment. These positive effects were associated with the activation of the tissue-repair pathways by the glycerophospholipids produced by R. mucosa from healthy individuals, which were not produced by the isolates from AD patients. 248
The alteration of vaginal flora has been shown to be associated with recurrent UTI, with the colonization of UTI-causing E. coli being derepressed due to the lower abundance of hydrogen peroxide-producing Lactobacillus in the vagina. 249 L. crispatus CTV-05 is a vaginal isolate that can produce hydrogen peroxide and adhere to the vaginal epithelial layer, 250 thus making it an ideal probiotic candidate for the treatment of recurrent UTI. In a randomized, placebo-controlled Phase 2 trial comprising 100 women with recurrent UTI, the patients were given a placebo or L. crispatus CTV-05 intravaginally for 10 weeks. 251 Recurrent UTI occurred in only 15% of the patients receiving the probiotic treatment compared to 27% in the placebo group. High levels of vaginal L. crispatus were observed in both groups, suggesting the expansion of the endogenous L. crispatus population in the placebo group. However, it was not associated with significant therapeutic advantages, unlike the L. crispatus CTV-05 isolate, which is able to outcompete E. coli in the vagina. 251
3.1.1.2. Prebiotic Supplementation
An alternative to the exogenous supplementation of probiotics is the administration of prebiotics, which are nondigestible substrates that can be utilized by members of the host–microbiome to confer health benefits. 252 Prebiotics are usually oligosaccharides that stimulate the growth of one or more species of bacteria already present in the microbiome. As different prebiotics can selectively increase the abundance of the microbes that can utilize them, these substrates can be used to remodel the microbiome—transitioning it from a disease state to a relatively healthier state ( Figure Figure1 1 ). Some common prebiotics are fructo-oligosaccharides (FOS) derived from inulins, galacto-oligosaccharides (GOS), xylo-oligosaccharides (XOS), and lactulose. 253 FOS, GOS, and XOS have been shown to promote Bifidobacterium expansion in the human gut, although there are conflicting reports of their effect on other bacterial genera due to differences in intervention doses and duration. 254 − 256

Modulation of microbiome composition by probiotics and prebiotics. A dysbiotic microbiome can contribute toward obesity development, inflammation, and cancer. Administering probiotics and prebiotics can change microbiome composition to ameliorate diseases.
In a prospective study on European newborn infants for the first 2 years of age, a gut microbiome analysis using stool samples was performed on infants with and without atopic dermatitis or other skin allergies. 257 Lower counts of Bifidobacterium were observed in the first year of life in infants with allergies compared to healthy controls. A higher number of Clostridia at 3 months, higher S. aureus at 6 months, and lower Bacteroides at 12 months were also observed. 257 An increase in Bifidobacterium levels after prebiotics administration to prevent atopic dermatitis was also evaluated in a randomized, prospective, placebo-controlled clinical trial comprising 259 infants receiving either 8 g/L of prebiotics (a mixture of GOS and FOS) or placebo. 258 It was observed that the group that received prebiotics showed a lower incidence of atopic dermatitis at 6 months of age compared to the group that received the placebo. This was accompanied by an increase in the levels of bifidobacteria, but not lactobacilli, as determined by the colony-forming units from the stool samples. Other microbes in stool samples were not analyzed. A similar reduction in the incidence of allergies was reported in infants receiving prebiotics until 2 years of age, although changes in microbiome composition were not determined. 259 The bifidogenic effect of FOS was also shown in adults with CD, wherein treatment with 15 g of FOS for 3 weeks led to an improvement in disease activity, an increase in the fecal bifidobacteria, and the modification of mucosal dendritic cell functions, such as increased IL-10 expression. 260
The modulation of the gut microbiome by prebiotics also has applications in cancer therapy. In a study by Han et al., the administration of a colon-retentive inulin gel to a mice model of colorectal cancer improved the antitumor efficacy of the immune checkpoint blocker, antiprogrammed cell death protein-1 (α-PD-1). 261 Previous studies have shown that patients that respond to immune checkpoint blockers have a higher abundance of beneficial bacteria, such as Bifidobacterium , Akkermansia , Ruminococcaceae, and Faecalibacterium in their gut microbiome compared to nonresponsive patients. 262 − 264 Han et al. showed that the oral administration of the inulin gel to the mice led to the expansion of such beneficial bacteria, including Akkermansia , Lactobacillus , and Roseburia . This elicited a T cell response in the mice that worked in synergy with α-PD-1 for an enhanced antitumor effect. 261
3.1.2. Depletion of Specific Members of the Microbiome
Contrary to increasing the abundance of specific microbes in the microbiome, the selective depletion of certain members is another viable way of engineering the microbiome, particularly during infections. Since the discovery of penicillin in 1928, antibiotics have been the primary mode of defense against pathogens. However, most antibiotics currently in use are nonspecific in their antimicrobial activity and, consequently, cause a significant decrease in the diversity and richness of the human microbiome. Dethlefsen and Relman showed that there was a rapid shift in the composition and loss of diversity in the gut microbiome of individuals within 3–4 days after administration of ciprofloxacin. 265 Although some members of the gut microbiome recovered after the end of the antibiotic course, recovery was incomplete, and the final composition was altered compared to the initial state. The altered composition of the microbiome due to antibiotics is also associated with increased susceptibility to other pathogens, immune dysregulation, and the rise of resistance genes. 266 , 267 To negate the negative impact of antibiotics on the microbiome, targeted therapies against pathogens are required. Some small-molecule antibiotics are in clinical development and show promise against specific pathogens. For example, ridinilazole is a DNA-binding small molecule with highly targeted action against Clostridium difficile . 268 In a Phase II randomized, double-blind clinical trial, ridinilazole was found to provide a superior clinical cure with no infection recurrence, compared to the current standard-of-care vancomycin. 269 This was accompanied by a less disrupted microbiome in the case of ridinilazole. Vancomycin treatment led to a significant reduction of Firmicutes, Bacteroidetes, and Actinobacteria and the expansion of Proteobacteria, whereas ridinilazole showed only a modest reduction of the Firmicutes. 270 Similarly, targeted antibiotics against the skin pathogen S. aureus , pneumonia-causing P. aeruginosa , and Enterobacteriaceae are also in development. 271
In addition to the collateral damage to the microbiome, broad-spectrum antibiotics may also suffer from poor efficacy, such as in biofilms in which the pathogens remain impervious to the antibiotics. This is exemplified in the case of bacterial vaginosis, which is characterized by the displacement of beneficial Lactobacillus spp. with anaerobic bacteria, predominantly Gardnerella vaginalis , which forms a biofilm on the vaginal epithelium. 272 Broad-spectrum antimicrobials have shown high short-term curing rates but are unable to prevent the recurrence of vaginosis, partly due to biofilm formation. 273 Landlinger et al. developed a narrow-spectrum engineered endolysin, a peptidoglycan-degrading enzyme, by identifying and performing domain shuffling on 14 native endolysins present in Gardnerella . 274 Among the various candidates, PM-447 was selected based on its high antimicrobial activity against various Gardnerella spp. and negligible activity against Lactobacillus spp. and other vaginal microbes. Interestingly, PM-447 was also able to target Gardnerella in vaginal samples from 13 patients with bacterial vaginosis and disperse the biofilm without affecting the remaining vaginal microbiome. 274 Further evaluation of PM-447 in animal models is still awaited.
Vaccines also have the potential to eliminate pathogens in the human microbiome to prevent diseases. For example, the pneumococcal conjugate vaccine (PCV-7) against Streptococcus pneumoniae has significantly decreased the prevalence of invasive pneumococcal disease, particularly in young children. 275 PCV-7 was designed against the seven virulent serotypes of S. pneumoniae , a natural colonizer of the upper respiratory tract. However, studies have shown that the niche vacated by virulent serotypes of the bacteria after vaccination was occupied by nonvaccine serotypes. Furthermore, an increase in the abundance of S. aureus , an ecological competitor of S. pneumoniae , was also observed. 276 , 277 The use of a broader PCV-13 vaccine was found to increase the diversity and stability of the nasal microbiome, 278 likely due to the opening of a larger niche that was occupied by nonpneumococcal bacteria. 279
As an alternative to antibiotics and vaccines, bacteriophages have also been used as natural predators that target pathogenic bacteria and their associated diseases. The main advantage of using phages is their narrow host range, enabling precise elimination of the pathogen. 280 Phages specific against S. aureus , Enterococcus faecium , Vibrio parahaemolyticus , Acinetobacter baumannii , and Mycobacterium tuberculosis have been previously isolated and found to be effective in targeting even antibiotic-resistant variants of the pathogens, at least in in vitro and in vivo models. 281
Beyond infections, bacteriophages have also been used as therapies against other disorders by modulating the microbiome. Duan et al. showed that patients with alcoholic hepatitis have a higher number of fecal E. faecalis compared to nonalcoholic individuals or patients with other alcohol-use disorders. 282 The authors identified cytolysin, an exotoxin produced by E. faecalis in the gut, to be responsible for liver injury. Upon administration of a bacteriophage targeting E. faecalis in mice inoculated with bacteria from patients with alcoholic hepatitis, a significant decrease in cytolysin levels was observed, accompanied by reduced liver injury. The fecal count of Enterococcus was also reduced, but no significant change in the microbiome composition was observed, indicating the targeted elimination of E. faecalis . 282
In another study, Zheng et al. used a phage isolated from human saliva against the pro-tumoral Fusobacterium nucleatum to develop a novel therapy for colorectal cancer (CRC). 283 Previous studies have shown that a high proliferation of F. nucleatum causes chemoresistance in CRC. 284 Therefore, by using a phage targeting F. nucleatum in combination with nanoparticles loaded with a chemotherapy drug, the authors demonstrated superior efficacy of this method in different CRC mice models, compared to the chemotherapy drug alone or an antibiotic cocktail. A significant reduction of F. nucleatum and an increase in the abundance of antitumoral SCFA-producing bacteria were also seen in the gut of the mice. 283
Bacteriophages have also been used to treat acne vulgaris, one of the most common dermatological disorders worldwide. One contributing factor to the development of the disease is the higher abundance of Propionibacterium acnes , although the exact mechanism is still debatable. 285 To reduce the number of P. acnes , Brown et al. formulated an aqueous cream comprising a cocktail of bacteriophages isolated from human skin microflora against P. acnes . 286 The cream was found to be effective in lysing P. acnes in vitro but has yet to be evaluated in animal models.
Engineered bacteria, both commensal and probiotic, are also emerging as robust therapies for targeted pathogen eradication. Although probiotic bacterial strains are known to prevent infection through the competitive exclusion of the pathogen and native production of antimicrobial agents, 287 these strategies often show poor clinical efficacy. With the aid of synthetic biology, novel functionalities can be incorporated into the bacteria of choice to enable highly efficient and selective pathogen elimination. This is achievable due to the integration of biosensors in the bacteria that detect quorum signaling molecules produced by the target pathogen, such as acyl homoserine lactone (AHL) by P. aeruginosa ( 288 ) and autoinducing peptides (AIP) by S. aureus , 289 which in turn activates the production and secretion of an antibacterial agent. Due to the high species specificity of quorum signaling molecules, the precise delivery of the therapeutic molecule becomes feasible—potentiating the use of engineered bacteria as viable alternative therapies for infections. Such strategies have been previously used to target P. aeruginosa , 288 S. aureus , 289 and V. cholerae . 290 Although these studies demonstrated the efficacy of the engineered bacteria in eliminating the target pathogen in either in vitro or in vivo models, the extent to which the microbiome is perturbed due to the administration of the engineered bacteria was not evaluated. Depending upon the chassis used, the engineered bacteria might rapidly transit through the microbiome or persist for a prolonged period. Although the former is unlikely to significantly alter the composition of the microbiome due to its inherent resistance to change, 291 the latter might have a considerable impact, and future studies designed to evaluate this will have to be conducted.
In addition to the engineered bacteria, synthetic biology has also been used to engineer bacteriophages, repurposing them as antimicrobial delivery vehicles. Various studies have engineered bacteriophages to deliver CRISPR-Cas9 systems into their host. 292 − 294 The CRISPR-Cas9 system is designed such that it recognizes a gene in the genome of the host, upon which it introduces double-stranded breaks in the genome, resulting in cell death. This approach is more specific compared to the use of wild-type bacteriophages because CRISPR-Cas9 is unable to induce cell death in the absence of the target gene. This strategy also enables the selective elimination of bacteria carrying antibiotic-resistance genes by programming CRISPR-Cas9 to identify these targets. 293 However, the escape of target bacteria from the killing activity of CRISPR-Cas9 is a major drawback for this method, and escapees, either by chromosomal deletions or loss of CRISPR arrays, have been reported previously. 294
To improve upon these drawbacks, Ting et al. devised a novel strategy for targeted bacterial depletion by programmed inhibitor cells (PICs), which are engineered bacteria carrying the type-6 secretion system (T6SS) with a nanobody displayed on the surface ( Figure Figure2 2 ). 295 The nanobody recognizes an antigen on the surface of Gram-negative bacteria, such as outer-membrane proteins BamA and intimin, enabling cell–cell adhesion. T6SS is an antagonistic system through which the engineered bacteria can deliver antibacterial toxins into the target bacterial cell. The engineered bacteria protect themselves from the toxins by expressing immunity proteins. Due to the fluidic nature of the gut environment, T6SS alone is likely to be inefficient in delivering the toxins. Thus, the authors incorporated the nanobody into the bacteria for enhanced antibacterial activity. 295 The authors showed that an intimin-expressing E. coli spiked into the fecal samples from mice was successfully depleted by 90% by using an engineered Enterobacter cloacae expressing an anti-intimin antibody on the surface with a native T6SS. This depletion was highly specific as an E. coli strain expressing partial intimin was unaltered. Analysis of the other bacteria in the fecal samples revealed only minor changes in microbiome composition, indicating the potential of PICs to be used as highly specific antimicrobials.

Strategies to deplete members of the microbiome. Nonspecific depletion can be mediated by broad-spectrum antibiotics. Specific depletion can be achieved by targeted antibiotics, bacteriophages, and engineered bacteria. QS, quorum signaling; PICs, programmed inhibitor cells; Nb, nanobody; T6SS, type-6 secretion system.
3.2. Changing the Functionality of the Microbiome
The previous section describes how microbiome composition is important in understanding its association with disease development and how it can be subsequently modulated for disease treatment. However, perhaps even more crucial than composition in understanding the influence that the microbiome exerts on its host is the microbiome’s function, corresponding to the active genes, proteins, and metabolites produced by its members. The significance of microbiome function stems from the likelihood that microbiomes with different compositions might still exhibit similar functions due to functional redundancy. This was observed in a study on the gut microbiome of obese and lean female twins that shared >93% of functional genes, comprising a core microbiome, despite having limited similarity at the phyla level. 296 A similar relative abundance of microbial clades associated with clinically varied diseases also suggests that the function of the microbiome, and not its composition, has a stronger influence and association with human health. 297
Morgan et al. demonstrated the importance of studying microbiome function in their study of microbiome samples from 231 IBD patients and healthy individuals, which were analyzed by 16S RNA sequencing and metagenomics. 298 In addition to the expected changes in microbiome composition, there was a more consistent shift in microbial functions, such as decreased carbohydrate metabolism and amino acid biosynthesis, as well as increased nutrient transport and uptake. Similarly, the enrichment of lipopolysaccharide biosynthesis, pathogenic processes, and inflammatory pathways and the depletion of amino acids and energy metabolism have been reported in patients infected with HIV on antiretroviral therapy. 299 To elucidate the exact mechanism by which microbiome function influences host health, multiomics studies beyond metagenomics are needed. These include transcriptomics, proteomics, and metabolomics to characterize the microbiome at the functional level in both healthy and diseased states. Although there are limited examples of such studies, some rationally designed interventions for modulating microbiome function have been reported before and are discussed later.
3.2.1. DNA Conjugation-Mediated Engineering
DNA conjugation is a method of in situ engineering of the microbiome by delivering a genetic payload to the target microbe via a donor bacterium. These payloads can be mobile genetic elements carrying genes to incorporate novel functionalities into the target bacteria. Such an engineering approach is advantageous because this enables the modification of even unculturable bacteria with complex phenotypes that may not be adequately replicated in non-native bacterial strains. 300 In a study by Brophy et al., the authors used a B. subtilis strain to transfer a miniaturized integrative and conjugative element (mini-ICE Bs1 ) efficiently to various Gram-positive bacteria isolated from the human skin and gut microbiome. 301 The conjugative transfer was placed under the control of an inducible promoter, and the genes that enable further propagation of the ICE Bs1 beyond the initial recipient were deleted as a safety feature. Because new donor strains carrying the DNA to be transferred can be created with ease, this strategy will be useful in rapidly engineering microbiomes with synthetic programs and modulating their function.
Another DNA conjugation strategy was developed by Ronda et al. wherein an E. coli donor strain was engineered to transfer mobile plasmids, either replicative or integrative, into recipient Gram-positive and Gram-negative strains. 302 The authors demonstrated that this method can be applied to engineer the microbiome by using the donor E. coli to deliver green fluorescent protein (GFP) into members of the gut microbiome in mice. By using a library of mobile plasmids, up to 5% of the bacteria were found to receive the plasmid 6 h postadministration of E. coli . The recipients belonged to all four major phyla in the gut microbiome, namely, Firmicutes, Bacteroides, Actinobacteria, and Proteobacteria. 302 Interestingly, the transconjugants persisted only for 72 h postadministration of the E. coli donor, suggesting unstable plasmid maintenance.
Jin et al. developed a genetic manipulation pipeline through which they were able to genetically modify 27 of the nonmodal bacteria belonging to the Firmicutes/Clostridia class. 303 The authors identified the culture conditions that can support bacterial growth followed by a library of genetic tools, including the origin of replications and antibiotic-resistance markers with potent promoters that are functional in the target bacteria. By utilizing these tools and further optimizing the conjugation protocol, the successful delivery of the deactivated Cpf1-based CRISPRi system for regulating gene expression was achieved. In this study, the authors demonstrated the application of their pipeline to modulate metabolites produced by the gut microbiome, such as the bile acid pool in mice, which has numerous implications on host health. 303
The studies described earlier indicate that DNA conjugation is a viable method for modulating microbiome function, although the area is still in infancy and needs further development, particularly an assessment of safety in humans. This is pertinent in the case of mobile genetic elements that are used to deliver the payloads, as such elements have a high tendency to propagate further into nontarget members of the microbiome by horizontal gene transfer. 304 In addition, limited studies on nonmodal members of the microbiome remain a bottleneck in their genetic engineering because it is difficult to reliably predict the functionality of the payload introduced into these microorganisms.
3.2.2. Use of Enzyme Inhibitors
The metabolic activity of microbial enzymes is an important process through which the microbiome can influence host health. Apart from playing a role in the normal functioning of the microbe itself, these enzymes can also metabolize drugs, prodrugs, and xenobiotics administered to the host, leading to unintended and potentially adverse outcomes. 305 This can be mitigated by using inhibitory chemicals that act on the specific microbial enzyme. For example, SN-38 is an anticancer drug used in colon cancer and against lung and brain tumors formed from the intravenously administered prodrug, CPT-11, in the liver. Subsequently, it is glucuronidated by UDP-glucuronosyltransferase in the liver into SN-38G, which is excreted into the GI tract. 306 Here, it is again converted to SN-38 by the bacterial β-glucuronidase enzymes—causing diarrhea and prohibiting an escalation in chemotherapy drug dosage. Although eliminating gut bacteria by antibiotics can potentially prevent SN-38 toxicity, this method has several drawbacks, as discussed in section 3.1.2 . Instead, Wallace et al. employed high-throughput screening to identify potent inhibitors of the bacterial β-glucuronidase that do not target orthologous mammalian enzymes. 307 In addition, these inhibitors neither killed the bacteria nor harmed mammalian cells. In a mice model, the inhibitor was found to protect mice from the chemotherapy drug’s toxicity, although improvements in drug efficacy remain to be studied.
Inhibitors for the gut microbiome-dependent production of trimethylamine (TMA) N -oxide (TMAO), which is associated with cardiovascular risks in humans, have also been reported. 308 , 309 Dietary choline, phosphatidylcholine, and carnitine are converted into TMA by the microbial choline-TMA lyase enzymes, which is subsequently converted to TMAO by hepatic flavin monooxygenase. 310 , 311 Wang et al. identified an analogue of choline, 3,3-dimethyl-1-butanol (DMB), that was observed to inhibit multiple microbial TMA lyases without killing the microbes. 308 The treatment of mice fed a high choline or l -carnitine diet with DMB resulted in lower plasma TMAO levels, the attenuation of atherosclerotic lesions, and the formation of macrophage foam cells. The same group also developed two other TMA lyase inhibitors, fluoromethylcholine and iodomethylcholine, that are nonlethal and able to accumulate within the microbe, resulting in a sustained decrease in TMAO levels in mice for 3 days after a single oral dose. 309 Treatment with these inhibitors also prevented thrombus formation by reducing platelet adherence to the collagen matrix in the arteries in mice fed choline. Interestingly, the inhibitors caused a change in gut microbiome composition despite being nonlethal, suggesting additional selection pressure on the microbes due to the inhibitors, which might eventually lead to resistance development.
The development of enzymatic inhibitors to modulate microbiome function faces two major challenges. First, the inhibitor should be able to act on all related microbial enzymes. In the absence of broad-spectrum inhibition, noninhibited microbial species may compensate, resulting in no net change in microbiome function. Second, the presence of human enzymes with similar functions as the microbial enzyme will necessitate the screening of a large library of chemicals to find targets that are inhibitory toward microbes and not mammalian cells, which might not be achievable in some cases.
3.2.3. Microbiome Metabolite Modulation by Engineered Microorganisms
Among the various strategies to modulate microbiome function, the most advanced is the administration of exogenous engineered bacteria that are either commensal or probiotic. Contrary to the administration of wild-type bacteria that are primarily used to change microbiome composition, engineered bacteria are equipped with novel functionalities that enable the regulation of microbiome function. By using the large repertoire of synthetic biology tools, bacteria can be reprogrammed to specifically target a disease, resulting in a highly precise autonomous therapy. Such therapies have been developed to target metabolic diseases, 312 − 314 prevent cancer, 315 − 317 inhibit pathogens, 318 , 319 and address other conditions 320 − 322 (for recent reviews, see refs ( 323 − 326 )). An increasing number of such studies have been reported thus far. However, in this section, we will only present studies that show how the microbiome has been implicated to play a major role in disease development through its metabolites, thus necessitating its functional modulation for therapy.
There is an intense interplay of metabolites between the human microbiome and its host, with microbiome metabolic pathways significantly associated with 34% of blood and 95% of fecal metabolites. 327 One such metabolite is ammonia, which is primarily produced by the metabolism of amino acids in our food by gut microbes. The human body regulates the levels of ammonia via the liver’s urea cycle. 328 However, in the case of liver failure, the metabolite accumulates in the blood, which can act as a neurotoxin at high concentrations.
To develop a therapy that reduces ammonia levels, E. coli Nissle 1917 (EcN) was genetically modified to convert gut ammonia into l -arginine, boosting the urea cycle. 329 The metabolic pathway was placed under the control of the anaerobic-inducible fnrS promoter (PfnrS) such that metabolic conversion was initiated only in the gut’s low-oxygen environment. In addition, the bacterial strain was made auxotrophic for thymidine as a biocontainment strategy. This strain was found to reduce ammonia levels in mice given a high-protein diet or administered with thioacetamide, a liver toxin. The engineered bacterium was also found to be safe to use in healthy volunteers at a dose of 5 × 10 11 CFU three times a day for 14 days. 329 However, in a Phase 2 clinical trial, the engineered EcN failed to significantly reduce blood ammonia in patients with cirrhosis compared to placebo. 330 The engineered EcN has since been repurposed for anticancer therapy in conjunction with immune checkpoint inhibitors by metabolizing waste ammonia from tumors into l -arginine, increasing T cell response against cancer ( Figure Figure3 3 ). 316

Modulation of microbiome functionality by DNA conjugation, enzyme inhibitors, and engineered bacteria.
SCFAs produced by the fermentation of dietary fibers by the gut microbiome have anti-inflammatory activity and play an important role in various host processes, such as improving intestinal barrier integrity and reducing colon cancer risk. 331 SCFAs, particularly butyrate, are also able to regulate appetite, decrease insulin resistance, promote fat oxidation, and stimulate the release of insulinotropic hormones. 332 , 333 Due to these effects on host metabolic activity, butyrate is likely to have a therapeutic effect on patients with obesity and diabetes. Oral delivery of butyrate is challenging due to its poor bioavailability and disagreeable odor and taste. Thus, different bacteria have been metabolically engineered to produce butyrate in the gut as an alternative treatment for obesity and diabetes. Bacillus subtilis SCK6 naturally produces butyrate at very low levels and thus was engineered to produce butyrate via the butyryl CoA:acetic acid CoA transferase pathway that is present in the gut’s butyrogenic microbes. 334 Further deletion of competing metabolic pathways yielded a strain that can produce 1.5 g/L of butyrate in vitro. When evaluated in mice given a high-fat diet, the engineered bacteria were able to retard weight gain, reduce visceral fat accumulation, and improve glucose tolerance. In addition to the decrease of Firmicutes and increase in Bacteroidetes, analysis of the metabolic pathways of the gut microbiome revealed a significant enhancement of the genes involved in carbohydrate, amino acid, vitamins, and energy metabolism. 334 EcN has also been engineered to produce 0.5–1 g/L butyrate in vitro but has yet to be evaluated in in vivo models. 335 , 336
Extracellular adenosine triphosphate (eATP) is an IBD-associated metabolite produced by activated immune cells and the gut microbiome. eATP is known to promote pro-inflammatory cytokine production and neuron apoptosis, as well as to suppress anti-inflammatory responses. A probiotic yeast, S. cerevisiae , was genetically modified to sense eATP in the gut and respond by producing ATP-degrading enzymes that convert eATP to AMP. In turn, AMP is further broken down to immunosuppressive adenosine. 337 To enable S. cerevisiae to respond to eATP, a human P2Y2 receptor evolved to sense eATP with 1 000-fold higher sensitivity was integrated into the probiotic strain. This was combined with a potent ATPase from potato to create an IBD treatment. In mice models of colitis, the engineered probiotic ameliorated inflammation, as observed by the lower expression of pro-inflammatory cytokines, reduced colon shortening, and improved histological scores. 337 The engineered probiotic performed better than the probiotic with constitutively expressed ATPase and even standard-of-care IBD therapies, suggesting the therapy’s potency against inflammatory disorders.
3.3. Natural and Synthetic Microbial Consortia
Microbial communities, such as those in the human microbiome, perform complex functions shaped by dynamic interactions within the community and with the environment. Such intricate functions are unlikely to be recapitulated by individual populations, which has resulted in heightened interest in microbial consortia. Additionally, increased microbial diversity within the consortia might also impart resilience against environmental changes, such as nutrient limitation. 338 Currently, two types of microbial consortia are being used for therapeutic applications: naturally occurring with undefined composition, such as the gut microbiome, which can be used as a fecal microbiota transplant (FMT); and synthetic, comprising a predetermined cocktail of probiotics or commensal microbes. Here, we will discuss some examples of these microbial consortia being used to target a variety of diseases and the challenges faced by these strategies.
3.3.1. Fecal Microbiota Transplantation
FMT involves the administration of dried feces, either the patient’s own or from a healthy donor to the patient ( Figure Figure4 4 ). The route of administration might vary, ranging from oral consumption of freeze-dried capsules and small/large intestine infusion to enema. 339 The aim is to reconstitute the native disease-associated microbiome into a healthier version to provide a positive health impact. FMT has shown the most promise in recurrent C. difficile infection with a resolution rate of 85–90% and is highly recommended for both mild and severe cases. 340 After initial vancomycin treatment for patients with C. difficile in a randomized clinical trial, FMT showed higher efficacy than a continued vancomycin regimen, accompanied by an increase in Bacteroidetes and Clostridium clusters and a decrease in Proteobacteria. 341 In mice and humans given broad-spectrum antibiotics, autologous FMT enabled the rapid and complete recovery of the dysbiotic microbiome within days and was the most effective intervention. 342 Surprisingly, the administration of a cocktail of probiotics significantly delayed the recovery of the microbiome compared to spontaneous recovery, with the recovery being incomplete. This was attributed to the soluble factors produced by the probiotics that caused the inhibition of the indigenous microbiome. 342

Engineering the microbiome by fecal microbiota transplantation (FMT) and synthetic microbial consortia. The main features of both strategies are presented along with unknown parameters (marked by “?”).
Apart from C. difficile infection, FMT has been used to treat ulcerative colitis, 343 , 344 type 2 diabetes, 345 irritable bowel syndrome, 346 and cancer; 347 however, only limited case studies and clinical trials have been reported to date. Further well-designed clinical trials are required to confirm FMT’s efficacy for indications beyond C. difficile infection.
There are certain aspects of FMT that must be considered and studied before it can be broadly applied in clinical settings. The selection of an appropriate donor is one of the key components for FMT success. Although autologous FMT is likely to provide superior compatibility, it may not always be feasible, thus necessitating a stool donor. Meticulous screening of the donor’s gut microbiome for potentially pathogenic species is required prior to FMT to ensure procedural safety. This has especially become relevant in the COVID-19 pandemic due to the detection of the SARS-CoV-2 virus in individual fecal samples. 348 Additionally, studies have been undertaken to identify the donors whose stool samples are most likely to result in successful engraftment of the gut microbiome. A high taxonomic diversity and the presence of specific bacterial families in the donor stool have been proposed to be key to disease-specific restoration of gut homeostasis in recipients. 349 Beyond donor selection, the recipients’ genetics, diet, and lifestyle are also likely to play a role in FMT maintenance. 339
As an alternative to FMT, Seres Therapeutics developed SER-109, a natural consortium of bacterial spores from healthy donors to treat recurrent C. difficile infection. 350 The spores are primarily from bacteria belonging to Firmicutes, as other phyla are not spore-formers. This is advantageous as previous studies have shown Firmicutes to be associated with a relatively higher concentration of secondary bile acids, which inhibits vegetative growth of C. difficile . 350 Moreover, these bile acids also prevent C. difficile spore germination, which is a major cause of recurrent infection. 351 The use of a purified spore consortium allows for oral delivery because the spores are resistant to gastric acid, reducing the risk of pathogen transmission seen in FMT. In a randomized, double-blind, placebo-controlled Phase 3 clinical trial comprising 182 patients, the group of patients receiving oral SER-109 after standard antibiotic regime showed a reduced recurrence of C. difficile infection compared to the patients receiving placebo after antibiotics (12% vs 40%). 352 Patients receiving SER-109 showed an engraftment of the dosed species within 1 week, which persisted for the study duration (8 weeks) with an increased abundance of Firmicutes and decreased proinflammatory Enterobacteriaceae. A higher concentration of secondary bile acids in the fecal samples from patients given SER-109 was also observed. 352
3.3.2. Synthetic Microbial Consortia
Due to the undefined community structure in naturally occurring microbiomes, it is challenging to quantify the contributions of individual members toward a specific function, making it unsuitable for further optimization. Synthetic microbial consortia can fulfill this unmet need as they can be rationally designed with reduced microbial complexity to perform functions that can predictably impart a therapeutic benefit to the host. For example, Tanoue et al. identified a consortium of 11 bacterial strains isolated from the feces of healthy human donors that can robustly induce interferon-γ-producing CD8 + T cells in the intestine. 353 The 11 strains are low-abundance members of the microbiome including those belonging to Bacteroides spp. and Parabacteroides spp., Eubacterium limosum , Ruminococcaceae bacterium cv2 , Phascolarctobacterium faecium , and Fusobacterium ulcerans . 354 Upon colonization in mice, the microbial consortium provided resistance to infection by Listeria monocytogenes and enhanced the efficacy of immune checkpoint inhibitors in tumor models. 353
GUT-108, a rationally designed consortium of 11 bacterial strains, was also shown to reverse colitis in a mice model. 355 The consortium comprises strains that can perform functions that are reduced in the gut microbiome of IBD patients. These include the production of SCFAs such as butyrate and propionate, secondary bile acids, deoxycholic acid, and lithocholic acid, as well as indole and its derivatives. 356 , 357 Additionally, several strains in GUT-108 produce antimicrobial factors that prevent the growth of opportunistic pathogens, which may further exacerbate the inflammatory response. 355 The administration of GUT-108 to a mice model of experimental colitis prevented the expansion of pathogenic bacteria belonging to the Enterobacteriaceae family, which was observed in mice receiving phosphate buffered saline (PBS). The successful engraftment of 10 strains of GUT-108 was observed in mice, which led to reduced inflammatory cytokines, induced IL-10 production, and increased the levels of metabolites that promote mucosal healing and immunoregulatory responses. 355
Beyond the synthetic consortia comprising wild-type bacterial strains, engineered bacteria with defined characteristics can also be used to develop synthetic communities. For example, Kong et al. created six two-strain consortia with unique interactions ranging from commensalism to predation. 358 They used these strains to further develop three- and four-strain member communities with predictable behavior, demonstrating the successful engineering of social interactions in a bacterial community. This approach may enable the design of synthetic consortia with more complex behavior that can impart beneficial effects to the host.
The design of microbial consortia presents unique challenges compared to single microbial populations. Similar to natural microbiomes, synthetic consortia should be able to maintain homeostasis and prevent certain members from outcompeting others even in different nutritional environments. However, this is difficult to achieve because microbes often show varied abilities to metabolize different resources, making the long-term prediction of homeostasis unfeasible. Applying synthetic biology to incorporate genetic circuits, such as the oscillatory predator–prey system, 359 into the microbes may potentially mitigate competition between consortium members. Another challenge is predicting the behavior of the microbial consortia, which can be attributed to the lack of omics-level understanding of metabolic interactions between the microbes. The knowledge gained through multiomics studies combined with computational modeling will ultimately aid in our ability to design synthetic microbial consortia with predictable and controllable functions. 360
3.4. Current Challenges and Limitations of Microbiome Engineering
The examples presented earlier (summarized in Table 2 ) demonstrate that it is feasible to engineer the microbiome for various therapeutic outcomes in the host. So far, in vitro and in vivo evaluations of different methods to modulate microbiome composition and function have shown promising results. With the exception of FMT, most therapeutics are still awaiting successful clinical translation. There are unique challenges to the development of different therapeutics based on a rationally engineered microbiome, which have been alluded to earlier. However, to further accelerate microbiome engineering to develop safe and effective therapeutic products, there is a need to bridge the knowledge gaps pertaining to microbe–microbe and microbe–host interactions and build novel tools to broaden the scope of microbiome engineering, as discussed later.
3.4.1. Inadequate Use of Multiomics Studies
As part of the Human Microbiome Project (HMP), different microbial communities in the human body have been comprehensively characterized by 16S rRNA gene sequencing to decipher the microbiome’s taxonomic complexity. Meanwhile, metagenomic whole-genome shotgun sequencing provides insight into the pathways present in the microbiome and their functions. 361 However, these data sets do not shed light on intramicrobial community interactions, how the microbiome interacts with the host, and how the host responds to its resident microbiome. A thorough understanding of these interactions is necessary for determining the causal role that the microbiome plays in disease development, which will eventually lead to novel therapies through microbiome engineering. To achieve this, healthy and diseased cohorts will have to be subjected to various multiomics assays encompassing not only the microbiome but also the host. Such studies are ongoing for preterm birth, IBD, and type 2 diabetes as part of the second phase of the HMP. 362 In the case of IBD, stool samples will be collected from patients with IBD and healthy controls. These will be used for multiomics assays such as 16S rRNA gene sequencing, metagenomic and metatranscriptomic sequencing, protein profiling, and metabolomics. In addition, the corresponding changes in the host will be determined by performing RNA-seq on colon biopsy samples, studying DNA methylation of the host genome, and interrogating the host cells with different metabolites. Importantly, the study will also include a survey of yeast and viruses found in the microbiome. 362
3.4.2. Spatiotemporal Control of the Engineered Microbiome
For the engineered microbiome to safely impart health benefits to the host, it should exhibit predictable spatiotemporal behavior. Environmental perturbations and spatial organization are major variables that can influence the complex and dynamic interactions of the microbiome. 363 , 364 For example, Sheth et al. studied the microbial biogeography of the mouse intestine and showed both positive and negative associations between individual taxa. 365 Additionally, the authors showed that mice given a low-fat or high-fat diet led to changes in the species richness and altered spatial organization of the colon microbiome. Therefore, the engineered microbiome should be resilient to any perturbations and be able to adapt to its community structure over different time scales to continue providing the intended therapeutic effect to the host. This is particularly important in the case of engineered microbiomes with an associated fitness cost, which might lead to evolutionary adaptations through random mutations and horizontal gene transfer. Similar adaptations have been observed in the commensal microbe Bacteroides fragilis , leading to its long-term prevalence in the human gut. 366 Moreover, mechanisms to safeguard the host against unintended microbiome functions should be built-in as a safety feature.
A potential way to achieve this level of dynamic control of the composition and function of the microbiome is through synthetic biology. Genetic functionalities, such as biosensors, can be incorporated into the microbiomes such that they can be controlled through external stimuli. Additionally, genetic circuits can be designed for autonomous feedback control of the microbiome.
3.4.3. Genetically Intractable Microorganisms
The majority of microbiome members most relevant to human health, such as Clostridium and other anaerobic Firmicutes, remain poorly cultivable and genetically intractable. 367 This has hindered our ability to interrogate these microorganisms for mechanisms by which they modulate human health. Elucidating these mechanisms will not only deepen our understanding of host–microbiome and intramicrobiome interactions but also lead to more robust microbiome-based therapeutics that include these health-promoting bacteria. Thus, there is a great need for the development of novel genetic engineering tools that can be used in such genetically intractable microorganisms. These tools can range from well-characterized promoters, ribosome binding sites, terminators, and reporter genes to more complex genomic manipulation systems, such as CRISPR-Cas and homologous recombination.
In the following section, we will discuss how these challenges can be overcome with the aid of enabling technologies, advancing our understanding of microbiome–host interactions and the development of robust microbiome-based therapeutics.
4. Enabling Technologies for Microbiome Research and Engineering
Many studies including the examples mentioned in section 3 support the feasibility of engineered microbe therapy for microbiome-related diseases. 368 − 371 However, despite many proof-of-concept studies, several clinical trials using engineered microbes could not show efficacy or were terminated due to a lack of efficacy in the Phase 2 trials (e.g., {"type":"clinical-trial","attrs":{"text":"NCT03447730","term_id":"NCT03447730"}} NCT03447730 , {"type":"clinical-trial","attrs":{"text":"NCT03234465","term_id":"NCT03234465"}} NCT03234465 , and {"type":"clinical-trial","attrs":{"text":"NCT03447730","term_id":"NCT03447730"}} NCT03447730 ). One contributing factor toward the lack of efficacy in these engineered microbes may be the absence of regulatory mechanisms. Typically, these mechanisms control the expression of exogenous genes under stable promoters in the microbiome to enhance efficacy or reduce side effects. One of the advantages of utilizing microbes as therapeutics is their high programmability compared to conventional chemical medicine. Synthetic biology approaches are expected to enhance efficacies and reduce side effects to leverage on the high programmability bestowed by the implementation of precise spatial/temporal control into engineered microbes.
To fully harness engineered probiotics for therapy, it is essential to keep in mind engineering approaches and designs based on a more sophisticated knowledge of the mechanistic insights of microbiome-associated diseases and the microbiome itself. As mentioned in section 3.1.1 , the administration of pasteurized A. muciniphila to overweight/obese individuals improved insulin sensitivity and reduced cholesterol and body weight without changing the gut microbiome composition. Beyond reinforcing the importance of monitoring microbiome activities, this result suggests that the microbiome’s function or activity shifts can be more important compared to microbiome composition. Accordingly, functional meta-omics such as metabolomics, metatranscriptomics, metaproteomics, and multimeta-omics are gaining attention for investigating the causality of microbiome-associated diseases at the molecular, gene, and pathway levels.
In this section, we will review various meta-omics approaches for understanding molecular insights in the microbiome community and host interactions. We will also touch upon the many synthetic biology tools that can facilitate the reprogramming of microbes to potentially develop robust therapeutics with unique advantages over other strategies for microbiome engineering.
4.1. Functional Omics Approach
Metagenomics is a powerful tool for investigating the dynamics of microbiota, having revealed the association between microbiota composition and many diseases. Yet, metagenomic data provides limited mechanistic insights into microbiome-linked health states. To address this gap, applying functional meta-omics approaches such as metabolomics, metatranscriptomics, and metaproteomics to microbiota are expected to provide further insights. Here we will review the current progress in deploying functional omics to microbiota for investigating critical metabolites, microbiome activity, and interactions of host/diseases. Furthermore, we will review the microbiome and host genetics interactions.
4.1.1. Discovery of Novel Metabolites and Biosynthesis
Interestingly, metabolites from microbiota play a more critical role in host–microbe interaction rather than the commensal microbe itself. However, the metabolites and biosynthesis pathways that underlie host–microbe interactions are still unclear. Several studies have shown that microbes interact with the host by modulating signal pathways via metabolites. 372 For instance, SCFAs such as acetate, butyrate, and propionate are fermented in the colon from dietary fibers. These SCFAs are known to modulate the differentiation and accumulation of regulatory T cell (T reg cell) by activating the G-protein coupled receptor. 373 , 374 In turn, activated T reg cells produce the anti-inflammation factor IL-10, which is assumed to suppress gut inflammation diseases like IBD. The link between microbiome composition and metabolites is known to be indirect due to the functional redundancy of metabolic pathways, which suggests the interchangeability of some species. 375 Thus, analyzing differences in microbial composition may not reflect functional metabolite differences. In fact, phylogeny prediction from metabolomic data has been so far unsuccessful, indicating the difficulty of linking microbiome and metabolome data. 376 For this reason, identifying the metabolites associated with disease can provide more straightforward data for helping implement synthetic approaches for therapeutic purposes.
Untargeted metabolomics using mass spectrometry has been used for the discovery of key disease-associated metabolites. 377 , 378 Koh et al. employed untargeted metabolome analysis toward type 2 diabetes and discovered that imidazole propionate was present at higher concentrations among patients with type 2 diabetes. 377 Imidazole propionate is produced from histidine by gut microbes and impairs insulin signaling through mTORC1. Previously, researchers identified the UrdA gene that produces imidazole propionate in vitro, finding that the UrdA gene was more abundant in subjects with type 2 diabetes. 377 Another example is phenylacetylglutamine (PAGln), which was identified through untargeted metabolome analysis as a biomarker associated with a higher risk of cardiovascular diseases among type 2 diabetes patients. In the gut, PAGln is converted from dietary phenylalanine by the microbial porA gene, where it reportedly enhanced platelet activation-related phenotypes by stimulating G-protein coupled receptors. 378
Because untargeted mass spectrometry identifies molecules by comparing the spectrum patterns of chemicals of interest to chemicals in a reference database, metabolite identification solely relies on the references used in the analysis. This means that untargeted identification is unable to pinpoint unknown chemicals. In fact, it is estimated that >90% of metabolites in the microbiome lack matches in public databases. 379 Such uncharacterized metabolites are called “dark matter”. 379 To tackle this problem, machine learning-based reference generation is gaining prominence. Machine learning is a method for automatically building mathematical models for classification or prediction, wherein an algorithm learns patterns from training data sets. Machine learning has been used across a wide variety of applications, proving its versatility. 380 Currently, machine learning is being used for identifying microbiome metabolites during data preprocessing (peak detection, alignment, and identification), data processing (structure identification and compound quantification), and biological interpretation. 381 For instance, DarkChem used a deep learning approach to generate MS/MS libraries for predicting chemical properties in metabolomics and chemical identification. 382
4.1.2. Metatranscriptomics and Metaproteomics
In metatranscriptomics, the transcriptional activities of microbiota are analyzed using RNA sequencing. Unlike metagenomics, metatranscriptomics allows for the identification of active microbes, genes, and pathways in microbial communities. Metatranscriptomics has since been applied to a number of different types of microbiotas, 383 including those from seawater, 384 , 385 soils, 386 , 387 and human microbiota. 388 Likewise, metatranscriptomics approaches in human microbiota have enabled a deeper understanding of host–microbiota interactions, active microbes and their pathways, and expression changes in disease progression. 389 , 390 Nowicki et al. demonstrated how metatranscriptomics was applied to subgingival plaque from gingivitis patients. The study observed a significant shift of microbiota composition and increased virulence genes expression as gingivitis progressed, 389 demonstrating the importance of transcriptomics analysis for understanding molecular mechanisms during disease progression. Meanwhile, Schirmer et al. performed metatranscriptomics on a longitudinal IBD cohort to elucidate gene expression and their differences among healthy and IBD patients. 390 In the study, they detected species-specific biases in transcriptional activity. One example is the methylerythritol phosphate pathway (MEP) genes. They showed that Bacteroides vulgatus became the main transcriptional contributor of MEP at severe IBD stages. This study highlighted how metatranscriptomics analyses are a powerful tool for monitoring microbiome activities and gaining further insights into the role of the microbiome in diseases.
Although RNA expression can be a good indicator of gene expression, it does not always reflect protein abundances. Alternatively, metaproteomics can be engaged as an alternative approach for monitoring gene activity in microbiota. Metaproteomics was initially applied to investigate microbial function in environmental 391 and gut microbiome samples from twins in a 2009 study. 392 So far, multiple studies have demonstrated how metaproteomics analysis can be deployed for human microbiome samples. 393 − 395 Although metaproteomics is not as common as metatranscriptomics because of its lower throughput compared to deep sequencer-based analysis, metaproteomics can provide information on the post-translational modifications of proteins 396 , 397 and the expression of proteins secreted from the host cell, 398 , 399 both of which cannot be monitored through metatranscriptomics.
Another study by Zhang et al. analyzed lysine acetylation (Kac) changes in proteins in the gut microbiome of patients with Crohn’s diseases and negative control subjects. 400 In the study, they employed a peptide immune-affinity enrichment strategy followed by mass spectrometry to characterize Kac peptides and their changes in the human gut microbiome. Using the strategy, they identified Kac sites of 52 host and 136 microbial proteins that were differentially modified between Crohn’s disease patients and nonpatients. Likewise, Lobel et al. investigated the effect of diet on the post-translational modifications of proteins in the gut microbiome in chronic kidney disease (CKD) model mice. 397 They discovered that a high sulfur amino acid-containing diet resulted in the post-translational modification of microbial tryptophanase. The protein modification reduced the production of uremic toxin in CKD model mice. These studies show that microbiome function can be altered via post-translational modifications without changing their composition, underlying the importance of metaproteomics for investigating mechanistic insights of microbiome-related phenotypes.
Given the advantages and disadvantages associated with meta-omics approaches, multimeta-omics approaches have been employed to comprehensively understand microbiota gene activities as well as interactions within microbiota or between the microbiota and host. One Human Microbiome Project team conducted a multiomics analysis on a longitudinal IBD cohort to elucidate the molecular profiles of the host and microbiome activity. 401 In their study, they conducted metagenomics, metatranscriptomics, and metaproteomics on stool and serum samples from 132 subjects for 1 year. This study provided a comprehensive description of host and microbial activities, which helped identify microbial, biochemical, and host factors that contributed to dysregulation. Mills et al. 402 combined metagenomics, metapeptidomics, metaproteomics, and metabolomics approaches from 250 fecal samples for ulcerative colitis (UC) studies. They discovered that proteinase activity derived from B. vulgatus was associated with UC severity. In vitro and in vivo experiments also showed that treatment with a proteinase inhibitor could suppress UC symptoms, highlighting the potential of a multiomics approach in identifying causal genes from complicated microbiomes.
4.1.3. Microbiome Genome-Wide Association Study
Conventionally, it was held that interindividual variations in the microbiome composition were mainly influenced by environmental factors rather than the host’s genetic factors. 403 However, evidence from twin 404 , 405 and family 406 studies have indicated the presence of interactions between the microbiome and host genetics. In a U.K. twin study, the relative abundances of gut microbiota were more highly correlated within monozygotic twins than dizygotic twins, suggesting that the interactions between the microbiome and host genetics influenced gut microbiota composition. A better understanding of host genetics and microbiome interactions can assist precision medicine approaches and enhance the efficacy of engineered microbe therapeutics.
A larger proportion of identified genetic loci linked to microbiome variance are related to the dietary preferences of hosts or their immunity. One of the most replicated genomic loci is the LCT locus. Studies across U.K., Dutch, Canadian, and Finnish populations have shown an association between the LCT locus and Actinobacteria or Bifidobacterium . LCT encodes lactase, which digests lactate in the gut. One of the strongest associations with Bifidobacteria in the LCT locus is the functional SNP rs4988235. This SNP is known to be strongly associated with lactose intolerance and lactase expression. Therefore, lactose intolerance is the inability to digest lactose caused by the lower expression of lactase. Bifidobacteria are known to have the ability to digest lactose, 407 indicating that they compensate for reduced lactase activity in the host. Another well-replicated locus in microbiome composition is ABO. The association of the microbiome to the ABO locus was reported in studies conducted among German, Finnish, and Dutch populations. However, the bacteria associated with the ABO locus differs among the three countries. In addition to the ABO loci, FUT2 is also known to be associated with the microbiome, with FUT2 genes determining the ABO antigen on mucosal cells. The functional association between ABO and FUT2 suggests that the ABO locus is a contributing factor to microbiome composition. However, the mechanistic insights behind the microbiome changes have yet to be elucidated. Although dozens of genomics loci were reportedly associated with microbiota composition, most of the loci were not replicated in other studies.
Finally, host genetic variance can influence the host’s health states. One well-known example is the ATG16L1 locus. ATG16L1 encodes a subunit of the autophagy-related ATG12-ATG5/ATG16L1 complex and is involved in autophagosome formation. However, its function is not limited to autophagy; it is also involved in immune responses such as inflammation. 408 Several genome-wide association studies (GWASs) have shown the association between IBD and the ATG16L1 locus. 409 , 410 Chu et al. revealed that ATG16L1 is essential for immunomodulation by B. fragilis , which is known to secrete outer membrane vesicle (OMV) including immunomodulatory molecules that induce regulatory T cells. 411 They showed that the risk allele of ATG16L1 (A300) did not show OMV-mediated regulatory T cell induction for mucosal inflammation suppression and also displayed a deficiency of ATG16L1. These results indicate host variant–microbiota interaction and the importance of considering host genetic factors for therapeutic purposes.
4.2. Synthetic Biology and Cellular Reprogramming of Microbes
Synthetic biology aims to design and engineer organisms with desired functions that are predictable and consistent. To achieve this aim, synthetic biology introduces into biology engineering principles such as modularization, logic gate, and circuit design. 412 , 413 These efforts enable researchers to choose optimal genetic parts (e.g., promoter, ribosome binding site, terminator, peptide tag, and biosensor) as well as construct all types of logic gates 414 (AND, OR, NOR, NOT, XOR, and NAND) and synthetic devices that can control microbes similar to machines like the oscillator 415 and genetic toggle switch. 416 In turn, these well-characterized and tunable parts allow the assembly of more functional systems through an optimization cycle called the design–build–test–learn cycle (DBTL cycle). In this subsection, we will review how synthetic biology can be used for cellular reprogramming. We will also discuss how synthetic biology allows the spatiotemporal regulation of engineered microbes and how such techniques can be applied to microbiome environments.
4.2.1. Regulating Microbe Behavior Using Genetic Logic Circuits
A logic gate is an electronic device that performs a basic logical function by processing binary inputs to binary outputs. Most logic gates process two inputs into one output following the truth table ( Figure Figure5 5 A). For example, AND gate outputs 1 only when both inputs are 1 and OR gate outputs 1 when either input is 1. Each logic gate can operate a simple task. However, devices with multiple logic gates can process complicated tasks like a computer. Synthetic biology has enabled the development and implementation of genetic logic gates by mainly connecting transcription networks using transcription factors. In synthetic logic gates, expression represents 1 and no expression represents 0. An AND gate can be constructed by using a promoter that requires two transcription activators for its transcription ( Figure Figure5 5 B). The AND gate can be a very powerful and useful tool for modulating inputs and an output. For example, Merk et al. constructed a genetic AND gate that expresses output genes when both ITPG and tetrathionate exist. 418 Because IPTG is an artificial inducer and tetrathionate is gut inflammation marker, this AND gate allows for the temporal and spatial regulation of the expression of specific genes. While GFP was used as an output in their proof-of-concept study, the AND gate can be used to temporally or spatially control the in situ secretion of medicine by changing the output accordingly.

(A) Logic gates and their truth tables. (B) One example of the transcriptional AND gates. 417 The output gene expression is activated only when both A and B are induced. (C) Application of synthetic logic gates for the spatiotemporal control of engineered microbes. (D) Transcriptional network found in repressilator. TetR represses cI, cI represses LacI, and LacI represses TetR.
In addition, such logic gates are scalable and can accommodate an increase in the number of outputs and inputs by connecting multiple logic gates that can process more complicated environments. Taketani et al. developed a 2-input and 3-output logic circuit by combining three NOR gates. This circuit was then implemented in Bacteroides thetaiotaomicron , enabling the bacteria to express different genes in an environment-dependent manner such as the bioreactor stage, the gut stage, and after release from the host. 417 In this study, they employed anhydrotetracycline (aTc) and the bile acid deoxycholic acid (DCA) as inputs and demonstrated how the engineered B. thetaiotaomicron changed its behavior in an input-dependent manner in a human gastrointestinal model. These proof-of-concept studies show that scalable logic gates allow for the spatial and temporal regulation of engineered microbes under complicated environments. Therefore, administering standalone engineered microbes that behave in an environment-dependent manner improves the specificity and localization of their activity, improving overall efficacy.
An oscillator is an electronic device that outputs a periodic signal. Elowitz and Leibler constructed a genetic oscillator that expresses a marker gene periodically. 415 In the original genetic oscillator, three transcription repressors, LacI, TetR, and cI, were encoded in one plasmid called repressilator, and GFP was encoded under pLtetO1 in the other plasmid. Because TetR represses cI, cI represses LacI, and LacI represses TetR expression, the expression of each gene changed periodically. The GFP reporter gene was regulated by TetR, and its expression also changed periodically. Although the original repressilator showed periodic GFP expression, only 40% of cells were found to behave properly. Accordingly, the experiment was found to not be robustly designed—causing error propagation by stochastic effects. A follow-up study done by Potvin-Trottier et al. improved the original by removing the degradation tags attached to the repressors and introducing a “sponge” sequence that soaked up TetR molecules. 419 These changes reduced stochastic effects and improved the robustness of the genetic oscillator. Recently, an updated version of the oscillator was tested by Riglar et al. in a mouse gut environment to quantify bacterial dynamics in vivo. 420 They developed the RINGS (repressilator-based inference of growth at single-cell level) method to estimate bacterial generations after synchronization. By using the RINGS method, they succeeded in doing so in the mouse gut environment to understand in vivo bacterial dynamics. In the study, an oscillation system was used to monitor bacterial dynamics in vivo, but the oscillation system could be utilized for the periodic administration of drugs in situ as well. Moreover, these studies highlighted how synthetic genetic circuits can function properly in microbiome environments through the DBTL cycle.
The examples of genetic circuits presented earlier demonstrate how microbes can be reprogrammed to exhibit desired behavior using different genetic parts, such as promoters, repressors, and activators. In the following sections, we will review different types of cellular reprogramming that can potentially improve the efficacy of microbial therapies for microbiome-associated diseases.
4.2.2. Biosensors and Quorum Sensing
To process environmental information, microbes can be equipped with biosensors that can detect pH, temperature, light, metals, and chemical and biological compounds, among others. 421 In synthetic biology, biosensors are integrated into synthetic genetic circuits to detect and process environmental information for downstream processing, which allows engineered microbes to change their behaviors in an environment-dependent manner and to communicate among bacterial communities.
To engineer therapeutic microbes, implementing biosensors into genetic circuits can provide several advantages. First, biosensor-based expression can reduce genetic burden and improve the genetic stability of engineered microbes. It is well-known that implementing a synthetic genetic circuit can exert a burden on microbes, 422 resulting in genetic mutation, a loss of engineered function, and growth defects in the engineered microbes. 420 Most of the genetic burden arises from the consumption of cellular resources, reducing cellular fitness. 423 Therefore, silencing genetic circuit activity through biosensors can reduce genetic burden and keep engineered microbes functional. Another approach for reducing genetic burden is by cooperating multiple engineered microbes via quorum sensing (QS).
Second, biosensor-based expression can reduce the risks of off-target and resultant side effects. All medications have side effects mainly due to their dosage or unwanted targeting. Biosensor-based expression control has often been utilized for cancer therapy development, which requires specific targeting to reduce severe side effects. One common strategy is to express anticancer products under a hypoxia promoter from bacteria that can colonize tumor microenvironments, such as Salmonella typhimurium , Clostridium novyi , and EcN. He et al. engineered EcN to express Tum-5 or p53 under oxygen-dependent P vhb promoters, 424 , 425 which activates transcription under hypoxic areas such as the tumor microenvironment. In the study by He’s team, engineered EcN was injected into mice bearing tumors. They confirmed that the engineered EcN could repress tumor growth and that no obvious side effects could potentially occur from nonspecific targeting. More research regarding reprogramming microbes for cancer treatment has been reviewed elsewhere. 426 Another promising target for engineered microbes with biosensors is pathogens. As discussed in section 3.1.2 , employing QS machineries and antimicrobial agents enable the engineering of microbes that sense and eliminate specific bacteria secreting QS signal molecules. Third, biosensors can be used for diagnostic tools by combining memory systems, which will be reviewed in the next section.
Biosensor specificity is crucial for the proper function of engineered microbes, especially in complex heterogeneous environments, such as the human gut, which contains several structurally similar ligands. However, wild-type biosensors are often nonspecific and react to several structurally similar molecules. Hence, it is necessary to develop biosensors that can differentiate between such ligands in vivo to ensure an accurate response to the disease. Meyer et al. employed directed evolution to engineer 12 highly specific biosensors with lower cross-reactivity. 427 Recently, Rottinghaus et al. engineered EcN for the specific sensing of aromatic amino acids or neurochemicals through the rational improvement of biosensor specificity based on the protein structures. 428 Such studies are expanding the toolbox of high-quality biosensors for therapeutics purposes.
4.2.3. Memory Systems
Cellular memory is a phenomenon wherein transient signals are converted into a prolonged response. Cellular memory is common in most organisms and is used widely in biological events such as differentiation, 429 epigenetics, 430 and immunity. 431 Due to its potential applications, many types of synthetic memory circuits have been constructed based on various mechanisms 432 such as transcription factors, 416 DNA recombination, 433 and RNAi. 434
Memory systems can be classified as either reversible or irreversible. Reversible memory systems can be turned off upon the detection of another signal. Gardner et al. constructed a genetic toggle switch that can function as a reversible memory system by implementing LacI and cI or TetR to mutually inhibit their expression ( Figure Figure6 6 A). 416 Because repressors mutually inhibit expression, coexpression is at an unstable steady state and the expression of either repressor becomes randomly dominant in each cell without any stimulus. Gardner et al. also demonstrated that temporal exposure to inhibitors of the repressors could switch the cellular state and that the state lasts long after inhibitor withdrawal. On the other hand, an irreversible memory system cannot be turned off once a signal is detected. O’Gorman et al. developed an irreversible memory system utilizing Flippase that excises the reporter’s cis-element after a specific stimulus and keeps reporter gene expression on in a mammalian cell. 435

(A) Gene circuit of the genetic toggle switch developed by Gardner et al. 416 TetR and lacI each repress transcription. Either tetR or lacI expression is dominant without inducer. Each inducer (aTc or IPTG) inhibits a repressor and induces expression. Once cells are exposed to an inducer, either lacI or tetR become dominant. (B) Toggle switch-based memory circuit for a gut inflammation diagnosis tool. Cro is activated upon the detection of the inflammation marker tetrathionate by the ttrS/ttrR component. Once the circuit has detected tetrathionate, expression of cro becomes dominant even after tetrathionate withdrawal.
Synthetic memory circuits have proven to be a useful research tool for investigating fundamental biological mechanisms and as a potential tool in medicine and industry. For instance, the Cre-loxP system for creating tissue-specific knockout strains is an irreversible memory system 436 for investigating gene function in specific organs and tissue because it does not perturb gene function in other organs and tissues. The system is also used to trace the developmental lineage of cells. 437 For industrial applications, synthetic memory circuits can be used to reduce the cost of inducers for constant induction to produce biochemicals of interest. 432 By combining biosensors, synthetic memory systems can be used as noninvasive diagnosis tools for gut microbiome-associated diseases. Kotula et al. demonstrated that a synthetic memory circuit using a cI/cro bistable genetic switch could function in vivo. 438 In a follow-up study done by Riglar et al., 420 they developed a diagnosis tool for gut inflammation by implementing a memory system and a biosensor to detect the inflammation marker tetrathionate in the E. coli strain NGF-1 ( Figure Figure6 6 B). They used the TtrR/TtrS two-component system to sense tetrathionate and trigger the memory device and cI/cro bistable genetic switch for a memory device. This may allow the detection of disease onset in intestinal organs before symptoms worsen.
Biosensors are an essential building block for processing environmental cues in synthetic biology approaches. However, the number of biosensors that can monitor the inner states of the microbiome are still limited. To overcome this problem, Naydich et al. developed a high-throughput memory system than can be used to screen for biosensors that function in murine gut. 439 They implemented a cI/cro bistable toggle switch as a memory device. In the memory off state, the cI repressor is dominant, while cro and downstream lacZ expression is off. The trigger device is composed of a dominant negative mutant of cI (cI DN ) under a candidate promoter that is triggered by the condition of interest. cI DN , which has an N55K mutation in its DNA-binding region, forms a dimer with wild-type (WT) cI and derepresses cro and lacZ expression. Once cro is expressed highly enough, cro can keep repressing cI even without a stimulus. Hence, by generating a trigger device library, a high-throughput memory system can be used to screen for promoters that are active during the condition of interest. They tested this high-throughput memory system to screen for promoters with an increased response to an inflamed murine gut environment. This research exemplifies how synthetic biology approaches can be used as an investigation tool.
4.2.4. Kill Switches for Biocontainment and Drug Delivery
As research on developing microbes as therapeutic agents has progressed, issues relating to the biosafety of genetically modified organisms have emerged, raising concerns over the increased risk of spreading potentially hazardous biological materials to environments. Hence, the implementation of effective biocontainment systems is essential for real world usage, especially in cases where engineered wild-type commensal microbes are used due to their resilience in wild environments compared to commonly used laboratory strains. Many biocontainment strategies have already been developed through synthetic biology approaches. 440 The most readily used strategy is to introduce auxotrophic mutations, in which microbes are engineered to be dependent on specific nutrients for growth. However, auxotrophic strains may survive in natural environments that provide the nutrient. Moreover, this strategy can be used only for microbes that can be isolated and cultured in vitro. Therefore, the kill switch can be an alternative strategy for biocontainment.
Kill switches have long been used in synthetic biology. In 1987, Molin et al. developed a conditional suicide switch by expressing the Hok gene under the Trp promoter repressed by tryptophan. 441 The Hok gene causes the depolarization of the cellular membrane and results in cell death. They showed that Hok can work in broad ranges of both Gram-positive and Gram-negative bacteria. Contreras et al. utilized the nuclease gene from Serratia marcescens for a suicide gene combined with a thermo-induction promoter. 442 In 2016, Chan et al. developed the “deadman” and “passcode” kill switches, 443 which are passively activated. The deadman switch activates the toxin gene and inactivates an essential gene in the absence of a signal (ATc). To increase robustness, a genetic toggle switch was implemented in the deadman switch ( Figure Figure7 7 A). On the other hand, the passcode death switch employed the hybrid LacI-GalR family TFs 444 , 445 to allow multiple input molecules that control cell death ( Figure Figure7 7 B). They showed that the loss of IS1 and IS5, which caused a large percentage of inactivating mutations in the passcode circuit after long-term culturing, increased the passcode’s stability.

(A) Genetic circuit of deadman kill switch. Output of the toggle switch activates a toxin and inactivates an essential gene to kill cells, which can be induced by IPTG. Mf-lon proteinase then degrades lacI and essential genes to increase circuit stability. (B) Genetic circuit of passcode kill switch. A, B, and C represent repressor genes. The loss of input a or b or the addition of input c activates the toxin gene expression.
Due to the kill switch’s lethality, engineered cells always have a selection pressure to eliminate the kill switch. 443 Hence, improving the genetic stability of the kill switch is essential for proper and effective function. It was reported that mutagenesis is one of the major reasons why an engineered circuit loses function. 443 Thus, restoring the mutation to its original sequence may help increase stability. Chavez et al. developed a mutation-restoring system using CRISPR/Cas9. 446 In their system, gRNAs were designed to recognize specific mutations and convert the mutations back to their original functional sequences. They demonstrated that this system worked in a murine gut environment and reduced mutation frequencies drastically. Although this system can prevent specific mutations only, this method can be potentially applied to prevent hotspot mutations causing the loss of function of kill switches.
Another approach to improve the kill switch’s stability is functional redundancy within the circuit. Rottinghaus et al. engineered EcN with an aTc-inducible kill switch, which induces the expression of gRNA and Cas9 to cut the EcN genomic DNA. 447 They demonstrated that ∼10% and ∼80% of loss-of-function mutations in the kill switch were accumulated at the gRNA and the Cas9 cassettes, respectively. To decrease the probability of losing Cas9 and gRNA functions, they employed four functionally redundant Cas9 cassettes and two gRNA cassettes to achieve a 10 –8.6 killing efficiency, which surpassed the recommended killing efficiency of 10 –8 by the National Institutes of Health.
Another application of the kill switch is in the release of functional compounds from cells to target cells, e.g., pathogens, inflammation, and cancer cells in situ. Although proteins can be designed to be secreted by adding a secretion signal, nonpermeable small compounds require a specific transporter or channel to be exported from cells. Because those transporters and channels are not available in many cases, the kill switch can be a versatile alternative method to secrete any compounds, including large and small molecules. Saeidi et al. 448 demonstrated the feasibility of using a kill switch for secretion purposes. In the study, a sensor device that sensed P. aeruginosa through quorum sensing by the LasR protein activated cell lysis and released the Pyocin S5 protein to kill P. aeruginosa . However, this method still carries the risk of releasing the genetic material introduced into the engineered microbes to the environment
4.3. Chassis Engineering
To facilitate cellular reprogramming of the desired microbe, the relevant genetic circuit has to be introduced into the microbe. Such microbes are typically referred to as the “chassis”, with the term based on the structural framework of equipment and machines like automobiles. Accordingly, the microbe is usually transformed with plasmids to introduce new functions without changing its genomic DNA. In fact, many early proof-of-concept studies were done through plasmid-based approaches. 415 , 416 Still, problems with the plasmid-based approach include the lower stability of plasmid DNA as well as expression noise caused by copy number differences among cells. Hence, the integration of genetic parts or devices into the chassis’ genomic DNA is preferable, especially for therapeutic purposes. Moreover, chassis engineering enables the removal or enhancement of the organisms’ features to engineer a more suitable chassis for therapeutic purposes. Despite the advantages of chassis development, the genetic manipulation of commensal microbes still lags behind model organisms such as E. coli and S. cerevisiae —both of which are not major members of the human microbiome. In this subsection, we will review current developments in CRISPR-based gene manipulation of microbes and in situ DNA transfer methodologies, which allow for the genetic modification of genetically intractable microorganisms.
4.3.1. CRISPR-Based Gene-Editing/Manipulation Tools
CRISPR was originally discovered as an immune system in archaea, although nowadays it is recognized as a genetics tool. CRISPR is mainly applied for gene editing through the introduction of DNA breaks, followed by homologous recombination using donor DNAs. CRISPR-directed homologous recombination has accelerated genome engineering in many organisms including those with genomes previously considered difficult to manipulate. 449 Currently, CRISPR tools are available in a wide range of commensal bacteria and yeast such as E. coli , 450 Lactobacillus , 451 Clostridium , 452 Bacteroides , 453 Staphylococcus , 454 Bacillus , 455 Saccharomyces , 456 and Candida ( 457 ) for site-directed mutagenesis and gene deletion/insertion. CRISPR has also been used to engineer the microbiome and commensal microbes for characterizing the gene function of microbiome-related phenotypes. Guo et al. employed CRISPR/Cas9 to demonstrate the potential link between host immunity response and SCFAs produced by Clostridium . 367 In the study, Guo et al. developed a CRISPR/Cas9 system for manipulating Clostridium sporogenes and deleted SCFA-related genes, resulting in the decreased SCFA production. By comparing immune responses between mice administered with wild-type strain and knockout strains, they showed that the loss of SCFA increased IgA plasma cells. This supports the immunomodulation function of SCFA. Ultimately, the study showed that CRISPR-based microbiome genetics can help identify causal genes and their interactions, which are required in synthetic biology approaches.
Although CRISPR-driven gene editing is widely available for many organisms, DNA breaks caused by CRISPR/Cas9 tend to lead to cell death rather than gene editing in a majority of commensal microbes that have limited homologous recombination activity. Hence, CRISPR/Cas9 cannot be used for the majority of nonmodel commensal bacteria. For these microbes, CRISPRi, CRISPRa, or base editors can be less-toxic alternatives. CRISPRi and CRISPRa are tools that modulate transcription activity rather than edit genes. Both tools employ dCas9, which has DNA binding activity rather than DNase activity, fused to a transcription repressor or activator, respectively, to modulate transcription near the loci sgRNA recognizes. Hence, CRISPRi and CRISPRa function as programmable transcription factors that ideally can target promoters of any gene. Their programmability allows knockdown screening for bacteria using CRISPRi libraries. 458 Accordingly, Peters et al. constructed a CRISPRi library that covers all of the essential genes of B. subtilis for functional screening and identifies the mechanism of action of antibiotics. 458
Due to the high programmability of CRISPRi and CRISPRa, they can be used as custom transcription factors for constructing genetic circuits. A number of CRISPR-based synthetic gene circuits have since been constructed. 459 Many logic gates, buffers, NOT, 460 AND, 436 , 461 , 462 OR, 462 NAND, 462 NOR, 436 , 462 , 463 XOR, 436 and NIMPLY 436 were successfully implemented using CRISPRi and CRISPRa. In addition to synthetic logic gates, synthetic gene circuits such as the bistable toggle switch, 464 oscillator, 464 and stripe pattern generation 464 were also implemented using CRISPRi and CRISPRa. So far, while most genetic circuits constructed by CRISPRi and CRISPRa are in E. coli or S. cerevisiae , this approach can also help construct genetic circuits in nonmodel organisms.
While CRISPR/Cas9 gene editing introduces mutations through DNA strand breaks and subsequent homologous recombination, base editors mutate DNA using deaminase. Base editors employ dCas9 or nickase Cas9 fused to a deaminase that can convert nucleotides. 465 , 466 For instance, the cytosine base editor converts C to G, 466 while the adenine base editor facilitates A to T conversion. 465 Because base editing is quite new, there is no study yet utilizing it for microbiome engineering. However, microbiome editing using base editors is expected to soon be applied in therapeutics due to its lower toxicity compared to using bacteria.
Another promising CRISPR-related tool for microbiome engineering is the CRISPR-associated Tn7 transposon (CAST). 467 , 468 CAST was first reported in 2017 by Peters et al. They reported that some bacteria carry a Tn7-like transposon, including Cas effector proteins of the CRISPR subtype I-F. The Tn7-like transposon lacks TnsE, which mediates target insertion of the Tn7 transposon. Hence, they hypothesized that this type of transposon hijacked and utilized the CRISPR system for DNA recognition, 467 with CAST transposing through a CRISPR-mediated manner. In 2019, two groups proved that CAST inserted into the target site in a CRISPR-mediated manner in E. coli . 469 , 470 Both showed that the target site can be reprogrammed by changing the gRNA sequence. Strecker et al. showed that DNA insertion efficiency can reach up to 80% without any selection, with the frequency of off-target insertion being <1%. According to studies, insertion efficiency is highly dependent on target sites. Strecker’s group also detected on-target insertion in 29 loci out of the 48 sites tested. In 2022, Rubin et al. applied CAST to develop the DNA-editing all-in-one RNA-guided CRISPR–Cas transposase (DART) system, which was site- and species-specific in a mouse gut microbial community. 471 This will be reviewed in the next section.
All in all, CRISPR enables the manipulation of a wide variety of genomic DNA. DNA delivery remains the first step in experimental manipulation for downstream processes, yet the majority of commensal microbes are not culturable. Consequently, in the next section, we will review the current progress of gene manipulation of microbiota in situ.
4.3.2. Genetic Manipulation of Microbes In Situ
Multiomics studies have uncovered how microbiota genes are associated with many human diseases and health states, as mentioned in section 2 . However, elucidating the causal relationship of microbiota genes to host disease remains tricky mainly due to the difficulty of genetic manipulation in nonmodel microbiota as well as the challenges involved in culturing them in vitro. 472 Consider how conventional DNA delivery methodologies such as chemical transformation and electroporation can only be applied in vitro. Therefore, alternative technologies for transferring DNA into microbiota in situ are gaining traction in microbiome engineering. In this section, we will review the current progress of DNA delivery technologies and commensal bacteria manipulation methods in situ.
To adapt to various conditions, environmental bacteria are known to actively exchange their plasmid DNAs among different species—a process called horizontal gene transfer (HGT). 473 One of the most widely used HGT methods is bacterial conjugation, where plasmid DNA is transferred from a donor bacteria to a recipient bacteria through a type IV secretion system. 474 The gut microbiota is considered a fertile environment for conjugative gene transfer. It has been reported that bacterial conjugation can be utilized to manipulate gut microbiota from various donors in situ. 475 , 476 Recently, bacterial conjugation has become increasingly prominent as a microbiome engineering tool. Ronda et al. developed a technique called metagenomic alteration of gut microbiome by in situ conjugation (MAGIC), which enables the transfer of plasmid DNAs from an E. coli donor strain using the Inc.Palpha-family RP4 conjugation system to gut microbiota in situ. 477 This system allowed them to introduce DNA to both Gram-positive and Gram-negative bacteria. The conjugation plasmid encoding the transposon and transposable cassette allows the integration of DNA into genomic DNA in situ. Fluorescence-activated cell sorting (FACS) and 16S RNA analysis showed that at least 5% of gut bacteria were successfully modified in situ. However, transconjugants were no longer detectable after 72 h, likely due to toxicity or vector instability. Consequently, further improvements are needed for MAGIC to be used in the stable genome manipulation of gut microbiota for the investigation and identification of causal genes in microbiome-associated diseases.
Another method for transferring DNA into bacteria in complex communities in situ is by using phages. Phages are viruses that infect specific bacteria and transfer their genomic DNA into bacterial cells. After infection, phage-derived plasmid DNA is integrated into host genomic DNA or replicated in the host. By cloning a desired DNA fragment into the phage’s genomic DNA, exogenous genes can be transferred to bacterial cells, where they confer new functions with a fairly high efficiency. Notably, the transduction efficiency of the P2 bacteriophage can reach nearly 100%, 478 far beyond other DNA delivery methods like chemical transformation or electroporation. Because of target specificity, high efficiency, and activity in situ, phages are now being applied in microbiome engineering. 479 , 480 For instance, Citorik et al. utilized a phage to deliver CRISPR/Cas9 with a gRNA targeting the antibiotic resistance gene in a synthetic E. coli population in waxworms and changed bacteria composition. 481 In addition, Lam et al. demonstrated that phages could deliver a CRISPR/Cas9 cassette to E. coli in the murine gut, 294 showing that CRISPR/Cas9 delivered by a phage in situ could cause a chromosomal large deletion.
Knowing genetically tractable microbes and choosing an optimal DNA transfer method for microbes of interest in situ are crucial because there is no versatile DNA transfer method for all commensal microbes. Rubin et al. 471 developed the environmental transformation sequencing (ET-seq) and DNA-editing all-in-one RNA-guided CRISPR–Cas transposase (DART) systems, techniques that allowed the identification of genetically tractable bacteria in microbial communities and organism- and locus-specific genetic manipulation in situ. In ET-seq, DNA containing a nontargeted mariner transposon was transferred to microbial communities by conjugation, electroporation, or natural transformation, after which transposon-integrated loci were identified by deep sequencing. Following the identification of tractable bacteria and an optimal DNA delivery method, organism- and locus-specific CRISPR-associated transposase plasmids were designed and introduced into soil and infant gut microbiota. Using DART, they targeted strain-specific genomic loci of E. coli and demonstrated that DART could change E. coli strain composition. Another example of the optimization of DNA manipulation was done by Jin et al. They developed a pipeline to manipulate nonmodel gut microbiota ( Clostridia ) in vitro and in the host. 303 Their pipeline included the identification of compatible rep and ori combinations for the vector, antibody selection for both E. coli and Clostridia , and, finally, the reduction of restriction modifications to increase stability for stable conjugation and genome modification.
5. Challenges and Limitations
In section 2 , we reviewed microbiome studies that showed the link between the microbiome and disease/health states. These studies suggested that the microbiome plays a crucial role in human health through microbiome–host interactions. Meanwhile, in section 3 , we reviewed how compositional and functional alterations of the microbiome could affect human health states, indicating that the microbiome can be a contributing factor to several diseases. Revealing the causality of microbiomes in diseases enables its modulation by various strategies. However, microbiome engineering therapies have not yet yielded a viable commercial product. Although we are awaiting the results of clinical evaluation in some cases, other therapies, particularly engineered bacteria therapies, have been unable to perform well in clinical trials so far due to lack of efficacy.
Accordingly, we propose three limitations hindering the feasibility of leveraging engineered microbiome therapy for diseases: (1) the lack of mechanistic understanding underlying microbiome-associated diseases at multiple levels; (2) the challenges involved in modifying genetically intractable organisms; and (3) the spatiotemporal regulation of the engineered microbiome. In section 4 , we reviewed the current progress of meta-omics studies and synthetic biology tools that can help resolve these limitations. In particular, metabolomics, metatranscriptomics, metaproteomics, and multimeta-omics approaches enable association studies at the metabolite, gene, and gene interaction levels—deepening our knowledge of the molecular mechanisms of microbiome-associated diseases and allowing the design of reprogrammed microbes with predictable and regulated behavior against specific targets. Moreover, CRISPR and in situ DNA transfer technologies using bacterial conjugation and phages permits the manipulation of genetic intractable microbes both in vitro and in situ. Advances in such new DNA manipulation technologies will help in the identification of responsible genes and the engineering of commensal microbes in vitro and in situ.
We also reviewed how common design principles in synthetic biology taken from engineering fields facilitate spatiotemporal regulation in engineered microbes with synthetic genetic circuits. Although most experiments were performed in in vitro conditions, some were applied in the gut microbiome, proving the feasibility of spatiotemporal regulation within the microbiome environment.
As mentioned earlier, functional meta-omics and synthetic biology approaches help resolve the limitations hindering the feasibility of engineered microbe therapy. However, experimental tools must still be specifically tuned for studying the microbiome, as synthetic biology emerged independently of microbiome studies. Hence, most available tools are designed for E. coli to work under constant laboratory conditions. To date, a collection of standardized genetic parts for the nonmodel commensal microbiome remains limited or is unavailable. For instance, E. coli genetic parts were originally developed for in vitro purposes; hence, most of their functionalities in microbiome environments are not well-evaluated and may cause the loss of robustness in genetic circuits. A robust design is crucial for therapeutic applications due to the diversity of the microbiomes found in individuals. Therefore, the optimization of genetic parts and gene circuits for in vivo environments is set to be accelerated by developments in in vitro platforms such as organs-on-a-chip and organoids.
Acknowledgments
We thank Kamila Isabelle Alabado Navarro for the comments made on the manuscript. We acknowledge the financial support from Investigatorship of the National Research Foundation of Singapore (NRF-NRFI05-2019-0004), NUS Medicine Synthetic Biology Translational Research Programme (NUHSRO/2020/077/MSC/02/SB), the Summit Research Programme of the National University Health System (NUHSRO/2016/053/SRP/05), the Synthetic Biology Initiative of the National University of Singapore (DPRT/943/09/14), ISF-NRF Joint Program of the National Research Foundation of Singapore (NRF2019-NRF-ISF003-3208), the Ministry of Education of Singapore (NUHSRO/2020/046/T1/3), the U.S. Air Force Office of Scientific Research–Asian Office of Aerospace Research and Development (FA2386-18-1-4058), and the Singapore Economic Development Board (S18-139S-IPP-1I). All figures were created using Biorender.com .
Biographies
Nikhil Aggarwal is currently a Research Fellow in Prof. Matthew Chang’s group at the National University of Singapore. He received his Ph.D. degree from the National University of Singapore in 2020 under the supervision of Prof. Matthew Chang, during which he worked on developing new genetic tools and engineering Lactobacillus spp. His research focuses on genetically engineering probiotic bacterial strains to develop novel therapeutic modalities by rewiring host–microbiome interactions.
Shohei Kitano obtained his Ph.D. degree from the Tokyo Institute of Technology in 2018. He is currently working in synthetic genomics in the Chang Lab in SynCTI at the National University of Singapore. His research interest lies in genomes and phenotypes and phenomena elicited by genomes and their interactions including the microbiome field. He is keen to employ synthetic approaches to omics studies.
Ginette Puah obtained her Bachelor’s and Master’s degrees in Chemistry and Biological Chemistry from Nanyang Technological University. She is currently a Ph.D. candidate at the National University of Singapore and is also a trainee in the WIL@NUS Corporate Laboratory supported by the Singapore Economic Development Board (EDB) industrial programme. Under the guidance of A/Prof. Matthew Chang and Dr. Sandra Kittelmann, she is applying synthetic biology to beneficial microorganisms for the production of exotic fatty acids.
Sandra Kittelmann received her Ph.D. from the Philipps University of Marburg (Max Planck Institute for Terrestrial Microbiology), Germany, in 2007. In the Janssen Lab at AgResearch, Ltd., Palmerston North, New Zealand, she developed new tools for microbiome research and identified rumen-dwelling taxa indicative of lower greenhouse gas emissions in sheep, work that contributed significantly towards the successful breeding of low methane-emitting sheep in New Zealand. In 2018, she joined Wilmar International, Ltd., WIL@NUS Corporate Laboratory, Singapore, and was appointed Team Lead (Wilmar Fellow) in 2020. Her scientific interests are in dissecting the structure and function of food, feed, and host-associated microbiomes to elucidate microbe–microbe and microbe–host interactions and in characterizing novel species and enzymes for application in bioprocesses and bioproduction.
In Young Hwang, Ph.D., is an Associate Professor at the Singapore Institute of Technology and a principal investigator at the NUS Synthetic Biology for Clinical and Technological Innovation (SynCTI). She has an adjunct appointment at National University of Singapore. She received her B.Sc. in Biomedical Science in 2005 and her Ph.D. in 2010 from University of Auckland. Her current research focuses on reprogramming microbes to perform novel functionalities that are relevant to therapeutic and industrial applications.
Matthew Wook Chang is Dean’s Chair Associate Professor of Biochemistry and Synthetic Biology at the Yong Loo Lin School of Medicine at the National University of Singapore (NUS). He is also the Director of the Singapore Consortium for Synthetic Biology (SINERGY), Wilmar-NUS Corporate Laboratory (WIL@NUS), NUS Synthetic Biology for Clinical and Technological Innovation (SynCTI), and NUS Medicine Synthetic Biology Translational Research Programme (Syn Bio TRP). His research focuses on studying the engineering of biology to develop autonomous, programmable cells for biomedical and biomanufacturing applications across various industries.
Author Contributions
∇ N.A., S.K., and G.R.Y.P. contributed equally.
The authors declare no competing financial interest.
- Hooper L. V.; Gordon J. I. Commensal Host-Bacterial Relationships in the Gut . Science 2001, 292 , 1115–1118. 10.1126/science.1058709. [ PubMed ] [ CrossRef ] [ Google Scholar ]
- Theis K. R.; Dheilly N. M.; Klassen J. L.; Brucker R. M.; Baines J. F.; Bosch T. C.; Cryan J. F.; Gilbert S. F.; Goodnight C. J.; Lloyd E. A.; et al. Getting the Hologenome Concept Right: An Eco-Evolutionary Framework for Hosts and Their Microbiomes . mSystems 2016, 1 , e00028-16. 10.1128/mSystems.00028-16. [ PMC free article ] [ PubMed ] [ CrossRef ] [ Google Scholar ]
- Simon J. C.; Marchesi J. R.; Mougel C.; Selosse M. A. Host-Microbiota Interactions: From Holobiont Theory to Analysis . Microbiome 2019, 7 , 5. 10.1186/s40168-019-0619-4. [ PMC free article ] [ PubMed ] [ CrossRef ] [ Google Scholar ]
- Berg G.; Rybakova D.; Fischer D.; Cernava T.; Verges M. C.; Charles T.; Chen X.; Cocolin L.; Eversole K.; Corral G. H.; et al. Microbiome Definition Re-Visited: Old Concepts and New Challenges . Microbiome 2020, 8 , 103. 10.1186/s40168-020-00875-0. [ PMC free article ] [ PubMed ] [ CrossRef ] [ Google Scholar ]
- Kim S. A.; Kim B. R.; Chun M. Y.; Youn S. W. Relation between Ph in the Trunk and Face: Truncal Ph Can Be Easily Predicted from Facial Ph . Ann. Dermatol. 2016, 28 , 216–221. 10.5021/ad.2016.28.2.216. [ PMC free article ] [ PubMed ] [ CrossRef ] [ Google Scholar ]
- Herath M.; Hosie S.; Bornstein J. C.; Franks A. E.; Hill-Yardin E. L. The Role of the Gastrointestinal Mucus System in Intestinal Homeostasis: Implications for Neurological Disorders . Front. Cell. Infect. Microbiol. 2020, 10 , 248. 10.3389/fcimb.2020.00248. [ PMC free article ] [ PubMed ] [ CrossRef ] [ Google Scholar ]
- Yatsunenko T.; Rey F. E.; Manary M. J.; Trehan I.; Dominguez-Bello M. G.; Contreras M.; Magris M.; Hidalgo G.; Baldassano R. N.; Anokhin A. P.; et al. Human Gut Microbiome Viewed across Age and Geography . Nature 2012, 486 , 222–227. 10.1038/nature11053. [ PMC free article ] [ PubMed ] [ CrossRef ] [ Google Scholar ]
- Guigoz Y.; Dore J.; Schiffrin E. J. The Inflammatory Status of Old Age Can Be Nurtured from the Intestinal Environment . Curr. Opin. Clin. Nutr. Metab. Care 2008, 11 , 13–20. 10.1097/MCO.0b013e3282f2bfdf. [ PubMed ] [ CrossRef ] [ Google Scholar ]
- Aas J. A.; Paster B. J.; Stokes L. N.; Olsen I.; Dewhirst F. E. Defining the Normal Bacterial Flora of the Oral Cavity . J. Clin. Microbiol. 2005, 43 , 5721–5732. 10.1128/JCM.43.11.5721-5732.2005. [ PMC free article ] [ PubMed ] [ CrossRef ] [ Google Scholar ]
- Wade W. G. The Oral Microbiome in Health and Disease . Pharmacol. Res. 2013, 69 , 137–143. 10.1016/j.phrs.2012.11.006. [ PubMed ] [ CrossRef ] [ Google Scholar ]
- Kumaraswamy K. L.; Vidhya M. Human Papilloma Virus and Oral Infections: An Update . J. Cancer. Res. Ther. 2011, 7 , 120–127. 10.4103/0973-1482.82915. [ PubMed ] [ CrossRef ] [ Google Scholar ]
- Wantland W. W.; Wantland E. M.; Remo J. W.; Winquist D. L. Studies on Human Mouth Protozoa . J. Dent. Res. 1958, 37 , 949–950. 10.1177/00220345580370052601. [ PubMed ] [ CrossRef ] [ Google Scholar ]
- Wescombe P. A.; Heng N. C.; Burton J. P.; Chilcott C. N.; Tagg J. R. Streptococcal Bacteriocins and the Case for Streptococcus Salivarius as Model Oral Probiotics . Future Microbiol. 2009, 4 , 819–835. 10.2217/fmb.09.61. [ PubMed ] [ CrossRef ] [ Google Scholar ]
- Loesche W. Dental Caries and Periodontitis: Contrasting Two Infections That Have Medical Implications . Infect. Dis. Clin. North. Am. 2007, 21 , 471–502. 10.1016/j.idc.2007.03.006. [ PubMed ] [ CrossRef ] [ Google Scholar ] vii
- Parahitiyawa N. B.; Scully C.; Leung W. K.; Yam W. C.; Jin L. J.; Samaranayake L. P. Exploring the Oral Bacterial Flora: Current Status and Future Directions . Oral Dis. 2010, 16 , 136–145. 10.1111/j.1601-0825.2009.01607.x. [ PubMed ] [ CrossRef ] [ Google Scholar ]
- Fejerskov O. Changing Paradigms in Concepts on Dental Caries: Consequences for Oral Health Care . Caries Res. 2004, 38 , 182–191. 10.1159/000077753. [ PubMed ] [ CrossRef ] [ Google Scholar ]
- Filoche S.; Wong L.; Sissons C. H. Oral Biofilms: Emerging Concepts in Microbial Ecology . J. Dent. Res. 2010, 89 , 8–18. 10.1177/0022034509351812. [ PubMed ] [ CrossRef ] [ Google Scholar ]
- Zarco M. F.; Vess T. J.; Ginsburg G. S. The Oral Microbiome in Health and Disease and the Potential Impact on Personalized Dental Medicine . Oral Dis. 2012, 18 , 109–120. 10.1111/j.1601-0825.2011.01851.x. [ PubMed ] [ CrossRef ] [ Google Scholar ]
- Horz H. P.; Conrads G. Diagnosis and Anti-Infective Therapy of Periodontitis . Expert Rev. Anti. Infect. Ther. 2007, 5 , 703–715. 10.1586/14787210.5.4.703. [ PubMed ] [ CrossRef ] [ Google Scholar ]
- Williams R. C.; Barnett A. H.; Claffey N.; Davis M.; Gadsby R.; Kellett M.; Lip G. Y.; Thackray S. The Potential Impact of Periodontal Disease on General Health: A Consensus View . Curr. Med. Res. Opin. 2008, 24 , 1635–1643. 10.1185/03007990802131215. [ PubMed ] [ CrossRef ] [ Google Scholar ]
- Li X.; Kolltveit K. M.; Tronstad L.; Olsen I. Systemic Diseases Caused by Oral Infection . Clin. Microbiol. Rev. 2000, 13 , 547–558. 10.1128/CMR.13.4.547. [ PMC free article ] [ PubMed ] [ CrossRef ] [ Google Scholar ]
- Vieira A. T.; Castelo P. M.; Ribeiro D. A.; Ferreira C. M. Influence of Oral and Gut Microbiota in the Health of Menopausal Women . Front. Microbiol. 2017, 8 , 1884. 10.3389/fmicb.2017.01884. [ PMC free article ] [ PubMed ] [ CrossRef ] [ Google Scholar ]
- Do L. G.; Ha D. H.; Bell L. K.; Devenish G.; Golley R. K.; Leary S. D.; Manton D. J.; Thomson W. M.; Scott J. A.; Spencer A. J. Study of Mothers’ and Infants’ Life Events Affecting Oral Health (Smile) Birth Cohort Study: Cohort Profile . BMJ. Open 2020, 10 , e041185 10.1136/bmjopen-2020-041185. [ PMC free article ] [ PubMed ] [ CrossRef ] [ Google Scholar ]
- Craig S. J. C.; Blankenberg D.; Parodi A. C. L.; Paul I. M.; Birch L. L.; Savage J. S.; Marini M. E.; Stokes J. L.; Nekrutenko A.; Reimherr M.; et al. Child Weight Gain Trajectories Linked to Oral Microbiota Composition . Sci. Rep. 2018, 8 , 14030. 10.1038/s41598-018-31866-9. [ PMC free article ] [ PubMed ] [ CrossRef ] [ Google Scholar ]
- Jensen E. D.; Selway C. A.; Allen G.; Bednarz J.; Weyrich L. S.; Gue S.; Pena A. S.; Couper J. Early Markers of Periodontal Disease and Altered Oral Microbiota Are Associated with Glycemic Control in Children with Type 1 Diabetes . Pediatr. Diabetes 2021, 22 , 474–481. 10.1111/pedi.13170. [ PubMed ] [ CrossRef ] [ Google Scholar ]
- Willis J. R.; Gabaldon T. The Human Oral Microbiome in Health and Disease: From Sequences to Ecosystems . Microorganisms 2020, 8 , 308. 10.3390/microorganisms8020308. [ PMC free article ] [ PubMed ] [ CrossRef ] [ Google Scholar ]
- Xue L.; Zou X.; Yang X. Q.; Peng F.; Yu D. K.; Du J. R. Chronic Periodontitis Induces Microbiota-Gut-Brain Axis Disorders and Cognitive Impairment in Mice . Exp. Neurol. 2020, 326 , 113176. 10.1016/j.expneurol.2020.113176. [ PubMed ] [ CrossRef ] [ Google Scholar ]
- Lin D.; Hutchison K. E.; Portillo S.; Vegara V.; Ellingson J. M.; Liu J.; Krauter K. S.; Carroll-Portillo A.; Calhoun V. D. Association between the Oral Microbiome and Brain Resting State Connectivity in Smokers . Neuroimage 2019, 200 , 121–131. 10.1016/j.neuroimage.2019.06.023. [ PMC free article ] [ PubMed ] [ CrossRef ] [ Google Scholar ]
- Yang I.; Arthur R. A.; Zhao L.; Clark J.; Hu Y.; Corwin E. J.; Lah J. The Oral Microbiome and Inflammation in Mild Cognitive Impairment . Exp. Gerontol. 2021, 147 , 111273. 10.1016/j.exger.2021.111273. [ PubMed ] [ CrossRef ] [ Google Scholar ]
- Cunha F. A.; Cota L. O. M.; Cortelli S. C.; Miranda T. B.; Neves F. S.; Cortelli J. R.; Costa F. O. Periodontal Condition and Levels of Bacteria Associated with Periodontitis in Individuals with Bipolar Affective Disorders: A Case-Control Study . J. Periodontal Res. 2019, 54 , 63–72. 10.1111/jre.12605. [ PubMed ] [ CrossRef ] [ Google Scholar ]
- Shafquat A.; Joice R.; Simmons S. L.; Huttenhower C. Functional and Phylogenetic Assembly of Microbial Communities in the Human Microbiome . Trends Microbiol. 2014, 22 , 261–266. 10.1016/j.tim.2014.01.011. [ PMC free article ] [ PubMed ] [ CrossRef ] [ Google Scholar ]
- Baker J. L.; Bor B.; Agnello M.; Shi W.; He X. Ecology of the Oral Microbiome: Beyond Bacteria . Trends Microbiol. 2017, 25 , 362–374. 10.1016/j.tim.2016.12.012. [ PMC free article ] [ PubMed ] [ CrossRef ] [ Google Scholar ]
- Knight R.; Callewaert C.; Marotz C.; Hyde E. R.; Debelius J. W.; McDonald D.; Sogin M. L. The Microbiome and Human Biology . Annu. Rev. Genomics Hum. Genet. 2017, 18 , 65–86. 10.1146/annurev-genom-083115-022438. [ PubMed ] [ CrossRef ] [ Google Scholar ]
- Yang I.; Nell S.; Suerbaum S. Survival in Hostile Territory: The Microbiota of the Stomach . FEMS Microbiol. Rev. 2013, 37 , 736–761. 10.1111/1574-6976.12027. [ PubMed ] [ CrossRef ] [ Google Scholar ]
- Wu W. M.; Yang Y. S.; Peng L. H. Microbiota in the Stomach: New Insights . J. Dig. Dis. 2014, 15 , 54–61. 10.1111/1751-2980.12116. [ PubMed ] [ CrossRef ] [ Google Scholar ]
- Oren A.; Garrity G. M. Valid Publication of the Names of Forty-Two Phyla of Prokaryotes . Int. J. Syst. Evol. Microbiol. 2021, 71 , 005056 10.1099/ijsem.0.005056. [ PubMed ] [ CrossRef ] [ Google Scholar ]
- Bik E. M.; Eckburg P. B.; Gill S. R.; Nelson K. E.; Purdom E. A.; Francois F.; Perez-Perez G.; Blaser M. J.; Relman D. A. Molecular Analysis of the Bacterial Microbiota in the Human Stomach . Proc. Natl. Acad. Sci. U. S. A. 2006, 103 , 732–737. 10.1073/pnas.0506655103. [ PMC free article ] [ PubMed ] [ CrossRef ] [ Google Scholar ]
- Delgado S.; Cabrera-Rubio R.; Mira A.; Suarez A.; Mayo B. Microbiological Survey of the Human Gastric Ecosystem Using Culturing and Pyrosequencing Methods . Microb. Ecol. 2013, 65 , 763–772. 10.1007/s00248-013-0192-5. [ PubMed ] [ CrossRef ] [ Google Scholar ]
- Liu X.; Shao L.; Liu X.; Ji F.; Mei Y.; Cheng Y.; Liu F.; Yan C.; Li L.; Ling Z. Alterations of Gastric Mucosal Microbiota across Different Stomach Microhabitats in a Cohort of 276 Patients with Gastric Cancer . EBioMedicine 2019, 40 , 336–348. 10.1016/j.ebiom.2018.12.034. [ PMC free article ] [ PubMed ] [ CrossRef ] [ Google Scholar ]
- Nardone G.; Compare D. The Human Gastric Microbiota: Is It Time to Rethink the Pathogenesis of Stomach Diseases? . United European Gastroenterol. J. 2015, 3 , 255–260. 10.1177/2050640614566846. [ PMC free article ] [ PubMed ] [ CrossRef ] [ Google Scholar ]
- Sung J.; Kim N.; Kim J.; Jo H. J.; Park J. H.; Nam R. H.; Seok Y. J.; Kim Y. R.; Lee D. H.; Jung H. C. Comparison of Gastric Microbiota between Gastric Juice and Mucosa by Next Generation Sequencing Method . J. Cancer. Prev. 2016, 21 , 60–65. 10.15430/JCP.2016.21.1.60. [ PMC free article ] [ PubMed ] [ CrossRef ] [ Google Scholar ]
- Zilberstein B.; Quintanilha A. G.; Santos M. A. A.; Pajecki D.; Moura E. G.; Alves P. R A.; Filho F. M.; de Souza J. A. U.; Gama-Rodrigues J. Digestive Tract Microbiota in Healthy Volunteers . Clinics 2007, 62 , 47–56. 10.1590/S1807-59322007000100008. [ PubMed ] [ CrossRef ] [ Google Scholar ]
- Yu G.; Torres J.; Hu N.; Medrano-Guzman R.; Herrera-Goepfert R.; Humphrys M. S.; Wang L.; Wang C.; Ding T.; Ravel J.; et al. Molecular Characterization of the Human Stomach Microbiota in Gastric Cancer Patients . Front. Cell Infect. Microbiol. 2017, 7 , 302. 10.3389/fcimb.2017.00302. [ PMC free article ] [ PubMed ] [ CrossRef ] [ Google Scholar ]
- Mitchell D. R.; Derakhshan M. H.; Wirz A. A.; Orange C.; Ballantyne S. A.; Going J. J.; McColl K. E. L. The Gastric Acid Pocket Is Attenuated in H. Pylori Infected Subjects . Gut 2017, 66 , 1555–1562. 10.1136/gutjnl-2016-312638. [ PubMed ] [ CrossRef ] [ Google Scholar ]
- Li T. H.; Qin Y.; Sham P. C.; Lau K. S.; Chu K. M.; Leung W. K. Alterations in Gastric Microbiota after H. Pylori Eradication and in Different Histological Stages of Gastric Carcinogenesis . Sci. Rep. 2017, 7 , 44935. 10.1038/srep44935. [ PMC free article ] [ PubMed ] [ CrossRef ] [ Google Scholar ]
- Arumugam M.; Raes J.; Pelletier E.; Le Paslier D.; Yamada T.; Mende D. R.; Fernandes G. R.; Tap J.; Bruls T.; Batto J. M.; et al. Enterotypes of the Human Gut Microbiome . Nature 2011, 473 , 174–180. 10.1038/nature09944. [ PMC free article ] [ PubMed ] [ CrossRef ] [ Google Scholar ]
- Eggesbo M.; Moen B.; Peddada S.; Baird D.; Rugtveit J.; Midtvedt T.; Bushel P. R.; Sekelja M.; Rudi K. Development of Gut Microbiota in Infants Not Exposed to Medical Interventions . APMIS 2011, 119 , 17–35. 10.1111/j.1600-0463.2010.02688.x. [ PMC free article ] [ PubMed ] [ CrossRef ] [ Google Scholar ]
- Karlsson C. L.; Molin G.; Cilio C. M.; Ahrne S. The Pioneer Gut Microbiota in Human Neonates Vaginally Born at Term-a Pilot Study . Pediatr. Res. 2011, 70 , 282–286. 10.1203/PDR.0b013e318225f765. [ PubMed ] [ CrossRef ] [ Google Scholar ]
- Biasucci G.; Rubini M.; Riboni S.; Morelli L.; Bessi E.; Retetangos C. Mode of Delivery Affects the Bacterial Community in the Newborn Gut . Early Hum. Dev. 2010, 86 , 13–15. 10.1016/j.earlhumdev.2010.01.004. [ PubMed ] [ CrossRef ] [ Google Scholar ]
- Huurre A.; Kalliomaki M.; Rautava S.; Rinne M.; Salminen S.; Isolauri E. Mode of Delivery - Effects on Gut Microbiota and Humoral Immunity . Neonatology 2008, 93 , 236–240. 10.1159/000111102. [ PubMed ] [ CrossRef ] [ Google Scholar ]
- Fallani M.; Young D.; Scott J.; Norin E.; Amarri S.; Adam R.; Aguilera M.; Khanna S.; Gil A.; Edwards C. A.; et al. Intestinal Microbiota of 6-Week-Old Infants across Europe: Geographic Influence Beyond Delivery Mode, Breast-Feeding, and Antibiotics . J. Pediatr. Gastroenterol. Nutr. 2010, 51 , 77–84. 10.1097/MPG.0b013e3181d1b11e. [ PubMed ] [ CrossRef ] [ Google Scholar ]
- Klaassens E. S.; Boesten R. J.; Haarman M.; Knol J.; Schuren F. H.; Vaughan E. E.; de Vos W. M. Mixed-Species Genomic Microarray Analysis of Fecal Samples Reveals Differential Transcriptional Responses of Bifidobacteria in Breast- and Formula-Fed Infants . Appl. Environ. Microbiol. 2009, 75 , 2668–2676. 10.1128/AEM.02492-08. [ PMC free article ] [ PubMed ] [ CrossRef ] [ Google Scholar ]
- Harmsen H. J.; Wildeboer-Veloo A. C.; Raangs G. C.; Wagendorp A. A.; Klijn N.; Bindels J. G.; Welling G. W. Analysis of Intestinal Flora Development in Breast-Fed and Formula-Fed Infants by Using Molecular Identification and Detection Methods . J. Pediatr. Gastroenterol. Nutr. 2000, 30 , 61–67. 10.1097/00005176-200001000-00019. [ PubMed ] [ CrossRef ] [ Google Scholar ]
- Roger L. C.; McCartney A. L. Longitudinal Investigation of the Faecal Microbiota of Healthy Full-Term Infants Using Fluorescence in Situ Hybridization and Denaturing Gradient Gel Electrophoresis . Microbiology 2010, 156 , 3317–3328. 10.1099/mic.0.041913-0. [ PubMed ] [ CrossRef ] [ Google Scholar ]
- Favier C. F.; Vaughan E. E.; De Vos W. M.; Akkermans A. D. Molecular Monitoring of Succession of Bacterial Communities in Human Neonates . Appl. Environ. Microbiol. 2002, 68 , 219–226. 10.1128/AEM.68.1.219-226.2002. [ PMC free article ] [ PubMed ] [ CrossRef ] [ Google Scholar ]
- Fallani M.; Amarri S.; Uusijarvi A.; Adam R.; Khanna S.; Aguilera M.; Gil A.; Vieites J. M.; Norin E.; Young D.; et al. Determinants of the Human Infant Intestinal Microbiota after the Introduction of First Complementary Foods in Infant Samples from Five European Centres . Microbiology 2011, 157 , 1385–1392. 10.1099/mic.0.042143-0. [ PubMed ] [ CrossRef ] [ Google Scholar ]
- O’Toole P. W.; Claesson M. J. Gut Microbiota: Changes Throughout the Lifespan from Infancy to Elderly . Int. Dairy J. 2010, 20 , 281–291. 10.1016/j.idairyj.2009.11.010. [ CrossRef ] [ Google Scholar ]
- Woodmansey E. J. Intestinal Bacteria and Ageing . J. Appl. Microbiol. 2007, 102 , 1178–1186. 10.1111/j.1365-2672.2007.03400.x. [ PubMed ] [ CrossRef ] [ Google Scholar ]
- Claesson M. J.; Cusack S.; O’Sullivan O.; Greene-Diniz R.; de Weerd H.; Flannery E.; Marchesi J. R.; Falush D.; Dinan T.; Fitzgerald G.; et al. Composition, Variability, and Temporal Stability of the Intestinal Microbiota of the Elderly . Proc. Natl. Acad. Sci. U. S. A. 2011, 108 , 4586–4591. 10.1073/pnas.1000097107. [ PMC free article ] [ PubMed ] [ CrossRef ] [ Google Scholar ]
- Flint H. J.; Scott K. P.; Louis P.; Duncan S. H. The Role of the Gut Microbiota in Nutrition and Health . Nat. Rev. Gastroenterol. Hepatol. 2012, 9 , 577–589. 10.1038/nrgastro.2012.156. [ PubMed ] [ CrossRef ] [ Google Scholar ]
- Islam K. B.; Fukiya S.; Hagio M.; Fujii N.; Ishizuka S.; Ooka T.; Ogura Y.; Hayashi T.; Yokota A. Bile Acid Is a Host Factor That Regulates the Composition of the Cecal Microbiota in Rats . Gastroenterology 2011, 141 , 1773–1781. 10.1053/j.gastro.2011.07.046. [ PubMed ] [ CrossRef ] [ Google Scholar ]
- Booijink C. C.; El-Aidy S.; Rajilic-Stojanovic M.; Heilig H. G.; Troost F. J.; Smidt H.; Kleerebezem M.; De Vos W. M.; Zoetendal E. G. High Temporal and Inter-Individual Variation Detected in the Human Ileal Microbiota . Environ. Microbiol. 2010, 12 , 3213–3227. 10.1111/j.1462-2920.2010.02294.x. [ PubMed ] [ CrossRef ] [ Google Scholar ]
- Zoetendal E. G.; Raes J.; van den Bogert B.; Arumugam M.; Booijink C. C.; Troost F. J.; Bork P.; Wels M.; de Vos W. M.; Kleerebezem M. The Human Small Intestinal Microbiota Is Driven by Rapid Uptake and Conversion of Simple Carbohydrates . ISME J. 2012, 6 , 1415–1426. 10.1038/ismej.2011.212. [ PMC free article ] [ PubMed ] [ CrossRef ] [ Google Scholar ]
- Rinninella E.; Raoul P.; Cintoni M.; Franceschi F.; Miggiano G. A. D.; Gasbarrini A.; Mele M. C. What Is the Healthy Gut Microbiota Composition? A Changing Ecosystem across Age, Environment, Diet, and Diseases . Microorganisms 2019, 7 , 14. 10.3390/microorganisms7010014. [ PMC free article ] [ PubMed ] [ CrossRef ] [ Google Scholar ]
- Gill S. R.; Pop M.; Deboy R. T.; Eckburg P. B.; Turnbaugh P. J.; Samuel B. S.; Gordon J. I.; Relman D. A.; Fraser-Liggett C. M.; Nelson K. E. Metagenomic Analysis of the Human Distal Gut Microbiome . Science 2006, 312 , 1355–1359. 10.1126/science.1124234. [ PMC free article ] [ PubMed ] [ CrossRef ] [ Google Scholar ]
- Khosravi A.; Mazmanian S. K. Disruption of the Gut Microbiome as a Risk Factor for Microbial Infections . Curr. Opin. Microbiol. 2013, 16 , 221–227. 10.1016/j.mib.2013.03.009. [ PMC free article ] [ PubMed ] [ CrossRef ] [ Google Scholar ]
- Walker A. W.; Duncan S. H.; McWilliam Leitch E. C.; Child M. W.; Flint H. J. Ph and Peptide Supply Can Radically Alter Bacterial Populations and Short-Chain Fatty Acid Ratios within Microbial Communities from the Human Colon . Appl. Environ. Microbiol. 2005, 71 , 3692–3700. 10.1128/AEM.71.7.3692-3700.2005. [ PMC free article ] [ PubMed ] [ CrossRef ] [ Google Scholar ]
- Louis P.; Scott K. P.; Duncan S. H.; Flint H. J. Understanding the Effects of Diet on Bacterial Metabolism in the Large Intestine . J. Appl. Microbiol. 2007, 102 , 1197–1208. 10.1111/j.1365-2672.2007.03322.x. [ PubMed ] [ CrossRef ] [ Google Scholar ]
- Kho Z. Y.; Lal S. K. The Human Gut Microbiome - a Potential Controller of Wellness and Disease . Front. Microbiol. 2018, 9 , 1835. 10.3389/fmicb.2018.01835. [ PMC free article ] [ PubMed ] [ CrossRef ] [ Google Scholar ]
- Barnaba V.; Sinigaglia F. Molecular Mimicry and T Cell-Mediated Autoimmune Disease . J. Exp. Med. 1997, 185 , 1529–1531. 10.1084/jem.185.9.1529. [ PMC free article ] [ PubMed ] [ CrossRef ] [ Google Scholar ]
- Bartlett J. G. Antimicrobial Agents Implicated in Clostridium Difficile Toxin-Associated Diarrhea of Colitis . Johns Hopkins Med. J. 1981, 149 , 6–9. [ PubMed ] [ Google Scholar ]
- Song H. J.; Shim K. N.; Jung S. A.; Choi H. J.; Lee M. A.; Ryu K. H.; Kim S. E.; Yoo K. Antibiotic-Associated Diarrhea: Candidate Organisms Other Than Clostridium Difficile . Korean J. Int. Med. 2008, 23 , 9–15. 10.3904/kjim.2008.23.1.9. [ PMC free article ] [ PubMed ] [ CrossRef ] [ Google Scholar ]
- Pear S. M.; Williamson T. H.; Bettin K. M.; Gerding D. N.; Galgiani J. N. Decrease in Nosocomial Clostridium Difficile-Associated Diarrhea by Restricting Clindamycin Use . Ann. Int. Med. 1994, 120 , 272–277. 10.7326/0003-4819-120-4-199402150-00003. [ PubMed ] [ CrossRef ] [ Google Scholar ]
- Lennard-Jones J. E. Classification of Inflammatory Bowel Disease . Scand. J. Gastroenterol. 1989, 24 , 2–6. 10.3109/00365528909091339. [ PubMed ] [ CrossRef ] [ Google Scholar ]
- Nagao-Kitamoto H.; Shreiner A. B.; Gillilland M. G. 3rd; Kitamoto S.; Ishii C.; Hirayama A.; Kuffa P.; El-Zaatari M.; Grasberger H.; Seekatz A. M.; et al. Functional Characterization of Inflammatory Bowel Disease-Associated Gut Dysbiosis in Gnotobiotic Mice. . Cell Mol. Gastroenterol. Hepatol. 2016, 2 , 468–481. 10.1016/j.jcmgh.2016.02.003. [ PMC free article ] [ PubMed ] [ CrossRef ] [ Google Scholar ]
- Sokol H.; Pigneur B.; Watterlot L.; Lakhdari O.; Bermudez-Humaran L. G.; Gratadoux J. J.; Blugeon S.; Bridonneau C.; Furet J. P.; Corthier G.; et al. Faecalibacterium Prausnitzii Is an Anti-Inflammatory Commensal Bacterium Identified by Gut Microbiota Analysis of Crohn Disease Patients . Proc. Natl. Acad. Sci. U. S. A. 2008, 105 , 16731–16736. 10.1073/pnas.0804812105. [ PMC free article ] [ PubMed ] [ CrossRef ] [ Google Scholar ]
- Willing B. P.; Dicksved J.; Halfvarson J.; Andersson A. F.; Lucio M.; Zheng Z.; Jarnerot G.; Tysk C.; Jansson J. K.; Engstrand L. A Pyrosequencing Study in Twins Shows That Gastrointestinal Microbial Profiles Vary with Inflammatory Bowel Disease Phenotypes . Gastroenterology 2010, 139 , 1844–1854. 10.1053/j.gastro.2010.08.049. [ PubMed ] [ CrossRef ] [ Google Scholar ]
- Machiels K.; Joossens M.; Sabino J.; De Preter V.; Arijs I.; Eeckhaut V.; Ballet V.; Claes K.; Van Immerseel F.; Verbeke K.; et al. A Decrease of the Butyrate-Producing Species Roseburia Hominis and Faecalibacterium Prausnitzii Defines Dysbiosis in Patients with Ulcerative Colitis . Gut 2014, 63 , 1275–1283. 10.1136/gutjnl-2013-304833. [ PubMed ] [ CrossRef ] [ Google Scholar ]
- Peng L.; He Z.; Chen W.; Holzman I. R.; Lin J. Effects of Butyrate on Intestinal Barrier Function in a Caco-2 Cell Monolayer Model of Intestinal Barrier . Pediatr. Res. 2007, 61 , 37–41. 10.1203/01.pdr.0000250014.92242.f3. [ PubMed ] [ CrossRef ] [ Google Scholar ]
- Carroll I. M.; Chang Y. H.; Park J.; Sartor R. B.; Ringel Y. Luminal and Mucosal-Associated Intestinal Microbiota in Patients with Diarrhea-Predominant Irritable Bowel Syndrome . Gut Pathog. 2010, 2 , 19. 10.1186/1757-4749-2-19. [ PMC free article ] [ PubMed ] [ CrossRef ] [ Google Scholar ]
- Bhattarai Y.; Muniz Pedrogo D. A.; Kashyap P. C. Irritable Bowel Syndrome: A Gut Microbiota-Related Disorder? . Am. J. Physiol. Gastrointest. Liver Physiol. 2017, 312 , G52–G62. 10.1152/ajpgi.00338.2016. [ PMC free article ] [ PubMed ] [ CrossRef ] [ Google Scholar ]
- Salonen A.; de Vos W. M.; Palva A. Gastrointestinal Microbiota in Irritable Bowel Syndrome: Present State and Perspectives . Microbiology 2010, 156 , 3205–3215. 10.1099/mic.0.043257-0. [ PubMed ] [ CrossRef ] [ Google Scholar ]
- Greenblum S.; Turnbaugh P. J.; Borenstein E. Metagenomic Systems Biology of the Human Gut Microbiome Reveals Topological Shifts Associated with Obesity and Inflammatory Bowel Disease . Proc. Natl. Acad. Sci. U. S. A. 2012, 109 , 594–599. 10.1073/pnas.1116053109. [ PMC free article ] [ PubMed ] [ CrossRef ] [ Google Scholar ]
- Schloissnig S.; Arumugam M.; Sunagawa S.; Mitreva M.; Tap J.; Zhu A.; Waller A.; Mende D. R.; Kultima J. R.; Martin J.; et al. Genomic Variation Landscape of the Human Gut Microbiome . Nature 2013, 493 , 45–50. 10.1038/nature11711. [ PMC free article ] [ PubMed ] [ CrossRef ] [ Google Scholar ]
- Faith J. J.; Guruge J. L.; Charbonneau M.; Subramanian S.; Seedorf H.; Goodman A. L.; Clemente J. C.; Knight R.; Heath A. C.; Leibel R. L.; et al. The Long-Term Stability of the Human Gut Microbiota . Science 2013, 341 , 1237439. 10.1126/science.1237439. [ PMC free article ] [ PubMed ] [ CrossRef ] [ Google Scholar ]
- Yan M.; Pamp S. J.; Fukuyama J.; Hwang P. H.; Cho D. Y.; Holmes S.; Relman D. A. Nasal Microenvironments and Interspecific Interactions Influence Nasal Microbiota Complexity and S. Aureus Carriage . Cell Host Microbe 2013, 14 , 631–640. 10.1016/j.chom.2013.11.005. [ PMC free article ] [ PubMed ] [ CrossRef ] [ Google Scholar ]
- Biswas K.; Hoggard M.; Jain R.; Taylor M. W.; Douglas R. G. The Nasal Microbiota in Health and Disease: Variation within and between Subjects . Front. Microbiol. 2015, 9 , 134. 10.3389/fmicb.2015.00134. [ PMC free article ] [ PubMed ] [ CrossRef ] [ Google Scholar ]
- Bassis C. M.; Tang A. L.; Young V. B.; Pynnonen M. A. The Nasal Cavity Microbiota of Healthy Adults . Microbiome 2014, 2 , 27. 10.1186/2049-2618-2-27. [ PMC free article ] [ PubMed ] [ CrossRef ] [ Google Scholar ]
- Zhou Y.; Mihindukulasuriya K. A.; Gao H.; La Rosa P. S.; Wylie K. M.; Martin J. C.; Kota K.; Shannon W. D.; Mitreva M.; Sodergren E.; et al. Exploration of Bacterial Community Classes in Major Human Habitats . Genome Biol. 2014, 15 , R66. 10.1186/gb-2014-15-5-r66. [ PMC free article ] [ PubMed ] [ CrossRef ] [ Google Scholar ]
- Ramakrishnan V. R.; Feazel L. M.; Gitomer S. A.; Ir D.; Robertson C. E.; Frank D. N. The Microbiome of the Middle Meatus in Healthy Adults . PLoS One 2013, 8 , e85507. 10.1371/journal.pone.0085507. [ PMC free article ] [ PubMed ] [ CrossRef ] [ Google Scholar ]
- Zhang Z.; Adappa N. D.; Doghramji L. J.; Chiu A. G.; Cohen N. A.; Palmer J. N. Different Clinical Factors Associated with Staphylococcus Aureus and Pseudomonas Aeruginosa in Chronic Rhinosinusitis . Int. Forum Allergy. Rhinol. 2015, 5 , 724–733. 10.1002/alr.21532. [ PubMed ] [ CrossRef ] [ Google Scholar ]
- Abreu N. A.; Nagalingam N. A.; Song Y.; Roediger F. C.; Pletcher S. D.; Goldberg A. N.; Lynch S. V. Sinus Microbiome Diversity Depletion and Corynebacterium Tuberculostearicum Enrichment Mediates Rhinosinusitis . Sci. Transl. Med. 2012, 4 , 151ra124. 10.1126/scitranslmed.3003783. [ PMC free article ] [ PubMed ] [ CrossRef ] [ Google Scholar ]
- Huang Y. J.; Nelson C. E.; Brodie E. L.; DeSantis T. Z.; Baek M. S.; Liu J.; Woyke T.; Allgaier M.; Bristow J.; Wiener-Kronish J. P.; et al. Airway Microbiota and Bronchial Hyperresponsiveness in Patients with Suboptimally Controlled Asthma . J. Allergy Clin. Immunol. 2011, 127 , 372–381. 10.1016/j.jaci.2010.10.048. [ PMC free article ] [ PubMed ] [ CrossRef ] [ Google Scholar ]
- Marri P. R.; Stern D. A.; Wright A. L.; Billheimer D.; Martinez F. D. Asthma-Associated Differences in Microbial Composition of Induced Sputum . J. Allergy Clin. Immunol. 2013, 131 , 346–352. 10.1016/j.jaci.2012.11.013. [ PMC free article ] [ PubMed ] [ CrossRef ] [ Google Scholar ]
- Bisgaard H.; Hermansen M. N.; Buchvald F.; Loland L.; Halkjaer L. B.; Bonnelykke K.; Brasholt M.; Heltberg A.; Vissing N. H.; Thorsen S. V.; et al. Childhood Asthma after Bacterial Colonization of the Airway in Neonates . N. Engl. J. Med. 2007, 357 , 1487–1495. 10.1056/NEJMoa052632. [ PubMed ] [ CrossRef ] [ Google Scholar ]
- Kraft M. The Role of Bacterial Infections in Asthma . Clin. Chest. Med. 2000, 21 , 301–313. 10.1016/S0272-5231(05)70268-9. [ PubMed ] [ CrossRef ] [ Google Scholar ]
- Sethi S.; Evans N.; Grant B. J.; Murphy T. F. New Strains of Bacteria and Exacerbations of Chronic Obstructive Pulmonary Disease . N. Engl. J. Med. 2002, 347 , 465–471. 10.1056/NEJMoa012561. [ PubMed ] [ CrossRef ] [ Google Scholar ]
- Dickson R. P.; Erb-Downward J. R.; Martinez F. J.; Huffnagle G. B. The Microbiome and the Respiratory Tract . Annu. Rev. Physiol. 2016, 78 , 481–504. 10.1146/annurev-physiol-021115-105238. [ PMC free article ] [ PubMed ] [ CrossRef ] [ Google Scholar ]
- Bassis C. M.; Erb-Downward J. R.; Dickson R. P.; Freeman C. M.; Schmidt T. M.; Young V. B.; Beck J. M.; Curtis J. L.; Huffnagle G. B. Analysis of the Upper Respiratory Tract Microbiotas as the Source of the Lung and Gastric Microbiotas in Healthy Individuals . mBio 2015, 6 , e00037-15 10.1128/mBio.00037-15. [ PMC free article ] [ PubMed ] [ CrossRef ] [ Google Scholar ]
- Hanada S.; Pirzadeh M.; Carver K. Y.; Deng J. C. Respiratory Viral Infection-Induced Microbiome Alterations and Secondary Bacterial Pneumonia . Front. Immunol. 2018, 9 , 2640. 10.3389/fimmu.2018.02640. [ PMC free article ] [ PubMed ] [ CrossRef ] [ Google Scholar ]
- Soret P.; Vandenborght L. E.; Francis F.; Coron N.; Enaud R.; Avalos M.; Schaeverbeke T.; Berger P.; Fayon M.; Thiebaut R.; et al. Respiratory Mycobiome and Suggestion of Inter-Kingdom Network During Acute Pulmonary Exacerbation in Cystic Fibrosis . Sci. Rep. 2020, 10 , 3589. 10.1038/s41598-020-60015-4. [ PMC free article ] [ PubMed ] [ CrossRef ] [ Google Scholar ]
- Worlitzsch D.; Tarran R.; Ulrich M.; Schwab U.; Cekici A.; Meyer K. C.; Birrer P.; Bellon G.; Berger J.; Weiss T.; et al. Effects of Reduced Mucus Oxygen Concentration in Airway Pseudomonas Infections of Cystic Fibrosis Patients . J. Clin. Invest. 2002, 109 , 317–325. 10.1172/JCI0213870. [ PMC free article ] [ PubMed ] [ CrossRef ] [ Google Scholar ]
- Schmidt A.; Belaaouaj A.; Bissinger R.; Koller G.; Malleret L.; D’Orazio C.; Facchinelli M.; Schulte-Hubbert B.; Molinaro A.; Holst O.; et al. Neutrophil Elastase-Mediated Increase in Airway Temperature During Inflammation . J. Cyst. Fibros. 2014, 13 , 623–631. 10.1016/j.jcf.2014.03.004. [ PubMed ] [ CrossRef ] [ Google Scholar ]
- Casadevall A.; Pirofski L. A. The Damage-Response Framework of Microbial Pathogenesis . Nat. Rev. Microbiol. 2003, 1 , 17–24. 10.1038/nrmicro732. [ PMC free article ] [ PubMed ] [ CrossRef ] [ Google Scholar ]
- Freestone P. P.; Hirst R. A.; Sandrini S. M.; Sharaff F.; Fry H.; Hyman S.; O’Callaghan C. Pseudomonas Aeruginosa-Catecholamine Inotrope Interactions: A Contributory Factor in the Development of Ventilator-Associated Pneumonia? . Chest 2012, 142 , 1200–1210. 10.1378/chest.11-2614. [ PubMed ] [ CrossRef ] [ Google Scholar ]
- Kanangat S.; Meduri G. U.; Tolley E. A.; Patterson D. R.; Meduri C. U.; Pak C.; Griffin J. P.; Bronze M. S.; Schaberg D. R. Effects of Cytokines and Endotoxin on the Intracellular Growth of Bacteria . Infect. Immun. 1999, 67 , 2834–2840. 10.1128/IAI.67.6.2834-2840.1999. [ PMC free article ] [ PubMed ] [ CrossRef ] [ Google Scholar ]
- Kaza S. K.; McClean S.; Callaghan M. Il-8 Released from Human Lung Epithelial Cells Induced by Cystic Fibrosis Pathogens Burkholderia Cepacia Complex Affects the Growth and Intracellular Survival of Bacteria . Int. J. Med. Microbiol. 2011, 301 , 26–33. 10.1016/j.ijmm.2010.06.005. [ PubMed ] [ CrossRef ] [ Google Scholar ]
- Lyte M.; Ernst S. Catecholamine Induced Growth of Gram Negative Bacteria . Life Sci. 1992, 50 , 203–212. 10.1016/0024-3205(92)90273-R. [ PubMed ] [ CrossRef ] [ Google Scholar ]
- Dickson R. P.; Martinez F. J.; Huffnagle G. B. The Role of the Microbiome in Exacerbations of Chronic Lung Diseases . Lancet 2014, 384 , 691–702. 10.1016/S0140-6736(14)61136-3. [ PMC free article ] [ PubMed ] [ CrossRef ] [ Google Scholar ]
- Byrd A. L.; Belkaid Y.; Segre J. A. The Human Skin Microbiome . Nat. Rev. Microbiol. 2018, 16 , 143–155. 10.1038/nrmicro.2017.157. [ PubMed ] [ CrossRef ] [ Google Scholar ]
- Adamczyk K.; Garncarczyk A.; Antonczak P.; Wcislo-Dziadecka D. The Foot Microbiome . J. Cosmet. Dermatol. 2020, 19 , 1039–1043. 10.1111/jocd.13368. [ PubMed ] [ CrossRef ] [ Google Scholar ]
- Costello E. K.; Lauber C. L.; Hamady M.; Fierer N.; Gordon J. I.; Knight R. Bacterial Community Variation in Human Body Habitats across Space and Time . Science 2009, 326 , 1694–1697. 10.1126/science.1177486. [ PMC free article ] [ PubMed ] [ CrossRef ] [ Google Scholar ]
- Grice E. A.; Kong H. H.; Conlan S.; Deming C. B.; Davis J.; Young A. C.; Bouffard G. G.; Blakesley R. W.; Murray P. R.; et al. Topographical and Temporal Diversity of the Human Skin Microbiome . Science 2009, 324 , 1190–1192. 10.1126/science.1171700. [ PMC free article ] [ PubMed ] [ CrossRef ] [ Google Scholar ]
- Grice E. A.; Segre J. A. The Skin Microbiome . Nat. Rev. Microbiol. 2011, 9 , 244–253. 10.1038/nrmicro2537. [ PMC free article ] [ PubMed ] [ CrossRef ] [ Google Scholar ]
- Oh J.; Byrd A. L.; Deming C.; Conlan S.; Kong H. H.; Segre J. A. Biogeography and Individuality Shape Function in the Human Skin Metagenome . Nature 2014, 514 , 59–64. 10.1038/nature13786. [ PMC free article ] [ PubMed ] [ CrossRef ] [ Google Scholar ]
- Findley K.; Oh J.; Yang J.; Conlan S.; Deming C.; Meyer J. A.; Schoenfeld D.; Nomicos E.; Park M.; et al. Topographic Diversity of Fungal and Bacterial Communities in Human Skin . Nature 2013, 498 , 367–370. 10.1038/nature12171. [ PMC free article ] [ PubMed ] [ CrossRef ] [ Google Scholar ]
- Oh J.; Byrd A. L.; Park M.; Kong H. H.; Segre J. A. Temporal Stability of the Human Skin Microbiome . Cell 2016, 165 , 854–866. 10.1016/j.cell.2016.04.008. [ PMC free article ] [ PubMed ] [ CrossRef ] [ Google Scholar ]
- Feng H.; Shuda M.; Chang Y.; Moore P. S. Clonal Integration of a Polyomavirus in Human Merkel Cell Carcinoma . Science 2008, 319 , 1096–1100. 10.1126/science.1152586. [ PMC free article ] [ PubMed ] [ CrossRef ] [ Google Scholar ]
- Hannigan G. D.; Meisel J. S.; Tyldsley A. S.; Zheng Q.; Hodkinson B. P.; SanMiguel A. J.; Minot S.; Bushman F. D.; Grice E. A. The Human Skin Double-Stranded DNA Virome: Topographical and Temporal Diversity, Genetic Enrichment, and Dynamic Associations with the Host Microbiome . mBio 2015, 6 , e01578-15. 10.1128/mBio.01578-15. [ PMC free article ] [ PubMed ] [ CrossRef ] [ Google Scholar ]
- Scharschmidt T. C.; Fischbach M. A. What Lives on Our Skin: Ecology, Genomics and Therapeutic Opportunities of the Skin Microbiome . Drug Discovery Today Dis. Mech. 2013, 10 , e83–e89. 10.1016/j.ddmec.2012.12.003. [ PMC free article ] [ PubMed ] [ CrossRef ] [ Google Scholar ]
- Holland K. T.; Greenman J.; Cunliffe W. J. Growth of Cutaneous Propionibacteria on Synthetic Medium; Growth Yields and Exoenzyme Production . J. Appl. Bacteriol. 1979, 47 , 383–394. 10.1111/j.1365-2672.1979.tb01198.x. [ PubMed ] [ CrossRef ] [ Google Scholar ]
- Bruggemann H.; Henne A.; Hoster F.; Liesegang H.; Wiezer A.; Strittmatter A.; Hujer S.; Durre P.; Gottschalk G. The Complete Genome Sequence of Propionibacterium Acnes, a Commensal of Human Skin . Science 2004, 305 , 671–673. 10.1126/science.1100330. [ PubMed ] [ CrossRef ] [ Google Scholar ]
- Marples R. R.; Downing D. T.; Kligman A. M. Control of Free Fatty Acids in Human Surface Lipids by Corynebacterium Acnes . J. Invest. Dermatol. 1971, 56 , 127–131. 10.1111/1523-1747.ep12260695. [ PubMed ] [ CrossRef ] [ Google Scholar ]
- Ingham E.; Holland K. T.; Gowland G.; Cunliffe W. J. Partial Purification and Characterization of Lipase (Ec 3.1.1.3) from Propionibacterium Acnes . J. Gen. Microbiol. 1981, 124 , 393–401. [ PubMed ] [ Google Scholar ]
- Gribbon E. M.; Cunliffe W. J.; Holland K. T. Interaction of Propionibacterium Acnes with Skin Lipids in Vitro . J. Gen. Microbiol. 1993, 139 , 1745–1751. 10.1099/00221287-139-8-1745. [ PubMed ] [ CrossRef ] [ Google Scholar ]
- Mukherjee S.; Mitra R.; Maitra A.; Gupta S.; Kumaran S.; Chakrabortty A.; Majumder P. P. Sebum and Hydration Levels in Specific Regions of Human Face Significantly Predict the Nature and Diversity of Facial Skin Microbiome . Sci. Rep. 2016, 6 , 36062. 10.1038/srep36062. [ PMC free article ] [ PubMed ] [ CrossRef ] [ Google Scholar ]
- Oh J.; Conlan S.; Polley E. C.; Segre J. A.; Kong H. H. Shifts in Human Skin and Nares Microbiota of Healthy Children and Adults . Genome Med. 2012, 4 , 77. 10.1186/gm378. [ PMC free article ] [ PubMed ] [ CrossRef ] [ Google Scholar ]
- Jo J. H.; Deming C.; Kennedy E. A.; Conlan S.; Polley E. C.; Ng W. I.; Segre J. A.; Kong H. H. Diverse Human Skin Fungal Communities in Children Converge in Adulthood . J. Invest. Dermatol. 2016, 136 , 2356–2363. 10.1016/j.jid.2016.05.130. [ PMC free article ] [ PubMed ] [ CrossRef ] [ Google Scholar ]
- Jo J. H.; Kennedy E. A.; Kong H. H. Topographical and Physiological Differences of the Skin Mycobiome in Health and Disease . Virulence 2017, 8 , 324–333. 10.1080/21505594.2016.1249093. [ PMC free article ] [ PubMed ] [ CrossRef ] [ Google Scholar ]
- Kyriakis K. P.; Terzoudi S.; Palamaras I.; Pagana G.; Michailides C.; Emmanuelides S. Pityriasis Versicolor Prevalence by Age and Gender . Mycoses 2006, 49 , 517–518. 10.1111/j.1439-0507.2006.01285.x. [ PubMed ] [ CrossRef ] [ Google Scholar ]
- Havlickova B.; Czaika V. A.; Friedrich M. Epidemiological Trends in Skin Mycoses Worldwide . Mycoses 2008, 51 , 2–15. 10.1111/j.1439-0507.2008.01606.x. [ PubMed ] [ CrossRef ] [ Google Scholar ]
- Seebacher C.; Bouchara J. P.; Mignon B. Updates on the Epidemiology of Dermatophyte Infections . Mycopathologia 2008, 166 , 335–352. 10.1007/s11046-008-9100-9. [ PubMed ] [ CrossRef ] [ Google Scholar ]
- Tomida S.; Nguyen L.; Chiu B. H.; Liu J.; Sodergren E.; Weinstock G. M.; Li H. Pan-Genome and Comparative Genome Analyses of Propionibacterium Acnes Reveal Its Genomic Diversity in the Healthy and Diseased Human Skin Microbiome . mBio 2013, 4 , e00003-13. 10.1128/mBio.00003-13. [ PMC free article ] [ PubMed ] [ CrossRef ] [ Google Scholar ]
- Fitz-Gibbon S.; Tomida S.; Chiu B. H.; Nguyen L.; Du C.; Liu M.; Elashoff D.; Erfe M. C.; Loncaric A.; Kim J.; et al. Propionibacterium Acnes Strain Populations in the Human Skin Microbiome Associated with Acne . J. Invest. Dermatol. 2013, 133 , 2152–2160. 10.1038/jid.2013.21. [ PMC free article ] [ PubMed ] [ CrossRef ] [ Google Scholar ]
- Kang D.; Shi B.; Erfe M. C.; Craft N.; Li H. Vitamin B12 Modulates the Transcriptome of the Skin Microbiota in Acne Pathogenesis . Sci. Transl. Med. 2015, 7 , 293. 10.1126/scitranslmed.aab2009. [ PMC free article ] [ PubMed ] [ CrossRef ] [ Google Scholar ]
- Picardo M.; Ottaviani M.; Camera E.; Mastrofrancesco A. Sebaceous Gland Lipids . Dermatoendocrinol. 2009, 1 , 68–71. 10.4161/derm.1.2.8472. [ PMC free article ] [ PubMed ] [ CrossRef ] [ Google Scholar ]
- Jahns A. C.; Lundskog B.; Ganceviciene R.; Palmer R. H.; Golovleva I.; Zouboulis C. C.; McDowell A.; Patrick S.; Alexeyev O. A. An Increased Incidence of Propionibacterium Acnes Biofilms in Acne Vulgaris: A Case-Control Study . Br. J. Dermatol. 2012, 167 , 50–58. 10.1111/j.1365-2133.2012.10897.x. [ PubMed ] [ CrossRef ] [ Google Scholar ]
- Leyden J. J.; Marples R. R.; Kligman A. M. Staphylococcus Aureus in the Lesions of Atopic Dermatitis . Br. J. Dermatol. 1974, 90 , 525–530. 10.1111/j.1365-2133.1974.tb06447.x. [ PubMed ] [ CrossRef ] [ Google Scholar ]
- Salava A.; Lauerma A. Role of the Skin Microbiome in Atopic Dermatitis . Clin. Transl. Allergy 2014, 4 , 33. 10.1186/2045-7022-4-33. [ PMC free article ] [ PubMed ] [ CrossRef ] [ Google Scholar ]
- Drago L.; De Grandi R.; Altomare G.; Pigatto P.; Rossi O.; Toscano M. Skin Microbiota of First Cousins Affected by Psoriasis and Atopic Dermatitis . Clin. Mol. Allergy 2016, 14 , 2. 10.1186/s12948-016-0038-z. [ PMC free article ] [ PubMed ] [ CrossRef ] [ Google Scholar ]
- Gonzalez M. E.; Schaffer J. V.; Orlow S. J.; Gao Z.; Li H.; Alekseyenko A. V.; Blaser M. J. Cutaneous Microbiome Effects of Fluticasone Propionate Cream and Adjunctive Bleach Baths in Childhood Atopic Dermatitis . J. Am. Acad. Dermatol. 2016, 75 , 481–493. 10.1016/j.jaad.2016.04.066. [ PMC free article ] [ PubMed ] [ CrossRef ] [ Google Scholar ]
- Zasloff M. Antimicrobial Peptides, Innate Immunity, and the Normally Sterile Urinary Tract . J. Am. Soc. Nephrol. 2007, 18 , 2810–2816. 10.1681/ASN.2007050611. [ PubMed ] [ CrossRef ] [ Google Scholar ]
- Wolfe A. J.; Brubaker L. “Sterile Urine” and the Presence of Bacteria . Eur. Urol. 2015, 68 , 173–174. 10.1016/j.eururo.2015.02.041. [ PMC free article ] [ PubMed ] [ CrossRef ] [ Google Scholar ]
- Pearce M. M.; Hilt E. E.; Rosenfeld A. B.; Zilliox M. J.; Thomas-White K.; Fok C.; Kliethermes S.; Schreckenberger P. C.; Brubaker L.; Gai X.; et al. The Female Urinary Microbiome: A Comparison of Women with and without Urgency Urinary Incontinence . mBio 2014, 5 , e01283-14. 10.1128/mBio.01283-14. [ PMC free article ] [ PubMed ] [ CrossRef ] [ Google Scholar ]
- Mansour B.; Monyok A.; Makra N.; Gajdacs M.; Vadnay I.; Ligeti B.; Juhasz J.; Szabo D.; Ostorhazi E. Bladder Cancer-Related Microbiota: Examining Differences in Urine and Tissue Samples . Sci. Rep. 2020, 10 , 11042. 10.1038/s41598-020-67443-2. [ PMC free article ] [ PubMed ] [ CrossRef ] [ Google Scholar ]
- Modena B. D.; Milam R.; Harrison F.; Cheeseman J. A.; Abecassis M. M.; Friedewald J. J.; Kirk A. D.; Salomon D. R. Changes in Urinary Microbiome Populations Correlate in Kidney Transplants with Interstitial Fibrosis and Tubular Atrophy Documented in Early Surveillance Biopsies . Am. J. Transplant. 2017, 17 , 712–723. 10.1111/ajt.14038. [ PMC free article ] [ PubMed ] [ CrossRef ] [ Google Scholar ]
- Fouts D. E.; Pieper R.; Szpakowski S.; Pohl H.; Knoblach S.; Suh M. J.; Huang S. T.; Ljungberg I.; Sprague B. M.; Lucas S. K.; et al. Integrated Next-Generation Sequencing of 16s Rdna and Metaproteomics Differentiate the Healthy Urine Microbiome from Asymptomatic Bacteriuria in Neuropathic Bladder Associated with Spinal Cord Injury . J. Transl. Med. 2012, 10 , 174. 10.1186/1479-5876-10-174. [ PMC free article ] [ PubMed ] [ CrossRef ] [ Google Scholar ]
- Ruiz-Gomez M. L.; Martin-Way D. A.; Perez-Ramirez M. D.; Gutierrez-Fernandez J. Male Deep Infections by Gardnerella Vaginalis. A Literature Review and a Case Report . Rev. Esp. Quimioter. 2019, 32 , 469–472. [ PMC free article ] [ PubMed ] [ Google Scholar ]
- Gottschick C.; Deng Z. L.; Vital M.; Masur C.; Abels C.; Pieper D. H.; Wagner-Dobler I. The Urinary Microbiota of Men and Women and Its Changes in Women During Bacterial Vaginosis and Antibiotic Treatment . Microbiome 2017, 5 , 99. 10.1186/s40168-017-0305-3. [ PMC free article ] [ PubMed ] [ CrossRef ] [ Google Scholar ]
- Bajic P.; Van Kuiken M. E.; Burge B. K.; Kirshenbaum E. J.; Joyce C. J.; Wolfe A. J.; Branch J. D.; Bresler L.; Farooq A. V. Male Bladder Microbiome Relates to Lower Urinary Tract Symptoms . Eur. Urol. Focus 2020, 6 , 376–382. 10.1016/j.euf.2018.08.001. [ PubMed ] [ CrossRef ] [ Google Scholar ]
- Groah S. L.; Perez-Losada M.; Caldovic L.; Ljungberg I. H.; Sprague B. M.; Castro-Nallar E.; Chandel N. J.; Hsieh M. H.; Pohl H. G. Redefining Healthy Urine: A Cross-Sectional Exploratory Metagenomic Study of People with and without Bladder Dysfunction . J. Urol. 2016, 196 , 579–587. 10.1016/j.juro.2016.01.088. [ PubMed ] [ CrossRef ] [ Google Scholar ]
- Thomas-White K. J.; Gao X.; Lin H.; Fok C. S.; Ghanayem K.; Mueller E. R.; Dong Q.; Brubaker L.; Wolfe A. J. Urinary Microbes and Postoperative Urinary Tract Infection Risk in Urogynecologic Surgical Patients . Int. Urogynecol. J. 2018, 29 , 1797–1805. 10.1007/s00192-018-3767-3. [ PMC free article ] [ PubMed ] [ CrossRef ] [ Google Scholar ]
- Komesu Y. M.; Dinwiddie D. L.; Richter H. E.; Lukacz E. S.; Sung V. W.; Siddiqui N. Y.; Zyczynski H. M.; Ridgeway B.; Rogers R. G.; Arya L. A.; et al. Defining the Relationship between Vaginal and Urinary Microbiomes . Am. J. Obstet. Gynecol. 2020, 222 , 154.E1–154.E10. 10.1016/j.ajog.2019.08.011. [ PMC free article ] [ PubMed ] [ CrossRef ] [ Google Scholar ]
- Grine G.; Lotte R.; Chirio D.; Chevalier A.; Raoult D.; Drancourt M.; Ruimy R. Co-Culture of Methanobrevibacter Smithii with Enterobacteria During Urinary Infection . EBioMedicine 2019, 43 , 333–337. 10.1016/j.ebiom.2019.04.037. [ PMC free article ] [ PubMed ] [ CrossRef ] [ Google Scholar ]
- Johnson G.; Wolfe A. J.; Putonti C. Characterization of the φCTX-Like Pseudomonas Aeruginosa Phage Dobby Isolated from the Kidney Stone Microbiota . Access Microbiol. 2019, 1 , e000002 10.1099/acmi.0.000002. [ PMC free article ] [ PubMed ] [ CrossRef ] [ Google Scholar ]
- Malki K.; Sible E.; Cooper A.; Garretto A.; Bruder K.; Watkins S. C.; Putonti C. Seven Bacteriophages Isolated from the Female Urinary Microbiota . Genome Announce. 2016, 4 , e01003-16. 10.1128/genomeA.01003-16. [ PMC free article ] [ PubMed ] [ CrossRef ] [ Google Scholar ]
- Khasriya R.; Sathiananthamoorthy S.; Ismail S.; Kelsey M.; Wilson M.; Rohn J. L.; Malone-Lee J. Spectrum of Bacterial Colonization Associated with Urothelial Cells from Patients with Chronic Lower Urinary Tract Symptoms . J. Clin. Microbiol. 2013, 51 , 2054–2062. 10.1128/JCM.03314-12. [ PMC free article ] [ PubMed ] [ CrossRef ] [ Google Scholar ]
- Paalanne N.; Husso A.; Salo J.; Pievilainen O.; Tejesvi M. V.; Koivusaari P.; Pirttila A. M.; Pokka T.; Mattila S.; Jyrkas J.; et al. Intestinal Microbiome as a Risk Factor for Urinary Tract Infections in Children . Eur. J. Clin. Microbiol. Infect. Dis. 2018, 37 , 1881–1891. 10.1007/s10096-018-3322-7. [ PubMed ] [ CrossRef ] [ Google Scholar ]
- Magruder M.; Sholi A. N.; Gong C.; Zhang L.; Edusei E.; Huang J.; Albakry S.; Satlin M. J.; Westblade L. F.; Crawford C.; et al. Gut Uropathogen Abundance Is a Risk Factor for Development of Bacteriuria and Urinary Tract Infection . Nat. Commun. 2019, 10 , 5521. 10.1038/s41467-019-13467-w. [ PMC free article ] [ PubMed ] [ CrossRef ] [ Google Scholar ]
- Garretto A.; Miller-Ensminger T.; Ene A.; Merchant Z.; Shah A.; Gerodias A.; Biancofiori A.; Canchola S.; Canchola S.; Castillo E.; et al. Genomic Survey of E. Coli from the Bladders of Women with and without Lower Urinary Tract Symptoms . Front. Microbiol. 2020, 11 , 2094. 10.3389/fmicb.2020.02094. [ PMC free article ] [ PubMed ] [ CrossRef ] [ Google Scholar ]
- Lavigne J. P.; Nicolas-Chanoine M. H.; Bourg G.; Moreau J.; Sotto A. Virulent Synergistic Effect between Enterococcus Faecalis and Escherichia Coli Assayed by Using the Caenorhabditis Elegans Model . PLoS One 2008, 3 , e3370. 10.1371/journal.pone.0003370. [ PMC free article ] [ PubMed ] [ CrossRef ] [ Google Scholar ]
- Croxall G.; Weston V.; Joseph S.; Manning G.; Cheetham P.; McNally A. Increased Human Pathogenic Potential of Escherichia Coli from Polymicrobial Urinary Tract Infections in Comparison to Isolates from Monomicrobial Culture Samples . J. Med. Microbiol. 2011, 60 , 102–109. 10.1099/jmm.0.020602-0. [ PubMed ] [ CrossRef ] [ Google Scholar ]
- Stapleton A. E.; Au-Yeung M.; Hooton T. M.; Fredricks D. N.; Roberts P. L.; Czaja C. A.; Yarova-Yarovaya Y.; Fiedler T.; Cox M.; Stamm W. E. Randomized, Placebo-Controlled Phase 2 Trial of a Lactobacillus Crispatus Probiotic Given Intravaginally for Prevention of Recurrent Urinary Tract Infection . Clin. Infect. Dis. 2011, 52 , 1212–1217. 10.1093/cid/cir183. [ PMC free article ] [ PubMed ] [ CrossRef ] [ Google Scholar ]
- Sumati A. H.; Saritha N. K. Association of Urinary Tract Infection in Women with Bacterial Vaginosis . J. Glob. Infect. Dis. 2009, 1 , 151–152. 10.4103/0974-777X.56254. [ PMC free article ] [ PubMed ] [ CrossRef ] [ Google Scholar ]
- Gilbert N. M.; O’Brien V. P.; Lewis A. L. Transient Microbiota Exposures Activate Dormant Escherichia Coli Infection in the Bladder and Drive Severe Outcomes of Recurrent Disease . PLoS Pathog. 2017, 13 , e1006238. 10.1371/journal.ppat.1006238. [ PMC free article ] [ PubMed ] [ CrossRef ] [ Google Scholar ]
- Ravel J.; Gajer P.; Abdo Z.; Schneider G. M.; Koenig S. S.; McCulle S. L.; Karlebach S.; Gorle R.; Russell J.; Tacket C. O.; et al. Vaginal Microbiome of Reproductive-Age Women . Proc. Natl. Acad. Sci. U. S. A. 2011, 108 , 4680–4687. 10.1073/pnas.1002611107. [ PMC free article ] [ PubMed ] [ CrossRef ] [ Google Scholar ]
- Antonio M. A.; Hawes S. E.; Hillier S. L. The Identification of Vaginal Lactobacillus Species and the Demographic and Microbiologic Characteristics of Women Colonized by These Species . J. Infect. Dis. 1999, 180 , 1950–1956. 10.1086/315109. [ PubMed ] [ CrossRef ] [ Google Scholar ]
- Zhou X.; Bent S. J.; Schneider M. G.; Davis C. C.; Islam M. R.; Forney L. J. Characterization of Vaginal Microbial Communities in Adult Healthy Women Using Cultivation-Independent Methods . Microbiology 2004, 150 , 2565–2573. 10.1099/mic.0.26905-0. [ PubMed ] [ CrossRef ] [ Google Scholar ]
- Boskey E. R.; Telsch K. M.; Whaley K. J.; Moench T. R.; Cone R. A. Acid Production by Vaginal Flora in Vitro Is Consistent with the Rate and Extent of Vaginal Acidification . Infect. Immun. 1999, 67 , 5170–5175. 10.1128/IAI.67.10.5170-5175.1999. [ PMC free article ] [ PubMed ] [ CrossRef ] [ Google Scholar ]
- O’Hanlon D. E.; Moench T. R.; Cone R. A. Vaginal Ph and Microbicidal Lactic Acid When Lactobacilli Dominate the Microbiota . PLoS One 2013, 8 , e80074. 10.1371/journal.pone.0080074. [ PMC free article ] [ PubMed ] [ CrossRef ] [ Google Scholar ]
- Aldunate M.; Srbinovski D.; Hearps A. C.; Latham C. F.; Ramsland P. A.; Gugasyan R.; Cone R. A.; Tachedjian G. Antimicrobial and Immune Modulatory Effects of Lactic Acid and Short Chain Fatty Acids Produced by Vaginal Microbiota Associated with Eubiosis and Bacterial Vaginosis . Front. Physiol. 2015, 6 , 164. 10.3389/fphys.2015.00164. [ PMC free article ] [ PubMed ] [ CrossRef ] [ Google Scholar ]
- Delgado-Diaz D. J.; Tyssen D.; Hayward J. A.; Gugasyan R.; Hearps A. C.; Tachedjian G. Distinct Immune Responses Elicited from Cervicovaginal Epithelial Cells by Lactic Acid and Short Chain Fatty Acids Associated with Optimal and Non-Optimal Vaginal Microbiota . Front. Cell. Infect. Microbiol. 2020, 9 , 446. 10.3389/fcimb.2019.00446. [ PMC free article ] [ PubMed ] [ CrossRef ] [ Google Scholar ]
- Zhou X.; Brown C. J.; Abdo Z.; Davis C. C.; Hansmann M. A.; Joyce P.; Foster J. A.; Forney L. J. Differences in the Composition of Vaginal Microbial Communities Found in Healthy Caucasian and Black Women . ISME J. 2007, 1 , 121–133. 10.1038/ismej.2007.12. [ PubMed ] [ CrossRef ] [ Google Scholar ]
- Zhou X.; Hansmann M. A.; Davis C. C.; Suzuki H.; Brown C. J.; Schutte U.; Pierson J. D.; Forney L. J. The Vaginal Bacterial Communities of Japanese Women Resemble Those of Women in Other Racial Groups . FEMS Immunol. Med. Microbiol. 2010, 58 , 169–181. 10.1111/j.1574-695X.2009.00618.x. [ PMC free article ] [ PubMed ] [ CrossRef ] [ Google Scholar ]
- Verstraelen H.; Verhelst R.; Claeys G.; Temmerman M.; Vaneechoutte M. Culture-Independent Analysis of Vaginal Microflora: The Unrecognized Association of Atopobium Vaginae with Bacterial Vaginosis . Am. J. Obstet. Gynecol. 2004, 191 , 1130–1132. 10.1016/j.ajog.2004.04.013. [ PubMed ] [ CrossRef ] [ Google Scholar ]
- Peebles K.; Velloza J.; Balkus J. E.; McClelland R. S.; Barnabas R. V. High Global Burden and Costs of Bacterial Vaginosis: A Systematic Review and Meta-Analysis . Sex Transm. Dis. 2019, 46 , 304–311. 10.1097/OLQ.0000000000000972. [ PubMed ] [ CrossRef ] [ Google Scholar ]
- Allsworth J. E.; Peipert J. F. Prevalence of Bacterial Vaginosis: 2001–2004 National Health and Nutrition Examination Survey Data . Obstet. Gynecol. 2007, 109 , 114–120. 10.1097/01.AOG.0000247627.84791.91. [ PubMed ] [ CrossRef ] [ Google Scholar ]
- Brotman R. M.; Klebanoff M. A.; Nansel T. R.; Yu K. F.; Andrews W. W.; Zhang J.; Schwebke J. R. Bacterial Vaginosis Assessed by Gram Stain and Diminished Colonization Resistance to Incident Gonococcal, Chlamydial, and Trichomonal Genital Infection . J. Infect. Dis. 2010, 202 , 1907–1915. 10.1086/657320. [ PMC free article ] [ PubMed ] [ CrossRef ] [ Google Scholar ]
- Brotman R. M.; Bradford L. L.; Conrad M.; Gajer P.; Ault K.; Peralta L.; Forney L. J.; Carlton J. M.; Abdo Z.; Ravel J. Association between Trichomonas Vaginalis and Vaginal Bacterial Community Composition among Reproductive-Age Women . Sex Transm. Dis. 2012, 39 , 807–812. 10.1097/OLQ.0b013e3182631c79. [ PMC free article ] [ PubMed ] [ CrossRef ] [ Google Scholar ]
- Martin H. L.; Richardson B. A.; Nyange P. M.; Lavreys L.; Hillier S. L.; Chohan B.; Mandaliya K.; Ndinya-Achola J. O.; Bwayo J.; Kreiss J. Vaginal Lactobacilli, Microbial Flora, and Risk of Human Immunodeficiency Virus Type 1 and Sexually Transmitted Disease Acquisition . J. Infect. Dis. 1999, 180 , 1863–1868. 10.1086/315127. [ PubMed ] [ CrossRef ] [ Google Scholar ]
- Gosmann C.; Anahtar M. N.; Handley S. A.; Farcasanu M.; Abu-Ali G.; Bowman B. A.; Padavattan N.; Desai C.; Droit L.; Moodley A.; et al. Lactobacillus-Deficient Cervicovaginal Bacterial Communities Are Associated with Increased Hiv Acquisition in Young South African Women . Immunity 2017, 46 , 29–37. 10.1016/j.immuni.2016.12.013. [ PMC free article ] [ PubMed ] [ CrossRef ] [ Google Scholar ]
- Feehily C.; Crosby D.; Walsh C. J.; Lawton E. M.; Higgins S.; McAuliffe F. M.; Cotter P. D. Shotgun Sequencing of the Vaginal Microbiome Reveals Both a Species and Functional Potential Signature of Preterm Birth . npj Biofilms Microbiomes 2020, 6 , 50. 10.1038/s41522-020-00162-8. [ PMC free article ] [ PubMed ] [ CrossRef ] [ Google Scholar ]
- Brown R. G.; Al-Memar M.; Marchesi J. R.; Lee Y. S.; Smith A.; Chan D.; Lewis H.; Kindinger L.; Terzidou V.; Bourne T.; et al. Establishment of Vaginal Microbiota Composition in Early Pregnancy and Its Association with Subsequent Preterm Prelabor Rupture of the Fetal Membranes . Transl. Res. 2019, 207 , 30–43. 10.1016/j.trsl.2018.12.005. [ PMC free article ] [ PubMed ] [ CrossRef ] [ Google Scholar ]
- Elovitz M. A.; Gajer P.; Riis V.; Brown A. G.; Humphrys M. S.; Holm J. B.; Ravel J. Cervicovaginal Microbiota and Local Immune Response Modulate the Risk of Spontaneous Preterm Delivery . Nat. Commun. 2019, 10 , 1305. 10.1038/s41467-019-09285-9. [ PMC free article ] [ PubMed ] [ CrossRef ] [ Google Scholar ]
- Brown R. G.; Marchesi J. R.; Lee Y. S.; Smith A.; Lehne B.; Kindinger L. M.; Terzidou V.; Holmes E.; Nicholson J. K.; Bennett P. R.; et al. Vaginal Dysbiosis Increases Risk of Preterm Fetal Membrane Rupture, Neonatal Sepsis and Is Exacerbated by Erythromycin . BMC Med. 2018, 16 , 9. 10.1186/s12916-017-0999-x. [ PMC free article ] [ PubMed ] [ CrossRef ] [ Google Scholar ]
- Freitas A. C.; Bocking A.; Hill J. E.; Money D. M.; Increased Richness and Diversity of the Vaginal Microbiota and Spontaneous Preterm Birth . Microbiome 2018, 6 , 117. 10.1186/s40168-018-0502-8. [ PMC free article ] [ PubMed ] [ CrossRef ] [ Google Scholar ]
- McKinnon L. R.; Achilles S. L.; Bradshaw C. S.; Burgener A.; Crucitti T.; Fredricks D. N.; Jaspan H. B.; Kaul R.; Kaushic C.; Klatt N.; et al. The Evolving Facets of Bacterial Vaginosis: Implications for Hiv Transmission . AIDS Res. Hum. Retroviruses 2019, 35 , 219–228. 10.1089/aid.2018.0304. [ PMC free article ] [ PubMed ] [ CrossRef ] [ Google Scholar ]
- Han L.; Taub R.; Jensen J. T. Cervical Mucus and Contraception: What We Know and What We Don’t . Contraception 2017, 96 , 310–321. 10.1016/j.contraception.2017.07.168. [ PubMed ] [ CrossRef ] [ Google Scholar ]
- Lacroix G.; Gouyer V.; Gottrand F.; Desseyn J. L. The Cervicovaginal Mucus Barrier . Int. J. Mol. Sci. 2020, 21 , 8266. 10.3390/ijms21218266. [ PMC free article ] [ PubMed ] [ CrossRef ] [ Google Scholar ]
- Domino S. E.; Hurd E. A.; Thomsson K. A.; Karnak D. M.; Holmen Larsson J. M.; Thomsson E.; Backstrom M.; Hansson G. C. Cervical Mucins Carry Alpha(1,2)Fucosylated Glycans That Partly Protect from Experimental Vaginal Candidiasis . Glycoconj. J. 2009, 26 , 1125–1134. 10.1007/s10719-009-9234-0. [ PMC free article ] [ PubMed ] [ CrossRef ] [ Google Scholar ]
- Cone R. A. Barrier Properties of Mucus . Adv. Drug. Delivery Rev. 2009, 61 , 75–85. 10.1016/j.addr.2008.09.008. [ PubMed ] [ CrossRef ] [ Google Scholar ]
- Agarwal K.; Lewis A. L. Vaginal Sialoglycan Foraging by Gardnerella Vaginalis: Mucus Barriers as a Meal for Unwelcome Guests? . Glycobiology 2021, 31 , 667–680. 10.1093/glycob/cwab024. [ PMC free article ] [ PubMed ] [ CrossRef ] [ Google Scholar ]
- Vagios S.; Mitchell C. M. Mutual Preservation: A Review of Interactions between Cervicovaginal Mucus and Microbiota . Front. Cell. Infect. Microbiol. 2021, 11 , 676114. 10.3389/fcimb.2021.676114. [ PMC free article ] [ PubMed ] [ CrossRef ] [ Google Scholar ]
- Gipson I. K.; Moccia R.; Spurr-Michaud S.; Argueso P.; Gargiulo A. R.; Hill J. A. 3rd; Offner G. D.; Keutmann H. T. The Amount of Muc5b Mucin in Cervical Mucus Peaks at Midcycle . J. Clin. Endocrinol. Metab. 2001, 86 , 594–600. 10.1210/jc.86.2.594. [ PubMed ] [ CrossRef ] [ Google Scholar ]
- Mirmonsef P.; Hotton A. L.; Gilbert D.; Gioia C. J.; Maric D.; Hope T. J.; Landay A. L.; Spear G. T. Glycogen Levels in Undiluted Genital Fluid and Their Relationship to Vaginal Ph, Estrogen, and Progesterone . PLoS One 2016, 11 , e0153553 10.1371/journal.pone.0153553. [ PMC free article ] [ PubMed ] [ CrossRef ] [ Google Scholar ]
- Farage M.; Maibach H. Lifetime Changes in the Vulva and Vagina . Arch. Gynecol Obstet. 2006, 273 , 195–202. 10.1007/s00404-005-0079-x. [ PubMed ] [ CrossRef ] [ Google Scholar ]
- Seidman J. D.; Cho K. R.; Ronnett B. M.; Kurman R. J.. Surface Epithelial Tumors of the Ovary . In Blaustein’s Pathology of the Female Genital Tract ; Springer: 2011; pp 679–784. [ Google Scholar ]
- Tester R.; Al-Ghazzewi F. H. Intrinsic and Extrinsic Carbohydrates in the Vagina: A Short Review on Vaginal Glycogen . Int. J. Biol. Macromol. 2018, 112 , 203–206. 10.1016/j.ijbiomac.2018.01.166. [ PubMed ] [ CrossRef ] [ Google Scholar ]
- Mirmonsef P.; Hotton A. L.; Gilbert D.; Burgad D.; Landay A.; Weber K. M.; Cohen M.; Ravel J.; Spear G. T. Free Glycogen in Vaginal Fluids Is Associated with Lactobacillus Colonization and Low Vaginal Ph . PLoS One 2014, 9 , e102467. 10.1371/journal.pone.0102467. [ PMC free article ] [ PubMed ] [ CrossRef ] [ Google Scholar ]
- Muhleisen A. L.; Herbst-Kralovetz M. M. Menopause and the Vaginal Microbiome . Maturitas 2016, 91 , 42–50. 10.1016/j.maturitas.2016.05.015. [ PubMed ] [ CrossRef ] [ Google Scholar ]
- McClelland R. S.; Lingappa J. R.; Srinivasan S.; Kinuthia J.; John-Stewart G. C.; Jaoko W.; Richardson B. A.; Yuhas K.; Fiedler T. L.; Mandaliya K. N.; et al. Evaluation of the Association between the Concentrations of Key Vaginal Bacteria and the Increased Risk of Hiv Acquisition in African Women from Five Cohorts: A Nested Case-Control Study . Lancet Infect. Dis. 2018, 18 , 554–564. 10.1016/S1473-3099(18)30058-6. [ PMC free article ] [ PubMed ] [ CrossRef ] [ Google Scholar ]
- Lee J. E.; Lee S.; Lee H.; Song Y. M.; Lee K.; Han M. J.; Sung J.; Ko G. Association of the Vaginal Microbiota with Human Papillomavirus Infection in a Korean Twin Cohort . PLoS One 2013, 8 , e63514. 10.1371/journal.pone.0063514. [ PMC free article ] [ PubMed ] [ CrossRef ] [ Google Scholar ]
- Norenhag J.; Du J.; Olovsson M.; Verstraelen H.; Engstrand L.; Brusselaers N. The Vaginal Microbiota, Human Papillomavirus and Cervical Dysplasia: A Systematic Review and Network Meta-Analysis . BJOG 2020, 127 , 171–180. 10.1111/1471-0528.15854. [ PubMed ] [ CrossRef ] [ Google Scholar ]
- Lev-Sagie A.; Goldman-Wohl D.; Cohen Y.; Dori-Bachash M.; Leshem A.; Mor U.; Strahilevitz J.; Moses A. E.; Shapiro H.; Yagel S.; et al. Vaginal Microbiome Transplantation in Women with Intractable Bacterial Vaginosis . Nat. Med. 2019, 25 , 1500–1504. 10.1038/s41591-019-0600-6. [ PubMed ] [ CrossRef ] [ Google Scholar ]
- Gilbert J. A.; Quinn R. A.; Debelius J.; Xu Z. Z.; Morton J.; Garg N.; Jansson J. K.; Dorrestein P. C.; Knight R. Microbiome-Wide Association Studies Link Dynamic Microbial Consortia to Disease . Nature 2016, 535 , 94–103. 10.1038/nature18850. [ PubMed ] [ CrossRef ] [ Google Scholar ]
- Manor O.; Dai C. L.; Kornilov S. A.; Smith B.; Price N. D.; Lovejoy J. C.; Gibbons S. M.; Magis A. T. Health and Disease Markers Correlate with Gut Microbiome Composition across Thousands of People . Nature Commun. 2020, 11 , 5206. 10.1038/s41467-020-18871-1. [ PMC free article ] [ PubMed ] [ CrossRef ] [ Google Scholar ]
- Falony G.; Joossens M.; Vieira-Silva S.; Wang J.; Darzi Y.; Faust K.; Kurilshikov A.; Bonder M. J.; Valles-Colomer M.; Vandeputte D.; et al. Population-Level Analysis of Gut Microbiome Variation . Science 2016, 352 , 560–564. 10.1126/science.aad3503. [ PubMed ] [ CrossRef ] [ Google Scholar ]
- Schnorr S. L.; Candela M.; Rampelli S.; Centanni M.; Consolandi C.; Basaglia G.; Turroni S.; Biagi E.; Peano C.; Severgnini M.; et al. Gut Microbiome of the Hadza Hunter-Gatherers . Nature Commun. 2014, 5 , 3654. 10.1038/ncomms4654. [ PMC free article ] [ PubMed ] [ CrossRef ] [ Google Scholar ]
- Koliada A.; Syzenko G.; Moseiko V.; Budovska L.; Puchkov K.; Perederiy V.; Gavalko Y.; Dorofeyev A.; Romanenko M.; Tkach S.; et al. Association between Body Mass Index and Firmicutes/Bacteroidetes Ratio in an Adult Ukrainian Population . BMC Microbiology 2017, 17 , 120. 10.1186/s12866-017-1027-1. [ PMC free article ] [ PubMed ] [ CrossRef ] [ Google Scholar ]
- Verdam F. J.; Fuentes S.; de Jonge C.; Zoetendal E. G.; Erbil R.; Greve J. W.; Buurman W. A.; de Vos W. M.; Rensen S. S. Human Intestinal Microbiota Composition Is Associated with Local and Systemic Inflammation in Obesity . Obesity 2013, 21 , E607–E615. 10.1002/oby.20466. [ PubMed ] [ CrossRef ] [ Google Scholar ]
- Kasai C.; Sugimoto K.; Moritani I.; Tanaka J.; Oya Y.; Inoue H.; Tameda M.; Shiraki K.; Ito M.; Takei Y.; et al. Comparison of the Gut Microbiota Composition between Obese and Non-Obese Individuals in a Japanese Population, as Analyzed by Terminal Restriction Fragment Length Polymorphism and Next-Generation Sequencing . BMC Gastroenterol. 2015, 15 , 100. 10.1186/s12876-015-0330-2. [ PMC free article ] [ PubMed ] [ CrossRef ] [ Google Scholar ]
- Crovesy L.; Masterson D.; Rosado E. L. Profile of the Gut Microbiota of Adults with Obesity: A Systematic Review . Eur. J. Clin. Nutr. 2020, 74 , 1251–1262. 10.1038/s41430-020-0607-6. [ PubMed ] [ CrossRef ] [ Google Scholar ]
- Manichanh C.; Rigottier-Gois L.; Bonnaud E.; Gloux K.; Pelletier E.; Frangeul L.; Nalin R.; Jarrin C.; Chardon P.; Marteau P.; et al. Reduced Diversity of Faecal Microbiota in Crohn’s Disease Revealed by a Metagenomic Approach . Gut 2006, 55 , 205. 10.1136/gut.2005.073817. [ PMC free article ] [ PubMed ] [ CrossRef ] [ Google Scholar ]
- Vester-Andersen M. K.; Mirsepasi-Lauridsen H. C.; Prosberg M. V.; Mortensen C. O.; Träger C.; Skovsen K.; Thorkilgaard T.; Nøjgaard C.; Vind I.; Krogfelt K. A.; et al. Increased Abundance of Proteobacteria in Aggressive Crohn’s Disease Seven Years after Diagnosis . Sci. Rep. 2019, 9 , 13473. 10.1038/s41598-019-49833-3. [ PMC free article ] [ PubMed ] [ CrossRef ] [ Google Scholar ]
- Huang T.-T.; Lai J.-B.; Du Y.-L.; Xu Y.; Ruan L.-M.; Hu S.-H. Current Understanding of Gut Microbiota in Mood Disorders: An Update of Human Studies . Front. Genet. 2019, 10 , 98. 10.3389/fgene.2019.00098. [ PMC free article ] [ PubMed ] [ CrossRef ] [ Google Scholar ]
- Giloteaux L.; Goodrich J. K.; Walters W. A.; Levine S. M.; Ley R. E.; Hanson M. R. Reduced Diversity and Altered Composition of the Gut Microbiome in Individuals with Myalgic Encephalomyelitis/Chronic Fatigue Syndrome . Microbiome 2016, 4 , 30. 10.1186/s40168-016-0171-4. [ PMC free article ] [ PubMed ] [ CrossRef ] [ Google Scholar ]
- Golfetto L.; de Senna F. D.; Hermes J.; Beserra B. T.; França F. d. S.; Martinello F. Lower Bifidobacteria Counts in Adult Patients with Celiac Disease on a Gluten-Free Diet . Arq. Gastroenterol. 2014, 51 , 139–143. 10.1590/S0004-28032014000200013. [ PubMed ] [ CrossRef ] [ Google Scholar ]
- Kerckhoffs A. P.; Samsom M.; van der Rest M. E.; de Vogel J.; Knol J.; Ben-Amor K.; Akkermans L. M. Lower Bifidobacteria Counts in Both Duodenal Mucosa-Associated and Fecal Microbiota in Irritable Bowel Syndrome Patients . World J. Gastroenterol. 2009, 15 , 2887–2892. 10.3748/wjg.15.2887. [ PMC free article ] [ PubMed ] [ CrossRef ] [ Google Scholar ]
- Lee H.-J.; Lee K.-E.; Kim J.-K.; Kim D.-H. Suppression of Gut Dysbiosis by Bifidobacterium Longum Alleviates Cognitive Decline in 5xfad Transgenic and Aged Mice . Sci. Rep. 2019, 9 , 11814. 10.1038/s41598-019-48342-7. [ PMC free article ] [ PubMed ] [ CrossRef ] [ Google Scholar ]
- Kong H. H.; Oh J.; Deming C.; Conlan S.; Grice E. A.; Beatson M. A.; Nomicos E.; Polley E. C.; Komarow H. D.; et al. Temporal Shifts in the Skin Microbiome Associated with Disease Flares and Treatment in Children with Atopic Dermatitis . Genome Res. 2012, 22 , 850–859. 10.1101/gr.131029.111. [ PMC free article ] [ PubMed ] [ CrossRef ] [ Google Scholar ]
- Takahashi Y.; Saito A.; Chiba H.; Kuronuma K.; Ikeda K.; Kobayashi T.; Ariki S.; Takahashi M.; Sasaki Y.; Takahashi H. Impaired Diversity of the Lung Microbiome Predicts Progression of Idiopathic Pulmonary Fibrosis . Respir. Res. 2018, 19 , 34. 10.1186/s12931-018-0736-9. [ PMC free article ] [ PubMed ] [ CrossRef ] [ Google Scholar ]
- Thomas-White K. J.; Gao X.; Lin H.; Fok C. S.; Ghanayem K.; Mueller E. R.; Dong Q.; Brubaker L.; Wolfe A. J. Urinary Microbes and Postoperative Urinary Tract Infection Risk in Urogynecologic Surgical Patients . Int.Urogynecol. J. 2018, 29 , 1797–1805. 10.1007/s00192-018-3767-3. [ PMC free article ] [ PubMed ] [ CrossRef ] [ Google Scholar ]
- Curtiss N.; Balachandran A.; Krska L.; Peppiatt-Wildman C.; Wildman S.; Duckett J. A Case Controlled Study Examining the Bladder Microbiome in Women with Overactive Bladder (Oab) and Healthy Controls . Eur. J. Obstet. Gynecol. Reprod. Biol. 2017, 214 , 31–35. 10.1016/j.ejogrb.2017.04.040. [ PubMed ] [ CrossRef ] [ Google Scholar ]
- Joung H.; Chu J.; Kim B.-K.; Choi I.-S.; Kim W.; Park T.-S. Probiotics Ameliorate Chronic Low-Grade Inflammation and Fat Accumulation with Gut Microbiota Composition Change in Diet-Induced Obese Mice Models . Appl. Microbiol. Biotechnol. 2021, 105 , 1203–1213. 10.1007/s00253-020-11060-6. [ PubMed ] [ CrossRef ] [ Google Scholar ]
- Cheng Y.-C.; Liu J.-R. Effect of Lactobacillus Rhamnosus Gg on Energy Metabolism, Leptin Resistance, and Gut Microbiota in Mice with Diet-Induced Obesity . Nutrients 2020, 12 , 2557. 10.3390/nu12092557. [ PMC free article ] [ PubMed ] [ CrossRef ] [ Google Scholar ]
- Borgeraas H.; Johnson L. K.; Skattebu J.; Hertel J. K.; Hjelmesæth J. Effects of Probiotics on Body Weight, Body Mass Index, Fat Mass and Fat Percentage in Subjects with Overweight or Obesity: A Systematic Review and Meta-Analysis of Randomized Controlled Trials . Obes. Rev. 2018, 19 , 219–232. 10.1111/obr.12626. [ PubMed ] [ CrossRef ] [ Google Scholar ]
- Larsen N.; Vogensen F. K.; Gøbel R. J.; Michaelsen K. F.; Forssten S. D.; Lahtinen S. J.; Jakobsen M. Effect of Lactobacillus Salivarius Ls-33 on Fecal Microbiota in Obese Adolescents . Clin. Nutr. 2013, 32 , 935–940. 10.1016/j.clnu.2013.02.007. [ PubMed ] [ CrossRef ] [ Google Scholar ]
- Gøbel R. J.; Larsen N.; Jakobsen M.; Mølgaard C.; Michaelsen K. F. Probiotics to Adolescents with Obesity: Effects on Inflammation and Metabolic Syndrome . J. Pediatr. Gastroenterol. Nutr. 2012, 55 , 673–678. 10.1097/MPG.0b013e318263066c. [ PubMed ] [ CrossRef ] [ Google Scholar ]
- Cani P. D.; de Vos W. M. Next-Generation Beneficial Microbes: The Case of Akkermansia Muciniphila . Front. Microbiol. 2017, 8 , 1765. 10.3389/fmicb.2017.01765. [ PMC free article ] [ PubMed ] [ CrossRef ] [ Google Scholar ]
- Depommier C.; Everard A.; Druart C.; Plovier H.; Van Hul M.; Vieira-Silva S.; Falony G.; Raes J.; Maiter D.; Delzenne N. M.; et al. Supplementation with Akkermansia Muciniphila in Overweight and Obese Human Volunteers: A Proof-of-Concept Exploratory Study . Nat. Med. 2019, 25 , 1096–1103. 10.1038/s41591-019-0495-2. [ PMC free article ] [ PubMed ] [ CrossRef ] [ Google Scholar ]
- Plovier H.; Everard A.; Druart C.; Depommier C.; Van Hul M.; Geurts L.; Chilloux J.; Ottman N.; Duparc T.; Lichtenstein L.; et al. A Purified Membrane Protein from Akkermansia Muciniphila or the Pasteurized Bacterium Improves Metabolism in Obese and Diabetic Mice . Nat. Med. 2017, 23 , 107–113. 10.1038/nm.4236. [ PubMed ] [ CrossRef ] [ Google Scholar ]
- Wang Y.; Guo Y.; Chen H.; Wei H.; Wan C. Potential of Lactobacillus Plantarum Zdy2013 and Bifidobacterium Bifidum Wbin03 in Relieving Colitis by Gut Microbiota, Immune, and Anti-Oxidative Stress . Can. J. Microbiol. 2018, 64 , 327–337. 10.1139/cjm-2017-0716. [ PubMed ] [ CrossRef ] [ Google Scholar ]
- Jang Y. J.; Kim W.-K.; Han D. H.; Lee K.; Ko G. Lactobacillus Fermentum Species Ameliorate Dextran Sulfate Sodium-Induced Colitis by Regulating the Immune Response and Altering Gut Microbiota . Gut Microbes 2019, 10 , 696–711. 10.1080/19490976.2019.1589281. [ PMC free article ] [ PubMed ] [ CrossRef ] [ Google Scholar ]
- Bjarnason I.; Sission G.; Hayee B. A Randomised, Double-Blind, Placebo-Controlled Trial of a Multi-Strain Probiotic in Patients with Asymptomatic Ulcerative Colitis and Crohn’s Disease . Inflammopharmacology 2019, 27 , 465–473. 10.1007/s10787-019-00595-4. [ PMC free article ] [ PubMed ] [ CrossRef ] [ Google Scholar ]
- Tamaki H.; Nakase H.; Inoue S.; Kawanami C.; Itani T.; Ohana M.; Kusaka T.; Uose S.; Hisatsune H.; Tojo M.; et al. Efficacy of Probiotic Treatment with Bifidobacterium Longum 536 for Induction of Remission in Active Ulcerative Colitis: A Randomized, Double-Blinded, Placebo-Controlled Multicenter Trial . Dig. Endosc. 2016, 28 , 67–74. 10.1111/den.12553. [ PubMed ] [ CrossRef ] [ Google Scholar ]
- Martyniak A.; Medyńska-Przęczek A.; Wędrychowicz A.; Skoczeń S.; Tomasik P. J. Prebiotics, Probiotics, Synbiotics, Paraprobiotics and Postbiotic Compounds in Ibd . Biomolecules 2021, 11 , 1903. 10.3390/biom11121903. [ PMC free article ] [ PubMed ] [ CrossRef ] [ Google Scholar ]
- Glassner K. L.; Abraham B. P.; Quigley E. M. M. The Microbiome and Inflammatory Bowel Disease . J. Allergy Clin. Immunol. 2020, 145 , 16–27. 10.1016/j.jaci.2019.11.003. [ PubMed ] [ CrossRef ] [ Google Scholar ]
- Cao Y.; Shen J.; Ran Z. H. Association between Faecalibacterium Prausnitzii Reduction and Inflammatory Bowel Disease: A Meta-Analysis and Systematic Review of the Literature . Gastroenterol. Res. Pract. 2014, 2014 , 872725. 10.1155/2014/872725. [ PMC free article ] [ PubMed ] [ CrossRef ] [ Google Scholar ]
- Zhao H.; Xu H.; Chen S.; He J.; Zhou Y.; Nie Y. Systematic Review and Meta-Analysis of the Role of Faecalibacterium Prausnitzii Alteration in Inflammatory Bowel Disease . J. Gastroenterol. Hepatol. 2021, 36 , 320–328. 10.1111/jgh.15222. [ PubMed ] [ CrossRef ] [ Google Scholar ]
- Qiu X.; Zhang M.; Yang X.; Hong N.; Yu C. Faecalibacterium Prausnitzii Upregulates Regulatory T Cells and Anti-Inflammatory Cytokines in Treating Tnbs-Induced Colitis . J. Crohns Colitis 2013, 7 , e558–e568. 10.1016/j.crohns.2013.04.002. [ PubMed ] [ CrossRef ] [ Google Scholar ]
- Martín R.; Chain F.; Miquel S.; Lu J.; Gratadoux J.-J.; Sokol H.; Verdu E. F.; Bercik P.; Bermúdez-Humarán L. G.; Langella P. The Commensal Bacterium Faecalibacterium Prausnitzii Is Protective in Dnbs-Induced Chronic Moderate and Severe Colitis Models . Inflamm. Bowel Dis. 2014, 20 , 417–430. 10.1097/01.MIB.0000440815.76627.64. [ PubMed ] [ CrossRef ] [ Google Scholar ]
- He X.; Zhao S.; Li Y. Faecalibacterium Prausnitzii: A Next-Generation Probiotic in Gut Disease Improvement . Can. J. Infect. Dis. Med. Microbiol. 2021, 2021 , 6666114. 10.1155/2021/6666114. [ CrossRef ] [ Google Scholar ]
- Sokol H.; Leducq V.; Aschard H.; Pham H.-P.; Jegou S.; Landman C.; Cohen D.; Liguori G.; Bourrier A.; Nion-Larmurier I.; et al. Fungal Microbiota Dysbiosis in Ibd . Gut 2017, 66 , 1039. 10.1136/gutjnl-2015-310746. [ PMC free article ] [ PubMed ] [ CrossRef ] [ Google Scholar ]
- Limon J. J.; Tang J.; Li D.; Wolf A. J.; Michelsen K. S.; Funari V.; Gargus M.; Nguyen C.; Sharma P.; Maymi V. I.; et al. Malassezia Is Associated with Crohn’s Disease and Exacerbates Colitis in Mouse Models . Cell Host Microbe 2019, 25 , 377–388. 10.1016/j.chom.2019.01.007. [ PMC free article ] [ PubMed ] [ CrossRef ] [ Google Scholar ]
- Norman J. M.; Handley S. A.; Baldridge M. T.; Droit L.; Liu C. Y.; Keller B. C.; Kambal A.; Monaco C. L.; Zhao G.; Fleshner P.; et al. Disease-Specific Alterations in the Enteric Virome in Inflammatory Bowel Disease . Cell 2015, 160 , 447–460. 10.1016/j.cell.2015.01.002. [ PMC free article ] [ PubMed ] [ CrossRef ] [ Google Scholar ]
- Kelesidis T.; Pothoulakis C. Efficacy and Safety of the Probiotic Saccharomyces Boulardii for the Prevention and Therapy of Gastrointestinal Disorders . Therap. Adv. Gastroenterol. 2012, 5 , 111–125. 10.1177/1756283X11428502. [ PMC free article ] [ PubMed ] [ CrossRef ] [ Google Scholar ]
- Myles I. A.; Williams K. W.; Reckhow J. D.; Jammeh M. L.; Pincus N. B.; Sastalla I.; Saleem D.; Stone K. D.; Datta S. K. Transplantation of Human Skin Microbiota in Models of Atopic Dermatitis . JCI Insight 2016, 1 , e86955. 10.1172/jci.insight.86955. [ PMC free article ] [ PubMed ] [ CrossRef ] [ Google Scholar ]
- Myles I. A.; Castillo C. R.; Barbian K. D.; Kanakabandi K.; Virtaneva K.; Fitzmeyer E.; Paneru M.; Otaizo-Carrasquero F.; Myers T. G.; Markowitz T. E.; et al. Therapeutic Responses to Roseomonas Mucosa in Atopic Dermatitis May Involve Lipid-Mediated Tnf-Related Epithelial Repair . Sci. Transl. Med. 2020, 12 , eaaz8631. 10.1126/scitranslmed.aaz8631. [ PMC free article ] [ PubMed ] [ CrossRef ] [ Google Scholar ]
- Gupta K.; Stapleton A. E.; Hooton T. M.; Roberts P. L.; Fennell C. L.; Stamm W. E. Inverse Association of H2o2-Producing Lactobacilli and Vaginal Escherichia Coli Colonization in Women with Recurrent Urinary Tract Infections . J. Infect. Dis. 1998, 178 , 446–450. 10.1086/515635. [ PubMed ] [ CrossRef ] [ Google Scholar ]
- Kwok L.; Stapleton A. E.; Stamm W. E.; Hillier S. L.; Wobbe C. L.; Gupta K. Adherence of Lactobacillus Crispatus to Vaginal Epithelial Cells from Women with or without a History of Recurrent Urinary Tract Infection . J. Urol. 2006, 176 , 2050–2054. 10.1016/j.juro.2006.07.014. [ PubMed ] [ CrossRef ] [ Google Scholar ]
- Sanders M. E.; Merenstein D. J.; Reid G.; Gibson G. R.; Rastall R. A. Probiotics and Prebiotics in Intestinal Health and Disease: From Biology to the Clinic . Nat. Rev. Gastroenterol. Hepatol. 2019, 16 , 605–616. 10.1038/s41575-019-0173-3. [ PubMed ] [ CrossRef ] [ Google Scholar ]
- Macfarlane S.; Macfarlane G. T.; Cummings J. H. Review Article: Prebiotics in the Gastrointestinal Tract . Aliment Pharmacol Ther. 2006, 24 , 701–714. 10.1111/j.1365-2036.2006.03042.x. [ PubMed ] [ CrossRef ] [ Google Scholar ]
- Liu F.; Li P.; Chen M.; Luo Y.; Prabhakar M.; Zheng H.; He Y.; Qi Q.; Long H.; Zhang Y.; et al. Fructooligosaccharide (FOS) and Galactooligosaccharide (GOS) Increase Bifidobacterium but Reduce Butyrate Producing Bacteria with Adverse Glycemic Metabolism in Healthy Young Population . Sci. Rep. 2017, 7 , 11789. 10.1038/s41598-017-10722-2. [ PMC free article ] [ PubMed ] [ CrossRef ] [ Google Scholar ]
- Tandon D.; Haque M. M.; Gote M.; Jain M.; Bhaduri A.; Dubey A. K.; Mande S. S. A Prospective Randomized, Double-Blind, Placebo-Controlled, Dose-Response Relationship Study to Investigate Efficacy of Fructo-Oligosaccharides (Fos) on Human Gut Microflora . Sci. Rep. 2019, 9 , 5473. 10.1038/s41598-019-41837-3. [ PMC free article ] [ PubMed ] [ CrossRef ] [ Google Scholar ]
- Finegold S. M.; Li Z.; Summanen P. H.; Downes J.; Thames G.; Corbett K.; Dowd S.; Krak M.; Heber D. Xylooligosaccharide Increases Bifidobacteria but Not Lactobacilli in Human Gut Microbiota . Food Funct. 2014, 5 , 436–445. 10.1039/c3fo60348b. [ PubMed ] [ CrossRef ] [ Google Scholar ]
- Björkstén B.; Sepp E.; Julge K.; Voor T.; Mikelsaar M. Allergy Development and the Intestinal Microflora During the First Year of Life . J. Allergy. Clin. Immunol. 2001, 108 , 516–520. 10.1067/mai.2001.118130. [ PubMed ] [ CrossRef ] [ Google Scholar ]
- Moro G.; Arslanoglu S.; Stahl B.; Jelinek J.; Wahn U.; Boehm G. A Mixture of Prebiotic Oligosaccharides Reduces the Incidence of Atopic Dermatitis During the First Six Months of Age . Arch. Dis. Child 2006, 91 , 814–819. 10.1136/adc.2006.098251. [ PMC free article ] [ PubMed ] [ CrossRef ] [ Google Scholar ]
- Arslanoglu S.; Moro G. E.; Schmitt J.; Tandoi L.; Rizzardi S.; Boehm G. Early Dietary Intervention with a Mixture of Prebiotic Oligosaccharides Reduces the Incidence of Allergic Manifestations and Infections During the First Two Years of Life . J. Nut. 2008, 138 , 1091–1095. 10.1093/jn/138.6.1091. [ PubMed ] [ CrossRef ] [ Google Scholar ]
- Lindsay J. O.; Whelan K.; Stagg A. J.; Gobin P.; Al-Hassi H. O.; Rayment N.; Kamm M. A.; Knight S. C.; Forbes A. Clinical, Microbiological, and Immunological Effects of Fructo-Oligosaccharide in Patients with Crohn’s Disease . Gut 2006, 55 , 348–355. 10.1136/gut.2005.074971. [ PMC free article ] [ PubMed ] [ CrossRef ] [ Google Scholar ]
- Han K.; Nam J.; Xu J.; Sun X.; Huang X.; Animasahun O.; Achreja A.; Jeon J. H.; Pursley B.; Kamada N.; et al. Generation of Systemic Antitumour Immunity Via the in Situ Modulation of the Gut Microbiome by an Orally Administered Inulin Gel . Nat. Biomed. Eng. 2021, 5 , 1377–1388. 10.1038/s41551-021-00749-2. [ PMC free article ] [ PubMed ] [ CrossRef ] [ Google Scholar ]
- Routy B.; Le Chatelier E.; Derosa L.; Duong C. P. M.; Alou M. T.; Daillère R.; Fluckiger A.; Messaoudene M.; Rauber C.; Roberti M. P.; et al. Gut Microbiome Influences Efficacy of Pd-1–Based Immunotherapy against Epithelial Tumors . Science 2018, 359 , 91–97. 10.1126/science.aan3706. [ PubMed ] [ CrossRef ] [ Google Scholar ]
- Gopalakrishnan V.; Spencer C. N.; Nezi L.; Reuben A.; Andrews M. C.; Karpinets T. V.; Prieto P. A.; Vicente D.; Hoffman K.; Wei S. C.; et al. Gut Microbiome Modulates Response to Anti–Pd-1 Immunotherapy in Melanoma Patients . Science 2018, 359 , 97–103. 10.1126/science.aan4236. [ PMC free article ] [ PubMed ] [ CrossRef ] [ Google Scholar ]
- Matson V.; Fessler J.; Bao R.; Chongsuwat T.; Zha Y.; Alegre M.-L.; Luke J. J.; Gajewski T. F. The Commensal Microbiome Is Associated with Anti–Pd-1 Efficacy in Metastatic Melanoma Patients . Science 2018, 359 , 104–108. 10.1126/science.aao3290. [ PMC free article ] [ PubMed ] [ CrossRef ] [ Google Scholar ]
- Dethlefsen L.; Relman D. A. Incomplete Recovery and Individualized Responses of the Human Distal Gut Microbiota to Repeated Antibiotic Perturbation . Proc. Natl. Acad. Sci. U.S.A. 2011, 108 , 4554–4561. 10.1073/pnas.1000087107. [ PMC free article ] [ PubMed ] [ CrossRef ] [ Google Scholar ]
- Francino M. P. Antibiotics and the Human Gut Microbiome: Dysbioses and Accumulation of Resistances . Front. Microbiol. 2016, 6 , 1543. 10.3389/fmicb.2015.01543. [ PMC free article ] [ PubMed ] [ CrossRef ] [ Google Scholar ]
- Raymond F.; Ouameur A. A.; Déraspe M.; Iqbal N.; Gingras H.; Dridi B.; Leprohon P.; Plante P.-L.; Giroux R.; Bérubé È.; et al. The Initial State of the Human Gut Microbiome Determines Its Reshaping by Antibiotics . ISME J. 2016, 10 , 707–720. 10.1038/ismej.2015.148. [ PMC free article ] [ PubMed ] [ CrossRef ] [ Google Scholar ]
- Vickers R. J.; Tillotson G.; Goldstein E. J. C.; Citron D. M.; Garey K. W.; Wilcox M. H. Ridinilazole: A Novel Therapy for Clostridium Difficile Infection . Int. J. Antimicrob. Agents 2016, 48 , 137–143. 10.1016/j.ijantimicag.2016.04.026. [ PubMed ] [ CrossRef ] [ Google Scholar ]
- Vickers R. J.; Tillotson G. S.; Nathan R.; Hazan S.; Pullman J.; Lucasti C.; Deck K.; Yacyshyn B.; Maliakkal B.; Pesant Y.; et al. Efficacy and Safety of Ridinilazole Compared with Vancomycin for the Treatment of Clostridium Difficile Infection: A Phase 2, Randomised, Double-Blind, Active-Controlled, Non-Inferiority Study . Lancet Infect. Dis. 2017, 17 , 735–744. 10.1016/S1473-3099(17)30235-9. [ PMC free article ] [ PubMed ] [ CrossRef ] [ Google Scholar ]
- Thorpe C. M.; Kane A. V.; Chang J.; Tai A.; Vickers R. J.; Snydman D. R. Enhanced Preservation of the Human Intestinal Microbiota by Ridinilazole, a Novel Clostridium Difficile-Targeting Antibacterial, Compared to Vancomycin . PLoS One 2018, 13 , e0199810 10.1371/journal.pone.0199810. [ PMC free article ] [ PubMed ] [ CrossRef ] [ Google Scholar ]
- Avis T.; Wilson F. X.; Khan N.; Mason C. S.; Powell D. J. Targeted Microbiome-Sparing Antibiotics . Drug Discovery 2021, 26 , 2198–2203. 10.1016/j.drudis.2021.07.016. [ PubMed ] [ CrossRef ] [ Google Scholar ]
- Machado A.; Cerca N. Influence of Biofilm Formation by Gardnerella Vaginalis and Other Anaerobes on Bacterial Vaginosis . J. Infect. Dis. 2015, 212 , 1856–1861. 10.1093/infdis/jiv338. [ PubMed ] [ CrossRef ] [ Google Scholar ]
- Bradshaw C. S.; Sobel J. D. Current Treatment of Bacterial Vaginosis—Limitations and Need for Innovation . J. Infect. Dis. 2016, 214 , S14–S20. 10.1093/infdis/jiw159. [ PMC free article ] [ PubMed ] [ CrossRef ] [ Google Scholar ]
- Landlinger C.; Tisakova L.; Oberbauer V.; Schwebs T.; Muhammad A.; Latka A.; Van Simaey L.; Vaneechoutte M.; Guschin A.; Resch G.; et al. Engineered Phage Endolysin Eliminates Gardnerella Biofilm without Damaging Beneficial Bacteria in Bacterial Vaginosis Ex Vivo . Pathogens 2021, 10 , 54. 10.3390/pathogens10010054. [ PMC free article ] [ PubMed ] [ CrossRef ] [ Google Scholar ]
- Whitney C. G.; Farley M. M.; Hadler J.; Harrison L. H.; Bennett N. M.; Lynfield R.; Reingold A.; Cieslak P. R.; Pilishvili T.; Jackson D.; et al. Decline in Invasive Pneumococcal Disease after the Introduction of Protein–Polysaccharide Conjugate Vaccine . N. Engl. J. Med. 2003, 348 , 1737–1746. 10.1056/NEJMoa022823. [ PubMed ] [ CrossRef ] [ Google Scholar ]
- Biesbroek G.; Wang X.; Keijser B. J.; Eijkemans R. M.; Trzciński K.; Rots N. Y.; Veenhoven R. H.; Sanders E. A.; Bogaert D. Seven-Valent Pneumococcal Conjugate Vaccine and Nasopharyngeal Microbiota in Healthy Children . Emerg. Infect. Dis. 2014, 20 , 201–210. 10.3201/eid2002.131220. [ PMC free article ] [ PubMed ] [ CrossRef ] [ Google Scholar ]
- Blaser M. J.; Falkow S. What Are the Consequences of the Disappearing Human Microbiota? . Nat. Rev. Microbiol. 2009, 7 , 887–894. 10.1038/nrmicro2245. [ PMC free article ] [ PubMed ] [ CrossRef ] [ Google Scholar ]
- Mika M.; Maurer J.; Korten I.; Allemann A.; Aebi S.; Brugger S. D.; Qi W.; Frey U.; Latzin P.; Hilty M. Influence of the Pneumococcal Conjugate Vaccines on the Temporal Variation of Pneumococcal Carriage and the Nasal Microbiota in Healthy Infants: A Longitudinal Analysis of a Case–Control Study . Microbiome 2017, 5 , 85. 10.1186/s40168-017-0302-6. [ PMC free article ] [ PubMed ] [ CrossRef ] [ Google Scholar ]
- Relman D. A.; Lipsitch M. Microbiome as a Tool and a Target in the Effort to Address Antimicrobial Resistance . Proc. Natl. Acad. Sci. U.S.A. 2018, 115 , 12902–12910. 10.1073/pnas.1717163115. [ PMC free article ] [ PubMed ] [ CrossRef ] [ Google Scholar ]
- Brown R.; Lengeling A.; Wang B. Phage Engineering: How Advances in Molecular Biology and Synthetic Biology Are Being Utilized to Enhance the Therapeutic Potential of Bacteriophages . Quant. Biol. 2017, 5 , 42–54. 10.1007/s40484-017-0094-5. [ CrossRef ] [ Google Scholar ]
- Zhang Y.; Li C.-X.; Zhang X.-Z. Bacteriophage-Mediated Modulation of Microbiota for Diseases Treatment . Adv. Drug Delivery Rev. 2021, 176 , 113856. 10.1016/j.addr.2021.113856. [ PubMed ] [ CrossRef ] [ Google Scholar ]
- Duan Y.; Llorente C.; Lang S.; Brandl K.; Chu H.; Jiang L.; White R. C.; Clarke T. H.; Nguyen K.; Torralba M.; et al. Bacteriophage Targeting of Gut Bacterium Attenuates Alcoholic Liver Disease . Nature 2019, 575 , 505–511. 10.1038/s41586-019-1742-x. [ PMC free article ] [ PubMed ] [ CrossRef ] [ Google Scholar ]
- Zheng D.-W.; Dong X.; Pan P.; Chen K.-W.; Fan J.-X.; Cheng S.-X.; Zhang X.-Z. Phage-Guided Therapeutic System for Cancer Therapy by Modulating Gut Microbiota . Nat. Biomed. Eng. 2019, 3 , 717–728. 10.1038/s41551-019-0423-2. [ PubMed ] [ CrossRef ] [ Google Scholar ]
- Yu T.; Guo F.; Yu Y.; Sun T.; Ma D.; Han J.; Qian Y.; Kryczek I.; Sun D.; Nagarsheth N. Fusobacterium Nucleatum Promotes Chemoresistance to Colorectal Cancer by Modulating Autophagy . Cell 2017, 170 , 548–563. 10.1016/j.cell.2017.07.008. [ PMC free article ] [ PubMed ] [ CrossRef ] [ Google Scholar ]
- Castillo D. E.; Nanda S.; Keri J. E. Propionibacterium (Cutibacterium) Acnes Bacteriophage Therapy in Acne: Current Evidence and Future Perspectives . Dermatol. Ther. 2019, 9 , 19–31. 10.1007/s13555-018-0275-9. [ PMC free article ] [ PubMed ] [ CrossRef ] [ Google Scholar ]
- Brown T. L.; Petrovski S.; Dyson Z. A.; Seviour R.; Tucci J. The Formulation of Bacteriophage in a Semi Solid Preparation for Control of Propionibacterium Acnes Growth . PLoS One 2016, 11 , e0151184 10.1371/journal.pone.0151184. [ PMC free article ] [ PubMed ] [ CrossRef ] [ Google Scholar ]
- Bermudez-Brito M.; Plaza-Díaz J.; Muñoz-Quezada S.; Gómez-Llorente C.; Gil A. Probiotic Mechanisms of Action . Ann. Nutr. Metab. 2012, 61 , 160–174. 10.1159/000342079. [ PubMed ] [ CrossRef ] [ Google Scholar ]
- Hwang I. Y.; Koh E.; Wong A.; March J. C.; Bentley W. E.; Lee Y. S.; Chang M. W. Engineered Probiotic Escherichia Coli Can Eliminate and Prevent Pseudomonas Aeruginosa Gut Infection in Animal Models . Nat. Commun. 2017, 8 , 15028. 10.1038/ncomms15028. [ PMC free article ] [ PubMed ] [ CrossRef ] [ Google Scholar ]
- Lubkowicz D.; Ho C. L.; Hwang I. Y.; Yew W. S.; Lee Y. S.; Chang M. W. Reprogramming Probiotic Lactobacillus Reuteri as a Biosensor for Staphylococcus Aureus Derived Aip-I Detection . ACS Synth. Biol. 2018, 7 , 1229–1237. 10.1021/acssynbio.8b00063. [ PubMed ] [ CrossRef ] [ Google Scholar ]
- Jayaraman P.; Holowko M. B.; Yeoh J. W.; Lim S.; Poh C. L. Repurposing a Two-Component System-Based Biosensor for the Killing of Vibrio Cholerae . ACS Synth. Biol. 2017, 6 , 1403–1415. 10.1021/acssynbio.7b00058. [ PubMed ] [ CrossRef ] [ Google Scholar ]
- Sommer F.; Anderson J. M.; Bharti R.; Raes J.; Rosenstiel P. The Resilience of the Intestinal Microbiota Influences Health and Disease . Nat. Rev. Microbiol. 2017, 15 , 630–638. 10.1038/nrmicro.2017.58. [ PubMed ] [ CrossRef ] [ Google Scholar ]
- Bikard D.; Euler C. W.; Jiang W.; Nussenzweig P. M.; Goldberg G. W.; Duportet X.; Fischetti V. A.; Marraffini L. A. Exploiting Crispr-Cas Nucleases to Produce Sequence-Specific Antimicrobials . Nat. Biotechnol. 2014, 32 , 1146–1150. 10.1038/nbt.3043. [ PMC free article ] [ PubMed ] [ CrossRef ] [ Google Scholar ]
- Citorik R. J.; Mimee M.; Lu T. K. Sequence-Specific Antimicrobials Using Efficiently Delivered Rna-Guided Nucleases . Nat. Biotechnol. 2014, 32 , 1141–1145. 10.1038/nbt.3011. [ PMC free article ] [ PubMed ] [ CrossRef ] [ Google Scholar ]
- Lam K. N.; Spanogiannopoulos P.; Soto-Perez P.; Alexander M.; Nalley M. J.; Bisanz J. E.; Nayak R. R.; Weakley A. M.; Yu F. B.; Turnbaugh P. J. Phage-Delivered Crispr-Cas9 for Strain-Specific Depletion and Genomic Deletions in the Gut Microbiome . Cell Rep. 2021, 37 , 109930. 10.1016/j.celrep.2021.109930. [ PMC free article ] [ PubMed ] [ CrossRef ] [ Google Scholar ]
- Ting S.-Y.; Martínez-García E.; Huang S.; Bertolli S. K.; Kelly K. A.; Cutler K. J.; Su E. D.; Zhi H.; Tang Q.; Radey M. C.; et al. Targeted Depletion of Bacteria from Mixed Populations by Programmable Adhesion with Antagonistic Competitor Cells . Cell Host Microbe 2020, 28 , 313–321. 10.1016/j.chom.2020.05.006. [ PMC free article ] [ PubMed ] [ CrossRef ] [ Google Scholar ]
- Turnbaugh P. J.; Hamady M.; Yatsunenko T.; Cantarel B. L.; Duncan A.; Ley R. E.; Sogin M. L.; Jones W. J.; Roe B. A.; Affourtit J. P.; et al. A Core Gut Microbiome in Obese and Lean Twins . Nature 2009, 457 , 480–484. 10.1038/nature07540. [ PMC free article ] [ PubMed ] [ CrossRef ] [ Google Scholar ]
- Moya A.; Ferrer M. Functional Redundancy-Induced Stability of Gut Microbiota Subjected to Disturbance . Trends Microbiol. 2016, 24 , 402–413. 10.1016/j.tim.2016.02.002. [ PubMed ] [ CrossRef ] [ Google Scholar ]
- Morgan X. C.; Tickle T. L.; Sokol H.; Gevers D.; Devaney K. L.; Ward D. V.; Reyes J. A.; Shah S. A.; LeLeiko N.; Snapper S. B.; et al. Dysfunction of the Intestinal Microbiome in Inflammatory Bowel Disease and Treatment . Genome Biol. 2012, 13 , R79. 10.1186/gb-2012-13-9-r79. [ PMC free article ] [ PubMed ] [ CrossRef ] [ Google Scholar ]
- Vázquez-Castellanos J. F.; Serrano-Villar S.; Latorre A.; Artacho A.; Ferrús M. L.; Madrid N.; Vallejo A.; Sainz T.; Martínez-Botas J.; Ferrando-Martínez S.; et al. Altered Metabolism of Gut Microbiota Contributes to Chronic Immune Activation in Hiv-Infected Individuals . Mucosal Immunol. 2015, 8 , 760–772. 10.1038/mi.2014.107. [ PubMed ] [ CrossRef ] [ Google Scholar ]
- Sheth R. U.; Cabral V.; Chen S. P.; Wang H. H. Manipulating Bacterial Communities by in Situ Microbiome Engineering . Trends Genet. 2016, 32 , 189–200. 10.1016/j.tig.2016.01.005. [ PMC free article ] [ PubMed ] [ CrossRef ] [ Google Scholar ]
- Brophy J. A. N.; Triassi A. J.; Adams B. L.; Renberg R. L.; Stratis-Cullum D. N.; Grossman A. D.; Voigt C. A. Engineered Integrative and Conjugative Elements for Efficient and Inducible DNA Transfer to Undomesticated Bacteria . Nat. Microbiol. 2018, 3 , 1043–1053. 10.1038/s41564-018-0216-5. [ PubMed ] [ CrossRef ] [ Google Scholar ]
- Ronda C.; Chen S. P.; Cabral V.; Yaung S. J.; Wang H. H. Metagenomic Engineering of the Mammalian Gut Microbiome in Situ . Nat. Methods 2019, 16 , 167–170. 10.1038/s41592-018-0301-y. [ PMC free article ] [ PubMed ] [ CrossRef ] [ Google Scholar ]
- Jin W.-B.; Li T.-T.; Huo D.; Qu S.; Li X. V.; Arifuzzaman M.; Lima S. F.; Shi H.-Q.; Wang A.; Putzel G. G.; et al. Genetic Manipulation of Gut Microbes Enables Single-Gene Interrogation in a Complex Microbiome . Cell 2022, 185 , 547–562. 10.1016/j.cell.2021.12.035. [ PMC free article ] [ PubMed ] [ CrossRef ] [ Google Scholar ]
- Liu L.; Chen X.; Skogerbø G.; Zhang P.; Chen R.; He S.; Huang D.-W. The Human Microbiome: A Hot Spot of Microbial Horizontal Gene Transfer . Genomics 2012, 100 , 265–270. 10.1016/j.ygeno.2012.07.012. [ PubMed ] [ CrossRef ] [ Google Scholar ]
- Wilson I. D.; Nicholson J. K. Gut Microbiome Interactions with Drug Metabolism, Efficacy, and Toxicity . Transl. Res. 2017, 179 , 204–222. 10.1016/j.trsl.2016.08.002. [ PMC free article ] [ PubMed ] [ CrossRef ] [ Google Scholar ]
- Smith N. F.; Figg W. D.; Sparreboom A. Pharmacogenetics of Irinotecan Metabolism and Transport: An Update . Toxicol. In Vitro 2006, 20 , 163–175. 10.1016/j.tiv.2005.06.045. [ PubMed ] [ CrossRef ] [ Google Scholar ]
- Wallace B. D.; Wang H.; Lane K. T.; Scott J. E.; Orans J.; Koo J. S.; Venkatesh M.; Jobin C.; Yeh L.-A.; Mani S.; et al. Alleviating Cancer Drug Toxicity by Inhibiting a Bacterial Enzyme . Science 2010, 330 , 831–835. 10.1126/science.1191175. [ PMC free article ] [ PubMed ] [ CrossRef ] [ Google Scholar ]
- Wang Z.; Roberts A. B.; Buffa J. A.; Levison B. S.; Zhu W.; Org E.; Gu X.; Huang Y.; Zamanian-Daryoush M.; Culley M. K.; et al. Non-Lethal Inhibition of Gut Microbial Trimethylamine Production for the Treatment of Atherosclerosis . Cell 2015, 163 , 1585–1595. 10.1016/j.cell.2015.11.055. [ PMC free article ] [ PubMed ] [ CrossRef ] [ Google Scholar ]
- Roberts A. B.; Gu X.; Buffa J. A.; Hurd A. G.; Wang Z.; Zhu W.; Gupta N.; Skye S. M.; Cody D. B.; Levison B. S.; et al. Development of a Gut Microbe–Targeted Nonlethal Therapeutic to Inhibit Thrombosis Potential . Nat. Med. 2018, 24 , 1407–1417. 10.1038/s41591-018-0128-1. [ PMC free article ] [ PubMed ] [ CrossRef ] [ Google Scholar ]
- Craciun S.; Balskus E. P. Microbial Conversion of Choline to Trimethylamine Requires a Glycyl Radical Enzyme . Proc. Natl. Acad. Sci. U. S. A. 2012, 109 , 21307–21312. 10.1073/pnas.1215689109. [ PMC free article ] [ PubMed ] [ CrossRef ] [ Google Scholar ]
- Wang Z.; Klipfell E.; Bennett B. J.; Koeth R.; Levison B. S.; DuGar B.; Feldstein A. E.; Britt E. B.; Fu X.; Chung Y.-M.; et al. Gut Flora Metabolism of Phosphatidylcholine Promotes Cardiovascular Disease . Nature 2011, 472 , 57–63. 10.1038/nature09922. [ PMC free article ] [ PubMed ] [ CrossRef ] [ Google Scholar ]
- Isabella V. M.; Ha B. N.; Castillo M. J.; Lubkowicz D. J.; Rowe S. E.; Millet Y. A.; Anderson C. L.; Li N.; Fisher A. B.; West K. A.; et al. Development of a Synthetic Live Bacterial Therapeutic for the Human Metabolic Disease Phenylketonuria . Nat. Biotechnol. 2018, 36 , 857. 10.1038/nbt.4222. [ PubMed ] [ CrossRef ] [ Google Scholar ]
- Bai L.; Gao M.; Cheng X.; Kang G.; Cao X.; Huang H. Engineered Butyrate-Producing Bacteria Prevents High Fat Diet-Induced Obesity in Mice . Microb. Cell Fact. 2020, 19 , 94. 10.1186/s12934-020-01350-z. [ PMC free article ] [ PubMed ] [ CrossRef ] [ Google Scholar ]
- Lubkowicz D.; Horvath N. G.; James M. J.; Cantarella P.; Renaud L.; Bergeron C. G.; Shmueli R. B.; Anderson C.; Gao J.-R.; Kurtz C. B.; et al. An Engineered Bacterial Therapeutic Lowers Urinary Oxalate in Preclinical Models and in Silico Simulations of Enteric Hyperoxaluria . Mol. Syst. Biol. 2022, 18 , e10539. 10.15252/msb.202110539. [ PMC free article ] [ PubMed ] [ CrossRef ] [ Google Scholar ]
- Ho C. L.; Tan H. Q.; Chua K. J.; Kang A.; Lim K. H.; Ling K. L.; Yew W. S.; Lee Y. S.; Thiery J. P.; Chang M. W. Engineered Commensal Microbes for Diet-Mediated Colorectal-Cancer Chemoprevention . Nat. Biomed. Eng. 2018, 2 , 27–37. 10.1038/s41551-017-0181-y. [ PubMed ] [ CrossRef ] [ Google Scholar ]
- Canale F. P.; Basso C.; Antonini G.; Perotti M.; Li N.; Sokolovska A.; Neumann J.; James M. J.; Geiger S.; Jin W.; et al. Metabolic Modulation of Tumours with Engineered Bacteria for Immunotherapy . Nature 2021, 598 , 662–666. 10.1038/s41586-021-04003-2. [ PubMed ] [ CrossRef ] [ Google Scholar ]
- Abedi M. H.; Yao M. S.; Mittelstein D. R.; Bar-Zion A.; Swift M. B.; Lee-Gosselin A.; Barturen-Larrea P.; Buss M. T.; Shapiro M. G. Ultrasound-Controllable Engineered Bacteria for Cancer Immunotherapy . Nat. Commun. 2022, 13 , 1585. 10.1038/s41467-022-29065-2. [ PMC free article ] [ PubMed ] [ CrossRef ] [ Google Scholar ]
- López-Igual R.; Bernal-Bayard J.; Rodríguez-Patón A.; Ghigo J.-M.; Mazel D. Engineered Toxin–Intein Antimicrobials Can Selectively Target and Kill Antibiotic-Resistant Bacteria in Mixed Populations . Nat. Biotechnol. 2019, 37 , 755–760. 10.1038/s41587-019-0105-3. [ PubMed ] [ CrossRef ] [ Google Scholar ]
- Tscherner M.; Giessen T. W.; Markey L.; Kumamoto C. A.; Silver P. A. A Synthetic System That Senses Candida Albicans and Inhibits Virulence Factors . ACS Synth. Biol. 2019, 8 , 434–444. 10.1021/acssynbio.8b00457. [ PubMed ] [ CrossRef ] [ Google Scholar ]
- Cubillos-Ruiz A.; Alcantar M. A.; Donghia N. M.; Cárdenas P.; Avila-Pacheco J.; Collins J. J. An Engineered Live Biotherapeutic for the Prevention of Antibiotic-Induced Dysbiosis . Nat. Biomed. Eng. 2022, 6 , 910–921. 10.1038/s41551-022-00871-9. [ PubMed ] [ CrossRef ] [ Google Scholar ]
- Vågesjö E.; Öhnstedt E.; Mortier A.; Lofton H.; Huss F.; Proost P.; Roos S.; Phillipson M. Accelerated Wound Healing in Mice by on-Site Production and Delivery of Cxcl12 by Transformed Lactic Acid Bacteria . Proc. Natl. Acad. Sci. U. S. A. 2018, 115 , 1895. 10.1073/pnas.1716580115. [ PMC free article ] [ PubMed ] [ CrossRef ] [ Google Scholar ]
- Praveschotinunt P.; Duraj-Thatte A. M.; Gelfat I.; Bahl F.; Chou D. B.; Joshi N. S. Engineered E. Coli Nissle 1917 for the Delivery of Matrix-Tethered Therapeutic Domains to the Gut . Nat. Commun. 2019, 10 , 5580. 10.1038/s41467-019-13336-6. [ PMC free article ] [ PubMed ] [ CrossRef ] [ Google Scholar ]
- Aggarwal N.; Breedon A. M. E.; Davis C. M.; Hwang I. Y.; Chang M. W. Engineering Probiotics for Therapeutic Applications: Recent Examples and Translational Outlook . Curr. Opin. Biotechnol. 2020, 65 , 171–179. 10.1016/j.copbio.2020.02.016. [ PubMed ] [ CrossRef ] [ Google Scholar ]
- Zhou Z.; Chen X.; Sheng H.; Shen X.; Sun X.; Yan Y.; Wang J.; Yuan Q. Engineering Probiotics as Living Diagnostics and Therapeutics for Improving Human Health . Microb. Cell Fact. 2020, 19 , 56. 10.1186/s12934-020-01318-z. [ PMC free article ] [ PubMed ] [ CrossRef ] [ Google Scholar ]
- Barra M.; Danino T.; Garrido D. Engineered Probiotics for Detection and Treatment of Inflammatory Intestinal Diseases . Front. Bioeng. Biotechnol. 2020, 8 , 265. 10.3389/fbioe.2020.00265. [ PMC free article ] [ PubMed ] [ CrossRef ] [ Google Scholar ]
- Shen H.; Aggarwal N.; Wun K. S.; Lee Y. S.; Hwang I. Y.; Chang M. W. Engineered Microbial Systems for Advanced Drug Delivery . Adv. Drug Delivery Rev. 2022, 187 , 114364. 10.1016/j.addr.2022.114364. [ PubMed ] [ CrossRef ] [ Google Scholar ]
- Visconti A.; Le Roy C. I.; Rosa F.; Rossi N.; Martin T. C.; Mohney R. P.; Li W.; de Rinaldis E.; Bell J. T.; Venter J. C.; et al. Interplay between the Human Gut Microbiome and Host Metabolism . Nat. Commun. 2019, 10 , 4505. 10.1038/s41467-019-12476-z. [ PMC free article ] [ PubMed ] [ CrossRef ] [ Google Scholar ]
- Strong A.; Gold J.; Gold N. B.; Yudkoff M. Hepatic Manifestations of Urea Cycle Disorders . Clin. Liver Dis. 2021, 18 , 198–203. 10.1002/cld.1115. [ PMC free article ] [ PubMed ] [ CrossRef ] [ Google Scholar ]
- Kurtz C. B.; Millet Y. A.; Puurunen M. K.; Perreault M.; Charbonneau M. R.; Isabella V. M.; Kotula J. W.; Antipov E.; Dagon Y.; Denney W. S.; et al. An Engineered E. Coli Nissle Improves Hyperammonemia and Survival in Mice and Shows Dose-Dependent Exposure in Healthy Humans . Sci. Transl. Med. 2019, 11 , eaau7975. 10.1126/scitranslmed.aau7975. [ PubMed ] [ CrossRef ] [ Google Scholar ]
- Synlogic . Synlogic Discontinues Development of Synb1020 to Treat Hyperammonemia . https://investor.synlogictx.com/news-releases/news-release-details/synlogic-discontinues-development-synb1020-treat-hyperammonemia (accessed May 7, 2022).
- Silva Y. P.; Bernardi A.; Frozza R. L. The Role of Short-Chain Fatty Acids from Gut Microbiota in Gut-Brain Communication . Front. Endocrinol. 2020, 11 , 25. 10.3389/fendo.2020.00025. [ PMC free article ] [ PubMed ] [ CrossRef ] [ Google Scholar ]
- Li Z.; Yi C.-X.; Katiraei S.; Kooijman S.; Zhou E.; Chung C. K.; Gao Y.; van den Heuvel J. K.; Meijer O. C.; Berbée J. F. P.; et al. Butyrate Reduces Appetite and Activates Brown Adipose Tissue Via the Gut-Brain Neural Circuit . Gut 2018, 67 , 1269. 10.1136/gutjnl-2017-314050. [ PubMed ] [ CrossRef ] [ Google Scholar ]
- Puddu A.; Sanguineti R.; Montecucco F.; Viviani G. L. Evidence for the Gut Microbiota Short-Chain Fatty Acids as Key Pathophysiological Molecules Improving Diabetes . Mediators Inflammation 2014, 2014 , 162021. 10.1155/2014/162021. [ PMC free article ] [ PubMed ] [ CrossRef ] [ Google Scholar ]
- Wang L.; Cheng X.; Bai L.; Gao M.; Kang G.; Cao X.; Huang H. Positive Interventional Effect of Engineered Butyrate-Producing Bacteria on Metabolic Disorders and Intestinal Flora Disruption in Obese Mice . Microbiol. Spectr. 2022, 10 , e0114721 10.1128/spectrum.01147-21. [ PMC free article ] [ PubMed ] [ CrossRef ] [ Google Scholar ]
- Bai Y.; Mansell T. J. Production and Sensing of Butyrate in a Probiotic E. Coli Strain . Int. J. Mol. Sci. 2020, 21 , 3615. 10.3390/ijms21103615. [ PMC free article ] [ PubMed ] [ CrossRef ] [ Google Scholar ]
- Hwang I. Y.; Kim H. R.; De Sotto R.; Chang M. W. Engineered Probiotics Modulate the Endocannabinoid System . Biotechnology Notes 2021, 2 , 33–38. 10.1016/j.biotno.2021.08.001. [ CrossRef ] [ Google Scholar ]
- Scott B. M.; Gutiérrez-Vázquez C.; Sanmarco L. M.; da Silva Pereira J. A.; Li Z.; Plasencia A.; Hewson P.; Cox L. M.; O’Brien M.; Chen S. K.; et al. Self-Tunable Engineered Yeast Probiotics for the Treatment of Inflammatory Bowel Disease . Nat. Med. 2021, 27 , 1212–1222. 10.1038/s41591-021-01390-x. [ PubMed ] [ CrossRef ] [ Google Scholar ]
- Brenner K.; You L.; Arnold F. H. Engineering Microbial Consortia: A New Frontier in Synthetic Biology . Trends Biotechnol. 2008, 26 , 483–489. 10.1016/j.tibtech.2008.05.004. [ PubMed ] [ CrossRef ] [ Google Scholar ]
- Hanssen N. M. J.; de Vos W. M.; Nieuwdorp M. Fecal Microbiota Transplantation in Human Metabolic Diseases: From a Murky Past to a Bright Future? . Cell Metab. 2021, 33 , 1098–1110. 10.1016/j.cmet.2021.05.005. [ PubMed ] [ CrossRef ] [ Google Scholar ]
- Cammarota G.; Ianiro G.; Tilg H.; Rajilić-Stojanović M.; Kump P.; Satokari R.; Sokol H.; Arkkila P.; Pintus C.; Hart A.; et al. European Consensus Conference on Faecal Microbiota Transplantation in Clinical Practice . Gut 2017, 66 , 569. 10.1136/gutjnl-2016-313017. [ PMC free article ] [ PubMed ] [ CrossRef ] [ Google Scholar ]
- van Nood E.; Vrieze A.; Nieuwdorp M.; Fuentes S.; Zoetendal E. G.; de Vos W. M.; Visser C. E.; Kuijper E. J.; Bartelsman J. F. W. M.; Tijssen J. G. P.; et al. Duodenal Infusion of Donor Feces for Recurrent Clostridium Difficile . N. Engl. J. Med. 2013, 368 , 407–415. 10.1056/NEJMoa1205037. [ PubMed ] [ CrossRef ] [ Google Scholar ]
- Suez J.; Zmora N.; Zilberman-Schapira G.; Mor U.; Dori-Bachash M.; Bashiardes S.; Zur M.; Regev-Lehavi D.; Ben-Zeev Brik R.; Federici S.; et al. Post-Antibiotic Gut Mucosal Microbiome Reconstitution Is Impaired by Probiotics and Improved by Autologous Fmt . Cell 2018, 174 , 1406–1423. 10.1016/j.cell.2018.08.047. [ PubMed ] [ CrossRef ] [ Google Scholar ]
- Costello S. P.; Hughes P. A.; Waters O.; Bryant R. V.; Vincent A. D.; Blatchford P.; Katsikeros R.; Makanyanga J.; Campaniello M. A.; Mavrangelos C.; et al. Effect of Fecal Microbiota Transplantation on 8-Week Remission in Patients with Ulcerative Colitis: A Randomized Clinical Trial . JAMA 2019, 321 , 156–164. 10.1001/jama.2018.20046. [ PMC free article ] [ PubMed ] [ CrossRef ] [ Google Scholar ]
- Shen Z.-H.; Zhu C.-X.; Quan Y.-S.; Yang Z.-Y.; Wu S.; Luo W.-W.; Tan B.; Wang X.-Y. Relationship between Intestinal Microbiota and Ulcerative Colitis: Mechanisms and Clinical Application of Probiotics and Fecal Microbiota Transplantation . World J. Gastroenterol. 2018, 24 , 5–14. 10.3748/wjg.v24.i1.5. [ PMC free article ] [ PubMed ] [ CrossRef ] [ Google Scholar ]
- Ng S. C.; Xu Z.; Mak J. W. Y.; Yang K.; Liu Q.; Zuo T.; Tang W.; Lau L.; Lui R. N.; Wong S. H. Microbiota Engraftment after Faecal Microbiota Transplantation in Obese Subjects with Type 2 Diabetes: A 24-Week, Double-Blind, Randomised Controlled Trial . Gut 2022, 71 , 716–723. 10.1136/gutjnl-2020-323617. [ PubMed ] [ CrossRef ] [ Google Scholar ]
- Xu D.; Chen V. L.; Steiner C. A.; Berinstein J. A.; Eswaran S.; Waljee A. K.; Higgins P. D. R.; Owyang C. Efficacy of Fecal Microbiota Transplantation in Irritable Bowel Syndrome: A Systematic Review and Meta-Analysis . Am. J. Gastroenterol. 2019, 114 , 1043–1050. 10.14309/ajg.0000000000000198. [ PMC free article ] [ PubMed ] [ CrossRef ] [ Google Scholar ]
- Chen D.; Wu J.; Jin D.; Wang B.; Cao H. Fecal Microbiota Transplantation in Cancer Management: Current Status and Perspectives . Int. J. Cancer 2019, 145 , 2021–2031. 10.1002/ijc.32003. [ PMC free article ] [ PubMed ] [ CrossRef ] [ Google Scholar ]
- Park S.-K.; Lee C.-W.; Park D.-I.; Woo H.-Y.; Cheong H. S.; Shin H. C.; Ahn K.; Kwon M.-J.; Joo E.-J. Detection of Sars-Cov-2 in Fecal Samples from Patients with Asymptomatic and Mild Covid-19 in Korea . Clin. Gastroenterol. Hepatol. 2021, 19 , 1387–1394. 10.1016/j.cgh.2020.06.005. [ PMC free article ] [ PubMed ] [ CrossRef ] [ Google Scholar ]
- Wilson B. C.; Vatanen T.; Cutfield W. S.; O’Sullivan J. M. The Super-Donor Phenomenon in Fecal Microbiota Transplantation . Front. Cell. Infect. Microbiol. 2019, 9 , 2. 10.3389/fcimb.2019.00002. [ PMC free article ] [ PubMed ] [ CrossRef ] [ Google Scholar ]
- McGovern B. H.; Ford C. B.; Henn M. R.; Pardi D. S.; Khanna S.; Hohmann E. L.; O’Brien E. J.; Desjardins C. A.; Bernardo P.; Wortman J. R.; et al. Ser-109, an Investigational Microbiome Drug to Reduce Recurrence after Clostridioides Difficile Infection: Lessons Learned from a Phase 2 Trial . Clin. Infect. Dis. 2021, 72 , 2132–2140. 10.1093/cid/ciaa387. [ PMC free article ] [ PubMed ] [ CrossRef ] [ Google Scholar ]
- Weingarden A. R.; Dosa P. I.; DeWinter E.; Steer C. J.; Shaughnessy M. K.; Johnson J. R.; Khoruts A.; Sadowsky M. J. Changes in Colonic Bile Acid Composition Following Fecal Microbiota Transplantation Are Sufficient to Control Clostridium Difficile Germination and Growth . PLoS One 2016, 11 , e0147210 10.1371/journal.pone.0147210. [ PMC free article ] [ PubMed ] [ CrossRef ] [ Google Scholar ]
- Feuerstadt P.; Louie T. J.; Lashner B.; Wang E. E. L.; Diao L.; Bryant J. A.; Sims M.; Kraft C. S.; Cohen S. H.; Berenson C. S.; et al. Ser-109, an Oral Microbiome Therapy for Recurrent Clostridioides Difficile Infection . N. Engl. J. Med. 2022, 386 , 220–229. 10.1056/NEJMoa2106516. [ PubMed ] [ CrossRef ] [ Google Scholar ]
- Tanoue T.; Morita S.; Plichta D. R.; Skelly A. N.; Suda W.; Sugiura Y.; Narushima S.; Vlamakis H.; Motoo I.; Sugita K.; et al. A Defined Commensal Consortium Elicits Cd8 T Cells and Anti-Cancer Immunity . Nature 2019, 565 , 600–605. 10.1038/s41586-019-0878-z. [ PubMed ] [ CrossRef ] [ Google Scholar ]
- Hofer U. The Next Step Towards Anticancer Microbiota Therapeutics . Nature Rev. Microbiol. 2019, 17 , 125–125. 10.1038/s41579-019-0152-2. [ PubMed ] [ CrossRef ] [ Google Scholar ]
- van der Lelie D.; Oka A.; Taghavi S.; Umeno J.; Fan T.-J.; Merrell K. E.; Watson S. D.; Ouellette L.; Liu B.; Awoniyi M.; et al. Rationally Designed Bacterial Consortia to Treat Chronic Immune-Mediated Colitis and Restore Intestinal Homeostasis . Nat. Commun. 2021, 12 , 3105. 10.1038/s41467-021-23460-x. [ PMC free article ] [ PubMed ] [ CrossRef ] [ Google Scholar ]
- Lloyd-Price J.; Arze C.; Ananthakrishnan A. N.; Schirmer M.; Avila-Pacheco J.; Poon T. W.; Andrews E.; Ajami N. J.; Bonham K. S.; Brislawn C. J.; et al. Multi-Omics of the Gut Microbial Ecosystem in Inflammatory Bowel Diseases . Nature 2019, 569 , 655–662. 10.1038/s41586-019-1237-9. [ PMC free article ] [ PubMed ] [ CrossRef ] [ Google Scholar ]
- Alexeev E. E.; Lanis J. M.; Kao D. J.; Campbell E. L.; Kelly C. J.; Battista K. D.; Gerich M. E.; Jenkins B. R.; Walk S. T.; Kominsky D. J.; et al. Microbiota-Derived Indole Metabolites Promote Human and Murine Intestinal Homeostasis through Regulation of Interleukin-10 Receptor . Am. J. Pathol. 2018, 188 , 1183–1194. 10.1016/j.ajpath.2018.01.011. [ PMC free article ] [ PubMed ] [ CrossRef ] [ Google Scholar ]
- Kong W.; Meldgin D. R.; Collins J. J.; Lu T. Designing Microbial Consortia with Defined Social Interactions . Nature Chem. Biol. 2018, 14 , 821–829. 10.1038/s41589-018-0091-7. [ PubMed ] [ CrossRef ] [ Google Scholar ]
- Balagaddé F. K.; Song H.; Ozaki J.; Collins C. H.; Barnet M.; Arnold F. H.; Quake S. R.; You L. A Synthetic Escherichia Coli Predator–Prey Ecosystem . Mol. Syst. Biol. 2008, 4 , 187. 10.1038/msb.2008.24. [ PMC free article ] [ PubMed ] [ CrossRef ] [ Google Scholar ]
- Lindemann S. R.; Bernstein H. C.; Song H.-S.; Fredrickson J. K.; Fields M. W.; Shou W.; Johnson D. R.; Beliaev A. S. Engineering Microbial Consortia for Controllable Outputs . ISME J. 2016, 10 , 2077–2084. 10.1038/ismej.2016.26. [ PMC free article ] [ PubMed ] [ CrossRef ] [ Google Scholar ]
- Lloyd-Price J.; Mahurkar A.; Rahnavard G.; Crabtree J.; Orvis J.; Hall A. B.; Brady A.; Creasy H. H.; McCracken C.; Giglio M. G.; et al. Strains, Functions and Dynamics in the Expanded Human Microbiome Project . Nature 2017, 550 , 61–66. 10.1038/nature23889. [ PMC free article ] [ PubMed ] [ CrossRef ] [ Google Scholar ]
- The Integrative Human Microbiome Project: Dynamic Analysis of Microbiome-Host Omics Profiles During Periods of Human Health and Disease . Cell Host Microbe 2014, 16 , 276–289. 10.1016/j.chom.2014.08.014. [ PMC free article ] [ PubMed ] [ CrossRef ] [ Google Scholar ]
- Mark Welch J. L.; Hasegawa Y.; McNulty N. P.; Gordon J. I.; Borisy G. G. Spatial Organization of a Model 15-Member Human Gut Microbiota Established in Gnotobiotic Mice . Proc. Natl. Acad. Sci. U. S. A. 2017, 114 , E9105–E9114. 10.1073/pnas.1711596114. [ PMC free article ] [ PubMed ] [ CrossRef ] [ Google Scholar ]
- Cao X.; Hamilton J. J.; Venturelli O. S. Understanding and Engineering Distributed Biochemical Pathways in Microbial Communities . Biochem. 2019, 58 , 94–107. 10.1021/acs.biochem.8b01006. [ PMC free article ] [ PubMed ] [ CrossRef ] [ Google Scholar ]
- Sheth R. U.; Li M.; Jiang W.; Sims P. A.; Leong K. W.; Wang H. H. Spatial Metagenomic Characterization of Microbial Biogeography in the Gut . Nat. Biotechnol. 2019, 37 , 877–883. 10.1038/s41587-019-0183-2. [ PMC free article ] [ PubMed ] [ CrossRef ] [ Google Scholar ]
- Zhao S.; Lieberman T. D.; Poyet M.; Kauffman K. M.; Gibbons S. M.; Groussin M.; Xavier R. J.; Alm E. J. Adaptive Evolution within Gut Microbiomes of Healthy People . Cell Host Microbe 2019, 25 , 656–667. 10.1016/j.chom.2019.03.007. [ PMC free article ] [ PubMed ] [ CrossRef ] [ Google Scholar ]
- Guo C.-J.; Allen B. M.; Hiam K. J.; Dodd D.; Van Treuren W.; Higginbottom S.; Nagashima K.; Fischer C. R.; Sonnenburg J. L.; Spitzer M. H.; et al. Depletion of Microbiome-Derived Molecules in the Host Using Clostridium Genetics . Science 2019, 366 , eaav1282. 10.1126/science.aav1282. [ PMC free article ] [ PubMed ] [ CrossRef ] [ Google Scholar ]
- Backhed F.; Manchester J. K.; Semenkovich C. F.; Gordon J. I. Mechanisms Underlying the Resistance to Diet-Induced Obesity in Germ-Free Mice . Proc. Natl. Acad. Sci. U. S. A. 2007, 104 , 979–984. 10.1073/pnas.0605374104. [ PMC free article ] [ PubMed ] [ CrossRef ] [ Google Scholar ]
- Turnbaugh P. J.; Ley R. E.; Mahowald M. A.; Magrini V.; Mardis E. R.; Gordon J. I. An Obesity-Associated Gut Microbiome with Increased Capacity for Energy Harvest . Nature 2006, 444 , 1027–1031. 10.1038/nature05414. [ PubMed ] [ CrossRef ] [ Google Scholar ]
- Ridaura V. K.; Faith J. J.; Rey F. E.; Cheng J.; Duncan A. E.; Kau A. L.; Griffin N. W.; Lombard V.; Henrissat B.; Bain J. R.; et al. Gut Microbiota from Twins Discordant for Obesity Modulate Metabolism in Mice . Science 2013, 341 , 1241214. 10.1126/science.1241214. [ PMC free article ] [ PubMed ] [ CrossRef ] [ Google Scholar ]
- Alang N.; Kelly C. R. Weight Gain after Fecal Microbiota Transplantation . Open Forum Infect. Dis. 2015, 2 , ofv004. 10.1093/ofid/ofv004. [ PMC free article ] [ PubMed ] [ CrossRef ] [ Google Scholar ]
- Donia M. S.; Fischbach M. A. Small Molecules from the Human Microbiota . Science 2015, 349 , 1254766. 10.1126/science.1254766. [ PMC free article ] [ PubMed ] [ CrossRef ] [ Google Scholar ]
- Smith P. M.; Howitt M. R.; Panikov N.; Michaud M.; Gallini C. A.; Bohlooly-Y Y. M.; Glickman J. N.; Garrett W. S. The Microbial Metabolites, Short-Chain Fatty Acids, Regulate Colonic Treg Cell Homeostasis . Science 2013, 341 , 569–573. 10.1126/science.1241165. [ PMC free article ] [ PubMed ] [ CrossRef ] [ Google Scholar ]
- Arpaia N.; Campbell C.; Fan X.; Dikiy S.; van der Veeken J.; deRoos P.; Liu H.; Cross J. R.; Pfeffer K.; Coffer P. J.; et al. Metabolites Produced by Commensal Bacteria Promote Peripheral Regulatory T-Cell Generation . Nature 2013, 504 , 451–455. 10.1038/nature12726. [ PMC free article ] [ PubMed ] [ CrossRef ] [ Google Scholar ]
- O’Connell T. M. The Application of Metabolomics to Probiotic and Prebiotic Interventions in Human Clinical Studies . Metabolites 2020, 10 , 120. 10.3390/metabo10030120. [ PMC free article ] [ PubMed ] [ CrossRef ] [ Google Scholar ]
- Han S.; Van Treuren W.; Fischer C. R.; Merrill B. D.; DeFelice B. C.; Sanchez J. M.; Higginbottom S. K.; Guthrie L.; Fall L. A.; Dodd D.; et al. A Metabolomics Pipeline for the Mechanistic Interrogation of the Gut Microbiome . Nature 2021, 595 , 415–420. 10.1038/s41586-021-03707-9. [ PMC free article ] [ PubMed ] [ CrossRef ] [ Google Scholar ]
- Koh A.; Molinaro A.; Stahlman M.; Khan M. T.; Schmidt C.; Manneras-Holm L.; Wu H.; Carreras A.; Jeong H.; Olofsson L. E.; et al. Microbially Produced Imidazole Propionate Impairs Insulin Signaling through Mtorc1 . Cell 2018, 175 , 947–961. 10.1016/j.cell.2018.09.055. [ PubMed ] [ CrossRef ] [ Google Scholar ]
- Nemet I.; Saha P. P.; Gupta N.; Zhu W.; Romano K. A.; Skye S. M.; Cajka T.; Mohan M. L.; Li L.; Wu Y.; et al. A Cardiovascular Disease-Linked Gut Microbial Metabolite Acts Via Adrenergic Receptors . Cell 2020, 180 , 862–877. 10.1016/j.cell.2020.02.016. [ PMC free article ] [ PubMed ] [ CrossRef ] [ Google Scholar ]
- Peisl B. Y. L.; Schymanski E. L.; Wilmes P. Dark Matter in Host-Microbiome Metabolomics: Tackling the Unknowns-a Review . Anal. Chim. Acta 2018, 1037 , 13–27. 10.1016/j.aca.2017.12.034. [ PubMed ] [ CrossRef ] [ Google Scholar ]
- Greener J. G.; Kandathil S. M.; Moffat L.; Jones D. T. A Guide to Machine Learning for Biologists . Nat. Rev. Mol. Cell. Biol. 2022, 23 , 40–55. 10.1038/s41580-021-00407-0. [ PubMed ] [ CrossRef ] [ Google Scholar ]
- Pomyen Y.; Wanichthanarak K.; Poungsombat P.; Fahrmann J.; Grapov D.; Khoomrung S. Deep Metabolome: Applications of Deep Learning in Metabolomics . Comput. Struct. Biotechnol. J. 2020, 18 , 2818–2825. 10.1016/j.csbj.2020.09.033. [ PMC free article ] [ PubMed ] [ CrossRef ] [ Google Scholar ]
- Colby S. M.; Nunez J. R.; Hodas N. O.; Corley C. D.; Renslow R. R. Deep Learning to Generate in Silico Chemical Property Libraries and Candidate Molecules for Small Molecule Identification in Complex Samples . Anal. Chem. 2020, 92 , 1720–1729. 10.1021/acs.analchem.9b02348. [ PubMed ] [ CrossRef ] [ Google Scholar ]
- Shakya M.; Lo C. C.; Chain P. S. G. Advances and Challenges in Metatranscriptomic Analysis . Front. Genet. 2019, 10 , 904. 10.3389/fgene.2019.00904. [ PMC free article ] [ PubMed ] [ CrossRef ] [ Google Scholar ]
- Berg C.; Dupont C. L.; Asplund-Samuelsson J.; Celepli N. A.; Eiler A.; Allen A. E.; Ekman M.; Bergman B.; Ininbergs K. Dissection of Microbial Community Functions During a Cyanobacterial Bloom in the Baltic Sea Via Metatranscriptomics . Front. Mar. Sci. 2018, 5 , 55. 10.3389/fmars.2018.00055. [ CrossRef ] [ Google Scholar ]
- Moniruzzaman M.; Wurch L. L.; Alexander H.; Dyhrman S. T.; Gobler C. J.; Wilhelm S. W. Virus-Host Relationships of Marine Single-Celled Eukaryotes Resolved from Metatranscriptomics . Nat. Commun. 2017, 8 , 16054. 10.1038/ncomms16054. [ PMC free article ] [ PubMed ] [ CrossRef ] [ Google Scholar ]
- White R. A. 3rd; Bottos E. M.; Roy Chowdhury T.; Zucker J. D.; Brislawn C. J.; Nicora C. D.; Fansler S. J.; Glaesemann K. R.; Glass K.; Jansson J. K. Moleculo Long-Read Sequencing Facilitates Assembly and Genomic Binning from Complex Soil Metagenomes . mSystems 2016, 1 , e00045-16. 10.1128/mSystems.00045-16. [ PMC free article ] [ PubMed ] [ CrossRef ] [ Google Scholar ]
- Damon C.; Lehembre F.; Oger-Desfeux C.; Luis P.; Ranger J.; Fraissinet-Tachet L.; Marmeisse R. Metatranscriptomics Reveals the Diversity of Genes Expressed by Eukaryotes in Forest Soils . PLoS One 2012, 7 , e28967. 10.1371/journal.pone.0028967. [ PMC free article ] [ PubMed ] [ CrossRef ] [ Google Scholar ]
- Franzosa E. A.; Morgan X. C.; Segata N.; Waldron L.; Reyes J.; Earl A. M.; Giannoukos G.; Boylan M. R.; Ciulla D.; Gevers D.; et al. Relating the Metatranscriptome and Metagenome of the Human Gut . Proc. Natl. Acad. Sci. U. S. A. 2014, 111 , E2329–2338. 10.1073/pnas.1319284111. [ PMC free article ] [ PubMed ] [ CrossRef ] [ Google Scholar ]
- Nowicki E. M.; Shroff R.; Singleton J. A.; Renaud D. E.; Wallace D.; Drury J.; Zirnheld J.; Colleti B.; Ellington A. D.; Lamont R. J.; et al. Microbiota and Metatranscriptome Changes Accompanying the Onset of Gingivitis . mBio 2018, 9 , e00575-18. 10.1128/mBio.00575-18. [ PMC free article ] [ PubMed ] [ CrossRef ] [ Google Scholar ]
- Schirmer M.; Franzosa E. A.; Lloyd-Price J.; McIver L. J.; Schwager R.; Poon T. W.; Ananthakrishnan A. N.; Andrews E.; Barron G.; Lake K.; et al. Dynamics of Metatranscription in the Inflammatory Bowel Disease Gut Microbiome . Nat. Microbiol. 2018, 3 , 337–346. 10.1038/s41564-017-0089-z. [ PMC free article ] [ PubMed ] [ CrossRef ] [ Google Scholar ]
- Wilmes P.; Bond P. L. Metaproteomics: Studying Functional Gene Expression in Microbial Ecosystems . Trends Microbiol. 2006, 14 , 92–97. 10.1016/j.tim.2005.12.006. [ PubMed ] [ CrossRef ] [ Google Scholar ]
- Verberkmoes N. C.; Russell A. L.; Shah M.; Godzik A.; Rosenquist M.; Halfvarson J.; Lefsrud M. G.; Apajalahti J.; Tysk C.; Hettich R. L.; et al. Shotgun Metaproteomics of the Human Distal Gut Microbiota . ISME J. 2009, 3 , 179–189. 10.1038/ismej.2008.108. [ PubMed ] [ CrossRef ] [ Google Scholar ]
- Tanca A.; Abbondio M.; Palomba A.; Fraumene C.; Manghina V.; Cucca F.; Fiorillo E.; Uzzau S. Potential and Active Functions in the Gut Microbiota of a Healthy Human Cohort . Microbiome 2017, 5 , 79. 10.1186/s40168-017-0293-3. [ PMC free article ] [ PubMed ] [ CrossRef ] [ Google Scholar ]
- Long S.; Yang Y.; Shen C.; Wang Y.; Deng A.; Qin Q.; Qiao L. Metaproteomics Characterizes Human Gut Microbiome Function in Colorectal Cancer . npj Biofilms Microbiomes 2020, 6 , 14. 10.1038/s41522-020-0123-4. [ PMC free article ] [ PubMed ] [ CrossRef ] [ Google Scholar ]
- Issa Isaac N.; Philippe D.; Nicholas A.; Raoult D.; Eric C. Metaproteomics of the Human Gut Microbiota: Challenges and Contributions to Other Omics . Clin. Mass. Spectrom. 2019, 14 , 18–30. 10.1016/j.clinms.2019.06.001. [ PMC free article ] [ PubMed ] [ CrossRef ] [ Google Scholar ]
- Breton J.; Legrand R.; Achamrah N.; Chan P.; do Rego J. L.; do Rego J. C.; Coeffier M.; Dechelotte P.; Fetissov S. O. Proteome Modifications of Gut Microbiota in Mice with Activity-Based Anorexia and Starvation: Role in Atp Production . Nutrition 2019, 67–68 , 110557. 10.1016/j.nut.2019.110557. [ PubMed ] [ CrossRef ] [ Google Scholar ]
- Lobel L.; Cao Y. G.; Fenn K.; Glickman J. N.; Garrett W. S. Diet Posttranslationally Modifies the Mouse Gut Microbial Proteome to Modulate Renal Function . Science 2020, 369 , 1518–1524. 10.1126/science.abb3763. [ PMC free article ] [ PubMed ] [ CrossRef ] [ Google Scholar ]
- Zhang X.; Chen W.; Ning Z.; Mayne J.; Mack D.; Stintzi A.; Tian R.; Figeys D. Deep Metaproteomics Approach for the Study of Human Microbiomes . Anal. Chem. 2017, 89 , 9407–9415. 10.1021/acs.analchem.7b02224. [ PubMed ] [ CrossRef ] [ Google Scholar ]
- Zhang X.; Deeke S. A.; Ning Z.; Starr A. E.; Butcher J.; Li J.; Mayne J.; Cheng K.; Liao B.; Li L.; et al. Metaproteomics Reveals Associations between Microbiome and Intestinal Extracellular Vesicle Proteins in Pediatric Inflammatory Bowel Disease . Nat. Commun. 2018, 9 , 2873. 10.1038/s41467-018-05357-4. [ PMC free article ] [ PubMed ] [ CrossRef ] [ Google Scholar ]
- Zhang X.; Ning Z.; Mayne J.; Yang Y.; Deeke S. A.; Walker K.; Farnsworth C. L.; Stokes M. P.; Couture J.-F.; Mack D.; et al. Widespread Protein Lysine Acetylation in Gut Microbiome and Its Alterations in Patients with Crohn’s Disease . Nat. Commun. 2020, 11 , 4120. 10.1038/s41467-020-17916-9. [ PMC free article ] [ PubMed ] [ CrossRef ] [ Google Scholar ]
- Mills R. H.; Dulai P. S.; Vazquez-Baeza Y.; Sauceda C.; Daniel N.; Gerner R. R.; Batachari L. E.; Malfavon M.; Zhu Q.; Weldon K.; et al. Multi-Omics Analyses of the Ulcerative Colitis Gut Microbiome Link Bacteroides Vulgatus Proteases with Disease Severity . Nat. Microbiol. 2022, 7 , 262–276. 10.1038/s41564-021-01050-3. [ PMC free article ] [ PubMed ] [ CrossRef ] [ Google Scholar ]
- Rothschild D.; Weissbrod O.; Barkan E.; Kurilshikov A.; Korem T.; Zeevi D.; Costea P. I.; Godneva A.; Kalka I. N.; Bar N.; et al. Environment Dominates over Host Genetics in Shaping Human Gut Microbiota . Nature 2018, 555 , 210–215. 10.1038/nature25973. [ PubMed ] [ CrossRef ] [ Google Scholar ]
- Goodrich J. K.; Davenport E. R.; Beaumont M.; Jackson M. A.; Knight R.; Ober C.; Spector T. D.; Bell J. T.; Clark A. G.; Ley R. E. Genetic Determinants of the Gut Microbiome in Uk Twins . Cell Host Microbe 2016, 19 , 731–743. 10.1016/j.chom.2016.04.017. [ PMC free article ] [ PubMed ] [ CrossRef ] [ Google Scholar ]
- Goodrich J. K.; Waters J. L.; Poole A. C.; Sutter J. L.; Koren O.; Blekhman R.; Beaumont M.; Van Treuren W.; Knight R.; Bell J. T.; et al. Human Genetics Shape the Gut Microbiome . Cell 2014, 159 , 789–799. 10.1016/j.cell.2014.09.053. [ PMC free article ] [ PubMed ] [ CrossRef ] [ Google Scholar ]
- Turpin W.; Espin-Garcia O.; Xu W.; Silverberg M. S.; Kevans D.; Smith M. I.; Guttman D. S.; Griffiths A.; Panaccione R.; Otley A.; et al. Association of Host Genome with Intestinal Microbial Composition in a Large Healthy Cohort . Nat. Genet. 2016, 48 , 1413–1417. 10.1038/ng.3693. [ PubMed ] [ CrossRef ] [ Google Scholar ]
- Hove H.; Norgaard H.; Brøbech Mortensen P. Lactic Acid Bacteria and the Human Gastrointestinal Tract . Eur. J. Clin. Nutr. 1999, 53 , 339–350. 10.1038/sj.ejcn.1600773. [ PubMed ] [ CrossRef ] [ Google Scholar ]
- Hamaoui D.; Subtil A. Atg16l1 Functions in Cell Homeostasis Beyond Autophagy . FEBS J. 2022, 289 , 1779–1800. 10.1111/febs.15833. [ PubMed ] [ CrossRef ] [ Google Scholar ]
- Hampe J.; Franke A.; Rosenstiel P.; Till A.; Teuber M.; Huse K.; Albrecht M.; Mayr G.; De La Vega F. M.; Briggs J.; et al. A Genome-Wide Association Scan of Nonsynonymous Snps Identifies a Susceptibility Variant for Crohn Disease in Atg16l1 . Nat. Genet. 2007, 39 , 207–211. 10.1038/ng1954. [ PubMed ] [ CrossRef ] [ Google Scholar ]
- Rioux J. D.; Xavier R. J.; Taylor K. D.; Silverberg M. S.; Goyette P.; Huett A.; Green T.; Kuballa P.; Barmada M. M.; Datta L. W.; et al. Genome-Wide Association Study Identifies New Susceptibility Loci for Crohn Disease and Implicates Autophagy in Disease Pathogenesis . Nat. Genet. 2007, 39 , 596–604. 10.1038/ng2032. [ PMC free article ] [ PubMed ] [ CrossRef ] [ Google Scholar ]
- Chu H.; Khosravi A.; Kusumawardhani I. P.; Kwon A. H.; Vasconcelos A. C.; Cunha L. D.; Mayer A. E.; Shen Y.; Wu W. L.; Kambal A.; et al. Gene-Microbiota Interactions Contribute to the Pathogenesis of Inflammatory Bowel Disease . Science 2016, 352 , 1116–1120. 10.1126/science.aad9948. [ PMC free article ] [ PubMed ] [ CrossRef ] [ Google Scholar ]
- Endy D. Foundations for Engineering Biology . Nature 2005, 438 , 449–453. 10.1038/nature04342. [ PubMed ] [ CrossRef ] [ Google Scholar ]
- Moe-Behrens G. H. The Biological Microprocessor, or How to Build a Computer with Biological Parts . Comput. Struct. Biotechnol. J. 2013, 7 , e201304003. 10.5936/csbj.201304003. [ PMC free article ] [ PubMed ] [ CrossRef ] [ Google Scholar ]
- Singh V. Recent Advances and Opportunities in Synthetic Logic Gates Engineering in Living Cells . Syst. Synth. Biol. 2014, 8 , 271–282. 10.1007/s11693-014-9154-6. [ PMC free article ] [ PubMed ] [ CrossRef ] [ Google Scholar ]
- Elowitz M. B.; Leibler S. A Synthetic Oscillatory Network of Transcriptional Regulators . Nature 2000, 403 , 335–338. 10.1038/35002125. [ PubMed ] [ CrossRef ] [ Google Scholar ]
- Gardner T. S.; Cantor C. R.; Collins J. J. Construction of a Genetic Toggle Switch in Escherichia Coli . Nature 2000, 403 , 339–342. 10.1038/35002131. [ PubMed ] [ CrossRef ] [ Google Scholar ]
- Taketani M.; Zhang J.; Zhang S.; Triassi A. J.; Huang Y. J.; Griffith L. G.; Voigt C. A. Genetic Circuit Design Automation for the Gut Resident Species Bacteroides Thetaiotaomicron . Nat. Biotechnol. 2020, 38 , 962–969. 10.1038/s41587-020-0468-5. [ PMC free article ] [ PubMed ] [ CrossRef ] [ Google Scholar ]
- Merk L. N.; Shur A. S.; Pandey A.; Murray R. M.; Green L. N.. Engineering Logical Inflammation Sensing Circuit for Gut Modulation . bioRxiv 2020, 10.1101/2020.11.10.377085. [ CrossRef ] [ Google Scholar ]
- Potvin-Trottier L.; Lord N. D.; Vinnicombe G.; Paulsson J. Synchronous Long-Term Oscillations in a Synthetic Gene Circuit . Nature 2016, 538 , 514–517. 10.1038/nature19841. [ PMC free article ] [ PubMed ] [ CrossRef ] [ Google Scholar ]
- Riglar D. T.; Giessen T. W.; Baym M.; Kerns S. J.; Niederhuber M. J.; Bronson R. T.; Kotula J. W.; Gerber G. K.; Way J. C.; Silver P. A. Engineered Bacteria Can Function in the Mammalian Gut Long-Term as Live Diagnostics of Inflammation . Nat. Biotechnol. 2017, 35 , 653–658. 10.1038/nbt.3879. [ PMC free article ] [ PubMed ] [ CrossRef ] [ Google Scholar ]
- Del Valle I.; Fulk E. M.; Kalvapalle P.; Silberg J. J.; Masiello C. A.; Stadler L. B. Translating New Synthetic Biology Advances for Biosensing into the Earth and Environmental Sciences . Front. Microbiol. 2021, 11 , 618373. 10.3389/fmicb.2020.618373. [ PMC free article ] [ PubMed ] [ CrossRef ] [ Google Scholar ]
- Ceroni F.; Algar R.; Stan G. B.; Ellis T. Quantifying Cellular Capacity Identifies Gene Expression Designs with Reduced Burden . Nat. Methods. 2015, 12 , 415–418. 10.1038/nmeth.3339. [ PubMed ] [ CrossRef ] [ Google Scholar ]
- Ceroni F.; Boo A.; Furini S.; Gorochowski T. E.; Borkowski O.; Ladak Y. N.; Awan A. R.; Gilbert C.; Stan G. B.; Ellis T. Burden-Driven Feedback Control of Gene Expression . Nat. Methods. 2018, 15 , 387–393. 10.1038/nmeth.4635. [ PubMed ] [ CrossRef ] [ Google Scholar ]
- He L.; Yang H.; Tang J.; Liu Z.; Chen Y.; Lu B.; He H.; Tang S.; Sun Y.; Liu F.; et al. Intestinal Probiotics E. Coli Nissle 1917 as a Targeted Vehicle for Delivery of P53 and Tum-5 to Solid Tumors for Cancer Therapy . J. Biol. Eng. 2019, 13 , 58. 10.1186/s13036-019-0189-9. [ PMC free article ] [ PubMed ] [ CrossRef ] [ Google Scholar ]
- He L.; Yang H.; Liu F.; Chen Y.; Tang S.; Ji W.; Tang J.; Liu Z.; Sun Y.; Hu S.; et al. Escherichia Coli Nissle 1917 Engineered to Express Tum-5 Can Restrain Murine Melanoma Growth . Oncotarget 2017, 8 , 85772–85782. 10.18632/oncotarget.20486. [ PMC free article ] [ PubMed ] [ CrossRef ] [ Google Scholar ]
- Sieow B. F.; Wun K. S.; Yong W. P.; Hwang I. Y.; Chang M. W. Tweak to Treat: Reprograming Bacteria for Cancer Treatment . Trends Cancer 2021, 7 , 447–464. 10.1016/j.trecan.2020.11.004. [ PubMed ] [ CrossRef ] [ Google Scholar ]
- Meyer A. J.; Segall-Shapiro T. H.; Glassey E.; Zhang J.; Voigt C. A. Escherichia Coli ″Marionette″ Strains with 12 Highly Optimized Small-Molecule Sensors . Nat. Chem. Biol. 2019, 15 , 196–204. 10.1038/s41589-018-0168-3. [ PubMed ] [ CrossRef ] [ Google Scholar ]
- Rottinghaus A. G.; Xi C.; Amrofell M. B.; Yi H.; Moon T. S. Engineering Ligand-Specific Biosensors for Aromatic Amino Acids and Neurochemicals . Cell Syst. 2022, 13 , 204–214. 10.1016/j.cels.2021.10.006. [ PMC free article ] [ PubMed ] [ CrossRef ] [ Google Scholar ]
- Wang L.; Walker B. L.; Iannaccone S.; Bhatt D.; Kennedy P. J.; Tse W. T. Bistable Switches Control Memory and Plasticity in Cellular Differentiation . Proc. Natl. Acad. Sci. U. S. A. 2009, 106 , 6638–6643. 10.1073/pnas.0806137106. [ PMC free article ] [ PubMed ] [ CrossRef ] [ Google Scholar ]
- Dean C. What Holds Epigenetic Memory? . Nat. Rev. Mol. Cell. Biol. 2017, 18 , 140. 10.1038/nrm.2017.15. [ PubMed ] [ CrossRef ] [ Google Scholar ]
- Boraschi D.; Italiani P. Innate Immune Memory: Time for Adopting a Correct Terminology . Front. Immunol. 2018, 9 , 799. 10.3389/fimmu.2018.00799. [ PMC free article ] [ PubMed ] [ CrossRef ] [ Google Scholar ]
- Inniss M. C.; Silver P. A. Building Synthetic Memory . Curr. Biol. 2013, 23 , R812–816. 10.1016/j.cub.2013.06.047. [ PMC free article ] [ PubMed ] [ CrossRef ] [ Google Scholar ]
- Yang L.; Nielsen A. A.; Fernandez-Rodriguez J.; McClune C. J.; Laub M. T.; Lu T. K.; Voigt C. A. Permanent Genetic Memory with > 1-Byte Capacity . Nat. Methods 2014, 11 , 1261–1266. 10.1038/nmeth.3147. [ PMC free article ] [ PubMed ] [ CrossRef ] [ Google Scholar ]
- Deans T. L.; Cantor C. R.; Collins J. J. A Tunable Genetic Switch Based on Rnai and Repressor Proteins for Regulating Gene Expression in Mammalian Cells . Cell 2007, 130 , 363–372. 10.1016/j.cell.2007.05.045. [ PubMed ] [ CrossRef ] [ Google Scholar ]
- O’Gorman S.; Fox D. T.; Wahl G. M. Recombinase-Mediated Gene Activation and Site-Specific Integration in Mammalian Cells . Science 1991, 251 , 1351–1355. 10.1126/science.1900642. [ PubMed ] [ CrossRef ] [ Google Scholar ]
- Kim H.; Kim M.; Im S. K.; Fang S. Mouse Cre-Loxp System: General Principles to Determine Tissue-Specific Roles of Target Genes . Lab. Anim. Res. 2018, 34 , 147–159. 10.5625/lar.2018.34.4.147. [ PMC free article ] [ PubMed ] [ CrossRef ] [ Google Scholar ]
- He L.; Li Y.; Li Y.; Pu W.; Huang X.; Tian X.; Wang Y.; Zhang H.; Liu Q.; Zhang L.; et al. Enhancing the Precision of Genetic Lineage Tracing Using Dual Recombinases . Nat. Med. 2017, 23 , 1488–1498. 10.1038/nm.4437. [ PMC free article ] [ PubMed ] [ CrossRef ] [ Google Scholar ]
- Kotula J. W.; Kerns S. J.; Shaket L. A.; Siraj L.; Collins J. J.; Way J. C.; Silver P. A. Programmable Bacteria Detect and Record an Environmental Signal in the Mammalian Gut . Proc. Natl. Acad. Sci. U. S. A. 2014, 111 , 4838–4843. 10.1073/pnas.1321321111. [ PMC free article ] [ PubMed ] [ CrossRef ] [ Google Scholar ]
- Naydich A. D.; Nangle S. N.; Bues J. J.; Trivedi D.; Nissar N.; Inniss M. C.; Niederhuber M. J.; Way J. C.; Silver P. A.; Riglar D. T. Synthetic Gene Circuits Enable Systems-Level Biosensor Trigger Discovery at the Host-Microbe Interface . mSystems 2019, 4 , e00125-19. 10.1128/mSystems.00125-19. [ PMC free article ] [ PubMed ] [ CrossRef ] [ Google Scholar ]
- Lee J. W.; Chan C. T. Y.; Slomovic S.; Collins J. J. Next-Generation Biocontainment Systems for Engineered Organisms . Nat. Chem. Biol. 2018, 14 , 530–537. 10.1038/s41589-018-0056-x. [ PubMed ] [ CrossRef ] [ Google Scholar ]
- Molin S.; Klemm P.; Poulsen L. K.; Biehl H.; Gerdes K.; Andersson P. Conditional Suicide System for Containment of Bacteria and Plasmids . Bio/Technology 1987, 5 , 1315–1318. 10.1038/nbt1287-1315. [ CrossRef ] [ Google Scholar ]
- Contreras A.; Molin S.; Ramos J. L. Conditional-Suicide Containment System for Bacteria Which Mineralize Aromatics . Appl. Environ. Microbiol. 1991, 57 , 1504–1508. 10.1128/aem.57.5.1504-1508.1991. [ PMC free article ] [ PubMed ] [ CrossRef ] [ Google Scholar ]
- Chan C. T.; Lee J. W.; Cameron D. E.; Bashor C. J.; Collins J. J. Deadman’ and ’Passcode’ Microbial Kill Switches for Bacterial Containment . Nat. Chem. Biol. 2016, 12 , 82–86. 10.1038/nchembio.1979. [ PMC free article ] [ PubMed ] [ CrossRef ] [ Google Scholar ]
- Meinhardt S.; Swint-Kruse L. Experimental Identification of Specificity Determinants in the Domain Linker of a Laci/Galr Protein: Bioinformatics-Based Predictions Generate True Positives and False Negatives . Proteins 2008, 73 , 941–957. 10.1002/prot.22121. [ PMC free article ] [ PubMed ] [ CrossRef ] [ Google Scholar ]
- Meinhardt S.; Manley M. W. Jr; Becker N. A.; Hessman J. A.; Maher L. J. 3rd; Swint-Kruse L. Novel Insights from Hybrid Laci/Galr Proteins: Family-Wide Functional Attributes and Biologically Significant Variation in Transcription Repression . Nucleic Acids Res. 2012, 40 , 11139–11154. 10.1093/nar/gks806. [ PMC free article ] [ PubMed ] [ CrossRef ] [ Google Scholar ]
- Chavez A.; Pruitt B. W.; Tuttle M.; Shapiro R. S.; Cecchi R. J.; Winston J.; Turczyk B. M.; Tung M.; Collins J. J.; Church G. M. Precise Cas9 Targeting Enables Genomic Mutation Prevention . Proc. Natl. Acad. Sci. U. S. A. 2018, 115 , 3669–3673. 10.1073/pnas.1718148115. [ PMC free article ] [ PubMed ] [ CrossRef ] [ Google Scholar ]
- Rottinghaus A. G.; Ferreiro A.; Fishbein S. R. S.; Dantas G.; Moon T. S. Genetically Stable Crispr-Based Kill Switches for Engineered Microbes . Nat. Commun. 2022, 13 , 672. 10.1038/s41467-022-28163-5. [ PMC free article ] [ PubMed ] [ CrossRef ] [ Google Scholar ]
- Saeidi N.; Wong C. K.; Lo T. M.; Nguyen H. X.; Ling H.; Leong S. S.; Poh C. L.; Chang M. W. Engineering Microbes to Sense and Eradicate Pseudomonas Aeruginosa, a Human Pathogen . Mol. Syst. Biol. 2011, 7 , 521. 10.1038/msb.2011.55. [ PMC free article ] [ PubMed ] [ CrossRef ] [ Google Scholar ]
- Reardon S. Crispr Gene-Editing Creates Wave of Exotic Model Organisms . Nature 2019, 568 , 441–442. 10.1038/d41586-019-01300-9. [ PubMed ] [ CrossRef ] [ Google Scholar ]
- Jiang Y.; Chen B.; Duan C.; Sun B.; Yang J.; Yang S. Multigene Editing in the Escherichia Coli Genome Via the Crispr-Cas9 System . Appl. Environ. Microbiol. 2015, 81 , 2506–2514. 10.1128/AEM.04023-14. [ PMC free article ] [ PubMed ] [ CrossRef ] [ Google Scholar ]
- Oh J. H.; van Pijkeren J. P. Crispr-Cas9-Assisted Recombineering in Lactobacillus Reuteri . Nucleic Acids Res. 2014, 42 , e131. 10.1093/nar/gku623. [ PMC free article ] [ PubMed ] [ CrossRef ] [ Google Scholar ]
- Wang Y.; Zhang Z. T.; Seo S. O.; Lynn P.; Lu T.; Jin Y. S.; Blaschek H. P. Bacterial Genome Editing with Crispr-Cas9: Deletion, Integration, Single Nucleotide Modification, and Desirable ″Clean″ Mutant Selection in Clostridium Beijerinckii as an Example . ACS Synth. Biol. 2016, 5 , 721–732. 10.1021/acssynbio.6b00060. [ PubMed ] [ CrossRef ] [ Google Scholar ]
- Zheng L.; Tan Y.; Hu Y.; Shen J.; Qu Z.; Chen X.; Ho C. L.; Leung E. L.; Zhao W.; Dai L. Crispr/Cas-Based Genome Editing for Human Gut Commensal Bacteroides Species . ACS Synth. Biol. 2022, 11 , 464–472. 10.1021/acssynbio.1c00543. [ PubMed ] [ CrossRef ] [ Google Scholar ]
- Chen W.; Zhang Y.; Yeo W. S.; Bae T.; Ji Q. Rapid and Efficient Genome Editing in Staphylococcus Aureus by Using an Engineered Crispr/Cas9 System . J. Am. Chem. Soc. 2017, 139 , 3790–3795. 10.1021/jacs.6b13317. [ PubMed ] [ CrossRef ] [ Google Scholar ]
- Westbrook A. W.; Moo-Young M.; Chou C. P. Development of a Crispr-Cas9 Tool Kit for Comprehensive Engineering of Bacillus Subtilis . Appl. Environ. Microbiol. 2016, 82 , 4876–4895. 10.1128/AEM.01159-16. [ PMC free article ] [ PubMed ] [ CrossRef ] [ Google Scholar ]
- DiCarlo J. E.; Norville J. E.; Mali P.; Rios X.; Aach J.; Church G. M. Genome Engineering in Saccharomyces Cerevisiae Using Crispr-Cas Systems . Nucleic Acids Res. 2013, 41 , 4336–4343. 10.1093/nar/gkt135. [ PMC free article ] [ PubMed ] [ CrossRef ] [ Google Scholar ]
- Vyas V. K.; Barrasa M. I.; Fink G. R. A Candida Albicans CRISPR System Permits Genetic Engineering of Essential Genes and Gene Families . Sci. Adv. 2015, 1 , e1500248. 10.1126/sciadv.1500248. [ PMC free article ] [ PubMed ] [ CrossRef ] [ Google Scholar ]
- Peters J. M.; Colavin A.; Shi H.; Czarny T. L.; Larson M. H.; Wong S.; Hawkins J. S.; Lu C. H. S.; Koo B. M.; Marta E.; et al. A Comprehensive, Crispr-Based Functional Analysis of Essential Genes in Bacteria . Cell 2016, 165 , 1493–1506. 10.1016/j.cell.2016.05.003. [ PMC free article ] [ PubMed ] [ CrossRef ] [ Google Scholar ]
- Santos-Moreno J.; Schaerli Y. Crispr-Based Gene Expression Control for Synthetic Gene Circuits . Biochem. Soc. Trans. 2020, 48 , 1979–1993. 10.1042/BST20200020. [ PMC free article ] [ PubMed ] [ CrossRef ] [ Google Scholar ]
- Nielsen A. A.; Voigt C. A. Multi-Input Crispr/Cas Genetic Circuits That Interface Host Regulatory Networks . Mol. Syst. Biol. 2014, 10 , 763. 10.15252/msb.20145735. [ PMC free article ] [ PubMed ] [ CrossRef ] [ Google Scholar ]
- Liu Y.; Zhan Y.; Chen Z.; He A.; Li J.; Wu H.; Liu L.; Zhuang C.; Lin J.; Guo X.; et al. Directing Cellular Information Flow Via Crispr Signal Conductors . Nat. Methods 2016, 13 , 938–944. 10.1038/nmeth.3994. [ PubMed ] [ CrossRef ] [ Google Scholar ]
- Gao Y.; Xiong X.; Wong S.; Charles E. J.; Lim W. A.; Qi L. S. Complex Transcriptional Modulation with Orthogonal and Inducible Dcas9 Regulators . Nat. Methods 2016, 13 , 1043–1049. 10.1038/nmeth.4042. [ PMC free article ] [ PubMed ] [ CrossRef ] [ Google Scholar ]
- Gander M. W.; Vrana J. D.; Voje W. E.; Carothers J. M.; Klavins E. Digital Logic Circuits in Yeast with Crispr-Dcas9 nor Gates . Nat. Commun. 2017, 8 , 15459. 10.1038/ncomms15459. [ PMC free article ] [ PubMed ] [ CrossRef ] [ Google Scholar ]
- Santos-Moreno J.; Tasiudi E.; Stelling J.; Schaerli Y. Multistable and Dynamic Crispri-Based Synthetic Circuits . Nat. Commun. 2020, 11 , 2746. 10.1038/s41467-020-16574-1. [ PMC free article ] [ PubMed ] [ CrossRef ] [ Google Scholar ]
- Gaudelli N. M.; Komor A. C.; Rees H. A.; Packer M. S.; Badran A. H.; Bryson D. I.; Liu D. R. Programmable Base Editing of a*T to G*C in Genomic DNA without DNA Cleavage . Nature 2017, 551 , 464–471. 10.1038/nature24644. [ PMC free article ] [ PubMed ] [ CrossRef ] [ Google Scholar ]
- Komor A. C.; Kim Y. B.; Packer M. S.; Zuris J. A.; Liu D. R. Programmable Editing of a Target Base in Genomic DNA without Double-Stranded DNA Cleavage . Nature 2016, 533 , 420–424. 10.1038/nature17946. [ PMC free article ] [ PubMed ] [ CrossRef ] [ Google Scholar ]
- Peters J. E.; Makarova K. S.; Shmakov S.; Koonin E. V. Recruitment of Crispr-Cas Systems by Tn7-Like Transposons . Proc. Natl. Acad. Sci. U. S. A. 2017, 114 , E7358–E7366. 10.1073/pnas.1709035114. [ PMC free article ] [ PubMed ] [ CrossRef ] [ Google Scholar ]
- Faure G.; Shmakov S. A.; Yan W. X.; Cheng D. R.; Scott D. A.; Peters J. E.; Makarova K. S.; Koonin E. V. Crispr-Cas in Mobile Genetic Elements: Counter-Defence and Beyond . Nat. Rev. Microbiol. 2019, 17 , 513–525. 10.1038/s41579-019-0204-7. [ PubMed ] [ CrossRef ] [ Google Scholar ]
- Strecker J.; Ladha A.; Gardner Z.; Schmid-Burgk J. L.; Makarova K. S.; Koonin E. V.; Zhang F. Rna-Guided DNA Insertion with Crispr-Associated Transposases . Science 2019, 365 , 48–53. 10.1126/science.aax9181. [ PMC free article ] [ PubMed ] [ CrossRef ] [ Google Scholar ]
- Klompe S. E.; Vo P. L. H.; Halpin-Healy T. S.; Sternberg S. H. Transposon-Encoded Crispr-Cas Systems Direct Rna-Guided DNA Integration . Nature 2019, 571 , 219–225. 10.1038/s41586-019-1323-z. [ PubMed ] [ CrossRef ] [ Google Scholar ]
- Rubin B. E.; Diamond S.; Cress B. F.; Crits-Christoph A.; Lou Y. C.; Borges A. L.; Shivram H.; He C.; Xu M.; Zhou Z.; et al. Species- and Site-Specific Genome Editing in Complex Bacterial Communities . Nat. Microbiol. 2022, 7 , 34–47. 10.1038/s41564-021-01014-7. [ PMC free article ] [ PubMed ] [ CrossRef ] [ Google Scholar ]
- Marsh J. W.; Ley R. E. Microbiome Engineering: Taming the Untractable . Cell 2022, 185 , 416. 10.1016/j.cell.2021.12.034. [ PubMed ] [ CrossRef ] [ Google Scholar ]
- Soucy S. M.; Huang J.; Gogarten J. P. Horizontal Gene Transfer: Building the Web of Life . Nat. Rev. Genet. 2015, 16 , 472–482. 10.1038/nrg3962. [ PubMed ] [ CrossRef ] [ Google Scholar ]
- Neil K.; Allard N.; Rodrigue S. Molecular Mechanisms Influencing Bacterial Conjugation in the Intestinal Microbiota . Front. Microbiol. 2021, 12 , 673260. 10.3389/fmicb.2021.673260. [ PMC free article ] [ PubMed ] [ CrossRef ] [ Google Scholar ]
- Doucet-Populaire F.; Trieu-Cuot P.; Andremont A.; Courvalin P. Conjugal Transfer of Plasmid DNA from Enterococcus Faecalis to Escherichia Coli in Digestive Tracts of Gnotobiotic Mice . Antimicrob. Agents Chemother. 1992, 36 , 502–504. 10.1128/AAC.36.2.502. [ PMC free article ] [ PubMed ] [ CrossRef ] [ Google Scholar ]
- Igimi S.; Ryu C. H.; Park S. H.; Sasaki Y.; Sasaki T.; Kumagai S. Transfer of Conjugative Plasmid Pam Beta 1 from Lactococcus Lactis to Mouse Intestinal Bacteria . Lett. Appl. Microbiol. 1996, 23 , 31–35. 10.1111/j.1472-765X.1996.tb00023.x. [ PubMed ] [ CrossRef ] [ Google Scholar ]
- Ronda C.; Chen S. P.; Cabral V.; Yaung S. J.; Wang H. H. Metagenomic Engineering of the Mammalian Gut Microbiome in Situ . Nat. Methods. 2019, 16 , 167–170. 10.1038/s41592-018-0301-y. [ PMC free article ] [ PubMed ] [ CrossRef ] [ Google Scholar ]
- Tridgett M.; Ababi M.; Osgerby A.; Ramirez Garcia R.; Jaramillo A. Engineering Bacteria to Produce Pure Phage-Like Particles for Gene Delivery . ACS Synth. Biol. 2021, 10 , 107–114. 10.1021/acssynbio.0c00467. [ PubMed ] [ CrossRef ] [ Google Scholar ]
- Voorhees P. J.; Cruz-Teran C.; Edelstein J.; Lai S. K. Challenges & Opportunities for Phage-Based in Situ Microbiome Engineering in the Gut . J. Controlled Release 2020, 326 , 106–119. 10.1016/j.jconrel.2020.06.016. [ PubMed ] [ CrossRef ] [ Google Scholar ]
- Borodovich T.; Shkoporov A. N.; Ross R. P.; Hill C. Phage-Mediated Horizontal Gene Transfer and Its Implications for the Human Gut Microbiome . Gastroenterol. Rep. 2022, 10 , goac012. 10.1093/gastro/goac012. [ PMC free article ] [ PubMed ] [ CrossRef ] [ Google Scholar ]
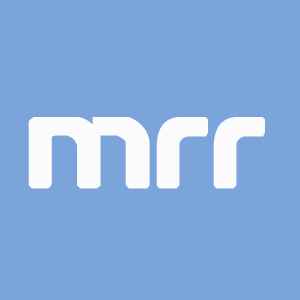
- About the Journal
- Aims and Scope
- Editorial Policies
- Editorial Board
- Author Instructions
- Article Processing Charges
- Editorial Process
- Manuscript Templates
- Submit a Manuscript
- Peer Review Guidelines
- All Articles
- Articles with Video Abstracts
- Video Abstract Guidelines
- All Special Issues
- Ongoing Special Issues
- Special Issue Ebooks
- Special Issue Guidelines
Partner's Meeting
The journal scope includes investigations of microbiomes present in one or various body compartments of human beings or other animals. Surveys on the establishment of microbial communities, microbe-microbe interactions, and microbe-host communication are welcome for publication. In particular, the journal emphasizes the identification and functional investigation of the role exerted by microbial taxa as well as microbial genes and microbial metabolites markers associated with health and disease. The journal focuses on studies that provide information on microbiome/microbiome composition in various animal-relevant niches and generate insights into the molecular mechanisms underlying microbiome function. Thus, the journal emphasizes functional interactions between microbes themselves, between microbes and their environment, and between microbes and their host.
The journal aims to publish high-quality research from scientists with a common interest in microbiome/microbiota research in all its multidisciplinary aspects. The main areas of interest include but are not limited to the following:
- the structure, activities and communal behavior of microbial communities
- microbial community genetics and evolutionary processes
- microbial symbioses, microbial interactions
- microbial metabolic and structural diversity
- microbial physiology, growth and survival
- microbes and surfaces, adhesion and biofouling
- modelling, theory development and new analysis pipelines
- viromes/phageome
- antimicrobials and competition in microbiomes
- fungal communities (mycobiomes)
- exploration of microbial dark matter
- culturomics approaches applied to microbiomes
- metabolomics and proteomic approaches applied to microbiomes
- metatranscriptomics applied to microbiomes
- new technological developments in the study of composition and activities of microbial communities, as-yet uncultured microorganisms
- gnotobiotic studies
- develoment of minimal microbial consortia and synthetic communities for functional microbe-host studies
- probiotics, prebiotics, synbiotics and postbiotics
- microbial-derived metabolites originating from food/feed and playing a role on host physiology
- effect of microbiota modulation in gastrointestinal disorders
- effect of microbiota modulation in metabolic disorders
- novel treatments and clinical trials
- impact of dietary components on the microbiota, and inter-individual variation in responses
- host-microbiome interaction in immune response
Interdisciplinary studies of fundamental problems are particularly welcome. The journal publishes a variety of formats including Original Research articles, Short Reports, Reviews and Commentaries, etc.
All published articles are preserved here permanently:
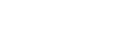
The current browser is not compatible
The following browsers are recommended for the best use experience
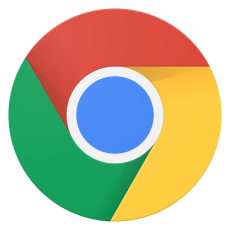
Only supports Mac
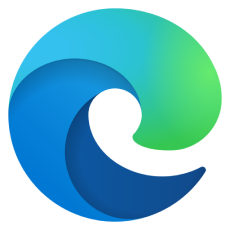
Submission guidelines
To facilitate the submission process, we are flexible with regard to the format, style and length of initial submissions. HOWEVER, data that supports the research within the manuscript MUST be made available at the time of submission.
For the purpose of peer review, please provide one editable manuscript document (such as .doc .docx or .RTF), with figures and tables placed in the body of the text at the point where they are referenced. Only LaTeX manuscripts should be submitted as a .pdf. You will be asked to provide .tex files at a later date.
Supplemental information and cover letters should be uploaded separately. Please refer to the submissions criteria regarding our policy on data.
Journal-specific formatting is required only at the revise stage after the first round of peer review.
Reviews - by invitation only
Reviews published in the journal are specially invited by the Editors of Microbiome .
- The key aims of reviews are to provide systematic and substantial coverage of mature subjects, evaluations of progress in specified areas, and/or critical assessments of emerging technologies.
- Reviews must be within the scope of Microbiome .
- They must be authored by leading experts in the field with a track record of publication in that specific field.
Unsolicited reviews and reviews submitted as research will not be considered in the journal.
Resubmissions policy
Please note, we may consider resubmissions of rejected manuscripts only if the handling Editor specifically states in the original decision letter that the manuscript will be reconsidered.
Cover Letter
Please include a cover letter as part of your submission explaining why your results meet the journal's scope, its impact and implications for the broad microbiome field.
Please also include suggestions for peer reviewers, their institutional email address and why these reviewers are suited to review your manuscript. Please also let us know if there are reviewers you would prefer us not to invite to review your manuscript.
Submit by section
During the submission process for Microbiome , we ask that authors submit their manuscript to a specified section as outlined below. This is to facilitate assessment and review of submitted manuscripts. The sections are headed by an Editor-in-Chief or Senior Editor , specializing in that subject.
Human microbiomes
- Gut: ecology, modulation, nutrition and probiotics
- Gut: bioinformatics, immunology, methods and pathology
- Respiratory microbiomes
- Genital microbiomes
- Skin microbiomes
- Oral microbiomes
Animal and Environmental
- Animal microbiomes
- Plant microbiomes
- Environmental: Water/ oceans
- Environmental microbiome: Soil
- Built environment microbiomes
Human, animal and environmental
- Bioinformatics, algorithms and software
- Resistomes
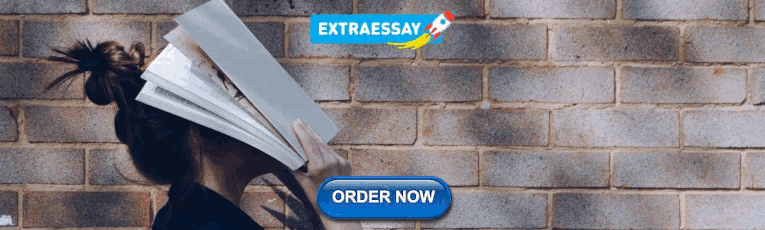
Our 3-step submission process
Before you submit.
Now you’ve identified a journal to submit to, there are a few things you should be familiar with before you submit.
- Make sure you are submitting to the most suitable journal - Aims and scope
- Understand the costs and funding options - Fees and funding
- Make sure your manuscript is accurate and readable - Language editing services
- Understand the copyright agreement - Copyright
Ready to submit
To give your manuscript the best chance of publication, follow these policies and formatting guidelines.
- Check the quality of your writing - Free Language check
- General formatting rules for all article types - Preparing your manuscript
- Make sure your submission is complete - Prepare supporting information
- Copyright and license agreement - Conditions of publication
- Read and agree to our Editorial Policies - Editorial policies
Submit and promote
After acceptance, we provide support so your article gains maximum impact in the scientific community and beyond.
Please note that manuscript can only be submitted by an author of the manuscript and may not be submitted by a third party.
- Who decides whether my work will be accepted? - Peer-review policy
- Want to submit to a different journal? - Manuscript transfers
- Spreading the word - Promoting your publication
Submit manuscript
- Editorial Board
- SNAPP Editor link
- Manuscript editing services
- Instructions for Editors
- Sign up for article alerts and news from this journal
Sister journals
Microbiome is a sister journal to
- Follow us on Twitter
Annual Journal Metrics
2022 Citation Impact 15.5 - 2-year Impact Factor 19.4 - 5-year Impact Factor 2.998 - SNIP (Source Normalized Impact per Paper) 3.709 - SJR (SCImago Journal Rank)
2023 Speed 41 days submission to first editorial decision for all manuscripts (Median) 196 days submission to accept (Median)
2023 Usage 3,219,809 downloads 7,051 Altmetric mentions
- More about our metrics
ISSN: 2049-2618
- General enquiries: [email protected]
Thank you for visiting nature.com. You are using a browser version with limited support for CSS. To obtain the best experience, we recommend you use a more up to date browser (or turn off compatibility mode in Internet Explorer). In the meantime, to ensure continued support, we are displaying the site without styles and JavaScript.
- View all journals
- My Account Login
- Explore content
- About the journal
- Publish with us
- Sign up for alerts
- Open access
- Published: 26 April 2024
Effects of microbiome-based interventions on neurodegenerative diseases: a systematic review and meta-analysis
- Zara Siu Wa Chui ORCID: orcid.org/0009-0001-3722-2374 1 ,
- Lily Man Lee Chan ORCID: orcid.org/0000-0003-3665-1449 2 ,
- Esther Wan Hei Zhang 3 ,
- Suisha Liang ORCID: orcid.org/0000-0003-0426-9827 4 , 6 ,
- Edmond Pui Hang Choi ORCID: orcid.org/0000-0002-8388-7342 2 ,
- Kris Yuet Wan Lok ORCID: orcid.org/0000-0002-3227-0799 2 ,
- Hein Min Tun ORCID: orcid.org/0000-0001-7597-5062 5 , 6 , 7 &
- Jojo Yan Yan Kwok ORCID: orcid.org/0000-0001-7444-6935 2 , 8
Scientific Reports volume 14 , Article number: 9558 ( 2024 ) Cite this article
11 Altmetric
Metrics details
- Neurodegenerative diseases
Neurodegenerative diseases (NDDs) are characterized by neuronal damage and progressive loss of neuron function. Microbiome-based interventions, such as dietary interventions, biotics, and fecal microbiome transplant, have been proposed as a novel approach to managing symptoms and modulating disease progression. Emerging clinical trials have investigated the efficacy of interventions modulating the GM in alleviating or reversing disease progression, yet no comprehensive synthesis have been done. A systematic review of the literature was therefore conducted to investigate the efficacy of microbiome-modulating methods. The search yielded 4051 articles, with 15 clinical trials included. The overall risk of bias was moderate in most studies. Most microbiome-modulating interventions changed the GM composition. Despite inconsistent changes in GM composition, the meta-analysis showed that microbiome-modulating interventions improved disease burden (SMD, − 0.57; 95% CI − 0.93 to − 0.21; I 2 = 42%; P = 0.002) with a qualitative trend of improvement in constipation. However, current studies have high methodological heterogeneity and small sample sizes, requiring more well-designed and controlled studies to elucidate the complex linkage between microbiome, microbiome-modulating interventions, and NDDs.
Similar content being viewed by others
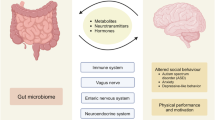
Microbiota–gut–brain axis and its therapeutic applications in neurodegenerative diseases
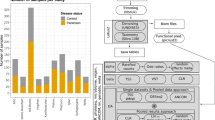
Meta-analysis of the Parkinson’s disease gut microbiome suggests alterations linked to intestinal inflammation
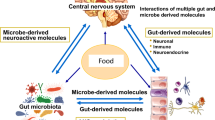
Role of diet and its effects on the gut microbiome in the pathophysiology of mental disorders
Introduction.
Neurodegenerative diseases (NDDs) are a diverse spectrum of disorders characterized by the progressive loss of neurons and deterioration in the central or peripheral nervous system, resulting in long-term motor and nonmotor impairments 1 . NDDs include Parkinson’s disease (PD), Alzheimer’s disease (AD), frontotemporal dementia and its variants, amyotrophic lateral sclerosis (ALS), and multiple sclerosis (MS). As the population ages, the incidence rate and prevalence of NDDs increase modestly, as demonstrated by an incidence estimated annual percentage changes of 0.52 for PD and 0.13 in men and 0.06 in women for AD 2 , 3 . Affecting millions of people worldwide, NDDs are a major public health concern; yet, despite decades of research effort, no effective treatments for curing or reversing their progression have been realized 3 . The exact pathophysiology of NDDs is also not fully elucidated owing to the heterogeneity and complexity of these diseases 4 , 5 , 6 . However, emerging evidence suggests that the gut microbiome (GM), the collection of microorganisms that inhabit the gastrointestinal (GI) tract, may play a role in modulating the risk and severity of NDDs.
GM, often called the second brain, harbors nearly 100 trillion bacteria, yeast, and other microorganisms, functioning symbiotically in day-to-day activities 7 . Host genetics, lifestyle, and environmental factors, such as diet, chemical exposure, infection, and host comorbidity, shape GM through the modulation of gut motility and secretion, which in turn affects various aspects of the host physiology, including immunomodulation, metabolic activity, and neuronal development and function. The connection between GM and metabolic and immune-related diseases is well established 8 . For example, obesity, as a complex metabolic disorder, is associated with decreased diversity and richness and altered composition in GM 9 . Wells et al. also identified that Prevotella correlates with the genetic risk and anticitrullinated protein antibody level of rheumatoid arthritis, suggesting the role of Prevotella as a potential mediator in disease progression 10 .
A growing body of evidence has suggested that GM also communicates bidirectionally via multiple pathways, which collectively is described by the gut–brain axis. The brain communicates with the gut through neuronal and hormonal pathways, including the hypothalamic pituitary adrenal axis (HPA) and sympathoadrenal axis 11 . The vagal nerves relay most signals from the brain to the gut 12 and coordinate stress and anti-inflammatory activities with HPA to regulate gut motility, intestinal permeability, and mucosal immune activity 13 . At the same time, GM can affect the brain by producing and releasing various molecules, such as metabolites, neurotransmitters, and cytokines; these molecules can reach the brain through multiple pathways and may be a key modulator in NDDs 14 .
Disruption of GM balance caused by host and environmental factors may lead to diseases or disorders 15 . Romano et al. performed a meta-analysis of 21 case–control studies to compare the GM composition of 1083 PD patients and 1213 healthy controls and revealed a lower abundance of Prevotellaceae and Lachnospiraceae families and higher abundance of Enterobacteriaceae and Akkermansiaceae families in patients than in controls 16 . Similarly, patterns of dysbiosis in other NDDs, including AD, MS, and ALS, have been reported in recent systematic review and meta-analysis 17 , 18 , 19 . Sampson et al. reported the requisite involvement of gut microbiota to elicit synucleinopathies in a PD model using wild-type and Thy1-α-synuclein genotype mice, in which the germ-free Thy1-α-synuclein genotype demonstrated limited motor and GI dysfunction compared with specific pathogen-free counterparts 20 . These findings suggest that GM may play a key role in the pathophysiology of NDDs, and modulating GM may be a potential strategy for preventing or treating NDDs.
However, many challenges and limitations remain in this research field. For example, most animal studies rely on germ-free or genetic models of NDDs, which may not fully recapitulate the human disease phenotypes or etiologies 21 . Standardized methods for assessing and manipulating GM across different studies are also lacking. Meanwhile, human studies, are mostly observational and cross-sectional, which cannot establish causality or directionality between GM and NDDs 22 .
In the recent decade, clinical trials have been conducted to investigate the efficacy of interventions modulating GM in alleviating or reversing disease progression. Yet, comprehensive synthesis of the available evidence in understanding microbiome-modulating methods is lacking. Therefore, this systematic review aims to summarize and critically appraise the current evidence regarding the effects of microbiome-modulating interventions on NDD-related clinical outcomes and to discuss the translatability and implementation potentials for future research and clinical application.
Inclusion criteria and search strategy
This protocol-based systematic review (PROSPERO ID: CRD42023437490) was conducted and reported in accordance with the Cochrane Handbook for Systematic Reviews of Interventions 23 and the Preferred Reporting Items for Systematic Reviews and Meta-Analyses statement 24 . Studies were selected in accordance with predefined inclusion and exclusion criteria. Randomized controlled trials (RCTs), quasi-experimental studies, single-arm studies, and pilot studies with microbiome-modulating intervention were included if studies (1) were conducted in adults (age > 18 years) with a diagnosis of NDDs, such as AD, PD, MS, and ALS, and (2) reported any microbiome outcomes. Microbiome-modulating intervention is defined as any treatment or intervention that alters the composition, diversity, or functionality of GM. The intervention can be, but not limited to, changes in diet or lifestyle, use of biotics, fecal microbiota transplantation (FMT), or other medications. The methods of microbiome analysis are not restricted, which may include, but not limited to, 16S ribosomal (r) RNA sequencing, shotgun metagenomic sequencing, and fluorescence in situ hybridization. Studies were excluded if they were not published in English.
PubMed, Ovid-Embase, and Web of Science were searched from inception to January 11, 2023. The search strategy was summarized as follows: [neurodegenerative diseases] AND [microbiome assessment] AND [microbiome-modulating methods: (diet) OR (supplement or biotics) OR (FMT)]. Online Resource 1 presents the full search strategy. Duplicate records were removed with EndNote and manually.
Data extraction and quality assessment
Two independent reviewers (ZSW Chui and EWH Zhang) extracted data from each trial using a pre-specific, standardized form and evaluated the risk of bias via the Cochrane Risk-of-Bias Tool 2 for RCTs 25 and the Risk Of Bias In Nonrandomized Studies of Interventions (ROBINS-I) for non-RCTs 26 . Discrepancies were identified and resolved by consensus with a third reviewer (LML Chan) and the supervisor (JYY Kwok).
A narrative synthesis was conducted for all trials to describe study design, country and setting of study, characteristics of participants and interventions, assessment time points, microbiota sequencing technique, main microbiota, and clinical outcomes (Table 1 ). Meta-analyses were performed among microbiota and clinical outcomes if they were reported by at least three studies. In view of wide variations in instruments used between trials to assess the primary outcomes, pooled effects were summarized as standardized mean differences (SMDs). SMD is a summary statistic used to report intervention effects in standardized units, rather than the original units of measurement for each scale. The total sample size, mean with standard deviation (SD) or median, and interquartile range of disease progression pre- and post-intervention were extracted to calculate SMD and SD. Twenty-five individual study results were corrected for directionality when appropriate. Considering the substantial variations in microbiome-modulating interventions and study design, we utilized a random-effect model to conduct the analysis using RevMan 5.4 27 , 28 . All significance tests were 2-tailed, and P value of < 0.05 was considered statistically significant. The heterogeneity among studies was assessed using I 2 statistic.
Study selection
The PRIMSA 2020 flow diagram (Fig. 1 ) shows the flow of the study selection process. A total of 7269 unique records identified from the search, of which 25 were deemed eligible for full review. Fourteen trials were included for qualitative synthesis. Seven trials were included in the meta-analyses.
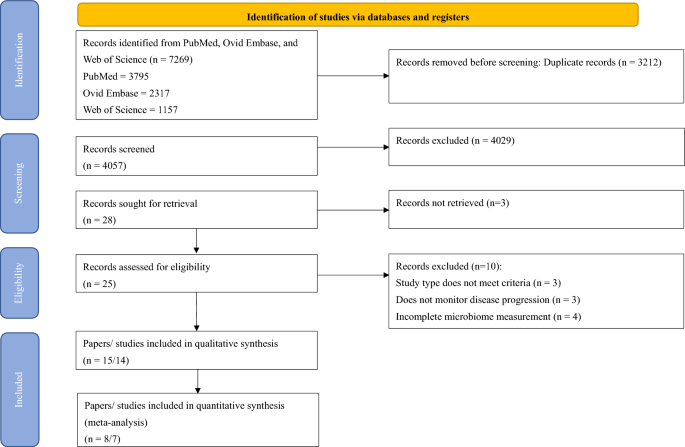
Preferred reporting items for systematic reviews and meta-analyses (PRISMA) flowchart.
Study characteristics
Table 1 shows a systematic presentation of information regarding the study characteristics. Fifteen articles comprised 14 trials that involved 445 patients: 5 trials/6 articles on sclerosis (n = 112), 6 trials on PD (n = 235), and 3 trials on AD and related disorders (n = 98). Of the 14 trials, 6 studies adopted single-arm design, 5 studies were RCT, and 3 were non-RCT.
Quality assessment
The quality of the methodology and risk of bias of the 15 articles were assessed in accordance with Cochrane RoB2 for randomized trials and ROBINS-I for nonrandomized trials 25 , 26 (Table 2 and 3 ).
Bias in the selection of reports is a common concern among studies; here, three out of five RCTs and seven out of nine non-RCTs were of moderate-to-high risk in the concerning domain owing to multiple measurements of disease progression. In general, RCTs had a lower risk of bias, in which no high-risk RCTs were included. The RCT performed by Al et al. was terminated early because of the sudden death of the principal investigator 22 ; nevertheless, results were analyzed in such a way that no directional bias toward or against the intervention exists, and this RCT was therefore assessed to have a moderate risk.
For non-RCTs, 66.78% of the studies were of serious risk of bias, and the remaining were of moderate risk 29 , 34 , 36 , 39 , 40 , 42 . Apart from reporting bias (D7), major concerns of bias included confounding bias (D1), selection bias (D2), and bias in data measurement (D6). Serious confounding bias mainly contributed to the lack of control of diet, which can affect the microbiome composition, leading to less conclusive results. In addition, many of the non-RCTs relied on self-reporting, while the participants were aware of the interventions. Becker et al. conducted an open-label study to modify GM in PD patients with resistance starch and collected subject-reported nonmotor data; consequently, the measurement could be inaccurate owing to subjective reporting 34 . Poor selection of participants and missing data were also common among nonrandomized clinical trials.
MS and amyotrophic lateral sclerosis
Five studies focusing on MS 22 , 29 , 30 , 31 , 32 and one study specifically on ALS 33 were included. These studies comprised three RCTs 22 , 32 , 33 and two single-arm studies 29 , 30 , 31 . The sample sizes of MS studies were small, ranging from 9 22 to 22 30 , 31 , whereas the study on ALS recruited 50 samples 33 . The study durations varied from 1 week 29 to 1 year 22 . A spectrum of microbiome-modulating interventions were used, ranging from probiotic supplementation 30 , 31 , 33 , dietary intervention (intermittent fasting) 32 , FMT 22 , and a multidimensional program consisting of dietary intervention and physical activities 29 . All studies used 16 s rRNA sequencing to analyze microbiome composition, covering V3 and/ or V4 regions. In addition to V3 and V4 regions, V13 region was also covered by Cignarella et al. to distinguish specific species of Lactobacillus , as well as V1 and V2 regions 32 .
MS is characterized with chronic inflammatory response in the central nervous system, which leads to pronounced Th1/Th17-mediated inflammation and increased proinflammatory cytokine concentration 43 , 44 . Therefore, improvement in MS progression can be evaluated by measuring inflammatory response and clinical functional and nonfunctional outcomes. Three out of four studies focusing on MS reported reduced inflammatory response or improved autoimmune response 29 , 30 , 31 , 32 , while the remaining underpowered study showed insignificant difference post-modulation 22 .
All of the studies had diverse microbiome patterns. The two studies on probiotic supplementation showed time-related changes in microbiome composition but had different microbiota outcomes despite using similar bacterial families, that is, Di Gioia et al. used Streptococcaceae and Lactobacillaceae families, while Tankou et al. used Bifidobacteriaceae family in addition to Streptococcaceae and Lactobacillaceae families 30 , 31 , 33 . Di Gioia et al. found no significant alterations in microbiota and probiotic supplementation, except for Rikenellaceae and trends of increase in Bateroidaceae and decrease in Prevotellaceae and Clostridiales , and no clinical improvement in ALS 33 . Tankou et al. reported enrichment of Lactobacillaceae , Streptococcaceae , and Bifidobacteriaceae and reduction of Akkermansia , Blautia , and Dorea , which were enriched in MS patients at baseline. They also observed reduced intermediate monocytes, increased effector memory CD8 T cells, and anti-inflammatory gene expression, with some association with microbiome changes, therefore suggesting an implication of synergistic effect with current therapies 30 , 31 .
Dietary interventions significantly improved disease progression and inflammatory tone in both studies 29 , 32 . Cignarella et al. reported increased Faecalibacterium, Lachnospiracea incertae sedis , and Blautia, improved Expanded Disability Status Scale (EDSS), and reduced serum leptin and peripheral blood leukocyte profile changes after 15 days of intermittent fasting in conjunction with corticosteroid treatment 32 . However, the difference in improvement of EDSS between the ad libitum control group and the intermittent fasting group was insignificant, and the MS Functional Composite was insignificantly different from the baseline in both groups, possibly due to the short intervention duration 32 . Barone et al. conducted a 1-week multidimensional program involving Mediterranean diet, neuromotor rehabilitation, and mindfulness 29 . They reported partial recovery of gut dysbiosis with reduced Collinsella , Actinobacteria , and Ruminococcus and increased Bacteroidetes and some short-chain fatty acid (SCFA) producers. They also observed reduced inflammatory tone and serum lipopolysaccharide, increased anti-inflammatory gene expression, and some associations with microbiome changes. Considering the significant improvement in the total score in the Modified Fatigue Impact Scale, the author concluded that the multidimensional approach may be effective in mitigating MS progression.
Al et al. conducted an RCT on FMT, in which they randomized participants into early (received FMT with 6-month follow-up, n = 4) or late intervention group (6-month observation, substantiated by FMT and 1-month follow-up, n = 5); however, the study was terminated early and underpowered because of the unexpected death of the principal investigator 22 . Preliminary results showed that FMT was well-tolerated without serious adverse events. Microbiome changes recapitulated the microbiome composition of the donor and had the potential to improve elevated intestinal permeability. Insignificant clinical changes were noted on disease severity measured by EDSS, without new MRI activity, and the inflammatory levels in terms of serum cytokines showed insignificant changes.
We identified six articles that fulfilled all criteria 34 , 35 , 36 , 37 , 38 , 39 . They included one RCT 38 , two non-RCT 34 , 36 , and three single-arm studies 35 , 37 , 39 , which used diversified microbiome-modulating methods, including prebiotics, FMT, dietary interventions, and probiotics. The sample size was in the range of 8–11 for non-RCTs and single-arm studies and 87–100 for RCTs. The study lasted 7 weeks to 1 year.
Two of the studies investigated GM with metagenomic sequencing 34 , 38 , while others used 16 s rRNA sequencing, despite sequencing different variable regions. Although different interventions were used, the alpha and beta diversities did not differ significantly in all studies. No consensus existed in terms of the change in a particular family, genus, or species.
Despite using different microbiome-modulating strategies, these studies showed a significant impact on alleviating disease burden. Motor functions, as measured by Unified PD Rating Scale (UPDRS), were significantly improved in four of the six studies that used FMT, probiotics, ovo-lacto diet, ovo-lacto diet with enema, and Mediterranean diet 35 , 36 , 38 , 39 , while others did not measure motor function. Apart from the study that used resistant starch 34 , constipation and GI-related symptoms were also improved in the three studies that used probiotics, FMT, and Mediterranean diet 35 , 37 , 38 . Other nonmotor symptoms, including anxiety and depression, were improved 34 , 35 , 38 , and inflammatory and PD-related fecal markers decreased 34 , 39 .
Sun et al. provided the only RCT that measured motor, nonmotor, and constipation symptoms, as well as microbiome-related metabolites 38 . Their study evaluated the synergistic effects of probiotics with conventional PD treatment (benserazide and dopamine agonist) by comparing it with a placebo group (placebo with conventional regimen) for 3 months. The probiotics led to increased Bifidobacterium animalis, Ruminococcaceae , and Lachnospira and decreased Lactobacillus fermentum and Klebsiella oxytoca , which might be related to changes in microbiome-related metabolites and neurotransmitter, consequently leading to a beneficial effect in PD patients.
Three included studies focused on AD or mild cognitive impairment, and the study designs were diversified: one single-arm 42 , RCT 41 , and non-RCT 40 . Changes in microbiome were measured by qPCR of designated microbial targets 42 , 16 s rRNA 41 , and histology and urease test of Helicobacter pyroli 40 . Given that the focus of the studies varied, they also modified GM with different approaches, including probiotic supplementation 42 , dietary treatment 41 , and antibiotic treatment 40 . The sample sizes ranged from 17 41 to 61 40 , and the studies lasted for 4 weeks 42 to 2 years 40 .
Leblhuber et al. investigated the effects of probiotic supplementation on immune activation 42 . They found that 4-week probiotic supplementation led to increased Faecalibacterium prausnitzii and altered tryptophan metabolites, yet no significant improvement in cognition was observed. Nagpal et al. 41 was the only cross-over study to compare the effects of Mediterranean-keto diet (MMKD) on AD markers. They found increased abundance of several bacterial families and genera, such as Enterobacteriaceae, Akkermansia , and Slackia , after modified MMKD compared with that after American Heart Association Diet (AHAD), which also altered the SCFA profile and was in association with CSF biomarkers, such as Ab40 and Ab42. Kountouras et al. focused on the impact of eradication of H. pylori in infected AD patients 45 . AD patients were significantly more susceptible to H. pylori infection, and the eradication therapy led to improved cognitive and functional status upon the 2-year clinical endpoint compared with infected patients. These studies suggested that modulating GM may alter AD progression via modifying SCFA and immune profile, leading to reduced AD marker and possibly improved status.
SCFA producers and fecal/serum SCFAs
Several included studies have explored the relationship between SCFA producers or fecal/serum SCFA concentration in NDDs. Of the eight studies that reported changes in SCFA producers or SCFA concentration, six reported improved outcomes, as measured by inflammatory tone, functional outcome, or GI symptoms, yet showed inconsistent changes in SCFA producers and SCFA concentration (Table 4 ).
Tankou et al. and Rusch et al. reported decreased Blautia after probiotic supplementation and Mediterranean diet, respectively 30 , 31 , 37 . Barone et al. reported a stable level of Blautia after multidimensional rehabilitation 29 . Kuai et al. and Cignarella et al. reported increased Blautia after FMT and intermittent fasting 32 , 35 . All of them, except Cignarella et al., reported improved clinical outcomes. Faecalibacterium was reported to have increased significantly in three studies after intermittent fasting, FMT, and probiotic supplementation. Sun et al. reported increased diversity of SGBs involved in SCFA synthesis after probiotic supplementation, in which the acetate and dopamine concentrations increased significantly, whereas the glutamine and tryptophan concentrations decreased 38 . The patients also exhibited improved clinical outcomes.
Meta-analysis: effect of microbiome modulation on clinical outcomes
We pooled all clinical trials to investigate the overall effectiveness of modulating the microbiome on motor symptom progression in NDD. Of the eight studies that assessed motor symptom progression, five (62.5%) reported statistically significantly improvements in motor symptom progression. We pulled all studies that reported motor symptom progression quantitatively before and after intervention and extracted the mean, interquartile range, or SD to calculate the standard mean differences of the studies, resulting in six studies. We did not include Al et al.’s study in meta-analysis, despite the reported EDSS, due to the study’s early termination, which resulted in incomplete and varied treatment conditions in the two groups 22 . Additionally, we excluded the study conducted by Cignarella et al. as it did not report numerical data for EDSS 32 . In the meta-analysis involving six studies with seven intervention groups (n = 249), microbiome-modulating interventions were significantly associated with a lower motor symptom burden (SMD, − 0.57; 95% CI − 0.93 to − 0.21; I 2 = 42%; P = 0.002; Fig. 2 ). They used different strategies, such as probiotics, antibiotics, FMT, and dietary changes, to alter the gut microbiota 33 , 35 , 36 , 38 , 39 , 40 . Four out of the six studies included patients with PD 30 , 31 , 35 , 36 , 39 , and the remaining involved patients with ALS 33 and AD 40 . The primary outcome measures were UPDRS for PD, ALS Functional Rating Scale-Revised for ALS, and Functional Rating Scale for Symptoms of Dementia for AD. Hegelmaier et al. compared the clinical outcomes of PD patients receiving ovo-lacto diet, with a subgroup receiving additional enema 36 . Considering that the aim of this meta-analysis was to study the pooled effect of microbiome-modulating methods, we compared the clinical outcomes before and after interventions and segregate the enema subgroup. We did not include studies on MS because they did not report numerical results on functional outcomes or were underpowered.
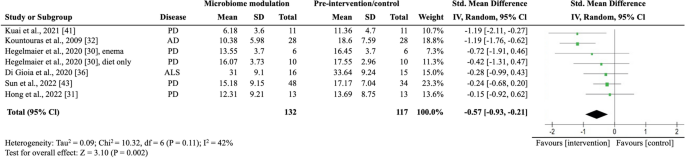
Random-effects meta-analysis of trials on the association between microbiome modulating intervention and clinical outcomes. AD Alzheimer’s disease; ALS amyotrophic lateral sclerosis; IV inverse variance; PD Parkinson’s disease; error bars represent 95% CIs; size of the shaded square indicates study weight; diamond represents pooled standardized mean difference and 95% CI.
Constipation and GI symptoms
Of the four trials that assessed constipation and GI-related symptoms 33 , 34 , 35 , 37 , three studies reported significant improvement compared with the baseline or placebo, whereas the remaining reported insignificant changes in bowel habits. The meta-analysis did not include Hegelmaier et al.’s study because they used the Bristol stool scale, a noncontinuous scale, in measuring constipation syndrome 36 . In the meta-analysis involving three trials (n = 76), microbiome-modulating interventions were insignificantly associated with improving constipation (SMD, − 1.01; 95% CI − 3.01 to 1.00; I 2 = 93%; P = 0.33; Fig. 3 ). The primary outcome measures included the Constipation Scoring System 34 , Wexner 35 , and GI Symptom Rating Scale 37 constipation scores.

Random-effects meta-analysis of trials on the association between microbiome modulating intervention and constipation symptoms. IV inverse variance; PD Parkinson’s disease; error bars represent 95% CIs; size of the shaded square indicates study weight; diamond represents pooled standardized mean difference and 95% CI.
This study is the first systematic review and meta-analysis to date synthesizing the current evidence from clinical trials that examined the effects of microbiome-modulating interventions on the disease burden of NDDs. Our meta-analysis demonstrated that microbiome-modulating interventions are significantly associated with reduction in motor symptom burden in NDDs, including PD, ALS, and AD. Findings from qualitative synthesis also suggested that microbiome-modulating interventions may reduce inflammation and alleviate GI symptoms, including constipation. Despite the promising effects of microbiome-modulating interventions, the relationships and mechanisms underpinning GM modulation and clinical outcomes remain inconclusive owing to the lack of high-quality clinical trials, the heterogeneity in study design, and the diverse nature of interventions among the included studies.
Microbiome modulation may improve motor symptoms and inflammatory tone
Our meta-analysis revealed that microbiome-modulating interventions can generally lower motor symptom burden in patients with NDDs. In addition, qualitative findings showed that inflammatory tone was generally improved in different NDDs by various microbiome-modulating interventions. NDDs are characterized by chronic inflammation, leaky gut, and decreased production of neuroactive substances, in which the degeneration and loss of neurons lead to long-term motor and nonmotor impairment 45 , 46 . The effect on alleviation on symptom burden might be explained by the restoration of GM to reduce inflammation 47 , re-establish intestinal permeability 22 , 37 , 48 , and enhance neuroactivity through the production of neurotransmitters 49 .
Xiang et al. performed systematic review and meta-analysis on the use of probiotics in AD and PD and suggested that probiotics improve AD possibly through anti-inflammatory pathways, as demonstrated by a decrease in the GSH level after probiotic supplementation 50 . In line with our study findings, microbiome modulation, not limited to probiotic supplementation, was found to reduce inflammation and thereby disease burden, which also applies to other NDDs including MS and ALS 22 , 29 , 30 , 31 , 32 , 33 , 39 .
Restoration of gut dysbiosis can reduce inflammation by multiple pathways, with many of the modulation methods focusing on increasing SCFA-producing bacteria, such as Roseburia spp., Blautia , and Prevotella spp., to increase serum or fecal SCFAs 30 , 31 , 34 , 38 , 42 or on reducing pathogenic bacteria, such as H. pyroli 39 , 40 . SCFAs, including butyrate, propionate, and acetate, exert anti-inflammatory effects by inhibiting the activation of nuclear factor-kappa B and the production of proinflammatory cytokines, such as tumor necrosis factor alpha and interleukin-6 51 , 52 . They can also promote the differentiation of regulatory T cells (Tregs) and suppress that of Th17 cells 53 . Apart from indirect homeostasis through SCFAs, some bacteria in the microbiome, such as Bacteroides fragilis , can directly induce Treg differentiation to maintain immune intolerance and prevent autoimmunity.
Along with consistent findings of decreased Lactobacillus in MS, Tankou et al. reported an enrichment of SCFA producers, including Akkermansia , Blautia , and Dorea , in MS patients at baseline . After probiotic supplementation, these SCFA producers decreased, but the expression of proinflammatory genes, such as HLA.DPA1 and MS risk allele HLA.DQA1, also decreased 30 , 31 . Our qualitative finding also showed that no consistency was established in the changes in SCFA producers or SCFA concentration, but clinical outcomes were improved in general (Table 4 ). In particular, while some studies suggested that certain species of Blautia and Dorea were associated with decreased levels of inflammatory markers 54 , 55 , others indicated that they had proinflammatory effects 56 , 57 . The activity of SCFA producers can vary depending on several factors and contribute differently in terms of SCFA production in the gut 58 , 59 . Given the complex nature of GM, additional studies are needed to elucidate other factors that influence its interactions with the immune system, such as its abundance, diversity, metabolites, or co-occurrence with other bacteria.
Microbiome modulation may alleviate constipation and GI symptoms
Constipation is common in NDDs and can affect the quality of life of patients. Constipation can be caused by the accumulation of pathological proteins in the GI tract, such as amyloid beta in AD, α-synuclein in PD, or myelin basic protein in MS, which induce dysfunction of the enteric nervous system (ENS) to affect gut motility and barrier 59 , 60 . Our study found that, qualitatively, microbiome-modulating interventions may also alleviate constipation. A reduction in constipation might be explained by the enhancement in the integrity and permeability of the intestinal barrier through restoring the microbiome 61 , 62 . In addition, SCFAs produced by bacteria can modulate intestinal peristalsis and upregulate the expression of tight junction proteins to strengthen the integrity of the gut barrier 63 . Secretion of neurotransmitters to stimulate ENS may also play a role in constipation 64 . However, available evidence remains inadequate, and the results have not reached statistical significance because of the lack of high-quality studies, which should ideally be blinded RCTs with appropriate sample size and statistical power.
Microbiome-modulating interventions and related GM changes
Although all of the included studies reported some degree of changes in GM composition, no consistent changes in GM were found in relation to the overall improvement in clinical outcomes. The inconsistency might be explained by the heterogeneity in interventions and disease nature, while other systematic reviews also observed diverging GM patterns 65 , 66 . In terms of studies that involved the use of probiotics, Lactobacillus and Bifidobacterium were commonly used, yet they resulted in different GM changes: one reported increased Lactobacillus and Bifidobacterium 30 , 31 , one reported increased B. animalis but decreased Lactobacillus fermentum 38 , and one reported an increase in F. prausnitzii only 42 . These observations are in line with other probiotic systematic reviews 67 . The exact relationship between GM and NDDs remains unknown, and further studies are needed to understand the impact of individual bacteria, the co-occurrence, and the molecular pathway in GM and diseases.
Strengths and limitations
This is the first comprehensive systematic review that examined the effects of a broad spectrum of microbiome-modulating interventions on NDDs, including MS, ALS, PD, and AD. NDDs represent a broad spectrum of disorders, and clinical microbiome trials remain lacking for some diseases, such as the Huntington disease. In addition, most included studies could only be synthesized qualitatively, and heterogeneity regarding the intervention type, outcome measures, and methodological differences was noted. Therefore, we adopted a random-effect model to account for the statistical heterogeneity among studies. Publication bias assessment was not possible given the limited number of available trials for quantitative synthesis, which may also result in minimal but statistically significant overestimation of effects 68 . Most of the included clinical trials had a small sample size and were of moderate risk of bias mainly subject to the selection of reported results. We also included nonrandomized and single-arm clinical trials, which might have a high risk of bias owing to the lack of comparison group. Studies should include a control group when possible and report the complete effect estimate on the basis of the P value, magnitude, or direction of results 69 , such as fold change in microbiome changes. When evaluating microbiome diversity, using multiple indices can provide a comprehensive and nuanced understanding of the microbiota diversity and composition 70 , yet all results of the chosen indices should be listed and interpreted to prevent reporting bias. The findings indicate that the relationship between microbiome-modulating interventions, GM composition, and clinical outcomes of NDDs has been poorly studied and skewed to certain NDDs, namely, PD.
A spectrum of microbiome-modulating components was identified, ranging from probiotic supplement to multidimensional lifestyle interventions consisting of diet modification, mindfulness, and physical activities. Owing to the large variation in methodology across the included studies, definitive conclusions on how microbiome-modulating interventions modulate the GM composition and clinical outcomes and affect the progression of NDDs were impossible to draw.
Most of the studies did not report on significant confounders, such as comorbidities, medication use, and lifestyle, which could affect microbial and clinical outcomes and thus might limit the transferability of our findings. Control conditions also differed between the studies, given that some control interventions comprised AHAD 41 , a placebo group that received conventional treatments 32 , 38 , or a group that received placebo for the first 3 months and probiotics for the next 3 months 33 , restricting the generalizability of our study findings.
Conclusions and implications
Microbiome-modulating interventions are likely to improve symptom burden, possibly through reducing inflammatory tone in NDD patients via increasing SCFA producers and reducing proinflammatory bacteria. However, the exact relationship remains unknown because no consistent changes in GM composition were identified. High-quality evidence of microbiome-modulating interventions for NDDs is still missing. This review underscores the need for rigorous large-scale studies to examine the effects of microbiome-modulating methods on NDDs.
Future clinical trials of microbiome-modulating methods on NDDs should (1) evaluate the changes in GM through microbiome modulation in terms of alpha and beta diversities and specific phylum, family, and species; (2) assess motor and nonmotor clinical outcomes and incorporate objective data in addition to self-reporting questionnaire; (3) account for confounding factors, including diet, age, medication record, lifestyle, and disease progression. Regarding the diverse methodology of existing GM modulation research, a standardized approach to GM evaluation, such as the STORMS checklist 71 , is necessary to understand the complex mechanisms and relationships between GM-modulating interventions, GM composition, and NDDs further.
Data availability
The dataset analyzed in this study is available from the corresponding author upon reasonable request.
Brettschneider, J. et al. Spreading of pathology in neurodegenerative diseases: A focus on human studies. Nat. Rev. Neurosci. 16 (2), 109–120 (2015).
Article CAS PubMed PubMed Central Google Scholar
Ou, Z. et al. Global trends in the incidence, prevalence, and years lived with disability of Parkinson’s disease in 204 countries/territories from 1990 to 2019. Front. Public Health 9 , 776847 (2021).
Article PubMed PubMed Central Google Scholar
Li, X. et al. Global, regional, and national burden of Alzheimer’s disease and other dementias, 1990–2019. Front. Aging Neurosci. 14 , 937486 (2022).
Young, A. L. et al. Uncovering the heterogeneity and temporal complexity of neurodegenerative diseases with Subtype and Stage Inference. Nat. Commun. 9 (1), 4273 (2018).
Article ADS PubMed PubMed Central Google Scholar
Twohig, D. & Nielsen, H. M. alpha-synuclein in the pathophysiology of Alzheimer’s disease. Mol. Neurodegener. 14 (1), 23 (2019).
Behl, T. et al. Multifaceted role of matrix metalloproteinases in neurodegenerative diseases: Pathophysiological and therapeutic perspectives. Int. J. Mol. Sci. 22 (3), 1413 (2021).
Backhed, F. et al. Host-bacterial mutualism in the human intestine. Science 307 (5717), 1915–1920 (2005).
Article ADS PubMed Google Scholar
Fan, Y. & Pedersen, O. Gut microbiota in human metabolic health and disease. Nat. Rev. Microbiol. 19 (1), 55–71 (2021).
Article CAS PubMed Google Scholar
Liu, B. N. et al. Gut microbiota in obesity. World J. Gastroenterol. 27 (25), 3837–3850 (2021).
Wells, P. M. et al. Associations between gut microbiota and genetic risk for rheumatoid arthritis in the absence of disease: A cross-sectional study. Lancet Rheumatol. 2 (7), e418–e427 (2020).
Carabotti, M. S. A., Maselli, M. A. & Severi, C. The gut-brain axis: Interactions between enteric microbiota, central and enteric nervous systems. Ann. Gastroenterol. 28 (2), 203 (2015).
PubMed PubMed Central Google Scholar
Fulling, C., Dinan, T. G. & Cryan, J. F. Gut microbe to brain signaling: What happens in Vagus. Neuron 101 (6), 998–1002 (2019).
Peterson, C. T. Dysfunction of the microbiota-gut-brain axis in neurodegenerative disease: The promise of therapeutic modulation with prebiotics, medicinal herbs, probiotics, and synbiotics. J. Evid. Based Integr. Med. 25 , 251569020X957225 (2020).
Article Google Scholar
Wu, S. et al. Roles and mechanisms of gut microbiota in patients with Alzheimer’s disease. Front. Aging Neurosci. 13 , 650047 (2021).
Article ADS CAS PubMed PubMed Central Google Scholar
Chen, Y., Xu, J. & Chen, Y. Regulation of neurotransmitters by the gut microbiota and effects on cognition in neurological disorders. Nutrients 13 (6), 2099 (2021).
Romano, S. et al. Meta-analysis of the Parkinson’s disease gut microbiome suggests alterations linked to intestinal inflammation. NPJ Parkinsons Dis. 7 (1), 27 (2021).
Paley, E. L. Discovery of gut bacteria specific to Alzheimer’s associated diseases is a clue to understanding disease Etiology: Meta-analysis of population-based data on human gut metagenomics and metabolomics. J. Alzheimers Dis. 72 (1), 319–355 (2019).
Sun, J. et al. Gut microbiome and amyotrophic lateral sclerosis: A systematic review of current evidence. J. Intern. Med. 290 (4), 758–788 (2021).
Article PubMed Google Scholar
Mirza, A. et al. The multiple sclerosis gut microbiota: A systematic review. Mult .Scler Relat. Disord. 37 , 101427 (2020).
Sampson, T. R. et al. Gut microbiota regulate motor deficits and neuroinflammation in a model of Parkinson’s disease. Cell 167 (6), 1469–1480 (2016).
Warner, B. B. The contribution of the gut microbiome to neurodevelopment and neuropsychiatric disorders. Pediatr. Res. 85 (2), 216–224 (2019).
Al, K. F. et al. Fecal microbiota transplantation is safe and tolerable in patients with multiple sclerosis: A pilot randomized controlled trial. Mult. Scler. J. Exp. Transl. Clin. 8 (2), 20552173221086664 (2022).
JPT, H., et al., Cochrane Handbook for Systematic Reviews of Interventions version 6.3 Cochrane, 2022.
Page, M. J. et al. The PRISMA 2020 statement: An updated guideline for reporting systematic reviews. BMJ 372 , n71 (2021).
Sterne, J. A. C. et al. RoB 2: A revised tool for assessing risk of bias in randomised trials. BMJ 366 , 14898 (2019).
Google Scholar
Sterne, J. A. et al. ROBINS-I: A tool for assessing risk of bias in non-randomised studies of interventions. BMJ 355 , i4919 (2016).
Hackenberger, B. K. Bayesian meta-analysis now - let’s do it. Croat Med. J. 61 (6), 564–568 (2020).
Team, J., JASP (Version 0.17.1)[Computer Software]. 2023.
Barone, M. et al. Influence of a high-impact multidimensional rehabilitation program on the gut microbiota of patients with multiple sclerosis. Int. J. Mol. Sci. 22 (13), 7173 (2021).
Tankou, S. K. et al. Investigation of probiotics in multiple sclerosis. Mult. Scler. 24 (1), 58–63 (2018).
Tankou, S. K. et al. A probiotic modulates the microbiome and immunity in multiple sclerosis. Ann. Neurol. 83 (6), 1147–1161 (2018).
Cignarella, F. et al. Intermittent fasting confers protection in CNS autoimmunity by altering the gut microbiota. Cell Metab. 27 (6), 1222–1235 (2018).
Di Gioia, D. et al. A prospective longitudinal study on the microbiota composition in amyotrophic lateral sclerosis. BMC Med. 18 (1), 153 (2020).
Article MathSciNet PubMed PubMed Central Google Scholar
Becker, A. et al. Effects of resistant starch on symptoms, fecal markers, and gut microbiota in Parkinson’s disease: The RESISTA-PD Trial. Genomics Proteomics Bioinform. 20 (2), 274–287 (2022).
Article CAS Google Scholar
Kuai, X. Y. et al. Evaluation of fecal microbiota transplantation in Parkinson’s disease patients with constipation. Microb. Cell Fact. 20 (1), 98 (2021).
Hegelmaier, T. et al. Interventional influence of the intestinal microbiome through dietary intervention and bowel cleansing might improve motor symptoms in Parkinson’s disease. Cells 9 (2), 376 (2020).
Rusch, C. et al. Mediterranean diet adherence in people with Parkinson’s disease reduces constipation symptoms and changes fecal microbiota after a 5-week single-arm pilot study. Front. Neurol. 12 , 794640 (2021).
Sun, H. et al. Probiotics synergized with conventional regimen in managing Parkinson’s disease. NPJ Parkinsons Dis. 8 (1), 62 (2022).
Hong, C. T. et al. Rifaximin modifies gut microbiota and attenuates inflammation in Parkinson’s disease: Preclinical and clinical studies. Cells 11 (21), 3468 (2022).
Kountouras, J. et al. Eradication of Helicobacter pylori may be beneficial in the management of Alzheimer’s disease. J. Neurol. 256 (5), 758–767 (2009).
Nagpal, R. et al. Modified Mediterranean-ketogenic diet modulates gut microbiome and short-chain fatty acids in association with Alzheimer’s disease markers in subjects with mild cognitive impairment. EBioMedicine 47 , 529–542 (2019).
Leblhuber, F. et al. Probiotic supplementation in patients with Alzheimer’s dementia: An explorative intervention study. Curr. Alzheimer Res. 15 (12), 1106–1113 (2018).
Bai, Z. et al. Cerebrospinal fluid and blood cytokines as biomarkers for multiple sclerosis: A systematic review and meta-analysis of 226 studies with 13,526 multiple sclerosis patients. Front Neurosci. 13 , 1026 (2019).
Lassmann, H. Multiple sclerosis pathology. Cold Spring Harb. Perspect. Med. 8 (3), e028936 (2018).
Gwak, M. G. & Chang, S. Y. Gut-brain connection: Microbiome, gut barrier, and environmental sensors. Immune Netw. 21 (3), e20 (2021).
Bhatt, A. P. et al. Nonsteroidal anti-inflammatory drug-induced leaky gut modeled using polarized monolayers of primary human intestinal epithelial cells. ACS Infect. Dis. 4 (1), 46–52 (2018).
Dixit, K. et al. Restoration of dysbiotic human gut microbiome for homeostasis. Life Sci. 278 , 119622 (2021).
Kowalski, K. & Mulak, A. Brain-gut-microbiota axis in Alzheimer’s disease. J. Neurogastroenterol. Motil. 25 (1), 48–60 (2019).
Zhang, H. et al. Implications of gut microbiota in neurodegenerative diseases. Front Immunol. 13 , 785644 (2022).
Xiang, S. et al. Efficacy and safety of probiotics for the treatment of Alzheimer’s disease, mild cognitive impairment, and parkinson’s disease: A systematic review and meta-analysis. Front. Aging Neurosci. 14 , 730036 (2022).
Omenetti, S. & Pizarro, T. T. The Treg/Th17 axis: A dynamic balance regulated by the gut microbiome. Front. Immunol. 6 , 639 (2015).
Cheng, H. et al. The Th17/Treg cell balance: A gut microbiota-modulated story. Microorganisms 7 (12), 583 (2019).
Zhou, W. et al. The gut microbe Bacteroides fragilis ameliorates renal fibrosis in mice. Nat. Commun. 13 (1), 6081 (2022).
Article ADS MathSciNet CAS PubMed PubMed Central Google Scholar
Tillett, B. J. & Hamilton-Williams, E. E. Microbiota derived factors as drivers of type 1 diabetes. Prog. Mol. Biol. Transl. Sci. 171 , 215–235 (2020).
Liu, X. et al. Blautia-a new functional genus with potential probiotic properties?. Gut Microbes 13 (1), 1–21 (2021).
Bolte, L. A. et al. Long-term dietary patterns are associated with pro-inflammatory and anti-inflammatory features of the gut microbiome. Gut 70 (7), 1287–1298 (2021).
Vacca, M. et al. The controversial role of human gut lachnospiraceae. Microorganisms 8 (4), 573 (2020).
Article MathSciNet CAS PubMed PubMed Central Google Scholar
Morrison, D. J. & Preston, T. Formation of short chain fatty acids by the gut microbiota and their impact on human metabolism. Gut Microbes 7 (3), 189–200 (2016).
Warnecke, T. et al. Gastrointestinal involvement in Parkinson’s disease: Pathophysiology, diagnosis, and management. NPJ Parkinsons Dis. 8 (1), 31 (2022).
Brudek, T. Inflammatory bowel diseases and Parkinson’s disease. J. Parkinsons Dis. 9 (s2), S331–S344 (2019).
Ohkusa, T. et al. Gut microbiota and chronic constipation: A review and update. Front Med. (Lausanne) 6 , 19 (2019).
Takiishi, T., Fenero, C. I. M. & Camara, N. O. S. Intestinal barrier and gut microbiota: Shaping our immune responses throughout life. Tissue Barriers 5 (4), e1373208 (2017).
Saleri, R. et al. Effects of different short-chain fatty acids (SCFA) on gene expression of proteins involved in barrier function in IPEC-J2. Porcine Health Manag. 8 (1), 21 (2022).
Nezami, B. G. & Srinivasan, S. Enteric nervous system in the small intestine: Pathophysiology and clinical implications. Curr. Gastroenterol. Rep. 12 (5), 358–365 (2010).
Attaye, I. et al. A systematic review and meta-analysis of dietary interventions modulating gut microbiota and cardiometabolic diseases-striving for new standards in microbiome studies. Gastroenterology 162 (7), 1911–1932 (2022).
Zhao, X. et al. Therapeutic and improving function of lactobacilli in the prevention and treatment of cardiovascular-related diseases: A novel perspective from gut microbiota. Front Nutr. 8 , 693412 (2021).
Alexander, G. E. Biology of Parkinson’s disease: Pathogenesis and pathophysiology of a multisystem neurodegenerative disorder. Dialogues Clin. Neurosci. 6 (3), 259–280 (2004).
van Aert, R. C. M., Wicherts, J. M. & van Assen, M. Publication bias examined in meta-analyses from psychology and medicine: A meta-meta-analysis. PLoS One 14 (4), e0215052 (2019).
Page, M. J. & Higgins, J. P. Rethinking the assessment of risk of bias due to selective reporting: A cross-sectional study. Syst. Rev. 5 (1), 108 (2016).
Risely, A. et al. Phylogeny- and abundance-based metrics allow for the consistent comparison of core gut microbiome diversity indices across host species. Front Microbiol. 12 , 659918 (2021).
Mirzayi, C. et al. Reporting guidelines for human microbiome research: The STORMS checklist. Nat. Med. 27 (11), 1885–1892 (2021).
Download references
Acknowledgements
We would like to acknowledge the matching and support received from the Research Platform Hong Kong Student Association of Neuroscience (HKSAN) programme. Dr Jojo Kwok served as the mentor for the programme, while Ms. Zara Chui and Ms. Esther Zhang were the elite students matched from the programme.
Author information
Authors and affiliations.
School of Biomedical Sciences, Li Ka Shing Faculty of Medicine, The University of Hong Kong, Pokfulam, Hong Kong SAR, China
Zara Siu Wa Chui
School of Nursing, Li Ka Shing Faculty of Medicine, The University of Hong Kong, Pokfulam, Hong Kong SAR, China
Lily Man Lee Chan, Edmond Pui Hang Choi, Kris Yuet Wan Lok & Jojo Yan Yan Kwok
Faculty of Medicine, The Chinese University of Hong Kong, Shatin, Hong Kong SAR, China
Esther Wan Hei Zhang
HKU-Pasteur Research Pole, School of Public Health, Li Ka Shing Faculty of Medicine, The University of Hong Kong, Pokfulam, Hong Kong SAR, China
Suisha Liang
The Jockey Club School of Public Health and Primary Care, Faculty of Medicine, The Chinese University of Hong Kong, Hong Kong SAR, China
Hein Min Tun
Microbiota I-Center (MagIC), Hong Kong SAR, China
Suisha Liang & Hein Min Tun
Li Ka Shing Institute of Health Sciences, Faculty of Medicine, The Chinese University of Hong Kong, Hong Kong SAR, China
Centre on Behavioral Health, The University of Hong Kong, Pokfulam, Hong Kong SAR, China
Jojo Yan Yan Kwok
You can also search for this author in PubMed Google Scholar
Contributions
Z.C.: Conceptualization (equal); data curation (lead); methodology (equal); writing—original draft preparation (lead); writing—review & editing (equal). L.C.: Conceptualization (equal); methodology (equal); writing— review & editing (equal). E.Z.: methodology (equal); data curation (equal). Suisha Liang: writing—review & editing (equal). E.C.: writing—review & editing (equal). K.L.: writing—review & editing (equal). J.K.: Writing—conceptualization (lead); methodology (equal); writing—review & editing (equal); supervision (lead). H.T.: Writing—review & editing (equal); supervision (equal).
Corresponding author
Correspondence to Jojo Yan Yan Kwok .
Ethics declarations
Competing interests.
The authors declare no competing interests.
Additional information
Publisher's note.
Springer Nature remains neutral with regard to jurisdictional claims in published maps and institutional affiliations.
Supplementary Information
Supplementary information., rights and permissions.
Open Access This article is licensed under a Creative Commons Attribution 4.0 International License, which permits use, sharing, adaptation, distribution and reproduction in any medium or format, as long as you give appropriate credit to the original author(s) and the source, provide a link to the Creative Commons licence, and indicate if changes were made. The images or other third party material in this article are included in the article's Creative Commons licence, unless indicated otherwise in a credit line to the material. If material is not included in the article's Creative Commons licence and your intended use is not permitted by statutory regulation or exceeds the permitted use, you will need to obtain permission directly from the copyright holder. To view a copy of this licence, visit http://creativecommons.org/licenses/by/4.0/ .
Reprints and permissions
About this article
Cite this article.
Chui, Z.S.W., Chan, L.M.L., Zhang, E.W.H. et al. Effects of microbiome-based interventions on neurodegenerative diseases: a systematic review and meta-analysis. Sci Rep 14 , 9558 (2024). https://doi.org/10.1038/s41598-024-59250-w
Download citation
Received : 07 December 2023
Accepted : 08 April 2024
Published : 26 April 2024
DOI : https://doi.org/10.1038/s41598-024-59250-w
Share this article
Anyone you share the following link with will be able to read this content:
Sorry, a shareable link is not currently available for this article.
Provided by the Springer Nature SharedIt content-sharing initiative
- Neurodegenerative disease
- Gut microbiome
- Multiple sclerosis
- Alzheimer’s disease
- Parkinson’s disease
- Microbiome modulating interventions
By submitting a comment you agree to abide by our Terms and Community Guidelines . If you find something abusive or that does not comply with our terms or guidelines please flag it as inappropriate.
Quick links
- Explore articles by subject
- Guide to authors
- Editorial policies
Sign up for the Nature Briefing newsletter — what matters in science, free to your inbox daily.

- All subject areas
- Agricultural and Biological Sciences
- Arts and Humanities
- Biochemistry, Genetics and Molecular Biology
- Business, Management and Accounting
- Chemical Engineering
- Computer Science
- Decision Sciences
- Earth and Planetary Sciences
- Economics, Econometrics and Finance
- Engineering
- Environmental Science
- Health Professions
- Immunology and Microbiology
- Materials Science
- Mathematics
- Multidisciplinary
- Neuroscience
- Pharmacology, Toxicology and Pharmaceutics
- Physics and Astronomy
- Social Sciences
- All subject categories
- Acoustics and Ultrasonics
- Advanced and Specialized Nursing
- Aerospace Engineering
- Agricultural and Biological Sciences (miscellaneous)
- Agronomy and Crop Science
- Algebra and Number Theory
- Analytical Chemistry
- Anesthesiology and Pain Medicine
- Animal Science and Zoology
- Anthropology
- Applied Mathematics
- Applied Microbiology and Biotechnology
- Applied Psychology
- Aquatic Science
- Archeology (arts and humanities)
- Architecture
- Artificial Intelligence
- Arts and Humanities (miscellaneous)
- Assessment and Diagnosis
- Astronomy and Astrophysics
- Atmospheric Science
- Atomic and Molecular Physics, and Optics
- Automotive Engineering
- Behavioral Neuroscience
- Biochemistry
- Biochemistry, Genetics and Molecular Biology (miscellaneous)
- Biochemistry (medical)
- Bioengineering
- Biological Psychiatry
- Biomaterials
- Biomedical Engineering
- Biotechnology
- Building and Construction
- Business and International Management
- Business, Management and Accounting (miscellaneous)
- Cancer Research
- Cardiology and Cardiovascular Medicine
- Care Planning
- Cell Biology
- Cellular and Molecular Neuroscience
- Ceramics and Composites
- Chemical Engineering (miscellaneous)
- Chemical Health and Safety
- Chemistry (miscellaneous)
- Chiropractics
- Civil and Structural Engineering
- Clinical Biochemistry
- Clinical Psychology
- Cognitive Neuroscience
- Colloid and Surface Chemistry
- Communication
- Community and Home Care
- Complementary and Alternative Medicine
- Complementary and Manual Therapy
- Computational Mathematics
- Computational Mechanics
- Computational Theory and Mathematics
- Computer Graphics and Computer-Aided Design
- Computer Networks and Communications
- Computer Science Applications
- Computer Science (miscellaneous)
- Computer Vision and Pattern Recognition
- Computers in Earth Sciences
- Condensed Matter Physics
- Conservation
- Control and Optimization
- Control and Systems Engineering
- Critical Care and Intensive Care Medicine
- Critical Care Nursing
- Cultural Studies
- Decision Sciences (miscellaneous)
- Dental Assisting
- Dental Hygiene
- Dentistry (miscellaneous)
- Dermatology
- Development
- Developmental and Educational Psychology
- Developmental Biology
- Developmental Neuroscience
- Discrete Mathematics and Combinatorics
- Drug Discovery
- Drug Guides
- Earth and Planetary Sciences (miscellaneous)
- Earth-Surface Processes
- Ecological Modeling
- Ecology, Evolution, Behavior and Systematics
- Economic Geology
- Economics and Econometrics
- Economics, Econometrics and Finance (miscellaneous)
- Electrical and Electronic Engineering
- Electrochemistry
- Electronic, Optical and Magnetic Materials
- Emergency Medical Services
- Emergency Medicine
- Emergency Nursing
- Endocrine and Autonomic Systems
- Endocrinology
- Endocrinology, Diabetes and Metabolism
- Energy Engineering and Power Technology
- Energy (miscellaneous)
- Engineering (miscellaneous)
- Environmental Chemistry
- Environmental Engineering
- Environmental Science (miscellaneous)
- Epidemiology
- Experimental and Cognitive Psychology
- Family Practice
- Filtration and Separation
- Fluid Flow and Transfer Processes
- Food Animals
- Food Science
- Fuel Technology
- Fundamentals and Skills
- Gastroenterology
- Gender Studies
- Genetics (clinical)
- Geochemistry and Petrology
- Geography, Planning and Development
- Geometry and Topology
- Geotechnical Engineering and Engineering Geology
- Geriatrics and Gerontology
- Gerontology
- Global and Planetary Change
- Hardware and Architecture
- Health Informatics
- Health Information Management
- Health Policy
- Health Professions (miscellaneous)
- Health (social science)
- Health, Toxicology and Mutagenesis
- History and Philosophy of Science
- Horticulture
- Human Factors and Ergonomics
- Human-Computer Interaction
- Immunology and Allergy
- Immunology and Microbiology (miscellaneous)
- Industrial and Manufacturing Engineering
- Industrial Relations
- Infectious Diseases
- Information Systems
- Information Systems and Management
- Inorganic Chemistry
- Insect Science
- Instrumentation
- Internal Medicine
- Issues, Ethics and Legal Aspects
- Leadership and Management
- Library and Information Sciences
- Life-span and Life-course Studies
- Linguistics and Language
- Literature and Literary Theory
- LPN and LVN
- Management Information Systems
- Management, Monitoring, Policy and Law
- Management of Technology and Innovation
- Management Science and Operations Research
- Materials Chemistry
- Materials Science (miscellaneous)
- Maternity and Midwifery
- Mathematical Physics
- Mathematics (miscellaneous)
- Mechanical Engineering
- Mechanics of Materials
- Media Technology
- Medical and Surgical Nursing
- Medical Assisting and Transcription
- Medical Laboratory Technology
- Medical Terminology
- Medicine (miscellaneous)
- Metals and Alloys
- Microbiology
- Microbiology (medical)
- Modeling and Simulation
- Molecular Biology
- Molecular Medicine
- Nanoscience and Nanotechnology
- Nature and Landscape Conservation
- Neurology (clinical)
- Neuropsychology and Physiological Psychology
- Neuroscience (miscellaneous)
- Nuclear and High Energy Physics
- Nuclear Energy and Engineering
- Numerical Analysis
- Nurse Assisting
- Nursing (miscellaneous)
- Nutrition and Dietetics
- Obstetrics and Gynecology
- Occupational Therapy
- Ocean Engineering
- Oceanography
- Oncology (nursing)
- Ophthalmology
- Oral Surgery
- Organic Chemistry
- Organizational Behavior and Human Resource Management
- Orthodontics
- Orthopedics and Sports Medicine
- Otorhinolaryngology
- Paleontology
- Parasitology
- Pathology and Forensic Medicine
- Pathophysiology
- Pediatrics, Perinatology and Child Health
- Periodontics
- Pharmaceutical Science
- Pharmacology
- Pharmacology (medical)
- Pharmacology (nursing)
- Pharmacology, Toxicology and Pharmaceutics (miscellaneous)
- Physical and Theoretical Chemistry
- Physical Therapy, Sports Therapy and Rehabilitation
- Physics and Astronomy (miscellaneous)
- Physiology (medical)
- Plant Science
- Political Science and International Relations
- Polymers and Plastics
- Process Chemistry and Technology
- Psychiatry and Mental Health
- Psychology (miscellaneous)
- Public Administration
- Public Health, Environmental and Occupational Health
- Pulmonary and Respiratory Medicine
- Radiological and Ultrasound Technology
- Radiology, Nuclear Medicine and Imaging
- Rehabilitation
- Religious Studies
- Renewable Energy, Sustainability and the Environment
- Reproductive Medicine
- Research and Theory
- Respiratory Care
- Review and Exam Preparation
- Reviews and References (medical)
- Rheumatology
- Safety Research
- Safety, Risk, Reliability and Quality
- Sensory Systems
- Signal Processing
- Small Animals
- Social Psychology
- Social Sciences (miscellaneous)
- Social Work
- Sociology and Political Science
- Soil Science
- Space and Planetary Science
- Spectroscopy
- Speech and Hearing
- Sports Science
- Statistical and Nonlinear Physics
- Statistics and Probability
- Statistics, Probability and Uncertainty
- Strategy and Management
- Stratigraphy
- Structural Biology
- Surfaces and Interfaces
- Surfaces, Coatings and Films
- Theoretical Computer Science
- Tourism, Leisure and Hospitality Management
- Transplantation
- Transportation
- Urban Studies
- Veterinary (miscellaneous)
- Visual Arts and Performing Arts
- Waste Management and Disposal
- Water Science and Technology
- All regions / countries
- Asiatic Region
- Eastern Europe
- Latin America
- Middle East
- Northern America
- Pacific Region
- Western Europe
- ARAB COUNTRIES
- IBEROAMERICA
- NORDIC COUNTRIES
- Afghanistan
- Bosnia and Herzegovina
- Brunei Darussalam
- Czech Republic
- Dominican Republic
- Netherlands
- New Caledonia
- New Zealand
- Papua New Guinea
- Philippines
- Puerto Rico
- Russian Federation
- Saudi Arabia
- South Africa
- South Korea
- Switzerland
- Syrian Arab Republic
- Trinidad and Tobago
- United Arab Emirates
- United Kingdom
- United States
- Vatican City State
- Book Series
- Conferences and Proceedings
- Trade Journals

- Citable Docs. (3years)
- Total Cites (3years)

Follow us on @ScimagoJR Scimago Lab , Copyright 2007-2024. Data Source: Scopus®

Cookie settings
Cookie Policy
Legal Notice
Privacy Policy
- Research article
- Open access
- Published: 23 April 2024
Meta-analysis of the human upper respiratory tract microbiome reveals robust taxonomic associations with health and disease
- Nick Quinn-Bohmann ORCID: orcid.org/0009-0001-5358-7322 1 , 2 ,
- Jose A. Freixas-Coutin 3 ,
- Jin Seo 3 ,
- Ruth Simmons ORCID: orcid.org/0000-0002-9303-639X 4 ,
- Christian Diener ORCID: orcid.org/0000-0002-7476-0868 1 &
- Sean M. Gibbons ORCID: orcid.org/0000-0002-8724-7916 1 , 2 , 5 , 6 , 7
BMC Biology volume 22 , Article number: 93 ( 2024 ) Cite this article
261 Accesses
9 Altmetric
Metrics details
The human upper respiratory tract (URT) microbiome, like the gut microbiome, varies across individuals and between health and disease states. However, study-to-study heterogeneity in reported case–control results has made the identification of consistent and generalizable URT-disease associations difficult.
In order to address this issue, we assembled 26 independent 16S rRNA gene amplicon sequencing data sets from case–control URT studies, with approximately 2–3 studies per respiratory condition and ten distinct conditions covering common chronic and acute respiratory diseases. We leveraged the healthy control data across studies to investigate URT associations with age, sex, and geographic location, in order to isolate these associations from health and disease states.
Conclusions
We found several robust genus-level associations, across multiple independent studies, with either health or disease status. We identified disease associations specific to a particular respiratory condition and associations general to all conditions. Ultimately, we reveal robust associations between the URT microbiome, health, and disease, which hold across multiple studies and can help guide follow-up work on potential URT microbiome diagnostics and therapeutics.
The human respiratory system is a complex structure, divided into the upper respiratory tract (URT) and the lower respiratory tract (LRT), and is primarily responsible for the exchange of oxygen and carbon dioxide with the atmosphere [ 1 ]. The upper respiratory tract, with an approximate surface area of 70 m 2 , is known to harbor a diverse microbial community [ 2 ]. Beginning at birth, colonization by microbes occurs through constant exposure to the surrounding environment via aspiration, inhalation, and direct contact [ 2 , 3 , 4 ]. A quasi-stable community develops over time, typically consisting of genera such as Corynebacterium and Dolosigranulum in young healthy children [ 5 ] and Corynebacterium and Staphylococcus in healthy adults [ 6 ] . The URT, consisting of the nares, nasal passages, mouth, sinuses, pharynx, and larynx, is the section of the respiratory tract most exposed to the environment and harbors the highest bacterial density [ 2 ]. Upsetting the balance of the URT microbiome may lead to opportunistic pathogen invasion and serious respiratory tract-related disease and infection [ 7 , 8 ]. Chronic respiratory diseases represent the largest disease burden worldwide, affecting over half a billion people in 2017 [ 9 ]. Pneumonia, an infection of the lungs, is a leading cause of mortality across the world, responsible for an estimated 3.2 million deaths in 2015 [ 10 ]. The likelihood of being infected by the influenza virus, another common respiratory pathogen that has caused recurrent epidemics over the past century, has been shown to be partially dependent on the composition of the URT microbiome [ 7 , 11 ]. Additional respiratory conditions, such as RSV, rhinosinusitis, and recurrent respiratory allergies, have all been linked with the disruption of the URT microbiome [ 12 , 13 , 14 ].
Maintaining a diverse commensal microbiome can be protective against the invasion of opportunistic pathogens [ 2 , 15 ]. Commensal bacteria can help to saturate metabolic niche space, preventing invasion and engraftment of potential pathogens [ 8 ]. Additionally, commensals have been shown to directly suppress viral infections through the activation of host immune responses [ 16 ]. Early exposure to certain commensal microbes can even lead to long-term immunomodulation, preventing autoimmune diseases and promoting tolerance to allergens [ 17 , 18 ]. Overall, the symbiotic relationship between the URT microbiome and the host appears critical for the maintenance of human health [ 2 , 19 ].
As with the gut microbiome, variability exists in the microbial composition of these URT communities across individuals. In addition to inter-individual heterogeneity and disease status, URT microbiome profiles may be shaped by other covariates known to impact community structure, such as age [ 1 , 7 ], and possibly others such as technical variation (e.g., sequencing methodologies), demographics, geographic location, and sex, although these associations are not well defined. Certain keystone or core taxa are well known to have a generally positive association with health, including the genera Dolosigranulum and Corynebacterium [ 20 , 21 , 22 ]. The sinonasal area is predominantly colonized by Corynebacterium and Staphylococcus [ 23 , 24 ], whereas the throat and tonsil areas are mostly colonized by Streptococcus , Fusobacterium , and Prevotella [ 25 , 26 ]. Certain species in the genera Streptococcus , Haemophilus , and Pseudomonas have been linked to negative health outcomes and disease [ 1 , 20 , 27 , 28 , 29 ]. However, respiratory illnesses are often polymicrobial, caused or facilitated by the presence of multiple organisms [ 30 ]. Identifying consistent signatures of URT health and disease has been hampered by the variability in reported results from individual case–control studies.
Here, we conducted a meta-analysis of the composition of the URT microbiome across health and disease states to identify consistent patterns that persist across independent studies in demographically and geographically divergent cohorts within and across multiple respiratory conditions. Using 16S rRNA amplicon sequencing data collected from the nasopharynx or the oropharynx across cases and controls from 26 independent studies representing 10 respiratory diseases and conditions, we observe robust associations between the relative abundance of specific genera and disease status. The diseases, conditions, or set of conditions included in the meta-analysis are as follows: asthma [ 31 , 32 , 33 ], chronic obstructive pulmonary disease (COPD) [ 34 ], COVID-19 [ 35 , 36 , 37 ], influenza [ 38 , 39 , 40 ], pneumonia [ 41 , 42 , 43 ], respiratory allergies [ 44 , 45 ], rhinosinusitis [ 46 , 47 , 48 ], respiratory syncytial virus (RSV, includes a range of conditions caused by the human respiratory syncytial virus) [ 49 , 50 , 51 ], respiratory tract infection (RTI, defined as a viral or bacterial infection of the upper or lower respiratory tract, including bronchitis) [ 52 , 53 , 54 ], and tonsillitis [ 55 , 56 ]. Knowledge of these consistent within- or across-disease associations may help guide the development of diagnostic tools and therapeutic interventions aimed at prevention or treatment of respiratory conditions.
Assembling case–control studies for a URT meta-analysis
To investigate the associations between the composition of the URT microbiome and disease susceptibility, we analyzed data collected from 26 independent case–control studies including 4706 total samples (study inclusion criteria outlined in the “ Methods ” section). Studies included in this meta-analysis had, at a minimum, publicly available 16S rRNA amplicon sequencing data and associated metadata on disease status, URT sampling site, sequencing method, and 16S rRNA hypervariable region used for amplicon sequencing. Unfortunately, additional metadata, such as age, gender, and other demographic data, were not uniformly available across all studies. Four studies included samples from both the nasopharynx and oropharynx; these samples were analyzed separately. For each study, raw data in FASTQ format were downloaded and processed through the same bioinformatic pipeline, defined in the “ Methods ” section below. All analyses were conducted at the genus level, given the phylogenetic resolution of partial 16S rRNA amplicon sequencing [ 57 ]. Details on each study included in this meta-analysis can be found in Additional file 1 : Table 1.
Alpha- and beta-diversity analyses show community-wide impacts of disease conditions
We compared URT microbiome alpha-diversity (Shannon index and Chao1 index) between disease cases and healthy controls at a per-study level. Prior to calculating diversity metrics, rarefaction to a sampling depth of 2000 reads was conducted. After rarefaction, 4536 samples remained, representing a loss of 170 samples. Due to the large compositional differences observed between the nasopharynx and oropharynx [ 40 ], diversity was investigated separately between these environments (Fig. 1 A, B). Across 20 studies sampling the nasopharynx, 7 showed significant differences in alpha-diversity as measured by the Shannon Index between cases and controls, spanning asthma, influenza, RSV, RTI, and respiratory allergies (two-tailed independent Student’s t -test, p < 0.05). All but one (Wen et al., Influenza) of these significant relationships showed significantly higher alpha-diversity in healthy vs unhealthy samples (Fig. 1 A). Across 10 studies sampling the oropharynx, four significant differences were observed between healthy and disease groups, for asthma, influenza, pneumonia, and RTI (two-tailed independent Student’s t -test, p < 0.05). Again, all but one (Wen et al., Influenza) showed significantly higher alpha-diversity in healthy vs unhealthy samples (Fig. 1 B). Similar relationships were observed when examining taxonomic richness (Chao1 index). Among studies sampling the nasopharyngeal microbiome, 10 of 20 showed significant differences between cases and controls, including six that were also significantly enriched in the same direction in the Shannon index (Fig. 1 C, two-tailed independent Student’s t -test, p < 0.05). For oropharyngeal samples, 8 of 10 studies showed significant enrichment between cases and controls (Fig. 1 D, two-tailed independent Student’s t -test, p < 0.05). It has not been well established whether or not alpha-diversity of the URT microbiome is associated with disease [ 58 ]. These results indicate that changes in alpha-diversity of the URT microbiome during respiratory disease are disease-specific, not wholly consistent across studies, and lean toward an overall decline in diversity in the disease state.
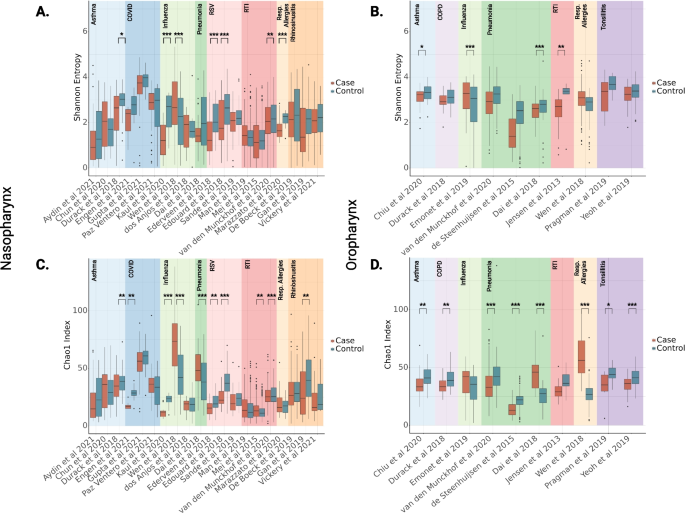
Alpha-diversity between disease cases and healthy controls for each respiratory condition. Alpha-diversity (Shannon Index or Chao1 index) is shown between cases and controls for each study. Shannon diversity for both samples from the nasopharynx ( N = 3223) ( A ) and the oropharynx ( N = 1313) ( B ) was calculated, as well as Chao1 richness for the nasopharynx ( N = 3223) ( C ) and the oropharynx ( N = 1313) ( D ). Significant differences between cases and controls were determined by independent Student’s t -test, two-tailed p -value * = p < 0.05, ** = p < 0.01, *** = p < 0.001
We calculated Bray–Curtis distances at the genus level, to investigate beta-diversity patterns across studies (Fig. 2 ). For these analyses, all samples from all studies were pooled after rarefaction, including samples from both URT sampling sites. Analysis by PERMANOVA showed significant differences in beta-diversity between samples collected from two different URT sites, the nasopharynx and the oropharynx (Fig. 2 A, PERMANOVA p < 0.05). This is consistent with findings that the nasopharyngeal and oropharyngeal microbiomes are compositionally distinct [ 59 ]. Additionally, a significant difference was observed between samples taken from different continents, which pushes against prior assertions that the URT microbiome is generally consistent across geographic regions [ 60 ] (Fig. 2 C, PERMANOVA p < 0.05). As expected, significant differences were observed in Bray–Curtis dissimilarity in cases relative to controls, as well as between disease conditions (Fig. 2 B, D, PERMANOVA p < 0.05). Finally, significant differences in beta-diversity were observed between sequencing methods, and 16S rRNA hypervariable region used for amplicon sequencing (Fig. 2 E, F, PERMANOVA p < 0.05). These results indicated that any further analysis would necessarily require consideration of these confounding variables.
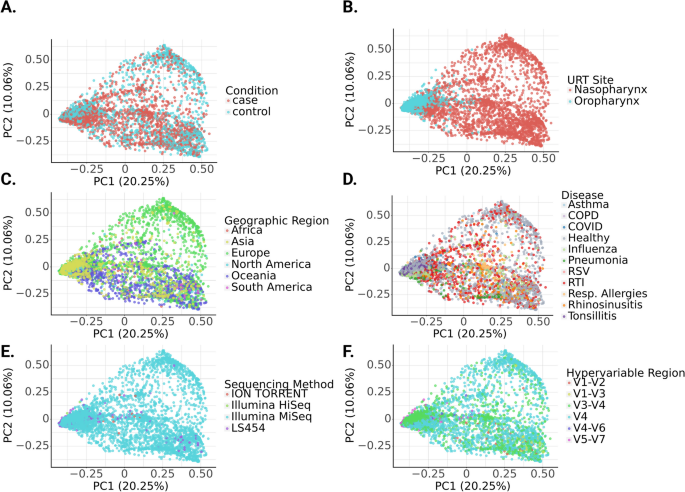
Principal coordinate analysis (PCoA) plots of genus-level Bray–Curtis distances along the first two principal coordinatess across all samples. Within subplots, each point represents a single sample ( N = 4536). Beta-diversity was significantly associated with disease status ( A ), URT sampling site ( B ), geographic region ( C ), disease type ( D ), sequencing method ( E ), and 16S rRNA hypervariable region used for amplicon sequencing ( F ). Significant differences in beta-diversity were observed for all six parameters, as determined by PERMANOVA, p < 0.001 in all cases
Covariates are significantly associated with URT microbiome composition
Next, we aimed to examine the influence of geographic regions on taxonomic composition in healthy URT samples. Using metadata on geographic regions available for all studies, multiple regression was run for each genus to estimate the effect of geographic region (Europe, N. America, S. America, Africa, Asia, or Oceania) on centered log-ratio transformed relative abundance data, correcting for URT sampling site, sequencing method, and hypervariable region. Ninety-eight genera showed significant association with at least one geographic region (Fig. 3 , multiple regression, FDR-corrected p -value < 0.05). FDR-corrected p -values and mean relative abundances of each taxon per geographic region can be found in the supplementary material (Additional file 1 : Table 2).
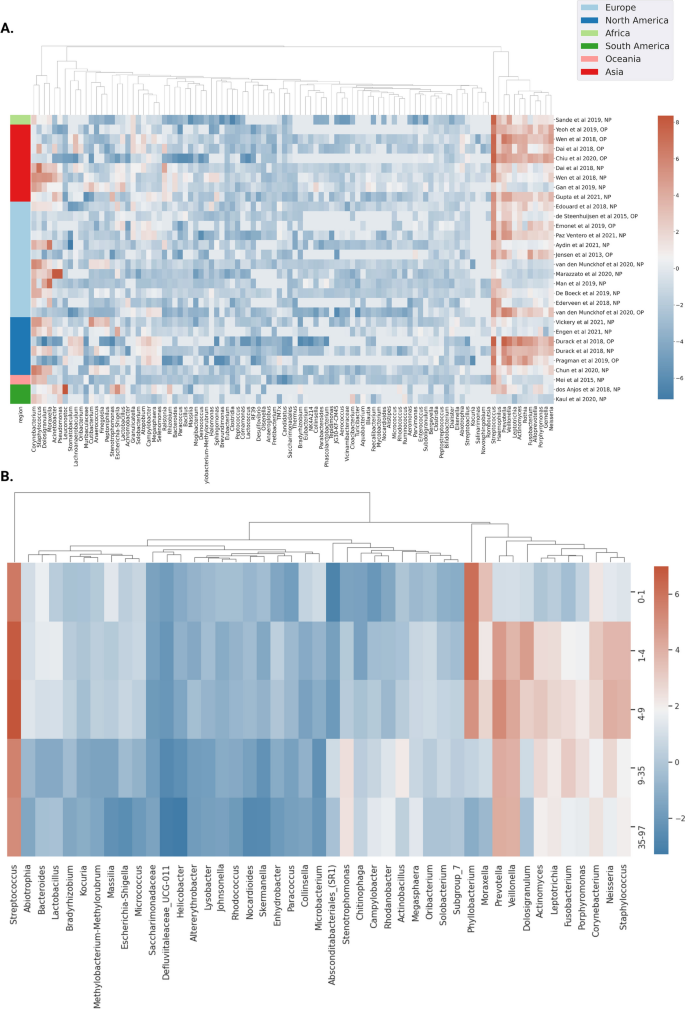
Significant differences in centered log-ratio (CLR) relative abundance of prevalent taxa between geographic regions and ages across healthy control samples. Heatmaps show significant taxonomic associations with geographic location and age in healthy controls. In both, mean CLR-transformed relative abundance is shown via color encoding, with red indicating higher CLR abundance and blue indicating lower CLR abundance. A Taxa displaying significant associations with geographic location in healthy controls are shown in each column ( N = 2387). Each row represents one study, with the URT sampling site annotated (NP = nasopharynx, OP = oropharynx). Geographic region per study is shown via the color bar to the left of the heatmap. Significance was determined by multiple regression, correcting for URT sampling site, sequencing method, and 16S hypervariable region, with FDR-corrected two-tailed p -value < 0.05. B Taxa significantly associated with age are shown, for samples with available metadata for age ( N = 554). Significance was determined by ANCOVA, treating age as a continuous variable, correcting for geographic region, URT sampling site, sequencing method, and 16S hypervariable region, with FDR-corrected two-tailed p -value < 0.05
To investigate how relative abundances of URT genera vary with age in healthy populations, ANCOVA analyses controlling for URT sampling site, geographic region, sequencing method, and 16S rRNA hypervariable region and treating age as a continuous variable were conducted. Overall, 45 genera were significantly associated with age (ANCOVA, FDR-corrected p -value < 0.05), based on ANCOVA containing a squared term for age to uncover potential non-linear relationships. Samples were grouped into age quantiles, in order to visualize mean CLR-transformed relative abundance across age groups for genera that showed significant associations (Fig. 3 ). FDR-corrected p -values associated with age and age^2, as well as mean relative abundances of each taxon per age quantile can be found in the supplementary material (Additional file 1 : Table 3). Using a multiple regression framework similar to the age analysis (i.e., with the same set of covariates), with sex as a categorical independent variable, no genera were found to be significantly associated with sex.
Within-study Random Forest Classifiers show how predictive URT microbiome profiles are in distinguishing cases from controls across disease types
Random forest classifiers were constructed on a per-study basis using genus-level URT relative abundance data, with fivefold cross-validation. The capacity of these classifiers to correctly discriminate cases from controls was assessed by calculating the area under the receiver-operating characteristic (AUROC, Fig. 4 ) from the results of cross-validation testing. Generally, moderate classification accuracy was observed, with an average per-study AUROC of 0.71. Higher AUROC values were observed for some disease conditions, such as influenza and pneumonia. Others showed less capacity to discriminate cases from controls, such as asthma and RTI. No strong correlation was observed between study sample count ( N ) and AUROC (Pearson correlation r = − 0.059, p = 0.75), nor between the URT sampling site and AUROC (two-tailed Student’s independent t -test, t = − 0.76, p = 0.45). These results indicate that URT composition contains information that can be leveraged to predict case versus control status, but that the predictive capacity can vary substantially across diseases.
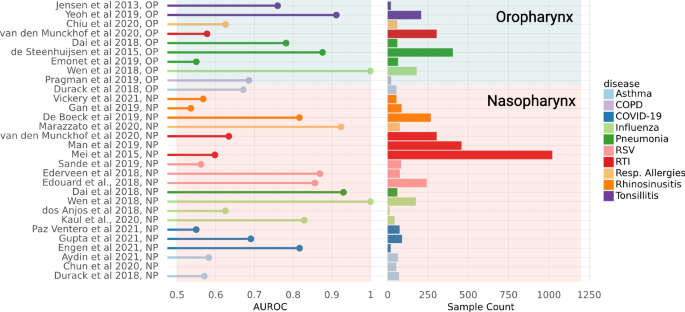
Area under the receiver-operating characteristic (AUROC) for classifying case versus control status from the URT microbiome profile across studies. AUROC values are shown for each study and sampling site ( N = 30 data sets, 4706 samples), based on random-forest classifiers constructed using fivefold cross-validation for data from each study, separately. Values less than 0.5 are not shown. Sample count for each study is shown (range = 12–1021). Per-study disease type is shown via color encoding. Shaded background indicates the URT sampling site of each study (nasopharynx = pink; oropharynx = blue)
URT microbiomes show distinct taxonomic associations across studies and disease states
We next investigated whether we could identify robust taxonomic patterns of URT microbiome disruption across disease conditions. We conducted logistic regression on a per-study basis, in order to avoid cross-study comparisons due to sparsity in available covariates, with disease status as the dependent variable, iterating through separate regressions for each genus (significant genera defined as those with FDR-corrected p < 0.05). Studies spanning 8 disease types showed significant enrichment in at least one taxon (Fig. 5 ). COPD, COVID-19, and asthma were the three respiratory conditions that showed no significant taxonomic enrichments in health or disease (all FDR-corrected p > 0.05). Several consistent enrichments, where a taxon showed significant enrichment in the same direction in at least two studies within a disease, were observed (Fig. 5 ; designated by black boxes drawn around cells in the heatmap). For instance, Pseudomonas was consistently enriched in cases of influenza, while Veillonella was consistently enriched in cases of influenza, pneumonia, and RSV. Overall consistent cross-disease associations with health or disease status were defined as those genera that showed significant enrichments in the same direction in at least three more studies across all diseases than in the opposing direction ( N same_direction − N opposite_direction ≥ 3). Following this heuristic , Corynebacterium , Veillonella , Fusobacterium , Rothia , and Gemella were all associated with health, although Corynebacterium , and Veillonella each showed enrichment in cases in one study. Pseudomonas and Acinetobacter were consistently associated with disease (Fig. 5 ). Influenza and pneumonia showed the largest number of significant enrichments among all the disease conditions analyzed. Streptococcus had the highest mean relative abundance of taxa with significant associations, at 17.2% ± 0.3%, followed by Corynebacterium , Staphylococcus , Dolosigranulum , Haemophilis , and Prevotella , all with mean relative abundances over 5% (Fig. 5 ). Effect sizes and FDR-corrected p values were recorded for each genus-disease pair (Additional file 1 : Table 4).
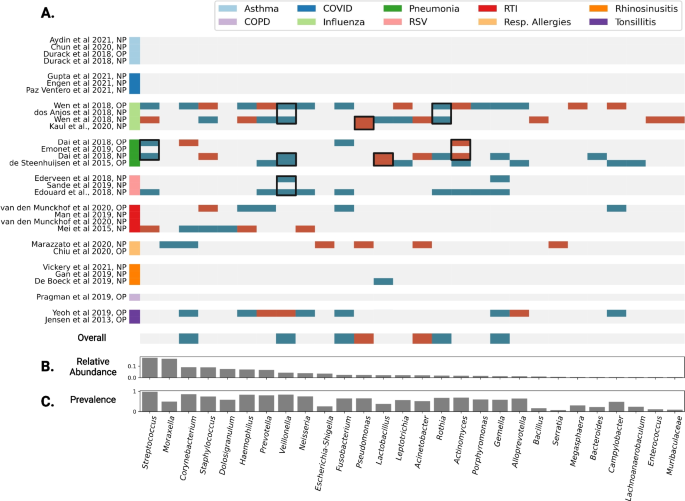
Within-study case vs. control logistic regression results at the genus-level. A Per-study taxonomic enrichment in cases is denoted in red, and enrichment in controls is denoted in blue ( N = 30 data sets, 4706 samples). Blank/gray spaces indicate no significant association. Only taxa with at least one significant association are shown. Significant associations are defined as having FDR-corrected two-tailed p -value < 0.05. Black boxes are shown around consistent enrichments within a disease, in which taxa are enriched in the same direction in at least two studies within a disease. Overall disease associations are shown in the last heatmap row, in which enrichment in the same direction in three or more studies than in the opposite direction ( N same_direction − N opposite_direction ≥ 3) are considered across-disease significant. B Mean relative abundance across all samples of each taxon shown in A . C Prevalence across all samples for each taxon shown in A
The results of this meta-analysis were consistent with prior findings regarding the composition of the URT microbiome in health and disease [ 1 ] and revealed novel compositional patterns within and across diseases and between healthy individuals across age and geography. They also underscore the importance of recognizing different types of dysbioses in the URT microbiome that can potentially contribute to disease.
URT microbiome samples showed a trend toward lower alpha-diversity in disease cases, as opposed to healthy controls, in at least one study representing asthma, RTI, influenza, respiratory allergies, RSV, and pneumonia (Fig. 2 A, B ). Previous studies have reported similar signatures in cases of bacterial or viral infection [ 61 , 62 ]. Influenza was the sole respiratory condition in which one study showed significantly higher alpha-diversity in disease cases, aligning with previous findings that alpha-diversity patterns vary depending on the disease context [ 43 ]. However, this finding will need further validation, as prior reports have found no association between URT alpha-diversity and susceptibility to influenza infection, and another study in this analysis showed an association in the opposite direction from what we report (likely due to methodological differences across analyses) [ 7 , 63 ].
Bray–Curtis dissimilarity between URT communities was associated with multiple covariates: case–control status, sampling site (nasopharynx or oropharynx), disease type, geographic region, sequencing method, and 16S rRNA hypervariable region used for amplicon sequencing (Fig. 2 ). Concordantly, prior work has shown significant beta-diversity differences between health and disease states [ 61 ] and separation between nasopharyngeal and oropharyngeal samples, with the oropharynx harboring a more diverse microbial population than the nasopharynx [ 40 ] (Fig. 2 ). The significant beta-diversity differences reported here between samples from distinct geographic regions were novel. Prior work has asserted a lack of geographic signal in the URT microbiome [ 60 ]. However, it is intuitive that variation in the surrounding environment could give rise to variation in URT composition (Fig. 2 ). Technical differences in sequencing methodology were significantly associated with beta-diversity, as one might expect (Fig. 2 ). These results underscore the need to account for relevant covariates when looking for associations between URT composition and diseases that are independent of these potentially confounding factors.
We next looked into how the covariates age, sex, and geographic location shaped the taxonomic composition of the URT microbiome in healthy individuals across studies, in order to identify and isolate these signals from health and disease associations, and further indicate which covariates should be considered in future analyses (Fig. 4 ). Relative abundances of several taxa ( N = 98) were observed to show significant associations with geography. Corynebacterium , a known health-associated taxon, showed higher mean relative abundance in samples from North America (12.0%), South America (15.2%), and Oceania (12.9%) than in samples from Africa (5.0%), Asia (4.2%), or Europe (8.6%). Conversely, Streptococcus showed much higher mean relative abundance in samples collected in Africa (35%) than in any other geographic region. Other taxa that show significant association with geographic region include Gemella, Pseudomonas, Rothia , and Veillonella , all of which show significant associations with health or disease via case–control analysis. Due to these significant differences in taxonomic composition, it is imperative to account for geographic location in the construction of diagnostic or therapeutic tools. Two keystone taxa, Dolosigranulum and Moraxella , were enriched in children as compared to adults, which was previously reported (Fig. 4 ) [ 5 ]. Additionally, we saw an increase in the health-associated taxon Veillonella in adults, when compared to children (Fig. 4 ). Due to the breadth of associations observed with age, and the purported inhibition of pathogenic invasion by some of these age-associated genera [ 2 ], we suggest that age should be included as a covariate when analyzing URT microbiome data, whenever possible. However, when age metadata are unavailable, we hope that the list of taxa provided here can be used to identify associations that may be driven by variation in age, rather than by disease. Sex showed no associations.
We ran case–control logistic regression analyses separately within each study and URT sampling site, to avoid pooling data across samples with very different demographic, biological, and methodological characteristics, similar to the approach taken in a prior meta-analysis of human gut microbiomes [ 64 ]. Robust taxonomic enrichments associated with case–control status were observed within 11 out of the 26 studies included in the meta-analysis (Fig. 5 ), including two studies that contained both nasopharyngeal and oropharyngeal samples. Studies from 7 of the 10 respiratory conditions included showed significant enrichment of at least one genus. Asthma, COPD, and COVID-19 were the three diseases that showed no significant URT genus-level associations, although previous URT studies have shown a microbial association with these diseases, such as with Rothia in COVID-19 patients [ 65 , 66 , 67 ].
Several consistent signatures were observed across studies within a disease. For instance, Veillonella was significantly enriched in controls for at least two independent studies within both pneumonia and RSV, and across OP and NP samples in the same study for influenza (Fig. 5 ). Two studies included in the meta-analysis, one in influenza and one in RSV, similarly report Veillonella enrichment in cases as compared to controls [ 49 , 62 ]. Conversely, Pseudomonas was significantly enriched in cases across two independent studies for influenza. This association was also reported in two influenza studies included in the meta-analysis [ 39 , 40 ]. Prevotella showed six significant enrichments across studies, but interestingly showed very inconsistent associations, with enrichment in controls in four studies and enrichment in cases in two. Here we see an example of putative dysbiosis taking many forms, and the health or disease associations of many taxa showing strong context-specificity. Across diseases, significant signatures were observed for several keystone taxa that were enriched in healthy individuals [ 2 ], like Corynebacterium, Veillonella , Fusobacterium , Rothia , and Gemella . Of these, Corynebacterium has been previously identified as a core taxon, putatively associated with health [ 1 ]. Additionally, these are largely abundant/prevalent taxa, with mean relative abundance above 5% for Corynebacterium , specifically (Fig. 5 ) . Conversely, a few genera known to harbor opportunistic pathogen species, including Pseudomonas and Acinetobacter , showed multiple associations with diseases. Acinetobacter baumannii and Pseudomonas aeruginosa are both known to cause disease in humans [ 27 , 28 , 29 , 68 ]. Understanding which taxa are strongly related to health or disease, and in which contexts, will further aid the development of effective microbial diagnostics and therapeutics.
There were several limitations to our study that are important to highlight. First, there were differences in amplicon sequencing methodologies across the 26 studies included in this analysis, which introduced substantial technical biases. For example, not all studies had paired-end reads available, so we elected to use only forward reads for all studies to mitigate potential bias. Using longer, merged reads for some studies and not others would impact the efficiency of taxonomic annotation across studies (i.e., even for studies with the same variable region sequenced). Furthermore, there are often a large number of paired-end reads that fail to merge, which can lead to a substantial drop in sequencing depth in a given sample, which is another layer of bias. Additionally, samples across studies showed differences in sequencing depth. To account for this, we elected to rarefy the data to normalize sampling depth across samples. While other options exist, the current consensus in the field is that rarefaction is still optimal for comparing point estimates of alpha- and beta-diversity across samples [ 69 ].
First, while we controlled for these technical variables in our statistical testing whenever possible, incomplete metadata on these differences across studies can skew the final results. Second, many studies were missing pertinent demographic metadata, such as sex or age, which limited our statistical power by preventing us from correcting for these covariates in regressions that pooled data across all studies. It was not possible to determine whether geographic region-related trends were consistent across age groups, due to age metadata not being available for a majority of samples. Third, some studies have nearly 100-fold more samples than others, which can skew regression results if samples were pooled across studies that differed substantially in cohort size. For these reasons, the case versus control genus enrichment analyses were conducted on a per-study basis, to avoid introducing these myriad biases into the regressions. Significant case–control hits from within-study regressions that were consistent across studies provided strong support for disease-specific associations that are independent of the aforementioned limitations.
Overall, these findings point to different flavors of dysbiosis that distinguish different disease states in the URT. In some cases, the disease state is characterized by a loss of putatively beneficial commensals, such as Veillonella in influenza, pneumonia, and RSV, and in other cases, it is characterized by the gain of putatively pathogenic taxa such as Pseudomonas in influenza, which mirrors what has been found across diseases in the human gut microbiome [ 64 ]. Future work should leverage these results to help guide the development of diagnostics and therapeutics for the URT.
Systematic review of relevant studies
A systematic review was conducted using two main search engines (PubMed and Embase) to retrieve all relevant publications describing microbiome sequencing in the human upper respiratory tract. A PRISMA flow chart (Additional file 2 : Fig. S1) shows how the publications were screened, identified as relevant, and finally selected based on inclusion and exclusion criteria. Briefly, a total of 153,586 reports were identified using relevant keywords such as “microbiome,” “16S rRNA,” “URT,” “oropharynx,” “nasopharynx,” and “larynx.” Of these, 37,083 were classified as conference abstracts, conference papers, short surveys, and book chapters and therefore were excluded from the analysis. Additional exclusion criteria included 16S rRNA studies from non-human URT, which filtered out 115,883 manuscripts, leaving only 620 manuscripts. Of these 620 manuscripts, a very strict and manual pre-selection was conducted to eliminate those with irrelevant topics or disease conditions, such as studies that involved interventions or those without healthy patient controls, as well as studies with unavailable sequencing data, incomplete metadata, or duplicate manuscripts that referred to the same clinical study. This pre-selection step reduced the number of manuscripts by approximately 90%, leaving only 68 manuscripts. The final selection step was conducted manually to ensure the public availability of well-curated metadata and corresponding raw sequencing data files. This step also excluded studies from overrepresented disease conditions, so that no more than 3 studies were selected per disease condition. At the end, a total of 26 peer-reviewed publications survived all inclusion and exclusion criteria, yielding a total of 10 URT-related conditions (asthma, chronic obstructive pulmonary disease, COVID-19, influenza, pneumonia, respiratory allergies, rhinosinusitis, RSV, respiratory tract infection, tonsillitis) with 1–3 studies per condition representing a total of 4,706 samples.
16S rRNA amplicon sequencing URT cohorts
All phylogenetic and read count data used in this study consisted of 16S rRNA gene amplicon sequencing data, with multiple hypervariable regions sequenced across studies, spanning the V1 to V7 regions. A full list of the 26 data sets analyzed in this study, along with links to SRA accession numbers and accompanying metadata, can be found in Additional file 1 : Table 1. The studies contained between 12 and 1021 subjects and varied in age from birth to 97 years old (in studies where age metadata was available), with more representation of young individuals. Studies were conducted in all six inhabited continents, with more representation from Europe and North America. 16S rRNA amplicon sequencing data consisting of FASTQ files, along with associated metadata, were downloaded from the NCBI SRA. While some studies included paired-end sequencing reads, only forward reads were used to maintain better analytical consistency across all studies and to avoid biases in the efficiency of taxonomic assignment between studies. Following data collection, all FASTQ data were imported into QIIME2 version 2022.8.3 [ 70 ] for further processing and analysis. Data were imported through the construction of a single-end Phred33v2 FASTQ manifest for each dataset. Following import, quality control and filtering in the QIIME2 DADA2 (v1.12.1) [ 71 ] plug-in removed chimeric sequences, trimmed left ends of all sequences by 10 bp to remove primers, truncated sequences uniformly at 200 bp, and identified amplicon sequence variants (ASVs). In total 623,507,314 reads were filtered, with 134,649,099 removed for poor quality or chimerism.
Data preprocessing and taxonomic classification
The Silva high-quality rRNA gene database version 138 was used to assign taxonomy to ASVs [ 72 ]. The full-length 16S rRNA classifier was used due to heterogeneity in the hypervariable region used for sequencing between studies. Mean classification at the genus level was 86.0% (Additional file 1 : Table 5; Additional file 2 : Fig. S2). At the species level, classification was unsuccessful, with a mean classification of 13.9%. As a result, all subsequent analyses were conducted at the genus level by binning ASV counts together based on their genus-level annotations. All subsequent data analysis was managed using pandas (v1.4.4) in Python (v3.8.13).
Alpha-diversity analyses
To investigate alpha-diversity, QIIME2 artifacts containing sequences for each study were merged into a single dataframe. Prior to calculation, algorithmic filtering removed any taxa with fewer than two reads per study, and any taxa present in less than 5% of samples across a study. This merged data frame was converted into a QIIME2 artifact and rarefied using the qiime feature-table rarefy function to a sampling depth of 2000. Alpha-diversity was calculated in QIIME2 via the alpha function within the diversity plugin. Shannon entropy and Chao1 index were used to estimate alpha-diversity for all samples included in the meta-analysis. Shannon entropy and Chao1 index for cases and controls within each disease were plotted and significant differences across groups were tested using two-tailed independent Student’s t -test ( p < 0.05) in SciPy (v1.8.1).
Beta-diversity analyses
To estimate beta-diversity, the filtered and rarefied genus count table constructed previously was used to construct a Bray–Curtis dissimilarity matrix using the beta function in the QIIME2 diversity plugin. Subsequently, principal coordinate analysis (PCoA) was used to analyze and visualize overall beta-diversity in scikit-bio version 0.5.7. Significant differences in beta-diversity were observed along multiple axes, including case vs. control status, disease type, geographic location, URT sampling site, sequencing method, and 16S rRNA hypervariable region as determined by PERMANOVA, using the adonis function within the diversity plugin for QIIME2.
URT compositional patterns across geographic regions
A genus-level abundance matrix was constructed using only healthy control samples, and taxa with fewer than two reads per study or those present in fewer than 5% of samples across a study were removed. To examine the association between geographic location and centered log-ratio (CLR) transformed relative abundance of common taxa, multiple regression was used to determine significant enrichments of taxa in each geographic region while correcting for URT sampling site, sequencing method, and 16S rRNA hypervariable region using the formula “clr ~ region + v_region + sequencing + URT” in statsmodels (v0.13.5) [ 73 ]. For the purpose of these analyses, the continents in which studies took place were used as the geographic regions, as too many countries were represented to have appropriate statistical power at smaller geographic scales. As sex and age metadata were not available for 61.5% of the studies, these covariates were not accounted for in this analysis. Multiple comparison correction for p -values was done using the Benjamini–Hochberg method for adjusting the false discovery rate (FDR) [ 74 ], using statsmodels (v0.14.1). Per-study mean CLR-transformed relative abundance of taxa identified to be significantly enriched in at least one geographic region (multiple regression, FDR-corrected p < 0.05) were added to a clustered heatmap, with color encoding the average CLR-transformed relative abundances in each context. Columns containing average CLR-transformed relative abundances were clustered via an agglomerative clustering algorithm using clustermap in seaborn (v0.12.2).
URT microbiome-age associations
Associations between age and CLR-transformed relative abundances was analyzed via ANCOVA in statsmodels. Using 10 studies for which age metadata was available, ANCOVA was conducted using the following formula “clr ~ age + age 2 + variable_region + sequencing + URT_site + region” that was used to determine significant associations with age, accounting for URT sampling site, geographic region, sequencing method, and 16S rRNA hypervariable region. The square term for age was included to determine if non-linear relationships existed between CLR and age. The p -values were corrected for multiple comparisons via the Benjamini–Hochberg FDR correction as previously described. Samples were split into quantiles by age for visualization. Significantly associated taxa (FDR-corrected p < 0.05) were added to a heatmap with color encoding the average CLR-transformed relative abundances.
URT microbiome associations with sex
Associations between sex and genus-level CLR abundances were determined via multiple regression. Using the 10 studies for which sex metadata was available, multiple regression were conducted using the following formula: “clr ~ sex + variable_region + sequencing + URT_site + region” in statsmodels. The resulting p -values were corrected for multiple comparisons via the Benjamini–Hochberg FDR correction. After correction, no taxa showed a significant association with sex.
Supervised classification of cases and control
Random forest classifiers were constructed for each study to classify cases and controls within each study using scikit-learn (v0.24.1) [ 74 , 75 ]. Classifiers were constructed with fivefold cross-validation, using the scikit-learn StratifiedKFold function to shuffle data. The RandomForestClassifier function within scikit-learn was used to construct classifiers with n_estimators = 100. Area under the curve of the receiver-operating characteristic was calculated using the results of cross-validation testing, using the cross_val_predict and roc_auc_score functions in scikit-learn .
URT microbiome-disease associations
To investigate the association between genera in the URT microbiome and disease, sample read counts were normalized using a CLR transformation, as above. Logistic regressions used case–control status as the dependent variable and CLR-transformed abundance as the independent variable, following the formula “case_control_status ~ clr” in statsmodels. Regressions were run separately within each study and sampling site. By running separate analyses within each study and sampling site, key confounders like geographic location, sampling site, 16S rRNA hypervariable region, and sequencing method were constant within a given regression analysis. Mean relative abundance of each taxon within a given study and sampling site found to be significant was calculated for visualizations. P -values were FDR-corrected as described above. Significance was assigned to any association with an FDR-corrected p -value less than 0.05. Results were plotted in a binary heatmap, with significant health-associated genera designated as blue and disease-associated genera designated as red. Heatmaps were constructed using seaborn.
Availability of data and materials
All data generated or analyzed during this study are included in this published article, its supplementary information files, and publicly available repositories. Additional supplementary data can be found in Additional file 1 : Tables 1–5. All original data are available on the NCBI SRA under accession codes provided in Additional file 1 : Table 1, with the exception of one study for which data is not publicly available. All intermediate data files for this analysis are available at Zenodo under DOI: 10.5281/zenodo.10962515. Analysis code can be found at the following GitHub repository: https://github.com/Gibbons-Lab/2023_URTmetaanalysis .
Abbreviations
- Upper respiratory tract
Nasopharynx
Respiratory tract infection
Respiratory syncytial virus
Chronic obstructive pulmonary disease
Coronavirus disease 2019
Amplicon sequence variant
False detection rates
Centered log-ratio
Area under the receiver-operating characteristic
Principal coordinate analysis
Analysis of covariance
National Center for Biotechnology Information Sequence Read Archive
Kumpitsch C, Koskinen K, Schöpf V, Moissl-Eichinger C. The microbiome of the upper respiratory tract in health and disease. BMC Biol. 2019;17:87.
Article PubMed PubMed Central Google Scholar
Man WH, de Steenhuijsen Piters WAA, Bogaert D. The microbiota of the respiratory tract: gatekeeper to respiratory health. Nat Rev Microbiol. 2017;15:259–70.
Article CAS PubMed PubMed Central Google Scholar
Lipinski JH, Moore BB, O’Dwyer DN. The evolving role of the lung microbiome in pulmonary fibrosis. Am J Physiol Lung Cell Mol Physiol. 2020;319:L675–82.
Siegel SJ, Weiser JN. Mechanisms of bacterial colonization of the respiratory tract. Annu Rev Microbiol. 2015;69:425–44.
Bosch AATM, Levin E, van Houten MA, Hasrat R, Kalkman G, Biesbroek G, et al. Development of upper respiratory tract microbiota in infancy is affected by mode of delivery. EBioMedicine. 2016;9:336–45.
Nesbitt H, Burke C, Haghi M. Manipulation of the upper respiratory microbiota to reduce incidence and severity of upper respiratory viral infections: a literature review. Front Microbiol. 2021;12:713703.
Lee KH, Gordon A, Shedden K, Kuan G, Ng S, Balmaseda A, et al. The respiratory microbiome and susceptibility to influenza virus infection. Plos One. 2019;14:e0207898.
Clark SE. Commensal bacteria in the upper respiratory tract regulate susceptibility to infection. Curr Opin Immunol. 2020;66:42–9.
GBD Chronic Respiratory Disease Collaborators. Prevalence and attributable health burden of chronic respiratory diseases, 1990–2017: a systematic analysis for the Global Burden of Disease Study 2017. Lancet Respir Med. 2020;8:585–96.
Article Google Scholar
Htun TP, Sun Y, Chua HL, Pang J. Clinical features for diagnosis of pneumonia among adults in primary care setting: a systematic and meta-review. Sci Rep. 2019;9:7600.
Moghadami M. A narrative review of influenza: a seasonal and pandemic disease. Iran J Med Sci. 2017;42:2–13.
PubMed PubMed Central Google Scholar
Rosas-Salazar C, Tang Z-Z, Shilts MH, Turi KN, Hong Q, Wiggins DA, et al. Upper respiratory tract bacterial-immune interactions during respiratory syncytial virus infection in infancy. J Allergy Clin Immunol. 2022;149:966–76.
Article CAS PubMed Google Scholar
Schenck LP, Surette MG, Bowdish DME. Composition and immunological significance of the upper respiratory tract microbiota. FEBS Lett. 2016;590:3705–20.
Psaltis AJ, Mackenzie BW, Cope EK, Ramakrishnan VR. Unraveling the role of the microbiome in chronic rhinosinusitis. J Allergy Clin Immunol. 2022;149:1513–21.
de SteenhuijsenPiters WAA, Sanders EAM, Bogaert D. The role of the local microbial ecosystem in respiratory health and disease. Philos Trans R Soc Lond B Biol Sci. 2015;370:20140294.
Li N, Ma W-T, Pang M, Fan Q-L, Hua J-L. The commensal microbiota and viral infection: a comprehensive review. Front Immunol. 2019;10:1551.
Olszak T, An D, Zeissig S, Vera MP, Richter J, Franke A, et al. Microbial exposure during early life has persistent effects on natural killer T cell function. Science. 2012;336:489–93.
Gollwitzer ES, Saglani S, Trompette A, Yadava K, Sherburn R, McCoy KD, et al. Lung microbiota promotes tolerance to allergens in neonates via PD-L1. Nat Med. 2014;20:642–7.
Li W, Ma ZS. The upper respiratory tract microbiome network impacted by SARS-CoV-2. Microb Ecol. 2023;86:1428–37.
Pettigrew MM, Laufer AS, Gent JF, Kong Y, Fennie KP, Metlay JP. Upper respiratory tract microbial communities, acute otitis media pathogens, and antibiotic use in healthy and sick children. Appl Environ Microbiol. 2012;78:6262–70.
Biesbroek G, Tsivtsivadze E, Sanders EAM, Montijn R, Veenhoven RH, Keijser BJF, et al. Early respiratory microbiota composition determines bacterial succession patterns and respiratory health in children. Am J Respir Crit Care Med. 2014;190:1283–92.
Article PubMed Google Scholar
Bomar L, Brugger SD, Yost BH, Davies SS, Lemon KP. Corynebacterium accolens releases antipneumococcal free fatty acids from human nostril and skin surface triacylglycerols. MBio. 2016;7:e01725-e1815.
Kim HJ, Jo A, Jeon YJ, An S, Lee K-M, Yoon SS, et al. Nasal commensal Staphylococcus epidermidis enhances interferon-λ-dependent immunity against influenza virus. Microbiome. 2019;7:80.
Menberu MA, Liu S, Cooksley C, Hayes AJ, Psaltis AJ, Wormald P-J, et al. Corynebacterium accolens has antimicrobial activity against Staphylococcus aureus and methicillin-resistant S. aureus pathogens isolated from the sinonasal niche of chronic rhinosinusitis patients. Pathogens. 2021;10:207.
Zaura E, Keijser BJF, Huse SM, Crielaard W. Defining the healthy “core microbiome” of oral microbial communities. BMC Microbiol. 2009;9:259.
Bach LL, Ram A, Ijaz UZ, Evans TJ, Lindström J. A longitudinal study of the human oropharynx microbiota over time reveals a common core and significant variations with self-reported disease. Front Microbiol. 2020;11:573969.
Harrison A, Mason KM. Pathogenesis of Haemophilus influenzae in humans. In: Emerging H, Infections R-E, editors. Hoboken. NJ, USA: John Wiley & Sons, Inc.; 2015. p. 517–33.
Google Scholar
Qin S, Xiao W, Zhou C, Pu Q, Deng X, Lan L, et al. Pseudomonas aeruginosa: pathogenesis, virulence factors, antibiotic resistance, interaction with host, technology advances and emerging therapeutics. Signal Transduct Target Ther. 2022;7:199.
Brouwer S, Rivera-Hernandez T, Curren BF, Harbison-Price N, De Oliveira DMP, Jespersen MG, et al. Pathogenesis, epidemiology and control of Group A Streptococcus infection. Nat Rev Microbiol. 2023;21:431–47.
Stearns JC, Davidson CJ, McKeon S, Whelan FJ, Fontes ME, Schryvers AB, et al. Culture and molecular-based profiles show shifts in bacterial communities of the upper respiratory tract that occur with age. ISME J. 2015;9:1246–59.
Aydin M, Weisser C, Rué O, Mariadassou M, Maaß S, Behrendt A-K, et al. The rhinobiome of exacerbated wheezers and asthmatics: insights from a German pediatric exacerbation network. Front Allergy. 2021;2:667562. NCBI SRA https://www.ncbi.nlm.nih.gov/bioproject/?term=PRJNA714100 (2021)
Chun Y, Do A, Grishina G, Grishin A, Fang G, Rose S, et al. Integrative study of the upper and lower airway microbiome and transcriptome in asthma. JCI Insight. 2020;5. NCBI SRA https://www.ncbi.nlm.nih.gov/bioproject/?term=PRJNA601757 (2020)
Durack J, Huang YJ, Nariya S, Christian LS, Ansel KM, Beigelman A, et al. Bacterial biogeography of adult airways in atopic asthma. Microbiome. 2018;6:104. NCBI SRA https://www.ncbi.nlm.nih.gov/bioproject/?term=PRJEB15534 (2016), https://www.ncbi.nlm.nih.gov/bioproject/?term=PRJEB22676 (2018)
Pragman AA, Knutson KA, Gould TJ, Isaacson RE, Reilly CS, Wendt CH. Chronic obstructive pulmonary disease upper airway microbiota alpha diversity is associated with exacerbation phenotype: a case-control observational study. Respir Res. 2019;20:114. NCBI SRA https://www.ncbi.nlm.nih.gov/bioproject/?term=PRJNA543785 (2019)
Ventero MP, Cuadrat RRC, Vidal I, Andrade BGN, Molina-Pardines C, Haro-Moreno JM, et al. Nasopharyngeal microbial communities of patients infected with SARS-CoV-2 that developed COVID-19. Front Microbiol. 2021;12:637430. NCBI SRA https://www.ncbi.nlm.nih.gov/bioproject/?term=PRJNA673585 (2020)
Gupta A, Karyakarte R, Joshi S, Das R, Jani K, Shouche Y, et al. Nasopharyngeal microbiome reveals the prevalence of opportunistic pathogens in SARS-CoV-2 infected individuals and their association with host types. Microbes Infect. 2022;24:104880. NCBI SRA https://www.ncbi.nlm.nih.gov/bioproject/?term=PRJNA707350 (2021)
Engen PA, Naqib A, Jennings C, Green SJ, Landay A, Keshavarzian A, et al. Nasopharyngeal microbiota in SARS-CoV-2 positive and negative patients. Biol Proced Online. 2021;23:10. NCBI SRA https://www.ncbi.nlm.nih.gov/bioproject/?term=PRJNA704967 (2021)
Borges LGDA, Giongo A, Pereira L de M, Trindade FJ, Gregianini TS, Campos FS, et al. Comparison of the nasopharynx microbiome between influenza and non-influenza cases of severe acute respiratory infections: a pilot study. Health Sci Rep. 2018;1:e47. NCBI SRA https://www.ncbi.nlm.nih.gov/bioproject/?term=PRJNA317701 (2016)
Kaul D, Rathnasinghe R, Ferres M, Tan GS, Barrera A, Pickett BE, et al. Microbiome disturbance and resilience dynamics of the upper respiratory tract during influenza A virus infection. Nat Commun. 2020;11:2537. NCBI SRA https://www.ncbi.nlm.nih.gov/bioproject/?term=PRJNA240559 (2014), https://www.ncbi.nlm.nih.gov/bioproject/240562 (2014)
Wen Z, Xie G, Zhou Q, Qiu C, Li J, Hu Q, et al. Distinct nasopharyngeal and oropharyngeal microbiota of children with influenza A virus compared with healthy children. Biomed Res Int. 2018;2018:6362716. NCBI SRA https://www.ncbi.nlm.nih.gov/bioproject/?term=PRJNA473282 (2018), https://www.ncbi.nlm.nih.gov/bioproject/?term=PRJNA344805 (2016)
Dai W, Wang H, Zhou Q, Feng X, Lu Z, Li D, et al. The concordance between upper and lower respiratory microbiota in children with Mycoplasma pneumoniae pneumonia. Emerg Microbes Infect. 2018;7:92. NCBI SRA https://www.ncbi.nlm.nih.gov/bioproject/?term=PRJNA344805 (2016), https://www.ncbi.nlm.nih.gov/bioproject/?term=PRJNA431097 (2018)
Emonet S, Lazarevic V, Leemann Refondini C, Gaïa N, Leo S, Girard M, et al. Identification of respiratory microbiota markers in ventilator-associated pneumonia. Intensive Care Med. 2019;45:1082–92. NCBI SRA https://www.ncbi.nlm.nih.gov/bioproject/?term=PRJEB20665 (2018)
de Steenhuijsen Piters WAA, Huijskens EGW, Wyllie AL, Biesbroek G, van den Bergh MR, Veenhoven RH, et al. Dysbiosis of upper respiratory tract microbiota in elderly pneumonia patients. ISME J. 2016;10:97–108. NCBI SRA https://www.ncbi.nlm.nih.gov/bioproject/?term=PRJNA276495 (2015)
Chiu C-Y, Chan Y-L, Tsai M-H, Wang C-J, Chiang M-H, Chiu C-C, et al. Cross-talk between airway and gut microbiome links to IgE responses to house dust mites in childhood airway allergies. Sci Rep. 2020;10:13449.
Marazzato M, Zicari AM, Aleandri M, Conte AL, Longhi C, Vitanza L, et al. 16S metagenomics reveals dysbiosis of nasal core microbiota in children with chronic nasal inflammation: role of adenoid hypertrophy and allergic rhinitis. Front Cell Infect Microbiol. 2020;10:458. NCBI SRA https://www.ncbi.nlm.nih.gov/bioproject/?term=PRJNA554533 (2019)
De Boeck I, Wittouck S, Martens K, Claes J, Jorissen M, Steelant B, et al. Anterior nares diversity and pathobionts represent sinus microbiome in chronic rhinosinusitis. mSphere. 2019;4. NCBI SRA https://www.ncbi.nlm.nih.gov/bioproject/?term=PRJEB30316 (2019), https://www.ncbi.nlm.nih.gov/bioproject/?term=PRJEB23057 (2017)
Gan W, Yang F, Tang Y, Zhou D, Qing D, Hu J, et al. The difference in nasal bacterial microbiome diversity between chronic rhinosinusitis patients with polyps and a control population. Int Forum Allergy Rhinol. 2019;9:582–92. NCBI SRA https://www.ncbi.nlm.nih.gov/bioproject/?term=PRJNA493980 (2018)
Vickery TW, Armstrong M, Kofonow JM, Robertson CE, Kroehl ME, Reisdorph NA, et al. Specialized pro-resolving mediator lipidome and 16S rRNA bacterial microbiome data associated with human chronic rhinosinusitis. Data Brief. 2021;36:107023. NCBI SRA https://www.ncbi.nlm.nih.gov/bioproject/?term=PRJNA678776 (2020)
Edouard S, Million M, Bachar D, Dubourg G, Michelle C, Ninove L, et al. The nasopharyngeal microbiota in patients with viral respiratory tract infections is enriched in bacterial pathogens. Eur J Clin Microbiol Infect Dis. 2018;37:1725–33. NCBI SRA https://www.ncbi.nlm.nih.gov/bioproject/?term=PRJEB14780 (2018)
Ederveen THA, Ferwerda G, Ahout IM, Vissers M, de Groot R, Boekhorst J, et al. Haemophilus is overrepresented in the nasopharynx of infants hospitalized with RSV infection and associated with increased viral load and enhanced mucosal CXCL8 responses. Microbiome. 2018;6:10. NCBI SRA https://www.ncbi.nlm.nih.gov/bioproject/?term=PRJEB20811 (2017)
Sande CJ, Njunge JM, Mwongeli Ngoi J, Mutunga MN, Chege T, Gicheru ET, et al. Airway response to respiratory syncytial virus has incidental antibacterial effects. Nat Commun. 2019;10:2218. NCBI SRA https://www.ncbi.nlm.nih.gov/bioproject/?term=PRJEB28053 (2018)
Man WH, van Houten MA, Mérelle ME, Vlieger AM, Chu MLJN, Jansen NJG, et al. Bacterial and viral respiratory tract microbiota and host characteristics in children with lower respiratory tract infections: a matched case-control study. Lancet Respir Med. 2019;7:417–26. NCBI SRA https://www.ncbi.nlm.nih.gov/bioproject/?term=PRJNA428382 (2018)
Teo SM, Mok D, Pham K, Kusel M, Serralha M, Troy N, et al. The infant nasopharyngeal microbiome impacts severity of lower respiratory infection and risk of asthma development. Cell Host Microbe. 2015;17:704–15. NCBI SRA https://www.ncbi.nlm.nih.gov/bioproject/?term=PRJNA275918 (2015)
van den Munckhof EHA, Hafkamp HC, de Kluijver J, Kuijper EJ, de Koning MNC, Quint WGV, et al. Nasal microbiota dominated by Moraxella spp. is associated with respiratory health in the elderly population: a case control study. Respir Res. 2020;21:181. NCBI SRA https://www.ncbi.nlm.nih.gov/bioproject/?term=PRJNA596902 (2019)
Jensen A, Fagö-Olsen H, Sørensen CH, Kilian M. Molecular mapping to species level of the tonsillar crypt microbiota associated with health and recurrent tonsillitis. Plos One. 2013;8:e56418.
Yeoh YK, Chan MH, Chen Z, Lam EWH, Wong PY, Ngai CM, et al. The human oral cavity microbiota composition during acute tonsillitis: a cross-sectional survey. BMC Oral Health. 2019;19:275. NCBI SRA https://www.ncbi.nlm.nih.gov/sra/?term=PRJNA559766 (2019)
Caudill MT, Brayton KA. The use and limitations of the 16S rRNA sequence for species classification of anaplasma samples. Microorganisms. 2022;10:605.
Avalos-Fernandez M, Alin T, Métayer C, Thiébaut R, Enaud R, Delhaes L. The respiratory microbiota alpha-diversity in chronic lung diseases: first systematic review and meta-analysis. Respir Res. 2022;23:214.
Lemon KP, Klepac-Ceraj V, Schiffer HK, Brodie EL, Lynch SV, Kolter R. Comparative analyses of the bacterial microbiota of the human nostril and oropharynx. MBio. 2010;1:e00129.
Gupta VK, Paul S, Dutta C. Geography, ethnicity or subsistence-specific variations in human microbiome composition and diversity. Front Microbiol. 2017;8:1162.
Abreu NA, Nagalingam NA, Song Y, Roediger FC, Pletcher SD, Goldberg AN, et al. Sinus microbiome diversity depletion and Corynebacterium tuberculostearicum enrichment mediates rhinosinusitis. Sci Transl Med. 2012;4:151ra124.
Li J, Jing Q, Li J, Hua M, Di L, Song C, et al. Assessment of microbiota in the gut and upper respiratory tract associated with SARS-CoV-2 infection. Microbiome. 2023;11:38.
Yildiz S, Mazel-Sanchez B, Kandasamy M, Manicassamy B, Schmolke M. Influenza A virus infection impacts systemic microbiota dynamics and causes quantitative enteric dysbiosis. Microbiome. 2018;6:9.
Duvallet C, Gibbons SM, Gurry T, Irizarry RA, Alm EJ. Meta-analysis of gut microbiome studies identifies disease-specific and shared responses. Nat Commun. 2017;8:1784.
Losol P, Park H-S, Song W-J, Hwang Y-K, Kim S-H, Holloway JW, et al. Association of upper airway bacterial microbiota and asthma: systematic review. Asia Pac Allergy. 2022;12:e32.
Hakansson AP, Orihuela CJ, Bogaert D. Bacterial-host interactions: physiology and pathophysiology of respiratory infection. Physiol Rev. 2018;98:781–811.
Watson RL, de Koff EM, Bogaert D. Characterising the respiratory microbiome. Eur Respir J. 2019;53(2):1801711. https://doi.org/10.1183/13993003.01711-2018 .
Asif M, Alvi IA, Rehman SU. Insight into Acinetobacter baumannii: pathogenesis, global resistance, mechanisms of resistance, treatment options, and alternative modalities. Infect Drug Resist. 2018;11:1249–60.
Weiss S, Xu ZZ, Peddada S, Amir A, Bittinger K, Gonzalez A, et al. Normalization and microbial differential abundance strategies depend upon data characteristics. Microbiome. 2017;5:27.
Bolyen E, Rideout JR, Dillon MR, Bokulich NA, Abnet CC, Al-Ghalith GA, et al. Reproducible, interactive, scalable and extensible microbiome data science using QIIME 2. Nat Biotechnol. 2019;37:852–7.
Callahan BJ, McMurdie PJ, Rosen MJ, Han AW, Johnson AJA, Holmes SP. DADA2: High-resolution sample inference from Illumina amplicon data. Nat Methods. 2016;13:581–3.
Quast C, Pruesse E, Yilmaz P, Gerken J, Schweer T, Yarza P, et al. The SILVA ribosomal RNA gene database project: improved data processing and web-based tools. Nucleic Acids Res. 2013;41(Database issue):D590-6.
CAS PubMed Google Scholar
Seabold S, Perktold J. Statsmodels: econometric and statistical modeling with python. In: Proceedings of the 9th Python in Science Conference. SciPy; 2010;57–61. https://doi.org/10.25080/Majora-92bf1922-011 .
Benjamini Y, Hochberg Y. Controlling the false discovery rate: a practical and powerful approach to multiple testing. J R Stat Soc. 1995;57:289–300.
Pedregosa F, Varoquaux G, Gramfort A, Michel V, Thirion B, Grisel O, Blondel M, et al. Scikit-Learn: Machine Learning in Python. J Mach Learn Res. 2011;12(85):2825–30.
Download references
Acknowledgements
Thanks to members of the Gibbons Lab for helpful comments on this work.
This work was funded by a research grant from Reckitt Health US LLC, by a Washington Research Foundation Distinguished Investigator Award, and by startup funds from the Institute for Systems Biology.
Author information
Authors and affiliations.
Institute for Systems Biology, Seattle, WA, 98109, USA
Nick Quinn-Bohmann, Christian Diener & Sean M. Gibbons
Molecular Engineering Graduate Program, University of Washington, Seattle, WA, 98195, USA
Nick Quinn-Bohmann & Sean M. Gibbons
Reckitt Health US LLC, 1 Philips Pkwy, Montvale, NJ, 07645, USA
Jose A. Freixas-Coutin & Jin Seo
Reckitt Benckiser Healthcare Ltd, 105 Bath Road, Slough, Berkshire, SL1 3UH, UK
Ruth Simmons
Department of Bioengineering, University of Washington, Seattle, WA, 98195, USA
Sean M. Gibbons
Department of Genome Sciences, University of Washington, Seattle, WA, 98195, USA
eScience Institute, University of Washington, Seattle, WA, 98195, USA
You can also search for this author in PubMed Google Scholar
Contributions
N.Q.B., S.M.G., and C.D. conceptualized the study. N.Q.B. ran the analyses, interpreted the results, and authored the first draft of the manuscript. S.M.G., C.D., and J.F.C. provided resources for the work and supervised the work. J.F.C. conducted the study selection for inclusion in the analysis. J.S. and R.S. provided support with the interpretation of results. All authors read and approved the final manuscript.
Corresponding authors
Correspondence to Nick Quinn-Bohmann or Sean M. Gibbons .
Ethics declarations
Ethics approval and consent to participate.
Not applicable.
Consent for publication
Competing interests.
This work was funded, in part, by Reckitt Health US LLC and co-authored by Reckitt employees: JFC, JS, and RS. The authors report no other competing interests.
Additional information
Publisher’s note.
Springer Nature remains neutral with regard to jurisdictional claims in published maps and institutional affiliations.
Supplementary Information
Additional file 1: table s1..
Studies Inclusion. Table S2. Taxonomic Classification Percentage. Table S3. Enrichment associated with geographic region in healthy controls. Table S4. Enrichment associated with age in healthy controls. Table S5. Results from case v. control logistic regression.
Additional file 2: Fig. S1.
Prisma Flowchart for Study Inclusion, An original search returned 153,586 studies. Filtering out conference abstracts, conference papers, short surveys and book chapters left 116,503 peer-reviewed publications. Additional screening removed 115,883 publications by screening for keywords “16S rRNA” and “human” and “upper respiratory” or “nasopharynx” or “oropharynx” or “larynx”, leaving 620 publications. Another phase of screening removed 552 publications for irrelevance (e.g., intervention studies or studies that lacked healthy controls), lack of sequencing data, unavailable, incomplete data/metadata, and duplicate studies reporting on the same cohort, leaving 68 publications. Of these, 42 were excluded due to overrepresentation of disease conditions in the final cohort, or problems with accessing the raw data and metadata. In the end, 26 publications remained, with 1-3 studies per disease. Fig. S2. Mean classification percentage for each study at each taxonomic level. Classification remained at or above 60% for all studies through the genus level. At the species level, a significant drop in classification percentage was observed.
Rights and permissions
Open Access This article is licensed under a Creative Commons Attribution 4.0 International License, which permits use, sharing, adaptation, distribution and reproduction in any medium or format, as long as you give appropriate credit to the original author(s) and the source, provide a link to the Creative Commons licence, and indicate if changes were made. The images or other third party material in this article are included in the article's Creative Commons licence, unless indicated otherwise in a credit line to the material. If material is not included in the article's Creative Commons licence and your intended use is not permitted by statutory regulation or exceeds the permitted use, you will need to obtain permission directly from the copyright holder. To view a copy of this licence, visit http://creativecommons.org/licenses/by/4.0/ . The Creative Commons Public Domain Dedication waiver ( http://creativecommons.org/publicdomain/zero/1.0/ ) applies to the data made available in this article, unless otherwise stated in a credit line to the data.
Reprints and permissions
About this article
Cite this article.
Quinn-Bohmann, N., Freixas-Coutin, J.A., Seo, J. et al. Meta-analysis of the human upper respiratory tract microbiome reveals robust taxonomic associations with health and disease. BMC Biol 22 , 93 (2024). https://doi.org/10.1186/s12915-024-01887-0
Download citation
Received : 02 September 2023
Accepted : 15 April 2024
Published : 23 April 2024
DOI : https://doi.org/10.1186/s12915-024-01887-0
Share this article
Anyone you share the following link with will be able to read this content:
Sorry, a shareable link is not currently available for this article.
Provided by the Springer Nature SharedIt content-sharing initiative
- Respiratory disease
- Case–control
- Meta-analysis
- Microbiology
BMC Biology
ISSN: 1741-7007
- Submission enquiries: [email protected]
- General enquiries: [email protected]
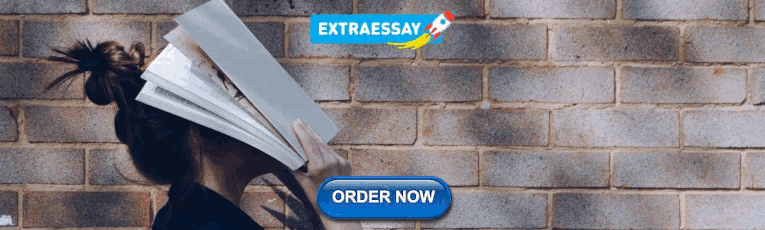
COMMENTS
Microbial Resource Reports, with Marco Ventura as Editor-in-Chief, focuses on research in the fields of microbiology such as bifidobacteria, intestinal microbiota, microbiome, probiotics, phages, and antibiotics. Published research on microbial ecology, intestinal microbiome, host-microbe interactions, intestinal fungi, metagenomics, microbial DNA sequencing, etc.
Microbiome Research Reports. Published by OAE Publishing Inc. Online ISSN: 2771-5965. Disciplines: Microbiology. Journal website Author guidelines. Top read articles. 54 reads in the past 30 days ...
At Microbiome, we are passionate about the dissemination of research.. We are pleased to announce that as of the 12th August 2019, all authors whose papers are accepted will receive a complimentary Video Byte. Created by our partners, Research Square, the fastest growing preprint platform in the world, a Video Byte is a 1-2 minute video summary of a paper that will be incorporated into the ...
MetaBIDx: a new computational approach to bacteria identification in microbiomes. Open Access Original Article 31 Mar 2024. DOI: 10.20517/mrr.2024.01. Diem-Trang Pham, Vinhthuy Phan. Views: 194 Downloads: 22. Download PDF 0. 1.
2022 Citation Impact 15.5 - 2-year Impact Factor 19.4 - 5-year Impact Factor 2.998 - SNIP (Source Normalized Impact per Paper) 3.709 - SJR (SCImago Journal Rank) 2023 Speed 41 days submission to first editorial decision for all manuscripts (Median) 196 days submission to accept (Median) 2023 Usage 3,219,809 downloads 7,051 Altmetric mentions
The central purpose of Microbiome is to unite investigators conducting microbiome research in environmental, agricultural, and biomedical arenas. Topics broadly addressing the study of microbial communities, such as, microbial surveys, bioinformatics, meta-omics approaches and community/host interaction modeling will be considered for ...
Fernandez-Julia et al. Microbiome Res Rep 2022;1:12 DOI: 10.20517/mrr.2021.05 Microbiome Research Reports ... it also highlights the need for further research into the phenomenon ... Changes in the composition of the gut microbiota can be influenced by various factors, such as type of diet, stress or environment, and may result in a so-called ...
2022 Citation Impact 15.5 - 2-year Impact Factor 19.4 - 5-year Impact Factor 2.998 - SNIP (Source Normalized Impact per Paper) 3.709 - SJR (SCImago Journal Rank) 2023 Speed 41 days submission to first editorial decision for all manuscripts (Median) 196 days submission to accept (Median) 2023 Usage 3,219,809 downloads 7,051 Altmetric mentions
The trends and growth of microbiome research over the past years. Data were extracted from Web of Science Core Collection database on March 29th, 2023. ... the number of citations that a journal has made to the publications × the journal impact factor (IF) in 2021. (A) is drawn by VOSviewer.
Microbiome research is a rapidly developing area of science and innovation, seeking to explore and exploit the complex communities of microbes associated with humans, animals, plants and other environments such as soils and oceans. Climate change. ... 5-year Impact Factor: The 5-year journal Impact Factor is the average number of times articles ...
Microbiome 2023-2024 Journal's Impact IF is 16.837. Check Out IF Ranking, Prediction, Trend & Key Factor Analysis. ... The central purpose of Microbiome is to unite investigators conducting microbiome research in environmental, agricultural, and biomedical arenas. ... Microbiome publishes reports - . Microbiome Key Factor Analysis. Coverage ...
Microbiome research has highlighted the importance of human-microbiota ecosystems in the promotion of health and various disease-causing processes. ... and the rise of resistance genes. 266,267 To negate the negative impact of antibiotics on the microbiome, targeted therapies against pathogens are required. Some small-molecule antibiotics are ...
Microbiome IF is decreased by a factor of 0.55 and approximate percentage change is -3.84% when compared to preceding year 2021, which shows a falling trend. The impact IF , also denoted as Journal impact score (JIS), of an academic journal is a measure of the yearly average number of citations to recent articles published in that journal.
impact of dietary components on the microbiota, and inter-individual variation in responses; host-microbiome interaction in immune response; Interdisciplinary studies of fundamental problems are particularly welcome. The journal publishes a variety of formats including Original Research articles, Short Reports, Reviews and Commentaries, etc.
Frontiers' impact; Progress Report 2022; All progress reports; Publishing model; How we publish; Open access; Fee policy; ... Start your submission and get more impact for your research by publishing with us. Author guidelines. ... Metabolism and the Microbiome susana fuentes. National Institute for Public Health and the Environment ...
Read the latest Research articles in Microbiome from Scientific Reports. ... Impact of the diet in the gut microbiota after an inter-species microbial transplantation in fish. ... Research articles
Strain et al. Microbiome Res Rep 2023;2:22 DOI: 10.20517/mrr.2022.14 Microbiome Research Reports ... The aim of this pilot study was to investigate the impact of dietary fibres on pathogen performance in the presence of gut microbiota. Methods: In an ex vivo gut model, pooled faecal samples were spiked with a cocktail of representative ...
2022 Citation Impact 15.5 - 2-year Impact Factor 19.4 - 5-year Impact Factor 2.998 - SNIP (Source Normalized Impact per Paper) 3.709 - SJR (SCImago Journal Rank) 2023 Speed 41 days submission to first editorial decision for all manuscripts (Median) 196 days submission to accept (Median) 2023 Usage 3,219,809 downloads 7,051 Altmetric mentions
Microbiome articles from across Nature Portfolio. ... Research Open Access 22 Apr 2024 npj Antimicrobials and Resistance. ... Research Open Access 18 Apr 2024 Scientific Reports. Volume: 14, P: 8963.
Scientific Reports - Effects of microbiome-based interventions on neurodegenerative diseases: a systematic review and meta-analysis ... and environmental factors, such as diet, chemical exposure ...
SCImago Journal Country & Rank SCImago Institutions Rankings SCImago Media Rankings SCImago Iber SCImago Research Centers Ranking SCImago Graphica Ediciones Profesionales de la ... Human Factors and Ergonomics; Human-Computer Interaction; ... Environmental Microbiome: journal: 1.713 Q1: 48: 83: 102: 6537: 725: 101: 6.30: 78.76: 23: Biology and ...
Advanced Gut & Microbiome Research - Journal Reports. Advanced Gut & Microbiome Research is an open access, peer-reviewed journal that publishes original research and review articles related to all aspects of fundamental and applied research on gastroenterology, microbiology and their interactions. ... Impact Factor-See full report. APC FREE ...
09 Jan 2024. 23 Dec 2023. Advanced Gut & Microbiome Research is an open access, peer-reviewed journal that publishes original research and review articles related to all aspects of fundamental and applied research on gastroenterology, microbiology and their interactions.
The pathogenesis of chronic wounds (CW) involves a multifaceted interplay of biochemical, immunological, hematological, and microbiological interactions. Biofilm development is a significant virulence trait which enhances microbial survival and pathogenicity and has various implications on the development and management of CW. Biofilms induce a prolonged suboptimal inflammation in the wound ...
The human upper respiratory tract (URT) microbiome, like the gut microbiome, varies across individuals and between health and disease states. However, study-to-study heterogeneity in reported case-control results has made the identification of consistent and generalizable URT-disease associations difficult. In order to address this issue, we assembled 26 independent 16S rRNA gene ...