- Bipolar Disorder
- Therapy Center
- When To See a Therapist
- Types of Therapy
- Best Online Therapy
- Best Couples Therapy
- Best Family Therapy
- Managing Stress
- Sleep and Dreaming
- Understanding Emotions
- Self-Improvement
- Healthy Relationships
- Student Resources
- Personality Types
- Verywell Mind Insights
- 2023 Verywell Mind 25
- Mental Health in the Classroom
- Editorial Process
- Meet Our Review Board
- Crisis Support
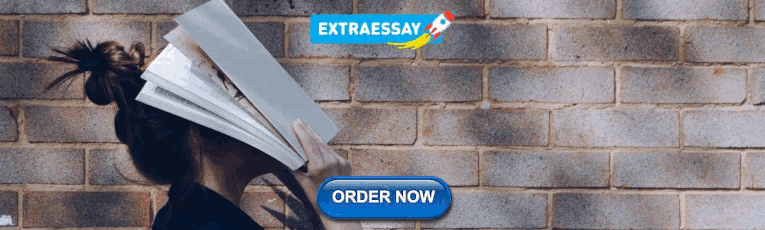
The Sapir-Whorf Hypothesis: How Language Influences How We Express Ourselves
Rachael is a New York-based writer and freelance writer for Verywell Mind, where she leverages her decades of personal experience with and research on mental illness—particularly ADHD and depression—to help readers better understand how their mind works and how to manage their mental health.
:max_bytes(150000):strip_icc():format(webp)/RachaelGreenProfilePicture1-a3b8368ef3bb47ccbac92c5cc088e24d.jpg)
Thomas Barwick / Getty Images
What to Know About the Sapir-Whorf Hypothesis
Real-world examples of linguistic relativity, linguistic relativity in psychology.
The Sapir-Whorf Hypothesis, also known as linguistic relativity, refers to the idea that the language a person speaks can influence their worldview, thought, and even how they experience and understand the world.
While more extreme versions of the hypothesis have largely been discredited, a growing body of research has demonstrated that language can meaningfully shape how we understand the world around us and even ourselves.
Keep reading to learn more about linguistic relativity, including some real-world examples of how it shapes thoughts, emotions, and behavior.
The hypothesis is named after anthropologist and linguist Edward Sapir and his student, Benjamin Lee Whorf. While the hypothesis is named after them both, the two never actually formally co-authored a coherent hypothesis together.
This Hypothesis Aims to Figure Out How Language and Culture Are Connected
Sapir was interested in charting the difference in language and cultural worldviews, including how language and culture influence each other. Whorf took this work on how language and culture shape each other a step further to explore how different languages might shape thought and behavior.
Since then, the concept has evolved into multiple variations, some more credible than others.
Linguistic Determinism Is an Extreme Version of the Hypothesis
Linguistic determinism, for example, is a more extreme version suggesting that a person’s perception and thought are limited to the language they speak. An early example of linguistic determinism comes from Whorf himself who argued that the Hopi people in Arizona don’t conjugate verbs into past, present, and future tenses as English speakers do and that their words for units of time (like “day” or “hour”) were verbs rather than nouns.
From this, he concluded that the Hopi don’t view time as a physical object that can be counted out in minutes and hours the way English speakers do. Instead, Whorf argued, the Hopi view time as a formless process.
This was then taken by others to mean that the Hopi don’t have any concept of time—an extreme view that has since been repeatedly disproven.
There is some evidence for a more nuanced version of linguistic relativity, which suggests that the structure and vocabulary of the language you speak can influence how you understand the world around you. To understand this better, it helps to look at real-world examples of the effects language can have on thought and behavior.
Different Languages Express Colors Differently
Color is one of the most common examples of linguistic relativity. Most known languages have somewhere between two and twelve color terms, and the way colors are categorized varies widely. In English, for example, there are distinct categories for blue and green .
Blue and Green
But in Korean, there is one word that encompasses both. This doesn’t mean Korean speakers can’t see blue, it just means blue is understood as a variant of green rather than a distinct color category all its own.
In Russian, meanwhile, the colors that English speakers would lump under the umbrella term of “blue” are further subdivided into two distinct color categories, “siniy” and “goluboy.” They roughly correspond to light blue and dark blue in English. But to Russian speakers, they are as distinct as orange and brown .
In one study comparing English and Russian speakers, participants were shown a color square and then asked to choose which of the two color squares below it was the closest in shade to the first square.
The test specifically focused on varying shades of blue ranging from “siniy” to “goluboy.” Russian speakers were not only faster at selecting the matching color square but were more accurate in their selections.
The Way Location Is Expressed Varies Across Languages
This same variation occurs in other areas of language. For example, in Guugu Ymithirr, a language spoken by Aboriginal Australians, spatial orientation is always described in absolute terms of cardinal directions. While an English speaker would say the laptop is “in front of” you, a Guugu Ymithirr speaker would say it was north, south, west, or east of you.
As a result, Aboriginal Australians have to be constantly attuned to cardinal directions because their language requires it (just as Russian speakers develop a more instinctive ability to discern between shades of what English speakers call blue because their language requires it).
So when you ask a Guugu Ymithirr speaker to tell you which way south is, they can point in the right direction without a moment’s hesitation. Meanwhile, most English speakers would struggle to accurately identify South without the help of a compass or taking a moment to recall grade school lessons about how to find it.
The concept of these cardinal directions exists in English, but English speakers aren’t required to think about or use them on a daily basis so it’s not as intuitive or ingrained in how they orient themselves in space.
Just as with other aspects of thought and perception, the vocabulary and grammatical structure we have for thinking about or talking about what we feel doesn’t create our feelings, but it does shape how we understand them and, to an extent, how we experience them.
Words Help Us Put a Name to Our Emotions
For example, the ability to detect displeasure from a person’s face is universal. But in a language that has the words “angry” and “sad,” you can further distinguish what kind of displeasure you observe in their facial expression. This doesn’t mean humans never experienced anger or sadness before words for them emerged. But they may have struggled to understand or explain the subtle differences between different dimensions of displeasure.
In one study of English speakers, toddlers were shown a picture of a person with an angry facial expression. Then, they were given a set of pictures of people displaying different expressions including happy, sad, surprised, scared, disgusted, or angry. Researchers asked them to put all the pictures that matched the first angry face picture into a box.
The two-year-olds in the experiment tended to place all faces except happy faces into the box. But four-year-olds were more selective, often leaving out sad or fearful faces as well as happy faces. This suggests that as our vocabulary for talking about emotions expands, so does our ability to understand and distinguish those emotions.
But some research suggests the influence is not limited to just developing a wider vocabulary for categorizing emotions. Language may “also help constitute emotion by cohering sensations into specific perceptions of ‘anger,’ ‘disgust,’ ‘fear,’ etc.,” said Dr. Harold Hong, a board-certified psychiatrist at New Waters Recovery in North Carolina.
As our vocabulary for talking about emotions expands, so does our ability to understand and distinguish those emotions.
Words for emotions, like words for colors, are an attempt to categorize a spectrum of sensations into a handful of distinct categories. And, like color, there’s no objective or hard rule on where the boundaries between emotions should be which can lead to variation across languages in how emotions are categorized.
Emotions Are Categorized Differently in Different Languages
Just as different languages categorize color a little differently, researchers have also found differences in how emotions are categorized. In German, for example, there’s an emotion called “gemütlichkeit.”
While it’s usually translated as “cozy” or “ friendly ” in English, there really isn’t a direct translation. It refers to a particular kind of peace and sense of belonging that a person feels when surrounded by the people they love or feel connected to in a place they feel comfortable and free to be who they are.
Harold Hong, MD, Psychiatrist
The lack of a word for an emotion in a language does not mean that its speakers don't experience that emotion.
You may have felt gemütlichkeit when staying up with your friends to joke and play games at a sleepover. You may feel it when you visit home for the holidays and spend your time eating, laughing, and reminiscing with your family in the house you grew up in.
In Japanese, the word “amae” is just as difficult to translate into English. Usually, it’s translated as "spoiled child" or "presumed indulgence," as in making a request and assuming it will be indulged. But both of those have strong negative connotations in English and amae is a positive emotion .
Instead of being spoiled or coddled, it’s referring to that particular kind of trust and assurance that comes with being nurtured by someone and knowing that you can ask for what you want without worrying whether the other person might feel resentful or burdened by your request.
You might have felt amae when your car broke down and you immediately called your mom to pick you up, without having to worry for even a second whether or not she would drop everything to help you.
Regardless of which languages you speak, though, you’re capable of feeling both of these emotions. “The lack of a word for an emotion in a language does not mean that its speakers don't experience that emotion,” Dr. Hong explained.
What This Means For You
“While having the words to describe emotions can help us better understand and regulate them, it is possible to experience and express those emotions without specific labels for them.” Without the words for these feelings, you can still feel them but you just might not be able to identify them as readily or clearly as someone who does have those words.
Rhee S. Lexicalization patterns in color naming in Korean . In: Raffaelli I, Katunar D, Kerovec B, eds. Studies in Functional and Structural Linguistics. Vol 78. John Benjamins Publishing Company; 2019:109-128. Doi:10.1075/sfsl.78.06rhe
Winawer J, Witthoft N, Frank MC, Wu L, Wade AR, Boroditsky L. Russian blues reveal effects of language on color discrimination . Proc Natl Acad Sci USA. 2007;104(19):7780-7785. 10.1073/pnas.0701644104
Lindquist KA, MacCormack JK, Shablack H. The role of language in emotion: predictions from psychological constructionism . Front Psychol. 2015;6. Doi:10.3389/fpsyg.2015.00444
By Rachael Green Rachael is a New York-based writer and freelance writer for Verywell Mind, where she leverages her decades of personal experience with and research on mental illness—particularly ADHD and depression—to help readers better understand how their mind works and how to manage their mental health.

An official website of the United States government
The .gov means it’s official. Federal government websites often end in .gov or .mil. Before sharing sensitive information, make sure you’re on a federal government site.
The site is secure. The https:// ensures that you are connecting to the official website and that any information you provide is encrypted and transmitted securely.
- Publications
- Account settings
Preview improvements coming to the PMC website in October 2024. Learn More or Try it out now .
- Advanced Search
- Journal List

The Sapir-Whorf Hypothesis and Probabilistic Inference: Evidence from the Domain of Color
Emily cibelli.
1 Department of Linguistics, Northwestern University, Evanston, IL 60208, United States of America
2 Department of Linguistics, University of California, Berkeley, CA 94720, United States of America
3 Cognitive Science Program, University of California, Berkeley, CA 94720, United States of America
Joseph L. Austerweil
4 Department of Psychology, University of Wisconsin, Madison, WI 53706, United States of America
Thomas L. Griffiths
5 Department of Psychology, University of California, Berkeley, CA 94720, United States of America
Terry Regier
Conceived and designed the experiments: EC YX JLA TLG TR. Performed the experiments: EC YX JLA. Analyzed the data: EC YX JLA. Wrote the paper: TR EC YX.
Associated Data
All relevant data are available within the paper and/or at: https://github.com/yangxuch/probwhorfcolor This GitHub repository is mentioned in the paper.
The Sapir-Whorf hypothesis holds that our thoughts are shaped by our native language, and that speakers of different languages therefore think differently. This hypothesis is controversial in part because it appears to deny the possibility of a universal groundwork for human cognition, and in part because some findings taken to support it have not reliably replicated. We argue that considering this hypothesis through the lens of probabilistic inference has the potential to resolve both issues, at least with respect to certain prominent findings in the domain of color cognition. We explore a probabilistic model that is grounded in a presumed universal perceptual color space and in language-specific categories over that space. The model predicts that categories will most clearly affect color memory when perceptual information is uncertain. In line with earlier studies, we show that this model accounts for language-consistent biases in color reconstruction from memory in English speakers, modulated by uncertainty. We also show, to our knowledge for the first time, that such a model accounts for influential existing data on cross-language differences in color discrimination from memory, both within and across categories. We suggest that these ideas may help to clarify the debate over the Sapir-Whorf hypothesis.
Introduction
The Sapir-Whorf hypothesis [ 1 , 2 ] holds that our thoughts are shaped by our native language, and that speakers of different languages therefore think about the world in different ways. This proposal has been controversial for at least two reasons, both of which are well-exemplified in the semantic domain of color. The first source of controversy is that the hypothesis appears to undercut any possibility of a universal foundation for human cognition. This idea sits uneasily with the finding that variation in color naming across languages is constrained, such that certain patterns of color naming recur frequently across languages [ 3 – 5 ], suggesting some sort of underlying universal basis. The second source of controversy is that while some findings support the hypothesis, they do not always replicate reliably. Many studies have found that speakers of a given language remember and process color in a manner that reflects the color categories of their language [ 6 – 13 ]. Reinforcing the idea that language is implicated in these findings, it has been shown that the apparent effect of language on color cognition disappears when participants are given a verbal [ 7 ] (but not a visual) interference task [ 8 , 11 , 12 ]; this suggests that language may operate through on-line use of verbal representations that can be temporarily disabled. However, some of these findings have a mixed record of replication [ 14 – 17 ]. Thus, despite the substantial empirical evidence already available, the role of language in color cognition remains disputed.
An existing theoretical stance holds the potential to resolve both sources of controversy. On the one hand, it explains effects of language on cognition in a framework that retains a universal component, building on a proposal by Kay and Kempton [ 7 ]. On the other hand, it has the potential to explain when effects of language on color cognition will appear, and when they will not—and why. This existing stance is that of the “category adjustment” model of Huttenlocher and colleagues [ 18 , 19 ]. We adopt this stance, and cast color memory as inference under uncertainty, instantiated in a category adjustment model, following Bae et al. [ 20 ] and Persaud and Hemmer [ 21 ]. The model holds that color memory involves the probabilistic combination of evidence from two sources: a fine-grained representation of the particular color seen, and the language-specific category in which it fell (e.g. English green ). Both sources of evidence are represented in a universal perceptual color space, yet their combination yields language-specific bias patterns in memory, as illustrated in Fig 1 . The model predicts that such category effects will be strongest when fine-grained perceptual information is uncertain. It thus has the potential to explain the mixed pattern of replications of Whorfian effects in the literature: non-replications could be the result of high perceptual certainty.

A stimulus is encoded in two ways: (1) a fine-grained representation of the stimulus itself, shown as a (gray) distribution over stimulus space centered at the stimulus’ location in that space, and (2) the language-specific category (e.g. English “green”) in which the stimulus falls, shown as a separate (green) distribution over the same space, centered at the category prototype. The stimulus is reconstructed by combining these two sources of information through probabilistic inference, resulting in a reconstruction of the stimulus (black distribution) that is biased toward the category prototype. Adapted from Fig 11 of Bae et al. (2015) [ 20 ].
In the category adjustment model, both the fine-grained representation of the stimulus and the category in which it falls are modeled as probability distributions over a universal perceptual color space. The fine-grained representation is veridical (unbiased) but inexact: its distribution is centered at the location in color space where the stimulus itself fell, and the variance of that distribution captures the observer’s uncertainty about the precise location of the stimulus in color space, with greater variance corresponding to greater uncertainty. Psychologically, such uncertainty might be caused by noise in perception itself, by memory decay over time, or by some other cause—and any increase in such uncertainty is modeled by a wider, flatter distribution for the fine-grained representation. The category distribution, in contrast, captures the information about stimulus location that is given by the named category in which the stimulus fell (e.g. green for an English-speaking observer). Because named color categories vary across languages, this category distribution is assumed to be language-specific—although the space over which it exists is universal. The model infers the original stimulus location by combining evidence from both of these distributions. As a result, the model tends to produce reconstructions of the stimulus that are biased away from the actual location of the stimulus and toward the prototype of the category in which it falls.
As illustrated in Fig 2 , this pattern of bias pulls stimuli on opposite sides of a category boundary in opposite directions, producing enhanced distinctiveness for such stimuli. Such enhanced distinctiveness across a category boundary is the signature of categorical perception, or analogous category effects in memory. On this view, language-specific effects on memory can emerge from a largely universal substrate when one critical component of that substrate is language-specific: the category distribution.

Model reconstructions tend to be biased toward category prototypes, yielding enhanced distinctiveness for two stimuli that fall on different sides of a category boundary. Categories are shown as distributions in green and blue; stimuli are shown as vertical black lines; reconstruction bias patterns are shown as arrows.
If supported, the category adjustment model holds the potential to clarify the debate over the Sapir-Whorf hypothesis in three ways. First, it would link that debate to independent principles of probabilistic inference. In so doing, it would underscore the potentially important role of uncertainty , whether originating in memory or perception, in framing the debate theoretically. Second, and relatedly, it would suggest a possible reason why effects of language on color memory and perception are sometimes found, and sometimes not [ 17 ]. Concretely, the model predicts that greater uncertainty in the fine-grained representation—induced for example through a memory delay, or noise in perception—will lead to greater influence of the category, and thus a stronger bias in reproduction. The mirror-image of this prediction is that in situations of relatively high certainty in memory or perception, there will be little influence of the category, to the point that such an influence may not be empirically detectable. Third, the model suggests a way to think about the Sapir-Whorf hypothesis without jettisoning the important idea of a universal foundation for cognition.
Closely related ideas appear in the literature on probabilistic cue integration [ 22 – 25 ]. For example, Ernst and Banks [ 24 ] investigated perceptual integration of cues from vision and touch in judging the height of an object. They found that humans integrate visual and haptic cues in a statistically optimal fashion, modulated by cue certainty. The category adjustment model we explore here can be seen as a form of probabilistic cue integration in which one of the cues is a language-specific category.
The category adjustment model has been used to account for category effects in various domains, including spatial location [ 18 , 26 ], object size [ 19 , 27 ], and vowel perception [ 28 ]. The category adjustment model also bears similarities to other theoretical accounts of the Sapir-Whorf hypothesis that emphasize the importance of verbal codes [ 7 , 8 ], and the interplay of such codes with perceptual representations [ 29 – 31 ]. Prior research has linked such category effects to probabilistic inference, following the work of Huttenlocher and colleagues [ 18 , 19 ]. Roberson and colleagues [ 32 ] invoked the category adjustment model as a possible explanation for categorical perception of facial expressions, but did not explore a formal computational model; Goldstone [ 33 ] similarly referenced the category adjustment model with respect to category effects in the color domain. Persaud and Hemmer [ 21 , 34 ] explored bias in memory for color, and compared empirically obtained memory bias patterns from English speakers with results predicted by a formally specified category adjustment model, but did not link those results to the debate over the Sapir-Whorf hypothesis, and did not manipulate uncertainty. More recently, a subsequent paper by the same authors and colleagues [ 35 ] explored category-induced bias in speakers of another language, Tsimané, and did situate those results with respect to the Sapir-Whorf hypothesis, but again did not manipulate uncertainty. Most recently, Bae et al. [ 20 ] extensively documented bias in color memory in English speakers, modeled those results with a category-adjustment computational model, and did manipulate uncertainty—but did not explore these ideas relative to the Sapir-Whorf hypothesis, or to data from different languages.
In what follows, we first present data and computational simulations that support the recent finding that color memory in English speakers is well-predicted by a category adjustment model, with the strength of category effects modulated by uncertainty. We then show, to our knowledge for the first time, that a category adjustment model accounts for influential existing cross-language data on color that support the Sapir-Whorf hypothesis.
In this section we provide general descriptions of our analyses and results. Full details are supplied in the section on Materials and Methods.
Study 1: Color reconstruction in English speakers
Our first study tests the core assumptions of the category adjustment model in English speakers. In doing so, it probes questions that were pursued by two studies that appeared recently, after this work had begun. Persaud and Hemmer [ 21 ] and Bae et al. [ 20 ] both showed that English speakers’ memory for a color tends to be biased toward the category prototype of the corresponding English color term, in line with a category adjustment model. Bae et al. [ 20 ] also showed that the amount of such bias increases when subjects must retain the stimulus in memory during a delay period, compared to when there is no such delay, as predicted by the principles of the category adjustment model. In our first study, we consider new evidence from English speakers that tests these questions, prior to considering speakers of different languages in our following studies.
English-speaking participants viewed a set of hues that varied in small steps from dark yellow to purple, with most hues corresponding to some variety of either green or blue. We collected two kinds of data from these participants: bias data and naming data. Bias data were based on participants’ non-linguistic reconstruction of particular colors seen. Specifically, for each hue seen, participants recreated that hue by selecting a color from a color wheel, either while the target was still visible ( Fig 3A : simultaneous condition), or from memory after a short delay ( Fig 3B : delayed condition). We refer to the resulting data as bias data, because we are interested in the extent to which participants’ reconstructions of the stimulus color are biased away from the original target stimulus. Afterwards, the same participants indicated how good an example of English green (as in Fig 3C ) and how good an example of English blue each hue was. We refer to these linguistic data as naming data.

Screenshots of example trials illustrating (A) simultaneous reconstruction, (B) delayed reconstruction, and (C) green goodness rating.
Fig 4 shows both naming and bias data as a function of target hue. The top panel of the figure shows the naming data and also shows Gaussian functions corresponding to the English color terms green and blue that we fitted to the naming data. Bias data were collected for only a subset of the hues for which naming data were collected, and the shaded region in the top panel of Fig 4 shows that subset, relative to the full range of hues for naming data. We collected bias data only in this smaller range because we were interested specifically in bias induced by the two color terms blue and green , and colors outside the shaded region seemed to us to clearly show some influence of neighboring categories such as yellow and purple . The bottom panel of the figure shows the bias data, plotted relative to the prototypes (means) of the fitted Gaussian functions for green and blue . It can be seen that reconstruction bias appears to be stronger in the delayed than in the simultaneous condition, as predicted, and that—especially in the delayed condition—there is an inflection in the bias pattern between the two category prototypes, suggesting that bias may reflect the influence of each of the two categories. The smaller shaded region in this bottom panel denotes the subset of these hues that we subsequently analyzed statistically, and to which we fit models. We reduced the range of considered hues slightly further at this stage, to ensure that the range was well-centered with respect to the two relevant category prototypes, for green and blue , as determined by the naming data.

In both top and bottom panels, the horizontal axis denotes target hue, ranging from yellow on the left to purple on the right. Top panel (naming data): The solid green and blue curves show, for each target hue, the average goodness rating for English green and blue respectively, as a proportion of the maximum rating possible. The dashed green and blue curves show Gaussian functions fitted to the naming goodness data. The dotted vertical lines marked at the bottom with green and blue squares denote the prototypes for green and blue , determined as the means of the green and blue fitted Gaussian functions, respectively. The shaded region in the top panel shows the portion of the spectrum for which bias data were collected. Bottom panel (bias data): Solid curves denote, for each target hue, the average reconstruction bias for that hue, such that positive values denote reconstruction bias toward the purple (here, right) end of the spectrum, and negative values denote reconstruction bias toward the yellow (here, left) end of the spectrum. Units for the vertical axis are the same as for the horizontal axis, which is normalized to length 1.0. The black and red curves show bias under simultaneous and delayed response, respectively. Blue stars at the top of the bottom panel mark hues for which there was a significant difference in the magnitude of bias between simultaneous and delayed conditions. The shaded region in the bottom panel shows the portion of the data that was analyzed statistically, and to which models were fit. In both panels, error bars represent standard error of the mean.
The absolute values (magnitudes) of the bias were analyzed using a 2 (condition: simultaneous vs. delayed) × 15 (hues) repeated measures analysis of variance. This analysis revealed significantly greater bias magnitude in the delayed than in the simultaneous condition. It also revealed that bias magnitude differed significantly as a function of hue, as well as a significant interaction between the factors of hue and condition. The blue stars in Fig 4 denote hues for which the difference in bias magnitude between the simultaneous and delayed conditions reached significance. The finding of greater bias magnitude in the delayed than in the simultaneous condition is consistent with the proposal that uncertainty is an important mediating factor in such category effects, as argued by Bae et al. [ 20 ]. It also suggests that some documented failures to find such category effects could in principle be attributable to high certainty, a possibility that can be explored by manipulating uncertainty.
We wished to test in a more targeted fashion to what extent these data are consistent with a category adjustment model in which a color is reconstructed based in part on English named color categories. To that end, we compared the performance of four models against these data; only one of these models considered both of the relevant English color categories, green and blue . As in Fig 1 , each model contains a fine-grained but inexact representation of the perceived stimulus, and (for most models) a representation of one or more English color categories. Each model predicts the reconstruction of the target stimulus from its fine-grained representation of the target together with any category information. Category information in the model is specified by the naming data. Each model has a single free parameter, corresponding to the uncertainty of the fine-grained representation; this parameter is fit to bias data.
- The null model is a baseline model that predicts hue reconstruction based only on the fine-grained representation of the stimulus, with no category component.
- The 1-category (green) model predicts hue reconstruction based on the fine-grained representation of the stimulus, combined with a representation of only the green category, derived from the green naming data.
- The 1-category (blue) model predicts hue reconstruction based on the fine-grained representation of the stimulus, combined with a representation of only the blue category, derived from the blue naming data.
- The 2-category model predicts hue reconstruction based on the fine-grained representation of the stimulus, combined with representations of both the green and blue categories.
If reproduction bias reflects probabilistic inference from a fine-grained representation of the stimulus itself, together with any relevant category, we would expect the 2-category model to outperform the others. The other models have access either to no category information at all (null model), or to category information for only one of the two relevant color categories (only one of green and blue ). The 2-category model in contrast combines fine-grained stimulus information with both of the relevant categories ( green and blue ); this model thus corresponds most closely to a full category adjustment model.
Fig 5 redisplays the data from simultaneous and delayed reconstruction, this time with model fits overlaid. The panels in the left column show data from simultaneous reconstruction, fit by each of the four models, and the panels in the right column analogously show data and model fits from delayed reconstruction. Visually, it appears that in the case of delayed reconstruction, the 2-category model fits the data at least qualitatively better than competing models: it shows an inflection in bias as the empirical data do, although not as strongly. For simultaneous reconstruction, the 2-category model fit is also reasonable but visually not as clearly superior to the others (especially the null model) as in the delayed condition.

Left column: Bias from simultaneous reconstruction, fit by each of the four models. The empirical data (black lines with error bars) in these four panels are the same, and only the model fits (red lines) differ. Within each panel, the horizontal axis denotes target hue, and the vertical axis denotes reconstruction bias. The green and blue prototypes are indicated as vertical lines with green and blue squares at the bottom. Right column: delayed reconstruction, displayed analogously.
Table 1 reports quantitative results of these model fits. The best fit is provided by the 2-category model, in both the simultaneous and delayed conditions, whether assessed by log likelihood (LL) or by mean squared errror (MSE). In line with earlier studies [ 20 , 21 ], these findings demonstrate that a category adjustment model that assumes stimulus reconstruction is governed by relevant English color terms provides a reasonable fit to data on color reconstruction by English speakers. The category adjustment model fits well both when the category bias is relatively slight (simultaneous condition), and when the bias is stronger (delayed condition).
LL = log likelihood (higher is better). MSE = mean squared error (lower is better). The best value in each row is shown in bold .
Study 2: Color discrimination across languages
The study above examined the categories of just one language, English, whereas the Sapir-Whorf hypothesis concerns cross-language differences in categorization, and their effect on cognition and perception. Empirical work concerning this hypothesis has not specifically emphasized bias in reconstruction, but there is a substantial amount of cross-language data of other sorts against which the category adjustment model can be assessed. One method that has been extensively used to explore the Sapir-Whorf hypothesis in the domain of color is a two-alternative forced choice (2AFC) task. In such a task, participants first are briefly shown a target color, and then shortly afterward are shown that same target color together with a different distractor color, and are asked to indicate which was the color originally seen. A general finding from such studies [ 8 – 10 ] is that participants exhibit enhanced discrimination for pairs of colors that would be named differently in their native language. For example, in such a 2AFC task, speakers of English show enhanced discrimination for colors from the different English categories green and blue , compared with colors from the same category (either both green or both blue ) [ 8 ]. In contrast, speakers of the Berinmo language, which has named color categories that differ from those of English, show enhanced discrimination across Berinmo category boundaries, and not across those of English [ 9 ]. Thus color discrimination in this task is enhanced at the boundaries of native language categories, suggesting an effect of those native language categories on the ability to discriminate colors from memory.
Considered informally, this qualitative pattern of results appears to be consistent with the category adjustment model, as suggested above in Fig 2 . We wished to determine whether such a model would also provide a good quantitative account of such results, when assessed using the specific color stimuli and native-language naming patterns considered in the empirical studies just referenced.
We considered cross-language results from two previous studies by Debi Roberson and colleagues, one that compared color memory in speakers of English and Berinmo, a language of Papua New Guinea [ 9 ], and another that explored color memory in speakers of Himba, a language of Namibia [ 10 ]. Berinmo and Himba each have five basic color terms, in contrast with eleven in English. The Berinmo and Himba color category systems are similar to each other in broad outline, but nonetheless differ noticeably. Following these two previous studies, we considered the following pairs of categories in these three languages:
- the English categories green and blue ,
- the Berinmo categories wor (covering roughly yellow, orange, and brown), and nol (covering roughly green, blue, and purple), and
- the Himba categories dumbu (covering roughly yellow and beige) and burou (covering roughly green, blue, and purple).
These three pairs of categories are illustrated in Fig 6 , using naming data from Roberson et al. (2000) [ 9 ] and Roberson et al. (2005) [ 10 ]. It can be seen that the English green - blue distinction is quite different from the Berinmo wor - nol and the Himba dumbu - burou distinctions, which are similar but not identical to each other. The shaded regions in this figure indicate specific colors that were probed in discrimination tasks. The shaded (probed) region that straddles a category boundary in Berinmo and Himba falls entirely within the English category green , and the shaded (probed) region that straddles a category boundary in English falls entirely within the Berinmo category nol and the Himba category burou , according to naming data in Fig 1 of Roberson et al. (2005) [ 10 ]. The empirical discrimination data in Fig 7 are based on those probed colors [ 9 , 10 ], and show that in general, speakers of a language tend to exhibit greater discrimination for pairs of colors that cross a category boundary in their native language, consistent with the Sapir-Whorf hypothesis.

The English categories green and blue (top panel), the Berinmo categories wor and nol (middle panel), and the Himba categories dumbu and burou (bottom panel), plotted against a spectrum of hues that ranges from dark yellow at the left, through green, to blue at the right. Colored squares mark prototypes: the shared prototype for Berinmo wor and Himba dumbu , and the prototypes for English green and blue ; the color of each square approximates the color of the corresponding prototype. For each language, the dotted-and-dashed vertical lines denote the prototypes for the two categories from that language, and the dashed vertical line denotes the empirical boundary between these two categories. Black curves show the probability of assigning a given hue to each of the two native-language categories, according to the category component of a 2-category model fit to each language’s naming data. The shaded regions mark the ranges of colors probed in discrimination tasks; these two regions are centered at the English green - blue boundary and the Berinmo wor - nol boundary. Data are from Roberson et al. (2000) [ 9 ] and Roberson et al. (2005) [ 10 ].

Top panels: Discrimination from memory by Berinmo and English speakers for pairs of colors across and within English and Berinmo color category boundaries. Empirical data are from Table 11 of Roberson et al. (2000:392). Empirical values show mean proportion correct 2AFC memory judgments, and error bars show standard error. Model values show mean model proportion correct 2AFC memory judgments after simulated reconstruction with native-language categories. Model results are range-matched to the corresponding empirical values, such that the minimum and maximum model values match the minimum and maximum mean values in the corresponding empirical dataset, and other model values are linearly interpolated. Bottom panels: Discrimination from memory by Himba and English speakers for pairs of colors across and within English and Himba color category boundaries, compared with model results based on native-language categories. Empirical data are from Table 6 of Roberson et al. (2005:400); no error bars are shown because standard error was not reported in that table.
We sought to determine whether the 2-category model explored above could account for these data. To that end, for each language, we created a version of the 2-category model based on the naming data for that language. Thus, we created an English model in which the two categories were based on empirical naming data for green and blue , a Berinmo model in which the two categories were based on empirical naming data for wor and nol , and a Himba model in which the two categories were based on empirical naming data for dumbu and burou . The black curves in Fig 6 show the probability of assigning a given hue to each of the two native-language categories, according to the category component of a 2-category model fit to each language’s naming data. Given this category information, we simulated color reconstruction from memory for the specific colors considered in the empirical studies [ 9 , 10 ] (the colors in the shaded regions in Fig 6 ). We did so separately for the cases of English, Berinmo, and Himba, in each case fitting a model based on naming data for a given language to discrimination data from speakers of that language. As in Study 1, we fit the model parameter corresponding to the uncertainty of fine-grained perceptual representation to the empirical non-linguistic (here discrimination) data, and we used a single value for this parameter across all three language models. The model results are shown in Fig 7 , beside the empirical data to which they were fit. The models provide a reasonable match to the observed cross-language differences in discrimination. Specifically, the stimulus pairs for which empirical performance is best are those that cross a native-language boundary—and these are stimulus pairs for which the corresponding model response is strongest.
Although not shown in the figure, we also conducted a followup analysis to test whether the quality of these fits was attributable merely to model flexibility, or to a genuine fit between a language’s category system and patterns of discrimination from speakers of that language. We did this by switching which language’s model was fit to which language’s discrimination data. Specifically, we fit the model based on Berinmo naming to the discrimination data from English speakers (and vice versa), and fit the model based on Himba naming to the discrimination data from English speakers (and vice versa), again adjusting the model parameter corresponding to the uncertainty of the fine-grained perceptual representation to the empirical discrimination data. The results are summarized in Table 2 . It can be seen that the discrimination data are fit better by native-language models (that is, models with a category component originally fit to that language’s naming data) than by other-language models (that is, models with a category component originally fit to another language’s naming data). These results suggest that cross-language differences in discrimination may result from category-induced reconstruction bias under uncertainty, guided by native-language categories.
The best value in each row is shown in bold . Data are fit better by native-language models than by other-language models.
Study 3: Within-category effects
Although many studies of categorical perception focus on pairs of stimuli that cross category boundaries, there is also evidence for category effects within categories. In a 2AFC study of categorical perception of facial expressions, Roberson and colleagues [ 32 ] found the behavioral signature of categorical perception (or more precisely in this case, categorical memory): superior discrimination for cross-category than for within-category pairs of stimuli. But in addition, they found an interesting category effect on within-category pairs, dependent on order of presentation. For each within-category pair they considered, one stimulus of the pair was always closer to the category prototype (the “good exemplar”) than the other (the “poor exemplar”). They found that 2AFC performance on within-category pairs was better when the target was the good exemplar (and the distractor was therefore the poor exemplar) than when the target was the poor exemplar (and the distractor was therefore the good exemplar)—even though the same stimuli were involved in the two cases. Moreover, performance in the former (good exemplar) case did not differ significantly from cross-category performance. Hanley and Roberson [ 36 ] subsequently reanalyzed data from a number of earlier studies that had used 2AFC tasks to explore cross-language differences in color naming and cognition, including those reviewed and modeled in the previous section. Across studies and across domains, including color, they found the same asymmetrical within-category effect originally documented for facial expressions.
This within-category pattern may be naturally explained in category-adjustment terms, as shown in Fig 8 , and as argued by Roberson and colleagues [ 32 ]. The central idea is that because the target is held in memory, it is subject to bias toward the prototype in memory, making discrimination of target from distractor either easier or harder depending on which of the two stimuli is the target. Although this connection with the category adjustment model has been made in the literature in general conceptual terms [ 32 ], followup studies have been theoretically focused elsewhere [ 31 , 36 ], and the idea has not to our knowledge been tested computationally using the specific stimuli and naming patterns involved in the empirical studies. We sought to do so.

The category adjustment model predicts: (top panel, good exemplar) easy within-category discrimination in a 2AFC task when the initially-presented target t is closer to the prototype than the distractor d is; (bottom panel, poor exemplar) difficult within-category discrimination with the same two stimuli when the initially-presented target t is farther from the prototype than the distractor d is. Category is shown as a distribution in blue; stimuli are shown as vertical black lines marked t and d; reconstruction bias patterns are shown as arrows.
The empirical data in Fig 9 illustrate the within-category effect with published results on color discrimination by speakers of English, Berinmo, and Himba. In attempting to account for these data, we considered again the English, Berinmo, and Himba variants of the 2-category model first used in Study 2, and also retained from that study the parameter value corresponding to the uncertainty of the fine-grained perceptual representation, in the case of native-language models. We simulated reconstruction from memory of the specific colors examined in Study 2. Following the empirical analyses, this time we disaggregated the within-category stimulus pairs into those in which the target was a good exemplar of the category (i.e. the target was closer to the prototype than the distractor was), vs. those in which the target was a poor exemplar of the category (i.e. the target was farther from the prototype than the distractor was). The model results are shown in Fig 9 , and match the empirical data reasonably well, supporting the informal in-principle argument of Fig 8 with a more detailed quantitative analysis.

Across: stimulus pair crosses the native-language boundary; GE: within-category pair, target is the good exemplar; PE: within-category pair, target is the poor exemplar. Empirical data are from Figs 2 (English: 10-second retention interval), 3 (Berinmo), and 4 (Himba) of Hanley and Roberson [ 36 ]. Empirical values show mean proportion correct 2AFC memory judgments, and error bars show standard error. Model values show mean model proportion correct 2AFC memory judgments after simulated reconstruction using native-language categories, range-matched as in Fig 7 . English model compared with English data: 0.00002 MSE; Berinmo model compared with Berinmo data: 0.00055 MSE; Himba model compared with Himba data: 0.00087 MSE.
Conclusions
We have argued that the debate over the Sapir-Whorf hypothesis may be clarified by viewing that hypothesis in terms of probabilistic inference. To that end, we have presented a probabilistic model of color memory, building on proposals in the literature. The model assumes both a universal color space and language-specific categorical partitionings of that space, and infers the originally perceived color from these two sources of evidence. The structure of this model maps naturally onto a prominent proposal in the literature that has to our knowledge not previously been formalized in these terms. In a classic early study of the effect of language on color cognition, Kay and Kempton [ 7 ] interpret Whorf [ 2 ] as follows:
Whorf […] suggests that he conceives of experience as having two tiers: one, a kind of rock bottom, inescapable seeing-things-as-they-are (or at least as human beings cannot help but see them), and a second, in which [the specific structures of a given language] cause us to classify things in ways that could be otherwise (and are otherwise for speakers of a different language).
Kay and Kempton argue that color cognition involves an interaction between these two tiers. The existence of a universal groundwork for color cognition helps to explain why there are constraints on color naming systems across languages [ 3 – 5 , 37 ]. At the same time, Kay and Kempton acknowledge a role for the language-specific tier in cognition, such that “there do appear to be incursions of linguistic categorization into apparently nonlinguistic processes of thinking” (p. 77). These two tiers map naturally onto the universal and language-specific components of the model we have explored here. This structure offers a straightforward way to think about effects of language on cognition while retaining the idea of a universal foundation underpinning human perception and cognition. Thus, this general approach, and our model as an instance of it, offer a possible resolution of one source of controversy surrounding the Sapir-Whorf hypothesis: taking that hypothesis seriously need not entail a wholesale rejection of important universal components of human cognition.
The approach proposed here also has the potential to resolve another source of controversy surrounding the Sapir-Whorf hypothesis: that some findings taken to support it do not replicate reliably (e.g. in the case of color: [ 15 – 17 ]). Framing the issue in terms of probabilistic inference touches this question by highlighting the theoretically central role of uncertainty , as in models of probabilistic cue integration [ 24 ]. We have seen stronger category-induced bias in color memory under conditions of greater delay and presumably therefore greater uncertainty (Study 1, and [ 20 ]). This suggests that in the inverse case of high certainty about the stimulus, any category effect could in principle be so small as to be empirically undetectable, a possibility that can be pursued by systematically manipulating uncertainty. Thus, the account advanced here casts the Sapir-Whorf hypothesis in formal terms that suggest targeted and quantitative followup tests. A related theoretical advantage of uncertainty is that it highlights an important level of generality: uncertainty could result from memory, as explored here, but it could also result from noise or ambiguity in perception itself, and on the view advanced here, the result should be the same.
The model we have proposed does not cover all aspects of language effects on color cognition. For example, there are documented priming effects [ 31 ] which do not appear to flow as naturally from this account as do the other effects we have explored above. However, the model does bring together disparate bodies of data in a simple framework, and links them to independent principles of probabilistic inference. Future research can usefully probe the generality and the limitations of the ideas we have explored here.
Materials and Methods
Code and data supporting the analyses reported here are available at https://github.com/yangxuch/probwhorfcolor.git .
The basic model we consider is shown in Fig 10 , which presents in graphical form the generative process behind Fig 1 above. Our model follows in general outline that of Bae et al. [ 20 ], but the formalization of inference within this structure more closely follows Feldman et al.’s [ 28 ] model of category effects in vowel perception. In our model, the perception of a stimulus S = s produces a fine-grained memory M , and a categorical code c . We wish to obtain a reconstruction s ^ of the original stimulus S = s , by combining evidence from the two internal representations M and c that s has produced. That reconstruction is derived as follows:
Because hue is a circular dimension, the components p ( M | S ) and p ( S | c ) could be modeled using circular normal or von Mises distributions, as was done by Bae et al. [ 20 ]. However each of our studies treats only a restricted subsection of the full hue circle, and for that reason we instead model these representations using normal distributions.

The perception of stimulus S = s produces a fine-grained memory M , and a categorical code c specifying the category in which s fell. We wish to reconstruct the original stimulus S = s , given M and c .
p ( M | S ) represents the fine-grained memory trace M of the original stimulus S = s . We model this as a normal distribution with mean μ m at the location of the original stimulus s , and with uncertainty captured by variance σ m 2 :
This is an unbiased representation of the original stimulus s because μ m = s .
p ( S | c ) captures the information about the location of stimulus S that is given by the categorical code c . We again model this as a normal distribution, this time centered at the prototype μ c of category c , with variance σ c 2 :
This assumes that there is a single categorical code c , and we use this assumption in some of our model variants below. However in other cases we will capture the fact that more than one category may be applicable to a stimulus. In such cases we assume that the perceiver knows, for each category c , the applicability π ( c ) of that category for the observed stimulus s . We model this as:
where p ( S = s | c ) is given by Eq (4) above, and p ( c ) is assumed to be uniform.
We consider three variants of this basic model, described below in order of increasing complexity: the null model, the 1-category model, and the 2-category model. For each model, we take the predicted reconstruction s ^ of a given stimulus S = s to be the expected value of the posterior distribution:
The null model assumes that reconstruction is based only on the fine-grained memory, with no category influence. This model is derived from Eq (2) by assuming that the memory component p ( M | S ) is as defined above, and the category component p ( S | c ) is uniform, yielding:
The predicted reconstruction for this model is given by the expected value of this distribution, namely:
where we have assumed μ m = s , the originally observed stimulus. This model predicts no category-induced bias: the reconstruction of the stimulus S = s is simply the value of the stimulus s itself.
1-category model
The 1-category model assumes that reconstruction is based both on fine-grained memory and on information from a single category, e.g. English green . This model is derived from Eq (2) by assuming that both the memory component p ( M | S ) and the category component p ( S | c ) are as defined above, yielding:
where we have assumed μ m = s , the originally observed stimulus. This equation parallels Eq (7) of Feldman et al. [ 28 ]. This model produces a reconstruction that is a weighted average of the original stimulus value s and the category prototype μ c , with weights determined by the relative certainty of each of the two sources of information. The same weighted average is also central to Ernst and Banks’ [ 24 ] study of cue integration from visual and haptic modalities. That study was based on the same principles we invoke here, and our model—like that of Feldman et al.—can be viewed as a probabilistic cue integration model in which one of the two cues being integrated is a category, rather than a cue from a different modality.
2-category model
The 2-category model is similar to the 1-category model, but instead of basing its reconstruction on a single category c , it bases its reconstruction on two categories c 1 and c 2 (e.g. English green and blue ). It does so by averaging together the reconstruction provided by the 1-category model for c 1 and the reconstruction provided by the 1-category model for c 2 , weighted by the applicability π ( c ) of each category c to the stimulus:
Here, p ( S | M , c ) inside the sum is given by the 1-category model specified in Eq (9) , and π ( c ) is the applicability of category c to the observed stimulus s as specified above in Eq (5) . Our equation here parallels Eq (9) of Feldman et al. [ 28 ] who similarly take a weighted average over 1-category models in their model of category effects in speech perception. The predicted reconstruction for this model is given by the expected value of this distribution, namely:
assuming as before that μ m = s , the original stimulus value. This equation follows Feldman et al. [ 28 ] Eq (10).
Fitting models to data
For each model, any category parameters μ c and σ c 2 are first fit to naming data. The single remaining free parameter σ m 2 , corresponding to the uncertainty of fine-grained memory, is then fit to non-linguistic bias or discrimination data, with no further adjustment of the category parameters. Although this two-step process is used in all of our studies, it is conducted in slightly different ways across studies; we supply study-specific details below in our presentation of each study. All model fits were done using fminsearch in Matlab.
Participants
Twenty subjects participated in the experiment, having been recruited at UC Berkeley. All subjects were at least 18 years of age, native English speakers, and reported normal or corrected-to-normal vision, and no colorblindness. All subjects received payment or course credit for participation.
Informed consent was obtained verbally; all subjects read an approved consent form and verbally acknowledged their willingness to participate in the study. Verbal consent was chosen because the primary risk to subjects in this study was for their names to be associated with their response; this approach allowed us to obtain consent and collect data without the need to store subjects’ names in any form. Once subjects acknowledged that they understood the procedures and agreed to participate by stating so to the experimenter, the experimenter recorded their consent by assigning them a subject number, which was anonymously linked to their data. All study procedures, including those involving consent, were overseen and approved by the UC Berkeley Committee for the Protection of Human Subjects.
Stimuli were selected by varying a set of hues centered around the blue - green boundary, holding saturation and lightness constant. Stimuli were defined in Munsell coordinate space, which is widely used in the literature we engage here (e.g. [ 9 , 10 ]). All stimuli were at lightness 6 and saturation 8. Hue varied from 5Y to 10P, in equal hue steps of 2.5. Colors were converted to xyY coordinate space following Table I(6.6.1) of Wyszecki and Stiles (1982) [ 38 ]. The colors were implemented in Matlab in xyY; the correspondence of these coordinate systems in the stimulus set, as well as approximate visualizations of the stimuli, are reported in Table 3 .
All stimuli were presented at lightness 6, saturation 8 in Munsell space.
We considered three progressively narrower ranges of these stimuli for different aspects of our analyses, in an attempt to focus the analyses on a region that is well-centered relative to the English color categories green and blue . We refer to these three progressively narrower ranges as the full range , the medium range , and the focused range . We specify these ranges below, together with the aspects of the analysis for which each was used.
- Full range: We collected naming data for green and blue relative to the full range, stimuli 1-27, for a total of 27 stimuli. We fit the category components of our models to naming data over this full range.
- Medium range: We collected bias data for a subset of the full range, namely the medium range, stimuli 5-23, for a total of 19 stimuli. We considered this subset because we were interested in bias induced by the English color terms green and blue , and we had the impression, prior to collecting naming or bias data, that colors outside this medium range had some substantial element of the neighboring categories yellow and purple .
- Focused range: Once we had naming data, we narrowed the range further based on those data, to the focused range, stimuli 5-19, for a total of 15 stimuli. The focused range extends between the (now empirically assessed) prototypes for green and blue , and also includes three of our stimulus hues on either side of these prototypes, yielding a range well-centered relative to those prototypes, as can be seen in the bottom panel of Fig 4 above. We considered this range in our statistical analyses, and in our modeling of bias patterns.
Experimental procedure
The experiment consisted of four blocks. The first two blocks were reconstruction (bias) tasks: one simultaneous block and one delay block. In the simultaneous block ( Fig 3A ), the subject was shown a stimulus color as a colored square (labeled as “Original” in the figure), and was asked to recreate that color in a second colored square (labeled as “Target” in the figure) as accurately as possible by selecting a hue from a color wheel. The (“Original”) stimulus color remained on screen while the subject selected a response from the color wheel; navigation of the color wheel would change the color of the response (“Target”) square. The stimulus square and response square each covered 4.5 degrees of visual angle, and the color wheel covered 11.1 degrees of visual angle. Target colors were drawn from the medium range of stimuli (stimuli 5—23 of Table 3 ). The color wheel was constructed based on the full range of stimuli (stimuli 1—27 of Table 3 ), supplemented by interpolating 25 points evenly in xyY coordinates between each neighboring pair of the 27 stimuli of the full range, to create a finely discretized continuum from yellow to purple, with 677 possible responses. Each of the 19 target colors of the medium range was presented five times per block in random order, for a total of 95 trials per block. The delay block ( Fig 3B ) was similar to the simultaneous block but with the difference that the stimulus color was shown for 500 milliseconds then disappeared, then a fixation cross was shown for 1000 milliseconds, after which the subject was asked to reconstruct the target color from memory, again using the color wheel to change the color of the response square. The one colored square shown in the final frame of Fig 3B is the response square that changed color under participant control. The order of the simultaneous block and delay block were counterbalanced by subject. Trials were presented with a 500 millisecond inter-trial interval.
Several steps were taken to ensure that responses made on the color wheel during the reconstruction blocks were not influenced by bias towards a particular spatial position. The position of the color wheel was randomly rotated up to 180 degrees from trial to trial. The starting position of the cursor was likewise randomly generated for each new trial. Finally, the extent of the spectrum was jittered one or two stimuli (2.5 or 5 hue steps) from trial to trial, which had the effect of shifting the spectrum slightly in the yellow or the purple direction from trial to trial. This was done to ensure that the blue - green boundary would not fall at a consistent distance from the spectrum endpoints on each trial.
The second two blocks were naming tasks. In each, subjects were shown each of the 27 stimuli of the full range five times in random order, for a total of 135 trials per block. On each trial, subjects were asked to rate how good an example of a given color name each stimulus was. In one block, the color name was green , in the other, the color name was blue ; order of blocks was counterbalanced by subject. To respond, subjects positioned a slider bar with endpoints “Not at all [green/blue]” and “Perfectly [green/blue]” to the desired position matching their judgment of each stimulus, as shown above in Fig 3C . Responses in the naming blocks were self-paced. Naming blocks always followed reconstruction blocks, to ensure that repeated exposure to the color terms green and blue did not bias responses during reconstruction.
The experiment was presented in Matlab version 7.11.0 (R2010b) using Psychtoolbox (version 3) [ 39 – 41 ]. The experiment was conducted in a dark, sound-attenuated booth on an LCD monitor that supported 24-bit color. The monitor had been characterized using a Minolta CS100 colorimeter. A chin rest was used to ensure that each subject viewed the screen from a constant position; when in position, the base of the subject’s chin was situated 30 cm from the screen.
As part of debriefing after testing was complete, each subject was asked to report any strategies they used during the delay block to help them remember the target color. Summaries of each response, as reported by the experimenter, are listed in Table 4 .
When subjects gave specific examples of color terms used as memory aids, they are reported here.
Color spectrum
We wished to consider our stimuli along a 1-dimensional spectrum such that distance between two colors on that spectrum approximates the perceptual difference between those colors. To this end, we first converted our stimuli to CIELAB color space. CIELAB is a 3-dimensional color space designed “in an attempt to provide coordinates for colored stimuli so that the distance between the coordinates of any two stimuli is predictive of the perceived color difference between them” (p. 202 of [ 42 ]). The conversion to CIELAB was done according to the equations on pp. 167-168 of Wyszecki and Stiles (1982) [ 38 ], assuming 2 degree observer and D65 illuminant. For each pair of neighboring colors in the set of 677 colors of our color wheel, we measured the distance (Δ E ) betwen these two colors in CIELAB space. We then arranged all colors along a 1-dimensional spectrum that was scaled to length 1, such that the distance between each pair of neighboring colors along that spectrum was proportional to the CIELAB Δ E distance between them. This CIELAB-based 1-dimensional spectrum was used for our analyses in Study 1, and an analogous spectrum for a different set of colors was used for our analyses in Studies 2 and 3.
Statistical analysis
As a result of the experiment detailed above, we obtained bias data from 20 participants, for each of 19 hues (the medium range), for 5 trials per hue per participant, in each of the simultaneous and delayed conditions. For analysis purposes, we restricted attention to the focused range of stimuli (15 hues), in order to consider a region of the spectrum that is well-centered with respect to green and blue , as we are primarily interested in bias that may be induced by these two categories. We wished to determine whether the magnitude of the bias differed as a function of the simultaneous vs. delayed condition, whether the magnitude of the bias varied as a function of hue, and whether there was an interaction between these two factors. To answer those questions, we conducted a 2 (condition: simultaneous vs. delayed) × 15 (hues) repeated measures analysis of variance (ANOVA), in which the dependent measure was the absolute value of the reproduction bias (reproduced hue minus target hue), averaged across trials for a given participant at a given target hue in a given condition. The ANOVA included an error term to account for across-subject variability. We found a main effect of condition, with greater bias magnitude in the delayed than in the simultaneous condition [ F (1, 19) = 61.61, p < 0.0001], a main effect of hue [ F (14, 266) = 4.565, p < 0.0001], and an interaction of hue and condition [ F (14, 266) = 3.763, p < 0.0001]. All hue calculations were relative to the CIELAB-based spectrum detailed in the preceding section.
We then conducted paired t-tests at each of the target hues, comparing each participant’s bias magnitude for that hue (averaged over trials) in the simultaneous condition vs. the delayed condition. Blue asterisks at the top of Fig 4 mark hues for which the paired t-test returned p < 0.05 when applying Bonferroni corrections for multiple comparisons.
Modeling procedure
We considered four models in accounting for color reconstruction in English speakers: the null model, a 1-category model for which the category was green , a 1-category model for which the category was blue , and a 2-category model based on both green and blue .
We fit these models to the data in two steps. We first fit any category parameters (the means μ c and variances σ c 2 for any categories c ) to the naming data. We then fit the one remaining free parameter ( σ m 2 ), which captures the uncertainty of fine-grained memory, to the bias data, without further adjusting the category parameters. We specify each of these two steps below.
We fit a Gaussian function to the goodness naming data for green , and another Gaussian function to the data for blue , using maximum likelihood estimation. The fitted Gaussian functions can be seen, together with the data to which they were fit, in the top panel of Fig 4 . This process determined values for the category means μ c and category variances σ c 2 for the two categories green and blue .
For each of the four variants of the category adjustment model outlined above (null, 1-category green, 1-category blue, and 2-category), we retained the category parameter settings resulting from the above fit to the naming data. We then obtained a value for the one remaining free parameter σ m 2 , corresponding to the uncertainty of fine-grained memory, by fitting the model to the bias data via maximum likelihood estimation, without further adjusting the category parameters.
Empirical data
The empirical data considered for this study were drawn from two sources: the study of 2AFC color discrimination by speakers of Berinmo and English in Experiment 6a of Roberson et al. (2000) [ 9 ], and the study of 2AFC color discrimination by speakers of Himba and English in Experiment 3b of Roberson et al. (2005) [ 10 ]. In both studies, two sets of color stimuli were considered, all at value (lightness) level 5, and chroma (saturation) level 8. Both sets varied in hue by increments of 2.5 Munsell hue steps. The first set of stimuli was centered at the English green - blue boundary (hue 7.5BG), and contained the following seven hues: 10G, 2.5BG, 5BG, 7.5BG, 10BG, 2.5B, 5B. The second set of stimuli was centered at the Berinmo wor - nol boundary (hue 5GY), and contained the following seven hues: 7.5Y, 10Y, 2.5GY, 5GY, 7.5GY, 10GY, 2.5G. Stimuli in the set that crossed an English category boundary all fell within a single category in Berinmo ( nol ) and in Himba ( burou ), and stimuli in the set that crossed a Berinmo category boundary also crossed a Himba category boundary ( dumbu - burou ) but all fell within a single category in English ( green ), according to naming data in Fig 1 of Roberson et al. (2005) [ 10 ]. Based on specifications in the original empirical studies [ 9 , 10 ], we took the pairs of stimuli probed to be those presented in Table 5 .
Any stimulus pair that includes a boundary color is considered to be a cross-category pair. All hues are at value (lightness) level 5, and chroma (saturation) level 8. 1s denotes a 1-step pair; 2s denotes a 2-step pair.
Based on naming data in Fig 1 of Roberson et al. 2005 [ 10 ], we took the prototypes of the relevant color terms to be:
English green prototype = 10GY
English blue prototype = 10B
Berinmo wor prototype = 5Y
Berinmo nol prototype = 5G
Himba dumbu prototype = 5Y
Himba burou prototype = 10G
Fig 6 above shows a spectrum of hues ranging from the Berinmo wor prototype (5Y) to the English blue prototype (10B) in increments of 2.5 Munsell hue steps, categorized according to each of the three languages we consider here. These Munsell hues were converted to xyY and then to CIELAB as above, and the positions of the hues on the spectrum were adjusted so that the distance between each two neighboring hues in the spectrum is proportional to the CIELAB Δ E distance between them. We use this CIELAB-based spectrum for our analyses below. The two shaded regions on each spectrum in Fig 6 denote the two target sets of stimuli identified above.
The discrimination data we modeled were drawn from Table 11 of Roberson et al. (2000:392) [ 9 ] and Table 6 of Roberson et al. (2005:400) [ 10 ].
We considered three variants of the 2-category model: an English blue - green model, a Berinmo wor - nol model, and a Himba dumbu - burou model. As in Study 1, we fit each model to the data in two steps. For each language’s model, we first fit the category component of that model to naming data from that language. Because color naming differs across these languages, this resulted in three models with different category components. For each model, we then retained and fixed the resulting category parameter settings, and fit the single remaining parameter, corresponding to memory uncertainty, to discrimination data. We detail these two steps below.
For the naming data, we modeled the probability of applying category name c to stimulus i as:
where p ( c ) is assumed to be uniform, and f ( i | c ) is a non-normalized Gaussian function corresponding to category c , with mean μ c and variance σ c 2 . There were two categories c for each model, e.g. wor and nol in the case of the Berinmo model. Category means μ c were set to the corresponding category prototypes shown above (e.g. μ c for Berinmo nol corresponded to 5G), and category variances σ c 2 were left as free parameters. We then adjusted these free category variances to reproduce the empirical boundary between the two categories c 1 and c 2 for that language, as follows. Sweeping from left ( c 1 ) to right ( c 2 ), we took the model’s boundary between c 1 and c 2 to be the first position i on the spectrum for which p ( c 1 | i ) ≤ p ( c 2 | i ); we refer to this as the model crossover point . We measured the distance in the CIELAB-based spectrum between the model crossover point and the empirical category boundary, and adjusted the category variances σ c 1 2 and σ c 2 2 so as to minimize that distance. This was done separately for each language’s model. Fig 6 shows the resulting fits of category components to naming data for each of the three languages.
We then simulated performance in the 2AFC discrimination task for each stimulus pair in Table 5 , by each model, as follows. Given a pair of stimuli, one stimulus was taken to be the target t and therefore held in memory, and the other taken to be the distractor d . We took the reconstruction r for the target stimulus t to be the expected value of the posterior for the 2-category model:
We then measured, along the hue spectrum in question, the distance dist ( r , t ) between the reconstruction r and the target t , and the distance dist ( r , d ) between the reconstruction r and the distractor d . We converted each of these two distances to a similarity score:
and modeled the proportion correct choice as:
These equations are based on Luce’s [ 43 ] (pp. 113-114) model of choice behavior. For each pair of stimuli, each stimulus was once taken to be the target, and once taken to be the distractor, and the results averaged to yield a mean discrimination score for that pair. Scores were then averaged across all pairs listed as within-category pairs, and separately for all pairs listed as cross-category pairs. These scores were range-matched to the empirical data, in an attempt to correct for other factors that could affect performance, such as familiarity with such tasks, etc.; such external factors could in principle differ substantially across participant pools for the three languages modeled. We measured MSE between the model output and the data so treated, and adjusted the remaining parameter σ m 2 , corresponding to memory uncertainty, so as to minimize this MSE. This entire process was conducted two times. The first time, each language’s model was fit to that same language’s discrimination data. Then, to test whether native-language categories allow a better fit than the categories of another language, we fit the Berinmo model to the English discrimination data (and vice versa), and the Himba model to the English discrimination data (and vice versa).
The empirical data considered for this study are those of Figs 2 (English green/blue , 10 second delay), 3 (Berinmo wor/nol ), and 4 (Himba dumbu/borou ) of Hanley and Roberson (2011) [ 36 ]. These data were originally published by Roberson and Davidoff (2000) [ 8 ], Roberson et al. (2000) [ 9 ], and Roberson et al. (2005) [ 10 ], respectively. The Berinmo and Himba stimuli and data were the same as in our Study 2, but the English stimuli and data reanalyzed by Hanley and Roberson (2011) [ 36 ] Fig 2 were instead drawn from Table 1 of Roberson and Davidoff (2000) [ 8 ], reproduced here in Table 6 , and used for the English condition of this study. These stimuli for English were at lightness (value) level 4, rather than 5 as for the other two languages. We chose to ignore this difference for modeling purposes.
Any stimulus pair that includes a boundary color is considered to be a cross-category pair. All hues are at value (lightness) level 4, and chroma (saturation) level 8. 1s denotes a 1-step pair; 2s denotes a 2-step pair.
All modeling procedures were identical to those of Study 2, with the exception that GE (target = good exemplar) and PE (target = poor exemplar) cases were disaggregated, and analyzed separately.
Acknowledgments
We thank Roland Baddeley, Paul Kay, Charles Kemp, Steven Piantadosi, and an anonymous reviewer for their comments.
Funding Statement
This research was supported by the National Science Foundation ( www.nsf.gov ) under grants DGE-1106400 (EC) and SBE-1041707 (YX, TR). Publication was made possible in part by support from the Berkeley Research Impact Initiative (BRII) sponsored by the UC Berkeley Library. The funders had no role in study design, data collection and analysis, decision to publish, or preparation of the manuscript.
Data Availability
The Sapir-Whorf Hypothesis Linguistic Theory
DrAfter123/Getty Images
- An Introduction to Punctuation
- Ph.D., Rhetoric and English, University of Georgia
- M.A., Modern English and American Literature, University of Leicester
- B.A., English, State University of New York
The Sapir-Whorf hypothesis is the linguistic theory that the semantic structure of a language shapes or limits the ways in which a speaker forms conceptions of the world. It came about in 1929. The theory is named after the American anthropological linguist Edward Sapir (1884–1939) and his student Benjamin Whorf (1897–1941). It is also known as the theory of linguistic relativity, linguistic relativism, linguistic determinism, Whorfian hypothesis , and Whorfianism .
History of the Theory
The idea that a person's native language determines how he or she thinks was popular among behaviorists of the 1930s and on until cognitive psychology theories came about, beginning in the 1950s and increasing in influence in the 1960s. (Behaviorism taught that behavior is a result of external conditioning and doesn't take feelings, emotions, and thoughts into account as affecting behavior. Cognitive psychology studies mental processes such as creative thinking, problem-solving, and attention.)
Author Lera Boroditsky gave some background on ideas about the connections between languages and thought:
"The question of whether languages shape the way we think goes back centuries; Charlemagne proclaimed that 'to have a second language is to have a second soul.' But the idea went out of favor with scientists when Noam Chomsky 's theories of language gained popularity in the 1960s and '70s. Dr. Chomsky proposed that there is a universal grammar for all human languages—essentially, that languages don't really differ from one another in significant ways...." ("Lost in Translation." "The Wall Street Journal," July 30, 2010)
The Sapir-Whorf hypothesis was taught in courses through the early 1970s and had become widely accepted as truth, but then it fell out of favor. By the 1990s, the Sapir-Whorf hypothesis was left for dead, author Steven Pinker wrote. "The cognitive revolution in psychology, which made the study of pure thought possible, and a number of studies showing meager effects of language on concepts, appeared to kill the concept in the 1990s... But recently it has been resurrected, and 'neo-Whorfianism' is now an active research topic in psycholinguistics ." ("The Stuff of Thought. "Viking, 2007)
Neo-Whorfianism is essentially a weaker version of the Sapir-Whorf hypothesis and says that language influences a speaker's view of the world but does not inescapably determine it.
The Theory's Flaws
One big problem with the original Sapir-Whorf hypothesis stems from the idea that if a person's language has no word for a particular concept, then that person would not be able to understand that concept, which is untrue. Language doesn't necessarily control humans' ability to reason or have an emotional response to something or some idea. For example, take the German word sturmfrei , which essentially is the feeling when you have the whole house to yourself because your parents or roommates are away. Just because English doesn't have a single word for the idea doesn't mean that Americans can't understand the concept.
There's also the "chicken and egg" problem with the theory. "Languages, of course, are human creations, tools we invent and hone to suit our needs," Boroditsky continued. "Simply showing that speakers of different languages think differently doesn't tell us whether it's language that shapes thought or the other way around."
- Definition and Discussion of Chomskyan Linguistics
- Generative Grammar: Definition and Examples
- Cognitive Grammar
- Universal Grammar (UG)
- Transformational Grammar (TG) Definition and Examples
- Linguistic Competence: Definition and Examples
- Definition and Examples of Case Grammar
- An Introduction to Semantics
- Construction Grammar
- Definition and Examples of Referents in English Grammar
- Pragmatics Gives Context to Language
- Figure of Thought in Rhetoric
- 10 Types of Grammar (and Counting)
- Where Did Language Come From? (Theories)
- What Is Lexicogrammar?
- Definition and Examples of Syntax

- Table of Contents
- Random Entry
- Chronological
- Editorial Information
- About the SEP
- Editorial Board
- How to Cite the SEP
- Special Characters
- Advanced Tools
- Support the SEP
- PDFs for SEP Friends
- Make a Donation
- SEPIA for Libraries
- Back to Entry
- Entry Contents
- Entry Bibliography
- Academic Tools
- Friends PDF Preview
- Author and Citation Info
- Back to Top
Supplement to Philosophy of Linguistics
Whorfianism.
Emergentists tend to follow Edward Sapir in taking an interest in interlinguistic and intralinguistic variation. Linguistic anthropologists have explicitly taken up the task of defending a famous claim associated with Sapir that connects linguistic variation to differences in thinking and cognition more generally. The claim is very often referred to as the Sapir-Whorf Hypothesis (though this is a largely infelicitous label, as we shall see).
This topic is closely related to various forms of relativism—epistemological, ontological, conceptual, and moral—and its general outlines are discussed elsewhere in this encyclopedia; see the section on language in the Summer 2015 archived version of the entry on relativism (§3.1). Cultural versions of moral relativism suggest that, given how much cultures differ, what is moral for you might depend on the culture you were brought up in. A somewhat analogous view would suggest that, given how much language structures differ, what is thinkable for you might depend on the language you use. (This is actually a kind of conceptual relativism, but it is generally called linguistic relativism, and we will continue that practice.)
Even a brief skim of the vast literature on the topic is not remotely plausible in this article; and the primary literature is in any case more often polemical than enlightening. It certainly holds no general answer to what science has discovered about the influences of language on thought. Here we offer just a limited discussion of the alleged hypothesis and the rhetoric used in discussing it, the vapid and not so vapid forms it takes, and the prospects for actually devising testable scientific hypotheses about the influence of language on thought.
Whorf himself did not offer a hypothesis. He presented his “new principle of linguistic relativity” (Whorf 1956: 214) as a fact discovered by linguistic analysis:
When linguists became able to examine critically and scientifically a large number of languages of widely different patterns, their base of reference was expanded; they experienced an interruption of phenomena hitherto held universal, and a whole new order of significances came into their ken. It was found that the background linguistic system (in other words, the grammar) of each language is not merely a reproducing instrument for voicing ideas but rather is itself the shaper of ideas, the program and guide for the individual’s mental activity, for his analysis of impressions, for his synthesis of his mental stock in trade. Formulation of ideas is not an independent process, strictly rational in the old sense, but is part of a particular grammar, and differs, from slightly to greatly, between different grammars. We dissect nature along lines laid down by our native languages. The categories and types that we isolate from the world of phenomena we do not find there because they stare every observer in the face; on the contrary, the world is presented in a kaleidoscopic flux of impressions which has to be organized by our minds—and this means largely by the linguistic systems in our minds. We cut nature up, organize it into concepts, and ascribe significances as we do, largely because we are parties to an agreement to organize it in this way—an agreement that holds throughout our speech community and is codified in the patterns of our language. The agreement is, of course, an implicit and unstated one, but its terms are absolutely obligatory ; we cannot talk at all except by subscribing to the organization and classification of data which the agreement decrees. (Whorf 1956: 212–214; emphasis in original)
Later, Whorf’s speculations about the “sensuously and operationally different” character of different snow types for “an Eskimo” (Whorf 1956: 216) developed into a familiar journalistic meme about the Inuit having dozens or scores or hundreds of words for snow; but few who repeat that urban legend recall Whorf’s emphasis on its being grammar, rather than lexicon, that cuts up and organizes nature for us.
In an article written in 1937, posthumously published in an academic journal (Whorf 1956: 87–101), Whorf clarifies what is most important about the effects of language on thought and world-view. He distinguishes ‘phenotypes’, which are overt grammatical categories typically indicated by morphemic markers, from what he called ‘cryptotypes’, which are covert grammatical categories, marked only implicitly by distributional patterns in a language that are not immediately apparent. In English, the past tense would be an example of a phenotype (it is marked by the - ed suffix in all regular verbs). Gender in personal names and common nouns would be an example of a cryptotype, not systematically marked by anything. In a cryptotype, “class membership of the word is not apparent until there is a question of using it or referring to it in one of these special types of sentence, and then we find that this word belongs to a class requiring some sort of distinctive treatment, which may even be the negative treatment of excluding that type of sentence” (p. 89).
Whorf’s point is the familiar one that linguistic structure is comprised, in part, of distributional patterns in language use that are not explicitly marked. What follows from this, according to Whorf, is not that the existing lexemes in a language (like its words for snow) comprise covert linguistic structure, but that patterns shared by word classes constitute linguistic structure. In ‘Language, mind, and reality’ (1942; published posthumously in Theosophist , a magazine published in India for the followers of the 19th-century spiritualist Helena Blavatsky) he wrote:
Because of the systematic, configurative nature of higher mind, the “patternment” aspect of language always overrides and controls the “lexation”…or name-giving aspect. Hence the meanings of specific words are less important than we fondly fancy. Sentences, not words, are the essence of speech, just as equations and functions, and not bare numbers, are the real meat of mathematics. We are all mistaken in our common belief that any word has an “exact meaning.” We have seen that the higher mind deals in symbols that have no fixed reference to anything, but are like blank checks, to be filled in as required, that stand for “any value” of a given variable, like …the x , y , z of algebra. (Whorf 1942: 258)
Whorf apparently thought that only personal and proper names have an exact meaning or reference (Whorf 1956: 259).
For Whorf, it was an unquestionable fact that language influences thought to some degree:
Actually, thinking is most mysterious, and by far the greatest light upon it that we have is thrown by the study of language. This study shows that the forms of a person’s thoughts are controlled by inexorable laws of pattern of which he is unconscious. These patterns are the unperceived intricate systematizations of his own language—shown readily enough by a candid comparison and contrast with other languages, especially those of a different linguistic family. His thinking itself is in a language—in English, in Sanskrit, in Chinese. [footnote omitted] And every language is a vast pattern-system, different from others, in which are culturally ordained the forms and categories by which the personality not only communicates, but analyzes nature, notices or neglects types of relationship and phenomena, channels his reasoning, and builds the house of his consciousness. (Whorf 1956: 252)
He seems to regard it as necessarily true that language affects thought, given
- the fact that language must be used in order to think, and
- the facts about language structure that linguistic analysis discovers.
He also seems to presume that the only structure and logic that thought has is grammatical structure. These views are not the ones that after Whorf’s death came to be known as ‘the Sapir-Whorf Hypothesis’ (a sobriquet due to Hoijer 1954). Nor are they what was called the ‘Whorf thesis’ by Brown and Lenneberg (1954) which was concerned with the relation of obligatory lexical distinctions and thought. Brown and Lenneberg (1954) investigated this question by looking at the relation of color terminology in a language and the classificatory abilities of the speakers of that language. The issue of the relation between obligatory lexical distinctions and thought is at the heart of what is now called ‘the Sapir-Whorf Hypothesis’ or ‘the Whorf Hypothesis’ or ‘Whorfianism’.
1. Banal Whorfianism
No one is going to be impressed with a claim that some aspect of your language may affect how you think in some way or other; that is neither a philosophical thesis nor a psychological hypothesis. So it is appropriate to set aside entirely the kind of so-called hypotheses that Steven Pinker presents in The Stuff of Thought (2007: 126–128) as “five banal versions of the Whorfian hypothesis”:
- “Language affects thought because we get much of our knowledge through reading and conversation.”
- “A sentence can frame an event, affecting the way people construe it.”
- “The stock of words in a language reflects the kinds of things its speakers deal with in their lives and hence think about.”
- “[I]f one uses the word language in a loose way to refer to meanings,… then language is thought.”
- “When people think about an entity, among the many attributes they can think about is its name.”
These are just truisms, unrelated to any serious issue about linguistic relativism.
We should also set aside some methodological versions of linguistic relativism discussed in anthropology. It may be excellent advice to a budding anthropologist to be aware of linguistic diversity, and to be on the lookout for ways in which your language may affect your judgment of other cultures; but such advice does not constitute a hypothesis.
2. The so-called Sapir-Whorf hypothesis
The term “Sapir-Whorf Hypothesis” was coined by Harry Hoijer in his contribution (Hoijer 1954) to a conference on the work of Benjamin Lee Whorf in 1953. But anyone looking in Hoijer’s paper for a clear statement of the hypothesis will look in vain. Curiously, despite his stated intent “to review and clarify the Sapir-Whorf hypothesis” (1954: 93), Hoijer did not even attempt to state it. The closest he came was this:
The central idea of the Sapir-Whorf hypothesis is that language functions, not simply as a device for reporting experience, but also, and more significantly, as a way of defining experience for its speakers.
The claim that “language functions…as a way of defining experience” appears to be offered as a kind of vague metaphysical insight rather than either a statement of linguistic relativism or a testable hypothesis.
And if Hoijer seriously meant that what qualitative experiences a speaker can have are constituted by that speaker’s language, then surely the claim is false. There is no reason to doubt that non-linguistic sentient creatures like cats can experience (for example) pain or heat or hunger, so having a language is not a necessary condition for having experiences. And it is surely not sufficient either: a robot with a sophisticated natural language processing capacity could be designed without the capacity for conscious experience.
In short, it is a mystery what Hoijer meant by his “central idea”.
Vague remarks of the same loosely metaphysical sort have continued to be a feature of the literature down to the present. The statements made in some recent papers, even in respected refereed journals, contain non-sequiturs echoing some of the remarks of Sapir, Whorf, and Hoijer. And they come from both sides of the debate.
3. Anti-Whorfian rhetoric
Lila Gleitman is an Essentialist on the other side of the contemporary debate: she is against linguistic relativism, and against the broadly Whorfian work of Stephen Levinson’s group at the Max Planck Institute for Psycholinguistics. In the context of criticizing a particular research design, Li and Gleitman (2002) quote Whorf’s claim that “language is the factor that limits free plasticity and rigidifies channels of development”. But in the claim cited, Whorf seems to be talking about the psychological topic that holds universally of human conceptual development, not claiming that linguistic relativism is true.
Li and Gleitman then claim (p. 266) that such (Whorfian) views “have diminished considerably in academic favor” in part because of “the universalist position of Chomskian linguistics, with its potential for explaining the striking similarity of language learning in children all over the world.” But there is no clear conflict or even a conceptual connection between Whorf’s views about language placing limits on developmental plasticity, and Chomsky’s thesis of an innate universal architecture for syntax. In short, there is no reason why Chomsky’s I-languages could not be innately constrained, but (once acquired) cognitively and developmentally constraining.
For example, the supposedly deep linguistic universal of ‘recursion’ (Hauser et al. 2002) is surely quite independent of whether the inventory of colour-name lexemes in your language influences the speed with which you can discriminate between color chips. And conversely, universal tendencies in color naming across languages (Kay and Regier 2006) do not show that color-naming differences among languages are without effect on categorical perception (Thierry et al. 2009).
4. Strong and weak Whorfianism
One of the first linguists to defend a general form of universalism against linguistic relativism, thus presupposing that they conflict, was Julia Penn (1972). She was also an early popularizer of the distinction between ‘strong’ and ‘weak’ formulations of the Sapir-Whorf Hypothesis (and an opponent of the ‘strong’ version).
‘Weak’ versions of Whorfianism state that language influences or defeasibly shapes thought. ‘Strong’ versions state that language determines thought, or fixes it in some way. The weak versions are commonly dismissed as banal (because of course there must be some influence), and the stronger versions as implausible.
The weak versions are considered banal because they are not adequately formulated as testable hypotheses that could conflict with relevant evidence about language and thought.
Why would the strong versions be thought implausible? For a language to make us think in a particular way, it might seem that it must at least temporarily prevent us from thinking in other ways, and thus make some thoughts not only inexpressible but unthinkable. If this were true, then strong Whorfianism would conflict with the Katzian effability claim. There would be thoughts that a person couldn’t think because of the language(s) they speak.
Some are fascinated by the idea that there are inaccessible thoughts; and the notion that learning a new language gives access to entirely new thoughts and concepts seems to be a staple of popular writing about the virtues of learning languages. But many scientists and philosophers intuitively rebel against violations of effability: thinking about concepts that no one has yet named is part of their job description.
The resolution lies in seeing that the language could affect certain aspects of our cognitive functioning without making certain thoughts unthinkable for us .
For example, Greek has separate terms for what we call light blue and dark blue, and no word meaning what ‘blue’ means in English: Greek forces a choice on this distinction. Experiments have shown (Thierry et al. 2009) that native speakers of Greek react faster when categorizing light blue and dark blue color chips—apparently a genuine effect of language on thought. But that does not make English speakers blind to the distinction, or imply that Greek speakers cannot grasp the idea of a hue falling somewhere between green and violet in the spectrum.
There is no general or global ineffability problem. There is, though, a peculiar aspect of strong Whorfian claims, giving them a local analog of ineffability: the content of such a claim cannot be expressed in any language it is true of . This does not make the claims self-undermining (as with the standard objections to relativism); it doesn’t even mean that they are untestable. They are somewhat anomalous, but nothing follows concerning the speakers of the language in question (except that they cannot state the hypothesis using the basic vocabulary and grammar that they ordinarily use).
If there were a true hypothesis about the limits that basic English vocabulary and constructions puts on what English speakers can think, the hypothesis would turn out to be inexpressible in English, using basic vocabulary and the usual repertoire of constructions. That might mean it would be hard for us to discuss it in an article in English unless we used terminological innovations or syntactic workarounds. But that doesn’t imply anything about English speakers’ ability to grasp concepts, or to develop new ways of expressing them by coining new words or elaborated syntax.
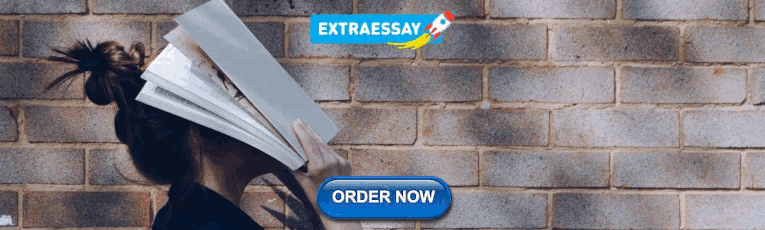
5. Constructing and evaluating Whorfian hypotheses
A number of considerations are relevant to formulating, testing, and evaluating Whorfian hypotheses.
Genuine hypotheses about the effects of language on thought will always have a duality: there will be a linguistic part and a non-linguistic one. The linguistic part will involve a claim that some feature is present in one language but absent in another.
Whorf himself saw that it was only obligatory features of languages that established “mental patterns” or “habitual thought” (Whorf 1956: 139), since if it were optional then the speaker could optionally do it one way or do it the other way. And so this would not be a case of “constraining the conceptual structure”. So we will likewise restrict our attention to obligatory features here.
Examples of relevant obligatory features would include lexical distinctions like the light vs. dark blue forced choice in Greek, or the forced choice between “in (fitting tightly)” vs. “in (fitting loosely)” in Korean. They also include grammatical distinctions like the forced choice in Spanish 2nd-person pronouns between informal/intimate and formal/distant (informal tú vs. formal usted in the singular; informal vosotros vs. formal ustedes in the plural), or the forced choice in Tamil 1st-person plural pronouns between inclusive (“we = me and you and perhaps others”) and exclusive (“we = me and others not including you”).
The non-linguistic part of a Whorfian hypothesis will contrast the psychological effects that habitually using the two languages has on their speakers. For example, one might conjecture that the habitual use of Spanish induces its speakers to be sensitive to the formal and informal character of the speaker’s relationship with their interlocutor while habitually using English does not.
So testing Whorfian hypotheses requires testing two independent hypotheses with the appropriate kinds of data. In consequence, evaluating them requires the expertise of both linguistics and psychology, and is a multidisciplinary enterprise. Clearly, the linguistic hypothesis may hold up where the psychological hypothesis does not, or conversely.
In addition, if linguists discovered that some linguistic feature was optional in two different languages, then even if psychological experiments showed differences between the two populations of speakers, this would not show linguistic determination or influence. The cognitive differences might depend on (say) cultural differences.
A further important consideration concerns the strength of the inducement relationship that a Whorfian hypothesis posits between a speaker’s language and their non-linguistic capacities. The claim that your language shapes or influences your cognition is quite different from the claim that your language makes certain kinds of cognition impossible (or obligatory) for you. The strength of any Whorfian hypothesis will vary depending on the kind of relationship being claimed, and the ease of revisability of that relation.
A testable Whorfian hypothesis will have a schematic form something like this:
- Linguistic part : Feature F is obligatory in L 1 but optional in L 2 .
- Psychological part : Speaking a language with obligatory feature F bears relation R to the cognitive effect C .
The relation R might in principle be causation or determination, but it is important to see that it might merely be correlation, or slight favoring; and the non-linguistic cognitive effect C might be readily suppressible or revisable.
Dan Slobin (1996) presents a view that competes with Whorfian hypotheses as standardly understood. He hypothesizes that when the speakers are using their cognitive abilities in the service of a linguistic ability (speaking, writing, translating, etc.), the language they are planning to use to express their thought will have a temporary online effect on how they express their thought. The claim is that as long as language users are thinking in order to frame their speech or writing or translation in some language, the mandatory features of that language will influence the way they think.
On Slobin’s view, these effects quickly attenuate as soon as the activity of thinking for speaking ends. For example, if a speaker is thinking for writing in Spanish, then Slobin’s hypothesis would predict that given the obligatory formal/informal 2nd-person pronoun distinction they would pay greater attention to the formal/informal character of their social relationships with their audience than if they were writing in English. But this effect is not permanent. As soon as they stop thinking for speaking, the effect of Spanish on their thought ends.
Slobin’s non-Whorfian linguistic relativist hypothesis raises the importance of psychological research on bilinguals or people who currently use two or more languages with a native or near-native facility. This is because one clear way to test Slobin-like hypotheses relative to Whorfian hypotheses would be to find out whether language correlated non-linguistic cognitive differences between speakers hold for bilinguals only when are thinking for speaking in one language, but not when they are thinking for speaking in some other language. If the relevant cognitive differences appeared and disappeared depending on which language speakers were planning to express themselves in, it would go some way to vindicate Slobin-like hypotheses over more traditional Whorfian Hypotheses. Of course, one could alternately accept a broadening of Whorfian hypotheses to include Slobin-like evanescent effects. Either way, attention must be paid to the persistence and revisability of the linguistic effects.
Kousta et al. (2008) shows that “for bilinguals there is intraspeaker relativity in semantic representations and, therefore, [grammatical] gender does not have a conceptual, non-linguistic effect” (843). Grammatical gender is obligatory in the languages in which it occurs and has been claimed by Whorfians to have persistent and enduring non-linguistic effects on representations of objects (Boroditsky et al. 2003). However, Kousta et al. supports the claim that bilinguals’ semantic representations vary depending on which language they are using, and thus have transient effects. This suggests that although some semantic representations of objects may vary from language to language, their non-linguistic cognitive effects are transitory.
Some advocates of Whorfianism have held that if Whorfian hypotheses were true, then meaning would be globally and radically indeterminate. Thus, the truth of Whorfian hypotheses is equated with global linguistic relativism—a well known self-undermining form of relativism. But as we have seen, not all Whorfian hypotheses are global hypotheses: they are about what is induced by particular linguistic features. And the associated non-linguistic perceptual and cognitive differences can be quite small, perhaps insignificant. For example, Thierry et al. (2009) provides evidence that an obligatory lexical distinction between light and dark blue affects Greek speakers’ color perception in the left hemisphere only. And the question of the degree to which this affects sensuous experience is not addressed.
The fact that Whorfian hypotheses need not be global linguistic relativist hypotheses means that they do not conflict with the claim that there are language universals. Structuralists of the first half of the 20th century tended to disfavor the idea of universals: Martin Joos’s characterization of structuralist linguistics as claiming that “languages can differ without limit as to either extent or direction” (Joos 1966, 228) has been much quoted in this connection. If the claim that languages can vary without limit were conjoined with the claim that languages have significant and permanent effects on the concepts and worldview of their speakers, a truly profound global linguistic relativism would result. But neither conjunct should be accepted. Joos’s remark is regarded by nearly all linguists today as overstated (and merely a caricature of the structuralists), and Whorfian hypotheses do not have to take a global or deterministic form.
John Lucy, a conscientious and conservative researcher of Whorfian hypotheses, has remarked:
We still know little about the connections between particular language patterns and mental life—let alone how they operate or how significant they are…a mere handful of empirical studies address the linguistic relativity proposal directly and nearly all are conceptually flawed. (Lucy 1996, 37)
Although further empirical studies on Whorfian hypotheses have been completed since Lucy published his 1996 review article, it is hard to find any that have satisfied the criteria of:
- adequately utilizing both the relevant linguistic and psychological research,
- focusing on obligatory rather than optional linguistic features,
- stating hypotheses in a clear testable way, and
- ruling out relevant competing Slobin-like hypotheses.
There is much important work yet to be done on testing the range of Whorfian hypotheses and other forms of linguistic conceptual relativism, and on understanding the significance of any Whorfian hypotheses that turn out to be well supported.
Copyright © 2024 by Barbara C. Scholz Francis Jeffry Pelletier < francisp @ ualberta . ca > Geoffrey K. Pullum < pullum @ gmail . com > Ryan Nefdt < ryan . nefdt @ uct . ac . za >
- Accessibility
Support SEP
Mirror sites.
View this site from another server:
- Info about mirror sites
The Stanford Encyclopedia of Philosophy is copyright © 2024 by The Metaphysics Research Lab , Department of Philosophy, Stanford University
Library of Congress Catalog Data: ISSN 1095-5054
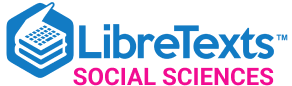
- school Campus Bookshelves
- menu_book Bookshelves
- perm_media Learning Objects
- login Login
- how_to_reg Request Instructor Account
- hub Instructor Commons
- Download Page (PDF)
- Download Full Book (PDF)
- Periodic Table
- Physics Constants
- Scientific Calculator
- Reference & Cite
- Tools expand_more
- Readability
selected template will load here
This action is not available.
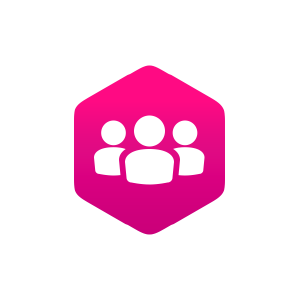
3.1: Linguistic Relativity- The Sapir-Whorf Hypothesis
- Last updated
- Save as PDF
- Page ID 75159
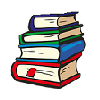
- Manon Allard-Kropp
- University of Missouri–St. Louis
Learning Objectives
After completing this module, students will be able to:
1. Define the concept of linguistic relativity
2. Differentiate linguistic relativity and linguistic determinism
3. Define the Sapir-Whorf Hypothesis (against more pop-culture takes on it) and situate it in a broader theoretical context/history
4. Provide examples of linguistic relativity through examples related to time, space, metaphors, etc.
In this part, we will look at language(s) and worldviews at the intersection of language & thoughts and language & cognition (i.e., the mental system with which we process the world around us, and with which we learn to function and make sense of it). Our main question, which we will not entirely answer but which we will examine in depth, is a chicken and egg one: does thought determine language, or does language inform thought?
We will talk about the Sapir-Whorf Hypothesis; look at examples that support the notion of linguistic relativity (pronouns, kinship terms, grammatical tenses, and what they tell us about culture and worldview); and then we will more specifically look into how metaphors are a structural component of worldview, if not cognition itself; and we will wrap up with memes. (Can we analyze memes through an ethnolinguistic, relativist lens? We will try!)
3.1 Linguistic Relativity: The Sapir-Whorf Hypothesis
In the 1920s, Benjamin Whorf was a graduate student studying with linguist Edward Sapir at Yale University in New Haven, Connecticut. Sapir, considered the father of American linguistic anthropology, was responsible for documenting and recording the languages and cultures of many Native American tribes, which were disappearing at an alarming rate. This was due primarily to the deliberate efforts of the United States government to force Native Americans to assimilate into the Euro-American culture. Sapir and his predecessors were well aware of the close relationship between culture and language because each culture is reflected in and influences its language. Anthropologists need to learn the language of the culture they are studying in order to understand the world view of its speakers. Whorf believed that the reverse is also true, that a language affects culture as well, by actually influencing how its speakers think. His hypothesis proposes that the words and the structures of a language influence how its speakers think about the world, how they behave, and ultimately the culture itself. (See our definition of culture in Part 1 of this document.) Simply stated, Whorf believed that human beings see the world the way they do because the specific languages they speak influence them to do so.
He developed this idea through both his work with Sapir and his work as a chemical engineer for the Hartford Insurance Company investigating the causes of fires. One of his cases while working for the insurance company was a fire at a business where there were a number of gasoline drums. Those that contained gasoline were surrounded by signs warning employees to be cautious around them and to avoid smoking near them. The workers were always careful around those drums. On the other hand, empty gasoline drums were stored in another area, but employees were more careless there. Someone tossed a cigarette or lighted match into one of the “empty” drums, it went up in flames, and started a fire that burned the business to the ground. Whorf theorized that the meaning of the word empty implied to the worker that “nothing” was there to be cautious about so the worker behaved accordingly. Unfortunately, an “empty” gasoline drum may still contain fumes, which are more flammable than the liquid itself.
Whorf ’s studies at Yale involved working with Native American languages, including Hopi. The Hopi language is quite different from English, in many ways. For example, let’s look at how the Hopi language deals with time. Western languages (and cultures) view time as a flowing river in which we are being carried continuously away from a past, through the present, and into a future. Our verb systems reflect that concept with specific tenses for past, present, and future. We think of this concept of time as universal, that all humans see it the same way. A Hopi speaker has very different ideas and the structure of their language both reflects and shapes the way they think about time. The Hopi language has no present, past, or future tense. Instead, it divides the world into what Whorf called the manifested and unmanifest domains. The manifested domain deals with the physical universe, including the present, the immediate past and future; the verb system uses the same basic structure for all of them. The unmanifest domain involves the remote past and the future, as well as the world of desires, thought, and life forces. The set of verb forms dealing with this domain are consistent for all of these areas, and are different from the manifested ones. Also, there are no words for hours, minutes, or days of the week. Native Hopi speakers often had great difficulty adapting to life in the English speaking world when it came to being “on time” for work or other events. It is simply not how they had been conditioned to behave with respect to time in their Hopi world, which followed the phases of the moon and the movements of the sun.
In a book about the Abenaki who lived in Vermont in the mid-1800s, Trudy Ann Parker described their concept of time, which very much resembled that of the Hopi and many of the other Native American tribes. “They called one full day a sleep, and a year was called a winter. Each month was referred to as a moon and always began with a new moon. An Indian day wasn’t divided into minutes or hours. It had four time periods—sunrise, noon, sunset, and midnight. Each season was determined by the budding or leafing of plants, the spawning of fish, or the rutting time for animals. Most Indians thought the white race had been running around like scared rabbits ever since the invention of the clock.”
The lexicon , or vocabulary, of a language is an inventory of the items a culture talks about and has categorized in order to make sense of the world and deal with it effectively. For example, modern life is dictated for many by the need to travel by some kind of vehicle—cars, trucks, SUVs, trains, buses, etc. We therefore have thousands of words to talk about them, including types of vehicles, models, brands, or parts.
The most important aspects of each culture are similarly reflected in the lexicon of its language. Among the societies living in the islands of Oceania in the Pacific, fish have great economic and cultural importance. This is reflected in the rich vocabulary that describes all aspects of the fish and the environments that islanders depend on for survival. For example, in Palau there are about 1,000 fish species and Palauan fishermen knew, long before biologists existed, details about the anatomy, behavior, growth patterns, and habitat of most of them—in many cases far more than modern biologists know even today. Much of fish behavior is related to the tides and the phases of the moon. Throughout Oceania, the names given to certain days of the lunar months reflect the likelihood of successful fishing. For example, in the Caroline Islands, the name for the night before the new moon is otolol , which means “to swarm.” The name indicates that the best fishing days cluster around the new moon. In Hawai`i and Tahiti two sets of days have names containing the particle `ole or `ore ; one occurs in the first quarter of the moon and the other in the third quarter. The same name is given to the prevailing wind during those phases. The words mean “nothing,” because those days were considered bad for fishing as well as planting.
Parts of Whorf ’s hypothesis, known as linguistic relativity , were controversial from the beginning, and still are among some linguists. Yet Whorf ’s ideas now form the basis for an entire sub-field of cultural anthropology: cognitive or psychological anthropology. A number of studies have been done that support Whorf ’s ideas. Linguist George Lakoff ’s work looks at the pervasive existence of metaphors in everyday speech that can be said to predispose a speaker’s world view and attitudes on a variety of human experiences. A metaphor is an expression in which one kind of thing is understood and experienced in terms of another entirely unrelated thing; the metaphors in a language can reveal aspects of the culture of its speakers. Take, for example, the concept of an argument. In logic and philosophy, an argument is a discussion involving differing points of view, or a debate. But the conceptual metaphor in American culture can be stated as ARGUMENT IS WAR. This metaphor is reflected in many expressions of the everyday language of American speakers: I won the argument. He shot down every point I made. They attacked every argument we made. Your point is right on target . I had a fight with my boyfriend last night. In other words, we use words appropriate for discussing war when we talk about arguments, which are certainly not real war. But we actually think of arguments as a verbal battle that often involve anger, and even violence, which then structures how we argue.
To illustrate that this concept of argument is not universal, Lakoff suggests imagining a culture where an argument is not something to be won or lost, with no strategies for attacking or defending, but rather as a dance where the dancers’ goal is to perform in an artful, pleasing way. No anger or violence would occur or even be relevant to speakers of this language, because the metaphor for that culture would be ARGUMENT IS DANCE.
3.1 Adapted from Perspectives , Language ( Linda Light, 2017 )
You can either watch the video, How Language Shapes the Way We Think, by linguist Lera Boroditsky, or read the script below.
Watch the video: How Language Shapes the Way We Think ( Boroditsky, 2018)
There are about 7,000 languages spoken around the world—and they all have different sounds, vocabularies, and structures. But do they shape the way we think? Cognitive scientist Lera Boroditsky shares examples of language—from an Aboriginal community in Australia that uses cardinal directions instead of left and right to the multiple words for blue in Russian—that suggest the answer is a resounding yes. “The beauty of linguistic diversity is that it reveals to us just how ingenious and how flexible the human mind is,” Boroditsky says. “Human minds have invented not one cognitive universe, but 7,000.”
Video transcript:
So, I’ll be speaking to you using language ... because I can. This is one these magical abilities that we humans have. We can transmit really complicated thoughts to one another. So what I’m doing right now is, I’m making sounds with my mouth as I’m exhaling. I’m making tones and hisses and puffs, and those are creating air vibrations in the air. Those air vibrations are traveling to you, they’re hitting your eardrums, and then your brain takes those vibrations from your eardrums and transforms them into thoughts. I hope.
I hope that’s happening. So because of this ability, we humans are able to transmit our ideas across vast reaches of space and time. We’re able to transmit knowledge across minds. I can put a bizarre new idea in your mind right now. I could say, “Imagine a jellyfish waltzing in a library while thinking about quantum mechanics.”
Now, if everything has gone relatively well in your life so far, you probably haven’t had that thought before.
But now I’ve just made you think it, through language.
Now of course, there isn’t just one language in the world, there are about 7,000 languages spoken around the world. And all the languages differ from one another in all kinds of ways. Some languages have different sounds, they have different vocabularies, and they also have different structures—very importantly, different structures. That begs the question: Does the language we speak shape the way we think? Now, this is an ancient question. People have been speculating about this question forever. Charlemagne, Holy Roman emperor, said, “To have a second language is to have a second soul”—strong statement that language crafts reality. But on the other hand, Shakespeare has Juliet say, “What’s in a name? A rose by any other name would smell as sweet.” Well, that suggests that maybe language doesn’t craft reality.
These arguments have gone back and forth for thousands of years. But until recently, there hasn’t been any data to help us decide either way. Recently, in my lab and other labs around the world, we’ve started doing research, and now we have actual scientific data to weigh in on this question.
So let me tell you about some of my favorite examples. I’ll start with an example from an Aboriginal community in Australia that I had a chance to work with. These are the Kuuk Thaayorre people. They live in Pormpuraaw at the very west edge of Cape York. What’s cool about Kuuk Thaayorre is, in Kuuk Thaayorre, they don’t use words like “left” and “right,” and instead, everything is in cardinal directions: north, south, east, and west. And when I say everything, I really mean everything. You would say something like, “Oh, there’s an ant on your southwest leg.” Or, “Move your cup to the north-northeast a little bit.” In fact, the way that you say “hello” in Kuuk Thaayorre is you say, “Which way are you going?” And the answer should be, “North-northeast in the far distance. How about you?”
So imagine as you’re walking around your day, every person you greet, you have to report your heading direction.
But that would actually get you oriented pretty fast, right? Because you literally couldn’t get past “hello,” if you didn’t know which way you were going. In fact, people who speak languages like this stay oriented really well. They stay oriented better than we used to think humans could. We used to think that humans were worse than other creatures because of some biological excuse: “Oh, we don’t have magnets in our beaks or in our scales.” No; if your language and your culture trains you to do it, actually, you can do it. There are humans around the world who stay oriented really well.
And just to get us in agreement about how different this is from the way we do it, I want you all to close your eyes for a second and point southeast.
Keep your eyes closed. Point. OK, so you can open your eyes. I see you guys pointing there, there, there, there, there ... I don’t know which way it is myself—
You have not been a lot of help.
So let’s just say the accuracy in this room was not very high. This is a big difference in cognitive ability across languages, right? Where one group—very distinguished group like you guys—doesn’t know which way is which, but in another group, I could ask a five-year-old and they would know.
There are also really big differences in how people think about time. So here I have pictures of my grandfather at different ages. And if I ask an English speaker to organize time, they might lay it out this way, from left to right. This has to do with writing direction. If you were a speaker of Hebrew or Arabic, you might do it going in the opposite direction, from right to left.
But how would the Kuuk Thaayorre, this Aboriginal group I just told you about, do it? They don’t use words like “left” and “right.” Let me give you hint. When we sat people facing south, they organized time from left to right. When we sat them facing north, they organized time from right to left. When we sat them facing east, time came towards the body. What’s the pattern? East to west, right? So for them, time doesn’t actually get locked on the body at all, it gets locked on the landscape. So for me, if I’m facing this way, then time goes this way, and if I’m facing this way, then time goes this way. I’m facing this way, time goes this way— very egocentric of me to have the direction of time chase me around every time I turn my body. For the Kuuk Thaayorre, time is locked on the landscape. It’s a dramatically different way of thinking about time.
Here’s another really smart human trait. Suppose I ask you how many penguins are there. Well, I bet I know how you’d solve that problem if you solved it. You went, “One, two, three, four, five, six, seven, eight.” You counted them. You named each one with a number, and the last number you said was the number of penguins. This is a little trick that you’re taught to use as kids. You learn the number list and you learn how to apply it. A little linguistic trick. Well, some languages don’t do this, because some languages don’t have exact number words. They’re languages that don’t have a word like “seven” or a word like “eight.” In fact, people who speak these languages don’t count, and they have trouble keeping track of exact quantities. So, for example, if I ask you to match this number of penguins to the same number of ducks, you would be able to do that by counting. But folks who don’t have that linguistic trait can’t do that.
Languages also differ in how they divide up the color spectrum—the visual world. Some languages have lots of words for colors, some have only a couple words, “light” and “dark.” And languages differ in where they put boundaries between colors. So, for example, in English, there’s a word for blue that covers all of the colors that you can see on the screen, but in Russian, there isn’t a single word. Instead, Russian speakers have to differentiate between light blue, goluboy , and dark blue, siniy . So Russians have this lifetime of experience of, in language, distinguishing these two colors. When we test people’s ability to perceptually discriminate these colors, what we find is that Russian speakers are faster across this linguistic boundary. They’re faster to be able to tell the difference between a light and a dark blue. And when you look at people’s brains as they’re looking at colors—say you have colors shifting slowly from light to dark blue—the brains of people who use different words for light and dark blue will give a surprised reaction as the colors shift from light to dark, as if, “Ooh, something has categorically changed,” whereas the brains of English speakers, for example, that don’t make this categorical distinction, don’t give that surprise, because nothing is categorically changing.
Languages have all kinds of structural quirks. This is one of my favorites. Lots of languages have grammatical gender; so every noun gets assigned a gender, often masculine or feminine. And these genders differ across languages. So, for example, the sun is feminine in German but masculine in Spanish, and the moon, the reverse. Could this actually have any consequence for how people think? Do German speakers think of the sun as somehow more female-like, and the moon somehow more male-like? Actually, it turns out that’s the case. So if you ask German and Spanish speakers to, say, describe a bridge, like the one here—“bridge” happens to be grammatically feminine in German, grammatically masculine in Spanish—German speakers are more likely to say bridges are “beautiful,” “elegant,” and stereotypically feminine words. Whereas Spanish speakers will be more likely to say they’re “strong” or “long,” these masculine words.
Languages also differ in how they describe events, right? You take an event like this, an accident. In English, it’s fine to say, “He broke the vase.” In a language like Spanish, you might be more likely to say, “The vase broke,” or “The vase broke itself.” If it’s an accident, you wouldn’t say that someone did it. In English, quite weirdly, we can even say things like, “I broke my arm.” Now, in lots of languages, you couldn’t use that construction unless you are a lunatic and you went out looking to break your arm—[laughter] and you succeeded. If it was an accident, you would use a different construction.
Now, this has consequences. So, people who speak different languages will pay attention to different things, depending on what their language usually requires them to do. So we show the same accident to English speakers and Spanish speakers, English speakers will remember who did it, because English requires you to say, “He did it; he broke the vase.” Whereas Spanish speakers might be less likely to remember who did it if it’s an accident, but they’re more likely to remember that it was an accident. They’re more likely to remember the intention. So, two people watch the same event, witness the same crime, but end up remembering different things about that event. This has implications, of course, for eyewitness testimony. It also has implications for blame and punishment. So if you take English speakers and I just show you someone breaking a vase, and I say, “He broke the vase,” as opposed to “The vase broke,” even though you can witness it yourself, you can watch the video, you can watch the crime against the vase, you will punish someone more, you will blame someone more if I just said, “He broke it,” as opposed to, “It broke.” The language guides our reasoning about events.
Now, I’ve given you a few examples of how language can profoundly shape the way we think, and it does so in a variety of ways. So language can have big effects, like we saw with space and time, where people can lay out space and time in completely different coordinate frames from each other. Language can also have really deep effects—that’s what we saw with the case of number. Having count words in your language, having number words, opens up the whole world of mathematics. Of course, if you don’t count, you can’t do algebra, you can’t do any of the things that would be required to build a room like this or make this broadcast, right? This little trick of number words gives you a stepping stone into a whole cognitive realm.
Language can also have really early effects, what we saw in the case of color. These are really simple, basic, perceptual decisions. We make thousands of them all the time, and yet, language is getting in there and fussing even with these tiny little perceptual decisions that we make. Language can have really broad effects. So the case of grammatical gender may be a little silly, but at the same time, grammatical gender applies to all nouns. That means language can shape how you’re thinking about anything that can be named by a noun. That’s a lot of stuff.
And finally, I gave you an example of how language can shape things that have personal weight to us—ideas like blame and punishment or eyewitness memory. These are important things in our daily lives.
Now, the beauty of linguistic diversity is that it reveals to us just how ingenious and how flexible the human mind is. Human minds have invented not one cognitive universe, but 7,000—there are 7,000 languages spoken around the world. And we can create many more—languages, of course, are living things, things that we can hone and change to suit our needs. The tragic thing is that we’re losing so much of this linguistic diversity all the time. We’re losing about one language a week, and by some estimates, half of the world’s languages will be gone in the next hundred years. And the even worse news is that right now, almost everything we know about the human mind and human brain is based on studies of usually American English-speaking undergraduates at universities. That excludes almost all humans. Right? So what we know about the human mind is actually incredibly narrow and biased, and our science has to do better.
I want to leave you with this final thought. I’ve told you about how speakers of different languages think differently, but of course, that’s not about how people elsewhere think. It’s about how you think. It’s how the language that you speak shapes the way that you think. And that gives you the opportunity to ask, “Why do I think the way that I do?” “How could I think differently?” And also, “What thoughts do I wish to create?”
Thank you very much.
Read the following text on what lexical differences between language can tell us about those languages’ cultures.
IResearchNet
Sapir-Whorf Hypothesis in Popular Psychology
The Sapir-Whorf Hypothesis or the linguistic relativity principle, formulated by Edward Sapir (1884–1936) and refined by his student, Benjamin Lee Whorf (1897–1941), states that human thinking is highly dependent on the language spoken by the individual thinker. As language is our main tool for organizing our experiences, the argument goes, the language that we use imposes limits both on what is experienced and on how it is expressed. The structure of the language spoken by a social group, in other words, influences their understanding of reality and therefore how they behave with respect to it.
Whorf was influenced by his study of the Hopi language, which does not contain any words or grammatical structures corresponding to notions of time; the language makes no reference to past, present, or future. From this, Whorf argues that the metaphysics embodied in the Hopi language is fundamentally different from that encoded in Western European languages such as English. This suggests that the fundamental worldview of the Hopi, and therefore their underlying thought processes, must be different from ours. Another favorite piece of evidence for the hypothesis involves the observation that the Inuit language has many different words for snow, depending on such characteristics as color, density, etc., whereas we in temperate climates merely call it by one word.
Academic Writing, Editing, Proofreading, And Problem Solving Services
Get 10% off with 24start discount code.
This hypothesis has been a favorite among opponents of Noam Chomsky’s ideas of a universal, deep structure to human languages and an innate language acquisition device; if different languages reflect or even determine different understandings of the world, then there is no underlying universal grammar. Evidence for this argument (also known as linguistic determinism—the idea that what we are capable of thinking is actually determined by the structure of our native language), however, is rather sparse, while countervailing evidence is all around. Consider the ability to learn foreign languages, for example. If the thought processes involved in the foreign languages were in fact alien to the mind of a speaker of another language, such learning should present almost insurmountable difficulties.
More difficult for Sapir-Whorf supporters, however, is the fact that their favorite pieces of evidence turn out simply not to be true. Ekkehart Malotki, an anthropologist who has studied the Hopi extensively, has shown that, contrary to Whorf’s claims, their language contains multiple tenses and words for units of time. Additionally, far from having no concept of time, they actually have fairly sophisticated methods for recording events. Regarding the Inuits, as far as anthropologists have been able to determine, there may be as many as a dozen words for snow, around the same number as in English: slush, melted snow, light powder, wet snow, dirty snow, or any of the other terms regularly heard during a New England winter. Outdoor enthusiasts may of course have more, as their activities may require them to distinguish among more varieties. Rather than suggesting that a cultural group’s language determines their worldview, the available evidence instead supports a weaker version of the Whorfian hypothesis: language does influence the way people perceive and remember their environment, and so it may predispose humans to look at the world in a certain way.
References:
- Hunter, E, and Agnoli, F. “The Whorfian Hypothesis: A Cognitive Psychology Perspective.” Psychological Review, 98(3) (1991): 377–390;
- Malotki, E. The Making of an Icon. Lincoln: University of Nebraska Press, 2000.
Click through the PLOS taxonomy to find articles in your field.
For more information about PLOS Subject Areas, click here .
Loading metrics
Open Access
Peer-reviewed
Research Article
The Sapir-Whorf Hypothesis and Probabilistic Inference: Evidence from the Domain of Color
Contributed equally to this work with: Emily Cibelli, Yang Xu
Affiliation Department of Linguistics, Northwestern University, Evanston, IL 60208, United States of America
Affiliations Department of Linguistics, University of California, Berkeley, CA 94720, United States of America, Cognitive Science Program, University of California, Berkeley, CA 94720, United States of America
Affiliation Department of Psychology, University of Wisconsin, Madison, WI 53706, United States of America
Affiliations Cognitive Science Program, University of California, Berkeley, CA 94720, United States of America, Department of Psychology, University of California, Berkeley, CA 94720, United States of America
* E-mail: [email protected]
- Emily Cibelli,
- Yang Xu,
- Joseph L. Austerweil,
- Thomas L. Griffiths,
- Terry Regier
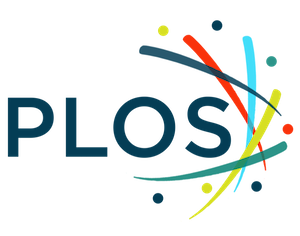
- Published: July 19, 2016
- https://doi.org/10.1371/journal.pone.0158725
- Reader Comments
16 Aug 2016: The PLOS ONE Staff (2016) Correction: The Sapir-Whorf Hypothesis and Probabilistic Inference: Evidence from the Domain of Color. PLOS ONE 11(8): e0161521. https://doi.org/10.1371/journal.pone.0161521 View correction
The Sapir-Whorf hypothesis holds that our thoughts are shaped by our native language, and that speakers of different languages therefore think differently. This hypothesis is controversial in part because it appears to deny the possibility of a universal groundwork for human cognition, and in part because some findings taken to support it have not reliably replicated. We argue that considering this hypothesis through the lens of probabilistic inference has the potential to resolve both issues, at least with respect to certain prominent findings in the domain of color cognition. We explore a probabilistic model that is grounded in a presumed universal perceptual color space and in language-specific categories over that space. The model predicts that categories will most clearly affect color memory when perceptual information is uncertain. In line with earlier studies, we show that this model accounts for language-consistent biases in color reconstruction from memory in English speakers, modulated by uncertainty. We also show, to our knowledge for the first time, that such a model accounts for influential existing data on cross-language differences in color discrimination from memory, both within and across categories. We suggest that these ideas may help to clarify the debate over the Sapir-Whorf hypothesis.
Citation: Cibelli E, Xu Y, Austerweil JL, Griffiths TL, Regier T (2016) The Sapir-Whorf Hypothesis and Probabilistic Inference: Evidence from the Domain of Color. PLoS ONE 11(7): e0158725. https://doi.org/10.1371/journal.pone.0158725
Editor: Daniel Osorio, University of Sussex, UNITED KINGDOM
Received: October 26, 2015; Accepted: June 21, 2016; Published: July 19, 2016
Copyright: © 2016 Cibelli et al. This is an open access article distributed under the terms of the Creative Commons Attribution License , which permits unrestricted use, distribution, and reproduction in any medium, provided the original author and source are credited.
Data Availability: All relevant data are available within the paper and/or at: https://github.com/yangxuch/probwhorfcolor This GitHub repository is mentioned in the paper.
Funding: This research was supported by the National Science Foundation ( www.nsf.gov ) under grants DGE-1106400 (EC) and SBE-1041707 (YX, TR). Publication was made possible in part by support from the Berkeley Research Impact Initiative (BRII) sponsored by the UC Berkeley Library. The funders had no role in study design, data collection and analysis, decision to publish, or preparation of the manuscript.
Competing interests: The authors have declared that no competing interests exist.
Introduction
The Sapir-Whorf hypothesis [ 1 , 2 ] holds that our thoughts are shaped by our native language, and that speakers of different languages therefore think about the world in different ways. This proposal has been controversial for at least two reasons, both of which are well-exemplified in the semantic domain of color. The first source of controversy is that the hypothesis appears to undercut any possibility of a universal foundation for human cognition. This idea sits uneasily with the finding that variation in color naming across languages is constrained, such that certain patterns of color naming recur frequently across languages [ 3 – 5 ], suggesting some sort of underlying universal basis. The second source of controversy is that while some findings support the hypothesis, they do not always replicate reliably. Many studies have found that speakers of a given language remember and process color in a manner that reflects the color categories of their language [ 6 – 13 ]. Reinforcing the idea that language is implicated in these findings, it has been shown that the apparent effect of language on color cognition disappears when participants are given a verbal [ 7 ] (but not a visual) interference task [ 8 , 11 , 12 ]; this suggests that language may operate through on-line use of verbal representations that can be temporarily disabled. However, some of these findings have a mixed record of replication [ 14 – 17 ]. Thus, despite the substantial empirical evidence already available, the role of language in color cognition remains disputed.
An existing theoretical stance holds the potential to resolve both sources of controversy. On the one hand, it explains effects of language on cognition in a framework that retains a universal component, building on a proposal by Kay and Kempton [ 7 ]. On the other hand, it has the potential to explain when effects of language on color cognition will appear, and when they will not—and why. This existing stance is that of the “category adjustment” model of Huttenlocher and colleagues [ 18 , 19 ]. We adopt this stance, and cast color memory as inference under uncertainty, instantiated in a category adjustment model, following Bae et al. [ 20 ] and Persaud and Hemmer [ 21 ]. The model holds that color memory involves the probabilistic combination of evidence from two sources: a fine-grained representation of the particular color seen, and the language-specific category in which it fell (e.g. English green ). Both sources of evidence are represented in a universal perceptual color space, yet their combination yields language-specific bias patterns in memory, as illustrated in Fig 1 . The model predicts that such category effects will be strongest when fine-grained perceptual information is uncertain. It thus has the potential to explain the mixed pattern of replications of Whorfian effects in the literature: non-replications could be the result of high perceptual certainty.
- PPT PowerPoint slide
- PNG larger image
- TIFF original image
A stimulus is encoded in two ways: (1) a fine-grained representation of the stimulus itself, shown as a (gray) distribution over stimulus space centered at the stimulus’ location in that space, and (2) the language-specific category (e.g. English “green”) in which the stimulus falls, shown as a separate (green) distribution over the same space, centered at the category prototype. The stimulus is reconstructed by combining these two sources of information through probabilistic inference, resulting in a reconstruction of the stimulus (black distribution) that is biased toward the category prototype. Adapted from Fig 11 of Bae et al. (2015) [ 20 ].
https://doi.org/10.1371/journal.pone.0158725.g001
In the category adjustment model, both the fine-grained representation of the stimulus and the category in which it falls are modeled as probability distributions over a universal perceptual color space. The fine-grained representation is veridical (unbiased) but inexact: its distribution is centered at the location in color space where the stimulus itself fell, and the variance of that distribution captures the observer’s uncertainty about the precise location of the stimulus in color space, with greater variance corresponding to greater uncertainty. Psychologically, such uncertainty might be caused by noise in perception itself, by memory decay over time, or by some other cause—and any increase in such uncertainty is modeled by a wider, flatter distribution for the fine-grained representation. The category distribution, in contrast, captures the information about stimulus location that is given by the named category in which the stimulus fell (e.g. green for an English-speaking observer). Because named color categories vary across languages, this category distribution is assumed to be language-specific—although the space over which it exists is universal. The model infers the original stimulus location by combining evidence from both of these distributions. As a result, the model tends to produce reconstructions of the stimulus that are biased away from the actual location of the stimulus and toward the prototype of the category in which it falls.
As illustrated in Fig 2 , this pattern of bias pulls stimuli on opposite sides of a category boundary in opposite directions, producing enhanced distinctiveness for such stimuli. Such enhanced distinctiveness across a category boundary is the signature of categorical perception, or analogous category effects in memory. On this view, language-specific effects on memory can emerge from a largely universal substrate when one critical component of that substrate is language-specific: the category distribution.
Model reconstructions tend to be biased toward category prototypes, yielding enhanced distinctiveness for two stimuli that fall on different sides of a category boundary. Categories are shown as distributions in green and blue; stimuli are shown as vertical black lines; reconstruction bias patterns are shown as arrows.
https://doi.org/10.1371/journal.pone.0158725.g002
If supported, the category adjustment model holds the potential to clarify the debate over the Sapir-Whorf hypothesis in three ways. First, it would link that debate to independent principles of probabilistic inference. In so doing, it would underscore the potentially important role of uncertainty , whether originating in memory or perception, in framing the debate theoretically. Second, and relatedly, it would suggest a possible reason why effects of language on color memory and perception are sometimes found, and sometimes not [ 17 ]. Concretely, the model predicts that greater uncertainty in the fine-grained representation—induced for example through a memory delay, or noise in perception—will lead to greater influence of the category, and thus a stronger bias in reproduction. The mirror-image of this prediction is that in situations of relatively high certainty in memory or perception, there will be little influence of the category, to the point that such an influence may not be empirically detectable. Third, the model suggests a way to think about the Sapir-Whorf hypothesis without jettisoning the important idea of a universal foundation for cognition.
Closely related ideas appear in the literature on probabilistic cue integration [ 22 – 25 ]. For example, Ernst and Banks [ 24 ] investigated perceptual integration of cues from vision and touch in judging the height of an object. They found that humans integrate visual and haptic cues in a statistically optimal fashion, modulated by cue certainty. The category adjustment model we explore here can be seen as a form of probabilistic cue integration in which one of the cues is a language-specific category.
The category adjustment model has been used to account for category effects in various domains, including spatial location [ 18 , 26 ], object size [ 19 , 27 ], and vowel perception [ 28 ]. The category adjustment model also bears similarities to other theoretical accounts of the Sapir-Whorf hypothesis that emphasize the importance of verbal codes [ 7 , 8 ], and the interplay of such codes with perceptual representations [ 29 – 31 ]. Prior research has linked such category effects to probabilistic inference, following the work of Huttenlocher and colleagues [ 18 , 19 ]. Roberson and colleagues [ 32 ] invoked the category adjustment model as a possible explanation for categorical perception of facial expressions, but did not explore a formal computational model; Goldstone [ 33 ] similarly referenced the category adjustment model with respect to category effects in the color domain. Persaud and Hemmer [ 21 , 34 ] explored bias in memory for color, and compared empirically obtained memory bias patterns from English speakers with results predicted by a formally specified category adjustment model, but did not link those results to the debate over the Sapir-Whorf hypothesis, and did not manipulate uncertainty. More recently, a subsequent paper by the same authors and colleagues [ 35 ] explored category-induced bias in speakers of another language, Tsimané, and did situate those results with respect to the Sapir-Whorf hypothesis, but again did not manipulate uncertainty. Most recently, Bae et al. [ 20 ] extensively documented bias in color memory in English speakers, modeled those results with a category-adjustment computational model, and did manipulate uncertainty—but did not explore these ideas relative to the Sapir-Whorf hypothesis, or to data from different languages.
In what follows, we first present data and computational simulations that support the recent finding that color memory in English speakers is well-predicted by a category adjustment model, with the strength of category effects modulated by uncertainty. We then show, to our knowledge for the first time, that a category adjustment model accounts for influential existing cross-language data on color that support the Sapir-Whorf hypothesis.
In this section we provide general descriptions of our analyses and results. Full details are supplied in the section on Materials and Methods.
Study 1: Color reconstruction in English speakers
Our first study tests the core assumptions of the category adjustment model in English speakers. In doing so, it probes questions that were pursued by two studies that appeared recently, after this work had begun. Persaud and Hemmer [ 21 ] and Bae et al. [ 20 ] both showed that English speakers’ memory for a color tends to be biased toward the category prototype of the corresponding English color term, in line with a category adjustment model. Bae et al. [ 20 ] also showed that the amount of such bias increases when subjects must retain the stimulus in memory during a delay period, compared to when there is no such delay, as predicted by the principles of the category adjustment model. In our first study, we consider new evidence from English speakers that tests these questions, prior to considering speakers of different languages in our following studies.
English-speaking participants viewed a set of hues that varied in small steps from dark yellow to purple, with most hues corresponding to some variety of either green or blue. We collected two kinds of data from these participants: bias data and naming data. Bias data were based on participants’ non-linguistic reconstruction of particular colors seen. Specifically, for each hue seen, participants recreated that hue by selecting a color from a color wheel, either while the target was still visible ( Fig 3A : simultaneous condition), or from memory after a short delay ( Fig 3B : delayed condition). We refer to the resulting data as bias data, because we are interested in the extent to which participants’ reconstructions of the stimulus color are biased away from the original target stimulus. Afterwards, the same participants indicated how good an example of English green (as in Fig 3C ) and how good an example of English blue each hue was. We refer to these linguistic data as naming data.
Screenshots of example trials illustrating (A) simultaneous reconstruction, (B) delayed reconstruction, and (C) green goodness rating.
https://doi.org/10.1371/journal.pone.0158725.g003
Fig 4 shows both naming and bias data as a function of target hue. The top panel of the figure shows the naming data and also shows Gaussian functions corresponding to the English color terms green and blue that we fitted to the naming data. Bias data were collected for only a subset of the hues for which naming data were collected, and the shaded region in the top panel of Fig 4 shows that subset, relative to the full range of hues for naming data. We collected bias data only in this smaller range because we were interested specifically in bias induced by the two color terms blue and green , and colors outside the shaded region seemed to us to clearly show some influence of neighboring categories such as yellow and purple . The bottom panel of the figure shows the bias data, plotted relative to the prototypes (means) of the fitted Gaussian functions for green and blue . It can be seen that reconstruction bias appears to be stronger in the delayed than in the simultaneous condition, as predicted, and that—especially in the delayed condition—there is an inflection in the bias pattern between the two category prototypes, suggesting that bias may reflect the influence of each of the two categories. The smaller shaded region in this bottom panel denotes the subset of these hues that we subsequently analyzed statistically, and to which we fit models. We reduced the range of considered hues slightly further at this stage, to ensure that the range was well-centered with respect to the two relevant category prototypes, for green and blue , as determined by the naming data.
In both top and bottom panels, the horizontal axis denotes target hue, ranging from yellow on the left to purple on the right. Top panel (naming data): The solid green and blue curves show, for each target hue, the average goodness rating for English green and blue respectively, as a proportion of the maximum rating possible. The dashed green and blue curves show Gaussian functions fitted to the naming goodness data. The dotted vertical lines marked at the bottom with green and blue squares denote the prototypes for green and blue , determined as the means of the green and blue fitted Gaussian functions, respectively. The shaded region in the top panel shows the portion of the spectrum for which bias data were collected. Bottom panel (bias data): Solid curves denote, for each target hue, the average reconstruction bias for that hue, such that positive values denote reconstruction bias toward the purple (here, right) end of the spectrum, and negative values denote reconstruction bias toward the yellow (here, left) end of the spectrum. Units for the vertical axis are the same as for the horizontal axis, which is normalized to length 1.0. The black and red curves show bias under simultaneous and delayed response, respectively. Blue stars at the top of the bottom panel mark hues for which there was a significant difference in the magnitude of bias between simultaneous and delayed conditions. The shaded region in the bottom panel shows the portion of the data that was analyzed statistically, and to which models were fit. In both panels, error bars represent standard error of the mean.
https://doi.org/10.1371/journal.pone.0158725.g004
The absolute values (magnitudes) of the bias were analyzed using a 2 (condition: simultaneous vs. delayed) × 15 (hues) repeated measures analysis of variance. This analysis revealed significantly greater bias magnitude in the delayed than in the simultaneous condition. It also revealed that bias magnitude differed significantly as a function of hue, as well as a significant interaction between the factors of hue and condition. The blue stars in Fig 4 denote hues for which the difference in bias magnitude between the simultaneous and delayed conditions reached significance. The finding of greater bias magnitude in the delayed than in the simultaneous condition is consistent with the proposal that uncertainty is an important mediating factor in such category effects, as argued by Bae et al. [ 20 ]. It also suggests that some documented failures to find such category effects could in principle be attributable to high certainty, a possibility that can be explored by manipulating uncertainty.
We wished to test in a more targeted fashion to what extent these data are consistent with a category adjustment model in which a color is reconstructed based in part on English named color categories. To that end, we compared the performance of four models against these data; only one of these models considered both of the relevant English color categories, green and blue . As in Fig 1 , each model contains a fine-grained but inexact representation of the perceived stimulus, and (for most models) a representation of one or more English color categories. Each model predicts the reconstruction of the target stimulus from its fine-grained representation of the target together with any category information. Category information in the model is specified by the naming data. Each model has a single free parameter, corresponding to the uncertainty of the fine-grained representation; this parameter is fit to bias data.
- The null model is a baseline model that predicts hue reconstruction based only on the fine-grained representation of the stimulus, with no category component.
- The 1-category (green) model predicts hue reconstruction based on the fine-grained representation of the stimulus, combined with a representation of only the green category, derived from the green naming data.
- The 1-category (blue) model predicts hue reconstruction based on the fine-grained representation of the stimulus, combined with a representation of only the blue category, derived from the blue naming data.
- The 2-category model predicts hue reconstruction based on the fine-grained representation of the stimulus, combined with representations of both the green and blue categories.
If reproduction bias reflects probabilistic inference from a fine-grained representation of the stimulus itself, together with any relevant category, we would expect the 2-category model to outperform the others. The other models have access either to no category information at all (null model), or to category information for only one of the two relevant color categories (only one of green and blue ). The 2-category model in contrast combines fine-grained stimulus information with both of the relevant categories ( green and blue ); this model thus corresponds most closely to a full category adjustment model.
Fig 5 redisplays the data from simultaneous and delayed reconstruction, this time with model fits overlaid. The panels in the left column show data from simultaneous reconstruction, fit by each of the four models, and the panels in the right column analogously show data and model fits from delayed reconstruction. Visually, it appears that in the case of delayed reconstruction, the 2-category model fits the data at least qualitatively better than competing models: it shows an inflection in bias as the empirical data do, although not as strongly. For simultaneous reconstruction, the 2-category model fit is also reasonable but visually not as clearly superior to the others (especially the null model) as in the delayed condition.
Left column: Bias from simultaneous reconstruction, fit by each of the four models. The empirical data (black lines with error bars) in these four panels are the same, and only the model fits (red lines) differ. Within each panel, the horizontal axis denotes target hue, and the vertical axis denotes reconstruction bias. The green and blue prototypes are indicated as vertical lines with green and blue squares at the bottom. Right column: delayed reconstruction, displayed analogously.
https://doi.org/10.1371/journal.pone.0158725.g005
Table 1 reports quantitative results of these model fits. The best fit is provided by the 2-category model, in both the simultaneous and delayed conditions, whether assessed by log likelihood (LL) or by mean squared errror (MSE). In line with earlier studies [ 20 , 21 ], these findings demonstrate that a category adjustment model that assumes stimulus reconstruction is governed by relevant English color terms provides a reasonable fit to data on color reconstruction by English speakers. The category adjustment model fits well both when the category bias is relatively slight (simultaneous condition), and when the bias is stronger (delayed condition).
LL = log likelihood (higher is better). MSE = mean squared error (lower is better). The best value in each row is shown in bold .
https://doi.org/10.1371/journal.pone.0158725.t001
Study 2: Color discrimination across languages
The study above examined the categories of just one language, English, whereas the Sapir-Whorf hypothesis concerns cross-language differences in categorization, and their effect on cognition and perception. Empirical work concerning this hypothesis has not specifically emphasized bias in reconstruction, but there is a substantial amount of cross-language data of other sorts against which the category adjustment model can be assessed. One method that has been extensively used to explore the Sapir-Whorf hypothesis in the domain of color is a two-alternative forced choice (2AFC) task. In such a task, participants first are briefly shown a target color, and then shortly afterward are shown that same target color together with a different distractor color, and are asked to indicate which was the color originally seen. A general finding from such studies [ 8 – 10 ] is that participants exhibit enhanced discrimination for pairs of colors that would be named differently in their native language. For example, in such a 2AFC task, speakers of English show enhanced discrimination for colors from the different English categories green and blue , compared with colors from the same category (either both green or both blue ) [ 8 ]. In contrast, speakers of the Berinmo language, which has named color categories that differ from those of English, show enhanced discrimination across Berinmo category boundaries, and not across those of English [ 9 ]. Thus color discrimination in this task is enhanced at the boundaries of native language categories, suggesting an effect of those native language categories on the ability to discriminate colors from memory.
Considered informally, this qualitative pattern of results appears to be consistent with the category adjustment model, as suggested above in Fig 2 . We wished to determine whether such a model would also provide a good quantitative account of such results, when assessed using the specific color stimuli and native-language naming patterns considered in the empirical studies just referenced.
We considered cross-language results from two previous studies by Debi Roberson and colleagues, one that compared color memory in speakers of English and Berinmo, a language of Papua New Guinea [ 9 ], and another that explored color memory in speakers of Himba, a language of Namibia [ 10 ]. Berinmo and Himba each have five basic color terms, in contrast with eleven in English. The Berinmo and Himba color category systems are similar to each other in broad outline, but nonetheless differ noticeably. Following these two previous studies, we considered the following pairs of categories in these three languages:
- the English categories green and blue ,
- the Berinmo categories wor (covering roughly yellow, orange, and brown), and nol (covering roughly green, blue, and purple), and
- the Himba categories dumbu (covering roughly yellow and beige) and burou (covering roughly green, blue, and purple).
These three pairs of categories are illustrated in Fig 6 , using naming data from Roberson et al. (2000) [ 9 ] and Roberson et al. (2005) [ 10 ]. It can be seen that the English green - blue distinction is quite different from the Berinmo wor - nol and the Himba dumbu - burou distinctions, which are similar but not identical to each other. The shaded regions in this figure indicate specific colors that were probed in discrimination tasks. The shaded (probed) region that straddles a category boundary in Berinmo and Himba falls entirely within the English category green , and the shaded (probed) region that straddles a category boundary in English falls entirely within the Berinmo category nol and the Himba category burou , according to naming data in Fig 1 of Roberson et al. (2005) [ 10 ]. The empirical discrimination data in Fig 7 are based on those probed colors [ 9 , 10 ], and show that in general, speakers of a language tend to exhibit greater discrimination for pairs of colors that cross a category boundary in their native language, consistent with the Sapir-Whorf hypothesis.
The English categories green and blue (top panel), the Berinmo categories wor and nol (middle panel), and the Himba categories dumbu and burou (bottom panel), plotted against a spectrum of hues that ranges from dark yellow at the left, through green, to blue at the right. Colored squares mark prototypes: the shared prototype for Berinmo wor and Himba dumbu , and the prototypes for English green and blue ; the color of each square approximates the color of the corresponding prototype. For each language, the dotted-and-dashed vertical lines denote the prototypes for the two categories from that language, and the dashed vertical line denotes the empirical boundary between these two categories. Black curves show the probability of assigning a given hue to each of the two native-language categories, according to the category component of a 2-category model fit to each language’s naming data. The shaded regions mark the ranges of colors probed in discrimination tasks; these two regions are centered at the English green - blue boundary and the Berinmo wor - nol boundary. Data are from Roberson et al. (2000) [ 9 ] and Roberson et al. (2005) [ 10 ].
https://doi.org/10.1371/journal.pone.0158725.g006
Top panels: Discrimination from memory by Berinmo and English speakers for pairs of colors across and within English and Berinmo color category boundaries. Empirical data are from Table 11 of Roberson et al. (2000:392). Empirical values show mean proportion correct 2AFC memory judgments, and error bars show standard error. Model values show mean model proportion correct 2AFC memory judgments after simulated reconstruction with native-language categories. Model results are range-matched to the corresponding empirical values, such that the minimum and maximum model values match the minimum and maximum mean values in the corresponding empirical dataset, and other model values are linearly interpolated. Bottom panels: Discrimination from memory by Himba and English speakers for pairs of colors across and within English and Himba color category boundaries, compared with model results based on native-language categories. Empirical data are from Table 6 of Roberson et al. (2005:400); no error bars are shown because standard error was not reported in that table.
https://doi.org/10.1371/journal.pone.0158725.g007
We sought to determine whether the 2-category model explored above could account for these data. To that end, for each language, we created a version of the 2-category model based on the naming data for that language. Thus, we created an English model in which the two categories were based on empirical naming data for green and blue , a Berinmo model in which the two categories were based on empirical naming data for wor and nol , and a Himba model in which the two categories were based on empirical naming data for dumbu and burou . The black curves in Fig 6 show the probability of assigning a given hue to each of the two native-language categories, according to the category component of a 2-category model fit to each language’s naming data. Given this category information, we simulated color reconstruction from memory for the specific colors considered in the empirical studies [ 9 , 10 ] (the colors in the shaded regions in Fig 6 ). We did so separately for the cases of English, Berinmo, and Himba, in each case fitting a model based on naming data for a given language to discrimination data from speakers of that language. As in Study 1, we fit the model parameter corresponding to the uncertainty of fine-grained perceptual representation to the empirical non-linguistic (here discrimination) data, and we used a single value for this parameter across all three language models. The model results are shown in Fig 7 , beside the empirical data to which they were fit. The models provide a reasonable match to the observed cross-language differences in discrimination. Specifically, the stimulus pairs for which empirical performance is best are those that cross a native-language boundary—and these are stimulus pairs for which the corresponding model response is strongest.
Although not shown in the figure, we also conducted a followup analysis to test whether the quality of these fits was attributable merely to model flexibility, or to a genuine fit between a language’s category system and patterns of discrimination from speakers of that language. We did this by switching which language’s model was fit to which language’s discrimination data. Specifically, we fit the model based on Berinmo naming to the discrimination data from English speakers (and vice versa), and fit the model based on Himba naming to the discrimination data from English speakers (and vice versa), again adjusting the model parameter corresponding to the uncertainty of the fine-grained perceptual representation to the empirical discrimination data. The results are summarized in Table 2 . It can be seen that the discrimination data are fit better by native-language models (that is, models with a category component originally fit to that language’s naming data) than by other-language models (that is, models with a category component originally fit to another language’s naming data). These results suggest that cross-language differences in discrimination may result from category-induced reconstruction bias under uncertainty, guided by native-language categories.
The best value in each row is shown in bold . Data are fit better by native-language models than by other-language models.
https://doi.org/10.1371/journal.pone.0158725.t002
Study 3: Within-category effects
Although many studies of categorical perception focus on pairs of stimuli that cross category boundaries, there is also evidence for category effects within categories. In a 2AFC study of categorical perception of facial expressions, Roberson and colleagues [ 32 ] found the behavioral signature of categorical perception (or more precisely in this case, categorical memory): superior discrimination for cross-category than for within-category pairs of stimuli. But in addition, they found an interesting category effect on within-category pairs, dependent on order of presentation. For each within-category pair they considered, one stimulus of the pair was always closer to the category prototype (the “good exemplar”) than the other (the “poor exemplar”). They found that 2AFC performance on within-category pairs was better when the target was the good exemplar (and the distractor was therefore the poor exemplar) than when the target was the poor exemplar (and the distractor was therefore the good exemplar)—even though the same stimuli were involved in the two cases. Moreover, performance in the former (good exemplar) case did not differ significantly from cross-category performance. Hanley and Roberson [ 36 ] subsequently reanalyzed data from a number of earlier studies that had used 2AFC tasks to explore cross-language differences in color naming and cognition, including those reviewed and modeled in the previous section. Across studies and across domains, including color, they found the same asymmetrical within-category effect originally documented for facial expressions.
This within-category pattern may be naturally explained in category-adjustment terms, as shown in Fig 8 , and as argued by Roberson and colleagues [ 32 ]. The central idea is that because the target is held in memory, it is subject to bias toward the prototype in memory, making discrimination of target from distractor either easier or harder depending on which of the two stimuli is the target. Although this connection with the category adjustment model has been made in the literature in general conceptual terms [ 32 ], followup studies have been theoretically focused elsewhere [ 31 , 36 ], and the idea has not to our knowledge been tested computationally using the specific stimuli and naming patterns involved in the empirical studies. We sought to do so.
The category adjustment model predicts: (top panel, good exemplar) easy within-category discrimination in a 2AFC task when the initially-presented target t is closer to the prototype than the distractor d is; (bottom panel, poor exemplar) difficult within-category discrimination with the same two stimuli when the initially-presented target t is farther from the prototype than the distractor d is. Category is shown as a distribution in blue; stimuli are shown as vertical black lines marked t and d; reconstruction bias patterns are shown as arrows.
https://doi.org/10.1371/journal.pone.0158725.g008
The empirical data in Fig 9 illustrate the within-category effect with published results on color discrimination by speakers of English, Berinmo, and Himba. In attempting to account for these data, we considered again the English, Berinmo, and Himba variants of the 2-category model first used in Study 2, and also retained from that study the parameter value corresponding to the uncertainty of the fine-grained perceptual representation, in the case of native-language models. We simulated reconstruction from memory of the specific colors examined in Study 2. Following the empirical analyses, this time we disaggregated the within-category stimulus pairs into those in which the target was a good exemplar of the category (i.e. the target was closer to the prototype than the distractor was), vs. those in which the target was a poor exemplar of the category (i.e. the target was farther from the prototype than the distractor was). The model results are shown in Fig 9 , and match the empirical data reasonably well, supporting the informal in-principle argument of Fig 8 with a more detailed quantitative analysis.
Across: stimulus pair crosses the native-language boundary; GE: within-category pair, target is the good exemplar; PE: within-category pair, target is the poor exemplar. Empirical data are from Figs 2 (English: 10-second retention interval), 3 (Berinmo), and 4 (Himba) of Hanley and Roberson [ 36 ]. Empirical values show mean proportion correct 2AFC memory judgments, and error bars show standard error. Model values show mean model proportion correct 2AFC memory judgments after simulated reconstruction using native-language categories, range-matched as in Fig 7 . English model compared with English data: 0.00002 MSE; Berinmo model compared with Berinmo data: 0.00055 MSE; Himba model compared with Himba data: 0.00087 MSE.
https://doi.org/10.1371/journal.pone.0158725.g009
Conclusions
We have argued that the debate over the Sapir-Whorf hypothesis may be clarified by viewing that hypothesis in terms of probabilistic inference. To that end, we have presented a probabilistic model of color memory, building on proposals in the literature. The model assumes both a universal color space and language-specific categorical partitionings of that space, and infers the originally perceived color from these two sources of evidence. The structure of this model maps naturally onto a prominent proposal in the literature that has to our knowledge not previously been formalized in these terms. In a classic early study of the effect of language on color cognition, Kay and Kempton [ 7 ] interpret Whorf [ 2 ] as follows:
Whorf […] suggests that he conceives of experience as having two tiers: one, a kind of rock bottom, inescapable seeing-things-as-they-are (or at least as human beings cannot help but see them), and a second, in which [the specific structures of a given language] cause us to classify things in ways that could be otherwise (and are otherwise for speakers of a different language).
Kay and Kempton argue that color cognition involves an interaction between these two tiers. The existence of a universal groundwork for color cognition helps to explain why there are constraints on color naming systems across languages [ 3 – 5 , 37 ]. At the same time, Kay and Kempton acknowledge a role for the language-specific tier in cognition, such that “there do appear to be incursions of linguistic categorization into apparently nonlinguistic processes of thinking” (p. 77). These two tiers map naturally onto the universal and language-specific components of the model we have explored here. This structure offers a straightforward way to think about effects of language on cognition while retaining the idea of a universal foundation underpinning human perception and cognition. Thus, this general approach, and our model as an instance of it, offer a possible resolution of one source of controversy surrounding the Sapir-Whorf hypothesis: taking that hypothesis seriously need not entail a wholesale rejection of important universal components of human cognition.
The approach proposed here also has the potential to resolve another source of controversy surrounding the Sapir-Whorf hypothesis: that some findings taken to support it do not replicate reliably (e.g. in the case of color: [ 15 – 17 ]). Framing the issue in terms of probabilistic inference touches this question by highlighting the theoretically central role of uncertainty , as in models of probabilistic cue integration [ 24 ]. We have seen stronger category-induced bias in color memory under conditions of greater delay and presumably therefore greater uncertainty (Study 1, and [ 20 ]). This suggests that in the inverse case of high certainty about the stimulus, any category effect could in principle be so small as to be empirically undetectable, a possibility that can be pursued by systematically manipulating uncertainty. Thus, the account advanced here casts the Sapir-Whorf hypothesis in formal terms that suggest targeted and quantitative followup tests. A related theoretical advantage of uncertainty is that it highlights an important level of generality: uncertainty could result from memory, as explored here, but it could also result from noise or ambiguity in perception itself, and on the view advanced here, the result should be the same.
The model we have proposed does not cover all aspects of language effects on color cognition. For example, there are documented priming effects [ 31 ] which do not appear to flow as naturally from this account as do the other effects we have explored above. However, the model does bring together disparate bodies of data in a simple framework, and links them to independent principles of probabilistic inference. Future research can usefully probe the generality and the limitations of the ideas we have explored here.
Materials and Methods
Code and data supporting the analyses reported here are available at https://github.com/yangxuch/probwhorfcolor.git .
The perception of stimulus S = s produces a fine-grained memory M , and a categorical code c specifying the category in which s fell. We wish to reconstruct the original stimulus S = s , given M and c .
https://doi.org/10.1371/journal.pone.0158725.g010
Null model.
1-category model.
2-category model.
Fitting models to data.
Participants.
Twenty subjects participated in the experiment, having been recruited at UC Berkeley. All subjects were at least 18 years of age, native English speakers, and reported normal or corrected-to-normal vision, and no colorblindness. All subjects received payment or course credit for participation.
Informed consent was obtained verbally; all subjects read an approved consent form and verbally acknowledged their willingness to participate in the study. Verbal consent was chosen because the primary risk to subjects in this study was for their names to be associated with their response; this approach allowed us to obtain consent and collect data without the need to store subjects’ names in any form. Once subjects acknowledged that they understood the procedures and agreed to participate by stating so to the experimenter, the experimenter recorded their consent by assigning them a subject number, which was anonymously linked to their data. All study procedures, including those involving consent, were overseen and approved by the UC Berkeley Committee for the Protection of Human Subjects.
Stimuli were selected by varying a set of hues centered around the blue - green boundary, holding saturation and lightness constant. Stimuli were defined in Munsell coordinate space, which is widely used in the literature we engage here (e.g. [ 9 , 10 ]). All stimuli were at lightness 6 and saturation 8. Hue varied from 5Y to 10P, in equal hue steps of 2.5. Colors were converted to xyY coordinate space following Table I(6.6.1) of Wyszecki and Stiles (1982) [ 38 ]. The colors were implemented in Matlab in xyY; the correspondence of these coordinate systems in the stimulus set, as well as approximate visualizations of the stimuli, are reported in Table 3 .
All stimuli were presented at lightness 6, saturation 8 in Munsell space.
https://doi.org/10.1371/journal.pone.0158725.t003
- Full range: We collected naming data for green and blue relative to the full range, stimuli 1-27, for a total of 27 stimuli. We fit the category components of our models to naming data over this full range.
- Medium range: We collected bias data for a subset of the full range, namely the medium range, stimuli 5-23, for a total of 19 stimuli. We considered this subset because we were interested in bias induced by the English color terms green and blue , and we had the impression, prior to collecting naming or bias data, that colors outside this medium range had some substantial element of the neighboring categories yellow and purple .
- Focused range: Once we had naming data, we narrowed the range further based on those data, to the focused range, stimuli 5-19, for a total of 15 stimuli. The focused range extends between the (now empirically assessed) prototypes for green and blue , and also includes three of our stimulus hues on either side of these prototypes, yielding a range well-centered relative to those prototypes, as can be seen in the bottom panel of Fig 4 above. We considered this range in our statistical analyses, and in our modeling of bias patterns.
Experimental procedure.
The experiment consisted of four blocks. The first two blocks were reconstruction (bias) tasks: one simultaneous block and one delay block. In the simultaneous block ( Fig 3A ), the subject was shown a stimulus color as a colored square (labeled as “Original” in the figure), and was asked to recreate that color in a second colored square (labeled as “Target” in the figure) as accurately as possible by selecting a hue from a color wheel. The (“Original”) stimulus color remained on screen while the subject selected a response from the color wheel; navigation of the color wheel would change the color of the response (“Target”) square. The stimulus square and response square each covered 4.5 degrees of visual angle, and the color wheel covered 11.1 degrees of visual angle. Target colors were drawn from the medium range of stimuli (stimuli 5—23 of Table 3 ). The color wheel was constructed based on the full range of stimuli (stimuli 1—27 of Table 3 ), supplemented by interpolating 25 points evenly in xyY coordinates between each neighboring pair of the 27 stimuli of the full range, to create a finely discretized continuum from yellow to purple, with 677 possible responses. Each of the 19 target colors of the medium range was presented five times per block in random order, for a total of 95 trials per block. The delay block ( Fig 3B ) was similar to the simultaneous block but with the difference that the stimulus color was shown for 500 milliseconds then disappeared, then a fixation cross was shown for 1000 milliseconds, after which the subject was asked to reconstruct the target color from memory, again using the color wheel to change the color of the response square. The one colored square shown in the final frame of Fig 3B is the response square that changed color under participant control. The order of the simultaneous block and delay block were counterbalanced by subject. Trials were presented with a 500 millisecond inter-trial interval.
Several steps were taken to ensure that responses made on the color wheel during the reconstruction blocks were not influenced by bias towards a particular spatial position. The position of the color wheel was randomly rotated up to 180 degrees from trial to trial. The starting position of the cursor was likewise randomly generated for each new trial. Finally, the extent of the spectrum was jittered one or two stimuli (2.5 or 5 hue steps) from trial to trial, which had the effect of shifting the spectrum slightly in the yellow or the purple direction from trial to trial. This was done to ensure that the blue - green boundary would not fall at a consistent distance from the spectrum endpoints on each trial.
The second two blocks were naming tasks. In each, subjects were shown each of the 27 stimuli of the full range five times in random order, for a total of 135 trials per block. On each trial, subjects were asked to rate how good an example of a given color name each stimulus was. In one block, the color name was green , in the other, the color name was blue ; order of blocks was counterbalanced by subject. To respond, subjects positioned a slider bar with endpoints “Not at all [green/blue]” and “Perfectly [green/blue]” to the desired position matching their judgment of each stimulus, as shown above in Fig 3C . Responses in the naming blocks were self-paced. Naming blocks always followed reconstruction blocks, to ensure that repeated exposure to the color terms green and blue did not bias responses during reconstruction.
The experiment was presented in Matlab version 7.11.0 (R2010b) using Psychtoolbox (version 3) [ 39 – 41 ]. The experiment was conducted in a dark, sound-attenuated booth on an LCD monitor that supported 24-bit color. The monitor had been characterized using a Minolta CS100 colorimeter. A chin rest was used to ensure that each subject viewed the screen from a constant position; when in position, the base of the subject’s chin was situated 30 cm from the screen.
As part of debriefing after testing was complete, each subject was asked to report any strategies they used during the delay block to help them remember the target color. Summaries of each response, as reported by the experimenter, are listed in Table 4 .
When subjects gave specific examples of color terms used as memory aids, they are reported here.
https://doi.org/10.1371/journal.pone.0158725.t004
Color spectrum.
We wished to consider our stimuli along a 1-dimensional spectrum such that distance between two colors on that spectrum approximates the perceptual difference between those colors. To this end, we first converted our stimuli to CIELAB color space. CIELAB is a 3-dimensional color space designed “in an attempt to provide coordinates for colored stimuli so that the distance between the coordinates of any two stimuli is predictive of the perceived color difference between them” (p. 202 of [ 42 ]). The conversion to CIELAB was done according to the equations on pp. 167-168 of Wyszecki and Stiles (1982) [ 38 ], assuming 2 degree observer and D65 illuminant. For each pair of neighboring colors in the set of 677 colors of our color wheel, we measured the distance (Δ E ) betwen these two colors in CIELAB space. We then arranged all colors along a 1-dimensional spectrum that was scaled to length 1, such that the distance between each pair of neighboring colors along that spectrum was proportional to the CIELAB Δ E distance between them. This CIELAB-based 1-dimensional spectrum was used for our analyses in Study 1, and an analogous spectrum for a different set of colors was used for our analyses in Studies 2 and 3.
Statistical analysis.
As a result of the experiment detailed above, we obtained bias data from 20 participants, for each of 19 hues (the medium range), for 5 trials per hue per participant, in each of the simultaneous and delayed conditions. For analysis purposes, we restricted attention to the focused range of stimuli (15 hues), in order to consider a region of the spectrum that is well-centered with respect to green and blue , as we are primarily interested in bias that may be induced by these two categories. We wished to determine whether the magnitude of the bias differed as a function of the simultaneous vs. delayed condition, whether the magnitude of the bias varied as a function of hue, and whether there was an interaction between these two factors. To answer those questions, we conducted a 2 (condition: simultaneous vs. delayed) × 15 (hues) repeated measures analysis of variance (ANOVA), in which the dependent measure was the absolute value of the reproduction bias (reproduced hue minus target hue), averaged across trials for a given participant at a given target hue in a given condition. The ANOVA included an error term to account for across-subject variability. We found a main effect of condition, with greater bias magnitude in the delayed than in the simultaneous condition [ F (1, 19) = 61.61, p < 0.0001], a main effect of hue [ F (14, 266) = 4.565, p < 0.0001], and an interaction of hue and condition [ F (14, 266) = 3.763, p < 0.0001]. All hue calculations were relative to the CIELAB-based spectrum detailed in the preceding section.
We then conducted paired t-tests at each of the target hues, comparing each participant’s bias magnitude for that hue (averaged over trials) in the simultaneous condition vs. the delayed condition. Blue asterisks at the top of Fig 4 mark hues for which the paired t-test returned p < 0.05 when applying Bonferroni corrections for multiple comparisons.
Modeling procedure.
We considered four models in accounting for color reconstruction in English speakers: the null model, a 1-category model for which the category was green , a 1-category model for which the category was blue , and a 2-category model based on both green and blue .
Empirical data.
The empirical data considered for this study were drawn from two sources: the study of 2AFC color discrimination by speakers of Berinmo and English in Experiment 6a of Roberson et al. (2000) [ 9 ], and the study of 2AFC color discrimination by speakers of Himba and English in Experiment 3b of Roberson et al. (2005) [ 10 ]. In both studies, two sets of color stimuli were considered, all at value (lightness) level 5, and chroma (saturation) level 8. Both sets varied in hue by increments of 2.5 Munsell hue steps. The first set of stimuli was centered at the English green - blue boundary (hue 7.5BG), and contained the following seven hues: 10G, 2.5BG, 5BG, 7.5BG, 10BG, 2.5B, 5B. The second set of stimuli was centered at the Berinmo wor - nol boundary (hue 5GY), and contained the following seven hues: 7.5Y, 10Y, 2.5GY, 5GY, 7.5GY, 10GY, 2.5G. Stimuli in the set that crossed an English category boundary all fell within a single category in Berinmo ( nol ) and in Himba ( burou ), and stimuli in the set that crossed a Berinmo category boundary also crossed a Himba category boundary ( dumbu - burou ) but all fell within a single category in English ( green ), according to naming data in Fig 1 of Roberson et al. (2005) [ 10 ]. Based on specifications in the original empirical studies [ 9 , 10 ], we took the pairs of stimuli probed to be those presented in Table 5 .
Any stimulus pair that includes a boundary color is considered to be a cross-category pair. All hues are at value (lightness) level 5, and chroma (saturation) level 8. 1s denotes a 1-step pair; 2s denotes a 2-step pair.
https://doi.org/10.1371/journal.pone.0158725.t005
Based on naming data in Fig 1 of Roberson et al. 2005 [ 10 ], we took the prototypes of the relevant color terms to be:
English green prototype = 10GY
English blue prototype = 10B
Berinmo wor prototype = 5Y
Berinmo nol prototype = 5G
Himba dumbu prototype = 5Y
Himba burou prototype = 10G
Fig 6 above shows a spectrum of hues ranging from the Berinmo wor prototype (5Y) to the English blue prototype (10B) in increments of 2.5 Munsell hue steps, categorized according to each of the three languages we consider here. These Munsell hues were converted to xyY and then to CIELAB as above, and the positions of the hues on the spectrum were adjusted so that the distance between each two neighboring hues in the spectrum is proportional to the CIELAB Δ E distance between them. We use this CIELAB-based spectrum for our analyses below. The two shaded regions on each spectrum in Fig 6 denote the two target sets of stimuli identified above.
The discrimination data we modeled were drawn from Table 11 of Roberson et al. (2000:392) [ 9 ] and Table 6 of Roberson et al. (2005:400) [ 10 ].
We considered three variants of the 2-category model: an English blue - green model, a Berinmo wor - nol model, and a Himba dumbu - burou model. As in Study 1, we fit each model to the data in two steps. For each language’s model, we first fit the category component of that model to naming data from that language. Because color naming differs across these languages, this resulted in three models with different category components. For each model, we then retained and fixed the resulting category parameter settings, and fit the single remaining parameter, corresponding to memory uncertainty, to discrimination data. We detail these two steps below.
The empirical data considered for this study are those of Figs 2 (English green/blue , 10 second delay), 3 (Berinmo wor/nol ), and 4 (Himba dumbu/borou ) of Hanley and Roberson (2011) [ 36 ]. These data were originally published by Roberson and Davidoff (2000) [ 8 ], Roberson et al. (2000) [ 9 ], and Roberson et al. (2005) [ 10 ], respectively. The Berinmo and Himba stimuli and data were the same as in our Study 2, but the English stimuli and data reanalyzed by Hanley and Roberson (2011) [ 36 ] Fig 2 were instead drawn from Table 1 of Roberson and Davidoff (2000) [ 8 ], reproduced here in Table 6 , and used for the English condition of this study. These stimuli for English were at lightness (value) level 4, rather than 5 as for the other two languages. We chose to ignore this difference for modeling purposes.
Any stimulus pair that includes a boundary color is considered to be a cross-category pair. All hues are at value (lightness) level 4, and chroma (saturation) level 8. 1s denotes a 1-step pair; 2s denotes a 2-step pair.
https://doi.org/10.1371/journal.pone.0158725.t006
All modeling procedures were identical to those of Study 2, with the exception that GE (target = good exemplar) and PE (target = poor exemplar) cases were disaggregated, and analyzed separately.
Acknowledgments
We thank Roland Baddeley, Paul Kay, Charles Kemp, Steven Piantadosi, and an anonymous reviewer for their comments.
Author Contributions
Conceived and designed the experiments: EC YX JLA TLG TR. Performed the experiments: EC YX JLA. Analyzed the data: EC YX JLA. Wrote the paper: TR EC YX.
- View Article
- Google Scholar
- 2. Whorf BL. Science and linguistics. In: Carroll JB, editor. Language, Thought, and Reality: Selected Writings of Benjamin Lee Whorf. MIT Press; 1956. p. 207–219.
- 3. Berlin B, Kay P. Basic color terms: Their universality and evolution. University of California Press; 1969.
- 5. Kay P, Berlin B, Maffi L, Merrifield WR, Cook R. The World Color Survey. CSLI Publications; 2009.
- PubMed/NCBI
- 21. Persaud K, Hemmer P. The influence of knowledge and expectations for color on episodic memory. In: Bello P, Guarini M, McShane M, Scassellati B, editors. Proceedings of the 36th Annual Meeting of the Cognitive Science Society. Cognitive Science Society; 2014. p. 1162–1167.
- 22. Yuille AL, Bülthoff HH. Bayesian decision theory and psychophysics. In: Knill DC, Richards W, editors. Perception as Bayesian Inference. Cambridge University Press; 1996. p. 123–162.
- 35. Hemmer P, Persaud K, Kidd C, Piantadosi S. Inferring the Tsimane’s use of color categories from recognition memory. In: Noelle DC, Dale R, Warlaumont AS, Yoshimi J, Matlock T, Jennings CD, et al., editors. Proceedings of the 37th Annual Meeting of the Cognitive Science Society. Cognitive Science Society; 2015. p. 896–901.
- 38. Wyszecki G, Stiles WS. Color science: Concepts and methods, quantitative data and formulae. Wiley; 1982.
- 42. Brainard DH. Color appearance and color difference specification. In: Shevell SK, editor. The science of color: Second edition. Elsevier; 2003. p. 191–216.
- 43. Luce RD. Detection and recognition. In: Luce RD, Bush RR, Galanter E, editors. Handbook of mathematical psychology. Wiley; 1963. p. 103–189.
Whorfian Hypothesis
- Reference work entry
- pp 1566–1567
- Cite this reference work entry
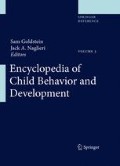
- Seongwon Yun 3 &
- Shelia M. Kennison 3
725 Accesses
This is a preview of subscription content, log in via an institution to check access.
Access this chapter
- Available as PDF
- Read on any device
- Instant download
- Own it forever
- Available as EPUB and PDF
- Durable hardcover edition
- Dispatched in 3 to 5 business days
- Free shipping worldwide - see info
Tax calculation will be finalised at checkout
Purchases are for personal use only
Institutional subscriptions
Berlin, B., & Kay, P. (1969). Basic color terms: Their universality and evolution . Berkeley, CA: University of California Press.
Google Scholar
Gordon, P. (2004). Numerical cognition without words: Evidence from Amazonia. Science, 306 , 496–499.
PubMed Google Scholar
Heider, E., & Olivier, D. (1972). The structure of the color space in naming and memory for two languages. Cognitive Psychology, 3 , 337–354.
Hunt, E., & Agnoli, F. (1991). The Whorfian hypothesis: A cognitive approach. Psychological Review, 98 , 377–389.
Pica, P., Lemer, C., & Izard, V. (2004). Exact and approximate calculation in an Amazonian indigene group with a reduced number lexicon. Science, 306 , 499–503.
Roberson, D., Davies, I., & Davidoff, J. (2000). Colour categories are not universal: Replications and new evidence from a Stone-Age culture. Journal of Experimental Psychology: Genera, 129 , 369–398.
Whorf, B. L. (1956). Language, thought, and reality . Cambridge, MA: MIT Press.
Download references
Author information
Authors and affiliations.
Department of Psychology, Oklahoma State University, 116 North Murray Hall, Stillwater, OK, 74078, USA
Seongwon Yun & Shelia M. Kennison
You can also search for this author in PubMed Google Scholar
Editor information
Editors and affiliations.
Neurology, Learning and Behavior Center, 230 South 500 East, Suite 100, Salt Lake City, Utah, 84102, USA
Sam Goldstein Ph.D.
Department of Psychology MS 2C6, George Mason University, Fairfax, VA, 22030, USA
Jack A. Naglieri Ph.D. ( Professor of Psychology ) ( Professor of Psychology )
Rights and permissions
Reprints and permissions
Copyright information
© 2011 Springer Science+Business Media, LLC
About this entry
Cite this entry.
Yun, S., Kennison, S.M. (2011). Whorfian Hypothesis. In: Goldstein, S., Naglieri, J.A. (eds) Encyclopedia of Child Behavior and Development. Springer, Boston, MA. https://doi.org/10.1007/978-0-387-79061-9_3087
Download citation
DOI : https://doi.org/10.1007/978-0-387-79061-9_3087
Publisher Name : Springer, Boston, MA
Print ISBN : 978-0-387-77579-1
Online ISBN : 978-0-387-79061-9
eBook Packages : Behavioral Science
Share this entry
Anyone you share the following link with will be able to read this content:
Sorry, a shareable link is not currently available for this article.
Provided by the Springer Nature SharedIt content-sharing initiative
- Publish with us
Policies and ethics
- Find a journal
- Track your research
- Subject List
- Take a Tour
- For Authors
- Subscriber Services
- Publications
- African American Studies
- African Studies
- American Literature
- Anthropology
- Architecture Planning and Preservation
- Art History
- Atlantic History
- Biblical Studies
- British and Irish Literature
- Childhood Studies
- Chinese Studies
- Cinema and Media Studies
- Communication
- Criminology
- Environmental Science
- Evolutionary Biology
- International Law
- International Relations
- Islamic Studies
- Jewish Studies
- Latin American Studies
- Latino Studies
Linguistics
- Literary and Critical Theory
- Medieval Studies
- Military History
- Political Science
- Public Health
- Renaissance and Reformation
- Social Work
- Urban Studies
- Victorian Literature
- Browse All Subjects
How to Subscribe
- Free Trials
In This Article Expand or collapse the "in this article" section Edward Sapir
Introduction, the essential sapir.
- The Collected Works of Edward Sapir Project
- Biography and Background
- American Linguistics, American Anthropology, and Sapir
- Time Perspective in Aboriginal American Culture : The Meeting of Linguistics and Ethnology
- Linguistic Relativity and the Sapir-Whorf Hypothesis
- Psychology and Culture
- Culture and Personality
- Work with Ishi, the “Last Yahi”
- Correspondence
- Sapir and His Circle
- Sapir the Humanist
- Appraisals and Legacies
- Miscellaneous
Related Articles Expand or collapse the "related articles" section about
About related articles close popup.
Lorem Ipsum Sit Dolor Amet
Vestibulum ante ipsum primis in faucibus orci luctus et ultrices posuere cubilia Curae; Aliquam ligula odio, euismod ut aliquam et, vestibulum nec risus. Nulla viverra, arcu et iaculis consequat, justo diam ornare tellus, semper ultrices tellus nunc eu tellus.
- Dene (Athabaskan) Languages
- Descriptive Grammar
- Endangered Languages
- Genealogical Classification
- History of Linguistics
- Indigenous Languages of the Americas
- Interface Between Phonology and Phonetics
- Linguistic Anthropology
- Linguistic Relativity
- The Dené-Yeniseian Hypothesis
Other Subject Areas
Forthcoming articles expand or collapse the "forthcoming articles" section.
- Cognitive Grammar
- Edward Sapir
- Teaching Pragmatics
- Find more forthcoming articles...
- Export Citations
- Share This Facebook LinkedIn Twitter
Edward Sapir by James Stanlaw LAST MODIFIED: 11 January 2024 DOI: 10.1093/obo/9780199772810-0318
Edward Sapir (b. 1884–d. 1939) is one of the most important figures in the history of American linguistics and anthropology before the Second World War. Both disciplines would likely be drastically different today had Sapir’s influence not been felt. He was recognized by his contemporaries as the finest Americanist (specialist on the languages of Native America) of his day. His investigations of Takelma, Paiute, Yana, and Wishram are definitive, and his work on other Native American languages—from Athabaskan to Uto-Aztecan—really have not been superseded. His work on phonetic transcription, and theoretical and practical phonemics, was seminal. His curiosity and wide-ranging explorations touched on almost all aspects of linguistics, and some of his ideas were quite prescient. For example, Sapir’s work on sound symbolism—largely neglected at the time (1929)—has recently received renewed attention. Sapir is also thought by anthropologists to be one of the discipline’s most original and profound theorists: He did groundbreaking work on culture and personality studies, cultural psychology, and the relationship between the individual and culture. He continues to be widely read. Together with Franz Boas—often said to be the father of American anthropology, and who instigated the field at the turn of the 20th century—Sapir insured that linguistics—especially how language relates to culture and thought—would be one of the new discipline’s four primary subfields. Indeed, debates on “linguistic relativity,” or the so-called Sapir-Whorf Hypothesis—the degrees and ways language may affect thought, experience, or perception—even today occupy the professional time of many in linguistics, anthropology, psychology, philosophy, and cognitive science. These issues are often contentious, and even have made their appearance in popular culture. Sapir was president of both the Linguistic Society of America (1933) and the American Anthropological Association (1938), an obvious indication of his stature in both fields.
To date, there has been no book-length secondary work on Sapir’s linguistics, anthropology, or psychology. Nonetheless, we are fortunate to have a good edited compilation of Sapir’s key articles in the form of Mandelbaum 1985 . Also of interest is a contemporary review of this collection, Harris 1951 . Darnell 2010 (cited under Biography and Background ) is an excellent, perhaps definitive, intellectual biography, and Sapir 2004 is an approachable book written by Sapir himself for a popular audience. Dinneen 1995 gives a brief introductory overview of Sapir’s technical style.
Dinneen, Francis. 1995. Edward Sapir. In General linguistics . By Francis Dinneen, 259–284. Washington, DC: Georgetown Univ. Press.
Gives a quick introductory summary of what Sapir was doing when he was engaged in formal linguistic analysis.
Harris, Zellig. 1951. Review of Selected writings of Edward Sapir in language, culture, and personality . Language 27.3: 288–333.
DOI: 10.2307/409757
A lengthy and technical review of the Selected Writings collection by one of the most important figures in American structural linguistics in the 1950s (and a teacher of Noam Chomsky). Though coming at things from a rather different angle than Sapir, Harris is nonetheless quite positive, saying things like Sapir’s writing was “often an artistic expression” (p. 330).
Mandelbaum, David, ed. 1985. Selected writings of Edward Sapir in language, culture, and personali ty. Rev. ed. Berkeley: Univ. of California Press.
First edition published 1949. Since then, it has been reprinted in paperback and is still readily available. For several generations of students, this was their main introduction to Sapir’s work, and this book does actually contains many classic pieces. Key articles here include: “The Psychological Reality of Phonemes,” “Time Perspective in Aboriginal American Culture,” and “The Unconscious Patterning of Behavior in Society” (some of these are discussed further under The Collected Works of Edward Sapir Project ). Included is a complete bibliography of Sapir’s published writings.
Sapir, Edward. 2004. Language : An introduction to the study of speech . New York: Dover.
Originally published in 1921 and reprinted by Harcourt Brace and World in 1949. In the 1960s it was reissued in paperback in several editions and has never gone out of print since—for good reason. This is exemplar Sapir: concise, erudite, and witty, showing Sapir’s breadth and depth, and his almost limitless knowledge of languages.
back to top
Users without a subscription are not able to see the full content on this page. Please subscribe or login .
Oxford Bibliographies Online is available by subscription and perpetual access to institutions. For more information or to contact an Oxford Sales Representative click here .
- About Linguistics »
- Meet the Editorial Board »
- Acceptability Judgments
- Acquisition, Second Language, and Bilingualism, Psycholin...
- Adpositions
- African Linguistics
- Afroasiatic Languages
- Algonquian Linguistics
- Altaic Languages
- Ambiguity, Lexical
- Analogy in Language and Linguistics
- Animal Communication
- Applicatives
- Applied Linguistics, Critical
- Arawak Languages
- Argument Structure
- Artificial Languages
- Australian Languages
- Austronesian Linguistics
- Auxiliaries
- Balkans, The Languages of the
- Baudouin de Courtenay, Jan
- Berber Languages and Linguistics
- Bilingualism and Multilingualism
- Biology of Language
- Borrowing, Structural
- Caddoan Languages
- Caucasian Languages
- Celtic Languages
- Celtic Mutations
- Chomsky, Noam
- Chumashan Languages
- Classifiers
- Clauses, Relative
- Clinical Linguistics
- Cognitive Linguistics
- Colonial Place Names
- Comparative Reconstruction in Linguistics
- Comparative-Historical Linguistics
- Complementation
- Complexity, Linguistic
- Compositionality
- Compounding
- Computational Linguistics
- Conditionals
- Conjunctions
- Connectionism
- Consonant Epenthesis
- Constructions, Verb-Particle
- Contrastive Analysis in Linguistics
- Conversation Analysis
- Conversation, Maxims of
- Conversational Implicature
- Cooperative Principle
- Coordination
- Creoles, Grammatical Categories in
- Critical Periods
- Cross-Language Speech Perception and Production
- Cyberpragmatics
- Default Semantics
- Definiteness
- Dementia and Language
- Dené-Yeniseian Hypothesis, The
- Dependencies
- Dependencies, Long Distance
- Derivational Morphology
- Determiners
- Dialectology
- Distinctive Features
- Dravidian Languages
- English as a Lingua Franca
- English, Early Modern
- English, Old
- Eskimo-Aleut
- Euphemisms and Dysphemisms
- Evidentials
- Exemplar-Based Models in Linguistics
- Existential
- Existential Wh-Constructions
- Experimental Linguistics
- Fieldwork, Sociolinguistic
- Finite State Languages
- First Language Attrition
- Formulaic Language
- Francoprovençal
- French Grammars
- Gabelentz, Georg von der
- Generative Syntax
- Genetics and Language
- Grammar, Categorial
- Grammar, Construction
- Grammar, Descriptive
- Grammar, Functional Discourse
- Grammars, Phrase Structure
- Grammaticalization
- Harris, Zellig
- Heritage Languages
- History of the English Language
- Hmong-Mien Languages
- Hokan Languages
- Humor in Language
- Hungarian Vowel Harmony
- Idiom and Phraseology
- Imperatives
- Indefiniteness
- Indo-European Etymology
- Inflected Infinitives
- Information Structure
- Interjections
- Iroquoian Languages
- Isolates, Language
- Jakobson, Roman
- Japanese Word Accent
- Jones, Daniel
- Juncture and Boundary
- Khoisan Languages
- Kiowa-Tanoan Languages
- Kra-Dai Languages
- Labov, William
- Language Acquisition
- Language and Law
- Language Contact
- Language Documentation
- Language, Embodiment and
- Language for Specific Purposes/Specialized Communication
- Language, Gender, and Sexuality
- Language Geography
- Language Ideologies and Language Attitudes
- Language in Autism Spectrum Disorders
- Language Nests
- Language Revitalization
- Language Shift
- Language Standardization
- Language, Synesthesia and
- Languages of Africa
- Languages of the Americas, Indigenous
- Languages of the World
- Learnability
- Lexical Access, Cognitive Mechanisms for
- Lexical Semantics
- Lexical-Functional Grammar
- Lexicography
- Lexicography, Bilingual
- Linguistic Accommodation
- Linguistic Areas
- Linguistic Landscapes
- Linguistic Prescriptivism
- Linguistic Profiling and Language-Based Discrimination
- Linguistics, Educational
- Listening, Second Language
- Literature and Linguistics
- Machine Translation
- Maintenance, Language
- Mande Languages
- Mass-Count Distinction
- Mathematical Linguistics
- Mayan Languages
- Mental Health Disorders, Language in
- Mental Lexicon, The
- Mesoamerican Languages
- Minority Languages
- Mixed Languages
- Mixe-Zoquean Languages
- Modification
- Mon-Khmer Languages
- Morphological Change
- Morphology, Blending in
- Morphology, Subtractive
- Munda Languages
- Muskogean Languages
- Nasals and Nasalization
- Niger-Congo Languages
- Non-Pama-Nyungan Languages
- Northeast Caucasian Languages
- Oceanic Languages
- Papuan Languages
- Penutian Languages
- Philosophy of Language
- Phonetics, Acoustic
- Phonetics, Articulatory
- Phonological Research, Psycholinguistic Methodology in
- Phonology, Computational
- Phonology, Early Child
- Policy and Planning, Language
- Politeness in Language
- Positive Discourse Analysis
- Possessives, Acquisition of
- Pragmatics, Acquisition of
- Pragmatics, Cognitive
- Pragmatics, Computational
- Pragmatics, Cross-Cultural
- Pragmatics, Developmental
- Pragmatics, Experimental
- Pragmatics, Game Theory in
- Pragmatics, Historical
- Pragmatics, Institutional
- Pragmatics, Second Language
- Prague Linguistic Circle, The
- Presupposition
- Psycholinguistics
- Quechuan and Aymaran Languages
- Reading, Second-Language
- Reciprocals
- Reduplication
- Reflexives and Reflexivity
- Register and Register Variation
- Relevance Theory
- Representation and Processing of Multi-Word Expressions in...
- Salish Languages
- Sapir, Edward
- Saussure, Ferdinand de
- Second Language Acquisition, Anaphora Resolution in
- Semantic Maps
- Semantic Roles
- Semantic-Pragmatic Change
- Semantics, Cognitive
- Sentence Processing in Monolingual and Bilingual Speakers
- Sign Language Linguistics
- Sociolinguistics
- Sociolinguistics, Variationist
- Sociopragmatics
- Sound Change
- South American Indian Languages
- Specific Language Impairment
- Speech, Deceptive
- Speech Perception
- Speech Production
- Speech Synthesis
- Switch-Reference
- Syntactic Change
- Syntactic Knowledge, Children’s Acquisition of
- Tense, Aspect, and Mood
- Text Mining
- Tone Sandhi
- Transcription
- Transitivity and Voice
- Translanguaging
- Translation
- Trubetzkoy, Nikolai
- Tucanoan Languages
- Tupian Languages
- Usage-Based Linguistics
- Uto-Aztecan Languages
- Valency Theory
- Verbs, Serial
- Vocabulary, Second Language
- Voice and Voice Quality
- Vowel Harmony
- Whitney, William Dwight
- Word Classes
- Word Formation in Japanese
- Word Recognition, Spoken
- Word Recognition, Visual
- Word Stress
- Writing, Second Language
- Writing Systems
- Zapotecan Languages
- Privacy Policy
- Cookie Policy
- Legal Notice
- Accessibility
Powered by:
- [66.249.64.20|195.158.225.244]
- 195.158.225.244
PSYCHOLOGY GCSE: LANG T&C - SAPIR WHORF HYPOTHESIS P1. The Study with Me Podcast
Hey guys, another episode out - woo!! So as promised, questions of the episode and answers to LAST WEEKS because I forgot to record them for this week, soz. Anyway answers here: 1. Piaget's theory refers to the idea that thought comes before language. In order to express a concept, an individual must have a schema of it. 2. Sapir Whorf 3. Schema refers to a mental structure that stores information and gets more complex with more information and experience 4. Preoperational 5. Face validity, child language development, but schemas are not possible to physically measure and so lack of scientific physical evidence, and lastly, the Sapir Whorf hypothesis opposes the theory and has more evidence to support. NOW THIS WEEKS: 1. What was the evidence to support the Sapir Whorf hypothesis? 2. What is language determinism 3. What is the weak version of the hypothesis? 4. What is a criticism of the hypothesis 5. How many words for snow did the Eskimos really have?
- More Episodes
- Malika Mamajonova
Top Podcasts In Education
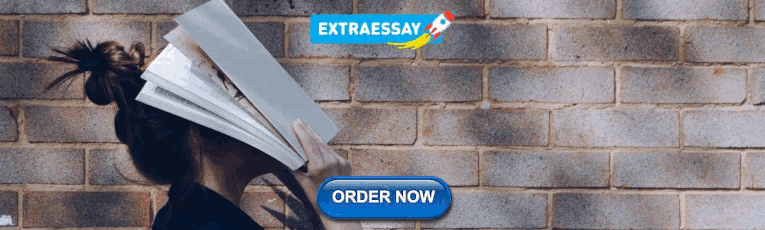
IMAGES
VIDEO
COMMENTS
Since the Sapir-Whorf hypothesis theorizes that our language use shapes our perspective of the world, people who speak different languages have different views of the world. In the 1920s, Benjamin Whorf was a Yale University graduate student studying with linguist Edward Sapir, who was considered the father of American linguistic anthropology.
Sapir-Whorf Hypothesis. The Sapir-Whorf hypothesis, also known as the linguistic relativity hypothesis, refers to the proposal that the particular language one speaks influences the way one thinks about reality. Linguistic relativity stands in close relation to semiotic-level concerns with the general relation of language and thought, and ...
Linguistic Relativity in Psychology. The Sapir-Whorf Hypothesis, also known as linguistic relativity, refers to the idea that the language a person speaks can influence their worldview, thought, and even how they experience and understand the world. While more extreme versions of the hypothesis have largely been discredited, a growing body of ...
The idea of linguistic relativity, also known as the Sapir-Whorf hypothesis (/ s ə ˌ p ɪər ˈ hw ɔːr f / sə-PEER WHORF), the Whorf hypothesis, or Whorfianism, is a principle suggesting that the structure of a language influences its speakers' worldview or cognition, and thus individuals' languages determine or shape their perceptions of the world.. The hypothesis has long been ...
The Sapir Whorf Hypothesis began as linguistic determinism. This concept states that our language determines how we think and perceive the world. There are different levels of linguistic determinism, some that are "stronger" than others. "Weaker" forms of this idea suggest that language simply influences the way we think.
The Sapir-Whorf hypothesis [ 1, 2] holds that our thoughts are shaped by our native language, and that speakers of different languages therefore think about the world in different ways. This proposal has been controversial for at least two reasons, both of which are well-exemplified in the semantic domain of color.
The Sapir-Whorf hypothesis is the linguistic theory that the semantic structure of a language shapes or limits the ways in which a speaker forms conceptions of the world. It came about in 1929. The theory is named after the American anthropological linguist Edward Sapir (1884-1939) and his student Benjamin Whorf (1897-1941).
The claim is very often referred to as the Sapir-Whorf Hypothesis (though this is a largely infelicitous label, as we shall see). ... In consequence, evaluating them requires the expertise of both linguistics and psychology, and is a multidisciplinary enterprise. Clearly, the linguistic hypothesis may hold up where the psychological hypothesis ...
After completing this module, students will be able to: 1. Define the concept of linguistic relativity. 2. Differentiate linguistic relativity and linguistic determinism. 3. Define the Sapir-Whorf Hypothesis (against more pop-culture takes on it) and situate it in a broader theoretical context/history. 4.
Introduction. The Sapir-Whorf hypothesis (a.k.a. the Whorfian hypothesis) concerns the relationship between language and thought. Neither the anthropological linguist Edward Sapir (b. 1884-d. 1939) nor his student Benjamin Whorf (b. 1897-d. 1941) ever formally stated any single hypothesis about the influence of language on nonlinguistic ...
The Sapir-Whorf hypothesis holds that language plays a powerful role in shaping human consciousness, affecting everything from private thought and perception to larger patterns of behavior in society—ultimately allowing members of any given speech community to arrive at a shared sense of social reality. This article starts with a brief ...
The Sapir-Whorf Hypothesis, also known as the linguistic relativity hypothesis, states that the language one knows affects how one thinks about the world. The hypothesis is most strongly associated with Benjamin Lee Whorf, a fire prevention engineer who became a scholar of language under the guidance of linguist and anthropologist Edward Sapir ...
The Sapir-Whorf Hypothesis or the linguistic relativity principle, formulated by Edward Sapir (1884-1936) and refined by his student, Benjamin Lee Whorf (1897-1941), states that human thinking is highly dependent on the language spoken by the individual thinker. As language is our main tool for organizing our experiences, the argument goes ...
The Sapir-Whorf hypothesis holds that the semantic categories of one's native language influence thought, and that as a result speakers of different languages think differently. This idea has captured the imaginations of many, and has inspired a large literature. However the hypothesis is also controversial, for
The Sapir-Whorf hypothesis holds that our thoughts are shaped by our native language, and that speakers of different languages therefore think differently. This hypothesis is controversial in part because it appears to deny the possibility of a universal groundwork for human cognition, and in part because some findings taken to support it have not reliably replicated.
The term Whorfian Hypothesis takes its name from Benjamin Lee Whorf (1876-1941) who claimed that the language one speaks influences one's thinking [ 7 ]. Whorf was an amateur linguist who studied with the anthropologist Edward Sapir in the 1920s and 1930s. The term Sapir-Whorf Hypothesis is also used to refer to their view that language ...
Theories in psychology and science are always changing and evolving. There are many different schools of thought that suggest how humans see the world, communicate, or make decisions. And when a theory or concept gets popular, it may spread like wildfire, bringing false information with it. ... The Sapir Whorf Hypothesis began as linguistic ...
The S apir-Whorf hypothesis, commonly referred to as the linguistic relativity hypothesis, explores the idea that the language one uses affects how one perceives reality. J.A. Luc y, ( 2001) [1].
The Essential Sapir. To date, there has been no book-length secondary work on Sapir's linguistics, anthropology, or psychology. Nonetheless, we are fortunate to have a good edited compilation of Sapir's key articles in the form of Mandelbaum 1985.Also of interest is a contemporary review of this collection, Harris 1951. Darnell 2010 (cited under Biography and Background) is an excellent ...
Benjamin Lee Whorf (born April 24, 1897, Winthrop, Mass., U.S.—died July 26, 1941, Wethersfield, Conn.) U.S. linguist noted for his hypotheses regarding the relation of language to thinking and cognition and for his studies of Hebrew and Hebrew ideas, of Mexican and Mayan languages and dialects, and of the Hopi language.
The Sapir-Whorf hypothesis holds that our thoughts are shaped by our native language, and that speakers of different languages therefore think differently. This hypothesis is controversial in part because it appears to deny the possibility of a universal groundwork for human cognition, and in part because some findings taken to support it have not reliably replicated.
Abstract. The history of empirical research on the Sapir-Whorf hypothesis is reviewed. A more sensitive test of the hypothesis is devised and a clear Whorfian effect is detected in the domain of color. A specific mechanism is proposed to account for this effect and a second experiment, designed to block the hypothesized mechanism, is performed.
The Sapir-Whorf Hypothesis is a widely used label for the linguistic relativity hypothesis, that is, the proposal that the particular language we speak shapes the way we think about the world. The label derives from the names of American anthropological linguists Edward Sapir and Benjamin Lee Whorf, who persuasively argued for this idea during ...
Hey guys, another episode out - woo!! So as promised, questions of the episode and answers to LAST WEEKS because I forgot to record them for this week, soz. Anyway answers here: 1. Piaget's theory refers to the idea that thought comes before language. In order to express a concept, an individ…