Site matching of wind turbine generators: a case study
Ieee account.
- Change Username/Password
- Update Address
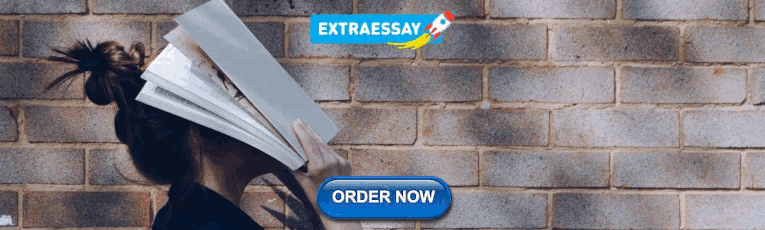
Purchase Details
- Payment Options
- Order History
- View Purchased Documents
Profile Information
- Communications Preferences
- Profession and Education
- Technical Interests
- US & Canada: +1 800 678 4333
- Worldwide: +1 732 981 0060
- Contact & Support
- About IEEE Xplore
- Accessibility
- Terms of Use
- Nondiscrimination Policy
- Privacy & Opting Out of Cookies
A not-for-profit organization, IEEE is the world's largest technical professional organization dedicated to advancing technology for the benefit of humanity. © Copyright 2024 IEEE - All rights reserved. Use of this web site signifies your agreement to the terms and conditions.
Browse Econ Literature
- Working papers
- Software components
- Book chapters
- JEL classification
More features
- Subscribe to new research
RePEc Biblio
Author registration.
- Economics Virtual Seminar Calendar NEW!
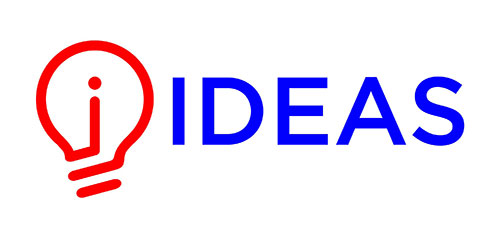
Optimal site matching of wind turbine generator: Case study of the Gulf of Suez region in Egypt
- Author & abstract
- 1 Reference
- 18 Citations
- Most related
- Related works & more
Corrections
- EL-Shimy, M.
Suggested Citation
Download full text from publisher, references listed on ideas.
Follow serials, authors, keywords & more
Public profiles for Economics researchers
Various research rankings in Economics
RePEc Genealogy
Who was a student of whom, using RePEc
Curated articles & papers on economics topics
Upload your paper to be listed on RePEc and IDEAS
New papers by email
Subscribe to new additions to RePEc
EconAcademics
Blog aggregator for economics research
Cases of plagiarism in Economics
About RePEc
Initiative for open bibliographies in Economics
News about RePEc
Questions about IDEAS and RePEc
RePEc volunteers
Participating archives
Publishers indexing in RePEc
Privacy statement
Found an error or omission?
Opportunities to help RePEc
Get papers listed
Have your research listed on RePEc
Open a RePEc archive
Have your institution's/publisher's output listed on RePEc
Get RePEc data
Use data assembled by RePEc
Advertisement
A new approximate capacity factor method for matching wind turbines to a site: case study of Humber region, UK
- Original Research
- Open access
- Published: 06 September 2019
- Volume 10 , pages 451–462, ( 2019 )
Cite this article
You have full access to this open access article
- Chidiebere Diyoke ORCID: orcid.org/0000-0002-2895-8179 1 , 2
8932 Accesses
29 Citations
14 Altmetric
Explore all metrics
Wind-based power is one of the renewable base power sources that are tipped to play a great role in decarbonising the globe. To achieve this potential, more wind farms are likely to be built. The capacity factor of a wind farm and hence its profitability is dependent on whether it is properly sized and sited. In fact, some wind power plants have failed wholly or underperformed, because the wind turbine plant installed did not match the wind site. In this paper, a new approximate capacity factor equation has been derived for matching wind turbines to potential site for optimum yield and profitability. The indexes of capacity factor and cost of electricity were used as metrics in the model. The proposed model was applied to the climatic conditions and wind turbine characteristics of Kappadagudda and Mailiao wind farms in India and Taiwan, respectively. The result obtained showed good agreement with measured data for the two wind farms. With respect to the Kappadagudda wind farm, the model computed CF of 38% is close to the Kappadagudda real wind farm annual CF of 36% representing an absolute error of 2% and a mean square error of 0.96%. In addition, it was found that the proposed model followed the same general trend with other six existing models compared.
Similar content being viewed by others
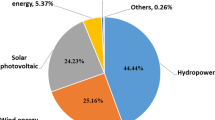
Cost, environmental impact, and resilience of renewable energy under a changing climate: a review
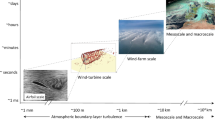
Wind-Turbine and Wind-Farm Flows: A Review
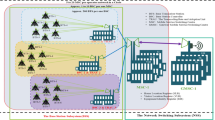
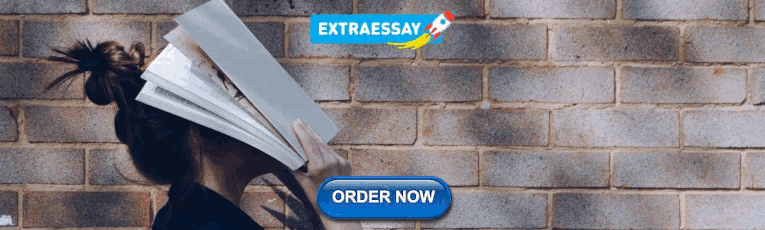
A review of renewable energy based power supply options for telecom towers
Avoid common mistakes on your manuscript.
Introduction
Global demand for energy has been increasing astronomically over the past years [ 1 , 2 ]. In a desperate bid to meet this rising energy demand as well as the target of producing a larger share of energy from renewables as required by the Kyoto protocol, the erection, capacity, and complexity of new wind farms has steadily increased in the recent past [ 2 ].
Global wind power capacity grew by 17% between 2014 and 2015 to 432.4 GW [ 2 ]. It is even forecasted to attain 2000 GW by 2030, and supply up to 17–19% of global electricity, and reduce CO 2 emissions by more than 3 billion tons per year. By 2050, it is forecasted to supply 25–30% of global electricity supply [ 3 ].
At European level, the trend is the same [ 2 ]. Yearly installations of wind power in the EU have increased over the last 14 years at a compound annual growth rate (CAGR) of 9% from 3.2 GW in 2000 to 12.8 GW in 2015 accounting for one-third of all new power installations since 2000 in the EU. In the UK, the wind capacity installation grew by 8% to 13.6 GW between 2014 and 2015 [ 1 ]. In the year 2030, 40 GW wind turbine capacity penetration representing 12% of total EU installed capacity is forecasted for the UK [ 1 ]. To meet the forecasted higher penetration of wind power, more wind farm sites will have to be erected.
The capacity factor of a wind farm and hence its profitability is dependent on whether it is properly sized and sited. Appropriate wind farm site planning and selection has main positive economic impacts for both wind farm investors and developers [ 4 ]. This is because the energy produced by a wind farm site depends upon many factors such as variation of the distribution of wind speed, wind turbine type, and characteristics speeds like the cut-in velocity ( v in ), cut-out velocity ( v o ), rated velocity ( v r ), hub height ( H h ), and the generator design [ 5 , 6 ].
So many different kinds of commercially available wind turbine plants (WTP) exist and the optimum selection of the appropriate wind turbine characteristics can improve the yield and profitability of a wind farm [ 7 ]. Moreover, as reported by Li and Chen [ 6 ], operational experience with some existing wind farms revealed that the main reason why some wind power plants have failed wholly or underperformed is the fact that the WTP installed did not match the wind site.
Therefore, a thorough matching of wind turbine generators to sites is required including the impact of the available turbine size and hub heights to guarantee both technical feasibility and financial competitiveness in terms of higher output and lower costs of generated electricity [ 4 , 6 ]. One of the ways of matching wind turbine to sites is through the determination of the CF, with wind turbines with higher CFs being preferred to ones with lower CFs.
So many CF methods have been cited in the literature for matching wind turbines to a potential wind farm site [ 7 , 8 , 9 , 10 ]. Manufacturer’s power curve can be used in combination with the site average velocity and the Weibull, Rayleigh, or Gamer probability density function (pdf) [ 8 , 11 ]. Under this method, the theoretical power curve of the chosen wind turbine is used to calculate the energy output. However, the use of manufacturer’s power curve can mis-capture the wind power potential at a site to a very large margin. This is because wind speed is region specific, and the manufacturer’s power curve is obtained through field measurements of wind speed and power usually averaged and normalized to a reference air density using normalized procedures [ 12 ]. Their use, therefore, will not represent a true potential of the wind power available in a site.
Another way to match wind turbines to a site is through approximate power curve method; whereby generic power curves are generated by means of fitting techniques like least squares or cubic spline interpolation [ 12 ]. In addition, wind turbines are matched to potential sites through modeling of the wind park efficiency [ 13 ], but the shortcoming of the model developed through this method is that it depends on some technical parameters which will be difficult to obtain from the manufacturers. Hu and Chen [ 14 ] applied the Simpsons 3/8 rule to a Weibull probability density function (pdf) to derive a six-parameter approximate cubic power curve. Although very good result was achieved, the resulting power curve equations are quite complex and require a lot of input data.
Other standard CF methods and models have also been developed in the literature for matching wind turbine to sites. These models include: linear, quadratic, and cubic models [ 12 ]. To match wind turbines accurately to a site, a model capturing all the wind regime of the site is paramount.
In this paper, a new approximate equation has been derived for matching wind turbines to site through estimating the CF. The effectiveness and applicability of the proposed model is first validated against a 1 year measured real wind farm power output of two different wind farms, and turbine parameters, namely Kappadagudda wind power station in India obtained from Jangamshetti and Rau [ 5 ] and Mailiao wind power station in Yunlin County, Taiwan. Then, a model comparison is done to show the relative performance of the model against other existing models. Using six commercial wind turbine makes of different manufacturers and 1 year wind speed characteristics data of Humber region in UK as a case study, the performance index of CF and cost of electricity per kWh (COE), is applied to select the most suitable and cost-effective WTP for the site.
Wind speed distribution and analysis
Figure 1 shows the 1 year hourly wind speed time-series used in the study. It is a 2005, 1 year hourly (10-min averaged) time-series wind speed data for Humber region UK obtained from Met office, UK. The average wind speed of the site is 4.21 m/s and standard deviation of 2.32 at 10 m height. A maximum wind speed of 6.54 m/s occurred in January, while a minimum wind speed of 3.19 occurred in March. The most probable wind speed and wind speed carrying maximum energy for the site are 4.14 and 5.56 m/s, respectively. Figure 2 shows the variation of wind speed and wind power density with height for the site. As can be observed, judging from the International Electro-technical Commission (IEC) standard classification for wind sites [ 15 ], the site is of low wind class and requires a class III wind turbine of large rotors and a min of 80 m mast to capture as much energy as possible from the lower wind speeds.
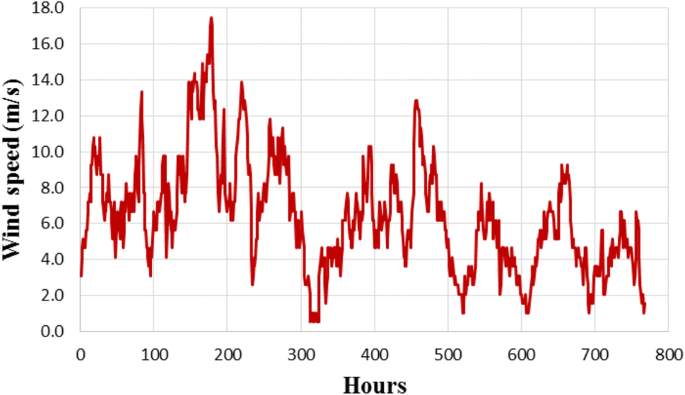
One year hourly wind speed time-series of Humber region, UK
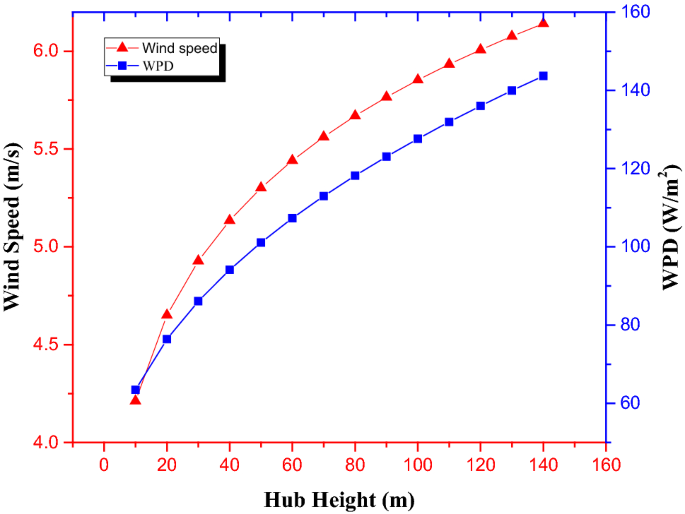
Variation of wind speed and wind power density with hub height
There are so many statistical models using pdf for describing the wind speed variation. However, commonly used pdf models in wind power studies are the Weibull, Rayleigh, and Gamma distributions [ 16 ]. The Weibull pdf is reported to best describe the wind speed variation [ 5 , 7 , 17 ]. In the work presented here, the Weibull pdf is used, since the Raleigh distribution is a subset of it. The Weibull pdf is represented by Eq. ( 1 ). It is a special case of Pearson type III or generalized two-parameter Gamma distribution [ 5 ]:
where \(f(v,k,c)\) is the probability of wind speed ( v ) at turbine hub height, c is a scale parameter which has the dimension of velocity, and k is a shape or location factor [ 18 ]. When the rate k = 2, the Weibull distribution results to the Rayleigh distributions with single parameter c .
The parameters of the Weibull pdf are obtained from the mean and standard deviation of the wind speed at the site of study using the standard deviation or moment method (MM). Other methods like the maximum-likelihood method (MLM), the modified maximum-likelihood method (MMLM), the energy pattern factor method (EPFM), and the graphical method can be used also [ 5 , 19 , 20 , 21 ]. Using the standard deviation method, c and k are determined from gamma function ( \(\varGamma\) ) expressions of Eqs. ( 2 ) and ( 3 ) iteratively [ 7 ]:
where the average wind speed, \(\bar{v}\) , is given as follows:
A satisfactory empirical method (EM) for estimating k is derived by Justus as follows [ 5 , 7 , 20 ]:
Figure 3 shows the monthly probability density function of wind speeds at 10 m height for one full year obtained using the EM computed statistical parameters. All the curves can be seen to have a similar tendency of wind speed. Hence, with respect to the computed statistical parameters for this location, wind speed is most uniform in June, while it is least uniform in Jan.
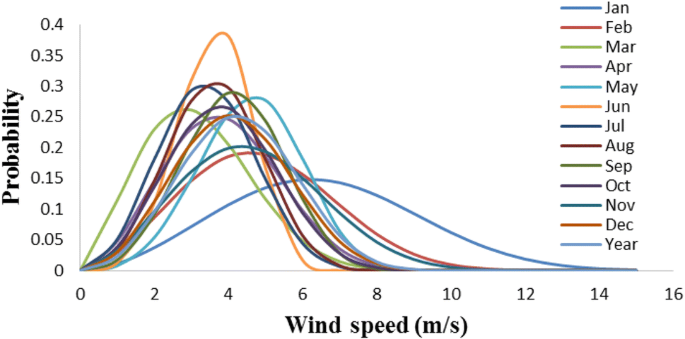
One year Weibull monthly pdf of wind speeds at 10 m height for the case study site
The wind speed at anemometer height h is extrapolated to the turbine hub height ( H ) using the relation:
where \(\alpha\) is the power law index assumed as 1/7 [ 17 , 22 , 23 ]. Applying Eqs. ( 6 ) to ( 2 ) gives an expression for calculating c at any hub height ( H ):
\(\alpha\) varies with local terrain conditions and is determined empirically [ 7 ]. Typical values of α for different terrain types can be found in Ref. [ 7 ].
The power of a wind turbine plant
A basic wind electrical system is shown in Fig. 4 . The electrical output ( P WTe ) of the wind turbine plant (WTP) is a function of area swept by the rotor blades ( A ), the wind velocity of the wind farm location, the WTP output power P WT , the generator input power P t , performance coefficient C p of the turbine, the transmission efficiency ( ɳ m ), and the generator efficiency ( ɳ g ), as shown in Eq. ( 8 ) [ 5 , 6 , 7 , 24 ].
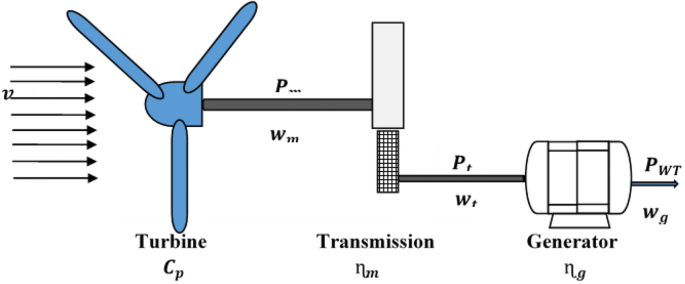
Typical diagram of a wind electrical system
The electrical power output is expressed as follows:
where \(\rho\) is density of air.
Given that WTP do operate at wind regimes between the cut-in speed ( \(v_{\text{in}}\) ), the rated speed ( \(v_{\text{r}}\) ), and cut-out speed ( \(v_{\text{o}}\) ), [ 25 ], a modified version of Eq. ( 8 ) is generally used to estimate the average power as in Eq. ( 9 ). P f is the wind turbine output characteristic as a percentage of rated power in the region between the cut-in wind speed and rated speed:
Existing CF models for matching wind turbines to site
Wind turbines are matched to a site by selecting from a range of potential wind turbines, the one with the highest wind power potential at a low cost for the site. One of the ways of measuring the wind power potential is by calculating the capacity factor (CF) of a selected wind turbine for the site, using the site climatic conditions.
The CF of a wind site is the fraction of rated output that is being outputted by the wind farm in the site. It is defined as the ratio of the average power output over a given period of time to the rated power output or the maximum possible amount of power it can produce over the same interval. The average power generated by a wind turbine can be estimated by integrating the power curve multiplied by the appropriate pdf. Thus, CF is a dimensionless quantity given generally by Eq. ( 10 ) [ 7 ]. For a given site wind regime, a WTP with higher CF is best suited to the site and will produce higher energy output:
The common models for estimating the CF are the linear power curve, quadratic power curve, exponential power curve, approximate cubic power curve, and the cubic power curve model [ 12 ].
Linear model
A linear power curve assumes that wind power output is linearly proportional to wind speed from cut-into rated wind speed, as shown in Eq. ( 11 ) [ 7 , 26 , 27 ]. This model is reported to overestimate the wind power potential at a site [ 7 , 27 , 28 ]:
Quadratic model
Quadratic model as represented by Eq. ( 12 ) is an improved version of the linear model. It is originally proposed by Justus et al. [ 20 ] and cited in [ 7 , 12 , 26 ]:
The model is complex, but has an advantage of requiring few parameters of the wind turbine and site to model. As reported in [ 28 ], the model predicts negative power output in parts of the partial power range if the cut-in wind speed is less than 26% of the rated wind speed.
Another version of the quadratic model exists that never predicts negative output power as wind machine design parameters are varied. This model proposed by [ 28 ] is reported to be more accurate than the simple linear model. The model is generally represented as follows:
where k is selected to be the shape parameter of the Weibull distribution describing the winds.
Thapar et al. [ 27 ] reported that the model lacks accuracy as it does not accurately represent the shape of actual power curve of the wind turbine in the range of cut-into rated speed. In another study, model Eq. ( 13 ) is applied to capacity factor Eq. ( 10 ) and integrated by change of variable technique to arrive at exponential model given as follows [ 29 ]:
The exponential model uses generic wind turbine characteristics such as the v in , v r , and v o , to show their effects on the average power production of a turbine [ 29 ]. For known values of the Weibull parameters c and k in a given wind regime, v in , v r , and v o can be selected to optimize the average power, and thereby maximize the total energy production [ 29 ].
For applications not concerned with Weibull wind models, k is set equal to 2 and model Eq. ( 15 ) results [ 28 ]. This model has been widely used by many authors [ 7 , 27 , 28 ] and seems to follow the power curve more closely than the linear curve as reported by [ 7 , 28 ]. With the model, it is possible to compute the average output power of a wind through an exact analytical integration [ 28 ]:
Cubic model
So many versions of the cubic model exist in the literature. In one model, the cut-in velocity is subtracted from the wind speed and rated speed of the turbine and the ratio raised to the third power. This model is given by the following formula [ 6 , 7 , 30 ]:
In another model as used in [ 7 ], the P f is estimated by raising to the third power, the ratio between the wind speed and rated speed of the turbine as in Eq. ( 17 ):
Chauhan and Saini [ 25 ] used another version of the cubic model in their analysis as shown below:
The proposed model
A typical wind turbine power curve is shown in Fig. 5 . As can be seen in the figure, a wind turbine does not produce its rated power ( P r ) at all wind speeds. Between the cut-in ( v in ) and rated speeds ( v r ), it produces a given fraction of its rated power denoted by P f .
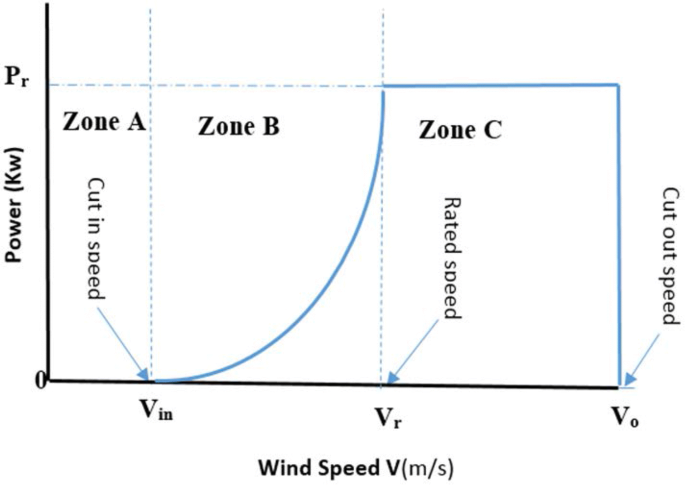
Typical wind turbine power curve
At this region between v in and v r , P f can be approximated with a linear curve ( a + bV ), quadratic curve ( av 2 + bv + c ), power curve ( ab v ), polynomial curve ( av n +, bv n −1 + cv n −2 +…..e), or even logistic function.
Let a polynomial of Eq. ( 19 ) represents the ascending part of the power curve between the cut-in speed ( v in ) and rated speed ( v r ):
Assuming that the coefficients c and d are negligible in the above equation, we arrive at an approximate polynomial given by Eq. ( 20 ) for the ascending segment of the power curve of the wind turbine:
At the rated velocity v r , the rated power can be expressed as follows:
Similarly, the power corresponding to the cut-in speed can be expressed as follows:
Letting \(\psi = {\raise0.7ex\hbox{${P_{\text{in}} }$} \!\mathord{\left/ {\vphantom {{P_{\text{in}} } {P_{\text{r}} }}}\right.\kern-0pt} \!\lower0.7ex\hbox{${P_{\text{r}} }$}}\) and solving the four equations leads to the proposed model as follows:
where \(\psi\) , the fraction of the rated power of the wind turbine that is being generated at the cut-in wind velocity and is estimated with Eq. ( 24 ), which represents the line of best fit of the scatter plot of the ratio \(\frac{{P_{\text{in}} }}{{P_{\text{r}} }}\) % of 190 commercially available WTG power curves of different capacities and manufacturers at various cut-in wind speeds in the range of 0–5 m/s:
The results of CF obtained using the proposed model (CF m ) are compared against a 1 year real wind farm measured CF (CF WF ) by means of Absolute Forecast Error (AFE), Mean Square Error (MSE), Mean Percentage Error (MPE), and Root Mean Square Error (RMSE).
The AFE is the positive difference between the wind farm CF and the model computed CF gives as follows:
MPE gives the average relative error between the models. For this, the relative differences between the model results and the wind farm are added and divided by the number of observations; thus:
where n is the number of observations.
Wind site matching: performance indicators
CF factor is one of the metrics often used to match wind turbines to site, since it indicates the fraction of the installed capacity of a WTP that can be generated in a given site. However, from investment perspective, investors are not just keen on the output but also the costs involved. Approximately 75–80% of the total investment cost (TIC) for a WTP are related to capital costs—that is the costs of the turbine, planning and project costs, civil works, electrical equipment, and grid connection [ 31 , 32 ]. As a result, the cost of capital (discount or interest rate) is a significant influence on wind electricity cost. This cost varies among countries and regions and should be factored in. Therefore, there is the need to factor in cost in the technique used to match wind turbines to a pottential wind farm site. To select the most suitable WTP characteristic for the site, turbine hub height ( H ) of 80 m and 2 MW rated is assumed as the base case and WTP of various capacity and model are matched to the site using two index of measurement; CF and COE in $/kWh.
The COE is calculated with Eq. ( 28 ) [ 33 ]:
where \({\text{TIC}}_{{{\text{MW}} - H}}\) is the TIC corrected for the variation of cost with height and capacity [ 7 ]. TIC at a given MW capacity at 80 m height is calculated with Eq. ( 29 ). At other heights, TIC is calculated with Eq. ( 30 ) [ 7 ]:
Here, \(\varphi\) is used to account for the effect of economies of scale on the TIC.
Other assumptions are;
TIC per kW of 1340–2330 $/kW, with an average of 1835 $/kW. These costs are based on data from IRENA and stated in 2013 prices [ 32 ];
O&M costs are assumed to be 5% over the lifetime of the turbine [ 33 , 34 , 35 ];
The lifetime of the turbine is set at 20 years as is the usual practice [ 32 ];
The discount rate is assumed 5.5% per annum.
Taxes, depreciation, and risk premiums are not taken into account and all calculations are based on fixed 2013 prices.
Base-case turbine of 2 MW with 80 m hub height is adopted.
TIC of WTP cost is linearly inversely proportional to capacity because of economies of scale effect [ 7 ].
TIC is linearly proportional to tower height [ 7 ].
Model validation and comparison
The model was validated against a representative real wind farm output data of a wind farm in India as documented in [ 5 ]. The technical characteristics of the wind turbine for the site and its specification are shown in Table 1 .
The annual capacity factor reported for the wind farm is 36%, while the model calculated annual capacity factor is 38% representing an MPE of about 5.5%. Figure 6 shows the comparison between estimated and measured monthly CF. Error analysis between the model and wind farm results showed the model recorded MSE, RMSE, and MPE of 0.96, 9.8, and 8%, respectively.
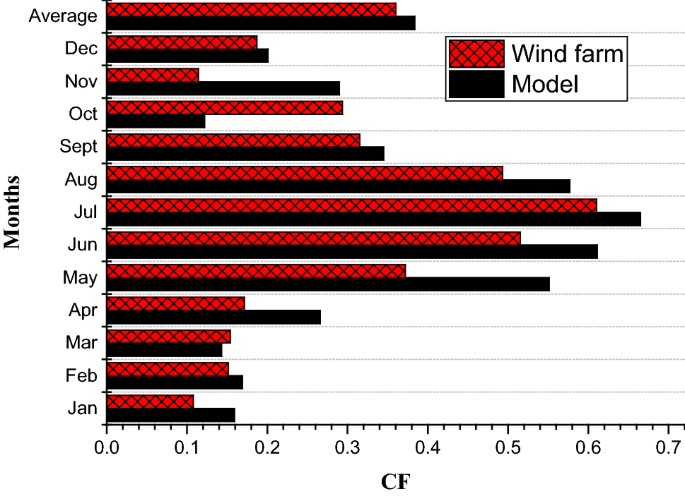
Comparison between CF estimated by model against actual wind farm measured CF
As can be seen, the model results are almost the same with that of the wind farm, with an overestimation MAE margin of not more than 10% except for the months of May and Oct where the error margin is more than 10%. The case for November is acceptable, since it was reported that there is a load shedding at the wind farm by the month of Nov [ 5 ]. In most of the months, the estimated CF is more than the measured value. This is to be expected, because as rightly pointed out by the authors [ 31 ], a model should estimate more energy than is obtainable in a wind farm, because in real life, power output or capacity factor will be impacted by so many factors, including array losses, or park effects that results as a result of wind turbines shadowing one another in a wind farm, leaving less energy in the wind downstream of each wind turbine [ 31 ]. Depending on the rotor of the turbines, these losses represent about 5–10% of the theoretical output described by the power curves [ 31 ]. In addition, since average and not real time-series wind speed of the wind farm location is used, lulls in wind speed and unavailability due to downtime like the one reported to have happened in Nov in the wind farm may occur that the model could not capture. Other losses that occur that the model cannot account for include rotor blade soiling losses, losses due to wind direction hysteresis, and grid losses typically in the range of 1–3%, that occur as a result of electrical (heat) losses in transformers and cabling within the collection grid inside the wind farm [ 31 ]. As reported by [ 31 ], wind farm developers assume energy losses in the rage of 10–15% below the theoretical power curves of wind turbines.
As a further validation of the model and to demonstrate its applicability to a different site and many years of measured wind farm data, the wind turbine parameters and three different years of measured climatic conditions of Mailiao power station in Taiwan are applied to the model to compute the CF of the wind farm for the years 2002, 2003, and 2005, respectively. The wind farm which is located in Yulin County in Taiwan started operation at about December 2002 as reported by Chang and Tu [ 36 ] and has four Vestas V47-660 kW turbines. The total capacity of the wind farm is 2.64 MW. The V47 turbines in the site have a hub height of 45 m. The cut in, rated, and cut-out speeds are 4, 15, and 25 m/s, respectively. Figure 7 shows the measured wind speed at the site for the years 2002, 2003, and 2005.
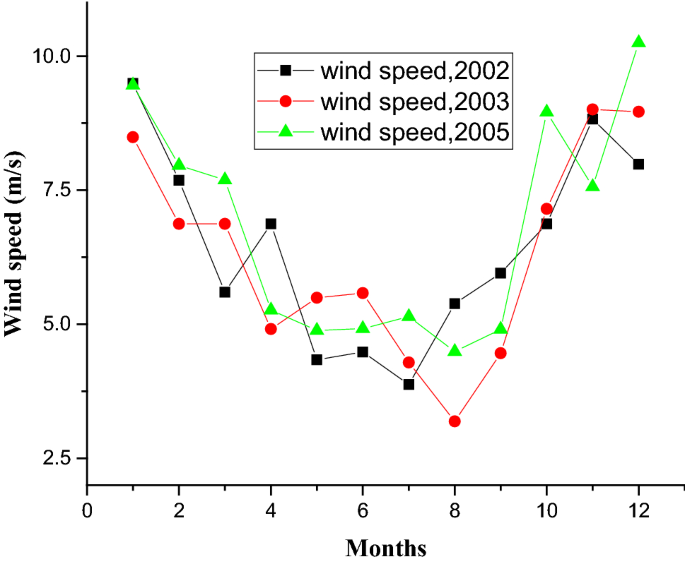
Measured monthly wind speed distribution of Mailiao wind farm site in Taiwan for years 2002, 2003, and 2005
The comparison of the wind farm measured CF with that calculated using the proposed model is shown in Fig. 8
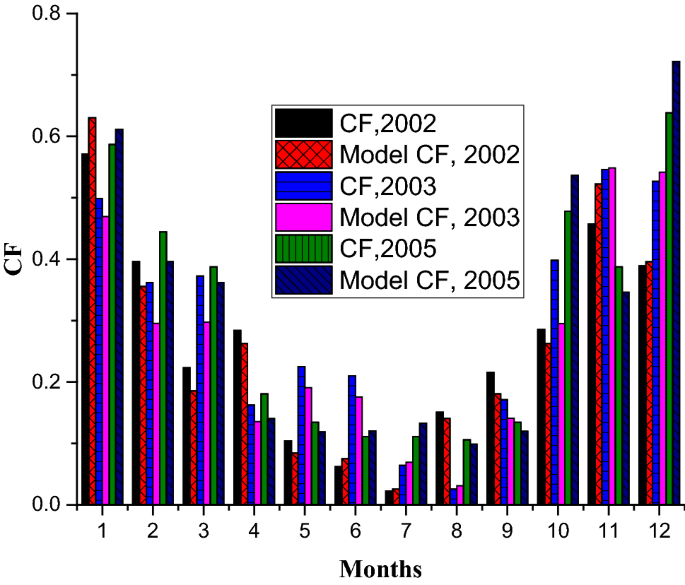
Comparison of monthly CFs obtained using proposed model against real wind farm measurement for years 2002, 2003, and 2005
It can be observed that the model predicted the CF of the wind farm to a good degree with an MPE of 12.14, 14.12, and 11.46% for years 2002, 2003, and 2005, respectively. In addition, the maximum and minimum MPE recorded by the model are 20.53 and 1.76 for year 2002, 25.9 and 0.43 for year 2003, and 22.32 and 4.20 for year 2005. There is a higher MPE error in the application of the model to the Taiwan wind farm when compared to the Kappadagudda wind power station. This is expected, because, for V47-660 kW Vestas wind turbine used for the Taiwan site, the best anemometer location for the best and accurate wind speed capture is in 2.5 rotor diameter upstream from the wind turbine. However, in the Mailiao wind farm, the anemometer of the Vestas V47-660 kW turbine was mounted on the nacelle cover of the turbine where it is 7 m behind the blade hub [ 36 ]. Hence, the measured wind speed for the site is unavoidably affected by the turbine wake leading to a velocity deficit error. The poor location of the anemometer, therefore, could have been responsible for the high MPE error recorded for the Mailiao wind farm site.
The performance of the proposed model relative to other existing models for estimating the CF is shown in Fig. 9 for the different wind regimes of the Kappadagudda wind farm from Jan to Dec.
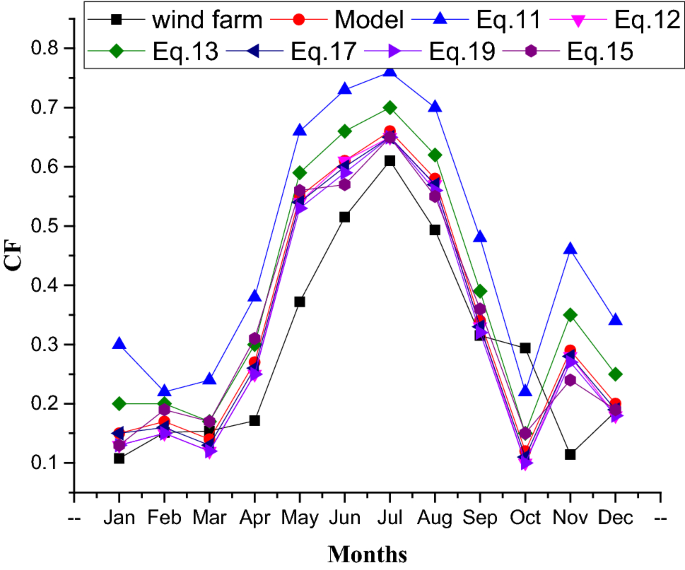
Comparison between CF obtained using the proposed model against other existing models for estimating CF
Clearly enough, the proposed model followed the same general trend with the wind farm and existing models. It recorded the same yearly CF estimation AFE of 2% with model Eqs. ( 13 , 14 ) and outperformed model Eqs. ( 11 , 12 and 17 , 18 ). Model equation ( 15 ) was adjudged by the authors of [ 34 ] as the best of all the existing models in terms of capturing the power output of a wind farm accurately. Comparison of the proposed model with the model shows that the proposed results followed closely model equation ( 15 ). Table 2 shows the absolute error between the wind farm output and the forecasts by the various models.
Case study of Humber region
The technical parameters of the selected WTP used in the case study are shown in Table 3 , while Table 4 depicts the monthly variation of Weibull wind distribution parameters for the site, computed using the different wind parameter estimation methods. For a more detailed analysis of the various methods, see the work of authors [ 21 ].
As can be seen from Table 4 , the values of the parameters computed by all the methods are close with a small variation. k and c values lie between minimum and maximum values of 1.09–4.17 and 3.59–7.43, respectively. k represents deviation of the wind speed from the average speed; in particular, the higher the value of k , the lower the deviation.
Using the annual values of the Weibull parameters, estimated using the empirical method (EM), the two performance indicators (CF and COE) are calculated for the selected commercial wind turbine generators at their designated operational heights (see Table 3 ). From the point of view of capacity factor and cost of generated electricity, WT6 is the first ranked turbine with highest capacity factor of 0.21 and lowest cost of energy of 0.08 $/kWh at a height of 105 m. The calculated COE lies in range of the weighted average COE in UK which ranges from 0.06 to USD 0.09 $/kWh [ 32 ]. WT11 ranked second with a CF of 0.19 and COE of 0.085 $/kWh at a height of 100 m. Figure 10 summarizes the results. WT2 of capacity 850 kW performed poorly in the site, because it has a high cut-in velocity of 4 m/s and a hub height of 65 m and the site being a low wind site means most of the energy was not captured by the turbine.
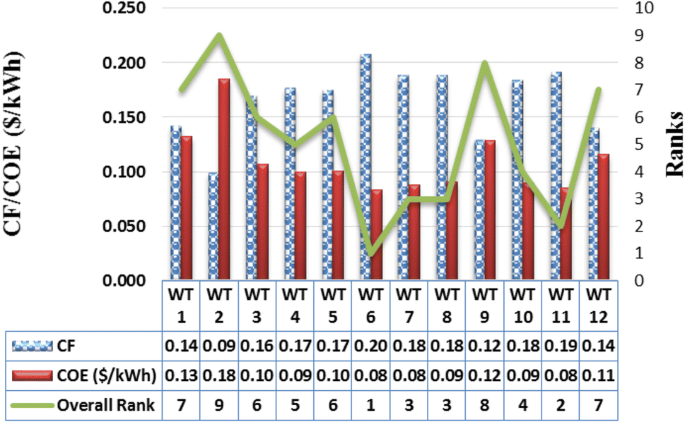
Performance indicators of analyzed potential commercial WTPs for the case study site
WT11 outperformed WT12, even though they are of the same capacity and WT12 has lower cut-in speed and higher cut-out speed, because WT11 has a bigger diameter and operated at a higher height which made it possible for it to convert more of the wind spectra of the site to power. In general, for a given height and capacity, the smaller the ratio of the area of the blades to the rated capacity of the wind turbine, the better its energy capture for the same wind characteristics.
The CF of all the WTPs increased with height, while the COE decreased with height. This is because at higher heights, the wind speed is higher and this means that more wind energy will be converted to power leading to a reduction in the COE.
At higher height also, the cost of the wind turbine increased due to the tower, but the increase in energy capture is more than the cost increase. Ordinarily, one expected the single capacity wind turbines of the order of 500–1500 kW would do well in the site. However, they generally did not do well, because the site is a low wind site and the hub heights they are available at could not let them capture more energy from the site. In addition, they costed higher, because the higher capacity WTPs enjoyed the benefits of economics of scale. Thus, WTP of capacity in the range of 1800–2.5 MW are the most suitable for the site, since they come in higher hub heights and bigger diameters that enable more conversion of wind speed to energy at higher hub heights. They also enjoy economics of scale.
A new approximate WTP CF model for matching WTP to potential site has been presented in this paper. Comparison of the model with a real wind farm data of Kappadagudda power station in India shows that the model computed CF of 38% is close to the real wind farm annual CF of 36% representing an absolute error of 2. Error analysis between the model and wind farm monthly CF results showed the model recorded MSE, RMSE, and MAE of 0.96, 9.8, and 8%, respectively. The proposed model followed the same general trend with other existing models compared. Out of six models compared, it recorded the same yearly CF estimation absolute error of 2% with two-model and outperformed other four models.
Within the limits of the assumptions made and using capacity factor and COE as indexes of site matching, it is found that the suitable commercial WTP to be placed in the case study site investigated for any future wind farm project is WT6 with a hub height of 105 m. It yielded the highest CF and lowest COE values of 0.21 and 0.083 $/kWh, respectively. The second and third ranked wind turbines are WT11 and WT7 with CF and COE values of 0.19, 0.0854 $/kWh and 0.189, 0.088 $/kWh, respectively.
Finally, it should be mentioned that the WTP TIC cost is roughly estimated, and the specific costs of the whole power conversion unit and constructions may vary depending on the country. These factors may have a significant effect on the COE results, so that the obtained indexes may not necessarily reflect exactly practical performances. However, the methods described above can be useful in the preliminary planning of wind power project. However, for a final investment decision, apart from a more rigorous technical analysis, optimized economic analysis may still be useful as a guide for investors wishing to invest in the site.
EWEA. Wind energy scenarios for 2030. A report by European Wind Energy Association, (2015). http://www.ewea.org/fileadmin/files/library/publications/scenarios/EWEA-Wind-energy-scenarios-2020.pdf (2015). Accessed 24 Mar 2018
Global Wind Energy Council (GWEC). Global Wind Report. Annual market update. https://www.researchgate.net/publication/301608267_Global_Wind_Report_-_Annual_Market_Update_2015 (2015). Accessed 24 Mar 2018
Global Wind Energy Council (GWEC). Global wind energy outlook 2014. An annual report by Global Wind Energy Council. https://gwec.net/publications/global-wind-energy-outlook/global-wind-energy-outlook-2014/ (2014). Accessed 18 Mar 2018
Herbert-Acero, J.F., Probst, O., Réthoré, P.E., Larsen, G.C., Castillo-Villar, K.K.: A review of methodological approaches for the design and optimization of wind farms. Energies 7 , 6930–7016 (2004)
Article Google Scholar
Jangamshetti, S.H., Rau, G.V.: Site matching of wind turbine generators: a case study. IEEE Trans. Energy Convers. 14 (4), 1537–1543 (1999)
Li, H., Chen, Z.: Design optimization and site matching of direct-drive permanent magnet wind power generator systems. Renew. Energy 34 , 1175–1184 (2009)
Albadi, M.H., El-Saadany, E.F.: Optimum turbine-site matching. Energy 35 , 3593–3602 (2010)
Lydia, M., Kumar, S.S., Selvakumar, A.I., Prem Kumar, G.E.: A comprehensive review on wind turbine power curve modeling techniques. Renew. Sustain. Energy Rev. 30 , 452–460 (2014)
Carta, J.A., Velázquez, S.: A new probabilistic method to estimate the long-term wind speed characteristics at a potential wind energy conversion site. Energy 36 , 2671–2685 (2011)
Ohunakin, O.S., Akinnawonu, O.O.: Assessment of wind energy potential and the economics of wind power generation in Jos, Plateau State, Nigeria. Energy Sustain. Dev. 16 , 78–83 (2012)
BoroumandJazi, G., Rismanchi, B., Saidur, R.: Technical characteristic analysis of wind energy conversion systems for sustainable development. Energy Convers. Manag. 69 , 87–94 (2013)
Carrillo, C., Obando Montaño, A.F., Cidrás, J., Díaz-Dorado, E.: Review of power curve modelling for wind turbines. Renew. Sustain. Energy Rev. 21 , 572–581 (2013)
Kiranoudis, C.T., Maroulis, Z.B.: Effective short-cut modelling of wind park efficiency. Renew. Energy 11 (4), 439–457 (1997)
Hu, S., Cheng, J.: Performance evaluation of pairing between sites and wind turbines. Renew. Energy 32 , 1934–1947 (2007)
IEC. Wind turbine generator systems: part 1, safety requirements. https://webstore.iec.ch/p-preview/info_iec61400-1%7Bed2.0%7Den.pdf (1999). Accessed 18 June 2018
Sathyajith, M., Geetha, S.P., Chee, M.L.: Analysis of Wind Regimes and Performance of Wind Turbines, pp. 71–83. Springer, New York (2011)
Google Scholar
Jowder, F.A.L.: Wind power analysis and site matching of wind turbine generators in Kingdom of Bahrain. Appl. Energy 86 , 538–545 (2009)
Akdağ, S.A., Dinler, A.: A new method to estimate Weibull parameters for wind energy applications. Energy Convers. Manag. 50 , 1761–1766 (2009)
Kwon, S.: Uncertainty analysis of wind energy potential assessment. Appl. Energy 87 , 856–865 (2010)
Justus, C., Hargraves, W.R., Yalcin, A.: Nationwide assessment of potential output from wind-powered generators. J. Appl. Meteorol. 15 , 367–678 (1976)
Costa Rocha, P.A., de Sousa, R.C., de Andrade, C.F., da Silva, M.E.V.: Comparison of seven numerical methods for determining Weibull parameters for wind energy generation in the northeast region of Brazil. Appl. Energy 89 , 395–400 (2012)
Hrayshat, E.S.: Wind resource assessment of the Jordanian southern region. Renew. Energy 32 , 1948–1960 (2007)
Khahro, S.F., Tabbassum, K., Soomro, A.M., Liao, X., Alvi, M.B., Dong, L., et al.: Techno-economical evaluation of wind energy potential and analysis of power generation from wind at Gharo, Sindh Pakistan. Renew. Sustain. Energy Rev. 35 , 460–474 (2014)
Jangamshetti, S.H.: Normalized power curves as a tool for identification of optimum wind turbine generator parameters. IEEE Trans. Energy Convers. 16 (3), 283–288 (2001)
Chauhan, A., Saini, R.P.: A review on integrated renewable energy system based power generation for stand-alone applications: configurations, storage options, sizing methodologies and control. Renew. Sustain. Energy Rev. 38 , 99–120 (2014)
Dialynas, E., Machias, A.: Reliability modelling interactive techniques of power systems including wind generating units. Arch. Fuer Elektrotech. 72 , 33–41 (1989)
Thapar, V., Agnihotri, G., Sethi, V.K.: Critical analysis of methods for mathematical modelling of wind turbines. Renew. Energy 36 , 3166–3177 (2011)
Powell, R.W.: An analytical expression for the average output power of a wind machine. Sol. Energy 26 , 77–80 (1981)
Johnson, G.L.: Wind Energy Systems. Prentice Hall, New York (2001)
Li, H., Chen, L., Han, L.: Comparison and evaluation of induction generator models in wind turbine systems for transient stability of power system. http://ee.cqu.edu.cn/myweb/upfile/20080626120806162.pdf . Accessed 02 Feb 2016
Søren, K., Poul-Erik, M., Shimon, A.: The Economics of Wind Energy. European Wind Energy Association, Brussels (2009)
IRENA. Renewable power generation costs in 2014. https://www.irena.org/documentdownloads/publications/irena_re_power_costs_2014_report.pdf (2015). Accessed 18 June 2019
Sathyajith M. Wind energy: fundamentals, resource analysis and economics. Springer online. https://www.dolcera.com/wiki/images/Wind_power_energy.pdf (2006). Accessed 17 Jan 2019
Diyoke, C., Idogwu, S., Ngwaka, U.C.: An economic assessment of biomass gasification for rural electrification in Nigeria. Int. J. Renew. Energy Technol. Res, 3 , 1–17 (2014)
Diyoke, C., Aneke, M., Wang, M., Wu, C.: Techno-economic analysis of wind power integrated with both compressed air energy storage (CAES) and biomass gasification energy storage (BGES) for power generation. RSC Adv. 8 , 22004–2202222 (2018)
Chang, T.J., Tu, Y.L.: Evaluation of monthly capacity factor of WECS using chronological and probabilistic wind speed data: a case study of Taiwan. Renew. Energy 32 (12), 1999–2010 (2007)
Download references
Acknowledgements
Chidiebere Diyoke is grateful to the Tertiary Education Trust Fund (TETFund) Nigeria. For the scholarship granted him through Enugu State University of Science and Technology (ESUT) for his Ph.D. research.
Author information
Authors and affiliations.
Department of Mechanical Engineering, Enugu State University of Science and Technology, Enugu, Nigeria
Chidiebere Diyoke
School of Engineering, Faculty of Science and Engineering, University of Hull, Hull, HU6 7RX, UK
You can also search for this author in PubMed Google Scholar
Corresponding author
Correspondence to Chidiebere Diyoke .
Ethics declarations
Conflict of interest.
We declare no conflict of interest whatsoever.
Additional information
Publisher's note.
Springer Nature remains neutral with regard to jurisdictional claims in published maps and institutional affiliations.
Rights and permissions
Open Access This article is distributed under the terms of the Creative Commons Attribution 4.0 International License ( http://creativecommons.org/licenses/by/4.0/ ), which permits unrestricted use, distribution, and reproduction in any medium, provided you give appropriate credit to the original author(s) and the source, provide a link to the Creative Commons license, and indicate if changes were made.
Reprints and permissions
About this article
Diyoke, C. A new approximate capacity factor method for matching wind turbines to a site: case study of Humber region, UK. Int J Energy Environ Eng 10 , 451–462 (2019). https://doi.org/10.1007/s40095-019-00320-5
Download citation
Received : 25 May 2019
Accepted : 31 August 2019
Published : 06 September 2019
Issue Date : December 2019
DOI : https://doi.org/10.1007/s40095-019-00320-5
Share this article
Anyone you share the following link with will be able to read this content:
Sorry, a shareable link is not currently available for this article.
Provided by the Springer Nature SharedIt content-sharing initiative
- Renewable energy
- Capacity factor
- Cost of electricity
- Wind power analysis
- Find a journal
- Publish with us
- Track your research
Academia.edu no longer supports Internet Explorer.
To browse Academia.edu and the wider internet faster and more securely, please take a few seconds to upgrade your browser .
Enter the email address you signed up with and we'll email you a reset link.
- We're Hiring!
- Help Center
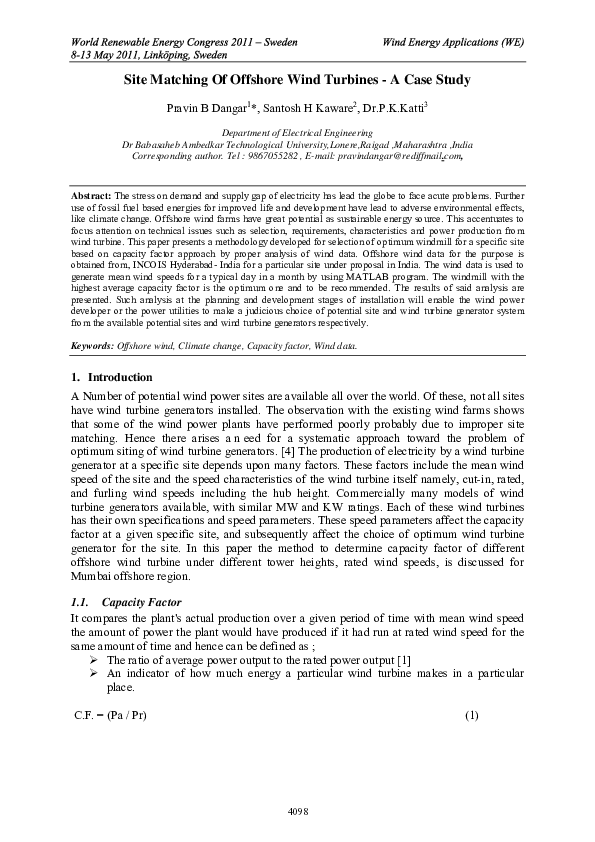
Site Matching Of Offshore Wind Turbines - A Case Study

2011, Proceedings of the World Renewable Energy Congress – Sweden, 8–13 May, 2011, Linköping, Sweden
RELATED TOPICS
- We're Hiring!
- Help Center
- Find new research papers in:
- Health Sciences
- Earth Sciences
- Cognitive Science
- Mathematics
- Computer Science
- Academia ©2024
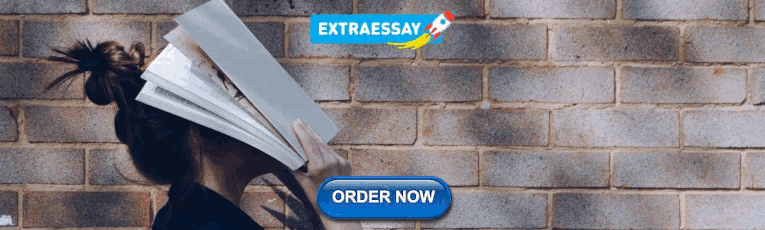
IMAGES
VIDEO
COMMENTS
Site matching of wind turbine generators is investigated based on the appropriate selection of statistical models and means of wind speed data. The wind speed means are computed using arithmetic mean, root mean square and cubic mean cuberoot. Wind speed frequency distributions are modelled using Weibull and Rayleigh probability density functions. Wind speed data of an existing wind power ...
Abstract. Site matching of wind turbine generators is investigated based on the appropriate selection of statistical models and means of wind speed data. The wind speed means are computed using ...
1.. IntroductionNumerous decisions are involved in the design of a wind turbine generator (WTG) for a specific site. Site-specific design optimization problem of a horizontal axis, variable-speed wind turbine was treated in Ref. [1].Factors affecting electricity production by a WTG include the mean wind speed of the site and more importantly, the characteristics of the windmill itself ...
During the last few years, Egypt has emerged as the leader of wind power in the Middle East and Africa. In the Gulf of Suez region, a continuously expanding large-scale grid-connected wind farm is available at Zafarana site. The Gulf of EL-Zayt site in the Gulf of Suez region is now under extensive studies related to wind power projects such as feasibility and bird migration studies.
The optimal site matching of wind turbine generators [16] as applied to the study site, shows that the rated wind speed of an optimal turbine is 4.27 m/s. This value is very low and no ...
Many types of wind turbines are available in current market. Table 1 lists the specifications of various commercial larger-scale wind turbine generators, including operation speeds, rated power, rotor diameter and hub height. For a pitch-controlled wind turbine, the typical power output curve can be shown as in Fig. 1 [11], [12].For wind speeds between cut-in speed (v c) and rated speed (v r ...
Site matching of wind turbine generators: a case study (PDF) Site matching of wind turbine generators: a case study | Suresh Jangamshetti - Academia.edu Academia.edu no longer supports Internet Explorer.
DOI: 10.1016/J.RENENE.2009.12.013 Corpus ID: 109615283; Optimal site matching of wind turbine generator: Case study of the Gulf of Suez region in Egypt @article{ELShimy2010OptimalSM, title={Optimal site matching of wind turbine generator: Case study of the Gulf of Suez region in Egypt}, author={M. EL-Shimy}, journal={Renewable Energy}, year={2010}, volume={35}, pages={1870-1878}, url={https ...
Downloadable (with restrictions)! During the last few years, Egypt has emerged as the leader of wind power in the Middle East and Africa. In the Gulf of Suez region, a continuously expanding large-scale grid-connected wind farm is available at Zafarana site. The Gulf of EL-Zayt site in the Gulf of Suez region is now under extensive studies related to wind power projects such as feasibility and ...
The U.S. Department of Energy's Office of Scientific and Technical Information Site matching of wind turbine generators: A case study (Journal Article) | OSTI.GOV skip to main content
1988. 7. Site matching of wind turbine generators is investigated based on the appropriate selection of statistical models and means of wind speed data. The wind speed means are computed using arithmetic mean, root mean square and cubic mean cuberoot. Wind speed frequency distributions are modelled using Weibull and Rayleigh probability density ...
has been derived for matching wind turbines to potential site f or optimum yield and profitability. The indexes of capacity ... generators: a case study. IEEE Trans. Energy Conv ers. 14 (4), 1537 ...
Janagmshetti SH, Rau VG. Site matching of wind turbine generators: a case study. IEEE Trans Energy Conv 1999;14(4):1537-43. [4] Janagmshetti SH, Rau VG. Optimum siting of wind turbine generators. IEEE Trans Energy Conv 2001;16(1):8-13. [5] Jowder FAL. Wind power analysis and site matching of wind turbine generators in Kingdom of Bahrain.
Wind-based power is one of the renewable base power sources that are tipped to play a great role in decarbonising the globe. To achieve this potential, more wind farms are likely to be built. The capacity factor of a wind farm and hence its profitability is dependent on whether it is properly sized and sited. In fact, some wind power plants have failed wholly or underperformed, because the ...
developer or the power utilities to make a judicious choice of potential site and wind turbine generator system from the available potential sites and wind turbine generators respectively. Keywords: Offshore wind, Climate change, Capacity factor, Wind data. 1. Introduction . A Number of potential wind power sites are available all over the world.
In addition, the site matching of wind turbine generators at 30 ... In order to study wind power in a particular site, the long-term records of wind speed have to be statistically analyzed. ... Site matching of wind turbines generator: a case study. IEEE Trans Energy Conv, 14 (4) (1999), pp. 1537-1543. Google Scholar [17]
There have been a number of attempts to match a specific site with a suitable turbine among existing ones, a process more commonly known as site matching of wind turbine generators [11] [12][13 ...
The stress on demand and supply gap of electricity has lead the globe to face acute problems. Further use of fossil fuel based energies for improved life and development have lead to adverse environmental effects, like climate change. Offshore wind farms have great potential as sustainable energy source. This accentuates to focus attention on technical issues such as selection, requirements ...
A new approximate capacity factor method for matching wind turbines to a site: case study of Humber region. 2019 • ... Suresh H. Jangamshetti, and Dr. V. Guruprasada Rau, Site matching of wind turbine Generators : A case study, IEEE Transactions on Energy Conversion, Vol. 14, N o. 4, December 1999 pp- 1537-1543 [7] Bogdas S.Borowy,Ziyad M ...
Finally, we used these formulations to evaluate the site location and the turbines needed for the production of synthetic methane from carbon dioxide and electrolytic hydrogen as a case study. Selecting the optimal turbine and site location results in competitive prices for synthetic methane, below 2€/MMBTU, and large savings in the ...
Wind turbine selection is an evaluation problem involving many factors, such as technology, economy, society, etc., and there exist internal dependencies and circular relationships among these factors. This increases the complexity of the selection problem. At the same time, with the development of wind power technology, the types of wind turbines on the market are increasing. Therefore, it is ...
S. H. Jangamshetti and V. G. Rau, "Site matching of wind turbine generators: A case study," PE-031-EC--08-1998, A paper recommended and approved by the IEEE Energy Development and Power ...
Site Matching Of Offshore Wind Turbines - A Case Study PK Katti 2011, Proceedings of the World Renewable Energy Congress - Sweden, 8-13 May, 2011, Linköping, Sweden