Graduate Programs

Our graduate programs provide a unique environment where linguistic theory, multiple methodologies, and computational research not only coexist, but interact in a highly synergistic fashion.
Our focus is on the Ph.D. degree. The department occasionally admits students already enrolled at Stanford for the M.A. degree. Ph.D. students in other departments at Stanford may also apply for the Ph.D. Minor.

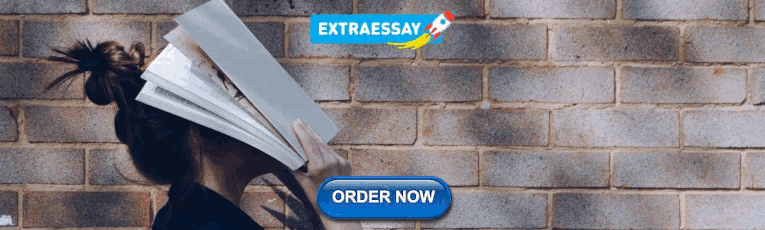
Doctoral Program
Our Ph.D. program emphasizes rigorous theoretical work that has at its base a firm empirical foundation in language data.

Ph.D. Minor
Our Ph.D. Minor allows Ph.D. students in other Stanford departments to develop a solid grounding in linguistics that can complement and enhance their studies and research in their home department.

M.A. for Stanford Graduate Students
We offer an MA degree for Stanford graduate students which develops students' knowledge of linguistics, preparing them for a professional career or doctoral study in linguistics or related disciplines.

Coterminal M.A. Program
Our Coterminal M.A. Program develops students' knowledge of linguistics, preparing them for a professional career or doctoral study in linguistics or related disciplines.

Ignacio Cases
Phd candidate in computational linguistics.
Stanford Linguistics and Stanford NLP Group
- Download Resume
How is the brain able to process an infinitude of expressions composed from a small number of elements? What are the computational principles underlying symbolic manipulation in the human mind? How does symbolic computation emerge from computations in neural networks? These questions are intimately related to our ability to learn and deeply understand natural language — arguably one of the key components of human intelligence, and most certainly a required step in our journey for brain-like AI.
As a PhD candidate at Stanford, advised by Dan Jurafsky , Chris Potts and Josh Greene , I study these research questions in the intersection between Computational Linguistics, Computer Science, and Cognitive Neuroscience.
In my work, I develop Deep Reinforcement Learning models that learn compositional semantics in a systematic way. In particular, I’m interested in the role that memory plays in these architectures as a catalyst in the emergence of symbolic computation from the underlying substratum.
Continual Learning and Meta-Learning
- Learning to Learn without Forgetting by Maximizing Transfer and Minimizing Interference with Matt Riemer, Robert Ajemian, Miao Liu, Irina Rish, Yuhai Tu, and Gerald Tesauro
- Continual Learning by Maximizing Transfer and Minimizing Interference with Matt Riemer, Robert Ajemian, Miao Liu, Irina Rish, Yuhai Tu, and Gerald Tesauro
- Learning to Compose with Scratchpad Memory , with Andrej Barbu, Yen-Ling Kuo, Diego Mendoza-Halliday and Boris Katz
Papers related to Computational Linguistics
- Cases, Ignacio, Atticus Geiger, Alex Tamkin, Kenny Xu, and Lauri Karttunen -->