Study Design 101: Case Control Study
- Case Report
- Case Control Study
- Cohort Study
- Randomized Controlled Trial
- Practice Guideline
- Systematic Review
- Meta-Analysis
- Helpful Formulas
- Finding Specific Study Types
A study that compares patients who have a disease or outcome of interest (cases) with patients who do not have the disease or outcome (controls), and looks back retrospectively to compare how frequently the exposure to a risk factor is present in each group to determine the relationship between the risk factor and the disease.
Case control studies are observational because no intervention is attempted and no attempt is made to alter the course of the disease. The goal is to retrospectively determine the exposure to the risk factor of interest from each of the two groups of individuals: cases and controls. These studies are designed to estimate odds.
Case control studies are also known as "retrospective studies" and "case-referent studies."
- Good for studying rare conditions or diseases
- Less time needed to conduct the study because the condition or disease has already occurred
- Lets you simultaneously look at multiple risk factors
- Useful as initial studies to establish an association
- Can answer questions that could not be answered through other study designs
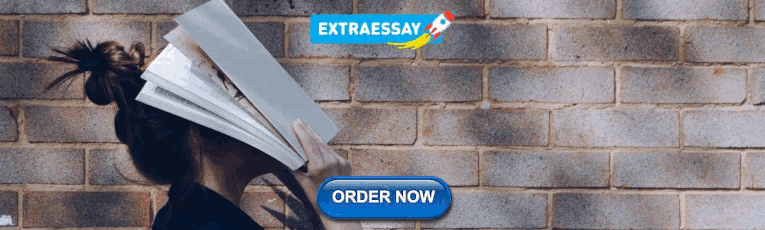
Disadvantages
- Retrospective studies have more problems with data quality because they rely on memory and people with a condition will be more motivated to recall risk factors (also called recall bias).
- Not good for evaluating diagnostic tests because it's already clear that the cases have the condition and the controls do not
- It can be difficult to find a suitable control group
Design pitfalls to look out for
Care should be taken to avoid confounding, which arises when an exposure and an outcome are both strongly associated with a third variable. Controls should be subjects who might have been cases in the study but are selected independent of the exposure. Cases and controls should also not be "over-matched."
Is the control group appropriate for the population? Does the study use matching or pairing appropriately to avoid the effects of a confounding variable? Does it use appropriate inclusion and exclusion criteria?
Fictitious Example
There is a suspicion that zinc oxide, the white non-absorbent sunscreen traditionally worn by lifeguards is more effective at preventing sunburns that lead to skin cancer than absorbent sunscreen lotions. A case-control study was conducted to investigate if exposure to zinc oxide is a more effective skin cancer prevention measure. The study involved comparing a group of former lifeguards that had developed cancer on their cheeks and noses (cases) to a group of lifeguards without this type of cancer (controls) and assess their prior exposure to zinc oxide or absorbent sunscreen lotions.
This study would be retrospective in that the former lifeguards would be asked to recall which type of sunscreen they used on their face and approximately how often. This could be either a matched or unmatched study, but efforts would need to be made to ensure that the former lifeguards are of the same average age, and lifeguarded for a similar number of seasons and amount of time per season.
Real-life Examples
Boubekri, M., Cheung, I., Reid, K., Wang, C., & Zee, P. (2014). Impact of windows and daylight exposure on overall health and sleep quality of office workers: a case-control pilot study. Journal of Clinical Sleep Medicine : JCSM : Official Publication of the American Academy of Sleep Medicine, 10 (6), 603-611. https://doi.org/10.5664/jcsm.3780
This pilot study explored the impact of exposure to daylight on the health of office workers (measuring well-being and sleep quality subjectively, and light exposure, activity level and sleep-wake patterns via actigraphy). Individuals with windows in their workplaces had more light exposure, longer sleep duration, and more physical activity. They also reported a better scores in the areas of vitality and role limitations due to physical problems, better sleep quality and less sleep disturbances.
Togha, M., Razeghi Jahromi, S., Ghorbani, Z., Martami, F., & Seifishahpar, M. (2018). Serum Vitamin D Status in a Group of Migraine Patients Compared With Healthy Controls: A Case-Control Study. Headache, 58 (10), 1530-1540. https://doi.org/10.1111/head.13423
This case-control study compared serum vitamin D levels in individuals who experience migraine headaches with their matched controls. Studied over a period of thirty days, individuals with higher levels of serum Vitamin D was associated with lower odds of migraine headache.
Related Formulas
- Odds ratio in an unmatched study
- Odds ratio in a matched study
Related Terms
A patient with the disease or outcome of interest.
Confounding
When an exposure and an outcome are both strongly associated with a third variable.
A patient who does not have the disease or outcome.
Matched Design
Each case is matched individually with a control according to certain characteristics such as age and gender. It is important to remember that the concordant pairs (pairs in which the case and control are either both exposed or both not exposed) tell us nothing about the risk of exposure separately for cases or controls.
Observed Assignment
The method of assignment of individuals to study and control groups in observational studies when the investigator does not intervene to perform the assignment.
Unmatched Design
The controls are a sample from a suitable non-affected population.
Now test yourself!
1. Case Control Studies are prospective in that they follow the cases and controls over time and observe what occurs.
a) True b) False
2. Which of the following is an advantage of Case Control Studies?
a) They can simultaneously look at multiple risk factors. b) They are useful to initially establish an association between a risk factor and a disease or outcome. c) They take less time to complete because the condition or disease has already occurred. d) b and c only e) a, b, and c
Evidence Pyramid - Navigation
- Meta- Analysis
- Case Reports
- << Previous: Case Report
- Next: Cohort Study >>

- Last Updated: Sep 25, 2023 10:59 AM
- URL: https://guides.himmelfarb.gwu.edu/studydesign101

- Himmelfarb Intranet
- Privacy Notice
- Terms of Use
- GW is committed to digital accessibility. If you experience a barrier that affects your ability to access content on this page, let us know via the Accessibility Feedback Form .
- Himmelfarb Health Sciences Library
- 2300 Eye St., NW, Washington, DC 20037
- Phone: (202) 994-2850
- [email protected]
- https://himmelfarb.gwu.edu
What Is A Case Control Study?
Julia Simkus
Editor at Simply Psychology
BA (Hons) Psychology, Princeton University
Julia Simkus is a graduate of Princeton University with a Bachelor of Arts in Psychology. She is currently studying for a Master's Degree in Counseling for Mental Health and Wellness in September 2023. Julia's research has been published in peer reviewed journals.
Learn about our Editorial Process
Saul Mcleod, PhD
Editor-in-Chief for Simply Psychology
BSc (Hons) Psychology, MRes, PhD, University of Manchester
Saul Mcleod, PhD., is a qualified psychology teacher with over 18 years of experience in further and higher education. He has been published in peer-reviewed journals, including the Journal of Clinical Psychology.
Olivia Guy-Evans, MSc
Associate Editor for Simply Psychology
BSc (Hons) Psychology, MSc Psychology of Education
Olivia Guy-Evans is a writer and associate editor for Simply Psychology. She has previously worked in healthcare and educational sectors.
On This Page:
A case-control study is a research method where two groups of people are compared – those with the condition (cases) and those without (controls). By looking at their past, researchers try to identify what factors might have contributed to the condition in the ‘case’ group.
Explanation
A case-control study looks at people who already have a certain condition (cases) and people who don’t (controls). By comparing these two groups, researchers try to figure out what might have caused the condition. They look into the past to find clues, like habits or experiences, that are different between the two groups.
The “cases” are the individuals with the disease or condition under study, and the “controls” are similar individuals without the disease or condition of interest.
The controls should have similar characteristics (i.e., age, sex, demographic, health status) to the cases to mitigate the effects of confounding variables .
Case-control studies identify any associations between an exposure and an outcome and help researchers form hypotheses about a particular population.
Researchers will first identify the two groups, and then look back in time to investigate which subjects in each group were exposed to the condition.
If the exposure is found more commonly in the cases than the controls, the researcher can hypothesize that the exposure may be linked to the outcome of interest.
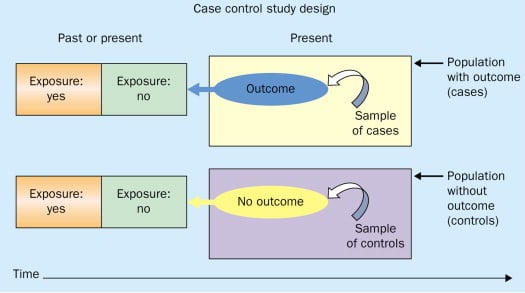
Figure: Schematic diagram of case-control study design. Kenneth F. Schulz and David A. Grimes (2002) Case-control studies: research in reverse . The Lancet Volume 359, Issue 9304, 431 – 434
Quick, inexpensive, and simple
Because these studies use already existing data and do not require any follow-up with subjects, they tend to be quicker and cheaper than other types of research. Case-control studies also do not require large sample sizes.
Beneficial for studying rare diseases
Researchers in case-control studies start with a population of people known to have the target disease instead of following a population and waiting to see who develops it. This enables researchers to identify current cases and enroll a sufficient number of patients with a particular rare disease.
Useful for preliminary research
Case-control studies are beneficial for an initial investigation of a suspected risk factor for a condition. The information obtained from cross-sectional studies then enables researchers to conduct further data analyses to explore any relationships in more depth.
Limitations
Subject to recall bias.
Participants might be unable to remember when they were exposed or omit other details that are important for the study. In addition, those with the outcome are more likely to recall and report exposures more clearly than those without the outcome.
Difficulty finding a suitable control group
It is important that the case group and the control group have almost the same characteristics, such as age, gender, demographics, and health status.
Forming an accurate control group can be challenging, so sometimes researchers enroll multiple control groups to bolster the strength of the case-control study.
Do not demonstrate causation
Case-control studies may prove an association between exposures and outcomes, but they can not demonstrate causation.
A case-control study is an observational study where researchers analyzed two groups of people (cases and controls) to look at factors associated with particular diseases or outcomes.
Below are some examples of case-control studies:
- Investigating the impact of exposure to daylight on the health of office workers (Boubekri et al., 2014).
- Comparing serum vitamin D levels in individuals who experience migraine headaches with their matched controls (Togha et al., 2018).
- Analyzing correlations between parental smoking and childhood asthma (Strachan and Cook, 1998).
- Studying the relationship between elevated concentrations of homocysteine and an increased risk of vascular diseases (Ford et al., 2002).
- Assessing the magnitude of the association between Helicobacter pylori and the incidence of gastric cancer (Helicobacter and Cancer Collaborative Group, 2001).
- Evaluating the association between breast cancer risk and saturated fat intake in postmenopausal women (Howe et al., 1990).
Frequently asked questions
1. what’s the difference between a case-control study and a cross-sectional study.
Case-control studies are different from cross-sectional studies in that case-control studies compare groups retrospectively while cross-sectional studies analyze information about a population at a specific point in time.
In cross-sectional studies , researchers are simply examining a group of participants and depicting what already exists in the population.
2. What’s the difference between a case-control study and a longitudinal study?
Case-control studies compare groups retrospectively, while longitudinal studies can compare groups either retrospectively or prospectively.
In a longitudinal study , researchers monitor a population over an extended period of time, and they can be used to study developmental shifts and understand how certain things change as we age.
In addition, case-control studies look at a single subject or a single case, whereas longitudinal studies can be conducted on a large group of subjects.
3. What’s the difference between a case-control study and a retrospective cohort study?
Case-control studies are retrospective as researchers begin with an outcome and trace backward to investigate exposure; however, they differ from retrospective cohort studies.
In a retrospective cohort study , researchers examine a group before any of the subjects have developed the disease, then examine any factors that differed between the individuals who developed the condition and those who did not.
Thus, the outcome is measured after exposure in retrospective cohort studies, whereas the outcome is measured before the exposure in case-control studies.
Boubekri, M., Cheung, I., Reid, K., Wang, C., & Zee, P. (2014). Impact of windows and daylight exposure on overall health and sleep quality of office workers: a case-control pilot study. Journal of Clinical Sleep Medicine: JCSM: Official Publication of the American Academy of Sleep Medicine, 10 (6), 603-611.
Ford, E. S., Smith, S. J., Stroup, D. F., Steinberg, K. K., Mueller, P. W., & Thacker, S. B. (2002). Homocyst (e) ine and cardiovascular disease: a systematic review of the evidence with special emphasis on case-control studies and nested case-control studies. International journal of epidemiology, 31 (1), 59-70.
Helicobacter and Cancer Collaborative Group. (2001). Gastric cancer and Helicobacter pylori: a combined analysis of 12 case control studies nested within prospective cohorts. Gut, 49 (3), 347-353.
Howe, G. R., Hirohata, T., Hislop, T. G., Iscovich, J. M., Yuan, J. M., Katsouyanni, K., … & Shunzhang, Y. (1990). Dietary factors and risk of breast cancer: combined analysis of 12 case—control studies. JNCI: Journal of the National Cancer Institute, 82 (7), 561-569.
Lewallen, S., & Courtright, P. (1998). Epidemiology in practice: case-control studies. Community eye health, 11 (28), 57–58.
Strachan, D. P., & Cook, D. G. (1998). Parental smoking and childhood asthma: longitudinal and case-control studies. Thorax, 53 (3), 204-212.
Tenny, S., Kerndt, C. C., & Hoffman, M. R. (2021). Case Control Studies. In StatPearls . StatPearls Publishing.
Togha, M., Razeghi Jahromi, S., Ghorbani, Z., Martami, F., & Seifishahpar, M. (2018). Serum Vitamin D Status in a Group of Migraine Patients Compared With Healthy Controls: A Case-Control Study. Headache, 58 (10), 1530-1540.
Further Information
- Schulz, K. F., & Grimes, D. A. (2002). Case-control studies: research in reverse. The Lancet, 359(9304), 431-434.
- What is a case-control study?

Leave a Comment Cancel reply
You must be logged in to post a comment.

- Hirsh Health Sciences
- Lilly Music
- Webster Veterinary
- Hirsh Health Sciences Library
Study Designs in the Health Sciences
- Case-Control
- Introduction
- Clinical Trials
- Randomized Controlled Trial (RCT)
- Systematic Reviews/Meta-Analysis
- Retrieving Articles by Study Design in PubMed
Case-Control Study
What is a case-control study.
“A study that compares patients who have a disease or outcome of interest (cases) with patients who do not have the disease or outcome (controls), and looks back retrospectively to compare how frequently the exposure to a risk factor is present in each group to determine the relationship between the risk factor and the disease.
Case control studies are observational because no intervention is attempted and no attempt is made to alter the course of the disease. The goal is to retrospectively determine the exposure to the risk factor of interest from each of the two groups of individuals: cases and controls. These studies are designed to estimate odds.
Case control studies are also known as "retrospective studies" and "case-referent studies.”[1]
Why use this type of study type?
- Good for studying rare conditions or diseases [1]
- Less time needed to conduct the study because the condition or disease has already occurred [1]
- Lets you simultaneously look at multiple risk factors [1]
- Useful as initial studies to establish an association [1]
- Can answer questions that could not be answered through other study designs [1]
Format and features
- Strengthening the Reporting of Observational Studies in Epidemiology (STROBE) Statement checklist for case-control studies [2]
References
- Himmelfarb Health Sciences Library (The George Washington University). Study Design 101: Case Control Study 2011; http://www.gwumc.edu/library/tutorials/studydesign101/casecontrols.html . Accessed March 1, 2013.
- Strengthening the Reporting of Observational Studies in Epidemiology (STROBE). STROBE checklist for case-control. [2007]; http://www.strobe-statement.org/fileadmin/Strobe/uploads/checklists/STROBE_checklist_v4_case-control.pdf . Accessed March 1, 2013.
A case-control study of readmission to the intensive care unit after cardiac surgery.
Benetis R, Sirvinskas E, Kumpaitiene B, Kinduris S.
Med Sci Monit. 2013 Feb 28; 19:148-52.
Background: The aim of this study was to identify predictors of repeated admission to the intensive care unit (ICU) of patients who underwent cardiac surgery procedures.
Material and Methods: This retrospective study analyzed 169 patients who underwent isolated coronary artery bypass grafting (CABG) between January 2009 and December 2010. The case group contained 54 patients who were readmitted to the ICU during the same hospitalization and the control group comprised 115 randomly selected patients.
Results: Logistic regression analysis revealed that independent predictors for readmission to the ICU after CABG were: older age of patients (odds ratio [OR] 1.04; CI 1.004-1.08); body mass index (BMI) >30 kg/m2 (OR 2.55; CI 1.31-4.97); EuroSCORE II >3.9% (OR 3.56; CI 1.59-7.98); non-elective surgery (OR 2.85; CI 1.37-5.95); duration of operation >4 h (OR 3.44; CI 1.54-7.69); bypass time >103 min (OR 2.5; CI 1.37-4.57); mechanical ventilation >530 min (OR 3.98; CI 1.82-8.7); and postoperative central nervous system (CNS) disorders (OR 3.95; CI 1.44-10.85). The hospital mortality of patients who were readmitted to the ICU was significantly higher compared to the patients who did not require readmission (17% vs. 3.8%, p=0.025). Conclusions: Identification of patients at risk of ICU readmission should focus on older patients, those who have higher BMI, who underwent non-elective surgery, whose operation time was more than 4 hours, and who have postoperative CNS disorders. Careful optimization of these high-risk patients and caution before discharging them from the ICU may help reduce the rate of ICU readmission, mortality, length of stay, and cost.
- << Previous: Introduction
- Next: Clinical Trials >>
- Last Updated: Dec 26, 2019 11:38 AM
- URL: https://researchguides.library.tufts.edu/study_design
Thank you for visiting nature.com. You are using a browser version with limited support for CSS. To obtain the best experience, we recommend you use a more up to date browser (or turn off compatibility mode in Internet Explorer). In the meantime, to ensure continued support, we are displaying the site without styles and JavaScript.
- View all journals
- Explore content
- About the journal
- Publish with us
- Sign up for alerts
- Published: 04 January 2019
Case-control studies: an efficient study design
- L. A. Harvey 1
Spinal Cord volume 57 , pages 1–2 ( 2019 ) Cite this article
3323 Accesses
3 Citations
4 Altmetric
Metrics details
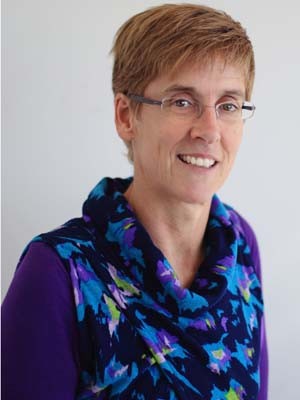
Case-control studies provide estimates of how much more likely an outcome is amongst people who are subject to a particular exposure than amongst people who are not [ 1 , 2 , 3 , 4 ]. So they are helpful for answering questions about the aetiology of a disease or condition (i.e. an outcome).
Case-control studies are particularly useful for studying the cause of an outcome that is rare and for studying the effects of prolonged exposure. For example, a case-control study could be used to determine whether long-term use of indwelling catheters (the exposure) causes bladder cancer (the outcome) in people with spinal cord injury. (This is an example of a study of prolonged exposure on risk of a rare disease. Incidentally, the causal link between catheters and bladder cancer is contentious [ 5 , 6 , 7 ]).
In this example, the cases would be people with spinal cord injury, from the study base, who develop bladder cancer. It is important that all cases, or a random sample of all cases, from the study base are identified; they should not merely be a sample of convenience. (The study base might be, for example, all of the people with a spinal cord injury in a geographical area, or all of the people who, if they developed the disease of interest, would present at a particular hospital.) The controls should be sampled from the same study base of people with spinal cord injury. Controls must be sampled in a way that is not influenced by whether they are or are not exposed. So in our example, the controls would be a randomly selected group of people with spinal cord injury drawn from the same study base as the cases, irrespective of whether they do or do not have bladder cancer and irrespective of whether they have or have not been exposed to indwelling catheters. Theoretically, a person could be both a case and a control, although this is unlikely to happen because bladder cancer is rare. Data must be collected on exposures and outcomes of every participant. In the current example, data must be collected on the use of indwelling catheters and presence of bladder cancer. From these data, it is possible to construct an odds ratio that depicts how much more likely a person who has used indwelling catheters is to develop bladder cancer than a person who has not used indwelling catheters. Case-control studies are observational studies, so even if cases and controls are sampled without regard to exposure, it is still necessary to rigorously adjust for confounding.
Often, researchers conduct a different sort of study and erroneously call it a case-control study. In that design, researchers sample controls from a population that does not develop the disease of interest. For example, they sample people with spinal cord injury who do not develop bladder cancer. When controls are sampled in this way, the odds ratios may provide biased estimates of the causal effect, even if confounding is rigorously controlled.
Matching may improve the efficiency of case-control designs. However, a common misunderstanding is that matched case-control studies need only involve collecting data on a convenient sample of cases and a convenient sample of people who are matched to the cases on a few variables [ 8 ]. This is not correct. As far back as 1986 Rothman said that:
''..because [case control studies] need not be expensive nor time-consuming to conduct….many studies have been conducted by would-be investigators who lack even a rudimentary appreciation for epidemiologic principles. Occasionally such haphazard research can produce fruitful or even extremely important results, but often the results are wrong because basic research principles have been violated ” (cited p. 431 [ 9 ]).
Importantly, a matched case-control design still requires that cases be people from the study base with the condition of interest and controls still need to be sampled from the same study base without regard to exposure. In a matched design, there is the additional complexity that cases and controls are matched on variables that are likely to confound estimates (e.g. time since injury or age). A well-conducted matched case-control design may be more efficient and therefore requires a smaller sample size than an unmatched study. However, matching on a variable that is not actually a confounder may reduce efficiency. Moreover, matching on a variable that is affected by the exposure (a mediator) or is affected by both the exposure and the outcome (a collider) may introduce bias. For example, while it might be tempting to match for smoking status because those who smoke are more likely to develop bladder cancer [ 5 , 6 ], this would only be necessary if smoking status influences the likelihood of using indwelling catheters (which would seem unlikely). It is therefore important to consider whether a variable is a true confounder before matching on it [ 10 ].
Spinal Cord values carefully designed case-control studies because they provide a very efficient way of estimating the causal effect of an exposure on the risk of developing a rare condition. However, they need to be grounded in key epidemiological principles to ensure that the results are trustworthy.
Pearce N. Analysis of matched case-control studies. BMJ. 2016;352:i969.
Article Google Scholar
Vandenbroucke JP, Pearce N. Case-control studies: basic concepts. Int J Epidemiol. 2012;41:1480–9.
Grobbee D, Hoes A. Clinical epidemiology. Principles, methods and applications for clinical research. Massachusetts: Jones and Bartlett Publishers; 2009.
Herbert R. Case-control studies. J Physiother. 2017;63:264–6.
Gui-Zhong L, Li-Bo M. Bladder cancer in individuals with spinal cord injuries: a meta-analysis. Spinal Cord. 2017;55:341–5.
Article CAS Google Scholar
Welk B, McIntyre A, Teasell R, Potter P, Loh E. Bladder cancer in individuals with spinal cord injuries. Spinal Cord. 2013;51:516–21.
Bothig R, Kurze I, Fiebag K, Kaufmann A, Schops W, Kadhum T, et al. Clinical characteristics of bladder cancer in patients with spinal cord injury: the experience from a single centre. Int Urol Nephrol. 2017;49:983–94.
Lewallen S, Courtright P. Epidemiology in practice: case-control studies. Community Eye Health. 1998;11:57–58.
CAS PubMed PubMed Central Google Scholar
Schulz KF, Grimes DA. Case-control studies: research in reverse. Lancet. 2002;359:431–4.
Pearl J, McKenzie D. The Book of Why. The New Science of Cause and Effect. New York: Basic Books; 2018.
Download references
Author information
Authors and affiliations.
University of Sydney, Sydney, Australia
L. A. Harvey
You can also search for this author in PubMed Google Scholar
Corresponding author
Correspondence to L. A. Harvey .
Additional information
Publisher’s note: Springer Nature remains neutral with regard to jurisdictional claims in published maps and institutional affiliations.
Rights and permissions
Reprints and permissions
About this article
Cite this article.
Harvey, L.A. Case-control studies: an efficient study design. Spinal Cord 57 , 1–2 (2019). https://doi.org/10.1038/s41393-018-0234-4
Download citation
Published : 04 January 2019
Issue Date : January 2019
DOI : https://doi.org/10.1038/s41393-018-0234-4
Share this article
Anyone you share the following link with will be able to read this content:
Sorry, a shareable link is not currently available for this article.
Provided by the Springer Nature SharedIt content-sharing initiative
This article is cited by
Traumatic spinal cord injury confers bladder cancer risk to patients managed without permanent urinary catheterization: lessons from a comparison of clinical data with the national database.
- Ralf Böthig
- Christian Tiburtius
- Klaus Golka
World Journal of Urology (2020)
Quick links
- Explore articles by subject
- Guide to authors
- Editorial policies

Have a language expert improve your writing
Run a free plagiarism check in 10 minutes, automatically generate references for free.
- Knowledge Base
- Methodology
- What Is a Case-Control Study? | Definition & Examples
What Is a Case-Control Study? | Definition & Examples
Published on 4 February 2023 by Tegan George .
A case-control study is an experimental design that compares a group of participants possessing a condition of interest to a very similar group lacking that condition. Here, the participants possessing the attribute of study, such as a disease, are called the ‘case’, and those without it are the ‘control’.
It’s important to remember that the case group is chosen because they already possess the attribute of interest. The point of the control group is to facilitate investigation, e.g., studying whether the case group systematically exhibits that attribute more than the control group does.
Table of contents
When to use a case-control study, examples of case-control studies, advantages and disadvantages of case-control studies, frequently asked questions.
Case-control studies are a type of observational study often used in fields like medical research, environmental health, or epidemiology. While most observational studies are qualitative in nature, case-control studies can also be quantitative , and they often are in healthcare settings. Case-control studies can be used for both exploratory and explanatory research , and they are a good choice for studying research topics like disease exposure and health outcomes.
A case-control study may be a good fit for your research if it meets the following criteria.
- Data on exposure (e.g., to a chemical or a pesticide) are difficult to obtain or expensive.
- The disease associated with the exposure you’re studying has a long incubation period or is rare or under-studied (e.g., AIDS in the early 1980s).
- The population you are studying is difficult to contact for follow-up questions (e.g., asylum seekers).
Retrospective cohort studies use existing secondary research data, such as medical records or databases, to identify a group of people with a common exposure or risk factor and to observe their outcomes over time. Case-control studies conduct primary research , comparing a group of participants possessing a condition of interest to a very similar group lacking that condition in real time.
Prevent plagiarism, run a free check.
Case-control studies are common in fields like epidemiology, healthcare, and psychology.
You would then collect data on your participants’ exposure to contaminated drinking water, focusing on variables such as the source of said water and the duration of exposure, for both groups. You could then compare the two to determine if there is a relationship between drinking water contamination and the risk of developing a gastrointestinal illness. Example: Healthcare case-control study You are interested in the relationship between the dietary intake of a particular vitamin (e.g., vitamin D) and the risk of developing osteoporosis later in life. Here, the case group would be individuals who have been diagnosed with osteoporosis, while the control group would be individuals without osteoporosis.
You would then collect information on dietary intake of vitamin D for both the cases and controls and compare the two groups to determine if there is a relationship between vitamin D intake and the risk of developing osteoporosis. Example: Psychology case-control study You are studying the relationship between early-childhood stress and the likelihood of later developing post-traumatic stress disorder (PTSD). Here, the case group would be individuals who have been diagnosed with PTSD, while the control group would be individuals without PTSD.
Case-control studies are a solid research method choice, but they come with distinct advantages and disadvantages.
Advantages of case-control studies
- Case-control studies are a great choice if you have any ethical considerations about your participants that could preclude you from using a traditional experimental design .
- Case-control studies are time efficient and fairly inexpensive to conduct because they require fewer subjects than other research methods .
- If there were multiple exposures leading to a single outcome, case-control studies can incorporate that. As such, they truly shine when used to study rare outcomes or outbreaks of a particular disease .
Disadvantages of case-control studies
- Case-control studies, similarly to observational studies, run a high risk of research biases . They are particularly susceptible to observer bias , recall bias , and interviewer bias.
- In the case of very rare exposures of the outcome studied, attempting to conduct a case-control study can be very time consuming and inefficient .
- Case-control studies in general have low internal validity and are not always credible.
Case-control studies by design focus on one singular outcome. This makes them very rigid and not generalisable , as no extrapolation can be made about other outcomes like risk recurrence or future exposure threat. This leads to less satisfying results than other methodological choices.
A case-control study differs from a cohort study because cohort studies are more longitudinal in nature and do not necessarily require a control group .
While one may be added if the investigator so chooses, members of the cohort are primarily selected because of a shared characteristic among them. In particular, retrospective cohort studies are designed to follow a group of people with a common exposure or risk factor over time and observe their outcomes.
Case-control studies, in contrast, require both a case group and a control group, as suggested by their name, and usually are used to identify risk factors for a disease by comparing cases and controls.
A case-control study differs from a cross-sectional study because case-control studies are naturally retrospective in nature, looking backward in time to identify exposures that may have occurred before the development of the disease.
On the other hand, cross-sectional studies collect data on a population at a single point in time. The goal here is to describe the characteristics of the population, such as their age, gender identity, or health status, and understand the distribution and relationships of these characteristics.
Cases and controls are selected for a case-control study based on their inherent characteristics. Participants already possessing the condition of interest form the “case,” while those without form the “control.”
Keep in mind that by definition the case group is chosen because they already possess the attribute of interest. The point of the control group is to facilitate investigation, e.g., studying whether the case group systematically exhibits that attribute more than the control group does.
The strength of the association between an exposure and a disease in a case-control study can be measured using a few different statistical measures , such as odds ratios (ORs) and relative risk (RR).
No, case-control studies cannot establish causality as a standalone measure.
As observational studies , they can suggest associations between an exposure and a disease, but they cannot prove without a doubt that the exposure causes the disease. In particular, issues arising from timing, research biases like recall bias , and the selection of variables lead to low internal validity and the inability to determine causality.
Sources for this article
We strongly encourage students to use sources in their work. You can cite our article (APA Style) or take a deep dive into the articles below.
George, T. (2023, February 04). What Is a Case-Control Study? | Definition & Examples. Scribbr. Retrieved 22 April 2024, from https://www.scribbr.co.uk/research-methods/case-control-studies/
Schlesselman, J. J. (1982). Case-Control Studies: Design, Conduct, Analysis (Monographs in Epidemiology and Biostatistics, 2) (Illustrated). Oxford University Press.
Is this article helpful?
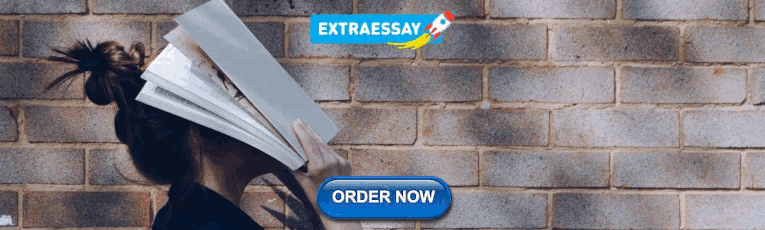
Tegan George
Other students also liked, what is an observational study | guide & examples, control groups and treatment groups | uses & examples, cross-sectional study | definitions, uses & examples.
Quantitative study designs: Case Control
Quantitative study designs.
- Introduction
- Cohort Studies
- Randomised Controlled Trial
Case Control
- Cross-Sectional Studies
- Study Designs Home
In a Case-Control study there are two groups of people: one has a health issue (Case group), and this group is “matched” to a Control group without the health issue based on characteristics like age, gender, occupation. In this study type, we can look back in the patient’s histories to look for exposure to risk factors that are common to the Case group, but not the Control group. It was a case-control study that demonstrated a link between carcinoma of the lung and smoking tobacco . These studies estimate the odds between the exposure and the health outcome, however they cannot prove causality. Case-Control studies might also be referred to as retrospective or case-referent studies.
Stages of a Case-Control study
This diagram represents taking both the case (disease) and the control (no disease) groups and looking back at their histories to determine their exposure to possible contributing factors. The researchers then determine the likelihood of those factors contributing to the disease.
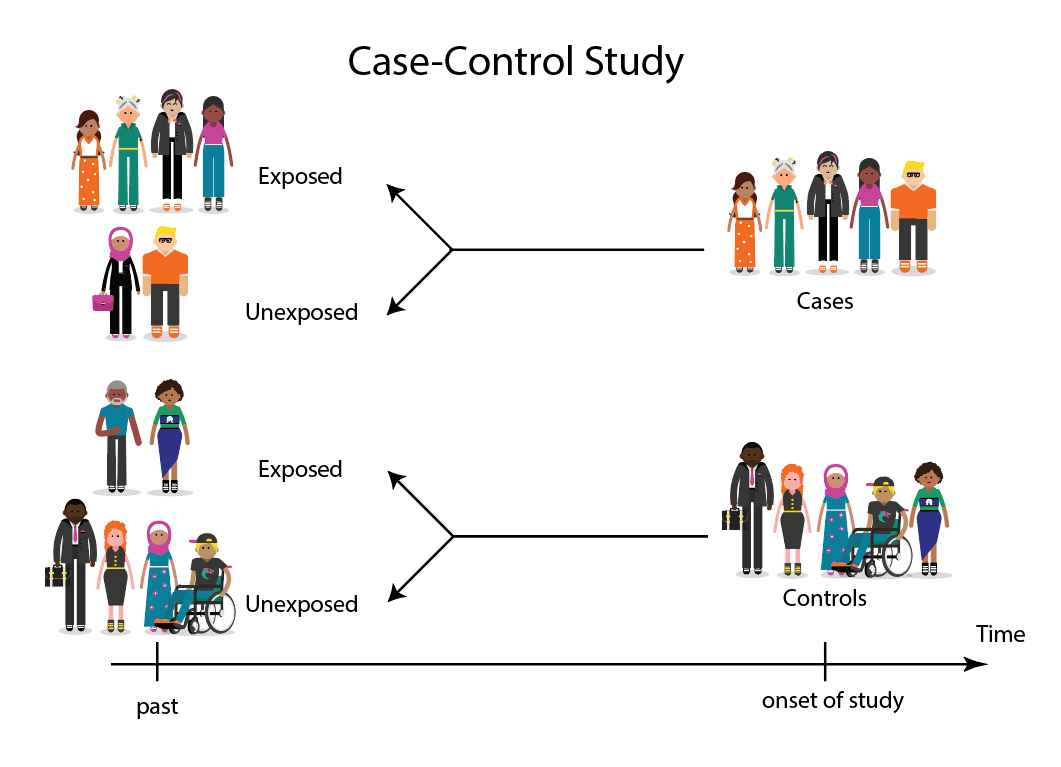
(FOR ACCESSIBILITY: A case control study is likely to show that most, but not all exposed people end up with the health issue, and some unexposed people may also develop the health issue)
Which Clinical Questions does Case-Control best answer?
Case-Control studies are best used for Prognosis questions.
For example: Do anticholinergic drugs increase the risk of dementia in later life? (See BMJ Case-Control study Anticholinergic drugs and risk of dementia: case-control study )
What are the advantages and disadvantages to consider when using Case-Control?
* Confounding occurs when the elements of the study design invalidate the result. It is usually unintentional. It is important to avoid confounding, which can happen in a few ways within Case-Control studies. This explains why it is lower in the hierarchy of evidence, superior only to Case Studies.
What does a strong Case-Control study look like?
A strong study will have:
- Well-matched controls, similar background without being so similar that they are likely to end up with the same health issue (this can be easier said than done since the risk factors are unknown).
- Detailed medical histories are available, reducing the emphasis on a patient’s unreliable recall of their potential exposures.
What are the pitfalls to look for?
- Poorly matched or over-matched controls. Poorly matched means that not enough factors are similar between the Case and Control. E.g. age, gender, geography. Over-matched conversely means that so many things match (age, occupation, geography, health habits) that in all likelihood the Control group will also end up with the same health issue! Either of these situations could cause the study to become ineffective.
- Selection bias: Selection of Controls is biased. E.g. All Controls are in the hospital, so they’re likely already sick, they’re not a true sample of the wider population.
- Cases include persons showing early symptoms who never ended up having the illness.
Critical appraisal tools
To assist with critically appraising case control studies there are some tools / checklists you can use.
CASP - Case Control Checklist
JBI – Critical appraisal checklist for case control studies
CEBMA – Centre for Evidence Based Management – Critical appraisal questions (focus on leadership and management)
STROBE - Observational Studies checklists includes Case control
SIGN - Case-Control Studies Checklist
NCCEH - Critical Appraisal of a Case Control Study for environmental health
Real World Examples
Smoking and carcinoma of the lung; preliminary report
- Doll, R., & Hill, A. B. (1950). Smoking and carcinoma of the lung; preliminary report. British Medical Journal , 2 (4682), 739–748. Retrieved from https://www.ncbi.nlm.nih.gov/pmc/articles/PMC2038856/
- Key Case-Control study linking tobacco smoking with lung cancer
- Notes a marked increase in incidence of Lung Cancer disproportionate to population growth.
- 20 London Hospitals contributed current Cases of lung, stomach, colon and rectum cancer via admissions, house-physician and radiotherapy diagnosis, non-cancer Controls were selected at each hospital of the same-sex and within 5 year age group of each.
- 1732 Cases and 743 Controls were interviewed for social class, gender, age, exposure to urban pollution, occupation and smoking habits.
- It was found that continued smoking from a younger age and smoking a greater number of cigarettes correlated with incidence of lung cancer.
Anticholinergic drugs and risk of dementia: case-control study
- Richardson, K., Fox, C., Maidment, I., Steel, N., Loke, Y. K., Arthur, A., . . . Savva, G. M. (2018). Anticholinergic drugs and risk of dementia: case-control study. BMJ , 361, k1315. Retrieved from http://www.bmj.com/content/361/bmj.k1315.abstract .
- A recent study linking the duration and level of exposure to Anticholinergic drugs and subsequent onset of dementia.
- Anticholinergic Cognitive Burden (ACB) was estimated in various drugs, the higher the exposure (measured as the ACB score) the greater likeliness of onset of dementia later in life.
- Antidepressant, urological, and antiparkinson drugs with an ACB score of 3 increased the risk of dementia. Gastrointestinal drugs with an ACB score of 3 were not strongly linked with onset of dementia.
- Tricyclic antidepressants such as Amitriptyline have an ACB score of 3 and are an example of a common area of concern.
Omega-3 deficiency associated with perinatal depression: Case-Control study
- Rees, A.-M., Austin, M.-P., Owen, C., & Parker, G. (2009). Omega-3 deficiency associated with perinatal depression: Case control study. Psychiatry Research , 166(2), 254-259. Retrieved from http://www.sciencedirect.com/science/article/pii/S0165178107004398 .
- During pregnancy women lose Omega-3 polyunsaturated fatty acids to the developing foetus.
- There is a known link between Omgea-3 depletion and depression
- Sixteen depressed and 22 non-depressed women were recruited during their third trimester
- High levels of Omega-3 were associated with significantly lower levels of depression.
- Women with low levels of Omega-3 were six times more likely to be depressed during pregnancy.
References and Further Reading
Doll, R., & Hill, A. B. (1950). Smoking and carcinoma of the lung; preliminary report. British Medical Journal, 2(4682), 739–748. Retrieved from https://www.ncbi.nlm.nih.gov/pmc/articles/PMC2038856/
Greenhalgh, Trisha. How to Read a Paper: the Basics of Evidence-Based Medicine, John Wiley & Sons, Incorporated, 2014. ProQuest Ebook Central, http://ebookcentral.proquest.com/lib/deakin/detail.action?docID=1642418 .
Himmelfarb Health Sciences Library. (2019). Study Design 101: Case-Control Study. Retrieved from https://himmelfarb.gwu.edu/tutorials/studydesign101/casecontrols.cfm
Hoffmann, T., Bennett, S., & Del Mar, C. (2017). Evidence-Based Practice Across the Health Professions (Third edition. ed.): Elsevier.
Lewallen, S., & Courtright, P. (1998). Epidemiology in practice: case-control studies. Community Eye Health, 11(28), 57. https://www.ncbi.nlm.nih.gov/pmc/articles/PMC1706071/
Pelham, B. W. a., & Blanton, H. (2013). Conducting research in psychology : measuring the weight of smoke /Brett W. Pelham, Hart Blanton (Fourth edition. ed.): Wadsworth Cengage Learning.
Rees, A.-M., Austin, M.-P., Owen, C., & Parker, G. (2009). Omega-3 deficiency associated with perinatal depression: Case control study. Psychiatry Research, 166(2), 254-259. Retrieved from http://www.sciencedirect.com/science/article/pii/S0165178107004398
Richardson, K., Fox, C., Maidment, I., Steel, N., Loke, Y. K., Arthur, A., … Savva, G. M. (2018). Anticholinergic drugs and risk of dementia: case-control study. BMJ, 361, k1315. Retrieved from http://www.bmj.com/content/361/bmj.k1315.abstract
Statistics How To. (2019). Case-Control Study: Definition, Real Life Examples. Retrieved from https://www.statisticshowto.com/case-control-study/
- << Previous: Randomised Controlled Trial
- Next: Cross-Sectional Studies >>
- Last Updated: Feb 29, 2024 4:49 PM
- URL: https://deakin.libguides.com/quantitative-study-designs

An official website of the United States government
The .gov means it’s official. Federal government websites often end in .gov or .mil. Before sharing sensitive information, make sure you’re on a federal government site.
The site is secure. The https:// ensures that you are connecting to the official website and that any information you provide is encrypted and transmitted securely.
- Publications
- Account settings
Preview improvements coming to the PMC website in October 2024. Learn More or Try it out now .
- Advanced Search
- Journal List
- Ann Indian Acad Neurol
- v.16(4); Oct-Dec 2013
Design and data analysis case-controlled study in clinical research
Sanjeev v. thomas.
Department of Neurology, Sree Chitra Tirunal Institute for Medical Sciences and Technology, Trivandrum, Kerala, India
Karthik Suresh
1 Department of Pulmonary and Critical Care Medicine, Johns Hopkins University School of Medicine, Louiseville, USA
Geetha Suresh
2 Department of Justice Administration, University of Louisville, Louiseville, USA
Clinicians during their training period and practice are often called upon to conduct studies to explore the association between certain exposures and disease states or interventions and outcomes. More often they need to interpret the results of research data published in the medical literature. Case-control studies are one of the most frequently used study designs for these purposes. This paper explains basic features of case control studies, rationality behind applying case control design with appropriate examples and limitations of this design. Analysis of sensitivity and specificity along with template to calculate various ratios are explained with user friendly tables and calculations in this article. The interpretation of some of the laboratory results requires sound knowledge of the various risk ratios and positive or negative predictive values for correct identification for unbiased analysis. A major advantage of case-control study is that they are small and retrospective and so they are economical than cohort studies and randomized controlled trials.
Introduction
Clinicians think of case-control study when they want to ascertain association between one clinical condition and an exposure or when a researcher wants to compare patients with disease exposed to the risk factors to non-exposed control group. In other words, case-control study compares subjects who have disease or outcome (cases) with subjects who do not have the disease or outcome (controls). Historically, case control studies came into fashion in the early 20 th century, when great interest arose in the role of environmental factors (such as pipe smoke) in the pathogenesis of disease. In the 1950s, case control studies were used to link cigarette smoke and lung cancer. Case-control studies look back in time to compare “what happened” in each group to determine the relationship between the risk factor and disease. The case-control study has important advantages, including cost and ease of deployment. However, it is important to note that a positive relationship between exposure and disease does not imply causality.
At the center of the case-control study is a collection of cases. [ Figure 1 ] This explains why this type of study is often used to study rare diseases, where the prevalence of the disease may not be high enough to permit for a cohort study. A cohort study identifies patients with and without an exposure and then “looks forward” to see whether or not greater numbers of patients with an exposure develop disease.

Comparison of cohort and case control studies
For instance, Yang et al . studied antiepileptic drug (AED) associated rashes in Asians in a case-control study.[ 1 ] They collected cases of confirmed anti-epileptic induced severe cutaneous reactions (such as Stevens Johnson syndrome) and then, using appropriate controls, analyzed various exposures (including type of [AED] used) to look for risk factors to developing AED induced skin disease.
Choosing controls is very important aspect of case-control study design. The investigator must weigh the need for the controls to be relevant against the tendency to over match controls such that potential differences may become muted. In general, one may consider three populations: Cases, the relevant control population and the population at large. For the study above, the cases include patients with AED skin disease. In this case, the relevant control population is a group of Asian patients without skin disease. It is important for controls to be relevant: In the anti-epileptic study, it would not be appropriate to choose a population across ethnicities since one of the premises of the paper revolves around particularly susceptibility to AED drug rashes in Asian populations.
One popular method of choosing controls is to choose patients from a geographic population at large. In studying the relationship between non-steroidal anti-inflammatory drugs and Parkinson's disease (PD), Wahner et al . chose a control population from several rural California counties.[ 2 ] There are other methods of choosing controls (using patients without disease admitted to the hospital during the time of study, neighbors of disease positive cases, using mail routes to identify disease negative cases). However, one must be careful not to introduce bias into control selection. For instance, a study that enrolls cases from a clinic population should not use a hospital population as control. Studies looking at geography specific population (e.g., Neurocysticercosis in India) cannot use controls from large studies done in other populations (registries of patients from countries where disease prevalence may be drastically different than in India). In general, geographic clustering is probably the easiest way to choose controls for case-control studies.
Two popular ways of choosing controls include hospitalized patients and patients from the general population. Choosing hospitalized, disease negative patients offers several advantages, including good rates of response (patients admitted to the hospital are generally already being examined and evaluated and often tend to be available to further questioning for a study, compared with the general population, where rates of response may be much lower) and possibly less amnestic bias (patients who are already in the hospital are, by default, being asked to remember details of their presenting illnesses and as such, may more reliably remember details of exposures). However, using hospitalized patients has one large disadvantage; these patients have higher severity of disease since they required hospitalization in the first place. In addition, patients may be hospitalized for disease processes that may share features with diseases under study, thus confounding results.
Using a general population offers the advantage of being a true control group, random in its choosing and without any common features that may confound associations. However, disadvantages include poor response rates and biasing based on geography. Administering long histories and questions regarding exposures are often hard to accomplish in the general population due to the number of people willing (or rather, not willing) to undergo testing. In addition, choosing cases from the general population from particular geographic areas may bias the population toward certain characteristics (such as a socio-economic status) of that geographic population. Consider a study that uses cases from a referral clinic population that draws patients from across socio-economic strata. Using a control group selected from a population from a very affluent or very impoverished area may be problematic unless the socio-economic status is included in the final analysis.
In case-controls studies, cases are usually available before controls. When studying specific diseases, cases are often collected from specialty clinics that see large numbers of patients with a specific disease. Consider for example, the study by Garwood et al .[ 3 ] which looked at patients with established PD and looked for associations between prior amphetamine use and subsequent development various neurologic disorders. Patients in this study were chosen from specialty clinics that see large numbers of patients with certain neurologic disorders. Case definitions are very important when planning to choose cases. For instance, in a hypothetical study aiming to study cases of peripheral neuropathy, will all patients who carry a diagnosis of peripheral neuropathy be included? Or, will only patients with definite electromyography evidence of neuropathy be included? If a disease process with known histopathology is being studied, will tissue diagnosis be required for all cases? More stringent case definitions that require multiple pieces of data to be present may limit the number of cases that can be used in the study. Less stringent criteria (for instance, counting all patients with the diagnosis of “peripheral neuropathy” listed in the chart) may inadvertently choose a group of cases that are too heterogeneous.
The disease history status of the chosen cases must also be decided. Will the cases being chosen have newly diagnosed disease, or will cases of ongoing/longstanding disease also be included? Will decedent cases be included? This is important when looking at exposures in the following fashion: Consider exposure X that is associated with disease Y. Suppose that exposure X negatively affects disease Y such that patients that are X + have more severe disease. Now, a case-control study that used only patients with long-standing or ongoing disease might miss a potential association between X and Y because X + patients, due to their more aggressive course of disease, are no longer alive and therefore were not included in the analysis. If this particular confounding effect is of concern, it can be circumvented by using incident cases only.
Selection bias occurs when the exposure of interest results in more careful screening of a population, thus mimicking an association. The classic example of this phenomenon was noted in the 70s, when certain studies noted a relationship between estrogen use and endometrial cancer. However, on close analysis, it was noted that patients who used estrogen were more likely to experience vaginal bleeding, which in turn is often a cause for close examination by physicians to rule out endometrial cancer. This is often seen with certain drug exposures as well. A drug may produce various symptoms, which lead to closer physician evaluation, thus leading to more disease positive cases. Thus, when analyzed in a retrospective fashion, more of the cases may have a particular exposure only insofar as that particular exposure led to evaluations that resulted in a diagnosis, but without any direct association or causality between the exposure and disease.
One advantage of case-control studies is the ability to study multiple exposures and other risk factors within one study. In addition, the “exposure” being studied can be biochemical in nature. Consider the study, which looked at a genetic variant of a kinase enzyme as a risk factor for development of Alzheimer's disease.[ 4 ] Compare this with the study mentioned earlier by Garwood et al .,[ 3 ] where exposure data was collected by surveys and questionnaires. In this study, the authors drew blood work on cases and controls in order to assess their polymorphism status. Indeed, more than one exposure can be assessed in the same study and with planning, a researcher may look at several variables, including biochemical ones, in single case-control study.
Matching is one of three ways (along with exclusion and statistical adjustment) to adjust for differences. Matching attempts to make sure that the control group is sufficiently similar to the cases group, with respects to variables such as age, sex, etc., Cases and controls should not be matched on variables that will be analyzed for possible associations to disease. Not only should exposure variables not be included, but neither should variables that are closely related to these variables. Lastly, overmatching should be avoided. If the control group is too similar to the cases group, the study may fail to detect the difference even if one exists. In addition, adding matching categories increases expense of the study.
One measure of association derived from case control studies are sensitivity and specificity ratios. These measures are important to a researcher, to understand the correct classification. A good understanding of sensitivity and specificity is essential to understand receiver operating characteristic curve and in distinguishing correct classification of positive exposure and disease with negative exposure and no disease. Table 1 explains a hypothetical example and method of calculation of specificity and sensitivity analysis.
Hypothetical example of sensitivity, specificity and predictive values

Interpretation of sensitivity, specificity and predictive values
Sensitivity and specificity are statistical measures of the performance of a two by two classification of cases and controls (sick or healthy) against positives and negatives (exposed or non-exposed).[ 5 ] Sensitivity measures or identifies the proportion of actual positives identified as the percentage of sick people who are correctly identified as sick. Specificity measures or identifies the proportion of negatives identified as the percentage of healthy people who are correctly identified as healthy. Theoretically, optimum prediction aims at 100% sensitivity and specificity with a minimum of margin of error. Table 1 also shows false positive rate, which is referred to as Type I error commonly stated as α “Alpha” is calculated using the following formula: 100 − specificity, which is equal to 100 − 90.80 = 9.20% for Table 1 example. Type 1 error is also known as false positive error is referred to as a false alarm, indicates that a condition is present when it is actually not present. In the above mentioned example, a false positive error indicates the percent falsely identified healthy as sick. The reason why we want Type 1 error to be as minimum as possible is because healthy should not get treatment.
The false negative rate, which is referred to as Type II error commonly stated as β “Beta” is calculated using the following formula: 100 − sensitivity which is equal to 100 − 73.30 = 26.70% for Table 1 example. Type II error is also known as false negative error indicates that a condition is not present when it should have been present. In the above mentioned example, a false negative error indicates percent falsely identified sick as healthy. A Type 1 error unnecessarily treats a healthy, which in turn increases the budget and Type II error would risk the sick, which would act against study objectives. A researcher wants to minimize both errors, which not a simple issue because an effort to decrease one type of error increases the other type of error. The only way to minimize both type of error statistically is by increasing sample size, which may be difficult sometimes not feasible or expensive. If the sample size is too low it lacks precision and it is too large, time and resources will be wasted. Hence, the question is what should be the sample size so that the study has the power to generalize the result obtained from the study. The researcher has to decide whether, the study has enough power to make a judgment of the population from their sample. The researcher has to decide this issue in the process of designing an experiment, how large a sample is needed to enable reliable judgment.
Statistical power is same as sensitivity (73.30%). In this example, large number of false positives and few false negatives indicate the test conducted alone is not the best test to confirm the disease. Higher statistical power increase statistical significance by reducing Type 1 error which increases confidence interval. In other words, larger the power more accurately the study can mirror the behavior of the study population.
The positive predictive values (PPV) or the precision rate is referred to as the proportion of positive test results, which means correct diagnoses. If the test correctly identifies all positive conditions then the PPV would be 100% and negative predictive value (NPV) would be 0. The calculative PPV in Table 1 is 11.8%, which is not large enough to predict cases with test conducted alone. However, the NPV 99.9% indicates the test correctly identifies negative conditions.
Clinical interpretation of a test
In a sample, there are two groups those who have the disease and those who do not have the disease. A test designed to detect that disease can have two results a positive result that states that the disease is present and a negative result that states that the disease is absent. In an ideal situation, we would want the test to be positive for all persons who have the disease and test to be negative for all persons who do not have the disease. Unfortunately, reality is often far from ideal. The clinician who had ordered the test has the result as positive or negative. What conclusion can he or she make about the disease status for his patient? The first step would be to examine the reliability of the test in statistical terms. (1) What is the sensitivity of the test? (2) What is the specificity of the test? The second step is to examine it applicability to his patient. (3) What is the PPV of the test? (4) What is the NPV of the test?
Suppose the test result had come as positive. In this example the test has a sensitivity of 73.3% and specificity of 90.8%. This test is capable of detecting the disease status in 73% of cases only. It has a false positivity of 9.2%. The PPV of the test is 11.8%. In other words, there is a good possibility that the test result is false positive and the person does not have the disease. We need to look at other test results and the clinical situation. Suppose the PPV of this test was close to 80 or 90%, one could conclude that most likely the person has the disease state if the test result is positive.
Suppose the test result had come as negative. The NPV of this test is 99.9%, which means this test gave a negative result in a patient with the disease only very rarely. Hence, there is only 0.1% possibility that the person who tested negative has in fact the disease. Probably no further tests are required unless the clinical suspicion is very high.
It is very important how the clinician interprets the result of a test. The usefulness of a positive result or negative result depends upon the PPV or NPV of the test respectively. A screening test should have high sensitivity and high PPV. A confirmatory test should have high specificity and high NPV.
Case control method is most efficient, for the study of rare diseases and most common diseases. Other measures of association from case control studies are calculation of odds ratio (OR) and risk ratio which is presented in Table 2 .
Different ratio calculation templates with sample calculation

Absolute risk means the probability of an event occurring and are not compared with any other type of risk. Absolute risk is expressed as a ratio or percent. In the example, absolute risk reduction indicates 27.37% decline in risk. Relative risk (RR) on the other hand compares the risk among exposed and non-exposed. In the example provided in Table 2 , the non-exposed control group is 69.93% less likely compared to exposed cases. Reader should keep in mind that RR does not mean increase in risk. This means that while a 100% likely risk among those exposed cases, unexposed control is less likely by 69.93%. RR does not explain actual risk but is expressed as relative increase or decrease in risk of exposed compared to non-exposed.
OR help the researcher to conclude whether the odds of a certain event or outcome are same for two groups. It calculates the odds of a health outcome when exposed compared to non-exposed. In our example an OR of. 207 can be interpreted as the non-exposed group is less likely to experience the event compared to the exposed group. If the OR is greater than 1 (example 1.11) means that the exposed are 1.11 times more likely to be riskier than the non-exposed.
Event rate for cases (E) and controls (C) in biostatistics explains how event ratio is a measure of how often a particular statistical exposure results in occurrence of disease within the experimental group (cases) of an experiment. This value in our example is 11.76%. This value or percent explains the extent of risk to patients exposed, compared with the non-exposed.
The statistical tests that can be used for ascertain an association depends upon the variable characteristics also. If the researcher wants to find the association between two categorical variables (e.g., a positive versus negative test result and disease state expressed as present or absent), Cochran-Armitage test, which is same as Pearson Chi-squared test can be used. When the objective is to find the association between two interval or ratio level (continuous) variables, correlation and regression analysis can be performed. In order to evaluate statistical significant difference between the means of cases and control, a test of group difference can be performed. If the researcher wants to find statically significant difference among means of more than two groups, analysis of variance can be performed. A detailed explanation and how to calculate various statistical tests will be published in later issues. The success of the research directly and indirectly depends on how the following biases or systematic errors, are controlled.
When selecting cases and controls, based on exposed or not-exposed factors, the ability of subjects to recall information on exposure is collected retrospectively and often forms the basis for recall bias. Recall bias is a methodological issue. Problems of recall method are: Limitations in human ability to recall and cases may remember their exposure with more accuracy than the controls. Other possible bias is the selection bias. In case-control studies, the cases and controls are selected from the same inherited characteristics. For instance, cases collected from referral clinics often exposed to selection bias cases. If selection bias is not controlled, the findings of association, most likely may be due to of chance resulting from the study design. Another possible bias is information bias, which arises because of misclassification of the level of exposure or misclassification of disease or other symptoms of outcome itself.
Case control studies are good for studying rare diseases, but they are not generally used to study rare exposures. As Kaelin and Bayona explains[ 6 ] if a researcher want to study the risk of asthma from working in a nuclear submarine shipyard, a case control study may not be a best option because a very small proportion of people with asthma might be exposed. Similarly, case-control studies cannot be the best option to study multiple diseases or conditions because the selection of the control group may not be comparable for multiple disease or conditions selected. The major advantage of case-control study is that they are small and retrospective and so they are economical than cohort studies and randomized controlled trials.
Source of Support: Nil
Conflict of Interest: Nil
- Open access
- Published: 07 January 2022
Identification of causal effects in case-control studies
- Bas B. L. Penning de Vries 1 &
- Rolf H. H. Groenwold 1 , 2
BMC Medical Research Methodology volume 22 , Article number: 7 ( 2022 ) Cite this article
5222 Accesses
2 Citations
8 Altmetric
Metrics details
Case-control designs are an important yet commonly misunderstood tool in the epidemiologist’s arsenal for causal inference. We reconsider classical concepts, assumptions and principles and explore when the results of case-control studies can be endowed a causal interpretation.
We establish how, and under which conditions, various causal estimands relating to intention-to-treat or per-protocol effects can be identified based on the data that are collected under popular sampling schemes (case-base, survivor, and risk-set sampling, with or without matching). We present a concise summary of our identification results that link the estimands to the (distribution of the) available data and articulate under which conditions these links hold.
The modern epidemiologist’s arsenal for causal inference is well-suited to make transparent for case-control designs what assumptions are necessary or sufficient to endow the respective study results with a causal interpretation and, in turn, help resolve or prevent misunderstanding. Our approach may inform future research on different estimands, other variations of the case-control design or settings with additional complexities.
Peer Review reports
Introduction
In causal inference, it is important that the causal question of interest is unambiguously articulated [ 1 ]. The causal question should dictate, and therefore be at the start of, investigation. When the target causal quantity, the estimand, is made explicit, one can start to question how it relates to the available data distribution and, as such, form a basis for estimation with finite samples from this distribution.
The counterfactual framework offers a language rich enough to articulate a wide variety of causal claims that can be expressed as what-if statements [ 1 ]. Another, albeit closely related, approach to causal inference is target trial emulation, an explicit effort to mitigate departures from a study (the ‘target trial’) that, if carried out, would enable one to readily answer the causal what-if question of interest [ 2 ]. While it may be too impractical or unethical to implement, making explicit what a target trial looks like has particular value in communicating the inferential goal and offers a reference against which to compare studies that have been or are to be conducted.
The counterfactual framework and emulation approach have become increasingly popular in observational cohort studies. Case-control studies, however, have not yet enjoyed this trend. A notable exception is given by Dickerman et al. [ 3 ], who recently outlined an application of trial emulation with case-control designs to statin use and colorectal cancer.
In this paper, we give an overview of how observational data obtained with case-control designs can be used to identify a number of causal estimands and, in doing so, recast historical case-control concepts, assumptions and principles in a modern and formal framework.
Preliminaries
Identification versus estimation.
An estimand is said to be identifiable if the distribution of the available data is compatible with exactly one value of the estimand, or therefore, if the estimand can be expressed as a functional of the available data distribution. Identifiability is a relative notion as it depends on which data are available as well as on the assumptions one is willing to make. Identification forms a basis for estimation with finite samples from the available data distribution [ 4 ]. Once the estimand has been made explicit and an identifying functional established, estimation is a purely statistical problem. While the identifying functional will often naturally translate into a plug-in estimator, there is, however, generally more than one way to translate an identifiability result into an estimator and different estimators may have important differences in their statistical properties. Moreover, while the estimand may be identifiable, there need not exist an estimator with the desired properties (see e.g. [ 5 ]). Here, our focus is on identification, so that the purely statistical issues of the next step in causal inference, estimation, can be momentarily put aside.
Case-control study nested in cohort study
To facilitate understanding, it is useful to consider every case-control study as being “nested” within a cohort study. A case-control study could be considered as a cohort study with missingness governed by the control sampling scheme. Therefore, when the observed data distribution of a case-control study is compatible with exactly one value of a given estimand, then so is the available or observed data distribution of the underlying cohort study. In other words, identifiability of an estimand with a case-control study implies identifiability of the estimand with the cohort study within which it is nested (conceptually). The converse is not evident and in fact may not be true. In this paper, the focus is on sets of conditions or assumptions that are sufficient for identifiability in case-control studies.
Set-up of underlying cohort study
Consider a time-varying exposure A k that can take one of two levels, 0 or 1, at K successive time points t k ( k =0,1,..., K −1), where t 0 denotes baseline (cohort entry or time zero). Study participants are followed over time until they sustain the event of interest or the administrative study end t K , whichever comes first. We denote by T the time elapsed from baseline until the event of interest and let Y k = I ( T < t k ) indicate whether the event has occurred by t k . The lengths between the time points are typically fixed at a constant (e.g., of one day, week, or month). Figure 1 depicts twelve equally spaced time points over, say, twelve months with several possible courses of follow-up of an individual. As the figure illustrates, individuals can switch between exposure levels during follow-up, as in any truly observational study. Apart from exposure and outcome data, we also consider a (vector of) covariate(s) L k , which describes time-fixed individual characteristics or time-varying characteristics typically relating to a time window just before exposure or non-exposure at t k , k =0,1,..., K −1.
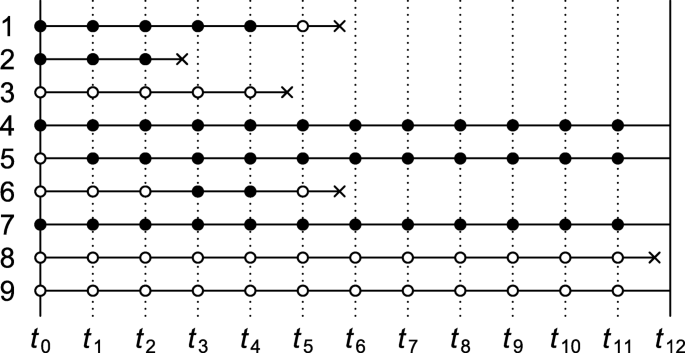
Illustration of possible courses of follow-up of an individual for a study with baseline t 0 and administrative study end t 12 . Solid bullets indicate ‘exposed’; empty bullets indicate ‘not exposed’. The incident event of interest is represented by a cross
Causal contrasts
Although there are many possible contrasts, particularly with time-varying exposures, for simplicity we consider only two pairs of mutually exclusive interventions: (1) setting baseline exposure A 0 to 1 versus 0; and (2) setting all of A 0 , A 1 ,..., A K −1 to 1 (‘always exposed’) versus all to 0 (‘never exposed’). For a =0,1, we let counterfactual outcome Y k ( a ) indicate whether the event has occurred by t k under the baseline-only intervention that sets A 0 to a . By convention, we write \(\overline {1}=(1,1,...,1)\) and \(\overline {0}=(0,0,...,0)\) , and let \(Y_{k}(\overline {1})\) and \(Y_{k}(\overline {0})\) indicate whether the event has occurred by t k under the intervention that sets all elements of ( A 0 , A 1 ,..., A K −1 ) to 1 and all to 0, respectively. Further details about the notation and set-up are given in Supplementary Appendix A.
Case-control sampling
The fact that each time-specific exposure variable can take only one value per time point means that at most one counterfactual outcome can be observed per individual. This type of missingness is common to all studies. Relative to the cohort studies within which they are nested, case-control studies have additional missingness, which is governed by the control sampling scheme. In this paper, we focus on three well-known sampling schemes: case-base sampling, survivor sampling, and risk-set sampling. The next sections give an overview of conditions under which intention-to-treat and always-versus-never-exposed per-protocol effects can be identified with the data that are observed under these sampling schemes.
Case-control studies without matching
Table 1 summarises a number of identification results for case-control studies without matching. Each result consists of one of the three aforementioned sampling schemes, an estimand, a set of assumptions, and an identification strategy. Under the conditions of the “Sampling scheme” and “Assumptions” columns, an identifying functional of the estimand of the “Estimand” column is obtained by following the steps of the “Identification strategy” column. More formal statements and proofs are given in Supplementary Appendix B.
In all case-control studies that we consider in this section, cases are compared with controls with regard to their exposure status via an odds ratio, even when an effect measure other than the odds ratio is targeted. An individual qualifies as a case if and only if they sustain the event of interest by the administrative study end (i.e., Y K =1) and adhered to one of the protocols of interest until the time of the incident event. In Fig. 1 , the individual represented by row 1 is therefore regarded as a case (an exposed case in particular) in our investigation of intention-to-treat effects but not in that of per-protocol effects. Whether an individual (also) serves as a control depends on the control sampling scheme.
Case-base sampling
The first result in Table 1 describes how to identify the intention-to-treat effect as quantified by the marginal risk ratio
under case-base sampling. (For identification of a conditional risk ratio, see Theorem 2 of Supplementary Appendix B.) Case-base sampling, also known as case-cohort sampling, means that no individual who is at risk at baseline of sustaining the event of interest is precluded from selection as a control. Selection as a control, S , is further assumed independent of baseline covariate L 0 and exposure A 0 . Selecting controls from survivors only (e.g., rows 4, 5, 7 and 9 in Fig. 1 ) violates this assumption when survival depends on L 0 or A 0 .
To account for baseline confounding, inverse probability weights could be derived from control data according to
We then compute the odds of baseline exposure among cases and among controls in the pseudopopulation that is obtained by weighting everyone by subject-specific values of W . The ratio of these odds coincides with the target risk ratio under the three key identifiability conditions of consistency, baseline conditional exchangeability and positivity [ 1 ]. Consistency here means that for a =0,1, Y K ( a )= Y K if A 0 = a , baseline conditional exchangeability that for a =0,1, A 0 is independent of Y K ( a ), and positivity that 0< Pr( A 0 =1| L 0 , S =1)<1.
The identification result for case-base sampling suggests a plug-in estimator: replace all functionals of the theoretical data distribution with sample analogues. For example, to obtain the weight for an individual with baseline covariate level l 0 , replace the theoretical propensity score Pr( A 0 =1| L 0 = l 0 , S =1) with an estimate \(\widehat {\Pr }(A_{0}=1|L_{0}=l_{0},S=1)\) derived from a fitted model (e.g., a logistic regression model) that imposes parametric constraints on the distribution of A 0 given L 0 among the controls.
Survivor sampling
With survivor (cumulative incidence or exclusive) sampling, a subject is eligible for selection as a control only if they reach the administrative study end event-free. To identify the conditional odds ratio of baseline exposure versus baseline non-exposure given L 0 ,
selection as a control, S , is assumed independent of baseline exposure A 0 given L 0 and survival until the end of study (i.e., Y K =0).
As is shown in Supplementary Appendix B, Theorem 3, the above odds ratio is identified by the ratio of the baseline exposure odds given L 0 among the cases versus controls, provided the key identifiability conditions of consistency, baseline conditional exchangeability, and positivity are met.
All estimands in Table 1 describe a marginal effect, except for the odds ratio, which is conditional on baseline covariates L 0 . The corresponding marginal odds ratio
is not identifiable from the available data distribution under the stated assumptions (see remark to Theorem 3, Supplementary Appendix B). However, approximate identifiability can be achieved by invoking the rare event assumption (or rare disease assumption), in which case the marginal odds ratio approximates the marginal risk ratio.
Risk-set sampling for intention-to-treat effect
With risk-set (or incidence density) sampling, for all time windows [ t k , t k +1 ), k =0,..., K −1, every subject who is event-free at t k is eligible for selection as a control for the period [ t k , t k +1 ). This means that study participants may be selected as a control more than once.
Consider the intention-to-treat effect quantified by the marginal (discrete-time) hazard ratio (or rate ratio)
(For identification of a conditional hazard ratio, see Theorem 5, Supplementary Appendix B.) For identification of the above marginal hazard ratio under risk-set sampling, it is assumed that selection as a control between t k and t k +1 , S k , is independent of the baseline covariates and exposure given eligibility at t k (i.e., Y k =0). It is also assumed that the sampling probability among those eligible, Pr( S k =1| Y k =0), is constant across time windows k =0,..., K −1. To this end, it suffices that the marginal hazard Pr( Y k +1 =1| Y k =0) remains constant across time windows and that every k th sampling fraction Pr( S k =1) is equal, up to a proportionality constant, to the probability Pr( Y k +1 =1, Y k =0) of an incident case in the k th window (see remark to Theorem 4, Supplementary Appendix B). For practical purposes, this suggests sampling a fixed number of controls for every case from among the set of eligible individuals. To illustrate, consider Fig. 1 and note first of all that the individual represented by row 1 trivially qualifies as a case, because the individual survived until the event occurred. Because the event was sustained between t 5 and t 6 , the proposed sampling suggests selecting a fixed number of controls from among those who are eligible at t 5 . Thus, rows (and only rows) 4 through 9 as well as row 1 itself in Fig. 1 qualify for selection as a control for this case. Even though the individual of row 1 is a case, the individual may also be selected as a control when the individuals of row 2, 3 and 6 (but not 8) sustain the event.
Once cases and controls are selected, we can start to derive inverse probability weights W according to Eq. 1 with S replaced with S 0 . We then compute the odds of baseline exposure among cases in the pseudopopulation that is obtained by weighting everyone by W and the odds of baseline exposure among controls weighted by W multiplied by the number of times the individual was selected as a control. The ratio of these odds coincides with the target hazard ratio under the three key identifiability conditions of consistency, baseline conditional exchangeability and positivity together with the assumption that the hazards in the numerator and denominator of the causal hazard ratio are constant across the time windows.
The consistency and exchangeability conditions are here slightly stronger than those of the previous subsections. Specifically, Theorem 4 (Supplementary Appendix B) requires consistency of the form: for all k =1,..., K and a =0,1, Y k ( a )= Y k if A 0 = a . The exchangeability condition requires, for a =0,1, that conditional on L 0 , the counterfactual outcomes Y 1 ( a ),..., Y K ( a ) are jointly independent of A 0 . The positivity condition takes the same form as in the previous subsections (i.e., 0< Pr( A 0 = a | L 0 , S 0 =1)<1).
Risk-set sampling for per-protocol effect
For the per-protocol effect quantified by the (discrete-time) hazard ratio (or rate ratio)
eligibility for selection as a control for the period [ t k , t k +1 ) again requires that the respective subject is event-free at t k (i.e., Y k =0). Selection as a control between t k and t k +1 , S k , is further assumed independent of covariate and exposure history up to t k given eligibility at t k (but see Supplementary Appendix B for a slightly weaker assumption). As for the intention-to-treat effect, it is also assumed that the probability to be selected as a control S k given eligibility is constant across time windows. This assumption is guaranteed to hold if the marginal hazard Pr( Y k +1 =1| Y k =0) remains constant across time windows and that every k th sampling fraction Pr( S k =1) is equal, up to a proportionality constant, to the probability of an incident case in the k th window. Figure 1 shows five incident events yet only three qualify as a case (rows 2, 3 and 8) when it concerns per-protocol effects. When the first case emerges (row 2), all rows meet the eligibility criterion for selection as a control. When the second emerges, the individual of row 2, who fails to survive event-free until t 4 , is precluded as a control. When the case of row 8 emerges, only the individuals of rows 4, 5, 7 and 9 are eligible as controls.
Once cases and controls are selected, we can start to derive time-varying inverse probability weights according to
It is important to note that the weights are derived from control information but are nonetheless used to weight both cases and controls [ 6 ]. The denominators of the weights describe the propensity to switch exposure level. However, once the weights are derived, every subject is censored from the time that they fail to adhere to one of the protocols of interest for all downstream analysis. The uncensored exposure levels are therefore constant over time. We then compute the baseline exposure odds among cases, weighted by the weights W k corresponding to the interval [ t k , t k +1 ) of the incident event (i.e., Y k =0, Y k +1 =1), as well as the baseline exposure odds among controls, weighted by \(\sum _{k=0}^{K-1}W_{k}S_{k}\) , the weighted number of times selected as control. The ratio of these odds equals the target hazard ratio under the three key identifiability conditions of consistency, sequential conditional exchangeability, and positivity together with the assumption that hazards in the numerator and denominator of the causal hazard ratio for the per-protocol effect are constant across the time windows. The consistency, exchangeability and positivity conditions take a somewhat different (stronger) form than in the previous subsections; we refer the reader to Supplementary Appendix A for further details.
Case-control studies with matching
Table 2 gives an overview of identification results for case-control studies with exact pair matching. Formal statements and proofs are given in Supplementary Appendix C, which also includes a generalisation of the results of Table 2 to exact 1-to- M matching. While the focus in this section is on exact covariate matching, for partial matching we refer the reader to Supplementary Appendix D, where we consider parametric identification by way of conditional logistic regression.
Pair matching involves assigning a single control exposure level, which we denote by A ′ , to every case. As for case-control studies without matching, in a case-control studies with matching an individual qualifies as a case if and only if they sustain the event of interest by the administrative study end (i.e., Y K =1) and adhered to one of the protocols of interest until the time of the incident event. How a matched control exposure is assigned is encoded in the sampling scheme and the assumptions of Table 2 . For example, for identification of the causal marginal risk ratio under case-base sampling, A ′ is sampled from all study participants whose baseline covariate value matches that of the case, independently of the participants’ baseline exposure value and whether they survive until the end of study. The matching is exact in the sense that the control exposure information is derived from an individual who has the same value for the baseline covariate as the case.
The identification strategy is the same for all results listed in Table 2 . Only the case-control pairs ( A 0 , A ′ ) with discordant exposure values (i.e., (1,0) or (0,1)) are used. Under the stated sampling schemes and assumptions, the respective estimands are identified by the ratio of discordant pairs.
This paper gives a formal account of how and when causal effects can be identified in case-control studies and, as such, underpins the case-control application of Dickerman et al. [ 3 ]. Like Dickerman et al., we believe that case-control studies should generally be regarded as being nested within cohort studies. This view emphasises that the threats to the validity of cohort studies should also be considered in case-control studies. For example, in case-control applications with risk-set sampling, researchers often consider the covariate and exposure status only at, or just before, the time of the event (for cases) or the time of sampling (for controls). However, where a cohort study would require information on baseline levels or the complete treatment and covariate history of participants, one should suspect that this holds for the nested case-control study too. To gain clarity, we encourage researchers to move away from using person-years, -weeks, or -days (rather than individuals) as the default units of inference [ 7 ], and to realise that inadequately addressed deviations from a target trial may lead to bias (or departure from identifiability), regardless of whether the study that attempts to emulate it is a case-control or a cohort study [ 3 ].
What is meant by a cohort study differs between authors and contexts [ 8 ]. The term ‘cohort’ may refer to either a ‘dynamic population’, or a ‘fixed cohort’, whose “membership is defined in a permanent fashion” and “determined by a single defining event and so becomes permanent” [ 9 ]. While it may sometimes be of interest to ask what would have happened with a dynamic cohort (e.g., the residents of a country) had it been subjected to one treatment protocol versus another, the results in this paper relate to fixed cohorts.
Like the cohort studies within which they are (at least conceptually) nested, case-control studies require an explicit definition of time zero, the time at which a choice is to be made between treatment strategies or protocols of interest [ 3 ]. Given a fixed cohort, time zero is generally determined by the defining event of the cohort (e.g., first diagnosis of a particular disease or having survived one year since diagnosis). This event may occur at different calendar times for different individuals. However, while a fixed cohort may be ‘open’ to new members relative to calendar time, it is always ‘closed’ along the time axis on which all subject-specific time zeros are aligned.
In this paper, time was regarded as discrete. Since we considered arbitrary intervals between time points and because, in real-world studies, time is never measured in a truly continuous fashion, this does not represent an important limitation for practical purposes. It is however important to note that the intervals between interventions and outcome assessments (in a target trial) are an intrinsic part of the estimand that lies at the start of investigation. Careful consideration of time intervals in the design of the conceptual target trial and of the actual cohort or case-control study is therefore warranted.
We emphasize that identification and estimation are distinct steps in causal inference. Although our focus was on the former, identifying functionals often naturally translate into estimators. The task of finding the estimator with the most appealing statistical properties is not necessarily straightforward, however, and is beyond the scope of this paper.
We specifically studied two causal contrasts (i.e., pairs of interventions), one corresponding to intention-to-treat effects and the other to always-versus-never per-protocol effects of a time-varying exposure. There are of course many more causal contrasts, treatment regimes and estimands conceivable that could be of interest. We argue that also for these estimands, researchers should seek to establish identifiability before they select an estimator.
The conditions under which identifiability is to be sought for practical purposes may well include more constraints or obstacles to causal inference, such as additional missingness (e.g., outcome censoring) and measurement error, than we have considered here. While some of our results assume that hazards or hazard ratios remain constant over time, in many cases these are likely time-varying [ 10 , 11 ]. There are also more case-control designs (e.g., the case-crossover design) to consider. These additional complexities and designs are beyond the scope of this paper and represent an interesting direction for future research.
The case-control family of study designs is an important yet often misunderstood tool for identifying causal relations [ 12 – 15 ]. Although there is much to be learned, we believe that the modern arsenal for causal inference, which includes counterfactual thinking, is well-suited to make transparent for these classical epidemiological study designs what assumptions are sufficient or necessary to endow the study results with a causal interpretation and, in turn, help resolve or prevent misunderstanding.
Availability of data and materials
Data sharing is not applicable to this article as no datasets were generated or analysed during the current study.
Hernán M, Robins J. Causal Inference: What If. Boca Raton: Chapman & Hall/CRC; 2020.
Google Scholar
Hernán MA, Robins JM. Using big data to emulate a target trial when a randomized trial is not available. Am J Epidemiol. 2016; 183(8):758–64.
Article Google Scholar
Dickerman BA, García-Albéniz X, Logan RW, Denaxas S, Hernán MA. Emulating a target trial in case-control designs: an application to statins and colorectal cancer. Int J Epidemiol. 2020; 49(5):1637–46.
Petersen ML, Van der Laan MJ. Causal models and learning from data: integrating causal modeling and statistical estimation. Epidemiol (Camb, Mass). 2014; 25(3):418.
Maclaren OJ, Nicholson R. Models, identifiability, and estimability in causal inference. In: 38th International Conference on Machine Learning. Workshop on the Neglected Assumptions in Causal Inference. ICML: 2021. https://sites.google.com/view/naci2021/home .
Robins JM. [Choice as an alternative to control in observational studies]: comment. Stat Sci. 1999; 14(3):281–93.
Hernán MA. Counterpoint: epidemiology to guide decision-making: moving away from practice-free research. Am J Epidemiol. 2015; 182(10):834–39.
Vandenbroucke JP, Pearce N. Incidence rates in dynamic populations. Int J Epidemiol. 2012; 41(5):1472–79.
Rothman KJ, Greenland S, Lash TL. Modern Epidemiology, Third edition. Philadelphia: Lippincott Williams & Wilkins; 2008.
Lefebvre G, Angers J-F, Blais L. Estimation of time-dependent rate ratios in case-control studies: comparison of two approaches for exposure assessment. Pharmacoepidemiol Drug Saf. 2006; 15(5):304–16.
Guess HA. Exposure-time-varying hazard function ratios in case-control studies of drug effects. Pharmacoepidemiol Drug Saf. 2006; 15(2):81–92.
Knol MJ, Vandenbroucke JP, Scott P, Egger M. What do case-control studies estimate? survey of methods and assumptions in published case-control research. Am J Epidemiol. 2008; 168(9):1073–81.
Pearce N. Analysis of matched case-control studies. BMJ. 2016; 352:i969.
Mansournia MA, Jewell NP, Greenland S. Case–control matching: effects, misconceptions, and recommendations. Eur J Epidemiol. 2018; 33(1):5–14.
Labrecque JA, Hunink MM, Ikram MA, Ikram MK. Do case-control studies always estimate odds ratios?. Am J Epidemiol. 2021; 190(2):318–21.
Download references
Acknowledgments
None declared.
RHHG was funded by the Netherlands Organization for Scientific Research (NWO-Vidi project 917.16.430). The content is solely the responsibility of the authors and does not necessarily represent the official views of the funding body.
Author information
Authors and affiliations.
Department of Clinical Epidemiology, Leiden University Medical Center, Leiden, PO Box 9600, 2300 RC, The Netherlands
Bas B. L. Penning de Vries & Rolf H. H. Groenwold
Department of Biomedical Data Sciences, Leiden University Medical Center, Leiden, The Netherlands
Rolf H. H. Groenwold
You can also search for this author in PubMed Google Scholar
Contributions
BBLPdV devised the project and wrote the manuscript and supplementary material with substantial input from RHHG, who supervised the project. The authors read and approved the final manuscript.
Corresponding author
Correspondence to Bas B. L. Penning de Vries .
Ethics declarations
Ethics approval and consent to participate.
Not applicable.
Consent for publication
Competing interests.
The authors declare that they have no competing interests.
Additional information
Publisher’s note.
Springer Nature remains neutral with regard to jurisdictional claims in published maps and institutional affiliations.
Supplementary Information
Additional file 1.
Supplementary material to ‘Identification of causal effects in case-control studies’.
Rights and permissions
Open Access This article is licensed under a Creative Commons Attribution 4.0 International License, which permits use, sharing, adaptation, distribution and reproduction in any medium or format, as long as you give appropriate credit to the original author(s) and the source, provide a link to the Creative Commons licence, and indicate if changes were made. The images or other third party material in this article are included in the article’s Creative Commons licence, unless indicated otherwise in a credit line to the material. If material is not included in the article’s Creative Commons licence and your intended use is not permitted by statutory regulation or exceeds the permitted use, you will need to obtain permission directly from the copyright holder. To view a copy of this licence, visit http://creativecommons.org/licenses/by/4.0/ . The Creative Commons Public Domain Dedication waiver ( http://creativecommons.org/publicdomain/zero/1.0/ ) applies to the data made available in this article, unless otherwise stated in a credit line to the data.
Reprints and permissions
About this article
Cite this article.
L. Penning de Vries, B.B., Groenwold, R.H.H. Identification of causal effects in case-control studies. BMC Med Res Methodol 22 , 7 (2022). https://doi.org/10.1186/s12874-021-01484-7
Download citation
Received : 26 August 2021
Accepted : 29 November 2021
Published : 07 January 2022
DOI : https://doi.org/10.1186/s12874-021-01484-7
Share this article
Anyone you share the following link with will be able to read this content:
Sorry, a shareable link is not currently available for this article.
Provided by the Springer Nature SharedIt content-sharing initiative
- Causal inference
- Case-control designs
- Identifiability
BMC Medical Research Methodology
ISSN: 1471-2288
- General enquiries: [email protected]
What is a Case-Control Study?
Affiliations.
- 1 Department of Neurosurgery, University of Alabama at Birmingham.
- 2 Department of Neurosurgery, Beth Israel Deaconess Medical Center, Boston, Massachusetts.
- 3 Department of Neurosurgery, Mobile Infirmary Medical Center, Mobile, Alabama.
- PMID: 30535401
- DOI: 10.1093/neuros/nyy590
Case-control (case-control, case-controlled) studies are beginning to appear more frequently in the neurosurgical literature. They can be more robust, if well designed, than the typical case series or even cohort study to determine or refine treatment algorithms. The purpose of this review is to define and explore the differences between case-control studies and other so-called nonexperimental, quasiexperimental, or observational studies in determining preferred treatments for neurosurgical patients.
Keywords: Case–control; Observational study; Study design.
Copyright © 2018 by the Congress of Neurological Surgeons.
- Biomedical Research
- Case-Control Studies*
- Research Design
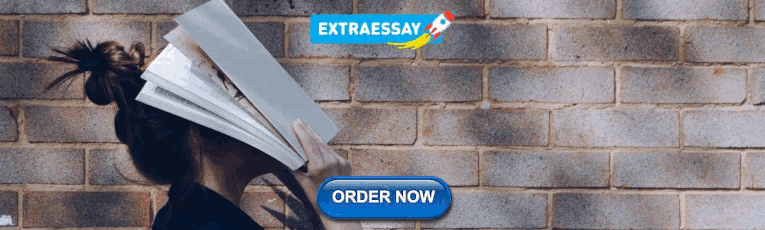
IMAGES
VIDEO
COMMENTS
Revised on June 22, 2023. A case-control study is an experimental design that compares a group of participants possessing a condition of interest to a very similar group lacking that condition. Here, the participants possessing the attribute of study, such as a disease, are called the "case," and those without it are the "control.".
Abstract. Case-control studies are observational studies in which cases are subjects who have a characteristic of interest, such as a clinical diagnosis, and controls are (usually) matched subjects who do not have that characteristic. After cases and controls are identified, researchers "look back" to determine what past events (exposures ...
A case-control study is a type of observational study commonly used to look at factors associated with diseases or outcomes.[1] The case-control study starts with a group of cases, which are the individuals who have the outcome of interest. The researcher then tries to construct a second group of individuals called the controls, who are similar to the case individuals but do not have the ...
Case-control studies are one of the major observational study designs for performing clinical research. The advantages of these study designs over other study designs are that they are relatively quick to perform, economical, and easy to design and implement. Case-control studies are particularly appropriate for studying disease outbreaks, rare diseases, or outcomes of interest. This article ...
A case control study is a retrospective, observational study that compares two existing groups. Researchers form these groups based on the existence of a condition in the case group and the lack of that condition in the control group. They evaluate the differences in the histories between these two groups looking for factors that might cause a ...
Case-Control study design is a type of observational study. In this design, participants are selected for the study based on their outcome status. Thus, some participants have the outcome of interest (referred to as cases), whereas others do not have the outcome of interest (referred to as controls). The investigator then assesses the exposure ...
Case control studies are observational because no intervention is attempted and no attempt is made to alter the course of the disease. The goal is to retrospectively determine the exposure to the risk factor of interest from each of the two groups of individuals: cases and controls. These studies are designed to estimate odds.
The case-control study design is often used in the study of rare diseases or as a preliminary study where little is known about the association between the risk factor and disease of interest. Compared to prospective cohort studies they tend to be less costly and shorter in duration. In several situations, they have greater statistical power ...
A case-control study is a research method where two groups of people are compared - those with the condition (cases) and those without (controls). By looking at their past, researchers try to identify what factors might have contributed to the condition in the 'case' group. ... Figure: Schematic diagram of case-control study design ...
A case-control study is a type of observational study commonly used to look at factors associated with diseases or outcomes. The case-control study starts with a group of cases, which are the individuals who have the outcome of interest. The researcher then tries to construct a second group of individuals called the controls, who are similar to ...
Case-control studies are particularly useful for studying the cause of an outcome that is rare and for studying the effects of prolonged exposure. For example, a case-control study could be used ...
classifications in research design,1 prospective and retrospective studies, cross-sectional and longitudinal studies,2 and cohort studies.3 This article considers a research design that is often used in present-day research in medicine and psychiatry: the case-control study. Case-Control Study: General Description
Case-control research is a vital tool used by epidemiologists, or researchers who look into the factors affecting health and illness of populations. Just one risk factor could be investigated for ...
Earlier articles in this series described classifications in research design, 1 prospective and retrospective studies, cross-sectional and longitudinal studies, 2 and cohort studies. 3 This article considers a research design that is often used in present-day research in medicine and psychiatry: the case-control study.
Case control studies are observational because no intervention is attempted and no attempt is made to alter the course of the disease. The goal is to retrospectively determine the exposure to the risk factor of interest from each of the two groups of individuals: cases and controls. These studies are designed to estimate odds.
Case-control studies are particularly useful for studying the cause of an outcome that is rare and for studying the effects of prolonged exposure. For example, a case-control study could be used ...
A case-control study is an experimental design that compares a group of participants possessing a condition of interest to a very similar group lacking that condition. Here, the participants possessing the attribute of study, such as a disease, are called the 'case', and those without it are the 'control'. It's important to remember ...
Case Control. In a Case-Control study there are two groups of people: one has a health issue (Case group), and this group is "matched" to a Control group without the health issue based on characteristics like age, gender, occupation. In this study type, we can look back in the patient's histories to look for exposure to risk factors that ...
Case control studies are observational because no intervention is attempted and no attempt is made to alter the course of the disease. The goal is to retrospectively determine the exposure to the risk factor of interest from each of the two groups of individuals: cases and controls. These studies are designed to estimate odds.
Introduction. Clinicians think of case-control study when they want to ascertain association between one clinical condition and an exposure or when a researcher wants to compare patients with disease exposed to the risk factors to non-exposed control group. In other words, case-control study compares subjects who have disease or outcome (cases ...
Case-control designs are an important yet commonly misunderstood tool in the epidemiologist's arsenal for causal inference. We reconsider classical concepts, assumptions and principles and explore when the results of case-control studies can be endowed a causal interpretation. We establish how, and under which conditions, various causal estimands relating to intention-to-treat or per ...
Abstract. Case-control studies are observational studies in which cases are subjects who have a characteristic of interest, such as a clinical diagnosis, and controls are (usually) matched ...
Abstract. Case-control (case-control, case-controlled) studies are beginning to appear more frequently in the neurosurgical literature. They can be more robust, if well designed, than the typical case series or even cohort study to determine or refine treatment algorithms. The purpose of this review is to define and explore the differences ...