Volume 21 Supplement 9
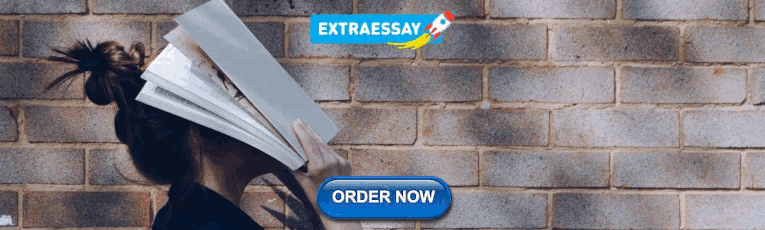
Selected Articles from the 20th International Conference on Bioinformatics & Computational Biology (BIOCOMP 2019)
- Introduction
- Open access
- Published: 03 December 2020
Current trend and development in bioinformatics research
- Yuanyuan Fu 1 ,
- Zhougui Ling 1 , 2 ,
- Hamid Arabnia 3 &
- Youping Deng 1
BMC Bioinformatics volume 21 , Article number: 538 ( 2020 ) Cite this article
10k Accesses
16 Citations
4 Altmetric
Metrics details
This is an editorial report of the supplements to BMC Bioinformatics that includes 6 papers selected from the BIOCOMP’19—The 2019 International Conference on Bioinformatics and Computational Biology. These articles reflect current trend and development in bioinformatics research.
The supplement to BMC Bioinformatics was proposed to launch during the BIOCOMP’19—The 2019 International Conference on Bioinformatics and Computational Biology held from July 29 to August 01, 2019 in Las Vegas, Nevada. In this congress, a variety of research areas was discussed, including bioinformatics which was one of the major focuses due to the rapid development and requirement of using bioinformatics approaches in biological data analysis, especially for omics large datasets. Here, six manuscripts were selected after strict peer review, providing an overview of the bioinformatics research trend and its application for interdisciplinary collaboration.
Cancer is one of the leading causes of morbidity and mortality worldwide. There exists an urgent need to identify new biomarkers or signatures for early detection and prognosis. Mona et al. identified biomarker genes from functional network based on the 407 differential expressed genes between lung cancer and healthy populations from a public Gene Expression Omnibus dataset. The lower expression of sixteen gene signature is associated with favorable lung cancer survival, DNA repair, and cell regulation [ 1 ]. A new class of biomarkers such as alternative splicing variants (ASV) have been studied in recent years. Various platforms and methods, for example, Affymetrix Exon-Exon Junction Array, RNA-seq, and liquid chromatography tandem mass spectrometry (LC–MS/MS), have been developed to explore the role of ASV in human disease. Zhang et al. have developed a bioinformatics workflow to combine LC–MS/MS with RNA-seq which provide new opportunities in biomarker discovery. In their study, they identified twenty-six alternative splicing biomarker peptides with one single intron event and one exon skipping event; further pathways indicated the 26 peptides may be involved in cancer, signaling, metabolism, regulation, immune system and hemostasis pathways which validated by the RNA-seq analysis [ 2 ].
Proteins serve crucial functions in essentially all biological processes and the function directly depends on their three-dimensional structures. Traditional approaches to elucidation of protein structures by NMR spectroscopy are time consuming and expensive, however, the faster and more cost-effective methods are critical in the development of personalized medicine. Cole et al. improved the REDRAFT software package in the important areas of usability, accessibility, and the core methodology which resulted in the ability to fold proteins [ 3 ].
The human microbiome is the aggregation of microorganisms that reside on or within human bodies. Rebecca et al. discussed the tissue-associated microbial detection in cancer using next generation sequencing (NGS). Various computational frameworks could shed light on the role of microbiota in cancer pathogenesis [ 4 ]. How to analyze the human microbiome data efficiently is a huge challenge. Zhang et al. developed a nonparametric test based on inter-point distance to evaluate statistical significance from a Bayesian point of view. The proposed test is more efficient and sensitive to the compositional difference compared with the traditional mean-based method [ 5 ].
Human disease is also considered as the cause of the interaction between genetic and environmental factors. In the last decades, there was a growing interest in the effect of metal toxicity on human health. Evaluating the toxicity of chemical mixture and their possible mechanism of action is still a challenge for humans and other organisms, as traditional methods are very time consuming, inefficient, and expensive, so a limited number of chemicals can be tested. In order to develop efficient and accurate predictive models, Yu et al. compared the results among a classification algorithm and identified 15 gene biomarkers with 100% accuracy for metal toxicant using a microarray classifier analysis [ 6 ].
Currently, there is a growing need to convert biological data into knowledge through a bioinformatics approach. We hope these articles can provide up-to-date information of research development and trend in bioinformatics field.
Availability of data and materials
Not applicable.
Abbreviations
The 2019 International Conference on Bioinformatics and Computational Biology
Liquid chromatography tandem mass spectrometry
Alternative splicing variants
Nuclear Magnetic Resonance
Residual Dipolar Coupling based Residue Assembly and Filter Tool
Next generation sequencing
Mona Maharjan RBT, Chowdhury K, Duan W, Mondal AM. Computational identification of biomarker genes for lung cancer considering treatment and non-treatment studies. 2020. https://doi.org/10.1186/s12859-020-3524-8 .
Zhang F, Deng CK, Wang M, Deng B, Barber R, Huang G. Identification of novel alternative splicing biomarkers for breast cancer with LC/MS/MS and RNA-Seq. Mol Cell Proteomics. 2020;16:1850–63. https://doi.org/10.1186/s12859-020-03824-8 .
Article Google Scholar
Casey Cole CP, Rachele J, Valafar H. Increased usability, algorithmic improvements and incorporation of data mining for structure calculation of proteins with REDCRAFT software package. 2020. https://doi.org/10.1186/s12859-020-3522-x .
Rebecca M, Rodriguez VSK, Menor M, Hernandez BY, Deng Y. Tissue-associated microbial detection in cancer using human sequencing data. 2020. https://doi.org/10.1186/s12859-020-03831-9 .
Qingyang Zhang TD. A distance based multisample test for high-dimensional compositional data with applications to the human microbiome . 2020. https://doi.org/10.1186/s12859-020-3530-x .
Yu Z, Fu Y, Ai J, Zhang J, Huang G, Deng Y. Development of predicitve models to distinguish metals from non-metal toxicants, and individual metal from one another. 2020. https://doi.org/10.1186/s12859-020-3525-7 .
Download references
Acknowledgements
This supplement will not be possible without the support of the International Society of Intelligent Biological Medicine (ISIBM).
About this supplement
This article has been published as part of BMC Bioinformatics Volume 21 Supplement 9, 2020: Selected Articles from the 20th International Conference on Bioinformatics & Computational Biology (BIOCOMP 2019). The full contents of the supplement are available online at https://bmcbioinformatics.biomedcentral.com/articles/supplements/volume-21-supplement-9 .
Publication of this supplement has been supported by NIH grants R01CA223490 and R01 CA230514 to Youping Deng and 5P30GM114737, P20GM103466, 5U54MD007601 and 5P30CA071789.
Author information
Authors and affiliations.
Department of Quantitative Health Sciences, John A. Burns School of Medicine, University of Hawaii at Manoa, Honolulu, HI, 96813, USA
Yuanyuan Fu, Zhougui Ling & Youping Deng
Department of Pulmonary and Critical Care Medicine, The Fourth Affiliated Hospital of Guangxi Medical University, Liuzhou, 545005, China
Zhougui Ling
Department of Computer Science, University of Georgia, Athens, GA, 30602, USA
Hamid Arabnia
You can also search for this author in PubMed Google Scholar
Contributions
YF drafted the manuscript, ZL, HA, and YD revised the manuscript. All authors read and approved the final manuscript.
Corresponding author
Correspondence to Youping Deng .
Ethics declarations
Ethics approval and consent to participate, consent for publication, competing interests.
The authors declare that they have no competing interests.
Additional information
Publisher's note.
Springer Nature remains neutral with regard to jurisdictional claims in published maps and institutional affiliations.
Rights and permissions
Open Access This article is licensed under a Creative Commons Attribution 4.0 International License, which permits use, sharing, adaptation, distribution and reproduction in any medium or format, as long as you give appropriate credit to the original author(s) and the source, provide a link to the Creative Commons licence, and indicate if changes were made. The images or other third party material in this article are included in the article's Creative Commons licence, unless indicated otherwise in a credit line to the material. If material is not included in the article's Creative Commons licence and your intended use is not permitted by statutory regulation or exceeds the permitted use, you will need to obtain permission directly from the copyright holder. To view a copy of this licence, visit http://creativecommons.org/licenses/by/4.0/ . The Creative Commons Public Domain Dedication waiver ( http://creativecommons.org/publicdomain/zero/1.0/ ) applies to the data made available in this article, unless otherwise stated in a credit line to the data.
Reprints and permissions
About this article
Cite this article.
Fu, Y., Ling, Z., Arabnia, H. et al. Current trend and development in bioinformatics research. BMC Bioinformatics 21 (Suppl 9), 538 (2020). https://doi.org/10.1186/s12859-020-03874-y
Download citation
Published : 03 December 2020
DOI : https://doi.org/10.1186/s12859-020-03874-y
Share this article
Anyone you share the following link with will be able to read this content:
Sorry, a shareable link is not currently available for this article.
Provided by the Springer Nature SharedIt content-sharing initiative
- Bioinformatics
- Human disease
BMC Bioinformatics
ISSN: 1471-2105
- General enquiries: [email protected]

An official website of the United States government
The .gov means it's official. Federal government websites often end in .gov or .mil. Before sharing sensitive information, make sure you're on a federal government site.
The site is secure. The https:// ensures that you are connecting to the official website and that any information you provide is encrypted and transmitted securely.
- Publications
- Account settings
- Browse Titles
NCBI Bookshelf. A service of the National Library of Medicine, National Institutes of Health.
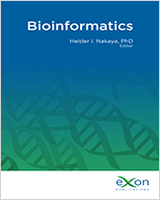
Bioinformatics
Editor: Nakaya Helder I. , PhD.
Affiliations
- Copyright and Permissions
Today, a single laboratory can generate a vast amount of biological data. There is a wealth of data already available in public databases, which makes the modern life sciences almost dependent on bioinformatics. This book brings together an international team of experts to discuss the state-of-the-art from several fields of bioinformatics, from the automatic identification and classification of viruses to the analysis of the transcriptome of single cells and plants, including artificial intelligence algorithms to discover biomarkers and text mining approaches to help in the interpretation of the findings. Machine learning, pattern discovery and analysis, error correction, Bayesian inference and novel computational techniques to discover chromosomal rearrangements continue to play crucial roles in biological discovery, and all of them are explored in chapters of this book. In sum, this book contains high-quality chapters that provide excellent views into key topics of current bioinformatics research, topics that should remain important for the next several years.
- Collapse All
- List of Contributors
- INTRODUCTION
- BIOINFORMATICS TOOLS FOR THE TEXT MINING OF GENE RANKING
- TEXT MINING GENE SELECTION FOR HYDATIDIFORM MOLES: A CASE STUDY
- UNDERSTANDING FOCAL SEGMENTAL GLOMERULOSCLEROSIS USING SINGLE CELL TRANSCRIPTOME OF A HEALTHY ADULT DONOR KIDNEY: PROOF OF CONCEPT
- ONLINE RESULT VISUALIZATION AND THE DEVELOPMENT OF AN INTERACTIVE WEB INTERFACE
- THE CAPTURE TECHNIQUE SIGNIFICANTLY DETERMINES THE QUANTITY OF MEASURABLE CELLS
- THE SEQUENCING TECHNIQUE DETERMINES THE OPTIONS FOR DATA ANALYSES
- HOW TO CHOOSE THE RIGHT APPROACH?
- COMPUTATIONAL ANALYSIS
- DOWNSTREAM ANALYSES
- NGS-BASED METHODS
- RNA-SEQ BASED METHODS
- HI-C BASED TECHNIQUES
- EVALUATION OF A BIOMARKER DISCOVERY METHOD
- COMPARISON OF BIOMARKER DISCOVERY METHODS
- ENSEMBLE OF MULTIPLE METHODS
- BUILDING BLOCKS OF BAYESIAN INFERENCE
- PROBABILISTIC MODELS FOR SINGLE GENE EXPRESSION
- BAYESIAN INFERENCE OF WHOLE TRANSCRIPTOME EXPRESSION
- MODELING GENE EXPRESSION ACROSS SAMPLES
- BAYESIAN DIFFERENTIAL GENE EXPRESSION: A STAN CASE STUDY
- EVALUATION STEPS
- EXPERIMENTS
- TRANSCRIPTOME ASSEMBLY STRATEGIES
- HOW TO EVALUATE TRANSCRIPTOME ASSEMBLY PERFORMANCE
- HOW TO GENERATE SIMULATED BENCHMARK TRANSCRIPTOME DATASETS
- PERFORMANCE COMPARISON AMONG TRANSCRIPTOME ASSEMBLERS
- THE WEMINE SYSTEM
- APPLICATIONS OF PATTERNS WITH VARYING ASSOCIATION RELATIONSHIPS
- DATABASES OF VIRAL PROFILE HMMS
- A ROADMAP FOR THE RATIONAL DESIGN OF PROFILE HMMS
- RATIONAL DESIGN OF PROFILE HMMS
- SCREENING SEQUENCING DATASETS WITH PROFILE HMMS
- USING PROFILE HMMS FOR TARGETED SEQUENCE RECONSTRUCTION
- FINDING MULTIGENE ELEMENTS IN CELLULAR ORGANISMS WITH PROFILE HMMS
- AN INTEGRATED APPROACH FOR VIRAL RESEARCH USING PROFILE HMMS
- MINIONDB - A DATABASE OF VIRAL PROFILE HMMS
- PATTERN DISCOVERY AND DISENTANGLEMENT
- CASE STUDIES
ISBN: 978-0-6450017-1-6
DOI: https://doi.org/10.36255/exonpublications.bioinformatics.2021
Helder I. Nakaya, PhD, Department of Clinical and Toxicological Analyses, School of Pharmaceutical Sciences, University of Sao Paulo, Sao Paulo, Brazil.
Published by
Exon Publications, Brisbane, Australia
Copyright© 2021 Exon Publications
Copyright of individual chapters belongs to the respective authors. The authors grant unrestricted publishing and distribution rights to the publisher. The electronic versions of the chapters are published under Creative Commons Attribution-NonCommercial 4.0 International (CC BY-NC 4.0). https://creativecommons.org/licenses/by-nc/4.0/ . Users are allowed to share and adapt the chapters for any non-commercial purposes as long as the authors and the publisher are explicitly identified and properly acknowledged as the original source. The book in its entirety is subject to copyright by the publisher. The reproduction, modification, replication and display of the book in its entirety, in any form, by anyone, for commercial purposes are strictly prohibited without the written consent of the publisher.
Notice to the user
The views and opinions expressed in this book are believed to be accurate at the time of publication. The publisher, editors or authors cannot be held responsible or liable for any errors, omissions or consequences arising from the use of the information contained in this book. The publisher makes no warranty, implicit or explicit, with respect to the contents of this book, or its use.
First Published in March 2021
Printed in Australia
Copyright of individual chapters belongs to the respective authors. The authors grant unrestricted publishing and distribution rights to the publisher. The electronic versions of the chapters are published under Creative Commons Attribution-NonCommercial 4.0 International (CC BY-NC 4.0). Users are allowed to share and adapt the chapters for any non-commercial purposes as long as the authors and the publisher are explicitly identified and properly acknowledged as the original source. The book in its entirety is subject to copyright by the publisher. The reproduction, modification, replication and display of the book in its entirety, in any form, by anyone, for commercial purposes are strictly prohibited without the written consent of the publisher.
- Cite this Page Helder I. N, editor. Bioinformatics [Internet]. Brisbane (AU): Exon Publications; 2021 Mar 20. doi: 10.36255/exonpublications.bioinformatics.2021
- PDF version of this title (54M)
Related Items in Bookshelf
- All Reference Works
- All Textbooks
Related information
- NLM Catalog Related NLM Catalog Entries
Similar articles in PubMed
- Protein Science Meets Artificial Intelligence: A Systematic Review and a Biochemical Meta-Analysis of an Inter-Field. [Front Bioeng Biotechnol. 2022] Protein Science Meets Artificial Intelligence: A Systematic Review and a Biochemical Meta-Analysis of an Inter-Field. Villalobos-Alva J, Ochoa-Toledo L, Villalobos-Alva MJ, Aliseda A, Pérez-Escamirosa F, Altamirano-Bustamante NF, Ochoa-Fernández F, Zamora-Solís R, Villalobos-Alva S, Revilla-Monsalve C, et al. Front Bioeng Biotechnol. 2022; 10:788300. Epub 2022 Jul 7.
- Machine learning: an indispensable tool in bioinformatics. [Methods Mol Biol. 2010] Machine learning: an indispensable tool in bioinformatics. Inza I, Calvo B, Armañanzas R, Bengoetxea E, Larrañaga P, Lozano JA. Methods Mol Biol. 2010; 593:25-48.
- Role of biological Data Mining and Machine Learning Techniques in Detecting and Diagnosing the Novel Coronavirus (COVID-19): A Systematic Review. [J Med Syst. 2020] Role of biological Data Mining and Machine Learning Techniques in Detecting and Diagnosing the Novel Coronavirus (COVID-19): A Systematic Review. Albahri AS, Hamid RA, Alwan JK, Al-Qays ZT, Zaidan AA, Zaidan BB, Albahri AOS, AlAmoodi AH, Khlaf JM, Almahdi EM, et al. J Med Syst. 2020 May 25; 44(7):122. Epub 2020 May 25.
- Review Promoting synergistic research and education in genomics and bioinformatics. [BMC Genomics. 2008] Review Promoting synergistic research and education in genomics and bioinformatics. Yang JY, Yang MQ, Zhu MM, Arabnia HR, Deng Y. BMC Genomics. 2008; 9 Suppl 1(Suppl 1):I1.
- Review Survey of Machine Learning Techniques in Drug Discovery. [Curr Drug Metab. 2019] Review Survey of Machine Learning Techniques in Drug Discovery. Stephenson N, Shane E, Chase J, Rowland J, Ries D, Justice N, Zhang J, Chan L, Cao R. Curr Drug Metab. 2019; 20(3):185-193.
Recent Activity
- Bioinformatics Bioinformatics
Your browsing activity is empty.
Activity recording is turned off.
Turn recording back on
Connect with NLM
National Library of Medicine 8600 Rockville Pike Bethesda, MD 20894
Web Policies FOIA HHS Vulnerability Disclosure
Help Accessibility Careers
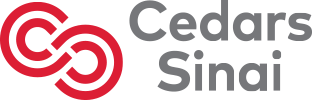
- Departments
- Anesthesiology
- Biomedical Sciences
- Cardiac Surgery
- Computational Biomedicine
- Neurosurgery
- Obstetrics & Gynecology
- Orthopaedics
- Pathology & Laboratory Medicine
- Physical Medicine & Rehabilitation
- Psychiatry & Behavioral Neurosciences
- Radiation Oncology
- Board of Governors Regenerative Medicine Institute
- F. Widjaja Inflammatory Bowel Disease Institute
- Samuel Oschin Comprehensive Cancer Institute
- Smidt Heart Institute
- Advanced Clinical Biosystems Research Institute
- Biomedical Imaging Research Institute
- Diabetes & Obesity Research Institute
- Geri & Richard Brawerman Nursing Institute
- Human Microbiome Research Institute
- Kao Autoimmunity Institute
- Maxine Dunitz Neurosurgical Institute
- Women's Guild Lung Institute
- Research Topics
- Laboratories
- Research Cores
- Clinical Trials
- Office of Research Administration
- Technology & Innovations
- Clinical & Translational Research Center
- News & Breakthroughs
- Graduate Medical Education
- Graduate School of Biomedical Sciences
- Continuing Medical Education
- Professional Training Programs
- Women's Guild Simulation Center
- Center for the Arts and Humanities in Medicine
- Medical Library
- Campus Life
- Office of the Dean
- Academic Calendar
- Back to Bioinformatics & Functional Genomics
Current Research
- Publications
- Research Team
The primary vision of the Center for Bioinformatics and Functional Genomics (CBFG) is to establish a research nexus that integrates leading-edge developments in computational sciences, next-generation genomics and epigenomics and functional biology to improve our understanding of disease etiology applied to personalized medicine. We utilize next-generation sequencing technologies, evolving computational methodologies, and functional assays to understand the underlying biological mechanisms driving cancer and other diseases, and apply these in the clinical setting to advance personalized health.
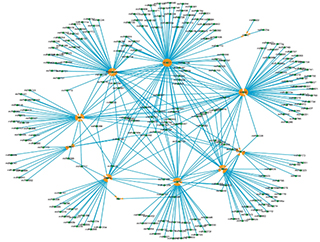
Bioinformatics
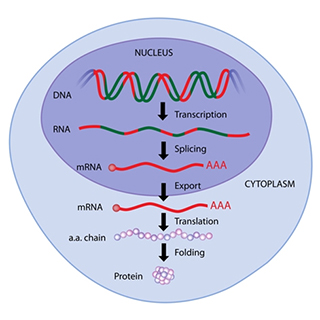
Functional Genomics
Emphasis on Collaborative Approach
- GWAS functional follow-up team
- Computational pipeline team
- Genetic epidemiology team
- Genomic-epigenomic tumor profiling team
- Single cell team
- Functional screening team
- IP-seq team
- Collaborative environment utilizing expertise to train collaborator laboratory staff on technologies and use of leading-edge technologies.
Driven by Technology Adoption and Development
- Separated pre- and post-PCR laboratories
- Virus production and downstream cell culture facilities
- NGS library QC suite
- Bioinformatics suite
- Genome-wide CRISPR and shRNA screening libraries
- Illumina miniseq
- Agilent bioanalyzer
- Olympic florescent microscope
- 10x genomics single cell controller
Education, Training and Outreach
- Weekly Omics seminar
- Single cell RNAseq
- ChIP sequencing/epigenomic profiling
- Whole genome sequencing
- Whole genome bisulfite sequencing
- Genome editing
- PhD student rotations
- Interdisciplinary mentorship of trainees
Have Questions or Need Help?
Contact us if you have questions, or wish to learn more about bioinformatics and functional genomics research at Cedars-Sinai .
Spielberg 8723 Alden Drive, Suite 100 Los Angeles, CA 90048
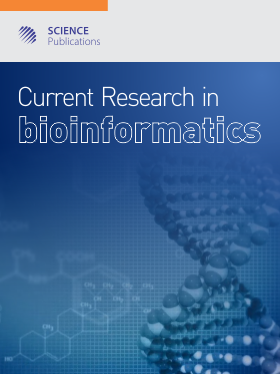
Current Research in Bioinformatics
Aims and scope.
Current Research in Bioinformatics, a peer reviewed international journal is launched to publish notable research on the application of information technology to the field of molecular biology. Researchers and scholars are invited to submit their original research / review articles in the field of creation and advancement of databases, algorithms, computational and statistical techniques, and theory to solve formal and practical problems arising from the management and analysis of biological data.
It is with great pleasure that we announce the SGAMR Annual Awards 2020. This award is given annually to Researchers and Reviewers of International Journal of Structural Glass and Advanced Materials Research (SGAMR) who have shown innovative contributions and promising research as well as others who have excelled in their Editorial duties.
This special issue "Neuroinflammation and COVID-19" aims to provide a space for debate in the face of the growing evidence on the affectation of the nervous system by COVID-19, supported by original studies and case series.
- Recently Published
- Most Viewed
- Most Downloaded
Thank you for visiting nature.com. You are using a browser version with limited support for CSS. To obtain the best experience, we recommend you use a more up to date browser (or turn off compatibility mode in Internet Explorer). In the meantime, to ensure continued support, we are displaying the site without styles and JavaScript.
- View all journals
- My Account Login
- Explore content
- About the journal
- Publish with us
- Sign up for alerts
- Review Article
- Open access
- Published: 01 April 2022
Current progress and open challenges for applying deep learning across the biosciences
- Nicolae Sapoval ORCID: orcid.org/0000-0002-0736-5075 1 na1 ,
- Amirali Aghazadeh 2 na1 ,
- Michael G. Nute 1 ,
- Dinler A. Antunes ORCID: orcid.org/0000-0001-7947-6455 3 ,
- Advait Balaji 1 ,
- Richard Baraniuk 4 ,
- C. J. Barberan 4 ,
- Ruth Dannenfelser 1 ,
- Chen Dun 1 ,
- Mohammadamin Edrisi ORCID: orcid.org/0000-0002-9738-1916 1 ,
- R. A. Leo Elworth 1 ,
- Bryce Kille 1 ,
- Anastasios Kyrillidis 1 ,
- Luay Nakhleh ORCID: orcid.org/0000-0003-3288-6769 1 ,
- Cameron R. Wolfe 1 ,
- Zhi Yan ORCID: orcid.org/0000-0003-2433-5553 1 ,
- Vicky Yao ORCID: orcid.org/0000-0002-3201-9983 1 &
- Todd J. Treangen ORCID: orcid.org/0000-0002-3760-564X 1 , 5
Nature Communications volume 13 , Article number: 1728 ( 2022 ) Cite this article
39k Accesses
77 Citations
207 Altmetric
Metrics details
- Computational biology and bioinformatics
- Computer science
- Machine learning
Deep Learning (DL) has recently enabled unprecedented advances in one of the grand challenges in computational biology: the half-century-old problem of protein structure prediction. In this paper we discuss recent advances, limitations, and future perspectives of DL on five broad areas: protein structure prediction, protein function prediction, genome engineering, systems biology and data integration, and phylogenetic inference. We discuss each application area and cover the main bottlenecks of DL approaches, such as training data, problem scope, and the ability to leverage existing DL architectures in new contexts. To conclude, we provide a summary of the subject-specific and general challenges for DL across the biosciences.
Similar content being viewed by others
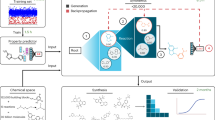
Generative AI for designing and validating easily synthesizable and structurally novel antibiotics
Kyle Swanson, Gary Liu, … Jonathan M. Stokes
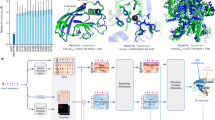
Highly accurate protein structure prediction with AlphaFold
John Jumper, Richard Evans, … Demis Hassabis
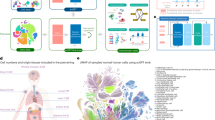
scGPT: toward building a foundation model for single-cell multi-omics using generative AI
Haotian Cui, Chloe Wang, … Bo Wang
Introduction
The recent success of AlphaFold2 1 in predicting the 3D structure of proteins from their sequences highlights one of the most effective applications of deep learning in computational biology to date. Deep learning (DL) allows for finding a representation of the data with multiple layers of abstraction using complex models that are composed of several layers of nonlinear computational units (Fig. 1 ). Observed through the success of DL in a broad variety of application domains, the efficacy of using DL depends on the development of specialized neural network architectures that can capture important properties of the data such as spatial locality (convolutional neural networks – CNNs), sequential nature (recurrent neural networks – RNNs), context dependence (Transformers), and data distribution (autoencoders – AEs). Figure 1 illustrates six DL architectures that have found the most applications within the realm of computational biology. We refer the reader to LeCun et al. 2 for a complete review of DL methods and architectures and keep the focus of the paper on computational biology applications. These DL models have revolutionized speech recognition, visual object recognition, and object detection and have lately played a key role in solving important problems in computational biology. The applications of DL in other areas of computational biology, such as functional biology, are only growing while other areas, such as phylogenetics, are in their infancy. Given the wide divide between the receptiveness of DL in different areas in computational biology, some key questions remain unanswered: (1) What makes an area prime for DL methods? (2) What are the potential limitations of DL for computational biology applications? (3) Which DL model is most appropriate for a specific application area in computational biology?
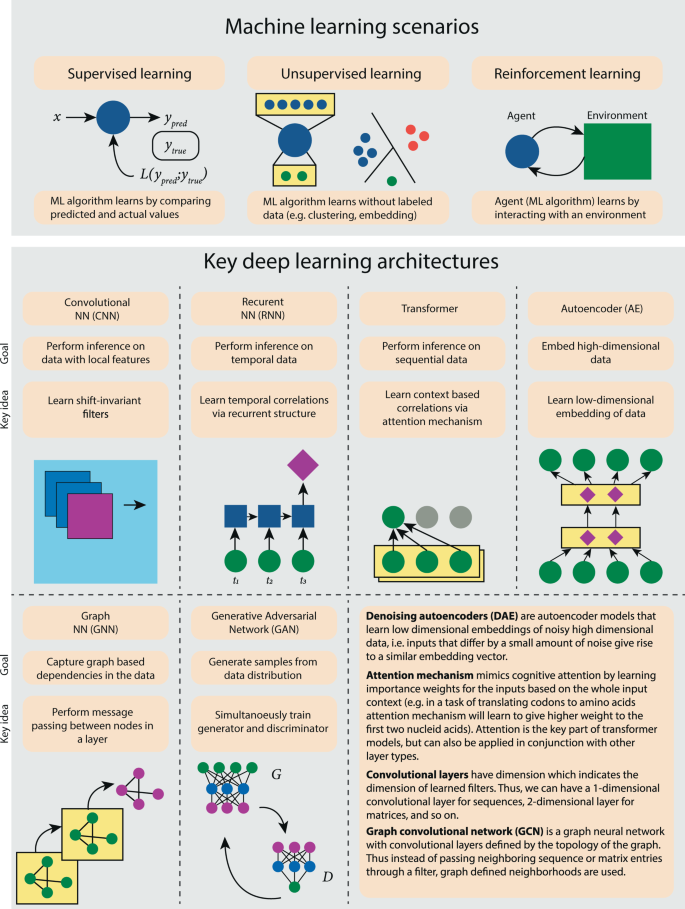
Top panel encapsulates the three most common paradigms of machine learning: supervised learning in which dataset contains ground truth labels, unsupervised learning in which dataset does not contain ground truth labels, and reinforcement learning in which an algorithmic agent interacts with a real or simulated environment. The bottom panels provide an overview of the most prevalent DL architecture ideas each designed to achieve specific highlighted goals. An additional set of short descriptions is provided for other common components of DL architectures mentioned in the manuscript.
In this paper, we aim to address these foundational questions from the lens of computational biology. The answers, however, are highly task specific and can only be addressed in the context of the corresponding applications. The pitfalls of applying machine learning (ML) in genomics have been discussed in Whalen et al. 3 , but our goal is to provide a perspective on the impact of DL across five distinct areas. While there are multiple areas of interest in the biosciences where DL has achieved notable successes (e.g. DeepVariant 4 , DeepArg 5 , metagenomic binning 6 , and lab-of-origin attribution 7 ), we aim to only focus on a few diverse and broad subtopics. In those areas we evaluate the improvements that DL has had over classical ML techniques in computational biology with varying levels of success to date (Fig. 2 ). For each area, we explore limitations of current approaches and opportunities for improvement, and include practical tips. We anchor our discussions around five broad, distinct areas in computational biology: protein structure prediction, protein function prediction, genome engineering, systems biology and data integration, and phylogenetic inference (Table 1 ). These areas provide a range of impact levels from major paradigm shifts (AlphaFold2) to DL applications in their infancy (phylogenetic inference); and collectively, they provide rich enough technical diversity to address the questions raised in this perspective. Over the next several subsections, we will review progress in each of the four computational biology topics, ordered from (i) paradigm shifting (where DL clearly outperforms other ML and classical approaches, and provides a field-wide impact), (ii) major success (where DL performance is typically higher than of that of other ML and classical approaches), (iii) moderate success (where DL performance is typically comparable to other ML and classical approaches) to (iv) minor successes (where DL methods are not widely adopted or underperform compared to other ML and classical approaches), and then discuss common challenges for DL in biosciences (Table 2) .
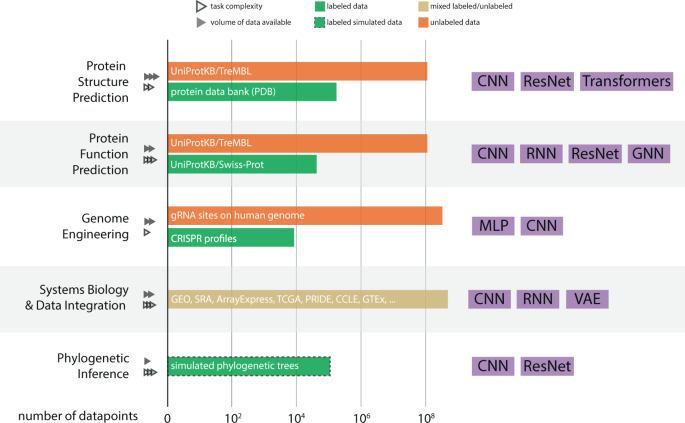
For each of the areas considered in this manuscript, it summarizes estimated sizes of key datasets and databases, as well as the projected growth rate of these. Additionally the rightmost column summarizes the most popular DL architectures applied to the corresponding areas in biosciences.
Paradigm shifting successes of DL
Protein structure prediction.
We start our discussion with protein structure prediction which is arguably one of the most successful applications of DL in computational biology; this success is what we refer to as a paradigm shift. It is largely known that the protein’s amino acid sequence determines its 3D structure, which is in turn directly related to its function (e.g., chemical reaction catalysis, signal transduction, scaffold, etc.) 8 , 9 . The history of protein structure prediction problem goes back to the determination of the 3D structure of myoglobin by John Kendrew in the 1950s which was a landmark in biochemistry and structural biology 10 . Since then, X-ray crystallography has become the gold-standard experimental method for protein structure determination 11 , 12 , as well as the reference to validate computational models for protein structure prediction. Considering the high cost and technical limitations of X-ray crystallography, and the growing access to biological sequences following the Human Genome Project, predicting the 3D structure of a protein from its sequence became the Mount Everest in computational biology 8 ; a challenge broadly known as the “protein folding problem”. Initial efforts concentrated on the use of biophysically-accurate energy functions and knowledge-based statistical reasoning, but faster progress was recently achieved with a greater focus on DL.
One of the key reasons for the recent success of DL in this area has been the wealth of unsupervised data in the form of multiple sequence alignment (MSA) 1 , 9 , 13 , 14 , 15 , 16 , 17 , which has enabled learning a nonlinear evolution-informed representation of proteins. Progress in the field has been accelerated by the creation of a bi-annual international competition, called the Critical Assessment of Protein Structure Prediction (CASP). Launched in 1994, CASP created the means to objectively test available methods through blind predictions, providing competing groups with a set of challenges (i.e., sequences of proteins with unknown structures), and evaluating their performances against the respective experimentally-determined structures. In their first participation in CASP13, AlphaFold, implemented by DeepMind group at Google, made the news by clearly outperforming the second best method 14 , and nearly twice beyond the projection based on previous editions 18 . Following recent trends in the field 13 , 16 , 19 , 20 , AlphaFold and AlphaFold2 leverage the combined use of DL and MSA 18 , 21 . This proved to be a winning strategy which was able to overcome the lack of large training datasets on protein structure. The Protein Data Bank (PDB) 22 is the reference database for experimentally-determined macromolecular structures, and currently hosts close to 180,000 entries. This is a small number of data points for a complex mapping involved in the problem, and these are further biased by technical constraints of the experimental methods. Protein sequence data, on the other hand, is available on a much larger scale. Therefore, MSA allows modeling methods to extract pairwise evolutionary correlations from this larger corpus of data, maximizing the learning on available structural data. Other key factors for the success of DL in this area include innovation in model design such as new attention strategies tuned towards invariances and symmetries in proteins, graph-based representations, and model recycling strategies.
The impact of AlphaFold2 on the field of structural biology is undeniable; it successfully demonstrated the use of a DL-based implementation for high accuracy protein structure prediction 21 . This achievement is already driving and accelerating further developments in the field, as highlighted by the remarkable number of early citations. In addition, DeepMind has partnered with the European Molecular Biology Laboratory (EMBL) 23 to create an open-access database of protein structures modeled with AlphaFold2 17 . The database already covers 98.5% of human proteins, for which at least 36% of the amino acid residues were predicted with high confidence. Finally, rather than retiring experimental methods, DL-based methods might augment the accuracy and reach of experimental methods as demonstrated by preliminary applications to solving challenging structures with data from X-ray crystallography and cryo-EM 1 , 15 . However, many caveats, limitations and open questions 8 , 9 remain. In particular, while AlphaFold2 successfully predicts the static structure of a protein, many key insights about protein’s biological function come from its dynamic conformations. Furthermore, dynamics of interaction of multiple proteins still present open challenges in the field. Moving forward, it will be important to monitor the application of DL to these follow up research areas.
Major successes of DL
Protein function prediction.
Predicting protein function is a natural next step after protein structure prediction. Protein function prediction involves mapping target proteins to curated ontologies, such as Gene Ontology (GO) terms, Biological Processes (BP), Molecular Functions (MF) and Cellular components (CC). Protein structure can convey a lot of information about these ontologies, however, there is no direct mapping between the two and the mapping is often very complex 24 . Despite the tremendous growth of protein sequences available in the UniProtKB database, functional annotations for the vast majority of proteins still remain partly or completely unknown 25 . Limited and imbalanced training examples, a large output space of possible functions and the hierarchical nature of the GO labels are some of the main bottlenecks associated with functional annotation of proteins 26 . To overcome some of the issues recent methods have leveraged features from different sources including sequence 27 , structure 22 , interaction networks 28 , scientific literature, homologies, domain information 29 and even incorporate one or a combination of DL architectures to handle different stages of prediction task (e.g. feature representation, feature selection, and classification).
One of the most successful DL approaches to the problem, DeepGO 30 incorporated CNN to learn sequence-level embeddings and combines it with knowledge graph embeddings for each protein obtained 31 from Protein-Protein Interaction (PPI) networks. DeepGO was one of the first DL based models to perform better than BLAST 32 and previous methods on functional annotation tasks over the three GO categories 30 . An improved version of the tool, DeepGOPlus 33 emerged as one of the top performers when compared to other tools in the CAFA3 challenge across the three GO categories 33 . DeepGOPlus used convolutional filters of different sizes with individual max-pooling to learn dense feature representations of protein sequences embedded in a one-hot encoding scheme. The authors showed that combining the outputs from CNN with homology-based predictions from DIAMOND 34 can result in better predictive accuracy.
Unsupervised methods such as DAEs also have been instrumental by learning dense, robust, and low-dimensional representations of proteins. Chicco et al. 35 developed a DAE to represent proteins for assigning missing GO annotations and showed 6% to 36% improvements compared to non-DL methods over six different GO datasets. Miranda and Hu 36 introduced the Stacked Denoising Autoencoders (sdAE) to learn more robust representation of proteins. Gilgorijevic et al. introduced deepNF 37 that uses Multimodal DAE (MDA) to extract features from multiple heterogeneous interaction networks which outperform methods based on matrix factorization and linear regression 37 . Methods for learning low-dimensional embeddings of proteins continue to grow.
Beyond just predicting Gene Ontology labels, studies have also focused on several other task-specific functional categories such as identifying specific enzyme functions 38 and potential post-translational modification sites 39 . These studies are a fundamental step towards developing novel proteins with specialized functions or modifying the efficacy of existing proteins as seen in the recent advances of DL in enzyme engineering 40 . Going forward, applications of deep learning in engineering proteins tailored to specific functions can help increase throughput of candidate proteins for pharmaceutical applications among other domains.
Besides these canonical architectures, there have been other approaches that have used a combination of the above methods for function classification 41 . Overall, previous results indicate that models integrating features from multi-modal data types (e.g., sequence, structure, PPI, etc) are more likely to outperform the ones that rely on a single datatype. Trends from literature indicate that relying on task-specific architectures could help greatly enhance the feature representation from respective data types. Future work in this direction could focus on combining DAEs and RNNs for sequence based representation, and Graph Convolutional Networks (GCNs) for structure based as well as PPI based information. Combining these representations in a hierarchical classifier such as the multi-task DNN with biologically-relevant regularization methods 42 , 43 could allow for an explainable and computationally feasible DL architecture for protein function prediction.
Genome engineering
Biomedical engineering, and in particular genome engineering, is an important area in biology where DL models have been increasingly employed. Among genome engineering technologies, clustered regularly interspaced short palindromic repeats (CRISPR), i.e., a family of DNA sequences found in the genomes of prokaryotic organisms, have been recently used as a guide to recognize and cleave specific locations on the human genome. In the CRISPR-associated protein 9 (Cas9) technology, a single-guide RNA (gRNA) steers the protein to a specific genomic target. When the 20-nucleotide gRNA sequence complements the genome, Cas9 creates a double-strand break (DSB) on the targets (an on-target event). Due to the ability to precisely target specific locations on the genome, we have observed enormous advancements in CRISPR-based editing technologies since the development of Cas9. However, recent studies have shown that multiple mismatches between the gRNA and the genomic targets are tolerated and, as a result, Cas9 can cut unwanted locations on the genome (an off-target event). Off-target edits have pathogenic effects on the functionality and integrity of the cell. Therefore, the full clinical deployment of Cas9 has been slow due to the insufficient efficiency, reliability, and controllability challenges for therapeutic purposes. As a result, reducing off-target while improving the on-target efficiency has been an important ultimate goal in genome engineering target by DL techniques.
The sheer complexity of the biological process involved in modeling the DNA repair process and the growing availability of labeled data caused by a rapid drop in the cost of CRISPR assays, have made DL-based methods particularly successful choices to find the root cause of these inefficiencies. The use of DL models was triggered by the observation that the on-target and off-target events and the DNA repair outcome 44 are predictable by the sequence around the DSB, its location on the genome, and the potential mistargeted sequences on the genome. Several computational tools have been successfully developed to design gRNAs with maximum on-target activity and minimum off-target effects 45 . DeepCas9 is among CNN-based models which learns functional gRNAs directly from their canonical sequence representation 46 , 47 . The success of DeepCRISPR, on the other hand, relies on extracting about half a billion unlabeled gRNA sequences from the human coding and non-coding regions and learning a low-dimensional representation of the gRNA 48 . DeepCRISPR also uses a data augmentation method to create less than a million sgRNAs with known knockout efficiencies to train a larger CNN model. CnnCrispr uses a language processing model to learn the representation of gRNA and then employs a combination of bidirectional LSTM and CNN 49 while RNNs have been the reason for the success of other models 50 . Attention mechanism has also been shown to improve the accuracy in predicting on and off target effects 51 , 52 . ADAPT 53 is another recent CNN-based method for fully-automated CRISPR design for vertebrate-infecting viral diagnostics which owes its success to the construction of a massive training CRISPR dataset. Recent methods for predicting the DNA repair outcome employ other strategies. SPROUT compensates the lack of labels on harder-to-collect human CD4+ T cells by predicting a summary statistics of the DNA repair outcome 54 . FORECasT employs a larger dataset from easier-to-collect human chronic myelogenous leukemia cell-line (K562) 55 . InDelphi creates hand-designed features of the input sequence including the length and GC content of the homologous sequences around the cut site 56 while CROTON avoids feature engineering and instead performs neural architecture search 57 . All these strategies help reducing the number of labeled data points required to learn the input-output mapping.
The future of DL is geared towards new editing technologies such as CRISPR-Cas12a (cpf1) 58 , base editors 59 , and prime editors 60 . While these methods do not introduce DSBs, their efficiency is still improving 61 ; in fact, DL has already shown promise in predicting the efficiency of Adenine base editors (ABEs) and Cytosine base editors (CBEs) 59 as well as prime editor 2 (PE2) activities in human cells 60 . The future challenges, however, are in understanding these models. CRISPRLand is a recent framework which takes the first step towards interpretation and visualization of DL models in terms of higher-order interactions 62 . Besides explainablity, we speculate that methods that enable an uncertainty estimate of the prediction outcome become more prevalent in genome editing. Further, due to the significant cell-type effects on the efficiency of the CRISPR experiments, it is critical to be aware of the distribution shifts in deploying DL models in genome engineering. The integration of domain adaptation 63 methods to limit the effect of such distribution shifts are among other important future directions.
Moderate successes of DL
Systems biology and data integration.
Systems biology takes a holistic view of modeling complex biological processes to ultimately unravel the link between genotype and phenotype. Integration of diverse -omics data is central in bridging this gap, enabling robust predictive models that have led to several recent breakthroughs, spanning from basic biology 64 to precision medicine. These data are now more accessible than ever, due to improvements in sequencing technologies and the establishment of open access public repositories where researchers can deposit their own studies, such as SRA 65 , GEO 65 , ArrayExpress 66 , and PRIDE 67 ; and large coordinated efforts with structured multi-omic datasets: TCGA 68 , CCLE 69 , GTEx 70 , and ENCODE 71 . Given recent successes and the prevalence of both single and co-assay data, the field is now focused on integrating different data types (e.g., genomics, transcriptomics, epigenomics, proteomics, metabolomics) on single individuals, across many individuals, within and between phenotypic groups, and across different organisms. Data integration tasks fall into two main categories: 1) integration across different platforms and studies of a single data type, at times with other non-omics data (e.g., protein-protein interactions, pathway annotations, motif presence) and 2) integration between different -omic data types (e.g., RNA-seq, ChIP-seq, ATAC-seq, BS-seq). Much progress has been made on integration within a single data type, especially transcriptomics data, with classical ML and statistical approaches developed for batch correction 72 , 73 , 74 , 75 , modeling global gene co-expression patterns 76 , Bayesian integration strategies for function prediction 77 , 78 , and phenotype classification 79 . More recently, the increasing prevalence of single-cell transcriptomics has given rise to a new host of classic ML 80 , 81 , 82 and DL 83 , 84 approaches for data integration across experiments. DL methods in this space have arisen out of the need for methods that scale well with the large number of cells and ability to model non-linear patterns of cell similarity 83 , 85 . Here, we have only skimmed the surface of methods being developed for expression data, but this trend is emerging for other -omics data types, similarly driven by the resolution of improved high-resolution experimental assays 86 , 87 . Broadly, data integration analyses that simultaneously combine data types together, either from different studies or different types, typically fall into one of three categories, given the stage at which the integration is performed 88 : concatenation-based, transformation-based, or model-based. While data integration across studies can be data of the same type, here we focus on methods that specifically integrate across different -omics types, as these questions introduce additional technical challenges and complexity.
Concatenation-based integration methods perform data integration early in the method pipeline by combining data, in raw or processed forms, before any joint modeling and analysis. Traditional ML concatenation-based methods are often unsupervised and typically use automatic feature extraction techniques such as lasso 89 , joint clustering schemes 90 , and dimensionality reduction 91 to find relevant signal. These methods are usually applied to well-curated, multi-omic datasets from large consortia (e.g., TCGA), and thus most often have been used to find meaningful patient subgroups characterized by distinct patterns across data modalities. More recently, autoencoders have been used as an initial data processing step to generate lower dimensional embeddings that are then concatenated together as features for downstream models 92 , 93 . These approaches have improved performance over existing methods likely due to the advantages autoencoders have in denoising tasks, as well as their abilities to model nonlinear latent structure, even without sample labels.
Instead of directly concatenating separate latent embeddings, some groups have pursued transformation-based integration methods by modeling data jointly by mapping to a common representation (e.g., graph or kernel matrix). Historically, classic transformation-based ML methods use known anchor references 94 , kernel 95 , or manifold methods 96 to align multi-omics data. This is a rapidly growing area in data integration, especially for DL methods. Building off of the use of anchors from classical ML methods, new state-of-the-art methods frequently train single modality autoencoders, followed by an alignment procedure across modalities 97 . This direction is exciting, because once trained, the models can be used to predict an unobserved modality given a single data type. Additional exciting developments harness the power of these embedding representations together with other DL methods, including CNNs and RNNs for wide ranging predictive tasks, including cell fate 98 , drug response 99 , survival 92 , 100 , and clinical disease features 101 .
Perhaps the most straightforward way to integrate multi-modal data is to train individual data modality models, then integrate them by combining the results from the individual models, termed model-based integration. To some degree, this is similar to ensemble approaches frequently used in classical ML. Methods in this space can take wide-ranging approaches, including building data modality-specific networks before fusing them using message-passing theory 102 or combining different data representations using a discriminative learning approach 103 . DL methods have yet to gain much momentum for model-based integration, likely because the very nature of most DL methods blurs the line between the transformation-based and model-based paradigms. Classical approaches here try to bridge data modalities by finding a common modeling space, while DL naturally can identify common representations and model them jointly, thus circumventing the need for separate modeling and integration steps. While it is clear that deep neural networks will likely lead to better performance in data integration tasks, it is also important to keep in mind the limitations of DL, as well as important areas for continued research. Specifically, it is known that DL has the tendency to overfit to data. On the other hand, in data integration tasks, batch effects can be prevalent and it is often easy to have “contamination” between the training and test sets, all of which can lead to inflated performance estimates. Thus, it is important to carefully set up truly independent evaluation sets and identify appropriate performance baselines 3 . Furthermore, while genome-wide and whole transcriptomics datasets have broad coverage across the genome and transcriptome, human data (and in some cases, model organism data) is often skewed towards a disproportional amount of sick individuals 104 , is sex-biased towards men 105 , and biased by race with an over-represented population of Europeans 106 . These biases can result in spurious associations that plague all ML methods, but may be particularly difficult to identify when using DL.
Minor successes of DL
Phylogenetics.
A phylogeny is an evolutionary tree that models the evolutionary history of a set of taxa. The phylogeny inference problem concerns building a phylogeny from data—often molecular sequences—obtained from the set of taxa under investigation 107 . Figure 3 illustrates the phylogeny inference problem on four taxa; in this case it can be viewed as a classification problem among three possible topologies. However, classification methods have a major limitation in that they cannot infer branch lengths, nor do they scale beyond a very small number of taxa because the number of possible topologies (classes) grows super-exponentially with this variable. But perhaps more importantly, classifiers like DL models require training data, and benchmark data where the true phylogeny is known is almost impossible to obtain in this field. Instead, simulations have been the method of choice for generating training data, but this is a major dependency and methods are known to have divergent performance on simulated and biological data 108 . For complex versions of the phylogeny inference problem, more realistic simulation protocols are needed. Finally, phylogenetic inference on a single gene is in one sense a simplified problem itself: inferring a single phylogeny from genome-wide data introduces the complication that different genes can have different histories, or the true phylogeny might be a network 109 , rather than a tree. For these reasons DL has either had limited success or been restricted to small sub-problems aside from the main inference task.
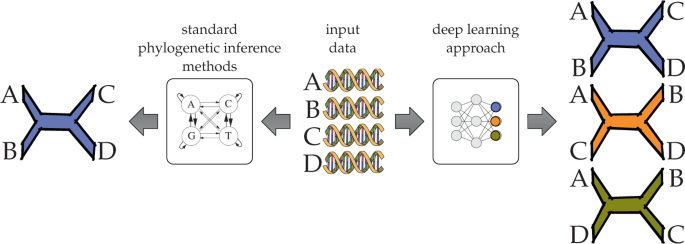
The input consists of sequences (DNA sequences in this illustration) obtained from the taxa of interest. Here, the taxa are A, B , C , and D . In standard approaches, such as maximum likelihood and maximum parsimony, a generative model in the form of a tree whose leaves are labeled by the four taxa is inferred. In the recently introduced DL approach to phylogenetic inference, the problem is viewed as a classification task where the network outputs correspond to the three possible tree topologies whose leaves are labeled by the taxa A , B , C , and D .
Nonetheless, there have been attempts to use DL for the classification task as described above. The Self-Organizing Tree (SOTA) algorithm 110 is a two-decades old unsupervised hierarchical clustering method based on a neural network to classify sequences and reconstruct phylogenetic trees from sequence data. SOTA follows the SOM (Self-Organizing Map) algorithm in growing cell structures from top to bottom dynamically until a desired (user-provided) taxonomic level is reached. Recently CNNs have been used to infer the unrooted phylogenetic tree on four taxa (called a quartet) 111 , 112 . Authors used simulated data for training a classifier which assigns sequences to their phylogenetic tree (Fig. 3) . But an analysis of the performance of the method of Zou et al. 112 by Zaharias et al. 113 shows that CNNs were not as accurate as other standard tree estimation methods, e.g., maximum likelihood, maximum parsimony, and neighbor joining, neither in terms of quartet estimation nor in terms of full tree estimation, especially when the sequence length was relatively short and/or rates of evolution were not sufficiently low. A potential workaround is to approach phylogeny inference as a graph generation problem, a more complex learning task.
Distance-based methods are another class of commonly used techniques for phylogenetic inference among which the neighbor joining method is the most common one, and DL has been applied to improve the distance representation. Jiang et al. 114 addressed the phylogenetic placement problem, i.e., the problem of adding a new taxon to a given tree without having to rebuild the tree from scratch, by training a CNN using a simulated backbone tree and sequences. Given the backbone tree with its associated and query sequences, the model outputs an embedding of the query and reference species which can be used as input to some distance-based phylogenetic placement tools, which then places the query sequences onto the reference tree. Bhattacharjee et al. 115 addressed the data imputation problem in the incomplete distance matrix using autoencoders. However, the key limitation of these methods is that trees cannot be reliably embedded into a Euclidean space of low dimensions 116 . Hyperbolic space, on the other hand, has been demonstrated to be more suitable for representing data with hierarchical latent structure 117 .
Other applications have used DL to aid in a more traditional inference pipeline. For example, the particular likelihood model to use for a maximum-likelihood search is often taken for granted as user decision, but a recent method used DL to optimize this decision 118 . In another case, DL was used to aid decision-making in the tree-search algorithm used in a traditional maximum likelihood heuristic. Finally, a very recent application uses a sparse learning model for something almost like the reverse process: given a phylogeny, it identifies the portions of a genome that most directly explain or relate to that model 119 . This can be used to validate phylogenetic inference as well as guide downstream analyses such as hypothesis generation and testing.
A traditional problem is the inference of perfect phylogeny where every site in the sequences mutates at most once along the branches of the tree. The problem of determining whether a perfect phylogeny exists and inferring it, if one exists, from binary data that is assumed to be correct is polynomially solvable. However, if the data is assumed to have errors, one approach to inferring a perfect phylogeny is by solving the minimum-flip problem: given a binary matrix of mutations - where each entry represents the presence (state 1) or absence (state 0) of mutation in a sample and a site - that does not admit a perfect phylogeny, the minimum number of “state flips" (from 0 to 1 or 1 to 0) to the data is sought so that a perfect phylogeny is admitted. Sadeqi Azer et al. 120 used an existing DL framework originally designed for solving the traveling salesman problem to tackle this problem 121 . Here, the input consists of the inferred single-nucleotide variations (SNVs) in single cells across different sites. The output is a matrix that admits a perfect phylogeny with the minimum number of state flips from the input matrix. The input matrix is flattened and passed through convolutional layers for encoding. The encoded data is fed to a Long Short Term Memory (LSTM) layer as a decoder. Then, an attention layer takes the outputs of the LSTM layer to score the entries of the mutation matrix according to the impact that flipping them might have on minimizing the overall number of state flips. This architecture results in a probability distribution on the entries of the input matrix that is used for flipping them. The model is trained using simulated data where the matrix and the number of flips to perform are provided. The key limitation of this approach is that there is no guarantee that the output admits a perfect phylogeny because the cost function might not be fully optimized.
Taken altogether, these related successes are impressive, but given the challenges outlined above it is difficult to conceive of an end-to-end DL model to directly estimate phylogenetic trees from raw data in the near future. And if one were to be developed, given its reliance on (likely simulated) training data, its applicability to actual biological sequences will need to be carefully validated before traditional phylogenetic methods are displaced.
General challenges for DL in the biosciences
Not all applications of DL have been equally successful in computational biology. While in some areas such as protein structure prediction and genome editing DL has found major success, in other areas like phylogenetic inference, DL has faced major hurdles (Table 1) . Most common issues faced by DL approaches stem from the lack of annotated data, inherent absence of the ground truth for non-simulated datasets, severe discrepancies between training data distribution and real-world test (e.g., clinical) data distribution, potential difficulties in result benchmarking and interpretation, and finally overcoming the biases and ethical issues in datasets and models. Additionally, with the growth of the data and DL models, training efficiency has become a major bottleneck for progress.
Specifically, the success of DL in different subareas in computational biology highly relies on the availability and diversity of standardized supervised and unsupervised datasets, ML benchmarks with clear biological impact, the computational nature of the problem, and the software engineering infrastructure to train the DL models. The remaining challenges of DL in computational biology are tied with improving model explainability, extracting actionable and human-understandable insights, boosting the efficiency and limiting the training costs, and finally mitigating the growing ethical issues of DL models; innovative solutions are emerging in DL and computational biology communities (Table 2 ). We will now review two key areas for improvement: (i) Explainability and (ii) Training efficiency.
Explainability
Perhaps one of the most critical limitations of DL models today, especially for biological and clinical applications, is that they are not as explainable as the simpler regression models in statistics; it is challenging to explain what each node of the network represents and how important it is to model performance. The highly nonlinear decision boundaries of DNNs and their overparameterized nature, which enable them to achieve high prediction accuracy, make them hard to explain as well. This lack of explanability becomes an important issue in computational biology, because trustworthiness of DNNs is arguably one of the most pressing problems in biological and sensitive clinical decision making applications. In fact, in biology often the question of why a model can predict well is as important as how accurately it can predict a phenomenon. For example in protein structure/function prediction we would like to know what rules in a predictive model govern the 3D geometry of a protein and its properties; in genome editing we aim to understand the biological DNA repair processes inferred from CRISPR models; in systems biology we aim to know the specific molecular differences that give rise to different phenotypes; in phylogenetics we aim to know the features that enable us to infer a phylogenetic tree. Addressing these questions are key in producing biological knowledge and creating actionable decisions in the clinical settings.
There have been some efforts in the ML community to develop methods to explain “black-box” DL models in the past few years 122 . Earlier works were developed in computer vision and biomedical applications, some of which have been applied to problems in computational biology as well. Activation maximization is a large class of algorithms which searches for an input which maximizes the model response typically by using gradient descent 123 , 124 ; the idea is to generate an input that best symbolizes an outcome. To make them human-interpretable, the input gets regularized using closed-form density functions of the data or GANs that mimic the data distribution. Methods that address the explainability question use more direct ways to gain insights from the NN function using their Taylor expansion 125 or Fourier transform 42 , 62 . The explanation takes the form of a heatmap which shows the importance of each input feature. Sensitivity analysis is another popular method of this sort which finds the input features to which the output is most sensitive to using backpropagation 126 ; this has been used for classification and diagnostic prediction of cancers using DNNs and gene expression profiling as well 127 . LIME 128 is a popular sensitivity analysis method which learns an interpretable model locally around the prediction. Simonyan et al. 124 proposed using the gradient of the output with respect to pixels of an input image to compute a saliency map of the image. To avoid the saturation effect in perturbation-based and gradient-based approaches, DeepLIFT 129 decomposes the output prediction of a neural network on a specific input by backpropagating the contributions of all neurons in the network to every feature of the input. SHAP 130 unifies these approaches using a theoretically grounded method which assigns each feature an importance value for a particular prediction. Finally, GNNExplainer 131 is a new approach among a family of methods which provide interpretable explanations for predictions of GNN-based models on graph-based DL tasks. Given an instance, GNNExplainer identifies a compact subgraph structure and a small subset of node features that have a crucial role in GNN’s prediction.
The efforts towards developing tools for explanation of DNNs are still in their infancy and are rapidly growing; challenges still abound towards a fully explainable systems in biology. The key problem is that the current general purpose methods to explain DL models are not sufficient especially in the clinical settings 132 . For the scientist and clinicians to trust these black box models they need to be able to explain themselves in a human-understandable fashion with quantifiable level of uncertainty, summarize the reasons for their behaviours, and suggest the additional steps (e.g., experiments, clinical studies, etc.) required to be able to reliably defend their decisions. We speculate that the new generation of explainable methods focus on helping these black-box models to transition from hypothesis generation machines into hypothesis testing ones which can communicate easier with medical practitioners.
Training efficiency
Despite the high accuracy of many DL approaches, their performance often comes at a high monetary and computational cost. For example, the monetary cost of consumed power and computation time is estimated to be up to hundreds of thousands of US dollars to train a single model 133 . The extreme costs of large DL models can prevent broader research community from reproducing and improving upon the current results. Thus, it is practical to consider lower-cost alternatives that are available and feasible for researchers with more modest resources. These issues are relevant for applying DL to computational biology. For instance, training the state-of-the-art protein structure prediction model AlphaFold2 requires computational resources equivalent to 100–200 GPUs running for a few weeks 21 . In the following paragraphs, we discuss common strategies utilized by the DL community to decrease the memory and computation cost in training, and potential directions for applying similar strategies to improve the efficiency of DL models in computational biology.
The most direct method of reducing the training cost of a DL method is to perform transfer learning on the available pretrained general model, instead of training the new model from scratch. It’s a common approach in training DL models for NLP tasks, and it has been shown that general language knowledge models are a good starting point for various different NLP tasks 134 . This approach can be adopted in computational biology, if all downstream tasks can start with a general model on biological data. For example, Zaheer et al. 135 trained a general human DNA sequence model based on human reference genome GRCh37, with self-supervised learning (masked DNA sequence prediction and next DNA sequence segment prediction). Subsequently, they have shown successful downstream task (Promoter Region Prediction) performance by solely applying transfer learning on the general model. Using pretrained models largely decreases (i) the size of task-specific datasets needed for training; and (ii) the total amount of local training needed for certain tasks that researchers are interested in. Thus creating general models that can be shared and used by the entire research community will greatly reduce the resources needed for training models on specific tasks by individual research groups. However, this approach will be less useful if the data distribution for different downstream tasks is drastically different compared with the data used by the general pretrained model. For instance, DeepVariant has limited applicability to non-human SNV calling due to the differences between diploid and haploid genomes, and nucleic acid distributions 4 . In these cases, we still need to train from scratch or spend a significant amount of resources on re-training the base model.
An alternative approach is to design DL model architectures with improved efficiency. As one of the most widely-studied architectures in DL, numerous low-cost variants of CNNs have been proposed. Some popular examples of efficient CNN architectures include the MobileNet family 136 , DenseNet 137 , EfficientNet 138 , and CSPNet 139 . Similarly, numerous efficiency-based architectural modifications have been proposed for the transformer model, many of which aim to reduce the quadratic computational complexity incurred by the self-attention mechanism 140 . Additionally, some transformer architectural variants explore the use of parameter sharing and factorization to reduce the memory cost of model training 141 . Going further, efficient architectural variants have been discovered for RNNs 142 and graph neural networks (GNNs) 143 , 144 , including specialized architectures that are tuned for better efficiency within the biological domain 145 .
For computational biology applications, one approach for boosting efficiency relies on exploiting inherent sparsity and locality of biological data (e.g. focusing only on the SNV calls rather than the whole genome 146 ). Researchers are also using transformers for DNA/RNA sequence modeling 135 , but transformer models have high training costs due to the expensive global attention mechanism. Prior domain expertise can be leveraged here to help prune attention neighborhoods, and subsequently improve training efficiency of the models. Finally, one can also change the model’s architecture during training to adaptively improve the training efficiency. The practice of model pruning, which removes unimportant parameters from the model, has become a popular method of deriving lightweight DL models 147 in deployment.
As the amount of biological data keeps increasing, the size of the neural networks will increase as well, and lead to a higher total number of training iterations required for convergence. Therefore it’s natural to explore dataset reduction strategies as one of solutions to the efficiency challenge. One feasible proposal is to construct coresets of the training dataset 148 . This can be done by using clustering methods on the dataset and choosing centroids as the representatives of the dataset. Alternatively, dataset condensation can be achieved by selecting the data samples that can best approximate the effect of training the model on the whole dataset. An orthogonal way of solving the high training cost problem for DL is to distribute the training on several cheap low-end devices. This step will decrease the total training time by distributing training, and decrease the total budget by using multiple cheap devices with less computation power. In general, the major distributed training methods are data parallelism, model parallelism and hybrid parallel training. Data parallel training splits and distributes parts of the dataset to each device 149 , where model parallel training splits and distributes parts of the model to each device 150 . As all above methods are task-agnostic, they can be readily applied to DL models for computational biology.
Concluding comments
In summary, while the success of DL in areas such as protein structure prediction is paradigm shifting, other areas such as function prediction, genome engineering, and multi-omics are also observing rapid gains in performance compared to traditional approaches. For other areas such as phylogenetics, classical computational approaches seem to have the upper hand in those areas. Additional advances specific to DL applied to challenges across the biosciences will further leverage domain-specific biological knowledge while striving for high explainability and improved efficiency.
Jumper, J. et al. Highly accurate protein structure prediction with AlphaFold. Nature 596, 583–589 (2021).
LeCun, Y., Bengio, Y. & Hinton, G. Deep learning. Nature 521 , 436–444 (2015).
Article CAS ADS PubMed Google Scholar
Whalen, S., Schreiber, J., Noble, W. S. & Pollard, K. S. Navigating the pitfalls of applying machine learning in genomics. Nat. Rev. Genet. 23 , 169–181 (2022).
Poplin, R. et al. A universal SNP and small-indel variant caller using deep neural networks. Nat. Biotechnol. 36, 983–987 (2018).
Arango-Argoty, G. et al. Deeparg: a deep learning approach for predicting antibiotic resistance genes from metagenomic data. Microbiome 6 , 1–15 (2018).
Article Google Scholar
Nissen, J. N. et al. Improved metagenome binning and assembly using deep variational autoencoders. Nat. Biotechnol. 39 , 555–560 (2021).
Article CAS PubMed Google Scholar
Nielsen, A. A. & Voigt, C. A. Deep learning to predict the lab-of-origin of engineered DNA. Nat. Commun. 9 , 1–10 (2018).
Article CAS Google Scholar
Pearce, R. & Zhang, Y. Toward the solution of the protein structure prediction problem. J. Biol. Chem. 297, 100870 (2021).
AlQuraishi, M. Machine learning in protein structure prediction. Curr. Opin. Chem. Biol. 65 , 1–8 (2021).
de Chadarevian, S. John Kendrew and myoglobin: Protein structure determination in the 1950s. Protein Sci. 27 , 1136–1143 (2018).
Article PubMed PubMed Central Google Scholar
Stollar, E. J. & Smith, D. P. Uncovering protein structure. Essays Biochem. 64 , 649–680 (2020).
Article CAS PubMed PubMed Central Google Scholar
Srivastava, A., Nagai, T., Srivastava, A., Miyashita, O. & Tama, F. Role of computational methods in going beyond X-ray crystallography to explore protein structure and dynamics. Int. J. Mol. Sci. 19 , 3401 (2018).
Article PubMed Central Google Scholar
Wang, S., Sun, S., Li, Z., Zhang, R. & Xu, J. Accurate de novo prediction of protein contact map by ultra-deep learning model. PLoS Computational Biol. 13 , e1005324 (2017).
Article ADS Google Scholar
Zheng, W. et al. Deep-learning contact-map guided protein structure prediction in CASP13. Proteins: Struct. Funct. Bioinforma. 87 , 1149–1164 (2019).
Baek, M. et al. Accurate prediction of protein structures and interactions using a three-track neural network. Science. 373 , 871–876 (2021).
Mirabello, C. & Wallner, B. RAWMSA: End-to-end deep learning using raw multiple sequence alignments. PloS One 14 , e0220182 (2019).
Tunyasuvunakool, K. et al. Highly accurate protein structure prediction for the human proteome. Nature https://doi.org/10.1038/s41586-021-03828-1 (2021).
AlQuraishi, M. AlphaFold at CASP13. Bioinformatics. 35 , 4862–4865 (2019).
Ingraham, J., Riesselman, A., Sander, C. & Marks, D. Learning protein structure with a differentiable simulator. In International Conference on Learning Representations (2018).
AlQuraishi, M. End-to-end differentiable learning of protein structure. Cell Syst. 8 , 292–301 (2019).
Senior, A. W. et al. Improved protein structure prediction using potentials from deep learning. Nature 577 , 706–710 (2020).
Article CAS PubMed ADS Google Scholar
Berman, H. M. et al. The protein data bank. Nucleic Acids Res. 28 , 235–242 (2000).
Article CAS PubMed PubMed Central ADS Google Scholar
Madeira, F. et al. The EMBL-EBI search and sequence analysis tools APIs in 2019. Nucleic Acids Res. 47 , W636–W641 (2019).
Bonetta, R. & Valentino, G. Machine learning techniques for protein function prediction. Proteins: Struct. Funct. Bioinforma. 88 , 397–413 (2020).
Huntley, R. P. et al. The GOA database: gene ontology annotation updates for 2015. Nucleic Acids Res. 43 , D1057–D1063 (2015).
Zhang, M.-L. & Zhou, Z.-H. A review on multi-label learning algorithms. IEEE Trans. Knowl. Data Eng. 26 , 1819–1837 (2013).
Consortium, U. UniProt: a worldwide hub of protein knowledge. Nucleic Acids Res. 47 , D506–D515 (2019).
Szklarczyk, D. et al. STRING v11: protein–protein association networks with increased coverage, supporting functional discovery in genome-wide experimental datasets. Nucleic Acids Res. 47 , D607–D613 (2019).
Mistry, J. et al. Pfam: The protein families database in 2021. Nucleic Acids Res. 49 , D412–D419 (2021).
Kulmanov, M., Khan, M. A. & Hoehndorf, R. DeepGO: predicting protein functions from sequence and interactions using a deep ontology-aware classifier. Bioinformatics. 34 , 660–668 (2018).
Alshahrani, M. et al. Neuro-symbolic representation learning on biological knowledge graphs. Bioinformatics. 33 , 2723–2730 (2017).
Altschul, S. F., Gish, W., Miller, W., Myers, E. W. & Lipman, D. J. Basic local alignment search tool. J. Mol. Biol. 215 , 403–410 (1990).
Kulmanov, M. & Hoehndorf, R. DeepGOPlus: improved protein function prediction from sequence. Bioinformatics. 36 , 422–429 (2020).
CAS PubMed Google Scholar
Buchfink, B., Xie, C. & Huson, D. H. Fast and sensitive protein alignment using DIAMOND. Nat. Methods. 12 , 59–60 (2015).
Chicco, D., Sadowski, P. & Baldi, P. Deep autoencoder neural networks for gene ontology annotation predictions. In Proceedings of the 5th ACM Conference On Bioinformatics, Computational Biology, and Health Informatics , 533–540 (2014).
Miranda, L. J. & Hu, J. A deep learning approach based on stacked denoising autoencoders for protein function prediction. In 2018 IEEE 42nd Annual Computer Software and Applications Conference (COMPSAC ), vol. 1, 480–485 (IEEE, 2018).
Gligorijević, V., Barot, M. & Bonneau, R. deepNF: deep network fusion for protein function prediction. Bioinformatics. 34 , 3873–3881 (2018).
Zou, Z., Tian, S., Gao, X. & Li, Y. mlDEEpre: multi-functional enzyme function prediction with hierarchical multi-label deep learning. Front. Genet. 9 , 714 (2019).
Li, S. et al. Deep learning-based prediction of species-specific protein S-glutathionylation sites. Biochim. Biophys. Acta Proteins Proteom. 1868 , 140422 (2020).
Mazurenko, S., Prokop, Z. & Damborsky, J. Machine learning in enzyme engineering. ACS Catal. 10 , 1210–1223 (2019).
Zhang, F. et al. Deepfunc: a deep learning framework for accurate prediction of protein functions from protein sequences and interactions. Proteomics. 19 , 1900019 (2019).
Aghazadeh, A. et al. Epistatic net allows the sparse spectral regularization of deep neural networks for inferring fitness functions. Nat. Commun. 12 , 1–10 (2021).
Brookes, D. H., Aghazadeh, A. & Listgarten, J. On the sparsity of fitness functions and implications for learning. In Proceedings of the National Academy of Sciences 119 (2022). https://www.pnas.org/content/119/1/e2109649118 .
van Overbeek, M. et al. DNA repair profiling reveals nonrandom outcomes at Cas9-mediated breaks. Mol. Cell. 63 , 633–646 (2016).
Article PubMed Google Scholar
Cui, Y., Xu, J., Cheng, M., Liao, X. & Peng, S. Review of CRISPR/Cas9 sgRNA design tools. Interdiscip. Sci. Computational Life Sci. 10 , 455–465 (2018).
Xue, L., Tang, B., Chen, W. & Luo, J. Prediction of CRISPR sgRNA activity using a deep convolutional neural network. J. Chem. Inf. Modeling. 59 , 615–624 (2018).
Kim, H. K. et al. SpCas9 activity prediction by DeepSpCas9, a deep learning–based model with high generalization performance. Sci. Adv. 5 , eaax9249 (2019).
Chuai, G. et al. DeepCRISPR: optimized CRISPR guide RNA design by deep learning. Genome Biol. 19 , 1–18 (2018).
Liu, Q., Cheng, X., Liu, G., Li, B. & Liu, X. Deep learning improves the ability of sgRNA off-target propensity prediction. BMC Bioinforma. 21 , 1–15 (2020).
Wang, D. et al. Optimized CRISPR guide RNA design for two high-fidelity Cas9 variants by deep learning. Nat. Commun. 10 , 1–14 (2019).
ADS Google Scholar
Liu, Q., He, D. & Xie, L. Prediction of off-target specificity and cell-specific fitness of CRISPR-Cas system using attention boosted deep learning and network-based gene feature. PLoS Computational Biol. 15 , e1007480 (2019).
Article CAS ADS Google Scholar
Zhang, G., Zeng, T., Dai, Z. & Dai, X. Prediction of CRISPR/Cas9 single guide RNA cleavage efficiency and specificity by attention-based convolutional neural networks. Computational Struct. Biotechnol. J. 19 , 1445–1457 (2021).
Metsky, H. C. et al. Designing sensitive viral diagnostics with machine learning. Nat. Biotechnol. https://doi.org/10.1038/s41587-022-01213-5 (2022).
Leenay, R. T. et al. Large dataset enables prediction of repair after CRISPR–Cas9 editing in primary T cells. Nat. Biotechnol. 37 , 1034–1037 (2019).
Allen, F. et al. Predicting the mutations generated by repair of Cas9-induced double-strand breaks. Nat. Biotechnol. 37 , 64–72 (2019).
Shen, M. W. et al. Predictable and precise template-free CRISPR editing of pathogenic variants. Nature . 563 , 646–651 (2018).
Li, V. R., Zhang, Z. & Troyanskaya, O. G. CROTON: an automated and variant-aware deep learning framework for predicting CRISPR/Cas9 editing outcomes. Bioinformatics. 37 , i342–i348 (2021).
Kim, H. K. et al. Deep learning improves prediction of CRISPR–Cpf1 guide RNA activity. Nat. Biotechnol. 36 , 239 (2018).
Song, M. et al. Sequence-specific prediction of the efficiencies of adenine and cytosine base editors. Nat. Biotechnol. 38 , 1037–1043 (2020).
Kim, H. K. et al. Predicting the efficiency of prime editing guide RNAs in human cells. Nat. Biotechnol. 39 , 198–206 (2021).
Anzalone, A. V., Koblan, L. W. & Liu, D. R. Genome editing with CRISPR–Cas nucleases, base editors, transposases and prime editors. Nat. Biotechnol. 38 , 824–844 (2020).
Aghazadeh, A., Ocal, O. & Ramchandran, K. CRISPRLand: Interpretable large-scale inference of DNA repair landscape based on a spectral approach. Bioinformatics. 36 , i560–i568 (2020).
Sun, B., Feng, J. & Saenko, K. Return of frustratingly easy domain adaptation. In Proceedings of the AAAI Conference on Artificial Intelligence, vol. 30 (2016).
Angermueller, C., Pärnamaa, T., Parts, L. & Stegle, O. Deep learning for computational biology. Mol. Syst. Biol. 12 , 878 (2016).
NCBI Resource Coordinators. Database resources of the National Center for Biotechnology Information. Nucleic Acids Res. 46 , D8-D13 (2018).
Athar, A. et al. ArrayExpress update - from bulk to single-cell expression data. Nucleic Acids Res. 47 , D711–D715 (2019).
Perez-Riverol, Y. et al. The PRIDE database and related tools and resources in 2019: improving support for quantification data. Nucleic Acids Res. 47 , D442–D450 (2019).
Grossman, R. L. et al. Toward a shared vision for cancer genomic data. N. Engl. J. Med. 375 , 1109–1112 (2016).
Barretina, J. et al. The Cancer cell line encyclopedia enables predictive modelling of anticancer drug sensitivity. Nature 483 , 603–607 (2012).
Lonsdale, J. et al. The genotype-tissue expression (GTEx) project. Nat. Genet. 45 , 580–585 (2013).
Consortium, E. P. et al. The ENCODE (ENCyclopedia of DNA elements) project. Science. 306 , 636–640 (2004).
Johnson, W. E., Li, C. & Rabinovic, A. Adjusting batch effects in microarray expression data using empirical Bayes methods. Biostatistics. 8 , 118–127 (2007).
Article PubMed MATH Google Scholar
Ritchie, M. E. et al. limma powers differential expression analyses for RNA-sequencing and microarray studies. Nucleic Acids Res. 43 , e47–e47 (2015).
Leek, J. T. Svaseq: removing batch effects and other unwanted noise from sequencing data. Nucleic Acids Res. 42 , e161–e161 (2014).
Risso, D., Ngai, J., Speed, T. P. & Dudoit, S. Normalization of RNA-seq data using factor analysis of control genes or samples. Nat. Biotechnol. 32 , 896–902 (2014).
Zhu, Q. et al. Targeted exploration and analysis of large cross-platform human transcriptomic compendia. Nat. Methods. 12 , 211–214 (2015).
Wong, A. K., Krishnan, A. & Troyanskaya, O. G. GIANT 2.0: genome-scale integrated analysis of gene networks in tissues. Nucleic Acids Res. 46 , W65–W70 (2018).
Yao, V. et al. An integrative tissue-network approach to identify and test human disease genes. Nat. Biotechnol. 36 , 1091–1099 (2018).
Ellis, S. E., Collado-Torres, L., Jaffe, A. & Leek, J. T. Improving the value of public RNA-seq expression data by phenotype prediction. Nucleic Acids Res. 46 , e54 (2018).
Hie, B., Bryson, B. & Berger, B. Efficient integration of heterogeneous single-cell transcriptomes using Scanorama. Nat. Biotechnol. 37 , 685–691 (2019).
Butler, A., Hoffman, P., Smibert, P., Papalexi, E. & Satija, R. Integrating single-cell transcriptomic data across different conditions, technologies, and species. Nat. Biotechnol. 36 , 411–420 (2018).
Korsunsky, I. et al. Fast, sensitive and accurate integration of single-cell data with Harmony. Nat. Methods. 16 , 1289–1296 (2019).
Lopez, R., Regier, J., Cole, M. B., Jordan, M. I. & Yosef, N. Deep generative modeling for single-cell transcriptomics. Nat. Methods. 15 , 1053–1058 (2018).
Amodio, M. et al. Exploring single-cell data with deep multitasking neural networks. Nat. Methods 16 , 1139–1145 (2019).
Eraslan, G., Simon, L. M., Mircea, M., Mueller, N. S. & Theis, F. J. Single-cell RNA-seq denoising using a deep count autoencoder. Nat. Commun. 10 , 1–14 (2019).
Angermueller, C., Lee, H. J., Reik, W. & Stegle, O. DeepCpG: accurate prediction of single-cell DNA methylation states using deep learning. Genome Biol. 18 , 1–13 (2017).
Google Scholar
Xiong, L. et al. SCALE method for single-cell ATAC-seq analysis via latent feature extraction. Nat. Commun. 10 , 1–10 (2019).
Ritchie, M. D., Holzinger, E. R., Li, R., Pendergrass, S. A. & Kim, D. Methods of integrating data to uncover genotype-phenotype interactions. Nat. Rev. Genet. 16 , 85–97 (2015).
Wang, H., Lengerich, B. J., Aragam, B. & Xing, E. P. Precision Lasso: accounting for correlations and linear dependencies in high-dimensional genomic data. Bioinformatics. 35 , 1181–1187 (2019).
Li, Z., Chang, C., Kundu, S. & Long, Q. Bayesian generalized biclustering analysis via adaptive structured shrinkage. Biostatistics. 21 , 610–624 (2020).
Article MathSciNet PubMed Google Scholar
Argelaguet, R. et al. Multi-omics factor analysis – a framework for unsupervised integration of multi-omics data sets. Mol. Syst. Biol. 14 , e8124 (2018).
Chaudhary, K., Poirion, O. B., Lu, L. & Garmire, L. X. Deep learning-based multi-omics integration robustly predicts survival in liver cancer. Clin. Cancer Res. 24 , 1248–1259 (2018).
Tong, L., Mitchel, J., Chatlin, K. & Wang, M. D. Deep learning based feature-level integration of multi-omics data for breast cancer patients survival analysis. BMC Med. Inform. Decis. Mak. 20 , 1–12 (2020).
Stuart, T. et al. Comprehensive integration of single-cell data. Cell. 177 , 1888–1902 (2019).
Mariette, J. & Villa-Vialaneix, N. Unsupervised multiple kernel learning for heterogeneous data integration. Bioinformatics. 34 , 1009–1015 (2018).
Welch, J. D., Hartemink, A. J. & Prins, J. F. MATCHER: manifold alignment reveals correspondence between single cell transcriptome and epigenome dynamics. Genome Biol. 18 , 1–19 (2017).
Wu, K. E., Yost, K. E., Chang, H. Y. & Zou, J. BABEL enables cross-modality translation between multiomic profiles at single-cell resolution. Proc Natl Acad. Sci. 118, e2023070118 (2021).
Buggenthin, F. et al. Prospective identification of hematopoietic lineage choice by deep learning. Nat. Methods. 14 , 403–406 (2017).
Sharifi-Noghabi, H., Zolotareva, O., Collins, C. C. & Ester, M. MOLI: multi-omics late integration with deep neural networks for drug response prediction. Bioinforma. 35 , i501–i509 (2019).
Ma, T. & Zhang, A. multi-view factorization autoencoder with network constraints for multi-omic integrative analysis. In 2018 IEEE International Conference on Bioinformatics and Biomedicine (BIBM) , 702-707 (IEEE, 2018).
Lee, G., Nho, K., Kang, B., Sohn, K.-A. & Kim, D. Predicting Alzheimer’s disease progression using multi-modal deep learning approach. Sci. Rep. 9 , 1952 (2019).
Article PubMed PubMed Central ADS Google Scholar
Wang, B. et al. Similarity network fusion for aggregating data types on a genomic scale. Nat. Methods. 11 , 333–337 (2014).
Keilwagen, J., Posch, S. & Grau, J. Accurate prediction of cell type-specific transcription factor binding. Genome Biol. 20 , 1–17 (2019).
Zollner, S. & Pritchard, J. K. Overcoming the winner’s curse: estimating penetrance parameters from case-control data. Am. J. Hum. Genet. 80 , 605–615 (2007).
Beery, A. K. & Zucker, I. Sex bias in neuroscience and biomedical research. Neurosci. Biobehav. Rev. 35 , 565–572 (2011).
Carlson, C. S. et al. Generalization and dilution of association results from European GWAS in populations of non-European ancestry: the PAGE study. PLoS Biol. 11 , e1001661 (2013).
Felsenstein, J. Inferring Phylogenies , vol. 2 (Sinauer Associates Sunderland, MA, 2004).
Nute, M., Saleh, E. & Warnow, T. Evaluating statistical multiple sequence alignment in comparison to other alignment methods on protein data sets. Syst. Biol. 68 , 396–411 (2018).
Nakhleh, L. In Problem solving handbook in computational biology and bioinformatics , 125–158 (Springer, 2010).
Dopazo, J. & Carazo, J. M. Phylogenetic reconstruction using an unsupervised growing neural network that adopts the topology of a phylogenetic tree. J. Mol. evolution. 44 , 226–233 (1997).
Suvorov, A., Hochuli, J. & Schrider, D. R. Accurate inference of tree topologies from multiple sequence alignments using deep learning. Syst. Biol. 69 , 221–233 (2020).
Zou, Z., Zhang, H., Guan, Y. & Zhang, J. Deep residual neural networks resolve quartet molecular phylogenies. Mol. Biol. Evolution 37 , 1495–1507 (2020).
Zaharias, P., Grosshauser, M. & Warnow, T. Re-evaluating deep neural networks for phylogeny estimation: the issue of taxon sampling J Comput Biol 29 , 74-89 (2021).
Jiang, Y., Balaban, M., Zhu, Q. & Mirarab, S. DEPP: Deep learning enables extending species trees using single genes. https://doi.org/10.1101/2021.01.22.427808 (2021).
Bhattacharjee, A. & Bayzid, M. S. Machine learning based imputation techniques for estimating phylogenetic trees from incomplete distance matrices. BMC genomics. 21 , 1–14 (2020).
Linial, N., London, E. & Rabinovich, Y. The geometry of graphs and some of its algorithmic applications. Combinatorica. 15 , 215–245 (1995).
Article MathSciNet MATH Google Scholar
Nickel, M. & Kiela, D. Poincaré embeddings for learning hierarchical representations. Adv. Neural Inf. Process. Syst. 30 , 6338–6347 (2017).
Abadi, S., Avram, O., Rosset, S., Pupko, T. & Mayrose, I. ModelTeller: model selection for optimal phylogenetic reconstruction using machine learning. Mol. Biol. Evolution 37 , 3338–3352 (2020).
Kumar, S. & Sharma, S. Evolutionary sparse learning for phylogenomics. Mol. Biol. Evolution. 38 , 4674–4682 (2021).
Azer, E. S., Ebrahimabadi, M. H., Malikić, S., Khardon, R. & Sahinalp, S. C. Tumor phylogeny topology inference via deep learning. iScience. 23 , 101655 (2020).
Bello, I., Pham, H., Le, Q. V., Norouzi, M. & Bengio, S. Neural combinatorial optimization with reinforcement learning. In Workshop at International Conference on Learning Representations, ICLR’17. (2017).
Montavon, G., Samek, W. & Müller, K.-R. Methods for interpreting and understanding deep neural networks. Digital Signal Process. 73 , 1–15 (2018).
Article MathSciNet Google Scholar
Berkes, P. & Wiskott, L. On the analysis and interpretation of inhomogeneous quadratic forms as receptive fields. Neural Comput. 18 , 1868–1895 (2006).
Article MathSciNet PubMed MATH Google Scholar
Simonyan, K., Vedaldi, A. & Zisserman, A. Deep inside convolutional networks: Visualising image classification models and saliency maps. In Workshop at International Conference on Learning Representations (2014).
Bach, S. et al. On pixel-wise explanations for non-linear classifier decisions by layer-wise relevance propagation. PloS One 10 , e0130140 (2015).
Zurada, J. M., Malinowski, A. & Cloete, I. Sensitivity analysis for minimization of input data dimension for feedforward neural network. In Proceedings of IEEE International Symposium on Circuits and Systems-ISCAS’94 , vol. 6, 447–450 (IEEE, 1994).
Khan, J. et al. Classification and diagnostic prediction of cancers using gene expression profiling and artificial neural networks. Nat. Med. 7 , 673–679 (2001).
Ribeiro, M. T., Singh, S. & Guestrin, C. "Why should I trust you?” explaining the predictions of any classifier. In Proceedings of the 22nd ACM SIGKDD International Conference on Knowledge Discovery and Data Mining , 1135–1144 (2016).
Shrikumar, A., Greenside, P. & Kundaje, A. Learning important features through propagating activation differences. In International Conference on Machine Learning, 3145–3153 (PMLR, 2017).
Lundberg, S. M. & Lee, S.-I. A unified approach to interpreting model predictions. In Proceedings of the 31st International Conference on Neural Information Processing Systems , 4768–4777 (2017).
Ying, R., Bourgeois, D., You, J., Zitnik, M. & Leskovec, J. GNNExplainer: Generating explanations for graph neural networks. Adv. Neural Inf. Process. Syst. 32 , 9240 (2019).
PubMed PubMed Central Google Scholar
Gilpin, L. H. et al. Explaining explanations: An overview of interpretability of machine learning. In 2018 IEEE 5th International Conference on Data Science and Advanced Analytics (DSAA) , 80–89 (IEEE, 2018).
Yang, Z. et al. Xlnet: Generalized autoregressive pretraining for language understanding. Adv. Neural Inf. Process. Syst. 32 (2019).
Devlin, J., Chang, M.-W., Lee, K. & Toutanova, K. BERT: Pre-training of deep bidirectional transformers for language understanding. In Proceedings of the 2019 Conference of the North American Chapter of the Association for Computational Linguistics: Human Language Technologies , Volume 1 (Long and Short Papers), 4171-4186 (Association for Computational Linguistics, Minneapolis, Minnesota, 2019). https://aclanthology.org/N19-1423 .
Zaheer, M. et al. Big bird: Transformers for longer sequences. In Advances in Neural Information Processing Systems ( NeurIPS ), 33 , 17283–17297 (2020).
Sandler, M., Howard, A., Zhu, M., Zhmoginov, A. & Chen, L.-C. Mobilenetv2: Inverted residuals and linear bottlenecks. In Proceedings of the IEEE Conference on Computer Vision and Pattern Recognition , 4510–4520 (2018).
Huang, G., Liu, Z., Van Der Maaten, L. & Weinberger, K. Q. Densely connected convolutional networks. In Proceedings of The IEEE Conference on Computer Vision and Pattern Recognition , 4700–4708 (2017).
Tan, M. & Le, Q. Efficientnet: Rethinking model scaling for convolutional neural networks. In International Conference on Machine Learning, 6105-6114 (PMLR, 2019).
Wang, C.-Y. et al. Cspnet: A new backbone that can enhance learning capability of CNN. In Proceedings of the IEEE/CVF Conference on Computer Vision and Pattern Recognition Workshops, 390–391 (2020).
Wu, Z., Liu, Z., Lin, J., Lin, Y. & Han, S. Lite transformer with long-short range attention. In International Conference on Learning Representations ( 2019).
Lan, Z. et al. ALBERT: A lite BERT for self-supervised learning of language representations. In 8th International Conference on Learning Representations, ICLR 2020, Addis Ababa, Ethiopia, April 26–30, 2020 (2020).
Kusupati, A. et al. Fastgrnn: A fast, accurate, stable and tiny kilobyte sized gated recurrent neural network. Adv. Neural Inf. Process. Syst. 31 , 9031–9042 (2018).
Chiang, W.-L. et al. Cluster-GCN: An efficient algorithm for training deep and large graph convolutional networks. In Proceedings of the 25th ACM SIGKDD International Conference on Knowledge Discovery & Data Mining , 257–266 (2019).
Zou, D. et al. Layer-dependent importance sampling for training deep and large graph convolutional networks. Adv. Neural Inf. Process. Syst. 32 , 11249–11259 (2019).
Pouladi, F., Salehinejad, H. & Gilani, A. M. Recurrent neural networks for sequential phenotype prediction in genomics. In 2015 International Conference on Developments of E-Systems Engineering (DeSE) , 225–230 (IEEE, 2015).
Ke, Z. & Vikalo, H. A convolutional auto-encoder for haplotype assembly and viral quasispecies reconstruction. Adv. Neural Inf. Process. Syst. 33 , 13493–13503 (2020).
Liu, Z., Sun, M., Zhou, T., Huang, G. & Darrell, T. Rethinking the value of network pruning. In International Conference on Learning Representations (2018).
Mirzasoleiman, B., Bilmes, J. & Leskovec, J. Coresets for data-efficient training of machine learning models. In International Conference on Machine Learning , 6950–6960 (PMLR, 2020).
Lin, T., Stich, S. U., Patel, K. K. & Jaggi, M. Don’t use large mini-batches, use local SGD. In International Conference on Learning Representations (2019).
Geng, J., Li, D. & Wang, S. Elasticpipe: An efficient and dynamic model-parallel solution to DNN training. In Proceedings of the 10th Workshop on Scientific Cloud Computing , 5–9 (2019).
Download references
Acknowledgements
A.A. is supported by the ARO (W911NF2110117). R.B. and CJ.B. are supported by NSF grants CCF-1911094, IIS-1838177, and IIS-1730574; ONR grants N00014-18-12571, N00014-20-1-2534, and MURI N00014-20-1-2787; AFOSR grant FA9550-18-1-0478; and a Vannevar Bush Faculty Fellowship, ONR grant N00014-18-1-2047. M.N and R.A.L.E. are supported by a training fellowship from the Gulf Coast Consortia, on the NLM Training Program in Biomedical Informatics & Data Science (T15LM007093). D.A.A. is partially supported by funds from the University of Houston. A.B., B.K., N.S., and T.J.T are partially supported by funds from the FunGCAT program from the Office of the Director of National Intelligence (ODNI), Intelligence Advanced Research Projects Activity (IARPA), via the Army Research Office (ARO) under Federal Award No. W911NF-17-2-0089. T.J.T is supported by NIH grant P01AI152999 and by NSF grant EF-212638. B.K. is supported by a fellowship from the National Library of Medicine Training Program in Biomedical Informatics and Data Science (5T15LM007093-30, PI: Kavraki). Z.Y., M.E., and L.N. are supported by NSF grants DBI-2030604 and IIS-2106837. R.D. and V.Y. are supported by Cancer Prevention & Research Institute of Texas (CPRIT) Award (RR190065). V.Y. is a CPRIT Scholar in Cancer Research and also supported by NIH grant RF1AG054564. A.K. is supported by NSF grants CCF-1907936, CNS-2003137.
Author information
These authors contributed equally: Nicolae Sapoval, Amirali Aghazadeh.
Authors and Affiliations
Department of Computer Science, Rice University, Houston, TX, USA
Nicolae Sapoval, Michael G. Nute, Advait Balaji, Ruth Dannenfelser, Chen Dun, Mohammadamin Edrisi, R. A. Leo Elworth, Bryce Kille, Anastasios Kyrillidis, Luay Nakhleh, Cameron R. Wolfe, Zhi Yan, Vicky Yao & Todd J. Treangen
Department of Electrical Engineering and Computer Sciences, University of California Berkeley, Berkeley, CA, USA
Amirali Aghazadeh
Department of Biology and Biochemistry, University of Houston, Houston, TX, USA
Dinler A. Antunes
Department of Electrical and Computer Engineering, Rice University, Houston, TX, USA
Richard Baraniuk & C. J. Barberan
Department of Bioengineering, Rice University, Houston, TX, USA
Todd J. Treangen
You can also search for this author in PubMed Google Scholar
Contributions
N.S. and A.A. designed figures and conceptualized the manuscript. N.S., A.A., R.A.L.E, and B.K. contributed text to the introduction and general challenges for deep learning in the biosciences sections. A.A. contributed text for the genome engineering section. D.A.A. contributed text for the protein structure prediction section. A.B. contributed text for the protein function prediction section. R.B., C.J.B., and A.A. contributed text for the explainability section. C.D., C.R.W., and A.K. contributed text for the training efficiency section. R.D. and V.Y. contributed text for the systems biology and data integration section. M.E., M.G.N., L.N., and Z.Y. contributed text and figures for the phylogenetics section. T.J.T. supervised the work and contributed to manuscript conceptualization. All authors have edited and reviewed the manuscript.
Corresponding author
Correspondence to Todd J. Treangen .
Ethics declarations
Competing interests.
The authors declare no competing interests.
Peer review
Peer review information.
Nature Communications thanks Bharath Ramsundar, Aurelien Tellier and the other, anonymous, reviewer(s) for their contribution to the peer review of this work.
Additional information
Publisher’s note Springer Nature remains neutral with regard to jurisdictional claims in published maps and institutional affiliations.
Rights and permissions
Open Access This article is licensed under a Creative Commons Attribution 4.0 International License, which permits use, sharing, adaptation, distribution and reproduction in any medium or format, as long as you give appropriate credit to the original author(s) and the source, provide a link to the Creative Commons license, and indicate if changes were made. The images or other third party material in this article are included in the article’s Creative Commons license, unless indicated otherwise in a credit line to the material. If material is not included in the article’s Creative Commons license and your intended use is not permitted by statutory regulation or exceeds the permitted use, you will need to obtain permission directly from the copyright holder. To view a copy of this license, visit http://creativecommons.org/licenses/by/4.0/ .
Reprints and permissions
About this article
Cite this article.
Sapoval, N., Aghazadeh, A., Nute, M.G. et al. Current progress and open challenges for applying deep learning across the biosciences. Nat Commun 13 , 1728 (2022). https://doi.org/10.1038/s41467-022-29268-7
Download citation
Received : 25 August 2021
Accepted : 09 March 2022
Published : 01 April 2022
DOI : https://doi.org/10.1038/s41467-022-29268-7
Share this article
Anyone you share the following link with will be able to read this content:
Sorry, a shareable link is not currently available for this article.
Provided by the Springer Nature SharedIt content-sharing initiative
This article is cited by
Data encoding for healthcare data democratization and information leakage prevention.
- Anshul Thakur
- Tingting Zhu
- David A. Clifton
Nature Communications (2024)
Strategies to increase the robustness of microbial cell factories
- Nuo-Qiao Lin
- Jian-Zhong Liu
Advanced Biotechnology (2024)
Classification and detection of natural disasters using machine learning and deep learning techniques: A review
- Kibitok Abraham
- Moataz Abdelwahab
- Mohammed Abo-Zahhad
Earth Science Informatics (2024)
Large sample size and nonlinear sparse models outline epistatic effects in inflammatory bowel disease
- Nora Verplaetse
- Antoine Passemiers
- Daniele Raimondi
Genome Biology (2023)
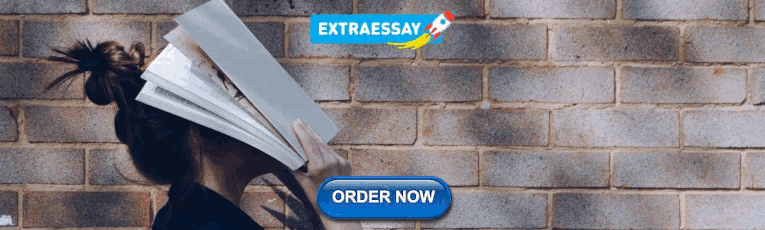
Extracellular vesicle-based liquid biopsy biomarkers and their application in precision immuno-oncology
- Karama Asleh
- Valerie Dery
- Rodney J. Ouellette
Biomarker Research (2023)
By submitting a comment you agree to abide by our Terms and Community Guidelines . If you find something abusive or that does not comply with our terms or guidelines please flag it as inappropriate.
Quick links
- Explore articles by subject
- Guide to authors
- Editorial policies
Sign up for the Nature Briefing newsletter — what matters in science, free to your inbox daily.

- What is Bioinformatics?
- MidSouth Bioinformatics Center
Academic Programs
- Minor in Bioinformatics
- M.S. in Bioinformatics
- Ph.D. in Bioinformatics
- Forms and Handbooks
- Topics and Tools
- Bioinformatics Classroom Resources
Drug Discovery and Development Conference 2024
Drug discovery and development colloquium 2024.
The goal of the colloquium is to showcase the synergistic interaction between chemistry, biology, pharmacology, and bioinformatics in the process of drug development. Our aim is to promote a professional dialogue between students and experts from different disciplines interested in the processes of drug discovery and development. This conference provides a platform for undergraduates, graduate students, and post-doctoral fellows to present their research. This conference will also include a professional development session, as well as providing great networking opportunities.
Virtual Conference Dates : July 22, 2022
Keynote Speakers
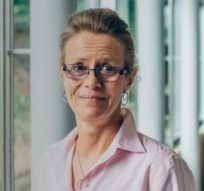
Current President of AAPS and Head of Advance Drug Delivery team at AstraZeneca
She has been instrumental in delivering new modality formulations in clinical studies with work experiences and partnerships across Sweden, UK and the US. Dr. Bak is also a great inspiration to women in science and an internationally known advocate of career development for women.
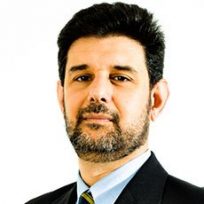
Associate Professor of Pharmacognosy and Natural Products Chemistry Department of Pharmacy of the National and Kapodistrian University Athens, Greece
He is a co-founder of the World Olive Center for Health, recipient of the inventor award in 2016, Greece and scientific coordinator of the Interreg MED project “ARISTOIL” which was recognized as the best INTERREG project in Europe for 2019.
- Bioinformatics
- EIT Building
- More contact information
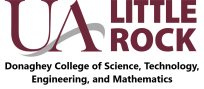
- Frontiers in Immunology
- Antigen Presenting Cell Biology
- Research Topics
Effect of steady state tissue imprinting on macrophages’ phenotype and inflammation resolution capacity
Total Downloads
Total Views and Downloads
About this Research Topic
Macrophages are highly abundant in all organs and play a vital role in tissue development, maintenance, and surveillance. They have been considered plastic and dynamic cells, rapidly adapting to their changing demands. Indeed, tissue-resident macrophages comprise a highly heterogeneous cell population with various phenotypes and functions according to their inter- and intra-organ-specific location. However, several recent studies have challenged the concept of plasticity by showing that the local tissue microenvironment strongly imprints macrophages with only limited genes being regulated by inflammation. What (micro-)environmental signals enforce tissue imprinting remains largely unknown. While tissue imprinting equips macrophages with the ability to safeguard tissue homeostasis, this may impair macrophages’ plasticity and ability to fully respond to and clear inflammation. Indeed, early during inflammation, tissue-resident macrophages have been found to be strongly depleted in mice and large numbers of monocytes are recruited that can give rise to macrophages, which replenish organs. These newly recruited macrophages may be necessary to react to inflammatory insults. While some of these newly recruited macrophages are only transient and lost upon inflammation resolution, others remain and become tissue resident. The latter, together with some remaining ‘original’ tissue-resident macrophages, may potentially retain an innate memory of the inflammatory event. If innate memory (trained immunity) affects macrophages capacity to resolve inflammation remains unknown. Similarly, it remains to be resolved which environmental clues signal to macrophages that inflammation has been resolved and that macrophages can return to their homeostatic phenotypes. The goal of this research topic is to expand the current knowledge regarding the tissue-specific regulation of macrophages in the steady state and inflammation resolution. An understanding of homeostatic tissue imprinting of macrophages may enable the discovery of novel therapeutic targets to promote chronic disease resolution. We welcome Original Research articles and Reviews addressing the following questions: (1) What are the mechanisms underlying tissue-specific metabolic (lipids, amino acids, sugars, iron) imprinting in steady state? (2) What are the mechanisms underlying tissue-specific ECM-driven (integrins, cytokines) imprinting in steady state? (3) What is the impact of tissue-specific imprinting on macrophage plasticity? (4) How does innate memory affect insult resolution? (5) Can tissue-specific clues reverse pro-disease phenotypes of macrophages in chronic diseases and can targeting these pathways enable inflammation resolution? Please Note: Studies on monocytes or other dendritic cells are also suitable for this topic. However, studies addressing how inflammation, cancer, microbiota, circadian rhythm, and central influences imprint myeloid cells are out of scope of this topic. We further do not accept research that only consists of bioinformatics analysis of either public databases or author-generated omics data
Keywords : macrophages, dendritic cells, monocytes, steady-state
Important Note : All contributions to this Research Topic must be within the scope of the section and journal to which they are submitted, as defined in their mission statements. Frontiers reserves the right to guide an out-of-scope manuscript to a more suitable section or journal at any stage of peer review.
Topic Editors
Topic coordinators, submission deadlines, participating journals.
Manuscripts can be submitted to this Research Topic via the following journals:
total views
- Demographics
No records found
total views article views downloads topic views
Top countries
Top referring sites, about frontiers research topics.
With their unique mixes of varied contributions from Original Research to Review Articles, Research Topics unify the most influential researchers, the latest key findings and historical advances in a hot research area! Find out more on how to host your own Frontiers Research Topic or contribute to one as an author.
Support Topics
Learn more about various research support topics that help shape the trajectory and success of your research.
Animal Subject Research
Regulation, compliance, and support for studies and experiments conducted on animals.
Biological Safety
Practices, policies, and procedures aimed at ensuring the safe handling, storage, and disposal of biological materials.
Chemical Safety
Practices, policies, and procedures aimed at ensuring the safe handling, storage, and disposal of chemical materials.
Clinical Trials
Regulation, compliance, and support for scientific studies or research investigations conducted with human participants.
Commercialization
Resources for translating discoveries and inventions from the lab into solutions with economic and societal impact.
Commercial Agreements & Contracts
Regulation, compliance, and support for research collaborations, projects, or partnerships with commercial and financial implications.
Community Outreach
Guidance and resources to engage communities in research endeavors.
Compliance for external consulting arrangements.
Corporate Engagement
Guidance on engaging with outside industry to perform research.
Data Management
Guidelines and related resources related to collecting, organizing, analyzing, and protecting research data.
Diversity, Equity, and Inclusion
Best practices and support for integrating diversity, equity, and inclusion into research topics and research management.
Export Compliance
Regulation and guidance for the international export of goods, technology, and information related to research.
Financial Conflicts of Interest
Regulation and compliance related to financial conflicts of interest (FCOI) and FCOI disclosure.
Funding Support
Resources and opportunities for research funding support.
Human Subject Research
Regulation, compliance, and support for studies and experiments involving human subjects.
Institutional Animal Care and Use
Policies and procedures related to the oversight of ethical treatment of animals used in research.
Institutional Review Board
Policies and procedures related to the oversight of ethical treatment of human participants in research.
Intellectual Property
Policies and procedures related to the legal rights of inventions produced by research.
International Research
Support for research involving international collaboration and partners.
Learning & Professional Development
Resources and support to enhance professional skills related to research.
Occupational Safety
Practices, policies, and regulations aimed at ensuring the health, safety, and well-being of employees and workers in their workplaces
Patent Policy
Policies and guidelines at Penn related to the creation, ownership, management, and use of patents.
Postdoctoral Affairs
Guidance, resources, and support for postdoctoral researchers and faculty mentors.
Post-Award Administration
Guidance and resources to ensure funded research is in compliance with the funding agency or organization.
Pre-Award Administration
Resources to support research activity and projects prior to award.
Proposal Preparation
Support and guidance in developing a research proposal.
Resources to support the publication process.
Radiation Safety
Practices, policies, and procedures aimed at ensuring the safe use of radiation and radiated materials in research.
Research Agreements
Resources and support for developing agreements governing a research project or collaboration.
Research Integrity
Policies and resources at Penn related to the ethical principles, values, and practices of research conduct and the dissemination of research findings.
Research Management
Guidance for planning and coordinating research activities and initiatives.
Research Misconduct
Policies and procedures related to potential cases of research misconduct.
Responsible Conduct of Research
Training and resources regarding ethical and professional standards for the responsible conduct of research.
Technology Licensing
Regulation and compliance related to the legal licensing of technology for use by an outside party.
Venture Formation
Guidance and support for the formation of a business or startup related to research activities.
Read our research on: Gun Policy | International Conflict | Election 2024
Regions & Countries
What’s it like to be a teacher in america today, public k-12 teachers are stressed about their jobs and few are optimistic about the future of education; many say poverty, absenteeism and mental health are major problems at their school.
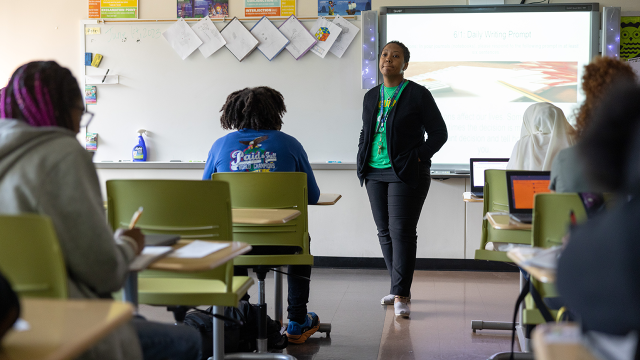
Pew Research Center conducted this study to better understand the views and experiences of public K-12 school teachers. The analysis in this report is based on an online survey of 2,531 U.S. public K-12 teachers conducted from Oct. 17 to Nov. 14, 2023. The teachers surveyed are members of RAND’s American Teacher Panel, a nationally representative panel of public K-12 school teachers recruited through MDR Education. Survey data is weighted to state and national teacher characteristics to account for differences in sampling and response to ensure they are representative of the target population.
Here are the questions used for this report , along with responses, and the survey methodology .
Low-poverty , medium-poverty and high-poverty schools are based on the percentage of students eligible for free and reduced-price lunch, as reported by the National Center for Education Statistics (less than 40%, 40%-59% and 60% or more, respectively).
Secondary schools include both middle schools and high schools.
All references to party affiliation include those who lean toward that party. Republicans include those who identify as Republicans and those who say they lean toward the Republican Party. Democrats include those who identify as Democrats and those who say they lean toward the Democratic Party.
Public K-12 schools in the United States face a host of challenges these days – from teacher shortages to the lingering effects of COVID-19 learning loss to political battles over curriculum .
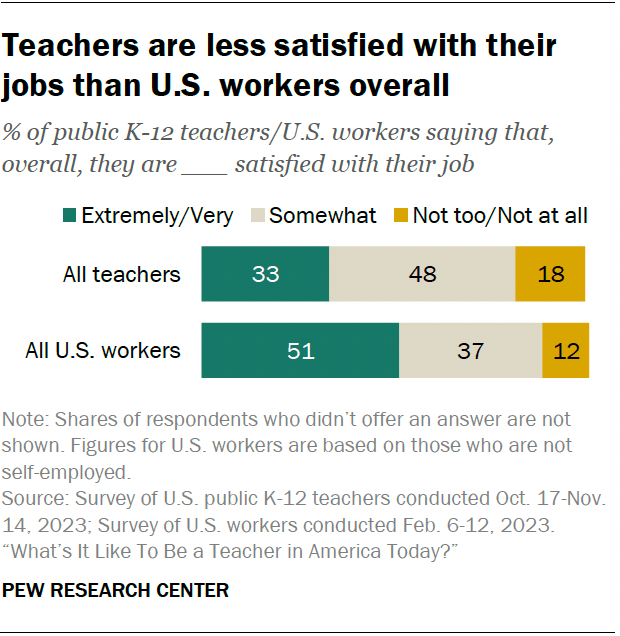
In the midst of all this, teachers express low levels of satisfaction with their jobs. In fact, they’re much less satisfied than U.S. workers overall.
Here’s how public K-12 teachers are feeling about their jobs:
- 77% say their job is frequently stressful.
- 68% say it’s overwhelming.
- 70% say their school is understaffed.
- 52% say they would not advise a young person starting out today to become a teacher.
When it comes to how their students are doing in school, teachers are relatively downbeat about both academic performance and behavior.
Here’s how public K-12 teachers rate academic performance and behavior at their school:
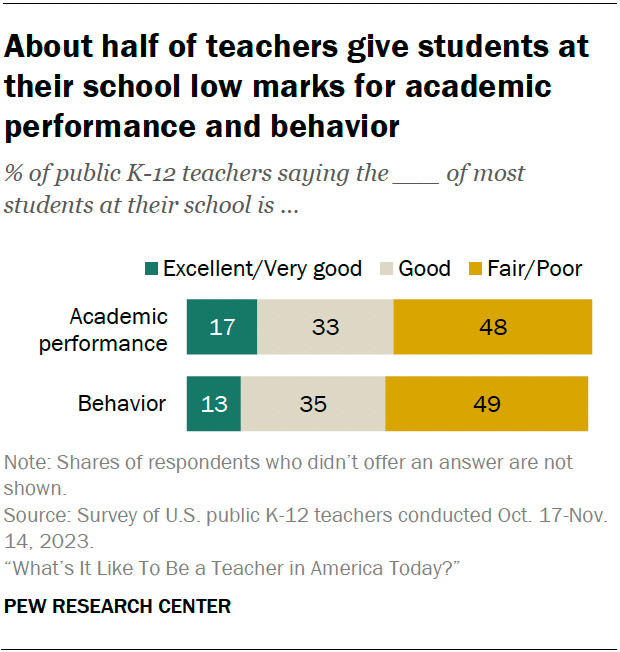
- 48% say the academic performance of most students at their school is fair or poor. A third say it’s good, and only 17% describe it as excellent or very good.
- 49% say the behavior of most students at their school is fair or poor; 35% say it’s good and 13% say it’s excellent or very good.
The COVID-19 pandemic likely compounded these issues. About eight-in-ten teachers (among those who have been teaching for at least a year) say the lasting impact of the pandemic on students’ behavior, academic performance and emotional well-being has been very or somewhat negative.
Assessments of student performance and behavior differ widely by school poverty level. 1 Teachers in high-poverty schools have a much more negative outlook. But feelings of stress and dissatisfaction among teachers are fairly universal, regardless of where they teach.
Related: What Public K-12 Teachers Want Americans To Know About Teaching
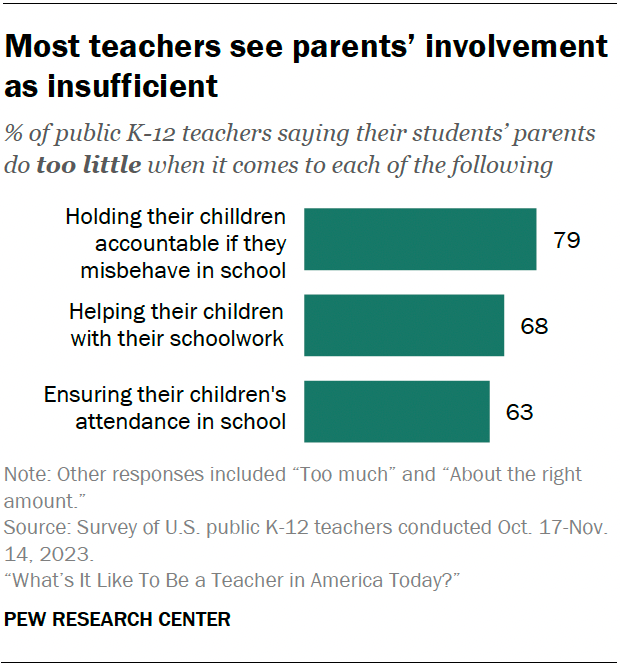
As they navigate these challenges, teachers don’t feel they’re getting the support or reinforcement they need from parents.
Majorities of teachers say parents are doing too little when it comes to holding their children accountable if they misbehave in school, helping them with their schoolwork and ensuring their attendance.
Teachers in high- and medium-poverty schools are more likely than those in low-poverty schools to say parents are doing too little in each of these areas.
These findings are based on a survey of 2,531 U.S. public K-12 teachers conducted Oct. 17-Nov. 14, 2023, using the RAND American Teacher Panel. 2 The survey looks at the following aspects of teachers’ experiences:
- Teachers’ job satisfaction (Chapter 1)
- How teachers manage their workload (Chapter 2)
- Problems students are facing at public K-12 schools (Chapter 3)
- Challenges in the classroom (Chapter 4)
- Teachers’ views of parent involvement (Chapter 5)
- Teachers’ views on the state of public K-12 education (Chapter 6)
Problems students are facing
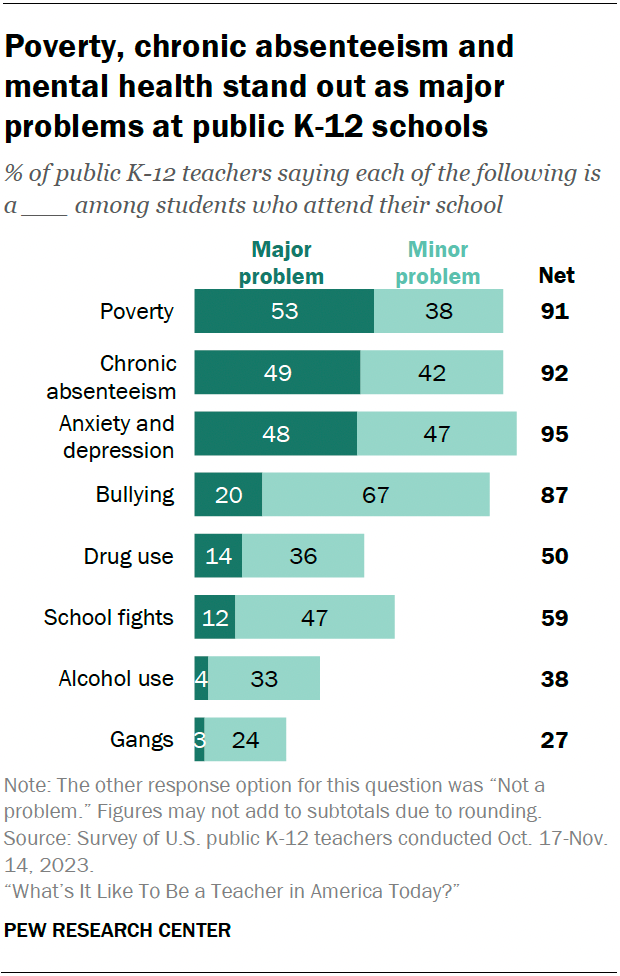
We asked teachers about some of the challenges students at their school are facing. Three problems topped the list:
- Poverty (53% say this is a major problem among students who attend their school)
- Chronic absenteeism (49%)
- Anxiety and depression (48%)
Chronic absenteeism (that is, students missing a substantial number of school days) is a particular challenge at high schools, with 61% of high school teachers saying this is a major problem where they teach. By comparison, 46% of middle school teachers and 43% of elementary school teachers say the same.
Anxiety and depression are viewed as a more serious problem at the secondary school level: 69% of high school teachers and 57% of middle school teachers say this is a major problem among their students, compared with 29% of elementary school teachers.
Fewer teachers (20%) view bullying as a major problem at their school, though the share is significantly higher among middle school teachers (34%).
A look inside the classroom
We also asked teachers how things are going in their classroom and specifically about some of the issues that may get in the way of teaching.
- 47% of teachers say students showing little or no interest in learning is a major problem in their classroom. The share rises to 58% among high school teachers.
- 33% say students being distracted by their cellphones is a major problem. This is particularly an issue for high school teachers, with 72% saying this is a major problem.
- About one-in-five teachers say students getting up and walking around when they’re not supposed to and being disrespectful toward them (21% each) are major problems. Teachers in elementary and middle schools are more likely than those in high schools to see these as challenges.
A majority of teachers (68%) say they’ve experienced verbal abuse from a student – such as being yelled at or threatened. Some 21% say this happens at least a few times a month.
Physical violence is less common. Even so, 40% of teachers say a student has been violent toward them , with 9% saying this happens at least a few times a month.
About two-thirds of teachers (66%) say that the current discipline practices at their school are very or somewhat mild. Only 2% say the discipline practices at their school are very or somewhat harsh, while 31% say they are neither harsh nor mild. Most teachers (67%) say teachers themselves don’t have enough influence in determining discipline practices at their school.
Behavioral issues and mental health challenges
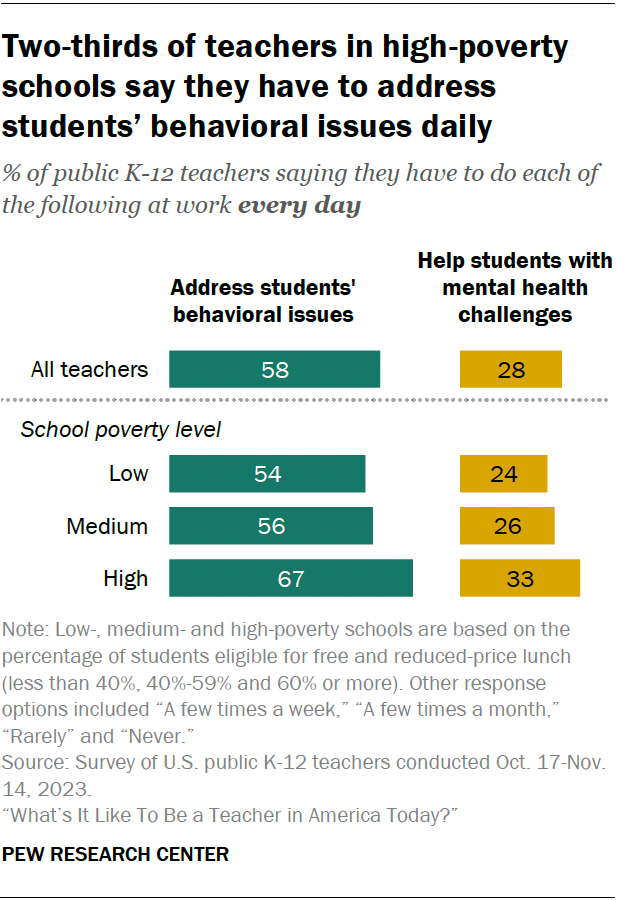
In addition to their teaching duties, a majority of teachers (58%) say they have to address behavioral issues in their classroom every day. About three-in-ten teachers (28%) say they have to help students with mental health challenges daily.
In each of these areas, elementary and middle school teachers are more likely than those at the high school level to say they do these things on a daily basis.
And teachers in high-poverty schools are more likely than those in medium- and low-poverty schools to say they deal with these issues each day.
Cellphone policies and enforcement
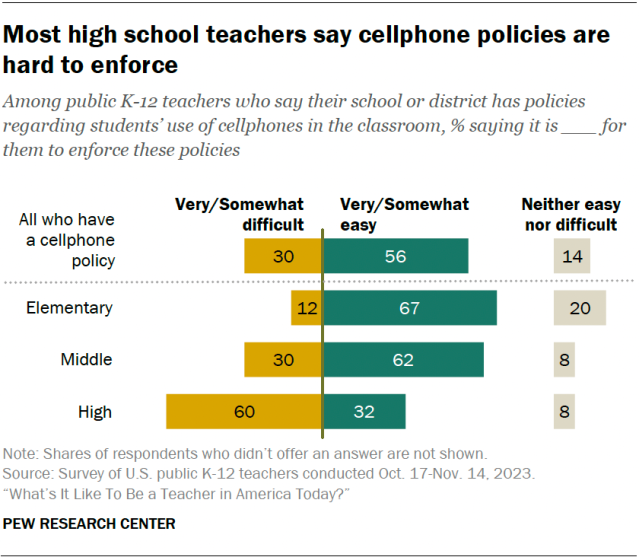
Most teachers (82%) say their school or district has policies regarding cellphone use in the classroom.
Of those, 56% say these policies are at least somewhat easy to enforce, 30% say they’re difficult to enforce, and 14% say they’re neither easy nor difficult to enforce.
Experiences with cellphone policies vary widely across school levels. High school teachers (60%) are much more likely than middle school (30%) and elementary school teachers (12%) to say the policies are difficult to enforce (among those who say their school or district has a cellphone policy).
How teachers are experiencing their jobs
Thinking about the various aspects of their jobs, teachers are most satisfied with their relationship with other teachers at their school (71% are extremely or very satisfied).
They’re least satisfied with how much they’re paid – only 15% are extremely or very satisfied with their pay, while 51% are not too or not at all satisfied.
Among teachers who don’t plan to retire or stop working this year, 29% say it’s at least somewhat likely they will look for a new job in the 2023-24 school year. Within that group, 40% say they would look for a job outside of education, 29% say they’d seek a non-teaching job in education, and only 18% say they’d look for a teaching job at another public K-12 school.
Do teachers find their work fulfilling and enjoyable?
Overall, 56% of teachers say they find their job to be fulfilling extremely often or often; 53% say their job is enjoyable. These are significantly lower than the shares who say their job is frequently stressful (77%) or overwhelming (68%).
Positive experiences are more common among newer teachers. Two-thirds of those who’ve been teaching less than six years say their work is fulfilling extremely often or often, and 62% of this group says their work is frequently enjoyable.
Teachers with longer tenures are somewhat less likely to feel this way. For example, 48% of those who’ve been teaching for six to 10 years say their work is frequently enjoyable.
Balancing the workload
Most teachers (84%) say there’s not enough time during their regular work hours to do tasks like grading, lesson planning, paperwork and answering work emails.
Among those who feel this way, 81% say simply having too much work is a major reason.
Many also point to having to spend time helping students outside the classroom, performing non-teaching duties like lunch duty, and covering other teachers’ classrooms as at least minor reasons they don’t have enough time to get all their work done.
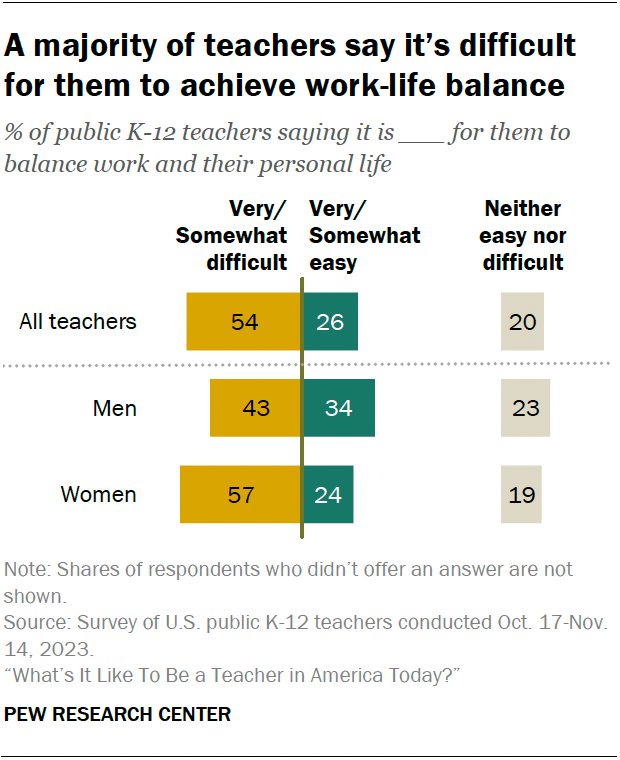
A majority of teachers (54%) say it’s very or somewhat difficult for them to balance work and their personal life. About one-in-four (26%) say it’s very or somewhat easy for them to balance these things, and 20% say it’s neither easy nor difficult.
Among teachers, women are more likely than men to say work-life balance is difficult for them (57% vs. 43%). Women teachers are also more likely to say they often find their job stressful or overwhelming.
How teachers view the education system
A large majority of teachers (82%) say the overall state of public K-12 education has gotten worse in the past five years.
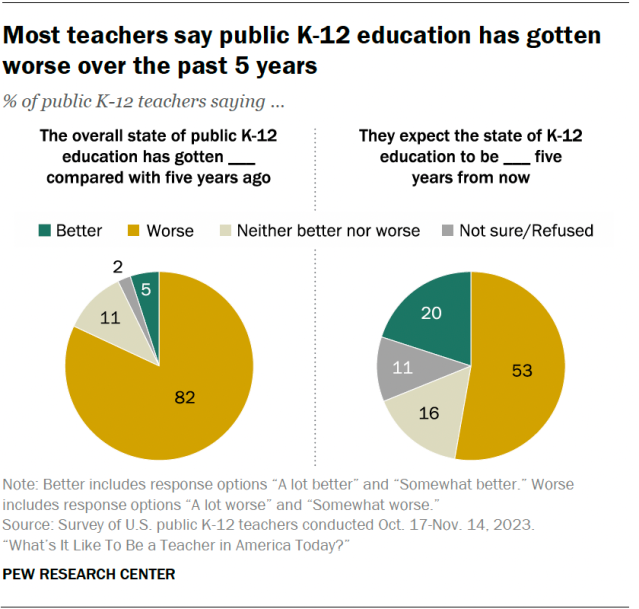
And very few are optimistic about the next five years: Only 20% of teachers say public K-12 education will be a lot or somewhat better five years from now. A narrow majority (53%) say it will be worse.
Among teachers who think things have gotten worse in recent years, majorities say the current political climate (60%) and the lasting effects of the COVID-19 pandemic (57%) are major reasons. A sizable share (46%) also point to changes in the availability of funding and resources.
Related: About half of Americans say public K-12 education is going in the wrong direction
Which political party do teachers trust more to deal with educational challenges?
On balance, more teachers say they trust the Democratic Party than say they trust the Republican Party to do a better job handling key issues facing the K-12 education system. But three-in-ten or more across the following issues say they don’t trust either party:
- Shaping school curriculum (42% say they trust neither party)
- Ensuring teachers have adequate pay and benefits (35%)
- Making schools safer (35%)
- Ensuring adequate funding for schools (33%)
- Ensuring all students have equal access to high-quality K-12 education (31%)
A majority of public K-12 teachers (58%) identify or lean toward the Democratic Party. This is higher than the share among the general public (47%).
- Poverty levels are based on the percentage of students in the school who are eligible for free and reduced-price lunch. ↩
- For details, refer to the Methodology section of the report. ↩
- Urban, suburban and rural schools are based on the location of the school as reported by the National Center for Education Statistics (rural includes town). Definitions match those used by the U.S. Census Bureau. ↩
Social Trends Monthly Newsletter
Sign up to to receive a monthly digest of the Center's latest research on the attitudes and behaviors of Americans in key realms of daily life
Report Materials
Table of contents, ‘back to school’ means anytime from late july to after labor day, depending on where in the u.s. you live, among many u.s. children, reading for fun has become less common, federal data shows, most european students learn english in school, for u.s. teens today, summer means more schooling and less leisure time than in the past, about one-in-six u.s. teachers work second jobs – and not just in the summer, most popular.
About Pew Research Center Pew Research Center is a nonpartisan fact tank that informs the public about the issues, attitudes and trends shaping the world. It conducts public opinion polling, demographic research, media content analysis and other empirical social science research. Pew Research Center does not take policy positions. It is a subsidiary of The Pew Charitable Trusts .
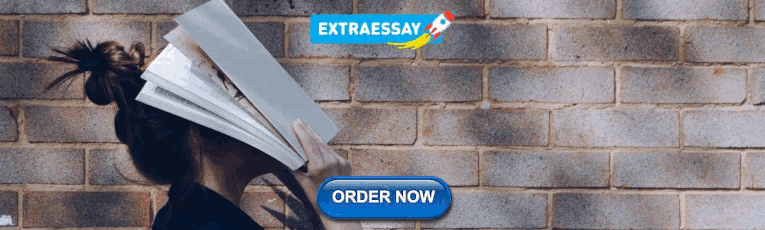
IMAGES
VIDEO
COMMENTS
Bioinformatics is a field of study that uses computation to extract knowledge from biological data. It includes the collection, storage, retrieval, manipulation and modelling of data for analysis ...
Researchers working in the scientific area always want to explore new and hot topics to make informed choices. In this article, all new, current, and demanding research topics in bioinformatics are mentioned. This article is helpful for the researchers who are looking for trends in bioinformatics to select a research topic of broad-spectrum. Since the […]
These articles reflect current trend and development in bioinformatics research. The supplement to BMC Bioinformatics was proposed to launch during the BIOCOMP'19—The 2019 International Conference on Bioinformatics and Computational Biology held from July 29 to August 01, 2019 in Las Vegas, Nevada. In this congress, a variety of research ...
This is an editorial report of the supplements to BMC Bioinformatics that includes 6 papers selected from the BIOCOMP'19—The 2019 International Conference on Bioinformatics and Computational Biology. These articles reflect current trend and development in bioinformatics research. Keywords: Bioinformatics, Biomarkers, Human disease, Microbiome.
Integration of computational high throughput sequencing, single-cell and spatial analysis of immune microenvironments regulation in cancers. An innovative journal that provides a forum for new discoveries in bioinformatics. It focuses on how new tools and applications can bring insights to specific biological problems.
This article is part of the Research Topic Artificial Intelligence in Bioinformatics and Drug ... Citation: Zhang X-M, Liang L, Liu L and Tang M-J (2021) Graph Neural Networks and Their Current Applications in Bioinformatics. Front. Genet. 12:690049. doi: 10.3389/fgene.2021.690049. Received: 02 April 2021; Accepted: 28 May 2021; Published: 29 ...
Machine Learning Applications in the Study of Parkinson's Disease: A Systematic Review. Jordi Martorell-Marugán, ... Pedro Carmona-Sáez. Read latest issue. Read the latest articles of Current Bioinformatics at ScienceDirect.com, Elsevier's leading platform of peer-reviewed scholarly literature.
Training in data science skills can unlock the potential of biomedical Big Data. To address the need for data science skills and knowledge, JAX offers a bioinformatics training program that equips researchers with the critical data science skills needed to move research forward.
In sum, this book contains high-quality chapters that provide excellent views into key topics of current bioinformatics research, topics that should remain important for the next several years. Today, a single laboratory can generate a vast amount of biological data. There is a wealth of data already available in public databases, which makes ...
The Harvard Bioinformatics Core (HBC) Current Topics in Bioinformatics series is excited to announce their 2023 schedule. These free, hands-on workshops are available to all Harvard affiliates and cover a variety of … Continue reading "HBC Current Topics in Bioinformatics 2023 Schedule"
Aims & Scope. Current Bioinformatics aims to publish all the latest and outstanding developments in bioinformatics.Each issue contains a series of timely, in-depth/mini-reviews, research papers and guest edited thematic issues written by leaders in the field, covering a wide range of the integration of biology with computer and information science.
The current field of neuroscience is increasingly using bioinformatics, which has provided new research avenues, thus enhancing our understanding of brain functions. Some well-known examples include high-throughput analysis of single-cell transcriptomics, comparative genomics, networks & neurophysiology, the latter encompassing neuronal modeling and high power computation. From focusing on a ...
Current Research. The primary vision of the Center for Bioinformatics and Functional Genomics (CBFG) is to establish a research nexus that integrates leading-edge developments in computational sciences, next-generation genomics and epigenomics and functional biology to improve our understanding of disease etiology applied to personalized medicine.
Aims and Scope. Current Research in Bioinformatics, a peer reviewed international journal is launched to publish notable research on the application of information technology to the field of molecular biology. Researchers and scholars are invited to submit their original research / review articles in the field of creation and advancement of ...
Bioinformatics and computational genomics have become increasingly important for biological and genomic research, and artificial intelligence (AI) has the potential to revolutionize these industries.
Deep Learning (DL) has recently enabled unprecedented advances in one of the grand challenges in computational biology: the half-century-old problem of protein structure prediction. In this paper ...
The goal of the colloquium is to showcase the synergistic interaction between chemistry, biology, pharmacology, and bioinformatics in the process of drug development. Our aim is to promote a professional dialogue between students and experts from different disciplines interested in the processes of drug discovery and development.
The goal of this research topic is to expand the current knowledge regarding the tissue-specific regulation of macrophages in the steady state and inflammation resolution. ... We further do not accept research that only consists of bioinformatics analysis of either public databases or author-generated omics data Keywords: macrophages ...
Best practices and support for integrating diversity, equity, and inclusion into research topics and research management. Export Compliance Regulation and guidance for the international export of goods, technology, and information related to research.
As more states pass laws legalizing marijuana for recreational use, Americans continue to favor legalization of both medical and recreational use of the drug.. An overwhelming share of U.S. adults (88%) say marijuana should be legal for medical or recreational use.. Nearly six-in-ten Americans (57%) say that marijuana should be legal for medical and recreational purposes, while roughly a third ...
The Harvard Bioinformatics Core (HBC) Current Topics in Bioinformatics series is excited to announce their 2023 schedule. These free, hands-on workshops are available to all Harvard affiliates and cover a variety of bioinformatics topics & related skills. Please find the topics in the table below and register on the HBC training website.All workshops are currently held remotely via Zoom.
Low-poverty, medium-poverty and high-poverty schools are based on the percentage of students eligible for free and reduced-price lunch, as reported by the National Center for Education Statistics (less than 40%, 40%-59% and 60% or more, respectively).. Secondary schools include both middle schools and high schools.. All references to party affiliation include those who lean toward that party.
The Harvard Chan Bioinformatics Core is pleased to announce the next session of the Harvard Chan Bioinformatics Core's (HBC) Current Topics in Bioinformatics 2023 series - Publication perfect I.. This free, hands-on workshop is available to all Harvard affiliates. The workshop is part of our series which covers a variety of bioinformatics topics & related skills.