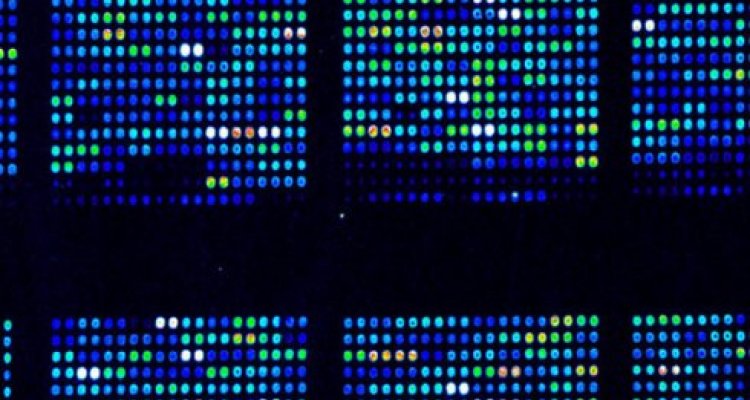
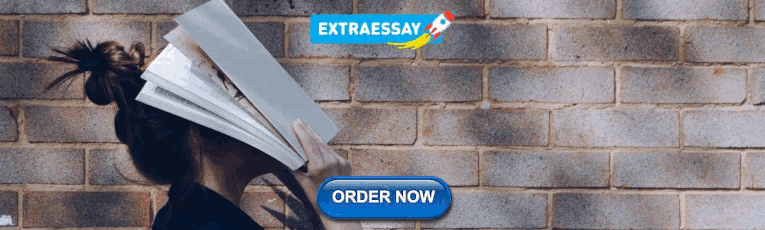
BSc and MSc Thesis Subjects of the Bioinformatics Group
On this page you can find an overview of the BSc and MSc thesis topics that are offered by our group. The procedure to find the right thesis project for you is described below.
MSc thesis: In the Bioinformatics group, we offer a wide range of MSc thesis projects, from applied bioinformatics to computational method development. Here is a list of available MSc thesis projects . Besides the fact that these topics can be pursued for a MSc thesis, they can also be pursued as part of a Research Practice .
BSc thesis: As a BSc student you will work as an apprentice alongside one of the PhD students or postdocs in the group. You will work on your own research project, closely guided by your supervisor. You will be expected to work with several tools and/or databases, be creative and potentially overcome technical challenges. Below you will find short descriptions of the research projects of our PhDs and Postdocs. In addition you can take a look at the list of MSc thesis projects above.
Procedure for WUR students:
- Request an intake meeting with one of our thesis coordinators by filling out the MSc intake form or BSc intake form and sending it to [email protected]
- Contact project supervisors to discuss specific projects that fit your background and interest
- Upon a match, take care of the required thesis administration together with your supervisor(s) and enroll in the thesis BrightSpace site to find more information on a thesis in the Bioinformatics group
Procedure for non-WUR students or students in other non-standard situations: We have limited space for interns from other institutes. If you are interested, please email our thesis coordinators at [email protected]; please attach your CV and indicate what are your main research interests.
BSc thesis topics
Integrative omics for the discovery of biosynthetic pathways in plants, molecular function prediction of natural products, linking the metabolome and genome, linking metagenomics and metatranscriptomics to study the endophytic root microbiome, exploiting variation in lettuce and its wild relatives.
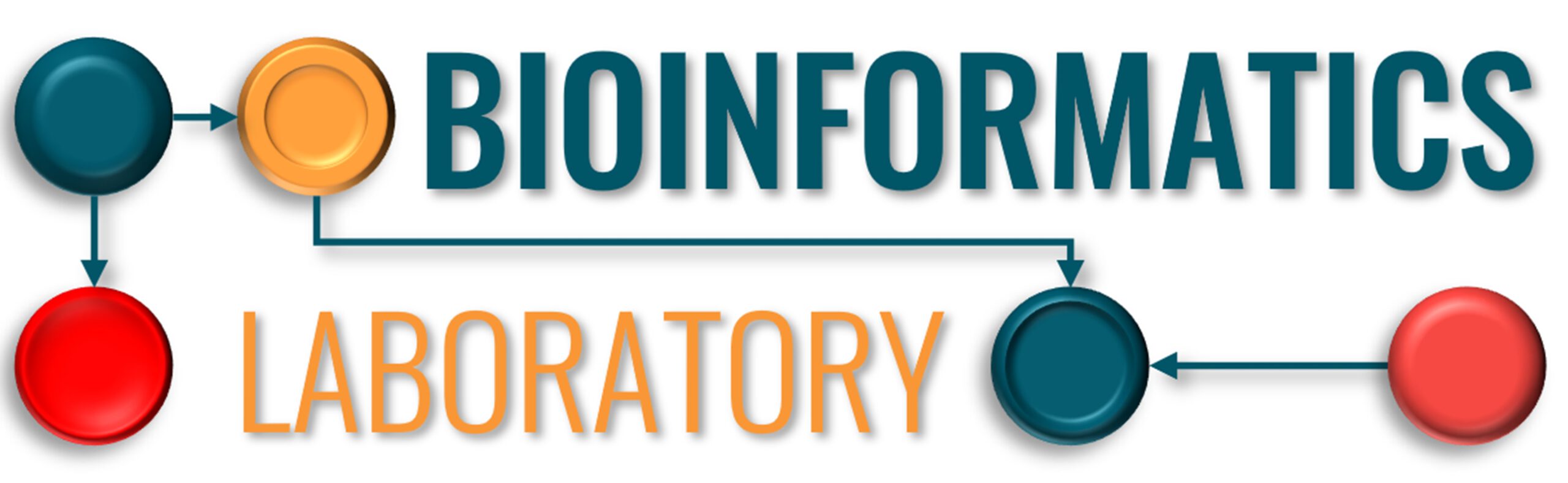
Medical Bioinformatics and Computational Modelling
PhD students at the Bioinformatics Laboratory
In Progress
- Balashova, D. Repertoire sequencing . University of Amsterdam, Amsterdam. ARCAID . Marie Curie COFUND, Horizon 2020. Van Kampen, A.H.C. (promotor), De Vries N. (promotor), Greiff V. (co-promotor).
- Lashgari, D. Kinetic maturation in the Germinal Center . University of Amsterdam, Amsterdam. Supported by AMC. Van Kampen, A.H.C. (promotor), Van Gils, M. (co-promotor), Hoefsloot, H. C. (co-promotor).
- Mahamune, U. Single Cell RNAseq and computational modelling . University of Amsterdam, Amsterdam. ARCAID . Marie Curie COFUND, Horizon 2020. Van Kampen, A.H.C. (promotor), Moerland, P.D. (co-promotor), E.G.M. van Baarsen (co-promotor).
- Valiente, R. G. Development of multiscale mathematical models of the germinal center (GC) to study its role in B-cell lymphoma (BCL) and/or rheumatoid arthritis (RA). (PhD thesis). University of Amsterdam, Amsterdam. COSMIC . Marie Curie ITN, Horizon 2020. Van Kampen, A.H.C. (promotor), De Vries, N. (promotor), Hoefsloot, H. C. (co-promotor), Guikema, J. E. (co-promotor).
- Stobbe, M. (2012). 18 October 2012. The road to knowledge: from biology to databases and back again. University of Amsterdam, Amsterdam. NBIC BioRange. Van Kampen, A.H.C. (promotor), Moerland, P. D. (co-promotor). [ UvA-DARE ]
- Shahand, S. (2015). 29 October 2015. Science gateways for biomedical big data analysis. University of Amsterdam, Amsterdam. COMMIT. Van Kampen, A. (promotor), Olabarriaga, S. (co-promotor). [ UvA-DARE ]
- Reshetova, P. (2017). 2 March 2017. Use of Prior Knowledge in Biological Systems Modelling. University of Amsterdam, Amsterdam. NBIC Biorange. Van Kampen, A.H.C (promotor), Smilde, A. (promotor), Westerhuis, J. (co-promotor). [ UvA-DARE ]
- Tejero Merino, E. (2022). 7 November 2022 Multiscale modelling of plasma cell differentiation in the Germinal Center. University of Amsterdam, Amsterdam. Supported by AMC. Van Kampen, A.H.C. (promotor), Guikema, J.E.J. (co-promotor), Hoefsloot, H. C. (co-promotor). [ PhD thesis] [ UvA-DARE ]
- Nandal, U. (2023). Computational approaches for biological data integration. University of Amsterdam, Amsterdam. NBIC BioRange. Van Kampen, A.H.C. (promotor), Moerland, P.D. (co-promotor). [ UvA-DARE ]
Co-supervised PhD students from other research groups
In Progress
- Balzaretti, G. Repertoire Sequencing . University of Amsterdam, Amsterdam. De Vries, N. (promotor), Van Kampen, A.H.C. (promotor).
- Lermo Jimenez, M. Epigenetics and breast cancer drug resistance . University of Amsterdam, Amsterdam. Verschure P. J. (promotor), Moerland, P.D. (co-promotor).
- Olivieri, A. Repertoire Sequencing. University of Amsterdam, Amsterdam. ARCAID , Marie Curie COFUND, Horizon 2020. De Vries, N. (promotor), Van Kampen, A.H.C. (promotor).
- Pollastro, S. Repertoire Sequencing . University of Amsterdam, Amsterdam. De Vries, N. (promotor), Van Kampen, A.H.C. (co-promotor).
- Stratigopoulou, M. Germinal Center and B-cell Lymphoma . University of Amsterdam, Amsterdam. COSMIC. Marie Curie ITN, Horizon 2020. Van Kampen, A.H.C. (promotor), Van Noesel, C. J. (promotor), De Vries, N. (co- promotor), Guikema, J. E. (co-promotor).
- Sontrop, H. (2015). 15 January 2015. A critical perspective on microarray breast cancer gene expression profiling. TU Delft, Delft. NBIC BioRange. Reinders, M. (promotor), Moerland, P. D. (co-promotor). [ Link ]
- Beckman, W. (2021). 17 August 2021. The Role of Epigenetics in Transcriptional Stochasticity and the Implications for Breast Cancer Drug Resistance . University of Amsterdam, Amsterdam. EpiPredict. Marie Curie ITN, Horizon 2016. Verschure P.J. (promotor), Van Kampen, A.H.C. (promotor). [ UvA-DARE ]
- Barros, R. S. (2022). 1 November 2022 High performance computing for clinical medical imaging . University of Amsterdam, Amsterdam. Henk Marquering (promotor), Van Kampen, A.H.C. (promotor), Olabarriaga, S. (co-promotor). [ UvA-DARE ]
- Anang, D. (2023) 6 November 2023. B and T Cell Immune Responses in Rheumatoid Arthritis and Myositis. In Search for the Immunological Drummers and Dancers . University of Amsterdam, Amsterdam. COSMIC . Marie Curie ITN, Horizon 2020. De Vries, N. (promotor), Van Kampen, A.H.C. (promotor), van Baarsen, E.G.M. (co-promotor). [ UvA-DARE ]
- Wegdam, W. (2024). In search of protein biomarkers in ovarian cancer and Gaucher disease. University of Amsterdam, Amsterdam. Aerts J.M.F.G. (promotor), Kenter, G.G. (promotor), Moerland, P.D. (co-promotor). [ UvA-DARE ]
Related Posts
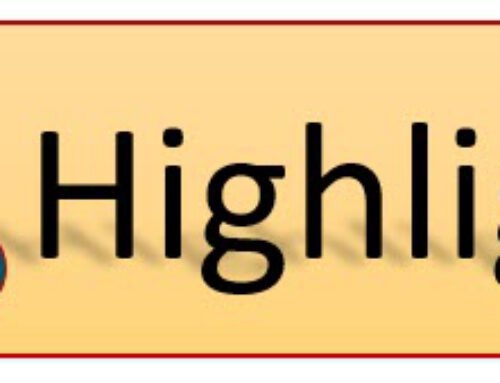
PhD thesis Umesh Nandal
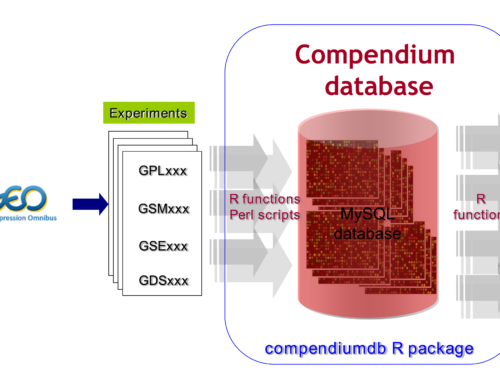
compendiumdb
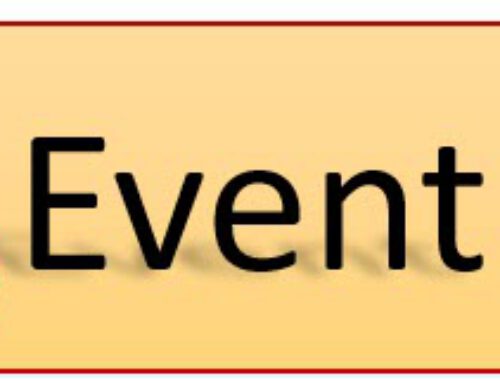
Participation in single-cell genomics 2022 conference
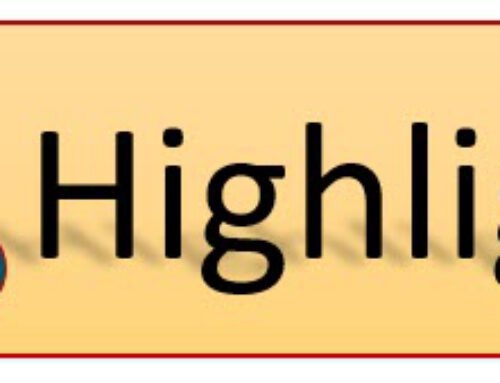
PhD thesis Elena Merino Tejero
Group Leader
Prof. dr. AHC van Kampen
[email protected] https://bioinformaticslaboratory.eu
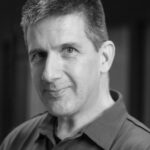
Amsterdam UMC – location AMC Department of Epidemiology and Data Science Bioinformatics Laboratory Meibergdreef 9 1105 AZ Amsterdam Zuidoost The Netherlands
How to get to us: [ pdf ] [ Google Maps ]
Epidemiology & Data Science
The Bioinformatics Laboratory is part of EDS
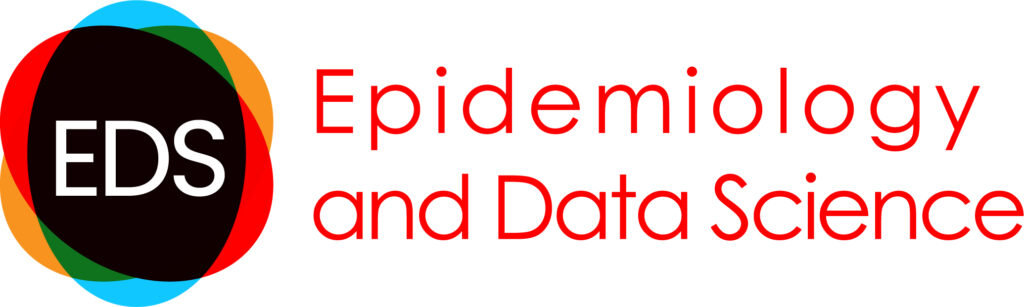
Read our Privacy Policy
Bioinformatics Laboratory – Your partner in bioinformatics and computational modelling since 1997
Privacy Overview
- Publications
- Software/Server
- Freiburg Galaxy
Diploma / Master / Bachelor theses and Projects
In the following we list available, currently processed, and finished theses and student projects. When looking for a topic, please check not only the available topics but also the processed and recently finished topics. There might be unannounced but available follow up theses or projects that are not yet announced. So if you find a topic interesting, please contact the corresponding supervisor for further information. Bioinformatics is a highly specialized application area of computer science and biology and to successfully solve research questions in this field, you require a lot of interdisciplinary knowledge. Therefore, to do a Master thesis with us, we have the minimum requirement that you have attended one of our teaching courses . We may also ask you to present an introductory talk about your chosen topic (given material provided by us) before we can accept you. This does not apply to Bachelor theses or projects.
Open Topics
Port a raw read pipeline for microbiome data analysis to galaxy.
Microbiome is the collection of all microbes, such as bacteria, fungi, viruses, along with their genes, which live inside and outside our bodies in all environments surrounding us. To investigate microbiomes, researchers use sequencing data and microbiome analyses. These analyses rely on sequencing data to investigate microbiomes. Such analysis relies on sophisticated computational approaches: assembly, binning, taxonomic classification, functional profiling etc. Analyzing microbiome data makes it possible to answer the two main questions for most microbiome analysis. Who (microorganisms) are there: by extracting the community from the microbiome reads What are they doing (and how): by extracting the gene/pathway abundance profile from the metagenomics reads and transcript abundance profiles from the metatranscriptomics reads and combining them These analyses rely on bioinformatics tools and also databases. Few workflows to process this data are available and most are not openly available, not transparent, or not easy to use by researchers. To tackle this problem, the Freiburg Galaxy team together with the microGalaxy community use Galaxy to build workflows to analyze microbiome sequencing data.
Project context: MGnify offers an automated pipeline for the analysis and archiving of microbiome data to help determine the taxonomic diversity and functional & metabolic potential of environmental samples. The pipeline even if documented is not really usable outside their resources. We would like to offer this pipeline for Galaxy users. This project aims to port the raw reads part of the pipeline into Galaxy. More information about the project can be found here: https://github.com/usegalaxy-eu/project-ideas/issues/31
CRISPR accessory proteins
The CRISPR-Cas system is an adaptive immune system in many archaea and bacteria, which provides resistance against invading genetic elements. The three major components of CRISPR-Cas systems are CRISPR-array, leader sequence and Cas genes. A recent study[1] demonstrated that there are proteins adjacent to the Cas proteins that help the CRISPR-Cas to switch targeting and degrading. This work aims to cluster/classify all the accessory proteins based on the associated Cas proteins. To do this, you will use the method from [1] to identify and analysis clusters. Project Outline - Start scanning all archaeal and bacterial genomes that have a CRISPR-Cas system. - Extract the up-and-downstream flanking genes of each CRISPR-Cas system. - Classify the genes according to different conditions and find clusters concerning locations and functions. [1] https://www.tandfonline.com/doi/full/10.1080/15476286.2018.1483685
Implementing new features for RNA-RNA interaction prediction
Our group develops the tool IntaRNA , which is one of the state-of-the-art programs for RNA-RNA interaction prediction. We are continously extending the tool (c++11, boost, autotools, openmp) that is hosted on Github BackofenLab/IntaRNA . Within the development process, we offer various student projects covering different aspects of the project. For a list of open topics, please refer to "student project" marked issues @github . If you are interested, please contact Martin Raden . Most topics can be adapted to be suitable for a student project, bachelor, or master thesis.
Docker based RNA-analysis workbench
You are interested in bleeding edge Linux-Kernel-Technologdy and virtualization? You want to help to distribute software packages in a OS-independend way? Than you can help us to solve the deployment problems of scientific software in a general way. That project will use Docker [1], an open source project that automates the deployment of applications, to produce self-contained images (containers). These containers are OS independent, versioned (like a git-history) and easy to use, which enables reproducibility of research results and easy deployment of entire software stacks. Prerequisites: Linux/Unix, Bash, autotools [1] https://www.docker.io Team-Project: can be combined with the "Graph visualization framework" and the "Galaxy Tool integration" project
Galaxy Tool integration
Galaxy is an open, web-based platform for data intensive research. The University of Freiburg is running a Galaxy server to serve all different needs of our researchers. In addition to the common Next-Gerneration-Sequencing Tools, we offer Tools for cheminformatics [1], proteomics and RNA bioinformatics. To integrate an apllication into Galaxy, a thin wrapper between the Galaxy API and the targeted application needs to be written. Here usability is key. Good wrappers are easy to use and abstracting complicated application details. As part of our Galaxy project we are permanently seeking for motivated tool-wrappers that are enthusiastic about usability, want to work with a vibrant community to make Bio- & Cheminformatik Tools accessible for more researchers. The overall aim is to put the developed wrapper in the Galaxy Tool Shed [2], a Galaxy Appstore, where everyone can get there favorite application with a few mouse clicks. Prerequisites: XML, Bash, autotools, Python [1] https://github.com/bgruening/galaxytools/tree/master/chemicaltoolbox [2] https://wiki.galaxyproject.org/Tool%20Shed Team-Project: can be combined with the "Graph visualization framework" and the "Docker" project
Interactive molecule design based on graph grammars
Further topics from the galaxy team.
The Freiburg Galaxy team is hosting further project ideas in its own GitHub repo. You want to work on NGS, big-data analysis, Cloud- or HPC-computing or develop complex front- end backends have a look at the topics in the link below: https://github.com/bgruening/project-ideas/issues
Further topics concerning CRISPR research
Further topics ....
Further Topics are available on request. If you have a suggestion for a topic you are interested in, do not hesitate to contact us. Otherwise, the completed theses may lead you.
Topics in Progress
Automated web crawling for publications using compensatory mutation experiments.
Compensatory mutation experiments provide the most reliable proof of specific inter-molecular base pairs formed by RNA-RNA interactions. They provide proof that specific base pairs are part of mechanism that is based on the formation of an RNA-RNA interaction. Such experiments are expensive both w.r.t. time and resources and thus often part of the methodology of research projects that try to unwind specific molecular biological mechanisms. Thus, the experiments are often "only" one step in a longer list of experiments to gather proof for a projects hypothesis and therefore only described within the main text of the manuscript. Since publication search engines typically only parse and index title and abstract information of published articles, identifying publications that involve compensatory mutations is not easily done. In order to better understand the details of RNA-RNA interactions, e.g. to improve prediction algorithms or to design new ones, compensatory mutation experiment data would be most beneficial. This projects aims at the development of a web crawling tool to systematically identify publications that provide such experimental details.
Design of a data base and respective user front ends to collect and browse compensatory mutation experiments
Compensatory mutation experiments provide the most reliable proof of specific inter-molecular base pairs formed by RNA-RNA interactions. They provide proof that specific base pairs are part of mechanism that is based on the formation of an RNA-RNA interaction. Such experiments are expensive both w.r.t. time and resources and thus often part of the methodology of research projects that try to unwind specific molecular biological mechanisms. Experimental details are often only presented in form of illustrative images or use non-uniform textual encodings. Thus, the extraction of such information is typically manually done. In order to better understand the details of RNA-RNA interactions, e.g. to improve prediction algorithms or to design new ones, compensatory mutation experiment data would be most beneficial. This projects aims at the development of a data base scheme suited to store experimental details and respective meta data. To help in the manual encoding and reviewing of compensatory mutations, an interactive user front end is to be developed.
Visualizing the effect of homo-dimerization on RNA-RNA interaction formation
The interactions formation between RNAs is key to many regulatory processes in life. Such RNA-RNA interactions (RRIs) are typically formed between regions of the molecules that are not involved in (intra-molecular) structure formation of the molecules themselves. Thus, in order to predict RRIs the structure of the interacting RNAs has to be taken into account. This is well modeled and done using accessibility-based RRI prediction tools like our in-house tool IntaRNA. Some regulatory molecules are produced in large amounts in order to fulfill their regulatory function via RRI formation. In such a scenario, it is quite likely that the molecules not only interact with their regulatory targets but also with molecules of their own type, which is called homo-dimerization. Dimerization can have multiple effects, e.g. (i) it might reduce the regulatory effect since many RNAs are bound and not available for interaction with the target molecule or (ii) it might change the structure of the dimerizing RNAs and thus "unlock" regions for RRI formation with the target that are otherwise blocked by intra-molecular structure. This project aims at studying such effects of homo-dimerization. To this end, a workflow is to be implemented that combines RRI prediction and constraint RNA structure prediction to model the effects of homo-dimerization. Furthermore, respective visualizations are to be developed and integrated into the workflow to simplify the study and interpretation of such effects.
Approximative iterative prediction of complex non-nested RNA structures
The structure of RNA molecules is typically studied in a simplified graph model that represents the formed intra-molecular interactions, i.e. base pairings. Due to computational complexity, such RNA structure models are typically restricted to nested base pairing models that can be visualized by a non-crossing planar graph. Such models were shown to cover the majority of structure defining base pairs and are thus often sufficient to do biologically relevant studies. Nevertheless, there is a large class of RNAs where the final structure is defined by the formation of non-nested base pairs, i.e. base pairs that have to be represented by crossing lines within a planar graph. Algorithms that consider such pairings often have a time complexity of O(n^5) or more depending on the imposed restrictions in which context crossing base pairs are considered. Thus, they are not feasible to be applied to long RNA molecules or in large scale studies. Within this project we want to tackle this problem with an iterative scheme of structure prediction. That is we will apply structure and interaction prediction approaches to predict nested and crossing structure elements in an hierarchical approach. While this will not necessarily identify the optimal crossing structure, it provides a most general model of crossing structure formation utilizing the speed of nested structure prediction approaches.
Genomic long range RNA-RNA interactions in flaviviruses
For the replication of flaviviruses, the formation of a specific long-range RNA-RNA interaction of the trailing untranslated regions of the virus genomes is crucial. This project aims at the prediction, comparison and modeling of these interactions using state-of-the-art tools for RNA-RNA interaction prediction and RNA alignment to identify common and species-specific details of these interactions.
Clustering SARS-CoV-2 spike protein sequences using autoencoder neural network
The aim of this project is to create a low-dimensional representation of SARS-CoV-2 spike protein sequences using an autoencoder neural network. Then, the low dimensional representation of sequences should be clustered using popular clustering algorithms such as TSNE and UMAP to explore if the original differences in sequences belonging to different clades (categories of sequences) are also maintained in lower dimensions. Related reading
Learn and predict nucleotide evolution in SARS-COV2 sequences using generative adversarial neural network
SARS-COV2 sequences mutate to multiple variants categorized into lineages and clades, some of which alter the pathogenicity of the virus making it more virulent. Using generative adversarial neural networks, artificial sequences can be generated using the knowledge of the evolution of SARS-COV2 sequences in the past. Ideally, the neural network should learn the 'edit' mechanism of the sequences that evolved in the past and should generate sequences based on the learned knowledge. The generated sequences should be compared with the true sequences to see how good the neural network performs.
Closed Topics
Integration of multi-modal omics analysis framework into galaxy.
Single-cell multimodal omics allows simultaneous profiling of different types information such as gene expression, DNA methylation, chromatin accessibility and surface protein levels of each individual cells. Such data enables cell characterization based on complex gene regulatory networks. Analysis of such datasets requires immense knowledge in programming languages such as R, python and statistics. To provide experimentalists with complex multimodal analysis workflows, this project aims to integrate computational workflows in Galaxy. We chose to integrate muon based workflows for such data analysis. The muon framework shares datatypes and features with an already Galaxy integrated framework called Scanpy. The objectives of this project are integration of muon multimodal analysis workflows into Galaxy and development of Galaxy training material based on the integrated workflows.
Creation of a tutorial for metagenomics data analysis
Emerging and powerful technologies like DNA sequencing are getting cheaper and therefore more accessible for many applications, e.g. in microbiome. This produces more data to analyze by scientists. Platforms like Galaxy help scientists to analyze their own (complex) data in a user friendly way. But they need to learn how to do that. The Galaxy Training Network (GTN) created an open-source e-learning infrastructure to provide a collection of tutorials developed and maintained by the worldwide Galaxy community ( https://training.galaxyproject.org ). Related to microbiome data analysis, the GTN currenlty offers 8 tutorials, built around a research story ( https://training.galaxyproject.org/training-material/topics/metagenomics/ ). The microGalaxy community aims to expand that catalog for whole-genome microbiome data analysis. The aim is this project is to create a tutorial using data from the Human Microbiome Project, tools an tutorials developed by the Hüttenhover lab to update the general overview tutorial.
Port an amplicon pipeline for microbiome data analysis to Galaxy
Project context: MGnify offers an automated pipeline for the analysis and archiving of microbiome data to help determine the taxonomic diversity and functional & metabolic potential of environmental samples. The pipeline even if documented is not really usable outside their resources. We would like to offer this pipeline for Galaxy users. This project aims to port the amplicon part of the pipeline into Galaxy. More information about the project can be found here: https://github.com/usegalaxy-eu/project-ideas/issues/31
Development of a Galaxy pipeline for detection of SARS-CoV-2 variants in wastewater samples
Nearly two years after the first report of SARS-CoV-2 in Wuhan, China, the COVID-19 pandemic has affected more than 485 million people. Wastewater surveillance has attracted extensive public attention during the SARS-CoV-2 pandemic, as a passive monitoring system to complement clinical and genomic surveillance activities. Several methods and protocols are already in place that effectively facilitate the detection and quantification of viral RNA in wastewater samples, and concentrations in wastewater have been shown to correlate with trends in reported cases. The Galaxy community has put a lot of efforts for continuous analysis of intra-host variation in SARS-CoV-2 ( https://galaxyproject.org/projects/covid19/ ), including development of workflows. The aim of this thesis are to: (i) Evaluate existing workflows for wastewater data analaysis; (ii) Expand and adaptat existing Galaxy workflows; (iii) Extensive test of workflows on mock and real data; (iv) Connect with existing data sources.
Machine-learning-based improvement of genome-wide target prediction of sRNAs
Identifying putative regulatory target regions of bacterial small (s)RNAs is still a challenging problem due to the high false positive rate of predictive methods. One way to greatly reduce false positives is to combine genome-wide predictions of related organisms, which is the core feature of the CopraRNA approach. This project aims at the identification and benchmarking of fast, simple but still sufficiently reliable target prediction workflows based on machine learning techniques to speedup CopraRNA.
Graph neural network based model for cancer driver prediction
- Python programming experience, Machine Learning
Gene prioritization based on pheotypes
Graph neural network-based method for single-cell rna-seq denoising, development of an automated scoring system for shared galaxy histories.
Due to the pandemic situation the interaction with the public face-to-face is not feasible. Therefore, we with the Street Science Community started the development of an online data analysis game ( http://streetscience.community/DNAnalyzer/ ). Within the game users will learn about the microbiome, DNA, sequencing and how to perform a data analysis. Galaxy provides the perfect platform to learn and later perform data analyses. To get scores for there data analysis gamer will share there histories. Within this project a tool will be developed where two shared galaxy history are compared and a score for the submitted history will be calculated. Further information about the topic can be found here: https://github.com/usegalaxy-eu/project-ideas/issues/28
Implementation of the infrastructure for an online & interactive game on DNA data analysis
Due to the pandemic situation the interaction with the public face-to-face is not feasible. Therefore the street science community started the development of an online data analysis game ( http://streetscience.community/DNAnalyzer/ ). Within the game users will learn about the microbiome, DNA, sequencing and how to perform a data analysis. Galaxy provides the perfect platform to learn and later perform data analyses. However, the gamer will register on a separate website connected with Galaxy and additionally tracks the successes and results of each gamer. The aim of this project is to implement a small webserver to register participants, display the videos, questions, puzzle, collect and display the score of the participants, and connect with the automated scoring system developed in an other master project. Further information about the topic can be found here: https://github.com/usegalaxy-eu/project-ideas/issues/22
Tool Resource Prediction for Genomic Datasets
The amount of CPU, RAM, and processing time for a tool to complete is dependent on the size of the input dataset and the complexity of the tool. By emulating these processing requirements with a benchmarking stress-testing tool such as stress-ng, we wish to accurately measure the footprint of the top set of tools on the UseGalaxy.eu workbench with repeated benchmarks, and try to predict their future footprint based on input data size and other extractable content, using machine-learning.
Integrating Multi-Omics Data and Pathway Structure with Explainable Graph Neural Network for Precision Medicine
Cancer is a disease that has afflicted the human species for ages, with each tumor possessing its own set of unique characteristics. As a result, people with comparable phenotypes respond to similar therapy in different ways. Largely unsolved, this area has started evolving over the past few decades owing to the availability of multi-omics data and large-scale data of cancer cell lines with different drugs approved for clinical trials. Consequently, a new area termed personalized tumor therapy has emerged. The goal of this research is to propose a novel method that aims at predicting drug response for cancer cell lines.
Analyzing miRNA processing patterns from single-cell small RNA sequening
Studying mRNA expression at single-cell resolution is a well established research area. There exists numerous experimental and computational methods to sequence and analyze the single-cell transcriptomcs. But all of them were designed and optimized to work with protein-coding genes only. Currently, there are only a very few experimental protocols to sequence small non-coding RNAs at single-cell level. It was shown that the existing computational methods that are used for single-cell mRNA-Seq can be used to cluster mature miRNAs and miRNAs also show cell-type specific expressions. In this project we aim to investigate whether miRNAs processing is cell-type specific. To achieve this, we use apply existing computational methods that were developed for bulk miRNA-Seq data to cluster individual cells based on miRNA processing patterns.
Analysis of CRISPR-Cas System in Marine Metagenomics
The 2020 Nobel Prize in Chemistry to Emmanuelle Charpentier and Jennifer A. Doudna for the discovery and development of CRISPR/Cas9 system highlight the importance of CRISPR-Cas systems. CRISPR-Cas system is an adaptive immune system found in prokaryotic lifeforms and is very diverse in nature. Cas proteins evolve rapidly. Here, we aim to analyse metagenomic data found in the marine ecosystem for the CRISPR-Cas proteins. The main focus is on class-2 type-V system, as the effector protein Cas12 from this system is a promising gene editing candidate. We used three databases for the analysis: Tara Oceans database with 2,631 draft metagenomes, MarRef dataset with 970 assembled metagenome, and IMG/VR dataset with above 90 percent completeness. We built four pipelines comprising different methods and tools for the whole analysis: pipeline 1 for detecting CRISPR-Cas systems and Cas12 proteins, pipeline 2 for transposons, pipeline 3 for repeats and their secondary structures, and pipeline 4 for the spacers and protospacer adjacent motifs (PAMs). We observed that the two tools (CRISPRCasIdentifier and CRISPRCasTyper) used for detecting CRISPR-Cas systems produce very different results, indicating the requirement for building a more accurate and robust tool for the identification of CRISPR-Cas systems. For different variants of Cas12 proteins, we detected different transposable elements. From the analysis of detected repeats, we identified 13 different secondary structures for the repeats found in type V systems and many having a conserved GAAAC or GAA sequence at the 3� terminus. During the spacer analysis, we detected different PAMs. Along with 5� T-rich PAMs, we also detected 5� A-rich PAMs along the upstream of detected spacer sequences. Our work shows that there is still a lot not known about Cas12 proteins, and further in-depth analysis can lead to a better understanding of Cas12 proteins and CRISPR-Cas systems.
Peak Calling und Workflow-Implementierung f�r das single cell Assay for Transposase-Accessible Chromatin Verfahren durch Sequenzierung
In der vorliegenden Bachelorarbeit wird das Verfahren scATAC-Seq und seine biologischen Hintergründe vorgestellt, welches offene Regionen im Chromatin des Genoms einzelner Zellen findet. Des Weiteren wird untersucht, wie die Daten von scATAC-Seq am besten verarbeitet werden, so dass mäglichst viele, hoch qualitative Informationen zu den offenen Chromatinregionen erhalten werden kännen. Dafür werden die Daten speziell vorverarbeitet, anschlie�end werden die Zellen teilweise gruppiert und schlussendlich die Peaks durch Peak Calling bestimmt. Im Anschluss werden die Peaks der einzelnen Zell-Gruppen wieder zusammengefügt, um sie schlussendlich zu vergleichen und auf verschiedene Qualitätskriterien zu überprüfen. In dieser Arbeit werden vier verschiedene Methoden vorgestellt, um diesen Ablauf, mit kleineren Änderungen, durchzuführen. Dazu werden ungefähr 3000, durch scATAC-Seq gewonnene, menschliche Zellen durch die verschiedenen Methoden bearbeitet und untersucht. Anschlie�end werden die Ergebnisse verglichen. Die Resultate zeigen Potential zur Feststellung von diesen Arten der Verarbeitung der Daten. Dabei kann in dieser Arbeit aber nicht eine Methode klar empfohlen werden, da es tiefere Untersuchung der gewonnenen Peaks benätigt, um ein abschlie�endes Urteil über die Qualität der Ergebnisse zu erhalten.
How genomes are shaped by direct and indirect selection pressure: a study in in silico experimental evolution
What are the different pressures that can shape genomes in evolution? The aim of this thesis is to focus particularly on the case of reductive genome evolution, i.e. the reduction of genome size over time as observed in some marine cyanobacteria. To address this topic, is used silico artificial evolution, a method in which genomes of virtual organisms evolve via computer simulations, and particularly the Aevol model. Several experiments have been conducted to test the effect of several parameters (population size, mutation rate, and selection strength) on the genome structures and other selection measures (e.g. fitness, robustness).
Drug repurposing and adverse event prediction through EHR knowledge graph completion
Drug repurposing is the process of discovering new indications of existing, approved drugs while the latter comprises identifying probable harmful effects of known or novel drugs. It is normally done by in vivo and vitro methods which are of high costs, slow results, and limited sample size besides some ethical issues. Therefore, effective computational methods are needed. In this project, we investigate EHR data and create a machine learning model using the relational graph attention network to predict the potential links between entities of interest link drugs, diagnoses, etc.
TAD detection in Hi-C data
Within the 3D space of a cell, DNA forms a structure resembling a ball of wool. The points of contacts of the DNA with itself, called DNA interactions, have "threads" within this "ball of wool" that form smaller loop-like structures called DNA loops. At a close genomic distance, these loops are called topological associated domains (TADs). A z-score based detection algorithm currently exists to detect these TADs, but lacks the ability to detect overlapping TADs and hierarchical structures. In this master project a new approach based on neural networks should be investigated and implemented.
CRISPR-Cas9 Off-Target Prediction Methods
- Python programming experience and Machine learning
Assigning tissues of action to genomic loci associated with kidney function
- R-Programming experience
Website for visualization and publishing of single-cell RNA-sequencing (scRNA-seq) datasets
Rri prediction ranking.
While IntaRNA is a state-of-the-art method to predict RNA-RNA interaction, it is not clear if this prediction will happen in nature. We are building a support vector machine model which should validate the in silico interaction on its occurrence in vivo and can therefore be use to post filter interaction predictions. RNA-RNA predictions can experimental be verified by mutation experiments. Based on this experimentally verified interactions we are building we are developing a positive and negative trainings set. This dataset is already discussed in CopomuS by Raden et al.
Machine Learning for Gene Discovery
Current approaches to finding new genes have a high false positive rate. Help us develop a tool to filter candidates in this straightforward Machine Learning project. You will expand on our Scikit-Learn python code and work with state of the art bioinformatics tools. The project covers feature extraction, filtering and classification on an annotated dataset of alignment files.
Binding Affinity Prediction of Protein-Ligand Complexes
This project predicts the binding affinities between the potential drugs (ligands) and the target proteins responsible for diseases or conditions.It uses the data of protein-ligand complexes stored in the PDBBind database to train a machine learning model.From every complex, features related to proteins are extracted by using the pocket-finding software fpocket. Four ML models were studied in this project - Simple Linear Regression, Random Forest Regression, Support Vector Regression, and Rotation Forest Regression.
Multi Protein-Ligand Interaction Prediction using Machine
In this thesis, a voxelization procedure was developed and applied to targets (or proteins) in the PCBA (PubChem BioAssay) dataset to create a three-dimensional image of the protein-ligand binding site. These voxelization data were used to train a neural network, more specifically a CNN autoencoder to featurize the binding site by keeping only the most relevant information. This information was then combined with ligand features (which have been calculated using the RDKit descriptor tool from the RDKit library) and finally using machine learning techniques, protein-ligand binding affinity was predicted for each protein-ligand pair.
BioBlend to Galaxy API extension and OpenAPI specification
BioBlend is a Python library to enable simple interaction with Galaxy via the command line or scripts.Galaxy is a data analysis platform for accessible, reproducible and transparent computational research. It includes a web interface through which users can design and perform tasks in a visual and interactive manner. The Galaxy server also exposes this functionality through its REST-based Application Programming Interface (API). In this project several important new features were introduced into BioBlend and the Galaxy API and a tutorial written for future developers.
Predicting Hi-C contact matrices using machine learning approaches
In recent years, many studies have shown that the three-dimensional conformation of genomes is a key factor for understanding several important mechanisms on the molecular biological level. However, the Hi-C experiments typically conducted to measure this 3D-structure are still expensive, so that computational methods for predicting the spatial chromatin organization from existing data have recently become subject to research. In this thesis, two machine learning approaches are investigated with regard to their usability for predicting chromosome conformation in form of Hi-C contact matrices from ChIP-seq data. Here, the first method adapts and extends an existing dense neural network architecture for Hi-C matrix predictions, while the novel second method, Hi-cGAN, leverages techniques from image synthesis, especially conditional generative adversarial networks (cGANs). While the dense neural network approach can neither produce satisfactory predictions for the Hi-C matrices of human cell lines GM12878 and K562, nor for Drosophila Melanogaster embry- onic cells in the chosen setting, Hi-cGAN yields encouraging outcomes in all three cases.
Within the 3D space of a cell, DNA forms a structure resembling a ball of wool. The points of contacts of the DNA with itself, called DNA interactions, have "threads" within this "ball of wool" that form smaller loop-like structures called DNA loops. At a close genomic distance, these loops are called topological associated domains (TADs). A z-score based detection algorithm currently exists to detect these TADs, but lacks the ability to detect overlapping TADs and hierarchical structures. In this master project a new approach based on machine learning classifiers should be investigated and implemented.
Hi-C interaction matrix prediction based on protein location
In the 3D space of a cell the DNA forms a structure that looks like a ball of wool. Obviously, many points of contacts of the DNA wire with itself, called DNA interactions, exists in this "ball of wool" and form a structure including DNA loops. These loops contribute to the stability of the DNA and do play an important role in gene regulation. Current research shows that proteins bind on the DNA at these loop locations and contribute to the formation of loops and therefore for the whole structure. The structure of the DNA can be read out with a technique called Hi-C and the resulting data is represented as an interaction matrix in the computer. However, Hi-C is an expensive technique and for many cell types no data is existing while at the same time the technique to read out the position of proteins on the DNA (ChIP-Seq) is quite cheap and a lot of data is online available. The goal of this master project is to use a random forest approach to predict Hi-C interaction matrices by learning the location of proteins. Based on the results of the master project from Andre Bajorat, possible optimizations for this model are investigated.
Hierarchical TAD detection in Hi-C data
Within the 3D space of a cell, DNA forms a structure resembling a ball of wool. The points of contacts of the DNA with itself, called DNA interactions, have "threads" within this "ball of wool" that form smaller loop-like structures called DNA loops. At a close genomic distance, these loops are called topological associated domains (TADs). A z-score based detection algorithm currently exists to detect these TADs, but lacks the ability to detect overlapping TADs and hierarchical structures. In this Bachelor thesis a method to detect these was developed and implemented.
Creating a linkage analysis workflow in Galaxy
Classical linkage analysis is the method of looking for genes that are inherited together in a family tree, which has been now superseded by variant analysis in the era of high-throughput sequencing, but is still relevant in rare disease studies. The Galaxy project is a free and open-source web-based platform for bioinformatic research, and offers users an interactive drag-and-drop avenue to perform their analyses. This project would involve wrapping tools into Galaxy, and chaining them together in a workflow for public user access. Optionally, training material can be written to guide users through the analysis. Applicants need only to know basic HTML/XML and Markdown.
Integrating a haplotype analysis visualization into Galaxy
The study of haplotypes is relevant to pedigree analysis, which looks for mutations inherited from founders that manifest only after many generations due to the semi-random/coalescent nature of inheritance. This project will be wrapping an existing haplotype visualization tool into Galaxy, an open source web-based bioinformatic analysis environment, in order to reach a greater number of users. Applicants must know basic Javascript and HTML/XML.
Multi-site RNA-RNA interaction prediction
Accessibility-based RNA-RNA interaction prediction methods are typically modelling a single block of consecutive inter-molecular base pairs. Thus, interaction pattern that consists of multiple concurrently formed blocks can not be predicted. Within this project, we are developing and testing possibilities to efficiently predict concurrent blocks of interaction within an accessibility-based prediction model. The approach will be based on IntaRNA , which is one of the state-of-the-art programs for RNA-RNA interaction prediction. The respective extensions of the IntaRNA package will be integrated into the main package for external use and further development.
Graph neural network-based method for disease gene prioritization
The uncovering of genes linked to human diseases is a pressing challenge in molecular biology and precision medicine. This task is often hindered by a large number of candidate genes and by the heterogeneity of the available information. Therefore, computational methods for the prioritization of candidate genes are needed to deal with these problems. A number of methods have been proposed and have shown potential results. However, there is still a need to develop more accurate disease gene prioritization methods. The aim of this project is to develop a graph neural network-based method for disease gene prioritization. This choice is supported by (1) graphs are a common and natural way to represent the gene relations, and (2) Neural network for graphs are now state-of-the-art in graph (graph node) classification problem.
A deep learning model to detect triple helices in genomics data
Triple helix formation has been known to interfere in the gene expression process by often modifying the transcription of targeted genes. Therefore, understanding how and where triple helices form is crucial to better understand gene expression. To identify regions where triple helices formed, wet-lab experiments and some computational methods are performed. However, non-existing methods are based on machine learning. Here we would like to propose a deep learning-based method to detect triple helices in genomic.
CRISPR/Cas9 is a unique and robust gene-editing method that has the ability to accurately edit target genes in a wide variety of organisms. However, experimental results indicate that the binding and cleavage of off-target sequences are a major concern for the application of CRISPR/Cas9 and the sgRNAs should be designed in such a way that the impact of off-targets is minimized. Several computational methods have been proposed as a substitute for expensive lab experiments to predict off-targets. Yet, powerful approaches need to be devised to make precise predictions. Here we aim at proposing a Graph Convolutional Network model to predict off-targets of CRISPR/Cas9. The proposed model is expected to overcome following typical challenges: data imbalance, robustness, prediction crossing different cell-types.
Ranking of mutations in RNA-RNA interactions
Point mutations are a common way to verify RNA-RNA interactions. So far, the selection of the position and the introduced mutation is done manually based on expert knowledge of the experimenter. Within this project, we are developing and testing possibilities to automatically evaluate and rank candidate mutations concerning their potential for interaction validation. The approach is based on IntaRNA , which is one of the state-of-the-art programs for RNA-RNA interaction prediction. The respective extensions of the IntaRNA package are integrated into the main package for external use and further development.
Benchmarking Big-Data Workflows Across European Academic Clouds to Evaluate Cloud Bursting Strategies
The Galaxy-Project, a web platform for big-data biomedical research, needs a lot of computational resources and cloud bursting, e.g. sending excess workloads to the cloud, may be a solution in high-demand situations. But how do the various academic clouds, spread across Europe, perform? May one be better suited than the other for a specific workload? Does physical distance and connectivity between data centers play a big enough role? What about the underlying infrastructure? Do they make a difference, even if the actual instance size is the same? In this work, where I benchmarked various academic clouds in Europe, I want to answer these questions and even offer a framework for future benchmarks, as the need for benchmarking more clouds in the future arise.
Base-pair probabilities for accessibility-based RNA-RNA interaction prediction
Computing base pair probabilities of RNA-RNA interactions allows for a number of useful applications, such as the creation of dot plots, which allow for easy and fast comparison between different base pairing patterns. A number of tools exist that already incorporate base pair probability calculation, such as RNAcofold and NUPACK. However these tools are limited to a specific algorithm for the optimal interaction computation that might lack in precision or computational efficiency depending on the application. IntaRNA on the other hand is a highly exible RNA-RNA interaction prediction tool that implements a large number of different prediction algorithms, including very efficient seed-constraint methods. This thesis explores the benefits and difficulties of introducing the computation of base pair probabilities into a number of IntaRNA predictors, including seed-based predictors. For this reason IntaRNA was extended with the ability to compute base pair probabilities, depending on the chosen prediction model. The output is provided as a dot plot to allow for easy investigation. Finally, a number of applications are presented that benet from base pair probabilities, including the comparison between verified and non-verified RNA-RNA interactions and the detection of multi-site RNA interactions. Based on these results, potential improvements for IntaRNA's prediction model are discussed, including different approaches for the accessibility computation and the incorporation of sequence conservation into the prediction estimation.
In the 3D space of a cell the DNA forms a structure that looks like a ball of wool. Obviously, many points of contacts of the DNA wire with itself, called DNA interactions, exists in this "ball of wool" and form a structure including DNA loops. These loops contribute to the stability of the DNA and do play an important role in gene regulation. Current research shows that proteins bind on the DNA at these loop locations and contribute to the formation of loops and therefore for the whole structure. The structure of the DNA can be read out with a technique called Hi-C and the resulting data is represented as an interaction matrix in the computer. However, Hi-C is an expensive technique and for many cell types no data is existing while at the same time the technique to read out the position of proteins on the DNA (ChIP-Seq) is quite cheap and a lot of data is online available. The goal of this master project is to use machine learning and neural network regression models/approaches to predict Hi-C interaction matrices by learning the location of proteins.
Hi-C interaction matrix correction
In the 3D space of a cell the DNA forms a structure that looks like a ball of wool. Obviously, many points of contacts of the DNA wire with itself, called DNA interactions, exists in this "ball of wool" and form a structure including DNA loops. However, many o f these contacts are random contacts or measurement errors and need to be corrected. A Python implementation is existing but comes to its limits for high resolution data caused by high memory usage. This master project should try out if a more efficient algorithm is existing and if an implementation in C++ is possible with less resources.
Statistical significance for RNA alignment predictions and an evaluation schema for multiple sequence alignments in local mode
To evaluate the predicted alignment of the RNA sequence-structure alignment tool LocARNA, so far the alignment score of the has been provided. The score is the optimal value of the objective function from the LocARNA optimization problem. However the scores are not very informative for the end-users, e.g. how well the predicted alignment is significant and likely to occur by chance. It would be desirable to have a statistical measure that not only rank the quality of a given alignment but also make it possible to compare the prediction to other alignment tools and the reference alignment. In this thesis an empirical p-value for LocARNA will be developed. Furthermore, to evaluate a multiple sequence alignment results a suitable scoring schema for multiple sequence alignments will be investigated.
p-value statistics of IntaRNA predictions
The RNA-RNA interaction prediction tool IntaRNA provides sophisticated and highly accurate results in terms of free energy minimization. Since it is non-trivial for users to interprete the provided free energy terms, this project investigates ways how energy statistics and respective p-values can be provided.
RNA-RNA interaction prediction via seed extension
Our group develops the tool IntaRNA , which is one of the state-of-the-art programs for RNA-RNA interaction prediction. We are continously extending the tool (C++, c++11, boost, doxygen, autotools, openmp) that is hosted on Github BackofenLab/IntaRNA . This project aims at the implementation and testing of seed-extension strategies to speedup and improve IntaRNA's predictions. The developed extensions to IntaRNA will be integrated into the main package for external use and further development.
Implementing bioinformatics algorithms for teaching
Within the last years, we have created interactive implementations of various algorithms discussed in our lectures. These are freely available at the Freiburg RNA tools - Teaching section of our public webserver. The algorithms are implemented in Javascript and are accompanied with according visualizations to better understand each approach.
Identifying and analysis of new anti-CRISPR proteins
CRISPR-Cas system of archaea and bacteria provides resistance against viruses and phages. Since phages have a constant battle against prokaryotic; recent discoveries show that have described phage genes that inhibit the CRISPR-Cas function. These are, however, likely to be quite diverse in function as they can interfere with the CRISPR-Cas response at different stages. This work aims to develop a new method of identifying a new family for anti-CRISPR proteins based on homology search.
Identification of CRISPR arrays using machine learning approach
Archaea and Bacteria are known to acquire immunity against viruses and plasmids through a widely conserved RNA-based gene silencing pathway. This mechanism involves non-coding RNA that originates from Clustered Regularly Interspaced Short Palindromic Repeats, and CRISPR-associated proteins (CRISPR-Cas system). CRISPRs consist of identical repeats that are between 20 to 47 base pairs in length, separated from each other by unique spacer sequences of similar length (27 to 72 base pairs). Most CRISPR arrays are flanked on the upstream (5') side by a leader sequence of 60 to 500 base pairs. These leaders often contain low complexity sequences and are rarely conserved between more distantly related species. Finally, there are the Cas genes, which are usually located directly up- or downstream of CRISPR array, however, they can also be found in very different locations. These genes encode protein complexes which work together with CRISPR arrays to confer the host cell with an adaptive immune system to fight invading viruses and plasmids. This work aims to develop a new tool to detect a CRISPR-Array using machine learning approaches.
Crossdating of wood samples using MICA-aligned density profiles
Our group develops the tool MICA , which enables Multiple Interval-based Curve Alignment of arbitrary curve/profile data. It is currently applied to derive meaningful consensus data of experimentally measured wood density samples. Within this project, we will use MICA density profile alignments to evaluate their potential for crossdating, i.e. the time annotation of wood samples. Given the increased information compared to standard methods based on ring widths, the approach should yield high precision even for small wood samples (few rings).
Modular benchmark pilot framework for evaluating RNA alignment tools
To benchmark the quality of RNA alignment algorithms, it is important to validate their performance and compare with similar tools. For this purpose a benchmark-pilot framework to automatically benchmark RNA alignment algorithms such as LocARNA and SPARSE will be developed. The aim is to have a modular and easily extendable framework to evaluate various range of tool for different computation platforms from PCs to High Performance Computing grid systems. The task of this project is focused on development of the benchmark-pilot code in python using SnakeMake workflow manager, to replace the previously deployed system.
RNA-RNA interaction prediction for long molecules
Our group develops the tool IntaRNA (see PhD-thesis A. Richter for details), which is one of the state-of-the-art programs for RNA-RNA interaction prediction. We are currently reimplementing and extending the tool (C++, c++11, boost, doxygen, autotools, openmp) that is hosted on Github BackofenLab/IntaRNA . This project aims at the implementation and testing of strategies to enable predictions for very long input molecules, for which the standard approach might break due to extreme memory consumption. The idea is to apply a window-based segmentation, which requires a special result handling to avoid duplications in the output. The developed extensions to IntaRNA will be integrated into the main package for external use and further development.
Constrained RNA-RNA interaction prediction
Our group develops the tool IntaRNA (see PhD-thesis A. Richter for details), which is one of the state-of-the-art programs for RNA-RNA interaction prediction. We are currently reimplementing and extending the tool (C++, c++11, boost, doxygen, autotools, openmp) that is hosted on Github BackofenLab/IntaRNA . This project aims at the implementation and testing of new prediction modi, which incorporate additional constraints to further improve prediction quality. To this end, an IntaRNA benchmark set and according protocol is compiled that is used in the course of the thesis to evaluate the newly integrated features. Furthermore, statistics on known interaction and single-molecule structures will provide the parameters for the new constraints. The developed extensions to IntaRNA will be integrated into the main package for external use and further development.
Within the last years, we have created interactive implementations of various algorithms discussed in our lectures. These are freely available at the Freiburg RNA tools - Teaching section of our public webserver. The algorithms are implemented in Javascript and are accompanied with according visualizations to better understand each approach. In the course of this project we are focusing on sequence alignment algorithms as taught in our Bioinformatics-1 and -2 lecture.
Prediction of non-consecutive RNA-RNA interactions
- exam in "RNA bioinformatics" lecture
- C++ experiences
Integration of BioJS into Galaxy
Galaxy is an open, web-based platform for data-intensive research. The University of Freiburg is running a Galaxy server to serve all different needs of our researchers. Visualization is a key aspect in the understanding of data analysis for medical and biological research. The Javascript library BioJS provides powerful visualization of multiple biological data. The overall aim is to integrate specific BioJS modules into Galaxy via its plugin architecture.
Large-scale clustering of non-coding RNAs in the Galaxy framework
Clustering of putative RNAs is currently the major approach for functional annotation of putative ncRNAs detected in genome-wide screens. GraphClust is one of the few approaches that can cluster hundreds of thousands putative ncRNAs as it is based on an alignment-free approach using an advanced graph kernel. The candidate clusters are iteratively retrieved and refined using RNA alignment tools. However the clustering pipeline requires in-depth knowledge as several tools have to be installed and configured. The goal of this project is an extension of the GraphClust tool using Galaxy framework that makes it possible to (a) perform the clustering of RNAs via a web interface, (b) run the computations on various operating systems and computation frameworks, (c) freely customize and extend the generic pipeline for specific needs. The project involves also attempts to apply the Galaxy workflow on a metatranscriptome dataset.
Characterization of ribosomal footprints with use of graph kernel based approaches
Ribosome profiling is an emerging technique that with use of deep sequencing methods, gives new insight to translation of proteins from single codon to genome scale. In comparison to former available methods microarrays and RNA-seq, Ribo-seq solely considers active mRNAs at translation phase in a cell which prepare information for protein synthesis. This novel charac- teristic of Ribo-seq provides new data with focus on translation level. The obtained patterns of ribosomal footprints may reveal new aspects in trans- lation field. The aim of this work is to classify Ribo-seq profiles according to different conditions and find clusters with respect to Ribo-seq profiles. This is done by a tool named BlockClust, which is based on a graph kernel method called Neighborhood fast graph kernel (NSPDK). BlockClust en- codes expression profiles data to graphs format and employ NSPDK method for achieving a high performance. Although BlockClust previously applied for clustering non-coding RNAs from their RNA-seq expression profiles, it can also be adapted to use for clustering and classification tasks on other types of data e.g. Ribosome profiling. Therefore, we have adapted Block- Clust by defining new attributes for finding patterns in Ribo-seq data and adding them to the former available set of attributes. Moreover, we per- formed an optimization by using different parameter sets. Furthermore, we showed that it is possible to employ BlockClust on Ribosome profiles. We achieved a good performance in classification of these profiles.
Approximate nearest neighbor query methods for large scale structured datasets
The task of efficiently finding the most similar representatives in a large set of entities is at the core of many problems in a variety of applications, ranging from chemoinformatics to recommendations systems; when the objects of interest are structured entities the problem becomes harder. In these cases structured instances are explicitly converted in sparse vectors that live in very high dimensional spaces (even millions of features). Exact algorithms have unfortunately a computational complexity that scales quadratically with the number of instances times the representation length of each instance, hence these approaches cannot be used when we have a large number of structured instances. A possible solution is to accept approximate results to gain efficiency. The candidate will extended one such approximate technique (the MinHash approximate nearest neighbor scheme) to efficiently solve the neighbor query in sub-linear time. The overall goals of the thesis were to provide an efficient and simple to use implementation for approximate nearest neighbor queries for large collections of high dimensional sparse vectors.
Learning to design RNA polymers with graph kernels
Graph data structures allow us to model complex entities in a natural and expressive way. In the machine learning literature, several types of discriminative systems that can deal with graphs in input are known (e.g. recursive neural networks, graph kernels, graphical models, etc), however, there are few generative approaches that can sample structures belonging to a desired distribution or class. The task of generating samples from a given distribution when this is accessible only via a finite number of examples is well developed when the domain of interest can be embedded in a vector space. The extension of these approaches to structured domains (i.e. where instances are strings, trees, graphs or hyper graphs) is however substantially less developed. One approach for learning constructive systems is based on a variant of the Metropolis Hastings (MH) algorithm guided by an efficient graph grammar, which, crucially, can be efficiently induced from an example set. Such a neighborhood graph based grammar is suitable when the feasibility constraints are local in nature. RNA polymers, which form structures comprising hundreds of nodes (nucleotides), exhibit however dependencies between distant portions of the structure. In order to extend the constructive system to the RNA domain, Mr. Mautner has introduced a multi level strategy based on a notion of graph minors, i.e. graphs obtained by edge contraction operations. An edge contraction is an operation which removes an edge from a graph while simultaneously merging the two vertices that it previously joined. By carefully defining a domain dependent contraction strategy, Mr. Maunter was able to operate on smaller graphs for which local rules are sufficient to capture the feasibility constraints.
Reinforcement learning techniques in RNA inverse folding
A non-coding RNA molecule functionality depends on its structure, which in turn, is determined by the specific arrangement of its nucleotides. The inverse folding of an RNA refers to the problem of designing an RNA sequence which will fold into a desired structure. This is a computationally complex problem. Algorithms which solve this problem take different approaches, but they share the following attitude: They start from an initial sequence or population and try to move it towards a desired product by performing normal or optimized search methods. RNA inverse folding programs are given different constraints such as GC-content ranges or basepair or nucleotide configurations. The output is normally one or more sequences which fold to the target structure. This work introduces a basic system that given a set of sample RNA secondary structures, produces models which generate structures similar to the sample set. The objectives and constraints are automatically extracted from samples. For doing this, a system is designed which generates models by performing learning on families of RNA sequences. This system consists of two subsystems: one responsible for decomposing secondary structures of sample RNAs into structural features and building a structural features corpus. It also extracts neighborhood connectivity models of structural features in the form of N-grams. The other subsystem is a reinforcement learning framework which uses the corpus and connectivity rules to produce models for generating structures which are similar to the samples. Results in this work show that the current system is able to produce models from RNA families which have a symmetric shape. To make the system capable of dealing with a broader range of RNA families and producing structures with functionalities identical to the sample structures, a refined feature extraction module has been added to the system. This module extracts the GC-content, size and local information of structural features and builds a refined feature corpus. This can provide the basis for a new set of experiments and a start point for producing models with practical applications.
Explorative Enumeration of large energy landscapes
- C++ implementation of the explorative energy landscape enumeration using the Vienna RNA package library.
- Parallelization, benchmarking and implementation tuning.
- Application of the developed program to large RNA molecules.
- Creation of a complete pipeline to study kinetics of RNA molecules including visualization.
Investigating LocARNA parameter search space by using automatic configuration methods
In recent years many novel RNA species have been discovered by new sequencing techniques. The correct classification of these RNAs into new and existing families heavily relies on accurate sequence-structure alignment tools, which makes it desirable to constantly improve their alignment quality. Therefore, having a high-performing RNA alignment tool is of fundamental importance in the field of computational biology. LocARNA implements an efficient heuristic version of Sankoff's accurate but computationally expensive algorithm for simultaneous sequence and structure alignment. The use of heuristics makes the algorithm applicable in practice, but also forces the inclusion of many additional parameters. Since the performance of an algorithm depends on the parameter setting, it is desirable to optimize these settings in order to improve alignment results. One way to find optimal parameter configurations is to use an automtic algorithm configuration technique. In this work the state of the art algorithm configuration tool SMAC is applied to improve LocARNA 's default parameter settings. The optimization focuses on fundamental parameters of the LocARNA algorithm. Both global and local alignment cases are covered, although for the local case this marks the first in-depth optimization attempt. Hence this work also introduces a complete local alignment parameter optimization pipeline for LocARNA. As a result, improved default parameter settings as well as different input scenario settings for both the global and local alignment cases are proposed. Notably, the average alignment quality of the local case on an extension of the Bralibase dataset was improved up to 26%. In conclusion, the presented work not only managed to optimize LocARNA 's local alignment but also provides a solid foundation for further works on parameter optimization using the implemented pipeline.
Graph-based clustering of CRISPR-Cas systems
- Find the best way to encode the CRISPR-Cas system as a graph that represents nature as realistically as possible.
- Use EDeN to perform unsupervised clustering of all available CRISPR-Cas systems in bacteria and archaea.
- Compare results to previous classification systems.
Learning to Construct Graphs with Real Vector Attributes Using Graph Kernels
Graph data structures allow us to model complex entities in a natural and expressive way. In the machine learning literature, several types of discriminative systems that can deal with graphs in input are known (e.g. recursive neural networks, graph kernels, graphical models, etc), however, there are few generative approaches that can sample structures belonging to a desired distribution or class. The task of generating samples from a given distribution when this is accessible only via a finite number of examples is well developed when the domain of interest can be embedded in a vector space. The extension of these approaches to structured domains (i.e. where instances are strings, trees, graphs or hyper-graphs) is however substantially less developed. While specialized applications exist, e.g. sampling phylogenetic trees, sampling dependency graphs for structural learning in graphical models, or sampling large Web like networks, data driven approaches that can deal with general types of graphs, are still in their infancy. Important applications of a successful generative graph system include the de-novo generation of molecular graphs for drugs and RNA biopolymers with user defined properties derived from prototypical natural examples. In these cases the spatial information of the atom arrangement becomes important for the determination of the associated physicochemical properties. There is therefore the necessity to upgrade these generative graph systems to deal with graphs that can encode spatial information in the form of multiple real valued attributes (e.g. 3D coordinates, distances, angles). In the Thesis the candidate will address the constructive learning problem using a variant of the Metropolis Hastings (MH) algorithm tailored for structural data types. She will upgraded the efficient graph grammar approach of a pre-existing code base to deal with graphs with real valued attributes.
A graph kernel approach to the identification and characterisation of structured noncoding RNAs using multiple sequence alignment information
Structured noncoding RNAs perform many functions that are essential for protein synthesis, RNA processing, and gene regulation. Structured RNAs can be detected by comparative genomics, in which homologous sequences are identified and inspected for mutations that conserve RNA secondary structure. To detect novel RNA classes in bacteria and archaea, a variety of bioinformatics strategies have been used, e.g. looking in upstream regions of protein coding genes for cis-regulatory RNAs. To identify ncRNAs independently from protein coding genes, Z. Weinberg has proposed a computational pipeline based on an initial BLAST clustering further refined by looking into secondary structures with CMfinder. The identified structures are then used in homology searches to find homologues that allow CMfinder to further refine its structural alignment. The resulting alignments are scored and then analysed manually to identify the most promising candidates and to infer possible biologic roles.
Interactive de novo molecular design
Synthesis of small molecules that improve on the curative properties of existing drugs or that are effective in previously untreatable illnesses is a very hard task, a task on which pharmaceutical companies are investing enormous amounts of resources. Computational methods become therefore an interesting solution when they can effectively replace the time consuming and expensive design, synthesis and test phases. Since de novo molecule-design systems have to explore a virtually infinite search space, exhaustive searching is infeasible, and they typically resort to local optimisation strategies. To date, one of the most critical aspects is the reliability of the evaluation function invoked to judge the quality of molecules that can be (and generally are) very different from those used in the function induction phase. One possible approach to overcome this difficulty is to integrate the expert knowledge of (medicinal) chemists in the evaluation loop. Doing so in an efficient way is not a trivial task, since one has to 1) minimise the number of times the system resorts to the expensive human oracle, and 2) use a form of interaction suitable for humans.
CRISPRloci visualization
- Find the best way to modify/customize the CGView tool in order to work for our purpose (Java).
- Integrate into CRISPRloci web server (JSP,Html,Java).
RNA energy landscapes with pseudoknot structures
Most studies of RNA kinetics use nested structure models to enable at least moderate sequence lengths. Nevertheless, there is evidence that pseudoknot structures are important for the function of some RNA molecules. Thus, ommitting them in kinetics fosters wrong results. This project will compare kinetics based on energy landscape with and without pseudoknot structures. Furthermore, new strategies have to be explored in order to face the vast increase of the landscape size to enable reasonable studies.
- C++ implementation of the explorative energy landscape enumeration strategies presented in our article in concert with the identified strategies by Bettina Hübner using the available algorithm implementations from the Energy Landscape Library (ELL) .
Similarity notions for RNA kinetics comparison
For larger RNA molecules it is often not computationally feasible to enumerate their whole energy landscape. Thus only partial fews of the landscapes are used to compute the kinetics of the respective molecule. Within this project, different strategies are explored to measure the similarity of kinetics, i.e. to evaluate how well the coarse grained model reflects the kinetics based on the complete energy landscape information.
Generating a local ncRNA benchmark set to evaluate local RNA alignment tools
Multiple local alignment of RNA sequences is by now still a challenging problem as parameters for already existing tools are not optimized yet for the local alignment case. The first step to solve this problem is the generation of a local benchmark set to be able to evaluate existing local RNA alignment tools. The main part of this work is the implementation of a pipeline to append genomic context of a given length to an already existing (global) benchmark set. A simple evaluation of LocARNA on the local ncRNA benchmark set and a random test set will be performed.
Differential Benchmarking of CopraRNA - Finding the optimal input for a specific question
- Generate an extensive dataset for differential benchmarking. (also non enteric bacteria)
- Write scripts that automatically run and evaluate the CopraRNA runs.
- Draw conclusions and develop guidelines for input organism selection.
Java GUI for Multiple Interval-based Curve Alignments (MICA)
- The MICA reimplementation of the core algorithm in Java.
- Development of a Graphical User Interface for MICA in Java.
- Application of the new tool on tree growth data and other data from literature, evaluating the new implementation.
Improving miRNA target prediction in humans using a highly descriptive graph-based, machine-learning model
- Compile training and test datasets of miRNA-mRNA interactions.
- Generate highly sensitive candidate interaction sites.
- Integrate all possible features into a novel graph model.
- Train and test machine learning model using different settings and parameters and use model to filter candidates.
- Compare results to existing tools.
Pruning strategies for large energy landscapes
The energy landscape framework enables the study of the folding kinetics of molecules. For instance the structure formation process of single RNA molecules or the interaction formation of two RNAs. To this end, transition probabilities of one structure to possible successive structures have to be identified. Unfortunately, there is an exponential growth of possible structures a molecule can adopt and accordingly an exponential growth of the energy landscape. One approach to face this problem is to group structures into "macro-states" and to consider only transitions between such structure ensembles. But their number is often still too large to enable kinetics computations. Within this project, different approaches to prune the macro-state energy landscape represenation are tested in order to reduce the according transition encoding to a feasible size open for kinetics computations. The pruning strategies are subject to quantitative and qualitative evaluations concerning reduced computational requirements and preserved kinetics quality.
RNA Barcodes for High-Throughput Sequencing Experiments
CLIP-seq is a method for genome-wide screening of interactions between RNAs and RNA-binding proteins. iCLIP is an extension of CLIP-seq that allows locating RNA-protein interactions with nuceleotide precision. iCLIP employs random sequence tags in to enable calculation of the number of binding events from PCR amplified source material. Errors introduced into these sequence tags during amplification or sequencing can lead to serious overestimation of binding events. This thesis examins the suitability of RNA barcodes developed for multiplex sequencing assays to prevent or mitigate this effect.
Graph-kernel based aromaticity prediction
- Data collection and preparation for training and testing of the SVMs.
- Evaluation of the NSPDK prediction using the available tools from the GGL- and NSPDK-package.
Atom mapping of chemical reactions via Constraint Programming
- C++ implementation of the CP-based atom mapping approach for even ITS rings presented in our article using the Gecode library.
- Extension of the CP-approach to odd rings.
- Evaluation of the approach using atom mappings of known chemical reactions.
Cluster based prediction of SH2 domain-peptide interactions using Graph Kernel
- Data colloection from several high-throughput experiments (e.g. microarray) and compile them to prepare the training and test sets.
- Optimise hyper-parameters for the NSPDK kernel.
- Use Support Vector Machine (SVM) based on NSPDK kernel for the classification.
Large Scale Activity Profile Induction for Small Molecules
- efficiency in the train and in the test phase: some bioassays with hundreds of thousands instances are available; in the test phase 30M compounds have to be screened;
- accuracy: the predicted activity profile has to be sufficiently close to the true activity profile to provide a reliable localization of compounds in activity space;
- semi-supervised mode of training should be possible: since many bioassays contain information only for few tens to hundreds compounds it is necessary to make the best use of the vast amount of unsupervised information available;
In this thesis the candidate will use a graph kernel (NSPDK) to train a linear max margin model via fast stochastic gradient descent technique. The candidate will set up the necessary infrastructure to perform and monitor the in-silico predictions and develop novel techniques for large scale semi-supervised problems in the chemoinformatics field.
Analysis of CLIP-seq and PARCLIP data for Argonaute to identify miRNA target sites
- Collect PARCLIP and HITS-CLIP data for mammals and identify the corresponding mRNA sequences to the CLIP sequences.
- Develop quality measures to map microRNA to each CLIP sequence.
- Explore general properties and uniqe characteristics of collected data. How do these datasets correspond to data found in microRNA databases?
- Optimise IntaRNA parameters to identify correct target sites so that the predictions are very sensitive.
Learning binding preferences of RNA-binding proteins using in vitro affinities and in vivo binding sites
Structural elements in long non-coding rnas.
Non-coding RNAs (ncRNAs) form a heterogeneous class of transcripts with little or no protein-coding capacity. Recently, it turned out that these molecules have a plethora of key regulatory roles in eukaryotic cells. NcRNAs directly act at the RNA level without ever being translated to protein. According to their length, one basically distinguishes small ( 200bp) ncRNAs. The function of a small RNA is typically determined by its secondary structure fold rather than underlying primary sequence. There are several ncRNA classes among small ncRNAs with well defined and well understood secondary structure motifs, examples include micro RNAs (usually forming stem-loop structures) or transfer RNAs (which exhibit the prominent cloverleaf motif). In contrast, it is unclear to which extent long non-coding RNAs contain and are determined by regions of conserved secondary structure. The aim of this work is to analyse secondary structures of long ncRNAs on a genome-wide scale with state-of-the-art bioinformatic techniques, to possibly identify and further characterise common structural elements shared by these transcripts. This may yield novel insights to the computational de novo prediction of long ncRNAs in recently sequenced eukayotic genomes, one of the open problems in current RNA bioinformatics.
De Novo Molecular Design Using Graph Kernels
Large scale multiple genome alignment via an efficient kernel method.
In order to make use of the large amount of genomic information that the sequencing experiments are making available, efficient algorithmic procedures are needed. One of the most fundamental type of processing for genomic data is that of genome alignment, whereby regions belonging to several related genomes are put in bi-univocal correspondence. As a result of the alignment procedure, information of biological relevance can be derived, such as the evolutionary conservation rate of given regions. The sequences in these regions are believed to be important and to correspond to functional biological entities like proteins and non-coding RNA. Correct alignments allow, in other terms, the (semi-)automatic discovery of biological objects (either belonging to known classes, or even to yet unknown classes). However, current genomic alignment techniques 1) are suitable for relatively closely related species, and 2) can process a relatively small number of genomes. In order to allow alignments for thousands of genomes, novel efficient techniques are needed. The choice of computational models suitable for this task has to take into consideration several requirements, such as a) efficiency, b) accuracy and c) flexibility.
Intersections of genomic intervals using interval trees
Testing to find overlaps between genomic features is an important task in genomics research. We know this feature as intersection. In this project I implement a fast and exible method to find intersections between two sets of genomic intervals by using interval trees. The implementation(unionBed) uses sets of features in BED format as input data and find overlaps between them. Then the unionBed results data is used to analyse three different secondary structure prediction hypotheses for co-transcriptional RNA folding and to compare them to each other.
hIntaRNA - Comparative prediction of sRNA targets in prokaryotes
The prediction of targets of bacterial sRNAs is a very challenging task, addressed by several approaches. The experimental testing and verification of sRNA targets is very costly and labour-intensive. Therefore, the reliable algorithmic prediction of putative sRNA targets could vastly reduce the amount of wet lab work. However, due to very short and often imperfect complementarity between the sRNA and its target the prediction is not a trivial task. The IntaRNA algorithm is one approach, which frequently, however, does not yield satisfying results yet and therefore demands improvement. It has been stated "that it is difficult to make significant target predictions when searching sequences from a single organism, and that targets should be predicted in a comparative analysis of multiple organisms". Eventhough this was stated for eukaryotes, the basic idea of this thesis also holds for bacteria. The task of improving the IntaRNA algorithm's prediction quality utilizes exactly this concept, also incorporating the individual phylogenetic distances between the organisms analyzed. For instance, there is compelling evidence, that the MicA and RybB sRNAs in E. coli and Salmonella each have homologous targets in both organisms, thus indicating a conservation on the regulatory level. Here, the implementation of the idea that overlapping target predictions for distinct organisms yield stronger evidence of correct functional prediction is presented.
Secondary structure motif determination in ncRNA via graph kernel based computational models
A partition function variant of rna base pair maximization in adp.
The goal of the project is to lay the foundations of computing RNA base pair probabilities, as done by the Mc Caskill algorithm, in the framework of Algebraic Dynamic Programming (ADP). In order to concentrate on the essential aspects of this problem, we simplify the scoring model of the algorithm to a Nussinov-style base pair maximization. The main challenge is to compute the outside part, which has no natural correspondence in the grammar parsing framework underlying ADP.
Generic JSP-based web frontend creation
Web frontends of terminal-based bioinformatics tools are important to ease their use for non-computer scientists and to enable ad hoc usage. The project aims at the development of a highly generic web frontend framework generalizing the currently available JSP-based frameworks of the CPSP-web-tools and Freiburg-RNA-tools . Main goals are to simplify the setup of new frontends for arbitrary terminal tools and to develop a robust generic framework. The integration is exemplified by creating a frontend for the recently developed program CARNA .
Alignmentverbesserungen mit Hilfe von Consensus-Dotplots
RNA-alignments are essential for identifying and characterising structured non-coding RNA. RNA-alignments are different to DNA or protein alignments in the fact that they not only align according to sequence similarity, but also take the base-pairing patterns of secondary structures into account. A common procedure to characterise the structure of non-coding RNAs is to predict the consensus structure of elements of the same family. The problem with this, is that any errors in the alignment are reflected directly in the quality of the predicted consensus structure. Therefore, it is of high importance to get the correct alignment of RNA families. The largest database of such family alignments is contained in Rfam. A common error in these alignments is that a small subset has been misaligned with respect to the structure, which results in some stems slightly offset to either the left or right in comparison to the others. The goal of this thesis is to develop a method to automatically detect and re-align these misaligned stems and to thus deliver a quick method to improve these common errors in the Rfam database. Furthermore, a key part of the work is to understand the state-of-the art in approaches to align RNA sequences and to perform benchmark experiments that compare current tools to the here developed method. It is also important to understand the complexity of measuring the "goodness" of one alignment and to develop and compare such measures.
Local sequence and structure features in long RNA sequences
There is much evidence in molecular biology that RNA plays an important role in living cells. Research results in the last decade have shown that protein coding sequences are only the tip of the iceberg w.r.t. genomic functional elements. Up to 90% of the genome is transcribed into RNA for which the function still remains largely unknown. The structure of an RNA is an important property for its correct function, e.g. the cloverleaf of a tRNA. However, the experimental determination of the structure is still a very challenging task, therefore we try to deduce the structure from the nucleotide sequence, which encodes it. Furthermore, we find evidence that long RNAs have local regions of functionality and that the entire sequence does not always contribute to a particular function. For example cis regulatory elements on mRNA such as SECIS elements and miRNA binding sites. In this project we want to analyse long RNA sequences in respect to different sequence and structure features. The project aims to identify signatures of natural RNAs and dependencies between RNA sequence and structure. Sequence features comprise the A,U,G and C content as well as di-nucleotide and tri-nucleotide content. In terms of structural features we want to consider accessibilities, base-pair probabilities, accuracies (MEA) and predictions from tools like RNALfold. Given these features, we want to identify dependencies between them and between different sequences. First, the project involves a graph visualisation for the raw data of single features and different combinations of features. Because of the huge amount of data, we need to be able to focus or zoom into regions of interest. Further, we want to reduce the feature information to only regions of high significance in comparison to a background model. Thus, a suitable background model needs to be defined for each feature. With the simplified view, it should be easier to visually spot correlations between several features at once. After an initial visual inspection, automatic methods shall be developed to analyse real datasets of different RNA classes to identify distinct sequence and/or structure signals. First we would like to concentrate on known cis regulatory elements within the UTRs of mRNAs and finally we would like to apply the automatic analysis developed in this thesis to find unknown signals in long non-coding RNAs.
RNA-Protein interaction prediction with Graph Kernels
The aim of this work is to help with the implementation and evaluation of the novel algorithm Exparna-P. This algorithm computes all exact pattern matchings between two RNA strands for the entire structure ensemble. In order to speed the algorithm up, a new method needs to be implemented which computes the probability that a position is unpaired under a loop. Then the already existing chaining algorithm has to be slightly modified in order to compute the best set of non-overlapping and non-crossing exact pattern matchings for Exparna-P. The third part of this bachelor thesis is the comparison of the performance of the Exparna-P tool compared to the Exparna tool.
Multiple sequence alignment methods of long non-coding RNAs
Long ncRNA is a rapidly advancing field of genetics, with yet only briely studied roles (in gene regulation), organization, conservation or medical implications. It is however expected that they will play a great role in further genetic studies and progress. Due to their (sometimes impressive) length (of up to several hundreds of kb) and other particularities, their sequences are rather difficult to align. However, valid sequence alignments are the essential pre-requisite for most subsequent bioinformatic studies of lncRNAs. Therefore, we analyse, compare and benchmark different alignment sets of vertebrate long ncRNAs, namely the Ensembl EPO alignmets, the Galaxy Multiz/TBA blocks and alignments generated by a self-developed pipeline and identify advantages and drawbacks of sequence alignments of lncRNAs.
Evaluating contaminations in genomic sequences
Despite continued advances in whole genome sequencing techniques and the development of powerful assembly algorithms, newly sequenced genomes still often suffer from contaminations during the sequencing process. The most common sources of contamination are accessory DNAs deliberately attached to the DNA/RNA under investigation, including vectors, adapters, linkers, and PCR primers. However, there are also unintended events, e.g. caused by transposon activity or simply impurities, leading to contaminated genomic sequences. These may then result in missassemblies of genomic sequences, meaningless analyses and potentially erroneous conclusions. However, noone knows to which extent publicly available genomes are contaminated. To encompass this unsatisfying situation we therefore plan to develop a comparative genomics approach to broadly identify contaminations in available genomic sequences. The project is not only open for bioinformaticians and computer scientists, it is also suitable for students with a background in biology.
A new heuristic algorithm for IntaRNA for improved RNA-RNA interaction prediction
The number of discovered ncRNAs(non-coding RNAs) that regulate target mRNAs by base pairing is growing fast. This demands for identification of the target mRNAs for those ncRNAs. Thus prediction of such interactions between ncRNAs and mRNAs became of great neccesity to help identify targets for known ncRNAs. A few computational algorithms for this purpose were developed to predict such interactions. While some of the algorithms were fast enough for genome-wide searches, they were not so accurate in predicting interactions between long RNAs. This is because they neglected an important factor for interaction formation which is the interacting site accessibility. IntaRNA considers site accessibility while maintaining the same time and space complexities of these fast algorithms. IntaRNA includes two algorithms, one that gives optimal results according to the Turner free energy model, but is time consuming with time complexity O(n 2 m 2 ). The second algorithm is heuristic with time complexity O(nm) only, but does not give optimal results for all input sequences. In this thesis we present improvements over both algorithms of IntaRNA. First we modified the non-heuristic algorithm to model more accurately how RNAs are actually forming an interaction. It simulates - in the same order - the sequence of events in which interaction formation is thought to happen in real. The new implementation allows to forbid high energy barriers that might be encountered during interaction formation and that are less likely to be overcome. Second we improved the accuracy of the heuristic algorithm of IntaRNA, making it more accurate and reliable for use in biological researches, without significantly increasing its runtime and space requirements.
Development and Implementation of an Alignment Program for Canonical Pseudoknots
At our lab, a general method to align various restricted classes of pseudoknots has been developed. The alignment scheme has also been implemented, but due to its generality, it is comparably slow and not suitable for many large scale practical applications. This work focuses on developing an efficient implementation of only one specialized instance of this scheme (The R&G pseudoknot class) that can be used in real practical scenarios. The topic is suitable for people interested in algorithms, datastructures, software development, and C++ programming.
RNA Consensus Interaction Prediction
RNA-RNA interaction is a subject of considerable biological relevance as the binding of ncRNA to mRNA can affect both the transcription and translation of the bound mRNA and hence regulate gene expression. The accuracy and reliability of single sequence RNA structure prediction has been shown to increase significantly when the structure of an aligned set of RNA homologs is computed. As such, it is posited that by augmenting an existing RNA-RNA interaction prediction algorithm, that determines an interaction structure based only on thermodynamics, with a phylogenetic component a structure prediction of improved quality can be obtained. This thesis presents the theory, implementation and evaluation of an algorithm that combines thermodynamic and phylogenetic information to predict a consensus interaction structure on a set of aligned mRNAs and ncRNAs.
Experimentelle und theoretische Untersuchungen zur Echtzeitanalyse Mikroarray-basierter RNA-Amplifikation
- Application of CDDM to a NASBA microarray with fixed amounts of target RNA
- Incorporation of NASBA amplification into the CDDM binding kinetics
Centroid-based identification of local RNA elements
In this thesis we try to tackle the problem of identifying local RNA elements in a genomewide scale. We employ a fast sparse algorithm to predict maximum expected accuracy structures based on base-pairing/unpairing probabilities. Moreover, we introduce a new locality definition and present an accuracy function reflecting this locality. Base-pairing and base-unpairing probabilities can be efficiently computed using RNAplfold included in the Vienna package. Based on these probabilities, we identify structured regions that have high probabilities of containing significant local RNA motifs. After that, we introduce our new program RNAMotid together with other included features that enables it to scan genome-wide sequences for structured regions. Moreover, we discuss how several modules were integrated together in our program to allow flexibility and optionality of the analysis. Finally, we evaluate the performance of RNAMotid in identifying local RNA motifs embedded in randomly shuffled context. Before that, we apply an overall parameter training followed by a family-based parameter training. Then we discuss the factors that affect the performance of RNAMotid.
Exploring structural characteristics of mRNA target sites using local folding
- Research and preparation of the topic: read about RNA secondary structure, local folding, accessibility, positional entropy, etc. Also gather information on what has previously been done in the structural analysis of target sites.
- Gather data: find experimentally validated target sites for different types of non-coding RNA (and proteins if possible).
- Apply existing local folding programs to the data and calculate the structural characteristics of the target sites.
- Implement a well-documented pipeline with Perl to be able to analyse arbitrary target sites in future.
- Written manuscript.
Folding simulations in side chain lattice protein models
Side chain lattice protein models are a reasonable and necessary extension of the widely used backbone lattice protein models. To enable folding simulations a structural neighborhood relation, a so called move set, has to be defined that is utilizes that enable e.g. Monte-Carlo simulations of the folding process. The thesis presents the K-local move set, a local move set defined generically for lattice protein models. The K-local move set is defined for both backbone and side-chain protein models via constraint satisfaction problems. The use of the constraint-based approach enabled its use for an arbitrary lattice. The K-local move set is then used for a simulation procedure for side-chain protein structures in the face-centered cubic lattice using real protein sequences and structures.
Infering RNA Stem-Loop descriptors from multiple sequence-structure alignments for an indexed-based RNA search method
RNA can be grouped into certain RNA families according structural and functional similarities. Currently, the Rfam 9.1 database ( http://rfam.sanger.ac.uk ) contains more than 1300 such families. We have already developed a fast index-based (with affix-trees) search method for RNAs. Here, the query is a descriptor and it consists of a stem-loop structure with possible wildcards at different positions. The more sequence information is given the faster is the underlying index-based search engine. On the other hand, if too much sequence information is given, related, but inexact matching stem-loop structure would not be found. Therefore, the goal of this bachelor thesis is to derive such descriptors from Rfam seed-alignments (or other multiple RNA sequence-structure alignments) too feed them into the search engine. If each necessary single descriptor gives a match within a certain region, one could infer a match of the underlying RNA family. A descriptor can been seen therefore as a necessary local motif of an RNA familiy.
Approximate pattern matching under generalised edit distance and extensions to suffix array library
The approximate pattern matching problem is the problem of finding all occurences of a certain pattern in a usually much longer text allowing for a fixed error threshold in the matching. The problem has been studied extensively and many very good solutions were found. However, general enough instances of the problem, namely those allowing for generalised error functions, remain with without satisfactory algorithms. This thesis is an attempt to provide such a solution. The new method provided relies on the suffix array data structure to preprocess the text linearly and allow later for fast queries. The new algorithm has the two desirable features of having a fairly simple explanation and implementation and having space and time bounds independent of the size of the alphabet, allowing for arbitrarily large alphabets. Furthermore, the new algorithm handles wildcards quite well while retaining the same time and space worst-case complexities. The algorithms are compared on genuine genetic data from Zebrafish genome and the results are presented. Finally, a parallelized version of the algorithm is presented on CREW-PRAM model of computation. In addition to presenting the new algorithm, several contributions were made to an existing affix array library.
A Library for Index-based Bidirectional Pattern Search with an Application to RNA Structural Motifs
In dieser Masterarbeit präsentieren wir sowohl bekannte, als auch neue Algorithmen zur effzienten Konstruktion und Verwendung von Indexdatenstrukturen. Diese Datenstrukturen haben mannigfaltige Anwendungsmöglichkeiten im Bereich des String-processings. Insbesondere können durch sie Mustersuchen in indexierten Texten beschleunigt werden, wodurch sie eine wichtige Rolle in der Analyse biomolekulare Sequenzen wie z.B. DNA- (Desoxyribonukleinsäure), RNA- (Ribonukleinsäure) und Protein-Sequenzen, spielen.
Variations of the Sankoff-Algorithm with a Focus on Heuristics
Aarhus University logo
Bioinformatics Research Centre
Master's thesis in bioinformatics.
In the Master’s program in bioinformatics, you must do a 30 ECTS Master’s thesis. You must start your 30 ECTS thesis no later than February 1 (or September 1 ) a year and a half after commencement of your studies (i.e. February 2021 for students admitted in summer 2019, or September 2021 for students admitted in winter 2020). You must complete your thesis (including the exam) no later than June 30 the same year, if you started on February 1 (or January 31 the following year, if you started on September 1).
You can read the course description for the MSc thesis project at:
kursuskatalog.au.dk/en/course/114372/Thesis-30-ECTS-Bioinformatics
You can read some general information and advice about Master’s thesis work at:
https://studerende.au.dk/en/studies/subject-portals/bioinformatics/masters-thesis/masters-thesis/
You can see abstracts of (some) Master's theses from BiRC at:
https://www.birc.au.dk/~cstorm/birc-msc/birc-msc.html
Thesis contract
Before you start your thesis, you must make a thesis contract. The thesis contract must be completed and approved by January 15 (or August 15 ). You can read about how to submit the contract on the above www page. As part of the thesis contract, you must attach a pdf file containing project description, project goals, activity plan, and supervision plan. This is very much like what you have to describe for a Project in Bioinformatics. At BiRC, you should use the following template for this description.
Problem statement, activity plan, and supervision plan (in docx format)
When formulating the thesis project, you should keep in mind that it should cover 30 ECTS of work, i.e. full-time work for the entire semester and the following exam period. Group projects should of course cover this for every group member.
Choosing a topic
Before you can make a thesis contract, and commence your thesis work, you must (of course) chose a topic and a supervisor. The supervisor must be a tenured researcher associated to BiRC, but you can also have one or more co-supervisors.
When choosing a thesis topic, it is a good idea to think about the classes and projects that you have done during your Master’s studies, and what kind of work do you like? Contact potential supervisors as early as possible to discuss your wishes and ideas. Remember that you are always welcome to come by our offices and discuss. You can also ask potential supervisors for examples of thesis’s that they have supervised in order to get a better idea of how a thesis can look.
Also, we plan an information meeting for students that focus on thesis and project work every Fall. Below are the slides from the last such information meeting.
Slides from MSc info meeting (November 2023)
Ten simple rules for writing a great MSc thesis at BiRC (November 2022)
The slides also contain good advice about how to organize your thesis work. The above www page also contains some advice.
Group projects: It is possible to do the thesis project as a group project. Each group member must fill out individual contracts stating the other groups members. A group hand in a single thesis, but each group member is examined individually. In general, we very much encourage group assignments as it for many students is motivating to work together in a group, and to have group member to discuss and solve the many the details of a thesis project together with.
Projects involving external collaborators: It is possible to do a project that involves external collaboration, e.g. with people from industry, or from other university departments. Such collaborators will be associated to your thesis as co-supervisors. In the thesis contract, it is possible to indicate that the thesis project is done in collaboration with an industrial partner, if an NDA has been signed, and if the final thesis report must be made public available.
The thesis report presents the completed work and can be written in Danish or English. The report must contain an English summary/abstract. The summary/abstract is included in the assessment, and the assessment places emphasis on the academic content, as well as the student’s spelling and writing skills. The extent of the thesis report is agreed with the supervisor, but is typically about 50-60 pages excluding frontpage, table of content and appendices. If the MSc thesis is done as a group project provided, the report must be done in such a way that the group members can be assessed individually. This means that you can either (1) do a joint report in which everyone is equally responsible for all parts of the report, or (2) do a joint report, where it is stated (fx in the table of content) who of you has done the individual parts of the report and is responsible for them. See https://studerende.au.dk/en/studies/subject-portals/bioinformatics/masters-thesis/masters-thesis/ under "Group assignment" for details.
In your thesis contract, you state the hand in date. This can between June 1 and 15 (or January 1 and 15 ), earlier dates are also possible. The exact date is (of course) decided in collaboration with your supervisor. You hand in your thesis via Digital Exam (like you are used to for Projects in Bioinformatics).
The thesis exam is 60 min oral exam. It starts with a 30 min presentation from you about your thesis work followed by a 30 min discussion between you, the examiner (your supervisor), and an external examiner. Your presentation is based upon a question that you get from your supervisor one week before the exam. The exam must be held before June 30 (or January 31 ). In principle, the exam can be held from the day after you hand in your thesis. The exact date is decided upon by your supervisor, and often depends on the availability of external examiners. The final grade reflects an overall assessment of your report, your presentation, and your discussion.
If you have any questions about thesis work, then you are always welcome to ask!
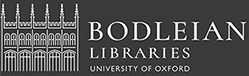
- Bodleian Libraries
- Oxford LibGuides
- Bioinformatics
- Theses & Dissertations
Bioinformatics: Theses & Dissertations
- Journals and Conference Proceedings
- Online resources
Links for Theses and Dissertations
- Proquest Dissertations and Theses Search US theses and dissertations. Accessed through OxLip+, search for 'dissertations and theses'.
- Oxford Research Archive (ORA) Search for and download recent Oxford DPhil theses. Also contains an archive of articles, papers and research posters produced by academics and researchers at Oxford University. more... less... ORA is freely available and does not require a log-in.
- EThOS Access to UK theses from the British Library. more... less... To use this service you will be required to set up an individual account.
- DART-Europe Search European E-theses.
Theses and Dissertations On-line
Electronic collections.
A number of recent theses and dissertations prepared at Oxford are available to download from the Oxford Research Archive (ORA) . The British Library provides access to UK theses through its EThOS service . Already digitised UK theses can be downloaded freely as PDF files. Requests can be made to digitise older theses, but there is a cost of around £40 and waiting time of 30 days for digitisation. The British Library no longer provides theses on microfilm.
Finding Oxford Theses
SOLO allows you to search for Theses in the Oxford collections.
1. Navigate to the SOLO homepage.
2. Click on the ' Advanced Search ' button
3. Click the ' Resource Type ' menu and choose the ' Theses ' option.
4. Type in the title or author of the thesis you are looking for and click the ' Search ' button.
Other Relevant Guides
- ORA: Oxford University Research Archive by Jason Partridge Last Updated Jan 9, 2024 1311 views this year
- << Previous: Online resources
- Last Updated: Apr 4, 2024 11:53 AM
- URL: https://libguides.bodleian.ox.ac.uk/bioinformatics
Website feedback
Accessibility Statement - https://visit.bodleian.ox.ac.uk/accessibility
Google Analytics - Bodleian Libraries use Google Analytics cookies on this web site. Google Analytics anonymously tracks individual visitor behaviour on this web site so that we can see how LibGuides is being used. We only use this information for monitoring and improving our websites and content for the benefit of our users (you). You can opt out of Google Analytics cookies completely (from all websites) by visiting https://tools.google.com/dlpage/gaoptout
© Bodleian Libraries 2021. Licensed under a Creative Commons Attribution 4.0 International Licence
- Research & Faculty
- Bioinformatics
Our Research Focus
Today’s data sets are of such magnitude and complexity that advanced bioinformatics methods are essential to their integration, management and dissemination. Our bioinformatics work incorporates data from both mouse and human genetic and genomic research and provides the annotations and interfaces necessary for delivering useful information to the research community.
Faculty & Staff
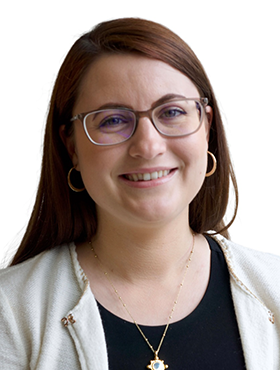
Tamar Abel, Ph.D.
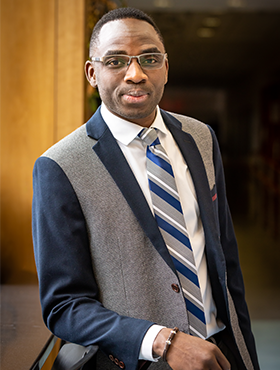
Rafiou Agoro, Ph.D.
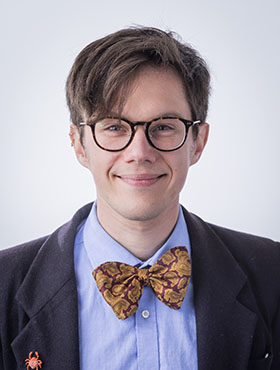
Joseph Albert, B.S.
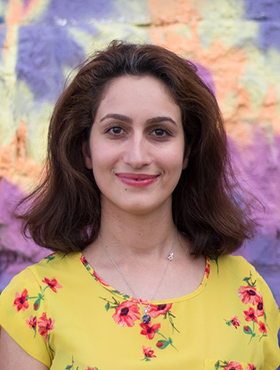
Elaheh Alizadeh, Ph.D.
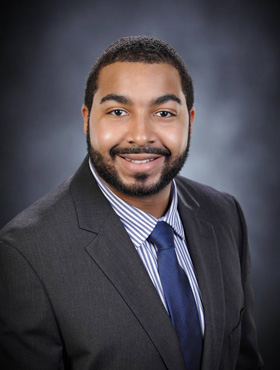
Kevin J. Anderson, Ph.D.
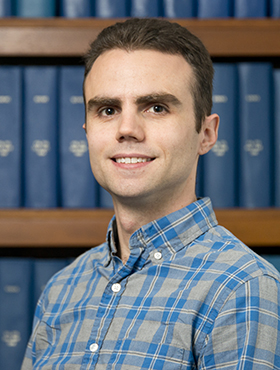
Peter Audano, III, Ph.D.
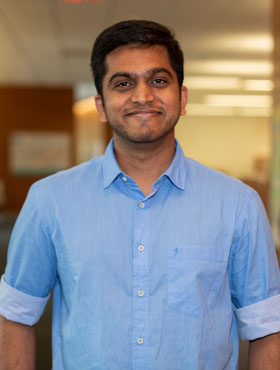
Parithi Balachandran, MS
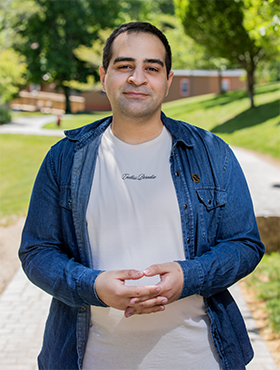
Yehya Barakat
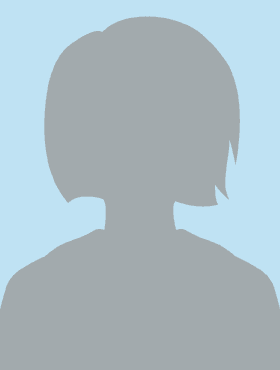
Susan Bello, Ph.D.
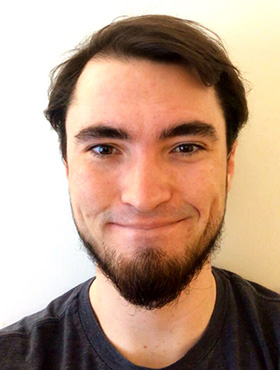
Daniel Berenzy
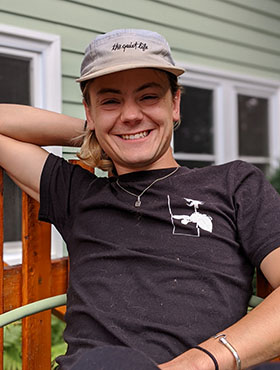
Alexander Berger, B.S.
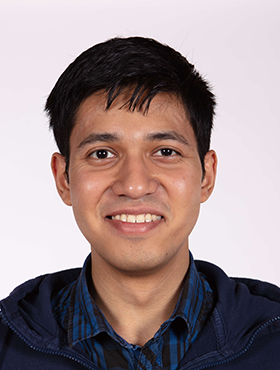
Redwan M Bhuiyan, BA
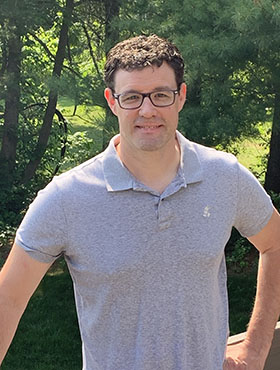
John Bluis, M.S.,B.S.
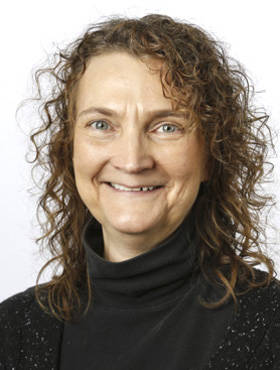
Molly Bogue, Ph.D.
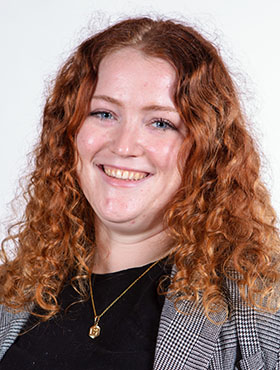
Samantha Bossé
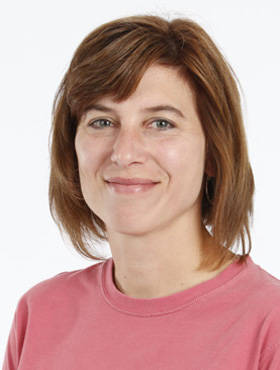
Rebecca Boumil, Ph.D.
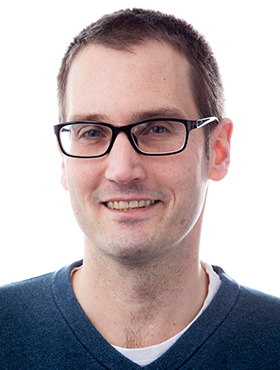
Jason Bubier, Ph.D.
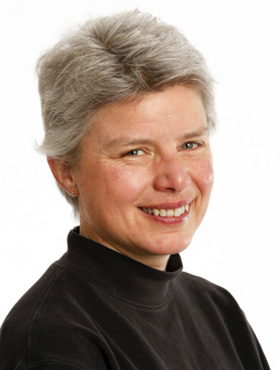
Carol Bult, Ph.D.
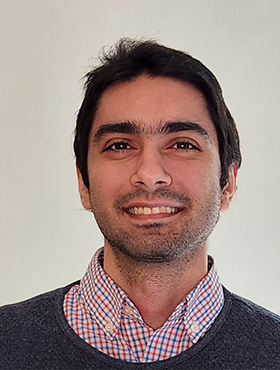
Arad Bustan, MSc
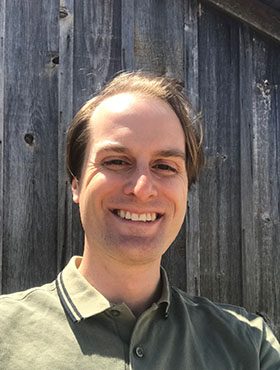
John Butts, MS
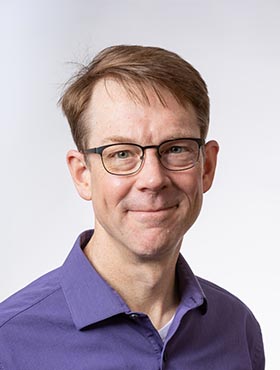
Gregory Carter, Ph.D.
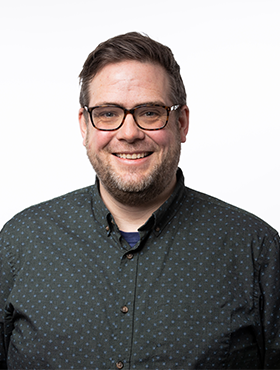
Gregory Cary, Ph.D.
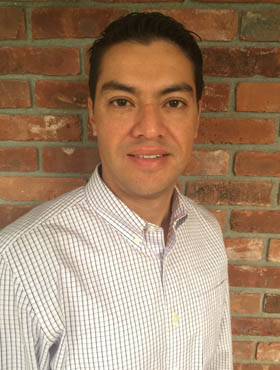
Francisco Castellanos, M.S.
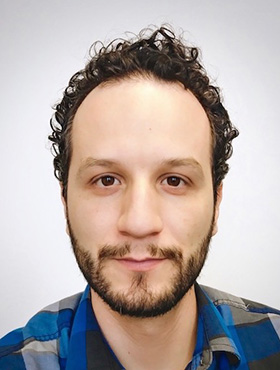
Rodrigo Castro, Ph.D.
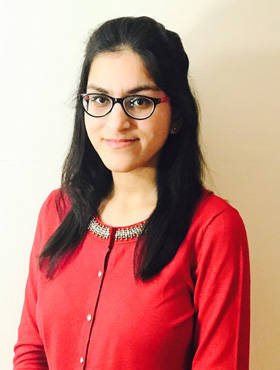
Harshpreet Chandok, M.S.
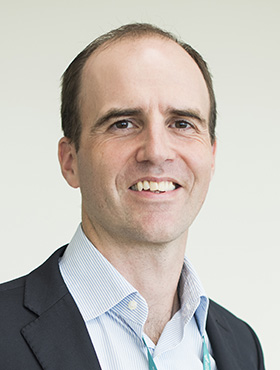
Damien Chaussabel, Ph.D.
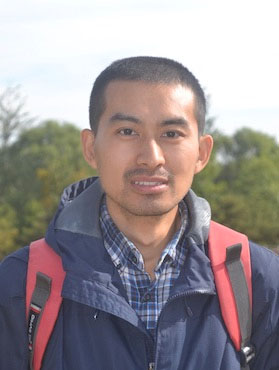
Yang Chen, Ph.D.
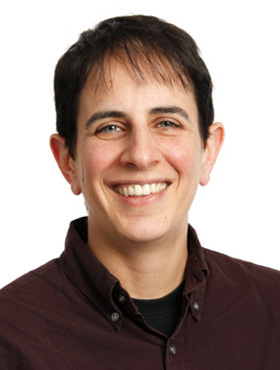
Elissa J. Chesler, Ph.D.
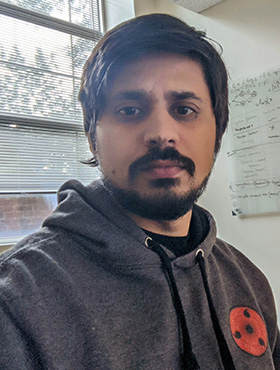
Anshul Choudhary, Ph.D.
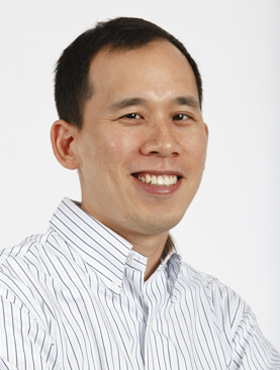
Jeffrey Chuang, Ph.D.
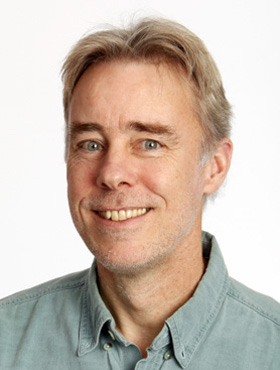
Gary Churchill, Ph.D.
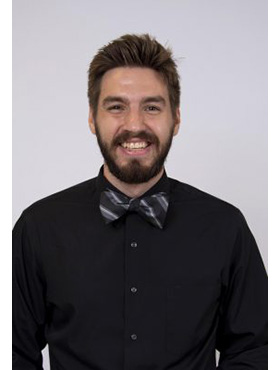
Ben Coleman
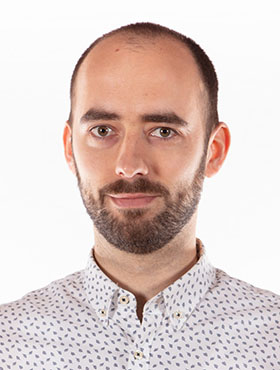
Daniel Danis, Ph.D.
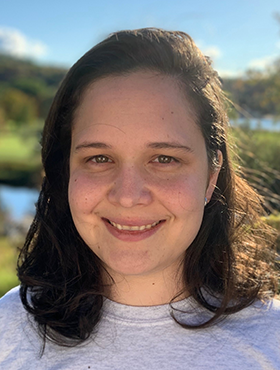
Georgia Doing, Ph.D.
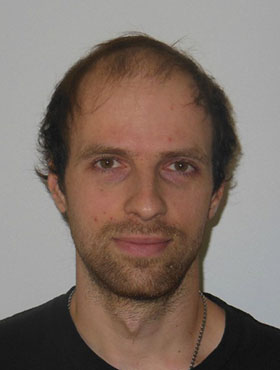
Sergii Domanskyi, Ph.D.
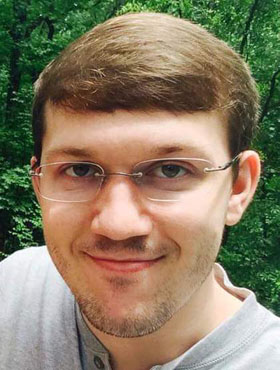
Matthew Dunn, B.S.
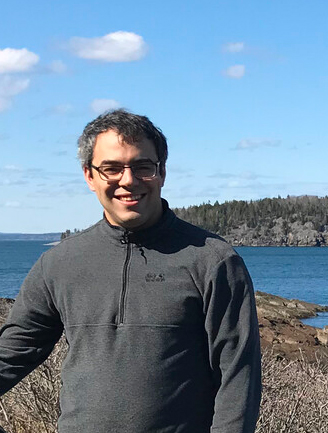
Baha El Kassaby, MSc
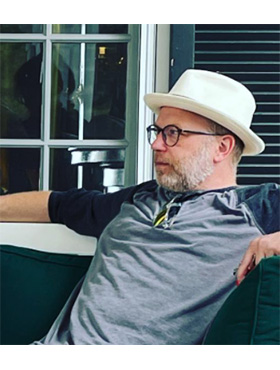
Jake Emerson, M.S.
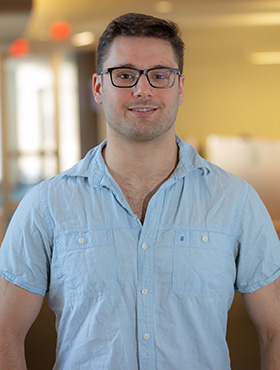
Ryan Englander
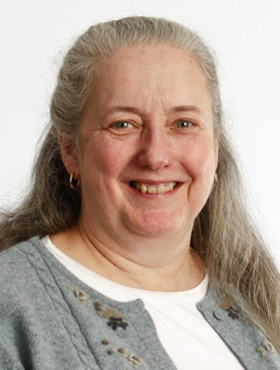
Janan Eppig, Ph.D.
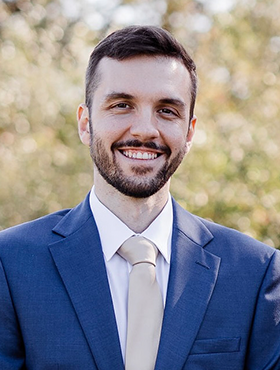
Ardian Ferraj, Ph.D.
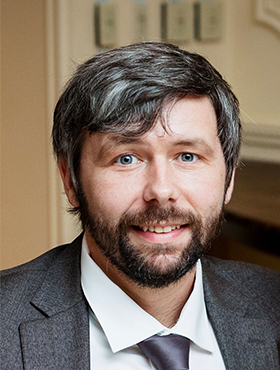
Peter Fields, Ph.D.
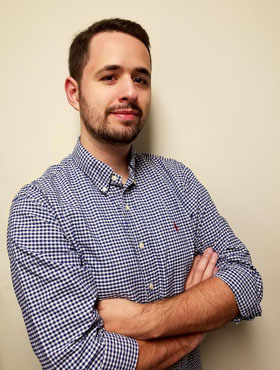
Bill Flynn, Ph.D.
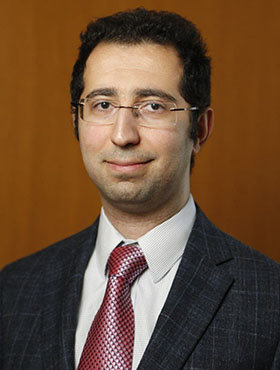
Ali Forougi Pour, Ph.D.
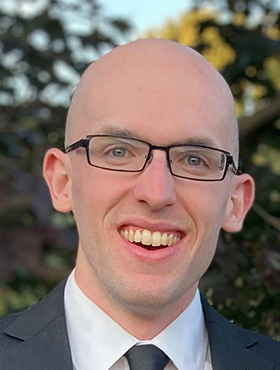
Zachary Frohock
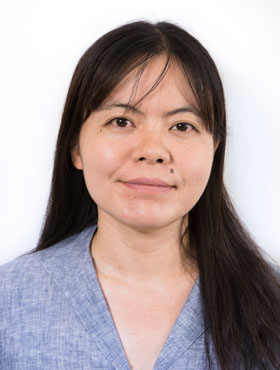
Mayuko Furuta, Ph.D.
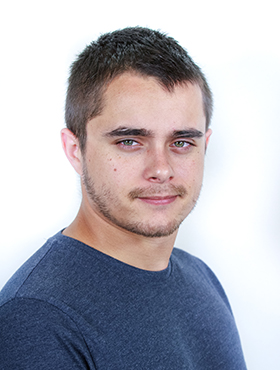
Michael Gargano, B.S.
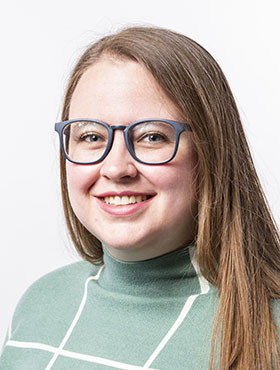
Alexis Garretson, MS
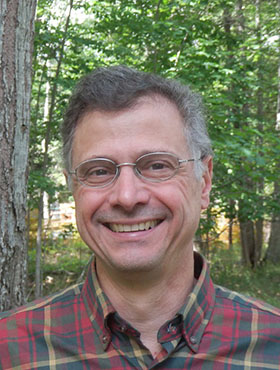
Daniel Gatti, Ph.D.
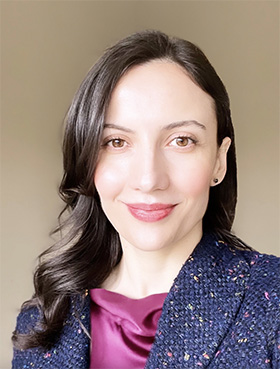
Eva Gega, M.S.
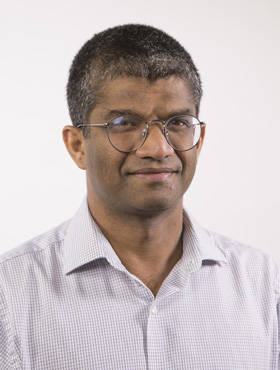
Joshy George, Ph.D.
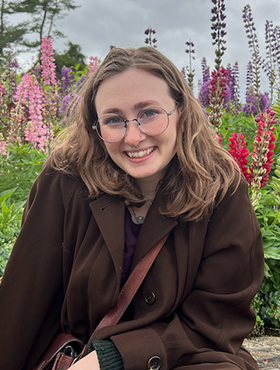
Orianna Goldberg
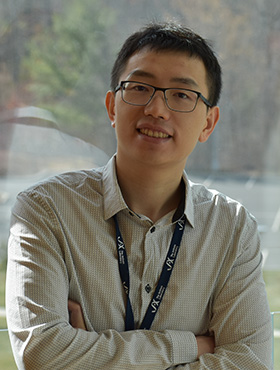
Minghao Gong, Ph.D.
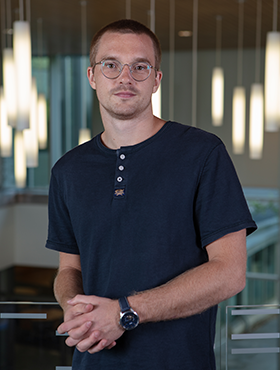
Titas Grabauskas
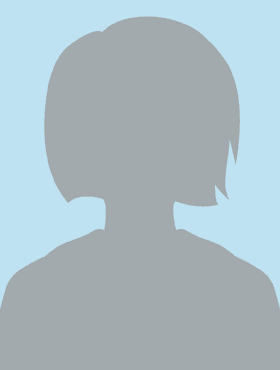
Annat Haber, Ph.D.
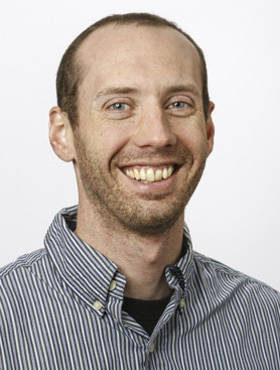
Jeffrey Harder, Ph.D.
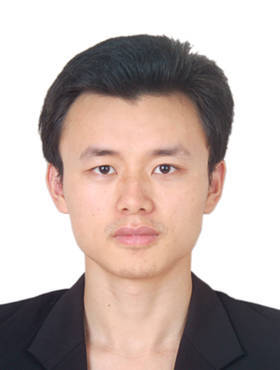
Hao He, Ph.D.
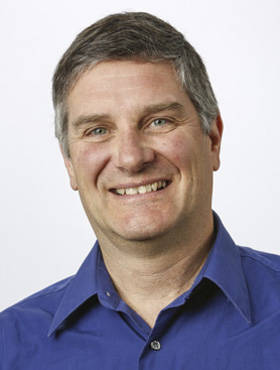
David Hill, Ph.D.
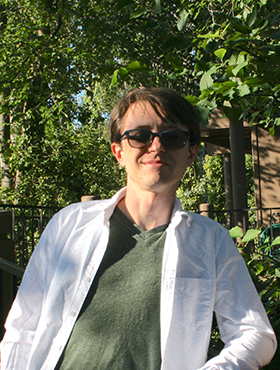
Tim Holland, M.S.
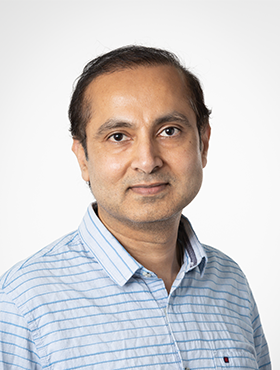
Vishnu Hosur, Ph.D.
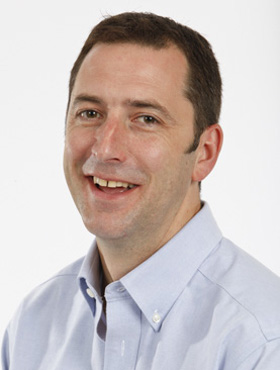
Gareth Howell, Ph.D.
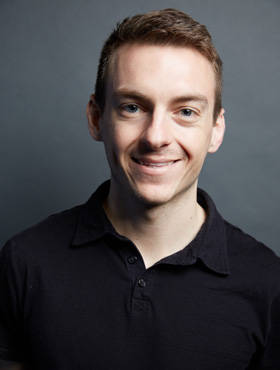
Kevin C. Johnson, Ph.D.
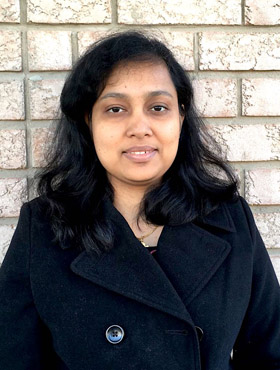
Beena Kadakkuzha, Ph.D.
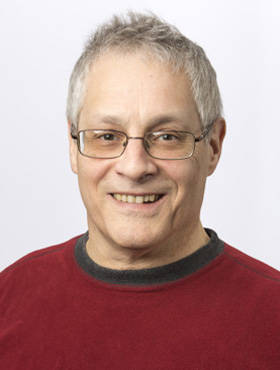
James Kadin, Ph.D.
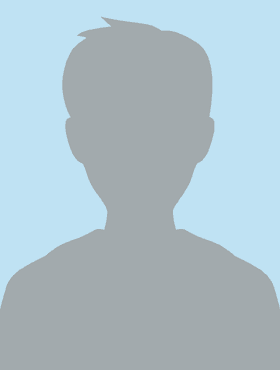
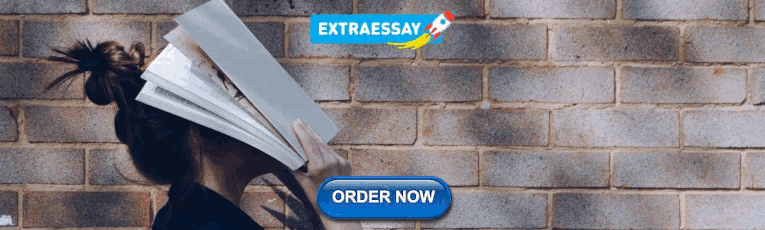
Hyeongu Kang, Ph.D.
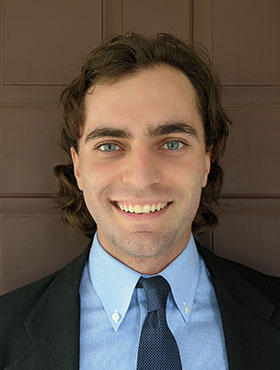
Stephen Keegan
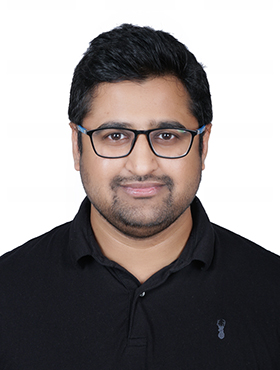
Taushif Khan, Ph.D.
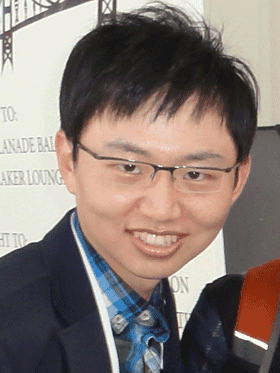
Ji Hun Kim, Ph.D.
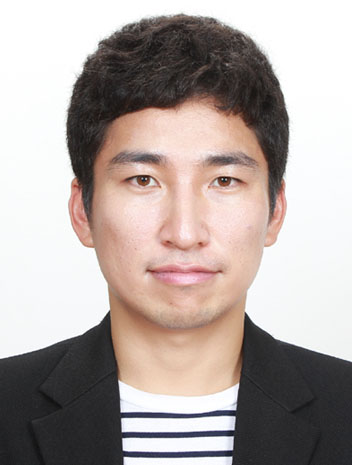
Kwondo Kim, Ph.D.
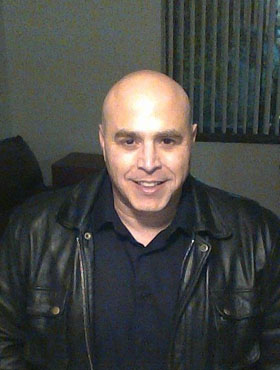
Neil Kindlon, M.S.
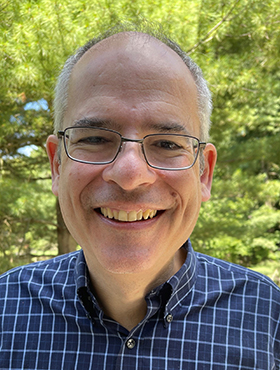
Mitch Kostich
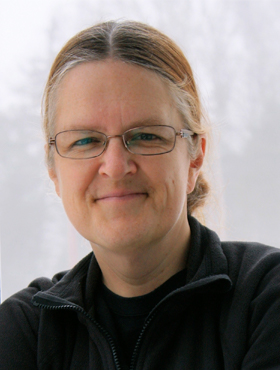
Debbie Krupke
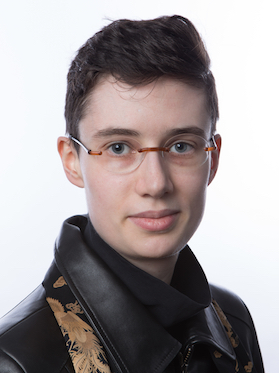
Lauren Kuffler
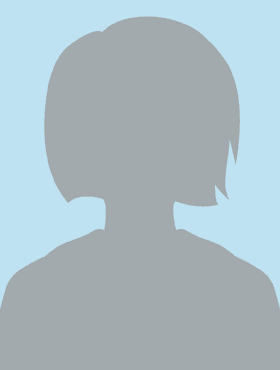
Sonal Kumar
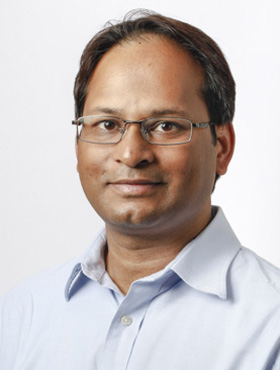
Vivek Kumar, Ph.D.
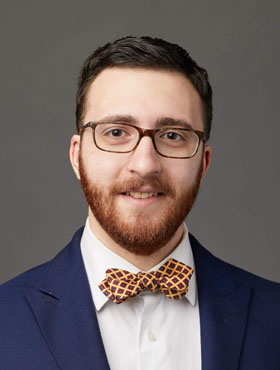
Peter Larson, BS
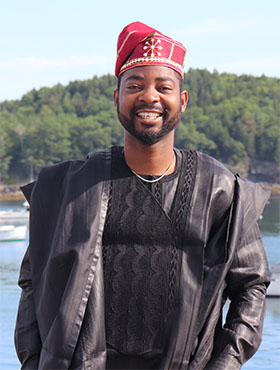
Raman Akinyanju Lawal, Ph.D.
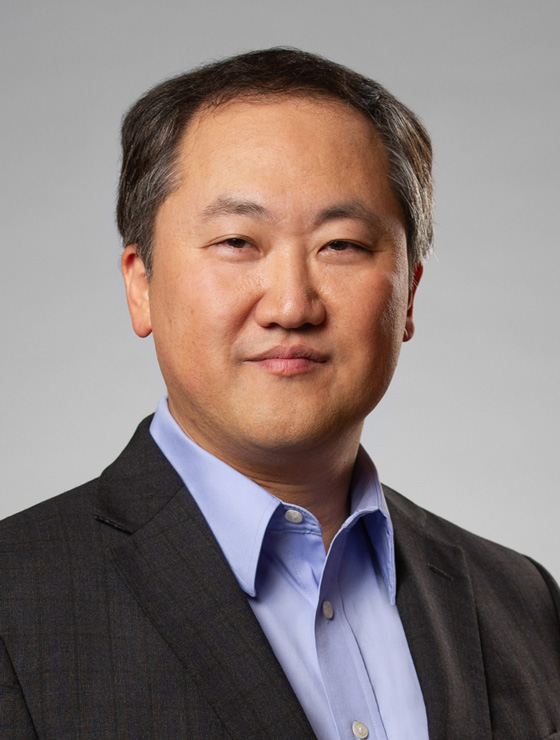
Charles Lee, Ph.D., FACMG
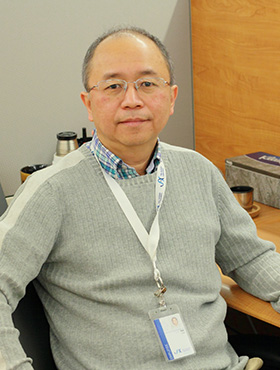
Shuzhao Li, Ph.D.
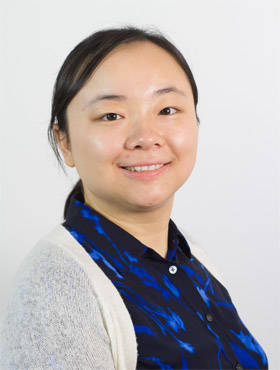
Sheng Li, Ph.D.
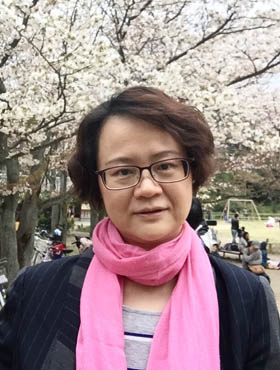
Yi Li, Ph.D.
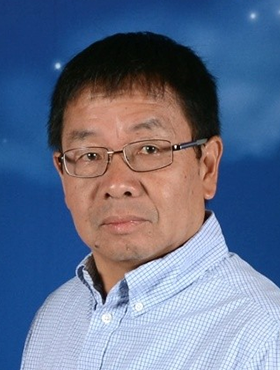
Hongping Liang, Ph.D.
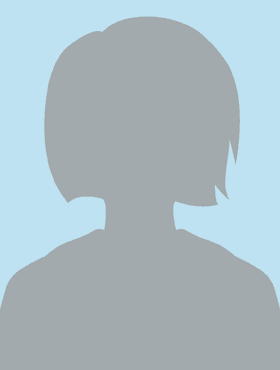
Yi Juin Liew, Ph.D.
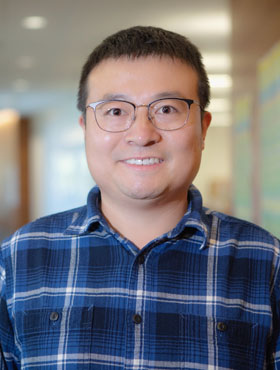
Yang Liu, Ph.D.
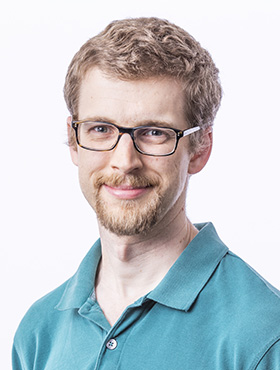
Michael Lloyd, Ph.D.
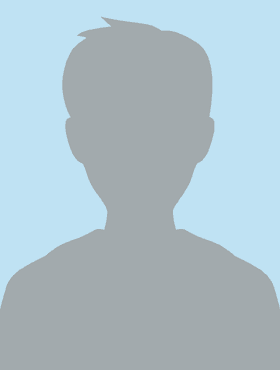
Matt Mahoney, Ph.D.
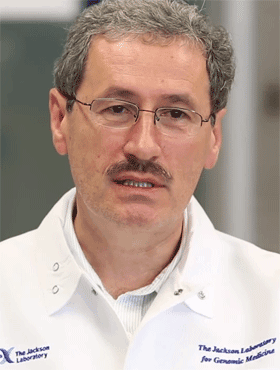
Radu Marches, Ph.D.
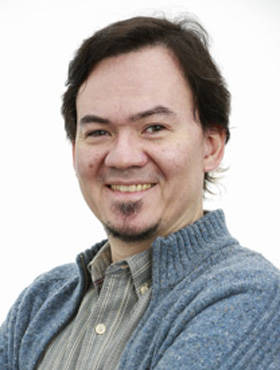
Eladio Marquez, Ph.D.
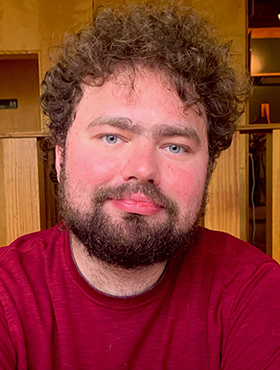
Seamus Mawe, B.A.
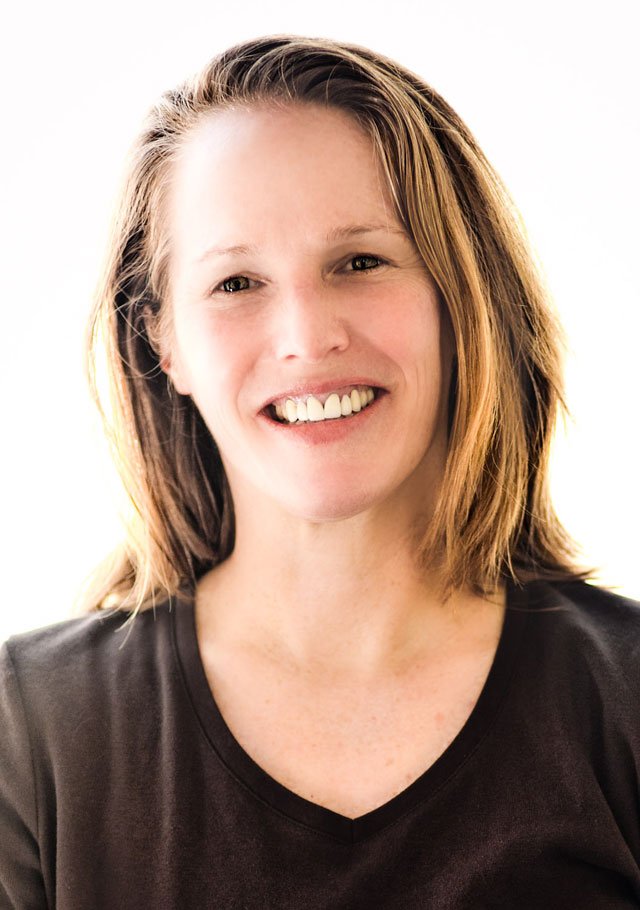
Susan McClatchy, M.S.
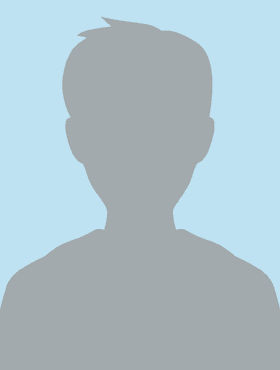
Dave Mellert, Ph.D.`
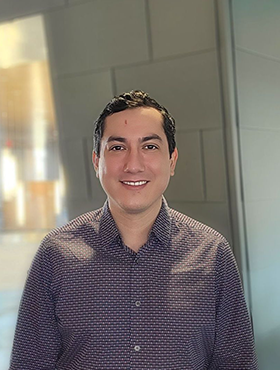
Adrian Melo Carrillo, Ph.D.
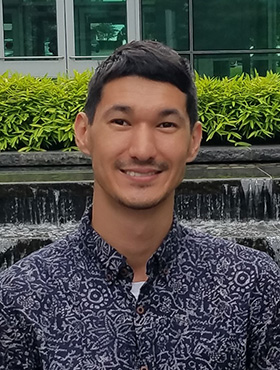
Nicholas Moskwa, Ph.D.
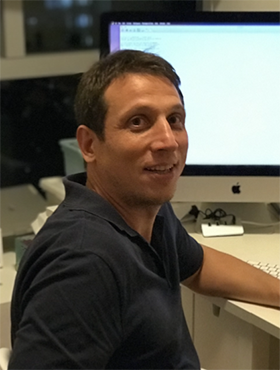
Efthymios Motakis, Ph.D.
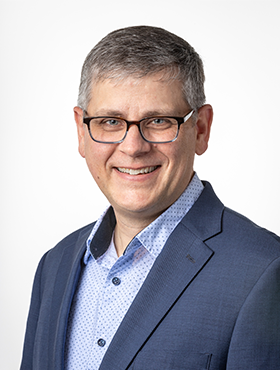
Steven Munger, Ph.D.
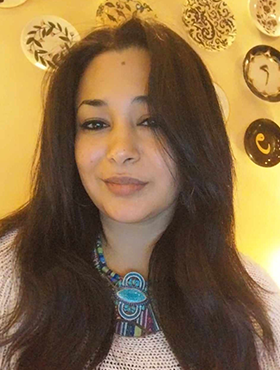
Lamis Naddaf, Ph.D.
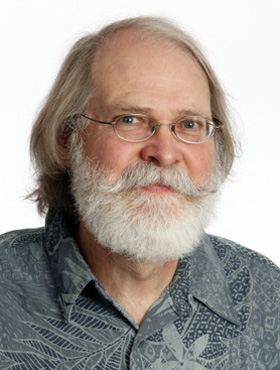
Jürgen Naggert, Ph.D.
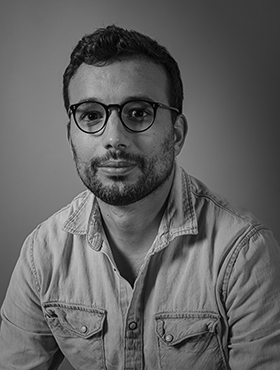
Djamel Nehar-Belaid, Ph.D.
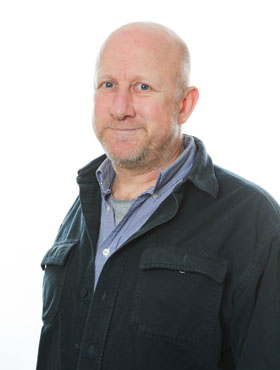
Steven Neuhauser
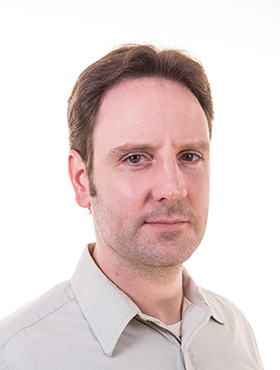
Francis O'Neill, M.D., M.A.
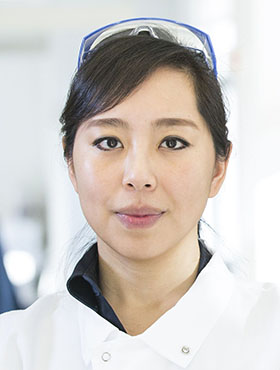
Julia Oh, Ph.D.
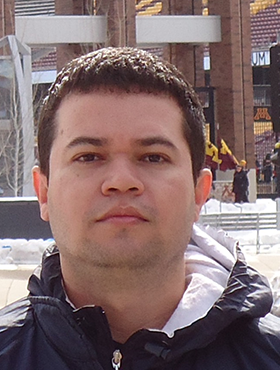
Nélio Oliveira
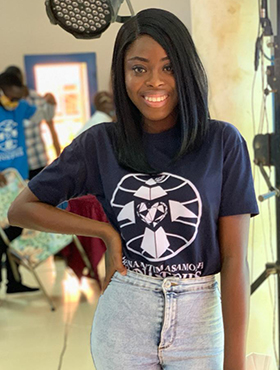
Hilda Opoku Frempong
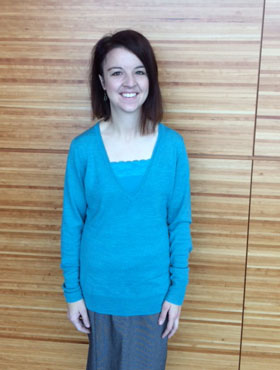
Carolyn Paisie, Ph.D.
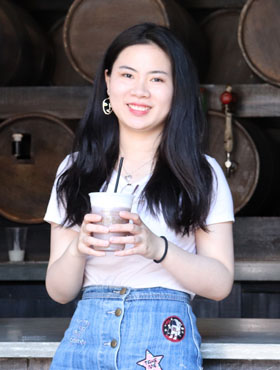
Ravi Pandey, Ph.D.
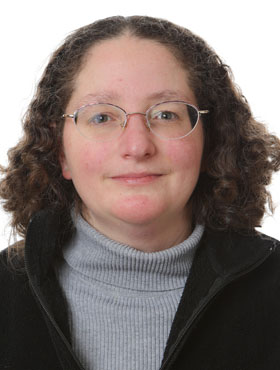
Michelle Perry
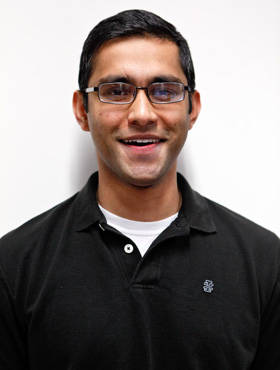
Vivek Philip, Ph.D.
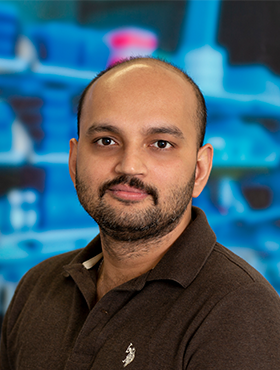
Avijit Podder, Ph.D.
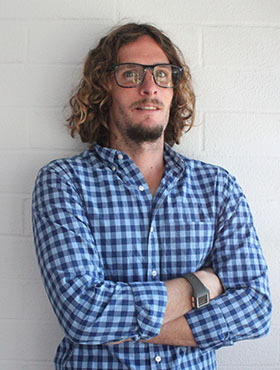
Olivier Bertrand Poirion, Ph.D.
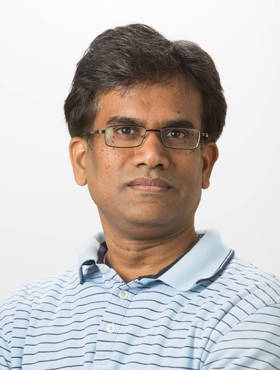
Narayanan Raghupathy, Ph. D.
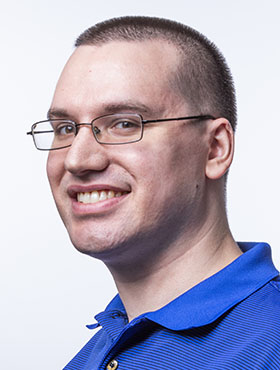
Daniel Rasicci
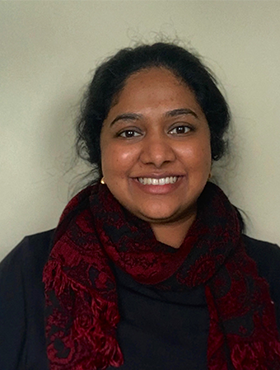
Sathyabaarathi Ravichandran, Ph.D.
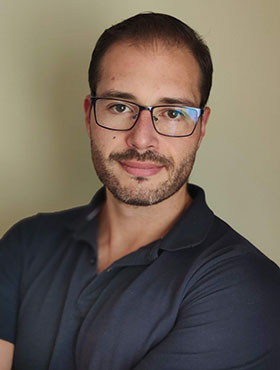
Gabriel E. Rech, Ph.D.
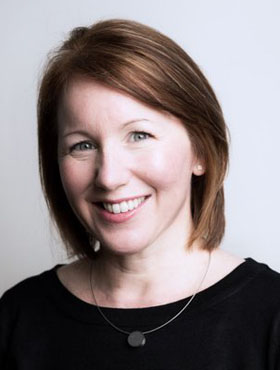
Laura Reinholdt, Ph.D.
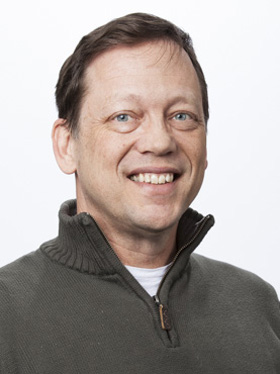
Joel Richardson, Ph.D.
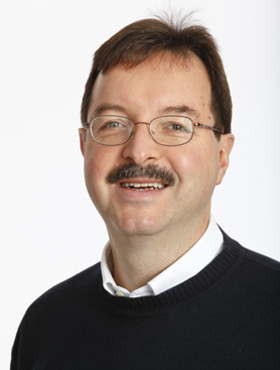
Martin Ringwald, Ph.D.
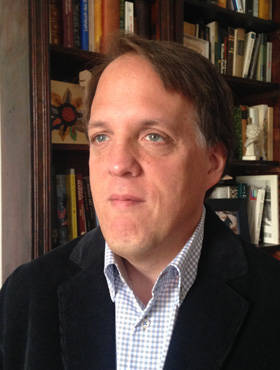
Peter Robinson, M.D., MSc.
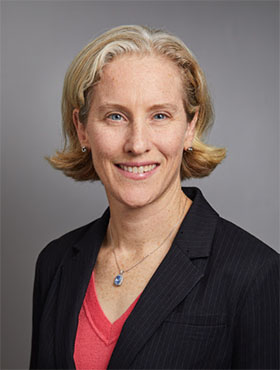
Jill Rubinstein, MD, Ph.D.
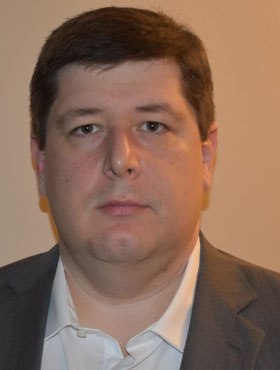
Shane Sanders, Ph.D.
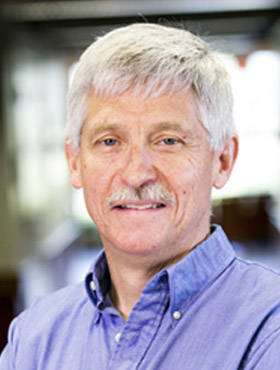
Michael Sasner, Ph.D.
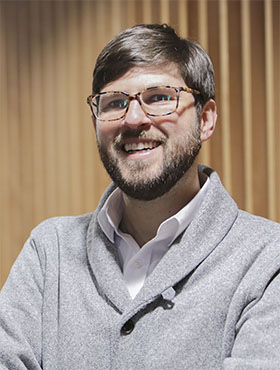
Michael Saul, Ph.D.
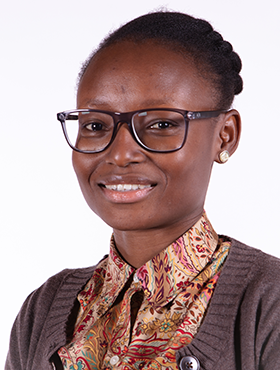
Martine Seignon
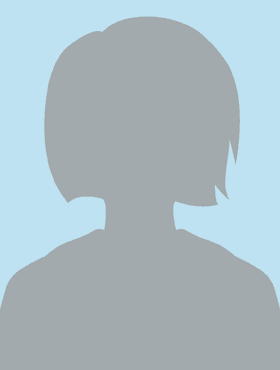
Jiayuan Shi
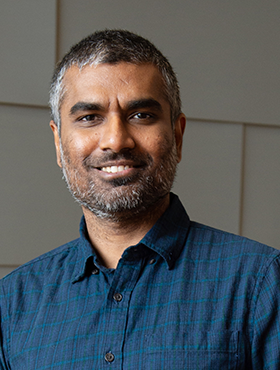
Santhosh Sivajothi, Ph.D.
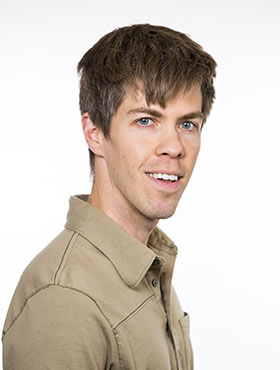
Daniel Skelly, Ph.D.
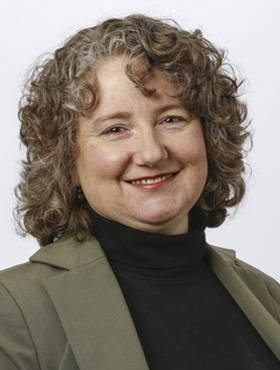
Cynthia Smith, Ph.D.
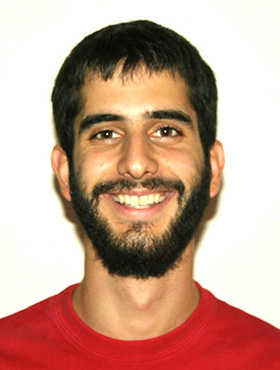
Antonios Somarakis
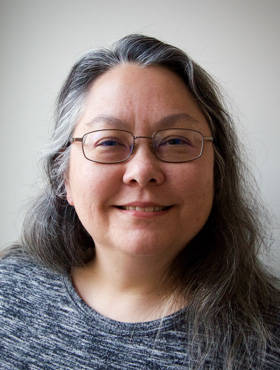
Grace Stafford, Ph.D.
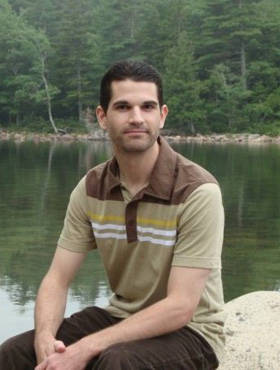
Timothy M. Stearns, B.S., M.S.
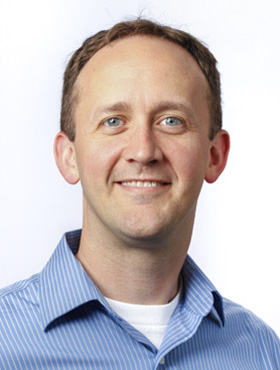
Michael Stitzel, Ph.D.
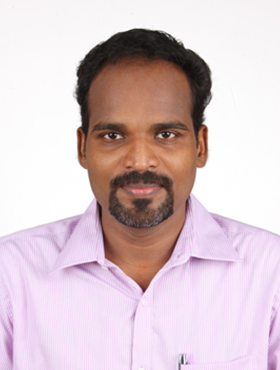
Jagadish Sundaramurthi, Ph.D.
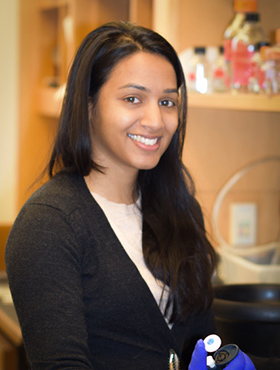
Sabriya Syed, Ph.D.
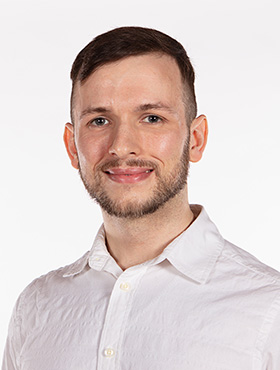
Aaron Taylor, Ph.D.
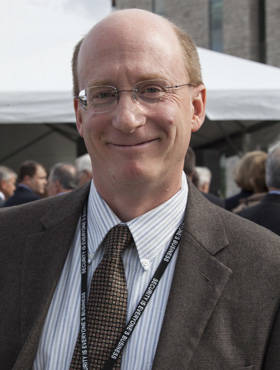
Gregg TeHennepe, B.A.
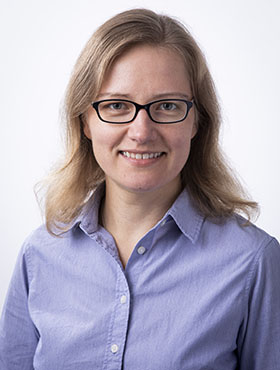
Maria Telpoukhovskaia, Ph.D.
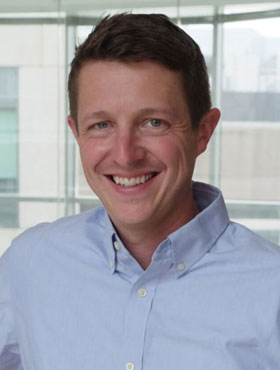
Ryan Tewhey, Ph.D.
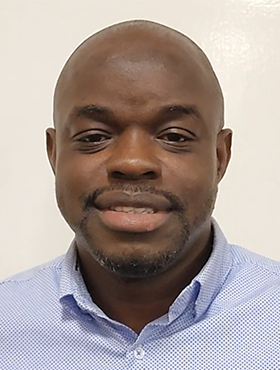
Abdulfatai Tijjani, DVM, Ph.D.
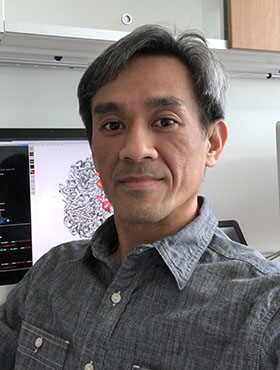
Harianto Tjong, Ph.D.
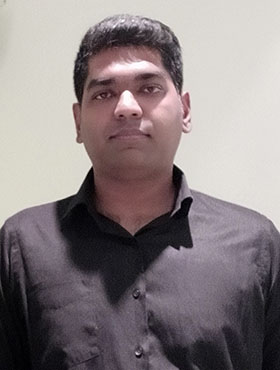
Mohammed Toufiq
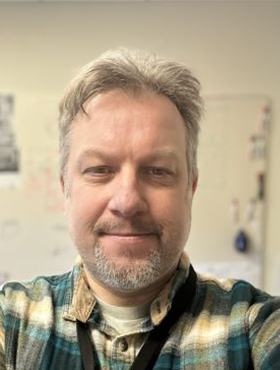
Jarek Trapszo
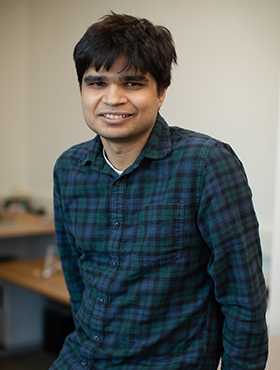
Rohit Tripathy
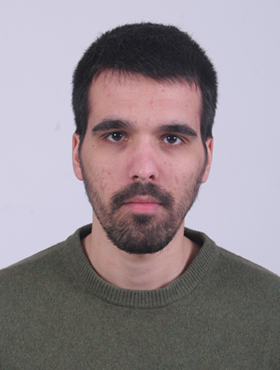
Fotios Tsetsos, Ph.D.
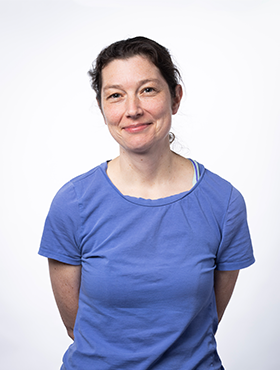
Anna Tyler, Ph.D.
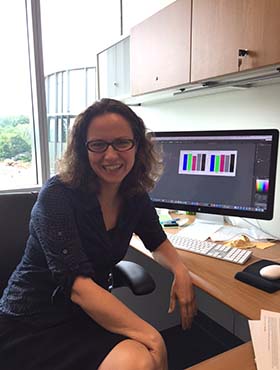
Duygu Ucar, Ph.D.
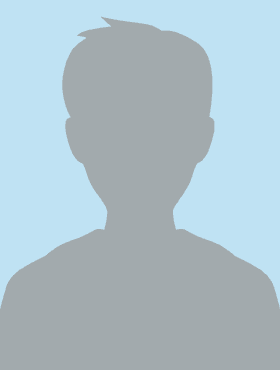
Robert Valenzuela
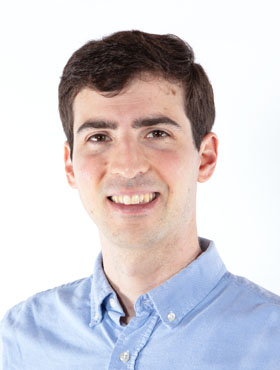
Frederick Varn, Ph.D.
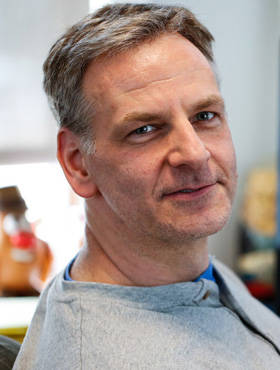
Dave Walton, B.A.
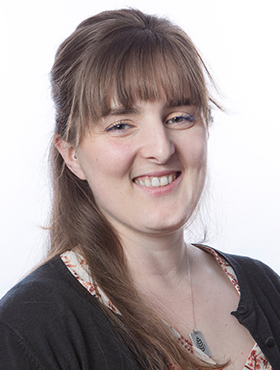
Ann Wells, Ph.D.
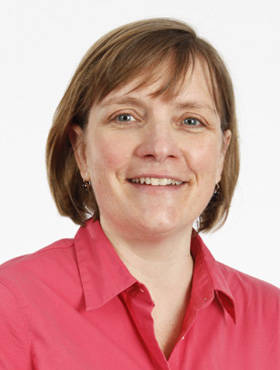
Julie Wells, Ph.D.
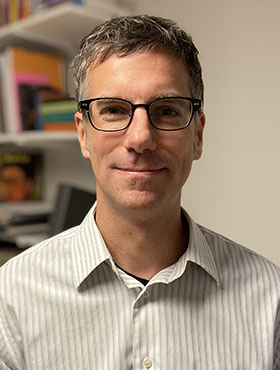
Brian White, Ph.D.
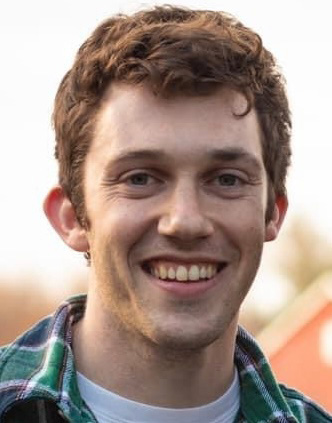
Sam Widmayer, Ph.D.
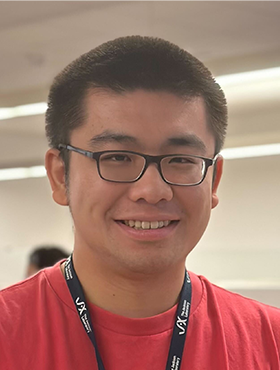
Ruoyu Yang, B.S.
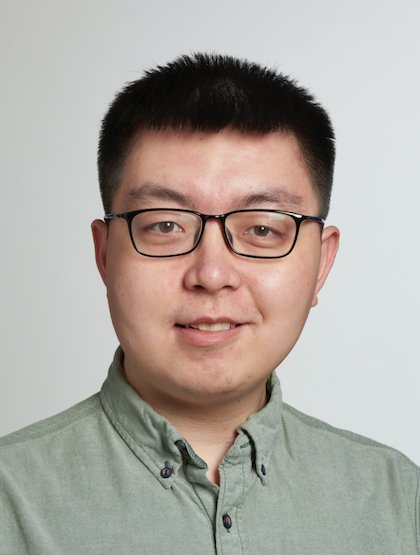
Miao Yu, Ph.D.
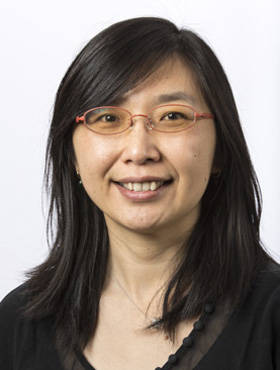
Chun Yu, Ph.D.
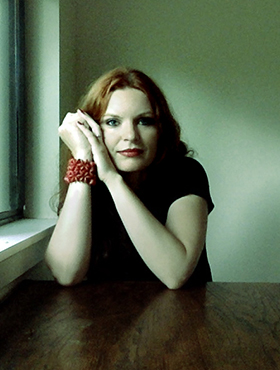
Marina Yurieva, Ph.D.
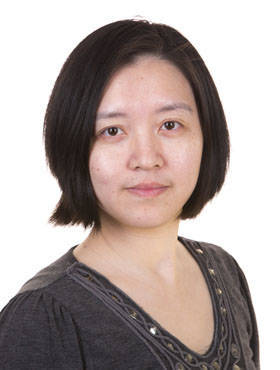
Y. Ada Zhan, Ph.D.
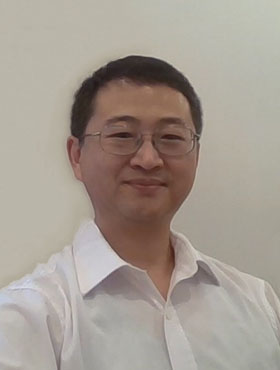
Ji-Gang Zhang, Ph.D.
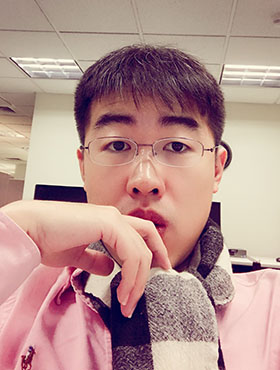
Zi-Ming Zhao, Ph.D.
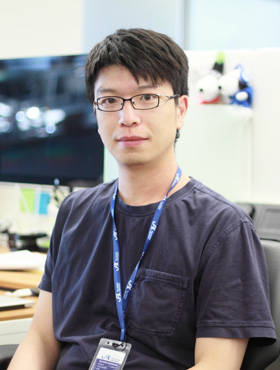
Wei Zhou, Ph.D.
Research centers & resources.
ATAC-seq is a new protocol to capture open chromatin sites by performing adaptor ligation and fragmentation of open chromatin regions. Due to its efficiency in requirement of...
Mouse Models of Human Cancer Database (MMHCdb)
The Mouse Models of Human Cancer Database (formerly, the Mouse Tumor Biology database) integrates data on the frequency, incidence, genetics, and pathology of neoplastic...
Computational Sciences
Computational Sciences supports computational approaches to complex problems; develops software applications and platforms that facilitate access, visualization and sharing...
Gene Ontology Consortium
The Gene Ontology (GO) project is a collaborative effort to address the need for consistent descriptions of gene products across databases.
Mouse Phenome Database
This resource is a collaborative standardized collection of measured data on laboratory mouse strains and populations. Includes baseline phenotype data sets as well as...
Genome Technologies
Genome Technologies (GT) offers sequencing services to The Jackson Laboratory investigators and collaborators.
Mouse Genome Informatics
International database resource for the laboratory mouse, providing integrated genetic, genomic, and biological data.
Random Forest Regression for Epigenetic Length prediction
QuIN (Query tool for Interaction Networks, available at quin.jax.org) is a tool for visualizing, annotating, and querying chromatin interactions derived from technologies...
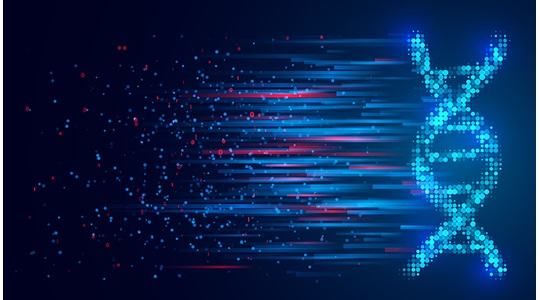
Applying AI and machine learning to complex research tasks
Machine learning: shining a light on genomic function.
- Precision medicine
- Computational Biology
- Genetics and Genomics
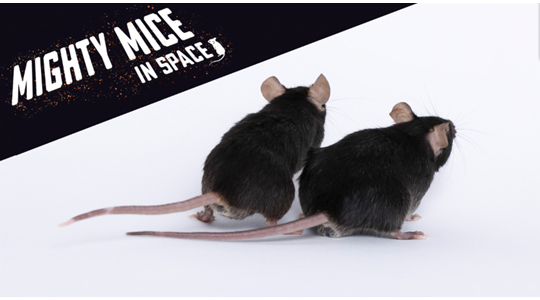
Mighty mice head to space on important health mission
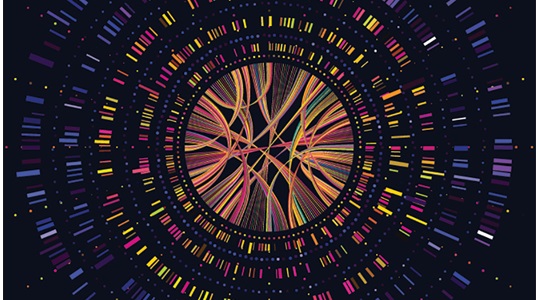
Addressing the urgent need for training in data science
- Genetic Tools
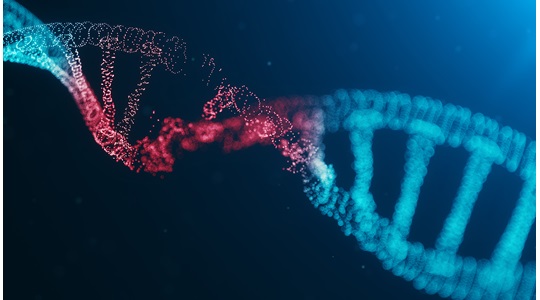
JAX center helps develop new genomic technology
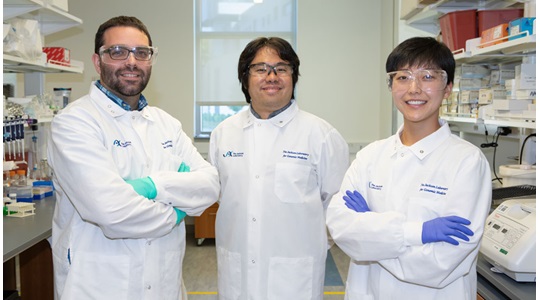
Using CRISPR technology to control RNA splicing
- CRISPR Cas9 system
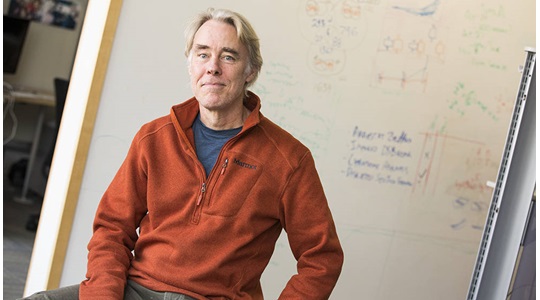
Gary Churchill elected as AAAS Fellow
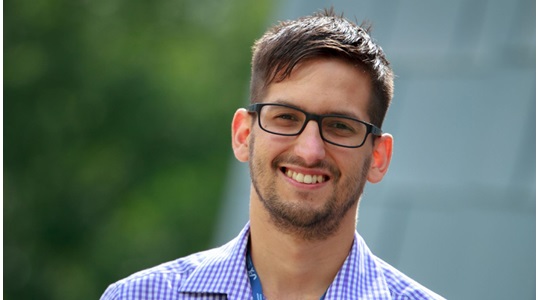
Making a community impact beyond the bench
Upcoming education, long-read sequencing workshop, the whole scientist regional short course, systems immunology in aging and complex diseases, human and mammalian genetics and genomics: the 65th mckusick short course, 33rd annual short course on experimental models of human cancer, the whole scientist, online courses and on-demand webinars, teaching the genome generation - new england, abdulfatai tijjani|postdoctoral associate.
Combines multi-omics technologies and statistical methods to study how genetic differences affect gene regulation, cell differentiation,...
Sathyabaarathi Ravichandran|Postdoctoral Associate
Focuses on understanding the age-associated changes in immune responses to vaccination, particularly to seasonal influenza and pneumococcal...
Mohammed Toufiq|Bioinformatics Analyst I
My research interests include Bioinformatics, Genomics, Immunology and Cancer Biology.
Fotios Tsetsos|Associate Computational Scientist
Investigating the impact of structural variation on complex disorders and their evolution. Studying the bilateral translation of human...
Harianto Tjong, Ph.D.|Computational Scientist
My primary research field is three-dimensional genome organization which includes development of computational analysis methods from data...
Rohit Tripathy|Associate Computational Scientist
I work on integrative cross-species data analysis and explainability of deep learning models in genomics.
Aaron Taylor, Ph.D.|Postdoctoral Associate
Multi-omic profiling of pediatric brain and bone cancer to discover novel prognostic biomarkers and therapeutic targets.
Carolyn Paisie, Ph.D.|Assistant Computational Scientist
My research interests are in bioinformatics, cancer biology, genetics, and genomics.
Vivek Philip, Ph.D.|Director, Computational Sciences
My interests lie in the application of statistical methodologies to the area of genetics, specifically quantitative trait genetics and...
Avijit Podder|Associate Computational Scientist
My research focuses on understanding the genetic complexity of human diseases using computational graph theory and multi-omics data...
Ravi Pandey|Bioinformatics Analyst II
Developing computational pipelines and employing computational strategies using genomic data to understand the genetics of...
Michelle Perry|Senior Scientific Curator
Developmental biology, genetics, disease models, nomenclature
Olivier Poirion, Ph.D.|Computational Scientist
Applies bioinformatics and machine learning algorithm methodologies to explore high-dimensional genomic datasets with an emphasis on...
We use cookies to personalize our website and to analyze web traffic to improve the user experience. You may decline these cookies although certain areas of the site may not function without them. Please refer to our privacy policy for more information.
Allow essential cookies
Required for basic site operations.
Allow analytics cookies
Used to analyze web traffic to improve the user experience.
Allow marketing cookies
Used to deliver personalized information and tailor communications.
- Director’s Welcome
- Participating Departments
- Frontiers in Computational Biosciences Seminar Series
- Current Ph.D. Students
- Current M.S. Students
- Bioinformatics Department Handbook
- B.I.G. Summer Institute
- The Collaboratory
- Diversity and Inclusiveness
- Helpful Information for Current Students
- Joint UCLA-USC Meeting
- Student Blog and Twitter Feed
- Social Gatherings
- Introduction to the Program
- Admissions Information
- Admissions FAQs
- Student Funding
- Curriculum and Graduate Courses
- Research Rotations
- Qualifying Exams
- Doctoral Dissertation
- Student Publications
- Capstone Project
- Undergraduate Courses
- Undergraduate and Masters Research
- Bioinformatics Minor Course Requirements
- Bioinformatics Minor FAQs
- Bioinformatics Minor End-of-Year Celebration
- For Engineering Students
Every master’s degree thesis plan requires the completion of an approved thesis that demonstrates the student’s ability to perform original, independent research.
Students must choose a permanent faculty adviser and submit a thesis proposal by the end of the third quarter of study. The proposal must be approved by the permanent adviser who served as the thesis adviser. The thesis is evaluated by a three-person committee that is nominated by the program and appointed by the Division of Graduate Education. Students must present the thesis in a public seminar.
Featured News
Researchers awarded $4.7 million to study genomic variation in stem cell production, dr. nandita garud recognized for her research on gut microbiome, ucla study reveals how immune cells can be trained to fight infections, ucla scientists decode the ‘language’ of immune cells, dr. eran halperin elected as fellow of international society for computational biology, upcoming events, barbara cheifet seminar, chongzhi zang seminar, mia levine seminar, omolola (lola) ogunyemi seminar, angela brooks seminar, recent student publications.
RECENT STUDENT PUBLICATIONS LINK-PLEASE CLICK!
Updates Coming Soon!
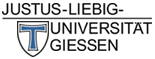
Direct Links
JLU von A-Z
Informationen für
- Schülerinnen & Schüler
- Studieninteressierte
- Studierende
- Menschen mit Fluchthintergrund
- Unternehmen
- Jobs & Karriere
- Wissenschaftler/innen
- Promovierende
- Weiterbildungsangebote für JLU-Angehörige
- Lehrerfortbildung
- Wissenschaftliche Weiterbildung
- Ehemalige (Alumni)
- E-Campus ( Stud.IP , ILIAS , FlexNow , eVV )
Studium & Campus
- Vor dem Studium
- Studienangebot
- Bewerbung/Einschreibung
- Information/Beratung
- Vorlesungsverzeichnis
- Studien- und Prüfungsordnungen (MUG)
- Hochschulrechenzentrum
- Universitätsbibliothek
- Campusplan | Geschosspläne/JLUmaps
- Raumvergabe (ZLIS)
- Studierendenwerk/Mensen
- Corporate Design, Leitfäden, Logos
- Bildergalerie Pressestelle
- Formulare | Rundschreiben
- SAP & JustOS (JLU-Online-Shop)
- Rechtliche Grundlagen (MUG)
- Störungsmeldung
- Datenschutz
Karriere, Kultur, Sport, Marketing
- Allgemeiner Hochschulsport (ahs)
- Botanischer Garten
- Career Services
- Gender & JLU
- Hochschuldidaktik
- Justus' Kinderuni
- Sammlungen der JLU
- Universitätsorchester
- Uni-Shop/Merchandising
- E-Mail-Kontakt
- Telefonbuch
- Wegbeschreibung
- Call Justus
Open thesis topics
Within our group we can offer various topics in the field of applied bioinformatics, high-throughput data analysis, genome and metagenome research as well as postgenomics and systems biology. Below you can find a list of suggested open topics for BSc and MSc theses and student projects. For further details on each topic or alternative projects please contact us.
Comparative genome analysis of Streptococcus agalactiae (GBS) from elephants (M.Sc.)
Background Group B Streptococci are fairly common. In livestock, they are the causative agent of an udder inflamation, most often seen in dairy cows.
In elephants, S. agalactiae is associated with Paronchya. Under human care, elephants are known to reach a high age. This comes with an age-related decline in their immune system, which can lead usually harmless skin- or foot diseases to become chronic. Gaining a better knowledge about the bacterial infections is a vital foundation for optimized treatments and therapeutic approaches.
In a newer study done by the "Hessische Landeslabor" (Hesse state labratory (LHL)), some S. agalactiae isolates were compared, using microbiological methods and had extensive biochemical profiles created. Noticable was the high number of isolates, for which the serotypes could not be determined. For this reason some isolates got sequenced, so a full comparative genome analysis could be done, using the latest methods in bioinformatics.
Thesis aims
- Implementation of typical bioinformatic analyses (Assembly, mapping, annotation...)
- Comparative analysis of GBS Isolates (ABR, pan- and coregenome, virulence factors...)
- Closer inspection of Genes for serotyping
Prerequisites
- Interested in solving biological/veterenary questions by usage of bioinformatics
- Extensive knowledge of the Linux command line
- Ability to work independently and methodical
Contact: Linda Fenske
Workflow Design (Nextflow) (M.Sc.)
Analysing (bacterial) sequence data for biological/medical questions means often repeating certain standard processes (QC, Assembly, Annotation etc.)
For better reproduceability and simplification of these processes, flexible pipelines with a wide palette of tools are used. Often Nextflow (of similar workflow tools) is used to enable support for a variety of enviroments or to simplify the installation.
With DSL2, Nextflow recently introduced a significant development of the Nextflow language, which promises a better scalability and modulariziation of pipelines, along with a better design of workflows.
- Revision and updating of an existing workflow for analysing bacerial data
- Transmission of the workflow from nf-DSL1 to DSL2
- Visualising the results (creating a GUI)
Prerequisites
- Knowledge of Nextflow or motivation to become acquainted with Nextflow
- Programming knowledge in Python, Groovy (Nextflow) or similar
- Knowledge and interest in visualisation and processing of data
Platon Bioinformatics Tool Enhancement for Faster Plasmid Identification (M.Sc.) - taken
Modern high-throughput sequencing devices enable the rapid determination of sequence data obtained from interacting microbial communities without a prior cultivation step. Hereby, access to genetic information from otherwise unculturable microbiota is easily achieved. (Computational) Interpretation of such data relies on either assignment of raw sequencing reads to corresponding source organisms in order to infer their taxonomic origin or gene-coding content, or, these metagenome datasets can be assembled, thereby recovering longer contiguous DNA stretches of the underlying microbial genomes.
Assembled metagenomic contigs are typically clustered (most often, depending on coverage or nucleotide composition), yielding individual draft or complete genomes of novel bacterial species. In this process, however, contigs of non-chromosomal origin such as plasmids are often overlooked.
Still, the analysis of plasmids is of utmost imoprtance, since they constitute a key mechanism of horizontal gene transfer between microbial hosts. They are known to harbor essential genes that are beneficial or important for microbial fittness or survival under certain environmental conditions (e.g. in the presence of certain antimicrobial agents) or perform metabolic processes that they otherwise wouldn‘t have been able to (e.g. degradation of novel substrates).
Several bioinformatics applications have been developed for the computational identification of plasmid-borne contigs, most typically focusing on the extraction of plasmid contigs from the assemblies of individual draft genomes. Among these tools are Platon (Schwengers et al., 2020), PlasClass (Pellow et al., 2020) and PlasFlow (Krawczyk et al., 2018), of which Platon exhibits excellent performance, but its runtime characteristics currently impede its application to potentially large metagenome assemblies.
- Overhaul of the Platon code base, switching from a contig-centered approach to one based on bulk data processing in order to significantly decrease overall runtime.
- Inlining of certain sub-analysis steps such as circularity testing into the python codebase instead of relying on the invocation of external tools: (Pyrodigal, pyHMMER, PyTrimal)
- Conditional tool execution: Do not invoke additional tools if preceding steps already exclude a sequence from being a plasmid
- Runtime and performance assessment with regard to the original implementation
Requirements
- Familiarity with Linux and (modular) python programming (incl. unit testing)
- Methodological way of working
- Able to work independently
Contact: Oliver Schwengers
Develop and Compare Curare Modules for Different DGE Libraries (M. Sc)
Differential gene expression analysis (DGE) is a commonly used method in RNA sequencing, in which the expressions of different genes in samples from different conditions are statistically compared to identify relevant genes in stress or defense situations. To simplify the execution of these analyses, the software Curare was developed.
Currently, the R library DESeq2 is used for the statistical evaluation of expression data, but there are also alternative libraries such as edgeR or Limma that pursue similar or completely different statistical approaches.
This Master's thesis aims to write, compare, and combine Curare modules for various DGE libraries. This requires working with different R libraries, integrating the evaluation into Curare (written in Snakemake), and visualizing the results in an HTML report.
- Write Curare modules for different DGE libraries and compare and combine them.
- Learn about different R libraries for statistical analysis of expression data.
- Integrate the analysis in Curare (written in Snakemake) and visualize the results in an HTML report.
Contact: Patrick Blumenkamp
Reconstruction and visualization of KEGG metabolic pathways in the EDGAR platform (M.Sc.)
EDGAR is a web-based platform for analyzing microbial data. It is developed by employees of the Bioinformatics and Systems Biology department at JLU Giessen and provides multifaceted methods for investigating genomes.
KEGG ( Kyoto Encyclopedia of Genes and Genomes) provides curated databases and resources for (among other things) the functional annotation and classification of genes. In previous projects, KEGG functional categories for all organisms and their corresponding genes were computed in the EDGAR platform. These are currently displayed directly in two analysis modules, in purely quantitative terms.
MinPath is a program for reconstructing biological/metabolic pathways. It attempts to infer a minimal biological metabolic network by excluding redundant metabolic pathways that can explain the genes found in a given dataset. The above-mentioned KEGG categories will be used as input for this program.
The goal of the project is to develop a comparative analysis module, based on KEGG pathway information, for the EDGAR platform.
Thesis Aims
- Parse the available KEGG data in a structured manner and compute KEGG metabolic pathways for all given genomes in EDGAR using MinPath.
- Design comparative visualizations for the EDGAR frontend using the resulting data, allowing users to interactively explore their data (see fig. 4 here as an example)
- Adjust the project scope in consultation with the student depending on the project status to accommodate shared ideas, as EDGAR incorporates a wide selection of data with potential for creative analysis methods.
Requirements
Programming skills in Python and JavaScript (can also be learned during the process)
Basic SQL database knowledge
PlasmidHunter: Validation of a metagenome-based plasmid search using public plasmid sequences (M.Sc.)
Plasmids play an important role in the genetic variability of organisms. They replicate independently and between organisms - within and between species. Therefore, plasmids are key drivers of horizontal gene transfer. Often, they are the effective and only difference between commensal and pathogenic bacterial strains. In recent years, it became obvious that plasmids belong to the main mechanisms for the dissemination of antimicrobial resistances and hence are of special interest in medical microbiology. Detecting plasmids and analyzing their dissemination is an important epidemiological and scientific topic that might help to detect current and prevent future outbreaks of antibiotic resistances.
One promising data source containing known and unknown plasmids are whole-metagenome datasets of samples from different sources (soil, waste water, the human gut). For many of these samples, sequencing data is freely accessible in public databases, often annotated with additional meta information such as date, source and location of each sample.
Our project processes these datasets from the MGnify database in a standardized way via modern cloud technologies and makes them accessible to users for a fast search of new plasmids within this huge amount of data.
This master thesis should validate this search via existing plasmid databases (such as PLSDB) and analyze search results including comprehensive visualizations.
- Implementation of a workflow to process PLSDB entries with our existing search workflow
- Statistical analysis of the results, and screen for potential interesting candidates for further analysis
- Visualization of the results
- Knowledge of command line tools and Python
- Interest in cloud technologies
- Prior experience with workflow systems, like Nextflow or Snakemake
Contact: Sebastian Beyvers
Webservice for searching gene families in plants (M. Sc.)
The input is a list of protein sequences. In step 1a, a Pfam search is performed with the sequences to find common domains. In step 1b, a multiple sequence alignment of the sequences is calculated. The conserved regions are automatically extracted from the alignment to calculate HMMs. In step 2, the HMMs of the domains from 1a and 1b are used to search a database of plant proteins.
- The results are visualized and made available for download
- Steps 1 and 2 are also provided as a command-line tool
- The programming language(s) and frameworks can be freely chosen
- Test data will be provided
Contact: Oliver Rupp
R ibosomal binding site prediction based on 16S-rRNA (M.Sc.)
Bacterial translation is initiated by the assembly of ribosomal proteins as part of the translation initiation complex at the coding sequence (CDS) start site. For most CDS, there is a ribosomal binding site (RBS) immediately upstream of the gene, consisting of a 5-10bp spacer and a (partial or complete) Shine-Dalgarno sequence (SD) 5’-AGGAGG-3’ to which the ribosome binds. However, some genes have neither an SD nor a known RBS and are still expressed (Omotajo, D. et al. , 2015) . The Shine-Dalgarno sequence was first described in E. coli but is found in many bacterial genomes and is complementary to the anti-SD sequence at the 3′-end of 16S-rRNA.
The exact Shine-Dalgarno and spacer sequences vary between bacterial species. However, because the anti-Shine-Dalgarno sequence is present in the 16S-rRNA of each bacterial genome, it can be used to predict RBS in a species-independent manner. Therefore, a deep learning approach using the 16S-rRNA sequences and the sequence upstream of the CDS is promising for accurately predicting the presence of RBS independent of species-specific variants.
- Design and implementation of a neural network for ribosomal binding site prediction in bacteria,
- evaluation of the features used by the neural network, and
- analysis of the presence of RBS in exemplary bacterial genomes
- Prior experience with deep learning frameworks such as Tensorflow/Keras, or willingness to learn them
- Prior experience in the development of documented code and dependency management or willingness to learn them
Contact: Julian Hahnfeld
Integrative Omics FAIR Workflow (M.Sc.) Background
Processing and analysing 'omics data often requires applying predefined building blocks of code, i.e. for performing quality control, statistical analysis or machine learning. However, biologists and ecologists are often overwhelmed with the technical complexity of programmatic approaches and interfaces. Hence, scientific workflows can not just automate, but also facilitate important re-occuring processes in high-throughput 'omics analysis.
The existing modularized iESTIMATE pipeline aims at automating and facilitating the complex analysis of ecological metabolomics data and the integration with other phenomics and preparation for sequencing and (meta-)genomics data. The central aim of the pipeline is to extract so called molecular traits that explain molecular mechanisms in plants or microorganisms. Thesis Aims
- Revision and modularisation of existing code to create the R package "iESTIMATE"
- Implementing a workflow in NextFlow or Common Workflow Language (CWL) using test data, implementing unit tests and capture provenance information
- Publish R package and the workflow following the FAIR principles
- Knowledge of R and a bit of Python
- Knowledge of Linux command line, containers, NextFlow (Groovy), YAML, or motivation to become acquainted with them
- Keen interest in analysis of integrative 'omics data and in topics in molecular ecology
Contact: Kristian Peters
THAIS NISENBAUM
Bioinformatics portfolio.
- Work Samples
INTRODUCTION
Hello, and welcome to my Bioinformatics portfolio. I am a highly motivated bioinformatics professional with a passion for solving complex biological problems using computational methods.I am currently completing my master’s degree in Biotechnology with a concentration in Bioinformatics from Johns Hopkins University. Throughout my studies, I have gained experience in various areas of bioinformatics, including genomics, transcriptomics, and proteomics. My thesis involves exploring the potential of DNA-Based digital twin technology for the advancement of precision medicine in tongue cancer.
My portfolio highlights my skills and expertise in bioinformatics, featuring projects and code snippets that demonstrate my ability to analyze, interpret, and visualize biological data. In addition, my portfolio includes examples of my proficiency in software development, as I have designed and implemented various bioinformatics tools and pipelines using programming languages such as Python, R, and SQL. I have also worked on several projects that involve the integration of multiple data types, including RNA-seq and ChIP-seq, and am experienced in using various bioinformatics databases and tools, including NCBI, Ensembl, and UCSC Genome Browser.
Overall, my bioinformatics portfolio is a testament to my dedication and passion for bioinformatics, demonstrating my eagerness to continue developing my skills and knowledge in the field. I am excited about the opportunities to collaborate with others in the field and contribute to advancing our understanding of the complex biological systems around us.
Thank you for taking the time to review my portfolio.
SKILLS SUMMARY
Programming languages.
Python, SQL, R, Javascript/JQuery
R/Bioconductor Packages
ggplot2, peptides, biomaRt, biostrings, seqinr, cn.mops, CNVtools, edgeR
Prokaryotic Gene Prediction and Annotation
Gene-Finding Programs: ORF Finder, Glimmer, FGENESB, GeneMark, EasyGene 1.2b, Prodigal
Promoter-Finding Programs: BROM, Neural Network Promoter Prediction
Eukaryotic Gene Prediction
Single Genome De Novo Gene Prediction: GENSCAN, HMMGene, FGENESH, Augustus
Expression-Based Prediction: Splign, Genomic BLAST, BLAT
Protein Prediction
PSORTb, Hierarchical Neural Network (HNN), PHD, Protein Homology/analogY Recognition Engine V 2.0 (PHYRE2)
Functional RNAs
PicTar, PPfold
Genomic Databases and Tools
NCBI, Genome Data Viewer (GDV), NCBI Variation Viewer, Ensembl, UCSC (Genome Browser, Table Browser), Galaxy, IGV, dbSNP, ClinVar, SNPEffect and SNPedia, Database of Genomic Variants (DGV), Genome Aggregation Database (GnomAD), InterPro, ENCODE, NONCODE, TargetScan, miRNEST 2.0, PolymiRTS, DIANA-TarBase v.8, miRDB, miRBase, Rfam, cneViewer, UCNEbase
Sequence Alignment
MUSCLE, Clustal Omega, MAFFT
Next Generation Sequencing Analysis
Velvet, BWA, Bowtie2, HISAT, FreeBayes, MACS2, Stringtie, DESeq2, BEDtools, SAMtools, GATK, SnpEff, ANNOVAR, TopHat, Cufflinks
Your browser is unsupported
We recommend using the latest version of IE11, Edge, Chrome, Firefox or Safari.
Richard and Loan Hill Department of Biomedical Engineering
Colleges of engineering and medicine, ms in bioinformatics.
Required Semester Hours: 36
Thesis track Heading link Copy link
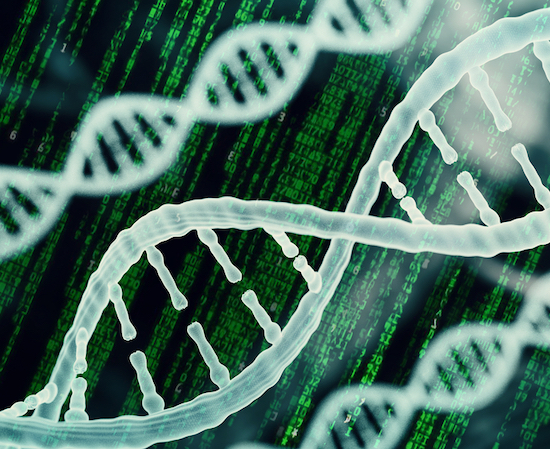
The thesis track is designed for MS in Bioinformatics students who are interested in conducting research. This track is strongly advised if you may be interested in pursuing a PhD in the future.
Researching and writing a master’s thesis is an academically intensive process that takes the place of 8 credits of traditional coursework. Students work with a faculty advisor to choose a topic of interest, engage in high-level study of that topic, and develop a paper that is suitable for presentation at a conference or submission to a journal.
The thesis experience provides definition to your master’s degree experience and can bolster your application for jobs or doctoral-level study by demonstrating your capabilities.
In the thesis option, you will earn 8 credits in BME 598 Master’s Thesis Research and at least 28 credit hours from coursework. At least 12 of your coursework credits must come from courses at the 500 level, excluding BME 595, BME 596, and BIOE 598. You may be allowed limited credit hours from BME 596 Independent Study with department approval. There is no comprehensive examination.
Recent UIC master’s thesis projects in bioinformatics include:
thesis titles Heading link Copy link
Nikita Dsouza
Strategies for Identification of Small Molecule Inhibitors of Ad2 E3-19K/HLA-A2 Binding Interaction
A Statistical Framework for GeneSet Enrichment Analysis based on DNA Methylation and Gene Expression
Navya Josyula
Identifying Ligand Binding Sites of Proteins using Crystallographic Bfactors and Relative Pocket Sizes
Non-thesis track Heading link Copy link
In the non-thesis track, you earn all of your required 36 credit hours from coursework. Of these, 16 must be from courses at the 500 level. There is no comprehensive examination.
Across-the-board requirements Heading link Copy link
- 1 hour of BME 595
- Present at least one seminar (BME 595) before graduation
- Students entering the program without an undergraduate degree in bioengineering or biomechanical engineering must also take BME 480, BME 481, and BME 530
MS alumni in their own words Heading link Copy link
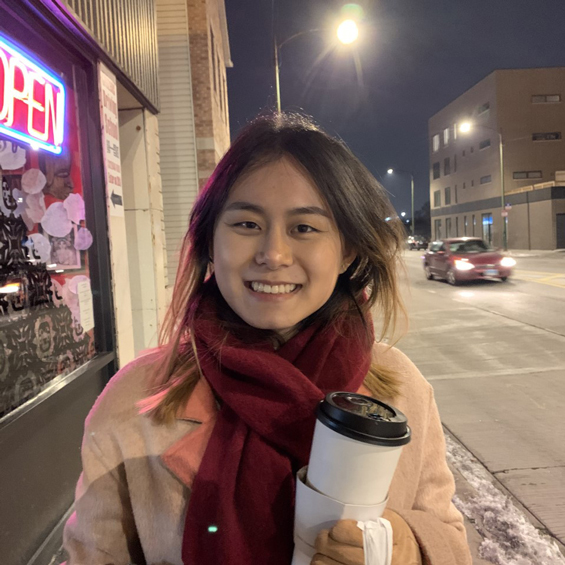
Daiqing Chen ’21 MS in Bioinformatics
What led you to choose bioinformatics for your MS degree? How do you think computational technology is changing biomedical engineering? I was doing molecular biology during my undergrad. Wet lab experiments are very time- and money-consuming. I have seen people using bioinformatics methods to solve biological questions, and I want to be able to use them. I actually don’t know much about engineering, but I believe a computational method can be useful for any field. The high efficiency allows people to do more things than ever before.
What are your plans for once you have completed your degree? I am planning on working as a research assistant in biological lab, most likely doing research about cancer. My time at UIC helped me get more familiar with American culture.
Have you worked in any labs? Yes, the Computational Functional Genomics Laboratory . I did a project to validate machine learning models that predict kidney function decline. I also worked on high-throughput single-cell sequence analysis.
Your primary hobby/outside interest: Playing badminton.
Favorite restaurant in Chicago: Minhin’s cuisine for the dim sum.
Additional information Heading link Copy link
- MS in Bioinformatics course checklist: thesis track
- MS in Bioinformatics course checklist: non-thesis track
- MS in Bioinformatics graduate catalog page
- UIC Graduate College admissions
- Important deadlines for BME graduate students
- Skip to Content
- Bulletin Home
Virginia Commonwealth University
Make it real..
- Academic Calendars
- Financial aid
- Maps and directions
- Technology Services
- Tuition and fees
- VCU Libraries
- Regulations
- Undergraduate
- Professional
- Bioinformatics, Master of Science (M.S.)
- Graduate Bulletin /
- VCU Life Sciences /
- Center for Biological Data Science /
- Graduate study
- Dual degree opportunities
- Dual degree and certificate opportunities
- College of Engineering
- College of Health Professions
- College of Humanities and Sciences
- School of the Arts
- School of Business
- School of Dentistry
- School of Education
- L. Douglas Wilder School of Government and Public Affairs
- School of Medicine
- School of Nursing
- School of Pharmacy
- School of Population Health
- School of Social Work
- Administration
- Graduate information
- Center for Environmental Studies
- Integrative Life Sciences, Doctor of Philosophy (Ph.D.)
- Integrative Life Sciences, Doctor of Philosophy (Ph.D.) with a concentration in behavioral and statistical genetics
- Integrative Life Sciences, Doctor of Philosophy (Ph.D.) with a concentration in bioinformatics and genome sciences
- da Vinci Center for Innovation
- Office of Research and Innovation
- VCU Health Sciences
- Graduate School
- Division of Strategic Enrollment Management and Student Success
- Division of Student Affairs
- Global Education Office
- VCU Continuing and Professional Education
- Office of Institutional Equity, Effectiveness and Success
This is the preliminary (or launch) version of the 2024-2025 VCU Bulletin. We may add courses that expose our students to cutting-edge content and transformative learning. We may also add content to the general education program that focuses on racial literacy and a racial literacy graduation requirement, and may receive notification of additional program approvals after the launch. The final edition and full PDF version will include these updates and will be available in August prior to the beginning of the fall semester.
Program goals
The VCU Center for Biological Data Science created and administers the Master of Science in Bioinformatics degree program to provide interested students with two options:
- The Master of Science in Bioinformatics thesis option is a traditional research- and thesis-oriented master’s degree. The mission of this research-oriented degree program is to prepare students for research careers in university, foundation or industry laboratories; and completion of a thesis provides problem-solving skills required for a research career. In addition to course work, students complete a substantial original research project with a VCU faculty mentor and write a thesis describing that work. This degree program prepares students for employment in an academic, industrial or government environment and for Ph.D. training programs.
The Master of Science in Bioinformatics non-thesis option is a Professional Science Master’s degree program. The mission of this professionally oriented program is to train graduates for leadership roles in bioinformatics, biotechnology, biomedicine and other sectors of the life sciences. The program imparts interdisciplinary knowledge, skills and experience in the biological, quantitative and information sciences, and engages students in the practices of business and entrepreneurship in collaboration with the commercial and public bioscience sectors. In addition to course work featuring both bioinformatics and business fundamentals, students participate in a summer externship at an industrial or government setting. This degree program not only prepares students with advanced training in bioinformatics, but also in workplace skills valued by employers.
Students enter the program from a variety of academic backgrounds (biology, chemistry, computer science, mathematics/statistics, etc.) assisted by flexible “bridge curricula” designed to help them meet program prerequisites. Students will have an effective exposure to the biotech industry and other career options and to real-life applications of their learning.
The Master of Science in Bioinformatics degree program will prepare students to:
- Synthesize and apply interdisciplinary subject matter: The M.S. in Bioinformatics degree program seeks to provide students with the skills and knowledge required to advance into Ph.D. training programs and research positions in universities, government labs or industry. The program provides a framework for the progressive development of a mastery of the interdisciplinary subject matter pertinent to bioinformatics and an ability to synthesize this information and apply it to key areas of investigation and experimentation in bioinformatics.
- Design, implement and interpret experimental approaches: The program relates the above framework to the development of the ability to design, implement and interpret experimental approaches.
- Develop communication skills: In addition, the program will develop skills in oral and written communication of interdisciplinary science concepts, experimental design, results and interpretation.
Student learning outcomes
- Oral communication skills: The candidate will demonstrate the achievement of an appropriate level of oral communication skills with respect to the content, organization, logical flow, presentation and appropriate use of language incorporating the use of visual aids, as measured by rubric.
- Written communication skills: The candidate will demonstrate the achievement of an appropriate level of written communication skill with respect to grammar, syntax, spelling and use of vocabulary to effectively present information, including the use of figures, tables and citations, as measured by rubric.
- Experimental design competency: The candidate will demonstrate the achievement of an appropriate level of competence in the ability to appraise, modify, and/or create and implement bioinformatics experimental protocols and to design and develop experiments, as measured by rubric.
- Problem-solving skills: The candidate will demonstrate an appropriate level of ability to analyze scientific problems including pertinent datasets and design and develop appropriate methods to solve said problems, as measured by rubric.
- Integrated knowledge of bioinformatics: The candidate will demonstrate an appropriate level of knowledge of fundamentals of molecular biology, computational science, statistics and a more detailed understanding of an individual area of internship research, including an appropriate familiarity with the research literature, as measured by rubric.
VCU Graduate Bulletin, VCU Graduate School and general academic policies and regulations for all graduate students in all graduate programs
The VCU Graduate Bulletin website documents the official admission and academic rules and regulations that govern graduate education for all graduate programs at the university. These policies are established by the graduate faculty of the university through their elected representatives to the University Graduate Council.
It is the responsibility of all graduate students, both on- and off-campus, to be familiar with the VCU Graduate Bulletin as well as the Graduate School website and academic regulations in individual school and department publications and on program websites. However, in all cases, the official policies and procedures of the University Graduate Council, as published on the VCU Graduate Bulletin and Graduate School websites, take precedence over individual program policies and guidelines.
Visit the academic regulations section for additional information on academic regulations for graduate students.
Degree candidacy requirements
A graduate student admitted to a program or concentration requiring a final research project, work of art, thesis or dissertation, must qualify for continuing master’s or doctoral status according to the degree candidacy requirements of the student’s graduate program. Admission to degree candidacy, if applicable, is a formal statement by the graduate student’s faculty regarding the student’s academic achievements and the student’s readiness to proceed to the final research phase of the degree program.
Graduate students and program directors should refer to the following degree candidacy policy as published in the VCU Graduate Bulletin for complete information and instructions.
Visit the academic regulations section for additional information on degree candidacy requirements.
Graduation requirements
As graduate students approach the end of their academic programs and the final semester of matriculation, they must make formal application to graduate. No degrees will be conferred until the application to graduate has been finalized.
Graduate students and program directors should refer to the following graduation requirements as published in the Graduate Bulletin for a complete list of instructions and a graduation checklist.
Visit the academic regulations section for additional information on graduation requirements.
Apply online today.
Admission requirements
Special requirements.
- International students requiring temporary U.S. visas should apply by April 1 for fall admission and Sept. 1 for spring admission.
In addition to the general admission requirements of the VCU Graduate School , applicants are encouraged to include in their personal statements, and request that their referees also discuss, one or more examples of creative and analytical contributions they have made to a recent research project (preferred) or to a challenging course assignment.
Because of the interdisciplinary nature of bioinformatics, applications are welcomed from students with various academic backgrounds, such as biology, biotechnology, molecular biology, computer science, mathematics or statistics. Our program provides “bridge curricula” to strengthen students’ preparation for graduate study in bioinformatics.
Degree requirements
Prerequisites and bridge curricula for master’s programs.
While an ideal preparation for the bioinformatics master’s programs would include substantial work in molecular biology, computer science, mathematics and statistics, the program has been designed to provide “bridge curricula” to accommodate academically strong students with majors in any one of these or related disciplines. These students would develop with the assistance of their advisers a “bridge curriculum” of largely undergraduate courses to meet the prerequisites for the program and prepare them for graduate-level work.
Program prerequisites are listed below. In general, students will not need to address the set corresponding to their undergraduate majors, but will usually need to address the other two sets. It is expected that all bridge course work will be completed during the first year. While bridge courses may be completed prior to initiating the graduate program, this is not required, and most students are able, through advising, to complete bridge courses alongside graduate course work during the first year of the program.
- Biology/genomic prerequisites: an introductory knowledge of biochemistry and molecular biology, one semester of organic chemistry (e.g. CHEM 301 ), cell biology (e.g. BIOL 300 ) and an undergraduate course in molecular biology or genetics
- Computational science prerequisites: an introductory knowledge of computer science, including at least one general computer programming language, met by taking structured programming (e.g. CMSC 255 ) and data structures and advanced programming (e.g. CMSC 256 )
- Quantitative/statistical prerequisites: an introductory knowledge of mathematics/statistics, met by taking calculus I (e.g. MATH 200 ) and at least one undergraduate course in statistics
Thesis research
In addition to general VCU Graduate School graduation requirements , students in the M.S. program must perform a credible original investigation under the supervision of their major advisers and the Graduate Advisory Committee. Students must develop and write short proposals in consultation with their major advisers and GAC. The project must be approved by the student’s GAC, based on a short (10-page) paper submitted by the student. This paper will include background on the project, including a review of the literature, the purpose, specific aims and rationale of the project, a statement about the specific hypothesis to be investigated, and proposed methods and statistical analyses.
Research projects will be based on ongoing research in the laboratories of faculty in the Center for Biological Data Science and across both campuses of VCU and the Virginia BioTechnology Research Park. Students in the program may perform research on the broad range of subjects, from molecules to ecosystems, studied by CBDS faculty.
Students shall prepare a written thesis describing the completed research performed during their tenure in the M.S. in Bioinformatics program following the format of the Graduate School Thesis and Dissertation Manual . An oral defense, consisting of a public presentation of the thesis and a committee meeting to discuss the thesis, under the direction of the GAC but open to all faculty members, shall be scheduled to examine the student’s research, thesis and underlying fundamental knowledge of the discipline encompassed by the student’s research. Announcement of the oral defense, including the candidate’s name, thesis title and the day, place and time of the defense, shall be made at least 10 working days in advance of the defense.
Non-thesis (professional science master’s) externship
In addition to general VCU Graduate School graduation requirements , students enrolled in the non-thesis, professional science master’s option complete a 10- to 12-week full-time externship at an industrial, government or academic site, usually during the summer between the first and second years of the bioinformatics program. In preparation for this externship, students will enroll in BNFO 620 and BNFO 621 in the semester preceding their externships. Upon initiating the externship, each student must develop and write a short proposal or prepare a PowerPoint presentation outlining the plans for the externship for review by the student’s GAC. Research projects will be based on ongoing research in the laboratories of the participating external advisers. Students in the program may perform computational research on a broad range of subjects, from molecules to ecosystems, encompassing the field of bioinformatics.
In the semester following the externship experience, non-thesis students shall prepare written papers (~10 pages) describing the completed research performed during their externships following the format of the Graduate School’s Thesis and Dissertation Manual . The paper should include background on the project, including a review of the literature, the purpose, specific aims and rationale of the project, the specific hypotheses investigated, description of the methods and statistical analyses implemented, results, discussion/conclusions, and a bibliography. An oral defense, consisting of a public presentation of the paper and a committee meeting to discuss the results, under the direction of the GAC but open to all faculty members and the adviser of the externship, shall be scheduled to examine the student’s underlying fundamental knowledge of the disciplines encompassed by the student’s externship. Announcement of the oral defense, including the candidate’s name, project title, and the day, place and time of the defense, shall be made at least 10 working days in advance of the defense.
Curriculum requirements
Thesis option.
The minimum number of graduate credit hours required for this degree is 34.
Recommended electives
Non-thesis option (professional science master’s), accelerated opportunities.
The department offers opportunities for qualified undergraduate students to earn both an undergraduate and graduate degree in a minimum of five years by completing approved graduate courses during the senior year of their undergraduate program. See the individual program page for concentrations in the Undergraduate Bulletin for details.
- B.S. in Bioinformatics with a concentration in biological/genomics sciences and M.S.
- B.S. in Bioinformatics with a concentration in computational sciences and M.S.
- B.S. in Bioinformatics with a concentration in quantitative/statistical sciences and M.S.
Contact Allison A. Johnson, Ph.D. Associate professor and graduate program director [email protected] (804) 828-6782
Program website: cbds.vcu.edu/academics/graduate
Virginia Commonwealth University Richmond, Virginia 23284 Phone: (804) 828-0100 [email protected]
All Bulletins © 2024-2025 Virginia Commonwealth University Wed Dec 27 2023 12:25:35 GMT-0600 (CST)
Print Options
Print this page.
The PDF will include all information unique to this page.
5 Machine Learning Projects in Bioinformatics For Practice
Explore Top Machine Learning Projects Ideas to Understand the Applications of Machine Learning in Bioinformatics| ProjectPro
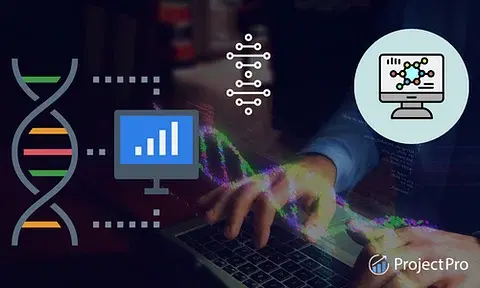
The term "bioinformatics" represents the use of computation and analysis methods to collect and analyze biological data. It's a multidisciplinary field that combines genetics, biology, statistics, mathematics, and computer science. Various branches of bioinformatics, including genomics, proteomics, and microarrays, extensively use machine learning for better outcomes.

Personalized Medicine: Redefining Cancer Treatment
Downloadable solution code | Explanatory videos | Tech Support
Top 5 Machine Learning Projects in Bioinformatics
Here are five exciting machine learning projects for bioinformatics to help you understand the application of machine learning in healthcare , mainly bioinformatics.
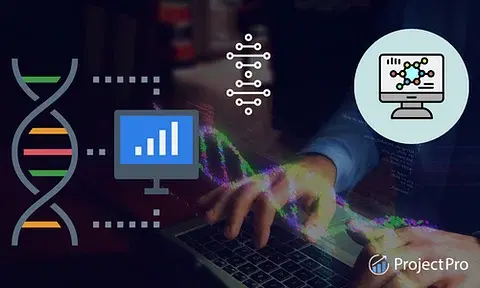
1. Anti-Cancer Drug Efficacy Prediction
Predicting which patients are likely to benefit or not from a specific therapy is a significant concern in cancer treatment because, generally speaking, not all patients will benefit from a particular medication. This enhances the efficacy of treatment and reduces the suffering and misery experienced by non-responders. Thus, there is an immediate need to find reliable biomarkers (i.e., genes or proteins) that can precisely predict which patients respond best to which medications. For this project, you will use fundamental data science techniques , such as data processing, integration, analysis, and visualization, to determine the most effective biomarkers for various cancer types.
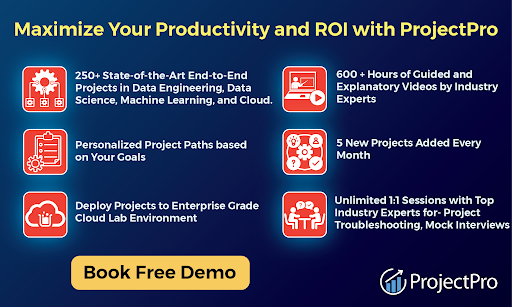
2. Autism Mutation Detection
In this machine learning project for bioinformatics, you will develop a deep-learning-based system that predicts the accurate regulatory effects and the harmful impacts of genetic variants to address the issue of detecting the impact of noncoding mutations on disease. This predictive genomics framework is likely relevant to complex human diseases, illustrates the significance of noncoding mutations in ASD [autism spectrum disorder], and identifies mutations with higher effects for further analysis. If you want to add some unique project to your machine learning portfolio , you must try working on this project.
Here's what valued users are saying about ProjectPro
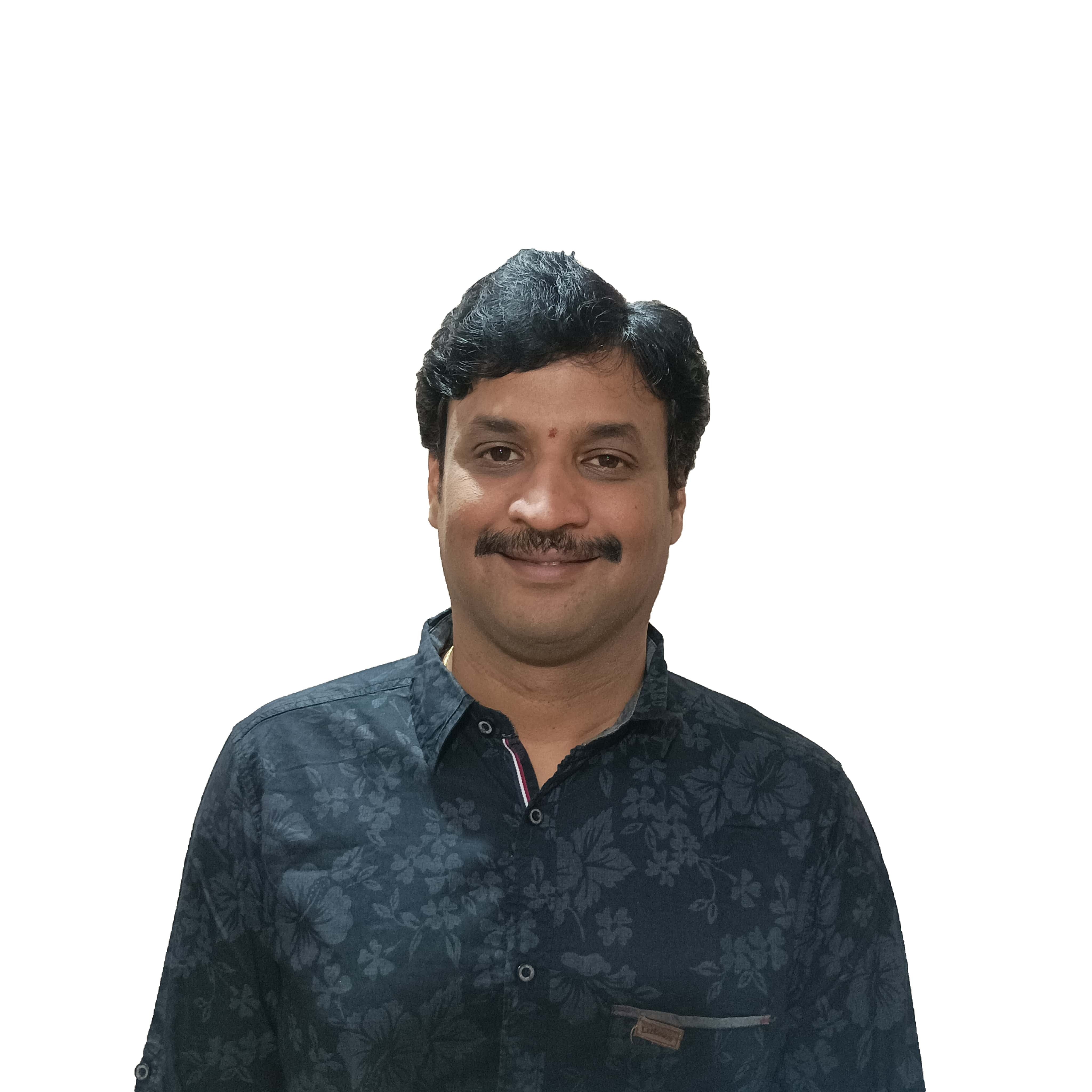
Anand Kumpatla
Sr Data Scientist @ Doubleslash Software Solutions Pvt Ltd
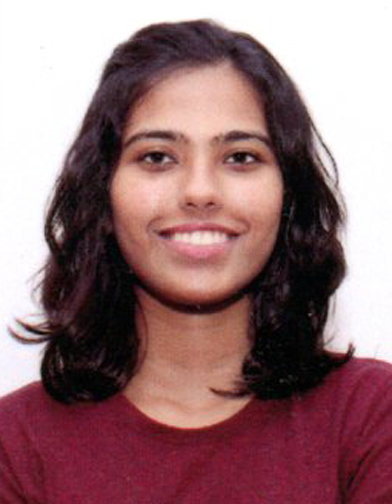
Savvy Sahai
Data Science Intern, Capgemini
Not sure what you are looking for?
3. Personalized Cancer Medication
This deep learning project can predict how different genetic variations affect a patient's health. You can use the MSKCC (Memorial Sloan Kettering Cancer Center) database, including thousands of mutations that top-notch scientists and physicians have thoroughly classified. For this machine learning project, you will create a machine learning algorithm using the Keras deep learning library and LSTM that automatically categorizes genetic variants utilizing this data set as a starting point. Additionally, this project entails using various NLP text processing techniques such as Lemmatization, Stemming, Tokenization, etc.
You don't have to remember all the machine learning algorithms by heart because of amazing libraries in Python. Work on these Machine Learning Projects in Python with code to know more!
4. Human Disease Genetic Basis Identification
Human genomes vary between individuals by.1%. Our genetic inclination to specific disorders, such as hypertension, is encoded within this small degree of variation. We can accurately define which gene variants belong to each disease by comparing populations of healthy and diseased people and their variations in the genes responsible for the diseases. In this bioinformatics, AI and machine learning project, strategies for finding the variation corresponding to disease are developed, along with statistics to support the predictions. Furthermore, this project develops methods for predicting how a gene mutation can alter the structure of the protein or the regulatory structure. You can also estimate the disease risk factor's history and evolution by recreating the genes' phylogeny.
5. Build a DNA Sequence Classifier
You will use a classification model in this project that can predict a gene's function just from the DNA sequence of the coding sequence. You will create a function that will extract from any sequence string all overlapping k-mers of a given length, count the k-mers and convert the k-mers list for each gene into string sequences using scikit-learn NLP tools.
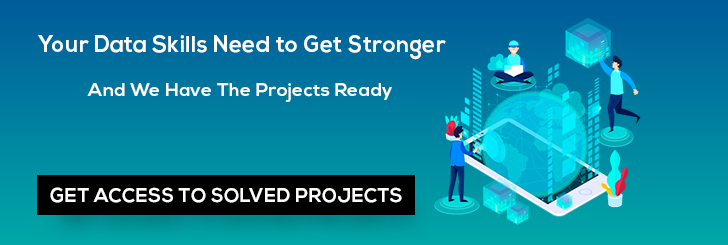
About the Author

Daivi is a highly skilled Technical Content Analyst with over a year of experience at ProjectPro. She is passionate about exploring various technology domains and enjoys staying up-to-date with industry trends and developments. Daivi is known for her excellent research skills and ability to distill

© 2024
© 2024 Iconiq Inc.
Privacy policy
User policy
Write for ProjectPro
at the Department of Biology: Biology, Molecular biology, Bioinformatics
Lund University
The browser you are using is not supported by this website. All versions of Internet Explorer are no longer supported, either by us or Microsoft (read more here: https://www.microsoft.com/en-us/microsoft-365/windows/end-of-ie-support ).
Please use a modern browser to fully experience our website, such as the newest versions of Edge, Chrome, Firefox or Safari etc.
Bioinformatics Master's degree project
BINP50, BINP51, BINP52
You can perform a Master's degree project in Bioinformatics if you are enrolled in the Master´s program in Bioinformatics at Lund University and have completed 45 credits of relevant advanced level courses within the programme.
You find detailed information about degree projects as well as registration forms on the learning platform in Canvas.
Before the start
Contact a supervisor within the area of your interest and discuss the general outline of the project. A 30 credit project should be about 20 weeks; 45 credits correspond to 30 weeks and 60 credits correspond to 40 weeks. Before proceeding, you should show your CV and LADOK excerpt to the supervisor. Note that the project can be conducted outside the university, at a company. If you do not find what you want, your Master’s programme coordinator will give advice.
Write a project plan together with your supervisor when you have decided about a project. The plan should be brief, but give a clear description of your specific project (2–3 A4 pages). It should contain:
- Project title
- Name and e-mail addresses to you and the supervisor
- Topic, time, and number of credits
- Introduction, with a theoretical background to the project and key references (5-10)
- The specific aim(s) of your project
- Time plan
If the project is carried out outside of Lund University , a contact person from within Lund University (Internal supervisor) has to be appointed.
During the project
Carefully document your work . You should document your work systematically in a README file. With the aid of the README file, you or another person should be able to recreate the current results from the original data. The README file may be used in the final examination and grading of your project.
You should participate in group meetings, seminars , and such that may be arranged in the group or at the department where you are working.
The project shall be presented as a scientific report in English. The format of your report should follow the instructions on how to write manuscripts for PLOS One. Your final report can be in manuscript form or you may choose a layout that is more similar to a printed paper.
Plagiarism . You are not allowed to present someone else’s work, such as text, figures or results, without giving proper reference. You may of course refer to the works of others, but you must write about it in your own words and refer to the source of information in a correct way. To prevent plagiarism, all Master's project reports are sent to "Ouriginal" (information to students on Urkund's website , a program that uses internet/databases to scan a report for plagiarised text. Your text is also added to the database, which prevents the contents of your report to be plagiarised by someone else in the future.
The examination
The examination committee consists of :
- Examiner. The programme coordinator will act as an examiner or appoint another examiner within the Department of Biology.
- External examiner: The external examiner is suggested by the supervisor and then appointed by the examiner.
Supervisor: Can take part in the discussion, but not in the decision (like at a PhD dissertation). If the supervisor is not present, the examiner will contact the supervisor for his or her opinion.
Seminar . At the examination, the student will give a presentation (about 20–30 min). After the presentation, the examination committee will ask questions and discuss the report and project, and thereafter the audience will be invited to ask questions. Finally, the examination committee has an internal discussion before the examiner decides about the grading.
Evaluation Criteria:
The Process is primarily evaluated by the Supervisor:
- Independence of the student
- Ability to carry out the project (knowledge and competence of the student)
- The student´s biological understanding of the project.
- The student´s ability to structure and write the report.
- The student´s commitment (is the student hard working and interested in the subject?)
The Product is primarily evaluated by the Examiner:
- Quality of the written report.
- Ability to explain the work and put it into a larger perspective.
- Quality of the presentation (including discussions during and after the presentation)
Application
Detailed information and application at Bioinformatics, MSc Projects in the learning platform Canvas, Lund University
Syllabus for Master´s Degree Projects in Bioinformatics:
- BINP50, 30 credits (pdf)
- BINP51, 45 credits (pdf))
- BINP52, 60 credits (pdf)
Project proposals
Project proposals in Bioinformatics at our blog
Find inspiration
Completed master's degree projects in bioinformatics in Lund University's student paper database
Lotta Persmark, Study advisor, biology and bioinformatics
Telephone : +46 46 222 37 28 Email : Lotta [dot] Persmark [at] biol [dot] lu [dot] se (Lotta[dot]Persmark[at]biol[dot]lu[dot]se)
Bioinformatics: A Tool for Sustainable Agriculture
- First Online: 26 August 2022
Cite this chapter
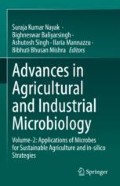
- Debi Prasad Mishra 6 ,
- J. Chandrakanta Badajena 6 ,
- Suraja Kumar Nayak 7 &
- Bighneswar Baliyarsingh 7
340 Accesses
Bioinformatics is a discipline of research that uses computational power for extraction of useful information from biological data. Bioinformatics utilizes computer technology for storage, analysis, and retrieval of genetic information which is achieved as a result of various scientific processes. In line with the research of biological bodies, keeping information of 100,000 genes of a human being without the availability of computational power would be beyond imagination. Biotechnology has become more efficient with the availability of highly reliable computer-assisted predictions. With the need for high amounts of agricultural products for ever-growing population, pesticides and chemical fertilizers were once successful in catering to the demands. After decades of practices of such products for agriculture, it was revealed that chemical fertilizers and pesticides leave a negative impact on the atmosphere which in turn is harmful for sustainable environment. Recent progress in computational software and computing tools along with modern bioinformatics analysis and genome analysis are proving to be successful in diminishing the effects of harmful agriculture and creating a better and sustainable agroatmosphere.
- Bioinformatics
- Sustainable agriculture
- Metabolomics
- Interactomics
This is a preview of subscription content, log in via an institution to check access.
Access this chapter
- Available as PDF
- Read on any device
- Instant download
- Own it forever
- Available as EPUB and PDF
- Compact, lightweight edition
- Dispatched in 3 to 5 business days
- Free shipping worldwide - see info
- Durable hardcover edition
Tax calculation will be finalised at checkout
Purchases are for personal use only
Institutional subscriptions
FAO (2010) The state of world fisheries and aquaculture. United Nations
Google Scholar
Agriculture Marketing (2008) https://india.gov.in . Accessed Feb 2008
Alonso R, Salavert F, Garcia-Garcia F et al (2015) Babelomics 5.0:functional interpretation for new generations of genomic data. Nucleic Acids Res 43(W1):W117–W121. https://doi.org/10.1093/nar/gkv384
Article CAS PubMed PubMed Central Google Scholar
Altschul SF, Madden TL, Schäffer AA et al (1997) Gapped BLAST and PSI-BLAST: a new generation of protein database search programs. Nucleic Acids Res 25(17):3389–3402. https://doi.org/10.1093/nar/25.17.3389
Ansorge WJ (2009) Next-generation DNA sequencing techniques. New Biotechnol 25(4):195–203. https://doi.org/10.1016/j.nbt.2008.12.009
Article CAS Google Scholar
Berners-Lee T, Hendler J, Lassila O (2001) The semantic web. Sci Am 284(5):34–43
Article Google Scholar
Blais A, Dynlacht BD (2005) Constructing transcriptional regulatory networks. Genes Dev 19(13):1499–1511. https://doi.org/10.1101/gad.1325605
Article CAS PubMed Google Scholar
Blazejczyk M, Miron M, Nadon R (2007) FlexArray: a statistical data analysis software for gene expression microarrays. Genome Quebec, Montreal, Canada. http://genomequebec.mcgill.ca/FlexArra
Brady SM, Provart NJ (2009) Web-queryable large-scale data sets for hypothesis generation in plant biology. Plant Cell 21(4):1034–1051. https://doi.org/10.1105/tpc.109.066050
Caicedo AL, Williamson SH, Hernandez RD et al (2007) Genome-wide patterns of nucleotide polymorphism in domesticated rice. PLoS Genet 3(9):1745–1756. https://doi.org/10.1371/journal.pgen.0030163
Chellappan P, Jin H (2009) Discovery of plant microRNAs and short-interfering RNAs by deep parallel sequencing. Methods Mol Biol 495:121–132. https://doi.org/10.1007/978-1-59745-477-3_11
Choi H, Pavelka N (2012) When one and one gives more than two: challenges and opportunities of integrative omics. Front Genet 2:105. https://doi.org/10.3389/fgene.2011.00105
Article PubMed PubMed Central Google Scholar
CIA Factbook (2021) India-Economy. https://www.cia.gov/the-world-factbook/countries/india/ . Accessed 20 July 2021
Durbin BP, Hardin JS, Hawkins DM et al (2002) A variance-stabilizing transformation for gene-expression microarray data. Bioinformatics 18(suppl_1):S105–S110. https://doi.org/10.1093/bioinformatics/18.suppl_1.S105
Article PubMed Google Scholar
Eisen MB, Spellman PT, Brown PO et al (1998) Cluster analysis and display of genome-wide expression patterns. Proc Natl Acad Sci 95(25):14863–14868
Esposito A, Colantuono C, Ruggieri V et al (2016) Bioinformatics for agriculture in the next-generation sequencing era. Chem Biol Technol Agric 3:9. https://doi.org/10.1186/s40538-016-0054-8
FAOSTAT (2014) Data. Faostat.fao.org . Accessed 17 Sept 2011
Harris MA, Clark J, Ireland A et al (2004) The Gene Ontology (GO) database and informatics resource. Nucleic Acids Res 32:D258–D261. https://doi.org/10.1093/nar/gkh036
He G, Zhu X, Elling AA et al (2010) Global epigenetic and transcriptional trends among two rice subspecies and their reciprocal hybrids. Plant Cell 22(1):17–33. https://doi.org/10.1105/tpc.109.072041
Heinrich R, Schuster S (2012) The regulation of cellular systems. Springer US. https://doi.org/10.1007/978-1-4613-1161-4
Book Google Scholar
Hoffmann R, Valencia A (2004) A gene network for navigating the literature. Nat Genet 36(7):664. https://doi.org/10.1038/ng0704-664
Hucka M, Finney A, Sauro HM et al (2003) The systems biology markup language (SBML): a medium for representation and exchange of biochemical network models. Bioinformatics 19(4):524–531. https://doi.org/10.1093/bioinformatics/btg015
IBEF (India Brand Equity Forum) (2021) Agriculture and food industry and exports. https://www.ibef.org/industry/agriculture-and-food-industry-india.aspx . Accessed June 2021
IBEF (India Brand Equity Forum) (2019) Agriculture in India: information about Indian Agriculture & Its Importance. https://www.ibef.org/industry/agriculture-india.aspx
Mishra BB, Nayak SK, Mohapatra S et al (eds) (2021) Environmental and agricultural microbiology: applications for sustainability. Wiley-Scrivener publisher, Beverly
Nayak SK, Dash B, Baliyarsingh B (2018) Microbial remediation of persistent agro-chemicals by soil bacteria: an overview. In: Patra J, Das G, Shin HS (eds) Microbial biotechnology. Springer, Singapore, pp 275–301. https://doi.org/10.1007/978-981-10-7140-9_13
Chapter Google Scholar
Flake L (2014) India’s Agricultural Exports Climb to Record High. International Agricultural Trade Report, US Department of Agriculture. https://www.fas.usda.gov/sites/default/files/2015-02/india_iatr_august_2014
Parray JA, Mir MY, Shameem N (2019) Advancement in sustainable agriculture: computational and bioinformatics tools. In: Sustainable agriculture: biotechniques in plant biology. Springer, Singapore, pp 465–547. https://doi.org/10.1007/978-981-13-8840-8_10
Sheng Q, Moreau Y, De Moor B (2003) Biclustering microarray data by Gibbs sampling. Bioinformatics 19(suppl_2):ii196–ii205. https://doi.org/10.1093/bioinformatics/btg1078
Vertovik S (1995) Indian indentured migration to the Caribbean. In: Cohen R (ed) The Cambridge survey of world migration. Cambridge University Press, Cambridge, New York, pp 57–68
Stuart JM, Segal E, Koller D et al (2003) A gene-coexpression network for global discovery of conserved genetic modules. Science 302(5643):249–255. https://doi.org/10.1126/science.1087447
Sundar S (2018) India economic survey 2018: farmers gain as agriculture mechanisation speeds up, but more R & D needed. In: The Financial Express. https://www.financialexpress.com/budget/india-economic-survey-2018-for-farmers-agriculture-gdp-msp/1034266/ . Accessed 29 Jan 2018
Xue J, Zhao S, Liang Y et al (2008) Bioinformatics and its applications in agriculture. In: Li D (ed) IFIP international federation for information processing, Volume 259; Computer and computing technologies in agriculture, vol 2. Springer, Boston, pp 977–982
Download references
Author information
Authors and affiliations.
Department of Information Technology, Odisha University of Technology and Research, Bhubaneswar, Odisha, India
Debi Prasad Mishra & J. Chandrakanta Badajena
Department of Biotechnology, Odisha University of Technology and Research, Bhubaneswar, Odisha, India
Suraja Kumar Nayak & Bighneswar Baliyarsingh
You can also search for this author in PubMed Google Scholar
Editor information
Editors and affiliations.
Department of Biotechnology, Odisha University of Technology and Research (formerly College of Engineering and Technology), Bhubaneswar, Odisha, India
Suraja Kumar Nayak
Bighneswar Baliyarsingh
School of Engineering, University of Guelph, Guelph, ON, Canada
Ashutosh Singh
Department of Agricultural Sciences, University of Sassari, Sassari, Sassari, Italy
Ilaria Mannazzu
Department of Microbiology, Odisha University of Agriculture and Technology, Bhubaneswar, Odisha, India
Bibhuti Bhusan Mishra
Rights and permissions
Reprints and permissions
Copyright information
© 2022 The Author(s), under exclusive license to Springer Nature Singapore Pte Ltd.
About this chapter
Mishra, D.P., Badajena, J.C., Nayak, S.K., Baliyarsingh, B. (2022). Bioinformatics: A Tool for Sustainable Agriculture. In: Nayak, S.K., Baliyarsingh, B., Singh, A., Mannazzu, I., Mishra, B.B. (eds) Advances in Agricultural and Industrial Microbiology. Springer, Singapore. https://doi.org/10.1007/978-981-16-9682-4_13
Download citation
DOI : https://doi.org/10.1007/978-981-16-9682-4_13
Published : 26 August 2022
Publisher Name : Springer, Singapore
Print ISBN : 978-981-16-9681-7
Online ISBN : 978-981-16-9682-4
eBook Packages : Biomedical and Life Sciences Biomedical and Life Sciences (R0)
Share this chapter
Anyone you share the following link with will be able to read this content:
Sorry, a shareable link is not currently available for this article.
Provided by the Springer Nature SharedIt content-sharing initiative
- Publish with us
Policies and ethics
- Find a journal
- Track your research
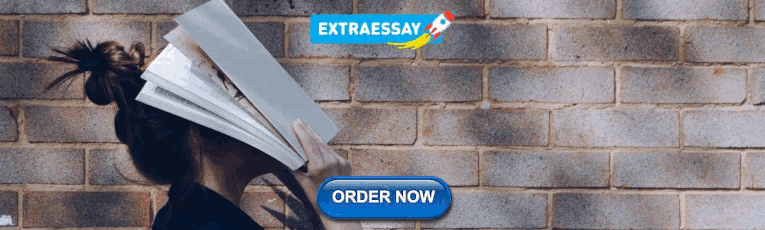
IMAGES
VIDEO
COMMENTS
MSc thesis: In the Bioinformatics group, we offer a wide range of MSc thesis projects, from applied bioinformatics to computational method development. Here is a list of available MSc thesis projects.Besides the fact that these topics can be pursued for a MSc thesis, they can also be pursued as part of a Research Practice.. BSc thesis: As a BSc student you will work as an apprentice alongside ...
This project will assess whether AMGs generally evolve into distinct shorter versions of the bacterial gene and whether the transfer of metabolic genes from phages to bacteria is a prevalent phenomenon. To this end, publicly available genomes of phages and bacteria will be scanned for metabolic genes (Shaffer et al. 2020).
Master`s Thesis Bioinformatics Masters Degree Programme, Institute of Biomedical Technology University of Tampere, Finland Tommi Rantapero May, 2012 . ii ACKNOWLEDGEMENTS This work has been done in the Genetic Predisposition to Prostate Cancer group lead ... opportunity to work with this interesting project. Her guidance and support has been
comments and for proofreading this thesis. My thanks to all the Functional Genomics Group members, in partic-ular to Mar Gonzalez-Porta, Gabriella Rustici and Johan Rung for all the helpful discussions, Mar and Gabriella for their companionship in teaching bioinformatics around the world and Lynn French for greatly
List of PhD theses produced at the Bioinformatics Laboratory or under co-supervision of the Bioinformatics Laboratory. ... EU projects. 3TR; ARCAID; COSMIC; EpiPredict (closed) Education. Overview; Introduction to Bioinformatics (ARCAID) ... (PhD thesis). University of Amsterdam, Amsterdam. COSMIC. Marie Curie ITN, Horizon 2020.
Bioinformatics and Systems Biology Graduate Program University of California, San Diego 9500 Gilman Drive, Dept. 0419 La Jolla, CA 92093-0419
Project example: Bioinformatic analyses of lipoproteins encoded in bacterial genomes. Bacterial lipoproteins are a family of proteins that can be readily identified by the bioinformatic analysis of conserved sequence motifs (Citation Sutcliffe and Harrington, 2002; Babu et al, 2006).Project work in this area was designed based on the authors experience of bacterial genome sequence analysis ...
Bioinformatics is a highly specialized application area of computer science and biology and to successfully solve research questions in this field, you require a lot of interdisciplinary knowledge. ... Within the Master thesis project of Bettina Hübner we have investigated and evaluated different guiding strategies for such an approach
Theses. Thesis Preparation and Filing: Staff from the University Archives and the UCLA Graduate Division present information on University regulations governing manuscript preparation and completion of degree requirements. Students should plan to attend at least one quarter before they plan to file a thesis or dissertation. More information is ...
In the Master's program in bioinformatics, you must do a 30 ECTS Master's thesis. You must start your 30 ECTS thesis no later than February 1 (or September 1) a year and a half after commencement of your studies (i.e. February 2021 for students admitted in summer 2019, or September 2021 for students admitted in winter 2020).
A number of recent theses and dissertations prepared at Oxford are available to download from the Oxford Research Archive (ORA). The British Library provides access to UK theses through its EThOS service. Already digitised UK theses can be downloaded freely as PDF files. Requests can be made to digitise older theses, but there is a cost of ...
Our bioinformatics work incorporates data from both mouse and human genetic and genomic research and provides the annotations and interfaces necessary for delivering useful information to the research community. ... (GO) project is a collaborative effort to address the need for consistent descriptions of gene products across databases. Mouse ...
Thesis. Every master's degree thesis plan requires the completion of an approved thesis that demonstrates the student's ability to perform original, independent research. Students must choose a permanent faculty adviser and submit a thesis proposal by the end of the third quarter of study. The proposal must be approved by the permanent ...
Open thesis topics. Within our group we can offer various topics in the field of applied bioinformatics, high-throughput data analysis, genome and metagenome research as well as postgenomics and systems biology. Below you can find a list of suggested open topics for BSc and MSc theses and student projects.
Integration of computational high throughput sequencing, single-cell and spatial analysis of immune microenvironments regulation in cancers. An innovative journal that provides a forum for new discoveries in bioinformatics. It focuses on how new tools and applications can bring insights to specific biological problems.
My thesis involves exploring the potential of DNA-Based digital twin technology for the advancement of precision medicine in tongue cancer. My portfolio highlights my skills and expertise in bioinformatics, featuring projects and code snippets that demonstrate my ability to analyze, interpret, and visualize biological data.
The thesis track is designed for MS in Bioinformatics students who are interested in conducting research. This track is strongly advised if you may be interested in pursuing a PhD in the future. Researching and writing a master's thesis is an academically intensive process that takes the place of 8 credits of traditional coursework.
The Master of Science in Bioinformatics thesis option is a traditional research- and thesis-oriented master's degree. ... A graduate student admitted to a program or concentration requiring a final research project, work of art, thesis or dissertation, must qualify for continuing master's or doctoral status according to the degree candidacy ...
A recent study has found that the interest of researchers in these topics plateaued over after the early 2000s [1]. Besides the above mentioned hot topics, the following topics are considered demanding in bioinformatics. Cloud computing, big data, Hadoop. Machine learning. Artificial intelligence.
Master Thesis. In the second course of the Master, students are required to complete a Master Thesis or Project. This internship offers the student the opportunity to become familiar with the real-world bioinformatics, integrating all the skills and knowledge acquired along the programme. Each academic course, the master coordinator opens an ...
Here are five exciting machine learning projects for bioinformatics to help you understand the application of machine learning in healthcare, mainly bioinformatics. 1. Anti-Cancer Drug Efficacy Prediction. Predicting which patients are likely to benefit or not from a specific therapy is a significant concern in cancer treatment because ...
BINP50, BINP51, BINP52. You can perform a Master's degree project in Bioinformatics if you are enrolled in the Master´s program in Bioinformatics at Lund University and have completed 45 credits of relevant advanced level courses within the programme. You find detailed information about degree projects as well as registration forms on the ...
Bioinformatics is a discipline of research that uses computational power for extraction of useful information from biological data. Bioinformatics utilizes computer technology for storage, analysis, and retrieval of genetic information which is achieved as a result of various scientific processes. In line with the research of biological bodies ...