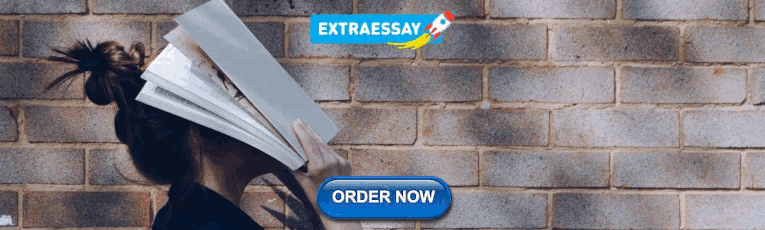
Understanding Clinical Data Analysis
Learning Statistical Principles from Published Clinical Research
- © 2017
- Ton J. Cleophas 0 ,
- Aeilko H. Zwinderman 1
Albert Schweitzer Hospital, Department Medicine Albert Schweitzer Hospital, Sliedrecht, The Netherlands
You can also search for this author in PubMed Google Scholar
Dept. Epidemiology and Biostatistics, Academic Medical Center Dept. Epidemiology and Biostatistics, Amsterdam, The Netherlands
The book uses the best-help-there-is for making the difficult issues understandable by using real data examples rather than hypothetical examples
Complementarily to real data examples, the book continually gives a philosophical treatise of the basics of the scientific method.
The book explains all of the novel issues of clinical data analysis from the past few years.
20k Accesses
4 Citations
1 Altmetric
This is a preview of subscription content, log in via an institution to check access.
Access this book
- Available as EPUB and PDF
- Read on any device
- Instant download
- Own it forever
- Compact, lightweight edition
- Dispatched in 3 to 5 business days
- Free shipping worldwide - see info
- Durable hardcover edition
Tax calculation will be finalised at checkout
Other ways to access
Licence this eBook for your library
Institutional subscriptions
Table of contents (10 chapters)
Front matter.
- Ton J. Cleophas, Aeilko H. Zwinderman
Randomized and Observational Research
Randomized clinical trials, history, designs, randomized clinical trials, analysis sets, statistical analysis, reporting issues, discrete data analysis, failure time data analysis, quantitative data analysis, subgroup analysis, interim analysis, multiplicity analysis, medical statistics: a discipline at the interface of biology and mathematics, back matter.
- Statistical Reasoning
- Hypothesis Testing
- Clinical data analysis
- Statistical methodologies
- Medical Statistics
About this book
This textbook consists of ten chapters, and is a must-read to all medical and health professionals, who already have basic knowledge of how to analyze their clinical data, but still, wonder, after having done so, why procedures were performed the way they were. The book is also a must-read to those who tend to submerge in the flood of novel statistical methodologies, as communicated in current clinical reports, and scientific meetings.
In the past few years, the HOW-SO of current statistical tests has been made much more simple than it was in the past, thanks to the abundance of statistical software programs of an excellent quality. However, the WHY-SO may have been somewhat under-emphasized. For example, why do statistical tests constantly use unfamiliar terms, like probability distributions, hypothesis testing, randomness, normality, scientific rigor, and why are Gaussian curves so hard, and do they make non-mathematicians getting lost all the time? The book will cover the WHY-SOs.
Authors and Affiliations
Ton J. Cleophas
Aeilko H. Zwinderman
About the authors
The authors are well-qualified in their field. Professor Zwinderman is past-president of the International Society of Biostatistics (2012-2015), and Professor Cleophas is past-president of the American College of Angiology (2000-2002). From their expertise they should be able to choose the best-help-there-is for making difficult issues understandable, that is real data examples from the global literature rather than hypothetical examples.
The authors have been working and publishing together for 18 years, and their research can be characterized as a continued effort to demonstrate that clinical data analysis is not mathematics but rather a discipline at the interface of philosophy, biology, and mathematics.
The authors, as professors and teachers in statistics at universities in The Netherlands and France for the most part of their lives, are convinced, that the scientific method of statistical reasoning and hypothesis testing is little used by physicians and other health workers, and they hope, that the current production will help them find the appropriate ways for answering their scientific questions.
Bibliographic Information
Book Title : Understanding Clinical Data Analysis
Book Subtitle : Learning Statistical Principles from Published Clinical Research
Authors : Ton J. Cleophas, Aeilko H. Zwinderman
DOI : https://doi.org/10.1007/978-3-319-39586-9
Publisher : Springer Cham
eBook Packages : Medicine , Medicine (R0)
Copyright Information : Springer International Publishing Switzerland 2017
Hardcover ISBN : 978-3-319-39585-2 Published: 31 August 2016
Softcover ISBN : 978-3-319-81917-4 Published: 14 June 2018
eBook ISBN : 978-3-319-39586-9 Published: 23 August 2016
Edition Number : 1
Number of Pages : X, 234
Number of Illustrations : 119 b/w illustrations, 92 illustrations in colour
Topics : Medicine/Public Health, general
- Publish with us
Policies and ethics
- Find a journal
- Track your research
Thank you for visiting nature.com. You are using a browser version with limited support for CSS. To obtain the best experience, we recommend you use a more up to date browser (or turn off compatibility mode in Internet Explorer). In the meantime, to ensure continued support, we are displaying the site without styles and JavaScript.
- View all journals
- My Account Login
- Explore content
- About the journal
- Publish with us
- Sign up for alerts
- Open access
- Published: 01 May 2024
A critical assessment of using ChatGPT for extracting structured data from clinical notes
- Jingwei Huang ORCID: orcid.org/0000-0003-2155-6107 1 ,
- Donghan M. Yang 1 ,
- Ruichen Rong 1 ,
- Kuroush Nezafati ORCID: orcid.org/0000-0002-6785-7362 1 ,
- Colin Treager 1 ,
- Zhikai Chi ORCID: orcid.org/0000-0002-3601-3351 2 ,
- Shidan Wang ORCID: orcid.org/0000-0002-0001-3261 1 ,
- Xian Cheng 1 ,
- Yujia Guo 1 ,
- Laura J. Klesse 3 ,
- Guanghua Xiao 1 ,
- Eric D. Peterson 4 ,
- Xiaowei Zhan 1 &
- Yang Xie ORCID: orcid.org/0000-0001-9456-1762 1
npj Digital Medicine volume 7 , Article number: 106 ( 2024 ) Cite this article
38 Altmetric
Metrics details
- Non-small-cell lung cancer
Existing natural language processing (NLP) methods to convert free-text clinical notes into structured data often require problem-specific annotations and model training. This study aims to evaluate ChatGPT’s capacity to extract information from free-text medical notes efficiently and comprehensively. We developed a large language model (LLM)-based workflow, utilizing systems engineering methodology and spiral “prompt engineering” process, leveraging OpenAI’s API for batch querying ChatGPT. We evaluated the effectiveness of this method using a dataset of more than 1000 lung cancer pathology reports and a dataset of 191 pediatric osteosarcoma pathology reports, comparing the ChatGPT-3.5 (gpt-3.5-turbo-16k) outputs with expert-curated structured data. ChatGPT-3.5 demonstrated the ability to extract pathological classifications with an overall accuracy of 89%, in lung cancer dataset, outperforming the performance of two traditional NLP methods. The performance is influenced by the design of the instructive prompt. Our case analysis shows that most misclassifications were due to the lack of highly specialized pathology terminology, and erroneous interpretation of TNM staging rules. Reproducibility shows the relatively stable performance of ChatGPT-3.5 over time. In pediatric osteosarcoma dataset, ChatGPT-3.5 accurately classified both grades and margin status with accuracy of 98.6% and 100% respectively. Our study shows the feasibility of using ChatGPT to process large volumes of clinical notes for structured information extraction without requiring extensive task-specific human annotation and model training. The results underscore the potential role of LLMs in transforming unstructured healthcare data into structured formats, thereby supporting research and aiding clinical decision-making.
Similar content being viewed by others
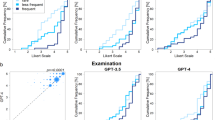
Systematic analysis of ChatGPT, Google search and Llama 2 for clinical decision support tasks
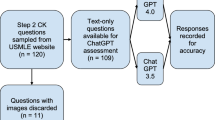
Assessing ChatGPT 4.0’s test performance and clinical diagnostic accuracy on USMLE STEP 2 CK and clinical case reports
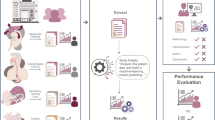
Large language models streamline automated machine learning for clinical studies
Introduction.
Large Language Models (LLMs) 1 , 2 , 3 , 4 , 5 , 6 , such as Generative Pre-trained Transformer (GPT) models represented by ChatGPT, are being utilized for diverse applications across various sectors. In the healthcare industry, early applications of LLMs are being used to facilitate patient-clinician communication 7 , 8 . To date, few studies have examined the potential of LLMs in reading and interpreting clinical notes, turning unstructured texts into structured, analyzable data.
Traditionally, the automated extraction of structured data elements from medical notes has relied on medical natural language processing (NLP) using rule-based or machine-learning approaches or a combination of both 9 , 10 . Machine learning methods 11 , 12 , 13 , 14 , particularly deep learning, typically employ neural networks and the first generation of transformer-based large language models (e.g., BERT). Medical domain knowledge needs to be integrated into model designs to enhance performance. However, a significant obstacle to developing these traditional medical NLP algorithms is the limited existence of human-annotated datasets and the costs associated with new human annotation 15 . Despite meticulous ground-truth labeling, the relatively small corpus sizes often result in models with poor generalizability or make evaluations of generalizability impossible. For decades, conventional artificial intelligence (AI) systems (symbolic and neural networks) have suffered from a lack of general knowledge and commonsense reasoning. LLMs, like GPT, offer a promising alternative, potentially using commonsense reasoning and broad general knowledge to facilitate language processing.
ChatGPT is the application interface of the GPT model family. This study explores an approach to using ChatGPT to extract structured data elements from unstructured clinical notes. In this study, we selected lung cancer pathology reports as the corpus for extracting detailed diagnosis information for lung cancer. To accomplish this, we developed and improved a prompt engineering process. We then evaluated the effectiveness of this method by comparing the ChatGPT output with expert-curated structured data and used case studies to provide insights into how ChatGPT read and interpreted notes and why it made mistakes in some cases.
Data and endpoints
The primary objective of this study was to develop an algorithm and assess the capabilities of ChatGPT in processing and interpreting a large volume of free-text clinical notes. To evaluate this, we utilized unstructured lung cancer pathology notes, which provide diagnostic information essential for developing treatment plans and play vital roles in clinical and translational research. We accessed a total of 1026 lung cancer pathology reports from two web portals: the Cancer Digital Slide Archive (CDSA data) ( https://cancer.digitalslidearchive.org/ ) and The Cancer Genome Atlas (TCGA data) ( https://cBioPortal.org ). These platforms serve as public data repositories for de-identified patient information, facilitating cancer research. The CDSA dataset was utilized as the “training” data for prompt development, while the TCGA dataset, after removing the overlapping cases with CDSA, served as the test data for evaluating the ChatGPT model performance.
From all the downloaded 99 pathology reports from CDSA for the training data, we excluded 21 invalid reports due to near-empty content, poor scanning quality, or missing report forms. Seventy-eight valid pathology reports were included as the training data to optimize the prompt. To evaluate the model performance, 1024 pathology reports were downloaded from cBioPortal. Among them, 97 overlapped with the training data and were excluded from the evaluation. We further excluded 153 invalid reports due to near-empty content, poor scanning quality, or missing report forms. The invalid reports were preserved to evaluate ChatGPT’s handling of irregular inputs separately, and were not included in the testing data for accuracy performance assessment. As a result, 774 valid pathology reports were included as the testing data for performance evaluation. These valid reports still contain typos, missing words, random characters, incomplete contents, and other quality issues challenging human reading. The corresponding numbers of reports used at each step of the process are detailed in Fig. 1 .
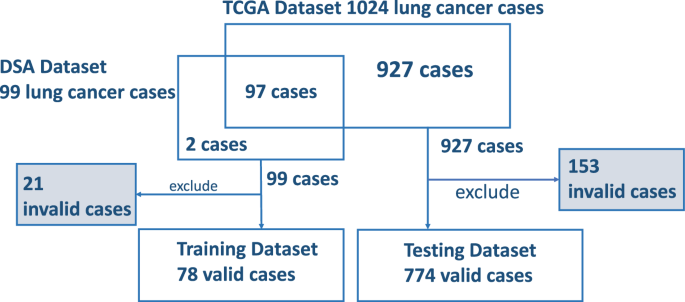
Exclusions are accounted for due to reasons such as empty reports, poor scanning quality, and other factors, including reports of stage IV or unknown conditions.
The specific task of this study was to identify tumor staging and histology types which are important for clinical care and research from pathology reports. The TNM staging system 16 , outlining the primary tumor features (T), regional lymph node involvement (N), and distant metastases (M), is commonly used to define the disease extent, assign prognosis, and guide lung cancer treatment. The American Joint Committee on Cancer (AJCC) has periodically released various editions 16 of TNM classification/staging for lung cancers based on recommendations from extensive database analyses. Following the AJCC guideline, individual pathologic T, N, and M stage components can be summarized into an overall pathologic staging score of Stage I, II, III, or IV. For this project, we instructed ChatGPT to use the AJCC 7 th edition Cancer Staging Manual 17 as the reference for staging lung cancer cases. As the lung cancer cases in our dataset are predominantly non-metastatic, the pathologic metastasis (pM) stage was not extracted. The data elements we chose to extract and evaluate for this study are pathologic primary tumor (pT) and pathologic lymph node (pN) stage components, overall pathologic tumor stage, and histology type.
Overall Performance
Using the training data in the CDSA dataset ( n = 78), we experimented and improved prompts iteratively, and the final prompt is presented in Fig. 2 . The overall performance of the ChatGPT (gpt-3.5-turbo-16k model) is evaluated in the TCGA dataset ( n = 774), and the results are summarized in Table 1 . The accuracy of primary tumor features (pT), regional lymph node involvement (pN), overall tumor stage, and histological diagnosis are 0.87, 0.91, 0.76, and 0.99, respectively. The average accuracy of all attributes is 0.89. The coverage rates for pT, pN, overall stage and histological diagnosis are 0.97, 0.94, 0.94 and 0.96, respectively. Further details of the accuracy evaluation, F1, Kappa, recall, and precision for each attribute are summarized as confusion matrices in Fig. 3 .
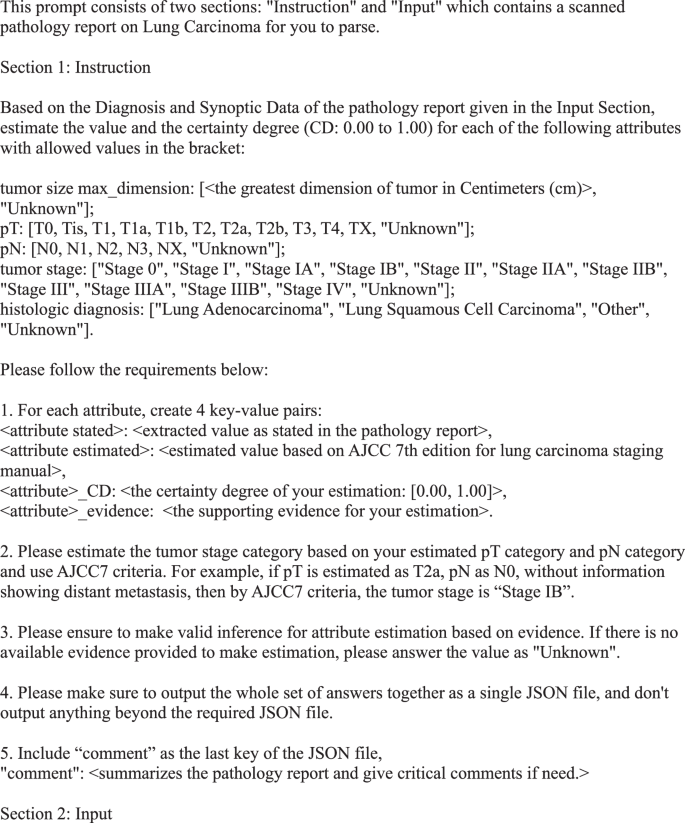
Final prompt for information extraction and estimation from pathology reports.
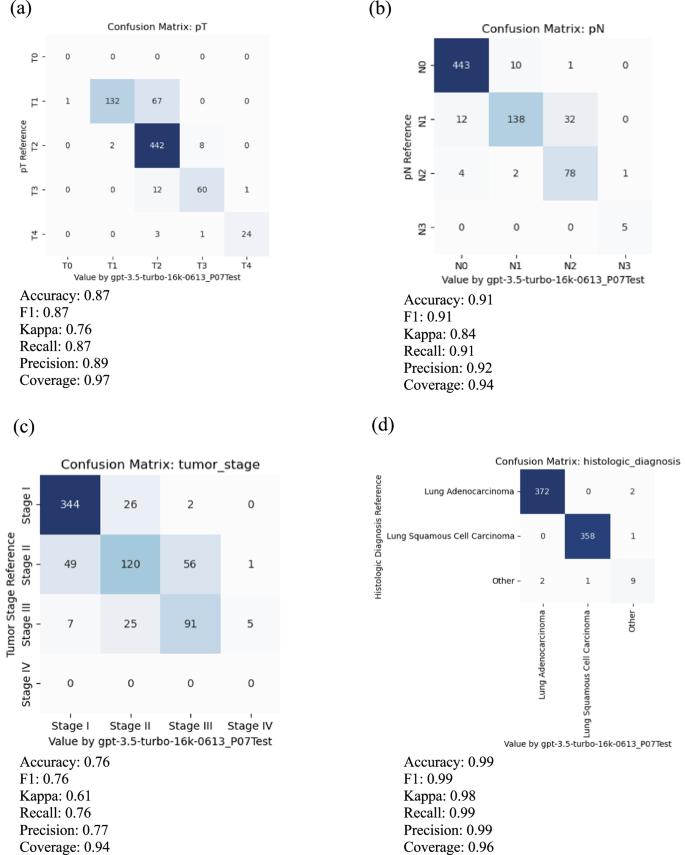
For meaningful evaluation, the cases with uncertain values, such as “Not Available”, “Not Specified”, “Cannot be determined”, “Unknown”, et al. in reference and prediction have been removed. a Primary tumor features (pT), b regional lymph node involvement (pN), c overall tumor stage, and d histological diagnosis.
Inference and Interpretation
To understand how ChatGPT reads and makes inferences from pathology reports, we demonstrated a case study using a typical pathology report in this cohort (TCGA-98-A53A) in Fig. 4a . The left panel shows part of the original pathology report, and the right panel shows the ChatGPT output with estimated pT, pN, overall stage, and histology diagnosis. For each estimate, ChatGPT gives the confidence level and the corresponding evidence it used for the estimation. In this case, ChatGPT correctly extracted information related to tumor size, tumor features, lymph node involvement, and histology information and used the AJCC staging guidelines to estimate tumor stage correctly. In addition, the confidence level, evidence interpretation, and case summary align well with the report and pathologists’ evaluations. For example, the evidence for the pT category was described as “The pathology report states that the tumor is > 3 cm and < 5 cm in greatest dimension, surrounded by lung or visceral pleura.” The evidence for tumor stage was described as “Based on the estimated pT category (T2a) and pN category (N0), the tumor stage is determined to be Stage IB according to AJCC7 criteria.” It shows that ChatGPT extracted relevant information from the note and correctly inferred the pT category based on the AJCC guideline (Supplementary Fig. 1 ) and the extracted information.
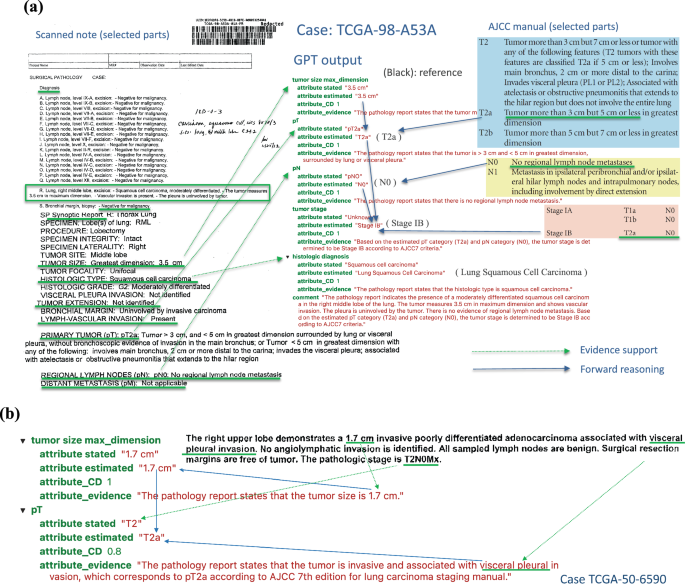
a TCGA-98-A53A. An example of a scanned pathological report (left panel) and ChatGPT output and interpretation (right panel). All estimations and support evidence are consistent with the pathologist’s evaluations. b The GPT model correctly inferred pT as T2a based on the tumor’s size and involvement according to AJCC guidelines.
In another more complex case, TCGA-50-6590 (Fig. 4b ), ChatGPT correctly inferred pT as T2a based on both the tumor’s size and location according to AJCC guidelines. Case TCGA-44-2656 demonstrates a more challenging scenario (Supplementary Fig. 2 ), where the report only contains some factual data without specifying pT, pN, and tumor stage. However, ChatGPT was able to infer the correct classifications based on the reported facts and provide proper supporting evidence.
Error analysis
To understand the types and potential reasons for misclassifications, we performed a detailed error analysis by looking into individual attributes and cases where ChatGPT made mistakes, the results of which are summarized below.
Primary tumor feature (pT) classification
In total, 768 cases with valid reports and reference values in the testing data were used to evaluate the classification performance of pT. Among them, 15 cases were reported with unknown or empty output by ChatGPT, making the coverage rate 0.97. For the remaining 753 cases, 12.6% of pT was misclassified. Among these misclassification cases, the majority were T1 misclassified as T2 (67 out of 753 or 8.9%) or T3 misclassified as T2 (12 out of 753, or 1.6%).
In most cases, ChatGPT extracted the correct tumor size information but used an incorrect rule to distinguish pT categories. For example, in the case TCGA-22-4609 (Fig. 5a ), ChatGPT stated, “Based on the tumor size of 2.0 cm, it falls within the range of T2 category according to AJCC 7th edition for lung carcinoma staging manual.” However, according to the AJCC 7 th edition staging guidelines for lung cancer, if the tumor is more than 2 cm but less than 3 cm in greatest dimension and does not invade nearby structures, pT should be classified as T1b. Therefore, ChatGPT correctly extracted the maximum tumor dimension of 2 cm but incorrectly interpreted this as meeting the criteria for classification as T2. Similarly, for case TCGA-85-A4JB, ChatGPT incorrectly claimed, “Based on the tumor size of 10 cm, the estimated pT category is T2 according to AJCC 7th edition for lung carcinoma staging manual.” According to the AJCC 7 th edition staging guidelines, a tumor more than 7 cm in greatest dimension should be classified as T3.
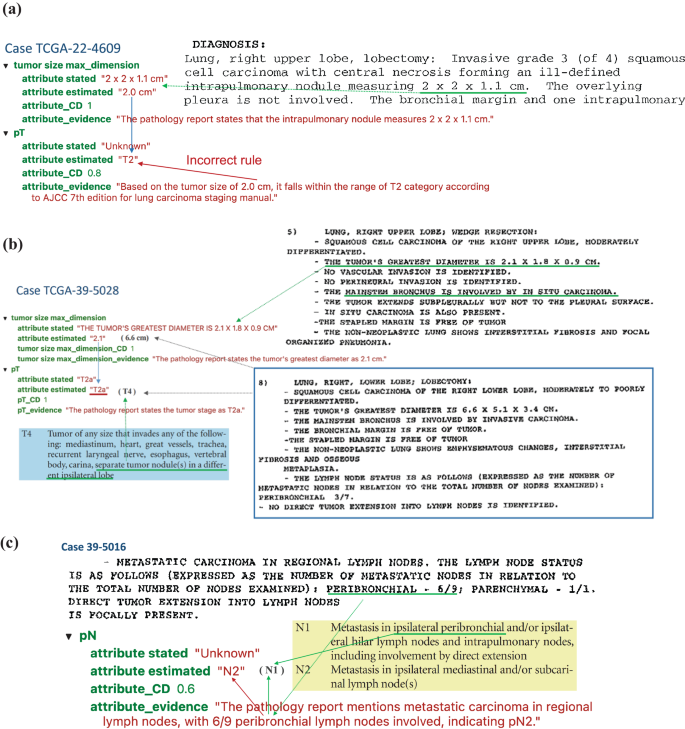
a TCGA-22-4609 illustrates a typical case where the GPT model uses a false rule, which is incorrect by AJCC guideline. b Case TCGA-39-5028 shows a complex case where there exist two tumors and the GPT model only capture one of them. c Case TCGA-39-5016 reveals a case where the GPT model made a mistake for getting confused with domain terminology.
Another challenging situation arose when multiple tumor nodules were identified within the lung. In the case of TCGA-39-5028 (Fig. 5b ), two separate tumor nodules were identified: one in the right upper lobe measuring 2.1 cm in greatest dimension and one in the right lower lobe measuring 6.6 cm in greatest dimension. According to the AJCC 7 th edition guidelines, the presence of separate tumor nodules in a different ipsilateral lobe results in a classification of T4. However, ChatGPT classified this case as T2a, stating, “The pathology report states the tumor’s greatest diameter as 2.1 cm”. This classification would be appropriated if the right upper lobe nodule were a single isolated tumor. However, ChatGPT failed to consider the presence of the second, larger nodule in the right lower lobe when determining the pT classification.
Regional lymph node involvement (pN)
The classification performance of pN was evaluated using 753 cases with valid reports and reference values in the testing data. Among them, 27 cases were reported with unknown or empty output by ChatGPT, making the coverage rate 0.94. For the remaining 726 cases, 8.5% of pN was misclassified. Most of these misclassification cases were N1 misclassified as N2 (32 cases). The AJCC 7th edition staging guidelines use the anatomic locations of positive lymph nodes to determine N1 vs. N2. However, most of the misclassification cases were caused by ChatGPT interpreting the number of positive nodes rather than the locations of the positive nodes. One such example is the case TCGA-85-6798. The report states, “Lymph nodes: 2/16 positive for metastasis (Hilar 2/16)”. Positive hilar lymph nodes correspond to N1 classification according to AJCC 7th edition guidelines. However, ChatGPT misclassifies this case as N2, stating, “The pathology report states that 2 out of 16 lymph nodes are positive for metastasis. Based on this information, the pN category can be estimated as N2 according to AJCC 7th edition for lung carcinoma staging manual.” This interpretation is incorrect, as the number of positive lymph nodes is not part of the criteria used to determine pN status according to AJCC 7th edition guidelines. The model misinterpreted pN2 predictions in 22 cases due to similar false assertions.
In some cases, the ChatGPT model made classification mistakes by misunderstanding the locations’ terminology. Figure 5c shows a case (TCGA-39-5016) where the ChatGPT model recognized that “6/9 peribronchial lymph nodes involved, “ corresponding with classification as N1, but ChatGPT misclassified this case as N2. By AJCC 7th edition guidelines, N2 is defined as “Metastasis in ipsilateral mediastinal and/or subcarinal lymph node(s)”. The ChatGPT model did not fully understand that terminology and made misclassifications.
Pathology tumor stage
The overall tumor stage classification performance was evaluated using 744 cases with valid reports and reference values as stage I, II and III in the testing data. Among them, 18 cases were reported as unknown or empty output by ChatGPT making the coverage rate as 0.94. For the remaining 726 cases, 23.6% of the overall stage was misclassified. Since the overall stage depends on individual pT and pN stages, the mistakes could come from misclassification of pT or pN (error propagation) or applying incorrect inference rules to determine the overall stage from pT and pN (incorrect rules). Looking into the 56 cases where ChatGPT misclassified stage II as stage III, 22 cases were due to error propagation, and 34 were due to incorrect rules. Figure 6a shows an example of error propagation (TCGA-MP-A4TK). ChatGPT misclassified the pT stage from T2a to T3, and then this mistake led to the incorrect classification of stage IIA to stage IIIA. Figure 6b illustrates a case (TCGA-49-4505) where ChatGPT made correct estimation of pT and pN but made false prediction about tumor stage by using a false rule. Among the 34 cases affected by incorrect rules, ChatGPT mistakenly inferred tumor stage as stage III for 26 cases where pT is T3 and pN is N0, respectively. For example, for case TCGA-55-7994, ChatGPT provided the evidence as “Based on the estimated pT category (T3) and pN category (N0), the tumor stage is determined to be Stage IIIA according to AJCC7 criteria”. According to AJCC7, tumors with T3 and N0 should be classified as stage IIB. Similarly, error analysis for other tumor stages shows that misclassifications come from both error propagation and applying false rules.
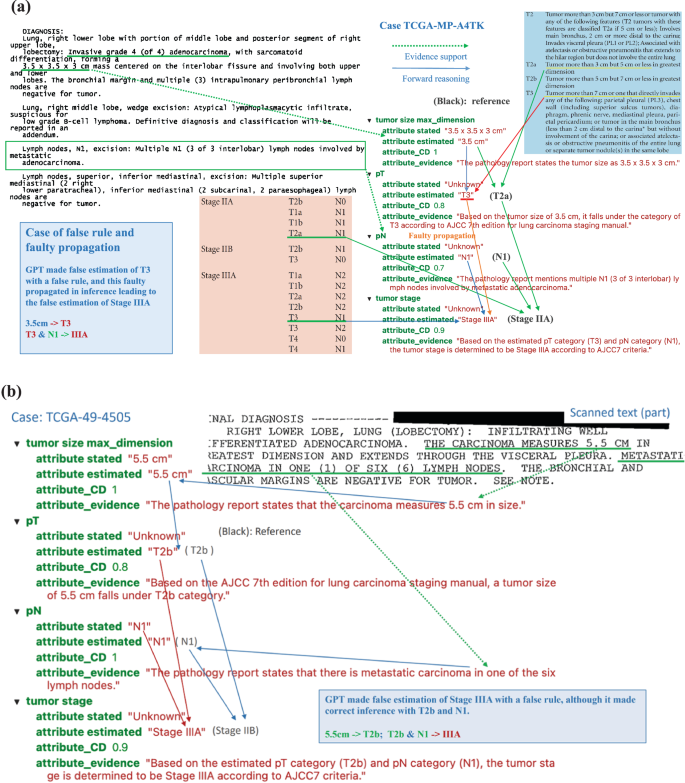
a Case TCGA-MP-A4TK: An example of typical errors GPT made in the experiments, i.e. GPT took false rule and further led to faulty propagation. b Case TCGA-49-4505: The GPT model made false estimation of Stage IIIA with a false rule, although it made correct inference with T2b and N1.
Histological diagnosis
The classification performance of histology diagnosis was evaluated using 762 cases with valid reports and reference values in the testing data. Among them, 17 cases were reported as either unknown or empty output by ChatGPT, making the coverage rate 0.96. For the remaining 745 cases, 6 ( < 1%) of histology types were misclassified. Among the mistakes that ChatGPT made for histology diagnosis, ChatGPT misclassified 3 of them as “other” type and 3 cases of actual “other” type (neither adenocarcinomas nor squamous cell carcinomas) as 2 adenocarcinomas and 1 squamous cell carcinoma. In TCGA-22-5485, two tumors exist: one squamous cell carcinoma and another adenocarcinoma, which should be classified as the ‘other’ type. However, ChatGPT only identified and extracted information for one tumor. In the case TCGA-33-AASB, which is the “other” type of histology, ChatGPT captured the key information and gave it as evidence: “The pathology report states the histologic diagnosis as infiltrating poorly differentiated non-small cell carcinoma with both squamous and glandular features”. However, it mistakenly estimated this case as “adenocarcinoma”. In another case (TCGA-86-8668) of adenocarcinoma, ChatGPT again captured key information and stated as evidence, “The pathology report states the histologic diagnosis as Bronchiolo-alveolar carcinoma, mucinous” but could not tell it is a subtype of adenocarcinoma. Both cases reveal that ChatGPT still has limitations in the specific domain knowledge in lung cancer pathology and the capability of correcting understanding its terminology.
Analyzing irregularities
The initial model evaluation and prompt-response review uncovered irregular scenarios: the original pathology reports may be blank, poorly scanned, or simply missing report forms. We reviewed how ChatGPT responded to these anomalies. First, when a report was blank, the prompt contained only the instruction part. ChatGPT failed to recognize this situation in most cases and inappropriately generated a fabricated case. Our experiments showed that, with the temperature set at 0 for blank reports, ChatGPT converged to a consistent, hallucinated response. Second, for nearly blank reports with a few random characters and poorly scanned reports, ChatGPT consistently converged to the same response with increased variance as noise increased. In some cases, ChatGPT responded appropriately to all required attributes but with unknown values for missing information. Last, among the 15 missing report forms in a small dataset, ChatGPT responded “unknown” as expected in only 5 cases, with the remaining 10 still converging to the hallucinated response.
Reproducibility evaluation
Since ChatGPT models (even with the same version) evolve over time, it is important to evaluate the stability and reproducibility of ChatGPT. For this purpose, we conducted experiments with the same model (“gpt-3.5-turbo-0301”), the same data, prompt, and settings (e.g., temperature = 0) twice in early April and the middle of May of 2023. The rate of equivalence between ChatGPT estimations in April and May on key attributes of interest (pT, pN, tumor stage, and histological diagnosis) is 0.913. The mean absolute error between certainty degrees in the two experiments is 0.051. Considering the evolutionary nature of ChatGPT models, we regard an output difference to a certain extent as reasonable and the overall ChatGPT 3.5 model as stable.
Comparison with other NLP methods
In order to have a clear perspective on how ChatGPT’s performance stands relative to established methods, we conducted a comparative analysis of the results generated by ChatGPT with two established methods: a keyword search algorithm and a deep learning-based Named Entity Recognition (NER) method.
Data selection and annotation
Since the keyword search and NER methods do not support zero-shot learning and require human annotations on the entity level, we carefully annotated our dataset for these traditional NLP methods. We used the same training and testing datasets as in the prompt engineering for ChatGPT. The training dataset underwent meticulous annotation by experienced medical professionals, adhering to the AJCC7 standards. This annotation process involved identifying and highlighting all relevant entities and text spans related to stage, histology, pN, and pT attributes. The detailed annotation process for the 78 cases required a few weeks of full-time work from medical professionals.
Keyword search algorithm using wordpiece tokenizer
For the keyword search algorithm, we employed the WordPiece tokenizer to segment words into subwords. We compiled an annotated entity dictionary from the training dataset. To assess the performance of this method, we calculated span similarities between the extracted spans in the validation and testing datasets and the entries in the dictionary.
Named Entity Recognition (NER) classification algorithm
For the NER classification algorithm, we designed a multi-label span classification model. This model utilized the pre-trained Bio_ClinicalBERT as its backbone. To adapt it for multi-label classification, we introduced an additional linear layer. The model underwent fine-tuning for 1000 epochs using the stochastic gradient descent (SGD) optimizer. The model exhibiting the highest overall F1 score on the validation dataset was selected as the final model for further evaluation in the testing dataset.
Performance evaluation
We evaluated the performance of both the keyword search and NER methods on the testing dataset. We summarized the predicted entities/spans and their corresponding labels. In cases where multiple related entities were identified for a specific category, we selected the most severe entities as the final prediction. Moreover, we inferred the stage information for corpora lacking explicit staging information by aggregating details from pN, pT, and diagnosis, aligning with the AJCC7 protocol. The overall predictions for stage, diagnosis, pN, and pT were compared against the ground truth table to gauge the accuracy and effectiveness of our methods. The results (Supplementary Table S1 ) show that the ChatGPT outperforms WordPiece tokenizer and NER Classifier. The average accuracy for ChatGPT, WordPiece tokenizer, and NER Classifier are 0.89, 0.51, and 0.76, respectively.
Prompt engineering process and results
Prompt design is a heuristic search process with many elements to consider, thus having a significantly large design space. We conducted many experiments to explore better prompts. Here, we share a few typical prompts and the performance of these prompts in the training data set to demonstrate our prompt engineering process.
Output format
The most straightforward prompt without special design would be: “read the pathology report and answer what are pT, pN, tumor stage, and histological diagnosis”. However, this simple prompt would make ChatGPT produce unstructured answers varying in format, terminology, and granularity across the large number of pathology reports. For example, ChatGPT may output pT as “T2” or “pT2NOMx”, and it outputs histological diagnosis as “Multifocal invasive moderately differentiated non-keratinizing squamous cell carcinoma”. The free-text answers will require a significant human workload to clean and process the output from ChatGPT. To solve this problem, we used a multiple choice answer format to force ChatGPT to pick standardized values for some attributes. For example, for pT, ChatGPT could only provide the following outputs: “T0, Tis, T1, T1a, T1b, T2, T2a, T2b, T3, T4, TX, Unknown”. For the histologic diagnosis, ChatGPT could provide output in one of these categories: Lung Adenocarcinoma, Lung Squamous Cell Carcinoma, Other, Unknown. In addition, we added the instruction, “Please make sure to output the whole set of answers together as a single JSON file, and don’t output anything beyond the required JSON file,” to emphasize the requirement for the output format. These requests in the prompt make the downstream analysis of ChatGPT output much more efficient. In order to know the certainty degree of ChatGPT’s estimate and the evidence, we asked ChatGPT to provide the following 4 outputs for each attribute/variable: extracted value as stated in the pathology report, estimated value based on AJCC 7th edition for lung carcinoma staging manual, the certainty degree of the estimation, and the supporting evidence for the estimation. The classification accuracy of this prompt with multiple choice output format (prompt v1) in our training data could achieve 0.854.
Evidence-based inference
One of the major concerns for LLM is that the results from the model are not supported by any evidence, especially when there is not enough information for specific questions. In order to reduce this problem, we emphasize the use of evidence for inference in the prompt by adding this instruction to ChatGPT: “Please ensure to make valid inferences for attribute estimation based on evidence. If there is no available evidence provided to make an estimation, please answer the value as “Unknown.” In addition, we asked ChatGPT to “Include “comment” as the last key of the JSON file.” After adding these two instructions (prompt v2), the performance of the classification in the training data increased to 0.865.
Chain of thought prompting by asking intermediate questions
Although tumor size is not a primary interest for diagnosis and clinical research, it plays a critical role in classifying the pT stage. We hypothesize that if ChatGPT pays closer attention to tumor size, it will have better classification performance. Therefore, we added an instruction in the prompt (prompt v3) to ask ChatGPT to estimate: “tumor size max_dimension: [<the greatest dimension of tumor in Centimeters (cm)>, ‘Unknown’]” as one of the attributes. After this modification, the performance of the classification in the training data increased to 0.90.
Providing examples
Providing examples is an effective way for humans to learn, and it should have similar effects for ChatGPT. We provided a specific example to infer the overall stage based on pT and pN by adding this instruction: “Please estimate the tumor stage category based on your estimated pT category and pN category and use AJCC7 criteria. For example, if pT is estimated as T2a and pN as N0, without information showing distant metastasis, then by AJCC7 criteria, the tumor stage is “Stage IB”.” After this modification (prompt v4), the performance of the classification in the training data increased to 0.936.
Although we can further refine and improve prompts, we decided to use prompt v4 as the final model and apply it to the testing data and get the final classification accuracy of 0.89 in the testing data.
ChatGPT-4 performance
LLM evolves rapidly and OpenAI just released the newest GPT-4 Turbo model (GPT-4-1106-preview) in November 2023. To compare this new model with GPT-3.5-Turbo, we applied this newest GPT model GPT-4-1106 to analyze all the lung cancer pathology notes in the testing data. The classification result and the comparison with the GPT-3.5-Turbo-16k are summarized in Supplementary Table 1 . The results show that GPT-4-turbo performs better in almost every aspect; overall, the GPT-4-turbo model increases performance by over 5%. However, GPT-4-Turbo is much more expensive than GPT-3.5-Turbo. The performance of GPT-3.5-Turbo-16k is still comparable and acceptable. As such, this study mainly focuses on assessing GPT-3.5-Turbo-16k, but highlights the fast development and promise of using LLM to extract structured data from clinical notes.
Analyzing osteosarcoma data
To demonstrate the broader application of this method beyond lung cancer, we collected and analyzed clinical notes from pediatric osteosarcoma patients. Osteosarcoma, the most common type of bone cancer in children and adolescents, has seen no substantial improvement in patient outcomes for the past few decades 18 . Histology grades and margin status are among the most important prognostic factors for osteosarcoma. We collected pathology reports from 191 osteosarcoma cases (approved by UTSW IRB #STU 012018-061). Out of these, 148 cases had histology grade information, and 81 had margin status information; these cases were used to evaluate the performance of the GPT-3.5-Turbo-16K model and our prompt engineering strategy. Final diagnoses on grade and margin were manually reviewed and curated by human experts, and these diagnoses were used to assess ChatGPT’s performance. All notes were de-identified prior to analysis. We applied the same prompt engineering strategy to extract grade and margin information from these osteosarcoma pathology reports. This analysis was conducted on our institution’s private Azure OpenAI platform, using the GPT-3.5-Turbo-16K model (version 0613), the same model used for lung cancer cases. ChatGPT accurately classified both grades (with a 98.6% accuracy rate) and margin status (100% accuracy), as shown in Supplementary Fig. 3 . In addition, Supplementary Fig. 4 details a specific case, illustrating how ChatGPT identifies grades and margin status from osteosarcoma pathology reports.
Since ChatGPT’s release in November 2022, it has spurred many potential innovative applications in healthcare 19 , 20 , 21 , 22 , 23 . To our knowledge, this is among the first reports of an end-to-end data science workflow for prompt engineering, using, and rigorously evaluating ChatGPT in its capacity of batch-processing information extraction tasks on large-scale clinical report data.
The main obstacle to developing traditional medical NLP algorithms is the limited availability of annotated data and the costs for new human annotations. To overcome these hurdles, particularly in integrating problem-specific information and domain knowledge with LLMs’ task-agnostic general knowledge, Augmented Language Models (ALMs) 24 , which incorporate reasoning and external tools for interaction with the environment, are emerging. Research shows that in-context learning (most influentially, few-shot prompting) can complement LLMs with task-specific knowledge to perform downstream tasks effectively 24 , 25 . In-context learning is an approach of training through instruction or light tutorial with a few examples (so called few-shot prompting; well instruction without any example is called 0-shot prompting) rather than fine-tuning or computing-intensive training, which adjusts model weights. This approach has become a dominant method for using LLMs in real-world problem-solving 24 , 25 , 26 . The advent of ALMs promises to revolutionize almost every aspect of human society, including the medical and healthcare domains, altering how we live, work, and communicate. Our study shows the feasibility of using ChatGPT to extract data from free text without extensive task-specific human annotation and model training.
In medical data extraction, our study has demonstrated the advantages of adopting ChatGPT over traditional methods in terms of cost-effectiveness and efficiency. Traditional approaches often require labor-intensive annotation processes that may take weeks and months from medical professionals, while ChatGPT models can be fine-tuned for data extraction within days, significantly reducing the time investment required for implementation. Moreover, our economic analysis revealed the cost savings associated with using ChatGPT, with processing over 900 pathology reports incurring a minimal monetary cost (less than $10 using GPT 3.5 Turbo and less than $30 using GPT-4 Turbo). This finding underscores the potential benefits of incorporating ChatGPT into medical data extraction workflows, not only for its time efficiency but also for its cost-effectiveness, making it a compelling option for medical institutions and researchers seeking to streamline their data extraction processes without compromising accuracy or quality.
A critical requirement for effectively utilizing an LLM is crafting a high-quality “prompt” to instruct the LLM, which has led to the emergence of an important methodology referred to as “prompt engineering.” Two fundamental principles guide this process: firstly, the provision of appropriate context, and secondly, delivering clear instructions about subtasks and the requirements for the desired response and how it should be presented. For a single query for one-time use, the user can experiment with and revise the prompt within the conversation session until a satisfactory answer is obtained. However, prompt design can become more complex when handling repetitive tasks over many input data files using the OpenAI API. In these instances, a prompt must be designed according to a given data feed while maintaining the generality and coverage for various input data features. In this study, we found that providing clear guidance on the output format, emphasizing evidence-based inference, providing chain of thought prompting by asking for tumor size information, and providing specific examples are critical in improving the efficiency and accuracy of extracting structured data from the free-text pathology reports. The approach employed in this study effectively leverages the OpenAI API for batch queries of ChatGPT services across a large set of tasks with similar input data structures, including but not limited to pathology reports and EHR.
Our evaluation results show that the ChatGPT (gpt-3.5-turbo-16k) achieved an overall average accuracy of 89% in extracting and estimating lung cancer staging information and histology subtypes compared to pathologist-curated data. This performance is very promising because some scanned pathology reports included in this study contained random characters, missing parts, typos, varied formats, and divergent information sections. ChatGPT also outperformed traditional NLP methods. Our case analysis shows that most misclassifications were due to a lack of knowledge of detailed pathology terminology or very specialized information in the current versions of ChatGPT models, which could be avoided with future model training or fine-tuning with more domain-specific knowledge.
While our experiments reveal ChatGPT’s strengths, they also underscore its limitations and potential risks, the most significant being the occasional “hallucination” phenomenon 27 , 28 , where the generated content is not faithful to the provided source content. For example, the responses to blank or near-blank reports reflect this issue, though these instances can be detected and corrected due to convergence towards an “attractor”.
The phenomenon of ‘hallucination’ in LLMs presents a significant challenge in the field. It is important to consider several key factors to effectively address the challenges and risks associated with ChatGPT’s application in medicine. Since the output of an LLM depends on both the model and the prompt, mitigating hallucination can be achieved through improvements in GPT models and prompting strategies. From a model perspective, model architecture, robust training, and fine-tuning on a diverse and comprehensive medical dataset, emphasizing accurate labeling and classification, can reduce misclassifications. Additionally, enhancing LLMs’ comprehension of medical terminology and guidelines by incorporating feedback from healthcare professionals during training and through Reinforcement Learning from Human Feedback (RLHF) can further diminish hallucinations. Regarding prompt engineering strategies, a crucial method is to prompt the GPT model with a ‘chain of thought’ and request an explanation with the evidence used in the reasoning. Further improvements could include explicitly requesting evidence from input data (e.g., the pathology report) and inference rules (e.g., AJCC rules). Prompting GPT models to respond with ‘Unknown’ when information is insufficient for making assertions, providing relevant context in the prompt, or using ‘embedding’ of relevant text to narrow down the semantic subspace can also be effective. Harnessing hallucination is an ongoing challenge in AI research, with various methods being explored 5 , 27 . For example, a recent study proposed “SelfCheckGPT” approach to fact-check black-box models 29 . Developing real-time error detection mechanisms is crucial for enhancing the reliability and trustworthiness of AI models. More research is needed to evaluate the extent, impacts, and potential solutions of using LLMs in clinical research and care.
When considering using ChatGPT and similar LLMs in healthcare, it’s important to thoughtfully consider the privacy implications. The sensitivity of medical data, governed by rigorous regulations like HIPAA, naturally raises concerns when integrating technologies like LLMs. Although it is a less concern to analyze public available de-identified data, like the lung cancer pathology notes used in this study, careful considerations are needed for secured healthcare data. More secured OpenAI services are offered by OpenAI security portal, claimed to be compliant to multiple regulation standards, and Microsoft Azure OpenAI, claimed could be used in a HIPAA-compliant manner. For example, de-identified Osteosarcoma pathology notes were analyzed by Microsoft Azure OpenAI covered by the Business Associate Agreement in this study. In addition, exploring options such as private versions of these APIs, or even developing LLMs within a secure healthcare IT environment, might offer good alternatives. Moreover, implementing strong data anonymization protocols and conducting regular security checks could further protect patient information. As we navigate these advancements, it’s crucial to continuously reassess and adapt appropriate privacy strategies, ensuring that the integration of AI into healthcare is both beneficial and responsible.
Despite these challenges, this study demonstrates our effective methodology in “prompt engineering”. It presents a general framework for using ChatGPT’s API in batch queries to process large volumes of pathology reports for structured information extraction and estimation. The application of ChatGPT in interpreting clinical notes holds substantial promise in transforming how healthcare professionals and patients utilize these crucial documents. By generating concise, accurate, and comprehensible summaries, ChatGPT could significantly enhance the effectiveness and efficiency of extracting structured information from unstructured clinical texts, ultimately leading to more efficient clinical research and improved patient care.
In conclusion, ChatGPT and other LLMs are powerful tools, not just for pathology report processing but also for the broader digital transformation of healthcare documents. These models can catalyze the utilization of the rich historical archives of medical practice, thereby creating robust resources for future research.
Data processing, workflow, and prompt engineering
The lung cancer data we used for this study are publicly accessible via CDSA ( https://cancer.digitalslidearchive.org/ ) and TCGA ( https://cBioPortal.org ), and they are de-identified data. The institutional review board at the University of Texas Southwestern Medical Center has approved this study where patient consent was waived for using retrospective, de-identified electronic health record data.
We aimed to leverage ChatGPT to extract and estimate structured data from these notes. Figure 7a displays our process. First, scanned pathology reports in PDF format were downloaded from TCGA and CDSA databases. Second, R package pdftools, an optical character recognition tool, was employed to convert scanned PDF files into text format. After this conversion, we identified reports with near-empty content, poor scanning quality, or missing report forms, and those cases were excluded from the study. Third, the OpenAI API was used to analyze the text data and extract structured data elements based on specific prompts. In addition, we extracted case identifiers and metadata items from the TCGA metadata file, which was used to evaluate the model performance.
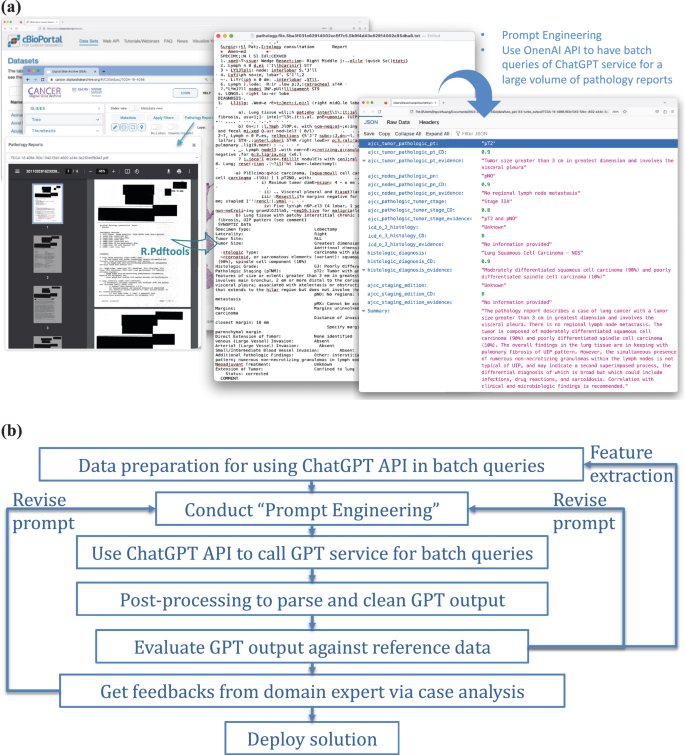
a Illustration of the use of OpenAI API for batch queries of ChatGPT service, applied to a substantial volume of clinical notes — pathology reports in our study. b A general framework for integrating ChatGPT into real-world applications.
In this study, we implemented a problem-solving framework rooted in data science workflow and systems engineering principles, as depicted in Fig. 7b . An important step is the spiral approach 30 to ‘prompt engineering’, which involves experimenting with subtasks, different phrasings, contexts, format specifications, and example outputs to improve the quality and relevance of the model’s responses. It was an iterative process to achieve the desired results. For the prompt engineering, we first define the objective: to extract information on TNM staging and histology type as structured attributes from the unstructured pathology reports. Second, we assigned specific tasks to ChatGPT, including estimating the targeted attributes, evaluating certainty levels, identifying key evidence of each attribute estimation, and generating a summary as output. The output was compiled into a JSON file. In this process, clinicians were actively formulating questions and evaluating the results.
Our study used the “gpt-3.5-turbo” model, accessible via the OpenAI API. The model incorporates 175 billion parameters and was trained on various public and authorized documents, demonstrating specific Artificial General Intelligence (AGI) capabilities 5 . Each of our queries sent to ChatGPT service is a “text completion” 31 , which can be implemented as a single round chat completion. All LLMs have limited context windows, constraining the input length of a query. Therefore, lengthy pathology reports combined with the prompt and ChatGPT’s response might exceed this limit. We used OpenAI’s “tiktoken” Python library to estimate the token count to ensure compliance. This constraint has been largely relaxed by the newly released GPT models with much larger context windows. We illustrate the pseudocode for batch ChatGPT queries on a large pathology report set in Supplementary Fig. 5 .
Model evaluation
We evaluated the performance of ChatGPT by comparing its output with expert-curated data elements provided in the TCGA structured data using the testing data set. Some staging records in the TCGA structured data needed to be updated; our physicians curated and updated those records. To mimic a real-world setting, we processed all reports regardless of data quality to collect model responses. For performance evaluation, we only used valid reports providing meaningful text and excluded the reports with near-empty content, poor scanning quality, and missing report forms, which were reported as irregular cases. We assessed the classification accuracy, F1, Kappa, recall, and precision for each attribute of interest, including pT, pN, overall stage, and histology types, and presented results as accuracy and confusion matrices. Missing data were excluded from the accuracy evaluation, and the coverage rate was reported for predicted values as ‘unknown’ or empty output.
Reporting summary
Further information on research design is available in the Nature Research Reporting Summary linked to this article.
Data availability
The lung cancer dataset we used for this study is “Pan-Lung Cancer (TCGA, Nat Genet2016)”, ( https://www.cbioportal.org/study/summary?id=nsclc_tcga_broad_2016 ) and the “luad” and “lusc” subsets from CDSA ( https://cancer.digitalslidearchive.org/ ). We have provided a reference regarding how to access the data 32 . We utilized the provided APIs to retrieve clinical information and pathology reports for the LUAD (lung adenocarcinoma) and LUSC (lung squamous cell carcinoma) cohorts. The pediatric data are the EHR data from UTSW clinic services. The data is available from the corresponding author upon reasonable request and IRB approval.
Code availability
All codes used in this paper were developed using APIs from OpenAI. The prompt for the API is available in Fig. 2 . Method-specific code is available from the corresponding author upon request.
Vaswani, A. et al. Attention is all you need. Adv. Neural Info. Processing Syst. 30 , (2017).
Devlin, J. et al. Bert: Pre-training of deep bidirectional transformers for language understanding . arXiv preprint arXiv:1810.04805, 2018.
Radford, A. et al. Improving language understanding by generative pre-training . OpenAI: https://cdn.openai.com/research-covers/language-unsupervised/language_understanding_paper.pdf (2018).
Touvron, H. et al. LLaMA: Open and efficient foundation language models . arXiv preprint arXiv:2302.13971 (2023).
OpenAi, GPT-4 Technical Report . arXiv:2303.08774: https://arxiv.org/pdf/2303.08774.pdf (2023).
Anil, R. et al. Palm 2 technical report . arXiv preprint arXiv:2305.10403 (2023).
Turner, B. E. W. Epic, Microsoft bring GPT-4 to EHRs .
Landi, H. Microsoft’s Nuance integrates OpenAI’s GPT-4 into voice-enabled medical scribe software .
Hao, T. et al. Health Natural Language Processing: Methodology Development and Applications. JMIR Med Inf. 9 , e23898 (2021).
Article Google Scholar
Pathak, J., Kho, A. N. & Denny, J. C. Electronic health records-driven phenotyping: challenges, recent advances, and perspectives. J. Am. Med. Inform. Assoc. 20 , e206–e211 (2013).
Article PubMed PubMed Central Google Scholar
Crichton, G. et al. A neural network multi-task learning approach to biomedical named entity recognition. BMC Bioinforma. 18 , 368 (2017).
Wang, J. et al. Document-Level Biomedical Relation Extraction Using Graph Convolutional Network and Multihead Attention: Algorithm Development and Validation. JMIR Med Inf. 8 , e17638 (2020).
Liu, Y. et al. Roberta: A robustly optimized BERT pretraining approach . arXiv preprint arXiv:1907.11692 (2019).
Rasmy, L. et al. Med-BERT: pretrained contextualized embeddings on large-scale structured electronic health records for disease prediction. npj Digit. Med. 4 , 86 (2021).
Wu, H. et al. A survey on clinical natural language processing in the United Kingdom from 2007 to 2022. npj Digit. Med. 5 , 186 (2022).
Amin, M. B. et al. AJCC cancer staging manual . 1024: Springer 2017.
Goldstraw, P. et al. The IASLC Lung Cancer Staging Project: Proposals for the Revision of the TNM Stage Groupings in the Forthcoming (Seventh) Edition of the TNM Classification of Malignant Tumours. J. Thorac. Oncol. 2 , 706–714 (2007).
Article PubMed Google Scholar
Yang, D. M. et al. Osteosarcoma Explorer: A Data Commons With Clinical, Genomic, Protein, and Tissue Imaging Data for Osteosarcoma Research. JCO Clin. Cancer Inform. 7 , e2300104 (2023).
The Lancet Digital, H., ChatGPT: friend or foe? Lancet Digital Health . 5 , e102 (2023).
Nature, Will ChatGPT transform healthcare? Nat. Med. 29 , 505–506 (2023).
Patel, S. B. & Lam, K. ChatGPT: the future of discharge summaries? Lancet Digit. Health 5 , e107–e108 (2023).
Article CAS PubMed Google Scholar
Ali, S. R. et al. Using ChatGPT to write patient clinic letters. Lancet Digit. Health 5 , e179–e181 (2023).
Howard, A., Hope, W. & Gerada, A. ChatGPT and antimicrobial advice: the end of the consulting infection doctor? Lancet Infect. Dis. 23 , 405–406 (2023).
Mialon, G. et al. Augmented language models: a survey . arXiv preprint arXiv:2302.07842 (2023).
Brown, T. et al. Language Models are Few-Shot Learners . Curran Associates, Inc. (2020).
Wei, J. et al. Chain of thought prompting elicits reasoning in large language models . Adv Neural Inf Processing Syst 35 , 24824–24837 (2022).
Ji, Z. et al. Survey of Hallucination in Natural Language Generation. ACM Comput. Surv. 55 , 1–38 (2023).
Alkaissi, H. & S. I. McFarlane, Artificial Hallucinations in ChatGPT: Implications in Scientific Writing. Cureus , (2023).
Manakul, P. A. Liusie, & M. J. F. Gales, SelfCheckGPT: Zero-Resource Black-Box Hallucination Detection for Generative Large Language Models . 2023.
Boehm, B. W. A spiral model of software development and enhancement. Computer 21 , 61–72 (1988).
OpenAi. OpenAI API Documentation . Available from: https://platform.openai.com/docs/guides/text-generation .
Gao, J. et al. Integrative analysis of complex cancer genomics and clinical profiles using the cBioPortal. Sci. Signal. 6 , 1–19 (2013).
Download references
Acknowledgements
This work was partially supported by the National Institutes of Health [P50CA70907, R35GM136375, R01GM140012, R01GM141519, R01DE030656, U01CA249245, and U01AI169298], and the Cancer Prevention and Research Institute of Texas [RP230330 and RP180805].
Author information
Authors and affiliations.
Quantitative Biomedical Research Center, Peter O’Donnell School of Public Health, University of Texas Southwestern Medical Center, 5323 Harry Hines Blvd, Dallas, TX, USA 75390, USA
Jingwei Huang, Donghan M. Yang, Ruichen Rong, Kuroush Nezafati, Colin Treager, Shidan Wang, Xian Cheng, Yujia Guo, Guanghua Xiao, Xiaowei Zhan & Yang Xie
Department of Pathology, University of Texas Southwestern Medical Center, 5323 Harry Hines Blvd, Dallas, TX, USA 75390, USA
Department of Pediatrics, University of Texas Southwestern Medical Center, 5323 Harry Hines Blvd, Dallas, TX, USA 75390, USA
Laura J. Klesse
Department of Internal Medicine, University of Texas Southwestern Medical Center, 5323 Harry Hines Blvd, Dallas, TX, USA 75390, USA
Eric D. Peterson
You can also search for this author in PubMed Google Scholar
Contributions
J.H., Y.X., X.Z. and G.X. designed the study. X.Z., K.N., C.T. and J.H. prepared, labeled, and curated lung cancer datasets. D.M.Y., X.C., Y.G., L.J.K. prepared, labeled, and curated osteosarcoma datasets. Z.C. provided critical inputs as pathologists. Y.X., G.X., E.P. provided critical inputs for the study. J.H. implemented experiments with ChatGPT. R.R. and K.N. implemented experiments with N.L.P. J.H., Y.X., G.X. and S.W. conducted data analysis. Y.X., G.X., J.H., X.Z., D.M.Y. and R.R. wrote the manuscript. All co-authors read and commented on the manuscript.
Corresponding authors
Correspondence to Xiaowei Zhan or Yang Xie .
Ethics declarations
Competing interests.
The authors declare no competing interests.
Additional information
Publisher’s note Springer Nature remains neutral with regard to jurisdictional claims in published maps and institutional affiliations.
Supplementary information
Supplemental figures and tables, reporting summary, rights and permissions.
Open Access This article is licensed under a Creative Commons Attribution 4.0 International License, which permits use, sharing, adaptation, distribution and reproduction in any medium or format, as long as you give appropriate credit to the original author(s) and the source, provide a link to the Creative Commons licence, and indicate if changes were made. The images or other third party material in this article are included in the article’s Creative Commons licence, unless indicated otherwise in a credit line to the material. If material is not included in the article’s Creative Commons licence and your intended use is not permitted by statutory regulation or exceeds the permitted use, you will need to obtain permission directly from the copyright holder. To view a copy of this licence, visit http://creativecommons.org/licenses/by/4.0/ .
Reprints and permissions
About this article
Cite this article.
Huang, J., Yang, D.M., Rong, R. et al. A critical assessment of using ChatGPT for extracting structured data from clinical notes. npj Digit. Med. 7 , 106 (2024). https://doi.org/10.1038/s41746-024-01079-8
Download citation
Received : 24 July 2023
Accepted : 14 March 2024
Published : 01 May 2024
DOI : https://doi.org/10.1038/s41746-024-01079-8
Share this article
Anyone you share the following link with will be able to read this content:
Sorry, a shareable link is not currently available for this article.
Provided by the Springer Nature SharedIt content-sharing initiative
Quick links
- Explore articles by subject
- Guide to authors
- Editorial policies
Sign up for the Nature Briefing newsletter — what matters in science, free to your inbox daily.

- SQL Cheat Sheet
- SQL Interview Questions
- MySQL Interview Questions
- PL/SQL Interview Questions
- Learn SQL and Database
- How to Design a Database for Real-Time Reporting?
- How to Design a Relational Database for Customer Reviews and Ratings Platform
- How to Design a Database for Mobile App Backend
- How to Design a Database for Event Management
- Design Patterns for Relational Databases
- Storing Hierarchical Data in a Relational Database
- How to Insert Dummy Data into Databases using Flask
- Top 7 Databases for Data Scientists in 2024
- How Does an API Work with A Database?
- Java Program to Insert Data from a Database to a Spread Sheet
- File and Database Storage Systems in System Design
- What are the Strategies for Data Migration in DBMS?
- What is Integration Databases in NoSQL?
- Schema Design and Relationship in NoSQL Document-Base Databases
- Sending data from a Flask app to PostgreSQL Database
- Difference between Data Science and Operations Research
- What is EII(Enterprise Information Integration)?
- How to Generating a Database-Backed API
- How to Use Google Sheets as a Database
- Points to remember for Database design Interview
- Integration of Heterogeneous Databases in Data Warehousing
- How to Import Data into Oracle Database ?
- How to Upload Excel Sheet Data to Firebase Realtime Database in Android?
- How to Import and Export Data to Database in MySQL Workbench?
- Differences between Operational Database Systems and Data Warehouse
- How to store XML data into a MySQL database using Python?
- Data Integration in Data Mining
- How to Choose The Right Database for Your Application?
- Different Sources of Data for Data Analysis
How to Design Database for Clinical Research Data Integration
Clinical research depends heavily on the effective integration and analysis of diverse datasets to find meaningful insights and drive scientific discoveries. A well-designed database architecture is fundamental to managing, integrating and analyzing clinical research data efficiently.
In this article, we will learn about How Database Design Principles for Clinical Research Data Integration by understanding various aspects of the article in detail.
Database Design Essentials for Clinical Research Data Integration
- Designing a robust database for clinical research data integration involves careful consideration of several critical factors, including data structure, interoperability, data standardization, security, and scalability.
- A well-structured database supports the fast integration of heterogeneous datasets by enabling comprehensive analysis and interpretation of clinical research data.
Features of Clinical Research Data Integration Systems
Clinical research data integration systems offer a range of features designed to speed up data collection, integration, analysis, and reporting. These features typically include:
- Data Standardization: Standardizing diverse data formats and terminologies to ensure interoperability and consistency across datasets.
- Data Mapping and Transformation: Mapping and transforming data from different sources into a unified format for integration and analysis.
- Data Quality Control: Implementing quality control measures to identify and address data inconsistencies, errors, and missing values.
- Security and Privacy: Incorporating robust security measures to protect sensitive patient data and ensure compliance with data protection regulations.
- Data Access and Sharing: Facilitating controlled access to integrated datasets for researchers while ensuring data privacy and confidentiality.
- Visualization and Reporting: Generating visualizations, dashboards, and reports to facilitate data interpretation and decision-making.
Entities and Attributes in Clinical Research Data Integration
Entities in a clinical research data integration database represent various aspects of research data, while attributes describe their characteristics. Common entities and their attributes include:
- StudyID (Primary Key): Unique identifier for each clinical study.
- Study Title: Title or name of the clinical study.
- Principal Investigator: Name of the principal investigator leading the study.
- Start Date/End Date: Dates when the study began and ended.
- PatientID (Primary Key): Unique identifier for each patient.
- Demographic Information: Patient demographics such as age, gender, ethnicity, etc.
- Medical History: Medical history and conditions relevant to the study.
Data Source:
- DataSourceID (Primary Key): Unique identifier for each data source.
- Data Type: Type of data source (e.g., electronic health records, genomic data, imaging data).
- Data Format: Format or schema of the data source.
Relationships in Clinical Research Data Integration:
In clinical research data integration databases, entities are interconnected through relationships that define the flow and associations of research data. Key relationships include:
Study-Patient Relationship:
- One-to-many relationship
- Each study involves multiple patients, while each patient can participate in multiple studies.
Study-Data Source Relationship:
- Many-to-many relationship
- Each study can utilize data from multiple sources, and each data source can contribute to multiple studies.
Entity Structures in SQL Format
Here’s how the entities mentioned above can be structured in SQL format:
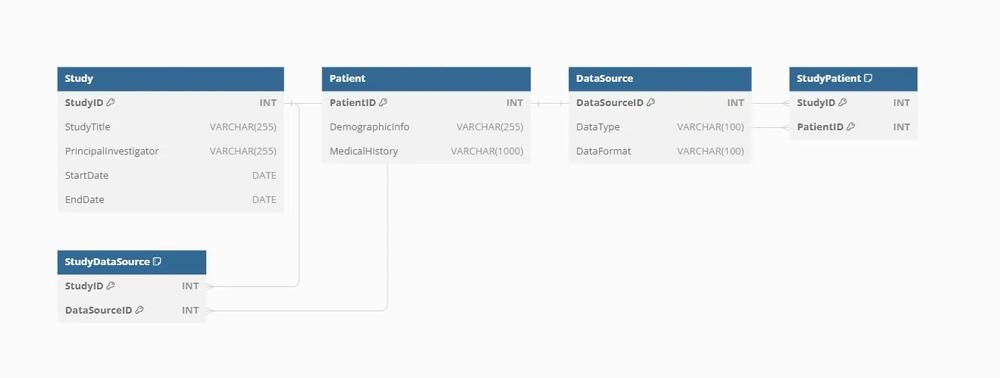
Tips & Best Practices for Enhanced Database Design
- Data Standardization: Implement standardized data formats and terminologies to ensure interoperability and consistency across integrated datasets.
- Interoperability: Use standardized protocols and interfaces for seamless integration with external data sources and systems.
- Data Governance: Establish data governance policies and procedures to maintain data quality, integrity, and security.
- Scalability: Design the database with scalability in mind to accommodate growing volumes of research data.
- Collaboration: Foster collaboration between researchers, data scientists, and IT professionals to ensure effective database design and implementation.
Designing a database for clinical research data integration requires a strategic approach focusing on data structure, interoperability, relationships, and security. By adhering to best practices and leveraging SQL effectively, developers can create a robust and scalable database schema to support the integration, analysis, and interpretation of diverse clinical research datasets. A well-designed database not only enhances research efficiency but also contributes to scientific discoveries and advancements in healthcare by enabling comprehensive analysis and interpretation of clinical research data.
Please Login to comment...
Similar reads.
- Database Design
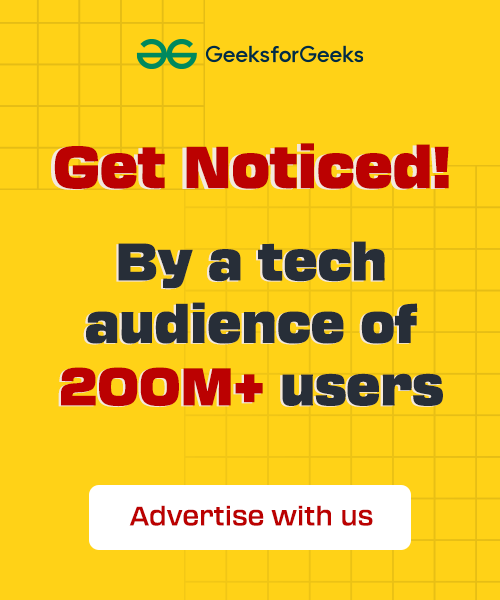
Improve your Coding Skills with Practice
What kind of Experience do you want to share?
- Top Courses
- Online Degrees
- Find your New Career
- Join for Free

Clinical Trials Analysis, Monitoring, and Presentation
This course is part of Clinical Trials Operations Specialization
Taught in English
Some content may not be translated

Instructors: Janet Holbrook, PhD, MPH +2 more
Instructors
Instructor ratings
We asked all learners to give feedback on our instructors based on the quality of their teaching style.
Financial aid available
4,791 already enrolled

(39 reviews)
Recommended experience
Beginner level
Learners should have some familiarity with basic scientific, statistical, and management concepts.
What you'll learn
Calculate clinical trial sample size
Monitor clinical trial performance
Analyze results from clinical trials
Communicate results from clinical trials
Skills you'll gain
- Research Methods
- Data Analysis
- Communication
- Sample Size Determination
Details to know

Add to your LinkedIn profile
See how employees at top companies are mastering in-demand skills
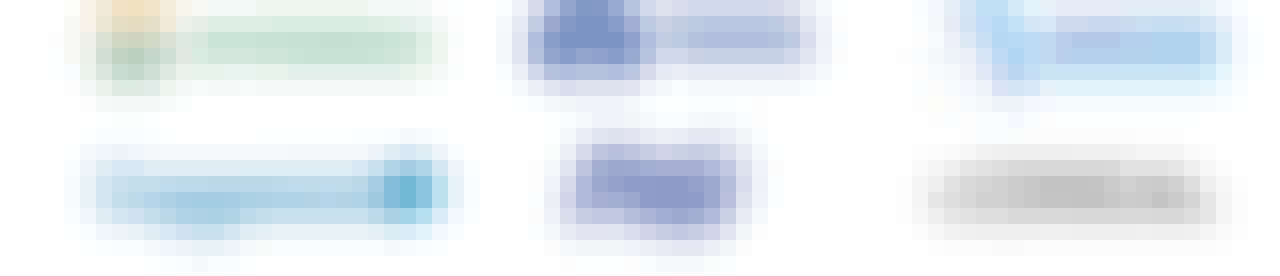
Build your subject-matter expertise
- Learn new concepts from industry experts
- Gain a foundational understanding of a subject or tool
- Develop job-relevant skills with hands-on projects
- Earn a shareable career certificate
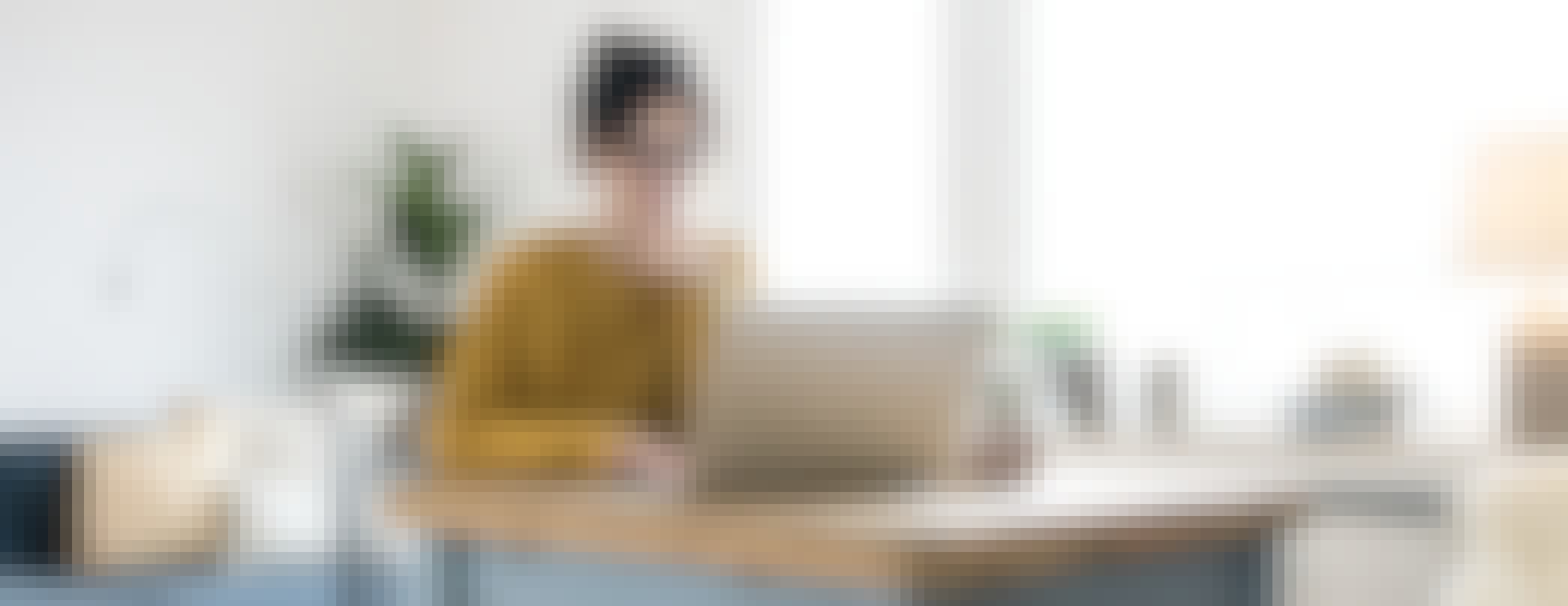
Earn a career certificate
Add this credential to your LinkedIn profile, resume, or CV
Share it on social media and in your performance review
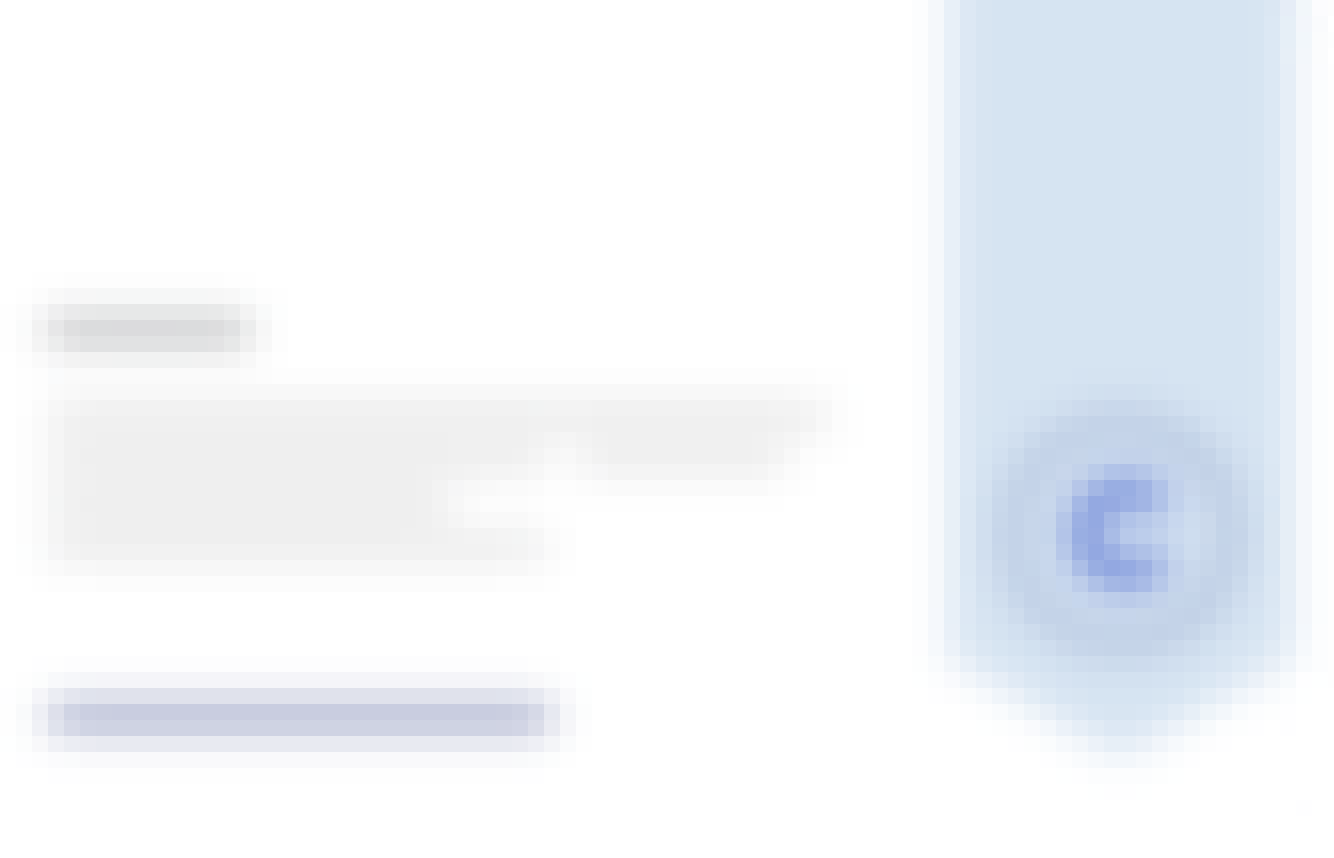
There are 5 modules in this course
In this course, you’ll learn more advanced operational skills that you and your team need to run a successful clinical trial. You’ll learn about the computation of sample size and how to develop a sample size calculation that’s suitable for your trial design and outcome measures. You’ll also learn to use statistical methods to monitor your trial for safety, integrity, and efficacy. Next, you’ll learn how to report the results from your clinical trials through both journal articles and data monitoring reports. Finally, we’ll discuss the role of the analyst throughout the trial process, plus a few additional topics such as simulations and adaptive designs.
Clinical Trial Sample Size
Sample size calculation in clinical trials refers to the process for determining how large a trial needs to be in order to have a reasonable expectation of detecting a difference between groups. The end result of the sample size calculation should be an estimate of the number of observations.
What's included
3 videos 1 reading 1 quiz
3 videos • Total 40 minutes
- Definitions and Introduction • 8 minutes • Preview module
- Sampling and Assumptions • 21 minutes
- Practicalities • 10 minutes
1 reading • Total 3 minutes
- Welcome to the course! • 3 minutes
1 quiz • Total 8 minutes
- Bias Control Randomization and Masking • 8 minutes
Trial Monitoring
In this module, you’ll learn about trial monitoring, which involves statistical methods to assess a trial while it is underway. These methods are used to assess safety, integrity, efficacy, recruitment, data collection, and data quality.
4 videos 1 quiz
4 videos • Total 34 minutes
- Goals and Responsibilities • 5 minutes • Preview module
- Interim Analyses • 13 minutes
- Safety Versus Efficacy Data • 6 minutes
- Statistical Monitoring • 10 minutes
1 quiz • Total 7 minutes
- Trial Monitoring • 7 minutes
Reporting Results From Randomized Clinical Trials (RCTs)
Skilled communication of your clinical trial results is critical to ensuring that your efforts have the intended impact. In this module, you’ll learn the best practices for reporting results in both journal publications and in data monitoring reports.
4 videos • Total 91 minutes
- Journal Articles • 22 minutes • Preview module
- Nuts and Bolts of Journal Articles • 17 minutes
- Tables and Figures • 23 minutes
- Data Monitoring Reports for RCTs • 27 minutes
1 quiz • Total 9 minutes
- Reporting Results from Randomized Clinical Trials • 9 minutes
Analyzing Trials
Analysts play an important role throughout the trial, not just at the end. In this module, you’ll learn about the analyst’s role, including how the analyst contributes to the trial at every stage of the process.
5 videos 1 quiz
5 videos • Total 35 minutes
- Role of the Analyst • 4 minutes • Preview module
- Statistical Analysis Plan • 2 minutes
- Analysis Population • 7 minutes
- Analysis Considerations • 10 minutes
- Types of Analyses • 10 minutes
- Analyzing Trials • 7 minutes
Advanced Topics
In this module, you’ll learn about some advanced operational functions that should be in your trial team’s toolkit, including simulations, adaptive designs, and Bayesian statistics.
3 videos • Total 39 minutes
- Simulations • 12 minutes • Preview module
- Adaptive Designs • 14 minutes
- Bayesian Approaches • 12 minutes
1 reading • Total 1 minute
- Closing Thoughts • 1 minute
1 quiz • Total 6 minutes
- Advanced Topics • 6 minutes

The mission of The Johns Hopkins University is to educate its students and cultivate their capacity for life-long learning, to foster independent and original research, and to bring the benefits of discovery to the world.
Recommended if you're interested in Public Health
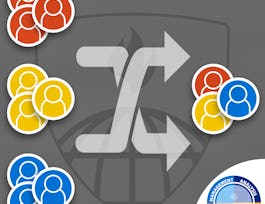
Johns Hopkins University
Design and Conduct of Clinical Trials
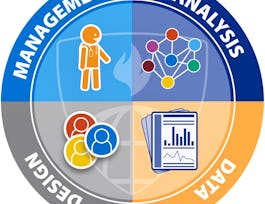
Clinical Trials Operations
Specialization
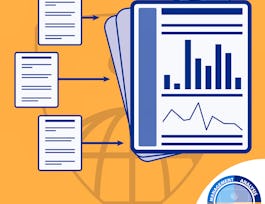
Clinical Trials Data Management and Quality Assurance
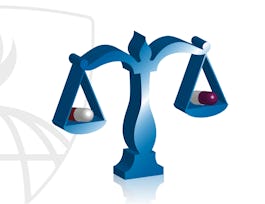
Design and Interpretation of Clinical Trials
Why people choose coursera for their career.

Learner reviews
Showing 3 of 39
Reviewed on Feb 15, 2024
It covers the process of clinical trial. I would prefer the beginners in clinical research should do this course.
Reviewed on Apr 25, 2023
Excellent Instructors! I learned a lot!
THank you very much!
New to Public Health? Start here.

Open new doors with Coursera Plus
Unlimited access to 7,000+ world-class courses, hands-on projects, and job-ready certificate programs - all included in your subscription
Advance your career with an online degree
Earn a degree from world-class universities - 100% online
Join over 3,400 global companies that choose Coursera for Business
Upskill your employees to excel in the digital economy
Frequently asked questions
When will i have access to the lectures and assignments.
Access to lectures and assignments depends on your type of enrollment. If you take a course in audit mode, you will be able to see most course materials for free. To access graded assignments and to earn a Certificate, you will need to purchase the Certificate experience, during or after your audit. If you don't see the audit option:
The course may not offer an audit option. You can try a Free Trial instead, or apply for Financial Aid.
The course may offer 'Full Course, No Certificate' instead. This option lets you see all course materials, submit required assessments, and get a final grade. This also means that you will not be able to purchase a Certificate experience.
What will I get if I subscribe to this Specialization?
When you enroll in the course, you get access to all of the courses in the Specialization, and you earn a certificate when you complete the work. Your electronic Certificate will be added to your Accomplishments page - from there, you can print your Certificate or add it to your LinkedIn profile. If you only want to read and view the course content, you can audit the course for free.
What is the refund policy?
If you subscribed, you get a 7-day free trial during which you can cancel at no penalty. After that, we don’t give refunds, but you can cancel your subscription at any time. See our full refund policy Opens in a new tab .
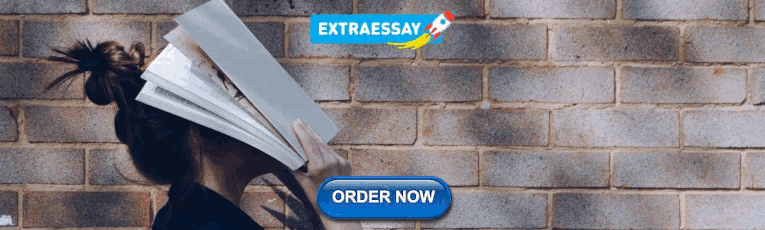
Is financial aid available?
Yes. In select learning programs, you can apply for financial aid or a scholarship if you can’t afford the enrollment fee. If fin aid or scholarship is available for your learning program selection, you’ll find a link to apply on the description page.
More questions
How Latinas Are Helping Shape Clinical Research
Upcoming events, revisiting the fda’s proposed single irb mandate: navigating changes and aligning for success, effective root cause analysis and capa investigations for drugs, devices and clinical trials, 2024 avoca quality consortium summit, maximizing trial success requires evolving feasibility and recruitment strategies, featured products.

Surviving an FDA GCP Inspection: Resources for Investigators, Sponsors, CROs and IRBs

Best Practices for Clinical Trial Site Management
Featured stories.

Thought Leadership: Remote Patient Monitoring Gives New View of Safety in Cardiac Clinical Trials

Ask the Experts: Applying Quality by Design to Protocols

Clinical Trials Need Greater Representation of Obese Patients, Experts Say
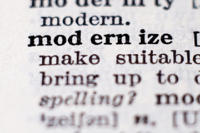
FDA IT Modernization Plan Prioritizes Data-Sharing, AI, Collaboration and More
Standard operating procedures for risk-based monitoring of clinical trials, the information you need to adapt your monitoring plan to changing times..
- - Google Chrome
Intended for healthcare professionals
- Access provided by Google Indexer
- My email alerts
- BMA member login
- Username * Password * Forgot your log in details? Need to activate BMA Member Log In Log in via OpenAthens Log in via your institution

Search form
- Advanced search
- Search responses
- Search blogs
- Efficacy of psilocybin...
Efficacy of psilocybin for treating symptoms of depression: systematic review and meta-analysis
Linked editorial.
Psilocybin for depression
- Related content
- Peer review
- Athina-Marina Metaxa , masters graduate researcher 1 ,
- Mike Clarke , professor 2
- 1 Nuffield Department of Primary Care Health Sciences, University of Oxford, Oxford OX2 6GG, UK
- 2 Northern Ireland Methodology Hub, Centre for Public Health, ICS-A Royal Hospitals, Belfast, Ireland, UK
- Correspondence to: A-M Metaxa athina.metaxa{at}hmc.ox.ac.uk (or @Athina_Metaxa12 on X)
- Accepted 6 March 2024
Objective To determine the efficacy of psilocybin as an antidepressant compared with placebo or non-psychoactive drugs.
Design Systematic review and meta-analysis.
Data sources Five electronic databases of published literature (Cochrane Central Register of Controlled Trials, Medline, Embase, Science Citation Index and Conference Proceedings Citation Index, and PsycInfo) and four databases of unpublished and international literature (ClinicalTrials.gov, WHO International Clinical Trials Registry Platform, ProQuest Dissertations and Theses Global, and PsycEXTRA), and handsearching of reference lists, conference proceedings, and abstracts.
Data synthesis and study quality Information on potential treatment effect moderators was extracted, including depression type (primary or secondary), previous use of psychedelics, psilocybin dosage, type of outcome measure (clinician rated or self-reported), and personal characteristics (eg, age, sex). Data were synthesised using a random effects meta-analysis model, and observed heterogeneity and the effect of covariates were investigated with subgroup analyses and metaregression. Hedges’ g was used as a measure of treatment effect size, to account for small sample effects and substantial differences between the included studies’ sample sizes. Study quality was appraised using Cochrane’s Risk of Bias 2 tool, and the quality of the aggregated evidence was evaluated using GRADE guidelines.
Eligibility criteria Randomised trials in which psilocybin was administered as a standalone treatment for adults with clinically significant symptoms of depression and change in symptoms was measured using a validated clinician rated or self-report scale. Studies with directive psychotherapy were included if the psychotherapeutic component was present in both experimental and control conditions. Participants with depression regardless of comorbidities (eg, cancer) were eligible.
Results Meta-analysis on 436 participants (228 female participants), average age 36-60 years, from seven of the nine included studies showed a significant benefit of psilocybin (Hedges’ g=1.64, 95% confidence interval (CI) 0.55 to 2.73, P<0.001) on change in depression scores compared with comparator treatment. Subgroup analyses and metaregressions indicated that having secondary depression (Hedges’ g=3.25, 95% CI 0.97 to 5.53), being assessed with self-report depression scales such as the Beck depression inventory (3.25, 0.97 to 5.53), and older age and previous use of psychedelics (metaregression coefficient 0.16, 95% CI 0.08 to 0.24 and 4.2, 1.5 to 6.9, respectively) were correlated with greater improvements in symptoms. All studies had a low risk of bias, but the change from baseline metric was associated with high heterogeneity and a statistically significant risk of small study bias, resulting in a low certainty of evidence rating.
Conclusion Treatment effects of psilocybin were significantly larger among patients with secondary depression, when self-report scales were used to measure symptoms of depression, and when participants had previously used psychedelics. Further research is thus required to delineate the influence of expectancy effects, moderating factors, and treatment delivery on the efficacy of psilocybin as an antidepressant.
Systematic review registration PROSPERO CRD42023388065.

- Download figure
- Open in new tab
- Download powerpoint
Introduction
Depression affects an estimated 300 million people around the world, an increase of nearly 20% over the past decade. 1 Worldwide, depression is also the leading cause of disability. 2
Drugs for depression are widely available but these seem to have limited efficacy, can have serious adverse effects, and are associated with low patient adherence. 3 4 Importantly, the treatment effects of antidepressant drugs do not appear until 4-7 weeks after the start of treatment, and remission of symptoms can take months. 4 5 Additionally, the likelihood of relapse is high, with 40-60% of people with depression experiencing a further depressive episode, and the chance of relapse increasing with each subsequent episode. 6 7
Since the early 2000s, the naturally occurring serotonergic hallucinogen psilocybin, found in several species of mushrooms, has been widely discussed as a potential treatment for depression. 8 9 Psilocybin’s mechanism of action differs from that of classic selective serotonin reuptake inhibitors (SSRIs) and might improve the treatment response rate, decrease time to improvement of symptoms, and prevent relapse post-remission. Moreover, more recent assessments of harm have consistently reported that psilocybin generally has low addictive potential and toxicity and that it can be administered safely under clinical supervision. 10
The renewed interest in psilocybin’s antidepressive effects led to several clinical trials on treatment resistant depression, 11 12 major depressive disorder, 13 and depression related to physical illness. 14 15 16 17 These trials mostly reported positive efficacy findings, showing reductions in symptoms of depression within a few hours to a few days after one dose or two doses of psilocybin. 11 12 13 16 17 18 These studies reported only minimal adverse effects, however, and drug harm assessments in healthy volunteers indicated that psilocybin does not induce physiological toxicity, is not addictive, and does not lead to withdrawal. 19 20 Nevertheless, these findings should be interpreted with caution owing to the small sample sizes and open label design of some of these studies. 11 21
Several systematic reviews and meta-analyses since the early 2000s have investigated the use of psilocybin to treat symptoms of depression. Most found encouraging results, but as well as people with depression some included healthy volunteers, 22 and most combined data from studies of multiple serotonergic psychedelics, 23 24 25 even though each compound has unique neurobiological effects and mechanisms of action. 26 27 28 Furthermore, many systematic reviews included non-randomised studies and studies in which psilocybin was tested in conjunction with psychotherapeutic interventions, 25 29 30 31 32 which made it difficult to distinguish psilocybin’s treatment effects. Most systematic reviews and meta-analyses did not consider the impact of factors that could act as moderators to psilocybin’s effects, such as type of depression (primary or secondary), previous use of psychedelics, psilocybin dosage, type of outcome measure (clinician rated or self-reported), and personal characteristics (eg, age, sex). 25 26 29 30 31 32 Lastly, systematic reviews did not consider grey literature, 33 34 which might have led to a substantial overestimation of psilocybin’s efficacy as a treatment for depression. In this review we focused on randomised trials that contained an unconfounded evaluation of psilocybin in adults with symptoms of depression, regardless of country and language of publication.
In this systematic review and meta-analysis of indexed and non-indexed randomised trials we investigated the efficacy of psilocybin to treat symptoms of depression compared with placebo or non-psychoactive drugs. The protocol was registered in the International Prospective Register of Systematic Reviews (see supplementary Appendix A). The study overall did not deviate from the pre-registered protocol; one clarification was made to highlight that any non-psychedelic comparator was eligible for inclusion, including placebo, niacin, micro doses of psychedelics, and drugs that are considered the standard of care in depression (eg, SSRIs).
Inclusion and exclusion criteria
Double blind and open label randomised trials with a crossover or parallel design were eligible for inclusion. We considered only studies in humans and with a control condition, which could include any type of non -active comparator, such as placebo, niacin, or micro doses of psychedelics.
Eligible studies were those that included adults (≥18 years) with clinically significant symptoms of depression, evaluated using a clinically validated tool for depression and mood disorder outcomes. Such tools included the Beck depression inventory, Hamilton depression rating scale, Montgomery-Åsberg depression rating scale, profile of mood states, and quick inventory of depressive symptomatology. Studies of participants with symptoms of depression and comorbidities (eg, cancer) were also eligible. We excluded studies of healthy participants (without depressive symptomatology).
Eligible studies investigated the effect of psilocybin as a standalone treatment on symptoms of depression. Studies with an active psilocybin condition that involved micro dosing (ie, psilocybin <100 μg/kg, according to the commonly accepted convention 22 35 ) were excluded. We included studies with directive psychotherapy if the psychotherapeutic component was present in both the experimental and the control conditions, so that the effects of psilocybin could be distinguished from those of psychotherapy. Studies involving group therapy were also excluded. Any non-psychedelic comparator was eligible for inclusion, including placebo, niacin, and micro doses of psychedelics.
Changes in symptoms, measured by validated clinician rated or self-report scales, such as the Beck depression inventory, Hamilton depression rating scale, Montgomery-Åsberg depression rating scale, profile of mood states, and quick inventory of depressive symptomatology were considered. We excluded outcomes that were measured less than three hours after psilocybin had been administered because any reported changes could be attributed to the transient cognitive and affective effects of the substance being administered. Aside from this, outcomes were included irrespective of the time point at which measurements were taken.
Search strategy
We searched major electronic databases and trial registries of psychological and medical research, with no limits on the publication date. Databases were the Cochrane Central Register of Controlled Trials via the Cochrane Library, Embase via Ovid, Medline via Ovid, Science Citation Index and Conference Proceedings Citation Index-Science via Web of Science, and PsycInfo via Ovid. A search through multiple databases was necessary because each database includes unique journals. Supplementary Appendix B shows the search syntax used for the Cochrane Central Register of Controlled Trials, which was slightly modified to comply with the syntactic rules of the other databases.
Unpublished and grey literature were sought through registries of past and ongoing trials, databases of conference proceedings, government reports, theses, dissertations, and grant registries (eg, ClinicalTrials.gov, WHO International Clinical Trials Registry Platform, ProQuest Dissertations and Theses Global, and PsycEXTRA). The references and bibliographies of eligible studies were checked for relevant publications. The original search was done in January 2023 and updated search was performed on 10 August 2023.
Data collection, extraction, and management
The results of the literature search were imported to the Endnote X9 reference management software, and the references were imported to the Covidence platform after removal of duplicates. Two reviewers (AM and DT) independently screened the title and abstract of each reference and then screened the full text of potentially eligible references. Any disagreements about eligibility were resolved through discussion. If information was insufficient to determine eligibility, the study’s authors were contacted. The reviewers were not blinded to the studies’ authors, institutions, or journal of publication.
The PRISMA (Preferred Reporting Items for Systematic Reviews and Meta-Analyses) flow diagram shows the study selection process and reasons for excluding studies that were considered eligible for full text screening. 36
Critical appraisal of individual studies and of aggregated evidence
The methodological quality of eligible studies was assessed using the Cochrane Risk of Bias 2 tool (RoB 2) for assessing risk of bias in randomised trials. 37 In addition to the criteria specified by RoB 2, we considered the potential impact of industry funding and conflicts of interest. The overall methodological quality of the aggregated evidence was evaluated using GRADE (Grading of Recommendations, Assessment, Development and Evaluation). 38
If we found evidence of heterogeneity among the trials, then small study biases, such as publication bias, were assessed using a funnel plot and asymmetry tests (eg, Egger’s test). 39
We used a template for data extraction (see supplementary Appendix C) and summarised the extracted data in tabular form, outlining personal characteristics (age, sex, previous use of psychedelics), methodology (study design, dosage), and outcome related characteristics (mean change from baseline score on a depression questionnaire, response rates, and remission rates) of the included studies. Response conventionally refers to a 50% decrease in symptom severity based on scores on a depression rating scale, whereas remission scores are specific to a questionnaire (eg, score of ≤5 on the quick inventory of depressive symptomatology, score of ≤10 on the Montgomery-Åsberg depression rating scale, 50% or greater reduction in symptoms, score of ≤7 on the Hamilton depression rating scale, or score of ≤12 on the Beck depression inventory). Across depression scales, higher scores signify more severe symptoms of depression.
Continuous data synthesis
From each study we extracted the baseline and post-intervention means and standard deviations (SDs) of the scores between comparison groups for the depression questionnaires and calculated the mean differences and SDs of change. If means and SDs were not available for the included studies, we extracted the values from available graphs and charts using the Web Plot Digitizer application ( https://automeris.io/WebPlotDigitizer/ ). If it was not possible to calculate SDs from the graphs or charts, we generated values by converting standard errors (SEs) or confidence intervals (CIs), depending on availability, using formulas in the Cochrane Handbook (section 7.7.3.2). 40
Standardised mean differences were calculated for each study. We chose these rather than weighted mean differences because, although all the studies measured depression as the primary outcome, they did so with different questionnaires that score depression based on slightly different items. 41 If we had used weighted mean differences, any variability among studies would be assumed to reflect actual methodological or population differences and not differences in how the outcome was measured, which could be misleading. 40
The Hedges’ g effect size estimate was used because it tends to produce less biased results for studies with smaller samples (<20 participants) and when sample sizes differ substantially between studies, in contrast with Cohen’s d. 42 According to the Cochrane Handbook, the Hedges’ g effect size measure is synonymous with the standardised mean difference, 40 and the terms may be used interchangeably. Thus, a Hedges’ g of 0.2, 0.5, 0.8, or 1.2 corresponds to a small, medium, large, or very large effect, respectively. 40
Owing to variation in the participants’ personal characteristics, psilocybin dosage, type of depression investigated (primary or secondary), and type of comparators, we used a random effects model with a Hartung-Knapp-Sidik-Jonkman modification. 43 This model also allowed for heterogeneity and within study variability to be incorporated into the weighting of the results of the included studies. 44 Lastly, this model could help to generalise the findings beyond the studies and patient populations included, making the meta-analysis more clinically useful. 45 We chose the Hartung-Knapp-Sidik-Jonkman adjustment in favour of more widely used random effects models (eg, DerSimonian and Laird) because it allows for better control of type 1 errors, especially for studies with smaller samples, and provides a better estimation of between study variance by accounting for small sample sizes. 46 47
For studies in which multiple treatment groups were compared with a single placebo group, we split the placebo group to avoid multiplicity. 48 Similarly, if studies included multiple primary outcomes (eg, change in depression at three weeks and at six weeks), we split the treatment groups to account for overlapping participants. 40
Prediction intervals (PIs) were calculated and reported to show the expected effect range of a similar future study, in a different setting. In a random effects model, within study measures of variability, such as CIs, can only show the range in which the average effect size could lie, but they are not informative about the range of potential treatment effects given the heterogeneity between studies. 49 Thus, we used PIs as an indication of variation between studies.
Heterogeneity and sensitivity analysis
Statistical heterogeneity was tested using the χ 2 test (significance level P<0.1) and I 2 statistic, and heterogeneity among included studies was evaluated visually and displayed graphically using a forest plot. If substantial or considerable heterogeneity was found (I 2 ≥50% or P<0.1), 50 we considered the study design and characteristics of the included studies. Sources of heterogeneity were explored by subgroup analysis, and the potential effects on the results are discussed.
Planned sensitivity analyses to assess the effect of unpublished studies and studies at high risk of bias were not done because all included studies had been published and none were assessed as high risk of bias. Exclusion sensitivity plots were used to display graphically the impact of individual studies and to determine which studies had a particularly large influence on the results of the meta-analysis. All sensitivity analyses were carried out with Stata 16 software.
Subgroup analysis
To reduce the risk of errors caused by multiplicity and to avoid data fishing, we planned subgroup analyses a priori and limited to: (1) patient characteristics, including age and sex; (2) comorbidities, such as a serious physical condition (previous research indicates that the effects of psilocybin may be less strong for such participants, compared with participants with no comorbidities) 33 ; (3) number of doses and amount of psilocybin administered, because some previous meta-analyses found that a higher number of doses and a higher dose of psilocybin both predicted a greater reduction in symptoms of depression, 34 whereas others reported the opposite 33 ; (4) psilocybin administered alongside psychotherapeutic guidance or as a standalone treatment; (5) severity of depressive symptoms (clinical v subclinical symptomatology); (6) clinician versus patient rated scales; and (7) high versus low quality studies, as determined by RoB 2 assessment scores.
Metaregression
Given that enough studies were identified (≥10 distinct observations according to the Cochrane Handbook’s suggestion 40 ), we performed metaregression to investigate whether covariates, or potential effect modifiers, explained any of the statistical heterogeneity. The metaregression analysis was carried out using Stata 16 software.
Random effects metaregression analyses were used to determine whether continuous variables such as participants’ age, percentage of female participants, and percentage of participants who had previously used psychedelics modified the effect estimate, all of which have been implicated in differentially affecting the efficacy of psychedelics in modifying mood. 51 We chose this approach in favour of converting these continuous variables into categorical variables and conducting subgroup analyses for two primary reasons; firstly, the loss of any data and subsequent loss of statistical power would increase the risk of spurious significant associations, 51 and, secondly, no cut-offs have been agreed for these factors in literature on psychedelic interventions for mood disorders, 52 making any such divisions arbitrary and difficult to reconcile with the findings of other studies. The analyses were based on within study averages, in the absence of individual data points for each participant, with the potential for the results to be affected by aggregate bias, compromising their validity and generalisability. 53 Furthermore, a group level analysis may not be able to detect distinct interactions between the effect modifiers and participant subgroups, resulting in ecological bias. 54 As a result, this analysis should be considered exploratory.
Sensitivity analysis
A sensitivity analysis was performed to determine if choice of analysis method affected the primary findings of meta-analysis. Specifically, we reanalysed the data on change in depression score using a random effects Dersimonian and Laird model without the Hartung-Knapp-Sidik-Jonkman modification and compared the results with those of the originally used model. This comparison is particularly important in the presence of substantial heterogeneity and the potential of small study effects to influence the intervention effect estimate. 55
Patient and public involvement
Research on novel depression treatments is of great interest to both patients and the public. Although patients and members of the public were not directly involved in the planning or writing of this manuscript owing to a lack of available funding for recruitment and researcher training, patients and members of the public read the manuscript after submission.
Figure 1 presents the flow of studies through the systematic review and meta-analysis. 56 A total of 4884 titles were retrieved from the five databases of published literature, and a further 368 titles were identified from the databases of unpublished and international literature in February 2023. After the removal of duplicate records, we screened the abstracts and titles of 875 reports. A further 12 studies were added after handsearching of reference lists and conference proceedings and abstracts. Overall, nine studies totalling 436 participants were eligible. The average age of the participants ranged from 36-60 years. During an updated search on 10 August 2023, no further studies were identified.

Flow of studies in systematic review and meta-analysis
After screening of the title and abstract, 61 titles remained for full text review. Native speakers helped to translate papers in languages other than English. The most common reasons for exclusion were the inclusion of healthy volunteers, absence of control groups, and use of a survey based design rather than an experimental design. After full text screening, nine studies were eligible for inclusion, and 15 clinical trials prospectively registered or underway as of August 2023 were noted for potential future inclusion in an update of this review (see supplementary Appendix D).
We sent requests for further information to the authors of studies by Griffiths et al, 57 Barrett, 58 and Benville et al, 59 because these studies appeared to meet the inclusion criteria but were only provided as summary abstracts online. A potentially eligible poster presentation from the 58th annual meeting of the American College of Neuropsychopharmacology was identified but the lead author (Griffiths) clarified that all information from the presentation was included in the studies by Davis et al 13 and Gukasyan et al 60 ; both of which we had already deemed ineligible.
Barrett 58 reported the effects of psilocybin on the cognitive flexibility and verbal reasoning of a subset of patients with major depressive disorder from Griffith et al’s trial, 61 compared with a waitlist group, but when contacted, Barrett explained that the results were published in the study by Doss et al, 62 which we had already screened and judged ineligible (see supplementary Appendix E). Benville et al’s study 59 presented a follow-up of Ross et al’s study 17 on a subset of patients with cancer and high suicidal ideation and desire for hastened death at baseline. Measures of antidepressant effects of psilocybin treatment compared with niacin were taken before and after treatment crossover, but detailed results are not reported. Table 1 describes the characteristics of the included studies and table 2 lists the main findings of the studies.
Characteristics of included studies
- View inline
Main findings of included studies
Side effects and adverse events
Side effects reported in the included studies were minor and transient (eg, short term increases in blood pressure, headache, and anxiety), and none were coded as serious. Cahart-Harris et al noted one instance of abnormal dreams and insomnia. 63 This side effect profile is consistent with findings from other meta-analyses. 30 68 Owing to the different scales and methods used to catalogue side effects and adverse events across trials, it was not possible to combine these data quantitatively (see supplementary Appendix F).
Risk of bias
The Cochrane RoB 2 tools were used to evaluate the included studies ( table 3 ). RoB 2 for randomised trials was used for the five reports of parallel randomised trials (Carhart-Harris et al 63 and its secondary analysis Barba et al, 64 Goodwin et al 18 and its secondary analysis Goodwin et al, 65 and von Rotz et al 66 ) and RoB 2 for crossover trials was used for the four reports of crossover randomised trials (Griffiths et al, 14 Grob et al, 15 and Ross et al 17 and its follow-up Ross et al 67 ). Supplementary Appendix G provides a detailed explanation of the assessment of the included studies.
Summary risk of bias assessment of included studies, based on domains in Cochrane Risk of Bias 2 tool
Quality of included studies
Confidence in the quality of the evidence for the meta-analysis was assessed using GRADE, 38 through the GRADEpro GDT software program. Figure 2 shows the results of this assessment, along with our summary of findings.

GRADE assessment outputs for outcomes investigated in meta-analysis (change in depression scores and response and remission rates). The risk in the intervention group (and its 95% CI) is based on the assumed risk in the comparison group and the relative effect of the intervention (and its 95% CI). BDI=Beck depression inventory; CI=confidence interval; GRADE=Grading of Recommendations, Assessment, Development and Evaluation; HADS-D=hospital anxiety and depression scale; HAM-D=Hamilton depression rating scale; MADRS=Montgomery-Åsberg depression rating scale; QIDS=quick inventory of depressive symptomatology; RCT=randomised controlled trial; SD=standard deviation
Meta-analyses
Continuous data, change in depression scores —Using a Hartung-Knapp-Sidik-Jonkman modified random effects meta-analysis, change in depression scores was significantly greater after treatment with psilocybin compared with active placebo. The overall Hedges’ g (1.64, 95% CI 0.55 to 2.73) indicated a large effect size favouring psilocybin ( fig 3 ). PIs were, however, wide and crossed the line of no difference (95% CI −1.72 to 5.03), indicating that there could be settings or populations in which psilocybin intervention would be less efficacious.

Forest plot for overall change in depression scores from before to after treatment. CI=confidence interval; DL=DerSimonian and Laird; HKSJ=Hartung-Knapp-Sidik-Jonkman
Exploring publication bias in continuous data —We used Egger’s test and a funnel plot to examine the possibility of small study biases, such as publication bias. Statistical significance of Egger’s test for small study effects, along with the asymmetry in the funnel plot ( fig 4 ), indicates the presence of bias against smaller studies with non-significant results, suggesting that the pooled intervention effect estimate is likely to be overestimated. 69 An alternative explanation, however, is that smaller studies conducted at the early stages of a new psychotherapeutic intervention tend to include more high risk or responsive participants, and psychotherapeutic interventions tend to be delivered more effectively in smaller trials; both of these factors can exaggerate treatment effects, resulting in funnel plot asymmetry. 70 Also, because of the relatively small number of included studies and the considerable heterogeneity observed, test power may be insufficient to distinguish real asymmetry from chance. 71 Thus, this analysis should be considered exploratory.

Funnel plot assessing publication bias among studies measuring change in depression scores from before to after treatment. CI=confidence interval; θ IV =estimated effect size under inverse variance random effects model
Dichotomous data
We extracted response and remission rates for each group when reported directly, or imputed information when presented graphically. Two studies did not measure response or remission and thus did not contribute data for this part of the analysis. 15 18 The random effects model with a Hartung-Knapp-Sidik-Jonkman modification was used to allow for heterogeneity to be incorporated into the weighting of the included studies’ results, and to provide a better estimation of between study variance accounting for small sample sizes.
Response rate —Overall, the likelihood of psilocybin intervention leading to treatment response was about two times greater (risk ratio 2.02, 95% CI 1.33 to 3.07) than with placebo. Despite the use of different scales to measure response, the heterogeneity between studies was not significant (I 2 =25.7%, P=0.23). PIs were, however, wide and crossed the line of no difference (−0.94 to 3.88), indicating that there could be settings or populations in which psilocybin intervention would be less efficacious.
Remission rate —Overall, the likelihood of psilocybin intervention leading to remission of depression was nearly three times greater than with placebo (risk ratio 2.71, 95% CI 1.75 to 4.20). Despite the use of different scales to measure response, no statistical heterogeneity was found between studies (I 2 =0.0%, P=0.53). PIs were, however, wide and crossed the line of no difference (0.87 to 2.32), indicating that there could be settings or populations in which psilocybin intervention would be less efficacious.
Exploring publication bias in response and remission rates data —We used Egger’s test and a funnel plot to examine whether response and remission estimates were affected by small study biases. The result for Egger’s test was non-significant (P>0.05) for both response and remission estimates, and no substantial asymmetry was observed in the funnel plots, providing no indication for the presence of bias against smaller studies with non-significant results.
Heterogeneity: subgroup analyses and metaregression
Heterogeneity was considerable across studies exploring changes in depression scores (I 2 =89.7%, P<0.005), triggering subgroup analyses to explore contributory factors. Table 4 and table 5 present the results of the heterogeneity analyses (subgroup analyses and metaregression, respectively). Also see supplementary Appendix H for a more detailed description and graphical representation of these results.
Subgroup analyses to explore potential causes of heterogeneity among included studies
Metaregression analyses to explore potential causes of heterogeneity among included studies
Cumulative meta-analyses
We used cumulative meta-analyses to investigate how the overall estimates of the outcomes of interest changed as each study was added in chronological order 72 ; change in depression scores and likelihood of treatment response both increased as the percentage of participants with past use of psychedelics increased across studies, as expected based on the metaregression analysis (see supplementary Appendix I). No other significant time related patterns were found.
We reanalysed the data for change in depression scores using a random effects Dersimonian and Laird model without the Hartung-Knapp-Sidik-Jonkman modification and compared the results with those of the original model. All comparisons found to be significant using the Dersimonian and Laird model with the Hartung-Knapp-Sidik-Jonkman adjustment were also significant without the Hartung-Knapp-Sidik-Jonkman adjustment, and confidence intervals were only slightly narrower. Thus, small study effects do not appear to have played a major role in the treatment effect estimate.
Additionally, to estimate the accuracy and robustness of the estimated treatment effect, we excluded studies from the meta-analysis one by one; no important differences in the treatment effect, significance, and heterogeneity levels were observed after the exclusion of any study (see supplementary Appendix J).
In our meta-analysis we found that psilocybin use showed a significant benefit on change in depression scores compared with placebo. This is consistent with other recent meta-analyses and trials of psilocybin as a standalone treatment for depression 73 74 or in combination with psychological support. 24 25 29 30 31 32 68 75 This review adds to those finding by exploring the considerable heterogeneity across the studies, with subsequent subgroup analyses showing that the type of depression (primary or secondary) and the depression scale used (Montgomery-Åsberg depression rating scale, quick inventory of depressive symptomatology, or Beck depression inventory) had a significant differential effect on the outcome. High between study heterogeneity has been identified by some other meta-analyses of psilocybin (eg, Goldberg et al 29 ), with a higher treatment effect in studies with patients with comorbid life threatening conditions compared with patients with primary depression. 22 Although possible explanations, including personal factors (eg, patients with life threatening conditions being older) or depression related factors (eg, secondary depression being more severe than primary depression) could be considered, these hypotheses are not supported by baseline data (ie, patients with secondary depression do not differ substantially in age or symptom severity from patients with primary depression). The differential effects from assessment scales used have not been examined in other meta-analyses of psilocybin, but this review’s finding that studies using the Beck depression inventory showed a higher treatment effect than those using the Montgomery-Åsberg depression rating scale and quick inventory of depressive symptomatology is consistent with studies in the psychological literature that have shown larger treatment effects when self-report scales are used (eg, Beck depression inventory). 76 77 This finding may be because clinicians tend to overestimate the severity of depression symptoms at baseline assessments, leading to less pronounced differences between before and after treatment identified in clinician assessed scales (eg, Montgomery-Åsberg depression rating scale, quick inventory of depressive symptomatology). 78
Metaregression analyses further showed that a higher average age and a higher percentage of participants with past use of psychedelics both correlated with a greater improvement in depression scores with psilocybin use and explained a substantial amount of between study variability. However, the cumulative meta-analysis showed that the effects of age might be largely an artefact of the inclusion of one specific study, and alternative explanations are worth considering. For instance, Studerus et al 79 identified participants’ age as the only personal variable significantly associated with psilocybin response, with older participants reporting a higher “blissful state” experience. This might be because of older people’s increased experience in managing negative emotions and the decrease in 5-hydroxytryptamine type 2A receptor density associated with older age. 80 Furthermore, Rootman et al 81 reported that the cognitive performance of older participants (>55 years) improved significantly more than that of younger participants after micro dosing with psilocybin. Therefore, the higher decrease in depressive symptoms associated with older age could be attributed to a decrease in cognitive difficulties experienced by older participants.
Interestingly, a clear pattern emerged for past use of psychedelics—the higher the proportion of study participants who had used psychedelics in the past, the higher the post-psilocybin treatment effect observed. Past use of psychedelics has been proposed to create an expectancy bias among participants and amplify the positive effects of psilocybin 82 83 84 ; however, this important finding has not been examined in other meta-analyses and may highlight the role of expectancy in psilocybin research.
Limitations of this study
Generalisability of the findings of this meta-analysis was limited by the lack of racial and ethnic diversity in the included studies—more than 90% of participants were white across all included trials, resulting in a homogeneous sample that is not representative of the general population. Moreover, it was not possible to distinguish between subgroups of participants who had never used psilocybin and those who had taken psilocybin more than a year before the start of the trial, as these data were not provided in the included studies. Such a distinction would be important, as the effects of psilocybin on mood may wane within a year after being administered. 21 85 Also, how psychological support was conceptualised was inconsistent within studies of psilocybin interventions; many studies failed to clearly describe the type of psychological support participants received, and others used methods ranging from directive guidance throughout the treatment session to passive encouragement or reassurance (eg, Griffiths et al, 14 Carhart-Harris et al 63 ). The included studies also did not gather evidence on participants’ previous experiences with treatment approaches, which could influence their response to the trials’ intervention. Thus, differences between participant subgroups related to past use of psilocybin or psychotherapy may be substantial and could help interpret this study’s findings more accurately. Lastly, the use of graphical extraction software to estimate the findings of studies where exact numerical data were not available (eg, Goodwin et al, 18 Grob et al 15 ), may have affected the robustness of the analyses.
A common limitation in studies of psilocybin is the likelihood of expectancy effects augmenting the treatment effect observed. Although some studies used low dose psychedelics as comparators to deal with this problem (eg, Carhart-Harris et al, 63 Goodwin et al, 18 Griffiths et al 14 ) or used a niacin placebo that can induce effects similar to those of psilocybin (eg, Grob et al, 15 Ross et al 17 ), the extent to which these methods were effective in blinding participants is not known. Other studies have, however, reported that participants can accurately identify the study groups to which they had been assigned 70-85% of the time, 84 86 indicating a high likelihood of insufficient blinding. This is especially likely for studies in which a high proportion of participants had previously used psilocybin and other hallucinogens, making the identification of the drug’s acute effects easier (eg, Griffiths et al, 14 Grob et al, 15 Ross et al 17 ). Patients also have expectations related to the outcome of their treatment, expecting psilocybin to improve their symptoms of depression, and these positive expectancies are strong predictors of actual treatment effects. 87 88 Importantly, the effect of outcome expectations on treatment effect is particularly strong when patient reported measures are used as primary outcomes, 89 which was the case in several of the included studies (eg, Griffiths et al, 14 Grob et al, 15 Ross et al 17 ). Unfortunately, none of the included studies recorded expectations before treatment, so it is not possible to determine the extent to which this factor affected the findings.
Implications for clinical practice
Although this review’s findings are encouraging for psilocybin’s potential as an effective antidepressant, a few areas about its applicability in clinical practice remain unexplored. Firstly, it is unclear whether the protocols for psilocybin interventions in clinical trials can be reliably and safely implemented in clinical practice. In clinical trials, patients receive psilocybin in a non-traditional medical setting, such as a specially designed living room, while they may be listening to curated calming music and are isolated from most external stimuli by wearing eyeshades and external noise-cancelling earphones. A trained therapist closely supervises these sessions, and the patient usually receives one or more preparatory sessions before the treatment commences. Standardising an intervention setting with so many variables is unlikely to be achievable in routine practice, and consensus is considerably lacking on the psychotherapeutic training and accreditations needed for a therapist to deliver such treatment. 90 The combination of these elements makes this a relatively complex and expensive intervention, which could make it challenging to gain approval from regulatory agencies and to gain reimbursement from insurance companies and others. Within publicly funded healthcare systems, the high cost of treatment may make psilocybin treatment inaccessible. The high cost associated with the intervention also increases the risk that unregulated clinics may attempt to cut costs by making alterations to the protocol and the therapeutic process, 91 92 which could have detrimental effects for patients. 92 93 94 Thus, avoiding the conflation of medical and commercial interests is a primary concern that needs to be dealt with before psilocybin enters mainstream practice.
Implications for future research
More large scale randomised trials with long follow-up are needed to fully understand psilocybin’s treatment potential, and future studies should aim to recruit a more diverse population. Another factor that would make clinical trials more representative of routine practice would be to recruit patients who are currently using or have used commonly prescribed serotonergic antidepressants. Clinical trials tend to exclude such participants because many antidepressants that act on the serotonin system modulate the 5-hydroxytryptamine type 2A receptor that psilocybin primarily acts upon, with prolonged use of tricyclic antidepressants associated with more intense psychedelic experiences and use of monoamine oxidase inhibitors or SSRIs inducing weaker responses to psychedelics. 95 96 97 Investigating psilocybin in such patients would, however, provide valuable insight on how psilocybin interacts with commonly prescribed drugs for depression and would help inform clinical practice.
Minimising the influence of expectancy effects is another core problem for future studies. One strategy would be to include expectancy measures and explore the level of expectancy as a covariate in statistical analysis. Researchers should also test the effectiveness of condition masking. Another proposed solution would be to adopt a 2×2 balanced placebo design, where both the drug (psilocybin or placebo) and the instructions given to participants (told they have received psilocybin or told they have received placebo) are crossed. 98 Alternatively, clinical trials could adopt a three arm design that includes both an inactive placebo (eg, saline) and active placebo (eg, niacin, lower psylocibin dose), 98 allowing for the effects of psilocybin to be separated from those of the placebo.
Overall, future studies should explore psilocybin’s exact mechanism of treatment effectiveness and outline how its physiological effects, mystical experiences, dosage, treatment setting, psychological support, and relationship with the therapist all interact to produce a synergistic antidepressant effect. Although this may be difficult to achieve using an explanatory randomised trial design, pragmatic clinical trial designs may be better suited to psilocybin research, as their primary objective is to achieve high external validity and generalisability. Such studies may include multiple alternative treatments rather than simply an active and placebo treatment comparison (eg, psilocybin v SSRI v serotonin-noradrenaline reuptake inhibitor), and participants would be recruited from broader clinical populations. 99 100 Although such studies are usually conducted after a drug’s launch, 100 earlier use of such designs could help assess the clinical effectiveness of psilocybin more robustly and broaden patient access to a novel type of antidepressant treatment.
Conclusions
This review’s findings on psilocybin’s efficacy in reducing symptoms of depression are encouraging for its use in clinical practice as a drug intervention for patients with primary or secondary depression, particularly when combined with psychological support and administered in a supervised clinical environment. However, the highly standardised treatment setting, high cost, and lack of regulatory guidelines and legal safeguards associated with psilocybin treatment need to be dealt with before it can be established in clinical practice.
What is already known on this topic
Recent research on treatments for depression has focused on psychedelic agents that could have strong antidepressant effects without the drawbacks of classic antidepressants; psilocybin being one such substance
Over the past decade, several clinical trials, meta-analyses, and systematic reviews have investigated the use of psilocybin for symptoms of depression, and most have found that psilocybin can have antidepressant effects
Studies published to date have not investigated factors that may moderate psilocybin’s effects, including type of depression, past use of psychedelics, dosage, outcome measures, and publication biases
What this study adds
This review showed a significantly greater efficacy of psilocybin among patients with secondary depression, patients with past use of psychedelics, older patients, and studies using self-report measures for symptoms of depression
Efficacy did not appear to be homogeneous across patient types—for example, those with depression and a life threatening illness appeared to benefit more from treatment
Further research is needed to clarify the factors that maximise psilocybin’s treatment potential for symptoms of depression
Ethics statements
Ethical approval.
This study was approved by the ethics committee of the University of Oxford Nuffield Department of Medicine, which waived the need for ethical approval and the need to obtain consent for the collection, analysis, and publication of the retrospectively obtained anonymised data for this non-interventional study.
Data availability statement
The relevant aggregated data and statistical code will be made available on reasonable request to the corresponding author.
Acknowledgments
We thank DT who acted as an independent secondary reviewer during the study selection and data review process.
Contributors: AMM contributed to the design and implementation of the research, analysis of the results, and writing of the manuscript. MC was involved in planning and supervising the work and contributed to the writing of the manuscript. AMM and MC are the guarantors. The corresponding author attests that all listed authors meet authorship criteria and that no others meeting the criteria have been omitted.
Funding: None received.
Competing interests: All authors have completed the ICMJE uniform disclosure form at https://www.icmje.org/disclosure-of-interest/ and declare: no support from any organisation for the submitted work; AMM is employed by IDEA Pharma, which does consultancy work for pharmaceutical companies developing drugs for physical and mental health conditions; MC was the supervisor for AMM’s University of Oxford MSc dissertation, which forms the basis for this paper; no other relationships or activities that could appear to have influenced the submitted work.
Transparency: The corresponding author (AMM) affirms that the manuscript is an honest, accurate, and transparent account of the study being reported; that no important aspects of the study have been omitted; and that any discrepancies from the study as registered have been explained.
Dissemination to participants and related patient and public communities: To disseminate our findings and increase the impact of our research, we plan on writing several social media posts and blog posts outlining the main conclusions of our paper. These will include blog posts on the websites of the University of Oxford’s Department of Primary Care Health Sciences and Department for Continuing Education, as well as print publications, which are likely to reach a wider audience. Furthermore, we plan to present our findings and discuss them with the public in local mental health related events and conferences, which are routinely attended by patient groups and advocacy organisations.
Provenance and peer review: Not commissioned; externally peer reviewed.
This is an Open Access article distributed in accordance with the Creative Commons Attribution Non Commercial (CC BY-NC 4.0) license, which permits others to distribute, remix, adapt, build upon this work non-commercially, and license their derivative works on different terms, provided the original work is properly cited and the use is non-commercial. See: http://creativecommons.org/licenses/by-nc/4.0/ .
- ↵ World Health Organization. Depressive Disorder (Depression); 2023. https://www.who.int/news-room/fact-sheets/detail/depression .
- GBD 2017 Disease and Injury Incidence and Prevalence Collaborators
- Cipriani A ,
- Furukawa TA ,
- Salanti G ,
- Trivedi MH ,
- Wisniewski SR ,
- Mitchell AJ
- Bockting CL ,
- Hollon SD ,
- Jarrett RB ,
- Nierenberg AA ,
- Petersen TJ ,
- Páleníček T ,
- Carbonaro TM ,
- Bradstreet MP ,
- Barrett FS ,
- Carhart-Harris RL ,
- Bolstridge M ,
- Griffiths RR ,
- Johnson MW ,
- Carducci MA ,
- Danforth AL ,
- Chopra GS ,
- Kraehenmann R ,
- Preller KH ,
- Scheidegger M ,
- Goodwin GM ,
- Aaronson ST ,
- Alvarez O ,
- Bogenschutz MP ,
- Podrebarac SK ,
- Roseman L ,
- Galvão-Coelho NL ,
- Gonzalez M ,
- Dos Santos RG ,
- Osório FL ,
- Crippa JA ,
- Zuardi AW ,
- Cleare AJ ,
- Martelli C ,
- Benyamina A
- Vollenweider FX ,
- Demetriou L ,
- Carhart-Harris RL
- Timmermann C ,
- Giribaldi B ,
- Goldberg SB ,
- Nicholas CR ,
- Raison CL ,
- Irizarry R ,
- Winczura A ,
- Dimassi O ,
- Dhillon N ,
- Griffiths RR
- Castro Santos H ,
- Gama Marques J
- Moreno FA ,
- Wiegand CB ,
- Taitano EK ,
- Liberati A ,
- Tetzlaff J ,
- Altman DG ,
- PRISMA Group
- Sterne JAC ,
- Savović J ,
- Guyatt GH ,
- Schünemann HJ ,
- Tugwell P ,
- Knottnerus A
- Sterne JA ,
- Sutton AJ ,
- Ioannidis JP ,
- Higgins JPT ,
- Chandler J ,
- Borenstein M ,
- Hedges LV ,
- Higgins JP ,
- Rothstein HR
- DerSimonian R ,
- ↵ Borenstein M, Hedges L, Rothstein H. Meta-analysis: Fixed effect vs. random effects. Meta-analysis. com. 2007;1-62.
- IntHout J ,
- Rovers MM ,
- Gøtzsche PC
- Spineli LM ,
- ↵ Higgins JP, Green S. Identifying and measuring heterogeneity. Cochrane handbook for systematic reviews of interventions. 2011;5(0).
- Austin PC ,
- O’Donnell KC ,
- Mennenga SE ,
- Bogenschutz MP
- Sander SD ,
- Berlin JA ,
- Santanna J ,
- Schmid CH ,
- Szczech LA ,
- Feldman HI ,
- Anti-Lymphocyte Antibody Induction Therapy Study Group
- ↵ Iyengar S, Greenhouse J. Sensitivity analysis and diagnostics. Handbook of research synthesis and meta-analysis. Russell Sage Foundation, 2009:417-33.
- McKenzie JE ,
- Bossuyt PM ,
- ↵ Griffiths R, Barrett F, Johnson M, Mary C, Patrick F, Alan D. Psilocybin-Assisted Treatment of Major Depressive Disorder: Results From a Randomized Trial. Proceedings of the ACNP 58th Annual Meeting: Poster Session II. In Neuropsychopharmacology. 2019;44:230-384.
- ↵ Barrett F. ACNP 58th Annual Meeting: Panels, Mini-Panels and Study Groups. [Abstract.] Neuropsychopharmacology 2019;44:1-77. doi: 10.1038/s41386-019-0544-z . OpenUrl CrossRef
- Benville J ,
- Agin-Liebes G ,
- Roberts DE ,
- Gukasyan N ,
- Hurwitz ES ,
- Považan M ,
- Rosenberg MD ,
- Carhart-Harris R ,
- Buehler S ,
- Kettner H ,
- von Rotz R ,
- Schindowski EM ,
- Jungwirth J ,
- Vargas AS ,
- Barroso M ,
- Gallardo E ,
- Isojarvi J ,
- Lefebvre C ,
- Glanville J
- Sukpraprut-Braaten S ,
- Narlesky M ,
- Strayhan RC
- Prouzeau D ,
- Conejero I ,
- Voyvodic PL ,
- Becamel C ,
- Lopez-Castroman J
- Więckiewicz G ,
- Stokłosa I ,
- Gorczyca P ,
- John Mann J ,
- Currier D ,
- Zimmerman M ,
- Friedman M ,
- Boerescu DA ,
- Attiullah N
- Borgherini G ,
- Conforti D ,
- Studerus E ,
- Kometer M ,
- Vollenweider FX
- Pinborg LH ,
- Rootman JM ,
- Kryskow P ,
- Turner EH ,
- Rosenthal R
- Bershad AK ,
- Schepers ST ,
- Bremmer MP ,
- Sepeda ND ,
- Hurwitz E ,
- Horvath AO ,
- Del Re AC ,
- Flückiger C ,
- Rutherford BR ,
- Pearson C ,
- Husain SF ,
- Harris KM ,
- George JR ,
- Michaels TI ,
- Sevelius J ,
- Williams MT
- Collins A ,
- Bonson KR ,
- Buckholtz JW ,
- Yamauchi M ,
- Matsushima T ,
- Coleshill MJ ,
- Colloca L ,
- Zachariae R ,
- Colagiuri B
- Heifets BD ,
- Pratscher SD ,
- Bradley E ,
- Sugarman J ,
- Open access
- Published: 30 April 2024
A comparative ethical analysis of the Egyptian clinical research law
- Sylvia Martin 1 ,
- Mirko Ancillotti 1 ,
- Santa Slokenberga 2 &
- Amal Matar 1 , 3
BMC Medical Ethics volume 25 , Article number: 48 ( 2024 ) Cite this article
98 Accesses
1 Altmetric
Metrics details
In this study, we examined the ethical implications of Egypt’s new clinical trial law, employing the ethical framework proposed by Emanuel et al. and comparing it to various national and supranational laws. This analysis is crucial as Egypt, considered a high-growth pharmaceutical market, has become an attractive location for clinical trials, offering insights into the ethical implementation of bioethical regulations in a large population country with a robust healthcare infrastructure and predominantly treatment-naïve patients.
We conducted a comparative analysis of Egyptian law with regulations from Sweden and France, including the EU Clinical Trials Regulation, considering ethical human subject research criteria, and used a directed approach to qualitative content analysis to examine the laws and regulations. This study involved extensive peer scrutiny, frequent debriefing sessions, and collaboration with legal experts with relevant international legal expertise to ensure rigorous analysis and interpretation of the laws.
On the rating of the seven different principles (social and scientific values, scientific validity, fair selection of participants, risk-benefit ratio, independent review, informed consent and respect for participants) Egypt, France, and EU regulations had comparable scores. Specific principles (Social Value, Scientific Value, and Fair selection of participants) were challenging to directly identify due to certain regulations embodying 'implicit' principles more than explicitly stated ones.
The analysis underscores Egypt's alignment with internationally recognized ethical principles, as outlined by Emanuel et al., through its comparison with French, Swedish, and EU regulations, emphasizing the critical need for Egypt to continuously refine its ethical regulations to safeguard participant protection and research integrity. Key issues identified include the necessity to clarify and standardize the concept of social value in research, alongside concerns regarding the expertise and impartiality of ethical review boards, pointing towards a broader agenda for enhancing research ethics in Egypt and beyond.
Peer Review reports
Introduction
Science relies on research to move forward and enhance knowledge. Different research areas deploy at different levels of human subjects’ involvement, from qualitative and non-interventional research methods to biomedical research and medical validation procedures. While virtually all research has ethical implications, clinical research calls for special attention, and it is widely agreed that it should be conducted according to more stringent ethical principles than other types of research [ 1 ]. To facilitate appropriate ethical implementation of research, it is imperative for ethical regulations to be receptive to the advancements in the field. The well-being of participants is a fundamental condition of research emphasized by the principles outlined worldwide in renowned ethical texts like the Belmont Report (cited in [ 2 ] Footnote 1 ), and the Helsinki Declaration (see [ 3 ] Footnote 2 ). These core principles include ensuring participants' entitlement to minimize harm and discomfort [ 2 ], as well as safeguarding their rights against exploitation [ 4 ].
For assessing whether the ethical requirements are fulfilled, and to ensure that international standards are respected, as it has been proposed by Artal & Rubenfeld [ 5 ], 2017, it is essential account for specific principles developed in the field. Research conduct that seemed legitimate to the men of science in the past is abhorrent to the contemporary conscience [ 6 ]. Ethical standards also depend on where they apply. Different societies, with their specific traditions and cultures, have systems of values and norms that may only partly coincide with research ethics principles informing international standards. Hence, there could be a gap between what is culturally acceptable and what is compliant with international ethics standards. However, the risk of ethical colonialism and its biases, may be difficult to avoid, as it can be considered factual that many international documents heavily rely on the Western perspective [ 7 ].
While cognizant of this, a few documents can be considered ethical reference points, such as the above-mentioned Belmont Report and the Helsinki Declaration for the protection of human participants in medical research [ 8 ]. Another influential example is the Ethical Framework for Biomedical Research from Emanuel et al. [ 9 , 10 ]. The Ethical Framework for Biomedical Research has heavily influenced the ethics work of leading institutions such as the Department of Health (DoH, South Africa Footnote 3 ) and Council for International Organizations of Medical Sciences (CIOMS). In research ethics, the framework has often been used to assess the functioning of ethical review committees and the ethical adequacy of legal regulations of research involving human subjects (see [ 11 , 12 , 13 ]). This ethical framework, rooted in major Western philosophical traditions but not explicitly aligned with any specific school of thought, enables the authors to formulate a set of principles that resonates with a broad consensus, accommodating diverse moral intuitions and beliefs.
Regulatory framework implementation in the new settings offers a great chance to explore what the most recent bioethical laws are (like for the BRICS countries in [ 14 ]). In this regard, the Egyptian Bioethical law from 2020 can be considered as an innovative example for other countries in the process of implementing bioethical regulations and improved bioethical education across the world [ 15 , 16 , 17 ].
As Egypt is considered an LMIC by the World Bank [ 18 ], yet a “high growth pharmaceutical market”, the country has become one of the most attractive locations for pharmaceutical companies to outsource their clinical trials. The country, with over 100 million inhabitants, provides a noteworthy example of implementing bioethical laws in a context with predominantly treatment-naive patients and a robust medical infrastructure encompassing public hospitals and healthcare professional representation.
The aim of the present paper is to analyze and discuss from an ethical perspective the new Egyptian clinical trial law. The Egyptian law is analyzed and discussed in relation to the Ethical Framework for Biomedical Research by Emanuel et al. [ 9 , 10 ], and in comparison to selected other national and supranational laws.
One of the recent countries adopting bioethical law to regulate clinical human subject research is Egypt, which enforced its first law on clinical trials in the official journal on December 23rd, 2020. The issuance of the law, which has long been in the making, was hastened by the COVID-19 pandemic and the urgency to carry out vaccine trials among the Egyptian population [ 19 ]. This regulation is part of a broader effort to enhance the respect for civil/human rights in the country. In 2022, the recent reports from the US Embassy still pointed out some issues [ 20 ], raising concern about fairness and equity in the whole society, and impacting ethical procedures in health and research. However, Egypt -as a United Nations member since 1945- has been participating in the global initiative to enhance human rights application [ 21 ]. Like other Arabic countries (Jordan, Saudi Arabia) registered in the UN Watch Database, it needed to justify their application of human rights [ 22 ]. Efforts are made to improve ethical skills among health care givers in Egypt. For example, EL-Khadry et al. [ 23 ], assessed the effect of educational intervention on knowledge and attitude towards research, research ethics, and biobanks among Egyptian paramedical and administrative teams. Egypt has witnessed exponential growth in medical research like in many developing countries, driven by the pressing need to improve healthcare [ 24 ]. Egypt held the 37th position in terms of publication volume in 2023 [ 25 ]. It is worth noting that in 2020, Egypt had only 838 researchers per million inhabitants, in stark contrast to the USA’s 4,821 researchers per million inhabitants (in 2019) and the United Arab Emirates’ 2,443 researchers per million inhabitants (Researchers in R&D (per million people) [ 26 ] representing the medium position compared to of BRICS countries like South Africa (484 researcher per million habitants) or China (1,585).
National examples: France and Sweden
On the national level, France and Sweden hold a long tradition of ethical regulation. French law influenced the structuring of the Egyptian legal system in 1875. Later, reforms were made to the Egyptian civil law under the guidance of a French legal expert Édouard Lambert in the 1930-the 1940s [ 27 ]. We selected France as a study focus due to its historical ties and influence on Egypt's regulatory framework. Additionally, for comparative analysis with another high-income Western nation, Sweden was chosen for its renowned status as a research leader, distinct from any historical connections with Egypt.
Northern European countries are still considered to be leading countries in research (Sweden is the 3 rd country in terms of research and development expenditures (% of GDP) after Israel and Korea in 2020 – [ 28 ] and have a long tradition of bioethics practices and reflections (e.g., Helsinki’s declaration in 2000 [ 29 ]). Specifically, Sweden is included in the study as an example of a Nordic country with an evidence-based culture of health policymaking [ 30 ] and constant interest for ethical inquiry in under-researched vulnerable populations [ 31 , 32 , 33 ]. In 2004, Sweden enforced “The Act concerning the Ethical Review of Research Involving Humans” (SFS nr: 2003:460) that sharpened ethical review procedures for biomedical research way earlier than other countries (e.g. Loi Jardé in France from 2012, and the Egyptian law 2020), introducing a reference that showcased innovative law in the early 2004 that remains in effect. In 2020, France had 4926 researchers per million inhabitants, quite comparable to the USA’s 4,821 researchers per million inhabitants (in 2019) and representing a European example of a “medium” score of researchers per million inhabitants. In comparison, Sweden counts for 7,930 researcher per a million inhabitant, Norway 6,699, Finland 7,527 or Denmark with 7,692 (Researchers in R&D (per million people) [ 34 ]. At the international level, France ranked at the 6 th position for publication volume, while Sweden ranked the 18 th [ 35 ].
Supra-national entity: the EU regulations to consider when considering France and Sweden
Supra-national European regulations play an important role in the legal system of EU countries even if such a supra-national level does not exist in Egypt. At the EU level, the EU Regulation on clinical trials on medicinal products for human use (CTR) governs the ethical review of clinical trials, however detailed aspects of ethics committees and ethical review depend on further regulation at Member State level. This means that even though the EU regulates ethical review of clinical trials in the CTR, there could be considerable divergences across Europe in how the committees are set up and perform their tasks. To begin with, the CTR requires that a clinical trial be subject to ethical review (Article 4), and it outlines several relevant aspects of the process of carrying out that review. However, modalities regarding ethical committee and its work are a question of the Member States’ regulation. Generally, the application for authorisation to conduct a clinical trial is divided into two parts. Part I focuses on the technical-scientific dimension, and part II on the ethical aspects which are reviewed by each member state concerned. An ethics committee, within the meaning of the CTR, is an independent body established in a Member State in accordance with the law of that Member State Footnote 4 . Under the national law, this body needs to be empowered to give opinions for the purposes of the CTR, considering the views of laypersons, in particular patients or patients’ organizations (Art. 2(2)(11)). The CTR prescribes in Recital 18 merely a guiding requirement that the member state needs to ensure that “the necessary expertise is available”. Member States should have a mechanism in place to ensure the involvement of laypersons, in particular patients or patients’ organisations. However, the effect of this involvement that the CTR requires is that their views are taken into account in the review (Art. 2(2)(11)). It is not uncommon that several ethics committees exist in a member state. How the involvement of an ethics committee is organized for the purposes of the tasks specified in the CTR is a question for the Member States to decide (recital 18). However, the process needs to be organized so that the relevant timelines of the clinical trials approvals set out in the CTR are met (Art. 4).
Design and data analysis
We examine the Egyptian law vis-à-vis France’s and Sweden’s framework, considering the obligations that stem in regards to clinical trials from the CTR. Furthermore, we examine these regulations in light of the Ethical Framework for Biomedical Research by Emanuel et al. [ 9 , 10 ]. Indeed, we will consider the EU implication into France and Sweden’s regulations.
A directed approach to qualitative content analysis was adopted using the seven principles informing the Ethical Framework for Biomedical Research as predetermined themes [ 36 ]. Two independent coders examined each selected regulation in their original version for French (MA, SM), Swedish (MA, AM), EU (MA, AM), and in an English translation for the Egyptian law (AM, SM). The coders discussed the results critically in debriefing sessions and their coding was discussed until consensus with a legal expert working with ethical regulations at the international level (SS) contributing the clarification of EU and both Swedish and French framework.
Theoretical framework
The seven principles that will serve as comparison criteria for our analysis are the following: “ (1) (Social) value - enhancements of health or knowledge must be derived from the research; (2) scientific validity- the research must be methodologically rigorous; (3) fair subject selection - scientific objectives, not vulnerability or privilege, and the potential for and distribution of risks and benefits, should determine communities selected as study sites and the inclusion criteria for individual subjects; (4) favorable risk-benefit ratio-within the context of standard clinical practice and the research protocol, risks must be minimized, potential benefits enhanced, and the potential benefits to individuals and knowledge gained for society must outweigh the risks; (5) independent review - unaffiliated individuals must review the research and approve, amend, or terminate it; (6) informed consent - individuals should be informed about the research and provide their voluntary consent; and (7) respect for enrolled subjects -subjects should have their privacy protected, the opportunity to withdraw, and their well-being monitored. ” (Emanuel et al., 2000, p2701 [ 9 ]).
For a thorough assessment, we exclusively examined the primary text of each law, excluding connections to other regulations (e.g., the "Code de la Santé" and "Code Penal" for French regulation or to “Law No. 151 of 2019, the Egyptian Medicines Authority” for the Egyptian text). Our analysis utilized the latest version of the law, including any amendments. These are:
Egypt’s law – no amendments December 23 rd , 2020, Law No. 214 of 2020 Regulating Clinical Medical Research.
French law : the “Loi Jardé” (LOI n° 2012-300 du 5 mars 2012 relative aux recherches impliquant la personne humaine) amended with the “Décret n° 2016-1537 du 16 novembre 2016 ”. We will consider the latest 2022 amendment for reference in our analysis.
Swedish law : Lag (2003:460) om etikprövning av forskning som avser människor with the following amendments: 2018:147, 2018:1092, 2019:1144, 2021:611, 2022:48.
Regulation (EU) No 536/2014 of the European Parliament and of the Council of 16 April 2014 on clinical trials on medicinal products for human use, and repealing Directive 2001/20/EC Text with EEA relevance OJ L 158, 27.5.2014, p. 1–76.
These laws have been identified for being the main relevant legal texts for biomedical research regulations and more specifically research involving human subjects. The selection of national and international regulations to assess was based on their presence within international pharmacological and biomedical research industry. Moreover, the EU regulation will be considered as an adjunct line of analysis to complement Swedish and French regulation examination as both countries are part of EU.
The full overview of coding procedure for all regulation is available as supplementary material (see Tables 1 , 2 and Appendix 1 ). A summary of this assessment is presented in Table 1 with a score system showing the compliance or absence of compliance to each principle. A score of 0 means there is little to no compliance with the criteria, while an X indicates satisfactory or complete compliance with the criteria.
- Social value
Egyptian law does not overtly address the social value of the research proposal. However this may be implied as the national Research Ethics Committee (REC Footnote 5 ) (Supreme Council) will take into account “national interest” when evaluating research protocols (Chapter (ch) 3, article (art) 7(2)).
Similarly, the Ethical Review Act, has no clauses that are focused on assessing the social value of research. However, the notion of research serving a social interest is implicitly demonstrated by the composition of the departmental REC, where five members out of 15 represent society’s interests (Section 25). In Section 8, it is indeed stated that the welfare of research participants must be prioritized over the needs of society.
France also lacks a clear statement on the social value. The law relates to “social” level as it often refers to the “Code de la santé” (CS) and to the “social security” system, but no clear points about social values per se. Social and scientific value is stated in the “Research organized and carried out on human beings to develop biological or medical knowledge shall be authorized“ (Art. L1121-1 CS).
Under the CTR, social value – enhancement of health – is the whole purpose, even if not expressis verbis stated, Art. 3 and Art. 6 ensure that as a general principle, (a) the rights, safety, dignity, and well-being of subjects are protected and prevail over all other interests; and (b) it is designed to generate reliable and robust data for example. Moreover, member state and Union inspections are envisaged (taking compliance with the EU regulation as a token of good research for society and for science). See Art. 78 and 79.
Scientific validity
Regarding Scientific validity, Egyptian law set up the responsibility of REC to ensure ethical quality (Art. 1, Art. 2, Art. 24) of the accepted protocols, but also set up standards for scientific quality (Art. 7; 2; Ch. 2 Art. 10) making sure that principal investigators have the required scientific competences (Ch. 5 Art. 22; Ch. 3 Art. 6 provided a detailed list of required competences §2, Ch. 4 Art. 9.).
The Swedish law emphasizes the importance of sound research. In Section 11, it is stated that research may only be approved if it is carried out by/under the supervision of a researcher with the necessary scientific competence. In Section 9, where the scientific value of the proposed research is weighed against, and if proportionate justifies, the risks to the health, safety, and personal integrity of research participants.
Article L1121_2 expresses the need for social and scientific validity. Art. L 1121-3 refers to “qualified personnel” for scientific validity and after the approval has been given by a REC. RECs have a regional organization and can be involved together with Commission nationale de l'informatique et des libertés (CNIL) for data security issues, and Committee of Experts for Research Study and Evaluation in Health domain. Any research is also regulated by EU rules (Article L1121-1 CS). Specific regulations for certain disciplines are stated in the CS (L1121-3) but not in the Loi Jardé.
At the EU level, Art. 4 requires prior authorization. In particular, a clinical trial shall be subject to scientific and ethical review and shall be authorized in accordance with the rules set out in the CTR. Under Article 6(1)(b)(i) the reliability and robustness of the data generated in the clinical trial, taking account of statistical approaches, design of the clinical trial and methodology, including sample size and randomization, comparator, and endpoints.
Fair selection of study population
The Egyptian law ensures the impartial selection of an appropriate number of research participants. There are specific recommendations for REC in regards to recruitment of specific sub-groups or vulnerable population. For example, prohibit research participants to enroll in simultaneous medical research and prohibit induced participation (Ch. 5 Art. 13; 14).
There are no definite clauses in the considered law that emphasize the requirement for fair selection of the study population. Nonetheless, protection of minors and individuals who cannot consent to research participation is described in Sections 18, 20, 21, and 22 (see Informed consent section).
French regulation requires that study participants be beneficiaries of the Social Security system (and if not, they will be considered as if they are). Fair selection of the participants is ensured by the CS (Articles L. 1121-5 to L. 1121-8). The main categories with stated protection are adults in coma, with dementia or for psychiatric conditions, or enfeebled patient, people deprived of their freedom, foreigners, minors, pregnant and nursing women. Moreover, situations such as “urgency” that may override any consent needed.
Art. 10 offers “specific considerations for vulnerable populations”, in particular, minors (see Art. 32), incapacitated subjects (see more Art. 31, 28, 29), pregnant or breastfeeding women (see Art. 33), the participation of specific groups or subgroups of subjects, where appropriate, specific consideration shall be given to the assessment of the application for authorization of that clinical trial on the basis of expertise in the population represented by the subjects concerned. Art. 34 also covers national measures for participants performing mandatory military service, persons deprived of liberty, persons who, due to a judicial decision, cannot take part in clinical trials, or persons in residential care institutions.
Favorable risk-benefit ratio
Clear specifications of the Principal investigator requires all the consideration about risk-benefit ratio (both at the physical and psychological level), ensuring dignity and health, adding a note for specific attention to reducing side effects (Art. 18; 6). Another layer of risk reduction is the provision to evaluate preclinical medical research (Ch. 5 Art. 10), the provision of health insurance coverage of any research participant (Ch. 7 Art. 18 §9), and ensuring that the research organization will be able to attend properly to research participants’ health needs in case adverse effects or health risks ensuing from the clinical trial (Ch. 11).
According to the Swedish legislation, the necessary condition for approving research is that fundamental personal freedoms, and human rights are respected. While Section 9 states that research may be approved if its scientific value outweighs the risks to research participants, Section 8 specifies that their welfare must be prioritized over the needs of society and science. Section 10 states that research should be conducted only if its expected result cannot be achieved in another way that involves less risk to the health, safety, and personal integrity of research participants.
Favorable benefit-risk ratio was refined with the inclusion of “new facts” issues that appeared with Loi Jardé. The most important point to emphasize is that the sponsor will be responsible for the care and necessary costs ensued from severe side effects, if they occur. These include both biomedical research (R1) as well as interventional minimal risk research (R2).
Art. 6 ensures that risks (minimization, safety measures) and inconveniences for the subject are considered and reduced for medicinal products and interventions compared to normal clinical practice. Suspected unexpected serious adverse reactions and annual reporting are strictly regulated at the EU level. Under the CTR, the committees are informed regarding suspected unexpected serious adverse reactions that are reported pursuant to the CTR as well as the annual report submitted to the European Medical Agency (Art. 44.3).
Independent review
Independent review will be implemented by a REC (Art. 1, 24), and it will protect the rights of participants, review the research protocol, decide on approval, amendments or renewal of the research, and lastly monitor the research (All this is in accordance to the executive regulations of law art 8). The specifics of the review process are detailed in several articles (Ch. 2 Art. 4: REC; Ch. 3 Art 1 to 4).
According to Section 6, independent review is mandatory whenever the research involves a physical intervention or involves affecting or risk harming the research participant physically or psychologically. Independent review is also required in the case of studies involving biological material taken from a living person and can be traced to that person. The same article emphasizes the principal investigator’s responsibility, who must take measures to prevent research from being carried out in violation of the law. Section 25 sets out the organization of the authority providing an independent review, i.e., the Ethics Review Authority. This is divided into operational regions, each composed of one or more departments according to their areas of expertise. Departments consist of a chairman, who is or has been an ordinary judge, and fifteen other members, of whom ten have scientific competence and five represent public interests, including at least one member who represents one or more patient organizations. The government appoints the chairman and its deputy, while the Ethics Review Authority chooses the other members and their deputies.
The independent review component is well established with the composition of the Committee for the Protection of Persons and the presence of 39 RECs across the 7 inter-regions committees. The repartition of REC into 2 colleges, one more scientific and the second more patient-related, support independent review, but the designation and recruitment of different REC members (depending on the national or local level, for example) is not clear. For the local levels (Art L1123-1), the text state that the Health Minister CPP for a fixed or undetermined duration and according to the needs. Their members are appointed by the Director General of the regional health agency in which the committee has its headquarters. The committees are completely independent in the performance of their duties. They have legal entity under public law. Committee resources are provided by the State. However, ethical approval can be obtained via institutional committees (in house at some hospitals and universities). Member of the National commission for research involving human need to declare their conflicts of interest (Art. L1123-1-1) which is not clarified for CPP (promotors of the same institution are – per definition- applying to their “in house” ethical committee).
Art. 4 refers to the need for prior authorization in accordance with the law of the Member State concerned Footnote 6 . The review by the ethics committee may encompass aspects addressed in Part I of the assessment report for the authorization of a clinical trial as referred to in Article 6 and in Part II of that assessment report as referred to in Article 7 as appropriate for each Member State concerned. Article 9 should ensure that the persons validating and assessing the application do not have conflicts of interest, are independent of the sponsor, of the clinical trial site and the investigators involved and of persons financing the clinical trial, as well as free of any other undue influence. A special mention explains that at least one layperson shall participate in the assessment.
Informed consent (IC)
Among the very first articles (Art. 1; 21), Egypt’s law provides a definition of IC, promoting its engagement into this ethical procedure “the written expression based on complete voluntary freewill of the person with full legal capacity, and it includes his explicit consent as a signature and a fingerprint to participate in clinical medical research, after all aspects of the research are explained to him, and in particular the potential effects or harms that may impact his/her decision to participate[…]”. The exception of obtaining IC is detailed in executive regulations (Ch. 5, Art. 12; 3). More specific consideration is also represented in other sections of the law: in Ch. 7 Art. 17 § 2: obtaining IC is mandatory. In Ch. 10, Art. 23 §2, IC is required for data usage and for further research. Furthermore it provides specification for consent of data usage.
Section 17 states that, in line of principle, research can only be performed if the research participant has voluntarily and explicitly consented in a documented way after receiving adequate and specific information. Section 16 describes what the fundamental pieces of information are. In cases where a research participant is in a dependent relationship with components of the research team or if the research participant has difficulties asserting their right, Section 14 states that issues of information and consent must be given special attention. Specific recommendations are provided in the case of minors or if the research participants turned 15 years (Section. 18). Sections 20, 21, and 22 list under what circumstances research can be performed without consent (illness, mental disorder, a weakened state of health, or any other similar condition of the research participant prevents their consent from being obtained).
IC regulations state that “Consent is free, informed and (voluntary) emphasizing the importance of individuals providing explicit agreement in various legal situations. It must be written for category 1 studies and may be oral for category 2 studies (but must be recorded in the medical file). For category 3 research and for studies on data collected in the course of normal care, the rule is that the patient “must not object.” No research mentioned in 1° of Art. L. 1121-1 may be carried out on a person without his or her free IC, given in writing after the person has been provided with the relevant information. Where it is impossible for the person concerned to express his or her consent in writing, it may be attested by the trusted support person provided for in Art. L. 1111-6, by a family member, or, failing this, by one of the person's close relations, provided that this trusted person, family member or close relation is independent of the investigator and the sponsor. Specific recommendations are provided for minors (under 18 solely). Article 4 is also providing details about the case where the participant cannot express consent and is not under guardianship. There are also options for “collective consent,” but they are only available for interventional research with minimal risk (epidemiologic search).
Art. 7 mentions the need for compliance with the requirements for IC as set out in Art. 29, explicating the regulations about written IC. A specific regulation has also been dedicated in Art. 30 for cluster trials. This specification states that “Where a clinical trial is to be conducted exclusively in one Member State, that Member State may, without prejudice to Art. 35, and by way of derogation from points (b), (c), and (g) of Art. 28(1), Art. 29(1), point (c) of Art. 29(2), 29(3), (4) and (5), points (a), (b) and (c) of Art. 31(1) and points (a), (b) and (c) of Art. 32(1), allow the investigator to obtain IC by the simplified means set out in paragraph 2 of this Article, provided that all of the conditions set out in paragraph 3 of this Article are fulfilled.”
Respect for participants
Egyptian law provides protection of privacy and data (Art. 12; 2), adequate information of researcher participants (Art,.15; 2. Ch. 5; Art 18:5), protection from publicity (Art. 15;3) together with a straightforward explanation of requirements to respect withdrawal of consent (Art. 2, 1) and compensation aspects (Art. 20:9, 10). The details of non-induced participation (for money or reward) could also be understood as a measure of respect for recruited participants (Art. 14).
Section 1 states that its purpose is to protect the individual and respect human dignity in research. This is reaffirmed under Section 7. Noteworthy, according to Section 40, some exceptions can be made with regards to requiring consent or processing of data if this is requested by the government or another authority. This is only possible if it is clear that the research does not entail any appreciable risk to an individual's health or safety or pose an infringement on an individual's integrity.
The respect for study participants was unclear in the text and focuses more on fair selection and risk protection of research participants. One specific element regarding the participant protection of “a deceased person, in a state of brain death, without his or her consent expressed during his or her lifetime or through the testimony of his or her family” (Art. L1125-13).
Article 28 prescribes general rules that must be met for a clinical trial to be lawfully conducted. This article clarifies that benefit to the participants, IC, right to mental and physical integrity, minimal pain or risk, guaranteed medical care, and no undue influence (including financial) are the basis for any medical research. EU regulation Article 28 of Regulation (EU) No 536/2014 of the European Parliament and of the Council, Article 2 make sure that withdrawal is free of constraints and will not have repercussions nor affect the participants' rights and care, but also makes sure that the withdrawal of consent does not affect the data collected prior to withdrawal.
Our results show that the Egyptian law fulfills the ethical requirements for human subject research and is comparable to the French, Swedish and EU regulations.
Detailing the results, we also observed that all regulations tended to have a very vague approach to “social values and scientific values” (principle 1). In terms of the fair selection of participants, the Swedish text was probably the vaguest (principle 3), but in general, this principle appeared to be well integrated. All other principles also were well represented in Egyptian law as in the French, Swedish, and EU’s laws (principles 4, 5, 6, 7). We explore in two separate points the results :1) Value and validity (in which principles 1, 2 and 5, with 5 as the way/procedure to reach value and validity), 2) Participant’s protection (principles 3, 4, 6, and 7).
Values and scientific validity
Principle 1, social values and scientific value.
Egyptian regulation suffers from the same issues in clarifying the social value aspect as French, Swedish, and EU regulation. The results from the assessment of social values reveal that most of the regulations struggle to clearly define under what specifications research should serve a social purpose. Social value is often envisioned at the level of cost-effectiveness measurements, and its definition may be difficult to normalize across states and cultures as it refers to “the general concept and practice of measuring social impacts, outcomes, and outputs through the lens of cost” [ 37 ]. In Emanuel et al’s vision, it is composed of the a) ensured benefit b) value for the prospective beneficiaries, c) dissemination of the results via long-term collaborative strategy d) avoiding to undermine the community’s existing healthcare [ 38 ]. Furthermore, these results question the importance of social value in research per se, for example, in specific areas where the social value aspect cannot be considered as an overarching guide. Recent debates questioned, for example, the justice and egalitarian arguments that can arise from questioning the social value of research [ 39 , 40 ] depending on how innovative and impactful on a societal level the research is.
Principle 2, Scientific validity
Egyptian law, French, Swedish, and EU regulation, tries to specify the scientific validity mostly via their REC members selection. The general implementation of a control mechanism and ethical review board’s competences question the real level of expertise or education that these members do have in order to review research protocols or scientific methods. For example, there is not a clear consensus about the need for specific competencies in order to have balanced and non-biased decisions in ethical vetting because [ 41 ] as different ethical reviewers will raise different concerns. In their results, they confirmed that the main influencing factor in readers-queries was the profession, with scientific validity issues being more frequently asked by scientific reviewers, whereas ethical issues were more frequently pointed out by ethicists.
Depending on the system and general community functioning, the selection of research ethics committees members can put into question the non-biased nature of assessment of scientific validity. For instance, in France, the members of the Nation Ethical Committee, who are responsible for offering direction to all REC, are "selected" or "designated" by the President of France. The same question could also be raised in Egypt were Central intelligence members are sitting at the National REC (the Supreme Council). The impact of politics and social politics in presumably non biased procedure is also rising with the use of preference studies to inform policy making and including patient’s advocacy in decisions boards as it can have a role in decision makings [ 42 ] providing advances in shared decision making but also leveraging non-biased decisions making as there is still not a unified definition of such processes [ 43 ] and for example, research shows that methodological standards are often downgraded to provide access to the co-researchers [ 44 ].
As it is sometimes defined in science in general, validity should be assessed in an adequate manner across medical field. Scientists refer to scientific quality measures (like in systematic literature review assessements scales for scientific quality) but validity interpretation can be difficult to apprenhend for an heterogeneous group of experts (like an REC). In its very classical definition “The validity of a research study refers to how well the results among the study participants represent true findings among similar individuals outside the study. This concept of validity applies to all types of clinical studies, including those about prevalence, associations, interventions, and diagnosis”, scientific validity could look easy to apprehend but even then, just having the precision “The validity of a research study includes two domains: internal and external validity” explain the layer of complexity that may not be represented in Emanuel’s principles definition [ 45 ]. Going further into the validity explanation and use in ethical consideration in biomedical research should be warranted. For example, some research look at different levels of validity to clarify what one considers as validity (scientific validity may be too vague to refer to permit clear assessment): congruence validity, criterion validities, etc [ 46 ]). Wages et al. in 2021 [ 47 ], showed the potential for using operating characteristics to inform design’s safety and accuracy in phase I clinical trials that could open the debate around a better definition of scientific validity checks in biomedical research. One part of the issue comes from scientific communities but the scientific validity should be also a matter of concerns for all REC members. The competencies of any participants should be addressed in the REC reviewer’s selection as some questions also arise from the medical field where shared decision-making has been implemented before and some debate remains about the representativeness of patients that do get involved in the medical decision-making [ 48 , 49 ].
Principle 5, Independent review
All ethical regulations, including Egypt’s, ensure that the review system is independent and thus RECs have the power to authorize, follow up and end any research to protect participants [ 29 ]. One of the major concerns still not addressed in the regulations is the difficulty to guarantee REC effectiveness in regards to some deficiencies in REC theory and structure [ 50 ]. The opportunities to enhance EC efficiency and effectiveness could also depend more on the researcher and the scientific community as Hickey et al., 2022 suggested [ 51 ]. Clarifying the collaborative approach across ethics committees and research can be the path to increased medical research efficiency.
Participant’s protection
Principle 3, fair selection.
In the Egyptian regulation, the fair selection of participants is pursued, which can be considered a positive development with respect to the previous regulation proposal, where protection of the rights and welfare of vulnerable subjects were not adequately considered [ 52 ]. Generally, one can wonder about the impact of fairness when recruiting for clinical trials. Ongoing discussions emphasize that the fair selection of participants could be a very ethically challenging issue as it is a ground for dilemmas [ 53 ], including the levels of “(1) fair inclusion; (2) fair burden sharing; (3) fair opportunity; and (4) fair distribution of third-party risks”. The equal opportunity issue also arose for example in 2022 when French law integrated EU requirements and shifted toward allowing research participants with no access to social security to be part of research [ 54 ], offering extended opportunities for participation but putting the question of fairness of selection into question.
Principle 4, Favorable risk-benefit ratio
Egyptian regulation, like the four other comparatives, consider “Risk-benefit” as a sine qua none principle and the clarity of Helsinki’s declaration [ 55 ]. However, no regulation mentioned the potential for under-reporting harms depending on what one considers to be “harm”. One of the aspects that are often under-considered is psychological harm, as even clinical trials in the field tend not to report psychological harm (compared to physical adverse effects of drugs in clinical trials) as can be noted in research by [ 56 , 57 ].
Principle 6, Informed consent
Informed consent is implemented overall, and as for principle 5, Egypt complies, like all comparatives to this standard practice. Even if a variety of informed consent exists, the law sticks to written consent without specifying the potential for renewed consent, broad consent, or other approaches [ 58 ] and the “blanket consent” potential.
Principle 7, Respect for recruited participants and study communities
Respect for participants reflects respecting autonomy across health care and research systems that appear to be consistent across the 4 regulations. Egyptian law places an equivalent emphasis on this principle as French law and is comparable to Swedish and EU regulation. All these regulatory frameworks effectively incorporate this principle into their bioethical laws. Heightened attention still needs to be paid to respect at different levels, such as for gender issues [ 59 ], or/and ethnicity [ 60 , 61 ].
Limitations
Our study contains limitations. The first one is that we looked for expressis verbis statements that limit the apprehension of the full corpus of laws application in a specific context. Indeed, some implicit references could counterbalance our conclusions. For instance, the CTR underscores the overarching objective of promoting the social value by enhancing health, even if not explicitly stated. Nevertheless, at the clinical level, practitioners may not have comprehensive access to all regulations and are likely to rely on referenced texts in the ethical application specific to their country. The lack of clarity (or complex implicit references) may hinder comprehension and result in a complex implementation process. Utilizing the principles proposed by Emanuel et al. may also be presented as a limitation as this analytical foundation may not comprehensively encapsulate the nuances inherent in the examined legal frameworks. Another limitation could be posited in the fact that the analysis may not fully reflect the influence of cultural and social variations among the three countries. Further research would need to also assess the overall structure of ethical procedure in each country and their organization (from Supreme councils, regional entities, national unified procedures, etc).
In conclusion, the Egyptian law in comparison to French, Swedish, and the connected EU regulations reveals its alignment with Emanuel et al.’s principles. However, several common challenges and areas of improvement can be sought with regards to each of the ethical principles and thus open the way for further research. The main topic identified via our analysis is the need to clarify and standardize the concept of social value of research, which often focuses on cost-effectiveness measurements and implicitly -not always directly- refers to a very difficult concept to apply [ 62 ]. Our second main discussion point highlights concern about the expertise and unbiased decision-making of ethical review boards. Further research is warranted to explore in more detail’s other principles. Overall, these findings highlight the need for continuous improvement and refinement of ethical regulations to ensure the protection of participants and the integrity of research in Egypt and other jurisdictions.
Based on the discussion, the following recommendations can be made for improving research ethics regulations in the countries in our analysis:
Clarify and Standardize Social Value: Develop clear guidelines and standards to define and measure the social value of research across different states and cultures. This should include a detailed framework for assessing research's contribution to societal benefits, cost-effectiveness, and its alignment with the long-term healthcare goals of the community.
Enhance Scientific Validity: Strengthen the criteria for the selection of Research Ethics Committee (REC) members to ensure they possess the necessary expertise and education to review research protocols and scientific methods effectively. This includes establishing more rigorous competency requirements and providing ongoing training to ensure balanced and non-biased decision-making in ethical approvals.
Improve Participant Protection: Emphasize the fair selection of participants by addressing ethical challenges and ensuring equitable opportunities for participation. This involves revising existing regulations to better protect the rights and welfare of vulnerable subjects and to promote fairness in participant selection.
Increase REC Effectiveness: Address deficiencies in REC theory and structure to enhance the effectiveness of research ethics committees. This could involve adopting more collaborative approaches between review boards and researchers, and ensuring that ethics committees have the authority, independence, competences and resources needed to oversee research effectively.
Promote Respect for Participants: Ensure that all research activities respect the autonomy and dignity of participants. This entails paying heightened attention to issues of gender, ethnicity, and other factors that may affect participants' experiences in research settings.
Further Research: Encourage further research into the nuances of ethical principles beyond those identified by Emanuel et al., to better understand the cultural and social variations that may affect the implementation of ethical guidelines in different jurisdictions and cultural contexts.
Availability of data and materials
All data generated or analyzed during this study are included in this published article [and its supplementary information files].
US Department of Health and Human Services. (1979). The Belmont Report: Office of the Secretary, Ethical Principles and Guidelines for the Protection of Human Subjects of Research, the National Commission for the Protection of Human Subjects of Biomedical and Behavioral Research.
« World Medical Association Declaration of Helsinki: Ethical Principles for Medical Research Involving Human Subjects », JAMA , vol. 310, n o 20, 27 novembre 2013, p. 2191–2194
Department of Health. (2015). Ethics in health research: Principles, processes and structures.
The composition of the ethics committees remains to be decided by a member state.
In France, the REC acronym coul refer more to the High National Ethical Committee, which issues recommendations on a societal scale. It is important to note that this committee is distinct from the day-to-day oversight of research ethical applications or clinical trials. The latter responsibility primarily falls within the purview of internal ethical committees situated within hospitals or universities, which have the authority to grant approvals. Externally, the (Comité de Protection des Personnes or CPP) serves as the most pertinent entity, akin to a Research Ethical Committee (REC); hence, we have opted to utilize the acronym REC for clarity.
The effect of the decision is, nonetheless, strong. Where an ethics committee has issued a negative opinion, on the condition that rules that are valid for the entire Member State apply, that Member State has a duty to refuse to authorise a clinical trial (Article 8.4, for extended authorisations see Article 14.10, for substantial modifications for the assessment report, see Articles 19, 20 and 23).
Abbreviations
Code de la santé / Health code
Research Ethics Committee
Clinical trial regulations
Low to medium income country
Commission nationale de l'informatique et des libertés / national commission for computer science and freedoms
French specific will stand for
Comité de Protection des personnes / person’s protection committee
Informed consent
Dooly M, Moore E, Vallejo C. Research ethics. Research-publishing net. 2017.
Google Scholar
Miracle VA. The Belmont Report: the triple crown of research ethics. Dimens Crit Care Nurs. 2016;35(4):223–8.
Article Google Scholar
Halonen JI, Erhola M, Furman E, Haahtela T, Jousilahti P, Barouki R, et al. The helsinki declaration 2020: Europe that protects. Lancet Planetary Health. 2020;4(11):e503–5.
Johansen MV, Aagaard-Hansen J, Riis P. Benefit–a neglected aspect of health research ethics. Dan Med Bull. 2008;55(4):216–8.
Artal R, Rubenfeld S. Ethical issues in research. Best Pract Re Clin Obstet Gynaecol. 2017;43:107–14.
Paul H. The scientific self: reclaiming its place in the history of research ethics. Sci Eng Ethics. 2018;24(5):1379–92.
Thaldar D, Shozi B, Kamwendo T. Culture and context: Why the global discourse on heritable genome editing should be broadened from the South African perspective. BioLaw Journal-Rivista Di BioDiritto. 2021;4:409–16.
World Medical Association Declaration of Helsinki: ethical principles for medical research involving human subjects. Jama. 2013;310(20):2191-4.
Emanuel EJ, Wendler D, Grady C. What makes clinical research ethical? Jama. 2000;283(20):2701–11.
Emanuel EJ, Wendler D, Grady C. An ethical framework for biomedical research. The Oxford textbook of clinical research ethics. 2008. p. 123–35.
Book Google Scholar
Rid A, Emanuel EJ. Ethical considerations of experimental interventions in the Ebola outbreak. Lancet. 2014;384(9957):1896–9.
Tsoka-Gwegweni JM, Wassenaar DR, Using the Emanuel, et al. framework to assess ethical issues raised by a biomedical research ethics committee in South Africa. J Empir Res Hum Res Ethics. 2014;9(5):36–45.
Mutenherwa F, Wassenaar DR, de Oliveira T. Ethical issues associated with HIV phylogenetics in HIV transmission dynamics research: a review of the literature using the Emanuel Framework. Dev World Bioeth. 2019;19(1):25–35.
Mantzaris E. Regulatory frameworks as a tool for ethical governance: drawing comparisons amongst the BRICS (Brazil, Russia, India, China and South Africa) countries. Afr J Public Affairs. 2017;9(8):91–104.
Sim JH, Ngan OMY, Ng HK. Bioethics education in the medical programme among Malaysian medical schools: where are we now? J Med Educ Curric Dev. 2019;6:2382120519883887.
de Lemos Tavares ACAL, Travassos AGA, Rego F, Nunes R. Bioethics curriculum in medical schools in Portuguese-speaking countries. BMC Med Educ. 2022;22(1):199.
Mukhamedzhanovna MZ, Akmalovna U, Nugmanovna M. The Uzbek Model of Bioethics: History and Modernity. Malim: jurnal pengajian umum asia tenggara (SEA Journal of General Studies). 2020;21.
Bank W. 2023a [Available from: https://datahelpdesk.worldbank.org/knowledgebase/articles/906519 .
Marzouk D, Sharawy I, Nakhla I, El Hodhod M, Gadallah H, El-Shalakany A, et al. Challenges during review of COVID-19 research proposals: experience of faculty of medicine, Ain Shams university research ethics committee, Egypt. Front Med. 2021;8:715796.
State USDo. 2022 [Available from: https://www.state.gov/reports/2022-country-reports-on-human-rights-practices/ .
Migiro. [Available from: https://www.worldatlas.com/articles/when-did-egypt-gain-itsindependence.html .
H S. United Nations Human Rights Council and Israel: Comparative Analysis with Egypt, Jordan, and Saudi Arabia (Doctoral dissertation, University Honors College Middle Tennessee State University). 2021.
EL-Khadry SW, Abdallah AR, Yousef MF, M. abdeldayem H, Ezzat S, Dorgham LS. Effect of educational intervention on knowledge and attitude towards research, research ethics, and biobanks among paramedical and administrative teams in the National Liver Institute, Egypt. Egyptian Liver Journal. 2020;10:1-8.
Normile D. The promise and pitfalls of clinical trials overseas. Science. 2008;322(5899):214–6.
SJR [Available from: https://www.scimagojr.com/countryrank.php .
Bank W. 2023b [Available from: https://data.worldbank.org/indicator/SP.POP.SCIE.RD.P6?end=2017&locations=EG&start=2007 .
Chaaban Y. Comparative law as a critical tool for legal research in Arab countries: a comparative study on contractual balance. Akkad J Law Public Policy. 2021;1(3):123–34.
Bank W. 2023c [Available from: https://data.worldbank.org/indicator/GB.XPD.RSDV.GD.ZS?locations=SE&most_recent_value_desc=true .
Guraya SY, London N, Guraya SS. Ethics in medical research. J Microscopy Ultrastructure. 2014;2(3):121–6.
Hansson MG, Dillner J, Bartram CR, Carlson JA, Helgesson G. Should donors be allowed to give broad consent to future biobank research? Lancet Oncol. 2006;7(3):266–9.
Norberg Wieslander K, Höglund AT, Frygner-Holm S, Godskesen T. Research ethics committee members’ perspectives on paediatric research: a qualitative interview study. Res Ethics. 2023;19(4):494–518.
Gallagher B, Berman AH, Bieganski J, Jones AD, Foca L, Raikes B, et al. National human research ethics: a preliminary comparative case study of Germany, Great Britain, Romania, and Sweden. Ethics Behavior. 2016;26(7):586–606.
Harcourt D, Quennerstedt A. Ethical guardrails when children participate in research: risk and practice in Sweden and Australia. Sage Open. 2014;4(3):2158244014543782.
Bank W. 2023d [Available from: https://data.worldbank.org/indicator/SP.POP.SCIE.RD.P6?end=2017&locations=EG&start=2007 .
Scimago Journal and Country Rank [Available from: https://www.scimagojr.com/countryrank.php .
Hsieh HF, Shannon SE. Three approaches to qualitative content analysis. Qual Health Res. 2005;15(9):1277-88.
Tuan MT. Measuring and/or estimating social value creation: Insights into eight integrated cost approaches: Bill & Melinda Gates Foundation Seattle, WA; 2008.
Emanuel EJ, Wendler D, Killen J, Grady C. What makes clinical research in developing countries ethical? The benchmarks of ethical research. J Infect Dis. 2004;189(5):930–7. https://doi.org/10.1086/381709 .
Juth N. For the sake of justice: should we prioritize rare diseases? Health Care Analysis. 2017;25:1–20.
Juth N, Henriksson M, Gustavsson E, Sandman L. Should we accept a higher cost per health improvement for orphan drugs? A review and analysis of egalitarian arguments. Bioethics. 2021;35(4):307–14.
Haaser T, Bouteloup V, Berdaï D, Saux M-C. The multidimensional nature of research ethics: letters issued by a French research ethics committee included similar proportions of ethical and scientific queries. J Empirical Res Hum Res Ethics. 2022;17(3):242–53.
Groot BC, Vink M, Haveman A, Huberts M, Schout G, Abma TA. Ethics of care in participatory health research: mutual responsibility in collaboration with co-researchers. Educ Action Res. 2019;27(2):286–302.
Bomhof-Roordink H, Gärtner FR, Stiggelbout AM, Pieterse AH. Key components of shared decision making models: a systematic review. BMJ Open. 2019;9(12):e031763.
Malterud K, Elvbakken KT. Patients participating as co-researchers in health research: a systematic review of outcomes and experiences. Scandinavian J Public Health. 2020;48(6):617–28.
Patino CM, Ferreira JC. Internal and external validity: can you apply research study results to your patients? Jornal brasileiro de pneumologia. 2018;44:183.
Larsen KR, Lukyanenko R, Mueller RM, Storey VC, VanderMeer D, Parsons J, et al., editors. Validity in design science research. Designing for Digital Transformation Co-Creating Services with Citizens and Industry: 15th International Conference on Design Science Research in Information Systems and Technology, DESRIST 2020, Kristiansand, Norway, December 2–4, 2020, Proceedings 15; 2020: Springer.
Wages NA, Horton BJ, Conaway MR, Petroni GR. Operating characteristics are needed to properly evaluate the scientific validity of phase I protocols. Contemp Clin Trials. 2021;108:106517.
Arora NK, McHorney CA. Patient preferences for medical decision making: who really wants to participate? Medical Care. 2000:335-41.
Lindsay SE, Alokozai A, Eppler SL, Fox P, Curtin C, Gardner M, et al. Patient preferences for shared decision making: not all decisions should be shared. J Am Acad Orthopaedic Surgeons. 2020;28(10):419.
Whitney SN. Institutional review boards: a flawed system of risk management. Res Ethics. 2016;12(4):182–200.
Hickey A, Davis S, Farmer W, Dawidowicz J, Moloney C, Lamont-Mills A, et al. Beyond criticism of ethics review boards: strategies for engaging research communities and enhancing ethical review processes. J Acad Ethics. 2021:1-19.
Silverman H, Edwards H, Shamoo A, Matar A. Enhancing research ethics capacity in the Middle East: experience and challenges of a Fogarty-sponsored training program. J Empir Res Hum Res Ethics. 2013;8(5):40–51.
MacKay D, Saylor KW. Four faces of fair subject selection. Am J Bioeth. 2020;20(2):5–19.
Pace C, Miller FG, Danis M. Enrolling the uninsured in clinical trials: an ethical perspective. Crit Care Med. 2003;31(3):S121–5.
Association WM. World Medical Association Declaration of Helsinki: ethical principles for medical research involving human subjects. Jama. 2013;310(20):2191–4.
Duggan C, Parry G, McMurran M, Davidson K, Dennis J. The recording of adverse events from psychological treatments in clinical trials: evidence from a review of NIHR-funded trials. Trials. 2014;15(1):1–9.
Jonsson U, Alaie I, Parling T, Arnberg FK. Reporting of harms in randomized controlled trials of psychological interventions for mental and behavioral disorders: a review of current practice. Contemp Clin Trials. 2014;38(1):1–8.
Hansson SO, Björkman B. Bioethics in Sweden. Cambridge Quarterly of Healthcare Ethics. 2006;15(3):285–93.
Cameron JJ, Stinson DA. Gender (mis) measurement: Guidelines for respecting gender diversity in psychological research. Soc Personal Psychol Compass. 2019;13(11):e12506.
Braddock CH III. Racism and bioethics: the myth of color blindness. Am J Bioeth. 2021;21(2):28–32.
Truong M, Sharif MZ. We’re in this together: a reflection on how bioethics and public health can collectively advance scientific efforts towards addressing racism. J Bioeth Inq. 2021;18(1):113–6.
Habets MG, van Delden JJ, Bredenoord AL. The social value of clinical research. BMC Med Ethics. 2014;15(1):1–7.
Download references
Acknowledgements
Sylvia Martin (SM) and Amal Matar (AM) conceived of the presented idea. SM, AM and Mirko Ancillotti (MA) developed the theory and performed the computations. SM, AM, MA, and Santa Slokenberga (SS) verified the analytical methods and discussed the results. All authors provided critical feedback and helped shape the research, final analysis and manuscript. None of the authors have competing interest to declare.
Open access funding provided by Uppsala University.
Author information
Authors and affiliations.
Center for Research and Bioethics, Uppsala University, Uppsala, Sweden
Sylvia Martin, Mirko Ancillotti & Amal Matar
Department of Law, Uppsala University, Uppsala, Sweden
Santa Slokenberga
Clinical Immunology and Transfusion Medicine Department, Uppsala University Hospital, Uppsala, Sweden
You can also search for this author in PubMed Google Scholar
Contributions
Sylvia Martin (SM) and Amal Matar (AM) conceived of the presented idea. SM, AM and Mirko Ancillotti (MA) developed the theory and performed the computations. SM, AM, MA, and Santa Slokenberga (SS) verified the analytical methods and discussed the results. All authors provided critical feedback and helped shape the research, final analysis and manuscript.
Corresponding author
Correspondence to Sylvia Martin .
Ethics declarations
Ethics approval and consent to participate.
Not applicable.
Consent for publication
Competing interests.
The authors declare no competing interests.
Additional information
Publisher’s note.
Springer Nature remains neutral with regard to jurisdictional claims in published maps and institutional affiliations.
Supplementary Information
Additional file 1: appendix 1..
Comparative table with references to law texts.
Rights and permissions
Open Access This article is licensed under a Creative Commons Attribution 4.0 International License, which permits use, sharing, adaptation, distribution and reproduction in any medium or format, as long as you give appropriate credit to the original author(s) and the source, provide a link to the Creative Commons licence, and indicate if changes were made. The images or other third party material in this article are included in the article's Creative Commons licence, unless indicated otherwise in a credit line to the material. If material is not included in the article's Creative Commons licence and your intended use is not permitted by statutory regulation or exceeds the permitted use, you will need to obtain permission directly from the copyright holder. To view a copy of this licence, visit http://creativecommons.org/licenses/by/4.0/ . The Creative Commons Public Domain Dedication waiver ( http://creativecommons.org/publicdomain/zero/1.0/ ) applies to the data made available in this article, unless otherwise stated in a credit line to the data.
Reprints and permissions
About this article
Cite this article.
Martin, S., Ancillotti, M., Slokenberga, S. et al. A comparative ethical analysis of the Egyptian clinical research law. BMC Med Ethics 25 , 48 (2024). https://doi.org/10.1186/s12910-024-01040-0
Download citation
Received : 15 November 2023
Accepted : 26 March 2024
Published : 30 April 2024
DOI : https://doi.org/10.1186/s12910-024-01040-0
Share this article
Anyone you share the following link with will be able to read this content:
Sorry, a shareable link is not currently available for this article.
Provided by the Springer Nature SharedIt content-sharing initiative
- Biomedical laws
- Ethical principles
- Clinical trials
BMC Medical Ethics
ISSN: 1472-6939
- General enquiries: [email protected]
Handbook home
- Search the Handbook
- Undergraduate courses
- Graduate courses
- Research courses
- Undergraduate subjects
- Graduate subjects
- Research subjects
- Breadth Tracks
- CAPS Login - Staff only
- Data Analysis in Clinical Research
Data Analysis in Clinical Research (CLRS90010)
Graduate coursework Points: 12.5 On Campus (Parkville)
View full page
About this subject
- Eligibility and requirements
- Dates and times
- Further information
- Timetable (login required) (opens in new window)
Contact information
Email: [email protected]
Phone: + 61 3 8344 0149
Contact hours : https://unimelb.edu.au/professional-development/contact-us
Data analysis methods are an integral part of modern clinical research. They are powerful techniques that enable researchers to draw meaningful conclusions from data collected through observation, survey, or experimentation.
However, data analysis is a huge discipline with different paradigms, schools of thought and alternative methodologies. Therefore consideration of the appropriate methods used must be undertaken when designing a study and selecting variables and groups.
This subject introduces students to the basic principles of qualitative and quantitative data analysis techniques. It will provide a functional grounding in the theoretical concepts behind each type of analysis, as well as exploration of the interpretation of data and the difference, where applicable, between clinical vs statistical significance.
Intended learning outcomes
On completion of this subject students should be able to:
- describe the theoretical concepts behind a range of qualitative and quantitative data analysis techniques
- compare and contrast the strengths and weaknesses of different qualitative and quantitative data analysis techniques
- describe a strategy for selecting an appropriate data analysis technique based on the study design selected and/or research data collected
- competently perform a range of basic data analysis techniques using appropriate analysis software and interpret analysis output/s
- provide a rationale for the importance of statistical power and perform power calculations
- identify and discuss the key elements associated with ensuring data integrity including storage, management, collation and coding
- critically compare and contrast statistical vs clinical significance and its relevance to clinical practice
- demonstrate confidence in discussing the validity of data analysis outcomes reported in the scientific literature.
Generic skills
- to engage with unfamiliar problems and identify relevant data analysis strategies
- to construct and express logical arguments and to work in abstract or general terms to increase the clarity and efficiency of data analysis
- communicate advanced data analysis concepts in written and oral form;
- the ability to comprehend complex data analysis information
- exercise responsibility for their own learning;
- manage their time effectively.
Last updated: 31 January 2024
- Open access
- Published: 30 April 2024
Clinical and epidemiological characteristics of 96 pediatric human metapneumovirus infections in Henan, China after COVID-19 pandemic: a retrospective analysis
- Wangquan Ji 1 , 2 ,
- Yu Chen 2 ,
- Shujie Han 2 ,
- Bowen Dai 2 ,
- Kang Li 2 ,
- Shuang Li 2 ,
- Zijie Li 2 ,
- Shouhang Chen 1 ,
- Yaodong Zhang 3 ,
- Xiaolong Zhang 4 ,
- Xiaolong Li 2 ,
- Qingmei Wang 1 ,
- Jiaying Zheng 1 ,
- Chenyu Wang 1 ,
- Qiujing Liang 1 ,
- Shujuan Han 1 ,
- Ruyu Zhang 1 ,
- Fang Wang 1 &
- Yuefei Jin 1 , 2
Virology Journal volume 21 , Article number: 100 ( 2024 ) Cite this article
48 Accesses
1 Altmetric
Metrics details
In the aftermath of the COVID-19 pandemic, there has been a surge in human metapneumovirus (HMPV) transmission, surpassing pre-epidemic levels. We aim to elucidate the clinical and epidemiological characteristics of HMPV infections in the post-COVID-19 pandemic era.
In this retrospective single-center study, participants diagnosed with laboratory confirmed HMPV infection through Targeted Next Generation Sequencing were included. The study encompassed individuals admitted to Henan Children's Hospital between April 29 and June 5, 2023. Demographic information, clinical records, and laboratory indicators were analyzed.
Between April 29 and June 5, 2023, 96 pediatric patients were identified as infected with HMPV with a median age of 33.5 months (interquartile range, 12 ~ 48 months). The majority (87.5%) of infected children were under 5 years old. Notably, severe cases were statistically younger. Predominant symptoms included fever (81.3%) and cough (92.7%), with wheezing more prevalent in the severe group (56% vs 21.1%). Coinfection with other viruses was observed in 43 patients, with Epstein–Barr virus (EBV) (15.6%) or human rhinovirus A (HRV type A) (12.5%) being the most common. Human respiratory syncytial virus (HRSV) coinfection rate was significantly higher in the severe group (20% vs 1.4%). Bacterial coinfection occurred in 74 patients, with Haemophilus influenzae (Hin) and Streptococcus pneumoniae (SNP) being the most prevalent (52.1% and 41.7%, respectively). Severe patients demonstrated evidence of multi-organ damage. Noteworthy alterations included lower concentration of IL-12p70, decreased lymphocytes percentages, and elevated B lymphocyte percentages in severe cases, with statistical significance. Moreover, most laboratory indicators exhibited significant changes approximately 4 to 5 days after onset.
Conclusions
Our data systemically elucidated the clinical and epidemiological characteristics of pediatric patients with HMPV infection, which might be instructive to policy development for the prevention and control of HMPV infection and might provide important clues for future HMPV research endeavors.
Introduction
Human metapneumovirus (HMPV), a member of paramyxovirus family, was first identified in 2001 [ 1 ]. It has since been commonly implicated in acute respiratory tract infections (ARTI) affecting both pediatric and adult populations worldwide. Despite efforts, a live-attenuated recombinant HMPV vaccine has demonstrated inadequate immunogenicity in children aged 6–59 months [ 2 ], and to date, no licensed vaccines or specific drugs are available for HMPV infections. Primary infections typically occur before the age of 5 years, with HMPV prevalence among this age group ranging from 1.1% to 86% globally [ 3 ]. In 2018, HMPV-associated hospital admissions among children under 5 years old globally amounted to 643,000, with 16,100 (hospital and community) HMPV-associated ARTI deaths [ 4 ]. These figures underscore the substantial socio-economic impact and disease burden associated with HMPV infection.
Over the past 3 years, the unprecedented implementation of non-pharmaceutical interventions during the COVID-19 pandemic has significantly impacted the epidemiology of various pediatric infectious diseases [ 5 , 6 , 7 ]. The return of respiratory virus circulation to pre-pandemic levels is anticipated as COVID-19 mitigation measures gradually ease [ 8 ]. The concept of “immunity debt” has been proposed to characterize the paucity of protective immunity resulting from prolonged decreased exposure to various pathogens [ 9 ]. In Western Australia, the incidence of HMPV infection surged threefold in 2021 compared to the period of 2017 ~ 2019. Moreover, the proportion of respiratory-coded admissions undergoing HMPV testing doubled in 2021 [ 10 , 11 ]. Similarly, in the United States, the number of HMPV infections suddenly spiked to record levels in the spring of 2023 ( https://www.cdc.gov/surveillance/nrevss/hmpv/region.html ). In China, several studies conducted before the COVID-19 pandemic have examined the prevalence and genotypic diversity of HPMV, as well as the epidemiological and clinical characteristics of hospitalized patients with HPMV infection [ 12 , 13 , 14 , 15 , 16 ]. A study conducted in the Netherlands demonstrated that the clinical impact of HMPV infection remained consistent between the non-COVID-19 period and the examined COVID-19 period, with no significant changes observed in terms of incidence and/or disease severity [ 17 ]. Nevertheless, the epidemiological and clinical characteristics of HMPV infections have shown disparities following the COVID-19 pandemic. Consequently, there is an urgent need for clinical investigations, particularly focusing on children hospitalized with HMPV infection, to provide a reference for clinical diagnosis and management. In this study, we present a detailed analysis of the clinical and epidemiological features of 96 pediatric patients in central China from April 29 to June 5, 2023. Our findings contribute to a comprehensive understanding of the characteristics of HMPV infections in the post-COVID-19 pandemic era.
Materials and methods
Study design and participants.
For this retrospective study, we included a total of 96 hospitalized pediatric patients diagnosed with HMPV infection who were admitted to Children’s Hospital Affiliated to Zhengzhou University between 29 April and 5 June 2023 (Fig. 1 ). Children’s Hospital Affiliated to Zhengzhou University (Henan Children’s Hospital) is the largest tertiary pediatric referral hospital in central China with 2,200 beds, which is located in Zhengzhou of Henan province. Respiratory specimens (including sputum, throat swab, or bronchoalveolar lavage fluid (BALF)) from the majority of hospitalized patients with respiratory illness underwent testing for respiratory pathogens using Targeted Next Generation Sequencing (tNGS) conducted by Guangzhou Kingmed Ctr for Clin Lab Co ltd. ( https://www.kingmed.com.cn/ ) [ 18 ]. All enrolled patients were categorized as either mild or severe based on the guidelines for the management of community-acquired pneumonia in children of the People’s Republic of China (2013 Edition) [ 19 , 20 ]. This observational study was approved by the Committee for Ethical Review of Zhengzhou University (ethical approval No: ZZUIRB2023-180), and written informed consent was obtained from the parents.
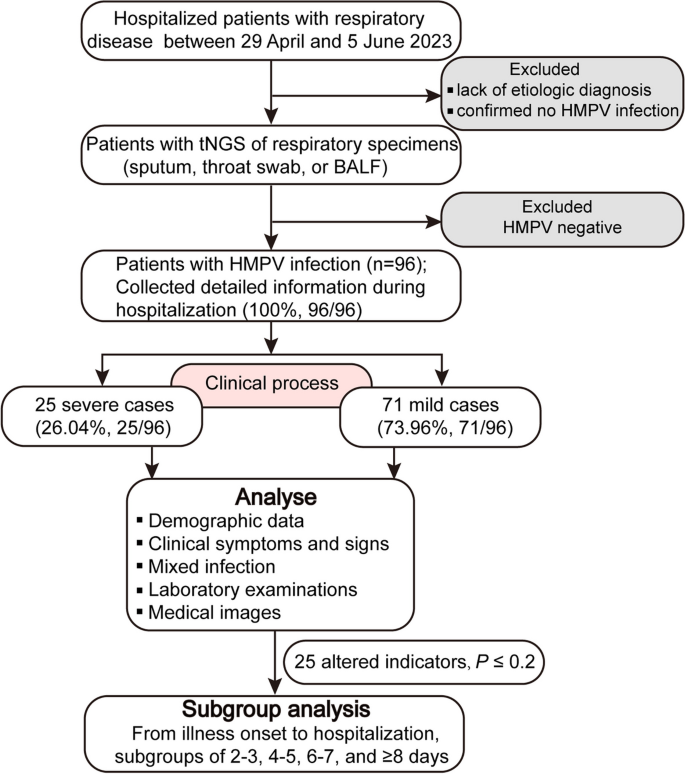
Flow chart of participants enrolment and data analysis procedure in the study. BALF, bronchoalveolar lavage fluid
Data collection
The electronic medical records data of the included patients underwent independently review and retrospective collection by trained researchers. To ensure quality control, two additional researchers cross-checked the data collection forms. Detailed information was extracted, encompassing sequencing data, demographic data, clinical symptoms and signs, laboratory examinations, medical images, and outcomes of treatment. Laboratory examinations comprised routine testing, coagulation function tests, lymphocyte subsets, inflammatory or infection-related biomarkers, analysis of immunological responses, and measurement of biomarkers for monitoring liver, myocardial, and renal functions.
Statistical analysis
The extracted data were initially entered into Microsoft Excel software (2016), and subsequently imported into SPSS 25.0 or GraphPad Prism 8.3 software for statistical analysis. Binomial or categorical variables were expressed as percentages, while clinical characteristics and laboratory findings (continuous variables) were presented as median with interquartile range (IQR). To compare variables across groups, the Mann–Whitney test was used for continuous variables, and Chi-squared test or Fisher exact test was employed for categorical variables. All statistical tests were two-sided, and a P -value < 0.05 was considered statistically significant.
Epidemiology and demographic characteristics of HPMV infections
HMPV infections were almost detected and hospitalized every day between April 29 and June 5, 2023. The number of infected patients under treatment is highest at May 30 (28 patients), and 16 infected patients remained hospitalized at June 5 (Fig. 2 A). In total, 96 pediatric patients (25 severe cases and 71 mild cases), were included in this study, with a median age of 33.5 months (IQR: 12–48 months). Sequencing data (partial M and N gene) from 9 patients were randomly selected and subjected to analysis. Detailed sequencing data are provided in Supplementary Fig. S 1 . Through multiple alignments with the nearest homologies from the NCBI databases, it was determined that all the selected viruses belong to subtype A2b. The majority (87.5%, 84/96) of infected children were under 5 years old, and more than half (65.63%, 63/96) were under 3 years old (Fig. 2 B). As shown in Table 1 , children in the severe group tended to be younger, with a median age of 1 year, compared to a median age of 3 years in the mild group. The age distribution significantly differed between the severe and mild groups ( P = 0.005). The male to female ratio was 1.53 (58/38). Although the difference was not significant ( P = 0.06, 77.5% vs 48%), a higher proportion of patients in the mild group resided in urban areas.
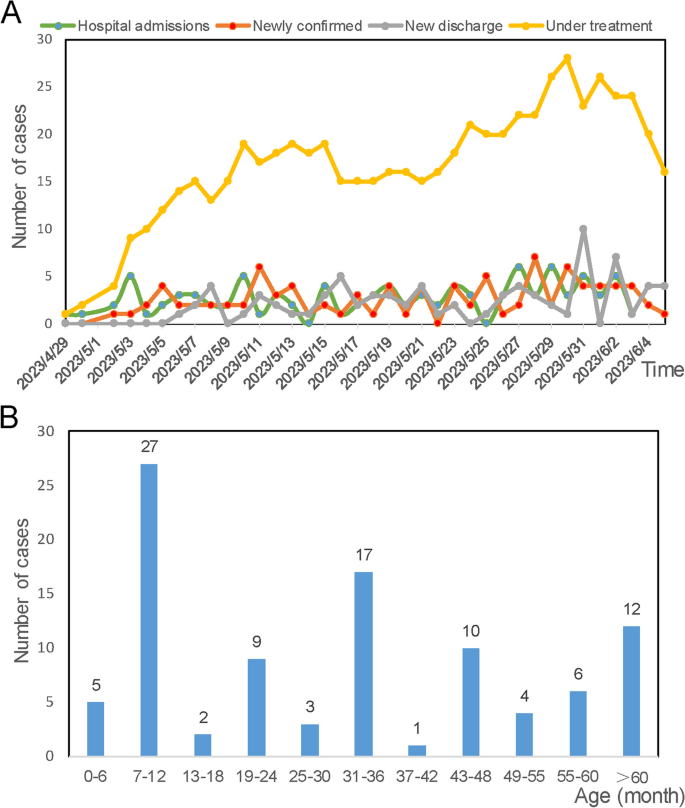
Time of onset and age distribution of laboratory-confirmed HMPV infections Clinical signs of HMPV infections
In Table 1 , we present a summary of the characteristics observed in HMPV infections. Upon admission, the majority of patients presented with fever (81.3%, 78/96) or cough (92.7%, 89/96), and almost one-third of patients (30.2%, 29/96) exhibited wheezing. The other 8 patients (8.3%, 9/96) also have exhibited fever, although this symptom was not recorded as the main complaint. Compared to mild cases, a significantly higher proportion of patients in the severe group exhibited wheezing symptoms ( P = 0.001, 21.1% vs 56%). Twelve patients presented with dyspnea on admission, all of whom were categorized into the severe group. Furthermore, 11 severe cases were admitted to the Pediatric Intensive Care Unit during their hospitalization. The majority of patients exhibited two or more respiratory symptoms. A higher proportion of patients in the mild group presented with symptoms of both cough and fever ( P = 0.003, 66.2% vs 32%). Patients with both cough and wheezing ( P = 0.018, 5.6% vs 24.0%), as well as those exhibiting concomitant fever, cough, wheezing ( P = 0.063, 12.7% vs 32.0%), were more severely ill. The respiratory rate and heart rate upon admission were significantly higher in severe patients compared to mild cases. Notably, severe patients experienced longer hospital stays, with a median duration of 8 days, in contrast to 6 days for mild patients ( P = 0.000321). Clinical respiratory symptoms resolved or disappeared by the time of discharge in almost all patients, except for one critically ill patient with a high suspicion of hemophagocytic syndrome who ultimately dropped out of treatment.
Coinfections with other causative agents based on tNGS
Among the 96 HMPV-infected patients, 91 (94.8%) were coinfected with other causative agents (Table 2 ). Correspondingly, 5 patients were solely infected with HMPV, presenting symptoms of fever and coughing. Additionally, forty-three patients were infected with another virus. Coinfections of HMPV and EBV (15.6%, 15/96) or HRV type A (12.5%, 12/96) were the most common. The rate of HRSV coinfections (20%) was significantly higher in the severe group compared to the mild group (1.4%). Bacterial coinfections were identified in 74 patients, with Hin detected in 50 children (52.1%), SNP in 40 children (41.7%), MC in 9 children (9.4%), KP in 8 children (8.3%), and SA in 7 children (7.3%). Regarding fungal coinfections, C. albicans infection in the upper respiratory tract was the most prevalent. Further details about the pathogens infecting all patients are shown in Fig. 3 .
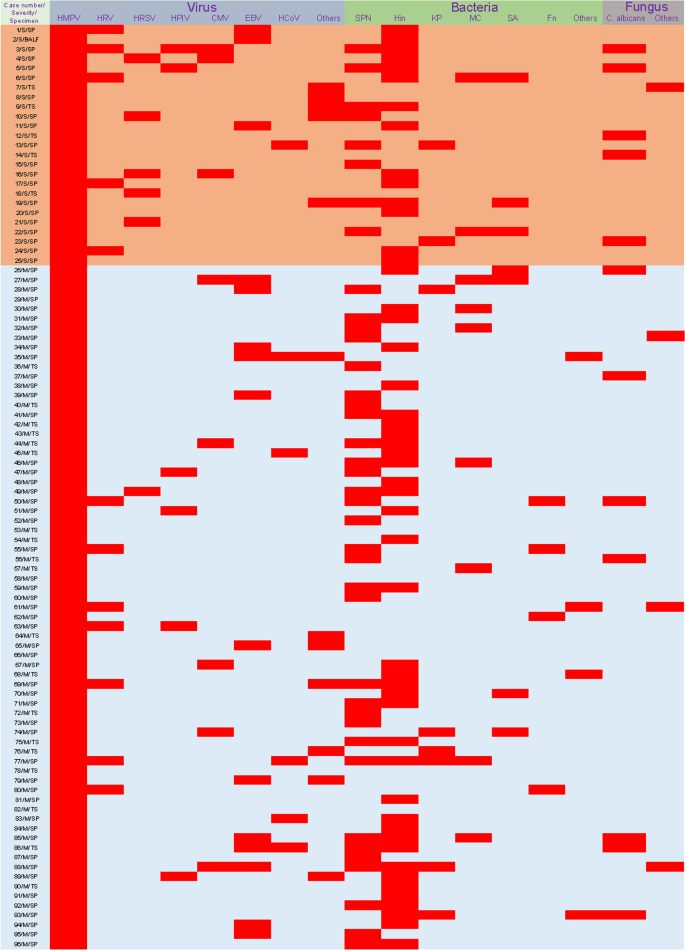
Coinfections in HMPV-infected patients. Red squares represent infection. Samples from case 44, 55 and 92 were subjected to multiple targeted sequencing of upper respiratory tract pathogens, including 105 pathogens. The others were subjected to targeted sequencing of multiple respiratory pathogens contained 198 pathogens. See Supplementary file 1 for more details of the targeted sequencing project. SP, sputum; TS, throat swab
Laboratory test findings
As shown in Table 3 , severe patients exhibited a significantly lower count of EOS ( P = 0.001), ESR count ( P = 0.013), percentage of BASO ( P = 0.013), percentage of EOS ( P = 0.000273), and percentage of LYMPH ( P = 0.000007), compared to mild group. Conversely, severe patients exhibited an increased percentage of NEUT ( P = 0.000022), and a higher count of NEUT ( P = 0.001), MONO ( P = 0.04). Additionally, severe patients exhibited more evidence of multiple-organ damage compared to mild cases, as indicated by higher levels of unconjugated bilirubin ( P = 0.038), alanine aminotransferase ( P = 0.004), aspartate aminotransferase ( P = 0.014), gamma-glutamyl transferase ( P = 0.000004), lactate dehydrogenase ( P = 0.004), and creatine kinase-MB ( P = 0.035). In contrast, levels of conjugated bilirubin ( P = 0.032), creatinine ( P = 0.014) and uric acid ( P = 0.04) decreased. Furthermore, several coagulation-related indices showed statistical significance between the two groups, including prolonged thrombin time, elevated prothrombin activity, decreased fibrinogen concentration, shortened prothrombin time and reduced international normalized ratio in severe group. Besides, decreased concentrations of Immunoglobulin G and Immunoglobulin A were observed in the severe group compared to the mild group. Other laboratory indices or organ damage biomarkers in the two groups were presented in Table 3 .
Inflammatory responses in HMPV-infected patients
To further elucidate the immune response, we analyzed the changes in lymphocyte subpopulations, serum cytokines, and chemokines levels were also analyzed (Fig. 4 ). We observed higher levels of cytokines, including interleukin (IL)-2, IL-4, IL-6, IL-10, tumor necrosis factor (TNF) -α, and SAA in severe patients compared to mild patients, although these differences did not reach statistical significance. Conversely, the levels of interferon (IFN)-γ, IL-17a, IL-12p70, were decreased in the severe patients, but only the lower levels of IL-12p70 exhibited statistical significance between the two groups ( P = 0.029). Regarding immune cells, the decline in lymphocytes percentage was more pronounced in severe patients ( P = 0.0402), while the percentage of B lymphocyte was significantly elevated ( P = 0.0309). There was no significant difference in the percentage of CD4 + T cells, CD8 + T cell, and NK cell, as well as the ratio of CD4 + T cells to CD8 + T cells between the two groups. Notably, severe patients exhibited more pronounced aggravated inflammatory responses, lymphopenia, and multiple-organ damage compared to those in mild cases.
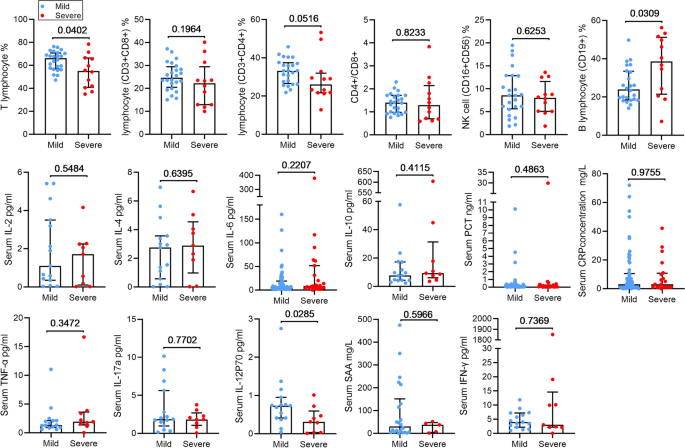
Inflammatory response in HMPV-infected patients. PCT, procalcitonin; SAA, serum amyloid A; The histogram represents the median and the whiskers represents the IQR
Dynamic change of several altered indicators
All subjects were stratified into different subsets based on the duration from illness onset to hospitalization, including subsets of 2–3, 4–5, 6–7, and ≥ 8 days. The altered indicators ( P ≤ 0.2) were analyzed within these subgroups. As shown in Fig. 5 , there was no statistically significant difference in PLT, percentage of BASO, uric acid, AST, CK-MB, ESR and C3 between the two groups. Obviously, most indicators exhibited significant changes after 4–5 days of illness onset. Compared to mild patients, severe cases exhibited significantly increased WBC count, NEUT count, MONO count, percentage of NEUT, and LDH, ALT, GGT in the peripheral blood at 4–5 days after illness onset. Conversely, LYMPH count, EOS count, percentage of LYMPH, percentage of LYMPH, and creatinine, IgG, and C4 in the serum were significantly reduced during the same period. The changes in percentage of LYMPH and NEUT remained consistent after 4–5 days after illness onset. Figure 5 showed specific changes in other indicators.
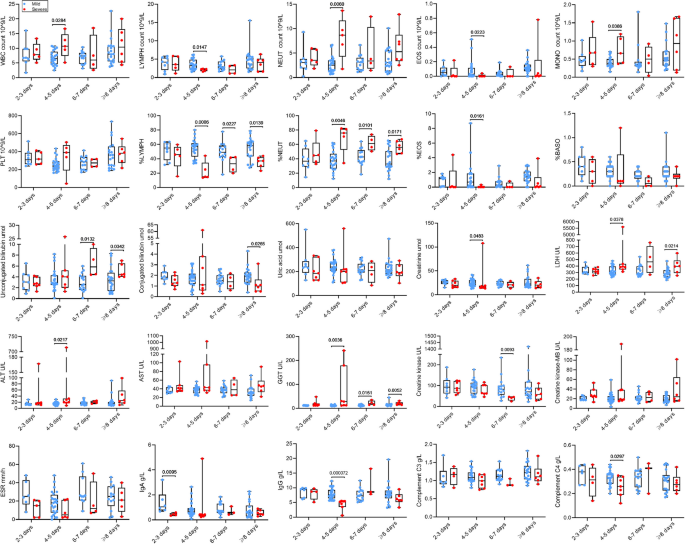
Subgroup analyses of laboratory indicators. The box plots show the medians (middle line) and first and third quartiles (boxes), and the whiskers show range of the measured values.
Abbreviations : WBC white blood cell, PLT platelet count, NEUT neutrophil, LYMPH lymphocyte, MONO monocyte, EOS eosinophilic granulocyte, BASO basophilic granulocyte, LDH lactate dehydrogenase, ALT alanine aminotransferase, AST aspartate aminotransferase, GGT gamma-glutamyl transferase, ESR erythrocyte sedimentation rate, Ig immunoglobulin
Radiologic findings
As mentioned previously, nearly all patients were coinfected with other causative agents, while only 5 patients were solely infected with HMPV. Among these, imaging examinations were conducted for three of the five patients. Despite presenting with mild clinical symptoms such as fever and cough, all three patients exhibited abnormal radiological findings, including focal ground-glass opacities and/or stripe shadows (Fig. 6 ).
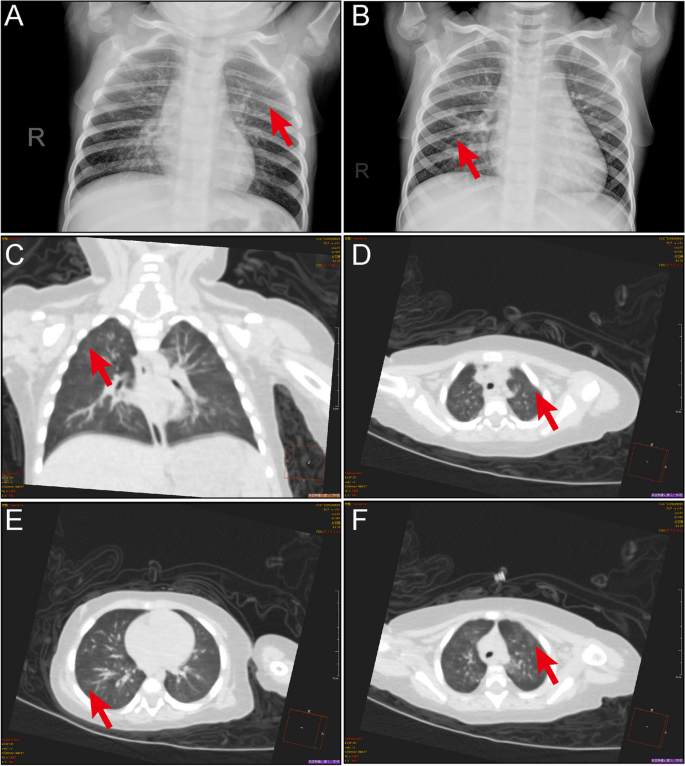
Pulmonary manifestations of HMPV infection. A CT scan of 1-year-old girl showing multiple ground-glass opacities in both lungs (arrow). B Chest radiograph of a 4-year-old girl displaying multiple ground-glass opacities (arrow). C-F Chest CT scans from a 7-month-old girl showing focal ground-glass opacities and stripes coexisting in the lung (arrows)
The etiological significance of HMPV in ARTI has been the focus of increasing attention worldwide [ 3 ]. HMPV infection among hospitalized children with ARTIs experienced a significant decrease during COVID-19 pandemic [ 14 ]. However, with the relaxation of COVID-19 mitigation measures, HMPV transmission has surpassed pre-epidemic levels [ 10 ]. The immunity debt [ 9 ] and the alterations in prevalent genetic subtypes [ 14 ] following the COVID-19 pandemic have raised our concerns. Hence, this study aimed to evaluate the epidemiological and clinical characteristics of HMPV infections in hospitalized children in Henan, China from April 29 to June 5, 2023.
In this study, we observed that the majority (87.5%) of infected children were under 5 years, with more than half (65.63%) being under 3 years old. Previous study has indicated that the hospital admission rate for HMPV-associated ARTI is notably higher among infants compared to older children, with approximately 58% of hospital admissions in children under 5 years occurring within the first year of life [ 4 , 21 ]. The elevated burden on infants may be attributed to the immaturity of their immune systems and the decline of maternal antibodies during the first months of life [ 22 ].
Upon admission, we observed that patients presenting with concurrent cough and wheezing, as well as those with simultaneous fever, cough, and wheezing, tended to be more severely ill. Generally, HMPV-associated respiratory diseases manifest along a spectrum from mild fever and cough to severe bronchiolitis and pneumonia [ 13 , 15 ]. A study involving hospitalized children in Beijing similarly reported that the majority of patients presented with cough and fever [ 14 ]. Wheezing is a common manifestation in children with HMPV infection, often progressing to life-threatening bronchiolitis and pneumonia [ 23 ]. A study has indicated that 13.0% ~ 60.7% of children infected with HMPV experience recurrent wheezing or receive a diagnosis of asthma [ 24 ]. However, distinguishing HMPV-associated pneumonia from infection caused by other pathogens based solely on clinical features remains challenging [ 25 , 26 , 27 ]. Several respiratory pathogens, including HMPV, exhibit similar incidence rates and clinical characteristics, thereby posing a diagnostic challenge for attending physicians.
A previous study revealed that HMPV was the third most common virus associated with coinfection [ 28 ]. Viral cocirculation can lead to competition among viruses, whereby the stronger virus may survive and/or mutate, potentially resulting in higher mortality and morbidity rates [ 29 ]. In our study, we identified 43 patients who were coinfected with another virus, with coinfections of HMPV with EBV (15.6%) or HRV type A (12.5%) being common. EBV infects over 95% of the global population and is often asymptomatic, typically occurring at a young age [ 30 ]. Unlike EBV, HRV type A is responsible for more than 50% of upper respiratory tract infections globally and it is the most common virus associated with wheezing in children aged between one and two years [ 31 ]. Previous studies have identified influenza, HRSV, adenoviral, and human bocavirus as the most common respiratory viruses involved in coinfections [ 14 , 15 , 23 , 32 ]. Our results findings diverged from previously published data, which could be attributed to the seasonality of different viruses [ 33 ], variations in sample types, and differences in sample sizes among studies. In our study, the rate of HRSV coinfections was significantly higher in the severe group compared to the mild group. Previous research has indicated that dual infections with HMPV and RSV are associated with severe bronchiolitis and an increased risk of Intensive Care Unit admission for mechanical ventilation [ 34 ]. Additionally, coinfections with bacterial pathogens often lead to a more severe course and increased mortality. While HMPV-associated pneumonia is generally less severe than bacterial pneumonia, it can be as severe as or even more severe than infections caused by other common pathogens [ 27 ]. In our study, Hin and SNP were the two most common bacterial coinfections, accounting for 52.1% and 41.7%, respectively. Therefore, further data are necessary to elucidate the cumulative clinical effects on disease severity resulting from coinfecting with other respiratory pathogens.
Significant alterations in several indicators among severe patients were notably observed at 4–5 days after the onset of illness, marking a critical time point for disease progression, and necessitating timely decisions regarding treatment interventions. Therefore, we should pay more attention to the diagnosis and intervention in the early stage of the disease. In our study, we observed a lower level of IL-12p70 in severe patients. However, previous research suggested that HMPV failed to induce production of IL-12p70 in BALF in a mouse model [ 35 ]. In terms of immune cells, we observed a more pronounced decline in the lymphocyte percentage among severe patients, while the percentage of B lymphocyte was significantly elevated. In other words, the function of the specific cellular immunity was decreased, while the function of humoral immune was increased. Nevertheless, the decreased concentrations of Immunoglobulin G and Immunoglobulin A in the severe group contradicted these results. In fact, interpreting these results is often challenging due to the complexities of the disease and the involvement of mixed infections. In clinical practice, patients with single HMPV infections are rare, emphasizing the necessity for further research on patients with mixed infections.
This study also had several limitations. Firstly, our focus was primarily on the epidemiological and clinical features of HMPV positive cases. Thus, other common respiratory pathogens were not excluded. Subsequently, our results more reflect the cumulative clinical effects of coinfection. Secondly, children with more severe symptoms are more likely to receive hospital care, which may affect our understanding of the clinical features of HMPV-positive patients. Despite these shortcomings, our results offer a valuable insight into the epidemiological and clinical characteristics of hospitalized patients with HMPV infection in Henan, China.
Our study elucidated important epidemiological and clinical characteristics of HMPV infection in Henan, China. These findings hold potential significance for informing policy development aimed at the prevention and control of HMPV infection. Additionally, our results may provide important insights for guiding HMPV research efforts in the post- COVID-19 pandemic era.
Availability of data and materials
No datasets were generated or analysed during the current study.
Abbreviations
Acute respiratory tract infections
Alanine aminotransferase
Aspartate aminotransferase
Alkaline phosphatase
α-hydroxybutyrate dehydrogenase
Bronchoalveolar lavage fluid
Basophilic granulocyte
Creatine kinase
Creatine kinase-MB
C-reactive protein
Cytomegalovirus
Canidia albicans
Eosinophilic granulocyte
Erythrocyte sedimentation rate
Epstein–Barr virus
Gamma-glutamyl transferase
Human rhinovirus A
Human respiratory syncytial virus
Human parainfluenza virus type 3
Human coronavirus
Haemophilus influenza
- Human metapneumovirus
Interquartile range
Immunoglobulin
Interleukin
Klebsiella pneumoniae
Lactate dehydrogenase
Moraxella catarrhalis
Platelet count
Procalcitonin
Red blood cell
Streptococcus pneumoniae
Serum amyloid A
Staphylococcus aureus
Tumor necrosis factor
Targeted Next Generation Sequencing
White blood cell
van den Hoogen BG, de Jong JC, Groen J, Kuiken T, de Groot R, Fouchier RA, et al. A newly discovered human pneumovirus isolated from young children with respiratory tract disease. Nat Med. 2001;7(6):719–24.
Article PubMed PubMed Central Google Scholar
Karron RA, San Mateo J, Wanionek K, Collins PL, Buchholz UJ. Evaluation of a Live Attenuated Human Metapneumovirus Vaccine in Adults and Children. J Pediatric Infect Dis Soc. 2018;7(1):86–9.
Article PubMed Google Scholar
Divarathna MVM, Rafeek RAM, Noordeen F. A review on epidemiology and impact of human metapneumovirus infections in children using TIAB search strategy on PubMed and PubMed Central articles. Rev Med Virol. 2020;30(1): e2090.
Wang X, Li Y, Deloria-Knoll M, Madhi SA, Cohen C, Ali A, et al. Global burden of acute lower respiratory infection associated with human metapneumovirus in children under 5 years in 2018: a systematic review and modelling study. Lancet Glob Health. 2021;9(1):e33–43.
Article CAS PubMed Google Scholar
Belingheri M, Paladino ME, Piacenti S, Riva MA. Effects of COVID-19 lockdown on epidemic diseases of childhood. J Med Virol. 2021;93(1):153–4.
Izu A, Nunes MC, Solomon F, Baillie V, Serafin N, Verwey C, et al. All-cause and pathogen-specific lower respiratory tract infection hospital admissions in children younger than 5 years during the COVID-19 pandemic (2020–22) compared with the pre-pandemic period (2015–19) in South Africa: an observational study. Lancet Infect Dis. 2023.
Perez A, Lively JY, Curns A, Weinberg GA, Halasa NB, Staat MA, et al. Respiratory Virus Surveillance Among Children with Acute Respiratory Illnesses - New Vaccine Surveillance Network, United States, 2016–2021. MMWR Morb Mortal Wkly Rep. 2022;71(40):1253–9.
Hatter L, Eathorne A, Hills T, Bruce P, Beasley R. Respiratory syncytial virus: paying the immunity debt with interest. Lancet Child Adolesc Health. 2021;5(12):e44–5.
Article CAS PubMed PubMed Central Google Scholar
Cohen R, Ashman M, Taha MK, Varon E, Angoulvant F, Levy C, et al. Pediatric Infectious Disease Group (GPIP) position paper on the immune debt of the COVID-19 pandemic in childhood, how can we fill the immunity gap? Infect Dis Now. 2021;51(5):418–23.
Foley DA, Yeoh DK, Minney-Smith CA, Shin C, Hazelton B, Hoeppner T, et al. A surge in human metapneumovirus paediatric respiratory admissions in Western Australia following the reduction of SARS-CoV-2 non-pharmaceutical interventions. J Paediatr Child Health. 2023;59(8):987–91.
Foley DA, Sikazwe CT, Minney-Smith CA, Ernst T, Moore HC, Nicol MP, et al. An Unusual Resurgence of Human Metapneumovirus in Western Australia Following the Reduction of Non-Pharmaceutical Interventions to Prevent SARS-CoV-2 Transmission. Viruses. 2022;14(10).
Zhao H, Feng Q, Feng Z, Zhu Y, Ai J, Xu B, et al. Clinical characteristics and molecular epidemiology of human metapneumovirus in children with acute lower respiratory tract infections in China, 2017 to 2019: A multicentre prospective observational study. Virol Sin. 2022;37(6):874–82.
Wang C, Wei T, Ma F, Wang H, Guo J, Chen A, et al. Epidemiology and genotypic diversity of human metapneumovirus in paediatric patients with acute respiratory infection in Beijing, China. Virol J. 2021;18(1):40.
Cong S, Wang C, Wei T, Xie Z, Huang Y, Tan J, et al. Human metapneumovirus in hospitalized children with acute respiratory tract infections in Beijing. China Infect Genet Evol. 2022;106: 105386.
Zeng SZ, Xiao NG, Zhong LL, Yu T, Zhang B, Duan ZJ. Clinical features of human metapneumovirus genotypes in children with acute lower respiratory tract infection in Changsha. China J Med Virol. 2015;87(11):1839–45.
Zhang C, Du LN, Zhang ZY, Qin X, Yang X, Liu P, et al. Detection and genetic diversity of human metapneumovirus in hospitalized children with acute respiratory infections in Southwest China. J Clin Microbiol. 2012;50(8):2714–9.
Jongbloed M, Leijte WT, Linssen CFM, van den Hoogen BG, van Gorp ECM, de Kruif MD. Clinical impact of human metapneumovirus infections before and during the COVID-19 pandemic. Infect Dis (Lond). 2021;53(7):488–97.
Li SY, Tong J, Liu Y, Shen W, Hu P. Targeted next generation sequencing is comparable with metagenomic next generation sequencing in adults with pneumonia for pathogenic microorganism detection. J Infection. 2022;85(5):E127–9.
Article CAS Google Scholar
Subspecialty Group of Respiratory Diseases TSoP, Chinese Medical Association The Editorial Board CJoP. [Guidelines for management of community acquired pneumonia in children(the revised edition of 2013) (II)]. Zhonghua Er Ke Za Zhi. 2013;51(11):856-62.
Subspecialty Group of Respiratory Diseases TSoPCMA, Editorial Board CJoP. [Guidelines for management of community acquired pneumonia in children (the revised edition of 2013) (I)]. Zhonghua Er Ke Za Zhi. 2013;51(10):745-52.
Wang X, Li Y, O’Brien KL, Madhi SA, Widdowson MA, Byass P, et al. Global burden of respiratory infections associated with seasonal influenza in children under 5 years in 2018: a systematic review and modelling study. Lancet Glob Health. 2020;8(4):e497–510.
Simon AK, Hollander GA, McMichael A. Evolution of the immune system in humans from infancy to old age. Proc Biol Sci. 1821;2015(282):20143085.
Google Scholar
Schildgen V, van den Hoogen B, Fouchier R, Tripp RA, Alvarez R, Manoha C, et al. Human Metapneumovirus: lessons learned over the first decade. Clin Microbiol Rev. 2011;24(4):734–54.
Coverstone AM, Wang L, Sumino K. Beyond Respiratory Syncytial Virus and Rhinovirus in the Pathogenesis and Exacerbation of Asthma: The Role of Metapneumovirus, Bocavirus and Influenza Virus. Immunol Allergy Clin North Am. 2019;39(3):391–401.
Wilkesmann A, Schildgen O, Eis-Hubinger AM, Geikowski T, Glatzel T, Lentze MJ, et al. Human metapneumovirus infections cause similar symptoms and clinical severity as respiratory syncytial virus infections. Eur J Pediatr. 2006;165(7):467–75.
Cui A, Xie Z, Xu J, Hu K, Zhu R, Li Z, et al. Comparative analysis of the clinical and epidemiological characteristics of human influenza virus versus human respiratory syncytial virus versus human metapneumovirus infection in nine provinces of China during 2009–2021. J Med Virol. 2022;94(12):5894–903.
Howard LM, Edwards KM, Zhu Y, Grijalva CG, Self WH, Jain S, et al. Clinical Features of Human Metapneumovirus-Associated Community-acquired Pneumonia Hospitalizations. Clin Infect Dis. 2021;72(1):108–17.
PubMed Google Scholar
Zhu Y, Xu B, Li C, Chen Z, Cao L, Fu Z, et al. A Multicenter Study of Viral Aetiology of Community-Acquired Pneumonia in Hospitalized Children in Chinese Mainland. Virol Sin. 2021;36(6):1543–53.
Nickbakhsh S, Mair C, Matthews L, Reeve R, Johnson PCD, Thorburn F, et al. Virus-virus interactions impact the population dynamics of influenza and the common cold. Proc Natl Acad Sci U S A. 2019;116(52):27142–50.
Damania B, Kenney SC, Raab-Traub N. Epstein-Barr virus: Biology and clinical disease. Cell. 2022;185(20):3652–70.
Vandini S, Biagi C, Fischer M, Lanari M. Impact of Rhinovirus Infections in Children. Viruses. 2019;11(6).
Du Y, Li W, Guo Y, Li L, Chen Q, He L, et al. Epidemiology and genetic characterization of human metapneumovirus in pediatric patients from Hangzhou China. J Med Virol. 2022;94(11):5401–8.
Moriyama M, Hugentobler WJ, Iwasaki A. Seasonality of Respiratory Viral Infections. Annu Rev Virol. 2020;7(1):83–101.
Esposito S, Daleno C, Prunotto G, Scala A, Tagliabue C, Borzani I, et al. Impact of viral infections in children with community-acquired pneumonia: results of a study of 17 respiratory viruses. Influenza Other Respir Viruses. 2013;7(1):18–26.
Guerrero-Plata A, Casola A, Garofalo RP. Human metapneumovirus induces a profile of lung cytokines distinct from that of respiratory syncytial virus. J Virol. 2005;79(23):14992–7.
Download references
Acknowledgements
Not applicable.
This work was supported by the National Natural Science Foundation of China (YJ., NO. 82002147 and NO. 82372229), China Postdoctoral Science Foundation (YJ., NO. 2019M662543), and by Open Research Fund of National Health Commission Key Laboratory of Birth Defects Prevention & Henan Key Laboratory of Population Defects Prevention (YJ., NO. ZD202301).
Author information
Authors and affiliations.
Department of Infectious Diseases, Children’s Hospital Affiliated to Zhengzhou University, Henan Children’s Hospital, Zhengzhou Children’s Hospital, Zhengzhou, 450018, Henan, China
Wangquan Ji, Shouhang Chen, Qingmei Wang, Jiaying Zheng, Chenyu Wang, Qiujing Liang, Shujuan Han, Ruyu Zhang, Fang Wang & Yuefei Jin
Department of Epidemiology, College of Public Health, Zhengzhou University, Zhengzhou, 450001, Henan, China
Wangquan Ji, Yu Chen, Shujie Han, Bowen Dai, Kang Li, Shuang Li, Zijie Li, Xiaolong Li & Yuefei Jin
Henan International Joint Laboratory of Children’s Infectious Diseases, Children’s Hospital Affiliated to Zhengzhou University, Henan Children’s Hospital, Zhengzhou Children’s Hospital, Zhengzhou, 450018, Henan, China
Yaodong Zhang
NHC Key Laboratory of Birth Defects Prevention; Henan Key Laboratory of Population Defects Prevention, Zhengzhou, 450002, Henan, China
Xiaolong Zhang
You can also search for this author in PubMed Google Scholar
Contributions
All authors contributed to the study design. Conceptualization: Wangquan Ji, Fang Wang, and Yuefei Jin; Methodology: Qingmei Wang, Jiaying Zheng, Chenyu Wang, Qiujing Liang, and Shujuan Han; Formal analysis and investigation: Yu Chen, Shujie Han, Bowen Dai, Kang Li, Shuang Li, Zijie Li, Qingmei Wang, Jiaying Zheng, Chenyu Wang, Qiujing Liang, Xiaolong Li, and Shujuan Han; Writing - original draft preparation: Wangquan Ji and Yuefei Jin; Writing - review and editing: Yuefei Jin, and Fang Wang; Funding acquisition: Yuefei Jin; Resources: Shouhang Chen, Yaodong Zhang, Xiaolong Zhang, and Ruyu Zhang; Supervision: Yuefei Jin, and Fang Wang. All authors commented on previous versions of the manuscript. All authors read and approved the final manuscript.
Corresponding authors
Correspondence to Fang Wang or Yuefei Jin .
Ethics declarations
Ethics approval and consent to participate.
This study received ethical approval from the Committee for Ethical Review of Zhengzhou University (ethical approval No: ZZUIRB2023-180) and written informed consent was obtained from the parents.
Consent for publication
The authors affirm that human research participants provided informed consent for publication of the images in Fig. 6 .
Competing interests
The authors declare no competing interests.
Additional information
Publisher's note.
Springer Nature remains neutral with regard to jurisdictional claims in published maps and institutional affiliations.
Supplementary Information
Supplementary material 1., supplementary material 2., rights and permissions.
Open Access This article is licensed under a Creative Commons Attribution 4.0 International License, which permits use, sharing, adaptation, distribution and reproduction in any medium or format, as long as you give appropriate credit to the original author(s) and the source, provide a link to the Creative Commons licence, and indicate if changes were made. The images or other third party material in this article are included in the article's Creative Commons licence, unless indicated otherwise in a credit line to the material. If material is not included in the article's Creative Commons licence and your intended use is not permitted by statutory regulation or exceeds the permitted use, you will need to obtain permission directly from the copyright holder. To view a copy of this licence, visit http://creativecommons.org/licenses/by/4.0/ . The Creative Commons Public Domain Dedication waiver ( http://creativecommons.org/publicdomain/zero/1.0/ ) applies to the data made available in this article, unless otherwise stated in a credit line to the data.
Reprints and permissions
About this article
Cite this article.
Ji, W., Chen, Y., Han, S. et al. Clinical and epidemiological characteristics of 96 pediatric human metapneumovirus infections in Henan, China after COVID-19 pandemic: a retrospective analysis. Virol J 21 , 100 (2024). https://doi.org/10.1186/s12985-024-02376-0
Download citation
Received : 14 December 2023
Accepted : 23 April 2024
Published : 30 April 2024
DOI : https://doi.org/10.1186/s12985-024-02376-0
Share this article
Anyone you share the following link with will be able to read this content:
Sorry, a shareable link is not currently available for this article.
Provided by the Springer Nature SharedIt content-sharing initiative
- Epidemiological characteristics
- Clinical characteristics
- Coinfection
Virology Journal
ISSN: 1743-422X
- Submission enquiries: [email protected]
Global Cancer Research Technologies Analysis Report 2024-2032: Key Players Striving for Innovation and Market Dominance in Genomics Technologies, Diagnostic Tools, and Therapeutic Interventions
April 30, 2024 03:09 ET | Source: Research and Markets Research and Markets
Dublin, April 30, 2024 (GLOBE NEWSWIRE) -- The "Cancer Research Technologies Market Size, Market Share, Application Analysis, Regional Outlook, Growth Trends, Key Players, Competitive Strategies and Forecasts, 2024 To 2032" report has been added to ResearchAndMarkets.com's offering. The cancer research technologies market is expected to grow at a CAGR of 7.5% during the forecast period of 2024 to 2032, propelled by key drivers, including the transformative impact of genomics and proteomics technologies, advancements in imaging for early detection, and the promise of liquid biopsy technologies.
This study report represents analysis of each segment from 2022 to 2032 considering 2023 as the base year. Compounded Annual Growth Rate (CAGR) for each of the respective segments estimated for the forecast period of 2024 to 2032.
The cancer research technologies market is characterized by intense competition among key players striving for innovation and market dominance. Leading companies such as Illumina Inc., Thermo Fisher Scientific, F. Hoffmann-La Roche AG, QIAGEN, PerkinElmer Inc., Roche Diagnostics, Agilent Technologies, Bio-Rad Laboratories, Becton, Dickinson and Company (BD), GE Healthcare, Siemens Healthineers, Abcam, GenScript Biotech Corporation, Merck KGaA, Cell Signaling Technology, and Sysmex Corporation are at the forefront, driving advancements in genomics technologies, diagnostic tools, and therapeutic interventions.
The cancer research technologies market exhibits geographical variations that significantly impact both revenue distribution and expected CAGR. North America dominates the market in terms of revenue, driven by robust investments in cancer research, advanced healthcare infrastructure, and a high prevalence of cancer cases.
The region's emphasis on translational research, strong collaborations between research institutions and industry players, and government initiatives supporting cancer research contribute to its leading revenue position in 2023. Meanwhile, Asia-Pacific is poised for the highest CAGR from 2024 to 2032, fueled by increasing awareness, rising cancer incidence, and a growing trend of outsourcing clinical trials and research activities to countries in this region. The strategic expansion of major market players and the establishment of research collaborations further contribute to the region's anticipated accelerated growth. Ethical considerations surrounding emerging technologies present a notable restraint. Market segmentation reveals the dominance of genomics technologies, cancer diagnosis as a leading research area, specific cancer types, and academic and research institutions as prominent end-users. North America stands out as the leading geographic segment, with a robust competitive landscape characterized by innovation and strategic collaborations among major players. The market is poised for continued growth, driven by technological advancements and a collective effort to unravel the complexities of cancer for improved diagnostics and treatment strategies. Key Market Drivers
Genomics Technologies Driving Precision Medicine The adoption of genomics technologies has revolutionized cancer research, offering insights into the genetic basis of cancers and paving the way for personalized treatment strategies. Advancements in next-generation sequencing (NGS) technologies, such as whole-genome sequencing and RNA sequencing, have enabled a comprehensive understanding of genetic alterations in tumors. This has significantly improved patient outcomes by tailoring treatments based on individual genetic profiles. Proteomics Technologies Unraveling Molecular Signatures Proteomics technologies play a pivotal role in deciphering the complex molecular signatures of cancers. Techniques like mass spectrometry and protein microarrays enable the identification and quantification of proteins involved in cancer pathways. The integration of proteomics data with genomics information enhances the understanding of disease mechanisms and facilitates the discovery of novel therapeutic targets. This contributes to advancing targeted therapies and improving the effectiveness of cancer treatments. Imaging Technologies Enhancing Early Detection Advancements in imaging technologies, including positron emission tomography (PET) and magnetic resonance imaging (MRI), have bolstered early cancer detection and monitoring. These technologies provide detailed insights into tumor characteristics and aid in assessing treatment response. Evidence-based research underscores the role of imaging technologies in reducing the time to diagnosis, enabling timely interventions, and ultimately improving patient outcomes. Restraint: Ethical Considerations in Emerging Technologies The ethical implications surrounding emerging technologies, particularly in areas like liquid biopsy and gene editing, pose a significant restraint in the cancer research technologies market. Issues related to patient consent, data privacy, and the potential misuse of genetic information raise ethical concerns among researchers, clinicians, and the broader public. While these technologies hold immense promise, addressing ethical challenges is crucial for responsible and equitable advancement in cancer research. Market Segmentation Analysis
Market by Technology: Genomics Dominate the Market Various cutting-edge technologies, including Genomics Technologies, Proteomics Technologies, Imaging Technologies, Liquid Biopsy Technologies, and others propel the cancer research technologies market. Genomics Technologies emerged as the revenue leader, leveraging its pivotal role in advancing precision medicine and tailoring cancer treatments based on individual genetic profiles.
The significant investments in genomic research, coupled with the increasing adoption of genomics in the research area of diagnosis and treatment, contribute to the high revenue in 2023. In terms of CAGR, Liquid Biopsy Technologies take the forefront, experiencing rapid growth due to their non-invasive nature and potential for early cancer detection, leading to a substantial expected increase in market share from 2024 to 2032. Market by Research Area: Cancer Diagnosis Dominate the Market Within the market's diverse research areas such as Cancer Diagnosis, Treatment Selection, Biomarker Discovery, Therapeutic Development, Cancer Epidemiology Research, Cancer Stem Cell Research, Radiation Oncology Advances, Functional Genomics in Cancer, Liquid Biopsy Applications, and Health Informatics in Cancer Research, Cancer Diagnosis claimed the highest revenue in 2023. This is primarily attributed to the critical role it plays in early detection and the subsequent personalized treatment of cancer patients.
The high prevalence of cancer and the emphasis on early intervention contribute to the robust revenue figures in 2023. In terms of CAGR, Therapeutic Development emerges as a significant growth driver, reflecting the continuous efforts to develop novel and effective cancer treatments, with an anticipated surge in CAGR during the forecast period. Market by Site of Cancer: Breast Cancer Dominates the Market The segmentation based on the site of cancer covers a multitude of cancer types, each with its unique characteristics and research focus. Breast cancer remains the dominant in terms of revenues in 2023, reflecting its prevalence and substantial research investments. The high incidence of breast cancer globally, coupled with extensive research efforts directed toward understanding and treating this malignancy, contributes to its leading position in generating revenue for the market in 2023. In terms of CAGR, Pancreatic cancer stands out with a notable growth rate, driven by increased attention to this challenging and often lethal cancer type, leading to a substantial projected rise in CAGR from 2024 to 2032. Market by End-User: Research Institutes Dominate the Market End-users in the cancer research technologies market, including Academic and Research Institutions, Pharmaceutical and Biotechnology Companies, Diagnostic Laboratories, and Hospitals, play pivotal roles in driving revenue. Academic and research institutions lead in revenue, emphasizing their central role in foundational research.
The substantial funding received by research institutions, often from government grants and collaborations, contributes significantly to the overall high revenue of the market in 2023. In terms of CAGR, Pharmaceutical and Biotechnology Companies emerge as key drivers, with a projected significant increase during the forecast period, highlighting the growing collaboration between research institutions and industry players. Market by Research Stage: Clinical Trials Stage Dominates the Market Different research stages, encompassing Pre-clinical Research, Clinical Trials, Public Health Research, Cross-disciplinary Research, and General Cancer Research, contribute to the overall revenue of the cancer research technologies market. Clinical Trials dominate in terms of revenue, highlighting the pivotal role of rigorous testing in bringing novel cancer therapies to market.
The high investment in clinical trial initiatives by pharmaceutical companies and research institutions contributes significantly to the market's robust revenue in 2023. In terms of CAGR, Cross-disciplinary Research exhibits a noteworthy growth rate, emphasizing the increasing importance of collaborative and interdisciplinary approaches to cancer research, with a projected surge in CAGR from 2024 to 2032.
Key questions answered in this report
- What are the key micro and macro environmental factors that are impacting the growth of Cancer Research Technologies market?
- What are the key investment pockets with respect to product segments and geographies currently and during the forecast period?
- Estimated forecast and market projections up to 2032.
- Which segment accounts for the fastest CAGR during the forecast period?
- Which market segment holds a larger market share and why?
- Are low and middle-income economies investing in the Cancer Research Technologies market?
- Which is the largest regional market for Cancer Research Technologies market?
- What are the market trends and dynamics in emerging markets such as Asia Pacific, Latin America, and Middle East & Africa?
- Which are the key trends driving Cancer Research Technologies market growth?
- Who are the key competitors and what are their key strategies to enhance their market presence in the Cancer Research Technologies market worldwide?
Companies Featured
- Illumina Inc.
- Thermo Fisher Scientific
- F. Hoffmann-La Roche AG
- PerkinElmer Inc.
- Roche Diagnostics
- Agilent Technologies
- Bio-Rad Laboratories
- Becton, Dickinson and Company (BD)
- GE Healthcare
- Siemens Healthineers
- GenScript Biotech Corporation
- Cell Signaling Technology
- Sysmex Corporation
For more information about this report visit https://www.researchandmarkets.com/r/7oryco
About ResearchAndMarkets.com ResearchAndMarkets.com is the world's leading source for international market research reports and market data. We provide you with the latest data on international and regional markets, key industries, the top companies, new products and the latest trends.

Related Links
- Tukysa (tucatinib) + Herceptin (trastuzumab) Emerging Drug Insight and Market Forecast - 2032
- Lenvatinib + pembrolizumab Emerging Drug Insight and Market Forecast - 2032
- Global Animal Model Market Size, Market Share, Application Analysis, Regional Outlook, Growth Trends, Key Players, Competitive Strategies and Forecasts, 2023-2031
Contact Data
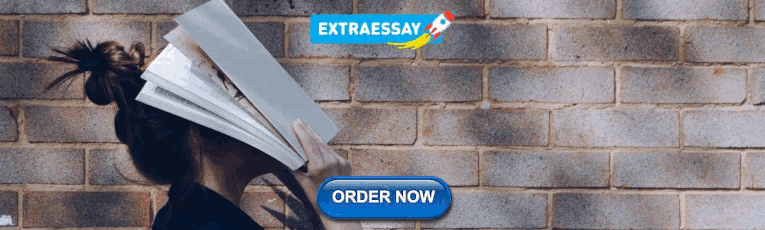
IMAGES
VIDEO
COMMENTS
Clinical research can be completed in two major steps: study designing and study reporting. Three study designs should be planned in sequence and iterated until properly refined: theoretical design, data collection design, and statistical analysis design.
This article is a practical guide to conducting data analysis in general literature reviews. The general literature review is a synthesis and analysis of published research on a relevant clinical issue, and is a common format for academic theses at the bachelor's and master's levels in nursing, physiotherapy, occupational therapy, public health and other related fields.
Here we'll provide an intuitive understanding of clinical research results. So this isn't a comprehensive statistics course - rather it offers a practical orientation to the field of medical research and commonly used statistical analysis. ... If a research question is evaluated through the collection of data points and statistical analysis ...
There are 6 modules in this course. This course presents critical concepts and practical methods to support planning, collection, storage, and dissemination of data in clinical research. Understanding and implementing solid data management principles is critical for any scientific domain. Regardless of your current (or anticipated) role in the ...
Four textbooks complementary to the current production and written by the same authors are Statistics applied to clinical studies 5th edition, 2012, Machine learning in medicine a complete overview, 2015, SPSS for starters and 2nd levelers 2nd edition, 2015, Clinical Data Analysis on a Pocket Calculator 2nd edition, 2016, all of them edited by ...
There are 4 modules in this course. This course will prepare you to complete all parts of the Clinical Data Science Specialization. In this course you will learn how clinical data are generated, the format of these data, and the ethical and legal restrictions on these data. You will also learn enough SQL and R programming skills to be able to ...
As a repercussion, the scientific process cycle is broken, leaving researchers who want to reuse prior results with three options: 1. Re-run the analysis if the code and original source data are ...
While data management has broad applications (and meaning) across many fields and industries, in clinical research the term data management is frequently used in the context of clinical trials. 1 ...
Abstract. Data are a key resource for modern societies and expected to improve quality, accessibility, affordability, safety, and equity of health care. Dental care and research are currently transforming into what we term data dentistry, with 3 main applications: 1) medical data analysis uses deep learning, allowing one to master unprecedented ...
Data analysis methods are an integral part of modern clinical research. They are powerful techniques that enable researchers to draw meaningful conclusions from data collected through observation, survey, or experimentation. However, data analysis is a huge discipline with different paradigms, schools of thought and alternative methodologies.
Clinical Data Science Specialization. Launch your career in Clinical Data Science. A six-course introduction to using clinical data to improve the care of tomorrow's patients. Taught in English. 21 languages available. Some content may not be translated. Instructors: Laura K. Wiley, PhD. +1 more. Enroll for Free.
7. Understand the reasons for performing research that is reproducible from data collection through publication of results. 9. Distinguish between variable types (e.g. continuous, binary, categorical) and understand the implications for selection of appropriate statistical methods. Extensively covered by required coursework.
approaches in clinical trial data analysis. Adaptive designs, Bayesian methods, and machine learning SJIF Impact Factor 6.222 Review Article ISSN 2394-3211 EJPMR ... flexible clinical research.[3] Data management and analysis in clinical trials are closely intertwined with regulatory requirements and compliance. Regulatory agencies, such as the ...
Integrative data analysis (IDA), a novel framework for conducting the simultaneous analysis of raw data pooled from multiple studies, offers many advantages including economy (i.e., reuse of extant data), power (i.e., large combined sample sizes), the potential to address new questions not answerable by a single contributing study (e.g., combining longitudinal studies to cover a broader swath ...
Data and endpoints. The primary objective of this study was to develop an algorithm and assess the capabilities of ChatGPT in processing and interpreting a large volume of free-text clinical notes.
Clinical research depends heavily on the effective integration and analysis of diverse datasets to find meaningful insights and drive scientific discoveries. A well-designed database architecture is fundamental to managing, integrating and analyzing clinical research data efficiently.. In this article, we will learn about How Database Design Principles for Clinical Research Data Integration by ...
This indicates that anemia is an independent risk factor for TD. Furthermore, we employed MR analysis to validate this causal relationship (IVW, OR = 1.045, 95% CI: 1.020-1.071, p < 0.001). The exact mechanisms underlying the testosterone-lowering effects of anemia remain unclear, as both clinical and basic research in this area is scarce.
Module 2 • 41 minutes to complete. In this module, you'll learn about trial monitoring, which involves statistical methods to assess a trial while it is underway. These methods are used to assess safety, integrity, efficacy, recruitment, data collection, and data quality. What's included. 4 videos 1 quiz.
212 Carnegie Center, Suite 301, Princeton, NJ 08540, USA. Phone 703.538.7600 - Toll free 888.838.5578. Cookie Settings
Objective To determine the efficacy of psilocybin as an antidepressant compared with placebo or non-psychoactive drugs. Design Systematic review and meta-analysis. Data sources Five electronic databases of published literature (Cochrane Central Register of Controlled Trials, Medline, Embase, Science Citation Index and Conference Proceedings Citation Index, and PsycInfo) and four databases of ...
Design and data analysis. We examine the Egyptian law vis-à-vis France's and Sweden's framework, considering the obligations that stem in regards to clinical trials from the CTR. Furthermore, we examine these regulations in light of the Ethical Framework for Biomedical Research by Emanuel et al. [9, 10]. Indeed, we will consider the EU ...
Data analysis methods are an integral part of modern clinical research. They are powerful techniques that enable researchers to draw meaningful conclusions from data collected through observation, survey, or experimentation. However, data analysis is a huge discipline with different paradigms, schools of thought and alternative methodologies.
Background In the aftermath of the COVID-19 pandemic, there has been a surge in human metapneumovirus (HMPV) transmission, surpassing pre-epidemic levels. We aim to elucidate the clinical and epidemiological characteristics of HMPV infections in the post-COVID-19 pandemic era. Methods In this retrospective single-center study, participants diagnosed with laboratory confirmed HMPV infection ...
Dublin, April 30, 2024 (GLOBE NEWSWIRE) -- The . Global Cancer Research Technologies Analysis Report 2024-2032: Key Players Striving for Innovation and Market Dominance in Genomics Technologies ...