
- SUGGESTED TOPICS
- The Magazine
- Newsletters
- Managing Yourself
- Managing Teams
- Work-life Balance
- The Big Idea
- Data & Visuals
- Reading Lists
- Case Selections
- HBR Learning
- Topic Feeds
- Account Settings
- Email Preferences
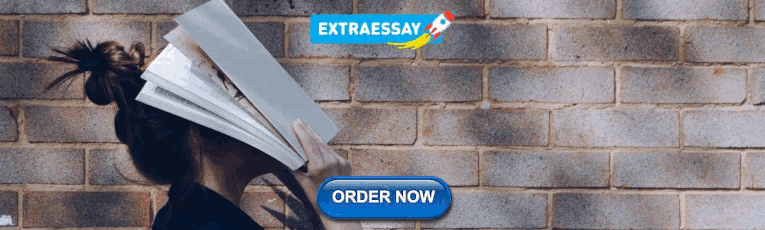
Companies Are Failing in Their Efforts to Become Data-Driven
- Thomas H. Davenport

According to a recent survey.
The percentage of firms identifying themselves as being data-driven has declined in each of the past 3 years — from 37.1% in 2017 to 32.4% in 2018 to 31.0% this year. These sobering results and declines come in spite of increasing investment in big data and AI initiatives. Whatever the reasons for the failure to achieve transformational results from data initiatives, the amount of data continues to rise in business and society. Analytical decisions and actions continue to be generally superior to those based on intuition and experience. The companies in the survey are investing heavily in big data and analytics. In short, the need for data-driven organizations and cultures isn’t going away.
Becoming “data-driven” has been a commonly professed objective for many firms over the past decade or so. Whether their larger goal is to achieve digital transformation, “compete on analytics,” or become “AI-first,” embracing and successfully managing data in all its forms is an essential prerequisite. Consistent with these goals, companies have attempted to treat data as an important asset, evolve their cultures in a more data-oriented direction, and adjust their strategies to emphasize data and analytics.

- Randy Bean is the author of Fail Fast, Learn Faster: Lessons in Data-Driven Leadership in an Age of Disruption, Big Data, and AI . He is a contributor to Harvard Business Review, Forbes, and MIT Sloan Management Review, and The Wall Street Journal, and is Innovation Fellow, Data Strategy with Paris-based Wavestone (EPA: WAVE) and Founder/CEO of NewVantage Partners, acquired by Wavestone in December 2021. You can contact Randy Bean at [email protected] and follow him on LinkedIn .
- Thomas H. Davenport is the President’s Distinguished Professor of Information Technology and Management at Babson College, a visiting scholar at the MIT Initiative on the Digital Economy, and a senior adviser to Deloitte’s AI practice. He is a coauthor of All-in on AI: How Smart Companies Win Big with Artificial Intelligence (Harvard Business Review Press, 2023).
Partner Center
- Trust Center
- Services Status
- NetApp On-premises
- Cloud Volumes ONTAP
- Amazon FSx for ONTAP
- Azure NetApp Files
- Google Cloud NetApp Volumes
- Copy and Sync
- Edge caching
- Backup and recovery
- Disaster recovery
- Replication
- Observability
- Classification
- AIOps & storage health
- Centralized File Storage
- Cyber Resilience
- Disaster Recovery
- Electronic Design Automation
- Google Cloud
- Workload Migration
- Cloud Storage
- Hybrid Cloud
- TCO Google Cloud
- TCO Cloud Tiering
- TCO Cloud Backup
- Cloud Volumes ONTAP Sizer
- Global File Cache ROI
- Azure NetApp Files Performance
- Cloud Insights ROI Calculator
- AVS/ANF TCO Estimator
- GCVE/CVS TCO Estimator
- VMC+FSx for ONTAP
- NetApp Keystone STaaS Cost Calculator
- CVS Google Cloud
- NetApp Community
- Success Stories
- Global Availability Map
- Resource Library
- Professional Services
- Cloud Solution Providers
- Data Protection
- AWS Big Data
- AWS Database
- AWS High Availability
- AWS Migration
- AWS Snapshots
- Infrastructure as Code AWS
- All Blog Posts
- Azure Backup
- Azure Big Data
- Azure Cost Management
- Azure Database
- Azure High Availability
- Azure Migration
- HPC on Azure
- Infrastructure as Code Azure
- Linux on Azure
- SAP on Azure
- Google Cloud Backup
- Google Cloud Database
- Google Cloud Migration
- Google Cloud Pricing
- Google Cloud Storage
- Cloud Backup
- Cloud Backup Services
- Backup Strategy
- Ransomware Protection
- Ransomware Recovery
- Kubernetes on AWS
- Kubernetes on Azure
- Kubernetes Storage
- Kubernetes Data Management
- CI/CD Pipeline
- Cloud Migration
- Desktop as a Service
- Elasticsearch
- Hybrid Cloud Management
- Muticloud Storage
- OpenShift Container Platform
- Virtual Desktop Infrastructure
- VMware Cloud
- Cloud Database
- Digital Transformation
- Cloud Automation
- Help Center
- Get Started
Bad Data Governance: A Real-Life Example (and How to Avoid It)
Subscribe to our blog.
Thanks for subscribing to the blog.
March 31, 2022
Topics: cloud data sense advanced data governance.
Bad data governance can pose significant risks to virtually any organization in any industry. But for an enterprise at global scale, the consequences can be huge.
That's the issue facing one multinational security company that left highly sensitive data in one of its Amazon S3 buckets exposed, allowing anyone to access nearly 3 TB of company data without authentication.
The company, which is a world leader in security products and services, used the misconfigured bucket to store approximately 1.5 million files containing information relating to its employees and business operations. Was there any way this exposure could have been avoided?
This post discusses the key facts of the story, the likely impact of the breach, and how data governance solutions like Cloud Data Sense can help bolster security postures to help avoid such crises.
Use the links below to jump down to the sections on:
What Information Was Exposed and When?
Impact of the breach, how cloud data sense reduces risk of exposure, multi-purpose data governance solution.
The data leak was discovered on 28 October 2021 by SafetyDetectives—a global group of cybersecurity experts, privacy researchers, tech reviewers and ethical hackers—following which the story was widely reported.
The organization found photographs of ID cards of airport and Securitas staff based in Colombia and Peru. These showed personal information about employees, such as their names, photos, job roles and national ID numbers.
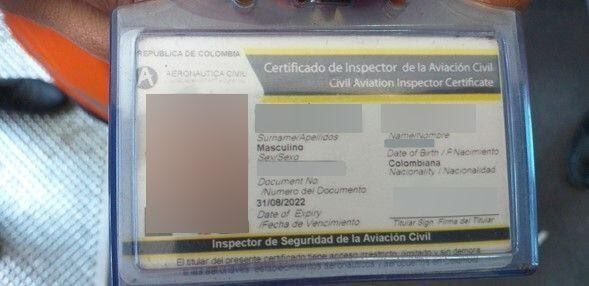
It also found other potentially sensitive information in photos of aircraft and luggage in baggage handling areas. Some also included sensitive data about other aviation industry companies associated with Securitas.
Furthermore, the photos contained exchangeable image file format (EXIF) metadata, such as the time, date and GPS locations of when and where the photos were taken.
In addition to data relating to airports in Colombia and Peru, SafetyDetectives believes sensitive information in connection with the security firm's services to numerous other industries has potentially been exposed.
SafetyDetectives said it reported the issue to both Securitas and Sweden's Computer Emergency Response Team (CERT) on 28 October 2021. Securitas then closed the bucket five days later.
However, SafetyDetectives said it didn't know how long the bucket had been left open before it discovered the misconfiguration. It was also unable to confirm how many people were affected by the leak or whether the contents of the bucket had fallen into the wrong hands.
It's therefore unclear as to what the full extent of the breach will be on the individuals concerned. Likewise, SafetyDetectives could not confirm how many organizations were affected beyond four identified airports in Colombia and Peru.
Nevertheless, the exposure poses a risk to travelers and airport staff, where security is of paramount concern. Criminals could also use the information to scam airline customers or create fake staff ID cards, through which they could impersonate airport employees in order to steal goods and possessions.
In addition to staff infiltration and reputational damage, Securitas could face sanctions for breaching data protection regulations in both Colombia and Peru, including financial penalties up to the equivalent of US$400,000 and US$122,000 in each of the countries respectively.
In a world where bad data governance can easily lead to leaked data and cyberattacks, companies need as much awareness of their data as possible. To improve data governance across any kind of storage environment, NetApp offers Cloud Data Sense .
Cloud Data Sense is a data discovery, classification, and optimization tool that's designed to help you maintain visibility and control over your information assets. It offers a range of features to support your data governance objectives, helping you:
- understand what types of data you store and where it resides
- set data retention policies
- identify and remove stale and duplicate data
- adjust permissions to data
- automatically generate reports on data
Check out this video to see Cloud Data Sense at work.
It is driven by powerful AI algorithms that provide contextual understanding of data, ensuring accurate discovery and classification of personal information. It supports both structured and unstructured data. And it natively integrates with a wide range of different storage repositories—including Amazon S3.
What makes Data Sense most relevant in this case, is a feature that can help you prevent accidental exposure of sensitive information. You can do this by simply searching for files with insecure file permissions. Alternatively, you can use the Investigation tab, which can show you where your sensitive data is located and whether it is stored in files that are open to the public or to anyone within your organization.
To help avoid a case such as the one discovered by SecrutiyDetectives detailed above, Data Sense provides the tooling it needed to spot such misconfigurations and help prevent exposure of the data in Amazon S3 buckets. Not only that, but it can do so in less than a minute—without the use of complex tooling or a command-line interface.
Cloud Data Sense provides a whole host of other capabilities to help you understand your data and manage it more effectively. Many of these could've played a similarly important role in helping avoid situations similar to the one Securitas found itself in through data governance shortcomings.
- Data discovery: Cloud Data Sense can help keep admins properly in the picture about the data owned and can reduce the scope for insecure information to slip through the net.
- Data classification: Through this capability Data Sense users can get clear insights into the types of data they store in S3 buckets and can bein a better position to take protective measures accordingly.
- Data ownership: Using the solution's file tagging capability , users are able to assign files to specific people within the company. That way, it’s possible to immediately establish lines of responsibility, allowing quick and efficient collaboration over file permission issues—without having to rely on just the names of files.
- Data optimization: Data Sense helps you identify stale and unnecessary data. This can help determine whether the information stored in S3 buckets is strictly necessary to retain. It can also streamline the data being stored, thereby reducing the impact of exposure.
The Securitas breach is a classic example of bad data governance and one of many high-profile cases of publicly exposed S3 buckets.
But it's important to remember that permission issues aren't just restricted to Amazon S3. They can put data at risk in virtually any type of storage repository.
That's why Cloud Data Sense provides off-the-shelf support for a wide range of different database management systems and storage environments, giving customers easy-to-use data governance capabilities across their entire data inventory.

Product Evangelist
Alation Help Center
Why Your Data Governance Strategy is Failing
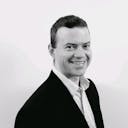
By Aaron Bradshaw
Published on October 5, 2021
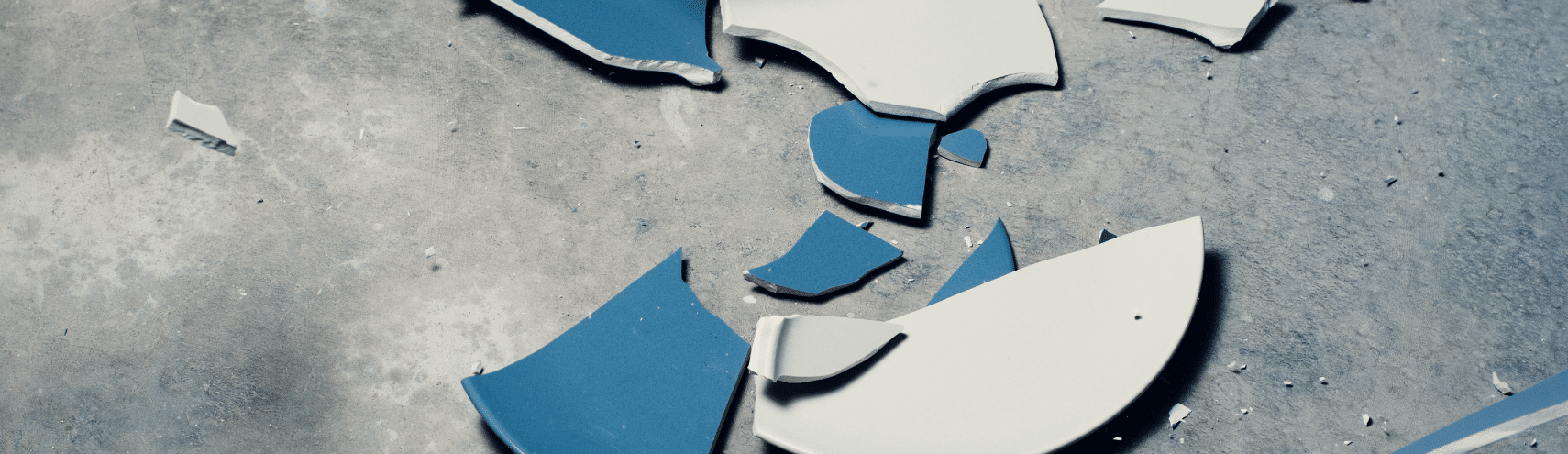
- What is data governance and how do you measure success?
Data governance is a system for answering core questions about data. It begins with establishing key parameters: What is data, who can use it, how can they use it, and why? Answers will differ widely depending upon a business’ industry and strategy for growth.
But what comes after these parameters are set? What does success look like? And how can leaders ensure their governance initiatives contribute to larger organizational goals?
The first step towards a successful data governance strategy is setting appropriate goals and milestones. These goals must also be SMART— Specific, Measurable, Attainable, Relevant, and Time-based. This best practice has been the foundation of the world’s most profitable organizations across all industries and business sectors for decades. Yet, so many companies today are still failing miserably in implementing data strategy and governance protocols.
- Why is your data governance strategy failing?
To measure failure vs. success, governance leaders must actively monitor all governance efforts. According to the Gartner report, The State of Data and Analytics Governance Is Worse Than You Think , approximately 80% of businesses readily acknowledge that high-quality data governance is essential to achieving long-term business goals, objectives, and outcomes.
However, about 50% of those surveyed also admit that they do not assess, monitor, or measure their data governance systems. Perhaps even more alarming: fewer than 33% expect to exceed their returns on investment for data analytics within the next two years.
Gartner further estimates that 60 to 85% of organizations fail in their big data analytics strategies annually (1). If this survey is trustworthy, then most business leaders are already fully aware of the critical importance of a detailed and well-thought-out data governance methodology.
So, why is YOUR data governance strategy failing? The answer may be more straightforward than you think.
- Common data governance challenges
The main reasons that a company’s data strategy and governance protocols fail to deliver are somewhat universal, regardless of the industry sector. For organizations to succeed in monitoring, managing, and optimizing their data resources, they must always keep the importance of their data-driven initiatives at the top of all strategic planning agendas.
Without a doubt, no company can achieve lasting profitability and sustainable growth with a poorly constructed data governance methodology . Today, all companies must pursue data analytics, Machine Learning & Artificial Intelligence (ML & AI) as an integral part of any standard business plan. The inevitable trend towards the digital economy leaves no room for companies that do not adhere to this core principle. Yet there is often lack of awareness of the trustworthiness (or lack thereof) of the data that these algorithms are being trained on.
It is commonplace for a company to create an enterprise data governance strategy that fails to even consider the end user. Most data governance initiatives focus on outcomes and compliance — rather than efficiency, scalability, or ease of use for the average human . These divergences of focus can lead to consumers feeling bogged down by overly complicated processes or leadership teams being unable to see initiative investments reap the desired rewards of their predictive business success metrics. (1).
Over time, this failure in strategy will lead end users to sidestep these practices completely. They may simply move and copy data without going through all of the necessary protocols related to data security and other best-practice standards. Below is a list of primary reasons covering why implementing good data governance policies can be so tricky.
Top 3 Roadblocks to Successful Data Governance
Roadblock #1: Governance Committee Challenges
Lack of focus on the right areas. In many cases, data strategy and governance protocols fail because the governance committee members focus more on their day-to-day tasks rather than the defining and monitoring of company-specific metrics, rules, and KPIs.
Lack of commitment. Governance strategies can fail when the company is more focused on talking up the ideals and objectives of the project without fully committing to its success. Management essentially talks the talk without walking the walk.
Improper priorities. When the committee faces one of two rather extreme scenarios, governance strategies fail. Either the program is so complex that substantive issues remain unaddressed, or the system becomes buried in trivial details that lack significance or relevance. One typical example of the latter is the use of “data cops” to police their lower-level end users.
Too much on their plates. It is also common for data governance strategies to fail because committee members are responsible for far too many projects. The committee often spends more time arguing than making progress towards creating favorable outcomes and results.
Incomplete data. If managers rely on incomplete or unproductive metrics, this can obstruct strategy. For example, it may be helpful to track specific daily activities or benchmarks for all data-related processes.
Too much time discussing the menial before getting to the meaningful. Numerous committees spend hours deliberating over every word in a Glossary definition, then 6 months down the line leaders complain there hasn’t been enough value shown
Roadblock #2: Data problems and inconsistencies
Inconsistencies in expectations can create enormous negative issues regarding data quality and governance. With so many missed signals and crossed wires, employees begin throwing their hands up in the air out of frustration.
A lack of a shared vocabulary across different departments within the organization can also lead to inconsistencies in data reporting, making it more difficult to launch or adhere to an effective data governance strategy. The negative feedback loop that follows creates even more confusion and generates even more insufficient insights.
A typical example might involve different employees using differing calculations for essential business metrics. The situation worsens when each employee performs these calculations in standalone none-systematic solutions that are not synced or disconnected from the company’s centralized information hub.
Roadblock #3: Silos Breed Misunderstanding
A data silo is an island of information that does not connect with other islands. Typically, these data silos will prevent two-way flows of data outside and inside of the organization. This disconnect often precludes the company from performing analytical reporting tasks efficiently and accurately.
Silos of understanding represent the same concern, but symbolize how people’s understanding and trust in the data suffer if they work in isolation. If the same piece of information means 5 things to 5 people, no BI report, Analysis or model will have the same meaning!
A big reason a data governance strategy fails is that it lacks a solid or robust knowledge hub to house its information and accumulated metadata. This disconnect between departments can lead to many different issues related to ineffective communication, misinformation, and poor decision-making.
- Data governance and AI
Absence of data governance is likely to lead to poor quality data. Bad data can spell trouble for AI systems . One common issue arises from the misinterpretation of raw data derived from different data sources — including those siloed in dispersed physical archives, cloud-based servers, and other digital databases.
This situation is also common when the company lacks a unified way to store its information, or if the company lacks data quality checks on data used to run AI and ML. The lower the quality of the data going into a model, the less reliable the output is going to be – and this can open up risk of regulatory fines for biased models .
The result of this scenario is that employees with different skills and tools will read the data differently, which leads to incorrect inferences and conclusions. These inconsistencies can then lead to poor decisions or even create security issues related to incorrect data usage in various reports and programs.
- Aligning data strategy and governance with business outcomes
The strategic goal of data governance is to align decisions-in-the-making with targeted business outcomes. This connection should be present at all levels of an organization, from a department and division level to the top-tier leadership. The entire organizational hierarchy must thoroughly understand how data initiatives tie to the company’s overall profitability and success. To achieve this objective, forward-thinking enterprises align data strategy with governance.
How to Align Data Strategy with Data Governance: Key Steps
Develop measurable KPIs.
Connect the KPIs to the relevant data resources to achieve the desired targets.
Ensure that both the metrics and the assets are linked clearly to stakeholder and company value.
Establish a data governance program that drives business value by aligning team roles to KPIs.
The data quality management team in coordination with the data governance committee will then familiarize the organizational hierarchy on how the enterprise data governance strategy will help each department achieve their related goals and objectives.
During these conversations, another important goal of the data quality management team is to nurture employee buy-in and recruit peer support. In doing so, the chances of long-term success rise exponentially.
The failure to govern data is hurting organizations of all sizes, industries, and business sectors. The irony is that most executives are acutely aware of the problem—but they aren’t doing much about it. Having a detailed plan of action from the very beginning streamlines the entire process.
- Picking the Right Data Governance Tools
Every enterprise will eventually face challenges regarding its data governance policies. Issues involving data quality, visibility, and security can be both highly complex and somewhat expected. Furthermore, many organizations in certain industries face a growing onslaught of regulatory scrutiny from organizations like HIPAA, SOX, GDPR, and CCPA. Is it any surprise that decision-makers are feeling overwhelmed?
At Alation, we help business leaders develop an effective data governance operating model and help them to better understand how data governance decisions should be made. We empower them to amplify the importance of an optimal data governance strategy throughout the organization while also providing guidance on how best to maintain this newfound enthusiasm for years to come.
With standardized, documented processes like the RACI matrix, data quality leaders will facilitate interdepartmental collaboration, communicate confidently about their data use protocols, and proactively resolve potential conflicts more quickly.
Alation’s active data governance framework delivers expertly governed data in real time by focusing on intelligent data policies, access controls, and privacy-enhancing technologies (PETs). By partnering with Alation, organizations will reap the following rewards:
Data discovery and classification: Alation’s automated data discovery platform saves time and effort by classifying and tagging global policies. These capabilities enable quick access to the information you need without going through a tedious manual inspection process — with faster turnaround times than ever before. Automated sensitive data discovery helps businesses find hidden assets of valuable insights quickly while also reducing their risk exposure.
Consistent data privacy: Alation has made it much easier for data teams to control the handling and employee access of sensitive data in a Snowflake data ecosystem. With one policy, they can apply the same settings across individual cloud instances without starting from scratch every time. This framework includes dynamic masking techniques, such as:
K-anonymization
Format-preserving masking
Joins on masked data
Differential privacy
Randomized response
And conditional logic capabilities that make implementing these controls easy while still protecting the most sensitive information.
Data monitoring and auditing: Data is a powerful tool for any company, but it can also be dangerous if sensitive information falls into the wrong hands. Alation’s automated data audit trails provide peace of mind for companies managing an overwhelming amount of valuable data. Our system helps track what data was accessed, by whom, when, and for what purpose . Meanwhile, data fingerprint capabilities can identify changes made over time in case anything questionable pops up down the line.
The reasons for data governance strategy failures are more commonplace than many business leaders might imagine. As the premium provider of professional data quality management and data governance consultancy services, Alation has the expertise and years of experience to help organizations of all industries and business sectors achieve success. For more information on developing an optimized data governance strategy, contact Alation today .
Gartner, “The State of Data and Analytics Governance Is Worse Than You Think” Saul Judah, Andrew White, 19 June 2020 GARTNER is a registered trademark and service mark of Gartner, Inc. and/or its affiliates in the U.S. and internationally and is used herein with permission
Tagged with
Related articles.
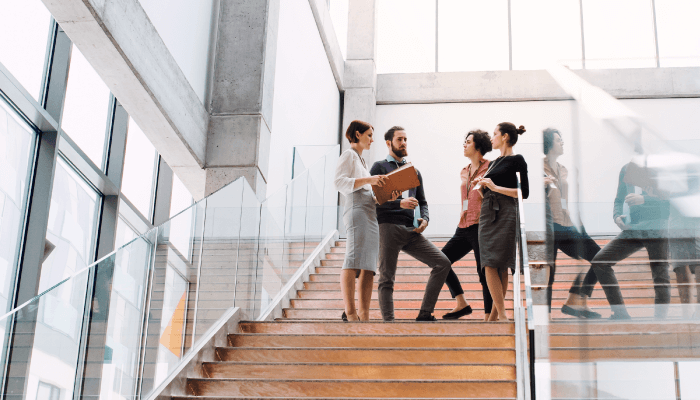
7 Benefits of Data Lineage to Businesses and How to Implement It
By Jesse Fröhling
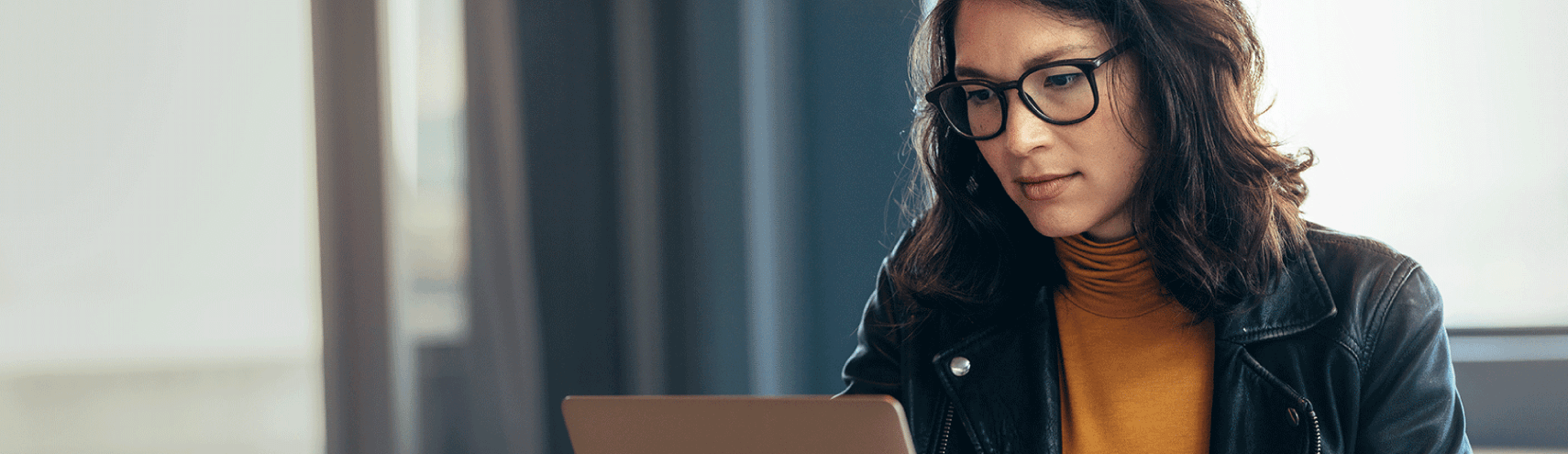
What is Active Data Governance?
By Talo Thomson
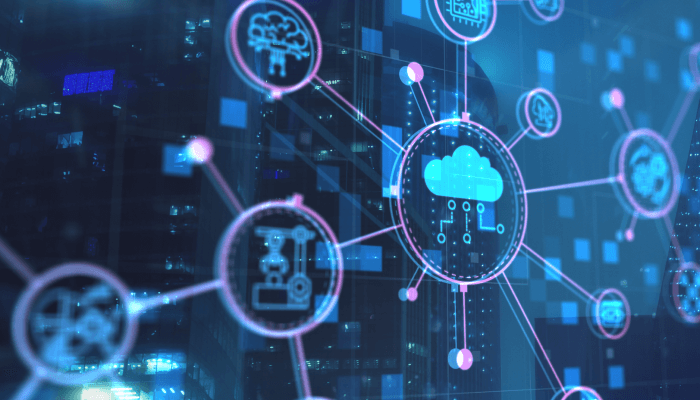
Unleashing the Power of Data Quality, Data Observability, and Data Governance on Alation With Snowflake, Anomalo, and dbt
By Mendelsohn Neil Chan
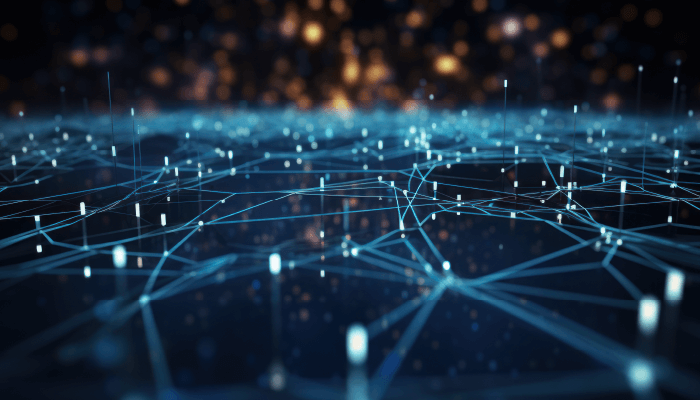
Maximizing Business Value with Data Products
By Michael Meyer
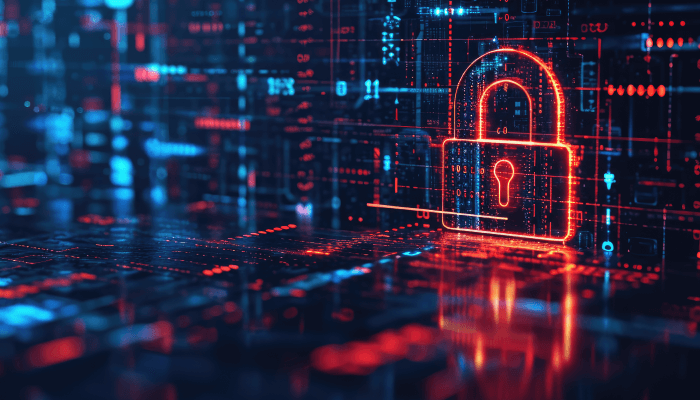
How to Create an Effective Data Retention Policy
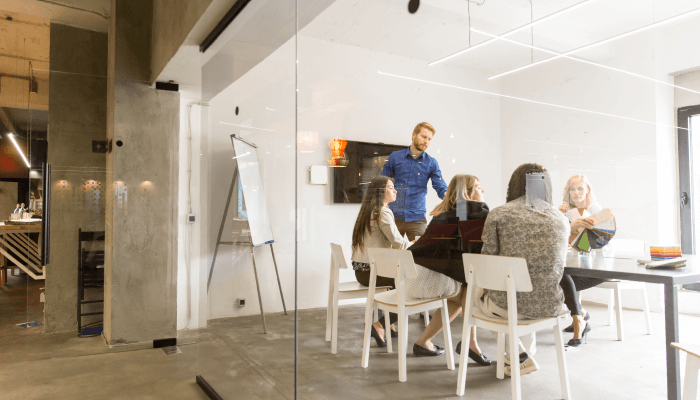
8 Reasons Why Startups Should Adopt a Modern Data Stack
By Jason Rushin
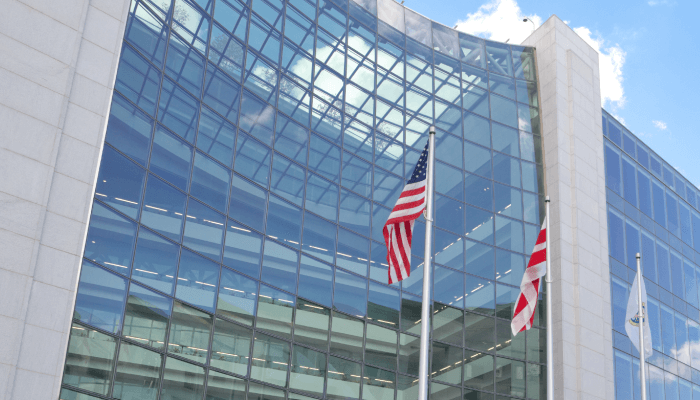
Federated Data Governance Explained
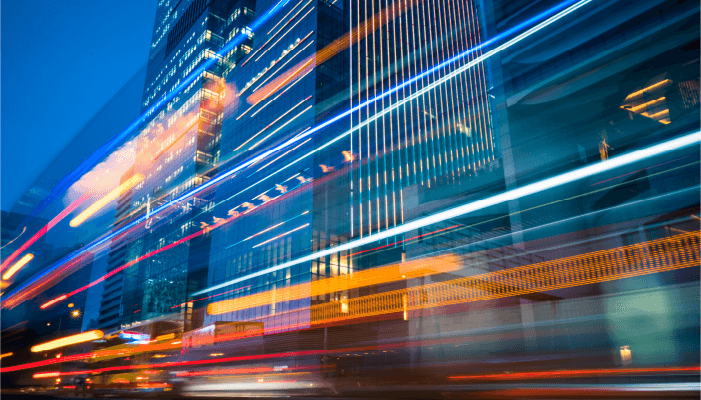
Your Data Culture Maturity Journey Starts With Knowing Where You Stand Today
By Nick Duce
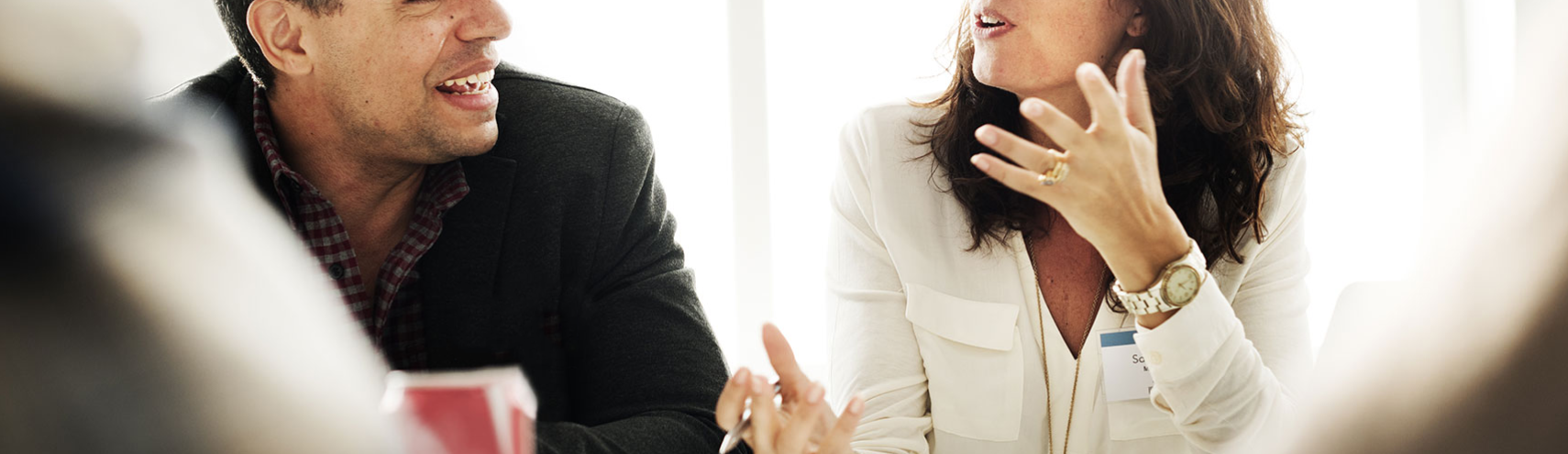
Data Governance for Dummies: Your Questions, Answered
By Jim Barker
- Questions? 877-634-9222
Why Companies Fail to Implement a Data Governance Strategy
Author: Bankim Sheth | | March 10, 2022
According to Gartner, only 20 percent of analytics insights will deliver business results through 2022. In other words, 80 percent of companies’ Big Data projects will fail and/or not deliver results.
There are many reasons for this failure, but poor (or a complete lack of) data governance strategies is most often to blame. This article discusses the importance of solid data governance implementation plans and why, despite its obvious benefits, many organizations find data governance implementation to be challenging.
Understanding Data Governance
Data governance (DG) should be the core element within the overall organizational data management plan. Proper implementation of data governance allows businesses to use their data resources to achieve desired business outcomes.
Ideally, a solid data governance program should include the data governance team, data stewards, and the governing body, often a steering committee (data governance council) comprising top organizational stakeholders. Together, this group creates policies/standards of data governance and the procedures implemented/enforced by data stewards and stakeholders of the organization.
What is Data Governance?
There are many complex definitions for data governance. Simply put, data governance is the “ set of processes/policies/strategies that manage the availability, usage/usability, security, and integrity of an organization’s enterprise business data .”
Data governance is critical in the present and future business landscape. It enables organizations to comply with ever-changing data privacy regulations and optimize their operations through sound, data-driven decision-making. An effective data governance program will ensure that the organization has trustworthy, single version of the truth, and consistent data, and prevents wrongful access/use and abuse.
Stakeholders of Organizational Data Governance
From business executives and IT professionals to data operators and end-users, several participants have key roles in ensuring data is collected, accessed, stored, and/or used appropriately. These are their specific roles:
- Chief Data Officer (CDO) – oversees the data governance implementation plan or program, and bears the highest responsibility for its success or failure.
- Data governance team – works on/coordinates the data governance program full time and reports to the DG manager/CDO, who also acts as DG manager.
- Data governance steering committee – business executives and representatives of data owners/operators who approve/govern DG policies and internal DG regulations and strategies.
- Data stewards – provide oversight of data sets to maintain data integrity and ensure implementation of policies from the committee and end-user compliance with the policies.
- Others – data modelers, data engineers, data architects, and data quality analysts also contribute to the DG process.
Steps to an Effective Data Governance Implementation Plan
A data governance program comprises the processes, rules, technologies, organizational structures, and policies that define how an organization implements its data governance. Every organization must document its DG framework and share/communicate it with all teams and data users.
Organizations will leverage DG software to automate various data governance and management tasks, called DG tools. These tools are often used together with tools that support master data management, metadata management, and data quality management.
Every data governance implementation plan must begin with the identification of custodians/owners of enterprise data assets. The CDO or another C-suite executive oversees creating the data governance program structure, including creating the DG team, identifying appropriate stewards, and convening the steering committee.
Once the structure is operational, the teams must develop policies and standards, authorization protocols, and protocols for access and usage. They must also implement rules that govern ongoing operations and define controls and audit procedures to ensure data consistency and operational compliance with internal and external regulations.
The data governance strategy should also define and document all data sources and procedures for its storage and security, i.e., how to protect it from unauthorized access, security attacks, and other mishaps.
Master Data Management vs. Data Governance
Data governance is often mistaken for Master Data Management (MDM), a closely related term. Master data refers to enterprise data that defines the subjects involved in business communications, events, and transactions–it defines the ‘who,’ ‘what,’ and ‘where’ of business subjects.
Therefore, MDM is a program that covers the operational processes and policies for manipulating master data to deliver understandably controlled, fit-for-purpose, and trusted master data. The rules implemented in MDM are defined within the data governance program, from creation and access of master data to its usage and eventual disposal.
Data governance does not operate as a separate process from master data management. However, it extends beyond MDM to govern and adjudicate all operational processes executed on enterprise data, master data, or otherwise. MDM requires data governance to function optimally.
The Benefits of an Effective Data Governance Program
The following are key benefits of having a solid data governance program:
- Breakdown data silos – data silos rise where teams operate without centralized coordination, resulting in different data sets that have undergone different manipulations.
- Harmonize organizational data – if all data in the organization is consistent, all operations will operate using the same data, increasing their efficacy and eventually improving performance.
- Ensure proper data usage – authorized users access and use the data as required to avoid errors and block potential abuse and misuse of personal, sensitive, or proprietary data.
- Balance privacy mandates with data collection practices – observe privacy regulations while collecting data to aid business decision-making.
- Harmonize data policies – create uniform policies and procedures to monitor and enforce adherence to said policies continuously.
- Improve data quality – this results in more accurate BI insights, eliminates data redundancy, decreases data management costs, and allows for stronger regulatory compliance.
There is a competitive advantage for the organization that has high-quality, uniform, and centralized data. Accurate insights result in accurate predictions/forecasts, and executives can work proactively to take advantage of opportunities or avert crises. This directly leads to increased ROI, revenues, and profits.
The Negative Impact of Data Governance Failures
Data governance failures can have disastrous consequences in the organization. The apparent result of non-existent, improper, or ineffective data governance is inconsistent, insecure, and illogically stored data. This makes it harder to merge, verify, and access data later. Incorrect data affects the quality of any analytics or business intelligence (BI) insights and reports gleaned from the data.
Weak data governance also impedes internal and external regulatory compliance. The company loses its customers’ trust after a disastrous breach. At worst, they have broken several laws and must pay hefty fines after the breach besides the lost revenue from defecting customers.
Other consequences of data governance failures include:
- Security breaches – the latest Identity Force report showed over 300 million personally identifiable information (PII) was breached in 2020. Poor DG can lead to breaches that expose customers to identity theft. Businesses take years to recover after such breaches.
- IP risks – businesses invest millions in research and development and can lose proprietary data on new and innovative products, services, and methods to external actors.
- Expensive storage – most companies don’t even know what data fills up their storage and end up storing irrelevant data from unknown sources. Effective DG enables organizations to store only valuable and business-critical information to decrease storage costs.
- Ransomware attacks – the worst outcome of a data breach is having the bad actors hold your company data hostage in an extortion scheme.
Common Reasons for Data Governance Issues
Many organizations don’t have a solid data governance implementation plan despite appreciating the importance of a solid data governance strategy. Some have documented their data governance strategy, but as their data ecosystem becomes more complex, the plan moves to exist only on paper.
There are several reasons for this:
Steering Committee
Implementing a data governance program may fail because the company does not wish to commit its resources to data governance efforts. Members of the DG steering committee often have other designations in the company, and their DG oversight role may play second fiddle to their primary job.
Business executives cannot be separated from the steering committee; understanding the business applications of data, they are best placed to define the rules, procedures, and metrics of the data governance strategy.
It is also easy for the steering committee to create a data governance program that is so high-level that no one in the organization can implement it. Conversely, they may become bogged down by the nitty-gritty of the program and fail to make progress building the foundational framework.
Unrepresentative Data
It is harder to implement a data governance strategy where different parts of the enterprise work with unexpected or unrepresentative data. End-users may apply data in ways the program makers didn’t expect because the strategy does not reflect the current business reality or other reasons.
Expectation gaps can result in data quality issues and disparate data sets that are more complex to integrate. Eventually, the business/stakeholders simply give up.
Inconsistent Data
Inconsistent data can result where different departments use different vocabulary to process their data. This creates confusion and leads to inaccurate/poor insights, and directly interferes with the initial data governance implementation plan.
Without a standardized process to define KPIs, the company will have disconnected reports, where the centralized assets don’t reflect the true health of the enterprise. There is no single version of truth to inform overall strategy, and the departments may end up undermining one another. In a vicious cycle, this makes it harder even to begin developing a data governance strategy.
Data silos are information repositories controlled by singular teams or departments and not visible across teams/departments in the organization. Isolated data leaves the organization unable to standardize its data repositories consistently, and other departments cannot benefit from the insights in the siloed data.
Business Value Demonstration
Many data professionals struggle right at the beginning – getting management to approve a data governance program. You can overcome this challenge by curating data quality disasters and connect how the program can prevent them while prioritizing the enterprise’s specific core objectives.
Data governance programs must be justified after implementation by developing quantifiable metrics that express how the data governance strategy adds value. It also helps to have the data governance implementation plan well-thought-out before approaching top executives.
Work with Datavail Experts to Implement Data Governance
Data governance failures cost businesses of all sizes billions of dollars every year. Just one company, Citigroup, was fined US $400 million in 2020 for ‘several longstanding deficiencies’ in their risk management and data governance practices.
Conversely, when implemented correctly, a data governance strategy can help to break down those pesky data silos, standardize and integrate your data, and provide accurate insight for business-wide action.
Do you want to learn more about creating and implementing a strong data governance program? Download our whitepaper to find solutions to the gnawing challenges of implementing your data governance strategy.
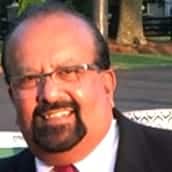
Blog Author
Bankim Sheth
Related Posts
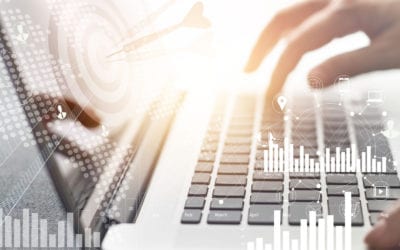
Oracle BI Publisher (BIP) Tips: Functions, Calculations & More
Check out these BI Publisher tips including functions & calculations so you can understand more about the production and support of BI Publisher reports.
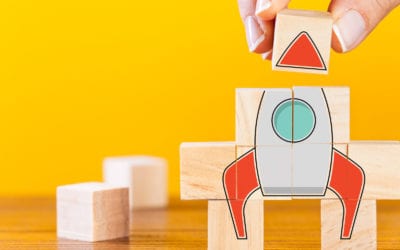
How to Index a Fact Table – A Best Practice
At the base of any good BI project is a solid data warehouse or data mart.
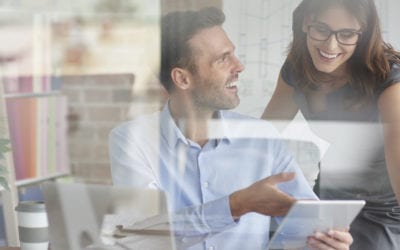
Qlik vs. Tableau vs. Power BI: Which BI Tool Is Right for You?
Tableau, Power BI, and Qlik each have their benefits. What are they and how do you choose? Read this blog post for a quick analysis.
Subscribe to Our Blog
Never miss a post! Stay up to date with the latest database, application and analytics tips and news. Delivered in a handy bi-weekly update straight to your inbox. You can unsubscribe at any time.
- content_search
Work with Us
Let’s have a conversation about what you need to succeed and how we can help get you there.
Work for Us
Where do you want to take your career? Explore exciting opportunities to join our team.
- What is data observability? Reliable data & AI delivered.
- Detect anomalies ML-enabled data anomaly detection.
- Triage incidents Get the right alert to the right team.
- Resolve the root cause Fix data issues in minutes.
- Measure data quality Measure what matters.
- Optimize cost and performance Rightsize runtime.
- Integrations The interoperability you need.

- Data quality monitoring and testing Deploy and manage monitors and tests on one platform.
- Report and dashboard integrity Produce reliable data your company can trusts.
- Data mesh and self serve data Empower data producers and consumers to self-serve.
- Customer-facing data products Launch and maintain performant and reliable products.
- Cloud migrations Deploy your warehouse/lake, transformation, and BI tools with confidence.
- Infrastructure and cost management Optimize your cloud storage and compute spend.
- Financial services
- Advertising, media, and entertainment
- Healthcare and life sciences

- Case studies
- DQ calculator
- GigaOm Data Observability Radar
- IMPACT Data Summit
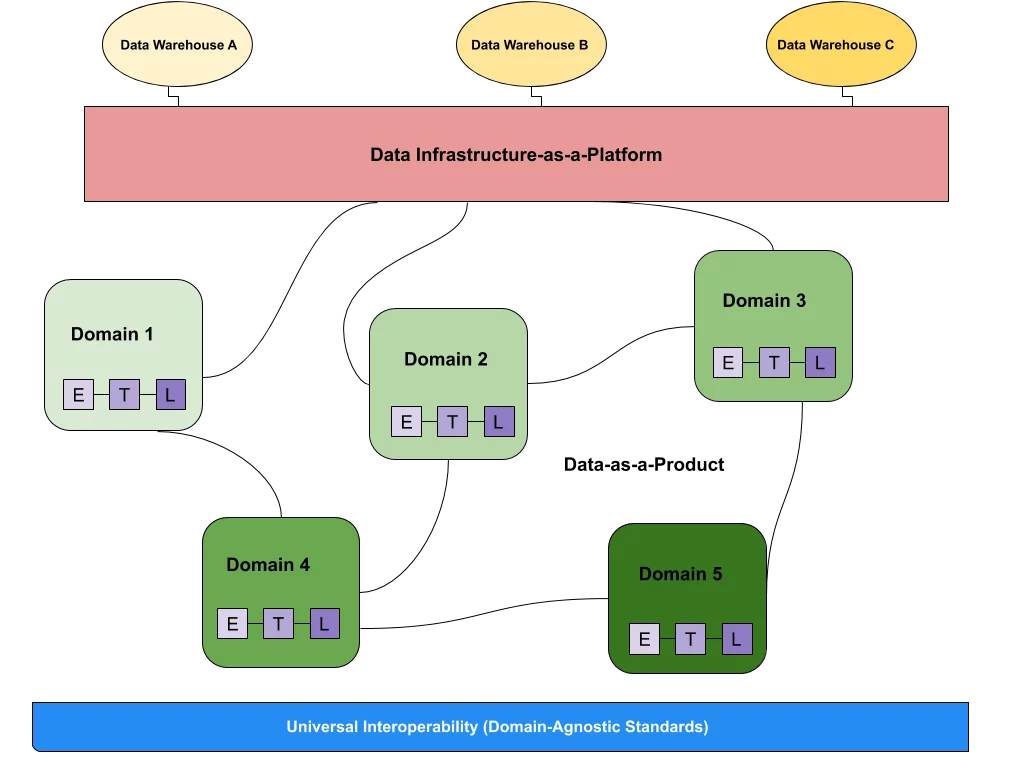
Updated Dec 19 2022
6 Reasons Why Companies Fail at Data Governance

Teresa Kovich
Teresa is a principal consultant at DAS42.

CEO and Co-founder, Monte Carlo. Proponent of data reliability and action movies.
Share this article
Everywhere we go in the cloud data space today, we’re hearing one message loud and clear: “you should be thinking about data governance”. It’s a sentiment that we wholeheartedly endorse, but we like to take it a little bit further – you should be thinking about data governance differently .
At DAS42, we offer services that help clients from retail startups to multinational media giants get value from their data quickly and scalably. Monte Carlo provides end-to-end data observability to help data teams discover and resolve their data issues faster—from ML-powered quality monitors and automatic field lineage to on-demand reliability insights and ultra-fast deployments. Together, we help data teams build and scale more reliable systems and data governance strategies.
In this article, we’ll share six of the top reasons that we have seen data governance initiatives fail for even some of the best data teams – and how you can avoid falling into the same traps.
You think data governance is about right and wrong
At a major ridesharing company, two teams had been struggling for months to reconcile their reporting. No matter what they did, they were never able to achieve the same results, even when they insisted that they were defining their metrics in the same way, having queried from the same tables and walked through the code line by line. DAS42 accepted the challenge, and what we found became one of our favorite examples of the criticality of data governance.
One team was using a filter on “mega-region = ‘US and Canada’”. The other team was using a filter on “country-code = 1 (US) OR 32 (Canada)”. These, we were told, were the same thing. But sure enough, we looked at the mapping table and found that country-code 1 included Puerto Rico, but the mega-region ‘US and Canada’ did not.
It wasn’t that one team was right and the other was wrong. It was that neither of them completely understood what was being included in or excluded from their metric .
This is one of the most common data governance errors we see, and it’s led to some of the most pernicious and disheartening data woes for our clients.
It is not enough to point at a query or output and say “this is the source of truth” – to say “this number is right, and any other number is wrong”. This is because fundamentally, data governance is not about right and wrong . Both of the teams mentioned above had numbers that would be valid, depending on the use case – depending on what the stakeholder meant when they said “we want these numbers for US and Canada”.
That’s not to say there’s no such thing as a “wrong” number. Of course, we’ve also seen analysts try to exclude test accounts with a clause like “last name does not contain ‘test’”, which is a terrible shame for real human beings named with surnames like Battesten or Contestanza. (We have yet to encounter a real person named Testy McTesterson, but stranger things have happened.)
But it is not useful to enshrine a number – a metric definition, for example – as right if you are not able to explain what differentiates it from the others.
Shift your mindset from “we need to know which of these numbers are right and wrong” to “we need to understand these numbers, what goes into them, what makes them different, and in what contexts it would be appropriate to use them”.
You over-emphasize executive buy-in
Don’t get us wrong – of course having respected senior team members as champions for your data governance initiatives is a powerful tool in your toolbox. But it’s also absolutely essential to have buy-in from the people handling the data on a day-to-day basis as well—your front-line staff, your engineers, your project managers—the people who will have to make changes to their processes in order for you to fully implement a governed framework.
Before you start trying to secure leadership and stakeholder buy-in, it’s important to be transparent about the current state of your data governance strategy. Consider how you might answer the following questions:
- How do you measure the data quality of the assets your company collects and stores?
- What are the KPIs or overall goals you’re going to hold your data governance strategy accountable for meeting?
- Do you have cross-functional involvement from leadership and data users in other parts of the company?
- Who at the company will be held accountable for meeting your strategy’s KPIs and goals?
- What checks and balances do you have to ensure KPIs are measured correctly and goals can be met?
In the same way that having visibility into your data pipelines makes it easy to ensure high data quality, transparency into both your data governance strategy and its incremental progress will be critical when it comes to keeping everyone informed and accountable on your leadership team.
You think of implementing data governance as a project
There are two primary mistakes data leaders make when it comes to implementing a data governance framework . The first is the “set it and forget it” mentality. They think of data governance as an initiative to be completed, and that once it’s done, they’ll be on to the next. The second mistake is similar, which is the inclination to over-govern. While understandable, both approaches miss the heart of what data governance is intended to be.
Just like data ingestion or quality assurance, data governance is a process. It may require more intentional effort on the front end, but data governance isn’t a project that’s ever truly completed. As your company grows and evolves, your metric definitions will evolve along with it. The problem with the approaches mentioned above is that they don’t leave room for change.
Remember that data governance is less about the right and wrong of your metrics and more about changing your company’s cultural approach to those metrics. Don’t think of data governance as something to be completed—think of data governance as something to be adopted.
You think a tool will do all the heavy lifting
The data landscape is crowded with tools, managed services, methodologies, and frameworks, and we don’t deny that many of them really can help you take your data to the next level. Data observability is one of those. But, as highlighted above, data governance isn’t a single tool, or a workflow you set up once and all your problems are solved—it’s an ongoing process that involves judgment, decision-making, and differentiation.
While data catalogs and other governance solutions often market themselves as the answer to all of your company’s data problems, many data leaders find these tools lacking in even the most rudimentary aspects when it comes to manual requirements.
There are tools and companies that can make things simpler, automate processes, and help you to step outside of your assumptions. But for all the innovation we’ve seen across the data landscape over the last decade, there’s still no technological replacement for the difficult (and immensely gratifying) work of talking about your company, your processes, your definitions, your measurements, and your goals.
You think you can focus on data governance alone
Some of the most earnest stakeholders we’ve seen profess their commitment to implementing data governance by putting it at the top of their priority list – and putting everything else on hold. They want to govern everything , and they want to do it now . This can cause several problems.
First, it can lead to frustration and disenchantment from stakeholders as they fail to see material gains in the form of new deliverables. (Of course, some of us think that data governance is tremendously exciting, but probably not everyone at your company is this particular variety of nerd.)
Second, if you fail to take an iterative approach, practical lessons might come too late. We’ve seen companies “govern everything” and then, a couple weeks after they’ve finished and everything is implemented, see something major that they missed… and they missed it across the board. We mentioned above the criticality of getting buy-in from stakeholders at all levels.
As you start on a data governance journey, choose a few key examples. These might be fundamental to your business – you may need to define your categories or product hierarchies, to determine your net operational revenue or availability rate, or you might need to define what you mean when you use the word “customer”. (How do you exclude those test accounts, anyway?) Choose two or three high-impact areas to govern. Build your governance muscles, and make it sustainable – something that you can do alongside your day-to-day work and “business-as-usual” deliverables.
And give yourself time to learn lessons as you move on to the next, and the next.
You don’t know what data actually matters
Once you’ve identified the first few domains you’d like to focus your energies on, the next step is to ensure that the data you’re governing is actually worth being governed. Not all data is created equal, and in today’s economic climate, it is not inaccurate to say that some data is worth more than others. For instance, data forecasting your company’s revenue next quarter is probably more worthy of your attention than a duplicate table sitting in a dusty corner of your Snowflake warehouse.
Before you roll out your governance strategy, identify what data actually matters most to your business and prioritize accordingly. Having visibility into your most critical assets – no matter what stage of the pipeline they’re in – can ensure that your team is a) spending time building a data governance program for data the business is actually using and b) tell you if the data is available, fresh, and, most importantly, accurate.
One way to do this? Set service-level agreements (SLAs) and service-level indicators (SLIs) for data assets with the most eyeballs, for instance, the table feeding your CFO’s quarterly metrics dashboard or that Salesforce data informing your ad campaigns.
Setting data reliability SLAs helps build trust and strengthen relationships between your data, your data team, and downstream consumers – whether that’s your customers or cross-functional teams at your company. Without these clearly defined metrics, consumers may make flawed assumptions or rely on anecdotal evidence about the reliability and trustworthiness of your data platform. In other words, data SLAs help your organization be more “data-driven” about data – and in turn, data governance.
So, let’s get started.
For most organizations, data governance is relegated to a few lone wolves responsible for convincing an entire team of “numbers people” to care about something that is inherently difficult to quantify. If it sounds like a trap, it’s because it is one – but it doesn’t have to be.
At the end of the day, the goal of your data governance strategy will be to ensure that teams across the entire company feel empowered to use data, and the only way to empower is to build trust and educate.
Our biggest suggestion: start your governance initiative on a few key functional areas (land and expand), tracking for a few key SLAs, and across a handful of critical data assets. In a world where bigger (data) is always better, sometimes it pays to start small.
Interested in learning more? Reach out to DAS42 or Monte Carlo to embark on your data governance journey.
Read more posts.

3 Takeaways from the 2019 Chief Data Officer Symposium
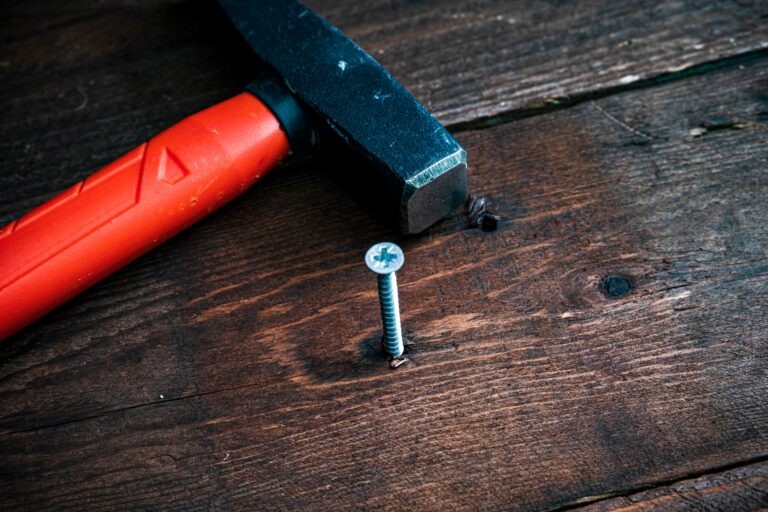
You’re (Finally) In The Cloud. Now, Stop Acting So On-Prem.
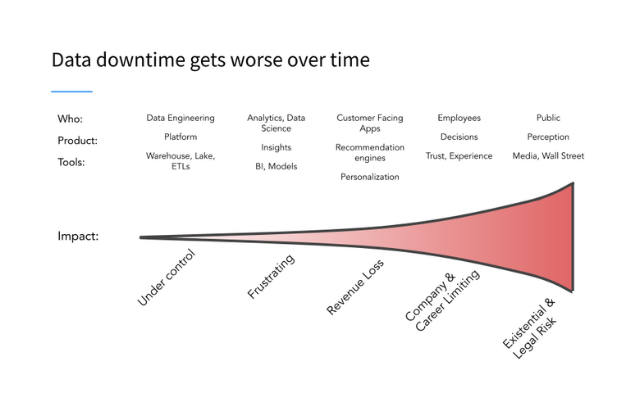
What is Data Downtime?
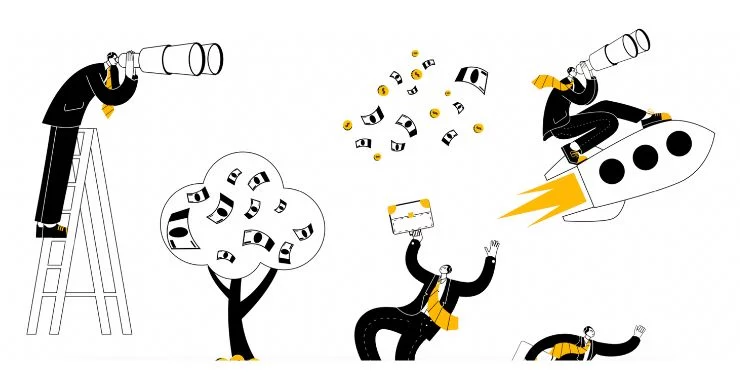
How Data and Finance Teams Can Be Friends (And Stop Being Frenemies)
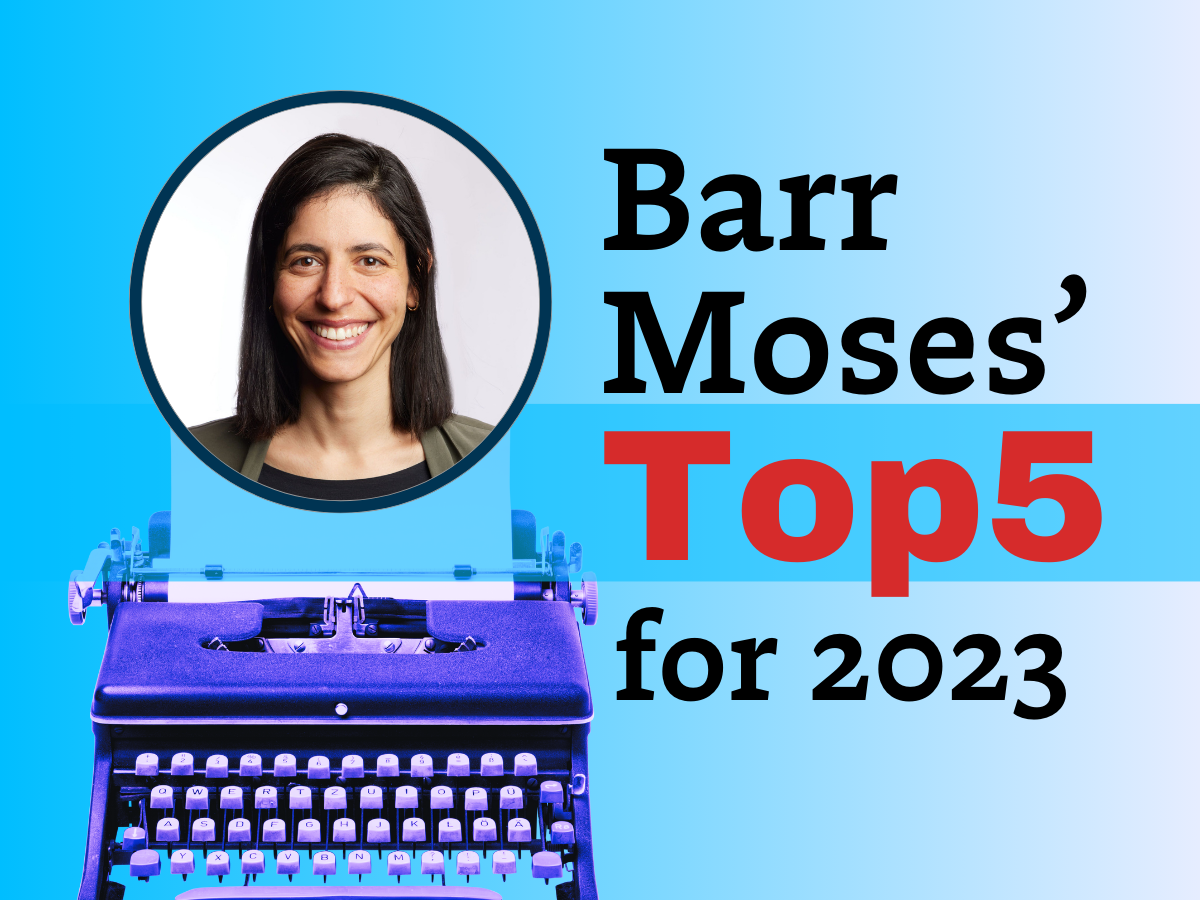
Barr’s Top 5 Articles of 2023
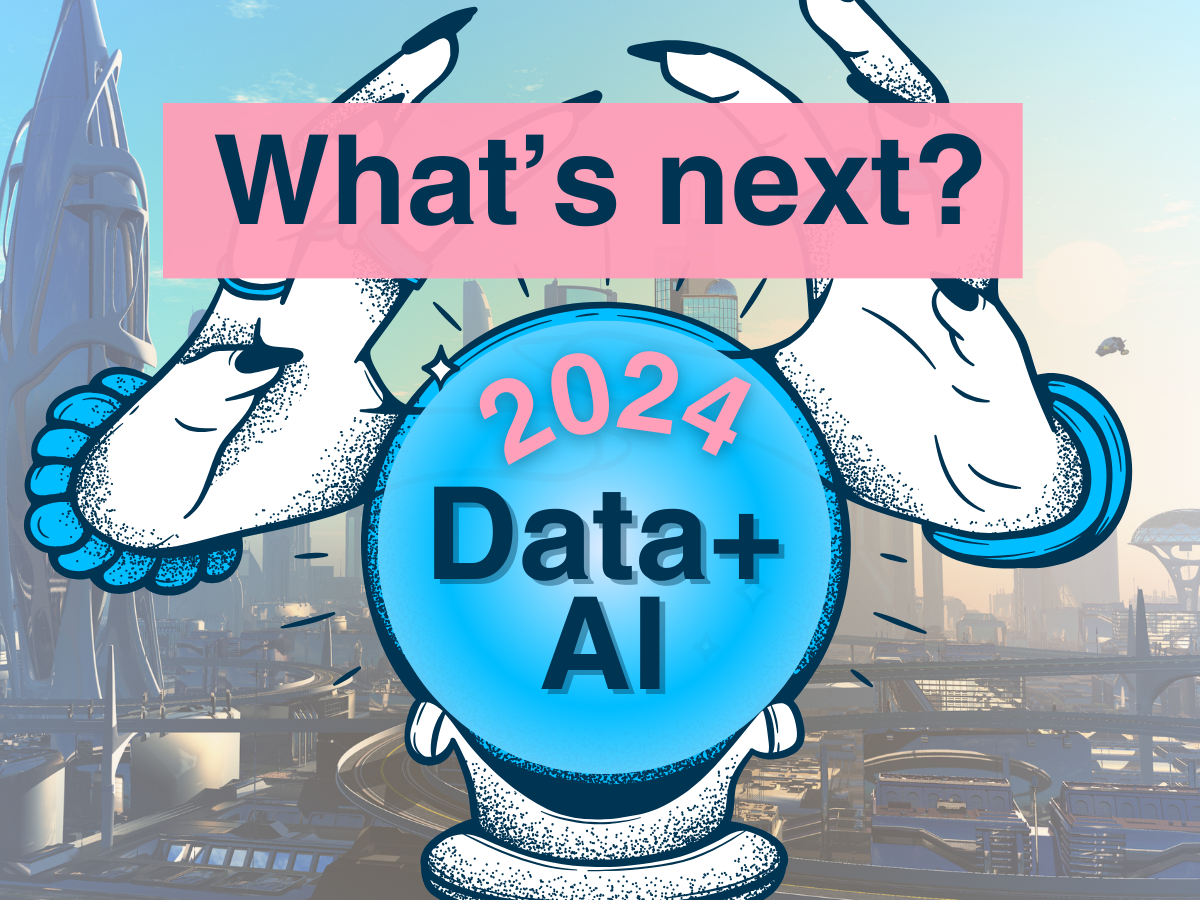
Top 10 Data Engineering & AI Trends for 2024
Designing data governance that delivers value
Executives in every industry know that data is important. Without it, there can be no digital transformation to propel the organization past competitors . There are no analytics driving new sources of revenue . Even running the basic business well isn’t possible. But for data to fuel these initiatives, it must be readily available, of high quality, and relevant. Good data governance ensures data has these attributes, which enable it to create value.
The problem is that most governance programs today are ineffective. The issue frequently starts at the top, with a C-suite that doesn’t recognize the value-creation potential in data governance. As a result, it becomes a set of policies and guidance relegated to a support function executed by IT and not widely followed—rendering the initiatives that data powers equally ineffective. In other cases, organizations try to use technology to solve the problem. While technology solutions such as data lakes and data-governance platforms can help, they aren’t a panacea.
Without quality-assuring governance, companies not only miss out on data-driven opportunities; they waste resources. Data processing and cleanup can consume more than half of an analytics team’s time, including that of highly paid data scientists, which limits scalability and frustrates employees. Indeed, the productivity of employees across the organization can suffer: respondents to our 2019 Global Data Transformation Survey reported that an average of 30 percent of their total enterprise time was spent on non-value-added tasks because of poor data quality and availability (Exhibit 1).
While it’s challenging to directly attribute value to data governance, there are multiple examples of its significant indirect value. Leading firms have eliminated millions of dollars in cost from their data ecosystems and enabled digital and analytics use cases worth millions or even billions of dollars. Data governance is one of the top three differences between firms that capture this value and firms that don’t. In addition, firms that have underinvested in governance have exposed their organizations to real regulatory risk , which can be costly.
Building the foundation for effective governance
While many organizations struggle to effectively scale data governance, some have excelled. For example, a leading global retailer, whose data governance was managed within IT, struggled to capture value from data for years. Then, as part of an enterprise-wide analytics transformation, it invested in educating and involving the entire senior-executive leadership team in data governance. It assigned to each executive leader (CFO, CMO, and so on) several data domains, or business-data subject areas, some of which, such as consumer transactions and employee data, spanned multiple functions or lines of business.
Once these leaders grasped the value of data governance, they became its champions. Within their domains, they selected representatives to act as data-domain owners and stewards and directly linked data-governance efforts to priority analytics use cases. They then worked in sprints to identify priority data based on the value they could deliver, checking in with the CEO and senior leadership team every few weeks. These efforts have begun to pay off, allowing the organization to stand up priority data domains over the course of a few months (versus years) and reduce the amount of time data scientists spend on data cleanup, accelerating analytics use-case delivery. The program continues to grow over time.
As the example demonstrates, effective data governance requires rethinking its organizational design. A typical governance structure includes three components:
- a central data management office (DMO), typically led by a chief data officer (CDO) , with a targeted data strategy and governance leaders who set the overall direction and standards
- governance roles organized by data domain where the day-to-day work is done
- a data council that brings domain leaders and the DMO together to connect the data strategy and priorities to the corporate strategy, approve funding, and address issues
This structure serves as the foundation for data governance, balancing central oversight, proper prioritization, and consistency while ensuring that the employees creating and using data are the ones leading its management (Exhibit 2).
Would you like to learn more about McKinsey Digital ?
Six ways to drive data-governance excellence.
The organizational foundation alone, however, is not enough. Six critical practices are needed to ensure data governance creates value.
1. Secure top management’s attention
As the aforementioned example highlights, success with data governance requires buy-in from business leadership. The first step is for the DMO to engage with the C-suite to understand their needs, highlight the current data challenges and limitations, and explain the role of data governance. The next step is to form a data-governance council within senior management (including, in some organizations, leaders from the C-suite itself), which will steer the governance strategy toward business needs and oversee and approve initiatives to drive improvement—for example, the appropriate design and deployment of an enterprise data lake—in concert with the DMO.
The DMO and the governance council should then work to define a set of data domains and select the business executives to lead them. These leaders drive governance efforts day-to-day by defining data elements and establishing quality standards. Companies need to invest the time to introduce these leaders to their new roles, which are typically added to their primary responsibilities. They should understand the value they will generate in these roles and be armed with the skills they need, including an understanding of the relevant regulations and core elements of the data architecture.
Critically, having top-down business-leadership buy-in will avoid the usual challenges around role clarity and empowerment. Data stewards on the business side will understand that the effort is an enterprise priority and make time to address it (which might be facilitated by a shift in their performance metrics or an adjustment in their other responsibilities). Top-down mandates also make it possible to immediately address conflicts over data ownership.
Leading organizations also create tangible ways to track progress and value creation. For example, they can measure the amount of time data scientists spend finding, curating, or enabling data for priority use cases, or the dollar losses associated with poor-quality data and associated business errors. Tracking impact metrics like these helps ensure the attention and continuing support of top management.
2. Integrate with primary transformation themes
To ensure that governance efforts create value, link them directly to continuing transformation efforts that already have CEO attention, such as digitization, omnichannel enablement, or enterprise-resource-planning modernization. These efforts typically depend on data availability and quality.
Linking governance to transformation themes simplifies senior leadership buy-in and changes the organizational construct. Rather than governance running on its own, such initiatives shift data responsibility and governance toward product teams, integrating it at the point of production and consumption.
For example, a European retailer embarked on a digital transformation of its core business and a rapid extension of its online business, which required significant redevelopment of the e-commerce stack, including back-end platforms. Data was identified as a critical enabler, and a DMO and a data council were set up to develop the core framing on the future ecosystem, as well as the structure of data domains, including the strategic goals on managing data in the future.
Lead product owners, who were heading several digital-transformation squads in dedicated functional areas, became data leaders within their area of responsibility. Product owners became data-domain owners. For example, the product owner working to drive process improvements around in-store checkout owned the sales and payment domains. This structure ensured that governance efforts were oriented primarily to enabling business needs and that the leaders creating and consuming data were actively shepherding it.
3. Prioritize data assets and focus data leadership accordingly
Many organizations approach data governance in a holistic manner, looking at all data assets at once. But such a large scope means slow relative progress in any given area and a risk that efforts aren’t linked directly to business needs. To succeed, data assets should be prioritized in two ways: by domains and by data within each domain.
The data council, supported by the DMO, should prioritize domains based on transformational efforts, regulatory requirements, and other inputs to create a road map for domain deployment. Then the organization should rapidly roll out priority domains, starting with two to three initially, and aim for each domain to be fully functional in several months.
For example, a North American retailer set a bold aspiration to transform the company over three years with advanced analytics. The company quickly realized that its current data would hold it back and established a DMO and data domains to scale governance. It identified ten domains across the enterprise and prioritized deployment of the first two—transactional data (logging in-store purchases) and product data (establishing a clear hierarchy of products and their details). This helped accelerate priority use cases around in-store assortment and inventory.
In addition to prioritizing domains, prioritize data assets within each domain by defining a level of criticality (and associated care) for each data element. Critical data typically represents no more than 10 to 20 percent of total data in most organizations. Critical elements, such as customer name or address, should receive a high level of care, including ongoing quality monitoring and clear tracking of flow across the organization, whereas for elements that are used less often in analytics, reporting, or business operations (such as a customer’s academic degree), ad hoc quality monitoring without tracking may suffice. This significantly narrows the scope of governance efforts and ensures that they are focused on the most important data.
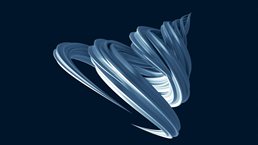
How chief data officers can navigate the COVID-19 response and beyond
4. apply the right level of governance.
Data-governance programs can vary dramatically across organizations and industries. Leading organizations take a “needs-based” approach, adopting the level of governance sophistication appropriate to their organization and then adjusting the level of rigor by data set.
It’s important to realize that data governance was largely first championed by banks under pressure from BCBS 239 1 Basel Committee on Banking Supervision’s standard number 239: “Principles for effective risk data aggregation and risk reporting.” and other regulations that required sophisticated governance models. Most other industries and organizations don’t face the same level of regulatory pressure, so the design of their programs should align with the level of regulation they uniquely face and the level of their data complexity. Organizations with multiple, distinct businesses spanning many geographies have more complex needs than those with a business in only one geography; similarly, a high pace of data change or low level of technology automation increases data complexity (Exhibit 3).
The most comprehensive governance model— say, for a global bank—will have a robust data-governance council (often with C-suite leaders involved) to drive it; a high degree of automation with metadata recorded in an enterprise dictionary or data catalog; data lineage tracked back to the source for many data elements; and a broader domain scope with ongoing prioritization as enterprise needs shift.
In contrast, targeted data governance for a regional technology company might have a data council that meets less frequently and includes C-suite leaders only periodically; metadata tracking that could even start in Excel; limited lineage tracking; and narrower domain scope, at least initially, to enable priority use cases.
In parallel with establishing the right level of governance for the organization as a whole, adjust the level of governance rigor across data sets. Many organizations’ legacy data standards set conservative restrictions on quality and access across the board. This minimizes risk but can stifle innovation. Leading organizations consciously balance opportunities and risks and differentiate governance by data set.
For example, organizations can apply light governance for data that is used only in an exploration setting and not beyond the boundaries of the science team. The team may also not need perfectly prepared and integrated data with full metadata available. Data masking may be appropriate to ensure privacy, together with strict internal non-disclosure agreements (NDAs). However, as soon as such data is used in a broader setting, such as in interactions with customers, stronger governance principles need to be applied.
An Asian financial institution took an aggressive approach to “free the data” using these principles. It agreed on the sensitivity level for each data set and was able to free the roughly 60 percent of enterprise data that was low risk, giving all employees access to use and explore it. On the other hand, highly sensitive data, such as personally identifiable information, was highly restricted both in terms of who could access it and how.
As organizations mature and their governance capabilities and technology continue to advance, scope becomes less important. A suite of tools is beginning to automate data-governance activities, and its coverage and cost-effectiveness will only improve over time. Both newer platforms, such as Octopai and erwin, and established organizations, such as Informatica and Collibra, are rolling out capabilities for automated metadata harvesting, lineage creation, data-quality management, and other governance functions.
5. Choose iterative and focused implementation
To ensure that data governance creates value fast, tailor governance priorities to the domain, and use iteration to adapt quickly. This goes beyond integrating governance with business needs, prioritizing use cases and domains, and applying needs-based governance; the key is to adopt iterative principles in day-to-day governance. For example, if there is a backlog of known data-quality issues, review and reprioritize daily, working to maximize the benefit to the business as priorities shift.
Push to enable priority use cases quickly even if the solution isn’t perfect. Longer-term development to make use cases production ready (by integrating with the core customer-relationship-management and operational customer master data) can occur once value has been demonstrated. For example, enhancing customer campaigns may not require a fully integrated set of data across the entire enterprise, but rather a tailored approach in a dedicated platform. Data governance should support and accelerate this tailored approach, focusing on solving issues around availability and quality in addition to establishing strong master-data management.
6. Generate excitement for data
When people are excited and committed to the vision of data enablement , they’re more likely to help ensure that data is high quality and safe. Leading organizations invest in change management to build data supporters and convert the skeptics. This can be the most difficult part of the program, as it requires motivating employees to use data and encouraging producers to share it (and ideally improve its quality at the source).
Successful organizations use a combination of interventions to drive the right behavior. These can include role modeling from the CEO and other senior leaders, recognition for high quality, responsive sources, and new demonstrated-use cases. Some organizations also offer training and qualifications, often as part of a larger academy approach , together with communicating about career opportunities in data jobs. Others have used successes in data and analytics to create excitement in the form of events, publications, or even data art. 2 TED compiled a series of talks on data art: ted.com/playlists/201/art_from_data. What works is highly dependent on the culture of the organization.
Getting started
Companies should begin their new data-governance approach by asking these six questions:
- What is the opportunity cost of not getting data governance right in terms of missed upside, extensive time lost in manually cleaning data, or incorrect and suboptimal business decisions?
- Who is leading governance efforts today, and what would it look like to elevate the conversation to the C-suite? Who should be involved?
- Where is governance most important? What domains and parts of domains does the organization most need right now?
- What governance archetype best fits the organization, and are current efforts aligned to that level of need?
- How can governance be accelerated by adjusting its focus and injecting iterative working concepts?
- Do you have the in-house capabilities to manage such a shift?
Data governance is critical to capturing value through analytics, digital, and other transformative opportunities. While many companies struggle to get it right, every company can succeed by shifting its mindset from thinking of data governance as frameworks and policies to embedding it strategically into the way the organization works every day.
Bryan Petzold is an associate partner in McKinsey’s Silicon Valley office, Matthias Roggendorf is a partner in the Berlin office, Kayvaun Rowshankish is a partner in the New York office, and Christoph Sporleder is a partner in the Frankfurt office.
Explore a career with us
Related articles.
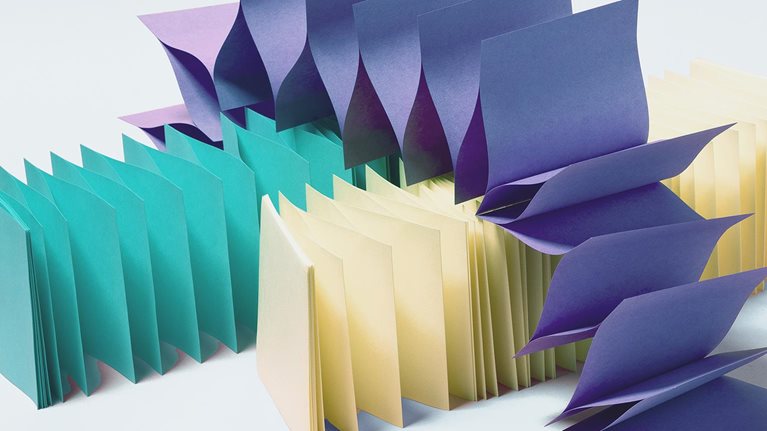
How to build a data architecture to drive innovation—today and tomorrow
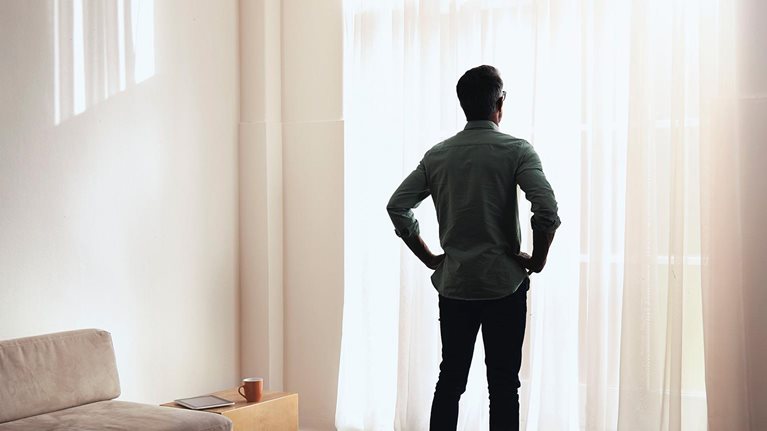
The digital-led recovery from COVID-19: Five questions for CEOs
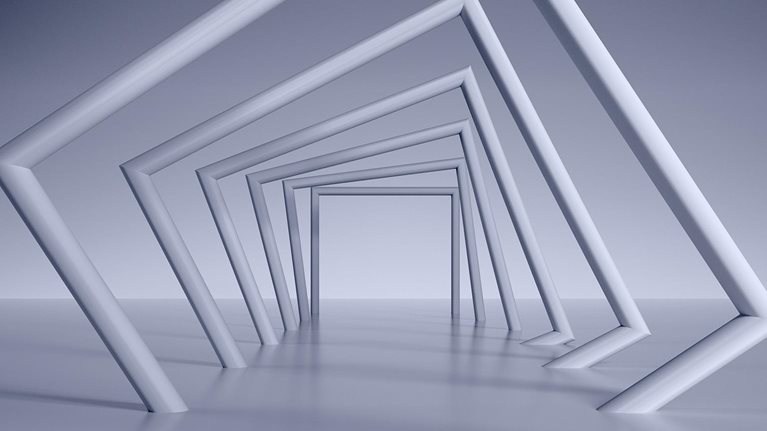
How to make the most of AI? Open up and share data
Your browser is not supported
Sorry but it looks as if your browser is out of date. To get the best experience using our site we recommend that you upgrade or switch browsers.
Find a solution
We use cookies to improve your experience on this website. To learn more, including how to block cookies, read our privacy policy .
- Skip to main content
- Skip to navigation
- Collaboration Platform
- Data Portal
- Reporting Tool
- PRI Academy
- PRI Applications

- Back to parent navigation item
- What are the Principles for Responsible Investment?
- PRI 2021-24 strategy
- The PRI work programme
- A blueprint for responsible investment
- About the PRI
- Annual report
- Public communications policy
- Financial information
- Procurement
- PRI sustainability
- Diversity, Equity & Inclusion for our employees
- Meet the team
- Board members
- Board committees
- 2023 PRI Board annual elections
- Signatory General Meeting (SGM)
- Signatory rights
- Serious violations policy
- Formal consultations
- Signatories
- Signatory resources
- Become a signatory
- Get involved
- Signatory directory
- Quarterly signatory update
- Multi-lingual resources
- Espacio Hispanohablante
- Programme Francophone
- Reporting & assessment
- R&A Updates
- Public signatory reports
- Progression pathways
- Showcasing leadership
- The PRI Leaders’ Group
- The PRI Awards
- News & events
- The PRI podcast
- News & press
- Upcoming events
- PRI in Person 2024
- All events & webinars
- Industry events
- Past events
- PRI in Person 2023 highlights
- PRI in Person & Online 2022 highlights
- PRI China Conference: Investing for Net-Zero and SDGs
- PRI Digital Forums
- Webinars on demand
- Investment tools
- Introductory guides to responsible investment
- Principles to Practice
- Stewardship
- Collaborative engagements
- Active Ownership 2.0
- Listed equity
- Passive investments
- Fixed income
- Credit risk and ratings
- Private debt
- Securitised debt
- Sovereign debt
- Sub-sovereign debt
- Private markets
- Private equity
- Real estate
- Climate change for private markets
- Infrastructure and other real assets
- Infrastructure
- Hedge funds
- Investing for nature: Resource hub
- Asset owner resources
- Strategy, policy and strategic asset allocation
- Mandate requirements and RfPs
- Manager selection
- Manager appointment
- Manager monitoring
- Asset owner DDQs
- Sustainability issues
- Environmental, social and governance issues
- Environmental issues
- Circular economy
- Social issues
- Social issues - case studies
- Social issues - podcasts
- Social issues - webinars
- Social issues - blogs
- Cobalt and the extractives industry
- Clothing and Apparel Supply Chain
- Human rights
- Human rights - case studies
- Modern slavery and labour rights
- Just transition
- Governance issues
- Tax fairness
- Responsible political engagement
- Cyber security
- Executive pay
- Corporate purpose
- Anti-corruption
- Whistleblowing
- Director nominations
- Climate change
- The PRI and COP28
- Inevitable Policy Response
- UN-convened Net-Zero Asset Owner Alliance
- Sustainability outcomes
- Sustainable Development Goals
- Sustainable markets
- Sustainable financial system
- Driving meaningful data
- Private retirement systems and sustainability
- Academic blogs
- Academic Seminar series
- Introduction to responsible investing academic research
- Our policy approach
- Policy reports
- Consultations and letters
- Global policy
- Policy toolkit
- Policy engagement handbook
- Regulation database
- A Legal Framework for Impact
- Fiduciary duty
- Australia policy
- Canada Policy
- China policy
- Stewardship in China
- EU taxonomy
- Japan policy
- SEC ESG-Related Disclosure
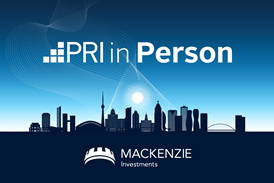
- More from navigation items
AI ethics and data governance
2020-11-01T06:00:00+00:00
Case study by EOS at Federated Hermes
In the spirit of showcasing leadership and raising standards of responsible investment among all our signatories, we are pleased to publish case studies of all the winning and shortlisted entries for the PRI Awards 2020 .
Give a brief overview of the initiative, its objectives, and why you decided to undertake it.
EOS at Federated Hermes (EOS) launched a combined thought leadership and public advocacy project in 2019 that aimed to help companies better understand the risks associated with artificial intelligence (AI) and data governance. The firm has created frameworks and tools that investors can use to address issues around freedom of speech, supply chains, data privacy, surveillance, user manipulation, bias and discrimination.
The cloud computing and analytics services offered by Big Tech companies such as
Alphabet (Google’s holding company), Facebook, Amazon, Microsoft and IBM can be incredibly useful for companies that want to use Big Data to profile their client base, develop predictive analytics and customise their services. However, a lack of awareness of the potential inherent biases in the data or analytical modelling, can lead to accusations of discrimination and unfair outcomes. Examples include the fine levied on Liberty Mutual 1 in the UK over its complaints procedure, and accusations of gender discrimination levelled at Goldman Sachs as a result of a credit card algorithm, which it denied. 2
EOS’s objective was to make companies aware of the risks related to privacy, bias and discrimination when using AI, and then to develop a risk‐aware culture at different levels within a firm. EOS has built its engagement strategy around two white papers:
1. Investors’ Expectations on Responsible Artificial Intelligence and Data Governance was published in April 2019, in conjunction with law firm Bryan Cave Leighton Paisner (BCLP), and addresses concerns from a legal and compliance, and a technical perspective.
2. Artificial Intelligence Applications in Financial Services was published in December 2019, in conjunction with Oliver Wyman, Marsh and BCLP, and addresses issues that affect asset managers, banks and insurers.
Describe how your project is aligned to Active Ownership 2.0, including:
a. The significance of the systemic, real‐world outcomes it seeks.
EOS wants to help companies become more transparent in how they use Big Data and machine learning. It expects companies to commit to overseeing the respect of all human rights and to publish AI principles, applicable use cases and white papers that highlight their challenges and limitations. For example, Google’s white papers on AI and on Disinformation are positive first steps. Although the material social issues may differ from sector to sector, the right to privacy, life, and equality and non‐discrimination will always apply, as these are fundamental human rights. The example of Alphabet raises a number of issues:
- Right to Privacy: Through its technologies and relationships, Alphabet currently collects sensitive personal data, which may include health, biometric, and real‐time location tracking. It deploys listening features on Google Home products, which was not disclosed when this was launched. Also, under Project Nightingale, which was revealed by a whistleblower and widely reported in the media in November 20193, Google allegedly collected the personal medical data of up to 50 million Americans from one of the largest healthcare providers in the US, without informing patients. This reportedly included individual names and medical histories, which could be accessed by Google staff. The allegation raises urgent questions about privacy, due process, and the civil rights of billions of individuals.
- Right to Political Participation: While Google has sought to limit microtargeting of political advertising, which risks the manipulation of specific groups or individuals, and sought to limit the spread of disinformation, political advertisers can still target using age, gender and area code.
- Right to Freedom of Opinion, Expression, and Information: The right to freedom of information is jeopardised by micro‐targeting and profiling, with no transparency on the algorithm that puts people into targeted buckets.
- Right to Health: Multiple sources have documented health and safety impacts on YouTube content moderators, especially mental health issuesarising from their work.
- Right to Equality & Non‐Discrimination, Right to Life & Security: YouTube was used to share scenes of the New Zealand mosque massacre in 2019. At present, the proliferation of hate speech and acts of violence still appear to occur on the platform.
b. The ambition, ingenuity or effort in the responsible investment tools/activities that were deployed.
EOS has engaged with 60 companies across the tech, banking and pharmaceutical sectors in the US, Europe and Asia. This included sending letters to companies outlining its concerns and requesting further information on their approach to AI and data governance risks. The firm also conducted an initial benchmarking of the management performance at banks on AI/data governance.
In 2019 EOS escalated its engagement at Alphabet, supporting a shareholder proposal calling for the establishment of a societal risk oversight committee, and speaking at its annual shareholder meeting, where the firm called for board directors to answer to shareholders.
Following the 2019 AGM, EOS stepped up the pressure on Alphabet.
Despite product‐level improvements, EOS still believes the company falls short on governance oversight on human rights‐related matters concerning the use of AI, including its overarching strategy on government‐related contracts and operations in non-democratic markets.
In November 2019, the firm sent a private letter to Alphabet signed by over 80 institutional investors representing nearly $10 trillion in assets under management and advice, raising concerns about the company’s lack of responsiveness on ESG-related issues and requesting a dialogue with Alphabet on human rights‐related issues.
EOS was a co‐lead filer with three other institutional investors who put together a shareholder proposal for Alphabet’s 2020 AGM. The proposal called for the establishment of a Human Rights Risk Oversight Committee to help anticipate and oversee management of the adverse human rights, and societal risks and impacts, associated with Alphabet’s technologies. With approximately 53% of Alphabet’s voting shares controlled by the company’s executive officers and board members, 16% support for the resolution translates to roughly 45% of the independent votes.
EOS has also carried out public policy and market best practice engagement, including:
- June 2019: presented at the Institute of Business Ethics on AI ethics
- July 2019: participated in a meeting with the OECD and Thun Group on AI and human rights in Geneva
- Sept 2019: HSBC invitation to speak on AI ethics. HSBC published its principles in February 2020.
- Feb 2020: PRI London Forum
- April 2020: contributed to ShareAction publication on responsible AI
- May 2020: UK All‐Party Parliamentary evidence session on corporate decision‐making and investment using AI
Subsequent to this project being reviewed as part of the PRI Awards 2020:
- August 2020: Moody’s seminar on AI and fintech
- September 2020: All-Parliamentary Group Corporate Responsibility Meeting on Responsible approach to AI. This discussed how data assets and predictive analytics are changing the way society functions. It explored ideas ranging from regulation to taxation, and raised awareness of supply chain issues given the concentration of cloud providers, plus the impact of AI on the most vulnerable segments of population.
c. The challenges associated with this initiative (e.g. free rider issues hindering first movers) how these were overcome, and what was learned.
The first challenge was to develop a foundational understanding of the long‐term sustainability issues relating to AI and data governance, and then translate this into an actionable engagement agenda.
EOS at Federated Hermes tackled this challenge by publishing the two reports mentioned above. It placed particular emphasis on the connection between AI ethics and the impact on human rights ‐ to help foster wider acceptance and understanding by stakeholders.
Challenges also remain in ranking the leadership of companies with trusted AI applications beyond the technology sector, as brand value is closely connected to product offering, customer services and other factors.
EOS developed a multi‐criteria appraisal system for scoring technology companies’ approach to AI and ethics, which can be used as an input to ESG integration, as well as monitoring the progress of engagement on this topic at individual companies.
Subsequent to this project being reviewed as part of the PRI Awards 2020:
In September 2020, EOS published the trusted AI assessment framework. This is a ‘three lines of defence’ model that it recommends to companies for trusted AI implementation. Each category of the assessment is mapped to the principles and analytical framework (legal and financial factor analysis, and salient social impact analysis) that EOS highlighted in the April 2019 paper.
Outline the results, including evaluation of its success against the objectives; were there any adjustments to the forward agenda; were there any insights learned from this project that can be applied more broadly?
Subsequent to engagement by EOS:
- Alphabet has made improvements in AI governance at the operational and product levels. (Although EOS continues to press for improvements at the oversight level.)
- Facebook has established a safety advisory board.
- Chinese insurance firm, Ping An, became one of the first major financial institutions globally to publish a set of AI ethical principles.
In addition to these successes, a European conglomerate sought EOS’s guidance on its AI ethics approach, and the board chairs of two other European financial conglomerates responded to EOS’s letters, detailing the progress made within their companies on AI ethics and data governance.
EOS has also expanded its engagement targets to go beyond financial services and cover healthcare and consumer companies, with positive feedback and insights gained from those engagements.
- HQ: Developed Markets
- PRI Awards 2020
- Stewardship project of the year
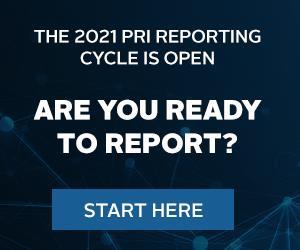
Related content
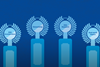
Winners of the PRI Awards 2020
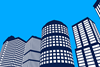
Stewardship in private equity: A guide for general partners
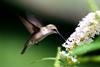
Investor initiatives
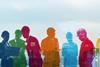
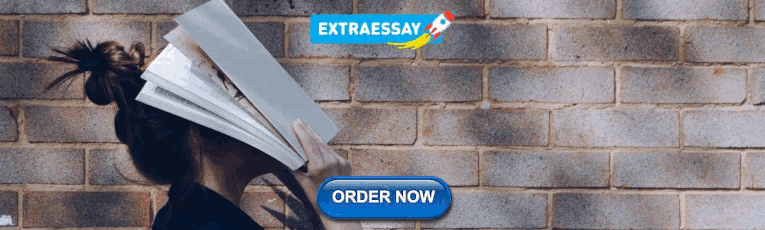
Delivery of the SDGs is off track. Investors are crucial in promoting human rights
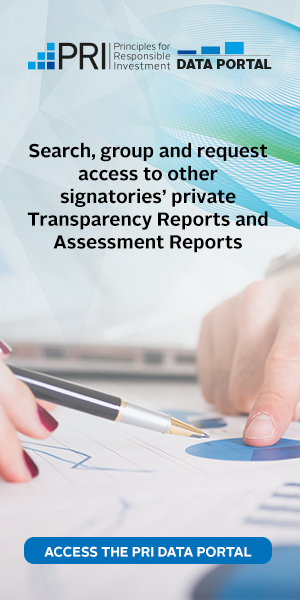
More from Showcasing leadership
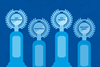
Shortlists for the PRI Awards 2022
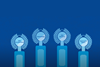
Judging panel for the PRI Awards 2022
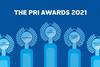
UniSuper’s Sustainable Path to 2050
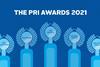
- News and press
- Annual Report
- PRI governance
- Privacy policy
- The PRI is an investor initiative in partnership with UNEP Finance Initiative and UN Global Compact .
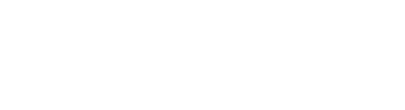
- PRI Association, 25 Camperdown Street, London, E1 8DZ, UK
- Company no: 7207947
- +44 (0)20 3714 3141
- [email protected]
- PRI DISCLAIMER The information contained on this website is meant for the purposes of information only and is not intended to be investment, legal, tax or other advice, nor is it intended to be relied upon in making an investment or other decision. All content is provided with the understanding that the authors and publishers are not providing advice on legal, economic, investment or other professional issues and services. PRI Association is not responsible for the content of websites and information resources that may be referenced. The access provided to these sites or the provision of such information resources does not constitute an endorsement by PRI Association of the information contained therein. PRI Association is not responsible for any errors or omissions, for any decision made or action taken based on information on this website or for any loss or damage arising from or caused by such decision or action. All information is provided “as-is” with no guarantee of completeness, accuracy or timeliness, or of the results obtained from the use of this information, and without warranty of any kind, expressed or implied. Content authored by PRI Association For content authored by PRI Association, except where expressly stated otherwise, the opinions, recommendations, findings, interpretations and conclusions expressed are those of PRI Association alone, and do not necessarily represent the views of any contributors or any signatories to the Principles for Responsible Investment (individually or as a whole). It should not be inferred that any other organisation referenced endorses or agrees with any conclusions set out. The inclusion of company examples does not in any way constitute an endorsement of these organisations by PRI Association or the signatories to the Principles for Responsible Investment. While we have endeavoured to ensure that information has been obtained from reliable and up-to-date sources, the changing nature of statistics, laws, rules and regulations may result in delays, omissions or inaccuracies in information. Content authored by third parties The accuracy of any content provided by an external contributor remains the responsibility of such external contributor. The views expressed in any content provided by external contributors are those of the external contributor(s) alone, and are neither endorsed by, nor necessarily correspond with, the views of PRI Association or any signatories to the Principles for Responsible Investment other than the external contributor(s) named as authors.
Site powered by Webvision Cloud
About Stanford GSB
- The Leadership
- Dean’s Updates
- School News & History
- Commencement
- Business, Government & Society
- Centers & Institutes
- Center for Entrepreneurial Studies
- Center for Social Innovation
- Stanford Seed
About the Experience
- Learning at Stanford GSB
- Experiential Learning
- Guest Speakers
- Entrepreneurship
- Social Innovation
- Communication
- Life at Stanford GSB
- Collaborative Environment
- Activities & Organizations
- Student Services
- Housing Options
- International Students
Full-Time Degree Programs
- Why Stanford MBA
- Academic Experience
- Financial Aid
- Why Stanford MSx
- Research Fellows Program
- See All Programs
Non-Degree & Certificate Programs
- Executive Education
- Stanford Executive Program
- Programs for Organizations
- The Difference
- Online Programs
- Stanford LEAD
- Stanford Innovation and Entrepreneurship Certificate
- Seed Transformation Program
- Aspire Program
- Seed Spark Program
- Faculty Profiles
- Academic Areas
- Awards & Honors
- Conferences
Faculty Research
- Publications
- Working Papers
- Case Studies
Research Hub
- Research Labs & Initiatives
- Business Library
- Data, Analytics & Research Computing
- Behavioral Lab
Research Labs
- Cities, Housing & Society Lab
- Golub Capital Social Impact Lab
Research Initiatives
- Corporate Governance Research Initiative
- Corporations and Society Initiative
- Policy and Innovation Initiative
- Rapid Decarbonization Initiative
- Stanford Latino Entrepreneurship Initiative
- Value Chain Innovation Initiative
- Venture Capital Initiative
- Career & Success
- Climate & Sustainability
- Corporate Governance
- Culture & Society
- Finance & Investing
- Government & Politics
- Leadership & Management
- Markets & Trade
- Operations & Logistics
- Opportunity & Access
- Organizational Behavior
- Political Economy
- Social Impact
- Technology & AI
- Opinion & Analysis
- Email Newsletter
Welcome, Alumni
- Communities
- Digital Communities & Tools
- Regional Chapters
- Women’s Programs
- Identity Chapters
- Find Your Reunion
- Career Resources
- Job Search Resources
- Career & Life Transitions
- Programs & Services
- Career Video Library
- Alumni Education
- Research Resources
- Volunteering
- Alumni News
- Class Notes
- Alumni Voices
- Contact Alumni Relations
- Upcoming Events
Admission Events & Information Sessions
- MBA Program
- MSx Program
- PhD Program
- Alumni Events
- All Other Events
- Operations, Information & Technology
- Classical Liberalism
- The Eddie Lunch
- Accounting Summer Camp
- Videos, Code & Data
- California Econometrics Conference
- California Quantitative Marketing PhD Conference
- California School Conference
- China India Insights Conference
- Homo economicus, Evolving
- Political Economics (2023–24)
- Scaling Geologic Storage of CO2 (2023–24)
- A Resilient Pacific: Building Connections, Envisioning Solutions
- Adaptation and Innovation
- Changing Climate
- Civil Society
- Climate Impact Summit
- Climate Science
- Corporate Carbon Disclosures
- Earth’s Seafloor
- Environmental Justice
- Operations and Information Technology
- Organizations
- Sustainability Reporting and Control
- Taking the Pulse of the Planet
- Urban Infrastructure
- Watershed Restoration
- Junior Faculty Workshop on Financial Regulation and Banking
- Ken Singleton Celebration
- Quantitative Marketing PhD Alumni Conference
- Presentations
- Theory and Inference in Accounting Research
- Stanford Closer Look Series
- Quick Guides
- Core Concepts
- Journal Articles
- Glossary of Terms
- Faculty & Staff
- Researchers & Students
- Research Approach
- Charitable Giving
- Financial Health
- Government Services
- Workers & Careers
- Short Course
- Adaptive & Iterative Experimentation
- Incentive Design
- Social Sciences & Behavioral Nudges
- Bandit Experiment Application
- Conferences & Events
- Get Involved
- Reading Materials
- Teaching & Curriculum
- Energy Entrepreneurship
- Faculty & Affiliates
- SOLE Report
- Responsible Supply Chains
- Current Study Usage
- Pre-Registration Information
- Participate in a Study
Corporate Governance Failure, Fraud, and Scandal: Data
This Data Spotlight provides data and statistics on unethical behavior in corporations and other negative outcomes including bankruptcy, litigation, and corruption in the United States. This data supplements in the issues introduced in the Quick Guide “ Introduction to Corporate Governance .”
- See the Current DEI Report
- Supporting Data
- Research & Insights
- Share Your Thoughts
- Search Fund Primer
- Affiliated Faculty
- Faculty Advisors
- Louis W. Foster Resource Center
- Defining Social Innovation
- Impact Compass
- Global Health Innovation Insights
- Faculty Affiliates
- Student Awards & Certificates
- Changemakers
- Dean Jonathan Levin
- Dean Garth Saloner
- Dean Robert Joss
- Dean Michael Spence
- Dean Robert Jaedicke
- Dean Rene McPherson
- Dean Arjay Miller
- Dean Ernest Arbuckle
- Dean Jacob Hugh Jackson
- Dean Willard Hotchkiss
- Faculty in Memoriam
- Stanford GSB Firsts
- Certificate & Award Recipients
- Dean’s Remarks
- Keynote Address
- Teaching Approach
- Analysis and Measurement of Impact
- The Corporate Entrepreneur: Startup in a Grown-Up Enterprise
- Data-Driven Impact
- Designing Experiments for Impact
- Digital Business Transformation
- The Founder’s Right Hand
- Marketing for Measurable Change
- Product Management
- Public Policy Lab: Financial Challenges Facing US Cities
- Public Policy Lab: Homelessness in California
- Lab Features
- Curricular Integration
- View From The Top
- Formation of New Ventures
- Managing Growing Enterprises
- Startup Garage
- Explore Beyond the Classroom
- Stanford Venture Studio
- Summer Program
- Workshops & Events
- The Five Lenses of Entrepreneurship
- Leadership Labs
- Executive Challenge
- Arbuckle Leadership Fellows Program
- Selection Process
- Training Schedule
- Time Commitment
- Learning Expectations
- Post-Training Opportunities
- Who Should Apply
- Introductory T-Groups
- Leadership for Society Program
- Certificate
- 2023 Awardees
- 2022 Awardees
- 2021 Awardees
- 2020 Awardees
- 2019 Awardees
- 2018 Awardees
- Social Management Immersion Fund
- Stanford Impact Founder Fellowships and Prizes
- Stanford Impact Leader Prizes
- Social Entrepreneurship
- Stanford GSB Impact Fund
- Economic Development
- Energy & Environment
- Stanford GSB Residences
- Environmental Leadership
- Stanford GSB Artwork
- A Closer Look
- California & the Bay Area
- Voices of Stanford GSB
- Business & Beneficial Technology
- Business & Sustainability
- Business & Free Markets
- Business, Government, and Society Forum
- Second Year
- Global Experiences
- JD/MBA Joint Degree
- MA Education/MBA Joint Degree
- MD/MBA Dual Degree
- MPP/MBA Joint Degree
- MS Computer Science/MBA Joint Degree
- MS Electrical Engineering/MBA Joint Degree
- MS Environment and Resources (E-IPER)/MBA Joint Degree
- Academic Calendar
- Clubs & Activities
- LGBTQ+ Students
- Military Veterans
- Minorities & People of Color
- Partners & Families
- Students with Disabilities
- Student Support
- Residential Life
- Student Voices
- MBA Alumni Voices
- A Week in the Life
- Career Support
- Employment Outcomes
- Cost of Attendance
- Knight-Hennessy Scholars Program
- Yellow Ribbon Program
- BOLD Fellows Fund
- Application Process
- Loan Forgiveness
- Contact the Financial Aid Office
- Evaluation Criteria
- GMAT & GRE
- English Language Proficiency
- Personal Information, Activities & Awards
- Professional Experience
- Letters of Recommendation
- Optional Short Answer Questions
- Application Fee
- Reapplication
- Deferred Enrollment
- Joint & Dual Degrees
- Entering Class Profile
- Event Schedule
- Ambassadors
- New & Noteworthy
- Ask a Question
- See Why Stanford MSx
- Is MSx Right for You?
- MSx Stories
- Leadership Development
- Career Advancement
- Career Change
- How You Will Learn
- Admission Events
- Personal Information
- Information for Recommenders
- GMAT, GRE & EA
- English Proficiency Tests
- After You’re Admitted
- Daycare, Schools & Camps
- U.S. Citizens and Permanent Residents
- Requirements
- Requirements: Behavioral
- Requirements: Quantitative
- Requirements: Macro
- Requirements: Micro
- Annual Evaluations
- Field Examination
- Research Activities
- Research Papers
- Dissertation
- Oral Examination
- Current Students
- Education & CV
- International Applicants
- Statement of Purpose
- Reapplicants
- Application Fee Waiver
- Deadline & Decisions
- Job Market Candidates
- Academic Placements
- Stay in Touch
- Faculty Mentors
- Current Fellows
- Standard Track
- Fellowship & Benefits
- Group Enrollment
- Program Formats
- Developing a Program
- Diversity & Inclusion
- Strategic Transformation
- Program Experience
- Contact Client Services
- Campus Experience
- Live Online Experience
- Silicon Valley & Bay Area
- Digital Credentials
- Faculty Spotlights
- Participant Spotlights
- Eligibility
- International Participants
- Stanford Ignite
- Frequently Asked Questions
- Founding Donors
- Location Information
- Participant Profile
- Network Membership
- Program Impact
- Collaborators
- Entrepreneur Profiles
- Company Spotlights
- Seed Transformation Network
- Responsibilities
- Current Coaches
- How to Apply
- Meet the Consultants
- Meet the Interns
- Intern Profiles
- Collaborate
- Research Library
- News & Insights
- Program Contacts
- Databases & Datasets
- Research Guides
- Consultations
- Research Workshops
- Career Research
- Research Data Services
- Course Reserves
- Course Research Guides
- Material Loan Periods
- Fines & Other Charges
- Document Delivery
- Interlibrary Loan
- Equipment Checkout
- Print & Scan
- MBA & MSx Students
- PhD Students
- Other Stanford Students
- Faculty Assistants
- Research Assistants
- Stanford GSB Alumni
- Telling Our Story
- Staff Directory
- Site Registration
- Alumni Directory
- Alumni Email
- Privacy Settings & My Profile
- Success Stories
- The Story of Circles
- Support Women’s Circles
- Stanford Women on Boards Initiative
- Alumnae Spotlights
- Insights & Research
- Industry & Professional
- Entrepreneurial Commitment Group
- Recent Alumni
- Half-Century Club
- Fall Reunions
- Spring Reunions
- MBA 25th Reunion
- Half-Century Club Reunion
- Faculty Lectures
- Ernest C. Arbuckle Award
- Alison Elliott Exceptional Achievement Award
- ENCORE Award
- Excellence in Leadership Award
- John W. Gardner Volunteer Leadership Award
- Robert K. Jaedicke Faculty Award
- Jack McDonald Military Service Appreciation Award
- Jerry I. Porras Latino Leadership Award
- Tapestry Award
- Student & Alumni Events
- Executive Recruiters
- Interviewing
- Land the Perfect Job with LinkedIn
- Negotiating
- Elevator Pitch
- Email Best Practices
- Resumes & Cover Letters
- Self-Assessment
- Whitney Birdwell Ball
- Margaret Brooks
- Bryn Panee Burkhart
- Margaret Chan
- Ricki Frankel
- Peter Gandolfo
- Cindy W. Greig
- Natalie Guillen
- Carly Janson
- Sloan Klein
- Sherri Appel Lassila
- Stuart Meyer
- Tanisha Parrish
- Virginia Roberson
- Philippe Taieb
- Michael Takagawa
- Terra Winston
- Johanna Wise
- Debbie Wolter
- Rebecca Zucker
- Complimentary Coaching
- Changing Careers
- Work-Life Integration
- Career Breaks
- Flexible Work
- Encore Careers
- D&B Hoovers
- Data Axle (ReferenceUSA)
- EBSCO Business Source
- Firsthand (Vault)
- Global Newsstream
- Market Share Reporter
- ProQuest One Business
- Student Clubs
- Entrepreneurial Students
- Stanford GSB Trust
- Alumni Community
- How to Volunteer
- Springboard Sessions
- Consulting Projects
- 2020 – 2029
- 2010 – 2019
- 2000 – 2009
- 1990 – 1999
- 1980 – 1989
- 1970 – 1979
- 1960 – 1969
- 1950 – 1959
- 1940 – 1949
- Service Areas
- ACT History
- ACT Awards Celebration
- ACT Governance Structure
- Building Leadership for ACT
- Individual Leadership Positions
- Leadership Role Overview
- Purpose of the ACT Management Board
- Contact ACT
- Business & Nonprofit Communities
- Reunion Volunteers
- Ways to Give
- Fiscal Year Report
- Business School Fund Leadership Council
- Planned Giving Options
- Planned Giving Benefits
- Planned Gifts and Reunions
- Legacy Partners
- Giving News & Stories
- Giving Deadlines
- Development Staff
- Submit Class Notes
- Class Secretaries
- Board of Directors
- Health Care
- Sustainability
- Class Takeaways
- All Else Equal: Making Better Decisions
- If/Then: Business, Leadership, Society
- Grit & Growth
- Think Fast, Talk Smart
- Spring 2022
- Spring 2021
- Autumn 2020
- Summer 2020
- Winter 2020
- In the Media
- For Journalists
- DCI Fellows
- Other Auditors
- Academic Calendar & Deadlines
- Course Materials
- Entrepreneurial Resources
- Campus Drive Grove
- Campus Drive Lawn
- CEMEX Auditorium
- King Community Court
- Seawell Family Boardroom
- Stanford GSB Bowl
- Stanford Investors Common
- Town Square
- Vidalakis Courtyard
- Vidalakis Dining Hall
- Catering Services
- Policies & Guidelines
- Reservations
- Contact Faculty Recruiting
- Lecturer Positions
- Postdoctoral Positions
- Accommodations
- CMC-Managed Interviews
- Recruiter-Managed Interviews
- Virtual Interviews
- Campus & Virtual
- Search for Candidates
- Think Globally
- Recruiting Calendar
- Recruiting Policies
- Full-Time Employment
- Summer Employment
- Entrepreneurial Summer Program
- Global Management Immersion Experience
- Social-Purpose Summer Internships
- Process Overview
- Project Types
- Client Eligibility Criteria
- Client Screening
- ACT Leadership
- Social Innovation & Nonprofit Management Resources
- Develop Your Organization’s Talent
- Centers & Initiatives
- Student Fellowships
Data Governance as a Collective Action Problem
- Published: 27 April 2019
- Volume 22 , pages 299–313, ( 2020 )
Cite this article
- Olivia Benfeldt ORCID: orcid.org/0000-0002-1261-3272 1 , 2 ,
- John Stouby Persson 2 &
- Sabine Madsen 1
5625 Accesses
47 Citations
3 Altmetric
Explore all metrics
While governing data as an organizational asset has clear benefits, mobilizing an organization to implement data governance remains elusive for practitioners. On that account, this paper examines why governing data is difficult in local government organizations. Based on a literature review and an empirical case study, we establish the inherent challenges and build on the notion of collective action to theorize the problem of data governance. Following an engaged scholarship approach, we collect empirical material through six group interviews with 34 representatives from 13 different Danish municipalities. We extend existing data governance research with our problem triangle that identifies and explicates the complex relations between six distinct challenges: value, collaboration, capabilities, overview, practices, and politics. We demonstrate the value in theorizing data governance as a collective action problem and argue for the necessity of ensuring researchers and practitioners achieve a common understanding of the inherent challenges, as a first step towards developing data governance solutions that are viable in practice.
This is a preview of subscription content, log in via an institution to check access.
Access this article
Price includes VAT (Russian Federation)
Instant access to the full article PDF.
Rent this article via DeepDyve
Institutional subscriptions
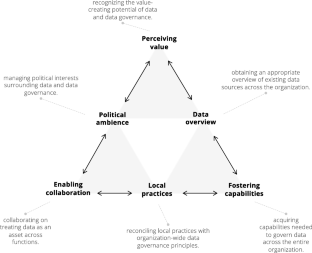
Similar content being viewed by others
Why Governing Data Is Difficult: Findings from Danish Local Government
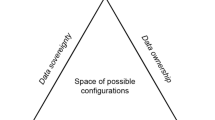
Data Governance in a Trilemma: A Qualitative Analysis of Rights, Values, and Goals in Building Data Commons
Jan Oleszczuk Zygmuntowski
Governance of Open Data Initiatives
Collecting personal data without a consented purpose is illegal according to GDPR but local government organizations also have vast amounts of non-personal data.
Agency for Digitisation. (2018). IT architecture. Danish Agency for Digitisation . https://digst.dk/data/it-arkitektur/ . Accessed 27 September 2018.
Alhassan, I., Sammon, D., & Daly, M. (2016). Data governance activities: An analysis of the literature. Journal of Decision Systems, 25 , 64–75. https://doi.org/10.1080/12460125.2016.1187397 .
Article Google Scholar
Alhassan, I., Sammon, D., & Daly, M. (2018). Data governance activities: A comparison between scientific and practice-oriented literature. Journal of Enterprise Information Management, 31 (2), 300–316. https://doi.org/10.1108/JEIM-01-2017-0007 .
Al-Ruithe, M., Benkhelifa, E., & Hameed, K. (2018). A systematic literature review of data governance and cloud data governance. Personal and Ubiquitous Computing , 1–21. https://doi.org/10.1007/s00779-017-1104-3 .
Baškarada, S., & Koronios, A. (2013). Data, information, knowledge, wisdom (DIKW): A semiotic theoretical and empirical exploration of the hierarchy and its quality dimension. Australasian Journal of Information Systems, 18 (1), 5–24. https://doi.org/10.3127/ajis.v18i1.748 .
Begg, C., & Caira, T. (2011). Data governance in practice: The SME quandary reflections on the reality of data governance in the small to medium Enterprise (SME) sector. 5th European Conference on Information Management and Evaluation (ECIME) , 75–83.
Begg, C., & Caira, T. (2012). Exploring the SME quandary: Data governance in Practise in the small to medium-sized Enterprise sector. The Electronic Journal Information Systems Evaluation, 15 (1), 3–13.
Google Scholar
Benfeldt Nielsen, O. (2017). A comprehensive review of data governance literature. In Selected Papers of the IRIS (Vol. 8).
Beynon-Davies, P. (2009). The “language” of informatics: The nature of information systems. International Journal of Information Management, 29 (2), 92–103. https://doi.org/10.1016/j.ijinfomgt.2008.11.002 .
Beynon-Davies, P. (2011). In-formation on the prairie: Signs, patterns, systems and prairie dogs. International Journal of Information Management, 31 (4), 307–316. https://doi.org/10.1016/j.ijinfomgt.2010.12.001 .
Brous, P., Janssen, M., & Vilminko-Heikkinen, R. (2016). Coordinating decision-making in data management activities: A systematic review of data governance principles. https://doi.org/10.1007/978-3-319-44421-5_9 .
Cheong, L., & Chang, V. (2007). The need for data governance: A case study. ACIS 2007 Proceedings , 99–1008.
Coleman, D. W., Hughes, A. A., & Perry, W. D. (2009). The role of data governance to relieve information sharing impairments in the federal government. 2009 WRI world congress on computer science and information engineering, CSIE 2009, 4 , 267–271. https://doi.org/10.1109/CSIE.2009.630 .
Constantinides, P., & Barrett, M. (2015). Information infrastructure development and governance as collective action. Information Systems Research, 26 (1), 40–56. https://doi.org/10.1287/isre.2014.0542 .
DalleMule, L., & Davenport, T. H. (2017). What’s your data strategy? Harvard Business Review, 11 .
Danish Ministry of Finance. (2016). A stronger and more secure Denmark: Digital strategy 2016–2020 . Danish Government.
Dewey, J. (1938). Logic: The theory of inquiry . New York: H. Holt and Company.
Dwivedi, Y. K., Janssen, M., Slade, E. L., Rana, N. P., Weerakkody, V., Millard, J., Hidders, J., & Snijders, D. (2017). Driving innovation through big open linked data (BOLD): Exploring antecedents using interpretive structural modelling. Information Systems Frontiers, 19 (2), 197–212. https://doi.org/10.1007/s10796-016-9675-5 .
Elbanna, A., Bunker, D., Levine, L., & Sleigh, A. (2019). Emergency management in the changing world of social media: Framing the research agenda with the stakeholders through engaged scholarship. International Journal of Information Management, 47 , 112–120.
European Commission. (2017). Europe’s digital Progress report - the digital economy and society index.
European Commission. (2018). 2018 reform of EU data protection rules. https://ec.europa.eu/commission/priorities/justice-and-fundamental-rights/data-protection/2018-reform-eu-data-protection-rules_en . Accessed 7 January 2019.
European Union. (2016). General data protection regulation. Official Journal of the European Union, L119 , 1–88.
Fontana, A., & Frey, J. H. (1994). Interviewing: The arts of science. Handbook of Qualitative Research, i, 120-121 , 361–376. https://doi.org/10.1016/j.jconhyd.2010.08.009 .
Gregor, S. (2006). The nature of theory in information systems. MIS Quarterly, 30 (3), 611–642.
Hardin, G. (1968). The tragedy of the commons. Science, 162 (3859), 1243–1248. https://doi.org/10.1126/science.162.3859.1243 .
Harvey, L. J., & Myers, M. D. (1995). Scholarship and practice: The contribution of ethnographic research methods to bridging the gap. Information Technology & People, 8 (3), 13–27. https://doi.org/10.1108/09593849510098244 .
Holahan, R., & Lubell, M. (2016). Collective Action Theory. In J. Torfing & C. K. Ansell (Eds.), Handbook on theories of governance (pp. 21–31). Cheltenham: Edward Elgar Publishing.
Chapter Google Scholar
Hsieh, H.-F., & Shannon, S. E. (2005). Three approaches to qualitative content analysis. Qualitative Health Research, 15 (9), 1277–1288. https://doi.org/10.1177/1049732305276687 .
Juell-Skielse, G., Lönn, C.-M., & Päivärinta, T. (2017). Modes of collaboration and expected benefits of inter-organizational E-government initiatives: A multi-case study. Government Information Quarterly, 34 (4), 578–590. https://doi.org/10.1016/j.giq.2017.10.008 .
Khatri, V., & Brown, C. V. (2010). Designing data governance. Communications of the ACM, 53 (1), 148. https://doi.org/10.1145/1629175.1629210 .
Kim, D., & Grant, G. (2010). E-government maturity model using the capability maturity model integration. Journal of Systems and Information Technology, 12 (3), 230–244. https://doi.org/10.1108/13287261011070858 .
Kiron, D. (2016). Lessons from becoming a data-driven organization. MIT Sloan Management Review, 58 (2).
Klievink, B., Romijn, B.-J., Cunningham, S., & de Bruijn, H. (2017). Big data in the public sector: Uncertainties and readiness. Information Systems Frontiers, 19 (2), 267–283. https://doi.org/10.1007/s10796-016-9686-2 .
Ladley, J. (2012). Data governance: How to design, deploy, and sustain an effective data governance program . Waltham: Morgan Kaufmann.
Lee, Y. W., Madnick, S. E., Wang, R. Y., Wang, F. L., & Zhang, H. (2014). A cubic framework for the chief data officer: Succeeding in a world of big data. MIS Quarterly Executive, 13 (1), 1–13.
Local Government Denmark. (2018). Municipal Responsibilities. Local Government Denmark . http://www.kl.dk/English/Municipal-Responsibilities/ . Accessed 27 September 2018.
Marchand, D. A., & Peppard, J. (2013). Why IT fumbles analytics. Harvard Business Review, 91 (1), 104–112.
Markus, M. L., Steinfield, C. W., & Wigand, R. T. (2006). Industry-Wide Information Systems Standardization as Collective Action: The Case of the U.S. Residential Mortgage Industry. MIS Quarterly, 30 , 439. https://doi.org/10.2307/25148768 .
Mathiassen, L. (2002). Collaborative practice research. Information Technology & People, 15 (4), 321–345. https://doi.org/10.1108/09593841111182250 .
Medaglia, R. (2012). eParticipation research: Moving characterization forward (2006–2011). Government Information Quarterly, 29 (3), 346–360.
Medaglia, R., Hedman, J., & Eaton, B. (2017). Public-private collaboration in the emergence of a National Electronic Identification Policy: The case of NemID in Denmark. Proceedings of the 50th Hawaii international conference on system sciences , 2782–2791.
Mindel, V., Mathiassen, L., & Rai, A. (2018). The sustainability of polycentric information commons. MIS Quarterly, 42 (2), 607–631. https://doi.org/10.25300/MISQ/2018/14015 .
Mingers, J., & Willcocks, L. (2014). An integrative semiotic framework for information systems: The social, personal and material worlds. Information and Organization, 24 (1), 48–70. https://doi.org/10.1016/j.infoandorg.2014.01.002 .
Monge, P. R., Fulk, J., Kalman, M. E., Flanagin, A. J., Parnassa, C., & Rumsey, S. (1998). Production of collective action in Alliance-based Interorganizational communication and information systems. Organization Science, 9 (3), 411–433.
Myers, M. D., & Newman, M. (2007). The qualitative interview in IS research: Examining the craft. Information and Organization, 17 (1), 2–26. https://doi.org/10.1016/j.infoandorg.2006.11.001 .
Nielsen, P. A., & Persson, J. S. (2016). Engaged problem formulation in IS research. Communications of the Association for Information Systems, 38 (1), 720–737.
Nielsen, O. B., Persson, J. S., & Madsen, S. (2019). Why governing data is difficult: Findings from Danish local government. In A. Elbanna, Y. K. Dwivedi, D. Bunker, & D. Wastell (Eds.), Smart Working, Living and Organising: IFIP WG 8.6 International Conference on Transfer and Diffusion of IT, TDIT 2018, Portsmouth, UK, June 25, 2018, Proceedings (Vol. 533, pp. 15–29). Springer International Publishing. https://doi.org/10.1007/978-3-030-04315-5 .
Olphert, W., & Damodaran, L. (2007). Citizen participation and engagement in the design of e-government services: The missing link in effective ICT design and delivery. Journal of the Association for Information Systems, 8 (9), 27.
Olson, M. (1965). The logic of collective action: public goods and the theory of groups (21. Printing.). Cambridge: Harvard University Press.
Ostrom, V. (1972). Polycentricity. Presented at the 1972 Annual meeting of the American Political Science Association, Washington, DC.
Ostrom, E. (1990). Governing the commons: The evolution of institutions for collective action. In Cambridge . New York: Cambridge University Press.
Ostrom, E. (2010). Analyzing collective action. Agricultural Economics, 41 , 155–166. https://doi.org/10.1111/j.1574-0862.2010.00497.x .
Otto, B. (2011a). Organizing data governance: Findings from the telecommunications industry and consequences for large service providers. Communications of the Association for Information Systems, 29 (1), 45–66.
Otto, B. (2011b). Data governance. Business and Information Systems Engineering, 3 (4), 241–244. https://doi.org/10.1007/s12599-011-0162-8 .
Otto, B. (2011c). A Morphology of the Organisation of Data Governance. ECIS 2011 Proceedings , 272. https://doi.org/10.1007/978-3-8348-9953-8 .
Pereira, G. V., Macadar, M. A., Luciano, E. M., & Testa, M. G. (2017). Delivering public value through open government data initiatives in a Smart City context. Information Systems Frontiers, 19 (2), 213–229. https://doi.org/10.1007/s10796-016-9673-7 .
Persson, J. S., Kaldahl, A., Skorve, E., & Nielsen, P. A. (2017). Value positions in E-government strategies: Something is (not) changing in the state of Denmark. Proceedings of the 25th European Conference on Information Systems , 904–917.
Pierce, E., Dismute, W. S., & Yonke, C. L. (2008). The state of information and data governance - understanding how organizations govern their information and data assets.
Porter, M. E., & Heppelmann, J. E. (2014). How smart, connected products are transforming competition. Harvard Business Review, 92 (11), 64–88.
Porter, M. E., & Heppelmann, J. E. (2015). How smart, connected products are transforming companies. Harvard Business Review, 93 (10), 96–114.
Ransbotham, S., & Kiron, D. (2017). Analytics as a source of business innovation. MIT Sloan Management Review, 19 .
Ransbotham, S., Kiron, D., & Prentice, P. K. (2016). Beyond the hype: The hard work behind analytics success. MIT Sloan Management Review, 19 .
Rose, J., Persson, J. S., Kræmmergaard, P., & Nielsen, P. A. (2012). IT Management in Local Government: The DISIMIT project .
Rose, J., Persson, J. S., & Heeager, L. T. (2015a). How e-government managers prioritise rival value positions: The efficiency imperative. Information polity, 20 (1), 35–59.
Rose, J., Persson, J. S., Heeager, L. T., & Irani, Z. (2015b). Managing e-government: Value positions and relationships. Information Systems Journal, 25 (5), 531–571. https://doi.org/10.1111/isj.12052 .
Soares, S. (2010). The IBM data governance unified process . USA : IBM Corporation.
Thompson, N., Ravindran, R., & Nicosia, S. (2015). Government data does not mean data governance: Lessons learned from a public sector application audit. Government Information Quarterly, 32 (3), 316–322. https://doi.org/10.1016/j.giq.2015.05.001 .
Torfing, J. (Ed.). (2012). Interactive governance: advancing the paradigm . Oxford: Oxford University Press.
Torfing, J., & Ansell, C. K. (Eds.). (2016). Handbook on theories of governance . Northampton: Edward Elgar Publishing.
Van de Ven, A. H. (2007). Engaged scholarship: A guide for organizational and social research . Oxford University Press on Demand.
Vilminko-Heikkinen, R. (2017). Data, technology, and people. Demystifying Master Data Management . Tampere University of Technology.
Vilminko-Heikkinen, R., Brous, P., & Pekkola, S. (2016). Paradoxes, conflicts and tensions in establishing master data management function. In 24th European Conference on Information Systems, ECIS 2016 .
Volkema, R. J. (1995). Creativity in MS/OR: Managing the process of formulating the problem. Interfaces, 25 (3), 81–87. https://doi.org/10.1287/inte.25.3.81 .
Wang, R. Y., & Strong, D. M. (1996). Beyond accuracy: What data quality means to data consumers. Journal of Management Information Systems, 12 (4), 5–33. https://doi.org/10.1080/07421222.1996.11518099 .
Weber, K., Otto, B., & Osterle, H. (2009). One size does not fit all — A contingency approach to data governance. ACM Journal of Data and Information Quality, 1 (1), 4 :1–4:27. https://doi.org/10.1145/1515693.1515696.http .
Weill, P., & Ross, J. W. (2004). IT governance on one page. CISR Working Paper, (349).
Winter, J. S., & Davidson, E. (2018). The healthcare AI juggernaut: Is PHI data governance possible? In Living with Monsters? Social Implications of Algorithmic Phenomena, Hybrid Agency and the Performativity of Technology . Presented at the IFIP WG 8.2 working conference 2018, San Francisco State University.
Download references
Author information
Authors and affiliations.
Aalborg University, Fibigerstræde 3, 9220, Aalborg, Denmark
Olivia Benfeldt & Sabine Madsen
Aalborg University, Selma Lagerlöfsvej 300, 9220, Aalborg, Denmark
Olivia Benfeldt & John Stouby Persson
You can also search for this author in PubMed Google Scholar
Corresponding author
Correspondence to Olivia Benfeldt .
Additional information
Publisher’s note.
Springer Nature remains neutral with regard to jurisdictional claims in published maps and institutional affiliations.
Rights and permissions
Reprints and permissions
About this article
Benfeldt, O., Persson, J.S. & Madsen, S. Data Governance as a Collective Action Problem. Inf Syst Front 22 , 299–313 (2020). https://doi.org/10.1007/s10796-019-09923-z
Download citation
Published : 27 April 2019
Issue Date : April 2020
DOI : https://doi.org/10.1007/s10796-019-09923-z
Share this article
Anyone you share the following link with will be able to read this content:
Sorry, a shareable link is not currently available for this article.
Provided by the Springer Nature SharedIt content-sharing initiative
- Data governance
- Collective action
- Local government
- Engaged scholarship
- Find a journal
- Publish with us
- Track your research
Data Topics
- Data Architecture
- Data Literacy
- Data Science
- Data Strategy
- Data Modeling
- Governance & Quality
- Data Education
- Enterprise Information Management
- Information Management Articles
Data Governance and Data Quality Use Cases
Given the high volume of erroneous data floating across the enterprise, it is increasingly difficult for industry operators to make business decisions or plan any course of action based on such poor-quality data. Moreover, what’s the point of applying advanced analytics or BI technologies on data that is flawed? A whitepaper titled Getting Ahead of […]
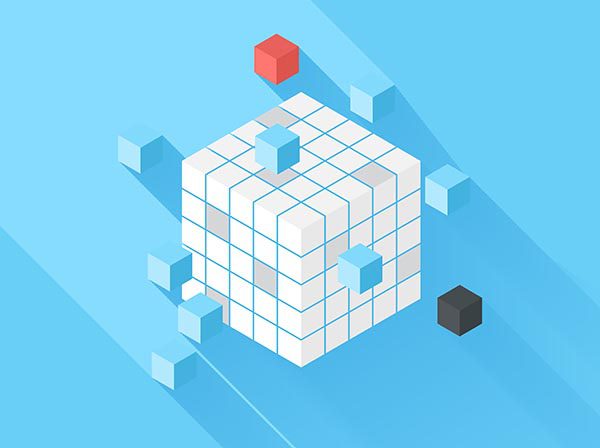
Given the high volume of erroneous data floating across the enterprise, it is increasingly difficult for industry operators to make business decisions or plan any course of action based on such poor-quality data. Moreover, what’s the point of applying advanced analytics or BI technologies on data that is flawed?

Data Quality and Data Governance: What’s the Connection?
Although Data Quality and Data Governance are often used interchangeably, they are very different, both in theory and practice. While Data Quality Management at an enterprise happens both at the front (incoming data pipelines) and back ends (databases, servers), the whole process is defined, structured, and implemented through a well-designed framework. This framework for managing enterprise data may be thought of as Data Governance framework , where rules and policies related to data ownership, data processes, and data technologies used in the framework are clearly defined. So, Data Governance provides the framework for managing Data Quality.
InCountry Launches Data Residency-As-A-Service for Multinational Organizations discusses a one-stop regulatory solution for all multinational or country-specific business operators grappling with newly emerging compliance laws and policies. This solution enables businesses to store data locally, thus avoiding across-the-border compliance issues. Use of DQ and DG Strategies in the Financial Services
Another Data Governance use case is sharply visible in financial services. In the digital-banking industry, DQ and DG have been exploited to transform entire business models. The banks that judiciously leveraged data platforms to reduce risks, streamline expenses, and boost revenues have impacted their bottom lines by 15 to 20 percent. The financial services industry leadership has now realized that a strong Data Strategy , which includes Data Quality and Data Governance, is the answer to developing efficient business models.
The major drivers of this transformation are, of course, explosive volume of data, dramatically reduced data-storage facilities, and high-speed processing. The increased focus on regulatory compliance of financial services has necessitated use of Data Quality and Data Governance strategies to re-invent the traditional financial services .
One of the SAS users group conducted a Case Study on National Bank of Canada, where the SAS system was used to design a credit-risk management system. National Bank of Canada’s Financial Group provides “financial services to retail, commercial, corporate and institutional clients.” They found the SAS System proactive, fast, and adaptable.
The Data Quality Dimension “Coverage” is the Most Prominent for AI Outcomes describes how “coverage” used as a DQ Dimension can prevent bad or wrong data to surface in ML use cases for the financial services sector.
Use Case for Data Governance: Risk Analysis
A widely used Data Governance application is risk management. Data breaches are common, and industry leaders are well aware of the adverse consequences of data breaches. The Top Five Data Governance Use Cases and Drivers describes how IT departments are proactively managing their “data-related risks” by adopting Data Governance 2.0 approach. According to the Trends in Data Stewardship and Data Governance Report , almost 98 percent of organizations have accepted the importance of Data Governance in assessing and managing data-driven risks. Data Governance for Avoiding Swamps in Data Lakes
The data lake has become a data-storage repository of choice as it can hold very high volumes of multi-format (structured, semi-structured, and unstructured) data. Data Governance allows the data to be “tagged,” which helps users uncover contexts very easily while searching for relevant data for a specific purpose. This tagging mechanism also helps verify the quality, view a sample, and get a historical account of past actions on the data. To avoid a swamp , the data also needs to be strictly governed in terms ownership, accountability, sharing, and usage.
Data Quality and Data Governance for AI Outcomes
In most AI systems, the efficiency and impact of the predictive models depend on the scale and diversity of the data as well as on cleanliness of the data. Even the most powerful AI system may fail to deliver the expected results if the used “data” is not adequately governed and passed through quality checks. Thus, all AI-enabled business analytics systems must also be exposed to sound Data Quality and Data Governance frameworks to operate at maximum efficiency levels.
Data Quality & Data Governance can Maximize Your AI Outcomes describes how Data Quality and Data Governance can enhance the “predictive efficiency” of ML algorithms.
Data Quality Use Cases A Talend blog post describes the use of Talend Data Quality solutions in six different industry verticals, which assure that in coming years, DQ platforms and tools will penetrate the global markets in a big way.
Common Applications of Data Quality Tools Data Quality Study Guide — A Review of Use Cases & Trends states that “It appears there is an abundance of data, but a scarcity of trust, and the need for data literacy .”
According to figures available from a Gartner , “C-Level executives believe that 33% of their data is inaccurate.” To gain the trust of both the employees and customers, these enterprises must turn to Data Quality tools.
A Syncsort blog post describes very common situations where Data Quality checks are performed without most people being aware of it
- Erroneous Addresses in Databases: In many cases, hardcopy forms may be used to collect the address data, which leads to handwritten and erroneous data. Sometimes, even online forms have many mistakes, generating a collection of low-quality data.
- Incomplete Phone Numbers: Phone numbers received directly from consumers are very often provided in haste and usually incomplete. This happens when the information provider does not know which components of the number (country code, area code, etc.) they are supposed to provide.
- Missing Field Entries: This happens very often while filling online forms. Users either miss certain field or enter field data in an incorrect format. Form designers have to take particular care to ensure that the fields provide information on entry format and that empty fields are flagged during form submission.
In the above cases, Data Quality tools are used to catch and rectify errors. Your Data Quality Situation is Unique (But it Really isn’t) talks about data profiling, which is how businesses choose to “organize, maintain, and utilize” their data. Data profiling makes every business’s DQ situation unique,and business users need to be aware of it. Data Quality Use Cases describes the SAS data-cleanup solution used in at least five different situations.
As global businesses continue to rely on data-driven solutions and AI systems for enhancing their competitiveness, Data Quality and Data Governance platforms will assume increased importance in the business landscape. The scale and volume of data-management solutions with a focus on quality and governance flooding the markets in the next few years may be surprising.
Image used under license from Shutterstock.com
Leave a Reply Cancel reply
You must be logged in to post a comment.
Official Sponsors of Mastering SAP for EAM and Supply Chain & Procurement – Gold Coast | Visit us at booth S5, Nov 13-14, 2023. Read more

1. No involvement of business right from the start
Oftentimes, people mistake data governance for a purely IT-led initiative. This stems from the belief that data quality, data analytics, and everything else data fall under the purview of IT. So, it’s commonplace to see the CIO and their IT team going it alone, even at the stage of formulating the strategy and plans.
True, they’ll have the technical experience and skills like choosing the suitable technology solution, integrating it with the existing system landscape, and implementing data-related activities.
But let’s take a step back and think for a moment about why we have data governance in the first place. To improve data quality, specify how data is used and accessed, and most of all, turn data into valuable insights. And who will benefit from all this? The business, of course!
So, for your business people to benefit from data governance, they’d need to be involved at the earliest stage to align the definition and application with their respective job functions. They can provide inputs on foundational matters like prioritisation of data areas to govern, data quality rules and validations, who should have access to which data sets, data retention strategy, etc.
Make no mistake—The involvement of your business people is crucial to add more meat (and teeth!) into the data governance strategy and planning, which in turn will determine its overall success.
2. Absence of clear goal-setting
Most people are aware that data governance isn’t the endgame; it’s a journey unique to each organisation. But this mindset might backfire if it’s set in motion with no ties to business and operational goals.
In the absence of goals, your data governance would become a corporate idealism, not producing tangible outcomes and in danger of being perceived as useless and inconsequential!
Just like any other goal, those you define must be SMART—Specific, Measurable, Achievable, Realistic, and Time-bound. For example, you can focus on governing Supply Chain master data as your first goal and define milestones leading up to it before moving on to other areas. By building a list of manageable and successive goals, you’ll have better control in producing desirable outcomes.
It’s also worth measuring and keeping track of the business KPIs that are in direct correlation with embedding governance. Once your Supply Chain master data is managed and classified, you’ll have better visibility of materials and spares in inventory and can work towards optimisation. You can then measure the resultant cost savings and productivity improvements and share them across your organisation.
By highlighting this success story, you can convince more people of its importance. What’s more—you can secure support and funding from your top management to proceed with the initiative.
3. Poor follow-through on execution
While it’s true that a carefully laid-out plan that engages the right people leads to smoother execution, there’s also the matter of sustaining it.
Data governance is embedded via refreshed mindset, modified behaviours, and new ways of working. Involving business users at the start of the journey is only half of the battle won.
But if they’re expected to see through the execution by just referring to pages and pages of guidance documents without a solid support and monitoring structure, you risk losing their interest. With people on the ground left to their own devices, your data governance initiative gets left behind too.
That’s why you need to have change management to ease the transition of your organisation towards embracing data governance.
This starts with well-thought-out communication and engagement plans to reach out to people from different organisation levels, prep them for what’s coming, address their doubts, and get them onboard.
To address the practical part, you need to identify suitable people for data-related roles; those with the right authority, experience, and skillsets. It’s wise to map the roles against a RACI matrix, a.k.a. Responsibility Assignment matrix. This way, you’ll have clear visibility of roles that should be held accountable and responsible for ongoing data governance and data quality efforts.
Data owners should be appointed for each business area to define the corresponding process and rules right from the start. Their involvement doesn’t stop there; they need to ensure that the rules and policies are updated to suit current business needs. Mapped against the RACI matrix, they are the accountable ones.
The role of data stewards is more operational where they execute the data quality rules and policies as well as cleanse data when the need arises. In RACI matrix, they’re those responsible.
Another thing that’s often taken lightly is documentation. While it’s widely understood that documentation tells you how to do your job, change management should take it a step further.
Documentation should elaborate on the rules of engagement, rationales for implementing business rules, deviations, and exceptions—essentially the whats and the whys. This helps ease your people into new ways of working, understand their roles better, and sustain the practices.
4. No awareness of the benefits
Building upon the previous point, people generally don’t like to be told what to do. It IS human nature!
You could end up having more disgruntled and disengaged employees despite side-stepping the earlier pitfalls. Part of your engagement plans should include focus groups to explain to people the importance of having data governance. While they may have a clear idea of the benefits to their organisation, it may not be enough to inspire action in them.
You should articulate benefits that resonate with them, ones that answer their inner burning question “What’s in it for me?”. You’ll be surprised that a personal reason such as ‘reducing stress and depression caused by bad data’ could be a legit motivating factor to imbibe data governance in day-to-day jobs.
5. No support and commitment from top management
Instilling any kind of organisational reform such as data governance should start from the top.
It’s not enough for top management to give the go-ahead and continue doing business as usual. They should walk the talk too.
People need to see first-hand that the executive leadership team uses data in everything they do—be it examining a single KPI metric or strategising the company’s future roadmap. By demonstrating this behaviour, it’s easier for them to rally people to get on the data governance bandwagon and apply it within their job functions.
It’s also a strategic step for top management to appoint data champions. They are responsible for promoting data governance and building awareness of its importance and relevance within the organisation. Most importantly, data champions should be able to identify and remove barriers that hinder its implementation.
Do NOT downplay the role of data technology
Yes, all these pitfalls allude to people and processes. But you shouldn’t underestimate the role of technology in solidifying the success of your data governance implementation and sustaining it.
Suitable data management and governance solution serve to facilitate and expedite data governance activities. Some examples are automating master data processes, doing data profiling and cleansing, and monitoring data status and health.
Without it, your people will have to spend time and resources doing these tasks by themselves. It’d be like a cottage industry of spreadsheets and in-house apps to manage and govern data. Over time, these siloed systems and disorganised ways of working will spiral out of control, creating chaos, stress, and unhealthy work environment.
Manual/semi-manual work will lead to more errors and people have to spend more time checking and rectifying them. This will divert their time and attention from doing strategic tasks such as encouraging data adoption and usage among their peers.
It’s hard for data governance to thrive in this situation.
Look no further than MDO
Master Data Online (MDO) is your ideal data management and governance platform. MDO doesn’t just automate and simplify your master data processes—it helps you implement data governance and execute the embedment activities with minimal human intervention.
It adopts a comprehensive governance framework that encompasses all data scenarios. Active governance ensures your data is cleansed, deduplicated, and enriched before entering your system. While passive governance supports ongoing validation and remediation of data within your system.
MDO helps you implement rules-based governance policies, enhanced with approval workflows and audit trails. This drives data ownership, builds trust in data, and increases its usage across your organisation—essentially the goals of data governance.
Look no further than MDO to complement and support your data governance journey.
Author: Shigim Yusof
Explore More
- 5 Reasons Why You Need a Master… August 29, 2023
- Master data management for material management in… July 21, 2023
- Why do companies need Business Process Management System June 20, 2023
- Where is master data management used in 2023 June 12, 2023
- How to select a master data management… June 12, 2023
Related Solutions
Data Governance Maturity Assessment: A Case Study Directorate General of Corrections
Ieee account.
- Change Username/Password
- Update Address
Purchase Details
- Payment Options
- Order History
- View Purchased Documents
Profile Information
- Communications Preferences
- Profession and Education
- Technical Interests
- US & Canada: +1 800 678 4333
- Worldwide: +1 732 981 0060
- Contact & Support
- About IEEE Xplore
- Accessibility
- Terms of Use
- Nondiscrimination Policy
- Privacy & Opting Out of Cookies
A not-for-profit organization, IEEE is the world's largest technical professional organization dedicated to advancing technology for the benefit of humanity. © Copyright 2024 IEEE - All rights reserved. Use of this web site signifies your agreement to the terms and conditions.

Table of Contents
Read more posts on.
- Data Governance
- Data Catalog
- Data Compliance
- Data Quality
- Data Lineage
- Data Access
- Business Glossary
View All Blog Posts
Share this blog post.
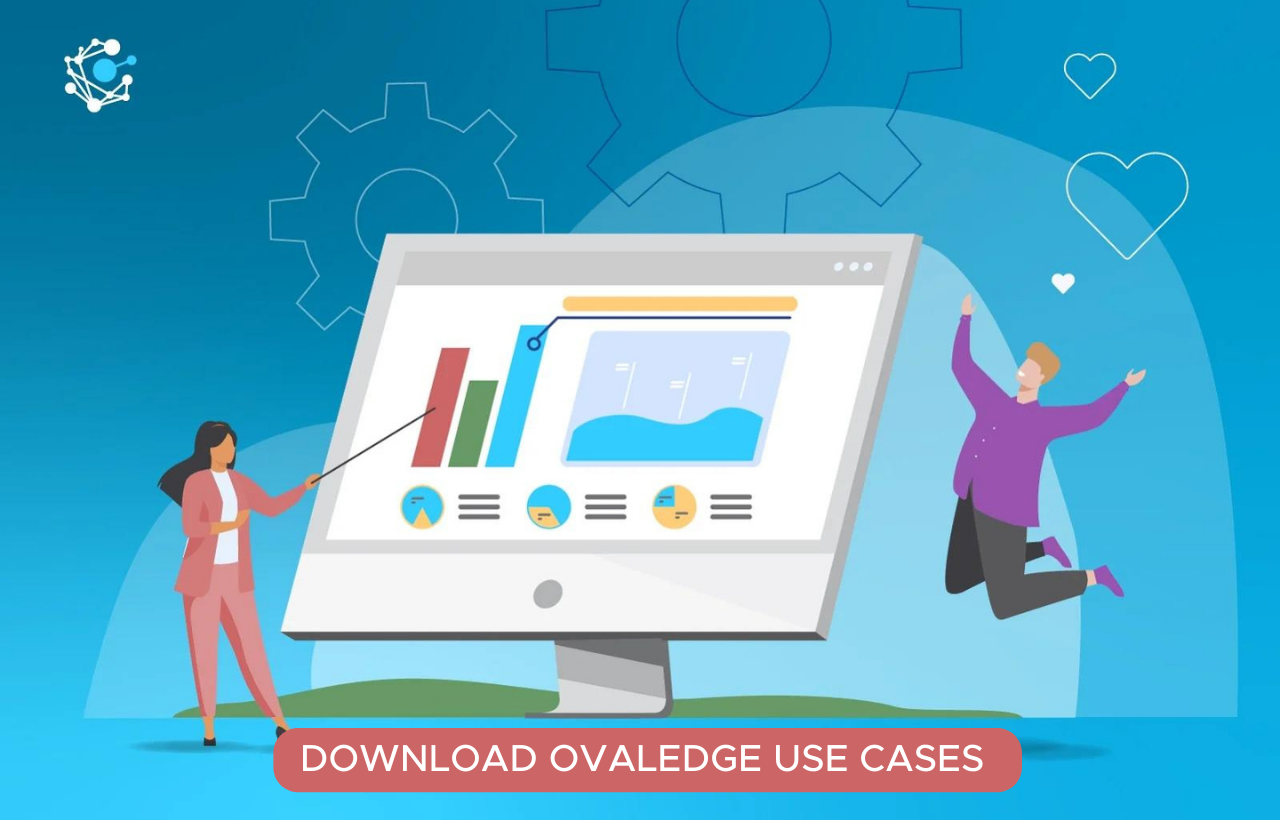
Top 5 Data Governance Use Cases
It’s almost impossible to emphasize how important data is to both people and industry. Not only is data at the heart of most business operations, but there is also increasing pressure from customers to have visibility of what’s being stored, how, and why.
Despite this, about 40 percent of enterprise data is “either inaccurate, incomplete, or unavailable,” which leads to an estimated annual loss of approximately $14 million.
This is why it’s so vital to have a clear understanding of what data governance is, and the different ways you should implement it.
If you’re unsure of what data governance is, you should read our Ultimate Guide , but for now here is how we define it:
Data governance is the process of organizing, securing, managing, and presenting data using methods and technologies that ensure it remains correct, consistent, and accessible to verified users.
Data governance isn’t as complicated as most people think, but it’s still important to have a clear idea of the primary use cases. This helps you effectively implement and improve your existing and future data initiatives.
In this article, we will cover the following Data Governance use cases:
Collaborative analytics or building new data products
Data privacy compliance, data discovery and data literacy provisions, create a centralized repository of all standardized business terms, centralized data access management.
When you’re building a new data product , you need your data to be easily accessible, not just to the data team, but also to other business users.
Often, this simply isn’t possible, because it requires specialist skills -such as SQL - to access and analyze the data.
Data governance removes this blocker by making it easy for anyone in the company to analyze the data meaningfully without needing someone else's help or technical training.
It also makes it significantly easier for the data team to collaborate on specific projects. Data governance tools make it simple to analyze data sets simultaneously, then share findings and results with key stakeholders.
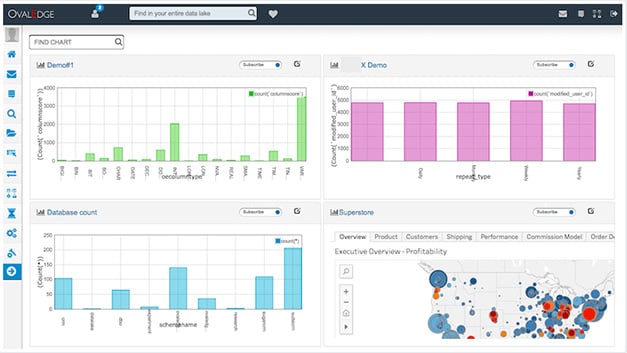
Fintech company Naranja X found that by implementing OvalEdge, they now get a much wider view of their product data:
Before OvalEdge integration, the Naranja X data team only governed a small percentage of the company’s data. Now 70% of the organization’s data warehouse is governed.
Over the last few years, there has been much more emphasis on how our personal data is stored, and what data is kept. And rightly so!
Companies have a duty to only capture the data they need, and only store the data they have permission to keep.
Regulations like GDPR, CCPA, and IAPP have been put in place to protect customers’ PII (Personally Identifiable Information), but they also create a lot of risk for organizations .
Management consulting company Gartner predicts that 75% of the global population will have its personal data covered under privacy regulations over the next couple of years.
With the expansion of privacy regulation efforts across dozens of jurisdictions in the next two years, many organizations will see the need to start their privacy program efforts now. In fact, Gartner predicts that large organizations’ average annual budget for privacy will exceed $2.5 million by 2024.
This can be a scary prospect for businesses, but it doesn’t need to be. Data governance gives you the tools you need to protect yourself from risk, while doing what’s best for your customers.
You can identify and report on where every piece of PII data is stored, even if it’s been masked and protected. Data governance tools also help you solve complex problems, like Right to Know and Right to Delete, which can feel almost impossible when you’ve got large amounts of data.
It also simplifies the auditing process, by helping you organize and report all your privacy terms quickly and easily. At the high level, companies should be able to:
- Identify where the PII is stored, where it came from, and its original source (if different).
- Provide PII data to customers, if requested.
- Delete customers PII data, if requested
Freelancing platform Upwork struggled to identify all the PII data stored across their various systems, until they used OvalEdge. Using our data governance tools, they found, classified and secured all their sensitive data in only a few weeks.
A key benefit to capturing and storing large amounts of data is that you’re able to identify critical information and will be able to build new data products. This can then be used to forecast, plan, and make decisions.
This isn’t always easy, though, and often the data you need is split across various data sources. This makes it difficult to identify and analyze your data, especially for anyone without a technical background.
This process is much more accessible using data governance. Tools like OvalEdge will crawl all your data sources, and index the metadata of every file, table and report.
This doesn’t just make it easier to access your data, but also empowers business users to carry out complex data discovery . Using the generated data catalog , they can search for individual records, research trends, and determine how to access critical information.
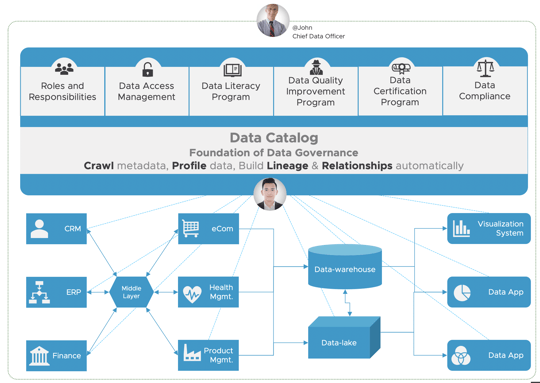
Making this data easier to access and understand also improves data literacy across your company. As Harvard Business Review reported in 2021, this is a huge problem for many businesses:
Ninety percent of business leaders cite data literacy as key to company success, but only 25% of workers feel confident in their data skills.
By giving people easier access to data using data governance, you will steadily improve data literacy across your organization. It also makes it much simpler to develop data standards, and communicate these standards company-wide.
A lot goes into building and running a successful business, but it’s fair to say that none of it would be possible without communication. Poor communication between colleagues, teams, and departments can cause countless problems, and sometimes be the difference between success and failure.
For example, Elon Musk’s attempted Twitter acquisition recently was shrouded in controversy , as neither party could agree on the definition of a ‘fake’ account.
Unfortunately, when you have lots of people, everyone with different backgrounds and education, miscommunication is inevitable. But that doesn’t mean there’s nothing you can do to improve it.
One thing you can do is ensure everyone is on the same page when they use certain words, terms and acronyms. Every business has specific ways of talking about what they do, and it’s important to define standard terminology in one place.
This might sound like a mammoth task, but it’s actually a very solvable problem.
Improve data literacy across your company with OvalEdge
Once your data governance tool has generated your data catalog, it’s easy to build a business glossary that everyone can access. This will include things like:
- Terms and definitions
- Data classification
- Reference data
- Technical metadata
Once this is done, you can share the glossary across the company, and continue updating it as the business evolves and grows.
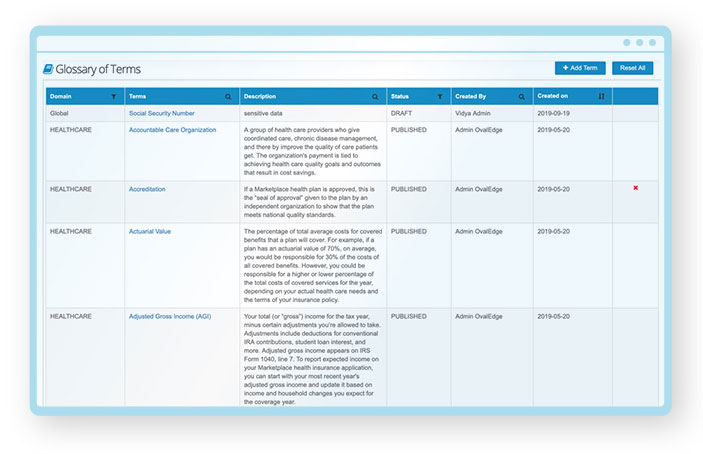
A Michigan-based healthca
re provider successfully grew its business by acquiring and merging with other companies, but quickly realized this had created a problem. Business terms were being used inconsistently, which made it hard for everyone to communicate.
Using OvalEdge, they were able to solve this by building a business glossary of standardized terminology, giving the whole company access. This has helped them communicate more effectively, and make better business decisions.
As we’ve already talked about, there are many benefits to storing large amounts of data, but there is also a lot of risk.
You need to be very careful with who can access what data. If you’re not, you can risk severe backlash from regulators and customers.
If your data is stored in multiple places, it’s hard to ensure everyone has access to the data they need, and it can be difficult to carry out audits.
But if your data is all stored in one place, this has its own challenges. The biggest of which is the complex task of managing permissions so that no-one sees anything they shouldn’t. This could be customers seeing another customers data, or audits missing key information.
This is why it’s so important to have effective data access governance . You can manage access strictly and carefully, wherever your data is stored.
Using automation, discoverability and workflows, you can classify the data in your catalog, then design access policies to suit your needs. This gives you full control, based on your own custom categorizations.
For example, a large accounting firm needed to store financial data for companies spanning more than 34 countries. The challenge is that every country has its own tax laws and regulations.
If their audits returned results from the wrong country, it would cause big issues for the accounting firm and their customers.
Instead, they used OvalEdge for their data access management to mitigate this risk, and can manage their thousands of clients with peace of mind.
Need help convincing stakeholders of the importance of data governance? Download our free Data Governance Business Case Builder
Download Our Trending White Papers
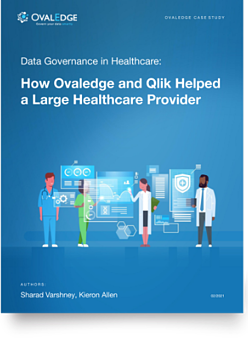
Schedule a Demo
Fill the information below to set up a demo.
OvalEdge Studied by Industry's Top Analyst Firms & Thought Leaders
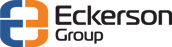
Are you ready to discover your competitive edge?
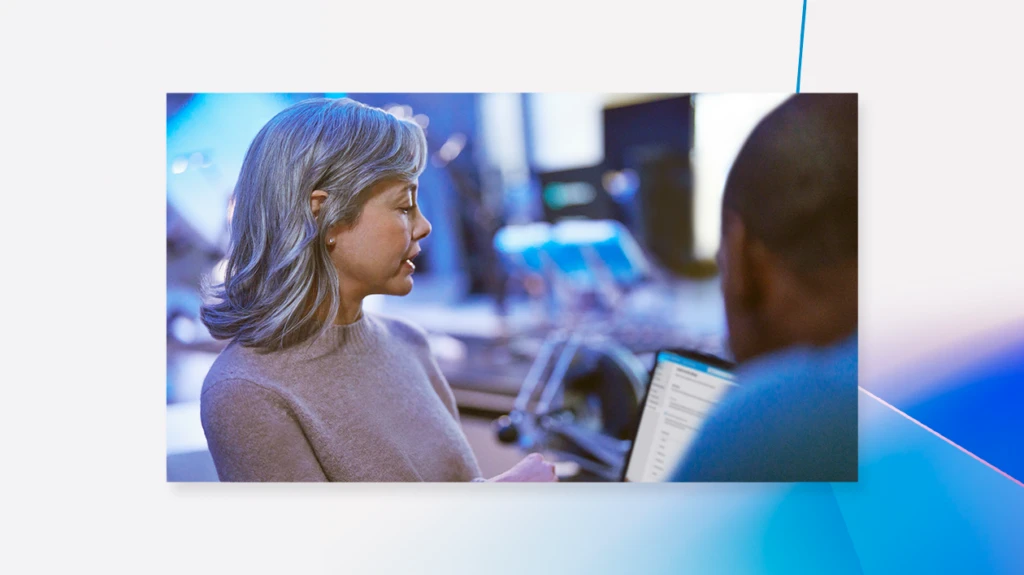
Analytics , Announcements , Microsoft Purview
Introducing modern data governance for the era of AI
By Karthik Ravindran General Manager, Data Governance for Microsoft Purview
Posted on March 26, 2024 6 min read
- Tag: Azure Marketplace
- Tag: Microsoft Fabric
The era of generative AI has arrived, offering new possibilities for every person, business, and industry. At the same time, the speed, scale, and sophistication of cyberattacks, increasing regulations, an ever-expanding data estate, and business demand for data insights are all converging. This convergence pressurizes business leaders to adopt a modern data governance and security strategy to confidently ensure AI readiness.
A modern data governance and security solution unifies data protection and governance capabilities, simplifies actions through business-friendly profiles and terminology with AI-powered business efficiency, and enables federated governance across a disparate multi-cloud data estate.
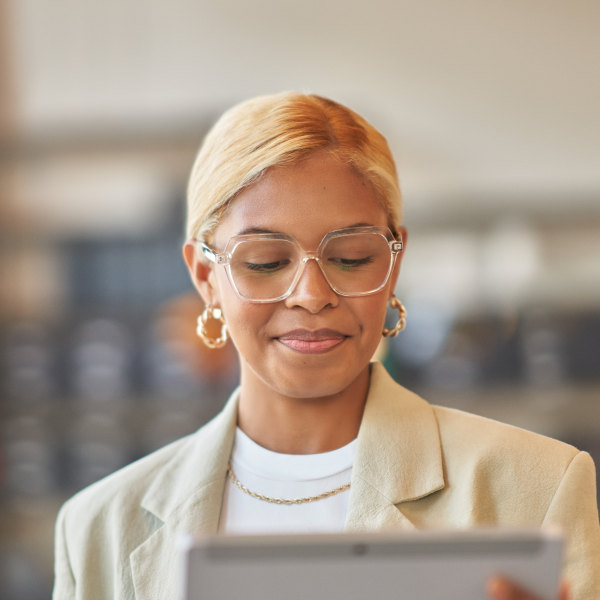
Microsoft Purview
Realize comprehensive data governance solutions for your business
Microsoft Purview is a comprehensive set of solutions that can help your organization govern, protect, and manage data, wherever it lives. Microsoft Purview provides integrated coverage and helps address the fragmentation of data across organizations, the lack of visibility that hampers data protection and governance, and the blurring of traditional IT management roles.
Today, we are excited to announce a reimagined data governance experience within Microsoft Purview, available in preview April 8, 2024. This new software-as-a-service (SaaS) experience offers sophisticated yet simple business-friendly interaction, integration across data sources, AI-enabled business efficiency, and actions and insights to help you put the ‘practice’ into your data governance practice.
“We are thrilled to leverage Microsoft Purview’s new innovative approach to activate the practice of federated data governance. These new capabilities enable us to responsibly scale our data strategy to drive business value, while enhancing our sophisticated data governance practice that meets the demands of our business and puts data insights at the core of our decision making.” Tony Bishop, Group Head of Data & Analytics, Aspen
Modern data governance with Microsoft Purview
I led Microsoft through our own modern data governance journey the past several years and this experience exposed the realities, challenges, and key ingredients of the modern data governance journey.
Our new Microsoft Purview data governance solution is grounded in years of applied learning and proven practices from navigating this data transformation journey along with the transformation journeys of our enterprise customers. To that end, our vision for a modern data governance solution is based on the following design principles:
Anchored on durable business concepts
The practice of data governance should enable an organization to accelerate the creation of responsible value from their data. By anchoring data governance investments to measurable business objectives and key results (OKRs), organizations can align their data governance practice to business priorities and demonstrate business value outcomes.
A unified, integrated, and extensible experience
A modern data governance solution should offer a single-pane-of-glass experience that integrates across multi-cloud data estate sources for data curation, management, health controls, discovery, and understanding, backed with compliant, self-serve data access. The unified experience reduces the need for laborious and costly custom-built or multiple-point solutions. This enables a focus on accelerating data governance practices, activating federated data governance across business units, and ensuring leaders have real-time insights into governance health.
Scale success with AI-enabled experiences
An ever-growing and changing data estate demands simplicity in how it is governed and to ensure business adoption and implementation efficiencies. Natural language interactions and machine learning (ML)-based recommendations across governance capabilities are critical to this simplification and accelerating data governance adoption.
A culture of data governance and protection
Data governance solutions must be built for the practice of federated data governance, unique to each organization. Just as adopting cloud solutions requires one to become a cloud company, adopting data governance requires one to become a data governance company. Modern data governance success requires C-Suite alignment and support, and must be simple, efficient, customizable, and flexible to activate your unique practice.
Introducing data governance for the business, by the business
We are thrilled to introduce the new Microsoft Purview data governance experience. Our new data governance capabilities will help any organization of any size to accelerate business value creation in the era of AI.
A business-friendly approach to govern multi-cloud data estates
Designed with the business in mind, the new governance experience supports different functions across the business with clear role definitions for governance administrators, business domain creators, data health owners, and data health readers.
Within Data Management, customers can easily define and assign business-friendly terminology (such as Finance and Claims). Business-friendly language follows the data governance experience through Data Products (a collection of data assets used for a business function), Business Domains (ownership of Data Products), Data Quality (assessment of quality), Data Access, Actions, and Data Estate Health (reports and insights).
This new data governance experience allows you to scan and search data across your data estate assets .
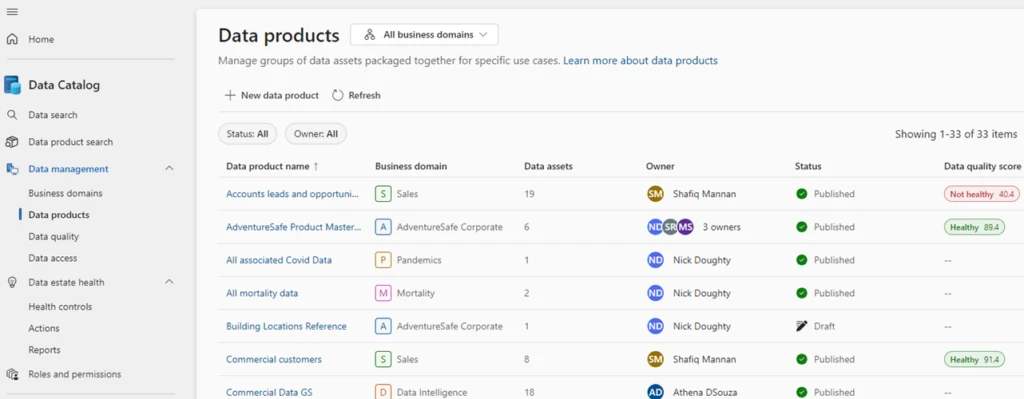
Built-in data quality capabilities and rules which follow the data
The new data quality model enables your organization to set rules top down with business domains, data products, and the data assets themselves. Policies can be set on a term or rule which flows through and helps save data stewards hours to days of manual work depending on the scale of your estate. Once rules and policies are applied, the data quality model will generate data quality scores at the asset, data product, or business domain level giving you snapshot insights into your data quality relative to your business rules.
Within the data quality model, there are two metadata analysis capabilities: 1) profiling—quick sample set insights 2) data quality scans—in-depth scans of full data sets. These profiling capabilities use your defined rules or built-in templates to reason over your metadata and give you data quality insights and recommendations .
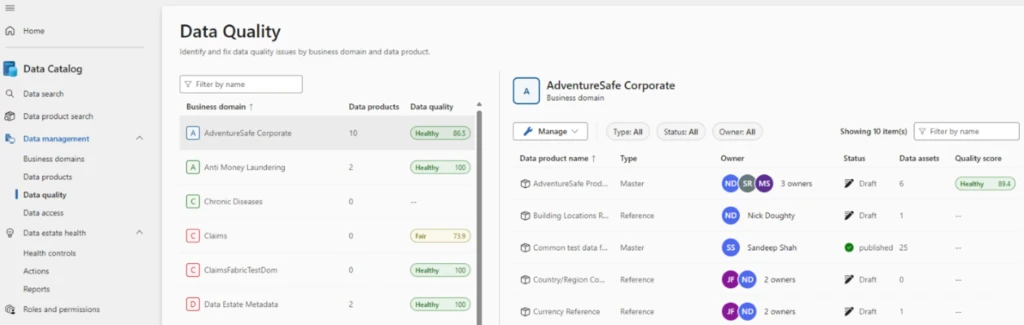
Apply industry standard controls in data estate health management
In partnership with EDM Council, new data health controls include a set of 14 standards for cloud data management controls. These standards govern how data is to be managed while controls create fidelity of how data assets are used/accessed . Examples are metadata completeness, cataloging, classification, access entitlement, and data quality. A data office can configure rules which determine the score and define what constitutes a red/yellow/green indicator score , ensuring your rules and indicators reflect the unique standards of your organization.
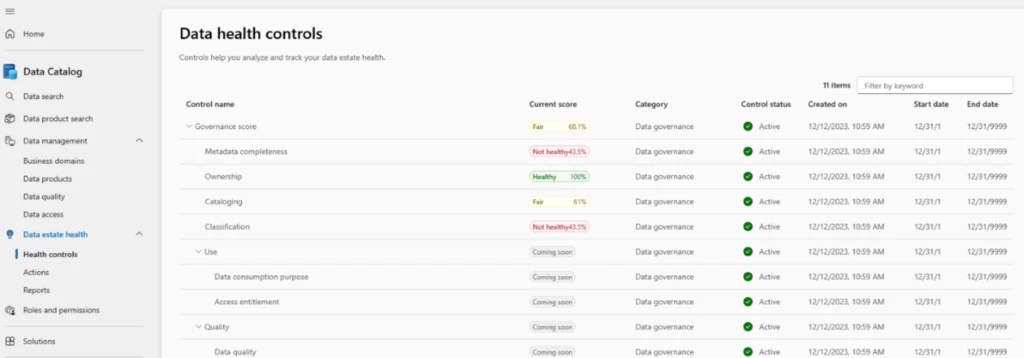
Summarized insights help activate and sustain your practice
Data governance is a practice which is nurtured over time. Aggregated insights help you put the “practice” into your data governance practice by showcasing the overall health of your governed data estate . Built-in reports surface deep insight across a variety of dimensions: assets, catalog adoption, classifications, data governance, data stewardship, glossary, and sensitivity labels.
The image below is the Data Governance report which can be filtered by business domain, data product, and status for deeper insights.
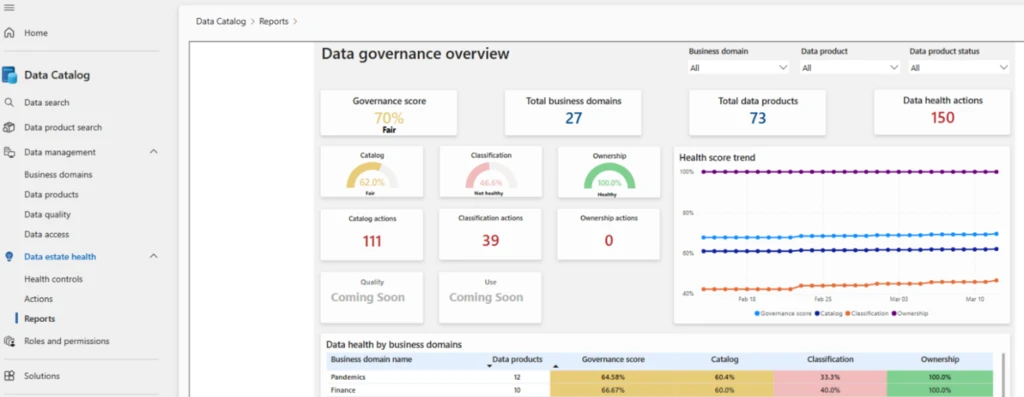
Stay on top of data governance health with aggregated actions
The new Actions center aggregates and summarizes governance-related actions by role, data product, or business domain . Actions stem from usage or implementation being out of alignment from defined controls. This interactive summary makes it easy for teams to manage and track actions—simply click on the action to make the change required. Cleaning up outstanding actions helps improve the overall posture of your data governance practice—key to making governance a team sport.
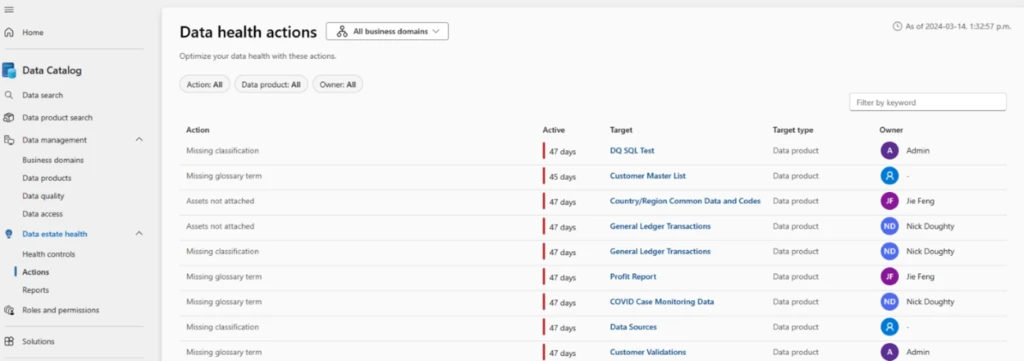
Announcing technology partnerships for even greater customer value
We are excited to announce a solution initiative with Ernst & Young LLP (EY US), who will bring their extensive experience in data solutions within financial services, to collaborate with Microsoft on producing data governance reports and playbooks purpose-built for US-oriented financial services customers. These reports and playbooks aim to accelerate the customer time to value for activating a governance practice that adheres to the unique regulation needs of the financial sector. These assets will be made available in Azure Marketplace over the course of preview and the learnings from this will also help inform future product roadmap.
Additionally, a modern data governance solution integrates and extends across your technology estate. With this new data governance experience, we are also excited to announce technology partnerships that will help seamlessly extend the value of Microsoft Purview to customers through pre-built integration. Integrations will light up over the course of preview and be available in Azure Marketplace.
Master Data Management
- CluedIn brings native Master Data Management and Data Quality functionality to Microsoft Fabric, Microsoft Purview, and the Azure stack. Learn more about CluedIn .
- Profisee Master Data Management is a complimentary and necessary piece of your data governance strategy. Learn more about Profisee .
- Semarchy combines master data management, data intelligence, and data integration into a singular application in any environment. Learn more about Semarchy .
Data Lineage
- Solidatus empowers data-rich enterprises to visualize, understand, and govern data like never before. Learn more about Solidatus .
Try the new experience
Available starting April 8, 2024, please log on to the Microsoft Purview portal and give the reimagined data governance experience within the “Data Catalog” tile a try. We’d love to hear your feedback!
Let us know what you think of Azure and what you would like to see in the future.
Provide feedback
Build your cloud computing and Azure skills with free courses by Microsoft Learn.
Explore Azure learning
Related posts
AI + Machine Learning , Analyst Reports , Azure AI , Azure Machine Learning , Azure Service Fabric , Microsoft Purview , Partners
Microsoft is a leader in the 2023 IDC MarketScape for AI Governance Platforms chevron_right
AI + Machine Learning , Analytics , Azure AI , Azure Machine Learning , Azure OpenAI Service , Best practices , Management and Governance , Microsoft Purview , Thought leadership
Building for the future: The enterprise generative AI application lifecycle with Azure AI chevron_right
AI + Machine Learning , Azure Cognitive Search , Azure Cosmos DB , Azure Machine Learning , Azure OpenAI Service , Events , Microsoft Purview , Text Analytics
What’s new in Azure Data & AI: Helping organizations manage the data deluge chevron_right
AI + Machine Learning , Automation , Azure OpenAI Service , Events , Management and Governance , Microsoft Purview
How Microsoft Azure helps drive agility and optimization for your business chevron_right
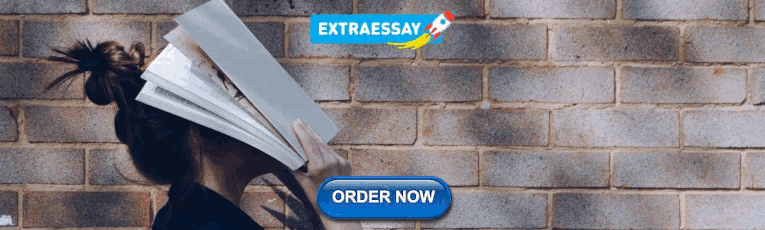
IMAGES
VIDEO
COMMENTS
The percentage of firms identifying themselves as being data-driven has declined in each of the past 3 years — from 37.1% in 2017 to 32.4% in 2018 to 31.0% this year. These sobering results and ...
The Global Data Governance Project: 52 case studies May 05, 2021 By: Thomas Struett and Susan Ariel Aaronson1 Executive Summary: Data is the most collected, analyzed, shared, and/or traded goods or services around the world. Despite its ubiquity, data governance is a relatively new governance responsibility for many countries.
5.2 Social and ethical tensions in the management and use of '-omics' data. This case study considers three areas at the confluence of data governance issues and '-omics' research and practice. The first surrounds how compatible these forms of data are and have been with open scientific inquiry.
The Securitas breach is a classic example of bad data governance and one of many high-profile cases of publicly exposed S3 buckets. But it's important to remember that permission issues aren't just restricted to Amazon S3. They can put data at risk in virtually any type of storage repository.
In many cases, data strategy and governance protocols fail because the governance committee members focus more on their day-to-day tasks rather than the defining and monitoring of company-specific metrics, rules, and KPIs. Lack of commitment. Governance strategies can fail when the company is more focused on talking up the ideals and objectives ...
The Negative Impact of Data Governance Failures. Data governance failures can have disastrous consequences in the organization. The apparent result of non-existent, improper, or ineffective data governance is inconsistent, insecure, and illogically stored data. This makes it harder to merge, verify, and access data later.
The first is the "set it and forget it" mentality. They think of data governance as an initiative to be completed, and that once it's done, they'll be on to the next. The second mistake is similar, which is the inclination to over-govern. While understandable, both approaches miss the heart of what data governance is intended to be.
Governance —" [S]tructures and processes that are designed to ensure accountability, transparency, responsiveness, rule of law, [and] stability…" 2. Risk —"The effect of uncertainty on [business] objectives." 3. Compliance —Acting in accordance with a wish or command. 4. Big Data —High-volume, high-velocity and high-variety ...
Tactic 1: Use a Guide. Using the Data Management Book of Knowledge (DMBOK) wheel, The Data Management Maturity Model from the CMMI Institute, the ten steps from the Data Governance Institute Data Governance Framework, and the First San Francisco Partners Data Governance Framework, she built a maturity model. That model was used to create a set ...
3. Prioritize data assets and focus data leadership accordingly. Many organizations approach data governance in a holistic manner, looking at all data assets at once. But such a large scope means slow relative progress in any given area and a risk that efforts aren't linked directly to business needs.
various stages of data governance, from collection to visualization and utilization. These case studies focus on the development of a data governance policy and the creation of systems for data use and visualization in Paterson Public Schools, Cleveland Metropolitan School District, Howard County Public Schools, and Baltimore County Public Schools.
This case study examines corporate governance issues at Wells Fargo and Company. The bank was embroiled in controversies due to its cross-selling tactics and the enormous pressure the management exerted on the employees to ensure its success.
EOS has built its engagement strategy around two white papers: 1. Investors' Expectations on Responsible Artificial Intelligence and Data Governance was published in April 2019, in conjunction with law firm Bryan Cave Leighton Paisner (BCLP), and addresses concerns from a legal and compliance, and a technical perspective. 2.
The Need for Data Governance: A Case Study. Lai Kuan Cheong, Vanessa Chang School of Information Systems Curtin Business School Curtin University of Technology Western Australia, Australia Email: [email protected]; [email protected].
The research looks at the need for Data Governance to manage data effectively. This study examines a large organisation that has adopted an ad-hoc Data Governance model to manage its data. It was ...
This paper reports on a single case study in a large telecommunications service provider in Saudi Arabia. The data are (1) gathered through semi-structured interviews that follow the CSF approach ...
September 2018. Accounting. Corporate Governance. Download. This Data Spotlight provides data and statistics on unethical behavior in corporations and other negative outcomes including bankruptcy, litigation, and corruption in the United States. This data supplements in the issues introduced in the Quick Guide " Introduction to Corporate ...
While governing data as an organizational asset has clear benefits, mobilizing an organization to implement data governance remains elusive for practitioners. On that account, this paper examines why governing data is difficult in local government organizations. Based on a literature review and an empirical case study, we establish the inherent challenges and build on the notion of collective ...
Although Data Quality and Data Governance are often used interchangeably, they are very different, both in theory and practice. While Data Quality Management at an enterprise happens both at the front (incoming data pipelines) and back ends (databases, servers), the whole process is defined, structured, and implemented through a well-designed ...
5 major Data Governance pitfalls. 1. No involvement of business right from the start. Oftentimes, people mistake data governance for a purely IT-led initiative. This stems from the belief that data quality, data analytics, and everything else data fall under the purview of IT.
The Stanford data governance maturity measurement tool in this study was used to measure the maturity of the data governance program. The results obtained are the maturity level of the data governance program with an average of 1.93 (initial), with the foundation component having an average value of 2. On the other hand, the project component ...
Data governance case study examples: Solving common data management management challenges. Here are hypothetical examples of each data governance use case and how to address them: 1. Data quality management. Problem. An insurance company has inconsistent and inaccurate customer address information, leading to delays in claims processing. Solution
This helps you effectively implement and improve your existing and future data initiatives. In this article, we will cover the following Data Governance use cases: Collaborative analytics or building new data products. Data privacy compliance. Data discovery and data literacy provisions. Create a centralized repository of all standardized ...
A modern data governance solution should offer a single-pane-of-glass experience that integrates across multi-cloud data estate sources for data curation, management, health controls, discovery, and understanding, backed with compliant, self-serve data access. The unified experience reduces the need for laborious and costly custom-built or ...