- Competition
- Inequality & Mobility
- Tax & Macroeconomics
- Value Added
- Elevating Research
- Connect with an Expert
- In the Media
- Terms of Use
- Privacy Policy
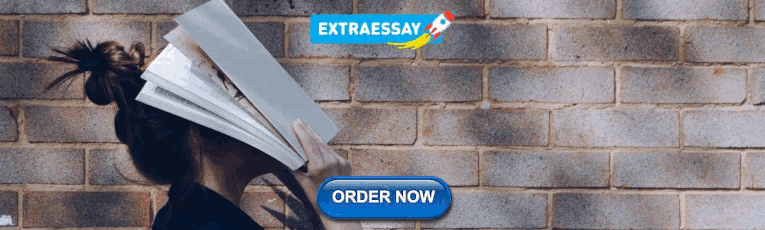
Connect with us
- Washington Center for Equitable Growth
- 740 15th St. NW 8th Floor
- Washington, D.C. 20005
- Phone: 202.545.6002
Income inequality affects our children’s educational opportunities
September 1, 2014.
Economic Inequality
Economic Wellbeing
One of the clearest manifestations of growing economic inequality in our nation today is the widening educational achievement gap between the children of the wealthiest and the children of everyone else . At first glance, this sounds like an obvious outcome. After all, wealthier families are able to afford expensive private schools , or homes in wealthy public school districts with more educational resources.
But a closer look at this education achievement gap over the past 50 years or so shows that the gap only began to widen in the 1970s, right about the time that wealth and income inequality in our nation also began to grow. The past 30 years have seen a sustained rise in inequality in wages, incomes, and wealth, leading to more and more income and wealth accruing to those at the top of the economic ladder, pulling the rich further away from those on the other rungs . 1
At the same time, the growing educational gap became ever more apparent. In the 1980s, the gap between the reading and math skills of the wealthiest 10 percent of kids and poorest 10 percent was about 90 points on an 800-point SAT-type scale. 2 Three decades later, the gap has grown to 125 points. This widening gap is largely due to differences in how well prepared children are for school before they enter kindergarten or even pre-kindergarten . 3 In this era of economic inequality, wealthier parents have far more resources, both in terms of time and money, to better prepare their children to succeed in school and later in life.
This widening educational achievement gap may threaten our future economic growth . With only a select few individuals receiving the best education and enrichment, we are not effectively developing the economic potential of our future workforce. To grow our economy we must provide educational and enrichment opportunities for children across the income spectrum, rather than only a select few at the top.
Wealth and income largely define the educational gap today, more so than race and ethnicity . In the 1950s and 1960s, the opposite was true. Back then, racial discrimination in all aspects of life led to deep racial inequality. Economic inequality, in contrast, was lower than at any time in U.S. history, according to extensive research done by economists Thomas Piketty at the Paris School of Economics and Emmanuel Saez at the University of California, Berkeley. 4 But anti-discrimination and civil rights legislation and school desegregation led to improved economic, social, and educational conditions for African Americans and other minorities beginning in the late 1960s. As a result, the gap today between white and black children is about 70 points on an 800-point SAT-type scale, 40 percent smaller than it was in the 1970s, and about half the size of the gap between rich and poor children, but still unacceptable. 5
The growth of the socioeconomic achievement gap appears to be largely because more affluent parents are increasingly investing more time and money in their kids’ educational enrichment—and at earlier periods in their children’s lives—than hard-pressed low-income and middle class families. 6 Indeed, surveys show that the amount of time and money parents invest in their children has grown sharply over the past four decades among both affluent and non-affluent parents. But the increase in these investments has been two to three times greater among high-income families. 7 Economists Richard Murnane of Harvard University and Greg Duncan at the University of California-Irvine find that between 1972 and 2006 the amount high-income families spent on their children’s enrichment activities grew by 150 percent, while the amount spent by low-income families grew by 57 percent. 8 In part, parents are spending more on their kids because they understand that educational success is increasingly important in today’s uncertain economic times, a point that sociologist Marianne Cooper at the Clayman Institute makes in her recent book “Cut Adrift.” 9 But low- and middle-income families can’t match the resources—both the money and flexible time—of the rich.
As a result, rich and poor children score very differently on school readiness tests before they enter kindergarten. Once they are in school, however, the gap grows very little—by less than 10 percent between kindergarten and high school. 10 Thus, it appears that the academic gap is widening because rich students are increasingly entering kindergarten much better prepared to succeed in school than low- and middle-class students. To be sure, there are important differences in the quality of schools serving low- and high-income students, but these differences do not appear to be as salient as the differences in children’s experiences prior to kindergarten.
The socioeconomic education gap is likely to affect us for decades to come . Think of it as a leading indicator of disparities in civic engagement, college enrollment, and adult success. Indeed, family income and wealth have become increasingly correlated with a variety of positive adolescent activities, such as sports participation, school leadership, extracurricular activities, and volunteer work, according to research conducted by Harvard University political scientist Robert D. Putnam and his colleagues. 11
Not only are the children of the rich doing better in elementary and high school than the children of the poor, they also are cornering the market on the seats in the best colleges. In a study that I conducted with several of my graduate students, we found that 15 percent of high-income students from the 2004 graduating class of high school enrolled in a highly selective college or university compared to only 5 percent of middle-income graduates and 2 percent of low-income graduates. 12 Because these colleges provide educational opportunities and access to social networks that often lead to high-paying jobs, children from low-income families risk are being locked out of the upper end of the economic spectrum. For low-income children, the American Dream is further out of reach.
This is bad news for our future economy and society because we need well-educated workers in order to sustainably boost economic productivity and grow the economy. So how can we prepare every child, not just those most affluent ones, to be productive members of society ? First of all, we must acknowledge that educational problems cannot be resolved by school alone. The achievement gap begins at an early age. To close it, we must invest in children’s early childhood educational opportunities. This means investing not only in preschool but also in parents. Specifically, we need to:
- Invest in high-quality early childhood education programs (pre-schools, day care) and make them affordable for all families.
- Invest in programs that help parents become their children’s first and best teacher.
- Provide policy solutions to help all parents have the time to be teachers through paid leave, paid sick days, workplace flexibility, and income support programs that ensure that families can focus on their children even in hard economic times.
In short, we can narrow the socioeconomic education gap through public policies that help parents of all incomes provide enriching educational opportunities for their children in the way that only affluent parents can do today.
1. Emmanuel Saez, Striking It Richer: The Evolution of Top Incomes in the United States (Updated with 2012 Preliminary Estimates ) (Berkeley, CA: University of California – Berkeley, September 3, 2013), http://elsa.berkeley.edu/~saez/saez-UStopincomes-2012.pdf .
2. Sean F. Reardon, “The Widening Academic Achievement Gap Between the Rich and the Poor: New Evidence and Possible Explanations,” in Whither Opportunity? Rising Inequality, Schools, and Children’s Life Chances , ed. Greg J. Duncan and Richard J. Murnane (New York, NY: Russell Sage Foundation, 2011).
4. Thomas Piketty and Emmanuel Saez, “Income Inequality in the United States, 1913–1998,” The Quarterly Journal of Economics 118, no. 1 (February 2003): 1–39.
5. Reardon, “The Widening Academic Achievement Gap Between the Rich and the Poor: New Evidence and Possible Explanations.”
6. Sabino Kornrich and Frank Furstenberg, “Investing in Children: Changes in Parental Spending on Children, 1972–2007,” Demography 50, no. 1 (February 1, 2013): 1–23, doi:10.1007/s13524-012-0146-4. See also, Ramey, G., & Ramey, V. A. (2009). The rug rat race. National Bureau of Economic Research Working Paper , 15284.
8. Greg J. Duncan and Richard J. Murnane, Whither Opportunity? Rising Inequality, Schools, and Children’s Life Changes (New York City: Russell Sage Foundation, 2012).
9. Marianne Cooper, Cut Adrift: Families in Insecure Times (University of California Press, 2014).
10. Sean F. Reardon, “The Widening Academic Achievement Gap Between the Rich and the Poor: New Evidence and Possible Explanations,” in Whither Opportunity? Rising Inequality, Schools, and Children’s Life Chances , ed. Greg J. Duncan and Richard J. Murnane (New York, NY: Russell Sage Foundation, 2011).
11. Robert D. Putnam, Carl B. Frederick, and Kaisa Snellman, Growing Class Gaps in Social Connectedness among American Youth (Cambridge, MA: Harvard Kennedy School of Government The Saguaro Seminar: Civic Engagement in America, August 8, 2012).
12. Sean F. Reardon, Rachel Baker, and Daniel Klasik, Race, Income, and Enrollment Patterns in Highly Selective Colleges, 1982-2004 (Stanford, CA: Center for Education Policy Analysis, August 3, 2012).
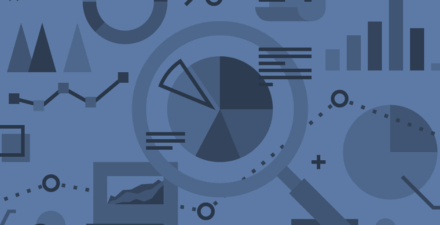
Short and medium run impacts of preschool education: Evidence from state pre-k programs
December 4, 2018
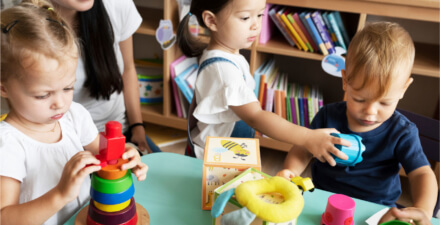
Economist Currie’s research on early childhood care and education highlights the need for a national childcare policy
April 2, 2019
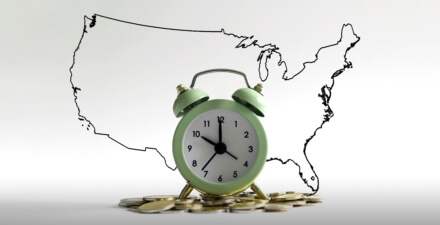
Paid Family and Medical Leave in the United States: A Research Agenda
October 22, 2018
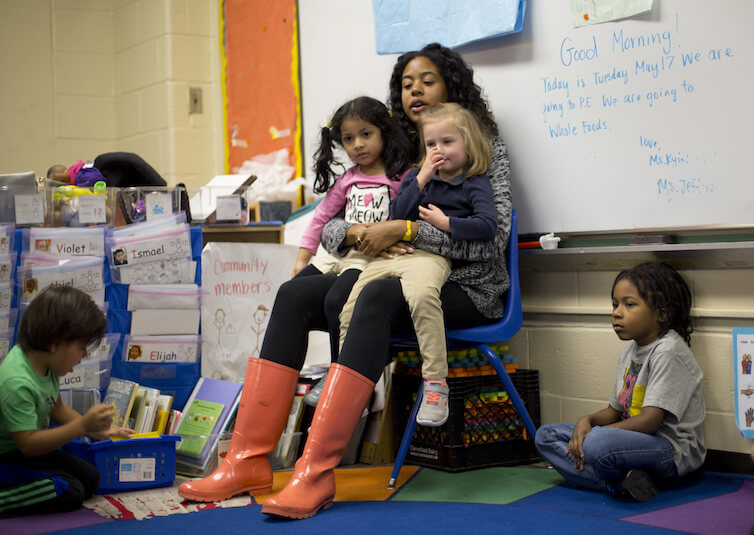
Does targeted or universal pre-Kindergarten better serve low-income kids?
March 13, 2017
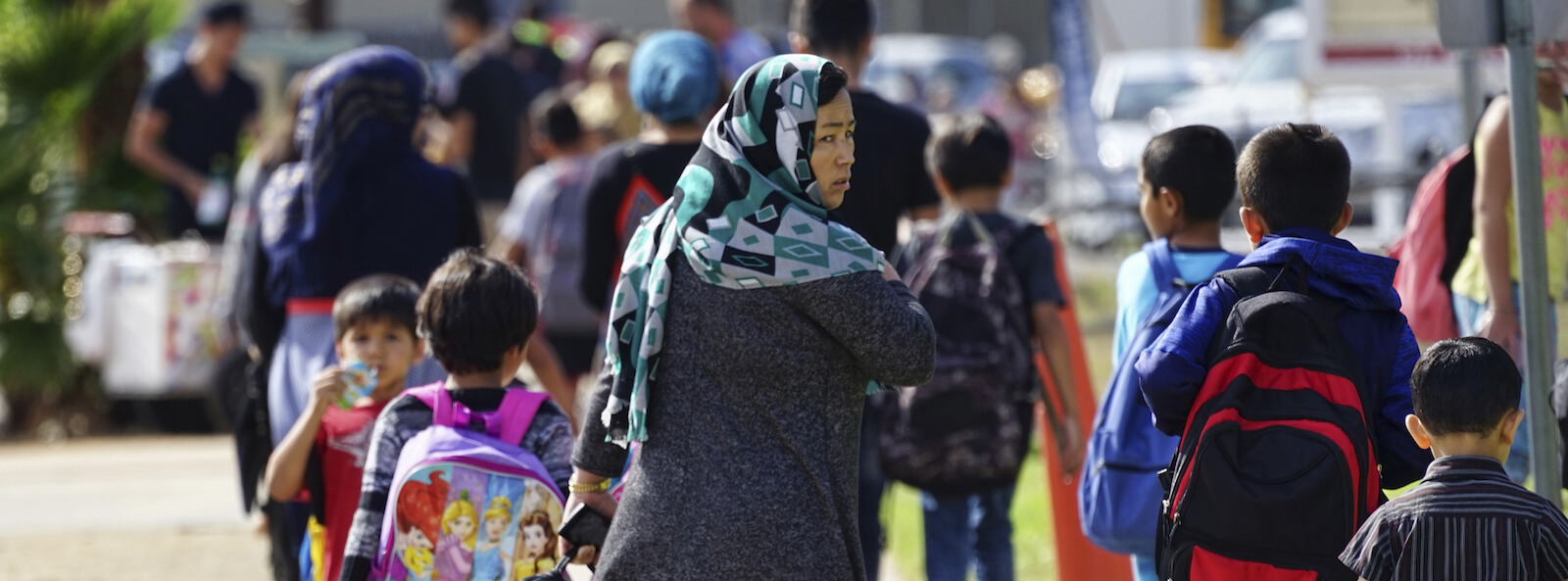
How economic inequality affects children’s outcomes
October 31, 2016
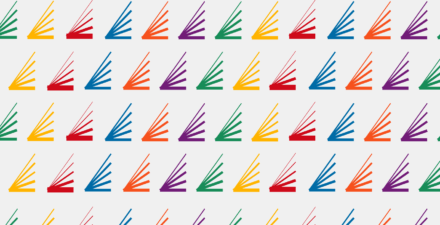
Are today’s inequalities limiting tomorrow’s opportunities?
October 3, 2018
Explore the Equitable Growth network of experts around the country and get answers to today's most pressing questions!
Growing Income Inequality Threatens American Education
- Share article
America has always taken pride in being the land of opportunity, a country in which hard work and sacrifice result in a better life for one’s children. Economic growth has made that dream a reality for generations of Americans, including many people who started out poor. The quarter century following World War II was a golden era for the U.S. economy, as high- and low-income families shared the benefits of substantial economic growth. But storm clouds began to gather in the 1970s. In particular, computer-driven technological changes favoring highly educated workers, plus demographic shifts such as the rise of single-parent families, have produced sharply growing income gaps among families.
In the past, America’s public schools have responded well to the challenges of a changing world. Indeed, America’s world leadership in education has fueled much of its prosperity and made the 20th century the “American Century” (Goldin & Katz, 2008). But technological changes, globalization, and rising income inequality have placed great strains on the decentralized American approach to public education. We are constantly reminded that the math, science, and language skills of our children and young adults lag far behind those of children in other countries. In international rankings, our college graduation rate has fallen from first to 12th.
In this article—the first of two appearing in consecutive months—we describe the origins and nature of growing income inequality and some of its consequences for American children. We document the increased family income inequality that’s occurred over the past 40 years. An increase in income disparity has been more than matched by an expanding gap between the money that low- and high-income parents spend on enrichment activities for their children.
Most distressingly, increasing gaps in academic achievement and educational attainments have accompanied the growth in income inequality. Differences in the reading and math achievement levels of low- and high-income children are much larger than several decades ago, as are differences in college graduation rates.
What accounts for these widening gaps? Drawing from the first part of our recent book, Restoring Opportunity: The Crisis of Inequality and the Challenge for American Education (Harvard Education Press and the Russell Sage Foundation, 2014), we explain that the evidence supports pathways operating through both families and schools. In addition to growing differences in the resources spent by poor and rich families on their children, declining real incomes for low-income families have affected maternal stress, mental health, and parenting. storing the educational opportunities that children from low-income families need if they are to lead productive and fulfilling lives.
Rising residential segregation by income has led to increasing concentrations of low- and high-income children attending separate schools. Peer problems, geographic mobility, and challenges in attracting and retaining good teachers have made it difficult to provide consistently high-quality learning experiences in schools serving a large proportion of low-income students.
Next month’s article draws from the second part of Restoring Opportunity to describe ideas based on proven policy approaches that will enable the country to make progress on the enormous task of re-storing the educational opportunities that children from low-income families need if they are to lead productive and fulfilling lives.
Widening Gaps
Based on U.S. Census Bureau data, the left-hand bar in each set of bars in Figure 1 shows the average income in a particular year (in 2012 dollars) for children at the 20th percentile of the nation’s family income distribution. This means that, in a given year, 20% of children lived in families with incomes below that level, while 80% had incomes above it. In 1970, the dividing line was drawn at $37,664.
The middle bar in each set shows the average family income in a given year at the 80th percentile of the distribution, which was about $100,000 (in 2012 dollars) in 1970. The right-hand bar in each set shows the average income for very high-income families — those with incomes exceeding those of 95% of U.S. families (a little more than about $150,000 in 1970).
In contrast to the two decades before 1970, when the incomes of these three groups grew at virtually identical rates, economic growth over the next four decades failed to lift all boats. In 2010, family income at the 20th percentile was more than 25% lower than the inflation-adjusted corresponding family income in 1970. In contrast, the real incomes of families at the 80th percentile grew by 23% to $125,000 over these four decades, while the incomes of the richest 5% of families rose even more. Census Bureau data also show that the decline of the incomes of families at the lower end of the spectrum is reflected in the nation’s child poverty rate: Over 16 million U.S. children—more than 20%—were living in poor families in 2012, up sharply from the 15% child poverty rate in 1970.
During this same time period, the gap between the average reading and mathematics skills of students from low- and high-income families increased substantially. As illustrated in Figure 2, among children who were adolescents in the late 1960s, test scores of low-income children lagged behind those of their better-off peers by four-fifths of a standard deviation — which represents about 80 points on the scale used to measure SAT scores. Forty years later, this gap was 50% larger, amounting to nearly 125 SAT-type points (Reardon, 2011). We were surprised to discover how much the income-based gap grew during this period in view of the decline in the racial gap in test scores in the decades following the 1954 U.S. Supreme Court decision in Brown vs. Board of Education .
Given the importance of academic preparation in determining educational success, it should come as no surprise that growth in the income gap in children’s reading and mathematics achievement has contributed to growth in the corresponding gap in the rate of college completion (Figure 3, which is based on Bailey & Dynarski, 2011). Among children growing up in relatively affluent families, the four-year college graduation rate of those who were teenagers in the mid-1990s was 18 percentage points higher than the rate for those who were teenagers in the late 1970s. In contrast, among children from low-income families, the graduation rate was only 4 percentage points higher for the later cohort than for the earlier one. Analysts differ in their assessments of the relative importance of college costs and academic preparation in explaining the increasing gulf between the college graduation rates of affluent and low-income children in our country. However, both cost burdens and academic performance are rooted, at least in part, in the growth in family income inequality.
Inequality Affects Skills Attainment
American society relies on its families to nurture its children and its schools to level the playing field for children born into different circumstances. More than any other institution, schools are charged with making equality of opportunity a reality. During a period of rising inequality, can schools play this critical role effectively? Or has growing income inequality affected families, neighborhoods, and schools in a manner that undercuts the effectiveness of schools serving disadvantaged populations?
Very young children tend to be completely dependent on their families to provide what they need for healthy development (Duncan & Magnuson, 2011). Children growing up in families with greater financial resources score higher on many dimensions of school readiness upon entering kindergarten. An obvious advantage of a higher family income is that it provides more resources to buy books, computers, high-quality childcare, summer camps, private schooling, and other enrichments. In the early 1970s, high-income families spent just under $3,000 more per year (in 2012 dollars) on child enrichment than low-income families (Figure 4; Duncan & Murnane, 2011). By 2006, this gap had nearly tripled, to $8,000. Spending differences are largest for enrichment activities such as music lessons, travel, and summer camps (Kaushal et al., 2011). Differential access to such activities may explain the gaps in background knowledge between children from high-income families and those from low-income families that are so predictive of reading skills in the middle and high school years (Snow, 2002).
Parents also spend different amounts and quality of time interacting with their children. High-income parents spend more time than low-income parents in literacy activities with their children. Most disparate is time spent in “novel” places — other than at home, school, or in the care of another parent or a childcare provider. Between birth and age six, children from high-income families spend an average of 1,300 more hours in novel contexts than children from low-income families (Phillips, 2011). These experiences, financed by the higher incomes of more affluent families, also contribute to the background knowledge that is so critical for comprehending science and social studies texts in middle school.
It is difficult to untangle the precise effects of a multitude of family-related factors — income and expenditures, family structure, time, and language use — on the disparities in children’s school readiness and success that have emerged over the past several decades. But the evidence linking income to children’s school achievement suggests that the sharp increase in the income gap between high- and low-income families since the 1970s and the concomitant increase in the income-based gap in children’s school success are hardly coincidental.
In particular, two experimental studies in the 1970s examined the overall effects on children of income supplements that boosted family income by as much as 50% (Maynard, 1977; Maynard & Murnane, 1979). At two of the three sites, researchers found that children in families randomly assigned to receive an income supplement did significantly better with respect to early academic achievement and school attendance than children in families that received no income supplement.
Still more evidence on policy-relevant effects of income increases comes from a study that takes advantage of the increasing generosity of the U.S. Earned Income Tax Credit (EITC) between 1993 and 1997 to compare children’s test scores before and after the credit was expanded (Dahl & Lochner, 2012). The authors found increases in low-income children’s achievement in middle childhood that coincided with the EITC expansion.
The strongest research evidence appears to indicate that money matters in a variety of ways for children’s long-term success in school. While some children always have enjoyed greater benefits and advantages than others, the income gap has widened dramatically over the past four decades and, as these research studies suggest, this has been a significant factor in widening the gap in children’s school success as well.
Researchers have long known that children attending schools with mostly low-income classmates have lower academic achievement and graduation rates than those attending schools with more affluent student populations. Less well understood are the ways in which student body composition shapes school functioning and children’s developmental trajectories and long-run outcomes.
In recent decades, it has been largely through an increase in income-based segregation of neighborhoods and schools that growing inequality of family income has affected the educational attainments of the nation’s children. Residential segregation by income has increased substantially in recent decades, as high-income families buy homes in neighborhoods where less-affluent families cannot afford to live, and poor families are increasingly surrounded by neighbors who are poor as well (Reardon & Bischoff, 2011). This reduces interactions between rich and poor in settings ranging from schools and child care centers to libraries and grocery stores. Without the financial and human resources and political clout of the wealthy, institutions in poorer neighborhoods, including schools, may decline in quality.
Perhaps most important, increasing residential segregation by income has led to increasing school segregation by income. From 1972 to 1988, schools became more economically segregated, and teenagers from affluent families were less and less likely to have classmates from low-income families (Altonji & Mansfield, 2011). As a result, a child from a poor family is two to four times as likely as a child from an affluent family to have classmates in either elementary or high school with behavioral problems and low skills. This sorting matters because the weak cognitive skills and behavioral issues of many low-income children have a negative effect on their classmates’ learning.
Student mobility resulting from these residential changes poses another threat to achievement. Urban families living in poverty move frequently, and, as a result of school sorting by socioeconomic status, children from poor families are especially likely to attend schools with relatively high numbers of new students arriving during the school year. Recent research has shown that children attending elementary schools with considerable student mobility make less progress in mathematics than do children in schools with less student turnover. Moreover, these negative effects apply to students who themselves are residentially stable as well as to those who are not and are likely to stem from disruption of instruction caused by the entry of new students into a class (Raudenbush, Jean, & Art, 2011).
Poor teacher quality, too, contributes to the weak performance of students in high-poverty schools. A substantial body of research has shown that schools serving high concentrations of poor, nonwhite, and low-achieving students find it difficult to attract and retain skilled teachers. In addition to preferring schools with relatively low proportions of low-achieving students, teachers favor schools in neighborhoods with higher-income residents and less violent crime (Boyd et al., 2011). In high-poverty schools, teacher commitment, parental involvement, and student achievement all tend to be lower.
Yet another challenge facing many of the nation’s schools concerns the school placements of new immigrants, many of whom speak little English. Today’s immigrants are more likely than their predecessors in the early 1970s to come from high-poverty countries. Black and Hispanic immigrants to New York City are much more likely to be poor than are white immigrants from Eastern Europe, and they are more likely to attend elementary and middle schools with native-born black and Hispanic students who are poor (Schwartz & Stiefel, 2011). Thus, while immigrants are not segregated from the native-born in New York City schools, their residential patterns contribute to segregation of schools by socioeconomic status and race.
Helping Low-income Children
By widening the gap in educational opportunities between children from low- and higher-income families, increasing income inequality jeopardizes the upward socioeconomic mobility that has long held our pluralistic democracy together. Improving educational outcomes for children growing up in low-income families is therefore critical to the nation’s future and requires a combination of policies that support low-income families and measures to improve the quality of schools that low-income children attend.
The United States has implemented a range of policies to raise the buying power of low-income families, including the Child Tax Credit, the Earned Income Tax Credit, cash assistance programs, and the Supplemental Nutrition Assistance Program (formerly Food Stamps). Recent studies show that the increases in family incomes produced by these programs result in improved educational outcomes for young children and health in adulthood (Hoynes, Schanzenbach, & Almond, 2013). Unfortunately, these programs are under attack as Congress seeks ways to reduce the federal budget deficit.
Improving the quality of schools attended by low-income children poses even more important and difficult challenges. As a nation, we have failed to appreciate the extent to which technological innovations have brought changes in the skills needed to succeed in today’s economy. Moreover, the rising economic and social inequality produced by technology and globalization has weakened neighborhoods and families in ways that make effective school reform all the more difficult. For a variety of historical reasons, our nation has not learned how to provide the consistent supports that schools—especially those serving large numbers of low-income children—must have to succeed.
Discussions of school reforms often center on simplistic silver bullets: more money, more accountability, more choice, new organizational structures. None of these reforms has turned the tide because none focuses directly on improving what matters most in education: the quality and consistency of the instruction and experiences offered to students. In our companion article, which will appear next month, we detail the building blocks that we consider essential for an “American solution” to the serious problems facing our nation’s schools.
- Altonji, J.G. & Mansfield, R. (2011). The role of family, school, and community characteristics in inequality in education and labor market outcomes. In G.J. Duncan & R.J. Murnane (Eds.), Whither opportunity? Rising inequality, schools, and children’s life chances (pp. 339-358). New York, NY: Russell Sage Foundation & Spencer Foundation.
- Bailey, M.J. & Dynarski, S.M. (2011). Inequality in postsecondary education. In G.J. Duncan & R.J. Murnane (Eds.), Whither opportunity? Rising inequality, schools, and children’s life chances (pp. 117-132). New York, NY: Russell Sage Foundation & Spencer Foundation.
- Boyd, D., Lankford, H., Loeb, S., Ronfeldt, M., & Wyckoff, J. (2011). The effect of school neighborhoods on teachers’ career decisions. In G.J. Duncan & R.J. Murnane (Eds.), Whither opportunity? Rising inequality, schools, and children’s life chances (pp. 377-396). New York, NY: Russell Sage Foundation & Spencer Foundation.
- Dahl, G.B. & Lochner, L. (2012). The impact of family income on child achievement: Evidence from the earned income tax credit. American Economic Review , 102 (5), 1927-1956.
- Duncan, G.J. & Magnuson, K. (2011). The nature and impact of early achievement skills, attention skills, and behavior problems. In G.J. Duncan & R.J. Murnane (Eds.), Whither opportunity? Rising inequality, schools, and children’s life chances (pp. 47-70). New York, NY: Russell Sage Foundation & Spencer Foundation.
- Duncan, G.J. & Murnane, R. J. (2011). Introduction: The American dream, then and now. In G.J. Duncan & R.J. Murnane (Eds.), Whither opportunity? Rising inequality, schools, and children’s life chances (pp. 3-26). New York, NY: Russell Sage Foundation & Spencer Foundation.
- Goldin, C.D. & Katz, L.F. (2008). The race between education and technology . Cambridge, MA.: Harvard University Press.
- Hoynes, H.W., Schanzenbach, D.W., & Almond, D. (2012). Long run impacts of childhood access to the safety net. Unpublished NBER Working Paper No. 18535.
- Kaushal, N., Magnuson, K., & Waldfogel, J. (2011). How is family income related to investments in children’s learning? In G.J. Duncan & R.J. Murnane (Eds.), Whither opportunity? Rising inequality, schools, and children’s life chances (pp. 187-206). New York, NY: Russell Sage Foundation & Spencer Foundation.
- Maynard, R.A. (1977). The effects of the rural income maintenance experiment on the school performance of children. American Economic Review, 67 (1), 370-375.
- Maynard, R.A. & Murnane, R.J. (1979). The effects of a negative income tax on school performance: Results of an experiment. Journal of Human Resources, 14 (4), 463-476.
- Phillips, M. (2011). Parenting, time use, and disparities in academic outcomes. In G.J. Duncan & R.J. Murnane (Eds.), Whither opportunity? Rising inequality, schools, and children’s life chances (pp. 207-228). New York, NY: Russell Sage Foundation & Spencer Foundation.
- Raudenbush, S.W., Jean, M., & Art, E. (2011). Year-by-year and cumulative impacts of attending a high-mobility elementary school on children’s mathematics achievement in Chicago, 1995-2005. In G.J. Duncan & R.J. Murnane (Eds.), Whither opportunity? Rising inequality, schools, and children’s life chances (pp. 359-376). New York, NY: Russell Sage Foundation & Spencer Foundation.
- Reardon, S.F. (2011). The widening academic achievement gap between the rich and the poor: New evidence and possible explanations. In G.J. Duncan & R.J. Murnane (Eds.), Whither opportunity? Rising inequality, schools, and children’s life chances (pp. 91-116). New York, NY: Russell Sage Foundation & Spencer Foundation.
- Reardon, S.F. & Bischoff, K. (2011). Income inequality and income segregation. American Journal of Sociology, 116 (4), 1092-1153.
- Schwartz, A.E. & Stiefel, L. (2011). Immigrants and inequality in public schools. In G.J. Duncan & R.J. Murnane (Eds.), Whither opportunity? Rising inequality, schools, and children’s life chances (pp. 419-442). New York, NY: Russell Sage Foundation & Spencer Foundation.
- Snow, C. (2002). Reading for understanding: Toward a research and development program in reading comprehension. Santa Monica, CA: Rand Corporation.
All articles published in Phi Delta Kappan are protected by copyright. For permission to use or reproduce Kappan articles, please e-mail [email protected] .
Sign Up for The Savvy Principal
Edweek top school jobs.

Sign Up & Sign In

Report | Children
Education inequalities at the school starting gate : Gaps, trends, and strategies to address them
Report • By Emma García and Elaine Weiss • September 27, 2017
Download PDF
Press release
Share this page:
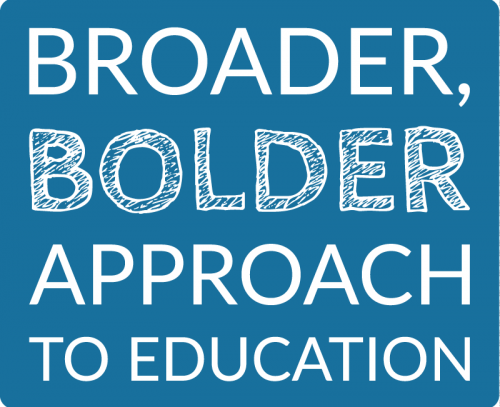
This report was produced in collaboration with the Broader, Bolder Approach to Education .
What this study finds: Extensive research has conclusively demonstrated that children’s social class is one of the most significant predictors—if not the single most significant predictor—of their educational success. Moreover, it is increasingly apparent that performance gaps by social class take root in the earliest years of children’s lives and fail to narrow in the years that follow. That is, children who start behind stay behind—they are rarely able to make up the lost ground.
Using data from two academic cohorts, the kindergarten classes of 1998 and 2010, this study examines the relationship between children’s socioeconomic status (SES) and their cognitive and noncognitive skills when starting school. We find that large performance gaps exist between children in the lowest and highest socioeconomic-status (SES) quintiles and that these gaps have persisted from the 1998 cohort to the 2010 cohort. The positive news is that the gaps have not grown, even as economic inequalities between these two groups of students have grown. The negative news is that the gaps have not narrowed, despite the fact that low-SES parents have substantially increased their engagement in their children’s early education.
Why it matters: These performance gaps reflect extensive unmet needs and thus untapped talents among low-SES children. The development of strong cognitive and noncognitive skills is essential for success in school and beyond. Low educational achievement leads to lowered economic prospects later in life, perpetuating a lack of social mobility across generations. It is also a loss to society when children’s talents are allowed to go fallow for lack of sufficient supports. The undeniable relationship between economic inequalities and education inequalities represents a societal failure that betrays the ideal of the “American dream.”
What can be done about it: Greater investments in pre-K programs can narrow the gaps between students at the start of school. And to ensure that these early gains are maintained, districts can provide continued comprehensive academic, health, nutrition, and emotional support for children through their academic years, including meaningful engagement of parents and communities. Such strategies have been successfully implemented in districts around the country, as described in this report, and can serve to mitigate the impact of economic inequalities on children’s educational achievement and improve their future life and work prospects.
For further discussion of policy solutions, see the companion to this report, Reducing and Averting Achievement Gaps: Key Findings from the Report ‘Education Inequalities at the School Starting Gate’ and Comprehensive Strategies to Mitigate Early Skills Gaps .
Executive summary
High and rising inequality is one of the United States’ most pressing economic and societal issues. Since the early 1980s, the total share of income claimed by the bottom 90 percent of Americans has steadily decreased, with the majority of income gains going to the top 1 percent. These trends would not be such a major concern if our education system compensated for these inequities by helping level the playing field and enabling children to rise above their birth circumstances.
But that is hardly the case. Rather, the fraction of children who earn more than their parents (absolute mobility) has fallen from approximately 90 percent for children born in 1940 to 50 percent for children born in the 1980s. And the tight links between economic inequalities and achievement gaps cast doubt on asserted equality of opportunity that promotes social mobility and puts the “American Dream” within viable reach.
Extensive research has conclusively demonstrated that children’s social class is one of the most significant predictors—if not the single most significant predictor—of their educational success. Moreover, it is increasingly apparent that performance gaps by social class take root in the earliest years of children’s lives and fail to narrow in the years that follow.
Much is known about the determinants and mechanisms that drive early skills gaps among children of different backgrounds, but our failure to narrow social-class-based skills gaps from one generation of students to the next calls for further analysis to determine the degree of influence these factors have and how interventions employed in recent years to address these factors have or have not worked and why. Moreover, shifting economic and demographic landscapes emphasize the need for more robust policy strategies to address the gaps. This three-part study thus combines a statistical analysis of early skills gaps among a recent cohort of children and changes in them over time with a qualitative study of multifaceted, school-district-level strategies to narrow them.
What we do: Questions, data and methodology
In this paper, we:
- Use data from the National Center for Education Statistics (NCES): the Early Childhood Longitudinal Study of the Kindergarten Classes of 1998–1999 and 2010–2011 to measure gaps in skills by social class. To measure gaps by social class, we use the socioeconomic status (SES) metric (primarily), a composite of information on parents’ educational attainment and job status as well as household income. We compare the average performance of children in the top fifth of the socioeconomic status distribution (high-SES) with the average performance of children in the bottom fifth (low-SES). Skills measured include reading and mathematics, as well as self-control and approaches to learning as reported by both teachers and parents.
- Examine SES-based gaps at kindergarten entry among the most recently surveyed cohort (the kindergarten class of 2010–2011). We study how gaps manifest in both cognitive and so-called noncognitive skills, as both skill types are important components of children’s development.
- Compare these SES gaps with those of an earlier cohort (1998–1999), with a focus on changes in the skills gaps between children in the high- and low-SES quintiles. We also analyze how sensitive gaps are to the inclusion of key determinants of student performance, such as family composition, children’s own characteristics, pre-K participation, and parental and educational practices at home.
- Review a set of 12 case studies of communities that have employed comprehensive educational strategies and wraparound supports to provide more children (especially low-income children) with strong early academic foundations, and to sustain and build on early gains throughout their K–12 school years.
- Based on examples from these diverse communities, we discuss implications: strategies that districts can employ and district and state policy changes to make those strategies easier to adopt and more sustainable. The report ends with conclusions and recommendations for further research, practice, and policy.
What we find
Our quantitative research produces a broad set of findings:
- Very large SES-based gaps in academic performance exist and have persisted across the two most recent cohorts of students when they start kindergarten. The estimated gaps between children in the highest and lowest fifths of the SES distribution are over a standard deviation (sd) in both reading and math in 2010 (unadjusted performance gaps are 1.2 and 1.3 sd respectively). Gaps in noncognitive skills such as self-control and approaches to learning are roughly between one-third and one-half as large (unadjusted performance gaps are about 0.4 sd in self-control, and slightly over 0.5 sd in approaches to learning in 2010).
- SES-based gaps across both types of skills among the 2010 kindergartners are virtually unchanged compared with the prior academic generation of students (the class of 1998). The only unadjusted cognitive skills gap between children in the high-SES and low-SES fifths that changed significantly over this period was the gap in reading skills, which increased by about a tenth of a standard deviation. Gaps in approaches to learning as reported by teachers and in self-control as reported by parents shrank between 1998 and 2010 by roughly the same amount (0.1 sd). Gaps in mathematics, in approaches to learning as reported by parents, and in self-control as reported by teachers did not change significantly.
- This means that though part of the SES gap is attributable to differences in these characteristics and in family investments between children in the high and low parts of the SES distribution, a substantial share of SES-related factors is not captured by these controls, but is important to explaining how and why gaps develop, and thus how to narrow them.
- Moreover, the capacity for these other factors to narrow gaps has decreased over time—as a whole, they accounted for a smaller share of the gaps in 2010 than they had in 1998. This suggests that, while such activities as parental time spent with children and center-based pre-K programs cushion the negative consequences of growing up in a low-SES household, they can do only so much, and that the consequences of poverty are increasingly hard to compensate for. This resistance of gaps to these controls is thus a matter of serious concern for researchers and policymakers alike.
- These children’s likelihood of attending center-based pre-K did not change significantly across generations (about 44 percent for both cohorts: 44.3 percent in 2010 vs. 43.7 percent in 1998). However, in 2010 their parents reported having a somewhat larger number of books at home for the children, and there was also an increase in both indices of activities (literacy/reading activities and other educational and engagement activities).
- In addition to doing more for their children, low-SES parents have greater expectations for their children’s educational attainment—a much smaller share saw them going no further than high school graduation, while a much greater share anticipated their children attaining bachelor’s and even advanced degrees in 2010.
- They were slightly more likely to live with two parents (the share not living with two parents decreased from 11.1 percent in 1998 to 9.6 percent) and to have attended center-based pre-K (the share in center-based pre-K increased from 65.8 in 1998 to 69.9 percent in 2010).
- The share of high-SES homes reporting having more than 200 children’s books slightly increased in 2010, as did parents’ expectations for their children’s educational attainment.
- Although research uses various indicators to measure individuals’ social class, from composite measures such as the socioeconomic status index we use to single indicators such as mother’s education or income, some sensitivity of the results to the indicator used is found. In our analyses, we find that all are equally reliable social-class proxies for the estimation of early achievement gaps, though absolute gaps and trends in them vary slightly depending on the indicator used.
Our qualitative review of community interventions also provides valuable information:
- A growing number of school districts across the country have embraced systems of comprehensive enrichment and supports for many or even all their students, based on the understanding that nurturing healthy child development requires leveraging the entire community. These districts took different approaches to enacting those comprehensive strategies, based on each community’s particular mix of needs and assets, ideological leaning, available sources of funding, and other factors. But all begin very early in children’s lives and align enriching school strategies with a targeted range of supports for children and their families.
- Moreover, school districts embracing what we refer to as “whole-child” approaches to education are seeing better outcomes for students, from improved readiness for kindergarten to higher test scores and graduation rates and narrower achievement gaps. They thus can provide guidance to other districts and to policymakers regarding how to implement such approaches, what to expect in terms of benefits, and which policies at the local and state levels can advance those approaches.
Conclusions
While the persistence of large skills gaps at kindergarten entry is troubling, the fact that, by and large, they did not grow in a generation—despite steadily increasing income inequality compounded by the worst economic crisis in many decades—is a good thing. But we must still be very concerned about these gaps. We would have liked to see evidence that parents’ increased dedication to and investments in their children’s early development, and increased investments in pre-K programs and other early education and economic supports, closed these gaps. However, the data suggest that these efforts simply contained them, and that these positive trends were insufficient to narrow the skills gaps at kindergarten entry. This failure to narrow gaps points to a lack of appropriate policy response at all levels of government, the neglect of decades of research across multiple disciplines on child development, and the resulting waste of critical opportunities to nurture an entire generation of children.
The policy recommendations of this report strengthen the idea that we need much greater investments in pre-K programs and continued comprehensive support for children through their academic years, including meaningful engagement of parents and communities, if we are to substantially improve the odds for disadvantaged children, in light of their extensive unmet needs and untapped talents.
Introduction: Facts about income inequality and its growth over time
One of today’s most pressing economic issues is the worrisome level of income inequality. Since 1979, the total share of income claimed by the bottom 90 percent of Americans has steadily decreased (Bivens 2016). In 1979, that 90 percent received about 67 percent of cash, market-based income (i.e., pretax income). By 2015, their share had decreased to about 52 percent of pretax income. The majority of income gains during this period went to the top 1 percent (EPI 2013; Mishel and Schieder 2016; Saez 2016). Polls reflect widespread concern about income and wage inequalities and associated trends and the desire for policies to address these inequalities ( New York Times 2015).
Rising inequality might not be such a major concern if our education, economic, and social protection systems acted as compensatory mechanisms, helping individuals, and especially children, rise above their birth circumstances and improve their mobility. But that is hardly the case. Rather, the fraction of children who earn more than their parents (a measure of what social scientists refer to as absolute mobility) has fallen from approximately 90 percent for children born in 1940 to 50 percent for children born in the 1980s (Chetty et al. 2016). Children of certain ethnic and racial minorities who are disproportionately likely to live in concentrated poverty are also more likely to do so over prolonged periods of time (Sharkey 2013). And the close connections between education inequalities and economic inequalities cast doubt on assertions that America provides “equality of opportunities” that promotes social mobility (Mishel 2015).
The influence of income inequality affects multiple aspects of society’s functioning, from health outcomes and even life expectancy to democratic ideals (Putnam 2015; Schanzenbach et al. 2016; Stringhini et al. 2017). In the education arena, children’s socioeconomic status (SES), of which income is a key component, is considered one of the most significant predictors—if not the most significant predictor—of educational success. A number of studies show the strong relationship between social class (of which socioeconomic status is a frequent measure) and test scores, educational attainment, and college attendance and completion (see Duncan, Morris, and Rodrigues 2011; García 2015; García and Weiss 2015; Lee and Burkam 2002; Mishel et al. 2012; Putnam 2015; among others).
As a result of these trends and associations, achievement gaps by social class have grown substantially since the 1960s, especially between children at the highest end of the income distribution and all of the others (Reardon 2011). Some researchers have identified a large increase in parental investment in education among high-SES parents as one driver of the divergence in education outcomes (Duncan and Murnane 2011), among other contributing factors, such as time parents spend with their children and time parents devote to education-enhancing activities (Morsy and Rothstein 2015; Van Voorhis et al. 2013): Spending on education-enhancing activities by parents in the top income fifth nearly tripled between the 1970s and the 2000s (from $3,500 in 1972 to $8,900 in 2006), while such spending by parents in the bottom income fifth remained low and changed much less (from $800 in 1972 to $1,300 in 2006) (Duncan and Murnane 2011). 1 More time can mean more frequent interactions during playtime, more time spent reading to children, and other parenting practices that contribute to children’s learning and development (Barbarin et al. 2010). In general, more leisure and educational time with children can promote their development and school readiness (Brooks-Gunn and Markman 2005; Hart and Risley 1995; Phillips 2011; Rothstein 2004; Van Voorhis et al. 2013; Waldfogel 2006). Given the evidence that parental engagement and spending directly and continuously translate into improvements in children’s achievement and preparation, the presence of the various achievement gaps are not surprising.
Education researchers and policymakers have long been attentive to issues related to equity—by race/ethnicity, SES, gender, and other characteristics. At least since the 1966 publication of the “Coleman Report” by sociologist James S. Coleman and coauthors, researchers and policymakers have understood the critical impacts of race, poverty, and segregation on educational attainment (Coleman et al. 1966). And educational inequities remain a major problem today. Rigorous research demonstrates that inequalities in both opportunity and outcomes along the lines of race and social class begin early and often persist throughout students’ K–12 years and beyond, and that they are much larger in the United States than in comparable countries (Bradbury et al. 2015; Putnam 2015). Some of the research carefully describes the specific contexts and challenges that minority and lower-social-class students face and how these challenges create early education gaps. Other studies illustrate the consequences of these gaps for children’s later learning and development (Duncan et al. 2007; Duncan and Magnuson 2011). 2 And though this body of research is smaller, a few studies have looked at trends in inequalities across cohorts (Carnoy and García 2017; Magnuson and Duncan 2016; Reardon 2011; Reardon and Portilla 2016), with mixed or inconclusive findings regarding the changes in the gaps. 3 In addition, these latter studies, however, do not address causes that could drive changes in the gaps over time. As such, there is a need both for a better understanding of these causes and for strategies to counter them.
In this paper, we describe recent skills gaps and trends in them by social class, as measured by socioeconomic status; analyze some of the major factors driving the gaps; and explore a set of diverse school district-level initiatives that are helping to narrow gaps. The paper is structured in three sections.
- First, we examine social-class-based gaps at kindergarten entry among the most recently surveyed kindergarten cohort (the kindergarten class of 2010–2011). We study how gaps manifest in both cognitive and so-called noncognitive skills, as both skill types are important components of children’s development.
- Next we compare these gaps with those of an earlier kindergarten cohort. We look at changes from 1998 to 2010 in the skills gaps between children in the top and bottom social-class quintiles (primarily using SES as the proxy for social class). We also analyze how sensitive gaps are to the inclusion of several key determinants of student performance, such as children’s own characteristics, family composition, and parental and education practices at home.
- Then we review a set of case studies of school districts that have employed comprehensive educational strategies to provide more children (especially low-income children) with strong early academic and life foundations, and to sustain and build on early gains throughout the K–12 school years.
- Finally, we look at the implications of our findings, and, based on the case study examples from diverse communities, we discuss strategies that districts can employ along with district and state policy changes that will make those strategies easier to adopt and more sustainable.
For the first two analyses, we use two nationally representative studies from the National Center for Education Statistics (NCES): the Early Childhood Longitudinal Study of the Kindergarten Classes of 1998–1999 and 2010–2011. These data provide information about children’s skills and about the children themselves, such as their race/ethnicity, socioeconomic status, language spoken at home, etc. The data also provide information on the children’s experiences in their early years, such as how actively their parents engaged them in enriching activities, whether they attended prekindergarten care, and the number of books the child has (see Appendix A). This information allows us to test the associations between children’s characteristics and their educational outcomes at school entry. For the second analysis, we draw on 12 case studies of community and school districts employing comprehensive educational strategies (Weiss 2016a–h). We explore the qualitative information provided on investments these districts have made in early childhood education, on both within-school and broader K–12 supports for children, and on evidence that these investments are delivering both improved academic achievement and broader gains for children. Based on this evidence, the report ends with conclusions and recommendations for further research, practice, and policy. Appendices A and B provide detailed discussions of the data and methodology used in this paper.
How large are recent performance gaps at kindergarten entry?
This section documents inequalities among the most recently tracked cohort of students as they entered kindergarten in 2010. It provides us with the most recently available view of the various aspects of gaps at the school starting gate, all of which are critically important for understanding the implications of those gaps. The findings below draw on the Early Childhood Longitudinal Study of the Kindergarten Class of 2010–2011, and we use data from the fall measurement in the kindergarten year. (This section partly builds on our previous work; see García 2015 and García and Weiss 2015. See Appendices A and B for details on the variables and methodology used.)
Our decision to examine performance in both cognitive and noncognitive skills reflects growing acceptance that children’s development is a complex process in which both skill types build on and interact with each other, and on evidence of the roles that both types of skills play in the education process and adulthood outcomes (see García 2015; García and Weiss 2016; Levin 2012a, 2012b). Traits and skills such as critical thinking, creativity, problem-solving, persistence, and self-control are vitally important to children’s full development, and are nurtured through life and school experiences. These skills, sometimes referred to as noncognitive or social and emotional skills, tend to develop—or lag—in tandem with cognitive skills. Noncognitive or social and emotional skills are thus linked to academic achievement, and also to outcomes in adult life, such as productivity and collegiality at work, good health, and civic participation.
For these analyses, we use a measure of socioeconomic status that has three components: the educational attainment of parents or guardians, parents’ occupational prestige (determined by a score), and household income (see more details about the SES construct in Tourangeau et al. 2013, 7-56 to 7-60). We divide children of the 2010–2011 kindergarten class into five groups based on SES quintile. To measure the gaps in performance by socioeconomic status, we compare the average performance of children in the top fifth of the SES distribution with the average performance of children in the bottom fifth. This provides an estimate of the relative advantage of a child in the top fifth of the SES distribution (referred to in this report as “high-SES”) with respect to a child in the bottom fifth (“low-SES”).
Children are not equally prepared for school when they enter kindergarten, and our analyses show that students’ social class strongly determines their relative position in the performance distribution. Most socioeconomically disadvantaged children lag substantially in both reading and math skills, and these skills levels rise along with socioeconomic status (sometimes referred to as socioeconomic gradients). Children in the highest socioeconomic group score significantly higher in reading and math than children in the lowest socioeconomic group. As Table 1 shows, the relative unadjusted gaps in reading and math, i.e., the advantages of high-SES children relative to low-SES children in 2010 are 1.17 and 1.25 sd, respectively (Table 1 also shows that, after controlling for clustered data, the gaps are 0.94 and 0.91 sd, respectively). 4 Reading and math skills advantages of children in the middle of the SES distribution relative to the lowest SES group are roughly half as large as the advantages of high-SES children to the lowest SES group. 5
Children in the lowest socioeconomic quintile also lag substantially in noncognitive skills, based on assessments by both parents and teachers, although these gaps are smaller than those in reading and math. Socioeconomic-based gaps in self-control and approaches to learning are approximately one-third to one-half as large as gaps in reading and math. 6 In 2010, children in the high-SES quintile scored 0.38 sd and 0.51 sd higher in self-control and approaches to learning as reported by teachers (0.36 sd and 0.56 sd after clustering; see Table 1) than children at the low-SES quintile (see Figure A ). Using parents’ assessments of the same skills, the gaps are 0.39 sd and 0.56 sd, respectively (0.33 sd and 0.46 sd after clustering; see Table 1).
Our analyses also document stark socioeconomic disparities in inputs, child and family characteristics, and other factors that can affect school readiness ( Table 2 ). Here too we find a correlation between socioeconomic status and other factors that impede educational development. Low-SES students are more likely than their high-SES peers to be immigrants and less likely to speak English at home, to live with two parents, to have participated in center-based pre-K care activities in the previous year, and to have engaged in early literacy practices at home. Among children in the low-SES group, half (50.4 percent) are Hispanic, 23.1 percent are white, 19.6 percent are black, and 2.5 percent are Asian. 7
Though these gaps in both cognitive and noncognitive skills are troubling and call for policy recommendations, better policy solutions can be designed if we understand how these gaps have changed over time and what factors have played a role in those changes. Education outcomes are the product of a combination of multiple factors, which can reinforce or mitigate relative advantages or disadvantages in a dynamic fashion. We examine these issues in the rest of the paper.
How do the performance gaps in the 2010–2011 kindergarten class compare with the gaps in the prior generation?
The analyses presented in this section compare the inequities in inputs and the performance gaps between high-SES and low-SES students who began kindergarten in 2010 with the gaps among high-SES and low-SES schoolchildren in the prior academic generation, the 1998 cohort. We also analyze factors that have had major influences on the changes in performance of kindergartners, and briefly discuss the research and policy implications of our findings.
How have the characteristics of the children in the lowest and highest SES groups changed in a generation?
We first analyze children’s characteristics by SES quintiles in the two cohorts. This enables us to identify differences in the characteristics of low-SES kindergartners in 2010 versus in 1998. These changes may help explain why the performance gaps we are studying grow or shrink (for example, if children in the low-SES quintile in 2010 were more likely than their 1998 peers to have access to public programs such as pre-K, they might be more prepared for kindergarten, and thus the relative advantage of high-SES children might shrink). 8
Table 2 shows the student and family characteristics of the kindergarten classes of 1998–1999 and of 2010–2011, by SES quintile. The table also includes pre-K care arrangements and two indices of developmental activities parents undertake with their children—indices of “literacy/reading activities” and “other activities”). 9 The table also summarizes parents’ expectations regarding their children’s educational attainment. To some extent, expectations are based on hope, but they can also respond to behavioral patterns children are exhibiting that hint at their future success. Expectations can also influence outcomes by representing how motivated parents are for their children’s education. The ECLS-K survey does not ask parents how their expectations (and changes in their expectations) affect their provision of educational activities or support, but their answers to the expectations question can be used as a reasonable proxy of the degree to which parents are aware of their children’s education and willing to support it. 10
The most significant changes in children’s characteristics by SES quintile are for children in the bottom of the distribution. In 2010, a greater share of children in this group are Hispanic (50.4 percent, an increase of 10.6 percentage points relative to the 1998 share of 39.8 percent), live in homes where the main language is not English (40.3 percent, an increase of 9.1 percentage points from 31.2 percent in 1998), and are immigrants (49.8 percent, an increase of 19.5 percentage points from 30.3 percent in 1998). In 2010, a greater share of children do not live with two parents (54.9 percent, an increase of 9.3 percentage points from 45.6 percent in 1998), and live in poverty (84.6 percent, an increase of 13.3 percentage points from 71.3 percent in 1998). These substantially greater disadvantages for children at the bottom of the SES scale could all be reflections of both the much weaker national economic context in 2010 versus 1998 and the growing inequality described above.
These children’s likelihood of attending center-based pre-K did not change significantly across generations (about 44 percent for both cohorts), but they were more likely to be looked after by parents or relatives (with the share increasing from 46.4 percent in 1998 to 50.9 percent in 2010). These children’s parents also reported having a somewhat larger number of books at home for the children, and there were increases in their indices of educational and engagement activities (two composite measures, with the literacy/reading index measuring how frequently parents read books to their child, tell stories, sing songs, and talk about nature and how frequently the child reads picture books and reads outside of school, and the “other” index measuring how frequently parents and children play games or do puzzles, play a sport or exercise together, and build something or play with construction toys; and how often parents help children do arts and crafts and involve children in household chores). These parents’ expectations about their children’s educational attainment also changed significantly: the share who expected their children to attain no more than a high school diploma decreased by more than half (from 24.1 percent in 1998 to 11.4 percent in 2010), and the share of parents who expected their children to attain at least a bachelor’s degree increased, markedly for those expecting their children to obtain an advanced degree (a master’s degree, Ph.D., or M.D.).
Among children in the high-SES quintile, the group in 2010 includes a lower share of white children (falling from 78.8 percent in 1998 to 71.3 percent) and a larger share of Asian children (increasing from 4.7 percent in 1998 to 8.7 percent). Children in the high-SES group became slightly more likely to live with their two parents (the share of children who lived with one parent decreased from 11.1 percent in 1998 to 9.6 percent), and to have attended center-based pre-K (65.8 percent in 1998 and 69.9 percent in 2010). We only see a small increase in the reported number of books at home. 11 The share of homes reporting having more than 200 books—the maximum—increased slightly in 2010, across all SES quintiles except for the middle quintile). As was true of low-SES parents, those in the highest quintile raised their expectations for their children’s educational attainment from 1998 to 2010. Compared with the 1998 cohort, a larger proportion of high-SES children in the 2010 cohort were expected by their parents to attain an advanced degree (master’s degree or higher), while a lower share expected their children to attain a bachelor’s degree only.
How did the performance gaps between the children in the lowest and highest SES groups change in a generation?
Changes over time in the input factors by socioeconomic status (child and family characteristics, early-education practices, and parents’ expectations) explored above have been found by researchers to have major impacts on the outcomes (test scores on reading and math, and measures of noncognitive skills) explored in this section. 12 In other words, we would expect that changes in the unadjusted skills gaps (gap measures that do not include controls for child and family characteristics, early-education practices, and parents’ expectations) would partially reflect the compositional differences between the class of 2010–2011 and the class of 1998–1999. For example, we would anticipate that if the more recent generation’s low-SES parents read to their children more frequently, helped them do more arts and crafts, or had higher expectations for them, these factors would correlate with narrowing skills gaps. Also, we would expect that the adjusted skills gaps (gap measures that are net of the influence of child and family characteristics, early-education practices, and parents’ expectations, and thus reflect the SES gaps) would be different for the two cohorts if the correlations between inputs and outcomes had changed over time or if the share of children’s outcomes the adjustments account for had changed over time.
To understand these factors’ potential influence on gaps, we examine both unadjusted and adjusted gaps in the tables in this section. We also examine gaps by some of the components of the SES index, such as household income or mother’s educational attainment, and by other variables that are sometimes used as proxies of the child’s socioeconomic background, such as number of books in the home. If the gaps by SES components and proxies somewhat differ, this tells us that researchers’ choices about how to divide children into groups and compare them matter—both for their findings and for their policy recommendations.
Table 3 shows the unadjusted and adjusted gaps between the standardized scores in reading and math of kindergarten children in the top SES quintile relative to the bottom SES quintile in 1998 and the change in that gap by 2010. 13 Table 4 performs the same analysis for gaps in measured noncognitive skills. The tables show two somewhat perplexing patterns. On the one hand, the cognitive and noncognitive skills gaps between high-SES and low-SES children are large and statistically significant in both cohorts. But while significant social-class-based performance gaps persist from one kindergarten generation to the next, there is not the same consistency in how the high-SES to low-SES gaps change. For some cognitive and noncognitive skills, the performance gaps grow, while for others the gaps shrink, or remain the same from one generation to the next (which may complicate the process of understanding why performance gaps have changed over time).
Beginning with our unadjusted model (data column one), the only substantial increase in the gap between high- and low-SES children from 1998 to 2010 was in reading skills, which increased by one-tenth of a standard deviation. There were no significant changes in gaps in math skills, which, as the literature indicates, are less sensitive than reading skills to parents’ activities at home (see Rothstein 2004, 2010). Similarly, gaps in approaches to learning as reported by parents and in self-control as reported by teachers did not change significantly, and gaps in approaches to learning as reported by teachers and in self-control as reported by parents shrank by roughly the same amount as the reading gap (about a tenth of a standard deviation—0.12 and 0.08 sd, respectively). Figure A provides a graphic illustration of the unadjusted gaps in cognitive and noncognitive skills of high- and low-SES children across the two cohorts.
The additional models estimated for each outcome and shown in Tables 3 and 4 offer other key findings. In Model 1, we used the full samples for the two cohorts but did not include any controls that capture characteristics of children or their parents or the early education practices in which families engage. Model 2 partitions the data into schools and classes, or clusters, so that the subjects in the clusters are more similar to one another than to those in other groups. Under this adjustment, the gaps shrink substantially, by between 15 and 25 percent across the skills, and the regression fit improves significantly (see increased adjusted R-squared, i.e., this model explains more of the total variation in the outcomes than the first model). This clustering takes into account school segregation, that is, that children are not randomly distributed but tend to concentrate in schools or classrooms with children of the same race, social class, etc. Clustered estimates provide a comparison of the skills gaps of peer students—those in the same schools and classrooms—rather than a comparison across schools. García (2015) and Magnuson and Duncan (2016) offer these estimates too.
How do child and family characteristics, activities, and expectations affect SES-based performance and performance gaps?
We next examine the contribution of the certain variables of interest to SES-based performance gaps. We approach this in two ways. First, we examine the changes in the gaps (Tables 3 and 4, Models 3 and 4) and the overall reduction in the gaps that results from controlling for children and their family characteristics, early literacy practices, and parental expectations of educational achievement ( Table 5 ). Second, we assess the influence of select early educational practices on performance and how that influence has changed over time by looking at the associations between these inputs and performance ( Table 6 ).
Models 3 and 4 in Tables 3 and 4 use the samples that result from removing observations without full information for the controls of interest. 14 Adding controls is important because performance gaps based on socioeconomic status may be explained by differences in variables other than the child’s socioeconomic status. In other words, we aim to determine which part of the gap is attributable to children’s SES, net of other factors that matter for performance. Thus, in the third data column (Model 3), we add controls for individual and family characteristics (gender, race/ethnicity, whether English is the primary language spoken at home, disability, age, whether children live with two parents) and early educational and play activities (center-based pre-K care, indices for literacy/reading activities and other activities, and total number of books the child has). Model 3 also includes the interactions between the early education variables with time. 15 In the fourth data column (Model 4), we control for the same factors as in Model 3 but add controls for parental expectations of children’s educational attainment (whether they expect their children’s highest level of education attained will be high school diploma or less, some college or vocational studies, bachelor’s degree, or advanced degree) and their interaction with time. 16 We describe these results in the next section.
Including covariates changes the estimates of SES-based skills gaps in various ways. First, the gaps between the top- and bottom-SES quintiles shrink, showing that SES-based gaps are partially explained by the variation in the controls (which is not visible in the tables). 17 Second, controls do not significantly change the SES-based gaps over time, in general; i.e., the coefficients associated with changes in the gaps between high- and low-SES children remain almost the same, or change very minimally, depending on the skill measured. The statistical significance of the SES-based skills gaps in 1998 is not affected by the inclusion of the controls (see rows “Gap in 1998–1999” in tables), but the statistical significance of the changes in the gaps between 1998 and 2010 (see rows “Change in gap by 2010–2011” in tables) is somewhat affected by the inclusion of the controls (note that the sizes of the coefficients measuring gaps in 1998 change after the inclusion of the controls, but that the sizes of the coefficients measuring changes in them between 1998 and 2010 do not change significantly). In reading, the change in the gap between 1998 and 2010 diminishes and becomes statistically insignificant in the last model (the relative gap increases by 0.08 sd but this change is not statistically significant), meaning that adding parental expectations of education accounts for some of the increase in the gap detected in Models 1 to 3. The only SES-based skills gap that shows a statistically significant increase from 1998 to 2010 once parental expectations are controlled for is the gap associated with parents’ assessment of approaches to learning, which increases by 0.11 sd. Gaps between high- and low-SES children in cognitive and noncognitive skills after adjustments are made are shown in Figure B .
As mentioned above, the fact that the skills gaps decrease after controls are taken into consideration affirms that SES-based gaps are due in part to variation in the controls among high- versus low-SES children. This trend can be seen in Table 5, which, as noted above, shows the overall reduction in gaps that results from controlling for child and family characteristics, early literacy practices, and parental expectations of educational achievement. With respect to cognitive skills, the 1998 gaps shrink by 46 percent and 53 percent, respectively, after the inclusion of the covariates. About half of the gaps are thus due to other factors that are associated both with SES status and with the outcomes themselves. The reduction in the 1998 gaps for noncognitive skills varies from 28 percent (approaches to learning as reported by teachers) to 74 percent (approaches to learning as reported by parents). (For self-control as reported by teachers, the reduction is 51 percent versus 35 percent when reported by parents.)
While the gaps hold after the inclusion of controls across outcomes, gaps in 2010 are less sensitive to the inclusion of the covariates than they were in 1998. This trend can also be seen in Table 5. 18 Declining values from 1998 to 2010 indicate that factors such as early literacy activities and other controls are not, as a group, explaining SES-based gaps as much as they had a decade prior. This change could be due to the failure of the index to fully capture parents’ efforts to nurture their children’s development and/or the index becoming somewhat out-of-date. In any event, the resistance of gaps to these controls should worry researchers and policymakers. The waning influence of these controls makes it harder to understand what drives SES gaps. It also suggests that the gaps may be growing more intractable or, at least are less easily narrowed via the enactment of known policy interventions.
Finally, we examine the association of performance outcomes (not performance gaps) with selected early educational practices, including having attended center-based pre-K, literacy/reading activities and other activities, and total number of children’s books in the home (Table 6). 19 We are mainly interested in two potential patterns: whether these factors are associated with outcomes (and, if so, how intense the associations are), and whether the relationships have changed over time.
In keeping with established research, having attended center-based pre-K is positively associated with children’s early reading and math skills. For 1998, the estimated coefficients are 0.11 sd for reading skills and 0.10 sd for math skills, substantial associations that do not change significantly over time. In other words, attending pre-K in 1998 improved kindergartners’ reading skills by 0.11 sd and improved kindergartners’ math skills by 0.10 sd relative to not attending pre-K. However, while center-based pre-K continues to reduce self-control as reported by teachers in 2010, the effect is less negative in 2010 (the 0.06 improvement from 1998 to 2010 shown in the bottom panel of the table shows us that the effect in 2010 was -0.07 [-0.13 plus 0.06], compared with -0.13 sd in 1998). We find no independent effect of center-based prekindergarten schooling (i.e., no effect in addition to SES, in addition to other individual and family characteristics, or in addition to other SES-mediated factors), on approaches to learning or on self-control as reported by parents. 20
The number of books children have at home likewise supports their skills at the beginning of kindergarten. Indeed, this factor is positively associated with all outcomes but self-control reported by parents. The coefficients are very small, of about 0.01 to 0.02 sd (associated with changes in outcomes for each 10 additional/fewer books the child has, as expressed by the continuous scale with which number of books in the home is measured, which is divided by 10 for the analyses (as mentioned in Appendix A), and these relationships do not change over the time period.
The two types of parenting activities that are summarized by the indices “reading/literacy activities” and “other activities” show interesting correlations with performance and patterns over time. On the one hand, the “reading/literacy activities” index (a composite of how frequently parents read books to their child, tell stories, sing songs, and talk about nature, and how frequently the child reads picture books and reads outside of school) is strongly and positively associated with all outcomes other than children’s self-control as reported by the teacher. The associations with cognitive skills, especially with reading, are strong and statistically significant—0.17 sd for reading performance and 0.07 sd for math—and these associations did not change significantly between 1998 and 2010. For noncognitive skills, the relationships are strong for those assessed by parents, though they shrink by about half over time: self-control is 0.14 sd in 1998 and decreases by 0.08 sd by 2010; approaches to learning is 0.32 sd in 1998 and decreases by 0.17 sd by 2010). The relationship is much weaker, though still statistically significant, for teachers’ assessed approaches to learning (it is 0.03 sd in 1998 and does not change significantly by 2010).
On the other hand, the index that measures other enrichment activities that parents do with their children (a composite of how frequently parents and children play games, do sports, build things, work on puzzles, do arts and crafts, and do chores) shows significant correlations with all of the skills, but they may be either positively correlated or negatively correlated, depending on the skill. For cognitive skills, the associations are statistically significant and negative, though stronger and somewhat more meaningful or more intense with reading achievement (-0.12 sd in 1998) than with math achievement (-0.04 sd). 21 These associations did not intensify nor weaken over time. For noncognitive skills the associations are highly positive and statistically significant, and very strong for parents’ assessment of approaches to learning (0.29 sd in 1998). As explained by García (2015), these correlations between “other activities” and noncognitive skills as assessed by parents could be bidirectional: engaging children in enrichment activities might enhance their noncognitive skills, but, at the same time, parents who are more inclined to participate in their children’s early play and educational time are probably more likely to perceive or judge that their engagement has an impact on their children’s skills. But the fact that both the frequency with which parents engage in most of these activities and the importance of this index for parent-assessed skills increased noticeably from 1998 to 2010 (by 0.22 sd for self-control and 0.27 sd for approaches to learning) suggests that parents are growing more informed and involved in their children’s early education over time. It also indicates that parents are increasingly acting on this knowledge and that this involvement will continue to grow, albeit potentially with decreasing marginal returns to time and resources invested. The association between “other activities” and teachers’ assessments of children’s noncognitive skills is also positive but weaker than that of parents’ assessments (about 0.03 sd for approaches to learning and 0.05 sd for self-control), and remained unchanged during the time period studied.
Finally, we find a strong association between parental expectations for their children’s educational attainment and all measured skills. In other words, net of socioeconomic status, the higher the expectations, the higher cognitive skills children have, and the higher the assessments by parents and teachers of children’s noncognitive skills. The parental expectations portion of the table measures children’s performance relative to children whose parents’ expectations are the lowest (high school diploma or less). While the expectation that a child will pursue some vocational education or complete college has a statistically positive influence on all skills measures except for reading, the expectation that their children will complete a bachelor’s degree or more education has a stronger influence, including on reading skills: between 0.11 to 0.16 sd higher in reading and between 0.17 to 0.22 sd higher in math in 1998. High expectations for children’s educational attainment also have a statistically positive effect on noncognitive skills. When the expectation is for an advanced degree (master’s or higher), coefficients vary from 0.12 sd in self-control by teachers to 0.38 sd in approaches to learning by parents in 1998. In addition, most of these associations—particularly the cognitive gradients—grow in 2010. Relative to children whose parents have low expectations, children whose parents have the highest expectations for their children’s attainment (graduate studies) perform much better in reading and math than in 1998 (relative gaps grow by 0.19 and 0.12 sd respectively). A similarly stronger association is noted for noncognitive skills assessed by teachers (though not for parents’ assessments of their children’s skills).
Sensitivity analyses: Do performance gaps vary based on which proxy for social class (socioeconomic status) is used?
Part of the challenge to making conclusive statements about trends in education gaps by social class is the existence of multiple valid proxies for measuring children’s social class or socioeconomic status. 22 Although researchers treat these proxies as equivalent, and even interchangeable, the lack of a comparison of results obtained using various indicators limits our capacity to extract major conclusions on social-class trends and their drivers, and hence hinders the plausibility and effectiveness of the policy recommendations that build on any specific indicator’s findings (net of other methodological and instrumental differences that may exist across studies).
We thus conduct analyses using several of the main proxies employed to measure socioeconomic status. The purpose of these analyses is twofold. The first purpose is to test the sensitivity of the estimated relative gaps, and of trends in them, to changes in the measurement of this key predictor of education performance. (In other words, if all the indicators are reliable proxies of SES, gaps and trends obtained using the various metrics should be similar.) The second purpose is to increase the comparability of the results of studies addressing trends in education inequalities that use various metrics of social class. This is an important issue; in addition to helping reconcile diverse results found in the literature, these analyses may reveal why patterns differ, and have significant policy implications.
As such, instead of the SES composite measure we use to estimate SES-based gaps in this report, we use three alternative indicators to run our analyses: mother’s educational attainment, household income, and number of books the child has in the home. Unlike the SES composite measure, two of these measures offer the advantage of being directly comparable over time. Both mother’s educational attainment and number of books the child has are objective categories. As a limitation, and mainly associated with the information that is available in the raw data, none of these categories can be transformed into a percentile-variable without major transformations. (The adjustments to ensure comparability over time are explained in Appendix A. See Reardon and Portilla 2016 for an analysis with a transformation of the income variable that offers a proper percentile comparison, based on the methodology developed by Reardon 2011.) Still, they are variables associated with social class and can be ordered in groups or categories that identify high- and low-social-class statuses. Thus, with the necessary caution when interpreting and using the findings, we offer this comparison of results as a sensitivity analysis.
We create five categories with these indicators, maintaining the structure of comparing “high-SES” (top quintile) with “low-SES” (bottom quintile) as in Tables 1–5 (note that we are using “SES” interchangeably with “social class” here). For simplicity, Tables 7–9 show only the results from two models: one without covariates (Model 1, baseline estimates) and one with all covariates (Model 4, fully adjusted estimates). We focus on the findings for the baseline relative gaps in 1998 and 2010 first ( Figures C–E ). The overall patterns found in the results suggest that all social-class gaps are statistically significant and sizable. However, the exact sizes of the gaps vary depending on the social-class indicator used and the outcome being assessed. Also, the changes in the gaps over time vary depending on the indicator used to capture children’s social class.
In addition to these general findings, we note some more detailed ones. For 1998, gaps by mother’s educational attainment (Figure C; Table 7) are the largest across all indicators (except for the gap in self-control as assessed by teachers, which is slightly smaller than the gaps as measured using household income and number of books the child has), while gaps by number of books (Figure E; Table 9) are the smallest across all indicators (except for the gap in approaches to learning as assessed by parents, which is slightly larger than the gap for household income). Again, according to the 1998 data, the coefficients of gaps by mother’s educational attainment are generally larger—and in three cases much larger—than those obtained using number of books in the home as the indicator of social class. For example, the relative gap is 1.29 sd in reading and 1.46 sd in math when mother’s education is the SES proxy, compared with gaps of 0.74 sd and 0.97 sd when number of books in the home is the SES proxy.
It is also important to note that gaps by mother’s educational attainment (Figure C; Table 7) and income (Figure D; Table 8)—two of the five components of the SES construct—are very close to the ones obtained by our SES composite measure (as shown in Figure A). All in all, results seem internally consistent as well as generally consistent with prior results on this topic (Reardon and Portilla 2016).
In terms of changes in the performance gaps over time (unadjusted), the findings vary depending on which indicators of social class are used, with mother’s education and household income being the indicators associated with the largest changes in the gaps. Changes in the performance gaps in cognitive skills between 1998 and 2010 by our composite SES measure and books are similar: an increase in the reading gap between children in the top and bottom quintiles of about a tenth of a standard deviation (0.10 sd with the composite SES measure [Figure A] and 0.08 sd if SES is proxied with books), and no significant change in mathematics (there are some differences in the noncognitive outcomes).
However, by mother’s educational attainment, there are no changes in relative reading and approaches to learning gaps reported by parents over time, and a significant reduction in the gaps in the remaining outcomes. Meanwhile, income-based gaps for the two cognitive skills—reading and math—decreased by -0.13 and -0.23 sd respectively, and for approaches to learning as reported by teachers by -0.13 sd. No significant changes occurred for the remaining noncognitive skills.
In sum, this sensitivity analysis demonstrates that all of the indicators are reliable proxies of SES for the estimation of early achievement gaps, though absolute gaps may vary slightly depending on the indicator used. However, the proxies are not equally reliable when we assess trends in the gaps by SES or their drivers. As such, aside from differences in the definitions and procedures used to construct each SES proxy, the proxies should not be treated as fully equivalent. The decomposition conducted here helps clarify the different weights that various components of SES may have in driving changes in gaps by social class. For example, variation in income across groups over time is associated with decreased performance gaps in the cognitive skills between 1998 and 2010, and variation in educational attainment quintiles or categories over time is associated with decreased performance gaps across cohorts in most noncognitive skills. But variation in books in the home over time and among groups is associated with increased gaps in reading and in parents’ assessed approaches to learning. Such findings also point to very different policy solutions: if mothers’ education is the main driver, enhancing that will improve children’s prospects. On the other hand, findings that indicate that income inequality is the larger culprit would point to the need for policies that reduce such inequalities. Future research should consider and look more closely into these questions.
What can we learn from these analyses?
The multiple factors and relationships examined in this section can now be examined from a policy perspective. If the aim is to increase equity, to improve children’s development across the board, and to improve our understanding of children’s development, there are two major policy recommendations:
- Directly support less-resourced families so that they have greater access to educational and economic resources (for the latter, see García and Weiss 2017). All the early educational and play activities measured, which include center-based pre-K care and literacy/reading and other activities, as well as the number of books a child has, are positively associated with children’s readiness, and in part account for social-class gaps, but are much less accessible to children of lower socioeconomic status. Virtually all of the associations between these factors and outcomes were strong and positive (with a handful of exceptions), and some even grew over time. A related research recommendation of particular interest would be to examine whether the intensity of these activities or practices has any threshold level of effectiveness (after which point they no longer affect children’s development). 23 Also, it would be helpful to understand why parents’ expectations of their children’s educational attainment increased so much and how this has affected children’s development. For example, do parents have a better understanding of the relationship between educational attainment and prospects for success in life and the workforce? Are children performing better because their parents expect more, or because parents who expect more are also delivering more in the form of enriching activities?
- Design and implement strategies that compensate at the community level for children’s lack of access to key foundational resources (economic and educational). These strategies can be considered indirect supports for less-resourced families that reduce inequities and complement the direct supports described above. Examples of communities that have enacted such comprehensive support initiatives provide a good starting point to explore how and why they emerge; the types of supports they provide (from preschool programs and home visits with parents to enriching summer programs, school-based health clinics, and more); the challenges of scaling them up and sustaining them; the benefits they deliver for students, and particularly for disadvantaged students; and their implications for policy at the local, state, and even federal levels. The next section of this report thus presents an analysis based on qualitative data from promising initiatives in a dozen school districts across the country (Weiss 2016a–h).
What are pioneering school districts doing to combat these inequities and resulting gaps?
This section of the report draws on a set of case studies published by the Broader, Bolder Approach to Education (BBA), a national campaign that advances evidence-based strategies to mitigate the impacts of poverty-related disadvantages on teaching and learning. 24 The case studies feature school districts that have employed comprehensive educational strategies to ensure that more children, especially low-income children, have strong early academic and life foundations, and that resulting early gains are sustained and built on through children’s K–12 years. (These strategies are often referred to as “whole-child” approaches to education, in reflection of their holistic nature.) We explore the premise that school districts that take a whole-child approach to education and a whole-community approach to delivering it are likely to enjoy larger gains in academic achievement and to narrow their race- and income-based achievement gaps. In doing so, we are building on evidence suggesting that consistent, strong supports for children and their families—both in and out of school—can avoid the “fade-out” seen among graduates of many pre-K programs and even enhance those programs’ early benefits.
This section is thus divided into four parts: (1) an introduction to the case study districts, followed by discussions of (2) how these districts invest in early childhood care and education, (3) how the districts’ investments in K–12 strategies sustain and boost the early childhood investments, and (4) how academic gains and narrowing achievement gaps indicate that the investments are paying off. Table 10 provides basic information on the 12 school districts/communities studied; Appendix E at the end of this report provides more information on key characteristics of these districts. 25
Introduction to the case studies: Why these districts enacted whole-child strategies
Large and growing disparities in the economic well-being of children in America and extensive evidence linking those disparities to widely diverging educational outcomes have prompted action among a growing number of communities and school districts. Heeding the evidence that out-of-school factors play even larger roles than school-based factors in school performance, these districts are seeking ways to mitigate the poverty-related impediments to effective teaching and learning.
These districts have benefited from a substantial body of research on strategies with promise to address core challenges that students and schools face—strategies that have been shown to shrink achievement gaps by narrowing major disparities in opportunity (Carter and Welner 2013). The first, and perhaps best-documented, of these strategies is high-quality early child care and education, especially when it engages parents early and in meaningful ways. High-quality early childhood education programs not only narrow achievement gaps at kindergarten entry but also deliver long-term benefits to children, their families, and society as a whole (Chaudry et al. 2017; Rolnick and Grunewald 2003).
Programs that support students’ physical and mental health and improve their nutrition are also known to reduce chronic absence and keep students focused and learning, and thus improve their academic performance (CDC 2016). Well-designed after-school and summer-enrichment programs likewise boost achievement, both directly and indirectly by enhancing students’ engagement in and attachment to school (Peterson 2013).
Whole-child approaches integrate these and other strategies into a comprehensive set of aligned interventions, leveraging the whole community’s resources to meet the broad range of student needs. While the impact of such comprehensive approaches has not been studied as extensively as the individual components, considerable theoretical and emerging empirical research point to the strong potential of such strategies to boost achievement and narrow gaps (Child Trends 2014; Oakes, Maier, and Daniel 2017; Weiss 2016i).
This section of the report seeks to add to that knowledge base by sharing qualitative information on how such comprehensive approaches have emerged and grown, what they look like when they are successfully implemented, and what types of outcomes and benefits result and how outcomes vary across diverse communities.
How are whole-child initiatives launched?
Each of the districts studied has distinct circumstances, and thus distinct reasons for coming to the conclusion, as a community, that it needed to take a comprehensive approach to education. At the same time, demographic trends that are affecting virtually every state—and many, if not most, school districts across the country—have played major roles in that decision in every case. 26 Indeed, community and school leaders in all of these districts cited students’ poverty (and, in some districts, demographic shifts) as posing challenges that required looking beyond the school walls to address.
How these factors triggered the initiative’s launch varied, but poverty was at the core in each community’s decision. For example, in 2008, community leaders identified East Durham as one of Durham, North Carolina’s, most distressed areas, based on a community risk assessment conducted by Duke University’s Children’s Environmental Health Initiative. The 120-block area’s 11,000 residents had a 40 percent poverty rate and a homeownership rate of just 19 percent, along with high rates of crime and unemployment, putting its 3,000 children and youth at high risk of academic failure (Weiss 2016e).
Across the country, in Vancouver, Washington, the share of children eligible for subsidized school meals rose from 39 percent to over 50 percent in less than a decade, such that, by 2015, in some central-city schools, more than four in five students qualified for subsidized school meals in 2015 (Weiss 2016b). In another distressed community, in north Minneapolis, median family income was just $18,000 in 2011, and fully one-fourth of the 5,500 Northside students were homeless or “highly mobile” (in such unstable housing that they were at risk of homelessness) (Weiss 2016d). In Pea Ridge, Arkansas, schools “had difficulty finding resources that met the needs of kids,” says superintendent Rick Neal. “We knew that we were not identifying all the needs that were there. I think that’s the way a lot of districts are” (Weiss 2016f). And in the early 1990s, the Tangelo Park neighborhood in Orlando, Florida—an isolated enclave of 3,000 residents, almost all low-income and African American—caught the attention of hotelier and philanthropist Harris Rosen, who was looking for a neighborhood in which to invest (Alvarez 2015).
Each of these districts took different approaches to enacting those comprehensive strategies, based on the community’s specific mix of needs and assets, ideological leaning, available sources of funding, and other factors. One of the most politically progressive of the districts studied, Montgomery County Public Schools (MCPS) in Maryland, paved the way for a whole-child approach in the early 1970s when it enacted housing policy that uses mixed-income residential developments to create communities with families of different income levels. In the 1990s, the county developed Linkages to Learning, a “community schools”–type approach targeted to engaging and partnering with low-income and immigrant parents and families and connecting them with a broad range of community resources (MCPS 2016). (Community schools are known for building partnerships with community agencies and private service providers to meet student and family needs.) Austin Independent School District (AISD), also in a politically progressive jurisdiction, began its whole-child efforts through parent- and community-organizing in schools. It has since invested in social and emotional learning and in a community schools strategy (CASEL 2017).
At the other end of the spectrum are whole-child approaches in Joplin, Missouri, and Pea Ridge, Arkansas, districts located in more politically conservative southern states. These districts operate under the umbrella of Bright Futures USA (a spinoff national nonprofit that began with Joplin’s Bright Futures initiative). The Bright Futures districts take a more individualistic angle, asserting that every member of the community has “time, talent, or treasure” to offer that can help children overcome disadvantage and ensure more equal opportunity (Weiss 2016a).
Two other districts have modeled their efforts on the Harlem Children’s Zone (HCZ). The Northside Achievement Zone in Minneapolis is funded through a grant from the federal Promise Neighborhoods initiative, enacted by the Obama Administration to help more communities dramatically improve the academic success for low-income children by adopting HCZ-like strategies. The East Durham Children’s Initiative in North Carolina is entirely privately funded so far (Weiss 2016e).
In both Kalamazoo, Michigan, and Orlando, Florida, pledges of “Promise” college scholarships have evolved into broader whole-child efforts (Alvarez 2015; Miller-Adams 2015).
Districts also take different approaches based on density. New York City—home to dozens of full-service community schools supported by the Children’s Aid Society and rapidly expanding to more—and Boston—home to the City Connects initiative—leverage a broad range of their respective cities’ arts and cultural offerings, along with health and nutrition and other social services (Weiss 2016g, 2016h). Cultural offerings to supplement other well-rounded services are also part of the full-service community schools district initiative in Vancouver, Washington. In contrast, Partners for Education, which serves the isolated region surrounding Berea College in Kentucky, was the first rural organization to receive a Promise Neighborhood grant and, thus, is a pioneer in exploring how well the model works outside the urban context (Berea College 2013).
What do whole-child initiatives do?
The sections below describe commonalities across these different approaches in terms of investments in children’s earliest years (before school starts), building on these investments throughout children’s K–12 years (both in and out of school), and the gains students and schools enjoy as a result of those investments. 27
How the case study districts invest in early childhood care and education
In keeping with their whole-child approaches to education policy and practice, every one of the 12 districts highlighted as a BBA case study has made investments in early childhood care and education, many of them substantial. These districts’ efforts begin long before children enter school and go beyond pre-K offerings to equip parents in the effort to ensure their children’s readiness for school.
One-on-one engagement with new parents
Investing in babies by engaging parents can include providing new parents with key information about child development and how to keep children healthy and safe. In Joplin, Missouri, Bright Futures Joplin partners with two of the area’s hospitals to deliver new baby “kits” with child development and early literacy information and is trying to raise funds to sustain the project long term and to expand it to reach every new parent (Weiss 2016a). In Vancouver, Washington, 6,000 “literacy packets” are delivered annually to families with children up to age five, providing child-development activities and lessons that families can complete at home (Weiss 2016b).
The districts leverage partnerships to connect parents with a range of school and community resources that support children from birth through kindergarten entry. In Eastern Kentucky, the whole-child program called Partners for Education works with Community Early Childhood Councils to host events such as Week of the Young Child, the Dolly Parton Imagination Library, and Kindergarten Transition Programs (Weiss 2016c). In Montgomery County, Maryland, “Judy Centers”—early child care and family education centers—leverage partnerships with social service agencies and local community nonprofits to increase parents’ access to mental health, nutrition, and other key services (Maryland State Department of Education 2017).
Educating and engaging parents early helps prepare children for school both academically and more broadly for healthy development. Those are the twin goals of the Minneapolis Northside Achievement Zone (NAZ), where currently only one in four preschoolers in the zone is ready for kindergarten based on standardized tests. To improve those odds, the zone has a team of “NAZ Navigators” who work with families to set and track progress toward goals in early childhood and to link this area of family support to goals in academics, housing, career and finance, and behavioral health (Weiss 2016d).
Parenting classes
Parents are children’s first and most important teachers. Like the one-on-one strategies described above, classes for parents provide information on child development, early literacy, health, and constructive disciplinary practices, and offer more specific guidance tailored to specific parents’ needs. Almost every district studied provides new-parent classes. The 1-2-3 Grow and Learn program is a weekly 90-minute literacy-rich program for young children and their parents offered at 12 elementary schools in high-poverty Vancouver neighborhoods. It lays the foundations for school readiness through social and education experiences. In addition, the district’s Family and Community Resource Centers offer parent workshops, groups, and courses to help parents support their children’s learning, while empowerment and skill-enhancement programs—such as job preparation, housing assistance, and parent leadership advisory groups—strengthen parents’ basic skills. Family Academy classes in the North Minneapolis Northside Achievement Zone include “College Bound Babies” (for parents of children up to three years old), which teaches early literacy, numeracy, and positive discipline skills, and “Foundations,” which empowers parents to feel confident talking with their children’s teachers and advocating for their children and their children’s schools.
In many cases, districts employ a combination of one-on-one and group supports, along the lines of Early Head Start. 28 The East Durham Children’s Initiative, a private program modeled loosely after the Harlem Children’s Zone, includes Durham Connects, a home visiting program that supports zone families with children up to age 3 and is followed by weekly or biweekly in-home parent education and support provided by two nonprofit social service providers, Healthy Families Durham and Jumpstart (Weiss 2016e). In Montgomery County, Maryland, family social workers collaborate with classroom teachers to help them develop Family Partnership Agreements, which are based on the strengths, needs, and personal goals of each family. A social worker–led team follows up by phone and with visits. In two of the district’s highest-poverty schools, these supports are complemented by early child care and family education centers (Judy Centers), which provide comprehensive early childhood education and support to children from birth to age five and their families (Marietta 2010).
Big investments in prekindergarten programs
Almost every state in the country now invests at least minimally in pre-K programs for disadvantaged children, and a growing share of states make these programs widely available. 29 Most of the districts we studied, however, have gone far beyond state programs through one or more strategies and funding mechanisms.
A few of these districts benefit from high-quality state pre-K programs that serve a large share of children, freeing the districts to invest in other aspects of early childhood enrichment. The Partners for Education initiative based in Berea, Kentucky, leverages the state pre-K program, which serves all three- and four-year olds who are either low-income or have other risk factors. This enables Partners for Education to use Promise Neighborhood grant funds to place early childhood specialists in pre-K classrooms throughout the four-county region (the region is a Promise Neighborhood region, which means that federal funds are available for a variety of education- and health-related investments). The specialists also provide coaching, professional development, and support for Head Start classrooms, as well as in-home tutoring over the summer.
In East Durham, North Carolina, strong state early education programs are supplemented by partner-led low-cost half-day preschool and a summer kindergarten readiness program, and home visits by parent advocates provide a range of supports, such as connections to state pre-K. In Kalamazoo, Michigan, the Pre-Kindergarten Early Education Program (PEEP) offers half- or full-day pre-K classes in elementary schools for four-year-olds at or below 250 percent of the federal poverty level, per state law, but it adds transportation and meals for those children. PEEP also works with other programs such as Head Start to provide families who are ineligible for PEEP with other options for low- or no-cost quality early education (KPS 2017).
Other districts with less comprehensive state support use federal resources to expand local options. For example, Vancouver draws on both state and federally funded early learning programs to provide pre-K in seven schools, along with district-supported programs for children in Title I schools. As of fall 2015, Vancouver’s new early learning center serves up to 100 additional children or more, with hot meals and playground space from an adjacent elementary school. Montgomery County also enhances state and federal programs with district-level investments: it provides the same literacy-rich curriculum in its Head Start classrooms as in district pre-K classrooms. And Montgomery County uses a blend of federal Title I and Head Start dollars to offer full-day Head Start in 18 of the poorest schools, serving 460 children (Marietta 2010). The Northside Achievement Zone in north Minneapolis uses federal Race to the Top Early Learning Fund money for scholarships for three- and four-year-olds to attend high-quality pre-K, serving 127 children in 2012–2013 and 156 in 2013–2014.
Local programs can also fill in where state programs are weak. Austin, Texas, uses local funds to provide enriching, hands-on full-day programs for the four-year-olds who would otherwise participate in lower-quality half-day state programs. Austin also provides a half-day program for three-year-olds who aren’t served by the state. Families who qualify for both state pre-K and Head Start also receive nutrition, health, and other services (AISD 2017).
Pea Ridge is another community using local resources to supplant state resources. A lack of available seats for children who are eligible for the state’s high-quality Arkansas Better Chance (ABC) pre-K program prompted Pea Ridge to seek a grant to open its own program, which serves 40 children: 20 at-risk children, who receive tuition scholarships, and 20 others whose parents can pay tuition (Weiss 2016f). Missouri’s pre-K program also has too few slots, so Bright Futures Joplin is building a new early childhood learning center that will be funded jointly by the district and the state.
Strengthening the transition to kindergarten
Featured districts also build on pre-K gains and help narrow school-readiness gaps with such programs as full-day kindergarten. Montgomery County Public Schools first started full-day kindergarten in “red zone schools,” those deemed to be most affected by high rates of student poverty, in 2000. Full-day kindergarten has since expanded to every school in the district (Marietta 2010). And Vancouver offers Kindergarten Jump Start, a school readiness program, at all 21 elementary schools, and full-day kindergarten; both programs seek to enhance the transition from pre-K into formal schooling.
Other investments in young children and their families
In addition to the above range of supports for infants, toddlers, and preschoolers and their parents, several of the districts studied by BBA have made additional investments in young children and their families. The Community Storywalk in Clay County, Kentucky, and the Born Learning Trail in Joplin, Missouri, provide opportunities for parents and paid caregivers to learn with their children in a hands-on way through outdoor and physical activities. In Eastern Kentucky, Partners for Education’s Promise Neighborhood grant supports work by national nonprofit Save the Children to improve the health and education outcomes of the region’s children through a literacy program that provides kids ages 5–12 with books and tools to develop strong reading skills. The Promise Neighborhood grant also allows Partners for Education to offer the Children’s Healthy Choices program, which provides healthy snacks and 30 minutes of daily physical activity for children in districts across Eastern Kentucky.
Joplin’s Little Blue Bookshelf program gives age-appropriate books to those children whose families cannot afford them, making the goal of 1,000 hours of reading by kindergarten a viable reality for every child. And the city’s Lend & Learn Libraries provide stimulating toys and socialization time for young children and their parents.
How the school districts invest in K–12 strategies to sustain and boost their early childhood investments
The whole-child approaches these communities embrace for children from birth to five years old continue as those children transition to kindergarten and through elementary, middle, and high school. This represents a sharp difference from most other districts, which focus heavily on narrow academic factors and assessments and thus neglect characteristics emphasized in pre-K, such as building strong teacher–student relationships and attending to the full range of children’s assets and needs. As these examples illustrate, students continue to benefit from a more comprehensive approach to education and there is an array of strategies school districts can use to deliver that comprehensive approach.
Enriching K–12 curricula and activities to sustain pre-K’s whole-child emphasis
A broad set of investments and activities can help sustain pre-K’s whole-child approach, including enhancing classroom experiences, aligning classroom lessons with out-of-school activities that expand children’s worldviews, and using targeted strategies to improve students’ readiness for college, careers, and civic engagement.
Schools that ensure hands-on learning both in and out of the classroom make the most of this opportunity. Joplin and Pea Ridge students and their teachers enjoy service learning projects that are a core component of the Bright Futures strategy. These range from kindergartners organizing coat drives and canned food drives for their neighbors to high school students designing and implementing water research projects and reporting on the health and safety of Joplin’s water supply to the city’s water management agency. In East Durham, partnerships with community agencies and nonprofits enable clubs, field trips to museums, and other enrichment activities.
After-school and summer programs help students build on what they learned during the school year, broaden students’ worldviews and skills, and reduce summer learning loss. In most of the districts studied, schools partner with organizations such as the YMCA, Boys and Girls Clubs, Boy Scouts, and Girl Scouts to provide out-of-school enrichment programs that range from organized sports and help with homework to math and book clubs, theater, and robotics. In addition to boosting student engagement, some focus in particular on academic and college preparatory help, and many also provide snacks or even full meals. Summer camps in Boston and East Durham and book deliveries and clubs in Pea Ridge and Eastern Kentucky—where online options help bridge long distances in rural areas—keep students reading, engaged, and on track for fall classes.
In several districts, the focus on nurturing not only students’ academic skills but also their social and emotional skills strengthens the transition to kindergarten and development throughout the K–12 years. Vancouver’s schools teach and model social and emotional learning in classrooms as part of the district’s work to improve school climate and track student data on engagement and mental health. Under City Connects—the whole-child collaboration among Boston College, Boston Public Schools, and community agencies—school coordinators meet at the start of the year with teachers to discuss the particular strengths and needs of each student and develop plans to support teachers with academic and enrichment activities and meet student needs with small-group sessions on healthy eating and dealing with bullies, referrals to mental health providers, and a range of other supports (Weiss 2016g).
Two districts have made social and emotional learning a particularly high priority. Austin is one of eight districts working with the Collaborative for Academic, Social, and Emotional Learning (CASEL) to comprehensively embed social and emotional learning in teacher training, teacher standards, curricula, and metrics for assessing student and school progress (CASEL 2017). In Montgomery County, former superintendent Joshua Starr drew on the Common Core’s emphasis on problem-solving and critical thinking to lead the design of a new curriculum and classroom practices that nurture social and emotional skills. These are complemented by enhanced support for teachers to nurture social and emotional learning in daily classroom practice, by standards-based report cards that track key social and emotional skills, and by constructive disciplinary policies that reengage students and build their soft skills instead of punishing them for infractions. 30
Several of the districts focus in particular on helping students—many of whom will be the first in their families to go to college—prepare for and make that leap. Strategies include middle-to-high-school transition programs in Joplin and Vancouver and clubs and specialized courses that advance students’ social and organizational skills in Vancouver and Montgomery County. In East Durham, three initiatives (Communities in Schools Durham, Student U, and Citizens in Schools) support youth who are preparing for graduation. They offer site-based mentoring from current undergraduates. Middle and high school students in the North Minneapolis Northside Achievement Zone receive similar assistance. And Vancouver’s GRADS Teen Parent program helps teen parents stay in school, graduate, and be more effective parents. De-tracking, an intentional decision to not separate students who are achieving at different levels into different classrooms or types of courses, which is the norm in Austin and in some Montgomery County high schools, helps ensure that college preparatory classes serve students of all income levels rather than just wealthier, nonminority students. 31
College readiness is also a high priority for many Bright Futures districts. In Joplin, programs such as Operation College Bound enhance students’ understanding of and access to postsecondary education, complementing initiatives that help students navigate transitions to higher education and other sensitive periods of their academic lives. And in Pea Ridge, specialized high schools such as the Manufacturing and Business Academy and Pea Ridge Academy provide targeted support for students who want to go straight to jobs and careers or need special academic supports.
Mentoring and tutoring to get and keep students engaged
In the case study districts, the whole-child approach includes understanding the critical importance of one-on-one relationships with caring adults who support children’s academic and broader needs. Strategies can be as simple as the car and bus “buddies” who greet children in Pea Ridge each morning as they arrive at school, or as intensive as the volunteer “lunch buddies” who meet regularly with Joplin and Pea Ridge students to eat with them, talk about their days, and offer guidance. Northside Achievement Zone in North Minneapolis partners with Big Brothers Big Sisters to connect students with mentors, and over 500 volunteer mentors in Vancouver, Washington, support students in Family and Community Resource Centers.
These relationships are key to efforts in large urban districts and remote rural ones. The Children’s Aid Society has partnered with the New York City Department of Education to integrate a strong school curriculum with out-of-school enrichment programming, as well as provide child and family support services designed to remove barriers to students’ learning (Weiss 2016h). Children’s Aid community schools offer both tutoring and mentoring among their after-school options, as do Boston’s City Connects schools. In Eastern Kentucky, to bridge the long distances between one school and community and another, mentors use Skype to connect with eighth- and ninth-graders in Promise Neighborhood area schools.
Supports for student health and family wellness as a tool for sustaining early gains
Several of the districts studied have established health clinics in some or all of their schools, including Montgomery County, Vancouver, and New York City. In some other districts, such as Austin, school coordinators can arrange for mobile clinics to come to schools. These clinics provide basic preventive care through immunizations and check-ups, along with prescriptions and other care for sick children, physical and mental health screenings, follow-up counseling, mental health care, and even crisis intervention when needed.
Nutrition is another critical factor that affects physical and mental health and thus learning. In East Durham, Back Pack Buddies and summer lunch programs prevent hunger and keep kids nourished. Food and clothing pantries plus social media outreach in Pea Ridge and Joplin enable counselors and teachers to meet targeted immediate needs so students can focus and learn. Montgomery County has expanded its breakfast-in-the-classroom program to serve all students in a growing share of schools (MCPS 2017).
Many of these districts look beyond meeting students’ basic health and nutrition needs to advancing their and their families’ wellness and strengthening their ties to the community. Vancouver’s GoReady! back-to-school festivals provide backpacks, school supplies, shoes and socks, immunizations and dental screenings, and even haircuts, plus resources from community partners . In Eastern Kentucky, physical and mental health supports provided through state-supported Family Resource and Youth Service Centers are complemented by school–community collaborative activities through a run/walk club, a summer fitness program, a Jump Start program, and gardening and food preservation activities. And the East Durham Children’s Initiative runs a Healthy Living Initiative that refers families to nutrition counseling programs, Zumba classes, cooking demonstrations, and walking groups; it also distributes children’s bicycles and partners with local farmers markets to provide families with fresh produce.
Though research has long affirmed the importance of parental engagement, many schools struggle to meaningfully engage parents. The case study districts show how it can be done. In the rural regions around Berea, Kentucky, where physical distance makes engagement difficult, Partners for Education’s Families and Schools Together project convenes parents, school staff, and local agency professionals to help parents build social networks. In the North Minneapolis Northside Achievement Zone (NAZ), a high-poverty heavily minority area, regular one-on-one meetings between parents and “connectors”—specialized social workers who grew up in the area, are familiar with its challenges, and are a core component of the NAZ strategy—provide opportunities to conduct family needs assessments and provide referrals to relevant services. These regular meetings lead to deeper parental engagement in their children’s schools.
And full-service community schools such as those in Vancouver and New York City specialize in parent outreach and engagement. Community schools in these districts draw on parental input to shape school policies and practices and provide parents with an opportunity to meet one another. For example, a “parents’ coffee room” in a New York City school with a large Dominican population evolved from simply providing a space for parents to hang out after student drop-off to a center for parent-led workshops, parent–student collaborative plays, and more.
Other targeted supports provide added help for the most vulnerable students and their families. In Vancouver, for example, student advocates conduct home visits to parents of kindergartners and first-graders who are at risk of chronic absenteeism. In these visits, the advocates emphasize the importance of attendance and brainstorm with parents ways to reduce specific barriers to attendance. Complementary in-school efforts reward strong attendance. High-risk Montgomery County Public Schools students benefit from an unusual, but very effective, system of targeted support. Specifically, the districts’ funding system redistributes money from wealthier schools to higher-poverty schools, enabling the latter to provide smaller classrooms, more individualized attention, and more specialists in English language learning, special education, and other areas (Elmore, Thomas, and Clayton 2006).
How academic gains, including smaller achievement gaps, indicate that the investments are paying off
Providing children from birth through 12th grade and their families with targeted supports both within and outside of school has enabled these communities to make progress toward a range of goals. First, compared with students in peer districts, these districts’ students tend to have better outcomes on traditional measures of academic achievement such as test scores and graduation rates. Second and just as, if not more, important, these districts have improved students’ kindergarten readiness, engagement, and health and well-being, and helped the students be better prepared for college, careers, and civic engagement. This is true in large part due to these districts’ intentional bucking of a growing trend of diverging practices in which students in high-poverty schools are subject to narrow academic drilling while students in wealthy schools benefit from a broader set of activities and learning experiences beyond a narrow focus on preparing for standardized tests. These districts ensure enrichment for all students, regardless of socioeconomic status. Finally, in contrast with the national trend in recent decades of rapidly growing achievement gaps between wealthy and poor students, these districts are also narrowing race- and income-based achievement gaps: while all students are gaining ground, those who started off behind tend to see the largest gains.
Most of the data presented in this section do not come from experimental studies; with a few exceptions (which are noted in the case studies), they rely on nonexperimental comparisons with a similar nontreatment group, such as other low-income children in the district or other high-poverty districts in the state. However, they are gathered from official district, state, or federal resources in all cases, except for the minority of cases in which such data are not publicly available. Perhaps most importantly, in contrast with many other programs that have reported substantially improved outcomes for very vulnerable groups of students, these programs do not cherry-pick students to get these results. Rather, these initiatives serve all students in the enrollment area for a school, a cluster of schools, or, in many cases, an entire district; as described above, they are serving some of the nation’s most vulnerable students and their families. 32 Moreover, many of these efforts are, for lack of a better term, “turnarounds.” That is, students in an existing system that is considered to be failing are offered a new approach in the same school building, making the large gains reported particularly striking given the notable lack of similar progress from much-larger-scale, more publicized attempts at employing other turnaround strategies. 33
Establishing more expansive goals and implementing ways to track progress toward those goals also offers timely guidance, given that the Every Student Succeeds Act (ESSA) asks states, districts, and schools to do just that. These districts have not only set broader goals, they are demonstrating real progress toward achieving these goals. Because of their success, many now serve as role models for other districts or entire regions, and a few are beginning to influence state policy as well.
Higher rates of kindergarten readiness predict school success
Some of the kindergarten readiness efforts described above have translated into improved readiness to learn and, thus, greater odds of success in kindergarten and throughout the K–12 years. In Eastern Kentucky, East Durham, and Minneapolis, children who participated in early learning programs significantly increased their rates of kindergarten readiness across a range of metrics and developmental domains. A study of Montgomery County Public Schools found much larger gains in reading for children in the full-day Head Start program than for children in the half-day program, with full-day students more than doubling their reading scores over the year and especially pronounced gains for the most vulnerable students: Hispanics and English language learners (Marietta 2010).
Rising test scores and narrowing gaps in core academic subjects are an important sign of sustained early gains
While only one of many indicators, rising test scores and narrowing gaps in core academic subjects are an important sign that schools in case study districts have sustained and enhanced early gains. Despite serving a higher percentage of low-income, black, Hispanic, and English language learner students than the district average, Austin’s Alliance Schools—schools in which community organizers have worked to empower parents in conjunction with teacher advocacy efforts—saw substantial gains in scores on the Texas Assessment of Academic Skills, the state’s main standardized test, in the three years after parent-organizing efforts began. Increases varied from four points to 15–19 points, with the latter increases occurring in schools with the highest levels of parental engagement (Henderson 2010). Subsequent rollout of social and emotional learning in district schools (some of which were also Alliance schools) produced gains in the share of students deemed proficient on the State of Texas Assessment of Academic Readiness (STAAR, the next-generation state assessments) in the years following that rollout, with students in the first set of schools with social and emotional learning programs scoring higher on state math and reading exams than those in later school cohorts. The small group of Minneapolis Northside Achievement Zone students who were tested increased their proficiency on the Minnesota Comprehensive Assessments (MCA) exam, with the share scoring as proficient rising from 14 percent in the 2012–2013 academic year to 22 percent in 2013–2014. 34 Students who had enrolled in the Northside Achievement Zone in 2013 had larger gains than those who enrolled in 2014, and, overall the largest proficiency gains were among first- and second-graders, with the smallest gains in middle schools.
Despite serving a much poorer and socially and economically isolated student body than in state schools overall, the Eastern Kentucky schools served by Partners for Education have seen substantially higher increases in test scores: from 2012 to 2015, math test scores in the Promise Neighborhood region rose 7.0 percentage points compared with 4.4 percentage points across the state, and reading scores rose 7.3 percentage points, compared with 5.8 percentage points statewide.
An independent study of middle school students who participated in the after-school programs run by Children’s Aid Society community schools in New York City had bigger gains in math and reading test scores than peers who did not participate. They also had higher relative increases in school attendance and in teacher-reported “motivation to learn.” And while the Children’s Aid Society did not make early childhood education investments a core component of its strategy, its Zero-to-Five program, which connects the federal Early Head Start and Head Start programs, produced relative test score gains among participants. Specifically, a study found that participants outperformed their peers 97 percent of the time on third-, fourth-, and fifth-grade standardized tests in math and reading, demonstrating a significant long-term positive effect (Caspe and Lorenzo Kennedy 2014).
Increases (or lack of decreases) in reading scores over the summer months (between the end of the school year and the start of the following year) can be an especially important indicator of sustainable academic achievement, since low-income students tend to lose substantial ground when they are out of school for the summer. Students who attended the North Minneapolis Northside Achievement Zone’s extended learning summer programs increased their reading test scores between the end of one school year and the beginning of the next, a period when scores normally decrease. And an evaluation of students who attended the East Durham Children’s Initiative’s summer camp in the summer of 2014 found that they lost no ground in literacy over those months.
Case study districts with more mature initiatives and those offering higher or more intensive doses of whole-child interventions are producing particularly large academic gains. Students enrolled in City Connects elementary schools in Boston score significantly higher on tests of both academic and noncognitive skills in elementary and secondary school, with the highest-risk students, such as English language learners, showing especially large gains. Scores of City Connects elementary school students on the Stanford Achievement Test version 9 increased between one-fourth and one-half a standard deviation greater than scores of their non–City Connects peers. And graduates of City Connects secondary schools are more likely to attend one of Boston’s three most selective public high schools.
Better student attendance and engagement are also predictors of academic gains
Chronic absenteeism depresses achievement, particularly among low-income students. A 2009 study found that New York City Children’s Aid Society’s community schools had “far higher” attendance than peer schools, and that schools with health centers tended to have higher attendance than those without health centers (Clark et al. 2009). Students attending City Connects high schools in Boston have significantly lower rates of chronic absenteeism than their peers (Boston College Center for Optimized Student Support 2012). In Joplin, Missouri, attendance rates among high school students increased 3.7 percentage points, rising from 91.3 percent in 2008 to 95.0 percent in 2012; black and Hispanic students closed gaps with their white peers over that period. At the same time, reportable disciplinary incidents—which keep students out of school and are found to drive at-risk students to disengage—dropped by over 1,000, from 3,648 in 2008 to 2,376 in 2012. 35
Every infant and toddler in East Durham whose family participated in the Healthy Families Durham home visiting program is up to date on immunizations; this helps at-risk children avoid missing school due to illness. In Pea Ridge, collaboration with one of the city’s doctors enabled the district to provide physical exams for high school students who would otherwise go without them. This not only improved their health but enabled them to participate in the kinds of extracurricular sports activities that boost student engagement. And City Connects’ practice of helping families draw on Medicaid coverage and of referring eligible students to insurance-eligible providers increases students’ access to both physical and mental health care. Given extensive evidence linking reduced absenteeism and improved physical and mental health to academic gains, these initiatives’ records of boosting both attendance and health represent another pathway to student success. 36
Increases in advanced coursework and completion of associated exams suggest improved college and career readiness
Because most of the initiatives studied have been in place for less than 10 years, and a few for five or fewer, there is less evidence of their impact on high school graduation and college enrollment. Nonetheless, the degree to which low-income and minority students in these districts perform better and have seen greater gains on these key indicators than their peers in comparable districts or across the state highlights the promise of comprehensive education approaches and, in some instances, their capacity to sustain and even boost children’s early gains.
Parent-organizing in Austin helped establish a program to get more low-income and minority middle school students into rigorous science and math programs, enabling them to successfully compete for slots in the prestigious LBJ High School Science Academy. From the 2007–2008 to the 2014–2015 academic year, the number of Kalamazoo Public School students taking Advanced Placement (AP) courses more than doubled, with low-income and African American students experiencing the largest absolute gains in participation and Hispanic students experiencing the largest percentage gains. Black and low-income students roughly quadrupled their participation in such courses; 263 black students and 193 low-income students took AP classes during the 2014–2015 academic year, up from 63 and 53 respectively in 2007–2008 (Miller-Adams 2015). Over the same period, the number of Hispanic students taking AP courses increased by a magnitude of 10—from just 8 to 78. And in Vancouver, which also made socioeconomic diversity of students in advanced courses a priority, enrollment in AP courses rose by 67 percent overall from 2007–2008 to 2013 –2014, and nearly three times as fast, by almost 200 percent, among low-income students.
Higher graduation rates and increasing college attendance of disadvantaged students are another measure of success of comprehensive strategies
In the early 2000s, the graduation rate at Austin’s Reagan High School fell below 50 percent and enrollment dropped to just 600 students. By 2015, with the benefit of a community schools strategy, the school was serving more than 1,200 students and had a graduation rate of 85 percent.
In the first six years of Bright Futures, Joplin’s graduation rate rose from 73 to 87 percent; from 2012 to 2015 it rose 13 percentage points, versus just 5 percentage points across the state as a whole. At the same time, the cohort dropout rate fell from 6.4 percent to 2.8 percent, with the dropout rate for black students falling slightly more. And in Kalamazoo, incentives to finish high school have proven to be powerful tools for disadvantaged students when combined with mentoring, tutoring, and after-school options. The district’s graduation rate rose from 64 percent in 2009 to 69 percent in 2014, with “five-year cohort graduation rates consistently higher than four-year rates, suggesting that some students may be opting to stay in school an extra year (or even just for the summer) to complete the credits necessary to get a high school diploma” (Miller-Adams 2015, 67). Moreover, African American girls in Kalamazoo graduate at higher rates than their peers across the state, and 85 percent of those graduates go to college.
Initiatives that have had time to mature have made particularly large gains. Montgomery County’s Linkages to Learning initiative began in 1993 and it substantially expanded its pre-K program around a decade later; a county policy responsible for improved racial integration has been in place even longer, since the early 1970s. Hispanic, low-income, and African American students in Montgomery County Public Schools are much more likely than their counterparts across the state to graduate from high school—80.0 vs. 77.5 percent, 81.0 vs. 77.8 percent, and 86.4 versus 80.5 percent, respectively. And from 2011 to 2014, a period when the share of students in poverty and the share of minority students rose in the district, overall graduation rates rose 2.9 percentage points, from 86.8 to 89.7 percent. There were much larger gains for Hispanic and black students, whose graduation rates rose (respectively) by 4.7 percentage points (from 75.3 to 80.0 percent) and 5.1 percentage points (from 81.3 to 86.4 percent), thus narrowing their gaps with their white peers by 3.4 and 3.8 percentage points, respectively (MCPS 2015). Participation in Boston’s City Connects program, which began in 2001, cuts a student’s odds of dropping out of high school nearly in half: 8.0 percent versus 15.2 percent for comparison students (Boston College Center for Optimized Student Support 2014). In Vancouver, the four-year graduation rate rose from 64 percent in 2010 to almost 80 percent in 2013, and the five-year rate rose from 69 percent in 2010 to over 80 percent in 2013. Vancouver’s Hispanic students had five-year graduation rate gains of over 15 percentage points.
Strong parent and community engagement is another sign of progress
The comprehensive, whole-child, whole-community approaches in the featured school districts have built strong school–community partnerships. Two indicators of the strength of the partnerships are the levels of parent and community engagement. In Joplin, 194 more adults are now serving as mentors and tutors than five years ago. And the American Association of School Administrators, National School Public Relations Association, and Blackboard Connected selected Vancouver Public Schools Superintendent Steve Webb and Chief of Staff Tom Hagley for their 2011 Leadership through Communication Award for their successful efforts to increase family engagement in high-poverty VPS schools.
Parental engagement boosts student achievement both directly and through other improvements to families’ situations. As they work actively with their “connectors,” Northside Achievement Zone parents in North Minneapolis become more likely to make academics a priority, to engage with their children’s schools, and to be focused on sending their children to college. The support also helps more families connect with stable housing, substantially reducing the number of times that some vulnerable families move. In 2014–2015, up to 300 Austin families benefited from help with legal, employment, health, and housing issues at the family resource center, which also provides classes for parents, including English language learning classes. And Montgomery County Public Schools social workers who specialize in early childhood education make an average of 200 home visits, 1,000 phone contacts, and 300 direct contacts with parents at school or conferences each month. These lead to roughly 1,000 monthly referrals to community services—many of them emergency interventions dealing with food, clothing, and housing—that help families meet their children’s basic needs and, thus, support their children’s education (Marietta 2010).
In some cases, engagement enhances school leadership. Through access to supports such as social services and adult education, parents of students in New York’s Children’s Aid Society community schools got more involved in their children’s schools, took more responsibility for their children’s schoolwork, reported feeling more welcome within the schools, and were observed to be a greater presence in the community schools than in comparison schools. And over 2,000 Kentucky parents have undergone training at the Berea Commonwealth Institute for Parent Leadership since its creation in 1997. Many of these parents have gone on to join school boards, serve on school councils, and engage in day-to-day educational advocacy.
Expansion of these initiatives shows that other districts, and even state policymakers, consider them successful
After City Connects succeeded in improving student achievement in over a dozen of Boston’s highest-poverty schools, the initiative caught the attention of state policymakers, who recruited City Connects to help turn around schools in Springfield, home to another large high-poverty urban district in Massachusetts. Aided by federal School Improvement Grant funds, City Connects has operated in Springfield since 2010, expanding from six to 13 schools in its first four years there. In New York City, the Children’s Aid Society played a central role in Mayor Bill de Blasio’s 2016 decision to employ a community schools strategy to turn around 100 of the city’s most struggling schools. And in both Vancouver and Austin, district leaders have led advocacy efforts to bring community schools to other communities in the region and to support the introduction of state-level legislation to enhance the work.
Bright Futures began in Joplin, Missouri, in 2009 but is now a national organization. Bright Futures USA has 50 affiliates in eight states, many of which—such as Pea Ridge—are just two or three years old. The newest affiliate, in Fairbanks, Alaska, has just been made official. In Virginia, Dave Sovine, superintendent of a second-year affiliate, Frederick County Public Schools, is reaching out to several of his counterparts across the region to create the first regional Bright Futures initiative (Gizriel 2016). If established, this would allow for the kind of cross-district collaboration identified by Bright Futures founder C.J. Huff as critical to breaking down the silos created by arbitrary boundaries that reflect political preferences rather than children’s daily realities. 37
As this report demonstrates, very large social-class-based gaps in academic performance exist and have persisted across the two most recently studied cohorts of students starting kindergarten. The estimated gap between children in the top fifth and the bottom fifth of the SES distribution is over a standard deviation in both reading and math in 2010 (unadjusted performance gaps are 1.17 and 1.25 sd respectively). Gaps in noncognitive skills such as self-control and approaches to learning—which are critical not only as foundations for academic achievement but also more broadly for children’s healthy development—are about half as large (about 0.4 sd in self-control, and slightly over 0.5 sd in approaches to learning in 2010).
Another important finding from our study is that gaps were not, on average, sensitive to the set of changes that may have occurred between 1998 and 2010: gaps across both types of skills are virtually unchanged compared with the prior generation of students—those who entered school in 1998. The only cognitive gap that changed substantially was in reading skills, which increased by about a tenth of a standard deviation. The gaps by SES in mathematics, in approaches to learning as reported by parents, and in self-control as reported by teachers did not change significantly. And relative gaps in approaches to learning as reported by teachers and in self-control as reported by parents shrank between 1998 and 2010, by about a tenth of a standard deviation. 38
We also find that, while taking into account children’s personal and family characteristics, parental activities, and other factors reduces the gaps somewhat, it does not come close to eliminating them. This means that there is a substantial set of SES-related factors that are not captured by the traditional covariates used in this study but that are important to understanding how and why gaps develop. Moreover, the capacity for these other factors—child and family characteristics, early education investments, and expectations—to narrow gaps has decreased over time. This suggests that, while such activities as parental time spent with children and center-based pre-K programs cushion the negative consequences of growing up in a low-social-class context, they can do only so much, and that the overall toxicity of lacking resources and supports is increasingly hard to compensate for. The resistance of gaps to these controls should thus be a matter of real concern for researchers and policymakers.
These troubling trends point to critical implications for policy and for our society: clearly, we are failing to provide the foundational experiences and opportunities that all children need to succeed in school and thrive in life. The failure to narrow gaps between 1998 and 2010 suggests, too, that investments in pre-K programs and other early education and economic supports were insufficient to counter rising rates of poverty and its increasing concentration in neighborhoods where black and Hispanic children tend to live and learn.
But there is also good news. The case study review in the previous section of this report explores district-level strategies to address these gaps, strategies that are being implemented in diverse communities across the country. The most effective ones begin very early in children’s lives and are sustained throughout their K–12 years and beyond. The communities studied all employ comprehensive educational approaches that align enriching school strategies with a range of supports for children and their families. Their implementation is often guided by holistic data and, to the extent possible, this report provides a summary, as well, of student outcomes, using both traditional academic measures and a broad range of other measures.
These findings also point to further research questions that need to be addressed, including why gaps changed or did not change, for whom they changed (or did not change), and what is the absolute change in children’s skills over time. 39
Parents are doing what they need to do, and a growing number of communities are, too, but as a society, we are still falling far short
Over the period studied, parents across all social class groups became more involved in their young children’s early education and development, with increases in involvement being especially pronounced among low-SES parents. Parents were more likely in 2010 than in 1998 to read regularly to their children; to sing to them; to play games with them; and to enroll them in center-based pre-K programs. Parents in 2010 also had significantly higher expectations for their children’s educational attainment, and mothers themselves were more highly educated—both factors that are associated with higher achievement for those children. In other words, parents’ actions show that they are doing more of what the brain science indicates they need to do, which either suggests that information about children’s needs during those years is more widely disseminated than it was for the prior cohort we studied, or that parenting styles have changed in a way that benefits the development in the early years.
And, as the case studies indicate, the number of communities that have embraced systems of comprehensive enrichment and supports (“Broader, Bolder Approaches to Education”) is growing. As these communities have shown, such comprehensive education policies are feasible; embedded in these policies is an understanding that children’s development involves nurturing a variety of competencies throughout the stages of development, that there are many individuals participating in these processes, and that coordinated efforts by various stakeholders are needed to put these processes to work. Key principles that span across the case studies include very early interventions and supports, parental engagement and education, pre-K, kindergarten transitions, whole-child approaches to curricula, and wraparound supports that are sustained through the K–12 years. Given the significant need for more such strategies, it is important to understand the factors that drove their enactment in a diverse set of communities, and to continue to monitor both the challenges these communities (and others like them) encounter and the outcomes/benefits of the initiatives.
However, despite the abundance of child development information available to researchers and parents—about the serious impacts of child poverty, about what works to counter those effects, about the importance of the first years of life for children, and about the value of education—our data indicate insufficient policy response at all levels of government. Pre-K programs have expanded incrementally and unevenly, with both access and quality still wildly disparate across states and overall availability severely insufficient. There is a dearth of home visiting programs and of quality child care (Bivens et al. 2016). Child poverty has increased (see Proctor, Semega, and Kollar 2016 for recent trends in child poverty rates). And the schools these children enter face increasing economic and racial segregation but with even fewer resources than they had in 1998 to deal with them (Adamson and Darling-Hammond 2012; Baker and Corcoran 2012; Carnoy and García 2017). And while a growing number of districts have embraced Broader, Bolder approaches, that number is failing to keep up with high and growing need.
In sum, it is actually positive, and somewhat impressive, that gaps by and large did not grow in the face of steadily increasing income inequality, compounded by the worst economic crisis in many decades (EPI 2012, 2013; Saez 2016). But it is disappointing and troubling that new policy investments made in the previous decade were insufficient to make even a dent in these stubborn gaps. We cannot ensure real opportunities for all our children unless we tackle the severe inequities underlying our findings. And while momentum to enact comprehensive and sustained strategies to close gaps is growing, such strategies are not being implemented nearly as quickly as children need them to be.
Next policy steps
These data on large, stubborn gaps across both traditional cognitive and noncognitive skills should guide the design of education policies at the federal, state, and local levels; the combined resources and support of government at all three levels are needed if we are to tackle these inequalities effectively. 40
Policymakers can begin by learning from the small-scale, district-level strategies presented in the review of case studies above (see the section “What are pioneering school districts doing to combat these inequities and resulting gaps?” above). Looking at these case studies, policymakers can ask: What are the key strategies these communities employed, what main components characterize these strategies, and how did these communities effectively implement the strategies? What challenges did these communities face, what was needed to overcome the challenges, and how can we shape policies that better support other communities’ abilities to respond to such challenges and, to the extent possible, avert them? The latter set of questions is particularly pertinent to issues of scalability, financing, and sustainability, all of which have posed significant challenges for the districts studied and others like them. Policymakers can further ask: What other sources or examples might we learn from? Obvious ones include other districts that employ “community schools” strategies (as Vancouver, New York City, and Austin do) and Promise Neighborhood initiatives beyond Berea/Eastern Kentucky and the Northside Achievement Zone. Bright Futures affiliates now exist in 50 districts across eight states—and the program continues to grow—offering another set of communities to look to.
Also, new opportunities under the Every Student Succeeds Act (ESSA)—from funding to expand and align early childhood education programs to broader and more supports-based educator- and school-accountability systems—provide another avenue for exploration and educational improvement. This is already the focus of states and districts across the country—as well as of education policy nonprofits and associations—and is a focus that has the potential to inspire viable larger-scale models (Cook-Harvey et al. 2016).
We must take action, in particular, in those areas of policy related to early education in which we have seen little or no progress over the past decade. These include child care: comprehensive supports that engage parents as partners in their children’s education must start early and be of high quality to prevent the emergence of gaps and provide time to close any gaps that emerge (Bivens et al. 2016, among others). Quality preschool, among the most-agreed-upon strategies to avert and narrow early gaps, continues to be much talked about but far too little invested in and far too infrequently and shoddily implemented. The advantages of preschool have been known for decades, and significant progress has been made in preschool enrollment over that time; however, preschool enrollment stagnated soon after 2000 (Barnett et al. 2017; U.S. ED 2015) and there continue to be significant inequities in access (see Table 2; García 2015) and, just as important, in quality (NIEER 2016). And the gains made through these early, whole-child-oriented supports must be sustained through children’s K–12 years, with attention to issues of funding levels and equity, racial and socioeconomic integration, and enriching opportunities in the hours after school and in the summer months.
Altogether, this report adds to the strong evidentiary base that identifies strategies to reduce the education consequences of economic inequality. It also sheds light on the need to conduct further research on the channels that drive or cushion changes in readiness. A close follow-up of these trends in the near future and of the measures adopted to really tackle inequities will not only determine what type of society we will be, but will also say a lot about what type of society we actually are. This study, affirming a growing number of other studies on these issues, points to an “American Dream” that is alive in public pronouncements but dormant and pale in reality.
About the authors
Emma García is an education economist at the Economic Policy Institute, where she specializes in the economics of education and education policy. Her areas of research include analysis of the production of education, returns to education, program evaluation, international comparative education, human development, and cost-effectiveness and cost-benefit analysis in education. Prior to joining EPI, García conducted research for the Center for Benefit-Cost Studies of Education and other research centers at Teachers College, Columbia University, and did consulting work for the National Institute for Early Education Research, MDRC, and the Inter-American Development Bank. García has a Ph.D. in economics and education from Teachers College, Columbia University.
Elaine Weiss served as the national coordinator for the Broader, Bolder Approach to Education (BBA) from 2011 to 2017, in which capacity she worked with four co-chairs, a high-level task force, and multiple coalition partners to promote a comprehensive, evidence-based set of policies to allow all children to thrive. Weiss came to BBA from the Pew Charitable Trusts, where she served as project manager for Pew’s Partnership for America’s Economic Success campaign. Weiss was previously a member of the Centers for Disease Control and Prevention’s task force on child abuse and served as volunteer counsel for clients at the Washington Legal Clinic for the Homeless. She holds a Ph.D. in public policy from the George Washington University and a J.D. from Harvard Law School.
Acknowledgments
An earlier version of this paper was prepared for “Strong Foundations: The Economic Futures of Kids and Communities,” the Federal Reserve System Community Development Research Conference, Washington, D.C., March 23–24, 2017. We appreciate the feedback we received from our discussant Richard Todd and from the audience. The authors gratefully acknowledge Rob Grunewald and Milagros Nores for their insightful comments and advice on earlier drafts of the paper. Special gratitude is expressed to Sean Reardon, for his advice and thorough guidance on the sensitivity analyses affecting the measurement of the cognitive skills and their implications for our study, and for sharing useful materials to help test our results. We thank Ben Zipperer and Yilin Pan for their advice on issues associated with multiple imputation of missing data. We are also grateful to Lora Engdahl and Krista Faries for editing this report, and to Margaret Poydock for her work preparing the tables and figures and formatting the report. Finally, we appreciate the assistance of communications staff at the Economic Policy Institute who helped to disseminate the study, especially Dan Crawford, Kayla Blado, and Elizabeth Rose.
Address correspondence to: Economic Policy Institute, 1225 Eye St. NW, Suite 600, Washington, D.C., 20005. Email: [email protected] ; [email protected] .
Figures and tables
Unadjusted cognitive and noncognitive skills gaps between high-ses and low-ses children at the beginning of kindergarten in 1998 and change in gaps by the beginning of kindergarten in 2010.
The data below can be saved or copied directly into Excel.
The data underlying the figure.
Notes: SES refers to socioeconomic status. The gaps are the baseline unadjusted standard deviation scores for high-SES children relative to low-SES children. The gap in 2010 equals the gap in 1998 plus the change in the gap from 1998 to 2010. For example, the gap in approaches to learning as reported by teachers in 2010 is 0.51 sd (0.63 – 0.12). For statistical significance of these numbers, see Tables 3 and 4, Model 1.
Source: EPI analysis of ECLS-K, kindergarten classes of 1998–1999 and 2010–2011 (National Center for Education Statistics)
Copy the code below to embed this chart on your website.
Fully adjusted cognitive and noncognitive skills gaps between high-SES and low-SES children at the beginning of kindergarten in 1998 and change in gaps by the beginning of kindergarten in 2010
Note: SES refers to socioeconomic status. The gaps are standard deviation scores for high-SES children relative to low-SES children after adjusting for all family and child characteristics, pre-K schooling, and enrichment activities with parents, and parental expectations for children’s educational attainment. The gap in 2010 equals the gap in 1998 plus the change in the gap from 1998 to 2010. For statistical significance of these numbers, see Tables 3 and 4, Model 4.
Unadjusted cognitive and noncognitive skills gaps between high-SES and low-SES children at the beginning of kindergarten in 1998 and change in gaps by the beginning of kindergarten in 2010, using mother's educational attainment as a proxy for socioeconomic status
Notes: The gaps are the baseline unadjusted standard deviation scores for high-SES children relative to low-SES children where high-SES children have mothers in the top quintile of the education distribution and low-SES children have mothers in bottom quintile of the education distribution. The gap in 2010 equals the gap in 1998 plus the change in the gap from 1998 to 2010. For statistical significance of these numbers, see Table 7, Model 1.
Unadjusted cognitive and noncognitive skills gaps between high-SES and low-SES children at the beginning of kindergarten in 1998 and change in gaps by the beginning of kindergarten in 2010, using household income as a proxy for socioeconomic status
Notes: The gaps are the baseline unadjusted standard deviation scores for high-SES children relative to low-SES children where high-SES children are in households with incomes in the top quintile of the income distribution and low-SES children are in households with incomes in bottom quintile of the income distribution. The gap in 2010 equals the gap in 1998 plus the change in the gap from 1998 to 2010. For statistical significance of these numbers, see Table 8, Model 1.
Unadjusted cognitive and noncognitive skills gaps between high-SES and low-SES children at the beginning of kindergarten in 1998 and change in gaps by the beginning of kindergarten in 2010, using number of books the child has in the home as a proxy for socioeconomic status
Notes: The gaps are the baseline unadjusted standard deviation scores for high-SES children relative to low-SES children where high-SES children have a number of books in the home in the top quintile of the books-in-the-home distribution and low-SES children have a number of books in the home in the bottom quintile of the books-in-the-home distribution. The gap in 2010 equals the gap in 1998 plus the change in the gap from 1998 to 2010. For statistical significance of these numbers, see Table 9, Model 1.
Reading and math achievement gaps, and principal noncognitive skills gaps between high-SES and low-SES children at the beginning of kindergarten in 2010–2011, under unadjusted and clustered models
Note: Using the full sample. For statistical significance, *** denotes p < 0.01, ** denotes p < 0.05, and * denotes p < 0.1. The number of observations is rounded to the nearest multiple of 10. Sizes may differ from those inferred from Tables 3–6, and from those in García 2015, due to differences in the sample sizes or to rounding.
Source: EPI analysis of ECLS-K, kindergarten class of 2010–2011 (National Center for Education Statistics)
Child and family characteristics, main developmental activities, and parental expectations for children, kindergarten classes of 1998–1999 and 2010–2011, by socioeconomic status (SES)
Note: SES refers to socioeconomic status.
Reading and math skills gaps between high-SES and low-SES children at the beginning of kindergarten in 1998 and change in gaps by the beginning of kindergarten in 2010, under unadjusted to fully adjusted models
Notes: Models 1 and 2 use the full sample; Models 3 and 4 use the complete cases sample. Robust standard errors are in parentheses. For statistical significance, *** denotes p < 0.01, ** denotes p < 0.05, and * denotes p < 0.1. The number of observations is rounded to the nearest multiple of 10. SES refers to socioeconomic status.
Noncognitive skills gaps between high-SES and low-SES children at the beginning of kindergarten in 1998 and change in gaps by the beginning of kindergarten in 2010, under unadjusted to fully adjusted models
Reductions in skills gaps between high-ses and low-ses children after accounting for missingness and covariates, 1998 and 2010.
Note: SES refers to socioeconomic status. Declining values from 1998 to 2010 indicate that factors such as early literacy activities and other controls were not as effective at shrinking SES-based gaps in 2010 as they were in 1998.
Summary of association between cognitive and noncognitive skills at kindergarten entry and selected early educational practices, fully adjusted differences (Model 4)
Notes: The robust standard errors are in parentheses. For statistical significance, *** denotes p < 0.01, ** denotes p < 0.05, and * denotes p < 0.1. The number of observations is rounded to the nearest multiple of 10.
Cognitive and noncognitive skills gaps between high-SES and low-SES children using mother's educational attainment as a proxy for socioeconomic status (SES), under unadjusted and fully adjusted models
Notes: Model 1 uses the full sample; Model 4 uses the complete cases sample. Robust standard errors are in parentheses. For statistical significance, *** denotes p < 0.01, ** denotes p < 0.05, and * denotes p < 0.1. The number of observations is rounded to the nearest multiple of 10.
Cognitive and noncognitive skills gaps between high-SES and low-SES children using household income as a proxy for socioeconomic status (SES), under unadjusted and fully adjusted models
Cognitive and noncognitive skills gaps between high-ses and low-ses children using number of books child has in the home as a proxy for socioeconomic status, under unadjusted and fully adjusted models, 'whole-child' case study initiatives, by service area.
*Indicates that while the initiative covers the entire county or region, a portion of the county or region receives more intensive services. **Indicates that the initiative will cover the entire school district under plans to expand.
Source: Case studies published on the Broader, Bolder Approach to Education website (www.boldapproach.org/case-studies)
1. Values are in 2008 dollars.
2. Early investments in education strongly predict adolescent and adult development (Cunha and Heckman 2007; Heckman 2008; Heckman and Kautz 2012). For instance, students with higher levels of behavioral skills learn more in school than peers whose attitudinal skills are less developed (Jennings and DiPrete 2010). In general, as Heckman asserted, “skills beget skills,” meaning that creating basic, foundational knowledge makes it easier to acquire skills in the future (Heckman 2008). Conversely, children who fail to acquire this early foundational knowledge may experience some permanent loss of opportunities to achieve to their full potential. Indeed, scholars have documented a correlation between lack of kindergarten readiness and not reading well at third grade, which is a key point at which failing to read well greatly reduces a child’s odds of completing high school (Fiester 2010; Hernandez 2011).
3. Research by Reardon (2011) had found systematic increases in income gaps among generations. Recent studies by Bassok and Latham (2016) and Reardon and Portilla (2016), however, show narrower achievement gaps at kindergarten entry between a recent cohort and the previous one, and thus a possible discontinuation or interruption of that trend. (Bassok et al. [2016] use an SES construct to compare relative teacher assessments of cognitive and behavioral skills among low-SES children versus all children, adjusted by various other characteristics; Reardon and Portilla [2016] look at relative performance of children in the 90th and 10th income percentiles, and use age-adjusted, standardized, outcome scores.) Research by Carnoy and García (2017) shows persistent social-class gaps, but no solid evidence regarding trends: their findings for students in the fourth and eighth grades, in math and reading, show that achievement gaps neither shrink nor grow consistently (they are a function of the social-class indicator, the grade level, or the subject).
4. Clustering takes into account the fact that children are not randomly distributed, but tend to be concentrated in schools or classrooms with children of the same race, social class, etc. These estimates offer an estimate of gaps within schools. See Appendix B for more details.
5. Results available upon request. See García 2015 for results for all SES-quintiles (the baseline or unadjusted gaps in that report correspond with Model 2 in this paper).
6. The Early Childhood Longitudinal Study asks both parents and teachers to rate children’s abilities across a range of these skills. The specific skills measured may vary between the home and classroom setting. Teachers likely evaluate their students’ skills levels relative to those of other children they teach. Parents, on the other hand, may be basing their expectations on family, community, culture, or other factors.
7. See García 2015 for a discussion of which factors in children’s early lives and their individual and family characteristics (in addition to social class) drive the gaps among children of the 2010 kindergarten class.
8. Note that the SES quintiles are constructed using each year’s distribution, and that changes in the overall and relative distribution may affect the characteristics of children in the different quintiles each year (i.e., there may be some groups who are relatively overrepresented in one or another quintile if changes in the SES components changed over time).
9. The detailed frequency with which parents develop or practice some activities with their children at home and others is available upon request.
10. Literature on expectations and on parental behaviors in the home find that they positively correlate with children’s cognitive development and outcomes (Simpkins, Davis-Kean, and Eccles 2005; Wentzel, Russell, and Baker 2016). This literature acknowledges the multiple pathways through which expectations and behaviors influence educational outcomes, as well as the importance of race, social class, and other factors as moderators of such associations (Davis-Kean 2005; Redd et al. 2004; Wentzel, Russell, and Baker 2016; Yamamoto and Holloway 2010).
11. This may be affected by the fact that the highest number of reported books in 1998 was “more than 200,” while in 2010 parents could choose from more categories, up to “more than 1,000.” We had to use 200 as our cap in order to compare data for the two kindergarten classes.
12. Evidence also points to many other factors that affect children’s school readiness, and these, too, likely changed over this time period. For example, access to prenatal care, health screenings, and nutritional programs could all have affected children’s development differently across these two cohorts, but we do not have access to these data and thus cannot control for them in our study. For links between school readiness, children’s health, and poverty, see AAP COCP 2016; Currie 2009; U.S. HHS and U.S. ED 2016.
13. Models include all quintiles in their specification. Tables that offer a comparison for all quintiles relative to the first quintile are available upon request. We focus the discussion on the gap between the top and bottom.
14. As a result, sample sizes become smaller (see Appendix Table C1). Assuming “missingness” (observations without full information) is completely at random, the findings are representative of the original sample and of the populations they represent. Analytic samples once missingness is accounted for are called the complete case samples. We tested to see whether the unadjusted gaps estimated above with the full sample remained the same when using the complete case samples. For Model 1, we found an average difference of 0.01 sd in the estimates of 1998 SES gaps, and an average difference of 0.02 sd in the estimates of the change in the gaps. For Model 2, the differences were 0.01 sd for the gaps’ estimates and 0.04 for changes in the gaps’ estimates. In terms of statistical significance, there are no significant changes in the estimates associated with the 1998 gaps, but there are two changes in the statistical significance of the estimates associated with the changes in the gaps by 2010 – 2011, and one change in the magnitude of the coefficient. The first change in the statistical significance of the estimates associated with the changes in the gaps by 2010 – 2011 is the change in the gap in approaches to learning as reported by parents, which is statistically significant when using the restricted sample (0.07 sd, at the 10 percent significance level, Model 1); and the second is the change in the gap in math which also becomes statistically significant when using the restricted sample (0.09, at the 10 percent significance level, Model 2). Finally, the one change in the magnitude of the coefficient, in this model, is the estimate of the change in the gap in reading, which increases when using the restricted sample (from 0.12 sd to 0.18 sd). Results are available upon request.
15. These interactions between inputs and time test for whether the influence of inputs in 2010 is smaller than, the same as, or larger than the influence of inputs in 1998. Also, although only the fully specified results are shown, as noted in Appendix B, these sets of controls are entered parsimoniously in order to determine how sensitive gaps and changes in gaps over time are to the inclusion of family characteristics only, to the added inclusion of family investments, and, finally, to the inclusion of parental expectations (for the inclusion of parental expectations, we incorporated interactions of the covariates with time parsimoniously as well). For all outcomes, and focusing on the models without interactions between covariates and time, we find that all gaps in 1998 continuously shrink as we add more controls. For example, in reading, adding family characteristics reduces the gap in 1998 by 11 percent, adding investments further reduces it by 15 percent, and adding expectations further reduces it by 9 percent. In math, these changes equal to 16 percent, 13 percent, and 10 percent. For changes in the gap by 2010–2011, for both reading and math, adding family characteristics and investments shrink the changes in the gaps, but adding expectations slightly increases the estimated coefficients (which are statistically significant for reading, but not for math in these models. For self-control (as reported by teachers) and approaches to learning (by parents), which are the only two noncognitive skills for which the change in the gap is statistically significant, adding family characteristics reduces the change in the “gap [by 2010–2011” coefficient], but adding investments increases it, and adding expectations further increases the changes in the gaps by 2010–2011. These results are not shown in the appendices, but are available upon request.
16. The interactions between parental expectations of children’s educational attainment and the time variable test for whether the influence of expectations in 2010 is smaller, the same, or larger, than the influence of expectations in 1998.
17. The change in the skills gaps by SES in 2010 due to the inclusion of the controls is not directly visible in the tables in this report. To see this, see the comparison of estimates of models MS1–MS3 in García 2015. The change in the skills gaps by SES in 1998 is directly observable in Tables 3 and 4 and is discussed below.
18. The numbers in the “Reduction” column in Table 5 (showing the shares of the SES-based skills gaps that are accounted for by controls) are always higher for 1998 than for 2010.
19. Please note that until this point in the report we have been concerned with SES gaps and not with performance directly (though SES gaps are the result of the influence of SES on performance, which leads to differential performance of children by SES and hence to a performance gap). The paragraphs above emphasize how controls mediate or explain some of the skills gaps by SES, so, in a way, controls inform our analysis of gaps because they reveal how changes in gaps may have been affected by changes in various factors’ capacity to influence performance. Now the focus is on exploring the independent effect of the covariates of interest on performance. In this report, because we address whether the education and selected practices affect outcomes, the main effect is measured for the 1998 cohort, and we measure how it changed between 1998 and 2010. The detailed discussion for the correlation between covariates and outcomes in 2010 is provided in Table 3 in García 2015.
20. This variable indicates whether the child was cared for in a center-based setting during the year prior to the kindergarten year, compared with other options (as explained in García 2015, these alternatives include no nonparental care arrangements; being looked after by a relative, a nonrelative, at home or outside; or a combination of options. Any finding associated with this variable may be interpreted as the association between attending prekindergarten programs, compared with other options, but must be interpreted with caution. In other words, the child may have attended a high-quality prekindergarten program, which could have been either private or public, or a low-quality one, which would have different impacts. He or she might have been placed in (noneducational) child care, either private or public, of high or low quality, for few or many hours per day, with very different implications for his or her development (Barnett 2008; Barnett 2011; Magnuson et al. 2004; Magnuson, Ruhm, and Waldfogel 2007; Nores and Barnett 2010). For the extensive literature explaining the benefits of pre-K schooling, see Camilli et al. 2010, and for a meta-analysis of results, see Duncan and Magnuson 2013. Thus, more detailed information on the characteristics of the nonparental care arrangements (type, quality, and quantity) would help researchers further disentangle the importance of this variable. This additional information would provide a much clearer picture of the effects of early childhood education on the different educational outcomes.
21. Because these associations seemed counterintuitive, we tested whether they were sensitive to the composition of the index. We removed one component of the index at a time and created five alternative measures of other enrichment activities that parents do with their children. The results indicate that the negative association between the index and reading is not sensitive to the components of the index (the coefficients for the main effect, i.e., for the effect in 1998 range between -0.14 and -0.09, are all statistically significant). For math, the associations lose some precision, but retain the negative sign (negative association) in four out of the five cases (minimum coefficient is -0.06). As a caveat, these components do not reflect whether the activities are undertaken by the child or guided by the adult, the time devoted to them, or how much they involve the use of vocabulary or math concepts. The associations could indicate that time spent on nonacademic activities detracts from parents’ time to spend on activities that are intended to boost their reading and math skills, among other possible explanations. These results are available upon request.
22. Note that in this section, “social class” and “socioeconomic status” (SES) are treated as equivalent terms; in the rest of the report, we refer to SES as a construct that is one measure of social class. See Appendices C and D for discussions of two other sensitivity analyses, one based on imputation of missing values for the main analysis in this paper, and the other on the utilization of various metrics of the cognitive variables. Overall, our findings were not sensitive to various multiple imputation tests. In terms of the utilization of different metrics for the cognitive variables, some sensitivity of the point estimates was detected.
23. With certain activities that are already so provided to high-SES children, there may be little room for doing more for them. For example, there are only 24 hours per day to read to your child, so there is a cap on reading from a cap on time. But perhaps there is still room to improve the influence of reading, if, for example, the way reading is done changes.
24. Eight of the 12 districts explored in this paper are the subjects of published case studies. Case studies for the other four are in progress and will be published later this year. When citing information from the published case studies, we cite the specific published study. For the four that are not yet published, we refer to the original sources being used to develop the case studies.
25. Missing or incomplete cells in the table indicate that data were not available on that aspect of student demographics or other characteristics. As per the source note, most data came either from the districts’ websites or from NCES.
26. In the country as a whole, poverty rates, which had been rising prior to 2007, sped up rapidly during the recession and in its aftermath (through 2011–2012), and minority students (mainly Hispanic and Asian) grew as a share of the U.S. public school student body. Between 2000 and 2013, even with a decline in the proportion of black students, the share of the student body that is minority (of black or Hispanic origin) increased from 30.0 percent to 40.5 percent, and the proportion of low-income students (those eligible for free or reduced-price lunch) also increased, up from 38.3 percent of all public school students in 2000 to 52.0 percent in 2013 (Carnoy and García 2017). The Southern Education Foundation revealed a troubling tipping point in 2013: for the first time since such data have been collected, over half of all public school students (51 percent) qualified for free or reduced-priced meals (i.e., over half of students were living in households at or below 185 percent of the federal poverty line). Across the South, shares were much higher, with the highest percentage, 71 percent—or nearly three in four students—in Mississippi (Southern Education Foundation 2015).
27. A full cross-cutting analysis of why and how these districts have employed whole-child/comprehensive educational approaches will be published as part of a book that draws on these case studies.
28. The federal Early Head Start (EHS) program includes both a home visiting and a center-based component, with many of the low-income infants and toddlers served benefiting from a combination of the two. Studies of EHS find improved cognitive, behavioral, and emotional skills for children as well as enhanced parenting behaviors.
29. According to one important source for data on access to and quality of state pre-K programs, the State of Preschool yearbook produced annually by the National Institute for Early Education Research (NIEER) at Rutgers University, as of 2015, 42 states and the District of Columbia were funding 57 programs. Moreover, programs continued to recover from cuts made during the Great Recession; enrollment, quality, and per-pupil spending were all up, on average, compared with the year before, albeit with the important caveat that two major states—Texas and Florida—lost ground, and that “[f]or the nation as a whole,…access to a high-quality preschool program remained highly unequal, and this situation is unlikely to change in the foreseeable future unless many more states follow the leaders” (NIEER 2016).
30. Elaine Weiss interview with Joshua Starr, June 2017.
31. Murnane and Levy 1996; Elaine Weiss interview with Joshua Starr, June 2017.
32. In recent years, a growing number of reports have emerged that some charter schools—which are technically public schools and often tout their successes in serving disadvantaged students—keep out students unlikely to succeed through complex application processes, fees, parent participation contracts, and other mechanisms, and then further winnow the student body of such students by pushing them out when they struggle academically or behaviorally. For more on this topic, see Burris 2017, PBS NewsHour 2015, and Simon 2013.
33. See AIR 2011 and Sparks 2017. The federal school improvement models, in order of severity (from lightest to most stringent) are termed “transformation,” “turnaround,” “restart,” and “closure” (AIR 2011, 3).
34. While the cut score on any given assessment/test needed for a student to be considered “proficient” is an arbitrary one, and, in Minnesota and many other states, changes from year to year and from one assessment to another, these gains are a helpful indicator of program effectiveness, as they are comparable over the time period described.
35. Joplin statistics are from internal data produced for the superintendent at that time that are no longer available.
36. Attendance Works , a national campaign to reduce chronic absence, points to a range of studies that document and explain the connections between chronic absenteeism, student physical and mental health, and student achievement. Areas of research include elementary school absenteeism, middle and high school absenteeism, health issues, and state and local data on how these problems play out, among others.
37. Elaine Weiss interview with C.J. Huff, June 2016.
38. See Appendix D for a discussion of results using other metrics for reading and math achievement. Results are not meaningfully different across metrics, though the point estimates differ slightly.
39. This last feature will be explored in a companion paper to this one, as soon as the necessary information is released by NCES. (As Tourangeau et al. [2013] note, the assessment scores for the 2010–2011 cohort are not directly comparable with those for the 1998–1999 cohort. We are waiting on the availability of this data to conduct a companion study that allows us to learn whether starting levels of knowledge rose over these years, and what the relative gains were for different demographic groups.)
40. We acknowledge that there are multiple noneducation public policy and economic policy areas to be called upon to address the problems studied in this report, namely, all the ones that ensure other factors that correlate with low-SES are attended, and, obviously, the ones that lead to fewer low-SES children. These other policies could help ensure that more children grow up in contexts with sufficient resources and healthy surroundings, or would leave fewer children without built-in supports at home that need to be compensated for afterwards. We made these points in two early studies, and in the policy brief companion to this study (García 2015; García and Weiss 2015; García and Weiss 2017). A similar comprehensive approach in terms of policy recommendations was used by Putnam (2015).
AAP Council on Community Pediatrics (AAP COCP). 2016. “Poverty and Child Health in the United States.” Pediatrics vol. 137, no. 4. pii:e20160339.
Adamson, Frank, and Linda Darling-Hammond. 2012. “Funding Disparities and the Inequitable Distribution of Teachers: Evaluating Sources and Solutions.” Education Policy Analysis Archives vol. 20 (November), 37.
Alvarez, Lizette. 2015. “ One Man’s Millions Turn a Community in Florida Around .” New York Times , May 25.
American Institutes for Research (AIR). 2011. School Turnaround: A Pocket Guide .
Austin Independent School District (AISD). 2017. “ Pre-K 4 ” (section on the AISD website).
Baker, Bruce D., and Sean P. Corcoran. 2012. The Stealth Inequities of School Funding . The Center for American Progress.
Barbarin, O.A., J. Downer, E. Odom, and D. Head. 2010. “Home–School Differences in Beliefs, Support, and Control during Public Pre-Kindergarten and Their Link to Children’s Kindergarten Readiness.” Early Childhood Research Quarterly vol. 25, no. 3, 358–72.
Barnett, W. Steven. 2008. Preschool Education and Its Lasting Effects: Research and Policy Implications . Great Lakes Center for Education Research and Practice.
Barnett, W. Steven. 2011. “Effectiveness of Early Educational Intervention.” Science vol. 333, no. 6045, 975–78. doi:10.1126/science.1204534.
Barnett, W. Steven, Elizabeth Votruba-Drzal, Eric Dearing, and Megan E. Carolan. 2017. “Publicly Supported Early Care and Education Programs.” In The Wiley Handbook of Early Childhood Development Programs, Practices, and Policies , Elizabeth Votruba-Drzal and Eric Dearing, eds. Malden, Mass., and Oxford: John Wiley.
Bassok, Daphna, Jenna E. Finch, RaeHyuck Lee, Sean F. Reardon, and Jane Waldfogel. 2016. “Socioeconomic Gaps in Early Childhood Experiences: 1998 to 2010.” AERA Open vol. 2, no. 3.
Bassok, Daphna, and Scott Latham. 2016. “ Kids Today: Changes in School-Readiness in an Early Childhood Era .” EdPolicyWorks Working Paper Series no. 35.
Berea College. 2013. “ U.S. Secretary of Education Visits First Rural Promise Neighborhood ” (news release). November 12.
Bradbury, Bruce, Miles Corak, Jane Waldfogel, and Elizabeth Washbrook. 2015. Too Many Children Left Behind: The U.S. Achievement Gap in Comparative Perspective. New York: Russell Sage Foundation.
Brooks-Gunn, Jeanne, and Lisa Markman. 2005. “The Contribution of Parenting to Ethnic and Racial Gaps in School Readiness.” Future of Children vol. 15, no. 1, 139–68.
Bivens, Josh. 2016. Progressive Redistribution without Guilt. Using Policy to Shift Economic Power and Make U.S. Incomes Grow Fairer and Faster . Economic Policy Institute.
Bivens, Josh, Emma García, Elise Gould, Elaine Weiss, and Valerie Wilson. 2016. It’s Time for an Ambitious National Investment in America’s Children: Investments in Early Childhood Care and Education Would Have Enormous Benefits for Children, Families, Society, and the Economy . Economic Policy Institute.
Boston College Center for Optimized Student Support. 2012. The Impact of City Connects: Progress Report 2012 .
Boston College Center for Optimized Student Support. 2014. The Impact of City Connects: Progress Report 2014 .
Burris, Carol. 2017. “ What the Public Isn’t Told about High Performing Charter Schools in Arizona .” Washington Post Answer Sheet blog, March 30.
Camilli, Gregory, Sadako Vargas, Sharon Ryan, and W. Steven Barnett. 2010. “Meta-Analysis of the Effects of Early Education Interventions on Cognitive and Social Development.” Teachers College Record vol. 112, no. 3, 579–620.
Carnoy, Martin, and Emma García. 2017. Five Key Trends in U.S. Student Performance. Progress by Blacks and Hispanics, the Takeoff of Asians, the Stall of Non-English Speakers, the Persistence of Socioeconomic Gaps, and the Damaging Effect of Highly Segregated Schools . Economic Policy Institute.
Carter, Prudence L., and Kevin G. Welner, eds. 2013. Closing the Opportunity Gap: What America Must Do to Give Every Child an Even Chance . New York: Oxford Univ. Press.
Caspe, Margaret, and Joy Lorenzo Kennedy. 2014. Sustained Success: The Long-Term Benefits of High Quality Early Childhood Education. New York: Children’s Aid Society.
Chaudry, Ajay, Taryn Morrissey, Christina Weiland, and Hirokazu Yoshikawa. 2017. Cradle to Kindergarten: A New Plan to Combat Inequality . New York: Russell Sage Foundation.
Chetty, Raj, David Grusky, Maximilian Hell, Nathaniel Hendren, Robert Manduca, and Jimmy Narang. 2016. “ The Fading American Dream: Trends in Absolute Income Mobility since 1940 .” NBER Working Paper no. 22910.
Child Trends. 2014. Making the Grade: Assessing the Evidence for Integrated Student Supports .
Clark, H., et al. 2009. Study Comparing Children’s Aid Society Community Schools to Other New York City Public Schools (All Schools and Peer Schools ). ActKnowledge.
Coleman, J.S., E. Campbell, C. Hobson, J. McPartland, A. Mood, F. Weinfeld, and R. York. 1966. Equality of Educational Opportunity . Washington, D.C.: U.S. Office of Education.
Collaborative for Academic, Social, and Emotional Learning (CASEL). 2017. “ Partner Districts: Austin ” (webpage). Accessed August 31, 2017.
Cook-Harvey, C.M., L. Darling-Hammond, L. Lam, C. Mercer, and M. Roc. 2016. Equity and ESSA: Leveraging Educational Opportunity Through the Every Student Succeeds Act . Palo Alto, Calif.: Learning Policy Institute.
Cunha, Flavio, and James J. Heckman. 2007. “The Technology of Skill Formation.” American Economic Review vol. 97, no. 2, 31–47.
Currie, Janet. 2009. “Healthy, Wealthy, and Wise: Socioeconomic Status, Poor Health in Childhood, and Human Capital Development.” Journal of Economic Literature vol. 47, no. 1, 87–122.
Davis-Kean, Pamela E. 2005. “The Influence of Parent Education and Family Income on Child Achievement: The Indirect Role of Parental Expectations and the Home Environment.” Journal of Family Psychology vol. 19, no. 2 (June 2005), 294–304. doi:10.1037/0893-3200.19.2.294.
Duncan, Greg J., Chantelle J. Dowsett, Amy Claessens, Katherine A. Magnuson, Aletha C. Huston, Pamela Klebanov, Linda S. Pagani, Leon Feinstein, Mimi Engel, and Jeanne Brooks-Gunn. 2007. “School Readiness and Later Achievement.” Developmental Psychology vol. 43, no. 6, 1428–46.
Duncan, Greg J., and Katherine A. Magnuson. 2011. “The Nature and Impact of Early Achievement Skills, Attention Skills, and Behavior Problems.” In Whither Opportunity?: Rising Inequality, Schools, and Children’s Life Chances , Greg J. Duncan and Richard Murnane, eds., 47–69. New York: Russell Sage Foundation.
Duncan, Greg J., and Katherine Magnuson. 2013. “Investing in Preschool Programs.” Journal of Economic Perspectives vol. 27, no. 2, 109–32.
Duncan, Greg J., Pamela A. Morris, and Chris Rodrigues. 2011. “Does Money Really Matter? Estimating Impacts of Family Income on Young Children’s Achievement with Data from Random-Assignment Experiments.” Developmental Psychology vol. 47, no. 5, 1263–79. doi:10.1037/a0023875.
Duncan, Greg J., and Richard Murnane. 2011. “Introduction: The American Dream, Then and Now.” In Whither Opportunity?: Rising Inequality, Schools, and Children’s Life Chances , Greg J. Duncan and Richard Murnane, eds. New York: Russell Sage Foundation.
Economic Policy Institute (EPI). 2012. “ The Great Recession .” State of Working America feature.
Economic Policy Institute (EPI). 2013. “ Inequality.is ” (interactive website).
Elmore, Richard, David Thomas, and Tonika Cheek Clayton. 2006. Differentiated Treatment in Montgomery County Public Schools . Public Education Leadership Project at Harvard University.
Fiester, Leila. 2010. Early Warning! Why Reading by the End of Third Grade Matters. KIDS COUNT Special Report . Annie E. Casey Foundation.
García, Emma. 2015. Inequalities at the Starting Gate: Cognitive and Noncognitive Skills Gaps between 2010–2011 Kindergarten Classmates . Economic Policy Institute.
García, Emma, and Elaine Weiss. 2015. Early Education Gaps by Social Class and Race Start U.S. Children Out on Unequal Footing. A Summary of the Major Findings in Inequalities at the Starting Gate . Economic Policy Institute.
García, Emma, and Elaine Weiss. 2016. Making Whole-Child Education the Norm: How Research and Policy Initiatives Can Make Social and Emotional Skills a Focal Point of Children’s Education . Economic Policy Institute.
García, Emma, and Elaine Weiss. 2017. Key Findings from the Report “Education Inequalities at the School Starting Gate” . Economic Policy Institute.
Hart, Betty, and Todd R. Risley. 1995. Meaningful Differences in the Everyday Experience of Young American Children . Baltimore, Md.: Brookes.
Heckman, James J. 2008. “Schools, Skills, and Synapses.” Economic Inquiry vol. 46, no. 3, 289–324.
Heckman, James J., and Tim Kautz. 2012. “Hard Evidence on Soft Skills.” Labour Economics vol. 19, no. 4, 451–64.
Henderson, Anne T. 2010. Community Organizing to Build Partnerships in Schools: The Alliance Schools Movement in Austin . Annenberg Institute for School Reform.
Hernandez, Donald J. 2011. Double Jeopardy: How Third-Grade Reading Skills and Poverty Influence High School Graduation . Annie E. Casey Foundation.
Gizriel, Sarah. 2016. “ Bright Futures Looking to Expand to Schools across Shenandoah Valley .” localDVM.com , December 9.
Jennings, J.L., and T.A. DiPrete. 2010. “Teacher Effects on Social and Behavioral Skills in Early Elementary School.” Sociology of Education vol. 83, no. 2, 135.
Kalamazoo Public Schools (KPS). 2017. “ PEEP Information and Applications ” (webpage). Accessed August 31, 2017.
Lee, Valerie E., and David T. Burkam. 2002. Inequality at the Starting Gate . Washington, D.C.: Economic Policy Institute.
Levin, Henry M. 2012a. “More Than Just Test Scores.” Prospects vol. 42, no. 3, 269–84.
Levin, Henry M. 2012b. “The Utility and Need for Incorporating Noncognitive Skills into Large-scale Educational Assessments.” In The Role of International Large-Scale Assessments: Perspectives from Technology, Economy, and Educational Research , Matthias von Davier et al., eds. Springer.
Magnuson, Katherine, and Greg J. Duncan. 2016. “Can Early Childhood Interventions Decrease Inequality of Economic Opportunity?” RSF: The Russell Sage Foundation Journal of the Social Sciences vol. 2, no. 2, 123–41.
Magnuson, Katherine A., M.K. Meyers, C.J. Ruhm, and Jane Waldfogel. 2004. “Inequality in Preschool Education and School Readiness.” American Educational Research Journal vol. 41, no. 1, 115–57.
Magnuson, Katherine A., Christopher Ruhm, and Jane Waldfogel. 2007. “Does Prekindergarten Improve School Preparation and Performance?” Economics of Education Review vol. 26, no. 1, 33–51.
Marietta, Geoff. 2010. Lessons for PreK-3rd from Montgomery County Public Schools: An FCD Case Study . Foundation for Child Development.
Maryland State Department of Education. 2017. “ Judy Centers ” (webpage). Accessed August 31, 2017.
Miller-Adams, Michelle. 2015. Promise Nation: Transforming Communities through Place-Based Scholarships . Kalamazoo, Mich.: W.E. Upjohn Institute for Employment Research.
Mishel, Lawrence. 2015. “ The Opportunity Dodge .” American Prospect , April 9.
Mishel, Lawrence, Josh Bivens, Elise Gould, and Heidi Shierholz. 2012. The State of Working America, 12th Edition , An Economic Policy Institute Book. Ithaca, N.Y.: Cornell Univ. Press.
Mishel, Lawrence, and Jessica Schieder. 2016. Stock Market Headwinds Meant Less Generous Year for Some CEOs . Economic Policy Institute.
Montgomery County Public Schools (MCPS). 2015. Graduation Rate Rises, Gap Narrows for MCPS Class of 2014 (public announcement). January 27.
Montgomery County Public Schools (MCPS). 2016. Linkages to Learning (brochure).
Montgomery County Public Schools (MCPS). 2017. “ Maryland Meals for Achievement ” (webpage). Accessed August 31, 2017.
Morsy, Leila, and Richard Rothstein. 2015. Five Social Disadvantages That Depress Student Performance: Why Schools Alone Can’t Close Achievement Gaps . Economic Policy Institute.
Murnane, Richard J., and Frank Levy. 1996. Teaching the New Basic Skills: Principles for Educating Children to Thrive in a Changing Economy . New York: The Free Press.
Murnane, Richard J., John B. Willett, Kristen L. Bub, and Kathleen McCartney. 2006. “Understanding Trends in the Black-White Achievement Gaps during the First Years of School.” Brookings-Wharton Papers on Urban Affairs.
Najarian, M., K. Tourangeau, C. Nord, K. Wallner-Allen, and J. Leggitt. Forthcoming. Early Childhood Longitudinal Study, Kindergarten Class of 2010–11 (ECLS-K:2011), First-Grade and Second-Grade Psychometric Report . Washington, D.C.: National Center for Education Statistics, Institute of Education Sciences, U.S. Department of Education.
National Center for Education Statistics (NCES) (U.S. Department of Education). Early Childhood Longitudinal Study, Kindergarten Class of 1998–99 (ECLS-K 1998–1999) .
National Center for Education Statistics (NCES) (U.S. Department of Education). Early Childhood Longitudinal Study, Kindergarten Class of 2010–11 (ECLS-K 2010–2011) .
National Institute for Early Education Research (NIEER). 2016. The State of Preschool 2015: State Preschool Yearbook .
New York Times /CBS News. 2015. “ Americans’ Views on Income Inequality and Workers’ Rights ” (poll results). June 3.
Nores, Milagros, and W. Steven Barnett. 2010. “Benefits of Early Childhood Interventions across the World: (Under) Investing in the Very Young.” Economics of Education Review vol. 29, no. 2, 271–82.
Nores, Milagros, and W. Steven Barnett. 2014. Access to High Quality Early Care and Education: Readiness and Opportunity Gaps in America . New Brunswick, N.J.: Center on Enhancing Early Learning Outcomes.
Nores, Milagros, and Emma García. 2014. “Language, Immigration and Hispanics. Understanding Achievement Gaps in the Early Years.” Paper presented at the Association for Public Policy Analysis and Management Fall Research Conference, November 6–8, Albuquerque, N.M.
Oakes, Jeannie, Anna Maier, and Julia Daniel. 2017. Community Schools: An Evidence-Based Strategy for Equitable School Improvement , Learning Policy Institute, June 5.
PBS NewsHour . 2015. “ In Reforming New Orleans, Have Charter Schools Left Some Students Out? ” (news segment).
Peterson, T.K., ed. 2013. Expanding Minds and Opportunities: Leveraging the Power of Afterschool and Summer Learning for Student Success . Washington, D.C.: Collaborative Communications Group.
Phillips, Meredith. 2011. “Parenting, Time Use, and Disparities in Academic Outcomes.” In Whither Opportunity?: Rising Inequality, Schools, and Children’s Life Chances , Greg J. Duncan and Richard Murnane, eds. New York: Russell Sage Foundation.
Proctor, Bernadette D., Jessica L. Semega, and Melissa A. Kollar. 2016. Income and Poverty in the United States: 2015 . U.S. Census Bureau, Current Population Reports, P60-256(RV).
Putnam, Robert. 2015. Our Kids: The American Dream in Crisis . New York: Simon and Schuster.
Ready, Douglas D. 2010. “Socioeconomic Disadvantage, School Attendance, and Early Cognitive Development.” Sociology of Education vol. 83, no. 4, 271–86.
Reardon, Sean F. 2007. “ Thirteen Ways of Looking at the Black-White Test Score Gap .” Working paper.
Reardon, Sean F. 2011. “The Widening Academic Achievement Gap between the Rich and the Poor: New Evidence and Possible Explanations.” In Whither Opportunity?: Rising Inequality, Schools, and Children’s Life Chances , Greg J. Duncan and Richard Murnane, eds. New York: Russell Sage Foundation.
Reardon, Sean F., and Ximena A. Portilla. 2016. “Recent Trends in Income, Racial, and Ethnic School Readiness Gaps at Kindergarten Entry.” AERA Open vol. 2, no. 3, 1–18. doi: 10.1177/2332858416657343.
Redd, Z., L. Guzman, L. Lippman, L. Scott, and G. Matthews. 2004. Parental Expectations for Children’s Educational Attainment: A Review of the Literature . Prepared by Child Trends for the National Center for Education Statistics.
Rolnick, Art, and Rob Grunewald. 2003. “Early Childhood Development: Economic Development with a High Public Return.” The Region vol. 17, no. 4, 6–12.
Rothstein, Richard. 2004. Class and Schools: Using Social, Economic, and Educational Reform to Close the Achievement Gap . Washington, D.C.: Economic Policy Institute; New York: Columbia University Teachers College.
Rothstein, Richard. 2010. “Family Environment in the Production of Schooling.” In International Encyclopedia of Education , Dominic J. Brewer, Patrick J. McEwan, eds. Oxford: Elsevier. doi: 10.1016/B978-0-08-044894-7.01233-1.
Saez, Emmanuel. 2016. Striking It Richer: The Evolution of Top Incomes in the United States (Updated with 2015 Preliminary Estimates) .
Schanzenbach, Diane, Megan Mumford, Ryan Nunn, and Lauren Bauer. 2016. Money Lightens the Load . The Hamilton Project, Brookings Institute.
Selzer, Michael H., Ken A. Frank, and Anthony S. Bryk. 1994. “The Metric Matters: The Sensitivity of Conclusions about Growth in Student Achievement to Choice of Metric.” Educational Evaluation and Policy Analysis vol. 16, 41–49.
Sharkey, Patrick. 2013. Stuck in Place: Urban Neighborhoods and the End of Progress toward Racial Equality . Chicago, Ill.: Univ. of Chicago Press.
Simon, Stephanie. 2013. “ Class Struggle: How Charter Schools Get Students They Want .” Reuters , February 15.
Simpkins, Sandra D., Pamela E. Davis-Kean, and Jacquelynne S. Eccles. 2005. “Parents’ Socializing Behavior and Children’s Participation in Math, Science, and Computer Out-of-School Activities.” Applied Developmental Science vol. 9, no. 1, 14–30. doi:10.1207/s1532480xads0901_3.
Southern Education Foundation. 2015. A New Majority: Low-Income Students Now a Majority in the Nation’s Public Schools . January.
Sparks, Sarah D. 2017. “Billions in School Improvement Spending but Not Much Student Improvement.” EdWeek , January 19.
StataCorp. 2015. Stata: Release 14 [statistical software]. College Station, Texas: StataCorp LP.
Stringhini, Silvia, et al. 2017. “Socioeconomic Status and the 25×25 Risk Factors as Determinants of Premature Mortality: A Multicohort Study and Meta-Analysis of 1.7 Million Men and Women.” The Lancet . Published online January 31, 2017. doi:10.1016/S0140-6736(16)32380-7.
Tourangeau, K., C. Nord, T. Lê, A.G. Sorongon, and M. Najarian. 2009. Early Childhood Longitudinal Study, Kindergarten Class of 1998–99 (ECLS-K): Combined User’s Manual for the ECLS-K Eighth-Grade and K–8 Full Sample Data Files and Electronic Codebooks (NCES 2009-004) . U.S. Department of Education. Washington, D.C.: National Center for Education Statistics.
Tourangeau, K., C. Nord, T. Lê, A.G. Sorongon, M.C. Hagedorn, P. Daly, and M. Najarian. 2013. Childhood Longitudinal Study, Kindergarten Class of 2010–11 (ECLS-K:2011), User’s Manual for the ECLS-K:2011 Kindergarten Data File and Electronic Codebook (NCES 2013-061). U.S. Department of Education. Washington, D.C.: National Center for Education Statistics.
Tourangeau, K., C. Nord, T. Lê, K. Wallner-Allen, M.C. Hagedorn, J. Leggitt, and M. Najarian. 2015. Early Childhood Longitudinal Study, Kindergarten Class of 2010–11 (ECLS-K:2011), User’s Manual for the ECLS-K:2011 Kindergarten–First Grade Data File and Electronic Codebook, Public Version (NCES 2015-078) . U.S. Department of Education. Washington, D.C.: National Center for Education Statistics.
Tourangeau, K., C. Nord, T. Lê, K. Wallner-Allen, N. Vaden-Kiernan, L. Blaker, and M. Najarian. 2017. Early Childhood Longitudinal Study, Kindergarten Class of 2010–11 (ECLS-K:2011) User’s Manual for the ECLS-K:2011 Kindergarten–Second Grade Data File and Electronic Codebook, Public Version (NCES 2017-285) . U.S. Department of Education. Washington, D.C.: National Center for Education Statistics.
U.S. Department of Education (U.S. ED). 2015. A Matter of Equity: Preschool in America .
U.S. Department of Health and Human Services (U.S. HHS) and U.S. Department of Education (U.S. ED). 2016. Policy Statement to Support the Alignment of Health and Early Learning Systems .
Van Voorhis, F.L., M.F. Maier, J.L. Epstein, C.M. Lloyd, and T. Leung. 2013. The Impact of Family Involvement on the Education of Children Ages 3 to 8: A Focus on Literacy and Math Achievement Outcomes and Social-Emotional Skills . MDRC.
Waldfogel, Jane. 2006. “What Do Children Need?” Public Policy Research vol. 13, no. 1, 26–34.
Weiss, Elaine. 2016a. Bright Futures in Joplin, Missouri . A Broader, Bolder Approach to Education.
Weiss, Elaine. 2016b. Vancouver Public Schools (Vancouver, WA) . A Broader, Bolder Approach to Education.
Weiss, Elaine. 2016c. Partners for Education at Berea College, Berea, Kentucky . A Broader, Bolder Approach to Education.
Weiss, Elaine. 2016d. Northside Achievement Zone (North Minneapolis, MN) . A Broader, Bolder Approach to Education.
Weiss, Elaine. 2016e. East Durham Children’s Initiative (East Durham, NC). A Broader, Bolder Approach to Education.
Weiss, Elaine. 2016f. Bright Futures (Pea Ridge, AR) . A Broader, Bolder Approach to Education.
Weiss, Elaine. 2016g. City Connects (Boston, MA) . A Broader, Bolder Approach to Education.
Weiss, Elaine. 2016h. The Children’s Aid Society Community Schools (New York, NY) . A Broader, Bolder Approach to Education.
Weiss, Elaine. 2016i. A Broader, Bolder Education Policy Framework . A Broader, Bolder Approach to Education.
Wentzel, Kathryn R., Shannon Russell, and Sandra Baker. 2016. “Emotional Support and Expectations from Parents, Teachers, and Peers Predict Adolescent Competence at School.” Journal of Educational Psychology vol. 108, no. 2, 242–255.
Yamamoto, Yoko, and Susan D. Holloway. 2010. “Parental Expectations and Children’s Academic Performance in Sociocultural Context.” Educational Psychology Review vol. 22, no. 3, 189–214. doi:10.1007/s10648-010-9121-z.
Appendix A. Data
Introduction.
Our research benefits from the existence of two companion studies conducted by the National Center for Education Statistics (NCES), the Early Childhood Longitudinal Study of the Kindergarten Class of 1998–1999 and the Early Childhood Longitudinal Study of the Kindergarten Class of 2010–2011 (hereafter, ECLS-K 1998–1999 and ECLS-K 2010–2011). The data from these studies come with multiple advantages and a few disadvantages.
The studies follow two nationally representative samples of children starting in their kindergarten year and continuing through their elementary school years (eighth grade for 1998–1999 cohort and fifth grade for the 2010–2011 cohort). The tracking of students over time is one of the most valuable features of the data. The studies include assessments of the children’s cognitive performance and knowledge as well as skills that belong in the category of noncognitive, or social and emotional, skills. The studies also include information on teachers and schools (provided by teachers and administrators) and interviews with parents.
Another valuable feature of the data is the availability of two ECLS-K studies (ECLS-K 1998–1999 and ECLS-K 2010–2011), which allows for cross-comparisons “of two nationally representative kindergarten classes experiencing different policy, educational, and demographic environments” (Tourangeau et al. 2013). The two studies are 12 years apart, or a full school cycle apart: when the 2010–2011 kindergarten class was starting school, the 1998–1999 class was starting the grade leading to their graduation. A comparison of the studies thus offers insightful information about the consequences of changes in the system that may have occurred during an entire cohort’s school life. For the 2010 study, the sample included 18,174 children in 968 schools. i The 1998 study sample included 21,409 children in 903 schools. ii
This existence of data from two cohorts is also a limitation to the current study, as explained by Tourangeau et al. (2013), who note that the assessment scores for the 2010–2011 class are not directly comparable with those developed for the class of 1998–1999. Although the IRT (Item Response Theory) procedures used in the analysis of data were similar across the two studies, each study incorporated different items, which means that the resulting scales are different. Tourangeau et al. (2013) state that “a subsequent release of the ECLS-K: 2010–2011 data will include IRT scores that are comparable with the ECLS-K 1998 cohort.” Up to the point of publication of the current study, this information had not yet been released, and we use standardized scores, instead of raw scores, for the outcomes examined. We can assess changes in the relative position in a distribution (i.e., how far apart high- and low-SES children are in 1998 and how far apart high- and low-SES children are in 2010), but not overall changes in their performance (i.e., it is not possible to ascertain whether performance has improved overall, or if gaps are smaller or larger due to an improvement in performance of children at the low end (specifically the lowest fifth) of the distribution or due to a decrease in the performance of children at the high end (highest fifth) of the distribution, etc.). A full comparison remains to be produced, upon data availability.
We use data for the first wave of each study, corresponding with fall kindergarten (or school entry).
For the analyses, we use the by-year standardized scores corresponding to the fall semester. (The 1998 IRT scale scores for reading and mathematics achievement and assessments of noncognitive skills are standardized using the 1998 distribution and its mean and sd; for 2010, we use the mean and sd of the 2010 distribution.)
Cognitive skills
Cognitive skills are assessed with instruments that measure each child’s:
- Reading skills: print familiarity, letter recognition, beginning and ending sounds, rhyming words, word recognition, vocabulary knowledge, and reading comprehension
- Math skills: conceptual knowledge, procedural knowledge, and problem-solving; number sense, properties, and operations; measurement; geometry and spatial sense; data analysis, statistics, and probability; and patterns, algebra, and functions
Principal noncognitive skills
We use the term “principal” to identify a set of noncognitive skills that are measured by both the ECLS-K 1998–1999 and 2010–2011 surveys, and that have been relatively extensively used in research.
Teachers are asked to assess each child’s:
- Self-control: ability to control behavior by respecting the property rights of others, controlling temper, accepting peer ideas for group activities, and responding appropriately to pressure from peers
- Approaches to learning: organizational skills (keeps belongings organized); curiosity (is eager to learn new things); independence (works independently); adaptability (easily adapts to changes in routine); persistence in completing tasks; focus (ability to pay attention); and ability to follow classroom rules
Parents are asked to assess their child’s:
- Self-control: ability to control behavior by refraining from fighting, arguing, throwing tantrums, and getting angry
- Approaches to learning: persistence (keeps working at something until finished); curiosity (shows interest in a variety of things); focus (concentrates on a task and ignores distractions); helpfulness (helps with chores); intellectual curiosity (is eager to learn new things); and creativity (in work and play)
For the analyses, we use the following set of covariates. The definitions, and the coding used for the covariates, by year, are shown in Appendix Table A1 .
Appendix B. Methodology
Gaps by socioeconomic status.
The expressions below show the specifications used to estimate the socioeconomic status–based (SES-based) performance gaps. For any achievement outcome A , we estimate four models:
- Model 1 shows the unadjusted (descriptive) differences for children belonging to different racial/ethnic groups or SES quintiles (the reference group is children in the lowest SES quintile, “low SES”).
- Model 2 adjusts for school clustering of students in different schools (i.e., gaps of students in the same schools). The purpose of this clustering is to account for school segregation (i.e., concentration of children of the same race, socioeconomic status, etc., in schools, which causes the raw average performance of students to differ from the adjusted-by-clustering average). It offers a comparison of the gaps shown by peer students in the same schools and classrooms (García 2015; Magnuson and Duncan 2016 offer these estimates as well).
These estimates build on all the available observations (i.e., only those children who have missing values in the outcome variables are eliminated from the analysis).
Because of lack of response in some of the covariates used as predictors of performance, we construct a common sample with observations with no missing information in any of the variables of interest (see information about missing data for each variable in Appendix Table C1 ). We estimate two more models: iii
- Model 3 shows gaps adjusted for child and family characteristics, prekindergarten care arrangements, number of books the child has, and early literacy practices at home iv
- Finally, Model 4 shows the fully adjusted differences (adjusted for child and family characteristics, prekindergarten care arrangements, early literacy practices at home, number of books the child has, and parental expectations)
The equation below shows the equation we estimate for Models 1 through 4.
Appendix C. Sensitivity analysis (I): Multiple imputation
Following standard approaches in this field, we use multiple imputation to impute missing values in both the independent and dependent variables, for the analysis of skills gaps and changes in them from 1998 to 2010 by socioeconomic status (main analysis). See share of missing data by variable in Appendix Table C1 . We use the mi commands in Stata 14, using chained equations, which jointly model all functional terms. The number of iterations was set up equal to 20. Imputation is performed by year.
Our functional form of the imputation model is specified using SES, gender, race, disability, age, type of family, number of books, educational activities, and parental expectations, as well as the original cognitive and noncognitive variables, as variables to be imputed. We use various specifications, combining different sets of auxiliary variables, mi impute methods, and other parameters, to capture any sensitivity of the results to the characteristics of the model. For example, income, family size, and ELL status are set as auxiliary variables and used in several of the imputation models. Another imputation option that was altered across models is the use of weights, as we ran out of imputation models using weights and not using them.
In the imputation model, in order to impute categorical variables’ missingness, we use the option augment, to prevent the large number of categorical variables to be imputed from causing problems of perfect prediction (StataCorp. 2015). The rest of the variables are first imputed as continuous variables. In a second exercise, we also impute SES and educational expectations as ordinal variables (also using the option augment).
In order to calculate the standardized dependent variables, we use the variables derived from the imputation variables (also known as passive imputation). This “fills in only the underlying imputation variables and computes the respective functional terms from the imputed variables” (StataCorp. 2015). In one case, we imputed the dependent variables directly as continuous variables (though we anticipated that the distribution of the scores imputed this way would not necessarily have a mean of 0 and a standard deviation of 1).
Using the imputed data, we estimate Models 1 through 4 following the specifications explained above (from no regressors to fully specified models).
The main findings of our analysis are not sensitive to missing data imputation. The estimates of the gaps in 1998 and the changes in the gaps from 1998 to 2010 are consistent across models in terms of statistical significance. There are some minor changes in the sizes of the estimated coefficients, especially those associated with the changes in the gaps (though all are statistically not different from 0, as discussed in the report using the results from the analysis with the complete cases). There are also some minor changes in the standard errors, though they are small enough to widen the coefficients’ statistical bandwidth to not include the 0.
Appendix D. Sensitivity analysis (II): The different scores available in ECLS-K and the sensitivity of the results to changing them
Children’s reading and mathematics skills are measured using several different metrics in ECLS-K. Among these, the best-known or more commonly used metrics in research are the IRT-based theta scores and the IRT-based scale scores (IRT stands for Item Response Theory). NCES provides data users with definitions of these metrics and recommendations on how to appropriately choose among the different metrics. NCES explains that both theta and IRT-based scale scores are valid indicators of ability. This makes them suitable for research purposes, even though each is expressed in its own unit of measurement. NCES recommends that analysts “consider the nature of their research questions, the type of statistical analysis to be conducted, the population of interest, and the audience” when choosing the appropriate score for analysis (see Tourangeau et al. 2013).
Although nothing would indicate that this could be the case, our work noted that results of analyses such as the one developed in this study are in some ways sensitive to the metrics used as dependent variables. v Thus, the purpose of this appendix is to illustrate the differences in the results associated with different analytic decisions in terms of the metrics used. As we will see, in essence, point estimates depend on the metric used, but the results do not change in a meaningful way and conclusions and implications remain unchanged. That is, although caution is required when interpreting the results obtained using different combinations of metrics, procedures (including standardization), and data waves, it is important to state that the main conclusions of this study— that social-class gaps in cognitive and noncognitive skills are large and have persisted over time — hold . So do the policy recommendations derived from those findings: sufficient, integrated, and sustained over-time efforts to tackle early gaps in a more effective manner.
The scores: Which one to use and definitions
NCES makes the following recommendations for researchers who are choosing among scales (see Tourangeau et al. 2013): vi
When choosing scores to use in analysis, researchers should consider the nature of their research questions, the type of statistical analysis to be conducted, the population of interest, and the audience. […] The IRT-based scale scores […] are overall measures of achievement. They are appropriate for both cross-sectional and longitudinal analyses. They are useful in examining differences in overall achievement among subgroups of children in a given data collection round or in different rounds, as well as in analysis looking at correlations between achievement and child, family, and school characteristics. […] Results expressed in terms of scale score points, scale score gains, or an average scale score may be more easily interpretable by a wider audience than results based on the theta scores. The IRT-based theta scores are overall measures of ability. They are appropriate for both cross-sectional and longitudinal analyses. They are useful in examining differences in overall achievement among subgroups of children in a given data collection round or across rounds, as well as in analysis looking at correlations between achievement and child, family, and school characteristics. […] The theta scores may be more desirable than the scale scores for use in a multivariate analysis because generally their distribution tends to be more normal than the distribution of the scale scores. However, for a broader audience of readers unfamiliar with IRT modeling techniques, the metric of the theta scores (from -6 to 6) may be less readily interpretable. […]
The two scores are defined as follows (see Tourangeau et al. 2013, section “3.1 Direct Cognitive Assessment: Reading, Mathematics, Science”):
The IRT-based scale score is an estimate of the number of items a child would have answered correctly in each data collection round if he or she had been administered all of the questions for that domain that were included in the kindergarten and first-grade assessments. To calculate the IRT-based overall scale score for each domain, a child’s theta is used to predict a probability for each assessment item that the child would have gotten that item correct. Then, the probabilities for all the items fielded as part of the domain in every round are summed to create the overall scale score. Because the computed scale scores are sums of probabilities, the scores are not integers. The IRT-based theta score is an estimate of a child’s ability in a particular domain (e.g., reading, mathematics, science, or SERS) based on his or her performance on the items he or she was actually administered. […] The theta scores are reported on a metric ranging from -6 to 6, with lower scores indicating lower ability and higher scores indicating higher ability. Theta scores tend to be normally distributed because they represent a child’s latent ability and are not dependent on the difficulty of the items included within a specific test.
Reardon (2007) describes the calculation of the theta scores in the following manner: vii
For each test [math and reading], a three-parameter IRT model was used to estimate each student’s latent ability…at each wave…. The IRT model assumes that each student’s probability of answering a given test item correctly is a function of the student’s ability and the characteristics [discrimination, difficulty, and guessability] of the item…. Given the pattern of students’ responses to the items on the test that they are given, the IRT model provides estimates of both the person-specific latent abilities at each wave… and the item parameters. (Reardon 2007, 10) viii
He also notes that “[b]ecause the ECLS-K tests contain many more ‘difficult’ items than ‘easy’ items, the relationship between theta and scale scores is not linear (a unit difference in theta corresponds to a larger difference in scale scores at theta=1 than at theta=-1, for example). The scale scores are difficult to interpret as an interval-scale metric (or are an interval-scaled metric only with respect to the specific set of items on the ECLS-K tests),” while he shows that the “theta scores are interval-scale metrics, in a behaviorally-meaningful sense” (Reardon 2007, 11, 13). ix
The analyses
For the analyses, both the scale and the theta scores need to be standardized by year (the original variables are not directly comparable because they rely on different instruments, as explained by NCES, and the resulting standardized variables have mean 0 and standard deviation 1). This is a common practice in the education field, as it allows researchers to use data that come from different studies and would not have a common scale otherwise. We need to take into consideration that the underlying units of measurement for each variable are different, but after standardization, the metrics are common, expressed in standard deviations and represent the population’s distribution of abilities.
The distributions of the scale and theta scores are shown in Appendix Figures D1 and D2 . In each figure, the plots reflect a more normally distributed pattern for the theta scores (right panel) than for the scale scores (left panel). The companion table, Appendix Table D1 , shows the range of variation for the four outcomes (mean and standard deviations are 0 and 1 as per construction).
We next offer a comparison of the results obtained when using the scale scores versus using the theta scores ( Appendix Table D2 ). We highlight the following main similarities and differences between the results obtained using the scale scores and the results using the theta scores.
- Gaps are all equally statistically significant and persistent.
- For example, looking at the unadjusted estimates in reading, the gap in 1998 between high- and low-SES children is 1.071 sd if using the scale scores and 1.233 sd if using the theta scores. In math, the gap between high- and low-SES children in 1998 is 1.258 sd if using the scale scores and 1.330 sd if using the theta scores.
- Looking at the adjusted estimates in reading, the 1998 gap between high- and low-SES children is 0.596 sd if using the scale scores and 0.684 sd if using the theta scores. In math, the gap between high- and low-SES children is 0.610 sd if using the scale scores and 0.632 sd if using the theta scores.
- For example, looking at the unadjusted estimates in reading, the change in the gap between 1998 and 2010 for high- and low-SES children is 0.098 sd if using the scale scores and -0.052 sd (not statistically significant) if using the theta scores. In math, the change in the gap between high- and low-SES children is -0.008 sd (not statistically significant) if using the scale scores and -0.078 sd if using the theta scores.
In Appendix Table D3 , we compare the results obtained using the different scales and the different proxies of socioeconomic status (our composite SES index, mother’s education, number of books, and household income).
- Gaps are larger, as mentioned above, when we use the theta scores than when we use the scale scores.
- Among the four social-class proxies, the largest gaps are associated with mother’s education, and the smallest gaps are associated with number of books. All are statistically significant.
- Looking at the unadjusted gaps, we note that trends are the same (and similar in size) if income is used as the proxy. For mother’s education, the change in the gap between 1998 and 2010 is -0.020 sd in reading (not statistically significant) and -0.154 sd in math if using the scale scores and -0.135 sd in reading and -0.218 sd in math if using the theta scores.
- With respect to the adjusted gaps, changes in the gaps are larger when using the theta scores both for household income and mother’s education as indicators of social class. Using the theta scores, the gaps in reading and math shrank over time, while using the scale scores, the only significant reduction was in math when mother’s education was the social class proxy.
Other considerations
There are two other significant pieces of information affecting the cognitive scores in more recent documentation released by NCES. In 2015, NCES announced in its ECLS-K User’s Manual that a
change in methodology required a re-calibration and re-reporting of the kindergarten reading scores since the release of the base-year file. Therefore, the kindergarten reading theta scores included in the K-1 data file are calculated differently than the previously released kindergarten theta scores and replace the kindergarten reading theta scores included in the base-year data file. The modeling approach stayed the same for mathematics and science, so the recalculation of kindergarten mathematics and science theta scores was not needed. (Tourangeau et al. 2015)
Following up on this, the most recent (2017) data user’s manual explains that
The method used to compute the theta scores allows for the calculation of theta for a given round that will not change based on later administrations of the assessments (which is not true for the scale scores, as described in the next section). Therefore, for any given child, the kindergarten, first-grade, and second-grade theta scores provided in subsequent data files will be the same as theta scores released in earlier data files , with one exception: the reading thetas provided in the base-year data file . After the kindergarten-year data collection, the methodology used to calibrate and compute reading scores changed; therefore, the reading thetas reported in the base-year file are not the same as the kindergarten reading thetas provided in the files with later-round data [emphasis added]. Any analysis involving kindergarten reading theta scores and reading theta scores from later rounds, for example an analysis looking at growth in reading knowledge and skills between the spring of kindergarten and the spring of first grade, should use the kindergarten reading theta scores from a data file released after the base year. The reading theta scores released in the kindergarten-year data file are appropriate for analyses involving only the kindergarten round data; analyses conducted with only data released in the base-year file are not incorrect, since those analyses do not compare kindergarten scores to scores in later rounds that were computed differently. However, now that the recomputed kindergarten theta scores are available in the kindergarten through first-grade and kindergarten through second-grade data files, it is recommended that researchers conduct any new analyses with the recomputed kindergarten reading theta scores. For more information on the methods used to calculate theta scores, see the ECLS-K: 2011 First-Grade and Second-Grade Psychometric Report (Najarian et al. forthcoming). (Tourangeau et al. 2017)
Therefore, because of these changes in NCES methodology and reporting, and in light of the comparisons in this appendix, one could expect additional slight changes in the estimates using the IRT-theta scores for reading for kindergarten if using rounds of data posterior to the first round (and probably if using the IRT-scale scores as well, as these values are derived from the theta scores), relative to the first data file of ECLS-K: 2010-2011 released by NCES in 2013. We would not necessarily expect, though, any changes when using the standardized transformation of those scores, because NCES’s documentation does not mention changes to the distribution of the scores, only to their values. We will explore these issues further upon the release of the scores that are comparable across the two ECLS-K studies without any transformation.
Appendix E. Descriptions of 12 community-level whole-child education initiatives
Initiatives that serve part of a school district, austin, texas.
The needs of children in Austin Independent School District (AISD) schools with the highest concentrations of poor, immigrant, and non-English-speaking families are supported through a combination of parent-organizing (schools with parent-organizing programs, led by the nonprofit Austin Interfaith, form a network of “Alliance Schools”), intensive embedding of social and emotional learning (SEL) in all aspects of school policy and practice, and the transformation of schools into “community schools” (i.e., schools that are hubs for the provision of academic, health, and social services).
- Organizing partners: Austin Interfaith (a nonprofit of congregations, public schools, and unions that is part of the national Industrial Areas Foundation [IAF]); the Collaborative for Academic, Social and Emotional Learning (CASEL); the American Federation of Teachers (AFT); and the National Education Association (NEA).
- Schools and students reached: The IAF/Alliance Schools network extended at its zenith into one-fourth of AISD elementary schools and one-half of AISD high-poverty elementary schools. CASEL worked in five high schools, and in the seven middle schools and 43 elementary schools that feed into these high schools, to embed social and emotional learning in school policies and practices. A middle school and a high school have been transformed into community schools and serve as the models for planned districtwide expansion of the “community schools” strategy into all AISD schools.
- General makeup of the student body: In the district overall, 60 percent of students qualify for subsidized meals, i.e., are eligible for free or reduced-price lunch (FRPL); 28 percent are English language learners (ELL); and 10 percent are special education students. In schools targeted for whole-child supports, relative to the general student body, students are poorer, more heavily minority and immigrant, and more likely to be living in single-parent households.
- Key features: Parent-organizing with teachers in Alliance Schools enables parents to partner with teachers to advocate for comprehensive supports for their children. Also, social and emotional learning (SEL) is embedded in all aspects of school efforts in the high schools and the feeder elementary and middle schools that worked with CASEL. Finally, health and other wraparound supports in high-needs middle and high schools, along with other community schools features, are expanding to additional district schools.
- Core funding: The district received a CASEL grant to embed social and emotional learning in school policies and practices, and also received in-kind support from the NoVo Foundation in the form of technical assistance. The United Way of Greater Austin provides funds for wraparound support, and AFT and NEA fund community schools work and expansion.
Boston, Massachusetts
The City Connects program provides targeted academic, social, emotional, and health supports to every child in 20 of the city’s schools with the highest shares of low-income, black, Hispanic, and immigrant students.
- Organizing partners: Boston College Center for Optimized Student Support, Boston Public Schools (BPS), and community agencies.
- Schools and students reached: The 20 BPS schools in the program serve more than 8,000 of the city’s most disadvantaged students (out of 125 BPS schools and 56,000 students).
- General makeup of the student body: The 20 urban schools serve neighborhoods that are poor and racially and ethnically diverse, with a heavy concentration of Hispanic English-language learners. Over 80 percent of the students in these schools are FRPL-eligible and roughly half do not speak English at home.
- Key features: School site coordinators in each school connect students with a tailored set of services and enrichment opportunities provided by a variety of public and private agencies. Universal state health care supports all students’ physical and mental health needs, and the city’s Universal Pre-Kindergarten (UPK) program now offers quality pre-K for all four-year-olds in Boston.
- Core funding: In addition to school district budget revenue, federal Race to the Top funds allocated to City Connects help defray costs. Several private foundations support various aspects of City Connects’ work.
Durham, North Carolina
The East Durham Children’s Initiative (EDCI) concentrates services and supports for the children and their families living in a 120-block, heavily distressed area of concentrated poverty and high crime within the city.
- Organizing partners: Community leaders launched EDCI and engaged the Duke University Center for Child and Family Health to grow capacity. EDCI is now a fully staffed nonprofit that runs the initiative.
- Schools and students reached: The 120-block area targeted by EDCI serves students in two neighborhood elementary schools, one middle school, one high school, and two charter schools.
- General makeup of the student body: The 120-block area is urban and poor with a predominantly black but very diverse student body. In Durham schools overall, 66 percent of students are FRPL-eligible, nearly half are black, almost one-third are Hispanic, and 18 percent are white.
- Key features: EDCI is a place-based initiative modeled on the Harlem Children’s Zone, providing a pipeline of high-quality cradle-to-college-or-career services. These include early childhood supports (that complement state pre-K programs), health and mental health services, and after-school and summer enrichment activities.
- Core funding: EDCI has an annual fund receiving contributions from individuals, corporations, fundraising events, and private foundations; it neither seeks nor receives public funding.
Minneapolis, Minnesota
The Northside Achievement Zone (NAZ) is a Promise Neighborhood, a designation awarded by the U.S. Department of Education Promise Neighborhoods program to some of the most distressed neighborhoods in the nation. Through the program, children and families who live in the 13-by-18 block NAZ receive individualized supports.
- Organizing partners: NAZ, the Promise Neighborhood grantee organization, is guided by a 20-member board of directors consisting of local leaders.
- Schools and students reached: The 13-by-18 block zone in North Minneapolis serves 5,500 students in 10 public, charter, and parochial K–12 schools, including one high school.
- General makeup of the student body: In this racially concentrated area of poverty, almost all residents are African American, and median family income is $18,000. One-third of children are homeless or “highly mobile” (not technically homeless but without stable housing).
- Key features: “Connectors” are in essence case managers who help families develop achievement plans, and “Navigators” connect families with community resources to move toward goals. The zone offers access to high-quality pre-K and parenting supports, as well as mentoring, enrichment, college preparatory support, and after-school and summer programs.
- Core funding: NAZ is anchored by a federal Promise Neighborhood grant. NAZ also receives private grants and is able to leverage federal Race to the Top Early Learning Challenge funds to support pre-K scholarship slots.
New York, New York
Through a collaboration between The Children’s Aid Society and the New York City Department of Education, 16 community schools in some of the most disadvantaged neighborhoods in three of the city’s five boroughs provide wraparound health, nutrition, mental health, and other services to students along with enriching in-and-out-of-school experiences, amplified by extensive parental and community engagement.
- Organizing partners: The Children’s Aid Society, the New York City Department of Education, the New York State Education Department, and other local and state agencies.
- Schools and students reached: Sixteen community schools in three boroughs serve some of the poorest immigrant and minority students in a school system of roughly one million students.
- General makeup of the student body: Students in Children’s Aid Society community schools are disadvantaged relative to the system overall, which serves a heavily low-income and minority student body: more than three quarters of New York City public school students are FRPL-eligible, 13 percent are English language learners, and nearly one in five receive special education services. These schools also have high concentrations of students of color: 27 percent are African American and 41 percent are Hispanic.
- Key features: Close coordination with local and state education, health, and other agencies along with community partnerships at each school enables wraparound health, mental health, and after-school and summer enrichment, as well as deep parental and community engagement.
- Core funding: A range of public dollars, including federal Elementary and Secondary Education Act (ESEA) Title I funds and funds from the federal 21st Century Community Learning Centers program, together with state and local funding for after-school and other programs, is supplemented by funds from individuals and foundations.
Orange County, Florida
The Tangelo Park Project (TPP) provides cradle-to-college support for all children residing in Orlando’s high-poverty, heavily African American Tangelo Park neighborhood.
- Organizing partners: The Tangelo Park Program board, along with Harris Rosen (the hotelier who envisioned and funds the program), work in close collaboration with the Tangelo Park Civic Association and the University of Central Florida.
- Schools and students reached: The program serves all children in the Tangelo Park neighborhood.
- General makeup of the student body: Virtually all residents in the low-income neighborhood are African American or Afro-Caribbean.
- Key features: Universal college scholarships—called “Promise” scholarships because they are guaranteed by an established fund—are supported by quality neighborhood-based early childhood education, health, counseling, and after-school and summer programs.
- Core funding: Harris Rosen funds early child care providers and universal college scholarships. Rosen also supports other services, such as a lifeguard at the YMCA, as needed.
Initiatives that serve all of a school district
Joplin, missouri.
Joplin’s Bright Futures initiative (which has spawned dozens of other Bright Futures affiliate districts under a Bright Futures USA umbrella since it launched in 2010) has a rapid response component that addresses children’s basic needs (within 24 hours of a need being reported), while strong school–community partnerships help meet students’ longer-term needs. Bright Futures also provides meaningful service learning opportunities in every school.
- Organizing partners: The Joplin School District’s superintendent and top leadership, in collaboration with parents and community, faith, business, and social service leaders.
- Schools and students reached: Bright Futures serves all of the district’s 7,874 students in all 17 schools.
- General makeup of the student body: Joplin is a heavily white community. As of 2015, nearly two-thirds (61 percent) of Joplin students are FRPL-eligible and 16 percent are classified as needing special education; just 3 percent are English language learners.
- Key features: The Bright Futures USA framework has three components. First, a rapid response system is designed to meet any student’s basic health, nutrition, or physical need within 24 hours of such a need being reported; this system is supported by combined resources from social service agencies, businesses, faith organizations, and individual community members. Second, school- and community-level councils build community leadership and partnerships with schools to meet longer-term needs and sustain systems. Third, service learning opportunities are embedded in all schools to help develop children as citizens. Teachers lead the service learning and receive training to do so. In addition to these three components, Joplin also provides pre-K for at-risk students, as well as tutoring, mentoring, and after-school and college preparatory programs based on student need.
- Core funding: Federally funded Americorps VISTA volunteers provide in-kind support; funds from the state departments of Elementary and Secondary Education and of Economic Development support Bright Futures work and conferences; and the regional Economic Security Corporation and a range of private funders supplement these federal and state funding sources.
Kalamazoo, Michigan
The “Kalamazoo Promise,” a guarantee by a group of anonymous local philanthropists to provide full college scholarships in perpetuity for graduates of the district’s public high schools brought Kalamazoo Public Schools (KPS), the city, and the community together to develop a set of comprehensive supports that enable more students to use the scholarships.
- Organizing partners: Kalamazoo Promise and Kalamazoo Public Schools, the local school district, in collaboration with Communities in Schools Kalamazoo (CIS) and other nonprofit entities.
- Schools and students reached: All KPS students (12,216 in 25 schools) who graduate from Kalamazoo public high schools are eligible for Promise scholarships. CIS works in all schools but to varying degrees and with varying levels of financial support.
- General makeup of the student body: In this combination urban–suburban district, a large majority of students (over 70 percent) are FRPL-eligible, 12 percent receive special education services, and 7 percent are English language learners. The share of African American students grew from less than one-third in 1987 to over half 30 years later; over this period the share of Hispanic students increased as well.
- Key features: The anchor for comprehensive supports is universal “Promise” college scholarships, which have spurred community leadership to provide quality pre-K programs and wraparound health, mental health, and other supports, and to launch a districtwide effort to create a college-going culture and resources to support that culture.
- Core funding: Anonymous donors have committed to funding Promise scholarships in perpetuity. CIS is supported by a combination of Title I funding, which helps support school coordinators; 21st Century Learning grants for after-school activities; and private individual and philanthropic donations.
Montgomery County, Maryland
All students in Montgomery County Public Schools (MCPS) benefit from zoning laws that advance integration and strong union–district collaboration on an enriching, equity-oriented curriculum. These efforts are bolstered by extra funding and wraparound supports for high-needs schools and communities.
- Organizing partners: MCPS, Montgomery County Education Association (the local teachers union), Montgomery County Council, and Linkages to Learning (a joint initiative of MCPS and the county council that provides an integrated focus on health, social services, community development, and engagement to support student learning, strong families, and healthy communities.)
- Schools and students reached: All 160,000 students in more than 200 schools are served via some services. Higher-poverty schools and their communities receive additional funds and supports that are broader and more intensive. For example, Linkages to Learning serves more than 5,400 individuals—students and their family members—per year at 29 schools. Over 3,700 of them receive comprehensive behavioral health or social wraparound services to mitigate the effects of poverty and reduce nonacademic barriers to learning.
- General makeup of student body: The MCPS school district as a whole is racially and socioeconomically diverse: 30 percent of students are Hispanic, 29 percent are white, 22 percent are African American, 14 percent are Asian, and 35 percent are FRPL-eligible (more than 40 percent of students have been FRPL-eligible at some point). On the poorer, Eastern side of the county, where more intensive whole-child supports are provided, the 10 highest-poverty schools have student bodies that are at least 80 percent FRPL-eligible.
- Key features: Mixed-use housing policies that enable racial and socioeconomic integration advance school-level integration that boosts low-income students’ learning, which the district enhances through various forms of support, including high-quality early childhood education, parent and community outreach, reallocation of funds to high-needs schools and students, nutrition and health services, and an emphasis on social and emotional learning.
- Core funding: MCPS is heavily locally funded, with almost no federal Title I dollars. The district’s whole-child approach draws on a combination of school district and county revenues, along with federal funding for Head Start programs, state pre-K dollars, and assorted other grants.
Pea Ridge, Arkansas
The Pea Ridge School District, a small suburban–rural district outside Fayetteville, Arkansas, is among the newer affiliates of Bright Futures USA, a national umbrella group that grew out of Bright Futures Joplin. As a Bright Futures affiliate, Pea Ridge is making good progress toward identifying and meeting students’ basic needs, engaging the community to meet longer-term needs, and making service learning a core component of school policy and practice.
- Organizing partners: Pea Ridge School District and Bright Futures USA.
- Schools and students reached: Eight hundred and fifty students are served in one primary school, one elementary school, one middle school, and one high school, as well as an alternative high school and a new career-tech charter high school.
- General makeup of the student body: The suburban–rural district is mostly white, with a small but growing Hispanic population, and predominantly middle-income with pockets of both higher-income families and families in poverty.
- Key features: The first component of the three-part Bright Futures USA framework is a rapid response system to meet every student’s basic health, nutrition, and physical needs within 24 hours through a combination of social service agency, business, faith, and individual community contributions. Other components include school- and community-level councils, which build community leadership and partnerships with schools to meet longer-term needs and sustain systems, and service learning embedded in all schools that is enhanced by supportive training for teachers. Pea Ridge also provides pre-K for at-risk students, as well as tutoring, mentoring, and after-school and college preparatory programs for students who need them.
- Core funding: State funds support meals and other needs for high-poverty schools, and Pea Ridge has secured a three-year private grant to support access to pre-K for low-income students.
Vancouver, Washington
Family and Community Resource Centers (FCRCs) currently serve 16 of the highest-needs Vancouver Public Schools (VPS) district schools, with mobile and lighter-touch support in other schools and plans to expand districtwide by 2020.
- Organizing partners: School district leaders coordinate the program with the support of six central-office staff (three of whom just support FCRCs). Technical and other assistance is provided by the Coalition for Community Schools.
- Schools and students reached: FCRCs serve 23,500 students in 16 VPS schools: 11 elementary schools, two middle schools, two high schools, and the Fruit Valley Learning Center (a combination elementary school and community center that also offers child care and Head Start programs). Plans are being made to expand FCRCs to all 35 VPS schools by 2020.
- General makeup of the student body: As of 2015, more than half of students were FRPL-eligible, with FRPL-eligibility rates in some central-city schools exceeding 80 percent. More than one in five students speak a language other than English at home and 12.5 percent of students are special education students; in FCRC schools, the shares of non–English speakers and special education students are even higher.
- Key services: VPS supports a range of early childhood education programs, including quality pre-K; middle and high school in-school enrichment; after-school and summer programs (provided by VPS partners); and help for parents and families through workshops, assistance, and referrals to a range of community resources.
- Core funding: District and Title I funds, which support basic FCRC needs, are supplemented by cash and in-kind donations from faith-based, social service, business, and association partners.
Initiative that serves multiple school districts
Eastern (appalachian) kentucky.
A federal Promise Neighborhood grant helps Berea College’s Partners for Education provide intensive supports for students and their families in four counties in the Eastern (Appalachian) region of Kentucky and provide lighter-touch supports in an additional 23 surrounding counties. (Berea College, which was established in 1855 by abolitionist education advocates, is unique among U.S. higher-education institutions. It admits only economically disadvantaged, academically promising students, most of whom are the first in their families to obtain postsecondary education, and it charges no tuition, so every student admitted can afford to enroll and graduates debt-free.)
- Organizing partners: Berea College launched Partners for Education (PfE), which is now a fully staffed nonprofit that runs the initiative.
- Schools and students reached: PfE serves 35,000 students in 22 schools in Clay, Jackson, Knox, and Owsley counties; tens of thousands more are served less intensively in an additional 23 counties in the region.
- General makeup of the student body: The Appalachian region is rural, very poor, and heavily white. The regional poverty rate is around 27 percent (in 2015), and reaches as high as 40 percent in some counties. About 80 percent of students are FRPL-eligible and 97 percent are white.
- Key features: Family engagement specialists meet directly with families and help coordinate services provided by a range of community partners. Other specialists provide basic academic, college preparatory, and health and other wraparound services to students.
- Core funding: Federal Promise Neighborhood, Full Service Community Schools, and Investing in Innovation grants are the most prominent sources of funding, but the initiative receives a range of other cash and in-kind supports.
Appendix tables and figures
Covariates from these models : ecls-k 1998--1999 and 2010--2011.
Source: ECLS-K, kindergarten classes of 1998–1999 and 2010–2011 (National Center for Education Statistics)
Missing data
Note: For detailed information about the construction of these variables, see Appendix Table A1.
Distribution of standardized scale and theta scores in mathematics, by year
Scale scores, 1998 (left) and 2010 (right).
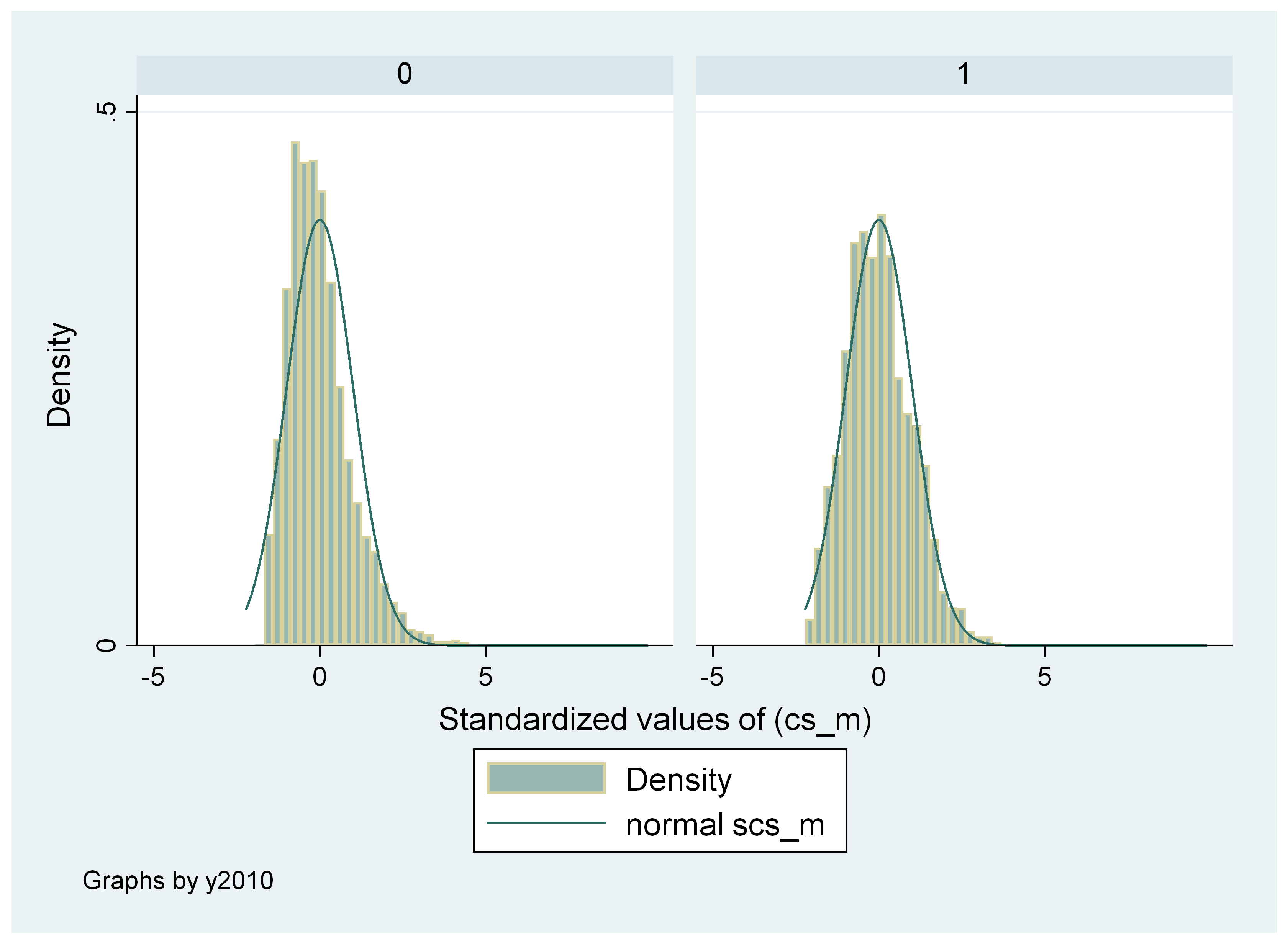
Theta scores, 1998 (left) and 2010 (right)
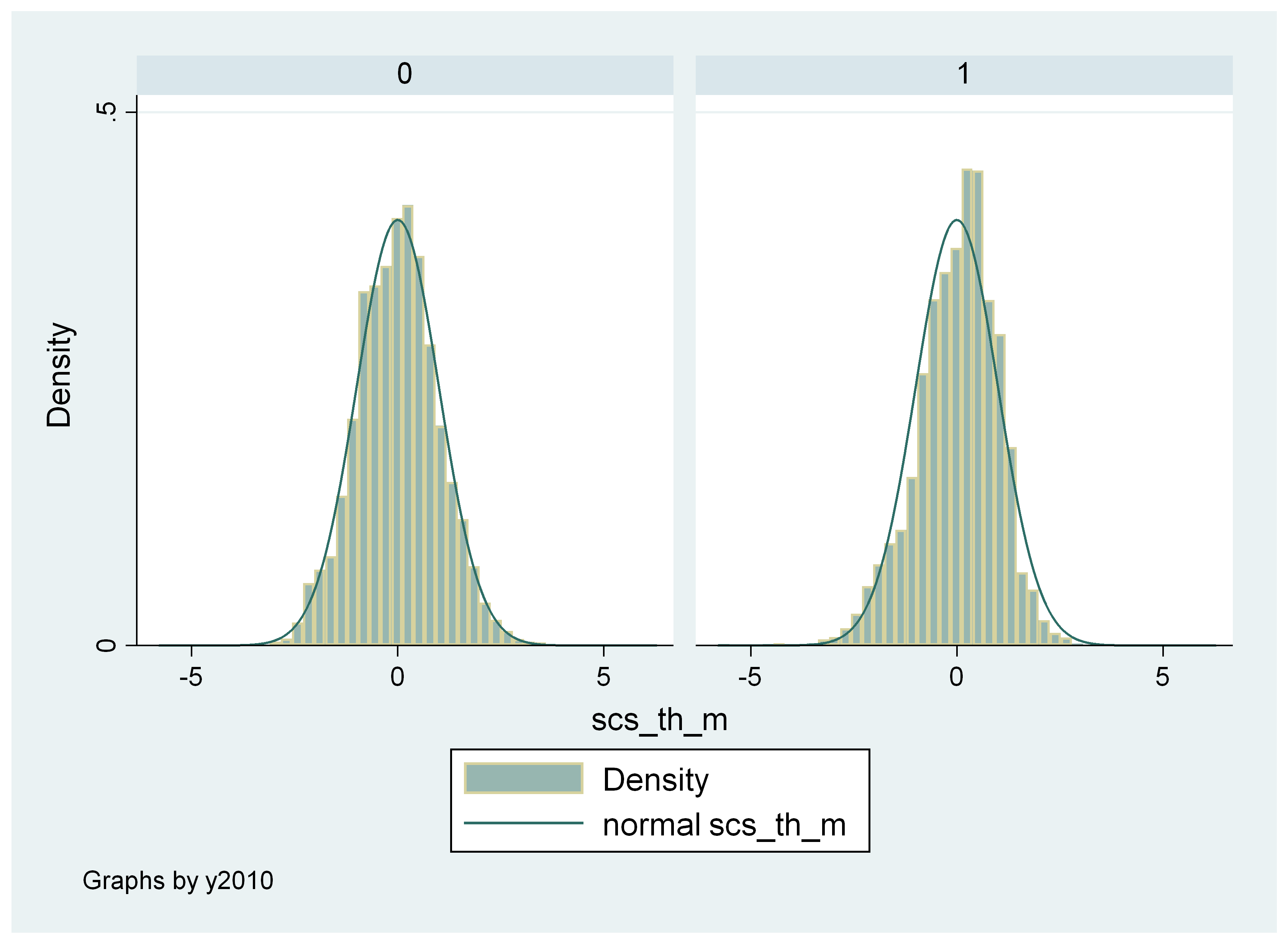
Distribution of standardized scale and theta scores in reading, by year
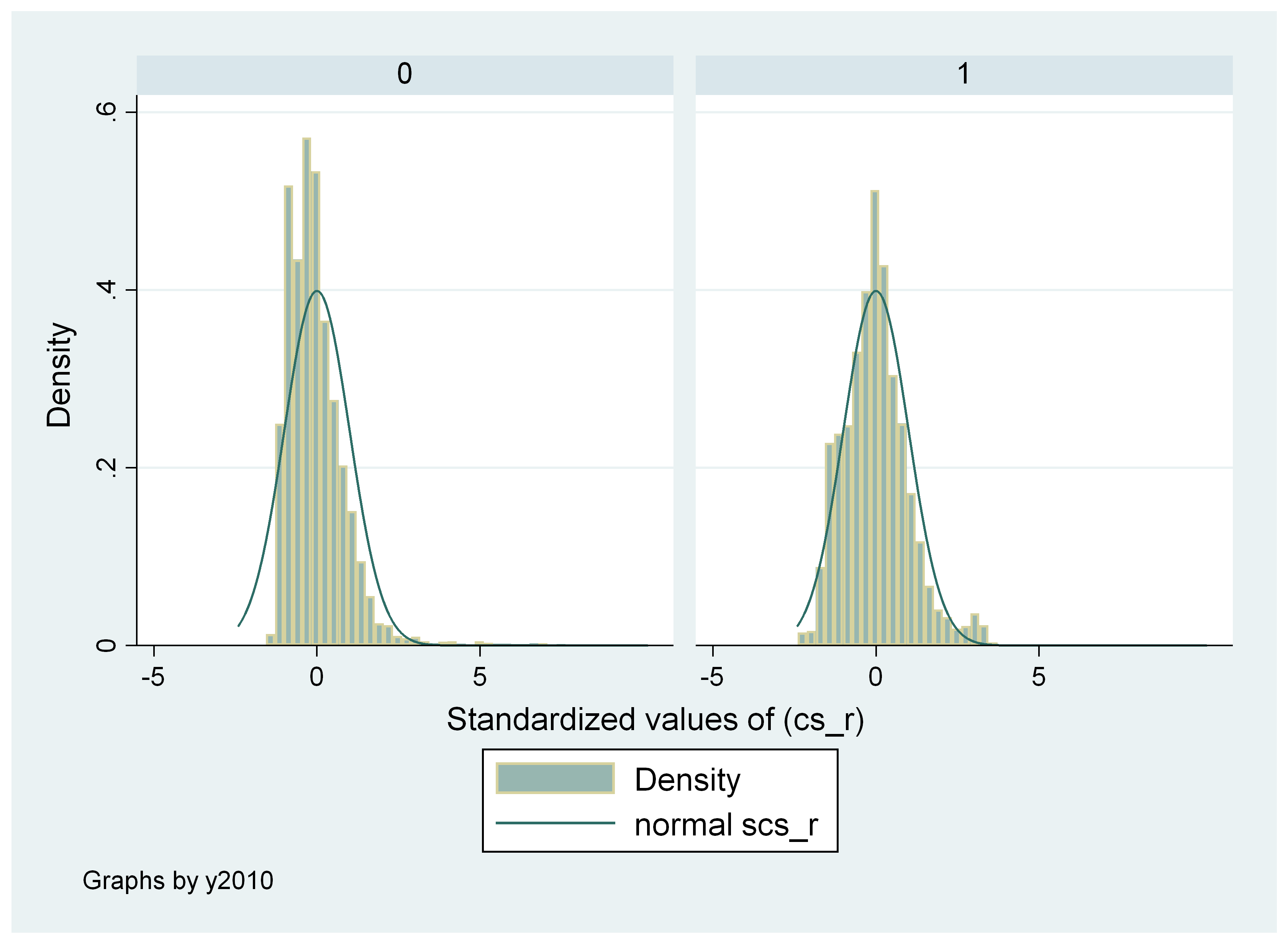
Descriptive statistics of standardized scale and theta scores, by year (not weighted)
Note: N is rounded to the nearest multiple of 10.
Reading and math skills gaps between high-SES and low-SES children at the beginning of kindergarten in 1998 and change in gaps by the beginning of kindergarten in 2010, using scale and theta scores as dependent variables
Notes: Standard errors are in the parentheses. N is rounded to the nearest multiple of 10. Asterisks denote statistical significance: *** p < 0.01, ** p < 0.05, * p < 0.1.
Source: ECLS-K, kindergarten classes of 1998-1999 and 2010–2011 (National Center for Education Statistics)
Reading and math skills gaps between high-social class and low-social class children at the beginning of kindergarten in 1998 and change in gaps by the beginning of kindergarten in 2010, using scale and theta scores as dependent variables
Notes: Standard errors are in parentheses. Asterisks denote statistical significance: *** p < 0.01, ** p < 0.05, * p < 0.1.
Endnotes to the appendices
i. The sample design used to select the individuals in the study was a three-stage process that involved using primary sampling units and schools with probabilities proportional to the number of children and the selection of a fixed number of children per school. In the last stage, children enrolled in kindergarten or ungraded schools were selected within each sampled school. A clustered design was used to limit the number of geographic areas and to minimize the number of schools and the costs of the study (Tourangeau et al. 2013, 4-1).
ii. The dataset in the first year followed a stratified design structure (Ready 2010, 274), in which the primary sampling units were geographic areas consisting of counties or groups of counties. About 1,000 schools — 903 for 1998 and 968 for 2010—were selected, and about 24 children per school were surveyed. Assessment of the children was performed by trained evaluators, while parents were surveyed over the telephone. Teachers and school administrators completed the questionnaires in their schools.
iii. As a sensitivity check, we estimate Models 1 and 2 using Models 1’s and Model 2’s specifications but using the restricted sample (these results are not shown here, but are available upon request).
iv. As a sensitivity check, we estimate Model 3 parsimoniously, by including family characteristics only, and then adding family investments (prekindergarten care arrangements, early literacy practices at home, and number of books the child has), and then adding parental expectations (with and without interactions with time); results of the sensitivity check are not shown, but are available upon request).
v. We refer to the fact that we are using the same data and that the scale and theta scores are based on the same instruments and are not independent from each other. Advice on this possibility is found in Reardon (2007), who cites work by Murnane et al. (2006) and Selzer, Frank, and Bryk (1994) that also warn about this option.
vi. From NCES: “IRT uses the pattern of right and wrong responses to the items actually administered in an assessment and the difficulty, discriminating ability, and guess-ability of each item to estimate each child’s ability on the same continuous scale. IRT has several advantages over raw number-right scoring. By using the overall pattern of right and wrong responses and the characteristics of each item to estimate ability, IRT can adjust for the possibility of a low-ability child guessing several difficult items correctly. If answers on several easy items are wrong, the probability of a correct answer on a difficult item would be quite low. Omitted items are also less likely to cause distortion of scores, as long as enough items have been answered to establish a consistent pattern of right and wrong answers. Unlike raw number-right scoring, which treats omitted items as if they had been answered incorrectly, IRT procedures use the pattern of responses to estimate the probability of a child providing a correct response for each assessment question” (Tourangeau et al. 2017, 3-2).
vii. The quoted text is abridged to remove variables and formulas specific to Reardon’s study and not central here.
viii. Also, “the estimated scale score is the estimated number of questions the student would have gotten correct if he or she had been asked all of the items on the test. The estimated scale score is obtained by summing the predicted probabilities of a correct response over all items, given the student’s estimated theta score and the estimated item parameters” (Reardon 2007, 11).
ix. They are equally spaced units along the scale without a predefined zero point.
See related work on Student achievement | Education | Educational inequity | Children | Economic inequality | Inequality and Poverty | Early childhood
See more work by Emma García and Elaine Weiss

- Previous Article
Contributor Notes
This paper presents new results on the relationship between income inequality and education expansion—that is, increasing average years of schooling and reducing inequality of schooling. When dynamic panel estimation techniques are used to address issues of persistence and endogeneity, we find a large, positive, statistically significant and stable relationship between inequality of schooling and income inequality, especially in emerging and developing economies and among older age cohorts. The relationship between income inequality and average years of schooling is positive, consistent with constant or increasing returns to additional years of schooling. While this positive relationship is small and not always statistically significant, we find a statistically significant negative relationship with years of schooling of younger cohorts. Statistical tests indicate that our dynamic estimators are consistent and that our identifying instruments are valid. Policy simulations suggest that education expansion will continue to be inequality reducing. This role will diminish as countries develop, but it could be enhanced through a stronger focus on reducing inequality in the quality of education.
- I. Introduction
The persistence of high and, in many countries, rising income inequality over recent decades is a growing concern for policy makers worldwide, and has received increasing attention both from economists and in public debate ( OECD, 2008 ; Clements and others, 2015; Dabla-Norris and others, 2015 ) . Rising inequality has been attributed to a range of factors, including the globalization and liberalization of factor and product markets; skill-biased technological change; increases in labor force participation by low-skilled workers; declining top marginal income tax rates; increasing bargaining power of high earners; and the growing share of high-income couples and single-parent households ( OECD, 2008 ; Alvaredo and others, 2013 ; Hoeller, Joumard, and Koske, 2014). However, many of these factors have also had beneficial effects on growth and poverty reduction both nationally and globally (Chen and Ravallion, 2010; Milanovic, 2012).
The focus of this paper is on the relationship between education expansion and income inequality . Expansion of education is often seen as an important policy instrument for combating rising income inequality over the medium term. Not only is education expansion viewed as being important for promoting economic growth ( Barro, 2013 ; Hanushek, 2013 ), but it can also help to break the intergenerational transmission of poverty and reduce inequality of opportunity ( Corak, 2013 ), which in turn reduces future income inequality. Reducing income inequality through education expansion would also reduce the need for fiscal redistribution through distortionary fiscal policies such as progressive income taxes or means-tested transfers. So, from this perspective, education expansion has a “win-win” potential to simultaneously achieve both efficiency and equity objectives.
The paper extends the existing empirical literature in a number of dimensions . First, it expands the econometric analysis to address key estimation challenges not addressed in the existing literature, more specifically the issues of the endogeneity of the education and income inequality relationship and the persistence of income inequality over time. Second, it uses a new database on income inequality that expands the period of analysis while recognizing the need to use comparable measures of income inequality. Thirdly, it allows for heterogeneity in the relationship between education expansion and income inequality across advanced and developing economies to capture possible differences in returns to education. Finally, it also allows for heterogeneity in the relationship between education expansion and income inequality across working-age groups since there is evidence that education and experience are complementary inputs in human capital formation so that returns to education, and thus income inequality, can be expected to increase with working age.
The structure of the paper is as follows . In Section 2 we briefly discuss the conceptual framework underpinning the analysis of the impact of education expansion on income inequality and outline our empirical strategy for estimating this relationship. Section 3 discusses the data used in the analysis. Section 4 presents results based on these data and estimation methods, and compares them with the existing literature. Based on these results, Section 5 uses simulation analysis to discuss the implications of past and future changes in education outcomes for income inequality. Section 6 concludes.
II. Economic Theory and Empirical Estimation
- A. Economic Theory
The standard theoretical framework for analyzing the relationship between education expansion and income inequality is the traditional human capital model . This model implies that the distribution of income (or earnings) is determined by both the level and distribution of education (or schooling) across the population. Using this model, earnings (Y) of an individual with S years of schooling can be approximated as 1 :
where Y 0 is the earnings of individuals with zero formal education, r is the rate of return to an additional year of schooling, and u captures other factors that influence earnings independent of education. The dispersion of earnings across individuals in a population can then be written as follows, with bar superscript denoting mean values:
Therefore, an increase in education inequality, Var(S), keeping the average level of schooling and other factors constant, unambiguously results in higher income inequality—i.e., the first two terms are unambiguously positive. However, the impact on income inequality of increasing the average level of schooling, S ¯ , keeping other factors constant, will depend on the relationship between r and S, i.e. Cov(r,S) —i.e., on the combined effect of the third and fourth terms. If the return to an extra year of schooling is constant across levels of schooling, so that Cov(r, S)=0, then an increase in the average level of schooling will unambiguously result in higher income inequality. Similarly, if the return to an extra year of schooling is higher at higher levels of schooling (Colclough and others, 2010; Castelló-Climent and Doménech, 2014 ), so that Cov(r, S)>0, then an increase in the average level of schooling will also unambiguously result in higher income inequality. However, if returns are lower at higher levels of education, as suggested by much of the empirical literature (Psacharopoulos and Patrinos, 2004), so that Cov(r, S)<0, then this will attenuate the increase in income inequality and, if sufficiently negative, may actually result in an increase in average schooling leading to a net decrease in income inequality. 2
- B. Empirical Estimation
To test the empirical relationship between income inequality and the average level of education and education inequality, we use the following country-panel specification :
where subscripts refer to country i and year t respectively, I is a measure of income inequality, E is average years of education, σ is a measure of education inequality, X denotes other variables that impact income inequality independently of education outcomes, α captures unobserved time-invariant country-fixed effects, and ε captures other unobserved determinants that can vary across countries and time periods.
The data sources for the key income inequality and education variables used in the analysis are as follows (see Appendix 1 for details on the other explanatory variables included in the regression):
Income Inequality (I): For our analysis we use the Gini coefficient for disposable income inequality as our dependent variable since this is the inequality index that is most widely available and used in the related literature. We use an updated Gini coefficient database based on that assembled by Bastagli, Coady and Gupta (2012), which emphasized the need for comparability of country inequality estimates across time. Gini estimates are taken at five-year intervals.
Average Education (E): The average level of education of a country is taken as the average years of school attainment for the population aged 25 and over from Barro and Lee (2013) . These data are collected from census and survey information in five-year intervals, as compiled by UNESCO, Eurostat, and other sources. Average education is constructed based on the distribution of education attainment in the population over age 25, by five-year age groups and, for most cases, in six attainment categories: no formal education, incomplete primary, complete primary, incomplete secondary, complete secondary, and complete tertiary.
Education Inequality (σ): The inequality of education in a country is taken as the Gini coefficient of years of education for a given five-year interval based on educational attainment data from Barro and Lee (2013) . Appendix 2 discusses the construction of this Gini in more detail.
Our point of departure is the papers by De Gregorio and Lee (2002), Castelló-Climent and Doménech (2014) , and Dabla-Norris and others (2015) —henceforth referred to as DGL, CCD, and DNO, respectively . DGL used country-panel data for around 70 advanced and developing economies covering the five-year periods from 1965 to 1990. They estimated the relationship between education outcomes and income inequality (as captured by the Gini coefficient for disposable income) using the technique of seemingly unrelated regressions (SURE). Exploiting only the cross-country variation, they assumed that coefficient estimates were common across panels and thus ignored country-fixed effects. If these assumptions are valid then SURE provides more efficient estimates than OLS. As with previous attempts to estimate the relationship between education and income inequality 3 , they found that income inequality increases with education inequality and decreases with the average level of education. However, the positive coefficient on inequality of education was statistically insignificant when controls for a country’s GDP per capita and the level of public social spending were added.
Similar results were found by CCD, who extended the database to 2010 and used a fixed-effects estimation model to control for unobserved factors (such as historical factors, institutions, or culture) specific to a country that affect the level of income inequality but do not change over time . They also used the Gini measure of inequality from the SWIID database (unlike DGL who used the WIID database). Since there are valid concerns about the use of these Gini coefficients, in the current paper we use the Gini database constructed by Bastagli, Coady and Gupta (2012), which emphasizes the importance of comparability of inequality measures over time. 4 CCD also controlled for other factors such as technological change (i.e, a time trend and the share of high-technology exports in total exports) and globalization (i.e., proxies for trade and financial openness). Reflecting their primary focus on “the race between education and technological change” they also include variables for the ratio of the average years of tertiary to primary education in the population 25 years and older as well as the Gini coefficient for education outcomes. When controlling for fixed effects, the authors find a positive relationship between inequality of education outcomes and income inequality, although this is not always significant. They also find a negative relationship between the relative supply of skills, which we interpret as their proxy for the level of education, and income inequality, although again this is not significant in all specifications.
DNO also used a fixed-effects estimation model with average years of education and education inequality as dependent variables along with other dependent variables similar to those used in CCD . Their analysis does not find any statistically significant relationship between the income Gini and education inequality and education levels. They find a negative but insignificant relationship between education inequality and income inequality and a positive (negative) but insignificant relationship for education level in advanced (emerging) countries. They do however find a significantly positive (negative) relationship between education level and the share of income accruing to the top (middle) income decile.
This paper extends the estimation strategy to address two remaining econometric issues, namely, persistence and endogeneity :
Persistence of Income Inequality : Income inequality tends to change only slowly over time with very little within-country variation over the sample period, suggesting that there may be some, possibly unobserved, slowly-changing factors that explain this persistence. For example, this state dependence could reflect factors that prevent intergenerational mobility so that it is harder for a person born poor to achieve social mobility than for a person born in the middle class ( Corak, 2013 ). If these unobserved factors are correlated with education outcomes, then the estimated OLS and fixed-effects coefficients can be biased.
Endogeneity of Education Outcomes : Any observed relationship between education outcomes and income inequality may reflect reverse causation, i.e., current income inequality also affects current educational attainment and its dispersion. Therefore, any unobserved factors that affect income inequality and also education outcomes can bias the estimated relationship between education outcomes and income inequality.
To address these two issues, we use dynamic panel estimation techniques . To control for persistence, it is common to include past income inequality levels as an additional independent variable. However, by construction, this implies that the exogeneity assumption in the fixed-effects estimator is violated so that fixed-effects estimates are then biased (Nickell, 1981). To address this problem, Arellano and Bond (1991) suggest using a first-differenced GMM (Diff-GMM) estimator that also deals with the endogeneity problem by first differencing the data and then deploying suitably lagged values of the independent and dependent variables as instruments. 5 However, Blundell and Bond (1998) show that the Diff-GMM estimator suffers from the weak instrument problem when the number of time periods is small and that this bias is exacerbated when the time series are persistent. Building on Arellano and Bover (1995), the system GMM estimator (Sys-GMM) developed by Blundell and Bond (1998) addresses this weak instrument problem by exploiting level restrictions which remain informative even in the presence of persistence. Thus, where the number of time periods is small and in the presence of persistence, Sys-GMM estimator can produce dramatic efficiency gains over the basic Diff-GMM estimator. 6 For this reason, our preferred model is the Sys-GMM estimator.
Recent research has shown that Sys-GMM may equally suffer from the weak instrument problem, particularly when the time series is large and when substantial unobserved heterogeneity exists (Hayakawa, 2006; Bun and Windmeijer, 2010) . We therefore complement our Sys-GMM estimates by using the approach adopted by Barro and Lee (2010), which instruments a cohort’s schooling with the educational attainment of its parents (Ins-GMM). Finally, to further test the robustness of our estimates, we employ the long-difference instrumental variables estimator proposed by Hahn, Hausman and Kuersteiner (2006), which has been shown to be much less biased and more efficient than conventional implementations of the Sys-GMM when the number of time periods is small and in the presence of persistence.
- III. Data Description
As indicated, the measure of income inequality used in the analysis is the Gini coefficient for disposable income, taken from the database constructed by Bastagli, Coady and Gupta (2012) . More specifically, we use Gini coefficients for five-year intervals from 1980 to 2010. Based on this, Figure 1 shows the profile of regional average income inequality over the last four decades. Whereas Gini coefficients are available from 1980 for advanced economies, for many other regions they are only available from 1990 onwards.

Disposable Income Inequality by Region, 1980–2010
Citation: IMF Working Papers 2017, 126; 10.5089/9781475595741.001.A001
- Download Figure
- Download figure as PowerPoint slide
Average income inequality is lowest in advanced countries (ADV) but has increased steadily over the last four decades . In Emerging European countries (EE), income inequality exhibits a step increase between 1990 and 1995, a period of substantial structural change associated with transition to market economies, but has stayed relatively stable (with a slight decrease) since then. Average income inequality in the Asian and Pacific (AP) and in the Middle East and North Africa (MENA) regions are higher than in ADV and EE and have also increased steadily over recent decades. More striking is the relatively large level of income inequality in countries in sub-Saharan Africa (SSA) and especially in Latin America and the Caribbean (LAC). 7 In SSA, while income inequality has been declining slowly it still remains among the highest in the world. Similarly, although average income inequality in LAC has declined sharply since its peak in 2000 it still has the most unequal income distribution in the world.
Figure 2 plots the inequality of education against average years of schooling for different country groupings . As expected, the data suggest an inverted-U relationship with education inequality increasing over lower levels of schooling before declining. However, the precise relationship seems to differ across country groups. Noticeable is the relatively sharp increase in education inequality in MENA and SSA countries as education expands from initial low levels. As a result, at around an average of 4 years of schooling, education inequality was substantially higher in MENA and SSA than in AP or LAC. Therefore, MENA and SSA appear to have experienced a much more unequal early expansion of education than in other regions, although there is some evidence that education inequality is beginning to decrease sharply as access to education expands further. 8

Education Levels and Education Inequality
- IV. Empirical Results
To facilitate comparison with the existing literature, we start by estimating the above equation using SURE and fixed-effects estimators . Table 1 (column 1) shows the correlations between both education levels and education inequality and income inequality based on an OLS estimator. Whereas income inequality is lower at higher education levels (consistent with decreasing returns to education), income inequality decreases with education inequality, although this relationship is insignificant. Together, variation in these variables explains 22 percent of the total variation in income inequality. Most of the variation in income inequality is explained by cross-country variation as opposed to changes over time. For instance, when time dummies are added (column 2) the explained variation increases to just 30 percent. However, when country-dummies are also added the explained variation increases to 87 percent. While both coefficients are negative and significant when time dummies are included, both are insignificant when country dummies are added highlighting the importance of controlling for country-fixed effects.
Education and Income Inequality: OLS, SURE and Fixed Effects Estimates
When other determinants of income inequality are added to the specification (column 4) , the coefficient signs on both education level and education inequality remain negative but the coefficients become smaller in absolute terms (compared to column 2) and insignificantly different from zero. The explained variation decreases to 79 percent reflecting the absence of country dummies. Estimating this relationship using SURE results in a smaller (in absolute terms) and still insignificantly negative coefficient on education inequality, but the negative coefficient on education level becomes larger in absolute terms and significant. Applying fixed effects results in the negative coefficient on education levels decreasing in absolute terms and remaining significant. Although the coefficient on education inequality now becomes positive it remains insignificant.
Table 2 presents the results when we attempt to control for endogeneity and persistence . With the first-difference estimator, the coefficient on education levels remains negative, decreases in absolute terms compared to the fixed effects estimate but now becomes insignificant. Consistent with theory, the coefficient on education inequality remains positive although still insignificant. Under both difference GMM (Diff-GMM) and system GMM (Sys-GMM) the positive coefficient on education inequality increases around six fold and becomes significant at the 10 and 5 percent levels respectively, while the coefficient on education levels becomes positive but remains insignificant. 9 When we instrument education levels and education inequality using parents’ schooling levels (Ins-GMM) the coefficient on education inequality remains positive, large and significant, while the positive coefficient on education level now becomes significant. Therefore, the coefficients on education inequality and levels remain quite stable across all the GMM estimators. The Arellano Bond tests for the serial correlation of the disturbances indicate that our GMM estimators are consistent. Moreover, both the Sargan and Hansen tests for over-identifying restrictions do not reject the hypothesis that our instruments are valid in our GMM estimations. Under the long-distance estimator, the positive coefficient on education inequality becomes smaller and insignificant while the coefficient on education levels remains positive but is smaller and insignificant. However, the insignificance found when running the long distance estimator could be explained by the reduction in the number of observations that we have to incur to implement this estimation.
Education and Income Inequality: Difference, GMM, Long Distance Estimates
When we allow these coefficients to differ between advanced and other countries, we find that the positive coefficient on education inequality is halved in advanced while the coefficient on education level remains positive and insignificant in both sets of countries . This result suggests that the impact of education inequality on income inequality is not homogeneous across different levels of development and shows that reducing education inequality is an even more important policy for developing countries while becoming less important as countries develop.
The literature on the returns to education levels points to a “fanning out” of these returns with age (a proxy for years of experience) consistent with the returns to education accruing later in an individual’s working career . Therefore, inequality in years of education is more likely to translate into higher income inequality among older workers than among younger workers ( Table 3 ). To test this, we add education inequality for older workers as an additional explanatory variable. Consistent with the literature on returns to education, we find that higher inequality in education levels among older workers (keeping inequality of education for all workers constant) is associated with a substantially larger level of income inequality. In addition, the acceleration of the skill-biased technological change means that higher education levels for the young could reduce the income gap between older and younger generations and thus income inequality. We test this by including average education for the young as an additional explanatory variable and find that higher education levels for the young are indeed associated with lower income inequality.
Education and Income Inequality: Cohort Effects
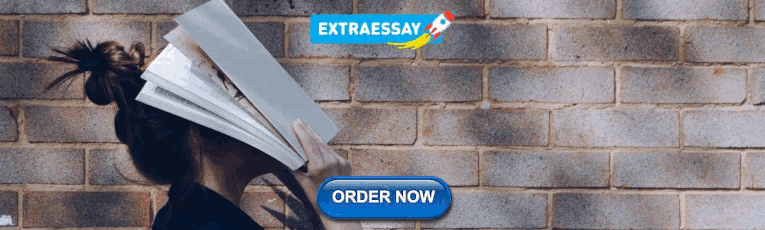
V. Policy Simulations
In this section, we use the results from our preferred Sys-GMM estimation to simulate the impacts of education inequality and level on income inequality to get a sense of their quantitative importance in determining income inequality . We start by analyzing how much of the change in income inequality over the last fifteen years from 1990 to 2005 can be attributed to changes in education outcomes. We then analyze how changing education outcomes over the subsequent two decades might impact income inequality.
- A. Education and Past Income Inequality
Trends in income inequality between 1990 and 2005 varied substantially across regions . On average in the sample, inequality decreased in both SSA and MENA countries while other regions experienced increases with the Gini increasing by over 5 points in both AP and EE ( Table 4 ). Across all regions, decreases in the inequality of education reduced income inequality, ranging from a 1.5 point decrease in ADV and EE to a 4.8 point decrease in MENA. However, increases in the level of education increased income inequality across all regions by 1.5 to 2.2 points. While the net effect of changing education outcomes was to slightly increase income inequality in ADV, the effect was negative in all other regions. These results highlight the important role education investments can play in mitigating increases in income inequality, in particular the key role played by education expansion strategies that emphasize more equal access to education
Implications of Changing Education Outcomes for Income Inequality, 1990–2005
- B. Education and Future Income Inequality
Table 5 presents projections of the impact of future changes in education levels and education inequality on income inequality from 2005 to 2025 . The average level of education is projected forward based on known education levels for current working cohorts while future working cohorts are assumed to have the same education outcomes of the youngest current working cohort (i.e., those aged 20–25 years in 2005). These changes in education levels are then used to project the impact on education inequality. The results indicate that changes in education outcomes will continue to have a dampening impact on income inequality over this period in most regions, with the exception of ADV, where it will increase income inequality by 0.1 points. Similar to the period 1990–2005, continued decreases in education inequality will have a dampening impact on income inequality while increasing education levels will increase income inequality, with both impacts being smaller than for the earlier shorter period. However, the results for ADV suggest that the income inequality reducing impact of education can be expected to decrease as the level of education increases, and the inequality of education decreases, in emerging and developing economies and converges to levels observed in advanced economies. This highlights the importance of also focusing on reducing the inequality of education quality (e.g., as captured by cognitive skills) to enhance the income inequality reducing impact of education expansion.
Implications of Changing Education Outcomes for Income Inequality, 2005–2025
VI. Conclusions
This paper presents new results on the relationship between education expansion and income inequality . It extends the existing literature in a number of dimensions. First, it addresses key econometric issues ignored in the existing literature related to the need to allow for the persistence of income inequality and the endogeneity of education and inequality outcomes, both of which require the use of dynamic panel analysis. Second, the analysis tests for heterogeneity in these relationships across country income groups as well as across different age cohorts. Finally, the paper uses a new database on income inequality that addresses concerns about the quality of the income inequality data currently widely used in the literature, and also extends the period of the analysis.
The analysis demonstrates clearly the importance of controlling for persistence, endogeneity and heterogeneity . When dynamic panel estimation techniques are applied, the positive relationship between education inequality and income inequality becomes substantially larger, statistically significant and stable across the various estimators. This is consistent with our theoretical insights based on the human capital model and confirms that education expansion reduces income inequality through decreasing the inequality of education. However, the relationship between income inequality and schooling levels is found to be positive but small and not always statistically significant. Statistical tests indicate that our dynamic estimators are consistent and that that our identifying instruments are valid.
Our policy simulations confirm that the net impact of education expansion over the last fifteen years has been to reduce income inequality, especially in emerging and developing economies . Although the magnitude of the net impact on income inequality varies across emerging and developing economies, it is always inequality reducing. This reduction reflects decreasing education inequality, which is only partly offset by the inequality-increasing effects of rising education levels. In advanced economies, education expansion is associated with a net increase in income inequality. This reflects the relatively smaller impact of decreasing education inequality at the lower levels of education inequality observed in advanced economies being offset by the income inequality-increasing impact of rising levels of education (consistent with constant or increasing returns to additional years of education).
Projections forward over two decades suggest that education expansion will continue to have an inequality-reducing impact in emerging and developing economies . Even though the inequality-reducing impact of falling education inequality is offset by the inequality-increasing impact of rising education levels, the net impact is still inequality reducing. The inequality-increasing impact of education expansion in advanced economies suggests that the inequality-reducing role of education expansion in emerging and developing economies will diminish as these countries develop. Therefore, other policies will also be needed to address rising income inequality. Among these, it is likely that focusing on reducing the inequality of education quality (e.g., improving cognitive skills) can help to enhance the role of education expansion as a force for reducing income inequality.
- Appendix 1. Explanatory Variables and their Sources
The explanatory variables used in this paper were selected based on factors identified in the literature as important determinants of income inequality. In this appendix, we briefly discuss the variables and their sources.
Level of income . We test the Kuznets inverted-U hypothesis. According to this hypothesis, there is a positive correlation between income inequality and per capita income at low levels of income, which eventually becomes negative after a country reaches a specific level of development. In order to test this hypothesis, we use the log of ppp per capita income and its square. We use the variable “GDP per capita, ppp” in the World Bank’s World Development Indicators (WDI).
Social public spending . Spending on social programs is expected to be progressively targeted and thus to reduce inequality. Government social expenditure is obtained from IMF, Government Finance Statistics Yearbook. It includes pension and other welfare benefits. For most countries data is available from 1990, but advanced economies have available data from 1970.
Trade openness . According to the Heckscher-Ohlin and the Stolper-Samuelson theoretical framework, greater trade openness should increase the relative demand and prices for unskilled labor and lead to a more equal distribution of wages in low-skilled-labor abundant countries and a more unequal distribution of wages in high-skilled labor abundant countries. We use the commonly used variable (exports + imports) / GDP to capture a country’s degree of trade openness.
Capital account openness . By relaxing credit supply constraints, capital openness is expected to reduce income inequality if institutions are strong ( LaGarda et al, 2016 ). In this paper, we use the variable “cap100” of the IMF’s Annual Report on Exchange Restrictions to reflect the degree of openness of the capital account. Higher values of the indices represent greater openness; lower values of the indices represent greater restrictiveness.
Credit to the private sector . Similar to capital account openness, greater availability of credit should minimize the amount of credit-constrained people in a country and reduce income inequality. In our paper, we use the variable “credit to the private sector/GDP” from the IMF’s Government Finance Statistics Yearbook.
Dependency ratio . Theoretical economic models posit that, all other things being equal, an ageing population and a rising dependency ratio tend to increase income inequality (Von Weizsäcker, 1995). We use population data from the World Bank’s WDI. Specifically, we use the proportion of the elderly (above 65 years old) and the children (below 16 years old) in the population.
Inflation . Several empirical and theoretical studies have analyzed the relationship between inflation and income inequality. The data on these studies have consistently shown a positive correlation between these two variables. In this paper, we use average annual CPI inflation as measured in the IMF’s Government Finance Statistics Yearbook.
Urban population . Reflecting the gap in income between rural and urban populations, different urbanization levels can lead to differences in income inequality. We use the percentage of the population living in urban areas in the World Bank’s WDI.
Summary Statistics
- Appendix 2. Constructing a Measure of Education Inequality
There is no measure of education inequality in Barro and Lee database, which is the source for our education data. Thus, we estimate each country’s education inequality using the standard method to calculate income inequality from a Lorenz curve.
In order to implement this method, we need a disaggregated measure of schooling attainment. The education attainment data provided in the Barro and Lee database is presented as the fraction of the population with no education, and with incomplete and complete primary, secondary and tertiary schooling. Thus, the first step in the estimation of the education Gini for education inequality is to assign an average education level to each of these categories of education achievement.
While assigning a specific average to these categories might be considered somehow arbitrary, the estimation results did not change much when we assigned slightly different numbers to these categories. Specifically, we assigned a value of 1 for the category no education, a value of 4 for incomplete and a 7 for complete primary education. We assigned a value of 10 for incomplete and a 13 for complete secondary education. Finally, we assigned a value of 16 for incomplete and 19 for complete tertiary education.
Finally, with these average numbers for each education category, we can calculate total number of years of education for different segments of each country’s population. Using these total years of education, we simply apply the concept of the Lorenz curve using the population of each segment as weights.
Acemoglu , Daron , 1997 , “ Matching, Heterogeneity, and the Evolution of Income Distribution ,” Journal of Economic Growth , Vol. 2 ( March ), pp. 61 – 92 .
- Search Google Scholar
- Export Citation
Adelman , Irma and Cynthia T. Morris , 1973 , Economic Growth and Social Equity in Developing Countries , Stanford University Press , Stanford, California .
Ahluwalia , Montek S. , 1976 , “ Income Distribution and Development: Some Stylized Facts ,” American Economic Review , Papers and Proceedings , Vo. 66 ( May ), pp. 128 – 35 .
Alvaredo , F. , A. Atkinson , T. Piketty , and E. Saez , 2013 , “ The Top 1 Percent in International and Historical Perspective ,” Journal of Economic Perspectives , Vol. 27 , No. 3 , pp. 3 – 20 .
Anand , Sudhir and Ravi Kanbur , 1993 , “ The Kuznets Process and the Inequality-Development Relationship ,” Journal of Development Economics , Vol. 40 ( February ), pp. 25 – 52 .
Atkinson , Anthony B. , 1997 , “ Bringing Income Distribution in from the Cold ,” Economic Journal , Vol. 107 ( March ), pp. 297 – 321 .
Barro , Robert and Jong-Wha Lee , 1994 , “ Sources of Economic Growth ,” Carnegie - Rochester Series on Public Policy , Vol. 40 ( June ), pp. 1 – 46 .
Barro , Robert and Jong-Wha Lee , 1996 , “ International Measures of Schooling Years and Schooling Quality ,” American Economic Review , Papers and Proceedings , Vol. 86 ( May ), pp. 218 – 23 .
Barro , Robert , 1999 , “ Inequality, Growth, and Investment ,” mimeo , Harvard University , Cambridge, Massachusetts .
Barro , Robert , 2013 , “ Education and Economic Growth”, Annals of Economics and Finance , Vol. 14 ( 2 ), pp. 301 – 28 .
Barro , Robert , and Jong-Wha Lee , 2015 , Education Matters: Global Schooling Gains from the 19th to the 21st Century , Chapter 2 ( London : Oxford University Press ).
Becker , Gary S. and Barry R. Chiswick , 1966 , “ Education and the Distribution of Earnings ,” American Economic Review , Papers and Proceedings , Vol. 56 ( May ), pp. 358 – 69 .
Becker , Gary S. and Nigel Tomes , 1986 , “ Human Capital and the Rise and Fall of Families ,” Journal of Labor Economics , Vol. 4 ( July ), S1–S39.
Benabou , Roland , 1994 , “ Education, Income Distribution, and Growth: The Local Connection ,” NBER Working Paper 4798 , National Bureau of Economic Research , Cambridge, Massachusetts .
Benabou , Roland , 1993 , “ Inequality and Growth ,” NBER Macroeconomics Annual , pp. 11 – 92 .
Bulir , Ales , 1998 , “ Income Inequality: Does Inflation Matter? ” IMF Working Paper WP/98/7 ( Washington : International Monetary Fund ).
Castelló-Climent , Amparo and Rafael Doménech , 2014 , Working Paper 12/28, BBVA Research .
Chenery , Hollis B. and Moshe Syrquin , 1975 , Patterns of Development , 1950–1970 , Oxford University Press for the World Bank , London .
Chiswick , Barry R. , 1971 , “ Earnings Inequality and Economic Development ,” Quarterly Journal of Economics , Vol. 85 , pp. 21 – 39 .
Corak , Miles , 2013 , “ Income Inequality, Equality of Opportunity, and Intergenerational Mobility ,” Journal of Economic Perspectives , Vol. 27 (No. 3 , Summer ), pp. 79 – 102 .
Dabla-Norris , Eras , Kalpana Kochhar , Nujin Suphaphiphat , Frantisek Ricka , and Evridiki Tsounta , 2015 , “ Causes and Consequences of Income Inequality: A Global Perspective ”, IMF Staff Discussion Paper SDN/15/13 ( Washington : International Monetary Fund ).
De Gregorio , Jose , 1995 , “ Comment ,” in Rudi Dornbusch and Sebastian Edwards (eds), Stabilization, Economic Reform and Growth ( Chicago : University of Chicago Press ).
De Gregorio , Jose and Se-Jik Kim , 2000 , “ Credit Markets with Differences in Abilities: Education, Distribution and Growth ,” International Economic Review , Vol. 41 ( August ), pp. 579 – 607 .
De Gregorio , Jose and Jong-Wha Lee , 1999 , “ Education and Income Distribution: New Evidence from Cross-Country Data ,” Development Discussion Papers No. 714 ( Cambridge, Massachusetts : Harvard Institute for International Development ), www.hiid.harvard.edu_pub_ddps.html ).
Deininger , Klaus W. and Lyn Squire , 1996 , “ A New Data Set Measuring Income Inequality ,” World Bank Economic Review , Vol. 10 ( September ), pp. 565 – 91 .
Deininger , Klaus W. and Lyn Squire , 1998 , “ New Ways of Looking at Old Issues: Inequality and Growth ,” Journal of Development Economics , Vol. 57 ( December ), pp. 259 – 87 .
Durlauf , Steven N. , 1996 , “ A Theory of Persistent Income Inequality ,” Journal of Economic Growth , Vol. 1 ( March ), pp. 75 – 93 .
Gallop , J. , Steven Radelet , and Andrew Warner , 1998 , “ Economic Growth and Income of the Poor,” mimeo ( Cambridge, Massachusetts : Harvard Institute for International Development ).
Galor , Oded and Daniel Tsiddon , 1997 , “ The Distribution of Human Capital and Economic Growth ,” Journal of Economic Growth , Vol. 2 ( March ), pp. 93 – 124 .
Galor , Oded and Joseph Zeira , 1993 , “ Income Distribution and Macroeconomics ,” Review of Economic Studies , Vol. 60 ( January ), pp. 35 – 53 .
Gottschalk , Peter and Timothy M. Smeeding , 1997 , “ Cross-National Comparisons of Earnings and Income Inequality ,” Journal of Economic Literature , Vol. 35 ( June ), pp. 633 – 87 .
Hanushek , E. , and L. Wobmann , 2010 , “ Education and Economic Growth”, International Encyclopedia of Education , Vol. 2 , pp. 245 – 52 .
Hanushek , E. , 2013 , “ Economic Growth in Developing Countries: The Role of Human Capital ”, Economics of Education Review , 37 , 204 – 212 .
Jenkins , Stephen , 2015 . “ World Income Inequality Databases: An Assessment of WIID and SWIID ,” Journal of Economic Inequality , Vol. 13 ( December ), pp. 629 – 71 .
Knight , John B. and Richard H. Sabot , 1983 , “ Educational Expansion and the Kuznets Effect ,” American Economic Review , Vol. 73 ( December ), pp. 1132 – 6 .
Kuznets , Simon , 1955 , “ Economic Growth and Income Inequality ,” American Economic Review , Vol. 45 ( March ), pp. 1 – 28 .
LaGarda , Guillermo and Kevin Gallagher , and Jennifer Linares , 2016 , “ Capital Openness and Income Inequality: A Cross-Country Study and The Role of CCT in Latin America ,” Munich Personal RePEc Archive papers (June) .
Li , Hongyi , Lyn Squire , and Heng-fu Zou , 1998 , “ Explaining International and Intertemporal Variations in Income Inequality ,” Economic Journal , Vol. 108 ( January ), pp. 26 – 43 .
Londoño , Juan Luis , 1996 , “ Poverty, Inequality, and Human Capital Development in Latin America, 1950–2025 ,” World Bank Latin American and Caribbean Studies 2 ( Washington : World Bank ).
Marin , A. and George Psacharopoulos , 1976 , “ Schooling and Income Distribution ,” Review of Economics and Statistics , Vol. 58 , pp. 332 – 8 .
Montenegro , Claudio and Harry Patrinos , 2014 , “ Comparable estimates of returns to schooling around the world ”, Policy Research Working Paper WPS 7020 . Washington, DC : World Bank Group .
Organisation for Economic Co-operation and Development (OECD) , 2008 , Growing Unequal? Income Distribution and Poverty in OECD Countries . Paris : OECD Publishing .
Psacharopoulos , George , 1977 , “ Unequal Access to Education and Income Distribution: An International Comparison ,” De Economist , Vol. 125 , pp. 383 – 92 .
Psacharopoulos , George and Maureen Woodhall , 1985 , Education for Development: An Analysis of Investment Choices , Oxford University Press , New York .
Ram , Rati , 1984 , “ Population Increase, Economic Growth, Educational Inequality, and Income Distribution: Some Recent Evidence ,” Journal of Development Economics , Vol. 14 ( April ), pp. 419 – 28 .
Ram , Rati , 1989 , “ Can Educational Expansion Reduce Income Inequality in Less-Developed Countries? ” Economics of Education Review , Vol. 8 ( 2 ), pp. 185 – 9 .
Sarel , Michael , 1997 , “ How Macroeconomic Factors Affect Income Distribution: The Cross-Country Evidence ,” IMF Working Paper WP_97_152 ( Washington : International Monetary Fund ).
Winegarden , C. R. , 1979 , “ Schooling and Income Distribution: Evidence from International Data ,” Economica , Vol. 46 , pp. 83 – 7 .
Schooling is taken here as a proxy for human capital. More generally, it can also be viewed as a proxy for other forms of human capital accumulation such as on-the-job training. For a discussion of the importance of schooling quality, see Barro (2013) and Hanushek and Wobmann (2010) . For a review of country-level estimates based on household survey data, see Montenegro and Patrinos (2014) .
Note that the total impact of education expansion on income inequality will depend on the relationship between average schooling and its dispersion. For instance, at the early stages of development, both the average level and dispersion of schooling are typically low. Expansion of education will initially tend to increase income inequality as a few more individuals gain higher education and earnings, but eventually lower the inequality of education and earnings as education becomes more widespread. The increase in the supply of high-education individuals will also tend to decrease the skilled wage premium and thus also income inequality. The net effect of education expansion on income inequality will therefore depend on the relative sign and magnitudes of these “composition” and “compression” effects, and is more likely to be positive at low levels of development and education attainment ( Knight and Sabot, 1983 ).
For reviews of past studies, see Psacharopoulos and Woodhall (1985 , pp264-70) and Ram (1989) .
The use of country fixed-effects estimators will control for time-invariant differences in income inequality measures across countries, e.g. due to the use of different household surveys based on incomes, expenditures or consumption. See Jenkins (2015) for a detailed and critical discussion of the issues that arise with the use of the WIID and SWIID income inequality databases.
This approach is typically seen as superior to that suggested by Anderson and Hsiao (1982) that includes the dependent variable lagged two periods as an independent variable in the differenced equation, which results in biased coefficients when the number of time periods is small.
Note also that the implicit assumption in Sys-GMM is that independent variables are predetermined (or weakly exogenous), depending only on past values of income inequality. For example, when a family decides on the choice of education in year t, it takes into account income developments up to this year and does not anticipate future income developments. This assumption can be tested with a Hausman test. The assumption of weak exogeneity also implies a lack of autocorrelation in the error terms. Testing for lack of second-order correlation in the difference equation is therefore equivalent to testing the validity of the weak exogeneity assumption. For this reason, in addition to the Hausman test, we also do the AR(2) test suggested by Arellano and Bond (1991).
Note that income inequality measures in both LAC and SSA are typically based on consumption or expenditure inequality, which tends to be lower than the income inequality measures typically used in ADV and EE. Therefore, the gap between ADV and other country groups may actually be higher.
Whereas in ADV and EE much of the expansion in education levels between 1950 and 2010 came from a decreasing share of the working population with primary education, in other regions, but especially in MENA and SSA, it has been driven by a decreasing share of the working population with no formal education.
We conducted a Wald test to assess the importance of keeping both education inequality and level in the regressions. We rejected the null of the test at 1 percent significance so decided to keep education level in the regressions.
Same Series
- Taking Down the Wall: Transition and Inequality
- Inequality and Growth: A Heterogeneous Approach
- Growing (Un)equal: Fiscal Policy and Income Inequality in China and BRIC+
- National Income and Its Distribution
- Inequality, Gender Gaps and Economic Growth: Comparative Evidence for Sub-Saharan Africa
- Rent Seeking and Endogenous Income Inequality
- Inequality of Opportunity, Inequality of Income and Economic Growth
- Measuring Income Inequality and Implications for Economic Transmission Channels
- Sharing the Growth Dividend: Analysis of Inequality in Asia
- Heterogeneity and Persistence in Returns to Wealth
Other IMF Content
- Fiscal Policy and Income Inequality
- Causes and Consequences of Income Inequality: A Global Perspective
- Income Inequality and Fiscal Policy
- Income Inequality and Fiscal Policy (2nd Edition)
- Chapter 1. Tackling Inequality
- Catalyst for Change: Empowering Women and Tackling Income Inequality
- Part I: Trends in Income Inequality and the Redistributive Role of Fiscal Policy
- For Whom the Bell Tolls: Climate Change and Inequality
- Chapter 1. Fiscal Policy and Income Inequality: An Overview
Other Publishers
Asian development bank.
- Trends and Drivers of Income Inequality in the Philippines, Thailand, and Viet Nam: A Decomposition Analysis
- ADB Economics Working Paper Series No. 529: Human Capital Spending, Inequality, and Growth in Middle-Income Asia
Inter-American Development Bank
- Inequality, Democracy, and Persistence: Is There a Political Kuznets Curve?
- The 1990s in Latin America: Another Decade of Persistent Inequality
- On Compulsory Voting and Income Inequality in a Cross-Section of Countries
- Foreign Aid, Income Inequality, and Poverty
- International Remittances and Income Inequality: An Empirical Investigation
- Education, Family Background and Racial Earnings Inequality in Brazil
- Can Financial Market Policies Reduce Income Inequality?
- Migration and Education Inequality in Rural Mexico
- Income Inequality and Economic Growth: Evidence from the American Data
- Capital Openness and Income Inequality: Smooth Sailing or Troubled Waters?
International Fund for Agricultural Development
- Asia's rural-urban disparity in the context of growing inequality.
International Labour Organization
- World of Work Report 2008: Income inequalities in the age of financial globalization
- Global Wage Report 2014/15: Wages and income inequality
The World Bank
- Informal Sector Heterogeneity and Income Inequality: Evidence from the Democratic Republic of Congo
- Persistent Misallocation and the Returns to Education in Mexico
- Top Incomes and the Measurement of Inequality in Egypt
- Egypt: Inequality of Opportunity in Education
- Global Inequality in a More Educated World
- Global income inequality: what it is and why it matters
- Mexico: two decades of the evolution of education and inequality
- Measuring education inequality: Gini coefficients of education
- Life satisfaction and income inequality
- Democracy and income inequality: an empirical analysis
Table of Contents
- Front Matter
- Income Inequality and Education Revisited
- View raw image
- Download Powerpoint Slide
International Monetary Fund Copyright © 2010-2021. All Rights Reserved.
- [66.249.64.20|81.177.182.159]
- 81.177.182.159
Character limit 500 /500
- Search Menu
- Author Guidelines
- Submission Site
- Open Access
- About International Studies Review
- About the International Studies Association
- Editorial Board
- Advertising and Corporate Services
- Journals Career Network
- Self-Archiving Policy
- Dispatch Dates
- Journals on Oxford Academic
- Books on Oxford Academic

Article Contents
Introduction, theoretical predictions, what the empirical evidence says, empirical challenges, acknowledgments.
- < Previous
On the Impact of Inequality on Growth, Human Development, and Governance

- Article contents
- Figures & tables
- Supplementary Data
Ines A Ferreira, Rachel M Gisselquist, Finn Tarp, On the Impact of Inequality on Growth, Human Development, and Governance, International Studies Review , Volume 24, Issue 1, March 2022, viab058, https://doi.org/10.1093/isr/viab058
- Permissions Icon Permissions
Inequality is a major international development challenge. This is so from an ethical perspective and because greater inequality is perceived to be detrimental to key socioeconomic and political outcomes. Still, informed debate requires clear evidence. This article contributes by taking stock and providing an up-to-date overview of the current knowledge on the impact of income inequality, specifically on three important outcomes: (1) economic growth; (2) human development, with a focus on health and education as two of its dimensions; and (3) governance, with emphasis on democracy. With particular attention to work in economics, which is especially developed on these topics, this article reveals that the existing evidence is somewhat mixed and argues for further in-depth empirical work across disciplines. It also points to explanations for the lack of consensus embedded in data quality and availability, measurement issues, and shortcomings of the different methods employed. Finally, we suggest promising future research avenues relying on experimental work for microlevel analysis and reiterate the need for more region- and country-specific studies and improvements in the availability and reliability of data.
La desigualdad es un desafío importante para el desarrollo internacional. Esto es así desde una perspectiva ética y debido a que la mayor desigualdad se percibe como perjudicial para los resultados políticos y socioeconómicos clave. Aun así, los debates informados requieren pruebas claras. Esta revisión contribuye estudiando la situación y ofreciendo un resumen actualizado del conocimiento actual sobre el impacto de la desigualdad de ingresos, específicamente en tres resultados importantes: (1) el crecimiento económico; (2) el desarrollo humano, con un enfoque en la salud y la educación como dos de sus dimensiones; y (3) la gobernanza, con énfasis en la democracia. Prestando especial atención al trabajo en economía que se desarrolla particularmente sobre estos temas, este ensayo demuestra que las pruebas existentes están mezcladas de alguna manera y argumenta a favor de promover el trabajo empírico en profundidad en todas las disciplinas. También señala las explicaciones para la falta de consenso que están integradas en la calidad y la disponibilidad de los datos, los problemas de medición y los defectos de los diferentes métodos empleados. Finalmente, sugerimos prometedoras vías de investigación para el futuro que dependen del trabajo experimental para el análisis a pequeña escala, y reiteramos la necesidad de realizar más estudios específicos de la región y el país, así como mejoras en la disponibilidad y la confiabilidad de los datos.
L'inégalité est un défi majeur du développement international. Il en est ainsi d'un point de vue éthique et parce qu'une plus grande inégalité est perçue comme allant au détriment des principaux résultats socio-économiques et politiques. Toutefois, des preuves claires sont nécessaires pour débattre en connaissance de cause. Cette analyse y contribue en faisant le bilan et en offrant une présentation à jour des connaissances actuelles sur l'impact de l'inégalité des revenus, en particulier sur trois résultats importants: (1) la croissance économique, (2) le développement humain, en se concentrant sur la santé et l’éducation en tant que deux de ses dimensions, et (3) la gouvernance, en mettant l'accent sur la démocratie. Cet essai accorde une attention particulière aux travaux en économie qui sont particulièrement développés sur ces sujets et révèle que les preuves existantes sont quelque peu mitigées et plaide pour un travail empirique plus approfondi dans toutes les disciplines. Il met également en évidence des explications du manque de consensus inhérent à la qualité et à la disponibilité des données, aux problèmes de mesure et aux lacunes des différentes méthodes employées. Enfin, nous suggérons des pistes de recherches futures prometteuses qui s'appuieraient sur des travaux expérimentaux pour l'analyse au niveau micro et nous réitérons la nécessité de réaliser davantage d’études spécifiques aux régions et aux pays et d'améliorer la disponibilité et la fiabilité des données.
Recent decades have witnessed sharp rises in inequality of income and wealth in many countries (though neither globally nor everywhere) as well as in the observed level of inequality of opportunities in access to basic services, such as health and education. Concern with these trends is paramount in Goal 10 of the Sustainable Development Goals approved by the United Nations General Assembly in 2015, aiming at “reducing inequality within and among countries.” The COVID-19 pandemic, which has both reflected and exacerbated inequalities, further spotlights this objective.
Pursuing this goal can obviously be justified from an ethical perspective. The case is also made in instrumental terms, with reference to potential negative effects of inequality on a variety of socioeconomic and political outcomes. The World Development Report (2006) drew attention to the implications of high levels of inequality for long-term development ( World Bank 2006 ). Indeed, economists in particular have long been concerned with the relationship between equity and efficiency 1 ; interestingly, the old classical view, contrary to the 2006 report, suggests a contradiction between equality and development.
Informed policy debate requires clear evidence on these impacts. This analytical essay provides a “state-of-art” on research on this big question. While recent reviews of the literature tend to focus on the impact of inequality on one specific outcome, we have a broader scope; we aim to bring new clarity to the debate by taking stock of the current knowledge on the effects on three important outcomes: (1) economic growth; (2) human development, with a focus on health and education as two of its dimensions; and (3) governance, with emphasis on democracy. While we start by highlighting how the various processes are connected, we address the impacts of inequality on these outcomes separately, developing an overview of the core arguments and underlying mechanisms, and of the existing evidence, with a particular focus on cross-country insights.
We draw in particular on the large and well-developed literature on these topics in economics while also taking key insights from other disciplines. 2 Our focus is on broad outcomes that are of particular importance for international development and that received great attention in studies examining the impact of inequality across disciplines. The effects of inequality on economic growth have been extensively debated in economics, the main disciplinary focus of this article. However, health and education—two important channels with high policy relevance—have also been the object of investigation in public health studies. Moreover, the field of political science has greatly contributed to the debate addressing the effects of inequality on political aspects, including those related to democratic governance. 3
Building on previous reviews focusing on specific outcomes (e.g., Voitchovsky 2011 ; Neves and Silva 2014 ; O'Donnell, van Doorslaer, and van Ourti 2015 ; Scheve and Stasavage 2017 ), but adopting the broader outlook of the seminal review by Thorbecke and Charumilind (2002) , this article provides an updated and comprehensive perspective on the consequences of inequality in three core areas of concern for international studies. 4
We combine the main theoretical arguments on the impact of inequality and underlying transmission channels in a general framework, providing a simplified view while emphasizing the connections between different processes. Overall, our review of an extensive body of work suggests there is no clear consensus emerging from the empirical evidence, and we argue there is room for additional in-depth work to uncover the effects through specific mechanisms of transmission. In particular, there is no consensus from the results of studies using reduced-form equations to examine the effect on growth, and less work has been dedicated to exploring the channels of transmission. Moreover, the negative link between inequality and secondary school enrolment is confirmed by the evidence, but further research is needed in terms of other education outcomes. The economic and public health literatures disagree on whether the negative effect of inequality on health is confirmed by the existing evidence, and there are mixed results emerging from political scientists for the effects of inequality on democracy and political participation. We advance the underlying explanations for this state of affairs, related to the challenges inherent in data quality and availability, measurement issues, and shortcomings of the different estimation methods employed, and suggest avenues for further research.
In the second section, we offer an outline of the main theoretical predictions of the effects of inequality on socioeconomic outcomes and on governance, presenting different channels of transmission. The third section follows the same structure and reviews the existing empirical evidence. We reflect on key empirical challenges of estimating the effects of inequality in the fourth section. The fifth section concludes.
Several theoretical explanations exist across disciplines for the effects of inequality on socioeconomic and political outcomes. Before we describe in more detail these channels of influence and the resulting outcomes, we highlight a broader set of arguments, which act as a roadmap for the rest of the section. Figure 1 provides a schematic overview.

Diagram with main outcomes of inequality
Source : Authors’ elaboration.
Starting from the left- to the right-hand side, the diagram represents different channels of transmission of the effects of higher levels of inequality, their intermediate effects, and the resulting positive or negative impact on our three outcomes of interest: growth, 5 human development, and democracy. We broadly divide these channels according to their underlying drivers: the poor, the population at large or the average, and the wealthy.
Overall, the diagram suggests that high inequality has predominantly harmful effects on our three outcomes of interest, according to theoretical explanations advanced in the literature. The dominant view then runs contra the expectations of the classical theorists, i.e., that inequality has a positive impact on growth, via savings and investment (shown at the top of figure 1 ). We highlight six main transmission channels.
First, inequality affects incentives for savings and investment and the overall level of institutional quality through its influence on policy making and increased political instability, and consequent effects on property rights and the regulatory framework. This has implications for growth both directly and indirectly via governance.
Second, by favoring private over public investment, inequality affects investment in public goods, namely health and education, with implications across the three outcomes. Third, and related, inequality results in underinvestment on human capital resulting from credit constraints, and high fertility, which affects education levels and overall economic growth.
Fourth, high taxation will be demanded by a well-endowed median voter and the likelihood of transition to and stability of democracy will also depend on the pressure for redistribution, which is higher with lower levels of equality. Moreover, and fifth, a small middle class will affect the demand not only for democracy but also for manufactures.
Finally, high levels of polarization will lead to weak social cohesion via their effects on social capital, as well as low trust and potential high levels in violent crime, which affect health directly and indirectly via investment in public health. Additionally, the concentration of power on the rich leads to increased probability of political violence and affects political engagement.
Some of these channels affect all of the outcomes. For instance, the effect through investment in public goods has detrimental effects on human development, and on growth and democracy. Moreover, the resulting polarization and social discontent, which increase the chances of political violence, again negatively impact the three outcomes. However, there is also some indication that, when it comes to growth, the effect might be ambiguous depending on the predominance of the effects of transmission mechanisms. The channel through savings (and investment) points to a potential positive effect, while the different effects through public investment, taxation, the structure of demand, imperfect credit markets, fertility, and social discontent suggest potential negative consequences for growth.
This section uncovers more details about these different theoretical predictions. It starts by introducing the main hypotheses advanced for the effects of inequality on growth. While the approach in this article considers the three outcomes separately, we recognize that they are not disjointed or orthogonal and refer to the links between them. Nevertheless, a full discussion of these interlinkages is beyond the scope of this article. As suggested in figure 1 and described in more detail below, some of these channels point to the impact of inequality on our remaining outcomes of interest, namely education and health, or governance. We return to them in the remaining two subsections, where we expand to consider the insights from other strands of literature.
How Inequality Affects Growth
An extensive literature examines the effects of inequality on growth, 6 highlighting multiple channels of transmission. 7 The early studies, referred to as the classical approach, argued that there is a positive effect of inequality on growth, explained via savings or incentives. However, subsequent work questioned this view, challenging some of its assumptions and proposing different channels of influence. Most of this work has predicted a negative effect of inequality. We briefly outline these channels in the next paragraphs and refer to Bourguignon (2015) , Neves and Silva (2014) , and Voitchovsky (2011) for complementary detail and reviews. 8
High inequality is growth enhancing
We start by drawing attention to the view of classical economists on income inequality, according to which there was a contradiction between equality and development (for a discussion of the trade-off between efficiency and equity, see Thorbecke 2016 ). Adam Smith defended that inequality had benefits based on arguments of (1) “trickle-down effects”—the increase in wealth will eventually benefit the poor, (2) incentive effects—inequality is necessary to encourage competition and to provide incentives for innovation, and (3) social stability—the different ranks in wealth distribution ensure peace and stability in society ( Walraevens 2021 , 3–6). The famous Kuznets curve ( Kuznets 1955 ), shaped like an inverted U-relationship between growth and inequality (as per capita income increases), seemed to reinforce this view. 9
Developed in the 1950s and 1960s, the so-called classical approach followed a similar line of thinking, based on arguments related to savings and incentives. The prominent work by Kaldor (1956) suggests a positive link between inequality and growth via saving rates, based on the assumption that the higher the level of income, the higher is the marginal propensity to save ( Aghion, Caroli, and García-Peñalosa 1999 , 1620). At the core of this assumption that the rich have a higher marginal propensity to save relative to the poor are two hypotheses: (1) consumption smoothing cannot occur unless the subsistence level of consumption is achieved, and therefore the poor cannot save, and (2) the possibility to save is conditioned by the previous generations, which leads to a concentration of savings in rich households ( Thorbecke and Charumilind 2002 , 1483).
Under this assumption, the redistribution of resources toward the rich leads to higher savings, which, in turn, improves growth via investment. This link is particularly important if one considers limited borrowing possibilities, initial setup costs, and the large investments involved in risky and high-return opportunities ( Aghion, Caroli, and García-Peñalosa 1999 , 1620; Voitchovsky 2011 , 558). Big investment projects involve large sunk costs, and therefore investment relies on the concentration of wealth in individuals to be able to afford them.
A second argument drew on the role of incentives and on the trade-off between efficiency and social justice mentioned earlier ( Aghion, Caroli, and García-Peñalosa 1999 , 1620). At the microlevel, in a simple moral hazard model, if output depends on unobserved effort, then setting a constant reward (in the form of wage) discourages effort, whereas linking the reward to output can be inefficient due to agents’ risk aversion. The same argument maintains at the aggregate level, assuming identical agents and/or perfect capital markets. As explained by Aghion, Caroli, and García-Peñalosa (1999 , 1620), redistribution will have a direct negative effect on growth as well as a negative indirect effect through the reduction in the incentives to accumulate wealth (resulting from redistribution through income tax).
High inequality has a negative effect on growth
Credit market imperfections and fertility.
The effects of inequality on growth via credit market imperfections and via fertility are linked by their focus on the circumstances of the poor and on human capital investment ( Voitchovsky 2011 ). The first channel addresses the impact of credit imperfections on investment decisions. If one considers the high fixed costs associated with, for instance, education, limitations on the access to credit may lead to underinvestment in human capital, which implies a negative impact on growth ( Neves and Silva 2014 , 3). This was the argument resulting from the Galor and Zeira (1993) model. Assuming that credit markets are imperfect and that investment in human capital is indivisible, they conclude that the distribution of wealth has an impact on aggregate investment in human capital and therefore on growth, both in the short and in the long run.
The reasoning behind the link between inequality and growth through fertility was similar. Poor families might not have the resources to invest in their children's education and, thus, their income depends on having bigger families; for richer families, it might be optimal to invest more in education and, consequently, to have fewer children ( Gründler and Scheuermeyer 2018 , 295). In this line of thinking, de la Croix and Doepke (2003) argued that a high fertility differential between the rich and the poor lowered average education. Thus, inequality leads to lower levels of human capital accumulation via the increased fertility differential and, therefore, to lower growth.
Taxation and regulatory policies
Seminal work by Alesina and Rodrik (1994) as well as Persson and Tabellini (1994) pointed to a negative link between inequality and growth through government expenditure and taxation, combining endogenous growth theory with political economy insights. They proposed two different mechanisms that Perotti (1996 , 151) termed “political” and “economic,” respectively. The Alesina and Rodrik (1994) model drew on the median voter theorem and considered tax revenues equally distributed among all individuals. Given that the tax rate is proportional to income, individuals with a lower share of capital income (relative to labor income) prefer higher taxes. Thus, the more equitable the distribution in the economy, the better endowed is the median voter, and the lower the equilibrium level of taxation. A lower rate of tax corresponds to a higher growth rate, which led them to conclude that there is an inverse relationship between inequality and subsequent economic growth.
Persson and Tabellini (1994) reached the same conclusion considering the role of incentives for productive accumulation and for growth. According to them, the incentives necessary for private savings and investment rely on individuals’ ability to “appropriate privately the fruits of their efforts” ( Persson and Tabellini 1994 , 600), which are in turn influenced by tax and regulatory policies. Inequality gives rise to policies that do not protect property rights or allow full appropriation of returns to investment and is therefore associated with lower economic growth.
Still, this result was defied by Li and Zou (1998) . They offered a more general framework than that proposed by Alesina and Rodrik (1994) , considering that government spending could be directed not only to production services—which entered the production function—but also to consumption services—which entered the utility function. Adding this extension, they showed that a more equal distribution could lead to lower growth via higher taxation and that the effect of income inequality on growth is, therefore, ambiguous.
The view outlined in Alesina and Rodrik (1994) and in Persson and Tabellini (1994) was also challenged by an alternative perspective suggesting that redistributive policies might also have a positive effect on growth in the presence of imperfect credit and insurance markets and that the popular support for these policies decreases with inequality ( Bénabou 2000 ). When combined, these two mechanisms could lead to multiple steady states, while the correlation with growth depends on the balance between incentive distortions and credit constraints ( Neves and Silva 2014 , 4). Voitchovsky (2011 , 556) lists the criticism toward the median voter argument and highlights how the channel through redistribution does not gather consensus.
The structure of demand
Zweimüller (2000) described the role of redistribution on growth through innovation. Building on the assumption of hierarchical preferences, the distribution of income affects the structure of demand: poor people spend mainly on basic needs whereas rich people spend on luxury goods. According to the author, inequality affects growth through its effect on the time path faced by an innovator. When a new and expensive good is introduced in the market, only rich consumers can afford it, until the increasing demand drives the price–wage ratio down (due to economies of scale), opening up the market to mass consumers ( Voitchovsky 2011 , 557). The optimal consumption levels of those affected by redistribution dictate the overall effect of changes in income inequality on long-run growth ( Zweimüller 2000 ). An earlier study by Murphy, Shleifer, and Vishny (1989) had already highlighted the importance of the middle class to the consumption of domestic manufactures and, therefore, to industrialization.
Sociopolitical instability and rent seeking
Another group of studies suggested a link between inequality and growth through sociopolitical instability, drawing attention to the effects on property rights. According to Alesina and Perotti (1996) , social unrest—resulting from social discontent caused by income inequality—can lead to an increasing probability of political violence as well as policy uncertainty and threats to property rights, which, in turn, have a negative impact on investment and thus on growth. Keefer and Knack (2002) claimed that income inequality leads to instability in government policies, namely those related to security of property rights, which affects the decisions of economic actors, and consequently slows the rate of growth. Relatedly, the Glaeser, Scheinkman, and Shleifer (2003) model showed a detrimental effect of inequality on property rights through the subversion of political regulatory and legal institutions by the rich for their own benefit.
The effect depends
Finally, we highlight contributions suggesting that different mechanisms might be present at different points. Galor and Moav (2004) proposed a unified theory between the credit market imperfections and the saving rate channels described earlier. According to them, the positive effect of inequality on growth suggested by classical theories corresponded to early stages of industrialization when physical capital accumulation is the primary driver of economic growth. However, at later stages, human capital accumulation becomes the main determinant of growth and credit constraints are largely binding, which explains the negative link between inequality and growth through credit market imperfections. As credit constraints become less binding due to wage increases, the aggregate effect of income distribution on growth is less significant.
A decade later, Halter, Oechslin, and Zweimüller (2014) presented a parsimonious theoretical model that takes into account both a short-term and a long-term effect of asset inequality. According to them, the short-term effect is positive and it occurs through an economic channel, whereas the long-term effect is negative and stems from a political economy channel.
How Inequality Affects Education and Health
Inequality can have both positive and negative effects on education.
While the literature examining the effects of education on inequality is extensive, the same is not true for studies looking at the other direction of causality. We distinguish between the arguments on the effects of inequality through expenditure on education and through school enrolment and attainment.
The provision of education depends on the willingness of citizens to redistribute resources via taxation, in line with Alesina and Rodrik (1994) and Perotti (1996 ). According to this political economy mechanism, increasing inequality will lead to lower availability of resources, as the rich will prefer not to contribute to public education, favoring private schools ( Mayer 2001 , 5). 10 Gutiérrez and Tanaka (2009) modeled the effect of inequality on school enrolment, and the preferred tax rate and expenditure per student focusing on parents’ decisions in developing countries. According to the authors, beyond a certain level of inequality, there is no longer support for public education. The model shows that, when considering the fact that parents can make a choice of sending their children either to work or to private or public schools, high inequality results in exiting public education, which has implications for the tax rate and expenditure per student. 11
According to the credit market imperfections’ channel discussed in section “How Inequality Affects Growth,” inequality creates obstacles in terms of access to education. In the presence of imperfect credit markets, the distribution of wealth affects the aggregate investment in human capital ( Galor and Zeira 1993 ; García-Peñalosa 1995 ). Additionally, inequality can affect enrolment by determining the number of poor who are able to substitute the return of child labor for school attendance ( Gutiérrez and Tanaka 2009 , 56). The Tanaka (2003) model shows that in contexts of high inequality, there is low support for public provision of schooling, which, in equilibrium, leads to a higher level of child labor.
The expected returns to the family from schooling will also affect the demand for education, as educated children are likely to have higher future income ( Birdsall 1999 , 17). If inequality is induced in part by increased returns to schooling, then there will be an incentive for children to stay in school and one could expect a positive relationship between an increase in inequality and educational attainment ( Mayer 2001 ; Thorbecke and Charumilind 2002 ; Dabla-Norris et al. 2015 ). 12
Inequality negatively affects health
The interest in understanding how income inequality affects health has instigated a broad range of work both in economics and in the fields of public health and sociology, 13 and different hypotheses are available. Generally, they suggest that inequality negatively affects health. Following O'Donnell, van Doorslaer, and van Ourti (2015) and Leigh, Jencks, and Smeeding (2011) , we distinguish between hypotheses that imply that the health of all individuals is affected and those that do not require that the health of every individual in society is under threat. 14
The first group of hypotheses proposes three different channels: public goods provision, social capital, and violent crime. 15 The effect through public goods provision can be negative or positive ( Leigh, Jencks, and Smeeding 2011 , 390). There will be a negative effect if inequality causes a reduction in the average value of publicly provided goods due to more heterogeneous preferences or if it enables the rich to acquire more political influence and, consequently, to pressure for a reduction in public spending on health. However, it can also be positive, given that as inequality increases among voters, the median voter will tend to support spending on health.
The effect through social capital builds on the assumption that income inequality leads to decreased social cohesion and, therefore, affects health through social 16 and psychosocial support, mechanisms of informal insurance, and diffusion of information ( O'Donnell, van Doorslaer, and van Ourti 2015 , 1501). Low trust can lead to disbelief about the improvements in health via public spending and links to higher mortality via smaller friendship networks as well ( Leigh, Jencks, and Smeeding 2011 , 390). Finally, although only a small percentage of deaths in developed countries results from violent crime, Leigh, Jencks, and Smeeding (2011 , 389) highlight the potentially larger secondary effects via increased stress about experiencing crime in the future. 17
In the second group of hypotheses, health depends on income at the individual level. The Wagstaff and van Doorslaer (2000) seminal review describes different interpretations. First, the absolute income hypothesis, which was also termed the “income artefact” hypothesis, suggests that the observed correlation between inequality and health is a result of the concave relationship between income and health; that is, the health gains of an additional unit of income are diminishing in an individual's income level. The term “artefact” applies to the fact that a redistribution of income leads to an increase in average population health even though there is no effect on the health of any individual, given their income. Second, the relative income hypothesis builds on the idea that psychosocial effects that result from individuals comparing their income with that of others (the mean income of the population or the community) affect health. Third, the deprivation hypothesis is a variation of the relative income hypothesis, and it argues that the crucial aspect is the extent of deprivation measured by the income gap. Fourth, and related, the relative position hypothesis states that what is important is the position of the individual in the income distribution.
How Inequality Affects Democratic Governance
In this section, we delve more deeply into the relationship between inequality and governance outcomes, democracy in particular, which have attracted considerable attention, especially within political economy and political science (see Bermeo 2009 ; Karl 2000 ). We start by focusing on the effects on democratic stability and democratic transition and then zoom in on the effects on political participation.
First, we refer back to the link between inequality and growth through political instability and social conflict described in section “High inequality has a negative effect on growth”. As highlighted by Fukuyama (2011 , 84), “[a] more likely reason why inequality is bad for growth is directly political: highly unequal countries are polarized between rich and poor, and the resulting social conflict destabilizes them, undermines democratic legitimacy, and reduces economic growth.” The summary in Thorbecke and Charumilind (2002 , 1486) suggests two main mechanisms: the relative deprivation hypothesis and resource mobilization. According to the first, discontent resulting from the gap between individual expected and achieved well-being leads to collective political violence. Inequality might deepen the grievances of certain groups or reduce the opportunity cost of engaging in violent conflict ( Dabla-Norris et al. 2015 , 9). Nevertheless, the second mechanism points to the ability of dissident groups to organize themselves as the key element.
The theoretical literature largely suggests negative effects of inequality on the likelihood of transition to and stability of democracy. It attributes an important role to democratic values and access to education, which are more likely to characterize citizens and the situation in equal societies, and to the middle class, which is more likely to promote tolerance and avoid extremist positions ( Houle 2015 , 145).
Two of the most prominent arguments for the link between inequality and democracy were presented in Boix (2003) and Acemoglu and Robinson (2006) . 18 The former argues that increasing levels of economic equality lead to a higher probability of democracy through redistribution. According to the theoretical predictions, the pressure for redistribution from the poor decreases with higher levels of equality, which means that a turn to democracy would be less costly for the holders of the most productive assets; that is, the payment of tax is less costly than repression.
The Acemoglu and Robinson (2006) predictions indicate a nonlinear, inverted U-shaped relationship. On the one hand, greater intergroup inequality increases the appeal of a revolution for citizens to increase their share in the income of the economy, thus increasing the likelihood of democracy. On the other hand, higher inequality also means higher aversion to democracy by elites as their tax burden is greater, thus discouraging democratization. Accordingly, the authors suggested that, for high levels of equality, there is no incentive for citizens to challenge the system and the interests of the elites are preserved. In societies with high levels of inequality, citizens try to rise up against the system, but this meets great repression from the elite, leading to a repressive non-democracy or a revolution, in certain cases. Therefore, the likelihood of democracy is higher for middle levels of inequality.
However, Houle (2009) highlighted three problems with these theories. First, they do not apply to transitions that are driven from above (e.g., from intra-elite competition). Second, the net effect of inequality is ambiguous because it makes redistribution more costly for the elites but, at the same time, it increases the population's demand for regime change. Finally, they ignore collective action problems and the challenges of mobilizing the population. More recently, Ansell and Samuels (2010) departed from Boix (2003) and Acemoglu and Robinson (2006) and proposed a contractarian approach that placed the focus on the citizens’ demand for protection against expropriation. According to these authors, democracy emerges from land equality and income inequality.
We briefly refer to a related group of studies examining the link from inequality to institutional quality and refer to Chong and Gradstein (2019) for details. Chong and Gradstein (2007 , 2019 ) argue that there is double causality: while inequality leads to subversion of institutions through the political power of the elite, poor institutional quality also causes a higher level of inequality. Furthermore, Kotschy and Sunde (2017) have proposed that inequality interacts with political institutions in shaping institutional quality. Some have also suggested that a link exists between inequality and corruption, via self-reinforcing mechanisms and social norms (e.g., Jong-sung and Khagram 2005 ) as well as via low trust (e.g., Rothstein and Uslaner 2005 ). 19
Finally, a strand of studies in political science has argued that there is a link between inequality and political participation. As reviewed in Solt (2008) , the theoretical predictions lead to different possible outcomes of economic inequality on political engagement 20 : a negative effect, a positive effect, or an effect that depends on the level of income of the individual. The first outcome is a result of the concentration of power: societies that are more unequal have a higher concentration of power, which has implications for how the issues that separate the rich from the poor are addressed in the political sphere. The rich will have a lower need to engage in the political process whereas the poor will feel removed from politics. The prediction of a positive effect results from the fact that the divergence in the views of the rich and the poor will be more apparent in societies with higher inequality, which should lead to higher participation in the political process. Finally, the last prediction hinges on the fact that political engagement entails the use of resources. Thus, with higher levels of inequality, one should expect greater engagement from the rich, who have more resources available, and lower political engagement from the poor. 21
We now move on to discuss the main insights from empirical analyses following the structure of the previous section. Although we focus here on cross-country analysis, which makes up a significant part of the evidence base, we also refer to studies examining these links at the regional level, especially in the United States.
Direct link
where |$g$| is the average annual growth rate, frequently measured as the log difference of gross domestic product (GDP) per capita; INEQ is a measure of income inequality (usually the Gini coefficient); Z m is a set of other variables commonly used in standard growth regressions; and u is the usual error term. This was then estimated, typically using basic ordinary least squares. To avoid reverse causation, inequality was measured at the beginning of the time span for growth, which usually considers a period of twenty to thirty years, and in some cases, authors employed instrumental variables to address endogeneity concerns.
Summary of results from selected empirical work testing the link between inequality and growth
Notes : DS, Deininger and Squire (1996) ; LIS, Luxemburg Income Study; OLS, ordinary least squares; 2SLS, two-stage least squares; WLS, weighted least squares; 3SLS, three-stage least squares; LSDV, least squares dummy variable; FE, fixed effects; RE, random effects; Sys-GMM, system GMM; Diff-GMM, difference GMM.
Source : Authors’ elaboration, inspired from Cingano (2014) and Neves and Silva (2014) .
The aim was to estimate the coefficient of the income inequality variable δ , and most of these studies found a negative effect of inequality on growth. Persson and Tabellini (1994) obtained evidence for this effect using historical panel data and postwar cross-sectional analysis. Both the studies by Alesina and Rodrik (1994) and Clarke (1995) confirm this relationship using data from, among others, Jain (1975) and Lecaillon et al. (1984) . Clarke (1995) showed that this was robust to different measures and empirical specifications.
Given the challenges imposed by scarce data, some authors turned to an analysis between states in the United States. Partridge (1997) tested the robustness of the Persson and Tabellini (1994) findings, and the results suggested a positive link between inequality and subsequent growth when considering either the Gini coefficient or the share of income of the middle quintile. 23 Using tax data at the state level for the period 1940–1980, Panizza (2002) warned that both the data and the methodology used led to significant differences in the estimated coefficients for the effect of inequality on growth.
While the quality and reliability of the data are important challenges pertaining to early studies ( Knowles 2005 ), the introduction of an improved and expanded dataset by Deininger and Squire (1996) led to a surge in new studies using panel estimators. In contrast with previous work, these studies found a positive link between inequality and growth. Li and Zou (1998) showed that the coefficient for lagged Gini has a positive sign and is significant in most growth regressions. Forbes (2000) confirmed this result using similar data and generalized method of moments (GMM) estimators. 24 Still, using the same dataset, Deininger and Squire (1998) found a negative effect of initial income inequality on growth, although the coefficient lost significance once they add regional dummies to the specification.
Offering a starting point to reconcile the differing views, some studies have argued that the relationship between inequality and growth depends on other factors. According to Barro (2000) , the effect of inequality on growth depends on the level of income of the country: panel evidence suggests growth-enhancing effects of inequality in richer countries (GDP per capita: above $2,000, 1985 US dollars) and negative effects in poorer countries (below $2,000). Moreover, Banerjee and Duflo (2003) have raised concerns about the functional form used in the literature, arguing against using a linear specification for the relationship between inequality and growth. Their empirical work suggests an inverted U-shaped function between changes in inequality and lower future growth rates. Using a small sample of industrialized countries, Voitchovsky (2005) showed empirical support for the hypothesis that the profile of inequality influenced its relationship with growth: top-end inequality seems to have a positive effect and bottom-end inequality a negative effect.
The debate has continued in the literature ever since. Cingano (2014) lends support to a negative effect of inequality on growth using data from the Organization for Economic Co-operation and Development (OECD) income distribution dataset. Additionally, the author suggests that reducing inequality by focusing on income disparities at the bottom of the income distribution has a greater positive effect on growth than by focusing on the top of the distribution. The Castelló-Climent (2010) results concur with this when considering the full sample of countries, but the results also find support for the argument of a differentiated effect according to the level of development. Halter, Oechslin, and Zweimüller (2014) argue that there is a time dimension to the link between inequality and growth, showing a positive coefficient for the current Gini coefficient and a negative coefficient for lagged Gini.
Some studies have used data from an additional dataset proposed by Solt (2009) , the Standardized World Income Inequality Database (SWIID). Yet, results also mirror the lack of consensus of earlier work. Applying system GMM, work from the International Monetary Fund finds a robust negative effect of inequality on growth ( Ostry, Berg, and Tsangarides 2014 ; Berg et al. 2018 ). While Gründler and Scheuermeyer (2018) concur with this result, Jäntti, Pirtillä, and Rönkkö (2020) raise concerns about the results in Berg et al. (2018) , resulting from the use of the SWIID dataset. El-Shagi and Shao (2019) criticize previous studies using system GMM and argue for the advantages of using a least-squares dummy variable estimation instead. In contrast, their results show a positive effect of inequality on growth over the medium term, primarily driven by market-based inequality.
Barro's (2000) view that the effect depends on the level of development in the country, confirmed in later analysis by the same author using the WIID dataset ( Barro 2008 ), has also been verified in some recent work. Gründler and Scheuermeyer (2018) see a negative and significant marginal effect of net inequality on growth in poor economies, which is, however, nonsignificant in high-income countries. 25
Channels of transmission
As discussed in section “How Inequality Affects Growth,” the theory proposes different channels through which inequality may affect growth. Although these specific mechanisms have received less attention in empirical work, we highlight the main findings, also summarized in table 2 .
Summary of empirical evidence on the different channels linking inequality and growth
Starting with the savings channel, while there is evidence of a positive link between inequality and personal savings when using household micro-data, studies based on cross-country aggregate data have found mixed results (see references in Thorbecke and Charumilind, 2002 ). Barro (2000) found that the investment ratio does not depend significantly on inequality. The channel via market imperfections and borrowing constraints found support in Deininger and Squire (1998) , who added that the effect through the investment in human capital seems more important than that via physical capital, as well as to some extent in Perotti (1996 ). 26 This channel also suggests that asset inequality matters for growth ( Ravallion 2001 , 1810), shown in both Birdsall and Londoño (1997) and Deininger and Olinto (2000) .
Moreover, there is published support for the channels related to sociopolitical instability ( Perotti 1996 ). Using data from a sample of seventy-one countries over the period 1960–1985, Alesina and Perotti (1996) found that a wealthy middle class is associated with lower levels of political instability, conducive to higher investment. Keefer and Knack (2002) showed evidence of a negative effect of inequality on growth and suggested that property rights are an important channel for this relationship.
Perotti (1996 ) confirmed the link between inequality and growth via fertility. Testing the same hypothesis, de la Croix and Doepke (2003) used Deininger and Squire's (1996) improved dataset and showed that the negative and significant effect of initial inequality on subsequent growth does not survive the inclusion of the differential fertility variable, which is negative and significant. They interpret this as meaning that the differential fertility is an important factor explaining the link between inequality and growth.
The fiscal policy channel received less support by Perotti (1996 ) while Persson and Tabellini (1994) also obtained coefficients with the expected sign but statistically insignificant for the links from inequality to redistributive policies and from redistribution to growth. Sylwester (2000) showed results from cross-country analysis that indicated that higher inequality is associated with higher subsequent expenditures for public education relative to GDP, which in turn has a negative effect on current growth but a long-term positive impact.
Recent studies have shown evidence that corroborates the theoretical effects via human capital accumulation ( Berg et al. 2018 ), via credit market imperfections ( Gründler and Scheuermeyer 2018 ), and via fertility ( Berg et al. 2018 ; Gründler and Scheuermeyer 2018 ) as channels through which inequality affects growth. Using data from twenty-one OECD countries over the period 1870–2011, Madsen, Islam, and Doucouliagos (2018) find support for the hypothesis that income inequality affects growth through different channels, namely savings, investment, education, and ideas production. Additionally, they concur with the arguments on differentiated effects. Although the negative impacts are significant in financially underdeveloped countries, there is little effect of inequality on the four outcomes in countries with highly developed financial markets.
Education and Health
In a recent paper, Castells-Quintana, Royuela, and Thiel (2019) estimated the effects of the Gini coefficient on the human development index (HDI) and found a negative effect in the long run, whereas in the short run the results change for different components of the index: a positive effect on income and a negative effect on educational outcomes. Moreover, they concur with the aforementioned studies that found distinct effects depending on the level of development. We are not aware of any other studies pursuing a similar analysis for the HDI, but in the remainder of this section, we discuss the empirical results on the link between inequality and education and health. We summarize the main conclusions in table 3 .
Summary of empirical evidence on the different hypotheses on the effects of inequality on education and health
Although there is an extensive body of empirical literature examining education as a determinant of income inequality, the evidence on the link from income inequality to educational outcomes is scarcer ( Thorbecke and Charumilind 2002 , 1488; Gutiérrez and Tanaka 2009 , 56). However, there is evidence that income inequality is reproduced in inequality in education, both in terms of achievements in primary and secondary school and in terms of access to tertiary education (see Buchmann and Hannum 2001 and references in Stewart 2016 ).
Regarding the links proposed in the theoretical work reviewed in the previous section, Sylwester (2000) reported a positive link between inequality and public expenditures on education. Considering the demand side, some studies have found a negative link between inequality and secondary school enrolment. Flug, Spilimbergo, and Wachtenheim (1998) and Easterly (2007) used cross-country analysis, while Esposito and Villaseñor (2018) used data from the 2010 Mexican Census. The study by Madsen, Islam, and Doucouliagos (2018) shows a negative impact of inequality on the combined primary, secondary, and tertiary school enrolment rate in financially underdeveloped countries (using a sample from OECD). Concurring with these findings, Berg et al. (2018) show a negative correlation between inequality and human capital, measured as the average years of primary and secondary schooling. Checchi (2003) provided support for the link between inequality and growth via borrowing constraints and showed evidence of a negative effect of inequality on access to secondary education. 27 Finally, using data from the United States for the period 1970–1990, Mayer (2001) found that the increase in inequality aggravates the gap in educational attainment between rich and poor children.
Given that the literature is extensive and stems from different fields of literature (including, public health), we summarize the main conclusions from different reviews, which distinguish between aggregate level and multilevel studies as well as cross-country and within-country empirical analyses. 28 Wagstaff and van Doorslaer (2000) highlighted that studies at the population level are limited in what they can reveal about the effects on individual health and that data at the individual level are required to disentangle the effects of the different hypotheses described in section “Inequality negatively affects health.” Still, existing evidence on these different channels remains inconclusive.
Lynch et al. (2004) found weak support for a direct effect of income inequality on health, although inequality contributes directly to some health outcomes (e.g., homicides). Furthermore, they underlined that the reduction of income inequality via income rises for the more disadvantaged contributes to improved health of these individuals and increases average population health. Rowlingson (2011) concludes that there is some evidence of an independent effect on health and social problems, but in line with Subramanian and Kawachi (2004) , also highlights the lack of consensus in the results and the need for further work. Still, from a systematic review of 155 published peer-review studies, Wilkinson and Pickett (2006) concluded that there is a link between greater income inequality and poorer health. Almost ten years later, the authors provided further support for the existence of a causal link between income inequality and health and reinforced their argument of the size of status and social class differences as an important mechanism ( Pickett and Wilkinson 2015 ).
The conclusions from the economics literature have pointed to no evidence of a causal relationship ( Nolan and Valenzuela 2019 ). From a detailed review of the literature, Deaton (2003 , 150) argued that “the stories about income inequality affecting health are stronger than the evidence” and that there is no robust evidence showing that income inequality in itself is an important determinant of population health, although it had effects through poverty. The review in Leigh, Jencks, and Smeeding (2011) concurred. However, they warned that given the data challenges and the limitations of the methods used to test the link between inequality and health, one should not jump to definite conclusions. Focusing on morbidity and mortality, the comprehensive review of empirical literature by O'Donnell, van Doorslaer, and van Ourti (2015) concludes that even though population health is negatively associated with income inequality, there is little evidence to support the hypothesis of a negative impact of income inequality on health.
We start this section by noting that the focus on voting underlying the political economy mechanism linking inequality and growth suggests that the effects should be observed in democracies ( Houle 2015 , 143). Thus, some of the early empirical literature on the relationship between inequality and growth also tested whether this effect was dependent on the regime type (e.g., see Alesina and Rodrik 1994 ; Persson and Tabellini 1994 ; Clarke 1995 ; Perotti 1996 ; Deininger and Squire 1998 ).
The results were mixed. Persson and Tabellini (1994) suggested that the negative link between inequality and growth is only present in democracies and that the transmission channel through government redistributive policies should be further investigated. However, Perotti (1996 ) counterargued that, although the data showed a stronger relationship between equality and growth in democracies, the effect of the democracy variable did not appear to be robust. Further criticism was advanced by Knack and Keefer (1997) , who, after some regime reclassification and deletion of doubtful observations, concluded that there is no evidence of a differential effect of inequality on growth in democracies and non-democracies. Østby (2013) and Stewart (2016) argued that there is compelling evidence for the link between horizontal inequality (i.e., inequality among groups) and civil conflict as well as other forms of group violence. However, more recent reviews suggest that the evidence on the link between inequality and political violence is mixed ( Lengfelder 2019 ).
We now turn to what the empirical evidence on the government outcomes described in section “How inequality affects democratic governance” shows, and summarize the main conclusions in table 4 . Using data from two panels on the periods 1950–1990 and 1850–1980, Boix (2003) showed empirical evidence for a positive link between equality (proxied by an adjusted Gini coefficient) and democratization and, particularly, democratic consolidation. In an extension of this analysis, Boix and Stokes (2003) concluded that economic equality, proxied by farm ownership (distribution of agricultural property) and literacy rates (quality of human capital), has a positive effect on both the probability of a democratic transition and the stability of democracy.
Summary of empirical evidence on the effects of inequality on different governance outcomes
Others found low support for a significant link between the two (e.g., Bollen and Jackman 1985 ). 29 Barro (1999) showed a negative, but only marginally significant coefficient for the effect of inequality on democracy, proxied as electoral rights and civil liberties, for the period 1972–1995. However, when entered alongside the share of income accruing to the middle class, the coefficient is nonsignificant. The empirical analysis in Houle (2009) went against previous results on the negative link between inequality and democracy and showed a weak positive and nonsignificant relationship. Using the capital share of the value added in the industrial sector as a measure of inequality to overcome the data limitations in previous studies, the author also did not find support for Acemoglu and Robinson (2006) ’s inverted U-shaped relationship but rather for a weakly U-shaped one.
More recently, Haggard and Kaufman (2012) used causal process observation to examine the association between inequality and transitions to and from democratic rule and found limited evidence supporting the link via distributive conflict between elites and masses. Additionally, the evidence in Scheve and Stasavage (2017) does not support the hypothesis of a link between wealth inequality and democracy. Dorsch and Maarek (2020) offer an explanation for the abundancy of null results found for the link between inequality and democratization, showing that higher levels of inequality are associated with higher probabilities of democratic improvements following economic downturns (“windows of opportunity”). However, following growth periods, the effect of inequality is null or small and negative.
Considering a broader approach to governance, we briefly refer to the literature linking inequality and institutional quality. 30 Both Easterly (2007) and Chong and Gradstein (2007 ) tested the causal relationship between these variables using an instrumental variables approach and system GMM methods, respectively, and found support for the effect of inequality on institutions. More recently, Kotschy and Sunde (2017) showed evidence of the importance of equality as a determinant of the effect of democratic institutions on institutional quality, measured by an index of economic freedom and an indicator of civil liberties. 31 It has also been shown that countries with more income inequality have more corruption ( Jong-Sung and Khagram 2005 ), and, in particular, survey evidence links perceptions of corruption and inequality to lower political trust ( Uslaner 2017 ).
Finally, there is evidence from advanced industrial democracies of a negative link between inequality and political participation ( Lengfelder 2019 ). Solt (2008) showed a negative effect of economic inequality on political engagement, namely political interest, the frequency of political discussion, and participation in elections among all citizens except the richest, using data from advanced industrial countries. Using cross-sectional data from OECD countries and within-country data for Germany and a range of methods, the recent study by Schäfer and Schwander (2019) finds support for the negative link between economic inequality and political participation. Relatedly, empirical work suggests that economic inequality harms support for democracy (e.g., Andersen 2012 ; Krieckhaus et al. 2014 ) and political inequality (e.g., Houle 2018 ). Still, there appears to be limited evidence of an effect of inequality on electoral turnout ( Stockemer and Scruggs 2012 ; Cancela and Geys 2016 ).
The lack of consensus in the literature, especially about the effect of inequality on growth, is notable. What explains this divergence, and what can be done to contribute to the existing knowledge? In this section, we discuss the key empirical challenges of estimating the effects of inequality: data quality and availability, conceptual and measurement issues, and the methodological difficulties of dealing with confounding variables and endogeneity.
Data quality and availability
Early studies drew on secondary datasets provided, for example, by the World Bank ( Jain 1975 ) or the International Labour Office ( Lecaillon et al. 1984 ). The expanded dataset proposed by Deininger and Squire (1996) was crucial in opening possibilities for panel methods. Additionally, databases offering secondary data compilations on income inequality provided by the United Nations University World Institute for Development Economics Research, WIID (based on household surveys), and SWIID, developed by Solt (2020) and resulting from multiple imputations of the WIID data, have been frequently used in empirical studies. The World Inequality Database ( WID.world 2017 ) has emerged as an additional database providing data on income shares captured by top income groups.
Atkinson and Brandolini (2001 , 2009 ) and Ferreira, Lustig, and Teles (2015) offer comprehensive analyses on secondary datasets on income distribution, drawing attention to issues of data quality and consistency linked to differences in the definitions used, sources of data, and the processing used to obtain “ready-made” income distribution statistics. 32 Atkinson and Brandolini (2001 ) focused mainly on the Deininger and Squire dataset and on data for OECD member countries. Jenkins (2015) follows a similar line of reasoning and compares the WIID and the SWIID, noting that for the latter it is also critical to consider issues relating to the quality of imputations. Jäntti, Pirtillä, and Rönkkö (2020) stress that, in most developing countries, the actual redistribution is only rarely measured, so figures in the SWIID reflect questionable imputations.
As demonstrated in Atkinson and Brandolini (2001 , 2009 ) and Jenkins (2015) , issues of noncomparability have consequences for econometric analysis and for trends over time. Voitchovsky (2011 , 566) warns that data scarcity and limitations in terms of data availability may lead to a trade-off between sources of bias and precision in inequality studies. Ravallion (2001 , 1809) notes, however, that measurement errors, including those resulting from comparability problems, will have a greater impact on analyses that allow for country fixed-effects rather than on standard growth regressions given that the signal-to-noise ratio is likely to be low for changes in measured inequality.
The challenges are even more striking for tests that require data at the individual level, namely those related to the relative hypotheses linking inequality to health. These hypotheses also lead to questions about the appropriate reference groups—how they are defined and formed—as well as in terms of endogeneity, as the position of the individual in relation to the reference may be affected by group membership ( O'Donnell, van Doorslaer, and van Ourti 2015 , 1505).
Concept and measurement of inequality
Issues of concept and measurement are also consequential. 33 Atkinson and Brandolini (2001 ) provide a useful summary of eight parameters to be chosen when defining an income distribution, among which are the unit of observation, concept of resource (e.g., income versus expenditure), and tax treatment of income. These closely link to measurement choices. Different mechanisms require a specific concept of inequality and this should be reflected in the measure of inequality used in the empirical analysis ( Voitchovsky 2011 , 567). Additionally, different parts of the distribution receive importance depending on the inequality measure used, and even the concept of income is open to measurement issues ( Deaton 2003 , 135).
Knowles's (2005) account of the relationship between inequality and growth illustrates these concerns. The author warns that the results in previous studies should be regarded with some degree of caution given that they failed to measure inequality in a consistent manner, mixing measures of the distributions of income before and after tax and the distribution of expenditure. Considering six different measures of inequality (three Gini coefficients and three top ten income shares), a recent study by Blotevogel et al. (2020) shows that the choice of the inequality indicator has important implications for the results obtained in empirical analysis, namely when considering different transmission channels between inequality and growth. In terms of the link between inequality and democratic governance, there is a concern that frequently used measures do not capture interclass inequality, which precludes the testing of theoretical hypotheses that hinge on this ( Houle 2015 , 147).
Criticism has also been directed at specific measures, in particular the widely used Gini coefficient. In light of the observations above, Gini coefficients will provide different information depending on how they are calculated, for example, if based on net income or on gross income ( Houle 2015 , 147). Moreover, some have argued that the use of absolute rather than relative measures might better capture perceptions of inequality on the ground (e.g., Bosmans et al. 2014 ; Atkinson and Brandolini 2004 ; Niño-Zarazúa, Roope, and Tarp 2017 ).
Estimation methods
A review of empirical studies on the inequality–growth link highlights contrasting findings between the early cross-country studies and those that employed panel estimation techniques, after the Deininger and Squire (1998) dataset became available. Some explanations have been advanced for this divergence.
Measurement error may affect the estimation results in cross-country estimation (country- or regional-specific measurement error), and also in panel data estimation, given that inequality tends to be persistent over time; thus, this method relies on more limited time-series variation in the data. The coefficients in cross-country studies may be biased due to time-invariant omitted variables ( Voitchovsky 2011 , 565), while if we consider that inequality is related to underlying determinants of development that are persistent, then fixed-effect estimates may be biased upward when considering long-run effects ( Castells-Quintana, Royuela, and Thiel 2019 , 454).
Additional explanations included the argument for the misspecification of the linearity in the effect of inequality and growth ( Banerjee and Duflo 2003 ) and the suggestion that the two methods capture different time effects, given the short- and long-term lag structures in panel and cross-country analyses, respectively ( Voitchovsky 2011 , 565).
Finally, several concerns have been raised regarding the use of different instruments to tackle reverse causality in the relationship between inequality and growth (see Easterly 2007 ) as well as health ( O'Donnell, van Doorslaer, and van Ourti 2015 , 1505) and democracy ( Houle 2015 , 147). While different attempts have been made using instrumental variable approaches, finding a valid instrument for inequality is certainly not straightforward. Furthermore, even if GMM has often been used to try to tackle these issues, Roodman (2009) warns about the risk of instrument proliferation and the possibility for generating false-positive results. As an illustration, he reexamined the analysis in Forbes (2000) and raised concerns over the positive effect of inequality on growth found in the original paper.
This review combined the different theoretical hypotheses concerning the impact of inequality on three core socioeconomic and political outcomes in a simplified framework and highlighted the mixed empirical evidence. We summarize the main conclusions as follows. First, in line with previous findings, the debate on whether there is a positive or a negative effect on growth remains open, with recent studies mirroring the disagreement in decades of empirical work. With the exception of the classical approach, most of the transmission channels between inequality and growth point to a negative effect of inequality. However, the evidence from reduced-form equations is not consensual and the channels of transmission have received less attention.
Second, while there seems to be some consensus in the evidence that there is a negative link between inequality and secondary school enrolment, there is need for further research in terms of other education outcomes. Although theory generally points toward a negative effect of inequality on health, the existing evidence does not provide clear support to this relationship, in the economic literature in particular, and there is a lot to be uncovered in terms of the mechanisms of transmission at the individual level. Third, theoretical predictions and empirical evidence show mixed results for the effects of inequality on democracy and political participation.
In understanding the diversity and divergence in theoretical and empirical results, a number of empirical challenges remain. Problems with data quality and availability are well understood in the literature, as are those related to the concept and measurement of inequality, and the shortcomings of different estimation methods.
In terms of potential avenues for future work, our review points for one to the value of further attention to different transmission channels (highlighted in figure 1 ). We first propose a methodological suggestion. While advances in econometric analysis will shed light on the analysis across countries, this could be complemented with the use of experimental work to understand specific channels in particular contexts. While not a substitute for empirical cross-country analysis, experiments can be employed to understand microlevel behavior. The controlled nature of this work avoids biases in econometric studies and mitigates issues of endogeneity and measurement errors.
The second avenue relates to the focus of the analysis. While this review mainly concentrated on cross-country analysis, there is indication that disaggregating the level of analysis might provide useful insights in terms of channels of transmission and underlying cases. For instance, it might be that in Africa, competition over natural resources is the main driver of inequality and in turn slower growth, while in Latin America, inequality may be the main driver for political instability. Furthering regional and country-specific analysis might help dig deeper into these effects.
Finally, despite the existing efforts to compile new—and improve on the existing—secondary datasets, problems persist with the available data. Thus, in light of the importance of data availability and reliability for the analysis of the trends and effects of inequality, we stress that earlier calls for more and better data continue to be both relevant and important for progress in our search for better understanding of the impact of inequality.
Equity here refers to equality of opportunities to pursue a life of one's choosing and protection from extreme deprivation in outcomes ( World Bank 2006 , 18–19). Efficiency refers to economic efficiency, underpinning economic growth ( Thorbecke 2016 ).
Given the multidimensionality of inequality and that its effects are in focus in different disciplines, we follow an interdisciplinary approach. Yet, in the empirical section, we focus on strands of work that employ similar (quantitative) methodologies.
We focus on the main arguments that have attracted attention in these disciplines and have made a concerted effort to address the gender citation gap that exists, for instance, in international relations scholarship (e.g., Maliniak, Powers, and Walter 2013 ).
Throughout, we refer to “income inequality” and “inequality” interchangeably. Although we recognize the multidimensionality of the concept, we focus on literature considering income inequality, which remains a dominant measure ( Stewart 2016 , 64), and refer to more extensive work on other aspects, in particular, the relevance of poverty rates (e.g., Ravallion 2012 ), inequality of opportunity (e.g., Marrero and Rodriguez 2013 ; Ferreira et al. 2018 ), gender inequality (e.g., Bandiera and Natraj 2013 ; Kabeer 2015 ), and horizontal inequalities ( Stewart 2005 ).
We use “growth” and “economic growth” interchangeably.
We highlight that there is expanding work on different facets of economic performance, such as growth volatility (e.g., Iyigun and Owen 2004 ) or the occurrence of crises (e.g., Morelli and Atkinson 2015 ).
Kuznets (1955) argued that the early stages of the development process would experience rising inequality, which would then fall as the country reached higher levels of per capita income. This relationship, known as the “Kuznets curve,” and other work looking at this direction of causality are not covered here.
See also a review of early studies in Bénabou (1996) and Aghion, Caroli, and García-Peñalosa (1999 ) and a more recent overview in Ehrhart (2009) .
Sandmo (2015) reviews the history of theories of income distribution, from Adam Smith until the 1970s.
For a summary of theoretical work on the choice between a public and a private education system, see García-Peñalosa (1995) .
Gutiérrez and Tanaka (2009) review previous theoretical models.
Additional mechanisms relate to social comparison and include relative deprivation and gratification in the context of neighborhood and school effects, and economic segregation ( Mayer 2001 ). The first refers to the fact that people compare themselves with those who are more disadvantaged, which in the case of children can lead to feeling less willing to study or stay in school and in the case of parents can cause stress and alienation. The second suggests that increases in inequality are likely to lead to more geographic segregation as the rich and poor have less in common. See Mayer (2001 , 4–7) for more details.
See Deaton (2003 ) and Lynch et al. (2004) for detailed descriptions of the emergence of debate on the link between income inequality and health.
We do not cover studies on the link between inequality and homicides and between inequality and life satisfaction and happiness ( Graham 2014 ).
Lynch et al. (2004 , 15–16) refer to additional nuances, related to the effects of inequality through psychosocial processes and through the differential accumulation of exposures deriving from material sources rather than from perceptions of disadvantage. They also mention the weak and strong versions of this hypothesis proposed by Mellor and Milyo (2002) .
For a study on the effects of inequality on group participation, see La Ferrara (2002) .
Thorbecke and Charumilind (2002) review the evidence and causal mechanisms linking inequality and crime.
For a review of the theoretical arguments developed earlier, see Bollen and Jackman (1985) .
This line of reasoning can be linked to the work by Glaeser, Scheinkman, and Shleifer (2003) mentioned in section “How inequality affects growth,” which discusses the negative effects of inequality on growth through institutional subversion (including corruption).
For further details, see Solt (2008 , 48–50).
It is also useful to refer here to studies examining the impact of inequality on electoral turnout (e.g., Stockemer and Scruggs 2012 ), support for democracy (e.g., Andersen 2012 ; Krieckhaus et al. 2014 ), and, more generally, political inequality (e.g., Houle 2018 ).
A more complete list of studies is available from the authors.
Studies in the 1990s also focus on determining whether there was a differential effect of inequality on growth in democracies and non-democracies ( Persson and Tabellini 1994 ; Alesina and Rodrik 1994 ; Perotti 1996 ; Clarke 1995 ; Deininger and Squire 1998 ). We discuss this in Section ”Governance.”
Two recent studies build on Forbes (2000) , attempting to overcome some of the remaining estimation challenges. Aiyar and Ebeke (2020) draw attention to the importance of considering equality of opportunity and find empirical support for their hypothesis that the negative effect of income inequality is greater in countries with low levels of equality of opportunity (measured by intergenerational mobility). Scholl and Klasen (2019) replicate Forbes’ (2000) finding but show that it disappears once they control for the experience of transition countries.
Islam and McGillivray (2020) highlight the increasing interest in wealth inequality and investigate its effect on growth using wealth data from Forbes Magazine and Credit Suisse over the period 2000–2012. The results suggest a negative effect.
Perotti (1996 ) empirically tested the channels of transmission, estimating different structural models: first, using each of these channels in a growth model and, then, estimating the effects of inequality on each of the channels.
With the exception of Flug, Spilimbergo, and Wachtenheim (1998) , all these studies employ the Gini coefficient as one of their measures of inequality. Flug, Spilimbergo, and Wachtenheim (1998) used the ratio of the income shares of the top quintile to the bottom two quintiles of the population, and the shares of income accruing to the top quintile and the lowest quintile were used, respectively, by Easterly (2007) and Checchi (2003) . In their robustness checks, Esposito and Villaseñor (2018) used the Atkinson and Theil indices.
We do not offer a comprehensive overview of the measures used in the literature. According to the review in Lynch et al. (2004) , the majority of the studies employ the Gini coefficient or different shares of income. In the list of studies reviewed by these authors, we counted sixty-nine out of ninety-eight using the Gini as (one of) the measure(s) of inequality.
The review of the initial studies in Bollen and Jackman (1985) argued that problems of specification, measurement, and sample composition led to inconclusive results in the existing empirical analyses.
Savoia, Easaw, and McKay (2010) reviewed the arguments linking inequality to institutional quality directly and via democracy and argued that the limited existing work suggests a negative link between inequality and institutions, noting there is a need for further research.
When considering the role of governance (using different indicators), the estimates in Islam and McGillivray (2020) indicate that improved governance may contribute to reduced wealth inequality and higher growth.
See also discussions of these shortcomings in Deaton (2003 ), Voitchovsky (2011) , and Houle (2015) .
As illustrated in section “What the empirical evidence says,” issues of concept and measurement for our outcome variables also matter to consideration of theories and hypothesis testing.
This study was prepared within the project “The impacts of inequality on growth, human development, and governance - @EQUAL.” Support by the Novo Nordisk Foundation Grant NNF19SA0060072 is acknowledged.
We are grateful to the editors and three anonymous referees for insightful and useful suggestions. We thank Anustup Kundu for excellent research assistance as well as Klarizze Puzon, Miguel Niño-Zarazúa, Carlos Gradín, and participants at an internal project workshop for valuable comments. The usual caveats apply.
Acemoglu Daron , Robinson James A. . 2006 . Economic Origins of Dictatorship and Democracy . Cambridge, MA : Cambridge University Press .
Google Scholar
Google Preview
Aghion Philippe , Caroli Eve , García-Peñalosa Cecilia . 1999 . “ Inequality and Economic Growth: The Perspective of the New Growth Theories .” Journal of Economic Literature 37 ( 4 ): 1615 – 60 .
Aiyar Shekhar , Ebeke Christian . 2020 . “ Inequality of Opportunity, Inequality of Income and Economic Growth .” World Development 136 : 105115 .
Alesina Alberto , Rodrik Dani . 1994 . “ Distributive Politics and Economic Growth .” The Quarterly Journal of Economics 109 ( 2 ): 465 – 90 .
Alesina Alberto , Perotti Roberto . 1996 . “ Income Distribution, Political Instability, and Investment .” European Economic Review 40 ( 6 ): 1203 – 28 .
Andersen Robert . 2012 . “ Support for Democracy in Cross-National Perspective: The Detrimental Effect of Economic Inequality .” Research in Social Stratification and Mobility 30 ( 4 ): 389 – 402 .
Ansell Ben , Samuels David . 2010 . “ Inequality and Democratization: A Contractarian Approach .” Comparative Political Studies 43 ( 12 ): 1543 – 74 .
Atkinson Anthony B. , Brandolini Andrea . 2001 . “ Promise and Pitfalls in the Use of ‘Secondary’ Data-Sets: Income Inequality in OECD Countries as a Case Study .” Journal of Economic Literature 39 ( 3 ): 771 – 99 .
Atkinson Anthony B. , Brandolini Andrea . 2004 . “ Global World Inequality: Absolute, Relative or Intermediate? ” Paper prepared for the 28th General Conference of The International Association for Research in Income and Wealth, Cork, Ireland, August 22–28, 2004 .
Atkinson Anthony B. , Brandolini Andrea . 2009 . “ On Data: A Case Study of the Evolution of Income Inequality across Time and across Countries .” Cambridge Journal of Economics 33 ( 3 ): 381 – 404 .
Bandiera Oriana , Natraj Ashwini . 2013 . “ Does Gender Inequality Hinder Development and Economic Growth? Evidence and Policy Implications .” The World Bank Research Observer 28 ( 1 ): 2 – 21 .
Banerjee Abhijit V. , Duflo Esther . 2003 . “ Inequality and Growth: What Can the Data Say? ” Journal of Economic Growth 8 ( 3 ): 267 – 99 .
Barro Robert J. 1999 . “ Determinants of Democracy .” Journal of Political Economy 107 ( S6 ): S158 – 83 .
Barro Robert J. . 2000 . “ Inequality and Growth in a Panel of Countries .” Journal of Economic Growth 5 : 5 – 32 .
Barro Robert J. . 2008 . “ Inequality and Growth Revisited .” ADB Working Paper Series on Regional Economic Integration 11. Asian Development Bank (ADB), Philippines. Accessed November 16, 2020. https://www.adb.org/sites/default/files/publication/28468/wp11-inequality-growth-revisited.pdf .
Bénabou Roland . 1996 . “ Inequality and Growth .” In NBER Macroeconomics Annual 1996 , Volume 11, edited by Bernanke Ben S. , Rotemberg Julio J. , 11 – 92 . Cambridge, MA : MIT Press .
Bénabou Roland . 2000 . “ Unequal Societies: Income Distribution and the Social Contract .” American Economic Review 90 ( 1 ): 96 – 129 .
Berg Andrew , Ostry Jonathan D. , Sangarides Charalambs G. , Yakhshilikov Yorbol . 2018 . “ Redistribution, Inequality, and Growth: New Evidence .” Journal of Economic Growth 23 ( 4 ): 259 – 305 .
Bermeo Nancy . 2009 . “ Poverty Inequality and Democracy (II): Does Electoral Democracy Boost Economic Equality? ” Journal of Democracy 20 ( 4 ): 21 – 35 .
Birdsall Nancy . 1999 . “ Education: The People's Asset .” Center on Social and Economic Dynamics Working Paper 5. Accessed August 13, 2020. https://www.researchgate.net/publication/5059764_Education_The_People's_Asset .
Birdsall Nancy , Londoño Juan Luis . 1997 . “ Asset Inequality Matters: An Assessment of the World Bank's Approach to Poverty Reduction .” The American Economic Review 87 ( 2 ): 32 – 37 .
Blotevogel Robert , Imamoglu Eslem , Moriyama Kenji , Sarr Babacar . 2020 . “ Measuring Income Inequality and Implications for Economic Transmission Channels .” IMF Working Paper WP/20/164 . Washington, DC : International Monetary Fund .
Boix Carles . 2003 . Democracy and Redistribution . Cambridge : Cambridge University Press .
Boix Carles , Stokes Susan C. . 2003 . “ Endogenous Democratization .” World Politics 55 ( 4 ): 517 – 49 .
Bollen Kenneth A. , Jackman Robert W. . 1985 . “ Political Democracy and the Size Distribution of Income .” American Sociological Review 50 ( 4 ): 438 – 57 .
Bosmans Kristof , Decanq Koen , Decoster André . 2014 . “ The Relativity of Decreasing Inequality between Countries .” Economica 81 ( 322 ): 276 – 92 .
Bourguignon Francois . 2015 . “ Revisiting the Debate on Inequality and Economic Development .” Revue d'économie politique 5 : 633 – 63 .
Buchmann Claudia , Hannum Emily . 2001 . “ Education and Stratification in Developing Countries: A Review of Theories and Research .” Annual Review of Sociology 27 ( 1 ): 77 – 102 .
Cancela Joao , Geys Benny . 2016 . “ Explaining Voter Turnout: A Meta-Analysis of National and Subnational Elections .” Electoral Studies 42 : 264 – 75 .
Castelló-Climent Amparo. 2010 . “ Inequality and Growth in Advanced Economies: An Empirical Investigation .” The Journal of Economic Inequality 8 : 293 – 321 .
Castells-Quintana David , Royuela Vicente , Thiel Fabian . 2019 . “ Inequality and Sustainable Development: Insights from an Analysis of the Human Development Index .” Sustainable Development 27 ( 3 ): 448 – 60 .
Checchi Daniele 2003 . “ Inequality in Incomes and Access to Education: A Cross-Country Analysis (1960–95) .” Labour 17 ( 2 ): 153 – 201 .
Chong Alberto , Gradstein Mark . 2007 . “ Inequality and Institutions .” Review of Economics and Statistics 89 ( 3 ): 454 – 65 .
Chong Alberto , Gradstein Mark . 2019 . “ Institutional Persistence, Income Inequality, and Individual Attitudes .” The Journal of Economic Inequality 17 ( 3 ): 401 – 13 .
Cingano Federico . 2014 . “ Trends in Income Inequality and Its Impact on Economic Growth .” OECD Social, Employment and Migration Working Papers 163 . Paris : OECD Publishing .
Clarke George R.G. 1995 . “ More Evidence on Income Distribution and Growth .” Journal of Development Economics 47 ( 2 ): 403 – 27 .
Dabla-Norris Era , Kochhar Kalpana , Suphaphiphat Nujin , Ricka Frantisek , Tsounta Evridiki . 2015 . “ Causes and Consequences of Income Inequality: A Global Perspective .” IMF Discussion Note SDN/15/13 . Washington, DC : International Monetary Fund .
De La Croix David , Doepke Matthias . 2003 . “ Inequality and Growth: Why Differential Fertility Matters .” American Economic Review 93 ( 4 ): 1091 – 113 .
Deaton Angus 2003 . “ Health, Inequality, and Economic Development .” Journal of Economic Literature 41 ( 1 ): 113 – 58 .
Deininger Klaus , Olinto Pedro . 2000 . “ Asset Distribution, Inequality, and Growth .” Policy Research Working Paper 2375 . Washington, DC : The World Bank .
Deininger Klaus , Squire Lyn . 1996 . “ A New Data Set Measuring Income Inequality .” The World Bank Economic Review 10 ( 3 ): 565 – 91 .
Deininger Klaus , Squire Lyn . 1998 . “ New Ways of Looking at Old Issues: Inequality and Growth .” Journal of Development Economics 57 ( 2 ): 259 – 87 .
Dorsch Michael T. , Maarek Paul . 2020 . “ Economic Downturns, Inequality, and Democratic Improvements .” European Journal of Political Economy 62 ( C ): 1 – 21 .
Easterly William . 2007 . “ Inequality Does Cause Underdevelopment: Insights from a New Instrument .” Journal of Development Economics 84 ( 2 ): 755 – 76 .
Ehrhart Christophe . 2009 . “ The Effects of Inequality on Growth: A Survey of the Theoretical and Empirical Literature .” ECINEQ Working Paper Series 2009-107. ECINEQ - Society for the Study of Economic Inequality. Accessed November 16, 2020. http://www.ecineq.org/milano/WP/ECINEQ2009-107.pdf .
El-Shagi Makram , Shao Liang . 2019 . “ The Impact of Inequality and Redistribution on Growth .” Review of Income and Wealth 65 ( 2 ): 239 – 63 .
Esposito Lucio , Villaseñor Adrián . 2018 . “ Wealth Inequality, Educational Environment and School Enrolment: Evidence from Mexico .” The Journal of Development Studies 54 ( 11 ): 2095 – 118 .
Ferreira Francisco H.G. , Lakner Christoph , Lugo Maria Ana , Özler Berk . 2018 . “ Inequality of Opportunity and Economic Growth: How Much Can Cross-Country Regressions Really Tell Us? ” Review of Income and Wealth 64 ( 4 ): 800 – 27 .
Ferreira Francisco H.G. , Lustig Nora , Teles Daniel . 2015 . “ Appraising Cross-National Income Inequality Databases: An Introduction .” The Journal of Economic Inequality 13 : 497 – 526 .
Fields Gary . 1989 . “ A Compendium of Data on Inequality and Poverty for the Developing World .” Unpublished manuscript, Cornell University .
Flug Karnit , Spilimbergo Antonio , Wachtenheim Erik . 1998 . “ Investment in Education: Do Economic Volatility and Credit Constraints Matter? ” Journal of Development Economics 55 ( 2 ): 465 – 81 .
Forbes Kristin J. 2000 . “ A Reassessment of the Relationship between Inequality and Growth .” American Economic Review 90 ( 4 ): 869 – 87 .
Fukuyama Francis . 2011 . “ Poverty, Inequality, and Democracy: Dealing with Inequality .” Journal of Democracy 22 ( 3 ): 79 – 89 .
Galor Oded , Zeira Joseph . 1993 . “ Income Distribution and Macroeconomics .” The Review of Economic Studies 60 ( 1 ): 35 – 52 .
Galor Oded , Moav Omer . 2004 . “ From Physical to Human Capital Accumulation: Inequality and the Process of Development .” Review of Economic Studies 71 ( 4 ): 1001 – 26 .
García-Peñalosa Cecilia . 1995 . “ The Paradox of Education or the Good Side of Inequality .” Oxford Economic Papers 47 ( 2 ): 265 – 85 .
Glaeser Edward , Scheinkman Jose , Shleifer Andrei . 2003 . “ The Injustice of Inequality .” Journal of Monetary Economics 50 ( 1 ): 199 – 222 .
Graham Carol . 2014 . “ Concluding Remarks. How Inequality Matters to Well-Being .” In Happiness and Economic Growth: Lessons from Developing Countries , edited by Clark Andrew E. , Senik Claudia , 249 – 66 . Oxford : Oxford University Press .
Gründler Klaus , Scheuermeyer Philipp . 2018 . “ Growth Effects of Inequality and Redistribution: What Are the Transmission Channels? ” Journal of Macroeconomics 55 : 293 – 313 .
Gutiérrez Catalina , Tanaka Ryuichi . 2009 . “ Inequality and Education Decisions in Developing Countries .” The Journal of Economic Inequality 7 : 55 – 81 .
Haggard Stephan , Kaufman Robert R. . 2012 . “ Inequality and Regime Change: Democratic Transitions and the Stability of Democratic Rule .” American Political Science Review 106 ( 3 ): 495 – 516 .
Halter Daniel , Oechslin Manuel , Zweimüller Josef . 2014 . “ Inequality and Growth: The Neglected Time Dimension .” Journal of Economic Growth 19 ( 1 ): 81 – 104 .
Houle Christian 2009 . “ Inequality and Democracy: Why Inequality Harms Consolidation But Does Not Affect Democratization .” World Politics 61 ( 4 ): 589 – 622 .
Houle Christian . 2015 . “ Does Inequality Harm Economic Development and Democracy?: Accounting for Missing Values, Noncomparable Observations, and Endogeneity .” In The Oxford Handbook of the Politics of Development , edited by Lancaster Carol , Walle Nicolas Van de , 140 – 61 . New York : Oxford University Press .
Houle Christian . 2018 . “ Does Economic Inequality Breed Political Inequality? ” Democratization 25 ( 8 ): 1500 – 18 .
Islam Md. Rabiuk , McGillivray Mark . 2020 . “ Wealth Inequality, Governance and Economic Growth .” Economic Modelling 88 ( C ): 1 – 13 .
Iyigun Murat F. , Owen Ann L. . 2004 . “ Income Inequality, Financial Development, and Macroeconomic Fluctuations .” The Economic Journal 114 ( 495 ): 352 – 76 .
Jain Shail . 1975 . The Size Distribution of Income: A Compilation of Data . Washington, DC : World Bank .
Jäntti Markus , Pirtillä Jukka , Rönkkö Risto . 2020 . “ Redistribution, Inequality, and Growth Revisited: Comment on ‘Redistribution, Inequality, and Growth: New Evidence’ .” WIDER Working Paper 2020/117 . Helsinki : UNU-WIDER .
Jenkins Stephen P. 2015 . “ World Income Inequality Databases: An Assessment of WIID and SWIID .” The Journal of Economic Inequality 13 : 629 – 71 .
Jong-sung You , Khagram Sanjeev . 2005 . “ A Comparative Study of Inequality and Corruption .” American Sociological Review 70 ( 1 ): 136 – 57 .
Kabeer Naila 2015 . “ Gender, Poverty and Inequality: A Brief History of Feminist Contributions in the Field of International Development .” Gender & Development 23 ( 2 ): 189 – 205 .
Kaldor Nicholas . 1956 . “ Theories of Distribution .” The Review of Economic Studies 23 ( 2 ): 83 – 100 .
Karl Terry Lynn . 2000 . “ Economic Inequality and Democracy Instability .” Journal of Democracy 11 ( 1 ): 149 – 56 .
Keefer Philip , Knack Stephen . 2002 . “ Polarization, Politics and Property Rights: Links between Inequality and Growth .” Public Choice 111 : 127 – 54 .
Knack Stephen , Keefer Philip . 1997 . “ Does Inequality Harm Growth Only in Democracies? A Replication and Extension .” American Journal of Political Science 41 ( 1 ): 323 – 32 .
Knowles Stephen . 2005 . “ Inequality and Economic Growth: The Empirical Relationship Reconsidered in the Light of Comparable Data .” Journal of Development Studies 41 ( 1 ): 135 – 59 .
Kotschy Rainer , Sunde Uwe . 2017 . “ Democracy, Inequality, and Institutional Quality .” European Economic Review 91 ( C ): 209 – 28 .
Krieckhaus Jonathan , Son Byunghwan , Bellinger Nisha Mukherjee , Wells Jason M. . 2014 . “ Economic Inequality and Democratic Support .” The Journal of Politics 76 ( 1 ): 139 – 51 .
Kuznets Simon . 1955 . “ Economic Growth and Income Inequality .” The American Economic Review 45 ( 1 ): 1 – 28 .
La Ferrara Eliana . 2002 . “ Inequality and Group Participation: Theory and Evidence from Rural Tanzania .” Journal of Public Economics 85 ( 2 ): 235 – 73 .
Lecaillon Jacques , Paukert Felix , Morrison Christian , Germadis Dimitri . 1984 . Income Distribution and Economic Development: An Analytical Survey . Geneva : International Labour Office .
Leigh Andrew , Christopher Jencks , Smeeding Timothy M. . 2011 . “ Health and Economic Inequality .” In The Oxford Handbook of Economic Inequality , edited by Nolan Brian , Salverda Wiemer , Smeeding Timothy M. , 384 – 405 . New York : Oxford University Press .
Lengfelder Christina 2019 . “ Exploring Dynamics of Inequality in Human Development .” 2019 UNDP Human Development Report, Background Paper NO. 3-2019. United Nations Development Programme (UNDP). Accessed June 6, 2020. http://hdr.undp.org/en/content/exploring-dynamics-inequality-human-development .
Li Hongyi , Zou Heng-fu . 1998 . “ Income Inequality Is Not Harmful for Growth: Theory and Evidence .” Review of Development Economics 2 ( 3 ): 318 – 34 .
Lynch John , Smith George Davey , Harper Sam , Hillemeier Marianne , Ross Nancy , Kaplan George A. , Wolfson Michael . 2004 . “ Is Income Inequality a Determinant of Population Health? Part 1. A Systematic Review .” The Milbank Quarterly 82 ( 1 ): 5 – 99 .
Madsen Jakob B. , Islam Md. Rabiuk , Doucouliagos Hristos . 2018 . “ Inequality, Financial Development and Economic Growth in the OECD, 1870–2011 .” European Economic Review 101 ( C ): 605 – 24 .
Maliniak Daniel , Powers Ryan , Walter Barbara F. . 2013 . “ The Gender Citation Gap in International Relations .” International Organization 67 ( 4 ): 889 – 922 .
Marrero Gustavo A. , Rodríguez Juan G. . 2013 . “ Inequality of Opportunity and Growth .” Journal of Development Economics 104 ( C ): 107 – 22 .
Mayer Susan E. 2001 . “ How Did the Increase in Economic Inequality between 1970 and 1990 Affect Children's Educational Attainment? ” American Journal of Sociology 107 ( 1 ): 1 – 32 .
Mellor Jennifer M. , Milyo Jeffrey . 2002 . “ Income Inequality and Health Status in the United States .” The Journal of Human Resources 37 ( 3 ): 510 – 39 .
Morelli Salvatore , Atkinson Anthony B. . 2015 . “ Inequality and Crises Revisited .” Economia Politica 32 : 31 – 51 .
Murphy Kevin M. , Shleifer Andrei , Vishny Robert . 1989 . “ Income Distribution, Market Size and Industrialization .” The Quarterly Journal of Economics 104 ( 3 ): 537 – 64 .
Neves Pedro Cunha , Silva Sandra Maria Tavares . 2014 . “ Inequality and Growth; Uncovering the Main Conclusions from the Empirics .” The Journal of Development Studies 50 ( 1 ): 1 – 21 .
Niño-Zarazúa Miguel , Roope Lawrence , Tarp Finn . 2017 . “ Global Inequality: Relatively Lower, Absolutely Higher .” Review of Income and Wealth 63 ( 4 ): 661 – 84 .
Nolan Brian , Valenzuela Luis . 2019 . “ Inequality and Its Discontents .” Oxford Review of Economic Policy 35 ( 3 ): 396 – 430 .
O'Donnell Owen , van Doorslaer Eddy , van Ourti Tom . 2015 . “ Health and Inequality .” In Handbook of Income Distribution , Volume 2B, edited by Atkinson Anthony B. , Francois Bourguignon , 1419 – 533 . Amsterdam : Elsevier .
Østby Gudrun . 2013 . “ Inequality and Political Violence: A Review of the Literature .” International Area Studies Review 16 ( 2 ): 206 – 31 .
Ostry Jonathan D. , Berg Andrew , Tsangarides Charalambos G. . 2014 . “ Redistribution, Inequality, and Growth .” IMF Staff Discussion Note SDN/14/02 . Washington, DC : International Monetary Fund .
Panizza Ugo . 2002 . “ Income Inequality and Economic Growth: Evidence from American Data .” Journal of Economic Growth 7 : 25 – 41 .
Partridge Mark D. 1997 . “ Is Inequality Harmful for Growth? Comment .” The American Economic Review 87 ( 5 ): 1019 – 32 .
Paukert Felix . 1973 . “ Income Distribution at Different Levels of Development: A Survey of the Evidence .” International Labour Review 108 : 97 – 125 .
Perotti Roberto . 1996 . “ Growth, Income Distribution, and Democracy: What the Data Say .” Journal of Economic Growth 1 : 149 – 87 .
Persson Torsten , Tabellini Guido . 1994 . “ Is Inequality Harmful for Growth? ” The American Economic Review 84 ( 3 ): 600 – 21 .
Pickett Kate E. , Wilkinson Richard G. . 2015 . “ Income Inequality and Health: A Causal Review .” Social Science & Medicine 128 : 316 – 26 .
Ravallion Martin 2001 . “ Growth, Inequality and Poverty: Looking Beyond Averages .” World Development 29 ( 11 ): 1803 – 15 .
Ravallion Martin . 2012 . “ Why Don't We See Poverty Convergence? ” American Economic Review 102 ( 1 ): 504 – 23 .
Roodman David . 2009 . “ A Note on the Theme of Too Many Instruments .” Oxford Bulletin of Economics and Statistics 71 ( 1 ): 135 – 58 .
Rothstein Bo , Uslaner Eric M. . 2005 . “ All for All: Equality, Corruption, and Social Trust .” World Politics 58 ( 1 ): 41 – 72 .
Rowlingson Karen 2011 . “ Does Income Inequality Cause Health and Social Problems? ” Joseph Rowntree Foundation, York. Accessed October 4, 2021. https://www.jrf.org.uk/file/41359/download?token=VV7qsS1J&filetype=full-report .
Sandmo Agnar . 2015 . “ The Principal Problem in Political Economy: Income Distribution in the History of Economic Thought .” In Handbook of Income Distribution , Volume 2, edited by Atkinson Anthony B. , Bourguignon Francois , 3 – 65 . Amsterdam : Elsevier .
Savoia Antonio , Easaw Joshy , McKay Andrew . 2010 . “ Inequality, Democracy, and Institutions: A Critical Review of Recent Research .” World Development 38 ( 2 ): 142 – 54 .
Schäfer Armin , Schwander Hanna . 2019 . “ ‘Don't Play If You Can't Win’: Does Economic Inequality Undermine Political Equality .” European Political Science Review 11 ( 3 ): 395 – 413 .
Scheve Kenneth , Stasavage David . 2017 . “ Wealth Inequality and Democracy .” Annual Review of Political Science 20 : 451 – 68 .
Scholl Nathalie , Klasen Stephan . 2019 . “ Re-Estimating the Relationship between Inequality and Growth .” Oxford Economic Papers 71 ( 4 ): 824 – 47 .
Solt Frederick . 2008 . “ Economic Inequality and Democratic Political Engagement .” American Journal of Political Science 52 ( 1 ): 48 – 60 .
Solt Frederick . 2009 . “ Standardizing the World Income Inequality Database .” Social Science Quarterly 90 ( 2 ): 231 – 42 .
Solt Frederick . 2020 . “ Measuring Income Inequality across Countries and over Time: The Standardized World Income Inequality Database .” Social Science Quarterly 101 ( 3 ): 1183 – 99 .
Stewart Frances . 2005 . “ Horizontal Inequalities: A Neglected Dimension of Development .” In Wider Perspectives on Global Development , edited by Shorrocks Anthony F. , 101 – 35 . London : Palgrave Macmillan .
Stewart Frances . 2016 . “ Changing Perspectives on Inequality and Development .” Studies in Comparative International Development 51 : 60 – 80 .
Stockemer Daniel , Scruggs Lyle . 2012 . “ Income Inequality, Development and Electoral Turnout—New Evidence on a Burgeoning Debate .” Electoral Studies 31 ( 4 ): 764 – 73 .
Subramanian S.V. , Kawachi Ichiro . 2004 . “ Income Inequality and Health: What Have We Learned So Far? ” Epidemiologic Reviews 26 ( 1 ): 78 – 91 .
Sylwester Kevin 2000 . “ Income Inequality, Education Expenditures, and Growth .” Journal of Development Economics 63 ( 2 ): 379 – 98 .
Tanaka Ryuichi 2003 . “ Inequality as a Determinant of Child Labor .” Economics Letters 80 ( 1 ): 93 – 97 .
Thorbecke Erik 2016 . “ Inequality and the Trade-Off between Efficiency and Equity .” Journal of Human Development and Capabilities 17 ( 3 ): 460 – 64 .
Thorbecke Erik , Charumilind Chutatong . 2002 . “ Economic Inequality and Its Socioeconomic Impact .” World Development 30 ( 9 ): 1477 – 95 .
Uslaner Eric M. 2017 . “ Political Trust, Corruption, and Inequality .” In Handbook on Political Trust , edited by Zmerli Sonja , van der Meer Tom W.G. , 302 – 15 . Chelton : Edward Elgar Publishing .
Voitchovsky Sarah 2005 . “ Does the Profile of Income Inequality Matter for Economic Growth? Distinguishing between the Effects of Inequality in Different Parts of the Income Distribution .” Journal of Economic Growth 10 : 273 – 96 .
Voitchovsky Sarah . 2011 . “ Inequality and Economic Growth .” In The Oxford Handbook of Economic Inequality , edited by Nolan Brian , Salverda Wiemer , Smeeding Timothy M. , 549 – 74 . New York : Oxford University Press .
Wagstaff Adam , van Doorslaer Eddy . 2000 . “ Income Inequality and Health: What Does the Literature Tell Us? ” Annual Review of Public Health 21 : 543 – 67 .
Walraevens Benoît . 2021 . “ Adam Smith's View of Economic Inequality .” Cambridge Journal of Economics 45 ( 1 ): 209 – 24 .
WID.world . 2017 . “ World Inequality Database .” Accessed January 26, 2021. https://wid.world .
Wilkinson Richard G. , Pickett Kate E. . 2006 . “ Income Inequality and Population Health: A Review and Explanation of the Evidence .” Social Science & Medicine 62 ( 7 ): 1768 – 84 .
World Bank . 2006 . World Development Report 2006: Equity and Development . Washington, DC : World Bank .
Zweimüller Josef . 2000 . “ Schumpeterian Entrepreneurs Meet Engel's Law: The Impact of Inequality on Innovation-Driven Growth .” Journal of Economic Growth 5 : 185 – 206 .
Email alerts
Citing articles via.
- Recommend to your Library
Affiliations
- Online ISSN 1468-2486
- Print ISSN 1521-9488
- Copyright © 2024 International Studies Association
- About Oxford Academic
- Publish journals with us
- University press partners
- What we publish
- New features
- Open access
- Institutional account management
- Rights and permissions
- Get help with access
- Accessibility
- Advertising
- Media enquiries
- Oxford University Press
- Oxford Languages
- University of Oxford
Oxford University Press is a department of the University of Oxford. It furthers the University's objective of excellence in research, scholarship, and education by publishing worldwide
- Copyright © 2024 Oxford University Press
- Cookie settings
- Cookie policy
- Privacy policy
- Legal notice
This Feature Is Available To Subscribers Only
Sign In or Create an Account
This PDF is available to Subscribers Only
For full access to this pdf, sign in to an existing account, or purchase an annual subscription.

- Chronicle Conversations
- Article archives
- Issue archives
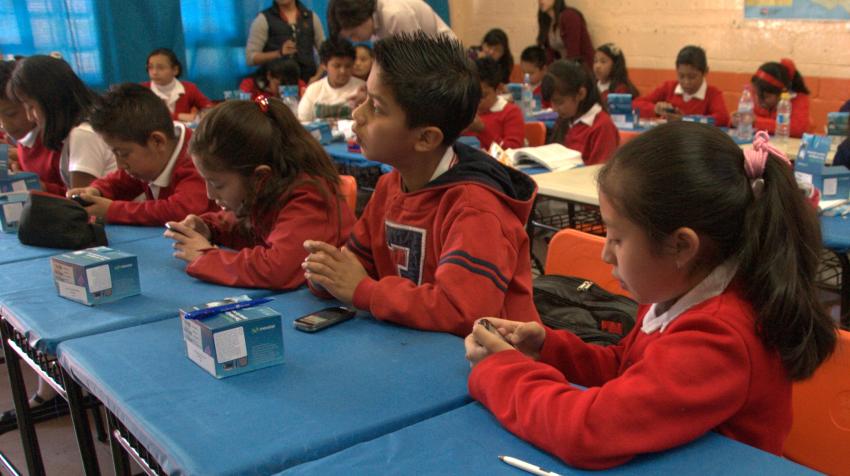
Recognizing and Overcoming Inequity in Education
About the author, sylvia schmelkes.
Sylvia Schmelkes is Provost of the Universidad Iberoamericana in Mexico City.
22 January 2020 Introduction
I nequity is perhaps the most serious problem in education worldwide. It has multiple causes, and its consequences include differences in access to schooling, retention and, more importantly, learning. Globally, these differences correlate with the level of development of various countries and regions. In individual States, access to school is tied to, among other things, students' overall well-being, their social origins and cultural backgrounds, the language their families speak, whether or not they work outside of the home and, in some countries, their sex. Although the world has made progress in both absolute and relative numbers of enrolled students, the differences between the richest and the poorest, as well as those living in rural and urban areas, have not diminished. 1
These correlations do not occur naturally. They are the result of the lack of policies that consider equity in education as a principal vehicle for achieving more just societies. The pandemic has exacerbated these differences mainly due to the fact that technology, which is the means of access to distance schooling, presents one more layer of inequality, among many others.
The dimension of educational inequity
Around the world, 258 million, or 17 per cent of the world’s children, adolescents and youth, are out of school. The proportion is much larger in developing countries: 31 per cent in sub-Saharan Africa and 21 per cent in Central Asia, vs. 3 per cent in Europe and North America. 2 Learning, which is the purpose of schooling, fares even worse. For example, it would take 15-year-old Brazilian students 75 years, at their current rate of improvement, to reach wealthier countries’ average scores in math, and more than 260 years in reading. 3 Within countries, learning results, as measured through standardized tests, are almost always much lower for those living in poverty. In Mexico, for example, 80 per cent of indigenous children at the end of primary school don’t achieve basic levels in reading and math, scoring far below the average for primary school students. 4
The causes of educational inequity
There are many explanations for educational inequity. In my view, the most important ones are the following:
- Equity and equality are not the same thing. Equality means providing the same resources to everyone. Equity signifies giving more to those most in need. Countries with greater inequity in education results are also those in which governments distribute resources according to the political pressure they experience in providing education. Such pressures come from families in which the parents attended school, that reside in urban areas, belong to cultural majorities and who have a clear appreciation of the benefits of education. Much less pressure comes from rural areas and indigenous populations, or from impoverished urban areas. In these countries, fewer resources, including infrastructure, equipment, teachers, supervision and funding, are allocated to the disadvantaged, the poor and cultural minorities.
- Teachers are key agents for learning. Their training is crucial. When insufficient priority is given to either initial or in-service teacher training, or to both, one can expect learning deficits. Teachers in poorer areas tend to have less training and to receive less in-service support.
- Most countries are very diverse. When a curriculum is overloaded and is the same for everyone, some students, generally those from rural areas, cultural minorities or living in poverty find little meaning in what is taught. When the language of instruction is different from their native tongue, students learn much less and drop out of school earlier.
- Disadvantaged students frequently encounter unfriendly or overtly offensive attitudes from both teachers and classmates. Such attitudes are derived from prejudices, stereotypes, outright racism and sexism. Students in hostile environments are affected in their disposition to learn, and many drop out early.
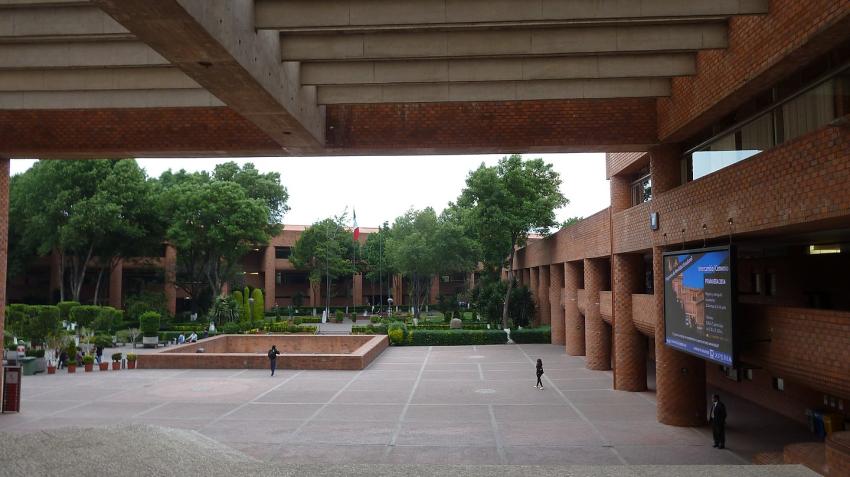
It doesn’t have to be like this
When left to inertial decision-making, education systems seem to be doomed to reproduce social and economic inequity. The commitment of both governments and societies to equity in education is both necessary and possible. There are several examples of more equitable educational systems in the world, and there are many subnational examples of successful policies fostering equity in education.
Why is equity in education important?
Education is a basic human right. More than that, it is an enabling right in the sense that, when respected, allows for the fulfillment of other human rights. Education has proven to affect general well-being, productivity, social capital, responsible citizenship and sustainable behaviour. Its equitable distribution allows for the creation of permeable societies and equity. The 2030 Agenda for Sustainable Development includes Sustainable Development Goal 4, which aims to ensure “inclusive and equitable quality education and promote lifelong learning opportunities for all”. One hundred eighty-four countries are committed to achieving this goal over the next decade. 5 The process of walking this road together has begun and requires impetus to continue, especially now that we must face the devastating consequences of a long-lasting pandemic. Further progress is crucial for humanity.
Notes 1 United Nations Educational, Scientific and Cultural Organization , Inclusive Education. All Means All , Global Education Monitoring Report 2020 (Paris, 2020), p.8. Available at https://en.unesco.org/gem-report/report/2020/inclusion . 2 Ibid., p. 4, 7. 3 World Bank Group, World Development Report 2018: Learning to Realize Education's Promise (Washington, DC, 2018), p. 3. Available at https://www.worldbank.org/en/publication/wdr2018 . 4 Instituto Nacional para la Evaluación de la Educación, "La educación obligatoria en México", Informe 2018 (Ciudad de México, 2018), p. 72. Available online at https://www.inee.edu.mx/wp-content/uploads/2018/12/P1I243.pdf . 5 United Nations Educational, Scientific and Cultural Organization , “Incheon Declaration and Framework for Action for the implementation of Sustainable Development Goal 4” (2015), p. 23. Available at https://iite.unesco.org/publications/education-2030-incheon-declaration-framework-action-towards-inclusive-equitable-quality-education-lifelong-learning/ The UN Chronicle is not an official record. It is privileged to host senior United Nations officials as well as distinguished contributors from outside the United Nations system whose views are not necessarily those of the United Nations. Similarly, the boundaries and names shown, and the designations used, in maps or articles do not necessarily imply endorsement or acceptance by the United Nations.

The LDC Future Forum: Accelerating the Attainment of the Sustainable Development Goals in the Least Developed Countries
The desired outcome of the LDC Future Forums is the dissemination of practical and evidence-based case studies, solutions and policy recommendations for achieving sustainable development.

From Local Moments to Global Movement: Reparation Mechanisms and a Development Framework
For two centuries, emancipated Black people have been calling for reparations for the crimes committed against them.
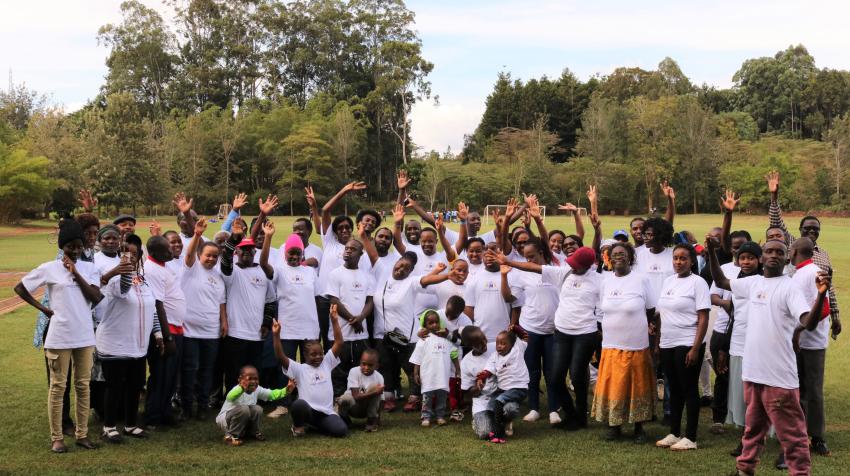
World Down Syndrome Day: A Chance to End the Stereotypes
The international community, led by the United Nations, can continue to improve the lives of people with Down syndrome by addressing stereotypes and misconceptions.
Documents and publications
- Yearbook of the United Nations
- Basic Facts About the United Nations
- Journal of the United Nations
- Meetings Coverage and Press Releases
- United Nations Official Document System (ODS)
- Africa Renewal
Libraries and Archives
- Dag Hammarskjöld Library
- UN Audiovisual Library
- UN Archives and Records Management
- Audiovisual Library of International Law
- UN iLibrary
News and media
- UN News Centre
- UN Chronicle on Twitter
- UN Chronicle on Facebook
The UN at Work
- 17 Goals to Transform Our World
- Official observances
- United Nations Academic Impact (UNAI)
- Protecting Human Rights
- Maintaining International Peace and Security
- The Office of the Secretary-General’s Envoy on Youth
- United Nations Careers
Informing and Advancing Effective Policy
- Newsletters
- Education Gap Grows Between Rich and Poor, Studies Say
By SABRINA TAVERNISE
Education was historically considered a great equalizer in American society, capable of lifting less advantaged children and improving their chances for success as adults. But a body of recently published scholarship suggests that the achievement gap between rich and poor children is widening, a development that threatens to dilute education’s leveling effects.
It is a well-known fact that children from affluent families tend to do better in school. Yet the income divide has received far less attention from policy makers and government officials than gaps in student accomplishment by race.

Now, in analyses of long-term data published in recent months, researchers are finding that while the achievement gap between white and black students has narrowed significantly over the past few decades, the gap between rich and poor students has grown substantially during the same period.
“We have moved from a society in the 1950s and 1960s, in which race was more consequential than family income, to one today in which family income appears more determinative of educational success than race,” said Sean F. Reardon, a Stanford University sociologist. Professor Reardon is the author of a study that found that the gap in standardized test scores between affluent and low-income students had grown by about 40 percent since the 1960s, and is now double the testing gap between blacks and whites.
In another study, by researchers from the University of Michigan , the imbalance between rich and poor children in college completion — the single most important predictor of success in the work force — has grown by about 50 percent since the late 1980s.
The changes are tectonic, a result of social and economic processes unfolding over many decades. The data from most of these studies end in 2007 and 2008, before the recession’s full impact was felt. Researchers said that based on experiences during past recessions, the recent downturn was likely to have aggravated the trend.
“With income declines more severe in the lower brackets, there’s a good chance the recession may have widened the gap,” Professor Reardon said. In the study he led, researchers analyzed 12 sets of standardized test scores starting in 1960 and ending in 2007. He compared children from families in the 90th percentile of income — the equivalent of around $160,000 in 2008, when the study was conducted — and children from the 10th percentile, $17,500 in 2008. By the end of that period, the achievement gap by income had grown by 40 percent, he said, while the gap between white and black students, regardless of income, had shrunk substantially.
The connection between income inequality among parents and the social mobility of their children has been a focus of President Obama as well as some of the Republican presidential candidates.
One reason for the growing gap in achievement, researchers say, could be that wealthy parents invest more time and money than ever before in their children (in weekend sports, ballet, music lessons, math tutors, and in overall involvement in their children’s schools), while lower-income families, which are now more likely than ever to be headed by a single parent, are increasingly stretched for time and resources. This has been particularly true as more parents try to position their children for college, which has become ever more essential for success in today’s economy.
A study by Sabino Kornrich, a researcher at the Center for Advanced Studies at the Juan March Institute in Madrid, and Frank F. Furstenberg, scheduled to appear in the journal Demography this year, found that in 1972, Americans at the upper end of the income spectrum were spending five times as much per child as low-income families. By 2007 that gap had grown to nine to one; spending by upper-income families more than doubled, while spending by low-income families grew by 20 percent.
“The pattern of privileged families today is intensive cultivation,” said Dr. Furstenberg, a professor of sociology at the University of Pennsylvania.
The gap is also growing in college. The University of Michigan study, by Susan M. Dynarski and Martha J. Bailey, looked at two generations of students, those born from 1961 to 1964 and those born from 1979 to 1982. By 1989, about one-third of the high-income students in the first generation had finished college; by 2007, more than half of the second generation had done so. By contrast, only 9 percent of the low-income students in the second generation had completed college by 2007, up only slightly from a 5 percent college completion rate by the first generation in 1989.
James J. Heckman, an economist at the University of Chicago, argues that parenting matters as much as, if not more than, income in forming a child’s cognitive ability and personality, particularly in the years before children start school.
“Early life conditions and how children are stimulated play a very important role,” he said. “The danger is we will revert back to the mindset of the war on poverty, when poverty was just a matter of income, and giving families more would improve the prospects of their children. If people conclude that, it’s a mistake.”
Meredith Phillips, an associate professor of public policy and sociology at the University of California, Los Angeles, used survey data to show that affluent children spend 1,300 more hours than low-income children before age 6 in places other than their homes, their day care centers, or schools (anywhere from museums to shopping malls). By the time high-income children start school, they have spent about 400 hours more than poor children in literacy activities, she found.
Charles Murray, a scholar at the American Enterprise Institute whose book, “Coming Apart: The State of White America, 1960-2010,” was published Jan. 31, described income inequality as “more of a symptom than a cause.”
The growing gap between the better educated and the less educated, he argued, has formed a kind of cultural divide that has its roots in natural social forces, like the tendency of educated people to marry other educated people, as well as in the social policies of the 1960s, like welfare and other government programs, which he contended provided incentives for staying single
“When the economy recovers, you’ll still see all these problems persisting for reasons that have nothing to do with money and everything to do with culture,” he said.
There are no easy answers, in part because the problem is so complex, said Douglas J. Besharov, a fellow at the Atlantic Council. Blaming the problem on the richest of the rich ignores an equally important driver, he said: two-earner household wealth, which has lifted the upper middle class ever further from less educated Americans, who tend to be single parents.
The problem is a puzzle, he said. “No one has the slightest idea what will work. The cupboard is bare.”
Mentioned Publications
The widening academic achievement gap between the rich and the poor: new evidence and possible explanations.
Sean F. Reardon
In this chapter I examine whether and how the relationship between family socioeconomic characteristics and academic achievement has changed during the last fifty years. In particular, I investigate the extent to which the rising income inequality of the last four decades has been paralleled by a similar increase in the income achievement gradient. As the income gap between high- and low-income families has widened, has the achievement gap between children in high- and low-income families also widened?
The answer, in brief, is yes. The achievement gap between children from high- and low-income families is roughly 30 to 40 percent larger among children born in 2001 than among those born twenty-five years earlier. In fact, it appears that the income achievement gap has been growing for at least fifty years, though the data are less certain for cohorts of children born before 1970. In this chapter, I describe and discuss these trends in some detail. In addition to the key finding that the income achievement gap appears to have widened substantially, there are a number of other important findings.
First, the income achievement gap (defined here as the average achievement difference between a child from a family at the 90th percentile of the family income distribution and a child from a family at the 10th percentile) is now nearly twice as large as the black-white achievement gap. Fifty years ago, in contrast, the black-white gap was one and a half to two times as large as the income gap. Second, as Greg Duncan and Katherine Magnuson note in chapter 3 of this volume, the income achievement gap is large when children enter kindergarten and does not appear to grow (or narrow) appreciably as children progress through school. Third, although rising income inequality may play a role in the growing income achievement gap, it does not appear to be the dominant factor. The gap appears to have grown at least partly because of an increase in the association between family income and children's academic achievement for families above the median income level: a given difference in family incomes now corresponds to a 30 to 60 percent larger difference in achievement than it did for children born in the 1970s. Moreover, evidence from other studies suggests that this may be in part a result of increasing parental investment in children's cognitive development. Finally, the growing income achievement gap does not appear to be a result of a growing achievement gap between children with highly and less-educated parents. Indeed, the relationship between parental education and children's achievement has remained relatively stable during the last fifty years, whereas the relationship between income and achievement has grown sharply. Family income is now nearly as strong as parental education in predicting children's achievement.
This chapter is now published in the book Whither Opportunity: https://www.russellsage.org/publications/whither-opportunity

You are here
- Sean Reardon
The Hechinger Report
Covering Innovation & Inequality in Education
A decade of research on the rich-poor divide in education

Share this:
- Click to share on LinkedIn (Opens in new window)
- Click to share on Pinterest (Opens in new window)
- Click to share on Reddit (Opens in new window)
- Click to share on WhatsApp (Opens in new window)
- Click to email a link to a friend (Opens in new window)
The Hechinger Report is a national nonprofit newsroom that reports on one topic: education. Sign up for our weekly newsletters to get stories like this delivered directly to your inbox. Consider supporting our stories and becoming a member today.

Get important education news and analysis delivered straight to your inbox
- Weekly Update
- Future of Learning
- Higher Education
- Early Childhood
- Proof Points
Americans like to believe that education can be a great equalizer, allowing even the poorest child who studies hard to enter the middle class. But when I looked at what academic researchers and federal data reports have said about the great educational divide between the rich and poor in our country, that belief turns out to be a myth. Basic education, from kindergarten through high school, only expands the disparities.
In 2015, during the Obama administration, the federal education department issued a report that showed how the funding gap between rich and poor schools grew 44 percent over a decade between 2001-2 and 2011-12. That meant that the richest 25 percent of school districts spent $1,500 more per student, on average, than the poorest 25 percent of school districts.
I wish I could have continued to track this data between rich and poor schools to see if school spending had grown more fair. But the Trump administration crunched the numbers differently. When it issued a report in 2018 , covering the 2014-15 school year, it found that the wealthiest 25 percent of districts spent $450 more per student than the poorest 25 percent.
That didn’t mean there was a giant 70 percent improvement from $1,500. The Trump administration added together all sources of funds, including federal funding, which amounts to 8 percent of total school spending, while the Obama administration excluded federal funds, counting only state and local dollars, which make up more than 90 percent of education funds. The Obama administration argued at the time that federal funds for poor students were intended to supplement local funds because it takes more resources to overcome childhood poverty, not to create a level playing field.
Rather than marking an improvement, there were signs in the Trump administration data that the funding gap between rich and poor had worsened during the Great Recession if you had compared the figures apples to apples, either including or excluding federal funds. In a follow-up report issued in 2019, the Trump administration documented that the funding gap between rich and poor schools had increased slightly to $473 per student between the 2014-15 and 2015-16 school years.
It’s not just a divide between rich and poor but also between the ultra rich and everyone else. In 2020, a Pennsylvania State University researcher documented how the wealthiest school districts in America — the top 1 percent — fund their schools at much higher levels than everyone else and are increasing their school spending at a faster rate. The school funding gap between a top 1 percent district (mostly white suburbs) and an average-spending school district at the 50th percentile widened by 32 percent between 2000 and 2015, the study calculated. Nassau County, just outside New York City on Long Island, has the highest concentration of students who attend the best funded public schools among all counties in the country. Almost 17 percent of all the top 1 percent students in the nation live in this one county.
Funding inequities are happening in a context of increased poverty in our schools. In 2013, I documented how the number of high poverty schools had increased by about 60 percent to one out of every five schools in 2011 from one out of every eight schools in 2000. To win this unwelcome designation, 75 percent or more of an elementary, middle or high school’s students lived in families poor enough to qualify for free or reduced-price lunch. It’s since gotten worse. In the most recent federal report , covering the 2016-17 school year, one out of every four schools in America was classified as high poverty.
It’s not just that poverty is becoming more concentrated in certain schools; more students in the school system are poor. In 2014, I documented a 40 percent jump in the number of school-aged children living in poverty between 2000 and 2012 from one out of every seven children to one out of every five students. In the most recent report, for the 2016-17 school year, the poverty rate declined from 21 percent in 2010 to 18 percent in 2017. About 13 million children under the age of 18 were in families living in poverty.
When you break the data down by race, there are other striking patterns. One third of all Black children under 18 were living in poverty in 2016-17, compared with a quarter of Hispanic children. White and Asian children have a similar poverty rate of 11 percent and 10 percent, respectively.
Sociologists like Sean Reardon at Stanford University and Ann Owens at the University of Southern California have built a body of evidence that school segregation by income is what’s really getting worse in America, not school segregation by race. But it’s a complicated argument because Black and Latino students are more likely to be poor and less likely to be rich. So the two things — race and poverty — are intertwined.
In 2019, Reardon studied achievement gaps in every school in America and found that the difference in poverty rates between predominantly Black and predominantly white schools explains the achievement gaps we see and why white schools tend to show higher test scores than Black schools. When white and Black schools have the same poverty rates, Reardon didn’t see a difference in academic achievement. The problem is that Black students are more often poor and attending schools with more poor students. And other than a handful of high-performing charter schools in a few cities, he couldn’t find examples of academic excellence among schools with a high-poverty student body.
“It doesn’t seem that we have any knowledge about how to create high-quality schools at scale under conditions of concentrated poverty,” said Reardon. “And if we can’t do that, then we have to do something about segregation. Otherwise we’re consigning Black and Hispanic and low-income students to schools that we don’t know how to make as good as other schools. The implication is that you have got to address segregation.”
Previous Proof Points columns cited in this column:
The number of high-poverty schools increases by about 60 percent
Poverty among school-age children increases by 40 percent since 2000
The gap between rich and poor schools grew 44 percent over a decade
Data show segregation by income (not race) is what’s getting worse in schools
In 6 states, school districts with the neediest students get less money than the wealthiest
An analysis of achievement gaps in every school in America shows that poverty is the biggest hurdle
Rich schools get richer: School spending analysis finds widening gap between top 1% and the rest of us
This story about education inequality in America written by Jill Barshay and produced by The Hechinger Report , a nonprofit, independent news organization focused on inequality and innovation in education. Sign up for the Hechinger newsletter .
Related articles
The Hechinger Report provides in-depth, fact-based, unbiased reporting on education that is free to all readers. But that doesn't mean it's free to produce. Our work keeps educators and the public informed about pressing issues at schools and on campuses throughout the country. We tell the whole story, even when the details are inconvenient. Help us keep doing that.
Join us today.
Jill Barshay SENIOR REPORTER
(212)... More by Jill Barshay
Letters to the Editor
At The Hechinger Report, we publish thoughtful letters from readers that contribute to the ongoing discussion about the education topics we cover. Please read our guidelines for more information. We will not consider letters that do not contain a full name and valid email address. You may submit news tips or ideas here without a full name, but not letters.
By submitting your name, you grant us permission to publish it with your letter. We will never publish your email address. You must fill out all fields to submit a letter.
Thanks to Jill Barshay for the excellent column reminding us that there is much more to the rich/poor divide in our public schools than just the availability of digital devices and wi-fi. The real problem with equity in education is the lack of equity in school funding, which is an issue both of inequity in society and the ways in which public schools are funded (i.e., primarily local tax revenues).
Other barriers that kept the “school door blocked” for many low income students during this season of remote learning — and, presumably, next school year, as well — include: 1. Some with access to devices and wi-fi have had service disconnected at times due to unpaid (unpayable) bills. 2. Many have no private space in their homes from where to participate in synchronous learning/Zoom calls 3. With loss of family income and no child care, some have work or baby-sitting responsibilities that interfere with participation 4. Deadening effects of online learning cause many low-income students to disconnect and/or “drop out”. 5. In ability to access teacher supports and specialized instruction, esp. for English language learners and children with special needs. 6. Parent inability to assist students with computer routines, glitches, log-ins, etc
As districts address equity in the coming school year, we must also address the modes of learning that we consider both effective and valuable. If the top priority is engaging all students we need high engagement models based in trauma-informed practices, social and racial justice curricula, service learning, interdisciplinary project- and place-based learning, outdoor learning and other innovative ways to make education relevant to all students, regardless of their zip codes. Relax the standards. Cancel high stakes testing. Trust teachers to use their creativity to connect with every student and family. Otherwise, “remote” or “hybrid” learning, regardless of the availability of technology, will only be widening the gaps that structural racism has already created.
Why are we NOT reaching out to the teaching programs started by Marva Collins in Chicago and Ron Clark in Atlanta? Why are we NOT looking at a book called Schools That Work and viewing the achievements and strategies followed by successful programs. Let’s follow successful schools, successful environments in urban, rural, and suburban locations. As an eductor who started teaching in the Ocean-Hill Brownsville area of Brooklyn, N,Y. in 1971, there was a wildcat strike happening and this area was the where decentralization took place in N.Y.C. Rev. Al Sharpton’s church was down the block from I.S. 271. It took 2 years before a no nonsense, BLACK principal, took control over the choas and the movement of 125 teachers going and then coming to this “high poverty” intermediate school. There was stability of staff and the message was, you’re here to learn. I taught there for 7 incredible years and grew to understand what it was like being a minority teacher and human being. I then moved to Columbia, MD. where I lived in a planned community where diversity of color, homes, religions and belief in humanity living together as ONE took place. I taught in a white disadvantaged area for 2 years and observed the same behaviors students exhibited except there was no leadership at the top of this school. Now I teach in a suburban area for the last 31 years with limited diversity and succeeds because of innovative leadership, extraordinary teachers, and pretty high achieving students. Yes, I know every students must have access to technology as a MUST. Yes, I know urban education, rural education, and suburban education do education diffferently. Yes, I know poverty sucks, and I know distant learning may be around for a while. Change must come from the top. Let’s follow the successful educators, the successful programs, the dynamic elected officials who can shake up things so our students, our kids, our educational systems can be the change that can bring poverty to it’s knees.
I live on Long Island and know that whatever is written here about us is true. The Freeport Public School waste millions of taxpayers dollars throwing out teaching equipment, devices books that could be just given to the less fortunate schools next door-Queens, Brooklyn and the Bronx; where we see children suffering because of lack of proper learning tools. I am from the Caribbean where l taught for years. Oh l wish we were as privileged as these children. Maybe one day the disparity will end. Hopefully.
I enjoy reading this post. I am currently doing my thesis and the research question is: Do California K-12 public schools in lower-income communities offer the same level of academic curriculum as those in middle-income and wealthy communities? Do you have the reference page for those studies or even any peer reviewers where you got the information? I would like to review those studies and use them for my thesis. Thank you
Your email address will not be published. Required fields are marked *
Save my name, email, and website in this browser for the next time I comment.
Sign me up for the newsletter!
Submit a letter
Featured Topics
Featured series.
A series of random questions answered by Harvard experts.
Explore the Gazette
Read the latest.
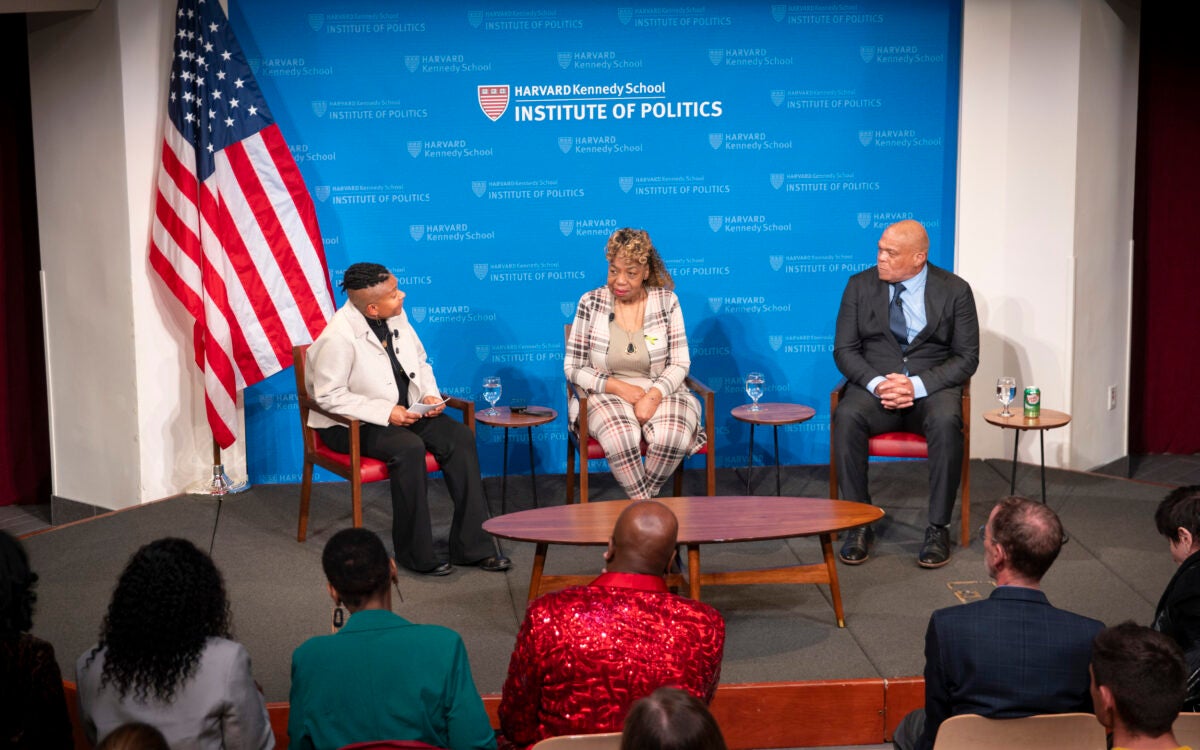
Remember Eric Garner? George Floyd?
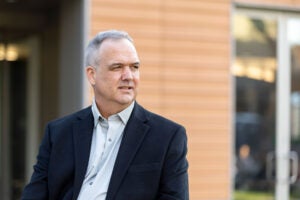
Lawyers reap big profits lobbying government regulators under the radar
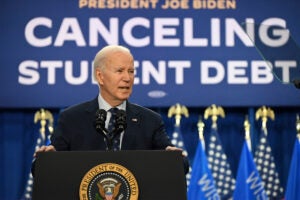
Younger votes still lean toward Biden — but it’s complicated
The costs of inequality: education’s the one key that rules them all.
When there’s inequity in learning, it’s usually baked into life, Harvard analysts say
Corydon Ireland
Harvard Correspondent
Third in a series on what Harvard scholars are doing to identify and understand inequality, in seeking solutions to one of America’s most vexing problems.
Before Deval Patrick ’78, J.D. ’82, was the popular and successful two-term governor of Massachusetts, before he was managing director of high-flying Bain Capital, and long before he was Harvard’s most recent Commencement speaker , he was a poor black schoolchild in the battered housing projects of Chicago’s South Side.
The odds of his escaping a poverty-ridden lifestyle, despite innate intelligence and drive, were long. So how did he help mold his own narrative and triumph over baked-in societal inequality ? Through education.
“Education has been the path to better opportunity for generations of American strivers, no less for me,” Patrick said in an email when asked how getting a solid education, in his case at Milton Academy and at Harvard, changed his life.
“What great teachers gave me was not just the skills to take advantage of new opportunities, but the ability to imagine what those opportunities could be. For a kid from the South Side of Chicago, that’s huge.”
If inequality starts anywhere, many scholars agree, it’s with faulty education. Conversely, a strong education can act as the bejeweled key that opens gates through every other aspect of inequality , whether political, economic , racial, judicial, gender- or health-based.
Simply put, a top-flight education usually changes lives for the better. And yet, in the world’s most prosperous major nation, it remains an elusive goal for millions of children and teenagers.
Plateau on educational gains
The revolutionary concept of free, nonsectarian public schools spread across America in the 19th century. By 1970, America had the world’s leading educational system, and until 1990 the gap between minority and white students, while clear, was narrowing.
But educational gains in this country have plateaued since then, and the gap between white and minority students has proven stubbornly difficult to close, says Ronald Ferguson, adjunct lecturer in public policy at Harvard Kennedy School (HKS) and faculty director of Harvard’s Achievement Gap Initiative. That gap extends along class lines as well.
“What great teachers gave me was not just the skills to take advantage of new opportunities, but the ability to imagine what those opportunities could be. For a kid from the South Side of Chicago, that’s huge.” — Deval Patrick
In recent years, scholars such as Ferguson, who is an economist, have puzzled over the ongoing achievement gap and what to do about it, even as other nations’ school systems at first matched and then surpassed their U.S. peers. Among the 34 market-based, democracy-leaning countries in the Organization for Economic Cooperation and Development (OECD), the United States ranks around 20th annually, earning average or below-average grades in reading, science, and mathematics.
By eighth grade, Harvard economist Roland G. Fryer Jr. noted last year, only 44 percent of American students are proficient in reading and math. The proficiency of African-American students, many of them in underperforming schools, is even lower.
“The position of U.S. black students is truly alarming,” wrote Fryer, the Henry Lee Professor of Economics, who used the OECD rankings as a metaphor for minority standing educationally. “If they were to be considered a country, they would rank just below Mexico in last place.”
Harvard Graduate School of Education (HGSE) Dean James E. Ryan, a former public interest lawyer, says geography has immense power in determining educational opportunity in America. As a scholar, he has studied how policies and the law affect learning, and how conditions are often vastly unequal.
His book “Five Miles Away, A World Apart” (2010) is a case study of the disparity of opportunity in two Richmond, Va., schools, one grimly urban and the other richly suburban. Geography, he says, mirrors achievement levels.
A ZIP code as predictor of success
“Right now, there exists an almost ironclad link between a child’s ZIP code and her chances of success,” said Ryan. “Our education system, traditionally thought of as the chief mechanism to address the opportunity gap, instead too often reflects and entrenches existing societal inequities.”
Urban schools demonstrate the problem. In New York City, for example, only 8 percent of black males graduating from high school in 2014 were prepared for college-level work, according to the CUNY Institute for Education Policy, with Latinos close behind at 11 percent. The preparedness rates for Asians and whites — 48 and 40 percent, respectively — were unimpressive too, but nonetheless were firmly on the other side of the achievement gap.
In some impoverished urban pockets, the racial gap is even larger. In Washington, D.C., 8 percent of black eighth-graders are proficient in math, while 80 percent of their white counterparts are.
Fryer said that in kindergarten black children are already 8 months behind their white peers in learning. By third grade, the gap is bigger, and by eighth grade is larger still.
According to a recent report by the Education Commission of the States, black and Hispanic students in kindergarten through 12th grade perform on a par with the white students who languish in the lowest quartile of achievement.
There was once great faith and hope in America’s school systems. The rise of quality public education a century ago “was probably the best public policy decision Americans have ever made because it simultaneously raised the whole growth rate of the country for most of the 20th century, and it leveled the playing field,” said Robert Putnam, the Peter and Isabel Malkin Professor of Public Policy at HKS, who has written several best-selling books touching on inequality, including “Bowling Alone: The Collapse and Revival of the American Community” and “Our Kids: The American Dream in Crisis.”
Historically, upward mobility in America was characterized by each generation becoming better educated than the previous one, said Harvard economist Lawrence Katz. But that trend, a central tenet of the nation’s success mythology, has slackened, particularly for minorities.
“Thirty years ago, the typical American had two more years of schooling than their parents. Today, we have the most educated group of Americans, but they only have about .4 more years of schooling, so that’s one part of mobility not keeping up in the way we’ve invested in education in the past,” Katz said.
As globalization has transformed and sometimes undercut the American economy, “education is not keeping up,” he said. “There’s continuing growth of demand for more abstract, higher-end skills” that schools aren’t delivering, “and then that feeds into a weakening of institutions like unions and minimum-wage protections.”
“The position of U.S. black students is truly alarming.” — Roland G. Fryer Jr.
Fryer is among a diffuse cohort of Harvard faculty and researchers using academic tools to understand the achievement gap and the many reasons behind problematic schools. His venue is the Education Innovation Laboratory , where he is faculty director.
“We use big data and causal methods,” he said of his approach to the issue.
Fryer, who is African-American, grew up poor in a segregated Florida neighborhood. He argues that outright discrimination has lost its power as a primary driver behind inequality, and uses economics as “a rational forum” for discussing social issues.
Better schools to close the gap
Fryer set out in 2004 to use an economist’s data and statistical tools to answer why black students often do poorly in school compared with whites. His years of research have convinced him that good schools would close the education gap faster and better than addressing any other social factor, including curtailing poverty and violence, and he believes that the quality of kindergarten through grade 12 matters above all.
Supporting his belief is research that says the number of schools achieving excellent student outcomes is a large enough sample to prove that much better performance is possible. Despite the poor performance by many U.S. states, some have shown that strong results are possible on a broad scale. For instance, if Massachusetts were a nation, it would rate among the best-performing countries.
At HGSE, where Ferguson is faculty co-chair as well as director of the Achievement Gap Initiative, many factors are probed. In the past 10 years, Ferguson, who is African-American, has studied every identifiable element contributing to unequal educational outcomes. But lately he is looking hardest at improving children’s earliest years, from infancy to age 3.
In addition to an organization he founded called the Tripod Project , which measures student feedback on learning, he launched the Boston Basics project in August, with support from the Black Philanthropy Fund, Boston’s mayor, and others. The first phase of the outreach campaign, a booklet, videos, and spot ads, starts with advice to parents of children age 3 or younger.
“Maximize love, manage stress” is its mantra and its foundational imperative, followed by concepts such as “talk, sing, and point.” (“Talking,” said Ferguson, “is teaching.”) In early childhood, “The difference in life experiences begins at home.”
At age 1, children score similarly
Fryer and Ferguson agree that the achievement gap starts early. At age 1, white, Asian, black, and Hispanic children score virtually the same in what Ferguson called “skill patterns” that measure cognitive ability among toddlers, including examining objects, exploring purposefully, and “expressive jabbering.” But by age 2, gaps are apparent, with black and Hispanic children scoring lower in expressive vocabulary, listening comprehension, and other indicators of acuity. That suggests educational achievement involves more than just schooling, which typically starts at age 5.
Key factors in the gap, researchers say, include poverty rates (which are three times higher for blacks than for whites), diminished teacher and school quality, unsettled neighborhoods, ineffective parenting, personal trauma, and peer group influence, which only strengthens as children grow older.
“Peer beliefs and values,” said Ferguson, get “trapped in culture” and are compounded by the outsized influence of peers and the “pluralistic ignorance” they spawn. Fryer’s research, for instance, says that the reported stigma of “acting white” among many black students is true. The better they do in school, the fewer friends they have — while for whites who are perceived as smarter, there’s an opposite social effect.
The researchers say that family upbringing matters, in all its crisscrossing influences and complexities, and that often undercuts minority children, who can come from poor or troubled homes. “Unequal outcomes,” he said, “are from, to a large degree, inequality in life experiences.”
Trauma also subverts achievement, whether through family turbulence, street violence, bullying, sexual abuse, or intermittent homelessness. Such factors can lead to behaviors in school that reflect a pervasive form of childhood post-traumatic stress disorder.
[gz_sidebar align=”left”]
Possible solutions to educational inequality:
- Access to early learning
- Improved K-12 schools
- More family mealtimes
- Reinforced learning at home
- Data-driven instruction
- Longer school days, years
- Respect for school rules
- Small-group tutoring
- High expectations of students
- Safer neighborhoods
[/gz_sidebar]
At Harvard Law School, both the Trauma and Learning Policy Initiative and the Education Law Clinic marshal legal aid resources for parents and children struggling with trauma-induced school expulsions and discipline issues.
At Harvard Business School, Karim R. Lakhani, an associate professor who is a crowdfunding expert and a champion of open-source software, has studied how unequal racial and economic access to technology has worked to widen the achievement gap.
At Harvard’s Project Zero, a nonprofit called the Family Dinner Project is scraping away at the achievement gap from the ground level by pushing for families to gather around the meal table, which traditionally was a lively and comforting artifact of nuclear families, stable wages, close-knit extended families, and culturally shared values.
Lynn Barendsen, the project’s executive director, believes that shared mealtimes improve reading skills, spur better grades and larger vocabularies, and fuel complex conversations. Interactive mealtimes provide a learning experience of their own, she said, along with structure, emotional support, a sense of safety, and family bonding. Even a modest jump in shared mealtimes could boost a child’s academic performance, she said.
“We’re not saying families have to be perfect,” she said, acknowledging dinnertime impediments like full schedules, rudimentary cooking skills, the lure of technology, and the demands of single parenting. “The perfect is the enemy of the good.”
Whether poring over Fryer’s big data or Barendsen’s family dinner project, there is one commonality for Harvard researchers dealing with inequality in education: the issue’s vast complexity. The achievement gap is a creature of interlocking factors that are hard to unpack constructively.
Going wide, starting early
With help from faculty co-chair and Jesse Climenko Professor of Law Charles J. Ogletree, the Achievement Gap Initiative is analyzing the factors that make educational inequality such a complex puzzle: home and family life, school environments, teacher quality, neighborhood conditions, peer interaction, and the fate of “all those wholesome things,” said Ferguson. The latter include working hard in school, showing respect, having nice friends, and following the rules, traits that can be “elements of a 21st-century movement for equality.”
In the end, best practices to create strong schools will matter most, said Fryer.
He called high-quality education “the new civil rights battleground” in a landmark 2010 working paper for the Handbook of Labor Economics called “Racial Inequality in the 21st Century: The Declining Significance of Discrimination.”
Fryer tapped 10 large data sets on children 8 months to 17 years old. He studied charter schools, scouring for standards that worked. He champions longer school days and school years, data-driven instruction, small-group tutoring, high expectations, and a school culture that prizes human capital — all just “a few simple investments,” he wrote in the working paper. “The challenge for the future is to take these examples to scale” across the country.
How long would closing the gap take with a national commitment to do so? A best-practices experiment that Fryer conducted at low-achieving high schools in Houston closed the gap in math skills within three years, and narrowed the reading achievement gap by a third.
“You don’t need Superman for this,” he said, referring to a film about Geoffrey Canada and his Harlem Children’s Zone, just high-quality schools for everyone, to restore 19th-century educator Horace Mann’s vision of public education as society’s “balance-wheel.”
Last spring, Fryer, still only 38, won the John Bates Clark medal, the most prestigious award in economics after the Nobel Prize. He was a MacArthur Fellow in 2011, became a tenured Harvard professor in 2007, was named to the prestigious Society of Fellows at age 25. He had a classically haphazard childhood, but used school to learn, grow, and prosper. Gradually, he developed a passion for social science that could help him answer what was going wrong in black lives because of educational inequality.
With his background and talent, Fryer has a dramatically unique perspective on inequality and achievement, and he has something else: a seemingly counterintuitive sense that these conditions will improve, once bad schools learn to get better. Discussing the likelihood of closing the achievement gap if Americans have the political and organizational will to do so, Fryer said, “I see nothing but optimism.”
Correction: An earlier version of this story inaccurately portrayed details of Dr. Fryer’s background.
Illustration by Kathleen M.G. Howlett. Harvard staff writer Christina Pazzanese contributed to this report.
Next Tuesday: Inequality in health care
Share this article
You might like.
Mother, uncle of two whose deaths at hands of police officers ignited movement talk about turning pain into activism, keeping hope alive
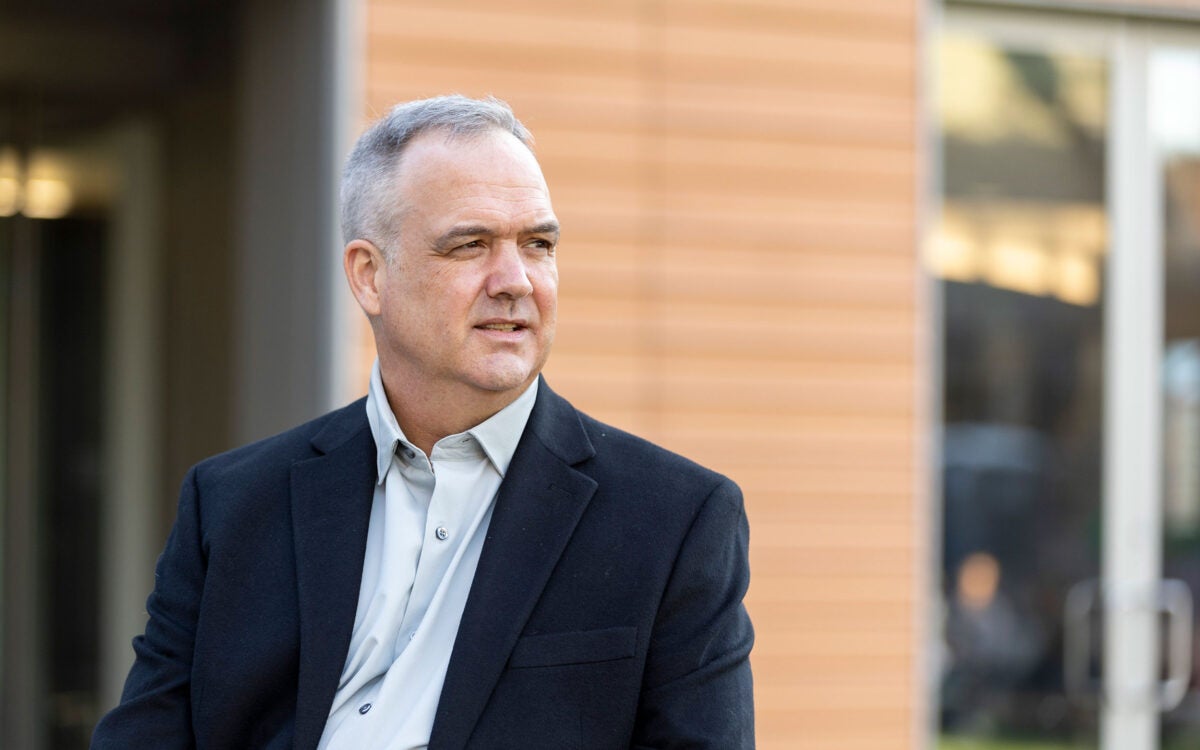
Study exposes how banks sway policy from shadows, by targeting bureaucrats instead of politicians
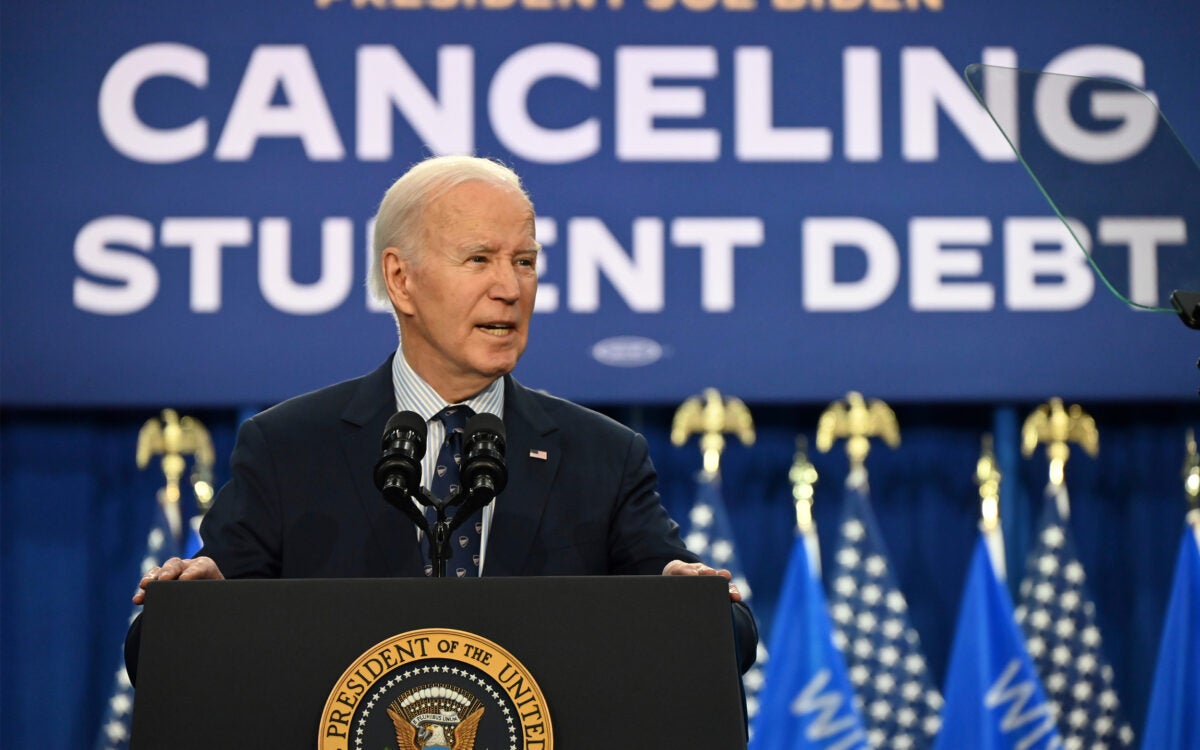
New IOP poll shows they still plan to show up to vote but are subject to ‘seismic mood swings’ over specific issues
Exercise cuts heart disease risk in part by lowering stress, study finds
Benefits nearly double for people with depression
So what exactly makes Taylor Swift so great?
Experts weigh in on pop superstar's cultural and financial impact as her tours and albums continue to break records.
Finding right mix on campus speech policies
Legal, political scholars discuss balancing personal safety, constitutional rights, academic freedom amid roiling protests, cultural shifts
- Architecture and Design
- Asian and Pacific Studies
- Business and Economics
- Classical and Ancient Near Eastern Studies
- Computer Sciences
- Cultural Studies
- Engineering
- General Interest
- Geosciences
- Industrial Chemistry
- Islamic and Middle Eastern Studies
- Jewish Studies
- Library and Information Science, Book Studies
- Life Sciences
- Linguistics and Semiotics
- Literary Studies
- Materials Sciences
- Mathematics
- Social Sciences
- Sports and Recreation
- Theology and Religion
- Publish your article
- The role of authors
- Promoting your article
- Abstracting & indexing
- Publishing Ethics
- Why publish with De Gruyter
- How to publish with De Gruyter
- Our book series
- Our subject areas
- Your digital product at De Gruyter
- Contribute to our reference works
- Product information
- Tools & resources
- Product Information
- Promotional Materials
- Orders and Inquiries
- FAQ for Library Suppliers and Book Sellers
- Repository Policy
- Free access policy
- Open Access agreements
- Database portals
- For Authors
- Customer service
- People + Culture
- Journal Management
- How to join us
- Working at De Gruyter
- Mission & Vision
- De Gruyter Foundation
- De Gruyter Ebound
- Our Responsibility
- Partner publishers

Your purchase has been completed. Your documents are now available to view.
Causes and Consequences of Income Inequality – An Overview
Rising income inequality is one of the greatest challenges facing advanced economies today. Income inequality is multifaceted and is not the inevitable outcome of irresistible structural forces such as globalisation or technological development. Instead, this review shows that inequality has largely been driven by a multitude of political choices. The embrace of neoliberalism since the 1980s has provided the key catalyst for political and policy changes in the realms of union regulation, executive pay, the welfare state and tax progressivity, which have been the key drivers of inequality. These preventable causes have led to demonstrable harmful outcomes that are not explicable solely by material deprivation. This review also shows that inequality has been linked on the economic front with reduced growth, investment and innovation, and on the social front with reduced health and social mobility, and greater violent crime.
1 Introduction
Income inequality has recently come to be viewed as one of the greatest challenges facing the world today. In recent years, the topic has dominated the agenda of the World Economic Forum (WEF), where the world’s top political and business leaders attend. Their global risks report, drawn from over 700 experts in attendance, pronounced inequality to be the greatest threat to the world economy in 2017 ( Elliott 2017 ). Likewise, the past decade has seen leading global figures such as former American President Barack Obama, Pope Francis, Chinese President Xi Jinping, and the former head of the International Monetary Fund (IMF), Christine Lagarde, all undertake speeches on the gravity of income inequality and the need to address its rise. This is because, as this research note shows, income inequality engenders harmful consequences that are not explicable solely by material deprivation.
The general dynamics of income inequality include a tendency to rise slowly and fluctuate over time. For instance, Japan had one of the highest rates in the world prior to the Second World War and the United States (US) one of the lowest, which has since completely reversed for both. The United Kingdom (UK) was also the second most equitable large European country in the 1970s but is now the most inequitable ( Dorling 2018 : 27–28).
High rates of inequality are rarely sustained for long periods because they tend to lead to or become punctuated by man-made disasters that lead to a levelling out. Scheidel (2017) posits that there in fact exists a violent ‘Four Horseman of Leveling’ (mass mobilisation warfare, transformation revolutions, state collapse, and lethal pandemics) for inequality, which have at times dramatically reduced inequalities because they can lead to the alteration of existing power structures or wipe out the wealth of elites and redistribute their resources. For instance, the pronounced shocks of the two world wars led to the ‘Great Compression’ of income throughout the West in the post-war years. There is already some evidence that the current global pandemic caused by the novel Coronavirus, has led to greater aversion to income inequality ( Asaria, Costa-Font, and Cowell 2021 ; Wiwad et al. 2021 ).
Thus, greater aversion to inequality has been able to reduce inequality in the past, this is because, as this review also shows, income inequality does not result exclusively from efficient market forces but arises out of a set of rules that is shaped by those with political power. Inequality’s rise is not inevitable, nor beyond the control of governments and policymakers, as they can affect distributional outcomes and inequality through public policy.
It is the purpose of this review to outline the causes and consequences of income inequality. The paper begins with an analysis of the key structural and institutional determinants of inequality, followed by an examination into the harmful outcomes of inequality. It then concludes with a discussion of what policymakers can do to arrest the rise of inequality.
2 Causes of Income Inequality
Broadly speaking, explanations for the increase in income inequality have largely been classified as either structural or institutional. Historically, economists emphasised structural causes of increasing income inequality, with globalisation and technological change at the forefront. However, in recent years opinion has shifted to emphasise more institutional political factors to do with the adoption of neoliberal reforms such as privatisation, deregulation and tax and welfare reductions since the early 1980s. They were first embraced and most heavily championed by the UK and US, spreading globally later, and which provide the crucial catalysts of rising income inequality ( Atkinson 2015 ; Brown 2017 ; Piketty 2020 ; Stiglitz 2013 ). I discuss each of these key factors in turn.
2.1 Globalisation
One of the earliest, and most prominent explanations for the rise of income inequality emphasised the role of globalisation ( Borjas, Freeman, and Katz 1992 ; Revenga 1992 ). Globalisation has led to the offshoring of many goods and services that used to be produced or completed domestically in the West, which has created downward pressures on the wages of lower skilled workers. According to the ‘market forces hypothesis,’ increasing inequality is a response to the rising demand for skills at the top, in which the spread of globalisation and technological progress have been facilitated through reduced barriers to trade and movement.
Proponents of globalisation as the leading cause of inequality have argued that globalisation has constrained domestic state choices and left governments collectively powerless to address inequality. Detractors admit that globalisation has indeed had deep structural effects on Western economies but its impact on the degree of agency available to domestic governments has been mediated by individual policy choices ( Thomas 2016 : 346). A key problem with attributing the cause of inequality to globalisation, is that the extent of the inequality increase has varied considerably across countries, even though they have all been exposed to the same effects of globalisation. The US also has the highest inequality amongst rich countries, but it is less reliant on international trade than most other developed countries ( Brown 2017 : 56). Moreover, a recent meta-analysis by Heimberger (2020) found that globalisation has a “small-to-moderate” inequality-increasing effect, with financial globalisation displaying the largest impact.
2.2 Technology
A related explanation for inequality draws attention to the impact of technology specifically. The advent of the digital age has placed a higher premium on the skills needed for non-routine work and reduced the value placed on lower skilled routine work, as it has enabled machines to replace jobs that could be routinised. This skill-biased technological change (SBTC) has led to major changes in the organisation of work, as many full-time permanent jobs with benefits have given way to part-time flexible work without benefits, that are often centred around the completion of short ‘gigs’ such as a car journey or food delivery. For instance, the Organisation for Economic Co-operation and Development (OECD) estimated in 2015 that since the 1990s, roughly 60% of all job creation has been in the form of non-standard work due to technological changes and that those employed in such jobs are more likely to be poor ( Brown 2017 : 60).
Relatedly, a prevailing doctrine in economics is ‘marginal productivity theory,’ which holds that people with greater productivity levels will earn higher incomes. This is due to the belief that a person’s productivity is equated to their societal contribution ( Stiglitz 2013 : 37). Since technology is a leading determinant in the productivity of different skills and SBTC has led to increased productivity, it has also become a justification for inequality. However, it is very difficult to separate any one person’s contribution to society from that of others, as even the most successful businessperson owes their success to the rule of law, good infrastructure, and a state educated workforce ( Stiglitz 2013 : 97–98).
Further criticisms of the SBTC explanation, are that there was still substantial SBTC when inequality first fell dramatically and then stabilised in the period from 1930 to 1980, and it has failed to explain the perpetuation of both the gender and racial wage gap, “or the dramatic rise in education-related wage gaps for younger versus older workers” ( Brown 2017 : 67). Although it is difficult to decouple globalisation and technology, as they each have compounding tendencies, it is most likely that globalisation and technology are important explanatory factors for inequality, but predominantly facilitate and underlie the following more determinant institutional factors that happen to be already present, such as reduced tax progressivity, rising executive pay, and union decline. It is to these factors that I now turn.
2.3 Tax Policy
Taxes overwhelmingly comprise the primary source of revenue that governments can use for redistribution, which is fundamental to alleviating income inequality. Redistribution is defended on economic grounds because the marginal utility of money declines as income rises, meaning that the benefit derived from extra income is much higher for the poor than the rich. However, since the late 1970s, a major rethinking surrounding redistributive policy occurred. This precipitated ‘trickle-down economics’ theory achieving prominence amongst American and British policymakers, whereby the benefits from tax cuts on the wealthy would trickle-down to everyone. Subsequently, expert opinion has determined that tax cuts do not actually spur economic growth ( CBPP 2017 ).
Personal income tax progressivity has declined sharply in the West, as the average top income tax rate for OECD members fell from 62% in 1981 to 35% in 2015 ( IMF 2017 : 11). However, the decline has been most pronounced in the UK and the US, which had top rates of around 90% in the 1960s and 1970s. Corporate tax rates have also plummeted by roughly one half across the OECD since 1980 ( Shaxson 2015 : 4). Recent International Monetary Fund (IMF) research found that between 1985 and 1995, redistribution through the tax system had offset 60% of the increase in market inequality but has since failed to respond to the continuing increase in inequality ( IMF 2017 ). Moreover, in a sample of 18 OECD countries encompassing 50 years, Hope and Limberg (2020) found that tax reforms even significantly increased pre-tax income inequality, while having no significant effect on economic growth.
This decline in tax progressivity has been a leading cause of rising income inequality, which has been compounded by the growing problem of tax avoidance. A complex global web of shell corporations has been constructed by international brokers in offshore tax havens that is able to keep wealth hidden from tax collectors. The total hidden amount in tax havens is estimated to be $7.6 trillion US dollars and rising, or roughly 8% of total global household wealth ( Zucman 2015 : 36). Recent research has revealed that tax havens are overwhelmingly used by the immensely rich ( Alstadsæter, Johannesen, and Zucman 2019 ), thus taxing this wealth would substantially reduce income inequality and increase revenue available for redistribution. The massive reduction in income tax progressivity in the Anglo world, after it had been amongst its leaders in the post-war years, also “probably explains much of the increase in the very highest earned incomes” since 1980 ( Piketty 2014 : 495–496).
2.4 Executive Pay
The enormous rising pay of executives since the 1980s, has also fuelled income inequality and more specifically the gap between executives and their employees. For example, the gap between Chief Executive Officers (CEO) and their workers at the 500 leading US companies in 2016, was 335 times, which is nearly 10 times larger than in 1980. It is a similar story in the UK, with a pay ratio of 131 for large British firms, which has also risen markedly since 1980 ( Dorling 2017 ).
Piketty (2014 : 335) posits that the dramatic reduction in top income tax has had an amplifying effect on top executives pay since it provides them with much greater incentive to seek larger remuneration, as far less is then taken in tax. It is difficult to objectively measure an individual’s contribution to a company and with the onset of trickle-down economics and accompanying business-friendly climate since the 1980s, top executives have found it relatively easy to convince boards of their monetary worth ( Gabaix and Landier 2008 ).
The rise in executive pay in both the UK and US, is far larger than the rest of the OECD. This may partially be explained by the English-speaking ‘superstar’ theory, whereby the global market demand for top CEOs is much higher for native English speakers due to English being the prime language of the global economy ( Deaton 2013 : 210). Saez and Veall (2005) provide support for the theory in a study of the top 1% of earners from the Canadian province of Quebec, which showed that English speakers were able to increase their income share over twice as much as their French-speaking counterparts from 1980 to 2000. This upsurge of income at the top of the labour market has been accompanied by stagnation or diminishing returns for the middle and lower parts of the labour market, which has been affected by the dramatic decline of union influence throughout the West.
2.5 Union Decline
Trade unions have typically been viewed as an important force for moderating income inequality. They “contribute to wage compression by restricting wage decline among low-wage earners” and restrain wage surges among high-wage earners ( Checchi and Visser 2009 : 249). The mere presence of unions can also drive up the wages of non-union employees in similar industries, as employers tend to give in to wage demands to keep unions out. Union density has also been proven to be strongly associated with higher redistribution both directly and indirectly, through its influence on left party governments ( Haddow 2013 : 403).
There had broadly existed a ‘social contract’ between labour and business, whereby collective bargaining establishes a wage structure in many industries. However, this contract was abandoned by corporate America in the mid-1970s when large-scale corporate donations influenced policymakers to oppose pro-union reform of labour law, leading to political defeats for unions ( Hacker and Pierson 2010 : 58–59). The crackdown of strikes culminating in the momentous Air Traffic Controllers’ strike (1981) in the US and coal miner’s strike (1984–85) in the UK, caused labour to become de-politicised, which was self-reinforcing, because as their political power dispersed, policymakers had fewer incentives to protect or strengthen union regulations ( Rosenfeld and Western 2011 ). Consequently, US union density has plummeted from around a third of the workforce in 1960, down to 11.9% last decade, with the steepest decline occurring in the 1980s ( Stiglitz 2013 : 81).
Although the decline in union density is not as steep cross-nationally, the pattern is still similar. Baccaro and Howell (2011 : 529) found that on average the unionisation rate decreased by 0.39% a year since 1974 for the 15 OECD members they surveyed. Increasingly, the decline in the fortunes of labour is being linked with the increase in inequality and the sharpest increases in income inequality have occurred in the two countries with the largest falls in union density – the UK and US. Recent studies have found that the weakening of organised unions accounts for between a third and a fifth of the total rise in income inequality in the US ( Rosenfeld and Western 2011 ), and nearly one half of the increase in both the Gini rate and the top 10%’s income share amongst OECD members ( Jaumotte and Buitron 2015 ).
To illustrate the changing relationship between inequality and unionisation, Figure 1 displays a local polynomial smoother scatter plot of union density by income inequality, for 23 OECD countries, 1980–2018. They are negatively correlated, as countries with higher union density have much lower levels of income inequality. Figure 2 further plots the time trends of both. Income inequality (as measured via the Gini coefficient) has climbed over 0.02 percentage points on average in these countries since 1980, which is roughly a one-tenth rise. Whereas union density has fallen on average from 44 to 35 percentage points, which is over one-fifth.

Gini coefficient by union density, OECD 1980–2018. Data on Gini coefficients from SWIID ( Solt 2020 ); data on union density from ICTWSS Database ( Visser 2019 ).

Gini coefficient by union density, 1980–2018. Data on Gini coefficients from SWIID ( Solt 2020 ); data on union density from ICTWSS Database ( Visser 2019 ).
In sum, income inequality is multifaceted and is not the inevitable outcome of irresistible structural forces such as globalisation or technological development. Instead, it has largely been driven by a multitude of political choices. Tridico (2018) finds that the increases in inequality from 1990 to 2013 in 26 OECD countries, was largely owing to increased financialisation, deepening labour flexibility, the weakening of trade unions and welfare state retrenchment. While Huber, Huo, and Stephens (2019) recently reveals that top income shares are unrelated to economic growth and knowledge-intensive production but is closely related to political and policy changes surrounding union density, government partisanship, top income tax rates, and educational investment. Lastly, Hager’s (2020) recent meta-analysis concludes that the “empirical record consistently shows that government policy plays a pivotal role” in shaping income inequality.
These preventable causes that have given rise to inequality have created socio-economic challenges, due to the demonstrably negative outcomes that inequality engenders. What follows is a detailed analysis of the significant mechanisms that income inequality induces, which lead to harmful outcomes.
3 Consequences of Income Inequality
Escalating income inequality has been linked with numerous negative outcomes. On the economic front, negative results transpire beyond the obvious poverty and material deprivation that is often associated with low incomes. Income inequality has also been shown to reduce growth, innovation, and investment. On the social front, Wilkinson and Pickett’s ground-breaking The Spirit Level ( 2009 ), found that societies that are more unequal have worse social outcomes on average than more egalitarian societies. They summarised an extensive body of research from the previous 30 years to create an Index of Health and Social Problems, which revealed a host of different health and social problems (measuring life expectancy, infant mortality, obesity, trust, imprisonment, homicide, drug abuse, mental health, social mobility, childhood education, and teenage pregnancy) as being positively correlated with the level of income inequality across rich nations and across states within the US. Figure 3 displays the cross-national findings via a sample of 21 OECD countries.

Index of health and social problems by Gini coefficient. Data on health and social problems index from The Equality Trust (2018) ; data on Gini coefficients from OECD (2020) .
3.1 Economic
Income inequality is predominantly an economic subject. Therefore, it is understandable that it can engender pervasive economic outcomes. Foremost economically speaking, it has been linked with reduced growth, investment and innovation. Leading international organisations such as the IMF, World Bank and OECD, pushed for neoliberal reforms beginning in the 1980s, although they have recently started to substantially temper their views due to their own research into inequality. A 2016 study by IMF economists, noted that neoliberal policies have delivered benefits through the expansion of global trade and transfers of technology, but the resulting increases in inequality “itself undercut growth, the very thing that the neo-liberal agenda is intent on boosting” ( Ostry, Loungani, and Furceri 2016 : 41). Cingano’s (2014) OECD cross-national study, found that once a country’s income inequality reaches a certain level it reduces growth. The growth rate in these countries would have been one-fifth higher had income inequality not increased, while the greater equality of the other countries included in the study helped to increase their growth rates.
Consumer spending is good for economic growth but rising income inequality shifts more money to the top of the income distribution, where higher income individuals have a much smaller propensity to consume than lower-income individuals. The wealthy save roughly 15–25% of their income, whereas low income individuals spend their entire income on consumer goods and services ( Stiglitz 2013 : 106). Therefore, greater inequality reduces demand in an economy and is a major contributor to the ‘secular stagnation’ (persistent insufficient demand relative to aggregate private savings) that the largest Western economies have been experiencing since the financial crisis. Inequality also increases the level of debt, as lower-income individuals borrow more to maintain their standard of living, especially in a climate of low interest rates. Combined with deregulation, greater debt increases instability and “was a major contributor to, if not the underlying cause of, the 2008 financial crash” ( Brown 2017 : 35–36).
Another key economic effect of income inequality is that it leads to reduced welfare spending and public investment. Since a greater share of the income distribution is earned by the very wealthy, governments have less income available to fund education, public amenities, and other services that the poor rely heavily on. This creates social separation, whereby the wealthy opt out in publicly funding services because their private equivalents are of better quality. This causes a cycle of increasing income inequality that is likely to eventually lead to a situation of “private affluence and public squalor” ( Marmot 2015 : 39).
Lastly, it has been proven that economic instability is a by-product of increasing inequality, which harms innovation. Both countries and American states with the highest inequality have been found to be the least innovative in terms of the amount of Intellectual Property (IP) patents they produce ( Dorling 2018 : 129–130). Although income inequality is predominantly an economic subject, its effects are so pervasive that it has also been linked to a host of negative health and societal outcomes.
Wilkinson and Pickett found key associations between income inequality for both physical and mental health. For example, they discovered that on average the life expectancy gap is more than four years between the least and most equitable richest nations (Japan and the US). Since their revelations, overall life expectancy has been reported to be declining in the US ( Case and Deaton 2020 ). It has held or declined every year since 2014, which has led to a cumulative drop of 1.13 years ( Andrasfay and Goldman 2021 ). Marmot (2015) has provided evidence that there exists a social gradient whereby differences in affluence translate into increasing health inequalities, which can be shown even down to the neighbourhood level, as more affluent areas have higher life expectancy on average than deprived areas, and a clear gradient appears where life expectancy increases in line with affluence.
Moreover, Marmot’s famous Whitehall studies, which are large-scale longitudinal studies of Whitehall employees of UK central government, found an inverse-relationship between salary grade and ill-health, whereby low-grade workers were four times as likely as high-grade workers to suffer from ill-health ( 2015 : 11). Health steadily improves with rank and the correlation is little affected by lifestyle controls such as tobacco and alcohol usage. However, the leading factor that seems to make the most difference in ill-health is job stress and a person’s sense of control over their work, including the variety of work and the use and development of skills ( Schrecker and Bambra 2015 : 54–55).
‘Psychosocial stresses,’ like those appearing in the Whitehall studies, have been found to be more common and frequent amongst low-income individuals, beyond just the workplace ( Jensen and van Kersbergen 2017 : 24). Wilkinson and Pickett (2019) posit that greater income inequality engenders low self-esteem, chronic stress and depression, stemming from status anxiety. This occurs because more importance is placed on where people fit in a hierarchy with greater inequality. For evidence, they outline a clear relationship of a much higher percentage of the population suffering from mental illness in more unequal countries. Meticulous research has shown that huge inequalities in income result in the poor having feelings of shame across a range of environments. Furthermore, Dickerson and Kemeny’s (2004) meta-analysis of 208 studies found that stress-hormone (cortisol) levels were raised particularly “when people felt that others were making negative judgements about them” ( Rowlingson 2011 : 24).
These effects on both mental and physical health can be best illustrated via the ‘absolute income’ and ‘relative income’ hypotheses ( Daly, Boyce, and Wood 2015 ). The relative income hypothesis posits that when an individual’s income is held constant, the relative income of others can affect a person’s health depending on how they view themselves in comparison to those above them ( Wilkinson 1996 ). This pattern also holds when income inequality increases at the societal level, because if such changes lead to increases in chronic stress, it can increase ill-health nationally. Whereas the absolute income hypothesis predicts that health gains from an extra unit of income diminish as an individual’s income rises ( Kawachi, Adler, and Dow 2010 ). A mean preserving transfer from a richer to poorer individual raises the health of the poorer individual more than it lowers the health of the richer person. This occurs because there is an optimum threshold of income required to maintain good health. Thus, when holding total income constant, a more equal distribution of income should improve overall population health. This pattern also applies at the country-wide level, as the “effect of income on health appears substantial as countries move from about $15,000 to 25,000 US dollars per capita,” but appears non-existent beyond that point ( Leigh, Jencks, and Smeeding 2009 : 386–387).
Income inequality also impacts happiness and wellbeing, as the happiest nations are routinely the ones with low inequality, such as Denmark and Norway. Happiness has been proven to be affected by the law of diminishing returns in economics. It states that higher income incrementally improves happiness but only up to a certain point, as any individual income earned beyond roughly $70,000 US dollars, does not bring about greater happiness ( Deaton 2013 : 53). The negative physical and mental health outcomes that income inequality provoke, also impact key societal areas such as crime, social mobility and education.
Rates of violent crime are lower in more equal countries ( Hsieh and Pugh 1993 ; Whitworth 2012 ). This is largely because more equal countries have less poverty, which leads to less people being desperate about their situation, as lower-income individuals have been shown to commit more crime. Relatedly, according to strain theory, more unequal societies place higher social value in achieving economic success, while providing lower means to achieve it ( Merton 1938 ). This generates strain, which may lead more individuals to pursue crime as a means of attaining financial success. At the opposite end of the income spectrum, the wealthy in more equal countries are also less likely to exploit others and commit fraud or exhibit other anti-social behaviour, partly because they feel less of a need to cut corners to get ahead, or to make money ( Dorling 2017 : 152–153). Homicides also tend to rise with inequality. Daly (2016) reveals that inequality predicts homicide rates better than any other variable and accounts for around half of the variance in murder rates between countries and American states. Roughly 90% of American homicides are committed by men, and since the majority of homicides occur over status, inequality raises the stakes of disputes over status amongst men.
Studies have also shown that there is a marked negative relationship between income inequality and social mobility. Utilising Intergenerational Earnings Elasticity data from Blanden, Gregg, and Machin (2005) , Wilkinson and Pickett (2009) first outline this relationship cross-nationally for eight OECD countries. Corak (2013) famously expanded on this with his ‘Great Gatsby Curve’ for 22 countries using the same measure. I update and expand on these studies in Figure 4 to include all 36 OECD members, utilising the WEF’s inaugural 2020 Social Mobility Index. It clearly shows that social mobility is much lower on average in more unequal countries across the entire OECD.

Index of social mobility by Gini coefficient. Data on social mobility index from World Economic Forum (2020) ; data on Gini coefficients from SWIID ( Solt 2020 ).
A primary driver for the negative relationship between inequality and social mobility, derives from the availability of resources during early childhood. Life chances have been shown to be determined in early childhood to a disproportionately large extent ( Jensen and van Kersbergen 2017 : 29). Children in more equitable regions such as Scandinavia, have better access to resources, as they go to similar schools, receive similar educational opportunities, and have access to a wider range of career options. Whereas in the UK and US, a greater number of jobs at the top are closed off to those at the bottom and affluent parents are far more likely to send their children to private schools and fund other ‘child enrichment’ goods and services ( Dorling 2017 : 26). Therefore, as income inequality rises, there is a greater disparity in the resources that rich and poor parents can invest in their children’s education, which has been shown to substantially affect “cognitive development and school achievement” ( Brown 2017 : 33–34).
4 Conclusions
The causes and consequences of income inequality are multifaceted. Income inequality is not the inevitable outcome of irresistible structural forces such as globalisation or technological development. Instead, it has largely been driven by a multitude of institutional political choices. These preventable causes that have given rise to inequality have created socio-economic challenges, due to the demonstrably negative outcomes that inequality engenders.
The neoliberal political consensus poses challenges for policymakers to arrest the rise of income inequality. However, there are many proven solutions that policymakers can enact if the appropriate will can be summoned. Restoring higher levels of labour protections would aid in reversing the declining trend of labour wage share. Similarly, government promotion and support for new corporate governance models that give trade unions and workers a seat at the table in ownership decisions through board memberships, would somewhat redress the increasing power imbalance between capital and labour that is generating more inequality. Greater regulation aimed at limiting the now dominant shareholder principle of maximising value through share buy-backs and instead offering greater incentives to pursue maximisation of stakeholder value, long-term financial stability and investment, can reduce inequality. Most importantly, tax policy can be harnessed to redress income inequality. Such policies include restoring higher marginal income and corporate tax rates, setting higher corporate tax rates for firms with higher ratios of CEO-to-worker pay, and establishing luxury taxes on spiralling compensation packages. Finally, a move away from austerity, which has gripped the West since the financial crisis, and a move towards much greater government investment and welfare state spending, would also lift growth and low-wages.
Alstadsæter, A., N. Johannesen, and G. Zucman. 2019. “Tax Evasion and Inequality.” American Economic Review 109 (6): 2073–103. Search in Google Scholar
Andrasfay, T., and N. Goldman. 2021. “Reductions in 2020 US Life Expectancy Due to COVID-19 and the Disproportionate Impact on the Black and Latino Populations.” Proceedings of the National Academy of Sciences 118 (5), https://doi.org/10.1073/pnas.2014746118 . Search in Google Scholar
Asaria, M., J. Costa-Font, and F. A. Cowell. 2021. “How Does Exposure to Covid-19 Influence Health and Income Inequality Aversion.” IZA Discussion Paper. no. 14103. Also available at https://ssrn.com/abstract=3785067 . Search in Google Scholar
Atkinson, A. B. 2015. Inequality: What Can Be Done? London: Harvard University Press. Search in Google Scholar
Baccaro, L., and C. Howell. 2011. “A Common Neoliberal Trajectory: The Transformation of Industrial Relations in Advanced Capitalism.” Politics & Society 39 (4): 521–63, https://doi.org/10.1177/0032329211420082 . Search in Google Scholar
Blanden, J., P. Gregg, and S. Machin. 2005. Intergenerational Mobility in Europe and North America . London: Centre for Economic Performance. Search in Google Scholar
Borjas, G. J., R. B. Freeman, and L. F. Katz. 1992. “On the Labor Market Effects of Immigration and Trade.” In Immigration and the Workforce , edited by G. J. Borjas, and R. B. Freeman, 213–44. Chicago: University of Chicago Press. Search in Google Scholar
Brown, R. 2017. The Inequality Crisis: The Facts and What We Can Do About It . Bristol: Polity Press. Search in Google Scholar
Case, A., and A. Deaton. 2020. Deaths of Despair and the Future of Capitalism . Princeton: Princeton University Press. Search in Google Scholar
Center on Budget and Policy Priorities (CBPP) . 2017. Tax Cuts for the Rich Aren’t an Economic Panacea – and Could Hurt Growth. Also available at https://www.cbpp.org/research/federal-tax/tax-cuts-for-the-rich-arent-an-economic-panacea-and-could-hurt-growth . Search in Google Scholar
Checchi, D., and J. Visser. 2009. “Inequality and the Labor Market: Unions.” In The Oxford Handbook of Economic Inequality , edited by B. Nolan, W. Salverda, and T. M. Smeeding, 230–56. Oxford: Oxford University Press. Search in Google Scholar
Cingano, F. 2014. Trends in Income Inequality and its Impact on Economic Growth . OECD Social, Employment and Migration Working Papers, No. 163. Paris: OECD Publishing. Search in Google Scholar
Corak, M. 2013. “Income Inequality, Equality of Opportunity, and Intergenerational Mobility.” Journal of Economic Perspectives 27 (3): 79–102, https://doi.org/10.1257/jep.27.3.79 . Search in Google Scholar
Daly, M. 2016. Killing the Competition: Economic Inequality and Homicide . Oxford: Routledge. Search in Google Scholar
Daly, M., C. Boyce, and A. Wood. 2015. “A Social Rank Explanation of How Money Influences Health.” Health Psychology 34 (3): 222–30, https://doi.org/10.1037/hea0000098 . Search in Google Scholar
Deaton, A. 2013. The Great Escape: Health, Wealth, and the Origins of Inequality . Princeton: Princeton University Press. Search in Google Scholar
Dickerson, S. S., and M. Kemeny. 2004. “Acute Stressors and Cortisol Responses: A Theoretical Integration and Synthesis of Laboratory Research.” Psychological Bulletin 130 (3): 355–91, https://doi.org/10.1037/0033-2909.130.3.355 . Search in Google Scholar
Dorling, D. 2017. The Equality Effect: Improving Life for Everyone . Oxford: New Internationalist Publications Ltd. Search in Google Scholar
Dorling, D. 2018. Do We Need Economic Inequality? Cambridge: Polity Press. Search in Google Scholar
Elliott, L. 2017. “Rising Inequality Threatens World Economy, Says WEF.” The Guardian. Also available at https://www.theguardian.com/business/2017/jan/11/inequality-world-economy-wef-brexit-donald-trump-world-economic-forum-risk-report . Search in Google Scholar
Gabaix, X., and A. Landier. 2008. “Why Has CEO Pay Increased So Much?” Quarterly Journal of Economics 123 (1): 49–100, https://doi.org/10.1162/qjec.2008.123.1.49 . Search in Google Scholar
Hacker, J. S., and P. Pierson. 2010. Winner-Take-All Politics: How Washington Made the Rich Richer – And Turned Its Back on the Middle Class . New York: Simon & Schuster. Search in Google Scholar
Haddow, R. 2013. “Labour Market Income Transfers and Redistribution.” In Inequality and the Fading of Redistributive Politics , edited by K. Banting, and J. Myles, 381–412. Vancouver: UBC Press. Search in Google Scholar
Hager, S. 2020. “Varieties of Top Incomes?” Socio-Economic Review 18 (4): 1175–98. Search in Google Scholar
Heimberger, P. 2020. “Does Economic Globalisation Affect Income Inequality? A Meta‐analysis.” The World Economy 43 (11): 2960–82, https://doi.org/10.1111/twec.13007 . Search in Google Scholar
Hope, D., and J. Limberg. 2020. The Economic Consequences of Major Tax Cuts for the Rich . London: London School of Economics and Political Science. Also available at http://eprints.lse.ac.uk/107919/ . Search in Google Scholar
Hsieh, C.-C., and M. D. Pugh. 1993. “Poverty, Inequality and Violent Crime: a Meta-Analysis of Recent Aggregate Data Studies.” Criminal Justice Review 18 (2): 182–202, https://doi.org/10.1177/073401689301800203 . Search in Google Scholar
Huber, E., J. Huo, and J. D. Stephens. 2019. “Power, Policy, and Top Income Shares.” Socio-Economic Review 17 (2): 231–53, https://doi.org/10.1093/ser/mwx027 . Search in Google Scholar
International Monetary Fund (IMF). 2017. Fiscal Monitor: Tackling Inequality . Washington: IMF. Search in Google Scholar
Jaumotte, F., and C. O. Buitron. 2015. “Power from the People.” Finance & Development 52 (1): 29–31. Search in Google Scholar
Jensen, C., and K. Van Kersbergen. 2017. The Politics of Inequality . London: Palgrave. Search in Google Scholar
Kawachi, I., N. E. Adler, and W. H. Dow. 2010. “Money, Schooling, and Health: Mechanisms and Causal Evidence.” Annals of the New York Academy of Sciences 1186 (1): 56–68, https://doi.org/10.1111/j.1749-6632.2009.05340.x . Search in Google Scholar
Leigh, A., C. Jencks, and T. Smeeding. 2009. “Health and Economic Inequality.” In The Oxford Book of Economic Equality , edited by W. Salverda, B. Nolan, and T. Smeeding, 384–405. Oxford: Oxford University Press. Search in Google Scholar
Marmot, M. 2015. The Health Gap: The Challenge of an Unequal World . London: Bloomsbury. Search in Google Scholar
Merton, R. 1938. “Social Structure and Anomie.” American Sociological Review 3 (5): 672–82, https://doi.org/10.2307/2084686 . Search in Google Scholar
Organisation for Economic Co-operation and Development (OECD) . 2020. “Income Inequality” (Indicator) . Paris: OECD Publishing. Also available at https://data.oecd.org/inequality/income-inequality.htm . Search in Google Scholar
Ostry, J. D., P. Loungani, and D. Furceri. 2016. “ Neoliberalism: Oversold? ” Finance and Development 532: 38–41. Also available at https://www.imf.org/external/pubs/ft/fandd/2016/06/ostry.htm . Search in Google Scholar
Piketty, T. 2014. Capital in the Twenty-First Century . Cambridge: Harvard University Press. Search in Google Scholar
Piketty, T. 2020. Capital and Ideology . Cambridge: Harvard University Press. Search in Google Scholar
Revenga, A. 1992. “Exporting Jobs? The Impact of Import Competition on Employment and Wages in U.S. Manufacturing.” Quarterly Journal of Economics 107 (1): 255–84, https://doi.org/10.2307/2118329 . Search in Google Scholar
Rosenfeld, J., and B. Western. 2011. “Unions, Norms, and the Rise in U.S. Wage Inequality.” American Sociological Review 78 (4): 513–37. Search in Google Scholar
Rowlingson, K. 2011. Does Income Inequality Cause Health and Social Problems? York: Joseph Rowntree Foundation. Search in Google Scholar
Saez, E., and M. Veall. 2005. “The Evolution of High Incomes in Northern America: Lessons from Canadian Evidence.” American Economic Review 95 (3): 831–49, https://doi.org/10.1257/0002828054201404 . Search in Google Scholar
Scheidel, W. 2017. The Great Leveller: Violence and the History of Inequality from the Stone Age to the Twenty-First Century . Princeton: Princeton University Press. Search in Google Scholar
Schrecker, T., and C. Bambra. 2015. How Politics Makes Us Sick: Neoliberal Epidemics . New York: Palgrave Macmillan. Search in Google Scholar
Shaxson, N. 2015. Ten Reasons to Defend the Corporation Tax . London: Tax Justice Network. Also available at http://www.taxjustice.net/wp-content/uploads/2013/04/Ten_Reasons_Full_Report.pdf . Search in Google Scholar
Solt, F. 2020. “Measuring Income Inequality across Countries and over Time: The Standardized World Income Inequality Database.” Social Science Quarterly 101 (3): 1183–99. Version 9.0, https://doi.org/10.1111/ssqu.12795 . Search in Google Scholar
Stiglitz, J. 2013. The Price of Inequality . London: Penguin Books. Search in Google Scholar
The Equality Trust . 2018. “The Spirit Level Data.” London. Also available at https://www.equalitytrust.org.uk/civicrm/contribute/transact?reset=1&id=5 . Search in Google Scholar
Thomas, A. 2016. Republic of Equals: Predistribution and Property-Owning Democracy . Oxford: Oxford University Press. Search in Google Scholar
Tridico, P. 2018. “The Determinants of Income Inequality in OECD Countries.” Cambridge Journal of Economics 42 (4): 1009–42, https://doi.org/10.1093/cje/bex069 . Search in Google Scholar
Visser, J. 2019. ICTWSS Database . Version 6.1. Amsterdam: Amsterdam Institute for Advanced Labour Studies (AIAS), University of Amsterdam. Search in Google Scholar
Whitworth, A. 2012. “Inequality and Crime across England: A Multilevel Modelling Approach.” Social Policy and Society 11 (1): 27–40, https://doi.org/10.1017/s1474746411000388 . Search in Google Scholar
Wilkinson, R. 1996. Unhealthy Societies: The Afflictions of Inequality . London: Routledge. Search in Google Scholar
Wilkinson, R., and K. Pickett. 2009. The Spirit Level: Why Equality is Better for Everyone . London: Penguin Books. Search in Google Scholar
Wilkinson, R., and K. Pickett. 2019. The Inner Level: How More Equal Societies Reduce Stress, Restore Sanity and Improve Everyone’s Well-Being . London: Penguin Books. Search in Google Scholar
Wiwad, D., B. Mercier, P. K. Piff, A. Shariff, and L. B. Aknin. 2021. “Recognizing the Impact of COVID-19 on the Poor Alters Attitudes towards Poverty and Inequality.” Journal of Experimental Social Psychology , https://doi.org/10.1016/j.jesp.2020.104083 . Search in Google Scholar
World Economic Forum. 2020. The Global Social Mobility Report 2020 . Geneva: World Economic Forum. Also available at https://www3.weforum.org/docs/Global_Social_Mobility_Report.pdf . Search in Google Scholar
Zucman, G. 2015. The Hidden Wealth of Nations: The Scourge of Tax Havens . Chicago: University of Chicago Press. Search in Google Scholar
© 2021 Walter de Gruyter GmbH, Berlin/Boston
- X / Twitter
Supplementary Materials
Please login or register with De Gruyter to order this product.
Journal and Issue
Articles in the same issue.

Page One Economics ®
Income and wealth inequality.

"For we, the people, understand that our country cannot succeed when a shrinking few do very well and a growing many barely make it. We believe that America's prosperity must rest upon the broad shoulders of a rising middle class."
—President Barack Obama 1
Introduction
There are many different types of inequality among people: educational attainment, work experience, and health—to name a few. This essay discusses economic inequality: its causes, measurement, and the potential impact of its growth in the U.S. economy.
Economists directly link differences in educational attainment and work experience, also known as human capital, to differences in economic outcomes. That is, formal education and job skills determine how likely a person is to find and hold a stable job that pays good wages. The flow of money from wages is the most important source of income for most people. Over time, regular income from employment allows people to own assets such as a home or a retirement financial portfolio. That stock of assets is called wealth .
Data collected by federal organizations such as the Census Bureau and the Board of Governors of the Federal Reserve System (BOG) allow us to measure how unequal the distributions of income and wealth are in the United States. Those data show that, since the 1970s, some individuals and families are earning much more income and accumulating much larger amounts of wealth than the typical family does.
Data reported by the World Bank allow us to compare the distribution of income across countries. As of 2018, the available data show large international differences in income inequality. Although not all countries in the world have data on income inequality, among those that do, the United States ranks among the top 25% most unequal.
What Causes Inequality?
The root cause of differences in income and wealth across individuals and households is a combination of personal and social factors. Personal factors are unique to individuals and include talent, effort, and luck. Such factors can be either nurtured or hindered by the family upbringing of the individual. Social factors affect groups of people and include education policies, labor market laws, tax codes, and financial regulations. At any moment in time, social factors can overpower personal factors to determine individual prosperity and increase inequality among people. 2
For example, as gradually more married women started working outside the home between 1960 and 2000, their family incomes increased and the differences in income between households became larger depending on whether they had one or two people earning wages. At the same time, differences in the types of jobs women and men tend to hold also contribute to income inequality between genders. 3
Because wealth is accumulated over time, older people are generally wealthier than younger people. For that reason, if there are many more young people than old people in the general population and the old hold more wealth than the young, overall wealth inequality will be high. 4
Finally, some people argue that the type of monetary policy used to ensure steady access to credit by households and businesses during recent economic contractions may contribute to higher levels of income inequality. However, that claim is hotly disputed. 5
How Is Income Inequality Measured?
There are different ways to measure how unequal income is in a country. The U.S. Census measures income inequality as the ratio of the mean, or average, income for the highest quintile (top 20 percent) of earners divided by the mean income of the lowest quintile (bottom 20 percent) of earners in a particular area. Let's say a small county has 500 people earning an income. To measure how unequal those incomes are, the Census surveys and sorts each person's income from highest to lowest, calculates the average income of the 100 people earning the most and the average income of the 100 people earning the least, and divides the first figure by the second figure.
Figure 1 Income Inequality by County
SOURCE: U.S. Census via FRED ® , Federal Reserve Bank of St. Louis; https://fred.stlouisfed.org/graph/?m=QRCJ , accessed June 23, 2021.
Figure 1 shows average county-level income inequality measured between 2016 and 2020. The Census considers the average income over a five-year period to account for the fact that peoples' income changes from year to year. Measured this way, income inequality can be as high as 130 or as low as 5. These measurements mean that the most affluent households in a particular county can earn as much as 130 times or as little as 5 times as much as the least affluent households do.
Another way to measure income inequality in a population is to calculate the Gini index . The World Bank uses that index to measure how much the distribution of income among households deviates from a perfectly equal distribution. The Gini index can take any value between 0 and 100. A value of 0 represents perfect equality: All households earn the same income. A value of 100 indicates perfect inequality: One household earns all the income, and all other households earn nothing.
Figure 2 Gini Index by Nation
SOURCE: World Bank via FRED ® , Federal Reserve Bank of St. Louis; https://fred.stlouisfed.org/graph/?m=QRFh , accessed April 6, 2021.
Figure 2 shows country-level income inequality measured with a Gini index in 2018. The highest value is 54, and the lowest value is 25. It is important to note that two countries can have very similar Gini indexes despite having very different distributions of income. For example, in 2018, the Gini index for the United States was 41.4 and for Bulgaria was 41.3, despite the fact that those two countries' economic and social histories are very different.
In the United States, since the 1970s, the Gini index has increased at a steady rate, indicating greater income inequality across families. 6 Some research suggests that this growing difference is related to the increased value of the stock market. Wealthier households hold more stocks than poorer households. So, when stock market prices rise, the income of wealthier households grows relatively more and overall income inequality increases. 7
How Is Wealth Inequality Measured?
The BOG combines information from two different surveys to measure how wealth is distributed among households: It takes the value of a household's assets (e.g., the current market price of a home) and liabilities (e.g., the unpaid part of a mortgage for a home) and calculates the difference between the two, which is called net worth . Next, the BOG sorts household wealth from highest to lowest and reports the net worth of four different groups: the wealthiest 1% of the population, the next 9%, the next 40%, and the bottom 50%.
Figure 3 Share of Total Net Worth Held by Population Groups
SOURCE: Board of Governors of the Federal Reserve System via FRED ® , Federal Reserve Bank of St. Louis; https://fred.stlouisfed.org/graph/?g=O2Kq , accessed April 6, 2021.
Figure 3 shows the share of total net worth held by each of those four groups. In 2021, the wealthiest 1% of the population (about 3.3 million households) held about one-third of total net worth; the next 9% (almost 30 million households) held a little more than one-third; the next 40% (about 133 million households) and the bottom 50% (about 166 million households) together held the rest—less than one-third of total net worth.
The data from the BOG show increasing wealth concentration since 1989, when the data first became available. 8 It is important to note that, over time, some individual households can move up or down between wealth groups, depending on the changing value of their assets. Also, some research suggests the particular nature of some economic fluctuations impacts some households' net worth more than others. For example, the real estate crash associated with the 2007-09 recession resulted in large losses for the poorest 50% of the population. 9
Does Inequality Matter?
The economic impact of growing income and wealth inequality in the United States is an intensely studied question. Economists are debating how to answer that question by analyzing data and creating mathematical models to study it. Because this is ongoing work, there is no single answer.
Some research shows that, in richer countries, more unequal income makes economic fluctuations more pronounced. 10 That finding means that the changes in overall income and employment known as business cycles become more dramatic. Moreover, statistical evidence suggests increased income inequality undermines economic growth due to lower educational achievements (and human capital) among poorer individuals and households. 11 As discussed earlier, education builds a person's human capital and is rewarded with higher income from employment. Finally, research suggests the increasing income and wealth inequality can undermine the use of monetary policy (as we know it) to maximize employment and ensure price stability. 12
Inequality in individual economic outcomes arises from a combination of personal traits and social conditions. The distributions of income and wealth in a society can be measured in multiple ways: comparing the highest to the lowest earners, calculating an index describing how unequal income is among all individuals, and assessing people's financial wellbeing according to the value of their wealth holdings. Regardless of how we measure income and wealth inequality, their distributions in the United States are becoming more unequal. This trend is likely to impact economic life as we know it. More research is needed to figure out precisely how that may happen.
1 Obama, Barack. "Inaugural Address." January 21, 2013; https://obamawhitehouse.archives.gov/the-press-office/2013/01/21/inaugural-address-president-barack-obama .
2 For an example of how the use of city maps to assess lending risk after the Great Depression influenced homeownership rates across population groups for decades afterward, see the following article: Mendez-Carbajo, Diego. "Neighborhood Redlining, Racial Segregation, and Homeownership." Federal Reserve Bank of St. Louis Page One Economics , September 2021; https://research.stlouisfed.org/publications/page1-econ/2021/09/01/neighborhood-redlining-racial-segregation-and-homeownership .
3 For more on gender and labor markets, see the following article: Mendez-Carbajo, Diego. "Gender and Labor Markets." Federal Reserve Bank of St. Louis Page One Economics , January 2022; https://research.stlouisfed.org/publications/page1-econ/2022/01/03/gender-and-labor-markets .
4 For more on aging and wealth inequality, see the following article: Vandenbroucke, Guillaume and Zhu, Heting. "Aging and Wealth Inequality." Federal Reserve Bank of St. Louis Economic Synopses , 2017, No. 2; https://research.stlouisfed.org/publications/economic-synopses/2017/02/24/aging-and-wealth-inequality/ .
5 For a contribution to the ongoing debate about the relationship between monetary policy and income inequality, see the following article: Bullard, James. "Income Inequality and Monetary Policy: A Framework with Answers to Three Questions." Presented at the C. Peter McColough Series on International Economics, Council on Foreign Relations, New York, June 26, 2014; http://research.stlouisfed.org/econ/bullard/pdf/Bullard_CFR_26June2014_Final.pdf .
6 The following FRED® graph shows the income Gini ratio of all families, reported by the U.S. Census Bureau since 1947: https://fred.stlouisfed.org/graph/?g=MKYg .
7 For more on income inequality and the stock market, see the following articles:
Bennett, Julie and Chien, YiLi. "The Large Gap in Stock Market Participation Between Black and White Households." Federal Reserve Bank of St. Louis Economic Synopses , 2022, No. 7; https://research.stlouisfed.org/publications/economic-synopses/2022/03/28/the-large-gap-in-stock-market-participation-between-black-and-white-households/ .
Owyang, Michael T. and Shell, Hannah G. "Taking Stock: Income Inequality and the Stock Market." Federal Reserve Bank of St. Louis Economic Synopses , 2016, No. 7; https://research.stlouisfed.org/publications/economic-synopses/2016/04/29/taking-stock-income-inequality-and-the-stock-market/ .
8 For more about the change in wealth distribution over time, see the following post: Federal Reserve Bank of St. Louis. "Comparing the Assets of the Rich, Poor, and Middle Class." FRED ® Blog , October 21, 2019; https://fredblog.stlouisfed.org/2019/10/comparing-the-assets-of-the-rich-poor-and-middle-class/ .
9 For more on how recessions impact household net worth, see the following article: Mendez-Carbajo, Diego. "How Recessions Have Affected Household Net Worth, 1990-2017: Uneven Experiences by Wealth Quantile." Federal Reserve Bank of St. Louis Economic Synopses , 2020, No. 38; https://research.stlouisfed.org/publications/economic-synopses/2020/08/07/how-recessions-have-affected-household-net-worth-1990-2017-uneven-experiences-by-wealth-quantile .
10 For more on the relationship between inequality and economic fluctuations, see the following article: Iyigun, Murat F. and Owen, Ann L. "Income Inequality and Macroeconomic Fluctuations." Board of Governors of the Federal Reserve System International Finance Discussion Papers , July 1997; https://www.federalreserve.gov/econres/ifdp/income-inequality-and-macroeconomic-fluctuations.htm .
11 For more on the relationship between income inequality and economic growth, see the following article: Cingano, Federico. "Trends in Income Inequality and its Impact on Economic Growth." Organisation for Economic Co-operation and Development OECD Social, Employment, and Migration Working Papers , 2014, No. 163; https://www.oecd.org/els/soc/trends-in-income-inequality-and-its-impact-on-economic-growth-sem-wp163.pdf .
12 For more on the relationship between income inequality and monetary policy, see the following article: Cairo, Isabel and Sim, Jae W. "Income Inequality, Financial Crises, and Monetary Policy." Board of Governors of the Federal Reserve System Finance and Economics Discussion Series , July 2018; https://www.federalreserve.gov/econres/feds/income-inequality-financial-crises-and-monetary-policy.htm .
© 2022, Federal Reserve Bank of St. Louis. The views expressed are those of the author(s) and do not necessarily reflect official positions of the Federal Reserve Bank of St. Louis or the Federal Reserve System.
Asset: A resource with economic value that an individual, corporation, or country owns with the expectation that it will provide future benefits.
Gini index: A statistical measure of income inequality in a population that ranges from 0 (indicating absolute income equality) to 100 (indicating a perfectly inequal income distribution).
Household: A group of people living in the same home, regardless of their relationship to one another.
Income: The payment people receive for providing resources in the marketplace. When people work, they provide human resources (labor) and in exchange they receive income in the form of wages or salaries. People also earn income in the forms of rent, profit, and interest.
Liability: A legal responsibility to pay back money from a loan or other type of debt.
Net worth: The value of a person's assets minus the value of his or her liabilities.
Quintile: Any of five equal groups into which a population can be divided according to the distribution of values of a particular variable.
Wealth: The value of a person's assets accumulated over time.
Cite this article

Subscribe to Our Newsletter
Stay current with brief essays, scholarly articles, data news, and other information about the economy from the Research Division of the St. Louis Fed.
SUBSCRIBE TO THE RESEARCH DIVISION NEWSLETTER
Research division.
- Legal and Privacy
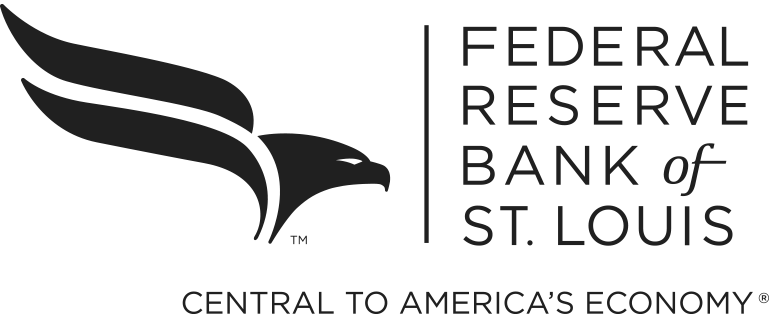
One Federal Reserve Bank Plaza St. Louis, MO 63102
Information for Visitors

Disaggregated Public Spending, Income Inequality and its Effect on Economic Growth: Empirical Evidence from Developing Countries
- Published: 22 April 2024
Cite this article
- Obaid Ullah 1 ,
- Zia Ur Rahman ORCID: orcid.org/0000-0001-5203-3881 2 , 3 ,
- Aijun Guo 1 &
- Ali Zeb 4
10 Accesses
Explore all metrics
This study aims to check the impact of disaggregated public spending and income inequality on economic growth within a panel of 24 developing countries from 1990 to 2017. We used second-generation methodologies to check the cointegration and cross-sectional dependency within the panel. The empirical estimations are being carried out using the augmented mean group (AMG) and common correlated effect (CCE) frameworks, acknowledged for their efficiency in accommodating heterogeneity and cross-sectional dependence. The empirical outcome discloses that income inequality is positively correlated with economic growth, though insignificantly. In contrast, the dissection of public spending indicates notable variations in their impact on economic growth. Specifically, expenditures directed toward health, agriculture, education, and overall spending exhibit a positive and statistically significant effect on economic growth. Conversely, government expenditures on defense, transport, social protection, and communication negatively link economic growth. In light of these findings, this study advocates for policy considerations emphasizing sectors positively correlated with economic growth, notably health, education, and agriculture. The assertion is grounded in the recognition that investments in these sectors foster economic development and contribute to cultivating a healthier and more skilled populace, thereby constituting key determinants of sustained economic growth. These nuanced empirical insights contribute to the ongoing discourse on effective public resource allocation strategies in developing countries.
This is a preview of subscription content, log in via an institution to check access.
Access this article
Price includes VAT (Russian Federation)
Instant access to the full article PDF.
Rent this article via DeepDyve
Institutional subscriptions
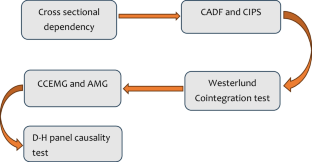
Similar content being viewed by others
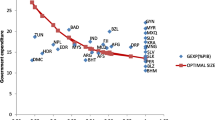
Public Sector Efficiency and Economic Growth in Developing Countries
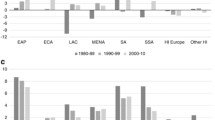
Trends and Composition of Public Expenditures: A Global and Regional Perspective
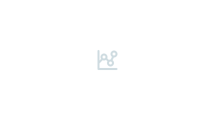
Productive government expenditure and its impact on income inequality: evidence from international panel data
Data availability.
Data will be available upon request.
Aban, M. P., & Garcia-Vigonte, F. (2022). A study on the effects of social spending on economic activity. Available at SSRN 4123354 .
Adrián Risso, W., & Sánchez Carrera, E. J. (2019). On the impact of innovation and inequality in economic growth. Economics of Innovation and New Technology, 28 (1), 64–81.
Article Google Scholar
Affandi, Y., Anugrah, D. F., & Bary, P. (2019). Human capital and economic growth across regions: A case study in Indonesia. Eurasian Economic Review, 9 , 331–347.
Ahmed, Z., Zafar, M. W., & Mansoor, S. (2020). Analyzing the linkage between military spending, economic growth, and ecological footprint in Pakistan: Evidence from cointegration and bootstrap causality. Environmental Science and Pollution Research, 27 , 41551–41567.
Aiyar, S., & Ebeke, C. (2020). Inequality of opportunity, inequality of income and economic growth. World Development, 136 , 105115.
Alam, F., Singh, H. P., & Singh, A. (2022). Economic growth in Saudi Arabia through sectoral reallocation of government expenditures. SAGE Open, 12 (4), 21582440221127160.
Albassam, B. A. (2022). Government spending and economic growth in the Middle East and North Africa region. International Review of Administrative Sciences, 88 (4), 1124–1140.
Alesina, A., Özler, S., Roubini, N., & Swagel, P. (1996). Political instability and economic growth. Journal of Economic Growth, 1 (2), 189–211.
Al-kasasbeh, O., Alzghoul, A., & Alhanatleh, H. (2022). The impact of fiscal policy and trade liberalization on economic growth: Evidence from structural breaks for Jordan. International Journal of Professional Business Review, 7 (6), e0850–e0850.
Alshammary, M. D., Khalid, N., Karim, Z. A., & Ahmad, R. (2022). Government expenditures and economic growth in the MENA region: A dynamic heterogeneous panel estimation. International Journal of Finance & Economics, 27 (3), 3287–3299.
Antolin-Diaz, J., & Surico, P. (2022). The long-run effects of government spending . Centre for Economic Policy Research.
Ayunku, P. E., & Etale, L. M. (2015). Effect of agriculture spending on economic growth in Nigeria: Empirical evidence. Research Journal of Finance and Accounting, 6 (2), 138–143.
Google Scholar
Balcilar, M., Gupta, R., Ma, W., & Makena, P. (2021). Income inequality and economic growth: A re-examination of theory and evidence. Review of Development Economics, 25 (2), 737–757.
Baltagi, B. H., Feng, Q., & Kao, C. (2012). A Lagrange multiplier test for cross-sectional dependence in a fixed effects panel data model. Journal of Econometrics, 170 (1), 164–177.
Barlas, A. W. (2020). The impact of government expenditure on economic growth in Afghanistan. Journal of Economics and Business , 3 (2).
Barro, R. J. (1999). Inequality, growth and investment. NBER , working pa .
Berg, A. G., & Ostry, J. D. (2017). Inequality and unsustainable growth: Two sides of the same coin? IMF Economic Review, 65 (4), 792–815.
Breusch, T. S., & Pagan, A. R. (1980). The Lagrange multiplier test and its applications to model specification in econometrics. The Review of Economic Studies, 47 (1), 239–253.
Bucci, A., Carbonari, L., Ranalli, M., & Trovato, G. (2021). Health and economic development: Evidence from non-OECD countries. Applied Economics, 53 (55), 6348–6375.
Chaudhuri, S., & Ravallion, M. (2003). Uneven growth in China and India. Dancing with Giants , 175–210.
Chen, H., Singh, B., & Aru, W. S. (2022). Relationship between government expenditure and economic growth: Evidence from Vanuatu. Journal of the Asia Pacific Economy, 27 (4), 640–659.
Chudik, A., Pesaran, M. H., & Tosetti, E. (2011). Weak and strong cross-section dependence and estimation of large panels . Oxford University Press.
Book Google Scholar
Davern, E. (2020). Trends and new developments in employment services to support transitions in sub-Saharan Africa, the Middle East and North Africa regions (Issue 19). ILO Working Paper.
Dissou, Y., Didic, S., & Yakautsava, T. (2016). Government spending on education, human capital accumulation, and growth. Economic Modelling, 58 , 9–21.
Dumitrescu, E. I., & Hurlin, C. (2012). Testing for Granger non-causality in heterogeneous panels. Economic Modelling, 29 (4), 1450–1460.
Eberhardt, M., & Bond, S. (2009). Cross-section dependence in nonstationary panel models: A novel estimator.
Eliasson, G. (2023). Bringing markets back into economics: On economy wide self-coordination by boundedly rational market agents. Journal of Economic Behavior & Organization, 216 , 686–710.
Fawaz, F., & Rahnama, M. (2022). The relationship between economic growth and income inequality in economies in transition. Problems of Economic Transition, 63 (7–9), 379–400.
Gnangoin, Y. T. B., Du, L., Assamoi, G., Edjoukou, A. J., & Kassi, D. F. (2019). Public spending, income inequality and economic growth in Asian countries: A panel GMM approach. Economies, 7 (4), 115.
Ifa, A., & Guetat, I. (2019). The short and long run causality relationship between public health spending and economic growth: Evidence from Tunisia and Morocco. Journal of Economic Development, 44 (3), 19–39.
Ikpesu, F. (2021). Banking sector credit, inflation and growth in sub-Saharan African countries. Journal of Transnational Management, 26 (3), 164–178.
Im, K. S., Pesaran, M. H., & Shin, Y. (2003). Testing for unit roots in heterogeneous panels. Journal of Econometrics, 115 (1), 53–74.
Jakovljevic, M., Timofeyev, Y., Ranabhat, C. L., Fernandes, P. O., Teixeira, J. P., Rancic, N., & Reshetnikov, V. (2020). Real GDP growth rates and healthcare spending–Comparison between the G7 and the EM7 countries. Globalization and Health, 16 (1), 1–13.
Johansson, Å. (2016). Public finance, economic growth and inequality: A survey of the evidence. OECD Economics Department Working Paper No. 1346 , 1346 .
Jorgenson, D. W., & Fraumeni, B. M. (2020). Investment in education and US economic growth. In The US savings challenge (pp. 114–149). Routledge.
Kapetanios, G., Pesaran, M. H., & Yamagata, T. (2011). Panels with non-stationary multifactor error structures. Journal of Econometrics, 160 (2), 326–348.
Khan, S. A. R., Zhang, Y., Kumar, A., Zavadskas, E., & Streimikiene, D. (2020). Measuring the impact of renewable energy, public health expenditure, logistics, and environmental performance on sustainable economic growth. Sustainable Development, 28 (4), 833–843.
Kuznets, S. (2019). Economic growth and income inequality. In The gap between rich and poor (pp. 25–37). Routledge.
Li, L., Rahman, Z. U., Khattak, S. I., & Hasan, M. M. (2022). Asymmetric effect of military expenditures on economic growth in Pakistan: A nonlinear-ARDL approach. Inzinerine Ekonomika-Engineering Economics, 33 (3), 316–325.
Mdingi, K., & Ho, S.-Y. (2021). Literature review on income inequality and economic growth. MethodsX, 8 , 101402.
Miró, J., Kyriazi, A., Natili, M., & Ronchi, S. (2023). Buffering national welfare states in hard times: The politics of EU capacity‐building in the social policy domain. Social Policy & Administration .
Mirrlees, J. A. (1971). An exploration in the theory of optimum income taxation. Welfare, Incentives, and Taxation, 38 (2), 175–208.
Mohmand, Y. T., Mehmood, F., Mughal, K. S., & Aslam, F. (2021). Investigating the causal relationship between transport infrastructure, economic growth and transport emissions in Pakistan. Research in Transportation Economics, 88 , 100972.
Musaba, E. C., Chilonda, P., & Matchaya, G. (2013). Impact of government sectoral expenditure on economic growth in Malawi, 1980–2007. Journal of Economics and Sustainable Development, 4 (2), 71–78.
Odhiambo, N. M. (2021). Health expenditure and economic growth in sub-Saharan Africa: An empirical investigation. Development Studies Research, 8 (1), 73–81.
Okoye, L. U., Omankhanlen, A. E., Okoh, J., Urhie, E., & Ahmed, A. (2019). Government expenditure and economic growth: The case of Nigeria. Proceedings of SOCIOINT , 1184–1194.
Onifade, S. T., Çevik, S., Erdoğan, S., Asongu, S., & Bekun, F. V. (2020). An empirical retrospect of the impacts of government expenditures on economic growth: New evidence from the Nigerian economy. Journal of Economic Structures, 9 (1), 1–13.
Parui, P. (2022). Government expenditure and economic growth: A post-Keynesian analysis. In Capitalism: An unsustainable future? (pp. 267–295). Routledge.
Pawlak, K., & Kołodziejczak, M. (2020). The role of agriculture in ensuring food security in developing countries: Considerations in the context of the problem of sustainable food production. Sustainability, 12 (13), 5488.
Pedroni, P. (1999). Critical values for cointegration tests in heterogeneous panels with multiple of economics and statistics, Vol. No. 0. Oxford Bulletin of Economics and Statistics, 61 , 653–670.
Pedroni, P. (2004). Panel cointegration: Asymptotic and finite sample properties of pooled time series tests with an application to the PPP hypothesis. Econometric Theory, 20 (3), 597–625.
Pesaran, M. H. (2004a). General diagnostic tests for cross section dependence in panels. IZA Discussion Paper , 6 (3).
Pesaran, M. H. (2004b). General diagnostic tests for cross section dependence in panels. Cambridge Working Papers. Economics , 1240 (1), 1.
Pesaran, M. H. (2006). Estimation and inference in large heterogeneous panels with a multifactor error structure. Econometrica, 74 (4), 967–1012.
Pesaran, M. H. (2007a). A simple panel unit root test in the presence of cross‐section dependence _ Enhanced Reader.pdf. JOURNAL OF APPLIED ECONOMETRICS .
Pesaran, M. H. (2007b). A simple panel unit root test in the presence of cross‐section dependence _ Enhanced Reader.pdf. JOURNAL OF APPLIED ECONOMETRICS .
Rahman, T., & Siddiqui, D. A. (2019). The effect of military spending on economic growth in the presence of arms trade: A global analysis. Available at SSRN 3401331 .
Ravallion, M., & Chen, S. (2022). Is that really a Kuznets curve? Turning points for income inequality in China. The Journal of Economic Inequality, 20 (4), 749–776.
Roca-Sagalés, O., & Sala, H. (2011). Government expenditures and the growth-inequality trade-off: The Swedish case. Journal of Income Distribution, 20 (2), 38–54.
Sabir, S., & Qamar, M. (2019). Fiscal policy, institutions and inclusive growth: Evidence from the developing Asian countries. International Journal of Social Economics, 46 (6), 822–837.
Sequeira, T. N. (2021). Inflation, economic growth and education expenditure. Economic Modelling, 99 , 105475.
Shahbaz, M., Song, M., Ahmad, S., & Vo, X. V. (2022). Does economic growth stimulate energy consumption? The role of human capital and R&D expenditures in China. Energy Economics, 105 , 105662.
Sharma, S., & Vidyapith, M. G. K. (2023). Wealth and income inequality in India. International Journal of Creative Research Thoughts, 11 (6), 416–428.
Solt, F. (2016). The Standardized World Income Inequality Database*. Social Science Quarterly, 97 (5), 1267–1281. https://doi.org/10.1111/ssqu.12295
Stefko, R., Gavurova, B., Ivankova, V., & Rigelsky, M. (2020). Gender inequalities in health and their effect on the economic prosperity represented by the GDP of selected developed countries—Empirical study. International Journal of Environmental Research and Public Health, 17 (10), 3555.
Tanzi, V. (2023). Monitoring the state or the market: From Laissez Faire to market fundamentalism . Cambridge University Press.
Teal, F., & Eberhardt, M. (2010). Productivity analysis in global manufacturing production .
Titumir, R. A. M., & Titumir, R. A. M. (2021). Poverty and inequality in Bangladesh. Numbers and narratives in Bangladesh’s economic development , 177–225.
Walker, C. C., Druckman, A., & Jackson, T. (2021). Welfare systems without economic growth: A review of the challenges and next steps for the field. Ecological Economics, 186 , 107066.
Westerlund, J. (2007). Testing for error correction in panel data. Oxford Bulletin of Economics and Statistics, 69 (6), 709–748.
Wolde, M., Sera, L., & Merra, T. M. (2022). Causal relationship between income inequality and economic growth in Ethiopia. Cogent Economics & Finance, 10 (1), 2087299.
Xia, W., Apergis, N., Bashir, M. F., Ghosh, S., Doğan, B., & Shahzad, U. (2022). Investigating the role of globalization, and energy consumption for environmental externalities: Empirical evidence from developed and developing economies. Renewable Energy, 183 , 219–228.
Yang, X. (2020). Health expenditure, human capital, and economic growth: An empirical study of developing countries. International Journal of Health Economics and Management, 20 (2), 163–176.
Download references
Author information
Authors and affiliations.
School of Economics, Lanzhou University, Lanzhou, 730000, Gansu, China
Obaid Ullah & Aijun Guo
School of Mathematical Sciences, Zhejiang Normal University, Jinhua, China
Zia Ur Rahman
School of Economics and Management, Zhejiang Normal University, Jinhua, China
School of Business, Zhengzhou University, Zhengzhou, Henan, China
You can also search for this author in PubMed Google Scholar
Corresponding author
Correspondence to Zia Ur Rahman .
Additional information
Publisher's note.
Springer Nature remains neutral with regard to jurisdictional claims in published maps and institutional affiliations.
Rights and permissions
Springer Nature or its licensor (e.g. a society or other partner) holds exclusive rights to this article under a publishing agreement with the author(s) or other rightsholder(s); author self-archiving of the accepted manuscript version of this article is solely governed by the terms of such publishing agreement and applicable law.
Reprints and permissions
About this article
Ullah, O., Rahman, Z.U., Guo, A. et al. Disaggregated Public Spending, Income Inequality and its Effect on Economic Growth: Empirical Evidence from Developing Countries. J Knowl Econ (2024). https://doi.org/10.1007/s13132-024-01991-0
Download citation
Received : 13 October 2022
Accepted : 10 April 2024
Published : 22 April 2024
DOI : https://doi.org/10.1007/s13132-024-01991-0
Share this article
Anyone you share the following link with will be able to read this content:
Sorry, a shareable link is not currently available for this article.
Provided by the Springer Nature SharedIt content-sharing initiative
- Public spending
- Income inequality
- Economic growth
- Find a journal
- Publish with us
- Track your research
Numbers, Facts and Trends Shaping Your World
Read our research on:
Full Topic List
Regions & Countries
- Publications
- Our Methods
- Short Reads
- Tools & Resources
Read Our Research On:
- Wealth Surged in the Pandemic, but Debt Endures for Poorer Black and Hispanic Families
2. Wealth gaps across racial and ethnic groups
Table of contents.
- The range of wealth within racial and ethnic groups
- The share of racial or ethnic groups’ wealth held by richer households, not counting the top 1%
- Households with no wealth or in debt
- How wealth varies by race and ethnicity
- The wealth of White households relative to the wealth of other households
- Households in the lower, middle or upper tiers of wealth
- The wealth of U.S. households by income tier
- Racial and ethnic wealth gaps by income tier
- Types of assets owned by U.S. households
- The value of assets owned by households
- The importance of home equity
- The debt level of households
- How high is household debt?
- Acknowledgments
- Data source
- Race and ethnicity
- Household head
- Adjusting for inflation
- Defining income tiers
- Statistical testing
Household wealth or net worth is the value of assets owned by every member of the household minus their debt. The terms are used interchangeably in this report. Assets include owned homes, vehicles, financial accounts, retirement accounts, stocks, bonds and mutual funds, and more. Debt refers to home mortgage loans, education loans, credit card balances, and any other loan or credit extended to the household. Net worth is negative when debt levels are greater than asset values. ( Refer to the methodology for more details.)
“Poorer,” “more solvent,” “wealthier” and “richer” refer to a household’s wealth status relative to other households of the same race and ethnicity . Poorer households rank in the bottom 25% when compared with other households of the same race and ethnicity; more solvent households place from the 25th up to and including the 50th percentile; wealthier households from the 50th up to and including the 75th percentile; and the richer are in the top 25%. The bottom 1% and the top 1% are excluded prior to the ranking so extreme values do not affect estimates for poorer and richer households.
Lower, middle and upper wealth tiers refer to a household’s wealth status relative to all other households in the U.S. The middle wealth tier consists of households whose wealth lies between one-quarter to four times as much as the median wealth of U.S. households – between $41,700 and $667,500 in 2021. Households in the lower wealth tier had wealth less than $41,700, and households in the upper wealth tier had wealth more than $667,500. The wealth tier boundaries vary across years. (Dollar amounts are expressed in December 2021 prices.)
Middle-income households are those with an income that is two-thirds to double that of the U.S. median household income, after incomes have been adjusted for household size and difference in the cost of living across areas. This was about $4,800 to $14,300 monthly in December 2021, for a household of three. Lower-income households had incomes less than $4,800, and upper-income households had incomes greater than $14,300. The income tier boundaries vary across years. (Dollar amounts are expressed in December 2021 prices.)
Households are grouped by the race and ethnicity of the survey reference person, or the household head. White, Black and Asian include those who report being only one race and are not Hispanic. Multiracial includes non-Hispanics of two or more races. Hispanics are of any race. American Indian or Pacific Islander households are not covered in our analysis because of small sample sizes.
The increase in the wealth of U.S. households from 2019 to 2021 was spread widely across racial and ethnic groups. Despite improved fortunes, Black, Hispanic and multiracial households trailed White and Asian households by large margins from the following perspectives, both before and after the pandemic:
- Their wealth relative to the wealth of White and Asian households.
- The shares of households that are in the middle or upper tiers of wealth.
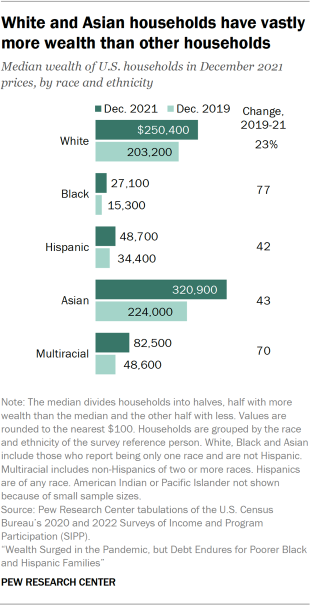
Asian households overall had more wealth than other households two years since the start of the pandemic. In 2021, Asian households had a median net worth of $320,900, compared with $250,400 for White households. The median net worth of Hispanic households ($48,700) and Black households ($27,100) was much less.
In dollar amounts, the wealth gap between White households and Black and Hispanic households increased from 2019 to 2021. For example, in 2019, the typical White household had $168,800 more in wealth than the typical Hispanic household. This gap increased to $201,700 in 2021.
In percentage terms, Black (77%), Hispanic (42%), Asian (43%) and White (23%) households all experienced strong gains in median wealth from 2019 to 2021.
What factors might explain the large wealth gaps across racial and ethnic groups that have endured for decades, if not longer ?
With respect to Black households, evidence points to the role of slavery and post-slavery practices, such as segregation, that created disparate opportunities for wealth accumulation . For Hispanic households, the relative youth of the population and immigration status play a role. In turn, these factors likely contribute to the lower rates and sizes of inheritances received by Black and Hispanic households in comparison with White households.
Asian households overall have more wealth than other households partly because of the higher level of education among Asian adults . In 2021, 61% of Asians ages 25 and older had a bachelor’s degree or higher level of education, compared with 42% of White adults. As a result, the typical Asian household has a much higher income than other households, with more potential for building wealth. But the Asian American population is very diverse, and income varies widely across Asian origin groups . As shown in the following chapter , lower-income Asian households had less wealth than lower-income White households in 2021.
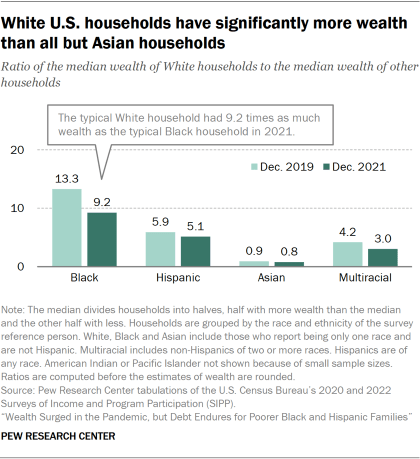
In 2021, the typical White household had 9.2 times as much wealth as the typical Black household – $250,400 vs. $27,100. This ratio stood at 13.3 in 2019, prior to the onset of the pandemic.
White households also had significantly more wealth than Hispanic households (5.1 times) and multiracial households (3.0 times) in 2021. But the median wealth of White households was about 20% less than that of Asian households.
The wealth gaps between White households and Black and Hispanic households in 2019 and 2021 are in line with recent history . But the apparent shrinking of racial and ethnic wealth gaps during the pandemic stands in contrast with what happened during the Great Recession of 2007-09 .
In the Great Recession, the median wealth of White households jumped to nearly 20 times the wealth of Black households and 15 times that of Hispanic households. The key factors then were a sharp decline in home prices and a steep rise in home foreclosures , non-events during the pandemic. Black and Hispanic households saw more harm from these developments during the Great Recession because housing is a more valuable part of their collection of assets .
How does the wealth of a household compare with all other U.S. households? What shares of households are in the middle tier of wealth, and what shares are in the lower or upper tiers?
To address these questions, we grouped U.S. households into three tiers of wealth. The middle tier consists of households whose wealth lies between one-quarter and four times the median wealth of U.S. households – between $41,700 and $667,500 in 2021. Households in the lower tier had wealth less than $41,700 in 2021, and households in the upper tier had wealth more than $667,500.
Fewer than half of U.S. households (44%, or 59 million) were in the middle tier in 2021. This was so despite the wide range of the middle tier – those near the top had 16 times as much wealth as those near the bottom. At the same time, about a third of households (32%, or 43 million) were in the lower wealth tier, and about a quarter (23%, or 31 million) were in the upper tier.
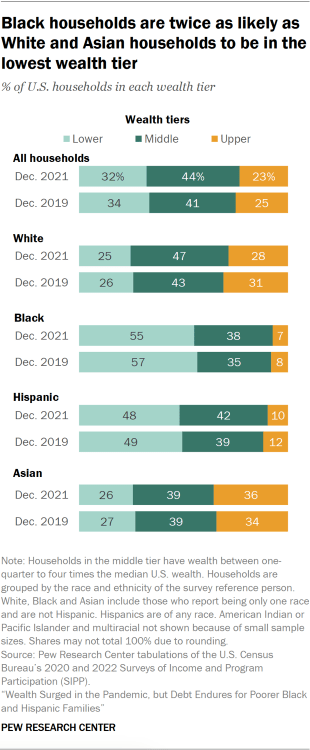
Only 45% of Black households were in either the middle or upper wealth tiers in 2021, the lowest share among the groups examined. The majority of Black households (55%) were in the lower wealth tier in 2021 – that is, they had less than $41,700 in wealth. A somewhat greater share of Hispanic households (52%) were in the middle or upper wealth tier, and 48% were in the lower wealth tier.
In contrast, more than 70% of White and Asian households were in either the middle or upper wealth tier. Roughly one-third of Asian households (36%) were in the upper tier alone, with a net worth of more than $667,500 in 2021. Slightly more than a quarter (28%) of White households were in the upper tier.
Increases in household wealth during the pandemic did lead to a more equal distribution of wealth. The share of households in the middle tier increased from 41% in 2019 to 44% in 2021. Meanwhile, the shares of households in the lower and upper wealth tiers edged down by 2 percentage points each.
A similar shift to the middle was seen in Hispanic and White households, whose shares in the middle wealth tier increased by 3 to 4 points from 2019 to 2021. However, no such change was experienced by Asian households, with their share in the middle wealth tier holding at 39%.
Sign up for our weekly newsletter
Fresh data delivery Saturday mornings
Sign up for The Briefing
Weekly updates on the world of news & information
- Asian Americans
- Black Americans
- COVID-19 & the Economy
- Economic Inequality
- Hispanics/Latinos
- Income & Wages
- Income, Wealth & Poverty
- Racial & Ethnic Groups
Key facts about Asian Americans living in poverty
Methodology: 2023 focus groups of asian americans, 1 in 10: redefining the asian american dream (short film), the hardships and dreams of asian americans living in poverty, key facts about asian american eligible voters in 2024, most popular, report materials.
1615 L St. NW, Suite 800 Washington, DC 20036 USA (+1) 202-419-4300 | Main (+1) 202-857-8562 | Fax (+1) 202-419-4372 | Media Inquiries
Research Topics
- Age & Generations
- Coronavirus (COVID-19)
- Economy & Work
- Family & Relationships
- Gender & LGBTQ
- Immigration & Migration
- International Affairs
- Internet & Technology
- Methodological Research
- News Habits & Media
- Non-U.S. Governments
- Other Topics
- Politics & Policy
- Race & Ethnicity
- Email Newsletters
ABOUT PEW RESEARCH CENTER Pew Research Center is a nonpartisan fact tank that informs the public about the issues, attitudes and trends shaping the world. It conducts public opinion polling, demographic research, media content analysis and other empirical social science research. Pew Research Center does not take policy positions. It is a subsidiary of The Pew Charitable Trusts .
Copyright 2024 Pew Research Center
Terms & Conditions
Privacy Policy
Cookie Settings
Reprints, Permissions & Use Policy
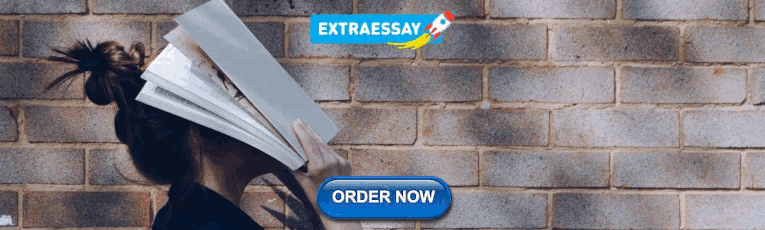
IMAGES
VIDEO
COMMENTS
ESSAYS ON EDUCATIONAL INEQUALITY 1 Essays on Educational Inequality: Learning Gaps, Social-Emotional Skills Gaps, and Parent Enrichment Outside of School Time An enduring question in sociology and education is how children's out-of-school environment contributes to educational inequality. In my dissertation, I shed fresh light
the top 1 percent of earners earn 22 percent of all income in the United States (Piketty & Saez 2013) is evidence of a distributional income inequality. A host of material, social, historical, and political conditions has increased the likelihood of disproportional access to opportunities, producing this distributional inequality.
This paper presents new evidence on the relationship between education and income inequality by drawing evidence from 145 countries between 1996 to 2016. Reviewing the economic theories of Simon ...
The past 30 years have seen a sustained rise in inequality in wages, incomes, and wealth, leading to more and more income and wealth accruing to those at the top of the economic ladder, pulling the rich further away from those on the other rungs. 1. At the same time, the growing educational gap became ever more apparent.
In 2010, family income at the 20th percentile was more than 25% lower than the inflation-adjusted corresponding family income in 1970. In contrast, the real incomes of families at the 80th ...
The influence of income inequality affects multiple aspects of society's functioning, from health outcomes and even life expectancy to democratic ideals (Putnam 2015; Schanzenbach et al. 2016; Stringhini et al. 2017). ... with mother's education and household income being the indicators associated with the largest changes in the gaps ...
Another significant factor that can impact income inequality is education, and specialized literature offers numerous works that address the subject such as Andrea Bergh's article Higher Education Policy, Enrollment, and Income Inequality or Catharine Hill's paper American Higher Education and Income Inequality . As we can easily notice ...
Essays on Higher Education and Inequality Abstract ... income students and students of color. In the second essay, I find admission to in-state four-year public colleges increases applicants' mean annual earnings by almost $8,000 eight to fourteen years after applying. Using student-level cost and aid data, I also find students experience large
A. Education and Past Income Inequality Trends in income inequality between 1990 and 2005 varied substantially across regions. On average in the sample, inequality decreased in both SSA and MENA countries while other regions experienced increases with the Gini increasing by over 5 points in both AP and EE (Table 4).
This paper presents new results on the relationship between income inequality and education expansion—that is, increasing average years of schooling and reducing inequality of schooling. ... Education Levels and Education Inequality. Citation: IMF Working Papers 2017, 126; 10.5089/9781475595741.001.A001. Note: Lines are based on non ...
Although there is an extensive body of empirical literature examining education as a determinant of income inequality, the evidence on the link from income inequality to educational outcomes is scarcer (Thorbecke and Charumilind 2002, 1488; Gutiérrez and Tanaka 2009, 56).
There are many explanations for educational inequity. In my view, the most important ones are the following: Equity and equality are not the same thing. Equality means providing the same resources ...
Professor Reardon is the author of a study that found that the gap in standardized test scores between affluent and low-income students had grown by about 40 percent since the 1960s, and is now double the testing gap between blacks and whites. In another study, by researchers from the University of Michigan, the imbalance between rich and poor ...
Lleras-Muney, 2005). Finally, obtaining more education than one's parents has been an important mechanism of upward socio-economic mobility for many generations of Americans. Educational Inequality There are large income-related gaps in educational attainments in the United States. For
This paper analyses the effects of innate ability, compulsory education (grades 1-9), and non-compulsory education (grades 10-12 and higher education) on inequality and intergenerational mobility of income, by constructing a four-period overlapping-generation model. We find that innate ability and family investment in early education play ...
An analysis of achievement gaps in every school in America shows that poverty is the biggest hurdle. Rich schools get richer: School spending analysis finds widening gap between top 1% and the rest of us. This story about education inequality in America written by Jill Barshay and produced by The Hechinger Report, a nonprofit, independent news ...
This paper presents new results on the relationship between income inequality and education expansion—that is, increasing average years of schooling and reducing inequality of schooling. When dynamic panel estimation techniques are used to address issues of persistence and endogeneity, we find a large, positive, statistically significant and stable relationship between inequality of ...
Third in a series on what Harvard scholars are doing to identify and understand inequality, in seeking solutions to one of America's most vexing problems.. Before Deval Patrick '78, J.D. '82, was the popular and successful two-term governor of Massachusetts, before he was managing director of high-flying Bain Capital, and long before he was Harvard's most recent Commencement speaker ...
The income inequality may affect the education competition. To see this, Han et al. (2012) attributes the rising income inequality observed after China's accession to WTO to higher returns to college. As a result, income inequality likely drives up the value of higher education, especially at the elite universities, thus reinforcing parents ...
This dissertation consists of three independent essays on income inequality. Chapter 1 (with Sydnee Caldwell) develops a method to estimate the outside employment opportunities available to each worker and to assess the impact of these outside options on wage inequality. We estimate a sufficient statistic, the "outside options index" (OOI ...
By contrast, those in the high-income group usually have fewer children and invest more in their education. Therefore, in the case of extreme income inequality, the high fertility differential has a negative impact on human capital, leading to a decline in economic growth. Income inequality and economic growth: Empirical evidence
The negative physical and mental health outcomes that income inequality provoke, also impact key societal areas such as crime, social mobility and education. 3.3 Social Rates of violent crime are lower in more equal countries ( Hsieh and Pugh 1993 ; Whitworth 2012 ).
Measured this way, income inequality can be as high as 130 or as low as 5. These measurements mean that the most affluent households in a particular county can earn as much as 130 times or as little as 5 times as much as the least affluent households do. Another way to measure income inequality in a population is to calculate the Gini index ...
According to the Congressional Budget Office, if you look at inequality based on post-tax-and-transfer income—a comprehensive view of income that reflects the full economic resources available ...
This study aims to check the impact of disaggregated public spending and income inequality on economic growth within a panel of 24 developing countries from 1990 to 2017. We used second-generation methodologies to check the cointegration and cross-sectional dependency within the panel. The empirical estimations are being carried out using the augmented mean group (AMG) and common correlated ...
In 2020, the median income of upper-income households was 7.3 times that of lower-income households, up from 6.3 in 1970. The median income of upper-income households was 2.4 times that of middle-income households in 2020, up from 2.2 in 1970. The share of aggregate U.S. household income held by the middle class has fallen steadily since 1970.
Abstract. This study explores the relationship between entrepreneurship and income inequality, positing that higher entrepreneurship contribute to reduced overall income inequality. Our empirical analysis reveals robust and statistically significant findings, with economic significance between -13.81% and -15.92%.
The gender gap in pay has remained relatively stable in the United States over the past 20 years or so. In 2022, women earned an average of 82% of what men earned, according to a new Pew Research Center analysis of median hourly earnings of both full- and part-time workers. These results are similar to where the pay gap stood in 2002, when ...
In 2021, Asian households had a median net worth of $320,900, compared with $250,400 for White households. The median net worth of Hispanic households ($48,700) and Black households ($27,100) was much less. In dollar amounts, the wealth gap between White households and Black and Hispanic households increased from 2019 to 2021.
Relation between crime and immigration, unemployment and income inequality in European countries: social issues and policy implications. Crime, Law and Social Change, n. 10144. 31 Pages Posted: 14 Apr 2024. ... PAPERS. 4,947. This Journal is curated by: Paul H. Robinson at University of Pennsylvania Carey Law School. Law & Society: Public Law ...