- Privacy Policy
Buy Me a Coffee

Home » Narrative Analysis – Types, Methods and Examples
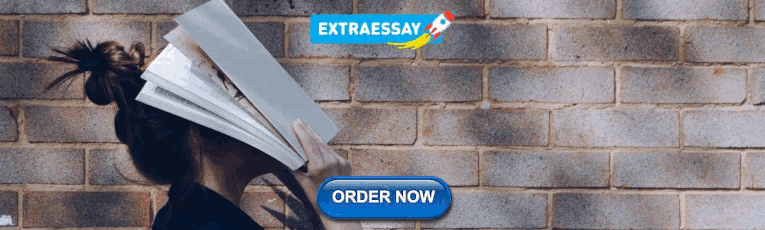
Narrative Analysis – Types, Methods and Examples
Table of Contents

Narrative Analysis
Definition:
Narrative analysis is a qualitative research methodology that involves examining and interpreting the stories or narratives people tell in order to gain insights into the meanings, experiences, and perspectives that underlie them. Narrative analysis can be applied to various forms of communication, including written texts, oral interviews, and visual media.
In narrative analysis, researchers typically examine the structure, content, and context of the narratives they are studying, paying close attention to the language, themes, and symbols used by the storytellers. They may also look for patterns or recurring motifs within the narratives, and consider the cultural and social contexts in which they are situated.
Types of Narrative Analysis
Types of Narrative Analysis are as follows:
Content Analysis
This type of narrative analysis involves examining the content of a narrative in order to identify themes, motifs, and other patterns. Researchers may use coding schemes to identify specific themes or categories within the text, and then analyze how they are related to each other and to the overall narrative. Content analysis can be used to study various forms of communication, including written texts, oral interviews, and visual media.
Structural Analysis
This type of narrative analysis focuses on the formal structure of a narrative, including its plot, character development, and use of literary devices. Researchers may analyze the narrative arc, the relationship between the protagonist and antagonist, or the use of symbolism and metaphor. Structural analysis can be useful for understanding how a narrative is constructed and how it affects the reader or audience.
Discourse Analysis
This type of narrative analysis focuses on the language and discourse used in a narrative, including the social and cultural context in which it is situated. Researchers may analyze the use of specific words or phrases, the tone and style of the narrative, or the ways in which social and cultural norms are reflected in the narrative. Discourse analysis can be useful for understanding how narratives are influenced by larger social and cultural structures.
Phenomenological Analysis
This type of narrative analysis focuses on the subjective experience of the narrator, and how they interpret and make sense of their experiences. Researchers may analyze the language used to describe experiences, the emotions expressed in the narrative, or the ways in which the narrator constructs meaning from their experiences. Phenomenological analysis can be useful for understanding how people make sense of their own lives and experiences.
Critical Analysis
This type of narrative analysis involves examining the political, social, and ideological implications of a narrative, and questioning its underlying assumptions and values. Researchers may analyze the ways in which a narrative reflects or reinforces dominant power structures, or how it challenges or subverts those structures. Critical analysis can be useful for understanding the role that narratives play in shaping social and cultural norms.
Autoethnography
This type of narrative analysis involves using personal narratives to explore cultural experiences and identity formation. Researchers may use their own personal narratives to explore issues such as race, gender, or sexuality, and to understand how larger social and cultural structures shape individual experiences. Autoethnography can be useful for understanding how individuals negotiate and navigate complex cultural identities.
Thematic Analysis
This method involves identifying themes or patterns that emerge from the data, and then interpreting these themes in relation to the research question. Researchers may use a deductive approach, where they start with a pre-existing theoretical framework, or an inductive approach, where themes are generated from the data itself.
Narrative Analysis Conducting Guide
Here are some steps for conducting narrative analysis:
- Identify the research question: Narrative analysis begins with identifying the research question or topic of interest. Researchers may want to explore a particular social or cultural phenomenon, or gain a deeper understanding of a particular individual’s experience.
- Collect the narratives: Researchers then collect the narratives or stories that they will analyze. This can involve collecting written texts, conducting interviews, or analyzing visual media.
- Transcribe and code the narratives: Once the narratives have been collected, they are transcribed into a written format, and then coded in order to identify themes, motifs, or other patterns. Researchers may use a coding scheme that has been developed specifically for the study, or they may use an existing coding scheme.
- Analyze the narratives: Researchers then analyze the narratives, focusing on the themes, motifs, and other patterns that have emerged from the coding process. They may also analyze the formal structure of the narratives, the language used, and the social and cultural context in which they are situated.
- Interpret the findings: Finally, researchers interpret the findings of the narrative analysis, and draw conclusions about the meanings, experiences, and perspectives that underlie the narratives. They may use the findings to develop theories, make recommendations, or inform further research.
Applications of Narrative Analysis
Narrative analysis is a versatile qualitative research method that has applications across a wide range of fields, including psychology, sociology, anthropology, literature, and history. Here are some examples of how narrative analysis can be used:
- Understanding individuals’ experiences: Narrative analysis can be used to gain a deeper understanding of individuals’ experiences, including their thoughts, feelings, and perspectives. For example, psychologists might use narrative analysis to explore the stories that individuals tell about their experiences with mental illness.
- Exploring cultural and social phenomena: Narrative analysis can also be used to explore cultural and social phenomena, such as gender, race, and identity. Sociologists might use narrative analysis to examine how individuals understand and experience their gender identity.
- Analyzing historical events: Narrative analysis can be used to analyze historical events, including those that have been recorded in literary texts or personal accounts. Historians might use narrative analysis to explore the stories of survivors of historical traumas, such as war or genocide.
- Examining media representations: Narrative analysis can be used to examine media representations of social and cultural phenomena, such as news stories, films, or television shows. Communication scholars might use narrative analysis to examine how news media represent different social groups.
- Developing interventions: Narrative analysis can be used to develop interventions to address social and cultural problems. For example, social workers might use narrative analysis to understand the experiences of individuals who have experienced domestic violence, and then use that knowledge to develop more effective interventions.
Examples of Narrative Analysis
Here are some examples of how narrative analysis has been used in research:
- Personal narratives of illness: Researchers have used narrative analysis to examine the personal narratives of individuals living with chronic illness, to understand how they make sense of their experiences and construct their identities.
- Oral histories: Historians have used narrative analysis to analyze oral histories to gain insights into individuals’ experiences of historical events and social movements.
- Children’s stories: Researchers have used narrative analysis to analyze children’s stories to understand how they understand and make sense of the world around them.
- Personal diaries : Researchers have used narrative analysis to examine personal diaries to gain insights into individuals’ experiences of significant life events, such as the loss of a loved one or the transition to adulthood.
- Memoirs : Researchers have used narrative analysis to analyze memoirs to understand how individuals construct their life stories and make sense of their experiences.
- Life histories : Researchers have used narrative analysis to examine life histories to gain insights into individuals’ experiences of migration, displacement, or social exclusion.
Purpose of Narrative Analysis
The purpose of narrative analysis is to gain a deeper understanding of the stories that individuals tell about their experiences, identities, and beliefs. By analyzing the structure, content, and context of these stories, researchers can uncover patterns and themes that shed light on the ways in which individuals make sense of their lives and the world around them.
The primary purpose of narrative analysis is to explore the meanings that individuals attach to their experiences. This involves examining the different elements of a story, such as the plot, characters, setting, and themes, to identify the underlying values, beliefs, and attitudes that shape the story. By analyzing these elements, researchers can gain insights into the ways in which individuals construct their identities, understand their relationships with others, and make sense of the world.
Narrative analysis can also be used to identify patterns and themes across multiple stories. This involves comparing and contrasting the stories of different individuals or groups to identify commonalities and differences. By analyzing these patterns and themes, researchers can gain insights into broader cultural and social phenomena, such as gender, race, and identity.
In addition, narrative analysis can be used to develop interventions that address social and cultural problems. By understanding the stories that individuals tell about their experiences, researchers can develop interventions that are tailored to the unique needs of different individuals and groups.
Overall, the purpose of narrative analysis is to provide a rich, nuanced understanding of the ways in which individuals construct meaning and make sense of their lives. By analyzing the stories that individuals tell, researchers can gain insights into the complex and multifaceted nature of human experience.
When to use Narrative Analysis
Here are some situations where narrative analysis may be appropriate:
- Studying life stories: Narrative analysis can be useful in understanding how individuals construct their life stories, including the events, characters, and themes that are important to them.
- Analyzing cultural narratives: Narrative analysis can be used to analyze cultural narratives, such as myths, legends, and folktales, to understand their meanings and functions.
- Exploring organizational narratives: Narrative analysis can be helpful in examining the stories that organizations tell about themselves, their histories, and their values, to understand how they shape the culture and practices of the organization.
- Investigating media narratives: Narrative analysis can be used to analyze media narratives, such as news stories, films, and TV shows, to understand how they construct meaning and influence public perceptions.
- Examining policy narratives: Narrative analysis can be helpful in examining policy narratives, such as political speeches and policy documents, to understand how they construct ideas and justify policy decisions.
Characteristics of Narrative Analysis
Here are some key characteristics of narrative analysis:
- Focus on stories and narratives: Narrative analysis is concerned with analyzing the stories and narratives that people tell, whether they are oral or written, to understand how they shape and reflect individuals’ experiences and identities.
- Emphasis on context: Narrative analysis seeks to understand the context in which the narratives are produced and the social and cultural factors that shape them.
- Interpretive approach: Narrative analysis is an interpretive approach that seeks to identify patterns and themes in the stories and narratives and to understand the meaning that individuals and communities attach to them.
- Iterative process: Narrative analysis involves an iterative process of analysis, in which the researcher continually refines their understanding of the narratives as they examine more data.
- Attention to language and form : Narrative analysis pays close attention to the language and form of the narratives, including the use of metaphor, imagery, and narrative structure, to understand the meaning that individuals and communities attach to them.
- Reflexivity : Narrative analysis requires the researcher to reflect on their own assumptions and biases and to consider how their own positionality may shape their interpretation of the narratives.
- Qualitative approach: Narrative analysis is typically a qualitative research method that involves in-depth analysis of a small number of cases rather than large-scale quantitative studies.
Advantages of Narrative Analysis
Here are some advantages of narrative analysis:
- Rich and detailed data : Narrative analysis provides rich and detailed data that allows for a deep understanding of individuals’ experiences, emotions, and identities.
- Humanizing approach: Narrative analysis allows individuals to tell their own stories and express their own perspectives, which can help to humanize research and give voice to marginalized communities.
- Holistic understanding: Narrative analysis allows researchers to understand individuals’ experiences in their entirety, including the social, cultural, and historical contexts in which they occur.
- Flexibility : Narrative analysis is a flexible research method that can be applied to a wide range of contexts and research questions.
- Interpretive insights: Narrative analysis provides interpretive insights into the meanings that individuals attach to their experiences and the ways in which they construct their identities.
- Appropriate for sensitive topics: Narrative analysis can be particularly useful in researching sensitive topics, such as trauma or mental health, as it allows individuals to express their experiences in their own words and on their own terms.
- Can lead to policy implications: Narrative analysis can provide insights that can inform policy decisions and interventions, particularly in areas such as health, education, and social policy.
Limitations of Narrative Analysis
Here are some of the limitations of narrative analysis:
- Subjectivity : Narrative analysis relies on the interpretation of researchers, which can be influenced by their own biases and assumptions.
- Limited generalizability: Narrative analysis typically involves in-depth analysis of a small number of cases, which limits its generalizability to broader populations.
- Ethical considerations: The process of eliciting and analyzing narratives can raise ethical concerns, particularly when sensitive topics such as trauma or abuse are involved.
- Limited control over data collection: Narrative analysis often relies on data that is already available, such as interviews, oral histories, or written texts, which can limit the control that researchers have over the quality and completeness of the data.
- Time-consuming: Narrative analysis can be a time-consuming research method, particularly when analyzing large amounts of data.
- Interpretation challenges: Narrative analysis requires researchers to make complex interpretations of data, which can be challenging and time-consuming.
- Limited statistical analysis: Narrative analysis is typically a qualitative research method that does not lend itself well to statistical analysis.
About the author
Muhammad Hassan
Researcher, Academic Writer, Web developer
You may also like

Cluster Analysis – Types, Methods and Examples

Discriminant Analysis – Methods, Types and...

MANOVA (Multivariate Analysis of Variance) –...

Documentary Analysis – Methods, Applications and...

ANOVA (Analysis of variance) – Formulas, Types...

Graphical Methods – Types, Examples and Guide
Using narrative analysis in qualitative research
Last updated
7 March 2023
Reviewed by
Jean Kaluza
After spending considerable time and effort interviewing persons for research, you want to ensure you get the most out of the data you gathered. One method that gives you an excellent opportunity to connect with your data on a very human and personal level is a narrative analysis in qualitative research.
Master narrative analysis
Analyze your qualitative data faster and surface more actionable insights
- What is narrative analysis?
Narrative analysis is a type of qualitative data analysis that focuses on interpreting the core narratives from a study group's personal stories. Using first-person narrative, data is acquired and organized to allow the researcher to understand how the individuals experienced something.
Instead of focusing on just the actual words used during an interview, the narrative analysis also allows for a compilation of data on how the person expressed themselves, what language they used when describing a particular event or feeling, and the thoughts and motivations they experienced. A narrative analysis will also consider how the research participants constructed their narratives.
From the interview to coding , you should strive to keep the entire individual narrative together, so that the information shared during the interview remains intact.
Is narrative analysis qualitative or quantitative?
Narrative analysis is a qualitative research method.
Is narrative analysis a method or methodology?
A method describes the tools or processes used to understand your data; methodology describes the overall framework used to support the methods chosen. By this definition, narrative analysis can be both a method used to understand data and a methodology appropriate for approaching data that comes primarily from first-person stories.
- Do you need to perform narrative research to conduct a narrative analysis?
A narrative analysis will give the best answers about the data if you begin with conducting narrative research. Narrative research explores an entire story with a research participant to understand their personal story.
What are the characteristics of narrative research?
Narrative research always includes data from individuals that tell the story of their experiences. This is captured using loosely structured interviews . These can be a single interview or a series of long interviews over a period of time. Narrative research focuses on the construct and expressions of the story as experienced by the research participant.
- Examples of types of narratives
Narrative data is based on narratives. Your data may include the entire life story or a complete personal narrative, giving a comprehensive account of someone's life, depending on the researched subject. Alternatively, a topical story can provide context around one specific moment in the research participant's life.
Personal narratives can be single or multiple sessions, encompassing more than topical stories but not entire life stories of the individuals.
- What is the objective of narrative analysis?
The narrative analysis seeks to organize the overall experience of a group of research participants' stories. The goal is to turn people's individual narratives into data that can be coded and organized so that researchers can easily understand the impact of a certain event, feeling, or decision on the involved persons. At the end of a narrative analysis, researchers can identify certain core narratives that capture the human experience.
What is the difference between content analysis and narrative analysis?
Content analysis is a research method that determines how often certain words, concepts, or themes appear inside a sampling of qualitative data . The narrative analysis focuses on the overall story and organizing the constructs and features of a narrative.
What is the difference between narrative analysis and case study in qualitative research?
A case study focuses on one particular event. A narrative analysis draws from a larger amount of data surrounding the entire narrative, including the thoughts that led up to a decision and the personal conclusion of the research participant.
A case study, therefore, is any specific topic studied in depth, whereas narrative analysis explores single or multi-faceted experiences across time.
What is the difference between narrative analysis and thematic analysis?
A thematic analysis will appear as researchers review the available qualitative data and note any recurring themes. Unlike narrative analysis, which describes an entire method of evaluating data to find a conclusion, a thematic analysis only describes reviewing and categorizing the data.
- Capturing narrative data
Because narrative data relies heavily on allowing a research participant to describe their experience, it is best to allow for a less structured interview. Allowing the participant to explore tangents or analyze their personal narrative will result in more complete data.
When collecting narrative data, always allow the participant the time and space needed to complete their narrative.
- Methods of transcribing narrative data
A narrative analysis requires that the researchers have access to the entire verbatim narrative of the participant, including not just the word they use but the pauses, the verbal tics, and verbal crutches, such as "um" and "hmm."
As the entire way the story is expressed is part of the data, a verbatim transcription should be created before attempting to code the narrative analysis.
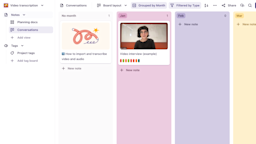
Video and audio transcription templates
- How to code narrative analysis
Coding narrative analysis has two natural start points, either using a deductive coding system or an inductive coding system. Regardless of your chosen method, it's crucial not to lose valuable data during the organization process.
When coding, expect to see more information in the code snippets.
- Types of narrative analysis
After coding is complete, you should expect your data to look like large blocks of text organized by the parts of the story. You will also see where individual narratives compare and diverge.
Inductive method
Using an inductive narrative method treats the entire narrative as one datum or one set of information. An inductive narrative method will encourage the research participant to organize their own story.
To make sense of how a story begins and ends, you must rely on cues from the participant. These may take the form of entrance and exit talks.
Participants may not always provide clear indicators of where their narratives start and end. However, you can anticipate that their stories will contain elements of a beginning, middle, and end. By analyzing these components through coding, you can identify emerging patterns in the data.
Taking cues from entrance and exit talk
Entrance talk is when the participant begins a particular set of narratives. You may hear expressions such as, "I remember when…," "It first occurred to me when…," or "Here's an example…."
Exit talk allows you to see when the story is wrapping up, and you might expect to hear a phrase like, "…and that's how we decided", "after that, we moved on," or "that's pretty much it."
Deductive method
Regardless of your chosen method, using a deductive method can help preserve the overall storyline while coding. Starting with a deductive method allows for the separation of narrative pieces without compromising the story's integrity.
Hybrid inductive and deductive narrative analysis
Using both methods together gives you a comprehensive understanding of the data. You can start by coding the entire story using the inductive method. Then, you can better analyze and interpret the data by applying deductive codes to individual parts of the story.
- How to analyze data after coding using narrative analysis
A narrative analysis aims to take all relevant interviews and organize them down to a few core narratives. After reviewing the coding, these core narratives may appear through a repeated moment of decision occurring before the climax or a key feeling that affected the participant's outcome.
You may see these core narratives diverge early on, or you may learn that a particular moment after introspection reveals the core narrative for each participant. Either way, researchers can now quickly express and understand the data you acquired.
- A step-by-step approach to narrative analysis and finding core narratives
Narrative analysis may look slightly different to each research group, but we will walk through the process using the Delve method for this article.
Step 1 – Code narrative blocks
Organize your narrative blocks using inductive coding to organize stories by a life event.
Example: Narrative interviews are conducted with homeowners asking them to describe how they bought their first home.
Step 2 – Group and read by live-event
You begin your data analysis by reading through each of the narratives coded with the same life event.
Example: You read through each homeowner's experience of buying their first home and notice that some common themes begin to appear, such as "we were tired of renting," "our family expanded to the point that we needed a larger space," and "we had finally saved enough for a downpayment."
Step 3 – Create a nested story structure
As these common narratives develop throughout the participant's interviews, create and nest code according to your narrative analysis framework. Use your coding to break down the narrative into pieces that can be analyzed together.
Example: During your interviews, you find that the beginning of the narrative usually includes the pressures faced before buying a home that pushes the research participants to consider homeownership. The middle of the narrative often includes challenges that come up during the decision-making process. The end of the narrative usually includes perspectives about the excitement, stress, or consequences of home ownership that has finally taken place.
Step 4 – Delve into the story structure
Once the narratives are organized into their pieces, you begin to notice how participants structure their own stories and where similarities and differences emerge.
Example: You find in your research that many people who choose to buy homes had the desire to buy a home before their circumstances allowed them to. You notice that almost all the stories begin with the feeling of some sort of outside pressure.
Step 5 – Compare across story structure
While breaking down narratives into smaller pieces is necessary for analysis, it's important not to lose sight of the overall story. To keep the big picture in mind, take breaks to step back and reread the entire narrative of a code block. This will help you remember how participants expressed themselves and ensure that the core narrative remains the focus of the analysis.
Example: By carefully examining the similarities across the beginnings of participants' narratives, you find the similarities in pressures. Considering the overall narrative, you notice how these pressures lead to similar decisions despite the challenges faced.
Divergence in feelings towards homeownership can be linked to positive or negative pressures. Individuals who received positive pressure, such as family support or excitement, may view homeownership more favorably. Meanwhile, negative pressures like high rent or peer pressure may cause individuals to have a more negative attitude toward homeownership.
These factors can contribute to the initial divergence in feelings towards homeownership.
Step 6 – Tell the core narrative
After carefully analyzing the data, you have found how the narratives relate and diverge. You may be able to create a theory about why the narratives diverge and can create one or two core narratives that explain the way the story was experienced.
Example: You can now construct a core narrative on how a person's initial feelings toward buying a house affect their feelings after purchasing and living in their first home.
Narrative analysis in qualitative research is an invaluable tool to understand how people's stories and ability to self-narrate reflect the human experience. Qualitative data analysis can be improved through coding and organizing complete narratives. By doing so, researchers can conclude how humans process and move through decisions and life events.
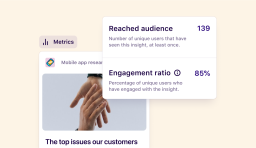
Learn more about qualitative transcription software
Get started today.
Go from raw data to valuable insights with a flexible research platform
Editor’s picks
Last updated: 21 December 2023
Last updated: 16 December 2023
Last updated: 6 October 2023
Last updated: 5 March 2024
Last updated: 25 November 2023
Last updated: 15 February 2024
Last updated: 11 March 2024
Last updated: 12 December 2023
Last updated: 6 March 2024
Last updated: 10 April 2023
Last updated: 20 December 2023
Latest articles
Related topics, log in or sign up.
Get started for free

Narrative Analysis 101
Everything you need to know to get started
By: Ethar Al-Saraf (PhD)| Expert Reviewed By: Eunice Rautenbach (DTech) | March 2023
If you’re new to research, the host of qualitative analysis methods available to you can be a little overwhelming. In this post, we’ll unpack the sometimes slippery topic of narrative analysis . We’ll explain what it is, consider its strengths and weaknesses , and look at when and when not to use this analysis method.
Overview: Narrative Analysis
- What is narrative analysis (simple definition)
- The two overarching approaches
- The strengths & weaknesses of narrative analysis
- When (and when not) to use it
- Key takeaways
What Is Narrative Analysis?
Simply put, narrative analysis is a qualitative analysis method focused on interpreting human experiences and motivations by looking closely at the stories (the narratives) people tell in a particular context.
In other words, a narrative analysis interprets long-form participant responses or written stories as data, to uncover themes and meanings . That data could be taken from interviews, monologues, written stories, or even recordings. In other words, narrative analysis can be used on both primary and secondary data to provide evidence from the experiences described.
That’s all quite conceptual, so let’s look at an example of how narrative analysis could be used.
Let’s say you’re interested in researching the beliefs of a particular author on popular culture. In that case, you might identify the characters , plotlines , symbols and motifs used in their stories. You could then use narrative analysis to analyse these in combination and against the backdrop of the relevant context.
This would allow you to interpret the underlying meanings and implications in their writing, and what they reveal about the beliefs of the author. In other words, you’d look to understand the views of the author by analysing the narratives that run through their work.
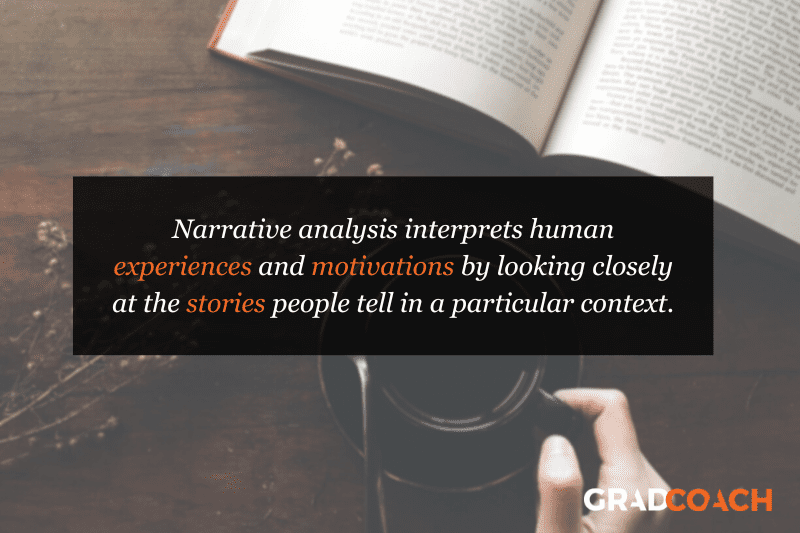
The Two Overarching Approaches
Generally speaking, there are two approaches that one can take to narrative analysis. Specifically, an inductive approach or a deductive approach. Each one will have a meaningful impact on how you interpret your data and the conclusions you can draw, so it’s important that you understand the difference.
First up is the inductive approach to narrative analysis.
The inductive approach takes a bottom-up view , allowing the data to speak for itself, without the influence of any preconceived notions . With this approach, you begin by looking at the data and deriving patterns and themes that can be used to explain the story, as opposed to viewing the data through the lens of pre-existing hypotheses, theories or frameworks. In other words, the analysis is led by the data.
For example, with an inductive approach, you might notice patterns or themes in the way an author presents their characters or develops their plot. You’d then observe these patterns, develop an interpretation of what they might reveal in the context of the story, and draw conclusions relative to the aims of your research.
Contrasted to this is the deductive approach.
With the deductive approach to narrative analysis, you begin by using existing theories that a narrative can be tested against . Here, the analysis adopts particular theoretical assumptions and/or provides hypotheses, and then looks for evidence in a story that will either verify or disprove them.
For example, your analysis might begin with a theory that wealthy authors only tell stories to get the sympathy of their readers. A deductive analysis might then look at the narratives of wealthy authors for evidence that will substantiate (or refute) the theory and then draw conclusions about its accuracy, and suggest explanations for why that might or might not be the case.
Which approach you should take depends on your research aims, objectives and research questions . If these are more exploratory in nature, you’ll likely take an inductive approach. Conversely, if they are more confirmatory in nature, you’ll likely opt for the deductive approach.
Need a helping hand?
Strengths & Weaknesses
Now that we have a clearer view of what narrative analysis is and the two approaches to it, it’s important to understand its strengths and weaknesses , so that you can make the right choices in your research project.
A primary strength of narrative analysis is the rich insight it can generate by uncovering the underlying meanings and interpretations of human experience. The focus on an individual narrative highlights the nuances and complexities of their experience, revealing details that might be missed or considered insignificant by other methods.
Another strength of narrative analysis is the range of topics it can be used for. The focus on human experience means that a narrative analysis can democratise your data analysis, by revealing the value of individuals’ own interpretation of their experience in contrast to broader social, cultural, and political factors.
All that said, just like all analysis methods, narrative analysis has its weaknesses. It’s important to understand these so that you can choose the most appropriate method for your particular research project.
The first drawback of narrative analysis is the problem of subjectivity and interpretation . In other words, a drawback of the focus on stories and their details is that they’re open to being understood differently depending on who’s reading them. This means that a strong understanding of the author’s cultural context is crucial to developing your interpretation of the data. At the same time, it’s important that you remain open-minded in how you interpret your chosen narrative and avoid making any assumptions .
A second weakness of narrative analysis is the issue of reliability and generalisation . Since narrative analysis depends almost entirely on a subjective narrative and your interpretation, the findings and conclusions can’t usually be generalised or empirically verified. Although some conclusions can be drawn about the cultural context, they’re still based on what will almost always be anecdotal data and not suitable for the basis of a theory, for example.
Last but not least, the focus on long-form data expressed as stories means that narrative analysis can be very time-consuming . In addition to the source data itself, you will have to be well informed on the author’s cultural context as well as other interpretations of the narrative, where possible, to ensure you have a holistic view. So, if you’re going to undertake narrative analysis, make sure that you allocate a generous amount of time to work through the data.
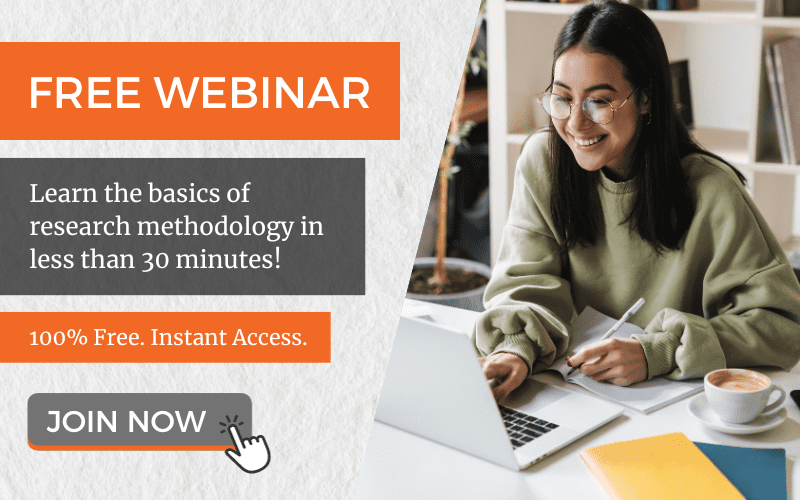
When To Use Narrative Analysis
As a qualitative method focused on analysing and interpreting narratives describing human experiences, narrative analysis is usually most appropriate for research topics focused on social, personal, cultural , or even ideological events or phenomena and how they’re understood at an individual level.
For example, if you were interested in understanding the experiences and beliefs of individuals suffering social marginalisation, you could use narrative analysis to look at the narratives and stories told by people in marginalised groups to identify patterns , symbols , or motifs that shed light on how they rationalise their experiences.
In this example, narrative analysis presents a good natural fit as it’s focused on analysing people’s stories to understand their views and beliefs at an individual level. Conversely, if your research was geared towards understanding broader themes and patterns regarding an event or phenomena, analysis methods such as content analysis or thematic analysis may be better suited, depending on your research aim .
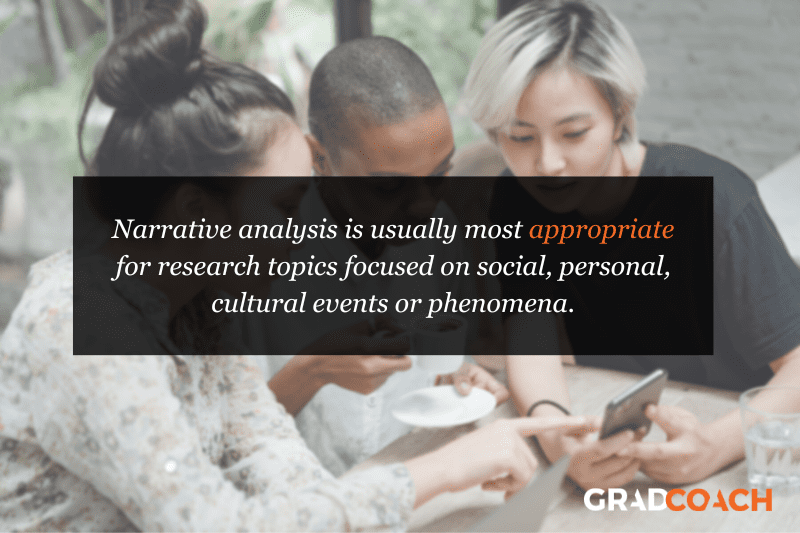
Let’s recap
In this post, we’ve explored the basics of narrative analysis in qualitative research. The key takeaways are:
- Narrative analysis is a qualitative analysis method focused on interpreting human experience in the form of stories or narratives .
- There are two overarching approaches to narrative analysis: the inductive (exploratory) approach and the deductive (confirmatory) approach.
- Like all analysis methods, narrative analysis has a particular set of strengths and weaknesses .
- Narrative analysis is generally most appropriate for research focused on interpreting individual, human experiences as expressed in detailed , long-form accounts.
If you’d like to learn more about narrative analysis and qualitative analysis methods in general, be sure to check out the rest of the Grad Coach blog here . Alternatively, if you’re looking for hands-on help with your project, take a look at our 1-on-1 private coaching service .
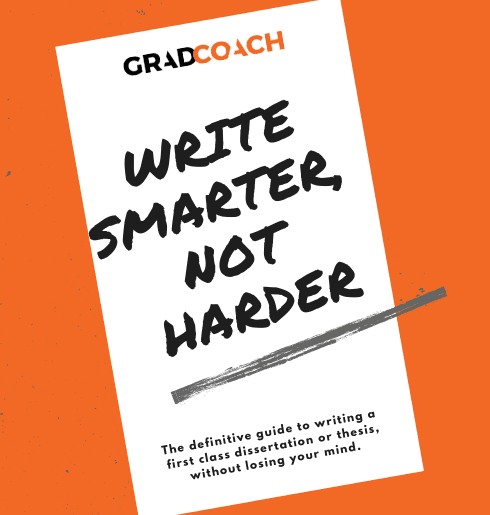
Psst… there’s more (for free)
This post is part of our dissertation mini-course, which covers everything you need to get started with your dissertation, thesis or research project.
You Might Also Like:
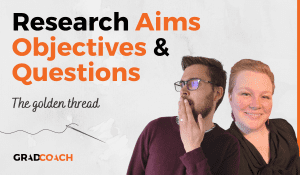
Thanks. I need examples of narrative analysis
Here are some examples of research topics that could utilise narrative analysis:
Personal Narratives of Trauma: Analysing personal stories of individuals who have experienced trauma to understand the impact, coping mechanisms, and healing processes.
Identity Formation in Immigrant Communities: Examining the narratives of immigrants to explore how they construct and negotiate their identities in a new cultural context.
Media Representations of Gender: Analysing narratives in media texts (such as films, television shows, or advertisements) to investigate the portrayal of gender roles, stereotypes, and power dynamics.
Where can I find an example of a narrative analysis table ?
Please i need help with my project,
how can I cite this article in APA 7th style?
please mention the sources as well.
My research is mixed approach. I use interview,key_inforamt interview,FGD and document.so,which qualitative analysis is appropriate to analyze these data.Thanks
Which qualitative analysis methode is appropriate to analyze data obtain from intetview,key informant intetview,Focus group discussion and document.
I’ve finished my PhD. Now I need a “platform” that will help me objectively ascertain the tacit assumptions that are buried within a narrative. Can you help?
Submit a Comment Cancel reply
Your email address will not be published. Required fields are marked *
Save my name, email, and website in this browser for the next time I comment.
- Print Friendly

Narrative Analysis: Methods and Examples
Narrative analysis is a powerful qualitative research tool. Narrative research can uncover behaviors, feelings and motivations that aren’t expressed explicitly….

Narrative analysis is a powerful qualitative research tool. Narrative research can uncover behaviors, feelings and motivations that aren’t expressed explicitly. It also provides rich linguistic data that may shed light on various aspects of cultural or social phenomena.
Narrative analysis provides researchers with detailed information about their subjects that they couldn’t get through other methods. Narrative analysis in qualitative research reveals hidden motivations that aren’t easy to perceive directly. This is especially true in research conducted with cultural subjects where the researcher must peel the many layers of a culture.
Let’s look at how narrative research is performed, what it can tell us about the subject, and some examples of narrative research.
What Is Narrative Research?
Examples of narrative research, difference between narrative analysis and case study, analyzing results in the narrative method.
Narrative analysis is a form of qualitative research in which the researcher focuses on a topic and analyzes the data collected from case studies, surveys, observations or other similar methods. The researchers write their findings, then review and analyze them.
To conduct narrative analysis, researchers must understand the background, setting, social and cultural context of the research subjects. This gives researchers a better idea of what their subjects mean in their narration. It’s especially true in context-rich research where there are many hidden layers of meaning that can only be uncovered by an in-depth understanding of the culture or environment.
Before starting narrative research, researchers need to know as much about their research subjects as possible. They interview key informants and collect large amounts of text from them. They even use other sources, such as existing literature and personal recollections.
From this large base of information, researchers choose a few instances they feel are good examples of what they want to talk about and then analyze them in depth.
Through this approach, researchers can gain a holistic view of the subject’s life and activities. It can show what motivates people and provide a better view of the society that the subjects live in by enabling researchers to see how individuals interact with one another.
- It’s been used by researchers to study indigenous peoples of various countries, such as the Maori in New Zealand.
- It can be used in medicine. Researchers, for instance, can study how doctors communicate with their patients during end-of-life care.
- The narrative model has been used to explore the relationship between music and social change in East Africa.
- Narrative research is being used to explore the differences in emotions experienced by different generations in Japanese society.
Through these examples of narrative research, we can see its nature and how it fills a gap left by other research methods.
Many people confuse narrative analysis in qualitative research with case studies. Here are some key differences between the two:
- A case study examines one context in depth, whereas narrative research explores how a subject has acted in various contexts across time
- Case studies are often longer and more detailed, but they rarely provide an overview of the subject’s life or experiences
- Narrative analysis implies that researchers are observing several instances that encompass the subject’s life, which is why it provides a richer view of things
Both tools can give similar results, but there are some differences that lead researchers to choose one or the other or, perhaps, even both in their research design.
Once the narratives have been collected, researchers notice certain patterns and themes emerging as they read and analyze the text. They note these down, compare them with other research on the subject, figure out how it all fits together and then find a theory that can explain these findings.
Many social scientists have used narrative research as a valuable tool to analyze their concepts and theories. This is mainly because narrative analysis is a more thorough and multifaceted method. It helps researchers not only build a deeper understanding of their subject, but also helps them figure out why people act and react as they do.
Storytelling is a central feature of narrative research. The narrative interview is an interactive conversation. This process can be very intimate and sometimes bring about powerful emotions from both parties. Therefore, this form of qualitative research isn’t suitable for everyone. The interviewer needs to be a good listener and must understand the interview process. The interviewee also needs to be comfortable to be able to provide authentic narratives.
Understanding what kind of research to use is a powerful tool for a manager. We can use narrative analysis in many ways. Narrative research is a multifaceted method that has the potential to show different results based on the researcher’s intentions for their study.
Learning how to use such tools will improve the productivity of teams. Harappa’s Thinking Critically course will show you the way. Learners will understand how to better process information and consider different perspectives in their analysis, which will allow for better-informed decision making. Our faculty will provide real-world insights to ensure an impactful learning experience that takes professionals at every stage of their careers to the next level.
Explore Harappa Diaries to learn more about topics such as Phenomenological Research , Types Of Survey Research , Examples Of Correlational Research and Tips to Improve your Analytical Skills to upgrade your knowledge and skills.
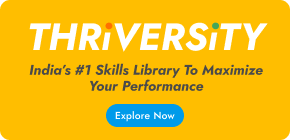
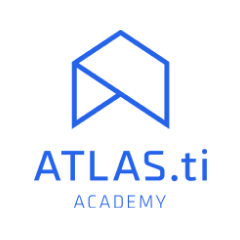
The Ultimate Guide to Qualitative Research - Part 2: Handling Qualitative Data
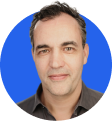
- Handling qualitative data
- Transcripts
- Field notes
- Survey data and responses
- Visual and audio data
- Data organization
- Data coding
- Coding frame
- Auto and smart coding
- Organizing codes
- Qualitative data analysis
- Content analysis
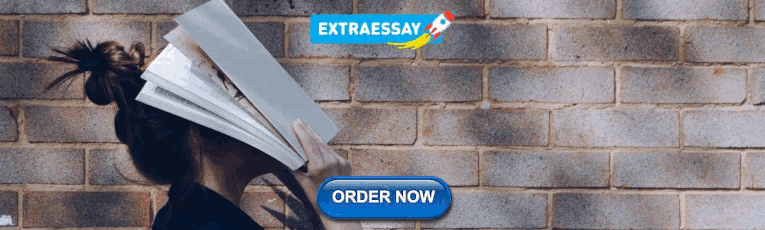
Thematic analysis
- Thematic analysis vs. content analysis
- Introduction
Types of narrative research
Research methods for a narrative analysis, narrative analysis, considerations for narrative analysis.
- Phenomenological research
- Discourse analysis
- Grounded theory
- Deductive reasoning
- Inductive reasoning
- Inductive vs. deductive reasoning
- Qualitative data interpretation
- Qualitative analysis software
Narrative analysis in research
Narrative analysis is an approach to qualitative research that involves the documentation of narratives both for the purpose of understanding events and phenomena and understanding how people communicate stories.
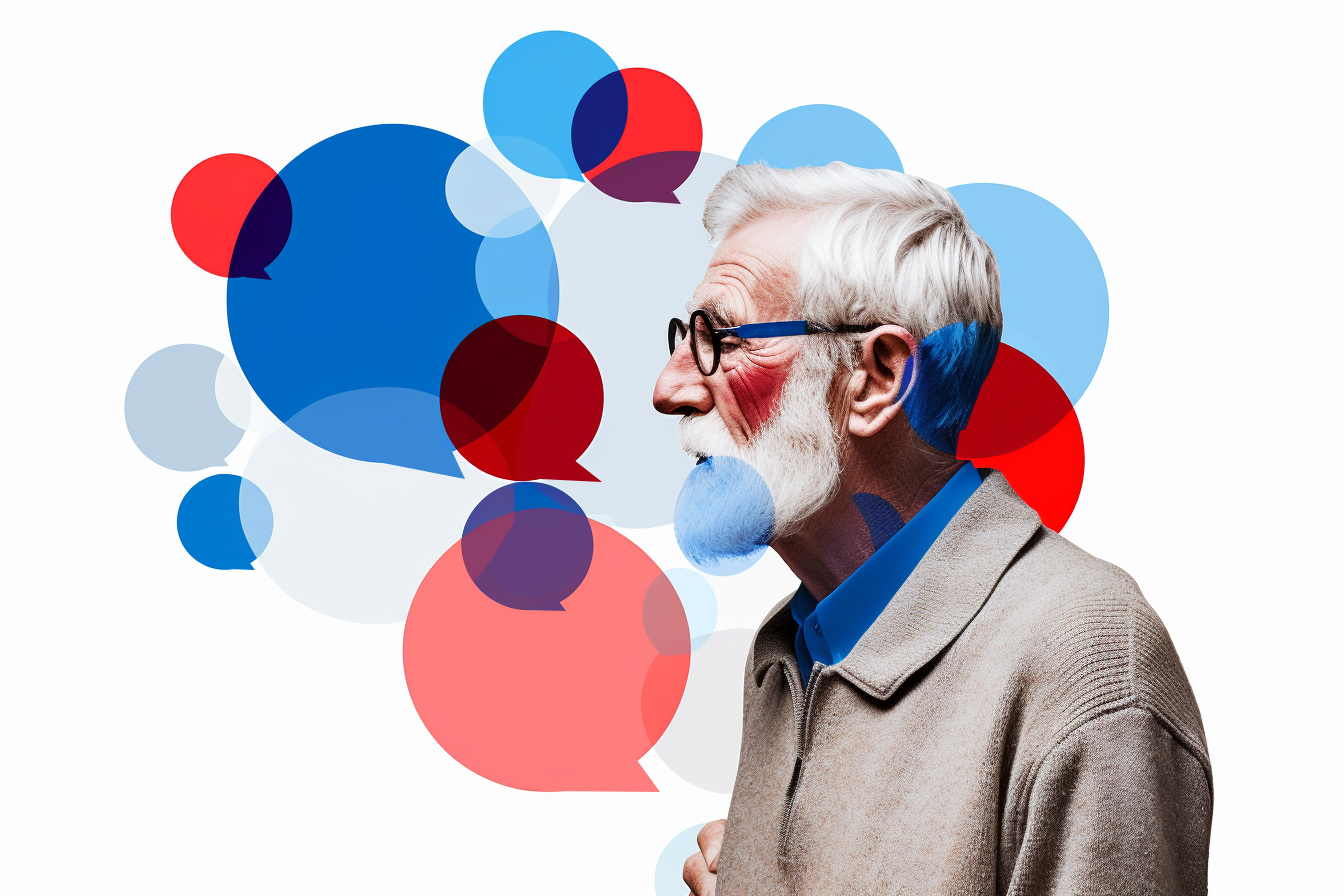
Let's look at the basics of narrative research, then examine the process of conducting a narrative inquiry and how ATLAS.ti can help you conduct a narrative analysis.
Qualitative researchers can employ various forms of narrative research, but all of these distinct approaches utilize perspectival data as the means for contributing to theory.
A biography is the most straightforward form of narrative research. Data collection for a biography generally involves summarizing the main points of an individual's life or at least the part of their history involved with events that a researcher wants to examine. Generally speaking, a biography aims to provide a more complete record of an individual person's life in a manner that might dispel any inaccuracies that exist in popular thought or provide a new perspective on that person’s history. Narrative researchers may also construct a new biography of someone who doesn’t have a public or online presence to delve deeper into that person’s history relating to the research topic.
The purpose of biographies as a function of narrative inquiry is to shed light on the lived experience of a particular person that a more casual examination of someone's life might overlook. Newspaper articles and online posts might give someone an overview of information about any individual. At the same time, a more involved survey or interview can provide sufficiently comprehensive knowledge about a person useful for narrative analysis and theoretical development.
Life history
This is probably the most involved form of narrative research as it requires capturing as much of the total human experience of an individual person as possible. While it involves elements of biographical research, constructing a life history also means collecting first-person knowledge from the subject through narrative interviews and observations while drawing on other forms of data , such as field notes and in-depth interviews with others.
Even a newspaper article or blog post about the person can contribute to the contextual meaning informing the life history. The objective of conducting a life history is to construct a complete picture of the person from past to present in a manner that gives your research audience the means to immerse themselves in the human experience of the person you are studying.
Oral history
While all forms of narrative research rely on narrative interviews with research participants, oral histories begin with and branch out from the individual's point of view as the driving force of data collection .
Major events like wars and natural disasters are often observed and described at scale, but a bird's eye view of such events may not provide a complete story. Oral history can assist researchers in providing a unique and perhaps unexplored perspective from in-depth interviews with a narrator's own words of what happened, how they experienced it, and what reasons they give for their actions. Researchers who collect this sort of information can then help fill in the gaps common knowledge may not have grasped.
The objective of an oral history is to provide a perspective built on personal experience. The unique viewpoint that personal narratives can provide has the potential to raise analytical insights that research methods at scale may overlook. Narrative analysis of oral histories can hence illuminate potential inquiries that can be addressed in future studies.
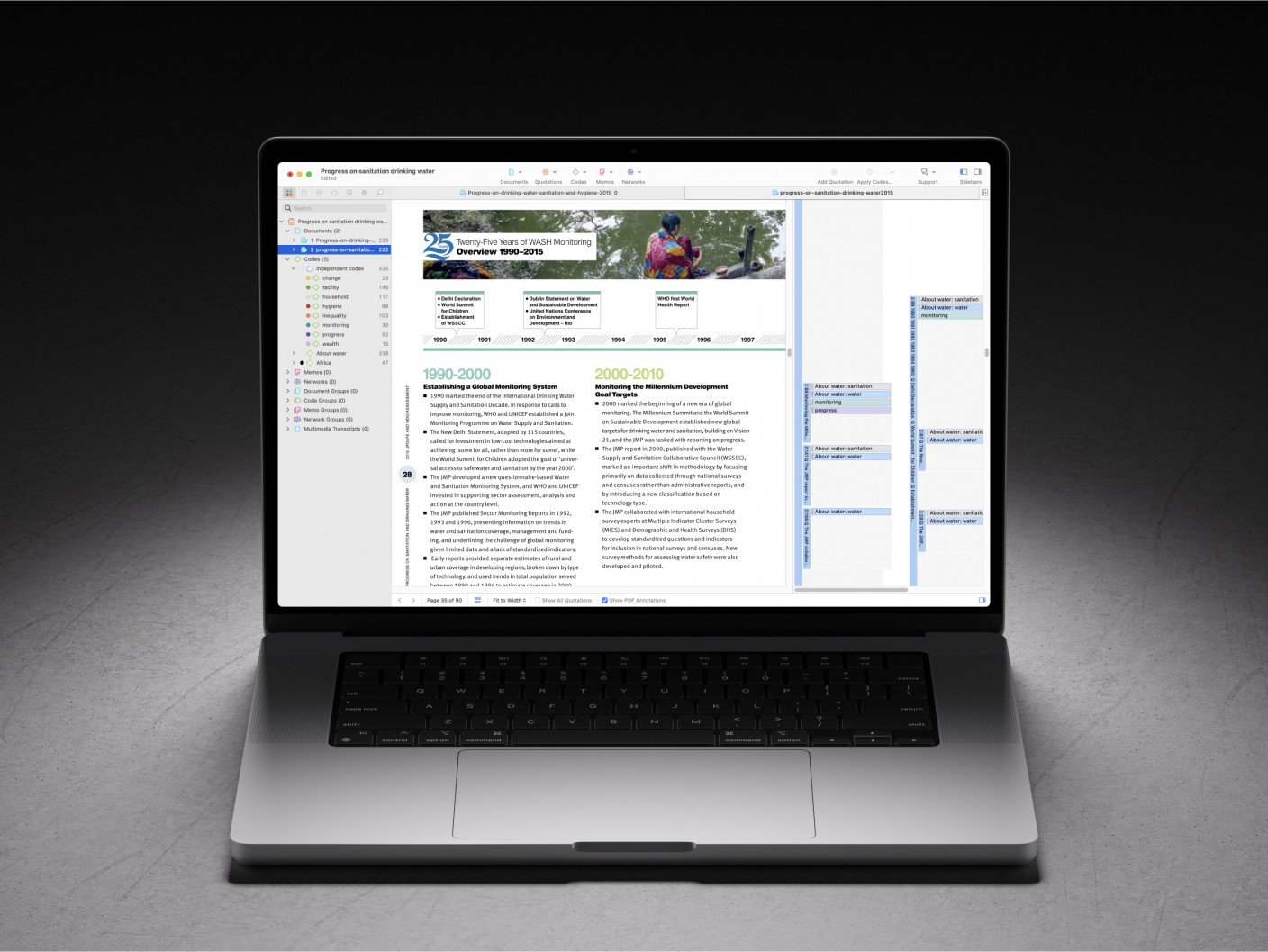
Whatever your research, get it done with ATLAS.ti.
From case study research to interviews, turn to ATLAS.ti for your qualitative research. Click here for a free trial.
To conduct narrative analysis, researchers need a narrative and research question . A narrative alone might make for an interesting story that instills information, but analyzing a narrative to generate knowledge requires ordering that information to identify patterns, intentions, and effects.
Narrative analysis presents a distinctive research approach among various methodologies , and it can pose significant challenges due to its inherent interpretative nature. Essentially, this method revolves around capturing and examining the verbal or written accounts and visual depictions shared by individuals. Narrative inquiry strives to unravel the essence of what is conveyed by closely observing the content and manner of expression.
Furthermore, narrative research assumes a dual role, serving both as a research technique and a subject of investigation. Regarded as "real-world measures," narrative methods provide valuable tools for exploring actual societal issues. The narrative approach encompasses an individual's life story and the profound significance embedded within their lived experiences. Typically, a composite of narratives is synthesized, intermingling and mutually influencing each other.
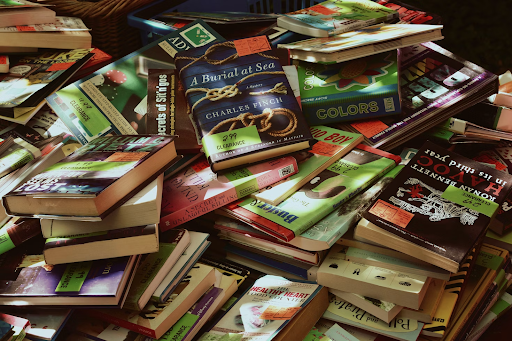
Designing a research inquiry
Sometimes, narrative research is less about the storyteller or the story they are telling than it is about generating knowledge that contributes to a greater understanding of social behavior and cultural practices. While it might be interesting or useful to hear a comedian tell a story that makes their audience laugh, a narrative analysis of that story can identify how the comedian constructs their narrative or what causes the audience to laugh.
As with all research, a narrative inquiry starts with a research question that is tied to existing relevant theory regarding the object of analysis (i.e., the person or event for which the narrative is constructed). If your research question involves studying racial inequalities in university contexts, for example, then the narrative analysis you are seeking might revolve around the lived experiences of students of color. If you are analyzing narratives from children's stories, then your research question might relate to identifying aspects of children's stories that grab the attention of young readers. The point is that researchers conducting a narrative inquiry do not do so merely to collect more information about their object of inquiry. Ultimately, narrative research is tied to developing a more contextualized or broader understanding of the social world.
Data collection
Having crafted the research questions and chosen the appropriate form of narrative research for your study, you can start to collect your data for the eventual narrative analysis.
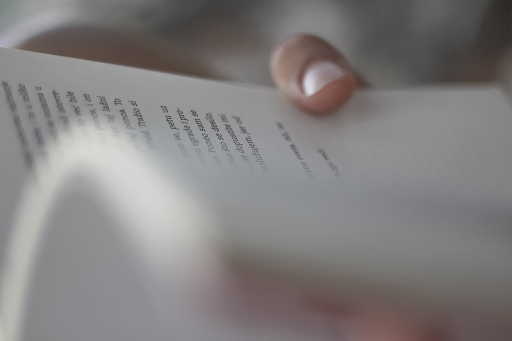
Needless to say, the key point in narrative research is the narrative. The story is either the unit of analysis or the focal point from which researchers pursue other methods of research. Interviews and observations are great ways to collect narratives. Particularly with biographies and life histories, one of the best ways to study your object of inquiry is to interview them. If you are conducting narrative research for discourse analysis, then observing or recording narratives (e.g., storytelling, audiobooks, podcasts) is ideal for later narrative analysis.
Triangulating data
If you are collecting a life history or an oral history, then you will need to rely on collecting evidence from different sources to support the analysis of the narrative. In research, triangulation is the concept of drawing on multiple methods or sources of data to get a more comprehensive picture of your object of inquiry.
While a narrative inquiry is constructed around the story or its storyteller, assertions that can be made from an analysis of the story can benefit from supporting evidence (or lack thereof) collected by other means.
Even a lack of supporting evidence might be telling. For example, suppose your object of inquiry tells a story about working minimum wage jobs all throughout college to pay for their tuition. Looking for triangulation, in this case, means searching through records and other forms of information to support the claims being put forth. If it turns out that the storyteller's claims bear further warranting - maybe you discover that family or scholarships supported them during college - your analysis might uncover new inquiries as to why the story was presented the way it was. Perhaps they are trying to impress their audience or construct a narrative identity about themselves that reinforces their thinking about who they are. The important point here is that triangulation is a necessary component of narrative research to learn more about the object of inquiry from different angles.
Conduct data analysis for your narrative research with ATLAS.ti.
Dedicated research software like ATLAS.ti helps the researcher catalog, penetrate, and analyze the data generated in any qualitative research project. Start with a free trial today.
This brings us to the analysis part of narrative research. As explained above, a narrative can be viewed as a straightforward story to understand and internalize. As researchers, however, we have many different approaches available to us for analyzing narrative data depending on our research inquiry.
In this section, we will examine some of the most common forms of analysis while looking at how you can employ tools in ATLAS.ti to analyze your qualitative data .
Qualitative research often employs thematic analysis , which refers to a search for commonly occurring themes that appear in the data. The important point of thematic analysis in narrative research is that the themes arise from the data produced by the research participants. In other words, the themes in a narrative study are strongly based on how the research participants see them rather than focusing on how researchers or existing theory see them.
ATLAS.ti can be used for thematic analysis in any research field or discipline. Data in narrative research is summarized through the coding process , where the researcher codes large segments of data with short, descriptive labels that can succinctly describe the data thematically. The emerging patterns among occurring codes in the perspectival data thus inform the identification of themes that arise from the collected narratives.
Structural analysis
The search for structure in a narrative is less about what is conveyed in the narrative and more about how the narrative is told. The differences in narrative forms ultimately tell us something useful about the meaning-making epistemologies and values of the people telling them and the cultures they inhabit.
Just like in thematic analysis, codes in ATLAS.ti can be used to summarize data, except that in this case, codes could be created to specifically examine structure by identifying the particular parts or moves in a narrative (e.g., introduction, conflict, resolution). Code-Document Analysis in ATLAS.ti can then tell you which of your narratives (represented by discrete documents) contain which parts of a common narrative.
It may also be useful to conduct a content analysis of narratives to analyze them structurally. English has many signal words and phrases (e.g., "for example," "as a result," and "suddenly") to alert listeners and readers that they are coming to a new step in the narrative.
In this case, both the Text Search and Word Frequencies tools in ATLAS.ti can help you identify the various aspects of the narrative structure (including automatically identifying discrete parts of speech) and the frequency in which they occur across different narratives.
Functional analysis
Whereas a straightforward structural analysis identifies the particular parts of a narrative, a functional analysis looks at what the narrator is trying to accomplish through the content and structure of their narrative. For example, if a research participant telling their narrative asks the interviewer rhetorical questions, they might be doing so to make the interviewer think or adopt the participant's perspective.
A functional analysis often requires the researcher to take notes and reflect on their experiences while collecting data from research participants. ATLAS.ti offers a dedicated space for memos , which can serve to jot down useful contextual information that the researcher can refer to while coding and analyzing data.
Dialogic analysis
There is a nuanced difference between what a narrator tries to accomplish when telling a narrative and how the listener is affected by the narrative. There may be an overlap between the two, but the extent to which a narrative might resonate with people can give us useful insights about a culture or society.
The topic of humor is one such area that can benefit from dialogic analysis, considering that there are vast differences in how cultures perceive humor in terms of how a joke is constructed or what cultural references are required to understand a joke.
Imagine that you are analyzing a reading of a children's book in front of an audience of children at a library. If it is supposed to be funny, how do you determine what parts of the book are funny and why?
The coding process in ATLAS.ti can help with dialogic analysis of a transcript from that reading. In such an analysis, you can have two sets of codes, one for thematically summarizing the elements of the book reading and one for marking when the children laugh.
The Code Co-Occurrence Analysis tool can then tell you which codes occur during the times that there is laughter, giving you a sense of what parts of a children's narrative might be funny to its audience.
Narrative analysis and research hold immense significance within the realm of social science research, contributing a distinct and valuable approach. Whether employed as a component of a comprehensive presentation or pursued as an independent scholarly endeavor, narrative research merits recognition as a distinctive form of research and interpretation in its own right.
Subjectivity in narratives
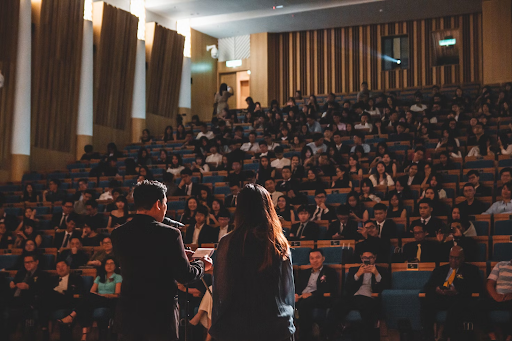
It is crucial to acknowledge that every narrative is intricately intertwined with its cultural milieu and the subjective experiences of the storyteller. While the outcomes of research are undoubtedly influenced by the individual narratives involved, a conscientious adherence to narrative methodology and a critical reflection on one's research can foster transparent and rigorous investigations, minimizing the potential for misunderstandings.
Rather than striving to perceive narratives through an objective lens, it is imperative to contextualize them within their sociocultural fabric. By doing so, an analysis can embrace the diverse array of narratives and enable multiple perspectives to illuminate a phenomenon or story. Embracing such complexity, narrative methodologies find considerable application in social science research.
Connecting narratives to broader phenomena
In employing narrative analysis, researchers delve into the intricate tapestry of personal narratives, carefully considering the multifaceted interplay between individual experiences and broader societal dynamics.
This meticulous approach fosters a deeper understanding of the intricate web of meanings that shape the narratives under examination. Consequently, researchers can uncover rich insights and discern patterns that may have remained hidden otherwise. These can provide valuable contributions to both theory and practice.
In summary, narrative analysis occupies a vital position within social science research. By appreciating the cultural embeddedness of narratives, employing a thoughtful methodology, and critically reflecting on one's research, scholars can conduct robust investigations that shed light on the complexities of human experiences while avoiding potential pitfalls and fostering a nuanced understanding of the narratives explored.
Turn to ATLAS.ti for your narrative analysis.
Researchers can rely on ATLAS.ti for conducting qualitative research. See why with a free trial.
Narrative Research
- Reference work entry
- First Online: 13 January 2019
- Cite this reference work entry
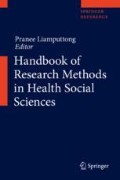
- Kayi Ntinda 2
2604 Accesses
11 Citations
13 Altmetric
Narrative research aims to unravel consequential stories of people’s lives as told by them in their own words and worlds. In the context of the health, social sciences, and education, narrative research is both a data gathering and interpretive or analytical framework. It meets these twin goals admirably by having people make sense of their lived health and well-being in their social context as they understand it, including their self-belief-oriented stories. Narrative research falls within the realm of social constructivism or the philosophy that people’s lived stories capture the complexities and nuanced understanding of their significant experiences. This chapter presents a brief overview of the narrative research approaches as forms of inquiry based on storytelling and premised on the truth value of the stories to best represent the teller’s life world. The chapter also discusses data collection, analysis, and presentation utilizing narrative analysis. In doing so, this chapter provides illustrative examples applying narrative-oriented approaches to research in the health and social sciences. The chapter concludes by outlining the importance of narrative research to person-centric investigations in which the teller-informant view matters to the resulting body of knowledge.
This is a preview of subscription content, log in via an institution to check access.
Access this chapter
- Available as PDF
- Read on any device
- Instant download
- Own it forever
- Available as EPUB and PDF
- Durable hardcover edition
- Dispatched in 3 to 5 business days
- Free shipping worldwide - see info
Tax calculation will be finalised at checkout
Purchases are for personal use only
Institutional subscriptions
Andrews M, Squire C, Tamboukou M, editors. Doing narrative research. London: Sage; 2013.
Google Scholar
Appel D, Papaikonomou M. Narratives on death and bereavement from three South African cultures: an exploratory study. J Psychol Afr. 2013;23(3):453–8.
Article Google Scholar
Atkinson P, Delamont S. Rescuing narrative from qualitative research. Narrat Inq. 2006;16(1):164–72.
Bleakley A. Writing with invisible ink: narrative, confessionalism and reflective practice. Reflective Pract. 2000;1(1):11–24.
Bochner AP. Notes toward an ethics of memory in autoethnographic inquiry. Ethical futures in qualitative research: decolonizing the politics of knowledge. 2007;197–208.
Bruner J. Life as narrative. Soc Res. 2004;71(3):691–710.
Caracciolo M. Narrative, meaning, interpretation: an enactivist approach. Phenomenol Cogn Sci. 2012;11:367–84.
Clandinin DJ. Engaging in narrative inquiry. Walnut Creek: Left Coast Press; 2013.
Clandinin DJ, Connelly FM. Narrative inquiry: experience and story in qualitative research. San Francisco: Jossey-Bass; 2000.
Clandinin DJ, Huber J. Narrative inquiry. In: McGaw B, Baker E, Peterson PP, editors. International encyclopaedia of education. 3rd ed. New York: Elsevier; in press.
Chapter Google Scholar
Clandinin DJ, Rosiek G. Mapping a landscape of narrative inquiry: borderland, spaces and tensions. In: Clandinin DJ, editor. Handbook of narrative inquiry: mapping a methodology. Thousand Oaks: Sage; 2007. p. 35–76.
Clandinin DJ, Murphy MS, Huber J, Orr AM. Negotiating narrative inquiries: living in a tension-filled midst. J Educ Res. 2009;103(2):81–90.
Cochran L. The promise of narrative career counselling. In: Maree K, editor. Shaping the story: a guide to facilitating narrative counselling. Pretoria: Van Schaik; 2007. p. 7–19.
Connelly FM, Clandinin DJ. Stories of experience and narrative inquiry. Educ Res. 1990;19(5):2–14.
Connelly FM, Clandinin DJ. Narrative inquiry. In: Green JL, Camilli G, Elmore P, editors. Handbook of complementary methods in education research. 3rd ed. Mahwah: Lawrence Erlbaum; 2006. p. 477–87.
Craig C, Huber J. Relational reverberation: shaping and reshaping narrative inquires in the midst of storied lives and contexts. In: Clandinin DJ, editor. Handbook of narrative inquiry: mapping a methodology. Thousand Oaks: Sage; 2007. p. 251–79.
Creswell JW. Educational research: planning, conducting, and evaluating quantitative. 4th ed. Upper Saddle River: Prentice Hall; 2012.
Crotty M. The foundations of social research: meaning and perspectives in research process. London: Sage; 1998.
Currie G. Narratives and narrators: a philosophy of stories. Oxford: Oxford University Press; 2010.
Book Google Scholar
Denzin NK, Lincoln Y. The landscape of qualitative research: theories and issues. Thousand Oaks: Sage; 2000.
Ellis C, Bochner AP. Autoethnography, personal narrative, reflexivity: researcher as subject. In: Denzin NK, Lincoln YS, editors. Handbook of qualitative research. 2nd ed. Thousand Oaks: Sage; 2000. p. 733–68.
Ellis C, Bochner AP. Autoethnography, personal narrative, reflexivity: researcher as subject. In: Denzin NK, Lincoln YS, editors. Handbook of qualitative research. 2nd ed. London: Sage; 2005. p. 733–68.
Eloff I. Narrative therapy as career counselling. In: Maree K, Ebersohn L, editors. Lifekills and career counselling. Sandton: Heinemann; 2002. p. 129–38.
Errante A. But sometimes you’re not part of the story: oral histories and ways of remembering and telling. Educ Res. 2000;29(2):16–27.
Frank AW. Illness and autobiographical work: dialogue as narrative destabilization. Qual Sociol. 2000;23(1):135–56.
Frank AW. Why study people’s stories? The dialogical ethics of narrative analysis. Int J Qual Methods. 2002;1(1):109–17.
Freeman M. Autobiographical understanding and narrative inquiry. In: Clandinin DJ, editor. Handbook of narrative inquiry: mapping a methodology. Thousand Oaks: Sage; 2007. p. 120–45.
Gadow S. Relational narrative: the postmodern turn in nursing ethics. Sch Inq Nurs Pract. 1999;13(1):57–70.
Gibson M. Narrative practice and social work education: using a narrative approach in social work practice education to develop struggling social work students. Practice. 2012;24(1):53–65.
Heidari F, Amiri A, Amiri Z. The effect of person-centered narrative therapy on happiness and death anxiety of elderly people. Asian Soc Sci. 2016;12(10):117–26. https://doi.org/10.5539/ass.v12n10p117 .
Herman D. Basic elements of narrative. Chichester: Wiley-Balckwell; 2009.
Jeon YH, Kraus SG, Jowsey T, Glasgow NJ. The experience of living with chronic heart failure: a narrative review of qualitative studies. BMC Health Serv Res. 2010;10(77):2–9. https://doi.org/10.1186/1472-6963-10-77 .
Kim JH. For whom the school bell toll: conflicting voices inside an alternative high school. Int J Educ Arts. 2006;7(6):1–19.
Kim JH, Latta MM. Narrative inquiry: seeking relations as modes of interactions. J Educ Res. 2009;103(2):69–71.
Kim SK, Park M. Effectiveness of person-centered care on people with dementia: a systematic review and meta-analysis. Clin Interv Aging. 2017;12:381–97. https://doi.org/10.2147/CIA.S117637 .
Maree JG, Ebersöhn L, Biagione-Cerone A. The effect of narrative career facilitation on the personal growth of a disadvantaged student – a case study. J Psychol Afr. 2010;20(3):403–11.
McMullen C, Braithwaite I. Narrative inquiry and the study of collaborative branding activity. Electron J Bus Res Methods. 2013;11(2):92–104.
Murphy N, Aquino-Russell C. Nurses practice beyond simple advocacy to engage in relational narratives: expanding opportunities for persons to influence the public space. Open Nurs J. 2008;2(40):40–7.
Newby P. Research methods for education. 2nd ed. New York: Routledge; 2014.
Ngazimbi EE, Hagedorn WB, Shillingford MA. Counselling caregivers of families affected by HIV/AIDS: the use of narrative therapy. J Psychol Afr. 2008;18(2):317–23.
Ntinda K. Constructing a framework for use of psychometric tests in schools: a consumer-oriented approach. Unpublished doctoral dissertation. Botswana: University of Botswana; 2012.
Nwoye A. A narrative approach to child and family therapy in Africa. Contemp Fam Ther. 2006;28(1):1–23.
Rhodes C, Brown AD. Narrative, organizations and research. Int J Manag Rev. 2005;7(3):167–88.
Riessman CK. Narrative methods for the human sciences. Los Angeles: Sage; 2008.
Riessman CK, Quinney L. Narrative in social work: a critical review. Qual Soc Work. 2005;4(4):391–412.
Savin-Baden M, Niekerk LV. Narrative inquiry: theory and practice. J Geogr High Educ. 2007;31(3):459–472.
Spector-Mersel G. Narrative research: time for the paradigm. Narrat Inq. 2010;20(1):204–24.
Trahar S. Beyond the story itself: narrative inquiry and autoethnography in intercultural research in higher education [41 paragraphs]. Forum Qual Soc Res. 2009;10(1):Art. 30. http://nbn-resolving.de/urn:nbn:de:0114-fqs0901308 .
Tsianakas V, Maben J, Wiseman T, Robert G, Richardson A, Madden P, Griffin M, Davies EA. Using patients’ experiences to identify priorities for quality improvement in breast cancer care: patient narratives, surveys or both? BMC Health Serv Res. 2012;12(271):2–11. https://doi.org/10.1186/1472-6963-12-271 .
Wang CC. Conversation with presence: a narrative inquiry into the learning experience of Chinese students studying nursing at Australian universities. Chin Nurs Res. 2017;4:43–50.
Wang CC, Geale SK. The power of story: narrative inquiry as a methodology in nursing research. Int J Nurs Sci. 2015;2(2):195–8.
Wood K, Chase E, Aggleton P. ‘Telling the truth is the best thing’: teenage orphans’ experiences of parental AIDS-related illness and bereavement in Zimbabwe. Soc Sci Med. 2006;63(7):1923–33.
Zulu NT, Munro N. “I am making it without you, dad”: resilient academic identities of black female university students with absent fathers: an exploratory multiple case study. J Psychol Afr. 2017;27(2):172–9.
Download references
Author information
Authors and affiliations.
Discipline of Educational counselling and Mixed-methods Inquiry Approaches, Faculty of Education, Office C.3.5, University of Swaziland, Kwaluseni Campus, Manzini, Swaziland
Kayi Ntinda
You can also search for this author in PubMed Google Scholar
Corresponding author
Correspondence to Kayi Ntinda .
Editor information
Editors and affiliations.
School of Science and Health, Western Sydney University, Penrith, NSW, Australia
Pranee Liamputtong
Rights and permissions
Reprints and permissions
Copyright information
© 2019 Springer Nature Singapore Pte Ltd.
About this entry
Cite this entry.
Ntinda, K. (2019). Narrative Research. In: Liamputtong, P. (eds) Handbook of Research Methods in Health Social Sciences. Springer, Singapore. https://doi.org/10.1007/978-981-10-5251-4_79
Download citation
DOI : https://doi.org/10.1007/978-981-10-5251-4_79
Published : 13 January 2019
Publisher Name : Springer, Singapore
Print ISBN : 978-981-10-5250-7
Online ISBN : 978-981-10-5251-4
eBook Packages : Social Sciences Reference Module Humanities and Social Sciences Reference Module Business, Economics and Social Sciences
Share this entry
Anyone you share the following link with will be able to read this content:
Sorry, a shareable link is not currently available for this article.
Provided by the Springer Nature SharedIt content-sharing initiative
- Publish with us
Policies and ethics
- Find a journal
- Track your research
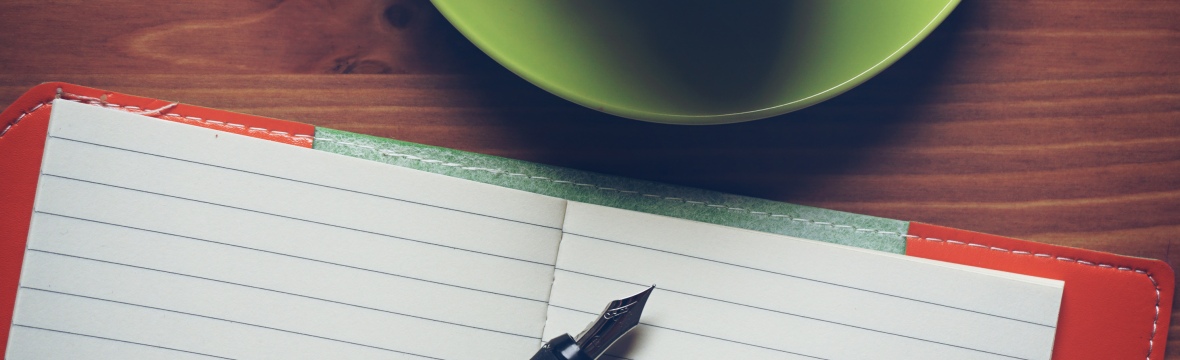
Dr Karen Lumsden
trainer / coach / consultant / researcher
Narrative Analysis
Narrative analysis is a valuable data analysis technique in qualitative research. It is typically used in those studies which have already employed narrative inquiry as a qualitative method. Narrative knowledge is created and constructed through the stories of lived experience and sense-making, the meanings people afford to them, and therefore offers valuable insight into the complexity of human lives, cultures, and behaviours. Narrative analysis uses the ‘story’ as the unit of analysis, in contrast to thematic and other forms of qualitative analysis.
Narrative analysis is useful for practitioners and researchers who wish to focus on individual experiences, e.g. via collected stories and unstructured interviews. Examples of possible applications include case studies; patients’ experiences of health care services or illness; life stories and experiences of social care clients; victims’ experiences of the criminal justice system.
This training benefits participants who wish to advance their knowledge of qualitative research methods. It explores the opportunities that narrative analysis offers in a range of applied and policy settings and contexts. It is relevant to researchers who have narrative data (or plan to collect narrative data) ready for analysis.
Share this:

An official website of the United States government
The .gov means it’s official. Federal government websites often end in .gov or .mil. Before sharing sensitive information, make sure you’re on a federal government site.
The site is secure. The https:// ensures that you are connecting to the official website and that any information you provide is encrypted and transmitted securely.
- Publications
- Account settings
Preview improvements coming to the PMC website in October 2024. Learn More or Try it out now .
- Advanced Search
- Journal List
- JMIR Res Protoc
- v.8(8); 2019 Aug

A Systematic Framework for Analyzing Patient-Generated Narrative Data: Protocol for a Content Analysis
Maryam zolnoori.
1 Department of Health Sciences Research, Mayo Clinic, Rochester, MN, United States
Joyce E Balls-Berry
2 College of Medicine and Science, Mayo Clinic, Rochester, MN, United States
Tabetha A Brockman
3 Community Engagement Program, Center for Clinical and Translational Science, Mayo Clinic, Rochester, MN, United States
4 Department of Psychiatry and Psychology, Mayo Clinic, Rochester, MN, United States
Christi A Patten
Associated data.
Definition of HTML, API, Web crawler, Python package, and XML.
Kinship terminology and UMLS (Unified Medical Language System).
Example of a formula for determining the sample size.
Examples of regular expression codes for reducing noise patterns in patients' posts.
Case study #2: strategies and solution of diabetes patients with financial difficulties for accessing medications and supplies.
Operational definitions for all predefined themes for the case study “identifying factors affecting patients’ attitudes towards antidepressants”.
New themes generated through inductive approach for the case study “identifying factors affecting patients’ attitudes towards antidepressants”.
Rules for refining the identified themes.
Guidelines for annotating the patients comments for the case study “diabetes patients solutions to access medications and supplies in the context of financial difficulties”.
Final analytical framework for the case study “identifying the underlying factors affecting patient attitudes toward antidepressants”.
Interpretation of the obtained results from the Cohen kappa.
Descriptive interpretation of the findings of the case study “access to diabetes medications”.
The major difference between the systematic framework and other content analysis frameworks.
Patient narrative data in online health care forums (communities) are receiving increasing attention from the scientific community for implementing patient-centered care. Natural language processing (NLP) methods are gaining more and more attention because of the enormous data volume. However, state-of-the-art NLP still cannot meet the need of high-resolution analysis of patients’ narratives. Manual qualitative analysis still plays a pivotal role in answering complicated research questions from analyzing patient narratives.
This study aimed to develop a systematic framework for qualitative analysis of patient-generated narratives in online health care forums.
Our systematic framework consists of 4 phases: (1) data collection, (2) data preparation, (3) content analysis, and (4) interpretation of the results. Data collection and data preparation phases are constructed based on text mining methods for identifying appropriate online health forums for data collection, differentiating posts of patients from other stakeholders, protecting patients’ privacy, sampling, and choosing the unit of analysis. Content analysis phase is built on the framework method, which facilitates and accelerates the identification of patterns and themes by an interdisciplinary research team. In the end, the focus of interpretation of the results phase is to measure the data quality and interpret the findings regarding the dimensions and aspects of patients’ experiences and concerns in their original contexts.
We demonstrated the usability of the proposed systematic framework using 2 case studies: one on determining factors affecting patients’ attitudes toward antidepressants and another on identifying the disease management strategies in patient with diabetes facing financial difficulties. The framework provides a clear step-by-step process for systematic content analysis of patient narratives and produces high-quality structured results that can be used for describing patterns or regularities in patients’ experiences, generating and testing hypotheses, and identifying areas of improvement in the health care systems.
Conclusions
The systematic framework is a rigorous and standardized method for qualitative analysis of patient narratives. Findings obtained through such a process indicate authentic dimensions and aspects of patient experiences and shed light on patients’ concerns, needs, preferences, and values, which are the core of patient-centered care.
International Registered Report Identifier (IRRID)
RR1-10.2196/13914
Introduction
Online health forums (communities) are increasingly accessible and convenient platforms for patients and caregivers to share health care experiences together with concerns of diagnosis, treatment, and outcomes. Almost 30% of the US population actively share and discuss health-related experiences on various online health forums, such as askapatient.com, patientslikeme.org, and dailystrength.org [ 1 ]. Patient-reported health care experiences in a narrative format on the internet are a valuable data source for implementing patient-centered care [ 2 ].
There are 2 methods for analyzing patient-generated narrative data: natural language processing (NLP) and qualitative content analysis. NLP is a set of methods and techniques to process human language components, such as identifying sentence structure and recognizing sentence meaning as humans do [ 3 ]. NLP algorithms build on statistical methods to infer patterns in data. The algorithms usually need an annotated dataset (train dataset) for learning meaningful patterns and concepts to make predictions about new data [ 4 ]. In the context of patients’ narratives, NLP is mostly used for clustering health-related messages [ 5 ], identifying the topics of concern [ 6 ], and recognizing the opinions and sentiments of patients toward different topics. With the fast-growing volume of patient-generated narratives in online health forums, NLP has been gaining increasing attention. However, the available NLP techniques are not able to provide a high-quality understanding of the text, such as identifying patients’ functional problems because of colloquial language, word ambiguity, and layperson terms [ 7 , 8 ].
The second method for analysis of patient-generated narratives is qualitative content analysis. Qualitative content analysis is a research method designed to identify the thematic structure of text documents by subjectively interpreting the context of the text [ 9 ]. This process is conducted through a systematic classification process of coding and identifying themes and patterns in the text. Unlike the NLP algorithms, this method can provide a deep insight into textual data for identifying patterns and various aspects of the textual content. However, this method is usually applied on a small sample of data because of the manual process of analysis. In the context of patient narrative data, qualitative content analysis has been used to identify meaningful patterns and themes in patients’ discussions in online health care forums to answer complex research questions such as factors affecting patients’ attitudes toward antidepressants and contributions of MD Anderson’s Facebook group to patient’s cancer experience.
In this study, we propose an efficient and cost-effective systematic framework built on text mining and qualitative content analysis approaches for analyzing patients’ narratives. This framework comprises 4 phases: (1) data collection, (2) data preparation, (3) content analysis, and (4) interpretation of findings. Data collection and data preparation phases utilize text mining methods to facilitate and accelerate the process of data collection and preparation for content analysis. The content analysis phase utilizes the framework method [ 10 ] and the deductive-inductive approach to facilitate the process of data analysis and interpretation of the findings. Overall, the proposed systematic framework offers a comprehensive approach to identify dimensions and aspects of patients’ narratives in online health care forums in an efficient manner.
In this study, we first provide a brief introduction to the framework method [ 10 ] and content analysis and theme generation approaches with examples from research conducted using patient-generated narratives. Next, we describe the proposed framework and demonstrate its applicability for analysis of patient narratives using 2 case studies: one on determining factors affecting patients’ attitudes toward antidepressants using data from askapatient.com [ 11 ] and another on identifying the disease management strategies in patients with diabetes facing financial difficulties using data from 4 diabetes-focused forums.
The Framework Method for Content Analysis
The framework method was developed in the qualitative research unit at the National Centre for Social Research in the United Kingdom in 1980 for analyzing narrative data related to policy [ 10 ] and later adopted in other fields, including health care. It identifies themes in data systematically, where each theme represents a semantic topic. More specifically, the framework method builds a matrix structure with each piece of narrative data (eg, a patient post or a sentence) stored in a row and each identified theme in a column. Using this structure, researchers can cluster narrative data around identified themes and identify the relationships between themes (see Figure 1 ). This framework provides a holistic, descriptive picture of a reasonably large sample of data.

Structure of the framework method. Sentences from patient posts (unit of analysis) organized in the rows and themes (adverse drug reactions) organized in the columns. Value “1” indicates to which theme a sentence was assigned.
Themes in the framework method are generated through deductive, inductive, or a combination of the deductive-inductive approach. The selected approach depends on the research question, the study’s aim, and the available knowledge about the phenomena under study. The deductive approach is used when the aim of the study is to retest the existing model, concept, or knowledge in a new context [ 12 ]. In the context of patient narratives, Latvala et al and Kasila et al used the inductive approach to identify meaningful concepts [ 13 , 14 ]. When the available knowledge about phenomena under study is limited, the inductive approach is used to identify new themes. For example, Zolnoori et al, Hilliard, and Sutton et al used the inductive approach to identify meaningful patterns and themes in patients’ discussions in online health care forums [ 15 - 17 ]. Finally, the deductive-inductive approach is used when researchers wish to test the existing knowledge in a new context and leave space to discover new aspects of the data that were not covered by existing knowledge. For example, Zolnoori et al used the deductive-inductive approach to test hypotheses concerning patients’ attitudes toward antidepressants using patients’ drug reviews in askapatient.com [ 11 ].
Building on our previous experiences and lessons learned from the content analysis of patients’ narratives, we propose a systematic framework to address the subjective nature of patients’ narratives [ 11 , 18 - 20 ]. This framework integrates text mining methods, the framework method, and deductive-inductive approach for content analysis and is composed of 4 main phases: (1) data collection, (2) data preparation, (3) content analysis, and (4) interpretation of the results. During the data collection phase, relevant health forums are selected regarding the aim of the study. The data preparation phase uses text mining methods for (1) distinguishing patients’ comments from other stakeholders (caregivers and clinicians), (2) sampling patients’ narratives, and (3) preparing the unit of analysis. The focus of content analysis phase is on the framework method and the deductive-inductive approach to generate a structured matrix that presents various aspects of patients’ narratives. Finally, the focus of interpretation phase is on measuring quality criteria, validation, and interpretation of findings. Figure 2 shows a schematic view of the systematic framework.

A schematic view of the systematic framework designed for content analysis of patient narrative data.
In the following sections, we demonstrate the implementation of each step of this systematic framework using 2 studies: (1) a case study that identified factors affecting patients attitudes toward antidepressants; we use the short title attitude to antidepressants to refer to this case study [ 11 ] and (2) a case study that analyzed the strategies and solutions of patients with diabetes facing financial difficulties to access medications and supplies; we use the short title access to diabetes medications to refer to this case study.
Phase I: Data Collection
The data collection phase of the systematic framework included selecting health forums, protecting patients’ privacy, and collecting data from these health forums.
Selection Health Forums for the Study
Health forums vary in covering patients’ experiences with the health care system. Researchers can select a health care forum for their study using (1) description of the forum or community, (2) initial analysis of randomly selected posts, and (3) analysis of medical concepts using text mining tools such as MetaMap [ 21 ] or clinical Text Analysis and Knowledge Extraction System [ 22 ]. Researchers may choose multiple forums for a study to reduce the risk of potential bias in the findings that may occur because of an unbalanced sampling of patient experiences in a health forum. However, determining the number of forums depends on the aim of the study and the level of heterogeneity in patient experiences. For example, for the study attitude to antidepressants , we used only 1 forum askapatient.com because our initial content analysis using MetaMap [ 17 ] showed that this forum covers a wide range of antidepressants’ side effects and potential factors affecting patients’ attitudes toward antidepressants.
Patients’ Privacy in the Health Forums
Although data in the forums are mostly anonymous and publicly available, further protection of patient’s privacy and requesting permission from owners of the data collection are recommended. Researchers need to submit the institutional review board’s (IRB’s) study approval to the affiliated institute. The IRB submission usually receives an exemption. In addition, to further protect patient privacy, deidentification of the data is recommended. For example, in both projects, we formulated regular expressions to eliminate emails, phone numbers, and URLs from posts. For the project attitude to antidepressants , we sought IRB approval through the University of Wisconsin-Milwaukee, and for the project access to diabetes medications , we sought IRB approval through the Mayo Clinic.
Data Collection From an Online Health Forum
Patient posts in the online health forums are mostly stored in the HTML format. To collect these data, the research team may use the application programming interface (API) specifically developed for the forum or community. If the API is not available, the research team may customize the existing open-source Web crawlers or develop a new one to collect data. For example, we used Beautiful Soup [ 23 ], a python package for parsing HTML and XML documents, to develop a Web crawler. Please see Multimedia Appendix 1 for the definition of HTML, API, Web crawler, Python package, and XML.
Phase II: Data Preparation
Data preparation phase consists of 3 steps: differentiating patient posts from other stakeholders, sampling, and defining the unit of analysis.
Differentiating Patient Posts From Other Stakeholders
Patients’ interests and perspectives on treatment are different from that of clinicians and caregivers who share their experiences and concerns for their patients in online health forums. Distinguishing patients’ experiences from other stakeholders can be achieved by utilizing text mining approaches such as unsupervised algorithms for text clustering [ 24 ] (eg, density-based spatial clustering of applications with noise, expectation-maximization, or agglomerative hierarchical clustering). For example, Lu et al leveraged the expectation-maximization algorithm for differentiation between patient posts from caregivers and clinicians [ 5 ]. To improve the performance of the clustering algorithms, selecting a proper feature such as writing style–based features (eg, lexical and syntactic) [ 25 , 26 ] and content-specific features (eg, kinship terminology, Unified Medical Language System [UMLS] semantic types and concept IDs, and n-grams) [ 26 , 27 ] is useful. See Multimedia Appendix 2 for more information about kinship terminology and UMLS.
Having a representative sample of online forums content is pivotal for statistical reliability and generalizability of the findings. To increase the likelihood of having a representative sample, the research team may utilize retrieval methods such as phrase-based vector space model [ 28 , 29 ] or knowledge-based query expansion method [ 30 ]. The retrieval methods are particularly useful when the forums do not have a built-in system for filtering specific information, and the content covers a wide range of patients’ experience.
If the size of retrieved relevant patient posts is extremely large, probability sampling methods (such as simple random sampling, stratified random sampling, or cluster sampling) are useful to obtain a robust sample size [ 31 ]. If the document retrieval methods do not retrieve relevant patient posts for the research question, the research team may use nonprobabilistic samplings, such as convenience sampling or judgmental sampling [ 31 ].
Determining the sample size is another concern in content analysis studies. There is no single formula for determining the sample size. The size of the sample is a factor of time and financial sources and data heterogeneity. Researchers may use the standard sampling formula for computing the sample size [ 32 ]. As an example of sample size calculation, please see Multimedia Appendix 3 [ 33 , 34 ].
Defining the Unit of Analysis
A unit of analysis is the smallest unit in the data sample containing information regarding the research question. Graneheim et al discussed that the unit of analysis should be large enough to convey a whole perspective and small enough to be kept in mind as a context for meaning unit during the analysis process [ 35 ].
For both case studies, the initial analysis showed that patients’ comments were composed of multiple sentences that covered various dimensions and aspects of experiences and concerns. Therefore, we used sentences as the unit of analysis. In addition, data analysis at the level of sentences ensured that no important segment of patient narratives was missed. Splitting patient posts into sentences is not an easy task because of colloquial language and grammatical and punctuation errors. Therefore, we preprocessed the data to remove noisy patterns and then split the patient posts into sentences using open-source Natural Language Toolkit [ 36 ]. Multimedia Appendix 4 shows examples of regular expression codes we used to handle the grammatical errors in patient posts.
Phase III: Content Analysis
After preparing the patient posts, the next step is on defining themes for content analysis. The framework method allows different approaches for generating themes: deductive, inductive, and combination of deductive-inductive. In this section, we illustrate the step-by-step procedure of generating themes using deductive-inductive approach for the 2 case studies. This approach allowed us to retest the available knowledge in the literature in the context of patient narratives while leaving space for discovering new aspects of the patient experiences in online health forums.
In this section, we explain the process of generating themes for the case study attitude to antidepressants . The process of generating themes for the case study access to diabetes medications is presented in Multimedia Appendix 5
Generating Themes Using Deductive Analysis
Our literature review showed that existing knowledge in the literature is useful for generating themes to analyze and summarize patient experiences with antidepressants in online forums. Accordingly, we conducted a systematic literature review to identify significant factors affecting patients’ attitudes toward antidepressants. We identified 5 main themes including pharmacological treatment, health care system, social-cognitive and psychological factors, patient-related factors, and depression that influence patients’ attitudes toward antidepressants. For each theme, we identified subthemes. Figure 3 shows the themes and subthemes generated using deductive analysis.

Generated themes using deductive approach for the case study attitudes to antidepressants.
To start coding patient posts using the predefined themes, developing guidelines with clear operational definitions for each theme is necessary. Operational definitions should include well-defined statements with explicit inclusion and exclusion criteria describing the segment of a text assigned to a theme. Each statement must accompany 1 or more examples extracted from patient posts. Table 1 provides an example of operational definitions of themes for the case study attitude to antidepressants . Multimedia Appendix 6 includes the operational definitions for all predefined themes of the study attitude to antidepressants .
Example of operational definition for the themes for the project patient attitudes toward antidepressants and category.
Themes generated in deductive approach were used for generating the initial analytical framework. We constructed the framework by organizing predefined themes in the columns and sentences of patient posts (unit of analysis) in the rows. Each patient post was split into sentences and identified using post ID and sentence index indicating the position of the sentence in the patient post. Figure 1 shows the structure of the analytical framework for the case study attitude to antidepressants.
Generating Themes Using Inductive Analysis
As patients in the forums have the freedom to anonymously share their experiences and concerns in the lay language without any limitations, it is likely that patient posts include information that may not fit into the predefined themes in the initial analytical framework. Therefore, in this step, although we coded sentences (of patient posts) using predefined themes, we used the inductive approach to generate new themes for sentences that could not be assigned to the predefined themes.
It is not necessary to use the whole sample for inductive analysis. Researchers may select a random portion of the sample (eg, 30%), regarding the availability of resources, size of a sample, and the level of heterogeneity in patient narratives. For example, in the study attitude to antidepressants , we created a subsample by randomly selecting 33.33% (300/900) of the posts for inductive analysis. To identify new themes, 4 coders read the sentences in the subsample and assigned them to proper themes by following the guidelines. The meaningful sentence that could not fit into predefined themes was discussed in our regular team meetings for new themes. For example, we could not assign this sentence I weaned slowly and I feel nauseous a lot to any predefined themes to any available themes, so we generated a new theme named withdrawal symptom . We created a theme named Not-applicable that contained sentences without any meaningful information related to the research question. For example, Feel free to email me about Effexor does not reflect any information about the patient’s attitude toward antidepressants.
Figure 4 shows new themes generated using the inductive approach for the project attitude to antidepressants. Multimedia Appendix 7 includes all the generated new themes with the examples from patient posts for this study.

Generated themes using inductive approach for the case study “attitude to antidepressants”.
Refining the Themes
Some of the themes generated in deductive approach may not fit into patient posts. For example, we could not find any sentences in the subsample of the study attitude to antidepressants to assign to the theme educational level . In addition, some of the new themes developed through inductive approach may fit into a few patient posts. For example, nearly 1.9% (17/892) of the patient posts in the subsample had information related to problem with financial support; therefore, we removed the theme from the list.
Themes generated using inductive and deductive approaches need to be refined before developing the final analytical framework. Theme refining can be conducted by creating rules such as setting a threshold on the number of sentences that should be assigned to a theme. For example, for the study attitude toward antidepressants , we set the threshold on 5%, that is, if a theme included less than 5% of sentences in the subsample, we excluded them from the list of themes. The value of the threshold depends on the importance of the rare or uncommon themes (patterns) in the dataset for the research team. For example, for the case study access to diabetes medications , we did not consider any threshold because of the importance of the rare information that patients reported to get access to medications. But for the case study attitude toward antidepressants , it was not possible to make any conclusion based on the rare themes in the dataset, that is, to measure the association between rare themes and patients’ attitudes. Therefore, our research team set the threshold on 5% after the initial analysis of 30% of the dataset.
Multimedia Appendix 8 includes all the rules generated to refine themes for the study attitude to antidepressants.
Developing the Guideline for Coding
To maintain consistency and uniformity of coding patient posts using the final themes across the sample, developing guidelines are necessary. Guidelines should include the aim of the project, operational definition of the themes with specific examples from patient posts, and inclusion and exclusion criteria for assigning a unit of analysis to themes. Operational definition for a theme should include a clear and precise statement that enables the annotators to recognize a segment of patient post that fit the theme. For example, theme adverse drug reaction (ADR) in the study attitude to antidepressants defined as any signs or symptoms that patients experienced after drug consumption and explicitly and certainly were linked to the drug consumption includes criteria to decide what is ADR by emphasizing on the time of occurrence of the sign or system, that is, after drug consumption . The definition also used the terms explicitly and certainly to exclude any vague or uncertain statements from the patient post. Vague or ambiguous operational definition increases the need for text interpretation, raises the risk of observational error in coding, and consequently results in low interannotator agreement (IAA).
The guidelines should also include instruction on coding the unit of analysis using themes. For example, whether the unit of analysis can be assigned to more than 1 theme or whether the unit of analysis should be interpreted in the context of the patient posts are important questions. Clear answers to these questions can certainly facilitate the process of coding and increase the quality of generated structured data. Finally, it would be useful if coding guidelines include the list of qualifications for hiring annotators and the estimated time for training. Multimedia Appendix 9 contains the guidelines that we developed for the study access to diabetes medications.
Selecting Appropriate Coding Environment
The research team should select a coding environment that facilitates construction of the analytical framework and the process of coding. For both case studies explained in this study, we used a spreadsheet to construct the analytical framework (see Figure 1 ). Annotators (coders) could assign a sentence to a theme by inserting 1 in the intersection of the sentence and the theme. However, if the unit of analysis is a phrase or a word, the spreadsheet may not be a convenient tool for constructing the analytical framework and annotating process. In this case, tools such as Brat [ 37 ] or MAE [ 38 ] are helpful. But if the unit of analysis can be defined as any segment of text (patient post), tools that were specifically designed for qualitative data analysis, such as NVivo (a qualitative data analysis (QDA) computer software package produced by QSR International) [ 39 ], would be more useful.
Developing the Final Analytical Framework
Overall, the final themes should meet the following criteria: (1) valid—themes should accurately reflect what is being measured, (2) mutually exclusive—themes should not overlap between operational definitions, and (3) exhaustive—themes should cover all the aspects of the data related to the research question. All aspect of the data means identifying all the dimensions of the dataset that provide meaningful information for the research question.
Figure 5 shows the final themes after refining them for the study attitude toward antidepressants . The refined themes were used for constructing the final analytical framework. Multimedia Appendix 10 presents the final analytical framework with all the themes and examples from patients’ drug reviews.

Final themes for the case study “attitude to antidepressants”.
Phase IV: Analysis of Findings
Before researchers summarize and interpret the data, they should evaluate the quality of the produced structured data. As assigning a certain observation of patient narratives to themes is a subjective process, a disagreement may happen between annotators (coders). In this section, we explain measures for computing IAA and then discuss summarizing and interpretation of the findings.
Computing Interannotator Agreement
Cohen kappa is the most popular method for computing IAA. It measures the agreement between 2 annotators who annotate N items (eg, 100 sentences) into M mutually exclusive themes (eg, 10 themes) and corrects the result for the agreement that would be expected by chance [ 40 , 41 ]. Possible values for the kappa coefficient ranges from –1 to 1, where 1 indicates complete agreement, 0 indicates completely random agreement, and –1 indicates complete disagreement. For the formula of Cohen kappa and detailed interpretation on the obtained values, see Multimedia Appendix 11 [ 42 , 43 ].
To improve the quality of produced structured data, researchers may decide to annotate each document (eg, patient post) by more than 2 annotators. In this case, Fleiss kappa (an adaptation of Cohen kappa for 3 or more raters) should be used for computing the IAA [ 41 ].
There are other methods for calculating IAA, such as pairwise agreement. If the annotation task requires identifying terms or phrases and determining their correct boundaries (eg, identifying sign or a symptom) in the patient’s posts, the pairwise agreement would be an appropriate measure. The kappa coefficient would not be suitable because the chance agreement is effectively 0 in this case. Please see Zolnoori et al [ 7 ] for the formula and examples related to computing pairwise agreement.
Interpretation of Findings
If the structured data developed during the process of content analysis is rich enough, it can provide substantial insight into patients’ concerns, needs, preferences, and attitudes. Interpretation of the result could start with a general description of the themes, followed by reporting the most frequent and infrequent identified patterns, and finally reporting the unexpected patterns in data. Multimedia Appendix 12 provides a descriptive interpretation of the findings of the case study access to diabetes medications.
Implication of Findings
The findings of content analysis can go beyond a simple description of themes. In fact, it can be used for describing patterns or regularities, generating and testing hypotheses, describing a phenomenon and the associated factors, identifying problematic areas in the health care systems, or even developing predictive models to predict a specific patient’s behavior, such as medication nonadherence behavior. For example, for the project attitude to antidepressants , we tested the association between themes (variables) and patient attitudes toward antidepressants using statistical methods (analysis of variance and chi-square test) [ 11 ].
Efficient Qualitative Research
Qualitative content analysis approaches are nonlinear, and iterative processes are more complicated than quantitative approaches because they are less structured and standardized. There are no single guidelines for content analysis. Selecting a specific approach strongly depends on the aim of the study, the research question, and the type of qualitative data. Researchers collecting and analyzing qualitative data, such as patient narratives, often wish to have a systematic approach including the detailed instruction on how to conduct qualitative research efficiently.
This study provided a systematic framework for the content analysis of patient-generated narratives in online health forums (communities). The systematic framework was built on text mining approaches for data collection and data preprocessing and qualitative content analysis using the framework method with the deductive-inductive approach for themes generation. We showed the feasibility and usefulness of the proposed systematic framework using 2 case studies: (1) a published study with a focus on identifying factors affecting patients’ attitudes toward antidepressants [ 11 ] and (2) an ongoing study with the focus on strategies and solutions of patients with diabetes facing financial difficulties to access medications and supplies. The data sources for these 2 studies were from online health forums. Using the systematic framework specified in this study, we could generate high-quality structured data that not only could identify the different dimensions and aspects of patients’ experiences but also could be used for testing hypotheses concerning the relationships between variables (themes) [ 11 ]. In addition, the structured data could also be used to train text mining algorithms to identify specific health-related information from patients’ narrative data [ 7 ].
The core component of the proposed framework (phase 3) is the framework method for qualitative content analysis [ 10 ]. Its prominent feature is the facilitation of constant comparative method through a matrix structure of the data. The matrix structure provides an intuitively structured overview of the summarized data that can facilitate and accelerate the identification of patterns and themes by highlighting the contradictory data and irregular cases. More importantly, it keeps a clear map between original data and themes in the analytical framework, indicating illustrative quotes for themes.
We used a combination of deductive-inductive approach to develop themes for both case studies in this study. However, the proposed systematic framework can be applied equally to studies aimed to use only inductive or deductive approach for data analysis. Our literature review showed that studies with focus on the qualitative content analysis of patient narratives in online health forums mostly used inductive approach for theme generation. But, we showed that the deductive analysis could accelerate the inductive analysis of patient narratives and identify new patterns and themes. There are major differences between this systematic framework proposed in this study and the framework of content analysis suggested by other papers. Please see Multimedia Appendix 13 for this difference [ 44 , 45 ].
Limitations
We acknowledge some limitations with our proposed framework:
- It is not appropriate for the analysis of very heterogeneous patients’ narratives, for example, if the patients’ experiences and the discussions in health forums are very diverse and cover a wide range of health topics. The systematic framework is most suitable for the studies with research questions targeting specific patient cohort with shared health concerns and experiences (eg, medications’ effects or difficulty in access to a health services).
- It is not suitable for qualitative studies aiming at developing a theory or analysis of the structure of the experiences or language or the social context associated with the language. The research team may adopt other qualitative approaches to achieve the aims, such as approaches for developing theories derived from the data (eg, grounded theory) [ 46 ], approaches with focus on identifying the structure of an individual’s experience (eg, phenomenology) [ 47 ], approaches with concern about the relationship between language and the social structure in which the language is used (eg, discourse analysis and ethnomethodology) [ 48 ], and approaches designed with the aim of investigating intention and language (eg, narrative analysis) [ 49 ].
- Although it provides a detailed instruction on analysis of patient posts, which may save time and resources similar to other qualitative analysis methods, it is still time consuming and resource intensive when involving time needed for developing guidelines and training annotators. This time needs to be factored into the study methods and approach.
Lessons Learned
Previous experiences with and lessons learned from the content analysis:
- Qualitative content analysis may seem confusing and complicated for novice researchers. They may find this process to be chaotic and grapple with the qualitative research terms and concepts, such as patterns, categories, and themes. But experiencing chaos during the analysis is normal. Qualitative researchers need to be open to the complexity of content analysis [ 50 ] and improve their experience for systematic thinking.
- During the content analysis process, it would be very helpful to review the research questions constantly. Frequently referring to the research question and aim of the study will help researchers to stay focused on only dimensions of the dataset that answer the research question. It is also very important to take a note of new ideas and identified themes during the whole process of analysis. If the data analysis is conducted in an Excel sheet, assigning a column to notes and ideas would be very useful.
- Content analysis is a very time-consuming process and unexpectedly challenging [ 12 ]. The research team should plan ahead to have sufficient time to think, reflect, and then conduct a review of the analysis.
- It is important to avoid any preunderstanding of the dataset to minimize the risk of bias during the process of content analysis and interpretation of the results [ 9 ].
- It is important to have a weekly meeting to discuss new ideas and identified patterns in the group. All team members should be open and receptive to new ideas. The research team should proceed with defining and updating analytical framework based on the summary of the meeting discussion each week.
- Creating a table or figure containing information about the process of analysis from the raw data to a meaningful unit of analysis, to the identified themes with examples from patients’ post would be very useful. Including the figure or table in the manuscript of the study will show the validity of the study and improve appreciation of reviewers and readers of the study’s findings.
Exploring patient-reported experiences and concerns in online health care forums (communities) and translating such content into meaningful concepts (themes) has become a challenge for health care researchers and health care providers. In this study, we introduced a systematic framework as a rigorous and standardized method to collect patient-reported experiences from online forums and convert their content to themes that are reliable and easily interpretable. The framework was built on the text mining approaches and the framework method with the deductive-inductive approach that benefit both researchers and clinicians by minimizing the cost, time, and human errors during the process of data processing and analysis. We showed the reliability and efficiency of this framework using 2 case studies: one identifying factors associated with patients’ attitude toward antidepressants and the other identifying solutions and strategies of patients with diabetes facing financial difficulties to access medications and supplies. Finding meaningful information through such a process indicates authentic dimensions and aspects of patient experiences and sheds light on patients’ concerns, needs, preferences, and values, which are the core of patient-centered care.
Acknowledgments
This publication was supported by CTSA grant number TL1 TR002380 from the National Center for Advancing Translational Science. Its contents are solely the responsibility of the authors and do not necessarily represent the official views of the National Institutes of Health.
Abbreviations
Multimedia appendix 1, multimedia appendix 2, multimedia appendix 3, multimedia appendix 4, multimedia appendix 5, multimedia appendix 6, multimedia appendix 7, multimedia appendix 8, multimedia appendix 9, multimedia appendix 10, multimedia appendix 11, multimedia appendix 12, multimedia appendix 13.
Conflicts of Interest: None declared.

- Section 2: Home
- Developing the Quantitative Research Design
- Qualitative Descriptive Design
- Design and Development Research (DDR) For Instructional Design
- Qualitative Narrative Inquiry Research
What is a Qualitative Narrative Inquiry Design?
Tips for using narrative inquiry in an applied manuscript, summary of the elements of a qualitative narrative inquiry design, sampling and data collection, resource videos.
- Action Research Resource
- Case Study Design in an Applied Doctorate
- SAGE Research Methods
- Research Examples (SAGE) This link opens in a new window
- Dataset Examples (SAGE) This link opens in a new window
- IRB Resource Center This link opens in a new window
Narrative inquiry is relatively new among the qualitative research designs compared to qualitative case study, phenomenology, ethnography, and grounded theory. What distinguishes narrative inquiry is it beings with the biographical aspect of C. Wright Mills’ trilogy of ‘biography, history, and society’(O’Tolle, 2018). The primary purpose for a narrative inquiry study is participants provide the researcher with their life experiences through thick rich stories. Narrative inquiry was first used by Connelly and Calandinin as a research design to explore the perceptions and personal stories of teachers (Connelly & Clandinin, 1990). As the seminal authors, Connelly & Clandinin (1990), posited:
Although narrative inquiry has a long intellectual history both in and out of education, it is increasingly used in studies of educational experience. One theory in educational research holds that humans are storytelling organisms who, individually and socially, lead storied lives. Thus, the study of narrative is the study of the ways humans experience the world. This general concept is refined into the view that education and educational research is the construction and reconstruction of personal and social stories; learners, teachers, and researchers are storytellers and characters in their own and other's stories. In this paper we briefly survey forms of narrative inquiry in educational studies and outline certain criteria, methods, and writing forms, which we describe in terms of beginning the story, living the story, and selecting stories to construct and reconstruct narrative plots.
Attribution: Reprint Policy for Educational Researcher: No written or oral permission is necessary to reproduce a tale, a figure, or an excerpt fewer that 500 words from this journal, or to make photocopies for classroom use. Copyright (1990) by the American Educational Research Association; reproduced with permission from the publisher.
- Example Qualitative Narrative Inquiry Design
First, the applied doctoral manuscript narrative inquiry researcher should recognize that they are earning a practical/professional based doctorate (Doctor of Education), rather than a research doctorate such as a Ph.D. Unlike a traditional Ph.D. dissertation oral defense where the candidates focus is on theory and research, the NU School of Education applied doctoral candidate presents their finding and contributions to practice to their doctoral committee as a conceptual professional conference level presentation that centers on how their study may resolve a complex problem or issue in the profession. When working on the applied doctoral manuscript keep the focus on the professional and practical benefits that could arise from your study. If the Applied Doctoral Experience (ADE) student is unsure as to whether the topic fits within the requirements of the applied doctoral program (and their specialization, if declared) they should reach out to their research course professor or dissertation chair for guidance. This is known as alignment to the topic and program, and is critical in producing a successful manuscript. Also, most applied doctoral students doing an educational narrative inquiry study will want to use a study site to recruit their participants. For example, the study may involve teachers or college faculty that the researcher will want to interview in order to obtain their stories. Permission may be need from not only the NU Institutional Review Board (IRB), but also the study site. For example, conducting interviews on campus, procuring private school district or college email lists, obtaining archival documents, etc.
The popularity of narrative inquiry in education is increasing as a circular and pedagogical strategy that lends itself to the practical application of research (Kim, 2016). Keep in mind that by and large practical and professional benefits that arise from a narrative inquiry study revolve around exploring the lived experiences of educators, education administrators, students, and parents or guardians. According to Dunne (2003),
Research into teaching is best served by narrative modes of inquiry since to understand the teacher’s practice (on his or her own part or on the part of an observer) is to find an illuminating story (or stories) to tell of what they have been involved with their student” (p. 367).
- Temporality – the time of the experiences and how the experiences could influence the future;
- Sociality – cultural and personal influences of the experiences; and;
- Spatiality – the environmental surroundings during the experiences and their influence on the experiences.
From Haydon and van der Riet (2017)
- Narrative researchers collect stories from individuals retelling of their life experiences to a particular phenomenon.
- Narrative stories may explore personal characteristics or identities of individuals and how they view themselves in a personal or larger context.
- Chronology is often important in narrative studies, as it allows participants to recall specific places, situations, or changes within their life history.
Sampling and Sample Size
- Purposive sampling is the most often used in narrative inquiry studies. Participants must meet a form of requirement that fits the purpose, problem, and objective of the study
- There is no rule for the sample size for narrative inquiry study. For a dissertation the normal sample size is between 6-10 participants. The reason for this is sampling should be terminated when no new information is forthcoming, which is a common strategy in qualitative studies known as sampling to the point of redundancy.
Data Collection (Methodology)
- Participant and researcher collaborate through the research process to ensure the story told and the story align.
- Extensive “time in the field” (can use Zoom) is spent with participant(s) to gather stories through multiple types of information including, field notes, observations, photos, artifacts, etc.
- Field Test is strongly recommended. The purpose of a field study is to have a panel of experts in the profession of the study review the research protocol and interview questions to ensure they align to the purpose statement and research questions.
- Member Checking is recommended. The trustworthiness of results is the bedrock of high-quality qualitative research. Member checking, also known as participant or respondent validation, is a technique for exploring the credibility of results. Data or results are returned to participants to check for accuracy and resonance with their experiences. Member checking is often mentioned as one in a list of validation techniques (Birt, et al., 2016).
Narrative Data Collection Essentials
- Restorying is the process of gathering stories, analyzing themes for key elements (e.g., time, place, plot, and environment) and then rewriting the stories to place them within a chronological sequence (Ollerenshaw & Creswell, 2002).
- Narrative thinking is critical in a narrative inquiry study. According to Kim (2016), the premise of narrative thinking comprises of three components, the storyteller’s narrative schema, his or her prior knowledge and experience, and cognitive strategies-yields a story that facilitates an understanding of the others and oneself in relation to others.
Instrumentation
- In qualitative research the researcher is the primary instrument.
- In-depth, semi-structured interviews are the norm. Because of the rigor that is required for a narrative inquiry study, it is recommended that two interviews with the same participant be conducted. The primary interview and a follow-up interview to address any additional questions that may arise from the interview transcriptions and/or member checking.
Birt, L., Scott, S., Cavers, D., Campbell, C., & Walter, F. (2016). Member checking: A tool to enhance trustworthiness or merely a nod to validation? Qualitative Health Research, 26 (13), 1802-1811. http://dx.doi.org./10.1177/1049732316654870
Cline, J. M. (2020). Collaborative learning for students with learning disabilities in inclusive classrooms: A qualitative narrative inquiry study (Order No. 28263106). Available from ProQuest Dissertations & Theses Global. (2503473076).
Connelly, F. M., & Clandinin, D. J. (1990). Stories of Experience and Narrative Inquiry. Educational Researcher, 19 (5), 2–14. https://doi.org/10.1080/03323315.2018.1465839
Dunne, J. (2003). Arguing for teaching as a practice: A reply to Alasdair Macintyre. Journal of Philosophy of Education . https://doi.org/10.1111/1467-9752.00331
Haydon, G., & der Riet, P. van. (2017). Narrative inquiry: A relational research methodology suitable to explore narratives of health and illness. Nordic Journal of Nursing Research , 37(2), 85–89. https://doi.org/10.1177/2057158516675217
Kim, J. H. (2016). Understanding Narrative Inquiry: The crafting and analysis of stories as research. Sage Publications.
Kim J. H. (2017). Jeong-Hee Kim discusses narrative methods [Video]. SAGE Research Methods Video https://www-doi-org.proxy1.ncu.edu/10.4135/9781473985179
O’ Toole, J. (2018). Institutional storytelling and personal narratives: reflecting on the value of narrative inquiry. Institutional Educational Studies, 37 (2), 175-189. https://doi.org/10.1080/03323315.2018.1465839
Ollerenshaw, J. A., & Creswell, J. W. (2002). Narrative research: A comparison of two restorying data analysis approaches. Qualitative Inquiry, 8 (3), 329–347.
- << Previous: Design and Development Research (DDR) For Instructional Design
- Next: Action Research Resource >>
- Last Updated: Jul 28, 2023 8:05 AM
- URL: https://resources.nu.edu/c.php?g=1013605

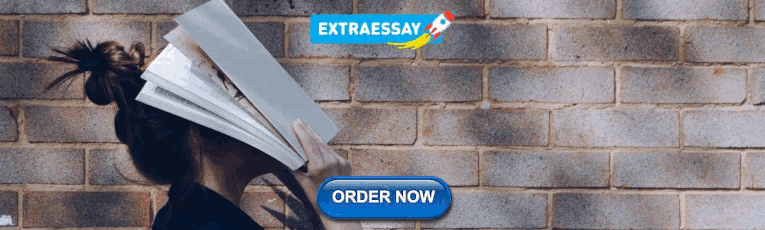
IMAGES
VIDEO
COMMENTS
Narrative analysis is a qualitative research methodology that involves examining and interpreting the stories or narratives people tell in order to gain insights into the meanings, experiences, and perspectives that underlie them. Narrative analysis can be applied to various forms of communication, including written texts, oral interviews, and ...
Narrative analysis is a type of qualitative data analysis that focuses on interpreting the core narratives from a study group's personal stories. Using first-person narrative, data is acquired and organized to allow the researcher to understand how the individuals experienced something. Instead of focusing on just the actual words used during ...
Simply put, narrative analysis is a qualitative analysis method focused on interpreting human experiences and motivations by looking closely at the stories (the narratives) people tell in a particular context. In other words, a narrative analysis interprets long-form participant responses or written stories as data, to uncover themes and meanings.
a sample narrative analysis. Narrative analysis is a method with a particular history and epistemology, and it is designed to answer certain types of research questions. As part of the growing recognition of the value and legitimacy of qualitative inquiry in psychology, narrative analysis is becoming increasingly articulated and refined.
Hence, understanding qualitative research analysis will greatly inform us, who are about to go into the details of narrative data analysis. I assume that you have taken one or two (or more) qualitative research courses as part of your program of study. As you know, data analysis in qualitative research in general is comprised of: examining raw ...
Narrative analysis is a form of qualitative research in which the researcher focuses on a topic and analyzes the data collected from case studies, surveys, observations or other similar methods. The researchers write their findings, then review and analyze them. To conduct narrative analysis, researchers must understand the background, setting ...
Narrative analysis in research. Narrative analysis is an approach to qualitative research that involves the documentation of narratives both for the purpose of understanding events and phenomena and understanding how people communicate stories. Collecting narrative data means focusing on individual research participants to understand particular ...
Oct 5, 2023. Qualitative data analysis varies by methodology, so there is no one approach that fits across different types of studies. Narrative research is focused on the elicitation and interpretation of people's narrative accounts of their experience. A method based on the use of diaries which have been created for the purposes of research.
In this book, Ruthellen Josselson and Phillip L. Hammack introduce readers to narrative analysis, a qualitative method that investigates how people make meaning of their lives and experiences in both social and cultural contexts. This method offers researchers a window into how individuals' stories are shaped by the categories they inhabit ...
Narrative inquiry has been defined as a methodology "in which stories are used to describe human action" (Polkinghorne 1995, p. 5).Schwandt further explains that stories are central to all aspects of narrative inquiry.Narrative inquiry includes not only generating data in the form of stories but is also a means of analyzing stories about life experiences and a method of representing and ...
Narrative inquiry appeared in the educational field in 1990 through the work of Connelly and Clandinin with a central focus on lived experience (Clandinin et al., 2007).The quest for knowledge in this qualitative research methodology intends to advance understanding of the experiences of people across place and time (Dewart et al., 2019). ...
strongly on narrative analysis as a qualitative research method, pointing toward possibilities for other research practices whenever appropriate. The Emergence of Narrative Analysis Having clarifi ed that narrative analysis is invested in both the means and the way these means are put to use to arrive at presentations and interpretations of
Learn the basics of narrative analysis in 10 minutes! Narrative analysis is a powerful qualitative analysis method that can help you understand the underlyin...
As previously noted, narrative research (also referred to as narrative analysis) is a family of approaches which focus on the stories that people use to understand and describe aspects of their lives from the stories they tell (Riessman and Quinney 2005; Kim and Latta 2009).The term "narrative" carries multiple meanings and is used in a variety of ways by different human or social science ...
Narrative analysis is a valuable data analysis technique in qualitative research. It is typically used in those studies which have already employed narrative inquiry as a qualitative method. Narrative knowledge is created and constructed through the stories of lived experience and sense-making, the meanings people afford to them, and therefore offers valuable insight into the…
For many researchers unfamiliar with qualitative research, determining how to conduct qualitative analyses is often quite challenging. Part of this challenge is due to the seemingly limitless approaches that a qualitative researcher might leverage, as well as simply learning to think like a qualitative researcher when analyzing data. From framework analysis (Ritchie & Spencer, 1994) to content ...
The second method for analysis of patient-generated narratives is qualitative content analysis. Qualitative content analysis is a research method designed to identify the thematic structure of text documents by subjectively interpreting the context of the text . This process is conducted through a systematic classification process of coding and ...
What is narrative analysis in qualitative research? Researchers use narrative analysis to understand how research participants construct story and narrative from their own personal experience. That means there is a dual layer of interpretation in narrative analysis. First the research participants interpret their own lives through narrative.
1. Provide an example of when narrative inquiry would be the most appropriate qualitative research approach. 2. Identify the activities involved in data collection. 3. Define and describe narrative thematic data analysis. 4. Discuss data verification and validation strategies used in qualitative research approaches.
This chapter is designed to help forge helpful pathways through the thickets of choices one have when engaging in narrative inquiry under the premise of qualitative inquiry as our overarching umbrella. It starts with a brief consideration of two questions: First, what forms do qualitative (narrative) data take, and where do one find that data? Second, having made decisions with regard to what ...
Data analysis in narrative research includes four stages: (1) prepar ing the data, (2) identifying basic units of data, (3) organizing data, and (4) interpretation of data as suggested by Newby ...
Narrative inquiry is relatively new among the qualitative research designs compared to qualitative case study, phenomenology, ethnography, and grounded theory. What distinguishes narrative inquiry is it beings with the biographical aspect of C. Wright Mills' trilogy of 'biography, history, and society' (O'Tolle, 2018).
Qualitative interviews as foundation for narrative analysis. The purpose of qualitative studies is to get in-depth-knowledge about certain contexts, to. achieve a comprehensive understanding of ...
Narrative analysis has developed especially strong in the field of organizational studies (Boje Citation 1991). Basic assumptions underlying most studies are that the human being is a story-telling animal, that narrative is important in making sense of experience (Boje Citation 1991). We can distinguish different approaches in narrative research.
Background . The goal of qualitative research is to learn more about the opinions and experiences of the subjects being studied in relation to a particular question. There is a paucity of information on opportunities and challenges encountered to conduct qualitative research among the academic staffs in the health sciences. The purpose of this study was to examine the opportunities and ...
The narrative findings in this qualitative study offer context-sensitive information for developing out-of-hospital overdose scenarios applicable to simulation training. These insights can serve as a valuable resource, aiding instructors and researchers in systematically creating evidence-based scenarios for both training and research purposes.