- Open supplemental data
- Reference Manager
- Simple TEXT file
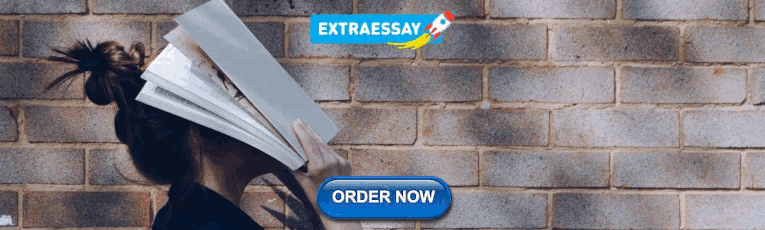
People also looked at
Original research article, selecting and justifying quantitative analysis techniques in single-case research through a user-friendly open-source tool.
- Department of Educational and Counseling Psychology, University at Albany, Albany, NY, United States
Certain quantification techniques may be more appropriate than others for single-case design analysis depending on the research questions, the data or graph characteristics, and other desired features. The purpose of this study was to introduce a newly developed and empirically validated user-friendly tool to assist researchers in choosing and justifying single-case design quantification techniques. A total of sixteen different quantification techniques and nine facets (research questions, data or graph characteristics, or desired features) that may affect the appropriateness of a single-case experimental design quantification technique were identified to be included in the tool. The resulting tool provides a ranking of recommended quantification techniques, from most to least appropriate, depending on the user input. A pretest and posttest design was utilized to test the effectiveness of the tool amongst 25 participants. The findings revealed that those who use single-case designs may need support in choosing and justifying their use of quantification techniques. Those who utilize single-case designs should use the developed tool (and other tools) to assist with their analyses. The open-source tool can be downloaded from osf.io/7usbj/ .
Introduction
A single-case experimental design 1 (SCED) is a research design that allows repeated measures of the dependent variable within one case ( What Works Clearinghouse, 2020 ). The dependent variable is measured at different sequential moments in time and across different levels of the independent variable (i.e., treatment indicator), which are also known as “phases” (e.g., baseline and treatment phase). The case serves as its own control rather than having multiple participants in an experimental or control group, as is typical for group comparison designs. The goal of using SCEDs is to find evidence for a functional relation (experimental control) between the researcher-manipulated independent variable and the dependent variable of interest. Two key features of SCEDs are replication and randomization, as these help establish internal and external validity ( Horner et al., 2005 ). Replication occurs when there are multiple opportunities to measure the effect of the independent variable on the dependent variable; this can occur within participants, between participants, or both. Randomization can enhance the internal validity of SCEDs by ensuring that any results are caused by the intervention and not caused by confounding or underlying variables. Randomization prevents experimenter bias because the introduction of the intervention is pre-determined.
Single-case experimental designs (SCEDs) have several benefits over group comparison designs, where inferences are made at the group level rather than at the individual level. SCEDs allow detailed information to emerge about a single case, which can be useful in fields such as behavior modification ( Shadish and Sullivan, 2011 ) when the problem of interest (e.g., the behavior) occurs at an infrequent rate and/or it is difficult to find a large number of participants ( Shadish, 2014 ). SCEDs can have a high degree of internal validity when they are well-designed ( What Works Clearinghouse, 2020 ). Although SCEDs can be time consuming, as repeated data points need to be taken over time, they can be more cost effective than group designs, which require a larger sample size ( Alnahdi, 2013 ). Similarly, they can be used for pilot studies before attempting a larger scale experiment using a group comparison design ( Shadish, 2014 ).
Due to the unique benefits of SCEDs, researchers have used this research design for over 60 years ( Horner et al., 2005 ). Especially since the turn of the century in 2000, there has been an increase in utilization of SCEDs for research in the field of psychology, as shown in Figure 1 .
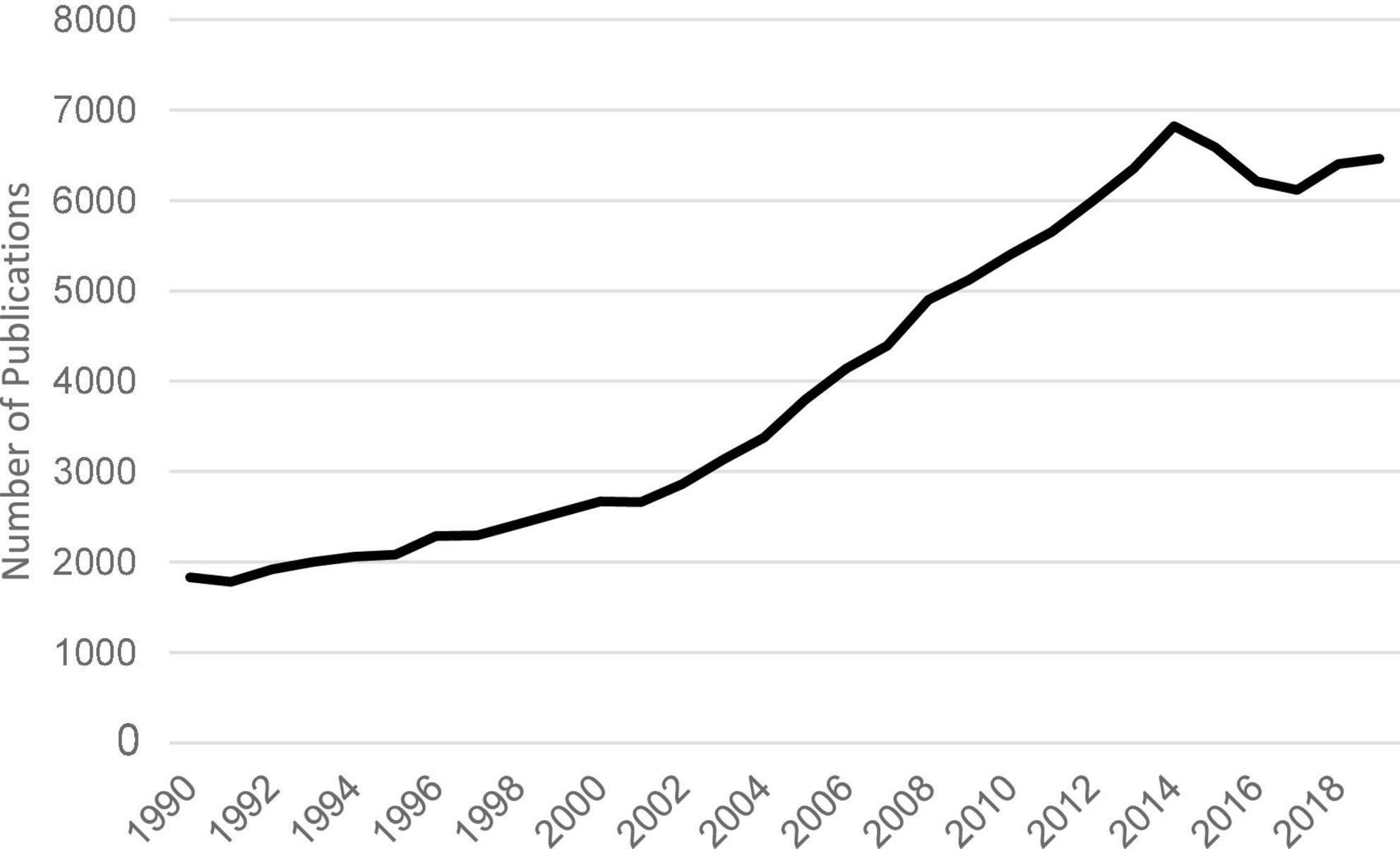
Figure 1. Number of SCED related publications between 1990 and 2019. Increase in the number of publications between 1990 and 2019 when searching terms “single subject” OR “single-subject” OR “single case” OR “single-case” OR “multiple baseline” OR “multiple-baseline” OR “reversal design” OR “ABAB design” OR “withdrawal design” OR “alternating treatment design” on PsychINFO database.
As SCEDs have become more popular, there has been an increased interest in the use of SCED quantitative analysis techniques to use complementary with visual analysis. Certain U.S. federal government laws [e.g., Individuals with Disabilities Education Act [IDEA], 2004 ] were enacted that called for the use of evidence-based practices, and so researchers who used SCEDs needed to ensure that they could quantitatively analyze the outcomes from the data ( Solomon et al., 2015 ). Furthermore, there has been an increase in outside pressure from evidence-based practice communities to use statistics that can also be understood by group comparison design researchers ( Shadish, 2014 ; What Works Clearinghouse, 2020 ). Shadish (2014) also acknowledged pressure from journals and publishing groups to quantify SCED results, and that editors from journals such as the Journal of School Psychology and School Psychology Quarterly have expressed the desire for more researchers to use quantitative analyses with SCEDs.
As a result of this increase in pressure to quantify SCED outcomes, many different statistical analysis techniques for SCEDs have been developed, including non-overlap indices (e.g., percent of non-overlapping data, Scruggs et al., 1987 ; improvement rate difference, Parker et al., 2009 ; non-overlap of all pairs, Parker and Vannest, 2009 ; Tau-U, Parker et al., 2011 ; baseline corrected Tau-U; Tarlow, 2017 ), regression-based effect sizes (e.g., Center et al., 1985-1986 ), the standardized mean difference ( Hedges et al., 2012 ), the log-response ratio and related statistics (e.g., percent of goal obtained, Ferron et al., 2020 ). 2 However, there remains a gap in knowledge as to how to select an appropriate quantification technique to reflect intervention effectiveness, as well as how to report these quantification techniques appropriately ( Solomon et al., 2015 ). Furthermore, researchers rarely report their justification for the quantification technique that they use ( Solomon et al., 2015 ), making it difficult to evaluate the appropriateness of the chosen technique. This can contribute to the improper use of these quantification techniques.
Many online calculators and other applications have been developed to assist in SCED calculations and selection (e.g., Baseline Corrected Tau Calculator, Tarlow, 2016 ; Single Case Research Calculator, Vannest et al., 2016 ; Tau Decision Chart, Fingerhut et al., 2021a ). Other researchers have focused on improving the way that the quality and outcomes of SCEDs are assessed (e.g., Single-Case Analysis and Review Framework; Ledford et al., 2016 ) and reported (Single-Case Reporting Guideline in Behavioral Interventions; Tate et al., 2016 ). However, researchers may still benefit from using a tool that helps them systematically determine the best quantification technique to use depending on the research questions, data characteristics, and desired features.
The first purpose of this study is to introduce an empirically validated and user-friendly tool to help applied SCED researchers choose and justify their use of appropriate SCED quantification techniques. The second purpose of this study is to test the effectiveness of the tool in assisting users to choose an appropriate SCED quantification technique and provide a justification for the quantification technique. A third aim of the study is to determine the social validity of the tool (i.e., acceptance amongst applied researchers). Thus, the research questions and hypotheses for this study are as follows:
1. Given an AB graph/data set and research question, is a tool for single-case quantification effective in assisting single-case researchers to choose an appropriate quantification technique? It was hypothesized that a tool for single-case quantification causes a statistically significant change in single-case researcher’s ability to select an appropriate quantification technique.
2. Given an AB graph/data set and research question, is a newly developed tool for single-case quantification effective in assisting single-case researchers to provide appropriate justifications for using a quantification technique? It was hypothesized that a tool for single-case quantification causes a statistically significant change in single-case researcher’s ability to provide appropriate justifications for using a quantification technique.
3. Will single-case researchers find a newly developed tool for single-case quantification technique selection to be socially valid? It was hypothesized that the tool for single-case quantification has high social validity (i.e., most single-case researchers report the tool to be at least “somewhat useful”).
Materials and methods
Sixteen different quantification techniques and nine different facets were identified to be included in a tool to help researchers choose and justify their use of SCED quantification techniques. These selected quantification techniques and components are based upon previous research about SCED quantification techniques (e.g., Manolov and Moeyaert, 2017 ; Manolov et al., 2022 ). Manolov and Moeyaert (2017) provided a comprehensive overview of the SCED quantification techniques available for use, along with various criterion that must be met for the technique to be considered both appropriate and useful. Manolov et al. (2022) built upon this review, while including more newly developed quantification techniques (e.g., log-response ratio; Pustejovsky, 2018 ). Manolov et al. (2022) proposed different dimensions and facets that can be used for justifying and selecting a proper quantification technique. Readers are advised to reference Table 1 of their article for details of these dimensions and facets.
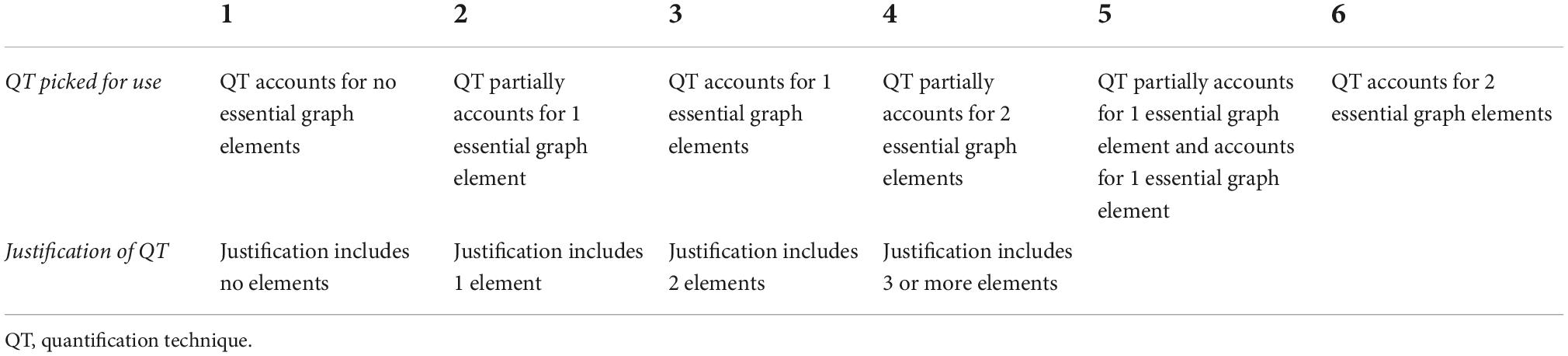
Table 1. Rubric with indicators.
The sixteen different quantification techniques that were identified for the tool based upon the previous research were: baseline-corrected Tau-U ( Tarlow, 2017 ), generalized least squares regression (e.g., Center et al., 1985-1986 ), Hedge’s g for single-case ( Hedges et al., 2012 ), improvement rate difference ( Parker et al., 2009 ), log-response ratio ( Pustejovsky, 2018 ), mean baseline reduction (e.g., Hershberger et al., 1999 ), mean phase difference ( Manolov and Solanas, 2013 ), non-overlap of all pairs ( Parker and Vannest, 2009 ), percent of goal obtained ( Ferron et al., 2020 ), percentage of all non-overlapping data ( Parker et al., 2007 ), percentage of data exceeding the median of the baseline phase ( Ma, 2006 ), percentage of non-overlapping data points ( Scruggs et al., 1987 ), percentage of data points exceeding the median trend ( Wolery et al., 2010 ), percentage of zero data ( Scotti et al., 1991 ), Tau-U ( Parker et al., 2011 ), and Tau-U Trend A ( Parker et al., 2011 ). The focus of the current study is to evaluate researchers’ ability to choose the most appropriate quantification technique for one case rather than across-cases, so between-case methods such as hierarchical linear modeling ( Van den Noortgate and Onghena, 2008 ; Moeyaert et al., 2014 ) and between-case standardized mean difference ( Pustejovsky et al., 2014 ) are not included.
Nine facets were identified for inclusion in the tool, based upon the previous research. These nine facets were identified in Manolov et al. (2022) as having an influence on the suitability of an analysis technique to quantify intervention effectiveness. These facets can be categorized within three broader categories, namely “research questions,” “data or graph characteristics,” and “desired features.” Although other facets exist, for the purpose of creating the tool the number of facets was limited to these nine. The four “research questions” included in the tool are: (a) What is the magnitude of change in level?, (b) What is the magnitude of change in linear trend?, (c) What is the magnitude of change in variability?, and (d) What is the amount of non-overlapping data points? The second category “data or graph characteristics” includes: (a) small number of data points in the baseline or treatment phase, (b) trend in baseline, (c) outliers, and (d) within-case variability. The third category, “desired features,” includes only one facet: a p -value to test for statistical significance. Although there are other research questions, data characteristics, and desired features that may affect appropriateness of quantification technique (e.g., autocorrelation, if the quantification technique produces a standardized or unstandardized outcome, etc.), other facets were not included because they are beyond the skill set of an applied researcher. Other facets may be added into a later version of the tool pending results from this current study (i.e., positive feedback indicating that all included facets were understood).
The tool was created in an Excel document. The user can input “yes” or “no” to each of these nine facets, and based on the user input, the tool determines the most appropriate quantification technique for use. For example, if the facet is “trend” (i.e., “Is there baseline trend?”), the user inputs “yes” if the user wants to find a quantification technique that can account for baseline trend/is robust against baseline trend. For example, referring to Figure 2 , the two facets are (a) research question: What is the amount of non-overlapping data points? and (b) data characteristic: trend in baseline. Thus, using the input (outlined in red in Figure 3 ), all inputs would be set to “no” except for “overlap/data separation between phases” and “baseline trend.” The input would be set to “yes” for these two facets. The output then automatically updates and displays the ranked quantification techniques in order of appropriateness. Referring to Figure 3 , baseline-corrected Tau-U is ranked first and thus most recommended, followed second by percentage of data exceeding the median trend and Tau-U Trend A , and the third most appropriate quantification techniques are found next (i.e., improvement rate difference, non-overlap of all pairs, and percentage of non-overlapping data).
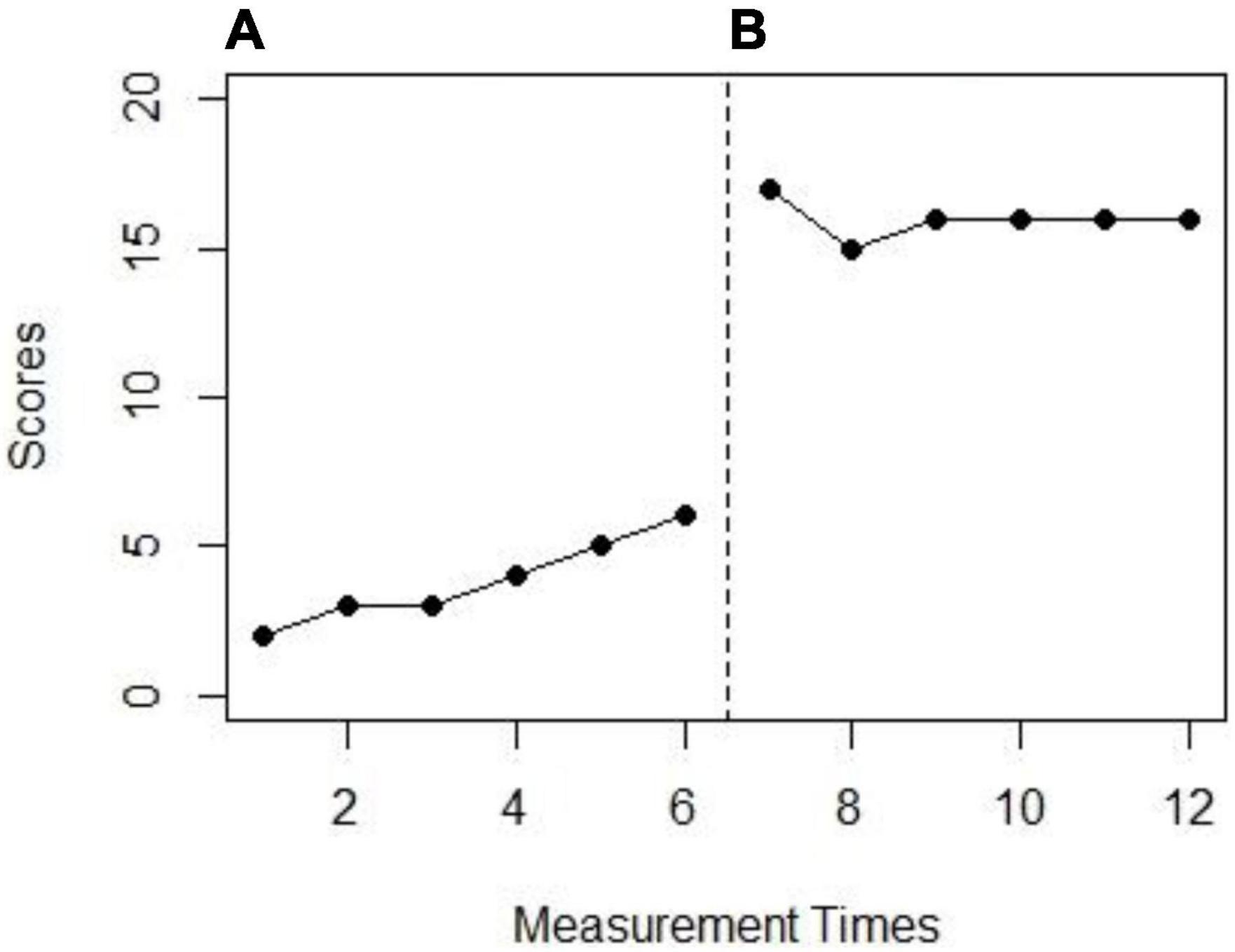
Figure 2. Example (A,B) graph with two facets (Data characteristic: baseline trend and research question: Non-overlapping data). Research question: What is the amount of non-overlapping data between phases (A,B) ?
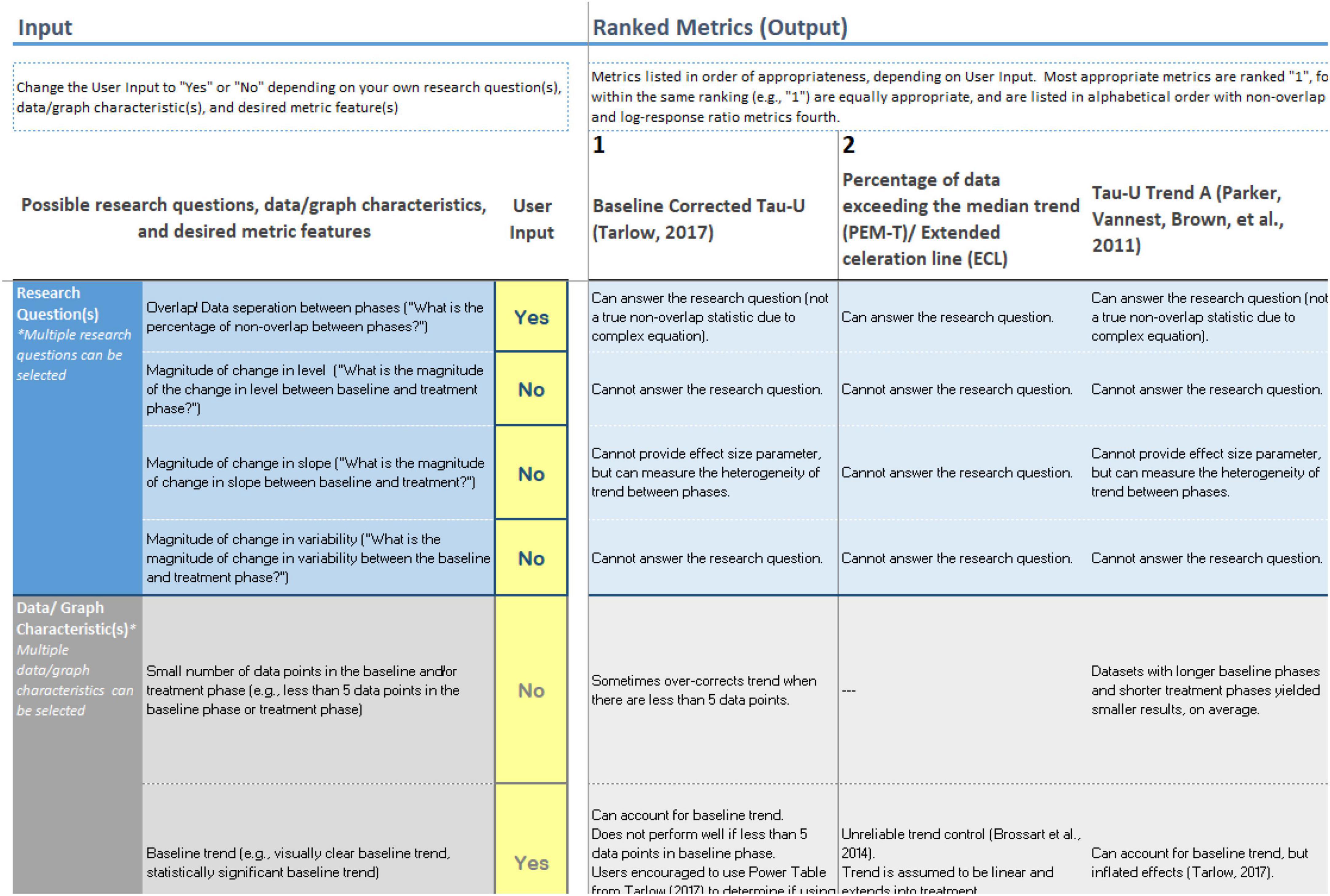
Figure 3. Tool input and output with ranked quantification techniques. As this is only a screenshot of the tool, readers are encouraged to download the tool themselves to read more details at: osf.io/7usbj/ .
Instructions (in both written and video form) for how to use the tool were added to the first sheet of the Excel document. The tool, as described in the previous section and displayed in Figure 3 , was added to the second sheet of the Excel document. On a third sheet, detailed information about each of the quantification techniques was added. On a fourth sheet, notes, references, and information about tool development were added. The complete tool with all four sheets can be downloaded from: osf.io/7usbj/
Five experts in the field of SCED validated the tool. These experts were systematically selected by searching “single-case” OR “single case” OR “single-subject” OR “single subject” on Web of Science starting from the year 2019, and sorting by Author/Creator. This ensured that the experts selected were familiar with the most recently developed SCED quantification techniques. First, the top five authors were contacted. After agreeing to review the tool, the experts were sent the tool and were asked to provide feedback regarding the accuracy of the information in the tool, the usability of the tool, and any other comments about the tool. Experts were also provided with a separate document that included five example graphs/data sets and research questions and were asked to evaluate the recommendations from the tool for each of the graphs/data sets and research questions. Next, the experts tested the tool and recorded their feedback. Three of the selected experts delivered feedback for the tool via writing (i.e., Word document) and two delivered feedback orally (i.e., Zoom meeting). There were several recommendations to adjust the tool that were made by multiple reviewers. Two reviewers recommended that the tool be explained carefully on the Instructions tab of the tool. Three reviewers recommended explaining in greater detail the definitions of the data characteristics. Two reviewers recommended clarifying that the tool is meant to be used for within-case estimates. All feedback was addressed, and the changes were made to the tool accordingly; the feedback along with the changes made were compiled into one document, which is found in Appendix A.
Participants
Institutional Review Board approval for the study was received from State University of New York at Albany. Anyone who conducted SCED research was eligible to participate. This was defined as someone who had interpreted the results of a SCED graph either with support (e.g., with help from an instructor or colleague) or independently within the last year. The participant needed to have conducted SCED analysis within the last year, and the participant also needed to be interested in quantifying the effect from a SCED graph. Snowball sampling was used to recruit participants. Participants were recruited from the Association for Behavior Analysis International list of colleges that offered applied behavior analysis courses; the professors who were part of the programs were contacted and asked to share information about the study with their students. Thus, potential participants were provided information about the study and then asked to provide other potential participants with information about the study.
A total of 25 participants participated in the study from a range of locations across several countries, with the majority of participants residing within the US. Two participants indicated Bachelor’s as their highest degree obtained, twelve participants indicated having a Master’s degree, and eleven participants indicated having a Ph.D. Seven participants identified with educational psychology department, six identified with school psychology, six identified with special education and/or applied behavior analysis, and six identified with a different department, such as psychology or prevention science. A total of two participants reported that they were very confident in their ability to choose appropriate quantification techniques, sixteen participants reported being somewhat confident, six participants were somewhat unconfident, and one participant reported being not at all confident. Similarly, one participant was very confident in their ability to justify using a quantification technique at the beginning of the study, sixteen participants were somewhat confident, seven participants were somewhat unconfident, and one participant was not at all confident. A total of ten participants had previously published SCED research in the past, thirteen participants had not yet published SCED research, but would like to in the future, and two participants had not and did not intend to publish SCED research.
The Excel tool included the four sheets (Instructions, Tool, Metric Details, and Notes and References).
Pre/posttest AB graphs/data sets and research questions
Five pre/posttest AB graphs/data sets and research questions were created to help determine the effectiveness of the tool. AB graphs were chosen for this study as these are the “building blocks” of more complex SCEDs (e.g., multiple-baseline designs, withdrawal designs, etc.). SCED quantification techniques that are used with AB designs can be extended for use with these more complex designs. The raw data for the graphs/data sets were simulated so that each of the five pre/posttest graphs/data sets clearly demonstrated two facets: one facet related to data characteristic or desired quantification technique feature and one related to the research question. These graphs are displayed in Appendix B and an example is displayed in Figure 2 .
A demographic survey and social validity survey were developed to help evaluate the effectiveness of the tool. The demographic survey includes questions regarding the participant’s academic department, education level, and confidence in choosing and justifying the use of quantification techniques. The social validity survey asks participants on a 4-point Likert scale how likely they would be to use the tool in the future and how helpful they find the tool to be for choosing and justifying the use of quantification techniques.
Rubric with indicators
A rubric with three indicators was developed to measure the appropriateness of the quantification technique chosen and the appropriateness of the justification provided by the participant. This rubric is displayed in Table 1 .
The elements used to evaluate the justification of the quantification technique are: “research question,” “quantification,” “number of observations,” “autocorrelation,” “outliers,” “missing data,” “baseline trend,” “variability,” “statistical properties,” and “sampling distribution.” These elements are founded upon the categories and facets identified by Manolov et al. (2022) .
Applied example
Referring to Table 1 , below is an example response and how it would be graded with the rubric.
Mean phase difference can be used for this graph and data set. MPD is a quantification technique that can estimate the magnitude of change in level between the baseline and treatment phase. The MPD estimate is 30.12.
This response is given a score of 5 for the indicator “quantification technique picked for use,” as MPD partially accounts for one of the essential elements and fully accounts for the other essential element. MPD can answer research questions related to the magnitude of change in level. MPD can be used when there are less than five data points in the baseline phase, but results are dependent on phase length, especially when the treatment phase is longer than the baseline phase ( Manolov and Solanas, 2013 ). For “justification of the quantification technique,” this response would be given a score of 2 since it includes only one essential element in the justification (related to “research question”).
After the tool was adjusted according to the expert feedback, participants were invited to participate in the study. Participants were given access to a Qualtrics link where they were able to read the consent form, located on the first page. After digitally signing the consent form, participants answered the screening questions. Next, the participants were shown the demographic survey and asked to complete the survey. After completing the demographic survey, participants were shown five pre/posttest AB graphs/data sets paired with the research questions. Participants were asked “Report the metric you would use to answer the research question(s). Provide a rationale for the metric(s) you would use. Optional: Complete the calculation.”
Next, the participants moved onto the next page where a link to the tool was provided. The participants read the instructions/watched the instructional video and could then access the tool. The participants were also provided with example graphs/data sets and research questions, and were allowed to practice using the tool for an unlimited amount of time. When participants were ready, they returned to the Qualtrics link. Participants were shown the same five pre/posttest AB graphs/data sets with research questions and given the same instructions as during pretest, but were instructed to use the tool to answer the questions. Once this was completed, participants moved onto the next page of Qualtrics and were asked to complete the demographic survey. The study completion time was recorded, and this marked the end of the study.
Statistical analysis
The participant received a score for each of the five pre/posttest AB graphs/data sets and research questions, as well as an accumulated score (i.e., across the five pre/posttest AB graphs/data sets and research questions) using the rubric in Table 1 , both before using the tool (pretest) and after accessing the tool (posttest). An alpha level of.05 was used, and Bonferroni corrections were applied for multiple testing to avoid inflated family-wise type I error. All analyses were conducted with IBM SPSS Statistics (Version 27.0.1.0; IBM Corp, 2017 ).
Two-tailed paired t -tests were conducted to compare the pretest and posttest scores in choice and justification of quantification technique for each of the five questions, as well as the accumulated score. These results were also supplemented with the Wilcoxon signed-rank test, which is the non-parametric version of the parametric paired t -test. A total of 30 participants are typically needed to make the assumption that the sampling distribution is normally distributed ( Brosamler, 1988 ; Fischer, 2010 ). If there are less than 30 participants and the pretest scores and change in scores are non-normal, then the sampling distribution will be non-normal. For this reason, all inferential results were supplemented with non-parametric tests, which do not make asymptotical assumptions. One-way ANOVAs were also conducted to determine if there were statistically significant differences in total (i.e., across the five questions) change in scores for choice and justification of quantification technique for participants depending on their academic background. The non-parametric equivalent of the ANOVA, Kruskal-Wallis test, was also conducted. Two-tailed independent paired t -tests and the non-parametric equivalent Mann-Whitney test were conducted to determine if there were statistically significant differences in total pretest scores and change in scores for choice and justification of quantification technique for participants depending on their education level. Descriptive analyses (i.e., averages and frequency) from the social validity survey were reported to determine the acceptability of the tool amongst the participants.
Descriptive statistics
Upon completing the study, seventeen of the twenty-five participants reported that they would be very likely to use the tool in the future, six participants reported that they would be somewhat likely, and two participants reported that they would be somewhat unlikely to use it in the future. A total of twenty-one participants rated the tool as being very helpful for choosing an appropriate quantification technique, three participants rated the tool as being somewhat helpful, and one participant rated the tool as somewhat unhelpful. A total of nineteen participants rated the tool as being very helpful for justifying the use of a quantification technique, four participants rated the tool as somewhat helpful, and two participants rated the tool as somewhat unhelpful. 3
Table 2 displays the pretest scores, posttest scores, and change in scores for choice and justification of quantification technique. There was a positive change in scores for each of the five questions for both choice and justification of quantification technique.
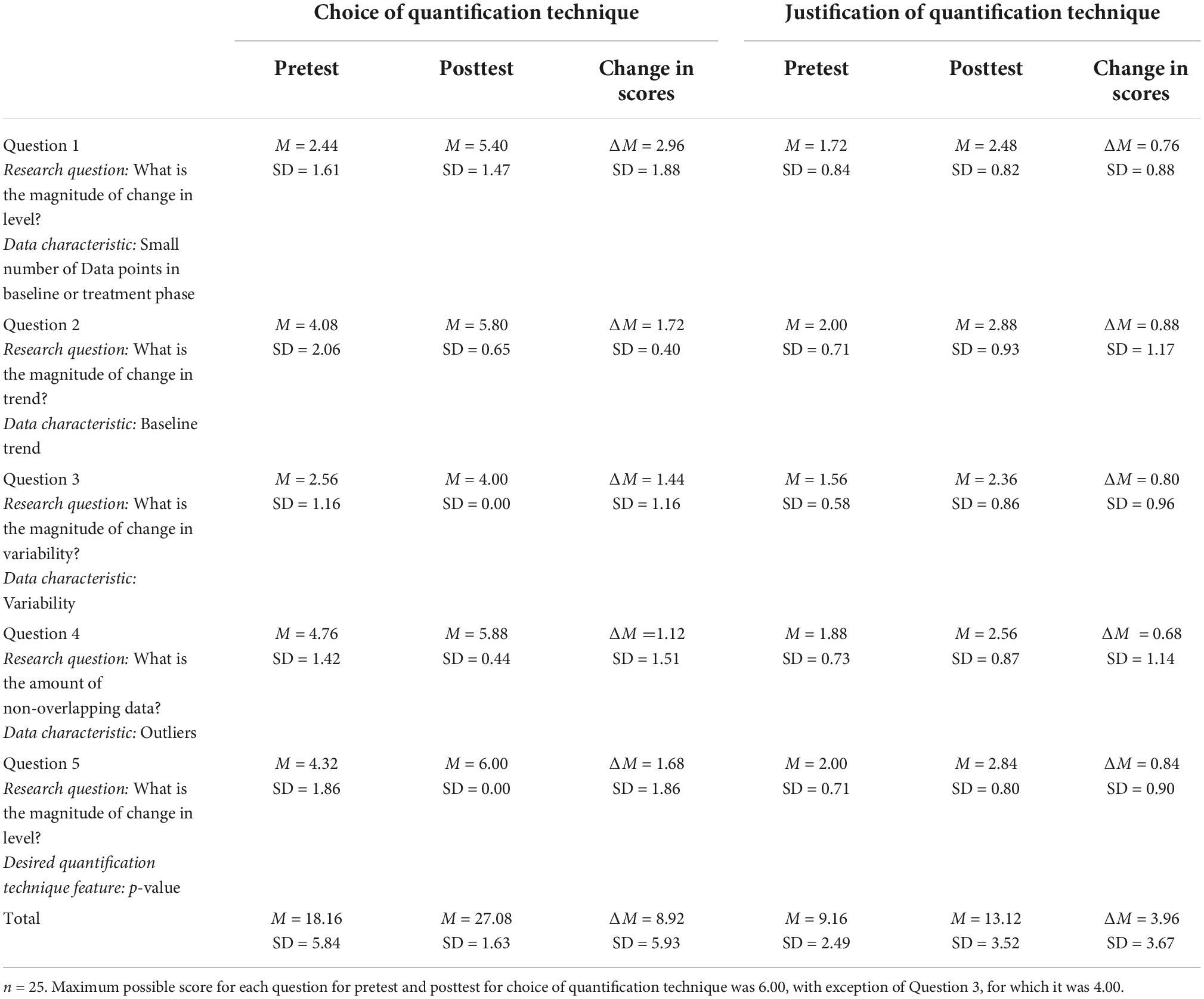
Table 2. Average scores for pretest and posttest questions 1–5.
Inferential analyses
Change in scores.
Six two-tailed paired t -tests were conducted to determine if the change in scores for (a) choosing and (b) justifying the quantification technique before and after using the tool was statistically significant for total (i.e., accumulated) change in scores and for each of the five individual questions.
The total average change in scores for choosing an appropriate quantification technique before ( M = 18.16, SE = 1.17) and after ( M = 27.08, SE = 0.33) using the tool was 8.92, d = 1.50, SE = 1.19, 95% CI (6.47, 11.37), t (24) = 7.52, p < 0.0083. This indicates a statistically and clinically significant change in total scores (i.e., across the five questions) after accessing the tool. The results of the non-parametric Wilcoxon signed-rank test were in line with the results of the parametric test ( Z = 4.28, p < 0.0083). The total change in scores for justifying the use of a quantification technique before ( M = 9.16, SE = 0.50) and after ( M = 13.12, SE = 0.70) using the tool was 3.96, d = 1.08, SE = 0.73, 95% CI [2.45, 5.47], t (24) = 5.40, p < 0.0083, indicating a statistically and clinically significant change in scores between the pretest and posttest. Similar results were obtained from the Wilcoxon signed-rank test ( Z = 3.69, p < 0.0083). There was a statistically significant change in scores for both choice and justification of quantification technique for each of the individual questions. These results are displayed in Table 3 and visually displayed in Figure 4 .
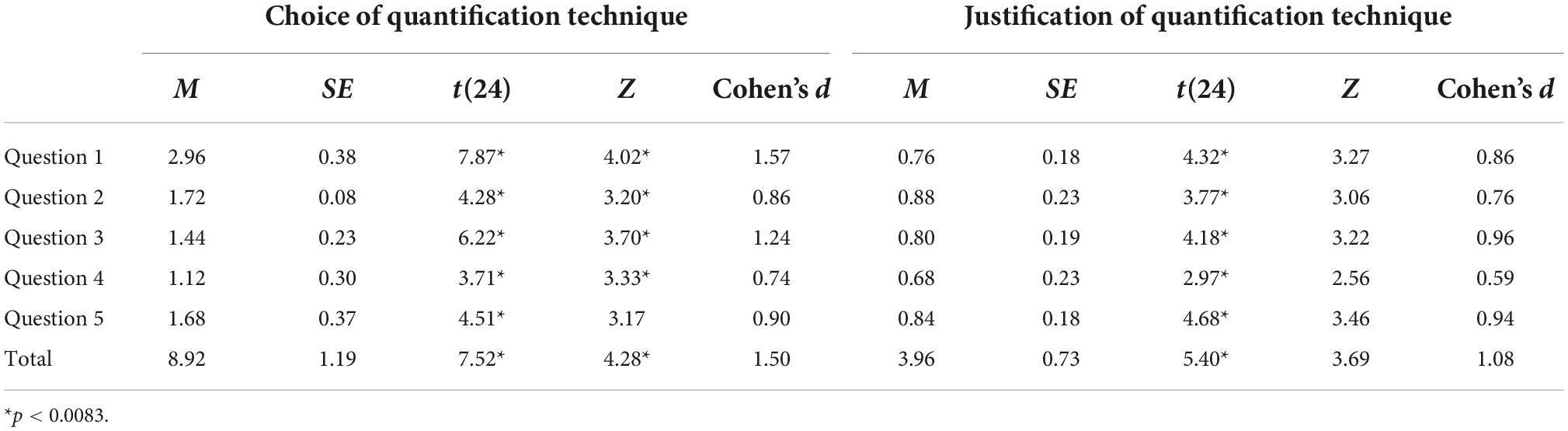
Table 3. Paired t -test results.
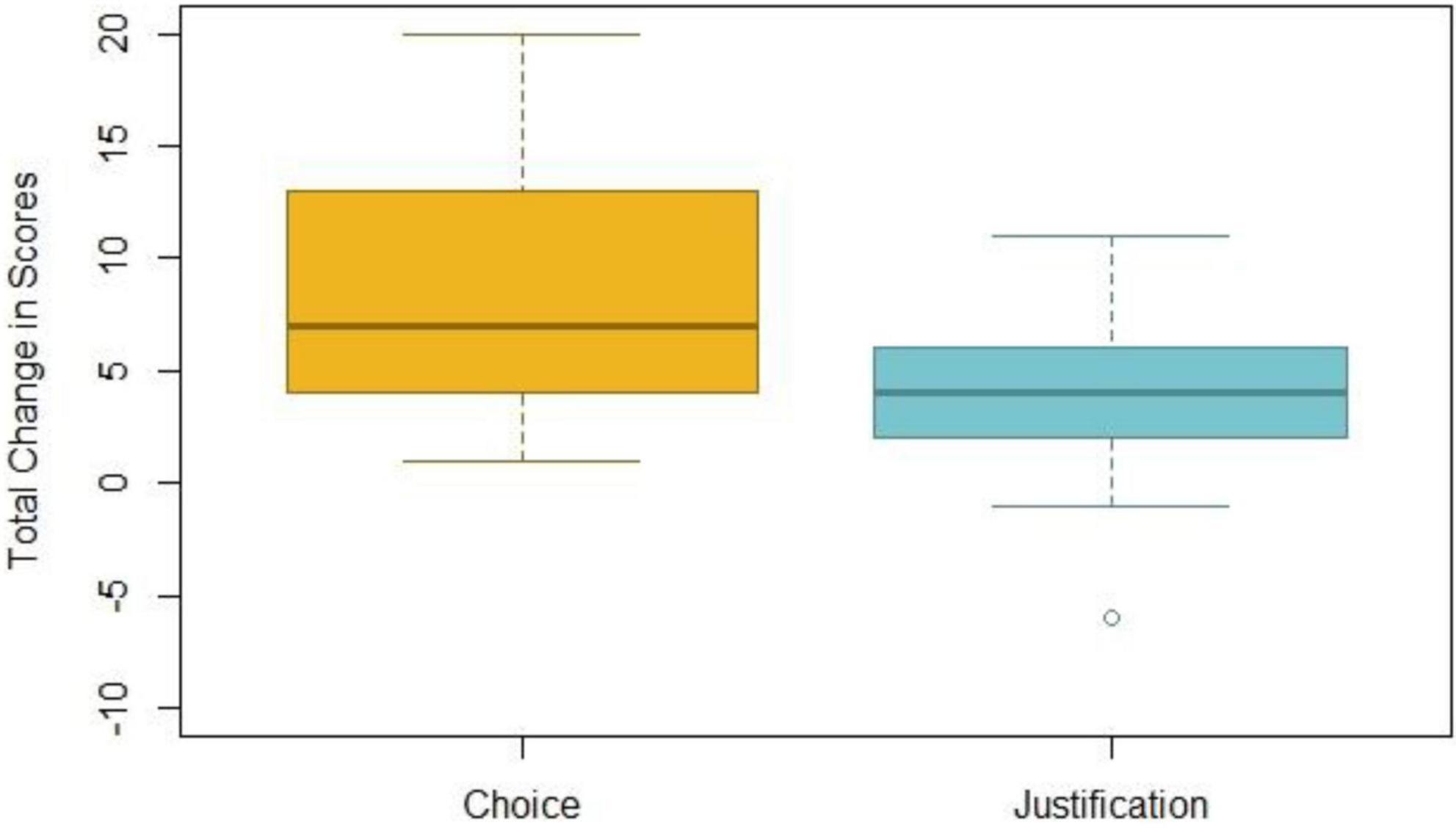
Figure 4. Total change in scores for choice and justification of quantification technique.
Academic department differences
Four one-way ANOVAs were conducted to determine if there was a statistically significant difference in total change in scores for choice and justification of quantification technique, depending on the participant’s academic department (special education, educational psychology, school psychology, and others). For the purpose of inferential analyses, participants who reported being a part of both special education and applied behavior analysis were coded as “special education” ( n = 2). Results of the ANOVAs are displayed in Table 4 and are visually displayed in Figure 5 .

Table 4. Analysis of variance results for academic department.
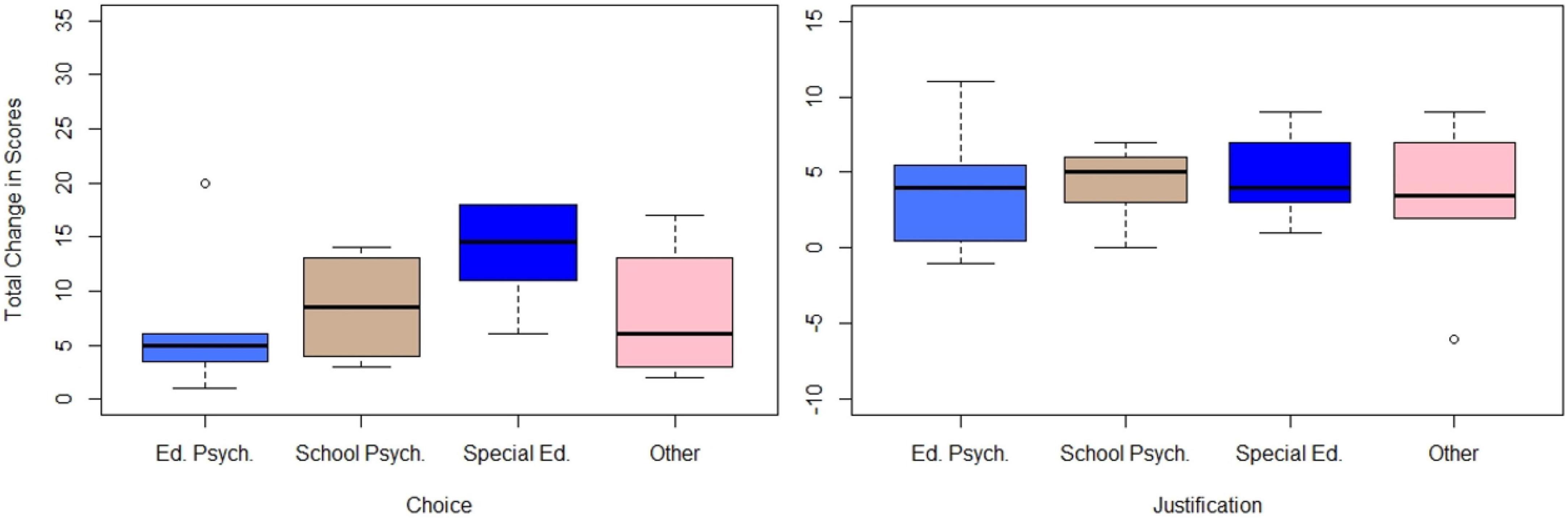
Figure 5. Total change in scores for choice and justification of quantification technique across academic departments.
The ANOVAs showed no statistically significant effect of academic department on total change in scores for choice of quantification technique [η 2 = 0.23, F (3, 21) = 2.14, p = 0.13,], nor for justification of quantification technique [η 2 = 0.02, F (3, 21) = 0.18, p = 0.91,]. A total of 23 and 2% of the variance in change in scores for choice and justification of quantification technique can be attributed to academic department, respectively. Although not statistically significant, 23% is a moderately large effect. The non-parametric Kruskal-Wallis test was also conducted and showed no effect of academic department on change in scores for choice of quantification technique [ H (3) = 5.84, p = 0.12], nor for justification of quantification technique [ H (3) = 0.44, p = 0.93].
Education level differences
Four independent two sample t -tests were conducted to determine if there was a difference in total change in scores for choice and justification of quantification technique depending on education level (Master’s Degree vs. Ph.D). Results of the independent t -tests are displayed in Table 5 and are visually displayed in Figure 6 .
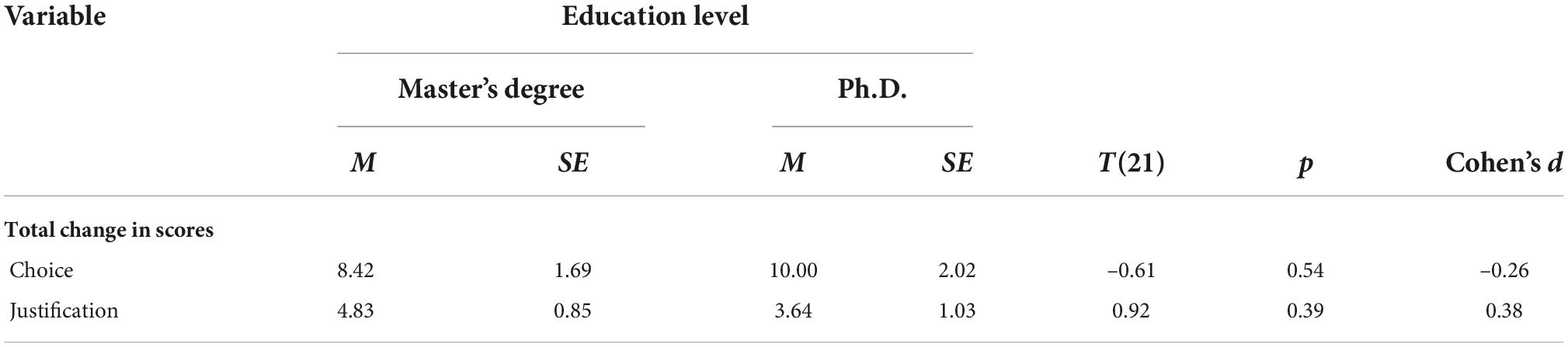
Table 5. Independent t -test results for education level.
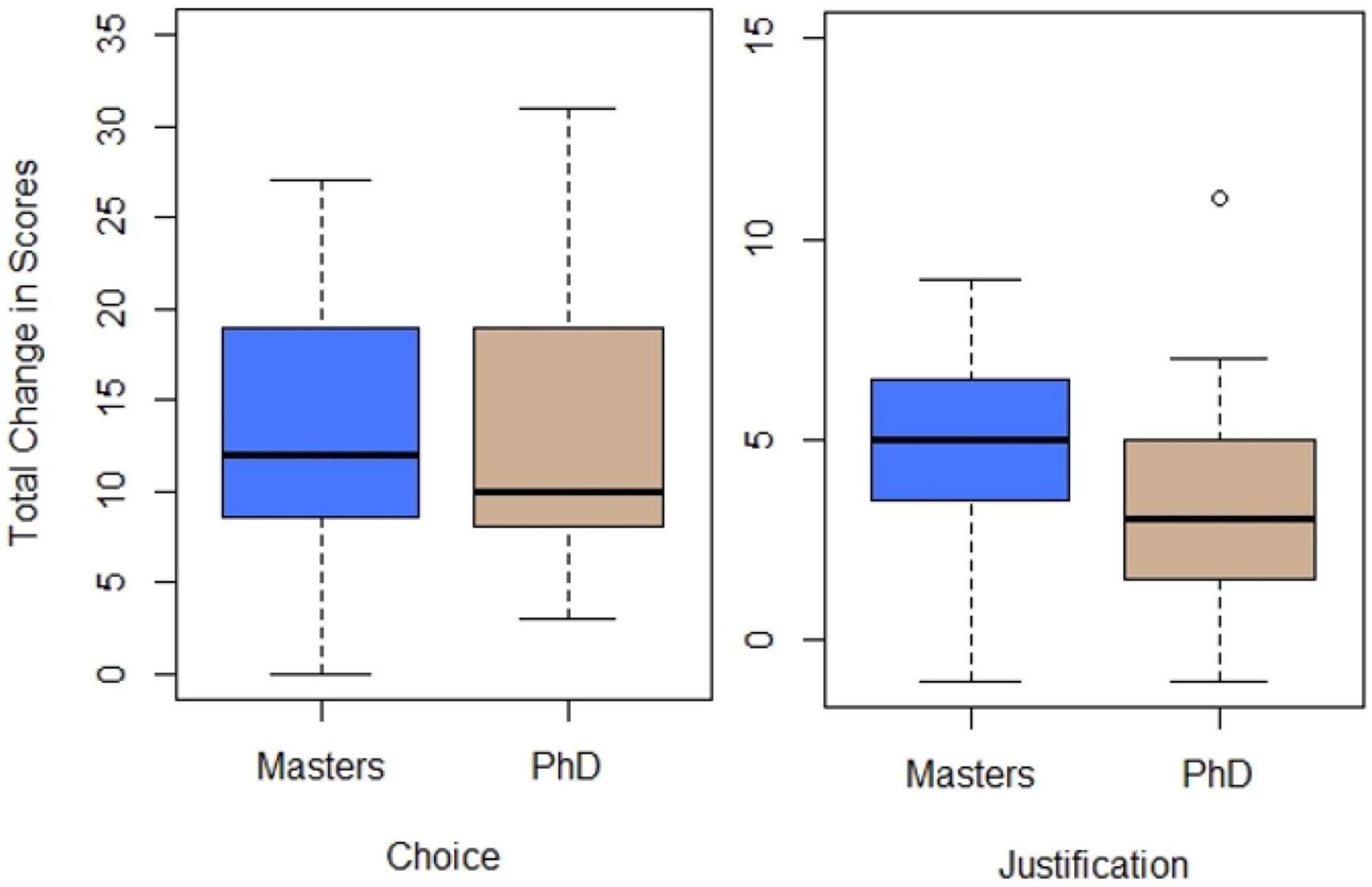
Figure 6. Total change in scores for choice and justification of quantification technique across education level.
The independent two sample t -test showed total change in score for quantification technique choice were not statistically significantly different for those with a Master’s Degree ( M = 8.42, SE = 1.69), d = –0.26, t (21) = –0.61, p = 0.54, than for those with a Ph.D ( M = 10.00, SE = 2.02). A Mann-Whitney test also indicated no difference in total change in scores for quantification technique choice between those with a Master’s degree than for those with a Ph.D ( U = 57.50, p = 0.60). The independent two sample t -test showed total change in scores for quantification technique justification were not statistically significantly different for those with a Master’s Degree ( M = 4.83, SE = 0.85), d = 0.38, t (21) = 0.92, p = 0.39 than for those with a Ph.D ( M = 3.64 SE = 1.03). A Mann-Whitney test also indicated no difference in total change in scores for quantification technique justification between those with a Master’s degree than for those with a Ph.D ( U = 47.00, p = 0.24).
This study had several purposes. First, a user-friendly tool was developed to help researchers and practitioners choose and justify their use of appropriate SCED quantification techniques. Secondly, the tool was formally tested with a pretest and posttest design. Furthermore, participant data was collected to determine the social validity of the tool (i.e., acceptance amongst users). Follow up analyses were also conducted to determine differences between academic departments and education levels.
The tool is highly effective in assisting participants to choose appropriate quantification techniques for various research questions, data characteristics, and desired quantification technique features. The effectiveness of the tool is demonstrated through the statistically significant positive change in scores between pretest and posttest for all five questions, as well as the change in variability of scores between pretest and posttest. The tool is also effective in improving the justifications provided for using quantification techniques. The mean change in score for justification of the quantification technique across the five questions was 0.79, indicating that the average participant is able to provide one additional justification after accessing the tool. The results from the social validity questions also demonstrate the effectiveness of the tool. The majority of participants ( n = 21, 84%) rated the tool as highly helpful for choosing an appropriate quantification technique to use. The majority of participants ( n = 19, 76%) also rated the tool as highly helpful for justifying use of the quantification technique. Seventeen (68%) participants reported that they would be very likely to use the tool in the future; most participants reported that they would be at least somewhat likely to use the tool in the future, demonstrating that the majority of participants found the tool to be practical.
The results of the study show that the tool is effective across different levels of education. It is unsurprising that education level was not found to be related to the effectiveness of the tool; those with various education levels could have various SCED experience. Results of this study showed no statistically significant difference in change in scores for choice or justification of the quantification technique across academic departments. However, it is worth noting that the effect size for the difference in change in scores for choice of quantification technique between academic departments is moderate (η 2 = 0.23), indicating that the lack of statistical significance could possibly be attributed to insufficient power as a result of a small sample size. Thus, it is possible that there is a true difference between the different academic departments. Although sampling across different departments to determine differences was beyond the scope of this study, future research could control for differences between academic departments, for example, to determine if there are true differences.
Implications for the field of single-case experimental design
The findings from this study have implications for the field of SCED. First, the low pretest scores demonstrate that the average SCED researcher, with varying education levels and from various academic departments, needs support in choosing and justifying SCED quantification techniques. For example, during pretest for Questions 1 4 and 3, 5 sixteen (64%) participants chose non-overlap techniques for use, although non-overlap techniques are unable to provide a quantification of the intervention effect that reflects the magnitude of the change in level, nor the magnitude of change in variability. Thus, such quantification techniques cannot provide a quantification of the intervention effect that answers either research question. These results reflect the misuse of non-overlap quantification techniques in particular, as similar pattern of mistakes are not evident for Question 4, for which the research question was about the amount of overlap/data separation between phases. SCED users may not realize that non-overlap quantification techniques cannot represent the magnitude of change/size of the effect ( Fingerhut et al., 2021a , b ). No longer using terms such as “metric” or “effect size” to refer to non-overlap measures may better help SCED researchers carefully consider the quantification techniques they use, and better understand what exactly the quantification of the intervention effects represent.
Similarly, participants of various education levels and academic departments chose quantification techniques that are inappropriate for use with the present graph/data characteristics. During pretest for Question 2, 6 four (16%) participants chose quantification techniques that are highly affected by baseline trend, even though trend was present in the baseline phase. Similarly, some participants chose quantification techniques that are not robust against outliers for Question 4 7 ( n = 14, 56%). Taken together, this inability to choose an appropriate quantification technique is especially concerning because certain quantification techniques may not yield reliable results if the quantification techniques are inappropriate for use with the data. For example, Fingerhut et al. (2021b) found that Tau estimates depend upon data characteristics such as baseline trend, within-case variability, and number of data points in the baseline phase. Pustejovsky (2019) found that procedural features such as the length of the observation sessions and the recording system used to collect measurements of behavior can influence the quantification of the intervention effect of certain SCED techniques. Vannest et al. (2018) and Manolov et al. (2022) explicitly call for researchers to choose SCED quantification techniques carefully in the context of their own research questions and data characteristics. Thus, it is concerning that pretest results highlight the use of inappropriate SCED quantification techniques.
Results of this study also show that SCED researchers with various education levels and from different academic departments need more support in justifying their use of quantification techniques. This is a logical finding, as people cannot justify their use of quantification techniques if they are unable to choose quantification techniques that are wholly appropriate for use in the first place. While it is encouraging that the average participant could provide one justification during pretest, the results of this study show that SCED users need more support in justifying their use of quantification techniques. The results of this study show that a user-friendly tool can help improve justifications provided.
Failure to take the research questions and data characteristics into account when choosing a quantification technique can ultimately result in the dissemination of findings that do not accurately represent actual intervention effects. Since the enactment of laws such as Every Student Succeeds Act in 2015, there has been a greater push to use evidence-based practices in the field of special education. Therefore, it has become more imperative that quantifications of the intervention effects from studies accurately reflect the effectiveness of interventions. If teachers, behaviorists, clinicians, school psychologists, and other practitioners rely on SCED research to determine which interventions to use with students, they may be using interventions that are not actually effective if the results are unreliable. Meta-analyses use and rely on primary level summary findings; if inappropriate quantification techniques are used in these primary studies, then biased or misleading results may be obtained at the meta-analytic level as well. Thus, it is imperative that appropriate SCED quantification techniques are used, and this study highlights that those conducting SCED research may currently be using inappropriate quantification techniques to analyze their data. However, the results of this study are encouraging in that this study provides evidence that teachers, practitioners, and researchers are able to improve the way that they understand and analyze SCED data with a self-paced and user-friendly tool.
This tool has several practical uses. It would be useful if those who review SCED studies, such as journal editors, could reference the authors of studies undergoing peer review to the SCED tool so that they can critically think about quantifying intervention effectiveness and provide a strong justification for the analysis used. This tool could easily be incorporated into a 1- or 2-h college course. This tool can be introduced and disseminated at special education, applied behavior analysis, or school psychology conferences. The Institute of Education Sciences hosts an advanced training session each summer for single-case research methods; this is another environment in which the tool can be introduced and disseminated.
Limitations of the study and tool
A total of 25 people participated in this study, and so generalizations beyond this sample are limited. It is possible that only those who were previously interested in learning about quantification techniques participated in the study, and so the results may be biased. The tool was not designed to aid in interpretations of the underlying scales of the different quantification techniques. Any calculations conducted and the resulting quantification of the intervention effect must be interpreted carefully, and assistance in understanding the clinical significance of the quantification of the intervention effect is not something that is provided by using this tool. It is possible that the tool is missing some quantification techniques, especially as the field of SCED continues to grow. However, the tool is posted through OSF and can be downloaded from osf.io/7usbj/ , and on the Notes and References sheet an email address is provided for users to send feedback ( [email protected] ). Thus, input of the broader research community is invited and can be used to further improve the tool. This tool is meant to be used for within-case estimates only. While some of the quantification techniques listed in the tool can be used for across-case estimates, there may be better options when conducting across-case estimates (see Notes and References sheet of the tool). Furthermore, as several of the experts who validated the tool pointed out, the tool is only appropriate for AB-based research designs, as the information listed in the tool assumes that the user is applying the quantification techniques to an AB-based design. Although the tool was tested with AB designs for the purpose of this study, researchers are encouraged to use the tool with stronger designs, such as withdrawal and reversal designs and multiple-baseline designs.
Future research
This study could be replicated with a larger sample. Prior SCED experience of participants could be a demographic question presented to participants, so that inferential results can be conducted to determine if there is a statistically significant relationship between length of SCED experience in years and the effectiveness of the tool. Future studies can focus on the effect of the tool on participants’ ability to calculate the quantification of the intervention effect correctly and interpret the quantification of the intervention effect as well. It would be helpful to conduct a formal study to determine if the tool is still helpful after it is made more complex. For example, more research questions (e.g., research questions about gradual effects or delayed effects) and data characteristics (e.g., autocorrelation) can be added into the tool. The tool can also be expanded so that it can be used for between-case estimates and with various research designs. It also can be converted into an R code or website.
Data availability statement
The original contributions presented in this study are included in the article/ Supplementary material , further inquiries can be directed to the corresponding author.
Ethics statement
The studies involving human participants were reviewed and approved by Institutional Review Board at University at Albany. The patients/participants provided their written informed consent to participate in this study.
Author contributions
Both authors listed have made a substantial, direct, and intellectual contribution to the work, and approved it for publication.
Conflict of interest
The authors declare that the research was conducted in the absence of any commercial or financial relationships that could be construed as a potential conflict of interest.
Publisher’s note
All claims expressed in this article are solely those of the authors and do not necessarily represent those of their affiliated organizations, or those of the publisher, the editors and the reviewers. Any product that may be evaluated in this article, or claim that may be made by its manufacturer, is not guaranteed or endorsed by the publisher.
Supplementary material
The Supplementary Material for this article can be found online at: https://www.frontiersin.org/articles/10.3389/feduc.2022.1064807/full#supplementary-material
- ^ Also known as a single case, single subject, single-subject, interrupted time series, small n , n of 1 trial, and n = 1.
- ^ Other popular analysis techniques include masked visual analysis ( Ferron and Jones, 2006 ), randomization tests ( Edgington, 1996 ), and the reliable change index ( Jacobson and Truax, 1991 ). While useful, these are not included in the present study because these methods primarily focus on p -values and statistical significance rather than quantifying the effect (i.e., producing an “effect size”).
- ^ Detailed participant data is available upon request from the first author.
- ^ Research question: What is the magnitude of change in level? Unique data characteristic: Small number of data points in baseline or treatment phase.
- ^ Research question: What is the magnitude of change in variability? Unique data characteristic: Variability.
- ^ Research question: What is the magnitude of change in trend? Unique data characteristic: Baseline trend.
- ^ Research question: What is the amount of non-overlapping data? Unique data characteristic: Outliers.
Alnahdi, G. H. (2013). Single-subject designs in special education: Advantages and limitations. J. Res. Spec. Educ. Needs 15, 257–265. doi: 10.1111/1471-3802.12039
CrossRef Full Text | Google Scholar
Brosamler, G. A. (1988). An almost everywhere central limit theorem. Math. Proc. Camb. Philos. Soc. 104, 561–574. doi: 10.1017/S0305004100065750
Center, B., Skiba, R., and Casey, A. (1985-1986). A methodology for the quantitative synthesis of intra-subject design research. J. Spec. Educ. 19, 387–400. doi: 10.1177/002246698501900404
Edgington, E. S. (1996). Randomized single-subject experimental designs. Behav. Res. Ther. 34, 567–574.
Google Scholar
Ferron, J., and Jones, P. K. (2006). Tests for the visual analysis of response-guided multiple-baseline design. J. Exp. Educ. 75, 66–81. doi: 10.3200/JEXE.75.1.66-81
Ferron, J., Goldstein, H., Olszewski, A., and Rohrer, L. (2020). Indexing effects in single-case experimental designs by estimating the percent of goal obtained. Evid. Based Commun. Assess. Interv. 14, 6–27. doi: 10.1080/17489539.2020.1732024
Fingerhut, J., Xu, X., and Moeyaert, M. (2021a). Selecting the proper Tau-U measure for single-case experimental designs: Development and application of a decision flowchart. Evid. Based Commun. Interv. 15, 99–114. doi: 10.1080/17489539.2021.1937851
Fingerhut, J., Xu, X., and Moeyaert, M. (2021b). Impact of within-case variability on Tau-U and regression-based effect size measures for single-case experimental data. Evid. Based Commun. Assess. Interv. 15, 115–141. doi: 10.3102/1583005
PubMed Abstract | CrossRef Full Text | Google Scholar
Fischer, H. (2010). A History of the Central Limit Theorem: From Classical to Modern Probability Theory. New York, NY: Springer. doi: 10.1007/978-0-387-87857-7_5
Hedges, L., Pustejovksy, J., and Shadish, W. (2012). A standardized mean difference effect size for single case designs. Res. Synth. Methods 3, 224–239. doi: 10.1002/jrsm.1052
Hershberger, S. L., Wallace, D. D., Green, S. B., and Marquis, J. G. (1999). “Meta-analysis of single-case designs,” in Statistical Strategies for Small Sample Research , ed. R. H. Hoyle (Thousand Oaks, CA: Sage), 109–132.
Horner, R. H., Carr, E. G., Halle, J., McGee, G., Odom, S., and Wolery, M. (2005). The use of single-subject research to identify evidence-based practice in special education. Except. Child. 71, 165–179. doi: 10.1177/001440290507100203
IBM Corp (2017). IBM SPSS Statistics for Windows. Armonk, NY: IBM Corp.
Individuals with Disabilities Education Act [IDEA] (2004). 20 U.S.C. §1400.
Jacobson, N. S., and Truax, P. (1991). Clinical significance: A statistical approach to defining meaningful change in psychotherapy research. J. Consult. Clin. Psychol. 59, 12–19. doi: 10.1037/0022-006X.59.1.12
Ledford, J. R., Lane, J. D., Zimmerman, K. N., Chazin, K. T., and Ayres, K. A. (2016). Single Case Analysis And Review Framework (SCARF). Available online at: http://ebip.vkcsites.org/scarf/ (accessed November 18, 2022).
Ma, H. (2006). An alternative method for quantitative synthesis of single-subject research: Percentage of data points exceeding the median. Behav. Modif. 30, 598–617. doi: 10.1177/0145445504272974
Manolov, R., and Moeyaert, M. (2017). Recommendations for choosing single-case data analytical techniques. Behav. Ther. 48, 97–114. doi: 10.1016/j.beth.2016.04.008
Manolov, R., and Solanas, A. (2013). A comparison of mean phase difference and generalized least squares for analyzing single-case data. J. Sch. Psychol. 51, 201–215. doi: 10.1016/j.jsp.2012.12.005
Manolov, R., Moeyaert, M., and Fingerhut, J. (2022). A priori metric justification for the quantitative analysis of single-case experimental data. Perspect. Behav. Sci. 45, 153–186. doi: 10.1007/s40614-021-00282-2
Moeyaert, M., Ferron, J. M., Beretvas, S. N., and Van den Noortgate, W. (2014). From a single-level analysis to a multilevel analysis of single-case experimental designs. J. Sch. Psychol. 52, 191–211. doi: 10.1016/j.jsp.2013.11.003
Parker, R. I., and Vannest, K. J. (2009). An improved effect size for single case research: Nonoverlap of all pairs (NAP). Behav. Ther. 40, 357–367. doi: 10.1016/j.beth.2008.10.006
Parker, R. I., Vannest, K. J., Davis, J. L., and Sauber, S. B. (2011). Combining nonoverlap and trend for single-case research: Tau-U. Behav. Ther. 42, 284–299. doi: 10.1016/j.beth.2010.08.006
Parker, R., Hagan-Burke, S., and Vannest, K. (2007). Percentage of all non-overlapping data (PAND): An alternative to PND. J. Spec. Educ. 40, 194–204. doi: 10.1177/00224669070400040101
Parker, R., Vannest, K., and Brown, L. (2009). The improvement rate difference for single case research. Except. Child. 75, 135–150. doi: 10.1177/001440290907500201
Pustejovsky, J. E. (2018). Using response ratios for meta-analyzing single-case designs with behavioral outcomes. J. Sch. Psychol. 68, 99–112. doi: 10.1016/j.jsp.2018.02.003
Pustejovsky, J. E. (2019). Procedural sensitivities of effect sizes for single-case designs with directly observed behavioral outcome measures. Psychol. Methods 24, 217–235. doi: 10.1037/met0000179
Pustejovsky, J. E., Hedges, L. V., and Shadish, W. R. (2014). Design-comparable effect sizes in multiple baseline designs: A general modeling framework. J. Educ. Behav. Stat. 39, 368–393. doi: 10.3102/1076998614547577
Scotti, J. R., Evans, I. M., Meyer, L. H., and Walker, P. (1991). A meta-analysis of intervention research with problem behavior: Treatment validity and standards of practice. Am. J. Ment. Retard. 96, 233–256.
Scruggs, T. E., Mastropieri, M. A., and Casto, G. (1987). The quantitative synthesis of single-subject research: Methodology and validation. Remedial Spec. Educ. 8, 24–33. doi: 10.1177/074193258700800206
Shadish, W. R. (2014). Statistical analyses of single-case designs: The shape of things to come. Curr. Direct. Psychol. Sci. 23, 139–146.
Shadish, W. R., and Sullivan, K. J. (2011). Characteristics of single-case designs used to assess intervention effects in 2008. Behav. Res. Methods 43, 971–980. doi: 10.3758/s13428-011-0111-y
Solomon, B. G., Howard, T. K., and Stein, B. L. (2015). Critical assumptions and distribution features pertaining to contemporary single-case effect sizes. J. Behav. Educ. 24, 438–458. doi: 10.1007/s10864-015-9221-4
Tarlow, K. R. (2016). Baseline Corrected Tau Calculator. Available online at: http://www.ktarlow.com/stats/tau
Tarlow, K. R. (2017). An improved rank correlation effect size statistic for single-case designs: Baseline corrected Tau. Behav. Modif. 41, 427–467. doi: 10.1177/0145445516676750
Tate, R. L., Perdices, M., Rosenkoetter, U., McDonald, S., Togher, L., Shadish, W., et al. (2016). The single-case reporting guideline in behavioural interventions (SCRIBE) 2016: Explanation and elaboration. Arch. Sci. Psychol. 4, 10–31. doi: 10.1037/arc0000027
Van den Noortgate, W., and Onghena, P. (2008). A multilevel meta-analysis of single-subject experimental design studies. Evid. Based Commun. Assess. Interv. 2, 142–158. doi: 10.1080/17489530802505362
Vannest, K. J., Parker, R. I., Gonen, O., and Adiguzel, T. (2016). Single Case Research: Web Based Calculators for SCR Analysis. (Version 2.0) [Web-based application]. College Station, TX: Texas A&M University.
Vannest, K., Peltier, C., and Haas, A. (2018). Results reporting in single case experiments and single case meta-analysis. Res. Dev. Disabil. 79, 10–18. doi: 10.1016/j.ridd.2018.04.029
What Works Clearinghouse (2020). What Works Clearinghouse Standards Handbook, Version 4.1. Washington, DC: U.S. Department of Education.
Wolery, M., Busick, M., Reichow, B., and Barton, E. E. (2010). Comparison of overlap methods for quantitatively synthesizing single-subject data. J. Spec. Educ. 44, 18–28. doi: 10.1177/0022466908328009
Keywords : single-case design, quantification techniques, evidence-based, open-source tool, decision-making, applied behavior analysis, practitioners, special education
Citation: Fingerhut J and Moeyaert M (2022) Selecting and justifying quantitative analysis techniques in single-case research through a user-friendly open-source tool. Front. Educ. 7:1064807. doi: 10.3389/feduc.2022.1064807
Received: 08 October 2022; Accepted: 11 November 2022; Published: 29 November 2022.
Reviewed by:
Copyright © 2022 Fingerhut and Moeyaert. This is an open-access article distributed under the terms of the Creative Commons Attribution License (CC BY) . The use, distribution or reproduction in other forums is permitted, provided the original author(s) and the copyright owner(s) are credited and that the original publication in this journal is cited, in accordance with accepted academic practice. No use, distribution or reproduction is permitted which does not comply with these terms.
*Correspondence: Joelle Fingerhut, [email protected]

Want to create or adapt books like this? Learn more about how Pressbooks supports open publishing practices.
10.1 Overview of Single-Subject Research
Learning objectives.
- Explain what single-subject research is, including how it differs from other types of psychological research.
- Explain what case studies are, including some of their strengths and weaknesses.
- Explain who uses single-subject research and why.
What Is Single-Subject Research?
Single-subject research is a type of quantitative research that involves studying in detail the behavior of each of a small number of participants. Note that the term single-subject does not mean that only one participant is studied; it is more typical for there to be somewhere between two and 10 participants. (This is why single-subject research designs are sometimes called small- n designs, where n is the statistical symbol for the sample size.) Single-subject research can be contrasted with group research , which typically involves studying large numbers of participants and examining their behavior primarily in terms of group means, standard deviations, and so on. The majority of this book is devoted to understanding group research, which is the most common approach in psychology. But single-subject research is an important alternative, and it is the primary approach in some areas of psychology.
Before continuing, it is important to distinguish single-subject research from two other approaches, both of which involve studying in detail a small number of participants. One is qualitative research, which focuses on understanding people’s subjective experience by collecting relatively unstructured data (e.g., detailed interviews) and analyzing those data using narrative rather than quantitative techniques. Single-subject research, in contrast, focuses on understanding objective behavior through experimental manipulation and control, collecting highly structured data, and analyzing those data quantitatively.
It is also important to distinguish single-subject research from case studies. A case study is a detailed description of an individual, which can include both qualitative and quantitative analyses. (Case studies that include only qualitative analyses can be considered a type of qualitative research.) The history of psychology is filled with influential cases studies, such as Sigmund Freud’s description of “Anna O.” (see Note 10.5 “The Case of “Anna O.”” ) and John Watson and Rosalie Rayner’s description of Little Albert (Watson & Rayner, 1920), who learned to fear a white rat—along with other furry objects—when the researchers made a loud noise while he was playing with the rat. Case studies can be useful for suggesting new research questions and for illustrating general principles. They can also help researchers understand rare phenomena, such as the effects of damage to a specific part of the human brain. As a general rule, however, case studies cannot substitute for carefully designed group or single-subject research studies. One reason is that case studies usually do not allow researchers to determine whether specific events are causally related, or even related at all. For example, if a patient is described in a case study as having been sexually abused as a child and then as having developed an eating disorder as a teenager, there is no way to determine whether these two events had anything to do with each other. A second reason is that an individual case can always be unusual in some way and therefore be unrepresentative of people more generally. Thus case studies have serious problems with both internal and external validity.
The Case of “Anna O.”
Sigmund Freud used the case of a young woman he called “Anna O.” to illustrate many principles of his theory of psychoanalysis (Freud, 1961). (Her real name was Bertha Pappenheim, and she was an early feminist who went on to make important contributions to the field of social work.) Anna had come to Freud’s colleague Josef Breuer around 1880 with a variety of odd physical and psychological symptoms. One of them was that for several weeks she was unable to drink any fluids. According to Freud,
She would take up the glass of water that she longed for, but as soon as it touched her lips she would push it away like someone suffering from hydrophobia.…She lived only on fruit, such as melons, etc., so as to lessen her tormenting thirst (p. 9).
But according to Freud, a breakthrough came one day while Anna was under hypnosis.
[S]he grumbled about her English “lady-companion,” whom she did not care for, and went on to describe, with every sign of disgust, how she had once gone into this lady’s room and how her little dog—horrid creature!—had drunk out of a glass there. The patient had said nothing, as she had wanted to be polite. After giving further energetic expression to the anger she had held back, she asked for something to drink, drank a large quantity of water without any difficulty, and awoke from her hypnosis with the glass at her lips; and thereupon the disturbance vanished, never to return.
Freud’s interpretation was that Anna had repressed the memory of this incident along with the emotion that it triggered and that this was what had caused her inability to drink. Furthermore, her recollection of the incident, along with her expression of the emotion she had repressed, caused the symptom to go away.
As an illustration of Freud’s theory, the case study of Anna O. is quite effective. As evidence for the theory, however, it is essentially worthless. The description provides no way of knowing whether Anna had really repressed the memory of the dog drinking from the glass, whether this repression had caused her inability to drink, or whether recalling this “trauma” relieved the symptom. It is also unclear from this case study how typical or atypical Anna’s experience was.
Figure 10.2
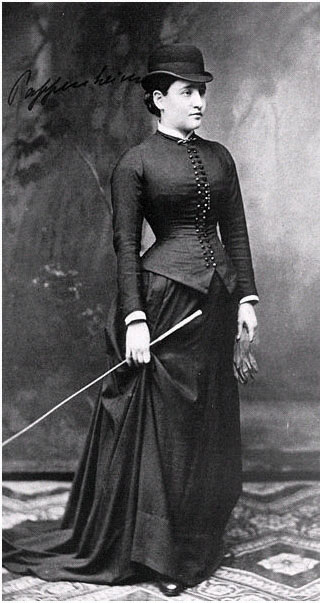
“Anna O.” was the subject of a famous case study used by Freud to illustrate the principles of psychoanalysis.
Wikimedia Commons – public domain.
Assumptions of Single-Subject Research
Again, single-subject research involves studying a small number of participants and focusing intensively on the behavior of each one. But why take this approach instead of the group approach? There are several important assumptions underlying single-subject research, and it will help to consider them now.
First and foremost is the assumption that it is important to focus intensively on the behavior of individual participants. One reason for this is that group research can hide individual differences and generate results that do not represent the behavior of any individual. For example, a treatment that has a positive effect for half the people exposed to it but a negative effect for the other half would, on average, appear to have no effect at all. Single-subject research, however, would likely reveal these individual differences. A second reason to focus intensively on individuals is that sometimes it is the behavior of a particular individual that is primarily of interest. A school psychologist, for example, might be interested in changing the behavior of a particular disruptive student. Although previous published research (both single-subject and group research) is likely to provide some guidance on how to do this, conducting a study on this student would be more direct and probably more effective.
A second assumption of single-subject research is that it is important to discover causal relationships through the manipulation of an independent variable, the careful measurement of a dependent variable, and the control of extraneous variables. For this reason, single-subject research is often considered a type of experimental research with good internal validity. Recall, for example, that Hall and his colleagues measured their dependent variable (studying) many times—first under a no-treatment control condition, then under a treatment condition (positive teacher attention), and then again under the control condition. Because there was a clear increase in studying when the treatment was introduced, a decrease when it was removed, and an increase when it was reintroduced, there is little doubt that the treatment was the cause of the improvement.
A third assumption of single-subject research is that it is important to study strong and consistent effects that have biological or social importance. Applied researchers, in particular, are interested in treatments that have substantial effects on important behaviors and that can be implemented reliably in the real-world contexts in which they occur. This is sometimes referred to as social validity (Wolf, 1976). The study by Hall and his colleagues, for example, had good social validity because it showed strong and consistent effects of positive teacher attention on a behavior that is of obvious importance to teachers, parents, and students. Furthermore, the teachers found the treatment easy to implement, even in their often chaotic elementary school classrooms.
Who Uses Single-Subject Research?
Single-subject research has been around as long as the field of psychology itself. In the late 1800s, one of psychology’s founders, Wilhelm Wundt, studied sensation and consciousness by focusing intensively on each of a small number of research participants. Herman Ebbinghaus’s research on memory and Ivan Pavlov’s research on classical conditioning are other early examples, both of which are still described in almost every introductory psychology textbook.
In the middle of the 20th century, B. F. Skinner clarified many of the assumptions underlying single-subject research and refined many of its techniques (Skinner, 1938). He and other researchers then used it to describe how rewards, punishments, and other external factors affect behavior over time. This work was carried out primarily using nonhuman subjects—mostly rats and pigeons. This approach, which Skinner called the experimental analysis of behavior —remains an important subfield of psychology and continues to rely almost exclusively on single-subject research. For excellent examples of this work, look at any issue of the Journal of the Experimental Analysis of Behavior . By the 1960s, many researchers were interested in using this approach to conduct applied research primarily with humans—a subfield now called applied behavior analysis (Baer, Wolf, & Risley, 1968). Applied behavior analysis plays an especially important role in contemporary research on developmental disabilities, education, organizational behavior, and health, among many other areas. Excellent examples of this work (including the study by Hall and his colleagues) can be found in the Journal of Applied Behavior Analysis .
Although most contemporary single-subject research is conducted from the behavioral perspective, it can in principle be used to address questions framed in terms of any theoretical perspective. For example, a studying technique based on cognitive principles of learning and memory could be evaluated by testing it on individual high school students using the single-subject approach. The single-subject approach can also be used by clinicians who take any theoretical perspective—behavioral, cognitive, psychodynamic, or humanistic—to study processes of therapeutic change with individual clients and to document their clients’ improvement (Kazdin, 1982).
Key Takeaways
- Single-subject research—which involves testing a small number of participants and focusing intensively on the behavior of each individual—is an important alternative to group research in psychology.
- Single-subject studies must be distinguished from case studies, in which an individual case is described in detail. Case studies can be useful for generating new research questions, for studying rare phenomena, and for illustrating general principles. However, they cannot substitute for carefully controlled experimental or correlational studies because they are low in internal and external validity.
- Single-subject research has been around since the beginning of the field of psychology. Today it is most strongly associated with the behavioral theoretical perspective, but it can in principle be used to study behavior from any perspective.
- Practice: Find and read a published article in psychology that reports new single-subject research. (A good source of articles published in the Journal of Applied Behavior Analysis can be found at http://seab.envmed.rochester.edu/jaba/jabaMostPop-2011.html .) Write a short summary of the study.
Practice: Find and read a published case study in psychology. (Use case study as a key term in a PsycINFO search.) Then do the following:
- Describe one problem related to internal validity.
- Describe one problem related to external validity.
- Generate one hypothesis suggested by the case study that might be interesting to test in a systematic single-subject or group study.
Baer, D. M., Wolf, M. M., & Risley, T. R. (1968). Some current dimensions of applied behavior analysis. Journal of Applied Behavior Analysis , 1 , 91–97.
Freud, S. (1961). Five lectures on psycho-analysis . New York, NY: Norton.
Kazdin, A. E. (1982). Single-case research designs: Methods for clinical and applied settings . New York, NY: Oxford University Press.
Skinner, B. F. (1938). The behavior of organisms: An experimental analysis . New York, NY: Appleton-Century-Crofts.
Watson, J. B., & Rayner, R. (1920). Conditioned emotional reactions. Journal of Experimental Psychology , 3 , 1–14.
Wolf, M. (1976). Social validity: The case for subjective measurement or how applied behavior analysis is finding its heart. Journal of Applied Behavior Analysis, 11 , 203–214.
Research Methods in Psychology Copyright © 2016 by University of Minnesota is licensed under a Creative Commons Attribution-NonCommercial-ShareAlike 4.0 International License , except where otherwise noted.
The Advantages and Limitations of Single Case Study Analysis
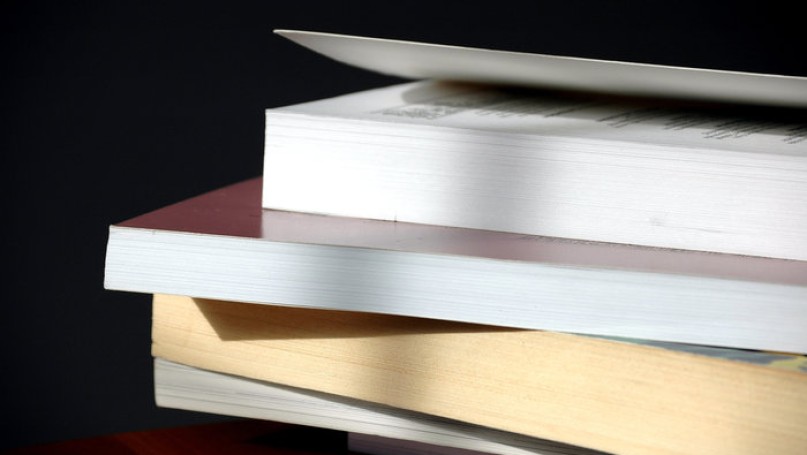
As Andrew Bennett and Colin Elman have recently noted, qualitative research methods presently enjoy “an almost unprecedented popularity and vitality… in the international relations sub-field”, such that they are now “indisputably prominent, if not pre-eminent” (2010: 499). This is, they suggest, due in no small part to the considerable advantages that case study methods in particular have to offer in studying the “complex and relatively unstructured and infrequent phenomena that lie at the heart of the subfield” (Bennett and Elman, 2007: 171). Using selected examples from within the International Relations literature[1], this paper aims to provide a brief overview of the main principles and distinctive advantages and limitations of single case study analysis. Divided into three inter-related sections, the paper therefore begins by first identifying the underlying principles that serve to constitute the case study as a particular research strategy, noting the somewhat contested nature of the approach in ontological, epistemological, and methodological terms. The second part then looks to the principal single case study types and their associated advantages, including those from within the recent ‘third generation’ of qualitative International Relations (IR) research. The final section of the paper then discusses the most commonly articulated limitations of single case studies; while accepting their susceptibility to criticism, it is however suggested that such weaknesses are somewhat exaggerated. The paper concludes that single case study analysis has a great deal to offer as a means of both understanding and explaining contemporary international relations.
The term ‘case study’, John Gerring has suggested, is “a definitional morass… Evidently, researchers have many different things in mind when they talk about case study research” (2006a: 17). It is possible, however, to distil some of the more commonly-agreed principles. One of the most prominent advocates of case study research, Robert Yin (2009: 14) defines it as “an empirical enquiry that investigates a contemporary phenomenon in depth and within its real-life context, especially when the boundaries between phenomenon and context are not clearly evident”. What this definition usefully captures is that case studies are intended – unlike more superficial and generalising methods – to provide a level of detail and understanding, similar to the ethnographer Clifford Geertz’s (1973) notion of ‘thick description’, that allows for the thorough analysis of the complex and particularistic nature of distinct phenomena. Another frequently cited proponent of the approach, Robert Stake, notes that as a form of research the case study “is defined by interest in an individual case, not by the methods of inquiry used”, and that “the object of study is a specific, unique, bounded system” (2008: 443, 445). As such, three key points can be derived from this – respectively concerning issues of ontology, epistemology, and methodology – that are central to the principles of single case study research.
First, the vital notion of ‘boundedness’ when it comes to the particular unit of analysis means that defining principles should incorporate both the synchronic (spatial) and diachronic (temporal) elements of any so-called ‘case’. As Gerring puts it, a case study should be “an intensive study of a single unit… a spatially bounded phenomenon – e.g. a nation-state, revolution, political party, election, or person – observed at a single point in time or over some delimited period of time” (2004: 342). It is important to note, however, that – whereas Gerring refers to a single unit of analysis – it may be that attention also necessarily be given to particular sub-units. This points to the important difference between what Yin refers to as an ‘holistic’ case design, with a single unit of analysis, and an ’embedded’ case design with multiple units of analysis (Yin, 2009: 50-52). The former, for example, would examine only the overall nature of an international organization, whereas the latter would also look to specific departments, programmes, or policies etc.
Secondly, as Tim May notes of the case study approach, “even the most fervent advocates acknowledge that the term has entered into understandings with little specification or discussion of purpose and process” (2011: 220). One of the principal reasons for this, he argues, is the relationship between the use of case studies in social research and the differing epistemological traditions – positivist, interpretivist, and others – within which it has been utilised. Philosophy of science concerns are obviously a complex issue, and beyond the scope of much of this paper. That said, the issue of how it is that we know what we know – of whether or not a single independent reality exists of which we as researchers can seek to provide explanation – does lead us to an important distinction to be made between so-called idiographic and nomothetic case studies (Gerring, 2006b). The former refers to those which purport to explain only a single case, are concerned with particularisation, and hence are typically (although not exclusively) associated with more interpretivist approaches. The latter are those focused studies that reflect upon a larger population and are more concerned with generalisation, as is often so with more positivist approaches[2]. The importance of this distinction, and its relation to the advantages and limitations of single case study analysis, is returned to below.
Thirdly, in methodological terms, given that the case study has often been seen as more of an interpretivist and idiographic tool, it has also been associated with a distinctly qualitative approach (Bryman, 2009: 67-68). However, as Yin notes, case studies can – like all forms of social science research – be exploratory, descriptive, and/or explanatory in nature. It is “a common misconception”, he notes, “that the various research methods should be arrayed hierarchically… many social scientists still deeply believe that case studies are only appropriate for the exploratory phase of an investigation” (Yin, 2009: 6). If case studies can reliably perform any or all three of these roles – and given that their in-depth approach may also require multiple sources of data and the within-case triangulation of methods – then it becomes readily apparent that they should not be limited to only one research paradigm. Exploratory and descriptive studies usually tend toward the qualitative and inductive, whereas explanatory studies are more often quantitative and deductive (David and Sutton, 2011: 165-166). As such, the association of case study analysis with a qualitative approach is a “methodological affinity, not a definitional requirement” (Gerring, 2006a: 36). It is perhaps better to think of case studies as transparadigmatic; it is mistaken to assume single case study analysis to adhere exclusively to a qualitative methodology (or an interpretivist epistemology) even if it – or rather, practitioners of it – may be so inclined. By extension, this also implies that single case study analysis therefore remains an option for a multitude of IR theories and issue areas; it is how this can be put to researchers’ advantage that is the subject of the next section.
Having elucidated the defining principles of the single case study approach, the paper now turns to an overview of its main benefits. As noted above, a lack of consensus still exists within the wider social science literature on the principles and purposes – and by extension the advantages and limitations – of case study research. Given that this paper is directed towards the particular sub-field of International Relations, it suggests Bennett and Elman’s (2010) more discipline-specific understanding of contemporary case study methods as an analytical framework. It begins however, by discussing Harry Eckstein’s seminal (1975) contribution to the potential advantages of the case study approach within the wider social sciences.
Eckstein proposed a taxonomy which usefully identified what he considered to be the five most relevant types of case study. Firstly were so-called configurative-idiographic studies, distinctly interpretivist in orientation and predicated on the assumption that “one cannot attain prediction and control in the natural science sense, but only understanding ( verstehen )… subjective values and modes of cognition are crucial” (1975: 132). Eckstein’s own sceptical view was that any interpreter ‘simply’ considers a body of observations that are not self-explanatory and “without hard rules of interpretation, may discern in them any number of patterns that are more or less equally plausible” (1975: 134). Those of a more post-modernist bent, of course – sharing an “incredulity towards meta-narratives”, in Lyotard’s (1994: xxiv) evocative phrase – would instead suggest that this more free-form approach actually be advantageous in delving into the subtleties and particularities of individual cases.
Eckstein’s four other types of case study, meanwhile, promote a more nomothetic (and positivist) usage. As described, disciplined-configurative studies were essentially about the use of pre-existing general theories, with a case acting “passively, in the main, as a receptacle for putting theories to work” (Eckstein, 1975: 136). As opposed to the opportunity this presented primarily for theory application, Eckstein identified heuristic case studies as explicit theoretical stimulants – thus having instead the intended advantage of theory-building. So-called p lausibility probes entailed preliminary attempts to determine whether initial hypotheses should be considered sound enough to warrant more rigorous and extensive testing. Finally, and perhaps most notably, Eckstein then outlined the idea of crucial case studies , within which he also included the idea of ‘most-likely’ and ‘least-likely’ cases; the essential characteristic of crucial cases being their specific theory-testing function.
Whilst Eckstein’s was an early contribution to refining the case study approach, Yin’s (2009: 47-52) more recent delineation of possible single case designs similarly assigns them roles in the applying, testing, or building of theory, as well as in the study of unique cases[3]. As a subset of the latter, however, Jack Levy (2008) notes that the advantages of idiographic cases are actually twofold. Firstly, as inductive/descriptive cases – akin to Eckstein’s configurative-idiographic cases – whereby they are highly descriptive, lacking in an explicit theoretical framework and therefore taking the form of “total history”. Secondly, they can operate as theory-guided case studies, but ones that seek only to explain or interpret a single historical episode rather than generalise beyond the case. Not only does this therefore incorporate ‘single-outcome’ studies concerned with establishing causal inference (Gerring, 2006b), it also provides room for the more postmodern approaches within IR theory, such as discourse analysis, that may have developed a distinct methodology but do not seek traditional social scientific forms of explanation.
Applying specifically to the state of the field in contemporary IR, Bennett and Elman identify a ‘third generation’ of mainstream qualitative scholars – rooted in a pragmatic scientific realist epistemology and advocating a pluralistic approach to methodology – that have, over the last fifteen years, “revised or added to essentially every aspect of traditional case study research methods” (2010: 502). They identify ‘process tracing’ as having emerged from this as a central method of within-case analysis. As Bennett and Checkel observe, this carries the advantage of offering a methodologically rigorous “analysis of evidence on processes, sequences, and conjunctures of events within a case, for the purposes of either developing or testing hypotheses about causal mechanisms that might causally explain the case” (2012: 10).
Harnessing various methods, process tracing may entail the inductive use of evidence from within a case to develop explanatory hypotheses, and deductive examination of the observable implications of hypothesised causal mechanisms to test their explanatory capability[4]. It involves providing not only a coherent explanation of the key sequential steps in a hypothesised process, but also sensitivity to alternative explanations as well as potential biases in the available evidence (Bennett and Elman 2010: 503-504). John Owen (1994), for example, demonstrates the advantages of process tracing in analysing whether the causal factors underpinning democratic peace theory are – as liberalism suggests – not epiphenomenal, but variously normative, institutional, or some given combination of the two or other unexplained mechanism inherent to liberal states. Within-case process tracing has also been identified as advantageous in addressing the complexity of path-dependent explanations and critical junctures – as for example with the development of political regime types – and their constituent elements of causal possibility, contingency, closure, and constraint (Bennett and Elman, 2006b).
Bennett and Elman (2010: 505-506) also identify the advantages of single case studies that are implicitly comparative: deviant, most-likely, least-likely, and crucial cases. Of these, so-called deviant cases are those whose outcome does not fit with prior theoretical expectations or wider empirical patterns – again, the use of inductive process tracing has the advantage of potentially generating new hypotheses from these, either particular to that individual case or potentially generalisable to a broader population. A classic example here is that of post-independence India as an outlier to the standard modernisation theory of democratisation, which holds that higher levels of socio-economic development are typically required for the transition to, and consolidation of, democratic rule (Lipset, 1959; Diamond, 1992). Absent these factors, MacMillan’s single case study analysis (2008) suggests the particularistic importance of the British colonial heritage, the ideology and leadership of the Indian National Congress, and the size and heterogeneity of the federal state.
Most-likely cases, as per Eckstein above, are those in which a theory is to be considered likely to provide a good explanation if it is to have any application at all, whereas least-likely cases are ‘tough test’ ones in which the posited theory is unlikely to provide good explanation (Bennett and Elman, 2010: 505). Levy (2008) neatly refers to the inferential logic of the least-likely case as the ‘Sinatra inference’ – if a theory can make it here, it can make it anywhere. Conversely, if a theory cannot pass a most-likely case, it is seriously impugned. Single case analysis can therefore be valuable for the testing of theoretical propositions, provided that predictions are relatively precise and measurement error is low (Levy, 2008: 12-13). As Gerring rightly observes of this potential for falsification:
“a positivist orientation toward the work of social science militates toward a greater appreciation of the case study format, not a denigration of that format, as is usually supposed” (Gerring, 2007: 247, emphasis added).
In summary, the various forms of single case study analysis can – through the application of multiple qualitative and/or quantitative research methods – provide a nuanced, empirically-rich, holistic account of specific phenomena. This may be particularly appropriate for those phenomena that are simply less amenable to more superficial measures and tests (or indeed any substantive form of quantification) as well as those for which our reasons for understanding and/or explaining them are irreducibly subjective – as, for example, with many of the normative and ethical issues associated with the practice of international relations. From various epistemological and analytical standpoints, single case study analysis can incorporate both idiographic sui generis cases and, where the potential for generalisation may exist, nomothetic case studies suitable for the testing and building of causal hypotheses. Finally, it should not be ignored that a signal advantage of the case study – with particular relevance to international relations – also exists at a more practical rather than theoretical level. This is, as Eckstein noted, “that it is economical for all resources: money, manpower, time, effort… especially important, of course, if studies are inherently costly, as they are if units are complex collective individuals ” (1975: 149-150, emphasis added).
Limitations
Single case study analysis has, however, been subject to a number of criticisms, the most common of which concern the inter-related issues of methodological rigour, researcher subjectivity, and external validity. With regard to the first point, the prototypical view here is that of Zeev Maoz (2002: 164-165), who suggests that “the use of the case study absolves the author from any kind of methodological considerations. Case studies have become in many cases a synonym for freeform research where anything goes”. The absence of systematic procedures for case study research is something that Yin (2009: 14-15) sees as traditionally the greatest concern due to a relative absence of methodological guidelines. As the previous section suggests, this critique seems somewhat unfair; many contemporary case study practitioners – and representing various strands of IR theory – have increasingly sought to clarify and develop their methodological techniques and epistemological grounding (Bennett and Elman, 2010: 499-500).
A second issue, again also incorporating issues of construct validity, concerns that of the reliability and replicability of various forms of single case study analysis. This is usually tied to a broader critique of qualitative research methods as a whole. However, whereas the latter obviously tend toward an explicitly-acknowledged interpretive basis for meanings, reasons, and understandings:
“quantitative measures appear objective, but only so long as we don’t ask questions about where and how the data were produced… pure objectivity is not a meaningful concept if the goal is to measure intangibles [as] these concepts only exist because we can interpret them” (Berg and Lune, 2010: 340).
The question of researcher subjectivity is a valid one, and it may be intended only as a methodological critique of what are obviously less formalised and researcher-independent methods (Verschuren, 2003). Owen (1994) and Layne’s (1994) contradictory process tracing results of interdemocratic war-avoidance during the Anglo-American crisis of 1861 to 1863 – from liberal and realist standpoints respectively – are a useful example. However, it does also rest on certain assumptions that can raise deeper and potentially irreconcilable ontological and epistemological issues. There are, regardless, plenty such as Bent Flyvbjerg (2006: 237) who suggest that the case study contains no greater bias toward verification than other methods of inquiry, and that “on the contrary, experience indicates that the case study contains a greater bias toward falsification of preconceived notions than toward verification”.
The third and arguably most prominent critique of single case study analysis is the issue of external validity or generalisability. How is it that one case can reliably offer anything beyond the particular? “We always do better (or, in the extreme, no worse) with more observation as the basis of our generalization”, as King et al write; “in all social science research and all prediction, it is important that we be as explicit as possible about the degree of uncertainty that accompanies out prediction” (1994: 212). This is an unavoidably valid criticism. It may be that theories which pass a single crucial case study test, for example, require rare antecedent conditions and therefore actually have little explanatory range. These conditions may emerge more clearly, as Van Evera (1997: 51-54) notes, from large-N studies in which cases that lack them present themselves as outliers exhibiting a theory’s cause but without its predicted outcome. As with the case of Indian democratisation above, it would logically be preferable to conduct large-N analysis beforehand to identify that state’s non-representative nature in relation to the broader population.
There are, however, three important qualifiers to the argument about generalisation that deserve particular mention here. The first is that with regard to an idiographic single-outcome case study, as Eckstein notes, the criticism is “mitigated by the fact that its capability to do so [is] never claimed by its exponents; in fact it is often explicitly repudiated” (1975: 134). Criticism of generalisability is of little relevance when the intention is one of particularisation. A second qualifier relates to the difference between statistical and analytical generalisation; single case studies are clearly less appropriate for the former but arguably retain significant utility for the latter – the difference also between explanatory and exploratory, or theory-testing and theory-building, as discussed above. As Gerring puts it, “theory confirmation/disconfirmation is not the case study’s strong suit” (2004: 350). A third qualification relates to the issue of case selection. As Seawright and Gerring (2008) note, the generalisability of case studies can be increased by the strategic selection of cases. Representative or random samples may not be the most appropriate, given that they may not provide the richest insight (or indeed, that a random and unknown deviant case may appear). Instead, and properly used , atypical or extreme cases “often reveal more information because they activate more actors… and more basic mechanisms in the situation studied” (Flyvbjerg, 2006). Of course, this also points to the very serious limitation, as hinted at with the case of India above, that poor case selection may alternatively lead to overgeneralisation and/or grievous misunderstandings of the relationship between variables or processes (Bennett and Elman, 2006a: 460-463).
As Tim May (2011: 226) notes, “the goal for many proponents of case studies […] is to overcome dichotomies between generalizing and particularizing, quantitative and qualitative, deductive and inductive techniques”. Research aims should drive methodological choices, rather than narrow and dogmatic preconceived approaches. As demonstrated above, there are various advantages to both idiographic and nomothetic single case study analyses – notably the empirically-rich, context-specific, holistic accounts that they have to offer, and their contribution to theory-building and, to a lesser extent, that of theory-testing. Furthermore, while they do possess clear limitations, any research method involves necessary trade-offs; the inherent weaknesses of any one method, however, can potentially be offset by situating them within a broader, pluralistic mixed-method research strategy. Whether or not single case studies are used in this fashion, they clearly have a great deal to offer.
References
Bennett, A. and Checkel, J. T. (2012) ‘Process Tracing: From Philosophical Roots to Best Practice’, Simons Papers in Security and Development, No. 21/2012, School for International Studies, Simon Fraser University: Vancouver.
Bennett, A. and Elman, C. (2006a) ‘Qualitative Research: Recent Developments in Case Study Methods’, Annual Review of Political Science , 9, 455-476.
Bennett, A. and Elman, C. (2006b) ‘Complex Causal Relations and Case Study Methods: The Example of Path Dependence’, Political Analysis , 14, 3, 250-267.
Bennett, A. and Elman, C. (2007) ‘Case Study Methods in the International Relations Subfield’, Comparative Political Studies , 40, 2, 170-195.
Bennett, A. and Elman, C. (2010) Case Study Methods. In C. Reus-Smit and D. Snidal (eds) The Oxford Handbook of International Relations . Oxford University Press: Oxford. Ch. 29.
Berg, B. and Lune, H. (2012) Qualitative Research Methods for the Social Sciences . Pearson: London.
Bryman, A. (2012) Social Research Methods . Oxford University Press: Oxford.
David, M. and Sutton, C. D. (2011) Social Research: An Introduction . SAGE Publications Ltd: London.
Diamond, J. (1992) ‘Economic development and democracy reconsidered’, American Behavioral Scientist , 35, 4/5, 450-499.
Eckstein, H. (1975) Case Study and Theory in Political Science. In R. Gomm, M. Hammersley, and P. Foster (eds) Case Study Method . SAGE Publications Ltd: London.
Flyvbjerg, B. (2006) ‘Five Misunderstandings About Case-Study Research’, Qualitative Inquiry , 12, 2, 219-245.
Geertz, C. (1973) The Interpretation of Cultures: Selected Essays by Clifford Geertz . Basic Books Inc: New York.
Gerring, J. (2004) ‘What is a Case Study and What Is It Good for?’, American Political Science Review , 98, 2, 341-354.
Gerring, J. (2006a) Case Study Research: Principles and Practices . Cambridge University Press: Cambridge.
Gerring, J. (2006b) ‘Single-Outcome Studies: A Methodological Primer’, International Sociology , 21, 5, 707-734.
Gerring, J. (2007) ‘Is There a (Viable) Crucial-Case Method?’, Comparative Political Studies , 40, 3, 231-253.
King, G., Keohane, R. O. and Verba, S. (1994) Designing Social Inquiry: Scientific Inference in Qualitative Research . Princeton University Press: Chichester.
Layne, C. (1994) ‘Kant or Cant: The Myth of the Democratic Peace’, International Security , 19, 2, 5-49.
Levy, J. S. (2008) ‘Case Studies: Types, Designs, and Logics of Inference’, Conflict Management and Peace Science , 25, 1-18.
Lipset, S. M. (1959) ‘Some Social Requisites of Democracy: Economic Development and Political Legitimacy’, The American Political Science Review , 53, 1, 69-105.
Lyotard, J-F. (1984) The Postmodern Condition: A Report on Knowledge . University of Minnesota Press: Minneapolis.
MacMillan, A. (2008) ‘Deviant Democratization in India’, Democratization , 15, 4, 733-749.
Maoz, Z. (2002) Case study methodology in international studies: from storytelling to hypothesis testing. In F. P. Harvey and M. Brecher (eds) Evaluating Methodology in International Studies . University of Michigan Press: Ann Arbor.
May, T. (2011) Social Research: Issues, Methods and Process . Open University Press: Maidenhead.
Owen, J. M. (1994) ‘How Liberalism Produces Democratic Peace’, International Security , 19, 2, 87-125.
Seawright, J. and Gerring, J. (2008) ‘Case Selection Techniques in Case Study Research: A Menu of Qualitative and Quantitative Options’, Political Research Quarterly , 61, 2, 294-308.
Stake, R. E. (2008) Qualitative Case Studies. In N. K. Denzin and Y. S. Lincoln (eds) Strategies of Qualitative Inquiry . Sage Publications: Los Angeles. Ch. 17.
Van Evera, S. (1997) Guide to Methods for Students of Political Science . Cornell University Press: Ithaca.
Verschuren, P. J. M. (2003) ‘Case study as a research strategy: some ambiguities and opportunities’, International Journal of Social Research Methodology , 6, 2, 121-139.
Yin, R. K. (2009) Case Study Research: Design and Methods . SAGE Publications Ltd: London.
[1] The paper follows convention by differentiating between ‘International Relations’ as the academic discipline and ‘international relations’ as the subject of study.
[2] There is some similarity here with Stake’s (2008: 445-447) notion of intrinsic cases, those undertaken for a better understanding of the particular case, and instrumental ones that provide insight for the purposes of a wider external interest.
[3] These may be unique in the idiographic sense, or in nomothetic terms as an exception to the generalising suppositions of either probabilistic or deterministic theories (as per deviant cases, below).
[4] Although there are “philosophical hurdles to mount”, according to Bennett and Checkel, there exists no a priori reason as to why process tracing (as typically grounded in scientific realism) is fundamentally incompatible with various strands of positivism or interpretivism (2012: 18-19). By extension, it can therefore be incorporated by a range of contemporary mainstream IR theories.
— Written by: Ben Willis Written at: University of Plymouth Written for: David Brockington Date written: January 2013
Further Reading on E-International Relations
- Identity in International Conflicts: A Case Study of the Cuban Missile Crisis
- Imperialism’s Legacy in the Study of Contemporary Politics: The Case of Hegemonic Stability Theory
- Recreating a Nation’s Identity Through Symbolism: A Chinese Case Study
- Ontological Insecurity: A Case Study on Israeli-Palestinian Conflict in Jerusalem
- Terrorists or Freedom Fighters: A Case Study of ETA
- A Critical Assessment of Eco-Marxism: A Ghanaian Case Study
Please Consider Donating
Before you download your free e-book, please consider donating to support open access publishing.
E-IR is an independent non-profit publisher run by an all volunteer team. Your donations allow us to invest in new open access titles and pay our bandwidth bills to ensure we keep our existing titles free to view. Any amount, in any currency, is appreciated. Many thanks!
Donations are voluntary and not required to download the e-book - your link to download is below.
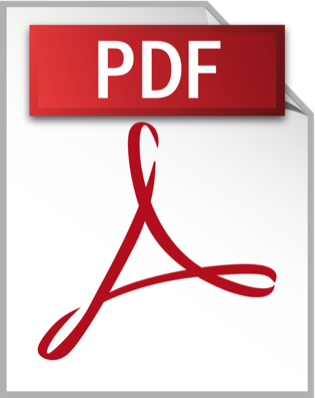
Have a language expert improve your writing
Run a free plagiarism check in 10 minutes, generate accurate citations for free.
- Knowledge Base
Methodology
- What Is a Case Study? | Definition, Examples & Methods
What Is a Case Study? | Definition, Examples & Methods
Published on May 8, 2019 by Shona McCombes . Revised on November 20, 2023.
A case study is a detailed study of a specific subject, such as a person, group, place, event, organization, or phenomenon. Case studies are commonly used in social, educational, clinical, and business research.
A case study research design usually involves qualitative methods , but quantitative methods are sometimes also used. Case studies are good for describing , comparing, evaluating and understanding different aspects of a research problem .
Table of contents
When to do a case study, step 1: select a case, step 2: build a theoretical framework, step 3: collect your data, step 4: describe and analyze the case, other interesting articles.
A case study is an appropriate research design when you want to gain concrete, contextual, in-depth knowledge about a specific real-world subject. It allows you to explore the key characteristics, meanings, and implications of the case.
Case studies are often a good choice in a thesis or dissertation . They keep your project focused and manageable when you don’t have the time or resources to do large-scale research.
You might use just one complex case study where you explore a single subject in depth, or conduct multiple case studies to compare and illuminate different aspects of your research problem.
Receive feedback on language, structure, and formatting
Professional editors proofread and edit your paper by focusing on:
- Academic style
- Vague sentences
- Style consistency
See an example
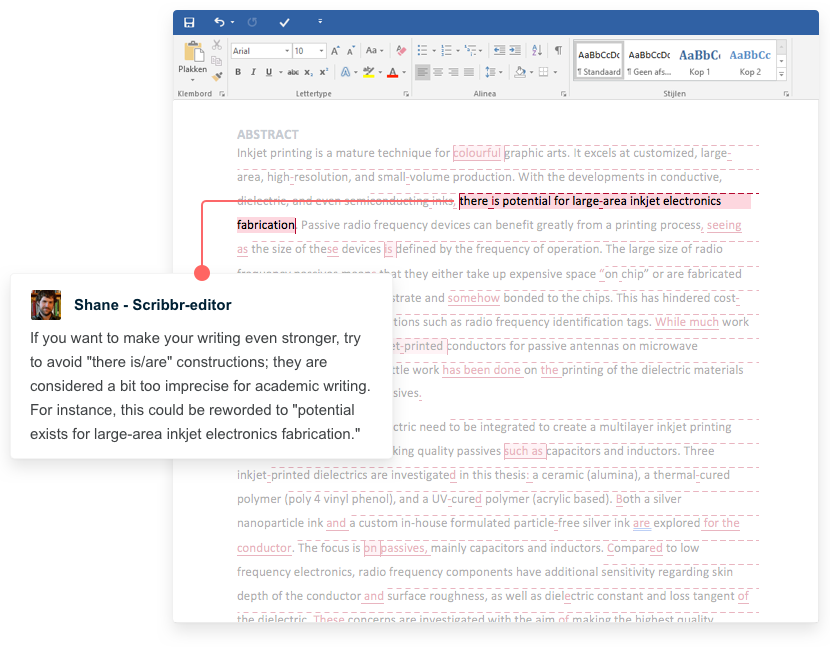
Once you have developed your problem statement and research questions , you should be ready to choose the specific case that you want to focus on. A good case study should have the potential to:
- Provide new or unexpected insights into the subject
- Challenge or complicate existing assumptions and theories
- Propose practical courses of action to resolve a problem
- Open up new directions for future research
TipIf your research is more practical in nature and aims to simultaneously investigate an issue as you solve it, consider conducting action research instead.
Unlike quantitative or experimental research , a strong case study does not require a random or representative sample. In fact, case studies often deliberately focus on unusual, neglected, or outlying cases which may shed new light on the research problem.
Example of an outlying case studyIn the 1960s the town of Roseto, Pennsylvania was discovered to have extremely low rates of heart disease compared to the US average. It became an important case study for understanding previously neglected causes of heart disease.
However, you can also choose a more common or representative case to exemplify a particular category, experience or phenomenon.
Example of a representative case studyIn the 1920s, two sociologists used Muncie, Indiana as a case study of a typical American city that supposedly exemplified the changing culture of the US at the time.
While case studies focus more on concrete details than general theories, they should usually have some connection with theory in the field. This way the case study is not just an isolated description, but is integrated into existing knowledge about the topic. It might aim to:
- Exemplify a theory by showing how it explains the case under investigation
- Expand on a theory by uncovering new concepts and ideas that need to be incorporated
- Challenge a theory by exploring an outlier case that doesn’t fit with established assumptions
To ensure that your analysis of the case has a solid academic grounding, you should conduct a literature review of sources related to the topic and develop a theoretical framework . This means identifying key concepts and theories to guide your analysis and interpretation.
There are many different research methods you can use to collect data on your subject. Case studies tend to focus on qualitative data using methods such as interviews , observations , and analysis of primary and secondary sources (e.g., newspaper articles, photographs, official records). Sometimes a case study will also collect quantitative data.
Example of a mixed methods case studyFor a case study of a wind farm development in a rural area, you could collect quantitative data on employment rates and business revenue, collect qualitative data on local people’s perceptions and experiences, and analyze local and national media coverage of the development.
The aim is to gain as thorough an understanding as possible of the case and its context.
Here's why students love Scribbr's proofreading services
Discover proofreading & editing
In writing up the case study, you need to bring together all the relevant aspects to give as complete a picture as possible of the subject.
How you report your findings depends on the type of research you are doing. Some case studies are structured like a standard scientific paper or thesis , with separate sections or chapters for the methods , results and discussion .
Others are written in a more narrative style, aiming to explore the case from various angles and analyze its meanings and implications (for example, by using textual analysis or discourse analysis ).
In all cases, though, make sure to give contextual details about the case, connect it back to the literature and theory, and discuss how it fits into wider patterns or debates.
If you want to know more about statistics , methodology , or research bias , make sure to check out some of our other articles with explanations and examples.
- Normal distribution
- Degrees of freedom
- Null hypothesis
- Discourse analysis
- Control groups
- Mixed methods research
- Non-probability sampling
- Quantitative research
- Ecological validity
Research bias
- Rosenthal effect
- Implicit bias
- Cognitive bias
- Selection bias
- Negativity bias
- Status quo bias
Cite this Scribbr article
If you want to cite this source, you can copy and paste the citation or click the “Cite this Scribbr article” button to automatically add the citation to our free Citation Generator.
McCombes, S. (2023, November 20). What Is a Case Study? | Definition, Examples & Methods. Scribbr. Retrieved April 17, 2024, from https://www.scribbr.com/methodology/case-study/
Is this article helpful?
Shona McCombes
Other students also liked, primary vs. secondary sources | difference & examples, what is a theoretical framework | guide to organizing, what is action research | definition & examples, what is your plagiarism score.
Quantitative study designs: Case Studies/ Case Report/ Case Series
Quantitative study designs.
- Introduction
- Cohort Studies
- Randomised Controlled Trial
- Case Control
- Cross-Sectional Studies
- Study Designs Home
Case Study / Case Report / Case Series
Some famous examples of case studies are John Martin Marlow’s case study on Phineas Gage (the man who had a railway spike through his head) and Sigmund Freud’s case studies, Little Hans and The Rat Man. Case studies are widely used in psychology to provide insight into unusual conditions.
A case study, also known as a case report, is an in depth or intensive study of a single individual or specific group, while a case series is a grouping of similar case studies / case reports together.
A case study / case report can be used in the following instances:
- where there is atypical or abnormal behaviour or development
- an unexplained outcome to treatment
- an emerging disease or condition
The stages of a Case Study / Case Report / Case Series
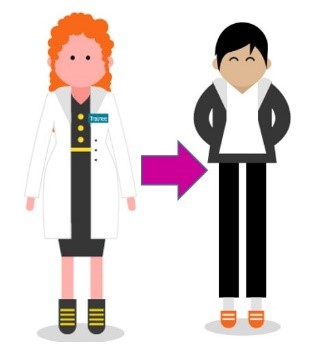
Which clinical questions does Case Study / Case Report / Case Series best answer?
Emerging conditions, adverse reactions to treatments, atypical / abnormal behaviour, new programs or methods of treatment – all of these can be answered with case studies /case reports / case series. They are generally descriptive studies based on qualitative data e.g. observations, interviews, questionnaires, diaries, personal notes or clinical notes.
What are the advantages and disadvantages to consider when using Case Studies/ Case Reports and Case Series ?
What are the pitfalls to look for.
One pitfall that has occurred in some case studies is where two common conditions/treatments have been linked together with no comprehensive data backing up the conclusion. A hypothetical example could be where high rates of the common cold were associated with suicide when the cohort also suffered from depression.
Critical appraisal tools
To assist with critically appraising Case studies / Case reports / Case series there are some tools / checklists you can use.
JBI Critical Appraisal Checklist for Case Series
JBI Critical Appraisal Checklist for Case Reports
Real World Examples
Some Psychology case study / case report / case series examples
Capp, G. (2015). Our community, our schools : A case study of program design for school-based mental health services. Children & Schools, 37(4), 241–248. A pilot program to improve school based mental health services was instigated in one elementary school and one middle / high school. The case study followed the program from development through to implementation, documenting each step of the process.
Cowdrey, F. A. & Walz, L. (2015). Exposure therapy for fear of spiders in an adult with learning disabilities: A case report. British Journal of Learning Disabilities, 43(1), 75–82. One person was studied who had completed a pre- intervention and post- intervention questionnaire. From the results of this data the exposure therapy intervention was found to be effective in reducing the phobia. This case report highlighted a therapy that could be used to assist people with learning disabilities who also suffered from phobias.
Li, H. X., He, L., Zhang, C. C., Eisinger, R., Pan, Y. X., Wang, T., . . . Li, D. Y. (2019). Deep brain stimulation in post‐traumatic dystonia: A case series study. CNS Neuroscience & Therapeutics. 1-8. Five patients were included in the case series, all with the same condition. They all received deep brain stimulation but not in the same area of the brain. Baseline and last follow up visit were assessed with the same rating scale.
References and Further Reading
Greenhalgh, T. (2014). How to read a paper: the basics of evidence-based medicine. (5th ed.). New York: Wiley.
Heale, R. & Twycross, A. (2018). What is a case study? Evidence Based Nursing, 21(1), 7-8.
Himmelfarb Health Sciences Library. (2019). Study design 101: case report. Retrieved from https://himmelfarb.gwu.edu/tutorials/studydesign101/casereports.cfm
Hoffmann T., Bennett S., Mar C. D. (2017). Evidence-based practice across the health professions. Chatswood, NSW: Elsevier.
Robinson, O. C., & McAdams, D. P. (2015). Four functional roles for case studies in emerging adulthood research. Emerging Adulthood, 3(6), 413-420.
- << Previous: Cross-Sectional Studies
- Next: Study Designs Home >>
- Last Updated: Feb 29, 2024 4:49 PM
- URL: https://deakin.libguides.com/quantitative-study-designs

Research Writing and Analysis
- NVivo Group and Study Sessions
- SPSS This link opens in a new window
- Statistical Analysis Group sessions
- Using Qualtrics
- Dissertation and Data Analysis Group Sessions
- Defense Schedule - Commons Calendar This link opens in a new window
- Research Process Flow Chart
- Research Alignment This link opens in a new window
- Step 1: Seek Out Evidence
- Step 2: Explain
- Step 3: The Big Picture
- Step 4: Own It
- Step 5: Illustrate
- Annotated Bibliography
- Literature Review This link opens in a new window
- Systematic Reviews & Meta-Analyses
- How to Synthesize and Analyze
- Synthesis and Analysis Practice
- Synthesis and Analysis Group Sessions
- Problem Statement
- Purpose Statement
- Quantitative Research Questions
- Qualitative Research Questions
- Trustworthiness of Qualitative Data
- Analysis and Coding Example- Qualitative Data
- Thematic Data Analysis in Qualitative Design
- Dissertation to Journal Article This link opens in a new window
- International Journal of Online Graduate Education (IJOGE) This link opens in a new window
- Journal of Research in Innovative Teaching & Learning (JRIT&L) This link opens in a new window
Writing a Case Study

What is a case study?

A Case study is:
- An in-depth research design that primarily uses a qualitative methodology but sometimes includes quantitative methodology.
- Used to examine an identifiable problem confirmed through research.
- Used to investigate an individual, group of people, organization, or event.
- Used to mostly answer "how" and "why" questions.
What are the different types of case studies?

Note: These are the primary case studies. As you continue to research and learn
about case studies you will begin to find a robust list of different types.
Who are your case study participants?

What is triangulation ?
Validity and credibility are an essential part of the case study. Therefore, the researcher should include triangulation to ensure trustworthiness while accurately reflecting what the researcher seeks to investigate.

How to write a Case Study?
When developing a case study, there are different ways you could present the information, but remember to include the five parts for your case study.

Was this resource helpful?
- << Previous: Thematic Data Analysis in Qualitative Design
- Next: Journal Article Reporting Standards (JARS) >>
- Last Updated: Apr 12, 2024 11:40 AM
- URL: https://resources.nu.edu/researchtools


An official website of the United States government
The .gov means it's official. Federal government websites often end in .gov or .mil. Before sharing sensitive information, make sure you're on a federal government site.
The site is secure. The https:// ensures that you are connecting to the official website and that any information you provide is encrypted and transmitted securely.
- Publications
- Account settings
- Browse Titles
NCBI Bookshelf. A service of the National Library of Medicine, National Institutes of Health.
Maben J, Griffiths P, Penfold C, et al. Evaluating a major innovation in hospital design: workforce implications and impact on patient and staff experiences of all single room hospital accommodation. Southampton (UK): NIHR Journals Library; 2015 Feb. (Health Services and Delivery Research, No. 3.3.)
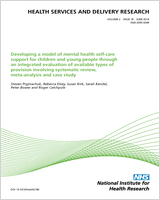
Evaluating a major innovation in hospital design: workforce implications and impact on patient and staff experiences of all single room hospital accommodation.
Chapter 5 case study quantitative data findings.
- Introduction
This chapter provides the results of the analysis of quantitative data from three different sources:
- Staff activity: task time distribution. Observations of staff activities were undertaken in each study ward to understand the types of tasks undertaken by staff and the proportion of time spent on each. Staff were shadowed by a researcher who logged their activities.
- Staff travel distances. These were collected by staff wearing pedometers. These data were collected before and after the shadowing sessions.
- Staff experience surveys. Staff surveys on each ward were conducted before and after the move to the new hospital and these data provide a comparison of perceptions of the ward environment in the old and new wards.
The survey probed perceptions of many aspects of the ward environment before and after the move. As discussed in Chapter 3 , the trust, the designers and stakeholders held various expectations about the benefits of the 100% single room design. We examined whether or not these expectations (or hypotheses about the effect of the move) were fulfilled. Specifically, the new hospital was designed to increase patient comfort, prevent infections, reduce numbers of patient falls, reduce patient stress, increase patient-centred care and increase the time spent by nurses on direct care (see Appendix 16 ). Concerns were raised about the possible reduction in staff observing and monitoring patients, increased travel distances and patient isolation.
This chapter primarily addresses the following two research questions:
- What are the advantages and disadvantages of a move to all single rooms for staff?
- Does the move to all single rooms affect staff experience and well-being and their ability to deliver effective and high-quality care?
- Staff activity: task time distribution results
Preliminary analysis showed that five activity categories accounted for 78% of observation data before the move and 83% of observation data after the move. This meant that numbers in the remaining categories were too low for analysis, so all subsequent analyses were confined to these five categories: direct care, indirect care, professional communication, medication tasks and ward-related activities. Proportion of time was derived by calculating the duration of each event from its start and end time, and then aggregating duration by activity for each observation session. The number of events for each activity was also counted ( Table 23 ).
Observations (events) per session before and after new build
Proportion of time spent in each type of activity was analysed using a general linear model with proportion of time as the dependent variable. The first model consisted of a single independent variable for before and after the new build and was used to ascertain the effect of the move to a new build, prior to adjusting for other variables. To this model were added ward (maternity, surgical, older people, AAU), staff group (midwife, RN, HCA) and day of the week. This second model was used to ascertain the effect of the move to the new build having adjusted for these variables.
Events were defined as a switch of activity (either to a new activity or to continue a previously interrupted activity) and were captured by a new entry in the PDA. The number of events (new or continuation of a previous activity) per hour was modelled in the same way except that a generalised linear model with a Poisson distribution and shift length in hours specified as offset (equivalent to modelling the hourly rate) was fitted to the data. An unadjusted analysis (before and after the new build only) and adjusted analysis (before and after the new build, ward, staff group and day of week) were performed.
Analysis of medication tasks was confined to RNs only. The fact that RMs work only on the postnatal ward means that it would not be possible to interpret whether any obtained results were due to the effect of the professional group or the ward. Therefore, staff group (i.e. midwives) was dropped from this model. On average the number of events (either new or continuations of previous activities) observed per session was higher before the move than after (177 vs. 153).
However, the move to the new build did not result in a significant change to the proportion of time spent on different activities ( Table 24 ). Although there was an increase in the proportion of direct care, indirect care, professional communication and medication tasks and a decrease in ward-related activities such as cleaning, bed making and stocking the utility room in adjusted analyses, none of these changes was statistically significant (see Table 24 ).
Mean proportion of time spent in each type of activity before and after move
Table 25 shows results for the analysis of the number of events per hour. The adjusted number of recorded events per hour decreased significantly for direct care ( p = 0.039) and professional communication ( p = 0.002), and increased significantly for medication tasks. A decrease in the number of events per hour for an activity, and no change in the proportion of time spent on that activity, suggests that there were fewer interruptions during these tasks and work was, therefore, less fragmented. This interpretation is supported by qualitative data showing that nurses could focus on direct care and communication tasks more easily in the single room environment. Staff had difficulty locating each other and also felt reluctant to interrupt a colleague providing direct care in a single room, and there were more frequent structured opportunities for professional communication within the small nursing teams.
Number of events per hour by type of activity before and after move
The number of events per hour increased significantly for medication tasks ( p = 0.001), showing increased fragmentation for this task. Again, this interpretation is supported by the qualitative data showing that when staff entered a patient room to administer medication they were likely to engage in other direct care activities; thus medication administration was not carried out in a single medication round, but integrated into patient care activities generally.
We also assessed the changes in patients’ contact time per patient-day to check if nurses spent more time with the patient instead of doing other activities. The analysis draws on day shift observation data (based on 118.5 hours of staff shadowing before the move and 254.5 hours after the move). The proportion of contact time was applied to the total NHPPD to provide an estimate of the patients’ contact time per patient-day (see Table 26 ).
Patients’ contact time per patient-day before and after move in the case study wards
After the move, the contact time per patient-days increased in all units, apart from surgery, where there was a decrease in direct care and an increase in indirect care activities, for example medication activities and professional communication, and essential ward/patient care activities.
These changes are the result of a combination of two factors: a change in the proportion of care (i.e. an increase/decrease in the time spent with the patient) and a change in NHPPD (i.e. an increase/decrease in the number of nurses working full-time during a day).
- Staff travel distances results
Statistical analysis
The data were analysed using a repeated measures general linear mixed model (GLMM) with steps per hour as the dependent variable and pre/post new build, ward (maternity, surgical, older people, AAU), observation session (repeated measure), staff group (midwife, RN, HCA) and day of the week as independent variables. The first GLMM analysis investigated the main effects of ward, pre/post move, staff group and day of the week. The second GLMM analysis investigated the interactions between pre/post move and ward, and between pre/post move and staff group. Because midwives were employed only on the maternity ward, there was potential confounding between the effects of ward and staff type. Initial analyses confirmed that removing maternity from the analyses improved the fit of the models. The first sensitivity analysis added a variable to the model that indicated whether or not a member of staff contributed to both the pre- and post-build samples. Only five staff contributed to both. The effect on the overall results was minor. A second sensitivity analysis fitted a model to first observation session data only, but allowed data to repeat across individual staff before and after the build. We report the results below, including where sensitivity analyses identified differences.
The data set contains information on 140 sessions collected on 53 staff (49%) prior to and 56 staff (51%) after the new build. A number of staff contributed more than one observation session: 85 provided one session, 18 provided two sessions, five provided three sessions and one provided four sessions. There were 73 sessions (52%) collected prior to the new build and 67 sessions (48%) after the new build. The average numbers of sessions per member of staff were 1.38 and 1.20, respectively. A small number of staff ( n = 5, 4%) were observed at both times (one RN and four HCAs). Table 27 shows descriptive data for ward and staff group.
Steps per hour before and after new build
The unadjusted means (see Table 27 ) show an increase in the number of steps per hour for all wards and staff groups. Staff working on the older people’s ward (from 664 to 845) and RNs (from 639 to 827) have seen the biggest increases.
Table 28 shows results for the main effects of ward, pre/post move, staff group and day of the week. The number of steps per hour increased significantly from a mean of 715 before the move to a mean of 839 [ F (1,83) = 10.36; p = 0.002] after the move. HCAs took significantly more steps per hour than nurses [ F (1,83) = 8.01; p = 0.006]. There were also significant differences between days of the week [ F (4,21) = 3.40; p = 0.027]. There was no significant difference between wards in the distances travelled ( Table 29 ).
F -tests on main effects
Mean steps per hour by wards, pre-/post move, staff group and day of the week
Table 30 shows results for the interactions between pre/post move and ward, and between pre/post move and staff group. Neither of the two interactions was statistically significant.
F -tests on interaction effects
The estimated marginal means ( Table 31 ) showed that there was an increase from pre to post build across all wards. Although the size of this increase did not differ significantly between wards, the increases in the surgical and older people’s wards were larger than for the AAU. RNs experienced a larger increase (from 624 to 811) in the number of steps per hour (from 3.74 to 4.86 miles) than HCAs (from 828 to 862 steps; from 4.96 to 5.17 miles).
Mean steps per hour for the interactions
The estimated marginal means from the second sensitivity analysis suggested a decrease in the number of steps per hour for the AAU from 901 to 836 and for HCAs from 876 to 855, rather than an increase as shown in Table 31 . The change in means for the remaining two wards and for RNs, from pre to post build, were in the same direction, and of the same order of magnitude (see Table 31 ).
- Staff experience survey
Because of staff leave, shift patterns and staff turnover during the course of the study, it was not possible to use a completely within-subjects design, in which the pre- and post-move surveys were completed by the same people. Despite this, 19 participants did complete surveys at both times, which meant a mixed within- and between-subjects design. One potential problem with this is that the subgroup who completed both surveys could have been sensitised to the research questions and, therefore, could have been more likely to report differences after the move than those who completed only one survey; that would bias our results. We addressed this by treating the design as a between-subjects design and checking for bias by comparing the results of our analyses for the whole group with separate within-subjects analyses on the subgroup who completed both surveys. The results were identical except for a small difference: perceptions of the effect of the accommodation on the delivery of care approached significance (0.099) in the within-subjects analysis whereas for the whole group this effect was significant (0.011). This can be attributed to lack of power in the subsample of 19. On this basis we proceeded with the analysis by treating the ‘before’ and ‘after’ samples as independent groups.
There were 152 items in the staff survey. Our approach to analysis was multifaceted. First, we explored the potential for grouping questions into subscales that would summarise a topic area. We thematically analysed the questions to determine those that were likely to be measuring attitudes to related aspects of the ward design, and then tested these subscales using statistical reliability analysis. Where reliability was not adequate we revised the items in the subscales until we had identified coherent subscales. These were then analysed using independent sample t -tests to determine if post-move responses were significantly different from the pre-move scores for each subscale. Similar analyses were undertaken for the teamwork and safety climate scales. Qualitative open-ended questions were analysed thematically using a content analytic approach. The well-being and stress items were compared before and after the move using the Pearson chi-squared test and Fisher’s exact test when expected frequencies were less than 5.
One of the aims of the study was to investigate if there were differences between the case study wards in their perceptions of the positives and negatives of the new single room accommodation. However, the relatively small number of staff in each of the case study wards meant that it was not possible to explore this question statistically. We therefore used correspondence analysis and perceptual mapping to examine the interaction between ward attributes and case study wards. Correspondence analysis is an exploratory mapping tool that allows visualisation of relationships in the data that would be difficult to identify if presented in a table. 114 It is related to other techniques such as factor analysis and multidimensional scaling. It does not rely on significance testing and is best viewed as an exploratory technique that provides insights into the similarities and differences between two variables. 115 Correspondence analysis does not address questions of whether or not there were differences in ratings between the attributes (e.g. whether or not privacy for patients was rated more highly than staff teamwork). Instead, it focuses on the differences between case study wards and the interaction between ratings and wards. It allows an examination of to what extent which wards are associated with particular ratings. In this way it allows us to qualitatively explore the quantitative data.
Ward environment survey subscales
Ten reliable subscales were formed. Table 32 shows the subscales and example items from each.
Description of subscales
Appendix 19 contains a complete list of all items used for each subscale.
Table 33 summarises the statistical analysis of the subscales showing means, Cronbach’s alpha and the number of items for each subscale before and after the move. According to accepted criteria, 115 alpha above 0.60 is acceptable for exploratory analyses, above 0.70 is acceptable for confirmatory purposes and above 0.80 is good for confirmatory purposes. Obtained coefficients were generally good, ranging mostly between 0.67 and 0.92. The lowest alpha, of 0.53, was obtained for the family/visitors subscale after the move, suggesting that this subscale is not internally consistent. However, the pre-move alpha was good (0.70), so it was decided to retain this subscale for exploratory purposes.
Mean subscale scores and reliability analysis before and after the move
Table 34 shows the results of independent sample t -tests comparing subscale scores before and after the move. Staff perceived significant improvements in the efficiency of the physical environment, the patient amenity, the effect of the environment on infection control, patient privacy, and family and visitors. The largest increases were found for perceptions of infection control and patient privacy. Perceptions of the effect of the ward environment on teamwork and care delivery were significantly more negative after the move. There were no significant differences in staff perceptions of staff facilities, patient safety and staff safety.
Results of t -tests comparing perceptions of the ward environment before and after the move
Although all subscales showed moderate to very good reliability, changes were not uniform for all items in every subscale; there were some exceptions to the overall trend. Overall ratings for the subscale ‘efficiency of physical environment’ increased, but ratings for the item ‘ward design/layout minimises walking distances for staff’ decreased. These perceptions were confirmed by our findings from the analysis of travel distances showing that staff took significantly more steps after than before the move. Some aspects of the design increased the amenity of the ward for staff but others did not. For example, staff toilet facilities, locker facilities and space at staff bases were rated more highly but ratings for social interaction and natural light decreased. These positive and negative aspects meant there was no significant difference in staff amenity before and after the move. The new ward was rated as much more positive for patients but there were reduced scores for three items after the move: social contact between patients, ability of patients to see staff and way finding. All aspects of teamwork and training were rated less positively, except for the item ‘discussing patient care with colleagues’, which increased. This finding is supported by our analysis of observation data showing that professional communication activities were less fragmented.
Although there were no significant differences in the effect of the ward layout on perceptions of patient safety, examination of the items showed that ratings for two items increased (‘minimising risk to patients of physical/verbal abuse from other patients/visitors’ and ‘minimising the risk of medication errors’) while ratings for two items decreased (‘responding to patient calls for assistance’ and ‘minimising the risk of falls/injury to patients’). This suggests that, although staff thought some risks to safety were reduced, they perceived an increased risk of falls and delays in responding to calls for assistance. Staff perceptions of a rise in risk of falls are detailed in Chapter 6 . Staff also reported being unable to hear calls for assistance when in a single room with a patient.
There were five items that did not fit into any of the subscales. These items were analysed singly using Fisher’s exact test and the results are shown in Table 35 . There was a significant relationship between the move and ratings for the number and location of hand basins, ease of keeping patient areas clean and quiet, and the overall comfort of patients, which all increased after the move. There was no relationship between the move and judgements of whether or not the location of the dirty utility room (where bedpans are stored and disposed of) reduces cross-contamination.
Results of single-item analyses
The distribution of responses for the four significant items showed that significantly more staff rated these aspects of single room accommodation as more positive after the move than before ( Tables 36 – 39 ).
Distribution of responses for the item ‘Number and location of CHWBs supports good hand hygiene’
Distribution of responses for the item ‘Easy to keep patient care areas clean’
Distribution of responses for the item ‘Overall comfort of patients’
Distribution of responses for the item ‘Easy to keep patient care areas quiet’
Expectations before the move and reality after the move
Before the move, staff were asked to rate on a five-point scale whether they thought single rooms would be better or worse for different aspects of clinical work (e.g. minimising the risk of patient falls, maintaining patient confidentiality, knowing when other staff might need help). After the move they again rated whether single rooms were better or worse for clinical work, thus providing a measure of whether or not their expectations about single rooms were met in reality. The questions were a subset of 23 questions from the first part of the survey and were analysed using Fisher’s exact test.
Results ( Table 40 ) showed that staff perceptions of whether or not single rooms were better than multibedded wards changed after the move for five items. Staff perceptions of whether or not single rooms were better for responding to calls for assistance, knowing when other staff might need help and minimising walking distances were rated as worse or much worse by significantly more staff after than before the move. Staff rated single rooms as positive for patient sleep and rest and for interactions between patients and visitors after the move.
Relationship between expectations before the move and reality after the move
Tables 41 – 45 show the distribution of significant responses.
Distribution of responses for the item ‘Responding to patient calls for assistance’
Distribution of responses for the item ‘Minimising staff walking distances’
Distribution of responses for the item ‘Patient sleep and rest’
Distribution of responses for the item ‘Knowing when other staff might need a helping hand’
Distribution of responses for the item ‘Patient interaction with visitors’
Teamwork and safety climate survey
To take into account our changes to the survey, we combined the four items about the quality of communication with doctors, nurses, nursing assistants and AHPs with the items in the information handover subscale to form a new subscale of seven items. Although this is different from the scales reported by Hutchinson et al. , 98 reliability analysis confirmed the original factor structure of the survey. There were two teamwork subscales and three safety climate subscales with good to high reliability ( Table 46 ). See Appendix 20 for a list of the items contained in each subscale.
Mean scores for all subscales decreased following the move. Independent sample t -tests showed that ratings for information handover and communication decreased significantly following the move [ t = 3.23, degrees of freedom (df) = 108, p = 0.002], indicating that information exchange and sharing within teams was perceived to be worse after the move. There were no other significant differences.
Correspondence analysis
Correspondence analysis transforms cross-tabulated data into a biplot showing distances between variables. In this study, case study ward was a column variable and mean questionnaire subscale score was a row variable (see Table 33 ). As appropriate when analysing mean scores, Euclidean distance was used and standardisation by removing row means was used. 114 , 116 This means that differences between the subscale means were not represented in the perceptual map, as we were not interested in whether or not, for example, infection control was rated more highly than privacy. Differences between wards, contained in the columns, were of interest and are represented in the perceptual map. Separate analyses were conducted for before and after the move and for the ward attributes and teamwork/safety climate survey.
Figure 11 shows perceptual maps of the association between ward attributes and wards before and after the move. The pre-move map shows that the points on the map were dispersed, indicating that the ratings were not strongly associated with particular wards. There was one exception in that ratings for the efficiency of the physical environment, privacy and infection control were higher for the older people’s ward than for the other wards. The post-move map shows that the highest ratings for the efficiency of the physical environment, the delivery of care, the staff facilities and teamwork were obtained in the older people’s and surgical wards, indicated by proximity on the map. Ratings for patient amenity, infection control, privacy and family/visitors were highest for the surgical ward. High ratings for patient safety were obtained in maternity and the surgical ward. Ratings for staff safety were similar in the older people’s, surgical and maternity wards. The acute assessment ward was not associated with any particular ward attributes, as was the case before the move.
Perceptual maps of (a) pre- and (b) post-move ward attributes by ward.
Figure 12 shows perceptual maps before and after the move of the association between teamwork/safety climate ratings and wards. The teamwork/safety climate survey consisted of two teamwork subscales – team input into decisions, and information handover and communication – and three safety climate subscales – attitudes to safety within own team, overall confidence in safety of organisation and perceptions of management attitudes to safety. The pre-move map shows that ratings of input into decisions, information and handover, and overall confidence in safety of the organisation were highest for the acute assessment ward. Ratings of safety attitudes within the team and management attitudes to safety were highest for the surgical ward. After the move, the surgical ward had the highest ratings for safety attitudes within the team, overall attitudes to safety and management; ratings for team input into decisions and information handover and communication were highest for the older people’s ward. Ratings for all safety climate subscales decreased in the acute assessment ward, which is indicated on the perceptual map by its location in a quadrant by itself. Maternity scores did not show a consistent pattern.
Perceptual map of (a) pre- and (b) post-move ratings of teamwork/safety climate by ward. Att., attitude; mgt., management.
These maps reveal some differences between wards in perceptions of the ward environment and show that perceptions were different before and after the move.
Staff ward preferences
Nursing staff were asked to indicate whether they would prefer single rooms, multibedded accommodation or a combination. There was a range of views ( Figure 13 ). In each phase, fewer than 18% of staff indicated a preference for 100% single rooms. The most common preference in each phase was a combination of 50% of beds in single rooms and 50% in bays (see Figure 13 ). In the pre-move survey, more staff reported a preference for more beds in bays ( n = 20) than in the post-move phase ( n = 12).
Nurse preferences for single room or multibedded accommodation.
Staff stress and well-being
There were five categorical questions about staff well-being that investigated whether or not they had experienced injuries and harassment in the previous 12 months ( Table 47 ). There were three items about job stress that asked participants to rate their stress on a five-point Likert scale ( Table 48 ) . Results showed no differences in staff well-being and stress before and after the move.
Relationship between move and staff well-being
Relationship between move and staff stress
Staff were asked 10 questions about their satisfaction with their own performance of various tasks during their last shift, and one question about their overall job satisfaction. Results ( Table 49 ) showed no significant effect for any of the job satisfaction items.
Relationship between job satisfaction and move
Qualitative survey data
Four open-ended questions were used to gain qualitative data about staff attitudes. The questions were:
- What two things do you think would most improve the current ward environment for staff?
- What two things do you think would most improve the current ward environment for patients?
- What two things are you most looking forward to in relation to the move to 100% single rooms in the new hospital?
- What two things are you most concerned about in relation to the move to 100% single rooms in the new hospital?
- What two things do you like the most about single room wards in the new hospital?
- What two things do you dislike most about single room wards in the new hospital?
In the following sections we present the results of the thematic analysis with frequency data (almost equal numbers of staff responded before and after the move, n = 55 and n = 54 respectively) and examples from participants’ written responses where appropriate. Table 50 shows that staff identified a number of things that would improve the ward accommodation for patients. The need for more space, improved patient facilities, privacy, and rest and sleep were largely met, since there were fewer people identifying these as needs after the move. However, the need for improved patient–staff ratios and a day room to provide patient social interaction were still reported after the move.
What would improve the current ward environment for patients ? Response frequencies
The need that staff perceived before the move for space around patient beds and staffing levels had decreased after the move ( Table 51 ). However, ventilation/heating/lighting, access to equipment and supplies and facilities for staff, including staff bases, were identified as needing improvement after move. In addition there was a need for improvements in monitoring patients, keeping track of colleagues, reducing isolation and reducing walking distances. These have all been identified by other parts of our results (see Chapter 6 ).
What would improve the current ward environment for staff ? Response frequencies
Staff were asked about the features of the ward they were most looking forward to in the pre-move phase, and most liked in the post-move phase ( Table 52 ). Results showed that staff most liked the increased patient privacy, patient sleep and rest, increased space, working in a modern environment and improved patient bathroom facilities.
What are you most looking forward to/do you most like about 100% single room accommodation? Response frequencies
Table 53 shows that staff were most concerned about being able to monitor patients, patient isolation and the risk of falls. Being unable to find staff and increased walking distances also emerged as features staff disliked about single rooms.
What are you most concerned about/do you most dislike about 100% single room accommodation? Response frequencies
- Most staff would prefer a mix of single rooms and multibedded rooms on wards.
- Staff activity events observed per session were higher after the move and direct care and professional communication events per hour decreased significantly, suggesting fewer interruptions and less fragmented care.
- A significant increase in medication tasks among recorded events suggests medication administration was integrated into patient care activities and was not undertaken as a medication ‘round’.
- Travel distances increased for all staff, with highest increases for staff in the older people’s ward and surgical wards and for RNs/RMs.
- efficiency in carrying out tasks
- patient amenity, including comfort, space, sleep, light and ventilation
- infection control
- patient privacy
- patient interaction with family/visitors and their involvement in care.
- In open comments, staff most liked the increased patient privacy, working in a modern environment, improved patient sleep and rest, and space around the bedside.
- delivery of care, including factors such as spending time with patients, communication with patients, monitoring patients and remaining close to patients, responding to calls for assistance, minimising the risks to staff, minimising walking distances and staff spending time with patients
- teamwork, including being able to locate staff, obtain assistance from colleagues, informal learning, keeping team members updated, discussing care with colleagues and knowing when other staff might need help.
- In addition, in open comments staff were most concerned about patient isolation, the risk of falls and staff isolation.
- There were no perceived differences in staff amenity and patient and staff safety.
- Ratings for information handover and communication decreased significantly following the move. This suggests that information exchange and sharing within teams – and between professions – was perceived to be worse after the move.
- Different wards valued different aspects of the ward environment.
- Ratings for staff toilet facilities, locker facilities and space at staff bases were rated more highly but ratings for social interaction and natural light decreased.
- No differences were found in staff job satisfaction, well-being or stress before and after the move.
- The need for improved patient–staff ratios and a day room to provide patient social interaction was still reported after the move.
Included under terms of UK Non-commercial Government License .
- Cite this Page Maben J, Griffiths P, Penfold C, et al. Evaluating a major innovation in hospital design: workforce implications and impact on patient and staff experiences of all single room hospital accommodation. Southampton (UK): NIHR Journals Library; 2015 Feb. (Health Services and Delivery Research, No. 3.3.) Chapter 5, Case study quantitative data findings.
- PDF version of this title (96M)
In this Page
Other titles in this collection.
- Health Services and Delivery Research
Recent Activity
- Case study quantitative data findings - Evaluating a major innovation in hospita... Case study quantitative data findings - Evaluating a major innovation in hospital design: workforce implications and impact on patient and staff experiences of all single room hospital accommodation
Your browsing activity is empty.
Activity recording is turned off.
Turn recording back on
Connect with NLM
National Library of Medicine 8600 Rockville Pike Bethesda, MD 20894
Web Policies FOIA HHS Vulnerability Disclosure
Help Accessibility Careers
- Open access
- Published: 17 April 2024
Dependence on visual information in patients with ACL injury for multi-joint coordination during single-leg squats: a case control study
- Minoru Toriyama 1 , 2 , 3 ,
- Atsuo Nakamae 1 ,
- Takumi Abe 4 ,
- Kazuhiko Hirata 1 , 5 &
- Nobuo Adachi 1
BMC Sports Science, Medicine and Rehabilitation volume 16 , Article number: 87 ( 2024 ) Cite this article
Metrics details
The influence of vision on multi-joint control during dynamic tasks in anterior cruciate ligament (ACL) deficient patients is unknown. Thus, the purpose of this study was to establish a new method for quantifying neuromuscular control by focusing on the variability of multi-joint movement under conditions with different visual information and to determine the cutoff for potential biomarkers of injury risk in ACL deficient individuals.
Twenty-three ACL deficient patients and 23 healthy subjects participated in this study. They performed single-leg squats under two different conditions: open eyes (OE) and closed eyes (CE). Multi-joint coordination was calculated with the coupling angle of hip flexion, hip abduction and knee flexion. Non-linear analyses were performed on the coupling angle. Dependence on vision was compared between groups by calculating the CE/OE index for each variable. Cutoff values were calculated using ROC curves with ACL injury as the dependent variable and significant variables as independent variables.
The sample entropy of the coupling angle was increased in all groups under the CE condition ( P < 0.001). The CE/OE index of coupling angle variability during the descending phase was higher in ACL deficient limbs than in the limbs of healthy participants ( P = 0.036). The CE/OE index of sample entropy was higher in the uninjured limbs of ACL deficient patients than in the limbs of healthy participants ( P = 0.027). The cutoff value of the CE/OE index of sample entropy was calculated to be 1.477 (Sensitivity 0.957, specificity 0.478).
ACL deficient patients depended on vision to control multiple joint movements not only on the ACL deficient side but also on the uninjured side during single leg squat task. These findings underscore the importance of considering visual dependence in the assessment and rehabilitation of neuromuscular control in ACL deficient individuals.
Peer Review reports
Anterior cruciate ligament (ACL) injury is the one of the most common injury of the knee in sports [ 1 ]. The incidence rate of ACL injuries remained relatively stable between 1990 and 2010, especially in females [ 2 ]. Moreover, patients who have undergone ACL reconstruction often require revision or suffer an ACL injury on the contralateral side. In the first 5 years after ACL reconstruction, the rate of new ACL injury is higher than the rate of primary ACL injury in the general population [ 3 ]. These high ACL injury rates may be due in part to the lack of effective prevention programs before injury and after ACL reconstruction.
Noncontact mechanisms account for 70–76% of all ACL injuries [ 4 ] and occur most commonly during dynamic activities involving rapid deceleration and landing [ 5 , 6 ]. Performing these movements with less risk of ACL injury requires more skillful neuromuscular control. In previous studies, neuromuscular control systems have often been represented by the movement of the centre of pressure (COP). Fernandes TL et al. observed that during single-leg standing and squat tasks, athletes with ACL injuries exhibited greater lateral shifts in the COP than did healthy subjects [ 7 ]. Similarly, Nematollahi M et al. reported that the trembling component of the COP, which reflects peripheral systems such as muscle activity, was significantly greater in individuals with ACL deficiency under both single-leg and double-leg conditions, indicating increased instability [ 8 ]. Bodkin SG et al. reported no significant difference in the average velocity of the COP during one-legged stance postural control between patients who had undergone ACL reconstruction and healthy subjects, suggesting that ACL reconstruction may restore some aspects of postural control to preinjury levels [ 9 ]. However, Steffen K et al. found no correlation between COP movement velocities, both anterior-posterior and lateral, during static and dynamic postural control and the risk of ACL injury in female elite handball and soccer players [ 10 ]. This indicates that poor movement specific to those at high risk of ACL injury is inadequately measured by COP and that it is difficult to identify features of the neuromuscular control system. Moreover, several studies suggest that noncontact ACL injury results from multi-plane joint moment caused by multi-directional ground reaction forces [ 5 , 11 , 12 ]. These studies show that controlling joint motion across multiple joints could lead to postural stabilization and prevention of ACL injury. The nonlinear analyses of motion variability associated with ACL injury or reconstruction have focused on two different joints or two joint motions [ 13 , 14 , 15 ]. On the other hand, it has been shown that more than three joint motions, including others in the knee joint, may be involved in the risk of noncontact ACL injury. Video analysis at the time of ACL injury reported low hip flexion angles [ 16 ], and weakness of the hip abductor muscle strength was a risk factor for non-contact ACL injuries [ 17 ]. In addition, a decrease in absorption in the lower extremity due to a smaller hip flexion angle motion [ 18 ], this is an energy absorption strategy that relies on distal joints such as the ankle joint and may increase the knee valgus motion [ 19 ]. It is speculated that the combined occurrence of these factors increases the risk of ACL injury. These joint movements can be controlled by muscles, unlike knee valgus motion which does not have a primary active muscle, so skillful control of these joint movements may be useful in preventing ACL injury.
Recent studies have shown that coordination patterns change depending on the availability of visual information [ 20 ]. Studies of stability control related to ACL injury have examined the influence of vision in static assessments such as quiet standing or one-legged standing. In several previous reports, COP deviation in patients after ACL reconstruction was greater with closed eyes than with open eyes, and these values showed a greater range of elevation than did those on the healthy or uninjured side [ 21 , 22 , 23 ].. However, prevention of ACL injuries or revision after ACL reconstruction requires stable postural control in more dynamic situations. Trulsson et al. reported deviations in muscular activity between the injured and noninjured sides in individuals with ACL injuries during single-leg squats, suggesting altered sensorimotor control [ 24 ]. Therefore, the influence of vision on the neuromuscular control system in more complex tasks, such as the single-leg squat, should be considered.
The purpose of this study was to reveal differences in neuromuscular control in ACL-deficient patients during single-leg squats with different visual information via nonlinear analysis for multiple joint movements. We hypothesized that ACL-deficient patients would exhibit more variability during movement in both of injured side and uninjured side, and that reduced visual information would further manifest their characteristic movements variability.
Participants
This study was approved by the Ethical Review Committee for Medical Research Involving Human Subjects in accordance with the Declaration of Hiroshima University (ID number: C-274-1). Twenty-three patients with non-contact ACL injury (23 affected knees) aged from 16 to 42 years (11 males and 12 females; mean age, 21.7 ± 6.9 years old) participated in this study. The recruitment period for this study was between July 2019 and June 2022. The inclusion criteria were as follows: outpatients of the Department of Orthopaedic Surgery, Hiroshima University Hospital, who completed junior high school or other courses, diagnosed by an experienced orthopaedic surgeon as having ACL injury based on MRI imaging findings and physical findings, requiring ACL reconstruction, and able to walk alone. The surgeon was trained to uniformly evaluate the physical examination findings, including the pivot shift test and the Lachman test. Patients were excluded if they had any of the following: under 16years old, bilateral ACL injury, history of lower limb injuries within 2 years, ligament reconstruction within 2 years, knee or hip joint arthroplasty or high tibial osteotomy, neuromuscular disorder, history of stroke or cardiovascular disease, or any other gait abnormalities. For comparison, 23 healthy subjects matching age and body size with no history of neuromuscular disorder or orthopaedic problems in the lower limbs participated. Participant characteristics are shown in Table 1 . All participants in this study gave informed consent using documentation and signed a consent form.
A prior power analysis for sample size was performed with G*Power (version 3.1; Franz Faul, Kiel University, Kiel, Germany); for an effect size of 0.3, power of 0.80, an α level of 0.05, and numerator degrees freedom of 1 and 2, number of groups of 6; a total of 90 and 111 samples for main effects, and 111 samples for interactions were needed, respectively. Therefore, there was a minimum of 23 samples for each condition and for each group considering possible dropout in this study.
Kinematic data on the patient motion were acquired using a three-dimensional motion analysis system (VICON NEXUS; Vicon Motion Systems, Oxford, UK) with 16 infrared cameras (Vicon Motion Systems, Oxford, UK) operating at 200 Hz. Before each measurement session, devices were calibrated, and the mean calibration residuals for trials were under 1.00 mm.
Infrared-reflecting markers 14 mm in diameter were attached to 45 landmarks including the left front head, right front head, left back head, right back head, 7th cervical vertebrae, 10th thoracic vertebrae, clavicle, sternum, right back, bilaterally acromion, lateral epicondyle approximating the elbow joint, wrist bar thumb side, and pinkie side, head of the 2nd metacarpus, anterior superior iliac spine, posterior superior iliac spine, great trochanter, lateral aspects of the thighs, lateral and medial epicondyles of the femur, lateral aspects of the shanks, lateral and medial condyles of the tibia, lateral and medial malleoli, head of the 2nd metatarsal heads, and the calcaneal tuberosity. Motion trials were captured as the participant performed single-leg squats (SLSs). Participants performed the actual task after completing a minimal set of fewer than five consecutive SLSs with their eyes open as a preliminary exercise. Participants were instructed to perform 12 SLSs with their hands on hips; requirements for the flexion angles of the joints and the depth of the squat were not specified. The SLSs were conducted in sync with a metronome set at 120 bpm, such that the metronome emitted a clicking sound once at the lowest position of the squat and once at the highest position. Participants performed the exercises under two randomized order conditions; the eyes-opened (OE) and eyes-closed (CE), with the supporting leg, which was defined as the lower limb on the supporting side when kicking a ball, in healthy subjects (Healthy) and both the ACL-deficient (ACLD) side and contralateral uninjured side (Uninjured) in patients with ACL injury. Successful trials were those in which the participants performed 12 repetitions without the opposite lower limb touching the ground and performed in rhythm. The first and last one each were excluded, and the 10 SLSs were analysed.
Data processing
The lower limb joint angles and centre of mass (COM) were calculated using the processing software Body-Builder (Vicon Motion Systems, Oxford, UK) based on collected marker coordinates. The centre of a participant’s ankle joint was estimated as the midpoint between the malleoli, while the knee joint centre was estimated as the midpoint between the lateral and medial epicondyles of the femur and the lateral and medial condyles of the tibia. The hip joint centre was estimated based on a previous study [ 25 ]. The collected marker coordinates were used to define the respective local coordinate systems of the fifteen-point body link model consisting of the head, thorax, both upper arms, both lower arms, hands, pelvis, both thighs, both shanks, and both feet. The position of the centre of mass position for each segment was calculated based on body inertia characteristics in a report by Okada et al. [ 26 ], and all composite centres of mass for all segments were used as the whole-body COM. A single squat was identified as the combined descending and ascending phases of a SLS indicated by the COM vertical displacement between the vertical maximum position.
Multiple joint coordination
Hip flexion-extension, hip abduction-adduction, and knee flexion-extension motions are associated with ACL injury [ 6 ]. The coordination of these three joint motions, hip flexion (+)–extension (-), hip abduction (+)–adduction (-) and knee flexion (+)–extension (-), and the coupling angle (CA) were obtained from the Appendix.
The COM data were divided into ascending and descending phases, and the coupling angle variability (CAV) was calculated for each phase by the Appendix, and the average of 10 SLSs. The sample entropy (SaEn) of the CA was calculated with embedding dimension, and tolerance was set to 2 and 0.2 × SD, respectively [ 27 ]. Non-linear analysis processing was conducted in open-source Python (version 3.9) under Jupyter Notebook with pandas, nolds, numpy, sklearn and Anaconda libraries.
Dependence of visual information
To examine the effect of visual acuity, the CE/OE index was calculated for each variable by dividing the CE value by the OE value. Values close to 1 suggest a minimal influence of visual information on balance, while values greater than 1 indicate a greater dependency on vision.
Statistical analysis
The statistical analyses were performed using IBM SPSS 25.0 (SPSS Inc., Chicago, IL, USA). Differences in physical characteristics between groups in the participants were tested with an unpaired t-test. A two-way factorial analysis of variance (ANOVA) was performed to assess the effects of group (Healthy vs. ACLD vs. Uninjured) and condition (eyes-open and eyes-closed) on COP values, CAV and SaEn. One-way ANOVA was performed for the CE/OE index for each variable. All variables are presented as the mean and SD. If significant main effects or interactions were identified using ANOVA, post hoc pairwise comparisons using the Tukey‒Kramer multiple comparisons test were then performed.
Finally, the capacities of dependence on visual information indicators for predicting ACL-injured risk were compared via area under the receiver-operating characteristics (ROC) curves (AUC) analysis. We analysed variables significantly different from healthy subjects for the uninjured side limb of ACL-injured individuals, rather than the injured leg, in order to identify the potential risk of ACL injury. The cutoff value was defined as the point at which the Youden Index of the ROC curve was the largest. All p values were two–sided and p < 0.05 was considered statistically significant.
Effects of ACL injury and condition on CAV and SaEn
Significant condition-specific effects were observed for ascending-phase CAV ( p < 0.001, F = 33.86), descending-phase CAV ( p = 0.048, F = 3.99) and SaEn CA ( p < 0.001, F = 52.02). Conversely, the main effect of group failed to reach statistical significance. All results of two-way ANOVA are shown in Table 2 .
Effects of visual information for CAV and SaEn
Group mean ± 95% confidence intervals, along with individual participant mean outcome measures are presented in Fig. 1 , with full statistical analysis reported in Table 3 . The CE/OE index of CAV during the descending phase in the ACLD was higher than that in healthy participants (95% CI 0.017-0.60; P = 0.036; Table 3 ). The CE/OE index of SaEn on the uninjured side was higher than that of healthy participants (95% CI 0.031–0.49; P = 0.027; Table 3 .)
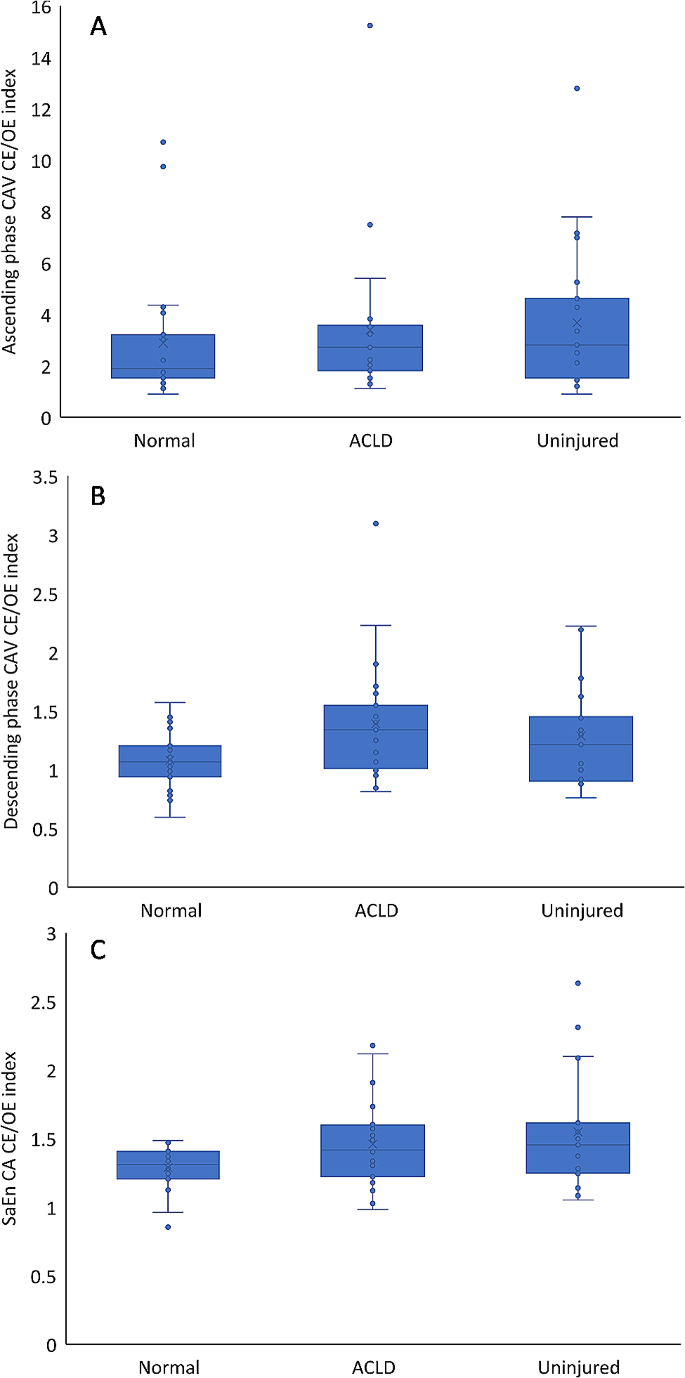
CE/OE index of CAV and SaEn. Group mean ± 95% confidence intervals and individual participant mean data. Data presented and abbreviations are as follows: Ascending phase CAV CE/OE index as ( A ), Descending phase CAV CE/OE index as ( B ), SaEn CA CE/OE index as( C )
ROC curve analysis
ROC curve analysis by CE/OE index of SaEn is shown in Fig. 2 . The cutoff of the CE/OE index of SaEn was calculated to be 1.477 (sensitivity 0.957, specificity 0.478), and AUC was 0.677(95% CI 0.513–0.84).
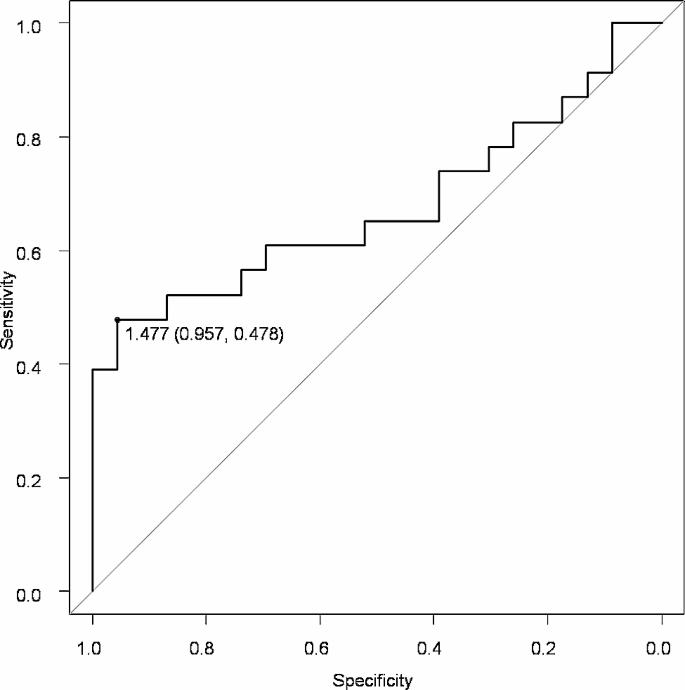
ROC curve of CE/OE index of SaEn. The ROC curves displayed CE/OE index of SaEn cutoff of 1.477 (sensitivity 0.957, specificity 0.478), and AUC was 0.677(95% CI 0.513–0.84)
The most important finding in this study is that patients with ACL injuries have contralateral neuromuscular control system dysfunction, indicating that stable and continuous joint motion is difficult at multiple joints. These results may quantify the potential risk of ACL injury in individuals with current ACL injuries and may be useful for preventing not only future reinjury of the reconstructed ACL but also new injury of the contralateral ACL.
Our study results showed that, in both healthy subjects and those with ACL injuries, the variability in postural control during SLSs was greater when the subjects’ eyes were closed than when they were open. Specifically, we observed an average increase in variability of 136.8% during the ascending phase and 117.3% during the descending phase, as measured by the CAV, and an average increase of 141.8% in the SaEn CA. This finding indicates greater variability in postural control during dynamic motor tasks with eyes closed. However, there was no difference in variability under both the open-eyes and closed-eyes conditions between the two groups. These results are consistent with the finding of Dingenen et al., who demonstrated no significant difference in single-leg stance COP stability among healthy, ACL-injured, and contralateral limbs under both open-eyes and closed-eyes conditions [ 28 ]. In contrast to our findings, prior studies have reported that compared with healthy subjects, individuals with ACL injuries exhibited impaired postural control on not only the injured side but also the contralateral side during static tasks such as static standing and single-leg standing [ 29 , 30 ]. We speculate that this difference is because we selected the SLS, which is a more dynamic task. Among several dynamic motor tasks, the SLS is more susceptible to dual-task effects [ 31 ]. Our study results indicate that it is not appropriate to compare the absolute values of the variability in the movement of SLSs between open and closed eyes. However, several reviews have indicated that a more dynamic assessment is needed for the prevention of noncontact ACL injuries and reinjury [ 32 , 33 ]. This shows that a novel dynamic postural control assessment index is needed to detect the risk that ACL-injured patients have.
In this study, CE/OE was evaluated to quantify the reliance on visual information in ACL-injured subjects. The results revealed greater variability on the ACLD limb than healthy subjects’ limb during the descending phase of CAV and on the uninjured limbs than healthy subjects’ limb during the entire SaEn. These results suggest that ACL-injured subjects use a postinjury adapted or preinjury potentially visually dependent movement strategy. ACL-injured patients are known to have different motor patterns on the contralateral side compared to healthy subjects [ 34 ], and lack of visual information promotes a more rigid movement pattern [ 35 ]. This might show that ACL-injured patients exhibit more rigid movement patterns when required to perform the dynamic task of collecting more sensory information due to the loss of visual information. In order to understand the clinical significance of these variables, we used ROC analysis to determine the cutoff risk of ACL injury on the uninjured side limb, which is less susceptible to changes in joint motion due to ACL injury. The cutoff value was 1.477, and the sensitivity was reasonably high, indicating the possibility of screening for ACL injury risk, and although the specificity is low, it is useful for clinically screening individuals for prevention programs. ACL-injured limbs demonstrated lower kinesthesia [ 36 ], fewer somatosensory evoked potentials than healthy subjects [ 37 ], and a lack of muscle coactivation modulation [ 38 ]. ACL-injured individuals might be implementing adaptations to the reduced afferent input at the knee joint due to ACL deficiency that increases afferent joint sensory input information by increasing joint motion variability [ 39 , 40 ]. In addition to these studies, the lack of visual information for the ACL-injured subjects may also increase variability in other multi-joint movements, including the hip joint that we evaluated, to increase dynamic afferent joint sensory input. Moreover, Diekfuss et al. evaluated altered brain connectivity that may have predisposed athletes to ACL injury and reported that those who went on to experience an ACL injury had decreased functional connectivity between the left primary sensory cortex and right posterior lobe of the cerebellum [ 41 ]. These previous reports suggest that ACL-injured patients have different neuromuscular control systems than healthy subjects even before ACL injury, which may have been highlighted by the visual deficits and dynamically unstable motor tasks in the present study. Future research exploring optimal variability in multiple joints will provide a better understanding of ACL injury prevention.
There are three limitations to this study. First, it is not clear when differences in motor control on the uninjured side occur in ACL-injured patients. The subjects with ACL injuries had lower Tegner activity scores than did the healthy subjects, which may indicate that inactivity affects postural control. In addition, it is known that ACL-injured individuals experience a variety of changes due to injury, one of which affects the contralateral lower extremity [ 42 ]. To verify this, a prospective cohort study is needed to determine if there are any differences in the contralateral lower extremity in future ACL injury survivors. Second, this study does not fully demonstrate whether ACL deficiency causes differences in movement characteristics on the ACL-injured side. Therefore, it is necessary to clarify the effects of afferent and efferent neuromuscular control system functions, including tests of proprioceptive function and evaluation of muscle activity. To investigate the effects of ACL deficiency, it is necessary to examine whether changes in movement variability occur when knee joint stability is improved through prospective studies after ACL reconstruction. The application of principal component analysis for dimensionality reduction in this study potentially constrained the multidimensional analysis of the data. Consequently, critical insights into the unique joint motion characteristics of individuals with ACL injuries, such as variability in motion during specific time intervals and the interplay between different conditions, might not have been fully captured. To overcome these limitations, future research should include analytical techniques capable of identifying variability across specific periods, such as Statistical Parametric Mapping [ 43 ], to provide a more comprehensive understanding of the factors contributing to ACL injury risk.
Subjects with ACL injuries exhibit increased variability and dependence on visual information during SLSs, as indicated by higher CE/OE indices in multiple joint CAV and SaEn than healthy subjects. These findings underscore the importance of considering visual dependence in the assessment and rehabilitation of neuromuscular control in ACL-deficient individuals.
Data availability
The data sets used and/or analyzed in this study are available from the corresponding author if reasonably requested.
Abbreviations
Anterior cruciate ligament
Closed eyes
Center of pressure
Body mass index
Magnetic resonance imaging
Single-leg squats
Anterior cruciate ligament deficient
Centre of mass
Coupling angle
Coupling angle variability
- Sample entropy
Area under the curve
Receiver-operating characteristic
Analysis of variance
Sun Z, Cieszczyk P, Huminska-Lisowska K, Michalowska-Sawczyn M, Yue S. Genetic determinants of the anterior cruciate ligament rupture in Sport: an Up-to-date systematic review. J Hum Kinet. 2023;87:105–17.
PubMed PubMed Central Google Scholar
Sanders TL, Maradit Kremers H, Bryan AJ, Larson DR, Dahm DL, Levy BA, Stuart MJ, Krych AJ. Incidence of anterior cruciate ligament tears and Reconstruction: a 21-Year Population-based study. Am J Sports Med. 2016;44(6):1502–7.
Article PubMed Google Scholar
Wright RW, Magnussen RA, Dunn WR, Spindler KP. Ipsilateral graft and contralateral ACL rupture at five years or more following ACL reconstruction: a systematic review. J Bone Joint Surg Am. 2011;93(12):1159–65.
Article PubMed PubMed Central Google Scholar
Agel J, Arendt EA, Bershadsky B. Anterior Cruciate Ligament Injury in National Collegiate Athletic Association Basketball and Soccer: a 13-Year review. Am J Sports Med. 2005;33(4):524–31.
Boden BP, Dean GS, Feagin JA, Garrett WE. Mechanisms of Anterior Cruciate Ligament Injury. Orthopedics. 2000;23(6):573–8.
Article CAS PubMed Google Scholar
Myer GD, Ford KR, Di Stasi SL, Foss KD, Micheli LJ, Hewett TE. High knee abduction moments are common risk factors for patellofemoral pain (PFP) and anterior cruciate ligament (ACL) injury in girls: is PFP itself a predictor for subsequent ACL injury? Br J Sports Med. 2015;49(2):118–22.
Fernandes TL, Felix EC, Bessa F, Luna NM, Sugimoto D, Greve JM, Hernandez AJ. Evaluation of static and dynamic balance in athletes with anterior cruciate ligament injury– A controlled study. Clinics. 2016;71(8):425–9.
Nematollahi M, Razeghi M, Tahayori B, Koceja D. The role of anterior cruciate ligament in the control of posture; possible neural contribution. Neurosci Lett. 2017;659:120–3.
Bodkin SG, Slater LV, Norte GE, Goetschius J, Hart JM. ACL reconstructed individuals do not demonstrate deficits in postural control as measured by single-leg balance. Gait Posture. 2018;66:296–9.
Steffen K, Nilstad A, Krosshaug T, Pasanen K, Killingmo A, Bahr R. No association between static and dynamic postural control and ACL injury risk among female elite handball and football players: a prospective study of 838 players. Br J Sports Med. 2017;51(4):253–9.
Quatman CE, Quatman-Yates CC, Hewett TE. A ‘Plane’ explanation of Anterior Cruciate Ligament Injury mechanisms a systematic review. Sports Med. 2010;40(9):729–46.
Serpell BG, Scarvell JM, Ball NB, Smith PN. Mechanisms and risk factors for noncontact ACL injury in age mature athletes who engage in field or court sports a summary of the literature since 1980. J Strength Conditioning Res. 2012;26(11):3160–76.
Article Google Scholar
Moraiti CO, Stergiou N, Vasiliadis HS, Motsis E, Georgoulis A. Anterior cruciate ligament reconstruction results in alterations in gait variability. Gait Posture. 2010;32(2):169–75.
Pollard CD, Stearns KM, Hayes AT, Heiderscheit BC. Altered lower extremity movement variability in female soccer players during side-step cutting after anterior cruciate ligament reconstruction. Am J Sports Med. 2015;43(2):460–5.
Davis K, Williams JL, Sanford BA, Zucker-Levin A. Assessing lower extremity coordination and coordination variability in individuals with anterior cruciate ligament reconstruction during walking. Gait Posture. 2019;67:154–9.
Olsen OE, Myklebust G, Engebretsen L, Bahr R. Injury mechanisms for anterior cruciate ligament injuries in team handball: a systematic video analysis. Am J Sports Med. 2004;32(4):1002–12.
Khayambashi K, Ghoddosi N, Straub RK, Powers CM. Hip muscle strength predicts Noncontact Anterior Cruciate Ligament Injury in male and female athletes: a prospective study. Am J Sports Med. 2016;44(2):355–61.
Decker MJ, Torry MR, Wyland DJ, Sterett WI, Steadman JR. Gender differences in lower extremity kinematics, kinetics and energy absorption during landing. Clin Biomech Elsevier Ltd. 2003;18(7):662–9.
Dadfar M, Soltani M, Novinzad MB, Raahemifar K. Lower extremity energy absorption strategies at different phases during single and double-leg landings with knee valgus in pubertal female athletes. Sci Rep. 2021;11(1):17516.
Article CAS PubMed PubMed Central Google Scholar
Busquets A, Ferrer-Uris B, Angulo-Barroso R, Federolf P. Gymnastics experience enhances the development of bipedal-stance multi-segmental coordination and control during proprioceptive reweighting. Front Psychol. 2021;12:661312.
Pahnabi G, Akbari M, Ansari NN, Mardani M, Ahmadi M, Rostami M. Comparison of the postural control between football players following ACL reconstruction and healthy subjects. Med J Islamic Repub Iran 2014, 28(1).
Henriksson M, Ledin T, Good L. Postural control after anterior cruciate ligament reconstruction and functional rehabilitation. Am J Sports Med. 2001;29(3):359–66.
Dauty M, Collon S, Dubois C. Change in posture control after recent knee anterior cruciate ligament reconstruction? Clin Physiol Funct Imaging. 2010;30(3):187–91.
Trulsson A, Miller M, Hansson GA, Gummesson C, Garwicz M. Altered movement patterns and muscular activity during single and double leg squats in individuals with anterior cruciate ligament injury. BMC Musculoskelet Disord. 2015;16:28.
Kurabayashi J, Mochimaru M, Kouchi M. Validation of the estimation methods for the hip joint center. J Soc Biomechanisms. 2003;27(1):29–36.
Okada H, Ae M, Fujii N, Morioka Y. Body segment Inertia properties of Japanese Elderly. Biomechanisms. 1996;13(0):125–39.
Richman JS, Moorman JR. Physiological time-series analysis using approximate entropy and sample entropy. Am J Physiol-Heart C. 2000;278(6):H2039–49.
Article CAS Google Scholar
Dingenen B, Janssens L, Luyckx T, Claes S, Bellemans J, Staes FF. Postural stability during the transition from double-leg stance to single-leg stance in anterior cruciate ligament injured subjects. Clin Biomech (Bristol Avon). 2015;30(3):283–9.
Sayuri Tookuni K, Neto RB, Augusto C, Pereira M, Rúbio D, Souza D, Maria DJ, Greve A, Ayala DA. Comparative Analysis of Postural Control in individuals with and without injuries on knee anterior cruciate ligament. Acta Ortop Bras. 2005;13(3):333–5403.
Google Scholar
Culvenor AG, Alexander BC, Clark RA, Collins NJ, Ageberg E, Morris HG, Whitehead TS, Crossley KM. Dynamic single-Leg Postural Control is impaired bilaterally following anterior Cruciate Ligament Reconstruction: implications for Reinjury Risk. J Orthop Sports Phys Ther. 2016;46(5):357–64.
Talarico MK, Lynall RC, Mauntel TC, Wasserman EB, Padua DA, Mihalik JP. Effect of single-Leg Squat speed and depth on dynamic Postural Control under single-Task and Dual-Task paradigms. J Appl Biomech. 2019;35(4):272–9.
Dai B, Cook RF, Meyer EA, Sciascia Y, Hinshaw TJ, Wang C, Zhu Q. The effect of a secondary cognitive task on landing mechanics and jump performance. Sports Biomech. 2018;17(2):192–205.
Alentorn-Geli E, Myer GD, Silvers HJ, Samitier G, Romero D, Lazaro-Haro C, Cugat R. Prevention of non-contact anterior cruciate ligament injuries in soccer players. Part 1: mechanisms of injury and underlying risk factors. Knee Surg Sports Traumatol Arthrosc. 2009;17(7):705–29.
Goerger BM, Marshall SW, Beutler AI, Blackburn JT, Wilckens JH, Padua DA. Anterior cruciate ligament injury alters preinjury lower extremity biomechanics in the injured and uninjured leg: the JUMP-ACL study. Br J Sports Med. 2015;49(3):188–95.
Abe T, Nakamae A, Toriyama M, Hirata K, Adachi N. Effects of limited previously acquired information about falling height on lower limb biomechanics when individuals are landing with limited visual input. Clin Biomech (Bristol Avon). 2022;96:105661.
Shidahara H, Deie M, Niimoto T, Shimada N, Toriyama M, Adachi N, Hirata K, Urabe Y, Ochi M. Prospective study of Kinesthesia after ACL Reconstruction. Int J Sports Med. 2011;32(5):386–92.
Ochi M, Iwasa J, Uchio Y, Adachi N, Sumen Y. The regeneration of sensory neurones in the reconstruction of the anterior cruciate ligament. J Bone Joint Surg Br. 1999;81(5):902–6.
Suarez T, Laudani L, Giombini A, Saraceni VM, Mariani PP, Pigozzi F, Macaluso A. Comparison in joint-position sense and muscle coactivation between anterior cruciate ligament-deficient and healthy individuals. J Sport Rehabil. 2016;25(1):64–9.
Srinivasan D, Tengman E, Hager CK. Increased movement variability in one-leg hops about 20years after treatment of anterior cruciate ligament injury. Clin Biomech (Bristol Avon). 2018;53:37–45.
Heidarnia E, Letafatkar A, Khaleghi-Tazji M, Grooms DR. Comparing the effect of a simulated defender and dual-task on lower limb coordination and variability during a side-cut in basketball players with and without anterior cruciate ligament injury. J Biomech. 2022;133:110965.
Diekfuss JA, Grooms DR, Nissen KS, Schneider DK, Foss KDB, Thomas S, Bonnette S, Dudley JA, Yuan W, Reddington DL, et al. Alterations in knee sensorimotor brain functional connectivity contributes to ACL injury in male high-school football players: a prospective neuroimaging analysis. Braz J Phys Ther. 2020;24(5):415–23.
Konishi Y, Aihara Y, Sakai M, Ogawa G, Fukubayashi T. Gamma loop dysfunction in the quadriceps femoris of patients who underwent anterior cruciate ligament reconstruction remains bilaterally. Scand J Med Sci Sports. 2007;17(4):393–9.
Pataky TC, Robinson MA, Vanrenterghem J, Donnelly CJW. Simultaneously assessing amplitude and temporal effects in biomechanical trajectories using nonlinear registration and statistical nonparametric mapping. J Biomech. 2022;136:111049.
Download references
Acknowledgements
We would like to thank Dr. Kunio SHIMIZU at School of Statistical Thinking for helpful analysis of circular statistics on the manuscript.
This work was supported by JSPS KAKENHI Grant Numbers JP17K18207 and JP 20K19394.
Author information
Authors and affiliations.
Department of Orthopaedic Surgery, Graduate School of Biomedical and Health Sciences, Hiroshima University, 1-2-3 Kasumi, Minami-ku, 734-8551, Hiroshima, Hiroshima, Japan
Minoru Toriyama, Atsuo Nakamae, Kazuhiko Hirata & Nobuo Adachi
Department of Rehabilitation, Faculty of Health Sciences, Nihon Fukushi University, Handa, Aichi, Japan
Minoru Toriyama
Department of Sports Medical Center, Hiroshima University Hospital, Hiroshima, Hiroshima, Japan
Department of Rehabilitation, Hiroshima Hiramatsu Hospital, Hiroshima, Hiroshima, Japan
Department of Rehabilitation, Division of Clinical Practice and Support, Hiroshima University Hospital, Hiroshima, Hiroshima, Japan
Kazuhiko Hirata
You can also search for this author in PubMed Google Scholar
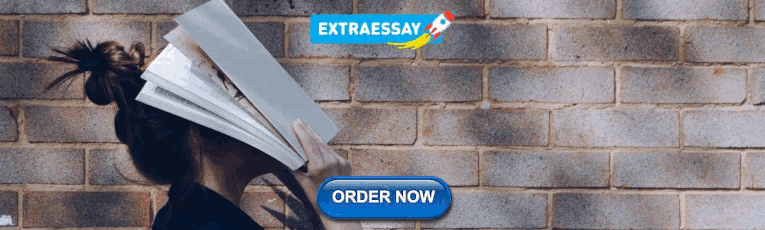
Contributions
MT: acquisition and analysis of data, statistical analysis, drafting the article. AN: conception and design, analysis and interpretation of data, drafting the article. TA: acquisition of data. KH: interpretation of data. NA: final approval of the manuscript.
Corresponding author
Correspondence to Atsuo Nakamae .
Ethics declarations
Competing interests.
The authors declare no competing interests.
Ethics approval and consent to participate
All methods were carried out in accordance with the Declaration of Helsinki. This study was approved by the Ethical Review Committee for Medical Research Involving Human Subjects in accordance with the Declaration of Hiroshima University (ID number: C-274-1). Written informed consent to be involved in the experimental procedures was obtained from all the participants before the start of this study.
Consent for publication
Additional information, publisher’s note.
Springer Nature remains neutral with regard to jurisdictional claims in published maps and institutional affiliations.
Electronic supplementary material
Below is the link to the electronic supplementary material.
Supplementary Material 1
Rights and permissions.
Open Access This article is licensed under a Creative Commons Attribution 4.0 International License, which permits use, sharing, adaptation, distribution and reproduction in any medium or format, as long as you give appropriate credit to the original author(s) and the source, provide a link to the Creative Commons licence, and indicate if changes were made. The images or other third party material in this article are included in the article’s Creative Commons licence, unless indicated otherwise in a credit line to the material. If material is not included in the article’s Creative Commons licence and your intended use is not permitted by statutory regulation or exceeds the permitted use, you will need to obtain permission directly from the copyright holder. To view a copy of this licence, visit http://creativecommons.org/licenses/by/4.0/ . The Creative Commons Public Domain Dedication waiver ( http://creativecommons.org/publicdomain/zero/1.0/ ) applies to the data made available in this article, unless otherwise stated in a credit line to the data.
Reprints and permissions
About this article
Cite this article.
Toriyama, M., Nakamae, A., Abe, T. et al. Dependence on visual information in patients with ACL injury for multi-joint coordination during single-leg squats: a case control study. BMC Sports Sci Med Rehabil 16 , 87 (2024). https://doi.org/10.1186/s13102-024-00875-9
Download citation
Received : 08 August 2023
Accepted : 02 April 2024
Published : 17 April 2024
DOI : https://doi.org/10.1186/s13102-024-00875-9
Share this article
Anyone you share the following link with will be able to read this content:
Sorry, a shareable link is not currently available for this article.
Provided by the Springer Nature SharedIt content-sharing initiative
- Anterior Cruciate Ligament(ACL)
- Movement variability
- Joint motion coordination
- Single-leg squat
- Visual information
BMC Sports Science, Medicine and Rehabilitation
ISSN: 2052-1847
- General enquiries: [email protected]
Thank you for visiting nature.com. You are using a browser version with limited support for CSS. To obtain the best experience, we recommend you use a more up to date browser (or turn off compatibility mode in Internet Explorer). In the meantime, to ensure continued support, we are displaying the site without styles and JavaScript.
- View all journals
- My Account Login
- Explore content
- About the journal
- Publish with us
- Sign up for alerts
- Open access
- Published: 12 April 2024
Inferring gene regulatory networks from single-cell multiome data using atlas-scale external data
- Qiuyue Yuan 1 &
- Zhana Duren ORCID: orcid.org/0000-0003-4685-811X 1
Nature Biotechnology ( 2024 ) Cite this article
8052 Accesses
78 Altmetric
Metrics details
- Dynamic networks
- Gene regulatory networks
Existing methods for gene regulatory network (GRN) inference rely on gene expression data alone or on lower resolution bulk data. Despite the recent integration of chromatin accessibility and RNA sequencing data, learning complex mechanisms from limited independent data points still presents a daunting challenge. Here we present LINGER (Lifelong neural network for gene regulation), a machine-learning method to infer GRNs from single-cell paired gene expression and chromatin accessibility data. LINGER incorporates atlas-scale external bulk data across diverse cellular contexts and prior knowledge of transcription factor motifs as a manifold regularization. LINGER achieves a fourfold to sevenfold relative increase in accuracy over existing methods and reveals a complex regulatory landscape of genome-wide association studies, enabling enhanced interpretation of disease-associated variants and genes. Following the GRN inference from reference single-cell multiome data, LINGER enables the estimation of transcription factor activity solely from bulk or single-cell gene expression data, leveraging the abundance of available gene expression data to identify driver regulators from case-control studies.
Similar content being viewed by others
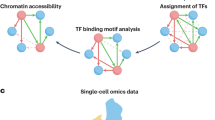
Gene regulatory network inference in the era of single-cell multi-omics
Pau Badia-i-Mompel, Lorna Wessels, … Julio Saez-Rodriguez
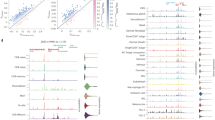
Single-cell multi-ome regression models identify functional and disease-associated enhancers and enable chromatin potential analysis
Sneha Mitra, Rohan Malik, … Christina S. Leslie
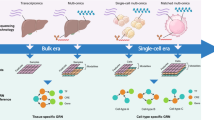
Gene regulatory network reconstruction: harnessing the power of single-cell multi-omic data
Daniel Kim, Andy Tran, … Pengyi Yang
GRNs 1 , 2 are collections of molecular regulators that interact with each other and determine gene activation and silencing in specific cellular contexts. A comprehensive understanding of gene regulation is fundamental to explain how cells perform diverse functions, how cells alter gene expression in response to different environments and how noncoding genetic variants cause disease. GRNs are composed of transcription factors (TFs) that bind DNA regulatory elements to activate or repress the expression of target genes.
Inference of GRNs is a central problem 2 , 3 , 4 , and there have been many attempts to approach this issue 2 , 5 , 6 , 7 , 8 , 9 , 10 , 11 , 12 , 13 . Co-expression-based methods such as WGCNA 14 , ARACNe 9 and GENIE3 (ref. 15 ) infer the TF–TG trans -regulation from gene expression by capturing the TF–TG covariation. Such networks have undirected edges, preventing distinction of direction from a TF A –TF B edge. Moreover, co-expressions are interpreted as correlations rather than causal regulations 16 . Genome-wide measurements of chromatin accessibility, such as DNase-seq 17 and assay for transposase-accessible chromatin sequencing (ATAC-seq) 18 , locate REs, enabling TF–RE connections by motif matching and connecting REs to their nearby TGs 19 . However, TF footprint approaches cannot distinguish within-family TFs sharing motifs. To overcome this limitation, we developed a statistical model, PECA 20 , to fit TG expression by TF expression and RE accessibility across a diverse panel of cell types. However, the problem still has not been fully resolved because heterogeneity of cell types in bulk data limits the accuracy of inference.
The advent of single-cell sequencing technology has enabled highly accurate regulatory analysis at the level of individual cell types. Single-cell RNA sequencing (scRNA-seq) data enables cell type-specific trans -regulation inference through co-expression analysis such as PIDC and SCENIC 21 , 22 , 23 , 24 , 25 , 26 , 27 , 28 , 29 , 30 . Single-cell sequencing assay for transposase-accessible chromatin (scATAC-seq) can be used to infer trans -regulation, as in DeepTFni 31 . Many methods integrate unpaired scRNA-seq and scATAC-seq data to infer trans -regulation. Those methods, including IReNA 32 , SOMatic 33 , UnpairReg 34 , CoupledNMF 35 , 36 , DC3 (ref. 36 ) and others 37 link TFs to REs by motif matching and link REs to TGs using the covariation of RE–TG or physical base pair distance. Recently, scJoint 38 was developed to transfer labels from scRNA-seq to scATAC-seq data, which may enable improved cell GRN inference. Despite extensive efforts, GRN inference accuracy has remained disappointingly low, marginally exceeding random predictions 39 .
Recent advances in single-cell sequencing 40 provide opportunities to address these challenges 41 , exemplified by SCENIC+ 42 . However, three major challenges persist in GRN inference. First, learning such a complex mechanism from limited data points remains a challenge. Although single-cell data offers a large number of cells, most of them are not independent. Second, incorporating prior knowledge such as motif matching into non-linear models is challenging. Third, inferred GRN accuracy assessed by experimental data is only marginally better than random prediction 39 .
To overcome these challenges, we propose a method called LINGER (Lifelong neural network for gene regulation). This research paper contributes to the field of GRN inference in multiple ways. First, LINGER uses lifelong learning, a previously defined concept 43 that incorporates large-scale external bulk data, mitigating the challenge of limited data but extensive parameters. Second, LINGER integrates TF–RE motif matching knowledge through manifold regularization, enabling prior knowledge incorporation into the model. Third, the accuracy of LINGER represents a fourfold to sevenfold relative increase. Fourth, LINGER enables the estimation of TF activity solely from gene expression data, identifying driver regulators.
LINGER: using bulk data to infer GRNs from single-cell multiome data
LINGER is a computational framework designed to infer GRNs from single-cell multiome data (Fig. 1 and Methods ). Using count matrices of gene expression and chromatin accessibility along with cell type annotation as input, it provides a cell population GRN, cell type-specific GRNs and cell-level GRNs. Each GRN contains three types of interactions, namely, trans -regulation (TF–TG), cis -regulation (RE–TG) and TF-binding (TF–RE). Note that TF–TF interactions are included in TF–TG pairs but TF self-regulation, which is challenging to model without additional data, is not considered. LINGER is distinguished by its ability to integrate the comprehensive gene regulatory profile from external bulk data. This is achieved through lifelong machine learning, also called continuous learning. The concept of lifelong learning is that the knowledge learned in the past helps us learn new things with little data or effort 44 . Lifelong learning has been proven to leverage the knowledge learned in previous tasks to learn the new task better 45 .
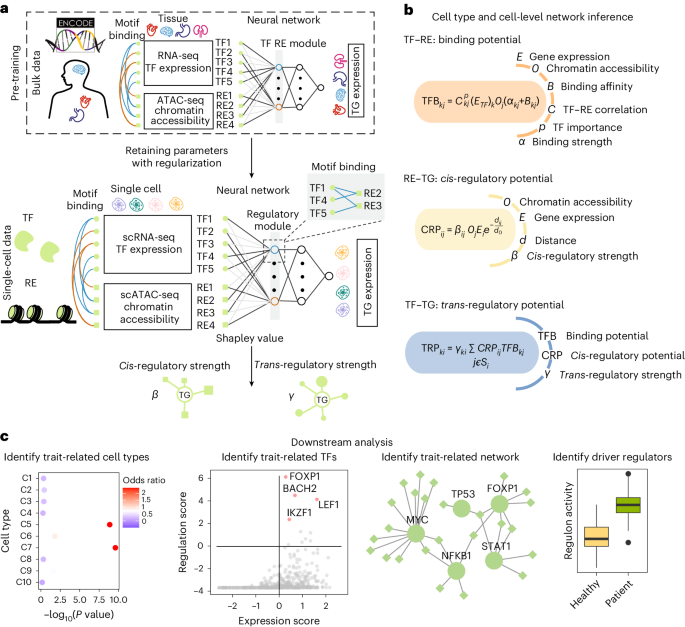
a , Schematic illustration of LINGER: a model predicting gene expression by TF expression and chromatin accessibility using a neural network model. LINGER pre-trains on the atlas-scale external bulk data and retains parameters by lifelong learning. The population-level GRN is generated from the neural network using the Shapley value. b , Strategy for constructing cell type-specific and cell-level GRNs. Cell type-specific and cell-level GRNs are inferred by an identical strategy, which combines consistent information across all cells, including regulatory strength, motif binding affinity and RE–TG distance, with context-specific information on gene expression and chromatin accessibility. c , Downstream analyses enabled by LINGER-inferred GRNs, including identifying complex regulatory landscape of GWAS traits and driver regulator identification.
LINGER leverages external data to enhance the inference from single-cell multiome data, incorporating three key steps: training on external bulk data, refining on single-cell data and extracting regulatory information using interpretable artificial intelligence techniques. In our approach, we use a neural network model to fit the expression of TGs, taking as input TF expression and the accessibility of REs. The second layer of the neural network model consists of weighted sums of TFs and REs, forming regulatory modules guided by TF–RE motif matching by incorporating manifold regularization. This leads to the enrichment of TF motifs binding to REs that belong to the same regulatory module. First, we pre-train using external bulk data obtained from the ENCODE project 46 , which contains hundreds of samples covering diverse cellular contexts, referred to as BulkNN.
For refinement on single-cell data, we apply elastic weight consolidation (EWC) loss, using bulk data parameters as a prior. The magnitude of parameter deviation is determined by the Fisher information, which reflects the sensitivity of the loss function to parameter changes. In the Bayesian context, knowledge gained from the bulk data is the prior distribution, forming our initial beliefs about the model parameters. As the model trains on new single-cell data, the posterior distribution is updated, combining the prior knowledge with the likelihood of the new data. EWC regularization encourages the posterior to remain close to the prior, retaining knowledge while adapting, preventing excessive changes and ensuring a more stable learning process 47 . After training the neural network model on single-cell data, we infer the regulatory strength of TF–TG and RE–TG interactions using the Shapley value, which estimates the contribution of each feature for each gene. The TF–RE binding strength is generated by the correlation of TF and RE parameters learned in the second layer (Fig. 1a ). LINGER then constructs the cell type-specific and cell-level GRNs based on the general GRN and the cell type-specific profiles (Fig. 1b and Methods ).
We will use independent datasets to validate the inference of GRN and then perform several downstream analyses: first, identification of the disease or trait-related cell type, TFs and GRN combining genome-wide association studies (GWAS) data; second, constructing regulon activity on external expression data and identifying driver regulators as differentially active TFs (Fig. 1c ).
LINGER improves the cellular population GRN inference
To assess the performance of LINGER, we used a public multiome dataset of peripheral blood mononuclear cells (PBMCs) from 10× Genomics (see Methods for details). To investigate whether a linear model is adequate for modeling gene expression or whether a non-linear model is necessary, we conducted a comparative analysis between two models. The first model employs an elastic net to predict the expression of TG by TFs and REs. The second model, single-cell neural network (scNN), is a three-layer neural network model sharing LINGER’s architecture. We assessed the gene expression prediction ability of the two models using fivefold cross-validation. We found that scNN modeled gene expression better than elastic net, with −log 10 P = 572.09, especially for those substantial proportions of genes that show negative Pearson’s correlation coefficient (PCC) in elastic net predictions (−log 10 P = 1,060.17; Fig. 2a ). This demonstrates that the three-layer neural network model scNN outperforms the elastic net model in predicting gene expression.
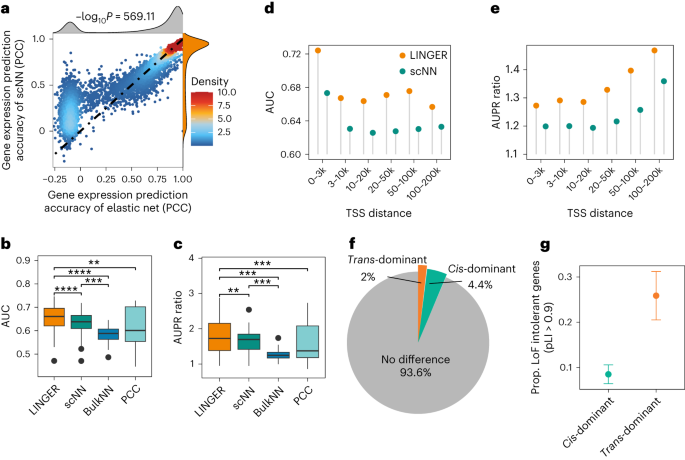
a , Correlation between predicted and real gene expression, showing higher accuracy for scNN than elastic net. The x axis represents the PCC of genes predicted by elastic net and real gene expression across cells, while the y axis gives the PCC for scNN. The points represent genes and the color of the points represents the density. The color of distribution in b – e indicates the different methods: orange, LINGER; gray, elastic net; dark green, scNN; blue, BulkNN; light blue, PCC. Null hypothesis testing results in a t -statistic with an effect size of 53.46, df = 15,659, −log 10 P = 572.09 and 95% confidence interval of [0.058, 0.063] from a two-sided paired t -test. b , Boxplot of the performance metric AUC for the predicted trans -regulatory strength across all ground truth data. The ground truth data for b and c are putative targets of TFs from 20 ChIP–seq data points from blood cells ( n = 20 independent samples). PCC denotes Pearson’s correlation coefficient between the chromatin accessibility of RE and the expression of TG. Note that all boxplots in this study present minima and maxima, the smallest and largest value that is not considered an outlier; center, median; bounds of box, 25th (Q1) to 75th (Q3) percentile; whiskers, 1.5 times the (Q3–Q1). In this study, we use the following convention for symbols indicating statistical significance: ns, P > 0.05; * P ≤ 0.05; ** P ≤ 0.01; *** P ≤ 0.001; **** P ≤ 0.0001. We hide the ns symbol when displaying significance levels. In detail, P = 8.32 × 10 −6 for LINGER and scNN, P = 8.57 × 10 −5 for LINGER and BulkNN and P = 1.24 × 10 −3 for LINGER and PCC. c , Boxplot of the performance metric AUPR ratio for the predicted trans -regulatory strength. P values in b and c are from one-sided paired t -tests. In detail, P = 3.49 × 10 −3 for LINGER and scNN, P = 2.13 × 10 −4 for LINGER and BulkNN and P = 4.53 × 10 −4 for LINGER and PCC. d , AUC for cis -regulatory strength inferred by LINGER. The ground truth data for d and e are the variant-gene links from eQTLGen. We divide RE–TG pairs into different groups based on the distance of the RE from the TSS of TG. e , AUPR ratio for cis -regulatory strength. f , Classification of the trans -dominant or cis -dominant gene. TFs contribute more to predicting the expression of trans -dominant genes, while REs contribute more to cis -dominant genes. g , Probability of trans -dominant and cis -dominant being loss-of-function (LoF)-intolerant genes. Points show estimated success probability from binomial distribution, at 0.26 and 0.09 for trans -dominant and cis -dominant, respectively. n = 317 and n = 693 independent sample size for trans -dominant and cis -dominant, respectively. Data are presented as means ± 1.96 × s.d.
To show the utility and effectiveness of integrating external bulk data, we compared LINGER to scNN, BulkNN and PCC. To evaluate the performance of trans -regulatory strength, we collected putative targets of TFs from chromatin immunoprecipitation followed by sequencing (ChIP–seq) data using a systematical standard ( Methods ) and, in total, obtained 20 data sets in blood cells as ground truth 48 (Supplementary Table 1 ). For each ground truth, we calculated the area under the receiver operating characteristic curve (AUC) and the area under the precision–recall curve (AUPR) ratio (see Methods ) by sliding the trans -regulatory predictions. Results show that scNN performs better than PCC and BulkNN. Compared to other methods, LINGER performs better, with a significantly higher AUC (Fig. 2b ) and AUPR ratio (Fig. 2c ) across all ground truth data.
To validate the cis -regulatory inference of LINGER, we calculated the consistency of the cis -regulatory coefficients with expression quantitative trait loci (eQTL) studies that link genotype variants to their TGs. We downloaded variant-gene links defined by eQTL in whole blood from GTEx 49 and eQTLGen 50 (Supplementary Table 2 ) as ground truth. As the distance between RE and TG is important for the prediction, we divided RE–TG pairs into different distance groups. LINGER achieved a higher AUC and AUPR ratio than scNN in all different distance groups in eQTLGen (Fig. 2d,e ) as well as GTEx (Extended Data Fig. 1a,b ). The above results show that LINGER improves the cis -regulatory and trans -regulatory strength inference by leveraging external data.
We next sought to investigate the dominant regulation for genes; that is, whether a gene is mainly regulated by cis -regulation or trans -regulation. To shed light on this question, we compared the average of cis -regulatory and trans -regulatory strength Shapley values by a two-sided unpaired t -test and performed Bonferroni P value correction. Our findings reveal that most genes exhibit no significant difference in cis -regulation and trans -regulation dominance. Specifically, 4.37% of genes are cis -regulation dominant, while 2.00% are trans -regulation dominant (Fig. 2f ). To discern evolutionary distinctions between trans -dominant and cis -dominant genes, we compared their strength of selection using pLI, which is an estimate of the ‘probability of being loss of function intolerant’ 51 . We observed that the percentage of selectively constrained genes with high pLI (>0.9) in the trans -dominant group was approximately three times higher than that in the cis -dominant group (Fig. 2g ). A previous study found that disease-associated genes from GWAS were enriched in selectively constrained genes, while eQTL genes were depleted in selectively constrained genes 52 . These observations highlight the importance of the trans -regulatory network in understanding complex diseases. Functional enrichment analysis 53 shows that the cis -regulatory dominant genes were significantly enriched in 38 GTEx aging signatures (Supplementary Table 3 ), which aligns with the conclusion that chromatin accessibility alterations occur in age-related macular degeneration 54 .
To gain an understanding of parameter sensitivity, we systematically evaluated the effects of TF–RE motif matching, cis -REs transcription start site (TSS) distance, activation function, number of nodes in hidden layers and metacell-generating method on the scNN. Note that the sigmoid activation function would not improve the gene expression prediction but would improve the GRN inference (Extended Data Fig. 2a ). Using motif matching information by manifold regularization loss properly by setting the weight will improve the performance. Compared to 0, weight 0.01 improved the performance on 100% (Extended Data Fig. 2c ) and 80% (Extended Data Fig. 2d ) of ground truth data based on the AUC and AUPR ratio, respectively. The performance of weight 10 decreases compared to 0.01 (Extended Data Fig. 2c,d ). To verify the robustness of our method to alternative metacell-generation approaches (see ‘PBMC 10× data’ in Methods ), we used metacells generated by the SEACells as a substitute for our original metacells. There were no significant differences in the performance between SEACells metacells and our original metacells (two-sided paired t -test, P = 0.89; Extended Data Fig. 2e ). Using REs within 1 Mb is the best across 200 kb, 500 kb, 1 Mb and 2 Mb (Extended Data Fig. 2f,g ).
We evaluated LINGER’s capability for lifelong learning by leveraging additional data sources. We split the ENCODE data into two batches (ENCODE1, ENCODE2) and applied two rounds of pre-training, then trained on PBMCs single-cell multiome data (ENCODE1+ENCODE2+sc). We compared the results with those obtained by using one batch of ENCODE data as pre-training (ENCODE1+sc). Extended Data Fig. 2h shows that compared to single pre-training, the addition of the second round of pre-training improved the performance of TF–TG inference for 85.5% (17 out of 20) and 75% (15 out of 20) of ChIP–seq data based on the AUC and AUPR ratio, respectively. This validates LINGER’s capability for continuous refinement through incremental learning from diverse datasets.
LINGER improves the cell type-specific GRN inference
We evaluated the cell type-specific GRN inference ( Methods ) of LINGER in PBMCs sc-multiome data as well as an in-silico mixture of H1, BJ, GM12878 and K562 cell lines from single-nucleus chromatin accessibility and mRNA expression sequencing (SNARE-seq) data 55 . To assess TF–RE binding prediction, we used ChIP–seq data as ground truth, including 20 TFs from four cell types within the blood and 33 TFs from the H1 cell line 48 (Supplementary Table 4 ). The putative target of TF from the ChIP–seq data serves as ground truth for the trans -regulatory potential. For the cis -regulatory potential, we incorporated promoter-capture Hi-C data of three primary blood cell types (Supplementary Table 5 ) 56 and single-cell eQTL 57 , including six immune cell types as ground truth for PBMCs.
To assess the TF–RE binding potential, we compared our method with TF–RE correlation (PCC) and motif binding affinity. For example, in naive CD4 T cells, LINGER achieves an AUC of 0.92 and an AUPR ratio of 5.17 for ETS1 , which is an improvement over PCC (AUC, 0.78; AUPR ratio, 2.71) and motif binding affinity (AUC, 0.70; AUPR ratio, 1.92) (Fig. 3a,e ). For binding sites of MYC in the H1 cell line, LINGER outperforms PCC and motif binding affinity-based predictions (Extended Data Fig. 3a,b ). For all 20 TFs in PBMCs, LINGER consistently exhibits the highest AUC and AUPR ratios, and the overall distributions are significantly higher than others in PBMCs ( P ≤ 8.72 × 10 −5 ; Fig. 3b,c and Supplementary Table 6 ). LINGER also outperforms other methods for H1 data ( P ≤ 6.68 × 10 −6 ; Extended Data Fig. 3c,d ). Furthermore, we compared LINGER with a state-of-the-art method, SCENIC+ 42 , which predicts TF–RE pairs from multiome single-cell data. Given that SCENIC+ does not provide a continuous score for all REs, we used the F1 score as a measure of accuracy. Fig. 3d shows that LINGER performs better for all 20 TFs binding site predictions.
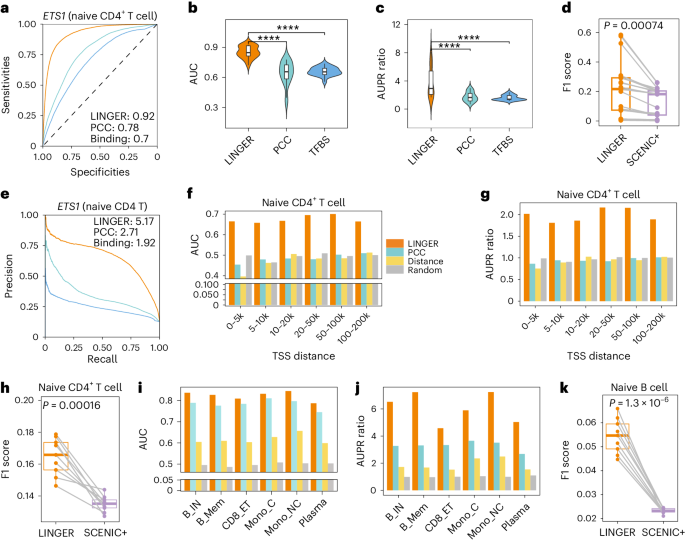
a , e , Receiver operating characteristic curve and precision–recall curve of binding potential for ETS1 in naive CD4 T cells. The ground truth for a and e is the ChIP–seq data of ETS1 in naive CD4 + T cells. The color in a – e represents the different methods used to predict TF–RE regulation. Orange, LINGER; green, PCC between the expression of TF and the chromatin accessibility of RE; blue, motif binding affinity of TF to RE. b , c , Violin plot of the AUC and AUPR ratio values of binding potential across diverse TFs and cell types. The ground truth is the ChIP–seq data for 20 TFs from different cell types in blood. The original data is in Supplementary Table 6 . The null hypothesis testing in b , comparing the AUC of LINGER with PCC and binding, results in t -statistics (one-sided paired t -test) with effect size, 8.99; df, 19; P = 1.42 × 10 −8 , 95% confidence intervals, [0.17, Inf] and effect size, 18.25; df, 19; P = 8.34 × 10 −14 ; 95% confidence intervals, [0.17, Inf], respectively. The null hypothesis testing in c , comparing the AUPR ratio of LINGER with PCC and binding, results in t-statistics (one-sided paired t -test) with effect size, 4.65; df, 19; P = 8.72 × 10 −5 ; 95% confidence intervals, [1.31, Inf] and effect size, 5.44, df, 19; P = 1.49 × 10 −5 ; 95% confidence intervals, [1.51, Inf], respectively. d , The performance metrics F1 score of binding potential. Each point represents ground truth data ( n = 20 independent samples). The P values for d , h and k are based on one-sided paired t -tests. f , g , AUC and AUPR ratio of cis -regulatory potential in naive CD4 + cells. The ground truth for f – h is promoter-capture Hi-C data. RE–TG pairs are divided into six distance groups ranging from 0–5 kb to 100–200 kb. PCC is calculated between the expression of TG and the chromatin accessibility of RE. Distance denotes the decay function of the distance to the TSS. Random denotes the uniform distribution from 0 to 1. h , F1 score of cis -regulatory in naive CD4 + cells for LINGER and SCENIC+ ( n = 9 independent samples). i , j , AUC and AUPR ratio of cis -regulatory potential. The ground truth is eQTL data from six immune cell types. k , F1 score of cis -regulatory potential in naive B cells. The ground truth is eQTL data from naive B cells ( n = 9 independent samples). This figure corresponds to the PBMC data.
To assess the cis -regulatory potential, we compared LINGER with four baseline methods, including distance-based methods, RE–TG correlation (PCC), random predictions, and SCENIC+. We divided RE–TG pairs of Hi-C data into six distance groups ranging from 0–5 kb to 100–200 kb. In naive CD4 T cells, LINGER achieves AUC ranging from 0.66 to 0.70 (Fig. 3f ) and AUPR ratio ranging from 1.81 to 2.16 (Fig. 3g ) across all distance groups, while other methods are close to random. In other cell types, LINGER exhibits consistent superiority over the baseline methods (Extended Data Fig. 3e–h ). All eQTL pairs were considered positive labels owing to the insufficient pairs available for division into distance groups. In all cell types, the AUC and AUPR ratio of LINGER are higher than the baseline methods (Fig. 3i,j ). We also compared our method with SCENIC+, which outputs predicted RE–TG pairs without importance scores. We selected the same number of top-ranking RE–TG pairs and calculated the F1 score using nine cutoffs corresponding to quantiles ranging from the 10th to the 90th percentile. As a result, LINGER attains significantly higher F1 scores than SCENIC+ in all cell types (Fig. 3h and Extended Data Fig. 3i,j ) based on Hi-C data. Taking eQTL as ground truth, the F1 score of LINGER is significantly higher than SCENIC+ (Fig. 3k ) and other cell types (Extended Data Fig. 3k–o ).
To evaluate the accuracy of trans -regulatory potential, we chose GENIE3 (ref. 15 ) and PIDC 21 for comparison based on the benchmarking literature of GRN inference from single-cell data 39 that we chose in previous work 58 (see Methods ). In addition, we compared LINGER with PCC and SCENIC+. For STAT1 in classical monocytes, LINGER improves the prediction performance, as evidenced by an AUC of 0.76 versus 0.57–0.59 and an AUPR ratio of 2.60 versus 1.26–1.36 (Fig. 4a,b ). A similar improvement is observed for CTCF in H1 (Extended Data Fig. 3p,q ). The average AUPR ratio across ground truth datasets for other methods was 1.17–1.29, 0.17–0.29 units above random prediction, whereas LINGER achieves 1.25 units above random prediction, indicating a fourfold to sevenfold relative increase (Fig. 4d ). Overall, LINGER consistently performs better than other methods for all 20 TFs in PBMCs, with a significantly higher AUC and AUPR ratio ( P ≤ 9.49 × 10 −9 ; Fig. 4c,d and Supplementary Table 7 ). LINGER outperforms other competitors in the H1 cell line ( P ≤ 3.00 × 10 −8 ; Extended Data Fig. 3r ). Unlike GENIE3 and PIDC, which solely use scRNA-seq data, our method effectively doubles the cell data by integrating both scRNA-seq and scATAC-seq. For a fairer comparison, we removed pre-training and used only half as many cells as input (scNN_half). Comparing to other competitors showed that scNN_half continued to significantly outperform all other methods (Extended Data Fig. 2b ). We also evaluated cell type-specific trans -regulatory potential to predict direct differentially expressed genes (DEGs) under perturbation of the TF, using perturbation experiment data as ground truth. We collected eight datasets for PBMCs (Supplementary Table 8 ) from the KnockTF database 59 . Extended Data Fig. 4a,b shows that LINGER outperforms all other methods ( P ≤ 3.72 × 10 −4 ).
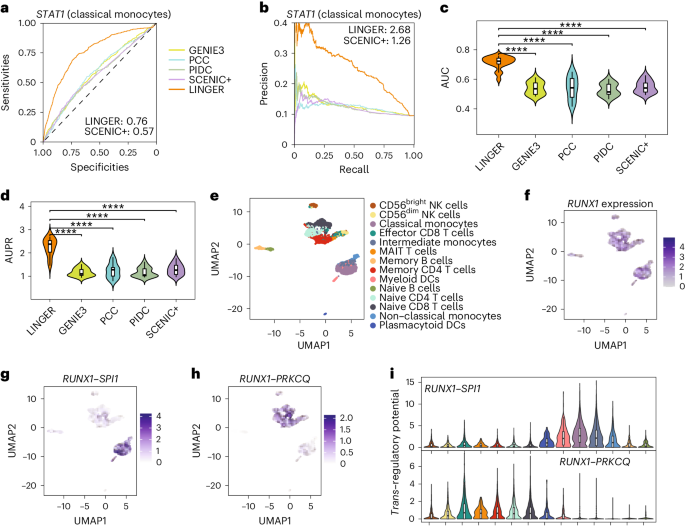
a , b , Receiver operating characteristic curve and precision–recall curve of trans -regulatory potential inference of STAT1 in classical monocytes. The ground truth data in a – d are putative targets of TFs from ChIP–seq data for the corresponding cell types in PBMCs. c , d , Violin plot of AUC and AUPR ratio values of trans -regulatory potential performance across diverse TFs and cell types. The original data is in Supplementary Table 7 . The sample size for the one-sided paired t -test is 20. For c , −log 10 ( P values) are 11.12, 7.72, 11,13 and 10.17 for GENIE3, PCC, PIDC and SCENIC+, respectively. For d , −log 10 ( P values) are 9.59, 8.02, 9.22 and 8.47, respectively. e , Uniform manifold approximation and projection (UMAP) of PBMCs including 14 cell types. NK cells, natural killer cells; MAIT, mucosal-associated invariant T cells; DCs; dendritic cells. f , UMAP of RUNX1 expression across PBMCs. g , UMAP of cell level trans -regulatory potential for RUNX1 (TF) –SPI1 (TG) across PBMCs. h , UMAP of cell level trans -regulatory potential for RUNX1 (TF) –PRKCQ (TG) across PBMCs. i , Violin plot of cell level trans -regulatory potential from different cell types. The sample size for each boxplot is the number of cells of each cell type, ranging from 98 to 1,848. This figure corresponds to the PBMCs.
The rationale for constructing a single-cell-level GRN is the same as a cell type-specific GRN, replacing the cell type-specific term with the single-cell term ( Methods ). We show the result of trans -regulation, taking RUNX1 as an example. RUNX1 is critical for establishing definitive hematopoiesis 60 and expresses at high levels in almost all PBMC cell types (Fig. 4e,f ). RUNX1 regulates SPI1 in monocytes (classical, non-classical and intermediate) and myeloid dendritic cells (Fig. 4g,i ), while regulates PRKCQ in CD56 dim natural killer cells, effector CD8 T cells, mucosal-associated invariant T cells, memory CD4 T cells, naive CD4 T cells and naive CD8 T cells (Fig. 4h,i ). This example illustrates the capability of LINGER to visualize gene regulation at the single-cell level.
LINGER reveals the regulatory landscape of GWAS traits
GWASs have identified thousands of disease variants, but the active cells and functions involving variant-regulated genes remain largely unknown 61 . We integrate GWAS summary statistics and cell type-specific GRN to identify the relevant cell types, key TFs and sub-GRN ( Methods ). We define a trait regulation score for TFs in each cell type, measuring the enrichment of GWAS genes downstream of TFs. In trait-relevant cell types, TFs with high trait regulation scores should be expressed to perform their function. We identify the trait-relevant cell types by assessing the concordance between TF expression and the trait regulation score.
In our specific study on inflammatory bowel disease (IBD), we collected the risk loci based on a GWAS meta-analysis of about 330,000 individuals from the NHGRI-EBI GWAS catalog 62 for study GCST90225550 63 . Figure 5a shows that in classical monocytes, trait regulation scores for the top-expressed TF are significantly higher than randomly selected TFs ( P = 8.9 × 10 −29 , one-sided unpaired t -test), while there is no significant difference for non-relevant cell types such as CD56 dim natural killer cells. The most relevant cell types in PBMCs are monocytes and myeloid dendritic cells (Fig. 5b ). These findings align with previous studies linking monocytes to the pathogenesis of IBD 64 , 65 .
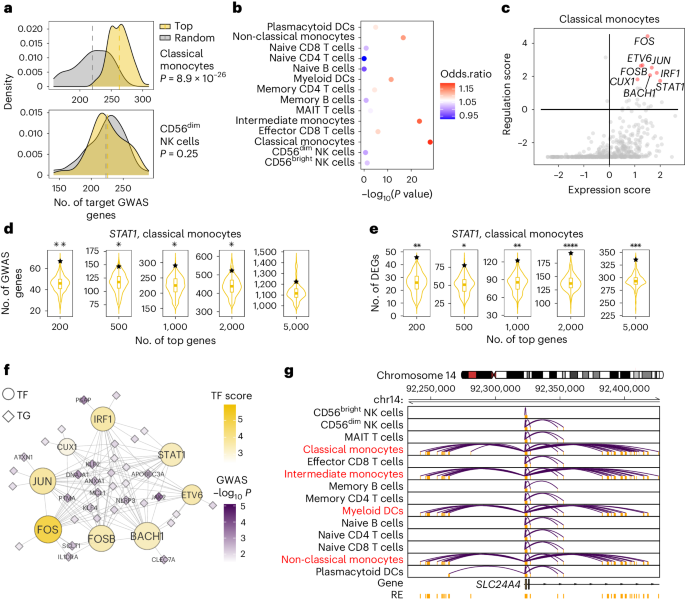
a , Distribution of the number of TGs for top expression TFs and randomly selected TFs in classical monocytes (top) and CD56 dim NK cells (bottom). The 100 top-expression TFs and 100 randomly selected TFs are used to generate the distribution. b , Enrichment of IBD GWAS to cell types in PBMCs. The color of the bubbles corresponds to the odds ratio of the number of TGs between top expression and randomly selected TFs. The x axis is the −log 10 ( P value) from the one-sided unpaired t -test for the number of TGs between top expression and randomly selected TFs. c , Key IBD-associated regulators in classical monocytes. The x axis is the z -score of the expression of TFs across all TFs. The y axis is the regulation score of TFs. The TFs in red are the top-ranked TFs according to the summation of the expression level and regulation score. d , Enrichment of GWAS IBD genes among STAT1 targets in classical monocytes. The violin plot is generated by randomly choosing 1,000 TFs; the number of overlapping genes for STAT1 is marked by a star. The different violin plots correspond to taking the top 200–5,000 genes as the TG for each TF, respectively. e , Enrichment of DEGs between inflamed biopsies and non-inflamed biopsies among STAT1 targets in classical monocytes. The details are the same as in d . f , Sub-network of IBD-relevant TFs from classical monocytes trans -regulatory network. The size of the TF or TG nodes corresponds to their degree in the network. The color of TF denotes the trait-relevant score, and the color of TG denotes the −log 10 ( P value) of GWAS SNP assigned to the gene. g , Cis -regulatory network at locus around SLC24A4 . The interaction denotes significant RE–TG links, and we use the location of the promoter to represent the gene.
We next identified key TFs by the sum of the expression level and trait regulation score. Figure 5c lists the top eight candidate TFs in classical monocytes. These TFs have been previously reported to be associated with IBD in the literature. FOS can increase the risk of recurrence of IBD 66 ; one variant identified in the IBD cohort is located at the exon of ETV6 ; IRF1 and ETV6 are key TFs with activity differences in IBD 67 ; genes FOS , FOSB and JUN encode potent mediators of IBD 68 ; CUX1 is induced in IBD 69 ; and STAT1 epigenetically contribute to the pathogenesis of IBD 70 .
To investigate the downstream targets of key TFs, we chose STAT1 as an example. Among the top 200 TGs regulated by STAT1 in classical monocytes, 67 of them overlap with the GWAS genes, which is statistically significant with a P value of less than 0.01 based on a background distribution from a random selection of TFs (one-sided bootstrap hypothesis testing). The numbers of overlapped TGs are all significant for the top 500, 1,000, 2,000 and 5,000 TGs (Fig. 5d ). Apart from GWAS-relevant genes, we collected the DEGs between inflamed biopsies and non-inflamed biopsies 71 and we found that these DEGs significantly overlapped with the top-ranked TGs of STAT1 (one-sided bootstrap hypothesis testing; Fig. 5e ). The lack of significant overlap between DEGs and GWAS genes ( P = 0.15, two-sided Fisher’s exact test) but the significant overlap of both DEGs and GWAS with the top-ranked TGs of STAT1 indicates the robustness and unbiased nature of our method.
Finally, we extracted the sub-network of the eight candidate TFs from the classical monocyte trans -regulatory network for IBD (Fig. 5f ). We also observed that the cis -regulatory network of SLC24A4 (Fig. 5g ), 46 kb from a risk single nucleotide polymorphism (SNP) rs11626366 ( P = 7.4 × 10 −3 ), is specifically dense in the IBD-relevant cell types, which shows the complex regulatory landscape of disease genes across different cell types.
Identify driver regulators based on transcription profiles
Researchers often identify DEGs between cases and controls using bulk or single-cell expression data, but the underlying regulatory drivers remain elusive. TF activity, focusing on the DNA-binding component of TF proteins, is a more reliable metric than mRNA for identifying driver regulators. One feasible approach is to estimate TF activity based on the expression patterns of downstream TGs, which necessitates the availability of an accurate GRN. Assuming that the GRN structure is consistent for the same cell type across individuals, we employed LINGER-inferred GRNs from single-cell multiome data of a single individual to estimate the TF activity of other individuals using gene expression data alone from the same cell type. By comparing TF activity between cases and controls, we identified driver regulators. This approach is valuable, as it leverages limited single-cell multiome data to estimate TF activity in multiple individuals using only gene expression data (see Methods ). We present two illustrative examples showcasing its utility.
Example 1: We collected the bulk gene expression data from 26 patients with acute myeloid leukemia (AML) and 38 healthy donors 72 . We calculated the TF activity for these samples based on the LINGER-inferred cell population GRN from PBMCs and found that FOXN1 is significantly less active in patients with AML than in healthy donors, and it is not differentially expressed (Fig. 6a,b ). In addition, we calculated the TF activity of the transcriptome profile (bulk RNA-seq data) of 671 individuals with AML 73 and performed survival analysis, which indicated that individuals with high FOXN1 activity level tend to have a higher survival probability (Fig. 6c ). Furthermore, FOXN1 has been reported as a tumor suppressor 74 .
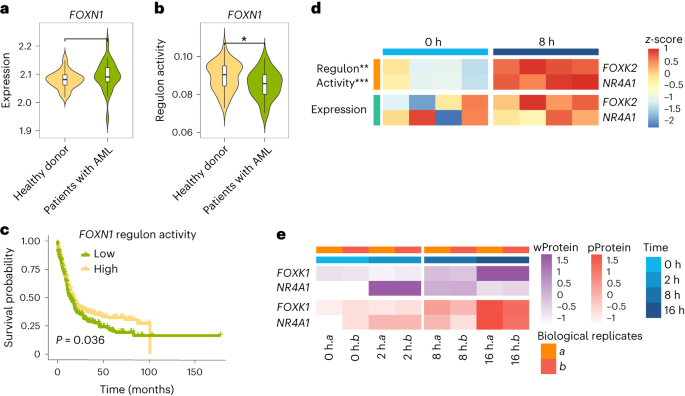
a , Violin plot of FOXN1 expression across healthy donors ( n = 38 independent samples) and patients with AML ( n = 26 independent samples), respectively. There is no significant difference in the mean expression (two-sided unpaired t -test). b , Violin plot of regulon activity of FOXN1 across healthy donors ( n = 38 independent samples) and patients with AML ( n = 26 independent samples), respectively (two-sided unpaired t -test, P = 0.035). c , AML survival by the regulon activity of FOXN1 ( P value is from a two-sided log-rank test). d , The heatmap of regulon activity and gene expression in response to TCR stimulation at 0 h and 8 h. Two-sided unpaired t -test for the difference in regulon activity, P = 0.0057 and P = 0.00081 for FOXK1 and NR4A1 , respectively; the P value for gene expression is >0.05. Heatmap is scaled by row. e , Heatmap of whole protein (wProtein) and phosphoproteomics (pProtein) expression in response to TCR stimulation at 0 h, 2 h, 8 h and 16 h. There are two biological replicates, represented by a and b . The wProtein and pProtein expression of FOXK1 and NR4A1 is higher at 8 h than at 0 h. The heatmap is scaled by row.
Example 2: We also present an example of the naive CD4 + T cell response upon T cell receptor (TCR) stimulation 75 , which induces T cell differentiation into various effector cells and activates T lymphocytes. We calculated the TF activity based on the GRN of naive CD4 + T cells and identified differentially active regulators in response to TCR stimulation at 8 h versus 0 h. FOXK2 and NR4A1 are activated at 8 h based on regulon activity (Fig. 6d ), which is consistent with the whole proteomics and phosphoproteomics data (Fig. 6e ) 76 . Other studies have also shown that FOXK2 affects the activation of T lymphocytes 77 , 78 and revealed the essential roles of NR4A1 in regulatory T cell differentiation 79 , 80 , suggesting that the identified TFs have important roles in naive CD4 + T cell response upon TCR stimulation. However, FOXK2 and NR4A1 show no significant differences in expression at 8 h versus 0 h (Fig. 6d ).
In silico perturbation
We performed in silico perturbation to predict the gene expression after knocking out TFs. To do so, we changed the expression of an individual TF or combinations of TFs to zero and used the predicted gene expression as the in silico perturbation gene expression. We used the expression difference before and after in silico perturbation to infer the TG. To assess the performance of the prediction, we collected perturbation data for eight TFs in blood cells from the KnockTF 59 database (Supplementary Table 8 ) as ground truth. We performed the in silico individual TF perturbation of the eight TFs using LINGER. As a comparison, we performed identical computational perturbation experiments using the CellOracle 81 and SCENIC+ 42 methods. The results, shown in Extended Data Fig. 4c,d , demonstrate that LINGER is more accurate than the alternative approaches ( P ≤ 3.72 × 10 −4 ).
To assess LINGER’s capability to infer differentiation behavior, we leveraged CellOracle 81 as a downstream analytical tool. We used the LINGER-inferred GRN as an input to CellOracle. This allowed us to investigate the capacity of LINGER-derived networks to recapitulate differentiation responses. Examining bone marrow mononuclear cell data 82 , which contains progenitor populations, we performed an in silico knockout of GATA1 , a known key regulator of erythroid and megakaryocytic differentiation 83 . CellOracle predictions based on the LINGER GRN showed that GATA1 knockout shifted proerythroblasts to a megakaryocytic or erythroid progenitor state (Extended Data Fig. 4e ), consistent with the functional role of GATA1 in inhibiting erythroblast maturation. These results demonstrate that LINGER can not only predict gene expression under perturbation but also enable downstream characterizations of differentiation trajectories through integration with complementary analytical frameworks like CellOracle.
Conclusions and discussions
LINGER is an neural network-based method that infers GRNs from paired single-cell multiomic data by incorporating bulk datasets and knowledge of TF–RE motif matching. Compared to existing tools, LINGER achieves substantially higher GRN inference accuracy. A key innovation is lifelong machine learning to leverage diverse cellular contexts, continually updating the model as new data emerge. This addresses historic challenges from limited single-cell datasets and vast parameter spaces hindering complex model fitting. LINGER’s lifelong learning approach has the advantage of pre-training on bulk collections, allowing users to easily retrain the model for their own studies while capitalizing on publicly available resources without direct access. Traditionally, GRN inference performance is assessed by gene expression prediction. However, the use of lifelong learning to leverage external data does not lead to improved gene expression prediction but does improve the GRN inference. This finding challenges the traditional strategy of evaluating GRN inference solely based on gene expression prediction and highlights the importance of considering the overall network structure and regulatory interactions.
The lifelong learning mechanism will encourage the model to retain prior knowledge from the bulk data when adapting to the new single-cell data. It is a tradeoff between retaining prior knowledge and fitting new data. The flexibility of the variation in prior knowledge is not constrained when fitting the new data. The extent to which the final result deviates from the prior knowledge depends on the loss incurred in fitting the new data. LINGER will learn this tradeoff automatically to obtain a maximized usage of the information from both datasets.
GRN inference by lifelong learning
LINGER is a computational framework to infer GRNs—pairwise regulation among TGs, REs and TFs—from single-cell multiome data. Overall, LINGER predicts gene expression by the TF expression and chromatin accessibility of REs based on neural network models. The contribution of each feature is estimated by the Shapley value of the neural network models, enabling the inference of the GRNs. To capture key information from the majority of tissue lineages, LINGER uses lifelong machine learning (continuous learning). Moreover, LINGER integrates motif binding data by incorporating a manifold regularization into the loss function.
The inputs for full training of LINGER are external bulk and single-cell paired gene expression and chromatin accessibility data. However, we provided a bulk data pre-trained LINGER model so that users can retrain it for their own single-cell data without accessing external bulk data. We collected paired bulk data—gene expression profiles and chromatin accessibility matrices—from 201 samples from diverse cellular contexts 84 from the ENCODE project 46 . Single-cell data are raw count matrices of multiome single-cell data (gene counts for RNA-seq and RE counts for ATAC-seq). LINGER trains individual models for each gene using a neural network architecture that includes an input layer and two fully connected hidden layers. The input layer has dimensions equal to the number of features, containing all TFs and REs within 1 Mb of the TSS for the gene to be predicted. The first hidden layer has 64 neurons with rectified linear unit activation that can capture regulatory modules, each of which contains multiple TFs and REs. These regulatory modules are characterized by enriched motifs of the TFs on the corresponding REs. The second hidden layer has 16 neurons with rectified linear unit activation. The output layer is a single neuron, which outputs a real value for gene expression prediction.
We first construct neural network models based on bulk data, using the same architecture described above. We extract the TF expression matrix \({\widetilde{E}}_{{\rm{TF}}}\in {{\mathbb{R}}}^{{N}_{{\rm{TF}}}\times {N}_{b}}\) from the bulk gene expression matrix \(\widetilde{E}\in {{\mathbb{R}}}^{{N}_{{\rm{TG}}}\times {N}_{b}}\) , with \({N}_{{\rm{TG}}}\) representing the number of genes, \({N}_{{\rm{TF}}}\) representing the number of TFs and \({N}_{b}\) representing the number of tissues. The loss function consists of mean squared error (MSE) and L1 regularization, which, for the i th gene is:
where \(\widetilde{O}\in {{\mathbb{R}}}^{{N}_{{\rm{RE}}}^{(i)}\times {N}_{b}}\) represents the chromatin accessibility matrix, with \({N}_{{\rm{RE}}}^{(i)}\) REs within 1 Mb of the TSS of the i th gene, and \(f\left({\left({\widetilde{E}}_{{\rm{TF}}}\right)}_{\bullet ,n},{{\widetilde{O}}^{(i)}}_{\bullet ,n},{\theta }_{b}^{(i)}\right)\) is the predicted gene expression from the neural network of sample n . The neural network is parametrized by a set of weights and biases, collectively denoted by \({\theta }_{b}^{(i)}\) . The weight λ 0 is a tuning parameter.
The loss function of LINGER is composed of MSE, L1 regularization, manifold regularization and EWC loss: \({{\mathcal{L}}}_{{\rm{LINGER}}}={{{\lambda }}_{1}{\mathcal{L}}}_{{\rm{MSE}}}\) \(+{{\lambda }}_{2}{{\mathcal{L}}}_{L1}+{{\lambda }}_{3}{{\mathcal{L}}}_{{\rm{Laplace}}}+{{{\lambda }}_{4}{\mathcal{L}}}_{{\rm{EWC}}}\) . \({{\mathcal{L}}}_{{\rm{Laplace}}}\) represents the manifold regularization because a Laplacian matrix is used to generate this regularization term. The loss function terms correspond to gene i , and for simplicity, we omit subscripts \((i)\) for the chromatin accessibility matrix ( \(O\) ), parameters for the bulk model ( \({\theta }_{b}\) ) and parameters for LINGER ( \({\theta }_{l}\) ).
Here, \({E}_{{\rm{TF}}}\in {{\mathbb{R}}}^{{N}_{{\rm{TF}}}\times {N}_{{\rm{sc}}}}\) represents the TF expression matrix from the single-cell RNA-seq data, consisting of \({N}_{{\rm{sc}}}\) cells; \(O\in {{\mathbb{R}}}^{{N}_{{\rm{RE}}}^{(i)}\times {N}_{{\rm{sc}}}}\) represents the RE chromatin accessibility matrix of the single-cell ATAC-seq data; \(E\in {{\mathbb{R}}}^{{{N}_{{\rm{TG}}}\times N}_{{\rm{sc}}}}\) represents the expression of the genes across cells; and \({\theta }_{l}\) represents the parameters in the neural network. We use metacells to train the models; therefore, \({N}_{{\rm{sc}}}\) is the number of cells from metacell data.
L1 regularization
Laplacian loss (manifold regularization)
We generate the adjacency matrix as: \({B}^{* }\in {{\mathbb{R}}}^{\left({N}_{{\rm{TF}}}+{N}_{{\rm{RE}}}^{(i)}\right)}\) \({\times \left({N}_{{\rm{TF}}}+{N}_{{\rm{RE}}}^{(i)}\right)}\) , where \({B}_{k,{N}_{{\rm{TF}}}+j}^{* }\) and \({B}_{{N}_{{\rm{TF}}}+j,k}^{* }\) represent the binding affinity of the TF \(k\) and the RE \(j\) , which is elaborated in the following sections. \({L}^{{\rm{Norm}}}\in {{\mathbb{R}}}^{\left({N}_{{\rm{TF}}}+{N}_{{\rm{RE}}}^{(i)}\right)\times \left({N}_{{\rm{TF}}}+{N}_{{\rm{RE}}}^{(i)}\right)}\) is the normalized Laplacian matrix based on the adjacency matrix.
where \({\theta }_{l}^{\left(1\right)}\in {{\mathbb{R}}}^{\left({N}_{{\rm{TF}}}+{N}_{{\rm{RE}}}^{(i)}\right)\times 64}\) is the parameter matrix of the first hidden layer, which can capture the densely connected TF–RE modules.
EWC loss. EWC constrains the parameters of the first layer to stay in a region of \({\theta }_{b}^{\left(1\right)}\) , which is previously learned from the bulk data 45 . To do so, EWC uses MSE between the parameters \({\theta }_{l}^{\left(1\right)}\) and \({\theta }_{b}^{\left(1\right)}\) , weighted by the Fisher information, a metric of how important the parameter is, allowing the model to protect the performance, both for single-cell data and bulk data 45 .
where \(F\) is the fisher information matrix, which is detailed below, and \({\theta }_{l}^{\left(1\right)}\in {{\mathbb{R}}}^{\left({N}_{{\rm{TF}}}+K\right)\times 64}\) is the parameter matrix of the first hidden layer.
To construct a normalized Laplacian matrix, we first generate the TF–RE binding affinity matrix for all REs from the single-cell ATAC-seq data. We extract the REs 1 Mb from the TSS for the gene to be predicted. Let \({N}_{{\rm{RE}}}^{(i)}\) be the number of these REs and \(B\in {{\mathbb{R}}}^{{N}_{{\rm{TF}}}\times {N}_{{\rm{RE}}}^{(i)}}\) be the TF–RE binding affinity matrix, where \({B}_{{kj}}\) represents the binding affinity for the TF \(k\) and RE \(j\) . We construct a graph, taking TFs as the first \({N}_{{\rm{TF}}}\) nodes, REs as the remaining \({N}_{{\rm{RE}}}^{\;(i)}\) nodes and binding affinity as the edge weight between TF and RE. The edge weights of TF–TF and RE–RE are set to zero. Then the adjacency matrix \({B}^{* }\in {{\mathbb{R}}}^{\left({N}_{{\rm{TF}}}+{N}_{{\rm{RE}}}^{(i)}\right)\times \left({N}_{{\rm{TF}}}+{N}_{{\rm{RE}}}^{(i)}\right)}\) is defined as:
The Fisher information matrix is calculated based on the neural network trained on bulk data:
GRN inference by Shapley value
The Shapley value measures the contribution of features in a machine-learning model and is widely used in algorithms such as deep learning, graphical models and reinforcement learning 85 . We use the average of absolute Shapley values across samples to infer the regulation strength of TF and RE to TGs, generating the RE–TG cis -regulatory strength and the TF–TG trans -regulatory strength. Let \({\beta }_{{ij}}\) represent the cis -regulatory strength of RE \(j\) and TG i , and \({\gamma }_{{ki}}\) represent the trans -regulatory strength. To generate the TF–RE binding strength, we use the weights from the input layer (TFs and REs) to all nodes in the second layer of the neural network model to embed the TF or RE. The TF–RE binding strength is calculated by the PCC between the TF and RE based on this embedding. \({\alpha }_{{kj}}\) represents the TF–RE binding strength.
Constructing cell type-specific GRNs
The TF–RE regulatory potential for a certain cell type is given by:
where \({\rm{TFB}}_{{kj}}\) is the TF–RE regulation potential of TF \(k\) and RE \(j\) ; \({s}_{k}\) is an importance score of TF \(k\) in the cell type to measure the preference of TF for activating cell type-specific open chromatin regions (which will be described in ‘TF importance score’ below); \({C}_{{kj}}\) is the PCC of TF \(k\) and RE \(j\) ; \({O}_{j}\) is the average chromatin accessibility across cells in the cell type; \({B}_{{kj}}\) is the binding affinity between TF \(k\) and RE \(j\) ; and \({\alpha }_{{kj}}\) is the TF–RE binding strength.
The RE–TG cis -regulatory potential is defined as:
where \({\rm{CRP}}_{{ij}}\) is the cis -regulatory potential of TG i and RE \(j\) ; \({\beta }_{{ij}}\) is the cis -regulatory strength of RE \(j\) and TG i ; \({O}_{j}\) is the average chromatin accessibility; \({E}_{i}\) is the average gene expression across cells in the cell type; \({d}_{{ij}}\) is the distance between genomic locations of TG i and RE \(j\) ; and \({d}_{0}\) is a fixed value used to scale the distance, which is set to 25,000 in this paper.
The TF–TG trans -regulatory potential is defined as the cumulative effect of corresponding REs on the TG:
where \({\gamma }_{{ki}}\) is the TF–TG trans -regulatory strength of TF \(k\) and TG i ; \({S}_{i}\) is the set of REs within 1 Mb from the TSS for TG i ; \({\rm{CRP}}_{{ij}}\) is the cis -regulatory potential of TG i and RE \(j\) ; and \({\rm{TFB}}_{{kj}}\) is the TF–RE regulation potential of TF \(k\) and RE \(j\) .
Constructing cell-level GRNs
Cell-level GRNs are inferred by integrating information consistent across all cells, such as regulatory strength, binding affinity and RE–TG distance, with cell-level information, such as gene expression and chromatin accessibility. This approach is similar to inferring cell type-specific GRNs, with the key difference that cell-level GRNs use cell-level TF expression \({E}_{{\rm{TF}}}\) , chromatin accessibility \(O\) and gene expression \(E\) rather than cell type-averaged data. This allows us to infer the network for each individual cell based on its specific characteristics rather than grouping cells into predefined types.
TF importance score
To systematically identify TFs playing a pivotal role in controlling the chromatin accessibility of cell type, we introduce a TF importance score. The score is designed to measure the preference of TFs for activating cell type-specific REs. The input is multiome single-cell data with known cell type annotations. There are four steps to generate the TF importance score:
Motif enrichment. We perform the motif enrichment analysis 86 to identify the motifs significantly enriched in the binding sites of the top 5,000 cell type-specific REs. We use the P value to measure the significant level of motif enrichment.
TF–RE correlation. To avoid dropouts in single-cell data, we recover the original count matrix by an average of the observed count of nearby cells. We calculate PCC between the TF expression and cell type-specific RE chromatin accessibility, with \({r}_{{kj}}\) representing the PCC of the TF \(k\) and the RE \(j\) . To mitigate the bias in the distribution of TF expression and REs chromatin accessibility so that the PCC is comparable across different TF–RE pairs, we permute the cell barcode in the gene expression data and then calculate, generating a background PCC distribution for each TF–RE pair. We generate a z -score for \({r}_{{kj}}\) ,
where \({\mu }_{{kj}}\) and \({\sigma }_{{kj}}^{2}\) are the mean and the variance of the background PCC distribution between \({\rm{TF}}_{k}\) and \({\rm{RE}}_{j}\) .
The co-activity score of the TF-motif pair. To pair TFs with their motifs, we match 713 TFs and 1,331 motifs, yielding 8,793 TF-motif pairs 84 . Let \(\left(k,m\right)\) denote the TF-motif pair of TF \(k\) and motif \(m\) . We then calculate a co-activity score for a TF-motif pair for \(\left(k,m\right)\) , defined as the average z -score across cell type-specific REs with at least one motif binding site. That is \({z}_{k,m}^{\;{co}}=\frac{1}{{N}_{m}}\sum _{j\in {\left\{{\rm{RE}}\;\right\}}_{m}}{z}_{{kj}}\) , where \({\left\{{\rm{RE}}\right\}}_{m}\) is the set of REs with the \(m\) -th motif binding; and \({N}_{m}=\left|{\left\{{\rm{RE}}\right\}}_{m}\right|\) is the number of REs in \({\left\{{\rm{RE}}\right\}}_{m}\) .
TF importance score. The score of the TF-motif pair, \(\left(k,m\right)\) , is given by:
where \({p}_{m}\) is the P value of the \(m\) th motif from the motif-enrichment analysis and \({s}_{(k,m)}\) is the importance score of the TF-motif pair \((k,m)\) . The TF importance score for the TF \(k\) is the average TF-motif pair TF importance score across motifs, omitting NA:
where \({N}_{(k,m)}=\left|\{{{m|s}}_{(k,m)}\ne {\rm{NA}}\}\right|\) is the number of the TF-motif pair of the TF \(k\) , whose CECI score is not NA.
TF–RE binding affinity matrix
We download 713 TF position weight matrices for the known motifs from GitHub page of PECA2 84 , which is collected from widely used databases including JASPAR, TRANSFAC, UniPROBE and Taipale. Given a list of REs, we calculate the binding affinity score for each TF by motif scan using Homer 86 , as a quantitative measure of the strength of the interaction between TF and RE 20 .
Identify motif-binding REs
We identify the REs with motif binding by motif scan using Homer 86 .
ChIP–seq-based validation
Given that the choice of TFs for benchmarking may affect the final results, we use the following standard to collect all ChIP–seq data from the Cistrome database that satisfies the following criteria.
The procedure for choosing ChIP–seq data for PBMC is as follows.
We downloaded all human TF ChIP–seq information, including 11,349 datasets.
We filtered samples that did not pass quality control, and 4,657 datasets remained.
We chose samples in blood tissue, and 609 datasets remained.
We filtered the cell line data that is not consistent with PBMC cell types, and 63 datasets remained.
We chose the TF expressed in single-cell data and with known motifs available, and 39 datasets remained.
We chose the experiments that were done in one of the 14 cell types detected in the PBMC data, and 20 datasets remained.
The procedure for choosing ChIP–seq data for the H1 cell line is as follows:
We chose the H1 cell line, and 42 datasets remained.
We chose the TF expressed in single-cell data and with known motifs available, and 33 datasets remained.
Perturbation-based validation
The criteria for choosing ground truth from the KnockTF database is similar to ChIP–seq data.
The procedure for choosing knockdown data for PBMC is as follows.
We selected the molecular type as ‘TF’ and chose the ‘Peripheral_blood’ tissue type, with 21 cases remaining.
There are 11 datasets included in the PBMCs cell type in the single-cell data.
We chose the TF expressed in single-cell data and with known motifs available, and 8 datasets remained.
PBMC 10× data
We download the PBMC 10K data from the 10× Genomics website ( https://support.10xgenomics.com/single-cell-multiome-atac-gex/datasets ). Note that it contains 11,909 cells, and the granulocytes were removed by cell sorting of this dataset. We use the filtered cells by features matrix from the output of 10× Genomics software Cell Ranger ARC as input and perform the downstream analysis. First, we perform weighted nearest neighbor analysis in Seurat (version 4.0) 87 , and it removes 1,497 cells. We also remove the cells that do not have surrogate ground truth and it results in 9,543 cells. We generate metacells data by randomly selecting the square root of the number of cells in each cell type and averaging the expression levels and chromatin accessibility of the 100 nearest cells to produce the gene expression and chromatin accessibility values of the selected cells. The metacells data were directly input into LINGER for analysis.
To measure the accuracy of a predictor, we defined the AUPR ratio as the ratio of the AUPR of a method to that of a random predictor. For a random predictor, the AUPR equals the fraction of positive samples in the dataset. The AUPR ratio is defined as \({\rm{AUPR}\frac{\#\,{sample}}{\#\,{real}\,{positive}}}\) , representing the fold change of the accuracy of a predictor compared to the random prediction.
We propose a method to integrate GWAS summary statistics data and cell type-specific GRNs to identify the relevant cell types, key TFs and sub-GRNs responsible for GWAS variants. To identify relevant cell types, we first project the risk SNP identified from GWAS summary data to a gene. We then link the gene within the 200 kb region centering on the SNP and assign the most significant P value of linked SNPs to each gene. In this study, the trait-related genes are defined as those with P < 0.01 after multiple testing adjustments. We then calculate a trait regulation score for each TF in each cell type, measuring the enrichment of GWAS genes downstream of the TF based on the cell type-specific GRN. We choose 1,000 top-ranked genes according to the trans -regulation as the TG of each TF and count the number of overlapping genes with trait-related genes. The enrichment of cell types to the GWAS traits is measured by a t -test comparing the number of overlapping genes between the 100 top-expressed and 100 randomly chosen TFs.
To identify key TFs of GWAS traits, we combine the trait regulation score and the gene expression level of TFs in each cell type. The trait regulation score is the z -score of the number of overlapping genes of a TF across all TFs. The expression level is also transformed to a z -score based on the gene expression. The final importance of key TFs is the summation of the expression level and trait regulation score.
To measure the activity of each TF on the independent transcriptional profiles, we first constructed a TG set for each TF based on the corresponding GRN. We perform quantile normalization to the trans -regulation score of each gene across all TFs. We then rank the genes for each TF and choose the top 1,000 genes as the target. Next, we use the R package AUCell 22 to calculate whether the TGs are enriched within the expressed genes for each sample, which defines the TF activity.
Benchmark the trans -regulatory potential
We compare LINGER’s performance of the trans -regulation prediction using PCC, SCENIC+, GENIE3 and PIDC as competitors to LINGER. Owing to the time-consuming nature of PIDC’s mutual information-based algorithm, we used the 5,000 most variable genes as input. As a result, there are 9 TFs and 14 TFs in ground truth data left for PBMCs and the H1 cell line, respectively.
Reporting summary
Further information on research design is available in the Nature Portfolio Reporting Summary linked to this article.
Data availability
The PBMC data used during this study was downloaded from the 10× Genomics website ( https://s3-us-west-2.amazonaws.com/10x.files/samples/cell-arc/1.0.0/pbmc_granulocyte_sorted_10k/pbmc_granulocyte_sorted_10k_fastqs.tar ) 40 . SNARE-seq was downloaded from NCBI Gene Expression Omnibus ( https://www.ncbi.nlm.nih.gov/geo/ ) under accession number GSE126074 (ref. 55 ).
Code availability
The software is available at GitHub 88 ( https://github.com/Durenlab/LINGER ) and the Zenodo repository under the GPLv3 license 89 . We used Python and R for this study.
Jacob, F. & Monod, J. On the regulation of gene activity. Cold Spring Harb. Symp. Quant. Biol. 26 , 193–211 (1961).
Article CAS Google Scholar
Hecker, M., Lambeck, S., Toepfer, S., van Someren, E. & Guthke, R. Gene regulatory network inference: data integration in dynamic models—a review. BioSystems 96 , 86–103 (2009).
Article CAS PubMed Google Scholar
Thieffry, D., Huerta, A. M., Perez-Rueda, E. & Collado-Vides, J. From specific gene regulation to genomic networks: a global analysis of transcriptional regulation in Escherichia coli . BioEssays 20 , 433–440 (1998).
Badia-i-Mompel, P. et al. Gene regulatory network inference in the era of single-cell multi-omics. Nat. Rev. Genet. 24 , 739–754 (2023).
Bansal, M., Gatta, D. G. & di Bernardo, D. Inference of gene regulatory networks and compound mode of action from time course gene expression profiles. Bioinformatics 22 , 815–822 (2006).
Wang, Y., Joshi, T., Zhang, X. S., Xu, D. & Chen, L. Inferring gene regulatory networks from multiple microarray datasets. Bioinformatics 22 , 2413–2420 (2006).
Iyer, A. S., Osmanbeyoglu, H. U. & Leslie, C. S. Computational methods to dissect gene regulatory networks in cancer. Curr. Opin. Syst. Biol. 2 , 115–122 (2017).
Article Google Scholar
Hempel, S., Koseska, A., Kurths, J. & Nikoloski, Z. Inner composition alignment for inferring directed networks from short time series. Phys. Rev. Lett. 107 , 054101 (2011).
Margolin, A. A. et al. ARACNE: an algorithm for the reconstruction of gene regulatory networks in a mammalian cellular context. BMC Bioinf. 7 , S7 (2006).
Zou, M. & Conzen, S. D. A new dynamic Bayesian network (DBN) approach for identifying gene regulatory networks from time course microarray data. Bioinformatics 21 , 71–79 (2005).
Perrin, B. E. et al. Gene networks inference using dynamic Bayesian networks. Bioinformatics 19 , 138–148 (2003).
Zhang, X. & Moret, B. M. E. Refining transcriptional regulatory networks using network evolutionary models and gene histories. Algorithms Mol. Biol. 5 , 1 (2010).
Article PubMed PubMed Central Google Scholar
Zhong, W. et al. Inferring regulatory networks from mixed observational data using directed acyclic graphs. Front. Genet. 11 , 8 (2020).
Article CAS PubMed PubMed Central Google Scholar
Fuller, T. F. et al. Weighted gene coexpression network analysis strategies applied to mouse weight. Mammalian Genome 18 , 463–472 (2007).
Huynh-Thu, V. A., Irrthum, A., Wehenkel, L. & Geurts, P. Inferring regulatory networks from expression data using tree-based methods. PLoS One 5 , e12776 (2010).
Wang, Y. X. R. & Huang, H. Review on statistical methods for gene network reconstruction using expression data. J. Theor. Biol. 362 , 53–61 (2014).
Article PubMed Google Scholar
Boyle, A. P. et al. High-resolution mapping and characterization of open chromatin across the genome. Cell 132 , 311–322 (2008).
Buenrostro, J. D., Giresi, P. G., Zaba, L. C., Chang, H. Y. & Greenleaf, W. J. Transposition of native chromatin for fast and sensitive epigenomic profiling of open chromatin, DNA-binding proteins and nucleosome position. Nat. Methods 10 , 1213–1218 (2013).
Neph, S. et al. Circuitry and dynamics of human transcription factor regulatory networks. Cell 150 , 1274–1286 (2012).
Duren, Z., Chen, X., Jiang, R., Wang, Y. & Wong, W. H. Modeling gene regulation from paired expression and chromatin accessibility data. Proc. Natl Acad. Sci. USA 114 , E4914–E4923 (2017).
Chan, T. E., Stumpf, M. P. H. & Babtie, A. C. Gene regulatory network inference from single-cell data using multivariate information measures. Cell Syst . 5 , 251–267.e3 (2017).
Aibar, S. et al. SCENIC: single-cell regulatory network inference and clustering. Nat. Methods 14 , 1083–1086 (2017).
Matsumoto, H. et al. SCODE: an efficient regulatory network inference algorithm from single-cell RNA-seq during differentiation. Bioinformatics 33 , 2314–2321 (2017).
Papili Gao, N., Ud-Dean, S. M. M., Gandrillon, O. & Gunawan, R. SINCERITIES: inferring gene regulatory networks from time-stamped single cell transcriptional expression profiles. Bioinformatics 34 , 258–266 (2018).
Sanchez-Castillo, M., Blanco, D., Tienda-Luna, I. M., Carrion, M. C. & Huang, Y. A Bayesian framework for the inference of gene regulatory networks from time and pseudo-time series data. Bioinformatics 34 , 964–970 (2018).
Hu, Y., Peng, T., Gao, L. & Tan, K. CytoTalk: de novo construction of signal transduction networks using single-cell transcriptomic data. Sci. Adv. 7 , eabf1356 (2021).
Frankowski, P. C. A. & Vert, J. P. Gene regulation inference from single-cell RNA-seq data with linear differential equations and velocity inference. Bioinformatics 36 , 4774–4780 (2020).
Specht, A. T. & Li, J. LEAP: constructing gene co-expression networks for single-cell RNA-sequencing data using pseudotime ordering. Bioinformatics 33 , 764–766 (2017).
Moerman, T. et al. GRNBoost2 and Arboreto: efficient and scalable inference of gene regulatory networks. Bioinformatics 35 , 2159–2161 (2019).
Zhang, S. et al. Inference of cell type-specific gene regulatory networks on cell lineages from single cell omic datasets. Nat. Commun. 14 , 3064 (2023).
Li, H. et al. Inferring transcription factor regulatory networks from single-cell ATAC-seq data based on graph neural networks. Nat. Mach. Intell. 4 , 389–400 (2022).
Jiang, J. et al. IReNA: integrated regulatory network analysis of single-cell transcriptomes and chromatin accessibility profiles. iScience 25 , 105359 (2022).
Jansen, C. et al. Building gene regulatory networks from scATAC-seq and scRNA-seq using linked self organizing maps. PLoS Comput. Biol. 15 , e1006555 (2019).
Yuan, Q. & Duren, Z. Integration of single-cell multi-omics data by regression analysis on unpaired observations. Genome Biol. 23 , 160 (2022).
Duren, Z. et al. Integrative analysis of single-cell genomics data by coupled nonnegative matrix factorizations. Proc. Natl Acad. Sci. USA 115 , 7723–7728 (2018).
Zeng, W. et al. DC3 is a method for deconvolution and coupled clustering from bulk and single-cell genomics data. Nat. Commun. 10 , 4613 (2019).
Wang, Z. et al. Cell-type-specific gene regulatory networks underlying murine neonatal heart regeneration at single-cell resolution. Cell Rep. 33 , 108472 (2020).
Lin, Y. et al. scJoint integrates atlas-scale single-cell RNA-seq and ATAC-seq data with transfer learning. Nat. Biotechnol. 40 , 703–710 (2022).
Pratapa, A., Jalihal, A. P., Law, J. N., Bharadwaj, A. & Murali, T. M. Benchmarking algorithms for gene regulatory network inference from single-cell transcriptomic data. Nat. Methods 17 , 147–154 (2020).
10× Genomics. PBMCs from C57BL/6 mice (v1, 150×150) ; single cell immune profiling dataset by Cell Ranger 3.1.0 (2019).
Duren, Z. et al. Regulatory analysis of single cell multiome gene expression and chromatin accessibility data with scREG. Genome Biol. 23 , 114 (2022).
González-Blas, C. B. et al. SCENIC+: single-cell multiomic inference of enhancers and gene regulatory networks. Nat. Methods 20 , 1355–1367 (2023).
Thrun, S. & Mitchell, T. M. Lifelong robot learning. Rob. Auton. Syst. 15 , 25–46 (1995).
Chaudhri, Z. & Liu, B. Lifelong Machine Learning (Springer International Publishing, 2022).
Parisi, G. I., Kemker, R., Part, J. L., Kanan, C. & Wermter, S. Continual lifelong learning with neural networks: a review. Neural Netw. 113 , 54–71 (2019).
ENCODE Project Consortium. An integrated encyclopedia of DNA elements in the human genome. Nature 489 , 57–74 (2012).
Kirkpatrick, J. et al. Overcoming catastrophic forgetting in neural networks. Proc. Natl Acad. Sci. USA 114 , 3521–3526 (2017).
Liu, T. et al. Cistrome: an integrative platform for transcriptional regulation studies. Genome Biol. 12 , R83 (2011).
Fairfax, B. P. et al. Innate immune activity conditions the effect of regulatory variants upon monocyte gene expression. Science 343 , 1246949 (2014).
Võsa, U. et al. Large-scale cis - and trans -eQTL analyses identify thousands of genetic loci and polygenic scores that regulate blood gene expression. Nat. Genet. 53 , 1300–1310 (2021).
Lek, M. et al. Analysis of protein-coding genetic variation in 60,706 humans. Nature 536 , 285–291 (2016).
Mostafavi, H., Spence, J. P., Naqvi, S. & Pritchard, J. K. Systematic differences in discovery of genetic effects on gene expression and complex traits. Nat. Genet. 55 , 1866–1875 (2023).
Kuleshov, M. V. et al. Enrichr: a comprehensive gene set enrichment analysis web server 2016 update. Nucleic Acids Res. 44 , W90–W97 (2016).
Wang, J. et al. ATAC-seq analysis reveals a widespread decrease of chromatin accessibility in age-related macular degeneration. Nat. Commun. 9 , 1364 (2018).
Chen, S., Lake, B. B. & Zhang, K. High-throughput sequencing of the transcriptome and chromatin accessibility in the same cell. Nat. Biotechnol. 37 , 1452–1457 (2019).
Javierre, B. M. et al. Lineage-specific genome architecture links enhancers and non-coding disease variants to target gene promoters. Cell 167 , 1369–1384.e19 (2016).
Yazar, S. et al. Single-cell eQTL mapping identifies cell type-specific genetic control of autoimmune disease. Science 376 , eabf3041 (2022).
Duren, Z. et al. Sc-compReg enables the comparison of gene regulatory networks between conditions using single-cell data. Nat. Commun. 12 , 4763 (2021).
Feng, C. et al. KnockTF: a comprehensive human gene expression profile database with knockdown/knockout of transcription factors. Nucleic Acids Res. 48 , D93–D100 (2020).
Satpathy, A. T. et al. Runx1 and Cbfβ regulate the development of Flt3 + dendritic cell progenitors and restrict myeloproliferative disorder. Blood 123 , 2968–2977 (2014).
Jagadeesh, K. A. et al. Identifying disease-critical cell types and cellular processes by integrating single-cell RNA-sequencing and human genetics. Nat. Genet. 54 , 1479–1492 (2022).
Sollis, E. et al. The NHGRI-EBI GWAS Catalog: knowledgebase and deposition resource. Nucleic Acids Res. 51 , D977–D985 (2023).
Mize, T.J. & Evans, L. M. Examination of a novel expression-based gene-SNP annotation strategy to identify tissue-specific contributions to heritability in multiple traits. Eur. J. Hum. Genet. 263 , 32 (2024).
Google Scholar
Anderson, A. et al. Monocytosis is a biomarker of severity in inflammatory bowel disease: analysis of a 6-year prospective natural history registry. Inflamm. Bowel Dis. 28 , 70–78 (2022).
Aschenbrenner, D. et al. Deconvolution of monocyte responses in inflammatory bowel disease reveals an IL-1 cytokine network that regulates IL-23 in genetic and acquired IL-10 resistance. Gut 70 , 1023–1036 (2021).
Wang, X., Guo, R., Lv, Y. & Fu, R. The regulatory role of Fos related antigen-1 in inflammatory bowel disease. Mol. Med. Rep. 17 , 1979–1985 (2018).
CAS PubMed Google Scholar
Nowak, J. K. et al. Characterisation of the circulating transcriptomic landscape in inflammatory bowel disease provides evidence for dysregulation of multiple transcription factors including NFE2, SPI1, CEBPB, and IRF2. J. Crohns Colitis 16 , 1255–1268 (2022).
Broom, O. J., Widjaya, B., Troelsen, J., Olsen, J. & Nielsen, O. H. Mitogen activated protein kinases: A role in inflammatory bowel disease? Clin. Exp. Immunol. 158 , 272–280 (2009).
Darsigny, M., St-Jean, S. & Boudreau, F. Cux1 transcription factor is induced in inflammatory bowel disease and protects against experimental colitis. Inflamm. Bowel Dis. 16 , 1739–1750 (2010).
Yu, Y. L. et al. STAT1 epigenetically regulates LCP2 and TNFAIP2 by recruiting EP300 to contribute to the pathogenesis of inflammatory bowel disease. Clin. Epigenetics 13 , 127 (2021).
Hu, S. et al. Inflammation status modulates the effect of host genetic variation on intestinal gene expression in inflammatory bowel disease. Nat. Commun. 12 , 1122 (2021).
Stirewalt, D. L. et al. Identification of genes with abnormal expression changes in acute myeloid leukemia. Genes Chromosomes Cancer 47 , 8–20 (2008).
Bottomly, D. et al. Integrative analysis of drug response and clinical outcome in acute myeloid leukemia. Cancer Cell 40 , 850–864.e9 (2022).
Ji, X., Ji, Y., Wang, W. & Xu, X. Forkhead box N1 inhibits the progression of non-small cell lung cancer and serves as a tumor suppressor. Oncology Lett. 15 , 7221–7230 (2018).
Yang, K. et al. T Cell exit from quiescence and differentiation into Th2 cells depend on raptor-mTORC1-mediated metabolic reprogramming. Immunity 39 , 1043–1056 (2013).
Tan, H. et al. Integrative proteomics and phosphoproteomics profiling reveals dynamic signaling networks and bioenergetics pathways underlying T cell activation. Immunity 46 , 488–503 (2017).
Blanchett, S., Boal-Carvalho, I., Layzell, S. & Seddon, B. NF-κB and extrinsic cell death pathways—entwined do-or-die decisions for T cells. Trends Immunol. 42 , 76–88 (2021).
Oh, H. & Ghosh, S. NF-κB: roles and regulation in different CD4 + T-cell subsets. Immunol. Rev. 252 , 41–51 (2013).
Sekiya, T. et al. Essential roles of the transcription factor NR4A1 in regulatory T cell differentiation under the influence of immunosuppressants. J. Immunol. 208 , 2122–2130 (2022).
Fassett, M. S., Jiang, W., D’Alise, A. M., Mathis, D. & Benoist, C. Nuclear receptor Nr4a1 modulates both regulatory T-cell (T reg ) differentiation and clonal deletion. Proc. Natl Acad. Sci. USA 109 , 3891–3896 (2012).
Kamimoto, K. et al. Dissecting cell identity via network inference and in silico gene perturbation. Nature 614 , 742–751 (2023).
Lance, C. et al. Multimodal single cell data integration challenge: results and lessons learned. Preprint at bioRxiv https://doi.org/10.1101/2022.04.11.487796 (2022).
Shivdasani, R. A. Molecular and transcriptional regulation of megakaryocyte differentiation. Stem Cells 19 , 397–407 (2001).
Duren, Z., Chen, X., Xin, J., Wang, Y. & Wong, W. H. Time course regulatory analysis based on paired expression and chromatin accessibility data. Genome Res. 30 , 622–634 (2020).
Rozemberczki, B. et al. The Shapley value in machine learning. Preprint at https://doi.org/10.48550/arXiv.2202.05594 (2022).
Heinz, S. et al. Simple combinations of lineage-determining transcription factors prime cis -regulatory elements required for macrophage and B cell identities. Mol. Cell 38 , 576–589 (2010).
Hao, Y. et al. Integrated analysis of multimodal single-cell data. Cell 184 , 3573–3587 (2021).
Qiuyue Y. & Duren Z. Predicting gene regulatory networks from single cell multiome data using atlas-scale external data. GitHub https://github.com/Durenlab/LINGER (2022).
Qiuyue Y. & Duren Z. Predicting gene regulatory networks from single cell multiome data using atlas-scale external data. Zendo https://zenodo.org/records/10639041 (2024).
Download references
Acknowledgements
The authors are supported by National Institutes of Health grants P20 GM139769 and R35 GM150513. The language in the text has been polished by GPT-3.5 and Grammarly.
Author information
Authors and affiliations.
Center for Human Genetics, Department of Genetics and Biochemistry, Clemson University, Greenwood, SC, USA
Qiuyue Yuan & Zhana Duren
You can also search for this author in PubMed Google Scholar
Contributions
Z.D. conceived the LINGER method. Z.D. and Q.Y. designed the analytical approach. Q.Y. performed the data analysis. Q.Y. wrote the software. Q.Y. and Z.D. wrote, revised and contributed to the final manuscript. The authors read and approved the final manuscript.
Corresponding author
Correspondence to Zhana Duren .
Ethics declarations
Competing interests.
The authors declare no competing interests.
Peer review
Peer review information.
Nature Biotechnology thanks Marc Sturrock, Ricard Argelaguet and Olivier Gandrillon for their contribution to the peer review of this work.
Additional information
Publisher’s note Springer Nature remains neutral with regard to jurisdictional claims in published maps and institutional affiliations.
Extended data
Extended data fig. 1 assessing the performance of cis -regulatory strength inferred by linger taking eqtl data for gtex as ground truth..
A . AUC for cis -regulatory strength inferred by LINGER. The ground truth for A and B is the variant-gene links from GTEx. We divide RE-TG pairs into different groups based on the distance of RE and the TSS of TG. B . AUPR ratio for cis -regulatory strength.
Extended Data Fig. 2 Parameter sensitivity.
A . Sensitivity of neural network structure and active function. B . Violin plot of AUC and AUPR ratio values of trans -regulatory potential performance across diverse TFs and cell types (n=20 independent sample). One-sided paired t-test result in -log 10 P-value 10.73, 7.11, 10.85, and 9.61 compared with GENIE3, PCC, PIDC, and SCENIC+ in terms of AUC, respectively. For AUPR ratio, -log 10 P-values are 8.94, 7.03, 8.48, and 7.57, respectively. C , D . Bar plot of AUC and AUPR ratio difference of different motif matching weight. The upper and lower figures refer to the difference in weight 0.01 to 0 and 0.01 to 10. The x-axis of C , D , and H refers to the ground truth data named by the TF name and Cistrome database ID. E . Scatter plot of AUC of original metacells and SEACells metacells as input. Each point refers to each ChIP-seq ground truth data. F, G . Box plot of AUPR ratio and AUC of defining regulatory element within different TSS distances from 200 Kb to 2 Mb (n = 20 independent sample). Two-sided paired t-test result in p-value 0.055(2 Mb and 1 Mb), 0.088(2 Mb and 500 Kb), 0.028(2 Mb and 200 Kb), 0.025(1 Mb and 500 Kb), 0.0056(1 Mb and 200 Kb), and 0.70(500 Kb and 200 Kb) in terms of AUC. For AUPR ratio, p-values are 0.0017(2 Mb and 1 Mb), 0.093(2 Mb and 500 Kb), 0.12(2 Mb and 200 Kb), 0.00048(1 Mb and 500 Kb), 0.00075(1 Mb and 200 Kb), and 0.64(500 Kb and 200 Kb). H . Bar plot of AUC and AUPR ratio difference of two rounds pre-train and single round pre-train.
Extended Data Fig. 3 Systematic benchmarking of cell type-specific GRN.
A, B . ROC curve and PR curve of binding potential for MYC in H1 cell line. The ground truth for A to D is the ChIP-seq data of MYC in the H1 cell line. The color in A to D represents the different competitors to predict TF-RE regulation. Orange represents LINGER, green represents PCC between the expression of TF and the chromatin accessibility of RE, and blue represents motif binding affinity of TF to RE. C, D . Violin plot of AUC and AUPR ratio values of binding potential across diverse TFs. The ground truth is ChIP-seq data for 33 TFs (n=33 independent sample). One-sided paired t-test is performed to test whether there is significant difference. In C , -log 10 P-values are 11.36 and 12.27 compared with PCC and TFBS, respectively. In D , -log P-values are 6.21 and 5.18, respectively. E , F . AUC and AUPR ratio of cis -regulatory potential in naïve CD8 T cells. The ground truth for E to J is promoter capture HiC data. RE-TG pairs are divided into six distance groups ranging from 0-5k to 100-200 kb. PCC is calculated between the expression of TG and the chromatin accessibility of RE. Distance denotes the decay function of the distance to the TSS. Random denotes the uniform distribution. G , H . AUC and AUPR ratio of cis -regulatory potential in naïve B cells. I , J . F1 score of cis -regulatory in naïve CD8 T cells and naïve B cells for LINGER and SCENIC+. P-values are from one-sided paired t-test with n=9 independent sample. K to O , F1 score of cis -regulatory potential in classical monocytes, effector CD8 T cells, memory B cells, non-classical monocytes, and plasmacytoid DC cells for LINGER and SCENIC+. The ground truth is eQTL data (n=9 independent sample). P-values are from one-sided paired t-test. P , Q . ROC curve and PR curve of trans -regulatory potential inference of CTCF in H1 cell line. The ground truth of P to R is putative targets of TFs from ChIP-seq data in the H1 cell line. R Violin plot of AUC and AUPR ratio values of trans -regulatory potential performance across diverse TFs in H1 cell line (n=33 independent sample). One-sided unpaired t-test result in -log 10 P-value 15.89, 15.64, 16.36, and 15.54 compared with GENIE3, PCC, PIDC, and SCENIC+ in terms of AUC, respectively. For AUPR ratio, -log 10 P-values are 11.01, 10.64, 11.20, and 11.17, respectively.
Extended Data Fig. 4 In silico perturbation.
A , B . Violin plot of AUC and AUPR ratio values of trans -regulatory potential performance across diverse TFs and cell types for PBMCs. The ground truth of A to D is 8 experimental perturbation data from KnockTF database (n=8 independent sample). One-sided paired t-test are performed to test the difference. For AUC, -log 10 P-values are 3.74, 3.43, 3.64, and 3.86 compared with GENIE3, PCC, PIDC, and SCENIC+, respectively. For AUPR ratio, -log 10 P-values are 3.36, 2.14, 1.69 and 1.80, respectively. C , D . Box plot of AUC and AUPR ratio values of in silico perturbation predicted target gene. P-values are from one-sided paired t-test with 8 independent samples. E . Differentiation behavior prediction on BMMC data after knocking out GATA1.
Supplementary information
Reporting summary, supplementary tables 1–8.
Table 1: Information of ground truth data for the trans -regulation and TF–RE binding potential for PBMC data. Table 2: Details of eQTL data as ground truth for the cis -regulation for PBMCs. Table 3: Functional enrichment of cis -regulatory dominant gene. Table 4: Ground truth data for the trans- regulation and TF-RE binding potential for H1 cell line. Table 5: Details of Hi-C data as ground truth for the cis -regulation for PBMCs. Table 6: Details of Fig. 3b,c Table 7: Details of Fig. 4c,d. Table 8: Ground truth data information of the trans -regulation for PBMC data from the KnockTF database.
Rights and permissions
Open Access This article is licensed under a Creative Commons Attribution 4.0 International License, which permits use, sharing, adaptation, distribution and reproduction in any medium or format, as long as you give appropriate credit to the original author(s) and the source, provide a link to the Creative Commons licence, and indicate if changes were made. The images or other third party material in this article are included in the article’s Creative Commons licence, unless indicated otherwise in a credit line to the material. If material is not included in the article’s Creative Commons licence and your intended use is not permitted by statutory regulation or exceeds the permitted use, you will need to obtain permission directly from the copyright holder. To view a copy of this licence, visit http://creativecommons.org/licenses/by/4.0/ .
Reprints and permissions
About this article
Cite this article.
Yuan, Q., Duren, Z. Inferring gene regulatory networks from single-cell multiome data using atlas-scale external data. Nat Biotechnol (2024). https://doi.org/10.1038/s41587-024-02182-7
Download citation
Received : 04 August 2023
Accepted : 26 February 2024
Published : 12 April 2024
DOI : https://doi.org/10.1038/s41587-024-02182-7
Share this article
Anyone you share the following link with will be able to read this content:
Sorry, a shareable link is not currently available for this article.
Provided by the Springer Nature SharedIt content-sharing initiative
Quick links
- Explore articles by subject
- Guide to authors
- Editorial policies
Sign up for the Nature Briefing newsletter — what matters in science, free to your inbox daily.

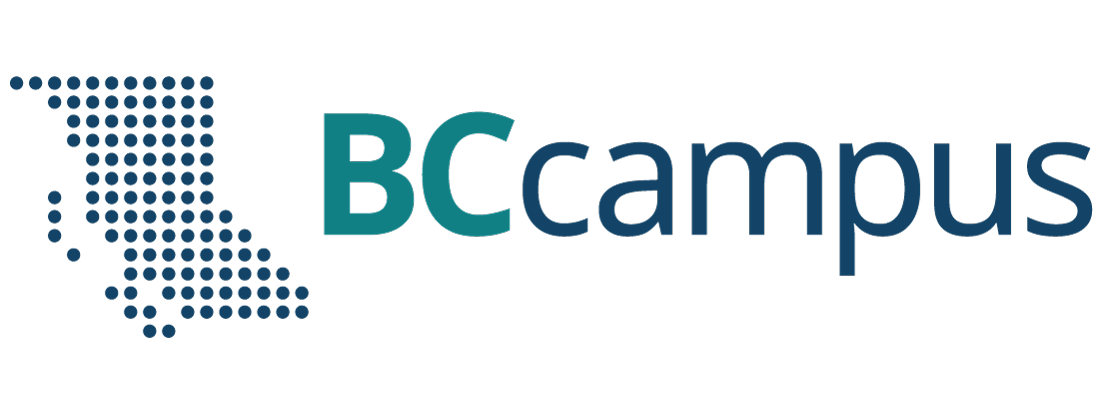
Want to create or adapt books like this? Learn more about how Pressbooks supports open publishing practices.
Chapter 10: Single-Subject Research
Overview of Single-Subject Research
Learning Objectives
- Explain what single-subject research is, including how it differs from other types of psychological research.
- Explain what case studies are, including some of their strengths and weaknesses.
- Explain who uses single-subject research and why.
What Is Single-Subject Research?
Single-subject research is a type of quantitative research that involves studying in detail the behaviour of each of a small number of participants. Note that the term single-subject does not mean that only one participant is studied; it is more typical for there to be somewhere between two and 10 participants. (This is why single-subject research designs are sometimes called small- n designs, where n is the statistical symbol for the sample size.) Single-subject research can be contrasted with group research , which typically involves studying large numbers of participants and examining their behaviour primarily in terms of group means, standard deviations, and so on. The majority of this textbook is devoted to understanding group research, which is the most common approach in psychology. But single-subject research is an important alternative, and it is the primary approach in some areas of psychology.
Before continuing, it is important to distinguish single-subject research from two other approaches, both of which involve studying in detail a small number of participants. One is qualitative research, which focuses on understanding people’s subjective experience by collecting relatively unstructured data (e.g., detailed interviews) and analyzing those data using narrative rather than quantitative techniques. Single-subject research, in contrast, focuses on understanding objective behaviour through experimental manipulation and control, collecting highly structured data, and analyzing those data quantitatively.
It is also important to distinguish single-subject research from case studies. A case study is a detailed description of an individual, which can include both qualitative and quantitative analyses. (Case studies that include only qualitative analyses can be considered a type of qualitative research.) The history of psychology is filled with influential cases studies, such as Sigmund Freud’s description of “Anna O.” (see Note 10.5 “The Case of “Anna O.””) and John Watson and Rosalie Rayner’s description of Little Albert (Watson & Rayner, 1920) [1] , who learned to fear a white rat—along with other furry objects—when the researchers made a loud noise while he was playing with the rat. Case studies can be useful for suggesting new research questions and for illustrating general principles. They can also help researchers understand rare phenomena, such as the effects of damage to a specific part of the human brain. As a general rule, however, case studies cannot substitute for carefully designed group or single-subject research studies. One reason is that case studies usually do not allow researchers to determine whether specific events are causally related, or even related at all. For example, if a patient is described in a case study as having been sexually abused as a child and then as having developed an eating disorder as a teenager, there is no way to determine whether these two events had anything to do with each other. A second reason is that an individual case can always be unusual in some way and therefore be unrepresentative of people more generally. Thus case studies have serious problems with both internal and external validity.
The Case of “Anna O.”
Sigmund Freud used the case of a young woman he called “Anna O.” to illustrate many principles of his theory of psychoanalysis (Freud, 1961) [2] . (Her real name was Bertha Pappenheim, and she was an early feminist who went on to make important contributions to the field of social work.) Anna had come to Freud’s colleague Josef Breuer around 1880 with a variety of odd physical and psychological symptoms. One of them was that for several weeks she was unable to drink any fluids. According to Freud,
She would take up the glass of water that she longed for, but as soon as it touched her lips she would push it away like someone suffering from hydrophobia.…She lived only on fruit, such as melons, etc., so as to lessen her tormenting thirst. (p. 9)
But according to Freud, a breakthrough came one day while Anna was under hypnosis.
[S]he grumbled about her English “lady-companion,” whom she did not care for, and went on to describe, with every sign of disgust, how she had once gone into this lady’s room and how her little dog—horrid creature!—had drunk out of a glass there. The patient had said nothing, as she had wanted to be polite. After giving further energetic expression to the anger she had held back, she asked for something to drink, drank a large quantity of water without any difficulty, and awoke from her hypnosis with the glass at her lips; and thereupon the disturbance vanished, never to return. (p.9)
Freud’s interpretation was that Anna had repressed the memory of this incident along with the emotion that it triggered and that this was what had caused her inability to drink. Furthermore, her recollection of the incident, along with her expression of the emotion she had repressed, caused the symptom to go away.
As an illustration of Freud’s theory, the case study of Anna O. is quite effective. As evidence for the theory, however, it is essentially worthless. The description provides no way of knowing whether Anna had really repressed the memory of the dog drinking from the glass, whether this repression had caused her inability to drink, or whether recalling this “trauma” relieved the symptom. It is also unclear from this case study how typical or atypical Anna’s experience was.
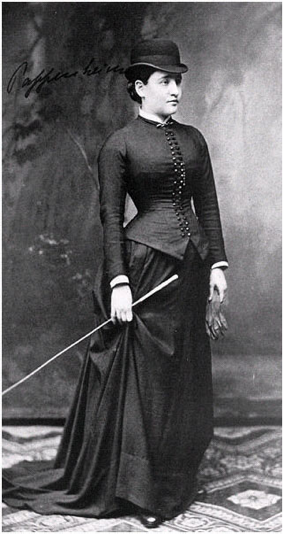
Assumptions of Single-Subject Research
Again, single-subject research involves studying a small number of participants and focusing intensively on the behaviour of each one. But why take this approach instead of the group approach? There are several important assumptions underlying single-subject research, and it will help to consider them now.
First and foremost is the assumption that it is important to focus intensively on the behaviour of individual participants. One reason for this is that group research can hide individual differences and generate results that do not represent the behaviour of any individual. For example, a treatment that has a positive effect for half the people exposed to it but a negative effect for the other half would, on average, appear to have no effect at all. Single-subject research, however, would likely reveal these individual differences. A second reason to focus intensively on individuals is that sometimes it is the behaviour of a particular individual that is primarily of interest. A school psychologist, for example, might be interested in changing the behaviour of a particular disruptive student. Although previous published research (both single-subject and group research) is likely to provide some guidance on how to do this, conducting a study on this student would be more direct and probably more effective.
A second assumption of single-subject research is that it is important to discover causal relationships through the manipulation of an independent variable, the careful measurement of a dependent variable, and the control of extraneous variables. For this reason, single-subject research is often considered a type of experimental research with good internal validity. Recall, for example, that Hall and his colleagues measured their dependent variable (studying) many times—first under a no-treatment control condition, then under a treatment condition (positive teacher attention), and then again under the control condition. Because there was a clear increase in studying when the treatment was introduced, a decrease when it was removed, and an increase when it was reintroduced, there is little doubt that the treatment was the cause of the improvement.
A third assumption of single-subject research is that it is important to study strong and consistent effects that have biological or social importance. Applied researchers, in particular, are interested in treatments that have substantial effects on important behaviours and that can be implemented reliably in the real-world contexts in which they occur. This is sometimes referred to as social validity (Wolf, 1976) [3] . The study by Hall and his colleagues, for example, had good social validity because it showed strong and consistent effects of positive teacher attention on a behaviour that is of obvious importance to teachers, parents, and students. Furthermore, the teachers found the treatment easy to implement, even in their often-chaotic elementary school classrooms.
Who Uses Single-Subject Research?
Single-subject research has been around as long as the field of psychology itself. In the late 1800s, one of psychology’s founders, Wilhelm Wundt, studied sensation and consciousness by focusing intensively on each of a small number of research participants. Herman Ebbinghaus’s research on memory and Ivan Pavlov’s research on classical conditioning are other early examples, both of which are still described in almost every introductory psychology textbook.
In the middle of the 20th century, B. F. Skinner clarified many of the assumptions underlying single-subject research and refined many of its techniques (Skinner, 1938) [4] . He and other researchers then used it to describe how rewards, punishments, and other external factors affect behaviour over time. This work was carried out primarily using nonhuman subjects—mostly rats and pigeons. This approach, which Skinner called the experimental analysis of behaviour —remains an important subfield of psychology and continues to rely almost exclusively on single-subject research. For excellent examples of this work, look at any issue of the Journal of the Experimental Analysis of Behaviour . By the 1960s, many researchers were interested in using this approach to conduct applied research primarily with humans—a subfield now called applied behaviour analysis (Baer, Wolf, & Risley, 1968) [5] . Applied behaviour analysis plays an especially important role in contemporary research on developmental disabilities, education, organizational behaviour, and health, among many other areas. Excellent examples of this work (including the study by Hall and his colleagues) can be found in the Journal of Applied Behaviour Analysis .
Although most contemporary single-subject research is conducted from the behavioural perspective, it can in principle be used to address questions framed in terms of any theoretical perspective. For example, a studying technique based on cognitive principles of learning and memory could be evaluated by testing it on individual high school students using the single-subject approach. The single-subject approach can also be used by clinicians who take any theoretical perspective—behavioural, cognitive, psychodynamic, or humanistic—to study processes of therapeutic change with individual clients and to document their clients’ improvement (Kazdin, 1982) [6] .
Key Takeaways
- Single-subject research—which involves testing a small number of participants and focusing intensively on the behaviour of each individual—is an important alternative to group research in psychology.
- Single-subject studies must be distinguished from case studies, in which an individual case is described in detail. Case studies can be useful for generating new research questions, for studying rare phenomena, and for illustrating general principles. However, they cannot substitute for carefully controlled experimental or correlational studies because they are low in internal and external validity.
- Single-subject research has been around since the beginning of the field of psychology. Today it is most strongly associated with the behavioural theoretical perspective, but it can in principle be used to study behaviour from any perspective.
- Practice: Find and read a published article in psychology that reports new single-subject research. ( An archive of articles published in the Journal of Applied Behaviour Analysis can be found at http://www.ncbi.nlm.nih.gov/pmc/journals/309/) Write a short summary of the study.
- Describe one problem related to internal validity.
- Describe one problem related to external validity.
- Generate one hypothesis suggested by the case study that might be interesting to test in a systematic single-subject or group study.
Media Attributions
- Pappenheim 1882 by unknown is in the Public Domain .
- Watson, J. B., & Rayner, R. (1920). Conditioned emotional reactions. Journal of Experimental Psychology, 3 , 1–14. ↵
- Freud, S. (1961). Five lectures on psycho-analysis . New York, NY: Norton. ↵
- Wolf, M. (1976). Social validity: The case for subjective measurement or how applied behaviour analysis is finding its heart. Journal of Applied Behaviour Analysis, 11 , 203–214. ↵
- Skinner, B. F. (1938). T he behaviour of organisms: An experimental analysis . New York, NY: Appleton-Century-Crofts. ↵
- Baer, D. M., Wolf, M. M., & Risley, T. R. (1968). Some current dimensions of applied behaviour analysis. Journal of Applied Behaviour Analysis, 1 , 91–97. ↵
- Kazdin, A. E. (1982). Single-case research designs: Methods for clinical and applied settings . New York, NY: Oxford University Press. ↵
A type of quantitative research that involves studying the behaviour of each small number of participants in detail.
The study of large numbers of participants and examining their behaviour primarily in terms of group means, standard deviations, and so on.
A detailed description of an individual, which can include both qualitative and quantitative analyses.
The study of strong and consistent effects that can be implemented reliably in the real-world contexts in which they occur.
Laboratory methods that rely on single-subject research; based upon B. F. Skinner’s philosophy of behaviourism which posits that everything organisms do is behaviour.
Starting in the 1960s, researchers began using single-subject techniques to conduct applied research with human subjects.
Research Methods in Psychology - 2nd Canadian Edition Copyright © 2015 by Paul C. Price, Rajiv Jhangiani, & I-Chant A. Chiang is licensed under a Creative Commons Attribution-NonCommercial-ShareAlike 4.0 International License , except where otherwise noted.
Share This Book
Read our research on: Gun Policy | International Conflict | Election 2024
Regions & Countries
Political typology quiz.
Notice: Beginning April 18th community groups will be temporarily unavailable for extended maintenance. Thank you for your understanding and cooperation.
Where do you fit in the political typology?
Are you a faith and flag conservative progressive left or somewhere in between.

Take our quiz to find out which one of our nine political typology groups is your best match, compared with a nationally representative survey of more than 10,000 U.S. adults by Pew Research Center. You may find some of these questions are difficult to answer. That’s OK. In those cases, pick the answer that comes closest to your view, even if it isn’t exactly right.
About Pew Research Center Pew Research Center is a nonpartisan fact tank that informs the public about the issues, attitudes and trends shaping the world. It conducts public opinion polling, demographic research, media content analysis and other empirical social science research. Pew Research Center does not take policy positions. It is a subsidiary of The Pew Charitable Trusts .
From a Psychoanalytic Narrative Case Study to Quantitative Single-Case Research
- First Online: 13 November 2014
Cite this chapter
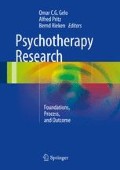
- Horst Kächele 4 , 5 ,
- Cornelia Albani 6 &
- Dan Pokorny 7
4283 Accesses
5 Citations
Narrative case studies concerning the psychoanalytic process date back more than a century; Breuer and Freud were the pioneers of this research path. A great shift in methodology occurred after the development of computers that could work with both text and numbers. On the one hand, it became possible to store detailed, verbatim protocols of therapy sessions. On the other hand, it was possible to analyze derived quantitative data by using sophisticated statistical procedures. This is exemplified in three different methods that analyze different psychoanalytical cases. We conclude by mentioning that research on the psychoanalytic process has to start with clinical experience, which can be used when introducing new observational tools to check for the appropriateness of each tool. This is made possible by the synergetic work of people and processes that were mentioned above.
This is a preview of subscription content, log in via an institution to check access.
Access this chapter
- Available as PDF
- Read on any device
- Instant download
- Own it forever
- Available as EPUB and PDF
- Compact, lightweight edition
- Dispatched in 3 to 5 business days
- Free shipping worldwide - see info
- Durable hardcover edition
Tax calculation will be finalised at checkout
Purchases are for personal use only
Institutional subscriptions
Throughout this text, the patients are named in concordance with our procedure that is explained by Thomä and Kächele ( 1987 ).
The PEP study group that was directed by Klaus Grawe and Horst Kächele “Psychotherapeutische Einzelfallprozessforschun” investigated two cases, one from Ulm (The Student) and one from Berne (The Forward) with quite a variety of process methods.
Albani C, Kühnast B, Pokorny D, Blaser G, Kächele H (2001a) Beziehungsmuster in Träumen und Geschichten über Beziehungen in einem psychoanalytischen Prozeß (Relationship patterns in dreams and stories about relationships in a psychoanalytic process). ForumPsychoanal 17:287–296
Google Scholar
Albani C, Pokorny D, Blaser G, König S, Geyer M, Thomä H, Kächele H (2001b) Zur empirischen Erfassung von Übertragung und Beziehungsmustern - eine Einzelfallanalyse (On the empirical study of transference and relationship patterns – a single case study). Psychother Psychol Med 51:1–10
Article Google Scholar
Albani C, Pokorny D, Blaser G, König S, Thomä H, Kächele H (2003) Study of a psychoanalytic process using the core conflictual relationship theme (CCRT) method according to the Ulm process model. Eur Psychother 4:11–32
Albani C, Pokorny D, Blaser G, Kächele H (2008) Beziehungsmuster und Beziehungskonflikte. Theorie, Klinik und Forschung. Vandenhoeck & Ruprecht, Göttingen
Book Google Scholar
Breuer J (1893–1895) Case I. Fräulein Anna O. In: Breuer J, Freud S (eds) Studies on hysteria. In J. Strachey (eds and trans) The standard edition of the complete psychological works of Sigmund Freud, vol 2. Hogarth Press, London, pp 21–47
Bucci W (1988) Converging evidence for emotional structures: theory and method. In: Dahl H, Kächele H, Thomä H (eds) Psychoanalytic process research strategies. Springer, Berlin, pp 29–49
Chapter Google Scholar
Bucci W (1997) Pattern of discourse in good and troubled hours. J Am Psychoanal Assoc 45:155–188
Article CAS PubMed Google Scholar
Compton A (1990) Psychoanalytic process. Psychoanal Q 59:585–598
CAS PubMed Google Scholar
Dahl H (1983) On the definition and measurement of wishes. In: Masling J (ed) Empirical studies of psychoanalytical theories. The Analytic Press, Hillsdale
Dahl H (1988) Frames of mind. In: Dahl H, Kächele H, Thomä H (eds) Psychoanalytic process research strategies. Springer, Berlin, pp 51–66
Dahl H, Kächele H, Thomä H (eds) (1988) Psychoanalytic process research strategies. Springer, Berlin
Dewald P (1972) The psychoanalytic process. A case illustration. Basic Books, New York, NY
Ferenczi S (1927 [1964]) Bausteine zur Psychoanalyse (Fundamentals of psychoanalysis), vol 2, Praxis (Practice). Internationaler Psychoanalytischer [Huber], Wien [Stuttgart – Bern]
Fonagy P, Moran G (1993) Selecting single case research designs for clinicians. In: Miller N, Luborsky L, Barber J, Docherty J (eds) Handbook of psychodynamic treatment research. Basic Books, New York, NY, pp 62–95
Forrester J (1980) Language and the origins of psychoanalysis. Macmillan, London
French TM (1952) The integration of behaviour. Basic postulates, vol 1. University of Chicago Press, Chicago, IL
Freud S (1895) Studies on hysteria. In: Strachey J (ed and trans) The standard edition of the complete psychological works of Sigmund Freud, vol 2. Hogarth Press, London
Freud S (1912) The dynamics of transference. SE XII: 97–108
Frommer J, Rennie DL (eds) (2001) Qualitative psychotherapy research. Methods and methodology. Pabst, Lengerich
Hilliard RB (1993) Single case methodology in psychotherapy process and outcome research. J Consult Clin Psychol 61:373–380
Iwakabe S, Gazzola N (2009) From single-case studies to practice-based knowledge: aggregating and synthesizing case studies. Psychother Res 19(4–5):601–611
Article PubMed Google Scholar
Jones EE, Windholz M (1990) The psychoanalytic case study: toward a method for systematic inquiry. J Am Psychoanal Assoc 38:985–1016
Kächele H (1981) Zur Bedeutung der Krankengeschichte in der klinisch-psychoanalytischen Forschung (On the relevance of case history in clinical psychoanalytic research). Jahrb Psychoanal 12:118–177
Kächele H (1986) Die Maschinelle Inhaltsanalyse in der Psychoanalytischen Prozessforschung (Computer-based content analysis in psychoanalytic process research). PSZ, Ulm
Kächele H (1992) Narration and observation in psychotherapy research. Reporting on a 20 year long journey from qualitative case reports to quantitative studies on the psychoanalytic process. Psychother Res 2:1–15
Kächele H (2009) Psychanalytische Prozesse. Methodische Illustrationen und methodologische Reflexionen (Psychoanalytic processes. Methodical illustrations and methodological reflections). Munich Electronic Database, München. http://edoc.ub.uni-muenchen.de/10558/
Kächele H (2010) Distinguishing psychoanalysis from psychotherapy. Int J Psychoanal 91:35–43
Kächele H, Albani C (2001) Die Arbeit an einem zentralen Beziehungsmuster als Musterbeispiel klinisch relevanter Empirie (Work on a core conflictual relationship pattern as a sample of clinically relevant research). In: Cierpka M, Buchheim P (eds) Psychodynamische Konzepte. Springer, Berlin, pp 169–190
Kächele H, Mergenthaler E (1983) Computer-aided analysis of psychotherapeutic discourse – a workshop. In: Minsel W-R, Herff W (eds) Methodology in psychotherapy research. Proceedings of the 1st European conference on psychotherapy research. Lang, Frankfurt a. M., pp 116–161
Kächele H, Thomä H, Ruberg W, Grünzig H-J (1988) Audio-recordings of the psychoanalytic dialogue: scientific, clinical and ethical problems. In: Dahl H, Kächele H, Thomä H (eds) Psychoanalytic process research strategies. Springer, Berlin, pp 179–194
Kächele H, Dengler D, Eckert R, Schnekenburger S (1990a) Veränderung des zentralen Beziehungskonfliktes durch eine Kurztherapie (Change of the core conflictual relationship conflict by a short term therapy). Psychother Psychol Med 40:178–185
Kächele H, Heldmaier H, Scheytt N (1990b) Fokusformulierungen als katamanestische Leitlinien (Focal formulations as guidelines for a follow-up study). Psychother Psychol Med 35:205–216
Kächele H, Scheytt N, Schwendele W (1999) Der STUDENT – eine detaillierte Verlaufsbeschreibung (THE STUDENT – a detailed description of a treatment process). In: Kächele H, Grawe K (eds) PEP-Projekt. http://www.horstkaechele.de/plib/index.php?id=1_23_22 (Unpublished manuscript)
Kächele H, Schachter J, Thomä H (eds) (2009) From psychoanalytic narrative to empirical single case research. Implications for Psychoanalytic Practice. Routledge, New York
Kazdin AE (1982) Single case research designs: methods for clinical and applied settings. Oxford University Press, Oxford
Klein M (1961) Narrative of a child analysis. Hogarth Press, London
Leuzinger-Bohleber M (1989) Veränderung kognitiver Prozesse in Psychoanalysen. Bd 2: Eine gruppen-statistische Untersuchung (Change of cognitive processes in psychoanalyses, vol 2: A group-statistical study). PSZ/Springer, Berlin
Leuzinger-Bohleber M (1995) Die Einzelfallstudie als psychoanalytisches Forschungsinstrument (The single case study as a psychoanalytic research instrument). Psyche – Z Psychoanal 49:434–480
CAS Google Scholar
Luborsky L (1995) The first trial of P-technique in psychotherapy research. A still-lively legacy. J Consult Clin Psychol 63:6–14
Luborsky L, Crits-Christoph P (eds) (1998) Understanding transference: the core conflictual relationship theme method, 2nd edn. American Psychological Association, Washington, DC
Luborsky L, Spence DP (1971) Quantitative research on psychoanalytic therapy. In: Bergin AE, Garfield SL (eds) Handbook of psychotherapy and behavior change, 1st edn. Wiley, New York, NY, pp 408–438
Luyten P, Blatt SJ, Corveleyn J (2006) Minding the gap between positivism and hermeneutics in psychoanalytic research. J Am Psychoanal Assoc 54:571–610
Malan DH (1963) A study of brief psychotherapy. Tavistock, London
Mergenthaler E (1985) Textbank systems: computer science in psychoanalysis. Springer, Heidelberg
Mergenthaler E, Kächele H (1988) The Ulm textbank management system: a tool for psychotherapy research. In: Dahl H, Kächele H, Thomä H (eds) Psychoanalytic process research strategies. Springer, Berlin, pp 195–212
Michels R (2000) The case history. With commentaries by S. Pulver, S. B. Bernstein, P. Rubovits-Seitz, I. Szecsödy, D. Tuckett, A. Wilson. J Am Psychoanal Assoc 48:355–375
Pokorny D (2008) Datenanalyse mit ZBKT-LU (Data analysis with the CCRT-LU). In: Albani C, Pokorny D, Blaser G, Kächele H (eds) Beziehungsmuster und Beziehungskonflikte. Theorie, Klinik und Forschung. Vandenhoeck & Ruprecht, Göttingen, pp 174–214
Propp WJ (1928) Morfologia delle fiabe. Einandi, Torino
Russell RL, Czogalik D (1989) Strategies for analyzing conversation: frequencies, sequences or rules. J Soc Behav Pers 3:221–236
Schachter J, Kächele H (2010) The couch in psychoanalysis. Contemp Psychoanal 46:439–459
Seitz P (1966) The consensus problem in psychoanalysis. In: Gottschalk LA, Auerbach AH (eds) Methods of research in psychotherapy. Appleton Century Crofts, New York, NY, pp 209–225
Spence DP (1982) Narrative truth and historical truth: meaning and interpretation in psychoanalysis. Norton, New York, NY
Stuhr U (2004) Klinische Fallstudien (Clinical case studies). In: Hau S, Leuzinger-Bohleber M (eds) Psychoanalytische Therapie. Eine Stellungnahme für die wissenschaftliche Öffentlichkeit und für den Wissenschaftlichen Beirat Psychotherapie. Forum Psychoanal 20:63–66
Thomä H (1961) Anorexia nervosa. Geschichte, Klinik und Theorie der Pubertätsmagersucht. Huber/Klett, Bern/Stuttgart; English (1967) Anorexia nervosa. Yale University Press, New Haven
Thomä H (1975) Prozessbeschreibung mit dem Topic Index (Description of a treatment process using the Topic Index). Vortrag an der Psychosomatischen Klinik, Universität Heidelberg
Thomä H, Kächele H (1987) Psychoanalytic practice, vol 1. Principles. Springer, Berlin; paperback. Aronson, New York, 1994
von Zeppelin I (1987) A process model of psychoanalytic therapy. In: Cheshire N, Thomä H (eds) The self and its symptoms. Wiley, New York, NY, pp 149–165
Weiss J, Sampson H, Group TMZpr (eds) (1986) The psychoanalytic process: theory, clinical observation, and empirical research. Guilford Press, New York
Winnicott DW (1972) Fragment of an analysis. In: Giovaccini PL (ed) Tactics and techniques in psychoanalytic therapy. Hogarth Press, London, pp 455–693
Wittgenstein L (1921/2014) Tractatus logico-philosophicus. Logisch-philosophische Abhandlung. Suhrkamp, Berlin
Download references
Author information
Authors and affiliations.
International Psychoanalytic University Berlin, StromstraÔe 3, 10555, Berlin, Germany
Horst Kächele
Sonnenweg 25, 89081, Ulm, Germany
SINOVA Hospital Aulendorf, Aulendorf, Germany
Cornelia Albani
Department Psychosomatic Medicine and Psychotherapy, Ulm University, Ulm, Germany
Dan Pokorny
You can also search for this author in PubMed Google Scholar
Corresponding author
Correspondence to Horst Kächele .
Editor information
Editors and affiliations.
University of Salento, Lecce, Italy, and Sigmund Freud University, Vienna, Austria
Omar C.G. Gelo
Sigmund Freud University, Vienna, Austria
Alfred Pritz
Bernd Rieken
Rights and permissions
Reprints and permissions
Copyright information
© 2015 Springer-Verlag Wien
About this chapter
Kächele, H., Albani, C., Pokorny, D. (2015). From a Psychoanalytic Narrative Case Study to Quantitative Single-Case Research. In: Gelo, O., Pritz, A., Rieken, B. (eds) Psychotherapy Research. Springer, Vienna. https://doi.org/10.1007/978-3-7091-1382-0_19
Download citation
DOI : https://doi.org/10.1007/978-3-7091-1382-0_19
Published : 13 November 2014
Publisher Name : Springer, Vienna
Print ISBN : 978-3-7091-1381-3
Online ISBN : 978-3-7091-1382-0
eBook Packages : Medicine Medicine (R0)
Share this chapter
Anyone you share the following link with will be able to read this content:
Sorry, a shareable link is not currently available for this article.
Provided by the Springer Nature SharedIt content-sharing initiative
- Publish with us
Policies and ethics
- Find a journal
- Track your research
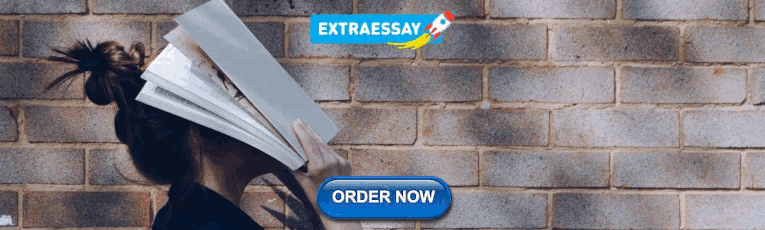
IMAGES
VIDEO
COMMENTS
Single-case studies can provide a viable alternative to large group studies such as randomized clinical trials. Single case studies involve repeated measures, and manipulation of and independent variable. They can be designed to have strong internal validity for assessing causal relationships between interventions and outcomes, and external ...
Single-case experimental designs (SCEDs) are a useful tool for evaluating the effects of interventions at an individual level and can play an important role in the development and validation of evidence-based practices. Historically, researchers relied on visual analysis of SCED data and eschewed statistical approaches. Although researchers increasingly include quantitative analysis in SCED ...
Introduction. A single-case experimental design 1 (SCED) is a research design that allows repeated measures of the dependent variable within one case (What Works Clearinghouse, 2020).The dependent variable is measured at different sequential moments in time and across different levels of the independent variable (i.e., treatment indicator), which are also known as "phases" (e.g., baseline ...
The single-case experiment has a storied history in psychology dating back to the field's founders: Fechner (1889), Watson (1925), and Skinner (1938).It has been used to inform and develop theory, examine interpersonal processes, study the behavior of organisms, establish the effectiveness of psychological interventions, and address a host of other research questions (for a review, see ...
Abstract. This chapter addresses the peculiarities, characteristics, and major fallacies of single case research designs. A single case study research design is a collective term for an in-depth analysis of a small non-random sample. The focus on this design is on in-depth.
The application of single-case research methods is entering a new phase of scientific relevance. Researchers in an increasing array of disciplines are finding single-case methods useful for the questions they are asking and the clinical needs in their fields (Kratochwill et al., 2010; Maggin et al., 2017; Maggin & Odom, 2014; Riley-Tillman, Burns, & Kilgus, 2020).
The majority of methods in psychology rely on averaging group data to draw conclusions. In this Perspective, Nickels et al. argue that single case methodology is a valuable tool for developing and ...
Increased use and dissemination of single-case intervention research has prompted efforts to clarify professional standards to guide the design, conduct, analysis, and reporting of research using single-case designs (SCDs; Ganz & Ayres, 2018; Kratochwill et al., 2013).The most recent proposal for SCD standards was released by the Institute of Education Sciences (IES) What Works Clearinghouse ...
A case study is a detailed description of an individual, which can include both qualitative and quantitative analyses. (Case studies that include only qualitative analyses can be considered a type of qualitative research.) ... Single-case research designs: Methods for clinical and applied settings. New York, NY: Oxford University Press.
Single case study analyses offer empirically-rich, context-specific, holistic accounts and contribute to both theory-building and, to a lesser extent, theory-testing. ... Exploratory and descriptive studies usually tend toward the qualitative and inductive, whereas explanatory studies are more often quantitative and deductive (David and Sutton ...
Within a case study research, one may study a single case or multiple cases. Single case studies are most common in case study researches. Yin (2014, p. 59) says that single cases are 'eminently justifiable' under certain conditions: (a) when the case under study is unique or atypical, and hence, its study is revelatory, (b) when the case ...
Revised on November 20, 2023. A case study is a detailed study of a specific subject, such as a person, group, place, event, organization, or phenomenon. Case studies are commonly used in social, educational, clinical, and business research. A case study research design usually involves qualitative methods, but quantitative methods are ...
A case study, also known as a case report, is an in depth or intensive study of a single individual or specific group, while a case series is a grouping of similar case studies / case reports together. A case study / case report can be used in the following instances: where there is atypical or abnormal behaviour or development.
A Case study is: An in-depth research design that primarily uses a qualitative methodology but sometimes includes quantitative methodology. Used to examine an identifiable problem confirmed through research. Used to investigate an individual, group of people, organization, or event. Used to mostly answer "how" and "why" questions.
Summary of Key Points: Single-case studies can provide a viable alternative to large group studies such as randomized clinical trials. Single-case studies involve repeated measures and manipulation of an independent variable. They can be designed to have strong internal validity for assessing causal relationships between interventions and outcomes, as well as external validity for ...
A multiple case studies approach was adopted that spanned over 2 years, as it is difficult to investigate all the aspects of a phenomenon in a single case study (Cruzes, Dybå, Runeson, & Höst, 2015). The purpose here is to suggest, help, and guide future research students based on what authors have learned while conducting an in-depth case ...
Page 5 of 7 Embedded Case Study Methods: TYPES OF CASE STUDIES Explanatory case studies can also serve to test cause-and-effect relationships. Clearly, according to conventional understanding of theory testing, a single case can only falsify a theory. However, a case may also be used for theory testing, either if the case is
The survey probed perceptions of many aspects of the ward environment before and after the move. As discussed in Chapter 3, the trust, the designers and stakeholders held various expectations about the benefits of the 100% single room design. We examined whether or not these expectations (or hypotheses about the effect of the move) were fulfilled. Specifically, the new hospital was designed to ...
The influence of vision on multi-joint control during dynamic tasks in anterior cruciate ligament (ACL) deficient patients is unknown. Thus, the purpose of this study was to establish a new method for quantifying neuromuscular control by focusing on the variability of multi-joint movement under conditions with different visual information and to determine the cutoff for potential biomarkers of ...
This chapter addresses single-case research designs' peculiarities, characteristics, and significant fallacies. A single case research design is a collective term for an in-depth analysis of a small non-random sample. The focus of this design is in-depth. ... possibly even significantly higher than pure quantitative research and other ...
LINGER: using bulk data to infer GRNs from single-cell multiome data. LINGER is a computational framework designed to infer GRNs from single-cell multiome data (Fig. 1 and Methods).Using count ...
of quantitative analysis, and whether there are dierences in attitudes toward quanti-tative by research discipline and years of experience. Method Participants To identify participants, the authors used reference lists from meta-analyses and sys-tematic reviews of single-case studies published between January 2014 and Decem-
In this study, in order to clarify the impact of the use of domestic timber for wooden chairs on greenhouse gas (hereinafter referred to as GHG) emissions from the product life cycle, chairs made of foreign hardwoods (referred to as Product A and Product B) manufactured by two wooden furniture manufacturers (referred to as Companies A and B) in the Hida-Takayama region of Gifu Prefecture ...
A case study is a detailed description of an individual, which can include both qualitative and quantitative analyses. (Case studies that include only qualitative analyses can be considered a type of qualitative research.) ... Single-case research designs: Methods for clinical and applied settings. New York, NY: Oxford University Press. ...
Take our quiz to find out which one of our nine political typology groups is your best match, compared with a nationally representative survey of more than 10,000 U.S. adults by Pew Research Center. You may find some of these questions are difficult to answer. That's OK. In those cases, pick the answer that comes closest to your view, even if ...
Single-case research (SCR) is characterized as a quantitative experimental methodology allowing for the establishment of a functional relation between an independent variable and a dependent variable (Ledford & Gast, 2018).The term functional relation is generally comparable to the term causal relation as used across other research methodologies. The utility of SCR is that functional relations ...
logR quantitative identification of high frequency sequences of fine sediment - A case study of the Chaoyanggou Terrace in the Songliao Basin. Author: Linru Yao. Institute of Geology, Yushulin Oilfield Development Limited liability company, China.
However, the relative decrease in the COP is substantially less than the relative increase in the capacity of the system compared with Heat Pumps with a single borehole. The quantitative relations between the amount and quality energy produced by Heat Pumps with parallel- and series-connected wells show that the amount of energy increases by ...
Narrative case studies concerning the psychoanalytic process date back more than a century; Breuer and Freud were the pioneers of this research path. A great shift in methodology occurred after the development of computers that could work with both text and numbers. On the one hand, it became possible to store detailed, verbatim protocols of ...