- Skip to main content
- Skip to primary sidebar
- Skip to footer
- QuestionPro

- Solutions Industries Gaming Automotive Sports and events Education Government Travel & Hospitality Financial Services Healthcare Cannabis Technology Use Case NPS+ Communities Audience Contactless surveys Mobile LivePolls Member Experience GDPR Positive People Science 360 Feedback Surveys
- Resources Blog eBooks Survey Templates Case Studies Training Help center

Home Market Research Research Tools and Apps
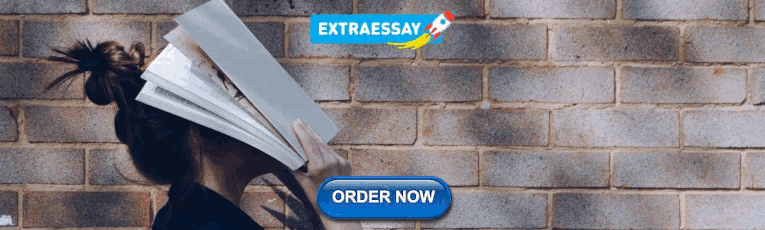
Analytical Research: What is it, Importance + Examples
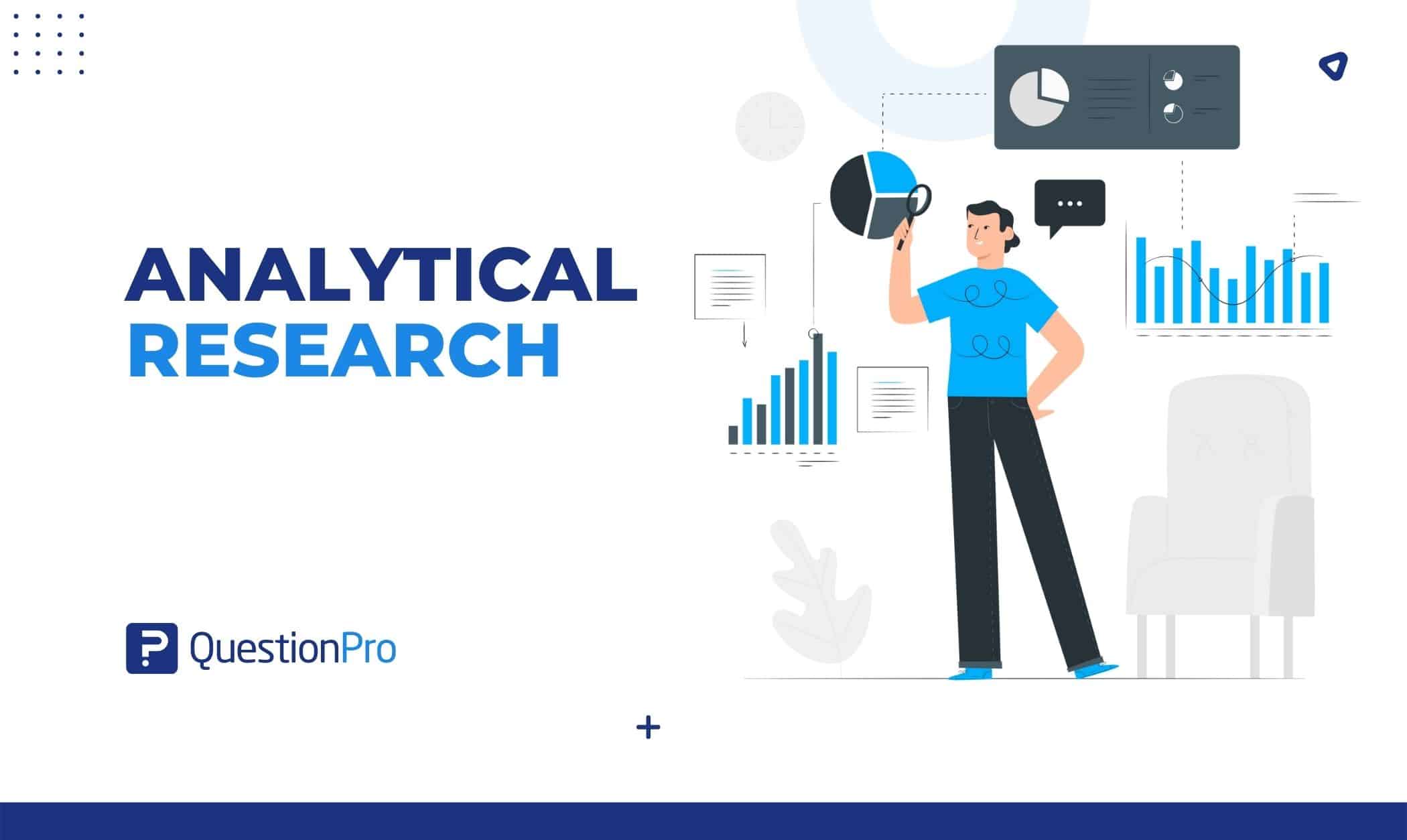
Finding knowledge is a loose translation of the word “research.” It’s a systematic and scientific way of researching a particular subject. As a result, research is a form of scientific investigation that seeks to learn more. Analytical research is one of them.
Any kind of research is a way to learn new things. In this research, data and other pertinent information about a project are assembled; after the information is gathered and assessed, the sources are used to support a notion or prove a hypothesis.
An individual can successfully draw out minor facts to make more significant conclusions about the subject matter by using critical thinking abilities (a technique of thinking that entails identifying a claim or assumption and determining whether it is accurate or untrue).
What is analytical research?
This particular kind of research calls for using critical thinking abilities and assessing data and information pertinent to the project at hand.
Determines the causal connections between two or more variables. The analytical study aims to identify the causes and mechanisms underlying the trade deficit’s movement throughout a given period.
It is used by various professionals, including psychologists, doctors, and students, to identify the most pertinent material during investigations. One learns crucial information from analytical research that helps them contribute fresh concepts to the work they are producing.
Some researchers perform it to uncover information that supports ongoing research to strengthen the validity of their findings. Other scholars engage in analytical research to generate fresh perspectives on the subject.
Various approaches to performing research include literary analysis, Gap analysis , general public surveys, clinical trials, and meta-analysis.
Importance of analytical research
The goal of analytical research is to develop new ideas that are more believable by combining numerous minute details.
The analytical investigation is what explains why a claim should be trusted. Finding out why something occurs is complex. You need to be able to evaluate information critically and think critically.
This kind of information aids in proving the validity of a theory or supporting a hypothesis. It assists in recognizing a claim and determining whether it is true.
Analytical kind of research is valuable to many people, including students, psychologists, marketers, and others. It aids in determining which advertising initiatives within a firm perform best. In the meantime, medical research and research design determine how well a particular treatment does.
Thus, analytical research can help people achieve their goals while saving lives and money.
Methods of Conducting Analytical Research
Analytical research is the process of gathering, analyzing, and interpreting information to make inferences and reach conclusions. Depending on the purpose of the research and the data you have access to, you can conduct analytical research using a variety of methods. Here are a few typical approaches:
Quantitative research
Numerical data are gathered and analyzed using this method. Statistical methods are then used to analyze the information, which is often collected using surveys, experiments, or pre-existing datasets. Results from quantitative research can be measured, compared, and generalized numerically.
Qualitative research
In contrast to quantitative research, qualitative research focuses on collecting non-numerical information. It gathers detailed information using techniques like interviews, focus groups, observations, or content research. Understanding social phenomena, exploring experiences, and revealing underlying meanings and motivations are all goals of qualitative research.
Mixed methods research
This strategy combines quantitative and qualitative methodologies to grasp a research problem thoroughly. Mixed methods research often entails gathering and evaluating both numerical and non-numerical data, integrating the results, and offering a more comprehensive viewpoint on the research issue.
Experimental research
Experimental research is frequently employed in scientific trials and investigations to establish causal links between variables. This approach entails modifying variables in a controlled environment to identify cause-and-effect connections. Researchers randomly divide volunteers into several groups, provide various interventions or treatments, and track the results.
Observational research
With this approach, behaviors or occurrences are observed and methodically recorded without any outside interference or variable data manipulation . Both controlled surroundings and naturalistic settings can be used for observational research . It offers useful insights into behaviors that occur in the actual world and enables researchers to explore events as they naturally occur.
Case study research
This approach entails thorough research of a single case or a small group of related cases. Case-control studies frequently include a variety of information sources, including observations, records, and interviews. They offer rich, in-depth insights and are particularly helpful for researching complex phenomena in practical settings.
Secondary data analysis
Examining secondary information is time and money-efficient, enabling researchers to explore new research issues or confirm prior findings. With this approach, researchers examine previously gathered information for a different reason. Information from earlier cohort studies, accessible databases, or corporate documents may be included in this.
Content analysis
Content research is frequently employed in social sciences, media observational studies, and cross-sectional studies. This approach systematically examines the content of texts, including media, speeches, and written documents. Themes, patterns, or keywords are found and categorized by researchers to make inferences about the content.
Depending on your research objectives, the resources at your disposal, and the type of data you wish to analyze, selecting the most appropriate approach or combination of methodologies is crucial to conducting analytical research.
Examples of analytical research
Analytical research takes a unique measurement. Instead, you would consider the causes and changes to the trade imbalance. Detailed statistics and statistical checks help guarantee that the results are significant.
For example, it can look into why the value of the Japanese Yen has decreased. This is so that an analytical study can consider “how” and “why” questions.
Another example is that someone might conduct analytical research to identify a study’s gap. It presents a fresh perspective on your data. Therefore, it aids in supporting or refuting notions.
Descriptive vs analytical research
Here are the key differences between descriptive research and analytical research:
The study of cause and effect makes extensive use of analytical research. It benefits from numerous academic disciplines, including marketing, health, and psychology, because it offers more conclusive information for addressing research issues.
QuestionPro offers solutions for every issue and industry, making it more than just survey software. For handling data, we also have systems like our InsightsHub research library.
You may make crucial decisions quickly while using QuestionPro to understand your clients and other study subjects better. Make use of the possibilities of the enterprise-grade research suite right away!
LEARN MORE FREE TRIAL
MORE LIKE THIS
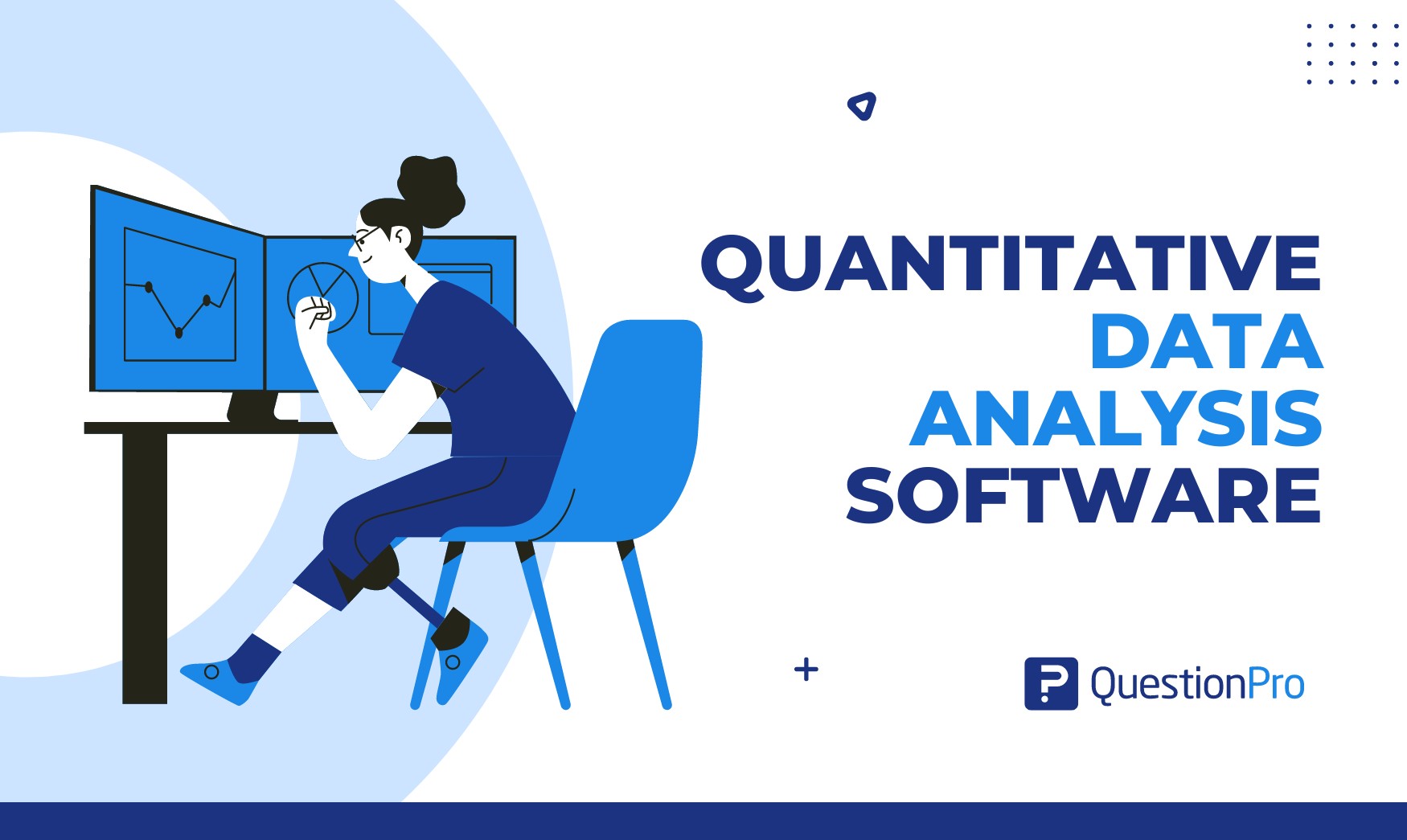
10 Quantitative Data Analysis Software for Every Data Scientist
Apr 18, 2024
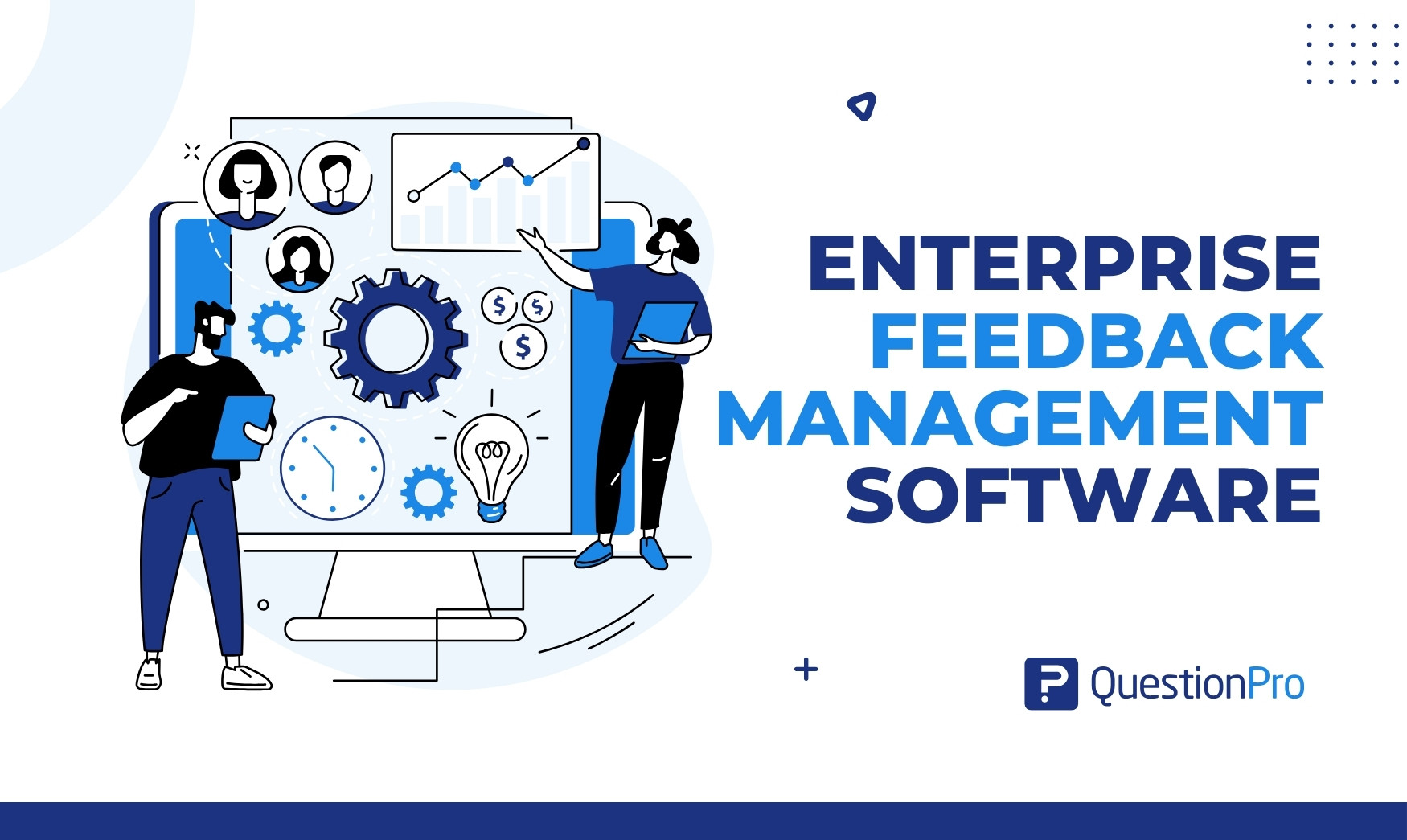
11 Best Enterprise Feedback Management Software in 2024
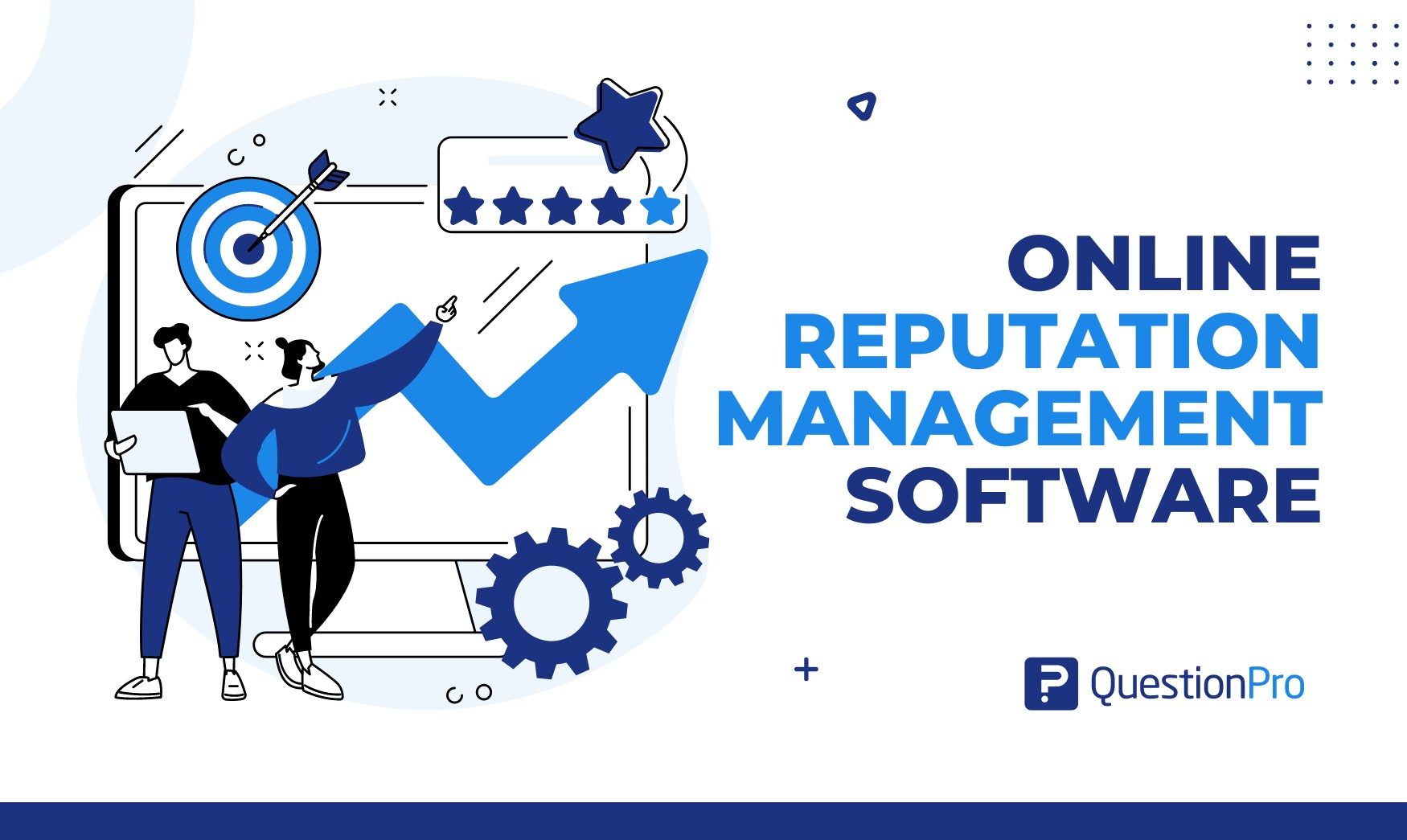
17 Best Online Reputation Management Software in 2024
Apr 17, 2024
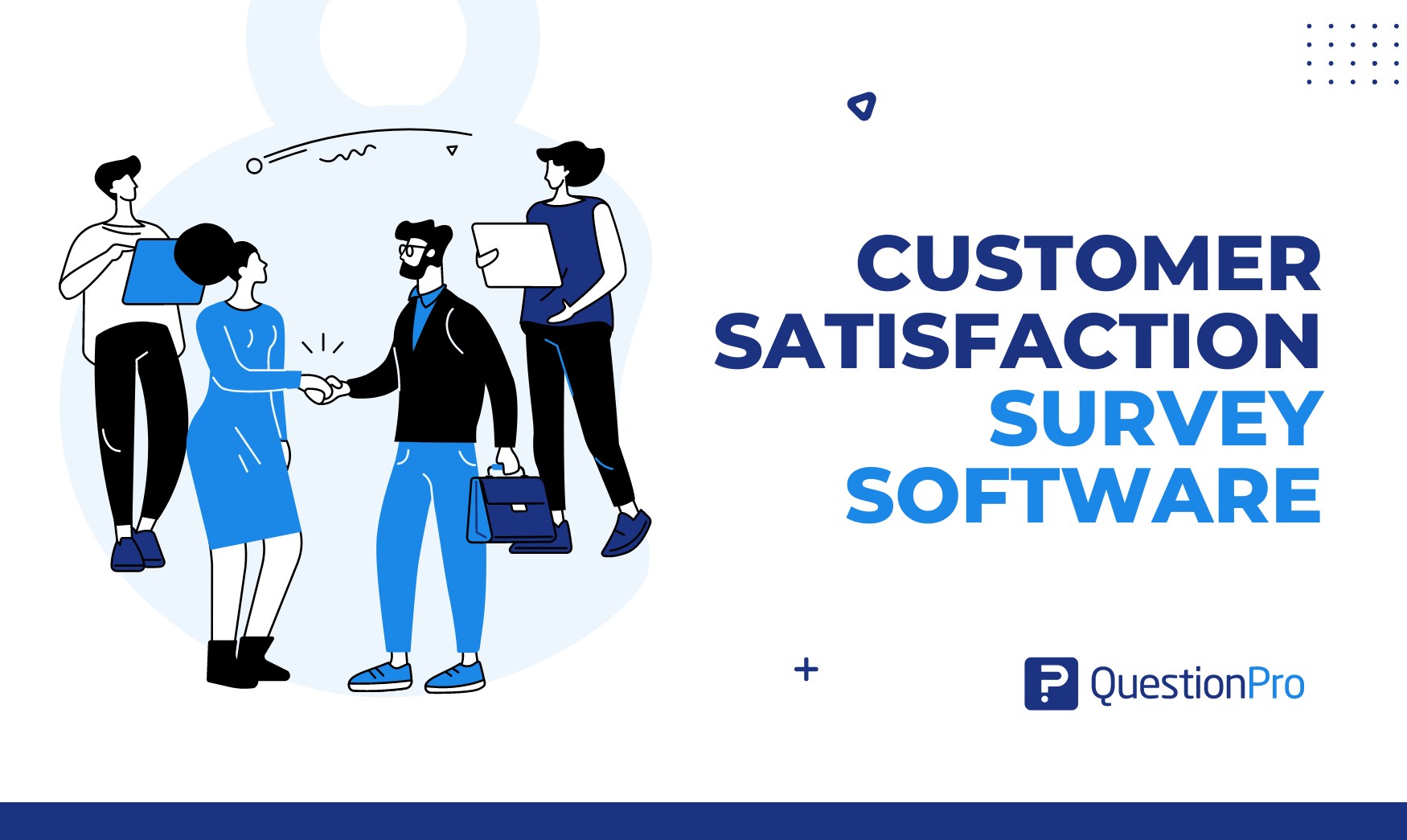
Top 11 Customer Satisfaction Survey Software in 2024
Other categories.
- Academic Research
- Artificial Intelligence
- Assessments
- Brand Awareness
- Case Studies
- Communities
- Consumer Insights
- Customer effort score
- Customer Engagement
- Customer Experience
- Customer Loyalty
- Customer Research
- Customer Satisfaction
- Employee Benefits
- Employee Engagement
- Employee Retention
- Friday Five
- General Data Protection Regulation
- Insights Hub
- Life@QuestionPro
- Market Research
- Mobile diaries
- Mobile Surveys
- New Features
- Online Communities
- Question Types
- Questionnaire
- QuestionPro Products
- Release Notes
- Research Tools and Apps
- Revenue at Risk
- Survey Templates
- Training Tips
- Uncategorized
- Video Learning Series
- What’s Coming Up
- Workforce Intelligence
Marketing Research
21 analytical models.
Marketing models consists of
- Analytical Model: pure mathematical-based research
- Empirical Model: data analysis.
“A model is a representation of the most important elements of a perceived real-world system”.
Marketing model improves decision-making
Econometric models
- Description
Optimization models
- maximize profit using market response model, cost functions, or any constraints.
Quasi- and Field experimental analyses
Conjoint Choice Experiments.
“A decision calculus will be defined as a model-based set of procedures for processing data and judgments to assist a manager in his decision making” ( Little 1976 ) :
- easy to control
- as complete as possible
- easy to communicate with
( K. S. Moorthy 1993 )
Mathematical Theoretical Models
Logical Experimentation
An environment as a model, specified by assumptions
Math assumptions for tractability
Substantive assumptions for empirical testing
Decision support modeling describe how things work, and theoretical modeling present how things should work.
Compensation package including salaries and commission is a tradeoff between reduced income risk and motivation to work hard.
Internal and External Validity are questions related to the boundaries conditions of your experiments.
“Theories are tested by their predictions, not by the realism of their super model assumptions.” (Friedman, 1953)
( McAfee and McMillan 1996 )
Competition is performed under uncertainty
Competition reveals hidden information
Independent-private-values case: selling price = second highest valuation
It’s always better for sellers to reveal information since it reduces chances of cautious bidding that is resulted from the winner’s curse
Competition is better than bargaining
- Competition requires less computation and commitment abilities
Competition creates effort incentives
( Leeflang et al. 2000 )
Types of model:
Predictive model
Sales model: using time series data
Trial rate: using exponential growth.
Product growth model: Bass ( 1969 )
Descriptive model
Purchase incidence and purchase timing : use Poisson process
Brand choice: Markov models or learning models.
Pricing decisions in an oligopolistic market Howard and Morgenroth ( 1968 )
Normative model
- Profit maximization based on price, adverting and quality ( Dorfman and Steiner 1976 ) , extended by ( H. V. Roberts, Ferber, and Verdoorn 1964 ; Lambin 1970 )
Later, Little ( 1970 ) introduced decision calculus and then multinomial logit model ( Peter M. Guadagni and Little 1983 )
Potential marketing decision automation:
Promotion or pricing programs
Media allocation
Distribution
Product assortment
Direct mail solicitation
( K. S. Moorthy 1985 )
Definitions:
Rationality = maximizing subjective expected utility
Intelligence = recognizing other firms are rational.
Rules of the game include
feasible set of actions
utilities for each combination of moves
sequence of moves
the structure of info (who knows what and when?)
Incomplete info stems from
unknown motivations
unknown ability (capabilities)
different knowledge of the world.
Pure strategy = plan of action
A mixed strategy = probability dist of pure strategies.
Strategic form representation = sets of possible strategies for every firm and its payoffs.
Equilibrium = a list of strategies in which “no firm would like unilaterally to change its strategy.”
Equilibrium is not outcome of a dynamic process.
Equilibrium Application
Oligopolistic Competition
Cournot (1838): quantities supplied: Cournot equilibrium. Changing quantities is more costly than changing prices
Bertrand (1883): Bertrand equilibrium: pricing.
Perfect competition
Product Competition: Hotelling (1929): Principle of Minimum Differentiation is invalid.
first mover advantage
deterrent strategy
optimal for entrants or incumbents
Perfectness of equilibria
Subgame perfectness
Sequential rationality
Trembling-hand perfectness
Application
Product and price competition in Oligopolies
Strategic Entry Deterrence
Dynamic games
Long-term competition in oligopolies
Implicit Collusion in practice : price match from leader firms
Incomplete Information
Durable goods pricing by a monopolist
predatory pricing and limit pricing
reputation, product quality, and prices
Competitive bidding and auctions
21.1 Building An Analytical Model
Notes by professor Sajeesh Sajeesh
Step 1: Get “good” idea (either from literature or industry)
Step 2: Assess the feasibility of the idea
Is it interesting?
Can you tell a story?
Who is the target audience?
Opportunity cost
Step 3: Don’t look at the literature too soon
- Even when you have an identical model as in the literature, it’s ok (it allows you to think)
Step 4: BUild the model
Simplest model first: 1 period, 2 product , linear utility function for consumers
Write down the model formulation
Everything should be as simple as possible .. but no simpler
Step 5: Generalizing the model
- Adding complexity
Step 6: Searching the literature
- If you find a paper, you can ask yourself why you didn’t do what the author has done.
Step 7: Give a talk /seminar
Step 8: Write the paper
21.2 Hotelling Model
( KIM and SERFES 2006 ) : A location model with preference variety
( Hotelling 1929 )
Stability in competition
Duopoly is inherently unstable
Bertrand disagrees with Cournot, and Edgeworth elaborates on it.
- because Cournot’s assumption of absolutely identical products between firms.
seller try to \(p_2 < p_1 c(l-a-b)\)
the point of indifference
\[ p_1 + cx = p_2 + cy \]
c = cost per unit of time in each unit of line length
q = quantity
x, y = length from A and B respectively
\[ a + x + y + b = l \]
is the length of the street
Hence, we have
\[ x = 0.5(l - a - b + \frac{p_2- p_1}{c}) \\ y = 0.5(l - a - b + \frac{p_1- p_2}{c}) \]
Profits will be
\[ \pi_1 = p_1 q_1 = p_1 (a+ x) = 0.5 (l + a - b) p_1 - \frac{p_1^2}{2c} + \frac{p_1 p_2}{2c} \\ \pi_2 = p_2 q_2 = p_2 (b+ y) = 0.5 (l + a - b) p_2 - \frac{p_2^2}{2c} + \frac{p_1 p_2}{2c} \]
To set the price to maximize profit, we have
\[ \frac{\partial \pi_1}{\partial p_1} = 0.5 (l + a - b) - \frac{p_1}{c} + \frac{p_2}{2c} = 0 \\ \frac{\partial \pi_2}{\partial p_2} = 0.5 (l - a + b) - \frac{p_2}{c} + \frac{p_1}{2c} = 0 \]
which equals
\[ p_1 = c(l + \frac{a-b}{3}) \\ p_2 = c(l - \frac{a-b}{3}) \]
\[ q_1 = a + x = 0.5 (l + \frac{a -b}{3}) \\ q_2 = b + y = 0.5 (l - \frac{a-b}{3}) \]
with the SOC satisfied
In case of deciding locations, socialism works better than capitalism
( d’Aspremont, Gabszewicz, and Thisse 1979 )
- Principle of Minimum Differentiation is invalid
\[ \pi_1 (p_1, p_2) = \begin{cases} ap_1 + 0.5(l-a-b) p_1 + \frac{1}{2c}p_1 p_2 - \frac{1}{2c}p_1^2 & \text{if } |p_1 - p_2| \le c(l-a-b) \\ lp_1 & \text{if } p_1 < p_2 - c(l-a-b) \\ 0 & \text{if } p_1 > p_2 + c(l-a-b) \end{cases} \]
\[ \pi_2 (p_1, p_2) = \begin{cases} bp_2 + 0.5(l-a-b) p_2 + \frac{1}{2c}p_1 p_2 - \frac{1}{2c}p_2^2& \text{if } |p_1 - p_2| \le c(l-a-b) \\ lp_2 & \text{if } p_2 < p_1 - c(l-a-b) \\ 0 & \text{if } p_2 > p_1 + c(l-a-b) \end{cases} \]
21.3 Positioning Models
Tabuchi and Thisse ( 1995 )
Relax Hotelling’s model’s assumption of uniform distribution of consumers to non-uniform distribution.
Assumptions:
Consumers distributed over [0,1]
\(F(x)\) = cumulative distribution of consumers where \(F(1) = 1\) = total population
2 distributions:
Traditional uniform density: \(f(x) =1\)
New: triangular density: \(f(x) = 2 - 2|2x-1|\) which represents consumer concentration
Transportation cost = quadratic function of distance.
Hence, marginal consumer is
\[ \bar{x} = (p_2 - p_1 + x^2_2-x_1^2)/2(x_2-x_1) \]
then when \(x_1 < x_2\) the profit function is
\[ \Pi_1 = p_1 F(\bar{x}) \]
\[ \Pi_2 = p_2[1-F(\bar{x})] \]
and vice versa for \(x_1 >x_2\) , and Bertrand game when \(x_1 = x_2\)
If firms pick simultaneously their locations, and then simultaneously their prices, and consumer density function is log-concave, then there is a unique Nash price equilibrium
Under uniform distribution, firms choose to locate as far apart as possible (could be true when observing shopping centers are far away from cities), but then consumers have to buy products that are far away from their ideal.
Under triangular density, no symmetric location can be found, but two asymmetric Nash location equilibrium can still be possible (decrease in equilibrium profits of both firms)
If firms pick sequentially their locations, and pick their prices simultaneously,
- Under both uniform and triangular, first entrant will locate at the market center
Sajeesh and Raju ( 2010 )
Model satiation (variety-seeking) as a relative reduction in the willingness to pay of the previously purchased brand. also known as negative state dependence
Previous studies argue that in the presence of variety seeking consumers, firms should enjoy higher prices and profits, but this paper argues that average prices and profits are lower.
- Firms should charge lower prices in the second period to prevent consumers from switching.
Period 0, choose location simultaneously
Period 1, choose prices simultaneously
Period 2, firms choose prices simultaneously
- K. S. Moorthy ( 1988 )
- 2 (identical) firms pick product (quality) first, then price.
Tyagi ( 2000 )
Extending Hotelling ( 1929 ) Tyagi ( 1999b ) Tabuchi and Thisse ( 1995 )
Two firms enter sequentially , and have different cost structures .
Paper shows second mover advantage
KIM and SERFES ( 2006 )
Consumers can make multiple purchases.
Some consumers are loyal to one brand, and others consume more than one product.
Shreay, Chouinard, and McCluskey ( 2015 )
- Quantity surcharges from different sizes of the same product (i.e., imperfect substitute or differentiated products) can be led by consumer preferences.
21.4 Market Structure and Framework
Basic model utilizing aggregate demand
Bertrand Equilibrium: Firms compete on price
Cournot Market structure: Firm compete on quantity
Stackelberg Market structure: Leader-Follower model
Because we start with the quantity demand function, it is important to know where it’s derived from Richard and Martin ( 1980 )
- studied how two firms compete on product quality and price (both simultaneous and sequential)
21.4.1 Cournot - Simultaneous Games
\[ TC_i = c_i q_i \text{ where } i= 1,2 \\ P(Q) = a - bQ \\ Q = q_1 +q_2 \\ \pi_1 = \text{price} \times \text{quantity} - \text{cost} = [a - b(q_1 +q_2)]q_1 - c_1 q_1 \\ \pi_2 = \text{price} \times \text{quantity} - \text{cost} = [a - b(q_1 +q_2)]q_1 - c_2 q_2 \\ \]
From (21.1)
is called reaction function, for best response function
From (21.2)
\[ q_1 = \frac{a-c_1}{2b} - \frac{a-c_2}{4b} + \frac{q_1}{4} \]
\[ q_1^* = \frac{a-2c_1+ c_2}{3b} \\ q_2^* = \frac{a-2c_2 + c_1}{3b} \]
Total quantity is
\[ Q = q_1 + q_2 = \frac{2a-c_1 -c_2}{3b} \]
\[ a-bQ = \frac{a+c_1+c_2}{3b} \]
21.4.2 Stackelberg - Sequential games
also known as leader-follower games
Stage 1: Firm 1 chooses quantity
Stage 2: Firm 2 chooses quantity
\[ c_2 = c_1 = c \]
Stage 2: reaction function of firm 2 given quantity firm 1
\[ R_2(q_1) = \frac{a-c}{2b} - \frac{q_1}{2} \]
\[ \pi_1 = [a-b(q_1 + \frac{a-c}{2b} - \frac{q_1}{2})]q_1 - cq_1 \\ = [a-b( \frac{a-c}{2b} + \frac{q_1}{2}]q_1 + cq_1 \]
\[ \frac{d \pi_1}{d q_1} = 0 \]
\[ \frac{a+c}{2} - b q_1 -c =0 \]
The Stackelberg equilibrium is
\[ q_1^* = \frac{a-c}{2b} \\ q_2^* = \frac{a-c}{4b} \]
Under same price (c), Cournot =
\[ q_1 = q_2 = \frac{a-c}{3b} \]
Leader produces more whereas the follower produces less compared to Cournot
\[ \frac{d \pi_W^*}{d \beta} <0 \]
for the entire quantity range \(d < \bar{d}\)
As \(\beta\) increases in \(\pi_W^*\) Firm W wants to reduce \(\beta\) .
Low \(\beta\) wants more independent
Firms W want more differentiated product
On the other hand,
\[ \frac{d \pi_S^*}{d \beta} <0 \]
for a range of \(d < \bar{d}\)
Firm S profit increases as \(\beta\) decreases when d is small
Firm S profit increases as \(\beta\) increases when d is large
Firm S profit increases as as product are more substitute when d is large
Firm S profit increases as products are less differentiated when d is large
21.5 More Market Structure
Dixit ( 1980 )
Based on Bain-Sylos postulate: incumbents can build capacity such that entry is unprofitability
Investment in capacity is not a credibility threat if incumbents can change their capacity.
Incumbent cannot deter entry
Tyagi ( 1999a )
More retailers means greater competition, which leads to lower prices for customers.
Effect of \((n+1)\) st retailer entry
Competition effect (lower prices)
Effect on price (i.e., wholesale price), also known as input cost effect
Manufacturers want to increase wholesale price because now manufacturers have higher bargaining power, which leads other retailers to reduce quantity (bc their choice of quantity is dependent on wholesale price), and increase in prices.
Jerath, Sajeesh, and Zhang ( 2016 )
Organized Retailer enters a market
Inefficient unorganized retailers exit
Remaining unorganized retailers increase their prices. Thus, customers will be worse off.
Amaldoss and Jain ( 2005 )
consider desire for uniqueness and conformism on pricing conspicuous goods
Two routes:
higher desire for uniqueness leads to higher prices and profits
higher desire for conformity leads to lower prices and profits
Under the analytical model and lab text, consumers’ desire for unique is increased from price increases, not the other way around.
\[ U_A = V - p_A - \theta t_s - \lambda_s(n_A) \\ U_B = V - p_B - (1-\theta) t_s - \lambda_s(n_B) \]
\(\lambda_s\) = sensitivity towards externality.
\(\theta\) is the position in the Hotelling’s framework.
\(t_s\) is transportation cost.
\[ U_A = V - p_A - \theta t_s + \lambda_c(n_A) \\ U_B = V - p_B - (1-\theta) t_s + \lambda_c(n_B) \]
Rational Expectations Equilibrium
If your expectations are rational, then your expectation will be realized in equilibrium
Say, Marginal Snob = \(\theta_s\) and \(\beta\) = number of snob in the market
\[ U_A^c \equiv U_B^c = \theta_s \]
Conformists
\[ U_A^c =U_B^c = \theta_c \]
Then, according to rational expectations equilibrium, we have
\[ \beta \theta_s +( 1- \beta) \theta_c = n_A \\ \beta (1-\theta_s) +( 1- \beta) (1-\theta_c) = n_B \]
\(\beta \theta_s\) = Number of snobs who buy from firm A
\((1-\beta)\theta_c\) = Number of conformists who buy from firm B
\(\beta(1-\theta_s)\) = Number of snobs who buy from firm B
\((1-\beta)(1-\theta_c)\) = Number of conformists who buy from firm B
which is the rational expectations equilibrium (whatever we expect happens in reality).
In other words, expectation are realized in equilibrium.
The number of people expected to buy the product is endogenous in the model, which will be the actual number of people who will buy it in the market.
We should not think of the expected value here in the same sense as expected value in empirical research ( \(E(.)\) ) because the expected value here is without any errors (specifically, measurement error).
- The utility function for snobs is such that overall when price increase for one product, snob will like to buy the product more. When price increases, conformist will reduce the purchase.
Balachander and Stock ( 2009 )
Adding a Limited edition product has a positive effect on profits (via increased willingness of consumers to pay for such a product), but negative strategic effect (via increasing price competition between brands)
Under quality differentiation, high-quality brand gain from LE products
Under horizontal taste differentiation, negative strategic effects lead to lower equilibrium profits for both brands, but they still have to introduce LE products because of prisoners’ dilemma
Sajeesh, Hada, and Raju ( 2020 )
two consumer segments:
functionality-oriented
exclusivity-oriented
Firm increase value enhancements when functionality-oriented consumers perceive greater product differentiation
Firms decrease value enhancements if exclusivity-oriented perceive greater product differentiation
21.6 Market Response Model
Marketing Inputs:
- Selling effort
- advertising spending
- promotional spending
Marketing Outputs:

Give phenomena for a good model:
- P1: Dynamic sales response involves a sales growth rate and a sales decay rate that are different
- P2: Steady-state response can be concave or S-shaped . Positive sales at 0 adverting.
- P3: Competitive effects
- P4: Advertising effectiveness dynamics due to changes in media, copy, and other factors.
- P5: Sales still increase or fall off even as advertising is held constant.
Saunder (1987) phenomena
- P1: Output = 0 when Input = 0
- P2: The relationship between input and output is linear
- P3: Returns decrease as the scale of input increases (i.e., additional unit of input gives less output)
- P4: Output cannot exceed some level (i.e., saturation)
- P5: Returns increase as scale of input increases (i.e., additional unit of input gives more output)
- P6: Returns first increase and then decrease as input increases (i.e., S-shaped return)
- P7: Input must exceed some level before it produces any output (i.e., threshold)
- P8: Beyond some level of input, output declines (i.e., supersaturation point)

Aggregate Response Models
Linear model: \(Y = a + bX\)
Through origin
can only handle constant returns to scale (i.e., can’t handle concave, convex, and S-shape)
The Power Series/Polynomial model: \(Y = a + bX + c X^2 + dX^3 + ...\)
- can’t handle saturation and threshold
Fraction root model/ Power model: \(Y = a+bX^c\) where c is prespecified
c = 1/2, called square root model
c = -1, called reciprocal model
c can be interpreted as elasticity if a = 0.
c = 1, linear
c <1, decreasing return
c>1, increasing returns
Semilog model: \(Y = a + b \ln X\)
- Good when constant percentage increase in marketing effort (X) result in constant absolute increase in sales (Y)
Exponential model: \(Y = ae^{bX}\) where X >0
b > 0, increasing returns and convex
b < 0, decreasing returns and saturation
Modified exponential model: \(Y = a(1-e^{-bX}) +c\)
Decreasing returns and saturation
upper bound = a + c
lower bound = c
typically used in selling effort
Logistic model: \(Y = \frac{a}{a+ e^{-(b+cX)}}+d\)
increasing return followed by decreasing return to scale, S-shape
saturation = a + d
good with saturation and s-shape
Gompertz model
ADBUDG model ( Little 1970 ) : \(Y = b + (a-b)\frac{X^c}{d + X^c}\)
c > 1, S-shaped
0 < c < 1
saturation effect
upper bound at a
lower bound at b
typically used in advertising and selling effort.
can handle, through origin, concave, saturation, S-shape
Additive model for handling multiple Instruments: \(Y = af(X_1) + bg(X_2)\)
Multiplicative model for handling multiple instruments: \(Y = aX_1^b X_2^c\) where c and c are elasticities. More generally, \(Y = af(X_1)\times bg(X_2)\)
Multiplicative and additive model: \(Y = af(X_1) + bg(X_2) + cf(X_1) g(X_2)\)
Dynamic response model: \(Y_t = a_0 + a_1 X_t + \lambda Y_{t-1}\) where \(a_1\) = current effect, \(\lambda\) = carry-over effect
Dynamic Effects
Carry-over effect: current marketing expenditure influences future sales
- Advertising adstock/ advertising carry-over is the same thing: lagged effect of advertising on sales
Delayed-response effect: delays between when marketing investments and their impact
Customer holdout effects
Hysteresis effect
New trier and wear-out effect
Stocking effect
Simple Decay-effect model:
\[ A_t = T_t + \lambda T_{t-1}, t = 1,..., \]
- \(A_t\) = Adstock at time t
- \(T_t\) = value of advertising spending at time t
- \(\lambda\) = decay/ lag weight parameter
Response Models can be characterized by:
The number of marketing variables
whether they include competition or not
the nature of the relationship between the input variables
- Linear vs. S-shape
whether the situation is static vs. dynamic
whether the models reflect individual or aggregate response
the level of demand analyzed
- sales vs. market share
Market Share Model and Competitive Effects: \(Y = M \times V\) where
Y = Brand sales models
V = product class sales models
M = market-share models
Market share (attraction) models
\[ M_i = \frac{A_i}{A_1 + ..+ A_n} \]
where \(A_i\) attractiveness of brand i
Individual Response Model:
Multinomial logit model representing the probability of individual i choosing brand l is
\[ P_{il} = \frac{e^{A_{il}}}{\sum_j e^{A_{ij}}} \]
- \(A_{ij}\) = attractiveness of product j for individual i \(A_{ij} = \sum_k w_k b_{ijk}\)
- \(b_{ijk}\) = individual i’s evaluation of product j on product attribute k, where the summation is over all the products that individual i is considering to purchase
- \(w_k\) = importance weight associated with attribute k in forming product preferences.
21.7 Technology and Marketing Structure and Economics of Compatibility and Standards
21.8 conjoint analysis and augmented conjoint analysis.
More technical on 27.1
Jedidi and Zhang ( 2002 )
- Augmenting Conjoint Analysis to Estimate Consumer Reservation Price
Using conjoint analysis (coefficients) to derive at consumers’ reservation prices for a product in a category.
Can be applied in the context of
product introduction
calculating customer switching effect
the cannibalization effect
the market expansion effect
\[ Utility(Rating) = \alpha + \beta_i Attribute_i \]
where \(\alpha\)
Netzer and Srinivasan ( 2011 )
Break conjoint analysis down to a sequence of constant-sum paired comparison questions.
Can also calculate the standard errors for each attribute importance.
21.9 Distribution Channels
McGuire and Staelin ( 1983 )
- Two manufacturing (wholesaling) firms differentiated and competing products: Upstream firms (manufacturers) and downstream channel members (retailers)
3 types of structure:
- Both manufacturers with privately owned retailers (4 players: 2 manufacturers, 2 retailers)
- Both vertically integrated (2 manufacturers)
- Mix: one manufacturer with a private retailer, and one manufacturer with vertically integrated company store (3 players)
Each retail outlet has a downward sloping demand curve:
\[ q_i = f_i(p_1,p_2) \]
Under decentralized system (4 players), the Nash equilibrium demand curve is a function of wholesale prices:
\[ q_i^* = g_i (w_1, w_2) \]
More rules:
- Assume 2 retailers respond, but not the competing manufacturer
And unobserved wholesale prices and market is not restrictive, and Nash equilibrium whole prices is still possible.
Under mixed structure , the two retailers compete, and non-integrated firm account for all responses in the market
Under integrated structure , this is a two-person game, where each chooses the retail price
Decision variables are prices (not quantities)
Under what conditions a manufacturer want to have intermediaries
Retail demand functions are assumed to be linear in prices
Demand functions are
\[ q_1' = \mu S [ 1 - \frac{\beta}{1 - \theta} p_1' + \frac{\beta \theta}{1- \theta}p_2'] \]
\[ q_2' = (1- \mu) S [ 1+ \frac{\beta \theta}{1- \theta} p_1' - \frac{\beta}{1- \theta} p_2'] \]
\(0 \le \mu , \theta \le 1; \beta, S >0\)
S is a scale factor, which equals industry demand ( \(q' \equiv q_1' + q_2'\) ) when prices are 0.
\(\mu\) = absolute difference in demand
\(\theta\) = substutability of products (reflected by the cross elasticities), or the ratio of the rate of change of quantity with respect to the competitor’s price to the rate of change of quantity with respect to own price.
\(\theta = 0\) means independent demands (firms are monopolists)
\(\theta \to 1\) means maximally substitutable
3 more conditions:
\[ P = \{ p_1', p_2' | p_i' -m' - s' \ge 0, i = 1,2; (1-\theta) - \beta p_1' \beta \theta p_2' \ge 0, (1- \theta) + \beta \theta p_1' - \beta p_2' \ge 0 \} \]
where \(m', s'\) are fixed manufacturing and selling costs per unit
To have a set of \(P\) , then
\[ \beta \le \frac{1}{m' + s'} \]
and to have industry demand no increase with increases in either price then
\[ \frac{\theta}{1 + \theta} \le \mu \le \frac{1}{1 + \theta} \]
After rescaling, the industry demand is
\[ q = 2 (1- \theta) (p_1+ p_2) \]
When each manufacturer is a monopolist ( \(\theta = 0\) ), it’s twice as profitable for each to sell through its own channel
When demand is maximally affected by the actions of the competing retailers ( \(\theta \to 1\) ), it’s 3 times as profitable to have private dealers.
The breakeven point happens at \(\theta = .708\)
In conclusion, the optimal distribution system depends of the degree of substitubability at the retail level.
Jeuland and Shugan ( 2008 )
Quantity discounts is offered because
Cost-based economies of scale
Demand based - large purchases tend to be more price sensitive
Strategic reason- single sourcing
Channel Coordination (this is where this paper contributes to the literature
K. S. Moorthy ( 1987 )
- Price discrimination - second degree
Geylani, Dukes, and Srinivasan ( 2007 )
Jerath and Zhang ( 2010 )
21.10 Advertising Models
Three types of advertising:
- Informative Advertising: increase overall demand of your brand
- Persuasive Advertising: demand shifting to your brand
- Comparison: demand shifting away from your competitor (include complementary)
n customers distributed uniformly along the Hotelling’s line (more likely for mature market where demand doesn’t change).
\[ U_A = V - p_A - tx \\ U_B = V - p_B - t(1-x) \]
For Persuasive advertising (highlight the value of the product to the consumer):
\[ U_A = A_A V - p_A - tx \]
or increase value (i.e., reservation price).
\[ U_A = \sqrt{Ad_A} V - p_A - tx \]
or more and more customers want the product (i.e., more customers think firm A product closer to what they want)
\[ U_A = V - p_A - \frac{tx}{\sqrt{Ad_A}} \]
Comparison Advertising:
\[ U_A = V - p_A - t\sqrt{Ad_{B}}x \\ U_B = V - p_B - t \sqrt{Ad_A}(1 - x) \]
Find marginal consumers
\[ V - p_A - t\sqrt{Ad_{B}}x = V - p_B - t \sqrt{Ad_A}(1 - x) \]
\[ x = \frac{1}{t \sqrt{Ad_A} + t \sqrt{Ad_B}} (-p_A + p_B + t \sqrt{Ad_A}) \]
then profit functions are (make sure the profit function is concave)
\[ \pi_A = p_A x n - \phi Ad_A \\ \pi_B = p_B (1-x) n - \phi Ad_B \]
\(\phi\) = per unit cost of advertising (e.g., TV advertising vs. online advertising in this case, TV advertising per unit cost is likely to be higher than online advertising per unit cost)
t can also be thought of as return on advertising (traditional Hotelling’s model considers t as transportation cost)
Equilibrium prices conditioned on advertising
\[ \frac{d \pi_A}{p_A} = - \frac{d}{p_A} () \\ \frac{d \pi_B}{p_B} = \frac{d}{p_B} \]
Then optimal pricing solutions are
\[ p_A = \frac{2}{3} t \sqrt{Ad_A} + \frac{1}{3} t \sqrt{Ad_B} \\ p_B = \frac{1}{3} t \sqrt{Ad_A} + \frac{2}{3} t \sqrt{Ad_B} \]
Prices increase with the intensities of advertising (if you invest more in advertising, then you charge higher prices). Each firm price is directly proportional to their advertising, and you will charger higher price when your competitor advertise as well.
Then, optimal advertising (with the optimal prices) is
\[ \frac{d \pi_A}{d Ad_A} \\ \frac{d \pi_B}{d Ad_B} \]
Hence, Competitive equilibrium is
\[ Ad_A = \frac{25 t^2 n^2}{576 \phi^2} \\ Ad_B = \frac{25t^2 n^2}{576 \phi^2} \\ p_A = p_B = \frac{5 t^2 n }{24 \phi} \]
As cost of advertising ( \(\phi\) ), firms spend less on advertising
Higher level of return on advertising ( \(t\) ), firms benefit more from advertising
With advertising in the market, the equilibrium prices are higher than if there were no advertising.
Since colluding on prices are forbidden, and colluding on advertising is hard to notice, firms could potential collude on advertising (e.g., pulsing).
Assumption:
- Advertising decision before pricing decision (reasonable because pricing is earlier to change, while advertising investment is determined at the beginning of each period).
Collusive equilibrium (instead of using \(Ad_A, Ad_B\) , use \(Ad\) - set both advertising investment equal):
\[ Ad_A = Ad_B = \frac{t^2 n^2}{16 \phi^2} > \frac{25t^2 n^2}{576 \phi^2} \]
Hence, collusion can be make advertising investment equilibrium higher, which makes firms charge higher prices, and customers will be worse off. (more reference Aluf and Shy - check Modeling Seminar Folder - Advertising).
Combine both Comparison and Persuasive Advertising
\[ U_A = V - p_A - tx \frac{\sqrt{Ad_B}}{\sqrt{Ad_A}} \\ U_B = V - p_B - t(1-x) \frac{\sqrt{Ad_A}}{\sqrt{Ad_B}} \]
Informative Advertising
- Increase number of n customers (more likely for new products where the number of potential customers can change)
How do we think about customers, how much to consume. People consume more when they have more availability, and less when they have less in stock ( Ailawadi and Neslin 1998 )
Villas-Boas ( 1993 )
- Under monopoly, firms would be better off to pulse (i.e., alternate advertising between a minimum level and efficient amount of advertising) because of the S-shaped of the advertising response function.
Model assumptions:
- The curve of the advertising response function is S-shaped
- Markov strategies: what firms do in this period depends on what might affect profits today or in the future (independent of the history)
Propositions:
- “If the loss from lowering the consideration level is larger than the efficient advertising expenditures, the unique Markov perfect equilibrium is for firms to advertise, whatever the consideration levels of both firms are.”
Nelson ( 1974 )
Quality of a brand is determined before a purchase of a brand is “search qualities”
Quality that is not determined before a purchase is “experience qualities”
Brand risks credibility if it advertises misleading information, and pays the costs of processing nonbuying customers
There is a reverse association between quality produced and utility adjusted price
Firms that want to sell more advertise more
Firms advertise to their appropriate audience, i.e., “those whose tastes are best served by a given brand are those most likely to see an advertisement for that brand” (p. 734).
Advertising for experience qualities is indirect information while advertising for search qualities is direct information . (p. 734).
Goods are classified based on quality variation (i.e., whether the quality variation was based on searhc of experience).
3 types of goods
experience durable
experience nondurable
search goods
Experience goods are advertised more than search goods because advertisers increase sales via increasing the reputability of the sellers.
The marginal revenue of advertisement is greater for search goods than for experience goods (p. 745). Moreover, search goods will concentrate in newspapers and magazines while experience goods are seen on other media.
For experience goods, WOM is better source of info than advertising (p. 747).
Frequency of purchase moderates the differential effect of WOM and advertising (e.g., for low frequency purchases, we prefer WOM) (p. 747).
When laws are moderately enforced, deceptive advertising will happen (too little law, people would not trust, too much enforcement, advertisers aren’t incentivized to deceive, but moderate amount can cause consumers to believe, and advertisers to cheat) (p. 749). And usually experience goods have more deceptive advertising (because laws are concentrated here).
Iyer, Soberman, and Villas-Boas ( 2005 )
Firms advertise to their targeted market (those who have a strong preference for their products) than competitor loyalists, which endogenously increase differentiation in the market, and increases equilibrium profits
Targeted advertising is more valuable than target pricing. Target advertising leads to higher profits regardless whether firms have target pricing. Target pricing increased competition for comparison shoppers (no improvement in equilibrium profits). (p. 462 - 463).
Comparison shoppers size:
\[ s = 1 - 2h \]
where \(h\) is the market size of each firm’s consumers (those who prefer to buy product from that firm). Hence, \(h\) also represents the differentiation between the two firms
See table 1 (p. 469).
\(A\) is the cost for advertising the entire market
\(r\) is the reservation price
Yuxin Chen et al. ( 2009 )
Combative vs. constructive advertising
Informative complementary and persuasive advertising
Informative: increase awareness, reduce search costs, increase product differentiation
Complementary (under comparison): increase utility by signaling social prestige
Persuasive: decrease price sensitivity (include combative)
Consumer response moderates the effect of combative adverting on price competition:
It decreases price competition
It increases price competition when (1) consumers preferences are biased (firms that advertise have their products favored by the consumers), (2) disfavor firms can’t advertise and only respond with price. because advertising war leads to a price war (when firms want to increase their own profitability while collective outcome is worse off).
21.11 Product Differentiation
Horizontal differentiation: different consumers prefer different products
Vertical differentiation: where you can say one good is “better” than the other.
Characteristics approach: products are the aggregate of their characteristics.
21.12 Product Quality, Durability, Warranties
Horizontal Differentiation
\[ U = V -p - t (\theta - a)^2 \]
Vertical Differentiation
\[ U_B = \theta s_B - p_B \\ U_A = \theta s_A - p_A \]
Assume that product B has a higher quality
\(\theta\) is the position of any consumer on the vertical differentiation line.
When \(U_A < 0\) then customers would not buy
Point of indifference along the vertical quality line
\[ \theta s_B - p_B = \theta s_A - p_A \\ \theta(s_B - s_A) = p_B - p_A \\ \bar{\theta} = \frac{p_B - p_A}{s_B - s_A} \]
If \(p_B = p_A\) for every \(\theta\) , \(s_B\) is preferred to \(s_A\)
\[ \pi_A = (p_A - c s_A^2) (Mktshare_A) \\ \pi_B = (p_B - cs_B^2) (Mktshare_B) \\ U_A = \theta s_A - p_A = 0 \\ \bar{\theta}_2 = \frac{p_A}{s_A} \]
- Wauthy ( 1996 )
\(\frac{b}{a}\) = such that market is covered, then
\[ 2 \le \frac{b}{a} \le \frac{2s_2 + s_1}{s_2 - s_1} \]
for the market to be covered
In vertical differentiation model, you can’t have both \(\theta \in [0,1]\) and full market coverage.
Alternatively, you can also specify \(\theta \in [1,2]; [1,4]\)
\[ \theta \in \begin{cases} [1,4] & \frac{b}{a} = 4 \\ [1,2] & \frac{b}{a} = 2 \end{cases} \]
Under Asymmetric Information
Adverse Selection: Before contract: Information is uncertain
Moral Hazard: After contract, intentions are unknown to at least one of the parties.
Alternative setup of Akerlof’s (1970) paper
Used cars quality \(\theta \in [0,1]\)
Seller - car of type \(\theta\)
Buyer = WTP = \(\frac{3}{2} \theta\)
Both of them can be better if the transaction occurs because buyer’s WTP for the car is greater than utility received by seller.
- Assume quality is observable (both sellers and buyers do know the quality of the cars):
Price as a function of quality \(p(\theta)\) where \(p(\theta) \in [\theta, 3/2 \theta]\) both parties can be better off
- Assume quality is unobservable (since \(\theta\) is uniformly distributed) (sellers and buyers do not know the quality of the used cars):
\[ E(\theta) = \frac{1}{2} \]
then \(E(\theta)\) for sellers is \(1/2\)
\(E(\theta)\) for buyer = \(3/2 \times 1/2\) = 3/4
then market happens when \(p \in [1/2,3/4]\)
- Asymmetric info (if only the sellers know the quality)
Seller knows \(\theta\)
Buyer knows \(\theta \sim [0,1]\)
From seller perspective, he must sell at price \(p \ge \theta\) and
From buyer perspective, quality of cars on sale is between \([0, p]\) . Then, you will have a smaller distribution than \([0,1]\)
If \(E[(\theta) | \theta \le p] = 0.5 p\)
Buyers’ utility is \(3/4 p\) but the price he has to pay is \(p\) (then market would not happen)
21.12.1 Akerlof ( 1970 )
- This paper is on adverse selection
- The relationship between quality and uncertainty (in automobiles market)
- 2 x 2 (used vs. new, good vs. bad)
\(q\) = probability of getting a good car = probability of good cars produced
and \((1-q)\) is the probability of getting a lemon
Used car sellers have knowledge about the probability of the car being bad, but buyers don’t. And buyers pay the same price for a lemon as for a good car (info asymmetry).
Gresham’s law for good and bad money is not transferable (because the reason why bad money drives out goo d money because of even exchange rate, while buyers of a car cannot tell if it is good or bad).
21.12.1.1 Asymmetrical Info
Demand for used automobiles depends on price quality:
\[ Q^d = D(p, \mu) \]
Supply for used cars depends on price
\[ S = S(p) \]
and average quality depends on price
\[ \mu = \mu(p) \]
In equilibrium
\[ S(p) = D(p, \mu(p)) \]
At no price will any trade happen
Assume 2 groups of graders:
First group: \(U_1 = M = \sum_{i=1}^n x_i\) where
\(M\) is the consumption of goods other than cars
\(x_i\) is the quality of the i-th car
n is the number of cars
Second group: \(U_2 = M + \sum_{i=1}^n \frac{3}{2} x_i\)
Group 1’s income is \(Y_1\)
Group 2’s income is \(Y_2\)
Demand for first group is
\[ \begin{cases} D_1 = \frac{Y_1}{p} & \frac{\mu}{p}>1 \\ D_1 = 0 & \frac{\mu}{p}<1 \end{cases} \]
Assume we have uniform distribution of automobile quality.
Supply offered by first group is
\[ S_2 = \frac{pN}{2} ; p \le 2 \]
with average quality \(\mu = p/2\)
Demand for second group is
\[ \begin{cases} D_2 = \frac{Y_2}{p} & \frac{3 \mu}{2} >p \\ D_2 = 0 & \frac{3 \mu}{2} < p \end{cases} \]
and supply by second group is \(S_2 = 0\)
Thus, total demand \(D(p, \mu)\) is
\[ \begin{cases} D(p, \mu) = (Y_2 + Y_1) / p & \text{ if } p < \mu \\ D(p, \mu) = (Y_2)/p & \text{ if } \mu < p < 3\mu /2 \\ D(p, \mu) = 0 & \text{ if } p > 3 \mu/2 \end{cases} \]
With price \(p\) , average quality is \(p/2\) , and thus at no price will any trade happen
21.12.1.2 Symmetric Info
Car quality is uniformly distributed \(0 \le x \le 2\)
\[ \begin{cases} S(p) = N & p >1 \\ S(p) = 0 \end{cases} \]
\[ \begin{cases} D(p) = (Y_2 + Y_1) / p & p < 1 \\ D(p) = Y_2/p & 1 < p < 3/2 \\ D(p) = 0 & p > 3/2 \end{cases} \]
\[ \begin{cases} p = 1 & \text{ if } Y_2< N \\ p = Y_2/N & \text{ if } 2Y_2/3 < N < Y_2 \\ p = 3/2 & \text{ if } N < 2 Y_2 <3 \end{cases} \]
This model also applies to (1) insurance case for elders (over 65), (2) the employment of minorities, (3) the costs of dishonesty, (4) credit markets in underdeveloped countries
To counteract the effects of quality uncertainty, we can have
- Brand-name good
- Licensing practices
21.12.2 Spence ( 1973 )
Built on ( Akerlof 1970 ) model
Consider 2 employees:
Employee 1: produces 1 unit of production
Employee 2: produces 2 units of production
We have \(\alpha\) people of type 1, and \(1-\alpha\) people of type 2
Average productivity
\[ E(P) = \alpha + 2( 1- \alpha) = 2- \alpha \]
You can signal via education.
To model cost of education,
Let E to be the cost of education for type 1
E/2 to be the cost education for type 2
If type 1 signals they are high-quality worker, then they have to go through the education and cost is E, and net utility of type 1 worker
\[ 2 - E < 1 \\ E >1 \]
If type 2 signals they are high-quality worker, then they also have to go through the education and cost is E/2 and net utility of type 2 worker is
\[ 2 - E/2 > 1 \\ E< 2 \]
If we keep \(1 < E < 2\) , then we have separating equilibrium (to have signal credible enough of education )
21.12.3 S. Moorthy and Srinivasan ( 1995 )
Money-back guarantee signals quality
Transaction cost are those the seller or buyer has to pay when redeeming a money-back guarantee
Money-back guarantee does not include product return (buyers have to incur expense), but guarantee a full refund of the purchase price.
If signals are costless, there is no difference between money-back guarantees and price
But signal are costly,
Under homogeneous buyers, low-quality sellers cannot mimic high-quality sellers’ strategy (i.e., money-back guarantee)
Under heterogeneous buyers,
when transaction costs are too high, the seller chooses either not to use money-back guarantee strategy or signal through price.
When transaction costs are moderate, there is a critical value of seller transaction costs where
below this point, the high-quality sellers’ profits increase with transaction costs
above this point, the high-quality sellers’ profits decrease with transaction costs
Uninformative advertising (“money-burning”) is defined as expenditures that do not affect demand directly. is never needed
Moral hazard:
- Consumers might exhaust consumption within the money-back guarantee period
Model setup
21.13 Bargaining
Abhinay Muthoo - Bargaining Theory with Applications (1999) (check books folder)
Josh Nash - Nash Bargaining (1950)
Allocation of scare resources
Allocations of
Determining the share before game-theoretic bargaining
Use a judge/arbitrator
Meet-in-the-middle
Forced Final: If an agreement is not reached, one party will use take it or leave it
Art: Negotiation
Science: Bargaining
Game theory’s contribution: to the rules for the encounter
Area that is still fertile for research
21.13.1 Non-cooperative
Outline for non-cooperative bargaining
Take-it-or-leave-it Offers
Bargain over a cake
If you accept, we trade
If you reject, no one eats
Under perfect info, there is a simple rollback equilibrium
In general, bargaining takes on a “take-it-or-counteroffer” procedure
If time has value, both parties prefer to trade earlier to trade later
- E.g., labor negotiations - later agreements come at a price of strikes, work stoppages
Delays imply less surplus left to be shared among the parties
Two-stage bargaining
I offer a proportion, \(p\) , of the cake to you
If rejected, you may counteroffer (and \(\delta\) of the cake melts)
In the first period: 1-p, p
In second period: \((1-\delta) (1-p),(1-\delta)p\)
Since period 2 is the final period, this is just like a take-it-or-leave-it offer
- You will offer me the smallest piece that I will accept, leaving you with all of \(1-\delta\) and leaving me with almost 0
Rollback: then in the first period: I am better off by giving player B more than what he would have in period 2 (i.e., give you at least as much surplus)
You surplus if you accept in the first period is \(p\)
Accept if: your surplus in first period greater than your surplus in second period \(p \ge 1 - \delta\)
IF there is a second stage, you get \(1 - \delta\) and I get 0
You will reject any offer in the first stage that does not offer you at least \(1 - \delta\)
In the first period, I offer you \(1 - \delta\)
Note: the more patient you are (the slower the cake melts) the more you receive now
Whether first or second mover has the advantage depends on \(\delta\) .
If \(\delta\) is high (melting fast), then first mover is better.
If \(\delta\) is low (melting slower), then second mover is better.
Either way - if both players think, agreement would be reached in the first period
In any bargaining setting, strike a deal as early as possible.
Why doesn’t this happen in reality?
reputation building
lack of information
Why bargaining doesn’t happen quickly? Information asymmetry
- Likelihood of success (e.g., uncertainty in civil lawsuits)
Rules of the bargaining game uniquely determine the bargain outcome
which rules are better for you depends on patience, info
What is the smallest acceptable piece? Trust your intuition
delays are always less profitable: Someone must be wrong
Non-monetary Utility
each side has a reservation price
- LIke in civil suit: expectation of wining
The reservation price is unknown
probabilistically determine best offer
but - probability implies a chance that non bargain will take place
Company negotiates with a union
Two types of bargaining:
Union makes a take-it-or-leave-it offer
Union makes a n offer today. If it’s rejected, the Union strikes, then makes another offer
- A strike costs the company 10% of annual profits.
Probability that the company is “highly profitable”, ie., 200k is \(p\)
If offer wage of $150k
Definitely accepted
Expected wage = $150K
If offer wage of $200K
Accepted with probability \(p\)
Expected wage = $200k(p)
\(p = .9\) (90% chance company is highly profitable
best offer: ask for $200K wage
Expected value of offer: \(.9 *200= 180\)
\(p = .1\) (10% chance company is highly profitable
Expected value of offer: \(.1 *200= 20\)
If ask for $10k, get $150k
not worth the risk to ask for more
If first-period offer is rejected: A strike costs the company 10% of annual profits
Strike costs a high-value company more than a low value company
Use this fact to screen
What if the union asks for $170k in the first period?
Low profit firms ($150k) rejects - as can’t afford to take
HIgh profit firm must guess what will happen if it rejects
Best case: union strikes and then asks for only $140k (willing to pay for some cost of strike), but not all)
In the mean time: strike cost the company $20K
High-profit firm accepts
Separating equilibrium
only high-profit firms accept the first period
If offer is rejected, Union knows that it is facing a low-profit firm
Ask for $140k
What’s happening
Union lowers price after a rejection
Looks like giving in
looks like bargaining
Actually, the union is screening its bargaining partner
Different “types” of firms have different values for the future
Use these different values to screen
Time is used as a screening device
21.13.2 Cooperative
two people diving cash
If they do not agree, they each get nothing
They cant divide up more than the whole thing
21.13.3 Nash ( 1950 )
Bargaining, bilateral monopoly (nonzero-sum two -person game).
Non action taken by one individual (without the consent of the other) can affect the other’s gain.
Rational individuals (maximize gain)
Full knowledge: tastes and preferences are known
Transitive Ordering: \(A>C\) when \(A>B\) , \(B>C\) . Also related to substitutability if two events are of equal probability
Continuity assumption
Properties:
\(u(A) > u(B)\) means A is more desirable than B where \(u\) is a utility function
Linearity property: If \(0 \le p \le 1\) , then \(u(pA + (1-p)B) = pu(A) + (1-p)u(B)\)
- For two person: \(p[A,B] + (1-p)[C,D] = [pA - (1-p)C, pB + (1-p)D]\)
Anticipation = \(p A - (1-p) B\) where
\(p\) is the prob of getting A
A and B are two events.
\(u_1, u_2\) are utility function
\(c(s)\) is the solution point in a set S (compact, convex, with 0)
If \(\alpha \in S\) s.t there is \(\beta \in S\) where \(u_1(\beta) > u_2(\alpha)\) and \(u_2(\beta) > u_2(\alpha)\) then \(\alpha \neq c(S)\)
- People try to maximize utility
If \(S \in T\) , c(T) is in S then \(c(T) = c(S)\)
If S is symmetric with respect to the line \(u_1 = u_2\) , then \(c(S)\) is on the line \(u_1 = u_2\)
- Equality of bargaining
21.13.4 Iyer and Villas-Boas ( 2003 )
- Presence of a powerful retailer (e.g., Walmart) might be beneficial to all channel members.
21.13.5 Desai and Purohit ( 2004 )
2 customers segment: hagglers, nonhagglers.
When the proportion of nonhagglers is sufficient high, a haggling policy can be more profitable than a fixed-price policy
21.14 Pricing and Search Theory
21.14.1 varian and purohit ( 1980 ).
From Stigler’s seminar paper ( Stiglitz and Salop 1982 ; Salop and Stiglitz 1977 ) , model of equilibrium price dispersion is born
Spatial price dispersion: assume uninformed and informed consumers
- Since consumers can learn from experience, the result does not hold over time
Temporal price dispersion: sales
This paper is based on
Stiglitz: assume informed (choose lowest price store) and uninformed consumers (choose stores at random)
Shilony ( Shilony 1977 ) : randomized pricing strategies
\(I >0\) is the number of informed consumers
\(M >0\) is the number of uninformed consumers
\(n\) is the number of stores
\(U = M/n\) is the number of uninformed consumers per store
Each store has a density function \(f(p)\) indicating the prob it charges price \(p\)
Stores choose a price based on \(f(p)\)
Succeeds if it has the lowest price among n prices, then it has \(I + U\) customers
Fails then only has \(U\) customers
Stores charge the same lowest price will share equal size of informed customers
\(c(q)\) is the cost curve
\(p^* = \frac{c(I+U)}{(I+U}\) is the average cost with the maximum number of customers a store can get
Prop 1: \(f(p) = 0\) for \(p >r\) or \(p < p^*\)
Prop 2: No symmetric equilibrium when stores charge the same price
Prop 3: No point masses in the equilibrium pricing strategies
Prop 4: If \(f(p) >0\) , then
\[ \pi_s(p) (1-F(p))^{n-1} + \pi_f (p) [1-(1-F(p))^{n-1}] =0 \]
Prop 5: \(\pi_f (p) (\pi_f(p) - \pi_s (p))\) is strictly decreasing in \(p\)
Prop 6: \(F(p^* _ \epsilon) >0\) for any \(\epsilon> 0\)
Prop 7: \(F(r- \epsilon) <1\) for any \(\epsilon > 0\)
Prop 8: No gap \((p_1, p_2)\) where \(f(p) \equiv 0\)
Decision to be informed can be endogenous, and depends on the “full price” (search costs + fixed cost)
21.14.2 Lazear ( 1984 )
Retail pricing and clearance sales
Goods’ characteristics affect pricing behaviors
Market’s thinness can affect price volatility
Relationship between uniqueness of a goods and its price
Price reduction policies as a function of shelf time
Single period model
\(V\) = the price of the only buyer who is willing to purchase the product
\(f(V)\) is the density of V (firm’s prior)
\(F(V)^2\) is its distribution function
Firms try to
\[ \underset{R}{\operatorname{max}} R[1 - F(R)] \]
where \(R\) is the price
\(1 - F(R)\) is the prob that \(V > R\)
Assume \(V\) is uniform \([0,1]\) then
\(F(R) = R\) so that the optimum is \(R = 0.5\) with expected profits of \(0.25\)
Two-period model
Failures in period 1 implies \(V<R_1\) .
Hence, based on Bayes’ theorem, the posterior distribution in period 2 is \([0, R_1]\)
\(F_2(V) = V/R_1\) (posterior distribution)
\(R_1\) affect (1) sales in period 1, (2) info in period 2
Then, firms want to choose \(R_1, R_2\) .Firms try to
\[ \underset{R_1, R_2}{\operatorname{max}} R_1[1 - F(R_1)] + R_2 [1-F_2(R_2)]F(R_1) \]
Then, in period 2, the firms try to
\[ \underset{R_2}{\operatorname{max}} R_2[1 - F_2(R_2)] \]
Based on Bayes’ Theorem
\[ F_2(R_2) = \begin{cases} F(R_2)/ F(R_1) & \text{for } R_2 < R_1 \\ 1 & \text{otherwise} \end{cases} \]
Due to FOC, second period price is always lower than first price price
Expected profits are higher than that of one-period due to higher expected probability of a sale in the two-period problem.
But this model assume
no brand recognition
no contagion or network effects
In thin markets and heterogeneous consumers
we have \(N\) customers examine the good with the prior probability \(P\) of being shoppers, and \(1-P\) being buyers who are willing to buy at \(V\)
There are 3 types of people
- customers = all those who inspect the good
- buyers = those whose value equal \(V\)
- shoppers = those who value equal \(0\)
An individual does not know if he or she is a buyer or shopper until he or she is a customer (i.e., inspect the goods)
Then, firms try to
\[ \begin{aligned} \underset{R_1, R_2}{\operatorname{max}} & R_1(\text{prob sale in 1}) + R_2 (\text{Posterior prob sale in 2})\times (\text{Prob no sale in 1}) \\ & R_1 \times (- F(R_1))(1-P^N) + R_2 \{ (1-F_2(R_2))(1- P^N) \} \times \{ 1 - [(1 - F(R_1))(1-P^N)] \} \end{aligned} \]
Based on Bayes’ Theorem, the density for period 2 is
\[ f_2(V) = \begin{cases} \frac{1}{R_1 (1- P^N) + P^N} \text{ for } V \le R_1 \\ \frac{P^N}{R_1 (1- P^N) + P^N} \text{ for } V > R_1 \end{cases} \]
Conclusion:
As \(P^N \to 1\) (almost all customers are shoppers), there is not much info to be gained. Hence, 2-period is no different than 2 independent one-period problems. Hence, the solution in this case is identical to that of one-period problem.
When \(P^N\) is small, prices start higher and fall more rapid as time unsold increases
When \(P^N \to 1\) , prices tend to be constant.
\(P^N\) can also be thought of as search cost and info.
Observable Time patterns of price and quantity
Pricing is a function of
The number of customers \(N\)
The proportion of shoppers \(P\)
The firm’s beliefs about the market (parameterized through the prior on \(V\) )
Markets where prices fall rapidly as time passes, the probability that the good will go unsold is low.
Goods with high initial price are likely to sell because high initial price reflects low \(P^N\) - low shoppers
Heterogeneity among goods
The more disperse prior leads to a higher expected price for a given mean. And because of longer time on shelf, expected revenues for such a product can be lower.
Fashion, Obsolescence, and discounting the future
The more obsolete, the more anxious is the seller
Goods that are “classic”, have a higher initial price, and its price is less sensitive to inventory (compared to fashion goods)
Discounting is irrelevant to the pricing condition due to constant discount rate (not like increasing obsolescence rate)
For non-unique good, the solution is identical to that of the one-period problem.
Simple model
Customer’s Valuation \(\in [0,1]\)
Firm’s decision is to choose a price \(p\) (label - \(R_1\) )
One-period model
Buy if \(V >R_1\) prob = \(1-R_1\)
Not buy if \(V<R_1\) probability = \(R_1\)
\(\underset{R_1}{\operatorname{max}} [R_1][1-R_1]\) hence, FOC \(R_1 = 1/2\) , then total \(\pi = 1/2-(1/2)^2 = 1/4\)
Two prices \(R_1, R_2\)
\(R_1 \in [0,1]\)
\(R_2 \in [0, R_1]\)
\[ \underset{R_1}{\operatorname{max}} [R_1][1-R_1] + R_2 (1 - R_2)(R_1) \]
\[ \underset{R_1}{\operatorname{max}} [R_2][\frac{R_1 - R_2}{R_1}] \]
FOC \(R_2 = R_1/2\)
\[ \underset{R_1}{\operatorname{max}} R_1(1-R_1) + \frac{R_1}{2}(1 - \frac{R_1}{2}) (R_1) \]
FOC: \(R_1 = 2/3\) then \(R_2 = 1/3\)
\(N\) customers
Each customer could be a
shopper with probability p with \(V <0\)
buyer with probability \(1-p\) with \(V > \text{price}\)
Modify equation 1 to incorporate types of consumers
\[ R_1(1 - R_1)(1- p^N) + R_2 (1- R_2) R_1 (1-p^N) [ 1 - (1-R_1)(1-p^N)] \]
Reduce costs by
Economy of scale \(c(\text{number of units})\)
Economy of scope \(c(\text{number of types of products})\) (typically, due to the transfer of knowledge)
Experience effect \(c(\text{time})\) (is a superset of economy of scale)
Lal and Sarvary ( 1999 )
Conventional idea: lower search cost (e.g., Internet) will increase price competition.
Demand side: info about product attributes:
digital attributes (those can be communicated via the Internet)
nondigital attributes (those can’t)
Supply side: firms have both traditional and Internet stores.
Monopoly pricing can happen when
high proportion of Internet users
Not overwhelming nondigital attributes
Favor familiar brands
destination shopping
Monopoly pricing can lead to higher prices and discourage consumer from searching
Stores serve as acquiring tools, while Internet maintain loyal customers.
Kuksov ( 2004 )
For products that cannot be changed easily (design),lower search costs lead to higher price competition
For those that can be easily changed, lower search costs lead to higher product differentiation, which in turn decreases price competition , lower social welfare, higher industry profits.
( Salop and Stiglitz 1977 )
21.15 Pricing and Promotions
Extensively studied
Issue of Everyday Low price vs Hi/Lo pricing
Short-term price discounts
offering trade-deals
consumer promotions
shelf-price discounts (used by everybody)
cents-off coupons (some consumers whose value of time is relatively low)
Loyalty is similar to inform under analytic modeling:
Uninformed = loyal
Informed = non-loyal
30 years back, few companies use price promotions
Effects of Short-term price discounts
measured effects (84%) ( Gupta 1988 )
Brand switching (14%)
purchase acceleration (2%)
quantity purchased
elasticity of ST price changes is an order of magnitude higher
Other effects:
general trial (traditional reason)
encourages consumers to carry inventory hence increase consumption
higher sales of complementary products
small effect on store switching
Asymmetric effect (based on brand strength) (bigger firms always benefit more)
- expect of store brands
Negative Effects
Expectations of future promotions
Lowering of Reference Price
Increase in price sensitivity
Post-promotional dip
Trade Discounts
Short-term discounts offered to the trade:
Incentivize the trade to push our product
gets attention of sales force
Disadvantages
might not be passed onto the consumer
trade forward buys (hurts production plans)
hard to forecast demand
trade expects discounts in the future (cost of doing business)
Scanback can help increase retail pass through (i.e., encourage retailers to have consumer discounts)
Determinants of pass through
Higher when
Consumer elasticity is higher
promoting brand is stronger
shape of the demand function
lower frequency of promotions
(Online) Shelf-price discounts ( Raju, Srinivasan, and Lal 1990 )
- If you are a stronger brand you can discount infrequently because the weaker brands cannot predict when the stronger brands will promote. Hence, it has to promote more frequently
Little over 1% get redeemed each year
The ability of cents-off coupons to price distribution has reduced considerably because of their very wide availability
Sales increases required to make free-standing-insert coupons profitable are not attainable
Coupon Design
Expiration dates
- Long vs short expiration dates: Stronger brands should have shorter windows (because a lot more of your loyalty customer base will utilize the coupons).
Method of distribution
In-store (is better)
Through the package
Targeted promotions
Package Coupons acquisition and retention trade-offs
3 types of package coupons:
Peel-off (lots more use the coupons) lowest profits for the firm
in-packs (fewer customers will buy the products in the first period)
on-packs (customers buy the products and they redeem in the next period) best approach
Trade and consumer promotion are necessary
Consumer promotion (avoid shelf price discount/news paper coupons, use package coupons
strong interaction between advertising and promotion (area for more research)
3 degrees price discrimination
- First-degree: based on willingness to pay
- Second-degree: based on quantity
- Third-degree: based on memberships
- Fourth-degree: based on cost to serve
21.15.1 Narasimhan ( 1988 )
Marketing tools to promote products:
Advertising
Trade promotions
Consumer promotions
Pricing promotions:
Price deals
Cents-off labels
Brand loyalty can help explain the variation in prices (in competitive markets)
Firms try to make optimal trade-off between
attracting brand switchers
loss of profits from loyal customers.
Deviation from the maximum price = promotion
Firms with identical products, and cost structures (constant or declining). Non-cooperative game.
Same reservation price
Three consumer segments:
Loyal to firm 1 with size \(\alpha_1 (0<\alpha_1<1)\)
Loyal to firm 2 with size \(\alpha_2(0 < \alpha_2 < \alpha_1)\) (asymmetric firm)
Switchers with size \(\beta (0 < \beta = 1 - \alpha_1 - \alpha_2)\)
Costless price change, no intertemporal effects (in quantity or loyalty)
To model \(\beta\) either
- \(d \in (-b, a)\) is switch cost (individual parameter)
\[ \begin{cases} \text{buy brand 1} & \text{if } P_1 \le P_2 - d \\ \text{buy brand 2} & \text{if } P_1 > P_2 - d \end{cases} \]
- Identical switchers (same d)
- \(d = 0\) (extremely price sensitive)
For case 1, there is a pure strategy, while case 2 and 3 have no pure strategies, only mixed strategies
Details for case 3:
Profit function
\[ \Pi_i (P_i, P_j) = \alpha_i P_i + \delta_{ij} \beta P_i \]
\[ \delta_{ij} = \begin{cases} 1 & \text{ if } P_i < P_j \\ 1/2 & \text{ if } P_i = P_j \\ 0 & \text{ if } P_i > P_j \end{cases} \]
and \(i = 1,2, i \neq j\)
Prop 1: no pure Nash equilibrium
Mixed Strategy profit function
\[ \Pi_i (P_i) = \alpha_i P_i + Prob(P_j > P_i) \beta P_i + Prob (P_j = P_i) \frac{\beta}{2} P_i \]
where \(P_i \in S_i^*, i \neq j; i , j = 1, 2\)
Then the expected profit functions of the two-player game is
\[ \underset{F_i}{\operatorname{max}} E(\Pi_i) = \int \Pi_i (P_i) d F_i (P_i) \]
\(P_i \in S_i^*\)
\[ \Pi_i \ge \alpha_i r \\ \int dF_i (P_i) = 1 \\ P_i \in S_i^* \]
21.15.2 Balachander, Ghosh, and Stock ( 2010 )
- Bundle discounts can be more profitable than price promotions (in a competitive market) due to increased loyalty (which will reduce promotional competition intensity).
21.15.3 Goić, Jerath, and Srinivasan ( 2011 )
Cross-market discounts, purchases in a source market can get you a price discounts redeemable in a target market.
- Increase prices and sales in the source market.
21.16 Market Entry Decisions and Diffusion
Peter N. Golder and Tellis ( 1993 )
Peter N. Golder and Tellis ( 2004 )
Boulding and Christen ( 2003 )
Van den Bulte and Joshi ( 2007 )
21.17 Principal-agent Models and Salesforce Compensation
21.17.1 gerstner and hess ( 1987 ), 21.17.2 basu et al. ( 1985 ), 21.17.3 raju and srinivasan ( 1996 ).
Compare to ( Basu et al. 1985 ) , basic quota plan is superior in terms of implementation
Different from ( Basu et al. 1985 ) , basic quota plan has
- Shape-induced nonoptimality: not a general curvilinear form
- Heterogeneity-induced nonoptimality: common rate across salesforce
However, only 1% of cases in simulation shows up with nonoptimality. Hence, minimal loss in optimality
Basic quota plan is a also robust against changes in
salesperson switching territory
territorial changes (e.g., business condition)
Heterogeneity stems from
Salesperson: effectiveness, risk level, disutility for effort, and alternative opportunity
Territory: Sales potential and volatility
Adjusting quotas can accommodate the heterogeneity
To assess nonoptimality, following Basu and Kalyanaram ( 1990 )
Moral hazard: cannot assess salesperson’s true effort.
The salesperson reacts to the compensation scheme by deciding on an effort level that maximizes his overall utility, i.e., the expected utility from the (stochastic) compensation minus the effort distuility.
Firm wants to maximize its profit
compensation is greater than saleperson’s alternative.
Dollar sales \(x_i \sim Gamma\) (because sales are non-negative and standard deviation getting proportionately larger as the mean increases) with density \(f_i(x_i|t_i)\)
Expected sales per period
\[ E[x_i |t_i] = h_i + k_i t_i , (h_i > 0, k_i >0) \]
- \(h_i\) = base sales level
- \(k_i\) = effectiveness of effort
and \(1/\sqrt{c}\) = uncertainty in sales (coefficient of variation) = standard deviation / mean where \(c \to \infty\) means perfect certainty
salesperson’s overall utility
\[ U_i[s_i(x_i)] - V_i(t_i) = \frac{1}{\delta_i}[s_i (x_i)]^{\delta_i} - d_i t_i^{\gamma_i} \] where
- \(0 < \delta_i <1\) (greater \(\delta\) means less risk-averse salesperson)
- \(\gamma_i >1\) (greater \(\gamma\) means more effort)
- \(V_i(t_i) = d_i t_i^{\gamma_i}\) is the increasing disutility function (convex)
21.17.4 Lal and Staelin ( 1986 )
A menu of compensation plans (salesperson can select, which depends on their own perspective)
Proposes conditions when it’s optimal to offer a menu
Under ( Basu et al. 1985 ) , they assume
Salespeople have identical risk characteristics
identical reservation utility
identical information about the environment
When this paper relaxes these assumptions, menu of contract makes sense
If you cannot distinguish (or have a selection mechanisms) between high performer and low performer, a menu is recommended. but if you can, you only need 1 contract like ( Basu et al. 1985 )
21.17.5 Simester and Zhang ( 2010 )
21.18 branding.
Wernerfelt ( 1988 )
- Umbrella branding
W. Chu and Chu ( 1994 )
retailer reputation
21.19 Marketing Resource Allocation Models
This section is based on ( Mantrala, Sinha, and Zoltners 1992 )
21.19.1 Case study 1
Concave sales response function
- Optimal vs. proportional at different investment levels
- Profit maximization perspective of aggregate function
\[ s_i = k_i (1- e^{-b_i x_i}) \]
- \(s_i\) = current-period sales response (dollars / period)
- \(x_i\) = amount of resource allocated to submarket i
- \(b_i\) = rate at which sales approach saturation
- \(k_i\) = sales potential
Allocation functions
Fixed proportion
\(R_i\) = Investment level (dollars/period)
\(w_i\) = fixed proportion or weights
\[ \hat{x}_i = w_i R; \\ \sum_{t=1}^2 w_t = 1; 0 < w_t < 1 \]
Informed allocator
- optimal allocations via marginal analysis (maximize profits)
\[ max C = m \sum_{i = 1}^2 k_i (1- e^{-b_i x_i}) \\ x_1 + x_2 \le R; x_i \ge 0 \text{ for } i = 1,2 \\ x_1 = \frac{1}{(b_1 + b_2)(b_2 R + \ln(\frac{k_1b_1}{k_2b_2})} \\ x_2 = \frac{1}{(b_1 + b_2)(b_2 R + \ln(\frac{k_2b_2}{k_1b_1})} \]
21.19.2 Case study 2
S-shaped sales response function:
21.19.3 Case study 3
Quadratic-form stochastic response function
- Optimal allocation only with risk averse and risk neutral investors.
21.20 Mixed Strategies
Games with finite number, and finite strategy for each player, there will always be a Nash equilibrium (might not be pure Nash, but always mixed)
Extended game
Suppose we allow each player to choose randomizing strategies
For example, the server might serve left half of the time, and right half of the time
In general, suppose the server serves left a fraction \(p\) of the time
What is the receiver’s best response?
Best Responses
If \(p = 1\) , the receiver should defend to the left
\(p = 0\) , the receiver should defend to the right
The expected payoff to the receiver is
\(p \times 3/4 + (1-p) \times 1/4\) if defending left
\(p \times 1/4 + (1-p) \times 3/4\) if defending right
Hence, she should defend left if
\(p \times 3/4 - (1-p)\times 1/4 > p \times 1/4 + (1-p) \times 3/4\)
We said to defend left whenever
\[ p \times 3/4 - (1-p)\times 1/4 > p \times 1/4 + (1-p) \times 3/4 \]
Server’s Best response
Suppose that the receiver goes left with probability \(q\)
if \(q = 1\) , the server should serve right
If \(q = 0\) , the server should server left
Hence, serve left if \(1/4 \times q + 3/4 \times (1-q) > 3/4\times q + 1/4 \times (1-q)\)
Simplifying, he should serve left if \(q < 1/2\)
Mixed strategy equilibrium:
A mixed strategy equilibrium is a pair of mixed strategies that are mutual best responses
In the tennis example, this occurred when each play chose a 50-50 mixture of left and right
Your best strategy is when you make the option given to your opponent is obsolete.
A player chooses his strategy to make his rival indifferent
A player earns the same expected payoff for each pure strategy chosen with positive probability
Important property: When player’s own payoff form a pure strategy goes up (or down), his mixture does not change.
21.21 Bundling
Say we have equal numbers of type 1 and type 2, then you would like to charge $5,000 for the equipment and $2,000 for installation when considering equipment and installment equally . If you price it separately, then your total profit is 14,000.
But you bundle, you get $16,000.
If we know that bundles work. But we don’t see every company does ti?
Because it depends on the number of type 1 and 2 customers, and negative correlation in willingness to pay .
For example:
Information Products
margin cost is close to 0.
Bundling of info products is very easy
hence always bundle
21.22 Market Entry and Diffusion
Product Life Cycle model
Bass ( 1969 )
Discussion of sales has 2 types
\(p\) = coefficient of innovation (fraction of innovators of the untapped market who buy in that period)
\(q\) = coefficient of imitation (fraction of the interaction which lead to sales in that period)
\(M\) = market potential
\(N(t)\) = cumulative sales till time \(t\)
\(M - N(t)\) = the untapped market
Sales in any time is People buying because of the pure benefits of the product, plus people buy the product after interacting with people who owned the product.
\[ S(t) = p(M- N(t)) + q \frac{N(t)}{M} [M-N(t)] \\ = pM + (q-p) N(t) - \frac{q}{M} [N(t)]^2 \]
one can estimate \(p,q,M\) from data
\(q > p\) (coefficient of imitation > coefficient of innovation) means that you have life cycle (bell-shaped curve)
Previous use
limited databases (PIM and ASSESOR) ( Urban et al. 1986 )
exclusion of nonsurvivors
single-informant self-report
New dataset overcomes these limitations and show 50% of the market pioneers fail, and their mean share is much lower
Early market leaders have greater long-term success and enter on average 13 years after pioneers.
Definitions (p. 159)
- Inventor: firms that develop patent or important technologies in a new product category
- Product pioneer: the first firm to develop a working model or sample in a new product category
- Market pioneer is the first firm to sell in a new product category
- Product category: a group of close substitutes
At the business level, being the leader can give you long-term profit disadvantage from the samples of consumer and industrial goods.
First-to-market leads to an initial profit advantage, which last about 12 to 14 years before becoming long-term disadvantage.
Consumer learning (education), market position (strong vs. weak) and patent protection can moderate the effect of first-mover on profit.
Research on product life cycle (PLC)
Consumer durables typically grow 45 per year over 8 years, then slowdown when sales decline by 15%, and stay below those of the previous peak for 5 years.
Slowdown typically happens when the product penetrates 35-50% of the market
large sales increases (at first) will have larger sales declines (at slowdown).
Leisure-enhancing products tend to have higher growth rate and shorter growth stages than non leisure-enhancing products
Time-saving products have lower growth rates and longer growth stages than non time-saving products
Lower likelihood of slowdown correlates with steeper price reduction, lower penetration, and higher economic growth
A hazard model gives reasonable prediction of the slowdown and takeoff.
Innovations market have two segments:
Influentials: aware of new developments an affect imitators
Imitators: model after influentials.
This market structure is reasonable because it exhibits consistent evidence with the prior research and market (e.g., dip between the early and later parts fo the diffusion curve).
” Erroneously specifying a mixed-influence model to a mixture process where influentials act independently from each other can generate systematic changes in the parameter values reported in earlier research.”
Two-segments model performs better than the standard mixed-influence, the Gamma/Shifted Gompertz, the Weibull-Gamma models, and similar to the Karmeshu-Goswami mixed influence model.
21.23 Principal-Agent Models and Salesforce Compensation
Key Question:
- Ensuring agents exert effort
- Design compensation plans such that workers exert high effort?
Designing contracts:
Effort can be monitored
Monitoring costs are too high
- Manger designs the construct
- manager offers the construct and worker chooses to accept
- Worker decides the extent of effort
- Outcome is observed and wage is given to the worker
Scenario 1 : Certainty
e = effort put in by worker
2 levels of e
- 2 if he works hard
- 0 if he shirks
Reservation utility = 10 (other alternative: can work somewhere else, or private money allows them not to work)
Agent’s Utility
\[ U = \begin{cases} w - e & \text{if he exerts effort e} \\ 10 & \text{if he works somewhere else} \end{cases} \]
Revenue is a function of effort
\[ R(e) = \begin{cases} H & \text{if } e = 2 \\ L & \text{if } e = 0 \end{cases} \]
\(w^H\) = wage if \(R(e) = H\)
\(w^L\) = wage if \(R(e) = L\)
Constraints:
Worker has to participate in this labor market - participation constraint \(w^H - 2 \ge 10\)
Incentive compatibility constraint (ensure that the works always put in the effort and the manager always pay for the higher wage): \(w^H - 2 \ge w^L -0\)
\[ w^H = 12 \\ w^L = 10 \]
Thus, contract is simple because of monitoring
Scenario 2 : Under uncertainty
\[ R(2) = \begin{cases} H & \text{w/ prob 0.8} \\ L & \text{w/ prob 0.2} \end{cases} \\ R(0) = \begin{cases} H & \text{w/ prob 0.4} \\ L & \text{w/ prob 0.6} \end{cases} \]
Agent Utility
\[ U = \begin{cases} E(w) - e & \text{if effort e is put} \\ 10 & \text{if they choose outside option} \end{cases} \]
Participation Constraint: \(0.8w^H + 0.2w^L -2 \ge 10\)
Incentive compatibility constraint: \(0.8w^H + 0.2w^L - 2 \ge 0.4 w^H + 0.6w^L - 0\)
\[ w^H = 13 \\ w^L = 8 \]
Expected wage bill that the manager has to pay:
\[ 13\times 0.8 + 8 \times 0.2 = 12 \]
Hence, the expected money the manager has to pay is the same for both cases (certainty vs. uncertainty)
Scenario 3 : Asymmetric Information
Degrees of risk aversion
Manger perspective
\[ R(2) = \begin{cases} H & \text{w/ prob 0.8} \\ L & \text{w/ prob 0.2} \end{cases} \]
Worker perspective (the number for worker is always lower, because they are more risk averse, managers are more risk neural) (the manager also knows this).
\[ R(2) = \begin{cases} H & \text{w/ prob 0.7} \\ L & \text{w/ prob 0.3} \end{cases} \]
Participation Constraint
\[ 0.7w^H + 0.3w^L - 2 \ge 10 \]
Incentive Compatibility Constraint
\[ 0.6 w^H + 0.3 w^L - 2 \ge 0.4 w^H + 0.6 w^L - 0 \]
(take R(0) from scenario 2)
\[ 0.7 w^H + 0.3 w^L = 12 \\ 0.3w^H - 0.3w^L = 2 \]
\[ w^H = 14 \\ w^L = 22/3 \]
Expected wage bill for the manager is
\[ 14 * 0.8 + 22/3*0.2 = 12.66 \]
Hence, expected wage bill is higher than scenario 2
Risk aversion from the worker forces the manager to pay higher wage
Grossman and Hart ( 1986 )
- landmark paper for principal agent model
21.23.1 Basu et al. ( 1985 )
Types of compensation plan:
Independent of salesperson’s performance (e.g., salary only)
Partly dependent on output (e.g., salary with commissions)
In comparison to others (e.g., sales contests)
Options for salesperson to choose the compensation plan
In the first 2 categories, the 3 major schemes:
- Straight salary
- Straight commissions
- Combination of base salary and commission
Dimensions that affect the proportion of salary tot total pay (p. 270, table 1)
Previous research assumes deterministic relationship between sales and effort, but this study says otherwise (stochastic relationship between sales and effort).
Firm: Risk neutral: maximize expected profits
Salesperson: Risk averse . Hence, diminishing marginal utility for income \(U(s) \ge 0; U'(s) >0, U''(s) <0\)
Expected utility of the salesperson for this job > alternative
Utility function of the salesperson: additively separable: \(U(s) - V(t)\) where \(s\) = salary, and \(t\) = effort (time)
Marginal disutility for effort increases with effort \(V(t) \ge 0, V'(t)>0, V''(t) >0\)
Constant marginal cost of production and distribution \(c\)
Known utility function and sales-effort response function (both principal and agent)
dollar sales \(x \sim Gamma, Binom\)
Expected profit for the firm
\[ \pi = \int[(1-c)x - s(x)]f(x|t)dx \]
Objective of the firm is to
\[ \underset{s(x)}{\operatorname{max}} \int[(1-c)x - s(x)]f(x|t)dx \]
subject to (agent’s best alternative e.g., other job offer - \(m\) )
\[ \int [U(s(x))]f(x|t) dx - V(t) \ge m \]
and the agent wants to maximize the the utility
\[ \underset{t}{\operatorname{max}} \int [U(s(x))]f(x|t)dx - V(t) \]
21.23.2 Lal and Staelin ( 1986 )
21.23.3 raju and srinivasan ( 1996 ).
Compare quota-based compensation with ( Basu et al. 1985 ) curvilinear compensation, the basic quota plan is simpler, and only in specical cases (about 1% in simulation) that differs from ( Basu et al. 1985 ) . And it’s easier to adapt to changes in moving salesperson and changing territory, unlike ( Basu et al. 1985 ) ’s plan where the whole commission rate structure needs to be changed.
Heterogeneity stems from:
Salesperson: disutility effort level, risk level, effectiveness, alternative opportunity
Territory: Sales potential an volatility
Adjusting the quota (per territory) can accommodate the heterogeneity
Quota-based < BLSS (in terms of profits)
- quota-based from curve (between total compensation and sales) (i.e., shape-induced nonoptimality)
- common salary and commission rate across salesforce (i.e., heterogeneity-induced nonoptimality)
To assess the shape-induced nonoptimality following
21.23.4 Joseph and Thevaranjan ( 1998 )
21.23.5 simester and zhang ( 2010 ).
- Tradeoff: Motivating manager effort and info sharing.
21.24 Meta-analyses of Econometric Marketing Models
21.25 dynamic advertising effects and spending models, 21.26 marketing mix optimization models.
Check this post for implementation in Python
21.27 New Product Diffusion Models
21.28 two-sided platform marketing models.
Example of Marketing Mix Model in practice: link
Understanding and solving intractable resource governance problems.
- In the Press
- Conferences and Talks
- Exploring models of electronic wastes governance in the United States and Mexico: Recycling, risk and environmental justice
- The Collaborative Resource Governance Lab (CoReGovLab)
- Water Conflicts in Mexico: A Multi-Method Approach
- Past projects
- Publications and scholarly output
- Research Interests
- Higher education and academia
- Public administration, public policy and public management research
- Research-oriented blog posts
- Stuff about research methods
- Research trajectory
- Publications
- Developing a Writing Practice
- Outlining Papers
- Publishing strategies
- Writing a book manuscript
- Writing a research paper, book chapter or dissertation/thesis chapter
- Everything Notebook
- Literature Reviews
- Note-Taking Techniques
- Organization and Time Management
- Planning Methods and Approaches
- Qualitative Methods, Qualitative Research, Qualitative Analysis
- Reading Notes of Books
- Reading Strategies
- Teaching Public Policy, Public Administration and Public Management
- My Reading Notes of Books on How to Write a Doctoral Dissertation/How to Conduct PhD Research
- Writing a Thesis (Undergraduate or Masters) or a Dissertation (PhD)
- Reading strategies for undergraduates
- Social Media in Academia
- Resources for Job Seekers in the Academic Market
- Writing Groups and Retreats
- Regional Development (Fall 2015)
- State and Local Government (Fall 2015)
- Public Policy Analysis (Fall 2016)
- Regional Development (Fall 2016)
- Public Policy Analysis (Fall 2018)
- Public Policy Analysis (Fall 2019)
- Public Policy Analysis (Spring 2016)
- POLI 351 Environmental Policy and Politics (Summer Session 2011)
- POLI 352 Comparative Politics of Public Policy (Term 2)
- POLI 375A Global Environmental Politics (Term 2)
- POLI 350A Public Policy (Term 2)
- POLI 351 Environmental Policy and Politics (Term 1)
- POLI 332 Latin American Environmental Politics (Term 2, Spring 2012)
- POLI 350A Public Policy (Term 1, Sep-Dec 2011)
- POLI 375A Global Environmental Politics (Term 1, Sep-Dec 2011)
Writing theoretical frameworks, analytical frameworks and conceptual frameworks
Three of the most challenging concepts for me to explain are the interrelated ideas of a theoretical framework, a conceptual framework, and an analytical framework. All three of these tend to be used interchangeably. While I find these concepts somewhat fuzzy and I struggle sometimes to explain the differences between them and clarify their usage for my students (and clearly I am not alone in this challenge), this blog post is an attempt to help discern these analytical categories more clearly.
A lot of people (my own students included) have asked me if the theoretical framework is their literature review. That’s actually not the case. A theoretical framework , the way I define it, is comprised of the different theories and theoretical constructs that help explain a phenomenon. A theoretical framework sets out the various expectations that a theory posits and how they would apply to a specific case under analysis, and how one would use theory to explain a particular phenomenon. I like how theoretical frameworks are defined in this blog post . Dr. Cyrus Samii offers an explanation of what a good theoretical framework does for students .
For example, you can use framing theory to help you explain how different actors perceive the world. Your theoretical framework may be based on theories of framing, but it can also include others. For example, in this paper, Zeitoun and Allan explain their theoretical framework, aptly named hydro-hegemony . In doing so, Zeitoun and Allan explain the role of each theoretical construct (Power, Hydro-Hegemony, Political Economy) and how they apply to transboundary water conflict. Another good example of a theoretical framework is that posited by Dr. Michael J. Bloomfield in his book Dirty Gold, as I mention in this tweet:
In Chapter 2, @mj_bloomfield nicely sets his theoretical framework borrowing from sociology, IR, and business-strategy scholarship pic.twitter.com/jTGF4PPymn — Dr Raul Pacheco-Vega (@raulpacheco) December 24, 2017
An analytical framework is, the way I see it, a model that helps explain how a certain type of analysis will be conducted. For example, in this paper, Franks and Cleaver develop an analytical framework that includes scholarship on poverty measurement to help us understand how water governance and poverty are interrelated . Other authors describe an analytical framework as a “conceptual framework that helps analyse particular phenomena”, as posited here , ungated version can be read here .
I think it’s easy to conflate analytical frameworks with theoretical and conceptual ones because of the way in which concepts, theories and ideas are harnessed to explain a phenomenon. But I believe the most important element of an analytical framework is instrumental : their purpose is to help undertake analyses. You use elements of an analytical framework to deconstruct a specific concept/set of concepts/phenomenon. For example, in this paper , Bodde et al develop an analytical framework to characterise sources of uncertainties in strategic environmental assessments.
A robust conceptual framework describes the different concepts one would need to know to understand a particular phenomenon, without pretending to create causal links across variables and outcomes. In my view, theoretical frameworks set expectations, because theories are constructs that help explain relationships between variables and specific outcomes and responses. Conceptual frameworks, the way I see them, are like lenses through which you can see a particular phenomenon.
A conceptual framework should serve to help illuminate and clarify fuzzy ideas, and fill lacunae. Viewed this way, a conceptual framework offers insight that would not be otherwise be gained without a more profound understanding of the concepts explained in the framework. For example, in this article, Beck offers social movement theory as a conceptual framework that can help understand terrorism . As I explained in my metaphor above, social movement theory is the lens through which you see terrorism, and you get a clearer understanding of how it operates precisely because you used this particular theory.
Dan Kaminsky offered a really interesting explanation connecting these topics to time, read his tweet below.
I think this maps to time. Theoretical frameworks talk about how we got here. Conceptual frameworks discuss what we have. Analytical frameworks discuss where we can go with this. See also legislative/executive/judicial. — Dan Kaminsky (@dakami) September 28, 2018
One of my CIDE students, Andres Ruiz, reminded me of this article on conceptual frameworks in the International Journal of Qualitative Methods. I’ll also be adding resources as I get them via Twitter or email. Hopefully this blog post will help clarify this idea!
You can share this blog post on the following social networks by clicking on their icon.
Posted in academia .
Tagged with analytical framework , conceptual framework , theoretical framework .
By Raul Pacheco-Vega – September 28, 2018
7 Responses
Stay in touch with the conversation, subscribe to the RSS feed for comments on this post .
Thanks, this had some useful clarifications for me!
I GOT CONFUSED AGAIN!
No need to be confused!
Thanks for the Clarification, Dr Raul. My cluttered mind is largely cleared, now.
Thanks,very helpful
I too was/am confused but this helps 🙂
Thank you very much, Dr.
Leave a Reply Cancel Some HTML is OK
Name (required)
Email (required, but never shared)
or, reply to this post via trackback .
About Raul Pacheco-Vega, PhD
Find me online.
My Research Output
- Google Scholar Profile
- Academia.Edu
- ResearchGate
My Social Networks
- Polycentricity Network
Recent Posts
- “State-Sponsored Activism: Bureaucrats and Social Movements in Brazil” – Jessica Rich – my reading notes
- Reading Like a Writer – Francine Prose – my reading notes
- Using the Pacheco-Vega workflows and frameworks to write and/or revise a scholarly book
- On framing, the value of narrative and storytelling in scholarly research, and the importance of asking the “what is this a story of” question
- The Abstract Decomposition Matrix Technique to find a gap in the literature
Recent Comments
- Hazera on On framing, the value of narrative and storytelling in scholarly research, and the importance of asking the “what is this a story of” question
- Kipi Fidelis on A sequential framework for teaching how to write good research questions
- Razib Paul on On framing, the value of narrative and storytelling in scholarly research, and the importance of asking the “what is this a story of” question
- Jonathan Wilcox on An improved version of the Drafts Review Matrix – responding to reviewers and editors’ comments
- Catherine Franz on What’s the difference between the Everything Notebook and the Commonplace Book?
Follow me on Twitter:
Proudly powered by WordPress and Carrington .
Carrington Theme by Crowd Favorite
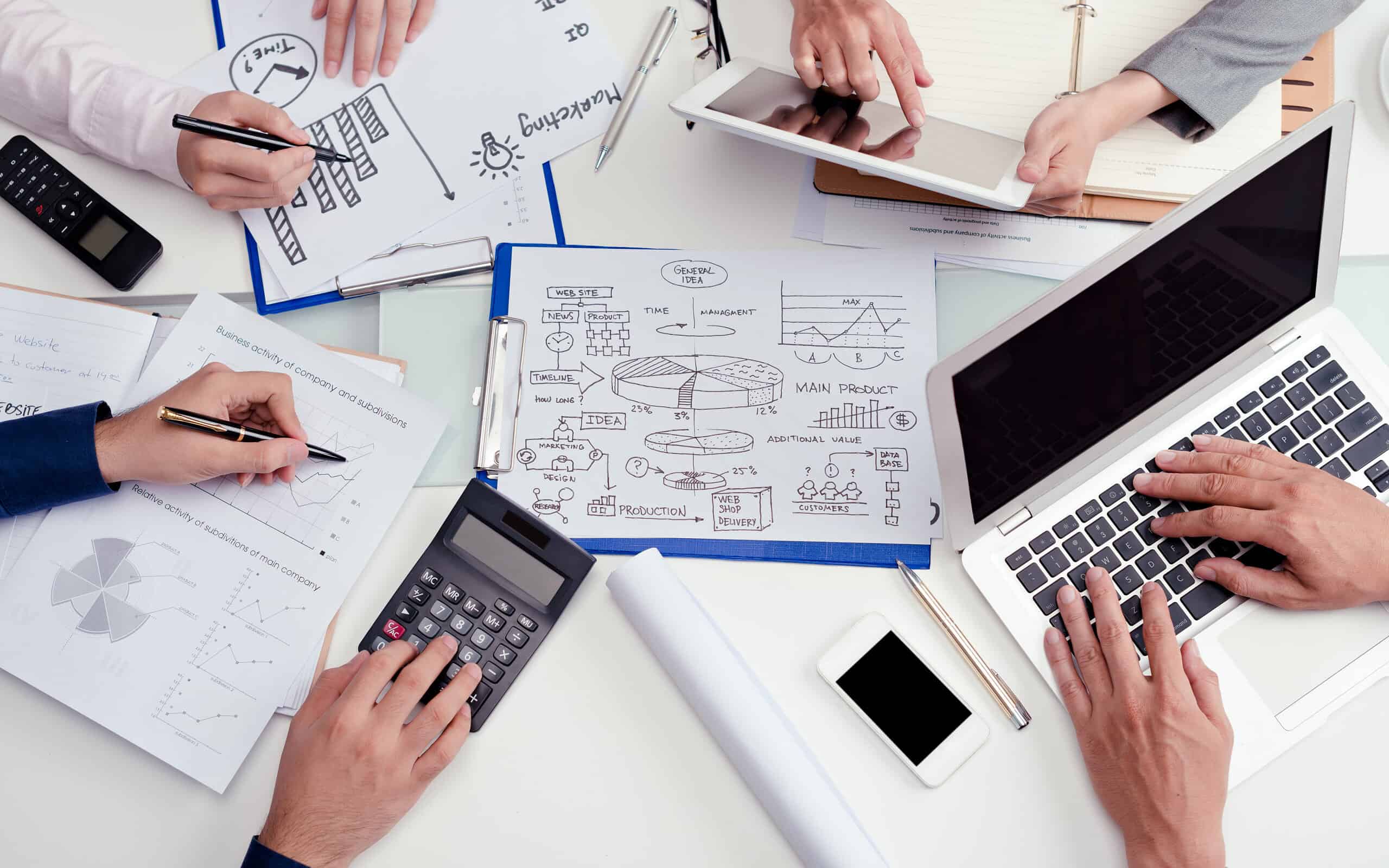
Analytical Modeling: Turning Complex Data into Simple Solutions
Updated: January 28, 2024 by iSixSigma Staff
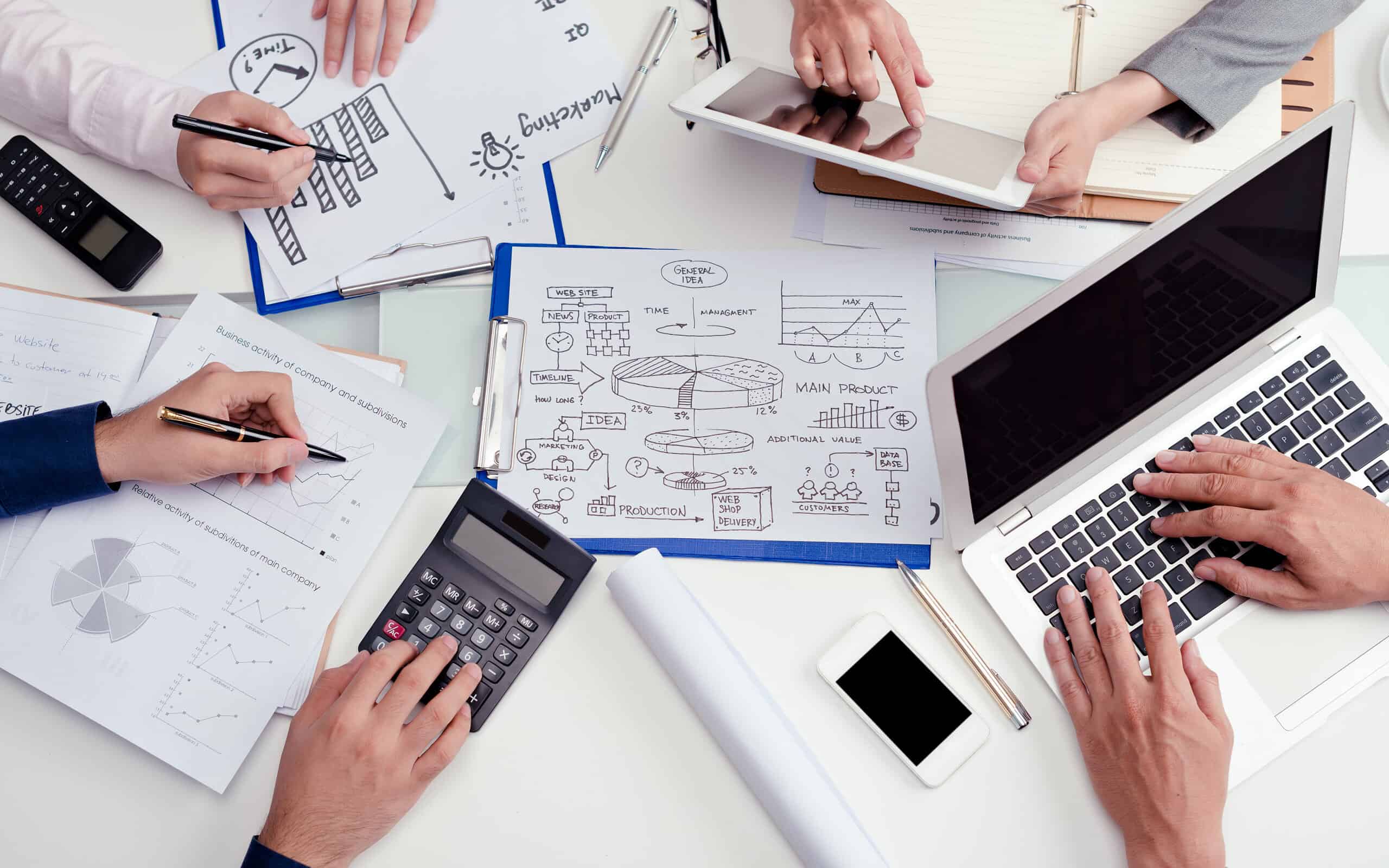
Not everything in business is quantifiable, but most of it is. Understanding the relationships between dozens of different factors and forces influencing a specific outcome can seem impossible, but it’s not. Analytical modeling is an effective and reliable technique for turning a mess of different variables and conditions into information you can actually use to make decisions.
Overview: What is analytical modeling?
Analytical modeling is a mathematical approach to business analysis that uses complex calculations that often involve numerous variables and factors. This type of analysis can be a powerful tool when seeking solutions to specific problems when used with proper technique and care.
3 benefits of analytical modeling
It’s hard to overstate the value of strong analytics. Mathematical analysis is useful at any scale and for almost every area of business management.
1. Data-driven decisions
The primary benefit of leveraging analytical modeling is the security of making data-driven decisions. Leaders don’t have to take a shot in the dark. They can use analytics to accurately define problems , develop solutions and anticipate outcomes.
2. Logical information structure
Analytical modeling is all about relating and structuring information in a sensible way. This means you can use the results to trace general outcomes to specific sources.
3. Can be shared and improved
The objective nature of analytical modeling makes it a perfect way to establish a common foundation for discussion among a diverse group. Rather than trying to get everyone on the same page through personal and subjective theorizing, using analytical data establishes a singular framework for universal reference within an organization.
Why is analytical modeling important to understand?
Like any other business practice, it’s important to understand this kind of analysis so you know what it can and can’t do. Even though it’s a powerful tool in the right hands, it’s not a magic solution that’s guaranteed to fix your problems.
Information requires interpretation
Information can be invaluable or completely worthless depending on how you use it. You should always carefully examine the factors and implications of the data in question before basing major decisions on it.
Analytics needs good data
Accurate, complete and relevant information are essential for a useful outcome. If poor data is put into a model, poor results will come out. Ensuring quality of data collection techniques is just as important as the modeling itself.
Various applications and approaches
Analytical modeling tends to focus on specific issues, questions or problems. There are several different types of models that can be used, which means you need to figure out the one that best fits each situation.
An industry example of analytical modeling
A barbecue restaurant serves customers every day of the week from lunch through dinner. To increase overall profit, management wants to reduce losses from waste and cut down on missed sales. Since they need to start preparing meat days in advance and any leftovers are discarded, the establishment needs to find a way to accurately predict how many customers they will have each day.
The restaurant hires outside contractors to create a predictive analytics model to address this need. The modelers examine various relevant factors, including historical customer attendance in previous weeks, weather predictions and upcoming specials or events of nearby restaurants. They create an initial model and start comparing actual results against predicted results until they’ve reached 90 percent accuracy, which is enough to meet the restaurant’s goals.
3 best practices when thinking about analytical modeling
Think about analytical modeling as a starting point for decisions and a tool that can be continually improved as you use it.
1. Start with a goal
Analytical modeling can’t answer a question that isn’t asked. It’s easy to make the mistake of looking for answers or patterns in general data. This kind of modeling is best used by created calculations to answer a specific initial question, like: “How can we turn more visitors into customers?” or “How can we make this process less wasteful.”
2. Continue to refine parameters
Think of the first model as a rough draft. Once you have an initial model delivering results, it’s important to compare it to reality and find ways to make the results even better.
3. Be consistent
Don’t just turn to analytics when faced with an urgent problem. If you make data mining and analysis a part of your daily operations, you’ll be in a much better position to actually leverage this strategy when the time comes.
Frequently Asked Questions (FAQ) about analytical modeling
What are the common forms of analytical models.
There are four main types of models: descriptive, diagnostic, predictive and prescriptive. The right one to use depends on the kind of question you need an answer to.
How do you make an analytical model?
Modeling requires access to a full set of relevant data points, relationship conditions and project objectives. For example, when trying to predict the outcome of a certain situation, modelers need to account for every factor that can impact this outcome and understand how each one of those factors influences the results as well as other variables in the calculation in a quantifiable way.
What is the purpose of analytical models?
The purpose of analytical modeling is to make sense of a process or situation that has too many variables to estimate accurately. It’s particularly important when dealing with larger operations and processes.
Managing with models
Companies survived for hundreds of years without computing technology to help them do complex modeling. However, that doesn’t mean you will be fine without it. The data revolution has already happened and the capabilities it offers companies can’t be ignored. Business leaders in every industry should be moving modeling to the center of their management practices if they are serious about growing in the years ahead.
About the Author
iSixSigma Staff
Have a language expert improve your writing
Run a free plagiarism check in 10 minutes, generate accurate citations for free.
- Knowledge Base
Methodology
Research Methods | Definitions, Types, Examples
Research methods are specific procedures for collecting and analyzing data. Developing your research methods is an integral part of your research design . When planning your methods, there are two key decisions you will make.
First, decide how you will collect data . Your methods depend on what type of data you need to answer your research question :
- Qualitative vs. quantitative : Will your data take the form of words or numbers?
- Primary vs. secondary : Will you collect original data yourself, or will you use data that has already been collected by someone else?
- Descriptive vs. experimental : Will you take measurements of something as it is, or will you perform an experiment?
Second, decide how you will analyze the data .
- For quantitative data, you can use statistical analysis methods to test relationships between variables.
- For qualitative data, you can use methods such as thematic analysis to interpret patterns and meanings in the data.
Table of contents
Methods for collecting data, examples of data collection methods, methods for analyzing data, examples of data analysis methods, other interesting articles, frequently asked questions about research methods.
Data is the information that you collect for the purposes of answering your research question . The type of data you need depends on the aims of your research.
Qualitative vs. quantitative data
Your choice of qualitative or quantitative data collection depends on the type of knowledge you want to develop.
For questions about ideas, experiences and meanings, or to study something that can’t be described numerically, collect qualitative data .
If you want to develop a more mechanistic understanding of a topic, or your research involves hypothesis testing , collect quantitative data .
You can also take a mixed methods approach , where you use both qualitative and quantitative research methods.
Primary vs. secondary research
Primary research is any original data that you collect yourself for the purposes of answering your research question (e.g. through surveys , observations and experiments ). Secondary research is data that has already been collected by other researchers (e.g. in a government census or previous scientific studies).
If you are exploring a novel research question, you’ll probably need to collect primary data . But if you want to synthesize existing knowledge, analyze historical trends, or identify patterns on a large scale, secondary data might be a better choice.
Descriptive vs. experimental data
In descriptive research , you collect data about your study subject without intervening. The validity of your research will depend on your sampling method .
In experimental research , you systematically intervene in a process and measure the outcome. The validity of your research will depend on your experimental design .
To conduct an experiment, you need to be able to vary your independent variable , precisely measure your dependent variable, and control for confounding variables . If it’s practically and ethically possible, this method is the best choice for answering questions about cause and effect.
Receive feedback on language, structure, and formatting
Professional editors proofread and edit your paper by focusing on:
- Academic style
- Vague sentences
- Style consistency
See an example
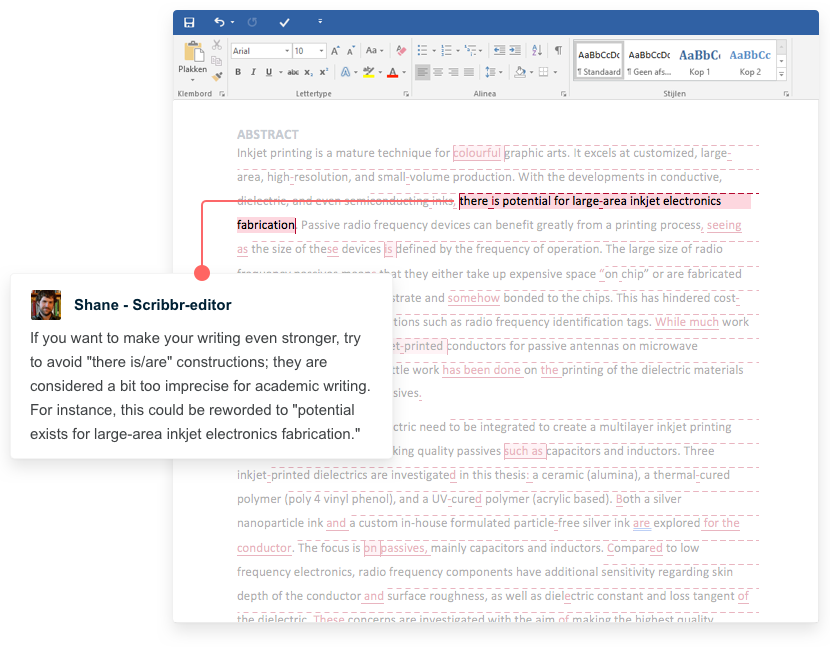
Your data analysis methods will depend on the type of data you collect and how you prepare it for analysis.
Data can often be analyzed both quantitatively and qualitatively. For example, survey responses could be analyzed qualitatively by studying the meanings of responses or quantitatively by studying the frequencies of responses.
Qualitative analysis methods
Qualitative analysis is used to understand words, ideas, and experiences. You can use it to interpret data that was collected:
- From open-ended surveys and interviews , literature reviews , case studies , ethnographies , and other sources that use text rather than numbers.
- Using non-probability sampling methods .
Qualitative analysis tends to be quite flexible and relies on the researcher’s judgement, so you have to reflect carefully on your choices and assumptions and be careful to avoid research bias .
Quantitative analysis methods
Quantitative analysis uses numbers and statistics to understand frequencies, averages and correlations (in descriptive studies) or cause-and-effect relationships (in experiments).
You can use quantitative analysis to interpret data that was collected either:
- During an experiment .
- Using probability sampling methods .
Because the data is collected and analyzed in a statistically valid way, the results of quantitative analysis can be easily standardized and shared among researchers.
Prevent plagiarism. Run a free check.
If you want to know more about statistics , methodology , or research bias , make sure to check out some of our other articles with explanations and examples.
- Chi square test of independence
- Statistical power
- Descriptive statistics
- Degrees of freedom
- Pearson correlation
- Null hypothesis
- Double-blind study
- Case-control study
- Research ethics
- Data collection
- Hypothesis testing
- Structured interviews
Research bias
- Hawthorne effect
- Unconscious bias
- Recall bias
- Halo effect
- Self-serving bias
- Information bias
Quantitative research deals with numbers and statistics, while qualitative research deals with words and meanings.
Quantitative methods allow you to systematically measure variables and test hypotheses . Qualitative methods allow you to explore concepts and experiences in more detail.
In mixed methods research , you use both qualitative and quantitative data collection and analysis methods to answer your research question .
A sample is a subset of individuals from a larger population . Sampling means selecting the group that you will actually collect data from in your research. For example, if you are researching the opinions of students in your university, you could survey a sample of 100 students.
In statistics, sampling allows you to test a hypothesis about the characteristics of a population.
The research methods you use depend on the type of data you need to answer your research question .
- If you want to measure something or test a hypothesis , use quantitative methods . If you want to explore ideas, thoughts and meanings, use qualitative methods .
- If you want to analyze a large amount of readily-available data, use secondary data. If you want data specific to your purposes with control over how it is generated, collect primary data.
- If you want to establish cause-and-effect relationships between variables , use experimental methods. If you want to understand the characteristics of a research subject, use descriptive methods.
Methodology refers to the overarching strategy and rationale of your research project . It involves studying the methods used in your field and the theories or principles behind them, in order to develop an approach that matches your objectives.
Methods are the specific tools and procedures you use to collect and analyze data (for example, experiments, surveys , and statistical tests ).
In shorter scientific papers, where the aim is to report the findings of a specific study, you might simply describe what you did in a methods section .
In a longer or more complex research project, such as a thesis or dissertation , you will probably include a methodology section , where you explain your approach to answering the research questions and cite relevant sources to support your choice of methods.
Is this article helpful?
Other students also liked, writing strong research questions | criteria & examples.
- What Is a Research Design | Types, Guide & Examples
- Data Collection | Definition, Methods & Examples
More interesting articles
- Between-Subjects Design | Examples, Pros, & Cons
- Cluster Sampling | A Simple Step-by-Step Guide with Examples
- Confounding Variables | Definition, Examples & Controls
- Construct Validity | Definition, Types, & Examples
- Content Analysis | Guide, Methods & Examples
- Control Groups and Treatment Groups | Uses & Examples
- Control Variables | What Are They & Why Do They Matter?
- Correlation vs. Causation | Difference, Designs & Examples
- Correlational Research | When & How to Use
- Critical Discourse Analysis | Definition, Guide & Examples
- Cross-Sectional Study | Definition, Uses & Examples
- Descriptive Research | Definition, Types, Methods & Examples
- Ethical Considerations in Research | Types & Examples
- Explanatory and Response Variables | Definitions & Examples
- Explanatory Research | Definition, Guide, & Examples
- Exploratory Research | Definition, Guide, & Examples
- External Validity | Definition, Types, Threats & Examples
- Extraneous Variables | Examples, Types & Controls
- Guide to Experimental Design | Overview, Steps, & Examples
- How Do You Incorporate an Interview into a Dissertation? | Tips
- How to Do Thematic Analysis | Step-by-Step Guide & Examples
- How to Write a Literature Review | Guide, Examples, & Templates
- How to Write a Strong Hypothesis | Steps & Examples
- Inclusion and Exclusion Criteria | Examples & Definition
- Independent vs. Dependent Variables | Definition & Examples
- Inductive Reasoning | Types, Examples, Explanation
- Inductive vs. Deductive Research Approach | Steps & Examples
- Internal Validity in Research | Definition, Threats, & Examples
- Internal vs. External Validity | Understanding Differences & Threats
- Longitudinal Study | Definition, Approaches & Examples
- Mediator vs. Moderator Variables | Differences & Examples
- Mixed Methods Research | Definition, Guide & Examples
- Multistage Sampling | Introductory Guide & Examples
- Naturalistic Observation | Definition, Guide & Examples
- Operationalization | A Guide with Examples, Pros & Cons
- Population vs. Sample | Definitions, Differences & Examples
- Primary Research | Definition, Types, & Examples
- Qualitative vs. Quantitative Research | Differences, Examples & Methods
- Quasi-Experimental Design | Definition, Types & Examples
- Questionnaire Design | Methods, Question Types & Examples
- Random Assignment in Experiments | Introduction & Examples
- Random vs. Systematic Error | Definition & Examples
- Reliability vs. Validity in Research | Difference, Types and Examples
- Reproducibility vs Replicability | Difference & Examples
- Reproducibility vs. Replicability | Difference & Examples
- Sampling Methods | Types, Techniques & Examples
- Semi-Structured Interview | Definition, Guide & Examples
- Simple Random Sampling | Definition, Steps & Examples
- Single, Double, & Triple Blind Study | Definition & Examples
- Stratified Sampling | Definition, Guide & Examples
- Structured Interview | Definition, Guide & Examples
- Survey Research | Definition, Examples & Methods
- Systematic Review | Definition, Example, & Guide
- Systematic Sampling | A Step-by-Step Guide with Examples
- Textual Analysis | Guide, 3 Approaches & Examples
- The 4 Types of Reliability in Research | Definitions & Examples
- The 4 Types of Validity in Research | Definitions & Examples
- Transcribing an Interview | 5 Steps & Transcription Software
- Triangulation in Research | Guide, Types, Examples
- Types of Interviews in Research | Guide & Examples
- Types of Research Designs Compared | Guide & Examples
- Types of Variables in Research & Statistics | Examples
- Unstructured Interview | Definition, Guide & Examples
- What Is a Case Study? | Definition, Examples & Methods
- What Is a Case-Control Study? | Definition & Examples
- What Is a Cohort Study? | Definition & Examples
- What Is a Conceptual Framework? | Tips & Examples
- What Is a Controlled Experiment? | Definitions & Examples
- What Is a Double-Barreled Question?
- What Is a Focus Group? | Step-by-Step Guide & Examples
- What Is a Likert Scale? | Guide & Examples
- What Is a Prospective Cohort Study? | Definition & Examples
- What Is a Retrospective Cohort Study? | Definition & Examples
- What Is Action Research? | Definition & Examples
- What Is an Observational Study? | Guide & Examples
- What Is Concurrent Validity? | Definition & Examples
- What Is Content Validity? | Definition & Examples
- What Is Convenience Sampling? | Definition & Examples
- What Is Convergent Validity? | Definition & Examples
- What Is Criterion Validity? | Definition & Examples
- What Is Data Cleansing? | Definition, Guide & Examples
- What Is Deductive Reasoning? | Explanation & Examples
- What Is Discriminant Validity? | Definition & Example
- What Is Ecological Validity? | Definition & Examples
- What Is Ethnography? | Definition, Guide & Examples
- What Is Face Validity? | Guide, Definition & Examples
- What Is Non-Probability Sampling? | Types & Examples
- What Is Participant Observation? | Definition & Examples
- What Is Peer Review? | Types & Examples
- What Is Predictive Validity? | Examples & Definition
- What Is Probability Sampling? | Types & Examples
- What Is Purposive Sampling? | Definition & Examples
- What Is Qualitative Observation? | Definition & Examples
- What Is Qualitative Research? | Methods & Examples
- What Is Quantitative Observation? | Definition & Examples
- What Is Quantitative Research? | Definition, Uses & Methods
Unlimited Academic AI-Proofreading
✔ Document error-free in 5minutes ✔ Unlimited document corrections ✔ Specialized in correcting academic texts
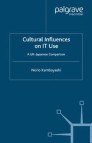
Cultural Influences on IT Use pp 35–73 Cite as
The Analytical Model and Research Methods
- Norio Kambayashi
18 Accesses
The preceding chapter has shown how the concepts of IT use and national culture have been used in an organisational context in previous studies. It has illuminated to what degree previous studies have clarified these concepts and what kinds of drawbacks have been involved in them. Based on this review, this chapter provides explanations for the analytical framework and the research methods used in our study. I will begin by investigating what kinds of research approach are available in the discipline of IT/IS and which is appropriate for the purpose of this study. My analysis will make clear that the survey approach is well-suited for the topic being pursued. I will discuss how I approach IT use in an organisational setting and the analytical levels of IT use which are to be examined, and in the following section focus on how to operationalise national culture in the study including a review and discussion of some previous studies on conceptualisation and operationalisation of national culture. Then, specifying patterns of IT use which are sensitive to cultural influences, I develop a model of cultural influences on organisational IT use. The whole analytical framework used for the study is presented, and explanations of methodological details follow. Some preliminary work for the field study and the pilot study done before the survey are also given and the chosen techniques of data collection and the structure of the collected data are illustrated in the final section.
This is a preview of subscription content, log in via an institution .
Buying options
- Available as PDF
- Read on any device
- Instant download
- Own it forever
- Compact, lightweight edition
- Dispatched in 3 to 5 business days
- Free shipping worldwide - see info
- Durable hardcover edition
Tax calculation will be finalised at checkout
Purchases are for personal use only
Unable to display preview. Download preview PDF.
You can also search for this author in PubMed Google Scholar
Copyright information
© 2003 Norio Kambayashi
About this chapter
Cite this chapter.
Kambayashi, N. (2003). The Analytical Model and Research Methods. In: Cultural Influences on IT Use. Palgrave Macmillan, London. https://doi.org/10.1057/9780230511118_3
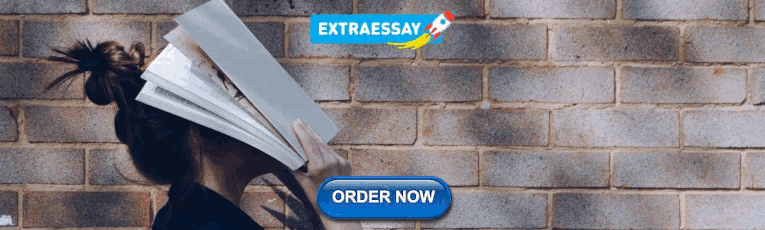
Download citation
DOI : https://doi.org/10.1057/9780230511118_3
Publisher Name : Palgrave Macmillan, London
Print ISBN : 978-1-349-50772-6
Online ISBN : 978-0-230-51111-8
eBook Packages : Palgrave Business & Management Collection Business and Management (R0)
Share this chapter
Anyone you share the following link with will be able to read this content:
Sorry, a shareable link is not currently available for this article.
Provided by the Springer Nature SharedIt content-sharing initiative
- Publish with us
Policies and ethics
- Find a journal
- Track your research

An official website of the United States government
The .gov means it’s official. Federal government websites often end in .gov or .mil. Before sharing sensitive information, make sure you’re on a federal government site.
The site is secure. The https:// ensures that you are connecting to the official website and that any information you provide is encrypted and transmitted securely.
- Publications
- Account settings
Preview improvements coming to the PMC website in October 2024. Learn More or Try it out now .
- Advanced Search
- Journal List
- Springer Nature - PMC COVID-19 Collection

Data Science and Analytics: An Overview from Data-Driven Smart Computing, Decision-Making and Applications Perspective
Iqbal h. sarker.
1 Swinburne University of Technology, Melbourne, VIC 3122 Australia
2 Department of Computer Science and Engineering, Chittagong University of Engineering & Technology, Chittagong, 4349 Bangladesh
The digital world has a wealth of data, such as internet of things (IoT) data, business data, health data, mobile data, urban data, security data, and many more, in the current age of the Fourth Industrial Revolution (Industry 4.0 or 4IR). Extracting knowledge or useful insights from these data can be used for smart decision-making in various applications domains. In the area of data science, advanced analytics methods including machine learning modeling can provide actionable insights or deeper knowledge about data, which makes the computing process automatic and smart. In this paper, we present a comprehensive view on “Data Science” including various types of advanced analytics methods that can be applied to enhance the intelligence and capabilities of an application through smart decision-making in different scenarios. We also discuss and summarize ten potential real-world application domains including business, healthcare, cybersecurity, urban and rural data science, and so on by taking into account data-driven smart computing and decision making. Based on this, we finally highlight the challenges and potential research directions within the scope of our study. Overall, this paper aims to serve as a reference point on data science and advanced analytics to the researchers and decision-makers as well as application developers, particularly from the data-driven solution point of view for real-world problems.
Introduction
We are living in the age of “data science and advanced analytics”, where almost everything in our daily lives is digitally recorded as data [ 17 ]. Thus the current electronic world is a wealth of various kinds of data, such as business data, financial data, healthcare data, multimedia data, internet of things (IoT) data, cybersecurity data, social media data, etc [ 112 ]. The data can be structured, semi-structured, or unstructured, which increases day by day [ 105 ]. Data science is typically a “concept to unify statistics, data analysis, and their related methods” to understand and analyze the actual phenomena with data. According to Cao et al. [ 17 ] “data science is the science of data” or “data science is the study of data”, where a data product is a data deliverable, or data-enabled or guided, which can be a discovery, prediction, service, suggestion, insight into decision-making, thought, model, paradigm, tool, or system. The popularity of “Data science” is increasing day-by-day, which is shown in Fig. Fig.1 1 according to Google Trends data over the last 5 years [ 36 ]. In addition to data science, we have also shown the popularity trends of the relevant areas such as “Data analytics”, “Data mining”, “Big data”, “Machine learning” in the figure. According to Fig. Fig.1, 1 , the popularity indication values for these data-driven domains, particularly “Data science”, and “Machine learning” are increasing day-by-day. This statistical information and the applicability of the data-driven smart decision-making in various real-world application areas, motivate us to study briefly on “Data science” and machine-learning-based “Advanced analytics” in this paper.

The worldwide popularity score of data science comparing with relevant areas in a range of 0 (min) to 100 (max) over time where x -axis represents the timestamp information and y -axis represents the corresponding score
Usually, data science is the field of applying advanced analytics methods and scientific concepts to derive useful business information from data. The emphasis of advanced analytics is more on anticipating the use of data to detect patterns to determine what is likely to occur in the future. Basic analytics offer a description of data in general, while advanced analytics is a step forward in offering a deeper understanding of data and helping to analyze granular data, which we are interested in. In the field of data science, several types of analytics are popular, such as "Descriptive analytics" which answers the question of what happened; "Diagnostic analytics" which answers the question of why did it happen; "Predictive analytics" which predicts what will happen in the future; and "Prescriptive analytics" which prescribes what action should be taken, discussed briefly in “ Advanced analytics methods and smart computing ”. Such advanced analytics and decision-making based on machine learning techniques [ 105 ], a major part of artificial intelligence (AI) [ 102 ] can also play a significant role in the Fourth Industrial Revolution (Industry 4.0) due to its learning capability for smart computing as well as automation [ 121 ].
Although the area of “data science” is huge, we mainly focus on deriving useful insights through advanced analytics, where the results are used to make smart decisions in various real-world application areas. For this, various advanced analytics methods such as machine learning modeling, natural language processing, sentiment analysis, neural network, or deep learning analysis can provide deeper knowledge about data, and thus can be used to develop data-driven intelligent applications. More specifically, regression analysis, classification, clustering analysis, association rules, time-series analysis, sentiment analysis, behavioral patterns, anomaly detection, factor analysis, log analysis, and deep learning which is originated from the artificial neural network, are taken into account in our study. These machine learning-based advanced analytics methods are discussed briefly in “ Advanced analytics methods and smart computing ”. Thus, it’s important to understand the principles of various advanced analytics methods mentioned above and their applicability to apply in various real-world application areas. For instance, in our earlier paper Sarker et al. [ 114 ], we have discussed how data science and machine learning modeling can play a significant role in the domain of cybersecurity for making smart decisions and to provide data-driven intelligent security services. In this paper, we broadly take into account the data science application areas and real-world problems in ten potential domains including the area of business data science, health data science, IoT data science, behavioral data science, urban data science, and so on, discussed briefly in “ Real-world application domains ”.
Based on the importance of machine learning modeling to extract the useful insights from the data mentioned above and data-driven smart decision-making, in this paper, we present a comprehensive view on “Data Science” including various types of advanced analytics methods that can be applied to enhance the intelligence and the capabilities of an application. The key contribution of this study is thus understanding data science modeling, explaining different analytic methods for solution perspective and their applicability in various real-world data-driven applications areas mentioned earlier. Overall, the purpose of this paper is, therefore, to provide a basic guide or reference for those academia and industry people who want to study, research, and develop automated and intelligent applications or systems based on smart computing and decision making within the area of data science.
The main contributions of this paper are summarized as follows:
- To define the scope of our study towards data-driven smart computing and decision-making in our real-world life. We also make a brief discussion on the concept of data science modeling from business problems to data product and automation, to understand its applicability and provide intelligent services in real-world scenarios.
- To provide a comprehensive view on data science including advanced analytics methods that can be applied to enhance the intelligence and the capabilities of an application.
- To discuss the applicability and significance of machine learning-based analytics methods in various real-world application areas. We also summarize ten potential real-world application areas, from business to personalized applications in our daily life, where advanced analytics with machine learning modeling can be used to achieve the expected outcome.
- To highlight and summarize the challenges and potential research directions within the scope of our study.
The rest of the paper is organized as follows. The next section provides the background and related work and defines the scope of our study. The following section presents the concepts of data science modeling for building a data-driven application. After that, briefly discuss and explain different advanced analytics methods and smart computing. Various real-world application areas are discussed and summarized in the next section. We then highlight and summarize several research issues and potential future directions, and finally, the last section concludes this paper.
Background and Related Work
In this section, we first discuss various data terms and works related to data science and highlight the scope of our study.
Data Terms and Definitions
There is a range of key terms in the field, such as data analysis, data mining, data analytics, big data, data science, advanced analytics, machine learning, and deep learning, which are highly related and easily confusing. In the following, we define these terms and differentiate them with the term “Data Science” according to our goal.
The term “Data analysis” refers to the processing of data by conventional (e.g., classic statistical, empirical, or logical) theories, technologies, and tools for extracting useful information and for practical purposes [ 17 ]. The term “Data analytics”, on the other hand, refers to the theories, technologies, instruments, and processes that allow for an in-depth understanding and exploration of actionable data insight [ 17 ]. Statistical and mathematical analysis of the data is the major concern in this process. “Data mining” is another popular term over the last decade, which has a similar meaning with several other terms such as knowledge mining from data, knowledge extraction, knowledge discovery from data (KDD), data/pattern analysis, data archaeology, and data dredging. According to Han et al. [ 38 ], it should have been more appropriately named “knowledge mining from data”. Overall, data mining is defined as the process of discovering interesting patterns and knowledge from large amounts of data [ 38 ]. Data sources may include databases, data centers, the Internet or Web, other repositories of data, or data dynamically streamed through the system. “Big data” is another popular term nowadays, which may change the statistical and data analysis approaches as it has the unique features of “massive, high dimensional, heterogeneous, complex, unstructured, incomplete, noisy, and erroneous” [ 74 ]. Big data can be generated by mobile devices, social networks, the Internet of Things, multimedia, and many other new applications [ 129 ]. Several unique features including volume, velocity, variety, veracity, value (5Vs), and complexity are used to understand and describe big data [ 69 ].
In terms of analytics, basic analytics provides a summary of data whereas the term “Advanced Analytics” takes a step forward in offering a deeper understanding of data and helps to analyze granular data. Advanced analytics is characterized or defined as autonomous or semi-autonomous data or content analysis using advanced techniques and methods to discover deeper insights, predict or generate recommendations, typically beyond traditional business intelligence or analytics. “Machine learning”, a branch of artificial intelligence (AI), is one of the major techniques used in advanced analytics which can automate analytical model building [ 112 ]. This is focused on the premise that systems can learn from data, recognize trends, and make decisions, with minimal human involvement [ 38 , 115 ]. “Deep Learning” is a subfield of machine learning that discusses algorithms inspired by the human brain’s structure and the function called artificial neural networks [ 38 , 139 ].
Unlike the above data-related terms, “Data science” is an umbrella term that encompasses advanced data analytics, data mining, machine, and deep learning modeling, and several other related disciplines like statistics, to extract insights or useful knowledge from the datasets and transform them into actionable business strategies. In [ 17 ], Cao et al. defined data science from the disciplinary perspective as “data science is a new interdisciplinary field that synthesizes and builds on statistics, informatics, computing, communication, management, and sociology to study data and its environments (including domains and other contextual aspects, such as organizational and social aspects) to transform data to insights and decisions by following a data-to-knowledge-to-wisdom thinking and methodology”. In “ Understanding data science modeling ”, we briefly discuss the data science modeling from a practical perspective starting from business problems to data products that can assist the data scientists to think and work in a particular real-world problem domain within the area of data science and analytics.
Related Work
In the area, several papers have been reviewed by the researchers based on data science and its significance. For example, the authors in [ 19 ] identify the evolving field of data science and its importance in the broader knowledge environment and some issues that differentiate data science and informatics issues from conventional approaches in information sciences. Donoho et al. [ 27 ] present 50 years of data science including recent commentary on data science in mass media, and on how/whether data science varies from statistics. The authors formally conceptualize the theory-guided data science (TGDS) model in [ 53 ] and present a taxonomy of research themes in TGDS. Cao et al. include a detailed survey and tutorial on the fundamental aspects of data science in [ 17 ], which considers the transition from data analysis to data science, the principles of data science, as well as the discipline and competence of data education.
Besides, the authors include a data science analysis in [ 20 ], which aims to provide a realistic overview of the use of statistical features and related data science methods in bioimage informatics. The authors in [ 61 ] study the key streams of data science algorithm use at central banks and show how their popularity has risen over time. This research contributes to the creation of a research vector on the role of data science in central banking. In [ 62 ], the authors provide an overview and tutorial on the data-driven design of intelligent wireless networks. The authors in [ 87 ] provide a thorough understanding of computational optimal transport with application to data science. In [ 97 ], the authors present data science as theoretical contributions in information systems via text analytics.
Unlike the above recent studies, in this paper, we concentrate on the knowledge of data science including advanced analytics methods, machine learning modeling, real-world application domains, and potential research directions within the scope of our study. The advanced analytics methods based on machine learning techniques discussed in this paper can be applied to enhance the capabilities of an application in terms of data-driven intelligent decision making and automation in the final data product or systems.
Understanding Data Science Modeling
In this section, we briefly discuss how data science can play a significant role in the real-world business process. For this, we first categorize various types of data and then discuss the major steps of data science modeling starting from business problems to data product and automation.
Types of Real-World Data
Typically, to build a data-driven real-world system in a particular domain, the availability of data is the key [ 17 , 112 , 114 ]. The data can be in different types such as (i) Structured—that has a well-defined data structure and follows a standard order, examples are names, dates, addresses, credit card numbers, stock information, geolocation, etc.; (ii) Unstructured—has no pre-defined format or organization, examples are sensor data, emails, blog entries, wikis, and word processing documents, PDF files, audio files, videos, images, presentations, web pages, etc.; (iii) Semi-structured—has elements of both the structured and unstructured data containing certain organizational properties, examples are HTML, XML, JSON documents, NoSQL databases, etc.; and (iv) Metadata—that represents data about the data, examples are author, file type, file size, creation date and time, last modification date and time, etc. [ 38 , 105 ].
In the area of data science, researchers use various widely-used datasets for different purposes. These are, for example, cybersecurity datasets such as NSL-KDD [ 127 ], UNSW-NB15 [ 79 ], Bot-IoT [ 59 ], ISCX’12 [ 15 ], CIC-DDoS2019 [ 22 ], etc., smartphone datasets such as phone call logs [ 88 , 110 ], mobile application usages logs [ 124 , 149 ], SMS Log [ 28 ], mobile phone notification logs [ 77 ] etc., IoT data [ 56 , 11 , 64 ], health data such as heart disease [ 99 ], diabetes mellitus [ 86 , 147 ], COVID-19 [ 41 , 78 ], etc., agriculture and e-commerce data [ 128 , 150 ], and many more in various application domains. In “ Real-world application domains ”, we discuss ten potential real-world application domains of data science and analytics by taking into account data-driven smart computing and decision making, which can help the data scientists and application developers to explore more in various real-world issues.
Overall, the data used in data-driven applications can be any of the types mentioned above, and they can differ from one application to another in the real world. Data science modeling, which is briefly discussed below, can be used to analyze such data in a specific problem domain and derive insights or useful information from the data to build a data-driven model or data product.
Steps of Data Science Modeling
Data science is typically an umbrella term that encompasses advanced data analytics, data mining, machine, and deep learning modeling, and several other related disciplines like statistics, to extract insights or useful knowledge from the datasets and transform them into actionable business strategies, mentioned earlier in “ Background and related work ”. In this section, we briefly discuss how data science can play a significant role in the real-world business process. Figure Figure2 2 shows an example of data science modeling starting from real-world data to data-driven product and automation. In the following, we briefly discuss each module of the data science process.
- Understanding business problems: This involves getting a clear understanding of the problem that is needed to solve, how it impacts the relevant organization or individuals, the ultimate goals for addressing it, and the relevant project plan. Thus to understand and identify the business problems, the data scientists formulate relevant questions while working with the end-users and other stakeholders. For instance, how much/many, which category/group, is the behavior unrealistic/abnormal, which option should be taken, what action, etc. could be relevant questions depending on the nature of the problems. This helps to get a better idea of what business needs and what we should be extracted from data. Such business knowledge can enable organizations to enhance their decision-making process, is known as “Business Intelligence” [ 65 ]. Identifying the relevant data sources that can help to answer the formulated questions and what kinds of actions should be taken from the trends that the data shows, is another important task associated with this stage. Once the business problem has been clearly stated, the data scientist can define the analytic approach to solve the problem.
- Understanding data: As we know that data science is largely driven by the availability of data [ 114 ]. Thus a sound understanding of the data is needed towards a data-driven model or system. The reason is that real-world data sets are often noisy, missing values, have inconsistencies, or other data issues, which are needed to handle effectively [ 101 ]. To gain actionable insights, the appropriate data or the quality of the data must be sourced and cleansed, which is fundamental to any data science engagement. For this, data assessment that evaluates what data is available and how it aligns to the business problem could be the first step in data understanding. Several aspects such as data type/format, the quantity of data whether it is sufficient or not to extract the useful knowledge, data relevance, authorized access to data, feature or attribute importance, combining multiple data sources, important metrics to report the data, etc. are needed to take into account to clearly understand the data for a particular business problem. Overall, the data understanding module involves figuring out what data would be best needed and the best ways to acquire it.
- Data pre-processing and exploration: Exploratory data analysis is defined in data science as an approach to analyzing datasets to summarize their key characteristics, often with visual methods [ 135 ]. This examines a broad data collection to discover initial trends, attributes, points of interest, etc. in an unstructured manner to construct meaningful summaries of the data. Thus data exploration is typically used to figure out the gist of data and to develop a first step assessment of its quality, quantity, and characteristics. A statistical model can be used or not, but primarily it offers tools for creating hypotheses by generally visualizing and interpreting the data through graphical representation such as a chart, plot, histogram, etc [ 72 , 91 ]. Before the data is ready for modeling, it’s necessary to use data summarization and visualization to audit the quality of the data and provide the information needed to process it. To ensure the quality of the data, the data pre-processing technique, which is typically the process of cleaning and transforming raw data [ 107 ] before processing and analysis is important. It also involves reformatting information, making data corrections, and merging data sets to enrich data. Thus, several aspects such as expected data, data cleaning, formatting or transforming data, dealing with missing values, handling data imbalance and bias issues, data distribution, search for outliers or anomalies in data and dealing with them, ensuring data quality, etc. could be the key considerations in this step.
- Machine learning modeling and evaluation: Once the data is prepared for building the model, data scientists design a model, algorithm, or set of models, to address the business problem. Model building is dependent on what type of analytics, e.g., predictive analytics, is needed to solve the particular problem, which is discussed briefly in “ Advanced analytics methods and smart computing ”. To best fits the data according to the type of analytics, different types of data-driven or machine learning models that have been summarized in our earlier paper Sarker et al. [ 105 ], can be built to achieve the goal. Data scientists typically separate training and test subsets of the given dataset usually dividing in the ratio of 80:20 or data considering the most popular k -folds data splitting method [ 38 ]. This is to observe whether the model performs well or not on the data, to maximize the model performance. Various model validation and assessment metrics, such as error rate, accuracy, true positive, false positive, true negative, false negative, precision, recall, f-score, ROC (receiver operating characteristic curve) analysis, applicability analysis, etc. [ 38 , 115 ] are used to measure the model performance, which can guide the data scientists to choose or design the learning method or model. Besides, machine learning experts or data scientists can take into account several advanced analytics such as feature engineering, feature selection or extraction methods, algorithm tuning, ensemble methods, modifying existing algorithms, or designing new algorithms, etc. to improve the ultimate data-driven model to solve a particular business problem through smart decision making.
- Data product and automation: A data product is typically the output of any data science activity [ 17 ]. A data product, in general terms, is a data deliverable, or data-enabled or guide, which can be a discovery, prediction, service, suggestion, insight into decision-making, thought, model, paradigm, tool, application, or system that process data and generate results. Businesses can use the results of such data analysis to obtain useful information like churn (a measure of how many customers stop using a product) prediction and customer segmentation, and use these results to make smarter business decisions and automation. Thus to make better decisions in various business problems, various machine learning pipelines and data products can be developed. To highlight this, we summarize several potential real-world data science application areas in “ Real-world application domains ”, where various data products can play a significant role in relevant business problems to make them smart and automate.
Overall, we can conclude that data science modeling can be used to help drive changes and improvements in business practices. The interesting part of the data science process indicates having a deeper understanding of the business problem to solve. Without that, it would be much harder to gather the right data and extract the most useful information from the data for making decisions to solve the problem. In terms of role, “Data Scientists” typically interpret and manage data to uncover the answers to major questions that help organizations to make objective decisions and solve complex problems. In a summary, a data scientist proactively gathers and analyzes information from multiple sources to better understand how the business performs, and designs machine learning or data-driven tools/methods, or algorithms, focused on advanced analytics, which can make today’s computing process smarter and intelligent, discussed briefly in the following section.

An example of data science modeling from real-world data to data-driven system and decision making
Advanced Analytics Methods and Smart Computing
As mentioned earlier in “ Background and related work ”, basic analytics provides a summary of data whereas advanced analytics takes a step forward in offering a deeper understanding of data and helps in granular data analysis. For instance, the predictive capabilities of advanced analytics can be used to forecast trends, events, and behaviors. Thus, “advanced analytics” can be defined as the autonomous or semi-autonomous analysis of data or content using advanced techniques and methods to discover deeper insights, make predictions, or produce recommendations, where machine learning-based analytical modeling is considered as the key technologies in the area. In the following section, we first summarize various types of analytics and outcome that are needed to solve the associated business problems, and then we briefly discuss machine learning-based analytical modeling.
Types of Analytics and Outcome
In the real-world business process, several key questions such as “What happened?”, “Why did it happen?”, “What will happen in the future?”, “What action should be taken?” are common and important. Based on these questions, in this paper, we categorize and highlight the analytics into four types such as descriptive, diagnostic, predictive, and prescriptive, which are discussed below.
- Descriptive analytics: It is the interpretation of historical data to better understand the changes that have occurred in a business. Thus descriptive analytics answers the question, “what happened in the past?” by summarizing past data such as statistics on sales and operations or marketing strategies, use of social media, and engagement with Twitter, Linkedin or Facebook, etc. For instance, using descriptive analytics through analyzing trends, patterns, and anomalies, etc., customers’ historical shopping data can be used to predict the probability of a customer purchasing a product. Thus, descriptive analytics can play a significant role to provide an accurate picture of what has occurred in a business and how it relates to previous times utilizing a broad range of relevant business data. As a result, managers and decision-makers can pinpoint areas of strength and weakness in their business, and eventually can take more effective management strategies and business decisions.
- Diagnostic analytics: It is a form of advanced analytics that examines data or content to answer the question, “why did it happen?” The goal of diagnostic analytics is to help to find the root cause of the problem. For example, the human resource management department of a business organization may use these diagnostic analytics to find the best applicant for a position, select them, and compare them to other similar positions to see how well they perform. In a healthcare example, it might help to figure out whether the patients’ symptoms such as high fever, dry cough, headache, fatigue, etc. are all caused by the same infectious agent. Overall, diagnostic analytics enables one to extract value from the data by posing the right questions and conducting in-depth investigations into the answers. It is characterized by techniques such as drill-down, data discovery, data mining, and correlations.
- Predictive analytics: Predictive analytics is an important analytical technique used by many organizations for various purposes such as to assess business risks, anticipate potential market patterns, and decide when maintenance is needed, to enhance their business. It is a form of advanced analytics that examines data or content to answer the question, “what will happen in the future?” Thus, the primary goal of predictive analytics is to identify and typically answer this question with a high degree of probability. Data scientists can use historical data as a source to extract insights for building predictive models using various regression analyses and machine learning techniques, which can be used in various application domains for a better outcome. Companies, for example, can use predictive analytics to minimize costs by better anticipating future demand and changing output and inventory, banks and other financial institutions to reduce fraud and risks by predicting suspicious activity, medical specialists to make effective decisions through predicting patients who are at risk of diseases, retailers to increase sales and customer satisfaction through understanding and predicting customer preferences, manufacturers to optimize production capacity through predicting maintenance requirements, and many more. Thus predictive analytics can be considered as the core analytical method within the area of data science.
- Prescriptive analytics: Prescriptive analytics focuses on recommending the best way forward with actionable information to maximize overall returns and profitability, which typically answer the question, “what action should be taken?” In business analytics, prescriptive analytics is considered the final step. For its models, prescriptive analytics collects data from several descriptive and predictive sources and applies it to the decision-making process. Thus, we can say that it is related to both descriptive analytics and predictive analytics, but it emphasizes actionable insights instead of data monitoring. In other words, it can be considered as the opposite of descriptive analytics, which examines decisions and outcomes after the fact. By integrating big data, machine learning, and business rules, prescriptive analytics helps organizations to make more informed decisions to produce results that drive the most successful business decisions.
In summary, to clarify what happened and why it happened, both descriptive analytics and diagnostic analytics look at the past. Historical data is used by predictive analytics and prescriptive analytics to forecast what will happen in the future and what steps should be taken to impact those effects. In Table Table1, 1 , we have summarized these analytics methods with examples. Forward-thinking organizations in the real world can jointly use these analytical methods to make smart decisions that help drive changes in business processes and improvements. In the following, we discuss how machine learning techniques can play a big role in these analytical methods through their learning capabilities from the data.
Various types of analytical methods with examples
Machine Learning Based Analytical Modeling
In this section, we briefly discuss various advanced analytics methods based on machine learning modeling, which can make the computing process smart through intelligent decision-making in a business process. Figure Figure3 3 shows a general structure of a machine learning-based predictive modeling considering both the training and testing phase. In the following, we discuss a wide range of methods such as regression and classification analysis, association rule analysis, time-series analysis, behavioral analysis, log analysis, and so on within the scope of our study.

A general structure of a machine learning based predictive model considering both the training and testing phase
Regression Analysis
In data science, one of the most common statistical approaches used for predictive modeling and data mining tasks is regression techniques [ 38 ]. Regression analysis is a form of supervised machine learning that examines the relationship between a dependent variable (target) and independent variables (predictor) to predict continuous-valued output [ 105 , 117 ]. The following equations Eqs. 1 , 2 , and 3 [ 85 , 105 ] represent the simple, multiple or multivariate, and polynomial regressions respectively, where x represents independent variable and y is the predicted/target output mentioned above:
Regression analysis is typically conducted for one of two purposes: to predict the value of the dependent variable in the case of individuals for whom some knowledge relating to the explanatory variables is available, or to estimate the effect of some explanatory variable on the dependent variable, i.e., finding the relationship of causal influence between the variables. Linear regression cannot be used to fit non-linear data and may cause an underfitting problem. In that case, polynomial regression performs better, however, increases the model complexity. The regularization techniques such as Ridge, Lasso, Elastic-Net, etc. [ 85 , 105 ] can be used to optimize the linear regression model. Besides, support vector regression, decision tree regression, random forest regression techniques [ 85 , 105 ] can be used for building effective regression models depending on the problem type, e.g., non-linear tasks. Financial forecasting or prediction, cost estimation, trend analysis, marketing, time-series estimation, drug response modeling, etc. are some examples where the regression models can be used to solve real-world problems in the domain of data science and analytics.
Classification Analysis
Classification is one of the most widely used and best-known data science processes. This is a form of supervised machine learning approach that also refers to a predictive modeling problem in which a class label is predicted for a given example [ 38 ]. Spam identification, such as ‘spam’ and ‘not spam’ in email service providers, can be an example of a classification problem. There are several forms of classification analysis available in the area such as binary classification—which refers to the prediction of one of two classes; multi-class classification—which involves the prediction of one of more than two classes; multi-label classification—a generalization of multiclass classification in which the problem’s classes are organized hierarchically [ 105 ].
Several popular classification techniques, such as k-nearest neighbors [ 5 ], support vector machines [ 55 ], navies Bayes [ 49 ], adaptive boosting [ 32 ], extreme gradient boosting [ 85 ], logistic regression [ 66 ], decision trees ID3 [ 92 ], C4.5 [ 93 ], and random forests [ 13 ] exist to solve classification problems. The tree-based classification technique, e.g., random forest considering multiple decision trees, performs better than others to solve real-world problems in many cases as due to its capability of producing logic rules [ 103 , 115 ]. Figure Figure4 4 shows an example of a random forest structure considering multiple decision trees. In addition, BehavDT recently proposed by Sarker et al. [ 109 ], and IntrudTree [ 106 ] can be used for building effective classification or prediction models in the relevant tasks within the domain of data science and analytics.

An example of a random forest structure considering multiple decision trees
Cluster Analysis
Clustering is a form of unsupervised machine learning technique and is well-known in many data science application areas for statistical data analysis [ 38 ]. Usually, clustering techniques search for the structures inside a dataset and, if the classification is not previously identified, classify homogeneous groups of cases. This means that data points are identical to each other within a cluster, and different from data points in another cluster. Overall, the purpose of cluster analysis is to sort various data points into groups (or clusters) that are homogeneous internally and heterogeneous externally [ 105 ]. To gain insight into how data is distributed in a given dataset or as a preprocessing phase for other algorithms, clustering is often used. Data clustering, for example, assists with customer shopping behavior, sales campaigns, and retention of consumers for retail businesses, anomaly detection, etc.
Many clustering algorithms with the ability to group data have been proposed in machine learning and data science literature [ 98 , 138 , 141 ]. In our earlier paper Sarker et al. [ 105 ], we have summarized this based on several perspectives, such as partitioning methods, density-based methods, hierarchical-based methods, model-based methods, etc. In the literature, the popular K-means [ 75 ], K-Mediods [ 84 ], CLARA [ 54 ] etc. are known as partitioning methods; DBSCAN [ 30 ], OPTICS [ 8 ] etc. are known as density-based methods; single linkage [ 122 ], complete linkage [ 123 ], etc. are known as hierarchical methods. In addition, grid-based clustering methods, such as STING [ 134 ], CLIQUE [ 2 ], etc.; model-based clustering such as neural network learning [ 141 ], GMM [ 94 ], SOM [ 18 , 104 ], etc.; constrained-based methods such as COP K-means [ 131 ], CMWK-Means [ 25 ], etc. are used in the area. Recently, Sarker et al. [ 111 ] proposed a hierarchical clustering method, BOTS [ 111 ] based on bottom-up agglomerative technique for capturing user’s similar behavioral characteristics over time. The key benefit of agglomerative hierarchical clustering is that the tree-structure hierarchy created by agglomerative clustering is more informative than an unstructured set of flat clusters, which can assist in better decision-making in relevant application areas in data science.
Association Rule Analysis
Association rule learning is known as a rule-based machine learning system, an unsupervised learning method is typically used to establish a relationship among variables. This is a descriptive technique often used to analyze large datasets for discovering interesting relationships or patterns. The association learning technique’s main strength is its comprehensiveness, as it produces all associations that meet user-specified constraints including minimum support and confidence value [ 138 ].
Association rules allow a data scientist to identify trends, associations, and co-occurrences between data sets inside large data collections. In a supermarket, for example, associations infer knowledge about the buying behavior of consumers for different items, which helps to change the marketing and sales plan. In healthcare, to better diagnose patients, physicians may use association guidelines. Doctors can assess the conditional likelihood of a given illness by comparing symptom associations in the data from previous cases using association rules and machine learning-based data analysis. Similarly, association rules are useful for consumer behavior analysis and prediction, customer market analysis, bioinformatics, weblog mining, recommendation systems, etc.
Several types of association rules have been proposed in the area, such as frequent pattern based [ 4 , 47 , 73 ], logic-based [ 31 ], tree-based [ 39 ], fuzzy-rules [ 126 ], belief rule [ 148 ] etc. The rule learning techniques such as AIS [ 3 ], Apriori [ 4 ], Apriori-TID and Apriori-Hybrid [ 4 ], FP-Tree [ 39 ], Eclat [ 144 ], RARM [ 24 ] exist to solve the relevant business problems. Apriori [ 4 ] is the most commonly used algorithm for discovering association rules from a given dataset among the association rule learning techniques [ 145 ]. The recent association rule-learning technique ABC-RuleMiner proposed in our earlier paper by Sarker et al. [ 113 ] could give significant results in terms of generating non-redundant rules that can be used for smart decision making according to human preferences, within the area of data science applications.
Time-Series Analysis and Forecasting
A time series is typically a series of data points indexed in time order particularly, by date, or timestamp [ 111 ]. Depending on the frequency, the time-series can be different types such as annually, e.g., annual budget, quarterly, e.g., expenditure, monthly, e.g., air traffic, weekly, e.g., sales quantity, daily, e.g., weather, hourly, e.g., stock price, minute-wise, e.g., inbound calls in a call center, and even second-wise, e.g., web traffic, and so on in relevant domains.
A mathematical method dealing with such time-series data, or the procedure of fitting a time series to a proper model is termed time-series analysis. Many different time series forecasting algorithms and analysis methods can be applied to extract the relevant information. For instance, to do time-series forecasting for future patterns, the autoregressive (AR) model [ 130 ] learns the behavioral trends or patterns of past data. Moving average (MA) [ 40 ] is another simple and common form of smoothing used in time series analysis and forecasting that uses past forecasted errors in a regression-like model to elaborate an averaged trend across the data. The autoregressive moving average (ARMA) [ 12 , 120 ] combines these two approaches, where autoregressive extracts the momentum and pattern of the trend and moving average capture the noise effects. The most popular and frequently used time-series model is the autoregressive integrated moving average (ARIMA) model [ 12 , 120 ]. ARIMA model, a generalization of an ARMA model, is more flexible than other statistical models such as exponential smoothing or simple linear regression. In terms of data, the ARMA model can only be used for stationary time-series data, while the ARIMA model includes the case of non-stationarity as well. Similarly, seasonal autoregressive integrated moving average (SARIMA), autoregressive fractionally integrated moving average (ARFIMA), autoregressive moving average model with exogenous inputs model (ARMAX model) are also used in time-series models [ 120 ].
In addition to the stochastic methods for time-series modeling and forecasting, machine and deep learning-based approach can be used for effective time-series analysis and forecasting. For instance, in our earlier paper, Sarker et al. [ 111 ] present a bottom-up clustering-based time-series analysis to capture the mobile usage behavioral patterns of the users. Figure Figure5 5 shows an example of producing aggregate time segments Seg_i from initial time slices TS_i based on similar behavioral characteristics that are used in our bottom-up clustering approach, where D represents the dominant behavior BH_i of the users, mentioned above [ 111 ]. The authors in [ 118 ], used a long short-term memory (LSTM) model, a kind of recurrent neural network (RNN) deep learning model, in forecasting time-series that outperform traditional approaches such as the ARIMA model. Time-series analysis is commonly used these days in various fields such as financial, manufacturing, business, social media, event data (e.g., clickstreams and system events), IoT and smartphone data, and generally in any applied science and engineering temporal measurement domain. Thus, it covers a wide range of application areas in data science.

An example of producing aggregate time segments from initial time slices based on similar behavioral characteristics
Opinion Mining and Sentiment Analysis
Sentiment analysis or opinion mining is the computational study of the opinions, thoughts, emotions, assessments, and attitudes of people towards entities such as products, services, organizations, individuals, issues, events, topics, and their attributes [ 71 ]. There are three kinds of sentiments: positive, negative, and neutral, along with more extreme feelings such as angry, happy and sad, or interested or not interested, etc. More refined sentiments to evaluate the feelings of individuals in various situations can also be found according to the problem domain.
Although the task of opinion mining and sentiment analysis is very challenging from a technical point of view, it’s very useful in real-world practice. For instance, a business always aims to obtain an opinion from the public or customers about its products and services to refine the business policy as well as a better business decision. It can thus benefit a business to understand the social opinion of their brand, product, or service. Besides, potential customers want to know what consumers believe they have when they use a service or purchase a product. Document-level, sentence level, aspect level, and concept level, are the possible levels of opinion mining in the area [ 45 ].
Several popular techniques such as lexicon-based including dictionary-based and corpus-based methods, machine learning including supervised and unsupervised learning, deep learning, and hybrid methods are used in sentiment analysis-related tasks [ 70 ]. To systematically define, extract, measure, and analyze affective states and subjective knowledge, it incorporates the use of statistics, natural language processing (NLP), machine learning as well as deep learning methods. Sentiment analysis is widely used in many applications, such as reviews and survey data, web and social media, and healthcare content, ranging from marketing and customer support to clinical practice. Thus sentiment analysis has a big influence in many data science applications, where public sentiment is involved in various real-world issues.
Behavioral Data and Cohort Analysis
Behavioral analytics is a recent trend that typically reveals new insights into e-commerce sites, online gaming, mobile and smartphone applications, IoT user behavior, and many more [ 112 ]. The behavioral analysis aims to understand how and why the consumers or users behave, allowing accurate predictions of how they are likely to behave in the future. For instance, it allows advertisers to make the best offers with the right client segments at the right time. Behavioral analytics, including traffic data such as navigation paths, clicks, social media interactions, purchase decisions, and marketing responsiveness, use the large quantities of raw user event information gathered during sessions in which people use apps, games, or websites. In our earlier papers Sarker et al. [ 101 , 111 , 113 ] we have discussed how to extract users phone usage behavioral patterns utilizing real-life phone log data for various purposes.
In the real-world scenario, behavioral analytics is often used in e-commerce, social media, call centers, billing systems, IoT systems, political campaigns, and other applications, to find opportunities for optimization to achieve particular outcomes. Cohort analysis is a branch of behavioral analytics that involves studying groups of people over time to see how their behavior changes. For instance, it takes data from a given data set (e.g., an e-commerce website, web application, or online game) and separates it into related groups for analysis. Various machine learning techniques such as behavioral data clustering [ 111 ], behavioral decision tree classification [ 109 ], behavioral association rules [ 113 ], etc. can be used in the area according to the goal. Besides, the concept of RecencyMiner, proposed in our earlier paper Sarker et al. [ 108 ] that takes into account recent behavioral patterns could be effective while analyzing behavioral data as it may not be static in the real-world changes over time.
Anomaly Detection or Outlier Analysis
Anomaly detection, also known as Outlier analysis is a data mining step that detects data points, events, and/or findings that deviate from the regularities or normal behavior of a dataset. Anomalies are usually referred to as outliers, abnormalities, novelties, noise, inconsistency, irregularities, and exceptions [ 63 , 114 ]. Techniques of anomaly detection may discover new situations or cases as deviant based on historical data through analyzing the data patterns. For instance, identifying fraud or irregular transactions in finance is an example of anomaly detection.
It is often used in preprocessing tasks for the deletion of anomalous or inconsistency in the real-world data collected from various data sources including user logs, devices, networks, and servers. For anomaly detection, several machine learning techniques can be used, such as k-nearest neighbors, isolation forests, cluster analysis, etc [ 105 ]. The exclusion of anomalous data from the dataset also results in a statistically significant improvement in accuracy during supervised learning [ 101 ]. However, extracting appropriate features, identifying normal behaviors, managing imbalanced data distribution, addressing variations in abnormal behavior or irregularities, the sparse occurrence of abnormal events, environmental variations, etc. could be challenging in the process of anomaly detection. Detection of anomalies can be applicable in a variety of domains such as cybersecurity analytics, intrusion detections, fraud detection, fault detection, health analytics, identifying irregularities, detecting ecosystem disturbances, and many more. This anomaly detection can be considered a significant task for building effective systems with higher accuracy within the area of data science.
Factor Analysis
Factor analysis is a collection of techniques for describing the relationships or correlations between variables in terms of more fundamental entities known as factors [ 23 ]. It’s usually used to organize variables into a small number of clusters based on their common variance, where mathematical or statistical procedures are used. The goals of factor analysis are to determine the number of fundamental influences underlying a set of variables, calculate the degree to which each variable is associated with the factors, and learn more about the existence of the factors by examining which factors contribute to output on which variables. The broad purpose of factor analysis is to summarize data so that relationships and patterns can be easily interpreted and understood [ 143 ].
Exploratory factor analysis (EFA) and confirmatory factor analysis (CFA) are the two most popular factor analysis techniques. EFA seeks to discover complex trends by analyzing the dataset and testing predictions, while CFA tries to validate hypotheses and uses path analysis diagrams to represent variables and factors [ 143 ]. Factor analysis is one of the algorithms for unsupervised machine learning that is used for minimizing dimensionality. The most common methods for factor analytics are principal components analysis (PCA), principal axis factoring (PAF), and maximum likelihood (ML) [ 48 ]. Methods of correlation analysis such as Pearson correlation, canonical correlation, etc. may also be useful in the field as they can quantify the statistical relationship between two continuous variables, or association. Factor analysis is commonly used in finance, marketing, advertising, product management, psychology, and operations research, and thus can be considered as another significant analytical method within the area of data science.
Log Analysis
Logs are commonly used in system management as logs are often the only data available that record detailed system runtime activities or behaviors in production [ 44 ]. Log analysis is thus can be considered as the method of analyzing, interpreting, and capable of understanding computer-generated records or messages, also known as logs. This can be device log, server log, system log, network log, event log, audit trail, audit record, etc. The process of creating such records is called data logging.
Logs are generated by a wide variety of programmable technologies, including networking devices, operating systems, software, and more. Phone call logs [ 88 , 110 ], SMS Logs [ 28 ], mobile apps usages logs [ 124 , 149 ], notification logs [ 77 ], game Logs [ 82 ], context logs [ 16 , 149 ], web logs [ 37 ], smartphone life logs [ 95 ], etc. are some examples of log data for smartphone devices. The main characteristics of these log data is that it contains users’ actual behavioral activities with their devices. Similar other log data can be search logs [ 50 , 133 ], application logs [ 26 ], server logs [ 33 ], network logs [ 57 ], event logs [ 83 ], network and security logs [ 142 ] etc.
Several techniques such as classification and tagging, correlation analysis, pattern recognition methods, anomaly detection methods, machine learning modeling, etc. [ 105 ] can be used for effective log analysis. Log analysis can assist in compliance with security policies and industry regulations, as well as provide a better user experience by encouraging the troubleshooting of technical problems and identifying areas where efficiency can be improved. For instance, web servers use log files to record data about website visitors. Windows event log analysis can help an investigator draw a timeline based on the logging information and the discovered artifacts. Overall, advanced analytics methods by taking into account machine learning modeling can play a significant role to extract insightful patterns from these log data, which can be used for building automated and smart applications, and thus can be considered as a key working area in data science.
Neural Networks and Deep Learning Analysis
Deep learning is a form of machine learning that uses artificial neural networks to create a computational architecture that learns from data by combining multiple processing layers, such as the input, hidden, and output layers [ 38 ]. The key benefit of deep learning over conventional machine learning methods is that it performs better in a variety of situations, particularly when learning from large datasets [ 114 , 140 ].
The most common deep learning algorithms are: multi-layer perceptron (MLP) [ 85 ], convolutional neural network (CNN or ConvNet) [ 67 ], long short term memory recurrent neural network (LSTM-RNN) [ 34 ]. Figure Figure6 6 shows a structure of an artificial neural network modeling with multiple processing layers. The Backpropagation technique [ 38 ] is used to adjust the weight values internally while building the model. Convolutional neural networks (CNNs) [ 67 ] improve on the design of traditional artificial neural networks (ANNs), which include convolutional layers, pooling layers, and fully connected layers. It is commonly used in a variety of fields, including natural language processing, speech recognition, image processing, and other autocorrelated data since it takes advantage of the two-dimensional (2D) structure of the input data. AlexNet [ 60 ], Xception [ 21 ], Inception [ 125 ], Visual Geometry Group (VGG) [ 42 ], ResNet [ 43 ], etc., and other advanced deep learning models based on CNN are also used in the field.

A structure of an artificial neural network modeling with multiple processing layers
In addition to CNN, recurrent neural network (RNN) architecture is another popular method used in deep learning. Long short-term memory (LSTM) is a popular type of recurrent neural network architecture used broadly in the area of deep learning. Unlike traditional feed-forward neural networks, LSTM has feedback connections. Thus, LSTM networks are well-suited for analyzing and learning sequential data, such as classifying, sorting, and predicting data based on time-series data. Therefore, when the data is in a sequential format, such as time, sentence, etc., LSTM can be used, and it is widely used in the areas of time-series analysis, natural language processing, speech recognition, and so on.
In addition to the most popular deep learning methods mentioned above, several other deep learning approaches [ 104 ] exist in the field for various purposes. The self-organizing map (SOM) [ 58 ], for example, uses unsupervised learning to represent high-dimensional data as a 2D grid map, reducing dimensionality. Another learning technique that is commonly used for dimensionality reduction and feature extraction in unsupervised learning tasks is the autoencoder (AE) [ 10 ]. Restricted Boltzmann machines (RBM) can be used for dimensionality reduction, classification, regression, collaborative filtering, feature learning, and topic modeling, according to [ 46 ]. A deep belief network (DBN) is usually made up of a backpropagation neural network and unsupervised networks like restricted Boltzmann machines (RBMs) or autoencoders (BPNN) [ 136 ]. A generative adversarial network (GAN) [ 35 ] is a deep learning network that can produce data with characteristics that are similar to the input data. Transfer learning is common worldwide presently because it can train deep neural networks with a small amount of data, which is usually the re-use of a pre-trained model on a new problem [ 137 ]. These deep learning methods can perform well, particularly, when learning from large-scale datasets [ 105 , 140 ]. In our previous article Sarker et al. [ 104 ], we have summarized a brief discussion of various artificial neural networks (ANN) and deep learning (DL) models mentioned above, which can be used in a variety of data science and analytics tasks.
Real-World Application Domains
Almost every industry or organization is impacted by data, and thus “Data Science” including advanced analytics with machine learning modeling can be used in business, marketing, finance, IoT systems, cybersecurity, urban management, health care, government policies, and every possible industries, where data gets generated. In the following, we discuss ten most popular application areas based on data science and analytics.
- Business or financial data science: In general, business data science can be considered as the study of business or e-commerce data to obtain insights about a business that can typically lead to smart decision-making as well as taking high-quality actions [ 90 ]. Data scientists can develop algorithms or data-driven models predicting customer behavior, identifying patterns and trends based on historical business data, which can help companies to reduce costs, improve service delivery, and generate recommendations for better decision-making. Eventually, business automation, intelligence, and efficiency can be achieved through the data science process discussed earlier, where various advanced analytics methods and machine learning modeling based on the collected data are the keys. Many online retailers, such as Amazon [ 76 ], can improve inventory management, avoid out-of-stock situations, and optimize logistics and warehousing using predictive modeling based on machine learning techniques [ 105 ]. In terms of finance, the historical data is related to financial institutions to make high-stakes business decisions, which is mostly used for risk management, fraud prevention, credit allocation, customer analytics, personalized services, algorithmic trading, etc. Overall, data science methodologies can play a key role in the future generation business or finance industry, particularly in terms of business automation, intelligence, and smart decision-making and systems.
- Manufacturing or industrial data science: To compete in global production capability, quality, and cost, manufacturing industries have gone through many industrial revolutions [ 14 ]. The latest fourth industrial revolution, also known as Industry 4.0, is the emerging trend of automation and data exchange in manufacturing technology. Thus industrial data science, which is the study of industrial data to obtain insights that can typically lead to optimizing industrial applications, can play a vital role in such revolution. Manufacturing industries generate a large amount of data from various sources such as sensors, devices, networks, systems, and applications [ 6 , 68 ]. The main categories of industrial data include large-scale data devices, life-cycle production data, enterprise operation data, manufacturing value chain sources, and collaboration data from external sources [ 132 ]. The data needs to be processed, analyzed, and secured to help improve the system’s efficiency, safety, and scalability. Data science modeling thus can be used to maximize production, reduce costs and raise profits in manufacturing industries.
- Medical or health data science: Healthcare is one of the most notable fields where data science is making major improvements. Health data science involves the extrapolation of actionable insights from sets of patient data, typically collected from electronic health records. To help organizations, improve the quality of treatment, lower the cost of care, and improve the patient experience, data can be obtained from several sources, e.g., the electronic health record, billing claims, cost estimates, and patient satisfaction surveys, etc., to analyze. In reality, healthcare analytics using machine learning modeling can minimize medical costs, predict infectious outbreaks, prevent preventable diseases, and generally improve the quality of life [ 81 , 119 ]. Across the global population, the average human lifespan is growing, presenting new challenges to today’s methods of delivery of care. Thus health data science modeling can play a role in analyzing current and historical data to predict trends, improve services, and even better monitor the spread of diseases. Eventually, it may lead to new approaches to improve patient care, clinical expertise, diagnosis, and management.
- IoT data science: Internet of things (IoT) [ 9 ] is a revolutionary technical field that turns every electronic system into a smarter one and is therefore considered to be the big frontier that can enhance almost all activities in our lives. Machine learning has become a key technology for IoT applications because it uses expertise to identify patterns and generate models that help predict future behavior and events [ 112 ]. One of the IoT’s main fields of application is a smart city, which uses technology to improve city services and citizens’ living experiences. For example, using the relevant data, data science methods can be used for traffic prediction in smart cities, to estimate the total usage of energy of the citizens for a particular period. Deep learning-based models in data science can be built based on a large scale of IoT datasets [ 7 , 104 ]. Overall, data science and analytics approaches can aid modeling in a variety of IoT and smart city services, including smart governance, smart homes, education, connectivity, transportation, business, agriculture, health care, and industry, and many others.
- Cybersecurity data science: Cybersecurity, or the practice of defending networks, systems, hardware, and data from digital attacks, is one of the most important fields of Industry 4.0 [ 114 , 121 ]. Data science techniques, particularly machine learning, have become a crucial cybersecurity technology that continually learns to identify trends by analyzing data, better detecting malware in encrypted traffic, finding insider threats, predicting where bad neighborhoods are online, keeping people safe while surfing, or protecting information in the cloud by uncovering suspicious user activity [ 114 ]. For instance, machine learning and deep learning-based security modeling can be used to effectively detect various types of cyberattacks or anomalies [ 103 , 106 ]. To generate security policy rules, association rule learning can play a significant role to build rule-based systems [ 102 ]. Deep learning-based security models can perform better when utilizing the large scale of security datasets [ 140 ]. Thus data science modeling can enable professionals in cybersecurity to be more proactive in preventing threats and reacting in real-time to active attacks, through extracting actionable insights from the security datasets.
- Behavioral data science: Behavioral data is information produced as a result of activities, most commonly commercial behavior, performed on a variety of Internet-connected devices, such as a PC, tablet, or smartphones [ 112 ]. Websites, mobile applications, marketing automation systems, call centers, help desks, and billing systems, etc. are all common sources of behavioral data. Behavioral data is much more than just data, which is not static data [ 108 ]. Advanced analytics of these data including machine learning modeling can facilitate in several areas such as predicting future sales trends and product recommendations in e-commerce and retail; predicting usage trends, load, and user preferences in future releases in online gaming; determining how users use an application to predict future usage and preferences in application development; breaking users down into similar groups to gain a more focused understanding of their behavior in cohort analysis; detecting compromised credentials and insider threats by locating anomalous behavior, or making suggestions, etc. Overall, behavioral data science modeling typically enables to make the right offers to the right consumers at the right time on various common platforms such as e-commerce platforms, online games, web and mobile applications, and IoT. In social context, analyzing the behavioral data of human being using advanced analytics methods and the extracted insights from social data can be used for data-driven intelligent social services, which can be considered as social data science.
- Mobile data science: Today’s smart mobile phones are considered as “next-generation, multi-functional cell phones that facilitate data processing, as well as enhanced wireless connectivity” [ 146 ]. In our earlier paper [ 112 ], we have shown that users’ interest in “Mobile Phones” is more and more than other platforms like “Desktop Computer”, “Laptop Computer” or “Tablet Computer” in recent years. People use smartphones for a variety of activities, including e-mailing, instant messaging, online shopping, Internet surfing, entertainment, social media such as Facebook, Linkedin, and Twitter, and various IoT services such as smart cities, health, and transportation services, and many others. Intelligent apps are based on the extracted insight from the relevant datasets depending on apps characteristics, such as action-oriented, adaptive in nature, suggestive and decision-oriented, data-driven, context-awareness, and cross-platform operation [ 112 ]. As a result, mobile data science, which involves gathering a large amount of mobile data from various sources and analyzing it using machine learning techniques to discover useful insights or data-driven trends, can play an important role in the development of intelligent smartphone applications.
- Multimedia data science: Over the last few years, a big data revolution in multimedia management systems has resulted from the rapid and widespread use of multimedia data, such as image, audio, video, and text, as well as the ease of access and availability of multimedia sources. Currently, multimedia sharing websites, such as Yahoo Flickr, iCloud, and YouTube, and social networks such as Facebook, Instagram, and Twitter, are considered as valuable sources of multimedia big data [ 89 ]. People, particularly younger generations, spend a lot of time on the Internet and social networks to connect with others, exchange information, and create multimedia data, thanks to the advent of new technology and the advanced capabilities of smartphones and tablets. Multimedia analytics deals with the problem of effectively and efficiently manipulating, handling, mining, interpreting, and visualizing various forms of data to solve real-world problems. Text analysis, image or video processing, computer vision, audio or speech processing, and database management are among the solutions available for a range of applications including healthcare, education, entertainment, and mobile devices.
- Smart cities or urban data science: Today, more than half of the world’s population live in urban areas or cities [ 80 ] and considered as drivers or hubs of economic growth, wealth creation, well-being, and social activity [ 96 , 116 ]. In addition to cities, “Urban area” can refer to the surrounding areas such as towns, conurbations, or suburbs. Thus, a large amount of data documenting daily events, perceptions, thoughts, and emotions of citizens or people are recorded, that are loosely categorized into personal data, e.g., household, education, employment, health, immigration, crime, etc., proprietary data, e.g., banking, retail, online platforms data, etc., government data, e.g., citywide crime statistics, or government institutions, etc., Open and public data, e.g., data.gov, ordnance survey, and organic and crowdsourced data, e.g., user-generated web data, social media, Wikipedia, etc. [ 29 ]. The field of urban data science typically focuses on providing more effective solutions from a data-driven perspective, through extracting knowledge and actionable insights from such urban data. Advanced analytics of these data using machine learning techniques [ 105 ] can facilitate the efficient management of urban areas including real-time management, e.g., traffic flow management, evidence-based planning decisions which pertain to the longer-term strategic role of forecasting for urban planning, e.g., crime prevention, public safety, and security, or framing the future, e.g., political decision-making [ 29 ]. Overall, it can contribute to government and public planning, as well as relevant sectors including retail, financial services, mobility, health, policing, and utilities within a data-rich urban environment through data-driven smart decision-making and policies, which lead to smart cities and improve the quality of human life.
- Smart villages or rural data science: Rural areas or countryside are the opposite of urban areas, that include villages, hamlets, or agricultural areas. The field of rural data science typically focuses on making better decisions and providing more effective solutions that include protecting public safety, providing critical health services, agriculture, and fostering economic development from a data-driven perspective, through extracting knowledge and actionable insights from the collected rural data. Advanced analytics of rural data including machine learning [ 105 ] modeling can facilitate providing new opportunities for them to build insights and capacity to meet current needs and prepare for their futures. For instance, machine learning modeling [ 105 ] can help farmers to enhance their decisions to adopt sustainable agriculture utilizing the increasing amount of data captured by emerging technologies, e.g., the internet of things (IoT), mobile technologies and devices, etc. [ 1 , 51 , 52 ]. Thus, rural data science can play a very important role in the economic and social development of rural areas, through agriculture, business, self-employment, construction, banking, healthcare, governance, or other services, etc. that lead to smarter villages.
Overall, we can conclude that data science modeling can be used to help drive changes and improvements in almost every sector in our real-world life, where the relevant data is available to analyze. To gather the right data and extract useful knowledge or actionable insights from the data for making smart decisions is the key to data science modeling in any application domain. Based on our discussion on the above ten potential real-world application domains by taking into account data-driven smart computing and decision making, we can say that the prospects of data science and the role of data scientists are huge for the future world. The “Data Scientists” typically analyze information from multiple sources to better understand the data and business problems, and develop machine learning-based analytical modeling or algorithms, or data-driven tools, or solutions, focused on advanced analytics, which can make today’s computing process smarter, automated, and intelligent.
Challenges and Research Directions
Our study on data science and analytics, particularly data science modeling in “ Understanding data science modeling ”, advanced analytics methods and smart computing in “ Advanced analytics methods and smart computing ”, and real-world application areas in “ Real-world application domains ” open several research issues in the area of data-driven business solutions and eventual data products. Thus, in this section, we summarize and discuss the challenges faced and the potential research opportunities and future directions to build data-driven products.
- Understanding the real-world business problems and associated data including nature, e.g., what forms, type, size, labels, etc., is the first challenge in the data science modeling, discussed briefly in “ Understanding data science modeling ”. This is actually to identify, specify, represent and quantify the domain-specific business problems and data according to the requirements. For a data-driven effective business solution, there must be a well-defined workflow before beginning the actual data analysis work. Furthermore, gathering business data is difficult because data sources can be numerous and dynamic. As a result, gathering different forms of real-world data, such as structured, or unstructured, related to a specific business issue with legal access, which varies from application to application, is challenging. Moreover, data annotation, which is typically the process of categorization, tagging, or labeling of raw data, for the purpose of building data-driven models, is another challenging issue. Thus, the primary task is to conduct a more in-depth analysis of data collection and dynamic annotation methods. Therefore, understanding the business problem, as well as integrating and managing the raw data gathered for efficient data analysis, may be one of the most challenging aspects of working in the field of data science and analytics.
- The next challenge is the extraction of the relevant and accurate information from the collected data mentioned above. The main focus of data scientists is typically to disclose, describe, represent, and capture data-driven intelligence for actionable insights from data. However, the real-world data may contain many ambiguous values, missing values, outliers, and meaningless data [ 101 ]. The advanced analytics methods including machine and deep learning modeling, discussed in “ Advanced analytics methods and smart computing ”, highly impact the quality, and availability of the data. Thus understanding real-world business scenario and associated data, to whether, how, and why they are insufficient, missing, or problematic, then extend or redevelop the existing methods, such as large-scale hypothesis testing, learning inconsistency, and uncertainty, etc. to address the complexities in data and business problems is important. Therefore, developing new techniques to effectively pre-process the diverse data collected from multiple sources, according to their nature and characteristics could be another challenging task.
- Understanding and selecting the appropriate analytical methods to extract the useful insights for smart decision-making for a particular business problem is the main issue in the area of data science. The emphasis of advanced analytics is more on anticipating the use of data to detect patterns to determine what is likely to occur in the future. Basic analytics offer a description of data in general, while advanced analytics is a step forward in offering a deeper understanding of data and helping to granular data analysis. Thus, understanding the advanced analytics methods, especially machine and deep learning-based modeling is the key. The traditional learning techniques mentioned in “ Advanced analytics methods and smart computing ” may not be directly applicable for the expected outcome in many cases. For instance, in a rule-based system, the traditional association rule learning technique [ 4 ] may produce redundant rules from the data that makes the decision-making process complex and ineffective [ 113 ]. Thus, a scientific understanding of the learning algorithms, mathematical properties, how the techniques are robust or fragile to input data, is needed to understand. Therefore, a deeper understanding of the strengths and drawbacks of the existing machine and deep learning methods [ 38 , 105 ] to solve a particular business problem is needed, consequently to improve or optimize the learning algorithms according to the data characteristics, or to propose the new algorithm/techniques with higher accuracy becomes a significant challenging issue for the future generation data scientists.
- The traditional data-driven models or systems typically use a large amount of business data to generate data-driven decisions. In several application fields, however, the new trends are more likely to be interesting and useful for modeling and predicting the future than older ones. For example, smartphone user behavior modeling, IoT services, stock market forecasting, health or transport service, job market analysis, and other related areas where time-series and actual human interests or preferences are involved over time. Thus, rather than considering the traditional data analysis, the concept of RecencyMiner, i.e., recent pattern-based extracted insight or knowledge proposed in our earlier paper Sarker et al. [ 108 ] might be effective. Therefore, to propose the new techniques by taking into account the recent data patterns, and consequently to build a recency-based data-driven model for solving real-world problems, is another significant challenging issue in the area.
- The most crucial task for a data-driven smart system is to create a framework that supports data science modeling discussed in “ Understanding data science modeling ”. As a result, advanced analytical methods based on machine learning or deep learning techniques can be considered in such a system to make the framework capable of resolving the issues. Besides, incorporating contextual information such as temporal context, spatial context, social context, environmental context, etc. [ 100 ] can be used for building an adaptive, context-aware, and dynamic model or framework, depending on the problem domain. As a result, a well-designed data-driven framework, as well as experimental evaluation, is a very important direction to effectively solve a business problem in a particular domain, as well as a big challenge for the data scientists.
- In several important application areas such as autonomous cars, criminal justice, health care, recruitment, housing, management of the human resource, public safety, where decisions made by models, or AI agents, have a direct effect on human lives. As a result, there is growing concerned about whether these decisions can be trusted, to be right, reasonable, ethical, personalized, accurate, robust, and secure, particularly in the context of adversarial attacks [ 104 ]. If we can explain the result in a meaningful way, then the model can be better trusted by the end-user. For machine-learned models, new trust properties yield new trade-offs, such as privacy versus accuracy; robustness versus efficiency; fairness versus robustness. Therefore, incorporating trustworthy AI particularly, data-driven or machine learning modeling could be another challenging issue in the area.
In the above, we have summarized and discussed several challenges and the potential research opportunities and directions, within the scope of our study in the area of data science and advanced analytics. The data scientists in academia/industry and the researchers in the relevant area have the opportunity to contribute to each issue identified above and build effective data-driven models or systems, to make smart decisions in the corresponding business domains.
In this paper, we have presented a comprehensive view on data science including various types of advanced analytical methods that can be applied to enhance the intelligence and the capabilities of an application. We have also visualized the current popularity of data science and machine learning-based advanced analytical modeling and also differentiate these from the relevant terms used in the area, to make the position of this paper. A thorough study on the data science modeling with its various processing modules that are needed to extract the actionable insights from the data for a particular business problem and the eventual data product. Thus, according to our goal, we have briefly discussed how different data modules can play a significant role in a data-driven business solution through the data science process. For this, we have also summarized various types of advanced analytical methods and outcomes as well as machine learning modeling that are needed to solve the associated business problems. Thus, this study’s key contribution has been identified as the explanation of different advanced analytical methods and their applicability in various real-world data-driven applications areas including business, healthcare, cybersecurity, urban and rural data science, and so on by taking into account data-driven smart computing and decision making.
Finally, within the scope of our study, we have outlined and discussed the challenges we faced, as well as possible research opportunities and future directions. As a result, the challenges identified provide promising research opportunities in the field that can be explored with effective solutions to improve the data-driven model and systems. Overall, we conclude that our study of advanced analytical solutions based on data science and machine learning methods, leads in a positive direction and can be used as a reference guide for future research and applications in the field of data science and its real-world applications by both academia and industry professionals.
Declarations
The author declares no conflict of interest.
This article is part of the topical collection “Advances in Computational Approaches for Artificial Intelligence, Image Processing, IoT and Cloud Applications” guest edited by Bhanu Prakash K N and M. Shivakumar.
Publisher's Note
Springer Nature remains neutral with regard to jurisdictional claims in published maps and institutional affiliations.

Qualitative Data Analysis Methods 101:
The “big 6” methods + examples.
By: Kerryn Warren (PhD) | Reviewed By: Eunice Rautenbach (D.Tech) | May 2020 (Updated April 2023)
Qualitative data analysis methods. Wow, that’s a mouthful.
If you’re new to the world of research, qualitative data analysis can look rather intimidating. So much bulky terminology and so many abstract, fluffy concepts. It certainly can be a minefield!
Don’t worry – in this post, we’ll unpack the most popular analysis methods , one at a time, so that you can approach your analysis with confidence and competence – whether that’s for a dissertation, thesis or really any kind of research project.
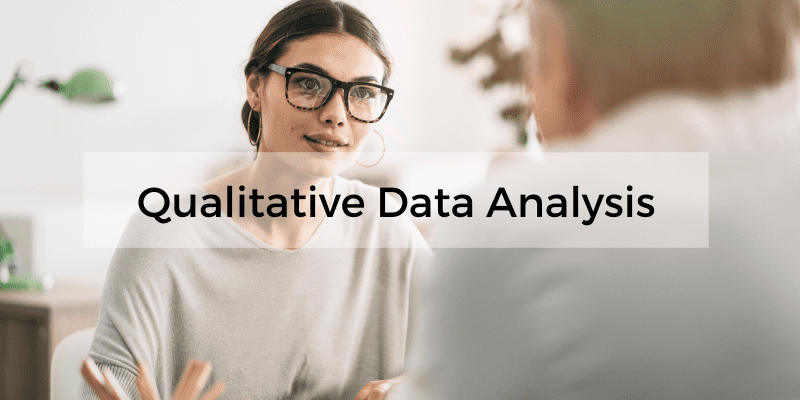
What (exactly) is qualitative data analysis?
To understand qualitative data analysis, we need to first understand qualitative data – so let’s step back and ask the question, “what exactly is qualitative data?”.
Qualitative data refers to pretty much any data that’s “not numbers” . In other words, it’s not the stuff you measure using a fixed scale or complex equipment, nor do you analyse it using complex statistics or mathematics.
So, if it’s not numbers, what is it?
Words, you guessed? Well… sometimes , yes. Qualitative data can, and often does, take the form of interview transcripts, documents and open-ended survey responses – but it can also involve the interpretation of images and videos. In other words, qualitative isn’t just limited to text-based data.
So, how’s that different from quantitative data, you ask?
Simply put, qualitative research focuses on words, descriptions, concepts or ideas – while quantitative research focuses on numbers and statistics . Qualitative research investigates the “softer side” of things to explore and describe , while quantitative research focuses on the “hard numbers”, to measure differences between variables and the relationships between them. If you’re keen to learn more about the differences between qual and quant, we’ve got a detailed post over here .
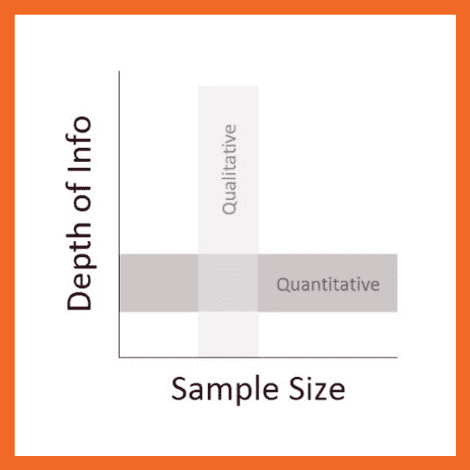
So, qualitative analysis is easier than quantitative, right?
Not quite. In many ways, qualitative data can be challenging and time-consuming to analyse and interpret. At the end of your data collection phase (which itself takes a lot of time), you’ll likely have many pages of text-based data or hours upon hours of audio to work through. You might also have subtle nuances of interactions or discussions that have danced around in your mind, or that you scribbled down in messy field notes. All of this needs to work its way into your analysis.
Making sense of all of this is no small task and you shouldn’t underestimate it. Long story short – qualitative analysis can be a lot of work! Of course, quantitative analysis is no piece of cake either, but it’s important to recognise that qualitative analysis still requires a significant investment in terms of time and effort.
Need a helping hand?
In this post, we’ll explore qualitative data analysis by looking at some of the most common analysis methods we encounter. We’re not going to cover every possible qualitative method and we’re not going to go into heavy detail – we’re just going to give you the big picture. That said, we will of course includes links to loads of extra resources so that you can learn more about whichever analysis method interests you.
Without further delay, let’s get into it.
The “Big 6” Qualitative Analysis Methods
There are many different types of qualitative data analysis, all of which serve different purposes and have unique strengths and weaknesses . We’ll start by outlining the analysis methods and then we’ll dive into the details for each.
The 6 most popular methods (or at least the ones we see at Grad Coach) are:
- Content analysis
- Narrative analysis
- Discourse analysis
- Thematic analysis
- Grounded theory (GT)
- Interpretive phenomenological analysis (IPA)
Let’s take a look at each of them…
QDA Method #1: Qualitative Content Analysis
Content analysis is possibly the most common and straightforward QDA method. At the simplest level, content analysis is used to evaluate patterns within a piece of content (for example, words, phrases or images) or across multiple pieces of content or sources of communication. For example, a collection of newspaper articles or political speeches.
With content analysis, you could, for instance, identify the frequency with which an idea is shared or spoken about – like the number of times a Kardashian is mentioned on Twitter. Or you could identify patterns of deeper underlying interpretations – for instance, by identifying phrases or words in tourist pamphlets that highlight India as an ancient country.
Because content analysis can be used in such a wide variety of ways, it’s important to go into your analysis with a very specific question and goal, or you’ll get lost in the fog. With content analysis, you’ll group large amounts of text into codes , summarise these into categories, and possibly even tabulate the data to calculate the frequency of certain concepts or variables. Because of this, content analysis provides a small splash of quantitative thinking within a qualitative method.
Naturally, while content analysis is widely useful, it’s not without its drawbacks . One of the main issues with content analysis is that it can be very time-consuming , as it requires lots of reading and re-reading of the texts. Also, because of its multidimensional focus on both qualitative and quantitative aspects, it is sometimes accused of losing important nuances in communication.
Content analysis also tends to concentrate on a very specific timeline and doesn’t take into account what happened before or after that timeline. This isn’t necessarily a bad thing though – just something to be aware of. So, keep these factors in mind if you’re considering content analysis. Every analysis method has its limitations , so don’t be put off by these – just be aware of them ! If you’re interested in learning more about content analysis, the video below provides a good starting point.
QDA Method #2: Narrative Analysis
As the name suggests, narrative analysis is all about listening to people telling stories and analysing what that means . Since stories serve a functional purpose of helping us make sense of the world, we can gain insights into the ways that people deal with and make sense of reality by analysing their stories and the ways they’re told.
You could, for example, use narrative analysis to explore whether how something is being said is important. For instance, the narrative of a prisoner trying to justify their crime could provide insight into their view of the world and the justice system. Similarly, analysing the ways entrepreneurs talk about the struggles in their careers or cancer patients telling stories of hope could provide powerful insights into their mindsets and perspectives . Simply put, narrative analysis is about paying attention to the stories that people tell – and more importantly, the way they tell them.
Of course, the narrative approach has its weaknesses , too. Sample sizes are generally quite small due to the time-consuming process of capturing narratives. Because of this, along with the multitude of social and lifestyle factors which can influence a subject, narrative analysis can be quite difficult to reproduce in subsequent research. This means that it’s difficult to test the findings of some of this research.
Similarly, researcher bias can have a strong influence on the results here, so you need to be particularly careful about the potential biases you can bring into your analysis when using this method. Nevertheless, narrative analysis is still a very useful qualitative analysis method – just keep these limitations in mind and be careful not to draw broad conclusions . If you’re keen to learn more about narrative analysis, the video below provides a great introduction to this qualitative analysis method.
QDA Method #3: Discourse Analysis
Discourse is simply a fancy word for written or spoken language or debate . So, discourse analysis is all about analysing language within its social context. In other words, analysing language – such as a conversation, a speech, etc – within the culture and society it takes place. For example, you could analyse how a janitor speaks to a CEO, or how politicians speak about terrorism.
To truly understand these conversations or speeches, the culture and history of those involved in the communication are important factors to consider. For example, a janitor might speak more casually with a CEO in a company that emphasises equality among workers. Similarly, a politician might speak more about terrorism if there was a recent terrorist incident in the country.
So, as you can see, by using discourse analysis, you can identify how culture , history or power dynamics (to name a few) have an effect on the way concepts are spoken about. So, if your research aims and objectives involve understanding culture or power dynamics, discourse analysis can be a powerful method.
Because there are many social influences in terms of how we speak to each other, the potential use of discourse analysis is vast . Of course, this also means it’s important to have a very specific research question (or questions) in mind when analysing your data and looking for patterns and themes, or you might land up going down a winding rabbit hole.
Discourse analysis can also be very time-consuming as you need to sample the data to the point of saturation – in other words, until no new information and insights emerge. But this is, of course, part of what makes discourse analysis such a powerful technique. So, keep these factors in mind when considering this QDA method. Again, if you’re keen to learn more, the video below presents a good starting point.
QDA Method #4: Thematic Analysis
Thematic analysis looks at patterns of meaning in a data set – for example, a set of interviews or focus group transcripts. But what exactly does that… mean? Well, a thematic analysis takes bodies of data (which are often quite large) and groups them according to similarities – in other words, themes . These themes help us make sense of the content and derive meaning from it.
Let’s take a look at an example.
With thematic analysis, you could analyse 100 online reviews of a popular sushi restaurant to find out what patrons think about the place. By reviewing the data, you would then identify the themes that crop up repeatedly within the data – for example, “fresh ingredients” or “friendly wait staff”.
So, as you can see, thematic analysis can be pretty useful for finding out about people’s experiences , views, and opinions . Therefore, if your research aims and objectives involve understanding people’s experience or view of something, thematic analysis can be a great choice.
Since thematic analysis is a bit of an exploratory process, it’s not unusual for your research questions to develop , or even change as you progress through the analysis. While this is somewhat natural in exploratory research, it can also be seen as a disadvantage as it means that data needs to be re-reviewed each time a research question is adjusted. In other words, thematic analysis can be quite time-consuming – but for a good reason. So, keep this in mind if you choose to use thematic analysis for your project and budget extra time for unexpected adjustments.
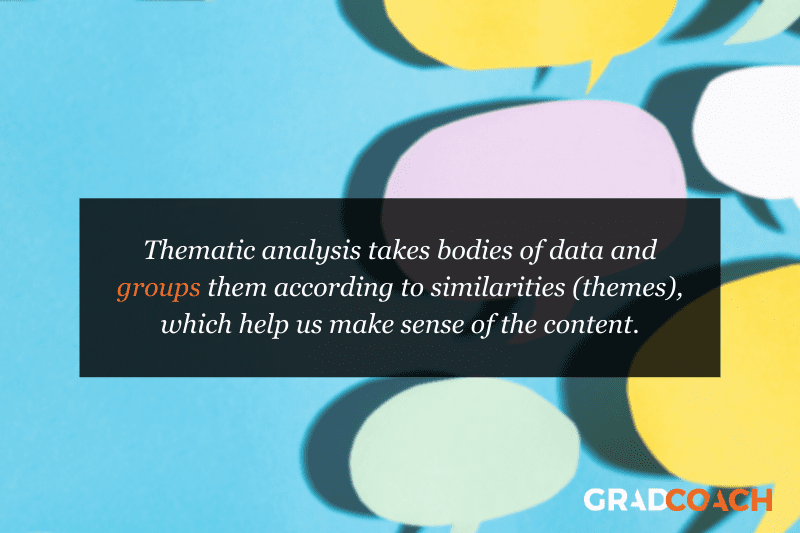
QDA Method #5: Grounded theory (GT)
Grounded theory is a powerful qualitative analysis method where the intention is to create a new theory (or theories) using the data at hand, through a series of “ tests ” and “ revisions ”. Strictly speaking, GT is more a research design type than an analysis method, but we’ve included it here as it’s often referred to as a method.
What’s most important with grounded theory is that you go into the analysis with an open mind and let the data speak for itself – rather than dragging existing hypotheses or theories into your analysis. In other words, your analysis must develop from the ground up (hence the name).
Let’s look at an example of GT in action.
Assume you’re interested in developing a theory about what factors influence students to watch a YouTube video about qualitative analysis. Using Grounded theory , you’d start with this general overarching question about the given population (i.e., graduate students). First, you’d approach a small sample – for example, five graduate students in a department at a university. Ideally, this sample would be reasonably representative of the broader population. You’d interview these students to identify what factors lead them to watch the video.
After analysing the interview data, a general pattern could emerge. For example, you might notice that graduate students are more likely to read a post about qualitative methods if they are just starting on their dissertation journey, or if they have an upcoming test about research methods.
From here, you’ll look for another small sample – for example, five more graduate students in a different department – and see whether this pattern holds true for them. If not, you’ll look for commonalities and adapt your theory accordingly. As this process continues, the theory would develop . As we mentioned earlier, what’s important with grounded theory is that the theory develops from the data – not from some preconceived idea.
So, what are the drawbacks of grounded theory? Well, some argue that there’s a tricky circularity to grounded theory. For it to work, in principle, you should know as little as possible regarding the research question and population, so that you reduce the bias in your interpretation. However, in many circumstances, it’s also thought to be unwise to approach a research question without knowledge of the current literature . In other words, it’s a bit of a “chicken or the egg” situation.
Regardless, grounded theory remains a popular (and powerful) option. Naturally, it’s a very useful method when you’re researching a topic that is completely new or has very little existing research about it, as it allows you to start from scratch and work your way from the ground up .
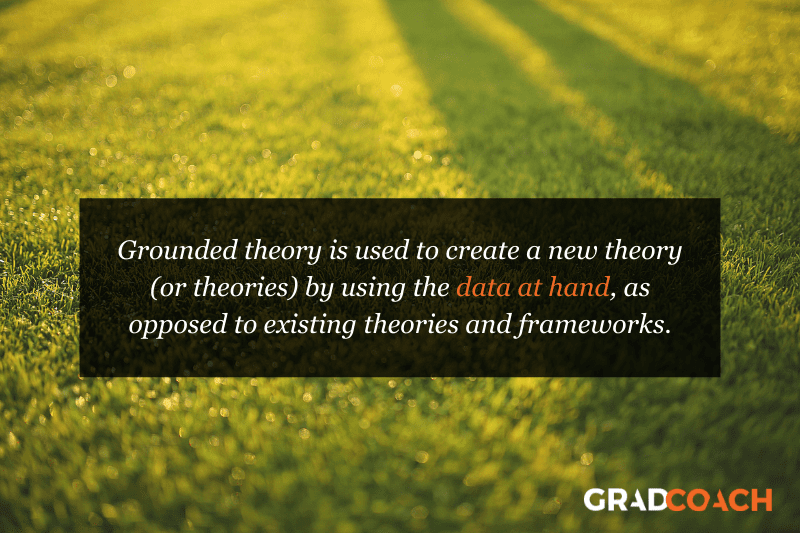
QDA Method #6: Interpretive Phenomenological Analysis (IPA)
Interpretive. Phenomenological. Analysis. IPA . Try saying that three times fast…
Let’s just stick with IPA, okay?
IPA is designed to help you understand the personal experiences of a subject (for example, a person or group of people) concerning a major life event, an experience or a situation . This event or experience is the “phenomenon” that makes up the “P” in IPA. Such phenomena may range from relatively common events – such as motherhood, or being involved in a car accident – to those which are extremely rare – for example, someone’s personal experience in a refugee camp. So, IPA is a great choice if your research involves analysing people’s personal experiences of something that happened to them.
It’s important to remember that IPA is subject – centred . In other words, it’s focused on the experiencer . This means that, while you’ll likely use a coding system to identify commonalities, it’s important not to lose the depth of experience or meaning by trying to reduce everything to codes. Also, keep in mind that since your sample size will generally be very small with IPA, you often won’t be able to draw broad conclusions about the generalisability of your findings. But that’s okay as long as it aligns with your research aims and objectives.
Another thing to be aware of with IPA is personal bias . While researcher bias can creep into all forms of research, self-awareness is critically important with IPA, as it can have a major impact on the results. For example, a researcher who was a victim of a crime himself could insert his own feelings of frustration and anger into the way he interprets the experience of someone who was kidnapped. So, if you’re going to undertake IPA, you need to be very self-aware or you could muddy the analysis.
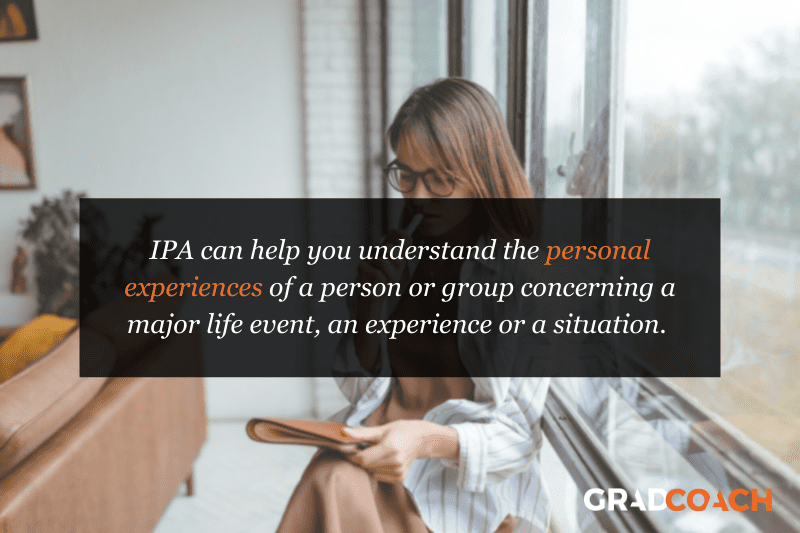
How to choose the right analysis method
In light of all of the qualitative analysis methods we’ve covered so far, you’re probably asking yourself the question, “ How do I choose the right one? ”
Much like all the other methodological decisions you’ll need to make, selecting the right qualitative analysis method largely depends on your research aims, objectives and questions . In other words, the best tool for the job depends on what you’re trying to build. For example:
- Perhaps your research aims to analyse the use of words and what they reveal about the intention of the storyteller and the cultural context of the time.
- Perhaps your research aims to develop an understanding of the unique personal experiences of people that have experienced a certain event, or
- Perhaps your research aims to develop insight regarding the influence of a certain culture on its members.
As you can probably see, each of these research aims are distinctly different , and therefore different analysis methods would be suitable for each one. For example, narrative analysis would likely be a good option for the first aim, while grounded theory wouldn’t be as relevant.
It’s also important to remember that each method has its own set of strengths, weaknesses and general limitations. No single analysis method is perfect . So, depending on the nature of your research, it may make sense to adopt more than one method (this is called triangulation ). Keep in mind though that this will of course be quite time-consuming.
As we’ve seen, all of the qualitative analysis methods we’ve discussed make use of coding and theme-generating techniques, but the intent and approach of each analysis method differ quite substantially. So, it’s very important to come into your research with a clear intention before you decide which analysis method (or methods) to use.
Start by reviewing your research aims , objectives and research questions to assess what exactly you’re trying to find out – then select a qualitative analysis method that fits. Never pick a method just because you like it or have experience using it – your analysis method (or methods) must align with your broader research aims and objectives.
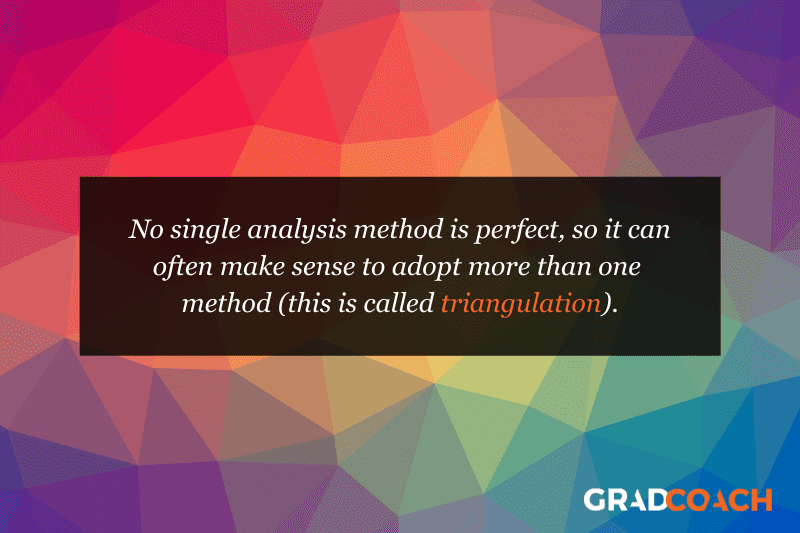
Let’s recap on QDA methods…
In this post, we looked at six popular qualitative data analysis methods:
- First, we looked at content analysis , a straightforward method that blends a little bit of quant into a primarily qualitative analysis.
- Then we looked at narrative analysis , which is about analysing how stories are told.
- Next up was discourse analysis – which is about analysing conversations and interactions.
- Then we moved on to thematic analysis – which is about identifying themes and patterns.
- From there, we went south with grounded theory – which is about starting from scratch with a specific question and using the data alone to build a theory in response to that question.
- And finally, we looked at IPA – which is about understanding people’s unique experiences of a phenomenon.
Of course, these aren’t the only options when it comes to qualitative data analysis, but they’re a great starting point if you’re dipping your toes into qualitative research for the first time.
If you’re still feeling a bit confused, consider our private coaching service , where we hold your hand through the research process to help you develop your best work.
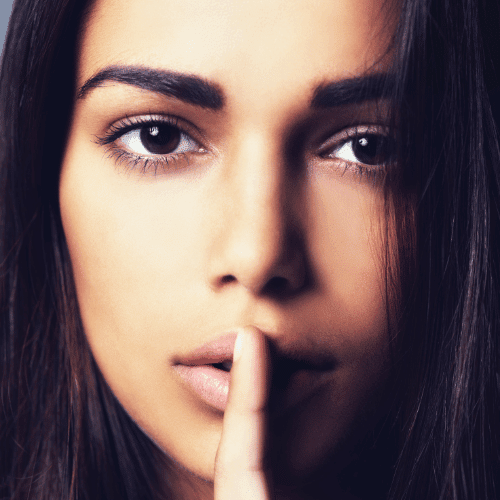
Psst... there’s more!
This post was based on one of our popular Research Bootcamps . If you're working on a research project, you'll definitely want to check this out ...
You Might Also Like:
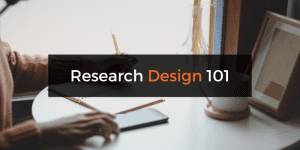
84 Comments
This has been very helpful. Thank you.
Thank you madam,
Thank you so much for this information
I wonder it so clear for understand and good for me. can I ask additional query?
Very insightful and useful
Good work done with clear explanations. Thank you.
Thanks so much for the write-up, it’s really good.
Thanks madam . It is very important .
thank you very good
This has been very well explained in simple language . It is useful even for a new researcher.
Great to hear that. Good luck with your qualitative data analysis, Pramod!
This is very useful information. And it was very a clear language structured presentation. Thanks a lot.
Thank you so much.
very informative sequential presentation
Precise explanation of method.
Hi, may we use 2 data analysis methods in our qualitative research?
Thanks for your comment. Most commonly, one would use one type of analysis method, but it depends on your research aims and objectives.
You explained it in very simple language, everyone can understand it. Thanks so much.
Thank you very much, this is very helpful. It has been explained in a very simple manner that even a layman understands
Thank nicely explained can I ask is Qualitative content analysis the same as thematic analysis?
Thanks for your comment. No, QCA and thematic are two different types of analysis. This article might help clarify – https://onlinelibrary.wiley.com/doi/10.1111/nhs.12048
This is my first time to come across a well explained data analysis. so helpful.
I have thoroughly enjoyed your explanation of the six qualitative analysis methods. This is very helpful. Thank you!
Thank you very much, this is well explained and useful
i need a citation of your book.
Thanks a lot , remarkable indeed, enlighting to the best
Hi Derek, What other theories/methods would you recommend when the data is a whole speech?
Keep writing useful artikel.
It is important concept about QDA and also the way to express is easily understandable, so thanks for all.
Thank you, this is well explained and very useful.
Very helpful .Thanks.
Hi there! Very well explained. Simple but very useful style of writing. Please provide the citation of the text. warm regards
The session was very helpful and insightful. Thank you
This was very helpful and insightful. Easy to read and understand
As a professional academic writer, this has been so informative and educative. Keep up the good work Grad Coach you are unmatched with quality content for sure.
Keep up the good work Grad Coach you are unmatched with quality content for sure.
Its Great and help me the most. A Million Thanks you Dr.
It is a very nice work
Very insightful. Please, which of this approach could be used for a research that one is trying to elicit students’ misconceptions in a particular concept ?
This is Amazing and well explained, thanks
great overview
What do we call a research data analysis method that one use to advise or determining the best accounting tool or techniques that should be adopted in a company.
Informative video, explained in a clear and simple way. Kudos
Waoo! I have chosen method wrong for my data analysis. But I can revise my work according to this guide. Thank you so much for this helpful lecture.
This has been very helpful. It gave me a good view of my research objectives and how to choose the best method. Thematic analysis it is.
Very helpful indeed. Thanku so much for the insight.
This was incredibly helpful.
Very helpful.
very educative
Nicely written especially for novice academic researchers like me! Thank you.
choosing a right method for a paper is always a hard job for a student, this is a useful information, but it would be more useful personally for me, if the author provide me with a little bit more information about the data analysis techniques in type of explanatory research. Can we use qualitative content analysis technique for explanatory research ? or what is the suitable data analysis method for explanatory research in social studies?
that was very helpful for me. because these details are so important to my research. thank you very much
I learnt a lot. Thank you
Relevant and Informative, thanks !
Well-planned and organized, thanks much! 🙂
I have reviewed qualitative data analysis in a simplest way possible. The content will highly be useful for developing my book on qualitative data analysis methods. Cheers!
Clear explanation on qualitative and how about Case study
This was helpful. Thank you
This was really of great assistance, it was just the right information needed. Explanation very clear and follow.
Wow, Thanks for making my life easy
This was helpful thanks .
Very helpful…. clear and written in an easily understandable manner. Thank you.
This was so helpful as it was easy to understand. I’m a new to research thank you so much.
so educative…. but Ijust want to know which method is coding of the qualitative or tallying done?
Thank you for the great content, I have learnt a lot. So helpful
precise and clear presentation with simple language and thank you for that.
very informative content, thank you.
You guys are amazing on YouTube on this platform. Your teachings are great, educative, and informative. kudos!
Brilliant Delivery. You made a complex subject seem so easy. Well done.
Beautifully explained.
Thanks a lot
Is there a video the captures the practical process of coding using automated applications?
Thanks for the comment. We don’t recommend using automated applications for coding, as they are not sufficiently accurate in our experience.
content analysis can be qualitative research?
THANK YOU VERY MUCH.
Thank you very much for such a wonderful content
do you have any material on Data collection
What a powerful explanation of the QDA methods. Thank you.
Great explanation both written and Video. i have been using of it on a day to day working of my thesis project in accounting and finance. Thank you very much for your support.
very helpful, thank you so much
Submit a Comment Cancel reply
Your email address will not be published. Required fields are marked *
Save my name, email, and website in this browser for the next time I comment.
- Print Friendly
- Privacy Policy
Buy Me a Coffee

Home » Descriptive Analytics – Methods, Tools and Examples
Descriptive Analytics – Methods, Tools and Examples
Table of Contents

Descriptive Analytics
Definition:
Descriptive analytics focused on describing or summarizing raw data and making it interpretable. This type of analytics provides insight into what has happened in the past. It involves the analysis of historical data to identify patterns, trends, and insights. Descriptive analytics often uses visualization tools to represent the data in a way that is easy to interpret.
Descriptive Analytics in Research
Descriptive analytics plays a crucial role in research, helping investigators understand and describe the data collected in their studies. Here’s how descriptive analytics is typically used in a research setting:
- Descriptive Statistics: In research, descriptive analytics often takes the form of descriptive statistics . This includes calculating measures of central tendency (like mean, median, and mode), measures of dispersion (like range, variance, and standard deviation), and measures of frequency (like count, percent, and frequency). These calculations help researchers summarize and understand their data.
- Visualizing Data: Descriptive analytics also involves creating visual representations of data to better understand and communicate research findings . This might involve creating bar graphs, line graphs, pie charts, scatter plots, box plots, and other visualizations.
- Exploratory Data Analysis: Before conducting any formal statistical tests, researchers often conduct an exploratory data analysis, which is a form of descriptive analytics. This might involve looking at distributions of variables, checking for outliers, and exploring relationships between variables.
- Initial Findings: Descriptive analytics are often reported in the results section of a research study to provide readers with an overview of the data. For example, a researcher might report average scores, demographic breakdowns, or the percentage of participants who endorsed each response on a survey.
- Establishing Patterns and Relationships: Descriptive analytics helps in identifying patterns, trends, or relationships in the data, which can guide subsequent analysis or future research. For instance, researchers might look at the correlation between variables as a part of descriptive analytics.
Descriptive Analytics Techniques
Descriptive analytics involves a variety of techniques to summarize, interpret, and visualize historical data. Some commonly used techniques include:
Statistical Analysis
This includes basic statistical methods like mean, median, mode (central tendency), standard deviation, variance (dispersion), correlation, and regression (relationships between variables).
Data Aggregation
It is the process of compiling and summarizing data to obtain a general perspective. It can involve methods like sum, count, average, min, max, etc., often applied to a group of data.
Data Mining
This involves analyzing large volumes of data to discover patterns, trends, and insights. Techniques used in data mining can include clustering (grouping similar data), classification (assigning data into categories), association rules (finding relationships between variables), and anomaly detection (identifying outliers).
Data Visualization
This involves presenting data in a graphical or pictorial format to provide clear and easy understanding of the data patterns, trends, and insights. Common data visualization methods include bar charts, line graphs, pie charts, scatter plots, histograms, and more complex forms like heat maps and interactive dashboards.
This involves organizing data into informational summaries to monitor how different areas of a business are performing. Reports can be generated manually or automatically and can be presented in tables, graphs, or dashboards.
Cross-tabulation (or Pivot Tables)
It involves displaying the relationship between two or more variables in a tabular form. It can provide a deeper understanding of the data by allowing comparisons and revealing patterns and correlations that may not be readily apparent in raw data.
Descriptive Modeling
Some techniques use complex algorithms to interpret data. Examples include decision tree analysis, which provides a graphical representation of decision-making situations, and neural networks, which are used to identify correlations and patterns in large data sets.
Descriptive Analytics Tools
Some common Descriptive Analytics Tools are as follows:
Excel: Microsoft Excel is a widely used tool that can be used for simple descriptive analytics. It has powerful statistical and data visualization capabilities. Pivot tables are a particularly useful feature for summarizing and analyzing large data sets.
Tableau: Tableau is a data visualization tool that is used to represent data in a graphical or pictorial format. It can handle large data sets and allows for real-time data analysis.
Power BI: Power BI, another product from Microsoft, is a business analytics tool that provides interactive visualizations with self-service business intelligence capabilities.
QlikView: QlikView is a data visualization and discovery tool. It allows users to analyze data and use this data to support decision-making.
SAS: SAS is a software suite that can mine, alter, manage and retrieve data from a variety of sources and perform statistical analysis on it.
SPSS: SPSS (Statistical Package for the Social Sciences) is a software package used for statistical analysis. It’s widely used in social sciences research but also in other industries.
Google Analytics: For web data, Google Analytics is a popular tool. It allows businesses to analyze in-depth detail about the visitors on their website, providing valuable insights that can help shape the success strategy of a business.
R and Python: Both are programming languages that have robust capabilities for statistical analysis and data visualization. With packages like pandas, matplotlib, seaborn in Python and ggplot2, dplyr in R, these languages are powerful tools for descriptive analytics.
Looker: Looker is a modern data platform that can take data from any database and let you start exploring and visualizing.
When to use Descriptive Analytics
Descriptive analytics forms the base of the data analysis workflow and is typically the first step in understanding your business or organization’s data. Here are some situations when you might use descriptive analytics:
Understanding Past Behavior: Descriptive analytics is essential for understanding what has happened in the past. If you need to understand past sales trends, customer behavior, or operational performance, descriptive analytics is the tool you’d use.
Reporting Key Metrics: Descriptive analytics is used to establish and report key performance indicators (KPIs). It can help in tracking and presenting these KPIs in dashboards or regular reports.
Identifying Patterns and Trends: If you need to identify patterns or trends in your data, descriptive analytics can provide these insights. This might include identifying seasonality in sales data, understanding peak operational times, or spotting trends in customer behavior.
Informing Business Decisions: The insights provided by descriptive analytics can inform business strategy and decision-making. By understanding what has happened in the past, you can make more informed decisions about what steps to take in the future.
Benchmarking Performance: Descriptive analytics can be used to compare current performance against historical data. This can be used for benchmarking and setting performance goals.
Auditing and Regulatory Compliance: In sectors where compliance and auditing are essential, descriptive analytics can provide the necessary data and trends over specific periods.
Initial Data Exploration: When you first acquire a dataset, descriptive analytics is useful to understand the structure of the data, the relationships between variables, and any apparent anomalies or outliers.
Examples of Descriptive Analytics
Examples of Descriptive Analytics are as follows:
Retail Industry: A retail company might use descriptive analytics to analyze sales data from the past year. They could break down sales by month to identify any seasonality trends. For example, they might find that sales increase in November and December due to holiday shopping. They could also break down sales by product to identify which items are the most popular. This analysis could inform their purchasing and stocking decisions for the next year. Additionally, data on customer demographics could be analyzed to understand who their primary customers are, guiding their marketing strategies.
Healthcare Industry: In healthcare, descriptive analytics could be used to analyze patient data over time. For instance, a hospital might analyze data on patient admissions to identify trends in admission rates. They might find that admissions for certain conditions are higher at certain times of the year. This could help them allocate resources more effectively. Also, analyzing patient outcomes data can help identify the most effective treatments or highlight areas where improvement is needed.
Finance Industry: A financial firm might use descriptive analytics to analyze historical market data. They could look at trends in stock prices, trading volume, or economic indicators to inform their investment decisions. For example, analyzing the price-earnings ratios of stocks in a certain sector over time could reveal patterns that suggest whether the sector is currently overvalued or undervalued. Similarly, credit card companies can analyze transaction data to detect any unusual patterns, which could be signs of fraud.
Advantages of Descriptive Analytics
Descriptive analytics plays a vital role in the world of data analysis, providing numerous advantages:
- Understanding the Past: Descriptive analytics provides an understanding of what has happened in the past, offering valuable context for future decision-making.
- Data Summarization: Descriptive analytics is used to simplify and summarize complex datasets, which can make the information more understandable and accessible.
- Identifying Patterns and Trends: With descriptive analytics, organizations can identify patterns, trends, and correlations in their data, which can provide valuable insights.
- Inform Decision-Making: The insights generated through descriptive analytics can inform strategic decisions and help organizations to react more quickly to events or changes in behavior.
- Basis for Further Analysis: Descriptive analytics lays the groundwork for further analytical activities. It’s the first necessary step before moving on to more advanced forms of analytics like predictive analytics (forecasting future events) or prescriptive analytics (advising on possible outcomes).
- Performance Evaluation: It allows organizations to evaluate their performance by comparing current results with past results, enabling them to see where improvements have been made and where further improvements can be targeted.
- Enhanced Reporting and Dashboards: Through the use of visualization techniques, descriptive analytics can improve the quality of reports and dashboards, making the data more understandable and easier to interpret for stakeholders at all levels of the organization.
- Immediate Value: Unlike some other types of analytics, descriptive analytics can provide immediate insights, as it doesn’t require complex models or deep analytical capabilities to provide value.
Disadvantages of Descriptive Analytics
While descriptive analytics offers numerous benefits, it also has certain limitations or disadvantages. Here are a few to consider:
- Limited to Past Data: Descriptive analytics primarily deals with historical data and provides insights about past events. It does not predict future events or trends and can’t help you understand possible future outcomes on its own.
- Lack of Deep Insights: While descriptive analytics helps in identifying what happened, it does not answer why it happened. For deeper insights, you would need to use diagnostic analytics, which analyzes data to understand the root cause of a particular outcome.
- Can Be Misleading: If not properly executed, descriptive analytics can sometimes lead to incorrect conclusions. For example, correlation does not imply causation, but descriptive analytics might tempt one to make such an inference.
- Data Quality Issues: The accuracy and usefulness of descriptive analytics are heavily reliant on the quality of the underlying data. If the data is incomplete, incorrect, or biased, the results of the descriptive analytics will be too.
- Over-reliance on Descriptive Analytics: Businesses may rely too much on descriptive analytics and not enough on predictive and prescriptive analytics. While understanding past and present data is important, it’s equally vital to forecast future trends and make data-driven decisions based on those predictions.
- Doesn’t Provide Actionable Insights: Descriptive analytics is used to interpret historical data and identify patterns and trends, but it doesn’t provide recommendations or courses of action. For that, prescriptive analytics is needed.
About the author
Muhammad Hassan
Researcher, Academic Writer, Web developer
You may also like

Digital Ethnography – Types, Methods and Examples

Predictive Analytics – Techniques, Tools and...

Big Data Analytics -Types, Tools and Methods

Diagnostic Analytics – Methods, Tools and...

Blockchain Research – Methods, Types and Examples

Social Network Analysis – Types, Tools and...

1. Introduction
4. discussion and conclusion, supporting information.

research papers \(\def\hfill{\hskip 5em}\def\hfil{\hskip 3em}\def\eqno#1{\hfil {#1}}\)

Ray-tracing analytical absorption correction for X-ray crystallography based on tomographic reconstructions
a Oxford e-Research Centre, Department of Engineering Science, University of Oxford, 7 Keble Road, Oxford OX1 3QG, United Kingdom, b Diamond Light Source, Harwell Science & Innovation Campus, Didcot OX11 0DE, United Kingdom, c Rosalind Franklin Institute, Harwell Science & Innovation Campus, Didcot OX11 0QX, United Kingdom, d Rutherford Appleton Laboratory, Research Complex at Harwell, Didcot OX11 0FA, United Kingdom, and e Department of Life Sciences, Imperial College London, Exhibition Road, London SW7 2AZ, United Kingdom * Correspondence e-mail: [email protected] , [email protected]
Processing of single-crystal X-ray diffraction data from area detectors can be separated into two steps. First, raw intensities are obtained by integration of the diffraction images, and then data correction and reduction are performed to determine structure-factor amplitudes and their uncertainties. The second step considers the diffraction geometry, sample illumination, decay, absorption and other effects. While absorption is only a minor effect in standard macromolecular crystallography (MX), it can become the largest source of uncertainty for experiments performed at long wavelengths. Current software packages for MX typically employ empirical models to correct for the effects of absorption, with the corrections determined through the procedure of minimizing the differences in intensities between symmetry-equivalent reflections; these models are well suited to capturing smoothly varying experimental effects. However, for very long wavelengths, empirical methods become an unreliable approach to model strong absorption effects with high fidelity. This problem is particularly acute when data multiplicity is low. This paper presents an analytical absorption correction strategy (implemented in new software AnACor ) based on a volumetric model of the sample derived from X-ray tomography. Individual path lengths through the different sample materials for all reflections are determined by a ray-tracing method. Several approaches for absorption corrections (spherical harmonics correction, analytical absorption correction and a combination of the two) are compared for two samples, the membrane protein OmpK36 GD, measured at a wavelength of λ = 3.54 Å, and chlorite dismutase, measured at λ = 4.13 Å. Data set statistics, the peak heights in the anomalous difference Fourier maps and the success of experimental phasing are used to compare the results from the different absorption correction approaches. The strategies using the new analytical absorption correction are shown to be superior to the standard spherical harmonics corrections. While the improvements are modest in the 3.54 Å data, the analytical absorption correction outperforms spherical harmonics in the longer-wavelength data ( λ = 4.13 Å), which is also reflected in the reduced amount of data being required for successful experimental phasing.
Keywords: absorption correction ; ray tracing ; long-wavelength crystallography ; X-ray tomography .
PDB references: OmpK36 GD NO, 8qur ; OmpK36 GD SH, 8quq ; OmpK36 GD AC, 8qvv ; OmpK36 GD ACSH, 8qvs ; chlorite dismutase NO, 8quv ; chlorite dismutase SH, 8quu ; chlorite dismutase AC, 8quz ; chlorite dismutase ACSH, 8qvb
2.1. Experiment workflow and data preparation
The diffraction experiment was immediately followed by tomography data collection at the same X-ray wavelength. One 180° tomography data set was collected for each crystal, with the kappa and phi axes set at 0° and a beam size of 700 × 700 µm and 100% transmission, using a propagation distance of 4.9 mm between scintillator and sample. For OmpK36 1800 projections, 30 flat-field images (without sample) and 30 dark images (without X-rays) were collected with an exposure of 0.15 s per 0.1° rotation. The measured flux for this data set was 1.5 × 10 12 photons s −1 , resulting in a total absorbed dose of 4.8 MGy. For the Cld crystal, 900 projections, 20 flat-field and 20 dark images were collected with an exposure of 0.28 s per 0.2° rotation and a measured flux of 4.3 × 10 11 photons s −1 , yielding a total absorbed dose of 0.8 MGy.
2.2. Analytical absorption correction
2.3. absorption coefficients, 2.4. implementation details.
Parallel computing is used by the built-in multiprocessing package in Python, and the calculations of all the reflections are evenly distributed to each CPU core. After applying sampling and parallel computing, on a cluster node with 48 CPU cores, the computational time for the analytical absorption correction of one data set of OmpK36 and Cld is about 40 and 30 min, respectively, with total RAM usage of around 200 GB.
The codes and further explanations of the algorithm are available at https://github.com/yishunlu-222/AnACor_public .
2.5. Absorption correction strategies
To evaluate the analytical absorption correction by ray-tracing in AnACor , four approaches are compared:
For Cld, the merging R factors, I / σ ( I ) and anomalous slopes are noticeably better for AC compared with SH. All merging statistics show further improvement for the combined ACSH correction. In contrast to OmpK36, where data quality indicators changed little between the SH and AC strategies, for Cld, the analytical absorption correction strategy (AC) gives substantially better data statistics compared with SH. For instance, in terms of the merging R factors, we observe a decrease of the R merge from 0.163 with SH to 0.112 with AC and a further decrease to 0.095 with the ACSH treatment. There is also an increase in the overall mean I / σ ( I ) from 20.22 for SH to 44.73 for the ACSH strategy with the high-resolution shell I / σ ( I ) following this trend. The anomalous slope value increases from 1.36 with SH to 2.48 and 2.5 for AC and ACSH, respectively. This indicates an impressive improvement in the anomalous signal as a result of applying analytical absorption corrections.
In this study we demonstrate the successful application of analytical absorption corrections based on 3D reconstructions from X-ray tomography implemented in AnACor . We describe the algorithm for calculating the path lengths from 3D models by a ray-tracing method. Two very long wavelength experiments from crystals of the proteins OmpK36 and Cld indicate that this approach substantially improves data quality and the success of experimental phasing compared with the standard scaling protocol based on spherical harmonics. Scaling without any absorption correction is presented as a control and unsurprisingly yields the poorest data quality statistics and anomalous peak heights, and for both samples experimental phasing is unsuccessful. This clearly indicates that data quality is severely affected by absorption effects, demonstrating the need for absorption corrections.
Data from OmpK36, which crystallizes in the monoclinic space group C 2, were collected at a wavelength of λ = 3.54 Å. A clear trend is visible: the analytical absorption correction (AC) is better than the spherical harmonics correction (SH) and the combination of the two (ACSH) improves the data even further. While the overall improvements on statistics are small, the fact that the OmpK36 structure could be solved after ACSH correction using only 2/3 of the data needed for the AC and SH strategies clearly highlights the importance of such an improvement. For the Cld data ( P 1, λ = 4.13 Å) the same trend is observed. However, while the difference between AC and ACSH is small, they outperform the spherical harmonics correction. This is in particular reflected in the outcome from experimental phasing, where two data sets are sufficient for both AC and ACSH, while three data sets are needed to solve the structure from data corrected by SH. In general, the combined approach of ACSH gives the best results for both samples/wavelengths, as it can model additional systematic effects present in the experimental data.
AnACor is able to correct data in multiple crystal orientations and for cases where the beam is smaller than the sample. Future work will allow the use of experimentally determined beam profiles and increase the efficiency and speed of the software. Currently, the bottleneck is the manual segmentation step to create the 3D models. The increased phase contrast at long wavelengths and limitations with the current beamline hardware, in particular the sphere of confusion of the goniometer, lead to blurred boundaries in the tomographic reconstructions. The resulting inaccuracies in the segmented 3D model can affect both the path length and the absorption coefficient calculations. The next stage of this work is therefore to understand, quantify and reduce these errors impacting the 3D model. Analytical absorption corrections are beneficial not only for long-wavelength macromolecular crystallography but also for highly absorbing samples in chemical crystallography. In this work the segmented 3D model is obtained by X-ray tomography on beamline I23 at Diamond Light Source. However, AnACor can also be used for analytical absorption corrections for data from other sources, as long as a file with annotated voxels is provided and the relation between the coordinate systems of the 3D model and the diffraction experiment is known.
Supporting information. DOI: https://doi.org/10.1107/S1600576724002243/yr5123sup1.pdf
‡ Joint first authors
Acknowledgements
The authors acknowledge the use of the University of Oxford Advanced Research Computing (ARC) facility in carrying out this work ( https://dx.doi.org/10.5281/zenodo.22558 ).
Funding information
AMO and JJAGK were supported by Diamond Light Source and the UK Science and Technology Facilities Council (STFC). AMO acknowledges the Biotechnology and Biological Sciences Research Council, and is the recipient of a Wellcome Investigator Award 210734/Z/18/Z and a Royal Society Wolfson Fellowship RSWF\R2\182017.
This is an open-access article distributed under the terms of the Creative Commons Attribution (CC-BY) Licence , which permits unrestricted use, distribution, and reproduction in any medium, provided the original authors and source are cited.
This paper is in the following e-collection/theme issue:
Published on 17.4.2024 in Vol 26 (2024)
Digital Interventions for Recreational Cannabis Use Among Young Adults: Systematic Review, Meta-Analysis, and Behavior Change Technique Analysis of Randomized Controlled Studies
Authors of this article:

- José Côté 1, 2, 3 , RN, PhD ;
- Gabrielle Chicoine 3, 4 , RN, PhD ;
- Billy Vinette 1, 3 , RN, MSN ;
- Patricia Auger 2, 3 , MSc ;
- Geneviève Rouleau 3, 5, 6 , RN, PhD ;
- Guillaume Fontaine 7, 8, 9 , RN, PhD ;
- Didier Jutras-Aswad 2, 10 , MSc, MD
1 Faculty of Nursing, Université de Montréal, Montreal, QC, Canada
2 Research Centre of the Centre Hospitalier de l’Université de Montréal, Montreal, QC, Canada
3 Research Chair in Innovative Nursing Practices, Montreal, QC, Canada
4 Knowledge Translation Program, Li Ka Shing Knowledge Institute, St. Michael’s Hospital, Toronto, ON, Canada
5 Department of Nursing, Université du Québec en Outaouais, Saint-Jérôme, QC, Canada
6 Women's College Hospital Institute for Health System Solutions and Virtual Care, Women's College Hospital, Toronto, ON, Canada
7 Ingram School of Nursing, Faculty of Medicine and Health Sciences, McGill University, Montreal, QC, Canada
8 Centre for Clinical Epidemiology, Lady Davis Institute for Medical Research, Sir Mortimer B. Davis Jewish General Hospital, Montreal, QC, Canada
9 Kirby Institute, University of New South Wales, Sydney, Australia
10 Department of Psychiatry and Addictology, Faculty of Medicine, Université de Montréal, Montreal, QC, Canada
Corresponding Author:
José Côté, RN, PhD
Research Centre of the Centre Hospitalier de l’Université de Montréal
850 Saint-Denis
Montreal, QC, H2X 0A9
Phone: 1 514 890 8000
Email: [email protected]
Background: The high prevalence of cannabis use among young adults poses substantial global health concerns due to the associated acute and long-term health and psychosocial risks. Digital modalities, including websites, digital platforms, and mobile apps, have emerged as promising tools to enhance the accessibility and availability of evidence-based interventions for young adults for cannabis use. However, existing reviews do not consider young adults specifically, combine cannabis-related outcomes with those of many other substances in their meta-analytical results, and do not solely target interventions for cannabis use.
Objective: We aimed to evaluate the effectiveness and active ingredients of digital interventions designed specifically for cannabis use among young adults living in the community.
Methods: We conducted a systematic search of 7 databases for empirical studies published between database inception and February 13, 2023, assessing the following outcomes: cannabis use (frequency, quantity, or both) and cannabis-related negative consequences. The reference lists of included studies were consulted, and forward citation searching was also conducted. We included randomized studies assessing web- or mobile-based interventions that included a comparator or control group. Studies were excluded if they targeted other substance use (eg, alcohol), did not report cannabis use separately as an outcome, did not include young adults (aged 16-35 y), had unpublished data, were delivered via teleconference through mobile phones and computers or in a hospital-based setting, or involved people with mental health disorders or substance use disorders or dependence. Data were independently extracted by 2 reviewers using a pilot-tested extraction form. Authors were contacted to clarify study details and obtain additional data. The characteristics of the included studies, study participants, digital interventions, and their comparators were summarized. Meta-analysis results were combined using a random-effects model and pooled as standardized mean differences.
Results: Of 6606 unique records, 19 (0.29%) were included (n=6710 participants). Half (9/19, 47%) of these articles reported an intervention effect on cannabis use frequency. The digital interventions included in the review were mostly web-based. A total of 184 behavior change techniques were identified across the interventions (range 5-19), and feedback on behavior was the most frequently used (17/19, 89%). Digital interventions for young adults reduced cannabis use frequency at the 3-month follow-up compared to control conditions (including passive and active controls) by −6.79 days of use in the previous month (95% CI −9.59 to −4.00; P <.001).
Conclusions: Our results indicate the potential of digital interventions to reduce cannabis use in young adults but raise important questions about what optimal exposure dose could be more effective, both in terms of intervention duration and frequency. Further high-quality research is still needed to investigate the effects of digital interventions on cannabis use among young adults.
Trial Registration: PROSPERO CRD42020196959; https://www.crd.york.ac.uk/prospero/display_record.php?RecordID=196959
Introduction
Cannabis use among young adults is recognized as a public health concern.
Young adulthood (typically the ages of 18-30 y) is a critical developmental stage characterized by a peak prevalence of substance use [ 1 , 2 ]. Worldwide, cannabis is a substance frequently used for nonmedical purposes due in part to its high availability in some regions and enhanced product variety and potency [ 3 , 4 ]. The prevalence of cannabis use (CU) among young adults is high [ 5 , 6 ], and its rates have risen in recent decades [ 7 ]. In North America and Oceania, the estimated past-year prevalence of CU is ≥25% among young adults [ 8 , 9 ].
While the vast majority of cannabis users do not experience severe problems from their use [ 4 ], the high prevalence of CU among young adults poses substantial global health concerns due to the associated acute and long-term health and psychosocial risks [ 10 , 11 ]. These include impairment of cognitive function, memory, and psychomotor skills during acute intoxication; increased engagement in behaviors with a potential for injury and fatality (eg, driving under the influence); socioeconomic problems; and diminished social functioning [ 4 , 12 - 14 ]. Importantly, an extensive body of literature reveals that subgroups engaging in higher-risk use, such as intensive or repeated use, are more prone to severe and chronic consequences, including physical ailments (eg, respiratory illness and reproductive dysfunction), mental health disorders (eg, psychosis, depression, and suicidal ideation or attempts), and the potential development of CU disorder [ 4 , 15 - 17 ].
Interventions to Reduce Public Health Impact of Young Adult CU
Given the increased prevalence of lifetime and daily CU among young adults and the potential negative impact of higher-risk CU, various prevention and intervention programs have been implemented to help users reduce or cease their CU. These programs primarily target young adults regardless of their CU status [ 2 , 18 ]. In this context, many health care organizations and international expert panels have developed evidence-based lower-risk CU guidelines to promote safer CU and intervention options to help reduce risks of adverse health outcomes from nonmedical CU [ 4 , 16 , 17 , 19 ]. Lower-risk guidance-oriented interventions for CU are based on concepts of health promotion [ 20 - 22 ] and health behavior change [ 23 - 26 ] and on other similar harm reduction interventions implemented in other areas of population health (eg, lower-risk drinking guidelines, supervised consumption sites and services, and sexual health) [ 27 , 28 ]. These interventions primarily aim to raise awareness of negative mental, physical, and social cannabis-related consequences to modify individual-level behavior-related risk factors.
Meta-analyses have shown that face-to-face prevention and treatment interventions are generally effective in reducing CU in young adults [ 18 , 29 - 32 ]. However, as the proportion of professional help seeking for CU concerns among young adults remains low (approximately 15%) [ 33 , 34 ], alternative strategies that consider the limited capacities and access-related barriers of traditional face-to-face prevention and treatment facilities are needed. Digital interventions, including websites, digital platforms, and mobile apps, have emerged as promising tools to enhance the accessibility and availability of evidence-based programs for young adult cannabis users. These interventions address barriers such as long-distance travel, concerns about confidentiality, stigma associated with seeking treatment, and the cost of traditional treatments [ 35 - 37 ]. By overcoming these barriers, digital interventions have the potential to have a stronger public health impact [ 18 , 38 ].
State of Knowledge of Digital Interventions for CU and Young Adults
The literature regarding digital interventions for substance use has grown rapidly in the past decade, as evidenced by several systematic reviews and meta-analyses of randomized controlled trial (RCT) studies on the efficacy or effectiveness of these interventions in preventing or reducing harmful substance use [ 2 , 39 - 41 ]. However, these reviews do not focus on young adults specifically. In addition, they combine CU-related outcomes with those of many other substances in their meta-analytical results. Finally, they do not target CU interventions exclusively.
In total, 4 systematic reviews and meta-analyses of digital interventions for CU among young people have reported mixed results [ 42 - 45 ]. In their systematic review (10 studies of 5 prevention and 5 treatment interventions up to 2012), Tait et al [ 44 ] concluded that digital interventions effectively reduced CU among adolescents and adults at the posttreatment time point. Olmos et al [ 43 ] reached a similar conclusion in their meta-analysis of 9 RCT studies (2 prevention and 7 treatment interventions). In their review, Hoch et al [ 42 ] reported evidence of small effects at the 3-month follow-up based on 4 RCTs of brief motivational interventions and cognitive behavioral therapy (CBT) delivered on the web. In another systematic review and meta-analysis, Beneria et al [ 45 ] found that web-based CU interventions did not significantly reduce consumption. However, these authors indicated that the programs tested varied significantly across the studies considered and that statistical heterogeneity was attributable to the inclusion of studies of programs targeting more than one substance (eg, alcohol and cannabis) and both adolescents and young adults. Beneria et al [ 45 ] recommend that future work “establish the effectiveness of the newer generation of interventions as well as the key ingredients” of effective digital interventions addressing CU by young people. This is of particular importance because behavior change interventions tend to be complex as they consist of multiple interactive components [ 46 ].
Behavior change interventions refer to “coordinated sets of activities designed to change specified behavior patterns” [ 47 ]. Their interacting active ingredients can be conceptualized as behavior change techniques (BCTs) [ 48 ]. BCTs are specific and irreducible. Each BCT has its own individual label and definition, which can be used when designing and reporting complex interventions and as a nomenclature system when coding interventions for their content [ 47 ]. The Behavior Change Technique Taxonomy version 1 (BCTTv1) [ 48 , 49 ] was developed to provide a shared, standardized terminology for characterizing complex behavior change interventions and their active ingredients. Several systematic reviews with meta-regressions that used the BCTTv1 have found interventions with certain BCTs to be more effective than those without [ 50 - 53 ]. A better understanding of the BCTs used in digital interventions for young adult cannabis users would help not only to establish the key ingredients of such interventions but also develop and evaluate effective interventions.
In the absence of any systematic review of the effectiveness and active ingredients of digital interventions designed specifically for CU among community-living young adults, we set out to achieve the following:
- conduct a comprehensive review of digital interventions for preventing, reducing, or ceasing CU among community-living young adults,
- describe the active ingredients (ie, BCTs) in these interventions from the perspective of behavior change science, and
- analyze the effectiveness of these interventions on CU outcomes.
Protocol Registration
We followed the Cochrane Handbook for Systematic Reviews of Interventions [ 54 ] in designing this systematic review and meta-analysis and the PRISMA (Preferred Reporting Items for Systematic Reviews and Meta-Analyses) 2020 guidelines in reporting our findings (see Multimedia Appendix 1 [ 55 ] for the complete PRISMA checklist). This review was registered in PROSPERO (CRD42020196959).
Search Strategy
The search strategy was designed by a health information specialist together with the research team and peer reviewed by another senior information specialist before execution using Peer Review of Electronic Search Strategies for systematic reviews [ 56 ]. The search strategy revolved around three concepts:
- CU (eg, “cannabis,” “marijuana,” and “hashish”)
- Digital interventions (eg, “telehealth,” “website,” “mobile applications,” and “computer”)
- Young adults (eg, “emerging adults” and “students”)
The strategy was initially implemented on March 18, 2020, and again on October 13, 2021, and February 13, 2023. The full, detailed search strategies for each database are presented in Multimedia Appendix 2 .
Information Sources
We searched 7 electronic databases of published literature: CINAHL Complete, Cochrane Database of Systematic Reviews, Cochrane Central Register of Controlled Trials, Embase, MEDLINE, PubMed, and PsycINFO. No publication date filters or language restrictions were applied. A combination of free-text keywords and Medical Subject Headings was tailored to the conventions of each database for optimal electronic searching. The research team also manually screened the reference lists of the included articles and the bibliographies of existing systematic reviews [ 18 , 31 , 42 - 45 ] to identify additional relevant studies (snowballing). Finally, a forward citation tracking procedure (ie, searching for articles that cited the included studies) was carried out in Google Scholar.
Inclusion Criteria
The population, intervention, comparison, outcome, and study design process is presented in Multimedia Appendix 3 . The inclusion criteria were as follows: (1) original research articles published in peer-reviewed journals; (2) use of an experimental study design (eg, RCT, cluster RCT, or pilot RCT); (3) studies evaluating the effectiveness (or efficacy) of digital interventions designed specifically to prevent, reduce, or cease CU as well as promote CU self-management or address cannabis-related harm and having CU as an outcome measure; (4) studies targeting young adults, including active and nonactive cannabis users; (5) cannabis users and nonusers not under substance use treatment used as controls in comparator, waitlist, or delayed-treatment groups offered another type of intervention (eg, pharmacotherapy or psychosocial) different from the one being investigated or participants assessed only for CU; and (6) quantitative CU outcomes (frequency and quantity) or cannabis abstinence. Given the availability of numerous CU screening and assessment tools with adequate psychometric properties and the absence of a gold standard in this regard [ 57 ], any instrument capturing aspects of CU was considered. CU outcome measures could be subjective (eg, self-reported number of CU days or joints in the previous 3 months) or objective (eg, drug screening test). CU had to be measured before the intervention (baseline) and at least once after.
Digital CU interventions were defined as web- or mobile-based interventions that included one or more activities (eg, self-directed or interactive psychoeducation or therapy, personalized feedback, peer-to-peer contact, and patient-to-expert communication) aimed at changing CU [ 58 ]. Mobile-based interventions were defined as interventions delivered via mobile phone through SMS text message, multimedia messaging service (ie, SMS text messages that include multimedia content, such as pictures, videos, or emojis), or mobile apps, whereas web-based interventions (eg, websites and digital platforms) were defined as interventions designed to be accessed on the web (ie, the internet), mainly via computers. Interventions could include self-directed and web-based interventions with human support. We defined young adults as aged 16 to 35 years and included students and nonstudents. While young adulthood is typically defined as covering the ages of 18 to 30 years [ 59 ], we broadened the range given that the age of majority and legal age to purchase cannabis differs across countries and jurisdictions. This was also in line with the age range targeted by several digital CU interventions (college or university students or emerging adults aged 15-24 years) [ 31 , 45 ]. Given the language expertise of the research team members and the available resources, only English- and French-language articles were retained.
Exclusion Criteria
Knowledge synthesis articles, study protocols, and discussion papers or editorials were excluded, as were articles with cross-sectional, cohort, case study or report, pretest-posttest, quasi-experimental, or qualitative designs. Mixed methods designs were included only if the quantitative component was an RCT. We excluded studies if (1) use of substances other than cannabis (eg, alcohol, opioids, or stimulants) was the focus of the digital intervention (though studies that included polysubstance users were retained if CU was assessed and reported separately); (2) CU was not reported separately as an outcome or only attitudes or beliefs regarding, knowledge of, intention to reduce, or readiness or motivation to change CU was measured; and (3) the data reported were unpublished (eg, conferences and dissertations). Studies of traditional face-to-face therapy delivered via teleconference on mobile phones and computers or in a hospital-based setting and informational campaigns (eg, web-based poster presentations or pamphlets) were excluded as well. Studies with samples with a maximum age of <15 years and a minimum age of >35 years were also excluded. Finally, we excluded studies that focused exclusively on people with a mental health disorder or substance use disorder or dependence or on adolescents owing to the particular health care needs of these populations, which may differ from those of young adults [ 1 ].
Data Collection
Selection of studies.
Duplicates were removed from the literature search results in EndNote (version X9.3.3; Clarivate Analytics) using the Bramer method for deduplication of database search results for systematic reviews [ 60 ]. The remaining records were uploaded to Covidence (Veritas Health Innovation), a web-based systematic review management system. A reviewer guide was developed that included screening questions and a detailed description of each inclusion and exclusion criterion based on PICO (population, intervention, comparator, and outcome), and a calibration exercise was performed before each stage of the selection process to maximize consistency between reviewers. Titles and abstracts of studies flagged for possible inclusion were screened first by 2 independent reviewers (GC, BV, PA, and GR; 2 per article) against the eligibility criteria (stage 1). Articles deemed eligible for full-text review were then retrieved and screened for inclusion (stage 2). Full texts were assessed in detail against the eligibility criteria again by 2 reviewers independently. Disagreements between reviewers were resolved through consensus or by consulting a third reviewer.
Data Extraction Process
In total, 2 reviewers (GC, BV, PA, GR, and GF; 2 per article) independently extracted relevant data (or informal evidence) using a data extraction form developed specifically for this review and integrated into Covidence. The form was pilot-tested on 2 randomly selected studies and refined accordingly. Data pertaining to the following domains were extracted from the included studies: (1) Study characteristics included information on the first and corresponding authors, publication year, country of origin, aims and hypotheses, study period, design (including details on randomization and blinding), follow-up times, data collection methods, and types of statistical analysis. (2) Participant characteristics included study target population, participant inclusion and exclusion criteria, sex or gender, mean age, and sample sizes at each data collection time point. (3) Intervention characteristics, for which the research team developed a matrix inspired by the template for intervention description and replication 12-item checklist [ 61 ] to extract informal evidence (ie, intervention descriptions) from the included studies under the headings name of intervention, purpose, underpinning theory of design elements, treatment approach, type of technology (ie, web or mobile) and software used, delivery format (ie, self-directed, human involvement, or both), provider characteristics (if applicable), intervention duration (ie, length of treatment and number of sessions or modules), material and procedures (ie, tools or activities offered, resources provided, and psychoeducational content), tailoring, and unplanned modifications. (4) Comparator characteristics were details of the control or comparison group or groups, including nature (passive vs active), number of groups or clusters (if applicable), type and length of the intervention (if applicable), and number of participants at each data collection time point. (5) Outcome variables, including the primary outcome variable examined in this systematic review, that is, the mean difference in CU frequency before and after the intervention and between the experimental and control or comparison groups. When possible, we examined continuous variables, including CU frequency means and SDs at the baseline and follow-up time points, and standardized regression coefficients (ie, β coefficients and associated 95% CIs). The secondary outcomes examined included other CU outcome variables (eg, quantity of cannabis used and abstinence) and cannabis-related negative consequences (or problems). Details on outcome variables (ie, definition, data time points, and missing data) and measurements (ie, instruments, measurement units, and scales) were also extracted.
In addition, data on user engagement and use of the digital intervention and study attrition rates (ie, dropouts and loss to follow-up) were extracted. When articles had missing data, we contacted the corresponding authors via email (2 attempts were made over a 2-month period) to obtain missing information. Disagreements over the extracted data were limited and resolved through discussion.
Data Synthesis Methods
Descriptive synthesis.
The characteristics of the included studies, study participants, interventions, and comparators were summarized in narrative and table formats. The template for intervention description and replication 12-item checklist [ 61 ] was used to summarize and organize intervention characteristics and assess to what extent the interventions were appropriately described in the included articles. As not all studies had usable data for meta-analysis purposes and because of heterogeneity, we summarized the main findings (ie, intervention effects) of the included studies in narrative and table formats for each outcome of interest in this review.
The BCTs used in the digital interventions were identified from the descriptions of the interventions (ie, experimental groups) provided in the articles as well as any supplementary material and previously published research protocols. A BCT was defined as “an observable, replicable, and irreducible component of an intervention designed to alter or redirect causal processes that regulate behavior” [ 48 ]. The target behavior in this review was the cessation or reduction of CU by young adults. BCTs were identified and coded using the BCTTv1 [ 48 , 49 ], a taxonomy of 93 BCTs organized into 16 hierarchical thematic clusters or categories. Applying the BCTTv1 in a systematic review allows for the comparison and synthesis of evidence across studies in a structured manner. This analysis allows for the identification of the explicit mechanisms underlying the reported behavior change induced by interventions, successful or not, and, thus, avoids making implicit assumptions about what works [ 62 ].
BCT coding was performed by 2 reviewers independently—BV coded all studies, and GC and GF coded a subset of the studies. All reviewers completed web-based training on the BCTTv1, and GF is an experienced implementation scientist who had used the BCTTv1 in prior work [ 63 - 65 ]. The descriptions of the interventions in the articles were read line by line and analyzed for the clear presence of BCTs using the guidelines developed by Michie et al [ 48 ]. For each article, the BCTs identified were documented and categorized using supporting textual evidence. They were coded only once per article regardless of how many times they came up in the text. Disagreements about including a BCT were resolved through discussion. If there was uncertainty about whether a BCT was present, it was coded as absent. Excel (Microsoft Corp) was used to compare the reviewers’ independent BCT coding and generate an overall descriptive synthesis of the BCTs identified. The BCTs were summarized by study and BCT cluster.
Statistical Analysis
Meta-analyses were conducted to estimate the size of the effect of the digital interventions for young adult CU on outcomes of interest at the posttreatment and follow-up assessments compared with control or alternative intervention conditions. The outcome variables considered were (1) CU frequency and other CU outcome variables (eg, quantity of cannabis used and abstinence) at baseline and the posttreatment time point or follow-up measured using standardized instruments of self-reported CU (eg, the timeline followback [TLFB] method) [ 66 ] and (2) cannabis-related negative consequences measured using standardized instruments (eg, the Marijuana Problems Scale) [ 67 ].
Under our systematic review protocol, ≥2 studies were needed for a meta-analysis. On the basis of previous systematic reviews and meta-analyses in the field of digital CU interventions [ 31 , 42 - 45 ], we expected between-study heterogeneity regarding outcome assessment. To minimize heterogeneity, we chose to pool studies with similar outcomes of interest based on four criteria: (1) definition of outcome (eg, CU frequency, quantity consumed, and abstinence), (2) type of outcome variable (eg, days of CU in the previous 90 days, days high per week in the previous 30 days, and number of CU events in the previous month) and measure (ie, instruments or scales), (3) use of validated instruments, and (4) posttreatment or follow-up time points (eg, 2 weeks or 1 month after the baseline or 3, 6, and 12 months after the baseline).
Only articles that reported sufficient statistics to compute a valid effect size with 95% CIs were included in the meta-analyses. In the case of articles that were not independent (ie, more than one published article reporting data from the same clinical trial), only 1 was included, and it was represented only once in the meta-analysis for a given outcome variable regardless of whether the data used to compute the effect size were extracted from the original paper or a secondary analysis paper. We made sure that the independence of the studies included in the meta-analysis of each outcome was respected. In the case of studies that had more than one comparator, we used the effect size for each comparison between the intervention and control groups.
Meta-analyses were conducted only for mean differences based on the change from baseline in CU frequency at 3 months after the baseline as measured using the number of self-reported days of use in the previous month. As the true value of the estimated effect size for outcome variables might vary across different trials and samples, we used a random-effects model given that the studies retained did not have identical target populations. The random-effects model incorporates between-study variation in the study weights and estimated effect size [ 68 ]. In addition, statistical heterogeneity across studies was assessed using I 2 , which measures the proportion of heterogeneity to the total observed dispersion; 25% was considered low, 50% was considered moderate, and 75% was considered high [ 69 ]. Because only 3 studies were included in the meta-analysis [ 70 - 72 ], publication bias could not be assessed. All analyses were completed using Stata (version 18; StataCorp) [ 73 ].
Risk-of-Bias Assessment
The risk of bias (RoB) of the included RCTs was assessed using the Cochrane RoB 2 tool at the outcome level [ 74 ]. Each distinct risk domain (ie, randomization process, deviations from the intended intervention, missing outcome data, measurement of the outcome, and selection of the reported results) was assessed as “low,” “some concerns,” or “high” based on the RoB 2 criteria. In total, 2 reviewers (GC and BV) conducted the assessments independently. Disagreements were discussed, and if not resolved consensually by the 2, the matter was left for a third reviewer (GF) to settle. The assessments were summarized by risk domain and outcome and converted into figures using the RoB visualization tool robvis [ 75 ].
Search Results
The database search generated a total of 13,232 citations, of which 7822 (59.11%) were from the initial search on March 18, 2020, and 2805 (21.2%) and 2605 (19.69%) were from the updates on October 13, 2021, and February 13, 2023, respectively. Figure 1 presents the PRISMA study flow diagram [ 76 ]. Of the 6606 unique records, 6484 (98.15%) were excluded based on title and abstract screening. Full texts of the remaining 1.85% (122/6606) of the records were examined, as were those of 25 more reports found through hand searching. Of these 147 records, 128 (87.1%) were excluded after 3 rounds of full-text screening. Of these 128 records, 39 (30.5%) were excluded for not being empirical research articles (eg, research protocols). Another 28.1% (36/128) were excluded for not meeting our definition of digital CU intervention. The remaining records were excluded for reasons that occurred with a frequency of ≤14%, including young adults not being the target population and the study not meeting our study design criteria (ie, RCT, cluster RCT, or pilot RCT). Excluded studies and reasons for exclusion are listed in Multimedia Appendix 4 . Finally, 19 articles detailing the results of 19 original studies were included.

Description of Studies
Study characteristics.
Multimedia Appendix 5 [ 70 - 72 , 77 - 92 ] describes the general characteristics of the 19 included studies. The studies were published between 2010 and 2023, with 58% (11/19) published in 2018 or later. A total of 53% (10/19) of the studies were conducted in the United States [ 77 - 86 ], 11% (2/19) were conducted in Canada [ 87 , 88 ], 11% (2/19) were conducted in Australia [ 71 , 89 ], 11% (2/19) were conducted in Germany [ 72 , 90 ], 11% (2/19) were conducted in Switzerland [ 70 , 91 ], and 5% (1/19) were conducted in Sweden [ 92 ]. A total of 79% (15/19) were RCTs [ 70 - 72 , 77 , 79 , 81 - 83 , 86 - 92 ], and 21% (4/19) were pilot RCTs [ 78 , 80 , 84 , 85 ].
Participant Characteristics
The studies enrolled a total of 6710 participants—3229 (48.1%) in the experimental groups, 3358 (50%) in the control groups, and the remaining 123 (1.8%) from 1 study [ 82 ] where participant allocation to the intervention condition was not reported. Baseline sample sizes ranged from 49 [ 81 ] to 1292 [ 72 ] (mean 352.89, SD 289.50), as shown in Multimedia Appendix 5 . Participant mean ages ranged from 18.03 (SD 0.31) [ 79 ] to 35.3 (SD 12.6) years [ 88 ], and the proportion of participants who identified as female ranged from 24.7% [ 91 ] to 84.1% [ 80 ].
Of the 19 included studies, 10 (53%) targeted adults aged ≥18 years, of which 7 (70%) studies focused on adults who had engaged in past-month CU [ 70 , 71 , 80 , 84 , 85 , 90 , 91 ], 2 (20%) studies included adults who wished to reduce or cease CU [ 72 , 89 ], and 1 (10%) study focused on noncollege adults with a moderate risk associated with CU [ 88 ]. Sinadinovic et al [ 92 ] targeted young adults aged ≥16 years who had used cannabis at least once a week in the previous 6 months. The remaining 8 studies targeted college or university students (aged ≥17 y) specifically, of which 7 (88%) studies focused solely on students who reported using cannabis [ 78 , 79 , 81 - 83 , 86 , 87 ] and 1 (12%) study focused solely on students who did not report past-month CU (ie, abstainers) [ 77 ].
Intervention Characteristics
The 19 included studies assessed nine different digital interventions: (1) 5 (26%) evaluated Marijuana eCHECKUP TO GO (e-TOKE), a commercially available electronic intervention used at colleges throughout the United States and Canada [ 77 , 78 , 81 - 83 ]; (2) 2 (11%) examined the internationally known CANreduce program [ 70 , 91 ]; (3) 2 (11%) evaluated the German Quit the Shit program [ 72 , 90 ]; (4) 2 (11%) assessed a social media–delivered, physical activity–focused cannabis intervention [ 84 , 85 ]; (5) 1 (5%) investigated the Swedish Cannabishjälpen intervention [ 92 ]; (6) 1 (5%) evaluated the Australian Grassessment: Evaluate Your Use of Cannabis website program [ 89 ]; (7) 1 (5%) assessed the Canadian Ma réussite, mon choix intervention [ 87 ]; (8) 1 (5%) examined the Australian Reduce Your Use: How to Break the Cannabis Habit program [ 71 ]; and (9) 4 (21%) each evaluated a unique no-name intervention described as a personalized feedback intervention (PFI) [ 79 , 80 , 86 , 88 ]. Detailed information regarding the characteristics of all interventions as reported in each included study is provided in Multimedia Appendix 6 [ 70 - 72 , 77 - 113 ] and summarized in the following paragraphs.
In several studies (8/19, 42%), the interventions were designed to support cannabis users in reducing or ceasing their consumption [ 70 , 72 , 80 , 87 , 89 - 92 ]. In 37% (7/19) of the studies, the interventions aimed at reducing both CU and cannabis-related consequences [ 79 , 81 - 85 , 88 ]. Other interventions focused on helping college students think carefully about the decision to use cannabis [ 77 , 78 ] and on reducing either cannabis-related problems among undergraduate students [ 86 ] or symptoms associated with CU disorder in young adults [ 71 ].
In 26% (5/19) of the studies, theory was used to inform intervention design along with a clear rationale for theory use. Of these 5 articles, only 1 (20%) [ 87 ] reported using a single theory of behavior change, the theory of planned behavior [ 114 ]. A total of 21% (4/19) of the studies selected only constructs of theories (or models) for their intervention design. Of these 4 studies, 2 (50%) evaluated the same intervention [ 72 , 90 ], which focused on principles of self-regulation and self-control theory [ 93 ]; 1 (25%) [ 70 ] used the concept of adherence-focused guidance enhancement based on the supportive accountability model of guidance [ 94 ]; and 1 (25%) [ 71 ] reported that intervention design was guided by the concept of self-behavioral management.
The strategies (or approaches) used in the delivery of the digital interventions were discussed in greater detail in 84% (16/19) of the articles [ 70 - 72 , 79 - 81 , 83 - 92 ]. Many of these articles (9/19, 47%) reported using a combination of approaches based on CBT or motivational interviewing (MI) [ 70 , 71 , 79 , 83 - 85 , 90 - 92 ]. PFIs were also often mentioned as an approach to inform intervention delivery [ 7 , 71 , 79 , 86 - 88 ].
More than half (13/19, 68%) of all the digital interventions were asynchronous and based on a self-guided approach without support from a counselor or therapist. The study by Côté et al [ 87 ] evaluated the efficacy of a web-based tailored intervention focused on reinforcing a positive attitude toward and a sense of control over cannabis abstinence through psychoeducational messages delivered by a credible character in short video clips and personalized reinforcement messages. Lee et al [ 79 ] evaluated a brief, web-based personalized feedback selective intervention based on the PFI approach pioneered by Marlatt et al [ 95 ] for alcohol use prevention and on the MI approach described by Miller and Rollnick [ 96 ]. Similarly, Rooke et al [ 71 ] combined principles of MI and CBT to develop a web-based intervention delivered via web modules, which were informed by previous automated feedback interventions targeting substance use. The study by Copeland et al [ 89 ] assessed the short-term effectiveness of Grassessment: Evaluate Your Use of Cannabis, a brief web-based, self-complete intervention based on motivational enhancement therapy that included personalized feedback messages and psychoeducational material. In the studies by Buckner et al [ 80 ], Cunningham et al [ 88 ], and Walukevich-Dienst et al [ 86 ], experimental groups received a brief web-based PFI available via a computer. A total of 16% (3/19) of the studies [ 77 , 78 , 82 ] applied a program called the Marijuana eCHECKUP TO GO (e-TOKE) for Universities and Colleges, which was presented as a web-based, norm-correcting, brief preventive and intervention education program designed to prompt self-reflection on consequences and consideration of decreasing CU among students. Riggs et al [ 83 ] developed and evaluated an adapted version of e-TOKE that provided participants with university-specific personalized feedback and normative information based on protective behavioral strategies for CU [ 97 ]. Similarly, Goodness and Palfai [ 81 ] tested the efficacy of eCHECKUP TO GO-cannabis, a modified version of e-TOKE combining personalized feedback, norm correction, and a harm and frequency reduction strategy where a “booster” session was provided at 3 months to allow participants to receive repeated exposure to the intervention.
In the remaining 32% (6/19) of the studies, which examined 4 different interventions, the presence of a therapist guide was reported. The intervention evaluated by Sinadinovic et al [ 92 ] combined principles of psychoeducation, MI, and CBT organized into 13 web-based modules and a calendar involving therapist guidance, recommendations, and personal feedback. In total, 33% (2/6) of these studies evaluated a social media–delivered intervention with e-coaches that combined principles of MI and CBT and a harm reduction approach for risky CU [ 84 , 85 ]. Schaub et al [ 91 ] evaluated the efficacy of CANreduce, a web-based self-help intervention based on both MI and CBT approaches, using automated motivational and feedback emails, chat with a counselor, and web-based psychoeducational modules. Similarly, Baumgartner et al [ 70 ] investigated the effectiveness of CANreduce 2.0, a modified version of CANreduce, using semiautomated motivational and adherence-focused guidance-based email feedback with or without a personal online coach. The studies by Tossman et al [ 72 ] and Jonas et al [ 90 ] used a solution-focused approach and MI to evaluate the effectiveness of the German Quit the Shit web-based program that involves weekly feedback provided by counselors.
In addition to using different intervention strategies or approaches, the interventions were diverse in terms of the duration and frequency of the program (eg, web-based activities, sessions, or modules). Of the 12 articles that provided details in this regard, 2 (17%) on the same intervention described it as a brief 20- to 45-minute web-based program [ 77 , 78 ], 2 (17%) on 2 different interventions reported including 1 or 2 modules per week for a duration of 6 weeks [ 71 , 92 ], and 7 (58%) on 4 different interventions described them as being available over a longer period ranging from 6 weeks to 3 months [ 70 , 72 , 79 , 84 , 85 , 87 , 90 , 91 ].
Comparator Types
A total of 42% (8/19) of the studies [ 72 , 77 - 80 , 85 , 87 , 92 ] used a passive comparator only, namely, a waitlist control group ( Multimedia Appendix 5 ). A total of 26% (5/19) of the studies used an active comparator only where participants were provided with minimal general health feedback regarding recommended guidelines for sleep, exercise, and nutrition [ 81 , 82 ]; strategies for healthy stress management [ 83 ]; educational materials about risky CU [ 88 ]; or access to a website containing information about cannabis [ 71 ]. In another 21% (4/19) of the studies, which used an active comparator, participants received the same digital intervention minus a specific component: a personal web-based coach [ 70 ], extended personalized feedback [ 89 ], web-based chat counseling [ 91 ], or information on risks associated with CU [ 86 ]. A total of 21% (4/19) of the studies had more than one control group [ 70 , 84 , 90 , 91 ].
Outcome Variable Assessment and Summary of Main Findings of the Studies
The methodological characteristics and major findings of the included studies (N=19) are presented in Multimedia Appendix 7 [ 67 , 70 - 72 , 77 - 92 , 115 - 120 ] and summarized in the following sections for each outcome of interest in this review (ie, CU and cannabis-related consequences). Of the 19 studies, 11 (58%) were reported as efficacy trials [ 7 , 77 , 79 , 81 - 83 , 86 - 88 , 91 , 92 ], and 8 (42%) were reported as effectiveness trials [ 70 - 72 , 78 , 84 , 85 , 89 , 90 ].
Across all the included studies (19/19, 100%), participant attrition rates ranged from 1.6% at 1 month after the baseline [ 77 , 78 ] to 75.1% at the 3-month follow-up [ 70 ]. A total of 37% (7/19) of the studies assessed and reported results regarding user engagement [ 71 , 78 , 84 , 85 , 90 - 92 ] using different types of metrics. In one article on the Marijuana eCHECKUP TO GO (e-TOKE) web-based program [ 78 ], the authors briefly reported that participation was confirmed for 98.1% (158/161) of participants in the intervention group. In 11% (2/19) of the studies, which were on a similar social media–delivered intervention [ 84 , 85 ], user engagement was quantified by tallying the number of comments or posts and reactions (eg, likes and hearts) left by participants. In both studies [ 84 , 85 ], the intervention group, which involved a CU-related Facebook page, displayed greater interactions than the control groups, which involved a Facebook page unrelated to CU. One article [ 84 ] reported that 80% of participants in the intervention group posted at least once (range 0-60) and 50% posted at least weekly. In the other study [ 85 ], the results showed that intervention participants engaged (ie, posting or commenting or clicking reactions) on average 47.9 times each over 8 weeks. In total, 11% (2/19) of the studies [ 90 , 91 ] on 2 different web-based intervention programs, both consisting of web documentation accompanied by chat-based counseling, measured user engagement either by average duration or average number of chat sessions. Finally, 16% (3/19) of the studies [ 71 , 91 , 92 ], which involved 3 different web-based intervention programs, characterized user engagement by the mean number of web modules completed per participant. Overall, the mean number of web modules completed reported in these articles was quite similar: 3.9 out of 13 [ 92 ] and 3.2 [ 91 ] and 3.5 [ 71 ] out of 6.
Assessment of CU
As presented in Multimedia Appendix 7 , the included studies differed in terms of how they assessed CU, although all used at least one self-reported measure of frequency. Most studies (16/19, 84%) measured frequency by days of use, including days of use in the preceding week [ 91 ] or 2 [ 80 ], days of use in the previous 30 [ 70 - 72 , 78 , 84 - 86 , 88 - 90 ] or 90 days [ 79 , 81 , 82 ], and days high per week [ 83 ]. Other self-reported measures of CU frequency included (1) number of CU events in the previous month [ 87 , 90 ], (2) cannabis initiation or use in the previous month (ie, yes or no) [ 77 ], and (3) days without CU in the previous 7 days [ 92 ]. In addition to measuring CU frequency, 42% (8/19) of the studies also assessed CU via self-reported measures of quantity used, including estimated grams consumed in the previous week [ 92 ] or 30 days [ 72 , 85 , 90 ] and the number of standard-sized joints consumed in the previous 7 days [ 91 ] or the previous month [ 70 , 71 , 89 ].
Of the 19 articles included, 10 (53%) [ 70 - 72 , 80 , 84 - 86 , 89 , 90 , 92 ] reported using a validated instrument to measure CU frequency or quantity, including the TLFB instrument [ 66 ] (n=9, 90% of the studies) and the Marijuana Use Form (n=1, 10% of the studies); 1 (10%) [ 79 ] reported using CU-related questions from an adaptation of the Global Appraisal of Individual Needs–Initial instrument [ 115 ]; and 30% (3/10) [ 81 , 82 , 91 ] reported using a questionnaire accompanied by a calendar or a diary of consumption. The 19 studies also differed with regard to their follow-up time measurements for assessing CU, ranging from 2 weeks after the baseline [ 80 ] to 12 months after randomization [ 90 ], although 12 (63%) of the studies included a 3-month follow-up assessment [ 70 - 72 , 79 , 81 , 82 , 84 , 85 , 88 , 90 - 92 ].
Of all studies assessing and reporting change in CU frequency from baseline to follow-up assessments (19/19, 100%), 47% (9/19) found statistically significant differences between the experimental and control groups [ 70 - 72 , 80 , 81 , 83 , 85 , 87 , 91 ]. Importantly, 67% (6/9) of these studies showed that participants in the experimental groups exhibited greater decreases in CU frequency 3 months following the baseline assessment compared with participants in the control groups [ 70 - 72 , 81 , 85 , 91 ], 22% (2/9) of the studies showed greater decreases in CU frequency at 6 weeks after the baseline assessment [ 71 , 83 ], 22% (2/9) of the studies showed greater decreases in CU frequency at 6 months following the baseline assessment [ 81 , 85 ], 11% (1/9) of the studies showed greater decreases in CU frequency at 2 weeks after the baseline [ 80 ], and 11% (1/9) of the studies showed greater decreases in CU frequency at 2 months after treatment [ 87 ].
In the study by Baumgartner et al [ 70 ], a reduction in CU days was observed in all groups, but the authors reported that the difference was statistically significant only between the intervention group with the service team and the control group (the reduction in the intervention group with social presence was not significant). In the study by Bonar et al [ 85 ], the only statistically significant difference between the intervention and control groups at the 3- and 6-month follow-ups involved total days of cannabis vaping in the previous 30 days. Finally, in the study by Buckner et al [ 80 ], the intervention group had less CU than the control group 2 weeks after the baseline; however, this was statistically significant only for participants with moderate or high levels of social anxiety.
Assessment of Cannabis-Related Negative Consequences
A total of 53% (10/19) of the studies also assessed cannabis-related negative consequences [ 78 - 84 , 86 , 88 , 92 ]. Of these 10 articles, 8 (80%) reported using a validated self-report instrument: 4 (50%) [ 81 , 82 , 86 , 88 ] used the 19-item Marijuana Problems Scale [ 67 ], 2 (25%) [ 78 , 79 ] used the 18-item Rutgers Marijuana Problem Index [ 121 , 122 ], and 2 (25%) [ 80 , 84 ] used the Brief Marijuana Consequences Questionnaire [ 116 ]. Only 10% (1/10) of the studies [ 92 ] used a screening tool, the Cannabis Abuse Screening Test [ 117 , 118 ]. None of these 10 studies demonstrated a statistically significant difference between the intervention and control groups. Of note, Walukevich-Dienst et al [ 86 ] found that women (but not men) who received an web-based PFI with additional information on CU risks reported significantly fewer cannabis-related problems than did women in the control group at 1 month after the intervention ( B =−1.941; P =.01).
Descriptive Summary of BCTs Used in Intervention Groups
After the 19 studies included in this review were coded, a total of 184 individual BCTs targeting CU in young adults were identified. Of these 184 BCTs, 133 (72.3% ) were deemed to be present beyond a reasonable doubt, and 51 (27.7%) were deemed to be present in all probability. Multimedia Appendix 8 [ 48 , 70 - 72 , 77 - 92 ] presents all the BCTs coded for each included study summarized by individual BCT and BCT cluster.
The 184 individual BCTs coded covered 38% (35/93) of the BCTs listed in the BCTTv1 [ 48 ]. The number of individual BCTs identified per study ranged from 5 to 19, with two-thirds of the 19 studies (12/19, 63%) using ≤9 BCTs (mean 9.68). As Multimedia Appendix 8 shows, at least one BCT fell into 13 of the 16 possible BCT clusters. The most frequent clusters were feedback monitoring , natural consequences , goal planning , and comparison of outcomes .
The most frequently coded BCTs were (1) feedback on behavior (BCT 2.2; 17/19, 89% of the studies; eg, “Once a week, participants receive detailed feedback by their counselor on their entries in diary and exercises. Depending on the involvement of each participant, up to seven feedbacks are given” [ 90 ]), (2) social support (unspecified) (BCT 3.1; 15/19, 79% of the studies; eg, “The website also features [...] blogs from former cannabis users, quick assist links, and weekly automatically generated encouragement emails” [ 71 ]), and (3) pros and cons (BCT 9.2; 14/19, 74% of the studies; eg, “participants are encouraged to state their personal reasons for and against their cannabis consumption, which they can review at any time, so they may reflect on what they could gain by successfully completing the program” [ 70 ]). Other commonly identified BCTs included social comparison (BCT 6.2; 12/19, 63% of the studies) and information about social and environmental consequences (BCT 5.3; 11/19, 58% of the studies), followed by problem solving (BCT 2.1; 10/19, 53% of the studies) and information about health consequences (BCT 5.1; 10/19, 53% of the studies).
RoB Assessment
Figure 2 presents the overall assessment of risk in each domain for all the included studies, whereas Figure 3 [ 70 - 72 , 77 - 92 ] summarizes the assessment of each study at the outcome level for each domain in the Cochrane RoB 2 [ 74 ].
Figure 2 shows that, of the included studies, 93% (27/29) were rated as having a “low” RoB arising from the randomization process (ie, selection bias) and 83% (24/29) were rated as having a “low” RoB due to missing data (ie, attrition bias). For bias due to deviations from the intended intervention (ie, performance bias), 72% (21/29) were rated as having a “low” risk, and for selective reporting of results, 59% (17/29) were rated as having a “low” risk. In the remaining domain regarding bias in measurement of the outcome (ie, detection bias), 48% (14/29) of the studies were deemed to present “some concerns,” mainly owing to the outcome assessment not being blinded (eg, self-reported outcome measure of CU). Finally, 79% (15/19) of the included studies were deemed to present “some concerns” or were rated as having a “high” RoB at the outcome level ( Figure 3 [ 70 - 72 , 77 - 92 ]). The RoB assessment for CU and cannabis consequences of each included study is presented in Multimedia Appendix 9 [ 70 - 72 , 77 - 92 ].

Meta-Analysis Results
Due to several missing data points and despite contacting the authors, we were able to carry out only 1 meta-analysis of our primary outcome, CU frequency. Usable data were retrieved from only 16% (3/19) [ 70 - 72 ] of the studies included in this review. These 3 studies provided sufficient information to calculate an effect size, including mean differences based on change-from-baseline measurements and associated 95% CIs (or SE of the mean difference) and sample sizes per intervention and comparison conditions. The reasons for excluding the other 84% (16/19) of the studies included heterogeneity in outcome variables or measurements, inconsistent results, and missing data ( Multimedia Appendix 10 [ 77 - 92 ]).
Figure 4 [ 70 - 72 ] illustrates the mean differences and associated 95% CIs of 3 unique RCTs [ 70 - 72 ] that provided sufficient information to allow for the measurement of CU frequency at 3 months after the baseline relative to a comparison condition in terms of the number of self-reported days of use in the previous month using the TLFB method. Overall, the synthesized effect of digital interventions for young adult cannabis users on CU frequency, as measured using days of use in the previous month, was −6.79 (95% CI −9.59 to −4.00). This suggests that digital CU interventions had a statistically significant effect ( P <.001) on reducing CU frequency at the 3-month follow-up compared with the control conditions (both passive and active controls). The results of the meta-analysis also showed low between-study heterogeneity ( I 2 =48.3%; P =.12) across the 3 included studies.

The samples of the 3 studies included in the meta-analysis varied in size from 225 to 1292 participants (mean 697.33, SD 444.11), and the mean age ranged from 24.7 to 31.88 years (mean 26.38, SD 3.58 years). These studies involved 3 different digital interventions and used different design approaches to assess intervention effectiveness. One study assessed the effectiveness of a web-based counseling program (ie, Quit the Shit) against a waitlist control [ 72 ], another examined the effectiveness of a fully self-guided web-based treatment program for CU and related problems (ie, Reduce Your Use: How to Break the Cannabis Habit) against a control condition website consisting of basic educational information on cannabis [ 71 ], and the third used a 3-arm RCT design to investigate whether the effectiveness of a minimally guided internet-based self-help intervention (ie, CANreduce 2.0) might be enhanced by implementing adherence-focused guidance and emphasizing the social presence factor of a personal e-coach [ 70 ].
Summary of Principal Findings
The primary aim of this systematic review was to evaluate the effectiveness of digital interventions in addressing CU among community-living young adults. We included 19 randomized controlled studies representing 9 unique digital interventions aimed at preventing, reducing, or ceasing CU and evaluated the effects of 3 different digital interventions on CU. In summary, the 3 digital interventions included in the meta-analysis proved superior to control conditions in reducing the number of days of CU in the previous month at the 3-month follow-up.
Our findings are consistent with those of 2 previous meta-analyses by Olmos et al [ 43 ] and Tait et al [ 44 ] and with the findings of a recently published umbrella review of systematic reviews and meta-analyses of RCTs [ 123 ], all of which revealed a positive effect of internet- and computer-based interventions on CU. However, a recent systematic review and meta-analysis by Beneria et al [ 45 ] found that web-based CU interventions did not significantly reduce CU. Beneria et al [ 45 ] included studies with different intervention programs that targeted diverse population groups (both adolescents and young adults) and use of more than one substance (eg, alcohol and cannabis). In our systematic review, a more conservative approach was taken—we focused specifically on young adults and considered interventions targeting CU only. Although our results indicate that digital interventions hold great promise in terms of effectiveness, an important question that remains unresolved is whether there is an optimal exposure dose in terms of both duration and frequency that might be more effective. Among the studies included in this systematic review, interventions varied considerably in terms of the number of psychoeducational modules offered (from 2 to 13), time spent reviewing the material, and duration (from a single session to a 12-week spread period). Our results suggest that an intervention duration of at least 6 weeks yields better results.
Another important finding of this review is that, although almost half (9/19, 47%) of the included studies observed an intervention effect on CU frequency, none reported a statistically significant improvement in cannabis-related negative consequences, which may be considered a more distal indicator. More than half (10/19, 53%) of the included studies investigated this outcome. It seems normal to expect to find an effect on CU frequency given that reducing CU is often the primary objective of interventions and because the motivation of users’ is generally focused on changing consumption behavior. It is plausible to think that the change in behavior at the consumption level must be maintained over time before an effect on cannabis-related negative consequences can be observed. However, our results showed that, in all the included studies, cannabis-related negative consequences and change in behavior (CU frequency) were measured at the same time point, namely, 3 months after the baseline. Moreover, Grigsby et al [ 124 ] conducted a scoping review of risk and protective factors for CU and suggested that interventions to reduce negative CU consequences should prioritize multilevel methods or strategies “to attenuate the cumulative risk from a combination of psychological, contextual, and social influences.”
A secondary objective of this systematic review was to describe the active ingredients used in digital interventions for CU among young adults. The vast majority of the interventions were based on either a theory or an intervention approach derived from theories such as CBT, MI, and personalized feedback. From these theories and approaches stem behavior change strategies or techniques, commonly known as BCTs. Feedback on behavior , included in the feedback monitoring BCT cluster, was the most common BCT used in the included studies. This specific BCT appears to be a core strategy in behavior change interventions [ 125 , 126 ]. In their systematic review of remotely delivered alcohol or substance misuse interventions for adults, Howlett et al [ 53 ] found that feedback on behavior , problem solving , and goal setting were the most frequently used BCTs in the included studies. In addition, this research group noted that the most promising BCTs for alcohol misuse were avoidance/reducing exposure to cues for behavior , pros and cons , and self-monitoring of behavior, whereas 2 very promising strategies for substance misuse in general were problem solving and self-monitoring of behavior . In our systematic review, in addition to feedback on behavior , the 6 most frequently used BCTs in the included studies were social support , pros and cons , social comparison , problem solving , information about social and environmental consequences , and information about health consequences . Although pros and cons and problem solving were present in all 3 studies of digital interventions included in our meta-analysis, avoidance/reducing exposure to cues for behavior was reported in only 5% (1/19) of the articles, and feedback on behavior was more frequently used than self-monitoring of behavior. However, it should be noted that the review by Howlett et al [ 53 ] examined digital interventions for participants with alcohol or substance misuse problems, whereas in this review, we focused on interventions that targeted CU from a harm reduction perspective. In this light, avoidance/reducing exposure to cues for behavior may be a BCT better suited to populations with substance misuse problems. Lending support to this, a meta-regression by Garnett et al [ 127 ] and a Cochrane systematic review by Kaner et al [ 128 ] both found interventions that used behavior substitution and credible source to be associated with greater reduction in excessive alcohol consumption compared with interventions that used other BCTs.
Beyond the number and types of BCTs used, reflecting on the extent to which each BCT in a given intervention suits (or does not suit) the targeted determinants (ie, behavioral and environmental causes) is crucial for planning intervention programs [ 26 ]. It is important when designing digital CU interventions not merely to pick a combination of BCTs that have been associated with effectiveness. Rather, the active ingredients must fit the determinants that the interventionists seek to influence. For example, action planning would be more relevant as a BCT for young adults highly motivated and ready to take action on their CU than would pros and cons , which aims instead to bolster motivation. Given that more than half of all digital interventions are asynchronous and based on a self-guided approach and do not offer counselor or therapist support, a great deal of motivation is required to engage in intervention and behavior change. Therefore, it is essential that developers consider the needs and characteristics of the targeted population to tailor intervention strategies (ie, BCTs) for successful behavior change (eg, tailored to the participant’s stage of change). In most of the digital interventions included in this systematic review, personalization was achieved through feedback messages about CU regarding descriptive norms, motives, risks and consequences, and costs, among other things.
Despite the high number of recent studies conducted in the field of digital CU interventions, most of the included articles in our review (17/19, 89%) reported on the development and evaluation of web-based intervention programs. A new generation of health intervention modalities such as mobile apps and social media has drawn the attention of researchers in the past decade and is currently being evaluated. In this regard, the results from a recently published scoping review [ 129 ], which included 5 studies of mobile apps for nonmedical CU, suggested that these novel modes of intervention delivery demonstrated adequate feasibility and acceptability. Nevertheless, the internet remains a powerful and convenient medium for reaching young adults with digital interventions intended to support safe CU behaviors [ 123 , 130 ].
Quality of Evidence
The GRADE (Grading of Recommendations Assessment, Development, and Evaluation) approach [ 131 - 133 ] was used to assess the quality of the evidence reviewed. It was deemed to be moderate for the primary outcome of this review, that is, CU frequency in terms of days of use in the previous month (see the summary of evidence in Multimedia Appendix 11 [ 70 , 72 ]). The direction of evidence was broadly consistent—in all 3 RCT studies [ 70 - 72 ] included in the meta-analysis, participants who received digital CU interventions reduced their consumption compared with those who received no or minimal interventions. The 3 RCTs were similar in that they all involved a web-based, multicomponent intervention program aimed at reducing or ceasing CU. However, the interventions did differ or vary in terms of several characteristics, including the strategies used, content, frequency, and duration. Given the small number of studies included in the meta-analysis, we could not conclude with certainty which intervention components, if any, contributed to the effect estimate observed.
Although inconsistency, indirectness, and imprecision were not major issues in the body of evidence, we downgraded the evidence from high to moderate quality on account of RoB assessments at the outcome level. The 3 RCT studies included in the meta-analysis were rated as having “some concerns” of RoB, mainly due to lack of blinding, which significantly reduced our certainty relative to subjective outcomes (ie, self-reported measures of CU frequency). A positive feature of these digital intervention trials is that most procedures are fully automated, and so there was typically a low RoB regarding randomization procedures, allocation to different conditions, and intervention delivery. It is impossible to blind participants to these types of behavior change interventions, and although some researchers have made attempts to counter the impact of this risk, performance bias is an inescapable issue in RCT studies of this kind. Blinding of intervention providers was not an issue in the 3 RCTs included in the meta-analysis because outcome data collection was automated. However, this same automated procedure made it very difficult to ensure follow‐up. Consequently, attrition was another source of bias in these RCT studies [ 70 - 72 ]. The participants lost to follow-up likely stopped using the intervention. However, there is no way of determining whether these people would have benefited more or less than the completers if they had seen the trial through.
The 3 RCTs included in the meta-analysis relied on subjective self-reported measures of CU at baseline and follow‐up, which are subject to recall and social desirability bias. However, all 3 studies used a well-validated instrument of measurement to determine frequency of CU, the TLFB [ 66 ]. This is a widely used, subjective self-report tool for measuring frequency (or quantity) of substance use (or abstinence). It is considered a reliable measure of CU [ 134 , 135 ]. Finally, it should be pointed out that any potential bias related to self‐reported CU frequency would have affected both the intervention and control groups (particularly in cases in which control groups received cannabis‐related information), and thus, it was unlikely to account for differential intervention effects. Moreover, we found RoB due to selective reporting in some studies owing mainly to the absence of any reference to a protocol. Ultimately, these limitations may have biased the results of the meta-analysis. Consequently, future research is likely to further undermine our confidence in the effect estimate we observed and report considerably different estimates.
Strengths and Limitations
Our systematic review and meta-analysis has a number of strengths: (1) we included only randomized controlled studies to ensure that the included studies possessed a rigorous research design, (2) we focused specifically on cannabis (rather than combining multiple substances), (3) we assessed the effectiveness of 3 different digital interventions on CU frequency among community-living young adults, and (4) we performed an exhaustive synthesis and comparison of the BCTs used in the 9 digital interventions examined in the 19 studies included in our review based on the BCTTv1.
Admittedly, this systematic review and meta-analysis has limitations that should be recognized. First, although we searched a range of bibliographic databases, the review was limited to articles published in peer-reviewed journals in English or French. This may have introduced publication bias given that articles reporting positive effects are more likely to be published than those with negative or equivocal results. Consequently, the studies included in this review may have overrepresented the statistically significant effects of digital CU interventions.
Second, only a small number of studies were included in the meta-analyses because many studies did not provide adequate statistical information for calculating and synthesizing effect sizes, although significant efforts were made to contact the authors in case of missing data. Because of the small sample size used in the meta-analysis, the effect size estimates may not be highly reflective of the true effects of digital interventions on CU frequency among young adults. Furthermore, synthesizing findings across studies that evaluated different modalities of web-based intervention programs (eg, fully self-guided vs with therapist guidance) and types of intervention approaches (eg, CBT, MI, and personalized feedback) may have introduced bias in the meta-analytical results due to the heterogeneity of the included studies, although heterogeneity was controlled for using a random-effects model and our results indicated low between-study heterogeneity.
Third, we took various measures to ensure that BCT coding was carried out rigorously throughout the data extraction and analysis procedures: (1) all coders received training on how to use the BCTTv1; (2) all the included articles were read line by line so that coders became familiar with intervention descriptions before initiating BCT coding; (3) the intervention description of each included article was double coded after a pilot calibration exercise with all coders, and any disagreements regarding the presence or absence of a BCT were discussed and resolved with a third party; and (4) we contacted the article authors when necessary and possible for further details on the BCTs they used. However, incomplete reporting of intervention content is a recognized issue [ 136 ], which may have resulted in our coding BCTs incorrectly as present or absent. Reliably specifying the BCTs used in interventions allows their active ingredients to be identified, their evidence to be synthesized, and interventions to be replicated, thereby providing tangible guidance to programmers and researchers to develop more effective interventions.
Finally, although this review identified the BCTs used in digital interventions, our approach did not allow us to draw conclusions regarding their effectiveness. Coding BCTs simply as present or absent does not consider the frequency, intensity, and quality with which they were delivered. For example, it is unclear how many individuals should self‐monitor their CU. In addition, the quality of BCT implementation may be critical in digital interventions where different graphics and interface designs and the usability of the BCTs used can have considerable influence on the level of user engagement [ 137 ]. In the future, it may be necessary to develop new methods to evaluate the dosage of individual BCTs in digital health interventions and characterize their implementation quality to assess their effectiveness [ 128 , 138 ]. Despite its limitations, this review suggests that digital interventions represent a promising avenue for preventing, reducing, or ceasing CU among community-living young adults.
Conclusions
The results of this systematic review and meta-analysis lend support to the promise of digital interventions as an effective means of reducing recreational CU frequency among young adults. Despite the advent and popularity of smartphones, web-based interventions remain the most common mode of delivery for digital interventions. The active ingredients of digital interventions are varied and encompass a number of clusters of the BCTTv1, but a significant number of BCTs remain underused. Additional research is needed to further investigate the effectiveness of these interventions on CU and key outcomes at later time points. Finally, a detailed assessment of user engagement with digital interventions for CU and understanding which intervention components are the most effective remain important research gaps.
Acknowledgments
The authors would like to thank Bénédicte Nauche, Miguel Chagnon, and Paul Di Biase for their valuable support with the search strategy development, statistical analysis, and linguistic revision, respectively. This work was supported by the Ministère de la Santé et des Services sociaux du Québec as part of a broader study aimed at developing and evaluating a digital intervention for young adult cannabis users. Additional funding was provided by the Research Chair in Innovative Nursing Practices. The views and opinions expressed in this manuscript do not necessarily reflect those of these funding entities.
Data Availability
The data sets generated and analyzed during this study are available from the corresponding author on reasonable request.
Authors' Contributions
JC contributed to conceptualization, methodology, formal analysis, writing—original draft, supervision, and funding acquisition. GC contributed to conceptualization, methodology, formal analysis, investigation, data curation, writing—original draft, visualization, and project administration. BV contributed to conceptualization, methodology, formal analysis, investigation, data curation, writing—original draft, and visualization. PA contributed to conceptualization, methodology, formal analysis, investigation, data curation, writing—original draft, visualization, and project administration. GR contributed to conceptualization, methodology, formal analysis, investigation, data curation, and writing—review and editing. GF contributed to conceptualization, methodology, formal analysis, investigation, data curation, and writing—review and editing. DJA contributed to conceptualization, methodology, formal analysis, writing—review and editing, and funding acquisition.
Conflicts of Interest
None declared.
PRISMA (Preferred Reporting Items for Systematic Reviews and Meta-Analyses) checklist.
Detailed search strategies for each database.
Population, intervention, comparison, outcome, and study design strategy.
Excluded studies and reasons for exclusion.
Study and participant characteristics.
Description of intervention characteristics in the included articles.
Summary of methodological characteristics and major findings of the included studies categorized by intervention name.
Behavior change techniques (BCTs) coded in each included study summarized by individual BCT and BCT cluster.
Risk-of-bias assessment of each included study for cannabis use and cannabis consequences.
Excluded studies and reasons for exclusion from the meta-analysis.
Summary of evidence according to the Grading of Recommendations Assessment, Development, and Evaluation tool.
- Arnett JJ. The developmental context of substance use in emerging adulthood. J Drug Issues. 2005;35(2):235-254. [ CrossRef ]
- Stockings E, Hall WD, Lynskey M, Morley KI, Reavley N, Strang J, et al. Prevention, early intervention, harm reduction, and treatment of substance use in young people. Lancet Psychiatry. Mar 2016;3(3):280-296. [ CrossRef ] [ Medline ]
- ElSohly MA, Chandra S, Radwan M, Majumdar CG, Church JC. A comprehensive review of cannabis potency in the United States in the last decade. Biol Psychiatry Cogn Neurosci Neuroimaging. Jun 2021;6(6):603-606. [ CrossRef ] [ Medline ]
- Fischer B, Robinson T, Bullen C, Curran V, Jutras-Aswad D, Medina-Mora ME, et al. Lower-Risk Cannabis Use Guidelines (LRCUG) for reducing health harms from non-medical cannabis use: a comprehensive evidence and recommendations update. Int J Drug Policy. Jan 2022;99:103381. [ FREE Full text ] [ CrossRef ] [ Medline ]
- Rotermann M. What has changed since cannabis was legalized? Health Rep. Feb 19, 2020;31(2):11-20. [ FREE Full text ] [ CrossRef ] [ Medline ]
- Degenhardt L, Stockings E, Patton G, Hall WD, Lynskey M. The increasing global health priority of substance use in young people. Lancet Psychiatry. Mar 2016;3(3):251-264. [ CrossRef ] [ Medline ]
- Buckner JD, Bonn-Miller MO, Zvolensky MJ, Schmidt NB. Marijuana use motives and social anxiety among marijuana-using young adults. Addict Behav. Oct 2007;32(10):2238-2252. [ FREE Full text ] [ CrossRef ] [ Medline ]
- Carliner H, Brown QL, Sarvet AL, Hasin DS. Cannabis use, attitudes, and legal status in the U.S.: a review. Prev Med. Nov 2017;104:13-23. [ FREE Full text ] [ CrossRef ] [ Medline ]
- World drug report 2020. United Nations Office on Drugs and Crime. 2020. URL: https://wdr.unodc.org/wdr2020/index2020.html [accessed 2023-11-28]
- National Academies of Sciences, Engineering, and Medicine, Health and Medicine Division, Board on Population Health and Public Health Practice, Committee on the Health Effects of Marijuana: An Evidence Review and Research Agenda. The Health Effects of Cannabis and Cannabinoids: The Current State of Evidence and Recommendations for Research. Washington, DC. The National Academies Press; 2017.
- Hall WD, Patton G, Stockings E, Weier M, Lynskey M, Morley KI, et al. Why young people's substance use matters for global health. Lancet Psychiatry. Mar 2016;3(3):265-279. [ CrossRef ] [ Medline ]
- Cohen K, Weizman A, Weinstein A. Positive and negative effects of cannabis and cannabinoids on health. Clin Pharmacol Ther. May 2019;105(5):1139-1147. [ CrossRef ] [ Medline ]
- Memedovich KA, Dowsett LE, Spackman E, Noseworthy T, Clement F. The adverse health effects and harms related to marijuana use: an overview review. CMAJ Open. Aug 16, 2018;6(3):E339-E346. [ FREE Full text ] [ CrossRef ] [ Medline ]
- Teeters JB, Armstrong NM, King SA, Hubbard SM. A randomized pilot trial of a mobile phone-based brief intervention with personalized feedback and interactive text messaging to reduce driving after cannabis use and riding with a cannabis impaired driver. J Subst Abuse Treat. Nov 2022;142:108867. [ FREE Full text ] [ CrossRef ] [ Medline ]
- Chan GC, Becker D, Butterworth P, Hines L, Coffey C, Hall W, et al. Young-adult compared to adolescent onset of regular cannabis use: a 20-year prospective cohort study of later consequences. Drug Alcohol Rev. May 2021;40(4):627-636. [ CrossRef ] [ Medline ]
- Hall W, Stjepanović D, Caulkins J, Lynskey M, Leung J, Campbell G, et al. Public health implications of legalising the production and sale of cannabis for medicinal and recreational use. Lancet. Oct 26, 2019;394(10208):1580-1590. [ CrossRef ] [ Medline ]
- The health and social effects of nonmedical cannabis use. World Health Organization. 2016. URL: https://apps.who.int/iris/handle/10665/251056 [accessed 2023-11-28]
- Boumparis N, Loheide-Niesmann L, Blankers M, Ebert DD, Korf D, Schaub MP, et al. Short- and long-term effects of digital prevention and treatment interventions for cannabis use reduction: a systematic review and meta-analysis. Drug Alcohol Depend. Jul 01, 2019;200:82-94. [ FREE Full text ] [ CrossRef ] [ Medline ]
- Jutras-Aswad D, Le Foll B, Bruneau J, Wild TC, Wood E, Fischer B. Thinking beyond legalization: the case for expanding evidence-based options for cannabis use disorder treatment in Canada. Can J Psychiatry. Feb 2019;64(2):82-87. [ FREE Full text ] [ CrossRef ] [ Medline ]
- Garnett CV, Crane D, Brown J, Kaner EF, Beyer FR, Muirhead CR, et al. Behavior change techniques used in digital behavior change interventions to reduce excessive alcohol consumption: a meta-regression. Ann Behav Med. May 18, 2018;52(6):530-543. [ FREE Full text ] [ CrossRef ] [ Medline ]
- Glanz K, Rimer BK, Viswanath K. Health Behavior: Theory, Research, and Practice, 5th Edition. Hoboken, NJ. Jossey-Bass; Jul 2015.
- Prestwich A, Webb TL, Conner M. Using theory to develop and test interventions to promote changes in health behaviour: evidence, issues, and recommendations. Curr Opin Psychol. Oct 2015;5:1-5. [ CrossRef ]
- Webb TL, Sniehotta FF, Michie S. Using theories of behaviour change to inform interventions for addictive behaviours. Addiction. Nov 2010;105(11):1879-1892. [ CrossRef ] [ Medline ]
- Cilliers F, Schuwirth L, van der Vleuten C. Health behaviour theories: a conceptual lens to explore behaviour change. In: Cleland J, Durning SJ, editors. Researching Medical Education. Hoboken, NJ. Wiley; 2015.
- Davis R, Campbell R, Hildon Z, Hobbs L, Michie S. Theories of behaviour and behaviour change across the social and behavioural sciences: a scoping review. Health Psychol Rev. 2015;9(3):323-344. [ FREE Full text ] [ CrossRef ] [ Medline ]
- Eldredge LK, Markham CM, Ruiter RA, Fernández ME, Kok G, Parcel GS. Planning Health Promotion Programs: An Intervention Mapping Approach, 4th Edition. Hoboken, NJ. John Wiley & Sons; Feb 2016.
- Marlatt GA, Blume AW, Parks GA. Integrating harm reduction therapy and traditional substance abuse treatment. J Psychoactive Drugs. 2001;33(1):13-21. [ CrossRef ] [ Medline ]
- Adams A, Ferguson M, Greer AM, Burmeister C, Lock K, McDougall J, et al. Guideline development in harm reduction: considerations around the meaningful involvement of people who access services. Drug Alcohol Depend Rep. Aug 12, 2022;4:100086. [ FREE Full text ] [ CrossRef ] [ Medline ]
- Davis ML, Powers MB, Handelsman P, Medina JL, Zvolensky M, Smits JA. Behavioral therapies for treatment-seeking cannabis users: a meta-analysis of randomized controlled trials. Eval Health Prof. Mar 2015;38(1):94-114. [ FREE Full text ] [ CrossRef ] [ Medline ]
- Gates PJ, Sabioni P, Copeland J, Le Foll B, Gowing L. Psychosocial interventions for cannabis use disorder. Cochrane Database Syst Rev. May 05, 2016;2016(5):CD005336. [ FREE Full text ] [ CrossRef ] [ Medline ]
- Halladay J, Scherer J, MacKillop J, Woock R, Petker T, Linton V, et al. Brief interventions for cannabis use in emerging adults: a systematic review, meta-analysis, and evidence map. Drug Alcohol Depend. Nov 01, 2019;204:107565. [ CrossRef ] [ Medline ]
- Imtiaz S, Roerecke M, Kurdyak P, Samokhvalov AV, Hasan OS, Rehm J. Brief interventions for cannabis use in healthcare settings: systematic review and meta-analyses of randomized trials. J Addict Med. 2020;14(1):78-88. [ CrossRef ] [ Medline ]
- Standeven LR, Scialli A, Chisolm MS, Terplan M. Trends in cannabis treatment admissions in adolescents/young adults: analysis of TEDS-A 1992 to 2016. J Addict Med. 2020;14(4):e29-e36. [ CrossRef ] [ Medline ]
- Montanari L, Guarita B, Mounteney J, Zipfel N, Simon R. Cannabis use among people entering drug treatment in europe: a growing phenomenon? Eur Addict Res. 2017;23(3):113-121. [ FREE Full text ] [ CrossRef ] [ Medline ]
- Kerridge BT, Mauro PM, Chou SP, Saha TD, Pickering RP, Fan AZ, et al. Predictors of treatment utilization and barriers to treatment utilization among individuals with lifetime cannabis use disorder in the United States. Drug Alcohol Depend. Dec 01, 2017;181:223-228. [ FREE Full text ] [ CrossRef ] [ Medline ]
- Gates P, Copeland J, Swift W, Martin G. Barriers and facilitators to cannabis treatment. Drug Alcohol Rev. May 2012;31(3):311-319. [ CrossRef ] [ Medline ]
- Hammarlund RA, Crapanzano KA, Luce L, Mulligan L, Ward KM. Review of the effects of self-stigma and perceived social stigma on the treatment-seeking decisions of individuals with drug- and alcohol-use disorders. Subst Abuse Rehabil. Nov 23, 2018;9:115-136. [ FREE Full text ] [ CrossRef ] [ Medline ]
- Bedrouni W. On the use of digital technologies to reduce the public health impacts of cannabis legalization in Canada. Can J Public Health. Dec 2018;109(5-6):748-751. [ FREE Full text ] [ CrossRef ] [ Medline ]
- Perski O, Hébert ET, Naughton F, Hekler EB, Brown J, Businelle MS. Technology-mediated just-in-time adaptive interventions (JITAIs) to reduce harmful substance use: a systematic review. Addiction. May 2022;117(5):1220-1241. [ FREE Full text ] [ CrossRef ] [ Medline ]
- Kazemi DM, Borsari B, Levine MJ, Li S, Lamberson KA, Matta LA. A systematic review of the mHealth interventions to prevent alcohol and substance abuse. J Health Commun. May 2017;22(5):413-432. [ FREE Full text ] [ CrossRef ] [ Medline ]
- Nesvåg S, McKay JR. Feasibility and effects of digital interventions to support people in recovery from substance use disorders: systematic review. J Med Internet Res. Aug 23, 2018;20(8):e255. [ FREE Full text ] [ CrossRef ] [ Medline ]
- Hoch E, Preuss UW, Ferri M, Simon R. Digital interventions for problematic cannabis users in non-clinical settings: findings from a systematic review and meta-analysis. Eur Addict Res. 2016;22(5):233-242. [ FREE Full text ] [ CrossRef ] [ Medline ]
- Olmos A, Tirado-Muñoz J, Farré M, Torrens M. The efficacy of computerized interventions to reduce cannabis use: a systematic review and meta-analysis. Addict Behav. Apr 2018;79:52-60. [ CrossRef ] [ Medline ]
- Tait RJ, Spijkerman R, Riper H. Internet and computer based interventions for cannabis use: a meta-analysis. Drug Alcohol Depend. Dec 01, 2013;133(2):295-304. [ CrossRef ] [ Medline ]
- Beneria A, Santesteban-Echarri O, Daigre C, Tremain H, Ramos-Quiroga JA, McGorry PD, et al. Online interventions for cannabis use among adolescents and young adults: systematic review and meta-analysis. Early Interv Psychiatry. Aug 2022;16(8):821-844. [ CrossRef ] [ Medline ]
- Craig P, Dieppe P, Macintyre S, Michie S, Nazareth I, Petticrew M. Developing and evaluating complex interventions: the new Medical Research Council guidance. Int J Nurs Stud. May 2013;50(5):587-592. [ FREE Full text ] [ CrossRef ] [ Medline ]
- Michie S, Abraham C, Eccles MP, Francis JJ, Hardeman W, Johnston M. Strengthening evaluation and implementation by specifying components of behaviour change interventions: a study protocol. Implement Sci. Feb 07, 2011;6:10. [ FREE Full text ] [ CrossRef ] [ Medline ]
- Michie S, Richardson M, Johnston M, Abraham C, Francis J, Hardeman W, et al. The behavior change technique taxonomy (v1) of 93 hierarchically clustered techniques: building an international consensus for the reporting of behavior change interventions. Ann Behav Med. Aug 2013;46(1):81-95. [ FREE Full text ] [ CrossRef ] [ Medline ]
- Michie S, Johnston M, Francis J, Hardeman W, Eccles M. From theory to intervention: mapping theoretically derived behavioural determinants to behaviour change techniques. Appl Psychol. Oct 2008;57(4):660-680. [ CrossRef ]
- Scott C, de Barra M, Johnston M, de Bruin M, Scott N, Matheson C, et al. Using the behaviour change technique taxonomy v1 (BCTTv1) to identify the active ingredients of pharmacist interventions to improve non-hospitalised patient health outcomes. BMJ Open. Sep 15, 2020;10(9):e036500. [ FREE Full text ] [ CrossRef ] [ Medline ]
- Dombrowski SU, Sniehotta FF, Avenell A, Johnston M, MacLennan G, Araújo-Soares V. Identifying active ingredients in complex behavioural interventions for obese adults with obesity-related co-morbidities or additional risk factors for co-morbidities: a systematic review. Health Psychol Rev. 2012;6(1):7-32. [ CrossRef ]
- Michie S, Abraham C, Whittington C, McAteer J, Gupta S. Effective techniques in healthy eating and physical activity interventions: a meta-regression. Health Psychol. Nov 2009;28(6):690-701. [ CrossRef ] [ Medline ]
- Howlett N, García-Iglesias J, Bontoft C, Breslin G, Bartington S, Freethy I, et al. A systematic review and behaviour change technique analysis of remotely delivered alcohol and/or substance misuse interventions for adults. Drug Alcohol Depend. Oct 01, 2022;239:109597. [ FREE Full text ] [ CrossRef ] [ Medline ]
- Higgins JP, Thomas J, Chandler J, Cumpston M, Li T, Page MJ, et al. Cochrane Handbook for Systematic Reviews of Interventions Version 6.4. London, UK. The Cochrane Collaboration; 2023.
- Page MJ, Moher D, Bossuyt PM, Boutron I, Hoffmann TC, Mulrow CD, et al. PRISMA 2020 explanation and elaboration: updated guidance and exemplars for reporting systematic reviews. BMJ. Mar 29, 2021;372:n160. [ FREE Full text ] [ CrossRef ] [ Medline ]
- McGowan J, Sampson M, Salzwedel DM, Cogo E, Foerster V, Lefebvre C. PRESS peer review of electronic search strategies: 2015 guideline statement. J Clin Epidemiol. Jul 2016;75:40-46. [ FREE Full text ] [ CrossRef ] [ Medline ]
- Halladay J, Petker T, Fein A, Munn C, MacKillop J. Brief interventions for cannabis use in emerging adults: protocol for a systematic review, meta-analysis, and evidence map. Syst Rev. Jul 25, 2018;7(1):106. [ FREE Full text ] [ CrossRef ] [ Medline ]
- Michie S, van Stralen MM, West R. The behaviour change wheel: a new method for characterising and designing behaviour change interventions. Implement Sci. Apr 23, 2011;6:42. [ FREE Full text ] [ CrossRef ] [ Medline ]
- Arnett JJ. Emerging adulthood. A theory of development from the late teens through the twenties. Am Psychol. May 2000;55(5):469-480. [ Medline ]
- Bramer WM, Giustini D, de Jonge GB, Holland L, Bekhuis T. De-duplication of database search results for systematic reviews in EndNote. J Med Libr Assoc. Jul 2016;104(3):240-243. [ FREE Full text ] [ CrossRef ] [ Medline ]
- Hoffmann TC, Glasziou PP, Boutron I, Milne R, Perera R, Moher D, et al. Better reporting of interventions: template for intervention description and replication (TIDieR) checklist and guide. BMJ. Mar 07, 2014;348:g1687. [ FREE Full text ] [ CrossRef ] [ Medline ]
- Presseau J, Ivers NM, Newham JJ, Knittle K, Danko KJ, Grimshaw JM. Using a behaviour change techniques taxonomy to identify active ingredients within trials of implementation interventions for diabetes care. Implement Sci. Apr 23, 2015;10:55. [ FREE Full text ] [ CrossRef ] [ Medline ]
- Fontaine G, Cossette S, Maheu-Cadotte MA, Deschênes MF, Rouleau G, Lavallée A, et al. Effect of implementation interventions on nurses' behaviour in clinical practice: a systematic review, meta-analysis and meta-regression protocol. Syst Rev. Dec 05, 2019;8(1):305. [ FREE Full text ] [ CrossRef ] [ Medline ]
- Fontaine G, Cossette S. A theory-based adaptive E-learning program aimed at increasing intentions to provide brief behavior change counseling: randomized controlled trial. Nurse Educ Today. Dec 2021;107:105112. [ CrossRef ] [ Medline ]
- Fontaine G, Cossette S. Development and design of E_MOTIV: a theory-based adaptive e-learning program to support nurses' provision of brief behavior change counseling. Comput Inform Nurs. Mar 01, 2023;41(3):130-141. [ CrossRef ] [ Medline ]
- Sobell LC, Sobell MB. Timeline follow-back: a technique for assessing self-reported alcohol consumption. In: Litten RZ, Allen JP, editors. Measuring Alcohol Consumption. Totowa, NJ. Humana Press; 1992.
- Stephens RS, Roffman RA, Simpson EE. Treating adult marijuana dependence: a test of the relapse prevention model. J Consult Clin Psychol. 1994;62(1):92-99. [ CrossRef ]
- Harris RJ, Deeks JJ, Altman DG, Bradburn MJ, Harbord RM, Sterne JA. Metan: fixed- and random-effects meta-analysis. Stata J. 2008;8(1):3-28. [ CrossRef ]
- Higgins JP, Thompson SG, Deeks JJ, Altman DG. Measuring inconsistency in meta-analyses. BMJ. Sep 06, 2003;327(7414):557-560. [ FREE Full text ] [ CrossRef ] [ Medline ]
- Baumgartner C, Schaub MP, Wenger A, Malischnig D, Augsburger M, Walter M, et al. CANreduce 2.0 adherence-focused guidance for internet self-help among cannabis users: three-arm randomized controlled trial. J Med Internet Res. Apr 30, 2021;23(4):e27463. [ FREE Full text ] [ CrossRef ] [ Medline ]
- Rooke S, Copeland J, Norberg M, Hine D, McCambridge J. Effectiveness of a self-guided web-based cannabis treatment program: randomized controlled trial. J Med Internet Res. Feb 15, 2013;15(2):e26. [ FREE Full text ] [ CrossRef ] [ Medline ]
- Tossmann HP, Jonas B, Tensil MD, Lang P, Strüber E. A controlled trial of an internet-based intervention program for cannabis users. Cyberpsychol Behav Soc Netw. Nov 2011;14(11):673-679. [ CrossRef ] [ Medline ]
- StataCorp. Stata statistical software: release 18. StataCorp LLC. College Station, TX. StataCorp LLC; 2023. URL: https://www.stata.com/ [accessed 2023-11-28]
- Sterne JA, Savović J, Page MJ, Elbers RG, Blencowe NS, Boutron I, et al. RoB 2: a revised tool for assessing risk of bias in randomised trials. BMJ. Aug 28, 2019;366:l4898. [ FREE Full text ] [ CrossRef ] [ Medline ]
- McGuinness LA, Higgins JP. Risk-of-bias VISualization (robvis): an R package and Shiny web app for visualizing risk-of-bias assessments. Res Synth Methods. Jan 2021;12(1):55-61. [ CrossRef ] [ Medline ]
- Haddaway NR, Page MJ, Pritchard CC, McGuinness LA. PRISMA2020: an R package and Shiny app for producing PRISMA 2020-compliant flow diagrams, with interactivity for optimised digital transparency and open synthesis. Campbell Syst Rev. Mar 27, 2022;18(2):e1230. [ FREE Full text ] [ CrossRef ] [ Medline ]
- Elliott JC, Carey KB. Correcting exaggerated marijuana use norms among college abstainers: a preliminary test of a preventive intervention. J Stud Alcohol Drugs. Nov 2012;73(6):976-980. [ CrossRef ] [ Medline ]
- Elliott JC, Carey KB, Vanable PA. A preliminary evaluation of a web-based intervention for college marijuana use. Psychol Addict Behav. Mar 2014;28(1):288-293. [ CrossRef ] [ Medline ]
- Lee CM, Neighbors C, Kilmer JR, Larimer ME. A brief, web-based personalized feedback selective intervention for college student marijuana use: a randomized clinical trial. Psychol Addict Behav. Jun 2010;24(2):265-273. [ FREE Full text ] [ CrossRef ] [ Medline ]
- Buckner JD, Zvolensky MJ, Lewis EM. On-line personalized feedback intervention for negative affect and cannabis: a pilot randomized controlled trial. Exp Clin Psychopharmacol. Apr 2020;28(2):143-149. [ CrossRef ] [ Medline ]
- Goodness TM, Palfai TP. Electronic screening and brief intervention to reduce cannabis use and consequences among graduate students presenting to a student health center: a pilot study. Addict Behav. Jul 2020;106:106362. [ CrossRef ] [ Medline ]
- Palfai TP, Saitz R, Winter M, Brown TA, Kypri K, Goodness TM, et al. Web-based screening and brief intervention for student marijuana use in a university health center: pilot study to examine the implementation of eCHECKUP TO GO in different contexts. Addict Behav. Sep 2014;39(9):1346-1352. [ FREE Full text ] [ CrossRef ] [ Medline ]
- Riggs NR, Conner BT, Parnes JE, Prince MA, Shillington AM, George MW. Marijuana eCHECKUPTO GO: effects of a personalized feedback plus protective behavioral strategies intervention for heavy marijuana-using college students. Drug Alcohol Depend. Sep 01, 2018;190:13-19. [ CrossRef ] [ Medline ]
- Bonar EE, Chapman L, Pagoto S, Tan CY, Duval ER, McAfee J, et al. Social media interventions addressing physical activity among emerging adults who use cannabis: a pilot trial of feasibility and acceptability. Drug Alcohol Depend. Jan 01, 2023;242:109693. [ CrossRef ] [ Medline ]
- Bonar EE, Goldstick JE, Chapman L, Bauermeister JA, Young SD, McAfee J, et al. A social media intervention for cannabis use among emerging adults: randomized controlled trial. Drug Alcohol Depend. Mar 01, 2022;232:109345. [ FREE Full text ] [ CrossRef ] [ Medline ]
- Walukevich-Dienst K, Neighbors C, Buckner JD. Online personalized feedback intervention for cannabis-using college students reduces cannabis-related problems among women. Addict Behav. Nov 2019;98:106040. [ CrossRef ] [ Medline ]
- Côté J, Tessier S, Gagnon H, April N, Rouleau G, Chagnon M. Efficacy of a web-based tailored intervention to reduce cannabis use among young people attending adult education centers in Quebec. Telemed J E Health. Nov 2018;24(11):853-860. [ CrossRef ] [ Medline ]
- Cunningham JA, Schell C, Bertholet N, Wardell JD, Quilty LC, Agic B, et al. Online personalized feedback intervention to reduce risky cannabis use. Randomized controlled trial. Internet Interv. Nov 14, 2021;26:100484. [ FREE Full text ] [ CrossRef ] [ Medline ]
- Copeland J, Rooke S, Rodriquez D, Norberg MM, Gibson L. Comparison of brief versus extended personalised feedback in an online intervention for cannabis users: short-term findings of a randomised trial. J Subst Abuse Treat. May 2017;76:43-48. [ CrossRef ] [ Medline ]
- Jonas B, Tensil MD, Tossmann P, Strüber E. Effects of treatment length and chat-based counseling in a web-based intervention for cannabis users: randomized factorial trial. J Med Internet Res. May 08, 2018;20(5):e166. [ FREE Full text ] [ CrossRef ] [ Medline ]
- Schaub MP, Wenger A, Berg O, Beck T, Stark L, Buehler E, et al. A web-based self-help intervention with and without chat counseling to reduce cannabis use in problematic cannabis users: three-arm randomized controlled trial. J Med Internet Res. Oct 13, 2015;17(10):e232. [ FREE Full text ] [ CrossRef ] [ Medline ]
- Sinadinovic K, Johansson M, Johansson AS, Lundqvist T, Lindner P, Hermansson U. Guided web-based treatment program for reducing cannabis use: a randomized controlled trial. Addict Sci Clin Pract. Feb 18, 2020;15(1):9. [ FREE Full text ] [ CrossRef ] [ Medline ]
- Kanfer FH. Implications of a self-regulation model of therapy for treatment of addictive behaviors. In: Miller WR, Heather N, editors. Treating Addictive Behaviors. Boston, MA. Springer; 1986;29-47.
- Mohr DC, Cuijpers P, Lehman K. Supportive accountability: a model for providing human support to enhance adherence to eHealth interventions. J Med Internet Res. Mar 10, 2011;13(1):e30. [ FREE Full text ] [ CrossRef ] [ Medline ]
- Marlatt GA, Baer JS, Kivlahan DR, Dimeff LA, Larimer ME, Quigley LA, et al. Screening and brief intervention for high-risk college student drinkers: results from a 2-year follow-up assessment. J Consult Clin Psychol. Aug 1998;66(4):604-615. [ CrossRef ]
- Miller WR, Rollnick S. Motivational Interviewing: Preparing People for Change. New York, NY. Guilford Press; 2002.
- Prince MA, Carey KB, Maisto SA. Protective behavioral strategies for reducing alcohol involvement: a review of the methodological issues. Addict Behav. Jul 2013;38(7):2343-2351. [ CrossRef ] [ Medline ]
- Lundqvist TN. Cognitive Dysfunctions in Chronic Cannabis Users Observed During Treatment: An Integrative Approach. Stockholm, Sweden. Almqvist & Wiksell; 1997.
- Kanter JW, Puspitasari AJ, Santos MM, Nagy GA. Behavioural activation: history, evidence and promise. Br J Psychiatry. May 2012;200(5):361-363. [ CrossRef ] [ Medline ]
- Jaffee WB, D'Zurilla TJ. Personality, problem solving, and adolescent substance use. Behav Ther. Mar 2009;40(1):93-101. [ CrossRef ] [ Medline ]
- Miller W, Rollnick S. Motivational Interviewing: Preparing People to Change Addictive Behavior. New York, NY. The Guilford Press; 1991.
- Gordon JR, Marlatt GA. Relapse Prevention: Maintenance Strategies in the Treatment of Addictive Behaviors. 2nd edition. New York, NY. The Guilford Press; 2005.
- Platt JJ, Husband SD. An overview of problem-solving and social skills approaches in substance abuse treatment. Psychotherapy (Chic). 1993;30(2):276-283. [ FREE Full text ]
- Steinberg KL, Roffman R, Carroll K, McRee B, Babor T, Miller M. Brief counseling for marijuana dependence: a manual for treating adults. Center for Substance Abuse Treatment, Substance Abuse and Mental Health Services Administration, US Department of Health and Human Services. URL: https://store.samhsa.gov/product/brief-counseling-marijuana-dependence-manual-treating-adults/sma15-4211 [accessed 2024-03-23]
- de Shazer S, Dolan Y. More Than Miracles: The State of the Art of Solution-Focused Brief Therapy. Oxfordshire, UK. Routledge; 2007.
- Copeland J, Swift W, Roffman R, Stephens R. A randomized controlled trial of brief cognitive-behavioral interventions for cannabis use disorder. J Subst Abuse Treat. Sep 2001;21(2):55-65. [ CrossRef ] [ Medline ]
- Linke S, McCambridge J, Khadjesari Z, Wallace P, Murray E. Development of a psychologically enhanced interactive online intervention for hazardous drinking. Alcohol Alcohol. 2008;43(6):669-674. [ FREE Full text ] [ CrossRef ] [ Medline ]
- Wang ML, Waring ME, Jake-Schoffman DE, Oleski JL, Michaels Z, Goetz JM, et al. Clinic versus online social network-delivered lifestyle interventions: protocol for the get social noninferiority randomized controlled trial. JMIR Res Protoc. Dec 11, 2017;6(12):e243. [ FREE Full text ] [ CrossRef ] [ Medline ]
- Sepah SC, Jiang L, Peters AL. Translating the diabetes prevention program into an online social network: validation against CDC standards. Diabetes Educ. Jul 2014;40(4):435-443. [ FREE Full text ] [ CrossRef ] [ Medline ]
- Cunningham JA, van Mierlo T. The check your cannabis screener: a new online personalized feedback tool. Open Med Inform J. May 07, 2009;3:27-31. [ FREE Full text ] [ CrossRef ] [ Medline ]
- Bertholet N, Cunningham JA, Faouzi M, Gaume J, Gmel G, Burnand B, et al. Internet-based brief intervention for young men with unhealthy alcohol use: a randomized controlled trial in a general population sample. Addiction. Nov 2015;110(11):1735-1743. [ FREE Full text ] [ CrossRef ] [ Medline ]
- Walker DD, Roffman RA, Stephens RS, Wakana K, Berghuis J, Kim W. Motivational enhancement therapy for adolescent marijuana users: a preliminary randomized controlled trial. J Consult Clin Psychol. Jun 2006;74(3):628-632. [ FREE Full text ] [ CrossRef ] [ Medline ]
- Miller MB, Leffingwell T, Claborn K, Meier E, Walters S, Neighbors C. Personalized feedback interventions for college alcohol misuse: an update of Walters and Neighbors (2005). Psychol Addict Behav. Dec 2013;27(4):909-920. [ FREE Full text ] [ CrossRef ] [ Medline ]
- Ajzen I. From intentions to actions: a theory of planned behavior. In: Kuhl J, Beckmann J, editors. Action Control. Berlin, Germany. Springer; 1985;11-39.
- Dennis M, Titus JC, Diamond G, Donaldson J, Godley SH, Tims FM, et al. The Cannabis Youth Treatment (CYT) experiment: rationale, study design and analysis plans. Addiction. Dec 11, 2002;97 Suppl 1(s1):16-34. [ CrossRef ] [ Medline ]
- Simons JS, Dvorak RD, Merrill JE, Read JP. Dimensions and severity of marijuana consequences: development and validation of the Marijuana Consequences Questionnaire (MACQ). Addict Behav. May 2012;37(5):613-621. [ FREE Full text ] [ CrossRef ] [ Medline ]
- Legleye S. The Cannabis Abuse Screening Test and the DSM-5 in the general population: optimal thresholds and underlying common structure using multiple factor analysis. Int J Methods Psychiatr Res. Jun 10, 2018;27(2):e1597. [ FREE Full text ] [ CrossRef ] [ Medline ]
- Legleye S, Karila LM, Beck F, Reynaud M. Validation of the CAST, a general population Cannabis Abuse Screening Test. J Subst Use. Jul 12, 2009;12(4):233-242. [ CrossRef ]
- Sobell LC, Sobell MB. Timeline follow back. In: Litten RZ, Allen JP, editors. Measuring Alcohol Consumption: Psychosocial and Biochemical Methods. Totowa, NJ. Humana Press; 1992;41-72.
- White HR, Labouvie EW, Papadaratsakis V. Changes in substance use during the transition to adulthood: a comparison of college students and their noncollege age peers. J Drug Issues. Aug 03, 2016;35(2):281-306. [ CrossRef ]
- White HR, Labouvie EW. Towards the assessment of adolescent problem drinking. J Stud Alcohol. Jan 1989;50(1):30-37. [ CrossRef ] [ Medline ]
- Cloutier RM, Natesan Batley P, Kearns NT, Knapp AA. A psychometric evaluation of the Marijuana Problems Index among college students: confirmatory factor analysis and measurement invariance by gender. Exp Clin Psychopharmacol. Dec 2022;30(6):907-917. [ FREE Full text ] [ CrossRef ] [ Medline ]
- Guo H, Yang H, Yuan G, Zhu Z, Zhang K, Zhang X, et al. Effectiveness of information and communication technology (ICT) for addictive behaviors: an umbrella review of systematic reviews and meta-analysis of randomized controlled trials. Comput Hum Behav. Oct 2023;147:107843. [ CrossRef ]
- Grigsby TJ, Lopez A, Albers L, Rogers CJ, Forster M. A scoping review of risk and protective factors for negative cannabis use consequences. Subst Abuse. Apr 07, 2023;17:11782218231166622. [ FREE Full text ] [ CrossRef ] [ Medline ]
- Harkin B, Webb TL, Chang BP, Prestwich A, Conner M, Kellar I, et al. Does monitoring goal progress promote goal attainment? A meta-analysis of the experimental evidence. Psychol Bull. Feb 2016;142(2):198-229. [ FREE Full text ] [ CrossRef ] [ Medline ]
- Samdal GB, Eide GE, Barth T, Williams G, Meland E. Effective behaviour change techniques for physical activity and healthy eating in overweight and obese adults; systematic review and meta-regression analyses. Int J Behav Nutr Phys Act. Mar 28, 2017;14(1):42. [ FREE Full text ] [ CrossRef ] [ Medline ]
- Garnett C, Crane D, Brown J, Kaner E, Beyer F, Muirhead C. Behavior Change Techniques Used in Digital Behavior Change Interventions to Reduce Excessive Alcohol Consumption: A Meta-regression. Ann Behav Med May 18. 2018;52(6):A. [ CrossRef ]
- Kaner EF, Beyer FR, Muirhead C, Campbell F, Pienaar ED, Bertholet N, et al. Effectiveness of brief alcohol interventions in primary care populations. Cochrane Database Syst Rev. Feb 24, 2018;2(2):CD004148. [ FREE Full text ] [ CrossRef ] [ Medline ]
- Sedrati H, Belrhiti Z, Nejjari C, Ghazal H. Evaluation of mobile health apps for non-medical cannabis use: a scoping review. Procedia Comput Sci. 2022;196:581-589. [ CrossRef ]
- Curtis BL, Ashford RD, Magnuson KI, Ryan-Pettes SR. Comparison of smartphone ownership, social media use, and willingness to use digital interventions between generation Z and millennials in the treatment of substance use: cross-sectional questionnaire study. J Med Internet Res. Apr 17, 2019;21(4):e13050. [ FREE Full text ] [ CrossRef ] [ Medline ]
- Schünemann H, Brożek J, Guyatt G, Oxman A. The GRADE Handbook. London, UK. The Cochrane Collaboration; 2013.
- Guyatt GH, Oxman AD, Schünemann HJ, Tugwell P, Knottnerus A. GRADE guidelines: a new series of articles in the Journal of Clinical Epidemiology. J Clin Epidemiol. Apr 2011;64(4):380-382. [ CrossRef ] [ Medline ]
- Guyatt GH, Oxman AD, Vist GE, Kunz R, Falck-Ytter Y, Alonso-Coello P, et al. GRADE: an emerging consensus on rating quality of evidence and strength of recommendations. BMJ. Apr 26, 2008;336(7650):924-926. [ FREE Full text ] [ CrossRef ] [ Medline ]
- Hjorthøj CR, Hjorthøj AR, Nordentoft M. Validity of Timeline Follow-Back for self-reported use of cannabis and other illicit substances--systematic review and meta-analysis. Addict Behav. Mar 2012;37(3):225-233. [ CrossRef ] [ Medline ]
- Robinson SM, Sobell LC, Sobell MB, Leo GI. Reliability of the Timeline Followback for cocaine, cannabis, and cigarette use. Psychol Addict Behav. Mar 2014;28(1):154-162. [ CrossRef ] [ Medline ]
- Abraham C, Michie S. A taxonomy of behavior change techniques used in interventions. Health Psychol. May 2008;27(3):379-387. [ CrossRef ] [ Medline ]
- Garrett JJ. The Elements of User Experience: User-Centered Design for the Web and Beyond. London, UK. Pearson Education; 2010.
- Lorencatto F, West R, Bruguera C, Brose LS, Michie S. Assessing the quality of goal setting in behavioural support for smoking cessation and its association with outcomes. Ann Behav Med. Apr 24, 2016;50(2):310-318. [ FREE Full text ] [ CrossRef ] [ Medline ]
Abbreviations
Edited by T Leung, G Eysenbach; submitted 30.11.23; peer-reviewed by H Sedrati; comments to author 02.01.24; revised version received 09.01.24; accepted 08.03.24; published 17.04.24.
©José Côté, Gabrielle Chicoine, Billy Vinette, Patricia Auger, Geneviève Rouleau, Guillaume Fontaine, Didier Jutras-Aswad. Originally published in the Journal of Medical Internet Research (https://www.jmir.org), 17.04.2024.
This is an open-access article distributed under the terms of the Creative Commons Attribution License (https://creativecommons.org/licenses/by/4.0/), which permits unrestricted use, distribution, and reproduction in any medium, provided the original work, first published in the Journal of Medical Internet Research, is properly cited. The complete bibliographic information, a link to the original publication on https://www.jmir.org/, as well as this copyright and license information must be included.
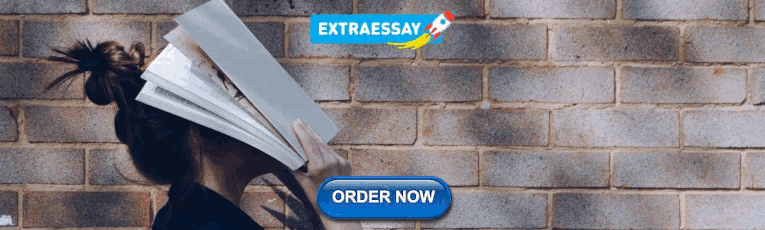
IMAGES
VIDEO
COMMENTS
Research summary Analytic models are a powerful approach for developing theory, yet are often poorly understood in the strategy and organizations community. Our goal is to enhance the influence of the method by clarifying for consumers of modeling research how to understand and appreciate analytic modeling and use modeling results to enhance ...
For example, it can look into why the value of the Japanese Yen has decreased. This is so that an analytical study can consider "how" and "why" questions. Another example is that someone might conduct analytical research to identify a study's gap. It presents a fresh perspective on your data.
21 Analytical Models. 21. Analytical Models. Marketing models consists of. Analytical Model: pure mathematical-based research. Empirical Model: data analysis. "A model is a representation of the most important elements of a perceived real-world system". Marketing model improves decision-making. Econometric models.
The analytical model may be solved via a closed form solution such as the position of a point mass given an initial position, velocity, and constant acceleration. Other solutions require numerical analysis methods to determine the change in state of the system as a function of time, space, and other parameters. ...
An analytical framework is, the way I see it, a model that helps explain how a certain type of analysis will be conducted. For example, in this paper, Franks and Cleaver develop an analytical framework that includes scholarship on poverty measurement to help us understand how water governance and poverty are interrelated.
Decision analysis is a systematic, quantitative, and transparent approach to making decisions under uncertainty. The fundamental tool of decision analysis is a decision-analytic model, most often a decision tree or a Markov model. A decision model provides a way to visualize the sequences of events that can occur following alternative decisions (or actions) in a logical framework, as well as ...
3 benefits of analytical modeling. It's hard to overstate the value of strong analytics. Mathematical analysis is useful at any scale and for almost every area of business management. 1. Data-driven decisions. The primary benefit of leveraging analytical modeling is the security of making data-driven decisions.
Request PDF | Analytic Models In Strategy, Organizations, And Management Research: A Guide For Consumers | Research summary Analytic models are a powerful approach for developing theory, yet are ...
Research methods are specific procedures for collecting and analyzing data. Developing your research methods is an integral part of your research design. When planning your methods, there are two key decisions you will make. First, decide how you will collect data. Your methods depend on what type of data you need to answer your research question:
Descriptive research classifies, describes, compares, and measures data. Meanwhile, analytical research focuses on cause and effect. For example, take numbers on the changing trade deficits between the United States and the rest of the world in 2015-2018. This is descriptive research.
In the model presented here (Fig. 1), the sources of production are grouped into six top-tier, or alpha, variables: investments and ongoing funding; investigator experience and training; efficiency of the research environment; the research mix of novelty, incremental advancement, and confirmatory studies; analytic accuracy; and passion ...
Analytical skills are essential for conducting and evaluating research, whether in academia, business, or policy. One of the tools that can help you develop and apply analytical skills is modeling ...
Based on this review, this chapter provides explanations for the analytical framework and the research methods used in our study. I will begin by investigating what kinds of research approach are available in the discipline of IT/IS and which is appropriate for the purpose of this study. My analysis will make clear that the survey approach is ...
This article explores the four main analytical models that organisations can deploy. The four main analytical models organisations can deploy are: descriptive. diagnostic. predictive. prescriptive. As you move from descriptive to prescriptive analytics, each model offers increasing value to an organisation. But, at the same time, they increase ...
Based on this, we finally highlight the challenges and potential research directions within the scope of our study. ... (AI), is one of the major techniques used in advanced analytics which can automate analytical model building . This is focused on the premise that systems can learn from data, recognize trends, and make decisions, ...
QDA Method #3: Discourse Analysis. Discourse is simply a fancy word for written or spoken language or debate. So, discourse analysis is all about analysing language within its social context. In other words, analysing language - such as a conversation, a speech, etc - within the culture and society it takes place.
Descriptive Analytics. Definition: Descriptive analytics focused on describing or summarizing raw data and making it interpretable. This type of analytics provides insight into what has happened in the past. It involves the analysis of historical data to identify patterns, trends, and insights. Descriptive analytics often uses visualization ...
Lifetime Customer Value. Eric Benjamin Seufert, in Freemium Economics, 2014. Implementing an analytics model. An analytics model, defined here as a model that is executed as a process within the analytics stack and not a model that is merely built on analytics output, is rolled out in two phases using a combination of statistical software and programmatic design.
The review reveals that further research is essential to develop analytical models using EVA metrics to forecast project performance. It also suggests that DSS should be model driven, function as ...
Abstract. This exposition on the Analytic Hierarchy Process (AHP) has the following objectives: (1) to discuss why AHP is a general methodology for a wide variety of decision and other applications, (2) to present brief descriptions of successful applications of the AHP, and (3) to elaborate on academic discourses relevant to the efficacy and ...
This paper presents an experimental investigation on the stress-strain behavior of steel fiber reinforced concrete (SFRC) subjected to uniaxial monotonic and cyclic tension. A total of 42 samples are fabricated and tested for various fiber volume fractions and aspect ratios. The entire cyclic tensile stress-strain curves are captured and the ...
The proposed article addresses pressing sustainability challenges, advocating for a profound transformation of existing development models, particularly emphasizing sustainable production and lifestyles. Utilizing a research method grounded in a comprehensive international knowledge base, the study explores the evolution of design for sustainability (DfS) approaches. Its significant ...
The analytical model, which specifies the energy transfer for cavity production as equivalent to the energy dissipated by velocity-dependent drag on the projectile, provides accurate estimates for ...
This research aims to develop a comprehensive simulation model for rainfall-interception-infiltration-runoff under vegetation cover conditions. The model integrates three key components: a vegetation interception model, Philip's infiltration model, and a kinematic wave model. ... The analytical model was calibrated using numerical solutions and ...
a Oxford e-Research Centre, Department of Engineering Science, University of Oxford, 7 Keble Road, ... quantify and reduce these errors impacting the 3D model. Analytical absorption corrections are beneficial not only for long-wavelength macromolecular crystallography but also for highly absorbing samples in chemical crystallography. In this ...
Background: The high prevalence of cannabis use among young adults poses substantial global health concerns due to the associated acute and long-term health and psychosocial risks. Digital modalities, including websites, digital platforms, and mobile apps, have emerged as promising tools to enhance the accessibility and availability of evidence-based interventions for young adults for cannabis ...