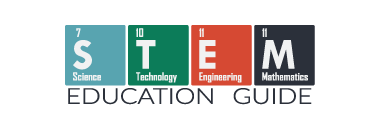
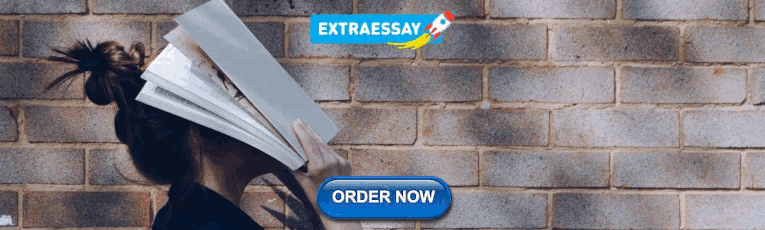
What is STEM? What You Need to Know
Krystal DeVille
March 21, 2024
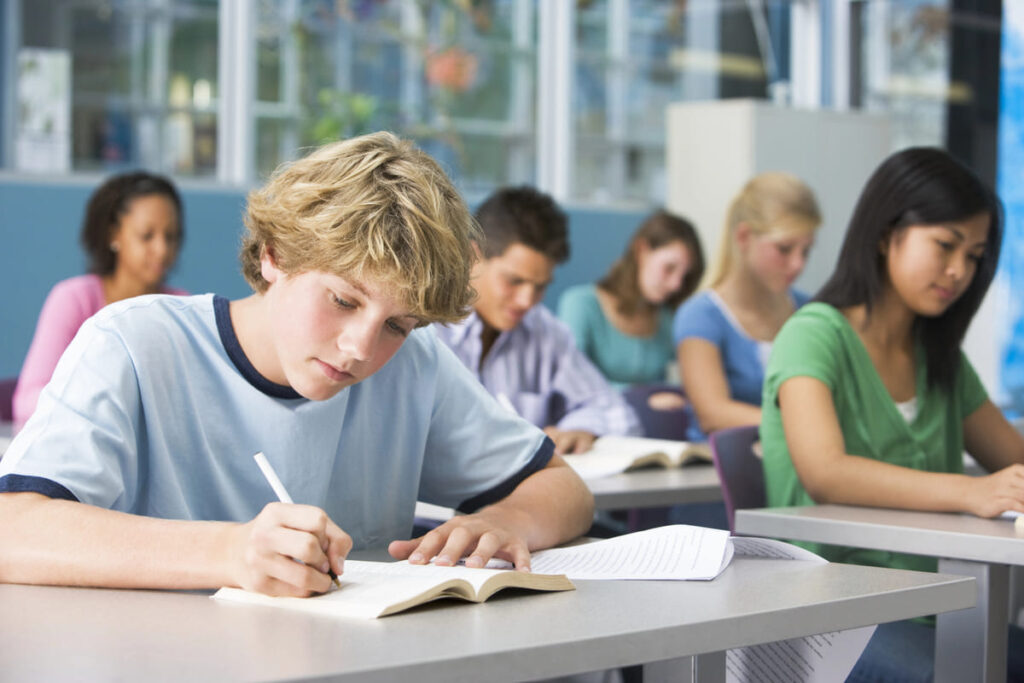
STEM, which stands for Science, Technology, Engineering, and Mathematics, is more than just a group of subjects. It’s a way of integrating these crucial areas into a holistic approach to learning and problem-solving.
As I explore STEM, I envision it as a fusion recipe that blends four basic ingredients to prepare students for the jobs of tomorrow. This educational framework aims to develop not only knowledge but also the ability to apply that knowledge in real-world scenarios.
Table of Contents
Key Takeaways:
- STEM intertwines science, tech, engineering, and math for integrated learning.
- A quality STEM education encourages problem-solving and real-world application.
- STEM fields are known for their significant growth and lucrative job opportunities.
Fundamentals of STEM
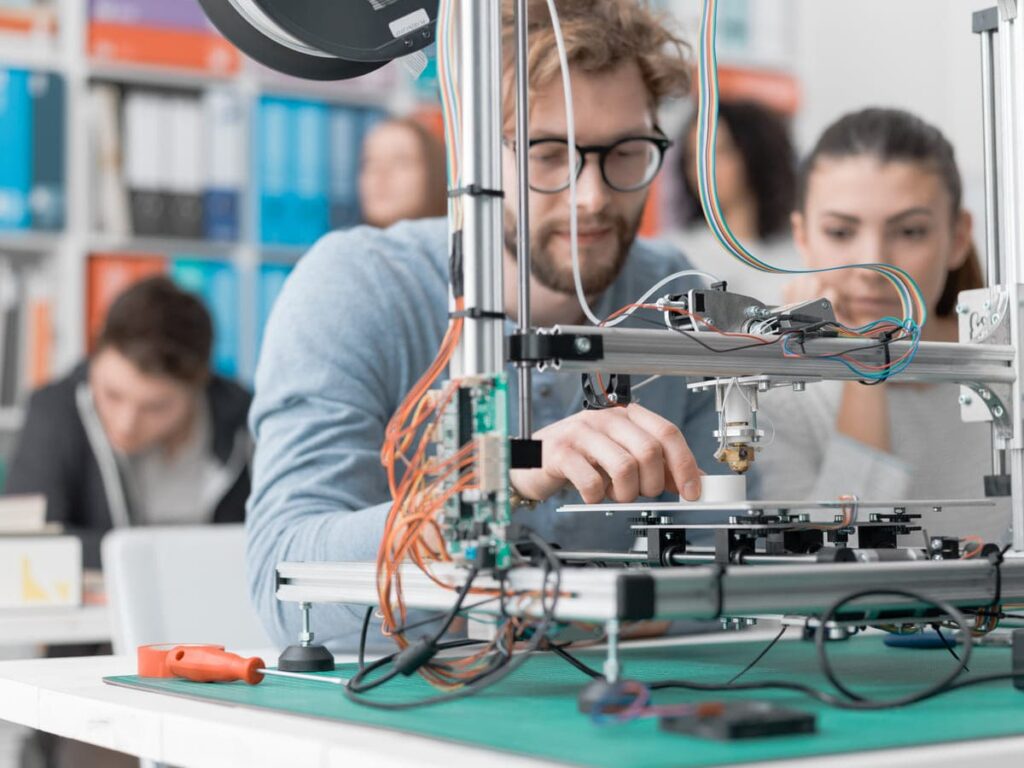
STEM education is genuinely at the forefront of preparing students for the tech-savvy job market that awaits them, or really, any job they would like to pursue.
Definitions and Components of STEM
STEM, the acronym, rolls off the tongue a bit easier than saying science, technology, engineering, and math each time, right? These four pillars are more pivotal than they have ever been. You see how fast the world changes.
I don’t think I’m that old, but I do remeber my teacher telling me I won’t always have a cacular in my pocket, (jokes on her right!?)
STEM is not just a collection of subjects, but an interdisciplinary approach. That’s really what sets it apart from “just learning.” It’s about interconnecting these fields to solve real-world problems rather than studying them in isolation.
Science explores the natural world, from atoms to ecosystems. Technology is all about gadgets and software – basically anything to make our lives easier and more connected.
Engineering is where design and utility meet, crafting everything from bridges to circuit boards. And let’s not forget math, the language that underlies it all, where we crunch numbers and patterns to predict outcomes. Where we have to prove it on paper to show that the “math works.”
History and Evolution of STEM
Back in the early 2000s, educators coined the term “SMET” but let’s be honest, it wasn’t catchy. Thankfully, Winona State University President Judith Ramaley had a lightbulb moment and switched the letters around to STEM — score one for marketing!
This idea wasn’t just a fresh coat of paint on an old concept; it signified a shift in thinking. Educators recognized the need for students to engage with these subjects cooperatively.
They revamped curricula to reflect this, realizing that the challenges of tomorrow require people who don’t just memorize facts but understand how to apply knowledge creatively and collaboratively. Facts don’t matter; if there is nothing practical, you get out of them.
This shift also led to the introduction of STEAM, where the ‘A’ stands for the Arts, acknowledging that creativity is just as crucial in innovation.
If you’d like to read more about STEAM, please take a look at our article: STEM vs. STEAM , Making Room for The Arts.
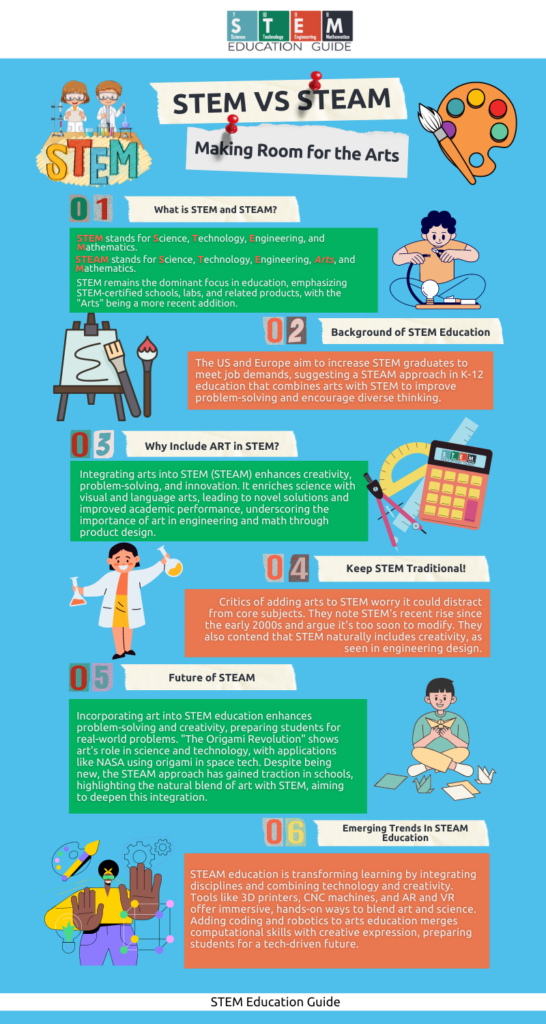
STEM Education
STEM education isn’t just a bunch of subjects thrown together; it’s about blending science, technology, engineering, and math in a way that gets students ready for a future where these skills will be in high demand.
Let’s get into what makes STEM education so important in schools and how it’s taught beyond the classroom walls.
Importance of STEM in Schools
STEM education is critical for young minds in elementary, middle, and high school. It’s not just about prepping U.S. students for the workforce. It’s about building literacy in STEM fields that sets a foundation for any career path they might choose later on.
I see firsthand how essential STEM skills are for development. When students get a taste of project-based learning, they’re building bridges to the future.
Curriculum and Learning Models
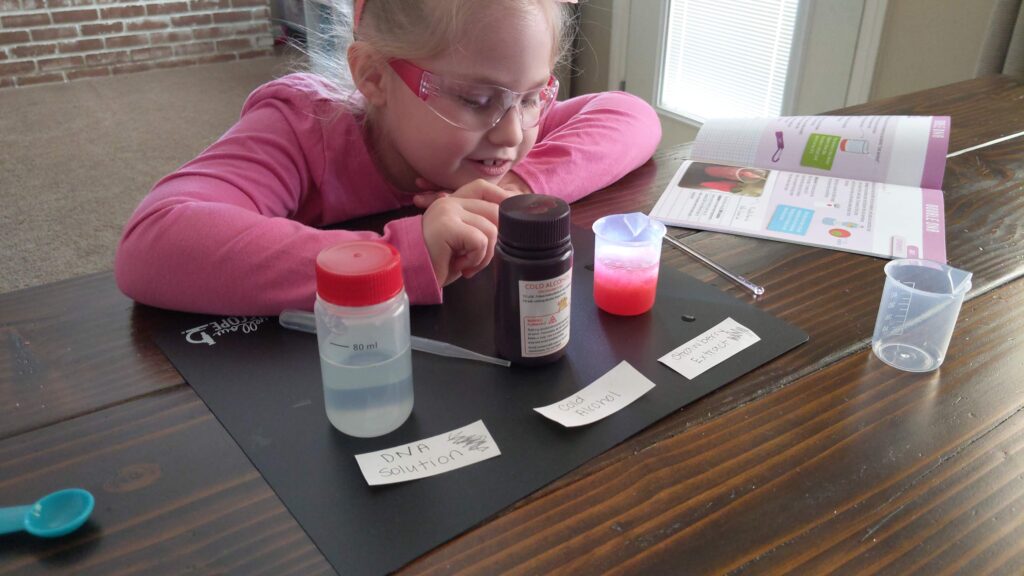
At its best, it incorporates a variety of learning models.
Blended learning is an excellent example, where students spin their gears online and hands-on. Doing research online or on the computer is fine, but students need to get away from the screen and get their hands on something to understand it fully.
Special shout-out to the interdisciplinary nature of STEM that bonds different subjects coherently.
Imagine it: A high school programming task suddenly throws in a curveball from physics, sparking a lightbulb moment for a student. It’s all about making connections, much like piecing together a puzzle that reveals a bigger picture.
STEM Beyond the Classroom
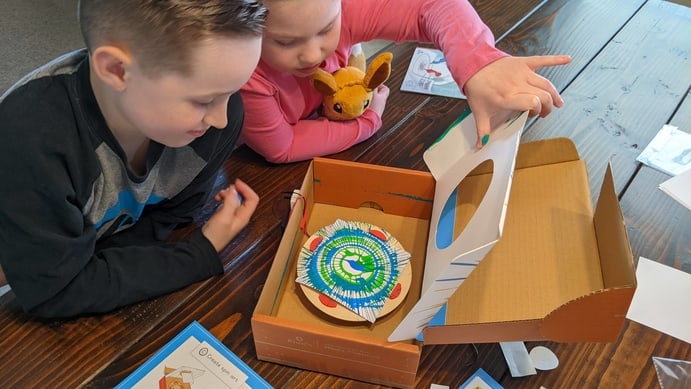
The magic of STEM doesn’t vanish when the school bell rings and the kids leave.
STEM literacy is an ongoing journey that extends to after-school programs, coding boot camps, and DIY science kits at home . High school students often roll up their sleeves in science fairs or internships that provide hands-on experience with real-world applications.
Seeing K-12 students approach everyday problems with a STEM mindset proves how valuable these skills are outside the traditional learning environment.
It’s a testament to the adaptability and relevance of STEM education that it doesn’t restrict itself to classroom corners.
It spills out, influencing how young minds perceive and interact with the world around them.
Understanding the basics of stem is just the beginning. Let’s go a little deeper and read our article on ‘ How can STEM education shape the future ’ and discover its pivotal role in molding tomorrow’s leaders.
Key Areas of Focus in STEM
Let’s get into the core components of STEM.
Science and Mathematics
Science is where curiosity meets experimentation. From physics to biology and chemistry , science encompasses various disciplines that allow us to understand the natural world.
Think of biology as studying life, chemistry as exploring substances, and physics as the foundation of natural phenomena.
It’s the blend of these natural sciences that provides us the canvas to paint our understanding of life, matter, and the forces that bind them.
Then there’s mathematics . The language of logic, it runs through the veins of STEM like a binding melody.
From basic algebra and geometry to brain-bending calculus and statistics , math provides tools for solving problems big and small.
Whether you find yourself calculating the area of complex shapes or crunching big data through statistical analysis, mathematics is the trusty sidekick to the sciences, making sense of patterns and quantifying our discoveries.
Technology and Engineering
Now, for technology and engineering – they’re the builders of our modern world that we always see.
Both fields rely on applying what we learn from science and math to create tangible solutions. Engineering is the practical application of those disciplines to design everything from bridges and gadgets to the device you’re using right now, with subdivisions like electronics and robotics .
Speaking of gadgets, Technology is the umbrella under which those gadgets dance in the rain of progress.
It includes information systems like computer science , which basically allows us to chat, share, and store information instantly.
Engineering and tech are the forces driving us forward, and they’re constantly evolving, so staying on top of the latest developments is as exciting as essential.
With each area interlacing closely with the others, STEM creates an intricate dance of knowledge that pushes the boundaries of what we can achieve.
It’s not just about individual brilliance, like that of mathematicians or scientists, but about collective progress in these interdependent fields.
Career Perspectives in STEM
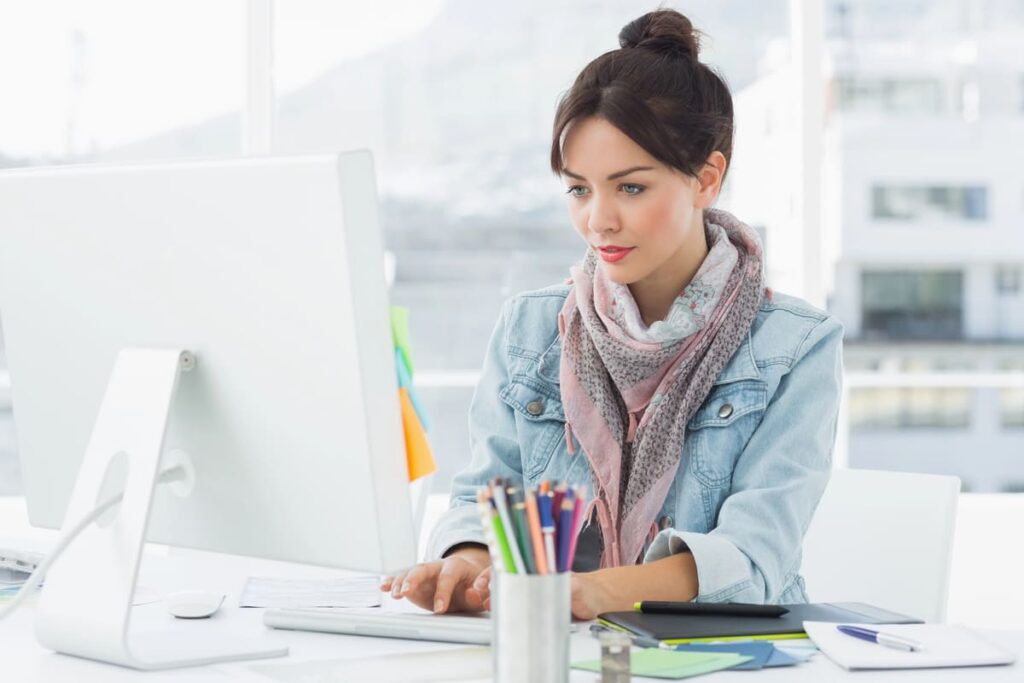
In STEM fields, the job landscape is vibrant, with plenty of room for newcomers like me to hop in.
Job Market and Demand
Isn’t it something? Data points to a 79% employment growth in STEM fields over three decades. What’s more, they peg an 11% boom from 2020 to 2030.
It’s not just IT and computer science; areas like electrical and mechanical engineering are also on fire.
As a STEM enthusiast, I can barely contain my excitement over these spirited demands in the job market.
STEM Professions and Skills
I’ve seen how STEM majors queue up to get into roles that require not just technical prowess but also an analytical mindset and the agility to navigate an economy fueled by continuous research and development.
The National Science Foundation says we STEM professionals are the backbone of innovation and economic growth, and who am I to argue?
High salary prospects sweeten the deal, especially in roles like systems managers where numbers can bubble up to six figures.
Here’s what’s trending in skills and roles:
- Computer Science & IT : Coding, cybersecurity, and data analytics are gold.
- Engineering : Both electrical and mechanical engineering demand creative problem-solving.
- Mathematics : Skills in analysis and modeling can weave through various sectors.
Broadening Participation
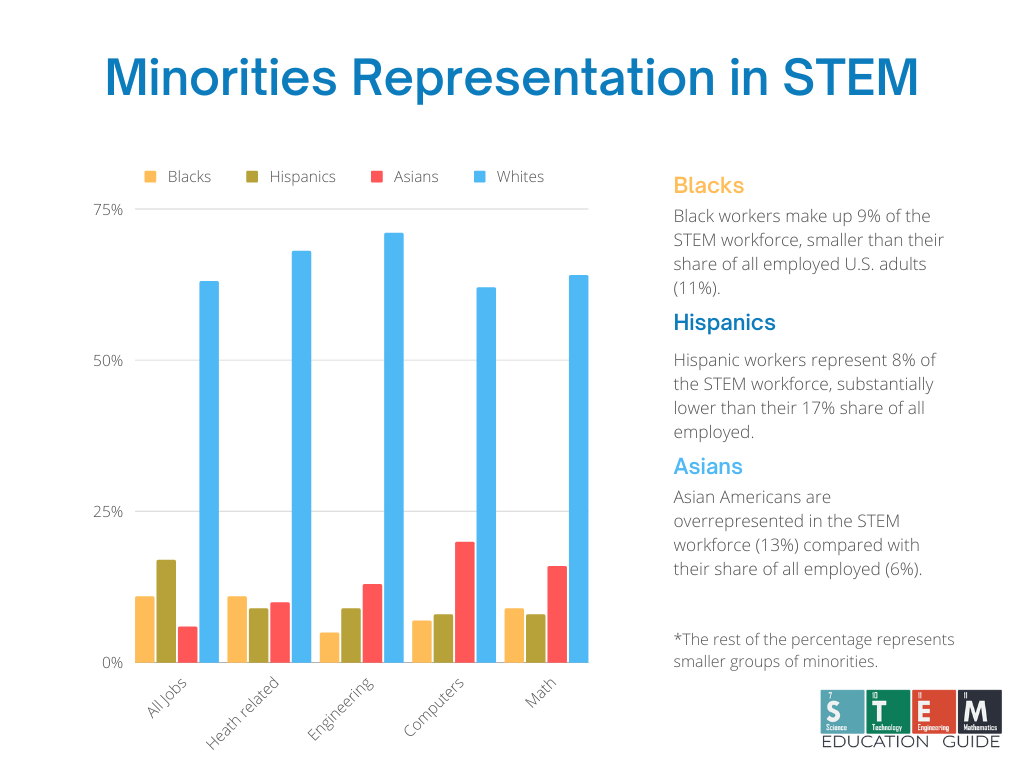
Diversity and Inclusion in STEM
Initiatives: Bold steps are being taken by organizations like the National Science Foundation (NSF) to involve a more diverse population in the sciences.
They recognize the importance of nurturing talent from underrepresented groups such as black and hispanic communities, and have developed initiatives aimed at encouraging their participation in STEM.
The numbers: Surprisingly, only a sliver of NSF funding goes towards such initiatives, but it’s a growing priority.
With schemes like the INCLUDES program , the goal is to dramatically shift the needle on this.
Education: Let’s not forget the folks standing in front of the classroom.
STEM teachers hail from all different backgrounds and are critical in shaping young minds.
The U.S. Department of Education understands this; hence, it pours resources into training a workforce of educators that mirrors the diversity of their students.
It’s about relatability and the light bulb moments that happen when students see themselves in their mentors.
Women and Minorities in STEM
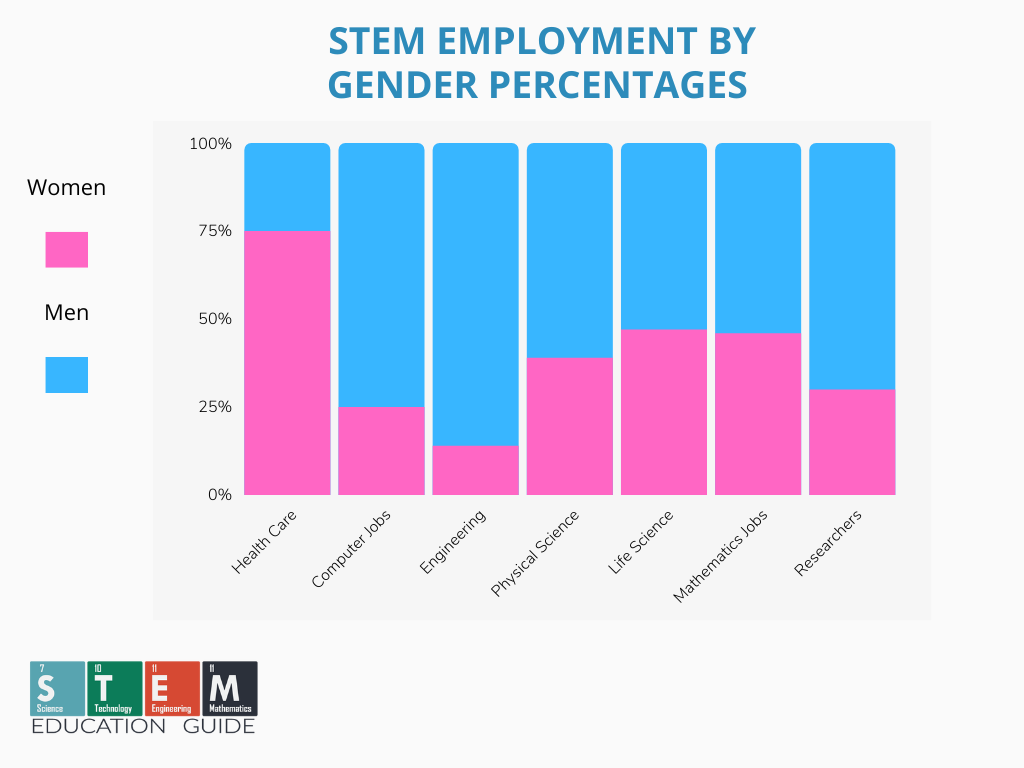
Statistics today: Fasten your seatbelts because the stats are in, and they might rattle you.
Women and minorities are still vastly underrepresented in STEM careers.
Change is on the horizon: But change doesn’t come from just sitting back.
Groups like the Society of Hispanic Professional Engineers (SHPE) and initiatives from the White House aim to rewrite this stale narrative by creating environments where everyone gets a fair shot at success.
Community and Support: It’s all about building a community now, isn’t it?
For women and minorities, this is a game changer.
These initiatives provide both a shoulder to lean on and a springboard to soar from – figuratively speaking. They’re creating a sense of belonging in places where it was scarce – that’s the magic ingredient for a thriving career in STEM.
International Perspective
Stem around the world.
In Australia , students are embracing STEM to become pivotal players in the global economy.
Their education system focuses on innovation and practical applications, pushing students to think beyond the textbook.
On the other hand, China is sprinting forward in STEM.
With a considerable push from the government, Chinese students often outshine others in international rankings like PISA. This shows that they aren’t just good at taking tests — they’re also becoming champions of innovation.
France and the United Kingdom are no slouches either.
They link STEM closely to economics, ensuring their citizens are equipped for future markets. Both nations believe in starting STEM education early, fostering a sense of intrigue and creativity in young minds.
Comparative Education Systems
Let’s get down to the nitty-gritty. How do education systems stack up?
The U.S. government has been a formidable force in promoting STEM, yet there’s room for improvement.
This is especially true when I peek at PISA scores , which show that American students often lag behind their peers in places like East Asia.
Comparing these systems feels like flipping through a kaleidoscope of methodologies.
Some countries stress rote learning, while places like the United Kingdom emphasize a more hands-on approach.
Every country I look at has its way of doing things, but no matter the method, the aim is the same: to equip students with the skills needed for a tech-driven future.
Advancements and Future of STEM
I’m about to walk you through a maze of brainy breakthroughs and a sneak peek at the skills you’ll need to thrive in the fast-moving STEM job market.
Innovations in STEM Education
In my journey through the world of STEM, I’ve seen some real game-changers in education.
We’re not just talking about learning science and math anymore. It’s how these subjects swirl together with technology and engineering that really spices things up.
We’ve moved beyond the classroom walls, with long-distance learning making a serious splash.
And you bet, arts are getting into the mix too—hello, STEAM! This creative buddy brings a whole new layer of imagination and innovation .
- Integration : Subjects are interlocking like pieces of a puzzle, making learning a whole scene and not just scattered bits.
- Creativity : Ditch the yawn-worthy lectures. Educators are crafting courses that light fires under our seats with exciting projects.
- Communication : It’s not a one-way street anymore. Students talk back, brainstorm, and swap ideas like Pokémon cards.
Industry Growth and Future Skills
Move over, old-school careers; the STEM industry’s growth is like popcorn at the movies—fast and massive.
My best guess is a rise in jobs across computer science , health , medicine , and robotics .
But wait, there’s more. We can’t ignore the hefty role of computing across other sectors, like economics , spurring on development and fattening up the economy .
- Computing : From writing code to cybersecurity—basically anything that makes you feel like a wizard.
- Data Analysis : It’s all the rage, like the avocado on toast of skills.
- Adaptability : Tech’s sprinting, not strolling, and keeping up means lacing up those flexible thinking shoes.
STEM’s trajectory is clear: innovate, integrate, and keep learning fun while polishing up the skills that’ll keep you ahead of the game.
From quantum computing to bionic limbs, the advancements we’re seeing are just the trailer of what’s to come. I’m stoked to see where it all leads—aren’t you?
Frequently Asked Questions
Let’s unravel some common curiosities about STEM education that might be buzzing in your head.
How does STEM education impact high school students?
I’ve noticed high schoolers who get into STEM programs often get a leg-up on critical thinking, problem-solving, and team collaboration.
It’s not just homework; they’re solving real-world puzzles.
What are the key skills developed in STEM programs?
In my experience, STEM hones in on problem-solving and innovation. You learn to tackle challenges with creativity, which is sort of like flexing your brain muscles in new ways.
Can you tell me about the career paths for STEM graduates?
STEM grads often land in diverse fields, from app development to renewable energy. There’s a ton of options, whether you fancy coding or crafting things.
What types of activities are included in STEM for younger kids?
Let me paint you a picture: it’s less about the ‘sit still and listen’ and more ‘let’s build a volcano!’ Kids get their hands dirty with experiments and interactive projects that make learning a blast.
Author: Krystal DeVille
Title: stem education guide founder, expertise: homeschooling, kids education, parenting.
Krystal DeVille is an accomplished journalist and homeschooling mother who created STEM Education Guide, a site that revolutionizes learning in science, technology, engineering, and math (STEM) for children. It makes complex subjects engaging and understandable with innovative, hands-on approaches.
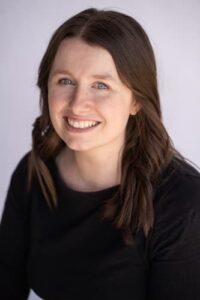
Leave a Comment Cancel reply
Save my name, email, and website in this browser for the next time I comment.
most recent
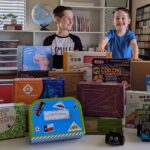
Activities and Games , Toy Gift Guides
Best stem subscription boxes for kids: hands-on reviews.
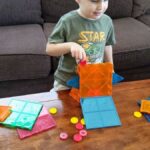
Activities and Games
Magnetic tiles vs traditional blocks: a comparative analysis.
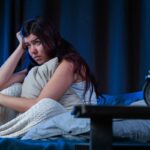
Why Can’t I Sleep? How Insomnia Is Plaguing the World
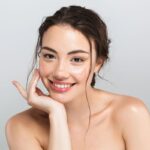
8 DIY Natural Moisturizers That Will Leave Your Skin Feeling Amazing
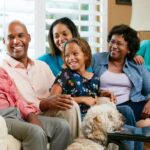
47 Influential Black History Month Quotes To Inspire and Empower
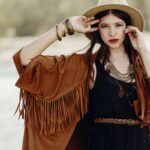
Embracing Boho Chic: A Guide to Boho Style for Women
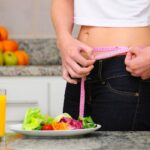
Losing Weight While Nourishing Your Body: A Breakdown of 11 Diet Plans
STEM Education Guide
[email protected] STEM Education Guide 9125 SVL BOX Victorville, CA 92395
Your Compass for STEM Discovery
© 2024 STEM Education Guide
What is STEM Education?
STEM education, now also know as STEAM, is a multi-discipline approach to teaching.
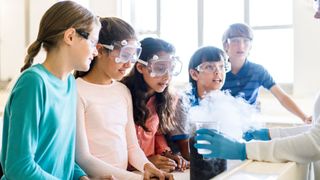
- Importance of STEAM education
STEAM blended learning
- Inequalities in STEAM
Additional resources
Bibliography.
STEM education is a teaching approach that combines science, technology, engineering and math . Its recent successor, STEAM, also incorporates the arts, which have the "ability to expand the limits of STEM education and application," according to Stem Education Guide . STEAM is designed to encourage discussions and problem-solving among students, developing both practical skills and appreciation for collaborations, according to the Institution for Art Integration and STEAM .
Rather than teach the five disciplines as separate and discrete subjects, STEAM integrates them into a cohesive learning paradigm based on real-world applications.
According to the U.S. Department of Education "In an ever-changing, increasingly complex world, it's more important than ever that our nation's youth are prepared to bring knowledge and skills to solve problems, make sense of information, and know how to gather and evaluate evidence to make decisions."
In 2009, the Obama administration announced the " Educate to Innovate " campaign to motivate and inspire students to excel in STEAM subjects. This campaign also addresses the inadequate number of teachers skilled to educate in these subjects.
The Department of Education now offers a number of STEM-based programs , including research programs with a STEAM emphasis, STEAM grant selection programs and general programs that support STEAM education.
In 2020, the U.S. Department of Education awarded $141 million in new grants and $437 million to continue existing STEAM projects a breakdown of grants can be seen in their investment report .
The importance of STEM and STEAM education
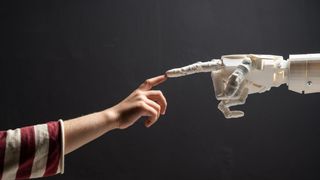
STEAM education is crucial to meet the needs of a changing world. According to an article from iD Tech , millions of STEAM jobs remain unfilled in the U.S., therefore efforts to fill this skill gap are of great importance. According to a report from the U.S. Bureau of Labor Statistics there is a projected growth of STEAM-related occupations of 10.5% between 2020 and 2030 compared to 7.5% in non-STEAM-related occupations. The median wage in 2020 was also higher in STEAM occupations ($89,780) compared to non-STEAM occupations ($40,020).
Between 2014 and 2024, employment in computer occupations is projected to increase by 12.5 percent between 2014 and 2024, according to a STEAM occupation report . With projected increases in STEAM-related occupations, there needs to be an equal increase in STEAM education efforts to encourage students into these fields otherwise the skill gap will continue to grow.
STEAM jobs do not all require higher education or even a college degree. Less than half of entry-level STEAM jobs require a bachelor's degree or higher, according to skills gap website Burning Glass Technologies . However, a four-year degree is incredibly helpful with salary — the average advertised starting salary for entry-level STEAM jobs with a bachelor's requirement was 26 percent higher than jobs in the non-STEAM fields.. For every job posting for a bachelor's degree recipient in a non-STEAM field, there were 2.5 entry-level job postings for a bachelor's degree recipient in a STEAM field.
What separates STEAM from traditional science and math education is the blended learning environment and showing students how the scientific method can be applied to everyday life. It teaches students computational thinking and focuses on the real-world applications of problem-solving. As mentioned before, STEAM education begins while students are very young:
Elementary school — STEAM education focuses on the introductory level STEAM courses, as well as awareness of the STEAM fields and occupations. This initial step provides standards-based structured inquiry-based and real-world problem-based learning, connecting all four of the STEAM subjects. The goal is to pique students' interest into them wanting to pursue the courses, not because they have to. There is also an emphasis placed on bridging in-school and out-of-school STEAM learning opportunities.
– Best microscopes for kids
– What is a scientific theory?
– Science experiments for kids
Middle school — At this stage, the courses become more rigorous and challenging. Student awareness of STEAM fields and occupations is still pursued, as well as the academic requirements of such fields. Student exploration of STEAM-related careers begins at this level, particularly for underrepresented populations.
High school — The program of study focuses on the application of the subjects in a challenging and rigorous manner. Courses and pathways are now available in STEAM fields and occupations, as well as preparation for post-secondary education and employment. More emphasis is placed on bridging in-school and out-of-school STEAM opportunities.
Much of the STEAM curriculum is aimed toward attracting underrepresented populations. There is a significant disparity in the female to male ratio when it comes to those employed in STEAM fields, according to Stem Women . Approximately 1 in 4 STEAM graduates is female.
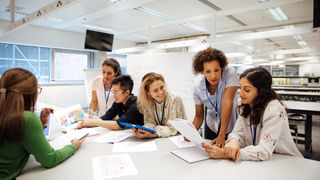
Inequalities in STEAM education
Ethnically, people from Black backgrounds in STEAM education in the UK have poorer degree outcomes and lower rates of academic career progression compared to other ethnic groups, according to a report from The Royal Society . Although the proportion of Black students in STEAM higher education has increased over the last decade, they are leaving STEAM careers at a higher rate compared to other ethnic groups.
"These reports highlight the challenges faced by Black researchers, but we also need to tackle the wider inequalities which exist across our society and prevent talented people from pursuing careers in science." President of the Royal Society, Sir Adrian Smith said.
Asian students typically have the highest level of interest in STEAM. According to the Royal Society report in 2018/19 18.7% of academic staff in STEAM were from ethnic minority groups, of these groups 13.2% were Asian compared to 1.7% who were Black.
If you want to learn more about why STEAM is so important check out this informative article from the University of San Diego . Explore some handy STEAM education teaching resources courtesy of the Resilient Educator . Looking for tips to help get children into STEAM? Forbes has got you covered.
- Lee, Meggan J., et al. ' If you aren't White, Asian or Indian, you aren't an engineer': racial microaggressions in STEM education. " International Journal of STEM Education 7.1 (2020): 1-16.
- STEM Occupations: Past, Present, And Future . Stella Fayer, Alan Lacey, and Audrey Watson. A report. 2017.
- Institution for Art Integration and STEAM What is STEAM education?
- Barone, Ryan, ' The state of STEM education told through 18 stats ', iD Tech.
- U.S. Department of Education , Science, Technology, Engineering, and Math, including Computer Science.
- ' STEM sector must step up and end unacceptable disparities in Black staff ', The Royal Society. A report, March 25, 2021.
- 'Percentages of Women in STEM Statistics' Stemwomen.com
Sign up for the Live Science daily newsletter now
Get the world’s most fascinating discoveries delivered straight to your inbox.
Low tides reveal Bronze Age fortress that likely defended against Irish mainland
Lab-grown 'minibrains' help reveal why traumatic brain injury raises dementia risk
Hundreds of mysterious 'rogue' planets discovered by James Webb telescope may finally have an explanation
Most Popular
By Anna Gora December 27, 2023
By Anna Gora December 26, 2023
By Anna Gora December 25, 2023
By Emily Cooke December 23, 2023
By Victoria Atkinson December 22, 2023
By Anna Gora December 16, 2023
By Anna Gora December 15, 2023
By Anna Gora November 09, 2023
By Donavyn Coffey November 06, 2023
By Anna Gora October 31, 2023
By Anna Gora October 26, 2023
- 2 April 8 solar eclipse: What time does totality start in every state?
- 3 Giant coyote killed in southern Michigan turns out to be a gray wolf — despite the species vanishing from region 100 years ago
- 4 James Webb telescope confirms there is something seriously wrong with our understanding of the universe
- 5 Co-sleeping with pet dogs — but not cats — linked to poorer sleep in study
- 2 Hundreds of mysterious 'rogue' planets discovered by James Webb telescope may finally have an explanation
- 3 NASA engineers discover why Voyager 1 is sending a stream of gibberish from outside our solar system
- 4 Giant coyote killed in southern Michigan turns out to be a gray wolf — despite the species vanishing from region 100 years ago
- 5 Longest eclipse ever: How scientists rode the supersonic Concorde jet to see a 74-minute totality
An official website of the United States government
Here's how you know
Official websites use .gov A .gov website belongs to an official government organization in the United States.
Secure .gov websites use HTTPS. A lock ( Lock Locked padlock ) or https:// means you've safely connected to the .gov website. Share sensitive information only on official, secure websites.
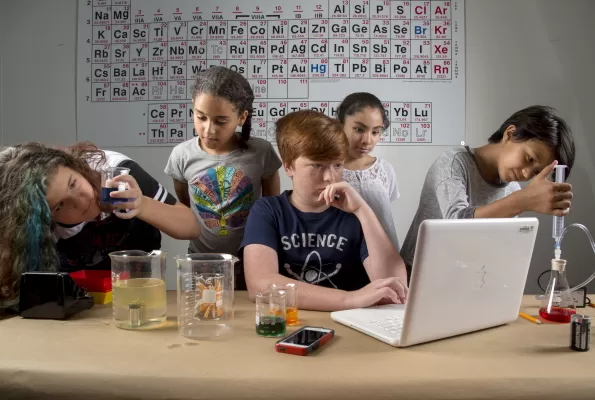
What do the data say about the current state of K-12 STEM education in the US?
A conversation with Julia Phillips of the National Science Board on the state of elementary and secondary STEM education in the nation.
The importance of a diverse STEM-educated workforce to the nation's prosperity, security and competitiveness grows every year. Preparing this future workforce must begin in the earliest grades, but the latest report from the National Science Board finds that the performance of U.S. students in STEM education continues to lag that of students from other countries.
Julia Phillips is a physicist and materials science researcher who chairs NSB's Committee on National Science and Engineering Policy, which oversees the congressionally mandated Science and Engineering Indicators report, also known as Indicators, in collaboration with NSF's National Center for Science and Engineering Statistics .
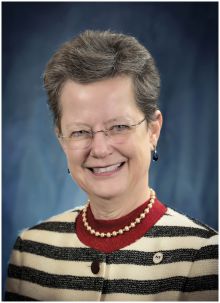
The latest Elementary and Secondary STEM Education report , the first of the 2022 Indicators reports, raises more concern about the state of STEM education in the nation and its potential impact on the economy and the U.S. standing in the world. Phillips discusses the key trends and their implications for science and education policy in the U.S.
Note: some of the conversation has been condensed and edited for clarity.
What does the report tell us about K-12 STEM education?
What we see is that the performance of children in the U.S. has not kept pace with the performance of students from other countries in science and mathematics for a decade or more. We have pretty much stayed steady, and other countries have improved dramatically. When you look at the closest economic competitors to the U.S., our scores are in last place in mathematics and in the middle of the pack in science. Math scores have not improved for more than a decade, and they're not good when you compare them to other countries.
This is just not something that we can be comfortable about. Our economy depends on math and science literacy. This is not only a concern for those with careers in those topics but also for the public at large.
You've said before that performance is "lumpy," with some groups of students performing very well and improving over time and others remaining stagnant or falling back. Where are the trouble spots?
I think it ought to be extremely disturbing to everyone in the U.S. that science and math performance is not equally distributed across the country. You see huge differences in performance based on race and ethnicity, so that Asian and white students do much better on these standardized tests than students of color. And you also see that there is a huge difference based on the socioeconomic background of students – students that are from higher socioeconomic backgrounds do much better than students from low socioeconomic backgrounds.
Data also show that the situation has only been exacerbated by the pandemic. We have a multi-year gap to pull out of just from COVID, and we were already in a weak position to begin with.
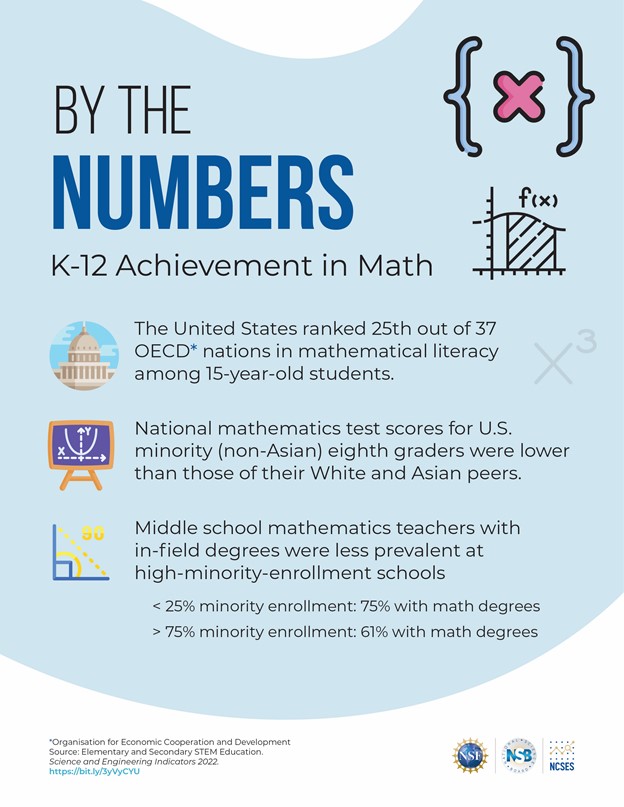
Why are the educational results so unevenly distributed?
We don't know exactly. But we can notice that certain things tend to occur at the same time.
For example, students of lower socioeconomic status or those from certain demographic groups tend to be in schools where teachers have less experience in teaching. There's separate evidence that teachers tend to get better as they get more experience.
Students from low socioeconomic status and minority backgrounds also tend to have teachers who are not originally educated in the fields that they teach, and that's particularly true in science.
Why should people care about these numbers?
Every parent should care, because careers in science and engineering are some of the best careers that a young person can pursue in terms of opportunities for making a really good living, from a certificate or associate degree all the way up through a Ph.D. You don't have to have the highest degree to make a really good living in a science and engineering field.
The second thing is that science and engineering is increasingly important for driving the U.S. economy. Many of the industries that we depend upon – including the auto industry, construction, all the way up through vaccine development – depend to an increasing level on literacy in math and science. If the U.S. is going to continue to have the wealth and prosperity that it has come to enjoy, being in the lead in many of these industries is going to be very important.
Julia Phillips on U.S. leadership in science
What can be done to turn these statistics around and improve STEM scores?
There has to be an all-hands-on-deck approach to emphasizing the importance of high-quality math and science education, beginning in the elementary grades and continuing all the way through as much education as a student gets. Communication is needed to say why it is important to have good math and science education.
NSF has prioritized programs that address this issue as well, like INCLUDES , which uses a collective approach to help broaden participation in STEM. Perhaps we could also be encouraging individuals with math and science backgrounds to go into teaching if they are drawn to that. We also need to increase the level of respect for the teaching profession.
How do you think education changed in recent decades, or even from when you were a student yourself and became interested in science?
In my own personal experience growing up in a small town in the middle of a bunch of cornfields in Illinois, I don't think I knew any practicing research scientists. But having teachers who were able to make science come alive with the things around us – whether it was nature, the stars, the gadgets in our house, whatever – they were able to make it interesting, relevant and exciting, and we were able to get a little taste for what we might be able to do. Teacher education programs must incorporate more STEM education so that elementary school teachers have the skills and comfort level they need to nurture young children's natural curiosity. NSF has funded some great research on STEM education that could be applied in the classroom, including work on teaching critical thinking, problem-solving, creativity and digital literacy.
With the internet, it is now possible for students to talk to practicing scientists and engineers, even if they don't live close to where the student is. Perhaps one good thing that the pandemic has taught us is that – if done correctly – virtual connectivity can augment educational opportunities in a very dramatic way.
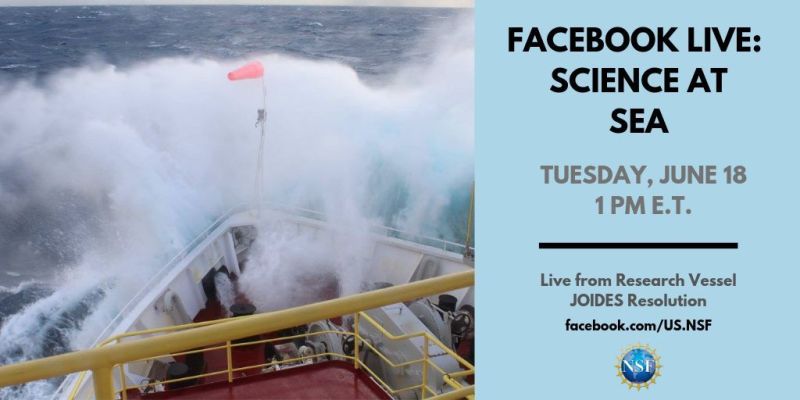
I also think there needs to be communication between the various groups that are responsible for K-12 education. For the most part that happens at the local school district, and standards are often set by the state. There needs to be communication between the federal level – which is where much science and math policy is established – and the very local level where the education policy is set and the requirements for education are carried out. It is a big problem, and a big challenge. But also, a big opportunity.
When Sputnik was launched, the attention of the entire nation was riveted. We need to get a spirit of curiosity and drive to do something to change the world into every school district, both at the administration and teacher level but also on the part of the kids and their parents.
About the Author
Related stories.
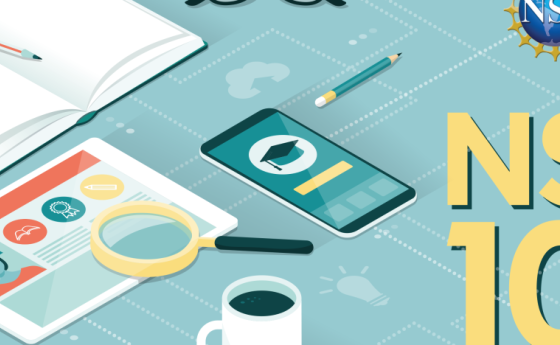
NSF 101: The NSF brand identity
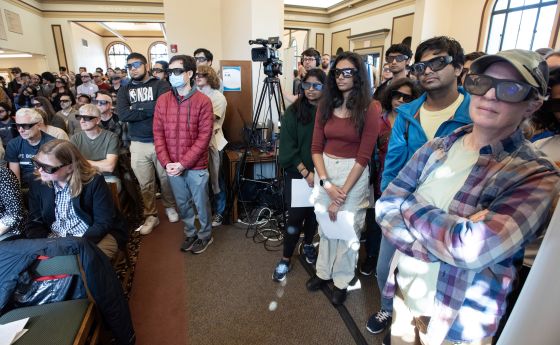
Bringing quantum entanglement to the people
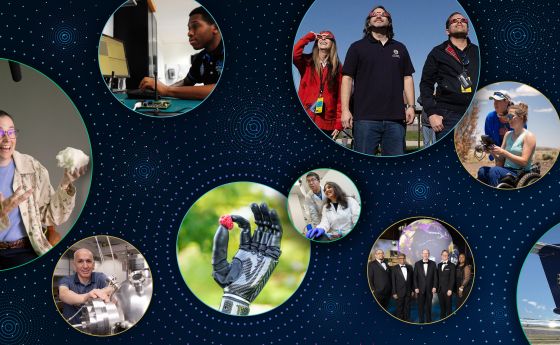
2023: A tremendous year for science that sets stage for an exciting 2024 and beyond
Featured Topics
Featured series.
A series of random questions answered by Harvard experts.
Explore the Gazette
Read the latest.
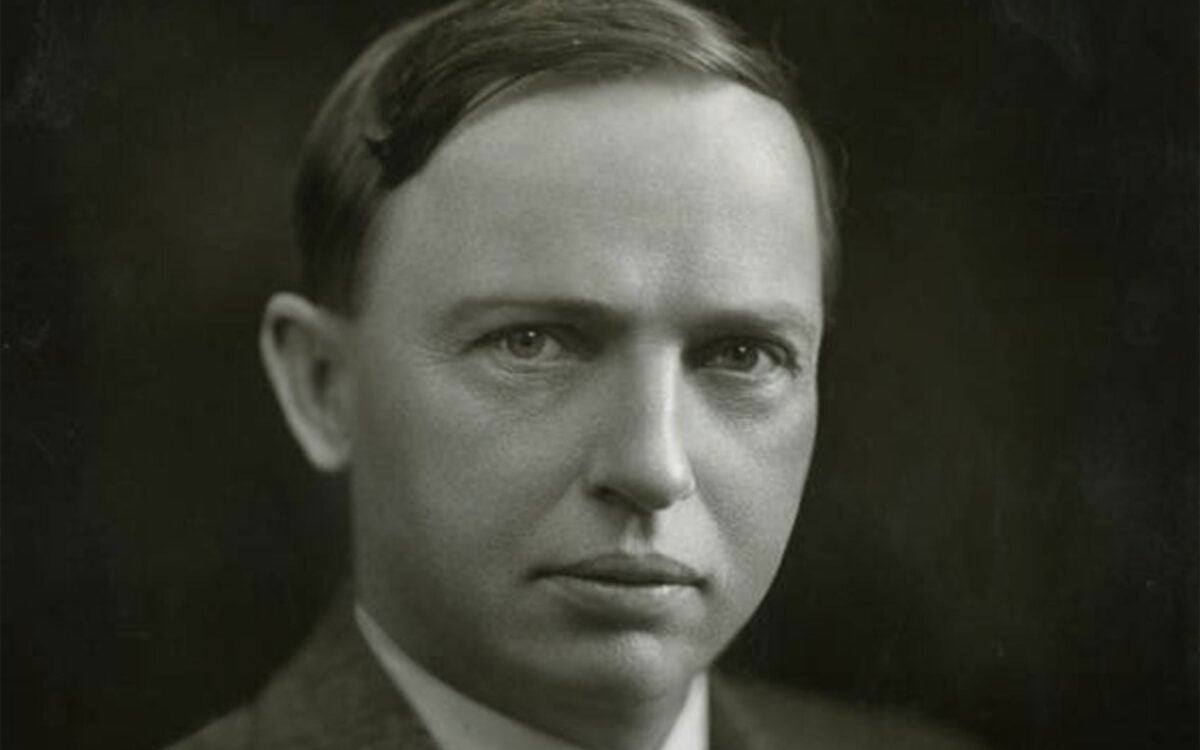
Harvard stargazer whose humanity still burns bright
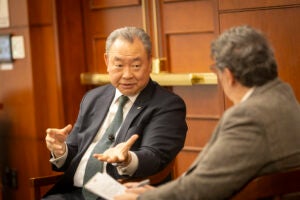
Taiwan sees warning signs in weakening congressional support for Ukraine
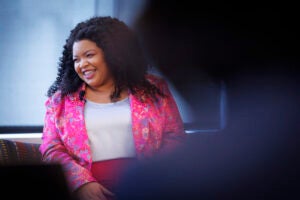
How dating sites automate racism
Employment in STEM occupations has grown 79 percent in the past three decades, according to U.S. Bureau of Labor Statistics.
iStock by Getty Images
Growing gap in STEM supply and demand
Brigid O’Rourke
Harvard Correspondent
Experts cite online learning, digital tools as ways to build inclusive and equitable STEM workforce
The evolution and impact of STEM education and its accompanying career opportunities reflect a positive in the fields of science, technology, engineering, and mathematics. But as the need grows for a specialized STEM-focused workforce, it’s becoming clear that not everyone has an equal opportunity.
During the Harvard-sponsored talk, “New Pathways to STEM,” panelists cited a large subset of students who are not being fully prepared for STEM careers. They then discussed ways the gap could be closed, pointing to online learning and the rapid advancement of new digital tools as ways to make STEM education more readily available. These new ways of learning, they said, can ultimately expand access to STEM education and create a more inclusive and equitable STEM workforce.
The need for a vast, talented workforce in STEM-related fields has never been more necessary, said Bridget Long, dean of the Harvard Graduate School of Education. Long cited the U.S. Bureau of Labor Statistics, which shows employment in STEM occupations has grown 79 percent in the past three decades. In addition, STEM jobs are projected to grow an additional 11 percent from 2020 to 2030. In Massachusetts alone, “40 percent of all employment revolves around innovation industries, such as clean energy, information technology, defense and advanced manufacturing,” said Long.
But, she added, “the importance of STEM education is about so much more than just jobs. STEM fields demand curious individuals eager to solve the world’s most pressing problems.”
“We need to have a new vision of how we prepare students to think critically about the world … as well as educating a society such that it has scientific literacy,” said Joseph L. Graves Jr., (upper left). Joining Graves were Brigid Long, Mike Edmonson, Amanda Dillingham, and Martin West.
The study of STEM subjects, she continued, teaches critical-thinking skills, and instills a mindset that will help students find success across numerous areas and disciplines. However, Long said, “too often the opportunity to learn and to be inspired by STEM is not available.
“Only 20 percent of high school graduates are prepared for college-level coursework in STEM majors,” she cited, adding, “fewer than half of high schools in the United States even offer computer science classes. So that begs the question — are kids going to be ready to meet the evolving and growing landscape of STEM professions?”
While STEM education opportunities are often scarce for high school students across the board, it’s even more pervasive when you consider how inequitably access is distributed by income, race, ethnicity, or gender. For example, Long said, “Native American, Black and Latinx students are the least likely to attend schools that teach computer science, as are students from rural areas, and [those with] economically disadvantaged backgrounds.
“It’s not surprising that these differences in educational opportunities lead to very large differences in what we see in the labor force. We are shutting students out of opportunity,” she said.
So what can be done to ensure more students from all backgrounds are exposed to a wide variety of opportunities? According to Graduate School of Education Academic Dean Martin West, who is also a member of the Massachusetts Board of Elementary and Secondary Education, a concerted effort is being made at the state level to work with — and through — teachers to convey to students the breadth of STEM opportunities and to assure them that “it’s not all sitting in front of a computer, or being in a science lab, but showing them that there are STEM opportunities in a wide range of fields.”
The relatively recent emergence of digital platforms, such as LabXchange, are helping to bridge the gap. LabXchange is a free online learning tool for science education that allows students, educators, scientists, and researchers to collaborate in a virtual community. The initiative was developed by Harvard University’s Faculty of Arts and Sciences and the Amgen Foundation . It offers a library of diverse content, includes a biotechnology learning resource available in 13 languages, and applies science to real-world issues. Teachers and students from across the country and around the world can access the free content and learn from wherever they are.
Many of the panelists also pointed to the need for steady funding in helping to address the inequities.
“Bottom line, if this nation wants to be a competitive leader in STEM, it has to revitalize its vision of what it needs to do, particularly in the public schools where most Black and brown people are, with regard to producing the human and physical infrastructure to teach STEM,” said Joseph L. Graves Jr., professor of biological sciences, North Carolina Agricultural and Technical State University. Graves is also a member of the Faculty Steering Committee, LabXchange’s Racial Diversity, Equity, and Inclusion in Science Education Initiative.
The panel noted how LabXchange is partnering with scholars from several historically Black colleges and universities to develop new digital learning resources on antiracism in education, science, and public health. The content, which will be freely available and translated into Spanish, is being funded by a $1.2 million grant from the Amgen Foundation. Aside from the highly successful LabXchange program, Mike Edmondson, vice president, Global Field Excellence and Commercial, Diversity Inclusion & Belonging at Amgen, noted the Amgen Biotech Experience and the Amgen Scholars program — both of which help to ensure that everyone has the opportunity to engage in science and to see themselves in a STEM career.
We also have to do a better job at helping people understand that that we cannot afford to fall behind in STEM education, Graves argued. “That means it’s going to cost us some money. So, America needs to be willing to pay … to build out STEM education infrastructure, so that we can produce the number of STEM professionals we need going forward,” he said. “We need to have a new vision of how we prepare students to think critically about the world … as well as educating a society such that it has scientific literacy.”
Amanda Dillingham, the program director of science and biology at East Boston High School, is on the front lines of this challenge, and says she believes that supporting teachers is one of the most critical steps that can be taken to address the issue in the immediate future.
When more funding is brought to the table, teachers “are able to coordinate networks … and build biotech labs in our classrooms and build robotics labs in our classrooms …. and are actually able to introduce students to [these fields and these careers] at a very early age,” said Dillingham.
Long and the panel also paid tribute to Rob Lue, the brainchild behind LabXchange, who passed away a year ago.
“Rob challenged science learners, scientists and educators to commit to ending racial inequity,” Long said. “Access was at the core of all of Rob’s many contributions to education at Harvard and beyond. He envisioned a world without barriers and where opportunity was available to anyone, especially in science. In everything that he did, he created an environment in which learners of all ages of diverse backgrounds could come together to imagine, learn, and achieve live exchange. Rob’s free online learning platform for science was his most expansive vision, and one that continues to inspire educators and learners around the world.”
Share this article
You might like.
Seminar foregrounds Harlow Shapley, who helped scholars escape Nazi rule
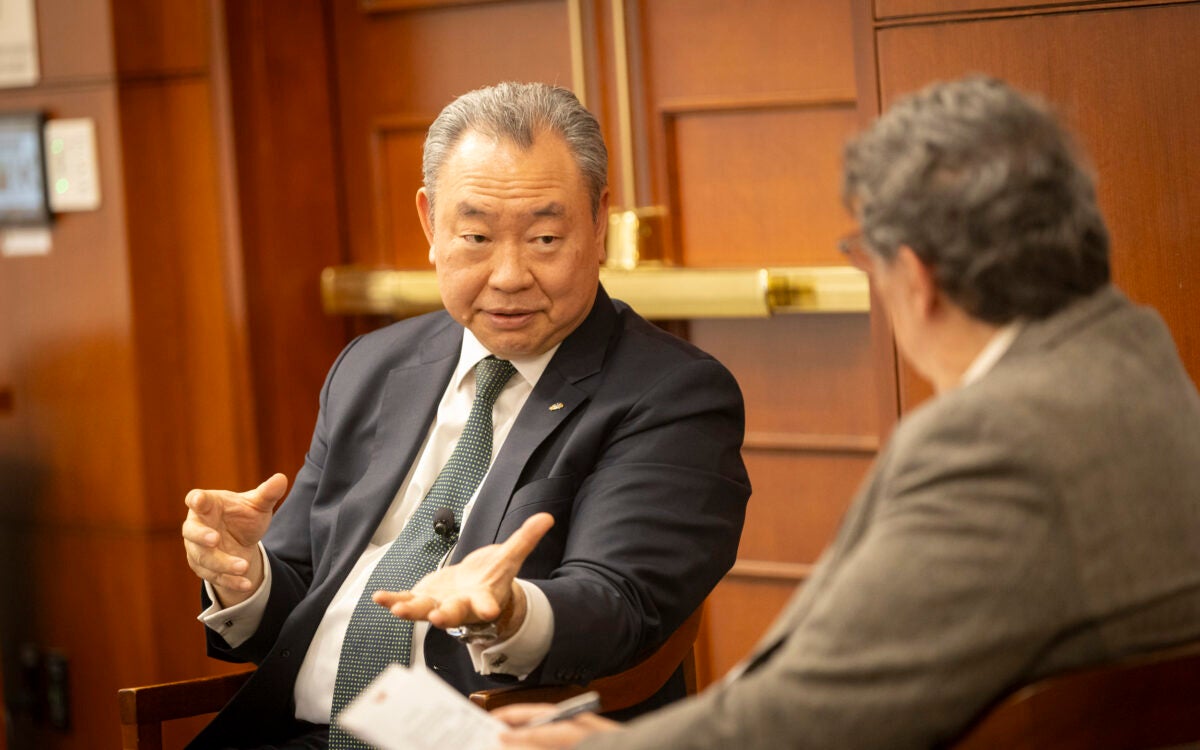
Ambassador says if Russia is allowed to take over sovereign nation, China may try to do same
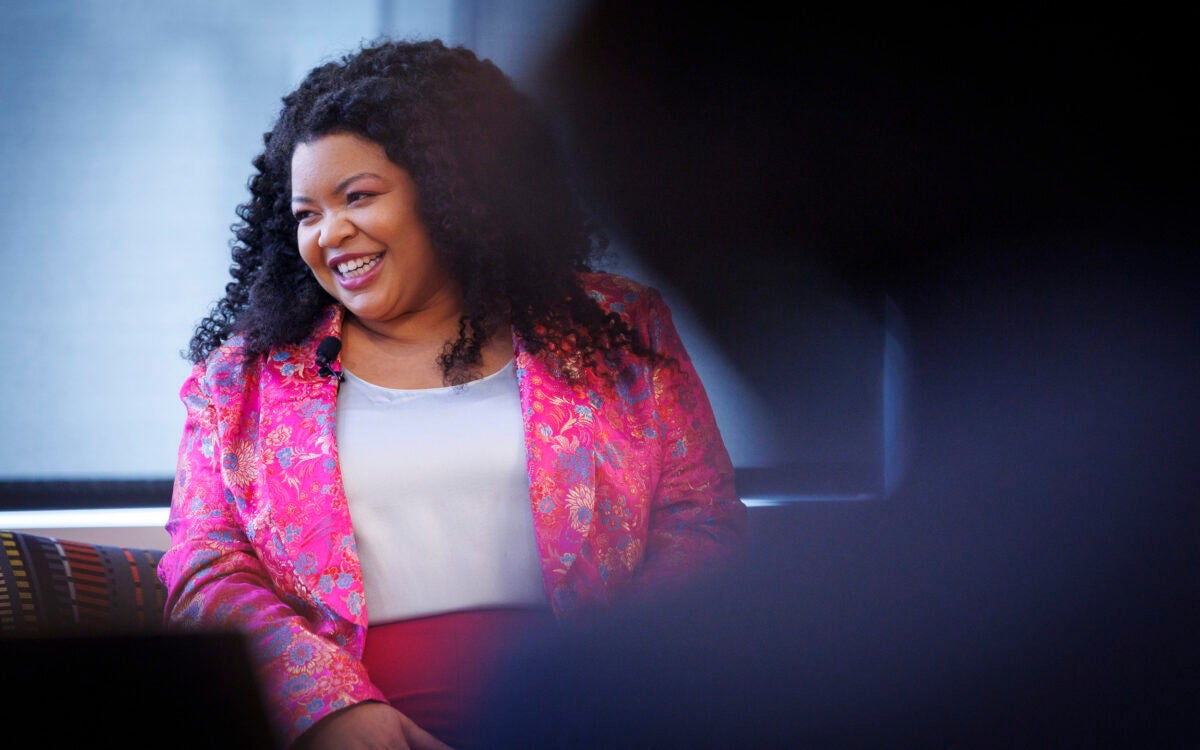
Sociologist’s new book finds algorithms that suggest partners often reflect stereotypes, biases
Yes, it’s exciting. Just don’t look at the sun.
Lab, telescope specialist details Harvard eclipse-viewing party, offers safety tips
Forget ‘doomers.’ Warming can be stopped, top climate scientist says
Michael Mann points to prehistoric catastrophes, modern environmental victories
We’re sorry, something doesn't seem to be working properly.
Please try refreshing the page. If that doesn't work, please contact support so we can address the problem.
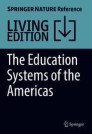
The Education Systems of the Americas pp 1–28 Cite as
The Education System of the United States: STEM Education in the United States – Progress without a Plan
- Christopher Reimann 3
- Living reference work entry
- First Online: 29 August 2020
206 Accesses
1 Citations
Given the highly decentralized nature of education in the United States, it is not surprising that STEM education in the United States has no uniformly embraced set of goals for STEM education nor strategies to attain them. A long history of local control over schools, including curriculum and instruction, means that most decisions concerning education are made by elected, volunteer members of the community who serve on more than ten thousand school boards across the country. When everyone is in charge, no one is in charge. This decentralization results in a disjointed policy and instructional environment with which efforts to improve STEM education must contend, including more recently a fixation on reading and mathematics at the elementary level, and more generally a legitimate questioning of the focus on STEM at the cost of other subjects that contribute to well-rounded students. Extensive opportunities for students to learn about STEM exist throughout the system, although these opportunities are not evenly distributed. For all of its limitations, decentralization may actually allow progress in STEM education to take place, in that it provides opportunities for new voices to be heard, new coalitions to form, and new approaches to be introduced and gain traction.
- The Education System of the United States: STEM Education in the United States – Progress without a Plan: STEM Education, Science Education
- Mathematics Teaching
- Teacher Preparation
This is a preview of subscription content, log in via an institution .
Achieve, Inc. (2004). Ready or not: Creating a high school diploma that counts . Washington, DC. Available at https://www.achieve.org/files/ReadyorNot.pdf . Last accessed 16 Jan 2019.
Alliance for Excellent Education. (n.d.). The Every Student Succeeds Act: Replacing No Child Left Behind. Available at https://all4ed.org/ESEA/ . Last accessed 4 Aug 2019.
American Enterprise Institute. (2015). Is the ‘new’ education philanthropy good for schools? Examining foundation-funded school reform. February 5, 2015 conference. Available at http://www.aei.org/events/new-education-philanthropy-good-schools-examining-foundation-funded-school-reform/ . Last accessed 11 Nov 2019.
Association of American Publishers. (n.d.). AAP Pre-K12 Adoption Schedule – April 2019. Available at https://publishers.org/sites/default/files/uploads/PreK-12/prek-12_textbook_adoption_schedule_4.2019.pdf . Last accessed 6 June 2019.
Bettinger, E. P., & Long, B. T. (2009). Addressing the needs of underprepared students in higher education: Does college remediation work? The Journal of Human Resources, 44 (3), 736–771.
Article Google Scholar
Bill & Melinda Gates Foundation. (n.d.). Professional Learning Partnership application. Available at http://k12education.gatesfoundation.org/school-network-rfp-spring-2019/ . Last accessed 1 May 2019.
California Department of Education. (n.d.). Instructional materials FAQ. Available at https://www.cde.ca.gov/ci/cr/cf/imfrpfaq1.asp . Last accessed 6 June 2019.
Danziger, S., Levav, J., & Avnaim-Pesso, L. (2011). Extraneous factors in judicial decisions. Proceedings of the National Academy of Sciences, 108 (17), 6889–6892. https://doi.org/10.1073/pnas.1018033108 .
Davies, P. (2014). Getting to the root of STEM. Fedgazette, Federal Reserve Bank of Minneapolis, April 30. Available at https://www.minneapolisfed.org/publications/fedgazette/getting-to-the-root-of-stem . Last accessed 5 Aug 2019.
Defense Activities for Non-Traditional Education Support (DANTES). (n.d.). Troops to teachers: Proud to serve again. Available at https://proudtoserveagain.com/ . Last accessed 5 Aug 2019.
Economist. (2006). The Birth of philanthrocapitalism. Feb 25. Available online at https://www.economist.com/weeklyedition/2006-02-25 .
Education Commission of the States. (n.d.). VitalSigns. Available at http://vitalsigns.ecs.org/state/United-States/teachers . Last accessed 4 Aug 2019.
Education Commission of the States (Julie Rowland). (2015). Trends in teacher certification. Available at https://www.ecs.org/clearinghouse/01/16/81/11681.pdf . Last accessed 1 Apr 2019.
Every Student Succeeds Act of 2015, Pub. L. No. 114-95 § 114 Stat. 1177 (2015–2016).
Google Scholar
Goldhaber, D., Krieg, J., Theobald, R., & Brown, N. (2015). Refueling the STEM and special education teacher pipelines. Phi Delta Kappan, 97 (4), 56–62.
Hahnel, C., & Jackson, O. (2012). Learning denied: The case for equitable access to effective teaching in California’s largest School District. The education trust-west. Available at https://edtrust.org/wp-content/uploads/2013/10/ETW-Learning-Denied-Report_0.pdf . Last accessed August 5, 2019.
Hegji, A. (2017). An overview of accreditation of higher education in the United States. Congressional research service. Available at https://fas.org/sgp/crs/misc/R43826.pdf . Last accessed 4 Aug 2019.
Heitin, L. H. (2015). When did Science education become STEM? Education week, April 2, 2015. Online at: https://blogs.edweek.org/edweek/curriculum/2015/04/when_did_science_education_become_STEM.html
Heitin, L. (2016). 2 in 5 high schools don’t offer physics, analysis finds. Education Week, 36 (1), 6. Available at https://www.edweek.org/ew/articles/2016/08/24/2-in-5-high-schools-dont-offer.html . Last accessed 4 Aug 2019.
Holmlund, T. D., Lesseig, K., & Slavit, D. (2018). Making sense of “STEM education” in K-12 contexts. Int J STEM Educ, 5 , Article Nr. 32. https://doi.org/10.1186/s40594-018-0127-2 .
Ivory, D., Green, E. L., & Eder, S. (2018). Education department unwinds unit investigating fraud at for-profits. New York Times , May 13. Available at https://www.nytimes.com/2018/05/13/business/education-department-for-profit-colleges.html . Last accessed 4 Aug 2019.
Kozol, J. (1991). Savage inequalities . New York: Random House.
Linked Learning Alliance. (n.d.). About the Linked Learning Alliance. Available at https://www.linkedlearning.org/linked-learning-alliance/ . Last accessed 23 June 2020.
Malzahn, K. A. (2019). 2018 NSSME+: Status of elementary school mathematics. Chapel Hill, NC: Horizon Research, Inc.
Meltzer, D. E., Plisch, M., & Vokos, S. (Eds.). (2012). Transforming the preparation of physics teachers: A call to action. A report by the task force on teacher education in physics (T-TEP) . College Park: American Physical Society.
Michigan Department of Education OER portal. (n.d.). #GoOpen Michigan. Available at https://www.michigan.gov/mde/0,4615,7-140-28753_80670%2D%2D-,00.html . Last accessed 23 June 2020.
Muniz, J. (2018). Diversifying the teacher workforce with ‘grow your own’ programs. New America, February 28. Available at https://www.newamerica.org/education-policy/edcentral/diversifying-teacher-workforce-grow-your-own-pathways/ . Last accessed 5 Aug 2019.
National Center for Educational Statistics (NCES). (n.d.-a). Schools and Staffing Survey. Retrieved 12 Available at https://nces.ed.gov/surveys/sass/ . Last accessed 12 Feb 2019.
National Center for Educational Statistics (NCES). (n.d.-b). National Assessment of Educational Progress. Available at https://nces.ed.gov/nationsreportcard/about/assessmentsched.aspx#f2 . Last Accessed 12 Feb 2019.
National Center for Educational Statistics (NCES). (n.d.-c). Public School Revenue Sources. The Condition of Education at a Glance. Available at https://nces.ed.gov/programs/coe/indicator_cma.asp . Last accessed 12 Feb 2019.
National Center for Educational Statistics (NCES). (n.d.-d). Public School enrollment. Available at https://nces.ed.gov/programs/coe/indicator_cga.asp . Last accessed 12 Feb 2019.
National Center for Educational Statistics (NCES). (n.d.-e). Back to School Statistics. Available at https://nces.ed.gov/fastfacts/display.asp?id=372 . Last accessed 12 Feb 2019.
National Center for Educational Statistics (NCES). (n.d.-f). Characteristics of degree-granting postsecondary institutions. Available at https://nces.ed.gov/programs/coe/indicator_csa.asp . Last accessed 4 Aug 2019.
National Center for Educational Statistics (NCES). (n.d.-g). Private School Enrollment. at https://nces.ed.gov/programs/coe/indicator_cgc.asp . Last accessed February 12, 2019.
National Center for Educational Statistics (NCES). (n.d.-h). Condition of Education. Public High School Graduation Rates. Available at https://nces.ed.gov/programs/coe/indicator_coi.asp . Last accessed 12 Feb 2019.
National Commission on Excellence in Education. (1983). A nation at risk: The imperative for education reform . Washington, DC: U.S. Department of Education.
National Conference of State Legislatures. (2019). Third-Grade Reading Legislation. at http://www.ncsl.org/research/education/third-grade-reading-legislation.aspx . Last accessed 4 Aug 2019.
National Council on Teacher Quality. (2019). A fair chance: Simple steps to strengthen and diversify the teacher workforce. Available at https://www.nctq.org/publications/A-Fair-Chance . Last accessed 4 Aug 2019.
National Research Council. (2012). A framework for K-12 science education: Practices, crosscutting concepts, and core ideas. Committee on a conceptual framework for new K-12 Science education standards. Board on Science education, division of Behavioral and social sciences and education . Washington, DC: The National Academies Press.
National Science and Technology Council, Committee on STEM Education. (2013). Federal science, technology, engineering, and mathematics (STEM) education 5-Year strategic plan. Available at https://obamawhitehouse.archives.gov/sites/default/files/microsites/ostp/stem_stratplan_2013.pdf . Last accessed 1 Apr 2020.
National Science Foundation. (1999). Teacher enhancement program. Available at www.nsf.gov/pubs/1999/nsf9992/teacher.htm . Last accessed 10 Apr 2019.
National Science Foundation. (2004). Interagency Educational Research Initiative (IERI) Program Solicitation. Available at https://www.nsf.gov/pubs/2004/nsf04553/nsf04553.htm . Last accessed 4 June 2019.
National Science Teaching Association. (n.d.). About NSTA: How many science teachers are teaching out of field? Available at https://www.nsta.org/about/clpa/faq.aspx . Last accessed 4 Aug 2019.
NGSS Lead States. (2013). Next generation science standards: For states, by states . Washington, DC: The National Academies Press.
Noonan, Ryan. Office of the Chief Economist, Economics and Statistics Administration, U.S. Department of Commerce. (2017). STEM Jobs: 2017 Update (ESA Issue Brief # 02–17). Available at http://www.esa.gov/reports/stem-jobs-2017-update . Last accessed 14 Apr 2019.
OECD. (2011). Viewing education in the United States through the prism of PISA. In lessons from PISA for the United States . Paris: OECD Publishing. https://doi.org/10.1787/9789264096660-3-en .
Book Google Scholar
Ogan-Bekiroglu, F., & Caner, F. (2018). STEM integrations and teachers’ role in this process. Education research highlights in mathematics, Science and Technology. The International Society for Research in Education and Science. Available at https://www.isres.org/books/chapters/STEM%20Integrations%20and%20Teachers%E2%80%99%20Role%20in%20This%20Process_26-12-2018.pdf . Last accessed 6 June 2019.
Oleson, A., Hora, M. T., & Benbow, R. J. (2014). STEM: How a poorly defined acronym is shaping education and workforce development policy in the United States (WCER working paper 2014–2). Wisconsin: University of Wisconsin–Madison, Wisconsin Center for Education Research. Available at http://www.wcer.wisc.edu/publications/workingPapers/papers.php . Last accessed 12 Feb 2019.
Padilla, A. M. (1991). English Only Vs. Bilingual Education: Ensuring a Language-Competent Society. Journal of Education, 173 (2), 38–51. https://doi.org/10.1177/002205749117300205 . Last accessed 4 Aug 2019.
Prensky, M. (2001). Digital natives, digital immigrants. On the Horizon 9(5). Available at https://www.marcprensky.com/writing/Prensky%20-%20Digital%20Natives,%20Digital%20Immigrants%20-%20Part1.pdf . Last accessed 1 Apr 2020.
Ronald Reagan Presidential Foundation & Institute. (n.d.). News conference, August 12, 1986. http://www.reaganfoundation.org . Last accessed 4 Aug 2019.
Rothwell, J. (2013). The hidden STEM economy. Metropolitan Policy Program . Washington, DC: Brookings Institute. Available at https://www.brookings.edu/wp-content/uploads/2016/06/TheHiddenSTEMEconomy610.pdf . Last accessed 4 Aug 2019.
Schmidt, W., McKnight, C., & Raizen, S. (2002). A splintered vision: An investigation of U.S. science and mathematics education . Netherlands: Springer.
Schmidt, W., Zoido, P., & Cogan, L. (2012). Schooling matters: Opportunity to learn in PISA 2012. OECD Education Working Papers, No. 95. https://doi.org/10.1787/5k3v0hldmchl-en .
Texas Education Agency. (n.d.). Instructional materials review and adoption process. Available at https://tea.texas.gov/Academics/Instructional_Materials/Review_and_Adoption_Process/The_Review_and_Adoption_Process/ . Last accessed 5 Jun 2019.
U.S. Army. (n.d.). ARMY STEM: Careers and jobs. Available at https://www.goarmy.com/careers-and-jobs/about-army-stem.html . Last accessed 10 Apr 2019.
U.S. Department of Education. (n.d.-a). Institute of Education Sciences, National Center for Education Statistics. Schools and staffing survey. Available at https://nces.ed.gov/surveys/sass/ . Last accessed 1 Apr 2020.
U.S. Department of Education. (n.d.-b). Institute of Education Sciences, National Center for Education Statistics, National Assessment of Educational Progress. The nation’s report card: 2015 science at grades 4, 8 and 12. Available at https://www.nationsreportcard.gov/ndecore/xplore/NDE . Last accessed 1 Apr 2020.
U.S. Department of Education. (n.d.-c). The federal role in education. Available at https://www2.ed.gov/about/overview/fed/role.html . Last accessed 1 Apr 2020.
U.S. Department of Education. (n.d.-d). Institute of Education Sciences, National Center for Education Statistics. Indicator 6: Elementary and secondary enrollment. Available at https://nces.ed.gov/programs/raceindicators/indicator_rbb.asp . Last accessed 1 Apr 2020.
U.S. Department of Education. (n.d.-e). Institute of Education Sciences, National Center for Education Statistics. Public high school graduation rates. Available at https://nces.ed.gov/programs/coe/indicator_coi.asp . Last accessed 1 Apr 2020.
U.S. Department of Education. (n.d.-f). Institute of Education Sciences, National Center for Education Statistics. Undergraduate retention and graduation rates. Available at https://nces.ed.gov/programs/coe/indicator_ctr.asp . Last accessed 1 Apr 2020.
U.S. Department of Education. (n.d.-g). Institute of Education Sciences, National Center for Education Statistics. Indicator 26: STEM degrees. Available at https://nces.ed.gov/programs/raceindicators/indicator_REG.asp#info . Last accessed 1 Apr 2020.
U.S. Department of Education. (n.d.-h). Institute of Education Sciences, National Center for Education Statistics, Digest of Education Statistics. Acceptance rates; number of applications, admissions, and enrollees; and enrollees’ SAT and ACT scores for degree-granting postsecondary institutions with first-year undergraduates, by control and level of institution: 2017–18. Available at https://nces.ed.gov/programs/digest/d18/tables/dt18_305.40.asp . Last accessed 1 Apr 2020.
U.S. Department of Education. (n.d.-i). Institute of Education Sciences, National Center for Education Statistics, Digest of Education Statistics. Number of degree-granting postsecondary institutions and enrollment in these institutions, by enrollment size, control, and classification of institution: Fall 2017. Available at https://nces.ed.gov/programs/digest/d18/tables/dt18_317.40.asp . Last accessed 1 Apr 2020.
U.S. Department of Education. (n.d.-j). Institute of Education Sciences, National Center for Education Statistics. Public and private school comparison. Available at https://nces.ed.gov/fastfacts/display.asp?id=55 . Last accessed 1 Apr 2020.
U.S. Department of Education, Institute of Education Sciences, National Center for Education Statistics, National Assessment of Educational Progress (NAEP). (n.d.). 2015 Fourth-Grade Science Assessment; 2015 Eighth-Grade Science Assessment. Available at https://www.nationsreportcard.gov/math_2017/nation/scores?grade=4 . Last accessed 1 Apr 2020.
U.S. Department of Education. Institute of Education Sciences, National Center for Education Statistics, National Assessment of Educational Progress (NAEP). (n.d.). 2017. Mathematics Assessments. Available at https://nces.ed.gov/nationsreportcard/ . Last accessed 1 Apr 2020.
U.S. Department of Education. Office of Educational Technology. (n.d.). #GoOpenStates. Available at https://tech.ed.gov/open/states/ . Last accessed 14 Apr 2019.
U.S. Department of Education. Office of Postsecondary Education. (2016). Teacher shortage areas nationwide listing 1990–1991 through 2016–2017. Available at https://www2.ed.gov/about/offices/list/ope/pol/tsa.pdf . Last accessed 4 Aug 2019.
U.S. Securities and Exchange Commission. (n.d.). Fast answers. Available at https://www.sec.gov/fast-answers/answersmperfhtm.html . Last accessed 5 Aug 2019.
Zakaria, F. (2015). In defense of a liberal education . New York: W.W. Norton &.
Download references
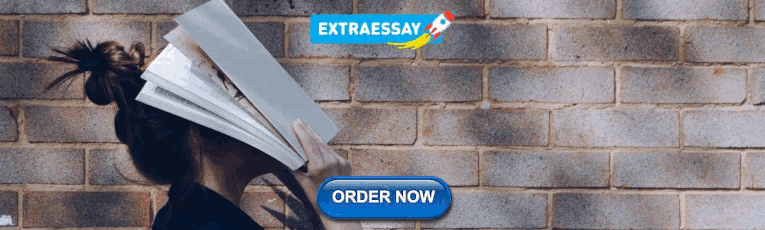
Author information
Authors and affiliations.
CREATE for STEM Institute, Michigan State University, East Lansing, MI, USA
Christopher Reimann
You can also search for this author in PubMed Google Scholar
Corresponding author
Correspondence to Christopher Reimann .
Editor information
Editors and affiliations.
Deutsches Institut für Internationa Pädagogische Forschung, Frankfurt, Germany
Sieglinde Jornitz
Institute of Education, University of Münster, Münster, Germany
Marcelo Parreira do Amaral
Rights and permissions
Reprints and permissions
Copyright information
© 2020 Springer Nature Switzerland AG
About this entry
Cite this entry.
Reimann, C. (2020). The Education System of the United States: STEM Education in the United States – Progress without a Plan. In: Jornitz, S., Parreira do Amaral, M. (eds) The Education Systems of the Americas. Global Education Systems. Springer, Cham. https://doi.org/10.1007/978-3-319-93443-3_24-1
Download citation
DOI : https://doi.org/10.1007/978-3-319-93443-3_24-1
Received : 02 April 2020
Accepted : 08 April 2020
Published : 29 August 2020
Publisher Name : Springer, Cham
Print ISBN : 978-3-319-93443-3
Online ISBN : 978-3-319-93443-3
eBook Packages : Springer Reference Education Reference Module Humanities and Social Sciences Reference Module Education
- Publish with us
Policies and ethics
- Find a journal
- Track your research
- Extended University
- UTEP Connect
- December 2021
You’ve probably heard about STEM. The integration of science, technology, engineering and mathematics has been a central focus both within and well outside of education.
In fact, it’s such a powerful concept that it has been hailed as critical to the future — for children, diversity, the workforce and the economy, among other areas. That’s why STEM education has received hundreds of millions of dollars in support from the U.S. government and remains one of the biggest priorities at all levels of the educational system. UTEP also offers a master's degree and a graduate certificate in STEM Education.
But what actually is STEM education, and why is it so important? Here’s what you need to know and how you can help.

What Is STEM Education?
It would be inaccurate to assume that STEM education is merely instruction in the STEM subjects of science, technology, engineering and mathematics. Rather, the idea is taken a step further.
STEM education refers to the integration of the four subjects into a cohesive, interdisciplinary and applied learning approach. This isn’t academic theory—STEM education includes the appropriate real-world application and teaching methods.
As a result, students in any subject can benefit from STEM education. That’s exactly why some educators and organizations refer to it as STEAM, which adds in arts or other creative subjects. They recognize just how powerful the philosophy behind STEM education can be for students.
Why Is STEM Education Important?
There are several layers to explore in discovering why STEM education is so important.
In 2018, the White House released the “Charting a Course for Success” report that illustrated how far the United States was behind other countries in STEM education.
It found that only 20% of high school grads were ready for the rigors of STEM majors. And how over the previous 15 years, the U.S. had produced only 10% of the world’s science and engineering grads.
Since the founding of the Nation, science, technology, engineering, and mathematics (STEM) have been a source of inspirational discoveries and transformative technological advances, helping the United States develop the world's most competitive economy and preserving peace through strength. The pace of innovation is accelerating globally, and with it the competition for scientific and technical talent. Now more than ever the innovation capacity of the United States — and its prosperity and securit — depends on an effective and inclusive STEM education ecosystem. - Charting a Course for Success
That was one of the most news-worthy developments in recent years. It set the stage for many arguments behind STEM in the context of the global economy and supporting it through education.
Job Outlook and Salary
One of the most direct and powerful arguments for the importance of STEM education is how relevant STEM is in the workforce. In 2018, the Pew Research Center found that STEM employment had grown 79% since 1990 (computer jobs increased 338%).
What about now? All occupations are projected to increase 7.7% by 2030, according to the Bureau of Labor Statistics (BLS). Non-STEM occupations will increase 7.5% while STEM occupations will increase 10.5% .
The findings are even more pronounced in terms of salary. The median annual wage for all occupations is $41, 950. Those in non-STEM occupations earn $40,020 and those in STEM occupations earn $89,780.
Even areas like entrepreneurship see the same types of results. A report from the Information Technology and Innovation Foundation (ITIF) found that tech-based startups pay more than double the national average wage and nearly three times the average overall startup wage. They only make up 3.8% of businesses but capture a much larger share of business research and development investment (70.1%), research and development jobs (58.7%) and wages (8.1%), among other areas.
Diversity and Skills
An important detail in the passage from “Charting a Course for Success” comes toward the end of the final sentence: “Now more than ever the innovation capacity of the United States—and its prosperity and security—depends on an effective and inclusive STEM education ecosystem.”
Being inclusive is incredibly important once you understand how STEM occupations are such high-demand, high-paying positions. Unfortunately, however, diversity is a significant issue here.
- The Pew Research Center noted how women account for the majority of healthcare practitioners and technicians but are underrepresented across many other STEM fields, especially in computer jobs and engineering. Black and Hispanic workers are also underrepresented in the STEM workforce.
- In the International Journal of STEM Education, authors noted how women are significantly underrepresented in STEM occupations. They make up less than a quarter of those working in STEM occupations and for women of color, representation is much lower — Hispanic, Asian and Black women receive less than 5% of STEM bachelor’s degrees in the U.S. Authors also pointed out how people of color overall are underrepresented in U.S.-based STEM leadership positions across industry, academia and the federal workforce.
These issues are troubling when you consider how it undermines students’ opportunities to pursue high-demand, high-paying roles. Yet, it’s more than that. STEM education is about a teaching philosophy that naturally integrates critical thinking and language skills in a way that enriches any subject. Perhaps you’ve experienced or can imagine an education that integrates problem solving and engineering practices into any subject, where technology is seamlessly integrated throughout. Any subject—art, language, social studies, health—can benefit.
So when students don’t receive an effective STEM education, they’re not only receiving less instruction in STEM subjects. They miss out on the universal application that high-level skills in STEM subjects can bring.
How You Can Make a Difference
Take the opportunity to encourage young minds in STEM education. Whether that means volunteering a little bit of your time at a local school or finding age-appropriate STEM literature and activities for your children, you can have an impact.
You can also consider pursuing a career or enhancing your career as a teacher or leader in STEM education, which represents a major problem right now in education. Researchers in Economic Development Quarterly noted how the current shortage of teachers in the U.S. is “ especially acute ” among STEM educators.
In just five courses, you can earn an online graduate certificate in STEM education and learn how you can increase STEM literacy through formal and informal learning opportunities across a variety of settings. Or there’s the 100% online M.A. in Education with a Concentration in STEM Education , which helps you to be a leader in STEM education. You’ll be prepared for advancement in roles across public and private schools, community-based organizations, research, nonprofits and nongovernmental organizations.
UTEP’s programs are focused on preparing today and tomorrow’s educators for working with modern students in multicultural settings who need to find motivation and engagement in their learning. And again, this is especially important. A study in Education Journal found that while students of all races enter into STEM majors at equal rates, minority students leave their major at nearly twice the rate of white students.
UTEP is one of only 17 Hispanic-Serving Institutions (HSIs) in the country to be designated as an R1 top tier research university. Interested in learning more about how you can engage and inspire students in STEM education? You can discuss that and more with a one-on-one consultation with an enrollment counselor.
LEARN MORE GET STARTED

Connect With Us
The University of Texas at El Paso Extended University UTEP Connect Online Programs 500 W University Ave. El Paso, Texas 79968
E: [email protected] P: 1-800-684-UTEP

Programs submenu
Regions submenu, topics submenu, leadership 2024, csis-csds transatlantic dialogue on the indo-pacific, chinese interference in taiwan's 2024 elections and lessons learned, the political pressure behind scotland on the ballot.
- Abshire-Inamori Leadership Academy
- Aerospace Security Project
- Africa Program
- Americas Program
- Arleigh A. Burke Chair in Strategy
- Asia Maritime Transparency Initiative
- Asia Program
- Australia Chair
- Brzezinski Chair in Global Security and Geostrategy
- Brzezinski Institute on Geostrategy
- Chair in U.S.-India Policy Studies
- China Power Project
- Chinese Business and Economics
- Defending Democratic Institutions
- Defense-Industrial Initiatives Group
- Defense 360
- Defense Budget Analysis
- Diversity and Leadership in International Affairs Project
- Economics Program
- Emeritus Chair in Strategy
- Energy Security and Climate Change Program
- Europe, Russia, and Eurasia Program
- Freeman Chair in China Studies
- Futures Lab
- Geoeconomic Council of Advisers
- Global Food and Water Security Program
- Global Health Policy Center
- Hess Center for New Frontiers
- Human Rights Initiative
- Humanitarian Agenda
- Intelligence, National Security, and Technology Program
- International Security Program
- Japan Chair
- Kissinger Chair
- Korea Chair
- Langone Chair in American Leadership
- Middle East Program
- Missile Defense Project
- Project on Fragility and Mobility
- Project on Nuclear Issues
- Project on Prosperity and Development
- Project on Trade and Technology
- Renewing American Innovation Project
- Scholl Chair in International Business
- Smart Women, Smart Power
- Southeast Asia Program
- Stephenson Ocean Security Project
- Strategic Technologies Program
- Transnational Threats Project
- Wadhwani Center for AI and Advanced Technologies
- All Regions
- Australia, New Zealand & Pacific
- Middle East
- Russia and Eurasia
- American Innovation
- Civic Education
- Climate Change
- Cybersecurity
- Defense Budget and Acquisition
- Defense and Security
- Energy and Sustainability
- Food Security
- Gender and International Security
- Geopolitics
- Global Health
- Human Rights
- Humanitarian Assistance
- Intelligence
- International Development
- Maritime Issues and Oceans
- Missile Defense
- Nuclear Issues
- Transnational Threats
- Water Security
The U.S. Should Strengthen STEM Education to Remain Globally Competitive
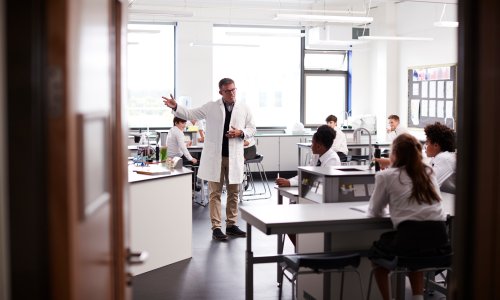
Photo: Monkey Business/Adobe Stock
Blog Post by Gabrielle Athanasia
Published April 1, 2022
Gabrielle Athanasia is a Program Coordinator and Research Assistant with the Renewing American Innovation Project at the Center for Strategic and International Studies in Washington, DC.
Jillian Cota is a research intern with the Renewing American Innovation Project at the Center for Strategic and International Studies in Washington, DC.
The Perspectives on Innovation Blog is produced by the Renewing American Innovation Project at the Center for Strategic and International Studies (CSIS), a private, tax-exempt institution focusing on international public policy issues. Its research is nonpartisan and nonproprietary. CSIS does not take specific policy positions. Accordingly, all views, positions, and conclusions expressed in this publication should be understood to be solely those of the author(s).
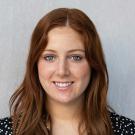
Gabrielle Athanasia
Programs & projects.
- Inclusive Innovation
- Renewing the Nation’s Innovation Ecosystem
WHAT IS STEM EDUCATION, AND WHAT IS THE DEFINITION OR MEANING OF STEM?
- STEM EDUCATION ARTICLES & OPINIONS
- What is STEM Education? STEM Definition and Meaning
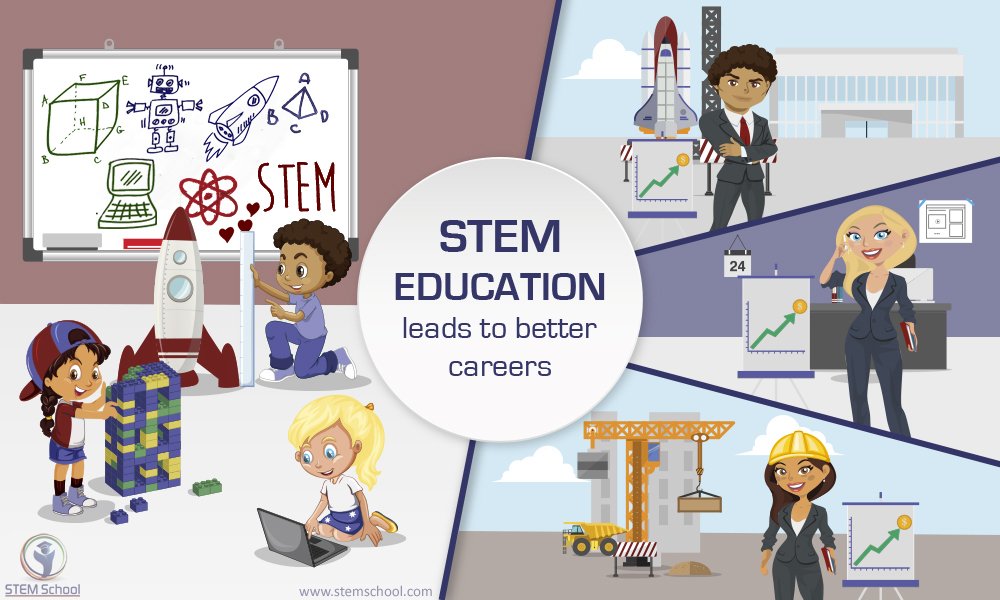
WHAT IS STEM EDUCATION? STEM DEFINITION AND MEANING
What is stem.
PISA Evaluations of STEM Subjects
National attention to stem, public and private funding of stem education.
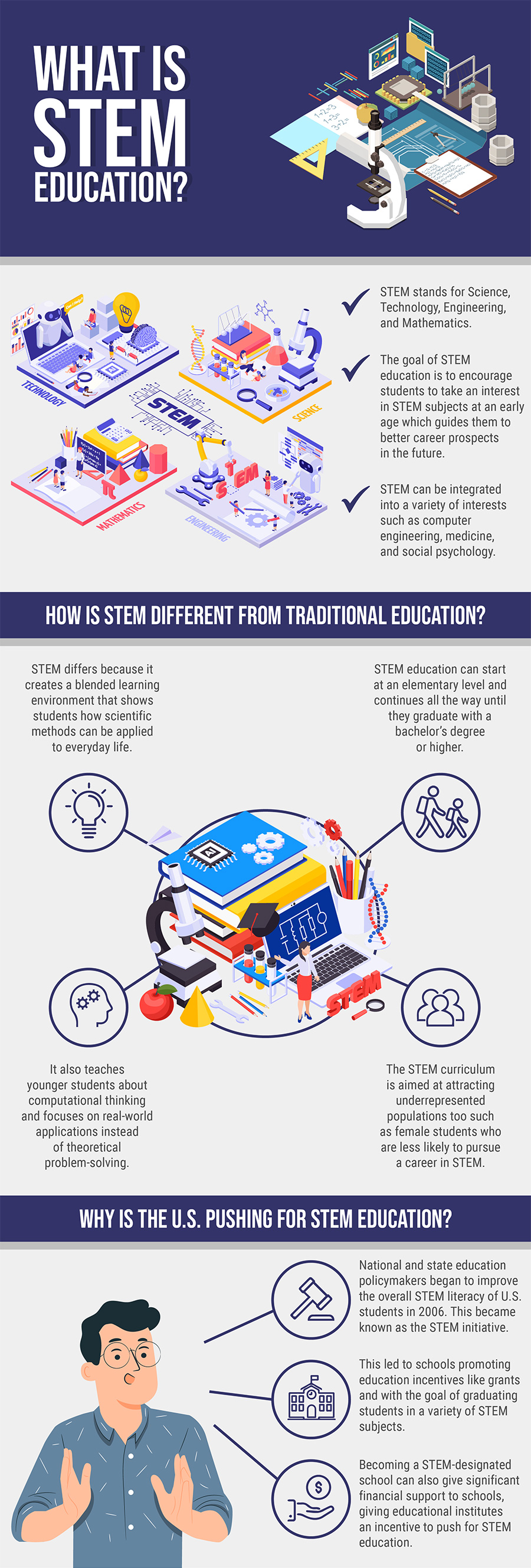
- References:
- https://nces.ed.gov/surveys/pisa/pisa2018/pdf/PISA2018_compiled.pdf
- http://www.nsf.gov/news/speeches/marrett/11/cm112005_drexelnrcreport.jsp
This article was reviewed and updated on January 8, 2022
Reset Password
- Open access
- Published: 19 September 2022
The application of AI technologies in STEM education: a systematic review from 2011 to 2021
- Weiqi Xu 1 &
- Fan Ouyang ORCID: orcid.org/0000-0002-4382-1381 1
International Journal of STEM Education volume 9 , Article number: 59 ( 2022 ) Cite this article
55k Accesses
45 Citations
12 Altmetric
Metrics details
The application of artificial intelligence (AI) in STEM education (AI-STEM), as an emerging field, is confronted with a challenge of integrating diverse AI techniques and complex educational elements to meet instructional and learning needs. To gain a comprehensive understanding of AI applications in STEM education, this study conducted a systematic review to examine 63 empirical AI-STEM research from 2011 to 2021, grounded upon a general system theory (GST) framework.
The results examined the major elements in the AI-STEM system as well as the effects of AI in STEM education. Six categories of AI applications were summarized and the results further showed the distribution relationships of the AI categories with other elements (i.e., information, subject, medium, environment) in AI-STEM. Moreover, the review revealed the educational and technological effects of AI in STEM education.
Conclusions
The application of AI technology in STEM education is confronted with the challenge of integrating diverse AI techniques in the complex STEM educational system. Grounded upon a GST framework, this research reviewed the empirical AI-STEM studies from 2011 to 2021 and proposed educational, technological, and theoretical implications to apply AI techniques in STEM education. Overall, the potential of AI technology for enhancing STEM education is fertile ground to be further explored together with studies aimed at investigating the integration of technology and educational system.
Introduction
Artificial intelligence in education (AIEd) is an emerging interdisciplinary field that applies AI technologies in education to transform and promote the instructional and learning design, process and assessment (Chen et al., 2020 ; Holmes et al., 2019 ; Hwang et al., 2020 ). The application of AI in STEM education (referred to AI-STEM in this paper), as a sub-branch of AIEd, focuses on the design and implementation of AI applications to support STEM education. Automated AI technologies, e.g., intelligence tutoring, automated assessment, data mining and learning analytics, have been used in STEM education to enhance the instruction and learning quality (Chen et al., 2020 ; Hwang et al., 2020 ; McLaren et al., 2010 ). STEM education is a complex system, from a system perspective, consisting of interdependent elements, including subject, information, medium, and environment (Rapoport, 1986 ; Von Bertalanffy, 1968 ). The application of AI, as a critical technology element, should take careful consideration of these complex factors, to achieve a high-quality STEM education (Byrne & Callaghan, 2014 ; Krasovskiy, 2020 ; Xu & Ouyang, 2022 ). This systematic review aims to examine the different elements, including AI technology, subject, information, medium, environment in the AI-STEM system to gain a holistic understanding of the application and integration of AI technologies in the STEM education contexts. Specifically, we collected and reviewed empirical AI-STEM research from 2011 to 2021, summarized the AI techniques and applications, the characteristics of other system elements (i.e., information, subject, medium, environment), the distribution of AI in these elements, and the effects of AI in STEM education. Based on the results, this systematic review provided educational and technological implications for the practice and research in the AI-STEM education.
Literature review
With the development of computer science and computational technologies, automatic, adaptive, and efficient AI technologies have been widely applied in various academic fields. Artificial Intelligence in Education (AIEd), as an interdisciplinary field, emphasizes applying AI to assist instructor’s instructional process, empower student’s learning process, and promote the transformation of educational system (Chen et al., 2020 ; Holmes et al., 2019 ; Hwang et al., 2020 ; Ouyang & Jiao, 2021 ). First, AIEd has potential to enhance instructional design and pedagogical development in the teaching processes, such as accessing students’ performance automatically (Wang et al., 2011 ; Zampirolli et al., 2021 ), monitoring and tracking students’ learning (Berland et al., 2015 ; Ji & Han, 2019 ), and predicting at-risk students (Hellings & Haelermans, 2020 ; Lamb et al., 2021 ). Second, AIEd is beneficial for improving student-centered learning, such as providing adaptive tutoring (Kose & Arslan, 2017 ; Myneni et al., 2013 ), recommending personalized learning resources (Ledesma & García, 2017 ; Zhang et al., 2020 ), and diagnosing students’ learning gaps (Liu et al., 2017 ). Third, AIEd also brings opportunities to transform the educational system by highlighting the essential role of technology (Hwang et al., 2020 ), enriching the mediums of knowledge delivery (Holstein et al., 2019 ; Yannier et al., 2020 ), and changing the instructor–student relationship (Xu & Ouyang, 2022 ). Overall, different AI technologies (e.g., machine learning, deep learning) have been deployed in the field of education to enhance instructional and learning process.
The development of AIEd also brought transformations to the field of science, technology, engineering and mathematics (STEM) education, as a sub-branch of AIEd named AI-STEM. STEM education aims to improve students’ interdisciplinary knowledge inquiry and application, as well as their higher-order thinking, critical thinking and problem-solving ability (Bybee, 2013 ; Pimthong & Williams, 2018 ). The application of AI in STEM education has advantages to provide adaptive and personalized learning environments or resources, and aid instructors to understand students’ learning behavioral patterns, and automatically assess STEM learning performances (Alabdulhadi & Faisal, 2021 ; Walker et al., 2014 ). However, STEM education is a complex system, consisting of interdependent elements, including subject (e.g., instructor, student), information, medium, and environment (Rapoport, 1986 ; Von Bertalanffy, 1968 ). Achieving a high quality of STEM education requires a careful consideration of the complex social, pedagogical, environmental factors, rather than merely applying AI technologies in education (Krasovskiy, 2020 ; Xu & Ouyang, 2022 ). Therefore, a major challenge in AI-STEM is how to appropriately select and apply AI techniques to adapt to the multiple elements (e.g., subject, information, environment) in STEM education with a goal of high-quality instruction and learning (Castañeda & Selwyn, 2018 ; Selwyn, 2016 ). To gain a holistic understanding of the integration of AI technologies in the STEM education contexts, it is crucial to systematically review and examine the complex elements in AI-STEM from a system perspective.
During the past decade, the emerging field of AIEd has gained great attention (Chen et al., 2020 ; Holmes et al., 2019 ; Hwang et al., 2020 ; Ouyang et al., 2022 ). But existing literature review of AIEd has mainly focused on the trends, applications, and effects of AIEd from a technological perspective (Chen et al., 2020 ; Tang et al., 2021 ; Zawacki-Richter et al., 2019 ). Specifically, we located 18 literature review articles of AIEd published from 2011 to 2021 (see Fig. 1 ). These AIEd reviews focused on different educational levels, fields, and contexts, including higher education (Zawacki-Richter et al., 2019 ), e-learning (Tang et al., 2021 ), mathematics education (Hwang & Tu, 2021 ), language education (Liang et al., 2021 ), medical education (Khandelwal et al., 2019 ; Lee et al., 2021 ), programming education (Le et al., 2013 ), and special education (Drigas & Ioannidou, 2012 ). For example, Zawacki-Richter et. al. ( 2019 ) reviewed AIEd in the higher education context and four AI technical applications were classified, namely intelligent tutoring systems, adaptive systems and personalization, profiling and prediction, and assessment and evaluation. Liang et. al. ( 2021 ) focused on the application of AI in language education and investigated the roles and research foci (e.g., research methods, research sample groups) of AI techniques in language education. Drigas and Ioannidou ( 2012 ) explored AIEd in special education and summarized AI applications based on the student’s disorders, including reading, writing and spelling difficulties, dyslexia, autistic spectrum disorder, etc.
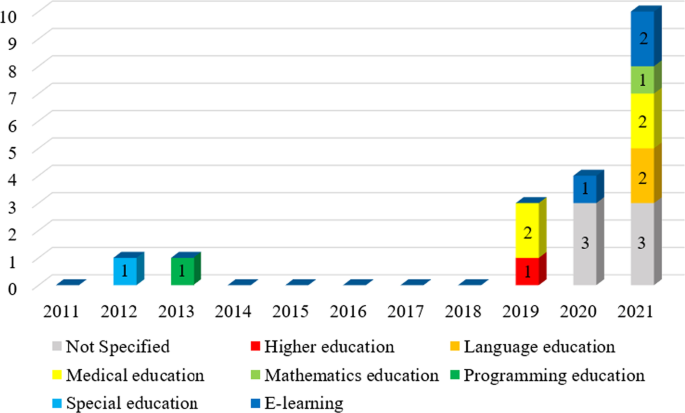
Existing literature review of AIEd articles, ranging from 2011 to 2021
Although various reviews were conducted to understand the field of AIEd, few of them focused on STEM education. Among these 18 literature review articles, we only located two works exploring the application of AI in STEM education. Le et. al. ( 2013 ) reviewed the AI-supported tutoring approaches in computer programming education and found that AI techniques were mainly applied to support feedback-based programming tutoring during the student’s individual learning. Hwang and Tu ( 2021 ) conducted a bibliometric mapping analysis to systematically review the roles of AI in mathematics education. The results clarified the role of AI in mathematics education into three main types, including intelligent tutoring systems, profiling and prediction, and adaptive systems and personalization. Although some review examined AI in computer science and mathematics education, there is a lack of literature review to investigate the application of AI in general STEM education context. More importantly, due to the complexity of AI-STEM, it is essential to systematically review multiple elements in AI-STEM as well as the effects of AI in the STEM education system.
To fill this gap, this systematic review aims to gain a comprehensive understanding of the integration of AI technologies in the STEM education contexts. Specifically, this review examined and summarized the applications and categories of AI element in the AI-STEM system, the characteristics of other system elements in AI-STEM except AI, the distribution of AI in these elements, and the effects of AI in STEM education. Three research questions (RQs) were proposed:
RQ1: What are the categories of the AI element in the AI-STEM system?
Rq2: what are the characteristics of other system elements (i.e., information, subject, medium, environment element) as well as the distribution of ai in these elements, rq3: what are the effects of ai in stem education.
In order to map the state-of-art of the application of AI techniques in STEM education, we conducted a systematic review from 2011 to 2021, following the Preferred Reporting Items for Systematic Reviews and Meta-Analyses (PRISMA) principles (Moher et al., 2009 ).
Database search
To locate the empirical studies of AI application in STEM education, the following major publisher databases were selected: Web of Science, Science Direct, Scopus, IEEE, EBSCO, ACM, Taylor & Francis, and Wiley (Guan et al., 2020 ). Filters were used to the empirical research and peer-reviewed articles in the field of education and educational research from January 2011 to December 2021. After the preliminary screening of articles, snowballing was conducted (Wohlin, 2014 ) to find the articles that were not extracted using the search strings.
Identification of search terms
Based on the specific requirements of bibliographic databases, we proposed the searching strategies. In terms of the research questions, three types of keywords were used as the search terms. First, keywords related to AIEd and specific AI applications were added (i.e., “artificial intelligence” OR “AI” OR “AIED” OR “machine learning” OR “intelligent tutoring system” OR “expert system” OR “recommended system” OR “recommendation system” OR “feedback system” OR “personalized learning” OR “adaptive learning” OR “prediction system” OR “student model” OR “learner model” OR “data mining” OR “learning analytics” OR “prediction model” OR “automated evaluation” OR “automated assessment” OR “robot” OR “virtual agent” OR “algorithm”). Second, keywords related to STEM were added (i.e., “STEM” OR “science” OR “technology” OR “math” OR “physics” OR “chemistry” OR “biology” OR “geography” OR “engineering” OR “programming” OR “lab”). Third, keywords related to education were added (i.e., “education” OR “learning” OR “course” OR “class” OR “teaching”).
Searching criteria
The search criteria were designed to locate the articles that focused on the applications of AI in STEM education. According to the research objectives, inclusion and exclusion criteria were adopted (see Table 1 ).
The screening process
The screening process involved the following procedures: (1) removing the duplicated articles; (2) reading the titles and abstracts and removing the articles according to the inclusion and exclusion criteria; (3) reading the full texts and removing the articles according to the inclusion and exclusion criteria; (4) using the snowballing to further locate the articles in Google Scholar, and (5) extracting data from the final filtered articles (see Fig. 2 ). All articles were imported into Mendeley software for screening.
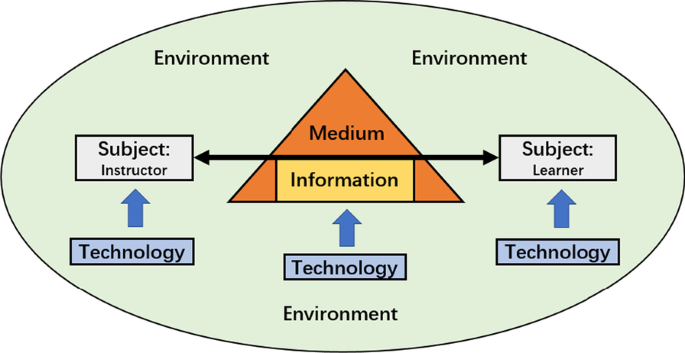
The selection flowchart used based on PRISMA (Moher et al., 2009 )
3373 articles were located as the result of the first round of searching. Among these records, 777 duplicates were removed and then 1879 records were excluded because they were not classified under Education & Educational research or journal article. By reviewing the titles and abstracts, the number of articles was reduced to 717 based on the criteria (see Table 1 ). The selected articles were examined by the first author to determine whether they were suitable for the purpose of this systematic review. The second author independently reviewed approximately 30% of the articles to confirm the reliability. The inter-rater agreement was 92%. Then, the full-text of articles were reviewed by the first author to verify that the articles met all the criteria for inclusion in the review. Finally, a total of 63 articles that met the criteria were identified for the systematic review.
Theoretical framework and analysis procedure
General system theory (GST) is a theoretical framework, arguing that the world is composed of different organic systems, which contain dynamically interacting elements and mutual relationships between them (Rapoport, 1986 ; Von Bertalanffy, 1950 ). The main principle of GST is that a system is not simply equal to the sum of its elements, but greater than the sum of its parts (Drack & Pouvreau, 2015 ; Von Bertalanffy, 1968 ). To deeply understand the complex nature and general rules of systems, GST highlights the system’s holistic principle to identify the internal elements, functional relationships of them, as well as the external influences upon a system (Crawford, 1974 ). The theoretical framework of GST has been widely applied in various fields to analyze different types of systems, such as physical, biological, social and educational systems (Drack & Pouvreau, 2015 ; Kitto, 2014 ). For example, Chen and Stroup ( 1993 ) suggested applying GST as an underpinned theoretical framework to guide the reform of science education and highlighted the integration of science curriculum to avoid the compartmentalized learning of physics, biology, and chemistry. Following this philosophy, we argue that GST can provide a new, holistic perspective to understand the integration of AI technologies and STEM education.
From the perspective of GST, AI-STEM can be viewed as an organic system, which mainly contains five basic elements, namely subject , information , medium , environment , and technology (Von Bertalanffy, 1968 ) (see Fig. 3 ). First, subject is defined as people in an educational system and different subjects of people (e.g., instructor, student) can take agency to interact with each other constantly and adaptively. Second, information refers to knowledge spread and constructed between subjects in an educational system, such as learning contents, course materials, knowledge artifacts, etc. Third, medium is the way or carrier to convey information and connect subjects in the system. Fourth, environment serves as an underlying context in an educational system, which influences the function of the whole educational system. Fifth, technology (e.g., AI techniques) is usually appeared as an external element to impact the functions of the educational system. Grounded upon GST, the integration of AI, as an external technology element, in an educational system (such as STEM) is a complex process, that has influences on other system elements (i.e., subject, information, medium, environment) and on the relationships between them. In summary, the framework of GST (see Fig. 3 ) highlights the multiple elements as well as their mutual relationships in AI-STEM system, which provides us a holistic view for applying AI technologies in STEM education.
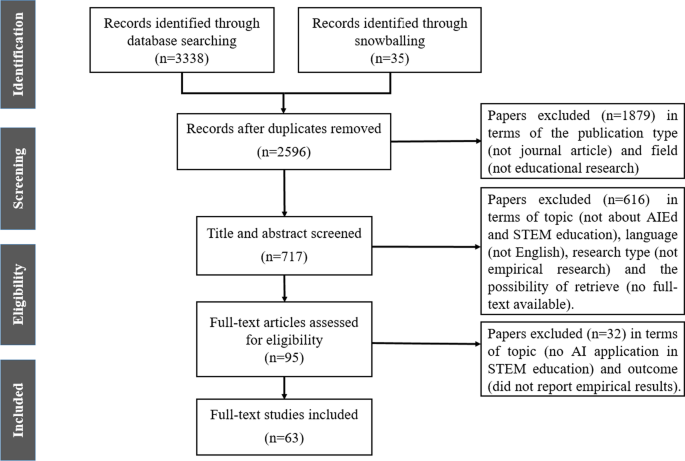
The integration of technology in an educational system from the GST perspective
We used content analysis method (Cohen et al., 2005 ; Zupic & Čater, 2015 ) to classify 63 AI-STEM articles in order to answer the research questions. Based on GST, a coding scheme of educational system elements was developed to systematically examine AI-STEM articles (see Table 2 ). This coding scheme included the subject of instructor (including instructor involvement and instructor strategy), the subject of learner (including educational level, sample size, and learning outcome), information (i.e., learning content), medium (i.e., educational medium), environment (i.e., educational context), and technology (i.e., AI technique).
63 articles were coded by two raters. The same article can be coded more than one code in one dimension. First, 20% of articles were coded by two coders independently in order to calculate coding reliability. Krippendorff’s ( 2004 ) alpha reliability was 0.91 among two raters at this phase. The remaining articles were coded independently by two raters after the reliability was ensured. Consensus was reached by two raters on conflicting coding results. We provided details and examples below to demonstrate how the coding results represented the review data (Graneheim & Lundman, 2004 ).
To answer the research questions, the results section presents the following three main topics: (1) the categories of the AI element in the AI-STEM system; (2) the characteristics of other system elements (i.e., information, subject, medium, and environment) as well as the distribution of AI in these elements, and (3) the effects and findings of the application of AI in STEM education.
Figure 4 demonstrates the trends of empirical studies by year. According to the distribution, the number of publication generally increased along the years. In addition, a majority of reviewed articles ( N = 42) were published in the last 4 years from 2018 to 2021. Only 9 of 63 reviewed articles were published in the first 4 years from 2011 to 2014.
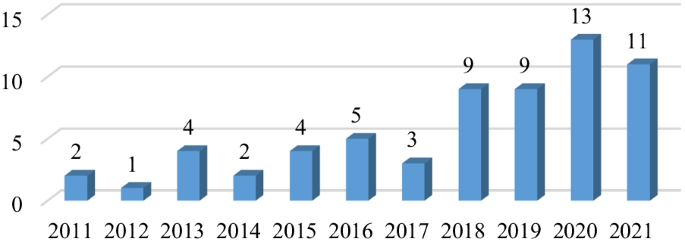
Distribution of the articles by year ( N = 63)
Regarding the element of AI technology in AI-STEM, six types of AI applications were identified, namely learning prediction ( N = 18, percentage = 29%), intelligent tutoring system ( N = 16, percentage = 25%), student behavior detection ( N = 13, percentage = 21%), automation ( N = 8, percentage = 13%), educational robots ( N = 6, percentage = 9%), and others ( N = 2, percentage = 3%) (see Fig. 5 and Table 3 ).
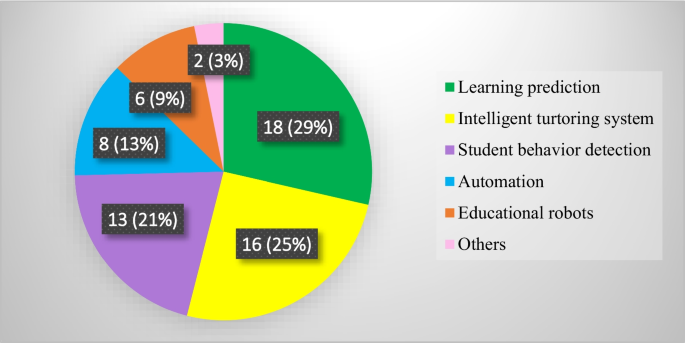
The application categories of AI techniques in STEM education ( N = 63)
Learning prediction
The first category of the AI applications in STEM education was learning prediction , illustrating should be something like systems which predict student learning performance or status in advance through AI algorithms and modeling approaches (Agrawal & Mavani, 2015 ; Lee et al., 2017 ). 18 of 63 reviewed articles (29%) focused on learning prediction in STEM education (see Fig. 5 ). Two sub-categories were summarized under learning prediction: learning performance prediction ( N = 14) and at-risk student prediction ( N = 4) (see Table 3 ). First, in the sub-category of learning performance prediction, AI algorithms and modeling techniques were employed in STEM education to help instructors adjust the instructional processes by predicting students’ learning performance (Deo et al., 2020 ; Hellings & Haelermans, 2020 ). For example, Buenaño-Fernández et. al. ( 2019 ) applied educational data mining and machine learning technique (i.e., decision tree) in computer engineering courses to predict students’ final performance based on their historical grades. Zabriskie et. al. ( 2019 ) utilized the random forest model and logistic regression model to predict the physics course outcomes. Another sub-category was predicting at-risk students and dropout factors in STEM education to help instructors intervene in student learning (Vyas et al., 2021 ; Yang et al., 2020 ). For example, Lacave et. al. ( 2018 ) used Bayesian networks techniques to investigate dropout factors of computer science students in higher education. Yang et. al. ( 2020 ) utilized random forest classification to create and examine the prediction models of identifying at-risk students in introductory physics classes. In summary, AI algorithms had been used in STEM education to help instructors or researchers predict students’ final academic performances and learning risks.
Intelligent tutoring system
The second category of the AI applications in STEM education was the intelligent tutoring system (ITS), defined as an AI-enabled system that was designed to provide customized instruction or feedback to students and promote personalized, adaptive learning (Chen et al., 2020 ; Hooshyar et al., 2015 ; Murray, 2003 ). Among the 63 reviewed articles, 16 articles (25%) focused on the applications of ITSs in promoting instruction and learning in STEM education (see Fig. 4 ). Three sub-categories were identified: instructional content delivery ( N = 9), recommendation of personalized learning path ( N = 4), and resource recommendation ( N = 3) (see Table 3 ). The first sub-category was using ITSs to deliver instructional content in STEM education. For example, Myneni et. al. ( 2013 ) introduced an interactive and intelligent learning system in physics education, where a virtual agent delivered physics concepts to students and decision algorithms were utilized to determine the support level of the virtual agent. Hooshyar et. al. ( 2018 ) proposed a novel flowchart-based ITS based on Bayesian networks techniques, which imitated a human instructor to conduct one-to-one instruction with students. The second sub-category of ITS was recommendations of personalized learning path based on student’s profile in STEM education. For example, De-Marcos et. al. ( 2015 ) combined genetic algorithm and parliamentary optimization algorithm to create personalized courseware sequencing paths in online STEM learning. Saito and Watanobe ( 2020 ) proposed an approach of recommending learning paths that applied recurrent neural network and sequential prediction model to create students’ ability charts and learning paths in programming learning based on their submission history. The third sub-category of ITS was recommending learning resource according to student’s needs in STEM education. For example, Ledesma and García ( 2017 ) introduced an expert system as a support tool to tackle mathematical topics, by recommending appropriate mathematical problems in accordance with a student’s learning style. Lin and Chen ( 2020 ) proposed a deep learning recommendation based system in programming learning that recommended learning tasks, learning missions and materials according to students’ learning processes and levels. In summary, AI technologies were widely applied in ITSs to enhance personalized and adaptive learning in STEM education through providing one-to-one tutoring and recommending personalized learning paths and resources.
Student behavior detection
The third category of the AI applications in STEM education was student behavior detection , which referred to systems to exploit and track students’ learning behaviors, patterns, and characteristics with AI-enabled data mining and learning analytics in the instructional and learning processes (Chrysafiadi & Virvou, 2013 ; Ji & Han, 2019 ; Zheng et al., 2020 ). Among the 63 reviewed articles, 13 articles (21%) focused on the applications of AI techniques to detect student behaviors in STEM education (see Fig. 5 ). Two sub-categories were summarized under student behavior detection: student behavior analysis ( N = 8) and student behavior monitoring ( N = 5) (see Table 3 ). First, the sub-category of student behavior detection, was applied in STEM education to analyze and reveal students’ latent behaviors. For example, Hsiao et. al. ( 2020 ) collected students’ learning data from programming learning platform and examined their learning behaviors through hidden Markov model, and the results revealed the reviewing patterns and reflecting strategies of students in learning programming. Pereira et. al. ( 2020 ) used data mining techniques including k-means and association rule algorithm to understand students’ behavior in introductory programming, to help novice programmers promote their learning. Another sub-category of student behavior monitoring was applied to help instructors track students’ learning in STEM education. For example, Balakrishnan ( 2018 ) helped instructors to motivate engineering students’ learning through monitoring their learning behaviors such as preferred learning materials and self-directed learning performance. Yannier et. al. ( 2020 ) introduced a mixed-reality AI system supported with computer vision algorithms to track children’s active learning behaviors in science education. In summary, student behavior detection had great potential to aid instructors and researchers to analyze, understand, and monitor students’ behaviors in STEM education.
The fourth category of the AI applications in STEM education was automation , which utilized AI technologies to automatically assess students’ performances and generate questions or tasks for instructors (Aldabe & Maritxalar, 2014 ; Wang et al., 2011 ; Zampirolli et al., 2021 ). Among 63 reviewed articles, 8 articles (13%) focused on the AI-supported automated techniques in STEM education (see Fig. 5 ). Two sub-categories were summarized under automation: automated assessment ( N = 7) and automated questions generation ( N = 1) (see Table 3 ). The first sub-category of automated assessment provided instructors and students with convenient assistance in STEM education. For example, Wang et. al. ( 2011 ) developed an automated assessment system, AutoLEP, to help novice programmers gain programming skills by providing syntactic and structural checking and immediate feedback automatically. García-Gorrostieta et. al. ( 2018 ) introduced a system for automatic argument assessment of computer engineering students’ final reports, to help them improve the abilities of statement and justification in science argumentation. Another sub-category of automated questions generation had potential to reduce instructors’ instructional burdens in STEM education. For example, Aldabe and Maritxalar ( 2014 ) proposed an approach to help instructors automatically create multiple-choice tests in science courses through the use of corpora and natural language processing techniques. In summary, AI techniques were used in STEM education to aid instructors and students through automatically generating questions and assessing academic performances.
Educational robots
The fifth category of the AI applications in STEM education was educational robots , which was the adoption of robots in STEM education to facilitate students’ learning experience as well as allow them to acquire knowledge in interactive ways (Atman Uslu et al., 2022 ; Cao et al., 2021 ; Yang & Zhang, 2019 ). It is worth noting that robots are applications that contain various techniques (e.g., mechanical manufacturing, electronic sensors, AI); therefore, considering the research topic, only AI-supported robots were included in this review. Among the 63 reviewed articles, 6 articles (9%) focused on the application of educational robots in STEM education (see Fig. 5 ). Two sub-categories were identified under educational robots: programming robots ( N = 3) and social robots ( N = 3) (see Table 3 ). The first sub-category, programming robots, were specifically designed as learning tools that engaged students to design and operate them with programming languages (Atman Uslu et al., 2022 ). For example, Rodríguez Corral et. al. ( 2016 ) applied a specific ball-shaped robot with sensing, wireless communication and output capabilities in computer courses to teach students object-oriented programming languages. Cao et. al. ( 2021 ) introduced an artificial intelligence robot called LEGO MINDSTORMS EV3, to implement instructional tasks in information technology courses to promote students’ innovation and operational ability. Another sub-category of social robots was a kind of intelligent humanoid robots, which could serve as tutors, tutees or learning companions to students and allow students to interact with them orally and physically (Belpaeme et al., 2018 ; Xu & Ouyang, 2022 ). For example, Verner et. al. ( 2020 ) employed RoboThespian, a life-size humanoid robot, as a tutor to convey science knowledge and concepts to elementary school students. In summary, AI-based educational robots were used in STEM education as instructional tools or educational subjects (e.g., tutor, tutee, companion) to convey knowledge, promote students’ operational skills, and enhance their learning experience.
Among 63 reviewed articles, 2 articles (3%) focused on other applications of AI techniques in STEM education, including AI textbook and group formation. Tehlan et. al. ( 2020 ) utilized a genetic algorithm‐based approach to form student groups in collaborative learning based on their skills and personality traits in a programming course. Koć-Januchta et. al. ( 2020 ) introduced AI-enriched textbook in biology course to improve students’ engagement by encouraging them to ask questions and receive suggested questions.
To further understand how AI techniques have been integrated in STEM education, we examined the other system elements, including information, subject (i.e., instructor, student), medium, and environment in AI-STEM research. In addition, we explored the distribution of AI categories in these elements, to reveal the relationships between AI techniques and these elements.
Information in AI-STEM research
Information (referred to learning content in this study) was described as the subject knowledge and learning contents conveyed in AI-STEM system. In the reviewed 63 studies, all of them mentioned learning content in STEM education, including science, technology, engineering, mathematics, and cross-disciplinary (i.e., more than one discipline) (see Table 4 ). Among 63 articles, 24 studies focused on technology, followed by articles that focused on science ( N = 22) and engineering ( N = 7). Mathematics ( N = 3) attracted the least attention. In addition, 7 studies contained interdisciplinary subjects, such as computer engineering (Buenaño-Fernández et al., 2019 ; Tehlan et al., 2020 ), engineering mathematics (Deo et al., 2020 ), and integrated STEM education (Suh et al., 2019 ; Wang, 2016 ).
Figure 6 shows the frequency of AI application categories in different learning contents. Among all the AI categories, student behavior detection was most frequently applied in the technology domain ( N = 8), followed by learning prediction in science ( N = 6), and learning prediction in engineering ( N = 5) (see Fig. 6 ).
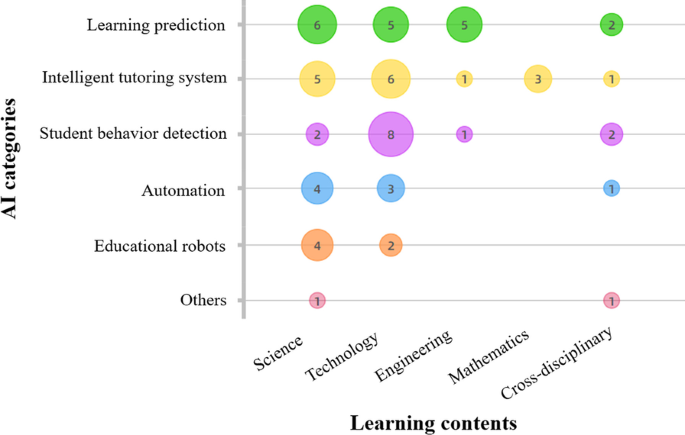
AI categories under learning content ( N = 63)
Instructor in AI-STEM research
Instructor , as a component of subject element in AI-STEM system, played a critical role in conducting instruction, conveying knowledge, and utilizing technologies. In the reviewed 63 studies, 50 of them mentioned the instructor involvement and 50 of them mentioned the instructional strategies, including traditional lecture, problem-based learning, project-based learning, game-based learning, self-learning, and collaborative learning (see Table 5 ). Regarding the instructor involvement, a majority of instructors would engage in the instructional and learning processes to support students ( N = 42), while some studies were conducted without instructors’ involvement and support ( N = 8). Additionally, among 50 articles, the traditional lecturing strategy was most frequently used by instructor ( N = 27), followed by problem-based learning ( N = 10). Also, some studies were carried out through project-based learning ( N = 5), self-learning ( N = 5), game-based learning ( N = 4), and collaborative learning ( N = 4).
All AI application categories were mainly applied with the instructor’s support, in which the most frequently used AI category were ITS ( N = 11) and learning prediction ( N = 11) (see Fig. 7 a). In addition, automation was only applied in lecture ( N = 8). Learning prediction was most frequently applied in lecture ( N = 8) and educational robots were most frequently applied in problem-based learning ( N = 4). Compared to other AI technologies, ITS and student behavior detection were integrated with more types of instructional strategies (see Fig. 7 b).
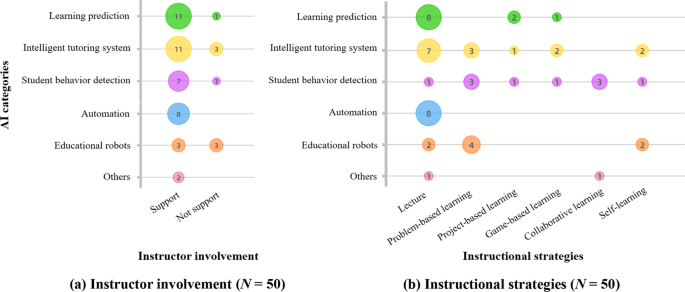
AI categories under instructor involvement and instructional strategies
Learner in AI-STEM research
Learner , as another component of subject element, could take agency to actively participate in the learning process as to influence the AI-STEM system. In the reviewed 63 studies, 59 of them mentioned the educational levels of learners, from kindergarten to higher education, and 55 of them mentioned sample sizes (see Table 6 ). Among all the educational levels, 43 focused on higher education ( N = 43), followed by elementary school ( N = 7), high school ( N = 5), and middle school ( N = 4). Only one study was conducted in kindergarten. In addition, the number of AI-STEM studies with the medium scale of learners ( N = 24) and the large scale of learners ( N = 21) were larger than the small-scale study ( N = 10).
AI application categories except educational robots were frequently applied in higher education (learning prediction: N = 13, ITS: N = 12, student behavior detection: N = 8, automation: N = 7). The educational robots were frequently applied in elementary school ( N = 3) (see Fig. 8 a). Moreover, regarding the sample size, learning prediction was most frequently used with a large scale ( N = 11), followed by ITS with a medium scale ( N = 9), and student behavior detection with a medium scale ( N = 7). Additionally, the categories of educational robots and others were not applied in large scale; the category of student behavior detection was not applied in small scale (see Fig. 8 b).
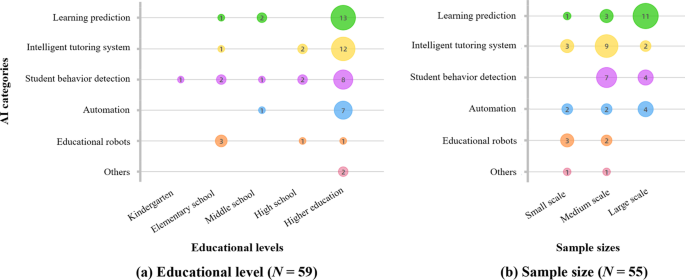
AI categories under educational levels and sample sizes
Medium in AI-STEM research
Medium (referred to educational medium in this study) was viewed as the way to convey information and connect subjects AI-STEM system. In the reviewed 63 studies, 50 of them mentioned the educational medium, including paper resource, entity resource (i.e., the material object in reality), computer system resource, web open resource, mobile phone resource and E-book resource (see Table 7 ). Among all educational mediums, computer system was the most frequently used in AI-STEM studies ( N = 28), followed by entity resource ( N = 10) and web open resource ( N = 9). Additionally, mobile phone resource ( N = 3), traditional paper resource ( N = 1), and E-book resource ( N = 1) was the infrequent medium to convey knowledge.
Among all the AI categories, ITS was most frequently used through computer system resource ( N = 15), followed by educational robots through entity resource ( N = 6), automation through computer system resource ( N = 5), and learning prediction through web open resource (see Fig. 9 ).
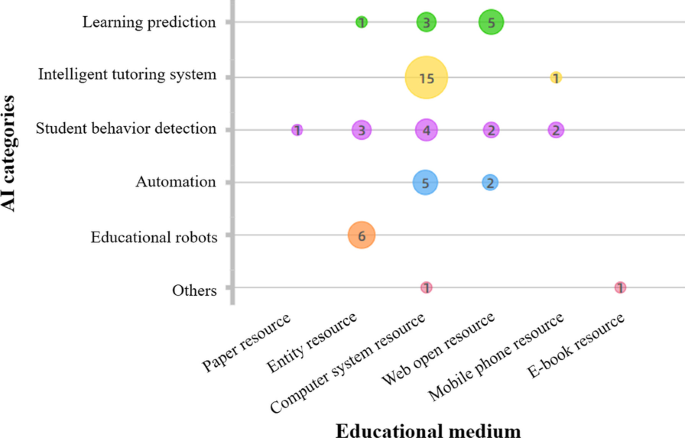
AI categories under educational medium ( N = 50)
Environment in AI-STEM research
Environment (referred to educational context in this study) served as an underlying context to influence the whole AI-STEM system. In the reviewed 63 studies, 51 of them mentioned the educational environment, including face-to-face environment, experimental learning environment, informal learning environment, web-based environment and augmented/virtual reality (see Table 8 ). Among the 51 studies, 33 studies were implemented in face-to-face environment, followed by web-based environment ( N = 11) and experimental environment ( N = 6). Two studies conducted in informal learning environment (McLurkin et al., 2013 ; Verner et al., 2020 ) and only one study conducted in augmented reality (Lin & Chen, 2020 ).
All categories of AI techniques were commonly applied in face-to-face environment, in which the most frequently used AI technology category was learning prediction ( N = 10), followed by automation ( N = 7), ITS ( N = 6), and educational robots ( N = 5). Moreover, compared to other AI categories, ITS was the most frequently used technique in the web-based environment ( N = 7) (see Fig. 10 ).
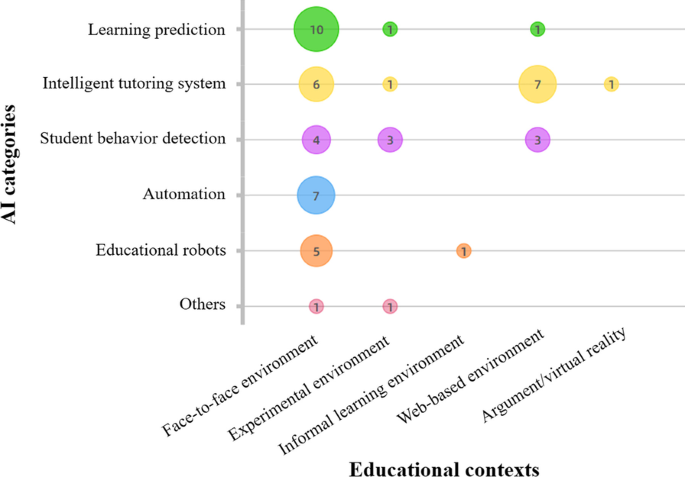
AI categories under educational contexts ( N = 51)
This review summarized the educational and technological effects of AI applications in AI-STEM research.
Educational effects and findings
From the educational perspective, 42 of the 63 reviewed articles reported the educational effects and findings when applying AI techniques in STEM education. Specifically, 30 out of the 42 articles reported the instruction and learning effects (e.g., learning performance, affective perception, higher-order thinking) of the application of AI techniques in STEM education. 12 articles out of 42 reported students’ learning behaviors and patterns by using AI-enabled data mining and learning analytics techniques.
The effect of learning performance
Among all the reviewed articles, 22 studies revealed the educational effects of AI technologies on students’ learning performance. Most of them showed significantly positive influence of AI techniques on the improvement of students’ learning performances ( N = 20). For example, Wu et. al. ( 2013 ) investigated the effect of a context-aware ubiquitous learning system in a geosciences course and the results showed that context-aware ubiquitous learning system had significantly positive effects on the learning achievements of students. Thai et. al. ( 2021 ) conducted a cluster randomized study to examine the effect of My Math Academy, a digital game-based learning environment that provided personalized content on kindergarten students; the results revealed the significant improvement of learning gains, especially for the moderate-level students. Tehlan et. al. ( 2020 ) used a quasi-experiment approach to examine the effects of genetic algorithm-supported pair programming in a programming course; the results found that the students’ learning performances were significantly higher in pair programming than individual programming. Two articles reported insignificant results of the learning performance effects. Koć-Januchta et. al. ( 2020 ) used a quasi-experiment to compare the effect of AI-enabled E-book and common E-book in students’ biology learning, and the results showed that there was no significant difference of students’ learning gains between these two types of books. Also, Hellings and Haelermans ( 2020 ) conducted a randomized experiment to examine the effect of a learning analytics dashboard with predictive function in a computer programming course, but no significant improvement was found on student performance in the final exam.
The effect of affective perception
Among all the reviewed articles, a majority of studies revealed the educational effects of AI technologies on students’ affective perception, such as attitude, interest, and motivation ( N = 17). On the one hand, students showed satisfaction and positive attitude towards the integration of AI technologies and STEM education. For example, Azcona et. al. ( 2019 ) used a questionnaire to find students’ positive feedbacks and attitudes towards the application of learning analytics in computer programming classes to detect and warn learning risks. Gavrilović et. al. ( 2018 ) evaluated student’s satisfaction of an AI-supported adaptive learning system in Java programming learning through the survey approach; the results revealed the positive feedbacks of students. On the other hand, the application of AI technologies also arouses students’ interests and motivation in STEM learning. For example, Balakrishnan ( 2018 ) used a mixed-method approach (i.e., questionnaire and interview) to examine the impact of a computer-based personalized learning environment (PLE) on engineering students’ motivation, and the results revealed the potential of PLE to engage students in learning with a strong sense of interest and motivation. Verner et. al. ( 2020 ) investigated students’ perceptions and attitudes towards an interactive robot tutor in science classes and found that the human–robot interaction fostered students’ active learning, maintain their attention and interest in the learning processes.
The effect of higher-order thinking
Among all the reviewed articles, some studies revealed the educational effects of AI technologies on students’ higher-order thinking ( N = 7), such as problem-solving ability, computational thinking, and self-regulated learning skills. For example, Hooshyar et. al. ( 2015 ) employed a quasi-experimental design to examine the impact of a flowchart-based intelligent tutoring system (FITS) on students’ programming learning and found better improvement of problem-solving abilities in the FITS group than the control group. Lin and Chen ( 2020 ) found that students who used a deep learning-based AR system performed significantly better in computational thinking than those using an AR system without deep learning recommendation. Jones and Castellano ( 2018 ) utilized adaptive robotic tutors to promote students’ self-regulated learning skills and found that when a robotic tutor provided scaffoldings adaptively, more self-regulated learning behaviors were observed from students over the control condition without scaffoldings. García-Gorrostieta et. al. ( 2018 ) used experimental evaluation to test the effect of the automatic argument assessment on students’ computer engineering writing, and the results revealed that the argument assessment system helped students improve argumentation ability in their writing.
The effect of student learning pattern and behavior
Among 42 reviewed articles that mentioned educational effects and findings, 12 articles revealed students’ learning patterns and behaviors in STEM education by using AI-enabled data mining and learning analytics approaches. For example, Sapounidis et. al. ( 2019 ) detected 48 children’s preference profiles on tangible and graphical programming through latent class modeling; results found that the graphical programming was preferred by a majority of children, especially children in younger ages. Pereira et. al. ( 2020 ) used learning analytics (i.e., k-means, association rule algorithms) in the Amazonas to understand students’ behavior in introductory programming courses and found high heterogeneity among them. Three clusters of novice programmers were detected to explain how student behaviors during programming influenced the learning outcomes. Wang ( 2016 ) utilized data mining and learning analytics techniques (i.e., association rule, decision tree) to investigate college students’ course-taking patterns in STEM learning; the results found that the most viable course-taking trajectories is taking mathematics courses after initial exposure to subject courses in STEM.
Technological effects and findings
From the technological perspective, 24 of the 63 reviewed articles reported the technological effect and findings (e.g., efficiency of technology, accuracy of algorithm) when applying AI techniques in STEM education. For example, Çınar et. al. ( 2020 ) utilized multiple machine learning algorithms, including Support Vector Machines (SVM), Gini, k-Nearest Neighbors (KNN), Breiman’s Bagging, Freund and Schapire’s Adaboost.M1 algorithms, to automatically grade open-ended physics questions; the results reported that AdaBoost.M1 had the best performance with the highest accuracy of prediction models among all machine learning algorithms. Nehm et. al. ( 2012 ) used a corpus of biology evolutionary explanations written by 565 undergraduates to test the efficacy of an automated assessment program, Summarization Integrated Development Environment (SIDE); the results showed that, compared to human expert scoring, SIDE had better performance when scoring models were built and tested at the individual item level, and the performance degraded when suites of items or entire instruments were used to build and test scoring models. Bertolini et. al. ( 2021 ) employed five machine learning methods to quantify predictive efficacy of predictive modeling in undergraduate students’ outcome in biology. Results found that individual machine learning methods, especially logistic regression achieved a poor prediction performance while ensemble machine learning methods, in particular the generalized linear model with elastic net (GLMNET), achieved the high accuracy. Deo et. al. ( 2020 ) adopted a computationally efficient AI model called extreme learning machines (ELM) to predict weighted score and the examination score in engineering mathematics courses; the results showed that ELM outperformed in prediction with respect to random forest and Volterra.
Discussion and implications
Addressing research questions.
Although AIEd has attracted wide attention in educational research and practice, few research works have investigated the applications of AI in STEM education context. To gain a comprehensive understanding of the integration of AI in STEM education, this study conducted a systematic review of AI-STEM empirical research from 2011 to 2021. Grounded upon GST, we examined the AI technologies and applications in STEM education, the characteristics of other system elements (i.e., information, subject, medium, environment), the distribution of AI in these elements, and the effects of AI applications in STEM education. To answer the first question, we found a gradually increasing trend of AI applications in STEM education in the past decade. Furthermore, six categories of AI applications were located, namely learning prediction, ITS, student behavior detection, automation, educational robots, and others (i.e., AI text book, group formation). Regarding the characteristics of elements and the distribution of AI in these elements, first, we found all categories of AI techniques, especially student behavior detection, ITS, and learning prediction, were frequently applied in the learning contents of science and technology. Second, instructors usually involved in STEM education to support students and they used lecturing strategy the most frequently, followed by problem-based learning. Automation was only applied in the lecturing instruction mode and educational robots were most frequently applied in the problem-based learning mode. Third, a majority of AI techniques (except educational robots) were applied in higher education with medium and large scale of learners. The most frequently used AI in higher education were learning prediction and ITSs. Fourth, computer system resource was the most frequently used medium to convey knowledge, particularly when it was applied in ITSs and automation, while paper, mobile phone, and E-book resources were seldom used in AI-STEM research. Fifth, the face-to-face environment was mainly utilized to support all categories of AI applications, and web-based environment was most frequently used supported with ITSs.
Regarding the third question, this review summarized the educational and technological effects and findings of AI applications in STEM. From the educational perspective, the results showed that most of the AI applications had positive effects on students’ academic performance. However, insignificant improvements of learning outcomes were also found in two empirical studies (Hellings & Haelermans, 2020 ; Koć-Januchta et al., 2020 ). Moreover, most students held positive attitudes towards the use of AI technology in STEM education, and AI technologies aroused their interest and motivation as well. In other words, the AI applications are beneficial for fostering student’s active learning in STEM education. Moreover, the applications of AI techniques also contributed to the development of students’ higher-order thinking, e.g., computational thinking, problem-solving ability. In addition, AI techniques have great potential to assist instructors by detecting students’ learning patterns and behaviors in STEM education. From the technological perspective, the reviewed articles mainly reported a good efficiency and algorithm accuracy when applying AI in STEM education. Specifically, AI algorithms, especially ensemble machine learning methods, performed well in learning prediction, automation, and personalized recommendation. Overall, underpinned by the GST framework, this review presented an overview of recent trends of the field of AI-STEM, which guided the following educational, technological, and theoretical implications.
Educational implications
The emergence of AI indirectly influences the subject elements (e.g., instructor, learner) in STEM education, which in turn would eventually influence the educational practices and effects. First, AI has potential to transform the instructor–student relationships in STEM education from the instructor-directed to student-centered learning (Cviko et al., 2014 ). When AI is applied in STEM education, the role of instructor is expected to shift from a leader to a collaborator or a facilitator under the AI-empowered, learner-as-leader paradigm (Ouyang & Jiao, 2021 ). However, this review found that the instructor-centered lecturing mode was the most frequently used instructional strategy in AI-STEM studies, while other student-centered instructional strategies (e.g., the project-based learning, collaborative learning, game-based learning) appeared infrequently. One of the reasons centers on the complexity of integrating technology and pedagogy in STEM education (Castañeda & Selwyn, 2018 ; Jiao et al., 2022 ; Loveless, 2011 ). For example, ITS and automation techniques are usually designed based on behaviorism (Skinner, 1953 ) to support instructor’s knowledge delivery and exam evaluation, which may be challenging for instructors to use when integrating it in the student-centered instructional strategies. Recent research has started to balance pedagogical design and technological application in educational practices in order to achieve the goal of AI–instructor collaboration and student-centered learning when AI is integrated (Baker & Smith, 2019 ; Holmes et al., 2019 ; Roll & Wylie, 2016 ). Furthermore, another critical question is: would AI replace instructor responsibilities and roles in STEM education (Segal, 2019 )? In this review, we found that the role of instructor was still irreplaceable, because the instructor’s involvement existed in most of the AI-STEM research. Even though AI can free instructors from redundant tasks in STEM education, it still lacks the human ability to convey social emotion, solve critical problems, and implement creative activities (Collinson, 1996 ; Gary, 2019 ; Muhisn et al., 2019 ). Therefore, although AI techniques can bring opportunities to develop STEM education (Hwang et al., 2020 ), we cannot overstate the role of technology and overlook the essential role of instructor (Selwyn, 2016 ). Overall, instructors, as important subjects in the educational system, need to take agency to promote the pedagogical designs and strategies when applying AI technologies, in order to achieve a high quality of AI-STEM education (Cantú-Ortiz et al., 2020 ).
Technological implications
Although AI has the potential to enhance the instruction and learning in STEM education (Chen et al., 2020 ; Holmes et al., 2019 ), the development of AI-STEM requires a better fit between AI technologies and other system elements in STEM education. First, regarding the relationships between AI and information element, the results showed that most of the AI applications were used in science and technology learning contents, and educational robots and automation were not applied in engineering and mathematics learning contents. Since STEM education contains interdisciplinary knowledge and learning contents from different subjects, AI is usually restricted in specific learning contents or courses (Douce et al., 2005 ). Therefore, one of the future directions is to expand the commonality and accessibility of AI techniques in different STEM subjects and courses. Second, the range of AI applications was mainly located in higher education, while few of AI techniques were applied in other educational levels, especially in kindergarten. To some extent, due to the complex function and feedback mechanisms, most of the AI techniques (e.g., ITS, learning prediction) might be appropriate for adult learners. Hence, some interactive AI techniques, e.g., social robots, AI-enabled games, can be designed and developed to support young children’s STEM learning (Belpaeme et al., 2018 ; Zapata-Cáceres & Martin-Barroso, 2021 ). Therefore, the ease of use is also one of the important considerations in future development of AI technologies (Law, 2019 ; Xu & Ouyang, 2022 ). Third, most of the AI-STEM research was conducted through the traditional mediums (e.g., computer system resource) and contexts (e.g., face-to-face learning environment). A future direction is to create AI-empowered STEM learning environment through combining the advanced educational mediums (e.g., E-book) and contexts (AR/VR), in order to better represent and convey knowledge (Mystakidis et al., 2021 ).
Theoretical implications
Due to the complexity of AI-STEM system, this research used a theoretical framework based on GST to examine the multiple elements (i.e., AI technology, information, subject, medium, environment) in AI-STEM research. Compared to previous AIEd reviews that mainly focused on the technological perspective, GST provides a holistic view for us to consider the complex human, pedagogical, environment factors when applying AI in STEM education (Kitto, 2014 ; Von Bertalanffy, 1968 ). For example, we found that sometimes instructors did not engage in STEM education to support students, especially when applying ITSs and educational robots. It might reveal a new trend that some AI technologies (e.g., social robot, virtual agent) might have the potential to replace the original role of instructor and work as a new subject to individually convey knowledge (Xu & Ouyang, 2022 ). Additionally, the results showed the different characteristics of learner’s sample size when applying different AI techniques. Learning prediction was more likely to applied with a large scale of students, and educational robots were inclined to be applied with a small scale of students. The features of AI technologies might explain this phenomenon. For example, a data training process is necessary before learning prediction, which requires the support of algorithmic modeling techniques and large data sets (Agrawal & Mavani, 2015 ; Lee et al., 2017 ), while educational robots, as human–machine interaction technologies, seem more suitable and practicable for small-scale STEM learning (Atman Uslu et al., 2022 ; Belpaeme et al., 2018 ). Overall, the current study utilized the GST framework to examine the multiple elements in the complex AI-STEM system; it is suggested that different stakeholders, e.g., educators, technical developers, and researchers, can adopt the GST framework as a guide to comprehensively consider the complex elements when applying AI techniques in STEM education (Kitto, 2014 ; Von Bertalanffy, 1950 ).
Conclusion, limitation, and future direction
The application of AI technology in STEM education is an emerging trend, which is confronted with the challenge of integrating diverse AI techniques in the complex STEM educational system. Grounded upon a GST framework, this research reviewed the empirical AI-STEM studies from 2011 to 2021. Specifically, this systematic review examined (1) the categories of the AI element in the AI-STEM system; (2) the characteristics of other system elements (i.e., information, subject, medium, and environment) as well as the distribution of AI in these elements, and (3) the effects of AI in STEM education. Based on the results, the current work proposed educational, technological, and theoretical implications for future AI-STEM research, to better aid the educators, researchers and technical developers to integrate the AI techniques and STEM education.
There are three limitations in this systematic review, which lead to future research directions. First, although we searched the best-known scholar databases with the keywords relevant to AI-STEM, some biases might exist in the searching and screening process. Since AI-STEM is a highly technology-dependent field, some studies might only highlight the technology rather than the education context. Therefore, future studies can adjust the searching criteria to solve these problems. Second, from a system perspective, we used a GST framework to examine the multiple elements in the complex AI-STEM system, but we did not investigate the mutual relationships between elements. Therefore, the complex relationships between different elements (e.g., instructor–learner, learner–learner relationship) in AI-STEM system need to be further explored in order to gain a deep understanding of the application of AI in STEM education (e.g., Xu & Ouyang, 2022 ). Third, the current study only implemented a systematic review, a meta-analysis could be conducted in the future to report the effect sizes of recent empirical studies to gain a deeper understanding of the effects of the AI-STEM integration in an educational system. Overall, the potential of AI technology for enhancing STEM education is fertile ground to be further explored together with studies aimed at investigating the integration of technology and educational system.
Availability of data and materials
The data are available upon request from the corresponding author.
*Reviewed articles ( N = 63)
Agrawal, H., & Mavani, H. (2015). Student performance prediction using machine learning. International Journal of Engineering Research and Technology, 4 (03), 111–113. https://doi.org/10.17577/IJERTV4IS030127
Article Google Scholar
Alabdulhadi, A., & Faisal, M. (2021). Systematic literature review of STEM self-study related ITSs. Education and Information Technologies, 26 , 1549–1588. https://doi.org/10.1007/s10639-020-10315-z
*Aldabe, I., & Maritxalar, M. (2014). Semantic similarity measures for the generation of science tests in Basque. IEEE Transactions on Learning Technologies, 7 (4), 375–387. https://doi.org/10.1109/TLT.2014.2355831
*Alemán, J. L. F. (2011). Automated assessment in a programming tools course. IEEE Transactions on Education, 54 (4), 576–581. https://doi.org/10.1109/TE.2010.2098442
Atman Uslu, N., Yavuz, G. Ö., & KoçakUsluel, Y. (2022). A systematic review study on educational robotics and robots. Interactive Learning Environments . https://doi.org/10.1080/10494820.2021.2023890
*Azcona, D., Hsiao, I. H., & Smeaton, A. F. (2019). Detecting students-at-risk in computer programming classes with learning analytics from students’ digital footprints. User Modeling and User-Adapted Interaction, 29 (4), 759–788. https://doi.org/10.1007/s11257-019-09234-7
Baker, T., & Smith, L. (2019). Educ-AI-tion rebooted? Exploring the future of artificial intelligence in schools and colleges. https://media.nesta.org.uk/documents/Future_of_AI_and_education_v5_WEB.Pdf
*Balakrishnan, B. (2018). Motivating engineering students learning via monitoring in personalized learning environment with tagging system. Computer Applications in Engineering Education, 26 (3), 700–710. https://doi.org/10.1002/cae.21924
Belpaeme, T., Kennedy, J., Ramachandran, A., Scassellati, B., & Tanaka, F. (2018). Social robots for education: A review. Science Robotics, 3 (21), eaat5954. https://doi.org/10.1126/scirobotics.aat5954
*Berland, M., Davis, D., & Smith, C. P. (2015). AMOEBA: Designing for collaboration in computer science classrooms through live learning analytics. International Journal of Computer-Supported Collaborative Learning, 10 (4), 425–447. https://doi.org/10.1007/s11412-015-9217-z
*Bertolini, R., Finch, S. J., & Nehm, R. H. (2021). Testing the impact of novel assessment sources and machine learning methods on predictive outcome modeling in undergraduate biology. Journal of Science Education and Technology, 30 (2), 193–209. https://doi.org/10.1007/s10956-020-09888-8
*Blikstein, P., Worsley, M., Piech, C., Sahami, M., Cooper, S., & Koller, D. (2014). Programming pluralism: Using learning analytics to detect patterns in the learning of computer programming. Journal of the Learning Sciences, 23 (4), 561–599. https://doi.org/10.1080/10508406.2014.954750
*Buenaño-Fernández, D., Gil, D., & Luján-Mora, S. (2019). Application of machine learning in predicting performance for computer engineering students: A case study. Sustainability, 11 (10), 1–18. https://doi.org/10.3390/su11102833
Bybee, R. W. (2013). The case for STEM education: Challenges and opportunities . NSTA Press.
Google Scholar
Byrne, D., & Callaghan, G. (2014). Complexity theory and the social sciences . Routledge.
Cantú-Ortiz, F. J., Galeano Sánchez, N., Garrido, L., Terashima-Marin, H., & Brena, R. F. (2020). An artificial intelligence educational strategy for the digital transformation. International Journal on Interactive Design and Manufacturing, 14 (4), 1195–1209. https://doi.org/10.1007/s12008-020-00702-8
*Cao, X., Li, Z., & Zhang, R. (2021). Analysis on academic benchmark design and teaching method improvement under artificial intelligence robot technology. International Journal of Emerging Technologies in Learning, 16 (5), 58–72. https://doi.org/10.3991/ijet.v16i05.20295
Castañeda, L., & Selwyn, N. (2018). More than tools? Making sense of the ongoing digitizations of higher education. International Journal of Educational Technology in Higher Education, 15 (1), 1–10. https://doi.org/10.1186/s41239-018-0109-y
Chen, D., & Stroup, W. (1993). General system theory: Toward a conceptual framework for science and technology education for all. Journal of Science Education and Technology, 2 (3), 447–459. https://doi.org/10.1007/BF00694427
Chen, L., Chen, P., & Lin, Z. (2020). Artificial intelligence in education: A review. IEEE Access, 8 , 75264–75278. https://doi.org/10.1109/ACCESS.2020.2988510
*Chrysafiadi, K., & Virvou, M. (2013). PeRSIVA: An empirical evaluation method of a student model of an intelligent e-learning environment for computer programming. Computers & Education, 68 , 322–333. https://doi.org/10.1016/j.compedu.2013.05.020
*Çınar, A., Ince, E., Gezer, M., & Yılmaz, Ö. (2020). Machine learning algorithm for grading open-ended physics questions in Turkish. Education and Information Technologies, 25 , 3821–3844. https://doi.org/10.1007/s10639-020-10128-0
Cohen, L., Manion, L., & Morrison, K. (2005). Research methods in education . Routledge Falmer.
Collinson, V. (1996). Reaching students: Teachers ways of knowing . Corwin Press.
Crawford, J. L. (1974). A systems approach model for the application of general systems theory principles to education [Doctoral dissertation, University of Houston]. The University of Houston Institutional Repository. https://hdl.handle.net/10657/10661
Cviko, A., McKenney, S., & Voogt, J. (2014). Teacher roles in designing technology-rich learning activities for early literacy: A cross-case analysis. Computers & Education, 72 , 68–79. https://doi.org/10.1016/j.compedu.2013.10.014
*De-Marcos, L., Garcia-Cabot, A., Garcia-Lopez, E., & Medina, J. A. (2015). Parliamentary optimization to build personalized learning paths: Case study in web engineering curriculum. International Journal of Engineering Education, 31 (4), 1092–1105.
*Deo, R. C., Yaseen, Z. M., Al-Ansari, N., Nguyen-Huy, T., Langlands, T. A. M., & Galligan, L. (2020). Modern artificial intelligence model development for undergraduate student performance prediction: An investigation on engineering mathematics courses. IEEE Access, 8 , 136697–136724. https://doi.org/10.1109/ACCESS.2020.3010938
Douce, C., Livingstone, D., & Orwell, J. (2005). Automatic test-based assessment of programming: A review. Journal on Educational Resources in Computing, 5 (3), 4-es. https://doi.org/10.1145/1163405.1163409
Drack, M., & Pouvreau, D. (2015). On the history of Ludwig von Bertalanffy’s “general systemology”, and on its relationship to cybernetics—Part III: Convergences and divergences. International Journal of General Systems, 44 (5), 523–571. https://doi.org/10.1080/03081079.2014.1000642
Drigas, A. S., & Ioannidou, R. E. (2012). Artificial intelligence in special education: A decade review. International Journal of Engineering Education, 28 (6), 1366. http://imm.demokritos.gr/publications/AI_IJEE.pdf
*Ferrarelli, P., & Iocchi, L. (2021). Learning Newtonian physics through programming robot experiments. Technology, Knowledge and Learning, 26 , 789–824. https://doi.org/10.1007/s10758-021-09508-3
*Figueiredo, M., Esteves, L., Neves, J., & Vicente, H. (2016). A data mining approach to study the impact of the methodology followed in chemistry lab classes on the weight attributed by the students to the lab work on learning and motivation. Chemistry Education Research and Practice, 17 (1), 156–171. https://doi.org/10.1039/c5rp00144g
*García-Gorrostieta, J. M., López-López, A., & González-López, S. (2018). Automatic argument assessment of final project reports of computer engineering students. Computer Applications in Engineering Education, 26 (5), 1217–1226. https://doi.org/10.1002/cae.21996
Gary, K. (2019). Pragmatic standards versus saturated phenomenon: Cultivating a love of learning. Journal of Philosophy of Education, 53 (3), 477–490. https://doi.org/10.1111/1467-9752.12377
*Gavrilović, N., Arsić, A., Domazet, D., & Mishra, A. (2018). Algorithm for adaptive learning process and improving learners’ skills in Java programming language. Computer Applications in Engineering Education, 26 (5), 1362–1382. https://doi.org/10.1002/cae.22043
Graneheim, U. H., & Lundman, B. (2004). Qualitative content analysis in nursing research: Concepts, procedures and measures to achieve trustworthiness. Nurse Education Today, 24 (2), 105–112. https://doi.org/10.1016/j.nedt.2003.10.001
Guan, C., Mou, J., & Jiang, Z. (2020). Artificial intelligence innovation in education: A twenty-year data-driven historical analysis. International Journal of Innovation Studies, 4 (4), 134–147. https://doi.org/10.1016/j.ijis.2020.09.001
*Hellings, J., & Haelermans, C. (2020). The effect of providing learning analytics on student behaviour and performance in programming: A randomised controlled experiment. Higher Education, 83 (1), 1–18. https://doi.org/10.1007/s10734-020-00560-z
Holmes, W., Bialik, M., & Fadel, C. (2019). Artificial intelligence in education: Promises and implications for teaching and learning . Center for Curriculum Redesign.
Holstein, K., McLaren, B. M., & Aleven, V. (2019). Co-designing a real-time classroom orchestration tool to support teacher—AI complementarity. Journal of Learning Analytics, 6 (2), 27–52. https://doi.org/10.18608/jla.2019.62.3
*Hooshyar, D., Ahmad, R. B., Yousefi, M., Yusop, F. D., & Horng, S. (2015). A flowchart-based intelligent tutoring system for improving problem-solving skills of novice programmers. Journal of Computer Assisted Learning, 31 , 345–361. https://doi.org/10.1111/jcal.12099
*Hooshyar, D., Binti Ahmad, R., Wang, M., Yousefi, M., Fathi, M., & Lim, H. (2018). Development and evaluation of a game-based Bayesian intelligent tutoring system for teaching programming. Journal of Educational Computing Research, 56 (6), 775–801. https://doi.org/10.1177/0735633117731872
*Hsiao, I. H., Huang, P. K., & Murphy, H. (2020). Integrating programming learning analytics across physical and digital space. IEEE Transactions on Emerging Topics in Computing, 8 (1), 206–217. https://doi.org/10.1109/TETC.2017.2701201
Hwang, G. J., & Tu, Y. F. (2021). Roles and research trends of artificial intelligence in mathematics education: A bibliometric mapping analysis and systematic review. Mathematics, 9 (6), 584. https://doi.org/10.3390/math9060584
Hwang, G. J., Xie, H., Wah, B. W., & Gašević, D. (2020). Vision, challenges, roles and research issues of artificial intelligence in education. Computers and Education: Artificial Intelligence, 1 , 100001. https://doi.org/10.1016/j.caeai.2020.100001
*Ji, Y., & Han, Y. (2019). Monitoring indicators of the flipped classroom learning process based on data mining—Taking the course of “virtual reality technology” as an example. International Journal of Emerging Technologies in Learning, 14 (3), 166–176. https://doi.org/10.3991/ijet.v14i03.10105
Jiao, P., Ouyang, F., Zhang, Q., & Alavi, A. H. (2022). Artificial intelligence-enabled prediction model of student academic performance in online engineering education. Artificial Intelligence Review . https://doi.org/10.1007/s10462-022-10155-y
*Jones, A., Bull, S., & Castellano, G. (2018). “I know that now, I’ m going to learn this next” Promoting self-regulated learning with a robotic tutor. International Journal of Social Robotics, 10 (4), 439–454. https://doi.org/10.1007/s12369-017-0430-y
*Jones, A., & Castellano, G. (2018). Adaptive robotic tutors that support self-regulated learning: A longer-term investigation with primary school children. International Journal of Social Robotics, 10 (3), 357–370. https://doi.org/10.1007/s12369-017-0458-z
*Khan, I., Ahmad, A. R., Jabeur, N., & Mahdi, M. N. (2021). Machine learning prediction and recommendation framework to support introductory programming course. International Journal of Emerging Technologies in Learning, 16 (17), 42–59. https://doi.org/10.3991/ijet.v16i17.18995
Khandelwal, P., Srinivasan, K., & Roy, S. S. (2019). Surgical education using artificial intelligence, augmented reality and machine learning: A review. In A. Sengupta & P. Eng (Eds.), 2019 IEEE international conference on consumer electronics—Taiwan (pp. 1–2). IEEE.
*Kinnebrew, J. S., Killingsworth, S. S., Clark, D. B., Biswas, G., Sengupta, P., Minstrell, J., Martinez-garza, M., & Krinks, K. (2017). Contextual markup and mining in digital games for science learning: Connecting player behaviors to learning goals. IEEE Transactions on Learning Technologies, 10 (1), 93–103. https://doi.org/10.1109/TLT.2016.2521372
Kitto, K. (2014). A contextualised general systems theory. Systems, 2 (4), 541–565. https://doi.org/10.3390/systems2040541
*Koć-Januchta, M. M., Schönborn, K. J., Tibell, L. A. E., Chaudhri, V. K., & Heller, H. C. (2020). Engaging with biology by asking questions: Investigating students’ interaction and learning with an artificial intelligence-enriched textbook. Journal of Educational Computing Research, 58 (6), 1190–1224. https://doi.org/10.1177/0735633120921581
*Kose, U., & Arslan, A. (2017). Optimization of self-learning in computer engineering courses: An intelligent software system supported by artificial neural network and vortex optimization algorithm. Computer Applications in Engineering Education, 25 (1), 142–156. https://doi.org/10.1002/cae.21787
*Krämer, N. C., Karacora, B., Lucas, G., Dehghani, M., Rüther, G., & Gratch, J. (2016). Closing the gender gap in STEM with friendly male instructors? On the effects of rapport behavior and gender of a virtual agent in an instructional interaction. Computers & Education, 99 , 1–13. https://doi.org/10.1016/j.compedu.2016.04.002
Krasovskiy, D. (2020). The challenges and benefits of adopting AI in STEM education. https://upjourney.com/the-challenges-and-benefits-of-adopting-ai-in-stem-education
Krippendorff, K. (2004). Reliability in content analysis: Some common misconceptions and recommendations. Human Communication Research, 30 (3), 411–433. https://doi.org/10.1093/hcr/30.3.411
*Lacave, C., Molina, A. I., & Cruz-Lemus, J. A. (2018). Learning analytics to identify dropout factors of computer science studies through Bayesian networks. Behaviour and Information Technology, 37 (10–11), 993–1007. https://doi.org/10.1080/0144929X.2018.1485053
*Lamb, R., Hand, B., & Kavner, A. (2021). Computational modeling of the effects of the science writing heuristic on student critical thinking in science using machine learning. Journal of Science Education and Technology, 30 (2), 283–297. https://doi.org/10.1007/s10956-020-09871-3
Law, N. W. Y. (2019). Human development and augmented intelligence. In The 20th international conference on artificial intelligence in education (AIED 2019), Chicago, IL, USA.
Le, N. T., Strickroth, S., Gross, S., & Pinkwart, N. (2013). A review of AI-supported tutoring approaches for learning programming. In N. Nguyen, T. Van Do, & H. Le Thi (Eds.), Advanced computational methods for knowledge engineering (pp. 267–279). Springer. https://doi.org/10.1007/978-3-319-00293-4_20
Chapter Google Scholar
*Ledesma, E. F. R., & García, J. J. G. (2017). Selection of mathematical problems in accordance with student’s learning style. International Journal of Advanced Computer Science and Applications, 8 (3), 101–105. https://doi.org/10.14569/IJACSA.2017.080316
Lee, J., Jang, D., & Park, S. (2017). Deep learning-based corporate performance prediction model considering technical capability. Sustainability, 9 (6), 899. https://doi.org/10.3390/su9060899
Lee, J., Wu, A. S., Li, D., & Kulasegaram, K. M. (2021). Artificial intelligence in undergraduate medical education: A scoping review. Academic Medicine, 96 (11S), S62–S70. https://doi.org/10.1097/ACM.0000000000004291
Liang, J. C., Hwang, G. J., Chen, M. R. A., & Darmawansah, D. (2021). Roles and research foci of artificial intelligence in language education: An integrated bibliographic analysis and systematic review approach. Interactive Learning Environments . https://doi.org/10.1080/10494820.2021.1958348
*Lin, P. H., & Chen, S. Y. (2020). Design and evaluation of a deep learning recommendation based augmented reality system for teaching programming and computational thinking. IEEE Access, 8 , 45689–45699. https://doi.org/10.1109/ACCESS.2020.2977679
Liu, M., Li, Y., Xu, W., & Liu, L. (2017). Automated essay feedback generation and its impact on revision. IEEE Transactions on Learning Technologies, 10 (4), 502–513. https://doi.org/10.1109/TLT.2016.2612659
Loveless, A. (2011). Technology, pedagogy and education: Reflections on the accomplishment of what teachers know, do and believe in a digital age. Technology, Pedagogy and Education, 20 (3), 301–316. https://doi.org/10.1080/1475939X.2011.610931
*Maestrales, S., Zhai, X., Touitou, I., Baker, Q., Schneider, B., & Krajcik, J. (2021). Using machine learning to score multi-dimensional assessments of chemistry and physics. Journal of Science Education and Technology, 30 (2), 239–254. https://doi.org/10.1007/s10956-020-09895-9
*Mahboob, K., Ali, S. A., & Laila, U. E. (2020). Investigating learning outcomes in engineering education with data mining. Computer Applications in Engineering Education, 28 (6), 1652–1670. https://doi.org/10.1002/cae.22345
*Matthew, F. T., Adepoju, A. I., Ayodele, O., Olumide, O., Olatayo, O., Adebimpe, E., Bolaji, O., & Funmilola, E. (2018). Development of mobile-interfaced machine learning-based predictive models for improving students’ performance in programming courses. International Journal of Advanced Computer Science and Applications, 9 (5), 105–115. https://doi.org/10.14569/IJACSA.2018.090514
McLaren, B. M., Scheuer, O., & Mikšátko, J. (2010). Supporting collaborative learning and e-discussions using artificial intelligence techniques. International Journal of Artificial Intelligence in Education, 20 (1), 1–46. https://doi.org/10.3233/JAI-2010-0001
McLurkin, J., Rykowski, J., John, M., Kaseman, Q., & Lynch, A. J. (2013). Using multi-robot systems for engineering education: Teaching and outreach with large numbers of an advanced, low-cost robot. IEEE Transactions on Education, 56 (1), 24-33. https://doi.org/10.1109/TE.2012.2222646
Moher, D., Liberati, A., Tetzlaff, J., Altman, D. G., Prisma Group. (2009). Preferred reporting items for systematic reviews and meta-analyses: The PRISMA statement. PLoS Medicine, 6 (7), e1000097. https://doi.org/10.1371/journal.pmed.1000097.t001
Muhisn, Z. A. A., Ahmad, M., Omar, M., & Muhisn, S. A. (2019). The Impact of socialization on collaborative learning method in e-learning management system (eLMS). International Journal of Emerging Technologies in Learning, 14 (20), 137–148.
Murray, T. (2003). An overview of intelligent tutoring system authoring tools: Updated analysis of the state of the art. In T. Murray, S. B. Blessing, & S. Ainsworth (Eds.), Authoring tools for advanced technology learning environments (pp. 491–544). Springer. https://doi.org/10.1007/978-94-017-0819-7_17
*Myneni, L. S., Narayanan, N. H., & Rebello, S. (2013). An interactive and intelligent learning system for physics education. IEEE Transactions on Learning Technologies, 6 (3), 228–239. https://doi.org/10.1109/TLT.2013.26
Mystakidis, S., Christopoulos, A., & Pellas, N. (2021). A systematic mapping review of augmented reality applications to support STEM learning in higher education. Education and Information Technologies, 27 , 1883–1927. https://doi.org/10.1007/S10639-021-10682-1/FIGURES/10
*Nehm, R. H., Ha, M., & Mayfield, E. (2012). Transforming biology assessment with machine learning: Automated scoring of written evolutionary explanations. Journal of Science Education and Technology, 21 (1), 183–196. https://doi.org/10.1007/s10956-011-9300-9
Ouyang, F., & Jiao, P. (2021). Artificial intelligence in education: The three paradigms. Computers and Education: Artificial Intelligence, 2 , 100020. https://doi.org/10.1016/j.caeai.2021.100020
Ouyang, F., Zheng, L., & Jiao, P. (2022). Artificial intelligence in online higher education: A systematic review of empirical research from 2011 to 2020. Education and Information Technologies . https://doi.org/10.1007/s10639-022-10925-9
*Pereira, F. D., Oliveira, E. H. T., Oliveira, D. B. F., Cristea, A. I., Carvalho, L. S. G., Fonseca, S. C., Toda, A., & Isotani, S. (2020). Using learning analytics in the Amazonas: Understanding students’ behaviour in introductory programming. British Journal of Educational Technology, 51 (4), 955–972. https://doi.org/10.1111/bjet.12953
Pimthong, P., & Williams, J. (2018). Preservice teachers’ understanding of STEM education. Kasetsart Journal of Social Sciences, 41 (2), 1–7. https://doi.org/10.1016/j.kjss.2018.07.017
Rapoport, A. (1986). General system theory: Essential concepts & applications . CRC Press.
*Rodríguez Corral, J. M., Morgado-Estévez, A., Cabrera Molina, D., Pérez-Peña, F., Amaya Rodríguez, C. A., & CivitBalcells, A. (2016). Application of robot programming to the teaching of object-oriented computer languages. International Journal of Engineering Education, 32 (4), 1823–1832.
Roll, I., & Wylie, R. (2016). Evolution and revolution in artificial intelligence in education. International Journal of Artificial Intelligence in Education, 26 (2), 582–599. https://doi.org/10.1007/s40593-016-0110-3
*Saito, T., & Watanobe, Y. (2020). Learning path recommendation system for programming education based on neural networks. International Journal of Distance Education Technologies, 18 (1), 36–64. https://doi.org/10.4018/IJDET.2020010103
*Sapounidis, T., Stamovlasis, D., & Demetriadis, S. (2019). Latent class modeling of children’s preference profiles on tangible and graphical robot programming. IEEE Transactions on Education, 62 (2), 127–133. https://doi.org/10.1109/TE.2018.2876363
Segal, M. (2019). A more human approach to artificial intelligence. Nature, 571 (7766), S18–S18. https://doi.org/10.1038/d41586-019-02213-3
Selwyn, N. (2016). Is technology good for education? Polity Press.
Skinner, B. F. (1953). Science and human behavior . Macmillan.
*Spikol, D., Ruffaldi, E., Dabisias, G., & Cukurova, M. (2018). Supervised machine learning in multimodal learning analytics for estimating success in project-based learning. Journal of Computer Assisted Learning, 34 (4), 366–377. https://doi.org/10.1111/jcal.12263
*Suh, S. C., Anusha Upadhyaya, B. N., & Ashwin Nadig, N. V. (2019). Analyzing personality traits and external factors for stem education awareness using machine learning. International Journal of Advanced Computer Science and Applications, 10 (5), 1–4. https://doi.org/10.14569/ijacsa.2019.0100501
Tang, K. Y., Chang, C. Y., & Hwang, G. J. (2021). Trends in artificial intelligence supported e-learning: A systematic review and co-citation network analysis (1998–2019). Interactive Learning Environments . https://doi.org/10.1080/10494820.2021.1875001
*Tehlan, K., Chakraverty, S., Chakraborty, P., & Khapra, S. (2020). A genetic algorithm-based approach for making pairs and assigning exercises in a programming course. Computer Applications in Engineering Education, 28 (6), 1708–1721. https://doi.org/10.1002/cae.22349
*Thai, K. P., Bang, H. J., & Li, L. (2021). Accelerating early math learning with research-based personalized learning games: A cluster randomized controlled trial. Journal of Research on Educational Effectiveness, 15 (1), 28–51. https://doi.org/10.1080/19345747.2021.1969710
*Troussas, C., Krouska, A., & Sgouropoulou, C. (2021). A novel teaching strategy through adaptive learning activities for computer programming. IEEE Transactions on Education, 64 (2), 103–109. https://doi.org/10.1109/TE.2020.3012744
*Tüfekçi, A., & Köse, U. (2013). Development of an artificial intelligence based software system on teaching computer programming and evaluation of the system. Hacettepe Üniversitesi Eğitim Fakültesi Dergisi, 28 (2), 469–481.
*Verner, I. M., Cuperman, D., Gamer, S., & Polishuk, A. (2020). Exploring affordances of robot manipulators in an introductory engineering course. International Journal of Engineering Education, 36 (5), 1691–1707.
Von Bertalanffy, L. (1950). An outline of general system theory. British Journal for the Philosophy of Science, 1 , 134–165. https://doi.org/10.1093/bjps/I.2.134
Von Bertalanffy, L. (1968). General system theory: Foundations, development, applications . George Braziller.
*Vyas, V. S., Kemp, B., & Reid, S. A. (2021). Zeroing in on the best early-course metrics to identify at-risk students in general chemistry: An adaptive learning pre-assessment vs. traditional diagnostic exam. International Journal of Science Education, 43 (4), 552–569. https://doi.org/10.1080/09500693.2021.1874071
Walker, E., Rummel, N., & Koedinger, K. R. (2014). Adaptive intelligent support to improve peer tutoring in algebra. International Journal of Artificial Intelligence in Education, 24 (1), 33–61. https://doi.org/10.1007/s40593-013-0001-9
*Wang, T., Su, X., Ma, P., Wang, Y., & Wang, K. (2011). Ability-training-oriented automated assessment in introductory programming course. Computers & Education, 56 (1), 220–226. https://doi.org/10.1016/j.compedu.2010.08.003
*Wang, X. (2016). Course-taking patterns of community college students beginning in STEM: Using data mining techniques to reveal viable STEM transfer pathways. Research in Higher Education, 57 (5), 544–569. https://doi.org/10.1007/s11162-015-9397-4
Wohlin, C. (2014). Guidelines for snowballing in systematic literature studies and a replication in software engineering. In C. Wohlin (Ed.), Proceedings of the 18th international conference on evaluation and assessment in software engineering (pp. 1–10). ACM Press. https://doi.org/10.1145/2601248.2601268
*Wu, P., Hwang, G., & Tsai, W. (2013). An expert system-based context-aware ubiquitous learning approach for conducting science learning activities. Journal of Educational Technology & Society, 16 (4), 217–230.
*Xing, W., Pei, B., Li, S., Chen, G., & Xie, C. (2019). Using learning analytics to support students’ engineering design: The angle of prediction. Interactive Learning Environments . https://doi.org/10.1080/10494820.2019.1680391
Xu, W., & Ouyang, F. (2022). A systematic review of AI role in the educational system based on a proposed conceptual framework. Education and Information Technologies, 27 , 4195–4223. https://doi.org/10.1007/s10639-021-10774-y
*Yahya, A. A., & Osman, A. (2019). A data-mining-based approach to informed decision-making in engineering education. Computer Applications in Engineering Education, 27 (6), 1402–1418. https://doi.org/10.1002/cae.22158
*Yang, J., Devore, S., Hewagallage, D., Miller, P., Ryan, Q. X., & Stewart, J. (2020). Using machine learning to identify the most at-risk students in physics classes. Physical Review Physics Education Research, 16 (2), 20130. https://doi.org/10.1103/PhysRevPhysEducRes.16.020130
Yang, J., & Zhang, B. (2019). Artificial intelligence in intelligent tutoring robots: A systematic review and design guidelines. Applied Sciences, 9 (10), 2078. https://doi.org/10.3390/app9102078
*Yannier, N., Hudson, S. E., & Koedinger, K. R. (2020). Active learning is about more than hands-on: A mixed-reality AI system to support STEM education. International Journal of Artificial Intelligence in Education, 30 (1), 74–96. https://doi.org/10.1007/s40593-020-00194-3
*Yu, Y. (2017). Teaching with a dual-channel classroom feedback system in the digital classroom environment. IEEE Transactions on Learning Technologies, 10 (3), 391–402. https://doi.org/10.1109/TLT.2016.2598167
*Zabriskie, C., Yang, J., Devore, S., & Stewart, J. (2019). Using machine learning to predict physics course outcomes. Physical Review Physics Education Research, 15 (2), 20120. https://doi.org/10.1103/PhysRevPhysEducRes.15.020120
*Zampirolli, F. A., BorovinaJosko, J. M., Venero, M. L. F., Kobayashi, G., Fraga, F. J., Goya, D., & Savegnago, H. R. (2021). An experience of automated assessment in a large-scale introduction programming course. Computer Applications in Engineering Education, 29 (5), 1284–1299. https://doi.org/10.1002/cae.22385
*Zapata-Cáceres, M., & Martin-Barroso, E. (2021). Applying game learning analytics to a voluntary video game: Intrinsic motivation, persistence, and rewards in learning to program at an early age. IEEE Access, 9 , 123588–123602. https://doi.org/10.1109/ACCESS.2021.3110475
Zawacki-Richter, O., Marín, V. I., Bond, M., & Gouverneur, F. (2019). Systematic review of research on artificial intelligence applications in higher education—Where are the educators? International Journal of Educational Technology in Higher Education, 16 (39), 1–27. https://doi.org/10.1186/s41239-019-0171-0
*Zhang, Z., Liu, H., Shu, J., Nie, H., & Xiong, N. (2020). On automatic recommender algorithm with regularized convolutional neural network and IR technology in the self-regulated learning process. Infrared Physics and Technology, 105 , 103211. https://doi.org/10.1016/j.infrared.2020.103211
Zheng, R., Jiang, F., & Shen, R. (2020). Intelligent student behavior analysis system for real classrooms. In P. C. Center (Ed.), 2020 IEEE international conference on acoustics, speech and signal processing (pp. 9244–9248). IEEE.
*Zulfiani, Z., Suwarna, I. P., & Miranto, S. (2018). Science education adaptive learning system as a computer-based science learning with learning style variations. Journal of Baltic Science Education, 17 (4), 711–727. https://doi.org/10.33225/jbse/18.17.711
Zupic, I., & Čater, T. (2015). Bibliometric methods in management and organization. Organizational Research Methods, 18 (3), 429–472. https://doi.org/10.1177/1094428114562629
Download references
Acknowledgements
The authors would like to thank Luyi Zheng for her help on preliminary data analysis.
This work was supported by National Natural Science Foundation of China, No. 62177041.
Author information
Authors and affiliations.
College of Education, Zhejiang University, #866, Yuhangtang Rd., Hangzhou, 310058, Zhejiang, China
Weiqi Xu & Fan Ouyang
You can also search for this author in PubMed Google Scholar
Contributions
WX conducted data collection and analysis as well as writing of the first draft of this manuscript. FO designed the research, designed and facilitated data analysis and revised the manuscript. Both authors read and approved the final manuscript.
Corresponding author
Correspondence to Fan Ouyang .
Ethics declarations
Competing interests.
The authors declare that they have no competing interests.
Additional information
Publisher's note.
Springer Nature remains neutral with regard to jurisdictional claims in published maps and institutional affiliations.
Rights and permissions
Open Access This article is licensed under a Creative Commons Attribution 4.0 International License, which permits use, sharing, adaptation, distribution and reproduction in any medium or format, as long as you give appropriate credit to the original author(s) and the source, provide a link to the Creative Commons licence, and indicate if changes were made. The images or other third party material in this article are included in the article's Creative Commons licence, unless indicated otherwise in a credit line to the material. If material is not included in the article's Creative Commons licence and your intended use is not permitted by statutory regulation or exceeds the permitted use, you will need to obtain permission directly from the copyright holder. To view a copy of this licence, visit http://creativecommons.org/licenses/by/4.0/ .
Reprints and permissions
About this article
Cite this article.
Xu, W., Ouyang, F. The application of AI technologies in STEM education: a systematic review from 2011 to 2021. IJ STEM Ed 9 , 59 (2022). https://doi.org/10.1186/s40594-022-00377-5
Download citation
Received : 11 June 2022
Accepted : 09 September 2022
Published : 19 September 2022
DOI : https://doi.org/10.1186/s40594-022-00377-5
Share this article
Anyone you share the following link with will be able to read this content:
Sorry, a shareable link is not currently available for this article.
Provided by the Springer Nature SharedIt content-sharing initiative
- Artificial intelligence
- Artificial intelligence in education
- STEM education
- General system theory
- Educational system
- Side Hustles
- Power Players
- Young Success
- Save and Invest
- Become Debt-Free
- Land the Job
- Closing the Gap
- Science of Success
- Pop Culture and Media
- Psychology and Relationships
- Health and Wellness
- Real Estate
- Most Popular
Related Stories
- Earn The 16 highest-paying college majors, 5 years after graduation
- Earn The 10 public colleges and universities where graduates earn the most
- Earn The best- and worst-paying college majors, 5 years after graduation
- Earn The 16 worst-paying college majors, five years after graduation
- Earn 10 U.S. cities where a $100,000 salary goes the furthest—half are in Texas
10 college majors to check out if you want to make a lot of money—without becoming an engineer

If you're looking for a high-paying college major , engineering degrees, including electrical and computer engineering, consistently rank as some of the options that can earn you the most money.
But engineering isn't for everyone.
You shouldn't force yourself into any major — or career path — you're not interested in just for the money. You may not always be able to do a job that you love, but why set yourself up for a career that bores you?
Broader science, technology and math majors earn a decent amount, too. Pharmacy and computer science are the best-paying nonengineering college degrees, according to a recent Bankrate analysis , which found those degree holders earn median salaries of $100,000 a year.
Using the latest American Community Survey data from the U.S. Census Bureau, Bankrate ranked majors by median salary among bachelor's degree holders between the ages of 18 and 77 who were in the labor force and are either employed or seeking work.
Here are the 10 highest-paying college degrees that aren't engineering:
1. TIE: Pharmacy, pharmaceutical sciences and administration
- Median salary: $100,000
1. TIE: Computer science
- Median salary: $90,000
4. Management information systems and statistics
- Median salary: $89,000
5. Health and medical preparatory programs
- Median salary: $87,000
6. TIE: Applied mathematics
- Median salary: $85,000
6. TIE: Economics
6. tie: transportation sciences and technologies, 9. tie: statistics and decision science.
- Median salary: $84,000
9. TIE: Information sciences
While you have to complete a doctoral degree to work as a full-fledged pharmacist, a bachelor's degree in pharmacy, pharmaceutical sciences and administration may still land you a well-paying job in research, clinical trials or another part of the field.
You could wind up in a software engineering position after earning a computer science degree, but you'll likely be a good fit for other nonengineering roles as well, such as a data scientist or IT specialist.
Your major doesn't have to define your career path . But if you have your heart set on a certain job or industry, it's wise to explore common job requirements to see if a certain major is ideal or if there are other ways to acquire the necessary skills.
DON'T MISS: Want to be smarter and more successful with your money, work & life? Sign up for our new newsletter!
Get CNBC's free Warren Buffett Guide to Investing , which distills the billionaire's No. 1 best piece of advice for regular investors, do's and don'ts, and three key investing principles into a clear and simple guidebook.

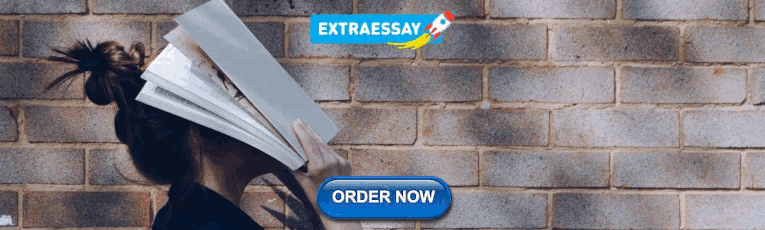
IMAGES
COMMENTS
STEM, field and curriculum centred on education in the disciplines of science, technology, engineering, and mathematics (STEM). The STEM acronym was introduced in 2001 by scientific administrators at the U.S. National Science Foundation (NSF). The organization previously used the acronym SMET when referring to the career fields in those disciplines or a curriculum that integrated knowledge and ...
Their education system focuses on innovation and practical applications, pushing students to think beyond the textbook. On the other hand, China is sprinting forward in STEM. With a considerable push from the government, Chinese students often outshine others in international rankings like PISA.
STEM education, now also know as STEAM, is a multi-discipline approach to teaching. STEM education is a teaching approach that combines science, technology, engineering and math. Its recent ...
Today, the U.S. Department of Education (Department) will host the YOU Belong in STEM National Coordinating Conference in Washington, D.C. as a key initiative for the Biden-Harris Administration. The Raise the Bar: STEM Excellence for All Students initiative is designed to strengthen Science, Technology, Engineering and Mathematics (STEM) education nationwide.
The Future Is STEM. In contrast with more traditional approaches, STEM education aims to provide instructional environments for blended learning and is committed to showing students how the scientific method can be applied in an everyday context. Indeed, STEM education is focused on the practical applications of problem-solving in the real world.
Create opportunities for all students to receive an accessible and high-quality STEM education and help them foster a love and curiosity for science and mathematics from an early age. • We must challenge our beliefs about the STEM education system and look for structural changes that make it both cost-accessible and sustainable.
Julia Phillips, NSB. The latest Elementary and Secondary STEM Education report, the first of the 2022 Indicators reports, raises more concern about the state of STEM education in the nation and its potential impact on the economy and the U.S. standing in the world. Phillips discusses the key trends and their implications for science and education policy in the U.S.
The need for a vast, talented workforce in STEM-related fields has never been more necessary, said Bridget Long, dean of the Harvard Graduate School of Education. Long cited the U.S. Bureau of Labor Statistics, which shows employment in STEM occupations has grown 79 percent in the past three decades. In addition, STEM jobs are projected to grow ...
Elementary and secondary STEM education is the foundation for student entry into postsecondary STEM majors and occupations. This report presents national trends in K-12 student achievement in STEM and compares U.S. STEM achievement with that of other nations. It explores mathematics and computer science achievement, by student characteristics.
The real-world knowledge and skills built in STEM education support a variety of career paths and outcomes. This only scratches the surface of defining STEM education and why it is important. Keep reading to learn more about STEM education as well as its value to the modern economy and students just like you.
Around the world, STEM education, that is Science, Technology, Engineering, and Mathematics (STEM), has become an important issue for educators and policy-makers alike (Holmlund et al. 2018).In spite of this global focus, this chapter argues that STEM education in the United States can best be understood as a steady change amid seemingly bewildering incoherence - in short, progress without a ...
Today, the U.S. Department of Education (Department) will host the YOU Belong in STEM National Coordinating Conference in Washington, D.C. as a key initiative for the Biden-Harris Administration. The Raise the Bar: STEM Excellence for All Students initiative is designed to strengthen Science, Technology, Engineering and Mathematics (STEM) education nationwide.
STEM education is about a teaching philosophy that naturally integrates critical thinking and language skills in a way that enriches any subject. Perhaps you've experienced or can imagine an education that integrates problem solving and engineering practices into any subject, where technology is seamlessly integrated throughout.
STEM education emphasizes the value of a rigorous, interdisciplinary approach to education, allowing students to compete and succeed in a modern global marketplace. Learn more and get access to ...
Accessible learning activities that invite intentional play and risk. STEM 2026 empha-sizes the benefits of inviting intentional play into the learning process in P-12 and at the postsecondary level. Activities that are designed to incorporate intentional play are applica-ble at all levels of the education continuum.
The STEM community responded vigorously to produce the Sputnik-spurred education reforms of the 1960s. Likewise, the United States needs a bold new federal strategy for improving education that includes the creation of high-quality, integrated instruction and materials, as well as the placement of problems associated with grand challenges of society at the center of study.
They call for renewed investment in the nation's STEM education system. Early STEM education provides the necessary foundation for those pursuing degrees and careers in the field later on. STEM education centers around programs designed to help students gain skills required to succeed in the innovation-focused twenty-first century job market ...
The challenges of implementing STEM education into colleges are like those seen when incorporating STEM education into any public or private school system. Resources and funding are perhaps the biggest hurdle, as a quality STEM program requires a fair share of materials, resources, and technologies that can be costly to implement and keep up to ...
Introduction. The movement that seeks to give new impetus to the development of Science, Technology, Engineering and Mathematics (STEM) began in the United States in the 1990s, sponsored by the National Science Foundation. After a few years of little social and educational impact (Friedman, Citation 2005), the STEM movement has experienced a global expansion in the current century, especially ...
Although the United States was key in the rapid development of COVID-19 vaccines, the data in this report show the U.S. S&E enterprise could be strengthened. The data reveal capacity-building areas in the U.S. S&E enterprise in the STEM education system and STEM workforce, R&D investment, and innovation activities.
The simplest definition of STEM acronym is what it stands for, which is science, technology, engineering, and mathematics. There are many organizations that are dedicated to this topic and their meaning of it is defined based on their own objectives. The ultimate goal of STEM education is to encourage students to take an interest in STEM ...
The application of artificial intelligence (AI) in STEM education (AI-STEM), as an emerging field, is confronted with a challenge of integrating diverse AI techniques and complex educational elements to meet instructional and learning needs. To gain a comprehensive understanding of AI applications in STEM education, this study conducted a systematic review to examine 63 empirical AI-STEM ...
Elementary and secondary STEM education is the foundation for student entry into postsecondary STEM majors and occupations. This report presents national trends in K-12 student achievement in STEM and compares U.S. STEM achievement with that of other nations. The report shows that student achievement in mathematics, which had already plateaued for the past decade, has now regressed ...
STEM stands for Science, Technology, Engineering, and Mathematics. This education system is the future of the coming generation. Before STEM, the organization used the SMET acronym but later, in 2001 the scientific administrators at the U.S. National Science Foundation (NSF) introduced the acronym STEM. STEM is one of the most researched topics ...
Teacher Vincent Squatrito was named Rural STEM Teacher of the Year by the Governor's Office of Science, Innovation and Technology and the Nevada Department of Education. Jackpot Combined Schools was
Earlier this month, the ACES Institute and the UH STEM Center hosted Day of Discovery, a hands-on STEM learning experience for middle school students. Students in the Middle School Postsecondary Readiness Project participated in various interactive learning opportunities across the University of Houston's campus. Students also received an amazing public art tour from Public Art of the ...
Here are the 10 highest-paying college degrees that aren't engineering: 1. TIE: Pharmacy, pharmaceutical sciences and administration. Median salary: $100,000. 1.