Click through the PLOS taxonomy to find articles in your field.
For more information about PLOS Subject Areas, click here .
Loading metrics
Open Access
Peer-reviewed
Research Article
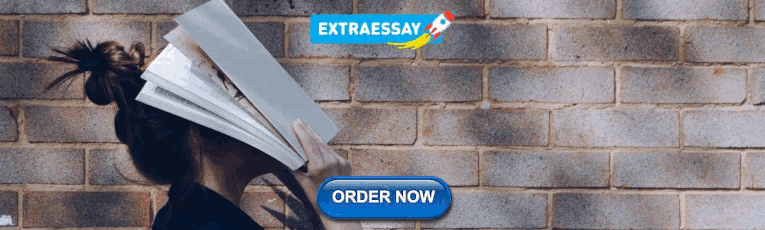
Differentiating the learning styles of college students in different disciplines in a college English blended learning setting
Roles Conceptualization, Formal analysis, Funding acquisition, Methodology, Supervision, Writing – original draft, Writing – review & editing
* E-mail: [email protected]
Affiliations Department of Linguistics, School of International Studies, Zhejiang University, Hangzhou City, Zhejiang Province, China, Center for College Foreign Language Teaching, Zhejiang University, Hangzhou City, Zhejiang Province, China, Institute of Asian Civilizations, Zhejiang University, Hangzhou City, Zhejiang Province, China

Roles Formal analysis, Project administration, Writing – review & editing
Affiliation Department of Linguistics, School of International Studies, Zhejiang University, Hangzhou City, Zhejiang Province, China
Roles Formal analysis, Writing – original draft
Roles Writing – review & editing
- Jie Hu,
- Yi Peng,
- Xueliang Chen,
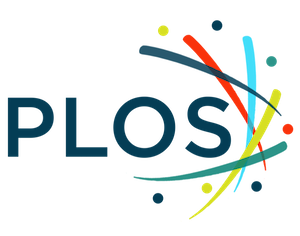
- Published: May 20, 2021
- https://doi.org/10.1371/journal.pone.0251545
- Peer Review
- Reader Comments
Learning styles are critical to educational psychology, especially when investigating various contextual factors that interact with individual learning styles. Drawing upon Biglan’s taxonomy of academic tribes, this study systematically analyzed the learning styles of 790 sophomores in a blended learning course with 46 specializations using a novel machine learning algorithm called the support vector machine (SVM). Moreover, an SVM-based recursive feature elimination (SVM-RFE) technique was integrated to identify the differential features among distinct disciplines. The findings of this study shed light on the optimal feature sets that collectively determined students’ discipline-specific learning styles in a college blended learning setting.
Citation: Hu J, Peng Y, Chen X, Yu H (2021) Differentiating the learning styles of college students in different disciplines in a college English blended learning setting. PLoS ONE 16(5): e0251545. https://doi.org/10.1371/journal.pone.0251545
Editor: Haoran Xie, Lingnan University, HONG KONG
Received: May 15, 2020; Accepted: April 29, 2021; Published: May 20, 2021
Copyright: © 2021 Hu et al. This is an open access article distributed under the terms of the Creative Commons Attribution License , which permits unrestricted use, distribution, and reproduction in any medium, provided the original author and source are credited.
Data Availability: All relevant data are within the paper and its Supporting Information files.
Funding: This research was supported by the Philosophical and Social Sciences Planning Project of Zhejiang Province in 2020 [grant number 20NDJC01Z] with the recipient Jie Hu, Second Batch of 2019 Industry-University Collaborative Education Project of Chinese Ministry of Education [grant number 201902016038] with the recipient Jie Hu, SUPERB College English Action Plan with the recipient Jie Hu, and the Fundamental Research Funds for the Central Universities of Zhejiang University with the recipient Jie Hu.
Competing interests: The authors have declared that no competing interests exist.
Introduction
Research background.
Learning style, as an integral and vital part of a student’s learning process, has been constantly discussed in the field of education and pedagogy. Originally developed from the field of psychology, psychological classification, and cognitive research several decades ago [ 1 ], the term “learning style” is generally defined as the learner’s innate and individualized preference for ways of participation in learning practice [ 2 ]. Theoretically, learning style provides a window into students’ learning processes [ 3 , 4 ], predicts students’ learning outcomes [ 5 , 6 ], and plays a critical role in designing individualized instruction [ 7 ]. Knowing a student’s learning style and personalizing instruction to students’ learning style could enhance their satisfaction [ 8 ], improve their academic performance [ 9 ], and even reduce the time necessary to learn [ 10 ].
Researchers in recent years have explored students’ learning styles from various perspectives [ 11 – 13 ]. However, knowledge of the learning styles of students from different disciplines in blended learning environments is limited. In an effort to address this gap, this study aims to achieve two major objectives. First, it investigates how disciplinary background impacts students’ learning styles in a blended learning environment based on data collected in a compulsory college English course. Students across 46 disciplines were enrolled in this course, providing numerous disciplinary factor resources for investigating learning styles. Second, it introduces a novel machine learning method named the SVM to the field of education to identify an optimal set of factors that can simultaneously differentiate students of different academic disciplines. Based on data for students from 46 disciplines, this research delves into the effects of a massive quantity of variables related to students’ learning styles with the help of a powerful machine learning algorithm. Considering the convergence of a wide range of academic disciplines and the detection of latent interactions between a large number of variables, this study aims to provide a clear picture of the relationship between disciplinary factors and students’ learning styles in a blended learning setting.
Literature review
Theories of learning styles..
Learning style is broadly defined as the inherent preferences of individuals as to how they engage in the learning process [ 2 ], and the “cognitive, affective and physiological traits” of students have received special attention [ 14 ]. To date, there has been a proliferation of learning style definitions proposed to explain people’s learning preferences, each focusing on different aspects. Efforts to dissect learning style have been contested, with some highlighting the dynamic process of the learner’s interaction with the learning environment [ 14 ] and others underlining the individualized ways of information processing [ 15 ]. One vivid explication involved the metaphor of an onion, pointing out the multilayer nature of learning styles. It was proposed that the outermost layer of the learning style could change in accordance with the external environment, while the inner layer is relatively stable [ 16 , 17 ]. In addition, a strong concern in this field during the last three decades has led to a proliferation of models that are germane to learning styles, including the Kolb model [ 18 ], the Myers-Briggs Type Indicator model [ 19 ] and the Felder-Silverman learning style model (FSLSM) [ 20 ]. These learning style models have provided useful analytical lenses for analyzing students’ learning styles. The Kolb model focuses on learners’ thinking processes and identifies four types of learning, namely, diverging, assimilating, converging, and accommodating [ 18 ]. The Myers-Briggs Type Indicator model classifies learners into extraversion and introversion types, with the former preferring to learn from interpersonal communication and the latter inclining to benefit from personal experience [ 19 ]. As the most popular available model, the FSLSM identifies eight categories of learners according to the four dimensions of perception, input, processing and understanding [ 20 ]. In contrast to other learning style models that divided students into only a few groups, the FSLSM describes students’ learning styles in a more detailed manner. The four paired dimensions delicately distinguish students’ engagement in the learning process, providing a solid basis for a steady and reliable learning style analysis [ 21 ]. In addition, it has been argued that the FSLSM is the most appropriate model for a technology-enhanced learning environment because it involves important theories of cognitive learning behaviors [ 22 , 23 ]. Therefore, a large number of scholars have based their investigations of students’ learning styles in the e-learning/computer-aided learning environment on FSLSM [ 24 – 28 ].
Learning styles and FSLSM.
Different students receive, process, and respond to information with different learning styles. A theoretical model of learning style can be used to categorize people according to their idiosyncratic learning styles. In this study, the FSLSM was adopted as a theoretical framework to address the collective impacts of differences in students’ learning styles across different disciplines (see Fig 1 ).
- PPT PowerPoint slide
- PNG larger image
- TIFF original image
This model specifies the four dimensions of the construct of learning style: visual/verbal, sensing/intuitive, active/reflective, and sequential/global. These four dimensions correspond to four psychological processes: input, perception, processing, and understanding.
https://doi.org/10.1371/journal.pone.0251545.g001
The FSLSM includes learning styles scattered among four dimensions.
Visual learners process information best when it is presented as graphs, pictures, etc., while verbal learners prefer spoken cues and remember best what they hear. Sensory learners like working with facts, data, and experimentation, while intuitive learners prefer abstract principles and theories. Active learners like to try things and learn through experimentation, while reflective learners prefer to think things through before taking action. Sequential learners absorb knowledge in a linear fashion and make progress step by step, while global learners tend to grasp the big picture before filling in all the details.
Learning styles and academic disciplines.
Learning styles vary depending on a series of factors, including but not limited to age [ 29 ], gender [ 30 ], personality [ 2 , 31 ], learning environment [ 32 ] and learning experience [ 33 ]. In the higher education context, the academic discipline seems to be an important variable that influences students’ distinctive learning styles, which echoes a multitude of investigations [ 29 , 34 – 41 ]. One notable study explored the learning styles of students from 4 clusters of disciplines in an academic English language course and proposed that the academic discipline is a significant predictor of students’ learning styles, with students from the soft-pure, soft-applied, hard-pure and hard-applied disciplines each favoring different learning modes [ 42 ]. In particular, researchers used the Inventory of Learning Styles (ILS) questionnaire and found prominent disparities in learning styles between students from four different disciplinary backgrounds in the special educational field of vocational training [ 43 ]. These studies have found significant differences between the learning styles of students from different academic disciplines, thus supporting the concept that learning style could be domain dependent.
Learning styles in an online/blended learning environment.
Individuals’ learning styles reflect their adaptive orientation to learning and are not fixed personality traits. Consequently, learning styles can vary among diverse contexts, and related research in different contexts is vital to understanding learning styles in greater depth. Web-based technologies eliminate barriers of space and time and have become integrated in individuals’ daily lives and learning habits. Online and blended learning have begun to pervade virtually every aspect of the education landscape [ 40 ], and this warrants close attention. In addition to a series of studies that reflected upon the application of information and communication technology in the learning process [ 44 , 45 ], recent studies have found a mixed picture of whether students in a web-based/blended learning environment have a typical preference for learning.
Online learning makes it possible for students to set their goals and develop an individualized study plan, equipping them with more learning autonomy [ 46 ]. Generally, students with a more independent learning style, greater self-regulating behavior and stronger self-efficacy are found to be more successful in an online environment [ 47 ]. For now, researchers have made substantial contributions to the identification and prediction of learning styles in an online learning environment [ 27 , 48 – 51 ]. For instance, an inspiring study focused on the manifestation of college students’ learning styles in a purely computer-based learning environment to evaluate the different learning styles of web-learners in the online courses, indicating that students’ learning styles were significantly related to online participation [ 49 ]. Students’ learning styles in interactive E-learning have also been meticulously investigated, from which online tutorials have been found to be contributive to students’ academic performance regardless of their learning styles [ 51 ].
As a flexible learning method, blended courses have combined the advantages of both online learning and traditional teaching methods [ 52 ]. Researchers have investigated students’ learning styles within this context and have identified a series of prominent factors, including perceived satisfaction and technology acceptance [ 53 ], the dynamics of the online/face-to-face environment [ 54 ], and curriculum design [ 55 ]. Based on the Visual, Aural, Reading or Write and Kinesthetic model, a comprehensive study scrutinized the learning styles of K12 students in a blended learning environment, elucidating the effect of the relationship between personality, learning style and satisfaction on educational outcomes [ 56 ]. A recent study underscored the negative effects of kinesthetic learning style, whereas the positive effects of visual or auditory learning styles on students’ academic performance, were also marked in the context of blended learning [ 57 ].
Considering that academic disciplines and learning environment are generally regarded as essential predictors of students’ learning styles, some studies have also concentrated on the effects of academic discipline in a blended learning environment. Focusing on college students’ learning styles in a computer-based learning environment, an inspiring study evaluated the different learning styles of web learners, namely, visual, sensing, global and sequential learners, in online courses. According to the analysis, compared with students from other colleges, liberal arts students, are more susceptible to the uneasiness that may result from remote teaching because of their learning styles [ 11 ]. A similar effort was made with the help of the CMS tool usage logs and course evaluations to explore the learning styles of disciplinary quadrants in the online learning environment. The results indicated that there were noticeable differences in tool preferences between students from different domains [ 12 ]. In comparison, within the context of blended learning, a comprehensive study employed chi-square statistics on the basis of the Community of Inquiry (CoI) presences framework, arguing that soft-applied discipline learners in the blended learning environment prefer the kinesthetic learning style, while no correlations between the learning style of soft-pure and hard-pure discipline students and the CoI presences were identified. However, it is noted that students’ blended learning experience depends heavily on academic discipline, especially for students in hard-pure disciplines [ 13 ].
Research gaps and research questions
Overall, the research seems to be gaining traction, and new perspectives are continually introduced. The recent literature on learning styles mostly focuses on the exploration of the disciplinary effects on the variation in learning styles, and some of these studies were conducted within the blended environment. However, most of the studies focused only on several discrete disciplines or included only a small group of student samples [ 34 – 41 ]. Data in these studies were gathered through specialized courses such as academic English language [ 42 ] rather than the compulsory courses available to students from all disciplines. Even though certain investigations indeed boasted a large number of samples [ 49 ], the role of teaching was emphasized rather than students’ learning style. In addition, what is often overlooked is that a large number of variables related to learning styles could distinguish students from different academic disciplines in a blended learning environment, whereas a more comprehensive analysis that takes into consideration the effects of a great quantity of variables related to learning styles has remained absent. Therefore, one goal of the present study is to fill this gap and shed light on this topic.
Another issue addressed in this study is the selection of an optimal measurement that can effectively identify and differentiate individual learning styles [ 58 ]. The effective identification and differentiation of individual learning styles can not only help students develop greater awareness of their learning but also provide teachers with the necessary input to design tailor-made instructions in pedagogical practice. Currently, there are two general approaches to identify learning styles: a literature-based approach and a data-driven approach. The literature-based approach tends to borrow established rules from the existing literature, while the data-driven approach tends to construct statistical models using algorithms from fields such as machine learning, artificial intelligence, and data mining [ 59 ]. Research related to learning styles has been performed using predominantly traditional instruments, such as descriptive statistics, Spearman’s rank correlation, coefficient R [ 39 ], multivariate analysis of variance [ 56 ] and analysis of variance (ANOVA) [ 38 , 43 , 49 , 57 ]. Admittedly, these instruments have been applied and validated in numerous studies, in different disciplines, and across multiple timescales. Nevertheless, some of the studies using these statistical tools did not identify significant results [ 36 , 53 , 54 ] or reached only loose conclusions [ 60 ]; this might be because of the inability of these methods to probe into the synergistic effects of variables. However, the limited functions of comparison, correlation, prediction, etc. are being complemented by a new generation of technological innovations that promise more varied approaches to addressing social and scientific issues. Machine learning is one such approach that has received much attention both in academia and beyond. As a subset of artificial intelligence, machine learning deals with algorithms and statistical models on computer systems, performing tasks based on patterns and inference instead of explicit instruction. As such, it can deal with high volumes of data at the same time, perform tasks automatically and independently, and continuously improve its performance based on past experience [ 54 ]. Similar machine learning approaches have been proposed and tested by different scholars to identify students’ learning styles, with varying results regarding the classification of learning styles. For instance, a study that examined the precision levels of four computational intelligence approaches, i.e., artificial neural network, genetic algorithm, ant colony system and particle swarm optimization, found that the average precision of learning style differentiation ranged between 66% and 77% [ 61 ]. Another study that classified learning styles through SVM reported accuracy levels ranging from 53% to 84% [ 62 ]. A comparison of the prediction performance of SVM and artificial neural networks found that SVM has higher prediction accuracy than the latter [ 63 ]. This was further supported by another study, which yielded a similar result between SVM and the particle swarm optimization algorithm [ 64 ]. Moreover, when complemented by a genetic algorithm [ 65 ] and ant colony system [ 66 ], SVM has also shown improved results. These findings across different fields point to the reliability of SVM as an effective statistical tool for identification and differentiation analysis.
Therefore, a comprehensive investigation across the four general disciplines in Biglan’s taxonomy using a strong machine learning approach is needed. Given the existence of the research gaps discussed above, this exploratory study seeks to address the following questions:
- Can students’ learning styles be applied to differentiate various academic disciplines in the blended learning setting? If so, what are the differentiability levels among different academic disciplines based on students’ learning styles?
- What are the key features that can be selected to determine the collective impact on differentiation by a machine learning algorithm?
- What are the collective impacts of optimal feature sets?
Materials and methods
This study adopted a quantitative approach for the analysis. First, a modified and translated version of the original ILS questionnaire was administered to collect scores for students’ learning styles. Then, two alternate data analyses were performed separately. One analysis involved a traditional ANOVA, which tested the main effect of discipline on students’ learning styles in each ILS dimension. The other analysis involved the support vector machine (SVM) technique to test its performance in classifying students’ learning styles in the blended learning course among 46 specializations. Then, SVM-based recursive feature elimination (SVM-RFE) was employed to specify the impact of students’ disciplinary backgrounds on their learning styles in blended learning. By referencing the 44 questions (operationalized as features in this study) in the ILS questionnaire, SVM-RFE could rank these features based on their relative importance in differentiating different disciplines and identify the key features that collectively differentiate the students’ learning style. These steps are intended to not only identify students’ learning style differences but also explain such differences in relation to their academic disciplinary backgrounds.
Participants
The participants included 790 sophomores taking the blended English language course from 46 majors at Z University. Sophomore students were selected for this study for two reasons. First, sophomores are one of the only two groups of students (the other group being college freshmen) who take a compulsory English language course, namely, the College English language course. Second, of these two groups of students, sophomores have received academic discipline-related education, while their freshmen counterparts have not had disciplinary training during the first year of college. In the College English language course, online activities, representing 55% of the whole course, include e-course teaching designed by qualified course teachers or professors, courseware usage for online tutorials, forum discussion and essay writing, and two online quizzes. Offline activities, which represent 45% of the whole course, include role-playing, ice-breaker activities, group presentations, an oral examination, and a final examination. Therefore, the effects of the academic discipline on sophomores’ learning styles might be sufficiently salient to warrant a comparison in a blended learning setting [ 67 ]. Among the participants, 420 were male, and 370 were female. Most participants were aged 18 to 19 years and had taken English language courses for at least 6 years. Based on Biglan’s typology of disciplinary fields, the students’ specializations were classified into the four broad disciplines of hard-applied (HA, 289/37.00%), hard-pure (HP, 150/19.00%), soft-applied (SA, 162/20.00%), and soft-pure (SP, 189/24.00%).
Biglan’s classification scheme of academic disciplines (hard (H) vs. soft (S) disciplines and pure (P) vs. applied (A) disciplines) has been credited as the most cited organizational system of academic disciplines in tertiary education [ 68 – 70 ]. Many studies have also provided evidence supporting the validity of this classification [ 69 ]. Over the years, research has indicated that Biglan’s typology is correlated with differences in many other properties and serves as an appropriate mechanism to organize discipline-specific knowledge or epistemologies [ 38 ] and design and deliver courses for students with different learning style preferences [ 41 ]. Therefore, this classification provides a convenient framework to explore differences across disciplinary boundaries. In general, HA disciplines include engineering, HP disciplines include the so-called natural sciences, SA disciplines include the social sciences, and SP disciplines include the humanities [ 41 , 68 , 71 ].
In learning style research, it is difficult to select an instrument to measure the subjects’ learning styles [ 72 ]. The criteria used for the selection of a learning style instrument in this study include the following: 1) successful use of the instrument in previous studies, 2) demonstrated validity and reliability, 3) a match between the purpose of the instrument and the aim of this study and 4) open access to the questionnaire.
The Felder and Soloman’s ILS questionnaire, which was built based on the FSLSM, was adopted in the present study to investigate students’ learning styles across different disciplines. First, the FSLSM is recognized as the most commonly used model for measuring individual learning styles on a general scale [ 73 ] in higher education [ 74 ] and has remained popular for many years across different disciplines in university settings and beyond. In the age of personalized instruction, this model has breathed new life into areas such as blended learning [ 75 ], online distance learning [ 76 ], courseware design [ 56 ], and intelligent tutoring systems [ 77 , 78 ]. Second, the FSLSM is based on previous learning style models; the FSLSM integrates all their advantages and is, thus, more comprehensive in delineating students’ learning styles [ 79 , 80 ]. Third, the FSLSM has a good predictive ability with independent testing sets (i.e., unknown learning style objects) [ 17 ], which has been repeatedly proven to be a more accurate, reliable, and valid model than most other models for predicting students’ learning performance [ 10 , 80 ]. Fourth, the ILS is a free instrument that can be openly accessed online (URL: https://www.webtools.ncsu.edu/learningstyles/ ) and has been widely used in the research context [ 81 , 82 ].
The modified and translated version of the original ILS questionnaire includes 44 questions in total, and 11 questions correspond to each dimension of the Felder-Silverman model as follows: questions 1–11 correspond to dimension 1 (active vs. reflective), questions 12–22 correspond to dimension 2 (sensing vs. intuitive), questions 23–33 correspond to dimension 3 (visual vs. verbal), and questions correspond 34–44 to dimension 4 (sequential vs. global). Each question is followed by five choices on a five-point Likert scale ranging from “strongly agree with A (1)”, “agree with A (2)”, “neutral (3)”, “agree with B (4)” and “strongly agree with B (5)”. Option A and option B represent the two choices offered in the original ILS questionnaire.
Ethics statements
The free questionnaires were administered in a single session by specialized staff who collaborated on the investigation. The participants completed all questionnaires individually. The study procedures were in accordance with the ethical standards of the Helsinki Declaration and were approved by the Ethics Committee of the School of International Studies, Zhejiang University. All participants signed written informed consent to authorize their participation in this research. After completion of the informed consent form, each participant was provided a gift (a pen) in gratitude for their contribution and participation.
Data collection procedure
Before the questionnaires were distributed, the researchers involved in this study contacted faculty members from various departments and requested their help. After permission was given, the printed questionnaires were administered to students under the supervision of their teachers at the end of their English language course. The students were informed of the purpose and importance of the study and asked to carefully complete the questionnaires. The students were also assured that their personal information would be used for research purposes only. All students provided written informed consent (see S2 File ). After the questionnaires were completed and returned, they were thoroughly examined by the researchers such that problematic questionnaires could be identified and excluded from further analysis. All questionnaires eligible for the data analysis had to meet the following two standards: first, all questions must be answered, and second, the answered questions must reflect a reasonable logic. Regarding the few missing values, the median number of a given individual’s responses on 11 questions per dimension included in the ILS questionnaire was used to fill the void in each case. In statistics, using the median number to impute missing values is common and acceptable because missing values represent only a small minority of the entire dataset and are assumed to not have a large impact on the final results [ 83 , 84 ].
In total, 850 questionnaires were administered to the students, and 823 of these questionnaires were retrieved. Of the retrieved questionnaires, the remaining 790 questionnaires were identified as appropriate for further use. After data screening, these questionnaires were organized, and their respective results were translated into an Excel format.
Data analysis method
During the data analysis, as a library of the SVM, the free package LIBSVM ( https://www.csie.ntu.edu.tw/~cjlin/libsvm/ ) was first applied as an alternative method of data analysis. Then, a traditional ANOVA was performed to examine whether there was a main effect of academic discipline on Chinese students’ learning styles. ANOVA could be performed using SPSS, a strong data analysis software that supports a series of statistical analyses. In regard to the examination of the effect of a single or few independent variables, SPSS ANOVA can produce satisfactory results. However, SVM, a classic data mining algorithm, outperforms ANOVA for dataset in which a large number of variables with multidimensions are intertwined and their combined/collective effects influence the classification results. In this study, the research objective was to efficiently differentiate and detect the key features among the 44 factors. Alone, a single factor or few factors might not be significant enough to discriminate the learning styles among the different disciplines. Selected by the SVM, the effects of multiple features may collectively enhance the classification performance. Therefore, the reason for selecting SVM over ANOVA is that in the latter case, the responses on all questions in a single dimension are summed instead of treated as individual scores; thus, the by-item variation is concealed. In addition, the SVM is especially suitable for statistical analysis with high-dimensional factors (usually > 10; 44-dimensional factors were included in this study) and can detect the effects collectively imposed by a feature set [ 85 ].
Originally proposed in 1992 [ 86 ], the SVM is a supervised learning model related to machine learning algorithms that can be used for classification, data analysis, pattern recognition, and regression analysis. The SVM is an efficient classification model that optimally divides data into two categories and is ranked among the top methods in statistical theory due to its originality and practicality [ 85 ]. Due to its robustness, accurate classification, and prediction performance [ 87 – 89 ], the SVM has high reproducibility [ 90 , 91 ]. Due to the lack of visualization of the computing process of the SVM, the SVM has been described as a “black box” method [ 92 ]; however, future studies in the emerging field of explainable artificial intelligence can help solve this problem and convert this approach to a “glass box” method [ 67 ]. This algorithm has proven to have a solid theoretical foundation and excellent empirical application in the social sciences, including education [ 93 ] and natural language processing [ 94 ]. The mechanism underlying the SVM is also presented in Fig 2 .
Hyperplanes 1 and 2 are two regression lines that divide the data into two groups. Hyperplane 1 is considered the best fitting line because it maximizes the distance between the two groups.
https://doi.org/10.1371/journal.pone.0251545.g002
The SVM contains the following two modules: one module is a general-purpose machine learning method, and the other module is a domain-specific kernel function. The SVM training algorithm is used to build a training model that is then used to predict the category to which a new sample instance belongs [ 95 ]. When a set of training samples is given, each sample is given the label of one of two categories. To evaluate the performance of SVM models, a confusion matrix, which is a table describing the performance of a classifier on a set of test data for which the true values are known, is used (see Table 1 ).
https://doi.org/10.1371/journal.pone.0251545.t001
ACC represents the proportion of true results, including both positive and negative results, in the selected population;
SPE represents the proportion of actual negatives that are correctly identified as such;
SEN represents the proportion of actual positives that are correctly identified as such;
AUC is a ranking-based measure of classification performance that can distinguish a randomly chosen positive example from a randomly chosen negative example; and
F-measure is the harmonic mean of precision (another performance indicator) and recall.
The ACC is a good metric frequently applied to indicate the measurement of classification performance, but the combination of the SPE, SEN, AUC, F-measure and ACC may be a measure of enhanced performance assessment and was frequently applied in current studies [ 96 ]. In particular, the AUC is a good metric frequently applied to validate the measurement of the general performance of models [ 97 ]. The advantage of this measure is that it is invariant to relative class distributions and class-specific error costs [ 98 , 99 ]. Moreover, to some extent, the AUC is statistically consistent and more discriminating than the ACC with balanced and imbalanced real-world data sets [ 100 ], which is especially suitable for unequal samples, such as the HA-HP model in this study. After all data preparations were completed, the data used for the comparisons were extracted separately. First, the processed data of the training set were run by using optimized parameters. Second, the constructed model was used to predict the test set, and the five indicators of the fivefold cross-validation and fivefold average were obtained. Cross-validation is a general validation procedure used to assess how well the results of a statistical analysis generalize to an independent data set, which is used to evaluate the stability of the statistical model. K-fold cross-validation is commonly used to search for the best hyperparameters of SVM to achieve the highest accuracy performance [ 101 ]. In particular, fivefold, tenfold, and leave-one-out cross-validation are typically used versions of k-fold cross-validation [ 102 , 103 ]. Fivefold cross-validation was selected because fivefold validation can generally achieve a good prediction performance [ 103 , 104 ] and has been commonly used as a popular rule of thumb supported by empirical evidence [ 105 ]. In this study, five folds (groups) of subsets were randomly divided from the entire set by the SVM, and four folds (training sample) of these subsets were randomly selected to develop a prediction model, while the remaining one fold (test sample) was used for validation. The above functions were all implemented with Python Programming Language version 3.7.0 (URL: https://www.python.org/ ).
Then, SVM-RFE, which is an embedded feature selection strategy that was first applied to identify differentially expressed genes between patients and healthy individuals [ 106 ], was adopted. SVM-RFE has proven to be more robust to data overfitting than other feature selection techniques and has shown its power in many fields [ 107 ]. This approach works by removing one feature each time with the smallest weight iteratively to a feature rank until a group of highly weighted features were selected. After this feature selection procedure, several SVM models were again constructed based on these selected features. The performance of the new models is compared to that of the original models with all features included. The experimental process is provided in Fig 3 for the ease of reference.
https://doi.org/10.1371/journal.pone.0251545.g003
The classification results produced by SVM and the ranking of the top 20 features produced by SVM-RFE were listed in Table 2 . Twenty variables have been selected in this study for two reasons: a data-based reason and a literature-based reason. First, it is clear that models composed of 20 features generally have a better performance than the original models. The performance of models with more than 20 is negatively influenced. Second, SVM-based studies in the social sciences have identified 20 to 30 features as a good number for an optimal feature set [ 108 ], and 20 features were selected for inclusion in the optimal feature set [ 95 ]. Therefore, in this study, the top 20 features were selected for subsequent analysis, as proposed in previous analyses that yielded accepted measurement rates. These 20 features retained most of the useful information from all 44 factors but with fewer feature numbers, which showed satisfactory representation [ 96 ].
https://doi.org/10.1371/journal.pone.0251545.t002
Results of RQ (1) What are the differentiability levels among different academic disciplines based on students’ learning styles?
To further measure the performance of the differentiability among students’ disciplines, the collected data were examined with the SVM algorithm. As shown in Table 2 , the five performance indicators, namely, the ACC, SPE, SEN, AUC and F-measure, were utilized to measure the SVM models. Regarding the two general performance indicators, i.e., the ACC value and AUC value, the HA-HP, HA-SA, and HA-SP-based models yielded a classification capacity of approximately 70.00%, indicating that the students in these disciplines showed a relatively large difference. In contrast, the models based on the H-S, A-P, HP-SA, HP-SP, and SA-SP disciplines only showed a moderate classification capacity (above 55.00%). This finding suggests that these five SVM models were not as effective as the other three models in differentiating students among these disciplines based on their learning styles. The highest ACC and AUC values were obtained in the model based on the HA-HP disciplines, while the lowest values were obtained in the model based on the HP-SA disciplines. As shown in Table 2 , the AUCs of the different models ranged from 57.76% (HP-SA) to 73.97% (HA-HP).
To compare the results of the SVM model with another statistical analysis, an ANOVA was applied. Prior to the main analysis, the students’ responses in each ILS dimension were summed to obtain a composite score. All assumptions of ANOVA were checked, and no serious violations were observed. Then, an ANOVA was performed with academic discipline as the independent variable and the students’ learning styles as the dependent variable. The results of the ANOVA showed that there was no statistically significant difference in the group means of the students’ learning styles in Dimension 1, F(3, 786) = 2.56, p = .054, Dimension 2, F(3, 786) = 0.422, p = .74, or Dimension 3, F(3, 786) = 0.90, p = .443. However, in Dimension 4, a statistically significant difference was found in the group means of the students’ learning styles, F (3, 786) = 0.90, p = .005. As the samples in the four groups were unbalanced, post hoc comparisons using Scheffé’s method were performed, demonstrating that the means of the students’ learning styles significantly differed only between the HA (M = 31.04, SD = 4.986) and SP (M = 29.55, SD = 5.492) disciplines, 95.00% CI for MD [0.19, 2.78], p = .016, whereas the other disciplinary models showed no significant differences. When compared with the results obtained from the SVM models, the three models (HA-HP, HA-SA, and HA-SP models) presented satisfactory differentiability capability of approximately 70.00% based on the five indicators.
In the case of a significant result, it was difficult to determine which questions were representative of the significant difference. With a nonsignificant result, it was possible that certain questions might be relevant in differentiating the participants. However, this problem was circumvented in the SVM, where each individual question was treated as a variable and a value was assigned to indicate its relative importance in the questionnaire. Using SVM also circumvented the inherent problems with traditional significance testing, especially the reliance on p-values, which might become biased in the case of multiple comparisons [ 109 ].
Results of RQ (2) What are the key features that can be selected to determine the collective impact on differentiation by a machine learning algorithm?
To examine whether the model performance improved as a result of this feature selection procedure, the 20 selected features were submitted to another round of SVM analysis. The same five performance indicators were used to measure the model performance (see Table 2 ). By comparing the performance of the SVM model and that of the SVM-RFE model presented in Table 2 , except for the HA-SP model, all other models presented a similar or improved performance after the feature selection process. In particular, the improvement in the HA-HP and HP-SA models was quite remarkable. For instance, in the HA-HP model, the ACC value increased from 69.32% in the SVM model to 82.59% in the SVM-RFE model, and the AUC score substantially increased from 73.97% in the SVM model to 89.13% in the SVM-RFE model. This finding suggests that the feature selection process refined the model’s classification accuracy and that the 20 features selected, out of all 44 factors, carry substantive information that might be informative for exploring disciplinary differences. Although results for the indicators of the 20 selected features were not very high, all five indicators above 65.00% showed that the model was still representative because only 20 of 44 factors could present the classification capability. Considering that there was a significant reduction in the number of questions used for the model construction in SVM-RFE (compared with those used for the SVM model), the newly identified top 20 features by SVM-RFE were effective enough to preserve the differential ability of all 44 questions. Thus, these newly identified top 20 factors could be recognized as key differential features for distinguishing two distinct disciplines.
To identify these top 20 features in eight models (see Table 2 ), SVM-RFE was applied to rank order all 44 features contained in the ILS questionnaire. To facilitate a detailed understanding of what these features represent, the questions related to the top 20 features in the HA-HP model are listed in Table 3 for ease of reference.
https://doi.org/10.1371/journal.pone.0251545.t003
Results of RQ (3) What are the collective impacts of optimal feature sets?
The collective impacts of optimal feature sets could be interpreted from four aspects, namely, the complexities of students’ learning styles, the appropriate choice of SVM, the ranking of SVM-RFE and multiple detailed comparisons between students from different disciplines. First, the FSLSM considers the fact that students’ learning styles are shaped by a series of factors during the growth process, which intertwine and interact with each other. Considering the complex dynamics of the learning style, selecting an approach that could detect the combined effects of a group of variables is needed. Second, recent years have witnessed the emergence of data mining approaches to explore students learning styles [ 28 , 48 – 50 , 110 ]. Specifically, as one of the top machine learning algorithms, the SVM excels in identifying the combined effects of high-order factors [ 87 ]. In this study, the SVM has proven to perform well in classifying students’ learning styles across different disciplines, with every indicator being acceptable. Third, the combination of SVM with RFE could enable the simultaneous discovery of multiple features that collectively determine classification. Notably, although SVM-FRE could rank the importance of the features, they should be regarded as an entire optimal feature set. In other words, the combination of these 20 features, rather than a single factor, could differentiate students’ learning styles across different academic disciplines. Last but not least, the multiple comparisons between different SVM models of discipline provide the most effective learning style factors, giving researchers clues to the nuanced differences between students’ learning styles. It can be seen that students from different academic disciplines understand, see and reflect things from individualized perspectives. The 20 most effective factors for all models scattered within 1 to 44, verifying students’ different learning styles in 4 dimensions. Therefore, the FSLSM provides a useful and effective tool for evaluating students’ learning styles from a rather comprehensive point of view.
The following discussions address the three research questions explored in the current study.
Levels of differentiability among various academic disciplines based on students’ learning styles with SVM
The results suggest that SVM is an effective approach for classification in the blended learning context in which students with diverse disciplinary backgrounds can be distinguished from each other according to their learning styles. All performance indicators presented in Tables 2 and 3 remain above the baseline of 50.00%, suggesting that between each two disciplines, students’ learning style differences can be identified. To some extent, these differences can be identified with a relatively satisfactory classification capability (e.g., 69.32% of the ACC and 73.97% of the AUC in the HA-HP model shown in Table 2 ). Further support for the SVM algorithm is obtained from the SVM-RFE constructed to assess the rank of the factors’ classification capacity, and all values also remained above the baseline value, while some values reached a relatively high classification capability (e.g., 82.59% of the ACC and 89.13% of the AUC in the HA-HP model shown in Table 2 ). While the results obtained mostly show a moderate ACC and AUC, they still provide some validity evidence supporting the role of SVM as an effective binary classifier in the educational context. However, while these differences are noteworthy, the similarities among students in different disciplines also deserve attention. The results reported above indicate that in some disciplines, the classification capacity is not relatively high; this was the case for the model based on the SA-SP disciplines.
Regarding low differentiability, one explanation might be the indistinct classification of some emerging “soft disciplines.” It was noted that psychology, for example, could be identified as “a discipline that can be considered predominantly ‘soft’ and slightly ‘purer’ than ‘applied’ in nature” [ 111 ] (p. 43–53), which could have blurred the line between the SA and SP disciplines. As there is now no impassable gulf separating the SA and SP disciplines, their disciplinary differences may have diminished in the common practice of lecturing in classrooms. Another reason comes from the different cultivation models of “soft disciplines” and “hard disciplines” for sample students. In their high school, sample students are generally divided into liberal art students and science students and are then trained in different environments of knowledge impartation. The two-year unrelenting and intensive training makes it possible for liberal art students to develop a similar thinking and cognitive pattern that is persistent. After the college entrance examination, most liberal art students select SA or SP majors. However, a year or more of study in university does not exert strong effects on their learning styles, which explains why a multitude of researchers have traditionally investigated the SA and SP disciplines together, calling them simply “social science” or “soft disciplines” compared with “natural science” or “hard disciplines”. There have been numerous contributions pointing out similarities in the learning styles of students from “soft disciplines” [ 37 , 112 – 114 ]. However, students majoring in natural science exhibit considerable differences in learning styles, demonstrating that the talent cultivation model of “hard disciplines” in universities is to some extent more influential on students’ learning styles than that of the “soft disciplines”. Further compelling interpretations of this phenomenon await only the development of a sufficient level of accumulated knowledge among scholars in this area.
In general, these results are consistent with those reported in many previous studies based on the Felder-Silverman model. These studies tested the precision of different computational approaches in identifying and differentiating the learning styles of students. For example, by means of a Bayesian network (BN), an investigation obtained an overall precision of 58.00% in the active/reflective dimension, 77.00% in the sensing/intuitive dimension and 63.00% in the sequential/global dimension (the visual/verbal dimension was not considered) [ 81 ]. With the help of the keyword attributes of learning objects selected by students, a precision of 70.00% in the active/reflective dimension, 73.30% in the sensing/intuitive dimension, 73.30% in the sequential/global dimension and 53.30% in the visual/verbal dimension was obtained [ 115 ].
These results add to a growing body of evidence expanding the scope of the application of the SVM algorithm. Currently, the applications of the SVM algorithm still reside largely in engineering or other hard disciplines despite some tentative trials in the humanities and social sciences [ 26 ]. In addition, as cross-disciplines increase in current higher education, it is essential to match the tailored learning styles of students and researchers studying interdisciplinary subjects, such as the HA, HP, SA and SP disciplines. Therefore, the current study is the first to incorporate such a machine learning algorithm into interdisciplinary blended learning and has broader relevance to further learning style-related theoretical or empirical investigations.
Verification of the features included in the optimal feature sets
Features included in the optimal feature sets provided mixed findings compared with previous studies. Some of the 20 identified features are verified and consistent with previous studies. A close examination of the individual questions included in the feature sets can offer some useful insights into the underlying psychological processes. For example, in six of the eight models constructed, Question 1 (“I understand something better after I try it out/think it through”) appears as the feature with the number 1 ranking, highlighting the great importance attached to this question. This question mainly reflects the dichotomy between experimentation and introspection. A possible revelation is that students across disciplines dramatically differ in how they process tasks, with the possible exception of the SA-SP disciplines. This difference has been supported by many previous studies. For example, it was found that technical students tended to be more tactile than those in the social sciences [ 116 ], and engineering students (known as HA in this study) were more inclined toward concrete and pragmatic learning styles [ 117 ]. Similarly, it was explored that engineering students prefer “a logical learning style over visual, verbal, aural, physical or solitary learning styles” [ 37 ] (p. 122), while social sciences (known as SA in this study) students prefer a social learning style to a logical learning style. Although these studies differ in their focus to a certain degree, they provide an approximate idea of the potential differences among students in their relative disciplines. In general, students in the applied disciplines show a tendency to experiment with tasks, while those in the pure disciplines are more inclined towards introspective practices, such as an obsession with theories. For instance, in Biglan’s taxonomy of academic disciplines, students in HP disciplines prefer abstract rules and theories, while students in SA disciplines favor application [ 67 ]. Additionally, Question 10 (“I find it easier to learn facts/to learn concepts”) is similar to Question 1, as both questions indicate a certain level of abstraction or concreteness. The difference between facts and concepts is closely related to the classification difference between declarative knowledge and procedural knowledge in cognitive psychology [ 35 , 38 ]. Declarative knowledge is static and similar to facts, while procedural knowledge is more dynamic and primarily concerned with operational steps. Students’ preferences for facts or concepts closely correspond to this psychological distinction.
In addition, Questions 2, 4, 7, and 9 also occur frequently in the 20 features selected for the different models. Question 2 (“I would rather be considered realistic/innovative”) concerns taking chances. This question reflects a difference in perspective, i.e., whether the focus should be on obtaining pragmatic results or seeking original solutions. This difference cannot be easily connected to the disciplinary factor. Instead, there are numerous factors, e.g., genetic, social and psychological factors, that may play a strong role in defining this trait. The academic discipline only serves to strengthen or diminish this difference. For instance, decades of research in psychology have shown that males are more inclined towards risk taking than females [ 118 – 121 ]. A careful examination of the current academic landscape reveals a gender difference; more females choose soft disciplines than males, and more males choose hard disciplines than females. This situation builds a disciplinary wall classifying students into specific categories, potentially strengthening the disciplinary effect. For example, Question 9 (“In a study group working on difficult material, I am more likely to jump in and contribute ideas/sit back and listen”) emphasizes the distinction between active participation and introspective thinking, reflecting an underlying psychological propensity in blended learning. Within this context, the significance of this question could also be explained by the psychological evaluation of “loss and gain”, as students’ different learning styles are associated with expected reward values and their internal motivational drives, which are determined by their personality traits [ 122 ]. When faced with the risk of “losing face”, whether students will express their ideas in front of a group of people depends largely on their risk and stress management capabilities and the presence of an appropriate motivation system.
The other two questions also convey similar messages regarding personality differences. Question 4 concerns how individuals perceive the world, while Question 7 concerns the preferred modality of information processing. Evidence of disciplinary differences in these respects was also reported [ 35 , 123 – 125 ]. The other questions, such as Questions 21, 27, and 39, show different aspects of potential personality differences and are mostly consistent with the previous discussion. This might also be a vivid reflection of the multi-faceted effects of blended learning, which may differ in their consonance with the features of each discipline. First, teachers from different domains use technology in different ways, and student from different disciplines may view blended learning differently. For instance, the characteristics of soft-applied fields entail specialized customization in blended courses, further broadening the gulf between different subjects [ 126 ]. Second, although blended learning is generally recognized as a stimulus to students’ innovation [ 127 ], some students who are used to an instructivist approach in which the educator acts as a ‘sage on the stage’ will find it difficult to adapt to a social constructivist approach in which the educator serves as a ‘guide on the side’ [ 128 ]. This difficulty might not only negatively affect students’ academic performance but also latently magnify the effects of different academic disciplines.
Interpretation of the collective impact of optimal feature sets
In each SVM model based on a two-discipline model, the 20 key features (collectively known as an optimal feature set) selected exert a concerted effect on students’ learning styles across different disciplines (see Table 2 ). A broad examination of the distribution of collective impact of each feature set with 20 features in the eight discipline models suggests that it is especially imperative considering the emerging cross-disciplines in academia. Current higher education often involves courses with crossed disciplines and students with diverse disciplinary backgrounds. In addition, with the rise of technology-enhanced learning, the design of personalized tutoring systems requires more nuanced information related to student attributes to provide greater adaptability [ 59 ]. By identifying these optimal feature sets, such information becomes accessible. Therefore, understanding such interdisciplinary factors and designing tailor-made instructions are essential for promoting learning success [ 9 ]. For example, in an English language classroom in which the students are a blend of HP and SP disciplines, instructors might consider integrating a guiding framework at the beginning of the course and stepwise guidelines during the process such that the needs of both groups are met. With the knowledge that visual style is dominant across disciplines, instructors might include more graphic presentations (e.g., Question 11) in language classrooms rather than continue to use slides or boards filled with words. Furthermore, to achieve effective communication with students and deliver effective teaching, instructors may target these students’ combined learning styles. While some methods are already practiced in real life, this study acts as a further reminder of the rationale underlying these practices and thus increases the confidence of both learners and teachers regarding these practices. Therefore, the practical implications of this study mainly concern classroom teachers and educational researchers, who may draw some inspiration for interdisciplinary curriculum design and the tailored application of learning styles to the instructional process.
Conclusions
This study investigated learning style differences among students with diverse disciplinary backgrounds in a blended English language course based on the Felder-Silverman model. By introducing a novel machine learning algorithm, namely, SVM, for the data analysis, the following conclusions can be reached. First, the multiple performance indicators used in this study confirm that it is feasible to apply learning styles to differentiate various disciplines in students’ blended learning processes. These disciplinary differences impact how students engage in their blended learning activities and affect students’ ultimate blended learning success. Second, some questions in the ILS questionnaire carry more substantive information about students’ learning styles than other questions, and certain underlying psychological processes can be derived. These psychological processes reflect students’ discipline-specific epistemologies and represent the possible interaction between the disciplinary background and learning style. In addition, the introduction of SVM in this study can provide inspiration for future studies of a similar type along with the theoretical significance of the above findings.
Despite the notable findings of this study, it is subject to some limitations that may be perfected in further research. First, the current analysis examined the learning styles without allowing for the effects of other personal or contextual factors. The educational productivity model proposed by Walberg underlines the significance of the collected influence of contextual factors on individuals’ learning [ 129 ]. For example, teachers from different backgrounds and academic disciplines are inclined to select various teaching methods and to create divergent learning environments [ 130 ], which should also be investigated thoroughly. The next step is therefore to take into account the effects of educational background, experience, personality and learning experience to gain a more comprehensive understanding of students’ learning process in the blended setting.
In conclusion, the findings of this research validate previous findings and offer new perspectives on students’ learning styles in a blended learning environment, which provides future implications for educational researchers, policy makers and educational practitioners (i.e., teachers and students). For educational researchers, this study not only highlights the merits of using machine learning algorithms to explore students’ learning styles but also provides valuable information on the delicate interactions between blended learning, academic disciplines and learning styles. For policy makers, this analysis provides evidence for a more inclusive but personalized educational policy. For instance, in addition to learning styles, the linkage among students’ education in different phases should be considered. For educational practitioners, this study plays a positive role in promoting student-centered and tailor-made teaching. The findings of this study can help learners of different disciplines develop a more profound understanding of their blended learning tendencies and assist teachers in determining how to bring students’ learning styles into full play pedagogically, especially in interdisciplinary courses [ 131 – 134 ].
Supporting information
https://doi.org/10.1371/journal.pone.0251545.s001
S2 File. Informed consent for participants.
https://doi.org/10.1371/journal.pone.0251545.s002
S1 Dataset.
https://doi.org/10.1371/journal.pone.0251545.s003
Acknowledgments
The authors would like to thank the anonymous reviewers for their constructive comments on this paper and Miss Ying Zhou for her suggestions during the revision on this paper.
- View Article
- Google Scholar
- 15. Dunn R, Dunn K, Perrin J. Teaching young children through their individual learning styles. Boston, MA: Allyn & Bacon, Inc;1994.
- 17. Curry L. Integrating concepts of cognitive or learning style: A review with attention to psychometric standards. Ottawa, ON: Canadian College of Health Service Executives;1987.
- 18. Kolb DA. Experiential learning: experience as the source of learning and development. Englewood Cliffs, NJ: Prentice-Hall;1984.
- 21. Anitha D, Deisy C, Lakshmi SB, Meenakshi MK. Proposing a Classification Methodology to Reduce Learning Style Combinations for Better Teaching and Learning. 2014 IEEE Sixth International Conference on Technology for Education, Amritapuri, India, 2014; 208–211. https://doi.org/10.1109/T4E.2014.5
- 23. Kuljis J, Liu F. A comparison of learning style theories on the suitability for e-learning. Web Technologies, Applications, and Services, 2005; 191–197. Retrieved from: https://www.mendeley.com/catalogue/da014340-bfb5-32d1-b144-73545a86d440/
- 30. Richardson JTE. Researching student learning. Buckingham: SRHE and Open University Press; 2000.
- 33. Marton F, Säljö R. Approaches to learning. Edinburgh: Scottish Academic Press; 1984.
- PubMed/NCBI
- 52. Thorne K. Blended learning: How to integrate online and traditional learning, London: Kogan Page; 2003.
- 59. Graf S. Adaptivity in learning management systems focusing on learning styles. Vienna, Austria: Vienna University of Technology;2007.
- 79. Crockett K, Latham A, Mclean D, Bandar Z, O’Shea J. On predicting learning styles in conversational intelligent tutoring systems using fuzzy classification trees. IEEE International Conference on Fuzzy Systems. 2011; 2481–2488. https://doi.org/10.1109/FUZZY.2011.6007514
- 82. Coffield, Ecclestone K, Moseley, Hall E. Learning styles and pedagogy in post 16 education: A critical and systematic review. London, UK: Learning and Skills Research Centre;2004.
- 86. Acuna E, Rodriguez C. The Treatment of Missing Values and its Effect on Classifier Accuracy. In Banks D, House L, McMorris FR, Arabie P, Gaul W, editors. Classification, Clustering, and Data Mining Applications. Springer Berlin Heidelberg;2004. p. 639–647.
- 88. Boser BE, Guyon IM, Vapnik VN. A training algorithm for optimal margin classifiers. A training algorithm for optimal margin classifiers. Proceedings of The Fifth Annual Workshop on Computational Learning Theory. New York: ACM Press, 1992: 144–152. https://doi.org/10.1145/130385.130401
- 100. Maloof MA. Learning when data sets are imbalanced and when costs are unequal and unknown. Proceedings of the 20th International Conference on Machine Learning (ICML-2003). 2003. Retrieved from: http://www.site.uottawa.ca/~nat/Workshop2003/maloof-icml03-wids.pdf
- 101. Yan L, Rodier R, Mozer M, Wolniewicz R. Optimizing classifier performance via the Wilcoxon-Mann-Withney statistics. Proceedings of the 20th International Conference on Machine Learning (ICML-2003). 2003. Retrieved from: http://citeseerx.ist.psu.edu/viewdoc/download?doi=10.1.1.324.1091&rep=rep1&type=pdf
- 105. James G, Witten D, Hastie T, Tibshirani R. An introduction to statistical learning: with applications in R (Springer Texts in Statistics). 1st ed. New York, NY: Springer Verlag;2013.
- 118. Anderson JR. Language, memory, and thought. Mahwah, NJ: Lawrence Erlbaum Associates;1976.
- 119. Anderson JR. The architecture of cognition. Cambridge, Massachusetts: Harvard University Press;1983.

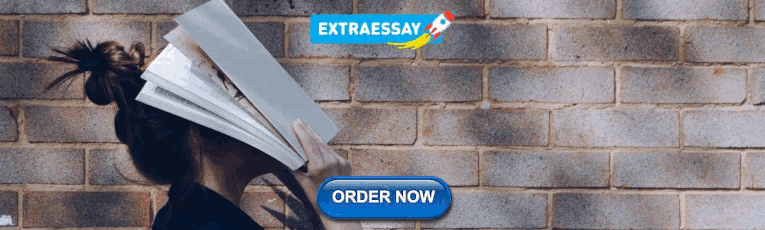
Thesis Statements
What this handout is about.
This handout describes what a thesis statement is, how thesis statements work in your writing, and how you can craft or refine one for your draft.
Introduction
Writing in college often takes the form of persuasion—convincing others that you have an interesting, logical point of view on the subject you are studying. Persuasion is a skill you practice regularly in your daily life. You persuade your roommate to clean up, your parents to let you borrow the car, your friend to vote for your favorite candidate or policy. In college, course assignments often ask you to make a persuasive case in writing. You are asked to convince your reader of your point of view. This form of persuasion, often called academic argument, follows a predictable pattern in writing. After a brief introduction of your topic, you state your point of view on the topic directly and often in one sentence. This sentence is the thesis statement, and it serves as a summary of the argument you’ll make in the rest of your paper.
What is a thesis statement?
A thesis statement:
- tells the reader how you will interpret the significance of the subject matter under discussion.
- is a road map for the paper; in other words, it tells the reader what to expect from the rest of the paper.
- directly answers the question asked of you. A thesis is an interpretation of a question or subject, not the subject itself. The subject, or topic, of an essay might be World War II or Moby Dick; a thesis must then offer a way to understand the war or the novel.
- makes a claim that others might dispute.
- is usually a single sentence near the beginning of your paper (most often, at the end of the first paragraph) that presents your argument to the reader. The rest of the paper, the body of the essay, gathers and organizes evidence that will persuade the reader of the logic of your interpretation.
If your assignment asks you to take a position or develop a claim about a subject, you may need to convey that position or claim in a thesis statement near the beginning of your draft. The assignment may not explicitly state that you need a thesis statement because your instructor may assume you will include one. When in doubt, ask your instructor if the assignment requires a thesis statement. When an assignment asks you to analyze, to interpret, to compare and contrast, to demonstrate cause and effect, or to take a stand on an issue, it is likely that you are being asked to develop a thesis and to support it persuasively. (Check out our handout on understanding assignments for more information.)
How do I create a thesis?
A thesis is the result of a lengthy thinking process. Formulating a thesis is not the first thing you do after reading an essay assignment. Before you develop an argument on any topic, you have to collect and organize evidence, look for possible relationships between known facts (such as surprising contrasts or similarities), and think about the significance of these relationships. Once you do this thinking, you will probably have a “working thesis” that presents a basic or main idea and an argument that you think you can support with evidence. Both the argument and your thesis are likely to need adjustment along the way.
Writers use all kinds of techniques to stimulate their thinking and to help them clarify relationships or comprehend the broader significance of a topic and arrive at a thesis statement. For more ideas on how to get started, see our handout on brainstorming .
How do I know if my thesis is strong?
If there’s time, run it by your instructor or make an appointment at the Writing Center to get some feedback. Even if you do not have time to get advice elsewhere, you can do some thesis evaluation of your own. When reviewing your first draft and its working thesis, ask yourself the following :
- Do I answer the question? Re-reading the question prompt after constructing a working thesis can help you fix an argument that misses the focus of the question. If the prompt isn’t phrased as a question, try to rephrase it. For example, “Discuss the effect of X on Y” can be rephrased as “What is the effect of X on Y?”
- Have I taken a position that others might challenge or oppose? If your thesis simply states facts that no one would, or even could, disagree with, it’s possible that you are simply providing a summary, rather than making an argument.
- Is my thesis statement specific enough? Thesis statements that are too vague often do not have a strong argument. If your thesis contains words like “good” or “successful,” see if you could be more specific: why is something “good”; what specifically makes something “successful”?
- Does my thesis pass the “So what?” test? If a reader’s first response is likely to be “So what?” then you need to clarify, to forge a relationship, or to connect to a larger issue.
- Does my essay support my thesis specifically and without wandering? If your thesis and the body of your essay do not seem to go together, one of them has to change. It’s okay to change your working thesis to reflect things you have figured out in the course of writing your paper. Remember, always reassess and revise your writing as necessary.
- Does my thesis pass the “how and why?” test? If a reader’s first response is “how?” or “why?” your thesis may be too open-ended and lack guidance for the reader. See what you can add to give the reader a better take on your position right from the beginning.
Suppose you are taking a course on contemporary communication, and the instructor hands out the following essay assignment: “Discuss the impact of social media on public awareness.” Looking back at your notes, you might start with this working thesis:
Social media impacts public awareness in both positive and negative ways.
You can use the questions above to help you revise this general statement into a stronger thesis.
- Do I answer the question? You can analyze this if you rephrase “discuss the impact” as “what is the impact?” This way, you can see that you’ve answered the question only very generally with the vague “positive and negative ways.”
- Have I taken a position that others might challenge or oppose? Not likely. Only people who maintain that social media has a solely positive or solely negative impact could disagree.
- Is my thesis statement specific enough? No. What are the positive effects? What are the negative effects?
- Does my thesis pass the “how and why?” test? No. Why are they positive? How are they positive? What are their causes? Why are they negative? How are they negative? What are their causes?
- Does my thesis pass the “So what?” test? No. Why should anyone care about the positive and/or negative impact of social media?
After thinking about your answers to these questions, you decide to focus on the one impact you feel strongly about and have strong evidence for:
Because not every voice on social media is reliable, people have become much more critical consumers of information, and thus, more informed voters.
This version is a much stronger thesis! It answers the question, takes a specific position that others can challenge, and it gives a sense of why it matters.
Let’s try another. Suppose your literature professor hands out the following assignment in a class on the American novel: Write an analysis of some aspect of Mark Twain’s novel Huckleberry Finn. “This will be easy,” you think. “I loved Huckleberry Finn!” You grab a pad of paper and write:
Mark Twain’s Huckleberry Finn is a great American novel.
You begin to analyze your thesis:
- Do I answer the question? No. The prompt asks you to analyze some aspect of the novel. Your working thesis is a statement of general appreciation for the entire novel.
Think about aspects of the novel that are important to its structure or meaning—for example, the role of storytelling, the contrasting scenes between the shore and the river, or the relationships between adults and children. Now you write:
In Huckleberry Finn, Mark Twain develops a contrast between life on the river and life on the shore.
- Do I answer the question? Yes!
- Have I taken a position that others might challenge or oppose? Not really. This contrast is well-known and accepted.
- Is my thesis statement specific enough? It’s getting there–you have highlighted an important aspect of the novel for investigation. However, it’s still not clear what your analysis will reveal.
- Does my thesis pass the “how and why?” test? Not yet. Compare scenes from the book and see what you discover. Free write, make lists, jot down Huck’s actions and reactions and anything else that seems interesting.
- Does my thesis pass the “So what?” test? What’s the point of this contrast? What does it signify?”
After examining the evidence and considering your own insights, you write:
Through its contrasting river and shore scenes, Twain’s Huckleberry Finn suggests that to find the true expression of American democratic ideals, one must leave “civilized” society and go back to nature.
This final thesis statement presents an interpretation of a literary work based on an analysis of its content. Of course, for the essay itself to be successful, you must now present evidence from the novel that will convince the reader of your interpretation.
Works consulted
We consulted these works while writing this handout. This is not a comprehensive list of resources on the handout’s topic, and we encourage you to do your own research to find additional publications. Please do not use this list as a model for the format of your own reference list, as it may not match the citation style you are using. For guidance on formatting citations, please see the UNC Libraries citation tutorial . We revise these tips periodically and welcome feedback.
Anson, Chris M., and Robert A. Schwegler. 2010. The Longman Handbook for Writers and Readers , 6th ed. New York: Longman.
Lunsford, Andrea A. 2015. The St. Martin’s Handbook , 8th ed. Boston: Bedford/St Martin’s.
Ramage, John D., John C. Bean, and June Johnson. 2018. The Allyn & Bacon Guide to Writing , 8th ed. New York: Pearson.
Ruszkiewicz, John J., Christy Friend, Daniel Seward, and Maxine Hairston. 2010. The Scott, Foresman Handbook for Writers , 9th ed. Boston: Pearson Education.
You may reproduce it for non-commercial use if you use the entire handout and attribute the source: The Writing Center, University of North Carolina at Chapel Hill
Make a Gift

Education Thesis Statement
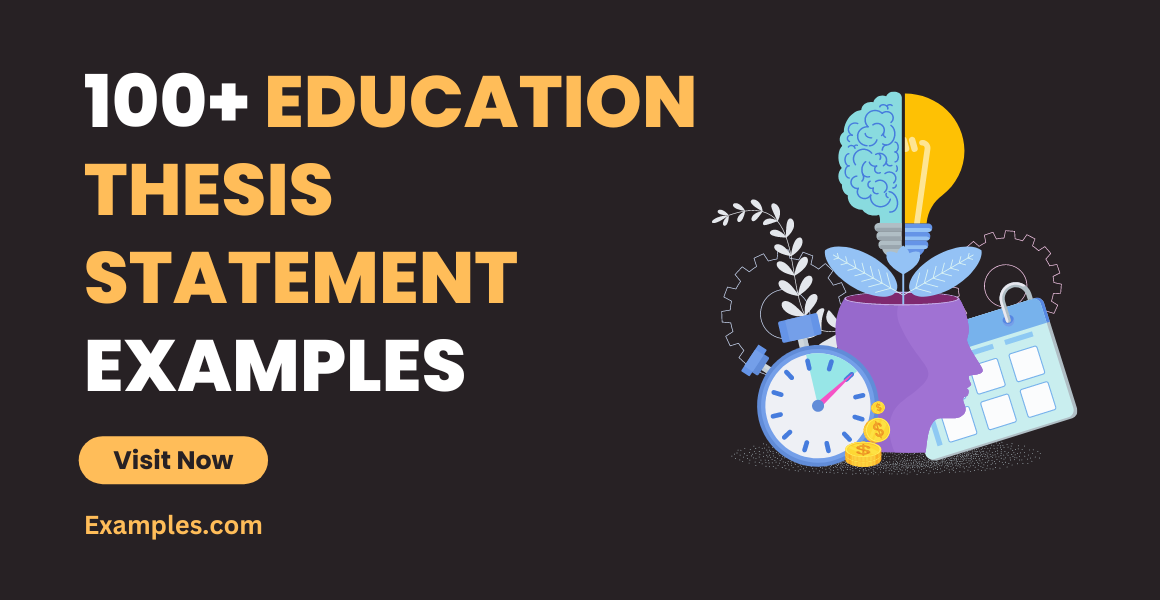
Crafting a strong thesis statement is essential for any successful educational essay or research paper. This one or two-sentence assertion forms the backbone of your argument, providing a concise summary of the point you intend to make. Whether you’re exploring the impact of technology in classrooms or analyzing the effectiveness of early childhood education, a well-structured thesis statement serves as a roadmap, guiding both.
What is Education Thesis Statement – Definition
An education thesis statement is a concise, focused, and arguable statement that presents the main idea or argument of an essay, research paper, or academic work related to the field of education. It outlines the scope of the study and provides a roadmap for the reader to understand the purpose and direction of the paper.
What is a Good Thesis Statement about Education
A comprehensive integration of technology in classrooms enhances students’ engagement, knowledge retention, and critical thinking skills, ultimately transforming traditional educational paradigms.”
What is an Example of an Education Topic Thesis Statement
“Implementing inclusive education policies in primary schools leads to improved academic outcomes for students with disabilities, fostering a more diverse and supportive learning environment.”
Remember, a good thesis statement is specific, debatable, and gives a clear indication of the focus of your paper. It should also be supported by evidence and analysis throughout the essay.
100 Education Statement Examples
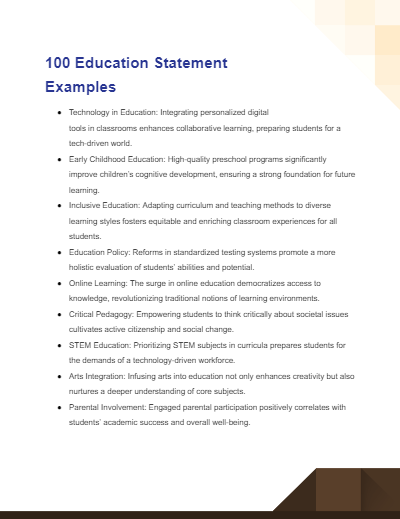
Size: 220 KB
Crafting effective education thesis statements is pivotal in academic writing. These succinct sentences encapsulate the core concepts of your research, guiding your paper’s trajectory. From dissecting teaching methodologies to examining education’s societal impacts, a well-structured thesis statement is a beacon that illuminates your scholarly journey.
- Technology in Education : Integrating personalized digital tools in classrooms enhances collaborative learning, preparing students for a tech-driven world.
- Early Childhood Education : High-quality preschool programs significantly improve children’s cognitive development, ensuring a strong foundation for future learning.
- Inclusive Education : Adapting curriculum and teaching methods to diverse learning styles fosters equitable and enriching classroom experiences for all students.
- Education Policy : Reforms in standardized testing systems promote a more holistic evaluation of students’ abilities and potential.
- Online Learning : The surge in online education democratizes access to knowledge, revolutionizing traditional notions of learning environments.
- Critical Pedagogy : Empowering students to think critically about societal issues cultivates active citizenship and social change.
- STEM Education : Prioritizing STEM subjects in curricula prepares students for the demands of a technology-driven workforce.
- Arts Integration : Infusing arts into education not only enhances creativity but also nurtures a deeper understanding of core subjects.
- Parental Involvement : Engaged parental participation positively correlates with students’ academic success and overall well-being.
- Higher Education Costs : Exploring alternative funding models is crucial to make higher education accessible and affordable for all.
- Global Education : Fostering cross-cultural awareness in schools cultivates tolerance, empathy, and a broader worldview among students.
- Special Education : Tailoring teaching strategies to the needs of students with disabilities empowers them to achieve their full potential.
- Motivation and Learning : Understanding motivational factors improves teaching methods and student engagement in the classroom.
- Physical Education : Incorporating regular physical activity into the curriculum promotes not only fitness but also cognitive and emotional development.
- Education and Employment : Analyzing the relationship between education levels and job prospects reveals the role of education in economic mobility.
- Bilingual Education : Studying the effects of bilingual instruction on cognitive development highlights the benefits of multilingualism in education.
- Gender Disparities in Education : Addressing gender biases in curricula and teaching practices contributes to more equitable educational experiences.
- Teacher Training : Enhancing teacher preparation programs leads to more effective classroom management and student engagement.
- Education and Social Media : Analyzing the impact of social media on students’ learning habits reveals new avenues for interactive and self-directed learning.
- Education and Mental Health : Integrating mental health education into the curriculum helps reduce stigma and promotes students’ psychological well-being.
- Education and Sustainability : Incorporating environmental education empowers students to become responsible stewards of the planet.
- Literacy Development : Investigating early literacy interventions highlights the importance of foundational reading skills in later academic success.
- Civic Education : Teaching civics fosters active participation in democratic processes and shapes informed and responsible citizens.
- Education for Special Needs Students : Creating individualized education plans (IEPs) enhances the learning experience for students with diverse abilities.
- Globalization and Education : Exploring how globalization affects educational policies and practices prepares students for a globalized world.
- Education and Poverty : Investigating the link between education and poverty reduction underscores the role of education in breaking the cycle of disadvantage.
- Character Education : Nurturing qualities like empathy, integrity, and resilience in students contributes to holistic personal and ethical development.
- Standardized Curriculum vs. Personalized Learning : Evaluating the benefits and drawbacks of standardized versus personalized learning approaches in classrooms.
- Education Technology Ethics : Examining the ethical implications of using student data in educational technology applications.
- Education and Immigration : Studying the educational challenges and opportunities faced by immigrant students in host countries.
- Critical Thinking Education : Integrating critical thinking skills into curricula prepares students to analyze and evaluate information independently.
- Education and Cultural Heritage : Incorporating cultural heritage education preserves traditions and fosters cultural pride among students.
- Education Funding Allocation : Investigating the impact of equitable distribution of funding on educational outcomes in different communities.
- Education and Neurodiversity : Creating inclusive classrooms that accommodate neurodiverse students promotes a more accepting society.
- Sexual Education : Implementing comprehensive sexual education equips students with vital knowledge for making informed decisions.
- Education and Democracy : Understanding the role of education in nurturing informed citizenship and active participation in democratic processes.
- Education and Indigenous Knowledge : Integrating indigenous knowledge systems into curricula honors diverse worldviews and promotes cultural understanding.
- Home Schooling vs. Public Schooling : Comparing the academic and social outcomes of students educated at home versus traditional schools.
- Peer-to-Peer Learning : Exploring the effectiveness of peer mentoring programs in enhancing students’ academic performance and social skills.
- Education and Artificial Intelligence : Analyzing the potential of AI to personalize learning experiences and address individual student needs.
- Vocational Education : Promoting vocational education as a viable pathway to skill development and successful career opportunities.
- Education and Ethical Dilemmas : Investigating ethical challenges faced by educators and students in modern educational settings.
- Education and LGBTQ+ Inclusivity : Creating safe and inclusive environments for LGBTQ+ students through policy changes and awareness programs.
- Education and Aging Population : Adapting educational strategies to meet the learning needs of an aging workforce.
- Assessment Methods : Exploring innovative assessment techniques that provide a more comprehensive understanding of student learning.
- Outdoor Education : Utilizing outdoor and experiential learning to enhance students’ practical skills and environmental awareness.
- Education and Artificial Reality : Harnessing the potential of virtual and augmented reality in creating immersive educational experiences.
- Emotional Intelligence in Education : Integrating emotional intelligence training in schools contributes to students’ interpersonal skills and emotional well-being.
- Education and Gifted Students : Tailoring instruction to meet the unique learning needs of gifted students supports their intellectual growth.
- Education and Nutrition : Recognizing the link between proper nutrition and cognitive development for optimal student learning.
- Education and Language Acquisition : Examining strategies for effective language acquisition among non-native speakers in educational settings.
- Education and Political Socialization : Investigating how education shapes students’ political beliefs and participation in civic activities.
- Education and Political Socialization : Investigating how education shapes students’ political beliefs and participation in civic activities
- Education and Digital Literacy : Evaluating the importance of teaching digital literacy skills to navigate the information-rich online world.
- Teacher-Student Relationships : Investigating the impact of positive teacher-student relationships on academic motivation and achievement.
- Education and Social Justice : Analyzing the role of education in addressing societal inequalities and promoting social justice.
- Education and Multilingualism : Exploring the benefits of a multilingual approach in education for cognitive development and cultural awareness.
- Education and Learning Disabilities : Implementing tailored strategies to support students with learning disabilities enhances their academic success.
- Education and Environmental Awareness : Integrating environmental education fosters a generation of environmentally conscious citizens.
- Education and Entrepreneurship : Promoting entrepreneurial education equips students with skills for innovation and economic contribution.
- Student Engagement Strategies : Investigating effective methods to enhance student engagement and participation in the learning process.
- Education and Artificial Intelligence Ethics : Examining ethical considerations when using AI in educational settings to ensure data privacy and equity.
- Education and Emotional Well-being : Creating emotionally supportive environments positively impacts students’ mental health and academic performance.
- Education and Cultural Assimilation : Analyzing how education can either preserve or dilute cultural heritage among immigrant communities.
- Distance Learning Challenges : Exploring the challenges and benefits of distance learning, especially in the context of global events.
- Education and Creativity : Fostering creative thinking skills in students through innovative teaching approaches and curricular design.
- Education and Student Autonomy : Investigating the benefits of allowing students more autonomy in their learning processes.
- Education and Gaming : Exploring the potential of educational games in enhancing learning outcomes and student engagement.
- Teacher Burnout : Examining the factors contributing to teacher burnout and strategies to promote educator well-being.
- Global Education Disparities : Analyzing the disparities in access to quality education across different regions of the world.
- Education and Learning Styles : Tailoring instruction to accommodate diverse learning styles enhances students’ comprehension and retention.
- Education and Brain Development : Studying the correlation between educational experiences and brain development in children and adolescents.
- Education and Ethics Education : Integrating ethics education cultivates morally responsible decision-making among students.
- Education and Socioeconomic Mobility : Examining how education can be a catalyst for upward social mobility in disadvantaged communities.
- Education and Peer Influence : Investigating how peer interactions shape students’ attitudes, behaviors, and academic choices.
- Education and Indigenous Language Revival : Promoting the revitalization of indigenous languages through education preserves cultural heritage.
- Teacher Evaluation Methods : Exploring effective methods for evaluating teacher performance and their impact on educational quality.
- Education and Critical Media Literacy : Developing media literacy skills equips students to critically analyze and navigate the digital information landscape.
- Education and Online Privacy : Raising awareness about online privacy and digital citizenship in educational settings.
- Education and Parental Expectations : Analyzing the effects of parental expectations on students’ academic motivation and achievements.
- Education and Gender Stereotypes : Exploring how education can challenge or reinforce traditional gender stereotypes and roles.
- Education and Mindfulness : Incorporating mindfulness practices in schools enhances students’ focus, emotional regulation, and well-being.
- Education and Aging Workforce : Adapting teaching methods to address the unique learning needs of mature students in continuing education.
- Education and Postcolonialism : Analyzing the influence of colonial history on education systems and curriculum development.
- Education and Lifelong Learning : Promoting the idea of education as a continuous process that extends beyond formal schooling.
Education Thesis Statement Examples for Argumentative Essay
Education is the cornerstone of societal progress, and an argumentative essay thesis statement can explore its multifaceted impact. A thesis statement could be: “Mandatory financial literacy education in schools should be implemented to empower students with essential life skills, promoting responsible financial decision-making.
- Mandatory Financial Literacy Education : “Mandatory financial literacy education in schools should be implemented to empower students with essential life skills, promoting responsible financial decision-making.”
- Comprehensive Sex Education : “The integration of comprehensive sex education into curricula is imperative to address the rising rates of teenage pregnancies and sexually transmitted infections.”
- Bilingual Education : “Bilingual education programs positively contribute to cognitive development, cross-cultural understanding, and global communication skills among students.”
- Diverse Perspectives in History Education : “The inclusion of diverse perspectives in history education fosters critical thinking and promotes a more accurate understanding of past events.”
- Importance of Arts Education : “Arts education should remain a fundamental component of the curriculum, as it enhances creativity, cognitive abilities, and emotional intelligence.”
- Media Literacy Education : “Promoting media literacy education equips students to navigate the complexities of the digital age, fostering critical analysis of information sources.”
- Restorative Justice in Education : “Implementing restorative justice practices in schools nurtures conflict resolution skills, reduces disciplinary disparities, and creates a more inclusive learning environment.”
- Environmental Education : “Environmental education cultivates a sense of responsibility for ecological sustainability, preparing students to address pressing global environmental challenges.”
- Mental Health Education : “Education about mental health and emotional well-being should be integrated into curricula to reduce stigma, enhance self-awareness, and support student mental health.”
- Coding and Computer Science Education : “Teaching coding and computer science in primary education enhances problem-solving abilities, technological literacy, and prepares students for a technology-driven future.”
Importance of Education Thesis Statement Examples
Highlighting the significance of education, a thesis statement like, “Access to quality education equips individuals with the tools to break the cycle of poverty, fosters critical thinking, and cultivates informed citizens essential for a thriving democracy.”
- Access to Quality Education : “Access to quality education equips individuals with the tools to break the cycle of poverty, fosters critical thinking, and cultivates informed citizens essential for a thriving democracy.”
- Education and Innovation : “Education empowers individuals to challenge societal norms, fostering innovation and progress through the exploration of new ideas and perspectives.”
- Early Childhood Education : “Investing in early childhood education yields lifelong benefits, promoting cognitive development, emotional intelligence, and academic success.”
- Education for Social Cohesion : “Education plays a pivotal role in promoting social cohesion, bridging cultural divides, and fostering mutual respect and understanding among diverse communities.”
- Education and Economic Growth : “An educated workforce drives economic growth by fostering innovation, increasing productivity, and attracting investment in a knowledge-based economy.”
- Empowerment through Education : “Education is the foundation of personal empowerment, enabling individuals to make informed decisions about their health, finances, and overall well-being.”
- Education in a Technological Era : “Quality education equips individuals with the skills to adapt to rapid technological changes, ensuring they remain competitive in a dynamic job market.”
- Education and Social Mobility : “Education serves as a catalyst for social mobility, enabling individuals to transcend their socioeconomic backgrounds and achieve upward mobility.”
- Education and Public Health : “In societies with higher levels of education, there is a positive correlation with improved public health outcomes, lower crime rates, and overall well-being.”
- Right to Education : “Education is a fundamental human right that should be accessible to all, regardless of gender, ethnicity, socioeconomic status, or geographical location.”
Lack of Education Thesis Statement Examples
Examining the consequences of inadequate education, a concise thesis statement might state: “The lack of accessible education perpetuates social inequality, limits economic mobility, and hinders personal and societal development, underscoring the urgent need for educational reforms.”
- Impact of Inaccessible Education : “The lack of accessible education perpetuates social inequality, limits economic mobility, and hinders personal and societal development, underscoring the urgent need for educational reforms.”
- Cycle of Poverty : “In regions with limited educational opportunities, there is a heightened risk of perpetuating cycles of poverty, resulting in diminished life prospects for generations.”
- Lack of Comprehensive Sex Education : “The absence of comprehensive sex education contributes to uninformed decisions, leading to higher rates of unintended pregnancies and the spread of sexually transmitted infections.”
- Educational Inequality and Civic Engagement : “Communities with inadequate educational infrastructure experience reduced civic engagement, hampering their ability to advocate for their rights and interests.”
- Challenges in Special Needs Education : “Without inclusive education practices, students with disabilities are often marginalized, denying them opportunities for holistic development and societal contribution.”
- Environmental Ignorance : “The lack of emphasis on environmental education results in a lack of awareness about sustainable practices, exacerbating environmental degradation and climate change.”
- Mental Health Education Gap : “A dearth of education around mental health perpetuates stigma, preventing individuals from seeking help and contributing to a global mental health crisis.”
- Gender Disparities in Education : “In societies where gender equity in education is not prioritized, women and girls face limited opportunities, reinforcing gender disparities in various sectors.”
- Education and Ignorance : “Communities without access to quality education struggle to break free from cycles of ignorance and misinformation, hindering progress and social cohesion.”
- Digital Literacy Divide : “The absence of education tailored to the digital age leaves individuals vulnerable to misinformation, cyber threats, and challenges presented by rapid technological advancements.”
Education Thesis Statement Examples for College
For a college-focused context, a thesis could be: “Integrating practical skills training into higher education curricula prepares students for real-world challenges, bridging the gap between academic knowledge and employability.”
- Practical Skills in Higher Education : “Integrating practical skills training into higher education curricula prepares students for real-world challenges, bridging the gap between academic knowledge and employability.”
- Interdisciplinary Learning in College : “College education should prioritize interdisciplinary learning, fostering a holistic understanding of complex global issues and encouraging innovative solutions.”
- Experiential Learning in College : “Promoting student engagement through experiential learning opportunities in college enhances critical thinking, problem-solving skills, and prepares students for lifelong learning.”
- Soft Skills Development in College : “Colleges should emphasize the development of soft skills such as communication, teamwork, and adaptability, essential for success in diverse professional environments.”
- Entrepreneurship Education in College : “Incorporating entrepreneurship education in college equips students with the mindset and skills needed to create and navigate their own career paths.”
- Cultural Competence in College : “College education should encourage cultural competence, promoting empathy and understanding in an increasingly interconnected and diverse world.”
- Technology-Enhanced Learning in College : “Embracing technology-enhanced learning methods in college empowers students to become digitally literate, adaptable, and well-prepared for the modern workforce.”
- Research-Oriented College Education : “Fostering a research-oriented approach in college education cultivates critical inquiry, creativity, and advances our understanding of various academic disciplines.”
- Mental Health Support in College : “Colleges should prioritize mental health and well-being services to support students during a transformative period, ensuring their holistic success.”
- Flexible Learning in College : “Offering flexible learning options, including online and hybrid courses, accommodates diverse student needs and promotes lifelong learning beyond traditional campus settings.”
Education Thesis Statement Examples for Students
Directing attention to students, a thesis might read: “Implementing personalized learning approaches in schools caters to diverse learning styles, enhances student engagement, and fosters a lifelong love for learning.”
- Personalized Learning for Students : “Implementing personalized learning approaches in schools caters to diverse learning styles, enhances student engagement, and fosters a lifelong love for learning.”
- Student-Centered Education : “Student-centered education that encourages curiosity, creativity, and critical thinking nurtures independent thought and prepares students for active citizenship.”
- Project-Based Learning for Students : “Incorporating project-based learning in schools develops problem-solving skills and empowers students to apply theoretical knowledge to real-world situations.”
- Student Agency in Education : “Encouraging student agency in educational decisions fosters a sense of ownership, boosting motivation, and promoting self-directed learning.”
- Learning from Failure for Students : “Education that emphasizes the value of failure as a stepping stone to success helps students develop resilience, adaptability, and a growth mindset.”
- Collaborative Learning for Students : “Promoting collaborative learning experiences in classrooms cultivates teamwork skills, enhances communication, and exposes students to diverse perspectives.”
- Extracurricular Involvement for Students : “Student involvement in extracurricular activities and community service fosters character development, empathy, and a sense of responsibility to society.”
- Arts and Creative Expression for Students : “Integrating arts and creative expression into education sparks imagination, enhances emotional intelligence, and encourages students to think outside the box.”
- Digital Literacy for Students : “Cultivating digital literacy skills equips students to navigate the digital landscape responsibly, critically evaluate information, and contribute positively online.”
- Mindfulness in Education for Students : “Education that incorporates mindfulness and well-being practices helps students manage stress, build emotional resilience, and maintain overall mental wellness.”
Education Thesis Statement Examples for Essay
In the context of an essay, a case study thesis statement could be: “Exploring the evolution of educational technology reveals its role as a transformative force in modern classrooms, reshaping traditional teaching methods and enhancing student outcomes.”
- Effective Study Habits : “Exploring effective study habits and time management strategies equips students with the tools to optimize their learning experience and achieve academic success.”
- Role of Teachers in Student Motivation : “Analyzing the pivotal role of teachers in motivating students through innovative teaching methods and supportive mentorship enhances the learning journey.”
- Educational Technology Integration : “Examining the integration of educational technology in classrooms highlights its potential to enhance engagement, collaboration, and personalized learning.”
- Impact of Standardized Testing : “Investigating the impact of standardized testing on curriculum, instruction, and student stress provides insights into the complexities of assessment-driven education systems.”
- Importance of Early Literacy : “Highlighting the significance of early literacy development in shaping future academic achievements emphasizes the need for targeted interventions and support.”
- Holistic Assessment Approaches : “Exploring alternative assessment methods beyond exams, such as project-based assessments and portfolios, offers a comprehensive view of student learning.”
- Cultural Competence in Education : “Analyzing the importance of cultural competence in educators for creating inclusive classrooms and fostering diverse student perspectives.”
- Critical Thinking in Education : “Investigating the cultivation of critical thinking skills through interdisciplinary learning encourages students to question, analyze, and form independent viewpoints.”
- Ethics Education : “Examining the integration of ethics education across disciplines prepares students to navigate ethical dilemmas and make informed moral decisions.”
- Education and Sustainable Development : “Exploring the role of education in promoting sustainable development addresses its contribution to environmental awareness, social responsibility, and global citizenship.”
Education Thesis Statement Examples about Online Learning
Regarding online learning, a thesis might state: “The rapid expansion of online education presents opportunities for global access to quality learning, yet challenges persist in ensuring equitable access and maintaining educational rigor.”
Education Thesis Statement Examples about Online Learning:
- Rise of Online Education : “The rapid expansion of online education presents opportunities for global access to quality learning, yet challenges persist in ensuring equitable access and maintaining educational rigor.”
- Hybrid Learning Models : “Examining the effectiveness of hybrid learning models highlights the potential of combining online and in-person elements to enhance engagement and flexibility in education.”
- Synchronous and Asynchronous Online Interactions : “Investigating the role of synchronous and asynchronous online interactions in virtual classrooms reveals their impact on student engagement, peer collaboration, and instructor feedback.”
- Online Assessment Methods : “Analyzing the role of online assessments in measuring student performance raises questions about the fairness, security, and authenticity of remote evaluation methods.”
- Digital Divide in Online Learning : “Exploring the digital divide’s impact on online learning access emphasizes the need for targeted interventions to bridge technological disparities among students.”
- Massive Open Online Courses (MOOCs) : “The rise of Massive Open Online Courses (MOOCs) challenges traditional education paradigms by offering large-scale, accessible learning experiences to diverse global audiences.”
- Artificial Intelligence in Online Education : “Examining the role of artificial intelligence in personalized online education sheds light on its potential to adapt content, pacing, and assessment to individual student needs.”
- Virtual Communities and Online Learning : “Investigating the social aspects of online learning environments explores the ways virtual communities, discussions, and collaborations contribute to a sense of belonging.”
- Online Simulations and Virtual Labs : “Analyzing the benefits of online simulations and virtual labs in science education showcases their role in providing experiential learning opportunities outside traditional labs.”
- Long-Term Effects of Online Learning : “The exploration of online learning’s long-term effects on students’ social skills, time management, and self-regulation offers insights into the broader impacts of digital education.”
Education Thesis Statement Examples for Parental Involvement
Focusing on parental involvement, a thesis could be: “Active parental engagement in a child’s education significantly impacts academic performance, creating a collaborative learning environment and fostering holistic development.”
- Active Parental Engagement : “Active parental engagement in a child’s education significantly impacts academic performance, creating a collaborative learning environment and fostering holistic development.”
- Early Childhood Parental Involvement : “Investigating the influence of parental involvement in early childhood education emphasizes its role in shaping cognitive, emotional, and social foundations for lifelong learning.”
- Parent-Teacher Partnerships : “Analyzing the impact of parent-teacher partnerships on student motivation and behavior management highlights the importance of consistent communication and shared goals.”
- Parental Involvement in Remote Learning : “Exploring strategies to involve parents in remote and online learning environments addresses the need for adaptable approaches to maintain strong home-school connections.”
- Parent-Led Initiatives in Schools : “Examining the impact of parent-led initiatives in schools reveals their potential to enhance school facilities, resources, and extracurricular opportunities for all students.”
- Challenges of Parental Involvement : “Investigating the challenges faced by parents from diverse backgrounds in engaging with school activities emphasizes the importance of culturally sensitive communication and support.”
- Parent Education Workshops : “Analyzing the role of parent education workshops in enhancing parenting skills, communication, and support systems contributes to positive student outcomes.”
- Parental Involvement and Absenteeism : “Exploring the impact of parental involvement on reducing absenteeism, dropout rates, and disciplinary issues underscores its potential as a preventive measure.”
- Parental Involvement in Curriculum Decisions : “Investigating the effects of parent participation in curriculum decisions and policy-making highlights their valuable insights and contributions to shaping educational priorities.”
- Technology and Parental Involvement : “Exploring the intersection of technology and parental involvement unveils the potential of digital platforms to facilitate communication, updates, and collaboration between parents and educators.”
Education Thesis Statement Examples for Special Needs
Addressing special needs education, a thesis might read: “Inclusive education practices empower students with diverse abilities by providing tailored support, promoting social integration, and challenging stigmas surrounding disabilities.”
- Inclusive Education Practices : “Inclusive education practices empower students with diverse abilities by providing tailored support, promoting social integration, and challenging stigmas surrounding disabilities.”
- Assistive Technology in Special Education : “Examining the impact of assistive technology in special education classrooms showcases its role in enhancing communication, learning experiences, and independence for students.”
- Individualized Education Programs (IEPs) : “Analyzing the effectiveness of Individualized Education Programs (IEPs) emphasizes their significance in providing personalized learning pathways for students with special needs.”
- Parental Experiences in Special Education : “Exploring the experiences of parents of children with disabilities within the education system sheds light on the challenges they face and the importance of collaborative partnerships.”
- Educator Training for Inclusive Classrooms : “Investigating the training and professional development needs of educators in inclusive classrooms addresses the necessity of equipping teachers with diverse teaching strategies.”
- Peer Support Programs : “Analyzing the benefits of peer support programs in fostering positive relationships between students with and without disabilities underscores their role in promoting empathy and understanding.”
- Accessible Learning Materials : “Examining the impact of accessible learning materials, such as Braille, a resources, and captioning, highlights their contribution to equitable educational experiences.”
- Sensory-Friendly Environments : “Investigating the role of sensory-friendly environments in schools demonstrates their ability to create inclusive spaces that accommodate the needs of students with sensory sensitivities.”
- Transition from School to Post-School Life : “Analyzing the transition process for students with special needs from school to post-school life underscores the importance of vocational training and community integration.”
- Mental Health Support in Special Education : “Exploring the intersection of mental health support and special education reveals the need for comprehensive strategies that address the unique emotional needs of students with disabilities.”
Education Thesis Statement Examples for Gender Equity
Exploring gender equity in education, a thesis statement could be: “Implementing gender-sensitive policies and curriculum reforms is essential to eliminate gender disparities in education, empowering all students to fulfill their potential regardless of gender.
- Gender-Sensitive Education : “Implementing gender-sensitive policies and curriculum reforms is essential to eliminate gender disparities in education, empowering all students to fulfill their potential regardless of gender.”
- Gender Bias in Educational Materials : “Examining the impact of gender bias in textbooks and educational materials underscores the importance of representation and accurate portrayals of diverse gender identities.”
- Gender-Responsive Pedagogy : “Analyzing the role of gender-responsive pedagogy in promoting equitable learning experiences challenges traditional teaching practices that perpetuate gender stereotypes.”
- Teacher Expectations and Gender : “Exploring the influence of teacher expectations on student performance highlights the need to address unconscious biases that can hinder gender-equitable educational outcomes.”
- Single-Sex Education vs. Coeducation : “Investigating the impact of single-sex education versus coeducation on academic achievement and personal development offers insights into the effects of different learning environments.”
- LGBTQ+ Students in Educational Settings : “Analyzing the experiences of LGBTQ+ students in educational settings emphasizes the importance of creating safe, inclusive spaces that respect and celebrate diverse identities.”
- Gender-Balanced Leadership : “Examining the impact of gender-balanced leadership and decision-making in schools addresses the need for role models and equitable representation at all levels of education.”
- Gender-Based Violence Prevention in Schools : “Investigating the effects of gender-based violence prevention programs in schools emphasizes their role in fostering respectful relationships and safe learning environments.”
- Parental Attitudes and Gender Roles : “Analyzing the influence of parental attitudes toward gender roles on children’s educational and career aspirations underscores the need for comprehensive family and community involvement.”
- Culture, Gender Equity, and Education : “Exploring the intersection of cultural norms, gender equity, and education in diverse societies reveals the complex factors that shape educational opportunities and challenges for different genders.”
What is a Good Thesis Statement about the Lack of Education?
A strong thesis statement about the lack of education should succinctly capture the essence of the issue while outlining its significance and potential consequences. Here’s a guide to crafting a powerful thesis statement on this topic:
Example Thesis Statement: “The pervasive lack of accessible education in underserved communities perpetuates cycles of poverty, limits economic mobility, and hampers societal progress, necessitating urgent reforms to ensure equitable learning opportunities for all.”
- Identify the Issue : Clearly state the problem you’re addressing – in this case, the lack of education.
- Highlight Significance : Express why the issue matters by emphasizing its impact on individuals and society as a whole.
- Show Consequences : Indicate the adverse effects of the lack of education, such as perpetuating poverty and hindering progress.
- Mention Urgency : Communicate the importance of addressing the issue promptly, as well as the need for reform.
What is an Example of a Thesis Statement in Inclusive Education?
A thesis statement on inclusive education should emphasize the importance of creating learning environments that cater to diverse learners’ needs. Here’s a guide to crafting such a thesis statement:
Example Thesis Statement: “Inclusive education, through its emphasis on diverse learning styles, individualized support, and community engagement, fosters a holistic and equitable learning experience that empowers all students to reach their fullest potential.”
- State Inclusion as a Goal : Clearly mention that the thesis is about inclusive education.
- Highlight Diverse Learning Styles : Emphasize the importance of accommodating various learning styles and needs.
- Emphasize Individualized Support : Stress the role of personalized assistance and adaptations in inclusive education.
- Mention Community Engagement : Indicate how involving the community contributes to a successful inclusive education environment.
- Discuss Empowerment : Express how inclusive education empowers all students to achieve their best outcomes.
How Do You Write a Thesis Statement for Education? – Step by Step Guide
- Identify Your Topic : Determine the specific aspect of education you want to address.
- Understand the Issue : Gain a deep understanding of the topic’s significance, challenges, and potential impact.
- Craft a Clear Idea : Develop a concise and focused main idea or argument related to education.
- Make It Debatable : Ensure your thesis statement presents an argument or perspective that can be debated or discussed.
- Address Significance : Highlight why the topic is important and relevant in the context of education.
- Consider Counterarguments : Acknowledge potential opposing viewpoints and consider incorporating counterarguments.
- Keep It Concise : Your thesis statement should be a single, clear, and well-structured sentence.
- Reflect Your Essay’s Scope : Make sure your thesis aligns with the scope of your essay or paper.
- Revise and Refine : Review and revise your thesis statement to ensure its clarity and accuracy.
- Seek Feedback : Share your thesis statement with peers or instructors for feedback and suggestions.
Tips for Writing a Thesis Statement on Education Topics
- Be Specific : Clearly state what your paper will address within the broad topic of education.
- Avoid Generalizations : Avoid overly broad or vague statements that lack focus.
- Express a Strong Position : Your thesis should convey a clear stance on the issue.
- Consider Your Audience : Tailor your thesis to resonate with your intended audience.
- Use Precise Language : Choose words that convey your message concisely and accurately.
- Make It Unique : Craft a thesis that sets your essay apart by presenting a unique perspective.
- Reflect Your Essay Structure : Your thesis should mirror the overall structure of your essay.
- Be Open to Revisions : Be willing to adjust your thesis as your research and writing progress.
- Proofread Carefully : Ensure your thesis statement is free of grammatical and typographical errors.
- Revise as Needed : It’s okay to revise your thesis as you refine your arguments and analysis.
Remember, a strong thesis statement sets the tone for your entire essay and guides your readers in understanding the focus and direction of your work. You may also be interested in our thesis statement for informative essay .
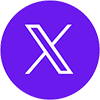
AI Generator
Text prompt
- Instructive
- Professional
Purdue Online Writing Lab Purdue OWL® College of Liberal Arts
Tips and Examples for Writing Thesis Statements
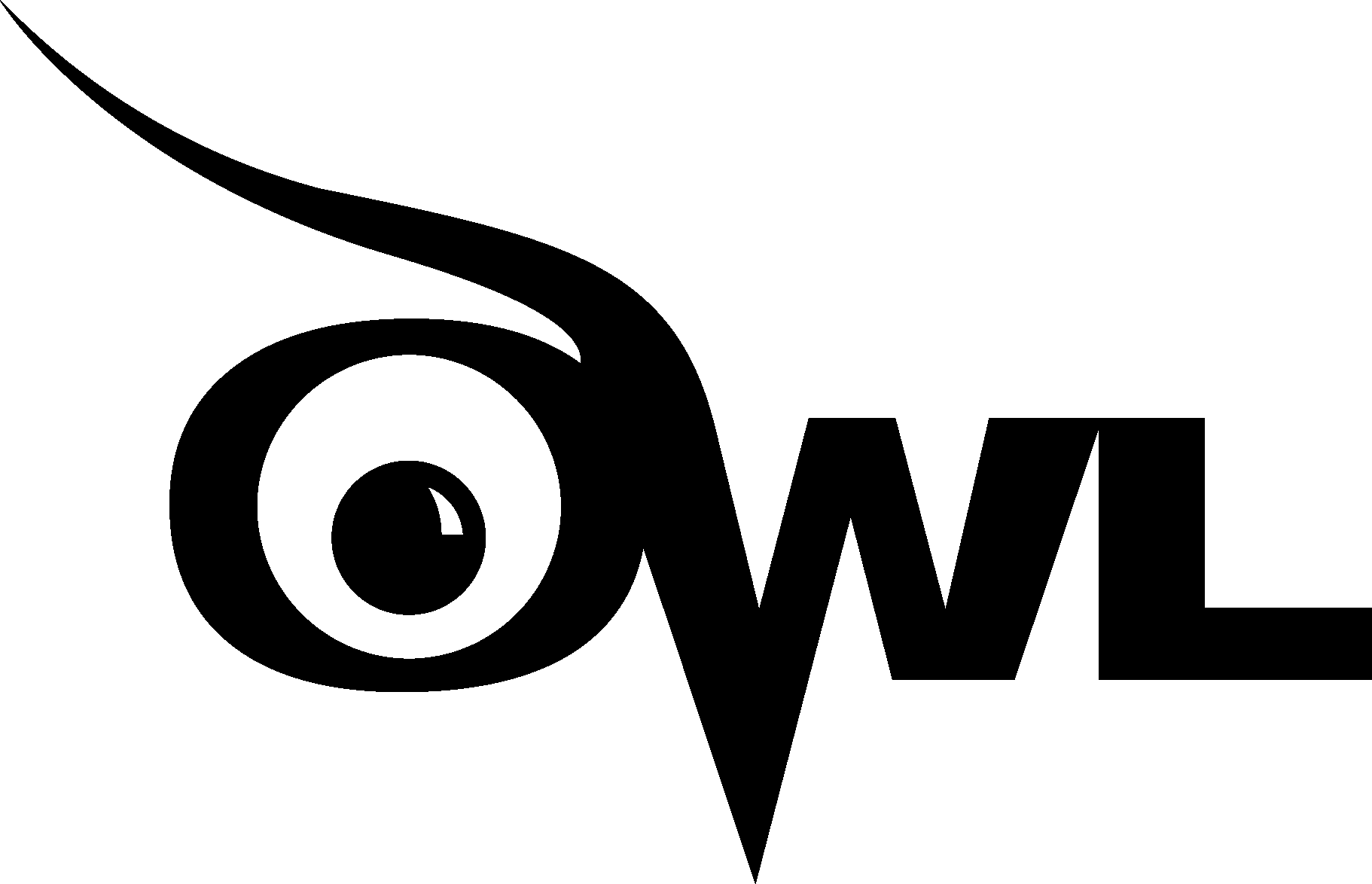
Welcome to the Purdue OWL
This page is brought to you by the OWL at Purdue University. When printing this page, you must include the entire legal notice.
Copyright ©1995-2018 by The Writing Lab & The OWL at Purdue and Purdue University. All rights reserved. This material may not be published, reproduced, broadcast, rewritten, or redistributed without permission. Use of this site constitutes acceptance of our terms and conditions of fair use.
Tips for Writing Your Thesis Statement
1. Determine what kind of paper you are writing:
- An analytical paper breaks down an issue or an idea into its component parts, evaluates the issue or idea, and presents this breakdown and evaluation to the audience.
- An expository (explanatory) paper explains something to the audience.
- An argumentative paper makes a claim about a topic and justifies this claim with specific evidence. The claim could be an opinion, a policy proposal, an evaluation, a cause-and-effect statement, or an interpretation. The goal of the argumentative paper is to convince the audience that the claim is true based on the evidence provided.
If you are writing a text that does not fall under these three categories (e.g., a narrative), a thesis statement somewhere in the first paragraph could still be helpful to your reader.
2. Your thesis statement should be specific—it should cover only what you will discuss in your paper and should be supported with specific evidence.
3. The thesis statement usually appears at the end of the first paragraph of a paper.
4. Your topic may change as you write, so you may need to revise your thesis statement to reflect exactly what you have discussed in the paper.
Thesis Statement Examples
Example of an analytical thesis statement:
The paper that follows should:
- Explain the analysis of the college admission process
- Explain the challenge facing admissions counselors
Example of an expository (explanatory) thesis statement:
- Explain how students spend their time studying, attending class, and socializing with peers
Example of an argumentative thesis statement:
- Present an argument and give evidence to support the claim that students should pursue community projects before entering college
- Welcome to MX Bikes Official Forum . Please login or sign up .
- MX Bikes Official Forum
Forgot your password?
- Help | Terms and Rules | Go Up ▲
- SMF 2.1 RC3 © 2020 , Simple Machines
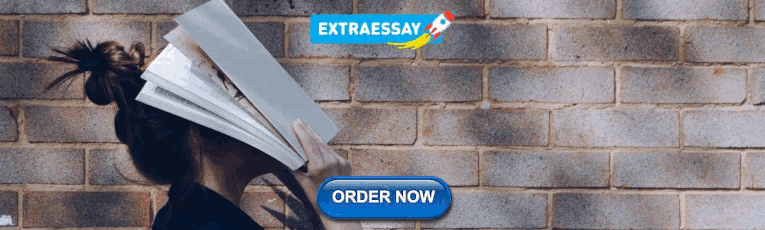
IMAGES
VIDEO
COMMENTS
Definitions of learning style generally focus on ways of learning. According to Fleming (2001) learning style is an individual's preferred way of gathering, organizing, and thinking about information. It is considered as the behaviors related to the psychological, cognitive, and affective domains of interaction with learning environments.
Thesis (Ed. D.)--Texas Tech University, 1999. Includes bibliographical references (leaves 108-116). ... Learning styles has been delineated as a sophisticated method within which the learner ought ...
Your thesis must be something that can be argued and proved by your research. I like the idea a previous poster had connecting the need to teach to learning styles even though standardized testing ...
Honors Thesis Lauren Hanzelka Learning Styles: A Viable Approach for Instruction? Introduction . For years, educators have been taught that students learn in different ways. One common instructional practice in the classroom is to identify the learning style of the student and then teach to that preferred mode.
One of the famous learning styles models is Grasha-Riechmann Learning Styles Model. This model integrates individual teaching and learning styles and demonstrates how the stylistic qualities of teachers and students can enhance the nature and quality of the learning experience (Grasha, 1996). It is based on the notion that to maximize learning ...
Universities strive to ensure quality education focused on the diversity of the student body. According to experiential learning theory, students display different learning preferences. This study has a three-fold objective: to compare learning styles based on personal and educational variables, to analyze the association between learning styles, the level of academic performance, and ...
Learning styles are critical to educational psychology, especially when investigating various contextual factors that interact with individual learning styles. Drawing upon Biglan's taxonomy of academic tribes, this study systematically analyzed the learning styles of 790 sophomores in a blended learning course with 46 specializations using a novel machine learning algorithm called the ...
Step 2: Write your initial answer. After some initial research, you can formulate a tentative answer to this question. At this stage it can be simple, and it should guide the research process and writing process. The internet has had more of a positive than a negative effect on education.
not the learning styles of the students have a bearing on their academic perfor-mance in English 3. FRAMEWORK There are a few selected theories that can support this study. The first theo - ry is Dunn and Dunn Learning Styles Models (Dunn and Burke, 2002). This theory states the following beliefs: that learning style is a biological and developmen-
Learning Styles and Their Relation to T eaching Styles. International Journal of Language and Linguistics. V ol. 2, No. 3, 2014, pp. 241-245. doi: 10.11648/j.ijll.20140203.23. Abstract: It is ...
A thesis statement: tells the reader how you will interpret the significance of the subject matter under discussion. is a road map for the paper; in other words, it tells the reader what to expect from the rest of the paper. directly answers the question asked of you. A thesis is an interpretation of a question or subject, not the subject itself.
her learning style preference. This questionnaire consists of thirty items that represent six learning style preferences, namely Visual, Auditory, Kinesthetic, Tactile, Group, and Individual. Five items for each learning style category were arranged randomly. Items 6,10,12,24,29 are Visual, items 1,7,9,17,20 are Auditory, items 2,8,15,19,26 are
Learning styles are an essential key for learning and perception towards learning and using modern technology tools. Kolb and Kolb pointed out that learning styles ... academic and other settings is learning styles" (p. 420). Problem Statement . This study was designed to explore the different learning styles of adult learners
The Learning Styles Inventory assessed students' reliance on Synthesis-Analysis, Elaborative Processing, Fact Retention, and Study Methods - the first two strategies reflect deeper, more involved ...
Example Thesis Statement: "The pervasive lack of accessible education in underserved communities perpetuates cycles of poverty, limits economic mobility, and hampers societal progress, necessitating urgent reforms to ensure equitable learning opportunities for all.".
Data generated from the LSMOC and Duration is corroborated to. formulate conclusions about the teacher's instructional strategies in Figures 1 and 2. Group A. Detailed descriptions of the learning styles were given to each of the. participants in Group A after they received their personal learning profile.
Tips for Writing Your Thesis Statement. 1. Determine what kind of paper you are writing: An analytical paper breaks down an issue or an idea into its component parts, evaluates the issue or idea, and presents this breakdown and evaluation to the audience.; An expository (explanatory) paper explains something to the audience.; An argumentative paper makes a claim about a topic and justifies ...
Satisfactory Essays. 1003 Words. 5 Pages. Open Document. Statement of problem: Learning style is an important to consideration in the educational process of the academic world which is also supported by literature. Among other variables, differences in learning styles may be influenced by language, culture, educational department and gender.
PROBLEM STATEMENT AND CONTEXT General Statement of the Problem Reaching all students with a variety of learning styles is necessary for full academic growth in school. Traditional teaching methods often do not address each type of learner. However, with the diverse needs of learners, matching instruction for diverse learning styles can be ...
Learning style thesis - Free download as Word Doc (.doc / .docx), PDF File (.pdf), Text File (.txt) or read online for free. The thesis is about the different learning style
a study of the preferred learning styles of students taking the english 1119 paper in smk tengku intan zaharah: are the teachers aware of these learning styles? November 2012 DOI: 10.13140/RG.2.1 ...
March 24, 2024, 06:44:59 PM. Vicente Carter from Reading was looking for thesis statement on learning styles. Jaquan Reed found the answer to a search query thesis statement on learning styles. ESSAYERUDITE.COM. thesis statement on learning styles. example of an introduction in a research proposal. write me culture report.