Applied Research on English Language
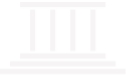
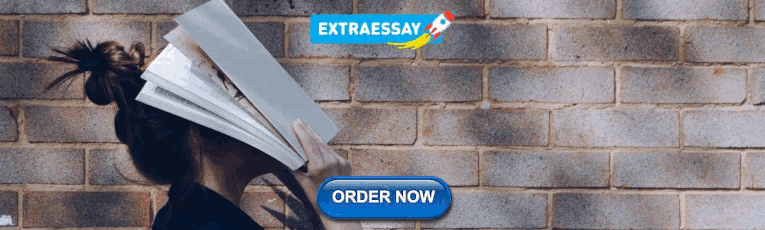
Subject Area and Category
- Linguistics and Language
University of Isfahan, Department of English
Publication type
22520198, 23225343
Information
How to publish in this journal
The set of journals have been ranked according to their SJR and divided into four equal groups, four quartiles. Q1 (green) comprises the quarter of the journals with the highest values, Q2 (yellow) the second highest values, Q3 (orange) the third highest values and Q4 (red) the lowest values.
The SJR is a size-independent prestige indicator that ranks journals by their 'average prestige per article'. It is based on the idea that 'all citations are not created equal'. SJR is a measure of scientific influence of journals that accounts for both the number of citations received by a journal and the importance or prestige of the journals where such citations come from It measures the scientific influence of the average article in a journal, it expresses how central to the global scientific discussion an average article of the journal is.
Evolution of the number of published documents. All types of documents are considered, including citable and non citable documents.
This indicator counts the number of citations received by documents from a journal and divides them by the total number of documents published in that journal. The chart shows the evolution of the average number of times documents published in a journal in the past two, three and four years have been cited in the current year. The two years line is equivalent to journal impact factor ™ (Thomson Reuters) metric.
Evolution of the total number of citations and journal's self-citations received by a journal's published documents during the three previous years. Journal Self-citation is defined as the number of citation from a journal citing article to articles published by the same journal.
Evolution of the number of total citation per document and external citation per document (i.e. journal self-citations removed) received by a journal's published documents during the three previous years. External citations are calculated by subtracting the number of self-citations from the total number of citations received by the journal’s documents.
International Collaboration accounts for the articles that have been produced by researchers from several countries. The chart shows the ratio of a journal's documents signed by researchers from more than one country; that is including more than one country address.
Not every article in a journal is considered primary research and therefore "citable", this chart shows the ratio of a journal's articles including substantial research (research articles, conference papers and reviews) in three year windows vs. those documents other than research articles, reviews and conference papers.
Ratio of a journal's items, grouped in three years windows, that have been cited at least once vs. those not cited during the following year.
Follow us on @ScimagoJR Scimago Lab , Copyright 2007-2024. Data Source: Scopus®

Cookie settings
Cookie Policy
Legal Notice
Privacy Policy
- Search Menu
- Advance articles
- Editor's Choice
- Key Concepts
- The View From Here
- Author Guidelines
- Submission Site
- Open Access
- Why Publish?
- About ELT Journal
- Editorial Board
- Advertising and Corporate Services
- Journals Career Network
- Self-Archiving Policy
- Dispatch Dates
- Terms and Conditions
- Journals on Oxford Academic
- Books on Oxford Academic
English Language Teaching Now and How It Could Be

- Article contents
- Figures & tables
- Supplementary Data
Michiko Weinmann, English Language Teaching Now and How It Could Be, ELT Journal , 2024;, ccae009, https://doi.org/10.1093/elt/ccae009
- Permissions Icon Permissions
English Language Teaching Now and How It Could Be , co-authored by Geoff Jordan and Michael H. Long (1945–2021), is a well-researched and provocative addition to the existing trove of English language teaching (ELT) books. Both authors are renowned in the ELT sphere: Jordan is a prominent ELT practitioner and teacher educator whose blog (https://applingtesol.wordpress.com/) provides commentary and critique on a range of ‘hot topics’ in second language acquisition (SLA) and second language teacher education (SLTE); Long is one of the most important SLA scholars, notably for his introduction of the concept of focus on form and his influential work on age effects, task-based language teaching (TBLT), and incidental learning.
The book’s premise is that ELT is highly skilled work that requires in-depth knowledge about how languages are learnt for teachers to best facilitate students’ language learning; as ‘experts on their own classrooms’ (p. 3), they are also required to invariably engage their experiences and practice in their pedagogical decision-making. In short, the aim should be ‘not just ELT, but first-rate, SLA-informed ELT ’ (p. 5; emphasis added). Accordingly, one of the book’s main goals is to provide ‘an up-to-date summary of SLA research and its implications for ELT, a critical review of current ELT practice, and suggestions for how it might be improved’ (p. xiii). The authors emphasise that while they ‘make many teaching recommendations’, these are presented as ‘ options, not recipes ’ (p. 3, emphasis in original). The second goal of the book is to examine ‘the often hidden political and economic interests’ (p. 1), e.g., ‘the powerful state interests and vastly profitable commercial enterprises that shape much of what goes on’ (p. 2). While a problematisation of ‘ELT’s dark side’ (p. 2) is not new (see for example, Block et al. 2012; O’Regan 2021), it is the bringing together of ‘a politically and SLA-informed treatment of ELT’ (p. 3, emphasis added) which provides the reader with a unique introduction to ‘pure’ SLA and other areas of Applied Linguistics research. The book seeks to target a broad audience, and is aimed at two main readerships: (1) undergraduate and Master’s students completing qualifications in TESOL or Applied Linguistics, and (2) practising teachers of English as an Additional, Second, or Foreign Language to adults.
Email alerts
Citing articles via.
- Recommend to Your Library
Affiliations
- Online ISSN 1477-4526
- Print ISSN 0951-0893
- Copyright © 2024 Oxford University Press
- About Oxford Academic
- Publish journals with us
- University press partners
- What we publish
- New features
- Open access
- Institutional account management
- Rights and permissions
- Get help with access
- Accessibility
- Advertising
- Media enquiries
- Oxford University Press
- Oxford Languages
- University of Oxford
Oxford University Press is a department of the University of Oxford. It furthers the University's objective of excellence in research, scholarship, and education by publishing worldwide
- Copyright © 2024 Oxford University Press
- Cookie settings
- Cookie policy
- Privacy policy
- Legal notice
This Feature Is Available To Subscribers Only
Sign In or Create an Account
This PDF is available to Subscribers Only
For full access to this pdf, sign in to an existing account, or purchase an annual subscription.
Recommended pages
- Undergraduate open days
- Postgraduate open days
- Accommodation
- Information for teachers
- Maps and directions
- Sport and fitness
English Language Research Journal
- ISSN 2057-4215
Aims and scope
English Language Research is a double-blind peer reviewed open access journal with an emphasis on original research into both theoretical and applied linguistic issues in context. The journal is based in the Department of English at the University of Birmingham, and has been the forum for many seminal works. It has been relaunched and expanded in 2013 as a journal committed to free online access, whilst maintaining the benchmarks of academic rigour and originality. The journal also actively welcomes submissions from postgraduate researchers and early career academics.
The next issue of the journal will be on a specific theme and a call for papers will be issued soon.
For enquiries about the suitability of studies for publication in English Language Research, prospective authors are welcome to contact the editors at: [email protected] .
- Issue 2: Special Issue on Metaphor
Editorial Board / Review Board
Editors, issue 2.
Sarah Turner, University of Birmingham
Editors, Issue 1
Phil Bennett, University of Birmingham Lee Oakley, University of Birmingham Sarah Turner, University of Birmingham
Advisory Board
Michael Barlow, University of Auckland Ylva Berglund-Prytz, University of Oxford Silvia Bernardini, University of Bologna Alice Deignan, University of Leeds Lynne Flowerdew, Hong Kong University of Science and Technology Nicholas Groom, University of Birmingham Susan Hunston, University of Birmingham Chris Kennedy, University of Birmingham Jeannette Littlemore, University of Birmingham Sofia Malamatidou, University of Birmingham Ute Römer , Georgia State University Gabriela Saldanha, University of Birmingham Michael Stubbs, Universität Trier Caroline Tagg, University of Birmingham Paul Thompson, University of Birmingham Geoffrey Williams, Université de Bretagne Sud

An official website of the United States government
The .gov means it’s official. Federal government websites often end in .gov or .mil. Before sharing sensitive information, make sure you’re on a federal government site.
The site is secure. The https:// ensures that you are connecting to the official website and that any information you provide is encrypted and transmitted securely.
- Publications
- Account settings
Preview improvements coming to the PMC website in October 2024. Learn More or Try it out now .
- Advanced Search
- Journal List
- Front Psychol
Trends and hot topics in linguistics studies from 2011 to 2021: A bibliometric analysis of highly cited papers
Associated data.
The datasets presented in this study can be found in online repositories. The names of the repository/repositories and accession number(s) can be found in the article/ supplementary material .
High citations most often characterize quality research that reflects the foci of the discipline. This study aims to spotlight the most recent hot topics and the trends looming from the highly cited papers (HCPs) in Web of Science category of linguistics and language & linguistics with bibliometric analysis. The bibliometric information of the 143 HCPs based on Essential Citation Indicators was retrieved and used to identify and analyze influential contributors at the levels of journals, authors, and countries. The most frequently explored topics were identified by corpus analysis and manual checking. The retrieved topics can be grouped into five general categories: multilingual-related , language teaching , and learning related , psycho/pathological/cognitive linguistics-related , methods and tools-related , and others . Topics such as bi/multilingual(ism) , translanguaging , language/writing development , models , emotions , foreign language enjoyment (FLE) , cognition , anxiety are among the most frequently explored. Multilingual and positive trends are discerned from the investigated HCPs. The findings inform linguistic researchers of the publication characteristics of the HCPs in the linguistics field and help them pinpoint the research trends and directions to exert their efforts in future studies.
1. Introduction
Citations, as a rule, exhibit a skewed distributional pattern over the academic publications: a few papers accumulate an overwhelming large citations while the majority are rarely, if ever, cited. Correspondingly, the highly cited papers (HCPs) receive the greatest amount of attention in the academia as citations are commonly regarded as a strong indicator of research excellence. For academic professionals, following HCPs is an efficient way to stay current with the developments in a field and to make better informed decisions regarding potential research topics and directions to exert their efforts. For academic institutions, government and private agencies, and generally the science policy makers, they keep a close eye on and take advantage of this visible indicator, citations, to make more informed decisions on research funding allocation and science policy formulation. Under the backdrop of ever-growing academic outputs, there is noticeable attention shift from publication quantity to publication quality. Many countries are developing research policies to identify “excellent” universities, research groups, and researchers ( Danell, 2011 ). In a word, HCPs showcase high-quality research, encompass significant themes, and constitute a critical reference point in a research field as they are “gold bullion of science” ( Smith, 2007 ).
2. Literature review
Bibliometrics, a term coined by Pritchard (1969) , refers to the application of mathematical methods to the analysis of academic publications. Essentially this is a quantitative method to depict publication patterns within a given field based on a body of literature. There are many bibliometric studies on natural and social sciences in general ( Hsu and Ho, 2014 ; Zhu and Lei, 2022 ) and on various specific disciplines such as management sciences ( Liao et al., 2018 ), biomass research ( Chen and Ho, 2015 ), computer sciences ( Xie and Willett, 2013 ), and sport sciences ( Mancebo et al., 2013 ; Ríos et al., 2013 ), etc. In these studies, researchers tracked developments, weighed research impacts, and highlighted emerging scientific fronts with bibliometric methods. In the field of linguistics, bibliometric studies all occurred in the past few years ( van Doorslaer and Gambier, 2015 ; Lei and Liao, 2017 ; Gong et al., 2018 ; Lei and Liu, 2018 , 2019 ). These bibliometric studies mostly examined a sub-area of linguistics, such as corpus linguistics ( Liao and Lei, 2017 ), translation studies ( van Doorslaer and Gambier, 2015 ), the teaching of Chinese as a second/foreign language ( Gong et al., 2018 ), academic journals like System ( Lei and Liu, 2018 ) or Porta Linguarum ( Sabiote and Rodríguez, 2015 ), etc. Although Lei and Liu (2019) took the entire discipline of linguistics under investigation, their research is exclusively focused on applied linguistics and restricted in a limited number of journals (42 journals in total), leaving publications in other linguistics disciplines and qualified journals unexamined.
Over the recent years, a number of studies have been concerned with “excellent” papers or HCPs. For example, Small (2004) surveyed the HCPs authors’ opinions on why their papers are highly cited. The strong interest, the novelty, the utility, and the high importance of the work were among the most frequently mentioned. Most authors also considered that their selected HCPs are indeed based on their most important work in their academic career. Aksnes (2003) investigated the characteristics of HCPs and found that they were generally authored by a large number of scientists, often involving international collaboration. Some researchers even attempted to predict the HCPs by building mathematical models, implying “the first mover advantage in scientific publication” ( Newman, 2008 , 2014 ). In other words, papers published earlier in a field generally are more likely to accumulate more citations than those published later. Although many papers addressed HCPs from different perspectives, they held a common belief that HCPs are very different from less or zero cited papers and thus deserve utmost attention in academic research ( Aksnes, 2003 ; Blessinger and Hrycaj, 2010 ; Yan et al., 2022 ).
Although an increased focus on research quality can be observed in different fields, opinions diverge on the range and the inclusion criterion of excellent papers. Are they ‘highly cited’, ‘top cited’, or ‘most frequently cited’ papers? Aksnes (2003) noted two different approaches to define a highly cited article, involving absolute or relative thresholds, respectively. An absolute threshold stipulates a minimum number of citations for identifying excellent papers while a relative threshold employs the percentile rank classes, for example, the top 10% most highly cited papers in a discipline or in a publication year or in a publication set. It is important to note that citations differ significantly in different fields and disciplines. A HCP in natural sciences generally accumulates more citations than its counterpart in social sciences. Thus, it is necessary to investigate HCPs from different fields separately or adopt different inclusion criterion to ensure a valid comparison.
The present study has been motivated by two considerations. First, the sizable number of publications of varied qualities in a scientific field makes it difficult or even impossible to conduct any reliable and effective literature research. Focusing on the quality publications, the HCPs in particular, might lend more credibility to the findings on trends. Second, HCPs can serve as a great platform to discover potentially important information for the development of a discipline and understand the past, present, and future of the scientific structure. Therefore, the present study aims to investigate the hot topics and publication trends in the Web of Science category of linguistics or language & linguistics (shortened as linguistics in later references) with bibliometric methods. The study aims to answer the following three questions:
- Who are the most productive and impactful contributors of the HCPs in WoS category of linguistics or language & linguistics in terms of publication venues, authors, and countries?
- What are the most frequently explored topics in HCPs?
- What are the general research trends revealed from the HCPs?
3. Materials and methods
Different from previous studies which used an arbitrary inclusion threshold (e.g., Blessinger and Hrycaj, 2010 ; Hsu and Ho, 2014 ), we rely on Essential Science Indicator (ESI) to identify the HCPs. Developed by Clarivate, a leading company in the areas of bibliometrics and scientometrics, ESI reveals emerging science trends as well as influential individuals, institutions, papers, journals, and countries in any scientific fields of inquiry by drawing on the complete WoS databases. ESI has been chosen for the following three reasons. First, ESI adopts a stricter inclusion criterion for HCPs identification. That is, a paper is selected as a HCP only when its citations exceed the top 1% citation threshold in each of the 22 ESI subject categories. Second, ESI is widely used and recognized for its reliability and authority in identifying the top-charting work, generating “excellent” metrics including hot and highly cited papers. Third, ESI automatically updates its database to generate the most recent HCPs, especially suitable for trend studies for a specified timeframe.
3.1. Data source
The data retrieval was completed at the portal of our university library on June 20, 2022. The methods to retrieve the data are described in Table 1 . The bibliometric indicators regarding the important contributors at journal/author/country levels were obtained. Specifically, after the research was completed, we clicked the “Analyze Results” bar on the result page for the detailed descriptive analysis of the retrieved bibliometric data.
Retrieval strategies.
Several points should be noted about the search strategies. First, we searched the bibliometric data from two sub-databases of WoS core collection: Social Science Citation Index (SSCI) and Arts & Humanities Citation Index (A&HCI). There is no need to include the sub-database of Science Citation Index Expanded (SCI-EXPANDED) because publications in the linguistics field are almost exclusively indexed in SSCI and A&HCI journals. WoS core collection was chosen as the data source because it boasts one of the most comprehensive and authoritative databases of bibliometric information in the world. Many previous studies utilized WoS to retrieve bibliometric data. van Oorschot et al. (2018) and Ruggeri et al. (2019) even indicated that WoS meets the highest standards in terms of impact factor and citation counts and hence guarantees the validity of any bibliometric analysis. Second, we do not restrict the document types as HCPs selection informed by ESI only considers articles and reviews. Third, we do not set the date range as the dataset of ESI-HCPs is automatically updated regularly to include the most recent 10 years of publications.
The aforementioned query obtained a total of 143 HCPs published in 48 journals contributed by 352 authors of 226 institutions. We then downloaded the raw bibliometric parameters of the 143 HCPs for follow-up analysis including publication years, authors, publication titles, countries, affiliations, abstracts, citation reports, etc. A complete list of the 143 HCPs can be found in the Supplementary Material . We collected the most recent impact factor (IF) of each journal from the 2022 Journal Citation Reports (JCR).
3.2. Data analysis
3.2.1. citation analysis.
A citation threshold is the minimum number of citations obtained by ranking papers in a research field in descending order by citation counts and then selecting the top fraction or percentage of papers. In ESI, the highly cited threshold reveals the minimum number of citations received by the top 1% of papers from each of the 10 database years. In other words, a paper has to meet the minimum citation threshold that varies by research fields and by years to enter the HCP list. Of the 22 research fields in ESI, Social Science, General is a broad field covering a number of WoS categories including linguistics and language & linguistics . We checked the ESI official website to obtain the yearly highly cited thresholds in the research field of Social Science , General as shown in Figure 1 ( https://esi.clarivate.com/ThresholdsAction.action ). As we can see, the longer a paper has been published, the more citations it has to receive to meet the threshold. We then divided the raw citation numbers of HCPs with the Highly Cited Thresholds in the corresponding year to obtain the normalized citations for each HCP.

Highly cited thresholds in the research field of Social Sciences, General.
3.2.2. Corpus analysis and manual checking
To determine the most frequently explored topics in these HCPs, we used both corpus-based analysis of word frequency and manual checking. Specifically, the more frequently a word or phrase occurs in a specifically designed corpus, the more likely it constitutes a research topic. In this study, we built an Abstract corpus with all the abstracts of the 143 HCPs, totaling 24,800 tokens. The procedures to retrieve the research topics in the Abstract corpus were as follows. First, the 143 pieces of abstracts were saved as separate .txt files in one folder. Second, AntConc ( Anthony, 2022 ), a corpus analysis tool for concordancing and text analysis, was employed to extract lists of n-grams (2–4) in decreasing order of frequency. We also generated a list of individual nouns because sometimes individual nouns can also constitute research topics. Considering our small corpus data, we adopted both frequency (3) and range criteria (3) for topic candidacy. That is, a candidate n-gram must occur at least 3 times and in at least 3 different abstract files. The frequency threshold guarantees the importance of the candidate topics while the range threshold guarantees that the topics are not overly crowded in a few number of publications. In this process, we actually tested the frequency and range thresholds several rounds for the inclusion of all the potential topics. In total, we obtained 531 nouns, 1,330 2-grams, 331 3-grams, and 81 4-grams. Third, because most of the retrieved n-grams cannot function as meaningful research topics, we manually checked all the candidate items and discussed extensively to decide their roles as potential research topics until full agreements were reached. Finally, we read all the abstracts of the 143 HCPs to further validate their roles as research topics. In the end, we got 118 topic items in total.
4.1. Main publication venues of HCPs
Of the 48 journals which published the 143 HCPs, 17 journals have contributed at least 3 HCPs ( Table 2 ), around 71.33% of the total examined HCPs (102/143), indicating that HCPs tend to be highly concentrated in a limited number of journals. The three largest publication outlets of HCPs are Bilingualism Language and Cognition (16), International Journal of Bilingual Education and Bilingualism (11), and Modern Language Journal (10). Because each journal varies greatly in the number of papers published per year and the number of HCPs is associated with journal circulations, we divided the total number of papers (TP) in the examined years (2011–2021) with the number of the HCPs to acquire the HCP percentage for each journal (HCPs/TP). The three journals with the highest HCPs/TP percentage are Annual Review of Applied Linguistics (2.26), Modern Language Journal (2.08), and Bilingualism Language and Cognition (1.74), indicating that papers published in these journals have a higher probability to enter the HCPs list.
Top 17 publication venues of HCPs.
N: the number of HCPs in each journal; N%: the percentage of HCPs in each journal in the total of 143 HCPs; TP: the total number of papers in the examined timespan (2011–2021); N/TP %: the percentage of HCPs in the total journal publications in the examined time span; TC/HCP: average citations of each HCP; R: journal ranking for the designated indicator; IF: Impact Factor in the year of 2022.
In terms of the general impact of the HCPs from each journal, we divided the number of HCPs with their total citations (TC) to obtain the average citations for each HCP (TC/HCP). The three journals with the highest TC/HCP are Journal of Memory and Language (837.86), Computational Linguistics (533.75), and Journal of Pragmatics (303.75). It indicates that even in the same WoS category, HCPs in different journals have strikingly different capability to accumulate citations. For example, the TC/HCP in System is as low as 31.73, which is even less than 4% of the highest TC/HCP in Journal of Memory and Language .
In regards to the latest journal impact factor (IF) in 2022, the top four journals with the highest IF are Computational Linguistics (7.778) , Modern Language Journal (7.5), Computer Assisted Language Learning (5.964), and Language Learning (5.24). According to the Journal Citation Reports (JCR) quantile rankings in WoS category of linguistics , all the journals on the list belong to the Q 1 (the top 25%), indicating that contributors are more likely to be attracted to contribute and cite papers in these prestigious high impact journals.
4.2. Authors of HCPs
A total of 352 authors had their names listed in the 143 HCPs, of whom 33 authors appeared in at least 2 HCPs as shown in Table 3 . We also provided in Table 3 other indicators to evaluate the authors’ productivity and impact including the total number of citations (TC), the number of citations per HCP, and the number of First author or Corresponding author HCPs (FA/CA). The reason we include the FA/CA indicator is that first authors and corresponding authors are usually considered to contribute the most and should receive greater proportion of credit in academic publications ( Marui et al., 2004 ; Dance, 2012 ).
Authors with at least 2 HCPs.
N: number of HCPs from each author; FA/CA: first author or corresponding author HCPs; TC: total citations of the HCPs from each author; C/HCP: average citations per HCP for each author.
In terms of the number of HCPs, Dewaele JM from Birkbeck Univ London tops the list with 7 HCPs with total citations of 492 (TC = 492), followed by Li C from Huazhong Univ Sci & Technol (#HCPs = 5; TC = 215) and Saito K from UCL (#HCPs = 5; TC = 576). It is to be noted that both Li C and Saito K have close academic collaborations with Dewaele JM . For example, 3 of the 5 HCPs by Li C are co-authored with Dewaele JM . The topics in their co-authored HCPs are mostly about foreign language learning emotions such as boredom , anxiety , enjoyment , the measurement , and positive psychology .
In regards to TC, Li, W . from UCL stands out as the most influential scholar among all the listed authors with total citations of 956 from 2 HCPs, followed by Norton B from Univ British Columbia (TC = 915) and Vasishth S from Univ Potsdam (TC = 694). The average citations per HCP from them are also the highest among the listed authors (478, 305, 347, respectively). It is important to note that Li, W.’ s 2 HCPs are his groundbreaking works on translanguaging which almost become must-reads for anyone who engages in translanguaging research ( Li, 2011 , 2018 ). Besides, Li, W. single authors his 2 HCPs, which is extremely rare as HCPs are often the results from multiple researchers. Norton B ’s HCPs are exploring some core issues in applied linguistics such as identity and investment , language learning , and social change that are considered the foundational work in its field ( Norton and Toohey, 2011 ; Darvin and Norton, 2015 ).
From the perspective of FA/CA papers, Li C from Huazhong Univ Sci and Technol is prominent because she is the first author of all her 5 HCPs. Her research on language learning emotions in the Chinese context is gaining widespread recognition ( Li et al., 2018 , 2019 , 2021 ; Li, 2019 , 2021 ). However, as a newly emerging researcher, most of her HCPs are published in the very recent years and hence accumulate relatively fewer citations (TC = 215). Mondada L from Univ Basel follows closely and single authors her 3 HCPs. Her work is mostly devoted to conversation analysis , multimodality , and social interaction ( Mondada, 2016 , 2018 , 2019 ).
We need to mention the following points regarding the productive authors of HCPs. First, when we calculated the number of HCPs from each author, only the papers published in the journals indexed in the investigated WoS categories were taken in account ( linguistics; language & linguistics ), which came as a compromise to protect the linguistics oriented nature of the HCPs. For example, Brysbaert M from Ghent University claimed a total of 8 HCPs at the time of the data retrieval, of which 6 HCPs were published in WoS category of psychology and more psychologically oriented, hence not included in our study. Besides, all the authors on the author list were treated equally when we calculated the number of HCPs, disregarding the author ordering. That implies that some influential authors may not be able to enter the list as their publications are comparatively fewer. Second, as some authors reported different affiliations at their different career stages, we only provide their most recent affiliation for convenience. Third, it is highly competitive to have one’s work selected as HCPs. The fact that a majority of the HCPs authors do not appear in our productive author list does not diminish their great contributions to this field. The rankings in Table 3 does not necessarily reflect the recognition authors have earned in academia at large.
4.3. Productive countries of HCPs
In total, the 143 HCPs originated from 33 countries. The most productive countries that contributed at least three HCPs are listed in Table 4 . The USA took an overwhelming lead with 59 HCPs, followed distantly by England with 31 HCPs. They also boasted the highest total citations (TC = 15,770; TC = 9,840), manifesting their high productivity and strong influence as traditional powerhouses in linguistics research. In regards to the average citations per HCP, Germany , England and the USA were the top three countries (TC/HCP = 281.67, 281.14, and 267.29, respectively). Although China held the third position with 19 HCPs published, its TC/HCP is the third from the bottom (TC/HCP = 66.84). One of the important reasons is that 13 out of the 19 HCPs contributed by scholars in China are published in the year of 2020 or 2021. The newly published HCPs may need more time to accumulate citations. Besides, 18 out of the 19 HCPs in China are first author and/or corresponding authors, indicating that scholars in China are becoming more independent and gaining more voice in English linguistics research.
Top 18 countries with at least 3 HCPs.
Two points should be noted here as to the productive countries. First, we calculated the HCP contributions from the country level instead of the region level. In other words, HCP contributions from different regions of the same country will be combined in the calculation. For example, HCPs from Scotland were added to the HCPs from England . HCPs from Hong Kong , Macau , and Taiwan are put together with the HCPs from Mainland China . In this way, a clear picture of the HCPs on the country level can be painted. Second, we manually checked the address information of the first author and corresponding author for each HCP. There are some cases where the first author or the corresponding author may report affiliations from more than one country. In this case, every country in their address list will be treated equally in the FA/CA calculation. In other word, a HCP may be classified into more than one country because of the different country backgrounds of the first and/or the corresponding author.
4.4. Top 20 HCPs
The top 20 HCPs with the highest normed citations are listed in decreasing order in Table 5 . The top cited publications can guide us to better understand the development and research topics in recent years.
Top 20 HCPs.
To save space, not full information about the HCPs is given. Some article titles have been abbreviated if they are too lengthy; for the authors, we report the first two authors and use “et al” if there are three authors or more; RC: raw citations; NC: normalized citations
By reading the titles and the abstracts of these top HCPs, we categorized the topics of the 20 HCPs into the following five groups: (i) statistical and analytical methods in (psycho)linguistics such as sentimental analysis, sentence simplification techniques, effect sizes, linear mixed models (#1, 3, 4, 6, 9, 14), (ii) language learning/teaching emotions such enjoyment, anxiety, boredom, stress (#11, 15, 16, 18, 19), (iii) translanguaging or multilinguilism (#5, 13, 20, 17), (iv) language perception (#2, 7, 10), (v) medium of instruction (#8, 12). It is no surprise that 6 out of the top 20 HCPs are about statistical methods in linguistics because language researchers aspire to employ statistics to make their research more scientific. Besides, we noticed that the papers on language teaching/learning emotions on the list are all published in the year of 2020 and 2021, indicating that these emerging topics may deserve more attention in future research. We also noticed two Covid-19 related articles (#16, 19) explored the emotions teachers and students experience during the pandemic, a timely response to the urgent need of the language learning and teaching community.
It is of special interest to note that papers from the journals indexed in multiple JCR categories seem to accumulate more citations. For example, Journal of Memory and Language , American Journal of Speech-Language Pathology , and Computational Linguistics are indexed both in SSCI and SCIE and contribute the top 4 HCPs, manifesting the advantage of these hybrid journals in amassing citations compared to the conventional language journals. Besides, different to findings from Yan et al. (2022) that most of the top HCPs in the field of radiology are reviews in document types, 19 out of the top 20 HCPs are research articles instead of reviews except Macaro et al. (2018) .
4.5. Most frequently explored topics of HCPs
After obtaining the corpus based topic items, we read all the titles and abstracts of the 143 HCPs to further validate their roles as research topics. Table 6 presents the top research topics with the observed frequency of 5 or above. We grouped these topics into five broad categories: bilingual-related, language learning/teaching-related, psycho/pathological/cognitive linguistics-related, methods and tools-related, and others . The observed frequency count for each topic in the abstract corpus were included in the brackets. We found that about 34 of the 143 HCPs are exploring bilingual related issues, the largest share among all the categorized topics, testifying its academic popularity in the examined timespan. Besides, 30 of the 143 HCPs are investigating language learning/teaching-related issues, with topics ranging from learners (e.g., EFL learners, individual difference) to multiple learning variables (e.g., learning strategy, motivation, agency). The findings here will be validated by the analysis of the keywords.
Categorization of the most explored research topics.
N: the number of the HCPs in each topic category; ELF: English as a lingua franca; CLIL: content and language integrated learning; FLE: foreign language enjoyment; FLCA: foreign language classroom anxiety
Several points should be mentioned regarding the topic candidacy. First, for similar topic expressions, we used a cover term and added the frequency counts. For example, multilingualism is a cover term for bilinguals, bilingualism, plurilingualism, and multilingualism . Second, for nouns of singular and plural forms (e.g., emotion and emotions ) or for items with different spellings (e.g., meta analysis and meta analyses ), we combined the frequency counts. Third, we found that some longer items (3 grams and 4 grams) could be subsumed to short ones (2 grams or monogram) without loss of essential meaning (e.g., working memory from working memory capacity ). In this case, the shorter ones were kept for their higher frequency. Fourth, some highly frequent terms were discarded because they were too general to be valuable topics in language research, for example, applied linguistics , language use , second language .
5. Discussion and implications
Based on 143 highly cited papers collected from the WoS categories of linguistics , the present study attempts to present a bird’s eye view of the publication landscape and the most updated research themes reflected from the HCPs in the linguistics field. Specifically, we investigated the important contributors of HCPs in terms of journals, authors and countries. Besides, we spotlighted the research topics by corpus-based analysis of the abstracts and a detailed analysis of the top HCPs. The study has produced several findings that bear important implications.
The first finding is that the HCPs are highly concentrated in a limited journals and countries. In regards to journals, those in the spheres of bilingualism and applied linguistics (e.g., language teaching and learning) are likely to accumulate more citations and hence to produce more HCPs. Journals that focus on bilingualism from a linguistic, psycholinguistic, and neuroscientific perspective are the most frequent outlets of HCPs as evidenced by the top two productive journals of HCPs, Bilingualism Language and Cognition and International Journal of Bilingual Education and Bilingualism . This can be explained by the multidisciplinary nature of bilingual-related research and the development of cognitive measurement techniques. The merits of analyzing publication venues of HCPs are two folds. One the one hand, it can point out which sources of high-quality publications in this field can be inquired for readers as most of the significant and cutting-edge achievements are concentrated in these prestigious journals. On the other hand, it also provides essential guidance or channels for authors or contributors to submit their works for higher visibility.
In terms of country distributions, the traditional powerhouses in linguistics research such as the USA and England are undoubtedly leading the HCP publications in both the number and the citations of the HCPs. However, developing countries are also becoming increasing prominent such as China and Iran , which could be traceable in the funding and support of national language policies and development policies as reported in recent studies ( Ping et al., 2009 ; Lei and Liu, 2019 ). Take China as an example. Along with economic development, China has given more impetus to academic outputs with increased investment in scientific research ( Lei and Liao, 2017 ). Therefore, researchers in China are highly motivated to publish papers in high-quality journals to win recognition in international academia and to deal with the publish or perish pressure ( Lee, 2014 ). These factors may explain the rise of China as a new emerging research powerhouse in both natural and social sciences, including English linguistics research.
The second finding is the multilingual trend in linguistics research. The dominant clustering of topics regarding multilingualism can be understood as a timely response to the multilingual research fever ( May, 2014 ). 34 out of the 143 HCPs have such words as bilingualism, bilingual, multilingualism , translanguaging , etc., in their titles, reflecting a strong multilingual tendency of the HCPs. Multilingual-related HCPs mainly involve three aspects: multilingualism from the perspectives of psycholinguistics and cognition (e.g., Luk et al., 2011 ; Leivada et al., 2020 ); multilingual teaching (e.g., Schissel et al., 2018 ; Ortega, 2019 ; Archila et al., 2021 ); language policies related to multilingualism (e.g., Shen and Gao, 2018 ). As a pedagogical process initially used to describe the bilingual classroom practice and also a frequently explored topic in HCPs, translanguaging is developed into an applied linguistics theory since Li’s Translanguaging as a Practical Theory of Language ( Li, 2018 ). The most common collocates of translanguaging in the Abstract corpus are pedagogy/pedagogies, practices, space/spaces . There are two main reasons for this multilingual turn. First, the rapid development of globalization, immigration, and overseas study programs greatly stimulate the use and research of multiple languages in different linguistic contexts. Second, in many non-English countries, courses are delivered through languages (mostly English) besides their mother tongue ( Clark, 2017 ). Students are required to use multiple languages as resources to learn and understand subjects and ideas. The burgeoning body of English Medium Instruction literature in higher education is in line with the rising interest in multilingualism. Due to the innate multidisciplinary nature, it is to be expected that, multilingualism, the topic du jour, is bound to attract more attention in the future.
The third finding is the application of Positive Psychology (PP) in second language acquisition (SLA), that is, the positive trend in linguistic research. In our analysis, 20 out of 143 HCPs have words or phrases such as emotions, enjoyment, boredom, anxiety , and positive psychology in their titles, which might signal a shift of interest in the psychology of language learners and teachers in different linguistic environments. Our study shows Foreign language enjoyment (FLE) is the most frequently explored emotion, followed by foreign language classroom anxiety (FLCA), the learners’ metaphorical left and right feet on their journey to acquiring the foreign language ( Dewaele and MacIntyre, 2016 ). In fact, the topics of PP are not entirely new to SLA. For example, studies of language motivations, affections, and good language learners all provide roots for the emergence of PP in SLA ( Naiman, 1978 ; Gardner, 2010 ). In recent years, both research and teaching applications of PP in SLA are building rapidly, with a diversity of topics already being explored such as positive education and PP interventions. It is to be noted that SLA also feeds back on PP theories and concepts besides drawing inspirations from it, which makes it “an area rich for interdisciplinary cross-fertilization of ideas” ( Macintyre et al., 2019 ).
It should be noted that subjectivity is involved when we decide and categorize the candidate topic items based on the Abstract corpus. However, the frequency and range criteria guarantee that these items are actually more explored in multiple HCPs, thus indicating topic values for further investigation. Some high frequent n-grams are abandoned because they are too general or not meaningful topics. For example, applied linguistics is too broad to be included as most of the HCPs concern issues in this research line instead of theoretical linguistics. By meaningful topics, we mean that the topics can help journal editors and readers quickly locate their interested fields ( Lei and Liu, 2019 ), as the author keywords such as bilingualism , emotions , and individual differences . The examination of the few 3/4-grams and monograms (mostly nouns) revealed that most of them were either not meaningful topics or they could be subsumed in the 2-grams. Besides, there is inevitably some overlapping in the topic categorizations. For example, some topics in the language teaching and learning category are situated and discussed within the context of multilingualism. The merits of topic categorizations are two folds: to better monitor the overlapping between the Abstract corpus-based topic items and the keywords; to roughly delineate the research strands in the HCPs for future research.
It should also be noted that all the results were based on the retrieved HCPs only. The study did not aim to paint a comprehensive and full picture of the whole landscape of linguistic research. Rather, it specifically focused on the most popular literature in a specified timeframe, thus generating the snapshots or trends in linguistic research. One of the important merits of this methodology is that some newly emerging but highly cited researchers can be spotlighted and gain more academic attention because only the metrics of HCPs are considered in calculation. On the contrary, the exclusion of some other highly cited researchers in general such as Rod Ellis and Ken Hyland just indicates that their highly cited publications are not within our investigated timeframe and cannot be interpreted as their diminishing academic influence in the field. Besides, the study does not consider the issue of collaborators or collaborations in calculating the number of HCPs for two reasons. First, although some researchers are regular collaborators such as Li CC and Dewaele JM, their individual contribution can never be undermined. Second, the study also provides additional information about the number of the FA/CA HCPs from each listed author, which may aid readers in locating their interested research.
We acknowledge that our study has some limitations that should be addressed in future research. First, our study focuses on the HCPs extracted from WoS SSCI and A&HCI journals, the alleged most celebrated papers in this field. Future studies may consider including data from other databases such as Scopus to verify the findings of the present study. Second, our Abstract corpus-based method for topic extraction involved human judgement. Although the final list was the result of several rounds of discussions among the authors, it is difficult or even impossible to avoid subjectivity and some worthy topics may be unconsciously missed. Therefore, future research may consider employing automatic algorithms to extract topics. For example, a dependency-based machine learning approach can be used to identify research topics ( Zhu and Lei, 2021 ).
Data availability statement
Author contributions.
SY: conceptualization and methodology. SY and LZ: writing-review and editing and writing-original draft. All authors contributed to the article and approved the submitted version.
This work was supported by Humanities and Social Sciences Youth Fund of China MOE under the grant 20YJC740076 and 18YJC740141.
Conflict of interest
The authors declare that the research was conducted in the absence of any commercial or financial relationships that could be construed as a potential conflict of interest.
Publisher’s note
All claims expressed in this article are solely those of the authors and do not necessarily represent those of their affiliated organizations, or those of the publisher, the editors and the reviewers. Any product that may be evaluated in this article, or claim that may be made by its manufacturer, is not guaranteed or endorsed by the publisher.
Supplementary material
The Supplementary material for this article can be found online at: https://www.frontiersin.org/articles/10.3389/fpsyg.2022.1052586/full#supplementary-material
- Aksnes D. W. (2003). Characteristics of highly cited papers . Res. Eval. 12 , 159–170. doi: 10.3152/147154403781776645 [ CrossRef ] [ Google Scholar ]
- Anthony L. (2022). AntConc (version 4.0.5) Tokyo, Japan: Waseda University. Available at: https://www.laurenceanthony.net/software (Accessed June 20, 2022).
- Archila P. A., Molina J., Truscott de Mejía A.-M. (2021). Fostering bilingual scientific writing through a systematic and purposeful code-switching pedagogical strategy . Int. J. Biling. Educ. Biling. 24 , 785–803. doi: 10.1080/13670050.2018.1516189 [ CrossRef ] [ Google Scholar ]
- Blessinger K., Hrycaj P. (2010). Highly cited articles in library and information science: an analysis of content and authorship trends . Libr. Inf. Sci. Res. 32 , 156–162. doi: 10.1016/j.lisr.2009.12.007 [ CrossRef ] [ Google Scholar ]
- Chen H., Ho Y. S. (2015). Highly cited articles in biomass research: a bibliometric analysis . Renew. Sust. Energ. Rev. 49 , 12–20. doi: 10.1016/j.rser.2015.04.060 [ CrossRef ] [ Google Scholar ]
- Clark S. (2017). Translanguaging in higher education: beyond monolingual ideologies . Int. J. Biling. Educ. Biling. 22 , 1048–1051. doi: 10.1080/13670050.2017.1322568 [ CrossRef ] [ Google Scholar ]
- Dance A. (2012). Authorship: Who’s on first? Nature 489 , 591–593. doi: 10.1038/nj7417-591a, PMID: [ PubMed ] [ CrossRef ] [ Google Scholar ]
- Danell R. (2011). Can the quality of scientific work be predicted using information on the author’s track record? J. Am. Soc. Inf. Sci. Technol. 62 , 50–60. doi: 10.1002/asi.21454 [ CrossRef ] [ Google Scholar ]
- Darvin R., Norton B. (2015). Identity and a model of Investment in Applied Linguistics . Annu. Rev. Appl. Linguist. 35 , 36–56. doi: 10.1017/S0267190514000191 [ CrossRef ] [ Google Scholar ]
- Dewaele J.-M., MacIntyre P. D. (2016). “ Foreign language enjoyment and foreign language classroom anxiety: the right and left feet of the language learner ” in Positive psychology in SLA . eds. Peter D. M., Tammy G., Sarah M. (Bristol, Blue Ridge Summit: Multilingual Matters; ), 215–236. [ Google Scholar ]
- Gardner R. (2010). Motivation and second language acquisition: The socio-educational model . New York: Peter Lang. [ Google Scholar ]
- Gong Y., Lyu B., Gao X. (2018). Research on teaching Chinese as a second or foreign language in and outside mainland China: a bibliometric analysis . Asia Pac. Educ. Res. 27 , 277–289. doi: 10.1007/s40299-018-0385-2 [ CrossRef ] [ Google Scholar ]
- Hsu Y., Ho Y. S. (2014). Highly cited articles in health care sciences and services field in science citation index Expanded . Methods Inf. Med. 53 , 446–458. doi: 10.3414/ME14-01-0022, PMID: [ PubMed ] [ CrossRef ] [ Google Scholar ]
- Lee I. (2014). Publish or perish: the myth and reality of academic publishing . Lang. Teach. 47 , 250–261. doi: 10.1017/S0261444811000504 [ CrossRef ] [ Google Scholar ]
- Lei L., Liao S. (2017). Publications in linguistics journals from mainland China, Hong Kong, Taiwan, and Macau (2003–2012): a bibliometric analysis . J. Quant. Ling. 24 , 54–64. doi: 10.1080/09296174.2016.1260274 [ CrossRef ] [ Google Scholar ]
- Lei L., Liu D. (2018). The research trends and contributions of System’s publications over the past four decades (1973–2017): a bibliometric analysis . System 80 , 1–13. doi: 10.1016/j.system.2018.10.003 [ CrossRef ] [ Google Scholar ]
- Lei L., Liu D. (2019). Research trends in applied linguistics from 2005 to 2016: a bibliometric analysis and its implications . Appl. Linguis. 40 , 540–561. doi: 10.1093/applin/amy003 [ CrossRef ] [ Google Scholar ]
- Leivada E., Westergaard M., Duabeitia J. A., Rothman J. (2020). On the phantom-like appearance of bilingualism effects on neurocognition: (how) should we proceed? Biling. Lang. Congn. 24 , 197–210. doi: 10.1017/S1366728920000358 [ CrossRef ] [ Google Scholar ]
- Li W. (2011). Moment analysis and translanguaging space: discursive construction of identities by multilingual Chinese youth in Britain . Energy Fuel 43 , 1222–1235. doi: 10.1016/j.pragma.2010.07.035 [ CrossRef ] [ Google Scholar ]
- Li W. (2018). Translanguaging as a practical theory of language . Appl. Linguis. 39 , 9–30. doi: 10.1093/applin/amx039 [ CrossRef ] [ Google Scholar ]
- Li C. (2019). A positive psychology perspective on Chinese EFL students’ trait emotional intelligence, foreign language enjoyment and EFL learning achievement . J. Multiling. Multicult. Dev. 41 , 246–263. doi: 10.1080/01434632.2019.1614187 [ CrossRef ] [ Google Scholar ]
- Li C. (2021). A control-value theory approach to boredom in English classes among university students in China . Mod. Lang. J. 105 , 317–334. doi: 10.1111/modl.12693 [ CrossRef ] [ Google Scholar ]
- Li C., Dewaele J. M., Hu Y. (2021). Foreign language learning boredom: conceptualization and measurement . Appl. Ling. Rev. doi: 10.1515/applirev-2020-0124 [ CrossRef ] [ Google Scholar ]
- Li C., Dewaele J. M., Jiang G. (2019). The complex relationship between classroom emotions and EFL achievement in China . Appl. Ling. Rev. 11 , 485–510. doi: 10.1515/applirev-2018-0043 [ CrossRef ] [ Google Scholar ]
- Li C., Jiang G., Jean-Marc D. (2018). Understanding Chinese high school students’ foreign language enjoyment: validation of the Chinese version of the foreign language enjoyment scale . System 76 , 183–196. doi: 10.1016/j.system.2018.06.004 [ CrossRef ] [ Google Scholar ]
- Liao S., Lei L. (2017). What we talk about when we talk about corpus: a bibliometric analysis of corpus-related research in linguistics (2000-2015) . Glottometrics 38 , 1–20. [ Google Scholar ]
- Liao H., Tang M., Li Z., Lev B. (2018). Bibliometric analysis for highly cited papers in operations research and management science from 2008 to 2017 based on essential science indicators . Omega 88 , 223–236. doi: 10.1016/j.omega.2018.11.005 [ CrossRef ] [ Google Scholar ]
- Luk G., Sa E. D., Bialystok E. (2011). Is there a relation between onset age of bilingualism and enhancement of cognitive control? Biling. Lang. Cogn. 14 , 588–595. doi: 10.1017/S1366728911000010 [ CrossRef ] [ Google Scholar ]
- Macaro E., Curle S., Pun J., Dearden J. (2018). A systematic review of English medium instruction in higher education . Lang. Teach. 51 , 36–76. doi: 10.1017/S0261444817000350 [ CrossRef ] [ Google Scholar ]
- Macintyre P., Gregersen T., Mercer S. (2019). Setting an agenda for positive psychology in SLA: theory, practice, and research . Mod. Lang. J. 103 , 262–274. doi: 10.1111/modl.12544 [ CrossRef ] [ Google Scholar ]
- Mancebo F. P., Sapena A. F., Herrera M. V., González L., Toca H., Benavent R. A. (2013). Scientific literature analysis of judo in web of science . Arch. Budo 9 , 81–91. doi: 10.12659/AOB.883883 [ CrossRef ] [ Google Scholar ]
- Marui M., Bozikov J., Katavi V., Hren D., Kljakovi-Gapi M., Marui A. (2004). Authorship in a small medical journal: a study of contributorship statements by corresponding authors . Sci. Eng. Ethics 10 , 493–502. doi: 10.1007/s11948-004-0007-7, PMID: [ PubMed ] [ CrossRef ] [ Google Scholar ]
- May S. (2014). The multilingual turn: Implications for SLA, TESOL and bilingual education . New York: Routledge. [ Google Scholar ]
- Mondada L. (2016). Challenges of multimodality: language and the body in social interaction . J. Socioling. 20 , 336–366. doi: 10.1111/josl.1_12177 [ CrossRef ] [ Google Scholar ]
- Mondada L. (2018). Multiple temporalities of language and body in interaction: challenges for transcribing multimodality . Res. Lang. Soc. Interact. 51 , 85–106. doi: 10.1080/08351813.2018.1413878 [ CrossRef ] [ Google Scholar ]
- Mondada L. (2019). Contemporary issues in conversation analysis: embodiment and materiality, multimodality and multisensoriality in social interaction . J. Pragmat. 145 , 47–62. doi: 10.1016/j.pragma.2019.01.016 [ CrossRef ] [ Google Scholar ]
- Naiman N. (1978). The good language learner . Clevedon, UK: Multilingual Matters. [ Google Scholar ]
- Newman M. (2008). The first-mover advantage in scientific publication . Eplasty 86 , 68001–68006. doi: 10.1209/0295-5075/86/68001 [ CrossRef ] [ Google Scholar ]
- Newman M. (2014). Prediction of highly cited papers . Eplasty 105 :28002. doi: 10.1209/0295-5075/105/28002 [ CrossRef ] [ Google Scholar ]
- Norton B., Toohey K. (2011). Identity, language learning, and social change . Lang. Teach. 44 , 412–446. doi: 10.1017/S0261444811000309 [ CrossRef ] [ Google Scholar ]
- Ortega L. (2019). SLA and the study of equitable multilingualism . Mod. Lang. J. 103 , 23–38. doi: 10.1111/modl.12525 [ CrossRef ] [ Google Scholar ]
- Ping Z., Thijs B., Glnzel W. (2009). Is China also becoming a giant in social sciences? Scientometrics 79 , 593–621. doi: 10.1007/s11192-007-2068-x [ CrossRef ] [ Google Scholar ]
- Pritchard A. (1969). Statistical bibliography or bibliometrics . J. Doc. 25 , 348–349. [ Google Scholar ]
- Ríos L. J. C., Tamao I. M., Olmos J. (2013). Bibliometric study (1922-2009) on rugby articles in research journals . South Afr. J. Res. Sport Phys. Educ. Rec. 17 , 313–109. doi: 10.3176/tr.2013.3.06 [ CrossRef ] [ Google Scholar ]
- Ruggeri G., Orsi L., Corsi S. (2019). A bibliometric analysis of the scientific literature on Fairtrade labelling . Int. IJC 43 , 134–152. doi: 10.1111/ijcs.12492 [ CrossRef ] [ Google Scholar ]
- Sabiote C. R., Rodríguez J. A. (2015). Bibliometric study and methodological quality indicators of the journal porta Linguarum during six year period 2008-2013 . Porta Ling. 24 , 135–150. doi: 10.30827/Digibug.53866 [ CrossRef ] [ Google Scholar ]
- Schissel J. L., De Korne H., López-Gopar M. E. (2018). Grappling with translanguaging for teaching and assessment in culturally and linguistically diverse contexts: teacher perspectives from Oaxaca, Mexico . Int. J. Biling. Educ. Biling. 24 , 340–356. doi: 10.1080/13670050.2018.1463965 [ CrossRef ] [ Google Scholar ]
- Shen Q., Gao X. (2018). Multilingualism and policy making in greater China: ideological and implementational spaces . Lang. Policy 18 , 1–16. doi: 10.1007/s10993-018-9473-7 [ CrossRef ] [ Google Scholar ]
- Small H. (2004). Why authors think their papers are highly cited . Scientometrics 60 , 305–316. doi: 10.1023/B:SCIE.0000034376.55800.18 [ CrossRef ] [ Google Scholar ]
- Smith D. R. (2007). The New Zealand timber economy, 1840–1935 . N. Z. Med. J. 120 , U2871–U2313. doi: 10.1016/0305-7488(90)90044-C, PMID: [ PubMed ] [ CrossRef ] [ Google Scholar ]
- van Doorslaer L., Gambier Y. (2015). Measuring relationships in translation studies. On affiliations and keyword frequencies in the translation studies bibliography . Perspectives 23 , 305–319. doi: 10.1080/0907676X.2015.1026360 [ CrossRef ] [ Google Scholar ]
- van Oorschot J. A. W. H., Hofman E., Halman J. (2018). A bibliometric review of the innovation adoption literature . Technol. Forecast. Soc. Chang. 134 , 1–21. doi: 10.1016/j.techfore.2018.04.032 [ CrossRef ] [ Google Scholar ]
- Xie Z., Willett P. (2013). The development of computer science research in the People’s republic of China 2000–2009: a bibliometric study . Inf. Dev. 29 , 251–264. doi: 10.1177/0266666912458515 [ CrossRef ] [ Google Scholar ]
- Yan S., Zhang H., Wang J. (2022). Trends and hot topics in radiology, nuclear medicine and medical imaging from 2011–2021: a bibliometric analysis of highly cited papers . Jpn. J. Radiol. 40 , 847–856. doi: 10.1007/s11604-022-01268-z, PMID: [ PMC free article ] [ PubMed ] [ CrossRef ] [ Google Scholar ]
- Zhu H., Lei L. (2021). A dependency-based machine learning approach to the identification of research topics: a case in COVID-19 studies . Lib. Hi Tech 40 , 495–515. doi: 10.1108/LHT-01-2021-0051 [ CrossRef ] [ Google Scholar ]
- Zhu H., Lei L. (2022). The research trends of text classification studies (2000–2020): a bibliometric analysis . SAGE Open 12 , 215824402210899–215824402210816. doi: 10.1177%2F21582440221089963 [ Google Scholar ]
This paper is in the following e-collection/theme issue:
Published on 16.4.2024 in Vol 26 (2024)
Adverse Event Signal Detection Using Patients’ Concerns in Pharmaceutical Care Records: Evaluation of Deep Learning Models
Authors of this article:

Original Paper
- Satoshi Nishioka 1 , PhD ;
- Satoshi Watabe 1 , BSc ;
- Yuki Yanagisawa 1 , PhD ;
- Kyoko Sayama 1 , MSc ;
- Hayato Kizaki 1 , MSc ;
- Shungo Imai 1 , PhD ;
- Mitsuhiro Someya 2 , BSc ;
- Ryoo Taniguchi 2 , PhD ;
- Shuntaro Yada 3 , PhD ;
- Eiji Aramaki 3 , PhD ;
- Satoko Hori 1 , PhD
1 Division of Drug Informatics, Keio University Faculty of Pharmacy, Tokyo, Japan
2 Nakajima Pharmacy, Hokkaido, Japan
3 Nara Institute of Science and Technology, Nara, Japan
Corresponding Author:
Satoko Hori, PhD
Division of Drug Informatics
Keio University Faculty of Pharmacy
1-5-30 Shibakoen
Tokyo, 105-8512
Phone: 81 3 5400 2650
Email: [email protected]
Background: Early detection of adverse events and their management are crucial to improving anticancer treatment outcomes, and listening to patients’ subjective opinions (patients’ voices) can make a major contribution to improving safety management. Recent progress in deep learning technologies has enabled various new approaches for the evaluation of safety-related events based on patient-generated text data, but few studies have focused on the improvement of real-time safety monitoring for individual patients. In addition, no study has yet been performed to validate deep learning models for screening patients’ narratives for clinically important adverse event signals that require medical intervention. In our previous work, novel deep learning models have been developed to detect adverse event signals for hand-foot syndrome or adverse events limiting patients’ daily lives from the authored narratives of patients with cancer, aiming ultimately to use them as safety monitoring support tools for individual patients.
Objective: This study was designed to evaluate whether our deep learning models can screen clinically important adverse event signals that require intervention by health care professionals. The applicability of our deep learning models to data on patients’ concerns at pharmacies was also assessed.
Methods: Pharmaceutical care records at community pharmacies were used for the evaluation of our deep learning models. The records followed the SOAP format, consisting of subjective (S), objective (O), assessment (A), and plan (P) columns. Because of the unique combination of patients’ concerns in the S column and the professional records of the pharmacists, this was considered a suitable data for the present purpose. Our deep learning models were applied to the S records of patients with cancer, and the extracted adverse event signals were assessed in relation to medical actions and prescribed drugs.
Results: From 30,784 S records of 2479 patients with at least 1 prescription of anticancer drugs, our deep learning models extracted true adverse event signals with more than 80% accuracy for both hand-foot syndrome (n=152, 91%) and adverse events limiting patients’ daily lives (n=157, 80.1%). The deep learning models were also able to screen adverse event signals that require medical intervention by health care providers. The extracted adverse event signals could reflect the side effects of anticancer drugs used by the patients based on analysis of prescribed anticancer drugs. “Pain or numbness” (n=57, 36.3%), “fever” (n=46, 29.3%), and “nausea” (n=40, 25.5%) were common symptoms out of the true adverse event signals identified by the model for adverse events limiting patients’ daily lives.
Conclusions: Our deep learning models were able to screen clinically important adverse event signals that require intervention for symptoms. It was also confirmed that these deep learning models could be applied to patients’ subjective information recorded in pharmaceutical care records accumulated during pharmacists’ daily work.
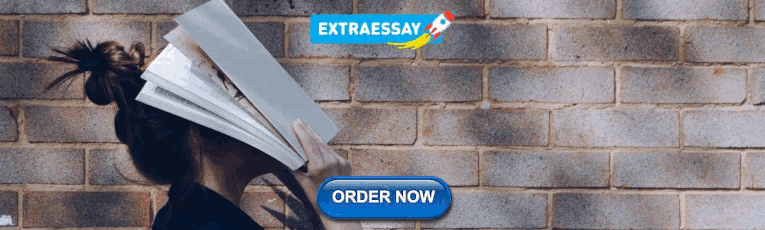
Introduction
Increasing numbers of people are expected to develop cancers in our aging society [ 1 - 3 ]. Thus, there is increasing interest in how to detect and manage the side effects of anticancer therapies in order to improve treatment regimens and patients’ quality of life [ 4 - 8 ]. The primary approaches for side effect management are “early signal detection and early intervention” [ 9 - 11 ]. Thus, more efficient approaches for this purpose are needed.
It has been recognized that patients’ voices concerning adverse events represent an important source of information. Several studies have indicated that the number, severity, and time of occurrence of adverse events might be underevaluated by physicians [ 12 - 15 ]. Thus, patient-reported outcomes (PROs) have recently received more attention in the drug evaluation process, reflecting patients’ real voices. Various kinds of PRO measures have been developed and investigated in different disease populations [ 16 , 17 ]. Health care authorities have also encouraged the pharmaceutical industry to use PROs for drug evaluation [ 18 , 19 ], and it is becoming more common to take PRO assessment results into consideration for drug marketing approval [ 20 , 21 ]. Similar trends can be seen in the clinical management of individual patients. Thus, health care professionals have an interest in understanding how to appropriately gather patients’ concerns in order to improve safety management and clinical decisions [ 22 - 24 ].
The applications of deep learning for natural language processing have expanded dramatically in recent years [ 25 ]. Since the development of a high-performance deep learning model in 2018 [ 26 ], attempts to apply cutting-edge deep learning models to various kinds of patient-generated text data for the evaluation of safety events or the analysis of unscalable subjective information from patients have been accelerating [ 27 - 31 ]. Most studies have been conducted to use patients’ narrative data for pharmacovigilance [ 27 , 32 - 35 ], while few have been aimed at improvement of real-time safety monitoring for individual patients. In addition, there have been some studies on adverse event severity grading based on health care records [ 36 - 39 ], but none has yet aimed to extract clinically important adverse event signals that require medical intervention from patients’ narratives. It is important to know whether deep learning models could contribute to the detection of such important adverse event signals from concern texts generated by individual patients.
To address this question, we have developed deep learning models to detect adverse event signals from individual patients with cancer based on patients’ blog articles in online communities, following other types of natural language processing–related previous work [ 40 , 41 ]. One deep learning model focused on the specific symptom of hand-foot syndrome (HFS), which is one of the typical side effects of anticancer treatments [ 42 ], and another focused on a broad range of adverse events that impact patients’ activities of daily living [ 43 ]. We showed that our models can provide good performance scores in targeting adverse event signals. However, the evaluation relied on patients’ narratives from the patients’ blog data used for deep learning model training, so further evaluation is needed to ensure the validity and applicability of the models to other texts regarding patients’ concerns. In addition, the blog data source did not contain medical information, so it was not feasible to assess whether the models could contribute to the extraction of clinically important adverse event signals.
To address these challenges, we focused on pharmaceutical care records written by pharmacists at community pharmacies. The gold standard format for pharmaceutical care records in Japan is the SOAP (subjective, objective, assessment, plan)-based document that follows the “problem-oriented system” concept proposed by Weed [ 44 ] in 1968. Pharmacists track patients’ subjective concerns in the S column, provide objective information or observations in the O column, give their assessment from the pharmacist perspective in the A column, and suggest a plan for moving forward in the P column [ 45 , 46 ]. We considered that SOAP-based pharmaceutical care records could be a unique data source suitable for further evaluation of our deep learning models because they contain both patients’ concerns and professional health care records by pharmacists, including the medication prescription history with time stamps. Therefore, this study was designed to assess whether our deep learning models could extract clinically important adverse event signals that require intervention by medical professionals from these records. We also aimed to evaluate the characteristics of the models when applied to patients’ subjective information noted in the pharmaceutical care records, as there have been only a few studies on the application of deep learning models to patients’ concerns recorded during pharmacists’ daily work [ 47 - 49 ].
Here, we report the results of applying our deep learning models to patients’ concern text data in pharmaceutical care records, focusing on patients receiving anticancer treatment.
Data Source
The original data source was 2,276,494 pharmaceutical care records for 303,179 patients, created from April 2020 to December 2021 at community pharmacies belonging to the Nakajima Pharmacy Group in Japan [ 50 ]. To focus on patients with cancer, records of patients with at least 1 prescription for an anticancer drug were retrieved by sorting individual drug codes (YJ codes) used in Japan (YJ codes starting with 42 refer to anticancer drugs). Records in the S column (ie, S records) were collected from the patients with cancer as the text data of patients’ concerns for deep learning model analysis.
Deep Learning Models
The deep learning models used for this research were those that we constructed based on patients’ narratives in blog articles posted in an online community and that showed the best performance score in each task in our previous work (ie, a Bidirectional Encoder Representations From Transformers [BERT]–based model for HFS signal extraction [ 42 ] and a T5-based model for adverse event signal extraction [ 43 ]). BERT [ 26 ] and T5 [ 51 ] both belong to a type of deep learning model that has recently shown high performance in several studies [ 29 , 52 ]. Hereafter, we refer to the deep learning model for HFS signals as the HFS model, the model for any adverse event signals as All AE (ie, all or any adverse events) model, and the model for adverse event signals limited to patients’ activities of daily living as the AE-L (adverse events limiting patients’ daily lives) model. It was also confirmed that these deep learning models showed similar or higher performance scores for the HFS, All AE, or AE-L identification tasks using 1000 S records randomly extracted from the data source of this study compared to the values obtained in our previous work [ 42 , 43 ] (the performance scores of sentence-level tasks from our previous work are comparable, as the mean number of words in the sentences in the data source in our previous work was 32.7 [SD 33.9], which is close to that of the S records used in this study, 38.8 [SD 29.4]). The method and results of the performance-level check are described in detail in Multimedia Appendix 1 [ 42 , 43 ]. We applied the deep learning models to all text data in this study without any adjustment in setting parameters from those used in constructing them based on patient-authored texts in our previous work [ 42 , 43 ].
Evaluation of Extracted S Records by the Deep Learning Models
In this study, we focused on the evaluation of S records that our deep learning models extracted as HFS or AE-L positive. Each positive S record was assessed as if it was a true adverse event signal, a sort of adverse event symptom, whether or not an intervention was made by health care professionals. We also investigated the kind of anticancer treatment prescription in connection with each adverse event signal identified in S records.
To assess whether an extracted positive S record was a true adverse event signal, we used the same annotation guidelines as in our previous work [ 43 ]. In brief, each S record was treated as an “adverse event signal” if any untoward medical occurrence happened to the patient, regardless of the cause. For the AE-L model only, if a positive S record was confirmed as an adverse event signal, it was further categorized into 1 or more of the following adverse event symptoms: “fatigue,” “nausea,” “vomiting,” “diarrhea,” “constipation,” “appetite loss,” “pain or numbness,” “rash or itchy,” “hair loss,” “menstrual irregularity,” “fever,” “taste disorder,” “dizziness,” “sleep disorder,” “edema,” or “others.”
For the assessment of interventions by health care professionals and anticancer treatment prescriptions, information from the O, A, and P columns and drug prescription history in the data source were investigated for the extracted positive S records. The interventions by health care professionals were categorized in any of the following: “adding symptomatic treatment for the adverse event signal,” “dose reduction or discontinuation of causative anticancer treatment,” “consultation with physician,” “others,” or “no intervention (ie, just following up the adverse event signal).” The actions categorized in “others” were further evaluated individually. For this assessment, we also randomly extracted 200 S records and evaluated them in the same way for comparison with the results from the deep learning model. Prescription history of anticancer treatment was analyzed by primary category of mechanism of action (MoA) with subcategories if applicable (eg, target molecule for kinase inhibitors).
Applicability Check to Other Text Data Including Patients’ Concerns
To check the applicability of our deep learning models to data from a different source, interview transcripts from patients with cancer were also evaluated. The interview transcripts were created by the Database of Individual Patient Experiences-Japan (DIPEx-Japan) [ 53 ]. DIPEx-Japan divides the interview transcripts into sections for each topic, such as “onset of disease” and “treatment,” and posts the processed texts on its website. Processing is conducted by accredited researchers based on qualitative research methods established by the University of Oxford [ 54 ]. In this study, interview text data created from interviews with 52 patients with breast cancer conducted from January 2008 to October 2018 were used to assess whether our deep learning models can extract adverse event signals from this source. In total, 508 interview transcripts were included with the approval of DIPEx-Japan.
Ethical Considerations
This study was conducted with anonymized data following approval by the ethics committee of the Keio University Faculty of Pharmacy (210914-1 and 230217-1) and in accordance with relevant guidelines and regulations and the Declaration of Helsinki. Informed consent specific to this study was waived due to the retrospective observational design of the study with the approval of the ethics committee of the Keio University Faculty of Pharmacy. To respect the will of each individual stakeholder, however, we provided patients and pharmacists of the pharmacy group with an opportunity to refuse the sharing of their pharmaceutical care records by posting an overview of this study at each pharmacy store or on their web page regarding the analysis using pharmaceutical care records. Interview transcripts from DIPEx-Japan were provided through a data sharing arrangement for using narrative data for research and education. Consent for interview transcription and its sharing from DIPEx-Japan was obtained from the participants when the interviews were recorded.
From the original data source of 2,180,902 pharmaceutical care records for 291,150 patients, S records written by pharmacists for patients with a history of at least 1 prescription of an anticancer drug were extracted. This yielded 30,784 S records for 2479 patients with cancer ( Table 1 ). The mean and median number of words in the S records were 38.8 (SD 29.4) and 32 (IQR 20-50), respectively. We applied our deep learning models, HFS, All AE, and AE-L, to these 30,784 S records for the evaluation of the deep learning models for adverse event signal detection.
For interview transcripts created by DIPEx-Japan, the mean and median number of words were 428.9 (SD 160.9) and 416 (IQR 308-526), respectively, in the 508 transcripts for 52 patients with breast cancer.
a SOAP: subjective, objective, assessment, plan.
b S: subjective.
Application of the HFS Model
First, we applied the HFS model to the S records for patients with cancer. The BERT-based model was used for this research as it showed the best performance score in our previous work [ 42 ].
S Records Extracted as HFS Positive
The S records extracted as HFS positive by the HFS model ( Table 2 ) amounted to 167 (0.5%) records for 119 (4.8%) patients. A majority of the patients had 1 HFS-positive record in their S records (n=91, 76.5%), while 2 patients had as many as 6 (1.7%) HFS-positive records. When we examined whether the extracted S records were true adverse event signals or not, 152 records were confirmed to be adverse event signals, while the other 15 records were false-positives. All the false-positive S records were descriptions about the absence of symptoms or confirmation of improving condition (eg, “no diarrhea, mouth ulcers, or limb pain so far” or “the skin on the soles of my feet has calmed down a lot with this ointment”). Some examples of S records that were predicted as HFS positive by the model are shown in Table S1 in Multimedia Appendix 2 .
The same examination was conducted with interview transcripts from DIPEx-Japan. Only 1 (0.2%) transcript was extracted as HFS positive by the HFS model, and it was a true adverse event signal (100%). The actual transcript extracted as HFS positive is shown in Table S2 in Multimedia Appendix 2 .
a S: subjective.
b HFS: hand-foot syndrome.
c All false-positive S records were denial of symptoms or confirmation of improving condition.
Interventions by Health Care Professionals
The 167 S records extracted as HFS positive as well as 200 randomly selected records were checked for interventions by health care professionals ( Figure 1 ). The proportion showing any action by health care professionals was 64.1% for 167 HFS-positive S records compared to 13% for the 200 random S records. Among the actions taken for HFS positives, “adding symptomatic treatment” was the most common, accounting for around half (n=79, 47.3%), followed by “other” (n=18, 10.8%). Most “other” actions were educational guidance from pharmacists, such as instructions on moisturizing, nail care, or application of ointment and advice on daily living (eg, “avoid tight socks”).

Anticancer Drugs Prescribed
The types of anticancer drugs prescribed for HFS-positive patients are summarized based on the prescription histories in Table 3 . For the 152 adverse event signals identified by the HFS model in the previous section, the most common MoA class of anticancer drugs used for the patients was antimetabolite (n=62, 40.8%), specifically fluoropyrimidines (n=59, 38.8%). Kinase inhibitors were next (n=49, 32.2%), with epidermal growth factor receptor (EGFR) inhibitors and multikinase inhibitors as major subgroups (n=28, 18.4% and n=14, 9.2%, respectively). The third and fourth most common MoAs were aromatase inhibitors (n=24, 15.8%) and antiandrogen or estrogen drugs (n=7, 4.6% each) for hormone therapy.
a EGFR: epidermal growth factor receptor.
b VEGF: vascular endothelial growth factor.
c HER2: human epidermal growth factor receptor-2.
d CDK4/6: cyclin-dependent kinase 4/6.
Application of the All AE or AE-L model
The All AE and AE-L models were also applied to the same S records for patients with cancer. The T5-based model was used for this research as it gave the best performance score in our previous work [ 43 ].
S Records Extracted as All AE or AE-L positive
The numbers of S records extracted as positive were 7604 (24.7%) for 1797 patients and 196 (0.6%) for 142 patients for All AE and AE-L, respectively. In the case of All AE, patients tended to have multiple adverse event positives in their S records (n=1315, 73.2% of patients had at least 2 positives). In the case of AE-L, most patients had only 1 AE-L positive (n=104, 73.2%), and the largest number of AE-L positives for 1 patient was 4 (2.8%; Table 4 ).
We focused on AE-L evaluation due to its greater importance from a medical viewpoint and lower workload for manual assessment, considering the number of positive S records. Of the 197 AE-L–positive S records, it was confirmed that 157 (80.1%) records accurately extracted adverse event signals, while 39 (19.9%) records were false-positives that did not include any adverse event signals ( Table 4 ). The contents of the 39 false-positives were all descriptions about the absence of symptoms or confirmation of improving condition, showing a similar tendency to the HFS false-positives (eg, “The diarrhea has calmed down so far. Symptoms in hands and feet are currently fine” and “No symptoms for the following: upset in stomach, diarrhea, nausea, abdominal pain, abdominal pain or stomach cramps, constipation”). Examples of S records that were predicted as AE-L positive are shown in Table S3 in Multimedia Appendix 2 .
The deep learning models were also applied to interview transcripts from DIPEx-Japan in the same manner. The deep learning models identified 84 (16.5%) and 18 (3.5%) transcripts as All AE or AE-L positive, respectively. Of the 84 All AE–positive transcripts, 73 (86.9%) were true adverse event signals. The false-positives of All AE (n=11, 13.1%) were categorized into any of the following 3 types: explanations about the disease or its prognosis, stories when their cancer was discovered, or emotional changes that did not include clear adverse event mentions. With regard to AE-L, all the 18 (100%) positives were true adverse event signals (Table S4 in Multimedia Appendix 2 ). Examples of actual transcripts extracted as All AE or AE-L positive are shown in Table S5 in Multimedia Appendix 2 .
b All AE: all (or any of) adverse event.
c AE-L: adverse events limiting patients’ daily lives.
d All false-positive S records were denial of symptoms or confirmation of improving condition.
Whether or not interventions were made by health care professionals was investigated for the 196 AE-L–positive S records. As in the HFS model evaluation, data from 200 randomly selected S records were used for comparison ( Figure 2 ). In total, 91 (46.4%) records in the 196 AE-L–positive records were accompanied by an intervention, while the corresponding figure in the 200 random records was 26 (13%) records. The most common action in response to adverse event signals identified by the AE-L model was “adding symptomatic treatment” (n=71, 36.2%), followed by “other” (n=11, 5.6%). “Other” included educational guidance from pharmacists, inquiries from pharmacists to physicians, or recommendations for patients to visit a doctor.

The types of anticancer drugs prescribed for patients with adverse event signals identified by the AE-L model were summarized based on the prescription histories ( Table 5 ). In connection with the 157 adverse event signals, the most common MoA of the prescribed anticancer drug was antimetabolite (n=62, 39.5%) and fluoropyrimidine (n=53, 33.8%), which accounted for the majority. Kinase inhibitor (n=31, 19.7%) was the next largest category with multikinase inhibitor (n=14, 8.9%) as the major subgroup. These were followed by antiandrogen (n=27, 17.2%), antiestrogen (n=10, 6.4%), and aromatase inhibitor (n=10, 6.4%) for hormone therapy.
b JAK: janus kinase.
c VEGF: vascular endothelial growth factor.
d BTK: bruton tyrosine kinase.
e FLT3: FMS-like tyrosine kinase-3.
f PARP: poly-ADP ribose polymerase.
g CDK4/6: cyclin-dependent kinase 4/6.
h CD20: cluster of differentiation 20.
Adverse Event Symptoms
For the 157 adverse event signals identified by the AE-L model, the symptoms were categorized according to the predefined guideline in our previous work [ 43 ]. “Pain or numbness” (n=57, 36.3%) accounted for the largest proportion followed by “fever” (n=46, 29.3%) and “nausea” (n=40, 25.5%; Table 6 ). Symptoms classified as “others” included chills, tinnitus, running tears, dry or peeling skin, and frequent urination. When comparing the proportion of the symptoms associated with or without interventions by health care professionals, a trend toward a greater proportion of interventions was observed in “fever,” “nausea,” “diarrhea,” “constipation,” “vomiting,” and “edema” ( Figure 3 , black boxes). On the other hand, a smaller proportion was observed in “pain or numbness,” “fatigue,” “appetite loss,” “rash or itchy,” “taste disorder,” and “dizziness” ( Figure 3 , gray boxes).

This study was designed to evaluate our deep learning models, previously constructed based on patient-authored texts posted in an online community, by applying them to pharmaceutical care records that contain both patients’ subjective concerns and medical information created by pharmacists. Based on the results, we discuss whether these deep learning models can extract clinically important adverse event signals that require medical intervention, and what characteristics they show when applied to data on patients’ concerns in pharmaceutical care records.
Performance for Adverse Event Signal Extraction
The first requirement for the deep learning models is to extract adverse event signals from patients’ narratives precisely. In this study, we evaluated the proportion of true adverse event signals in positive S records extracted by the HFS or AE-L model. True adverse event signals amounted to 152 (91%) and 157 (80.1%) for the HFS and AE-L models, respectively ( Tables 2 and 4 ). Given that the proportion of true adverse event signals in 200 randomly extracted S records without deep learning models was 54 (27%; categories other than “no adverse event” in Figures 1 and 2 ), the HFS and AE-L models were able to concentrate S records with adverse event mentions. Although 15 (9%) for the HFS model and 39 (19.9%) for the AE-L model were false-positives, it was confirmed all of the false-positive records described a lack of symptoms or confirmation of improving condition. We considered that such false-positives are due to the unique feature of pharmaceutical care records, where pharmacists might proactively interview patients about potential side effects of their medications. As the data set of blog articles we used to construct the deep learning models included few such cases (especially comments on lack of symptoms), our models seemed unable to exclude them correctly. Even though we confirmed that the proportion of true “adverse event” signals extracted from the S records by the HFS or AE-L model was more than 80%, the performance scores to extract true “HFS” or “AE-L” signals were not so high based on the performance check using 1000 randomly extracted S records ( F 1 -scores were 0.50 and 0.22 for true HFS and AE-L signals, respectively; Table S1 in Multimedia Appendix 1 ). It is considered that the performance to extract true HFS and AE-L signals was relatively low due to the short length of texts in the S records, providing less context to judge the impact on patients’ daily lives, especially for the AE-L model (the mean word number of the S records was 38.8 [SD 29.4; Table 1 ], similar to the sentence-level tasks in our previous work [ 42 , 43 ]). However, we consider a true adverse event signal proportion of more than 80% in this study represents a promising outcome, as this is the first attempt to apply our deep learning models to a different source of patients’ concern data, and the extracted positive cases would be worthy of evaluation by a medical professional, as the potential adverse events could be caused by drugs taken by the patients.
When the deep learning models were applied to DIPEx-Japan interview transcripts, including patients’ concerns, the proportion of true adverse event signals was also more than 80% (for All AE: n=73, 86.9% and for HFS and AE-L: n=18, 100%). The difference in the results between pharmaceutical care S records and DIPEx-Japan interview transcripts was the features of false-positives, descriptions about lack of symptoms or confirmation of improving condition in S records versus explanations about disease or its prognosis, stories about when their cancer was discovered, or emotional changes in interview transcripts. This is considered due to the difference in the nature of the data source; the pharmaceutical care records were generated in a real-time manner by pharmacists through their daily work, where adverse event signals are proactively monitored, while the interview transcripts were purely based on patients’ retrospective memories. Our deep learning models were able to extract true adverse event signals with an accuracy of more than 80% from both text data sources in spite of the difference in their nature. When looking at future implementation of the deep learning models in society (discussed in the Potential for Deep Learning Model Implementation in Society section), it may be desirable to further adjust deep learning models to reduce false-positives depending upon the features of the data source.
Identification of Important Adverse Events Requiring Medical Intervention
To assess whether the models could extract clinically important adverse event signals, we investigated interventions by health care professionals connected with the adverse event signals that are identified by our deep learning models. In the 200 randomly extracted S records, only 26 (13%) consisted of adverse event signals, leading to any intervention by health care professionals. On the other hand, the proportion of signals associated with interventions was increased to 107 (64.1%) and 91 (46.4%) in the S records extracted as positive by the HFS and AE-L models, respectively ( Figures 1 and 2 ). These results suggest that both deep learning models can screen clinically important adverse event signals that require intervention from health care professionals. The performance level in screening adverse event signals requiring medical intervention was higher in the HFS model than in the AE-L model (n=107, 64.1% vs n=91, 46.4%; Figures 1 and 2 ). Since the target events were specific and adverse event signals of HFS were narrowly defined, which is one of the typical side effects of some anticancer drugs, we consider that health care providers paid special attention to HFS-related signals and took action proactively. In both deep learning models, similar trends were observed in actions taken by health care professionals in response to extracted adverse event signals; common actions were attempts to manage adverse event symptoms by symptomatic treatment or other mild interventions, including educational guidance from pharmacists or recommendations for patients to visit a doctor. More direct interventions focused on the causative drugs (ie, “dose reduction or discontinuation of anticancer treatment”) amounted to less than 5%; 7 (4.2%) for the HFS model and 6 (3.1%) for the AE-L model ( Figures 1 and 2 ). Thus, it appears that our deep learning models can contribute to screening mild to moderate adverse event signals that require preventive actions such as symptomatic treatments or professional advice from health care providers, especially for patients with less sensitivity to adverse event signals or who have few opportunities to visit clinics and pharmacies.
Ability to Catch Real Side Effect Signals of Anticancer Drugs
Based on the drug prescription history associated with S records extracted as HFS or AE-L positive, the type and duration of anticancer drugs taken by patients experiencing the adverse event signals were investigated. For the HFS model, the most common MoA of anticancer drug was antimetabolite (fluoropyrimidine: n=59, 38.8%), followed by kinase inhibitors (n=49, 32.2%, of which EGFR inhibitors and multikinase inhibitors accounted for n=28, 18.4% and n=14, 9.2%, respectively) and aromatase inhibitors (n=24, 15.8%; Table 3 ). It is known that fluoropyrimidine and multikinase inhibitors are typical HFS-inducing drugs [ 55 - 58 ], suggesting that the HFS model accurately extracted HFS side effect signals derived from these drugs. Note that symptoms such as acneiform rash, xerosis, eczema, paronychia, changes in the nails, arthralgia, or stiffness of limb joints, which are common side effects of EGFR inhibitors or aromatase inhibitors [ 59 , 60 ], might be extracted as closely related expressions to those of HFS signals. When looking at the MoA of anticancer drugs for patients with adverse event signals identified by the AE-L model, antimetabolite (fluoropyrimidine) was the most common one (n=53, 33.8%), as in the case of those identified by the HFS model, followed by kinase inhibitors (n=31, 19.7%) and antiandrogens (n=27, 17.2%; Table 5 ). Since the AE-L model targets a broad range of adverse event symptoms, it is difficult to rationalize the relationship between the adverse event signals and types of anticancer drugs. However, the type of anticancer drugs would presumably closely correspond to the standard treatments of the cancer types of the patients. Based on the prescribed anticancer drugs, we can infer that a large percentage of the patients had breast or lung cancer, indicating that our study results were based on data from such a population. Thus, a possible direction for the expansion of this research would be adjusting the deep learning models by additional training with expressions for typical side effects associated with standard treatments of other cancer types. To interpret these results correctly, it should be noted that we could not investigate anticancer treatments conducted outside of the pharmacies (eg, the time-course relationship with intravenously administered drugs would be missed, as the administration will be done at hospitals). To further evaluate how useful this model is in side effect signal monitoring for patients with cancer, comprehensive medical information for the eligible patients would be required.
Suitability of the Deep Learning Models for Specific Adverse Event Symptoms
Among the adverse event signals identified by the AE-L model, the type of symptom was categorized according to a predefined annotation guideline that we previously developed [ 43 ]. The most frequently recorded adverse event signals identified by the AE-L model were “pain or numbness” (n=57, 36.3%), “fever” (n=46, 29.3%), and “nausea” (n=40, 25.5%; Table 6 ). Since the pharmaceutical care records had information about interventions by health care professionals, the frequency of the presence or absence of the interventions for each symptom was examined. A trend toward a greater proportion of interventions was observed in “fever,” “nausea,” “diarrhea,” “constipation,” “vomiting,” and “edema” ( Figure 3 , black boxes). There seem to be 2 possible explanations for this: these symptoms are of high importance and require early medical intervention or effective symptomatic treatments are available for these symptoms in clinical practice so that medical intervention is an easy option. On the other hand, a trend for a smaller proportion of adverse event signals to result in interventions was observed for “pain or numbness,” “fatigue,” “appetite loss,” “rash or itchy,” “taste disorder,” and “dizziness” ( Figure 3 , gray boxes). The reason for this may be the lack of effective symptomatic treatments or the difficulty of judging whether the severity of these symptoms justifies medical intervention by health care providers. In either case, there may be room for improvement in the quality of medical care for these symptoms. We expect that our research will contribute to a quality improvement in safety monitoring in clinical practice by supporting adverse event signal detection in a cost-effective manner.
Potential for Deep Learning Model Implementation in Society
Although we evaluated our deep learning models using pharmaceutical care records in this study, the main target of future implementation of our deep learning models in society would be narrative texts that patients directly write to record their daily experiences. For example, the application of these deep learning models to electronic media where patients record their daily experiences in their lives with disease (eg, health care–related e-communities and disease diary applications) could enable information about adverse event signal onset that patients experience to be provided to health care providers in a timely manner. Adverse event signals can automatically be identified and shared with health care providers based on the concern texts that patients post to any platform. This system will have the advantage that health care providers can efficiently grasp safety-related events that patients experience outside of clinic visits so that they can conduct more focused or personalized interactions with patients at their clinic visits. However, consideration should be given to avoid an excessive burden on health care providers. For instance, limiting the sharing of adverse event signals to those of high severity or summarizing adverse event signals over a week rather than sharing each one in a real-time manner may be reasonable approaches for medical staff. We also need to think about how to encourage patients to record their daily experiences using electronic tools. Not only technical progress and support but also the establishment of an ecosystem where both patients and medical staff can feel benefit will be required. Prospective studies with deep learning models to follow up patients in the long term and evaluate outcomes will be needed. We primarily looked at patient-authored texts as targets of implementation, but our deep learning models may also be worth using medical data including patients’ subjective concerns, such as pharmaceutical care S records. As this study confirmed that our deep learning models are applicable to patients’ concern texts tracked by pharmacists, it should be possible to use them to analyze other “patient voice-like” medical text data that have not been actively investigated so far.
Limitations
First, the major limitation of this study was that we were not able to collect complete medical information of the patients. Although we designed this study to analyze patients’ concerns extracted by the deep learning models and their relationship with medical information contained in the pharmaceutical care records, some information could not be tracked (eg, missing history of medical interventions or anticancer treatment at hospitals as well as diagnosis of patients’ primary cancers). Second, there might be a data creation bias in S records for patients’ concerns by pharmacists. For example, symptoms that have little impact on intervention decisions might less likely be recorded by them. It should be also noted that the characteristics of S records may not be consistent at different community pharmacies.
Conclusions
Our deep learning models were able to screen clinically important adverse event signals that require intervention by health care professionals from patients’ concerns in pharmaceutical care records. Thus, these models have the potential to support real-time adverse event monitoring of individual patients taking anticancer treatments in an efficient manner. We also confirmed that these deep learning models constructed based on patient-authored texts could be applied to patients’ subjective information recorded by pharmacists through their daily work. Further research may help to expand the applicability of the deep learning models for implementation in society or for analysis of data on patients’ concerns accumulated in professional records at pharmacies or hospitals.
Acknowledgments
This work was supported by Japan Society for the Promotion of Science, Grants-in-Aid for Scientific Research (KAKENHI; grant 21H03170) and Japan Science and Technology Agency, Core Research for Evolutional Science and Technology (CREST; grant JPMJCR22N1), Japan. Mr Yuki Yokokawa and Ms Sakura Yokoyama at our laboratory advised SN about the structure of pharmaceutical care records. This study would not have been feasible without the high quality of pharmaceutical care records created by many individual pharmacists at Nakajima Pharmacy Group through their daily work.
Data Availability
The data sets generated and analyzed during this study are available from the corresponding author on reasonable request.
Authors' Contributions
SN and SH designed the study. SN retrieved the subjective records of patients with cancer from the data source for the application of deep learning models and organized other data for subsequent evaluations. SN ran the deep learning models with the support of SW. SN, YY, and KS checked the adverse event signals for each subjective record that was extracted as positive by the models for hand-foot syndrome or adverse events limiting patients’ daily lives and evaluated the adverse event signal symptoms, details of interventions taken by health care professionals, and types of anticancer drugs prescribed for patients based on available data from the data source. HK and SI advised on the study concept and process. MS and RT provided pharmaceutical records at their community pharmacies along with advice on how to use and interpret them. SY and EA supervised the natural language processing research as specialists. SH supervised the study overall. SN drafted and finalized the paper. All authors reviewed and approved the paper.
Conflicts of Interest
SN is an employee of Daiichi Sankyo Co, Ltd. All other authors declare no conflicts of interest.
Performance evaluation of deep learning models.
Examples of S records and sample interview transcripts.
- Global cancer observatory: cancer over time. World Health Organization. URL: https://gco.iarc.fr/overtime/en [accessed 2023-07-02]
- Mattiuzzi C, Lippi G. Current cancer epidemiology. J Epidemiol Glob Health. 2019;9(4):217-222. [ FREE Full text ] [ CrossRef ] [ Medline ]
- Montazeri F, Komaki H, Mohebi F, Mohajer B, Mansournia MA, Shahraz S, et al. Editorial: disparities in cancer prevention and epidemiology. Front Oncol. 2022;12:872051. [ FREE Full text ] [ CrossRef ] [ Medline ]
- Lasala R, Santoleri F. Association between adherence to oral therapies in cancer patients and clinical outcome: a systematic review of the literature. Br J Clin Pharmacol. 2022;88(5):1999-2018. [ FREE Full text ] [ CrossRef ] [ Medline ]
- Pudkasam S, Polman R, Pitcher M, Fisher M, Chinlumprasert N, Stojanovska L, et al. Physical activity and breast cancer survivors: importance of adherence, motivational interviewing and psychological health. Maturitas. 2018;116:66-72. [ FREE Full text ] [ CrossRef ] [ Medline ]
- Markman M. Chemotherapy-associated neurotoxicity: an important side effect-impacting on quality, rather than quantity, of life. J Cancer Res Clin Oncol. 1996;122(9):511-512. [ CrossRef ] [ Medline ]
- Jitender S, Mahajan R, Rathore V, Choudhary R. Quality of life of cancer patients. J Exp Ther Oncol. 2018;12(3):217-221. [ Medline ]
- Di Nardo P, Lisanti C, Garutti M, Buriolla S, Alberti M, Mazzeo R, et al. Chemotherapy in patients with early breast cancer: clinical overview and management of long-term side effects. Expert Opin Drug Saf. 2022;21(11):1341-1355. [ FREE Full text ] [ CrossRef ] [ Medline ]
- Cuomo RE. Improving cancer patient outcomes and cost-effectiveness: a Markov simulation of improved early detection, side effect management, and palliative care. Cancer Invest. 2023;41(10):858-862. [ CrossRef ] [ Medline ]
- Pulito C, Cristaudo A, La Porta C, Zapperi S, Blandino G, Morrone A, et al. Oral mucositis: the hidden side of cancer therapy. J Exp Clin Cancer Res. 2020;39(1):210. [ FREE Full text ] [ CrossRef ] [ Medline ]
- Bartal A, Mátrai Z, Szûcs A, Liszkay G. Main treatment and preventive measures for hand-foot syndrome, a dermatologic side effect of cancer therapy. Magy Onkol. 2011;55(2):91-98. [ FREE Full text ] [ Medline ]
- Basch E, Jia X, Heller G, Barz A, Sit L, Fruscione M, et al. Adverse symptom event reporting by patients vs clinicians: relationships with clinical outcomes. J Natl Cancer Inst. 2009;101(23):1624-1632. [ FREE Full text ] [ CrossRef ] [ Medline ]
- Basch E. The missing voice of patients in drug-safety reporting. N Engl J Med. 2010;362(10):865-869. [ FREE Full text ] [ CrossRef ] [ Medline ]
- Fromme EK, Eilers KM, Mori M, Hsieh YC, Beer TM. How accurate is clinician reporting of chemotherapy adverse effects? A comparison with patient-reported symptoms from the Quality-of-Life Questionnaire C30. J Clin Oncol. 2004;22(17):3485-3490. [ FREE Full text ] [ CrossRef ] [ Medline ]
- Liu L, Suo T, Shen Y, Geng C, Song Z, Liu F, et al. Clinicians versus patients subjective adverse events assessment: based on patient-reported outcomes version of the common terminology criteria for adverse events (PRO-CTCAE). Qual Life Res. 2020;29(11):3009-3015. [ CrossRef ] [ Medline ]
- Churruca K, Pomare C, Ellis LA, Long JC, Henderson SB, Murphy LED, et al. Patient-reported outcome measures (PROMs): a review of generic and condition-specific measures and a discussion of trends and issues. Health Expect. 2021;24(4):1015-1024. [ FREE Full text ] [ CrossRef ] [ Medline ]
- Pérez-Alfonso KE, Sánchez-Martínez V. Electronic patient-reported outcome measures evaluating cancer symptoms: a systematic review. Semin Oncol Nurs. 2021;37(2):151145. [ FREE Full text ] [ CrossRef ] [ Medline ]
- Patient-reported outcome measures: use in medical product development to support labeling claims. U.S. Food & Drug Administration. 2009. URL: https://www.fda.gov/regulatory-information/search-fda-guidance-documents/patient-reported-outcome-measures-use-medical-product-development-support-labeling-claims [accessed 2023-11-26]
- Appendix 2 to the guideline on the evaluation of anticancer medicinal products in man—the use of patient-reported outcome (PRO) measures in oncology studies—scientific guideline. European Medicines Agency. 2016. URL: https://www.ema.europa.eu/en/appendix-2-guideline-evaluation-anticancer-medicinal-products-man-use-patient-reported-outcome-pro [accessed 2023-11-26]
- Weber SC. The evolution and use of patient-reported outcomes in regulatory decision making. RF Q. 2023;3(1):4-9. [ FREE Full text ]
- Teixeira MM, Borges FC, Ferreira PS, Rocha J, Sepodes B, Torre C. A review of patient-reported outcomes used for regulatory approval of oncology medicinal products in the European Union between 2017 and 2020. Front Med (Lausanne). 2022;9:968272. [ FREE Full text ] [ CrossRef ] [ Medline ]
- Newell S, Jordan Z. The patient experience of patient-centered communication with nurses in the hospital setting: a qualitative systematic review protocol. JBI Database System Rev Implement Rep. 2015;13(1):76-87. [ FREE Full text ] [ CrossRef ] [ Medline ]
- Yagasaki K, Takahashi H, Ouchi T, Yamagami J, Hamamoto Y, Amagai M, et al. Patient voice on management of facial dermatological adverse events with targeted therapies: a qualitative study. J Patient Rep Outcomes. 2019;3(1):27. [ FREE Full text ] [ CrossRef ] [ Medline ]
- Giardina TD, Korukonda S, Shahid U, Vaghani V, Upadhyay DK, Burke GF, et al. Use of patient complaints to identify diagnosis-related safety concerns: a mixed-method evaluation. BMJ Qual Saf. 2021;30(12):996-1001. [ FREE Full text ] [ CrossRef ] [ Medline ]
- Lauriola I, Lavelli A, Aiolli F. An introduction to deep learning in natural language processing: models, techniques, and tools. Neurocomputing. 2022;470:443-456. [ CrossRef ]
- Devlin J, Chang MW, Lee K, Toutanova K. BERT: pre-training of deep bidirectional transformers for language understanding. 2019. Presented at: 2019 Annual Conference of the North American Chapter of the Association for Computational Linguistics: Human Language Technologies; June 2-7, 2019;4171-4186; Minneapolis, MN, USA.
- Dreisbach C, Koleck TA, Bourne PE, Bakken S. A systematic review of natural language processing and text mining of symptoms from electronic patient-authored text data. Int J Med Inform. 2019;125:37-46. [ FREE Full text ] [ CrossRef ] [ Medline ]
- Sim JA, Huang X, Horan MR, Stewart CM, Robison LL, Hudson MM, et al. Natural language processing with machine learning methods to analyze unstructured patient-reported outcomes derived from electronic health records: a systematic review. Artif Intell Med. 2023;146:102701. [ CrossRef ] [ Medline ]
- Weissenbacher D, Banda JM, Davydova V, Estrada-Zavala D, Gascó Sánchez L, Ge Y, et al. Overview of the seventh social media mining for health applications (#SMM4H) shared tasks at COLING 2022. 2022. Presented at: Proceedings of the Seventh Workshop on Social Media Mining for Health Applications, Workshop & Shared Task; October 12-17, 2022;221-241; Gyeongju, Republic of Korea. URL: https://aclanthology.org/2022.smm4h-1.54/
- Matsuda S, Ohtomo T, Okuyama M, Miyake H, Aoki K. Estimating patient satisfaction through a language processing model: model development and evaluation. JMIR Form Res. 2023;7(1):e48534. [ FREE Full text ] [ CrossRef ] [ Medline ]
- Yu D, Vydiswaran VGV. An assessment of mentions of adverse drug events on social media with natural language processing: model development and analysis. JMIR Med Inform. 2022;10(9):e38140. [ FREE Full text ] [ CrossRef ] [ Medline ]
- Liu X, Chen H. A research framework for pharmacovigilance in health social media: identification and evaluation of patient adverse drug event reports. J Biomed Inform. 2015;58:268-279. [ FREE Full text ] [ CrossRef ] [ Medline ]
- Nikfarjam A, Sarker A, O'Connor K, Ginn R, Gonzalez G. Pharmacovigilance from social media: mining adverse drug reaction mentions using sequence labeling with word embedding cluster features. J Am Med Inform Assoc. 2015;22(3):671-681. [ FREE Full text ] [ CrossRef ] [ Medline ]
- Kakalou C, Dimitsaki S, Dimitriadis VK, Natsiavas P. Exploiting social media for active pharmacovigilance: the PVClinical social media workspace. Stud Health Technol Inform. 2022;290:739-743. [ CrossRef ] [ Medline ]
- Bousquet C, Dahamna B, Guillemin-Lanne S, Darmoni SJ, Faviez C, Huot C, et al. The adverse drug reactions from patient reports in social media project: five major challenges to overcome to operationalize analysis and efficiently support pharmacovigilance process. JMIR Res Protoc. 2017;6(9):e179. [ FREE Full text ] [ CrossRef ] [ Medline ]
- Young IJB, Luz S, Lone N. A systematic review of natural language processing for classification tasks in the field of incident reporting and adverse event analysis. Int J Med Inform. 2019;132:103971. [ CrossRef ] [ Medline ]
- Jacobsson R, Bergvall T, Sandberg L, Ellenius J. Extraction of adverse event severity information from clinical narratives using natural language processing. Pharmacoepidemiol Drug Saf. 2017;26(S2):37. [ FREE Full text ]
- Liang C, Gong Y. Predicting harm scores from patient safety event reports. Stud Health Technol Inform. 2017;245:1075-1079. [ CrossRef ] [ Medline ]
- Jiang G, Wang L, Liu H, Solbrig HR, Chute CG. Building a knowledge base of severe adverse drug events based on AERS reporting data using semantic web technologies. Stud Health Technol Inform. 2013;192(1-2):496-500. [ CrossRef ] [ Medline ]
- Usui M, Aramaki E, Iwao T, Wakamiya S, Sakamoto T, Mochizuki M. Extraction and standardization of patient complaints from electronic medication histories for pharmacovigilance: natural language processing analysis in Japanese. JMIR Med Inform. 2018;6(3):e11021. [ FREE Full text ] [ CrossRef ] [ Medline ]
- Watanabe T, Yada S, Aramaki E, Yajima H, Kizaki H, Hori S. Extracting multiple worries from breast cancer patient blogs using multilabel classification with the natural language processing model bidirectional encoder representations from transformers: infodemiology study of blogs. JMIR Cancer. 2022;8(2):e37840. [ FREE Full text ] [ CrossRef ] [ Medline ]
- Nishioka S, Watanabe T, Asano M, Yamamoto T, Kawakami K, Yada S, et al. Identification of hand-foot syndrome from cancer patients' blog posts: BERT-based deep-learning approach to detect potential adverse drug reaction symptoms. PLoS One. 2022;17(5):e0267901. [ FREE Full text ] [ CrossRef ] [ Medline ]
- Nishioka S, Asano M, Yada S, Aramaki E, Yajima H, Yanagisawa Y, et al. Adverse event signal extraction from cancer patients' narratives focusing on impact on their daily-life activities. Sci Rep. 2023;13(1):15516. [ FREE Full text ] [ CrossRef ] [ Medline ]
- Weed LL. Medical records that guide and teach. N Engl J Med. 1968;278(11):593-600. [ CrossRef ] [ Medline ]
- Podder V, Lew V, Ghassemzadeh S. SOAP Notes. Treasure Island, FL. StatPearls Publishing; 2023.
- Shenavar Masooleh I, Ramezanzadeh E, Yaseri M, Sahere Mortazavi Khatibani S, Sadat Fayazi H, Ali Balou H, et al. The effectiveness of training on daily progress note writing by medical interns. J Adv Med Educ Prof. 2021;9(3):168-175. [ FREE Full text ] [ CrossRef ] [ Medline ]
- Grothen AE, Tennant B, Wang C, Torres A, Sheppard BB, Abastillas G, et al. Application of artificial intelligence methods to pharmacy data for cancer surveillance and epidemiology research: a systematic review. JCO Clin Cancer Inform. 2020;4:1051-1058. [ FREE Full text ] [ CrossRef ] [ Medline ]
- Ohno Y, Kato R, Ishikawa H, Nishiyama T, Isawa M, Mochizuki M, et al. Using the natural language processing system MedNER-J to analyze pharmaceutical care records. medRxiv. Preprint posted online on October 2, 2023. [ CrossRef ]
- Ranchon F, Chanoine S, Lambert-Lacroix S, Bosson JL, Moreau-Gaudry A, Bedouch P. Development of artificial intelligence powered apps and tools for clinical pharmacy services: a systematic review. Int J Med Inform. 2023;172:104983. [ CrossRef ] [ Medline ]
- Nakajima Pharmacy. URL: https://www.nakajima-phar.co.jp/ [accessed 2023-12-07]
- Raffel C, Shazeer N, Roberts A, Lee K, Narang S, Matena M, et al. Exploring the limits of transfer learning with a unified text-to-text transformer. J Mach Learn Res. 2020;21(140):1-67. [ FREE Full text ]
- Pathak A. Comparative analysis of transformer based language models. Comput Sci Inf Technol. 2021.:165-176. [ FREE Full text ] [ CrossRef ]
- DIPEx Japan. URL: https://www.dipex-j.org/ [accessed 2024-02-04]
- Herxheimer A, McPherson A, Miller R, Shepperd S, Yaphe J, Ziebland S. Database of patients' experiences (DIPEx): a multi-media approach to sharing experiences and information. Lancet. 2000;355(9214):1540-1543. [ CrossRef ] [ Medline ]
- Lara PE, Muiño CB, de Spéville BD, Reyes JJ. Hand-foot skin reaction to regorafenib. Actas Dermosifiliogr. 2016;107(1):71-73. [ CrossRef ]
- Zaiem A, Hammamia SB, Aouinti I, Charfi O, Ladhari W, Kastalli S, et al. Hand-foot syndrome induced by chemotherapy drug: case series study and literature review. Indian J Pharmacol. 2022;54(3):208-215. [ FREE Full text ] [ CrossRef ] [ Medline ]
- McLellan B, Ciardiello F, Lacouture ME, Segaert S, Van Cutsem E. Regorafenib-associated hand-foot skin reaction: practical advice on diagnosis, prevention, and management. Ann Oncol. 2015;26(10):2017-2026. [ FREE Full text ] [ CrossRef ] [ Medline ]
- Ai L, Xu Z, Yang B, He Q, Luo P. Sorafenib-associated hand-foot skin reaction: practical advice on diagnosis, mechanism, prevention, and management. Expert Rev Clin Pharmacol. 2019;12(12):1121-1127. [ CrossRef ] [ Medline ]
- Tenti S, Correale P, Cheleschi S, Fioravanti A, Pirtoli L. Aromatase inhibitors-induced musculoskeletal disorders: current knowledge on clinical and molecular aspects. Int J Mol Sci. 2020;21(16):5625. [ FREE Full text ] [ CrossRef ] [ Medline ]
- Lacouture ME, Melosky BL. Cutaneous reactions to anticancer agents targeting the epidermal growth factor receptor: a dermatology-oncology perspective. Skin Therapy Lett. 2007;12(6):1-5. [ FREE Full text ] [ Medline ]
Abbreviations
Edited by G Eysenbach; submitted 25.12.23; peer-reviewed by CY Wang, L Guo; comments to author 24.01.24; revised version received 14.02.24; accepted 09.03.24; published 16.04.24.
©Satoshi Nishioka, Satoshi Watabe, Yuki Yanagisawa, Kyoko Sayama, Hayato Kizaki, Shungo Imai, Mitsuhiro Someya, Ryoo Taniguchi, Shuntaro Yada, Eiji Aramaki, Satoko Hori. Originally published in the Journal of Medical Internet Research (https://www.jmir.org), 16.04.2024.
This is an open-access article distributed under the terms of the Creative Commons Attribution License (https://creativecommons.org/licenses/by/4.0/), which permits unrestricted use, distribution, and reproduction in any medium, provided the original work, first published in the Journal of Medical Internet Research, is properly cited. The complete bibliographic information, a link to the original publication on https://www.jmir.org/, as well as this copyright and license information must be included.

Vol. 12, No. 2, 2023
Pages 1-200
10.22108/are.2023.27950
- View Article
- PDF 786.81 K
Research Article
The impact of synchronous online teaching on iraqi efl learners’ oral comprehension.
10.22108/are.2022.134671.1967
Salman Hintaw Abdulhussien

Encouraging EFL Students’ Willingness to Communicate inside Vietnamese High School Classrooms: Teachers’ Strategies and Students’ Beliefs
Pages 25-50
10.22108/are.2022.134674.1968
Viet Quoc Hoang; Hung Phu Bui
Google Translate in Foreign Language Learning: A Systematic Review
Pages 51-84
10.22108/are.2023.134264.1949
Vahid Reza Mirzaeian; Katayoun Oskoui
Construction and Validation of EFL Teachers’ Professional Identity Perception Inventory of Technology-Enhanced Instructions during the COVID-19 Pandemic
Pages 85-132
10.22108/are.2023.136213.2038
Masoumeh Dousti; Zahra Amirian
Intersectionality in Second Language Education and the Birth of a New Term
Pages 133-154
10.22108/are.2023.137020.2063
Tahmineh Khalili; Seyyed Mohammad Reza Adel
The Role of Data-Driven Learning in Developing Trait Emotional Intelligence
Pages 155-174
10.22108/are.2023.137132.2069
Javad Zare ; Khadijeh Aqajani Delavar
“I Don’t Like Working Hard to Materialize Another Person’s Dreams”: The Emotion Labor and Identity Dilemma of a Novice Iranian Female EFL Teacher at Private Language Institutes
Pages 175-200
10.22108/are.2023.137256.2070
Yasser Aminifard ; Rahman Sahragard ; Luis Javier Pentón Herrera

- Articles in Press
- Current Issue
Journal Archive
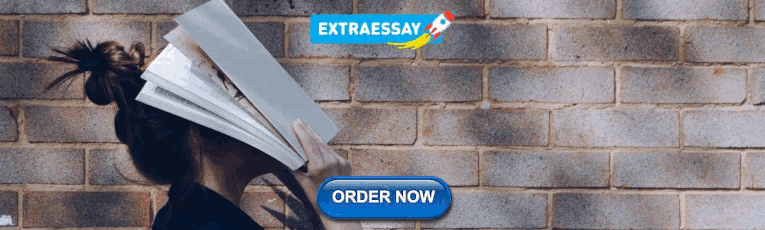
IMAGES
VIDEO
COMMENTS
Journal of Applied Research on English Language (AREL), formerly known as Applied Research in English, is published by the English department, University of Isfahan, Iran. The journal disseminates peer-reviewed original research studies in TEFL and Applied Linguistics, in open-access formats. AREL uses plagiarism detection services such as ...
A peer-reviewed, open access journal in education, teaching & english. ... Applied Research on English Language 2252-0198 (Print) / 2322-5343 (Online) ... Language and Literature: English language Keywords education teaching english. Added 29 July 2013 • Updated 3 July 2019 ...
About Journal. Applied Research on English Language is a peer reviewed international journal publishing high quality articles dedicated to all aspects of English language teaching and learning in Open Access Format. The Journal considers only manuscripts that have not been published (or submitted simultaneously), at any language, elsewhere.
Scope. Applied Research on English Language is an international, peer-reviewed journal publishing high-quality, original research. All submitted manuscripts are subject to initial appraisal by the Editor, and, if found suitable for further consideration, to peer review by independent, anonymous expert referees.
Aims and Scope. Aim and scope. Applied Research on English Language is an international, peer-reviewed journal publishing high-quality, original research. All submitted manuscripts are subject to initial appraisal by the Editor, and, if found suitable for further consideration, to peer review by independent, anonymous expert referees. Applied ...
International Association for Applied Linguistics (AILA) AILA (originally founded in 1964 in France) is an international federation of national and regional associations of Applied Linguistics. Publishes research into language with relevance to real-world problems. Connections are made between fields, theories, research methods, scholarly.
Publishes research for all those involved in English Language Teaching. Content links the everyday concerns of practitioners with insights gained from relevant academic disciplines such as applied linguistics, education, psychology, and sociology.
The book seeks to target a broad audience, and is aimed at two main readerships: (1) undergraduate and Master's students completing qualifications in TESOL or Applied Linguistics, and (2) practising teachers of English as an Additional, Second, or Foreign Language to adults. English Language Teaching Now and How It Could Be is organised in ...
ISSN 2057-4215; Aims and scope. English Language Research is a double-blind peer reviewed open access journal with an emphasis on original research into both theoretical and applied linguistic issues in context.The journal is based in the Department of English at the University of Birmingham, and has been the forum for many seminal works.
A peer-reviewed, open access journal in applied linguistics, english & english language. This website uses cookies to ensure you get the best experience. ... Journal of Research on English and Language Learning 2721-5024 (Print) / 2721-5016 (Online) Website ISSN Portal ...
The ISSN of Applied Research on English Language journal is 22520198, 23225343. An International Standard Serial Number (ISSN) is a unique code of 8 digits. It is used for the recognition of journals, newspapers, periodicals, and magazines in all kind of forms, be it print-media or electronic.
Applied Research on English Language (ARE) Are by University of Isfahan is licensed under a CreativCommons. Attribution-NonCommercial-NoDerivatives 4.0 International License
English Language and Linguistics, published four times a year, is an international journal which focuses on the description of the English language within the framework of contemporary linguistics.The journal is concerned equally with the synchronic and the diachronic aspects of English language studies and publishes articles of the highest quality which make a substantial contribution to our ...
Journals Articles. Search by keywords: In the field: Search. Applied Research on English Language 2252-0198 (Print) / 2322-5343 (Online) Website ISSN Portal About Articles Added 29 July 2013 • Updated 3 July 2019 ...
High citations most often characterize quality research that reflects the foci of the discipline. This study aims to spotlight the most recent hot topics and the trends looming from the highly cited papers (HCPs) in Web of Science category of linguistics and language & linguistics with bibliometric analysis. The bibliometric information of the 143 HCPs based on Essential Citation Indicators ...
The Modern Language Journal - Wiley Online Library. CONGRATULATIONS to winners of the 2023 American Association for Applied Linguistics Research Article Award for their article: "Land as Interlocutor: A Study of Ojibwe Learner Language in Interaction on and with Naturally Occurring 'Materials.'". S1, 86-105.
Her work appears in journals such as the International Journal of Corpus Linguistics, Corpus Linguistics and Linguistic Theory, and Research Methods in Applied Linguistics. She serves on several editorial boards (e.g., Journal of English for Academic Purposes) and is the Review Editor for the International Journal of Learner Corpus Research.
Get access to Applied Research on English Language details, impact factor, Journal Ranking, H-Index, ISSN, Citescore, Scimago Journal Rank (SJR). Check top authors, submission guidelines, Acceptance Rate, Review Speed, Scope, Publication Fees, Submission Guidelines at one place. Improve your chances of getting published in Applied Research on English Language with Researcher.Life.
After final acceptance issued by the journal, 570,000 Tomans should be paid electronically, only via AREL's operation system. ... Applied Research on English Language. Newsletter Subscription. Subscribe to the journal newsletter and receive the latest news and updates. Subscribe
Language Teaching Research is a peer-reviewed journal that publishes research within the area of second or foreign language teaching. Although articles are written in English, the journal welcomes studies dealing with the teaching of languages other … | View full journal description. This journal is a member of the Committee on Publication ...
Applied Research is a multidisciplinary journal for researchers across the physical sciences, natural sciences, life sciences and engineering fields.. Our goal is to bridge the gap between fundamental and applied research and highlight the path towards the application of ideas through experiments, protocols, software, instrumentation, and other approaches.
Overall Journal Statistics. Submission Statistics; Submission Count: 755: Accept Count: 82: Reject Count: 603: Published articles (From 2020) Number of Volumes: 5: ... Applied Research on English Language. Newsletter Subscription. Subscribe to the journal newsletter and receive the latest news and updates.
The aim is to provide a snapshot of some of the most exciting work published in the various research areas of the journal. ... The analysis was built upon previously developed strategies and applied to Meta ... Uthus, D.; Tanzer, G.; Georg, M. Youtube-asl: A large-scale, open-domain American sign language-English parallel corpus. In Proceedings ...
Our deep learning models were applied to the S records of patients with cancer, and the extracted adverse event signals were assessed in relation to medical actions and prescribed drugs. ... Journal of Medical Internet Research 8305 articles ... (174) Clinical Information and Decision Making (24) Natural Language Processing (24) Machine ...
Journal Archive. Volume 12 (2023) Issue 4. Issue 3. Issue 2. Issue 1. Volume 11 (2022) Volume 10 (2021) Volume 9 (2020) Volume 8 (2019) Volume 7 (2018) ... Applied Research on English Language. Newsletter Subscription. Subscribe to the journal newsletter and receive the latest news and updates.