Have a language expert improve your writing
Run a free plagiarism check in 10 minutes, generate accurate citations for free.
- Knowledge Base
Methodology
- How to Write a Strong Hypothesis | Steps & Examples
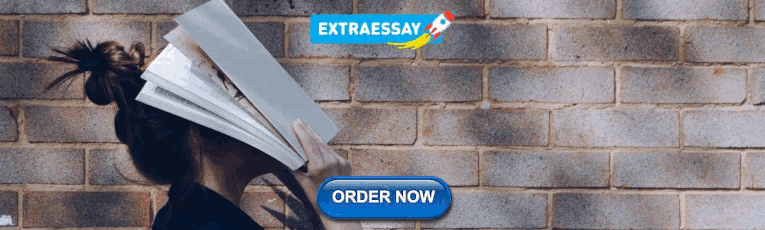
How to Write a Strong Hypothesis | Steps & Examples
Published on May 6, 2022 by Shona McCombes . Revised on November 20, 2023.
A hypothesis is a statement that can be tested by scientific research. If you want to test a relationship between two or more variables, you need to write hypotheses before you start your experiment or data collection .
Example: Hypothesis
Daily apple consumption leads to fewer doctor’s visits.
Table of contents
What is a hypothesis, developing a hypothesis (with example), hypothesis examples, other interesting articles, frequently asked questions about writing hypotheses.
A hypothesis states your predictions about what your research will find. It is a tentative answer to your research question that has not yet been tested. For some research projects, you might have to write several hypotheses that address different aspects of your research question.
A hypothesis is not just a guess – it should be based on existing theories and knowledge. It also has to be testable, which means you can support or refute it through scientific research methods (such as experiments, observations and statistical analysis of data).
Variables in hypotheses
Hypotheses propose a relationship between two or more types of variables .
- An independent variable is something the researcher changes or controls.
- A dependent variable is something the researcher observes and measures.
If there are any control variables , extraneous variables , or confounding variables , be sure to jot those down as you go to minimize the chances that research bias will affect your results.
In this example, the independent variable is exposure to the sun – the assumed cause . The dependent variable is the level of happiness – the assumed effect .
Here's why students love Scribbr's proofreading services
Discover proofreading & editing
Step 1. Ask a question
Writing a hypothesis begins with a research question that you want to answer. The question should be focused, specific, and researchable within the constraints of your project.
Step 2. Do some preliminary research
Your initial answer to the question should be based on what is already known about the topic. Look for theories and previous studies to help you form educated assumptions about what your research will find.
At this stage, you might construct a conceptual framework to ensure that you’re embarking on a relevant topic . This can also help you identify which variables you will study and what you think the relationships are between them. Sometimes, you’ll have to operationalize more complex constructs.
Step 3. Formulate your hypothesis
Now you should have some idea of what you expect to find. Write your initial answer to the question in a clear, concise sentence.
4. Refine your hypothesis
You need to make sure your hypothesis is specific and testable. There are various ways of phrasing a hypothesis, but all the terms you use should have clear definitions, and the hypothesis should contain:
- The relevant variables
- The specific group being studied
- The predicted outcome of the experiment or analysis
5. Phrase your hypothesis in three ways
To identify the variables, you can write a simple prediction in if…then form. The first part of the sentence states the independent variable and the second part states the dependent variable.
In academic research, hypotheses are more commonly phrased in terms of correlations or effects, where you directly state the predicted relationship between variables.
If you are comparing two groups, the hypothesis can state what difference you expect to find between them.
6. Write a null hypothesis
If your research involves statistical hypothesis testing , you will also have to write a null hypothesis . The null hypothesis is the default position that there is no association between the variables. The null hypothesis is written as H 0 , while the alternative hypothesis is H 1 or H a .
- H 0 : The number of lectures attended by first-year students has no effect on their final exam scores.
- H 1 : The number of lectures attended by first-year students has a positive effect on their final exam scores.
If you want to know more about the research process , methodology , research bias , or statistics , make sure to check out some of our other articles with explanations and examples.
- Sampling methods
- Simple random sampling
- Stratified sampling
- Cluster sampling
- Likert scales
- Reproducibility
Statistics
- Null hypothesis
- Statistical power
- Probability distribution
- Effect size
- Poisson distribution
Research bias
- Optimism bias
- Cognitive bias
- Implicit bias
- Hawthorne effect
- Anchoring bias
- Explicit bias
Prevent plagiarism. Run a free check.
A hypothesis is not just a guess — it should be based on existing theories and knowledge. It also has to be testable, which means you can support or refute it through scientific research methods (such as experiments, observations and statistical analysis of data).
Null and alternative hypotheses are used in statistical hypothesis testing . The null hypothesis of a test always predicts no effect or no relationship between variables, while the alternative hypothesis states your research prediction of an effect or relationship.
Hypothesis testing is a formal procedure for investigating our ideas about the world using statistics. It is used by scientists to test specific predictions, called hypotheses , by calculating how likely it is that a pattern or relationship between variables could have arisen by chance.
Cite this Scribbr article
If you want to cite this source, you can copy and paste the citation or click the “Cite this Scribbr article” button to automatically add the citation to our free Citation Generator.
McCombes, S. (2023, November 20). How to Write a Strong Hypothesis | Steps & Examples. Scribbr. Retrieved April 9, 2024, from https://www.scribbr.com/methodology/hypothesis/
Is this article helpful?
Shona McCombes
Other students also liked, construct validity | definition, types, & examples, what is a conceptual framework | tips & examples, operationalization | a guide with examples, pros & cons, what is your plagiarism score.
- Resources Home 🏠
- Try SciSpace Copilot
- Search research papers
- Add Copilot Extension
- Try AI Detector
- Try Paraphraser
- Try Citation Generator
- April Papers
- June Papers
- July Papers

The Craft of Writing a Strong Hypothesis

Table of Contents
Writing a hypothesis is one of the essential elements of a scientific research paper. It needs to be to the point, clearly communicating what your research is trying to accomplish. A blurry, drawn-out, or complexly-structured hypothesis can confuse your readers. Or worse, the editor and peer reviewers.
A captivating hypothesis is not too intricate. This blog will take you through the process so that, by the end of it, you have a better idea of how to convey your research paper's intent in just one sentence.
What is a Hypothesis?
The first step in your scientific endeavor, a hypothesis, is a strong, concise statement that forms the basis of your research. It is not the same as a thesis statement , which is a brief summary of your research paper .
The sole purpose of a hypothesis is to predict your paper's findings, data, and conclusion. It comes from a place of curiosity and intuition . When you write a hypothesis, you're essentially making an educated guess based on scientific prejudices and evidence, which is further proven or disproven through the scientific method.
The reason for undertaking research is to observe a specific phenomenon. A hypothesis, therefore, lays out what the said phenomenon is. And it does so through two variables, an independent and dependent variable.
The independent variable is the cause behind the observation, while the dependent variable is the effect of the cause. A good example of this is “mixing red and blue forms purple.” In this hypothesis, mixing red and blue is the independent variable as you're combining the two colors at your own will. The formation of purple is the dependent variable as, in this case, it is conditional to the independent variable.
Different Types of Hypotheses

Types of hypotheses
Some would stand by the notion that there are only two types of hypotheses: a Null hypothesis and an Alternative hypothesis. While that may have some truth to it, it would be better to fully distinguish the most common forms as these terms come up so often, which might leave you out of context.
Apart from Null and Alternative, there are Complex, Simple, Directional, Non-Directional, Statistical, and Associative and casual hypotheses. They don't necessarily have to be exclusive, as one hypothesis can tick many boxes, but knowing the distinctions between them will make it easier for you to construct your own.
1. Null hypothesis
A null hypothesis proposes no relationship between two variables. Denoted by H 0 , it is a negative statement like “Attending physiotherapy sessions does not affect athletes' on-field performance.” Here, the author claims physiotherapy sessions have no effect on on-field performances. Even if there is, it's only a coincidence.
2. Alternative hypothesis
Considered to be the opposite of a null hypothesis, an alternative hypothesis is donated as H1 or Ha. It explicitly states that the dependent variable affects the independent variable. A good alternative hypothesis example is “Attending physiotherapy sessions improves athletes' on-field performance.” or “Water evaporates at 100 °C. ” The alternative hypothesis further branches into directional and non-directional.
- Directional hypothesis: A hypothesis that states the result would be either positive or negative is called directional hypothesis. It accompanies H1 with either the ‘<' or ‘>' sign.
- Non-directional hypothesis: A non-directional hypothesis only claims an effect on the dependent variable. It does not clarify whether the result would be positive or negative. The sign for a non-directional hypothesis is ‘≠.'
3. Simple hypothesis
A simple hypothesis is a statement made to reflect the relation between exactly two variables. One independent and one dependent. Consider the example, “Smoking is a prominent cause of lung cancer." The dependent variable, lung cancer, is dependent on the independent variable, smoking.
4. Complex hypothesis
In contrast to a simple hypothesis, a complex hypothesis implies the relationship between multiple independent and dependent variables. For instance, “Individuals who eat more fruits tend to have higher immunity, lesser cholesterol, and high metabolism.” The independent variable is eating more fruits, while the dependent variables are higher immunity, lesser cholesterol, and high metabolism.
5. Associative and casual hypothesis
Associative and casual hypotheses don't exhibit how many variables there will be. They define the relationship between the variables. In an associative hypothesis, changing any one variable, dependent or independent, affects others. In a casual hypothesis, the independent variable directly affects the dependent.
6. Empirical hypothesis
Also referred to as the working hypothesis, an empirical hypothesis claims a theory's validation via experiments and observation. This way, the statement appears justifiable and different from a wild guess.
Say, the hypothesis is “Women who take iron tablets face a lesser risk of anemia than those who take vitamin B12.” This is an example of an empirical hypothesis where the researcher the statement after assessing a group of women who take iron tablets and charting the findings.
7. Statistical hypothesis
The point of a statistical hypothesis is to test an already existing hypothesis by studying a population sample. Hypothesis like “44% of the Indian population belong in the age group of 22-27.” leverage evidence to prove or disprove a particular statement.
Characteristics of a Good Hypothesis
Writing a hypothesis is essential as it can make or break your research for you. That includes your chances of getting published in a journal. So when you're designing one, keep an eye out for these pointers:
- A research hypothesis has to be simple yet clear to look justifiable enough.
- It has to be testable — your research would be rendered pointless if too far-fetched into reality or limited by technology.
- It has to be precise about the results —what you are trying to do and achieve through it should come out in your hypothesis.
- A research hypothesis should be self-explanatory, leaving no doubt in the reader's mind.
- If you are developing a relational hypothesis, you need to include the variables and establish an appropriate relationship among them.
- A hypothesis must keep and reflect the scope for further investigations and experiments.
Separating a Hypothesis from a Prediction
Outside of academia, hypothesis and prediction are often used interchangeably. In research writing, this is not only confusing but also incorrect. And although a hypothesis and prediction are guesses at their core, there are many differences between them.
A hypothesis is an educated guess or even a testable prediction validated through research. It aims to analyze the gathered evidence and facts to define a relationship between variables and put forth a logical explanation behind the nature of events.
Predictions are assumptions or expected outcomes made without any backing evidence. They are more fictionally inclined regardless of where they originate from.
For this reason, a hypothesis holds much more weight than a prediction. It sticks to the scientific method rather than pure guesswork. "Planets revolve around the Sun." is an example of a hypothesis as it is previous knowledge and observed trends. Additionally, we can test it through the scientific method.
Whereas "COVID-19 will be eradicated by 2030." is a prediction. Even though it results from past trends, we can't prove or disprove it. So, the only way this gets validated is to wait and watch if COVID-19 cases end by 2030.
Finally, How to Write a Hypothesis
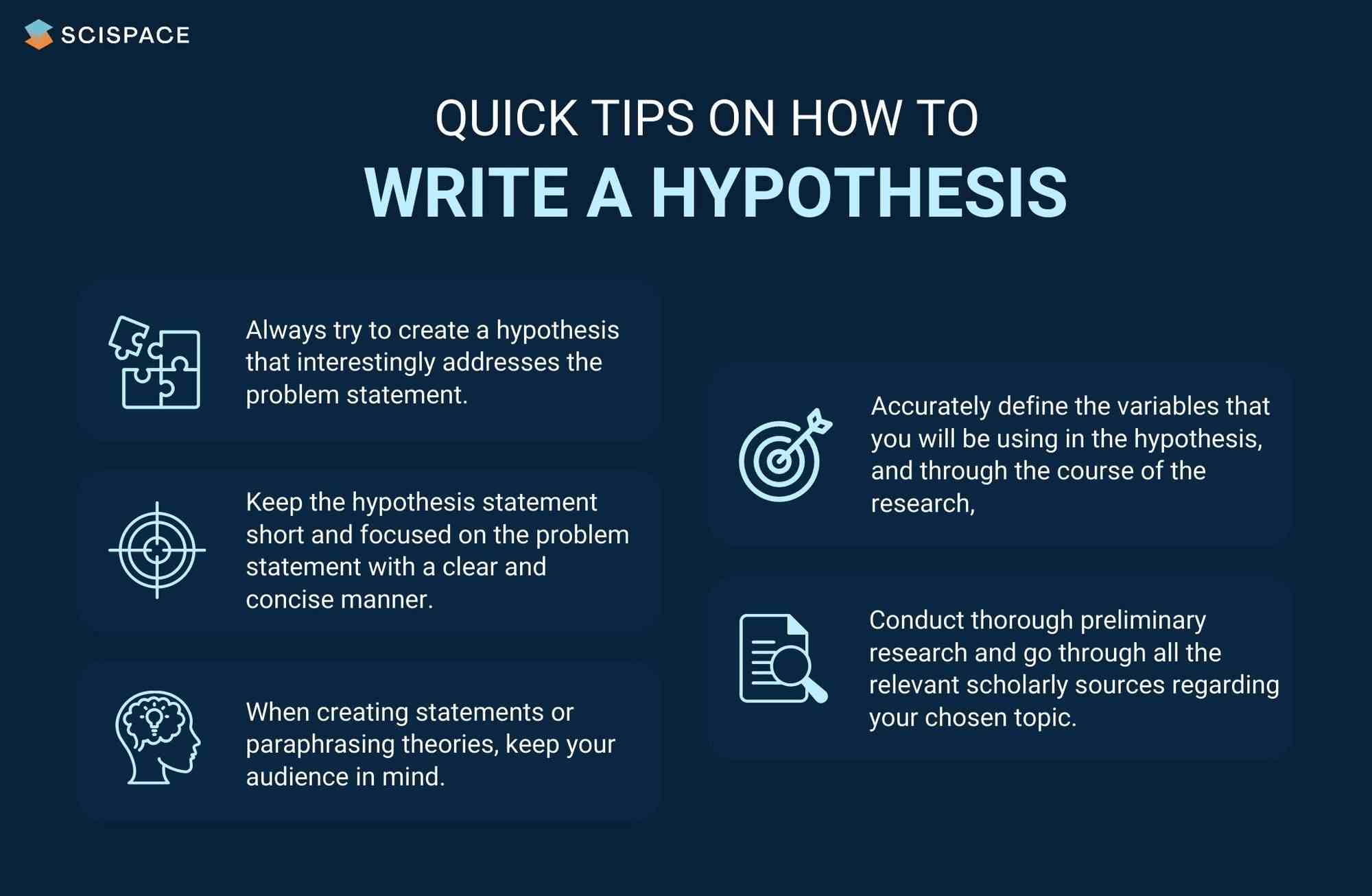
Quick tips on writing a hypothesis
1. Be clear about your research question
A hypothesis should instantly address the research question or the problem statement. To do so, you need to ask a question. Understand the constraints of your undertaken research topic and then formulate a simple and topic-centric problem. Only after that can you develop a hypothesis and further test for evidence.
2. Carry out a recce
Once you have your research's foundation laid out, it would be best to conduct preliminary research. Go through previous theories, academic papers, data, and experiments before you start curating your research hypothesis. It will give you an idea of your hypothesis's viability or originality.
Making use of references from relevant research papers helps draft a good research hypothesis. SciSpace Discover offers a repository of over 270 million research papers to browse through and gain a deeper understanding of related studies on a particular topic. Additionally, you can use SciSpace Copilot , your AI research assistant, for reading any lengthy research paper and getting a more summarized context of it. A hypothesis can be formed after evaluating many such summarized research papers. Copilot also offers explanations for theories and equations, explains paper in simplified version, allows you to highlight any text in the paper or clip math equations and tables and provides a deeper, clear understanding of what is being said. This can improve the hypothesis by helping you identify potential research gaps.
3. Create a 3-dimensional hypothesis
Variables are an essential part of any reasonable hypothesis. So, identify your independent and dependent variable(s) and form a correlation between them. The ideal way to do this is to write the hypothetical assumption in the ‘if-then' form. If you use this form, make sure that you state the predefined relationship between the variables.
In another way, you can choose to present your hypothesis as a comparison between two variables. Here, you must specify the difference you expect to observe in the results.
4. Write the first draft
Now that everything is in place, it's time to write your hypothesis. For starters, create the first draft. In this version, write what you expect to find from your research.
Clearly separate your independent and dependent variables and the link between them. Don't fixate on syntax at this stage. The goal is to ensure your hypothesis addresses the issue.
5. Proof your hypothesis
After preparing the first draft of your hypothesis, you need to inspect it thoroughly. It should tick all the boxes, like being concise, straightforward, relevant, and accurate. Your final hypothesis has to be well-structured as well.
Research projects are an exciting and crucial part of being a scholar. And once you have your research question, you need a great hypothesis to begin conducting research. Thus, knowing how to write a hypothesis is very important.
Now that you have a firmer grasp on what a good hypothesis constitutes, the different kinds there are, and what process to follow, you will find it much easier to write your hypothesis, which ultimately helps your research.
Now it's easier than ever to streamline your research workflow with SciSpace Discover . Its integrated, comprehensive end-to-end platform for research allows scholars to easily discover, write and publish their research and fosters collaboration.
It includes everything you need, including a repository of over 270 million research papers across disciplines, SEO-optimized summaries and public profiles to show your expertise and experience.
If you found these tips on writing a research hypothesis useful, head over to our blog on Statistical Hypothesis Testing to learn about the top researchers, papers, and institutions in this domain.
Frequently Asked Questions (FAQs)
1. what is the definition of hypothesis.
According to the Oxford dictionary, a hypothesis is defined as “An idea or explanation of something that is based on a few known facts, but that has not yet been proved to be true or correct”.
2. What is an example of hypothesis?
The hypothesis is a statement that proposes a relationship between two or more variables. An example: "If we increase the number of new users who join our platform by 25%, then we will see an increase in revenue."
3. What is an example of null hypothesis?
A null hypothesis is a statement that there is no relationship between two variables. The null hypothesis is written as H0. The null hypothesis states that there is no effect. For example, if you're studying whether or not a particular type of exercise increases strength, your null hypothesis will be "there is no difference in strength between people who exercise and people who don't."
4. What are the types of research?
• Fundamental research
• Applied research
• Qualitative research
• Quantitative research
• Mixed research
• Exploratory research
• Longitudinal research
• Cross-sectional research
• Field research
• Laboratory research
• Fixed research
• Flexible research
• Action research
• Policy research
• Classification research
• Comparative research
• Causal research
• Inductive research
• Deductive research
5. How to write a hypothesis?
• Your hypothesis should be able to predict the relationship and outcome.
• Avoid wordiness by keeping it simple and brief.
• Your hypothesis should contain observable and testable outcomes.
• Your hypothesis should be relevant to the research question.
6. What are the 2 types of hypothesis?
• Null hypotheses are used to test the claim that "there is no difference between two groups of data".
• Alternative hypotheses test the claim that "there is a difference between two data groups".
7. Difference between research question and research hypothesis?
A research question is a broad, open-ended question you will try to answer through your research. A hypothesis is a statement based on prior research or theory that you expect to be true due to your study. Example - Research question: What are the factors that influence the adoption of the new technology? Research hypothesis: There is a positive relationship between age, education and income level with the adoption of the new technology.
8. What is plural for hypothesis?
The plural of hypothesis is hypotheses. Here's an example of how it would be used in a statement, "Numerous well-considered hypotheses are presented in this part, and they are supported by tables and figures that are well-illustrated."
9. What is the red queen hypothesis?
The red queen hypothesis in evolutionary biology states that species must constantly evolve to avoid extinction because if they don't, they will be outcompeted by other species that are evolving. Leigh Van Valen first proposed it in 1973; since then, it has been tested and substantiated many times.
10. Who is known as the father of null hypothesis?
The father of the null hypothesis is Sir Ronald Fisher. He published a paper in 1925 that introduced the concept of null hypothesis testing, and he was also the first to use the term itself.
11. When to reject null hypothesis?
You need to find a significant difference between your two populations to reject the null hypothesis. You can determine that by running statistical tests such as an independent sample t-test or a dependent sample t-test. You should reject the null hypothesis if the p-value is less than 0.05.

You might also like

Consensus GPT vs. SciSpace GPT: Choose the Best GPT for Research
Literature Review and Theoretical Framework: Understanding the Differences

Types of Essays in Academic Writing - Quick Guide (2024)
- Privacy Policy
Buy Me a Coffee

Home » What is a Hypothesis – Types, Examples and Writing Guide
What is a Hypothesis – Types, Examples and Writing Guide
Table of Contents

Definition:
Hypothesis is an educated guess or proposed explanation for a phenomenon, based on some initial observations or data. It is a tentative statement that can be tested and potentially proven or disproven through further investigation and experimentation.
Hypothesis is often used in scientific research to guide the design of experiments and the collection and analysis of data. It is an essential element of the scientific method, as it allows researchers to make predictions about the outcome of their experiments and to test those predictions to determine their accuracy.
Types of Hypothesis
Types of Hypothesis are as follows:
Research Hypothesis
A research hypothesis is a statement that predicts a relationship between variables. It is usually formulated as a specific statement that can be tested through research, and it is often used in scientific research to guide the design of experiments.
Null Hypothesis
The null hypothesis is a statement that assumes there is no significant difference or relationship between variables. It is often used as a starting point for testing the research hypothesis, and if the results of the study reject the null hypothesis, it suggests that there is a significant difference or relationship between variables.
Alternative Hypothesis
An alternative hypothesis is a statement that assumes there is a significant difference or relationship between variables. It is often used as an alternative to the null hypothesis and is tested against the null hypothesis to determine which statement is more accurate.
Directional Hypothesis
A directional hypothesis is a statement that predicts the direction of the relationship between variables. For example, a researcher might predict that increasing the amount of exercise will result in a decrease in body weight.
Non-directional Hypothesis
A non-directional hypothesis is a statement that predicts the relationship between variables but does not specify the direction. For example, a researcher might predict that there is a relationship between the amount of exercise and body weight, but they do not specify whether increasing or decreasing exercise will affect body weight.
Statistical Hypothesis
A statistical hypothesis is a statement that assumes a particular statistical model or distribution for the data. It is often used in statistical analysis to test the significance of a particular result.
Composite Hypothesis
A composite hypothesis is a statement that assumes more than one condition or outcome. It can be divided into several sub-hypotheses, each of which represents a different possible outcome.
Empirical Hypothesis
An empirical hypothesis is a statement that is based on observed phenomena or data. It is often used in scientific research to develop theories or models that explain the observed phenomena.
Simple Hypothesis
A simple hypothesis is a statement that assumes only one outcome or condition. It is often used in scientific research to test a single variable or factor.
Complex Hypothesis
A complex hypothesis is a statement that assumes multiple outcomes or conditions. It is often used in scientific research to test the effects of multiple variables or factors on a particular outcome.
Applications of Hypothesis
Hypotheses are used in various fields to guide research and make predictions about the outcomes of experiments or observations. Here are some examples of how hypotheses are applied in different fields:
- Science : In scientific research, hypotheses are used to test the validity of theories and models that explain natural phenomena. For example, a hypothesis might be formulated to test the effects of a particular variable on a natural system, such as the effects of climate change on an ecosystem.
- Medicine : In medical research, hypotheses are used to test the effectiveness of treatments and therapies for specific conditions. For example, a hypothesis might be formulated to test the effects of a new drug on a particular disease.
- Psychology : In psychology, hypotheses are used to test theories and models of human behavior and cognition. For example, a hypothesis might be formulated to test the effects of a particular stimulus on the brain or behavior.
- Sociology : In sociology, hypotheses are used to test theories and models of social phenomena, such as the effects of social structures or institutions on human behavior. For example, a hypothesis might be formulated to test the effects of income inequality on crime rates.
- Business : In business research, hypotheses are used to test the validity of theories and models that explain business phenomena, such as consumer behavior or market trends. For example, a hypothesis might be formulated to test the effects of a new marketing campaign on consumer buying behavior.
- Engineering : In engineering, hypotheses are used to test the effectiveness of new technologies or designs. For example, a hypothesis might be formulated to test the efficiency of a new solar panel design.
How to write a Hypothesis
Here are the steps to follow when writing a hypothesis:
Identify the Research Question
The first step is to identify the research question that you want to answer through your study. This question should be clear, specific, and focused. It should be something that can be investigated empirically and that has some relevance or significance in the field.
Conduct a Literature Review
Before writing your hypothesis, it’s essential to conduct a thorough literature review to understand what is already known about the topic. This will help you to identify the research gap and formulate a hypothesis that builds on existing knowledge.
Determine the Variables
The next step is to identify the variables involved in the research question. A variable is any characteristic or factor that can vary or change. There are two types of variables: independent and dependent. The independent variable is the one that is manipulated or changed by the researcher, while the dependent variable is the one that is measured or observed as a result of the independent variable.
Formulate the Hypothesis
Based on the research question and the variables involved, you can now formulate your hypothesis. A hypothesis should be a clear and concise statement that predicts the relationship between the variables. It should be testable through empirical research and based on existing theory or evidence.
Write the Null Hypothesis
The null hypothesis is the opposite of the alternative hypothesis, which is the hypothesis that you are testing. The null hypothesis states that there is no significant difference or relationship between the variables. It is important to write the null hypothesis because it allows you to compare your results with what would be expected by chance.
Refine the Hypothesis
After formulating the hypothesis, it’s important to refine it and make it more precise. This may involve clarifying the variables, specifying the direction of the relationship, or making the hypothesis more testable.
Examples of Hypothesis
Here are a few examples of hypotheses in different fields:
- Psychology : “Increased exposure to violent video games leads to increased aggressive behavior in adolescents.”
- Biology : “Higher levels of carbon dioxide in the atmosphere will lead to increased plant growth.”
- Sociology : “Individuals who grow up in households with higher socioeconomic status will have higher levels of education and income as adults.”
- Education : “Implementing a new teaching method will result in higher student achievement scores.”
- Marketing : “Customers who receive a personalized email will be more likely to make a purchase than those who receive a generic email.”
- Physics : “An increase in temperature will cause an increase in the volume of a gas, assuming all other variables remain constant.”
- Medicine : “Consuming a diet high in saturated fats will increase the risk of developing heart disease.”
Purpose of Hypothesis
The purpose of a hypothesis is to provide a testable explanation for an observed phenomenon or a prediction of a future outcome based on existing knowledge or theories. A hypothesis is an essential part of the scientific method and helps to guide the research process by providing a clear focus for investigation. It enables scientists to design experiments or studies to gather evidence and data that can support or refute the proposed explanation or prediction.
The formulation of a hypothesis is based on existing knowledge, observations, and theories, and it should be specific, testable, and falsifiable. A specific hypothesis helps to define the research question, which is important in the research process as it guides the selection of an appropriate research design and methodology. Testability of the hypothesis means that it can be proven or disproven through empirical data collection and analysis. Falsifiability means that the hypothesis should be formulated in such a way that it can be proven wrong if it is incorrect.
In addition to guiding the research process, the testing of hypotheses can lead to new discoveries and advancements in scientific knowledge. When a hypothesis is supported by the data, it can be used to develop new theories or models to explain the observed phenomenon. When a hypothesis is not supported by the data, it can help to refine existing theories or prompt the development of new hypotheses to explain the phenomenon.
When to use Hypothesis
Here are some common situations in which hypotheses are used:
- In scientific research , hypotheses are used to guide the design of experiments and to help researchers make predictions about the outcomes of those experiments.
- In social science research , hypotheses are used to test theories about human behavior, social relationships, and other phenomena.
- I n business , hypotheses can be used to guide decisions about marketing, product development, and other areas. For example, a hypothesis might be that a new product will sell well in a particular market, and this hypothesis can be tested through market research.
Characteristics of Hypothesis
Here are some common characteristics of a hypothesis:
- Testable : A hypothesis must be able to be tested through observation or experimentation. This means that it must be possible to collect data that will either support or refute the hypothesis.
- Falsifiable : A hypothesis must be able to be proven false if it is not supported by the data. If a hypothesis cannot be falsified, then it is not a scientific hypothesis.
- Clear and concise : A hypothesis should be stated in a clear and concise manner so that it can be easily understood and tested.
- Based on existing knowledge : A hypothesis should be based on existing knowledge and research in the field. It should not be based on personal beliefs or opinions.
- Specific : A hypothesis should be specific in terms of the variables being tested and the predicted outcome. This will help to ensure that the research is focused and well-designed.
- Tentative: A hypothesis is a tentative statement or assumption that requires further testing and evidence to be confirmed or refuted. It is not a final conclusion or assertion.
- Relevant : A hypothesis should be relevant to the research question or problem being studied. It should address a gap in knowledge or provide a new perspective on the issue.
Advantages of Hypothesis
Hypotheses have several advantages in scientific research and experimentation:
- Guides research: A hypothesis provides a clear and specific direction for research. It helps to focus the research question, select appropriate methods and variables, and interpret the results.
- Predictive powe r: A hypothesis makes predictions about the outcome of research, which can be tested through experimentation. This allows researchers to evaluate the validity of the hypothesis and make new discoveries.
- Facilitates communication: A hypothesis provides a common language and framework for scientists to communicate with one another about their research. This helps to facilitate the exchange of ideas and promotes collaboration.
- Efficient use of resources: A hypothesis helps researchers to use their time, resources, and funding efficiently by directing them towards specific research questions and methods that are most likely to yield results.
- Provides a basis for further research: A hypothesis that is supported by data provides a basis for further research and exploration. It can lead to new hypotheses, theories, and discoveries.
- Increases objectivity: A hypothesis can help to increase objectivity in research by providing a clear and specific framework for testing and interpreting results. This can reduce bias and increase the reliability of research findings.
Limitations of Hypothesis
Some Limitations of the Hypothesis are as follows:
- Limited to observable phenomena: Hypotheses are limited to observable phenomena and cannot account for unobservable or intangible factors. This means that some research questions may not be amenable to hypothesis testing.
- May be inaccurate or incomplete: Hypotheses are based on existing knowledge and research, which may be incomplete or inaccurate. This can lead to flawed hypotheses and erroneous conclusions.
- May be biased: Hypotheses may be biased by the researcher’s own beliefs, values, or assumptions. This can lead to selective interpretation of data and a lack of objectivity in research.
- Cannot prove causation: A hypothesis can only show a correlation between variables, but it cannot prove causation. This requires further experimentation and analysis.
- Limited to specific contexts: Hypotheses are limited to specific contexts and may not be generalizable to other situations or populations. This means that results may not be applicable in other contexts or may require further testing.
- May be affected by chance : Hypotheses may be affected by chance or random variation, which can obscure or distort the true relationship between variables.
About the author
Muhammad Hassan
Researcher, Academic Writer, Web developer
You may also like

Data Collection – Methods Types and Examples

Delimitations in Research – Types, Examples and...

Research Process – Steps, Examples and Tips

Research Design – Types, Methods and Examples

Institutional Review Board – Application Sample...

Evaluating Research – Process, Examples and...
2.4 Developing a Hypothesis
Learning objectives.
- Distinguish between a theory and a hypothesis.
- Discover how theories are used to generate hypotheses and how the results of studies can be used to further inform theories.
- Understand the characteristics of a good hypothesis.
Theories and Hypotheses
Before describing how to develop a hypothesis it is imporant to distinguish betwee a theory and a hypothesis. A theory is a coherent explanation or interpretation of one or more phenomena. Although theories can take a variety of forms, one thing they have in common is that they go beyond the phenomena they explain by including variables, structures, processes, functions, or organizing principles that have not been observed directly. Consider, for example, Zajonc’s theory of social facilitation and social inhibition. He proposed that being watched by others while performing a task creates a general state of physiological arousal, which increases the likelihood of the dominant (most likely) response. So for highly practiced tasks, being watched increases the tendency to make correct responses, but for relatively unpracticed tasks, being watched increases the tendency to make incorrect responses. Notice that this theory—which has come to be called drive theory—provides an explanation of both social facilitation and social inhibition that goes beyond the phenomena themselves by including concepts such as “arousal” and “dominant response,” along with processes such as the effect of arousal on the dominant response.
Outside of science, referring to an idea as a theory often implies that it is untested—perhaps no more than a wild guess. In science, however, the term theory has no such implication. A theory is simply an explanation or interpretation of a set of phenomena. It can be untested, but it can also be extensively tested, well supported, and accepted as an accurate description of the world by the scientific community. The theory of evolution by natural selection, for example, is a theory because it is an explanation of the diversity of life on earth—not because it is untested or unsupported by scientific research. On the contrary, the evidence for this theory is overwhelmingly positive and nearly all scientists accept its basic assumptions as accurate. Similarly, the “germ theory” of disease is a theory because it is an explanation of the origin of various diseases, not because there is any doubt that many diseases are caused by microorganisms that infect the body.
A hypothesis , on the other hand, is a specific prediction about a new phenomenon that should be observed if a particular theory is accurate. It is an explanation that relies on just a few key concepts. Hypotheses are often specific predictions about what will happen in a particular study. They are developed by considering existing evidence and using reasoning to infer what will happen in the specific context of interest. Hypotheses are often but not always derived from theories. So a hypothesis is often a prediction based on a theory but some hypotheses are a-theoretical and only after a set of observations have been made, is a theory developed. This is because theories are broad in nature and they explain larger bodies of data. So if our research question is really original then we may need to collect some data and make some observation before we can develop a broader theory.
Theories and hypotheses always have this if-then relationship. “ If drive theory is correct, then cockroaches should run through a straight runway faster, and a branching runway more slowly, when other cockroaches are present.” Although hypotheses are usually expressed as statements, they can always be rephrased as questions. “Do cockroaches run through a straight runway faster when other cockroaches are present?” Thus deriving hypotheses from theories is an excellent way of generating interesting research questions.
But how do researchers derive hypotheses from theories? One way is to generate a research question using the techniques discussed in this chapter and then ask whether any theory implies an answer to that question. For example, you might wonder whether expressive writing about positive experiences improves health as much as expressive writing about traumatic experiences. Although this question is an interesting one on its own, you might then ask whether the habituation theory—the idea that expressive writing causes people to habituate to negative thoughts and feelings—implies an answer. In this case, it seems clear that if the habituation theory is correct, then expressive writing about positive experiences should not be effective because it would not cause people to habituate to negative thoughts and feelings. A second way to derive hypotheses from theories is to focus on some component of the theory that has not yet been directly observed. For example, a researcher could focus on the process of habituation—perhaps hypothesizing that people should show fewer signs of emotional distress with each new writing session.
Among the very best hypotheses are those that distinguish between competing theories. For example, Norbert Schwarz and his colleagues considered two theories of how people make judgments about themselves, such as how assertive they are (Schwarz et al., 1991) [1] . Both theories held that such judgments are based on relevant examples that people bring to mind. However, one theory was that people base their judgments on the number of examples they bring to mind and the other was that people base their judgments on how easily they bring those examples to mind. To test these theories, the researchers asked people to recall either six times when they were assertive (which is easy for most people) or 12 times (which is difficult for most people). Then they asked them to judge their own assertiveness. Note that the number-of-examples theory implies that people who recalled 12 examples should judge themselves to be more assertive because they recalled more examples, but the ease-of-examples theory implies that participants who recalled six examples should judge themselves as more assertive because recalling the examples was easier. Thus the two theories made opposite predictions so that only one of the predictions could be confirmed. The surprising result was that participants who recalled fewer examples judged themselves to be more assertive—providing particularly convincing evidence in favor of the ease-of-retrieval theory over the number-of-examples theory.
Theory Testing
The primary way that scientific researchers use theories is sometimes called the hypothetico-deductive method (although this term is much more likely to be used by philosophers of science than by scientists themselves). A researcher begins with a set of phenomena and either constructs a theory to explain or interpret them or chooses an existing theory to work with. He or she then makes a prediction about some new phenomenon that should be observed if the theory is correct. Again, this prediction is called a hypothesis. The researcher then conducts an empirical study to test the hypothesis. Finally, he or she reevaluates the theory in light of the new results and revises it if necessary. This process is usually conceptualized as a cycle because the researcher can then derive a new hypothesis from the revised theory, conduct a new empirical study to test the hypothesis, and so on. As Figure 2.2 shows, this approach meshes nicely with the model of scientific research in psychology presented earlier in the textbook—creating a more detailed model of “theoretically motivated” or “theory-driven” research.

Figure 2.2 Hypothetico-Deductive Method Combined With the General Model of Scientific Research in Psychology Together they form a model of theoretically motivated research.
As an example, let us consider Zajonc’s research on social facilitation and inhibition. He started with a somewhat contradictory pattern of results from the research literature. He then constructed his drive theory, according to which being watched by others while performing a task causes physiological arousal, which increases an organism’s tendency to make the dominant response. This theory predicts social facilitation for well-learned tasks and social inhibition for poorly learned tasks. He now had a theory that organized previous results in a meaningful way—but he still needed to test it. He hypothesized that if his theory was correct, he should observe that the presence of others improves performance in a simple laboratory task but inhibits performance in a difficult version of the very same laboratory task. To test this hypothesis, one of the studies he conducted used cockroaches as subjects (Zajonc, Heingartner, & Herman, 1969) [2] . The cockroaches ran either down a straight runway (an easy task for a cockroach) or through a cross-shaped maze (a difficult task for a cockroach) to escape into a dark chamber when a light was shined on them. They did this either while alone or in the presence of other cockroaches in clear plastic “audience boxes.” Zajonc found that cockroaches in the straight runway reached their goal more quickly in the presence of other cockroaches, but cockroaches in the cross-shaped maze reached their goal more slowly when they were in the presence of other cockroaches. Thus he confirmed his hypothesis and provided support for his drive theory. (Zajonc also showed that drive theory existed in humans (Zajonc & Sales, 1966) [3] in many other studies afterward).
Incorporating Theory into Your Research
When you write your research report or plan your presentation, be aware that there are two basic ways that researchers usually include theory. The first is to raise a research question, answer that question by conducting a new study, and then offer one or more theories (usually more) to explain or interpret the results. This format works well for applied research questions and for research questions that existing theories do not address. The second way is to describe one or more existing theories, derive a hypothesis from one of those theories, test the hypothesis in a new study, and finally reevaluate the theory. This format works well when there is an existing theory that addresses the research question—especially if the resulting hypothesis is surprising or conflicts with a hypothesis derived from a different theory.
To use theories in your research will not only give you guidance in coming up with experiment ideas and possible projects, but it lends legitimacy to your work. Psychologists have been interested in a variety of human behaviors and have developed many theories along the way. Using established theories will help you break new ground as a researcher, not limit you from developing your own ideas.
Characteristics of a Good Hypothesis
There are three general characteristics of a good hypothesis. First, a good hypothesis must be testable and falsifiable . We must be able to test the hypothesis using the methods of science and if you’ll recall Popper’s falsifiability criterion, it must be possible to gather evidence that will disconfirm the hypothesis if it is indeed false. Second, a good hypothesis must be logical. As described above, hypotheses are more than just a random guess. Hypotheses should be informed by previous theories or observations and logical reasoning. Typically, we begin with a broad and general theory and use deductive reasoning to generate a more specific hypothesis to test based on that theory. Occasionally, however, when there is no theory to inform our hypothesis, we use inductive reasoning which involves using specific observations or research findings to form a more general hypothesis. Finally, the hypothesis should be positive. That is, the hypothesis should make a positive statement about the existence of a relationship or effect, rather than a statement that a relationship or effect does not exist. As scientists, we don’t set out to show that relationships do not exist or that effects do not occur so our hypotheses should not be worded in a way to suggest that an effect or relationship does not exist. The nature of science is to assume that something does not exist and then seek to find evidence to prove this wrong, to show that really it does exist. That may seem backward to you but that is the nature of the scientific method. The underlying reason for this is beyond the scope of this chapter but it has to do with statistical theory.
Key Takeaways
- A theory is broad in nature and explains larger bodies of data. A hypothesis is more specific and makes a prediction about the outcome of a particular study.
- Working with theories is not “icing on the cake.” It is a basic ingredient of psychological research.
- Like other scientists, psychologists use the hypothetico-deductive method. They construct theories to explain or interpret phenomena (or work with existing theories), derive hypotheses from their theories, test the hypotheses, and then reevaluate the theories in light of the new results.
- Practice: Find a recent empirical research report in a professional journal. Read the introduction and highlight in different colors descriptions of theories and hypotheses.
- Schwarz, N., Bless, H., Strack, F., Klumpp, G., Rittenauer-Schatka, H., & Simons, A. (1991). Ease of retrieval as information: Another look at the availability heuristic. Journal of Personality and Social Psychology, 61 , 195–202. ↵
- Zajonc, R. B., Heingartner, A., & Herman, E. M. (1969). Social enhancement and impairment of performance in the cockroach. Journal of Personality and Social Psychology, 13 , 83–92. ↵
- Zajonc, R.B. & Sales, S.M. (1966). Social facilitation of dominant and subordinate responses. Journal of Experimental Social Psychology, 2 , 160-168. ↵

Share This Book
- Increase Font Size

How to Develop a Good Research Hypothesis
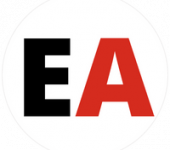
The story of a research study begins by asking a question. Researchers all around the globe are asking curious questions and formulating research hypothesis. However, whether the research study provides an effective conclusion depends on how well one develops a good research hypothesis. Research hypothesis examples could help researchers get an idea as to how to write a good research hypothesis.
This blog will help you understand what is a research hypothesis, its characteristics and, how to formulate a research hypothesis
Table of Contents
What is Hypothesis?
Hypothesis is an assumption or an idea proposed for the sake of argument so that it can be tested. It is a precise, testable statement of what the researchers predict will be outcome of the study. Hypothesis usually involves proposing a relationship between two variables: the independent variable (what the researchers change) and the dependent variable (what the research measures).
What is a Research Hypothesis?
Research hypothesis is a statement that introduces a research question and proposes an expected result. It is an integral part of the scientific method that forms the basis of scientific experiments. Therefore, you need to be careful and thorough when building your research hypothesis. A minor flaw in the construction of your hypothesis could have an adverse effect on your experiment. In research, there is a convention that the hypothesis is written in two forms, the null hypothesis, and the alternative hypothesis (called the experimental hypothesis when the method of investigation is an experiment).
Characteristics of a Good Research Hypothesis
As the hypothesis is specific, there is a testable prediction about what you expect to happen in a study. You may consider drawing hypothesis from previously published research based on the theory.
A good research hypothesis involves more effort than just a guess. In particular, your hypothesis may begin with a question that could be further explored through background research.
To help you formulate a promising research hypothesis, you should ask yourself the following questions:
- Is the language clear and focused?
- What is the relationship between your hypothesis and your research topic?
- Is your hypothesis testable? If yes, then how?
- What are the possible explanations that you might want to explore?
- Does your hypothesis include both an independent and dependent variable?
- Can you manipulate your variables without hampering the ethical standards?
- Does your research predict the relationship and outcome?
- Is your research simple and concise (avoids wordiness)?
- Is it clear with no ambiguity or assumptions about the readers’ knowledge
- Is your research observable and testable results?
- Is it relevant and specific to the research question or problem?
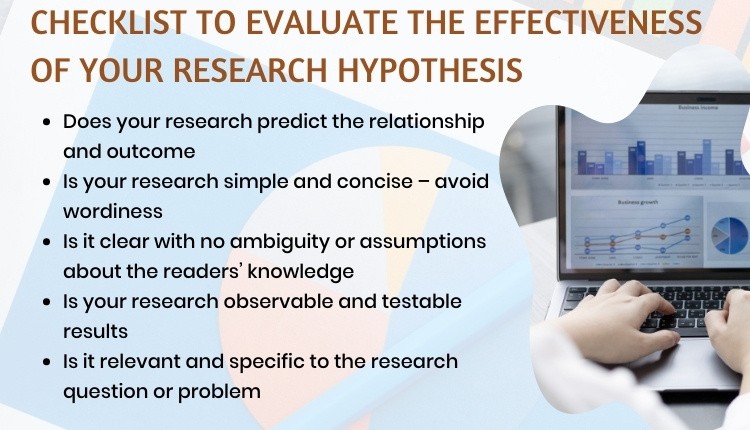
The questions listed above can be used as a checklist to make sure your hypothesis is based on a solid foundation. Furthermore, it can help you identify weaknesses in your hypothesis and revise it if necessary.
Source: Educational Hub
How to formulate a research hypothesis.
A testable hypothesis is not a simple statement. It is rather an intricate statement that needs to offer a clear introduction to a scientific experiment, its intentions, and the possible outcomes. However, there are some important things to consider when building a compelling hypothesis.
1. State the problem that you are trying to solve.
Make sure that the hypothesis clearly defines the topic and the focus of the experiment.
2. Try to write the hypothesis as an if-then statement.
Follow this template: If a specific action is taken, then a certain outcome is expected.
3. Define the variables
Independent variables are the ones that are manipulated, controlled, or changed. Independent variables are isolated from other factors of the study.
Dependent variables , as the name suggests are dependent on other factors of the study. They are influenced by the change in independent variable.
4. Scrutinize the hypothesis
Evaluate assumptions, predictions, and evidence rigorously to refine your understanding.
Types of Research Hypothesis
The types of research hypothesis are stated below:
1. Simple Hypothesis
It predicts the relationship between a single dependent variable and a single independent variable.
2. Complex Hypothesis
It predicts the relationship between two or more independent and dependent variables.
3. Directional Hypothesis
It specifies the expected direction to be followed to determine the relationship between variables and is derived from theory. Furthermore, it implies the researcher’s intellectual commitment to a particular outcome.
4. Non-directional Hypothesis
It does not predict the exact direction or nature of the relationship between the two variables. The non-directional hypothesis is used when there is no theory involved or when findings contradict previous research.
5. Associative and Causal Hypothesis
The associative hypothesis defines interdependency between variables. A change in one variable results in the change of the other variable. On the other hand, the causal hypothesis proposes an effect on the dependent due to manipulation of the independent variable.
6. Null Hypothesis
Null hypothesis states a negative statement to support the researcher’s findings that there is no relationship between two variables. There will be no changes in the dependent variable due the manipulation of the independent variable. Furthermore, it states results are due to chance and are not significant in terms of supporting the idea being investigated.
7. Alternative Hypothesis
It states that there is a relationship between the two variables of the study and that the results are significant to the research topic. An experimental hypothesis predicts what changes will take place in the dependent variable when the independent variable is manipulated. Also, it states that the results are not due to chance and that they are significant in terms of supporting the theory being investigated.
Research Hypothesis Examples of Independent and Dependent Variables
Research Hypothesis Example 1 The greater number of coal plants in a region (independent variable) increases water pollution (dependent variable). If you change the independent variable (building more coal factories), it will change the dependent variable (amount of water pollution).
Research Hypothesis Example 2 What is the effect of diet or regular soda (independent variable) on blood sugar levels (dependent variable)? If you change the independent variable (the type of soda you consume), it will change the dependent variable (blood sugar levels)
You should not ignore the importance of the above steps. The validity of your experiment and its results rely on a robust testable hypothesis. Developing a strong testable hypothesis has few advantages, it compels us to think intensely and specifically about the outcomes of a study. Consequently, it enables us to understand the implication of the question and the different variables involved in the study. Furthermore, it helps us to make precise predictions based on prior research. Hence, forming a hypothesis would be of great value to the research. Here are some good examples of testable hypotheses.
More importantly, you need to build a robust testable research hypothesis for your scientific experiments. A testable hypothesis is a hypothesis that can be proved or disproved as a result of experimentation.
Importance of a Testable Hypothesis
To devise and perform an experiment using scientific method, you need to make sure that your hypothesis is testable. To be considered testable, some essential criteria must be met:
- There must be a possibility to prove that the hypothesis is true.
- There must be a possibility to prove that the hypothesis is false.
- The results of the hypothesis must be reproducible.
Without these criteria, the hypothesis and the results will be vague. As a result, the experiment will not prove or disprove anything significant.
What are your experiences with building hypotheses for scientific experiments? What challenges did you face? How did you overcome these challenges? Please share your thoughts with us in the comments section.
Frequently Asked Questions
The steps to write a research hypothesis are: 1. Stating the problem: Ensure that the hypothesis defines the research problem 2. Writing a hypothesis as an 'if-then' statement: Include the action and the expected outcome of your study by following a ‘if-then’ structure. 3. Defining the variables: Define the variables as Dependent or Independent based on their dependency to other factors. 4. Scrutinizing the hypothesis: Identify the type of your hypothesis
Hypothesis testing is a statistical tool which is used to make inferences about a population data to draw conclusions for a particular hypothesis.
Hypothesis in statistics is a formal statement about the nature of a population within a structured framework of a statistical model. It is used to test an existing hypothesis by studying a population.
Research hypothesis is a statement that introduces a research question and proposes an expected result. It forms the basis of scientific experiments.
The different types of hypothesis in research are: • Null hypothesis: Null hypothesis is a negative statement to support the researcher’s findings that there is no relationship between two variables. • Alternate hypothesis: Alternate hypothesis predicts the relationship between the two variables of the study. • Directional hypothesis: Directional hypothesis specifies the expected direction to be followed to determine the relationship between variables. • Non-directional hypothesis: Non-directional hypothesis does not predict the exact direction or nature of the relationship between the two variables. • Simple hypothesis: Simple hypothesis predicts the relationship between a single dependent variable and a single independent variable. • Complex hypothesis: Complex hypothesis predicts the relationship between two or more independent and dependent variables. • Associative and casual hypothesis: Associative and casual hypothesis predicts the relationship between two or more independent and dependent variables. • Empirical hypothesis: Empirical hypothesis can be tested via experiments and observation. • Statistical hypothesis: A statistical hypothesis utilizes statistical models to draw conclusions about broader populations.

Wow! You really simplified your explanation that even dummies would find it easy to comprehend. Thank you so much.
Thanks a lot for your valuable guidance.
I enjoy reading the post. Hypotheses are actually an intrinsic part in a study. It bridges the research question and the methodology of the study.
Useful piece!
This is awesome.Wow.
It very interesting to read the topic, can you guide me any specific example of hypothesis process establish throw the Demand and supply of the specific product in market
Nicely explained
It is really a useful for me Kindly give some examples of hypothesis
It was a well explained content ,can you please give me an example with the null and alternative hypothesis illustrated
clear and concise. thanks.
So Good so Amazing
Good to learn
Thanks a lot for explaining to my level of understanding
Explained well and in simple terms. Quick read! Thank you
Rate this article Cancel Reply
Your email address will not be published.
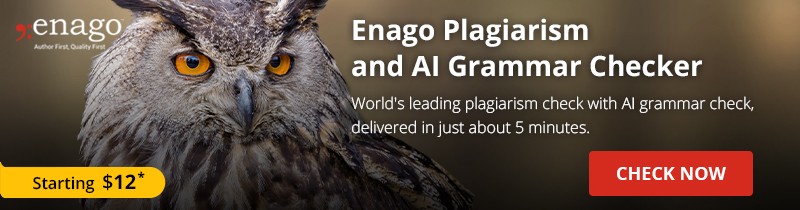
Enago Academy's Most Popular Articles
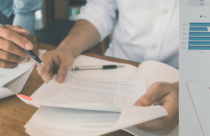
- Reporting Research
Choosing the Right Analytical Approach: Thematic analysis vs. content analysis for data interpretation
In research, choosing the right approach to understand data is crucial for deriving meaningful insights.…
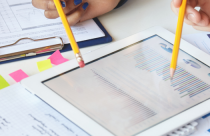
Comparing Cross Sectional and Longitudinal Studies: 5 steps for choosing the right approach
The process of choosing the right research design can put ourselves at the crossroads of…
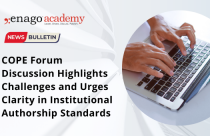
- Industry News
COPE Forum Discussion Highlights Challenges and Urges Clarity in Institutional Authorship Standards
The COPE forum discussion held in December 2023 initiated with a fundamental question — is…
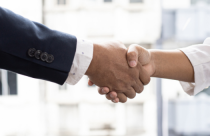
- Career Corner
Unlocking the Power of Networking in Academic Conferences
Embarking on your first academic conference experience? Fear not, we got you covered! Academic conferences…
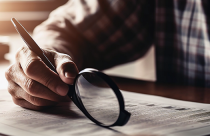
Research Recommendations – Guiding policy-makers for evidence-based decision making
Research recommendations play a crucial role in guiding scholars and researchers toward fruitful avenues of…
Choosing the Right Analytical Approach: Thematic analysis vs. content analysis for…
Comparing Cross Sectional and Longitudinal Studies: 5 steps for choosing the right…
How to Design Effective Research Questionnaires for Robust Findings

Sign-up to read more
Subscribe for free to get unrestricted access to all our resources on research writing and academic publishing including:
- 2000+ blog articles
- 50+ Webinars
- 10+ Expert podcasts
- 50+ Infographics
- 10+ Checklists
- Research Guides
We hate spam too. We promise to protect your privacy and never spam you.
I am looking for Editing/ Proofreading services for my manuscript Tentative date of next journal submission:
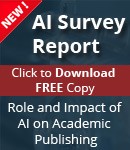
What should universities' stance be on AI tools in research and academic writing?

Want to create or adapt books like this? Learn more about how Pressbooks supports open publishing practices.
Developing a Hypothesis
Rajiv S. Jhangiani; I-Chant A. Chiang; Carrie Cuttler; and Dana C. Leighton
Learning Objectives
- Distinguish between a theory and a hypothesis.
- Discover how theories are used to generate hypotheses and how the results of studies can be used to further inform theories.
- Understand the characteristics of a good hypothesis.
Theories and Hypotheses
Before describing how to develop a hypothesis, it is important to distinguish between a theory and a hypothesis. A theory is a coherent explanation or interpretation of one or more phenomena. Although theories can take a variety of forms, one thing they have in common is that they go beyond the phenomena they explain by including variables, structures, processes, functions, or organizing principles that have not been observed directly. Consider, for example, Zajonc’s theory of social facilitation and social inhibition (1965) [1] . He proposed that being watched by others while performing a task creates a general state of physiological arousal, which increases the likelihood of the dominant (most likely) response. So for highly practiced tasks, being watched increases the tendency to make correct responses, but for relatively unpracticed tasks, being watched increases the tendency to make incorrect responses. Notice that this theory—which has come to be called drive theory—provides an explanation of both social facilitation and social inhibition that goes beyond the phenomena themselves by including concepts such as “arousal” and “dominant response,” along with processes such as the effect of arousal on the dominant response.
Outside of science, referring to an idea as a theory often implies that it is untested—perhaps no more than a wild guess. In science, however, the term theory has no such implication. A theory is simply an explanation or interpretation of a set of phenomena. It can be untested, but it can also be extensively tested, well supported, and accepted as an accurate description of the world by the scientific community. The theory of evolution by natural selection, for example, is a theory because it is an explanation of the diversity of life on earth—not because it is untested or unsupported by scientific research. On the contrary, the evidence for this theory is overwhelmingly positive and nearly all scientists accept its basic assumptions as accurate. Similarly, the “germ theory” of disease is a theory because it is an explanation of the origin of various diseases, not because there is any doubt that many diseases are caused by microorganisms that infect the body.
A hypothesis , on the other hand, is a specific prediction about a new phenomenon that should be observed if a particular theory is accurate. It is an explanation that relies on just a few key concepts. Hypotheses are often specific predictions about what will happen in a particular study. They are developed by considering existing evidence and using reasoning to infer what will happen in the specific context of interest. Hypotheses are often but not always derived from theories. So a hypothesis is often a prediction based on a theory but some hypotheses are a-theoretical and only after a set of observations have been made, is a theory developed. This is because theories are broad in nature and they explain larger bodies of data. So if our research question is really original then we may need to collect some data and make some observations before we can develop a broader theory.
Theories and hypotheses always have this if-then relationship. “ If drive theory is correct, then cockroaches should run through a straight runway faster, and a branching runway more slowly, when other cockroaches are present.” Although hypotheses are usually expressed as statements, they can always be rephrased as questions. “Do cockroaches run through a straight runway faster when other cockroaches are present?” Thus deriving hypotheses from theories is an excellent way of generating interesting research questions.
But how do researchers derive hypotheses from theories? One way is to generate a research question using the techniques discussed in this chapter and then ask whether any theory implies an answer to that question. For example, you might wonder whether expressive writing about positive experiences improves health as much as expressive writing about traumatic experiences. Although this question is an interesting one on its own, you might then ask whether the habituation theory—the idea that expressive writing causes people to habituate to negative thoughts and feelings—implies an answer. In this case, it seems clear that if the habituation theory is correct, then expressive writing about positive experiences should not be effective because it would not cause people to habituate to negative thoughts and feelings. A second way to derive hypotheses from theories is to focus on some component of the theory that has not yet been directly observed. For example, a researcher could focus on the process of habituation—perhaps hypothesizing that people should show fewer signs of emotional distress with each new writing session.
Among the very best hypotheses are those that distinguish between competing theories. For example, Norbert Schwarz and his colleagues considered two theories of how people make judgments about themselves, such as how assertive they are (Schwarz et al., 1991) [2] . Both theories held that such judgments are based on relevant examples that people bring to mind. However, one theory was that people base their judgments on the number of examples they bring to mind and the other was that people base their judgments on how easily they bring those examples to mind. To test these theories, the researchers asked people to recall either six times when they were assertive (which is easy for most people) or 12 times (which is difficult for most people). Then they asked them to judge their own assertiveness. Note that the number-of-examples theory implies that people who recalled 12 examples should judge themselves to be more assertive because they recalled more examples, but the ease-of-examples theory implies that participants who recalled six examples should judge themselves as more assertive because recalling the examples was easier. Thus the two theories made opposite predictions so that only one of the predictions could be confirmed. The surprising result was that participants who recalled fewer examples judged themselves to be more assertive—providing particularly convincing evidence in favor of the ease-of-retrieval theory over the number-of-examples theory.
Theory Testing
The primary way that scientific researchers use theories is sometimes called the hypothetico-deductive method (although this term is much more likely to be used by philosophers of science than by scientists themselves). Researchers begin with a set of phenomena and either construct a theory to explain or interpret them or choose an existing theory to work with. They then make a prediction about some new phenomenon that should be observed if the theory is correct. Again, this prediction is called a hypothesis. The researchers then conduct an empirical study to test the hypothesis. Finally, they reevaluate the theory in light of the new results and revise it if necessary. This process is usually conceptualized as a cycle because the researchers can then derive a new hypothesis from the revised theory, conduct a new empirical study to test the hypothesis, and so on. As Figure 2.3 shows, this approach meshes nicely with the model of scientific research in psychology presented earlier in the textbook—creating a more detailed model of “theoretically motivated” or “theory-driven” research.
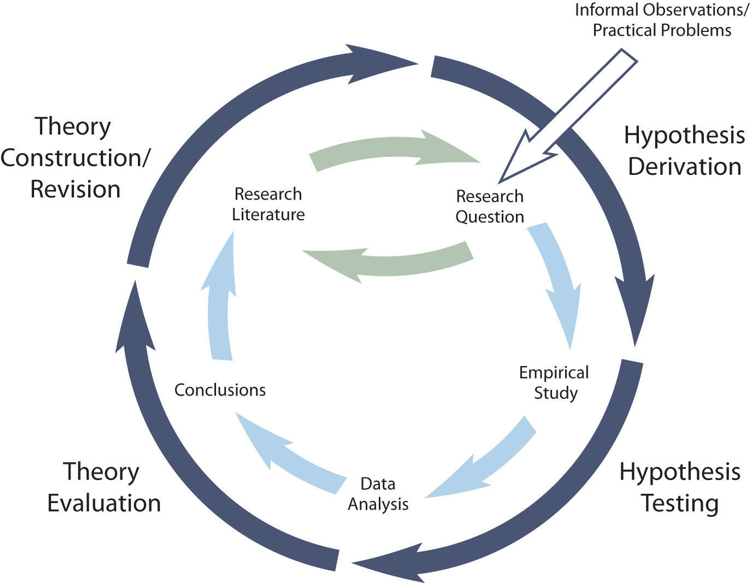
As an example, let us consider Zajonc’s research on social facilitation and inhibition. He started with a somewhat contradictory pattern of results from the research literature. He then constructed his drive theory, according to which being watched by others while performing a task causes physiological arousal, which increases an organism’s tendency to make the dominant response. This theory predicts social facilitation for well-learned tasks and social inhibition for poorly learned tasks. He now had a theory that organized previous results in a meaningful way—but he still needed to test it. He hypothesized that if his theory was correct, he should observe that the presence of others improves performance in a simple laboratory task but inhibits performance in a difficult version of the very same laboratory task. To test this hypothesis, one of the studies he conducted used cockroaches as subjects (Zajonc, Heingartner, & Herman, 1969) [3] . The cockroaches ran either down a straight runway (an easy task for a cockroach) or through a cross-shaped maze (a difficult task for a cockroach) to escape into a dark chamber when a light was shined on them. They did this either while alone or in the presence of other cockroaches in clear plastic “audience boxes.” Zajonc found that cockroaches in the straight runway reached their goal more quickly in the presence of other cockroaches, but cockroaches in the cross-shaped maze reached their goal more slowly when they were in the presence of other cockroaches. Thus he confirmed his hypothesis and provided support for his drive theory. (Zajonc also showed that drive theory existed in humans [Zajonc & Sales, 1966] [4] in many other studies afterward).
Incorporating Theory into Your Research
When you write your research report or plan your presentation, be aware that there are two basic ways that researchers usually include theory. The first is to raise a research question, answer that question by conducting a new study, and then offer one or more theories (usually more) to explain or interpret the results. This format works well for applied research questions and for research questions that existing theories do not address. The second way is to describe one or more existing theories, derive a hypothesis from one of those theories, test the hypothesis in a new study, and finally reevaluate the theory. This format works well when there is an existing theory that addresses the research question—especially if the resulting hypothesis is surprising or conflicts with a hypothesis derived from a different theory.
To use theories in your research will not only give you guidance in coming up with experiment ideas and possible projects, but it lends legitimacy to your work. Psychologists have been interested in a variety of human behaviors and have developed many theories along the way. Using established theories will help you break new ground as a researcher, not limit you from developing your own ideas.
Characteristics of a Good Hypothesis
There are three general characteristics of a good hypothesis. First, a good hypothesis must be testable and falsifiable . We must be able to test the hypothesis using the methods of science and if you’ll recall Popper’s falsifiability criterion, it must be possible to gather evidence that will disconfirm the hypothesis if it is indeed false. Second, a good hypothesis must be logical. As described above, hypotheses are more than just a random guess. Hypotheses should be informed by previous theories or observations and logical reasoning. Typically, we begin with a broad and general theory and use deductive reasoning to generate a more specific hypothesis to test based on that theory. Occasionally, however, when there is no theory to inform our hypothesis, we use inductive reasoning which involves using specific observations or research findings to form a more general hypothesis. Finally, the hypothesis should be positive. That is, the hypothesis should make a positive statement about the existence of a relationship or effect, rather than a statement that a relationship or effect does not exist. As scientists, we don’t set out to show that relationships do not exist or that effects do not occur so our hypotheses should not be worded in a way to suggest that an effect or relationship does not exist. The nature of science is to assume that something does not exist and then seek to find evidence to prove this wrong, to show that it really does exist. That may seem backward to you but that is the nature of the scientific method. The underlying reason for this is beyond the scope of this chapter but it has to do with statistical theory.
- Zajonc, R. B. (1965). Social facilitation. Science, 149 , 269–274 ↵
- Schwarz, N., Bless, H., Strack, F., Klumpp, G., Rittenauer-Schatka, H., & Simons, A. (1991). Ease of retrieval as information: Another look at the availability heuristic. Journal of Personality and Social Psychology, 61 , 195–202. ↵
- Zajonc, R. B., Heingartner, A., & Herman, E. M. (1969). Social enhancement and impairment of performance in the cockroach. Journal of Personality and Social Psychology, 13 , 83–92. ↵
- Zajonc, R.B. & Sales, S.M. (1966). Social facilitation of dominant and subordinate responses. Journal of Experimental Social Psychology, 2 , 160-168. ↵
A coherent explanation or interpretation of one or more phenomena.
A specific prediction about a new phenomenon that should be observed if a particular theory is accurate.
A cyclical process of theory development, starting with an observed phenomenon, then developing or using a theory to make a specific prediction of what should happen if that theory is correct, testing that prediction, refining the theory in light of the findings, and using that refined theory to develop new hypotheses, and so on.
The ability to test the hypothesis using the methods of science and the possibility to gather evidence that will disconfirm the hypothesis if it is indeed false.
Developing a Hypothesis Copyright © 2022 by Rajiv S. Jhangiani; I-Chant A. Chiang; Carrie Cuttler; and Dana C. Leighton is licensed under a Creative Commons Attribution-NonCommercial-ShareAlike 4.0 International License , except where otherwise noted.
Share This Book
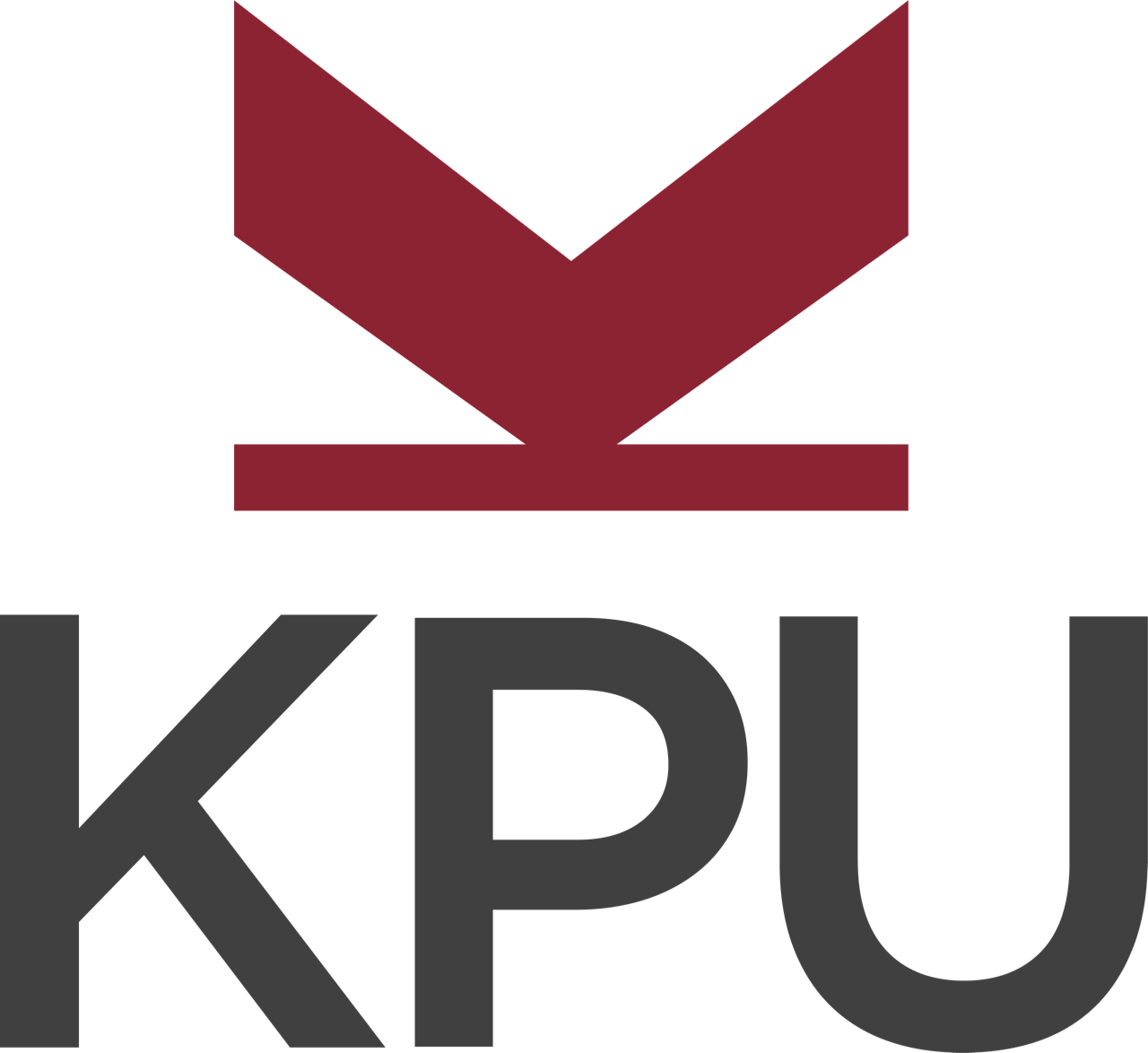
Want to create or adapt books like this? Learn more about how Pressbooks supports open publishing practices.
Overview of the Scientific Method
10 Developing a Hypothesis
Learning objectives.
- Distinguish between a theory and a hypothesis.
- Discover how theories are used to generate hypotheses and how the results of studies can be used to further inform theories.
- Understand the characteristics of a good hypothesis.
Theories and Hypotheses
Before describing how to develop a hypothesis, it is important to distinguish between a theory and a hypothesis. A theory is a coherent explanation or interpretation of one or more phenomena. Although theories can take a variety of forms, one thing they have in common is that they go beyond the phenomena they explain by including variables, structures, processes, functions, or organizing principles that have not been observed directly. Consider, for example, Zajonc’s theory of social facilitation and social inhibition (1965) [1] . He proposed that being watched by others while performing a task creates a general state of physiological arousal, which increases the likelihood of the dominant (most likely) response. So for highly practiced tasks, being watched increases the tendency to make correct responses, but for relatively unpracticed tasks, being watched increases the tendency to make incorrect responses. Notice that this theory—which has come to be called drive theory—provides an explanation of both social facilitation and social inhibition that goes beyond the phenomena themselves by including concepts such as “arousal” and “dominant response,” along with processes such as the effect of arousal on the dominant response.
Outside of science, referring to an idea as a theory often implies that it is untested—perhaps no more than a wild guess. In science, however, the term theory has no such implication. A theory is simply an explanation or interpretation of a set of phenomena. It can be untested, but it can also be extensively tested, well supported, and accepted as an accurate description of the world by the scientific community. The theory of evolution by natural selection, for example, is a theory because it is an explanation of the diversity of life on earth—not because it is untested or unsupported by scientific research. On the contrary, the evidence for this theory is overwhelmingly positive and nearly all scientists accept its basic assumptions as accurate. Similarly, the “germ theory” of disease is a theory because it is an explanation of the origin of various diseases, not because there is any doubt that many diseases are caused by microorganisms that infect the body.
A hypothesis , on the other hand, is a specific prediction about a new phenomenon that should be observed if a particular theory is accurate. It is an explanation that relies on just a few key concepts. Hypotheses are often specific predictions about what will happen in a particular study. They are developed by considering existing evidence and using reasoning to infer what will happen in the specific context of interest. Hypotheses are often but not always derived from theories. So a hypothesis is often a prediction based on a theory but some hypotheses are a-theoretical and only after a set of observations have been made, is a theory developed. This is because theories are broad in nature and they explain larger bodies of data. So if our research question is really original then we may need to collect some data and make some observations before we can develop a broader theory.
Theories and hypotheses always have this if-then relationship. “ If drive theory is correct, then cockroaches should run through a straight runway faster, and a branching runway more slowly, when other cockroaches are present.” Although hypotheses are usually expressed as statements, they can always be rephrased as questions. “Do cockroaches run through a straight runway faster when other cockroaches are present?” Thus deriving hypotheses from theories is an excellent way of generating interesting research questions.
But how do researchers derive hypotheses from theories? One way is to generate a research question using the techniques discussed in this chapter and then ask whether any theory implies an answer to that question. For example, you might wonder whether expressive writing about positive experiences improves health as much as expressive writing about traumatic experiences. Although this question is an interesting one on its own, you might then ask whether the habituation theory—the idea that expressive writing causes people to habituate to negative thoughts and feelings—implies an answer. In this case, it seems clear that if the habituation theory is correct, then expressive writing about positive experiences should not be effective because it would not cause people to habituate to negative thoughts and feelings. A second way to derive hypotheses from theories is to focus on some component of the theory that has not yet been directly observed. For example, a researcher could focus on the process of habituation—perhaps hypothesizing that people should show fewer signs of emotional distress with each new writing session.
Among the very best hypotheses are those that distinguish between competing theories. For example, Norbert Schwarz and his colleagues considered two theories of how people make judgments about themselves, such as how assertive they are (Schwarz et al., 1991) [2] . Both theories held that such judgments are based on relevant examples that people bring to mind. However, one theory was that people base their judgments on the number of examples they bring to mind and the other was that people base their judgments on how easily they bring those examples to mind. To test these theories, the researchers asked people to recall either six times when they were assertive (which is easy for most people) or 12 times (which is difficult for most people). Then they asked them to judge their own assertiveness. Note that the number-of-examples theory implies that people who recalled 12 examples should judge themselves to be more assertive because they recalled more examples, but the ease-of-examples theory implies that participants who recalled six examples should judge themselves as more assertive because recalling the examples was easier. Thus the two theories made opposite predictions so that only one of the predictions could be confirmed. The surprising result was that participants who recalled fewer examples judged themselves to be more assertive—providing particularly convincing evidence in favor of the ease-of-retrieval theory over the number-of-examples theory.
Theory Testing
The primary way that scientific researchers use theories is sometimes called the hypothetico-deductive method (although this term is much more likely to be used by philosophers of science than by scientists themselves). Researchers begin with a set of phenomena and either construct a theory to explain or interpret them or choose an existing theory to work with. They then make a prediction about some new phenomenon that should be observed if the theory is correct. Again, this prediction is called a hypothesis. The researchers then conduct an empirical study to test the hypothesis. Finally, they reevaluate the theory in light of the new results and revise it if necessary. This process is usually conceptualized as a cycle because the researchers can then derive a new hypothesis from the revised theory, conduct a new empirical study to test the hypothesis, and so on. As Figure 2.3 shows, this approach meshes nicely with the model of scientific research in psychology presented earlier in the textbook—creating a more detailed model of “theoretically motivated” or “theory-driven” research.
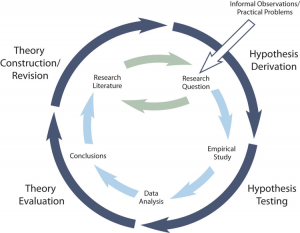
As an example, let us consider Zajonc’s research on social facilitation and inhibition. He started with a somewhat contradictory pattern of results from the research literature. He then constructed his drive theory, according to which being watched by others while performing a task causes physiological arousal, which increases an organism’s tendency to make the dominant response. This theory predicts social facilitation for well-learned tasks and social inhibition for poorly learned tasks. He now had a theory that organized previous results in a meaningful way—but he still needed to test it. He hypothesized that if his theory was correct, he should observe that the presence of others improves performance in a simple laboratory task but inhibits performance in a difficult version of the very same laboratory task. To test this hypothesis, one of the studies he conducted used cockroaches as subjects (Zajonc, Heingartner, & Herman, 1969) [3] . The cockroaches ran either down a straight runway (an easy task for a cockroach) or through a cross-shaped maze (a difficult task for a cockroach) to escape into a dark chamber when a light was shined on them. They did this either while alone or in the presence of other cockroaches in clear plastic “audience boxes.” Zajonc found that cockroaches in the straight runway reached their goal more quickly in the presence of other cockroaches, but cockroaches in the cross-shaped maze reached their goal more slowly when they were in the presence of other cockroaches. Thus he confirmed his hypothesis and provided support for his drive theory. (Zajonc also showed that drive theory existed in humans [Zajonc & Sales, 1966] [4] in many other studies afterward).
Incorporating Theory into Your Research
When you write your research report or plan your presentation, be aware that there are two basic ways that researchers usually include theory. The first is to raise a research question, answer that question by conducting a new study, and then offer one or more theories (usually more) to explain or interpret the results. This format works well for applied research questions and for research questions that existing theories do not address. The second way is to describe one or more existing theories, derive a hypothesis from one of those theories, test the hypothesis in a new study, and finally reevaluate the theory. This format works well when there is an existing theory that addresses the research question—especially if the resulting hypothesis is surprising or conflicts with a hypothesis derived from a different theory.
To use theories in your research will not only give you guidance in coming up with experiment ideas and possible projects, but it lends legitimacy to your work. Psychologists have been interested in a variety of human behaviors and have developed many theories along the way. Using established theories will help you break new ground as a researcher, not limit you from developing your own ideas.
Characteristics of a Good Hypothesis
There are three general characteristics of a good hypothesis. First, a good hypothesis must be testable and falsifiable . We must be able to test the hypothesis using the methods of science and if you’ll recall Popper’s falsifiability criterion, it must be possible to gather evidence that will disconfirm the hypothesis if it is indeed false. Second, a good hypothesis must be logical. As described above, hypotheses are more than just a random guess. Hypotheses should be informed by previous theories or observations and logical reasoning. Typically, we begin with a broad and general theory and use deductive reasoning to generate a more specific hypothesis to test based on that theory. Occasionally, however, when there is no theory to inform our hypothesis, we use inductive reasoning which involves using specific observations or research findings to form a more general hypothesis. Finally, the hypothesis should be positive. That is, the hypothesis should make a positive statement about the existence of a relationship or effect, rather than a statement that a relationship or effect does not exist. As scientists, we don’t set out to show that relationships do not exist or that effects do not occur so our hypotheses should not be worded in a way to suggest that an effect or relationship does not exist. The nature of science is to assume that something does not exist and then seek to find evidence to prove this wrong, to show that it really does exist. That may seem backward to you but that is the nature of the scientific method. The underlying reason for this is beyond the scope of this chapter but it has to do with statistical theory.
- Zajonc, R. B. (1965). Social facilitation. Science, 149 , 269–274 ↵
- Schwarz, N., Bless, H., Strack, F., Klumpp, G., Rittenauer-Schatka, H., & Simons, A. (1991). Ease of retrieval as information: Another look at the availability heuristic. Journal of Personality and Social Psychology, 61 , 195–202. ↵
- Zajonc, R. B., Heingartner, A., & Herman, E. M. (1969). Social enhancement and impairment of performance in the cockroach. Journal of Personality and Social Psychology, 13 , 83–92. ↵
- Zajonc, R.B. & Sales, S.M. (1966). Social facilitation of dominant and subordinate responses. Journal of Experimental Social Psychology, 2 , 160-168. ↵
A coherent explanation or interpretation of one or more phenomena.
A specific prediction about a new phenomenon that should be observed if a particular theory is accurate.
A cyclical process of theory development, starting with an observed phenomenon, then developing or using a theory to make a specific prediction of what should happen if that theory is correct, testing that prediction, refining the theory in light of the findings, and using that refined theory to develop new hypotheses, and so on.
The ability to test the hypothesis using the methods of science and the possibility to gather evidence that will disconfirm the hypothesis if it is indeed false.
Research Methods in Psychology Copyright © 2019 by Rajiv S. Jhangiani, I-Chant A. Chiang, Carrie Cuttler, & Dana C. Leighton is licensed under a Creative Commons Attribution-NonCommercial-ShareAlike 4.0 International License , except where otherwise noted.
Share This Book
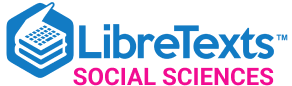
- school Campus Bookshelves
- menu_book Bookshelves
- perm_media Learning Objects
- login Login
- how_to_reg Request Instructor Account
- hub Instructor Commons
- Download Page (PDF)
- Download Full Book (PDF)
- Periodic Table
- Physics Constants
- Scientific Calculator
- Reference & Cite
- Tools expand_more
- Readability
selected template will load here
This action is not available.
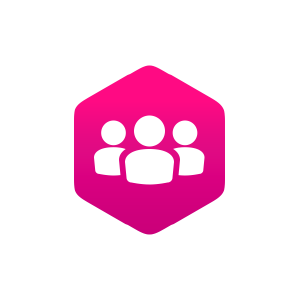
Developing Theories & Hypotheses
- Last updated
- Save as PDF
- Page ID 40843
2.5: Developing a Hypothesis
Learning objectives.
- Distinguish between a theory and a hypothesis.
- Discover how theories are used to generate hypotheses and how the results of studies can be used to further inform theories.
- Understand the characteristics of a good hypothesis.
Theories and Hypotheses
Before describing how to develop a hypothesis, it is important to distinguish between a theory and a hypothesis. A theory is a coherent explanation or interpretation of one or more phenomena. Although theories can take a variety of forms, one thing they have in common is that they go beyond the phenomena they explain by including variables, structures, processes, functions, or organizing principles that have not been observed directly. Consider, for example, Zajonc’s theory of social facilitation and social inhibition (1965) [1] . He proposed that being watched by others while performing a task creates a general state of physiological arousal, which increases the likelihood of the dominant (most likely) response. So for highly practiced tasks, being watched increases the tendency to make correct responses, but for relatively unpracticed tasks, being watched increases the tendency to make incorrect responses. Notice that this theory—which has come to be called drive theory—provides an explanation of both social facilitation and social inhibition that goes beyond the phenomena themselves by including concepts such as “arousal” and “dominant response,” along with processes such as the effect of arousal on the dominant response.
Outside of science, referring to an idea as a theory often implies that it is untested—perhaps no more than a wild guess. In science, however, the term theory has no such implication. A theory is simply an explanation or interpretation of a set of phenomena. It can be untested, but it can also be extensively tested, well supported, and accepted as an accurate description of the world by the scientific community. The theory of evolution by natural selection, for example, is a theory because it is an explanation of the diversity of life on earth—not because it is untested or unsupported by scientific research. On the contrary, the evidence for this theory is overwhelmingly positive and nearly all scientists accept its basic assumptions as accurate. Similarly, the “germ theory” of disease is a theory because it is an explanation of the origin of various diseases, not because there is any doubt that many diseases are caused by microorganisms that infect the body.
A hypothesis , on the other hand, is a specific prediction about a new phenomenon that should be observed if a particular theory is accurate. It is an explanation that relies on just a few key concepts. Hypotheses are often specific predictions about what will happen in a particular study. They are developed by considering existing evidence and using reasoning to infer what will happen in the specific context of interest. Hypotheses are often but not always derived from theories. So a hypothesis is often a prediction based on a theory but some hypotheses are a-theoretical and only after a set of observations have been made, is a theory developed. This is because theories are broad in nature and they explain larger bodies of data. So if our research question is really original then we may need to collect some data and make some observations before we can develop a broader theory.
Theories and hypotheses always have this if-then relationship. “ If drive theory is correct, then cockroaches should run through a straight runway faster, and a branching runway more slowly, when other cockroaches are present.” Although hypotheses are usually expressed as statements, they can always be rephrased as questions. “Do cockroaches run through a straight runway faster when other cockroaches are present?” Thus deriving hypotheses from theories is an excellent way of generating interesting research questions.
But how do researchers derive hypotheses from theories? One way is to generate a research question using the techniques discussed in this chapter and then ask whether any theory implies an answer to that question. For example, you might wonder whether expressive writing about positive experiences improves health as much as expressive writing about traumatic experiences. Although this question is an interesting one on its own, you might then ask whether the habituation theory—the idea that expressive writing causes people to habituate to negative thoughts and feelings—implies an answer. In this case, it seems clear that if the habituation theory is correct, then expressive writing about positive experiences should not be effective because it would not cause people to habituate to negative thoughts and feelings. A second way to derive hypotheses from theories is to focus on some component of the theory that has not yet been directly observed. For example, a researcher could focus on the process of habituation—perhaps hypothesizing that people should show fewer signs of emotional distress with each new writing session.
Among the very best hypotheses are those that distinguish between competing theories. For example, Norbert Schwarz and his colleagues considered two theories of how people make judgments about themselves, such as how assertive they are (Schwarz et al., 1991) [2] . Both theories held that such judgments are based on relevant examples that people bring to mind. However, one theory was that people base their judgments on the number of examples they bring to mind and the other was that people base their judgments on how easily they bring those examples to mind. To test these theories, the researchers asked people to recall either six times when they were assertive (which is easy for most people) or 12 times (which is difficult for most people). Then they asked them to judge their own assertiveness. Note that the number-of-examples theory implies that people who recalled 12 examples should judge themselves to be more assertive because they recalled more examples, but the ease-of-examples theory implies that participants who recalled six examples should judge themselves as more assertive because recalling the examples was easier. Thus the two theories made opposite predictions so that only one of the predictions could be confirmed. The surprising result was that participants who recalled fewer examples judged themselves to be more assertive—providing particularly convincing evidence in favor of the ease-of-retrieval theory over the number-of-examples theory.
Theory Testing
The primary way that scientific researchers use theories is sometimes called the hypothetico-deductive method (although this term is much more likely to be used by philosophers of science than by scientists themselves). Researchers begin with a set of phenomena and either construct a theory to explain or interpret them or choose an existing theory to work with. They then make a prediction about some new phenomenon that should be observed if the theory is correct. Again, this prediction is called a hypothesis. The researchers then conduct an empirical study to test the hypothesis. Finally, they reevaluate the theory in light of the new results and revise it if necessary. This process is usually conceptualized as a cycle because the researchers can then derive a new hypothesis from the revised theory, conduct a new empirical study to test the hypothesis, and so on. As Figure \(\PageIndex{1}\) shows, this approach meshes nicely with the model of scientific research in psychology presented earlier in the textbook—creating a more detailed model of “theoretically motivated” or “theory-driven” research.
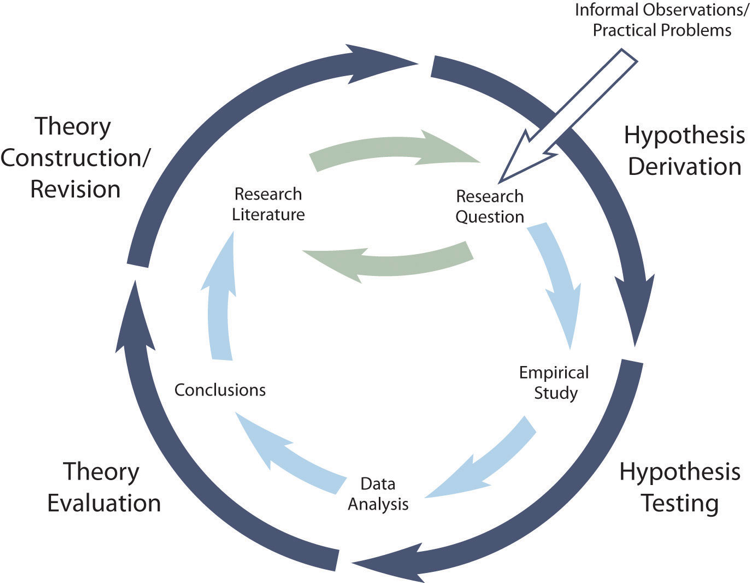
As an example, let us consider Zajonc’s research on social facilitation and inhibition. He started with a somewhat contradictory pattern of results from the research literature. He then constructed his drive theory, according to which being watched by others while performing a task causes physiological arousal, which increases an organism’s tendency to make the dominant response. This theory predicts social facilitation for well-learned tasks and social inhibition for poorly learned tasks. He now had a theory that organized previous results in a meaningful way—but he still needed to test it. He hypothesized that if his theory was correct, he should observe that the presence of others improves performance in a simple laboratory task but inhibits performance in a difficult version of the very same laboratory task. To test this hypothesis, one of the studies he conducted used cockroaches as subjects (Zajonc, Heingartner, & Herman, 1969) [3] . The cockroaches ran either down a straight runway (an easy task for a cockroach) or through a cross-shaped maze (a difficult task for a cockroach) to escape into a dark chamber when a light was shined on them. They did this either while alone or in the presence of other cockroaches in clear plastic “audience boxes.” Zajonc found that cockroaches in the straight runway reached their goal more quickly in the presence of other cockroaches, but cockroaches in the cross-shaped maze reached their goal more slowly when they were in the presence of other cockroaches. Thus he confirmed his hypothesis and provided support for his drive theory. (Zajonc also showed that drive theory existed in humans [Zajonc & Sales, 1966] [4] in many other studies afterward).
Incorporating Theory into Your Research
When you write your research report or plan your presentation, be aware that there are two basic ways that researchers usually include theory. The first is to raise a research question, answer that question by conducting a new study, and then offer one or more theories (usually more) to explain or interpret the results. This format works well for applied research questions and for research questions that existing theories do not address. The second way is to describe one or more existing theories, derive a hypothesis from one of those theories, test the hypothesis in a new study, and finally reevaluate the theory. This format works well when there is an existing theory that addresses the research question—especially if the resulting hypothesis is surprising or conflicts with a hypothesis derived from a different theory.
To use theories in your research will not only give you guidance in coming up with experiment ideas and possible projects, but it lends legitimacy to your work. Psychologists have been interested in a variety of human behaviors and have developed many theories along the way. Using established theories will help you break new ground as a researcher, not limit you from developing your own ideas.
There are three general characteristics of a good hypothesis. First, a good hypothesis must be testable and falsifiable . We must be able to test the hypothesis using the methods of science and if you’ll recall Popper’s falsifiability criterion, it must be possible to gather evidence that will disconfirm the hypothesis if it is indeed false. Second, a good hypothesis must be logical. As described above, hypotheses are more than just a random guess. Hypotheses should be informed by previous theories or observations and logical reasoning. Typically, we begin with a broad and general theory and use deductive reasoning to generate a more specific hypothesis to test based on that theory. Occasionally, however, when there is no theory to inform our hypothesis, we use inductive reasoning which involves using specific observations or research findings to form a more general hypothesis. Finally, the hypothesis should be positive. That is, the hypothesis should make a positive statement about the existence of a relationship or effect, rather than a statement that a relationship or effect does not exist. As scientists, we don’t set out to show that relationships do not exist or that effects do not occur so our hypotheses should not be worded in a way to suggest that an effect or relationship does not exist. The nature of science is to assume that something does not exist and then seek to find evidence to prove this wrong, to show that it really does exist. That may seem backward to you but that is the nature of the scientific method. The underlying reason for this is beyond the scope of this chapter but it has to do with statistical theory.
- Zajonc, R. B. (1965). Social facilitation. Science, 149 , 269–274 ↵
- Schwarz, N., Bless, H., Strack, F., Klumpp, G., Rittenauer-Schatka, H., & Simons, A. (1991). Ease of retrieval as information: Another look at the availability heuristic. Journal of Personality and Social Psychology, 61 , 195–202. ↵
- Zajonc, R. B., Heingartner, A., & Herman, E. M. (1969). Social enhancement and impairment of performance in the cockroach. Journal of Personality and Social Psychology, 13 , 83–92. ↵
- Zajonc, R.B. & Sales, S.M. (1966). Social facilitation of dominant and subordinate responses. Journal of Experimental Social Psychology, 2 , 160-168. ↵
Reviewing the Literature, Developing a Hypothesis, Study Design
- First Online: 30 November 2016
Cite this chapter
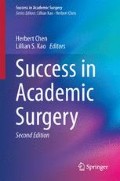
- Rosalie Carr M.D. 5 &
- C. Max Schmidt M.D., Ph.D., M.B.A., F.A.C.S. 5
Part of the book series: Success in Academic Surgery ((SIAS))
924 Accesses
Rigorous research investigation requires a thorough review of the literature on the topic of interest. This promotes development of an original, relevant and feasible hypothesis. Design of an optimal study to test the hypothesis then requires adequate power, freedom from bias, and conduct within a reasonable timeframe with resources available to the investigator.
This is a preview of subscription content, log in via an institution to check access.
Access this chapter
- Available as PDF
- Read on any device
- Instant download
- Own it forever
- Available as EPUB and PDF
- Compact, lightweight edition
- Dispatched in 3 to 5 business days
- Free shipping worldwide - see info
Tax calculation will be finalised at checkout
Purchases are for personal use only
Institutional subscriptions
Suggested Additional Reading
Friedman LM, et al. Fundamentals of clinical trials. 4th ed. 2010.
Google Scholar
Hulley SB. Designing clinical research. 4th ed. 2013.
Penson, Wei. Clinical research methods for surgeons. 2006.
Piantadosi S. Clinical trials: a methodological perspective. 2nd ed. 2005.
Download references
Acknowledgements
Carl Schmidt, MD, MSCI
Assistant Professor Surgery
The Ohio State University
Financial Support
Indiana Genomics Initiative (INGEN) of Indiana University. INGEN is supported in part by Lilly Endowment Inc. (CMS)
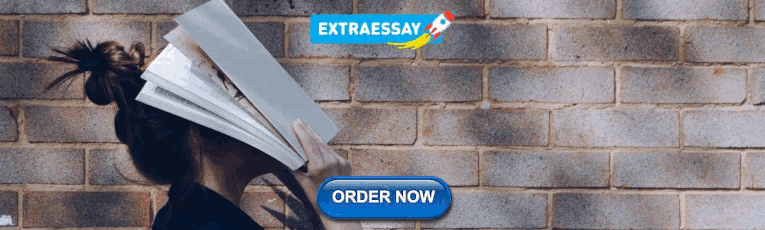
Author information
Authors and affiliations.
Department of Surgery, Biochemistry and Molecular Biology, Indiana University School of Medicine, 980 W Walnut St C522, Indianapolis, IN, 46202, USA
Rosalie Carr M.D. & C. Max Schmidt M.D., Ph.D., M.B.A., F.A.C.S.
You can also search for this author in PubMed Google Scholar
Editor information
Editors and affiliations.
Department of Surgery, University of Alabama at Birmingham, Birmingham, Alabama, USA
Herbert Chen
Department of Surgery, The University of Texas Health Science Centre, Houston, Texas, USA
Lillian S. Kao
Rights and permissions
Reprints and permissions
Copyright information
© 2017 Springer International Publishing Switzerland
About this chapter
Carr, R., Schmidt, C.M. (2017). Reviewing the Literature, Developing a Hypothesis, Study Design. In: Chen, H., Kao, L. (eds) Success in Academic Surgery. Success in Academic Surgery. Springer, Cham. https://doi.org/10.1007/978-3-319-43952-5_3
Download citation
DOI : https://doi.org/10.1007/978-3-319-43952-5_3
Published : 30 November 2016
Publisher Name : Springer, Cham
Print ISBN : 978-3-319-43951-8
Online ISBN : 978-3-319-43952-5
eBook Packages : Medicine Medicine (R0)
Share this chapter
Anyone you share the following link with will be able to read this content:
Sorry, a shareable link is not currently available for this article.
Provided by the Springer Nature SharedIt content-sharing initiative
- Publish with us
Policies and ethics
- Find a journal
- Track your research
- Work together
- Product development
- Ways of working
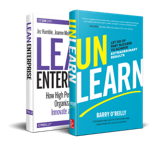
Have you read my two bestsellers, Unlearn and Lean Enterprise? If not, please do. If you have, please write a review!
- Read my story
- Get in touch
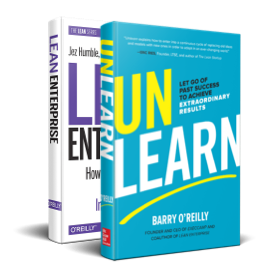
- Oval Copy 2 Blog
How to Implement Hypothesis-Driven Development
- Facebook__x28_alt_x29_ Copy
Remember back to the time when we were in high school science class. Our teachers had a framework for helping us learn – an experimental approach based on the best available evidence at hand. We were asked to make observations about the world around us, then attempt to form an explanation or hypothesis to explain what we had observed. We then tested this hypothesis by predicting an outcome based on our theory that would be achieved in a controlled experiment – if the outcome was achieved, we had proven our theory to be correct.
We could then apply this learning to inform and test other hypotheses by constructing more sophisticated experiments, and tuning, evolving, or abandoning any hypothesis as we made further observations from the results we achieved.
Experimentation is the foundation of the scientific method, which is a systematic means of exploring the world around us. Although some experiments take place in laboratories, it is possible to perform an experiment anywhere, at any time, even in software development.
Practicing Hypothesis-Driven Development [1] is thinking about the development of new ideas, products, and services – even organizational change – as a series of experiments to determine whether an expected outcome will be achieved. The process is iterated upon until a desirable outcome is obtained or the idea is determined to be not viable.
We need to change our mindset to view our proposed solution to a problem statement as a hypothesis, especially in new product or service development – the market we are targeting, how a business model will work, how code will execute and even how the customer will use it.
We do not do projects anymore, only experiments. Customer discovery and Lean Startup strategies are designed to test assumptions about customers. Quality Assurance is testing system behavior against defined specifications. The experimental principle also applies in Test-Driven Development – we write the test first, then use the test to validate that our code is correct, and succeed if the code passes the test. Ultimately, product or service development is a process to test a hypothesis about system behavior in the environment or market it is developed for.
The key outcome of an experimental approach is measurable evidence and learning. Learning is the information we have gained from conducting the experiment. Did what we expect to occur actually happen? If not, what did and how does that inform what we should do next?
In order to learn we need to use the scientific method for investigating phenomena, acquiring new knowledge, and correcting and integrating previous knowledge back into our thinking.
As the software development industry continues to mature, we now have an opportunity to leverage improved capabilities such as Continuous Design and Delivery to maximize our potential to learn quickly what works and what does not. By taking an experimental approach to information discovery, we can more rapidly test our solutions against the problems we have identified in the products or services we are attempting to build. With the goal to optimize our effectiveness of solving the right problems, over simply becoming a feature factory by continually building solutions.
The steps of the scientific method are to:
- Make observations
- Formulate a hypothesis
- Design an experiment to test the hypothesis
- State the indicators to evaluate if the experiment has succeeded
- Conduct the experiment
- Evaluate the results of the experiment
- Accept or reject the hypothesis
- If necessary, make and test a new hypothesis
Using an experimentation approach to software development
We need to challenge the concept of having fixed requirements for a product or service. Requirements are valuable when teams execute a well known or understood phase of an initiative and can leverage well-understood practices to achieve the outcome. However, when you are in an exploratory, complex and uncertain phase you need hypotheses. Handing teams a set of business requirements reinforces an order-taking approach and mindset that is flawed. Business does the thinking and ‘knows’ what is right. The purpose of the development team is to implement what they are told. But when operating in an area of uncertainty and complexity, all the members of the development team should be encouraged to think and share insights on the problem and potential solutions. A team simply taking orders from a business owner is not utilizing the full potential, experience and competency that a cross-functional multi-disciplined team offers.
Framing Hypotheses
The traditional user story framework is focused on capturing requirements for what we want to build and for whom, to enable the user to receive a specific benefit from the system.
As A…. <role>
I Want… <goal/desire>
So That… <receive benefit>
Behaviour Driven Development (BDD) and Feature Injection aims to improve the original framework by supporting communication and collaboration between developers, tester and non-technical participants in a software project.
In Order To… <receive benefit>
As A… <role>
When viewing work as an experiment, the traditional story framework is insufficient. As in our high school science experiment, we need to define the steps we will take to achieve the desired outcome. We then need to state the specific indicators (or signals) we expect to observe that provide evidence that our hypothesis is valid. These need to be stated before conducting the test to reduce the bias of interpretation of results.
If we observe signals that indicate our hypothesis is correct, we can be more confident that we are on the right path and can alter the user story framework to reflect this.
Therefore, a user story structure to support Hypothesis-Driven Development would be;
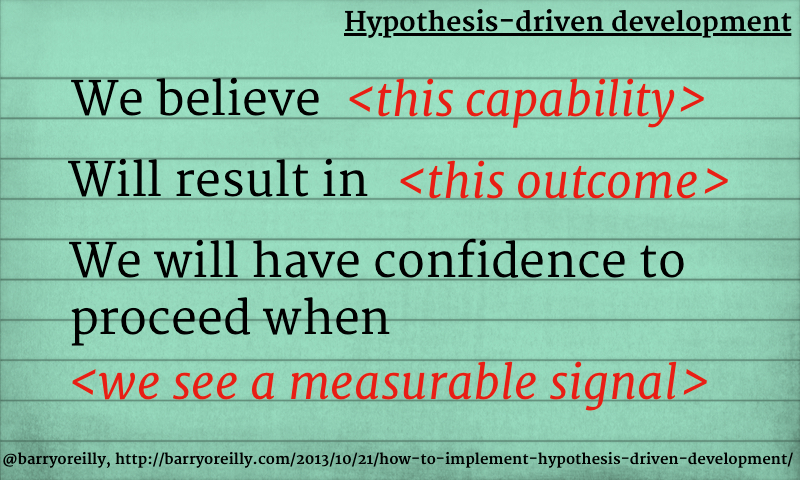
We believe < this capability >
What functionality we will develop to test our hypothesis? By defining a ‘test’ capability of the product or service that we are attempting to build, we identify the functionality and hypothesis we want to test.
Will result in < this outcome >
What is the expected outcome of our experiment? What is the specific result we expect to achieve by building the ‘test’ capability?
We will have confidence to proceed when < we see a measurable signal >
What signals will indicate that the capability we have built is effective? What key metrics (qualitative or quantitative) we will measure to provide evidence that our experiment has succeeded and give us enough confidence to move to the next stage.
The threshold you use for statistical significance will depend on your understanding of the business and context you are operating within. Not every company has the user sample size of Amazon or Google to run statistically significant experiments in a short period of time. Limits and controls need to be defined by your organization to determine acceptable evidence thresholds that will allow the team to advance to the next step.
For example, if you are building a rocket ship you may want your experiments to have a high threshold for statistical significance. If you are deciding between two different flows intended to help increase user sign up you may be happy to tolerate a lower significance threshold.
The final step is to clearly and visibly state any assumptions made about our hypothesis, to create a feedback loop for the team to provide further input, debate, and understanding of the circumstance under which we are performing the test. Are they valid and make sense from a technical and business perspective?
Hypotheses, when aligned to your MVP, can provide a testing mechanism for your product or service vision. They can test the most uncertain areas of your product or service, in order to gain information and improve confidence.
Examples of Hypothesis-Driven Development user stories are;
Business story.
We Believe That increasing the size of hotel images on the booking page Will Result In improved customer engagement and conversion We Will Have Confidence To Proceed When we see a 5% increase in customers who review hotel images who then proceed to book in 48 hours.
It is imperative to have effective monitoring and evaluation tools in place when using an experimental approach to software development in order to measure the impact of our efforts and provide a feedback loop to the team. Otherwise, we are essentially blind to the outcomes of our efforts.
In agile software development, we define working software as the primary measure of progress. By combining Continuous Delivery and Hypothesis-Driven Development we can now define working software and validated learning as the primary measures of progress.
Ideally, we should not say we are done until we have measured the value of what is being delivered – in other words, gathered data to validate our hypothesis.
Examples of how to gather data is performing A/B Testing to test a hypothesis and measure to change in customer behavior. Alternative testings options can be customer surveys, paper prototypes, user and/or guerilla testing.
One example of a company we have worked with that uses Hypothesis-Driven Development is lastminute.com . The team formulated a hypothesis that customers are only willing to pay a max price for a hotel based on the time of day they book. Tom Klein, CEO and President of Sabre Holdings shared the story of how they improved conversion by 400% within a week.
Combining practices such as Hypothesis-Driven Development and Continuous Delivery accelerates experimentation and amplifies validated learning. This gives us the opportunity to accelerate the rate at which we innovate while relentlessly reducing costs, leaving our competitors in the dust. Ideally, we can achieve the ideal of one-piece flow: atomic changes that enable us to identify causal relationships between the changes we make to our products and services, and their impact on key metrics.
As Kent Beck said, “Test-Driven Development is a great excuse to think about the problem before you think about the solution”. Hypothesis-Driven Development is a great opportunity to test what you think the problem is before you work on the solution.
We also run a workshop to help teams implement Hypothesis-Driven Development . Get in touch to run it at your company.
[1] Hypothesis-Driven Development By Jeffrey L. Taylor
More strategy insights
Say hello to venture capital 3.0, negotiation made simple with dr john lowry, how high performance organizations innovate at scale, read my newsletter.
Insights in every edition. News you can use. No spam, ever. Read the latest edition
We've just sent you your first email. Go check it out!
- Explore Insights
- Nobody Studios
- LinkedIn Learning: High Performance Organizations
- Bipolar Disorder
- Therapy Center
- When To See a Therapist
- Types of Therapy
- Best Online Therapy
- Best Couples Therapy
- Best Family Therapy
- Managing Stress
- Sleep and Dreaming
- Understanding Emotions
- Self-Improvement
- Healthy Relationships
- Student Resources
- Personality Types
- Guided Meditations
- Verywell Mind Insights
- 2023 Verywell Mind 25
- Mental Health in the Classroom
- Editorial Process
- Meet Our Review Board
- Crisis Support
7 Main Developmental Theories
Child Development Theories of Freud, Erickson, and More
Kendra Cherry, MS, is a psychosocial rehabilitation specialist, psychology educator, and author of the "Everything Psychology Book."
:max_bytes(150000):strip_icc():format(webp)/IMG_9791-89504ab694d54b66bbd72cb84ffb860e.jpg)
Amy Morin, LCSW, is a psychotherapist and international bestselling author. Her books, including "13 Things Mentally Strong People Don't Do," have been translated into more than 40 languages. Her TEDx talk, "The Secret of Becoming Mentally Strong," is one of the most viewed talks of all time.
:max_bytes(150000):strip_icc():format(webp)/VW-MIND-Amy-2b338105f1ee493f94d7e333e410fa76.jpg)
Verywell / JR Bee
- Top Theories
Child development theories focus on explaining how children change and grow over the course of childhood. These developmental theories center on various aspects of growth, including social, emotional, and cognitive development.
The study of human development is a rich and varied subject. We all have personal experience with development, but it is sometimes difficult to understand how and why people grow, learn, and act as they do.
Why do children behave in certain ways? Is their behavior related to their age, family relationships, or individual temperaments? Developmental psychologists strive to answer such questions as well as to understand, explain, and predict behaviors that occur throughout the lifespan.
In order to understand human development, a number of different theories of child development have arisen to explain various aspects of human growth.
History of Developmental Theories
Child development that occurs from birth to adulthood was largely ignored throughout much of human history. Children were often viewed simply as small versions of adults and little attention was paid to the many advances in cognitive abilities, language usage, and physical growth that occur during childhood and adolescence.
Interest in the field of child development finally began to emerge early in the 20th century, but it tended to focus on abnormal behavior. Eventually, researchers became increasingly interested in other topics including typical child development as well as the influences on development.
More recent theories outline the developmental stages of children and identify the typical ages at which these growth milestones occur.
Why Developmental Theories are Important
Developmental theories provide a framework for thinking about human growth and learning. But why do we study development? What can we learn from psychological theories of development? If you have ever wondered about what motivates human thought and behavior, understanding these theories can provide useful insight into individuals and society.
An understanding of child development is essential because it allows us to fully appreciate the cognitive, emotional, physical, social, and educational growth that children go through from birth and into early adulthood.
Why is it important to study how children grow, learn, and change? An understanding of child development is essential because it allows us to fully appreciate the cognitive, emotional, physical, social, and educational growth that children go through from birth and into early adulthood.
7 Best-Known Developmental Theories
There are many child development theories that have been proposed by theorists and researchers. Some of the major theories of child development are known as grand theories; they attempt to describe every aspect of development, often using a stage approach. Others are known as mini-theories; they instead focus only on a fairly limited aspect of development such as cognitive or social growth.
Freud's Psychosexual Developmental Theory
Psychoanalytic theory originated with the work of Sigmund Freud . Through his clinical work with patients suffering from mental illness, Freud came to believe that childhood experiences and unconscious desires influenced behavior.
According to Freud, conflicts that occur during each of these stages can have a lifelong influence on personality and behavior. Freud proposed one of the best-known grand theories of child development.
According to Freud’s psychosexual theory, child development occurs in a series of stages focused on different pleasure areas of the body. During each stage, the child encounters conflicts that play a significant role in the course of development.
His theory suggested that the energy of the libido was focused on different erogenous zones at specific stages. Failure to progress through a stage can result in fixation at that point in development, which Freud believed could have an influence on adult behavior.
So what happens as children complete each stage? And what might result if a child does poorly during a particular point in development? Successfully completing each stage leads to the development of a healthy adult personality.
Failing to resolve the conflicts of a particular stage can result in fixations that can then have an influence on adult behavior.
While some other child development theories suggest that personality continues to change and grow over the entire lifetime, Freud believed that it was early experiences that played the greatest role in shaping development. According to Freud, personality is largely set in stone by the age of five.
Erikson's Psychosocial Developmental Theory
Psychoanalytic theory was an enormously influential force during the first half of the twentieth century. Those inspired and influenced by Freud went on to expand upon Freud's ideas and develop theories of their own. Of these neo-Freudians, Erik Erikson's ideas have become perhaps the best known.
Erikson's eight-stage theory of psychosocial development describes growth and change throughout life, focusing on social interaction and conflicts that arise during different stages of development.
While Erikson’s theory of psychosocial development shared some similarities with Freud's, it is dramatically different in many ways. Rather than focusing on sexual interest as a driving force in development, Erikson believed that social interaction and experience played decisive roles.
His eight-stage theory of human development described this process from infancy through death. During each stage, people are faced with a developmental conflict that impacts later functioning and further growth.
Unlike many other developmental theories, Erik Erikson's psychosocial theory focuses on development across the entire lifespan. At each stage, children and adults face a developmental crisis that serves as a major turning point.
Successfully managing the challenges of each stage leads to the emergence of a lifelong psychological virtue.
Behavioral Child Development Theories
During the first half of the twentieth century, a new school of thought known as behaviorism rose to become a dominant force within psychology. Behaviorists believed that psychology needed to focus only on observable and quantifiable behaviors in order to become a more scientific discipline.
According to the behavioral perspective, all human behavior can be described in terms of environmental influences. Some behaviorists, such as John B. Watson and B.F. Skinner , insisted that learning occurs purely through processes of association and reinforcement.
Behavioral theories of child development focus on how environmental interaction influences behavior and is based on the theories of theorists such as John B. Watson, Ivan Pavlov, and B. F. Skinner. These theories deal only with observable behaviors. Development is considered a reaction to rewards, punishments, stimuli, and reinforcement.
This theory differs considerably from other child development theories because it gives no consideration to internal thoughts or feelings. Instead, it focuses purely on how experience shapes who we are.
Two important types of learning that emerged from this approach to development are classical conditioning and operant conditioning . Classical conditioning involves learning by pairing a naturally occurring stimulus with a previously neutral stimulus. Operant conditioning utilizes reinforcement and punishment to modify behaviors.
Piaget's Cognitive Developmental Theory
Cognitive theory is concerned with the development of a person's thought processes. It also looks at how these thought processes influence how we understand and interact with the world.
Theorist Jean Piaget proposed one of the most influential theories of cognitive development.
Piaget proposed an idea that seems obvious now, but helped revolutionize how we think about child development: Children think differently than adults .
His cognitive theory seeks to describe and explain the development of thought processes and mental states. It also looks at how these thought processes influence the way we understand and interact with the world.
Piaget then proposed a theory of cognitive development to account for the steps and sequence of children's intellectual development.
- Sensorimotor Stage: A period of time between birth and age two during which an infant's knowledge of the world is limited to his or her sensory perceptions and motor activities. Behaviors are limited to simple motor responses caused by sensory stimuli.
- Pre-Operational Stage: A period between ages 2 and 6 during which a child learns to use language. During this stage, children do not yet understand concrete logic, cannot mentally manipulate information, and are unable to take the point of view of other people.
- Concrete Operational Stage: A period between ages 7 and 11 during which children gain a better understanding of mental operations. Children begin thinking logically about concrete events but have difficulty understanding abstract or hypothetical concepts.
- Formal Operational Stage: A period between age 12 to adulthood when people develop the ability to think about abstract concepts. Skills such as logical thought, deductive reasoning, and systematic planning also emerge during this stage.
Bowlby's Attachment Theory
There is a great deal of research on the social development of children. John Bowbly proposed one of the earliest theories of social development. Bowlby believed that early relationships with caregivers play a major role in child development and continue to influence social relationships throughout life.
Bowlby's attachment theory suggested that children are born with an innate need to form attachments. Such attachments aid in survival by ensuring that the child receives care and protection. Not only that but these attachments are characterized by clear behavioral and motivational patterns.
In other words, both children and caregivers engage in behaviors designed to ensure proximity. Children strive to stay close and connected to their caregivers who in turn provide a safe haven and a secure base for exploration.
Researchers have also expanded upon Bowlby's original work and have suggested that a number of different attachment styles exist. Children who receive consistent support and care are more likely to develop a secure attachment style, while those who receive less reliable care may develop an ambivalent, avoidant, or disorganized style.
Bandura's Social Learning Theory
Social learning theory is based on the work of psychologist Albert Bandura . Bandura believed that the conditioning and reinforcement process could not sufficiently explain all of human learning.
For example, how can the conditioning process account for learned behaviors that have not been reinforced through classical conditioning or operant conditioning According to social learning theory, behaviors can also be learned through observation and modeling.
By observing the actions of others, including parents and peers, children develop new skills and acquire new information.
Bandura's child development theory suggests that observation plays a critical role in learning, but this observation does not necessarily need to take the form of watching a live model.
Instead, people can also learn by listening to verbal instructions about how to perform a behavior as well as through observing either real or fictional characters displaying behaviors in books or films.
Vygotsky's Sociocultural Theory
Another psychologist named Lev Vygotsky proposed a seminal learning theory that has gone on to become very influential, especially in the field of education. Like Piaget, Vygotsky believed that children learn actively and through hands-on experiences.
His sociocultural theory also suggested that parents, caregivers, peers, and the culture at large were responsible for developing higher-order functions. In Vygotsky's view, learning is an inherently social process. Through interacting with others, learning becomes integrated into an individual's understanding of the world.
This child development theory also introduced the concept of the zone of proximal development, which is the gap between what a person can do with help and what they can do on their own. It is with the help of more knowledgeable others that people are able to progressively learn and increase their skills and scope of understanding.
A Word From Verywell
As you can see, some of psychology's best-known thinkers have developed theories to help explore and explain different aspects of child development. While not all of these theories are fully accepted today, they all had an important influence on our understanding of child development.
Today, contemporary psychologists often draw on a variety of theories and perspectives in order to understand how kids grow, behave, and think. These theories represent just a few of the different ways of thinking about child development.
In reality, fully understanding how children change and grow over the course of childhood requires looking at many different factors that influence physical and psychological growth. Genes, the environment, and the interactions between these two forces determine how kids grow physically as well as mentally.
Bellman M, Byrne O, Sege R. Developmental assessment of children . BMJ. 2013;346:e8687. doi:10.1136/bmj.e8687
Marwaha S, Goswami M, Vashist B. Prevalence of Principles of Piaget's Theory Among 4-7-year-old Children and their Correlation with IQ . J Clin Diagn Res. 2017;11(8):ZC111-ZC115. doi:10.7860/JCDR/2017/28435.10513
Barnes GL, Woolgar M, Beckwith H, Duschinsky R. John Bowlby and contemporary issues of clinical diagnosis . Attachment (Lond). 2018;12(1):35-47.
Fryling MJ, Johnston C, Hayes LJ. Understanding observational learning: an interbehavioral approach . Anal Verbal Behav. 2011;27(1):191-203.
Esteban-guitart M. The biosocial foundation of the early Vygotsky: Educational psychology before the zone of proximal development . Hist Psychol. 2018;21(4):384-401. doi:10.1037/hop0000092
Berk, LE. Child Development. 8th ed. USA: Pearson Education, Inc; 2009.
Shute, RH & Slee, PT. Child Development Theories and Critical Perspectives, Second Edition. New York: Routledge; 2015.
By Kendra Cherry, MSEd Kendra Cherry, MS, is a psychosocial rehabilitation specialist, psychology educator, and author of the "Everything Psychology Book."
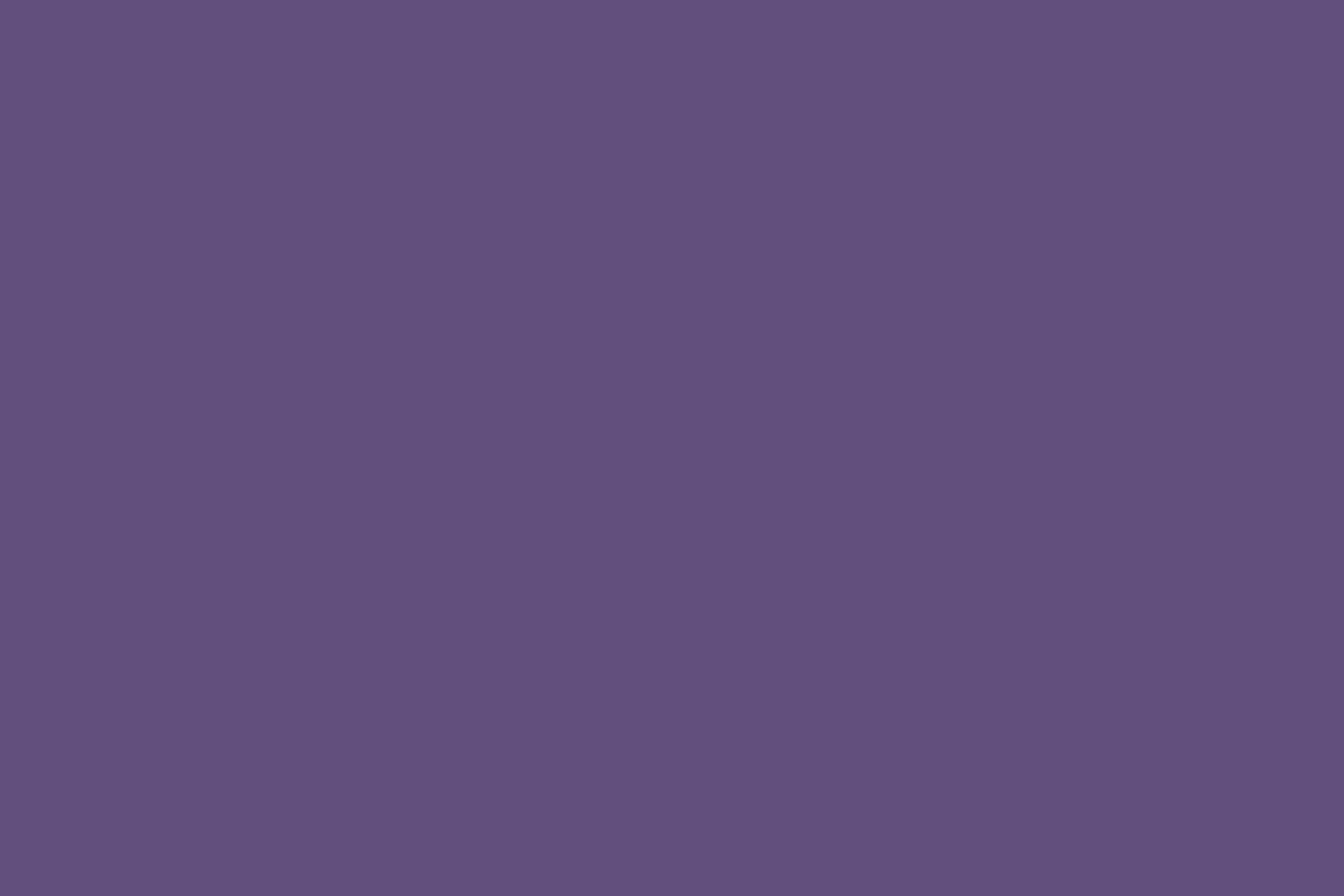
How to Implement Hypothesis-Driven Development
Remember back to the time when we were in high school science class. Our teachers had a framework for helping us learn – an experimental approach based on the best available evidence at hand. We were asked to make observations about the world around us, then attempt to form an explanation or hypothesis to explain what we had observed. We then tested this hypothesis by predicting an outcome based on our theory that would be achieved in a controlled experiment – if the outcome was achieved, we had proven our theory to be correct.
We could then apply this learning to inform and test other hypotheses by constructing more sophisticated experiments, and tuning, evolving or abandoning any hypothesis as we made further observations from the results we achieved.
Experimentation is the foundation of the scientific method, which is a systematic means of exploring the world around us. Although some experiments take place in laboratories, it is possible to perform an experiment anywhere, at any time, even in software development.
Practicing Hypothesis-Driven Development is thinking about the development of new ideas, products and services – even organizational change – as a series of experiments to determine whether an expected outcome will be achieved. The process is iterated upon until a desirable outcome is obtained or the idea is determined to be not viable.
We need to change our mindset to view our proposed solution to a problem statement as a hypothesis, especially in new product or service development – the market we are targeting, how a business model will work, how code will execute and even how the customer will use it.
We do not do projects anymore, only experiments. Customer discovery and Lean Startup strategies are designed to test assumptions about customers. Quality Assurance is testing system behavior against defined specifications. The experimental principle also applies in Test-Driven Development – we write the test first, then use the test to validate that our code is correct, and succeed if the code passes the test. Ultimately, product or service development is a process to test a hypothesis about system behaviour in the environment or market it is developed for.
The key outcome of an experimental approach is measurable evidence and learning.
Learning is the information we have gained from conducting the experiment. Did what we expect to occur actually happen? If not, what did and how does that inform what we should do next?
In order to learn we need use the scientific method for investigating phenomena, acquiring new knowledge, and correcting and integrating previous knowledge back into our thinking.
As the software development industry continues to mature, we now have an opportunity to leverage improved capabilities such as Continuous Design and Delivery to maximize our potential to learn quickly what works and what does not. By taking an experimental approach to information discovery, we can more rapidly test our solutions against the problems we have identified in the products or services we are attempting to build. With the goal to optimize our effectiveness of solving the right problems, over simply becoming a feature factory by continually building solutions.
The steps of the scientific method are to:
- Make observations
- Formulate a hypothesis
- Design an experiment to test the hypothesis
- State the indicators to evaluate if the experiment has succeeded
- Conduct the experiment
- Evaluate the results of the experiment
- Accept or reject the hypothesis
- If necessary, make and test a new hypothesis
Using an experimentation approach to software development
We need to challenge the concept of having fixed requirements for a product or service. Requirements are valuable when teams execute a well known or understood phase of an initiative, and can leverage well understood practices to achieve the outcome. However, when you are in an exploratory, complex and uncertain phase you need hypotheses.
Handing teams a set of business requirements reinforces an order-taking approach and mindset that is flawed.
Business does the thinking and ‘knows’ what is right. The purpose of the development team is to implement what they are told. But when operating in an area of uncertainty and complexity, all the members of the development team should be encouraged to think and share insights on the problem and potential solutions. A team simply taking orders from a business owner is not utilizing the full potential, experience and competency that a cross-functional multi-disciplined team offers.
Framing hypotheses
The traditional user story framework is focused on capturing requirements for what we want to build and for whom, to enable the user to receive a specific benefit from the system.
As A…. <role>
I Want… <goal/desire>
So That… <receive benefit>
Behaviour Driven Development (BDD) and Feature Injection aims to improve the original framework by supporting communication and collaboration between developers, tester and non-technical participants in a software project.
In Order To… <receive benefit>
As A… <role>
When viewing work as an experiment, the traditional story framework is insufficient. As in our high school science experiment, we need to define the steps we will take to achieve the desired outcome. We then need to state the specific indicators (or signals) we expect to observe that provide evidence that our hypothesis is valid. These need to be stated before conducting the test to reduce biased interpretations of the results.
If we observe signals that indicate our hypothesis is correct, we can be more confident that we are on the right path and can alter the user story framework to reflect this.
Therefore, a user story structure to support Hypothesis-Driven Development would be;
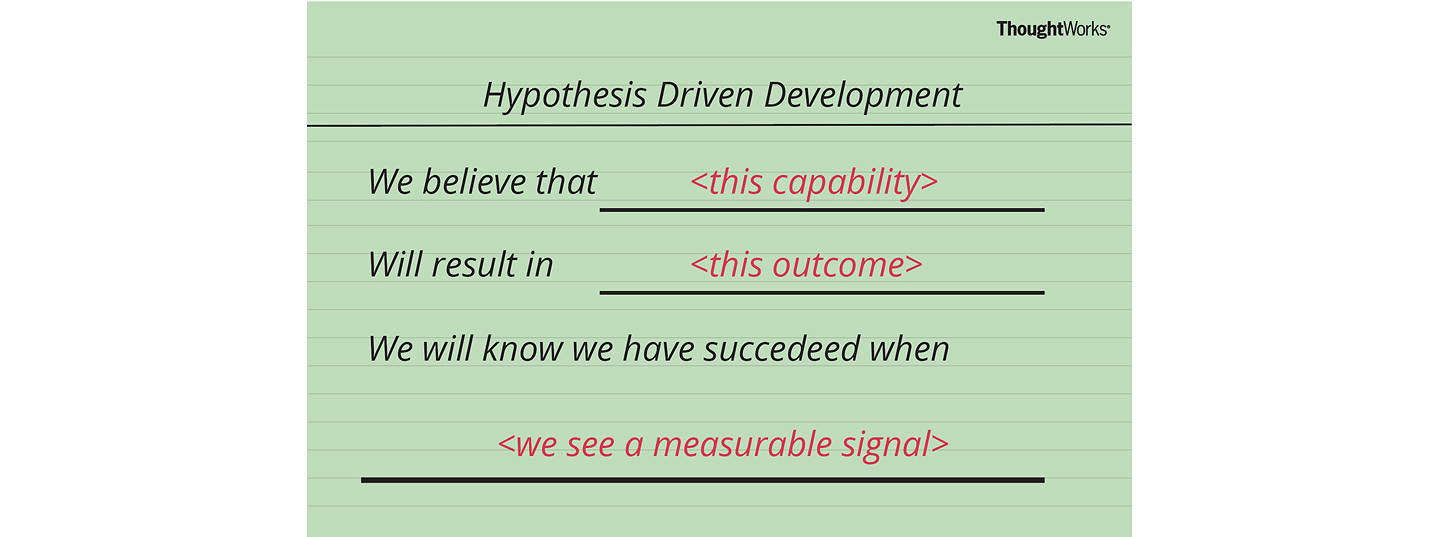
We believe < this capability >
What functionality we will develop to test our hypothesis? By defining a ‘test’ capability of the product or service that we are attempting to build, we identify the functionality and hypothesis we want to test.
Will result in < this outcome >
What is the expected outcome of our experiment? What is the specific result we expect to achieve by building the ‘test’ capability?
We will know we have succeeded when < we see a measurable signal >
What signals will indicate that the capability we have built is effective? What key metrics (qualitative or quantitative) we will measure to provide evidence that our experiment has succeeded and give us enough confidence to move to the next stage.
The threshold you use for statistically significance will depend on your understanding of the business and context you are operating within. Not every company has the user sample size of Amazon or Google to run statistically significant experiments in a short period of time. Limits and controls need to be defined by your organization to determine acceptable evidence thresholds that will allow the team to advance to the next step.
For example if you are building a rocket ship you may want your experiments to have a high threshold for statistical significance. If you are deciding between two different flows intended to help increase user sign up you may be happy to tolerate a lower significance threshold.
The final step is to clearly and visibly state any assumptions made about our hypothesis, to create a feedback loop for the team to provide further input, debate and understanding of the circumstance under which we are performing the test. Are they valid and make sense from a technical and business perspective?
Hypotheses when aligned to your MVP can provide a testing mechanism for your product or service vision. They can test the most uncertain areas of your product or service, in order to gain information and improve confidence.
Examples of Hypothesis-Driven Development user stories are;
Business story
We Believe That increasing the size of hotel images on the booking page
Will Result In improved customer engagement and conversion
We Will Know We Have Succeeded When we see a 5% increase in customers who review hotel images who then proceed to book in 48 hours.
It is imperative to have effective monitoring and evaluation tools in place when using an experimental approach to software development in order to measure the impact of our efforts and provide a feedback loop to the team. Otherwise we are essentially blind to the outcomes of our efforts.
In agile software development we define working software as the primary measure of progress.
By combining Continuous Delivery and Hypothesis-Driven Development we can now define working software and validated learning as the primary measures of progress.
Ideally we should not say we are done until we have measured the value of what is being delivered – in other words, gathered data to validate our hypothesis.
Examples of how to gather data is performing A/B Testing to test a hypothesis and measure to change in customer behaviour. Alternative testings options can be customer surveys, paper prototypes, user and/or guerrilla testing.
One example of a company we have worked with that uses Hypothesis-Driven Development is lastminute.com . The team formulated a hypothesis that customers are only willing to pay a max price for a hotel based on the time of day they book. Tom Klein, CEO and President of Sabre Holdings shared the story of how they improved conversion by 400% within a week.
Combining practices such as Hypothesis-Driven Development and Continuous Delivery accelerates experimentation and amplifies validated learning. This gives us the opportunity to accelerate the rate at which we innovate while relentlessly reducing cost, leaving our competitors in the dust. Ideally we can achieve the ideal of one piece flow: atomic changes that enable us to identify causal relationships between the changes we make to our products and services, and their impact on key metrics.
As Kent Beck said, “Test-Driven Development is a great excuse to think about the problem before you think about the solution”. Hypothesis-Driven Development is a great opportunity to test what you think the problem is, before you work on the solution.
How can you achieve faster growth?
Piaget’s Theory and Stages of Cognitive Development
Saul Mcleod, PhD
Editor-in-Chief for Simply Psychology
BSc (Hons) Psychology, MRes, PhD, University of Manchester
Saul Mcleod, PhD., is a qualified psychology teacher with over 18 years of experience in further and higher education. He has been published in peer-reviewed journals, including the Journal of Clinical Psychology.
Learn about our Editorial Process
Olivia Guy-Evans, MSc
Associate Editor for Simply Psychology
BSc (Hons) Psychology, MSc Psychology of Education
Olivia Guy-Evans is a writer and associate editor for Simply Psychology. She has previously worked in healthcare and educational sectors.
On This Page:
Key Takeaways
- Jean Piaget is famous for his theories regarding changes in cognitive development that occur as we move from infancy to adulthood.
- Cognitive development results from the interplay between innate capabilities (nature) and environmental influences (nurture).
- Children progress through four distinct stages , each representing varying cognitive abilities and world comprehension: the sensorimotor stage (birth to 2 years), the preoperational stage (2 to 7 years), the concrete operational stage (7 to 11 years), and the formal operational stage (11 years and beyond).
- A child’s cognitive development is not just about acquiring knowledge, the child has to develop or construct a mental model of the world, which is referred to as a schema .
- Piaget emphasized the role of active exploration and interaction with the environment in shaping cognitive development, highlighting the importance of assimilation and accommodation in constructing mental schemas.
Stages of Development
Jean Piaget’s theory of cognitive development suggests that children move through four different stages of intellectual development which reflect the increasing sophistication of children’s thought
Each child goes through the stages in the same order (but not all at the same rate), and child development is determined by biological maturation and interaction with the environment.
At each stage of development, the child’s thinking is qualitatively different from the other stages, that is, each stage involves a different type of intelligence.
Although no stage can be missed out, there are individual differences in the rate at which children progress through stages, and some individuals may never attain the later stages.
Piaget did not claim that a particular stage was reached at a certain age – although descriptions of the stages often include an indication of the age at which the average child would reach each stage.
The Sensorimotor Stage
Ages: Birth to 2 Years
The first stage is the sensorimotor stage , during which the infant focuses on physical sensations and learning to coordinate its body.
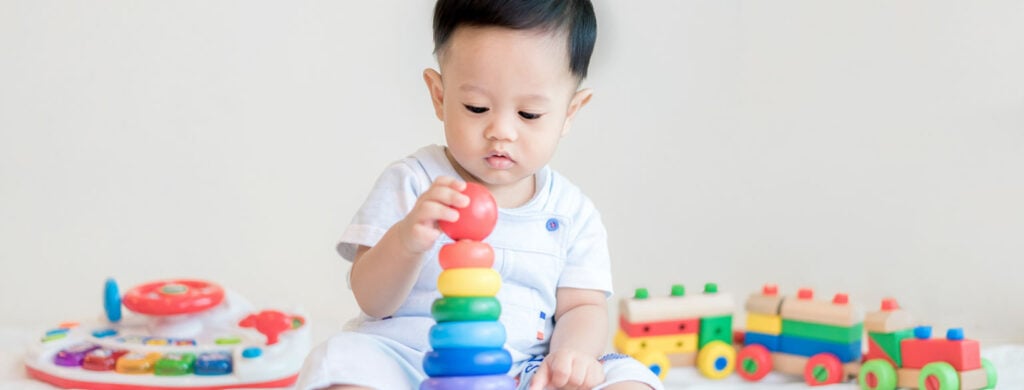
Major Characteristics and Developmental Changes:
- The infant learns about the world through their senses and through their actions (moving around and exploring their environment).
- During the sensorimotor stage, a range of cognitive abilities develop. These include: object permanence; self-recognition (the child realizes that other people are separate from them); deferred imitation; and representational play.
- They relate to the emergence of the general symbolic function, which is the capacity to represent the world mentally
- At about 8 months, the infant will understand the permanence of objects and that they will still exist even if they can’t see them and the infant will search for them when they disappear.
During the beginning of this stage, the infant lives in the present. It does not yet have a mental picture of the world stored in its memory therefore it does not have a sense of object permanence.
If it cannot see something, then it does not exist. This is why you can hide a toy from an infant, while it watches, but it will not search for the object once it has gone out of sight.
The main achievement during this stage is object permanence – knowing that an object still exists, even if it is hidden. It requires the ability to form a mental representation (i.e., a schema) of the object.
Towards the end of this stage the general symbolic function begins to appear where children show in their play that they can use one object to stand for another. Language starts to appear because they realise that words can be used to represent objects and feelings.
The child begins to be able to store information that it knows about the world, recall it, and label it.
Individual Differences
- Cultural Practices : In some cultures, babies are carried on their mothers’ backs throughout the day. This constant physical contact and varied stimuli can influence how a child perceives their environment and their sense of object permanence.
- Gender Norms : Toys assigned to babies can differ based on gender expectations. A boy might be given more cars or action figures, while a girl might receive dolls or kitchen sets. This can influence early interactions and sensory explorations.
Learn More: The Sensorimotor Stage of Cognitive Development
The Preoperational Stage
Ages: 2 – 7 Years
Piaget’s second stage of intellectual development is the preoperational stage . It takes place between 2 and 7 years. At the beginning of this stage, the child does not use operations, so the thinking is influenced by the way things appear rather than logical reasoning.
A child cannot conserve which means that the child does not understand that quantity remains the same even if the appearance changes.
Furthermore, the child is egocentric; he assumes that other people see the world as he does. This has been shown in the three mountains study.
As the preoperational stage develops, egocentrism declines, and children begin to enjoy the participation of another child in their games, and let’s pretend play becomes more important.
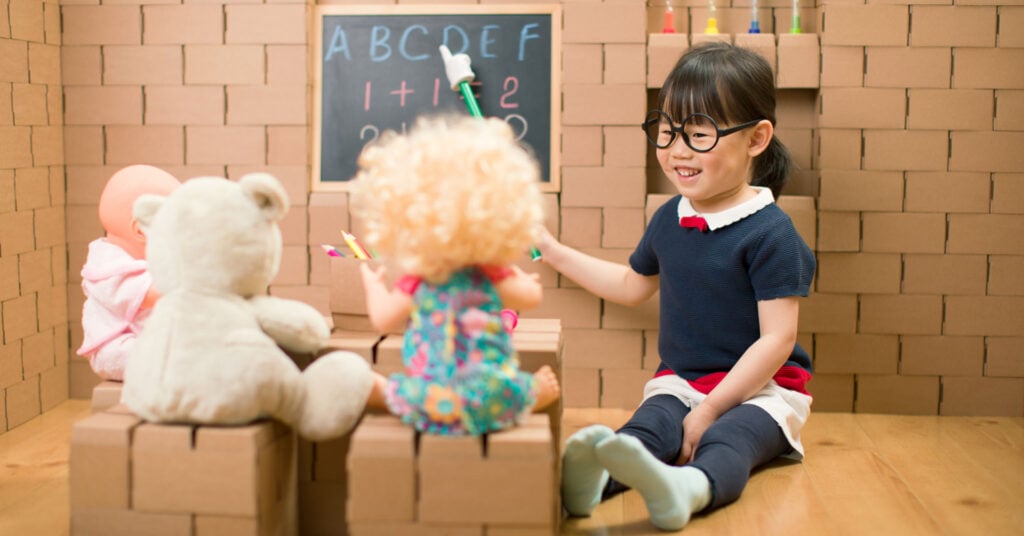
Toddlers often pretend to be people they are not (e.g. superheroes, policemen), and may play these roles with props that symbolize real-life objects. Children may also invent an imaginary playmate.
- Toddlers and young children acquire the ability to internally represent the world through language and mental imagery.
- During this stage, young children can think about things symbolically. This is the ability to make one thing, such as a word or an object, stand for something other than itself.
- A child’s thinking is dominated by how the world looks, not how the world is. It is not yet capable of logical (problem-solving) type of thought.
- Moreover, the child has difficulties with class inclusion; he can classify objects but cannot include objects in sub-sets, which involves classifying objects as belonging to two or more categories simultaneously.
- Infants at this stage also demonstrate animism. This is the tendency for the child to think that non-living objects (such as toys) have life and feelings like a person’s.
By 2 years, children have made some progress toward detaching their thoughts from the physical world. However, have not yet developed logical (or “operational”) thought characteristics of later stages.
Thinking is still intuitive (based on subjective judgments about situations) and egocentric (centered on the child’s own view of the world).
- Cultural Storytelling : Different cultures have unique stories, myths, and folklore. Children from diverse backgrounds might understand and interpret symbolic elements differently based on their cultural narratives.
- Race & Representation : A child’s racial identity can influence how they engage in pretend play. For instance, a lack of diverse representation in media and toys might lead children of color to recreate scenarios that don’t reflect their experiences or background.
Learn More: The Preoperational Stage of Cognitive Development
The Concrete Operational Stage
Ages: 7 – 11 Years
By the beginning of the concrete operational stage , the child can use operations (a set of logical rules) so they can conserve quantities, realize that people see the world in a different way (decentring), and demonstrate improvement in inclusion tasks. Children still have difficulties with abstract thinking.
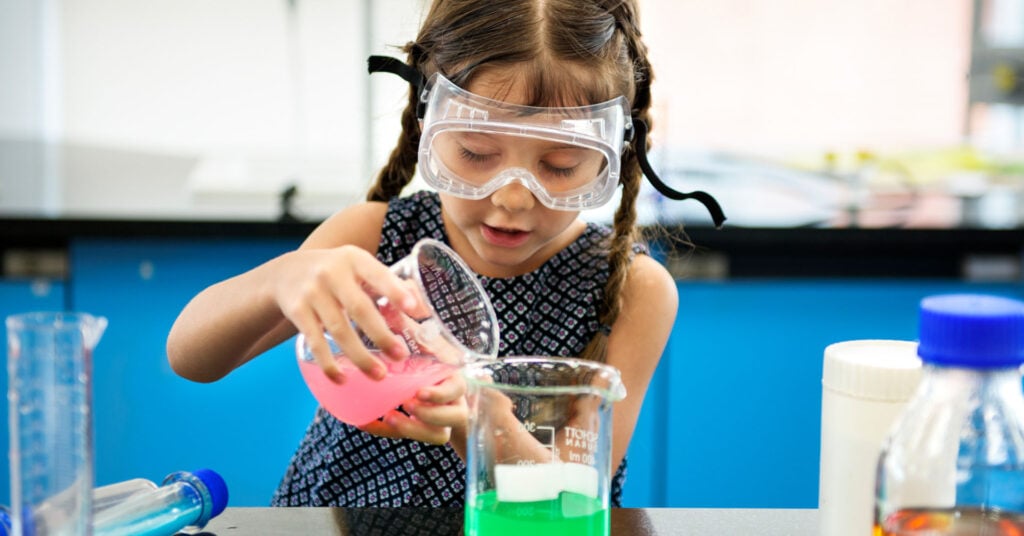
- During this stage, children begin to think logically about concrete events.
- Children begin to understand the concept of conservation; understanding that, although things may change in appearance, certain properties remain the same.
- During this stage, children can mentally reverse things (e.g., picture a ball of plasticine returning to its original shape).
- During this stage, children also become less egocentric and begin to think about how other people might think and feel.
The stage is called concrete because children can think logically much more successfully if they can manipulate real (concrete) materials or pictures of them.
Piaget considered the concrete stage a major turning point in the child’s cognitive development because it marks the beginning of logical or operational thought. This means the child can work things out internally in their head (rather than physically try things out in the real world).
Children can conserve number (age 6), mass (age 7), and weight (age 9). Conservation is the understanding that something stays the same in quantity even though its appearance changes.
But operational thought is only effective here if the child is asked to reason about materials that are physically present. Children at this stage will tend to make mistakes or be overwhelmed when asked to reason about abstract or hypothetical problems.
- Cultural Context in Conservation Tasks : In a society where resources are scarce, children might demonstrate conservation skills earlier due to the cultural emphasis on preserving and reusing materials.
- Gender & Learning : Stereotypes about gender abilities, like “boys are better at math,” can influence how children approach logical problems or classify objects based on perceived gender norms.
Learn More: The Concrete Operational Stage of Development
The Formal Operational Stage
Ages: 12 and Over
The formal operational period begins at about age 11. As adolescents enter this stage, they gain the ability to think in an abstract manner, the ability to combine and classify items in a more sophisticated way, and the capacity for higher-order reasoning.
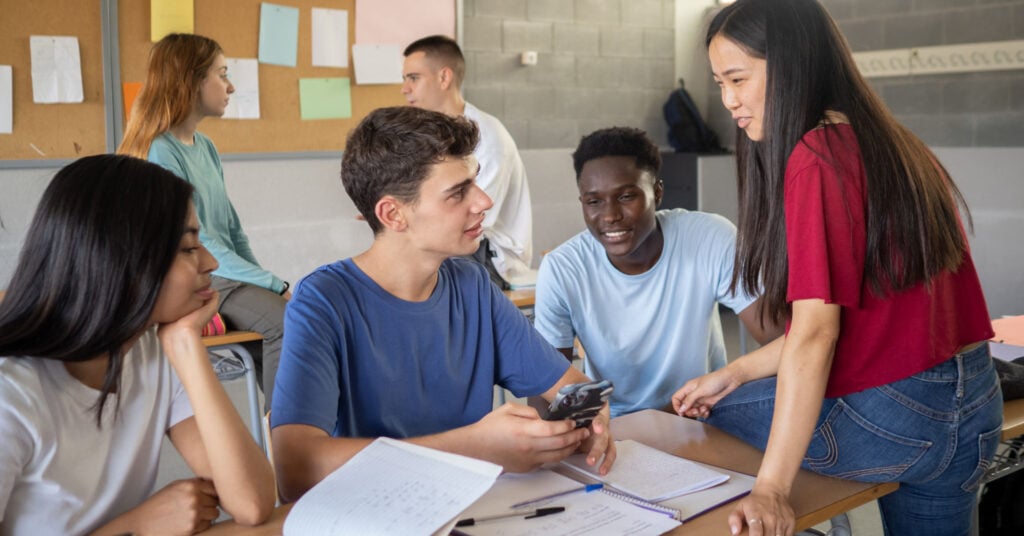
Adolescents can think systematically and reason about what might be as well as what is (not everyone achieves this stage). This allows them to understand politics, ethics, and science fiction, as well as to engage in scientific reasoning.
Adolescents can deal with abstract ideas: e.g. they can understand division and fractions without having to actually divide things up, and solve hypothetical (imaginary) problems.
- Concrete operations are carried out on things whereas formal operations are carried out on ideas. Formal operational thought is entirely freed from physical and perceptual constraints.
- During this stage, adolescents can deal with abstract ideas (e.g. no longer needing to think about slicing up cakes or sharing sweets to understand division and fractions).
- They can follow the form of an argument without having to think in terms of specific examples.
- Adolescents can deal with hypothetical problems with many possible solutions. E.g. if asked ‘What would happen if money were abolished in one hour’s time? they could speculate about many possible consequences.
From about 12 years children can follow the form of a logical argument without reference to its content. During this time, people develop the ability to think about abstract concepts, and logically test hypotheses.
This stage sees the emergence of scientific thinking, formulating abstract theories and hypotheses when faced with a problem.
- Culture & Abstract Thinking : Cultures emphasize different kinds of logical or abstract thinking. For example, in societies with a strong oral tradition, the ability to hold complex narratives might develop prominently.
- Gender & Ethics : Discussions about morality and ethics can be influenced by gender norms. For instance, in some cultures, girls might be encouraged to prioritize community harmony, while boys might be encouraged to prioritize individual rights.
Learn More: The Formal Operational Stage of Development
Piaget’s Theory
- Piaget’s theory places a strong emphasis on the active role that children play in their own cognitive development.
- According to Piaget, children are not passive recipients of information; instead, they actively explore and interact with their surroundings.
- This active engagement with the environment is crucial because it allows them to gradually build their understanding of the world.
1. How Piaget Developed the Theory
Piaget was employed at the Binet Institute in the 1920s, where his job was to develop French versions of questions on English intelligence tests. He became intrigued with the reasons children gave for their wrong answers to the questions that required logical thinking.
He believed that these incorrect answers revealed important differences between the thinking of adults and children.
Piaget branched out on his own with a new set of assumptions about children’s intelligence:
- Children’s intelligence differs from an adult’s in quality rather than in quantity. This means that children reason (think) differently from adults and see the world in different ways.
- Children actively build up their knowledge about the world . They are not passive creatures waiting for someone to fill their heads with knowledge.
- The best way to understand children’s reasoning is to see things from their point of view.
Piaget did not want to measure how well children could count, spell or solve problems as a way of grading their I.Q. What he was more interested in was the way in which fundamental concepts like the very idea of number , time, quantity, causality , justice , and so on emerged.
Piaget studied children from infancy to adolescence using naturalistic observation of his own three babies and sometimes controlled observation too. From these, he wrote diary descriptions charting their development.
He also used clinical interviews and observations of older children who were able to understand questions and hold conversations.
2. Piaget’s Theory Differs From Others In Several Ways:
Piaget’s (1936, 1950) theory of cognitive development explains how a child constructs a mental model of the world. He disagreed with the idea that intelligence was a fixed trait, and regarded cognitive development as a process that occurs due to biological maturation and interaction with the environment.
Children’s ability to understand, think about, and solve problems in the world develops in a stop-start, discontinuous manner (rather than gradual changes over time).
- It is concerned with children, rather than all learners.
- It focuses on development, rather than learning per se, so it does not address learning of information or specific behaviors.
- It proposes discrete stages of development, marked by qualitative differences, rather than a gradual increase in number and complexity of behaviors, concepts, ideas, etc.
The goal of the theory is to explain the mechanisms and processes by which the infant, and then the child, develops into an individual who can reason and think using hypotheses.
To Piaget, cognitive development was a progressive reorganization of mental processes as a result of biological maturation and environmental experience.
Children construct an understanding of the world around them, then experience discrepancies between what they already know and what they discover in their environment.
Piaget claimed that knowledge cannot simply emerge from sensory experience; some initial structure is necessary to make sense of the world.
According to Piaget, children are born with a very basic mental structure (genetically inherited and evolved) on which all subsequent learning and knowledge are based.
Schemas are the basic building blocks of such cognitive models, and enable us to form a mental representation of the world.
Piaget (1952, p. 7) defined a schema as: “a cohesive, repeatable action sequence possessing component actions that are tightly interconnected and governed by a core meaning.”
In more simple terms, Piaget called the schema the basic building block of intelligent behavior – a way of organizing knowledge. Indeed, it is useful to think of schemas as “units” of knowledge, each relating to one aspect of the world, including objects, actions, and abstract (i.e., theoretical) concepts.
Wadsworth (2004) suggests that schemata (the plural of schema) be thought of as “index cards” filed in the brain, each one telling an individual how to react to incoming stimuli or information.
When Piaget talked about the development of a person’s mental processes, he was referring to increases in the number and complexity of the schemata that a person had learned.
When a child’s existing schemas are capable of explaining what it can perceive around it, it is said to be in a state of equilibrium, i.e., a state of cognitive (i.e., mental) balance.
Operations are more sophisticated mental structures which allow us to combine schemas in a logical (reasonable) way.
As children grow they can carry out more complex operations and begin to imagine hypothetical (imaginary) situations.
Apart from the schemas we are born with schemas and operations are learned through interaction with other people and the environment.
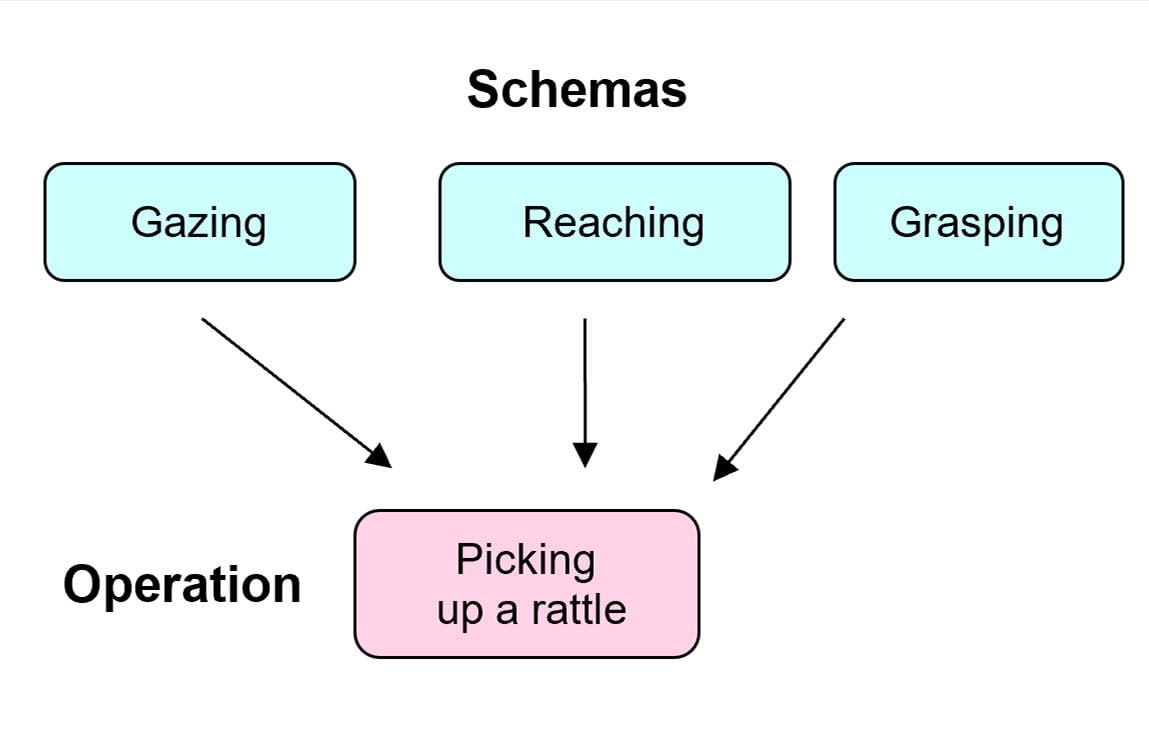
Piaget emphasized the importance of schemas in cognitive development and described how they were developed or acquired.
A schema can be defined as a set of linked mental representations of the world, which we use both to understand and to respond to situations. The assumption is that we store these mental representations and apply them when needed.
Examples of Schemas
A person might have a schema about buying a meal in a restaurant. The schema is a stored form of the pattern of behavior which includes looking at a menu, ordering food, eating it and paying the bill.
This is an example of a schema called a “script.” Whenever they are in a restaurant, they retrieve this schema from memory and apply it to the situation.
The schemas Piaget described tend to be simpler than this – especially those used by infants. He described how – as a child gets older – his or her schemas become more numerous and elaborate.
Piaget believed that newborn babies have a small number of innate schemas – even before they have had many opportunities to experience the world. These neonatal schemas are the cognitive structures underlying innate reflexes. These reflexes are genetically programmed into us.
For example, babies have a sucking reflex, which is triggered by something touching the baby’s lips. A baby will suck a nipple, a comforter (dummy), or a person’s finger. Piaget, therefore, assumed that the baby has a “sucking schema.”
Similarly, the grasping reflex which is elicited when something touches the palm of a baby’s hand, or the rooting reflex, in which a baby will turn its head towards something which touches its cheek, are innate schemas. Shaking a rattle would be the combination of two schemas, grasping and shaking.
4. The Process of Adaptation
Piaget also believed that a child developed as a result of two different influences: maturation, and interaction with the environment. The child develops mental structures (schemata) which enables him to solve problems in the environment.
Adaptation is the process by which the child changes its mental models of the world to match more closely how the world actually is.
Adaptation is brought about by the processes of assimilation (solving new experiences using existing schemata) and accommodation (changing existing schemata in order to solve new experiences).
The importance of this viewpoint is that the child is seen as an active participant in its own development rather than a passive recipient of either biological influences (maturation) or environmental stimulation.
When our existing schemas can explain what we perceive around us, we are in a state of equilibration . However, when we meet a new situation that we cannot explain it creates disequilibrium, this is an unpleasant sensation which we try to escape, and this gives us the motivation to learn.
According to Piaget, reorganization to higher levels of thinking is not accomplished easily. The child must “rethink” his or her view of the world. An important step in the process is the experience of cognitive conflict.
In other words, the child becomes aware that he or she holds two contradictory views about a situation and they both cannot be true. This step is referred to as disequilibrium .
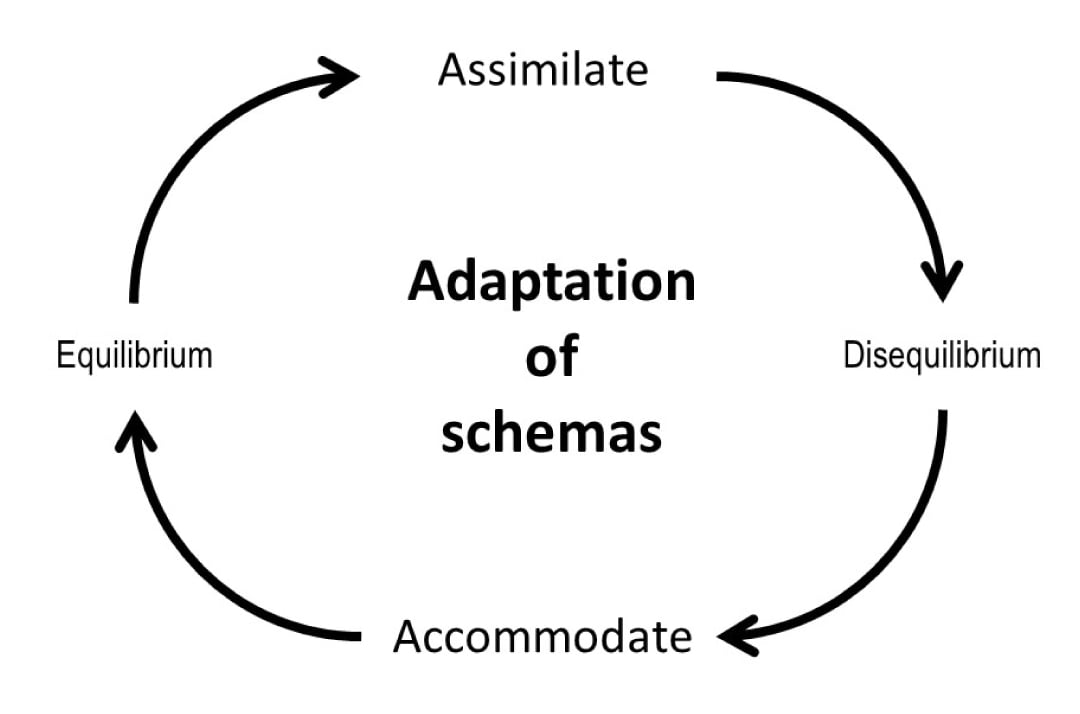
Jean Piaget (1952; see also Wadsworth, 2004) viewed intellectual growth as a process of adaptation (adjustment) to the world. This happens through assimilation, accommodation, and equilibration.
To get back to a state of equilibration, we need to modify our existing schemas to learn and adapt to the new situation.
This is done through the processes of accommodation and assimilation . This is how our schemas evolve and become more sophisticated. The processes of assimilation and accommodation are continuous and interactive.
5. Assimilation
Piaget defined assimilation as the cognitive process of fitting new information into existing cognitive schemas, perceptions, and understanding. Overall beliefs and understanding of the world do not change as a result of the new information.
Assimilation occurs when the new experience is not very different from previous experiences of a particular object or situation we assimilate the new situation by adding information to a previous schema.
This means that when you are faced with new information, you make sense of this information by referring to information you already have (information processed and learned previously) and trying to fit the new information into the information you already have.
- Imagine a young child who has only ever seen small, domesticated dogs. When the child sees a cat for the first time, they might refer to it as a “dog” because it has four legs, fur, and a tail – features that fit their existing schema of a dog.
- A person who has always believed that all birds can fly might label penguins as birds that can fly. This is because their existing schema or understanding of birds includes the ability to fly.
- A 2-year-old child sees a man who is bald on top of his head and has long frizzy hair on the sides. To his father’s horror, the toddler shouts “Clown, clown” (Siegler et al., 2003).
- If a baby learns to pick up a rattle he or she will then use the same schema (grasping) to pick up other objects.
6. Accommodation
Accommodation: when the new experience is very different from what we have encountered before we need to change our schemas in a very radical way or create a whole new schema.
Psychologist Jean Piaget defined accommodation as the cognitive process of revising existing cognitive schemas, perceptions, and understanding so that new information can be incorporated.
This happens when the existing schema (knowledge) does not work, and needs to be changed to deal with a new object or situation.
In order to make sense of some new information, you actually adjust information you already have (schemas you already have, etc.) to make room for this new information.
- A baby tries to use the same schema for grasping to pick up a very small object. It doesn’t work. The baby then changes the schema by now using the forefinger and thumb to pick up the object.
- A child may have a schema for birds (feathers, flying, etc.) and then they see a plane, which also flies, but would not fit into their bird schema.
- In the “clown” incident, the boy’s father explained to his son that the man was not a clown and that even though his hair was like a clown’s, he wasn’t wearing a funny costume and wasn’t doing silly things to make people laugh. With this new knowledge, the boy was able to change his schema of “clown” and make this idea fit better to a standard concept of “clown”.
- A person who grew up thinking all snakes are dangerous might move to an area where garden snakes are common and harmless. Over time, after observing and learning, they might accommodate their previous belief to understand that not all snakes are harmful.
7. Equilibration
Piaget believed that all human thought seeks order and is uncomfortable with contradictions and inconsistencies in knowledge structures. In other words, we seek “equilibrium” in our cognitive structures.
Equilibrium occurs when a child’s schemas can deal with most new information through assimilation. However, an unpleasant state of disequilibrium occurs when new information cannot be fitted into existing schemas (assimilation).
Piaget believed that cognitive development did not progress at a steady rate, but rather in leaps and bounds. Equilibration is the force which drives the learning process as we do not like to be frustrated and will seek to restore balance by mastering the new challenge (accommodation).
Once the new information is acquired the process of assimilation with the new schema will continue until the next time we need to make an adjustment to it.
Equilibration is a regulatory process that maintains a balance between assimilation and accommodation to facilitate cognitive growth. Think of it this way: We can’t merely assimilate all the time; if we did, we would never learn any new concepts or principles.
Everything new we encountered would just get put in the same few “slots” we already had. Neither can we accommodate all the time; if we did, everything we encountered would seem new; there would be no recurring regularities in our world. We’d be exhausted by the mental effort!
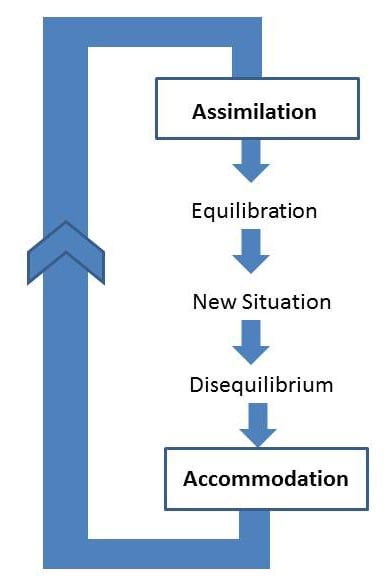
Applications to Education
Think of old black and white films that you’ve seen in which children sat in rows at desks, with ink wells, would learn by rote, all chanting in unison in response to questions set by an authoritarian old biddy like Matilda!
Children who were unable to keep up were seen as slacking and would be punished by variations on the theme of corporal punishment. Yes, it really did happen and in some parts of the world still does today.
Piaget is partly responsible for the change that occurred in the 1960s and for your relatively pleasurable and pain-free school days!
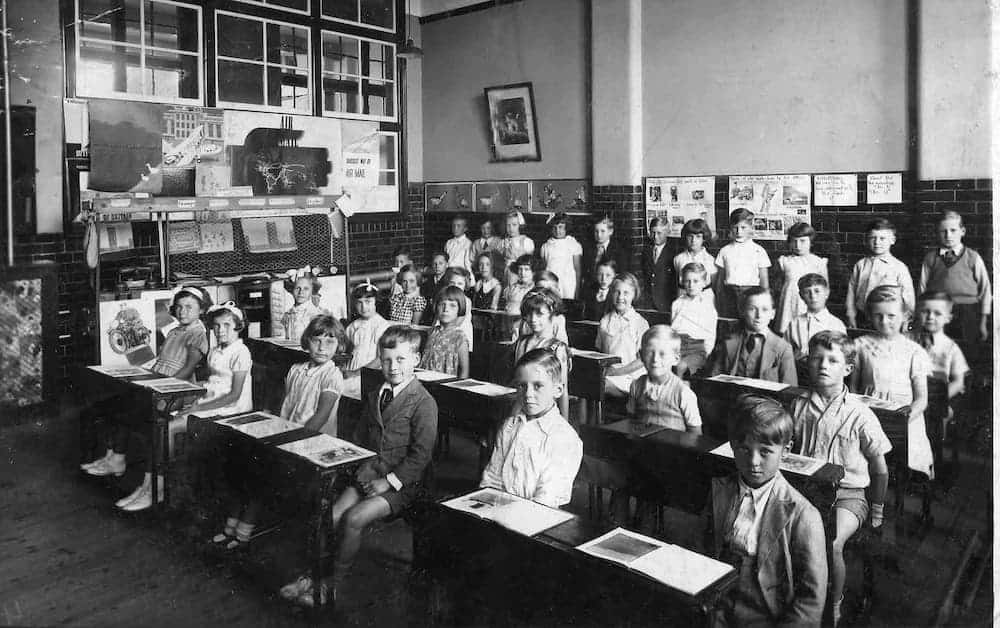
“Children should be able to do their own experimenting and their own research. Teachers, of course, can guide them by providing appropriate materials, but the essential thing is that in order for a child to understand something, he must construct it himself, he must re-invent it. Every time we teach a child something, we keep him from inventing it himself. On the other hand that which we allow him to discover by himself will remain with him visibly”. Piaget (1972, p. 27)
Plowden Report
Piaget (1952) did not explicitly relate his theory to education, although later researchers have explained how features of Piaget’s theory can be applied to teaching and learning.
Piaget has been extremely influential in developing educational policy and teaching practice. For example, a review of primary education by the UK government in 1966 was based strongly on Piaget’s theory. The result of this review led to the publication of the Plowden Report (1967).
In the 1960s the Plowden Committee investigated the deficiencies in education and decided to incorporate many of Piaget’s ideas into its final report published in 1967, even though Piaget’s work was not really designed for education.
The report makes three Piaget-associated recommendations:
- Children should be given individual attention and it should be realized that they need to be treated differently.
- Children should only be taught things that they are capable of learning
- Children mature at different rates and the teacher needs to be aware of the stage of development of each child so teaching can be tailored to their individual needs.
“The report’s recurring themes are individual learning, flexibility in the curriculum, the centrality of play in children’s learning, the use of the environment, learning by discovery and the importance of the evaluation of children’s progress – teachers should “not assume that only what is measurable is valuable.”
Discovery learning – the idea that children learn best through doing and actively exploring – was seen as central to the transformation of the primary school curriculum.
How to teach
Within the classroom learning should be student-centered and accomplished through active discovery learning. The role of the teacher is to facilitate learning, rather than direct tuition.
Because Piaget’s theory is based upon biological maturation and stages, the notion of “readiness” is important. Readiness concerns when certain information or concepts should be taught.
According to Piaget’s theory, children should not be taught certain concepts until they have reached the appropriate stage of cognitive development.
According to Piaget (1958), assimilation and accommodation require an active learner, not a passive one, because problem-solving skills cannot be taught, they must be discovered.
Therefore, teachers should encourage the following within the classroom:
- Educational programs should be designed to correspond to Piaget’s stages of development. Children in the concrete operational stage should be given concrete means to learn new concepts e.g. tokens for counting.
- Devising situations that present useful problems, and create disequilibrium in the child.
- Focus on the process of learning, rather than the end product of it. Instead of checking if children have the right answer, the teacher should focus on the student’s understanding and the processes they used to get to the answer.
- Child-centered approach. Learning must be active (discovery learning). Children should be encouraged to discover for themselves and to interact with the material instead of being given ready-made knowledge.
- Accepting that children develop at different rates so arrange activities for individual children or small groups rather than assume that all the children can cope with a particular activity.
- Using active methods that require rediscovering or reconstructing “truths.”
- Using collaborative, as well as individual activities (so children can learn from each other).
- Evaluate the level of the child’s development so suitable tasks can be set.
- Adapt lessons to suit the needs of the individual child (i.e. differentiated teaching).
- Be aware of the child’s stage of development (testing).
- Teach only when the child is ready. i.e. has the child reached the appropriate stage.
- Providing support for the “spontaneous research” of the child.
- Using collaborative, as well as individual activities.
- Educators may use Piaget’s stages to design age-appropriate assessment tools and strategies.
Classroom Activities
Sensorimotor stage (0-2 years):.
Although most kids in this age range are not in a traditional classroom setting, they can still benefit from games that stimulate their senses and motor skills.
- Object Permanence Games : Play peek-a-boo or hide toys under a blanket to help babies understand that objects still exist even when they can’t see them.
- Sensory Play : Activities like water play, sand play, or playdough encourage exploration through touch.
- Imitation : Children at this age love to imitate adults. Use imitation as a way to teach new skills.
Preoperational Stage (2-7 years):
- Role Playing : Set up pretend play areas where children can act out different scenarios, such as a kitchen, hospital, or market.
- Use of Symbols : Encourage drawing, building, and using props to represent other things.
- Hands-on Activities : Children should interact physically with their environment, so provide plenty of opportunities for hands-on learning.
- Egocentrism Activities : Use exercises that highlight different perspectives. For instance, having two children sit across from each other with an object in between and asking them what the other sees.
Concrete Operational Stage (7-11 years):
- Classification Tasks : Provide objects or pictures to group, based on various characteristics.
- Hands-on Experiments : Introduce basic science experiments where they can observe cause and effect, like a simple volcano with baking soda and vinegar.
- Logical Games : Board games, puzzles, and logic problems help develop their thinking skills.
- Conservation Tasks : Use experiments to showcase that quantity doesn’t change with alterations in shape, such as the classic liquid conservation task using different shaped glasses.
Formal Operational Stage (11 years and older):
- Hypothesis Testing : Encourage students to make predictions and test them out.
- Abstract Thinking : Introduce topics that require abstract reasoning, such as algebra or ethical dilemmas.
- Problem Solving : Provide complex problems and have students work on solutions, integrating various subjects and concepts.
- Debate and Discussion : Encourage group discussions and debates on abstract topics, highlighting the importance of logic and evidence.
- Feedback and Questioning : Use open-ended questions to challenge students and promote higher-order thinking. For instance, rather than asking, “Is this the right answer?”, ask, “How did you arrive at this conclusion?”
While Piaget’s stages offer a foundational framework, they are not universally experienced in the same way by all children.
Social identities play a critical role in shaping cognitive development, necessitating a more nuanced and culturally responsive approach to understanding child development.
Piaget’s stages may manifest differently based on social identities like race, gender, and culture:
- Race & Teacher Interactions : A child’s race can influence teacher expectations and interactions. For example, racial biases can lead to children of color being perceived as less capable or more disruptive, influencing their cognitive challenges and supports.
- Racial and Cultural Stereotypes : These can affect a child’s self-perception and self-efficacy . For instance, stereotypes about which racial or cultural groups are “better” at certain subjects can influence a child’s self-confidence and, subsequently, their engagement in that subject.
- Gender & Peer Interactions : Children learn gender roles from their peers. Boys might be mocked for playing “girl games,” and girls might be excluded from certain activities, influencing their cognitive engagements.
- Language : Multilingual children might navigate the stages differently, especially if their home language differs from their school language. The way concepts are framed in different languages can influence cognitive processing. Cultural idioms and metaphors can shape a child’s understanding of concepts and their ability to use symbolic representation, especially in the pre-operational stage.
Curriculum Development
According to Piaget, children’s cognitive development is determined by a process of maturation which cannot be altered by tuition so education should be stage-specific.
For example, a child in the concrete operational stage should not be taught abstract concepts and should be given concrete aid such as tokens to count with.
According to Piaget children learn through the process of accommodation and assimilation so the role of the teacher should be to provide opportunities for these processes to occur such as new material and experiences that challenge the children’s existing schemas.
Furthermore, according to this theory, children should be encouraged to discover for themselves and to interact with the material instead of being given ready-made knowledge.
Curricula need to be developed that take into account the age and stage of thinking of the child. For example there is no point in teaching abstract concepts such as algebra or atomic structure to children in primary school.
Curricula also need to be sufficiently flexible to allow for variations in the ability of different students of the same age. In Britain, the National Curriculum and Key Stages broadly reflect the stages that Piaget laid down.
For example, egocentrism dominates a child’s thinking in the sensorimotor and preoperational stages. Piaget would therefore predict that using group activities would not be appropriate since children are not capable of understanding the views of others.
However, Smith et al. (1998), point out that some children develop earlier than Piaget predicted and that by using group work children can learn to appreciate the views of others in preparation for the concrete operational stage.
The national curriculum emphasizes the need to use concrete examples in the primary classroom.
Shayer (1997), reported that abstract thought was necessary for success in secondary school (and co-developed the CASE system of teaching science). Recently the National curriculum has been updated to encourage the teaching of some abstract concepts towards the end of primary education, in preparation for secondary courses. (DfEE, 1999).
Child-centered teaching is regarded by some as a child of the ‘liberal sixties.’ In the 1980s the Thatcher government introduced the National Curriculum in an attempt to move away from this and bring more central government control into the teaching of children.
So, although the British National Curriculum in some ways supports the work of Piaget, (in that it dictates the order of teaching), it can also be seen as prescriptive to the point where it counters Piaget’s child-oriented approach.
However, it does still allow for flexibility in teaching methods, allowing teachers to tailor lessons to the needs of their students.
Social Media (Digital Learning)
Jean Piaget could not have anticipated the expansive digital age we now live in.
Today, knowledge dissemination and creation are democratized by the Internet, with platforms like blogs, wikis, and social media allowing for vast collaboration and shared knowledge. This development has prompted a reimagining of the future of education.
Classrooms, traditionally seen as primary sites of learning, are being overshadowed by the rise of mobile technologies and platforms like MOOCs (Passey, 2013).
The millennial generation, defined as the first to grow up with cable TV, the internet, and cell phones, relies heavily on technology.
They view it as an integral part of their identity, with most using it extensively in their daily lives, from keeping in touch with loved ones to consuming news and entertainment (Nielsen, 2014).
Social media platforms offer a dynamic environment conducive to Piaget’s principles. These platforms allow for interactions that nurture knowledge evolution through cognitive processes like assimilation and accommodation.
They emphasize communal interaction and shared activity, fostering both cognitive and socio-cultural constructivism. This shared activity promotes understanding and exploration beyond individual perspectives, enhancing social-emotional learning (Gehlbach, 2010).
A standout advantage of social media in an educational context is its capacity to extend beyond traditional classroom confines. As the material indicates, these platforms can foster more inclusive learning, bridging diverse learner groups.
This inclusivity can equalize learning opportunities, potentially diminishing biases based on factors like race or socio-economic status, resonating with Kegan’s (1982) concept of “recruitability.”
However, there are challenges. While the potential of social media in learning is vast, its practical application necessitates intention and guidance. Cuban, Kirkpatrick, and Peck (2001) note that certain educators and students are hesitant about integrating social media into educational contexts.
This hesitancy can stem from technological complexities or potential distractions. Yet, when harnessed effectively, social media can provide a rich environment for collaborative learning and interpersonal development, fostering a deeper understanding of content.
In essence, the rise of social media aligns seamlessly with constructivist philosophies. Social media platforms act as tools for everyday cognition, merging daily social interactions with the academic world, and providing avenues for diverse, interactive, and engaging learning experiences.
Applications to Parenting
Parents can use Piaget’s stages to have realistic developmental expectations of their children’s behavior and cognitive capabilities.
For instance, understanding that a toddler is in the pre-operational stage can help parents be patient when the child is egocentric.
Play Activities
Recognizing the importance of play in cognitive development, many parents provide toys and games suited for their child’s developmental stage.
Parents can offer activities that are slightly beyond their child’s current abilities, leveraging Vygotsky’s concept of the “Zone of Proximal Development,” which complements Piaget’s ideas.
- Peek-a-boo : Helps with object permanence.
- Texture Touch : Provide different textured materials (soft, rough, bumpy, smooth) for babies to touch and feel.
- Sound Bottles : Fill small bottles with different items like rice, beans, bells, and have children shake and listen to the different sounds.
- Memory Games : Using cards with pictures, place them face down, and ask students to find matching pairs.
- Role Playing and Pretend Play : Let children act out roles or stories that enhance symbolic thinking. Encourage symbolic play with dress-up clothes, playsets, or toy cash registers. Provide prompts or scenarios to extend their imagination.
- Story Sequencing : Give children cards with parts of a story and have them arranged in the correct order.
- Number Line Jumps : Create a number line on the floor with tape. Ask students to jump to the correct answer for math problems.
- Classification Games : Provide a mix of objects and ask students to classify them based on different criteria (e.g., color, size, shape).
- Logical Puzzle Games : Games that involve problem-solving using logic, such as simple Sudoku puzzles or logic grid puzzles.
- Debate and Discussion : Provide a topic and let students debate on pros and cons. This promotes abstract thinking and logical reasoning.
- Hypothesis Testing Games : Present a scenario and have students come up with hypotheses and ways to test them.
- Strategy Board Games : Games like chess, checkers, or Settlers of Catan can help in developing strategic and forward-thinking skills.
Critical Evaluation
- The influence of Piaget’s ideas on developmental psychology has been enormous. He changed how people viewed the child’s world and their methods of studying children.
He was an inspiration to many who came after and took up his ideas. Piaget’s ideas have generated a huge amount of research which has increased our understanding of cognitive development.
- Piaget (1936) was one of the first psychologists to make a systematic study of cognitive development. His contributions include a stage theory of child cognitive development, detailed observational studies of cognition in children, and a series of simple but ingenious tests to reveal different cognitive abilities.
- His ideas have been of practical use in understanding and communicating with children, particularly in the field of education (re: Discovery Learning). Piaget’s theory has been applied across education.
- According to Piaget’s theory, educational programs should be designed to correspond to the stages of development.
- Are the stages real? Vygotsky and Bruner would rather not talk about stages at all, preferring to see development as a continuous process. Others have queried the age ranges of the stages. Some studies have shown that progress to the formal operational stage is not guaranteed.
For example, Keating (1979) reported that 40-60% of college students fail at formal operation tasks, and Dasen (1994) states that only one-third of adults ever reach the formal operational stage.
The fact that the formal operational stage is not reached in all cultures and not all individuals within cultures suggests that it might not be biologically based.
- According to Piaget, the rate of cognitive development cannot be accelerated as it is based on biological processes however, direct tuition can speed up the development which suggests that it is not entirely based on biological factors.
- Because Piaget concentrated on the universal stages of cognitive development and biological maturation, he failed to consider the effect that the social setting and culture may have on cognitive development.
Cross-cultural studies show that the stages of development (except the formal operational stage) occur in the same order in all cultures suggesting that cognitive development is a product of a biological process of maturation.
However, the age at which the stages are reached varies between cultures and individuals which suggests that social and cultural factors and individual differences influence cognitive development.
Dasen (1994) cites studies he conducted in remote parts of the central Australian desert with 8-14-year-old Indigenous Australians. He gave them conservation of liquid tasks and spatial awareness tasks. He found that the ability to conserve came later in the Aboriginal children, between ages of 10 and 13 (as opposed to between 5 and 7, with Piaget’s Swiss sample).
However, he found that spatial awareness abilities developed earlier amongst the Aboriginal children than the Swiss children. Such a study demonstrates cognitive development is not purely dependent on maturation but on cultural factors too – spatial awareness is crucial for nomadic groups of people.
Vygotsky , a contemporary of Piaget, argued that social interaction is crucial for cognitive development. According to Vygotsky the child’s learning always occurs in a social context in cooperation with someone more skillful (MKO). This social interaction provides language opportunities and Vygotsky considered language the foundation of thought.
- Piaget’s methods (observation and clinical interviews) are more open to biased interpretation than other methods. Piaget made careful, detailed naturalistic observations of children, and from these, he wrote diary descriptions charting their development. He also used clinical interviews and observations of older children who were able to understand questions and hold conversations.
Because Piaget conducted the observations alone the data collected are based on his own subjective interpretation of events. It would have been more reliable if Piaget conducted the observations with another researcher and compared the results afterward to check if they are similar (i.e., have inter-rater reliability).
Although clinical interviews allow the researcher to explore data in more depth, the interpretation of the interviewer may be biased.
For example, children may not understand the question/s, they have short attention spans, they cannot express themselves very well, and may be trying to please the experimenter. Such methods meant that Piaget may have formed inaccurate conclusions.
- As several studies have shown Piaget underestimated the abilities of children because his tests were sometimes confusing or difficult to understand (e.g., Hughes , 1975).
Piaget failed to distinguish between competence (what a child is capable of doing) and performance (what a child can show when given a particular task). When tasks were altered, performance (and therefore competence) was affected. Therefore, Piaget might have underestimated children’s cognitive abilities.
For example, a child might have object permanence (competence) but still not be able to search for objects (performance). When Piaget hid objects from babies he found that it wasn’t till after nine months that they looked for it.
However, Piaget relied on manual search methods – whether the child was looking for the object or not.
Later, researchers such as Baillargeon and Devos (1991) reported that infants as young as four months looked longer at a moving carrot that didn’t do what it expected, suggesting they had some sense of permanence, otherwise they wouldn’t have had any expectation of what it should or shouldn’t do.
- The concept of schema is incompatible with the theories of Bruner (1966) and Vygotsky (1978). Behaviorism would also refute Piaget’s schema theory because is cannot be directly observed as it is an internal process. Therefore, they would claim it cannot be objectively measured.
- Piaget studied his own children and the children of his colleagues in Geneva to deduce general principles about the intellectual development of all children. His sample was very small and composed solely of European children from families of high socio-economic status. Researchers have, therefore, questioned the generalisability of his data.
- For Piaget, language is considered secondary to action, i.e., thought precedes language. The Russian psychologist Lev Vygotsky (1978) argues that the development of language and thought go together and that the origin of reasoning has more to do with our ability to communicate with others than with our interaction with the material world.
Piaget’s Theory vs Vygotsky
Piaget maintains that cognitive development stems largely from independent explorations in which children construct knowledge of their own.
Whereas Vygotsky argues that children learn through social interactions, building knowledge by learning from more knowledgeable others such as peers and adults. In other words, Vygotsky believed that culture affects cognitive development.
These factors lead to differences in the education style they recommend: Piaget would argue for the teacher to provide opportunities that challenge the children’s existing schemas and for children to be encouraged to discover for themselves.
Alternatively, Vygotsky would recommend that teachers assist the child to progress through the zone of proximal development by using scaffolding.
However, both theories view children as actively constructing their own knowledge of the world; they are not seen as just passively absorbing knowledge.
They also agree that cognitive development involves qualitative changes in thinking, not only a matter of learning more things.
What is cognitive development?
Cognitive development is how a person’s ability to think, learn, remember, problem-solve, and make decisions changes over time.
This includes the growth and maturation of the brain, as well as the acquisition and refinement of various mental skills and abilities.
Cognitive development is a major aspect of human development, and both genetic and environmental factors heavily influence it. Key domains of cognitive development include attention, memory, language skills, logical reasoning, and problem-solving.
Various theories, such as those proposed by Jean Piaget and Lev Vygotsky, provide different perspectives on how this complex process unfolds from infancy through adulthood.
What are the 4 stages of Piaget’s theory?
Piaget divided children’s cognitive development into four stages; each of the stages represents a new way of thinking and understanding the world.
He called them (1) sensorimotor intelligence , (2) preoperational thinking , (3) concrete operational thinking , and (4) formal operational thinking . Each stage is correlated with an age period of childhood, but only approximately.
According to Piaget, intellectual development takes place through stages that occur in a fixed order and which are universal (all children pass through these stages regardless of social or cultural background).
Development can only occur when the brain has matured to a point of “readiness”.
What are some of the weaknesses of Piaget’s theory?
Cross-cultural studies show that the stages of development (except the formal operational stage) occur in the same order in all cultures suggesting that cognitive development is a product of a biological maturation process.
However, the age at which the stages are reached varies between cultures and individuals, suggesting that social and cultural factors and individual differences influence cognitive development.
What are Piaget’s concepts of schemas?
Schemas are mental structures that contain all of the information relating to one aspect of the world around us.
According to Piaget, we are born with a few primitive schemas, such as sucking, which give us the means to interact with the world.
These are physical, but as the child develops, they become mental schemas. These schemas become more complex with experience.
Baillargeon, R., & DeVos, J. (1991). Object permanence in young infants: Further evidence . Child development , 1227-1246.
Bruner, J. S. (1966). Toward a theory of instruction. Cambridge, Mass.: Belkapp Press.
Cuban, L., Kirkpatrick, H., & Peck, C. (2001). High access and low use of technologies in high school classrooms: Explaining an apparent paradox. American Educational Research Journal , 38 (4), 813-834.
Dasen, P. (1994). Culture and cognitive development from a Piagetian perspective. In W .J. Lonner & R.S. Malpass (Eds.), Psychology and culture (pp. 145–149). Boston, MA: Allyn and Bacon.
Gehlbach, H. (2010). The social side of school: Why teachers need social psychology. Educational Psychology Review , 22 , 349-362.
Hughes, M. (1975). Egocentrism in preschool children . Unpublished doctoral dissertation. Edinburgh University.
Inhelder, B., & Piaget, J. (1958). The growth of logical thinking from childhood to adolescence . New York: Basic Books.
Keating, D. (1979). Adolescent thinking. In J. Adelson (Ed.), Handbook of adolescent psychology (pp. 211-246). New York: Wiley.
Kegan, R. (1982). The evolving self: Problem and process in human development . Harvard University Press.
Nielsen. 2014. “Millennials: Technology = Social Connection.” http://www.nielsen.com/content/corporate/us/en/insights/news/2014/millennials-technology-social-connecti on.html.
Passey, D. (2013). Inclusive technology enhanced learning: Overcoming cognitive, physical, emotional, and geographic challenges . Routledge.
Piaget, J. (1932). The moral judgment of the child . London: Routledge & Kegan Paul.
Piaget, J. (1936). Origins of intelligence in the child. London: Routledge & Kegan Paul.
Piaget, J. (1945). Play, dreams and imitation in childhood . London: Heinemann.
Piaget, J. (1957). Construction of reality in the child. London: Routledge & Kegan Paul.
Piaget, J., & Cook, M. T. (1952). The origins of intelligence in children . New York, NY: International University Press.
Piaget, J. (1981). Intelligence and affectivity: Their relationship during child development.(Trans & Ed TA Brown & CE Kaegi) . Annual Reviews.
Plowden, B. H. P. (1967). Children and their primary schools: A report (Research and Surveys). London, England: HM Stationery Office.
Siegler, R. S., DeLoache, J. S., & Eisenberg, N. (2003). How children develop . New York: Worth.
Vygotsky, L. S. (1978). Mind in society: The development of higher psychological processes . Cambridge, MA: Harvard University Press.
Wadsworth, B. J. (2004). Piaget’s theory of cognitive and affective development: Foundations of constructivism . New York: Longman.
Further Reading
- BBC Radio Broadcast about the Three Mountains Study
- Piagetian stages: A critical review
- Bronfenbrenner’s Ecological Systems Theory


An official website of the United States government
The .gov means it’s official. Federal government websites often end in .gov or .mil. Before sharing sensitive information, make sure you’re on a federal government site.
The site is secure. The https:// ensures that you are connecting to the official website and that any information you provide is encrypted and transmitted securely.
- Publications
- Account settings
Preview improvements coming to the PMC website in October 2024. Learn More or Try it out now .
- Advanced Search
- Journal List
- J Korean Med Sci
- v.36(50); 2021 Dec 27

Formulating Hypotheses for Different Study Designs
Durga prasanna misra.
1 Department of Clinical Immunology and Rheumatology, Sanjay Gandhi Postgraduate Institute of Medical Sciences, Lucknow, India.
Armen Yuri Gasparyan
2 Departments of Rheumatology and Research and Development, Dudley Group NHS Foundation Trust (Teaching Trust of the University of Birmingham, UK), Russells Hall Hospital, Dudley, UK.
Olena Zimba
3 Department of Internal Medicine #2, Danylo Halytsky Lviv National Medical University, Lviv, Ukraine.
Marlen Yessirkepov
4 Department of Biology and Biochemistry, South Kazakhstan Medical Academy, Shymkent, Kazakhstan.
Vikas Agarwal
George d. kitas.
5 Centre for Epidemiology versus Arthritis, University of Manchester, Manchester, UK.
Generating a testable working hypothesis is the first step towards conducting original research. Such research may prove or disprove the proposed hypothesis. Case reports, case series, online surveys and other observational studies, clinical trials, and narrative reviews help to generate hypotheses. Observational and interventional studies help to test hypotheses. A good hypothesis is usually based on previous evidence-based reports. Hypotheses without evidence-based justification and a priori ideas are not received favourably by the scientific community. Original research to test a hypothesis should be carefully planned to ensure appropriate methodology and adequate statistical power. While hypotheses can challenge conventional thinking and may be controversial, they should not be destructive. A hypothesis should be tested by ethically sound experiments with meaningful ethical and clinical implications. The coronavirus disease 2019 pandemic has brought into sharp focus numerous hypotheses, some of which were proven (e.g. effectiveness of corticosteroids in those with hypoxia) while others were disproven (e.g. ineffectiveness of hydroxychloroquine and ivermectin).
Graphical Abstract

DEFINING WORKING AND STANDALONE SCIENTIFIC HYPOTHESES
Science is the systematized description of natural truths and facts. Routine observations of existing life phenomena lead to the creative thinking and generation of ideas about mechanisms of such phenomena and related human interventions. Such ideas presented in a structured format can be viewed as hypotheses. After generating a hypothesis, it is necessary to test it to prove its validity. Thus, hypothesis can be defined as a proposed mechanism of a naturally occurring event or a proposed outcome of an intervention. 1 , 2
Hypothesis testing requires choosing the most appropriate methodology and adequately powering statistically the study to be able to “prove” or “disprove” it within predetermined and widely accepted levels of certainty. This entails sample size calculation that often takes into account previously published observations and pilot studies. 2 , 3 In the era of digitization, hypothesis generation and testing may benefit from the availability of numerous platforms for data dissemination, social networking, and expert validation. Related expert evaluations may reveal strengths and limitations of proposed ideas at early stages of post-publication promotion, preventing the implementation of unsupported controversial points. 4
Thus, hypothesis generation is an important initial step in the research workflow, reflecting accumulating evidence and experts' stance. In this article, we overview the genesis and importance of scientific hypotheses and their relevance in the era of the coronavirus disease 2019 (COVID-19) pandemic.
DO WE NEED HYPOTHESES FOR ALL STUDY DESIGNS?
Broadly, research can be categorized as primary or secondary. In the context of medicine, primary research may include real-life observations of disease presentations and outcomes. Single case descriptions, which often lead to new ideas and hypotheses, serve as important starting points or justifications for case series and cohort studies. The importance of case descriptions is particularly evident in the context of the COVID-19 pandemic when unique, educational case reports have heralded a new era in clinical medicine. 5
Case series serve similar purpose to single case reports, but are based on a slightly larger quantum of information. Observational studies, including online surveys, describe the existing phenomena at a larger scale, often involving various control groups. Observational studies include variable-scale epidemiological investigations at different time points. Interventional studies detail the results of therapeutic interventions.
Secondary research is based on already published literature and does not directly involve human or animal subjects. Review articles are generated by secondary research. These could be systematic reviews which follow methods akin to primary research but with the unit of study being published papers rather than humans or animals. Systematic reviews have a rigid structure with a mandatory search strategy encompassing multiple databases, systematic screening of search results against pre-defined inclusion and exclusion criteria, critical appraisal of study quality and an optional component of collating results across studies quantitatively to derive summary estimates (meta-analysis). 6 Narrative reviews, on the other hand, have a more flexible structure. Systematic literature searches to minimise bias in selection of articles are highly recommended but not mandatory. 7 Narrative reviews are influenced by the authors' viewpoint who may preferentially analyse selected sets of articles. 8
In relation to primary research, case studies and case series are generally not driven by a working hypothesis. Rather, they serve as a basis to generate a hypothesis. Observational or interventional studies should have a hypothesis for choosing research design and sample size. The results of observational and interventional studies further lead to the generation of new hypotheses, testing of which forms the basis of future studies. Review articles, on the other hand, may not be hypothesis-driven, but form fertile ground to generate future hypotheses for evaluation. Fig. 1 summarizes which type of studies are hypothesis-driven and which lead on to hypothesis generation.

STANDARDS OF WORKING AND SCIENTIFIC HYPOTHESES
A review of the published literature did not enable the identification of clearly defined standards for working and scientific hypotheses. It is essential to distinguish influential versus not influential hypotheses, evidence-based hypotheses versus a priori statements and ideas, ethical versus unethical, or potentially harmful ideas. The following points are proposed for consideration while generating working and scientific hypotheses. 1 , 2 Table 1 summarizes these points.
Evidence-based data
A scientific hypothesis should have a sound basis on previously published literature as well as the scientist's observations. Randomly generated (a priori) hypotheses are unlikely to be proven. A thorough literature search should form the basis of a hypothesis based on published evidence. 7
Unless a scientific hypothesis can be tested, it can neither be proven nor be disproven. Therefore, a scientific hypothesis should be amenable to testing with the available technologies and the present understanding of science.
Supported by pilot studies
If a hypothesis is based purely on a novel observation by the scientist in question, it should be grounded on some preliminary studies to support it. For example, if a drug that targets a specific cell population is hypothesized to be useful in a particular disease setting, then there must be some preliminary evidence that the specific cell population plays a role in driving that disease process.
Testable by ethical studies
The hypothesis should be testable by experiments that are ethically acceptable. 9 For example, a hypothesis that parachutes reduce mortality from falls from an airplane cannot be tested using a randomized controlled trial. 10 This is because it is obvious that all those jumping from a flying plane without a parachute would likely die. Similarly, the hypothesis that smoking tobacco causes lung cancer cannot be tested by a clinical trial that makes people take up smoking (since there is considerable evidence for the health hazards associated with smoking). Instead, long-term observational studies comparing outcomes in those who smoke and those who do not, as was performed in the landmark epidemiological case control study by Doll and Hill, 11 are more ethical and practical.
Balance between scientific temper and controversy
Novel findings, including novel hypotheses, particularly those that challenge established norms, are bound to face resistance for their wider acceptance. Such resistance is inevitable until the time such findings are proven with appropriate scientific rigor. However, hypotheses that generate controversy are generally unwelcome. For example, at the time the pandemic of human immunodeficiency virus (HIV) and AIDS was taking foot, there were numerous deniers that refused to believe that HIV caused AIDS. 12 , 13 Similarly, at a time when climate change is causing catastrophic changes to weather patterns worldwide, denial that climate change is occurring and consequent attempts to block climate change are certainly unwelcome. 14 The denialism and misinformation during the COVID-19 pandemic, including unfortunate examples of vaccine hesitancy, are more recent examples of controversial hypotheses not backed by science. 15 , 16 An example of a controversial hypothesis that was a revolutionary scientific breakthrough was the hypothesis put forth by Warren and Marshall that Helicobacter pylori causes peptic ulcers. Initially, the hypothesis that a microorganism could cause gastritis and gastric ulcers faced immense resistance. When the scientists that proposed the hypothesis themselves ingested H. pylori to induce gastritis in themselves, only then could they convince the wider world about their hypothesis. Such was the impact of the hypothesis was that Barry Marshall and Robin Warren were awarded the Nobel Prize in Physiology or Medicine in 2005 for this discovery. 17 , 18
DISTINGUISHING THE MOST INFLUENTIAL HYPOTHESES
Influential hypotheses are those that have stood the test of time. An archetype of an influential hypothesis is that proposed by Edward Jenner in the eighteenth century that cowpox infection protects against smallpox. While this observation had been reported for nearly a century before this time, it had not been suitably tested and publicised until Jenner conducted his experiments on a young boy by demonstrating protection against smallpox after inoculation with cowpox. 19 These experiments were the basis for widespread smallpox immunization strategies worldwide in the 20th century which resulted in the elimination of smallpox as a human disease today. 20
Other influential hypotheses are those which have been read and cited widely. An example of this is the hygiene hypothesis proposing an inverse relationship between infections in early life and allergies or autoimmunity in adulthood. An analysis reported that this hypothesis had been cited more than 3,000 times on Scopus. 1
LESSONS LEARNED FROM HYPOTHESES AMIDST THE COVID-19 PANDEMIC
The COVID-19 pandemic devastated the world like no other in recent memory. During this period, various hypotheses emerged, understandably so considering the public health emergency situation with innumerable deaths and suffering for humanity. Within weeks of the first reports of COVID-19, aberrant immune system activation was identified as a key driver of organ dysfunction and mortality in this disease. 21 Consequently, numerous drugs that suppress the immune system or abrogate the activation of the immune system were hypothesized to have a role in COVID-19. 22 One of the earliest drugs hypothesized to have a benefit was hydroxychloroquine. Hydroxychloroquine was proposed to interfere with Toll-like receptor activation and consequently ameliorate the aberrant immune system activation leading to pathology in COVID-19. 22 The drug was also hypothesized to have a prophylactic role in preventing infection or disease severity in COVID-19. It was also touted as a wonder drug for the disease by many prominent international figures. However, later studies which were well-designed randomized controlled trials failed to demonstrate any benefit of hydroxychloroquine in COVID-19. 23 , 24 , 25 , 26 Subsequently, azithromycin 27 , 28 and ivermectin 29 were hypothesized as potential therapies for COVID-19, but were not supported by evidence from randomized controlled trials. The role of vitamin D in preventing disease severity was also proposed, but has not been proven definitively until now. 30 , 31 On the other hand, randomized controlled trials identified the evidence supporting dexamethasone 32 and interleukin-6 pathway blockade with tocilizumab as effective therapies for COVID-19 in specific situations such as at the onset of hypoxia. 33 , 34 Clues towards the apparent effectiveness of various drugs against severe acute respiratory syndrome coronavirus 2 in vitro but their ineffectiveness in vivo have recently been identified. Many of these drugs are weak, lipophilic bases and some others induce phospholipidosis which results in apparent in vitro effectiveness due to non-specific off-target effects that are not replicated inside living systems. 35 , 36
Another hypothesis proposed was the association of the routine policy of vaccination with Bacillus Calmette-Guerin (BCG) with lower deaths due to COVID-19. This hypothesis emerged in the middle of 2020 when COVID-19 was still taking foot in many parts of the world. 37 , 38 Subsequently, many countries which had lower deaths at that time point went on to have higher numbers of mortality, comparable to other areas of the world. Furthermore, the hypothesis that BCG vaccination reduced COVID-19 mortality was a classic example of ecological fallacy. Associations between population level events (ecological studies; in this case, BCG vaccination and COVID-19 mortality) cannot be directly extrapolated to the individual level. Furthermore, such associations cannot per se be attributed as causal in nature, and can only serve to generate hypotheses that need to be tested at the individual level. 39
IS TRADITIONAL PEER REVIEW EFFICIENT FOR EVALUATION OF WORKING AND SCIENTIFIC HYPOTHESES?
Traditionally, publication after peer review has been considered the gold standard before any new idea finds acceptability amongst the scientific community. Getting a work (including a working or scientific hypothesis) reviewed by experts in the field before experiments are conducted to prove or disprove it helps to refine the idea further as well as improve the experiments planned to test the hypothesis. 40 A route towards this has been the emergence of journals dedicated to publishing hypotheses such as the Central Asian Journal of Medical Hypotheses and Ethics. 41 Another means of publishing hypotheses is through registered research protocols detailing the background, hypothesis, and methodology of a particular study. If such protocols are published after peer review, then the journal commits to publishing the completed study irrespective of whether the study hypothesis is proven or disproven. 42 In the post-pandemic world, online research methods such as online surveys powered via social media channels such as Twitter and Instagram might serve as critical tools to generate as well as to preliminarily test the appropriateness of hypotheses for further evaluation. 43 , 44
Some radical hypotheses might be difficult to publish after traditional peer review. These hypotheses might only be acceptable by the scientific community after they are tested in research studies. Preprints might be a way to disseminate such controversial and ground-breaking hypotheses. 45 However, scientists might prefer to keep their hypotheses confidential for the fear of plagiarism of ideas, avoiding online posting and publishing until they have tested the hypotheses.
SUGGESTIONS ON GENERATING AND PUBLISHING HYPOTHESES
Publication of hypotheses is important, however, a balance is required between scientific temper and controversy. Journal editors and reviewers might keep in mind these specific points, summarized in Table 2 and detailed hereafter, while judging the merit of hypotheses for publication. Keeping in mind the ethical principle of primum non nocere, a hypothesis should be published only if it is testable in a manner that is ethically appropriate. 46 Such hypotheses should be grounded in reality and lend themselves to further testing to either prove or disprove them. It must be considered that subsequent experiments to prove or disprove a hypothesis have an equal chance of failing or succeeding, akin to tossing a coin. A pre-conceived belief that a hypothesis is unlikely to be proven correct should not form the basis of rejection of such a hypothesis for publication. In this context, hypotheses generated after a thorough literature search to identify knowledge gaps or based on concrete clinical observations on a considerable number of patients (as opposed to random observations on a few patients) are more likely to be acceptable for publication by peer-reviewed journals. Also, hypotheses should be considered for publication or rejection based on their implications for science at large rather than whether the subsequent experiments to test them end up with results in favour of or against the original hypothesis.
Hypotheses form an important part of the scientific literature. The COVID-19 pandemic has reiterated the importance and relevance of hypotheses for dealing with public health emergencies and highlighted the need for evidence-based and ethical hypotheses. A good hypothesis is testable in a relevant study design, backed by preliminary evidence, and has positive ethical and clinical implications. General medical journals might consider publishing hypotheses as a specific article type to enable more rapid advancement of science.
Disclosure: The authors have no potential conflicts of interest to disclose.
Author Contributions:
- Data curation: Gasparyan AY, Misra DP, Zimba O, Yessirkepov M, Agarwal V, Kitas GD.
Chapter 6 - Hypothesis Development
Chapter 6 overview.
This chapter discusses the third step of the SGAM, highlighted below in gold, Hypothesis Development.
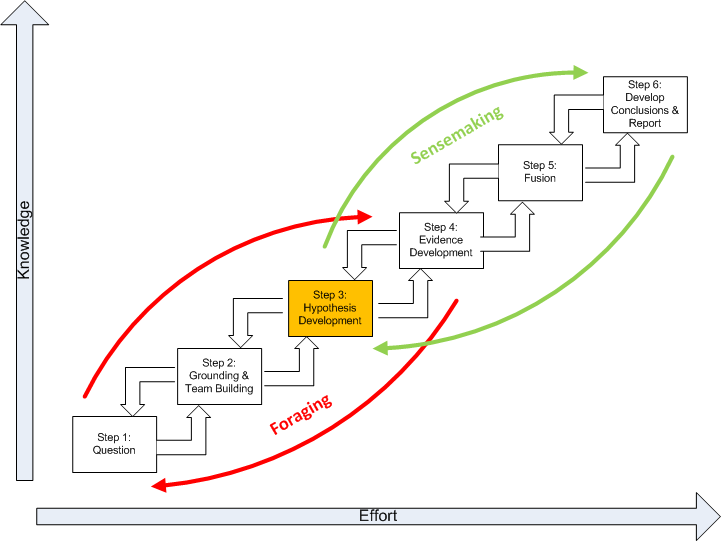
A hypothesis is often defined as an educated guess because it is informed by what you already know about a topic. This step in the process is to identify all hypotheses that merit detailed examination, keeping in mind that there is a distinction between the hypothesis generation and hypothesis evaluation .
If the analysis does not begin with the correct hypothesis, it is unlikely to get the correct answer. Psychological research into how people go about generating hypotheses shows that people are actually rather poor at thinking of all the possibilities. Therefore, at the hypothesis generation stage, it is wise to bring together a group of analysts with different backgrounds and perspectives for a brainstorming session. Brainstorming in a group stimulates the imagination and usually brings out possibilities that individual members of the group had not thought of. Experience shows that initial discussion in the group elicits every possibility, no matter how remote, before judging likelihood or feasibility. Only when all the possibilities are on the table, is the focus on judging them and selecting the hypotheses to be examined in greater detail in subsequent analysis.
When screening out the seemingly improbable hypotheses, it is necessary to distinguish hypotheses that appear to be disproved (i.e., improbable) from those that are simply unproven. For an unproven hypothesis, there is no evidence that it is correct. For a disproved hypothesis, there is positive evidence that it is wrong. Early rejection of unproven, but not disproved, hypotheses biases the analysis, because one does not then look for the evidence that might support them. Unproven hypotheses should be kept alive until they can be disproved. One example of a hypothesis that often falls into this unproven but not disproved category is the hypothesis that an opponent is trying to deceive us. You may reject the possibility of denial and deception because you see no evidence of it, but rejection is not justified under these circumstances. If deception is planned well and properly implemented, one should not expect to find evidence of it readily at hand. The possibility should not be rejected until it is disproved, or, at least, until after a systematic search for evidence has been made, and none has been found.
There is no "correct" number of hypotheses to be considered. The number depends upon the nature of the analytical problem and how advanced you are in the analysis of it. As a general rule, the greater your level of uncertainty, or the greater the impact of your conclusion, the more alternatives you may wish to consider. More than seven hypotheses may be unmanageable; if there are this many alternatives, it may be advisable to group several of them together for your initial cut at the analysis.
Developing Multiple Hypotheses
Developing good hypotheses requires divergent thinking to ensure that all hypotheses are considered. It also requires convergent thinking to ensure that redundant and irrational hypotheses are eliminated. A hypothesis is stated as an "if … then" statement. There are two important qualities about a hypothesis expressed as an "if … then" statement. These are:
- Is the hypothesis testable; in other words, could evidence be found to test the validity of the statement?
- Is the hypothesis falsifiable; in other words, could evidence reveal that such an idea is not true?
Hypothesis development is ultimately experience-based. In this experienced-based reasoning, new knowledge is compared to previous knowledge. New knowledge is added to this internal knowledge base. Before long, an analyst has developed an internal set of spatial rules. These rules are then used to develop possible hypotheses.
Looking Forward
Developing hypotheses and evidence is the beginning of the sensemaking and Analysis of Competing Hypotheses (ACH) process. ACH is a general purpose intelligence analysis methodology developed by Richards Heuer while he was an analyst at the Central Intelligence Agency (CIA). ACH draws on the scientific method, cognitive psychology, and decision analysis. ACH became widely available when the CIA published Heuer’s The Psychology of Intelligence Analysis . The ACH methodology can help the geospatial analyst overcome cognitive biases common to analysis in national security, law enforcement, and competitive intelligence. ACH forces analysts to disprove hypotheses rather than jump to conclusions and permit biases and mindsets to determine the outcome. ACH is a very logical step-by-step process that has been incorporated into our Structured Geospatial Analytical Method. A complete discussion of ACH is found in Chapter 8 of Heuer’s book.
General Approaches to Problem Solving Utilizing Hypotheses
Science follows at least three general methods of problem solving using hypotheses. These can be called the:
- method of the ruling theory
- method of the working hypothesis
- method of multiple working hypotheses
The first two are the most popular but they can lead to overlooking relevant perspectives, data, and encourage biases. It has been suggested that multiple hypotheses offers a more effective way of overcoming this problem.
Ruling Theories and Working Hypotheses
Our desire to reach an explanation commonly leads us to a tentative interpretation that is based on a single case. The explanation can blind us to other possibilities that we ignored at first glance. This premature explanation can become a ruling theory, and our research becomes focused on proving that ruling theory. The result is a bias to evidence that disproves the ruling theory or supports an alternate explanation. Only if the original hypothesis was by chance correct does our analysis lead to any meaningful intelligence work. The working hypothesis is supposed to be a hypothesis to be tested, not in order to prove the hypothesis, but as a stimulus for study and fact-finding. Nonetheless, the single working hypothesis can become a ruling theory, and the desire to prove the working hypothesis, despite evidence to the contrary, can become as strong as the desire to prove the ruling theory.
Multiple Hypotheses
The method of multiple working hypotheses involves the development, prior to our search for evidence, of several hypotheses that might explain what are attempting to explain. Many of these hypotheses should be contradictory, so that many will prove to be improbable. However, the development of multiple hypotheses prior to the intelligence analysis lets us avoid the trap of the ruling hypothesis and thus makes it more likely that our intelligence work will lead to meaningful results. We open-mindedly envision all the possible explanations of the events, including the possibility that none of the hypotheses are plausible and the possibility that more research and hypothesis development is needed. The method of multiple working hypotheses has several other beneficial effects on intelligence analysis. Human actions are often the result of several factors, not just one, and multiple hypotheses make it more likely that we will see the interaction of the several factors. The beginning with multiple hypotheses also promotes much greater thoroughness than analysis directed toward one hypothesis, leading to analytic lines that we might otherwise overlook, and thus to evidence and insights that might never have been considered. Thirdly, the method makes us much more likely to see the imperfections in our understanding and thus to avoid the pitfall of accepting weak or flawed evidence for one hypothesis when another provides a more possible explanation.
Drawbacks of Multiple Hypotheses
Multiple hypotheses have drawbacks. One is that it is difficult to express multiple hypotheses simultaneously, and therefore there is a natural tendency to favor one. Another problem is developing a large number of hypotheses that can be tested. A third possible problem is that of the indecision that arises as an analyst balances the evidence for various hypotheses, which is likely preferable to the premature rush to a false conclusion.
Actions That Help the Analyst Develop Hypotheses
Action 1: Brainstorming . Begin with a brainstorming session with your knowledge team to identify a set of alternative hypotheses. Focus on the hypotheses that are:
- logically consistent with the theories and data uncovered in your grounding;
- address the quality and relationships of spaces.
State the hypotheses stated in an "if ... then" format, for example:
- If the DC Shooter is a terrorist, then the geospatial pattern of events would be similar to other terrorist acts.
- If the DC Shooter is a serial killer, then the geospatial pattern of events would be similar to other serial killers.
Action 2: Review the hypotheses for testability , i.e., can evidence be could found to test the validity of the statement.
Action 3: Check the hypotheses for falsifiability , i.e., could evidence reveal that such an idea is not true.
Action 4: Combine redundant hypotheses.
Action 5:Consider the elimination of improbable and unproven hypotheses.
Thank you for visiting nature.com. You are using a browser version with limited support for CSS. To obtain the best experience, we recommend you use a more up to date browser (or turn off compatibility mode in Internet Explorer). In the meantime, to ensure continued support, we are displaying the site without styles and JavaScript.
- View all journals
- Explore content
- About the journal
- Publish with us
- Sign up for alerts
- Review Article
- Published: 10 May 2022
Understanding and shaping the future of work with self-determination theory
- Marylène Gagné ORCID: orcid.org/0000-0003-3248-8947 1 ,
- Sharon K. Parker ORCID: orcid.org/0000-0002-0978-1873 1 ,
- Mark A. Griffin ORCID: orcid.org/0000-0003-4326-7752 1 ,
- Patrick D. Dunlop ORCID: orcid.org/0000-0002-5225-6409 1 ,
- Caroline Knight ORCID: orcid.org/0000-0001-9894-7750 1 ,
- Florian E. Klonek ORCID: orcid.org/0000-0002-4466-0890 1 &
- Xavier Parent-Rocheleau ORCID: orcid.org/0000-0001-5015-3214 2
Nature Reviews Psychology volume 1 , pages 378–392 ( 2022 ) Cite this article
44k Accesses
55 Citations
35 Altmetric
Metrics details
- Human behaviour
- Science, technology and society
Self-determination theory has shaped our understanding of what optimizes worker motivation by providing insights into how work context influences basic psychological needs for competence, autonomy and relatedness. As technological innovations change the nature of work, self-determination theory can provide insight into how the resulting uncertainty and interdependence might influence worker motivation, performance and well-being. In this Review, we summarize what self-determination theory has brought to the domain of work and how it is helping researchers and practitioners to shape the future of work. We consider how the experiences of job candidates are influenced by the new technologies used to assess and select them, and how self-determination theory can help to improve candidate attitudes and performance during selection assessments. We also discuss how technology transforms the design of work and its impact on worker motivation. We then describe three cases where technology is affecting work design and examine how this might influence needs satisfaction and motivation: remote work, virtual teamwork and algorithmic management. An understanding of how future work is likely to influence the satisfaction of the psychological needs of workers and how future work can be designed to satisfy such needs is of the utmost importance to worker performance and well-being.
Similar content being viewed by others
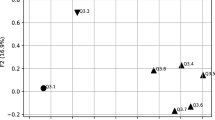
Structured multi-criteria model of self-managed motivation in organizations based on happiness at work: pandemic related study
Joanna Nieżurawska, Radosław A. Kycia, … Agnieszka Niemczynowicz
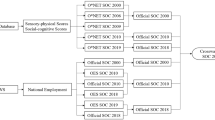
The future of the labor force: higher cognition and more skills
Wen Zhang, Kee-Hung Lai & Qiguo Gong
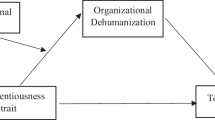
The level of conscientiousness trait and technostress: a moderated mediation model
Eva Ariño-Mateo, Matías Arriagada Venegas, … David Pérez-Jorge
Introduction
The nature of work is changing as technology enables new forms of automation and communication across many industries. Although the image of human-like robots replacing human jobs is vivid, it does not reflect the typical ways people will engage with automation and how technology will change job requirements in the future. A more relevant picture is one in which people interact over dispersed networks using continuously improving communication platforms mediated by artificial intelligence (AI). Examples include the acceleration of remote working arrangements caused by the COVID-19 pandemic and the increased use of remote control operations across many industries including mining, manufacturing, transport, education and health.
Historically, automation has replaced more routine physically demanding, dangerous or repetitive work in industries such as manufacturing, with little impact on professional and managerial occupations 1 . However, since the mid-2010s, automation has replaced many repetitive error-prone administrative tasks such as processing legal documents, directing service queries and employee selection screening 2 , 3 . Thus, work requirements for employees are increasingly encompassing tasks that cannot be readily automated, such as interpersonal negotiations and service innovations 4 : in other words, work that cannot be easily achieved through algorithms.
The role of motivation is often overlooked when designing and implementing technology in the workplace, even though technological changes can have a major impact on people’s motivation. Self-determination theory offers a useful multidimensional conceptualization of motivation that can help predict these impacts. According to self-determination theory 5 , 6 , three psychological needs must be fulfilled to adequately motivate workers and ensure that they perform optimally and experience well-being. Specifically, people need to feel that they are effective and masters of their environment (need for competence), that they are agents of their own behaviour as opposed to a ‘pawn’ of external pressures (need for autonomy), and that they experience meaningful connections with other people (need for relatedness) 5 , 7 . Meta-analytic evidence shows that satisfying these three needs is associated with better performance, reduced burnout, more organizational commitment and reduced turnover intentions 8 .
Self-determination theory also distinguishes between different types of motivation that workers might experience: intrinsic motivation (doing something for its own sake, out of interest and enjoyment), extrinsic motivation (doing something for an instrumental reason) and amotivation (lacking any reason to engage in an activity). Extrinsic motivation is subdivided according to the degree to which external influences are internalized (absorbed and transformed into internal tools to regulate activity engagement) 5 , 9 . According to meta-analytic evidence, more self-determined (that is, intrinsic or more internalized) motivation is more positively associated with key attitudinal and performance outcomes, such as job satisfaction, organizational commitment, job performance and proactivity than more controlled motivation (that is, extrinsic or less internalized) 10 . Consequently, researchers advocate the development and promotion of self-determined motivation across various life domains, including work 11 . Satisfaction of the three psychological needs described above is significantly related to more self-determined motivation 8 .
Given the impact of the needs proposed in self-determination theory on work motivation and consequently work outcomes (Fig. 1 ), it is important to find ways to satisfy these needs and avoid undermining them in the workplace. Organizational research has consequently focused on managerial and leadership behaviours that support or thwart these needs and promote different types of work motivation 12 , 13 , 14 , 15 , 16 , 17 , 18 , 19 , 20 , 21 , 22 , 23 (Fig. 2 ). There is also substantial research on the effects of work design (the nature and organization of people’s work tasks within a job or role, such as who makes what decisions, the extent to which people’s tasks are varied, or whether people work alone or in a team structure) and compensation systems on need satisfaction and work motivation 24 , 25 , 26 , 27 , 28 , 29 , 30 , 31 , 32 , 33 , 34 , 35 , 36 , 37 , and how individuals can seek to meet their needs and enhance their motivation through proactive efforts to craft their jobs 38 , 39 , 40 .
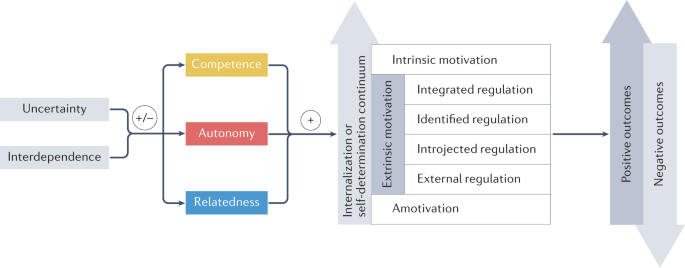
According to self-determination theory, satisfaction of three psychological needs (competence, autonomy and relatedness) influences work motivation, which influences outcomes. More intrinsic and internalized motivations are associated with more positive outcomes than extrinsic and less internalized motivations. These needs and motivations might be influenced by the increased uncertainty and interdependence that characterize the future of work.
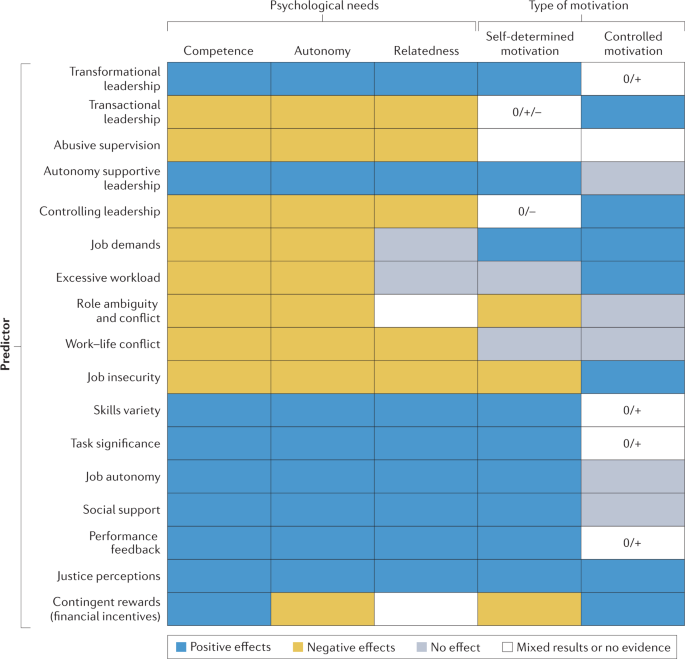
Summary of research findings 5 , 6 , 7 , 8 , 9 , 10 , 11 , 12 , 13 , 14 , 15 , 16 , 17 , 18 , 19 , 20 , 21 , 22 , 23 , 24 , 25 , 26 , 27 , 28 , 29 , 30 and available meta-analyses 8 , 10 . In cases where the evidence is mixed, a negative sign indicates a negative correlation, a positive sign indicates a positive correlation, and a zero indicates no statistically significant correlation.
Importantly, the work tasks that people are more likely to do in future work will require high-level cognitive and emotional skills that are more likely to be developed, used, and sustained when underpinned by self-determined motivation 40 , 41 , 42 , 43 , 44 , 45 , 46 , 47 , 48 , 49 , 50 . Therefore, if individuals are to be effective in future work, it is important to understand how future work might meet — or fail to meet — the psychological needs proposed by self-determination theory.
In this Review, we outline how work is changing and explain the consequences of these changes for satisfying workers’ psychological needs. We then focus on two areas where technology is already changing the worker experience: when workers apply for jobs and go through selection processes; and when the design of their work — what work they do, as well as how, when and where they do it — is transformed by technology. In particular, we focus on three domains where technology is already changing work design: remote work, virtual teams and algorithmic management. We conclude by discussing the importance of satisfying the psychological needs of workers when designing and implementing technologies in the workplace.
Future work requirements
The future workplace might evolve into one where psychological needs are better fulfilled, or one where they are neglected. In addition, there is growing concern that future work will meet the needs of people with adequate access to technology and the skills to use it, but will further diminish fulfillment for neglected and disadvantaged groups 51 (Box 1 ). To understand how future work might align with human needs, it is necessary to map key work features to core constructs of self-determination theory. Future work might be characterized by environmental uncertainty interdependence, complexity, volatility and ambiguity 52 . Here we focus on uncertainty and interdependence because these features capture core concerns about the future and its implication for connections among people in the changing context of work 53 . Higher levels of uncertainty require more adaptive behaviours, whereas higher levels of interdependence require more social, team-oriented and network-oriented behaviours 54 .
We first consider the increasing role of uncertainty in the workplace. Rapid changes in technology and global supply chains mean that the environment is more unpredictable and that there is increasing uncertainty about what activities are needed to be successful. Reducing uncertainty is central to most theories of human adaptation 55 and is a strong motivational basis for goals and behaviour 56 . If uncertainty becomes a defining and pervasive feature of organizational life, organizational leaders should think beyond reducing uncertainty and instead leverage and even create it 55 . In other words, in a highly dynamic context, it might be more functional and adaptive for employees and organizational leaders to consider more explorative approaches to coping with uncertainty, such as experimentation and improvization. All of these considerations imply that future effective work will require adaptive behaviours such as modifying the way work is done, and proactive behaviours such as innovating and creating new ways of working 54 .
Under higher levels of uncertainty, specific actions are difficult to define in advance. In contrast to action sequences that can be codified (for example, with algorithms) and repeated in predictable environments, the best action sequence is likely to involve flexibility and experimentation when the workplace is more uncertain. In this context, individuals must be motivated to explore new ideas, adjust their behaviour and engage with ongoing change. In stable and predictable environments, less self-determined forms of motivation might be sufficient to maintain the enactment of repetitive tasks and automation is more feasible as a replacement or support. However, under conditions of uncertainty, individuals will benefit from showing cognitive flexibility, creativity and proactivity, all behaviours that are more likely to emerge when people have self-determined motivation 40 , 41 , 44 , 46 , 47 , 48 , 49 , 57 .
Adaptive (coping with and responding to change) and proactive (initiating change) performance can be promoted by satisfying the needs for competence, autonomy and relatedness, and self-determined motivation 4 , 58 . For example, when individuals experience internalized motivation, they have a ‘reason to’ engage in the sometimes psychologically risky behaviour of proactivity 40 . Both adaptivity and proactivity depend on individuals having sufficient autonomy to work differently, try new ideas and negotiate multiple pathways to success. Hence, successful organizational functioning depends on people who can act autonomously to regulate their behaviour in response to a more unpredictable and changing environment 31 , 54 , 59 .
The second feature of the evolving workplace is an increasing level of interdependence among people, systems and technology. People will connect with each other in more numerous and complex ways as communication technologies become more reliable, deeply networked and faster. For example, medical teams from disparate locations might collaborate more easily in real time to support remote surgical procedures. They will also connect with automated entities such as cobots (robots that interact with humans) and decision-making aids supported by constantly updating algorithms. For example, algorithms might provide medical teams with predictive information about patient progress based on streaming data such as heart rate. As algorithms evolve in complexity and predictive accuracy, they will modify the work context and humans will need to adapt to work with the new information created 60 .
This interconnected and evolving future workplace requires individuals who can interact effectively across complex networks. The nature of different communication technologies can both increase and decrease feelings of relatedness depending on the extent to which they promote meaningful interactions. Typically, work technologies are developed to facilitate productivity and efficiency. However, given that human performance is also influenced by feelings of relatedness 8 , it is important to ensure that communication technologies and the way networks of people are managed by these technologies can fulfill this need.
The rapid growth of networks enabled by communication technologies (for example, Microsoft Teams, Slack and Webex) has produced positive and negative effects on performance and well-being. For example, these technologies can be a buffer against loneliness for remote workers or homeworkers 61 and enable stronger connections among distributed workers 62 . However, networking platforms lead some individuals to experience more isolation rather than more connectedness 63 . Workplace networks might also engender these contrasting effects by, for example, building a stronger understanding between individuals in a work group who do not usually get to interact or by limiting contact to more superficial communication that prevents individuals from building stronger relationships.
Both uncertainty and interdependence will challenge people’s feelings of competence. Uncertainty can lead to reduced access to predictable resources and less certainty about the success of work effort; the proliferation of networks and media can lead to feeling overwhelmed and to difficulties in managing communication and relationships. Moreover, technologies and automation can lead to the loss of human competencies as people stop using these skills 64 , 65 , 66 , 67 . For example, automating tasks that require humans to have basic financial skills diminishes opportunities for humans to develop expertise in financial skills.
Uncertainty and interdependence are likely to persist and increase in the future. This has implications for whether and how psychological needs will be satisfied or frustrated. In addition, because uncertainty and interdependence require people to behave in more adaptive and proactive ways, it is important to create future work that satisfies psychological needs.
Box 1 Inequalities caused by future work
Future work is likely to exacerbate inequalities. First, the digital divide (unequal access to, and ability to use, information communication technologies) 51 is likely to be exacerbated by technological advances that might become more costly and require more specialized skills. Moreover, the COVID-19 pandemic exacerbated work inequalities by providing better opportunities to those with digital access and skills 210 , 211 . The digital divide now also includes ‘algorithm awareness’ (knowing what algorithms do) which influences whether and how people are influenced by technology. Indeed, the degree to which algorithms influence attitudes and behaviours is negatively associated with the degree to which people are aware of algorithms and understand how they work 212 .
Second, future work is likely to require new technical and communication skills, as well as adaptive and proactive skills. Thus, people with such skills are more likely to find work than those who do not or who have fewer opportunities (for example, education access) to develop them. Even gig work requires that workers have access to relevant platforms and adequate skills for using them. These future work issues are therefore likely to increase gaps between skilled and non-skilled segments of the population, and consequently to increase societal pay disparities and poverty.
For example, workforce inequalities between mature and younger workers are likely to increase owing to real or perceived differences in technology-related skills, with increased disparities in the type of jobs these workers engage in 210 , 213 . Older workers might miss out on opportunities to upskill or might choose to leave the workforce early rather than face reskilling. This could decrease workforce diversity and strengthen negative stereotypes about mature workers (such as that they are not flexible, adaptable or motivated to keep up with changing times) 214 . Furthermore, inequalities in terms of pay have already been observed between men and women 215 . Increased robotization increases the gender pay gap 216 , and this gap is likely to be exacerbated as remote working becomes more common (as was shown during the pandemic) 217 . For example, one study found that salaries did not increase as much for women working flexibly compared to men 218 ; another study found that home workers tended to be employees with young children and these workers were 50% less likely to be promoted than those based in the office 140 .
To promote equality in future work and ensure that psychological needs are met, managers will need to adopt ‘meta-strategies’ to promote inclusivity (ensuring that all employees feel included in the workplace and are treated fairly, regardless of whether they are working remotely or not), individualization of work (ensuring that work is tailored to individual needs and desires) and employee integration (promoting interaction between employees of all ages, nationalities and backgrounds) 213 .
The future of employee selection
Changing economies are increasing demand for highly skilled labour, meaning that employers are forced to compete heavily for talent 68 . Meanwhile, technological developments, largely delivered online, have radically increased the reach, scalability and variety of selection methods available to employers 69 . Technology-based assessments also afford candidates the autonomy to interact with prospective employers at times and locations of their choosing 70 , 71 . Furthermore, video-based, virtual, gamified and AI-based assessment technologies 3 , 72 , 73 , 74 have improved the fidelity and immersion of the selection process. The fidelity of a selection assessment represents the extent to which it can reproduce the physical and psychological aspects of the work situation that the assessment is intended to simulate 75 . Virtual environments and video-based assessments can better reproduce working environments than traditional ‘paper and pencil’ assessments, and AI is being used to simulate social interactions in work or similar contexts 74 . Immersion represents how engrossing or absorbing an assessment experience is. Immersion is enhanced by richer media and gamified assessment elements 75 , 76 . These benefits have driven the widespread adoption of technology in recruitment practices 77 , but they have also attracted criticism. For example, the use of AI to analyse candidate data (such as CVs, social media profiles, text-based responses to interview questions, and videos) 78 raises concerns about the relevance of data being collected for selecting employees, transparency in how the data are used, and biases in selection based on these data 79 .
Candidates with a poor understanding of what data are being collected and how they are being used might experience a technology-based selection process as autonomy-thwarting. For example, the perceived job-relatedness of an assessment is associated with whether or not candidates view the assessment positively 69 , 80 . However, with today’s technology, assessments that appear typical or basic (such as a test or short recorded interview response) might also involve the collection of additional ‘trace’ data such as mouse movements and clicks (in the case of tests), or ancillary information such as ‘micro-expressions’ or candidates’ video backdrops 81 . We expect that it would be difficult for candidates to evaluate the job-relatedness of this information, unless provided with a rationale. Candidates may also feel increasing pressure to submit to employers’ requests to share personal information, such as social media profiles, which may further frustrate autonomy to the extent that candidates are reluctant to share this information 82 .
Furthermore, if candidates do not understand how technology-driven assessments work and are not able to receive feedback from assessment systems, their need for competence may be thwarted 83 . For example, initial research shows that people perceive fewer opportunities to demonstrate their strengths and capabilities in interviews they know will be evaluated by AI, compared to those evaluated by humans 83 .
Finally, because candidates are increasingly interacting with systems, rather than people, their opportunities to build relatedness with employers might be stifled. A notable exemplar is the use of asynchronous video interviews 70 , 71 , a type of video-based assessment where candidates log into an online system, are presented with a series of questions, and are asked to video-record their responses. Unlike a traditional or videoconference interview, candidates completing an asynchronous video interview do not interact directly with anyone from the employer organization, and they consequently often describe the experience as impersonal 84 . Absent any interventions, the use of asynchronous video interviews removes the opportunity for candidates to meet the employer and get a feel for what it might be like to work for the employer, or to ask questions of their own 84 .
Because technologies have changed rapidly, research on candidates’ reactions to these new selection methods has not kept up 69 . Nonetheless, to the extent that test-related and technology-related anxiety influences motivation and performance when completing an online assessment or a video interview, the performance of applicants might be adversely affected 85 . Furthermore, candidate experience can influence decisions to accept a job offer and how positively the candidate will talk about the organization to other potential candidates and even clients, thereby influencing brand reputation 86 . Thus, technology developments offer clear opportunities to improve the satisfaction of candidates’ needs and to assess them in richer environments that more closely resemble work settings. However, there are risks that technology that is needs-thwarting or is implemented in a needs-thwarting manner, will add to the uncertainty already inherent in competitive job applications. In the context of a globally competitive skills market, employers risk losing high-quality candidates.
The future of work design
Discussion in the popular press about the impact of AI and other forms of digitalization focuses on eradicating large numbers of jobs and mass unemployment. However, the reality is that tasks within jobs are being influenced by digitalization rather than whole jobs being replaced 87 . Most occupations in most industries have at least some tasks that could be replaced by AI, yet currently there is no occupation in which all tasks could be replaced 88 . The consequence of this observation is that people will need to increasingly interact with machines as part of their jobs. This raises work design questions, such as how people and machines should share tasks, and the consequences of different choices in this respect.
Work design theory is intimately connected to self-determination theory, with early scholars arguing that work arrangements should create jobs in which employees can satisfy their core psychological needs 89 . Core aspects of work design, including decision-making power, the opportunity to use skills and do a variety of tasks, the ability to ascertain the impact of one’s work, performance feedback 90 , social contact, time pressure, emotional demands and role conflict 91 are important predictors of job satisfaction, job performance 92 and work motivation 93 . Some evidence suggests that these motivating characteristics (considered ‘job resources’ according to the jobs demands–resources model) 94 are especially important for fostering motivation or reducing strain when job demands (aspects of a job that require sustained physical, emotional or mental effort) are high 93 , 95 . For example, autonomy and social support can reduce the effect of workload on negative outcomes such as exhaustion 96 .
Technology can potentially influence work design and therefore employee motivation in positive ways 1 . Increasing workers’ task variety and opportunities for more complex problem-solving should occur whenever technology takes over tasks (such as assembly line or mining work). Leaving the less routine and more interesting tasks for people to do 97 increases the opportunity for workers to fulfill their need for competence. For example, within manufacturing, complex production systems in which cyber-machines are connected in a factory-wide information network require strategic human decision-makers operating in complex, varied and high-level autonomy jobs 98 . Technology (such as social media) can also enhance social contact and support in some jobs and under some circumstances 86 , 87 (but see ref. 63 ), increasing opportunities for meeting relatedness needs.
However, new technologies can also undermine the design of motivating work, and thus reduce workers’ need satisfaction 1 . For example, in the aviation industry, manual flying skills can become degraded due to a lack of opportunity to practice when aircraft are highly automated 99 , decreasing the opportunity for pilots to meet their need for competence. As another example, technology has enabled the introduction of ‘microwork’ in which jobs are broken down into small tasks that are then carried out via information communication technologies 100 . Such jobs often lack variety, skill use and meaning 101 , again reducing the opportunity for the work to meet competence needs. In an analysis of robots in surgery, technology designed purely for ‘efficiency’ reduced the opportunities for trainee surgeons to engage in challenging tasks and resulted in impaired skill development 102 , and therefore probably reduced competence need satisfaction. Thus, poor work design might negatively influence work motivation through poor need satisfaction, especially the need for competence, owing to the lack of opportunity to maintain one’s skills or gain new ones 2 .
As the above examples show, the impact of new technologies on work design, and hence on need satisfaction, is powerful — but also mixed. That is, digital technologies can increase or decrease motivational work characteristics and can thereby influence need satisfaction (Fig. 3 ). The research shows that there is no deterministic relationship between technology and work design; instead, the effect of new technology on work design, and hence on motivation, depends on various moderating factors 1 . These moderating factors include individual aspects, such as the level of skill an individual has or the individual’s personality. Highly skilled individuals or those with proactive personalities might actively shape the technology and/or craft their work design to better meet their needs and increase their motivation 1 . For example, tech-savvy Uber drivers subject to algorithmic management sometimes resist or game the system, such as by cancelling rides to avoid negative ratings from passengers 103 .
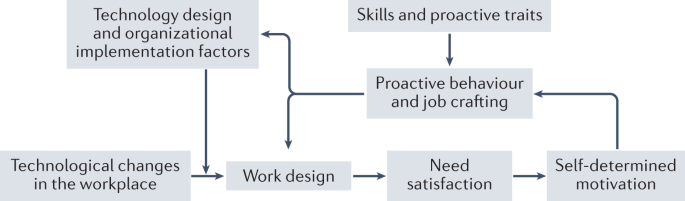
The causal relationships among the possible (but not exhaustive) variables implicated in the influence of technology on work design and work motivation discussed in this Review.
More generally, individuals proactively seek a better fit with their job through behaviours such as idiosyncratic deals (non-standard work arrangements negotiated between an employee and an employer) and job crafting (changing one’s work design to align one’s job with personal needs, goals and skills) 39 , 40 (Box 2 ). Consequently, although there is relatively little research on proactivity in work redesign through technology, it is important to recognize that individuals will not necessarily be passive in the face of negative technologies. Just as time pressure can stimulate proactivity 104 , we should expect that technology that creates poor work design will motivate job crafting and other proactive behaviours from workers seeking to meet their psychological needs better 105 . This perspective fits with a broader approach to technology that emphasizes human agency 106 .
Importantly, mitigating and managing the impact of technology on work is not the sole responsibility of individuals. Organizational implementation factors (for example, whether technology is selected, designed and implemented in a participatory way or how much training is given to support the introduction of technology) and technological design factors (for example, how much worker control is built into automated systems) are also fundamental in shaping the effect of technology on work design. Understanding these moderating factors is important because they provide potential ‘levers’ for creating more motivating work while still capitalizing on the advantages of technologies. For example, in one case study 107 , several new digital technologies such as cobots and digital paper flow (systems that integrate and automate different organizational functions, such as sales and purchasing with accounting, inventory control and dispatch) were implemented following a strong technocentric approach (that is, highly focused on engineering solutions) with little worker participation, and with limited attention to creating motivating work design. A more human-centred approach could have prevented the considerable negative outcomes that followed (including friction, reduced morale, loss of motivation, errors and impaired performance) 107 . Ultimately, how technology is designed and implemented should be proactively adapted to better meet human competencies, needs and values.
Box 2 The future of careers
Employment stability started to decline during the 1980s with the rise of public ownership and international trade, the increased use of performance-based incentives and contracts, and the introduction of new technologies. Employment stability is expected to continue to decline with the growth of gig work and continued technological developments 219 , 220 . Indeed, people will more frequently be asked to change career paths as work is transformed by technology, to use and ‘sell’ their transferrable skills in creative ways, and to reskill. The rise of more precarious work and new employment relationships (for example, in gig work) adds to these career challenges 221 . The current generation of workers is likely to experience career shocks (disruptive events that trigger a sensemaking process regarding one’s career) caused by rapid technological changes, and indeed many workers have already experienced career shocks from the pandemic 222 . Moreover, rapid technological change and increasing uncertainty pushes organizations to hire for skill sets rather than fitting people into set jobs, requiring people to be aware of their skills and to know how to market them.
In short, the careers of the current and future workforce will be non-linear and will require people to be more adaptive and proactive in crafting their career. For this reason, the concept of a protean career, whereby people have an adaptive and self-directed career, is likely to be increasingly important 223 . A protean career is a career that is guided by a search for self-fulfillment and is characterized by frequent learning cycles that push an individual into constant transformation; a successful protean career therefore requires a combination of adaptivity skills and identity awareness 224 , 225 . Adaptivity allows people to forge their career by using, or even creating, emerging opportunities. Having a solid sense of self helps individuals to make choices according to personal strengths and values. However, a protean career orientation might fit only a small segment of the labour market. Change-averse individuals might regard protean careers as career-destructive and the identity changes associated with a protean career might be regarded as stressful. In addition, overly frequent transitions might limit deep learning opportunities and achievements, and disrupt important support networks 221 .
Nonetheless, career-related adaptive and proactive behaviours can be encouraged by satisfying psychological needs. In fact, protean careers tend to flourish in environments that provide autonomy and allow for proactivity, with support for competence and learning 223 , 226 . Moreover, people have greater self-awareness when they feel autonomous. Indeed, self-awareness is a component of authenticity and mindfulness, both of which are linked to the satisfaction of the need for autonomy 227 , 228 . Thus, supporting psychological needs during training, development and career transitions is likely to assist people in crafting successful careers.
Applications
In what follows, we describe three specific cases where technology is already influencing work design (virtual and remote work, virtual teamwork, and algorithmic management), and consider the potential consequences for worker need satisfaction and motivation.
Virtual and remote work
Technologies have significantly altered when and where people can work, with the Covid-19 pandemic vastly accelerating the extent of working from home (Box 3 ). Remote work has persisted beyond the early stages of the Covid-19 pandemic with hybrid working — where people work from home some days a week and at the workplace on other days — becoming commonplace 108 . The development of information communication technologies (such as Microsoft Teams) has enabled workers to easily connect with colleagues, clients and patients remotely 105 , for example, via online patient ‘telehealth’ consultations, webinars and discussion forums. Technology has even enabled the remote control of other technologies, such as manufacturing machinery, vehicles and remote systems that monitor hospital ward patient vital signs through AI 1 . However, even when people are working on work premises (that is, not working remotely), an increasing amount of work in many jobs is done virtually (for example, online training or communicating with a colleague next door via email).
Working virtually is inherently tied to changes in uncertainty and interdependence. Virtual work engenders uncertainty because workplace and interpersonal cues are less available or reliable in providing virtual employees with role clarity and ensuring smooth interactions. Indeed, ‘screen’ interactions are more stressful and effortful than face-to-face interactions. It is more difficult to decipher and synchronize non-verbal behaviour on a screen than face-to-face, particularly given the lack of body language cues due to camera frame limitations, increasing the cognitive load for meeting attendees 109 , 110 , 111 , 112 . Non-verbal synchrony can be affected by the video streaming speed, which also increases cognitive load 109 , 110 , 111 , 112 . Virtual interactions involve ‘hyper gaze’ from seeing grids of staring faces, which the brain interprets as a threat 109 , 110 , 111 , 112 . Seeing oneself on screen increases self-consciousness during social interactions, which can cause anxiety, especially in women and those from minoritized groups 109 , 110 , 111 , 112 . Finally, reduced mobility from having to stay in the camera frame has been shown to reduce individual performance relative to face-to-face meetings 109 , 110 , 111 , 112 . Research on virtual interactions is still in its infancy. In one study, workers were randomly assigned to have their camera either on or off during their daily virtual meetings for a week. Those with the camera on during meetings experienced more daily fatigue and less daily work engagement than those with the camera off 113 .
Lower-quality virtual communication between managers and colleagues can leave individuals unclear about their goals and priorities, and how they should achieve them 114 . This calls for more self-regulation 115 because employees must structure their daily work activities and remind themselves of their work priorities and goals, without relying on the physical presence of colleagues or managers. If virtual workers must coordinate some of their work tasks with colleagues, it can be difficult to synchronize and coordinate actions, working schedules and breaks, motivate each other, and assist each other with timely information exchange 115 . This can make it harder for employees to acquire and share information 53 .
Virtual work also affects work design and changes how psychological needs can be satisfied and frustrated (Table 1 ), which has implications for both managers and employees. Physical workplace cues that usually guide work behaviours and routines in the office do not exist in virtual work, consequently demanding more autonomous regulation of work behaviours 116 , 117 . Some remote workers experience an increased sense of control and autonomy over their work environment 118 , 119 , 120 under these circumstances, resulting in lower family–work conflict, depression and turnover 121 , 122 . However, managers and organizations might rob workers of this autonomy by closely monitoring them, for example by checking their computer or phone usage 123 . This type of close monitoring reflects a lack of manager trust in individuals’ abilities or intentions to work effectively remotely. This lack of trust leads to decreased feelings of autonomy 124 , increased employee home–work conflict 105 and distress 125 , 126 . Surveillance has been shown to decrease self-determined motivation 127 . It is therefore important to train managers in managing remote workers in an autonomy-supportive way to avoid these negative consequences 128 . The negative effects of monitoring can also be reduced if monitoring is used constructively to help employees develop through feedback 129 , 130 , 131 , 132 , 133 , and when employees participate in the design and control of the monitoring systems 134 , 135 .
Information communication technology might satisfy competence needs by increasing access to global information and communication and the ability to analyse data 136 . For example, online courses, training and webinars can improve workers’ knowledge, skills and abilities, and can therefore help workers to carry out their work tasks more proficiently, which increases self-efficacy and a sense of competence. Furthermore, the internet allows people to connect rapidly and asynchronously with experts around the world, who may be able to provide information needed to solve a work problem that local colleagues cannot help with 136 . This type of remote work is increasingly occurring whether or not individuals themselves are based remotely, and can potentially enhance performance.
At the same time, technology might thwart competence needs, and increase fatigue and stress. For example, constant electronic messages (such as email or keeping track of online messaging platforms such as Slack or Microsoft Teams) are likely to increase in volume when working remotely, but can be distracting and prevent individuals from completing core tasks while they respond to incoming messages 136 . The frustration of the need for competence can increase if individuals are constantly switching tasks to deal with overwhelming correspondence and failing to finish tasks in a timely manner. In addition, information communication technology enables access to what some individuals might perceive as an overwhelming amount of information (for example, through the internet, email and messages) which can lead to a lot of time spent sifting and processing information. This can be interpreted as a job demand that might make individuals feel incompetent if it is not clear what information is most important. Individuals might also require training in the use of information communication technology, and even then, technology can malfunction, preventing workers from completing tasks, and causing frustration and distress 136 , 137 .
Finally, remote workers can suffer from professional isolation because there are fewer opportunities to meet or be introduced to connections that enable career development and progression 138 , which could influence their feelings of competence in the long run. Although some research suggests that those who work flexibly are viewed as less committed to their career 139 and might be overlooked for career progression 140 , other research has found no relationship between remote working and career prospects 119 .
Virtual work can also present challenges for meeting workers’ need for relatedness 141 . Remote workers can feel isolated from, and excluded by, colleagues and fail to gain the social support they might receive if co-located 142 , 143 , weakening their sense of belonging to a team or organization 144 and their job performance 145 . This effect will probably be accentuated in the future: if the current trend for working from home continues, more people will be dissociated from office social environments more often and indefinitely. Office social environments could be degraded permanently if fewer people frequent the office on a daily basis, such that workers may not be in the office at the same time as collaborators, and there might be fewer people to ask for help or talk with informally. We do not yet know the long-term implications of a degraded social environment, but some suggest that extended virtual working could create a society where people have poor communication skills and in which social isolation and anxiety are exacerbated 146 . Self-determination theory suggests that it will be critical to actively design hybrid and remote work that meets relatedness needs to prevent these long-term issues. When working remotely, simple actions could be effective, such as actively providing opportunities for connecting with others, for example, through ‘virtual coffee breaks’ 147 . Individuals could also be ‘buddied’ up into pairs who regularly check in with each other via virtual platforms.
Hybrid work seems to offer the best of both worlds, providing opportunities for connection and collaboration while in the workplace, and affording autonomy in terms of flexible working. Some research suggests that two remote workdays a week provides the optimum balance 148 . However, it is likely that this balance will be affected by individual characteristics and desires, as well as by differences in work roles and goals. For example, Israeli employees with autism who had to work from home during the COVID-19 pandemic experienced significantly lower competence and autonomy satisfaction than before the pandemic 149 . Yet remote workers high in emotional stability and job autonomy reported higher autonomy and relatedness satisfaction compared to those with low emotional stability 120 . These findings suggest that managers and individuals should consider the interplay between individual characteristics, work design and psychological need satisfaction when considering virtual and remote work.
Box 3 The ‘great resignation’
‘The great resignation’ refers to the massive wave of employee departures during the COVID-19 pandemic in several parts of the world, including North America, Europe and China 229 , 230 , that can be attributed in part to career shocks caused by the pandemic 222 . In the healthcare profession, the shock consisted of an exponential increase in workload and the resulting exhaustion, coupled with the disorganization caused by lack of resources and compounded by health fears 231 . In other industries, the pandemic caused work disruptions by forcing or allowing people to work from home, furloughing employees for varying periods of time, or lay-offs caused by an abrupt loss of business (such as in the tourism and hospitality industries).
Scholars have speculated that these shocks have resulted in a staggering number of people not wanting to go back to work or quitting their current jobs 232 . For example, the hospitality and tourism industries failed to attract employees back following lay-offs 233 . Career shocks can trigger a sensemaking process that can lead one to question how time is spent at work and the benefits one draws from it. For example, the transition to working from home made employees question how and why they work 234 . Frequent health and financial concerns, juggling school closures and complications in caring for dependents have compounded exhaustion and disorganization issues. Some have even renamed ‘the great resignation’ as ‘the great discontent’ to highlight that many people reported wanting to quit because of dissatisfaction with their work conditions 235 .
It might be helpful to understand ‘the great resignation’ through the lens of basic psychological need satisfaction. Being stretched to the limit might influence the need for competence and relatedness when workers feel they have suboptimal ways to connect with colleagues and insufficient time to balance work with other life activities that connect them to family and friends 128 , 236 . The sensemaking process that accompanies career shocks might highlight a lack of meaningful work that decreases the satisfaction of the need for autonomy. This lack of need satisfaction might lead people to take advantage of the disruption to ‘cut their losses’ by reorienting their life priorities and career goals, leading to resignation from their current jobs 237 , 238 .
Alternatively, the experiences gained from working differently during the COVID-19 pandemic might have made many workers aware of how work could be (for example, one does not have to commute), emboldening them to demand better work design and work conditions for themselves. Not surprisingly, barely a year after ‘the great resignation’ many are now talking about ‘the great reshuffle’, suggesting that many people who quit their jobs used this time to rethink their careers and find more satisfying work 239 . Generally, this has meant getting better pay and seeking work that aligns better with individual values and that provides a better work–life balance: in other words, work that better meets psychological needs for competence, autonomy and relatedness.
Virtual teamwork
Uncertainty and interconnectedness make work more complex, increasing the need for teamwork across many industries 150 . Work teams are groups of individuals that must both collaborate and work interdependently to achieve shared objectives 151 . Technology has created opportunities to develop work teams that operate virtually. Virtual teams are individuals working interdependently towards a common goal but who are geographically dispersed and who rely on electronic technologies to perform their work 152 , 153 . Thus, virtual teamwork is a special category of virtual work that also involves collective psychological experiences (that are shaped by and interact with virtual work) 154 . This adds another layer of complexity and therefore requires a separate discussion.
Most research conceptualizes team virtuality as a construct with two dimensions: geographical dispersion and reliance on technology 153 , 155 . Notably, these dimensions are not completely independent because team members require technology to communicate and coordinate tasks when working in different locations 156 , 157 . Virtuality differs between and within teams. Team members might be in different locations on some days and the same location on other days, which changes the level of team virtuality over time. Thus, teams are not strictly virtual or non-virtual. Team virtuality influences how team members coordinate tasks and share information 130 , which is critical for team effectiveness (usually assessed by a team’s tangible outputs, such as their productivity, and team member reactions, such as satisfaction with, or commitment to, the team) 158 .
Although individual team members might react differently to working in a virtual team, multi-level theory suggests that team members collectively develop shared experiences, called team emergent states 159 , 160 . Team emergent states include team cohesion (the bond among group members) 161 , team trust 162 , and team motivation and engagement 159 , 163 . These emergent states arise out of individual psychological behaviours and states 164 and are influenced by factors that are internal (for example, interactions between team members) and external (for example, organizational team rewards, organizational leadership and project deadlines) to the team, as well as team structure (for example, team size and composition). Team emergent states, particularly team trust, are critical for virtual team effectiveness because reliance on technology often brings uncertainties and fewer opportunities for social control 165 .
Team virtuality is likely to affect team functioning via its impact on psychological need satisfaction, in a fashion similar to remote work. However, the need for coordination and information sharing to achieve team goals is likely to be enhanced by how team members support and satisfy each other’s psychological needs 166 , which might be more difficult under virtual work conditions. In addition to affecting individual performance, need satisfaction within virtual teams can also influence collective-level team processes, such as coordination and trust, which ultimately affect team performance. For example, working in a virtual team might make it more difficult to feel meaningful connections because team members in different locations often have less contact than co-located team members. Virtual team members predominantly interact via technology, which — as described in the previous section — might influence the quality of relationships they can develop with their team members 141 , 167 , 168 and consequently the satisfaction of relatedness needs 169 .
Furthermore, virtual team members must master electronic communication technology (including virtual meeting and breakout rooms, internet connectivity issues, meeting across different time zones, and email overload), which can lead to frustrations and ‘technostress’ 170 . Frustrations with electronic communication might diminish the psychological need for competence because team members might feel ineffective in mastering their environment.
In sum, virtual team members might experience lower relatedness and competence need satisfaction. However, these needs are critical determinants of work motivation. Furthermore, virtual team members can also develop shared collective experiences around their need satisfaction. Thus, self-determination theory offers explanatory mechanisms (that is, team members’ need satisfaction, which influences work motivation) that are at play in virtual teams and that organizations should consider when implementing virtual teams.
Algorithmic management
Algorithmic management refers to the use of software algorithms to partially or completely execute workforce management functions (for example, hiring and firing, coordinating work, and monitoring performance) 2 , 123 , 171 , 172 . This phenomenon first appeared on gig economy platforms such as Uber, Instacart and Upwork, where all management is automated 173 . However, it is rapidly spreading to traditional work settings. Examples include monitoring the productivity, activity and emotions of remote workers 174 , the algorithmic determination of truck drivers’ routes and time targets 175 , and automated schedule creation in retail settings 176 . The constant updating of the algorithms as more data is collected and the opacity of this process makes algorithmic management unpredictable, which produces more uncertainty for workers 177 .
Algorithmic management has repercussions for work design. Specifically, whether algorithmic management systems consider human motivational factors in their design influences whether workers are given enough autonomy, skills usage, task variety, social contact, role clarity (including knowing the impact of one’s work) and a manageable workload 123 . So far, empirical evidence show that algorithmic management features predominantly reduce employees’ basic needs for autonomy, competence and relatedness because of how they influence work design (Fig. 4 ).
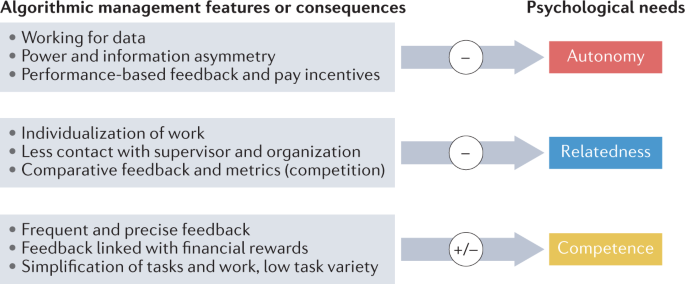
Summary of the features and consequences of algorithmic management on autonomy needs, relatedness needs and competence needs.
Algorithmic management tends to foster the ‘working-for-data’ phenomenon (or datafication of work) 172 , 178 , 179 , leading workers to focus their efforts on aspects of work that are being monitored and quantified at the expense of other tasks that might be more personally valued or meaningful. This tendency is reinforced by the fact that algorithms are updated with new incoming data, increasing the need for workers to pay close attention to what ‘pays off’ at any given moment. Monitoring and quantifying worker behaviours might reduce autonomy because it is experienced as controlling and narrows goal focus to only quantifiable results 127 , 180 ; there is some evidence that this is the case when algorithmic management systems are used to this end 172 , 178 , 181 . Rigid rules about how to carry out work often determine performance ratings (for example, imposing a route to deliver goods or prescribing how equipment and materials must be used) and even future task assignments and firing decisions, with little to no opportunity for employee input 182 , 183 , 184 . Thus, the combination of telling workers what to do to reach performance targets and how to get it done significantly limits their autonomy to make decisions based on their knowledge and skills.
Some algorithmic management platforms do not reveal all aspects of a given task (for example, not revealing the client destination before work is accepted) or penalize workers who decline jobs 185 , thereby severely restricting their choices. This encourages workers to either overwork to the point of exhaustion, find ways to game the system 184 , or misbehave 186 . Moreover, the technical complexity and opacity of algorithmic systems 187 , 188 , 189 deprives workers of the ability to understand and master the system that governs their work, which limits their voice and enpowerment 172 , 185 , 190 . Workers’ typical response to the lack of transparency is to organize themselves on social media to share any insights they have on what the algorithm ‘wants’ as a way to gain back some control over their work 183 , 191 .
Finally, algorithmic management usually provides comparative feedback (comparing one’s results to other workers’) and is linked to incentive pay structures, both of which reduce self-determined motivation as they are experienced as more controlling 26 , 192 . For instance, after algorithms estimated normal time standards for each ‘act’, algorithmic tracking and case allocation systems forced homecare nurses to reduce the ‘social’ time spent with patients because they were assigned more patients per day, thereby limiting nurses’ autonomy to decide how to perform their work 181 . Because these types of quantified metric are often directly linked to performance scores, pay incentives and future allocation of tasks or schedules (that is, getting future work), algorithmic management reduces workers’ freedom in decision-making related to their work, which can significantly reduce their self-determined motivation 123 .
Algorithmic management also tends to individualize work, which affects the need for relatedness. For example, algorithmic management inevitably transforms or reduces (sometimes even eliminates) contact with a supervisor 2 , 182 , 193 , leading to the feeling that the organization does not care about the worker and provides little social support 194 , 195 . ‘App-workers’, who obtain work through gig-work platforms such as Uber, reportedly crave more social interactions and networking opportunities 179 , 185 , 194 and often attempt to compensate for a lack of relatedness by creating support groups that connect virtually and physically 183 , 191 , 195 . Increased competitive climates due to comparative feedback or displaying team members’ individual rankings 175 , 196 can also hamper relatedness. Indeed, when workers have to compete against each other to rank highly (which influences their chances of getting future work and the financial incentives they receive), they are less likely to develop trusting and supportive relationships.
Researchers have formulated contradictory predictions about the potential implications of algorithmic management on competence satisfaction. On the one hand, using quantified metrics, algorithmic management systems can provide more frequent, unambiguous and performance-related feedback, often in the form of ratings and rankings 177 , and simultaneously link this feedback to financial rewards. Informational feedback can enhance intrinsic motivation because it provides information about one’s competence. At the same time, linking rewards to this feedback could decrease intrinsic motivation, because the contingency between work behaviour and pay limits worker discretion and therefore reduces their autonomy 26 . The evidence so far suggests that the mostly comparative feedback provided by algorithmic management is insufficiently informative because the value of the feedback is short-lived — continuously updating algorithms change what is required to perform well 177 , 183 , 185 . This short-lived feedback can undermine feelings of mastery or competence. In addition, algorithmic management is often associated with simplified tasks, and with lower problem-solving opportunities and job variety 123 . However, gamification features on some platforms might increase intrinsic motivation 179 , 183 .
The nascent research on the effects of algorithmic management on workers’ motivation indicates mostly negative effects on self-determined forms of motivation, because the way it is designed decreases the satisfaction of competence, autonomy and relatedness needs. Algorithmic management is being rapidly adopted across an increasing number of industries. Thus, technology developers and those who implement the technology in organizations will need to pay closer attention to how it changes work design to avoid negative effects on work motivation.
Summary and future directions
Self-determination theory can help predict the motivational consequences of future work and these motivational considerations should be taken into account when designing and implementing technology. More self-determined motivation will be needed to deal with the uncertainty and interdependence that will characterize future work. Thus, research examining how need satisfaction and work motivation influence people’s ability to adapt to uncertainty, or even leverage it, is needed. For example, future research could examine how different managerial styles influence adaptivity and proactivity in highly uncertain work environments 197 . Need-satisfying leadership, such as transformational leadership (charismatic or inspirational) 15 , can encourage job crafting and other proactive work behaviours 198 , 199 . Transactional leadership (focused on monitoring, rewarding and sanctioning) might promote self-determined motivation during organizational crises 23 . In addition, research on the quality of interconnectedness (the breadth and depth of interactions and networks) could provide insight on how to manage the increased interconnectedness workers are experiencing.
Technology can greatly assist in recruiting and selecting workers; self-determination theory can inform guidelines on how to design and use such technologies. It is important that the technology is easy to use and perceived as useful to the candidates for best representing themselves 200 , 201 . This can be done by ensuring that candidates have complete instructions before an assessment starts, even possibly getting a ‘practice run’, to improve their feelings of competence. It is also important for candidates to feel some amount of control and less pressure associated with online asynchronous assessments. Giving candidates some choice over testing platforms and the order of questions or settings, explaining how the results will be used, or allowing candidates to ask questions, could improve feelings of autonomy 70 . Finally, it is crucial to enhance perceptions that the organization cares about getting to know candidates and forging connections with them despite using these tools. For example, enhancing these tools with personalized videos of organizational members and providing candidates with feedback following selection decisions might increase feelings of relatedness. These suggestions need to be empirically tested 202 .
More research is also needed on how technology is transforming work design, and consequently influencing worker need satisfaction and motivation. Research in behavioural health has examined how digital applications that encourage healthy behaviours can be designed to fulfill the needs for competence, autonomy and relatedness 203 . Whether and how technology designed for other purposes (such as industrial robots, information communication technology, or automated decision-making systems) can be deliberately designed to meet these core human needs remains an open question. To date, little research has examined how work technologies are created, and what can be done to influence the process to create more human-centred designs. Collaborative research across social science and technical disciplines (such as engineering and computing) is needed.
In terms of implementation, although there is a long history of studies investigating the impact of technology on work design, current digital technologies are increasingly autonomous. This situation presents new challenges: a human-centred approach to automation in which the worker has transparent influence over the technical system has frequently been recommended as the optimal way to achieve high performance and to avoid automation failures 1 , 204 . But it is not clear that this work design strategy will be equally effective in terms of safety, productivity and meeting human needs when workers can no longer understand or control highly autonomous technology.
Given the likely persistence of virtual and remote work into the future, there is a critical need to understand how psychological needs can be satisfied when working remotely. Multi-wave studies that explore the boundary conditions of need satisfaction would advance knowledge around who is most likely to experience need satisfaction, when and why. Such knowledge can be leveraged to inform the design of interventions, such as supervisor training, to improve well-being and performance outcomes for virtual and remote workers. Similarly, no research to date has used self-determination theory to better understand how team virtuality affects how well team members support each other’s psychological needs. Within non-virtual teams, need satisfaction is influenced by the extent to which team members exhibit need-supportive behaviours towards each other 205 . For example, giving autonomy and empowering virtual teams is crucial for good team performance 206 . Studies that track team activities and interaction patterns, including virtual communication records, over time could be used to examine the effects of need support and thwarting between virtual team members 207 , 208 .
Finally, although most studies have shown negative effects of algorithmic management on workers’ motivation and work design characteristics, researchers should not view the effects of algorithmic management as predetermined and unchangeable. Sociotechnical aspects of the system 2 , 209 (such as transparency, privacy, accuracy, invasiveness and human control) and organizational policies surrounding their use could mitigate the motivational effects of algorithmic management. In sum, it is not algorithms that shape workers’ motivation, but how organizations design and use them 3 . Given that applications that use algorithmic management are developed mostly by computer and data scientists, sometimes with input from marketing specialists 185 , organizations would benefit from employing psychologists and human resources specialists to enhance the motivational potential of these applications.
Parker, S. K. & Grote, G. Automation, algorithms, and beyond: why work design matters more than ever in a digital world. Appl. Psychol . https://doi.org/10.1111/apps.12241 (2020).
Article PubMed PubMed Central Google Scholar
Jarrahi, M. H. et al. Algorithmic management in a work context. Big Data Soc . 8 , https://doi.org/10.1177/20539517211020332 (2021).
Langer, M. & Landers, R. N. The future of artificial intelligence at work: a review on effects of decision automation and augmentation on workers targeted by algorithms and third-party observers. Comput. Hum. Behav. 123 , 106878 (2021).
Article Google Scholar
Gagné, M., Parker, S. K. & Griffin, M. A. in Research Agenda for Employee Engagement in a Changing World of Work (eds Meyer, J. P. & Schneider, B.) 137–153 (Edward Elgar, 2021).
Deci, E. L. & Ryan, R. M. Intrinsic motivation and Self-Determination in Human Behavior (Plenum, 1985).
Gagné, M. & Deci, E. L. Self-determination theory and work motivation. J. Organ. Behav. 26 , 331–362 (2005).
Deci, E. L. & Ryan, R. M. The ‘what’ and ‘why’ of goal pursuits: human needs and the self-determination of behavior. Psychol. Inq. 11 , 227–268 (2000).
Van den Broeck, A., Ferris, D. L., Chang, C.-H. & Rosen, C. C. A review of self-determination theory’s basic psychological needs at work. J. Manag. 42 , 1195–1229 (2016).
Google Scholar
Howard, J., Gagné, M. & Morin, A. J. S. Putting the pieces together: reviewing the structural conceptualization of motivation within SDT. Motiv. Emot. 44 , 846–861 (2020).
Van den Broeck, A., Howard, J. L., Van Vaerenbergh, Y., Leroy, H. & Gagné, M. Beyond intrinsic and extrinsic motivation: a meta-analysis on self-determination theory’s multidimensional conceptualization of work motivation. Organ. Psychol. Rev. 11 , 240–273 (2021).
Ryan, R. M. & Deci, E. L. Self-Determination Theory: Basic Psychological Needs in Motivation, Development, and Wellness (Guilford, 2017).
Charbonneau, D., Barling, J. & Kelloway, E. K. Transformational leadership and sports performance: the mediating role of intrinsic motivation. J. Appl. Soc. Psychol. 31 , 1521–1534 (2001).
Eyal, O. & Roth, G. Principals’ leadership and teachers’ motivation: self-determination theory analysis. J. Educ. Adm. 49 , 256–275 (2011).
Fernet, C., Trépanier, S.-G., Austin, S., Gagné, M. & Forest, J. Transformational leadership and optimal functioning at work: on the mediating role of employees’ perceived job characteristics and motivation. Work Stress 29 , 11–31 (2015).
Hetland, H., Hetland, J., Andreassen, C. S., Pallessen, S. & Notelaers, G. Leadership and fulfillment of the three basic psychological needs at work. Career Dev. Int. 16 , 507–523 (2011).
Kovajnic, S., Schuh, S. C. & Jonas, K. Transformational leadership and performance: an experimental investigation of the mediating effects of basic needs satisfaction and work engagement. J. Occup. Organ. Psychol. 86 , 543–555 (2013).
Kovajnic, S., Schuh, S. C., Klaus, J., Quaquebeke, N. & Dick, R. How do transformational leaders foster positive employee outcomes? A self-determination-based analysis of employees’ needs as mediating links. J. Organ. Behav. 33 , 1031–1052 (2012).
Lian, H., Lance Ferris, D. & Brown, D. J. Does taking the good with the bad make things worse? How abusive supervision and leader–member exchange interact to impact need satisfaction and organizational deviance. Organ. Behav. Hum. Decis. Process. 117 , 41–52 (2012).
Slemp, G. R., Kern, M. L., Patrick, K. J. & Ryan, R. M. Leader autonomy support in the workplace: a meta-analytic review. Motiv. Emot. 42 , 706–724 (2018).
Tims, M., Bakker, A. B. & Xanthopoulou, D. Do transformational leaders enhance their followers’ daily work engagement? Leadersh. Q. 22 , 121–131 (2011).
Wang, Z. N. & Gagné, M. A Chinese–Canadian cross-cultural investigation of transformational leadership, autonomous motivation and collectivistic value. J. Leadersh. Organ. Stud. 20 , 134–142 (2013).
Bono, J. E. & Judge, T. A. Self-concordance at work: understanding the motivational effects of transformational leaders. Acad. Manage. J. 46 , 554–571 (2003).
Gagné, M. et al. Uncovering relations between leadership perceptions and motivation under different organizational contexts: a multilevel cross-lagged analysis. J. Bus. Psychol. 35 , 713–732 (2020).
Cerasoli, C. P., Nicklin, J. M. & Ford, M. T. Intrinsic motivation and extrinsic incentives jointly predict performance: a 40-year meta-analysis. Psychol. Bull. 140 , 980–1008 (2014).
Article PubMed Google Scholar
Cerasoli, C. P., Nicklin, J. M. & Nassrelgrgawi, A. S. Performance, incentives, and needs for autonomy, competence, and relatedness: a meta-analysis. Motiv. Emot. 40 , 781–813 (2016).
Deci, E. L., Koestner, R. & Ryan, R. M. A meta-analytic review of experiments examining the effects of extrinsic rewards on intrinsic motivation. Psychol. Bull. 125 , 627–668 (1999).
Kuvaas, B., Buch, R., Gagné, M., Dysvik, A. & Forest, J. Do you get what you pay for? Sales incentives and implications for motivation and changes in turnover intention and work effort. Motiv. Emot. 40 , 667–680 (2016).
Kuvaas, B., Shore, L. M., Buch, R. & Dysvik, A. Social and economic exchange relationships and performance contingency: differential effects of variable pay and base pay. Int. J. Hum. Resour. Manag. 31 , 408–431 (2020).
Nordgren Selar, A., Sverke, M., Falkenberg, H. & Gagné, M. It’s [not] all’bout the money: the relative importance of performance-based pay and support for psychological needs for job performance. Scand. J. Work Organ. Psychol. 5 , 1–14 (2020).
Olafsen, A. H., Halvari, H., Forest, J. & Deci, E. L. Show them the money? The role of pay, managerial need support, and justice in a self-determination theory model of intrinsic work motivation. Scand. J. Psychol. 56 , 447–457 (2015).
Parker, S. K., Wall, T. D. & Jackson, P. R. ‘That’s not my job’: developing flexible employee work orientations. Acad. Manage. J. 40 , 899–929 (1997).
Parker, S. L., Bell, K., Gagné, M., Carey, K. & Hilpert, T. Collateral damage associated with performance-based pay: the role of stress appraisals. Eur. J. Work Organ. Psychol. 28 , 691–707 (2019).
Thibault Landry, A., Forest, J., Zigarmi, D., Houson, D. & Boucher, É. The carrot or the stick? Investigating the functional meaning of cash rewards and their motivational power according to self-determination theory. Compens. Benefits Rev. 49 , 9–25 (2017).
Thibault Landry, A., Forest, J. & Zigarmi, D. Revisiting the use of cash rewards in the workplace: evidence of their differential impact on employees’ experiences in three samples using self-determination theory. Compens. Benefits Rev. 51 , 92–111 (2019).
Van den Broeck, A., Vansteenkiste, M., De Witte, H. & Lens, W. Explaining the relationships between job characteristics, burnout, and engagement: the role of basic psychological need satisfaction. Work Stress 22 , 277–294 (2008).
Weibel, A., Rost, K. & Osterloh, M. Pay for performance in the public sector — benefits and (hidden) costs. J. Public Adm. Res. Theory 20 , 387–412 (2010).
White, M. H. & Sheldon, K. M. The contract year syndrome in the NBA and MLB: a classic undermining pattern. Motiv. Emot. 38 , 196–205 (2014).
Bakker, A. B. & van Woerkom, M. Flow at work: a self-determination perspective. Occup. Health Sci. 1 , 47–65 (2017).
Tims, M., Bakker, A. B. & Derks, D. Development and validation of the job crafting scale. J. Vocat. Behav. 80 , 173–186 (2012).
Parker, S. K., Bindl, U. K. & Strauss, K. Making things happen: a model of proactive motivation. J. Manag. 36 , 827–856 (2010).
Amabile, T. M. Effects of external evaluations on artistic creativity. J. Pers. Soc. Psychol. 37 , 221–233 (1979).
Amabile, T. M. Motivation and creativity: effects of motivational orientation on creative writers. J. Pers. Soc. Psychol. 48 , 393–399 (1985).
Amabile, T. M., Hennessey, B. A. & Grossman, B. S. Social influences on creativity: the effects of contracted-for reward. J. Pers. Soc. Psychol. 50 , 14–23 (1986).
Boggiano, A. K., Flink, C., Shields, A., Seelbach, A. & Barrett, M. Use of techniques promoting students’ self-determination: effects on students’ analytic problem-solving skills. Motiv. Emot. 17 , 319–336 (1993).
Fabes, R. A., Moran, J. D. & McCullers, J. C. The hidden costs of reward and WAIS subscale performance. Am. J. Psychol. 94 , 387–398 (1981).
Koestner, R., Ryan, R. M., Bernieri, F. & Holt, K. Setting limits on children’s behavior: the differential effects of controlling vs informational styles on intrinsic motivation and creativity. J. Pers. 52 , 231–248 (1984).
McGraw, K. O. in The Hidden Costs of Reward (eds Lepper. M. R. & Greene, D.) 33–60 (Erlbaum, 1978).
McGraw, K. O. & McCullers, J. C. Evidence of a detrimental effect of extrinsic incentives on breaking a mental set. J. Exp. Soc. Psychol. 15 , 285–294 (1979).
Utman, C. H. Performance effects of motivational state: a meta-analysis. Personal. Soc. Psychol. Rev. 1 , 170–182 (1997).
Vansteenkiste, M., Simons, J., Lens, W., Sheldon, K. M. & Deci, E. L. Motivating, learning, performance, and persistence: the synergistic effects of intrinsic goal contents and autonomy-supportive contexts. J. Pers. Soc. Psychol. 87 , 246–260 (2004).
Van Dijk, J. The Digital Divide (Wiley, 2020).
Latham, G. P. & Ernst, C. T. Keys to motivating tomorrow’s workforce. Hum. Resour. Manag. Rev. 16 , 181–198 (2006).
Yang, L. et al. The effects of remote work on collaboration among information workers. Nat. Hum. Behav . 6 , 43–54 (2022).
Griffin, M. A., Neal, A. & Parker, S. K. A new model of work role performance: positive behavior in uncertain and interdependent contexts. Acad. Manage. J. 50 , 327–347 (2007).
Griffin, M. A. & Grote, G. When is more uncertainty better? A model of uncertainty regulation and effectiveness. Acad. Manage. Rev. 45 , 745–765 (2020).
Van Den Bos, K. & Lind, E. A. in Handbook of the Uncertain Self (eds Arkin, R. M., Oleson, K. C. & Carroll, P. J.) 122–141 (Routledge, 2013).
Amabile, T. M. The social psychology of creativity: a componential conceptualization. J. Pers. Soc. Psychol. 45 , 357–376 (1983).
Gagné, M., Forest, J., Vansteenkiste, M., Crevier-Braud, L. & Broeck, A. The multidimensional work motivation scale: validation evidence in seven languages and nine countries. Eur. J. Work Organ. Psychol. 24 , 178–196 (2015).
Wall, T. D. & Jackson, P. R. in The Changing Nature of Work (ed. Howard, A.) 139–174 (Jossey-Bass, 1995).
Sturm, T. et al. Coordinating human and machine learning for effective organizational learning. MIS Q. 45 , 1581–1602 (2021).
Hislop, D. et al. Variability in the use of mobile ICTs by homeworkers and its consequences for boundary management and social isolation. Inf. Organ. 25 , 222–232 (2015).
Kellogg, K. C., Orlikowski, W. J. & Yates, J. Life in the trading zone: structuring coordination across boundaries in postbureaucratic organizations. Organ. Sci. 17 , 22–44 (2006).
Lisitsa, E. et al. Loneliness among young adults during covid-19 pandemic: the mediational roles of social media use and social support seeking. J. Soc. Clin. Psychol. 39 , 708–726 (2020).
Bhardwaj, S., Bhattacharya, S., Tang, L. & Howell, K. E. Technology introduction on ships: the tension between safety and economic rationality. Saf. Sci. 115 , 329–338 (2019).
Rani, U. & Furrer, M. Digital labour platforms and new forms of flexible work in developing countries: algorithmic management of work and workers. Compet. Change 25 , 212–236 (2021).
Schörpf, P., Flecker, J., Schönauer, A. & Eichmann, H. Triangular love–hate: management and control in creative crowdworking. N. Technol. Work Employ. 32 , 43–58 (2017).
World Economic Forum. The future of jobs: employment, skills and workforce strategy for the fourth industrial revolution. WEF https://www.weforum.org/reports/the-future-of-jobs (2016).
World Economic Forum. The future of jobs report 2020. WEF https://www.weforum.org/reports/the-future-of-jobs-report-2020 (2020).
Woods, S. A., Ahmed, S., Nikolaou, I., Costa, A. C. & Anderson, N. R. Personnel selection in the digital age: a review of validity and applicant reactions, and future research challenges. Eur. J. Work Organ. Psychol. 29 , 64–77 (2020).
Lukacik, E.-R., Bourdage, J. S. & Roulin, N. Into the void: a conceptual model and research agenda for the design and use of asynchronous video interviews. Hum. Resour. Manag. Rev . 32 , 100789 (2020).
Dunlop, P. D., Holtrop, D. & Wee, S. How asynchronous video interviews are used in practice: a study of an Australian-based AVI vendor. Int. J. Sel. Assess . https://doi.org/10.1111/ijsa.12372 (2022).
Armstrong, M. B., Ferrell, J. Z., Collmus, A. B. & Landers, R. N. Correcting misconceptions about gamification of assessment: more than SJTs and badges. Ind. Organ. Psychol. 9 , 671–677 (2016).
Armstrong, M. B. Landers, R. N. & Collmus, A. B. in Emerging Research and Trends in Gamification (eds Gangadharbatla, H. & Davis, D. Z.) 140–165 (IGI Global, 2016).
Kotlyar, I. & Krasman, J. Virtual simulation: new method for assessing teamwork skills. Int. J. Sel. Assess . https://doi.org/10.1111/ijsa.12368 (2021).
Alexander, A. L., Brunyé, T. T., Sidman, J. & Weil, S. A. From Gaming to Training: A Review of Studies on Fidelity, Immersion, Presence, and Buy-in and Their Effects on Transfer in PC-Based Simulations and Games (DARWARS Training Impact Group, 2005).
Buil, I., Catalán, S. & Martínez, E. Understanding applicants’ reactions to gamified recruitment. J. Bus. Res. 110 , 41–50 (2020).
Basch, J. M. & Melchers, K. G. The use of technology-mediated interviews and their perception from the organization’s point of view. Int. J. Sel. Assess . 29 , 495–502 (2021).
Raghavan, M., Barocas, S., Kleinberg, J. & Levy, K. in Proc. 2020 Conf. Fairness Account. Transparency 469–481 (ACM, 2020).
Tippins, N. T., Oswald, F. L. & McPhail, S. M. Scientific, legal, and ethical concerns about AI-based personnel selection tools: a call to action. Pers. Assess. Decis . 7 , 1 (2021).
Hausknecht, J. P., Day, D. V. & Thomas, S. C. Applicant reactions to selection procedures: an updated model and meta-analysis. Pers. Psychol. 57 , 639–683 (2004).
Auer, E. M., Mersy, G., Marin, S., Blaik, J. & Landers, R. N. Using machine learning to model trace behavioral data from a game-based assessment. Int. J. Sel. Assess. 30 , 82–102 (2021).
Cook, R., Jones-Chick, R., Roulin, N. & O’Rourke, K. Job seekers’ attitudes toward cybervetting: scale development, validation, and platform comparison. Int. J. Sel. Assess. 28 , 383–398 (2020).
Langer, M., König, C. J. & Hemsing, V. Is anybody listening? The impact of automatically evaluated job interviews on impression management and applicant reactions. J. Manag. Psychol. 35 , 271–284 (2020).
Guchait, P., Ruetzler, T., Taylor, J. & Toldi, N. Video interviewing: a potential selection tool for hospitality managers — a study to understand applicant perspective. Int. J. Hosp. Manag. 36 , 90–100 (2014).
Vansteenkiste, M. et al. Autonomous and controlled regulation of performance-approach goals: their relations to perfectionism and educational outcomes. Motiv. Emot. 34 , 333–353 (2010).
McCarthy, J. M. et al. Applicant perspectives during selection: a review addressing “so what?,” “what’s new?,” and “where to next?” J. Manag. 43 , 1693–1725 (2017).
Jesuthasan, R. & Boudreau, J. W. Reinventing Jobs: A Four-Step Approach for Applying Automation to Work (Harvard Business, 2018).
Brynjolfsson, E., Mitchell, T. & Rock, D. What can machines learn, and what does it mean for occupations and the economy? AEA Pap. Proc. 108 , 43–47 (2018).
Hackman, J. R. & Lawler, E. E. Employee reactions to job characterisitics. J. Appl. Psychol. 55 , 259–286 (1971).
Hackman, J. R. & Oldham, G. R. Development of the job diagnostics survey. J. Appl. Psychol. 60 , 159–170 (1975).
Parker, S. K., Wall, T. D. & Cordery, J. L. Future work design research and practice: towards an elaborated model of work design. J. Occup. Organ. Psychol. 74 , 413–440 (2001).
Humphrey, S. E., Nahrgang, J. D. & Morgeson, F. P. Integrating motivational, social and contextual work design features: a meta-analytic summary and theoretical extension of the work design literature. J. Appl. Psychol. 92 , 1332–1356 (2007).
Gagné, M. & Panaccio, A. in Oxford Handbook of Employee Engagement, Motivation, and Self-Determination Theory (ed. Gagné, M.) 165–180 (Oxford Univ. Press, 2014).
Demerouti, E., Bakker, A. B., Nachreiner, F. & Schaufeli, W. B. The job demands–resources model of burnout. J. Appl. Psychol. 86 , 499–512 (2001).
Bakker, A. B. & Demerouti, E. Job demands–resources theory: taking stock and looking forward. J. Occup. Health Psychol. 22 , 273–285 (2017).
Bakker, A. B., Demerouti, E. & Euwema, M. C. Job resources buffer the impact of job demands on burnout. J. Occup. Health Psychol. 10 , 170–180 (2005).
Walsh, S. M. & Strano, M. S. Robotic Systems and Autonomous Platforms (Woodhead, 2019).
Waschull, S., Bokhorst, J. A. C., Molleman, E. & Wortmann, J. C. Work design in future industrial production: transforming towards cyber-physical systems. Comput. Ind. Eng. 139 , 105679 (2020).
Haslbeck, A. & Hoermann, H.-J. Flying the needles: flight deck automation erodes fine-motor flying skills among airline pilots. Hum. Factors 58 , 533–545 (2016).
Lehdonvirta, V. & Ernkvist, M. Knowledge Map of the Virtual Economy: Converting the Virtual Economy into Development Potential (World Bank, 2011).
Kittur, A. et al. in Proc. 2013 Conf. Comput. Support. Coop. Work 1301–1318 (ACM, 2013).
Beane, M. Shadow learning: building robotic surgical skill when approved means fail. Adm. Sci. Q. 64 , 87–123 (2019).
Mohlmann, M. & Zalmanson, L. in Proc. Int. Conf. Inf. Syst. 1–17 (ICIS, 2017).
Ohly, S. & Fritz, C. Work characteristics, challenge appraisal, creativity, and proactive behavior: a multi-level study. J. Organ. Behav. 31 , 543–565 (2010).
Wang, B., Liu, Y. & Parker, S. K. How does the use of information communication technology affect individuals? A work design perspective. Acad. Manag. Ann. 14 , 695–725 (2020).
Orlikowski, W. J. The duality of technology: rethinking the concept of technology in organizations. Organ. Sci. 3 , 398–427 (1992).
Kadir, B. A. & Broberg, O. Human-centered design of work systems in the transition to industry 4.0. Appl. Ergon. 92 , 103334 (2021).
Bloom, N. How working from home works out (Stanford Univ., 2020).
Bailenson, J. N. Nonverbal overload: a theoretical argument for the causes of Zoom fatigue. Technol. Mind Behav . https://doi.org/10.1037/tmb0000030 (2021).
Jun, H. & Bailenson, J. N. in International Handbook of Emotions and Media (eds Döveling, K. & Konijn, E. A.) 303–315 (Routledge, 2021).
Ratan, R., Miller, D. B. & Bailenson, J. N. Facial appearance dissatisfaction explains differences in zoom fatigue. Cyberpsychol. Behav. Soc. Netw. 25 , 124–129 (2021).
Riedl, R. On the stress potential of videoconferencing: definition and root causes of Zoom fatigue. Electron. Mark. https://doi.org/10.1007/s12525-021-00501-3 (2021).
Shockley, K. M. et al. The fatiguing effects of camera use in virtual meetings: a within-person field experiment. J. Appl. Psychol. 106 , 1137–1155 (2021).
Raghuram, S., Garud, R., Wiesenfeld, B. & Gupta, V. Factors contributing to virtual work adjustment. J. Manag. 27 , 383–405 (2001).
Raghuram, S., Wiesenfeld, B. & Garud, R. Technology enabled work: the role of self-efficacy in determining telecommuter adjustment and structuring behavior. J. Vocat. Behav. 63 , 180–198 (2003).
Muraven, M. Autonomous self-control is less depleting. J. Res. Personal. 42 , 763–770 (2008).
Muraven, M., Gagne, M. & Rosman, H. Helpful self-control: autonomy support, vitality, and depletion. J. Exp. Soc. Psychol. 44 , 573–585 (2008).
Feldman, D. C. & Gainey, T. W. Patterns of telecommuting and their consequences: framing the research agenda. Hum. Resour. Manag. Rev. 7 , 369–388 (1997).
Gajendran, R. S. & Harrison, D. A. The good, the bad, and the unknown about telecommuting: meta-analysis of psychological mediators and individual consequences. J. Appl. Psychol. 92 , 1524–1541 (2007).
Perry, S. J., Rubino, C. & Hunter, E. M. Stress in remote work: two studies testing the demand-control-person model. Eur. J. Work Organ. Psychol. 27 , 577–593 (2018).
Kossek, E. E., Lautsch, B. A. & Eaton, S. C. Telecommuting, control, and boundary management: correlates of policy use and practice, job control, and work–family effectiveness. J. Vocat. Behav. 68 , 347–367 (2006).
Johnson, A. et al. A review and agenda for examining how technology-driven changes at work will impact workplace mental health and employee well-being. Aust. J. Manag. 45 , 402–424 (2020).
Parent-Rocheleau, X. & Parker, S. K. Algorithms as work designers: how algorithmic management influences the design of jobs. Hum. Resour. Manag. Rev. https://doi.org/10.1016/j.hrmr.2021.100838 (2021).
Seppälä, T., Lipponen, J., Pirttila-Backman, A.-M. & Lipsanen, J. Reciprocity of trust in the supervisor–subordinate relationship: the mediating role of autonomy and the sense of power. Eur. J. Work Organ. Psychol. 20 , 755–778 (2011).
Parker, S. K., Knight, C. & Keller, A. Remote Managers Are Having Trust Issues (Harvard Business, 2020).
Staples, D. S. A study of remote workers and their differences from non-remote workers. J. End User Comput. 13 , 3–14 (2001).
Enzle, M. E. & Anderson, S. C. Surveillant intentions and intrinsic motivation. J. Pers. Soc. Psychol. 64 , 257–266 (1993).
Senécal, C., Vallerand, R. J. & Guay, F. Antecedents and outcomes of work-family conflict: toward a motivational model. Pers. Soc. Psychol. Bull. 27 , 176–186 (2001).
Aiello, J. R. & Shao, Y. in Human–Computer Interaction: Applications and Case Studies (eds Smith, M. J. & Salvendy, G.) 1011–1016 (Elsevier Science, 1993).
Griffith, T. L. Monitoring and performance: a comparison of computer and supervisor monitoring. J. Appl. Soc. Psychol. 23 , 549–572 (1993).
Stone, E. F. & Stone, D. L. in Research in Personnel and Human Resource Management Vol. 8 (eds Ferris, G. R. & Rowland, K. M.) 349–411 (JAI, 1990).
Wells, D. L., Moorman, R. H. & Werner, J. M. The impact of the perceived purpose of electronic performance monitoring on an array of attitudinal variables. Hum. Resour. Dev. Q. 18 , 121–138 (2007).
Ravid, D. M., Tomczak, D. L., White, J. C. & Behrend, T. S. EPM 20/20: a review, framework, and research agenda for electronic performance monitoring. J. Manag. 46 , 100–126 (2020).
De Tienne, K. B. & Abbott, N. T. Developing an employee-centered electronic monitoring system. J. Syst. Manag. 44 , 12–13 (1993).
Stanton, J. M. & Barnes-Farrell, J. L. Effects of electronic performance monitoring on personal control, task satisfaction, and task performance. J. Appl. Psychol. 81 , 738–745 (1996).
Day, A., Barber, L. K. & Tonet, J. in The Cambridge Handbook of Technology and Employee Behavior (ed. Landers, R. N.) 580–607 (Cambridge Univ. Press, 2019).
Day, A., Scott, N. & Kevin Kelloway, E. in New Developments in Theoretical and Conceptual Approaches to Job Stress Vol. 8 (eds Perrewé, P. L. & Ganster, D. C.) 317–350 (Emerald, 2010).
Cooper, C. D. & Kurland, N. B. Telecommuting, professional isolation, and employee development in public and private organizations. J. Organ. Behav. 23 , 511–532 (2002).
Coltrane, S., Miller, E. C., DeHaan, T. & Stewart, L. Fathers and the flexibility stigma. J. Soc. Issues 69 , 279–302 (2013).
Bloom, N., Liang, J., Roberts, J. & Ying, Z. J. Does working from home work? evidence from a Chinese experiment. Q. J. Econ. 130 , 165–218 (2014).
Charalampous, M., Grant, C. A., Tramontano, C. & Michailidis, E. Systematically reviewing remote e-workers’ well-being at work: a multidimensional approach. Eur. J. Work Organ. Psychol. 28 , 51–73 (2019).
Bloom, N. Don’t Let Employees Pick Their WFH Days (Harvard Business, 2021).
Schade, H. M., Digutsch, J., Kleinsorge, T. & Fan, Y. Having to work from home: basic needs, well-being, and motivation. Int. J. Environ. Res. Public Health 18 , 1–18 (2021).
Morganson, V. J., Major, D. A., Oborn, K. L., Verive, J. M. & Heelan, M. P. Comparing telework locations and traditional work arrangements: differences in work–life balance support, job satisfaction, and inclusion. J. Manag. Psychol. 25 , 578–595 (2010).
Golden, T. D., Veiga, J. F. & Dino, R. N. The impact of professional isolation on teleworker job performance and turnover intentions: does time spent teleworking, interacting face-to-face, or having access to communication-enhancing technology matter? J. Appl. Psychol. 93 , 1412–1421 (2008).
Peiperl, M. & Baruch, Y. Back to square zero: the post-corporate career. Organ. Dyn. 25 , 7–22 (1997).
Akkirman, A. D. & Harris, D. L. Organizational communication satisfaction in the virtual workplace. J. Manag. Dev. 24 , 397–409 (2005).
Golden, T. D. & Veiga, J. F. The impact of extent of telecommuting on job satisfaction: resolving inconsistent findings. J. Manag. 31 , 301–318 (2005).
Goldfarb, Y., Gal, E. & Golan, O. Implications of employment changes caused by COVID-19 on mental health and work-related psychological need satisfaction of autistic employees: a mixed-methods longitudinal study. J. Autism Dev. Disord . 52 , 89–102 (2022).
O’Neill, T. A. & Salas, E. Creating high performance teamwork in organizations. Hum. Resour. Manag. Rev. 28 , 325–331 (2018).
Hollenbeck, J. R., Beersma, B. & Schouten, M. E. Beyond team types and taxonomies: a dimensional scaling conceptualization for team description. Acad. Manage. Rev. 37 , 82–106 (2012).
Gilson, L. L., Maynard, M. T., Young, N. C. J., Vartiainen, M. & Hakonen, M. Virtual teams research: 10 years, 10 themes, and 10 opportunities. J. Manag. 41 , 1313–1337 (2015).
Raghuram, S., Hill, N. S., Gibbs, J. L. & Maruping, L. M. Virtual work: bridging research clusters. Acad. Manag. Ann. 13 , 308–341 (2019).
Handke, L., Klonek, F. E., Parker, S. K. & Kauffeld, S. Interactive effects of team virtuality and work design on team functioning. Small Group. Res. 51 , 3–47 (2020).
Foster, M. K., Abbey, A., Callow, M. A., Zu, X. & Wilbon, A. D. Rethinking virtuality and its impact on teams. Small Group. Res. 46 , 267–299 (2015).
Lautsch, B. A. & Kossek, E. E. Managing a blended workforce: telecommuters and non-telecommuters. Organ. Dyn. 40 , 10–17 (2011).
Mesmer-Magnus, J. R., DeChurch, L. A., Jimenez-Rodriguez, M., Wildman, J. & Shuffler, M. A meta-analytic investigation of virtuality and information sharing in teams. Organ. Behav. Hum. Decis. Process 115 , 214–225 (2011).
Mathieu, J. & Gilson, L. in Oxford Handbook of Organizational Psychology Vol. 2 (ed. Kozlowski, S. W.) 910–930 (Oxford Univ. Press, 2012).
Chen, G. & Kanfer, R. Toward a systems theory of motivated behavior in work teams. Res. Organ. Behav. 27 , 223–267 (2006).
Marks, M. A., Mathieu, J. E. & Zaccaro, S. J. A temporally based framework and taxonomy of team processes. Acad. Manage. Rev. 26 , 356–376 (2001).
Beal, D. J., Cohen, R. R., Burke, M. J. & McLendon, C. L. Cohesion and performance in groups: a meta-analytic clarification of construct relations. J. Appl. Psychol. 88 , 989–1004 (2003).
de Jong, B., Dirks, K. T. & Gillespie, N. M. Trust and team performance: a meta-analysis of main effects, moderators, and covariates. J. Appl. Psychol. 101 , 1134–1150 (2016).
Barrick, M. R., Thurgood, G. R., Smith, T. A. & Courtright, S. H. Collective organizational engagement: linking motivational antecedents, strategic implementation, and firm performance. Acad. Manage. J. 58 , 111–135 (2015).
Waller, M. J., Okhuysen, G. A. & Saghafian, M. Conceptualizing emergent states: a strategy to advance the study of group dynamics. Acad. Manag. Ann. 10 , 561–598 (2016).
Breuer, C., Hüffmeier, J. & Hertel, G. Does trust matter more in virtual teams? A meta-analysis of trust and team effectiveness considering virtuality and documentation as moderators. J. Appl. Psychol. 101 , 1151–1177 (2016).
Gagne, M. A model of knowledge-sharing motivation. Hum. Resour. Manage. 48 , 571–589 (2009).
Sewell, G. & Taskin, L. Out of sight, out of mind in a new world of work? Autonomy, control, and spatiotemporal scaling in telework. Organ. Stud. 36 , 1507–1529 (2015).
Tietze, S. & Nadin, S. The psychological contract and the transition from office-based to home-based work. Hum. Resour. Manag. J. 21 , 318–334 (2011).
Orsini, C. & Rodrigues, V. Supporting motivation in teams working remotely: the role of basic psychological needs. Med. Teach. 42 , 828–829 (2020).
Ragu-Nathan, T. S., Tarafdar, M., Ragu-Nathan, B. S. & Tu, Q. The consequences of technostress for end users in organizations: conceptual development and empirical validation. Inf. Syst. Res. 19 , 417–433 (2008).
Lee, M. K. Understanding perception of algorithmic decisions: fairness, trust, and emotion in response to algorithmic management. Big Data Soc. 5 , 2053951718756684 (2018).
Gal, U., Jensen, T. B. & Stein, M.-K. Breaking the vicious cycle of algorithmic management: a virtue ethics approach to people analytics. Inf. Organ. 30 , 100301 (2020).
Rosenblat, A. Uberland: How Algorithms are Rewriting the Rules of Work (Univ. California Press, 2018).
Charbonneau, É. & Doberstein, C. An empirical assessment of the intrusiveness and reasonableness of emerging work surveillance technologies in the public sector. Public Adm. Rev. 80 , 780–791 (2020).
Levy, K. E. C. The contexts of control: information, power, and truck-driving work. Inf. Soc. 31 , 160–174 (2015).
Vargas, T. L. Consumer redlining and the reproduction of inequality at dollar general. Qual. Sociol. 44 , 205–229 (2021).
Stark, D. & Pais, I. Algorithmic management in the platform economy. Sociologica 14 , 47–72 (2020).
Schafheitle, S. et al. No stone left unturned? Toward a framework for the impact of datafication technologies on organizational control. Acad. Manag. Discov. 6 , 455–487 (2020).
Norlander, P., Jukic, N., Varma, A. & Nestorov, S. The effects of technological supervision on gig workers: organizational control and motivation of Uber, taxi, and limousine drivers. Int. J. Hum. Resour. Manag. 32 , 4053–4077 (2021).
Carayon, P. Effects of electronic performance monitoring on job design and worker stress: results of two studies. Int. J. Human–Computer Interact. 6 , 177–190 (1994).
Moore, S. & Hayes, L. J. B. in Humans and Machines At Work: Dynamics of Virtual Work (eds. Moore, P. V., Upchurch, M. & Whittaker, X.) 101–124 (Palgrave Macmillan, 2018).
De Cremer, D. Leadership by Algorithm (Harriman House, 2020).
Goods, C., Veen, A. & Barratt, T. “Is your gig any good?” Analysing job quality in the Australian platform-based food-delivery sector. J. Ind. Relat. 61 , 502–527 (2019).
Wood, A. J., Graham, M., Lehdonvirta, V. & Hjorth, I. Good gig, bad gig: autonomy and algorithmic control in the global gig economy. Work Employ. Soc. 33 , 56–75 (2019).
Duggan, J., Sherman, U., Carbery, R. & McDonnell, A. Algorithmic management and app-work in the gig economy: a research agenda for employment relations and HRM. Hum. Resour. Manag. J. 30 , 114–132 (2020).
Reid-Musson, E., MacEachen, E. & Bartel, E. ‘Don’t take a poo!’: worker misbehaviour in on-demand ride-hail carpooling. N. Technol. Work Employ. 35 , 145–161 (2020).
Anthony, C. When knowledge work and analytical technologies collide: the practices and consequences of black boxing algorithmic technologies. Adm. Sci. Q . 66 , 1173–1212 (2021).
Zednik, C. Solving the black box problem: a normative framework for explainable artificial intelligence. Phil. Technol. 34 , 265–288 (2021).
Schlicker, N. et al. What to expect from opening up ‘black boxes’? Comparing perceptions of justice between human and automated agents. Comput. Hum. Behav. 122 , 106837 (2021).
Terry, E., Marks, A., Dakessian, A. & Christopoulos, D. Emotional labour and the autonomy of dependent self-employed workers: the limitations of digital managerial control in the home credit sector. Work Employ. Soc . https://doi.org/10.1177/0950017020979504 (2021).
Gregory, K. ‘My life is more valuable than this’: understanding risk among on-demand food couriers in Edinburgh. Work Employ. Soc. 35 , 316–331 (2021).
Dweck, C. S. & Leggett, E. L. A social-cognitive approach to motivation and personality. Psychol. Rev. 25 , 109–116 (1988).
Wesche, J. S. & Sonderegger, A. When computers take the lead: the automation of leadership. Comput. Hum. Behav. 101 , 197–209 (2019).
Duggan, J., Sherman, U., Carbery, R. & McDonnell, A. Boundaryless careers and algorithmic constraints in the gig economy. Int. J. Hum. Resour. Manag . https://doi.org/10.1080/09585192.2021.1953565 (2021).
Timko, P. & van Melik, R. Being a Deliveroo rider: practices of platform labor in Nijmegen and Berlin. J. Contemp. Ethnogr. 50 , 497–523 (2021).
Leclercq-Vandelannoitte, A. An ethical perspective on emerging forms of ubiquitous IT-based control. J. Bus. Ethics 142 , 139–154 (2017).
Cai, Z., Parker, S. K., Chen, Z. & Lam, W. How does the social context fuel the proactive fire? A multilevel review and theoretical synthesis. J. Organ. Behav. 40 , 209–230 (2019).
Hetland, J., Hetland, H., Bakker, A. B. & Demerouti, E. Daily transformational leadership and employee job crafting: the role of promotion focus. Eur. Manag. J. 36 , 746–756 (2018).
Schmitt, A., Den Hartog, D. N. & Belschak, F. D. Transformational leadership and proactive work behaviour: a moderated mediation model including work engagement and job strain. J. Occup. Organ. Psychol. 89 , 588–610 (2016).
Lee, Y., Lee, J. & Hwang, Y. Relating motivation to information and communication technology acceptance: self-determination theory perspective. Comput. Hum. Behav. 51 , 418–428 (2015).
Nikou, S. A. & Economides, A. A. Mobile-based assessment: integrating acceptance and motivational factors into a combined model of self-determination theory and technology acceptance. Comput. Hum. Behav. 68 , 83–95 (2017).
Basch, J. M. et al. A good thing takes time: the role of preparation time in asynchronous video interviews. Int. J. Sel. Assess. 29 , 378–392 (2021).
Peters, D., Calvo, R. A. & Ryan, R. M. Designing for motivation, engagement and wellbeing in digital experience. Front. Psychol. 9 , 797 (2018).
Grote, G., Ryser, C., Wäfler, T., Windischer, A. & Weik, S. KOMPASS: a method for complementary function allocation in automated work systems. Int. J. Hum. Comput. Stud. 52 , 267–287 (2000).
Jungert, T., Van den Broeck, A., Schreurs, B. & Osterman, U. How colleagues can support each other’s needs and motivation: an intervention on employee work motivation. Appl. Psychol. 67 , 3–29 (2018).
Kirkman, B. L., Rosen, B., Tesluk, P. E. & Gibson, C. B. The impact of team empowerment on virtual team performance: the moderating role of face-to-face interaction. Acad. Manage. J. 47 , 175–192 (2004).
Klonek, F., Gerpott, F. H., Lehmann-Willenbrock, N. & Parker, S. K. Time to go wild: how to conceptualize and measure process dynamics in real teams with high-resolution. Organ. Psychol. Rev. 9 , 245–275 (2019).
Waller, M. J., Uitdewilligen, S., Rico, R. & Thommes, M. S. in The Emerald Handbook of Group and Team Communication Research (eds Beck, S. J., Keyton, J. & Poole, M. S.) 135–153 (Emerald, 2021).
Makarius, E. E., Mukherjee, D., Fox, J. D. & Fox, A. K. Rising with the machines: a sociotechnical framework for bringing artificial intelligence into the organization. J. Bus. Res. 120 , 262–273 (2020).
Lythreatis, S., Singh, S. K. & El-Kassar, A.-N. The digital divide: a review and future research agenda. Technol. Forecast. Soc. Change 175 , 121359 (2021).
Lai, J. & Widmar, N. O. Revisiting the digital divide in the COVID-19 era. Appl. Econ. Perspect. Policy 43 , 458–464 (2021).
Gran, A.-B., Booth, P. & Bucher, T. To be or not to be algorithm aware: a question of a new digital divide? Inf. Commun. Soc. 24 , 1779–1796 (2021).
Parker, S. K. & Andrei, D. M. Include, individualize, and integrate: organizational meta-strategies for mature workers. Work Aging Retire. 6 , 1–7 (2019).
Petery, G. A., Iles, L. J. & Parker, S. K. Putting successful aging into context. Ind. Organ. Psychol. 13 , 377–382 (2020).
Graf, N., Brown, A. & Patten, E. The narrowing, but persistent, gender gap in pay. Pew Research Center http://www.www.pewresearch.org/ft_18-04-06_wage_gap/ (2018).
Aksoy, C. G., Özcan, B. & Philipp, J. Robots and the gender pay gap in Europe. Eur. Econ. Rev. 134 , 103693 (2021).
Madgavkar, A., White, O., Krishnan, M., Mahajan, D. & Azcue, X. COVID-19 and gender equality: countering the regressive effects. McKinsey https://www.mckinsey.com/featured-insights/future-of-work/covid-19-and-gender-equality-countering-the-regressive-effects (2020).
Glass, J. Blessing or curse? Work–family policies and mother’s wage growth over time. Work Occup. 31 , 367–394 (2004).
Valletta, R. G. Declining job security. J. Labor. Econ. 17 , S170–S197 (1999).
Givord, P. & Maurin, E. Changes in job security and their causes: an empirical analysis for France, 1982–2002. Eur. Econ. Rev. 48 , 595–615 (2004).
Baruch, Y. & Vardi, Y. A fresh look at the dark side of contemporary careers: toward a realistic discourse. Br. J. Manag. 27 , 355–372 (2016).
Akkermans, J., Richardson, J. & Kraimer, M. L. The Covid-19 crisis as a career shock: implications for careers and vocational behavior. J. Vocat. Behav. 119 , 103434 (2020).
Gubler, M., Arnold, J. & Coombs, C. Reassessing the protean career concept: empirical findings, conceptual components, and measurement. J. Organ. Behav. 35 , S23–S40 (2014).
Hall, D. T. Careers in Organizations (Scott Foresman, 1976).
Hall, D. T. Careers In And Out Of Organizations (SAGE, 2002).
Hall, D. T. & Mirvis, P. H. in The Career Is Dead — Long Live The Career: A Relational Approach To Careers (ed. Hall, D. T.) 15–45 (Jossey-Bass, 1996).
Kernis, M. H. & Goldman, B. M. A Multicomponent Conceptualization of Authenticity: Theory and Research Vol. 38 (Zana, M. P.) 283–357 (Academic, 2006).
Ryan, W. S. & Ryan, R. M. Toward a social psychology of authenticity: exploring within-person variation in autonomy, congruence, and genuineness using self-determination theory. Rev. Gen. Psychol. 23 , 99–112 (2019).
Klotz, A. The COVID vaccine means a return to work. And a wave of resignations. NBC https://www.nbcnews.com/think/opinion/covid-vaccine-means-return-work-wave-resignations-ncna1269018 (2021).
Tharoor, I. The ‘great resignation’ goes global. Washington Post https://www.washingtonpost.com/world/2021/10/18/labor-great-resignation-global/ (2021).
Sheather, J. & Slattery, D. The great resignation — how do we support and retain staff already stretched to the limit? BJM Opinion https://blogs.bmj.com/bmj/2021/09/21/the-great-resignation-how-do-we-support-and-retain-staff-already-stretched-to-their-limit/ (2021).
Hirsch, P. B. The great discontent. J. Bus. Strategy 42 , 439–442 (2021).
Williamson, I. O. The ‘great resignation’ is a trend that began before the pandemic — and bosses need to get used to it. The Conversation https://theconversation.com/the-great-resignation-is-a-trend-that-began-before-the-pandemic-and-bosses-need-to-get-used-to-it-170197 (2021).
Hopkins, J. C. & Figaro, K. A. The great resignation: an argument for hybrid leadership. Int. J. Bus. Manag. Res. 9 , 393–400 (2021).
Gandhi, V. & Robison, J. The ‘great resignation’ is really the ‘great discontent’. Gallup https://www.gallup.com/workplace/351545/great-resignation-really-great-discontent.aspx (2021).
Warner, M. A. & Hausdorf, P. A. The positive interaction of work and family roles: using need theory to further understand the work–family interface. J. Manag. Psychol. 24 , 372–385 (2009).
Richer, S. F., Blanchard, C. & Vallerand, R. J. A motivational model of work turnover. J. Appl. Soc. Psychol. 32 , 2089–2113 (2002).
Gillet, N., Gagné, M., Sauvagère, S. & Fouquereau, E. The role of supervisor autonomy support, organizational support, and autonomous and controlled motivation in predicting employees’ satisfaction and turnover intentions. Eur. J. Work Organ. Psychol. 22 , 450–460 (2013).
Christian, A. How the great resignation is turning into the great reshuffle. BBC https://www.bbc.com/worklife/article/20211214-great-resignation-into-great-reshuffle (2021).
Download references
Author information
Authors and affiliations.
Future of Work Institute, Curtin University, Perth, Western Australia, Australia
Marylène Gagné, Sharon K. Parker, Mark A. Griffin, Patrick D. Dunlop, Caroline Knight & Florian E. Klonek
Department of Human Resources Management, HEC Montréal, Montréal, Québec, Canada
Xavier Parent-Rocheleau
You can also search for this author in PubMed Google Scholar
Contributions
All authors researched data for the article. M.G. and S.K.P. contributed substantially to discussion of the content. All authors wrote the article. All authors reviewed or edited the manuscript before submission.
Corresponding author
Correspondence to Marylène Gagné .
Ethics declarations
Competing interests.
The authors declare no competing interests.
Peer review
Peer review information.
Nature Reviews Psychology thanks Arnold Bakker, Richard Ryan, and the other, anonymous, reviewer for their contribution to the peer review of this work.
Additional information
Publisher’s note.
Springer Nature remains neutral with regard to jurisdictional claims in published maps and institutional affiliations.
Rights and permissions
Springer Nature or its licensor (e.g. a society or other partner) holds exclusive rights to this article under a publishing agreement with the author(s) or other rightsholder(s); author self-archiving of the accepted manuscript version of this article is solely governed by the terms of such publishing agreement and applicable law.
Reprints and permissions
About this article
Cite this article.
Gagné, M., Parker, S.K., Griffin, M.A. et al. Understanding and shaping the future of work with self-determination theory. Nat Rev Psychol 1 , 378–392 (2022). https://doi.org/10.1038/s44159-022-00056-w
Download citation
Accepted : 06 April 2022
Published : 10 May 2022
Issue Date : July 2022
DOI : https://doi.org/10.1038/s44159-022-00056-w
Share this article
Anyone you share the following link with will be able to read this content:
Sorry, a shareable link is not currently available for this article.
Provided by the Springer Nature SharedIt content-sharing initiative
This article is cited by
Linking undergraduates’ future work self and employability: a moderated mediation model.
- Lingyan Hou
BMC Psychology (2024)
Optimizing work and off-job motivation through proactive recovery strategies
- Miika Kujanpää
- Anja H. Olafsen
Nature Reviews Psychology (2024)
Experience shapes non-linearities between team behavioral interdependence, team collaboration, and performance in massively multiplayer online games
- Carlos Carrasco-Farré
- Nancy Hakobjanyan
Scientific Reports (2024)
Improving Reactions to Forced-Choice Personality Measures in Simulated Job Application Contexts Through the Satisfaction of Psychological Needs
- Tristan C. Borman
- Patrick D. Dunlop
- Matthew Neale
Journal of Business and Psychology (2024)
Nurses’ Early Career Organizational and Occupational Commitment Trajectories: A Dual Target Growth Mixture Investigation
- Simon A. Houle
- Alexandre J. S. Morin
- Claude Fernet
Quick links
- Explore articles by subject
- Guide to authors
- Editorial policies
Sign up for the Nature Briefing newsletter — what matters in science, free to your inbox daily.

Jump to navigation
Call for Papers: ‘Global South Diasporic Voices: Rethinking Praxis and Theory in Communication for Development’
Call for Papers: Journal of Global Diaspora & Media
Special Issue: ‘Global South Diasporic Voices: Rethinking Praxis and Theory in Communication for Development’
View the full call here>>
https://www.intellectbooks.com/journal-of-global-diaspora-media#call-for-papers
Recent shifts in global migration patterns, particularly in the last two decades, and the advent of information and communication technologies (ICTs) have positioned Diasporas as significant actors in development narratives. However, the preponderance of western-centric voices in development discourse obscures Indigenous perspectives, thus positioning scholars who straddle the Global North and South as unique experts given their knowledge exchange and socio-political advocacy (Brinkerhoff 2009).
This issue invites contributions that explore how these diasporic interventions challenge and expand existing C4D paradigms, incorporating Indigenous knowledge systems and leveraging digital Diasporas for development (Karim 2003). We seek to explore the nuanced ways in which diaspora communities engage with and transform C4D practices. This encompasses a critical assessment of how traditional media and ICTs facilitate or hinder the diaspora's development contributions, the role of social media in creating transnational public spheres for development discourse (Castells 2008), and the potential for media to act as a catalyst for social change within Global South contexts (Manyozo 2012).
Contributions may address, but are not limited to, the following areas:
Theoretical reconceptualizations of C4D in the context of diaspora and transnationalism.
Case studies on the use of media and ICTs by the diaspora for development, including the impact of social media platforms.
Analyses of the challenges and opportunities presented by digital Diasporas in influencing development agendas.
Critical examinations of how Diasporas negotiate identity, representation and politics in media narratives related to development.
We encourage submissions that critique existing models and inform policy direction, present innovative approaches for integrating diaspora voices into development communication strategies and reflect diverse methodologies and interdisciplinary participation.
Submissions:
Submission of abstracts should include name, institutional affiliation, contact information, title and a 400-word abstract. Email your abstracts to all guest editors Carolyn Walcott [email protected] , Maha Bashri [email protected] and Farooq Kperogi [email protected] .
Publication deadlines and timeline:
Abstracts due: 26 April 2024
Confirmation of acceptance: 20 May 2024
Full manuscript due: 19 September 2024
Revisions sent out (peer review): 26 October 2024
Final submission: January 2025
Online First publication: June 2025
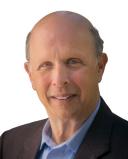
Left Brain - Right Brain
Developing extraordinary abilities through savant syndrome, personal perspective: a hypothesis regarding the etiology of savant abilities..
Posted April 8, 2024 | Reviewed by Kaja Perina
- Types of savant syndrome include congenital, acquired as a result of brain dysfunction, and sudden-onset.
- Savant abilities include five areas: art, calendar computation, mathematics, music, and visuospatial skills.
- The skills of prodigious savants are so outstanding that they would be spectacular in a non-impaired person.
The savant syndrome is a rare psychological phenomenon in which people manifest extraordinary skills beyond their usual abilities (Rudzinski et al., 2023). Here, we will discuss possible causes of this phenomenon, as well as how savant abilities might be elicited.
There are three recognized types of this syndrome:
Congenital. Affected individuals typically have an associated developmental mental disability, and approximately half of these have a diagnosis of autism . In 2015, there were 287 known cases of this type (Treffert & Rebedew, 2015).
Acquired. In 2015, there were 32 known cases of neurotypical individuals who developed savant abilities following a head injury , stroke, onset of dementia , or other central nervous system disorder (Treffert & Rebedew, 2015).
Sudden onset. In 2021 there were 11 reports of neurotypical individuals without developmental disabilities or a central nervous system disorder who suddenly developed transient or permanent savant abilities (Treffert & Ries, 2021).
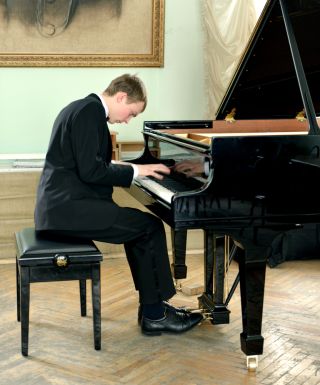
Savant abilities usually relate to five general areas (Rudzinski et al., 2023; Treffert, 2009):
Art. This has usually involved drawing, painting, or sculpting.
Calendar computation. Savants can quickly calculate the day of the week of any date in history.
Mathematics. Involves lightning calculation or the ability to compute prime numbers, sometimes in the absence of simple mathematical abilities.
Music. Usually, this has involved the performance of music, most often with the piano. Some have developed perfect pitch, and some have composed music once they have honed their abilities to perform.
Visuospatial/mechanical skills. These include the ability to measure distances precisely without instruments, to accurately construct complex structures, or to make maps.
Less commonly reported savant abilities include sudden extensive mastery of one or more foreign languages, unusual sensory discrimination in smell, perfect appreciation of passing time without access to a clock, and outstanding knowledge in specific fields such as neurophysiology, statistics, history, or navigation (Treffert, 2009).
Savant syndrome is typically associated with a single extraordinary ability in affected individuals.
Talented savants are considered those whose savant abilities are highly developed in comparison to the individual’s abilities in other areas of their life.
Prodigious savants are those whose skills are so outstanding that they would be spectacular even in a non-impaired person. In 2009, there were fewer than 100 known such people in the world. (Treffert, 2009).
Etiology of Savant Abilities
Speculated mechanisms for developing savant syndrome include that left brain dysfunction leads to the right brain developing new abilities to compensate for a loss of function on the left, or uncovering of pre-existing right brain abilities that had been suppressed by the left brain (Treffert, 2009). Evidence for these mechanisms include:
- The reporting of a child who developed savant abilities after a left brain injury (Brink, 1980).
- The painting skills of an artist improved dramatically after a left occipital stroke (Rudzinski et al., 2023).
- The loss of function in the left anterior frontal lobe or left temporal lobe in frontotemporal lobe dementia has been associated with the development of savant syndrome (Hou et al., 2000; Miller et al., 2000).
- Males outnumber females 6:1 in savant syndrome, as compared to a 4:1 ratio in autism spectrum disorders. Since the prenatal left brain hemisphere develops later than the right hemisphere, it is speculated that it is more likely to be exposed to detrimental prenatal influences. For instance, in male fetuses, testosterone can slow growth and impair neuronal development, which likely has a greater effect on the left brain (Geschwind & Galaburda, 2003).
- Stimulation of the left frontotemporal area with transcranial magnetic pulses can block left hemispheric function and has been demonstrated to be associated with developing skills similar to those seen in savant syndrome (Young et al., 2004).
My Hypothesis
From an evolutionary perspective, it never made sense to me that individuals would possess a latent extraordinary right-brain ability that would rarely be expressed in the absence of brain damage. What evolutionary advantage would be gained by the resource expenditure required to develop such an ability were it unlikely ever to manifest?

If the right brain acts to compensate for left brain dysfunction, why would this lead to the development of extraordinary abilities rather than the resumption of usual abilities?
Experiences from my work with hypnosis have helped shape a new hypothesis regarding the nature of the right brain ability that is usually suppressed by an intact left brain.
- With hypnosis , when the conscious mind is calmed, one of my 14-year-old patients has demonstrated a savant-like ability to communicate in writing in a foreign language that he had never studied. This patient told me that he did not understand the language, but rather that his subconscious transmitted the language from “God.”
- A 13-year-old patient demonstrated the ability to write sophisticated poetry while in hypnosis (Anbar, 2021), which his subconscious stated was the result of channeling the poetry from “muses.”
- Some of my school-age patients have reported, with or without hypnosis, that they saw “visions” of people who were not from this world and yet seemed “real.” I have discussed with them that when preschool children often talk about “imaginary friends” perhaps they are similarly seeing “visions.”
- In most people, the left brain hemisphere is dominant for language (Knecht, et al., 2000). Thus, when we talk about how we consciously think or feel, it is the left brain that is speaking.
- Some studies from people whose connection between the brain hemispheres is disrupted appear to demonstrate that each brain hemisphere can think independently, although this conclusion recently has been doubted (de Haan, 2020).
- When I have taught patients’ subconscious to speak with me, after returning to conscious awareness, patients often say they do not recall the interactions with their subconscious. Perhaps this is because the left brain is not fully aware of right brain content during a state of hypnosis.
- The subconscious of one of my 13-year-old patients said it was left-handed while the patient was right-handed. (Note that the left hand is controlled by the right brain.) This subconscious said that during sports, the patient played left-handed because it was better at sports than the conscious (Anbar, 2001).
From these observations, I formed the following hypothesis about an ability of the right brain that can be activated through hypnosis or because of savant syndrome: The right brain can serve as a conduit to information from outside of the individual brain, similar to psychologist Carl Jung’s concept of how we tap into a collective unconscious (Doyle, 2018).
I speculate that in neurotypical individuals the left brain suppresses the right brain conduit ability to prevent confusion between reality and information from outside of our usual reality. Such suppression may, in part, be the result of how preschoolers in the Western world are taught to think of “imaginary friends” (which may represent images from outside of our reality) as “pretend.”
When the left brain is damaged, perhaps the conduit activity is restored, which allows individuals access to extraordinary information including that leading to savant abilities.
The existence of savants pushes us to ask whether it is possible for neurotypical individuals to tap into extraordinary abilities. The use of hypnosis may be one such route for some individuals.
Anbar RD. (2001). Automatic word processing: A new forum for hypnotic expression. Am J Clin Hypnosis . 44:27-36.
Anbar RD. (2021). Changing Children’s Lives with Hypnosis: A Journey to the Center. Lanham, MD: Rowman & Littlefield.
Brink T. (1980). Idiot savant with unusual mechanical ability. Am J Psychiatry. 137:250-251.
de Haan EHF, Corballis PM, Hillyard SA, et al. (2020). Split brain: What we know now and why is it important for understanding consciousness. Neuropsychol Rev. 30:224-233.
Doyle, DJ. (2018). What Does it Mean to be Human? Life, Death, Personhood and the Transhumanist Movement. Cham, Switzerland: Springer.
Geschwind N, Galaburda AM. (2003). Cerebral Lateralization: Biological Mechanisms, Associations, and Pathology. Cambridge, MA: MIT Press.
Hou C, Miller BL, Cummings JL, Goldberg M, Mychack P, Bottino V, Benson DF. (2000). Autistic savants. Neuropsychiatry Neuropsychol Behav Neurol . 13:29-38.
Knecht S, Drager B, Deppe M, Lobe L, Lohmann H, Floel A, Ringelstein EB, Henningsen H. (2000). Handedness and hemispheric dominance in healthy humans. Brain. 123 pt. 12:2512-2518.
Rudzinkski G, Pozarowska K, Brzuszkiewicz K, Soroka E. (2023). Psychiatr Pol. Jun 17:1-11.
Treffert DA. (2009). The savant syndrome: an extraordinary condition. A synopsis: past, present, future. Phil Trans Soc B . 364:1351-1357.
Treffert DA, Rebedew DL. (2015). The savant syndrome registry: a preliminary report. WMJ . 114:158-162.
Treffert DA, Ries HJ. (2021). The sudden savant: a new form of extraordinary abilities. WMJ . 120:69-73.
Young RL, Ridding MC, Morrell TL. (2004). Switching skills on by turning off part of the brain. Neurocase . 10:215-222.
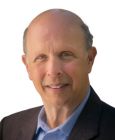
Ran D. Anbar, M.D., FAAP, is board-certified in both pediatric pulmonology and general pediatrics. He is the author of the new book Changing Children’s Lives with Hypnosis: A Journey to the Center .
- Find a Therapist
- Find a Treatment Center
- Find a Psychiatrist
- Find a Support Group
- Find Teletherapy
- United States
- Brooklyn, NY
- Chicago, IL
- Houston, TX
- Los Angeles, CA
- New York, NY
- Portland, OR
- San Diego, CA
- San Francisco, CA
- Seattle, WA
- Washington, DC
- Asperger's
- Bipolar Disorder
- Chronic Pain
- Eating Disorders
- Passive Aggression
- Personality
- Goal Setting
- Positive Psychology
- Stopping Smoking
- Low Sexual Desire
- Relationships
- Child Development
- Therapy Center NEW
- Diagnosis Dictionary
- Types of Therapy
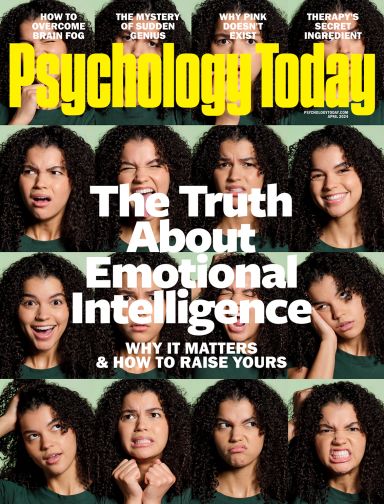
Understanding what emotional intelligence looks like and the steps needed to improve it could light a path to a more emotionally adept world.
- Coronavirus Disease 2019
- Affective Forecasting
- Neuroscience
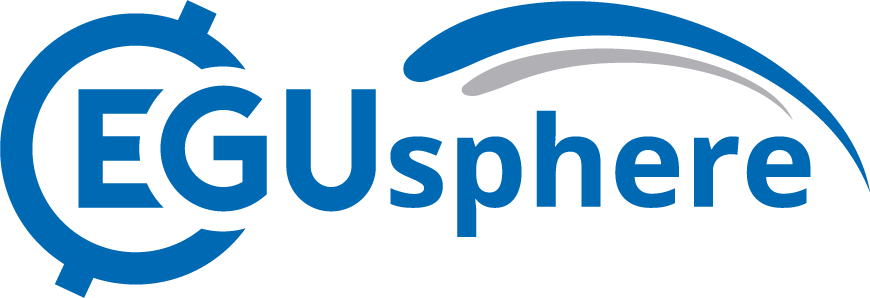
Preprint
- Preprint egusphere-2024-331
Evolution of crystallographic preferred orientations of ice sheared to high strains by equal-channel angular pressing
Abstract. Plastic deformation of polycrystalline ice 1 h induces crystallographic preferred orientations (CPOs), which give rise to anisotropy in the viscosity of ice, thereby exerting a strong influence on the flow of glaciers and ice sheets. The development of CPOs is governed by two pivotal mechanisms: recrystallization dominated by subgrain/lattice rotation and by strain-induced grain boundary migration (GBM). To examine the impact of strain on the transition of the dominant mechanism, synthetic ice (doped with ∼1 vol.% graphite) was deformed using equal-channel angular pressing technique, enabling multiple passes to accumulate substantial shear strains. Nominal shear strains up to 6.2, equivalent to a nominal von Mises strain of ε′ ≈ 3.6, were achieved in samples at a temperature of −5 °C. Cryo-electron backscatter diffraction analysis reveals a primary cluster of crystal c axes perpendicular to the shear plane in all samples, accompanied by a secondary cluster of c axes at an oblique angle to the primary cluster antithetic to the shear direction. With increasing strain, the primary c-axis cluster strengthens, while the secondary cluster weakens. The angle between the clusters remains within the range of 45° to 60°. The c-axis clusters are elongated perpendicular to the shear direction, with this elongation intensifying as strain increases. Subsequent annealing of the highest-strain sample reveals the same CPO patterns as observed prior to annealing, albeit slightly weaker. A synthesis of various experimental data suggest that the CPO pattern, including the orientation of the secondary cluster, results from a balance of two competing mechanisms: lattice rotation due to dislocation slip, which fortifies the primary cluster while rotating and weakening the secondary one, and grain growth by strain-induced GBM, which reinforces both clusters while rotating the secondary cluster in the opposite direction. As strain increases, GBM contributes progressively less. This investigation supports the previous hypothesis that a single cluster of c axes could be generated in high-strain experiments, while further refining our comprehension of CPO development in ice.
- Preprint (PDF, 9432 KB)
- Preprint (9432 KB)
- Metadata XML

Status : open (until 22 May 2024)
Report abuse
Please provide a reason why you see this comment as being abusive. You might include your name and email but you can also stay anonymous.
Please provide a reason why you see this comment as being abusive.
Please confirm reCaptcha.

Viewed (geographical distribution)
Daniel h. richards, rachel worthington, david j. prior.
Screen Rant
The rookie season 6, episode 6's tim & lucy twist has been building since season 5.
The Rookie season 6, episode 6 featured a significant development in Tim and Lucy's relationship, and it's ultimately for the best for the couple.
Spoiler alert: This article contains spoilers from The Rookie season 6, episode 6.
- Tim broke up with Lucy in The Rookie season 6, episode 6, as he is pushing her away to protect her from his own struggles.
- The Rookie has been foreshadowing Chenford's split since season 5.
- The breakup may lead to individual growth for both Tim and Lucy.
Tim Bradford and Lucy Chen have been The Rookie 's "it couple" since before they were even a couple, and while season 6's significant development in their romance is heartbreaking, it'll ultimately make their relationship stronger in the long run. The ABC procedural drama series' writers have clarified that they didn't plan for Tim and Lucy (affectionately dubbed "Chenford" by fans) to be the show's central romance when it premiered in 2018. However, the chemistry between Eric Winter and Melissa O'Neil was palpable and undeniable, so a slow burn commenced between their two characters until they got together in season 5.
When the series premiere of The Rookie aired, Lucy was a rookie cop alongside Nathan Fillion's John Nolan and Titus Makin Jr's Jackson West. Tim was Lucy's training officer, and the two got off to a rough start, as Lucy was a starry-eyed, naive rookie, and Tim was a hardened, no-nonsense veteran. Over time, Tim and Lucy became friends , but there were signs that their relationship could become something more. Eventually, after multiple other love interests, shared undercover kisses, and near-death experiences, Tim and Lucy started dating in The Rookie season 5. But it all came crashing down in season 6.
Tim Broke Up With Lucy In The Rookie Season 6, Episode 6
Tim is pushing lucy away.
Someone from Tim's past resurfaced in The Rookie season 6 , episode 5, and it sadly changed the course of his relationship with Lucy. David Dastmalchian guest starred as Ray, someone Tim served with in the military, and the audience learned that Tim previously made a pact with Mark Greer (one of Tim's Army buddies) that they would kill Ray if they ever saw him again. While he dealt with this new development, Tim completely iced Lucy out and refused to tell her anything about the matter. He was afraid that her involvement would ruin her career, but Lucy didn't care.
The Rookie season 6 is only expected to contain 10 episodes due to production delays related to the WGA and SAG-AFTRA strikes in 2023.
Lucy was frustrated that Tim wouldn't let her in, and she became even angrier when Ray threatened her in episode 6, resulting in her demanding that Tim tell her what was going on. As viewers were aware, Tim didn't want to kill Ray, but Ray was also a problem because of the false report Tim previously filed about him dying overseas. Despite settling the Ray problem and Tim finally opening up to Lucy, the tension between the couple hadn't been resolved by the end of The Rookie episode , and that's when Tim dropped a bombshell on Lucy.
While Tim and Lucy's split is devastating, The Rookie has been foreshadowing a breakup between the fan-favorite couple for a long time.
Tim was forced to lie about the report to protect himself and the people he loves. However, Tim's lies became too much for him to bear, and he felt as if he had lost himself. Lucy tried to comfort Tim, but he began to spiral and claimed that he couldn't return to the way things were (maybe never). Tim broke up with Lucy, claiming she deserved much better than him. She rebutted with "You don't get to lie to me, and then use that as an excuse to leave me," but Tim's mind was made up in The Rookie .
The Rookie Has Been Foreshadowing Chenford's Breakup Since Season 5
Tim & lucy's breakup has been a long time coming.
While Tim and Lucy's split is devastating, The Rookie has been foreshadowing a breakup between the fan-favorite couple for a long time. The first few season 5 episodes showcasing the early days of Chenford's relationship were exciting, but it soon became clear that miscommunication would be a major issue for Tim and Lucy. Lucy didn't inform Tim about her schemes to secure him a spot on Metro, and she wasn't upfront about how her actions would diminish her chances on the detectives' exam. Sadly, their problems only worsened during the season 6 premiere.
Some time apart will be good for Tim and Lucy's individual growth.
Lucy accused Tim of trying to sabotage her while studying for the exam, and Tim couldn't believe she would think he would do that to her. The couple eventually made up and even exchanged "I love you's" during The Rookie 's 100th episode . The next hour featured Lucy passing the test, but ranking low, meaning she probably wouldn't get promoted to detective. All of these developments (plus the Ray situation) spelled trouble for Chenford in The Rookie season 6, and it was only a matter of time before they broke up.
The Rookie Season 6 Theory Reveals Aaron's New Love Interest Is Actually The Villain
Tim & lucy need time apart to work on their own issues, the breakup can be good for chenford in the rookie season 6.
Of course, Tim and Lucy's breakup is a hard pill to swallow for those who waited years for them to admit their feelings. But on the other hand, some time apart will be good for Tim and Lucy's individual growth. Tim is clearly lost and has reached a breaking point, affecting his relationship with Lucy. He needs to work on himself before he can be the boyfriend that Lucy deserves. Meanwhile, Lucy's career is at a standstill, and she should take this time to figure out her path forward (as long as The Rookie season 6 ends with Chenford getting back together).
The Rookie season 6, episode 7, "Crushed," premieres on Tuesday, April 30, at 9pm ET.
*Availability in US
Not available
The Rookie is a police procedural television series that stars Nathan Fillion as police officer John Nolan. At 45 years old, John becomes the oldest rookie at the Los Angeles Police Department. The show premiered on ABC in 2018.
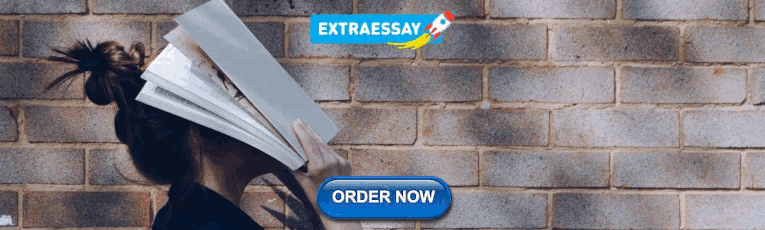
IMAGES
VIDEO
COMMENTS
5. Phrase your hypothesis in three ways. To identify the variables, you can write a simple prediction in if…then form. The first part of the sentence states the independent variable and the second part states the dependent variable. If a first-year student starts attending more lectures, then their exam scores will improve.
What is a hypothesis and how can you write a great one for your research? A hypothesis is a tentative statement about the relationship between two or more variables that can be tested empirically. Find out how to formulate a clear, specific, and testable hypothesis with examples and tips from Verywell Mind, a trusted source of psychology and mental health information.
3. Simple hypothesis. A simple hypothesis is a statement made to reflect the relation between exactly two variables. One independent and one dependent. Consider the example, "Smoking is a prominent cause of lung cancer." The dependent variable, lung cancer, is dependent on the independent variable, smoking. 4.
A research hypothesis, in its plural form "hypotheses," is a specific, testable prediction about the anticipated results of a study, established at its outset. It is a key component of the scientific method. Hypotheses connect theory to data and guide the research process towards expanding scientific understanding.
Definition: Hypothesis is an educated guess or proposed explanation for a phenomenon, based on some initial observations or data. It is a tentative statement that can be tested and potentially proven or disproven through further investigation and experimentation. Hypothesis is often used in scientific research to guide the design of experiments ...
A theory is broad in nature and explains larger bodies of data. A hypothesis is more specific and makes a prediction about the outcome of a particular study. Working with theories is not "icing on the cake." It is a basic ingredient of psychological research. Like other scientists, psychologists use the hypothetico-deductive method.
The steps to write a research hypothesis are: 1. Stating the problem: Ensure that the hypothesis defines the research problem 2. Writing a hypothesis as an 'if-then' statement: Include the action and the expected outcome of your study by following a 'if-then' structure. 3.
A cyclical process of theory development, starting with an observed phenomenon, then developing or using a theory to make a specific prediction of what should happen if that theory is correct, testing that prediction, refining the theory in light of the findings, and using that refined theory to develop new hypotheses, and so on. ...
Scientific hypotheses are essential for progress in rapidly developing academic disciplines. Proposing new ideas and hypotheses require thorough analyses of evidence-based data and predictions of the implications. ... In fact, hypothesis is usually formulated by referring to a few scientific facts or compelling evidence derived from a handful ...
A cyclical process of theory development, starting with an observed phenomenon, then developing or using a theory to make a specific prediction of what should happen if that theory is correct, testing that prediction, refining the theory in light of the findings, and using that refined theory to develop new hypotheses, and so on. ...
Theories and Hypotheses. Before describing how to develop a hypothesis, it is important to distinguish between a theory and a hypothesis. A theory is a coherent explanation or interpretation of one or more phenomena. Although theories can take a variety of forms, one thing they have in common is that they go beyond the phenomena they explain by including variables, structures, processes ...
Abstract. Rigorous research investigation requires a thorough review of the literature on the topic of interest. This promotes development of an original, relevant and feasible hypothesis. Design of an optimal study to test the hypothesis then requires adequate power, freedom from bias, and conduct within a reasonable timeframe with resources ...
Practicing Hypothesis-Driven Development[1] is thinking about the development of new ideas, products, and services - even organizational change - as a series of experiments to determine whether an expected outcome will be achieved. The process is iterated upon until a desirable outcome is obtained or the idea is determined to be not viable.
7 Main Developmental Theories. Child development theories focus on explaining how children change and grow over the course of childhood. These developmental theories center on various aspects of growth, including social, emotional, and cognitive development. The study of human development is a rich and varied subject.
Practicing Hypothesis-Driven Development is thinking about the development of new ideas, products and services - even organizational change - as a series of experiments to determine whether an expected outcome will be achieved. The process is iterated upon until a desirable outcome is obtained or the idea is determined to be not viable.
4. Formulate your hypothesis. After collecting background information and making a prediction based on your question, plan a statement that lays out your variables, subjects and predicted outcome. Whether you write it as an "if/then" or declarative statement, your hypothesis should include the prediction to be tested.
Piaget divided children's cognitive development into four stages; each of the stages represents a new way of thinking and understanding the world. He called them (1) sensorimotor intelligence, (2) preoperational thinking, (3) concrete operational thinking, and (4) formal operational thinking. Each stage is correlated with an age period of ...
Formulating Hypotheses for Different Study Designs. Generating a testable working hypothesis is the first step towards conducting original research. Such research may prove or disprove the proposed hypothesis. Case reports, case series, online surveys and other observational studies, clinical trials, and narrative reviews help to generate ...
Chapter 6 Overview. This chapter discusses the third step of the SGAM, highlighted below in gold, Hypothesis Development. Structured Geospatial Analytic Process, Step 3. A hypothesis is often defined as an educated guess because it is informed by what you already know about a topic. This step in the process is to identify all hypotheses that ...
Lev Semyonovich Vygotsky (Russian: Лев Семёнович Выготский, [vɨˈɡotskʲɪj]; Belarusian: Леў Сямёнавіч Выгоцкі; November 17 [O.S. November 5] 1896 - June 11, 1934) was a Russian psychologist, best known for his work on psychological development in children and creating the framework known as cultural-historical activity theory.
Developing a research hypothesis is a critical step in the business development process, serving as a foundation for further investigation and analysis. It's a statement that predicts a ...
According to self-determination theory, satisfaction of three psychological needs (competence, autonomy and relatedness) influences work motivation, which influences outcomes.
Developing mutually respectful research-practice partnerships while engaging young citizens Civics education in contentious times: Working with teachers to create locally-specific curricula in a post-truth world , by William Toledo, London, UK, Lexington Books, 2021, 143 pp., $105.00 (hardcover), $39.99 (paperback), $38.00 (ebook), ISBN 978-1 ...
This article examines the urban development of Moscow from 1992 to 2015, arguing that the city's recent transformation from grey asphalt jungle to a "city comfortable for life" is driven by a process of neoliberal restructuring. In particular, the study finds that a set of multi-scalar dynamics—namely, the global financial crisis, the ...
Special Issue: 'Global South Diasporic Voices: Rethinking Praxis and Theory in Communication for Development' ... However, the preponderance of western-centric voices in development discourse obscures Indigenous perspectives, thus positioning scholars who straddle the Global North and South as unique experts given their knowledge exchange ...
development programmes, we adopt a historical institutionalist approach to the study of policy and politics (Mahoney & Thelen 2010). Using the method of historical process-tracing, we seek to 'identify the causal mechanism between independent variables and the outcome of the dependent variable' (George & Bennett 2005, p. 206).
The savant syndrome is a rare psychological phenomenon in which people manifest extraordinary skills beyond their usual abilities (Rudzinski et al., 2023). Here, we will discuss possible causes of ...
Abstract. Plastic deformation of polycrystalline ice 1 h induces crystallographic preferred orientations (CPOs), which give rise to anisotropy in the viscosity of ice, thereby exerting a strong influence on the flow of glaciers and ice sheets. The development of CPOs is governed by two pivotal mechanisms: recrystallization dominated by subgrain/lattice rotation and by strain-induced grain ...
Tim Bradford and Lucy Chen have been The Rookie's "it couple" since before they were even a couple, and while season 6's significant development in their romance is heartbreaking, it'll ultimately make their relationship stronger in the long run. The ABC procedural drama series' writers have clarified that they didn't plan for Tim and Lucy (affectionately dubbed "Chenford" by fans) to be the ...
Fundamentally, urban development is linked to the issues of economic, spatial and housing development. Housing development is dominated by a widely shared basic understanding that housing is an important and problematic area that concerns everyone and is connected to demography. Below, we analyse the attitudes and ideas of the main actors involved.