- Age: 14-16 MYP Individuals and Societies
- Age: 14-16 GCSE / IGCSE Geography
- Natural Environments
- Economic Development
- IGCSE Geography Revision Question Bank
- 2.1 Earthquakes and volcanoes
- 2.4 Weather
- 2.5 Climate and natural vegetation
- Distribution
- Plate Tectonics
- Plate Boundaries | Plate Margins
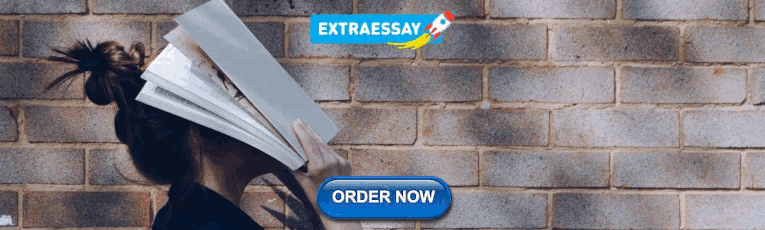
Volcano case study - Mount Etna (2002-2003), Italy
- Volcano case study - Mount Nyiragongo, Democratic Republic of Congo
- Volcanic hazard management - Mount Rainier, USA
- Earthquakes
- Earthquake case study - 2005 Kashmir
- Earthquake case study - Chuetsu Offshore Earthquake - 2007
- Why was the Haitian Earthquake so deadly?
- Earthquakes - Managing the hazard
Can you describe the location of Mount Etna? Could you draw a sketch map to locate Mount Etna?

Case study task
Use the resources and links that can be found on this page to produce a detailed case study of the 2002-2003 eruption of Mount Etna. You should use the 'Five W's" subheadings to give your case study structure.
What happened?
The Guardian - Sicilian city blanketed in ash [28 October 2002]
When did it happen?
Immediately before midnight on 26 October 2002 (local time=GMT+1), a new flank eruption began on Mount Etna. The eruption ended after three months and two days, on 28 January 2003.
Where did it happen?
The eruption occurred from fissures on two sides of the volcano: at about 2750 m on the southern flank and at elevations between 2500 and 1850 m on the northeastern flank.

Why did it happen?
Mount Etna is a volcano. The reasons why Mount Etna is located where it is are complex. Here are some of the theories:
- One theory envisages a hot spot or mantle-plume origin for this volcano, like those that produce the volcanoes in Hawaii.
- Another theory involves the subduction of the African plate under the Eurasian plate.
- Another group of scientists believes that rifting along the eastern coast of Sicily allows the uprise of magma.
Who was affected by it happening?
- The Italian Government declared a state of emergency in parts of Sicily, after a series of earthquakes accompanying the eruption of forced about 1,000 people flee their homes.
- A ship equipped with a medical clinic aboard was positioned off Catania - to the south of the volcano - to be ready in case of emergency.
- Emergency workers dug channels in the earth in an attempt to divert the northern flow away from the town of Linguaglossa.
- Schools in the town have been shut down, although the church has remained open for people to pray.
- Villagers also continued their tradition of parading their patron saint through the streets to the railway station, to try to ward off the lava flow.
- Civil protection officials in Catania, Sicily's second-biggest city, which sits in the shadow of Etna, surveyed the mountain by helicopter and were ready to send water-carrying planes into the skies to fight the fires.
- The tourist complex and skiing areas of Piano Provenzana were nearly completely devastated by the lava flows that issued from the NE Rift vents on the first day of the eruption.
- Heavy tephra falls caused by the activity on the southern flank occurred mostly in areas to the south of the volcano and nearly paralyzed public life in Catania and nearby towns.
- For more than two weeks the International Airport of Catania, Fontanarossa, had to be closed due to ash on the runways.
- Strong seismicity and ground deformation accompanied the eruption; a particularly strong shock (magnitude 4.4) on 29 October destroyed and damaged numerous buildings on the lower southeastern flank, in the area of Santa Venerina.
- Lava flows from the southern flank vents seriously threatened the tourist facilities around the Rifugio Sapienza between 23 and 25 November, and a few days later destroyed a section of forest on the southwestern flank.
- The eruption brought a heightened awareness of volcanic and seismic hazards to the Sicilian public, especially because it occurred only one year and three months after the previous eruption that was strongly featured in the information media.
Look at this video clip from an eruption on Mount Etna in November 2007. What sort of eruption is it?
There is no commentary on the video - could you add your own explaining what is happening and why?
You should be able to use the knowledge and understanding you have gained about 2002-2003 eruption of Mount Etna to answer the following exam-style question:
In many parts of the world, the natural environment presents hazards to people. Choose an example of one of the following: a volcanic eruption, an earthquake, or a drought. For a named area, describe the causes of the example which you have chosen and its impacts on the people living there. [7 marks]
- Comment on Twitter
- 0 Shopping Cart
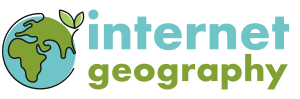
Europe’s most active volcano , Mount Etna, has been hitting the headlines recently after a series of spectacular eruptions. In Etna’s first eruption of 2021, explosive lava fountains reached over 1500 m in one of the most amazing eruptions in decades.
Mount Etna, located on the island of Sicily, has been largely dormant for the past two years. The stratovolcano (composite) dominates the skyline of the Italian island, where it sits on the eastern coast.
Located between the cities of Messina and Catania, it is the highest active volcano in Europe outside the Caucasus – a region between the Black Sea and the Caspian Sea – and the highest peak in Italy south of the Alps.
The recent activity is typical of a strombolian eruption among the normal activities of the more than 3,300-metre-high volcano. The recent eruption is the strongest explosion in the southern crater since it was discovered in 1971.
On Monday 22nd February 2021, at around 11 pm, the lava fountains, surrounded by gigantic clouds of smoke, exceeded 1,500 metres (4,900ft) in height, while thousands of rock fragments, some the size of fridges, were thrown from the crater into the sky for several kilometres.
Etna is a hyperactive volcano with over 3,500 years of historically documented eruptions. The volcano has been erupting on and off since September 2013. Since September 2019, it’s been erupting from its various summit craters virtually continuously. In December 2020, Etna’s explosive activity and lava output began to spike, and in February 2021, it has been launching fluid lava skywards.
Etna is an unusual volcano in that it can produce explosive eruptions of runny lava and release slower flowing, thick lava flows. Scientists are still trying to work out why this is the case.
The magma from the latest eruption appears to be coming up from deep within the mantle . Extremely hot, fluid magma is rapidly rising through the network of conduits within and below the volcano. However, there is another factor that is contributing to the current explosive eruptions.
There are high quantities of water vapour in Etna’s magma, which makes it explosive. The water does not cool the magma. As the molten magma approaches the surface, the pressure drops, and the bubble of water vapour expands violently, leading to lava being ejected out of the volcano.
Following each explosive lava fountain , less gassy magma lingers just below the vent. This is then cleared when a new volley of gassy magma rises from below. These explosive eruptions are known as volcanic paroxysms.
Authorities have reported no danger to the nearby towns, however, local airports have been temporarily closed, as has the airspace around the volcano. Etna’s last major eruption was in 1992. Despite the explosive nature of the recent eruption, there is no risk to the population, other than from the ash that covers buildings and smoke that can, after a few hours, cause breathing problems. In March 2017 vulcanologists, tourists and a BBC film crew were injured during an eruption when a flow of lava ran into snow, producing superheated steam that sent fragments of rock flying in all directions.
Further reading For a Volcanologist Living on Mount Etna, the Latest Eruption Is a Delight – Advisory – this article contains expletives (swear words).
In Pictures: Mount Etna eruption lights up Sicily’s night sky
Mount Etna: BBC crew caught up in volcano blast
Mount Etna illuminates night sky with 1,500-metre lava fountain
Share this:
- Click to share on Twitter (Opens in new window)
- Click to share on Facebook (Opens in new window)
- Click to share on Pinterest (Opens in new window)
- Click to email a link to a friend (Opens in new window)
- Click to share on WhatsApp (Opens in new window)
- Click to print (Opens in new window)
Leave a Reply
Leave a reply cancel reply.
Your email address will not be published. Required fields are marked *
Save my name, email, and website in this browser for the next time I comment.
I agree to the terms and conditions laid out in the Privacy Policy
Notify me of follow-up comments by email.
Notify me of new posts by email.
This site uses Akismet to reduce spam. Learn how your comment data is processed .
Please Support Internet Geography
If you've found the resources on this site useful please consider making a secure donation via PayPal to support the development of the site. The site is self-funded and your support is really appreciated.
Search Internet Geography
Top posts and pages.

Latest Blog Entries

Pin It on Pinterest
- Click to share
- Print Friendly
Thank you for visiting nature.com. You are using a browser version with limited support for CSS. To obtain the best experience, we recommend you use a more up to date browser (or turn off compatibility mode in Internet Explorer). In the meantime, to ensure continued support, we are displaying the site without styles and JavaScript.
- View all journals
- My Account Login
- Explore content
- About the journal
- Publish with us
- Sign up for alerts
- Open access
- Published: 25 October 2022
Energy threshold changes in volcanic activity at Mt. Etna (Italy) inferred from volcanic tremor
- Horst Langer ORCID: orcid.org/0000-0002-2508-8067 1 ,
- Susanna Falsaperla ORCID: orcid.org/0000-0002-1071-3958 1 ,
- Salvatore Spampinato ORCID: orcid.org/0000-0002-1954-1080 1 &
- Alfio Messina ORCID: orcid.org/0000-0002-3358-7210 1
Scientific Reports volume 12 , Article number: 17895 ( 2022 ) Cite this article
2236 Accesses
2 Citations
4 Altmetric
Metrics details
- Volcanology
From the 2010s on, pattern classification has proven an effective method for flagging alerts of volcano unrest before eruptive activity at Mt. Etna, Italy. The analysis has been applied online to volcanic tremor data, and has supported the surveillance activity of the volcano that provides timely information to Civil Protection and other authorities. However, after declaring an alert, no one knows how long the volcano unrest will last and if a climactic eruptive activity will actually begin. These are critical aspects when considering the effects of a prolonged state of alert. An example of longstanding unrest is related to the Christmas Eve eruption in 2018, which was heralded by several months of almost continuous Strombolian activity. Here, we discuss the usage of thresholds to detect conditions leading to paroxysmal activity, and the challenges associated with defining such thresholds, leveraging a dataset of 52 episodes of lava fountains occurring in 2021. We were able to identify conservative settings regarding the thresholds, allowing for an early warning of impending paroxysm in almost all cases (circa 85% for the first 4 months in 2021, and over 90% for the whole year). The chosen thresholds also proved useful to predict that a paroxysmal activity was about to end. Such information provides reliable numbers for volcanologists for their assessments, based on visual information, which may not be available in bad weather or cloudy conditions.
Similar content being viewed by others
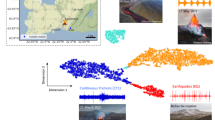
Tremor clustering reveals pre-eruptive signals and evolution of the 2021 Geldingadalir eruption of the Fagradalsfjall Fires, Iceland
Zahra Zali, S. Mostafa Mousavi, … Fabrice Cotton
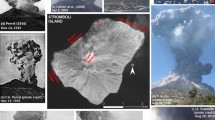
Major explosions and paroxysms at Stromboli (Italy): a new historical catalog and temporal models of occurrence with uncertainty quantification
Andrea Bevilacqua, Antonella Bertagnini, … Augusto Neri
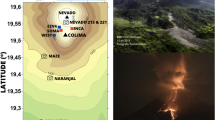
Tracking volcanic explosions using Shannon entropy at Volcán de Colima
Pablo Rey-Devesa, Janire Prudencio, … Jesús M. Ibáñez
Introduction
Etna is an active basaltic volcano, with frequent episodes of eruptive activity in the form of Strombolian explosions, lava fountains and lava flows 1 . For example, hundreds of lava fountains occurred from 2000 to 2021 alone 2 , 3 , 4 . They stem from the summit craters, which are located at ~ 3300 m a.s.l., far away from inhabited areas (Fig. 1 ). Nevertheless, lava fountains produce abundant fallout of ash and lapilli, causing air and road traffic disruption, with heavy social and economic impacts (e.g., 5 ).
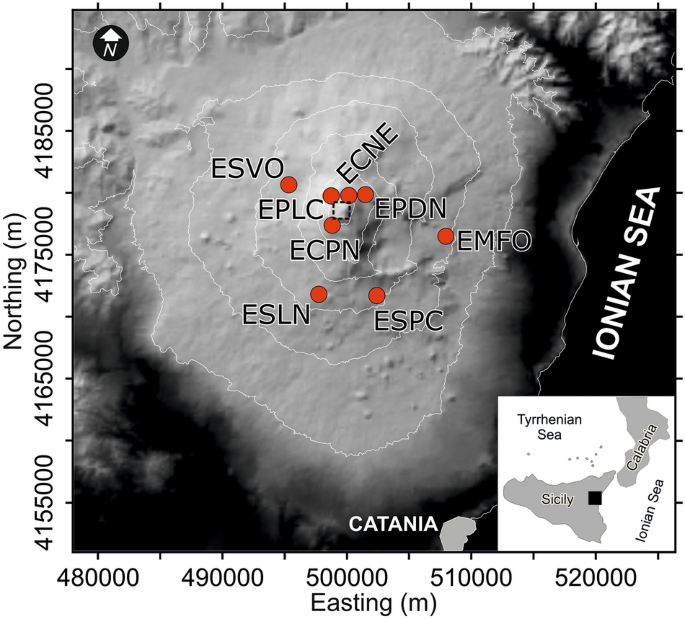
Digital elevation model (DEM) of Etna from TINITALY/01 by Tarquini et al. 26 . The DEM is referenced in the UTM WGS 84 zone 32 projection system, and is published with a CC BY 4.0 license ( https://doi.org/10.13127/TINITALY/1.0 ; last access 14 September 2022). Red circles mark the location of the permanent seismic stations we used in this study. The dashed square marks the area of Etna’s summit craters.
Early changes detected by a monitoring network of sensors are of paramount importance to highlight impending eruptions. In this perspective, volcano observatories around the world, such as at Kilauea (Hawaii 6 ), Soufrière Hills volcano (Montserrat 7 ), and Piton de la Fournaise (Réunion 8 ), have not only enhanced their monitoring systems, but have also exploited cutting-edge tools for automated data processing. Unglert et al. 6 study various unsupervised classification techniques with respect to their capacity to retrieve certain spectral patterns on Kilauea; Hammer et al. 7 propose a dynamic application of Hidden Markov Models for event classification on Montserrat; Hibert et al. 8 apply Random Forests to the discrimination of volcano-tectonic events from rockfalls on Piton de la Fournaise.
The monitoring of the background seismic radiation, which is mainly of volcanic origin and therefore called volcanic tremor (e.g., 9 ), focuses on the development over time of the amplitude and frequency content of the signal. It has become key for the surveillance of volcanic activity on Mt. Etna, where volcanic tremor is persistent 10 , 11 , 12 (Fig. 1 ). Amplitude-ratio based criteria, such as short- and long-time averages (STA/LTA trigger algorithm), are effective in short-lived lava fountains, but have limits in the presences of a gradual increase of activity. Also, amplitude-based criteria, such as RSAM 13 , do not account for changes in the spectral characteristics, which provide information for warning purposes 14 . On Mt. Etna, the application of pattern recognition techniques to spectral features (see “ Methods ” section) has enabled detecting impending unrests in their very early stages (e.g., 14 ). Consequently, the staff of Istituto Nazionale di Geofisica e Vulcanologia (INGV), who runs monitoring and surveillance activity, gains precious lead time for alerting government authorities. To this end, Spampinato et al. 15 designed a multi-station-alert system that follows the principles of voting a law in a parliament. The system counts each seismic station for which an anomaly in volcanic tremor is detected, applying triggering parameters at each single station as described by D’Agostino et al. 12 . Additional information beyond the binary decision “criticality: yes–no”, can be inferred from a voting scheme based on the number of stations where an anomaly is detected, and their weights. Even though the criticalities are flagged at each single station, the lead times vary from station to station allowing some insight into the development of an unrest. The voting essentially expresses some degree of certainty to which a criticality is declared (here, the detection of specific variations in the frequency content of the signal radiated by the volcanic system). However, a condition of volcano unrest may hold true for months and not just be short-lived. This is a serious drawback even during time spans with mild, long-lasting volcanic activity, as the voting scheme does not explicitly account for the signal energy at each station. A similar condition, hovering around low to moderate Strombolian activity, occurred at Etna more or less over the whole of 2018. Tremor amplitudes remained at an intermediate level, which is marked by a yellow band in Fig. 2 . As a consequence, many stations maintained an alert flag over long-time spans before a climactic eruption started on Christmas Eve. Multidisciplinary studies have actually documented prolonged magma replenishment from depth starting at least 6 months before the eruption (e.g., 16 ).
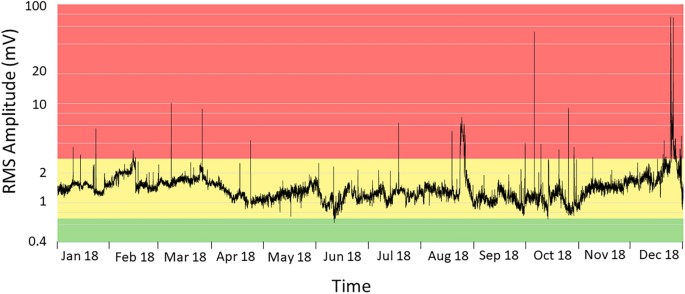
RMS amplitude of volcanic tremor at the ESLN station in 2018. The station is equipped with a velocimeter. The data are filtered between 0.5 and 2.5 Hz, and averaged over 30 min. The tremor amplitudes remained at an intermediate level, marked by the yellow band, concurrent with low to moderate Strombolian activity more or less throughout the year.
The concept to flag even modest signs of unrest was successful for the previous years, starting from the 2010s 14 ; however, the almost continuous state of alert in 2018 jeopardized confidence in the robustness of the system as if it were due to a malfunction. To overcome the drawback, we envisaged the use of thresholds of the flag alert not only for the detection of the unrest (such as weak to mild Strombolian activity in the context of Etna), but also for the onset of impending, (more energetic) eruptive activity.
Figure 3 refers to a lava fountain episode on February 19, 2021. In the figure, we depict the spectral amplitude of volcanic tremor (panel I); the spectrogram with frequency content up to 15 Hz (panel II); and the results from Self-Organizing Maps (SOM) (panel III) and fuzzy clustering (FC) (panel IV) where the membership of a pattern to a cluster is given by a membership vector (see " Methods " section). We exploit the possibility given by the SOM to represent the spectral characteristics as an RGB (Red–Green–Blue; see " Methods " section) color code, which allows us to visualize the development of pattern characteristics over time as a sequence of colored symbols. A simplified, yet effective representation consists in plotting the saturation degrees of the Red-Green components (R-G saturation in panel V of Fig. 3 ) in the SOM colors. The two R-G saturation curves can be easily used to define thresholds and trigger criteria, and we focus on their variation in the following sections.
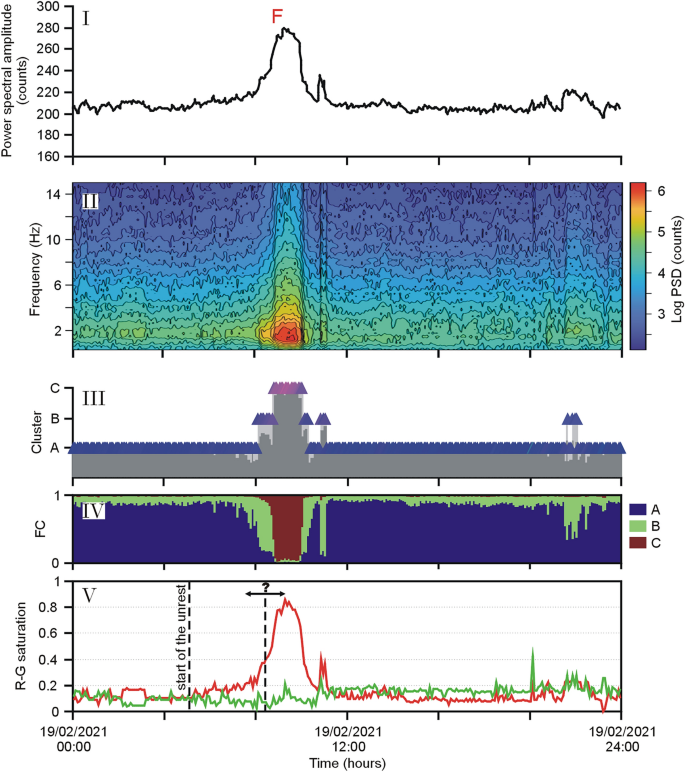
Episode of lava fountain (F) at Etna on February 19, 2021. Based on the analysis of volcanic tremor at the ESVO station, the diagrams cover 24 h: ( I ) spectral amplitude; ( II ) spectrogram; ( III ) results of the SOM in the form of colored triangles; ( IV ) fuzzy clustering (FC) considering three clusters; ( V ) normalized values of the saturation of the Red-Green (R-G) components in the SOM colors. The first dashed line marks the start of the unrest (warning flag of the multi-station system by Spampinato et al. 15 ). The second dashed line (with the question mark) refers to the possible range of transition to a paroxysmal activity.
The spectral amplitudes peaked at ~ 9:00 UTC (the climax of the lava fountain) and then sharply decreased, so that at 10:15 UTC the phenomenon was essentially over (Fig. 3 I–II). The development of the spectral characteristics is clearly mirrored in the SOM colors (Fig. 3 III). Before the onset of the eruption, the symbols had typically blue colors that changed to purple and intense red as the paroxysmal phase approached. The climax of the lava fountain had a clear regime during which cluster “C” showed up in the SOM (Fig. 3 III). The presence of this cluster has been reported as typical for eruptive activity with intense tremor radiation 14 , 15 . In the R-G curves, the saturation of the red component was also achieved during the climax of the volcanic activity, when the spectral amplitudes peaked (Fig. 3 V). We noticed that the trend of the R saturation (R hereafter) shown in Fig. 3 initially had a slow increase, which speeded up only when the paroxysm became impending. From a visual inspection of those values, a ‘critical’ threshold of R could be inferred somewhere between 0.55 or 0.6, which marked a sharp acceleration towards the value 1 (full red saturation) shortly after. We observed that such a trend also holds for the R-G values of the lava fountains in 2011 from Spampinato et al. 15 (see Supplementary Information ). Moving on from this evidence, we investigated the presence of ‘critical’ thresholds of the R-G values, leading eruptive activity towards a climax. Here, we focus on two time-intervals, namely the year 2018 with the December 24 climactic lava effusion, and the time span from January to April 1, 2021 with 19 paroxysmal lava fountains. The results of our analysis were then tested on a new dataset encompassing all the 33 lava fountain episodes that occurred from May to December 2021.
Fixing the threshold
The Christmas Eve eruption in 2018 was heralded by short-term (days) as well as long-term (months) changes in ground deformation, tectonic seismicity, and magma composition (e.g., 16 , 17 , 18 ). In Fig. 4 we show how many stations reached or topped three arbitrary threshold values of the R saturation, namely 0.5, 0.55 and 0.6, in 2018. Discarding peaks associated with the occurrence of earthquakes (mostly regional events or teleseisms marked with T in Fig. 4 ), we notice that R = 0.6 was reached at four stations on December 9. Only a lower number of stations reached synchronously this value from January to December 9, a timeframe during which volcanologists reported Strombolian activity and a few overflows at the summit craters 4 . On the other hand, all stations topped the value 0.6 ~ 2 ½ hours before the Christmas Eve eruption on December 24, and even peaked full saturation (R = 1) during the climax of the eruption.
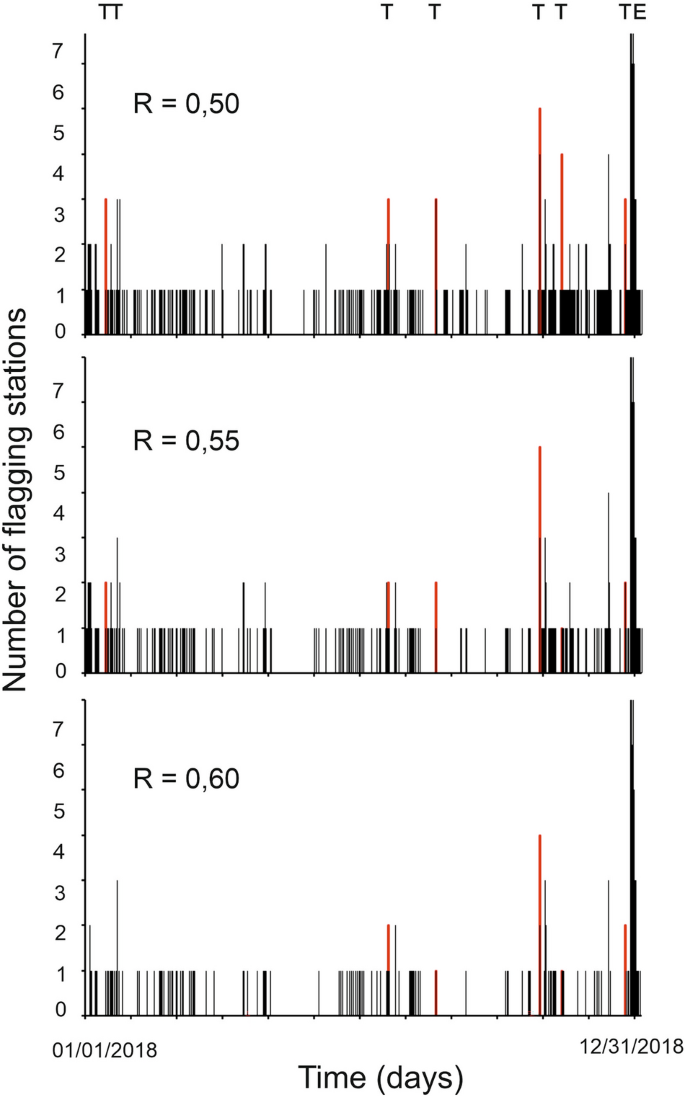
Number of stations that reached or topped three arbitrary threshold values (0.50, 0.55, and 0.60) of the R saturation in 2018. T and E stand for teleseisms and the Christmas Eve eruption, respectively. Red bars mark warnings caused by teleseisms.
So far, we have considered thresholds, such as 0.5, 0.55 or 0.6, for the R component moving on from rather generic considerations on Fig. 3 , the Supplementary Information , and somewhat following our intuition. However, each decision made on the basis of the information provided by volcanic observatories comes with costs and efforts. In this context, it is mandatory that criteria are defined following reproducible rules. Any rule for setting up criteria must deal with a principal dilemma: The greater the degree of confidence in our system, the more challenging the choice of thresholds and criteria will be. The major challenge comes from the risk of losing relevant eruptive activity for some reasons, such as peculiarities of the phenomenon or temporary technical failures of the seismic network. In other words, we have to appraise various criteria comparing the “true positives” (event correctly flagged) with respect to “false positives” (event flagged without having occurred). The so-called ‘Receiver Operation Characteristic’ (ROC hereafter) curves 19 are often used in binary decision problems, such as business decisions (e.g., launching a marketing campaign) or health care issues (for instance, whether to start a certain medical treatment). Here they allow us to establish rules for the identification of suitable criteria to evaluate the performance of our system. In ROC diagrams, we plot for each criterion the ‘True Positive Rate’ (TPR; the share of recognized events with respect to all those truly occurred) versus the ‘False Positive Rate’ (FPR), which is obtained from the ratio of all erroneously identified events with respect to all observations without the event of interest.
In the following, we discuss how to set up the threshold in the saturation of the red component (R) and the number of stations for which we require that the threshold is reached or topped in 2021. The year 2021 was mostly characterized by frequent episodes of paroxysmal volcanic activity, mainly lava fountains preceded and followed by longer time intervals with Strombolian activity and sporadic episodes of ash emission and lava effusion. The lava fountains were short-lived events with durations of several hours 20 . Their column height reached up to 10 km above ground, and formed a considerable threat to the air traffic for the fallout of pyroclastic material and ash over the municipalities located along the flanks of the volcano 4 .
Here, we start considering the time span from January 1st to April 1st, 2021, during which there were 19 lava fountains along with almost persistent Strombolian activity. To select the threshold, we consider five alternatives of the R value ranging from 0.5 to 0.6, counting the number of stations where the chosen saturation value is reached or topped. Our observations are evaluated every 5 min. This time span corresponds to the one for which the patterns, represented by the colored symbols, are obtained. Consequently, we have 288 evaluations per day, in total 26,208 patterns in the considered time span.
Keeping the threshold fixed, we count how many true and false positives (TP and FP) were declared accounting for the number of stations reaching that threshold. Figure 5 depicts the ROC curves obtained for R equal to 0.5, 0.52, 0.55, 0.58, and 0.6. Seven stations were functioning in the time span from January 1st to April 1st (Fig. 1 ), with occasional gaps at single stations. On the whole, there were 635 time intervals of 5 min concurrent with a paroxysm, which marked the highest value reachable for true positives, namely TPR = 1. In terms of the maximum false positive rate FPR = 1, we acquire a number of 25,573 (5-min) time intervals, whereas TPR = 1 is never reached. Figure 5 shows the ROC curves for various threshold values of the R saturation, with their major variations in the range 0 ≥ FPR ≤ 0.2.
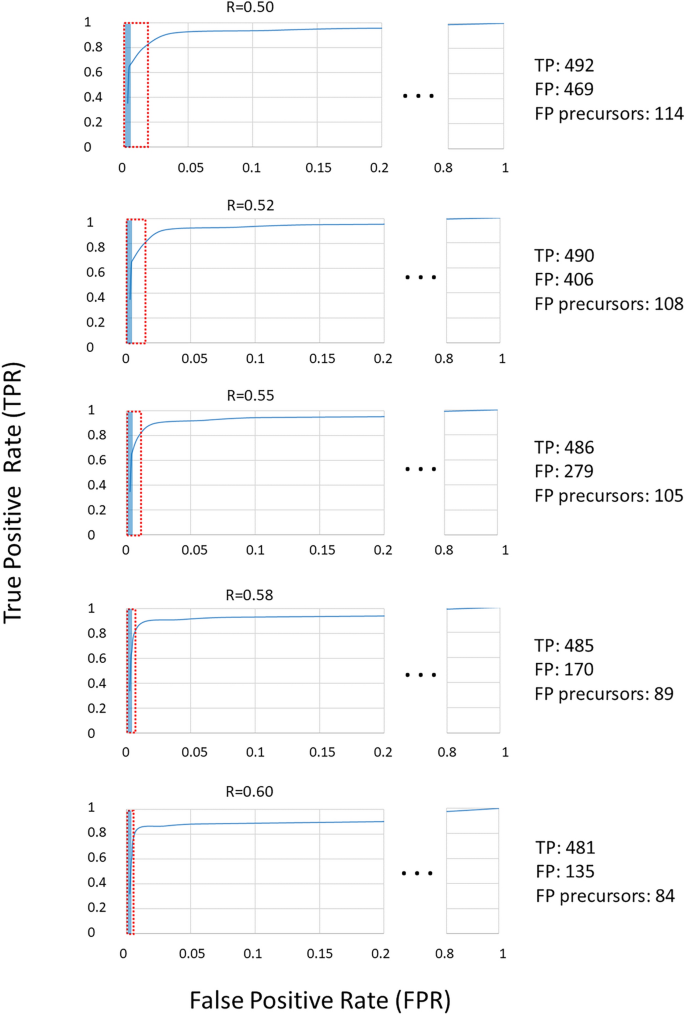
Receiver Operation Characteristic (ROC) curves for various threshold values of the R saturation. The number of True Positives (TP) and False Positives (FP) refers to a warning with at least four stations. The red and blue rectangles mark the range of FP for each threshold and the part of FP that comes as a precursor, respectively (the corresponding values are shown on the right side of the figure).
The interpretation of ROC curves can be guided by the so-called ‘Area Under the Curve’ (AUC), which is essentially the integral of an ROC curve. In case of a mere random result, then 0.5 < AUC < 0.6, namely TPR and FPR increase to the same degree, and the diagonal line across the ROC diagram has slope 1. The performance is good when 0.8 < AUC < 0.9 15 , 19 . Overall, all ROC curves shown in Fig. 5 have AUC ~ 0.95, a value indicative of an excellent performance of the system. The calculation of these AUCs, however, is ill-conditioned here, due to problems arising from the low number of TP (635) with respect to the maximum number of possible FP (i.e., ca. 26,000). This leads to errors in computing the integral of the ROC. Indeed, the tail of the ROC with FPR greater than 0.1 dominates the AUC, even though being supported by only one FPR/TPR pair. As mentioned above, we have no valid configuration achieving TPR = 1. Our discussion will therefore focus on the absolute values of TP and FP (see Table 1 ). In general, we get TPR ~ 0.8 (namely ~ 480 out of 635 true positives for all ROC) when requiring that the threshold for an alert flag is reached in at least 4 stations. In this case, the number of false positives is 135 for R = 0.6, 170 for R = 0.58 and reaches 469 when choosing R = 0.50. Based on the values in Table 1 , a configuration with a minimum of four flagging stations (called “Min4”) and thresholds such as R = 0.58 or 0.6 keeps the number of false positives well below the number of true positives (Fig. 6 ). With less conservative options (R = 0.5 and R = 0.52), more or less equal numbers of true and false positives are obtained.
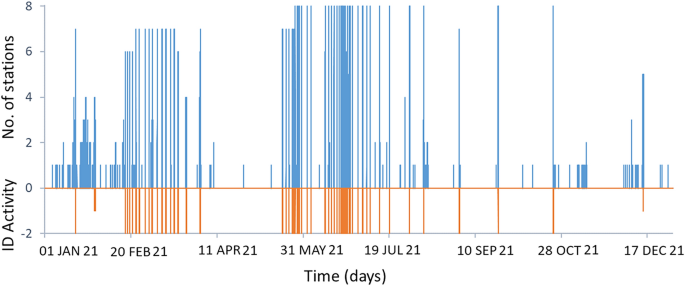
Number of stations reaching the threshold R = 0.58 (blue line, positive values) and volcanic activity (orange line) in 2021. The negative values in the y-axis are associated with the presence of strong effusive activity with ash emission (“− 1”) and lava fountains (“− 2”), respectively.
Requiring a rate close to 90% of detected true positives, we can opt for three flagging stations and all thresholds from R = 0.5 to R = 0.6. However, with the less conservative choices (R = 0.5 and R = 0.52) we encounter a considerable amount of FP (twice the number of TP for R = 0.5 and 1.5 fold the number of TP for R = 0.52). Again, the conservative choices may be preferred.
In many applications, for instance in medical disciplines, false positives are generally undesired. They can be the ruin of disease diagnostics, as the chance of belonging to a group of erroneously positive subjects tops the risk of being among the people actually affected by a disease. In those cases, at least a second test is recommended (e.g., 21 ). Such a rigid understanding of false positives is not feasible for our problem. In the context of eruption forecasting, volcanic observatories are interested in precursory phenomena, that is, peculiarities which may herald the unrest. Such precursors are intrinsically false positives, even though they are welcome for the needs of ‘lead times’, namely the timeframe between the moment when the first positive (precursor) is detected and the true positive is observed.
To be accepted as precursors in our system, we require that the positives detected form a chain longer than 5 min without gaps (no more than one positive missing in the sequence). As an example, we list in Table 2 the time difference between when the value 0.58 of the R component was reached and the moment in which the onset of each of the paroxysmal events from January to April 1, 2021 was reported (see also Fig. 6 ). Here the lead time is positive when the threshold R = 0.58 was reached before the onset given by the INGV timely communications to government authorities, such as Civil Protection, prefectures, and aviation authorities. As patterns are created every 5 min and the precision of the times reported is even less, the lead times of Table 2 are multiples of 5 min. In most cases, lead times are positive and vary from a few minutes to several hours depending on the dynamics of the eruptive event. The ECNE station, being one of the closest to the summit craters where the activity started, is the first to signal it at almost any time. On the other hand, the station ESVO is in most cases the last site where the threshold R = 0.58 was reached. The “Max” column in Table 2 shows the longest lead times among all operating stations, which are obtained requesting that only one station meets the threshold. The highest value (255 min, corresponding to 51 patterns) refers to the station ESPC for the paroxysm on April 1, 2021, whereas ECNE had on average the largest lead times. In fact, if we sum up all lead times (keeping the positives only), we obtain 213 patterns that fall in the lead time range, namely the patterns that can be considered as precursors (see row “Sum/5” in Table 3 ). Nonetheless, there are 2280 false positives for the same configuration (Table 1 ). In a more conservative configuration, where we request that the threshold is met at four or more stations, we still obtain positive lead times in 15 out of the 19 reported paroxysms (see Table 2 , column “Min4”); in one case (“nd” in the table) the number of operating stations was less than four.
Table 3 gives a summary of the lead times for various thresholds of the R value, namely 0.50, 0.52, 0.55, 0.58, and 0.60. Keeping R = 0.58 and increasing the minimum number of alert flags to four stations (Fig. 6 ), the resulting lead time is the value reported in the column “Min4” in Table 3 . As before, if we sum up the lead times in that column (keeping the positives only), we obtain 89 positive patterns as precursors. Comparing the 89 precursor patterns to the total of 170 false positives for the configuration R = 0.58 / four stations (see Table 1 ), we find this configuration more convenient than the previous choice with only one station. Focusing on the “Max” and “Min4” columns of this table, we find that also the configuration R = 0.60 / four stations provides a reasonable performance. With this configuration we have a total of 135 FP (see Table 1 ), 84 of which are actually precursors (Table 3 ).
In the choice of the most convenient configuration of our system, we may also consider the appearance of cluster C instead of the saturation in red. Cluster C shows up in the context of strong volcanic activity 14 (see panel III in Fig. 3 ). If we calculate the lead times replacing the R threshold with patterns prevalently belonging to cluster C, we obtain a similar picture as before (see Table 4 ). Again, in the majority of cases, the ECNE station is the one where cluster C appears first, and ESVO is typically the last one. The values in the “Max” and “Min4” columns resemble those found for R = 0.52 or 0.55 in Table 3 . Consequently, from a practical viewpoint, it may be sufficient to focus on the R thresholds, as the appearance of the cluster C does not really add new, independent evidence. Cluster C also appears due to sources which are not linked to the volcano, such as during teleseisms or strong stormy weather (see, e.g., 14 ).
Discussion and conclusions
In this paper, we calculate the ‘true’ value of thresholds at Etna that may lead to a climax after the detection of volcanic unrest. To this end, we have refined a previous alert system by Spampinato et al. 15 based on a multi-station voting scheme. This kind of voting provides information redundancy, which improves robustness against possible operational failures of a single station—a frequent phenomenon in harsh environmental conditions. A further point is the increased reliability of an alert issued from a group of stations, which is less affected by local disturbances, such as environmental noise or instrumental effects. However, after the scheme sums over all the positive votes and issues an alert, there is no indication of the intensity of the impending eruptive activity. The decision-based vote may also be insufficient when moderate anomalies become persistent over long-time spans. In the year 2018, for instance, the volcano fed Strombolian activity of low to modest intensity over several months, yielding a nearly uninterrupted alert flag. Such a continuous, longstanding switched-on red light may decrease the staff’s level of attention in a volcano observatory and can even be annoying for the authorities, who operate on an emergency basis. It is therefore important to mark ‘points of no return’, i.e., thresholds which, once topped, herald an impending change to a major increase of the eruptive activity. Analyzing the Supplementary Information (redrawn from Spampinato et al. 15 ), we surmised that such thresholds may indeed exist. Convincing evidence for their existence has been found from a visual inspection of the R-G curves, in which the red component (R = 0.6 or higher values) in the SOM appeared as a good candidate. We also tried to figure out at how many stations the threshold has to be reached or topped. In a new, in-depth analysis we focused on the period from Jan 1st to April 1st, 2021, when 19 lava fountains occurred. This data set has been used as a learning ensemble for the choice of a threshold, marking the non-return point, and the number of stations to consider. The choice was guided using Receiver Operation Characteristic (ROC) curves, which come as diagrams of true-positive vs. false-positive rates. In these diagrams we count, for each specific threshold and number of stations where the threshold is met, how many times a critical phenomenon—here a paroxysmal event—was truly identified by the spectral characteristics of the patterns (true positives; TP). We compare these TP to the number of cases where the phenomenon was flagged, without actually having occurred. A commonly used parameter describing the validity of a ROC curve is the Area Under the Curve (AUC). Unfortunately, the calculation of AUC is ill-conditioned in our context, as we have no configuration where we are able to recognize all true positives, even requiring that the threshold is reached at only one station. However, this should not be taken as a deficiency. In fact, a part of the ‘false positives’ belongs to the so-called lead time, the time interval leading to the occurrence of the phenomenon we are interested in. Those false positives are in reality precursors, thus welcome in the context of monitoring and alert. We carried out a systematic analysis of true and false positives, varying both the threshold of the red saturation (R = 0.5…0.6), as well as the number of stations where this should be reached or topped. We found a reasonable compromise for R = 0.58 or 0.6 together with the request that this R value should be reached at least in four stations (Fig. 6 ). In these configurations, a majority of the false positives fall into lead times. In other words, they are precursors rather than errors caused by noise or other problems.
Applying the same scheme, that is, considering saturation thresholds of the red component and a (minimum) number of stations, we essentially cancel out the phenomenon of a continuous alert we were facing before. Certainly, the volcano was in an almost persistent state of unrest in the year 2018 until December, when the climactic Christmas Eve eruption occurred. In Fig. 4 we recognize that, with the requirement of at least 3 stations reaching a threshold of R = 0.5, we acquire some false alarms mainly caused by the presence of regional earthquakes and teleseisms due to their low frequency content. Using a more conservative setting, i.e. R = 0.55 or higher, and requiring at least 4 stations to reach the threshold, we actually get no more than one false alarm, which occurred on October 25, 2018 during a teleseism.
To test if the system is up to the task with the chosen thresholds, we considered the 33 episodes of lava fountains from May to December 2021. We focused on the two conservative settings mentioned above, i.e. R = 0.58 or R = 0.6, always requiring the threshold to be reached or topped at a minimum of four stations. In both the configurations we almost always noticed positive lead times, i.e., the impending paroxysmal activity was detected before its occurrence was reported in the communications released by INGV-OE. In only two single cases was the detection delayed by 5 min (1 pattern length). On average, the lead times were ~ 40 min with R = 0.58 and ~ 35 min choosing R = 0.6. A further issue is the ‘closure’ of the activity, i.e., the prediction that the eruptive crisis is going to end. In both configurations, the closure was declared, on average, 20 min earlier than the end times reported in the communications (see Table 5 ). Occasionally, the closure by our revised system was delayed, producing a number of false positives. However, these were essentially the only false positives encountered in the tested period May-December, 2021.
In a recent paper published during the preparation of the present study, Calvari and Nunnari 20 re-analyzed the visual and infrared- images recorded by the INGV video cameras installed on the volcano. They considered 66 lava fountains occurring from December 2020 to February 2022, for each of which they report start and end times inferred from their analysis of the images. We decided to use their study as a further test of our system. We compared the times they report for the eruptive episodes in 2021 with the times we calculated, considering the configuration R = 0.58 and four or more stations reaching or topping this threshold. Table 6 gives a summary of the comparison. On average, our system had an additional positive lead time of ~ 13 min (the corresponding median is 8 min). The absolute discrepancies (counting negative and positive differences, all positive) are somewhat higher. On the other hand, end times were fairly close to each other. The tremor-based system marked the end of an episode on average 6.8 min later (median is 0); taking the absolute differences we obtain an average of 10 min (median 5 min).
The comparison of our results to those inferred by the detailed analysis of the video camera images highlights that the tremor-based system is a valid proxy for the automatic identification of impending paroxysmal events. Note that two of the episodes reported in 2021 by Calvari and Nunnari 20 , namely those on February 23 at 03:45 UTC and March 4 at 01:30 UTC, were among the weakest in terms of the ‘Time Averaged Discharge Rate’ given by the authors. Those episodes did not reach the energy levels of tremor needed to trigger the alert flag at four stations. As the transition of various states of volcanic activity is not always clear cut, the tremor-based criteria may provide a guide to help volcanologists to better distinguish the activity regimes.
We can therefore envisage using a two-stage warning system. The first, which has been operating so far remains active, as it effectively recognizes mild phenomena of unrest, such as Strombolian activity, or minor lava effusions, not always leading to a relevant threat to human facilities. In the presence of a first stage of warning, the observatory staff will follow the ongoing development. Once the second level of criticality is reached (e.g., R = 0.58 at four stations or more) little doubt is left that the situation is heading towards a larger unrest, such as a paroxysmal lava fountain or a major lava emission as happened during the Christmas Eve eruption in 2018. It is worth noting that such a two-stage warning system overcomes problems of methods based on STA/LTA strategies, which fail when there is a slow increase in energy of the seismic radiation. Indeed, in this case, LTA slowly creeps to high values, which cannot be easily topped by the STA.
The practical choice of the parameters R and the number of stations is partly a matter of the decision makers, who decide whether the actual risk of missing an event can be accepted, and what is the desired lead time. A comparison of the number of patterns, being precursors with respect to the total of false positives encountered for a configuration, can guide the choice. Here, using R = 0.58 or 0.6 and four stations, most of the false positives come as precursors, thus they are opportune rather than representing a deficiency of the system. Others occur shortly after a paroxysmal phenomenon dies out, as their occurrence is still linked to the fading volcanic activity.
Feature extraction
Langer et al. 14 developed a scheme for automatic feature extraction based on spectral analysis, which resembles the Seismic Spectral Amplitude Measurement (SSAM) proposed by Rogers and Stephen 22 . The first step is the calculation of the Short Time Fourier Transform (STFT), with a gliding window applied to the whole time series. Each window has a length of 1024 points (corresponding to 10.24 s) and is shifted by 500 points. Each short-time spectrum forms an element in a spectrogram. Frequency bins are averaged over the power spectral amplitudes in a selected frequency band to reduce the number of features. A further simplification is obtained by considering an ensemble of 60 short-time power spectra, i.e., a time span of 5 min. We preferred to study the 10% (bottom) percentile, focusing on the lowest amplitudes encountered in the 5-min time span, to largely eliminate the effect of short-lived transients (e.g., wind gusts or local earthquakes).
Self-organizing maps
SOM—or Kohonen maps 23 —are a type of artificial neural network. SOM form a mesh of nodes, which are small clusters with each one representing a number of patterns. The centroid of the clusters can be understood as a prototype of patterns. During the learning phase, the feature vectors W of the centroids are adjusted so that the sum of the distances between the original data and their representing prototype nodes converges to a minimum. Formally, during the training of SOM, one minimizes the sum of the distances
where Vj is the normalized input feature vector and Wi the weights stored in the nodes. V and W have the same dimension. A core step is the identification of the closest node to the actual input vector, i.e., the best matching unit (BMU) for the jth pattern. During the learning steps, neighboring nodes lying within a certain radius of influence are considered as well. Weights are gradually adjusted according to the so-called learning rate λ, which decreases with time t. A second parameter φ (called influence radius) describes the dependence on distance ∆ of the upgrade of a node. φ (∆,t) is maximum for the BMU, whereas nodes outside the radius of influence are not upgraded at all. Similar to the learning rate λ, also φ (∆,t) decreases with time. The upgrade of weights follows the relationship:
The introduction of the term φ (∆,t) has an important effect, known as ‘Topological fidelity’, i.e. patterns represented by neighboring nodes in the SOM are truly close to each other also in the original data space. SOM can be particularly instructive when the weights take the form of a color code. For this purpose, Principal Component Analysis is applied, projecting the multi-dimensional weights vector in a 2D representation space spanned by the principal axes z1 and z2. The coordinates of a node with respect to the axes are expressed in terms of a color code, such that saturation in red (“R”) stands for the first, saturation in green (“G”) for the second axis, and saturation in blue is just complementary to saturation in green. The coding allows identifying the position of a pattern on the map simply by an appropriate colored symbol. For more details, the reader can consult textbooks such as Kohonen 23 or Langer et al. 24 .
Spampinato et al. 15 probed Self-Organizing Maps (SOM) in the framework of a multi-station system aimed at early warning. They considered continuously recorded data from 11 permanent seismic stations. The seismic network was composed of two rings with the center at the summit craters: an inner ring of seven stations (within a radius of ∼ 3 km), and an outer ring with four stations placed at distances of up to ~ 8 km (Fig. 1 ). Following the strategy proposed in Spampinato et al. 15 , our trigger parameters are defined for each single station. These parameters are basically based on the RGB color codes, with “R” (red) and “G” (green) being independent, whereas “B” (blue) is complementary to “G”, i.e., B = 1 − G. The classifier has been tested on past data streams, encompassing various episodes of eruptive activity for which each of the 11 seismic stations issued a timely warning.
Fuzzy clustering
The cluster analysis follows a non-hierarchical, partitioning strategy of clustering. The measure of the heterogeneity of the k-th cluster is expressed as the sum of the squared distances of the patterns in each cluster from their centroid vector. Contrary to crisp clustering, where each pattern is exclusively assigned to one cluster, in fuzzy clustering 25 each pattern may belong to a certain degree to all possible classes. Consequently, the class membership of a pattern, rather than being a simple ID, is given by a vector. The partition is consequently described by a M × K matrix of class membership values, with M being the number of patterns in the entire data set, and K the number of clusters. Transitional regimes become evident in the gradual changes in the class membership vector. In our application, we calculate the fuzzy membership vectors on the original feature vectors and show them together with the SOM colors. In Fig. 3 (see panel IV) the patterns are assigned to the cluster with the highest membership degree.
Data availability
The raw seismic data analysed during the current study are available in the European Integrated Data Archives ( www.eida.ingv.it ). The software for the pattern recognition can be freely downloaded from https://earthref.org/ERDA/974/ . The dataset that we used to create the statistics of this article are freely available in https://doi.org/10.13127/etna/mavt2021 .
Doglioni, C., Innocenti, F. & Mariotti, G. Why Mt. Etna?. Terra Nova 13 , 25–31 (2001).
Article ADS CAS Google Scholar
Alparone, S., Andronico, D., Lodato, L. & Sgroi, T. Relationship between tremor and volcanic activity during the Southeast Crater eruption on Mount Etna in early 2000. J. Geophys. Res. 108 , 2241. https://doi.org/10.1029/2002JB001866 (2003).
ADS Google Scholar
Behncke, B. et al. The 2011–2012 summit activity of Mount Etna: Birth, growth and products of the new SE crater. J. Volcanol. Geotherm. Res. 270 , 10–21 (2014).
Andronico, D., Cannata, A., Di Grazia, G. & Ferrari, F. The 1986–2021 paroxysmal episodes at the summit craters of Mt. Etna: Insights into volcano dynamics and hazard. Earth-Sci. Rev. 220 , 103686. https://doi.org/10.1016/j.earscirev.2021.103686 (2021).
Article Google Scholar
Andronico, D., Scollo, S. & Cristaldi, A. Unexpected hazards from tephra fallouts at Mt Etna: The 23 November 2013 lava fountain. J. Volcanol. Geotherm. Res. 304 , 118–125. https://doi.org/10.1016/j.jvolgeores.2015.08.007 (2015).
Unglert, K., Radic, V. & Jellinek, A. M. Principal component analysis vs. self-organizing maps combined with hierarchical clustering for pattern recognition in volcano seismic spectra. J. Volcanol. Geotherm. Res. 320 , 58–74. https://doi.org/10.1016/j.jvolgeores.2016.04.014 (2016).
Hammer, C., Beyreuther, M. & Ohrnberger, M. A seismic-event spotting system for volcano fast-response systems. Bull. Seismol. Soc. Am. 102 (3), 948–960. https://doi.org/10.1785/0120110167 (2012).
Hibert, C. et al. Automatic identification of rockfalls and volcano-tectonic earthquakes at the Piton de la Fournaise volcano using a Random Forest algorithm. J. Volcanol. Geotherm. Res. 340 , 130–142 (2017).
McNutt, S. R. & Roman, D. C. Volcanic Seismicity. In The Encyclopaedia of Volcanoes 2nd edn (eds Sigurdsson, H. et al. ) 1011–1034 (Academic Press, 2015). https://doi.org/10.1016/B978-0-12-385938-9.00059-6 .
Chapter Google Scholar
Falsaperla, S. et al. Volcanic tremor at Mt. Etna, Italy, preceding and accompanying the eruption of July–August, 2001. Pure Appl. Geophys. 162 (11), 2111–2132 (2005).
Article ADS Google Scholar
Cannata, A. et al. Monitoring seismo-volcanic and infrasonic signals at volcanoes: Mt. Etna case study. Pure Appl. Geophys. 170 , 1751–1771 (2013).
D’Agostino, M. et al. Volcano Monitoring and Early Warning on Mt Etna, Sicily Based on Volcanic Tremor: Methods and Technical Aspects. In Complex Monitoring of Volcanic Activity: Methods and Results (ed. Zobin, V. M.) 53–91 (Nova Sci. Publ, 2013).
Google Scholar
Endo, E. T. & Murray, T. Real-time Seismic Amplitude Measurement (RSAM): A volcano monitoring and prediction tool. Bull. Volcanol. 53 , 533–545 (1991).
Langer, H. et al. Detecting imminent eruptive activity at Mt. Etna, Italy, in 2007–2008 through pattern classification of volcanic tremor data. J. Volcanol. Geotherm. Res. 200 , 1–17 (2011).
Spampinato, S. et al. Short-term detection of volcanic unrest at Mt. Etna by means of a multi-station warning system. Sci. Rep. 9 , 6506. https://doi.org/10.1038/s41598-019-42930-3 (2019).
Article ADS CAS PubMed PubMed Central Google Scholar
Borzì, A. M., Giuffrida, M., Zuccarello, F., Palano, M. & Viccaro, M. The Christmas 2018 eruption at Mount Etna: Enlightening how the volcano factory works through a multiparametric inspection. Geochem. Geophys. Geosyst. 21 , 10. https://doi.org/10.1029/2020GC009226 (2020).
Alparone, S. et al. Seismological constraints on the 2018 Mt. Etna (Italy) flank eruption and implications for the flank dynamics of the volcano. Terra Nova 32 , 334–344. https://doi.org/10.1111/ter.12463 (2020).
Mattia, M. et al. Combined seismic and geodetic analysis before, during, and after the 2018 Mount Etna eruption. Geochem. Geophys. Geosyst. 21 , e2020GC009218. https://doi.org/10.1029/2020GC009218 (2020).
Metz, C. E. Basic principles of ROC analysis. Semin. Nuclear Med. 8 , 283–298 (1978).
Article CAS Google Scholar
Calvari, S. & Nunnari, G. Comparison between automated and manual detection of lava fountains from fixed monitoring thermal cameras at Etna Volcano, Italy. Remote Sens. 14 , 2392. https://doi.org/10.3390/rs14102392 (2022).
Gigerenzer, G. Calculated Risks: How to Know When Numbers Deceive You 328 (Simon & Schuster, 2015) ( ISBN 978-0743205566 ).
Rogers, J. A. & Stephens, C. D. SSAM: Real-time seismic spectral amplitude measurement on a PC and its application to volcano monitoring. Bull. Seismol. Soc. Am. 85 (2), 632–639. https://doi.org/10.1785/BSSA0850020632 (1995).
Kohonen, T. Self-organizing Maps 3rd edn, 501 (Springer, 2001).
Book Google Scholar
Langer, H., Falsaperla, S. & Hammer, C. Advantages and Pitfalls of Pattern Recognition. In Computational Geophysics Vol. 3 (ed. Spichak, V.) (Elsevier B.V., 2020) ( ISBN: 9780128118429, ISSN: 2468-547X ).
Zadeh, L. A. Fuzzy sets. Inf. Control. 8 , 338–353 (1965).
Tarquini, S., Isola, I., Favalli, M. & Battistini, A. TINITALY, a Digital Elevation Model of Italy with a 10 Meters Cell Size (Version 1.0) (Istituto Nazionale di Geofisica e Vulcanologia (INGV), 2007). https://doi.org/10.13127/TINITALY/1.0 .
Download references
Acknowledgements
This work was undertaken within the project IMPACT (A multidisciplinary Insight on the kinematics and dynamics of Magmatic Processes at Mt. Etna Aimed at identifying preCursor phenomena and developing early warning sysTems). IMPACT belongs to the Progetti Dipartimentali INGV [DIP7], https://progetti.ingv.it/index.php/it/progetti-dipartimentali/vulcani/impact#informazioni-sul-progetto . We are grateful to Graziella Barberi for the preparation of Fig. 1 . We thank Stephen Conway for revising the English language. We also thank Wes Thelen and an anonymous reviewer for helpful comments, and Alessandro Aiuppa for editorial handling.
Author information
Authors and affiliations.
Istituto Nazionale di Geofisica e Vulcanologia, Sezione di Catania, Osservatorio Etneo, Piazza Roma 2, 95125, Catania, Italy
Horst Langer, Susanna Falsaperla, Salvatore Spampinato & Alfio Messina
You can also search for this author in PubMed Google Scholar
Contributions
S.F. and H.L. conceived and designed this study; S.S. and A.M. prepared the data; H.L. carried out the ROC test; S.F. and H.L. wrote the paper; S.F., H.L., A.M. and S.S. prepared the figures; S.F., H.L., A.M. and S.S. contributed to the discussion of the paper.
Corresponding author
Correspondence to Susanna Falsaperla .
Ethics declarations
Competing interests.
The authors declare no competing interests.
Additional information
Publisher's note.
Springer Nature remains neutral with regard to jurisdictional claims in published maps and institutional affiliations.
Supplementary Information
Supplementary information., rights and permissions.
Open Access This article is licensed under a Creative Commons Attribution 4.0 International License, which permits use, sharing, adaptation, distribution and reproduction in any medium or format, as long as you give appropriate credit to the original author(s) and the source, provide a link to the Creative Commons licence, and indicate if changes were made. The images or other third party material in this article are included in the article's Creative Commons licence, unless indicated otherwise in a credit line to the material. If material is not included in the article's Creative Commons licence and your intended use is not permitted by statutory regulation or exceeds the permitted use, you will need to obtain permission directly from the copyright holder. To view a copy of this licence, visit http://creativecommons.org/licenses/by/4.0/ .
Reprints and permissions
About this article
Cite this article.
Langer, H., Falsaperla, S., Spampinato, S. et al. Energy threshold changes in volcanic activity at Mt. Etna (Italy) inferred from volcanic tremor. Sci Rep 12 , 17895 (2022). https://doi.org/10.1038/s41598-022-20766-8
Download citation
Received : 06 July 2022
Accepted : 19 September 2022
Published : 25 October 2022
DOI : https://doi.org/10.1038/s41598-022-20766-8
Share this article
Anyone you share the following link with will be able to read this content:
Sorry, a shareable link is not currently available for this article.
Provided by the Springer Nature SharedIt content-sharing initiative
This article is cited by
Towards scientific forecasting of magmatic eruptions.
- Valerio Acocella
- Maurizio Ripepe
- Erouscilla Joseph
Nature Reviews Earth & Environment (2023)
By submitting a comment you agree to abide by our Terms and Community Guidelines . If you find something abusive or that does not comply with our terms or guidelines please flag it as inappropriate.
Quick links
- Explore articles by subject
- Guide to authors
- Editorial policies
Sign up for the Nature Briefing newsletter — what matters in science, free to your inbox daily.

- Open supplemental data
- Reference Manager
- Simple TEXT file
People also looked at
Original research article, mechanisms of ash generation at basaltic volcanoes: the case of mount etna, italy.
- 1 School of Earth and Environmental Sciences, University of Manchester, Manchester, United Kingdom
- 2 Istituto Nazionale di Geofisica e Vulcanologia, Sezione di Catania, Osservatorio Etneo, Catania, Italy
- 3 Istituto Nazionale di Geofisica e Vulcanologia, Sezione di Pisa, Pisa, Italy
- 4 Istituto Nazionale di Geofisica e Vulcanologia, Sezione di Roma1, Rome, Italy
Basaltic volcanism is the most widespread volcanic activity on Earth and planetary bodies. On Earth, eruptions can impact global and regional climate, and threaten populations living in their shadow, through a combination of ash, gas and lava. Ash emissions are a very typical manifestation of basaltic activity; however, despite their frequency of occurrence, a systematic investigation of basaltic ash sources is currently incomplete. Here, we revise four cases of ash emissions at Mount Etna linked with the most common style of eruptive activity at this volcano: lava fountains (4–5 September 2007), continuous Strombolian activity transitioning to pulsing lava fountaining (24 November 2006), isolated Strombolian explosions (8 April 2010), and continuous to pulsing ash explosions (last phase of 2001 eruption). By combining observations on the eruptive style, deposit features and ash characteristics, we propose three mechanisms of ash generation based on variations in the magma mass flow rate. We then present an analysis of magma residence time within the conduit for both cylindrical and dike geometry, and find that the proportion of tachylite magma residing in the conduit is very small compared to sideromelane, in agreement with observations of ash componentry for lava fountain episodes at Mount Etna. The results of this study are relevant to classify ash emission sources and improve hazard mitigation strategies at basaltic volcanoes where the explosive activity is similar to Mount Etna.
Introduction
Explosive volcanism is characterised by magma fragmenting into particles of different size varying from micrometre to metre. In the presence of an eruption column, ash particles are volcanic fragments up to 2 mm in size that are dispersed to large distances from the eruptive centre in comparison to coarser fragments (e.g., bombs and lapilli) that fall in more proximal areas. Abundant ash has characterised most of the explosive activity at Mount Etna, Italy, since 1995 ( Figure 1 ) (e.g., La Delfa et al., 2001 ; Alparone et al., 2003 ; Andronico et al., 2009a , 2015 and references therein), often deeply affecting people’s everyday life and the overall economy of Eastern Sicily (e.g., Barsotti et al., 2010 ; Andronico et al., 2014a ; Andronico and Del Carlo, 2016 ; Horwell et al., 2017 ). At Etna ash emissions accompany different eruptive styles, from mild to moderate Strombolian explosions to high energy lava fountain activity (e.g., Andronico et al., 2008a , 2015 ), from short-lasting ash explosions ( Andronico et al., 2013 ) to long-lasting explosive eruptions like those occurred in 2001 (20 days; e.g., Taddeucci et al., 2002 ; Scollo et al., 2007 ) and in 2002–2003 (∼2 months; e.g., Andronico et al., 2005 ). All the 1995–2019 explosive activity producing significant ash emissions in the atmosphere and involving summit craters or flank areas has been summarised in Table 1 .
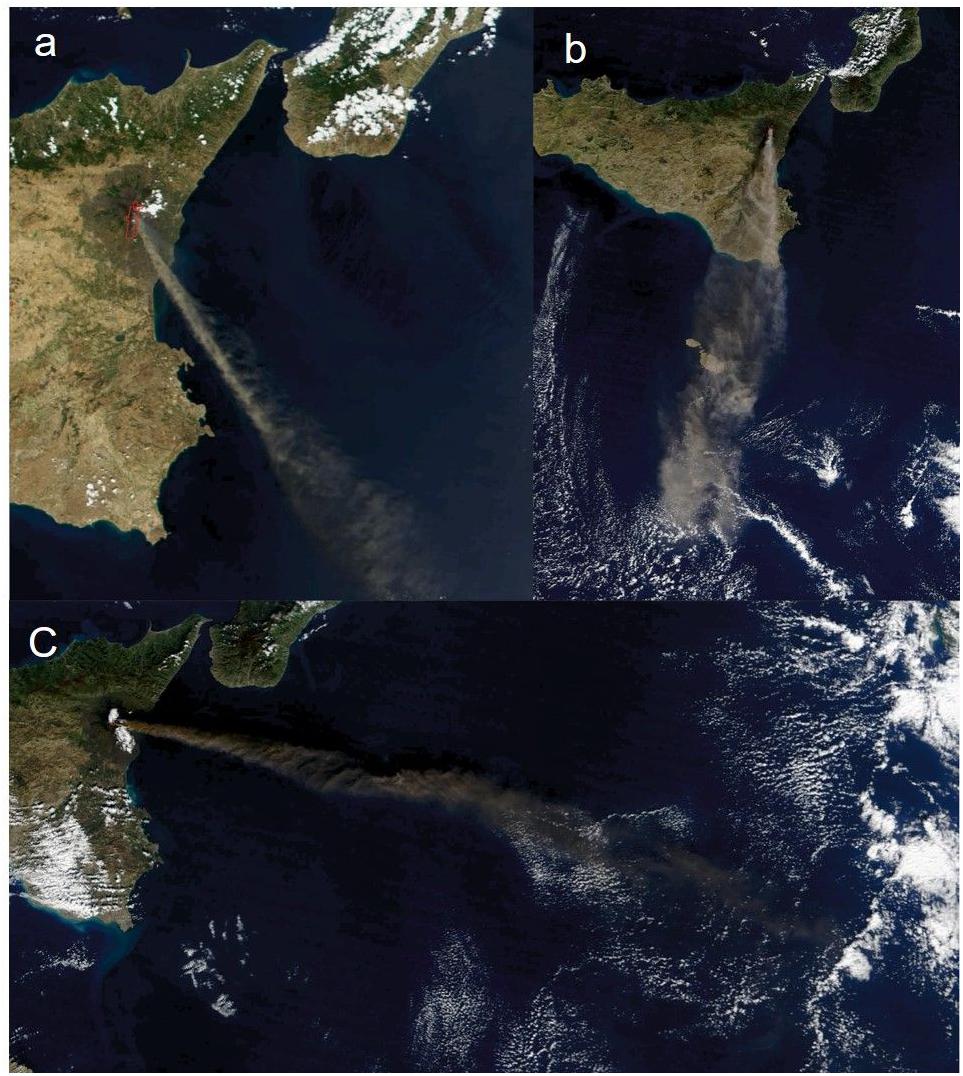
Figure 1. Satellite images of ash dispersal during the 2001 and 2002–2003 Mount Etna eruptions. (a) July 22, 2001; Image courtesy Jacques Descloitres, MODIS Land Rapid Response Team at https://visibleearth.nasa.gov/ view.php?id=56431 . (b) October 27, 2002; image courtesy Jacques Descloitres, MODIS Rapid Response Team at NASA GSFC, at https://visibleearth.nasa.gov/view.php?id=10376 . (c) November 12, 2002; image courtesy Jeff Schmaltz, MODIS Rapid Response Team, NASA GSFC at https://visibleearth.nasa.gov/view.php?id=10398 . Mount Etna coordinates 37°45′18′N, 14°59′42′E.
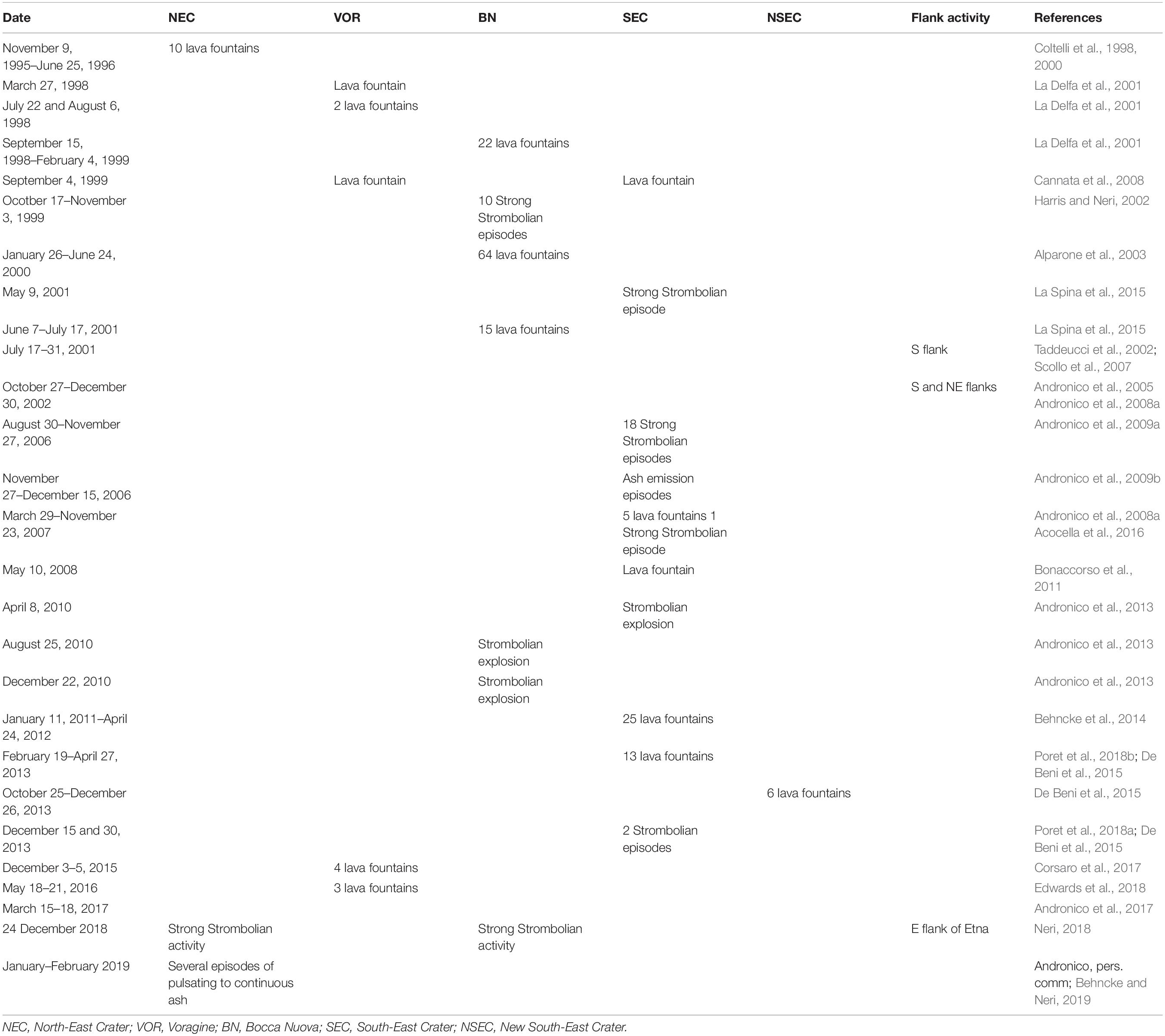
Table 1. Summary of the explosive activity (summit eruptions, i.e., lava fountains, strong Strombolian episodes, Strombolian explosions and sustained ash emissions, and flank eruptions) producing significant ash emission up to tens–hundreds of kilometres of distance away from Etna from 1995 to 2017.
Based on visual observations of the eruptive activity and textural and compositional features of ash samples, it was found that the characteristics of ash particles at Etna usually vary with the eruptive style. For example, ash emitted during Strombolian explosions and at the peak of lava fountain activity is more vesicular, less crystallised and with a less compositionally evolved groundmass than that erupted during less explosive events or at the end of a long-lasting explosive eruption, and it contains less lithic material ( Taddeucci et al., 2004 ; Andronico et al., 2008b ). Based on ground and satellite data, Andronico et al. (2009b) have attempted a first classification of ash-enriched Etnean volcanic plumes, subdividing ash emissions in the autumn of 2006 into five categories and demonstrating the utility of such classifications for volcanic hazard mitigation planners and civil protection purposes. It is worth specifying, however, that this classification is valid mostly for that period of activity in 2006 and it does not cover the whole range of explosive activity displayed by Etna.
Previous research on ash characteristics and the link between ash and eruption behaviour has improved our general knowledge on ash emissions at Etna ( Taddeucci et al., 2002 ; Andronico et al., 2013 ). A better understanding of ash formation has the potential of further improving hazard assessment and forecasting at this volcano. However, while the sources and features of ash particles at Etna have been investigated in several cases, a systematic comparison is still lacking. The present paper represents a concrete step forward in the attempt of classifying the most common mechanisms through which ash originates at Etna. In the following, first we briefly revise the characteristics of Etna ash, then we present four different case studies of ash emissions, each associated with a different eruptive style and marked by a different duration and intensity (i.e., mass eruption rate; MER) of the tephra emission. Ultimately, we link each ash emission case study to a different, peculiar mechanism of ash formation. Although based on case studies from Etna, the proposed mechanisms can explain ash generated by other basaltic volcanic systems [e.g., Paricutin, Mexico ( Pioli et al., 2008 ), and Villarrica, Chile ( Romero et al., 2018 )] which, during specific eruptive phases, resemble closely the Etna explosive activity in terms of duration, intensity and style of emission.
Characteristics of Ash at Etna
Textural and compositional features of ash particles erupted from explosive activity at Etna have been described in previous studies, and for details on the topic the reader is referred to those works (e.g., Taddeucci et al., 2002 , 2004 ; Andronico et al., 2009a , 2013 , 2014b ). Here we summarise the common aspects that can help extending from specific to general cases. Juvenile ash particles at Etna consist of two end-members: sideromelane and tachylite ( Figure 2 ). The former has fluidal to irregular morphology, is yellow to brown in colour, transparent, vesicular and generally glassy in the groundmass. The latter is blocky, grey to black, generally opaque (sometimes it can be shiny), poorly vesicular and crystallised in the groundmass. There is however, a continuous, progressive transition of textural features between the two ash types, mainly generated by the different extent of groundmass crystallisation, far more pronounced in tachylite, and by the higher content of (sub) spherical vesicles, as opposed to vesicles with complex and/or irregular shapes, in sideromelane ( Figure 2 ). As a general rule, glass composition of Etna ash overlaps with the compositional field of pyroclastic material erupted from this volcano since 1995 ( Corsaro et al., 2017 ; Pompilio et al., 2017 ). Within the same eruption, tachylite glass tends to be more compositionally differentiated in comparison to its sideromelane counterpart, with a higher silica, alkali and phosphorous content and lower magnesium and calcium ( Taddeucci et al., 2002 , 2004 ; Polacci et al., 2006 ). Compositional variations are mainly related to fractionation of a few tens of percent of crystal phases forming the groundmass. Lithic ash from Etna eruptions is not always easily distinguishable from tachylite juveniles, but is often marked by altered/oxidised surfaces and secondary minerals overgrowths.
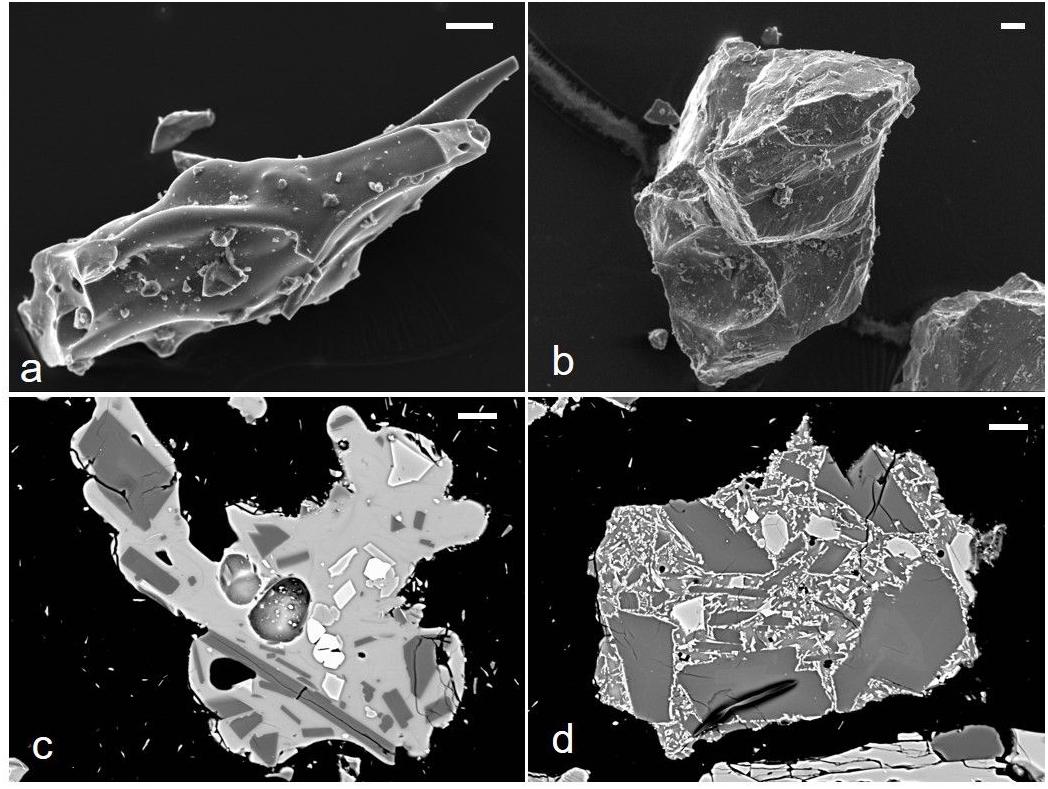
Figure 2. Secondary (top) and backscattered (bottom) scanning electron microscope images of typical sideromelane (a,c) and tachylite (b,d) ash particles at Mount Etna. Scale bar 20 μm in all images.
Visual observations of the eruptive activity integrated with investigations of ash componentry have revealed that the proportion of sideromelane ash increases with increasing eruption intensity ( Taddeucci et al., 2004 , and this work, section “Representative Episodes of Ash Emission at Etna”). Therefore, Strombolian and lava fountain activity generally produce more sideromelane and fewer tachylite than low energy, impulsive ash explosions ( Andronico et al., 2008b ). This correlation, together with textural and compositional observations, suggested a longer conduit residence time for tachylite with respect to sideromelane ash. Hence, several authors hypothesised that tachylite ash particles at Etna are generated by the fragmentation of a cooler, viscous, crystallised and degassed magma at the conduit walls, while sideromelane represents the hotter, less viscous and vesiculating magma rising in the central portion of the conduit ( Taddeucci et al., 2004 ; Polacci et al., 2006 ; Pompilio et al., 2017 ).
Ash dispersal at Etna is mostly controlled by eruption intensity and the ensuing plume height, as expected. For example, on 23 February and 23 November 2013, two lava fountain episodes characterised by relatively high eruption columns (at least for the most common explosive activity at Etna) of up to 9–10 km a.s.l. and high MERs, generated dispersal of ash particles up to 400 km from Etna, in Puglia (Italy) ( Poret et al., 2018a , b ). Lower intensity impulsive explosions and discontinuous explosive activity generally produce lower and intermittent eruption columns above the vent, producing minor ash dispersal. However, if the intensity is low but the duration of the activity is prolonged for days to weeks, the continuous injection of relatively fine-grained ash in the atmosphere is able to form a sustained tephra column feeding an eruption cloud spreading hundreds of km away from the volcano. This occurred, for example, during the 2001 and 2002–2003 eruptions (e.g., Andronico et al., 2005 ; Villani et al., 2006 ; Scollo et al., 2007 ) ( Figure 1 ).
Representative Episodes of Ash Emission at Etna
In the following we present and revise four case studies, in order of decreasing eruption intensity, during which ash was vented at Etna from either a single, short-lasting (minutes to tens of hours) explosive event (i.e., 24 November 2006, 4–5 September 2007, 8 April 2010) or a longer period of erupted activity that involved several successive explosive events over the course of a week (i.e., the last phase of the 2001 eruption). We have chosen these specific case studies because they cover the most common eruptive styles and intensities that have characterised the explosive activity at Etna in the last decades: sustained lava fountain activity (the 2007 event), continuous Strombolian activity transitioning to quasi-steady or pulsing lava fountain (the 2006 event), isolated Strombolian explosions (the 2010 event) and nearly continuous to pulsing ash explosions (the 2001 eruption). For each case study we provide a brief description of the event (or eruption), as well as a description of the associated deposit and ash particle features. This overall information represents a basic framework for classifying ash emissions at Etna and provides the constraints necessary to model mechanisms of ash formation.
Case Study No. 1: The 4–5 September 2007 Sustained Lava Fountain
This episode of lava fountain from the Southeast Crater (SEC) at Etna is one of the best studied lava fountains occurred in the last 40 years of eruptive history of the volcano for its long duration (about 10 h) and steady, sustained, violent jets of magma and gas resulting in a continuous 2 km-high eruption plume ( Andronico et al., 2008a ) ( Figure 3 ). For the high eruptive intensity and the textural features of the erupted ejecta, this activity compares well with the most energetic episodes of the well-studied paroxysmal, cyclic fountaining activity that characterised SEC in 2000 ( Alparone et al., 2003 ; Polacci et al., 2006 ) and the New Southeast Crater (NSEC) in 2011–2012 and 2013 (e.g., Behncke et al., 2014 ; De Beni et al., 2015 ). The paroxysmal sequences at NSEC, in particular, included also very high energy episodes characterised by high eruption columns (7–8 km above the vent; Scollo et al., 2014 ) and very large spreading of tephra up to 400 km from the vent (e.g., Poret et al., 2018a , b ). This suggests that the magnitude of lava fountains at Etna may range significantly, in terms of eruption column height and MER, from small- to large-scale events ( Andronico et al., 2015 ). This terminology also well describes the four lava fountains episodes which took place in December 2015 at the Voragine crater. These episodes were, in fact, characterised by large-scale eruption columns, reaching the troposphere-stratosphere boundary and forming relatively thick tephra deposits on the volcanic slopes ( Vulpiani et al., 2016 ; Corsaro et al., 2017 ; Pompilio et al., 2017 ; Cannata et al., 2018 ). The tephra deposit ( Figures 3B,C ), resulting from the juvenile material fallout from the Eastward directed plume of the 4–5 September lava fountain, blanketed an area on the mainland extending up to 19 km away from the vent before being subsequently dispersed in the Ionian sea ( Andronico et al., 2008a ). Investigations carried out on the ash collected in medial and distal areas reveal that the whole sample is completely made up of sideromelane particles containing large sub-spherical vesicles, with a minor proportion of sideromelane particles characterised by a high number of smaller vesicles ( Andronico et al., 2008a ).
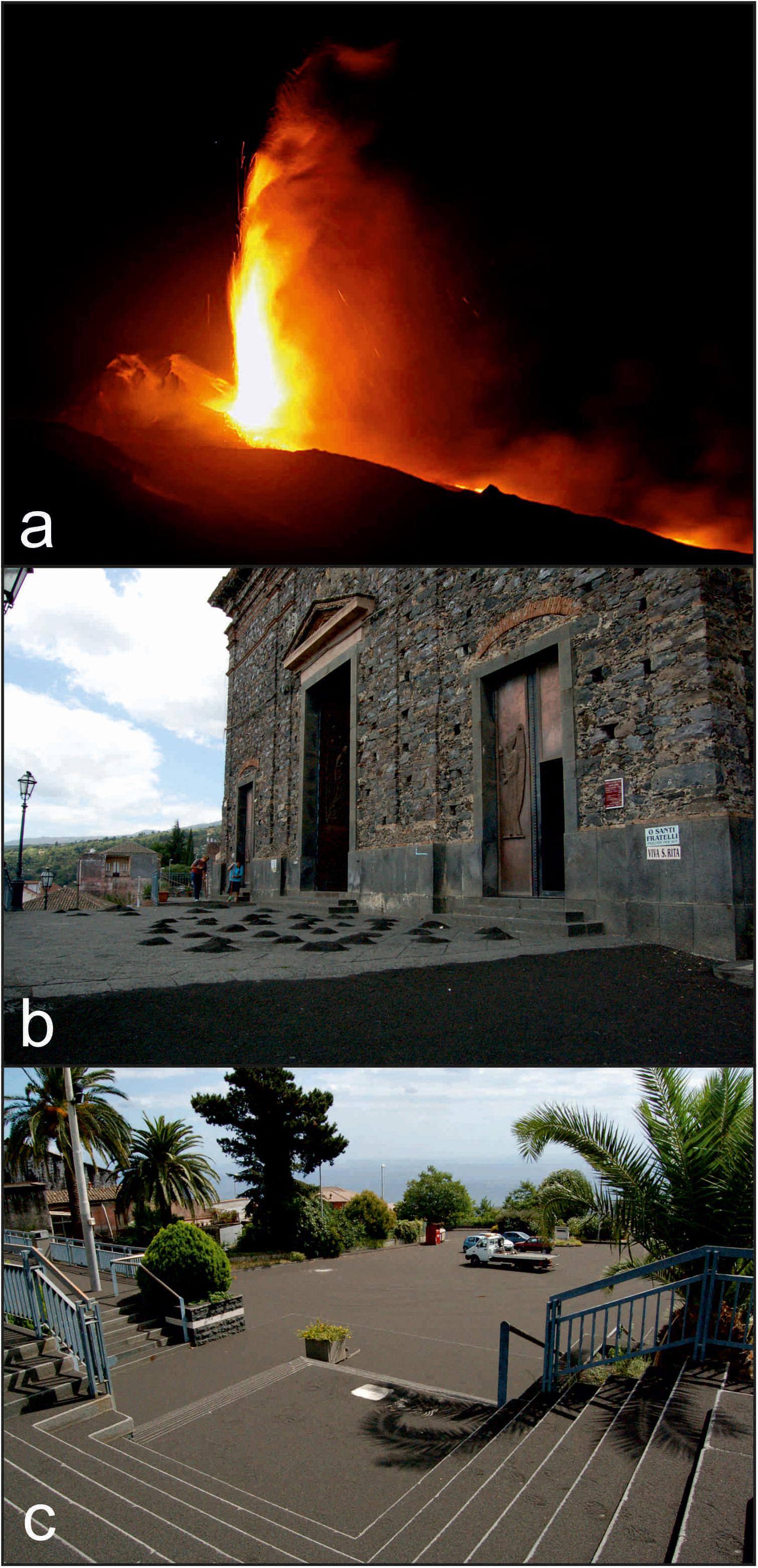
Figure 3. 4–5 September 2007 lava fountain at Mount Etna: (a) magma jets from the South-East Crater (photo at https://forum.meteonetwork.it/meteo-foto/62785-eruzione-etna-04-settembre-2007-a.html ); (b,c) images of the tephra deposit in the village of S. Alfio, ∼13 km from the vent (photo by Daniele Andronico). Reproduced with permission.
Case Study No. 2: The 24 November 2006 Continuous Strombolian Activity
The explosive activity that occurred at SEC on 24 November 2006 represents one of the paroxysmal episodes that took place at Etna during the August–December 2006 eruption. Such explosive activity developed high energy, continuous Strombolian explosions that transitioned to a quasi-sustained or pulsing lava fountain for about 13 h generating an ash plume up to 2 km high on the summit craters and rotating from SE to S ( De Beni et al., 2006 ; Nicotra and Viccaro, 2012 ; Andronico et al., 2014b ) ( Figure 4 ). Based on their steady supply, duration and large tephra fallout, Andronico et al. (2009b) classified plumes like the one generated by the 24 November 2006 explosive activity as middle eruptive intensity plumes , the most dangerous plume type, in terms of volcanic hazard, throughout the 2006 autumn Etna eruption. Long, though small-scale, eruptions lasting several hours like the 24 November 2006 event may cause greater anxiety and worry to the local population and authorities than shorter and higher intensity eruptions (e.g., 12 January 2011; Andronico et al., 2014a ). Indeed, the relatively low eruption intensity, combined with the long-lasting tephra fallout, was enough to produce a continuous deposit up to distances of 15 km from SEC. At greater distances (∼25–30 km), a discontinuous tephra layer formed in the city of Catania and surroundings ( Figure 4 ) where, although at a low sedimentation rate on the ground (5–6 g/m 2 h –1 ; Andronico et al., 2009b , 2014b ), the ash dispersal caused temporary closure of the airport infrastructures and disruption to the air traffic. During this activity, the emitted ash was composed mostly of lithic particles (typically subrounded and with weathered surfaces), while the juvenile fraction (sideromelane and tachylite) represented about a quarter or less of the ash componentry in most of the collected samples at different distances from the vent ( Andronico et al., 2014b ). Tachylite fragments were microcrystalline, blocky and characterised by fresh, angular surfaces, while sideromelane clasts were mainly glassy with irregular to elongate shape. Hybrid partially crystallised particles made of both sideromelane and tachylite were also present ( Andronico et al., 2014b ).
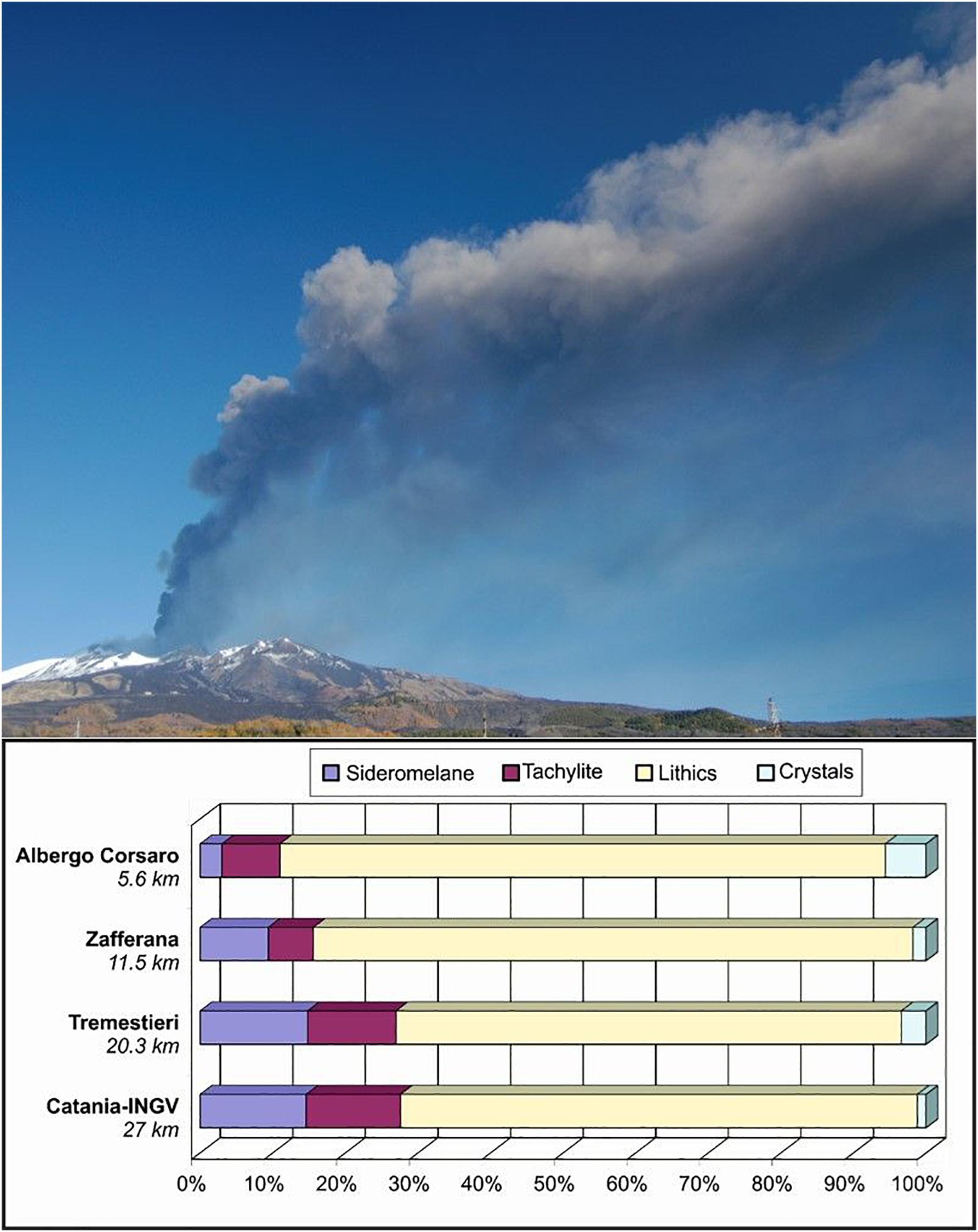
Figure 4. Plume of the 24 November 2006 activity (from Polacci et al., 2009 ) and diagrams illustrating the relative abundance of ash componentry at different distances from the vent. Reproduced with permission.
Case Study No. 3: The 8 April 2010 Single Strombolian Explosion
This case study is well representative of the explosive activity occurred in 2010, when the Etna summit craters produced several single or short sequences of impulsive ash release events, lasting usually less than 1 min. Most of them consisted of small failures involving the rims and/or the inner walls of the summit craters; however, some of them were much more intense and correlated with seismic and acoustic signals ( Andronico et al., 2013 ), suggesting some magmatic dynamics were involved in it. One of the major explosions took place on 8 April 2010 at SEC and produced a dark ash emission rising quickly up to 600–700 m of height above the eruptive vent, which formed a small eruption cloud dispersed to the NE ( Andronico et al., 2013 ) ( Figure 5 ). Light tephra fallout occurred at Linguaglossa, a village located 16 km from the vent, where the ash deposit had unimodal grain-size distribution (peaked at 0.25 mm) and a mass loading of 18 g/m 2 ( Andronico et al., 2013 ).
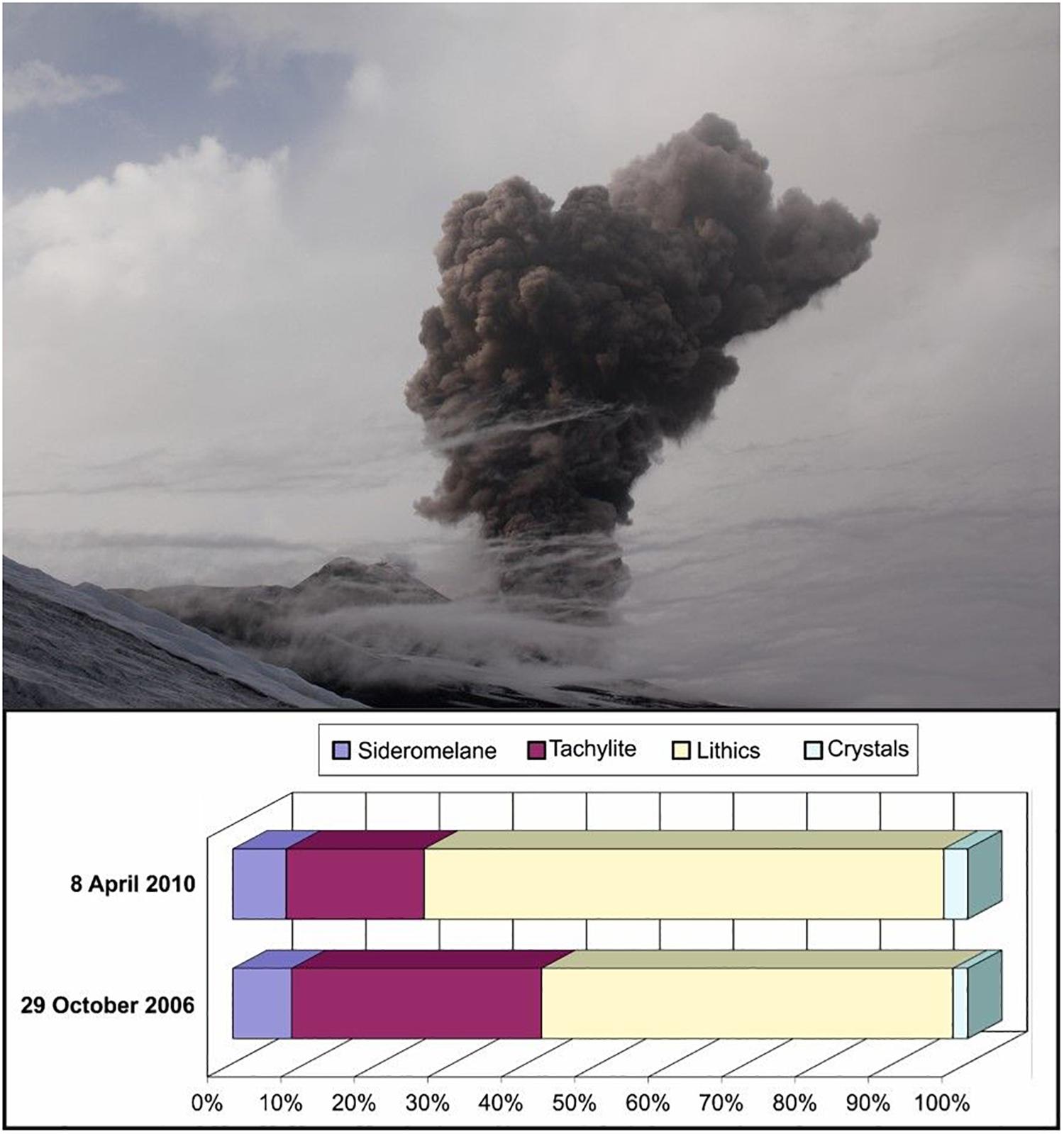
Figure 5. Image of the peak in activity of the 8 April 2010 explosion and comparison between the ash componentry of the 29 October 2006 explosion and the average value of 4 samples collected at increasing distance from the vent after the 8 April 2010 explosion (data Andronico et al., 2013 ). Photo from Thomas Bretscher at https://www.flickr.com/photos/bretscher/4508419186/in/album-72157623824783982/ . Reproduced with permission.
Another example of this activity is the ash emission that rose a few hundred metres above SEC on 29 October 2006, forming a diluted ash plume which generated modest tephra fallout on the southern slopes of the volcano. A thin, discontinuous ash layer was deposited up to Catania, reaching a maximum loading of 30 g/m 2 at Rifugio Sapienza (∼5 km from the vent) and a minimum of 5 g/m 2 in Catania (∼27 km from the vent) ( Cristaldi and Scollo, 2006 ). Lithic clasts dominated the ash componentry in both cases. On 8 April, furthermore, between 4.7 and 16 km from the vent, the ash consisted mainly of tachylite (12–27%), a variable, decreasing fraction of sideromelane particles (from 18 to 1%), abundant lithics including weathered volcanic fragments of glass and crystals (∼50–80%), and negligible amounts of crystals (2–5%) ( Figure 5 ). On 29 October the distal erupted ash mainly consisted of lithics (56%) and tachylite (34%) particles, with poor or negligible percentages of sideromelane (8%) and crystals (2%), respectively ( Figure 5 ) ( Cristaldi and Scollo, 2006 ).
Case Study No. 4: The Ash Explosions During the Last Phase of the 2001 Eruption
In comparison to case studies 1, 2, and 3, where the eruptive activity involved one of the summit craters, the July–August 2001 Etna eruption was marked by lateral activity and the ash was emitted mostly by two newly-formed vents located at 2550 and 2100 m a.s.l. on the south flank of the volcano. In the last phase of the July–August 2001 Etna eruption (1–7 August 2001), the activity at the 2550 m a.s.l. vent transitioned from spatter-forming Strombolian explosions to a series of sustained to pulsed, ash-rich explosions ( Taddeucci et al., 2002 , 2004 ). Ash erupted at first as an almost continuous plume; then the intensity and frequency of explosions decreased to isolated, cannon-like events producing discrete plumes. Plumes were easily bent by moderate wind and their height was in the 500–1500 m range. Ash from these plumes was deposited very locally, reaching a total thickness of about 20 cm at a distance of about 50 m eastward of the vent ( Taddeucci et al., 2002 ). Ash deposited in this time window presents a gradual but systematic increase (from about 64 to 73 particles %) of blocky, poorly vesicular to non-vesicular, microlite-rich tachylite particles at the expenses of sideromelane, and a small increase (from 1 to 7%) of lithic particles. These changes are accompanied by a concomitant decrease in the overall vesicularity of the ash particles ( Taddeucci et al., 2002 ) ( Figure 6 ).
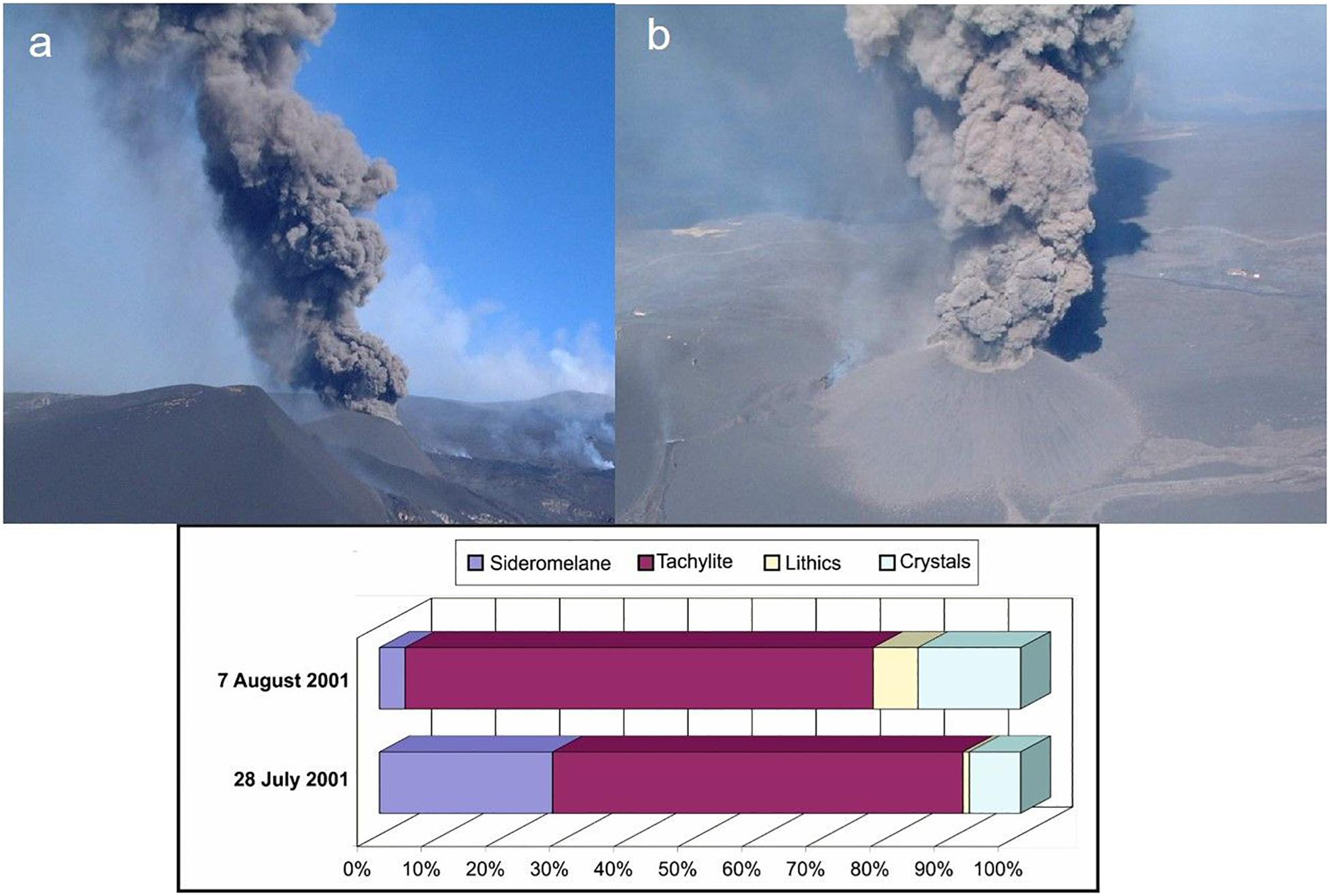
Figure 6. Images (a,b) of the pulsing ash plume generated during the final phase of activity of the vent at 2550 m a.s.l. in 2001, and diagrams of ash componentry at the beginning and at the end of this final phase of activity. Reproduced with permission.
Mechanisms of Ash Formation at Etna
We present here three mechanisms of ash formation that we link with the four different types of ash emission activity described in the previous section. We anticipate however, that such mechanisms may not be exhaustive to describe and explain mechanisms of ash formation at Etna or other basaltic volcanoes characterised by ash emission activity, and that other mechanisms may therefore be feasible. We propose that variations in the magma mass flow rate are a common, key-parameter in determining variations in the style of activity of erupted ash, translating into different mechanisms of ash generation.
Mechanism No. 1 (The Sustained 4–5 September 2007 Lava Fountain and the Continuous Strombolian Activity of 24 November 2006)
The first mechanism concerns ash accompanying lava fountain activity and generated by fragmentation of fast-rising (order of 10 to 40 m/s, La Spina et al., 2016 ; Giuffrida et al., 2018 ), vesiculating magma. Previous studies have well documented that lava fountaining from SEC at Etna is driven by the superposition of two distinct outgassing mechanisms, gas foam collapse and syn-eruptive vesiculation in the glassy pockets between bubble walls ( Figure 7 ) ( Allard et al., 2005 ; Polacci et al., 2006 , 2009 ; Vergniolle and Ripepe, 2008 ). Drawing from the model of Jaupart and Vergniolle (1988 , 1989 ), we assume here that gas bubbles are exsolved and accumulated in a foam layer at the roof of a storage area below the eruptive crater ( Figure 7 ). We also assume that an increase in the magma mass flow rate into the storage area increases the bubble supply to the foam, promoting bubble coalescence and enhancing the potential for the foam layer to collapse, ascend rapidly and fragment explosively at the top of a magma column that fills the conduit almost entirely. Considering a storage area depth of approximately 1.5–1.9 km (at least for SEC; Bonaccorso, 2006 ), the rapidity and intensity with which the fountain events take place do not allow the involved magma to reside in the conduit for longer than a few minutes (see section “Analysis of magma residence time in conduits”). This greatly limits the occurrence of magma cooling and crystallisation at the conduit walls, and, as a result, decreases the generation of tachylite and its presence in the deposited ash. We suggest that the combination of the two outgassing mechanisms described above, foam collapse and syn-eruptive vesiculation, results into a more efficient and rapid magma fragmentation, providing a constant supply of vesicular sideromelane ash to the erupted plume. These observations agree with the characteristics of the sideromelane ash produced by the lava fountain episode of the 4–5 September 2007 (see section “Representative Episodes of Ash Emission at Etna”).
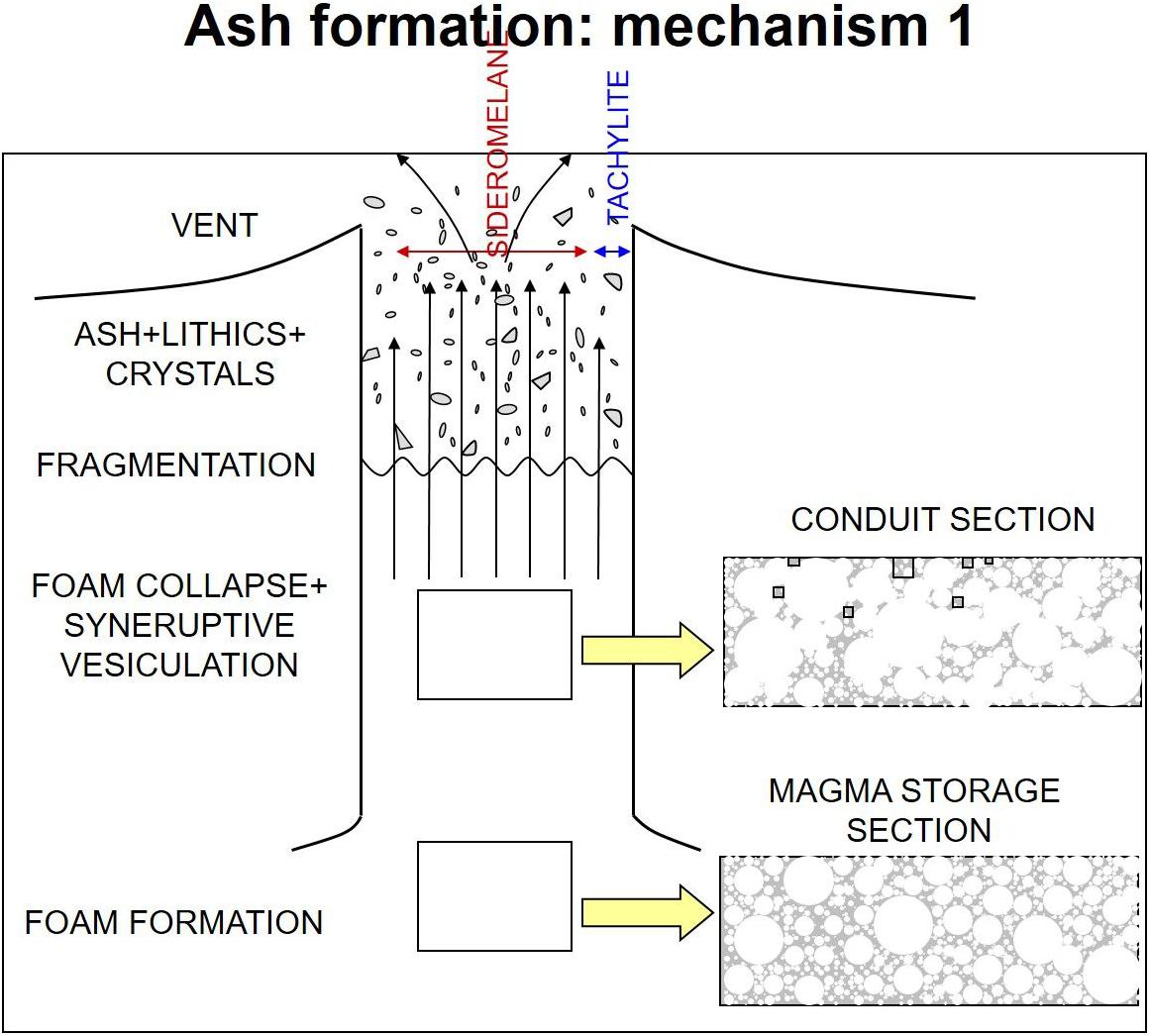
Figure 7. Sketch of ash formation by fragmentation of vesiculating magma (mechanism no. 1). Grey areas in conduit section and in magma storage section insets indicate liquid magma (plus crystals). Not to scale. See text for further explanation.
Tachylite ash was present in the ash samples collected after the continuous Strombolian activity that transitioned to pulsating lava fountain activity of the 24 November 2006. This episode was subdivided into three main eruption phases (resumption, paroxysmal and conclusive), similarly to what was proposed for the 2000 lava fountains by Alparone et al. (2003) . It started with high energy Strombolian explosions that increased in frequency and intensity with time originating a quasi-sustained lava fountain, where the pulsed behaviour may be explained in terms of oscillations in the magma mass flow rate, hence, in the bubble supply rate to the foam layer. Sustained lava fountaining lasts as long as the bubble supply is sufficient to sustain the critical thickness needed for the foam layer to collapse. If the bubble supply rate decreases, the foam layer critical thickness may be no longer supported and the steady fountain phase of the event ceases, progressively shifting to pulsed behaviour and/or less energetic Strombolian explosions till, eventually, the end of the activity. Potential consequences deriving from this series of events are sudden changes in the magma fragmentation depth, longer magma residence time in the conduit, increasing proportion of cooled, crystallised (tachylite-like) magma vs. vesiculating (sideromelane-like) magma, and variations in the proportion of sideromelane vs. tachylite ash particles in the deposited fragmented magma (section “Analysis of magma residence time in conduits”). Ultimately, the pressure decrease associated with the lack of a sustained magma column inside the conduit, occurring during the initial and waning phase of an eruption, can favour the collapse of conduit walls and the production of lithics ( Aravena et al., 2018 ). Similarly, pressure profiles associated with smaller conduits favour conduit walls instability and thus eruptions with a higher proportion of lithic fragments ( Aravena et al., 2017 ). This explains the high abundance of lithics in the ash componentry of the 24 November 2006 activity (see Figure 4 ).
Mechanism No. 2 (The Last Phase of the 2001 Eruption)
The second mechanism concerns ash produced by low energy explosive activity or by impulsive explosions that characterise the final period of a prolonged explosive eruption. This type of ash is generated by the fragmentation of a partially degassed magma column occupying the conduit and left from previous explosive activity. We envisage that slugs or pockets of gas rise across the magma and burst at the free magma surface progressively emptying a magma body that is increasingly cooler, crystallising and losing gas both vertically and horizontally from the conduit centre to the walls ( Figure 8 ). Here vesicles are deformed and collapsed by both magma strain rate and increasing crystallisation, eventually generating the complex vesicle shapes typical of tachylite-forming magma ( Taddeucci et al., 2004 ; Polacci et al., 2006 ). This mechanism of ash formation from a tachylite-rich magma well agrees with descriptions of the dynamics and tephra samples characterising the last phase of the 2001 Etna eruption. During this period of explosive activity, a decrease in the magma mass flow rate determined also a decrease in the eruption explosivity ( Taddeucci et al., 2004 ), allowing a proportion of magma to stagnate in the proximity of the conduit walls and promoting an increase of tachylite versus sideromelane ash in the related deposited fragmented magma ( Figure 8 and section “Analysis of magma residence time in conduits”). In comparison to the first mechanism, the ash fallout generated by mechanism no. 2 is fewer because the explosive events from which it is erupted are shorter in duration and significantly less energetic than lava fountain activity. In addition, the ash is finer than that erupted from a lava fountain because this mechanism of formation involves, besides ash from primary magmatic fragmentation, material eroded from the conduit walls and/or collapsed inside the crater, as well as recycled ash particles (e.g., ash particles that have been ejected and that fall back into the conduit; D’Oriano et al., 2014 ). This is supported by comparing grain-size data from the 4–5 September 2007 lava fountain ( Andronico et al., 2008a ) and 24 November 2006 pulsed lava fountain ( Andronico et al., 2014b ) with those from distal samples of the last phase of the 2001 eruption: the latter has a mode at 2.5–3 phi (Cristaldi and Andronico, pers. comm.), while the former have a coarser mode considering the grain-size distribution of both the total deposit and single samples collected at comparable distances. Given these particles were generated during a flank eruption, the different shallow feeding system may have affected ash generation and, consequently, componentry, changing for example the proportion of tachylite versus sideromelane with respect to the case where ash is vented from a central vent/crater along a well, already structured and relatively wider conduit.
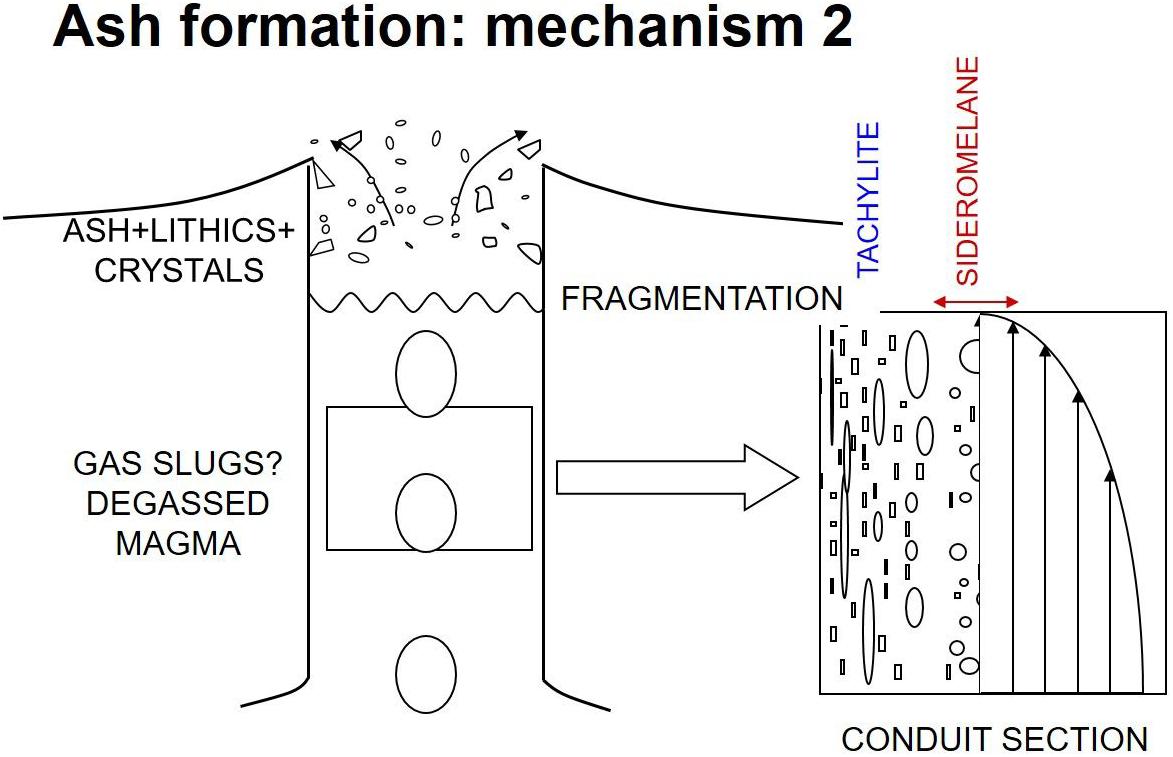
Figure 8. Sketch of ash formation by fragmentation of partially degassed magma (mechanism no. 2). In conduit section inset arrows indicate increasing velocity gradient from conduit centre (where sideromelane is) to walls (where tachylite is). Not to scale. See text for further explanation.
Mechanism No. 3 (The 8 April 2010 Single Strombolian Explosion)
The third mechanism highlighted in this study mainly concerns ash produced by collapses of intracrateric material and conduit lining ( Figure 9 ). Visual observations and images from video cameras as well as seismic and infrasonic signals suggest that this mechanism of ash formation is often promoted by intracrateric gas jets or isolated Strombolian bursts. Such mechanism usually occurs at the end of a high energy Strombolian explosive episode or between consecutive explosive events within an eruption or within a period of explosive activity, the latter case being nicely illustrated by the April 8 2010 explosion ( Andronico et al., 2013 ). In agreement with features displayed by ash erupted from this episode and from the 29 October 2006 event, ash generated by mechanism no. 3 mainly consists of lithic particles (weathered scoria and lava fragments from the conduit walls) and microlite-rich tachylite ash from the cooling, crystallising magma skin at the conduit walls and the very top of the magma column filling the conduit. Notwithstanding the prevalence of lithic clasts in the ash componentry during ash emissions in 2010, the presence of clear seismic and acoustic geophysical signals may help to detect the presence of a pressurised magma/gas trigger and thus to hypothesise the possible involvement not only of old and/or stagnating magma but also of fresh material ( Andronico et al., 2013 ). This mechanism is similar to type 2 Strombolian activity described for Stromboli volcano by Patrick et al. (2007) .
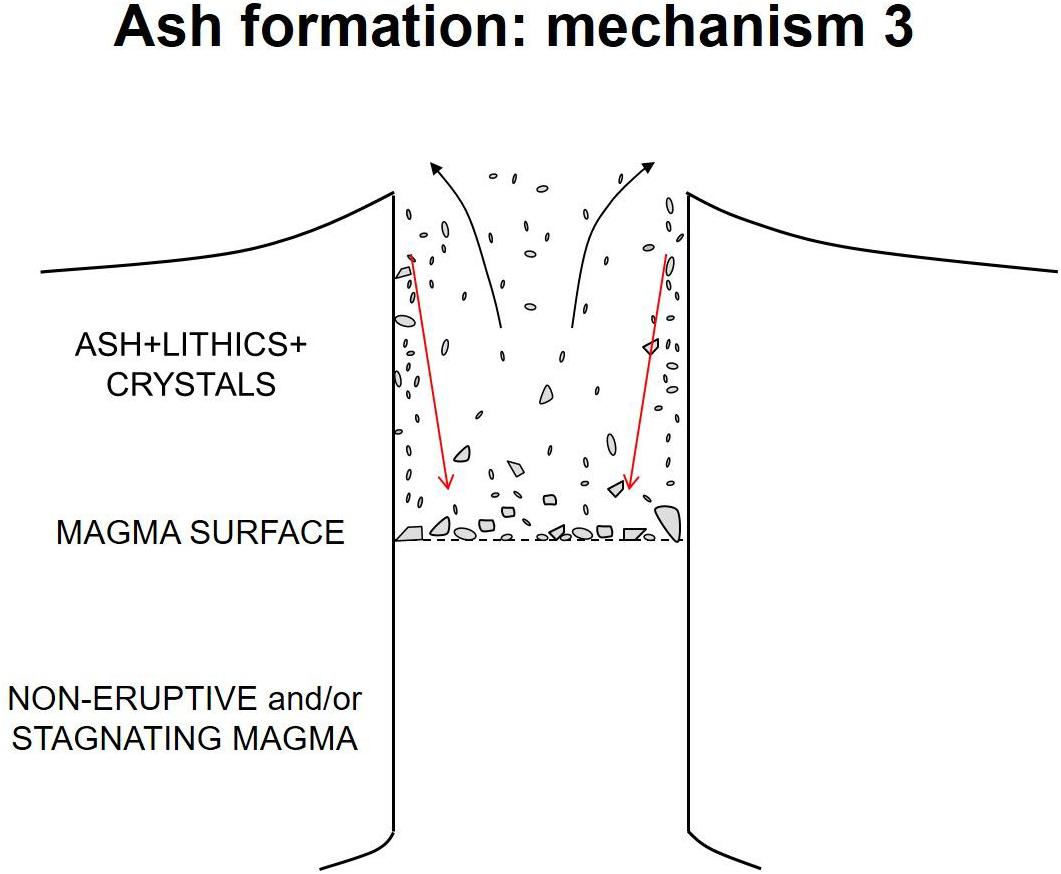
Figure 9. Sketch of ash formation by collapses of intracrateric material and conduit lining (mechanism no. 3). Red arrows indicate collapsing material. Not to scale. See text for further explanation.
Analysis of Magma Residence Time in Conduits
Processes occurring in volcanic conduits, the pathways through which magma travels from its storage region to the surface, have a fundamental control on the nature of eruptions and associated phenomena ( Polacci et al., 2017 ). In the previous sections we have seen how the relative amount of tachylite and sideromelane can be associated with different residence times of magma within the conduit, and thus with different ascent rates that can be associated with either a different average velocity, or different ascent velocities between the centre of the conduit and the portions close to conduit walls. In this section we present an analysis, for both cylindrical conduits and dikes, aimed at quantifying the fractions of erupted mass representative of different residence times within the conduit (and thus different ascent velocities).
First of all, we introduce some notation holding for both cylindrical conduits and dikes (for further details about this analysis please refer to the Supplementary Material ). We denote with r the distance from the axis of the conduit or the dike (see Figure 10 ), corresponding to the radius of the conduit (or the semi-width of the dike), and with u z the vertical velocity, function of r . If u z,avg is the average velocity, and assuming the flow being Newtonian with kinematic viscosity ν, we can introduce the Reynolds number
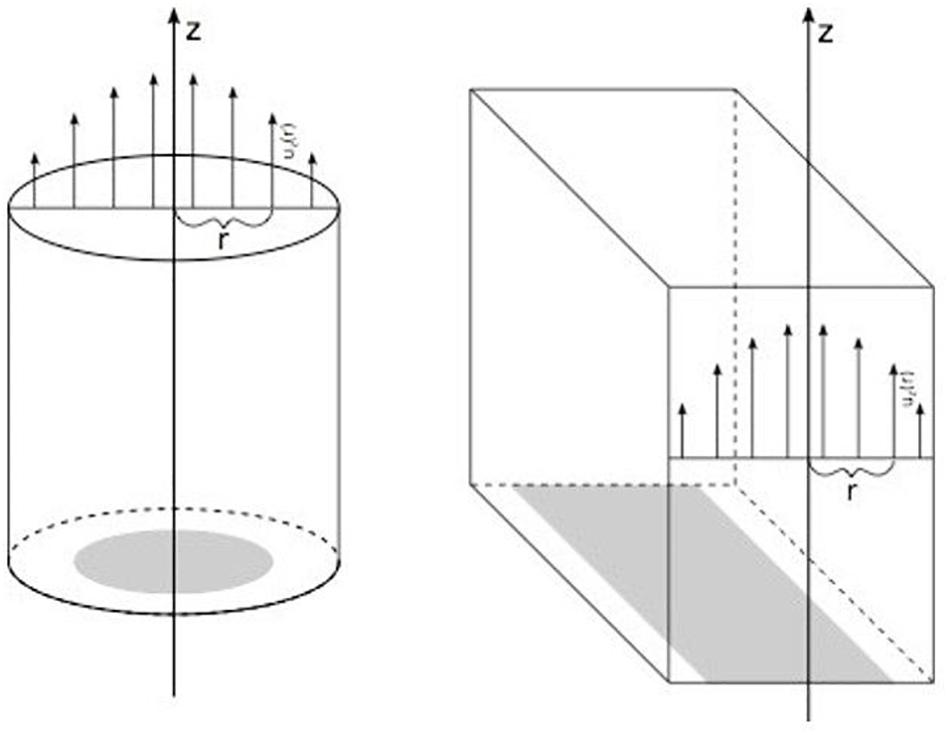
Figure 10. Sketch of the geometries investigated in the analysis of conduit residence times and the velocity profiles. For both geometries, r denotes the distance from the vertical axis.
The Reynolds number is a dimensionless quantity allowing to predict if the flow regime is laminar (Re < 2300 for a cylindrical conduit) or turbulent (Re > 4000 for a cylindrical conduit). We can imagine a laminar flow as a flow where all the fluid velocity vectors line up in the direction of the flow (the vertical axis z in Figure 10 ). Below the depth of fragmentation, for typical eruptions at Etna we have R = O (10 0 −10 1 ), ν = O (10 −1 −10 0 ), and u z , avg = O (10 0 ) ( La Spina et al., 2016 ), and laminar flow characterises nearly the entire conduit. Thus, assuming that the flow is fully developed and introducing the normalised radius r ¯ = r R and the normalised velocity u ¯ = u z ( r ) u z , max (where u z,max is the maximum velocity), we can write:
If we denote now with t ¯ = t ( r ) t min (where t min = 1/ u z , max is the ascent time per unit length associated with the maximum velocity) the normalised ascent time, we can also write:
It is important to observe that the two equations above are independent of viscosity, flow rate values and conduit size, and that they hold for both cylindrical and dike geometries.
Now, if Q is the volumetric flow rate through the whole cross section of the conduit, and if we denote with Q ( r ) the cumulative volumetric flow rate through the portion from the centre up to a distance r from the axis (represented in grey in Figure 10 ), we can also introduce a non-dimensional normalised radius, defined as Q ¯ ( r ) = Q ( r ) Q , with Q ¯ ( r ) = 0 for r = 0 and Q ¯ ( r ) = 1 for r=R .
For a cylindrical conduit it holds:
The last two equations allow us to quantify the volume of magma associated with different ascent velocities and with different ascent times. In particular, the last one gives, for a fixed value of t ¯ , the fraction of magma residing in the conduit during the rise for a time smaller than t ¯ times the minimum ascent time.
Similarly, for a dike geometry, we have the following equation defining the normalised cumulative volumetric flow as a function of the normalised radius:
and thus, using the equations introduced above relating normalised radius, velocity and time, we obtain:
Equations (1) and (2) are independent of ascent velocity and conduit size, and thus they allow to quantify the relative proportions of volumes rising with different times also for conduits of variable width and when velocity changes during the ascent.
The two relationships between normalised cumulative volumetric flow and ascent time are plotted in Figure 11 , where on the top axis of both the plots we have also reported a temporal scale in which we assumed an average ascent time of 300 s. We remark that the average velocity for a cylindrical conduit is half the maximum velocity, while for a dike is 2/3 of the maximum velocity. Thus, for a cylindrical conduit, the fastest ascent time within the conduit (at the centre) is 150 s, while for a dike it is 200 s. The plot allows us also to quantify the portion of magma residing in the conduit for a time 10 times larger than the minimum ascent time, which correspond to 1% of the total magma for a cylindrical conduit and less than 0.4% for a dike. This is consistent with the timescales presented for mechanism 1 and the very small amount of tachylite present in the related case studies (see section “Mechanisms of Ash Formation at Etna”). In order to have a steady ascent producing a significant amount of tachylite (∼50%) at the conduit walls during the rise, it is thus needed an average ascent time one order of magnitude larger, comparable to the characteristic crystallisation times. In addition, the relationships obtained and the plots show that if the same volume of magma is erupted with the same volumetric flow rate (i.e., with same average velocity) through a dike rather than through a cylinder, the ratio between tachylite and sideromelane will be smaller. This counterintuitive result is a consequence of the higher average velocity to maximum velocity ratio of the dike with respect to the cylinder.
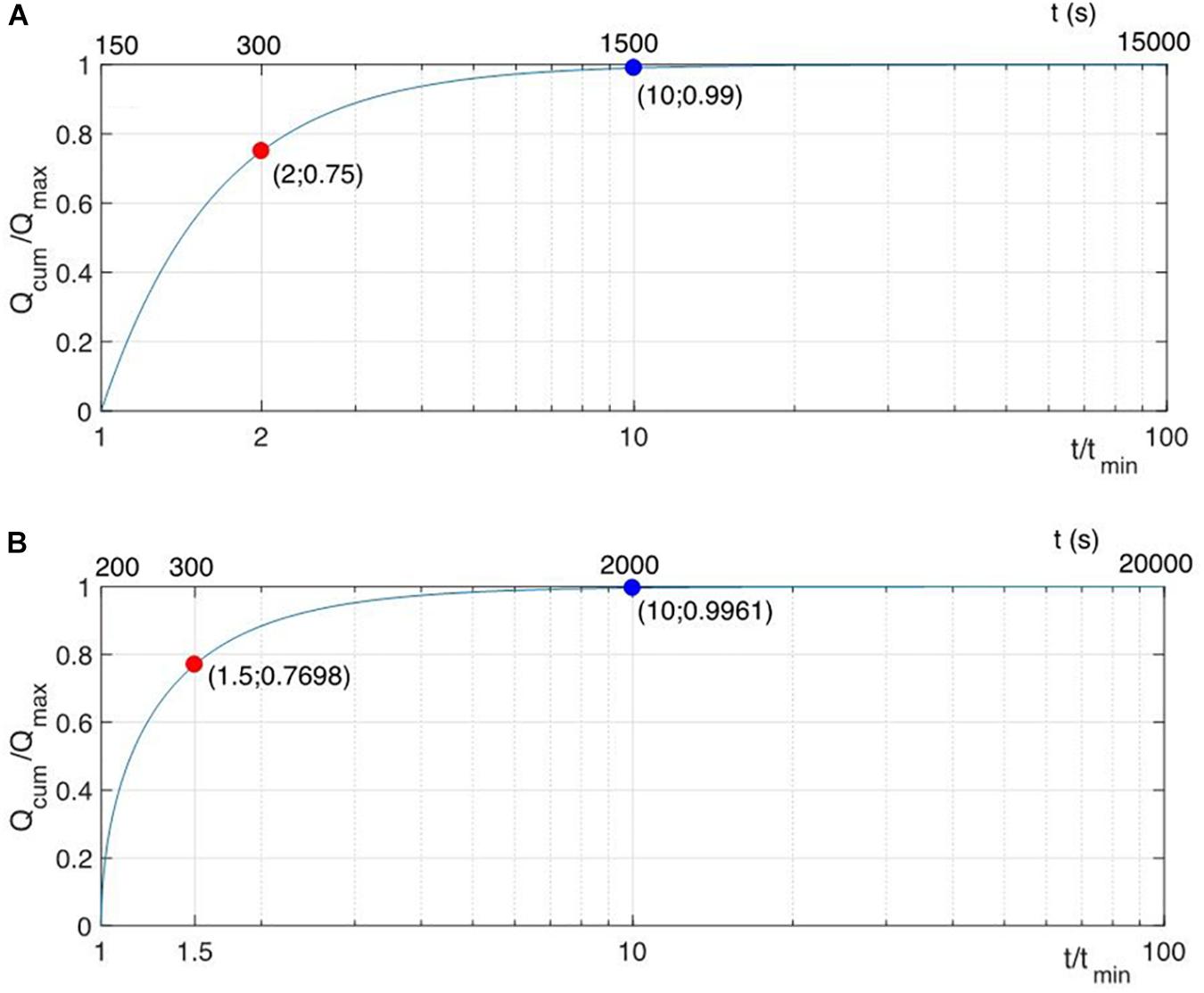
Figure 11. Relationships between normalised cumulative volumetric flow and normalised ascent time for a fully-developed laminar flow in a cylindrical conduit (A) and in a dike (B) . The red dots are plotted for the average ascent time, while the blue dots for a time 10 times larger than the minimum ascent time. A non-normalised time scale is also reported on the top of each plot, where the average ascent time has been fixed to 300 s.
Conclusions
Ash emissions are widespread during explosive basaltic activity, and often have a significant impact on people’s life and infrastructure. For example, between January and February 2019 Mount Etna North-East Crater produced several episodes of continuous to pulsing ash emissions, which, despite being characterised by a very low, uncommon sedimentation rate (a few g/m 2 during several hours of activity), were able nonetheless to cause disruption at the Fontanarossa International airport of Catania (29 km from the vent). To mitigate hazard at basaltic volcanoes, it is therefore imperative to improve knowledge on mechanisms of ash generation. In this study, we use Mount Etna as a general case study. Compositional and textural features of ash particles from Etna and other basaltic volcanoes have been well characterised and the explosive activity that produces them well studied. Yet, a systematic investigation of ash sources is still incomplete and mechanisms of ash generation poorly understood. With this study, we aim to fill this gap in knowledge. By revising four ash emission episodes that are representative of the most common explosive activity at Etna in the last decades, we propose three mechanisms of ash generation based on variations in the magma mass flow rate that apply to other basaltic volcanoes erupting ash and whose explosive activity is similar to Etna. Additionally, we provide an analysis of magma residence time in the volcanic conduit, which explains why different ash particles reside in the conduit for a shorter time than others. This analysis sheds light on the proportion of sideromelane and tachylite textures found in ash from lava fountaining and continuous Strombolian activity, in agreement with our first proposed ash generation mechanism. The main finding of this study is that, integrating field observations with magma residence time calculations, we are able to provide improved information on both ash sources and mechanisms of ash formation from basaltic volcanoes erupting ash. The broader implication of this investigation is that our results are significantly relevant to the wider volcanological community, particularly modellers of eruption dynamics and scientists involved with volcano monitoring and surveillance, and should be used to improve eruption forecasting and hazard assessment and to inform stakeholders on how to implement risk mitigation strategies in active volcanic areas.
Data Availability
The data generated for this study are available on request to the corresponding author.
Author Contributions
MP and DA conceived the study. DA, MP, and AC collated most of the literature data on the Etna activity discussed in the manuscript. JT provided data on the 2001 Etna eruption. MdMV provided the analysis of magma residence time in the conduit. MP wrote the manuscript, with contribution from all co-authors.
This research was funded by the RCUK NERC DisEqm project (NE/N018575/1). We also acknowledge the European Union’s Horizon 2020 Research and Innovation Programme under grant agreement no. 654182, which has partially supported this research.
Conflict of Interest Statement
The authors declare that the research was conducted in the absence of any commercial or financial relationships that could be construed as a potential conflict of interest.
The reviewer MV declared a shared affiliation, with no collaboration, with the authors, DA, AC, to the handling Editor at the time of review.
Acknowledgments
We thank all the INGV staff at the Osservatorio Etneo in Catania devoted to the maintenance of the camera network which allows us to study the eruptive activity at Etna in great detail. We also acknowledge F. Arzilli and G. La Spina for interesting discussions on magma residence time in basaltic systems.
Supplementary Material
The Supplementary Material for this article can be found online at: https://www.frontiersin.org/articles/10.3389/feart.2019.00193/full#supplementary-material
Acocella, V., Neri, M., Behncke, B., Bonforte, A., Del Negro, C., and Ganci, G. (2016). why does a mature volcano need new vents? the case of the New Southeast Crater at Etna. Front. Earth Sci. 4:67. doi: 10.3389/feart.2016.00067
CrossRef Full Text | Google Scholar
Allard, P., Burton, M. R., and Mure’, F. (2005). Spectroscopic evidence for a lava fountain driven by previously accumulated magmatic gas. Nature 433, 407–410. doi: 10.1038/nature03246
PubMed Abstract | CrossRef Full Text | Google Scholar
Alparone, S., Andronico, D., Lodato, L., and Sgroi, T. (2003). Relationship between tremor and volcanic activity during the Southeast Crater eruption on Mount Etna in early 2000. J. Geophys. Res. 108:B52241. doi: 10.1029/2002JB001866
Andronico, D., Branca, S., Calvari, S., Burton, M. R., Caltabiano, T., Corsaro, R. A., et al. (2005). A multi-disciplinary study of the 2002-03 Etna eruption: insights for a complex plumbing system. Bull. Volcanol. 67, 314–330 doi: 10.1007/s00445-004-0372-8
Andronico, D., Corsaro, R. A., Cristaldi, A., Lo Castro, M. D., Messina, L., Scollo, S., et al. (2017). L’attività Esplosiva del Cratere di SE tra il 15 e il 18 Marzo 2017: Dispersione Del Deposito Distale di Caduta e Caratteristiche Tessiturali Delle Ceneri er Uttate. Rapporto Interno N. 005/2017. Available at: http://www.ct.ingv.it (accessed May 2017).
Google Scholar
Andronico, D., Cristaldi, A., and Scollo, S. (2008a). The 4–5 september 2007 lava fountain at South-East Crater of Mt Etna, Italy. J. Volcanol. Geotherm. Res. 173, 325–328. doi: 10.1016/j.jvolgeores.2008.02.004
Andronico, D., Scollo, S., Caruso, S., and Cristaldi, A. (2008b). The 2002–03 Etna explosive activity: tephra dispersal and features of the deposits. J. Geophys. Res. 113:B04209. doi: 10.1029/2007JB005126
Andronico, D., and Del Carlo, P. (2016). PM10 measurements in urban settlements after lava fountain episodes at Mt. Etna, Italy: pilot test to assess volcanic ash hazard to human health. Nat. Hazards Earth Syst. Sci. 16, 29–40. doi: 10.5194/nhess-16-29-2016
Andronico, D., Lo Castro, M. D., Sciotto, M., and Spina, L. (2013). The 2010 ash emissions at the summit craters of Mt Etna: relationship with seismo-acoustic signals. J. Geophys. Res. Solid Earth 118, 51–70. doi: 10.1029/2012JB009895
Andronico, D., Scollo, S., and Cristaldi, A. (2015). ). Unexpected hazards from tephra fallouts at Mt Etna: the 23 november 2013 lava fountain. J. Volcanol. Geotherm. Res. 304, 118–125. doi: 10.1016/j.jvolgeores.2015.08.007
Andronico, D., Scollo, S., Cristaldi, A., and Ferrari, F. (2009a). Monitoring ash emission episodes at Mt. Etna: the 16 november 2006 case study. J. Volcanol. Geotherm. Res. 180, 123–134. doi: 10.1016/j.jvolgeores.2008.10.019
Andronico, D., Spinetti, C., Cristaldi, A., and Buongiorno, M. F. (2009b). Observations of Mt. Etna volcanic ash plumes in 2006: an integrated approach from ground-based and polar satellite NOAA-AVHRR monitoring system. J. Volcanol. Geotherm. Res. 180, 135–147. doi: 10.1016/j.jvolgeores.2008.11.013
Andronico, D., Scollo, S., Cristaldi, A., and Lo Castro, M. D. (2014a). Representivity of incompletely sampled fall deposits in estimating eruption source parameters: a test using the 12–13 January 2011 lava fountain deposit from Mt. Etna volcano, Italy. Bull. Volcanol. 76:861. doi: 10.1007/s00445-014-0861-3
Andronico, D., Scollo, S., Lo Castro, M. D., Cristaldi, A., Lodato, L., and Taddeucci, J. (2014b). Eruption dynamics and tephra dispersal from the 24 november 2006 paroxysm at South-East Crater, Mt Etna, Italy. J. Volcanol. Geotherm. Res. 274, 78–91. doi: 10.1016/j.jvolgeores.2014.01.009
Aravena, Á, Cioni, R., de’ Michieli Vitturi, M., and Neri, A. (2018). Conduit stability effects on intensity and steadiness of explosive eruptions. Sci. Rep. 8:4125. doi: 10.1038/s41598-018-22539-8
Aravena, Á, de’ Michieli Vitturi, M., Cioni, R., and Neri, A. (2017). Stability of volcanic conduits during explosive eruptions. J. Volcanol. Geotherm. Res. 339, 52–62. doi: 10.1016/j.jvolgeores.2017.05.003
Barsotti, S., Andronico, D., Neri, A., Del Carlo, P., Baxter, P. J., Aspinall, W. P., et al. (2010). Quantitative assessment of volcanic ash hazards for health and infrastructure at Mt Etna (Italy) by numerical simulation. J. Volcanol. Geotherm. Res. 192, 85–96. doi: 10.1016/j.jvolgeores.2010.02.011
Behncke, B., Branca, S., Corsaro, R. A., De Beni, E., Miraglia, L., and Proietti, C. (2014). The 2011–2012 summit activity of Mount Etna: birth, growth and products of the new SE crater. J. Volcanol. Geotherm. Res. 270, 10–21. doi: 10.1016/j.jvolgeores.2013.11.012
Behncke, B., and Neri, M. (2019). L’Etna Sbuffa Ed Emette Cenere: Nuova Eruzione o Attività di routine? Blog INGVvulcani, 19 Febbraio 2019. Available at: https://ingvvulcani.wordpress.com/2019/02/19/letna-sbuffa-ed-emette-cenere-nuova-eruzione-o-attivita-di-routine/ (accessed Feburary 19, 2019).
Bonaccorso, A. (2006). Explosive activity at Mt. Etna summit craters and source modeling by using high-precision continuous tilt. J. Volcanol. Geotherm. Res. 158, 221–234. doi: 10.1016/j.jvolgeores.2006.05.007
Bonaccorso, A., Cannata, A., Corsaro, R. A., Di Grazia, G., Gambino, S., Greco, F., et al. (2011). Multidisciplinary investigation on a lava fountain preceding a flank eruption: the 10 May 2008 Etna case, Geochem. Geophys. Geosyst. 12:Q07009. doi: 10.1029/2010GC003480
Cannata, A., Catania, A., Alparone, S., and Gresta, S. (2008). Volcanic tremor at Mt. Etna: inferences on magma dynamics during effusive and explosive activity. J. Volcanol. Geotherm. Res. 178, 19–31. doi: 10.1016/j.jvolgeores.2007.11.027
Cannata, A., Di Grazia, G., Giuffrida, M., Gresta, S., Palano, M., Sciotto, M., et al. (2018). Space-time evolution of magma storage and transfer at Mt. Etna volcano (Italy): the 2015-2016 reawakening of Voragine crater. Geochem. Geophys. Geosyst. 19, 471–495. doi: 10.1002/2017GC007296
Coltelli, M., Del Carlo, P., and Pompilio, M. (2000). Etna: eruptive activity in 1996. Acta Vulcanol. 1, 63–67.
Coltelli, M., Pompilio, M., Del Carlo, P., Calvari, S., Pannucci, S., and Scribano, V. (1998). Mt Etna – 1993–95 eruptive activity. Acta Vulcanol. 1, 141–148.
Corsaro, R. A., Andronico, D., Behncke, B., Branca, S., De Beni, E., Caltabiano, T., et al. (2017). Monitoring the December 2015 summit eruptions of Mt. Etna (Italy): implications on eruptive dynamics. J. Volcanol. Geotherm. Res. 341, 53–69. doi: 10.1016/j.jvolgeores.2017.04.018
Cristaldi, A., and Scollo, S. (2006). Rapporto Sull’emissione Di Cenere all’Etna Nei Giorni 29 e 31 ottobre 2006. Italy. INGV Internal Report No UFVG2006/128.
De Beni, E., Behncke, B., Branca, S., Nicolosi, I., Carluccio, R., D’Ajello Caracciolo, F., et al. (2015). The continuing story of Etna’s new southeast crater (2012–2014): evolution and volume calculations based on field surveys and aerophotogrammetry. J. Volcanol. Geotherm. Res. 303, 175–186. doi: 10.1016/j.jvolgeores.2015.07.021
De Beni, E., Norini, G., and Polacci, M. (2006). Aggiornamento Dell’attività Eruttiva (24 Novembre 2006, ore 13:00). Internal. Available at: http://www.ct.ingv.it/Report/ (accessed November 24, 2006).
D’Oriano, C., Bertagnini, A., Cioni, R., and Pompilio, M. (2014). Identifying recycled ash in basaltic eruptions. Sci. Rep. 4:5851. doi: 10.1038/srep05851
Edwards, M. J., Pioli, L., Andronico, D., Scollo, S., Ferrari, F., and Cristaldi, A. (2018). Shallow factors controlling the explosivity of basaltic magmas: the 17–25 May 2016 eruption of Etna Volcano (Italy). J. Volcanol. Geotherm. Res. 357, 425–436. doi: 10.1016/j.jvolgeores.2018.05.015
Giuffrida, M., Viccaro, M., and Ottolini, L. (2018). Ultrafast syn-eruptive degassing and ascent trigger high-energy basic eruptions. Sci. Rep. 8:147. doi: 10.1038/s41598-017-18580-8
Harris, A. J. L., and Neri, M. (2002). Volumetric observations during paroxysmal eruptions at Mount Etna: pressurized drainage of a shallow chamber or pulsed supply? J. Volcanol. Geotherm. Res. 116, 79–95. doi: 10.1016/s0377-0273(02)00212-3
Horwell, C. J., Sargent, P., Andronico, D., Lo Castro, M. D., Tomatis, M., Hillman, S. E., et al. (2017). The iron-catalysed surface reactivity and health-pertinent physical characteristics of explosive volcanic ash from Mt. Etna, Italy. J. Appl. Volcanol. 6:12. doi: 10.1186/s13617-017-0063-8
Jaupart, C., and Vergniolle, S. (1988). Laboratory models of hawaiian and strombolian eruptions. Nature 331, 58–60. doi: 10.1038/331058a0
Jaupart, C., and Vergniolle, S. (1989). The generation and collapse of a foam layer at the roof of a basaltic magma chamber. J. Fluid Mech. 203, 347–380. doi: 10.1017/S0022112089001497
La Delfa, S., Patane, G., Clocchiatti, R., Joron, J. L., and Tanguy, J. C. (2001). Activity of Mount Etna preceding the february 1999 fissure eruption: inferred mechanism from seismological andgeochemical data. J. Volcanol. Geotherm. Res. 105, 121–139. doi: 10.1016/S0377-0273(00)00249-243
La Spina, A., Burton, M., Allard, P., Alparone, S., and Muré, F. (2015). Open-path FTIR spectroscopy of magma degassing processes during eight lava fountains on Mount Etna. Earth Planet. Sci. Lett. 413, 123–134. doi: 10.1016/j.epsl.2014.12.038
La Spina, G., Burton, M., de’ Michieli Vitturi, M., and Arzilli, F. (2016). Role of syn-eruptive plagioclase disequilibrium crystallization in basaltic magma ascent dynamics. Nat. Commun. 7:13402. doi: 10.1038/ncomms13402
Neri, M. (2018). L’eruzione Laterale Etnea Iniziata il 24 Dicembre 2018. Blog INGVvulcani. Available at: https://ingvvulcani.wordpress.com/2018/12/25/leruzione-laterale-etnea-iniziata-il-24-dicembre-2018/ (accessed December 25, 2018).
Nicotra, E., and Viccaro, M. (2012). Transient uprise of gas and gas-rich magma batches fed the pulsating behaviour of the 2006 eruptive episodes at Mt. Etna volcano. J. Volcanol. Geotherm. Res. 227-228, 102–118. doi: 10.1016/j.jvolgeores.2012.03.004
Patrick, M. R., Harris, A. J. L., Ripepe, M., Dehn, J., Rothery, D. A., and Calvari, S. (2007). Strombolian explosive styles and source conditions: insights from thermal (FLIR) video. Bull. Volcanol. 69, 769–784. doi: 10.1007/s00445-006-0107-100
Pioli, L., Erlund, E., Johnson, E., Cashman, K., Wallace, P., Rosi, M., et al. (2008). Explosive dynamics of violent strombolian eruptions: the eruption of Parícutin Volcano 1943–1952 (Mexico). Earth. Planet. Sci. Lett. 271, 358–368. doi: 10.1016/j.epsl.2008.04.026
Polacci, M., Burton, M. R., La Spina, A., Murè, F., Favretto, S., and Zanini, F. (2009). The role of syn-eruptive vesiculation on explosive basaltic activity at Mt. Etna, Italy. J. Volcanol. Geotherm. Res. 179, 265–269. doi: 10.1016/j.jvolgeores.2008.11.026
Polacci, M., Corsaro, R. A., and Andronico, D. (2006). Coupled textural and compositional characterization of basaltic scoria: insights into the transition from strombolian to fire fountain activity at Mount Etna. Italy. Geology 34, 201–204. doi: 10.1130/G22318.1
Polacci, M., de’ Michieli Vitturi, M., Arzilli, F., Burton, M. R., and Carr, B. (2017). From magma ascent to ash generation: investigating volcanic conduit processes by integrating experiments, numerical modeling, and observations. Ann. Geophys. 60:S0666. doi: 10.4401/ag-7449
Pompilio, M., Bertagnini, A., Del Carlo, P., and Di Roberto, A. (2017). Magma dynamics within a basaltic conduit revealed by textural and compositional features of erupted ash: the December 2015 Mt. Etna paroxysms. Sci. Rep. 7:4805. doi: 10.1038/s41598-017-05065-x
Poret, M., Corradini, S., Merucci, L., Costa, A., Andronico, D., Montopoli, M., et al. (2018a). Reconstructing volcanic plume evolution integrating satellite and ground-based data: application to the 23 November 2013 Etna eruption. Atmos. Chem. Phys. 18, 4695–4714. doi: 10.5194/acp-18-4695-2018
Poret, M., Costa, A., Andronico, D., Scollo, S., Gouhier, M., and Cristaldi, A. (2018b). Modeling eruption source parameters by integrating field, ground-based, and satellite-based measurements: the case of the 23 February 2013 Etna paroxysm. J. Geophys. Res. Solid Earth 123, 5427–5450. doi: 10.1029/2017JB015163
Romero, J. E., Vera, F., Polacci, M., Morgavi, D., Arzilli, F., Ayaz Alam, M., et al. (2018). Tephra from the 3 March 2015 sustained column related to explosive lava fountain activity at Volcán Villarrica (Chile). Front. Earth Sci. 6:98. doi: 10.3389/feart.2018.00098
Scollo, S., Del Carlo, P., and Coltelli, M. (2007). Tephra fallout of 2001 Etna flank eruption: analysis of the deposit and plume dispersion. J. Volcanol. Geotherm. Res. 160, 147–164. doi: 10.1016/j.jvolgeores.2006.09.007
Scollo, S., Prestifilippo, M., Pecora, E., Corradini, S., Merucci, L., Spata, G., et al. (2014). Eruption column height 25 estimation of the 2011-2013 Etna lava fountains. Ann. Geophys. 57:S0214. doi: 10.4401/ag-6396
Taddeucci, J., Pompilio, M., and Scarlato, P. (2002). Monitoring the explosive activity of the july –august 2001 eruption of Mt. Etna (Italy) by ash characterization. Geophys. Res. Lett. 29, 1–4. doi: 10.1029/2001GL014372
Taddeucci, J., Pompilio, M., and Scarlato, P. (2004). Conduit processes during the july–august explosive activity of Mt. Etna (Italy): inferences from glass chemistry and crystal size distribution of ash particles. J. Volcanol. Geotherm. Res. 137, 33–54. doi: 10.1016/j.jvolgeores.2004.05.011
Vergniolle, S., and Ripepe, M. (2008). From strombolian explosions to fire fountains at Etna Volcano (Italy): what do we learn from acoustic measurements? Geol. Soc. Lond. Spec. Publ. 307: 103–124.
Villani, M. G., Mona, L., Maurizi, A., Pappalardo, G., Tiesi, A., Pandolfi, M., et al. (2006). Transport of volcanic aerosol in the troposphere: the case study of the 2002 Etna plume. J. Geophys. Res. 111:D21102. doi: 10.1029/2006JD007126
Vulpiani, G., Ripepe, M., and Valade, S. (2016). Mass discharge rate retrieval combining weather radar and thermal camera observations. J. Geophys. Res. Solid Earth. 121, 5679–5695. doi: 10.1002/2016jb013191
Keywords : basaltic volcanism, ash generation mechanisms, sideromelane and tachylite, conduit magma residence time, Mount Etna
Citation: Polacci M, Andronico D, de’ Michieli Vitturi M, Taddeucci J and Cristaldi A (2019) Mechanisms of Ash Generation at Basaltic Volcanoes: The Case of Mount Etna, Italy. Front. Earth Sci. 7:193. doi: 10.3389/feart.2019.00193
Received: 11 April 2019; Accepted: 10 July 2019; Published: 02 August 2019.
Reviewed by:
Copyright © 2019 Polacci, Andronico, de’ Michieli Vitturi, Taddeucci and Cristaldi. This is an open-access article distributed under the terms of the Creative Commons Attribution License (CC BY) . The use, distribution or reproduction in other forums is permitted, provided the original author(s) and the copyright owner(s) are credited and that the original publication in this journal is cited, in accordance with accepted academic practice. No use, distribution or reproduction is permitted which does not comply with these terms.
*Correspondence: Margherita Polacci, [email protected]
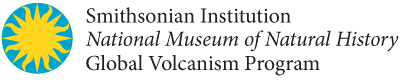
- Current Eruptions
- Smithsonian / USGS Weekly Volcanic Activity Report
- Bulletin of the Global Volcanism Network
- Weekly Report 20th Anniversary
- Holocene Volcano List
- Pleistocene Volcano List
- Country Volcano Lists
- Volcano Search
- Eruption Search
- Deformation Search
- Emission Search
- Webservices
- Database Information
- Image Collections
- Video Collections
- Theme Collections
- Keyword Collections
- St. Helens 40th Anniversary
- Frequent Questions
- Information Sources
- Google Earth Placemarks
- This Dynamic Planet
- Eruptions, Earthquakes & Emissions Application
- Volcano Numbers
- Volcano Naming
- How to Cite
- Terms of Use
Report on Etna (Italy) — November 2002
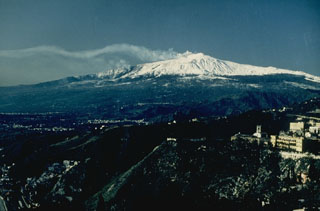
Bulletin of the Global Volcanism Network, vol. 27, no. 11 (November 2002) Managing Editor: Richard Wunderman. Etna (Italy) Witnesses saw N- and S-flank eruptions begin at around 0200 on 27 October
Please cite this report as: Global Volcanism Program, 2002. Report on Etna (Italy) (Wunderman, R., ed.). Bulletin of the Global Volcanism Network , 27:11. Smithsonian Institution. https://doi.org/10.5479/si.GVP.BGVN200211-211060
37.748°N, 14.999°E; summit elev. 3357 m
All times are local (unless otherwise noted).
After the violent flank eruption of July-August 2001, Mount Etna was rather calm for more than 10 months, except for usual fumes from the four summit craters [and minor ash emissions]. In the first days of July 2002 weak magmatic activity resumed sporadically at the NE Crater with ejection of bombs that fell on the outer slopes of the cone. On 12 September explosions occurred every 2 or 3 minutes and were violent enough to throw large spatter as far as the northern rim of the Voragine (Central Crater). However, there were many days without explosive activity and, at other times, the NE Crater emitted large clouds of brownish ash. Although a magnitude 3.7 earthquake had struck the northern flank of the volcano on 22 September, subsequent days were so calm that, to these contributors, the following events came as quite a surprise.
As the National Institute of Geophysics and Volcanology (INGV) previously reported ( BGVN 27:10), a seismic swarm began to shake Etna late during the evening of 26 October 2002. One observer, Maurice Aubert, happened to be in a hotel on the northern flank (at Piano Provenzana, 1,816 m elevation). There the seismic shocks were distinctly felt after midnight and rapidly reached hazardous levels. Hours later, at 0205 on 27 October, lava fountains began to play along a fissure 1-2 km up slope, but decreased at 0220 when lava flows expanded downwards.
The seismic intensity of earthquakes felt the night of the 26th ranged from II to VII or perhaps VIII. The approximate timing and seismic intensity was recorded as follows at 0030, II; at 0140, VI; at 0200, VI; at 0320, VII; and at 0343, VII or VIII. Maurice Aubert and his group hastily retreated shortly after 0320, exiting while cracks were developing through the mountain road. The last of the above-reported intensities was felt during their departure, when a strong earthquake shook their car.
Vents at ~2,700 m elevation on the southern flank (on the Piano del Lago) are here called the S2700 vents. These new S-flank vents lay just SE of the ancient cone of Monte Frumento Supino and ~800 m NW of the Laghetto cone, which appeared in 2001.
Watching the S2700 vents, Giuseppe Scarpinati saw two lava fountains develop after 0200, together with a large ash plume that drifted S. The eruptive phenomena were accompanied by strong detonations and rumblings together with continuous earthquakes that were felt in Acireale, a town at Etna's southeastern foot.
Lava flows from the northern vents invaded and over ran the flat area containing tourist facilities at Piano Provenzana and proceeded as two branches downwards through the pine trees towards Linguaglossa, a village ~10 km to the NE. The greatest damage was not the loss of all tourist facilities at Piano Provenzana, but was instead due to heavy ashfall S of the volcano, which led to closing of the Catania airport on the afternoon of 27 October.
On the morning of 28 October the S fissure had developed at least three explosive vents. A 100-to-200-m-high lava fountain, ~200 m downslope, fed lava flows that extended by more than 2 km toward the uninhabited area of Monte Nero degli Zappini (figures 97 and 98). During the day, however, the effusive activity significantly decreased, and on 29 October the lava fronts virtually stopped on the southern side, although violent degassing at the upper end of the fissure continued unabated. Sustained release of high pressure gas fed a voluminous SE-directed ash plume that reached to more than 5 km altitude. At the same time on the 29th, a large plume of white vapor was emitted at the summit from the central crater vents (Bocca Nuova, Voragine) and the NE Crater. The SE crater, the main site of the 2001 eruption, remained entirely calm.
Strong earthquakes on 29 October caused damage on the lower E flank of the mountain, particularly at Santa Venerina where some 1,000 people were left homeless. The main shock was recorded by Jean-Claude Tanguy in the SE region of the volcano (Trecastagni) at 17 seconds after 1102 (± 5 sec). Horizontal ground motions there lasted 7 to 8 seconds. The INGV reported the seismic event as M 4.4, located 8-9 km beneath Santa Venerina. Other strong shocks at 1739 and 1814 (M 4.0 and 4.1) caused walls to collapse along the road between Zafferana and Milo.
On 30 October soon after midday the Bocca Nuova vent began to emit large clouds of brownish ash. This activity culminated between 1310 and 1320, and the ash cloud merged into the still large, dark ash plume from the southern lateral vents. However, Strombolian explosive activity was still vigorous at the main explosive center, which included a group of about six vents near 2,000 m elevation (called the N2000 vents). These vents, which produced photogenic activity into the night (figure 99), lie just to the E of an old cinder cone known as Monte Ponte di Ferro (at 2,040 m elevation). Here the accumulation of pyroclasts had built a spatter rampart ~200 m long and 30 to 40 m high, the upper part of which reached 2,035 m elevation (± 5 m, measured from Mt. Ponte di Ferro using both altimeter and inclinometer).
On 31 October the wind gradually shifted from the N to the W and then SW, so that ashfall from S2700 vents affected localities NE of the volcano including Reggio di Calabria, whose airport also had to be closed. At the northern vents the lava effusion was on a waning stage, but violent explosions from the two upper vents of the N2000 group threw blocks of ancient material amid juvenile tephra (figure 100).
On 1 November all activity ceased on the northern side except for very small residual lava flows, but the S2700 upper vent appeared to enter a phase of sustained explosive activity resembling a small subplinian column that continued to cause disruptions around the volcano. It was not until 12 November at 1340 that the activity abruptly changed to typical Strombolian explosions of liquid lava clots with loud detonations. On 13 November at about 1600 a small lava flow began to trickle from the lower base of the S2700 cone. The lava effusion increased on 14 November, expanding downwards along the 27-28 October flows. Meanwhile ash emission recommenced at the S2700 crater.
This kind of eruption style is quite unusual at Mount Etna. The authors suggest that it could indicate that a considerable amount of magma has intruded into the S rift zone, which would account for strong degassing without any significant lava effusion between 2 and 13 November.
Geological Summary. Mount Etna, towering above Catania on the island of Sicily, has one of the world's longest documented records of volcanism, dating back to 1500 BCE. Historical lava flows of basaltic composition cover much of the surface of this massive volcano, whose edifice is the highest and most voluminous in Italy. The Mongibello stratovolcano, truncated by several small calderas, was constructed during the late Pleistocene and Holocene over an older shield volcano. The most prominent morphological feature of Etna is the Valle del Bove, a 5 x 10 km caldera open to the east. Two styles of eruptive activity typically occur, sometimes simultaneously. Persistent explosive eruptions, sometimes with minor lava emissions, take place from one or more summit craters. Flank vents, typically with higher effusion rates, are less frequently active and originate from fissures that open progressively downward from near the summit (usually accompanied by Strombolian eruptions at the upper end). Cinder cones are commonly constructed over the vents of lower-flank lava flows. Lava flows extend to the foot of the volcano on all sides and have reached the sea over a broad area on the SE flank.
Information Contacts: Jean-Claude Tanguy , University of Paris 6 & Institut de Physique du Globe, 94107 St. Maur des Fossés, France; Maurice Aubert , University of Clermont-Ferrand, Department of Geology, 63038 Clermont-Ferrand, France; Roberto Clocchiatti , CNRS-CEN Saclay, Lab. Pierre Süe, 91191 Gif sur Yvette, France; Santo La Delfa and Giuseppe Patané , University of Catania, Department of Geological Sciences, Corso Italia 55, 95129 Catania, Italy; Giuseppe Scarpinati ,via Muggia 7, 95024 Acireale, Italy.

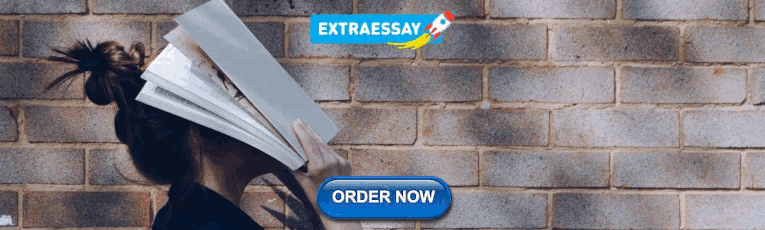
Mount Etna Puffs ‘Smoke Rings’ Into the Sky
The circular wisps are mostly condensed water vapor
/https://tf-cmsv2-smithsonianmag-media.s3.amazonaws.com/accounts/headshot/Will-Sullivan-photo.png)
Will Sullivan
Daily Correspondent
:focal(2304x1733:2305x1734)/https://tf-cmsv2-smithsonianmag-media.s3.amazonaws.com/filer_public/b5/6a/b56a6290-6345-49b5-8aca-162b3e8c49f8/gettyimages-2144166316.jpg)
Sicily’s Mount Etna, the tallest active volcano in Europe, drew attention last week, but not because of a volcanic eruption—it’s blowing circular rings of vapor from its top.
These “smoke rings," which are called volcanic vortex rings by scientists, are made up of gas and water vapor shot from a newly formed crater, writes the Washington Post ’s Leo Sands.
Researchers have previously observed volcanic vortex rings at a number of different volcanoes, according to a 2023 study on the rings’ dynamics in the journal Scientific Reports . Etna’s new rings were first spotted last Wednesday.
The cold atmosphere above the hot volcano causes the water vapor to condense, making the rings visible, per the study.
“On its own it is colorless, but once it reaches the condensation level then it becomes whitish—and that’s what we are seeing,” Ana Casas Ramos , a volcanologist at the Australian National University, tells the Australian Broadcasting Corporation (ABC). “The water vapor is coming out very hot and then once it reaches shallow levels, like atmospheric levels, it then encounters cold air and that’s when you get this condensation.”
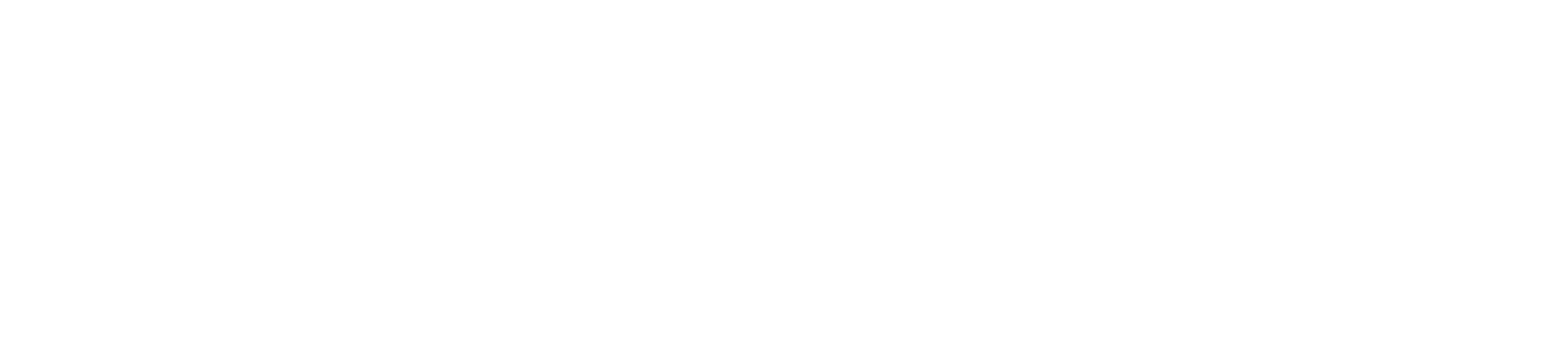
Etna stretches around 10,900 feet high and covers an area of about 600 square miles. The volcano has been active for the past 2.6 million years. It has erupted dozens of times in recorded history, including a particularly intense 1669 eruption that spewed 990 million cubic yards of lava and destroyed a dozen villages, per the Encyclopedia Britannica .
The volcano has had recurring periods of activity throughout the 20th and 21st centuries, including last November .
Observations of rings above Etna, as well as Italy’s Mount Vesuvius, destroyer of Pompeii, date back to 1724, according to the 2023 study. More recently, vortex rings have also been spotted at volcanoes in Alaska, Ecuador, Guatemala, Japan, Vanuatu, New Zealand and Nicaragua.
Scientists previously weren’t always sure how these rings were being made, and the 2023 study dove into the mechanism using computer simulations, according to National Geographic ’s Robin George Andrews.
Their results showed that in order for the rings to take shape, gas bubbles need to be released from the top of a channel carrying magma to the surface. “Imagine a very narrow, cylindrical conduit, within which, at a certain depth, there is magma,” Behncke writes via email to the Washington Post . “Every so often, a bubble forms at the surface of the magma, bursts, and sends a slug of gas at high speed through that conduit.”
/https://tf-cmsv2-smithsonianmag-media.s3.amazonaws.com/filer_public/71/a7/71a785e8-b4c2-41a1-8470-de2ecf93ff11/gettyimages-2144165266.jpg)
The rings’ white color indicates that they’re mostly water vapor, per the study. Behncke tells the Washington Post that the rings are about 80 percent water vapor, and the rest is mostly sulfur dioxide and carbon dioxide.
Casas Ramos tells the ABC that the degassing lowered the probability of explosive eruptions. “If it does culminate in an eruption, let’s say in the coming months or weeks, it’ll be just a couple of puffs, a bit of ash, maybe a bit of magma but nothing violent,” she says to the publication.
Get the latest stories in your inbox every weekday.
/https://tf-cmsv2-smithsonianmag-media.s3.amazonaws.com/accounts/headshot/Will-Sullivan-photo.png)
Will Sullivan | | READ MORE
Will Sullivan is a science writer based in Washington, D.C. His work has appeared in Inside Science and NOVA Next .
The Mount Etna case study: a multisensor view
Ieee account.
- Change Username/Password
- Update Address
Purchase Details
- Payment Options
- Order History
- View Purchased Documents
Profile Information
- Communications Preferences
- Profession and Education
- Technical Interests
- US & Canada: +1 800 678 4333
- Worldwide: +1 732 981 0060
- Contact & Support
- About IEEE Xplore
- Accessibility
- Terms of Use
- Nondiscrimination Policy
- Privacy & Opting Out of Cookies
A not-for-profit organization, IEEE is the world's largest technical professional organization dedicated to advancing technology for the benefit of humanity. © Copyright 2024 IEEE - All rights reserved. Use of this web site signifies your agreement to the terms and conditions.
Clustering activity at Mt Etna based on volcanic tremor: A case study
- Research Article
- Open access
- Published: 20 April 2021
- Volume 14 , pages 1121–1143, ( 2021 )
Cite this article
You have full access to this open access article
- Giuseppe Nunnari ORCID: orcid.org/0000-0002-7117-3174 1
1604 Accesses
5 Citations
Explore all metrics
This paper deals with the classification of volcanic activity into three classes, referred to as Quite, Strombolian and Paroxysm. The main purpose is to give a measure of the reliability with which such a classification, typically carried out by experts, can be performed by Machine Learning algorithms, by using the volcanic tremor as a feature. Both supervised and unsupervised methods are considered. It is experimentally shown that at least the Paroxysm activity can be reliably classified. Performances are rigorously assessed, in comparison with the classification made by expert volcanologists, in terms of popular indices such as the f 1 -score and the Area under the ROC curve ( AuC ). The work is basically a case study carried out on a dataset recorded in the area of the Mt Etna volcano. However, as volcanic tremor is a geophysical signal widely available, considered methods and strategies can be easily applied to similar volcanic areas.
Similar content being viewed by others
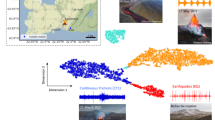
Tremor clustering reveals pre-eruptive signals and evolution of the 2021 Geldingadalir eruption of the Fagradalsfjall Fires, Iceland
Zahra Zali, S. Mostafa Mousavi, … Fabrice Cotton
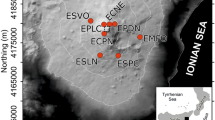
Energy threshold changes in volcanic activity at Mt. Etna (Italy) inferred from volcanic tremor
Horst Langer, Susanna Falsaperla, … Alfio Messina
Understanding the Cotopaxi Volcano Activity with Clustering-Based Approaches
Avoid common mistakes on your manuscript.
Introduction
Nowadays, classifying volcanic activity is delegated to human experts, as it requires a timely consultation of various kinds of instrumental data. This activity is then all the more demanding as the time interval between samples is smaller. It is therefore interesting to know to what extent Machine Learning (ML) approaches can help to solve this problem by automatically processing instrumental data. In particular, among several kinds of geophysical data, such as seismic, ground deformation, chemical, etc., in this paper we chose to consider the seismic tremor, which is widely available in areas of active volcanism. Although the use of volcanic tremor for classifying volcanic activity is not new in literature, see for instance (Langer et al. 2011 ) who applied Self-Organized Maps (SOM) and Fuzzy clustering, with the aim of detecting imminent eruptive activity at Mt Etna, we must consider that a clear measure of the reliability of ML algorithms to cluster volcanic activity, based on the use of volcanic tremor, is still incomplete and could benefit from additional contributions. In this work, we aim to contribute to this field, providing a reliable estimation of the performances of both supervised and unsupervised classifiers, adopting as metric the f 1 -score, which, as is known, represents the harmonic mean between Precision and Recall and in terms of Area under the ROC ( AuC ). Furthermore, we will compare the f 1 index obtained by using the unsupervised approaches against a supervised classification algorithm, in order to estimate the performance loss. Results presented in this paper can integrate those recently presented by Spampinato et al. ( 2019 ), who measured the reliability of a warning system, based on the use of seismic tremor measured on Etna, in terms of the AuC index, estimating \(AuC\simeq 0.8\) . The validity of the results shown in this work is based on a dataset, recorded at Mt Etna from January 2011 and December 2015, which contains a quite high number of paroxysm episodes (namely 48) and therefore allowing a reliable estimate of the classifiers performances.
Review papers concerning clustering approaches are provided by Liao ( 2005 ), Xu and Tian ( 2015 ), and Bano and Khan ( 2018 ), while some application of Machine Learning for the specific field of volcanic seismic signals processing has been recently provided by Malfante et al. ( 2018 ). Recent papers dealing with ML application in solid Earth geoscience and seismology have been provided by Bergen et al. ( 2019 ) and Kong et al. ( 2019 ). Other ML methods and applications in geophysics, with specific examples to classify tremor data can be found in the recent book (Langer et al. 2020 ).
The paper is organized as follows: in Section “ Statement of the problem ” the problem is stated. The considered dataset and pre-processing steps are described in Section “ Dataset and preliminary analysis ”. Considered supervised classification methods are listed in Section “ Supervised classification of volcanic activity ”, together with a description of the classification metrics. Numerical results concerning supervised methods are reported in Section “ Supervised classification ”. Unsupervised classification approaches considered and results, in comparison with the supervised approaches are reported in Section “ Numerical results concerning unsupervised classification ”. In Section “ Discussion and Conclusions ”, conclusions are drawn. A formal description of considered ML approaches and hints about their implementation is reported in Appendix A .
Statement of the problem
The problem of classifying volcanic activity consists in associating a label to each kind of activity observed at the summit crater area. In particular, in this work we will follow the line drawn by Cannavó et al. ( 2017 ) and subsequently continued by Hajian et al. ( 2019 ), who classify the volcanic activity into three classes based on the following description:
The class of Quite ( Q ) state, labeled also as 0, indicates quiet, i.e. no relevant activity or unknown activity, characterized by low amplitudes of all physical features that can be related with the volcanic activity. Representing classes with a double symbol, numerical and literal, make easier on one hand the visualization as a timeseries of classes (numeric representation) and the readability (literal representation).
the class of Strombolian ( S ) activity, labeled also as 1, indicates Strombolian activity, essentially characterized by a mildly explosive activity, with medium amplitude of seismic tremor RMS, shallower source of the seismic tremor, presence of clustered infrasonic events, no eruption column, but possible ash emissions.
the class of Paroxysm ( P ) activity, labeled also as 2, indicates paroxysm, i.e. an energetic activity with lava fountains, characterized by high amplitude of seismic tremor RMS, presence of clustered infrasonic events, shallower source of the seismic tremor, eruption column formation and ash emissions.
For example, Mt Etna would appear to an observer as represented in Fig. 1 , during a Strombolian activity and as in Fig. 2 during a paroxysmal. Comparing the two images it is evident that the ejected matter reaches even some thousands of meters for Paroxysm and only a few tens or some hundreds of meters for Strombolian activity. This is representative of the fact that the energy levels corresponding to the various kinds of volcanic activity can be quite different.
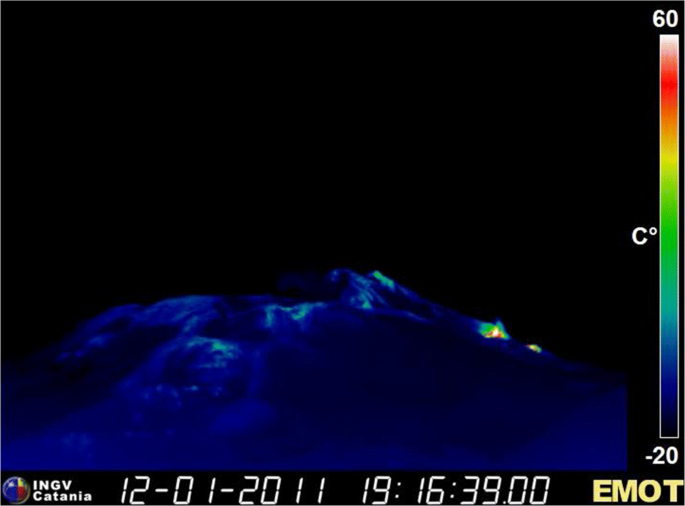
Example of Strombolian activity at Mount Etna. The image was kindly provided by the INGV-Sez. di Catania
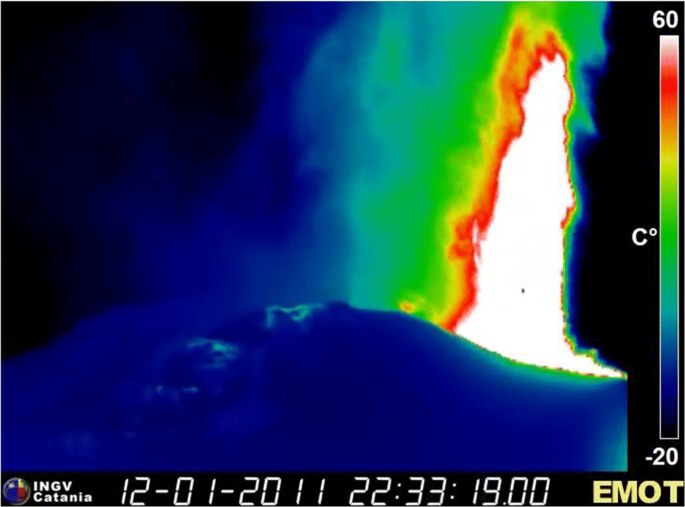
Example of Paroxysm activity at Mt Etna. The image was kindly provided by the INGV-Sez. di Catania
In a well-monitored volcanic area, such as Mt Etna, the onset of this type of activity can be detected by various instruments such as cameras or Doppler radars. However, in remote volcanoes, very often these kinds of sensors are not present, while seismic monitoring networks are more frequently installed.
The classification of volcanic activity at Mt Etna by using a supervised Bayesian Network (BN) classifier, which discriminate the three kinds of activity mentioned above, based on five features, namely the seismic RMS tremor, the infrasonic RMS tremor, the depth of the volcanic source, the radar Doppler and the tilt derivative, was first proposed by Cannavó et al. ( 2017 ). Other supervised classification approaches, namely Decision Tree (DT) and K-Nearest-Neighbor (KNN), on the same dataset have been applied by Hajian et al. ( 2019 ).
Dataset and preliminary analysis
The dataset taken into account in this study was recorded from January 1, 2011 to December 31, 2015 and after processing was sampled every 10 minutes. During this time interval the eruptive activity of Mt Etna was rich in episodes characterized by phases of degassing, alternating with explosive and effusive activities, to periodic eruptions from fissures opened on its flanks. A detailed description of the activity occurred is outside the scope of this work and the interested readers can refer to Cannavó et al. ( 2017 ) and references therein. For the purposes of this paper, it is enough to mention that expert volcanologists have labeled the 262944 samples into 213190 samples of Q activity, 49048 of S activity and 706 samples of P activity. Samples labeled as Q , S and P are the 81.08 % , 18,65 % and 0.27 % of the total, respectively. This time-series of classes, shown in Fig. 3 , will be, again, considered in this paper for evaluating to what extent classification of volcanic activity can be performed by unsupervised classifiers (i.e. clustered) and estimate the performance in comparison with supervised ones. Classification will be carried out considering the seismic tremor as the only feature.
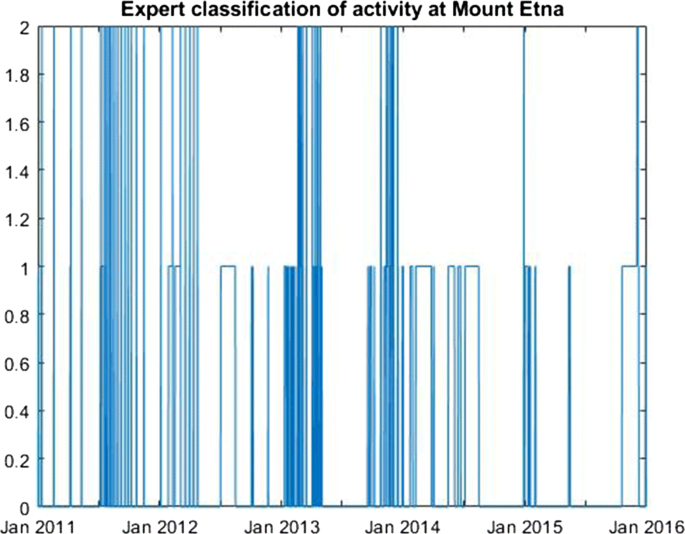
Time series of classes from January 2011 to December 2015, recorded at Mt Etna with 10 m sampling time: 0, 1 and 2 represents Quiet, Strombolian and Paroxysmal activity, respectively. The dataset was kindly provided by the INGV-Sez. di Catania
Volcanic seismic tremor
Volcanic seismic tremor, also referred to as harmonic tremor, is a sustained release of seismic and infrasonic energy typically associated with the underground movement of magma, the venting of volcanic gases from magma, or both. It is a long-duration release of seismic energy, with distinct spectral lines, that often precedes or accompanies volcanic activity. More generally, volcanic tremor is a long-duration continuous signal generated by a spatially distributed source, which contrasts distinctly with transient sources of seismic radiation, such as earthquakes and explosions. The relation between long-period events and an imminent eruption was first observed by Chouet ( 1996 ). As indicator of the level of volcanic seismic tremor, the root mean square (RMS) amplitudes of seismic signals in the tremor band have been routinely recorded at the Istituto Nazionale di Geofisica e Vulcanologia (INGV), Osservatorio Etneo, for real-time volcano monitoring, through the so-called permanent seismic network, equipped with broadband (40 s cutoff period), three-component seismometer, which records data in real time at a sampling rate of 100 Hz. The original signals are then filtered in the band [0.5,5.5] Hz, since, as suggested by (Chouet 1996 ), it concentrates the main part of released seismic energy. The geographic coordinates of most of the considered seismic stations are reported in Table 1 .
Regression analysis of tremor time series
RMS tremor recorded at different stations of Mt Etna are linear correlated. To illustrate this aspect, we have computed linear regression models of the form:
x ( t ) is the tremor value measured at time t in a given reference station, assumed as input;
y ( t ) is the tremor estimated by the linear model, into another station, assumed as output;
m and b are the slope and the offset coefficients, respectively.
Figure 4 , report, as an example, the scatter plots of tremor recorded at the couples ESLN-ECZM, assuming the tremor recorded at the ESLN station as input and that at ECZM as output; the strong linear correlation appears evident. In order to highlight that this is almost independent of the particular class of activity, samples belonging to different classes were represented by different colors. The regression coefficient R , the slope and the offset, for the whole set of stations are reported in Table 2 . It can be seen that almost all stations exhibit R close to 1. The lower R values corresponds to stations, namely ECNE, ECPN, EPDN, located very close to the active craters areas, which are, probably for this reason, characterized by very high tremor levels and/or other kinds of local phenomena. For classification purposes, the high linear correlation of tremor time series, implies that the dataset dimensionality can be significantly reduced, as it will be discussed in the next section. For now, we mention here that this property suggested to us a strategy for filling missing data at several stations. Indeed, in the considered time interval a not negligible quantity of missing data has plagued the network, as shown in the last column of Table 2 . It is possible to see that in the considered time interval, the percentage of missing data ranges between about 19 % and 38 % , and in particular, only two stations are below 20 % .
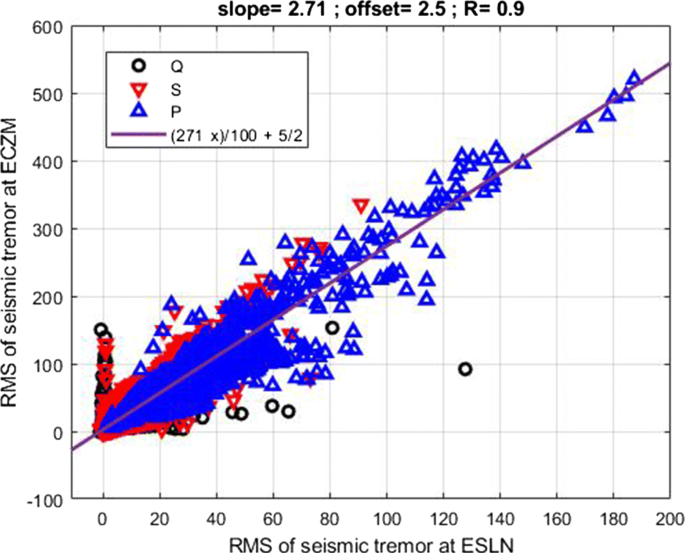
Scatter plot and corresponding linear regression model at the ECZM - ESLN couple of stations
In order to overcome this shortcoming, which would have involved the loss of many samples useful for classification, among the various candidate strategies, we have chosen to fill missing data by the average value of the tremor calculated using expression ( 2 ), learned from (Cannavó et al. 2017 ),
In this expression, the index, i refers to the n seismic stations, μ t represents the median operator over two years previous the time t , and σ t represents the interquartile range over the same period. An example of filled time series at the station EBCN, in comparison with the true one, is reported in Fig. 5 .
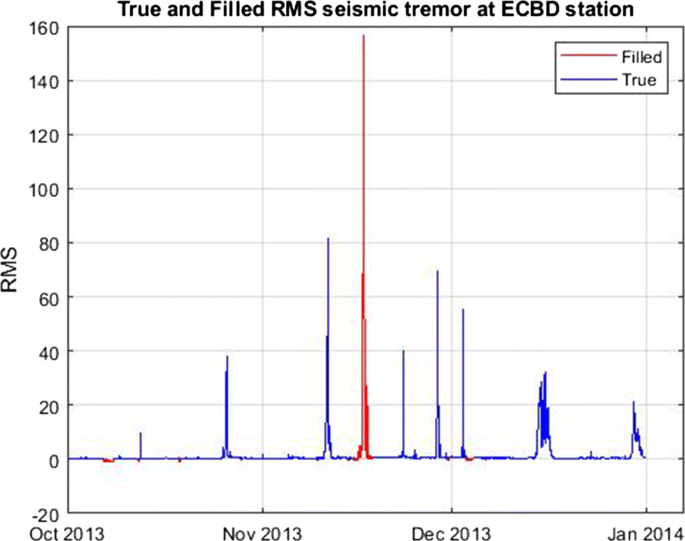
Filled and original tremor time series at the ECBD station
Supervised classification of volcanic activity
In this paper, to perform the supervised classification, of volcanic activity at Mount Etna, the six classification models listed below were considered:
A Fisher Discriminant model, here simply referred to as DISC, implemented by using the fitcdiscr function of the Matlab Statistical and Machine and Learning Toolbox, choosing a linear kernel. More details about this kind of classifiers are reported in Section A .
A Multiclass error-correcting output model. In particular, as ECOC, in this paper we refer to an Ensemble multiclass classifier implemented by using the fitcecoc Matlab function, using a Support Vector Machine (SVM) algorithm, with linear kernel and One-Versus-One (OVO) coding scheme. More details are reported in Section A .
An Ensemble model, here referred to as ENSE, implemented through the fitcensemble function of the Matlab Statistical Toolbox. We have adopted as basic learner a Adaboost2 algorithm, building a medium coarse tree with a maximum number of splits set to 20, a maximum number of cycles up to 30 and a learning rate set to 0.1. A short description of Ensemble models is reported in Appendix A .
A K-Nearest Neighbor model, here referred to as KNN, implemented through the fitcknn function of the Matlab library, setting Euclidean distance metrics and 10 samples as the maximum number of neighbors. More details in Section A .
A Naive-Bayes model, here referred to as NBYE, based on the fitcnb function of the Matlab library, with normal kernel distribution and unbounded support. More details in Section A .
A Decision Tree for multiclass classification, here simply referred to as TREE, implemented through the Matlab function fitctree , growing a medium coarse tree, with a maximum number of splits up to 20. The Gini’s index was adopted as a split criterion. More details in Section A .
There are no particular reasons behind the choice of these methods, other that are among the most popular. Obviously, many other supervised classification algorithms could have been chosen, including the popular neural networks. We want to stress here that the main aim of this work is not to compare classification algorithms, but to measure to what extent the described problem can be solved using widely available and easily implementable algorithms. More deep details concerning these approaches can be found in popular textbooks such as Bishop ( 2006 ), Hastie et al. ( 2008 ), and Goodfellow et al. ( 2016 ) or directly from the MATLAB Statistical and Machine Learning Toolbox (Inc 2017 ).
Reducing the dimensionality of the dataset
As well known, see for instance (Liu and Motoda 2008 ), performances of classification algorithms significantly depend on the number of features. Therefore, reducing the dimensionality of the dataset is a good practice (Mohamed 2020 ). We have performed this task by using the Principal Component Analysis (PCA), which consists of projecting each data point onto the main directions in the feature space, in order to obtain lower-dimensional data, while preserving as much of the data variability as possible. The first principal component can be defined as the direction that maximizes the variance of the projected data. Similarly, the i t h principal component is the direction orthogonal to the first ( i − 1) t h principal ones that maximizes the variance of the projected data.
For our data set, the cumulative variability explained, versus the number of principal components, is shown in Fig. 6 . It can be seen that taking the first two components it is possible to account for about 96 % of the dataset variability. This percentage rises to 99 % , if the first three principal components are considered. Therefore, the PCA analysis points out that the original 17-D dataset can be reduced to 2-D or 3-D. In this paper, in order to be more conservative, we have chosen to consider 3 principal components. However, the effectiveness of reducing the dataset dimensionality by using the PCA must be done with some caution, as a known drawback of the PCA approach for classification problems is that the variance is preserved regardless of the classes (see for instance (Lei et al. 2017 )). Thus, a subspace with large variance is not necessarily one in which classes are well separated. In addition to the reduction of the dataset dimensionality through the PCA, we have explored the possibility of performing this task by heuristically choosing two stations of the network and finally by averaging the seismic tremor among all the stations in the network through expression ( 2 ). Summarizing, the following three reduced data set were considered to perform the supervised classification:
The 3-D dataset represented by the first three PCA components;
a 2-D dataset consisting of tremor recorded at two selected stations of the network, namely ESLN and EBCN;
the 1-D dataset obtained averaging the RMS tremor over the whole network, by using expression ( 2 ).
The stations ESLN and EBCN, were selected according to the following criteria:
The time series recorded at these stations are not affected by very high tremor levels, as for instance observed at stations ECNE, EPDN and EPIT.
From trials performed, it seems that this couple of stations allow a good classification of class P . However, it is to be stressed, that other choices would be possible, without significantly affecting the classifier performances.
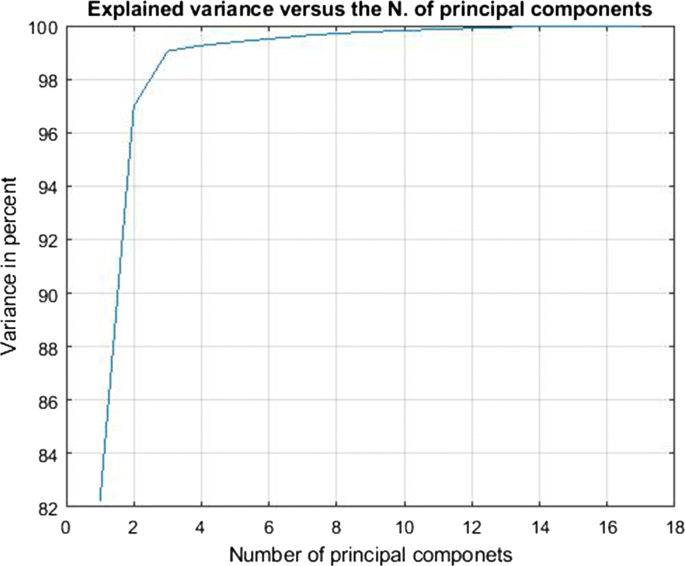
Cumulative explained variance versus the principal components
Classification metrics
Given a classification experiment, let us indicate as P ( i ) and N ( i ) the number of actual positive and actual negative cases in the i t h class, respectively. Moreover, let T P ( i ), T N ( i ), F P ( i ) and F N ( i ) the number of true positive, true negative, false positive and false negative cases, respectively, recognized by the classifier, for the i t h class. Referring to these quantities, the following rates can also be defined:
The meaning of the above indices can be expressed as follows:
The T P R ( i ) expresses the proportion of actual positives that are correctly classified by the model as belonging to the i t h class. Best values of TPR approach to 1, while in the worst case TPR approach 0. The TPR is referred to also as specificity or Recall ( r ).
The T N R ( i ) expresses the proportion of actual negatives that are correctly classified as not belonging to the i t h class. As for the TPR , best values of TNR approach 1, while worst values approaches 0. The TNR is referred to also as specificity of selectivity.
The F N R ( i ) expresses the proportion of false negatives in the i t h class, with respect to all actual positives in the same class. Of course in the best case FNR approaches 0, while in the worst case approaches 1.
The F P R ( i ) expresses the proportion of false positives in the i t h class with respect to the total number of actual negatives in the same class. Similar to the FNR in the best case FNR approaches 0, while in the worst case approaches 1.
Another useful index, is the Positive Predicted Value ( PPV ) or simply Precision , which, for the generic class i is defined as:
In expression ( 7 ), FDR stands for False Discovery Rate.
For the purposes of this paper we also make use of the f 1 -score, defined as:
It is easy to verify that indicating as r ( i ) the TPR for the i t h class and as p ( i ) the PPV , the f 1 can be also written as:
which highlights that it is the harmonic mean between p ( i ) and r ( i ). Therefore, f 1 is a more reliable measure of performance than the simple Precision and/or Recall . For this reason, in this paper we will always show f 1 , instead of the simple Precision and/or Recall .
A useful way to collect most of the performance indices shown above is the so-called Confusion Matrix (CM). On the CM, see for instance the next Section “ Numerical results concerning unsupervised classification ”, the rows correspond to the predicted class (Output Class) and the columns correspond to the true class (Target Class). The diagonal cells correspond to observations that are correctly classified. The off-diagonal cells correspond to incorrectly classified observations. Both the number of observations and the percentage of the total number of observations are shown in each cell. The column on the far right of the plot shows the percentages of all the examples predicted to belong to each class that are correctly and incorrectly classified, i.e the PPV and the FDR . The row at the bottom of the plot shows the percentages of all the examples belonging to each class that are correctly and incorrectly classified, i.e. the TPR and the FNR , respectively. The cell in the bottom right of the plot shows the overall Accuracy . The accuracy can formally be described by using expression ( 10 )
I ( g ) is a function that returns 1 if g is true and 0 otherwise,
C ( x n ) the class label assigned by the classifier to the sample x n
y n the true class label of the sample x n
N is the number of samples in the testing set.
Another useful index for evaluating the degree of accuracy and reliability of a statistical classification is Cohen’s Kappa, since it compensates for random hits. It can be computed (see for instance (Tallon-Ballesteros and Riquelme 2014 )) starting from the CM, by using expression ( 11 )
m is the dimension of the confusion matrix CM, i.e. the number of classes.
C M i i , i = 1,…, m the entries of the CM main diagonal.
C i t r u e the true number of labels of class i .
C i p r e d the predicted number of labels of class i .
The range of Kappa values extends from − 1 ≤ κ ≤ 1, with positive one indicating strong agreement, negative one indicating strong disagreement, and zero indicating chance-level agreement.
Another useful tool for evaluating the reliability of supervised classifiers is represented by the Receiver Operating Characteristic ( ROC ) metric and in particular the so-called area under curve AuC . ROC curves typically feature true positive rate on the Y axis, and false positive rate on the X axis. This means that the top left corner of the plot is the ideal point, since it is characterized by a false positive rate of zero, and a true positive rate of one. Even though this is not very realistic, it does mean that a larger area under the curve ( AUC ) is usually better. For a specific application of the ROC curves to evaluate the reliability of a warning system in volcanic areas, the readers can see the recent paper (Spampinato et al. 2019 ). For more general further details about metrics concerning classifiers, see Powers ( 2011 ).
- Supervised classification
Preliminary considerations
Before starting with the description of supervised classification results, it can be useful to make some preliminary considerations regarding the separability of the three considered classes, using the seismic tremor as the only feature. While in Section “ Reducing the dimensionality of the dataset ” we have drawn attention to the fact that the tremor recorded in the various stations is linearly correlated, which implies that the dimensionality of the dataset can be significantly reduced, here, we want to point out that classes, as labeled by the experts, are largely overlapping in the feature space of the RMS tremor. To justify this statement, we can look at Fig. 4 , from which it is easy to realize that the three classes are largely overlapping. This is more evident between classes Q and S which therefore, regardless of the classification technique, we may expect that will result poorly separable. Among the three classes, class P seems to have more chances to be correctly classified. To further confirm that the three classes are not easily separable, by using the seismic tremor as the only feature, we have applied the Silhouette criterion (Rouseeuw 1987 ). The test was performed by using the K-means algorithm and the search was extended in the range from 1 up to 6 clusters. Results reported in Fig. 7 show that that the highest Silhouette value occurs at 2 clusters, suggesting that this is the optimal number of clusters, regardless of the dataset, among the considered ones. It is also to be observed that the K-means operating on the 3-D data set was not able to contribute, since it indicates a silhouette value independent of the number of classes.
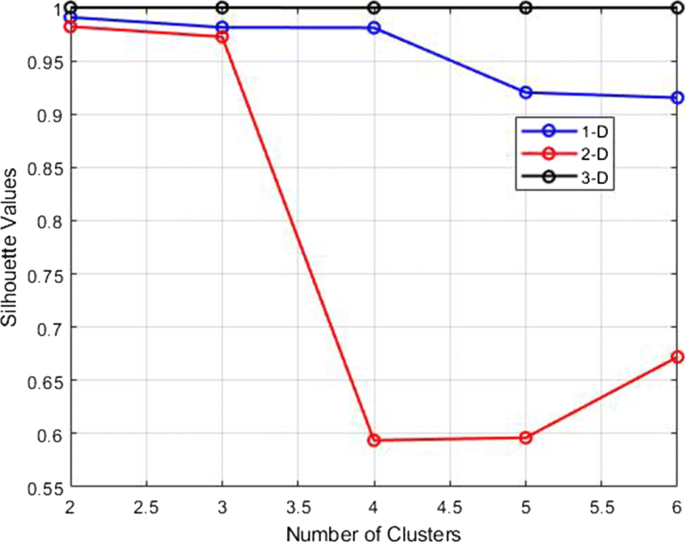
Silhouette values versus the number of clusters for the three reduced dataset
Assessing the generalization capabilities of classifiers
A popular strategy to estimate the generalization capabilities of a model is to divide the available samples in at least two subsets for training and for testing , respectively. The former is used for tuning the model parameters and the latter for assessing its performance on fresh data. However, sometimes the testing set is used in some way during the training, for instance to understand when the training must be stopped. In this case, a third set of data, referred to as the validation set is introduced, in order to be more confident that performances are evaluated on a really fresh dataset.
Since we have a limited number of samples in the P class, a validation set is not realistic. To overcome this drawback, we have adopted the k-fold cross validation strategy. This technique consists of splitting the dataset into k groups, after shuffling the samples randomly. Then for each unique group the following steps are performed:
A group is held out and used for testing.
The remaining groups are used for training a model.
The fitted model is evaluated on the testing set.
The obtained score is then retained while the model is discarded.
This means that each sample is given the opportunity to be used in the hold out set 1 time and used to train the model k − 1 times. At the end of the rotation process, the skill of the model is evaluated using the sample of model evaluation scores.
In the next section, we report validation accuracy computed for the three different reduced dataset, as above discussed.
Numerical results
We start with the global indices, i.e. computed averaging over the classes, namely the Accuracy, the f 1 index and Cohen’s K, which are shown in Fig. 8 .
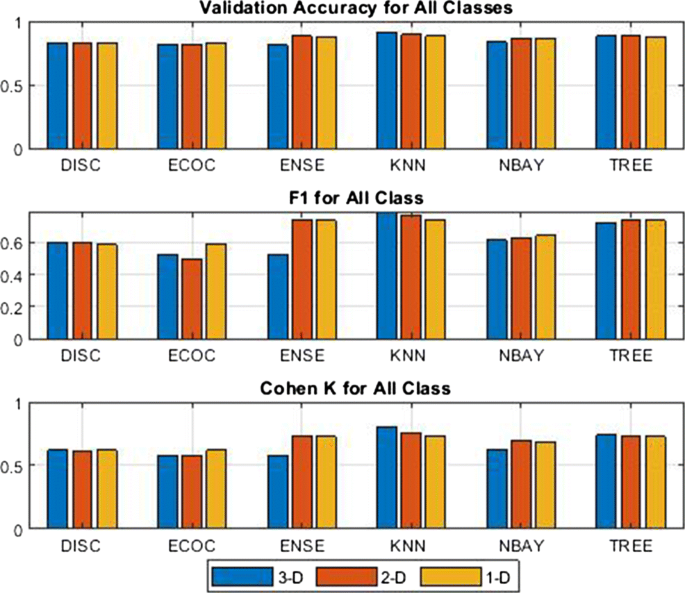
Global indices for the three options
Roughly speaking, it is possible to say that in terms of Accuracy , there are not significant differences between the various classifiers and to the use of a particular kind of reduced dataset. Instead, the global f 1 index points out a slight advantage using the ENSE and the KNN.
As for the Cohen K index, it is always higher than 0.6, thus meaning that the classification is globally enough reliable. Furthermore, also this index contributes to assign an advantage to the use of ENSE and KNN.
However, as stressed above, for our application, non all classes have the same importance. In order to evaluate the reliability in discriminating individual classes, we report in Fig. 9 , the single class f 1 indices. The figure highlights that this index, regardless of the classification method and the data set, is quite high for the Q class, but limited for the S class, which is therefore poorly discriminated. Another aspect, probably the most interesting for the purposes of this work, is that the f 1 index for the P class is about 0.7 for almost all the considered models, and in particular for the DISC, ENSE and KNN classifiers, regardless of the reduced dataset. However, even if it may seem trivial, with regard to the classification of class P , there is no substantial difference even to operate with the 1-D dataset.
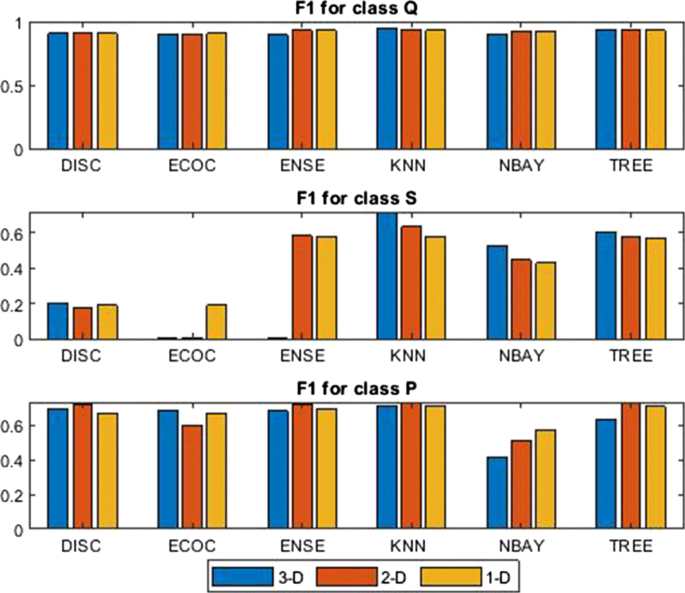
f 1 evaluated for each class and each classification approach
The ROC curves for the six classifiers are shown in Fig. 10 . It is possible to see that regardless for the considered classifiers the P class is always best classified among the others. In more detail, the Area under curve ( AuC ) for the six classifiers and for each class is reported in Fig. 11 . It is possible to see that almost all classifiers work well for classifying P class samples, but perform poorly for the Q and S classes. On average, for the P class the AuC index is 0.87, while for the Q and S class are 0.65 and 0.64, respectively.
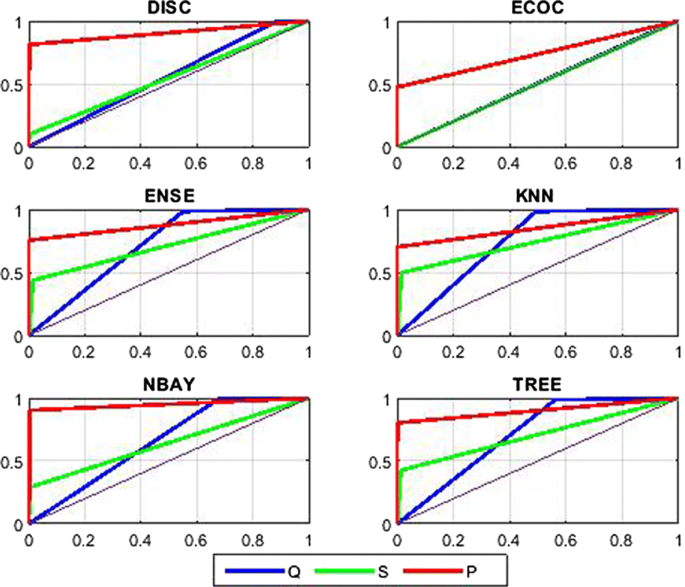
ROC curves for the six classifiers trained using the 2-D dataset
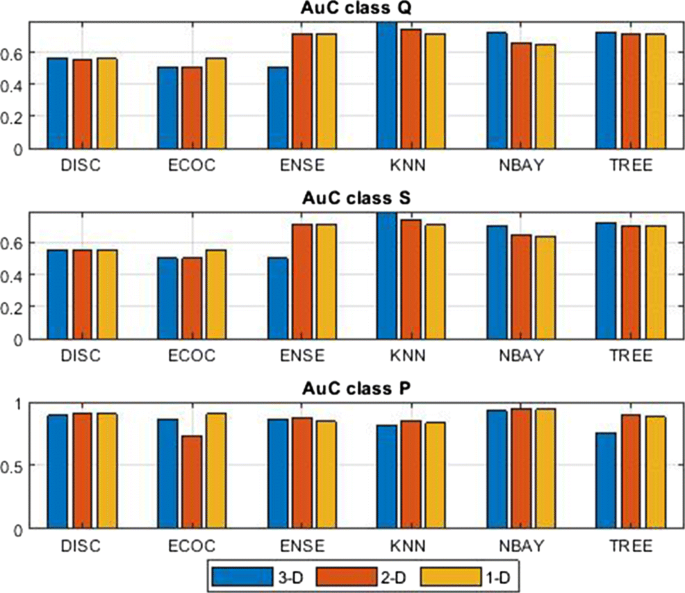
Area under the ROC for all methods and classes.
Operating with the 2-D dataset offers the possibility to visualize the boundary regions, as shown in Fig. 12 . The regions in red, green and blue colors represent couples of values classified as class Q , S and P , respectively. In most of the cases, the shape of these areas can be easily interpreted. For instance, looking at the DISC, which was trained as a classifier with linear boundaries, it is possible to see that low values of RMS at both the ESLN and EBCN are attributed to the Q class, low values at ESLN and high at EBCN are attributed to the S class and, finally, high values at both stations are attributed to the P class. An easy interpretation can also be found for the NBAY, ECOC, ENSE and TREE classifiers, while the interpretation of the KNN is a little more difficult. Indeed, the classification regions for this classifier exhibit, in the middle-upper part of the plan, an area assigned to class S , that the other classifiers definitely assign to class P . This is due to the fact that not only classes Q and S are partially overlapping in the expert classification, but also classes S and P .
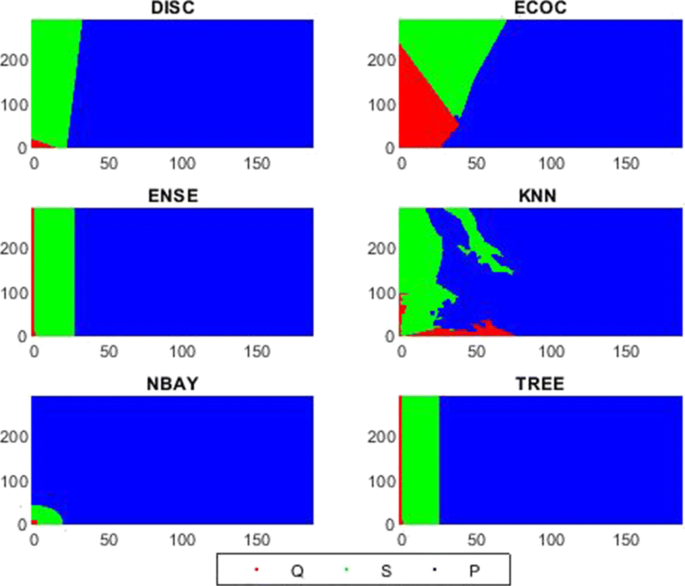
Classification regions computed for the six classifiers, trained by using the k-fold approach (axes units represent RMS of seismic tremor). The axes are graduated in RMS of seismic tremor; on the abscissa and ordinate we have the values measured at the ESLN and EBCN stations, respectively
Supervised classification by using a more balanced data set
Among the criticisms that can be made to the results shown in the previous section, there could be that of having carried out the classification on data set in which the number of samples in the various classes are strongly unbalanced, especially comparing class Q and class P . Indeed, as mentioned in Section “ Dataset and preliminary analysis ”, the original data set, from now referred as the Full dataset, contains 262944 samples labeled into 213190 samples of class Q , 49048 of class S and only 706 of class P , which in percent are the 81.08 % , 18,65 % and 0.27 % , respectively. Therefore, one might be led to think that reducing the weight of the Q class samples, which are overwhelmingly, there could be some advantage in terms of performance. To address this question, we carried out the classification experiment described below.
From the Full dataset we extracted a new dataset, in the following referred as the Subset, which includes all the P samples of the Full data set, almost all the S samples, but a smaller amount of samples of the Q class. In order to extract such a subset we have considered that the Full dataset contains 48 paroxysmal episodes occurred on Mount Etna during 2011-2015. With the aim of preserving all P samples, we extracted 48 data windows, centered on the starting date of each paroxysmal episode and spanning a prefixed number of samples before and after this date. The time series of classes obtained in this way is shown in Fig. 13 .
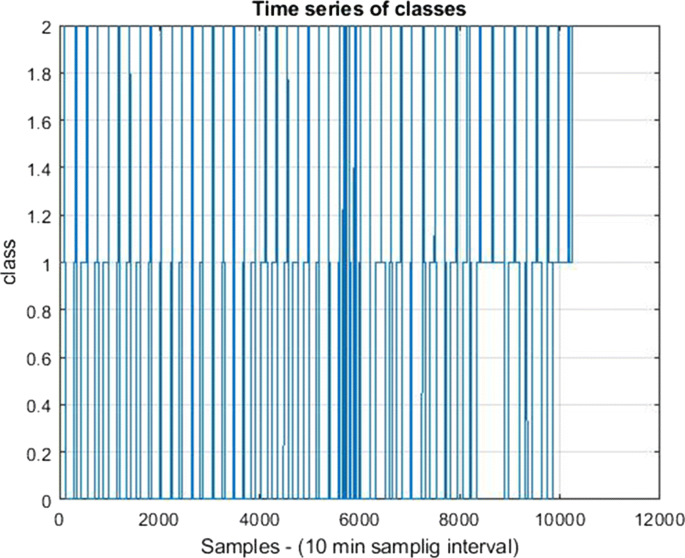
Time series of the classes for the Subset
Such a time series consists of 10278 samples, of which 5834 labeled as Q , 3738 as S and 706 as P. This means that in the Subset the weights of Q , S and P are 56.76 % , 36.37 % and 6.87 % respectively.
With the obtained Subset, the models of six supervised classifiers, were obtained, trained, by using the k-folding technique. These new classifiers were subjected to classifying the Full dataset and the performance indices were estimated and compared with those of the corresponding classifiers, trained and tested with the Full dataset. Such a comparison, in terms of the f 1 index, evaluated for the single classes, is shown in Fig. 14 .
The following considerations can be made:
The f 1 indices for the Q class are almost equal for the two intercompared cases, except that the ECOC model trained with the subset was not able to classify samples of this class.
As concerning the S class, the f 1 index is lower than 0.6 for all classifiers and in particular DISC and ECOC perform very poorly. It is to be stressed that the ECOC model trained with the Subset was not able to correctly classify any samples of the Full dataset assigned to the S class.
For the P class there are not substantial differences for the two inter-compared cases.
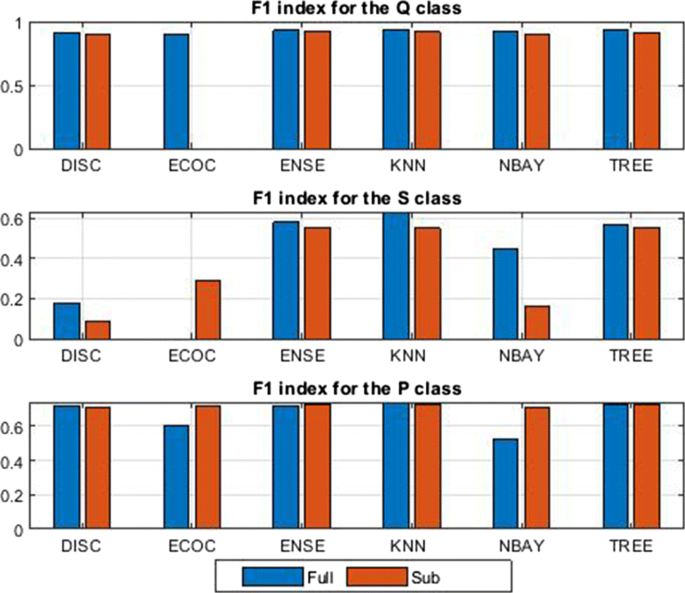
f 1 index obtained for the model trained and tested with the Full data set and the model trained with the Subset and tested with the Full set
In summary, from experimental results, it seems that there are no advantages training the classifiers with the Subset, if it is used for classifying samples of the Full dataset, which of course is what a user would like to do. On the contrary, some classes are worse classified compared to the case in which the training is carried out on the Full dataset.
Numerical results concerning unsupervised classification
In this section we report numerical results obtained by using the following three clustering approaches:
Fuzzy c-means.
Gaussian mixture models.
A short description of each algorithm is provided in Section A . The kmeans, fcm and fitgmdist functions of the Matlab Statistical and Machine Learning Toolbox, were considered for implementing the three approaches listed above. The criteria for setting the parameters, left to the user are indicated below. During the model fitting, in this case, dealing with unsupervised classification, the dataset was not split, i.e. the whole dataset was given as input to the classifiers.
Similarly to what was done for the supervised classification, we report results obtained performing the clustering on three dataset with reduced dimension, presented in Section “ Supervised classification ”. The performances of the unsupervised clustering were evaluated assuming as true the time series of classes shown in Fig. 3 . The global indices, in terms of Accuracy, f 1 and Cohen K, for the three dataset are shown in Fig. 15 . In the figure, in correspondence with the f 1 index relating to the 3-D dataset, the values should not be considered zero, but Not a Number . The reason is that, for all trials carried out, choosing different options for the tunable parameters, the considered clustering algorithms, were not able to correctly recognize any sample of the class P class. For this reason, in the following of this section we will report results for the 2-D and 1-D data sets, only.
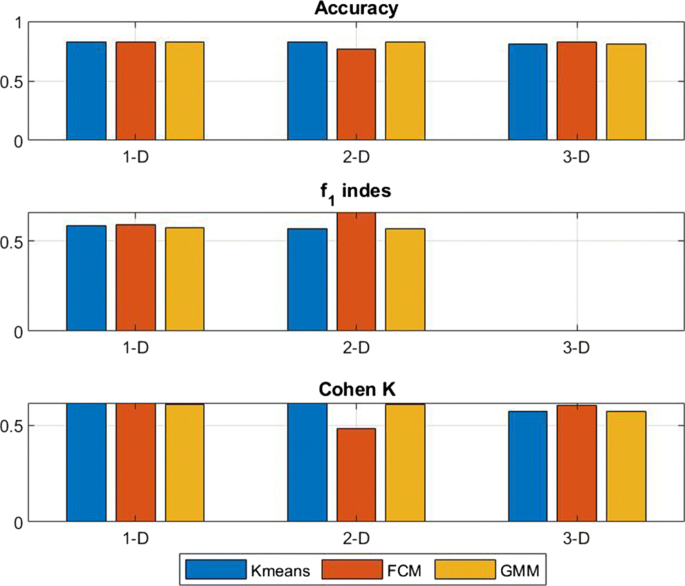
Global Accuracy, f 1 and Cohen K for the 1-D, 2-D and 3-D dataset and for the three considered clustering approaches
Results by using the k-means and the 2-D dataset
As explained in Section A.2.1 , using this approach it is necessary to choose the kind of distance. Based on trials performed, among the Euclidean , cityblock , cosine and correlation , we have chosen the former one. The classification regions of the K-means performed on 2-D dataset in the ESLN-EBCN feature plane, are shown in Fig. 16 . It can be seen that the algorithm determines well-distinct regions: the one in the lower corner in red color, characterized by low values of the tremor at both stations, represents the Q class, the intermediate one in green represents the S class and, finally, the region in blue color, characterized by high values of tremor at both the stations, represents the P class. For each class, the searched cluster centers are reported in Table 3 . Applying the classification mask shown in Fig. 16 to the 2-D dataset, we obtained the pattern distribution shown in Fig. 17 . Performances of the k-means classifier, in terms of Confusion Matrix, assuming as target the expert classification, are reported in detail in Fig. 18 .
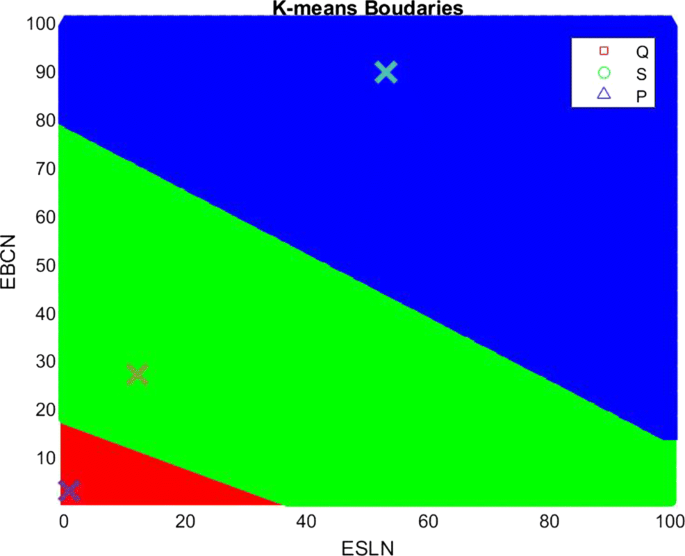
Classification regions obtained performing the K-means clustering; cluster centers for the three classes are also indicated by the × symbol
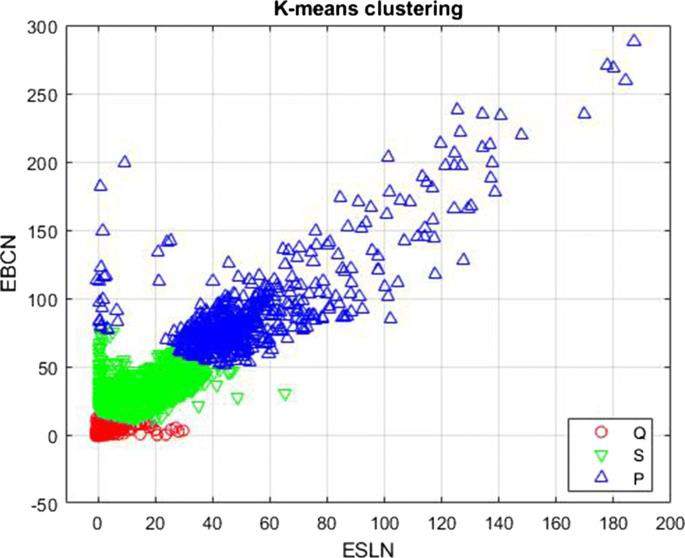
Samples classified by the K-means (axes units represent RMS of seismic tremor)
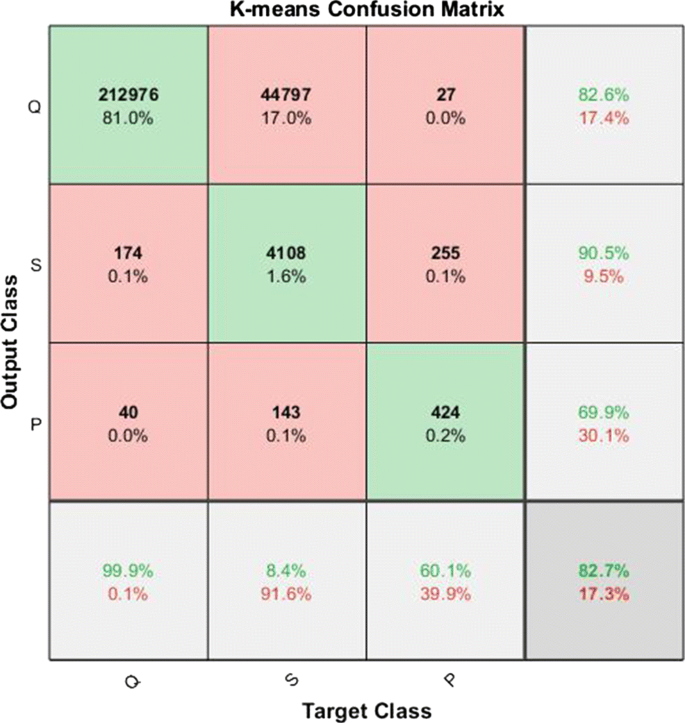
Confusion Matrix obtained classifying the 2-D dataset by using the K-means algorithm
The behavior of the K-means algorithm, referring to the P class, can be considered relatively satisfactory. Indeed, of the 706 samples labeled by the expert as belonging to P , 424 have been correctly attributed, while 255 were recognized as S and only 27 as Q , therefore giving TPR% = 60.1 and PPV % = 69.9. A weak point of the K-means classifier, for the considered dataset is the low value of TPR (8.4%) for the class S . Indeed, 44797 samples of the total 49048 assigned by the human expert as belonging to the S class, are instead recognized as Q . Obviously, this contributes to lowering the classifier’s performance also with respect to the Q class.
The ROC curves obtained applying the K-means algorithm to the 2-D dataset are reported in Fig. 19 . The figure confirms that in terms of ROC curves, the only class classified with acceptable accuracy by K-means is class P , being AuC = 0.81. For both the remaining classes Q and S we have AuC = 0.54, therefore indicating a behavior very close to a random classifier.
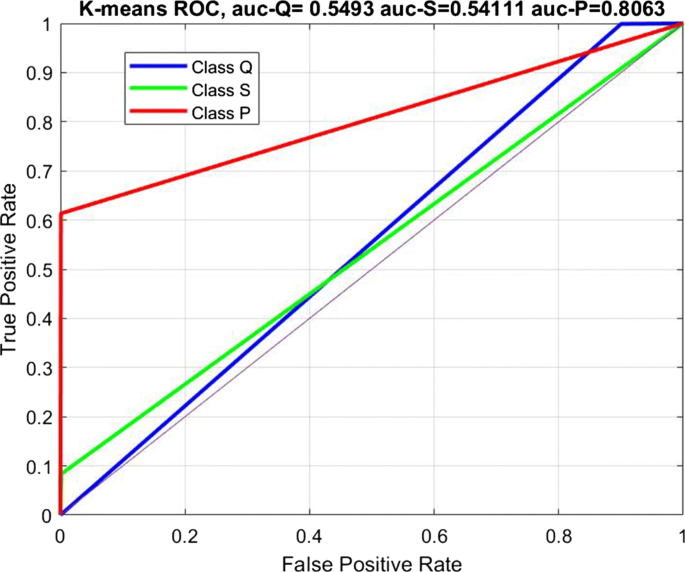
ROC curves of the K-means classifier for the three classes
Results by using the FCM and 2-D dataset
Working with the FCM algorithm, in addition to the choice of the metrics, which also in this case we have chosen as Euclidean, it is possible to tune the m parameter (see Section “ A.2.2 ”), which controls the degree of fuzzy overlap among the clusters. After some trials, we have experimentally found that best results were obtained by setting m = 2, which is also the default value assumed by the fcm Matlab function. The cluster centers obtained for the FCM model, reported in Table 4 , are a bit different from the ones searched by the K-means algorithm.
The classification regions provided by the FCM in the ESLN-EBCN feature plane are shown in Fig. 20 . Compared with the analogous mask obtained by the K-means it is possible to see that the area assigned to the Q -region is smaller, while that assigned to the P one is larger. The performances of this classifier are reported in Fig. 21 in terms of Confusion Matrix. It is possible to see the FCM, compared with the K-means, gives higher TPR for the P and S classes, but with a lower PPV. In particular, for the P class we have a TPR of 82.9%, but with a PPV = 56%, which in terms of f 1 means f 1 = 0.67. Therefore, by using the FCM, we get a higher number of samples correctly classified but at the price of a higher level of false positive.
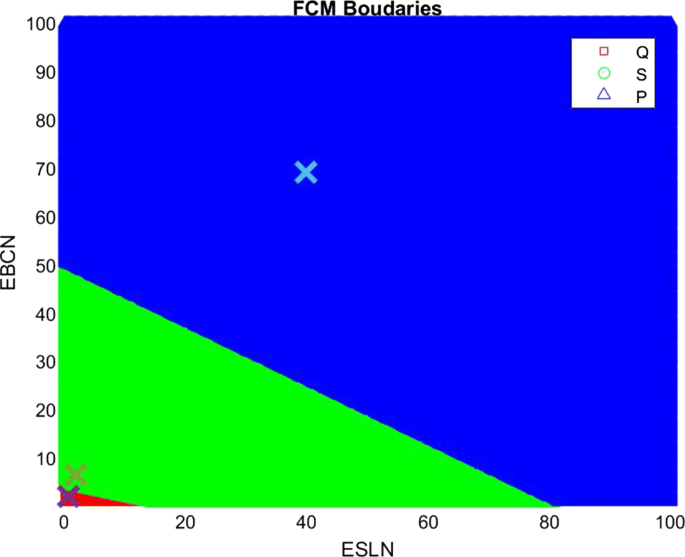
Classification regions obtained by using the FCM algorithm. The cluster centers are indicated by the × symbol
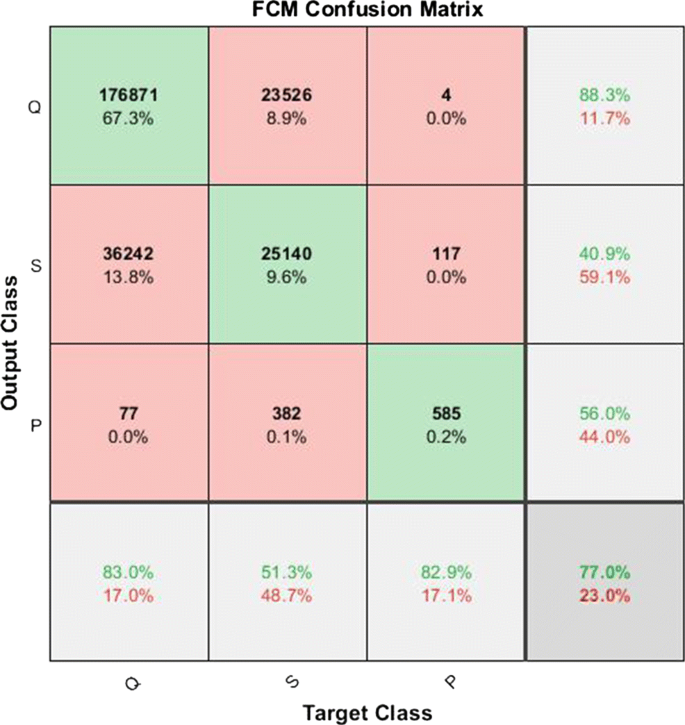
Confusion Matrix obtained classifying the 2-D dataset by using the FCM algorithm
The ROC curves obtained applying the FCM algorithm to the 2-D dataset are reported in Fig. 22 which shows that for this classifier the AuC assumes the values AuC = 0.91 for the P class and about 0.67 for both the S and Q classes. Therefore, while the P class is classified with an acceptable accuracy, the Q and S are poorly classified. However, in terms of ROC curves, the FCM performs better than the K-means.
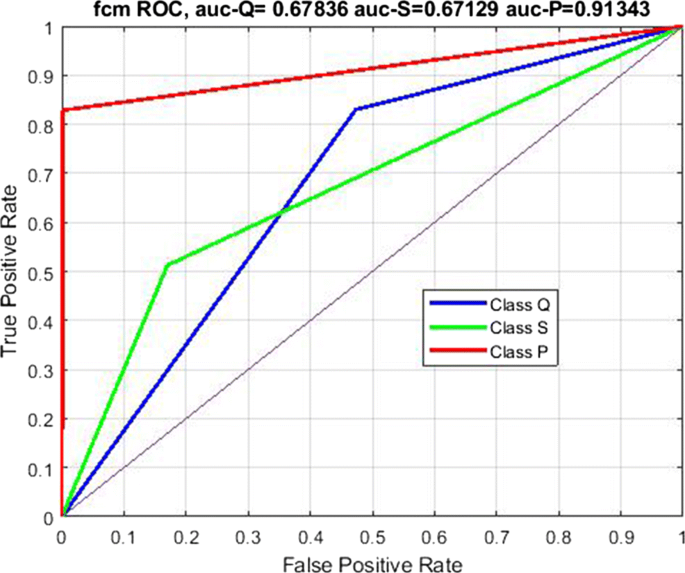
ROC curves for each class obtained by using the FCM algorithm
Results by using the GMM
Tuning a GMM model offers to the user the possibility of choosing different settings for the covariance matrices. Indeed, even if ultimately the covariance matrices are automatically computed by the software, the choice of their structure is left to the user. After some trials, with the aim of maximizing the model performances against the P class, we have found convenient to assume, diagonal covariance matrices, shared among the classes. The obtained mixing coefficients π i and mean μ i parameters of the GMM model are reported in Table 5 . The classification mask, in the feature plane ESLN-EBCN, is shown in Fig. 23 .
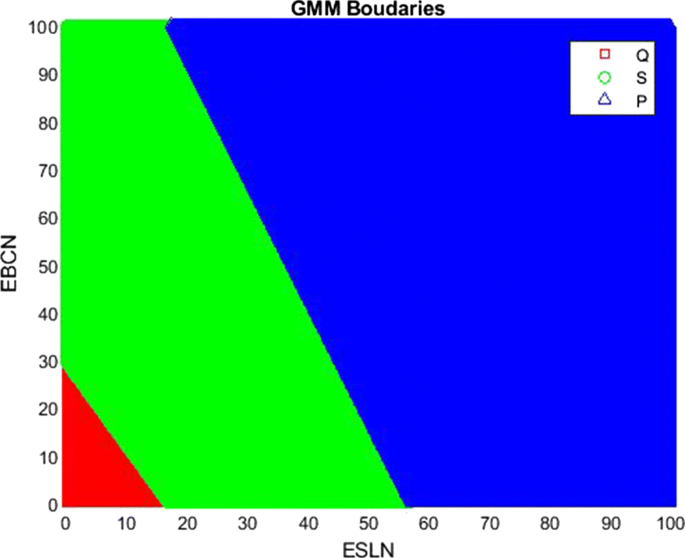
Classification regions obtained by using the GMM algorithm
It is possible to see that also this model, similar to the k-means and FCM ones, simply classifies as Q low RMS values recorded at both ESLN and ECBN, as S low RMS at ESLN and high at ECBN and, finally, as P high RMS values at both the stations. Obviously, the position of the boundary regions are different with respect to K-means and FCM.
The classier performances in terms of CM are reported in Fig. 24 , while the ROC curves are reported in Fig. 25 . This last figure shows that for this classifier the AuC is 0.80 for the P class and about 0.54 for both the S and Q classes. Therefore, while the P class is classified with an acceptable accuracy, the Q and S are poorly classified. In terms of AuC, the GMM performs worse than the FCM and similar to the Kmeans.
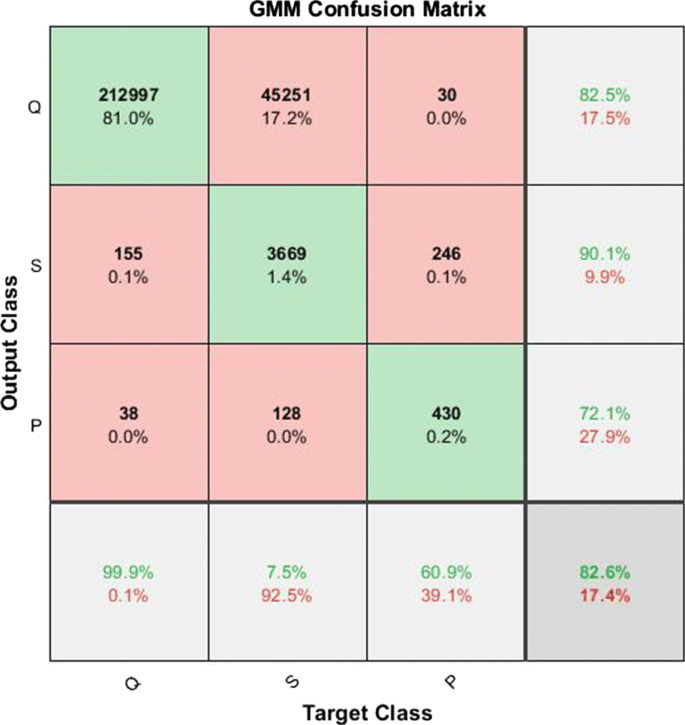
Confusion Matrix obtained by using the GMM model
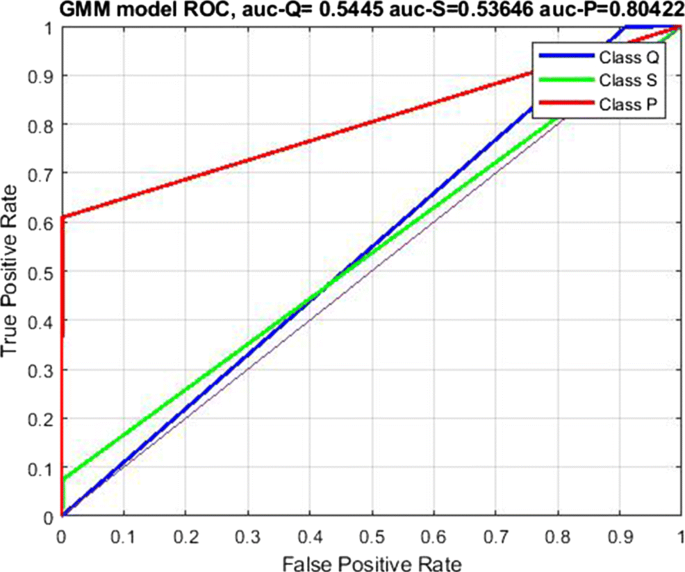
ROC curves for the three classes obtained by using the GMM algorithm
Comparison among the unsupervised classifiers for the 2-D data
A direct comparison among the three unsupervised classifiers, in terms of global Accuracy, f 1 and Cohen’s K, by using the 2-D dataset is reported in Fig. 26 . It is possible to see that Kmeans and GMM perform quite similar in terms of all the three considered global indices. In particular, while they outperform the FCM model in terms of Accuracy and Cohen’s K, the FCM performs better in terms of global f 1 .
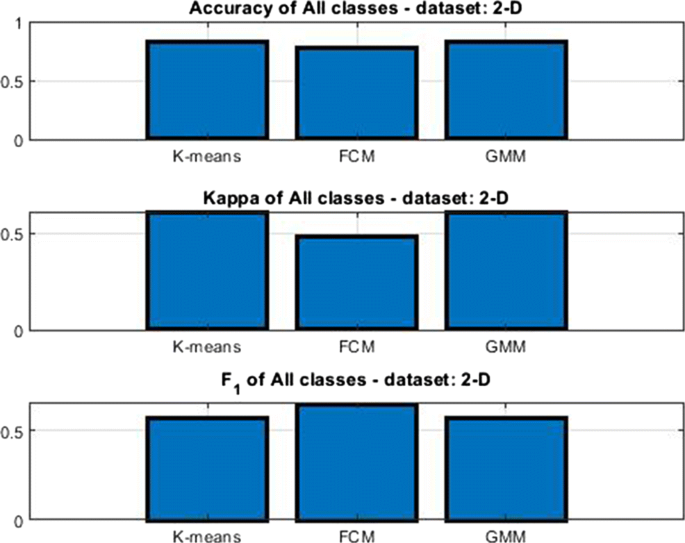
Comparison among the unsupervised classifiers in terms of global Accuracy, f 1 and Cohen’s K, by using the 2-D dataset
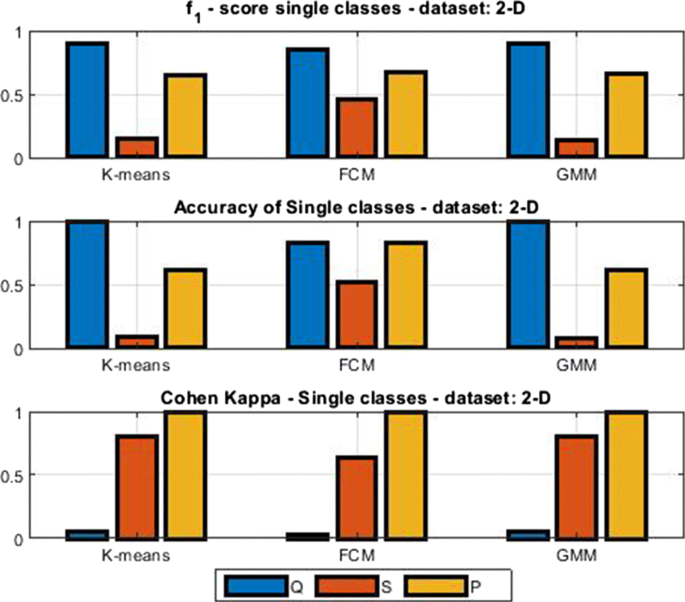
Comparison among the unsupervised classifiers in terms of f 1 for each individual class by using the 2-D dataset
The performance indices for each single class are shown in Fig. 27 . The figure shows that the f 1 score for class Q is quite high regardless of the clustering algorithm and so is the Accuracy. For class S both the f 1 and Accuracy indices are low. For class P , the f 1 index is about 0.7 regardless of the clustering method. Furthermore, it should be noted that the Cohen K index for class Q is very low, unlike the Accuracy index, thus indicating that the statistic on class Q is not very reliable. On the contrary, the statistic on class P is very reliable.
Models in 1-D and comparison with 2-D
Operating in 1-D, i.e. training the models by using the average seismic tremor, estimated model parameters are reported in Tables 6 and 7 for the K-means and FCM model, respectively. The parameters of the fitted GMM model are reported in Table 8 .
A comparison of the performance, in terms of f 1 and AuC, Accuracy and Cohen’s K indices, between models fitted by using the 1-D and 2-D dataset, is reported in Figs. 28 , 29 , As a general comment it is easy to say that there are not meaningful advantage in performing the unsupervised clustering operating on the 2-D with respect to the 1-D dataset. This result which may appear trivial, is essentially due to the fact that the seismic tremor timeseries at different stations are strongly correlated, as discussed in the previous Section “ Regression analysis of tremor time series ”.
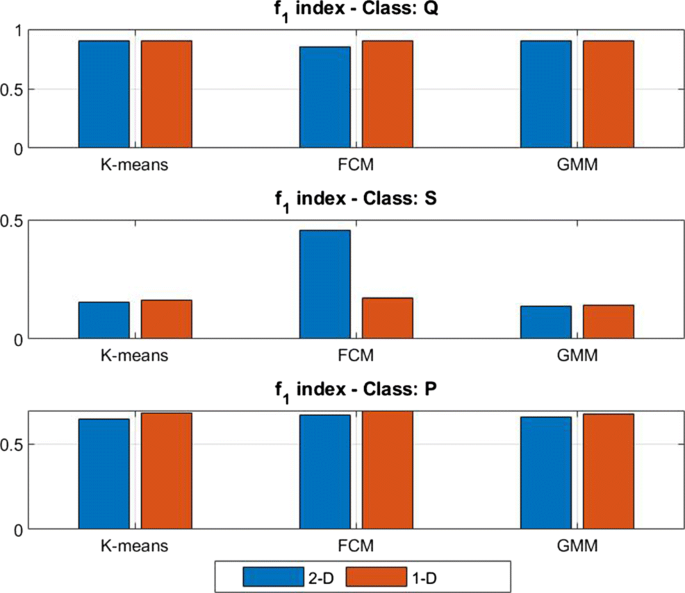
Performance in terms of the f 1 index by using the 2-D and the 1-D dataset
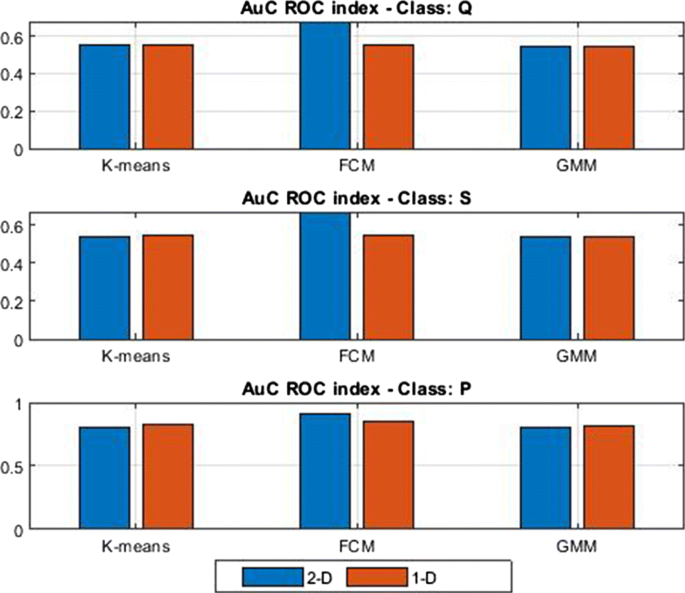
Comparison in terms of AuCindex by using the 2-D and the 1-D dataset
In more details, Fig. 28 shows that the f 1 index, averaged among the methods, is about 0.88 for the Q class, about 0.25 for the S class and about 0.66 for the P class. So it might seem that in terms of f 1 , both class Q and class P are well classified. In reality, if we look at the AuC index reported in Fig. 29 , we realize that class Q has an Auc index of 0.58 while class P has an AuC of 0.84. Bearing in mind that a random classifier has an AuC = 0.5, we can conclude that while the value of f 1 = 0.88 is not representative of the true performance of the classifier with respect to class Q , the value average value of f 1 = 0.66 for class P is representative of the true capabilities of the classifier. In a few words we can conclude that the unique class reliably discriminated is class P .
Another result that could be interesting to observe is that the value of Auc for class P , estimated in this paper, could agree with that obtained by Spampinato et al. ( 2019 ), operating with different methodologies on the same area, who computed a value \( AuC \sim 0.8\) .
Measuring the performance of the FCM classifier assuming as true the output of the ENSE supervised classifier
In this section we report a comparison between one of the best among the unsupervised classification algorithms, in our case the FCM operating on the 1-D dataset, and one of the best supervised classifiers presented in the previous section. For this comparison we have chosen the ENSE classifier. In order to perform this comparison, we have computed a CM, assuming as target the time series obtained by the ENSE classifier and as output the one provided by the FCM. Such a CM is shown in Fig. 30 . It is possible to see that:
Supervised and unsupervised algorithms almost fully agree in classifying samples of class Q , being TPR% = 100 and PPV % = 91.6.
The two kinds of classifiers almost fully agree also for the P class, being TPR% = 100 and PPV % = 97.6.
Supervised and unsupervised algorithms do not fully agree with samples of S class, exhibiting PPV = 100% but TPR = 18.5%.
This experimentally demonstrates that the unsupervised classification of RMS tremor can effectively replace the supervised one, limited to the clustering of paroxysm activity. In other terms, it is possible to reach the same level of performances, avoiding the laborious commitment of the experts or at least they could take the results provided the unsupervised classifier as a good starting point for further developments. Furthermore, without significant loss of performance, it is possible to carry out the classification on the 1-D dataset, that is, using the average RMS tremor time series, obtained using the expression ( 2 ).
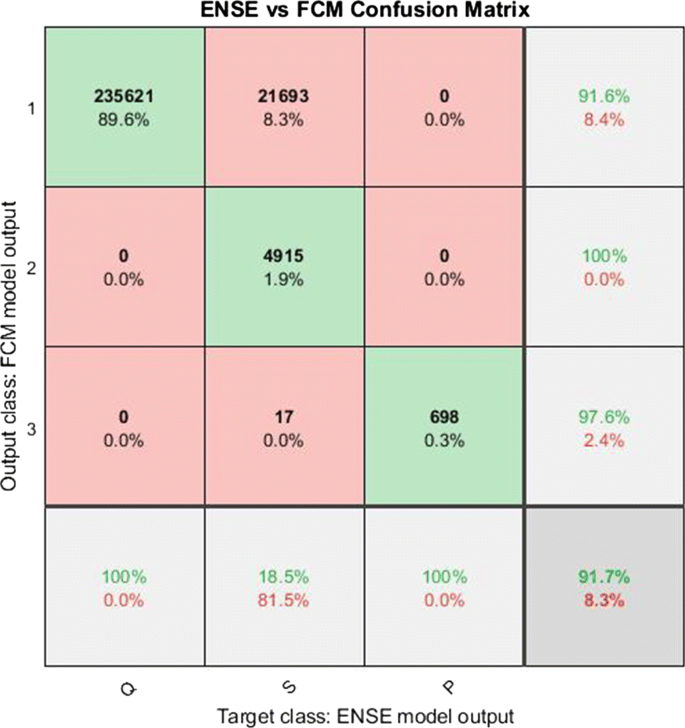
Confusion Matrix obtained assuming as target the output of the ENSE supervised classifier and as output class the output of the FCM clustering algorithm
Comparing the true and the unsupervised time series of class
The following discussion aims to show that the misclassified samples occur during the transition phases from quiet to paroxysm and vice versa. Indeed, the 706 samples classified by the experts as belonging to the P class, form actually 48 paroxysmal events (or patterns). A few of these episodes are shown in Fig. 31 . The time series of classes provided by the unsupervised classifier is reported in yellow color, the average RMS tremor is reported in red color, and the expert class in blue. The figures also show that paroxysm is always preceded by Strombolian and usually also followed. It is also possible to see that the yellow and the blue curves usually agree during paroxysm, while the major differences can be observed during the Strombolian activity. It is also interesting to observe the close correlation between the average level of the RMS tremor (red curve) and the time series of class provided by the unsupervised classifier (yellow curve). Therefore, the greatest uncertainties concern the classification of the S episodes, for which the average level of the RMS tremor is not particularly different from the level it assumes during the activity classified as Q .
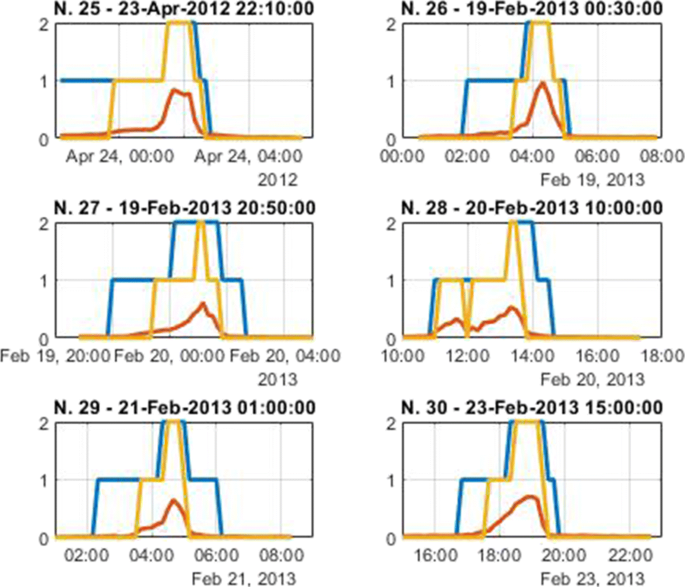
Comparison among the true (blue color) and clustered (yellow) time series of classes for a few paroxysm episodes; the red curve is the average RMS tremor
Discussion and Conclusions
In this work we have addressed both the problem of supervised and unsupervised clustering of volcanic activity, based on seismic tremor, a kind of geophysical signal widely available in many volcanoes around the world, in order to objectively evaluate to what extent popular clustering algorithms can automate this task, usually performed by human experts. Results achieved can be summarized as follows:
All the considered classification algorithms, both supervised and non, agree that by using the RMS of tremor as the only feature, only events belonging to class P can be classified with a reliability that, measured in terms of the f 1 index, is about 0.7 and in terms of Au C about 0.8.
Almost all the supervised classifiers considered are able to perform this task of classifying the class P events, but a limited superiority can be assigned to ENSE, KNN and TREE models. Similarly, among the considered unsupervised classifiers a limited superiority can be assigned to the FCM one.
The greatest uncertainties of classifiers concern the attribution of events belonging to class S . In this regard, the RMS level of tremor alone is not enough to safely discriminate whether these samples are to be attributed to class Q or to class S .
As a final remark, it is to stress that the expert classification is not necessarily based exclusively on the evaluation of physical signals and the dating of the events can therefore be imprecise. Therefore, a basic problem arises, concerning the establishment of objective criteria that experts must consider to classify volcanic activity. However, this problem is beyond the scope of this paper and is left for future developments.
Bano S, Khan MNA (2018) A survey of data clustering methods. Int J Adv Sc Technol 113:133–142. https://doi.org/10.14257/ijast.2018.113.14
Article Google Scholar
Bergen KJ, Johnson PA, de Hoop MV, Beroza GC (2019) Machine learning for datadriven discovery in solid Earth geoscience. Science 363:1–10. https://doi.org/10.1126/science.aau0323
Bezdec JC (1981) Algorithms, pattern recognition with fuzzy objective function. Plenum Press, New York, pp 1–272. https://doi.org/10.1007/978-1-4757-0450-1
Book Google Scholar
Bishop CM (2006) Pattern recognition and machine learning. Springer, Berlin, pp 1–758
Google Scholar
Breiman L, Friedman J, Olshen R, Stone C (1984) 11 Classification and regression trees. CRC Press, Boca Raton, pp 246–280
Cannavó F, Cannata A, Cassisi C, Di Grazia G, Montalto P, Prestifilippo M, et al. (2017) A multivariate probabilistic graphical model for real-time volcano monitoring on Mount Etna. J Geophys Res Solid Earth 122:3480–3496. https://doi.org/10.1002/2016JB013512
Chouet B (1996) Long-period volcano seismicity: its source and use in eruption forecasting. Nature 380(6572):309–316. https://doi.org/10.1038/380309a0
Dietterich TG, Bakiri G (1995) Solving multiclass learning problems via error-correcting output codes. J Artif Intell Res 2:263–286
Fisher RA (2008) The use of multiple measurements in taxonomic problems. Annals of Eugenics, Chapman - Hall/CRC. Taylor - Francis Group, LLC vol. 7, pp 179–188
Goodfellow I, Bengio Y, Courville A (2016) Deep Learning the Mit Press
Hajian A, Cannavó F, Greco F, Nunnari G (2019) Classification of mount etna (italy) volcanic activity by machine learning approaches. Annals of Geophisics 62(2, VO231):1–11. https://doi.org/10.4401/ag-8049
Hastie T, Tibshirani R, Friedman J (2008) The Elements of statistical learnings - data mining, inference, and prediction. Springer, Berlin, pp 1–764
Inc The MathWorks (2017) Statistical and machine learning toolbox user guide, R2017b, The MathWorks Inc 1–2070
Kong Q, Trugman TD, Ross ZE, Bianco MJ, Meade J, Gerstoft P (2019) Machine Learning in seismology: turning data into insights. Seismology Res Lett 90(1):3–14
Langer H, Falsaperla S, Hammer C (2020) Advantages and pitfalls of pattern recognition - selected cases in geophysics. Elsevier, Amsterdam, pp 1–331
Langer H, Falsaperla S, Messina A, Spampinato S, Behncke B (2011) Detecting imminent eruptive activity at Mt Etna, Italy, in 2007–2008 through pattern classification of volcanic tremor data. J Volcanol Geotherm Res 200:1–17
Lei B, Xu G, Feng M, Zou Y, Van der Heiden F, De Ridder D, Tax DMJ (2017) Classification, parameter estimation and state estimation - an engineering approach using MATLAB Wiley
Liao TW (2005) Clustering of time series data - a survey. Pattern Recogn 38:1857–1874
Liu H, Motoda H (2008) Less is more, (in Computational Methods of Feature selection, Liu and Motoda Eds, chap 1), Chapman-Hall, CRC Press, 3–15
Malfante M, Dalla Mura M, Mtaxian JP, Mars JI, Macedo O, Inza A (2018) Machine learning for volcano-seismic signals. IEEE Signal Process Mag 35(2):20–30. https://doi.org/10.1109/MSP.2017.2779166
Mohamed AA (2020) An effective dimension reduction algorithm for clustering Arabic text. Egyptian Inf J 21:1–5
Powers DMW (2011) Evaluation: from precision, recall and f-measure to ROC, informedeness, markedness and correlatio. J Mach Learn Technol 2(1):37–63
Rouseeuw PJ (1987) Silhouettes: a graphical aid to the interpretation and validation of cluster analysis. J Comput Appl Math 20(1):53–65
Spampinato S, Langer H, Messina A, Falsaperla S (2019) Short-term detection of volcanic unrest at Mt. Etna by means of a multi-station warning system, Scientific Reports
Tallon-Ballesteros AJ, Riquelme JC (2014) Data mining methods applied to a digital forensics task for supervised machine learning. In: Computational intelligence in digital forensics-forensic investigation and applications. https://doi.org/10.1007/978-3-319-05885-6-17 , pp 413–451
Xu L, Jordan MI (1996) On convergence properties of the EM algorithm for Gaussian mixtures. Neural Comput 8(1):129–151
Xu D, Tian D (2015) A comprehensive survey of clustering algorithms. Ann Data Sci 2 (2):165–193. https://doi.org/10.1007/s40745-015-0040-1
Download references
Acknowledgements
We are indebted to the Authors of the paper (Cannavó et al. 2017 ) for enabling data used in this paper. This work was supported by the Universitá degli Studi di Catania, under the project Piano della Ricerca 2020-2022.
Open access funding provided by Università degli Studi di Catania within the CRUI-CARE Agreement.
Author information
Authors and affiliations.
Dipartimento di Ingegneria Elettrica Elettronica e Informatica, Universitá degli Studi di Catania, Viale A. Doria, 6, 95125, Catania, Italy
Giuseppe Nunnari
You can also search for this author in PubMed Google Scholar
Corresponding author
Correspondence to Giuseppe Nunnari .
Additional information
Communicated by: H. Babaie
Publisher’s note
Springer Nature remains neutral with regard to jurisdictional claims in published maps and institutional affiliations.
The purpose of this section is to provide a formal description of the ML methods considered in this paper and to give some useful insights on how they have been implemented. All the software used in this work has been coded by the author by using the standard Matlab Statistical and Machine Learning Toolbox library (Inc 2017 ). In the following we report a brief description of each method and references to papers where these methods were originally introduced.
A.1.1 Supervised classification
In this section, we provide a description of the six supervised approaches considered in the paper, starting with the Discriminant Analysis.
Discriminant analysis
Discriminant analysis, here referred as DISCR, is the evolution of the original Fisher discriminant, named for its inventor (Fisher 2008 ). The model for discriminant analysis works as follows:
The class vector Y is generated assuming that the input data X can be reliably fitted assuming a Gaussian mixture distribution.
For linear discriminant analysis, the model assumes the same covariance matrix for each class, i.e. only the means vary. It could be easily demonstrated that assuming different covariance matrices for each class, the boundary curves become, in general, quadratic.
Predictions are performed according with the following expression:
\(\hat {y}\) is the predicted class.
K is the number of classes.
\(\hat {P}(k|x)\) is the posterior probability of class k for observation x .
C ( y | k ) is the cost of classifying an observation as y when its true class is k .
Therefore, the user can choose the type of boundary regions (linear or quadratic) based on the kind of assumption about the structure of covariance matrix (e.g equal or different for the various classes, diagonal or full etc).
Another choice left to the user is the cost function C . By default, the standard form of the cost matrix C , is C i , j = 1 if i ≠ j and C i , j = 0 if i = j . Entries for the C matrix can also be negative. In this paper we have assumed the standard form.
In the Matlab Statistical toolbox this method is implemented by the function fitcdiscr which allows several options. Among these we have chosen to operate with a linear discriminant type. This implies that we have assumed that all classes have the same covariance matrix. Furthermore the algorithm applies a regularization expression of the form
where \(\hat {\varSigma }\) is the empirical pooled covariance matrix and γ is the amount of regularization 0 ≤ γ ≤ 1. In this paper we have set γ = 0. Moreover, we have adopted a double logit score transformation of data.
A.1.2 Error-correcting output codes
Error-Correcting Output Codes (ECOC) (Dietterich and Bakiri 1995 ) is an ensemble method designed for multi-class classification problem (i.e. K > 2 ). Indeed, while some algorithms, such as Decision Tree, Naive Bayes can handle multi-class problems directly, ECOC is a meta method which combines L binary classifiers in order to solve the multi-class problem. All kinds of classifiers can be used as binary classifiers, such as Discriminant, KNN, Naive Bayes, SVM, Decision Trees etc. The number L depends on the kind of coding considered among several available, such as one-versus-all (OVA), one-versus-one (OVO), etc. For instance if the OVO coding is adopted the number of needed binary classifiers is L = K ( K − 1)/2, where as usual, K is the number of classes. The basic ingredients of the ECOC method are:
a coding design matrix M
a binary loss function g (⋅,⋅)
The coding design matrix is a matrix whose elements direct which classes are trained by each binary learner, that is, how the multiclass problem is reduced to a series of binary problems. Each row of the coding design matrix corresponds to a distinct class, and each column corresponds to a binary learner. In a ternary coding design, for a particular column (or binary learner):
a row containing 1 directs the binary learner to group all observations in the corresponding class into a positive class.
A row containing − 1 directs the binary learner to group all observations in the corresponding class into a negative class.
A row containing 0 directs the binary learner to ignore all observations in the corresponding class.
For instance, suppose that, as is the case in this paper, that the goal is to classify data into 3 classes, namely Q, S and P. Choosing the one-versus-one (OVO) coding, the coding matrix assumes the form shown in Table 9 .
In this ECOC model the Learner L 1 trains on observations in Class Q or Class S, and treats Class Q as the positive class and Class S as the negative class. The other learners are trained similarly.
In general, indicating as:
M the coding design matrix whose elements are m k , l ,
s l be the predicted classification score for the positive class of learner l
g (⋅,⋅) a binary loss is function
L the number of learners
the algorithm assigns a new observation to the class \(\hat {k}\) that minimizes the aggregation of the losses for the L binary learners. Different expressions can be used to compute the predicted class for the observation. For instance, in the loss-based decoding , the class producing the minimum sum of the binary losses over binary classifiers determines the predicted class of an observation, as expressed by Eq. 14
Another possible choice is the loss-weighted decoding , where the class producing the minimum average of the binary losses, over binary classifiers, determines the predicted class of an observation, that is Eq. 15 .
As concerning the loss function g (⋅,⋅) a number of possible choices are possible, such as the Hamming function, the exponential function, etc. Here, just to give an idea, we report the Hamming Loss function ( 16 ).
where, y j ∈{− 1, 1, 0} is a class label for a particular binary learner and as s j is the score for observation j . In the Matlab Statistical and Machine Learning Toolbox the ECOC model is implemented by the function fitcecoc , which allows several parameters to be chosen. In this application we have considered all default parameters which use K ( K − 1)/2 binary Support Vector Machine (SVM) models together with the one-versus-one coding system.
A.1.3 Ensemble methods
Under the name Ensemble Methods, here referred as ENSE, a wide range of algorithms can be recognized, based on the idea that the use of multiple learning algorithms allows to obtain better predictive performance than could be obtained from any of the constituent learning algorithms alone. This idea can be formalized as follows. Consider for example a set of k regression models. Suppose that each model makes an error 𝜖 i on each example, with the errors drawn from a zero-mean multivariate normal distribution with variances \(E[{\epsilon _{i}^{2}}]\) and covariance c = E [ 𝜖 i 𝜖 j ]. Then it is possible to demonstrate (Goodfellow et al. 2016 ) that the expected error of the ensemble predictor is
This means that in the case where the errors are perfectly correlated and c = v , the mean squared error reduces to v , so the model averaging does not help at all. In the case where the errors are perfectly uncorrelated and c = 0, the expected squared error of the ensemble is only \(\frac {1}{k}\) . This means that the expected squared error of the ensemble decreases linearly with the ensemble size. In other words, on average, the ensemble will perform at least as well as any of its members, and if the members make independent errors, the ensemble will perform significantly better than its members. Different approaches are available to build the ensemble of models. Among the most popular we have the Bagging and the Boosting approaches.
In the framework of the Matlab Statistical and Machine Learning Toolbox, the Ensemble method is implemented as the function fitcensemble that has been used in this paper. In particular we have adopted as basic learner the Adaboost2 algorithm building a medium coarse tree with a maximum number of splits set to 20, a maximum number of cycles up to 30 and a learning rate set to 0.1.
A.1.4 K-Nearest Neighbor K classifier
In classification problems a key point is that of estimating the posterior probability \(\hat {p}(y|x)\) , x ∈ R p and y ∈ 1,…, n c , i.e. the probability of having the class k ∈ [1,… n c ], after observing the feature vector x . To perform this estimation, let assume the following.
Suppose that we have a training data set T = {( x i , y i )}, i = 1,…, N , y ∈{1,…, n c } and let N k be the number of points x that belongs to the class k . Obviously, we must have \({\sum }_{k=1}^{n_{c}} N_{k}=N\) . If we wish to classify a new point x , we draw an hypersphere centered at x containing precisely K points irrespective of their class. Here, we are indicating for historic reasons as K this number which might be confused with the number of classes we want to discriminate, but the reader will understand the true meaning by the context.
Let us indicate as κ the number of points inside the sphere belonging to the class k . It is trivial to understand that:
the conditional density associated with each class y = k can be written as
the unconditional density p ( x ) can be written as
the priority for each class can be written as
In order to classify a new observation x , we can introduce the following estimation function
Now, in order to estimate p ( y = k | x ) we can use the Bayes’ rule
Substituting expressions ( 18 ),( 19 ) and ( 20 ) into ( 22 ), we obtain the following estimation function
The interpretation of Eq. 23 is simple: a new observation vector x will be assigned to the class corresponding to the samples that are in greater number in its neighborhood.
It is trivial to understand that the parameter κ controls the trade-off between the bias and the variance.
The K-Nearest Neighbor classifier is implemented in the Matlab Toolbox under the function fitcknn that we have used in this paper, setting the dimension of the neighbor to 10 and the Euclidean distance as metrics.
A.1.5 Naive-Bayes classifier
Naive Bayes classifiers, here referred to as NBYE, are probabilistic classifiers based on the Bayes theorem, with a strong (naive) independence assumption between the features. In more detail, indicating as X the features matrix, and as Y the output class, the generic feature x ∈ X is assigned to one of K class label y ∈ Y such that \(\hat {y}=\arg \max \limits _{y} p(y|x)\) , where p ( y | x ) is the class posterior probability density, which is computed by applying the Bayes rule. The assumption of independence among features greatly simplifies the computation of p ( y | x ). Naive Bayes classifiers are implemented in the Matlab Toolbox under the function fitcnb that we have used in this paper choosing a normal kernel distribution and unbounded support.
A.1.6 Decision learning tree
Decision Tree (DTs) algorithms, here referred as TREE, originally introduced by Breiman et al. ( 1984 )), belongs to the non-parametric supervised learning methods, used for both classification and regression problems. They are based on the use of a tree-like model of decisions which mimic the human level thinking so, in simple cases, they can be easily interpreted. The key idea underlying tree-based methods is that of partitioning the feature space into a set of cuboids, and then fit a simple model (like a constant) in each one. A DT consists of a tree in which each internal (i.e. non-leaf) node is labeled with an input feature. Therefore, a basic problem during the building of the tree is the choice of the feature to be associated to a splitting node. This problem is basically solved by invoking the concept of impurity . Several definition of this quantity are available, such as:
the Gini’s impurity or Gini’s Diversity Index (gdi), defined as
where the sum is over the classes i at the node, and p ( i ) is the observed fraction of classes with class i that reach the node. A node with just one class (a pure node) has a Gini index 0; otherwise the Gini index is positive. So the Gini index is a measure of node impurity.
The Deviance, defined as
where p ( i ) is defined as for the Gini index.
Decision trees are implemented in the Matlab Statistical and Machine Learning Toolbox under the function fitctree, that we have considered in this paper by using the Gini’s index as split criterion.
A.2. Unsupervised classification
Among the numerous unsupervised classification algorithms, in this work we have taken what are probably the simplest and most popular: K-means, Fuzzy C-Means (FCM) and Gaussian Mixture models (GMM).
A.2.1 K-means
The K-means is the prototype of distance based clustering algorithms. After indicating the number K of classes the user intends to classify, the algorithm searches for the cluster centers which best represent the classes, by optimizing an appropriate cost function. Formally, if we have a data set { x 1 ,…, x N } consisting of N observations of a random D -dimensional Euclidean variable x , let us indicate as μ k , k = 1,… K a set of prototypes , i.e. candidates as cluster centers, associated with the classes. Then, the following cost function is defined
which represents the sum of the squares of the distances of each data point to its assigned prototype vector μ k . In expression ( 26 ), r n k ∈{0, 1,} is a binary indicator variable describing which of the K clusters the data point x n is assigned to, so that if data point x n is assigned to cluster k then r n k = 1, and r n k = 0 for j ≠ k .
With these definitions, the clustering problem is to find values { r n k } and { μ k } which minimize J . This optimization problem can be solved with an iterative procedure in which each iteration involves two successive steps corresponding to successive optimizations with respect to the r n k and the μ k . In the first step J is minimized with respect to the r n k , keeping the μ k fixed. In the second phase J is minimized with respect to the μ k , keeping r n k fixed. These two steps of updating r n k and updating μ k correspond respectively to the E (expectation) and M (maximization) steps of the known EM algorithm that we encounter also in the Gaussian Mixture algorithm. Here, it must be stressed that the Euclidean distance in expression ( 26 ), which is usually adopted, for special purposes can be replaced. A list of allowed distance definitions is reported in (Xu and Tian 2015 ). For high dimensional dataset others definitions may be adopted, such as the L 1 distance (also known as cityblock ), the cosine distance, i.e. one minus the cosine of the included angle between points (treated as vectors), the correlation , i.e. one minus the sample correlation between points, and so on. In the Matlab toolbox the K-means is implemented under the function kmeans which was considered in this paper with the default parameters, in particular by using the squared Euclidean distance metric.
A.2.2 Fuzzy c-means
The Fuzzy c-means (FCM) (Bezdec 1981 ) can be considered an extension of the K-means, but while in this latter algorithm each point belongs exclusively to one class, the FCM allows each data point to belong to multiple clusters, with varying degrees of membership. In more detail the FCM algorithm is based on the minimization of the following cost function:
D is the number of data points.
N is the number of clusters.
m is the fuzzy partition matrix exponent for controlling the degree of fuzzy overlap, with m > 1. Fuzzy overlap refers to how fuzzy the boundaries between clusters are, that is the number of data points that have significant membership in more than one cluster.
x i is the ith data point.
c j is the center of the jth cluster.
μ i j is the degree of membership of x i in the jth cluster. For a given data point, x i , the sum of the membership values for all clusters is one.
The FCM algorithm performs the following steps:
Randomly initialize the cluster membership values, μ i j .
Calculate the cluster centers:
update μ i j according with the following expression
Calculate the objective function, J m .
Repeat steps 2 to 4 until J m improves by less than a specified minimum threshold or until after a specified maximum number of iterations.
The user can choose a fuzzy partition matrix exponent, indicted as m , being m > 1, for controlling the degree of fuzzy overlap.
In the Matlab toolbox the FCM algorithm is implemented under the function fcm and considered in this paper assuming the default parameter, which means that the fuzzy partition matrix exponent is set to m = 2 and the maximum number of iterations set to 100.
A.2.3 Gaussian mixture models
Gaussian mixture models are probabilistic clustering algorithms which can be useful for fitting multidimensional dataset by a superposition of K Gaussian distributions, as shown in expression ( 30 )
where π k are the so-called mixing coefficients, K the number of clusters the user would like to separate, N ( x | μ k ,Σ k ), normal gaussian distribution with mean μ k and covariance Σ k . Obviously, in a multidimensional space μ k are vectors and Σ k matrices. The mixing coefficients are themselves probabilities and must meet the condition: \({\sum }_{k=1}^{K}{\pi _{k}}=1\) .
One of the main advantages of using Gaussian mixtures is that it is possible to determine the shape of the distribution depending on the data covariance. Various options for the covariance matrix structures, represent the degree of freedom for the user. A Gaussian Mixture clustering model makes use of the iterative Expectation-Maximization (EM) algorithm (Xu and Jordan 1996 ). In the Matlab Toolbox, the training of a GMM model can be performed by using the fitgmdist function by which allows several parameters to be set, including other than the class number K, also the kind of assumptions concerning the covariance matrices (full or diagonal), the maximum number of iterations, the regularization value. In this paper we have set the Covariance Type to full, the Shared Covariance option to True and the Regularization Value to 0.01.
Rights and permissions
Open Access This article is licensed under a Creative Commons Attribution 4.0 International License, which permits use, sharing, adaptation, distribution and reproduction in any medium or format, as long as you give appropriate credit to the original author(s) and the source, provide a link to the Creative Commons licence, and indicate if changes were made. The images or other third party material in this article are included in the article's Creative Commons licence, unless indicated otherwise in a credit line to the material. If material is not included in the article's Creative Commons licence and your intended use is not permitted by statutory regulation or exceeds the permitted use, you will need to obtain permission directly from the copyright holder. To view a copy of this licence, visit http://creativecommons.org/licenses/by/4.0/ .
Reprints and permissions
About this article
Nunnari, G. Clustering activity at Mt Etna based on volcanic tremor: A case study. Earth Sci Inform 14 , 1121–1143 (2021). https://doi.org/10.1007/s12145-021-00606-5
Download citation
Received : 09 November 2020
Accepted : 11 March 2021
Published : 20 April 2021
Issue Date : September 2021
DOI : https://doi.org/10.1007/s12145-021-00606-5
Share this article
Anyone you share the following link with will be able to read this content:
Sorry, a shareable link is not currently available for this article.
Provided by the Springer Nature SharedIt content-sharing initiative
- Unsupervised clustering
- Fuzzy-c means
- Gaussian mixed models
- Volcanic activity
- Find a journal
- Publish with us
- Track your research
- Share full article
Advertisement
Supported by
Mount Etna Puffs Perfect Smoke Rings Into Sicilian Sky
The volcano, one of Europe’s most active, has been spewing volcanic vortex rings at a rapid pace. But that doesn’t mean a big explosion is on the way, one expert says.

By Elisabetta Povoledo
Reported from Rome
For just over a week, Mount Etna, one of Europe’s most active volcanoes, has been spewing circular, mostly white smoke rings into the skies over Sicily.
It’s not the first time Mount Etna has enchanted onlookers with its puffing (it’s been dubbed the Gandalf of volcanoes , after the pipe-puffing wizard in “Lord of the Rings.”) But experts there say this month Etna “has broken all previous records” with the frequency of the rings, according to Boris Behncke, a volcanologist at the National Institute of Geophysics and Volcanology of Catania, who posted about the phenomenon on Facebook .
The rings, known as volcanic vortex rings, appeared earlier this month after a small vent opened on the northwest border of the Southeast crater. The phenomenon occurs when enough pressure builds up so that magma inside the crater propels condensed gases, predominantly water vapor, through the vent.
In this case, the vent is perfectly circular, making for particularly perfect rings. “It is bellissimo,” said Simona Scollo, another volcanologist at the INGV Etna Observatory in Catania, using the Italian word for beautiful. Ms. Scollo copublished a study on the dynamics of volcanic vortex rings last year in the journal Scientific Reports .
But, she said, the activity does not mean that Mount Etna is going to erupt in a particularly spectacular way. “No, no, no,” she said.
During a telephone interview Tuesday, she said that the mechanism for the smoke rings was similar to how dolphins blow bubble rings . “They compress the water in their mouths, and using their tongue they push it out of their mouths and create such a pressure that it forms a ring,” she said.
Depending on weather conditions, the rings hang in the air anywhere from one to 10 minutes, according to the study. “If there is turbulence they fall apart more quickly,” she said.

Interviewed in The New York Times last year, Ms. Scollo said the study hoped to better understand how volcanoes functioned, “not only when they create a disaster for people or when they are very dangerous,” but in calmer times, too.
The new vent in the volcano has been spewing hundreds of rings, but another opening on the volcano has also been spewing rings, albeit more spaced out, since last year.
The phenomenon was first recorded on Etna in 1724, followed by periodic sightings, most recently last year , and quite spectacularly in 2000.
According to the description for its 2013 inscription as a UNESCO World Heritage site , Mount Etna is “the highest Mediterranean island mountain and the most active stratovolcano in the world.”
“No volcano on earth produces as many volcanic vortex rings as Etna, we knew this for some time,” noted Mr. Behncke.
Mount Etna isn’t the only volcano where the phenomenon has been registered. Volcanic vortex rings have been puffed by a number of volcanoes worldwide, from Momotombo in Nicaragua to ash-spewing plane stopper Eyjafjallajökul in Iceland, to Mount Redoubt in Alaska to another active Italian volcano, Stromboli .
Ms. Scollo said that the activity from the new vent was slowing down. And it could end altogether.
“It can stop because the properties of the conduit that allowed for the formation of these volcanic vortex rings can change, maybe with obstructions,” she said. Or the amount of gas within the conduit could decrease, she added.
Elisabetta Povoledo is a reporter based in Rome, covering Italy, the Vatican and the culture of the region. She has been a journalist for 35 years. More about Elisabetta Povoledo
- International
- Schools directory
- Resources Jobs Schools directory News Search
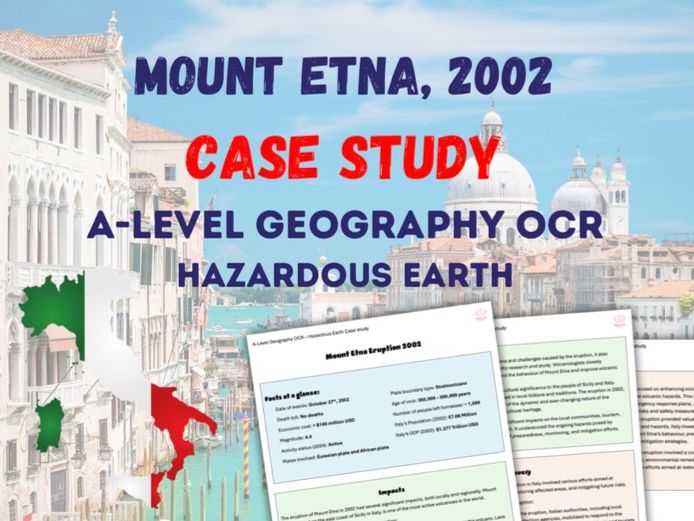
Mount Etna 2002 Case Study A-Level Geography OCR
Subject: Geography
Age range: 16+
Resource type: Lesson (complete)

Last updated
18 April 2024
- Share through email
- Share through twitter
- Share through linkedin
- Share through facebook
- Share through pinterest
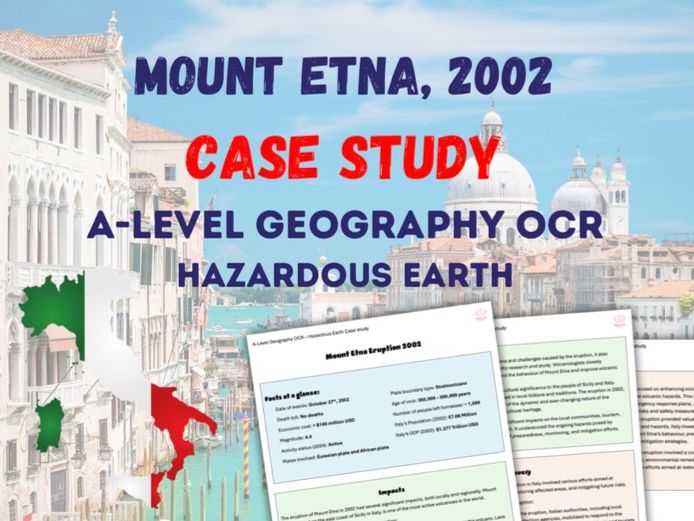
This 3 page case study consists of three sections (facts at a glance, impacts, and recovery), all of which are valuable knowledge to students studying A-Level Geography OCR. This particular Mount Etna case study is for the Hazard Earth topic.
This case study will help students get an understanding of the different ways in which volcanic eruptions can impact an area as well as the effects eruptions have socially, environmentally, and economically. This knowledge will be valuable to students in the summer exam series.
Italy’s Mount Etna volcano, which erupted in 2002 had many widespread impacts on the local economy as well as on the environment also, so acts as a good case study for students to learn about as part of the hazardous earth topic of the OCR specification.
A-Level geography is a great subject for A-Level students to learn. It teaches them a range of topics from different landscape systems, understanding food around the world, migration patterns, and more. All of which will be used by students throughout their careers and beyond.
Want a free resource? Simply leave a positive review on a resource you’ve bought and get one of equal value for FREE. To claim just email us at [email protected] with your name and the resource you would like.
Tes paid licence How can I reuse this?
Get this resource as part of a bundle and save up to 35%
A bundle is a package of resources grouped together to teach a particular topic, or a series of lessons, in one place.
6x Hazardous Earth Case Studies OCR A-Level Geography
Included is 6 case studies made for the hazardous earth topic of the A-Level Geography OCR specification. The six case studies include: - Chile Earthquake 2010 - China Earthquake 2008 - Haiti Earthquake 2010 - Iceland Volcanic eruption 2010 - Japan Earthquake and Tsunami 2011 - Mount Etna Eruption 2002 Each case study covers elements which would be useful for students to know for each case study that can be applied in the exam. Examples include recovery methods, reasons for vulnerability, impacts, causes, and more. Each case study is 2/3 pages and they all include a short header section which includes a list of facts for students to memorize to gain extra marks in the exam. A-Level geography is a great subject for A-Level students to learn. It teaches them a range of topics from different landscape systems, understanding food around the world, migration patterns, and more. All of which will be used by students throughout their careers and beyond. Who are Phillips Resources? At Phillips Resources we use your feedback to create the highest quality and most desired resources so that students can get the grades they desire and teachers are trusting in the resources they use and also are left stress free from not having to create their own resources as often.
Your rating is required to reflect your happiness.
It's good to leave some feedback.
Something went wrong, please try again later.
This resource hasn't been reviewed yet
To ensure quality for our reviews, only customers who have purchased this resource can review it
Report this resource to let us know if it violates our terms and conditions. Our customer service team will review your report and will be in touch.
Not quite what you were looking for? Search by keyword to find the right resource:
How to Hike Mt. Etna, an Active Volcano in Italy
M ount Etna loomed large over everything as we arrived in eastern Sicily that early January. From miles away, I could see the steam plumes rising from the snow-topped behemoth, like blown-out candles on a hastily iced birthday cake. Appropriately, I was on a milestone birthday road trip around Sicily, from Palermo to Catania. The second-to-last stop: Mount Etna, Europe's most active volcano and also its tallest, at 10,900 feet. Scientists believe Etna has been active for more than 500,000 years, presiding over Sicily long before humans arrived.
I'm no stranger to volcano hikes. I've summited several active stratovolcanoes (composite volcanoes) in my lifetime, from New Zealand's Tongariro to Nicaragua's Concepción. Italy is the only country on mainland Europe with active volcanoes, thanks largely to its location near two tectonic plates. Vesuvius, the most notorious among them, engulfed and preserved the ancient cities of Pompeii and Herculaneum after a violent eruption in 79 A.D. Stromboli has had regular minor eruptions for thousands of years. While Mount Etna hasn't had a catastrophic eruption since an infamous incident in 1669, its many eruptions in 2023 filled the news with jaw-dropping photos of lava vaulting into the atmosphere. When I thought of Sicily, I thought of turquoise-colored waters, stunning cathedrals, small villages, ancient ruins and, of course, the wine and food (pasta alla Norma in particular). I hadn't pictured an almost-constantly gurgling volcano that locals ski down in winter.
Now, one might ask, why voluntarily climb something that could blow at any minute? It's a perfectly reasonable question, and it sits at the smoldering heart of volcano tourism, which has exploded (no pun intended) in recent years as eruptions have captured the world-Kīlauea in Hawai'i and Fagradalsfjall in Iceland, for example-even as the volcano blowouts damage or disrupt local economies.
Volcano tourism brings thousands of visitors to Sicily each year. Ten municipalities surround Mount Etna, with several villages of 5,000 residents or fewer who depend heavily on tourism from volcano visitors. Since its 2013 designation as a UNESCO World Heritage Site, Etna has been one of Sicily's top-growing attractions. The Parco dell'Etna has taken steps to keep the crowds in check to maintain the volcano's integrity: specifically, limited parking, steep access fees, and the strong recommendation to take guided visits. The cable car to the start of the trails (8,202 feet) is 50 euros per person. Navigating Etna's unmarked paths and frequently shifting landscape requires the expertise of volcano guides. Visitors are permitted to hike up to about 9,000 feet alone, after which a guide is necessary, for both safety and environmental reasons.
A mountain of many moods
On the morning of our hike, my rental car begrudgingly changed gears on the snake-like winding drive from the town of Nicolosi to Rifugio Sapienza (6,266 feet above sea level) in Parco dell'Etna. As we approached the southern slope of the volcano along a twisting road, we spotted the glistening sea, villas with citrus trees poking over the gates, and small family vineyards making use of the area's fertile volcanic soil.
After acquiring gourmet deli sandwiches for our future lunch break at the meeting point (it's Italy, after all), we took the cable car up to meet our volcano guide, Vincenzo Greco . Vincenzo is a local volcanologist and second-generation Etna guide. He studied geology at the University of Catania and became the youngest person ever to achieve certification as a volcanology guide in Italy.
We started our ascent-excited to work off days of Sicilian cannoli-and trekked through the Valle del Bove. Winter-tanned Vincenzo introduced us to the volcano. He explained which eruption created which new landscape, noting how old this new rock ledge was or how this patch of ground has changed in the past decade. Six weeks before my arrival, in November, a new vent opened on the southeast crater at nearly 9,200 feet. A second vent opened three days later, effusing the new lava . In December, the lava flows reached Valle del Leone and the greater Valle del Bove, depressions on the eastern slope, creating a lava flow field and burping up occasional ash amid the steam and gasses. The lava flows would stop in February, once again reworking the landscape of Etna.
Everything here changes. I recalled the homes we passed on the way in, squarely in the line of Etna's fire if she ever got angry enough. With the windy road and altitude, there could be no escape if something happened. You really have to love the volcano, Vincenzo said, or at least love living by it or perhaps just be a bit stubborn.
Vincenzo and Etna have a passionate Italian relationship. He loves Etna, but their quarrels are, well . . . volcanic. He studies her moods and knows her mannerisms. He flew a drone over an eruption once and her heat melted his camera. It was an expensive mistake, but the few photos he got were spectacular.
About half an hour into the hike, Vincenzo ducked toward a large crevasse between two rocks. "Feel this!" he exclaimed excitedly. "But be careful." I inched forward, waving my hand around in the air aimlessly until a burst of boiling heat hit my skin. It was a small steam vent-Etna saying hello. The power of the invisible heat surprised me. It was a reminder of how easy it is to fall into a false sense of security up here. This isn't your average molehill. Thanks to unmarked paths and a disorienting amount of regular landscape shifts, Vincenzo and his fellow guides have had to rescue tourists when Etna gets moody.
Our hiking group turned a corner and came face-to-face with a lava tunnel formed by a previous eruption. We smushed into the narrowing pathway one at a time. The rock walls towered overhead on both sides, blocking out the sun above. Following the same path as a recent river of molten red lava was a stark reminder of Etna's power.
A crunchy layer of snow covered the black lava rocks throughout the journey, turning the scene into a black-and-white photo anytime the clouds rolled in and covered the bluebird sky. Typically, this time of year, hikers might need snowshoes or cross-country skis in addition to hiking boots. Instead, it was unseasonably warm across Sicily. We sat down for lunch as a crater pumped out billowing white puffs in the distance, our jackets off, the sun blazing. Up next, the final stop: one of Etna's four main craters, at more than 10,000 feet above sea level.
In some parts, the crater rim narrowed to the width of two footsteps side by side, on loose lava pebbles. The winds whipped the smell of sulfur away. I could see the clouds off in the distance, a white blanket over the valley. Above me, the sun beat down from a bright blue sky. The black lava rocks absorbed the light, but the white snow was blinding. For a brief moment, I grew disoriented. I felt like I could walk out onto the blanket of clouds, thousands of feet above sea level. Maybe it was the combination of altitude, sulfur, and adrenaline, but I felt briefly euphoric in this otherworldly atmosphere. In that moment, I realized Etna's pull. I brought myself back to earth by looking down into the crater, the bottom of which was so deep it wasn't visible. Halfway around the crater, a dizzyingly steep drop appeared. With a flourish, Vincenzo appeared to hop off the ledge. Was my guide supposed to jump off? Would I be stuck in Etna's clutches forever?
The descent was arguably more challenging than the climb. Vincenzo took off, hopping down the steep drop of loose lava pebbles. Others followed suit. Unwilling to body surf down thousands of jagged little rocks, I attempted to switchback. My boots sank deep into the ground with each sideways step, as if I had attempted to walk across a ball pit. I felt my shoes fill with lava rocks. Time to throw caution to the wind. Hiking poles in hand, I "skied" down Mount Etna, sending mini avalanches of lava rocks flying with each skip. The lava rocks felt springy at that speed.
Once we arrived at the cable car, the world changed. We had come back down through the clouds, and everything was gray. The seaside views were gone, covered by fog. I tried to catch one last glimpse of Etna from below. She was gone, off in her own world.
Know before you go
Getting there.
Sicily has two major airports: Palermo Airport (Falcone Borsellino Airport) on the west side and Catania Airport (Vincenzo Bellini Airport) on the east. Catania is about an hour from Mount Etna and Palermo about three.
Where to stay
The town of Nicolosi is the gateway to the southern entrance of Mount Etna.
Hotel Alle Pendici is a nine-room B&B dotted with framed photos of Mount Etna throughout the seasons. The rooms are basic but cozy with a ski chalet ambience.
Blanc Maison Etna is a five-room B&B next to a beautifully manicured park. The rooms have views of the pool, the garden, or the volcano.
Tour operators we love
The Society of Guides Vulcanologiche Etna Nord offers a variety of tours with licensed volcano guides, including ours, Vincenzo.
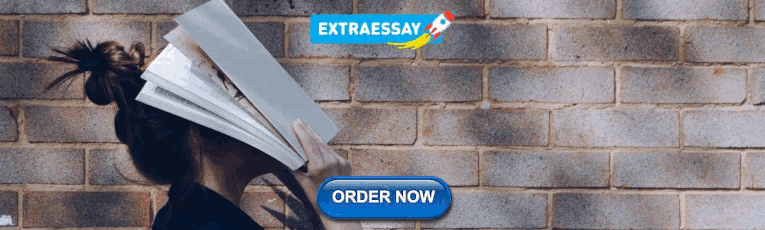
IMAGES
VIDEO
COMMENTS
Case study task. Use the resources and links that can be found on this page to produce a detailed case study of the 2002-2003 eruption of Mount Etna. You should use the 'Five W's" subheadings to give your case study structure. What happened? The Guardian - Sicilian city blanketed in ash [28 October 2002] When did it happen?
A case study of a sparsely populated area - Himalayan Mountains; A case study of a densely populated area - Greater London; What is a settlement? ... Mount Etna, located on the island of Sicily, has been largely dormant for the past two years. The stratovolcano (composite) dominates the skyline of the Italian island, where it sits on the ...
Mount etna is a highly active volcano, constantly erupting for the last seven years. the Volcano is slowly migrating to become more explosive. meaning all the hazard discussed will likely become more hazardous. ... Pyroclastic density currents at Etna volcano, Italy: The 11 February 2014 case study. Journal of Volcanology and Geothermal ...
The main characteristics of Mt. Etna summit and flank activities are summarized in Table 1 reporting the volumes of erupted deposits (lava and proximal tephra) that are published in the literature. The Table highlights that most of lava flows are estimated in terms of volume, whereas the proximal tephra emitted during the paroxysmal episodes are not estimated except for the 2011-2012 and ...
1. Introduction [2] The most destructive flank eruption of Mount Etna volcano occurred in 1669, when nine villages and a large part of the city of Catania were destroyed [Corsaro et al., 1996].More recently, the eruptions of 1923, 1928, 1971, 1981 and 1991-92 have threatened inhabited areas, although only that of 1928 produced significant damages.
Cannata, A. et al. Monitoring seismo-volcanic and infrasonic signals at volcanoes: Mt. Etna case study. Pure Appl. Geophys. 170 , 1751-1771 (2013). Article ADS Google Scholar
Mount Etna is generally viewed as an open conduit volcano in which magma differentiation takes place "en route" to the surface, in a continuous time-space interval comprised between magma arrival at crustal levels and eruption at the permanently active summit craters [1,2,3,4,5,6,7,8].Rift-related, lateral and sub-terminal eruptions are less frequent, and strictly connected to the tectonic ...
Monitoring Seismo-volcanic and Infrasonic Signals at Volcanoes: Mt. Etna Case Study ANDREA CANNATA, 1 GIUSEPPE DI GRAZIA,1 MARCO ALIOTTA,1 CARMELO CASSISI,2 PLACIDO MONTALTO,1 and DOMENICO PATANE` 1 Abstract—Volcanoes generate a broad range of seismo-volca-nic and infrasonic signals, whose features and variations are often closely related to ...
Here, we revise four cases of ash emissions at Mount Etna linked with the most common style of eruptive activity at this volcano: lava fountains (4-5 September 2007), continuous Strombolian activity transitioning to pulsing lava fountaining (24 November 2006), isolated Strombolian explosions (8 April 2010), and continuous to pulsing ash ...
Mount Etna, the largest continental volcano in the world, has a substantial environ? mental impact on the local area. Indeed, the Etna region is one of the most prosperous and densely populated parts of Sicily. This derives in large measure from the ample water supply from the porous lavas and the fertile volcanic soils. Nowhere on the slopes of the volcano, however, is free from the risk of ...
of Mt. Etna showing their spatial distributions of semblance. The concentric lines in b are altitude contour lines from 1.75 to 3.25 km a.s.l. with spacing of 0.25 km.
At Mt. Etna, volcanic tremor, LP events, VLP events, infrasonic events, and infrasonic tremor are recorded. As an example, Figs. 2 and 3 show the seismic and infrasonic signals recorded by EBEL seismic and infrasonic stations, respectively, on 11-12 May 2011. It is worth noting that, during the night between 11 and 12 May, a lava fountain took place at NSEC, accompanied by amplitude ...
Figure 98. Southern vents of Etna as photographed from the SW in the early afternoon of 28 October 2002. From left to right the image shows the summit craters emitting white vapor, the cone of Mt. Frumento Supino, the S2700 explosive vents giving off a dark column, the lower lava vent emitting a faint white plume, new lava flows (dark narrow band), 2001 cone, and Montagnola cone.
Guglielmino F, Bignami C, Bonforte A, Briole P, Obrizzo F, Puglisi G, Stramondo S, Wegmüller U (2011) Analysis of satellite and in situ ground deformation data integrated by the SISTEM approach: the April 3, 2010 earthquake along the Pernicana fault (Mt. Etna—Italy) case study. Earth Planet Sc Lett 312:327-336
Mount Etna is characterized principally by two types of eruptions ... 2010 earthquake along the Pernicana fault (Mt. Etna − Italy) case study. Earth Planet. Sci. Lett., 312 (3-4) (2011), pp. 327-336, 10.1016/j.epsl.2011.10.028 (ISSN 0012-821X) View PDF View article View in Scopus Google Scholar. Gvirtzman and Nur, 1999.
DOI: 10.1109/IGARSS.1997.615330 Corpus ID: 62151644; The Mount Etna case study: a multisensor view @article{Horn1997TheME, title={The Mount Etna case study: a multisensor view}, author={Ralf Horn and K.P. Papathanassiou and Andreas Reigber and Rolf Scheiber and P. Hauskneckt and Peter Strobl and R. Boehl and Megan Scheele and Ralf Reulke and Wolfgang Baerwald and Giuseppe Puglisi and Mauro ...
Mount Etna blows spectacular smoke rings into the sky. Watch on. Etna stretches around 10,900 feet high and covers an area of about 600 square miles. The volcano has been active for the past 2.6 ...
In this work, we analysed the seismic noise recorded at Mt. Etna by 18 stations during the interval 2007-2015 in the frequency band 0.1-0.3 Hz, chosen to avoid contamination from volcanic tremor.Variations in time of medium seismic velocity in the range − 0.8 to 0.8% were found, mostly affecting the stations located on the volcano summit and flanks.
Three DLR institutes, forming the cluster "Sensorik fuer Fernerkundung and Navigation", and two italian CNR institutes, I.R.E.C.E. and I.I.V., agreed on carrying out a joint airborne multisensor campaign in the Mt. Etna area in summer 1996. The site is of high geological interest. The deployment of an experimental SAR (E-SAR), a multispectral optical scanner (DAIS) and a wide angle stereo ...
The work is basically a case study carried out on a dataset recorded in the area of the Mt Etna volcano. However, as volcanic tremor is a geophysical signal widely available, considered methods and strategies can be easily applied to similar volcanic areas. ... Nunnari, G. Clustering activity at Mt Etna based on volcanic tremor: A case study ...
Scollo copublished a study on the dynamics of volcanic vortex rings last year in the journal Scientific Reports. But, she said, the activity does not mean that Mount Etna is going to erupt in a ...
Italy's Mount Etna volcano, which erupted in 2002 had many widespread impacts on the local economy as well as on the environment also, so acts as a good case study for students to learn about as part of the hazardous earth topic of the OCR specification. A-Level geography is a great subject for A-Level students to learn.
The island of Sicily, Italy. When did mount Etna erupt. 30th October 2002 & lasted 7 days. Magnitude of mount Etna. 3 - 4 on the Richter scale as minor earthquakes also occurred during the eruption. Impacts of mount Etna. Eruption completely destroyed the tourist area of piano provenzana & also damaged many villages on the east of Etna ...
Case studies all in one ... Mount St Helens 1980 volcano - Population of 2.5million who live near the area ... elk - Causing a magnitude of 4.2 earthquake shortly after - Killing 57 people Mont Etna 2002 volcano - No deaths - The Italian Government declared a 'state of emergency' in the eastern half of Sicily, after ...
Navigating Etna's unmarked paths and frequently shifting landscape requires the expertise of volcano guides. Visitors are permitted to hike up to about 9,000 feet alone, after which a guide is ...