Diagnosis and Management of Dementia: Review
Affiliations.
- 1 Rush Alzheimer's Disease Center, Rush University Medical Center, Chicago, Illinois.
- 2 Department of Neurological Sciences, Rush University Medical Center, Chicago, Illinois.
- 3 Department of Family Medicine, Rush University Medical Center, Chicago, Illinois.
- PMID: 31638686
- PMCID: PMC7462122
- DOI: 10.1001/jama.2019.4782
Importance: Worldwide, 47 million people live with dementia and, by 2050, the number is expected to increase to 131 million.
Observations: Dementia is an acquired loss of cognition in multiple cognitive domains sufficiently severe to affect social or occupational function. In the United States, Alzheimer disease, one cause of dementia, affects 5.8 million people. Dementia is commonly associated with more than 1 neuropathology, usually Alzheimer disease with cerebrovascular pathology. Diagnosing dementia requires a history evaluating for cognitive decline and impairment in daily activities, with corroboration from a close friend or family member, in addition to a thorough mental status examination by a clinician to delineate impairments in memory, language, attention, visuospatial cognition such as spatial orientation, executive function, and mood. Brief cognitive impairment screening questionnaires can assist in initiating and organizing the cognitive assessment. However, if the assessment is inconclusive (eg, symptoms present, but normal examination findings), neuropsychological testing can help determine whether dementia is present. Physical examination may help identify the etiology of dementia. For example, focal neurologic abnormalities suggest stroke. Brain neuroimaging may demonstrate structural changes including, but not limited to, focal atrophy, infarcts, and tumor, that may not be identified on physical examination. Additional evaluation with cerebrospinal fluid assays or genetic testing may be considered in atypical dementia cases, such as age of onset younger than 65 years, rapid symptom onset, and/or impairment in multiple cognitive domains but not episodic memory. For treatment, patients may benefit from nonpharmacologic approaches, including cognitively engaging activities such as reading, physical exercise such as walking, and socialization such as family gatherings. Pharmacologic approaches can provide modest symptomatic relief. For Alzheimer disease, this includes an acetylcholinesterase inhibitor such as donepezil for mild to severe dementia, and memantine (used alone or as an add-on therapy) for moderate to severe dementia. Rivastigmine can be used to treat symptomatic Parkinson disease dementia.
Conclusions and relevance: Alzheimer disease currently affects 5.8 million persons in the United States and is a common cause of dementia, which is usually accompanied by other neuropathology, often cerebrovascular disease such as brain infarcts. Causes of dementia can be diagnosed by medical history, cognitive and physical examination, laboratory testing, and brain imaging. Management should include both nonpharmacologic and pharmacologic approaches, although efficacy of available treatments remains limited.
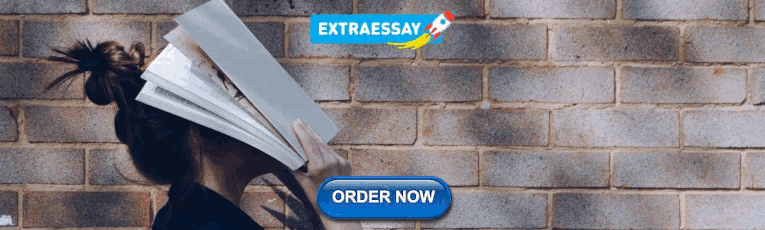
Publication types
- Research Support, N.I.H., Extramural
- Research Support, Non-U.S. Gov't
- Alzheimer Disease / diagnosis
- Alzheimer Disease / therapy
- Cholinesterase Inhibitors / adverse effects
- Cholinesterase Inhibitors / therapeutic use
- Dementia / diagnosis*
- Dementia / therapy*
- Excitatory Amino Acid Antagonists / adverse effects
- Excitatory Amino Acid Antagonists / therapeutic use
- Memantine / adverse effects
- Memantine / therapeutic use
- Neuroimaging
- Neuropsychological Tests
- Cholinesterase Inhibitors
- Excitatory Amino Acid Antagonists
Grants and funding
- P30 AG010161/AG/NIA NIH HHS/United States
- R01 AG040039/AG/NIA NIH HHS/United States
- R01 NS084965/NS/NINDS NIH HHS/United States
- RF1 AG059621/AG/NIA NIH HHS/United States
Advertisement
Machine Learning for Dementia Prediction: A Systematic Review and Future Research Directions
- Original Paper
- Open access
- Published: 01 February 2023
- Volume 47 , article number 17 , ( 2023 )
Cite this article
You have full access to this open access article
- Ashir Javeed 1 , 2 na1 ,
- Ana Luiza Dallora 2 na1 ,
- Johan Sanmartin Berglund 2 ,
- Arif Ali 3 ,
- Liaqata Ali 4 &
- Peter Anderberg 2 , 5
14k Accesses
24 Citations
47 Altmetric
Explore all metrics
Nowadays, Artificial Intelligence (AI) and machine learning (ML) have successfully provided automated solutions to numerous real-world problems. Healthcare is one of the most important research areas for ML researchers, with the aim of developing automated disease prediction systems. One of the disease detection problems that AI and ML researchers have focused on is dementia detection using ML methods. Numerous automated diagnostic systems based on ML techniques for early prediction of dementia have been proposed in the literature. Few systematic literature reviews (SLR) have been conducted for dementia prediction based on ML techniques in the past. However, these SLR focused on a single type of data modality for the detection of dementia. Hence, the purpose of this study is to conduct a comprehensive evaluation of ML-based automated diagnostic systems considering different types of data modalities such as images, clinical-features, and voice data. We collected the research articles from 2011 to 2022 using the keywords dementia, machine learning, feature selection, data modalities, and automated diagnostic systems. The selected articles were critically analyzed and discussed. It was observed that image data driven ML models yields promising results in terms of dementia prediction compared to other data modalities, i.e., clinical feature-based data and voice data. Furthermore, this SLR highlighted the limitations of the previously proposed automated methods for dementia and presented future directions to overcome these limitations.
Similar content being viewed by others
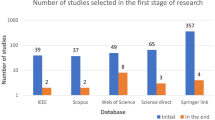
Early detection of dementia using artificial intelligence and multimodal features with a focus on neuroimaging: A systematic literature review
Ovidijus Grigas, Rytis Maskeliunas & Robertas Damaševičius
Data Mining and Machine Learning Methods for Dementia Research
A Comparative Analysis of Classification Algorithms for Dementia Prediction
Avoid common mistakes on your manuscript.
Introduction
Over a period of time, the advancements made in the field of medical science helped to increase the lifespan in the modern world [ 1 ]. This increased life expectancy raised the prevalence of neurocognitive disorders, affecting a significant part of the older population as well as global economies. In 2010, it was estimated that $604 billion have been spent on dementia patients in the USA alone[ 2 ]. The number of dementia patients is rapidly increasing worldwide, and statistical projections suggest that 135 million people might be affected by dementia by 2050 [ 3 ]. There are several risk factors that contribute to the development of dementia, including aging, head injury, and lifestyle. While age is the most prominent risk factor for dementia; figures suggest that a person at the age of 65 years old has 1–2% risk of developing dementia disease. By the age of 85 years old, this risk can reach to 30% [ 4 ].
Dementia is a mental disorder that is characterized by a progressive deterioration of cognitive functions that can affect daily life activities such as memory, problem solving, visual perception, and the ability to focus on a particular task. Usually, older adults are most vulnerable to dementia, and people take it as an inevitable consequence of aging, which is perhaps the wrong perception. Dementia is not a part of the normal ageing process; however, it should be considered a serious form of cognitive decline that affects your daily life. Actually, the primary cause for the development of dementia is the several diseases and injuries that affect the human brain [ 5 ]. Dementia is ranked on the seventh place in the leading causes of deaths in the world [ 6 ]. Furthermore, it is the major cause of disability and dependency among older people globally [ 6 ]. A change in the person’s ordinary mental functioning and obvious signs of high cognitive deterioration are required for a diagnosis of dementia [ 7 ]. Figure 1 presents the progression of dementia with age.
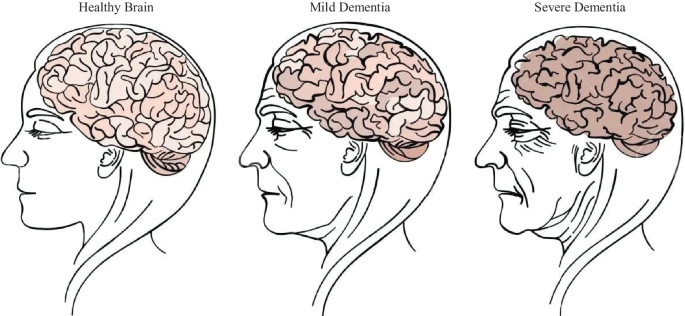
Progression of dementia disease with ageing
Types of dementia
Dementia is not a single disease, but, it is used as a generic term for several different cognitive disorders. Figure 2 provides the overview of different types of dementia along with the percentage of particular dementia type occurrence in the patients [ 8 ]. To have a better idea about dementia, we have studied common types of dementia for better problem awareness.
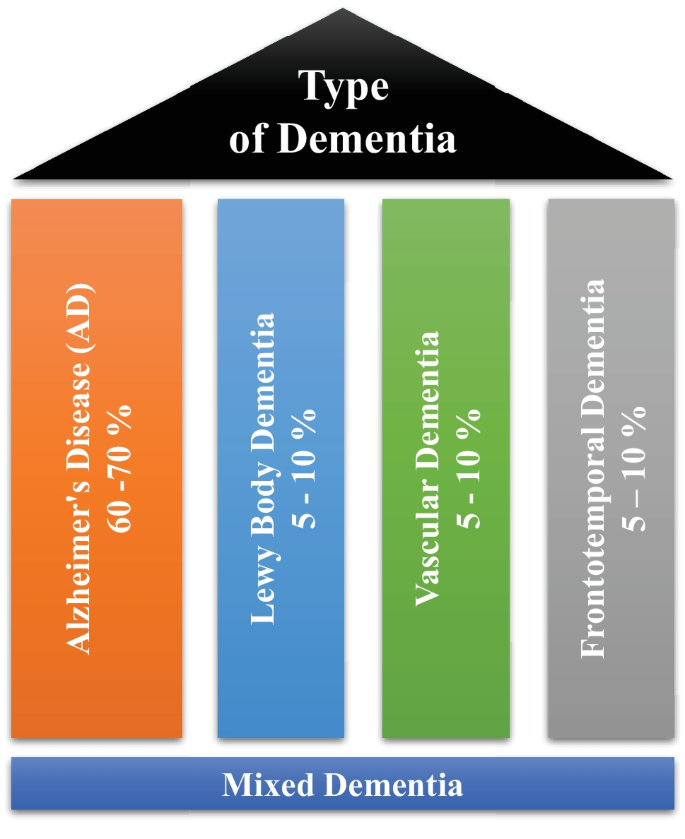
Types of dementia disease
Alzheimer’s disease
Alzheimer’s disease (AD) is thought to develop when abnormal amounts of amyloid beta (A \(\beta\) ) build up in the brain, either extracellularly as amyloid plaques, tau proteins or intracellularly as neurofibrillary tangles, affecting neuronal function, connectivity and leading to progressive brain function loss [ 9 ]. This diminished ability to eliminate proteins with ageing is regulated by brain cholesterol [ 10 ] and is linked to other neurodegenerative illnesses [ 11 ]. Except for 1–2% of cases where deterministic genetic anomalies have been discovered, the aetiology of the majority of Alzheimer’s patients remains unexplained [ 12 ]. The amyloid beta (A \(\beta\) ) hypothesis and the cholinergic hypothesis are two competing theories presented to explain the underlying cause of AD [ 13 ].
Vascular dementia
Vascular dementia (VaD) is a subtype of dementia caused by problems with the brain’s blood flow, generally in the form of a series of minor strokes, which results in a slow decline of cognitive capacity [ 14 ]. The VaD refers to a disorder characterized by a complicated mix of cerebrovascular illnesses that result in structural changes in the brain, as a result of strokes and lesions, which lead to cognitive impairment. A chronological relationship between stroke and cognitive impairments is necessary to make the diagnosis [ 15 ]. Ischemic or hemorrhagic infarctions in several brain areas, such as the anterior cerebral artery region, the parietal lobes, or the cingulate gyrus, are associated with VaD. In rare cases, infarcts in the hippocampus or thalamus might cause dementia [ 16 ]. A stroke increases the risk of dementia by 70%, whereas a recent stroke increases the risk by almost 120% [ 17 ]. Brain vascular lesions can also be caused by diffuse cerebrovascular disease, such as small vessel disease [ 18 ]. Risk factors for VaD include age, hypertension, smoking, hypercholesterolemia, diabetes mellitus, cardiovascular disease, and cerebrovascular sickness; geographic origin, genetic proclivity, and past strokes are also risk factors [ 19 ]. Cerebral amyloid angiopathy, which develops when beta amyloid accumulates in the brain, can occasionally lead to vascular dementia.
Lewy body dementia
Lewy body dementia (LBD) is a subtype of dementia characterized by abnormal deposits of the protein alpha-synuclein in the brain. These deposits, known as Lewy bodies, affect brain chemistry, causing problems with thinking, movement, behavior, and mood. Lewy body dementia is one of the most common causes of dementia [ 20 ]. Progressive loss of mental functions, visual hallucinations, as well as changes in alertness and concentration are prevalent in persons with LBD. Other adverse effects include tight muscles, delayed movement, difficulty walking, and tremors, all of which are also signs and symptoms of Parkinson’s disease [ 21 ]. LBD might be difficult to identify. Early LBD symptoms are commonly confused with those of other brain diseases or mental problems. Lewy body dementia can occur alone or in conjunction with other brain disorders [ 22 ]. It is a progressive disorder, which means that symptoms emerge gradually and worsen with time. A timespan of five to eight years is averaged, although it can last anywhere from two to twenty years for certain people [ 23 ]. The rate at which symptoms arise varies greatly from person to person, depending on overall health, age, and the severity of symptoms.
Frontotemporal dementia
Frontotemporal Dementia (FTD) is a subtype of dementia characterized by nerve cell loss in the frontal and temporal lobes of the brain [ 24 ]. As a result, the lobes contract. FTD can have an impact on behavior, attitude, language, and movement. This is one of the most common dementias in people under the age of 65. FTD most commonly affects persons between the ages of 40 and 65; however, it may also afflict young adults and older individuals [ 25 ]. The lobes decrease, and behavior, attitude, language, and mobility can all be affected by FTD. FTD affects both men and women equally. Dissociation from family, extreme oniomania, obscene speech, screaming, and the inability to regulate emotions, behavior, personality, and temperament are examples of social display patterns caused by FTD [ 26 ]. The symptoms of FTD appeared several years prior to visiting a neurologist [ 27 ].
Mixed Dementia (MD)
Mixed dementia occurs, when more than one kind of dementia coexists in a patient, and it is estimated to happen in around 10% of all dementia cases [ 6 ]. AD and VaD dementia are the two subtypes that are most common in MD [ 28 ]. This case is usually associated with factors such as old age, high blood pressure, and brain blood vessel damage [ 29 ]. Because one dementia subtype often predominates, MD is difficult to identify. As a result, the individuals affected by MD are rarely treated and miss out on potentially life-changing medicines. MD can cause symptoms to begin earlier than the actual diagnosis of the disease and spread swiftly to affect the most areas of the brain [ 30 ].
Recently, numerous automated methods have been developed based on machine learning for early the prediction of different diseases [ 31 , 32 , 33 , 34 , 35 , 36 , 37 , 38 , 39 , 40 , 41 , 42 , 43 , 44 , 45 , 46 , 47 , 48 ]. This systematic literature review (SLR) presented hereby, investigates machine learning-based automated diagnostic systems that are designed and developed by scientists to predict dementia and its subtypes, such as AD, VaD, LBD, FTD and MD. We used the Preferred Reporting Items for Systematic Reviews and Meta-Analysis (PRISMA) criteria to conduct this SLR [ 49 , 50 ]. A comprehensive search was conducted to retrieve the research articles that contain ML approaches to predict the development of dementia and its subtypes using three different types of data modalities (images, clinical-variables, voice).
Aim of the study
SLRs are done to synthesize current evidence, to identify gaps in the literature, and to provide the groundwork for future studies [ 51 ]. Previous, SLRs studies have been done on automated diagnostic systems for dementia prediction based on ML approaches, which focused on a single sort of data modality. These SLR investigations did not emphasize the limits of previously published automated approaches for dementia prediction. The SLR presented herein assesses the previously proposed automated diagnostic systems based on deep learning (DL) and ML algorithms for the prediction of dementia and its common subtypes (e.g. AD, VaD, FTD, MD). The aim of this SLR is to analyse and evaluate the performance of automated diagnostic systems for dementia prediction using different data modalities. The main question is decomposed in the following sub-research questions:
What types of ML and DL techniques have been used by researchers to diagnose dementia?
Examine the methods of feature extraction or selection used by the researchers.
Analyze the different performance evaluation measures that are adopted by the researcher to validate the effectiveness of the proposed diagnostic system for demetnia.
Analyze the performance of ML models on various data types.
Identification of weaknesses in previously proposed ML models for dementia prediction.
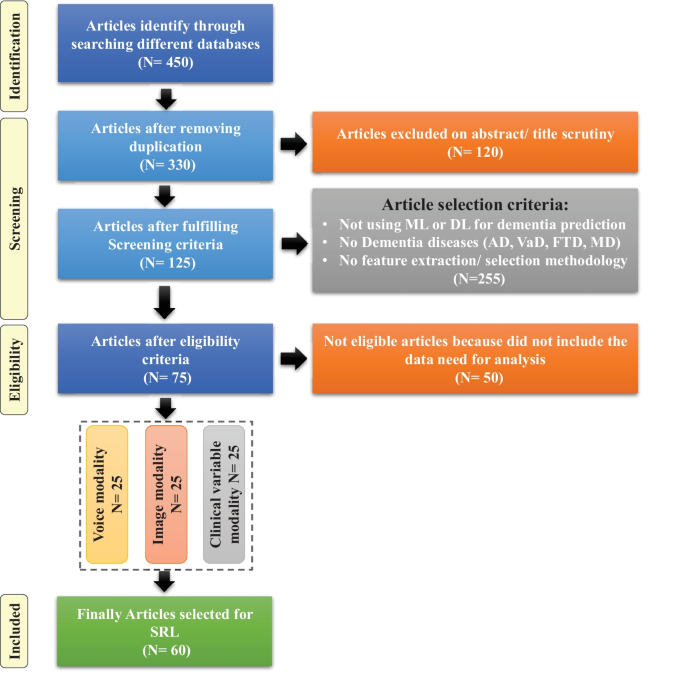
Flow diagram of PRISMA (Preferred Reporting Items for Systematic Reviews and Meta-analyses)
Article selection
For this SLR study, the research articles were selected based on keywords such as ML, DL, dementia and its subtypes (AD, VaD, FTD, and MD). For the collection of research articles, we conducted an electronic search from different online databases such as ScienceDirect, PubMed, IEEE Xplore Digital Library, Springer, Hindawi, and PLOs, which helped to gather 450 research studies on the specific topic. After reviewing the title and abstract in each study, 120 publications were found to be ineligible for processing, while 330 articles were selected for further processing. Following the deduplication of data, 125 full-text publications were retrieved for further processing after the screening phase of the article selection, with 205 of them being eliminated due to not satisfying the article selection criteria of the screening phase. Finally, 50 research articles were eliminated due to not fulfilling the eligibility criteria for article selection. The final set of selected papers consisted of 75 research papers, among these final selected articles, each of the data modalities (image, clinical-variables, voice) contained 25 papers. After rerunning the database searches in May 2022, no further suitable research article was found for the selection. Figure 3 presents the workflow for article selection, which includes the four PRISMA guidelines-recommended steps such as identification, screening, eligibility, and inclusion [ 49 , 50 ]. In recent years, ML scientists have shown a strong interest in designing and developing ML-based automated diagnostic systems for dementia prediction. Therefore, the number of research articles in this research area has been increased and it can be depicted from Fig. 4 where research articles are published years wise with regarding data modality. The publications utilized in this study were selected based on the following criteria:
Studies that present automated diagnostic systems for dementia and its common subtypes (AD,VaD, FTD, MD).
Studies published between 2011 and 2022.
Studies employing ML approaches for dementia diagnosis.
Studies which have utilized several data modalities.
Studies published in the English language.
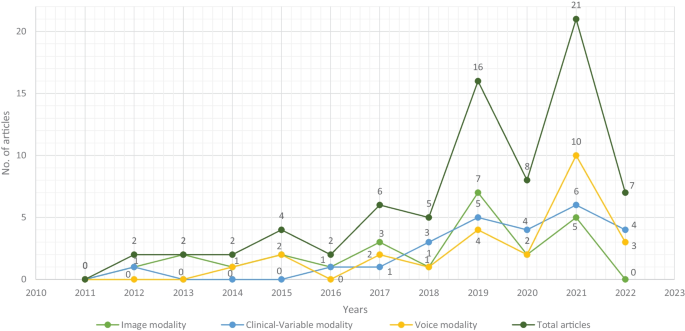
Selected research articles which are published from 2011 to 2022 regarding data modality
Machine learning for dementia
Over the years, the increasing use and availability of medical equipment has resulted in a massive collection of electronic health records (EHR) that might be utilized to identify dementia using developing technologies such as ML and DL [ 52 ]. These EHRs are one of the most widely available and used clinical datasets. They are a crucial component of contemporary healthcare delivery, providing rapid access to accurate, up-to-date, comprehensive patient information while also assisting with precise diagnosis and coordinated, efficient care [ 53 ]. Laboratory tests, vital signs, drugs, and other therapies, as well as comorbidities, can be used to identify the people at risk of dementia using the EHRs’ data [ 54 ]. In some situations, patients may also be subjected to costly and invasive treatments such as neuroimaging scans i.e., magnetic resonance imaging (MRI) and position emission tomography (PET)) and cerebrospinal fluid (CSF) collection for biomarker testing [ 55 , 56 , 57 ]. These tests’ findings may also be found in the EHR. According to researchers, such longitudinal clinical EHR data can be used to track the advancement of AD dementia over time [ 58 ]. Recently, several automated diagnostic systems for different diseases, such as Parkinson’s disease [ 59 ], hepatitis [ 47 ], carcinoma [ 41 ], and heart failure [ 60 , 61 , 62 ] prediction have been designed by employing ML and DL techniques. Inspired by this fact, the unmet demand for dementia knowledge, along with the availability of relevant huge datasets, has motivated scientists to investigate the utility of artificial intelligence (AI), which is gaining a prominent role in the area of healthcare innovation [ 63 ]. ML, a subset of AI, can model the relationship between input quantities and clinical outcomes, identify hidden patterns in enormous volumes of data, and draw conclusions or make decisions that help with more accurate clinical decision-making [ 51 ]. However, computational hypotheses generated by ML models must still be confirmed by subject matter experts in order to achieve enough precision for clinical decision-making [ 64 ].
In this SLR, we have included studies that have used ML predictive models (supervised and unsupervised) for dementia prediction and excluded studies that have used statistical methods for cohort summarization and hypothesis testing (e.g., odds ratio, chi-square distribution, Kruskal-Wallis test, and Kappa-Cohen test). Furthermore, we have referenced the data modality-based study [ 65 ] for this literature review, where we have categorized the three data modality types such as image, clinical-variable and voice. Thus, we have studied each modality-based automated diagnostic system for dementia prediction that has been proposed in the past using ML and DL.
This section explains the datasets that were used in the selected research papers for experiments and performance evaluation of the proposed automated diagnostic systems designed by the researchers using ML algorithms for dementia and its subtypes. A total of 61 datasets were studied from the selected research articles. These datasets are compiled from a wide range of organizations and hospitals throughout the world. Only a few datasets are openly available to the public, while others are compiled by researchers from various hospitals and healthcare institutes. We have only included datasets that have been used to diagnose AD, VaD, FTD, MD, and LBD using ML and DL techniques. On the basis of data modality, we have categorised the dataset into three types: images, clinical_variables and voice datasets. The datasets differ in terms of the number of variables (features) and samples. As a result, we examined each modality of the dataset one by one.
Image modality based datasets
There are several image datasets based on brain imaging, such as magnetic resonance imaging (MRI), collected by the researchers for the diagnosis of dementia. From the Table 1 , it can be depicted that Open Access Series of Imaging Studies (OASIS) and Alzheimer’s Disease Neuroimaging Initiative (ADNI) datasets are mostly used by the researchers for the experimental purpose. OASIS aims to make neuroimaging datasets available to the scientific community for free. By gathering and openly disseminating this multimodal dataset produced by the Knight ADRC and its related researchers, they had used different samples and variables of the datasets in their research work. ADNI researchers acquire, validate, and use data such as MRI, PET imaging, genetics, cognitive assessments, CSF, and blood biomarkers as disease predictors. The ADNI website contains research information and data from the North American ADNI project, which includes Alzheimer’s disease patients, people with mild cognitive impairment, and older controls. Table 1 provides us with the following information: dataset_id, dataset name, number of samples in the particular dataset, variables in the dataset, and finally, the type of dementia.
Clinical-variables modality based datasets
Throughout the course of time, the growing usage and availability of medical devices have resulted in an overwhelming collection of clinical EHR data. Furthermore, the patient’s medical history consists of medical tests and clinical records that can be used for the prediction of diseases. Thus, the importance of clinical data emerges as a vital tool for proactive management of disease. The dataset based on clinical variables for dementia consists of medical tests that are used by doctors to check the dementia status in patients, such as the Mini Mental Status Exam (MMSE), the Montreal Cognitive Assessment (MoCA), the Telephone Interview for Cognitive Status (TICS), and the Brief Interview for Mental Status (BIMS). Clinical-variables based datasets consist of information about these medical tests along with patient personal information, i.e., age, sex, and marital status. Hereby, Table 2 provides the information regarding clinical-variables modality-based datasets that are used by the researchers for the design and development of automated diagnostic systems for dementia patients based on ML. Table 2 presents the dataset_id, dataset name, number of samples in the particular dataset, variables in the dataset, and finally the type of dementia.
Voice modality based datasets
Speech analysis is a useful technique for clinical linguists in detecting various types of neurodegenerative disorders affecting the language processing areas. Individuals suffering from Parkinson’s disease (PD, deterioration of voice quality, unstable pitch), Alzheimer’s disease (AD, monotonous pitch), and the non-fluent form of Primary Progressive Aphasia (PPA-NF, hesitant, non-fluent speech) may experience difficulties with prosody, fluency, and voice quality. Besides imaging and clinical-variables data, the researchers employed voice recording data to identify dementia using ML and DL algorithms. The data collection process for voice data varies from dataset to dataset, for example, in a few datasets, patients were requested to answer a prepared set of questions (interview) in a specific time interval. In a few datasets, selected neuropsychological tests were carried out, the description of each neuropsychological test was played and was followed by an answering window. Table 3 presents the dataset_id, dataset name, number of samples in the particular dataset, variables in the dataset, and finally the subtype of dementia.
Data sharing challenges
In this digital era, public health decision-making has grown progressively complicated, and the utilization of data has become critical [ 66 ]. Data are employed at the local level to observe public health and target interventions; at the national scale for resource allocation, prioritization, and planning; and at the global scale for disease burden estimates, progress in health and development measurement, and the containment of evolving global health threats [ 67 , 68 ]. Van Panhuis et al. have adequately described the challenges to exchanging health data [ 69 ]. Based on our initial analysis, we built on this taxonomy to identify the hurdles related to data sharing in global public health, and we have highlighted how they may apply to each typology as given below.
Lack of complete data, lost data, restrictive as well as conflicting data formats, a lack of metadata and standards, a lack of interoperability of datasets (e.g., structure or “language”), and a lack of appropriate analytic solutions are examples of technical barriers encountered by health information management systems.
Individuals and organizations face motivational challenges when it comes to sharing data. These impediments include a lack of incentives, opportunity costs, apprehension about criticism, and disagreements over data usage and access.
The potential and present costs of sharing data are both economic hurdles.
Political obstacles are those that are built into the norms of local health governance and often emerge as regulations and guidelines. They can also entail trust and ownership difficulties.
Legal issues that arise as a result of data collection, analysis, and usage include questions regarding who owns or controls the data, transparency, informed permission, security, privacy, copyright, human rights, damage, and stigma.
Ethical constraints include a lack of perceived reciprocity (i.e., the other side will not disclose data) and proportionality (i.e., deciding not to share data based on an assessment of the risks and benefits). An overall concern is that frameworks, rules, and regulations have not kept up with technological changes that are transforming how data is collected, analyzed, shared, and used.
ML based diagnostic models for dementia: Image modality
In recent years, researchers have designed many ML and DL algorithms for the detection of dementia and its subtypes using MRI images of the brain. For example, Dashtipour et al. [ 70 ] proposed a ML based method for the prediction of Alzheimer’s disease. In their proposed model, they used DL techniques to extract the features from brain images, and for classification purposes, they deployed SVM and bidirectional long short-term memory (BiLSTM). Through their proposed model, they had reported the classification accuracy of 91.28%. Moreover, for early detection of the AD, a DL based approach was proposed by Helaly et al. In their proposed work, they employed convolutional neural networks (CNN). The Alzheimer’s disease spectrum is divided into four phases. Furthermore, different binary medical image classifications were used for each two-pair class of Alzheimer’s disease stages. Two approaches were used to categorize medical images and diagnose Alzheimer’s disease. The first technique employs basic CNN architectures based on 2D and 3D convolution to cope with 2D and 3D structural brain images from the ADNI dataset. They had achieved highly promising accuracies for 2D and 3D multi-class AD stage classification of 93.61% and 95.17%, respectively. The VGG19 pre-trained model had been fine-tuned and obtained an accuracy of 97% for multi-class AD stage classification [ 71 ]. Vandenberghe et al. had proposed a method for binary classification of 18F-flutemetramol PET using ML techniques for AD and mild cognitive impairment (MCI). They had tested whether support vector machines (SVM), a supervised ML technique, can duplicate the assignments made by blindfolded visual readers, as well as which image components had the highest diagnostic value according to SVM and how 18F-fluoromethylamol-based SVM classification compares to structural MRI-based SVM classification in the same cases. Their F-flutemetamol based classifier was able to replicate the assignments obtained by visual read with 100% accuracy [ 72 ]. Odusami et al. proposed a novel method for the detection of early-stage dementia from functional brain changes in MRI using a fine-tuned ResNet-18 network. Their research work presents a DL based technique for predicting MCI, early MCI, late MCI, and Alzheimer’s disease (AD). The ADNI fMRI dataset was used for analysis and consisted of 138 participants. On EMCI vs. AD, LMCI vs. AD, and MCI vs. AD, the fine-tuned ResNet18 network obtained classification accuracy of 99.99%, 99.95%, and 99.95%, respectively [ 73 ]. Zheng et al. had presented a ML based framework for differential diagnosis between VaD and AD using structural MRI features. The least absolute shrinkage and selection operator (LASSO) was then used to build a feature set that was fed into SVM for classification. To ensure unbiased evaluation of model performance, a comparative analysis of classification models was conducted using different ML algorithms to discover which one had better performance in the differential diagnosis between VaD and AD. The diagnostic performance of the classification models was evaluated using quantitative parameters derived from the receiver operating characteristic curve (ROC). The experimental finding had shown that the SVM with RBF performed well for the differential diagnosis of VaD and AD, with sensitivity (SEN), specificity (SPE), and accuracy (ACC) values of 82.65%, 87.17%, and 84.35%, respectively (AUC = 86.10–95%, CI = 0.820–0.902) [ 74 ]. Basheer et al. [ 75 ] had presented an innovative technique by making improvements in capsule network design for the best prediction outcomes. The study used the OASIS dataset with dimensions (373 X 15) to categorize the labels as demented or non-demented. To make the model swifter and more accurate, several optimization functions were performed on the variables, as well as the feature selection procedure. The claims were confirmed by demonstrating the correlation accuracy at various iterations and layers with an allowable accuracy of 92.39%. L. K. Leong and A. A. Abdullah had proposed a method for the prediction of AD based on ML techniques with the Boruta algorithm as a feature selection method. According to the Boruta algorithm, Random Forest Grid Search Cross Validation (RF GSCV) outperformed other 12 ML models, including conventional and fine-tuned models, with 94.39% accuracy, 88.24% sensitivity, 100.00% specificity, and 94.44% AUC even for the small OASIS-2 longitudinal MRI dataset [ 76 ]. Battineni et al. had presented a SVM based ML model for the prediction of dementia. Their proposed model had achieved an accuracy and precision of 68.75% and 64.18% using the OASIS-2 dataset [ 77 ]. Mathotaarachchi et al. had analyzed the amyloid imaging using ML approaches for the detection of dementia. To overcome the inherent unfavorable and imbalance proportions between persons with stable and progressing moderate cognitive impairment in a short observation period. The innovative method had achieved 84.00% accuracy and an AUC of 91.00% for the ROC [ 78 ]. Aruna and Chitra had presented a ML approach for the identification of dementia from MRI images, where they had deployed Independent Component Analysis (ICA) to extract the features from the images, and for classification purposes, SVM with different kernels is used. Through their proposed method, they had obtained an accuracy of 90.24% [ 79 ] (Fig. 5 ).
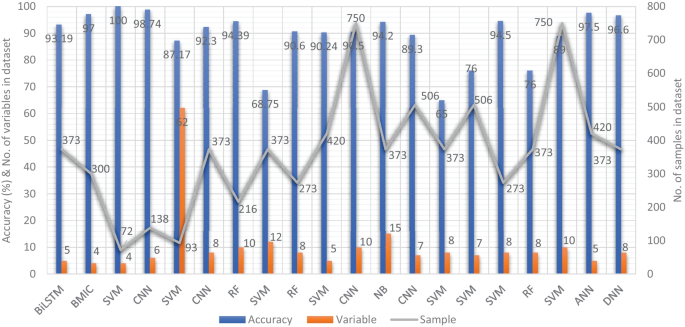
Accuracy comparison of different ML models based on image modality
Supervised ML techniques and CNNs were examined by Herzog and Magoulas. They had achieved the accuracy of 92.5% and 75.0% for NC vs EMCI, 93.0% and 90.5% for NC vs. AD, respectively [ 80 ]. Battineni et al. had comprehensive applied ML model on MRI to predict Alzheimer’s disease (AD) in older subjects, and they had proposed two ML models for AD detection. In the first trial, manual feature selection was utilized for model training, and ANN produced the highest AUC of 81.20% by ROC. The NB had earned the greatest AUC of 94.20% by ROC in the second trial, which included wrapping approaches for the automated feature selection procedure [ 81 ]. Ma et al. had conducted a study where they compared feature-engineered and non-feature-engineered ML methods for blinded clinical evaluation for dementia of Alzheimer’s type classification using FDG-PET. The highest accuracy of 84.20% was obtained through CNN’s [ 82 ]. Bidani et al. had presented a novel approach in the field of DL that combines both the deep convolutional neural network (DCNN) model and the transfer learning model to detect and classify dementia. When the features were retrieved, the dementia detection and classification strategy from brain MRI images using the DCNN model provided an improved classification accuracy of 81.94%. The transfer learning model, on the other hand, had achieved an accuracy of 68.13% [ 83 ].
Moscoso et al. had designed a predictive model for the prediction of Alzheimer’s disease using MRI images. Their proposed model had obtained the highest accuracy of 84.00% [ 84 ]. Khan and Zubair had presented an improved multi-modal based ML approach for the prognosis of AD. Their proposed model had a five-stage ML pipeline, where each stage was further categorized into different sub-levels. Their proposed model had reported the highest accuracy of 86.84% using RF [ 85 ]. Mohammed et al. had evaluated the two CNN models (AlexNet and ResNet-50) and hybrid DL/ML approaches (AlexNet+SVM and ResNet-50+SVM) for AD diagnosis using the OASIS dataset. They had found that RF algorithm had attained an overall accuracy of 94%, as well as precision, recall, and F1 scores of 93%, 98%, and 96%, respectively [ 86 ]. Salvatore et al. had developed a ML method for early AD diagnosis using magnetic resonance imaging indicators. In their proposed ML model, they used PCA for extracting features from the images and SVM for the classification of dementia. They had achieved a classification accuracy of 76% using a 20-fold cross validation scheme [ 87 ]. Katako et al. had identified the AD related FDGPET pattern that is also found in LBD and Parkinson’s disease dementia using ML approaches. They studied different ML algorithms, but SVM with an iterative single data algorithm produced the best performance, i.e., sensitivity 84.00%, specificity 95.00% through 10-fold cross-validation [ 88 ]. Gray et al. had presented a system in which RF proximities were utilized to learn a low-dimensional manifold from labelled training data and then infer the clinical labels of test data that translated to this space. Their proposed model, voxel-based (FDG-PET), obtained an accuracy of 87.9% using ten-fold cross-validation [ 89 ]. Table 4 provides the overall performance evaluation of the ML models that were presented by the researchers for the prediction of dementia and its subtypes by using image data as a modality.
ML based diagnostic models for dementia: Clinical-variable modality
Aside from image-based ML techniques for dementia prediction, several research studies have utilized clinical-variable data with ML algorithms to predict dementia and its subtypes. For instance, Chiu et al. had designed a screening instrument to detect MCI and dementia using ML techniques. They had developed a questionnaire to assist neurologists and neuropsychologists in the screening of MCI and dementia. The contribution of 45 items that matched the patient’s replies to questions was ranked using feature selection through information gain (IG). Among the 45 items, 12 were ranked the highest in feature selection. The ROC analysis showed that AUC in test group was 94.00% [ 96 ]. Stamate et al. had developed a framework for the prediction of MCI and dementia. Their proposed framework was based on the ReliefF approach paired with statistical permutation tests for feature selection, model training, tweaking, and testing using ML algorithms such as RF, SVM, Gaussian Processes, Stochastic Gradient Boosting, and eXtreme Gradient Boosting. The stability of model performances was studied using computationally expensive Monte Carlo simulations, and the results of their proposed framework were given as for dementia detection, the accuracy was 88.00%, sensitivity was 93.00%, and the specificity was 94.00%, whereas moderate cognitive impairment had a sensitivity of 86.00% and a specificity of 90% [ 97 ]. Stamate et al. developed a system for detecting dementia subtypes (AD) in blood utilizing DL and other supervised ML approaches such as RF and extreme gradient boosting. The AUC for the proposed DL method was 85% (0.80–0.89), for XGBoost it was 88% (0.86–0.89), and for RF it was 85% (0.83–0.87). In comparison, CSF measurements of amyloid, p-tau, and t-tau (together with age and gender) gave AUC values of 78%, 83%, and 87%, respectively, by using the XGBoost [ 98 ]. Bansal1 et al. had performed the comparative analysis of the different ML methods for the detection of dementia using clinical-variables. In their experiments, they exploited the performance of four ML models, such as J48, NB, RF, and multilayer perceptrons. From the results of experiments, they had concluded that j48 outperformed the rest of the ML models for the detection of dementia [ 99 ]. Nori et al. had experimented the lasso algorithm on a big dataset of patient and identify the 50 variables by ML model with an AUC of 69.30% [ 100 ]. Alam et al. [ 101 ]used signal processing on wearable sensor data streams (e.g., electrodermal activity (EDA), photoplethysmogram (PPG), and accelerometer (ACC)) and machine learning techniques to measure cognitive deficits and their relationship with functional health deterioration.
Gurevich et al. had used SVM and neuropsychological test for the classification of AD from other causes of cognitive impairment. The highest classification accuracy they had achieved through their proposed method was 89.00% [ 102 ]. Karaglani et al. had proposed a ML based automated diagnosis system for AD by using blood-based biosignatures. In their proposed method, they used mRNA-based statistically equivalent signatures for feature ranking and a RF model for classification. Their proposed automated diagnosis system had reported the accuracy of 84.60% using RF [ 103 ]. Ryzhikova et al. had analyzed cerebrospinal fluid for the diagnosis of AD by using ML algorithms. For classification purposes, artificial neural networks (ANN) and SVM discriminant analysis (SVM-DA) statistical methods were applied, with the best findings allowing for the distinguishing of AD and HC participants with 84.00% sensitivity and specificity. The proposed classification models have a high discriminative power, implying that the technique has a lot of potential for AD diagnosis [ 104 ]. Cho and Chen had designed a double layer dementia diagnosis system based on ML where fuzzy cognitive maps (FCMs) and probability neural networks (PNNs) were used to provide initial diagnoses at the base layer, and Bayesian networks (BNs) were used to provide final diagnoses at the top layer. Diagnosis results, “proposed treatment,” and “no treatment required” might be used to provide medical institutions with self-testing or secondary dementia diagnosis. The highest accuracy reported by their proposed system was 83.00% [ 105 ]. Facal et al. had studied the role of cognitive reserve in the conversion from MCI to dementia using ML. Nine ML classification algorithms were tried in their study, and seven relevant performance parameters were generated to assess the prediction accuracy for converted and non-converted individuals. The use of ML algorithms on socio-demographic, basic health, and CR proxy data allowed for the prediction of dementia conversion. The Gradient Boosting Classifier (ACC = 0.93; F1 = 0.86 and Cohen’s kappa = 0.82) and RF Classifier (ACC = 92%; F1 = 0.79 and Cohen’s kappa = 0.71) performed the best [ 106 ]. Jin et al. had proposed automatic classification of dementia from learning of clinical consensus diagnosis in India using ML techniques. All viable ML models exhibited remarkable discriminative skills (AUC >90%) as well as comparable accuracy and specificity (both around 95%). The SVM model beat other ML models by obtaining the highest sensitivity (0.81), F1 score (0.72), kappa (.70, showing strong agreement), and accuracy (second highest) (0.65). As a consequence, the SVM was chosen as the best model in their research work [ 107 ]. James et al. had evaluated the performance of ML algorithms for predicting the progression of dementia in memory clinic patients. According to their findings, ML algorithms outperformed humans in predicting incident all-cause dementia within two years. Using all 258 variables, the gradient-boosted trees approach had an overall accuracy of 92% , sensitivity of 0.45, specificity of 0.97, and an AUC of 0.92. Analysis of variable significance had indicated that just 6 variables were necessary for ML algorithms to attain an accuracy of 91% and an AUC of at least 89.00% [ 108 ]. Bougea et al. had investigated the effectiveness of logistic regression (LR), K-nearest neighbours (K-NNs), SVM, the Naive Bayes classifier, and the Ensemble Model to correctly predict PDD or DLB. The K-NN classification model exhibited an overall accuracy of 91.2% based on 15 top clinical and cognitive scores, with 96.42% sensitivity and 81% specificity in distinguishing between DLB and PDD. Based on the 15 best characteristics, the binomial logistic regression classification model had attained an accuracy of 87.5%, with 93.93% sensitivity and 87% specificity. Based on the 15 best characteristics, the SVM classification model had achieved an accuracy of 84.6% of overall instances, 90.62% sensitivity, and 78.58% specificity. A model based on NB classification obtained an accuracy of 82.05%, sensitivity of 93.10%, and a specificity of 74.41%. Finally, an ensemble model, which was constructed by combining the separate ones, attained 89.74% accuracy, 93.75% sensitivity, and 85.73% specificity [ 109 ] (Fig. 6 ).
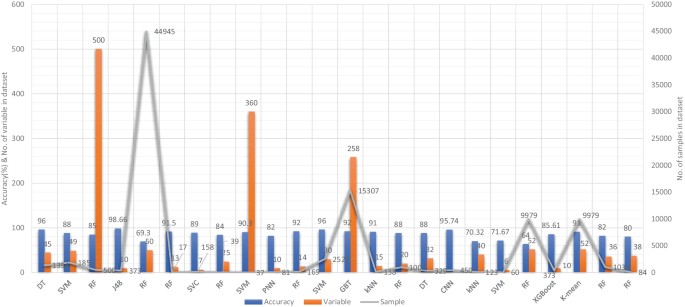
Accuracy comparison of different ML models based on clinical-variable modality
Salem et al. had presented a regression-based ML model for the prediction of dementia. In their proposed method, they had investigated ML approaches for unbalanced learning. In their suggested supervised ML approach, they started by intentionally oversampling the minority class and undersampling the majority class, in order to reduce the bias of the ML model to be trained on the dataset. Furthermore, they had deployed cost-sensitive strategies to penalize the ML models when an instance was misclassified in the minority class. According to their findings, the balanced RF was the most resilient probabilistic model (with just 20 features/variables) with an F1 score of 0.82, a G-Mean of 0.88, and an AUC of 0.88 using ROC. With a F1-score of 0.74 and an AUC of 0.80 by ROC, the calibrated-weighted SVM was their top classification model for the same number of features [ 110 ]. Gutierrez et al. had designed an automated diagnosis system for the detection of AD and FTD by using feature engineering and genetic algorithms. Their proposed system had obtained the accuracy of 84% [ 111 ]. Mirzaei and Adeli had analyzed the state-of-the-art ML techniques used for the detection and classification of AD [ 112 ]. Hsiu et al. had studied ML algorithms for early identification of cognitive impairment. Their proposed model had obtained the accuracy of 70.32% by threefold cross-validation scheme [ 113 ]. Several classification models were constructed using various ML and feature selection methodologies to automate MCI detection using gait biomarkers. They had demonstrated, however, that dual-task walking differentiated between MCI and CN individuals. The ML model used for MCI pre-screening based on inertial sensor-derived gait biomarkers achieved 71.67% accuracy and 83.33% sensitivity, respectively, as reported by Shahzad et al. [ 114 ]. Hane et al. investigated the use of deidentified clinical notes acquired from multiple hospital systems over a 10-year period to enhance retrospective ML models predicting the risk of developing AD. The AUC improved from 85.00% to 94.00% by utilizing clinical notes, and the positive predictive value (PPV) rose from 45.07% (25,245/56,018) to 68.32% (14,153/20,717) in the model at the beginning of disease [ 115 ]. Table 5 provides the overall performance evaluation of the ML models that were presented by the researchers for the prediction of dementia and its subtypes by using clinical-variable data as a modality.
ML based diagnostic models for dementia: Voice modality
Similar to the image and clinical-variable modalities, researchers had also developed automated diagnostic systems based on voice data for the prediction of dementia. Hereby, we have reviewed the research work done by the scientists in detail. For example, Chlasta and Wolk had worked on the computer-based automated screening of dementia patients by spontaneous speech analysis using DL and ML techniques. In their work, they used neural networks to extract the features from the voice data; the extracted features were then fed into a linear SVM for classification purposes. Their SVM model had obtained the accuracy of 59.1% while CNN based ML model had reported the accuracy of 63.6% [ 121 ]. Chien et al. had presented an ML model for the assessment of AD using speech data. Their suggested model included a feature sequence that was used to extract the features from the raw audio data, as well as a recurrent neural network (RNN) for classification. Their proposed ML model had reported an accuracy of 83.80% based on the ROC curve [ 122 ]. Shimoda et al. had designed an ML model that identified the risk of dementia based on the voice feature in telephone conversations. Extreme gradient boosting (XGBoost), RF, and LR based ML models were used, with each audio file serving as one observation. The predictive performance of the constructed ML models was tested by characterizing the ROC curve and determining the AUC, sensitivity, and specificity [ 123 ]. Nishikawa et al. had developed an ensemble discriminating system based on a classifier with statistical acoustic characteristics and a neural network of transformer models, with an F1-score of 90.70% [ 124 ]. Liu et al. had introduced a new technique for recognizing Alzheimer’s disease that used spectrogram features derived from speech data, which aided families in comprehending the illness development of patients at an earlier stage, allowing them to take preventive measures. They used ML techniques to diagnose AD using speech data collected from older adults who displayed the attributes described in the speech. Their proposed method had obtained the maximum accuracy of 84.40% based on LogisticRegressionCV [ 125 ]. Searle et al. had created a ML model to assess spontaneous speech, which might potentially give an efficient diagnostic tool for earlier AD detection. Their suggested model was a fundamental Term Frequency-Inverse Document Frequency (TF-IDF) vectorizer as input into an SVM model, and the top performing models were a pre-trained transformer-based model ’DistilBERT’ when used as an embedding layer into simple linear models. The proposed model had obtained the highest accuracy of 82.00% [ 126 ]. Zhu et al. had suggested an ML model that employed the speech pause as an effective biomarker in dementia detection, with the purpose of reducing the detection, model’s confidence levels by adding perturbation to the speech pauses of the testing samples. They next investigated the impact of the perturbation in training data on the detection model using an adversarial training technique. The proposed model had achieved an accuracy of 84.00% [ 127 ]. Ossewaarde et al. had proposed ML model based on SVM for the classification of spontaneous speech of individuals with dementia based on automatic prosody analysis. Their findings suggest that the classifier can distinguish some dementia types (PPA-NF, AD), but not others (PD) [ 128 ]. Xue et al. had developed an ML model based on DL for the detection of dementia by using voice recordings. In their ML model, long short-term memory (LSTM) network and the convolutional neural network (CNN) utilized audio recordings to categorize whether the recording contained a participant with either NC or only DE and to discriminate between recordings belonging to those with DE and those without DE (i.e., NDE (NC+MCI)) [ 129 ]. Weiner et al. had presented two pipelines of feature extraction for dementia detection: the manual pipeline used manual transcriptions, while the fully automatic pipeline used transcriptions created by automatic speech recognition (ASR). The acoustic and linguistic features that they had extracted need no language specific tools other than the ASR system. Using these two different feature extraction pipelines, they had automatically detect dementia [ 130 ] (Fig. 7 ).
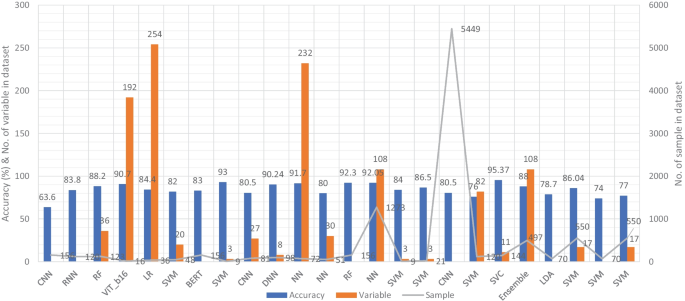
Accuracy comparison of different ML models based on voice modality
Furthermore, Sadeghian et al. had presented the empirical evidence that a combination of acoustic features from speech, linguistic features were extracted from an automatically determined transcription of the speech including punctuation, and results of a mini mental state exam (MMSE) had achieved strong discrimination between subjects with a probable AD versus matched normal controls [ 131 ]. Khodabakhsh et al. had evaluated the linguistic and prosodic characteristics in Turkish conversational language for the identification of AD. Their research suggested that prosodic characteristics outperformed linguistic features by a wide margin. Three of the prosodic features had helped to achieve a classification accuracy of more than 80%, However, their feature fusion experiments did not improve classification performance any more [ 132 ]. Edwards et al. had analyzed the text data at both the word level and phoneme level, which leads to the best-performing system in combination with audio features. Thus, the proposed system was both multi-modal (audio and text) and multi-scale (word and phoneme levels). Experiments with larger neural language models had not resulted in improvement, given the small amount of text data available [ 133 ]. Kumar et al. had identified speech features relevant in predicting AD based on ML. They had deployed neural network for the classification and obtained the accuracy of 92.05% [ 134 ]. Ossewaarde et al. had built ML model based on SVM for the classification from spontaneous speech of individuals with dementia by using automatic prosody [ 128 ]. Luz et al. had developed an ML approach for analyzing patient speech in dialogue for dementia identification. They had designed a prediction model, and the suggested strategy leveraged additive logistic regression (ML boosting method) on content-free data gathered through dialogical interaction. Their proposed model obtained the accuracy of 86.50% [ 135 ]. Sysed et al. had designed a multimodal system that identified linguistic and paralinguistic traits of dementia using an automated screening tool. Their proposed system had used bag-of-deep-feature for feature selection and ensemble model for classification [ 136 ]. Moreover, Sarawgi et al. had used multimodal inductive transfer learning for AD detection and severity. Their proposed system further achieved state-of-the-art AD classification accuracy of 88.0% when evaluated on the full benchmark DementiaBank Pitt database. Table 6 provides the overall performance evaluation of the ML models that were presented by the researchers for the prediction of dementia and its subtypes by using voice-modality data.
In this SLR, we examined the research work that employed ML and DL algorithms to analyze clinical data in order to identify variables that might help predict dementia. We studied 75 research articles that were published in the last 10 years that used image, clinical-variable, and voice data to predict dementia and its subtypes. Nowadays, the healthcare industry creates a vast quantity of data on patients’ health; this data is used by researchers to enhance individual health by utilizing developing technologies such as ML and DL. As a result, researchers can not only distinguish dementia patients from healthy people with high accuracy, but also forecast the disease progression of MCI patients.
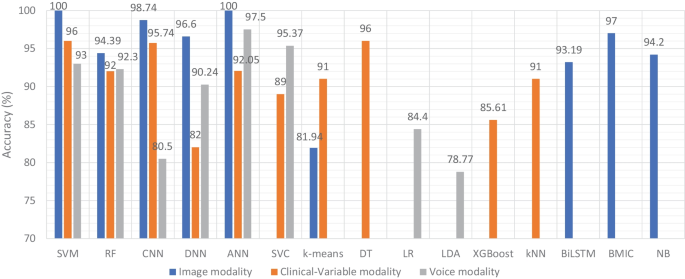
Accuracy comparison of ML models based on data modality
Therefore, researchers have expressed a strong interest in designing and developing automated diagnostic systems based on ML and DL techniques. As seen in Fig. 4 ., there has been an exponential increase in the number of such research publications that use ML algorithms for dementia prediction and detection in the previous four years. We investigated the selected papers using significant performance assessment criteria for ML and DL approaches such as data attributes, computational methodologies, and study emphasis. In this SLR, we have uncovered research gaps in the present literature as well as anticipated future research opportunities. Additionally, in Fig. 8 model comparison, we examined the performance of multiple ML algorithms for dementia prediction based on three types of data modalities: image, clinical-variable, and voice. The accuracy gained by image-based ML algorithms is higher when compared to clinical-variable and voice modalities, as shown in Fig. 8 model comparison. Moreover, the researchers’ suggested SVM, RF, and ANN-based ML techniques outperformed the rest of the ML algorithms in terms of performance. According to Fig. 8 model comparison, voice modality-based ML models show worse accuracy when compared to image and clinical-variable modality data. As a result, there is still a performance gap for researchers to close in order to improve the performance of ML algorithms for the prediction of dementia using voice data. Hence, researchers have shown a strong interest in the creation of automated diagnosis systems for dementia prediction utilizing speech data and ML algorithms, as illustrated in Fig. 4 .
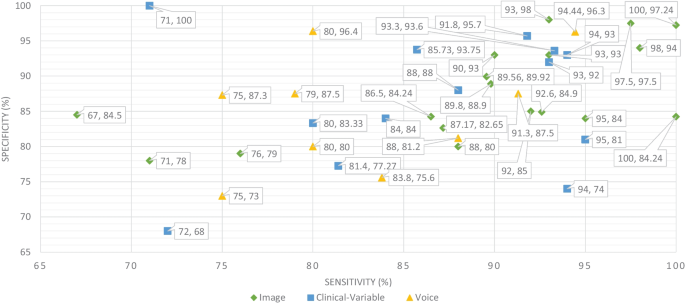
Sensitivity and specificity comparison of ML based on modality
The ML and DL models are likely prone to problems such as poor quality of data, poor selection of ML model, Bias Variance tradeoff and training too complex models. Thus, scientists have developed various evaluation metrics (i.e., ROC, AUC, MCC, F1-score, K-fold) and methods to avoid these problems. The data is a crucial element in ML because ML models work only with numeric data; therefore, poor data quality results in lower performance of ML models. Moreover, imbalance classes in the dataset also cause the bias results from the ML models. Thus, this problem can be overcome by oversampling or undersampling the training data. There are different techniques that are used by the AI engineers for oversampling, such as random oversampling and the synthetic minority oversampling technique (SMOTE). To evaluate the bias researchers’ work, use sensitivity and specificity as an evaluation metric to measure the bias of the ML model. Higher values of sensitivity and specificity means model is free from the biasness while having either one parameter value higher and other one is lower means there is biasness exist. Thus, we have also studied the sensitivity and specificity, along with the accuracy, of the previously proposed ML models for dementia prediction. Figure 9 Comparison provides a brief description of the sensitivity and specificity of the ML models for the detection of dementia based on different data modalities. From Fig. 9 , we can observe that ML models have higher values for sensitivity and specificity when using image data as compared to clinical-variable and voice modality data. In comparison to accuracy from Fig. 8 to sensitivity and specificity from Fig. 9 , we have noted that the results obtained from image based modality are more reliable and precise using ML and DL algorithms in spite of clinical and voice modality.
Furthermore, the correlation between sensitivity and specificity would help us understand the efficacy of the ML models, which are designed for automated disease prediction. The mathematical terms “sensitivity” and “specificity” indicate the accuracy of a test that reports the presence or absence of a disease. Individuals who meet the requirement are labelled “positive,” while those who do not are considered “negative”. The chance of a positive test, conditioned on being actually positive, is referred to as sensitivity (the true positive rate), while specificity (true negative rate) is the likelihood of a negative test if it is actually negative. Sensitivity and specificity are inversely proportional, which means that as sensitivity rises, specificity falls, and vice versa. Mathematically, sensitivity and specificity are given as:
On the other hand, accuracy is a ratio of number of correct assessments / number of all assessments. The proportion of genuine positive outcomes (both true positive and true negative) in the selected population is represented by the numerical value of accuracy. The test result is accurate 99% of the time, whether positive or negative. For the most part, this is right. However, it is worth noting that the equation of accuracy means that even if both sensitivity and specificity are high, say 99%, this does not imply that the test’s accuracy is also high. In addition to sensitivity and specificity, accuracy is determined by the prevalence of the illness in the target population. A diagnosis for a rare ailment in the target group may have high sensitivity and specificity but low accuracy. However, for a balanced dataset, ML models with higher sensitivity and specificity result in higher accuracy. Hence, accuracy must be interpreted carefully. The mathematical formula for accuracy is given as:
where, TP stands for the number of true positives, FP stands for the number of false positives, TN stands for the true negative, and FN stands for the false negative.
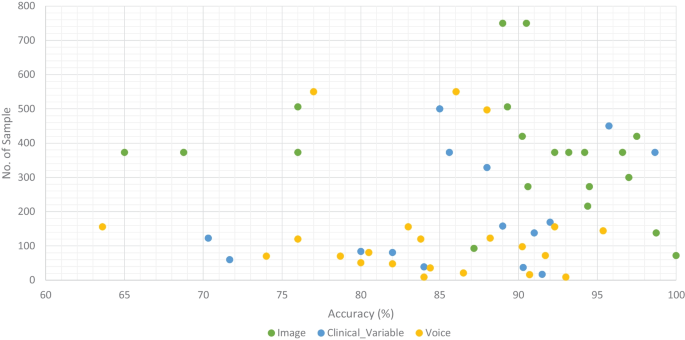
Accuracy comparison of ML models along with number of sample in the dataset based on data modality
We classified all datasets that were used by researchers to test the performance of their proposed ML models for the prediction of dementia (AD, VaD, MCI, and FTD) into three types: image, clinical-variable, and voice. A total of 61 datasets were examined in terms of the number of samples and variables in the datasets. In image modality datasets from the Table 1 , it can be observed that the ADNI dataset has a significant number of samples, which is 750, while the NINDS-AIREN dataset has more variables as compared to the rest of the datasets in the image modality data. Moreover, from the Table 2 of clinical-variable modality datasets, it can be noticed that the ADRD dataset has the highest number of samples (44945) as compared to the rest of the dataset, while the Raman spectral dataset has the highest number of variables (366). In the last, Table 3 of voice data modality elaborated the dataset of voice modality where FHS dataset has highest number of samples of 5449 while VBSD dataset had highest variables of 254 as compared to rest of the datasets in voice modality. The type of data and the size of the dataset are two important factors that have a significant influence on the performance of ML models. Thus, we have also studied this factor by comparing the accuracy along with the number of samples in the dataset with respect to data modalities. From Fig. 10 , it can be observed that the majority of the ML models that used image data have higher accuracy along with a higher number of samples in the dataset. There are few ML models that show poor performance when the number of samples in the dataset is large. While, clinical-variable and voice modalities show prominent performance when the number of samples in the dataset is small.
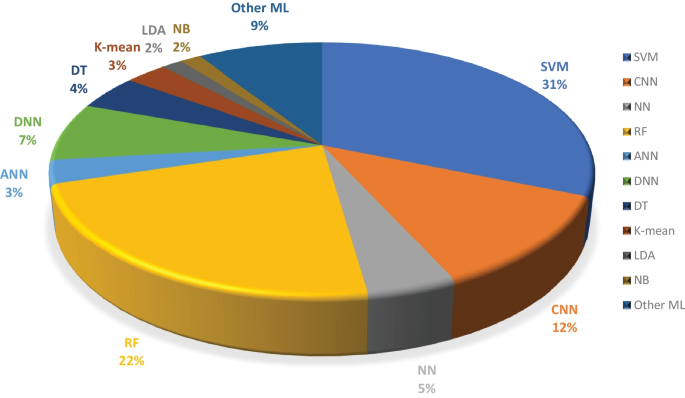
Overall percentage of ML models used in the selected research articles regardless of data modality
Moreover, we examined the effectiveness of ML classifiers utilized by the researchers in their proposed automated diagnostic systems for dementia prediction and classification. According to the selected studies of this SLR, SVM is the most commonly used ML classifier by researchers for the classification of patients and normal subjects using three data modalities (i.e., image, clinical-variable, voice), RF is the second most commonly used ML classifier by researchers, and CNN is the third most commonly used ML classifier by researchers. It can be observed from the Fig. 11 . SVMs are the most powerful tools for the binary classification task, along with RF. From Fig. 8 , we can see that SVM also obtained the highest average accuracy based on three types of data modalities. Hence, this factor also encourages the scientists to employ SVM as a binary classifier for dementia prediction or other disease prediction systems. From Fig. 11 , we can observe the percentage of other ML classifiers that were used by the researchers in selected research articles for the automated diagnosis of dementia.
There are several evaluation metrics that are used for the performance assessment of ML models, such as F1score, AUC, ROC, Matthew’s correlation coefficient (MCC), cross-validation, K-fold, specificity, sensitivity, and accuracy. Each evaluation metric has its own pros and cons. Thus, the selection of appropriate evaluation metrics for the assessment of the ML model is essential to understanding its efficiency and performance. For instance, when data plays a vital role in ML models for decision-making and a dataset has unbalanced classes, it may be possible that results from the ML predictive model might be biassed due to the unbalanced nature of the data in the dataset. Thus, here evaluation metrics help to eliminate the factor of biasness in the results, i.e., the k-fold. The F1-score evolution metric is suitable for the classification of multiple classes in the dataset. while ROC tells us how well the ML model can differentiate binary classes. As a result, AUC and ROC reveal how effectively the probabilities from the positive classes are separated from the probabilities from the negative classes. From Fig. 12 , it can be depicted that cross validation is mostly used in the studies that were selected for this SLR to evaluate the performance of proposed ML models. MCC is the second most used evaluation metric, while ROC is in third place. The proposition of other evaluation metrics used by the researchers to validate the efficiency of their proposed ML models can be observed from Fig. 12 .
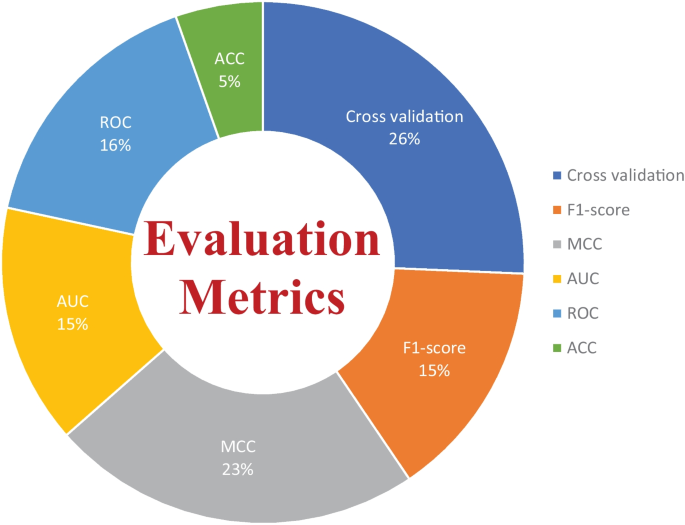
Overall percentage of evaluation metrics of ML models used by the researchers in the selected research articles
Limitations in the previously proposed ML models
ML algorithms have been effectively applied to a broad range of real-world challenges, including banking, cybersecurity, transportation, and robots. They do, however, have fundamental limitations that make them inappropriate for every problem. In the clinical domain, researchers have concentrated on the supervised learning approach, developing various automated diagnostics for AD, MCI, and dementia prediction using supervised machine algorithms. From the Figs. 8 and 11 , It can be noticed that supervised ML classifiers are mostly used by the researchers in the selected past research articles. Because supervised machine learning approaches have various limitations, automated diagnostic methods for dementia prediction based on supervised techniques suffer from some, if not all, of these constraints. In this part, we have examined the drawbacks of supervised ML-based techniques for dementia prediction, which are as follows:
The model overfitting problem affects the performance of ML models. As previously indicated, several researchers have used the k-fold cross-validation approach to evaluate the efficacy of their constructed diagnostic system. However, because of data leaks, it may result in highly biassed findings.
To deal with problem of imbalance classes in the dataset, Researchers and scientists had devised several techniques to eliminate the problem of imbalance classes such as random oversampling example (ROSE), synthetic minority over-sampling technique (SMOTE) and random over sampling (ROS) etc. Unfortunately, in the selected study, the researchers had not considered this factor to deal with the problem of imbalanced classes in the dataset that cause problems of bias.
Supervised ML models require training on a dataset; nevertheless, training on a large quantity of significant data is a hard and time-consuming job, especially for slow learning algorithms like kNN.
For training and testing of the ML models, researchers had used different data partitioning methods, which resulted in inconsistent comparisons of accuracy and other evaluation metrics among the proposed ML models for dementia prediction. Thus, standard data partition schemes should be adopted (holdout) for the comparison of ML models developed by the researcher for dementia prediction.
Another challenge with ML-based automated diagnostic systems for dementia is the time complexity of the proposed ML algorithms. The time complexity means the overall time require to complete all the computational tasks by the ML model for making a prediction. The ML model can forecast results only after it has been trained on the training data, which takes time to analyze. Furthermore, ML models include a large number of parameters that must be manually modified in the case of supervised learning. As a result, it takes a significant amount of effort and time to fine-tune the hyperparameters of the ML model in order to get higher performance.
DL technology has demonstrated cutting-edge performances for the prediction of various diseases in the recent years. However, DL technology needs a massive quantity of data for model training, which is a time-consuming and tough task. Due to the complexity of data models, training is quite costly. Furthermore, DL necessitates the use of pricey GPUs and hundreds of workstations, which are not effective in terms of economics.
Future research directions
In recent years, several ML models have been presented for the prediction of AD and MCI; nevertheless, there are still certain areas that need to be explored by academics and experts. In this section, we have discussed different research areas and the future prospects of ML algorithms for dementia detection. We infer from this study that the following major parameters have a role in the efficient identification of dementia and its forms.
Data is extremely important in the case of ML-based automated detection of dementia, especially when DL models are considered. Many of the publicly available datasets, however, are modest in size. But future research should concentrate on gathering a huge number of samples for the datasets. In this SLR, we studied ML-based automated diagnostic systems for dementia prediction using three different kinds of data modalities (image, clinical_variable, voice). From Fig. 10 , it can be observed that only the image modality based ML model obtained the higher accuracy along with the large size of the dataset, while the voice modality based ML model obtained the higher accuracy on a small dataset. Thus, for the researchers, there is still room available for designing and developing the automated prediction of dementia and its sub-types by using voice data. Therefore, the interest of researchers have been tremendously raised for the development of automated diagnostic systems for dementia prediction using voice data modality and this trend can be confirmed from the Fig. 4 . There is still a lot room available for the improvement in design and construction of automated diagnostic systems for the dementia using clinical-variable data modality for the researchers. Because, the ML model was developed in the past using clinical-variable data, it displays mix performance by using clinical_variable modality, i.e., when the number of samples is lower in the dataset, the ML shows lower accuracy. Thus. In the future, we need to increase the number of samples in the dataset so that we have larger datasets for experimental purposes and the designed ML model can be effectively evaluated.
In selected studies of this SLR, the majority of ML algorithms belong to the supervised category of learning. While few researchers used an unsupervised ML approach for the prediction of dementia and its subtypes, Altough, unspervsied learning approaches suffer from the limitation such as less accuracy, more expensive in term of computational etc. Therefore, it will encourage scientists and researchers to design and construct new techniques and methods using supervised ML algorithms that are more precise and accurate for the prediction of dementia and its subtypes. Moreover, in this SLR, we have analysed the various ML models based on three data modalities (image, clinical-variable, and voice), and we have comprehensively compared previously proposed ML-based systems in terms of various evaluation metrics, but with different data modalities, it would be suggested that multimodal processing techniques based on ML would provide more reliable and efficient results. Hence, in the future, researchers should exploit multimodal approaches based on ML for a better prediction of dementia and its subtypes.
In contrast to earlier SLR studies that examined numerous ML techniques proposed for the automated diagnosis of dementia and its subtypes (AD, VaD, FTD, and MCI) using one type of data modality, this study reviewed ML methods for dementia considering different types of data modalities such as image data, clinical variables, and voice data. The research articles published from 2011 to 2022 were gathered using different databases. It was pointed out that ML approaches based on image data modality has shown better performance compared with ML methods trained on clinical variables based data and voice data modality. Furthermore, this study critically evaluated the previously proposed methods and highlighted limitations in these methods. To overcome these limitations, this study presented future research directions in the domain of automated dementia prediction using ML approaches. We hope that this SLR will be helpful for AI and ML researchers and medical practitioners who are working in the domain of automated diagnostic systems for dementia prediction.
Data Availability
Not applicable.
Code Availability
Menéndez, G.: La revolución de la longevidad: cambio tecnológico, envejecimiento poblacional y transformación cultural. Revista de Ciencias Sociales 30 (41), 159–178 (2017)
Prince, M.J., Wimo, A., Guerchet, M.M., Ali, G.C., Wu, Y.-T., Prina, M.: World alzheimer report 2015-the global impact of dementia: An analysis of prevalence, incidence, cost and trends (2015)
Vrijsen, J., Matulessij, T., Joxhorst, T., de Rooij, S.E., Smidt, N.: Knowledge, health beliefs and attitudes towards dementia and dementia risk reduction among the dutch general population: a cross-sectional study. BMC public health 21 (1), 1–11 (2021)
Widiger, T.A., Costa, P.T., Association, A.P., et al : Personality Disorders and the Five-factor Model of Personality. JSTOR, (2013)
Lo, R.Y.: The borderland between normal aging and dementia. Tzu-Chi Medical Journal 29 (2), 65 (2017)
WHO: Dementia. World Health Organization. https://www.who.int/news-room/fact-sheets/detail/dementia
Budson, A.E., Solomon, P.R.: Memory Loss E-book: A Practical Guide for Clinicians. Elsevier Health Sciences, (2011)
friendly wyoming, D.: Types of dementia. Dementia friendly wyoming. https://www.dfwsheridan.org/types-dementia
Tackenberg, C., Kulic, L., Nitsch, R.M.: Familial alzheimer’s disease mutations at position 22 of the amyloid \(\beta\) -peptide sequence differentially affect synaptic loss, tau phosphorylation and neuronal cell death in an ex vivo system. PloS one 15 (9), 0239584 (2020)
Wang, H., Kulas, J.A., Wang, C., Holtzman, D.M., Ferris, H.A., Hansen, S.B.: Regulation of beta-amyloid production in neurons by astrocyte-derived cholesterol. Proceedings of the National Academy of Sciences 118 (33) (2021)
Vilchez, D., Saez, I., Dillin, A.: The role of protein clearance mechanisms in organismal ageing and age-related diseases. Nature communications 5 (1), 1–13 (2014)
Breijyeh, Z., Karaman, R.: Comprehensive review on alzheimer’s disease: Causes and treatment. Molecules 25 (24), 5789 (2020)
Long, J.M., Holtzman, D.M.: Alzheimer disease: an update on pathobiology and treatment strategies. Cell 179 (2), 312–339 (2019)
Román, G.C.: Vascular dementia may be the most common form of dementia in the elderly. Journal of the neurological sciences 203 , 7–10 (2002)
Gold, G., Bouras, C., Canuto, A., Bergallo, M.F., Herrmann, F.R., Hof, P.R., Mayor, P.-A., Michel, J.-P., Giannakopoulos, P.: Clinicopathological validation study of four sets of clinical criteria for vascular dementia. American Journal of Psychiatry 159 (1), 82–87 (2002)
Lina, R.: Atrial fibrillation in aging; methodological aspects and the relation to dementia and cerebral vascular disease (2022)
Kuźma, E., Lourida, I., Moore, S.F., Levine, D.A., Ukoumunne, O.C., Llewellyn, D.J.: Stroke and dementia risk: a systematic review and meta-analysis. Alzheimer’s & Dementia 14 (11), 1416–1426 (2018)
Tay, J., Morris, R.G., Tuladhar, A.M., Husain, M., de Leeuw, F.-E., Markus, H.S.: Apathy, but not depression, predicts all-cause dementia in cerebral small vessel disease. Journal of Neurology, Neurosurgery & Psychiatry 91 (9), 953–959 (2020)
Morton, R.E., St. John, P.D., Tyas, S.L.: Migraine and the risk of all-cause dementia, alzheimer’s disease, and vascular dementia: A prospective cohort study in community-dwelling older adults. International journal of geriatric psychiatry 34 (11), 1667–1676 (2019)
Sanford, A.M.: Lewy body dementia. Clinics in geriatric medicine 34 (4), 603–615 (2018)
Taylor, J.-P., McKeith, I.G., Burn, D.J., Boeve, B.F., Weintraub, D., Bamford, C., Allan, L.M., Thomas, A.J., T O’Brien, J.: New evidence on the management of lewy body dementia. The Lancet Neurology 19 (2), 157–169 (2020)
McKEITH, I.G., Galasko, D., Wilcock, G.K., Byrne, E.J.: Lewy body dementia–diagnosis and treatment. The British Journal of Psychiatry 167 (6), 709–717 (1995)
Surendranathan, A., Kane, J.P., Bentley, A., Barker, S.A., Taylor, J.-P., Thomas, A.J., Allan, L.M., McNally, R.J., James, P.W., McKeith, I.G., et al.: Clinical diagnosis of lewy body dementia. BJPsych open 6 (4) (2020)
Finger, E.C.: Frontotemporal dementias. Continuum: Lifelong Learning in Neurology 22 (2 Dementia), 464 (2016)
Rabinovici, G.D., Miller, B.L.: Frontotemporal lobar degeneration. CNS drugs 24 (5), 375–398 (2010)
Organization, W.H., et al.: The ICD-10 classification of mental and behavioural disorders. Clinical descriptions and diagnostic guidelines; 2004. Geneva: WHO (2021)
Ghetti, B., Buratti, E., Boeve, B., Rademakers, R.: Frontotemporal Dementias vol. 320. Springer, (2021)
Custodio, N., Montesinos, R., Lira, D., Herrera-Pérez, E., Bardales, Y., Valeriano-Lorenzo, L.: Mixed dementia: A review of the evidence. Dementia & neuropsychologia 11 , 364–370 (2017)
Garcia-Ptacek, S., Kåreholt, I., Cermakova, P., Rizzuto, D., Religa, D., Eriksdotter, M.: Causes of death according to death certificates in individuals with dementia: a cohort from the swedish dementia registry. Journal of the American Geriatrics Society 64 (11), 137–142 (2016)
Arvanitakis, Z., Shah, R.C., Bennett, D.A.: Diagnosis and management of dementia. Jama 322 (16), 1589–1599 (2019)
Ullah, H., Bin Heyat, M.B., AlSalman, H., Khan, H.M., Akhtar, F., Gumaei, A., Mehdi, A., Muaad, A.Y., Islam, M.S., Ali, A., et al.: An effective and lightweight deep electrocardiography arrhythmia recognition model using novel special and native structural regularization techniques on cardiac signal. Journal of Healthcare Engineering 2022 (2022)
Javeed, A., Ali, L., Mohammed Seid, A., Ali, A., Khan, D., Imrana, Y.: A clinical decision support system (cdss) for unbiased prediction of caesarean section based on features extraction and optimized classification. Computational Intelligence and Neuroscience 2022 (2022)
Imrana, Y., Xiang, Y., Ali, L., Abdul-Rauf, Z., Hu, Y.-C., Kadry, S., Lim, S.: \(\chi\) 2-bidlstm: A feature driven intrusion detection system based on \(\chi\) 2 statistical model and bidirectional lstm. Sensors 22 (5), 2018 (2022)
Ali, L., Zhu, C., Zhao, H., Zhang, Z., Liu, Y.: An integrated system for unbiased parkinson’s disease detection from handwritten drawings. In: Advances in Intelligent Systems and Computing, pp. 3–13. Springer, (2022)
Ali, L., Niamat, A., Khan, J.A., Golilarz, N.A., Xingzhong, X., Noor, A., Nour, R., Bukhari, S.A.C.: An optimized stacked support vector machines based expert system for the effective prediction of heart failure. IEEE Access 7 , 54007–54014 (2019)
Ali, L., Zhu, C., Zhou, M., Liu, Y.: Early diagnosis of parkinson’s disease from multiple voice recordings by simultaneous sample and feature selection. Expert Systems with Applications 137 , 22–28 (2019)
Ali, L., Khan, S.U., Golilarz, N.A., Yakubu, I., Qasim, I., Noor, A., Nour, R.: A feature-driven decision support system for heart failure prediction based on statistical model and gaussian naive bayes. Computational and Mathematical Methods in Medicine 2019 (2019)
Mehbodniya, A., Khan, I.R., Chakraborty, S., Karthik, M., Mehta, K., Ali, L., Nuagah, S.J.: Data mining in employee healthcare detection using intelligence techniques for industry development. Journal of Healthcare Engineering 2022 (2022)
Ali, L., Khan, S.U., Arshad, M., Ali, S., Anwar, M.: A multi-model framework for evaluating type of speech samples having complementary information about parkinson’s disease. In: 2019 International Conference on Electrical, Communication, and Computer Engineering (ICECCE), pp. 1–5 (2019). IEEE
Ahmad, F.S., Ali, L., Khattak, H.A., Hameed, T., Wajahat, I., Kadry, S., Bukhari, S.A.C., et al : A hybrid machine learning framework to predict mortality in paralytic ileus patients using electronic health records (ehrs). Journal of Ambient Intelligence and Humanized Computing 12 (3), 3283–3293 (2021)
Ali, L., Wajahat, I., Golilarz, N.A., Keshtkar, F., Bukhari, S.A.C.: Lda–ga–svm: improved hepatocellular carcinoma prediction through dimensionality reduction and genetically optimized support vector machine. Neural Computing and Applications 33 (7), 2783–2792 (2021)
Ali, L., Zhu, C., Zhang, Z., Liu, Y.: Automated detection of parkinson’s disease based on multiple types of sustained phonations using linear discriminant analysis and genetically optimized neural network. IEEE journal of translational engineering in health and medicine 7 , 1–10 (2019)
Ali, L., He, Z., Cao, W., Rauf, H.T., Imrana, Y., Heyat, M.B.B.: Mmdd-ensemble: A multimodal data–driven ensemble approach for parkinson’s disease detection. Frontiers in Neuroscience 15 (2021)
Rehman, A., Khan, A., Ali, M.A., Khan, M.U., Khan, S.U., Ali, L.: Performance analysis of pca, sparse pca, kernel pca and incremental pca algorithms for heart failure prediction. In: 2020 International Conference on Electrical, Communication, and Computer Engineering (ICECCE), pp. 1–5 (2020). IEEE
Ahmed, F.S., Ali, L., Joseph, B.A., Ikram, A., Mustafa, R.U., Bukhari, S.A.C.: A statistically rigorous deep neural network approach to predict mortality in trauma patients admitted to the intensive care unit. Journal of Trauma and Acute Care Surgery 89 (4), 736–742 (2020)
Imrana, Y., Xiang, Y., Ali, L., Abdul-Rauf, Z.: A bidirectional lstm deep learning approach for intrusion detection. Expert Systems with Applications 185 , 115524 (2021)
Akbar, W., Wu, W.-p., Saleem, S., Farhan, M., Saleem, M.A., Javeed, A., Ali, L.: Development of hepatitis disease detection system by exploiting sparsity in linear support vector machine to improve strength of adaboost ensemble model. Mobile Information Systems 2020 (2020)
Ali, L., Bukhari, S.: An approach based on mutually informed neural networks to optimize the generalization capabilities of decision support systems developed for heart failure prediction. Irbm 42 (5), 345–352 (2021)
McInnes, M.D., Moher, D., Thombs, B.D., McGrath, T.A., Bossuyt, P.M., Clifford, T., Cohen, J.F., Deeks, J.J., Gatsonis, C., Hooft, L., et al : Preferred reporting items for a systematic review and meta-analysis of diagnostic test accuracy studies: the prisma-dta statement. Jama 319 (4), 388–396 (2018)
Liberati, A., Altman, D.G., Tetzlaff, J., Mulrow, C., Gøtzsche, P.C., Ioannidis, J.P., Clarke, M., Devereaux, P.J., Kleijnen, J., Moher, D.: The prisma statement for reporting systematic reviews and meta-analyses of studies that evaluate health care interventions: explanation and elaboration. Journal of clinical epidemiology 62 (10), 1–34 (2009)
Martí-Juan, G., Sanroma-Guell, G., Piella, G.: A survey on machine and statistical learning for longitudinal analysis of neuroimaging data in alzheimer’s disease. Computer methods and programs in biomedicine 189 , 105348 (2020)
McGinnis, J.M., Olsen, L., Goolsby, W.A., Grossmann, C., et al : Clinical Data as the Basic Staple of Health Learning: Creating and Protecting a Public Good: Workshop Summary. National Academies Press, (2011)
Vaughn, V.M., Linder, J.A.: Thoughtless design of the electronic health record drives overuse, but purposeful design can nudge improved patient care. BMJ Publishing Group Ltd (2018)
Doody, R., Stevens, J., Beck, C., Dubinsky, R., Kaye, J., Gwyther, L., Mohs, R., Thal, L., Whitehouse, P., DeKosky, S., et al : Practice parameter: Management of dementia (an evidence-based review): Report of the quality standards subcommittee of the american academy of neurology. Neurology 56 (9), 1154–1166 (2001)
Chi, C.-L., Zeng, W., Oh, W., Borson, S., Lenskaia, T., Shen, X., Tonellato, P.J.: Personalized long-term prediction of cognitive function: Using sequential assessments to improve model performance. Journal of biomedical informatics 76 , 78–86 (2017)
Johnson, K.A., Minoshima, S., Bohnen, N.I., Donohoe, K.J., Foster, N.L., Herscovitch, P., Karlawish, J.H., Rowe, C.C., Carrillo, M.C., Hartley, D.M., et al : Appropriate use criteria for amyloid pet: a report of the amyloid imaging task force, the society of nuclear medicine and molecular imaging, and the alzheimer’s association. Alzheimer’s & Dementia 9 (1), 1–16 (2013)
Shaw, L.M., Arias, J., Blennow, K., Galasko, D., Molinuevo, J.L., Salloway, S., Schindler, S., Carrillo, M.C., Hendrix, J.A., Ross, A., et al : Appropriate use criteria for lumbar puncture and cerebrospinal fluid testing in the diagnosis of alzheimer’s disease. Alzheimer’s & Dementia 14 (11), 1505–1521 (2018)
Mills, K.L., Tamnes, C.K.: Methods and considerations for longitudinal structural brain imaging analysis across development. Developmental cognitive neuroscience 9 , 172–190 (2014)
Ali, L., Zhu, C., Golilarz, N.A., Javeed, A., Zhou, M., Liu, Y.: Reliable parkinson’s disease detection by analyzing handwritten drawings: construction of an unbiased cascaded learning system based on feature selection and adaptive boosting model. Ieee Access 7 , 116480–116489 (2019)
Javeed, A., Rizvi, S.S., Zhou, S., Riaz, R., Khan, S.U., Kwon, S.J.: Heart risk failure prediction using a novel feature selection method for feature refinement and neural network for classification. Mobile Information Systems 2020 (2020)
Javeed, A., Zhou, S., Yongjian, L., Qasim, I., Noor, A., Nour, R.: An intelligent learning system based on random search algorithm and optimized random forest model for improved heart disease detection. IEEE Access 7 , 180235–180243 (2019)
Ali, L., Rahman, A., Khan, A., Zhou, M., Javeed, A., Khan, J.A.: An automated diagnostic system for heart disease prediction based on chi2 statistical model and optimally configured deep neural network. IEEE Access 7 , 34938–34945 (2019)
Maddox, T.M., Rumsfeld, J.S., Payne, P.R.: Questions for artificial intelligence in health care. Jama 321 (1), 31–32 (2019)
Chen, P.-H.C., Liu, Y., Peng, L.: How to develop machine learning models for healthcare. Nature materials 18 (5), 410–414 (2019)
Javeed, A., Khan, S.U., Ali, L., Ali, S., Imrana, Y., Rahman, A.: Machine learning-based automated diagnostic systems developed for heart failure prediction using different types of data modalities: A systematic review and future directions. Computational and Mathematical Methods in Medicine 2022 (2022)
Thacker, S.B., Qualters, J.R., Lee, L.M., for Disease Control, C., Prevention, et al : Public health surveillance in the united states: evolution and challenges. MMWR Suppl 61 (3), 3–9 (2012)
Boerma, J.T., Stansfield, S.K.: Health statistics now: are we making the right investments? The Lancet 369 (9563), 779–786 (2007)
Walport, M., Brest, P.: Sharing research data to improve public health. The Lancet 377 (9765), 537–539 (2011)
Van Panhuis, W.G., Paul, P., Emerson, C., Grefenstette, J., Wilder, R., Herbst, A.J., Heymann, D., Burke, D.S.: A systematic review of barriers to data sharing in public health. BMC public health 14 (1), 1–9 (2014)
Dashtipour, K., Taylor, W., Ansari, S., Zahid, A., Gogate, M., Ahmad, J., Assaleh, K., Arshad, K., Imran, M.A., Abbai, Q.: Detecting alzheimer’s disease using machine learning methods. In: EAI (2021)
Helaly, H.A., Badawy, M., Haikal, A.Y.: Deep learning approach for early detection of alzheimer’s disease. Cognitive Computation, 1–17 (2021)
Vandenberghe, R., Nelissen, N., Salmon, E., Ivanoiu, A., Hasselbalch, S., Andersen, A., Korner, A., Minthon, L., Brooks, D.J., Van Laere, K., et al : Binary classification of 18f-flutemetamol pet using machine learning: comparison with visual reads and structural mri. Neuroimage 64 , 517–525 (2013)
Odusami, M., Maskeliūnas, R., Damaševičius, R., Krilavičius, T.: Analysis of features of alzheimer’s disease: Detection of early stage from functional brain changes in magnetic resonance images using a finetuned resnet18 network. Diagnostics 11 (6), 1071 (2021)
Zheng, Y., Guo, H., Zhang, L., Wu, J., Li, Q., Lv, F.: Machine learning-based framework for differential diagnosis between vascular dementia and alzheimer’s disease using structural mri features. Frontiers in Neurology, 1097 (2019)
Basheer, S., Bhatia, S., Sakri, S.B.: Computational modeling of dementia prediction using deep neural network: Analysis on oasis dataset. IEEE Access 9 , 42449–42462 (2021)
Leong, L.K., Abdullah, A.A.: Prediction of alzheimer’s disease (ad) using machine learning techniques with boruta algorithm as feature selection method. In: Journal of Physics: Conference Series, vol. 1372, p. 012065 (2019). IOP Publishing
Battineni, G., Chintalapudi, N., Amenta, F.: Machine learning in medicine: Performance calculation of dementia prediction by support vector machines (svm). Informatics in Medicine Unlocked 16 , 100200 (2019)
Mathotaarachchi, S., Pascoal, T.A., Shin, M., Benedet, A.L., Kang, M.S., Beaudry, T., Fonov, V.S., Gauthier, S., Rosa-Neto, P., Initiative, A.D.N., et al : Identifying incipient dementia individuals using machine learning and amyloid imaging. Neurobiology of aging 59 , 80–90 (2017)
Aruna, S., Chitra, S.: Machine learning approach for identifying dementia from mri images. International Journal of Computer and Information Engineering 9 (3), 881–888 (2016)
Herzog, N.J., Magoulas, G.D.: Brain asymmetry detection and machine learning classification for diagnosis of early dementia. Sensors 21 (3), 778 (2021)
Battineni, G., Chintalapudi, N., Amenta, F., Traini, E.: A comprehensive machine-learning model applied to magnetic resonance imaging (mri) to predict alzheimer’s disease (ad) in older subjects. Journal of Clinical Medicine 9 (7), 2146 (2020)
Ma, D., Yee, E., Stocks, J.K., Jenkins, L.M., Popuri, K., Chausse, G., Wang, L., Probst, S., Beg, M.F.: Blinded clinical evaluation for dementia of alzheimer’s type classification using fdg-pet: A comparison between feature-engineered and non-feature-engineered machine learning methods. Journal of Alzheimer’s Disease 80 (2), 715–726 (2021)
Bidani, A., Gouider, M.S., Travieso-González, C.M.: Dementia detection and classification from mri images using deep neural networks and transfer learning. In: International Work-Conference on Artificial Neural Networks, pp. 925–933 (2019). Springer
Moscoso, A., Silva-Rodríguez, J., Aldrey, J.M., Cortés, J., Fernández-Ferreiro, A., Gómez-Lado, N., Ruibal, Á., Aguiar, P., Initiative, A.D.N., et al : Prediction of alzheimer’s disease dementia with mri beyond the short-term: Implications for the design of predictive models. NeuroImage: Clinical 23 , 101837 (2019)
Khan, A., Zubair, S.: An improved multi-modal based machine learning approach for the prognosis of alzheimer’s disease. Journal of King Saud University-Computer and Information Sciences (2020)
Mohammed, B.A., Senan, E.M., Rassem, T.H., Makbol, N.M., Alanazi, A.A., Al-Mekhlafi, Z.G., Almurayziq, T.S., Ghaleb, F.A.: Multi-method analysis of medical records and mri images for early diagnosis of dementia and alzheimer’s disease based on deep learning and hybrid methods. Electronics 10 (22), 2860 (2021)
Salvatore, C., Cerasa, A., Battista, P., Gilardi, M.C., Quattrone, A., Castiglioni, I.: Magnetic resonance imaging biomarkers for the early diagnosis of alzheimer’s disease: a machine learning approach. Frontiers in neuroscience 9 , 307 (2015)
Katako, A., Shelton, P., Goertzen, A.L., Levin, D., Bybel, B., Aljuaid, M., Yoon, H.J., Kang, D.Y., Kim, S.M., Lee, C.S., et al : Machine learning identified an alzheimer’s disease-related fdg-pet pattern which is also expressed in lewy body dementia and parkinson’s disease dementia. Scientific reports 8 (1), 1–13 (2018)
Gray, K.R., Aljabar, P., Heckemann, R.A., Hammers, A., Rueckert, D.: Random forest-based manifold learning for classification of imaging data in dementia. In: International Workshop on Machine Learning in Medical Imaging, pp. 159–166 (2011). Springer
De Bruijne, M.: Machine learning approaches in medical image analysis: From detection to diagnosis. Elsevier (2016)
Tong, T., Wolz, R., Gao, Q., Hajnal, J.V., Rueckert, D.: Multiple instance learning for classification of dementia in brain mri. In: International Conference on Medical Image Computing and Computer-Assisted Intervention, pp. 599–606 (2013). Springer
Akhila, J., Markose, C., Aneesh, R.: Feature extraction and classification of dementia with neural network. In: 2017 International Conference on Intelligent Computing, Instrumentation and Control Technologies (ICICICT), pp. 1446–1450 (2017). IEEE
Chen, Y., Pham, T.D.: Development of a brain mri-based hidden markov model for dementia recognition. Biomedical engineering online 12 (1), 1–16 (2013)
Patil, M., Yardi, A.: Ann based dementia diagnosis using dct for brain mr image compression. In: 2013 International Conference on Communication and Signal Processing, pp. 451–454 (2013). IEEE
Gulhare, K.K., Shukla, S., Sharma, L.: Deep neural network classification method to alzheimer’s disease detection. International Journals of Advanced Research in Computer Science and Software Engineering 7 (6), 1–4 (2017)
Chiu, P.-Y., Tang, H., Wei, C.-Y., Zhang, C., Hung, G.-U., Zhou, W.: Nmd-12: A new machine-learning derived screening instrument to detect mild cognitive impairment and dementia. PloS one 14 (3), 0213430 (2019)
Stamate, D., Alghamdi, W., Ogg, J., Hoile, R., Murtagh, F.: A machine learning framework for predicting dementia and mild cognitive impairment. In: 2018 17th IEEE International Conference on Machine Learning and Applications (ICMLA), pp. 671–678 (2018). IEEE
Visser, P.J., Lovestone, S., Legido-Quigley, C.: A metabolite-based machine learning approach to diagnose alzheimer-type dementia in blood: Results from the european medical information framework for alzheimer disease biomarker discovery cohort (2019)
Bansal, D., Chhikara, R., Khanna, K., Gupta, P.: Comparative analysis of various machine learning algorithms for detecting dementia. Procedia computer science 132 , 1497–1502 (2018)
Nori, V.S., Hane, C.A., Martin, D.C., Kravetz, A.D., Sanghavi, D.M.: Identifying incident dementia by applying machine learning to a very large administrative claims dataset. PLoS One 14 (7), 0203246 (2019)
Alam, M.A.U., Roy, N., Holmes, S., Gangopadhyay, A., Galik, E.: Automated functional and behavioral health assessment of older adults with dementia. In: 2016 IEEE First International Conference on Connected Health: Applications, Systems and Engineering Technologies (CHASE), pp. 140–149 (2016). IEEE
Gurevich, P., Stuke, H., Kastrup, A., Stuke, H., Hildebrandt, H.: Neuropsychological testing and machine learning distinguish alzheimer’s disease from other causes for cognitive impairment. Frontiers in aging neuroscience 9 , 114 (2017)
Karaglani, M., Gourlia, K., Tsamardinos, I., Chatzaki, E.: Accurate blood-based diagnostic biosignatures for alzheimer’s disease via automated machine learning. Journal of clinical medicine 9 (9), 3016 (2020)
Ryzhikova, E., Ralbovsky, N.M., Sikirzhytski, V., Kazakov, O., Halamkova, L., Quinn, J., Zimmerman, E.A., Lednev, I.K.: Raman spectroscopy and machine learning for biomedical applications: Alzheimer’s disease diagnosis based on the analysis of cerebrospinal fluid. Spectrochimica Acta Part A: Molecular and Biomolecular Spectroscopy 248 , 119188 (2021)
Cho, P.-C., Chen, W.-H.: A double layer dementia diagnosis system using machine learning techniques. In: International Conference on Engineering Applications of Neural Networks, pp. 402–412 (2012). Springer
Facal, D., Valladares-Rodriguez, S., Lojo-Seoane, C., Pereiro, A.X., Anido-Rifon, L., Juncos-Rabadán, O.: Machine learning approaches to studying the role of cognitive reserve in conversion from mild cognitive impairment to dementia. International journal of geriatric psychiatry 34 (7), 941–949 (2019)
Jin, H., Chien, S., Meijer, E., Khobragade, P., Lee, J., et al : Learning from clinical consensus diagnosis in india to facilitate automatic classification of dementia: Machine learning study. JMIR Mental Health 8 (5), 27113 (2021)
James, C., Ranson, J.M., Everson, R., Llewellyn, D.J.: Performance of machine learning algorithms for predicting progression to dementia in memory clinic patients. JAMA network open 4 (12), 2136553–2136553 (2021)
Bougea, A., Efthymiopoulou, E., Spanou, I., Zikos, P.: A novel machine learning algorithm predicts dementia with lewy bodies versus parkinson’s disease dementia based on clinical and neuropsychological scores. Journal of geriatric psychiatry and neurology, 0891988721993556 (2021)
Salem, F.A., Chaaya, M., Ghannam, H., Al Feel, R.E., El Asmar, K.: Regression based machine learning model for dementia diagnosis in a community setting. Alzheimer’s & Dementia 17 , 053839 (2021)
Garcia-Gutierrez, F., Delgado-Alvarez, A., Delgado-Alonso, C., Díaz-Álvarez, J., Pytel, V., Valles-Salgado, M., Gil, M.J., Hernández-Lorenzo, L., Matías-Guiu, J., Ayala, J.L., et al.: Diagnosis of alzheimer’s disease and behavioural variant frontotemporal dementia with machine learning-aided neuropsychological assessment using feature engineering and genetic algorithms. International journal of geriatric psychiatry 37 (2) (2022)
Mirzaei, G., Adeli, H.: Machine learning techniques for diagnosis of alzheimer disease, mild cognitive disorder, and other types of dementia. Biomedical Signal Processing and Control 72 , 103293 (2022)
Hsiu, H., Lin, S.-K., Weng, W.-L., Hung, C.-M., Chang, C.-K., Lee, C.-C., Chen, C.-T.: Discrimination of the cognitive function of community subjects using the arterial pulse spectrum and machine-learning analysis. Sensors 22 (3), 806 (2022)
Shahzad, A., Dadlani, A., Lee, H., Kim, K.: Automated prescreening of mild cognitive impairment using shank-mounted inertial sensors based gait biomarkers. IEEE Access (2022)
Hane, C.A., Nori, V.S., Crown, W.H., Sanghavi, D.M., Bleicher, P.: Predicting onset of dementia using clinical notes and machine learning: case-control study. JMIR medical informatics 8 (6), 17819 (2020)
Aschwanden, D., Aichele, S., Ghisletta, P., Terracciano, A., Kliegel, M., Sutin, A.R., Brown, J., Allemand, M.: Predicting cognitive impairment and dementia: A machine learning approach. Journal of Alzheimer’s Disease 75 (3), 717–728 (2020)
Ryu, S.-E., Shin, D.-H., Chung, K.: Prediction model of dementia risk based on xgboost using derived variable extraction and hyper parameter optimization. IEEE Access 8 , 177708–177720 (2020)
de Langavant, L.C., Bayen, E., Yaffe, K., et al : Unsupervised machine learning to identify high likelihood of dementia in population-based surveys: development and validation study. Journal of medical Internet research 20 (7), 10493 (2018)
Fouladvand, S., Mielke, M.M., Vassilaki, M., Sauver, J.S., Petersen, R.C., Sohn, S.: Deep learning prediction of mild cognitive impairment using electronic health records. In: 2019 IEEE International Conference on Bioinformatics and Biomedicine (BIBM), pp. 799–806 (2019). IEEE
Balea-Fernandez, F.J., Martinez-Vega, B., Ortega, S., Fabelo, H., Leon, R., Callico, G.M., Bibao-Sieyro, C.: Analysis of risk factors in dementia through machine learning. Journal of Alzheimer’s Disease 79 (2), 845–861 (2021)
Chlasta, K., Wołk, K.: Towards computer-based automated screening of dementia through spontaneous speech. Frontiers in Psychology, 4091 (2021)
Chien, Y.-W., Hong, S.-Y., Cheah, W.-T., Yao, L.-H., Chang, Y.-L., Fu, L.-C.: An automatic assessment system for alzheimer’s disease based on speech using feature sequence generator and recurrent neural network. Scientific Reports 9 (1), 1–10 (2019)
Shimoda, A., Li, Y., Hayashi, H., Kondo, N.: Dementia risks identified by vocal features via telephone conversations: A novel machine learning prediction model. PloS one 16 (7), 0253988 (2021)
Nishikawa, K., Akihiro, K., Hirakawa, R., Kawano, H., Nakatoh, Y.: Machine learning model for discrimination of mild dementia patients using acoustic features. Cognitive Robotics (2021)
Liu, L., Zhao, S., Chen, H., Wang, A.: A new machine learning method for identifying alzheimer’s disease. Simulation Modelling Practice and Theory 99 , 102023 (2020)
Searle, T., Ibrahim, Z., Dobson, R.: Comparing natural language processing techniques for alzheimer’s dementia prediction in spontaneous speech. arXiv preprint arXiv:2006.07358 (2020)
Zhu, Y., Tran, B., Liang, X., Batsis, J.A., Roth, R.M.: Towards interpretability of speech pause in dementia detection using adversarial learning. arXiv preprint arXiv:2111.07454 (2021)
Ossewaarde, R., Jonkers, R., Jalvingh, F., Bastiaanse, R.: Classification of spontaneous speech of individuals with dementia based on automatic prosody analysis using support vector machines (svm). In: The Thirty-Second International Flairs Conference (2019)
Xue, C., Karjadi, C., Paschalidis, I.C., Au, R., Kolachalama, V.B.: Detection of dementia on voice recordings using deep learning: a framingham heart study. Alzheimer’s research & therapy 13 (1), 1–15 (2021)
Weiner, J., Engelbart, M., Schultz, T.: Manual and automatic transcriptions in dementia detection from speech. In: INTERSPEECH, pp. 3117–3121 (2017)
Sadeghian, R., Schaffer, J.D., Zahorian, S.A.: Speech processing approach for diagnosing dementia in an early stage (2017)
Khodabakhsh, A., Yesil, F., Guner, E., Demiroglu, C.: Evaluation of linguistic and prosodic features for detection of alzheimer’s disease in turkish conversational speech. EURASIP Journal on Audio, Speech, and Music Processing 2015 (1), 1–15 (2015)
Edwards, E., Dognin, C., Bollepalli, B., Singh, M.K., Analytics, V.: Multiscale system for alzheimer’s dementia recognition through spontaneous speech. In: INTERSPEECH, pp. 2197–2201 (2020)
Kumar, Y., Maheshwari, P., Joshi, S., Baths, V.: Ml-based analysis to identify speech features relevant in predicting alzheimer’s disease. arXiv preprint arXiv:2110.13023 (2021)
Luz, S., de la Fuente, S., Albert, P.: A method for analysis of patient speech in dialogue for dementia detection. arXiv preprint arXiv:1811.09919 (2018)
Syed, Z.S., Syed, M.S.S., Lech, M., Pirogova, E.: Automated recognition of alzheimer’s dementia using bag-of-deep-features and model ensembling. IEEE Access 9 , 88377–88390 (2021)
Orsulic-Jeras, S., Sanders, B., Powers, S., Ejaz, F., Cordell, A., Wilk, C.: Developing a reminiscence therapy platform-lifebio memorytm-with a novel machine-learning-based application that transfers speech to text and generates life stories for nursing home residents with dementia. Alzheimer’s & Dementia 17 , 052281 (2021)
Sarawgi, U., Zulfikar, W., Soliman, N., Maes, P.: Multimodal inductive transfer learning for detection of alzheimer’s dementia and its severity. arXiv preprint arXiv:2009.00700 (2020)
Calzà, L., Gagliardi, G., Favretti, R.R., Tamburini, F.: Linguistic features and automatic classifiers for identifying mild cognitive impairment and dementia. Computer Speech & Language 65 , 101113 (2021)
Haider, F., De La Fuente, S., Luz, S.: An assessment of paralinguistic acoustic features for detection of alzheimer’s dementia in spontaneous speech. IEEE Journal of Selected Topics in Signal Processing 14 (2), 272–281 (2019)
Lopez-de-Ipiña, K., Alonso, J.B., Solé-Casals, J., Barroso, N., Henriquez, P., Faundez-Zanuy, M., Travieso, C.M., Ecay-Torres, M., Martinez-Lage, P., Eguiraun, H.: On automatic diagnosis of alzheimer’s disease based on spontaneous speech analysis and emotional temperature. Cognitive Computation 7 (1), 44–55 (2015)
Orimaye, S.O., Wong, J.S.-M., Golden, K.J.: Learning predictive linguistic features for alzheimer’s disease and related dementias using verbal utterances. In: Proceedings of the Workshop on Computational Linguistics and Clinical Psychology: From Linguistic Signal to Clinical Reality, pp. 78–87 (2014)
Santander-Cruz, Y., Salazar-Colores, S., Paredes-García, W.J., Guendulain-Arenas, H., Tovar-Arriaga, S.: Semantic feature extraction using sbert for dementia detection. Brain Sciences 12 (2), 270 (2022)
Download references
Acknowledgements
The first author’s learning process was supported by the National E-Infrastructure for Aging Research (NEAR), Sweden. NEAR is working on improving the health condition of older adults in Sweden.
Open access funding provided by Blekinge Institute of Technology. This research received no external funding.
Author information
Ashir Javeed and Ana Luiza Dallora contributed equally to this work.
Authors and Affiliations
Aging Research Center, Karolinska Institutet, Tomtebodavagen, Stockholm, 17165, Solna, Sweden
Ashir Javeed
Department of Health, Blekinge Institute of Technology, Valhallavägen 1, Karlskrona, 37141, Blekinge, Sweden
Ashir Javeed, Ana Luiza Dallora, Johan Sanmartin Berglund & Peter Anderberg
Department of Computer Science, University of Science and Technology Bannu, Township, Bannu, 28100, Khyber-Pakhtunkhwa, Pakistan
Department of Electrical Engineering, University of Science and Technology Bannu, Township, Bannu, 28100, Khyber-Pakhtunkhwa, Pakistan
Liaqata Ali
School of Health Sciences, University of Skovde, Högskolevägen 1, Skövde, SE-541 28, Skövde, Sweden
Peter Anderberg
You can also search for this author in PubMed Google Scholar
Contributions
Conceptualization by Peter Anderber, Data curation by Liaqat ALi, Formal analysis by Ana Luiza Dallora, Write up and Methodology by Ashir Javeed, Proofread by Arif Ali, Supervised by Johan Sanmartin Berglund. If any of the sections are not relevant to your manuscript.
Corresponding author
Correspondence to Johan Sanmartin Berglund .
Ethics declarations
Ethics approval.
This study was carried out in accordance with the Declaration of Helsinki and was approved by the Research Ethics Committee at Blekinge Institute of Technology (BTH).
Consent to Participate
Consent for publication, conflict of interest.
The authors declare no conflict of interest.
Additional information
Publisher’s note.
Springer Nature remains neutral with regard to jurisdictional claims in published maps and institutional affiliations.
Rights and permissions
Open Access This article is licensed under a Creative Commons Attribution 4.0 International License, which permits use, sharing, adaptation, distribution and reproduction in any medium or format, as long as you give appropriate credit to the original author(s) and the source, provide a link to the Creative Commons licence, and indicate if changes were made. The images or other third party material in this article are included in the article's Creative Commons licence, unless indicated otherwise in a credit line to the material. If material is not included in the article's Creative Commons licence and your intended use is not permitted by statutory regulation or exceeds the permitted use, you will need to obtain permission directly from the copyright holder. To view a copy of this licence, visit http://creativecommons.org/licenses/by/4.0/ .
Reprints and permissions
About this article
Javeed, A., Dallora, A.L., Berglund, J.S. et al. Machine Learning for Dementia Prediction: A Systematic Review and Future Research Directions. J Med Syst 47 , 17 (2023). https://doi.org/10.1007/s10916-023-01906-7
Download citation
Received : 16 July 2022
Accepted : 03 January 2023
Published : 01 February 2023
DOI : https://doi.org/10.1007/s10916-023-01906-7
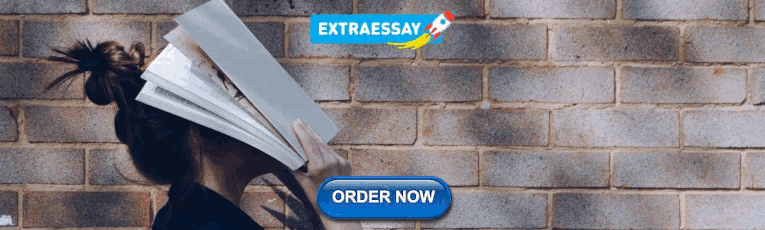
Share this article
Anyone you share the following link with will be able to read this content:
Sorry, a shareable link is not currently available for this article.
Provided by the Springer Nature SharedIt content-sharing initiative
- Dementia prediction
- Feature selection
- Machine learning
- Deep learning
- Find a journal
- Publish with us
- Track your research
Research highlights importance of support reciprocity during early-stage dementia care
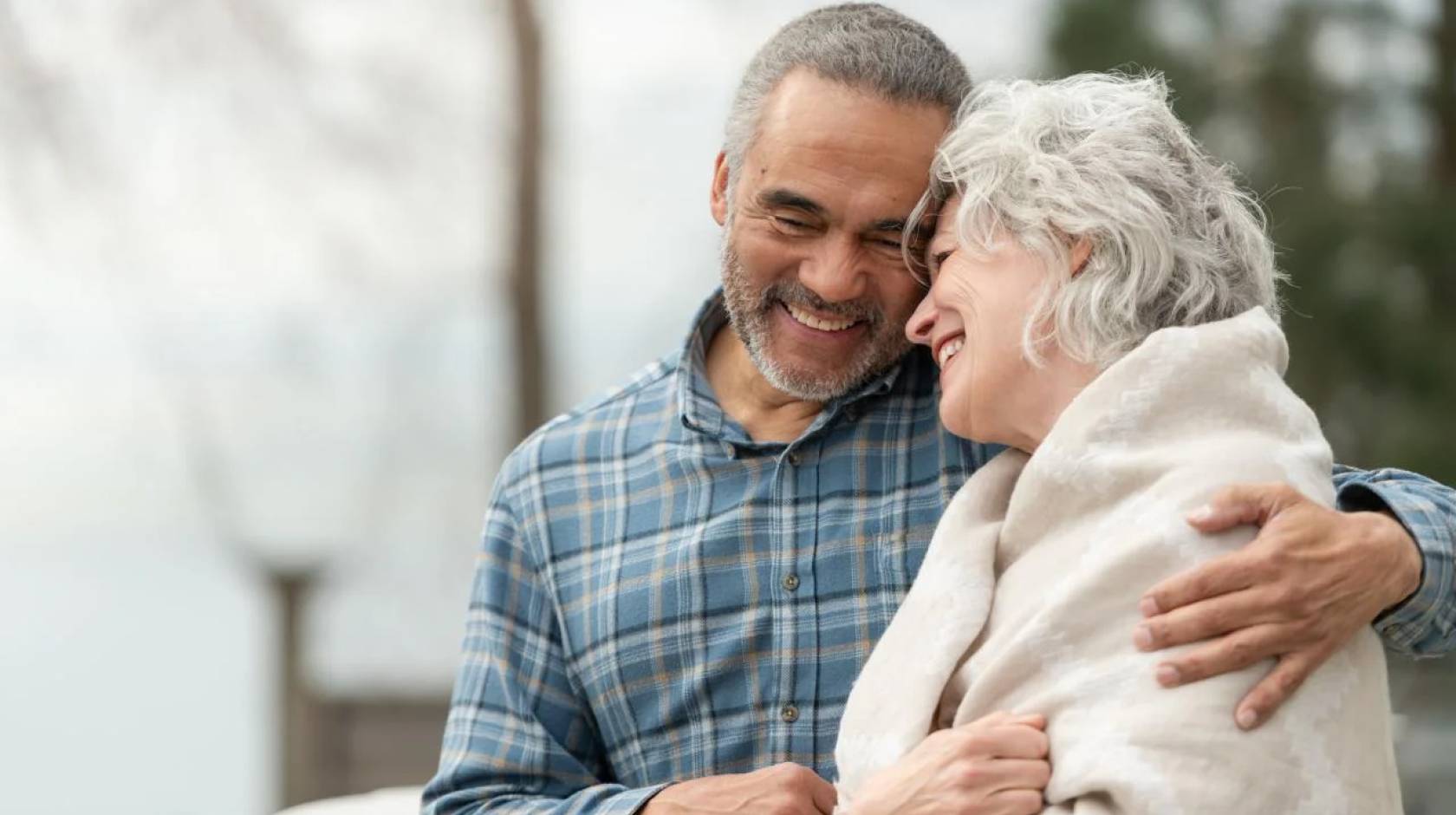
In cases of Alzheimer’s disease, it’s common for a spouse to take on the role of caregiver. Research led by the University of California, Davis, suggests that patients can also support their caretakers, and that reciprocated care has mutual benefits.
A new paper published in the journal Aging and Mental Health highlights that couples coping with dementia can navigate the challenges with greater resilience and well-being by working together and supporting each other.
“We know that dementia is degenerative, there’s no way back, and spousal caregivers typically face substantial burden,” said Meng Huo, assistant professor with the Department of Human Ecology and lead author of the study. “But having a mutually beneficial relationship in early stages may help reduce caregiver burden and even slow the progression of dementia symptoms.”
Nearly 7 million people aged 65 and older in the United States have Alzheimer’s disease. In this study, researchers surveyed 72 couples from Northern California and Nevada in which one person was living with early-stage Alzheimer’s disease and their spouse was the primary caregiver. They assessed the support that spouses provided to each other and found that people with dementia assisted their spousal caregivers often.
“Debunking the myth that dementia patients are only recipients of care is crucial,” Huo said. “Recognizing their potential to help will encourage them to be able to maintain their personal dignity. Dementia patients know they’re declining, but it’s important for them to also know that they can still do a lot of things.”
Practical and emotional support
Huo said the positive effects of empathy and support on emotional well-being, stress levels, communication and illness management can contribute to better overall health outcomes for individuals with dementia and their caregivers.
Researchers found that the support given by patients occurred in various ways, like practical help such as folding the laundry, dishwashing and cooking. Huo said the most common type of support was emotional, including showing appreciation and care.
“In one example, the caregiver had surgery and the dementia patient talked about how she brought flowers and food when she went to the hospital to see the caregiver, and she encouraged the caregiver to stay socially connected to make sure there’s companionship,” Huo said.
Caregiver, patient empathy
Researchers hope the findings can improve existing interventions that mostly target caregivers by including the dementia patients too.
“Dementia caregivers need support,” Huo said. “Support can occur within this ongoing relationship between dementia patients and caregivers. By maintaining the support reciprocity, we may be able promote effective illness management in the long run.”
This study was funded by the National Institute on Aging and the UC Davis Alzheimer’s Disease Research Center. Other listed authors on this paper are from Seoul National University in South Korea, the University of Texas at Austin and Pennsylvania State University.
Keep reading
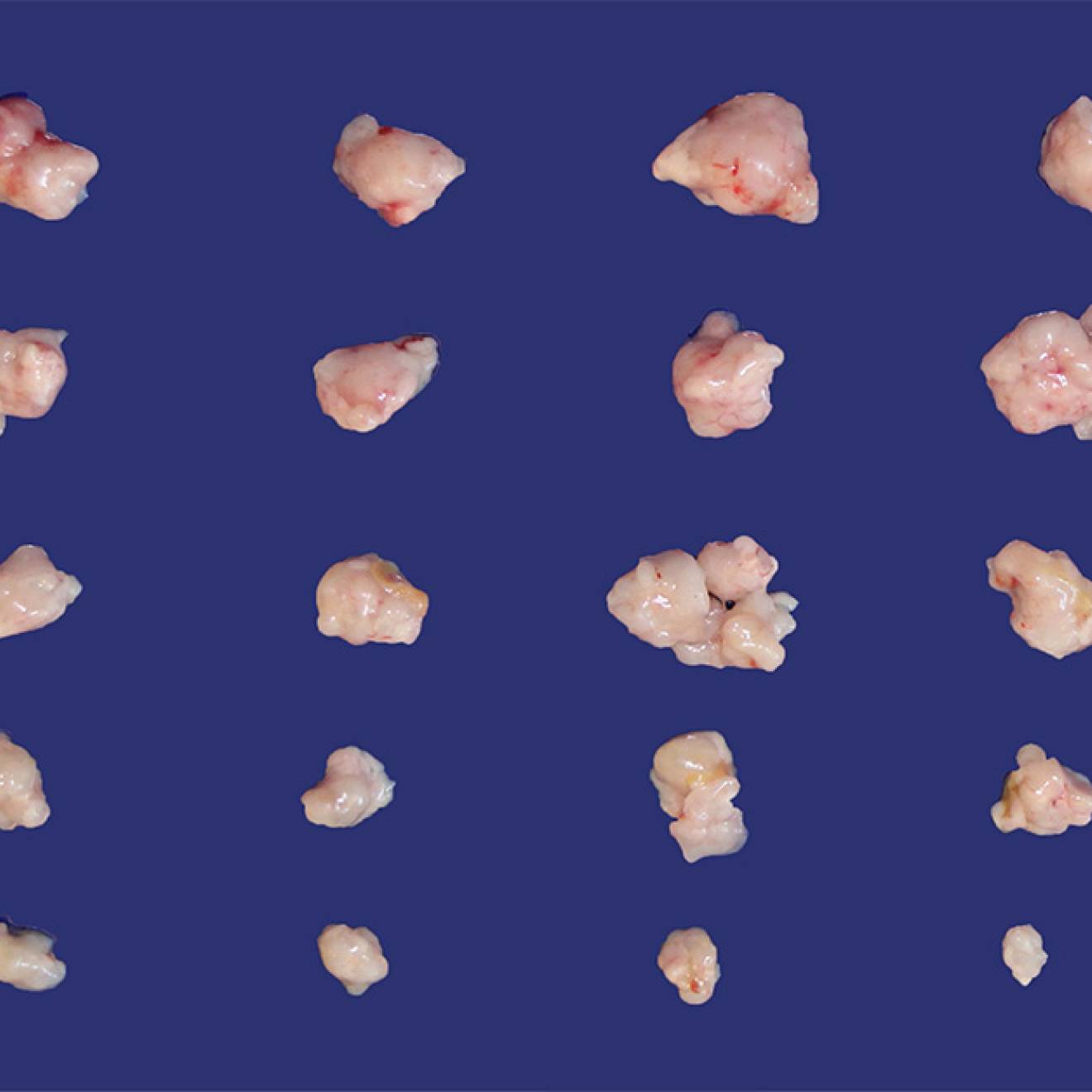
One-two punch: Novel drug pairing could beat pancreatic…
UC San Diego researchers find a combination of drugs outperformed other treatments in human and mouse models of pancreatic cancer; now urge clinical trial.
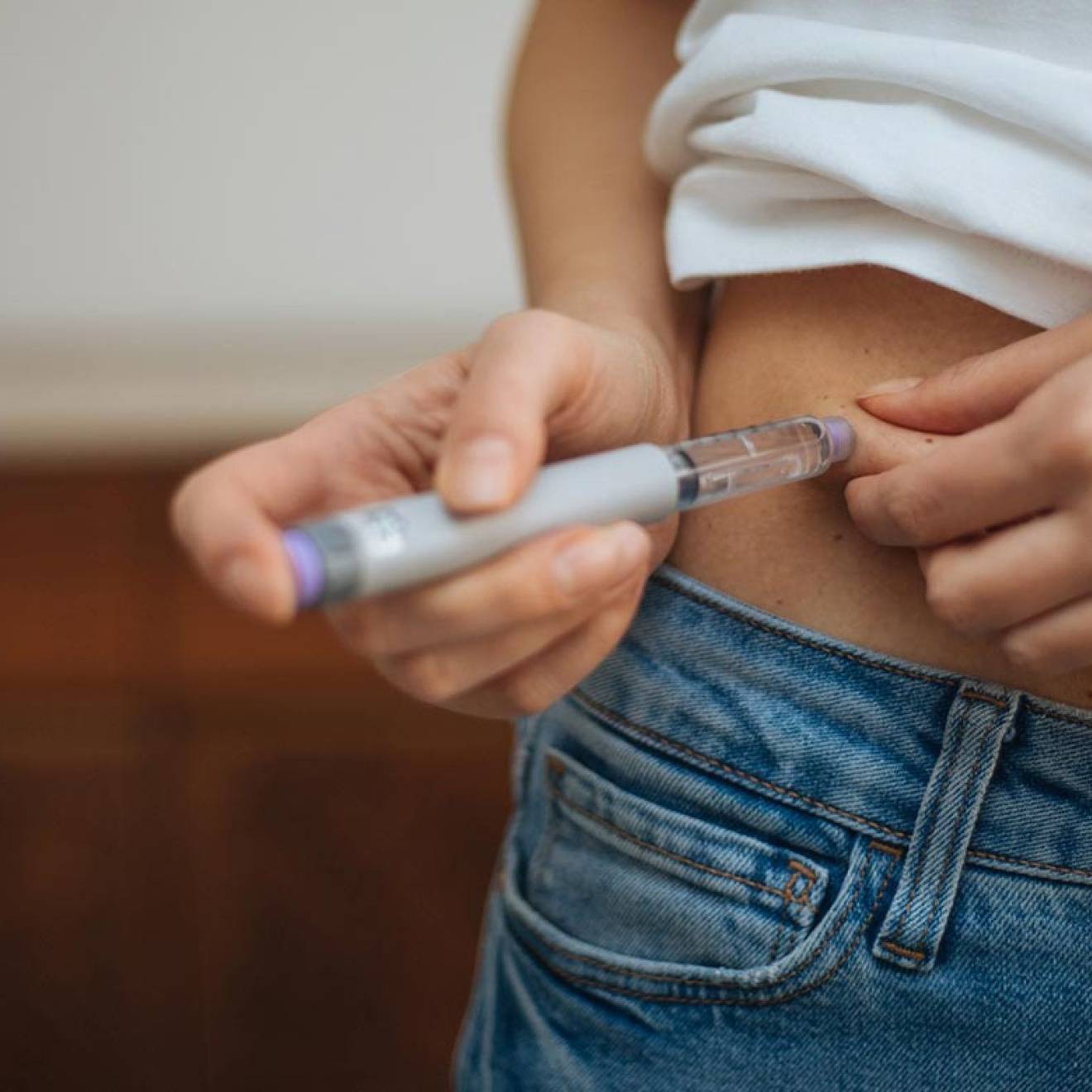
Are the newest weight loss drugs too good to be true?
What you need to know about Wegovy, Ozempic and Mounjaro

Risk factors for faster aging in the brain revealed in new study
Researchers from the Nuffield Department of Clinical Neurosciences at the University of Oxford have used data from UK Biobank participants to reveal that diabetes, traffic-related air pollution and alcohol intake are the most harmful out of 15 modifiable risk factors for dementia.
The researchers had previously identified a 'weak spot' in the brain, which is a specific network of higher-order regions that not only develop later during adolescence, but also show earlier degeneration in old age. They showed that this brain network is also particularly vulnerable to schizophrenia and Alzheimer's disease.
In this new study, published in Nature Communications , they investigated the genetic and modifiable influences on these fragile brain regions by looking at the brain scans of 40,000 UK Biobank participants aged over 45.
The researchers examined 161 risk factors for dementia, and ranked their impact on this vulnerable brain network, over and above the natural effects of age. They classified these so-called 'modifiable' risk factors -- as they can potentially be changed throughout life to reduce the risk of dementia -- into 15 broad categories: blood pressure, cholesterol, diabetes, weight, alcohol consumption, smoking, depressive mood, inflammation, pollution, hearing, sleep, socialisation, diet, physical activity, and education.
Prof. Gwenaëlle Douaud, who led this study, said: 'We know that a constellation of brain regions degenerates earlier in aging, and in this new study we have shown that these specific parts of the brain are most vulnerable to diabetes, traffic-related air pollution -- increasingly a major player in dementia -- and alcohol, of all the common risk factors for dementia.'
'We have found that several variations in the genome influence this brain network, and they are implicated in cardiovascular deaths, schizophrenia, Alzheimer's and Parkinson's diseases, as well as with the two antigens of a little-known blood group, the elusive XG antigen system, which was an entirely new and unexpected finding.'
Prof. Lloyd Elliott, a co-author from Simon Fraser University in Canada, concurs: 'In fact, two of our seven genetic findings are located in this particular region containing the genes of the XG blood group, and that region is highly atypical because it is shared by both X and Y sex chromosomes. This is really quite intriguing as we do not know much about these parts of the genome; our work shows there is benefit in exploring further this genetic terra incognita .'
Importantly, as Prof. Anderson Winkler, a co-author from the National Institutes of Health and The University of Texas Rio Grande Valley in the US, points out: 'What makes this study special is that we examined the unique contribution of each modifiable risk factor by looking at all of them together to assess the resulting degeneration of this particular brain 'weak spot'. It is with this kind of comprehensive, holistic approach -- and once we had taken into account the effects of age and sex -- that three emerged as the most harmful: diabetes, air pollution, and alcohol.'
This research sheds light on some of the most critical risk factors for dementia, and provides novel information that can contribute to prevention and future strategies for targeted intervention.
- Brain Tumor
- Healthy Aging
- Alzheimer's Research
- Nervous System
- Disorders and Syndromes
- Alzheimer's
- Brain Injury
- Air pollution
- Diabetic diet
- Multi-infarct dementia
- Environmental impact assessment
- Dementia with Lewy bodies
- Diabetes mellitus type 1
Story Source:
Materials provided by University of Oxford . Note: Content may be edited for style and length.
Journal Reference :
- Jordi Manuello, Joosung Min, Paul McCarthy, Fidel Alfaro-Almagro, Soojin Lee, Stephen Smith, Lloyd T. Elliott, Anderson M. Winkler, Gwenaëlle Douaud. The effects of genetic and modifiable risk factors on brain regions vulnerable to ageing and disease . Nature Communications , 2024; 15 (1) DOI: 10.1038/s41467-024-46344-2
Cite This Page :
Explore More
- Illuminating Oxygen's Journey in the Brain
- DNA Study IDs Descendants of George Washington
- Heart Disease Risk: More Than One Drink a Day
- Unlocking Supernova Stardust Secrets
- Why Do Some Memories Become Longterm?
- Cell Division Quality Control 'Stopwatch'
- What Controls Sun's Differential Rotation?
- Robot, Can You Say 'Cheese'?
- Researchers Turn Back the Clock On Cancer Cells
- Making Long-Term Memories: Nerve-Cell Damage
Trending Topics
Strange & offbeat.
6.9 million Americans have Alzheimer's disease: How to reduce your risk

A new report estimates 6.9 million older Americans are living with Alzheimer’s disease in 2024, an increase of about 200,000 cases of the mind-robbing disease from 2023 and "a significant public health crisis," according to an expert.
Another 5 million to 7 million adults have mild cognitive impairment, a set of early changes to memory and thinking linked to Alzheimer's, according to an Alzheimer's Association's annual facts and figures report released Wednesday.
The report also highlights good news. Other studies indicate that dementia rates have declined over the past 25 years as more adults are achieving higher levels of education, staying active and exercising, reducing their blood pressure, avoiding cigarettes and staying socially engaged.
Adults face a higher risk of Alzheimer's and other types of dementia as they age, and the number of Americans 65 and older is projected to swell from 58 million in 2022 to 82 million in 2050. In just six years, the youngest baby boomers will be 65.
The nation's aging population will create profound economic and social challenges. The annual cost of caring for people with Alzheimer’s or other types of dementia will be $360 billion in 2024, up $15 billion from a year ago, the report said.
Medicare and Medicaid will cover the bulk of that, spending $231 billion this year to care for people with Alzheimer’s and dementia. Public and private spending to take care of Alzheimer's and dementia patients will skyrocket to nearly $1 trillion in 2050, the report projects.
"Our population is aging, so we really need to address these issues," said Sam Fazio, the Alzheimer Association's senior director of quality care and psychosocial research. "Alzheimer's disease remains a significant public health crisis."
Lifestyle changes reduce risk
Other Alzheimer's experts not involved with the report said more Americans are taking steps to reduce their risk for Alzheimer's or dementia.
Research suggests up to 40% of dementia cases can be prevented through lifestyle changes, said Dr. Keith Vossel, a neurologist and director of the Mary S. Easton Center for Alzheimer’s Research and Care at the University of California, Los Angeles.
Vossel said people who exercise regularly, do not smoke and achieve higher levels of education tend to have lower risk. Reducing blood pressure in midlife, in particular, is linked to lower risk, he said.
Paying close attention to elevated blood pressure is especially important, Vossel said. "We know that lowering blood pressure among people with elevated blood pressure in middle life can lower risk of dementia or (mild cognitive impairment) later on."
Caregivers spend 31 hours a week on Alzheimer's, dementia patients
Families and other caregivers take on an array of tasks, scheduling appointments and feeding and caring for people with Alzheimer's or dementia. The report said 11.5 million relatives and caregivers provided more than 18 million hours of unpaid care last year.
That amounted to a full-time job for caregivers who spent an average of nearly 31 hours a week caring for a person with Alzheimer's or dementia.
In July, the Centers for Medicare & Medicaid Services will launch an initiative to improve the quality of life for people with dementia, allowing them to remain at home and reduce the strain on unpaid caregivers. The model, called Guiding an Improved Dementia Experience , will coordinate care and provide a 24/7 support line. Families also can access care navigators who can connect patients and caregivers to services and support. Doctors and clinics who participate will receive a monthly per-patient fee from Medicare.
Fazio said access to navigators is crucial because the report showed that families live through a great deal of stress and that workers in the field believe the health care system is not equipped to help people living with dementia. President Joe Biden recently expanded a similar navigator plan for cancer patients in which private health insurers will cover such services.
Families "really want help and need help to navigate the system," Fazio said.
New drugs, old target
Of the eight drugs approved for Alzheimer's patients, only two attempt to attack the disease and slow memory and cognitive decline. Biogen has discontinued one of those drugs, aducanumab, sold under the brand Aduhelm. The Food and Drug Administration approved the drug despite mixed clinical trial results . Biogen also faced withering criticism when it launched Aduhelm, initially priced at $56,000 a year.
In January 2023, Eisai won FDA approval for its amyloid beta-busting drug , lecanemab. Sold under the brand name Leqembi, the drug is intended for patients in the early stages of the disease, the population studied in clinical trials.
The Alzheimer's Association report notes that the benefits of lecanemab "in the short term may be imperceptible" because it's designed to slow the disease, not reverse cognitive decline. The report said the long-term results of the drug are not clear.
Earlier this month, the FDA delayed action for Eli Lilly's drug donanemab , the drug manufacturer said. The FDA expects to convene an advisory committee to discuss the treatment.
Clinical trials of all three amyloid-removing drugs have side effects visible on brain scans, such as brain swelling and bleeding. Some patients don't notice symptoms. Others have experienced headaches, dizziness, nausea, confusion and vision changes.
Though drugmakers largely have focused on drugs to target and clear amyloid from the brains of Alzheimer's patients, the report says, other studies are examining different methods of attacking the disease. Other potential drugs are being studied to limit the accumulation of tau protein, inflammation, altered cell metabolism and damage from toxic oxygen molecules, the report said.
Ken Alltucker is on Twitter at @kalltucker, or can be emailed at [email protected].
March 27, 2024
Does Long-Term Benadryl Use Increase Dementia Risk?
Benadryl, which contains diphenhydramine, is a drugstore mainstay and just one medication out of many that could possibly damage brain health
By Hannah Seo
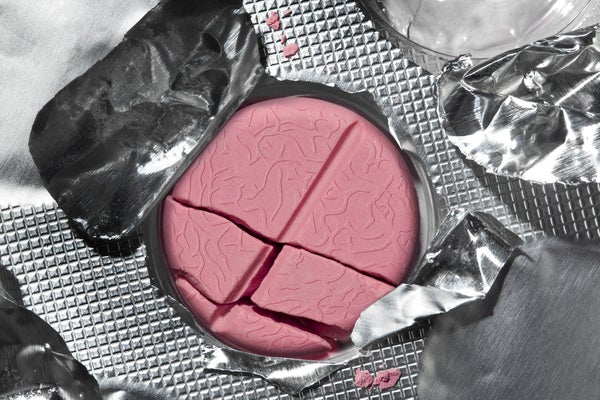
John Kuczala/Getty Images
In the past few months TikTok videos about the over-the-counter antihistamine Benadryl have gone viral because of research suggesting that long-term use of the popular drug is associated with an increased risk of developing dementia. And similar effects on memory and cognition have also been suggested for dozens of other common medications.
Benadryl is a brand-name medication that contains diphenhydramine, the active ingredient in many allergy, cold and anti-itch drugs. It can cause significant drowsiness and is also found in several sleep aids. Diphenhydramine has anticholinergic effects, meaning it blocks the action of a neurotransmitter called acetylcholine. Medical uses of anticholinergics go beyond allergy relief; drugs in this class have long been used as prescription tricyclic antidepressants and incontinence treatments, as well as over-the-counter sleep aids. But experts have been finding evidence that links anticholinergics to increased dementia risks. “That’s now clear, and we have plenty of data to back it up,” says Malaz Boustani, a geriatrician and neuroscientist at the Indiana University School of Medicine. He and other researchers are now trying to determine whether anticholinergics really are contributing to dementia development in any way—and if so, what exactly is happening in the brains of vulnerable adults.
Anticholinergics inhibit the parasympathetic nervous system , which regulates rest and stress responses. The drugs do this by binding to brain cell receptors and blocking acetylcholine, a neurotransmitter that plays a part in many bodily systems, including heart regulation, muscle contractions, urination and digestion. Acetylcholine also affects attention and memory—and a lack of the neurotransmitter in the brain has been linked to Alzheimer’s disease . When bound to receptors, anticholinergics prevent acetylcholine from acting on the parasympathetic nervous system, often causing effects such as dry mouth. These medications can also make people groggy by blocking histamine receptors, which are involved in alertness as well as allergies. Side effects should fade once the drug is out of someone’s system (which in Benadryl’s case would be within a couple of days). But Boustani says less is known about the effects of regular or heavy use.
On supporting science journalism
If you're enjoying this article, consider supporting our award-winning journalism by subscribing . By purchasing a subscription you are helping to ensure the future of impactful stories about the discoveries and ideas shaping our world today.
A 2015 paper in JAMA Internal Medicine offered some striking insight into possible long-term effects. The hallmark study—which became the basis for the recent viral TikToks—observed adults 65 years or older who had been taking various kinds and amounts of anticholinergic drugs for 10 years. The group reporting the highest amount of such use had a 54 percent increased risk of developing dementia. Since the study, a steady stream of new research has corroborated this link.
The studies show an association between dementia risk and the quantity and frequency of anticholinergic use. One capsule of Benadryl to relieve an allergy every once in a while probably won’t affect someone all that much, but repeated doses of anticholinergic drugs over months or years pose a greater risk, Boustani says. Taking multiple anticholinergics for multiple conditions may also increase your exposure and could raise the risk further, he adds. Another consideration is that some of these drugs have more potent effects than others. Boustani’s team created the Anticholinergic Cognitive Burden (ACB) Scale , a list of drugs with scores based on their potential negative effects on the brain. The scale goes from 1 (possibly an anticholinergic burden) to 3 (definitely a strong anticholinergic burden), Boustani says. For example, someone taking three anticholinergic drugs, all with a score of 1, will have a total anticholinergic cognitive burden score of 3. Boustani says that person may see roughly the same cumulative effects as someone who just takes one anticholinergic with a score of 3. There are currently no protocols or recommendations for doctors and pharmacists to consider a person’s ACB score when prescribing drugs. But the American Geriatrics Society maintains a list of drugs older people should avoid, and it does include many anticholinergic medications.
Researchers are still digging into the specific brain mechanisms that may be involved in elevated dementia risk from these drugs, Boustani says. A leading hypothesis suggests this might be related to how the drugs interact with neurotransmitter receptors. Long-term anticholinergic use might leave acetylcholine receptors perpetually blocked, triggering a series of reactions that either leads to the production of beta-amyloid—a toxic protein thought to be a main cause of dementia—or prevents the brain from clearing this protein out. Researchers have suggested these drugs might affect inflammation and blood flow in the brain, which may result in memory loss and weakened cognition. But so far this research is inconclusive.
Some experts are skeptical that anticholinergics play a role in developing dementia; they suspect the drugs may instead worsen underlying cognitive issues. Ariel Gildengers, a psychiatrist at the University of Pittsburgh, led a 2023 study that did not show a link between anticholinergics and developing dementia. But it did show a link between the drugs and mild cognitive impairment (MCI), a condition in which people have worse cognitive function than expected at their age but do not meet the criteria for dementia. And MCI does have a noticeable chance of turning into dementia. About 10 to 15 percent of people living with MCI develop dementia each year, according to the Alzheimer’s Association . “If you are in the earliest stages of dementia, then anticholinergics may kind of make the dementia more apparent without actually causing the dementia,” Gildengers says.
Research in this area has several major caveats, says Shelly Gray, a pharmacy professor at the University of Washington, who was the first author of the 2015 JAMA paper. “We cannot determine that anticholinergics actually cause dementia” because the 2015 study and others on the relationship have been observational, she says. Clinical trials are needed to confirm causation. Most of these studies are also done in adults aged 65 and older, who are more likely to develop dementia than younger adults and more likely to face medical conditions such as insomnia, which are often treated with anticholinergics. Gray, Boustani and Gildengers say that they keep these possible confounding factors in mind when they design and analyze their studies.
Because research has focused mostly on older adults, there is less evidence on whether younger people who take anticholinergics have increased risk of dementia later in life. Gray says it’s generally a good idea to avoid anticholinergics, however, especially if you’re older. “A big reason why we're seeing anticholinergic medications used by older adults is because they are available over the counter,” Gray says. Many readily available medications have anticholinergic active ingredients, including Benadryl, as well as the antihistamine Dimetapp (which contains the drug brompheniramine), the motion sickness medication Dramamine (dimenhydrinate) and the sleep aid Unisom (doxylamine).
Many anticholinergics can be swapped with other options, Gray says. For example, studies show that cognitive behavioral therapy is often effective for insomnia. "Second-generation antihistamines,” such as those in Claritin and Zyrtec, along with steroid-based nasal sprays such as Flonase, will counteract allergy symptoms without targeting acetylcholine receptors—says Jyothi Tirumalasetty, a clinical assistant professor of medicine at Stanford University, who specializes in allergy and immunology.
The Food and Drug Administration first approved Benadryl as a prescription antihistamine in 1946 and for over-the-counter sales in 1980s. Given the research on potential risks, Tirumalasetty says the FDA might evaluate the drug’s safety differently today and may consider making it available only through prescription. The FDA did not respond to a request for comment by the time of publication . In an e-mail to Scientific American, Kenvue (formerly a division of Johnson & Johnson and Benadryl’s current proprietor) wrote, “We are not aware of any studies that show a causal link between labeled use of diphenhydramine, and an increased risk of developing dementia. Diphenhydramine is an ingredient which is generally recognized as safe and effective by the FDA for [over-the-counter] use.”
Researchers are continuing to investigate anticholinergics and their possible long-term effects on brain health, hoping to help with future clinical and regulation guidance. Boustani says many questions persist, such as how the timing of taking or discontinuing these drugs affects the development of future memory problems.
For now, Boustani is focused on empowering his patients, especially older adults, with the ability to understand their medications and any attendant risks. He says people taking or considering an anticholinergic at any age should ask themselves a few questions: “Are there any alternatives? What symptoms am I addressing, and is alleviating them worth this risk? What is the smallest dose and time period I can take this medication for?”
“The brain is a precious organ,” Boustani says. “There is no health without brain health.”
Featured Clinical Reviews
- Screening for Atrial Fibrillation: US Preventive Services Task Force Recommendation Statement JAMA Recommendation Statement January 25, 2022
- Evaluating the Patient With a Pulmonary Nodule: A Review JAMA Review January 18, 2022
- Download PDF
- CME & MOC
- Share X Facebook Email LinkedIn
- Permissions
Skin Biopsy Detection of Phosphorylated α-Synuclein in Patients With Synucleinopathies
- 1 Department of Neurology, Beth Israel Deaconess Medical Center, Harvard Medical School, Boston, Massachusetts
- 2 HonorHealth Neurology, Scottsdale, Arizona
- 3 CND Life Sciences, Scottsdale, Arizona
- 4 Department of Neurology, Mayo Clinic College of Medicine, Scottsdale, Arizona
- 5 Evergreen Health, Kirkland, Washington
- 6 Department of Neurology, Carver College of Medicine, University of Iowa, Iowa City
- 7 Department of Neurology, Northshore University Health System, Glenview, Illinois
- 8 Movement Disorder Center of Arizona, Scottsdale
- 9 Department of Neurology, University of California, San Diego
- 10 Department of Neurology, University of California, San Franscisco
- 11 Department of Neurology, New York University Grossman School of Medicine, New York
- 12 Parkinson’s Disease Treatment Center of Southwest Florida, Port Charlotte
- 13 Department of Neurology, New York University Grossman Long Island School of Medicine, New York
- 14 Parkinson’s Disease and Movement Disorders Center of Boca Raton, Boca Raton, Florida
- 15 Department of Neurology, Swedish Medical Center, Seattle, Washington
- 16 Rocky Mountain Movement Disorders Center, Englewood, Colorado
- 17 Department of Neurology, University of Utah, Salt Lake City
- 18 Department of Neurology, Duke University School of Medicine, Durham, North Carolina
- 19 Department of Neurology, University of Florida Health Center, Gainesville
- 20 Department of Neurology, Stanford University Medical Center, Palo Alto, California
- 21 Center for Neurosciences, Tuscan, Arizona
- 22 The Neurology Center of Southern California, Carlsbad
- 23 Department of Neurology, Boston Medical Center, Boston, Massachusetts
- 24 Aventura Associates, Aventura, Florida
- 25 Department of Neurology, Mayo Clinic Rochester, Rochester, New York
- 26 Texas Movement Disorder Specialists, Georgetown
- 27 Department of Neurology, The University of Texas Southwestern Medical Center, Dallas
- 28 Department of Neurology, Lahey Clinic, Burlington, Massachusetts
Question Can skin biopsy detect phosphorylated α-synuclein in individuals with synucleinopathies such as Parkinson disease (PD), multiple system atrophy (MSA), dementia with Lewy bodies (DLB), and pure autonomic failure (PAF)?
Finding In this cross-sectional study of 428 participants, the proportions of individuals with cutaneous phosphorylated α-synuclein detected by skin biopsy were 92.7% with PD, 98.2% with MSA, 96.0% with DLB, 100% with PAF, and 3.3% with no history of synucleinopathy (controls).
Meaning A high proportion of individuals meeting clinical consensus criteria for PD, DLB, MSA, and PAF had a skin biopsy positive for phosphorylated α-synuclein in this study, although further research is needed to validate the findings in unselected clinical populations.
Importance Finding a reliable diagnostic biomarker for the disorders collectively known as synucleinopathies (Parkinson disease [PD], dementia with Lewy bodies [DLB], multiple system atrophy [MSA], and pure autonomic failure [PAF]) is an urgent unmet need. Immunohistochemical detection of cutaneous phosphorylated α-synuclein may be a sensitive and specific clinical test for the diagnosis of synucleinopathies.
Objective To evaluate the positivity rate of cutaneous α-synuclein deposition in patients with PD, DLB, MSA, and PAF.
Design, Setting, and Participants This blinded, 30-site, cross-sectional study of academic and community-based neurology practices conducted from February 2021 through March 2023 included patients aged 40 to 99 years with a clinical diagnosis of PD, DLB, MSA, or PAF based on clinical consensus criteria and confirmed by an expert review panel and control participants aged 40 to 99 years with no history of examination findings or symptoms suggestive of a synucleinopathy or neurodegenerative disease. All participants completed detailed neurologic examinations and disease-specific questionnaires and underwent skin biopsy for detection of phosphorylated α-synuclein. An expert review panel blinded to pathologic data determined the final participant diagnosis.
Exposure Skin biopsy for detection of phosphorylated α-synuclein.
Main Outcomes Rates of detection of cutaneous α-synuclein in patients with PD, MSA, DLB, and PAF and controls without synucleinopathy.
Results Of 428 enrolled participants, 343 were included in the primary analysis (mean [SD] age, 69.5 [9.1] years; 175 [51.0%] male); 223 met the consensus criteria for a synucleinopathy and 120 met criteria as controls after expert panel review. The proportions of individuals with cutaneous phosphorylated α-synuclein detected by skin biopsy were 92.7% (89 of 96) with PD, 98.2% (54 of 55) with MSA, 96.0% (48 of 50) with DLB, and 100% (22 of 22) with PAF; 3.3% (4 of 120) of controls had cutaneous phosphorylated α-synuclein detected.
Conclusions and Relevance In this cross-sectional study, a high proportion of individuals meeting clinical consensus criteria for PD, DLB, MSA, and PAF had phosphorylated α-synuclein detected by skin biopsy. Further research is needed in unselected clinical populations to externally validate the findings and fully characterize the potential role of skin biopsy detection of phosphorylated α-synuclein in clinical care.
Read More About
Gibbons CH , Levine T , Adler C, et al. Skin Biopsy Detection of Phosphorylated α-Synuclein in Patients With Synucleinopathies. JAMA. Published online March 20, 2024. doi:10.1001/jama.2024.0792
Manage citations:
© 2024
Artificial Intelligence Resource Center
Cardiology in JAMA : Read the Latest
Browse and subscribe to JAMA Network podcasts!
Others Also Liked
Select your interests.
Customize your JAMA Network experience by selecting one or more topics from the list below.
- Academic Medicine
- Acid Base, Electrolytes, Fluids
- Allergy and Clinical Immunology
- American Indian or Alaska Natives
- Anesthesiology
- Anticoagulation
- Art and Images in Psychiatry
- Artificial Intelligence
- Assisted Reproduction
- Bleeding and Transfusion
- Caring for the Critically Ill Patient
- Challenges in Clinical Electrocardiography
- Climate and Health
- Climate Change
- Clinical Challenge
- Clinical Decision Support
- Clinical Implications of Basic Neuroscience
- Clinical Pharmacy and Pharmacology
- Complementary and Alternative Medicine
- Consensus Statements
- Coronavirus (COVID-19)
- Critical Care Medicine
- Cultural Competency
- Dental Medicine
- Dermatology
- Diabetes and Endocrinology
- Diagnostic Test Interpretation
- Drug Development
- Electronic Health Records
- Emergency Medicine
- End of Life, Hospice, Palliative Care
- Environmental Health
- Equity, Diversity, and Inclusion
- Facial Plastic Surgery
- Gastroenterology and Hepatology
- Genetics and Genomics
- Genomics and Precision Health
- Global Health
- Guide to Statistics and Methods
- Hair Disorders
- Health Care Delivery Models
- Health Care Economics, Insurance, Payment
- Health Care Quality
- Health Care Reform
- Health Care Safety
- Health Care Workforce
- Health Disparities
- Health Inequities
- Health Policy
- Health Systems Science
- History of Medicine
- Hypertension
- Images in Neurology
- Implementation Science
- Infectious Diseases
- Innovations in Health Care Delivery
- JAMA Infographic
- Law and Medicine
- Leading Change
- Less is More
- LGBTQIA Medicine
- Lifestyle Behaviors
- Medical Coding
- Medical Devices and Equipment
- Medical Education
- Medical Education and Training
- Medical Journals and Publishing
- Mobile Health and Telemedicine
- Narrative Medicine
- Neuroscience and Psychiatry
- Notable Notes
- Nutrition, Obesity, Exercise
- Obstetrics and Gynecology
- Occupational Health
- Ophthalmology
- Orthopedics
- Otolaryngology
- Pain Medicine
- Palliative Care
- Pathology and Laboratory Medicine
- Patient Care
- Patient Information
- Performance Improvement
- Performance Measures
- Perioperative Care and Consultation
- Pharmacoeconomics
- Pharmacoepidemiology
- Pharmacogenetics
- Pharmacy and Clinical Pharmacology
- Physical Medicine and Rehabilitation
- Physical Therapy
- Physician Leadership
- Population Health
- Primary Care
- Professional Well-being
- Professionalism
- Psychiatry and Behavioral Health
- Public Health
- Pulmonary Medicine
- Regulatory Agencies
- Reproductive Health
- Research, Methods, Statistics
- Resuscitation
- Rheumatology
- Risk Management
- Scientific Discovery and the Future of Medicine
- Shared Decision Making and Communication
- Sleep Medicine
- Sports Medicine
- Stem Cell Transplantation
- Substance Use and Addiction Medicine
- Surgical Innovation
- Surgical Pearls
- Teachable Moment
- Technology and Finance
- The Art of JAMA
- The Arts and Medicine
- The Rational Clinical Examination
- Tobacco and e-Cigarettes
- Translational Medicine
- Trauma and Injury
- Treatment Adherence
- Ultrasonography
- Users' Guide to the Medical Literature
- Vaccination
- Venous Thromboembolism
- Veterans Health
- Women's Health
- Workflow and Process
- Wound Care, Infection, Healing
- Register for email alerts with links to free full-text articles
- Access PDFs of free articles
- Manage your interests
- Save searches and receive search alerts
Study finds 3 big risk factors for dementia

Diabetes, air pollution and alcohol consumption could be the biggest risk factors for dementia, a study has found.
Researchers compared modifiable risk factors for dementia — which is characterized by the impairment of memory, thinking and reasoning — and studied how these factors appear to affect certain brain regions that are already particularly vulnerable to Alzheimer’s disease and schizophrenia.
The research, based on brain scans of nearly 40,000 adults, between ages 44 and 82, in Britain was published Wednesday in Nature Communications.
These vulnerable regions of the brain develop during adolescence and help the brain process and integrate “bits of information across different modalities, across different senses,” said Gwenaëlle Douaud , an associate professor at the University of Oxford and co-author of the study. But “they’re the first ones to go when we start aging.”
“What we’re trying to do is say: What are the common risk factors for dementia that are affecting these regions?” Douaud said. “These are the three most harmful but then, obviously, the others, they have an effect.”
- Researchers investigated the genetic and modifiable risk factors that contribute to the vulnerability of the “most fragile parts of the brain” by studying the brain scans of nearly 40,000 relatively healthy participants from the U.K. Biobank.
- The study examined 161 modifiable risk factors, including blood pressure, cholesterol, diabetes, weight, alcohol consumption, smoking, mood, inflammation, pollution, hearing, sleep, socialization, diet, physical activity and education.
- A diagnosis of diabetes, the amount of nitrogen dioxide in the air and how often someone drinks alcohol — from never to daily, or nearly every day — were found to be the three most detrimental risk factors to these regions of the brain, Douaud said.
- Diabetes, air pollution and alcohol consumption each has an effect that is about twice as much as the other leading risk factors, Douaud said. The next major risk factors are sleep, weight, smoking and blood pressure.
- Researchers identified seven genetic clusters that affect these vulnerable parts of the brain, some of which are also associated with Parkinson’s and Alzheimer’s diseases. Douaud said the genetic and modifiable risk factors are not comparable.
More than 55 million people live with dementia around the world, and that figure is expected to increase to 153 million by 2050, according to the World Health Organization.
Get Well+Being tips straight to your inbox

Dementia is the loss of cognitive function, and symptoms result from brain neurons losing their connection to other brain cells and eventually dying, according to the National Institute on Aging . Everyone loses neurons over time, but the loss is more significant in dementia patients.
Diabetes and alcohol consumption “have been consistently shown to be associated with both cerebral and cognitive decline,” the researchers wrote in the Nature Communications study. And there is growing evidence that exposure to air pollution is a risk factor for cognitive decline and dementia.
A 2020 Lancet report on dementia found that a dozen modifiable risk factors such as hypertension, hearing impairment, smoking and obesity together account for up to 40 percent of dementia cases worldwide.
A person’s age, genes, family history, a traumatic brain injury or a stroke also are potential risk factors .
What other experts say
Gill Livingston , a professor of psychiatry of older people at University College London and the lead author of the 2020 Lancet report, said that the new study was “very interesting” but that the participants in the U.K. Biobank are a “much healthier” and highly motivated group. The findings may not be applicable to a broader population.
Still, they show that people can make decisions to reduce their risk of cognitive decline as they age, Livingston said.
“There’s quite a lot people can do in their everyday life to maintain cognitive health ,” she said. “This just reinforces it.”
What you can do about it
Try to eat a healthy, varied diet to help lower your blood sugar, take measures to protect against “traffic-related pollution” and drink alcohol in moderation, Douaud said in an email.
“Of course, some of these should not only be down to individuals, and the burden should also be shared with (local) governments devising helpful policies,” she said.
Livingston said social and physical activity — talking with friends and exercising — “make a huge difference.” And stimulating experiences, walking outside , “seeing different things” can be beneficial, she said.
Hearing loss , which is more likely as a person ages, can take away opportunities for conversations that lead to “rapid brain stimulation,” Livingston said.
“And if you smoke, stop,” she said.
Marlene Cimons contributed to this report.
Read more from Well+Being
Well+Being shares news and advice for living well every day. Sign up for our newsletter to get tips directly in your inbox.
Vitamin B12 for fatigue has no proven benefit unless you have a deficiency that causes anemia.
Flashes, shimmers and blind spots: Here’s what migraine aura looks like.
Sparkling water is a better choice for your teeth than most popular beverages.
You can help your brain form healthy habits and break the bad ones.
Our 7 best tips to build an exercise habit .


An official website of the United States government
The .gov means it’s official. Federal government websites often end in .gov or .mil. Before sharing sensitive information, make sure you’re on a federal government site.
The site is secure. The https:// ensures that you are connecting to the official website and that any information you provide is encrypted and transmitted securely.
- Publications
- Account settings
Preview improvements coming to the PMC website in October 2024. Learn More or Try it out now .
- Advanced Search
- Journal List
- Indian J Psychol Med
- v.43(5 Suppl); 2021 Sep
Ethical Issues in Dementia Research
Mina chandra.
1 Centre of Excellence in Mental Health, Atal Bihari Vajpayee Institute of Medical Sciences and Dr Ram Manohar Lohia Hospital, New Delhi, Delhi, India.
Vijay Harbishettar
2 Health Heal Geriatric Healthcare Services, Bengaluru, Karnataka, India.
Harbandna Sawhney
Shabbir amanullah.
3 Woodstock General Hospital, Woodstock, Ontario, Canada.
Dementia is a global public health issue with an urgent need for developing newer and more effective treatment strategies. Research in the area of dementia, however, poses unique ethical and legal challenges. Epidemiological studies, studies on pharmacological and non-pharmacological interventions have to deal with obtaining consent from persons with cognitive impairments, those from diverse cultural groups and need to contend with privacy and confidentiality issues. The caregiver support intervention research has not yet translated into policy change and effective clinical care. Biomedical research that involves invasive procedures may not translate into short- or long-term therapeutic benefits but is necessary research. Palliative care research in dementia has to deal with ethical issues involving people at end-of-life research. Proposed research may not receive approval, citing necessary safeguards to the vulnerable older people against invasive studies even when it is least invasive. This article aims to review the ethical aspects for safeguarding vulnerable older people with dementia and the potential challenges in conducting dementia research from a researcher’s perspective.
Some of the safeguards for ethical research include determining capacity to consent, obtaining advanced directives in early stages and proxy consent from caregivers, obtaining informed consent in cognitively impaired individuals. Future research policies need to consider the logistics of involving older people in research, enhancing caregiver support, and encouraging supportive decision-making. It will also need to address developing capacity assessment tools while addressing advanced care planning that will ensure the well-being of subjects in research.
Background:
Dementia has become a global public health issue, with hospitalization rates being 65% higher in seniors with dementia than others. 1 , 2 The pressures on healthcare systems mean an urgent need to develop robust preventive and treatment strategies for dementia, which requires multidisciplinary research. However, the patient’s stage of illness and ability to engage in discussions around the merits of participating in research and caregiver concerns is an important aspect of dementia research.
Hence, dementia research poses unique ethical challenges compared to populations with other diseases, which has led to the evolution of an ethical framework for dementia research. This article aims to review and give a viewpoint on the ethical aspects for safeguarding vulnerable older people with dementia and the potential challenges in conducting dementia research from a researcher’s perspective.
Materials and Methods
Systematic Review of PubMed was performed using the following search string to obtain studies on ethical issues in dementia research:
(dementia[Title] OR ncd[Title] OR neurocognitive[Title] OR alzheimer[Title] OR cognitive[Title] or OR cognition[Title] OR neuropsychology[Title] OR neuropsychological[Title] OR pick[Title] OR lewy[Title] OR frontotemporal[Title] OR huntington[Title] OR prion[Title]) AND (ethics[Title] OR ethical[Title]) AND (research[Title])
Seventy six results were obtained, which included publications from 1984 to 2021. Results were filtered for the availability of free full text and, subsequently, 15 studies were left. References within the articles were reviewed to obtain a comprehensive review.
These articles were reviewed for relevance, and all were found suitable for inclusion in this review.
These articles were evaluated in detail (authors MC and HS ) to create the first draft. The second draft incorporated the clinical and research experience of the authors who had varying levels of experience. An in-depth discussion was held with all authors regarding various aspects of the paper on a virtual platform. The inputs from all authors were combined to formulate a viewpoint on ethical issues in dementia research.
The methodology is illustrated in detail in Figure 1 .

Ethical Issues Across Specific Types of Dementia Research
Epidemiological studies in dementia.
Epidemiological studies have dealt with consent issues in cognitively impaired populations with low baseline educational attainment or culturally diverse populations. Furthermore, there are often privacy and confidentiality issues in field settings, issues around medical care, and rehabilitative measures for identified persons with dementia (PwD). This raises questions about basic ethical issues of beneficence and nonmaleficence. Another ethical aspect of dementia in low- and middle-income countries (LMIC) like India is the lack of culturally sensitive screening and evaluation instruments. Concerns about the validity of nosological criteria of dementia in uneducated, multiethnic, multilingual populations have not been adequately raised or addressed. There is a genuine concern that instruments designed for the Western population may not be appropriate and may end up mislabeling and stigmatizing some with only age-related cognitive impairment. This is especially significant given that the treatment gap in dementia in LMICs is more than 90%. 3
Dementia research has also investigated modifiable risk factors for dementia to identify targets for early intervention. Lower levels of educational attainment and decreased physical activity levels have been noted to be associated with Alzheimer’s disease (AD). 4 Hence, the population-level increase in educational status and physical activity levels can potentially reduce the risk of cognitive impairment.
Biomarker Research in Dementia
A major ethical issue in research on biomarkers in dementia has been a disproportionately excessive focus on AD, usually diagnosed as “probable AD” 5 even though other types of dementia commonly exist in clinical practice. Similarly, an emphasis on neuroimaging and biomarkers as indicators of dementia risk has not translated into clinical practice. 6 , 7 For example, it is known that up to 50% decrease in Cerebrospinal Fluid (CSF) Aβ 42 concentrations and twofold to threefold increase in CSF total tau increase in F2-isoprostanes occurs in AD or mild cognitive impairment. Still, these tests are not routinely performed in clinical practice either for diagnosis or prognosis. 8
This leads to a fundamental question on the nature of dementia research, which does not yield any diagnostic or therapeutic benefit to patients with dementia in the short or long term while subjecting cognitively impaired individuals for invasive and painful procedures.
Research on Pharmacological and Nonpharmacological Interventions for Dementia
Both pharmacological and nonpharmacological interventions have been studied for dementia. While pharmacological intervention can be standardized, nonpharmacological interventions may not use a standardized treatment manual, which is ethically questionable. There is also a need to develop standardized methodologies for assessing nonpharmacological interventions to reach a more robust conclusion.
Among pharmacological interventions, there have been extensive trials on acetylcholinesterase inhibitors and memantine. 9 However, since 2003, no new drug has been approved by the FDA to treat AD. More than 200 therapeutic agents have been assessed, and then either they are abandoned or have failed investigational programs. 10
Some Pharmaceutical companies like Pfizer have abandoned dementia research. 11
A pertinent ethical question is about the rights of the participants with dementia who participated in these failed trials in good faith.
Caregiver Support Interventions Research
Caregivers of patients of dementia face many challenges, with studies reporting 30%–55% of caregivers experience anxiety or depressive symptoms, which may adversely impact the quality of care provided to the patient. 12 – 14 Although studies have shown that psychosocial interventions may be effective in dementia caregivers to reduce their burden, they often face logistic barriers, stigma among caregivers, and a lack of structured instructional manuals. 15
The beneficial impact of psychosocial intervention for dementia includes delayed institutionalization of patients, improved symptomatology, and providing services that caregivers highly value. 16 However, despite robust evidence, caregiver interventions have not been translated into policy domain and clinical practice. This is not ethical considering caregivers have a significant burden because of caring for patients with dementia. The lack of translation of evidence-based, low-cost interventions for dementia in policy and clinical care across many nations globally raises important ethical questions for the future research.
Clinical Trials
For developing new drugs for the treatment of PwD, clinical trials are essential, but it requires well written informed consent assent process and rigorous documentation before recruitment. Participants will need more intense monitoring compared to cognitively intact individuals. 17
There is concern about the robustness of the informed consent process for clinical trials for dementia conducted in LMICs with a population with limited baseline educational attainment. In some situations, it may be necessary that an independent clinician may undertake capacity assessments and informed consent to get involved in research instead of those research staff involved in the study.
Genetic Testing
Genetic testing for dementia includes testings for symptomatic individuals (diagnostic testing) and asymptomatic at-risk individuals (predictive testing).
Advance directives may be useful in such cases. As genetic testing results may affect the patient and other family members, familial genetic counseling is essential before testing and for disclosure of results. 18
End of Life/Palliative Care Issues in Dementia Research
It may be difficult to determine the lifespan of patients in advanced stages of dementia, and many patients receive palliative care in hospices in high-income settings. 19 Dementia research may include some participants in palliative care or who enter palliative care during the research. This raises ethical dilemmas of prolonging life at the cost of quality of life to complete research. Transference of a patient in the end stages of life to an unfamiliar setting may also lead to ethical dilemmas. The ability to participate in research may vary based on the clinical condition, and this poses challenges for the researcher, having to reassess this every time. Many exclusions of research participants during research may not serve the purpose, and this poses an ethical question to those who are participating, whether the study serves its purpose.
Ethical Dilemmas in Multicentric International Research
The 10/66 Dementia Research Group found that even though 66% of people with dementia live in developing countries, less than 10% of dementia research is conducted. Underrepresentation of LMIC populations with large absolute numbers of dementia is a fundamental ethical issue in dementia research and must be addressed by policymakers and grant agencies.
Even within LMICs, ethnic, linguistic, and religious minorities are underrepresented, necessitating the development of novel strategies to improve the participation of minority groups. 20 Equitable partnership and participation in research across social groups is an important ethical goal in dementia research pertaining to rights of participation and justice.
Researchers lead most multicentric international dementia research in LMICs from high-income settings. It is often found that many such protocols contain research instruments that have not been culturally and linguistically validated for the target population resulting in underreporting or overreporting of cognitive impairment and dementia. In addition, the informed consent documents in multicentric international trials may not be adequately translated to cater to the informational needs of target populations with low educational attainment, as is often the case in LMICs. They may result in a lack of awareness of rights and compensation to participants.
Ethical Aspects of Data Sharing Agreement to Enhance Research Outputs
For dementia research to reach any breakthrough, it is important to use harmonized protocols and share anonymized data with strict precautions for confidentiality, privacy, and data stewardship for implementing big data analytics. Alzheimer Europe gave a report, “Data Sharing in Dementia Research,” which reviews the recent changes in research policy and gives recommendations to aid data sharing in dementia research. This is ethical utilization of limited resources for dementia research, but such initiatives are lacking in the Asian context. 21
Ethical Issues Specific to Dementia Research
Memory impairment, poor comprehension, hearing, or visual impairment at varying degrees is common in dementia patients. This poses a challenge for the investigators to obtain consent, and more participants with impaired ability to comprehend information will get excluded from the study. On the other hand, vulnerable PwD in institutional care may agree/disagree to participate in research due to possible coercion and fear. Some studies on PwD in advanced stages include PwD with sensory deficits, which are not likely to have competency. This makes such studies difficult due to ethical dilemmas and leaves a gap in the understanding of dementia and probable medical progress for the care of such patients. 22 – 24 The progressive and continuous nature of the illness may necessitate a periodic reassessment of capacity for consent to participate in the study.
If PwD cannot have impaired capacity to give consent, proxy consent from caregivers can be taken. 25
An ethical issue with dementia research is that persons without supportive family or carers may be excluded from the research (and its benefits) because they lack adequate representation. 26
In the case of proxy consent, the patient’s beliefs must be kept in mind while making a decision. 27 Advanced directive may help in this aspect. However, some ethical issues emerge when proxy decision-makers decide as per their beliefs about the right decision for the patient. 28 Some research has shown that the data provided by proxies may differ from data provided by PwD. 29 – 31 In the decision-making in dementia research by caregivers, it is essential for researchers or the ethical committees to ensure that there is no secondary gain by the caregiver considering the late stage of illness and issues around property, will, or any other form.
In dementia research, it is required to obtain both informed consent and assent. 32 Assent may be defined as “the agreement to participate in research-based upon less than full understanding.” 33 In contrast to informed consent, which requires an individual to understand the research protocol, 34 to give assent, an individual must only have a minimal level of understanding to make a meaningful choice. 35 An individual’s level of cooperation may be indicative of assent or dissent. 36 Frustration, discomfort, unhappiness, or passivity may indicate a lack of cooperation in research or dissent. 37 A study which audiotaped informed consent encounters for research concluded that any interpretation regarding assent should be made with caution for PwD. Also, along with the cognitive aspect, the emotional and social dimensions of informed consent warrant attention. 38
One of the main concerns for ethical issues in dementia is related to involving older people in research. Possible barriers may include physical and cognitive impairments, lack of transport, a lower threshold for burden, changes in routine, and negative beliefs about medication. 39 , 40 These factors may affect the risk–benefit ratio for participation in research. Issues of beneficence versus nonmaleficence may need to be addressed.
Recruitment and maintenance of older people in studies may also involve higher costs. 41
On the other hand, failure to include older people in studies could lead to inequities in healthcare and biased results. 42
A databases search (PubMed and CINAHL) has revealed that only 3%–6% of clinical trials were based on older populations. 40 Studies have shown that clinical trials may sometimes involve participants who are not representative of those for whom the medication is most likely to be used. Older individuals are often excluded from the trials. 43 , 44
Respect for autonomy may be difficult in PwDs on account of impaired cognition.
Researchers and Institutional Ethics Committees and Institutional Review Boards can uphold the ethical principles of respect, beneficence, and justice through the informed consent/assent process and objectively assess the risks and benefits of participation in research. 45
Higher involvement of caregivers in dementia research safeguards the rights of PwD.
Patient and caregiver should share a harmonious relationship. However, in some cases, PwD may object to being accompanied by a caregiver for participation in a study. 46 It has also been suggested that the involvement of the caretaker may amount to paternalism. 47 Table 1 highlights the ethical issues in dementia research.
Ethical Issues Specific to Dementia Research.
The progressive nature of the disease in dementia with poor interepisodic recovery, involvement of older age group, high caregiver burden, and issues around consent poses unique ethical challenges around dementia research. Developing decisional capacity assessment tools, advanced care planning, and a standardized approach to research would help in addressing the ethical barriers
- Involving the elderly population: Dementia research should include all age groups and all stages of severity so that results are not biased.
- Research tools: Standardized manuals for nonpharmacological interventions need to be developed for a more structured research approach. Furthermore, developing culturally and linguistically validated instruments and harmonized protocols would help in a uniform approach to research.
- Consent: Supportive decision/assent making should be done to ensure ethical safeguards. It should be assumed that PwD has capacity unless proved otherwise.
- Advanced care planning: In the early stages of dementia, advanced directives should be formed not just for clinical care but also for research participation. This would enhance research participation while upholding ethics.
Along with capacity assessment tools that check decision making in specific areas, knowledge of the patient’s hopes, beliefs, and personal history should also be used to document decision making and withdrawal of cons. 48
Data Safety Management Boards—Serious Adverse Events reporting as per established protocols enhances ethical conduct of dementia research. Scientists should make the study results accessible to the wider scientific community by sharing them in institutional repositories. This allows for secondary data research and avoids unnecessary research in a vulnerable population. At the same time, data sharing and attendant scrutiny increase confidence in research implementation as per ethical and legal regulatory framework.
Recommendations for the ethics committee and regulatory authority are illustrated in Table 2 .
Recommendations for Ethics Committee and Regulatory Authority
Declaration of Conflicting Interests: The authors declared no potential conflicts of interest with respect to the research, authorship, and/or publication of this article.
Funding: The authors received no financial support for the research, authorship, and/or publication of this article.
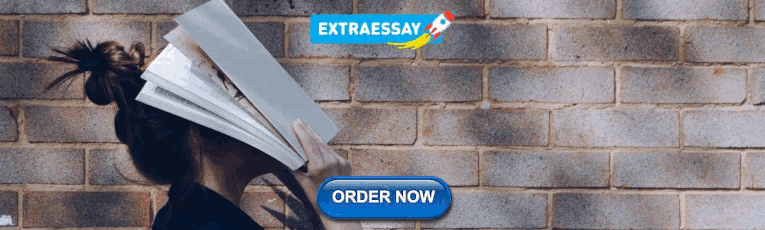
IMAGES
COMMENTS
INTRODUCTION. Dementia is a common public health problem. 1 Worldwide, approximately 47 million people have dementia and this number is expected to increase to 131 million by 2050. 1 Reductions in age-adjusted incidence of dementia have occurred in the United States (US) and other developed countries over the last 20 years, perhaps associated with increasing formal educational attainment.
Dementia Research Centre, UK Dementia Research Institute, University College London, London, UK ... to identify the evidence for advances likely to have the greatest impact since our 2017 paper and build on its work. Our interdisciplinary, international group of experts presented, debated, and agreed on the best available evidence. ...
1. Introduction. Alzheimer's disease (AD) (named after the German psychiatric Alois Alzheimer) is the most common type of dementia and can be defined as a slowly progressive neurodegenerative disease characterized by neuritic plaques and neurofibrillary tangles (Figure 1) as a result of amyloid-beta peptide's (Aβ) accumulation in the most affected area of the brain, the medial temporal ...
SUBMIT PAPER. Dementia is an international peer reviewed journal that acts as a major forum for social research of direct relevance to understanding lived experience and improving the quality of life, well-being, and quality of care for people with dementia, their families, and communities. View full journal description.
Dementia is a syndrome that involves severe loss of cognitive abilities as a result of disease or injury. Dementia caused by traumatic brain injury is often static, whereas dementia caused by ...
Importance Worldwide, 47 million people live with dementia and, by 2050, the number is expected to increase to 131 million.. Observations Dementia is an acquired loss of cognition in multiple cognitive domains sufficiently severe to affect social or occupational function. In the United States, Alzheimer disease, one cause of dementia, affects 5.8 million people.
The transforming landscape of dementia research. Rapid progress in the development of novel biomarkers and lifestyle interventions and the emergence of the first disease-modifying therapy have ...
On 4 October 2022, the WHO released a dementia research blueprint to support implementation of the Global action plan on the public health response to dementia in 2017-2025, representing a first ...
Observations: Dementia is an acquired loss of cognition in multiple cognitive domains sufficiently severe to affect social or occupational function. In the United States, Alzheimer disease, one cause of dementia, affects 5.8 million people. Dementia is commonly associated with more than 1 neuropathology, usually Alzheimer disease with ...
The randomization was stratified according to clinical subgroup (mild cognitive impairment due to Alzheimer's disease or mild Alzheimer's disease-related dementia on the basis of the ...
Modelling of the UK change suggests a 57% increase in the number of people with dementia from 2016 to 2040, 70% of that expected if age-specific incidence rates remained steady, 10 such that by 2040 there will be 1·2 million UK people with dementia.
Based on these procedures the clinical imaging has steered to a clever heading in finding of dementia. This paper is organized as follows: Section 2 discusses the importance of various neuroimaging techniques used for dementia detection using various neuroimaging modalities. ... Dementia research faces difficulties in determining the predictive ...
Abstract and Figures. Dementia is a neurodegenerative disorder characterized by progressive and continuous loss of cognitive functions. The neuropsychiatric symptoms include apathy; agitation and ...
The Alzheimer's Association is the largest non-profit funder of Alzheimer's disease research. Its mission is "to eliminate Alzheimer's disease through the advancement of research; to provide and enhance care and support for all affected; and to reduce the risk of dementia through the promotion of brain health". ISTAART. AAIC Conference.
Seven recent papers amplify advances in Alzheimer's research. New findings from big-data and open-science research are revealing clues about the molecular mechanisms of Alzheimer's disease and new ways to discover potential therapeutic targets and biomarkers. These new discoveries were made by six research teams participating in the ...
For etiological research, this calls for investigation of dementia without any assumptions of its cause, as excluding, for example, patients with stroke from studies about Alzheimer disease may falsely imply irrelevance of hypertension and alike in the cause of (clinical) Alzheimer disease. 65 This not only applies to dementia cases in which ...
Introduction. Alzheimer disease (AD) is one of the greatest medical care challenges of our century and is the main cause of dementia. In total, 40 million people are estimated to suffer from dementia throughout the world, and this number is supposed to become twice as much every 20 years, until approximately 2050. 1 Because dementia occurs mostly in people older than 60 years, the growing ...
Meta-analysis of genome-wide association studies on Alzheimer's disease and related dementias identifies new loci and enables generation of a new genetic risk score associated with the risk of ...
This research sheds light on some of the most critical risk factors for dementia, and provides novel information that can contribute to prevention and future strategies for targeted intervention. The paper 'The effects of genetic and modifiable risk factors on brain regions vulnerable to ageing and disease' is published in Nature ...
The final set of selected papers consisted of 75 research papers, among these final selected articles, each of the data modalities (image, clinical-variables, voice) contained 25 papers. ... Aside from image-based ML techniques for dementia prediction, several research studies have utilized clinical-variable data with ML algorithms to predict ...
Research led by the University of California, Davis, suggests that patients can also support their caretakers, and that reciprocated care has mutual benefits. A new paper published in the journal Aging and Mental Health highlights that couples coping with dementia can navigate the challenges with greater resilience and well-being by working ...
Acting now on dementia prevention, intervention, and . care will vastly improve living and dying for individuals with dementia and their families, and thus society. Introduction. Worldwide around 50 million people live with dementia, and this number is projected to increase to 152 million by 2050, 1. rising particularly in low-income and middle-
This research sheds light on some of the most critical risk factors for dementia, and provides novel information that can contribute to prevention and future strategies for targeted intervention. ...
Research suggests up to 40% of dementia cases can be prevented through lifestyle changes, said Dr. Keith Vossel, a neurologist and director of the Mary S. Easton Center for Alzheimer's Research ...
Increased research capacity is needed to address the important shortcomings of current dementia care. Good-quality care is of central importance throughout all phases of the illness, from ...
Research in this area has several major caveats, says Shelly Gray, a pharmacy professor at the University of Washington, who was the first author of the 2015 JAMA paper. "We cannot determine ...
Dementia is a clinical diagnosis requiring new functional dependence on the basis of progressive cognitive decline. It is estimated that 1.3% of the entire UK population, or 7.1% of those aged 65 or over, have dementia. Applying these to 2013 population estimates gives an estimated number of 19,765 people living with dementia in Northern Ireland.
Importance Finding a reliable diagnostic biomarker for the disorders collectively known as synucleinopathies (Parkinson disease [PD], dementia with Lewy bodies [DLB], multiple system atrophy [MSA], and pure autonomic failure [PAF]) is an urgent unmet need. Immunohistochemical detection of cutaneous phosphorylated α-synuclein may be a sensitive and specific clinical test for the diagnosis of ...
The research, based on brain scans of nearly 40,000 adults, between ages 44 and 82, in Britain was published Wednesday in Nature Communications. ... Dementia is the loss of cognitive function, ...
Abstract. Dementia is a global public health issue with an urgent need for developing newer and more effective treatment strategies. Research in the area of dementia, however, poses unique ethical and legal challenges. Epidemiological studies, studies on pharmacological and non-pharmacological interventions have to deal with obtaining consent ...