Global prevalence of autism: A systematic review update
Affiliations.
- 1 Montreal Neurological Institute-Hospital, Faculty of Medicine & Health Sciences, McGill University Montreal, Montreal, Canada.
- 2 Department of Psychiatry, Oregon Health and Science University, Portland, Oregon, USA.
- 3 Population Health Sciences, Department of Pediatrics, Waisman Center, University of Wisconsin-Madison, Madison, Wisconsin, USA.
- 4 Department of Global Health and Population, Harvard T H Chan School of Public Health, Boston, Massachusetts, USA.
- 5 Autism Speaks, New York City, New York, USA.
- PMID: 35238171
- PMCID: PMC9310578
- DOI: 10.1002/aur.2696
Prevalence estimates of autism are essential for informing public policy, raising awareness, and developing research priorities. Using a systematic review, we synthesized estimates of the prevalence of autism worldwide. We examined factors accounting for variability in estimates and critically reviewed evidence relevant for hypotheses about biological or social determinants (viz., biological sex, sociodemographic status, ethnicity/race, and nativity) potentially modifying prevalence estimates of autism. We performed the search in November 2021 within Medline for studies estimating autism prevalence, published since our last systematic review in 2012. Data were extracted by two independent researchers. Since 2012, 99 estimates from 71 studies were published indicating a global autism prevalence that ranges within and across regions, with a median prevalence of 100/10,000 (range: 1.09/10,000 to 436.0/10,000). The median male-to-female ratio was 4.2. The median percentage of autism cases with co-occurring intellectual disability was 33.0%. Estimates varied, likely reflecting complex and dynamic interactions between patterns of community awareness, service capacity, help seeking, and sociodemographic factors. A limitation of this review is that synthesizing methodological features precludes a quality appraisal of studies. Our findings reveal an increase in measured autism prevalence globally, reflecting the combined effects of multiple factors including the increase in community awareness and public health response globally, progress in case identification and definition, and an increase in community capacity. Hypotheses linking factors that increase the likelihood of developing autism with variations in prevalence will require research with large, representative samples and comparable autism diagnostic criteria and case-finding methods in diverse world regions over time. LAY SUMMARY: We reviewed studies of the prevalence of autism worldwide, considering the impact of geographic, ethnic, and socioeconomic factors on prevalence estimates. Approximately 1/100 children are diagnosed with autism spectrum disorder around the world. Prevalence estimates increased over time and varied greatly within and across sociodemographic groups. These findings reflect changes in the definition of autism and differences in the methodology and contexts of prevalence studies.
Keywords: autism; methodology; prevalence; risk factors; social determinants; time trends.
© 2022 The Authors. Autism Research published by International Society for Autism Research and Wiley Periodicals LLC.
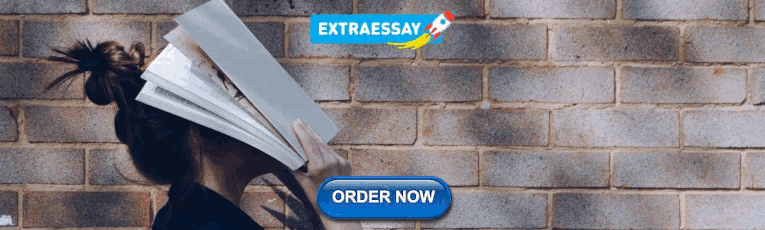
Publication types
- Systematic Review
- Research Support, Non-U.S. Gov't
- Autism Spectrum Disorder* / diagnosis
- Autistic Disorder* / epidemiology
- Population Surveillance / methods
Grants and funding
- CIHR/Canada
Thank you for visiting nature.com. You are using a browser version with limited support for CSS. To obtain the best experience, we recommend you use a more up to date browser (or turn off compatibility mode in Internet Explorer). In the meantime, to ensure continued support, we are displaying the site without styles and JavaScript.
- View all journals
- Explore content
- About the journal
- Publish with us
- Sign up for alerts
- Published: 29 June 2022
Incidence, prevalence, and global burden of autism spectrum disorder from 1990 to 2019 across 204 countries
- Marco Solmi ORCID: orcid.org/0000-0003-4877-7233 1 , 2 , 3 , 4 , 5 na1 ,
- Minjin Song 6 na1 ,
- Yon Dong Keon ORCID: orcid.org/0000-0003-1628-9948 7 , 8 na1 ,
- Seung Won Lee ORCID: orcid.org/0000-0001-5632-5208 9 , 10 na1 ,
- Eric Fombonne ORCID: orcid.org/0000-0002-8605-3538 11 , 12 ,
- Min Seo Kim 13 ,
- Seoyeon Park 6 ,
- Min Ho Lee 6 ,
- Jimin Hwang 14 ,
- Roberto Keller ORCID: orcid.org/0000-0002-6873-9827 15 ,
- Ai Koyanagi 16 , 17 ,
- Louis Jacob 16 , 18 ,
- Elena Dragioti ORCID: orcid.org/0000-0001-9019-4125 19 , 20 ,
- Lee Smith 21 ,
- Christoph U. Correll 22 , 23 , 24 , 25 ,
- Paolo Fusar-Poli ORCID: orcid.org/0000-0003-3582-6788 4 , 26 , 27 , 28 ,
- Giovanni Croatto ORCID: orcid.org/0000-0003-0591-3936 29 ,
- Andre F. Carvalho ORCID: orcid.org/0000-0002-2500-5671 30 ,
- Jae Won Oh 31 ,
- San Lee 31 , 32 ,
- Corentin J. Gosling 5 , 33 , 34 ,
- Keun-Ah Cheon 35 ,
- Dimitris Mavridis ORCID: orcid.org/0000-0003-1041-4592 36 , 37 ,
- Che-Sheng Chu 38 , 39 , 40 ,
- Chih-Sung Liang ORCID: orcid.org/0000-0003-1138-5586 41 , 42 ,
- Joaquim Radua 4 , 43 , 44 ,
- Laurent Boyer 45 ,
- Guillaume Fond ORCID: orcid.org/0000-0003-3249-2030 45 ,
- Jae Il Shin ORCID: orcid.org/0000-0003-2326-1820 46 &
- Samuele Cortese 5 , 47 , 48 , 49 , 50
Molecular Psychiatry volume 27 , pages 4172–4180 ( 2022 ) Cite this article
4897 Accesses
51 Citations
25 Altmetric
Metrics details
- Autism spectrum disorders
- Psychiatric disorders
Autism spectrum disorder (ASD) substantially contributes to the burden of mental disorders. Improved awareness and changes in diagnostic criteria of ASD may have influenced the diagnostic rates of ASD. However, while data on trends in diagnostic rates in some individual countries have been published, updated estimates of diagnostic rate trends and ASD-related disability at the global level are lacking. Here, we used the Global Burden of Diseases, Injuries, and Risk Factors Study data to address this gap, focusing on changes in prevalence, incidence, and disability-adjusted life years (DALYs) of ASD across the world. From 1990 to 2019, overall age-standardized estimates remained stable globally. Both prevalence and DALYs increased in countries with high socio-demographic index (SDI). However, the age-standardized incidence decreased in some low SDI countries, indicating a need to improve awareness. The male/female ratio decreased between 1990 and 2019, possibly accounted for by increasing clinical attention to ASD in females. Our results suggest that ASD detection in low SDI countries is suboptimal, and that ASD prevention/treatment in countries with high SDI should be improved, considering the increasing prevalence of the disorder. Additionally, growing attention is being paid to ASD diagnosis in females, who might have been left behind by ASD epidemiologic and clinical research previously. ASD burden estimates are underestimated as GBD does not account for mortality in ASD.
This is a preview of subscription content, access via your institution
Access options
Subscribe to this journal
Receive 12 print issues and online access
251,40 € per year
only 20,95 € per issue
Rent or buy this article
Prices vary by article type
Prices may be subject to local taxes which are calculated during checkout
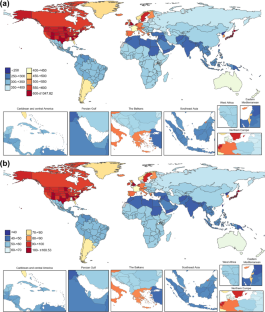
Similar content being viewed by others
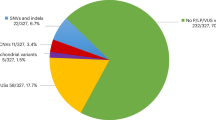
Comprehensive whole-genome sequence analyses provide insights into the genomic architecture of cerebral palsy
Darcy L. Fehlings, Mehdi Zarrei, … Stephen W. Scherer
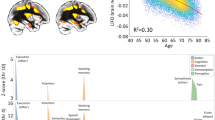
The effects of genetic and modifiable risk factors on brain regions vulnerable to ageing and disease
Jordi Manuello, Joosung Min, … Gwenaëlle Douaud
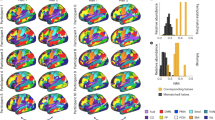
A precision functional atlas of personalized network topography and probabilities
Robert J. M. Hermosillo, Lucille A. Moore, … Damien A. Fair
Data availability
Data are publicly available at the Institute for Health Metrics and Evaluation (IHME) website ( http://www.ghdx.healthdata.org/gbd-results-tool ).
Steinhausen HC, Jakobsen H. Incidence rates of treated mental disorders in childhood and adolescence in a complete nationwide birth cohort. J Clin Psychiatry. 2019;80:17m12012.
Kim JY, Son MJ, Son CY, Radua J, Eisenhut M, Gressier F, et al. Environmental risk factors and biomarkers for autism spectrum disorder: an umbrella review of the evidence. Lancet Psychiatry. 2019;6:590–600.
PubMed Google Scholar
Kim JH, Kim JY, Lee J, Jeong GH, Lee E, Lee S, et al. Environmental risk factors, protective factors, and peripheral biomarkers for ADHD: an umbrella review. Lancet Psychiatry. 2020;7:955–70.
Solmi M, Radua J, Olivola M, Croce E, Soardo L, Salazar de Pablo G, et al. Age at onset of mental disorders worldwide: large-scale meta-analysis of 192 epidemiological studies. Mol Psychiatry. 2021. https://doi.org/10.1038/s41380-021-01161-7 .
American Psychiatric Association. Diagnostic and Statistical Manual of Mental Disorders, 5th edition (DSM-V). Washington: American Psychiatric Association; 2013.
Weye N, Santomauro DF, Agerbo E, Christensen MK, Iburg KM, Momen NC, et al. Register-based metrics of years lived with disability associated with mental and substance use disorders: a register-based cohort study in Denmark. Lancet Psychiatry. 2021;8:310–9.
Delobel-Ayoub M, Ehlinger V, Klapouszczak D, Maffre T, Raynaud J-P, Delpierre C, et al. Socioeconomic disparities and prevalence of autism spectrum disorders and intellectual disability. PLoS One. 2015;10:e0141964–e0141964.
PubMed PubMed Central Google Scholar
Collaborators IS-LDBIMD. The burden of mental disorders across the states of India: the Global Burden of Disease Study 1990-2017. Lancet Psychiatry. 2020;7:148–61.
Google Scholar
Mohammadi MR, Ahmadi N, Khaleghi A, Zarafshan H, Mostafavi S-A, Kamali K, et al. Prevalence of autism and its comorbidities and the relationship with maternal psychopathology: a national population-based study. Arch Iran Med. 2019;22:546–53.
Eapen V, Mabrouk AA, Zoubeidi T, Yunis F. Prevalence of pervasive developmental disorders in preschool children in the UAE. J Trop Pediatr. 2007;53:202–5.
Davidovitch M, Hemo B, Manning-Courtney P, Fombonne E. Prevalence and incidence of autism spectrum disorder in an israeli population. J Autism Dev Disord. 2013;43:785–93.
Qiu S, Lu Y, Li Y, Shi J, Cui H, Gu Y, et al. Prevalence of autism spectrum disorder in Asia: A systematic review and meta-analysis. Psychiatry Res. 2020;284:112679.
Yeargin-Allsopp M, Rice C, Karapurkar T, Doernberg N, Boyle C, Murphy C. Prevalence of Autism in a US metropolitan area. JAMA 2003;289:49–55.
Idring S, Lundberg M, Sturm H, Dalman C, Gumpert C, Rai D, et al. Changes in prevalence of autism spectrum disorders in 2001–11: findings from the Stockholm Youth Cohort. J Autism Dev Disord. 2015;45:1766–73.
Baxter AJ, Brugha TS, Erskine HE, Scheurer RW, Vos T, Scott JG. The epidemiology and global burden of autism spectrum disorders. Psychol Med. 2015;45:601–13.
CAS PubMed Google Scholar
Olusanya BO, Davis AC, Wertlieb D, Boo N-Y, Nair MKC, Halpern R, et al. Developmental disabilities among children younger than 5 years in 195 countries and territories, 1990–2016: a systematic analysis for the Global Burden of Disease Study 2016. Lancet Glob Heal. 2018;6:e1100–e1121.
Rubenstein E, Daniels J, Schieve LA, Christensen DL, Van Naarden Braun K, Rice CE, et al. Trends in special education eligibility among children with autism spectrum disorder, 2002–10. Public Health Rep. 2018;133:85–92.
Vos T, Lim SS, Abbafati C, Abbas KM, Abbasi M, Abbasifard M, et al. Global burden of 369 diseases and injuries in 204 countries and territories, 1990–2019: a systematic analysis for the Global Burden of Disease Study 2019. Lancet. 2020;396:1204–22.
Murray CJL, Abbafati C, Abbas KM, Abbasi M, Abbasi-Kangevari M, Abd-Allah F, et al. Five insights from the Global Burden of Disease Study 2019. Lancet 2020;396:1135–59.
Kim YS, Fombonne E, Koh Y-J, Kim S-J, Cheon K-A, Leventhal BL. A comparison of DSM-IV pervasive developmental disorder and DSM-5 autism spectrum disorder prevalence in an epidemiologic sample. J Am Acad Child Adolesc Psychiatry. 2014;53:500–8.
Maenner MJ, Rice CE, Arneson CL, Cunniff C, Schieve LA, Carpenter LA, et al. Potential impact of DSM-5 criteria on autism spectrum disorder prevalence estimates. JAMA Psychiatry. 2014;71:292–300.
Bent CA, Barbaro J, Dissanayake C. Change in autism diagnoses prior to and following the introduction of DSM-5. J Autism Dev Disord. 2017;47:163–71.
Tessari L, Angriman M, Díaz-Román A, Zhang J, Conca A, Cortese S. Association between exposure to pesticides and ADHD or Autism Spectrum Disorder: a systematic review of the literature. J Atten Disord. 2022;26:48–71.
Kyu HH, Abate D, Abate KH, Abay SM, Abbafati C, Abbasi N, et al. Global, regional, and national disability-adjusted life-years (DALYs) for 359 diseases and injuries and healthy life expectancy (HALE) for 195 countries and territories, 1990–2017: a systematic analysis for the Global Burden of Disease Study 2017. Lancet. 2018;392:1859–922.
Lai M-C, Lombardo MV, Ruigrok AN, Chakrabarti B, Auyeung B, Szatmari P, et al. Quantifying and exploring camouflaging in men and women with autism. Autism 2017;21:690–702.
Sasayama D, Kuge R, Toibana Y, Honda H. Trends in autism spectrum disorder diagnoses in Japan, 2009 to 2019. JAMA Netw Open. 2021;4:e219234.
Schendel DE, Thorsteinsson E. Cumulative incidence of autism into adulthood for birth cohorts in Denmark, 1980-2012. JAMA - J Am Med Assoc. 2018;320:1811–3.
Arango C, Dragioti E, Solmi M, Cortese S, Domschke K, Murray R, et al. Evidence-based atlas of risk and protective factors of mental disorders: meta-umbrella review. World Psychiatry. 2021; In press.
Dragioti E, Solmi M, Favaro A, Fusar-Poli P, Dazzan P, Thompson T, et al. Association of antidepressant use with adverse health outcomes: a systematic umbrella review. JAMA Psychiatry. 2019;76:1241–55.
Wu J, Morris JK. Trends in maternal age distribution and the live birth prevalence of Down’s syndrome in England and Wales: 1938-2010. Eur J Hum Genet. 2013;21:943–7.
Li YH, Wang YP, Dai L, Zhou GX, Liang J, Li Q, et al. The trend of national advanced maternal age woman proportion in hospital-based surveillance. Zhonghua Yu Fang Yi Xue Za Zhi. 2009;43:1073–6.
Oh Y, Bae J. Impact of changes in maternal age and parity distribution on the increasing trends in the low birth weight and very low birth weight rates in South Korea, 2005-15. J Prev Med Public Heal. 2019;52:123–30.
Mousiolis A, Baroutis G, Papantoniou N, Costalos C, Antsaklis A. Maternal age demographic trends in Greece from 1980 to 2008. J Reprod Med. 2013;58:246–55.
Wang W, Xie X, Yuan T, Wang Y, Zhao F, Zhou Z, et al. Epidemiological trends of maternal hypertensive disorders of pregnancy at the global, regional, and national levels: a population‐based study. BMC Pregnancy Childbirth. 2021;21:364.
Heslehurst N, Ells LJ, Simpson H, Batterham A, Wilkinson J, Summerbell CD. Trends in maternal obesity incidence rates, demographic predictors, and health inequalities in 36 821 women over a 15-year period. BJOG An. Int J Obstet Gynaecol. 2007;114:187–94.
CAS Google Scholar
Ogden CL, Carroll MD, Kit BK, Flegal KM. Prevalence of childhood and adult obesity in the United States, 2011-2. JAMA 2014;311:806–14.
CAS PubMed PubMed Central Google Scholar
Malik VS, Willet WC, Hu FB. Nearly a decade on—trends, risk factors and policy implications in global obesity. Nat Rev Endocrinol. 2020;16:615–6.
Mazza M, Pino MC, Keller R, Vagnetti R, Attanasio M, Filocamo A, et al. Qualitative differences in attribution of mental states to other people in autism and schizophrenia: what are the tools for differential diagnosis? J Autism Dev Disord. 2021. 2021. https://doi.org/10.1007/s10803-021-05035-3 .
Hyman SL, Levy SE, Myers SM. Council on Children with disabilities SONDANDBP Identification, evaluation, and management of children with autism spectrum disorder. Pediatrics. 2020;145:e20193447.
Stuart EA, McGinty EE, Kalb L, Huskamp HA, Busch SH, Gibson TB, et al. Increased service use among children with autism spectrum disorder associated with mental health parity law. Health Aff. 2017;36:337–45.
Toft G, Liu C, Menon J, Schendel D, Loss G, Ehrenstein V. Assessment of educational attainment and employment among individuals with autism spectrum disorder in Denmark. JAMA Pediatr. 2021;175:601–8.
Narzisi A, Posada M, Barbieri F, Chericoni N, Ciuffolini D, Pinzino M, et al. Prevalence of Autism Spectrum Disorder in a large Italian catchment area: a school-based population study within the ASDEU project. Epidemiol Psychiatr Sci. 2018;29:e5–e5.
Chiarotti F, Venerosi A. Epidemiology of autism spectrum disorders: a review of worldwide prevalence estimates since 2014. Brain Sci. 2020;10:274.
Delobel-Ayoub M, Saemundsen E, Gissler M, Ego A, Moilanen I, Ebeling H, et al. Prevalence of Autism Spectrum disorder in 7-9-year-old children in Denmark, Finland, France and Iceland: a population-based registries approach within the ASDEU Project. J Autism Dev Disord. 2020;50:949–59.
National Autistic Society. Benefits for autistic children. Natl Autistic Soc. 2020. https://www.autism.org.uk/advice-and-guidance/topics/benefits-and-money/benefits/benefits-you-can-get/benefits-for-autistic-children . Accessed 28 March 2022.
Sun X, Allison C, Wei L, Matthews FE, Auyeung B, Wu YY, et al. Autism prevalence in China is comparable to Western prevalence. Mol Autism. 2019;10:7.
Wan Y, Hu Q, Li T, Jiang L, Du Y, Feng L, et al. Prevalence of autism spectrum disorders among children in China: a systematic review. Shanghai Arch Psychiatry. 2013;25:70–80.
Cantor J, McBain RK, Kofner A, Stein BD, Yu H. Fewer than half of us mental health treatment facilities provide services for children with autism spectrum disorder. Health Aff. 2020;39:968–74.
Hirvikoski T, Mittendorfer-Rutz E, Boman M, Larsson H, Lichtenstein P, Bölte S. Premature mortality in autism spectrum disorder. Br J Psychiatry. 2016;208:232–8.
Global, regional, and national burden of 12 mental disorders in 204 countries and territories, 1990–2019: a systematic analysis for the Global Burden of Disease Study 2019. Lancet Psychiatry. 2022;9:137–50.
Ahmad OB, Boschi Pinto C, Lopez AD, Age Standardization of rates: a new WHO Standard. GPE Discuss Pap Ser No 31. 2001:10–12.
Fombonne E, MacFarlane H, Salem AC. Epidemiological surveys of ASD: advances and remaining challenges. J Autism Dev Disord. 2021. https://doi.org/10.1007/s10803-021-05005-9 .
Bastiaansen JA, Meffert H, Hein S, Huizinga P, Ketelaars C, Pijnenborg M, et al. Diagnosing autism spectrum disorders in adults: the use of Autism Diagnostic Observation Schedule (ADOS) module 4. J Autism Dev Disord. 2011;41:1256–66.
Grzadzinski R, Dick C, Lord C, Bishop S. Parent-reported and clinician-observed autism spectrum disorder (ASD) symptoms in children with attention deficit/hyperactivity disorder (ADHD): implications for practice under DSM-5. Mol Autism. 2016;7:7.
Havdahl KA, Hus Bal V, Huerta M, Pickles A, Øyen A-S, Stoltenberg C, et al. Multidimensional influences on autism symptom measures: implications for use in etiological research. J Am Acad Child Adolesc Psychiatry. 2016;55:1054–1063. e3
Matsuo J, Kamio Y, Takahashi H, Ota M, Teraishi T, Hori H, et al. Autistic-like traits in adult patients with mood disorders and schizophrenia. PLoS One. 2015;10:e0122711.
Turban JL, van Schalkwyk GI. ‘Gender Dysphoria’ and autism spectrum disorder: is the link real? J Am Acad Child Adolesc Psychiatry. 2018;57:8–9.e2
Download references
Author information
These authors contributed equally: Marco Solmi, Minjin Song, Dong Keon Yon, Seung Won Lee.
Authors and Affiliations
Department of Psychiatry, University of Ottawa, Ontario, ON, Canada
Marco Solmi
Department of Mental Health, The Ottawa Hospital, Ontario, ON, Canada
Ottawa Hospital Research Institute (OHRI) Clinical Epidemiology Program University of Ottawa, Ottawa, ON, Canada
Early Psychosis: Interventions and Clinical-Detection (EPIC) Lab, Department of Psychosis Studies, Institute of Psychiatry, Psychology, King’s College London, London, UK
Marco Solmi, Paolo Fusar-Poli & Joaquim Radua
Centre for Innovation in Mental Health (CIMH), School of Psychology, Faculty of Environmental and Life Sciences, University of Southampton, Southampton, UK
Marco Solmi, Corentin J. Gosling & Samuele Cortese
Yonsei University College of Medicine, Seoul, Republic of Korea
Minjin Song, Seoyeon Park & Min Ho Lee
Department of Pediatrics, Kyung Hee University College of Medicine, Seoul, Republic of Korea
Yon Dong Keon
Center for Digital Health, Medical Science Research Institute, Kyung Hee University College of Medicine, Seoul, Republic of Korea
Department of Data Science, Sejong University College of Software Convergence, Seoul, South Korea
Seung Won Lee
Sungkyunkwan University School of Medicine, Suwon, Republic of Korea
Department of Psychiatry, Oregon Health & Science University, Portland, OR, USA
Eric Fombonne
Department of Pediatrics, Oregon Health & Science University, Portland, OR, USA
Samsung Advanced Institute for Health Sciences & Technology (SAIHST), Sungkyunkwan University, Samsung Medical Center, Seoul, Republic of Korea
Min Seo Kim
Department of Epidemiology, Johns Hopkins Bloomberg School of Public Health, Baltimore, MD, USA
Jimin Hwang
Adult Autism Center, Mental Health Department, Health Unit ASL Città di Torino, 10138, Turin, Italy
Roberto Keller
Parc Sanitari Sant Joan de Déu/CIBERSAM/ISCIII, Universitat de Barcelona, Fundació Sant Joan de Déu, Sant Boi de Llobregat, 08830, Barcelona, Spain
Ai Koyanagi & Louis Jacob
ICREA, Pg. Lluis Companys 23, Barcelona, Spain
Ai Koyanagi
Faculty of Medicine, University of Versailles Saint-Quentin-en-Yvelines, Montigny-le-Bretonneux, 78180, France
Louis Jacob
Pain and Rehabilitation Centre, and Department of Health, Medicine and Caring Sciences, Linkoping University, SE-581 85, Linkoping, Sweden
Elena Dragioti
Research Laboratory Psychology of Patients, Families & Health Professionals, Department of Nursing, School of Health Sciences, University of Ioannina, Ioannina, 45500, Greece
Centre for Health, Performance, and Wellbeing, Anglia Ruskin University, Cambridge, CB1 1PT, UK
Department of Psychiatry, Zucker Hillside Hospital, Glen Oaks, New York City, NY, USA
Christoph U. Correll
Department of Psychiatry and Molecular Medicine, Donald and Barbara Zucker School of Medicine at Hofstra/Northwell, Hempstead, NY, USA
Center for Psychiatric Neuroscience, Feinstein Institute for Medical Research, Manhasset, NY, USA
Department of Child and Adolescent Psychiatry, Charité-Universitätsmedizin Berlin, Berlin, Germany
Department of Brain and Behavioral Sciences, University of Pavia, Pavia, Italy
Paolo Fusar-Poli
OASIS service, South London and Maudsley NHS Foundation Trust, London, UK
National Institute for Health Research, Maudsley Biomedical Research Centre, London, UK
Department of mental health, AULSS3 Serenissima, Veneto, Italy
Giovanni Croatto
IMPACT (Innovation in Mental and Physical Health and Clinical Treatment) Strategic Research Centre, School of Medicine, Barwon Health, Deakin University, Geelong, VIC, Australia
Andre F. Carvalho
Department of Psychiatry, Yongin Severance Hospital, Yonsei University College of Medicine, Yongin, Republic of Korea
Jae Won Oh & San Lee
Department of Psychiatry and Institute of Behavioral Science in Medicine, Yonsei University College of Medicine, Seoul, Republic of Korea
DysCo Lab, Paris Nanterre University, Nanterre, France
Corentin J. Gosling
Laboratoire de Psychopathologie et Processus de Santé, Université de Paris, Paris, France
Division of Child and Adolescent Psychiatry, Department of Psychiatry, Severance Hospital, Institute of Behavioral Science in Medicine, Yonsei University College of Medicine, Seoul, Korea
Keun-Ah Cheon
Department of Primary Education, Evidence Synthesis Methods Team, University of Ioannina, Ioannina, Greece
Dimitris Mavridis
Faculté de Médecine, Paris Descartes University, Sorbonne Paris Cité, Paris, France
Department of Psychiatry, Kaohsiung Veterans General Hospital, Kaohsiung, 813, Taiwan
Che-Sheng Chu
Center for Geriatric and Gerontology, Kaohsiung Veterans General Hospital, Kaohsiung, 813, Taiwan
Graduate Institute of Medicine, College of Medicine, Kaohsiung Medical University, Kaohsiung, 807, Taiwan
Department of Psychiatry, Beitou Branch, Tri-Service General Hospital, National Defense Medical Center, Taipei, 11490, Taiwan
Chih-Sung Liang
Graduate Institute of Medical Sciences, National Defense Medical Center, Taipei, 11490, Taiwan
Imaging of Mood- and Anxiety-Related Disorders (IMARD) Group, Institut d’Investigacions Biomèdiques August Pi I Sunyer (IDIBAPS), Centro de Investigación Biomédica en Red de Salud Mental (CIBERSAM), Barcelona, Spain
Joaquim Radua
Department of Clinical Neuroscience, Centre for Psychiatric Research and Education, Karolinska Institutet, Stockholm, Sweden
AP-HM, Aix-Marseille University, CEReSS-Health Service Research and Quality of Life Center, Fondation FondaMental, Marseille, France
Laurent Boyer & Guillaume Fond
Department of Pediatrics, Yonsei University College of Medicine, Seoul, Republic of Korea
Jae Il Shin
Clinical and Experimental Sciences (CNS and Psychiatry), Faculty of Medicine, University of Southampton, Southampton, UK
Samuele Cortese
Division of Psychiatry and Applied Psychology, School of Medicine, University of Nottingham, Nottingham, UK
Hassenfeld Children’s Hospital at NYU Langone, New York University Child Study Center, New York City, NY, USA
Solent NHS Trust, Child and Adolescent Mental Health Service (CAMHS), Southampton, UK
You can also search for this author in PubMed Google Scholar
Contributions
All authors contributed and approved the study’s protocol. MS and SC created the first draft of the manuscript. MS, DY and SL analysed data. MS, JS, and SC provided overall guidance. All authors read, edited, and approved the final version of the manuscript.
Corresponding author
Correspondence to Jae Il Shin .
Ethics declarations
Competing interests.
MS received honoraria/has been consultant for Angelini, Lundbeck. CUC has been a consultant and/or advisor to or has received honoraria from: AbbVie, Acadia, Alkermes, Allergan, Angelini, Aristo, Axsome, Damitsa, Gedeon Richter, Hikma, IntraCellular Therapies, Janssen/J&J, Karuna, LB Pharma, Lundbeck, MedAvante-ProPhase, MedInCell, Medscape, Merck, Mitsubishi Tanabe Pharma, Mylan, Neurocrine, Noven, Otsuka, Pfizer, Recordati, Rovi, Servier, SK Life Science, Sumitomo Dainippon, Sunovion, Supernus, Takeda, Teva, and Viatris. He provided expert testimony for Janssen and Otsuka. He served on a Data Safety Monitoring Board for Lundbeck, Rovi, Supernus, and Teva. He has received grant support from Janssen and Takeda. He received royalties from UpToDate and is also a stock option holder of LB Pharma. SC is supported by the following grants from the National Institute for Health and Care Research (NIHR): RP-PG-0618-20003, NIHR128472, NIHR130077, NIHR203035, NIHR203684. The views expressed are those of the author(s) and not necessarily those of the NIHR or the Department of Health and Social Care.
Ethics approval
We followed the standard procedure recommended to register additional publication from GBD2019 project after publication of capstone paper [ 18 ]. Our study was also approved by the Institutional Review Board at Yonsei University Health System for the data use.
Additional information
Publisher’s note Springer Nature remains neutral with regard to jurisdictional claims in published maps and institutional affiliations.
Supplementary information
Rights and permissions.
Springer Nature or its licensor (e.g. a society or other partner) holds exclusive rights to this article under a publishing agreement with the author(s) or other rightsholder(s); author self-archiving of the accepted manuscript version of this article is solely governed by the terms of such publishing agreement and applicable law.
Reprints and permissions
About this article
Cite this article.
Solmi, M., Song, M., Yon, D.K. et al. Incidence, prevalence, and global burden of autism spectrum disorder from 1990 to 2019 across 204 countries. Mol Psychiatry 27 , 4172–4180 (2022). https://doi.org/10.1038/s41380-022-01630-7
Download citation
Received : 27 December 2021
Revised : 07 May 2022
Accepted : 12 May 2022
Published : 29 June 2022
Issue Date : October 2022
DOI : https://doi.org/10.1038/s41380-022-01630-7
Share this article
Anyone you share the following link with will be able to read this content:
Sorry, a shareable link is not currently available for this article.
Provided by the Springer Nature SharedIt content-sharing initiative
This article is cited by
The burden of mental disorders in asian countries, 1990–2019: an analysis for the global burden of disease study 2019.
- Xiaowei Tang
Translational Psychiatry (2024)
A robust microbiome signature for autism spectrum disorder across different studies using machine learning
- Lucia N. Peralta-Marzal
- David Rojas-Velazquez
- Alejandro Lopez-Rincon
Scientific Reports (2024)
Molecular profiling of the hippocampus of children with autism spectrum disorder
- Lindsay E. Rexrode
- Joshua Hartley
- Harry Pantazopoulos
Molecular Psychiatry (2024)
Autism, gender, and identity in college students
- Jessica Cooper
Discover Psychology (2024)
Pain detection through facial expressions in children with autism using deep learning
- P. V. K. Sandeep
- N. Suresh Kumar
Soft Computing (2024)
Quick links
- Explore articles by subject
- Guide to authors
- Editorial policies

Featured Clinical Reviews
- Screening for Atrial Fibrillation: US Preventive Services Task Force Recommendation Statement JAMA Recommendation Statement January 25, 2022
- Evaluating the Patient With a Pulmonary Nodule: A Review JAMA Review January 18, 2022
- Download PDF
- Share X Facebook Email LinkedIn
- Permissions
Autism Prevalence Has Been on the Rise in the US for Decades—And That’s Progress
- Original Investigation Racial and Ethnic Disparities in Geographic Access to Autism Resources Across the US Bennett M. Liu; Kelley Paskov, PhD; Jack Kent, MM; Maya McNealis, BS; Soren Sutaria; Olivia Dods; Christopher Harjadi; Nate Stockham, PhD; Andrey Ostrovsky, MD; Dennis P. Wall, PhD JAMA Network Open
When Matthew Maenner, PhD, was in college, he started working with autistic children as a behavioral therapist. In those days—the early 2000s—autism was still a relatively new diagnosis, and there were few supports available for autistic people. “No one knew what to do to help,” Maenner recalled in a recent interview with JAMA . Parents were desperate to find ways to support their children, sometimes turning to unproven diets or pricey speech-generation devices.
Their challenges stuck with him. After graduating in 2003 with a degree in psychology, Maenner joined the Wisconsin branch of the Autism and Developmental Disabilities Monitoring (ADDM) Network, a multistate autism prevalence-tracking project run by the US Centers for Disease Control and Prevention (CDC).
Read More About
Harris E. Autism Prevalence Has Been on the Rise in the US for Decades—And That’s Progress. JAMA. 2023;329(20):1724–1726. doi:10.1001/jama.2023.6078
Manage citations:
© 2024
Artificial Intelligence Resource Center
Cardiology in JAMA : Read the Latest
Browse and subscribe to JAMA Network podcasts!
Others Also Liked
Select your interests.
Customize your JAMA Network experience by selecting one or more topics from the list below.
- Academic Medicine
- Acid Base, Electrolytes, Fluids
- Allergy and Clinical Immunology
- American Indian or Alaska Natives
- Anesthesiology
- Anticoagulation
- Art and Images in Psychiatry
- Artificial Intelligence
- Assisted Reproduction
- Bleeding and Transfusion
- Caring for the Critically Ill Patient
- Challenges in Clinical Electrocardiography
- Climate and Health
- Climate Change
- Clinical Challenge
- Clinical Decision Support
- Clinical Implications of Basic Neuroscience
- Clinical Pharmacy and Pharmacology
- Complementary and Alternative Medicine
- Consensus Statements
- Coronavirus (COVID-19)
- Critical Care Medicine
- Cultural Competency
- Dental Medicine
- Dermatology
- Diabetes and Endocrinology
- Diagnostic Test Interpretation
- Drug Development
- Electronic Health Records
- Emergency Medicine
- End of Life, Hospice, Palliative Care
- Environmental Health
- Equity, Diversity, and Inclusion
- Facial Plastic Surgery
- Gastroenterology and Hepatology
- Genetics and Genomics
- Genomics and Precision Health
- Global Health
- Guide to Statistics and Methods
- Hair Disorders
- Health Care Delivery Models
- Health Care Economics, Insurance, Payment
- Health Care Quality
- Health Care Reform
- Health Care Safety
- Health Care Workforce
- Health Disparities
- Health Inequities
- Health Policy
- Health Systems Science
- History of Medicine
- Hypertension
- Images in Neurology
- Implementation Science
- Infectious Diseases
- Innovations in Health Care Delivery
- JAMA Infographic
- Law and Medicine
- Leading Change
- Less is More
- LGBTQIA Medicine
- Lifestyle Behaviors
- Medical Coding
- Medical Devices and Equipment
- Medical Education
- Medical Education and Training
- Medical Journals and Publishing
- Mobile Health and Telemedicine
- Narrative Medicine
- Neuroscience and Psychiatry
- Notable Notes
- Nutrition, Obesity, Exercise
- Obstetrics and Gynecology
- Occupational Health
- Ophthalmology
- Orthopedics
- Otolaryngology
- Pain Medicine
- Palliative Care
- Pathology and Laboratory Medicine
- Patient Care
- Patient Information
- Performance Improvement
- Performance Measures
- Perioperative Care and Consultation
- Pharmacoeconomics
- Pharmacoepidemiology
- Pharmacogenetics
- Pharmacy and Clinical Pharmacology
- Physical Medicine and Rehabilitation
- Physical Therapy
- Physician Leadership
- Population Health
- Primary Care
- Professional Well-being
- Professionalism
- Psychiatry and Behavioral Health
- Public Health
- Pulmonary Medicine
- Regulatory Agencies
- Reproductive Health
- Research, Methods, Statistics
- Resuscitation
- Rheumatology
- Risk Management
- Scientific Discovery and the Future of Medicine
- Shared Decision Making and Communication
- Sleep Medicine
- Sports Medicine
- Stem Cell Transplantation
- Substance Use and Addiction Medicine
- Surgical Innovation
- Surgical Pearls
- Teachable Moment
- Technology and Finance
- The Art of JAMA
- The Arts and Medicine
- The Rational Clinical Examination
- Tobacco and e-Cigarettes
- Translational Medicine
- Trauma and Injury
- Treatment Adherence
- Ultrasonography
- Users' Guide to the Medical Literature
- Vaccination
- Venous Thromboembolism
- Veterans Health
- Women's Health
- Workflow and Process
- Wound Care, Infection, Healing
- Register for email alerts with links to free full-text articles
- Access PDFs of free articles
- Manage your interests
- Save searches and receive search alerts
- Help & FAQ
Global prevalence of autism: A systematic review update
Research output : Contribution to journal › Review article › peer-review
Prevalence estimates of autism are essential for informing public policy, raising awareness, and developing research priorities. Using a systematic review, we synthesized estimates of the prevalence of autism worldwide. We examined factors accounting for variability in estimates and critically reviewed evidence relevant for hypotheses about biological or social determinants (viz., biological sex, sociodemographic status, ethnicity/race, and nativity) potentially modifying prevalence estimates of autism. We performed the search in November 2021 within Medline for studies estimating autism prevalence, published since our last systematic review in 2012. Data were extracted by two independent researchers. Since 2012, 99 estimates from 71 studies were published indicating a global autism prevalence that ranges within and across regions, with a median prevalence of 100/10,000 (range: 1.09/10,000 to 436.0/10,000). The median male-to-female ratio was 4.2. The median percentage of autism cases with co-occurring intellectual disability was 33.0%. Estimates varied, likely reflecting complex and dynamic interactions between patterns of community awareness, service capacity, help seeking, and sociodemographic factors. A limitation of this review is that synthesizing methodological features precludes a quality appraisal of studies. Our findings reveal an increase in measured autism prevalence globally, reflecting the combined effects of multiple factors including the increase in community awareness and public health response globally, progress in case identification and definition, and an increase in community capacity. Hypotheses linking factors that increase the likelihood of developing autism with variations in prevalence will require research with large, representative samples and comparable autism diagnostic criteria and case-finding methods in diverse world regions over time. Lay Summary: We reviewed studies of the prevalence of autism worldwide, considering the impact of geographic, ethnic, and socioeconomic factors on prevalence estimates. Approximately 1/100 children are diagnosed with autism spectrum disorder around the world. Prevalence estimates increased over time and varied greatly within and across sociodemographic groups. These findings reflect changes in the definition of autism and differences in the methodology and contexts of prevalence studies.
- methodology
- risk factors
- social determinants
- time trends
ASJC Scopus subject areas
- General Neuroscience
- Clinical Neurology
- Genetics(clinical)
Access to Document
- 10.1002/aur.2696
Other files and links
- Link to publication in Scopus
- Link to the citations in Scopus
Fingerprint
- Autistic Disorder Medicine & Life Sciences 100%
- Systematic Reviews Medicine & Life Sciences 73%
- Cross-Sectional Studies Medicine & Life Sciences 11%
- Geography Medicine & Life Sciences 11%
- Social Welfare Medicine & Life Sciences 10%
- Public Policy Medicine & Life Sciences 10%
- Autism Spectrum Disorder Medicine & Life Sciences 9%
- Intellectual Disability Medicine & Life Sciences 8%
T1 - Global prevalence of autism
T2 - A systematic review update
AU - Zeidan, Jinan
AU - Fombonne, Eric
AU - Scorah, Julie
AU - Ibrahim, Alaa
AU - Durkin, Maureen S.
AU - Saxena, Shekhar
AU - Yusuf, Afiqah
AU - Shih, Andy
AU - Elsabbagh, Mayada
N1 - Publisher Copyright: © 2022 The Authors. Autism Research published by International Society for Autism Research and Wiley Periodicals LLC.
PY - 2022/5
Y1 - 2022/5
N2 - Prevalence estimates of autism are essential for informing public policy, raising awareness, and developing research priorities. Using a systematic review, we synthesized estimates of the prevalence of autism worldwide. We examined factors accounting for variability in estimates and critically reviewed evidence relevant for hypotheses about biological or social determinants (viz., biological sex, sociodemographic status, ethnicity/race, and nativity) potentially modifying prevalence estimates of autism. We performed the search in November 2021 within Medline for studies estimating autism prevalence, published since our last systematic review in 2012. Data were extracted by two independent researchers. Since 2012, 99 estimates from 71 studies were published indicating a global autism prevalence that ranges within and across regions, with a median prevalence of 100/10,000 (range: 1.09/10,000 to 436.0/10,000). The median male-to-female ratio was 4.2. The median percentage of autism cases with co-occurring intellectual disability was 33.0%. Estimates varied, likely reflecting complex and dynamic interactions between patterns of community awareness, service capacity, help seeking, and sociodemographic factors. A limitation of this review is that synthesizing methodological features precludes a quality appraisal of studies. Our findings reveal an increase in measured autism prevalence globally, reflecting the combined effects of multiple factors including the increase in community awareness and public health response globally, progress in case identification and definition, and an increase in community capacity. Hypotheses linking factors that increase the likelihood of developing autism with variations in prevalence will require research with large, representative samples and comparable autism diagnostic criteria and case-finding methods in diverse world regions over time. Lay Summary: We reviewed studies of the prevalence of autism worldwide, considering the impact of geographic, ethnic, and socioeconomic factors on prevalence estimates. Approximately 1/100 children are diagnosed with autism spectrum disorder around the world. Prevalence estimates increased over time and varied greatly within and across sociodemographic groups. These findings reflect changes in the definition of autism and differences in the methodology and contexts of prevalence studies.
AB - Prevalence estimates of autism are essential for informing public policy, raising awareness, and developing research priorities. Using a systematic review, we synthesized estimates of the prevalence of autism worldwide. We examined factors accounting for variability in estimates and critically reviewed evidence relevant for hypotheses about biological or social determinants (viz., biological sex, sociodemographic status, ethnicity/race, and nativity) potentially modifying prevalence estimates of autism. We performed the search in November 2021 within Medline for studies estimating autism prevalence, published since our last systematic review in 2012. Data were extracted by two independent researchers. Since 2012, 99 estimates from 71 studies were published indicating a global autism prevalence that ranges within and across regions, with a median prevalence of 100/10,000 (range: 1.09/10,000 to 436.0/10,000). The median male-to-female ratio was 4.2. The median percentage of autism cases with co-occurring intellectual disability was 33.0%. Estimates varied, likely reflecting complex and dynamic interactions between patterns of community awareness, service capacity, help seeking, and sociodemographic factors. A limitation of this review is that synthesizing methodological features precludes a quality appraisal of studies. Our findings reveal an increase in measured autism prevalence globally, reflecting the combined effects of multiple factors including the increase in community awareness and public health response globally, progress in case identification and definition, and an increase in community capacity. Hypotheses linking factors that increase the likelihood of developing autism with variations in prevalence will require research with large, representative samples and comparable autism diagnostic criteria and case-finding methods in diverse world regions over time. Lay Summary: We reviewed studies of the prevalence of autism worldwide, considering the impact of geographic, ethnic, and socioeconomic factors on prevalence estimates. Approximately 1/100 children are diagnosed with autism spectrum disorder around the world. Prevalence estimates increased over time and varied greatly within and across sociodemographic groups. These findings reflect changes in the definition of autism and differences in the methodology and contexts of prevalence studies.
KW - autism
KW - methodology
KW - prevalence
KW - risk factors
KW - social determinants
KW - time trends
UR - http://www.scopus.com/inward/record.url?scp=85125547426&partnerID=8YFLogxK
UR - http://www.scopus.com/inward/citedby.url?scp=85125547426&partnerID=8YFLogxK
U2 - 10.1002/aur.2696
DO - 10.1002/aur.2696
M3 - Review article
C2 - 35238171
AN - SCOPUS:85125547426
SN - 1939-3792
JO - Autism Research
JF - Autism Research
- Open access
- Published: 08 July 2022
The global prevalence of autism spectrum disorder: a comprehensive systematic review and meta-analysis
- Nader Salari 1 ,
- Shabnam Rasoulpoor 2 ,
- Shna Rasoulpoor 3 ,
- Shamarina Shohaimi 4 ,
- Sima Jafarpour 5 ,
- Nasrin Abdoli 6 ,
- Behnam Khaledi-Paveh 7 &
- Masoud Mohammadi ORCID: orcid.org/0000-0002-5722-8300 8
Italian Journal of Pediatrics volume 48 , Article number: 112 ( 2022 ) Cite this article
27k Accesses
91 Citations
9 Altmetric
Metrics details
Autism spectrum disorder (ASD) is one of the serious developmental disorders that is usually diagnosed below the age of three years. Although the severity of the disease’s symptoms varies from patient to patient, the ability to communicate with others is affected in all forms of ASD. This study aimed to determine the prevalence of ASD in high-risk groups by continent.
The present study was conducted by systematic review and meta-analysis from 2008 to July 2021. Databases such as Science Direct, PubMed, Scopus, SID, Magiran, Web of Science (WoS), and Google Scholar from 2008 to July 2021 were searched to find related studies. Data were analysed using Comprehensive Meta-Analysis software (Version 2).
A total of 74 studies with 30,212,757 participants were included in this study. The prevalence of ASD in the world was 0.6% (95% confidence interval: 0.4–1%). Subgroup analyses indicated that the prevalence of ASD in Asia, America, Europe, Africa and Australia was 0.4% (95% CI: 0.1–1), 1% (95% CI: 0.8–1.1), 0.5% (95% CI: 0.2–1), 1% (95% CI: 0.3–3.1), 1.7% (95% CI: 0.5–6.1) respectively.
ASD imposes a heavy health burden on communities around the world. Early detection of ASD can reduce the incidence of developmental disorders and improve patients’ communication skills. Therefore, health policymakers need to be aware of the prevalence and increasing trend of ASD to implement appropriate planning and interventions to reduce its consequences.
Autism Spectrum Disorder (ASD) is a neurological developmental disorder characterized by abnormalities in social relationships and repetitive or restricted behavioural patterns [ 1 ]. Numerous studies have been conducted on ASD, attributing the etiology of ASD to genetic, environmental, immunological, perinatal, neuroanatomical, and biochemical factors [ 2 ]. The autism spectrum encompasses a range of disorders, including Autistic disorder, Rett disorder, Asperger syndrome, and pervasive developmental disorder [ 3 , 4 ].
Patients with ASD have deficits in social interactions, verbal and nonverbal social communication skills, as well as intelligence and motor functions. These patients also exhibit unusual interests, repetitive behaviours, and unusual responses to sensory experiences [ 5 ]. Autism spectrum disorder is associated with high levels of anxiety, stress, and isolation in patients’ families [ 6 , 7 ]. Also, ASD imposes a heavy economic burden on society and the patients’ families [ 8 ]. These patients require considerable care, demanding significant financial resources. The direct and indirect costs of caring for children and adults with ASD in the United States in 2015 were estimated at $268.3 billion, which is more than the cost of stroke and hypertension. Overall, the cost of education, health care, and other lifelong services for an autistic patient varies from $ 1.4 million to $ 2.4 million per year [ 9 ].
Epidemiological surveys show an increasing trend in the annual prevalence of ASD. Besides the true increase in the prevalence of ASD, a variety of other reasons, such as a broader definition of ASD, changes in diagnostic criteria and screening tools, shifts in research methods, and increased awareness of ASD, have been suggested to contribute to this phenomenon [ 10 , 11 , 12 ].
Epidemiological studies have shown a rapid increase in the prevalence of ASD in recent years, with a prevalence of four to five times more in boys than girls. The average prevalence of autism spectrum disorder in Asia, Europe and North America is estimated at 1% [ 13 , 14 ]. According to the Centers for Disease Control and Prevention (CDC) report in the United States, the prevalence of ASD among 8-year-old children was 1 in 59 in 2014 and 1 in 54 in 2016 [ 15 ]. The prevalence of ASD in children and adolescents in the United States was reported at 2.5% in 2014–2016 [ 16 ]. In another study in Italy, the prevalence of ASD among 7–9-year-old children was 1.15% [ 17 ]. In Asia, the prevalence of ASD has been reported to be 3.9%, with a prevalence of 0.14 to 2.9% in the Arab countries around the Persian Gulf [ 18 , 19 ]. It is important to obtain an accurate estimation of the prevalence of autism to determine the economic burden and health services and allocate sufficient budget and services to autistic children or adults and their families [ 20 ]. In addition, by accurately determining the prevalence of ASD, vulnerable groups and geographical and environmental risk factors can be identified [ 21 , 22 ].
This article provided an overall estimate of the global prevalence of ASD by systematically reviewing available studies. An updated and comprehensive estimate of the prevalence of autism spectrum disorder helps health professionals develop public health strategies. Therefore, given the importance of autism spectrum disorder, we conducted a systematic and meta-analysis of ASD prevalence studies worldwide.
Search strategy
This study was performed according to the Preferred Reporting Items for Systematic Reviews and Meta-Analyses (PRISMA 2020) guidelines [ 23 ]. Electronic databases such as Science Direct, PubMed, Scopus, SID, Magiran, Web of Science, and Google Scholar from 2008 to July 2021 were searched to find related studies. A comprehensive search was performed using the following keywords: autism, autistic disorder, ASD, autism spectrum conditions, epidemiology, cross-sectional study, and prevalence. All related studies were identified and transferred to EndNote software for reference selection management. Reference lists of related studies were also examined manually to find other potentially eligible studies.
Inclusion and exclusion criteria
Studies were selected based on the following inclusion criteria: 1) Cross-sectional or cohort studies published from 2008 to 2021.2). 2) Articles were published in English and Persian. 4) Studies that used valid autism diagnostic tools such as: The Diagnostic and Statistical Manual of Mental Disorder,4th edition [DSM-IV], International Classification of Disease, 9th revision [ICD-9], Diagnostic and Statistical Manual of Mental Disorder text revision, 4th edition [DSM-IV TR], International Classification of Diseases, 10th revision [ICD-10], Diagnostic and Statistical Manual-5[DSM-5], or by tools (the Autism Diagnostic Interview Revised [ADI-R], Autism Diagnostic Observation Schedule [ADOS], Autism Behavior Checklist [ABC], Clancy Autism Behavior Scale [CABS], Children Autism Spectrum Test [CAST], and Checklist for Autism in Toddlers [CHAT]), Autism Spectrum Screening Questionnaire [ASSQ], Social Communication Disorder Checklist [SCDC], Modified Checklist for Autism in Toddlers [M-CHAT], Social Communication Questionnaire [SCQ], Indian Scale for Assessment of Autism [ISAA], Autism Quotient-10[AQ-10], Reporting Questionnaire for Children [RQC] 4) Studies that provided detailed information about participants and cases of autism spectrum disorder and its prevalence. The exclusion criteria were as follows: 1) Studies with duplicate or overlapping data; 2) studies without full text; 3) Studies with unknown detection methods.
Study selection and data extraction
Initially, all databases were searched based on search strategies and duplicate studies were excluded. Subsequently, a list of relevant articles was prepared for further evaluation. In the first stage, the title and abstract of the remaining articles were carefully screened based on the inclusion and exclusion criteria. In the second stage, by evaluating the suitability of the studies, the full text of relevant articles remaining was examined, and irrelevant studies were excluded. To avoid bias, all steps were reviewed by two reviewers independently, and reasons for deleting articles were mentioned. In cases where there was disagreement between the two researchers, the article was reviewed by a third reviewer.
Information and characteristics of included articles such as the name of the first author, year of publication, type of residence, the origin of study, gender, sample size, age, assessment tool, diagnostic criteria, Autism Spectrum Disorder and the prevalence of ASD were extracted. Finally, a total of 74 articles were selected for quality assessment.
Quality assessment
The methodological quality of studies was assessed according to the Reporting of Observational Studies in Epidemiology (STROBE) checklist. The STROBE checklist consisted of six scales / general sections, including title, abstract, introduction, methods, results, and discussion. Some of these scales had 32 items and included various methodological aspects of title, problem statement, study objectives, study type, the statistical population of the study, sampling method, determining the appropriate sample size, definition of variables and procedures, study data collection tools, statistical analysis methods and findings. The quality score ranged between 0 and 32; Studies with a score ≥ 16 were considered good and average methodological quality, and studies with a score <16 were identified as poor quality.
Statistical analysis
I 2 statistic test was used to evaluate the heterogeneity of selected studies. In order to assess the publication bias, due to the high volume of samples included in the studies, the Begg and Mazumdar test was used at a significance level of 0.1 and its corresponding Funnel plot. Data analysis was performed using Comprehensive Meta-Analysis software (Version 2).
Search results and study characteristics
A total of 3457 studies were collected in the initial literature search. After eliminating duplicate studies, 1834 eligible studies were selected by reviewing the titles and abstracts (Fig. 1 ). Of the 902 remaining studies in the screening stage, 821 articles were excluded by studying the full-text based on the inclusion and exclusion criteria. Seven articles were assessed as low quality and removed. Finally, a total of 74 articles were included in this meta-analysis. Studies were published from 2008 to 2021 in all countries of the world. A summary of the main findings and characteristics of the included studies are shown in Table 1 . Differences in methods, the definition of autism, screening tools, and diagnostic criteria between countries made it very difficult to compare studies (Table 1 and Fig. 1 ).
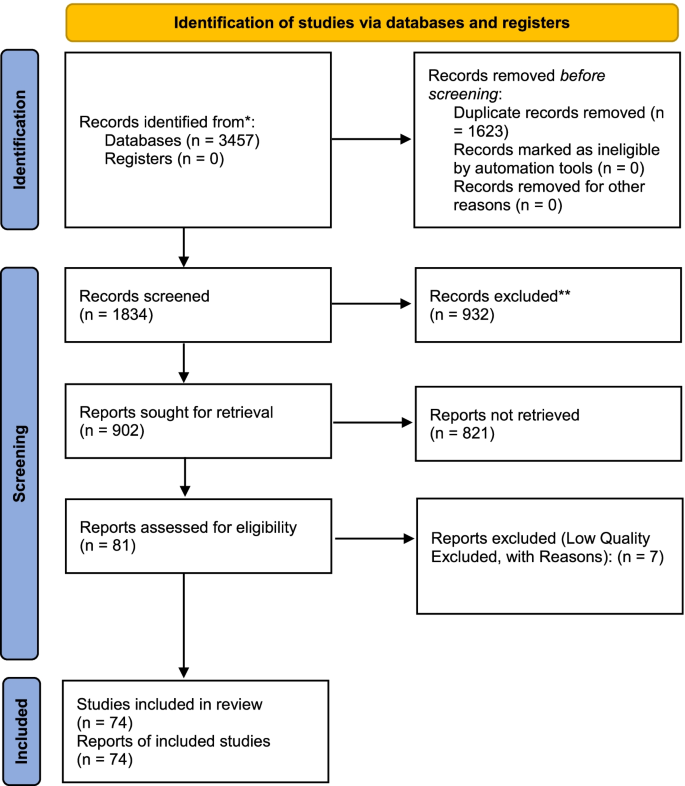
Flow chart indicating the stages of article selection in this review (PRISMA 2020)
Heterogeneity and publication bias
Egger’s and Begg’s tests were used to evaluate publication bias in the included studies. Results suggested no publication bias in the present study ( P = 0.109) (Fig. 2 ). Based on the I 2 test results (99.9%) and due to the heterogeneity of selected studies, the random-effects model was used to combine the reported results and estimate the prevalence of ASD. The potential reason for the heterogeneity between studies can be due to differences in the year of study, sample size, the origin of the study, and sampling error. The results were evaluated based on meta-regression.
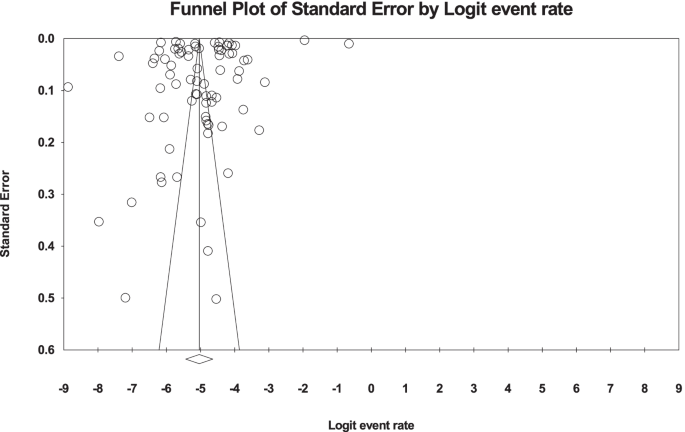
Funnel Plot Results related to the prevalence of ASD in the world
Based on the results of the present study, the global prevalence of ASD was 0.006 (95% CI: 0.004–0.01), or as a percentage of 0.6% (95% CI: 0.4–1),; the midpoint of each part shows the prevalence of each included study, and the diamond shape shows the prevalence of ASD in the population of all studies (Fig. 3 ).
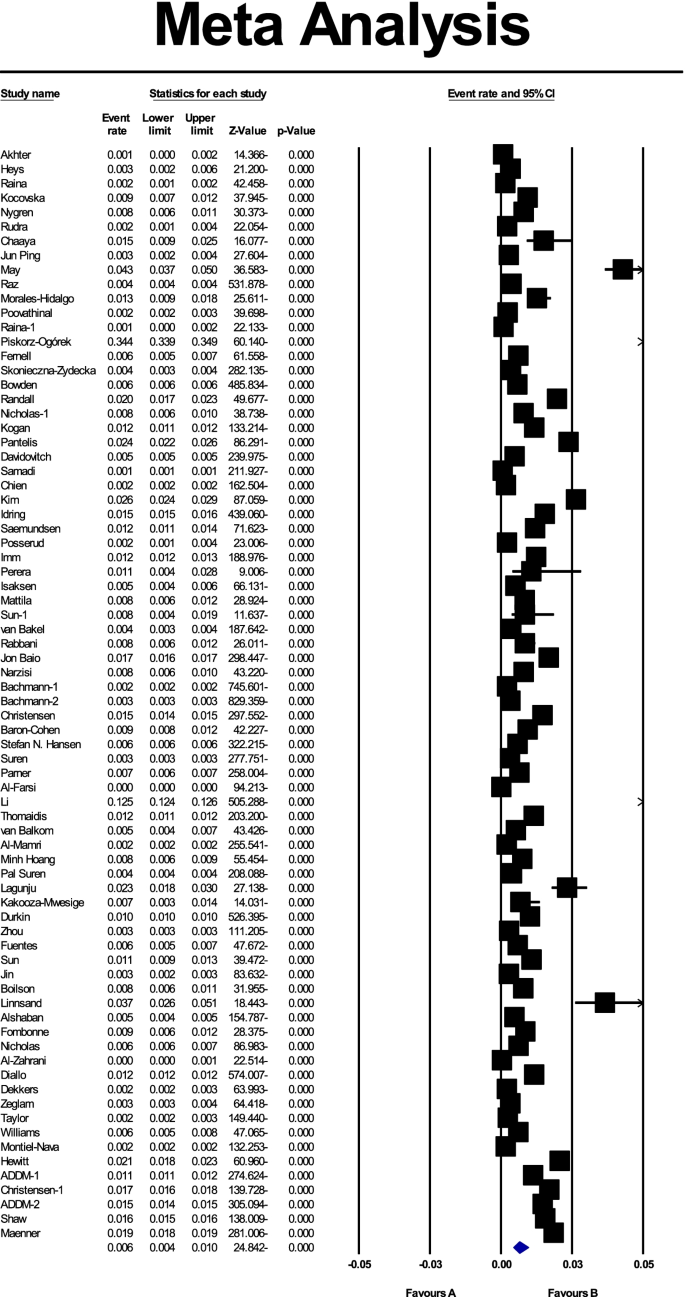
The prevalence of ASD in the world with 95% confidence interval
Meta-regression test
In order to investigate the effects of potential factors influencing the heterogeneity of the prevalence of ASD in the world, meta-regression was used for variables, including sample size and the year in which the study was conducted (Figs. 4 and 5 ). According to Fig. 4 , the prevalence of ASD globally decreases with increasing sample size, which was statistically significant ( P < 0.05) (Fig. 4 ). In addition, the prevalence of ASD in the world decreases with increasing the year in which the study was conducted (Fig. 5 ), and this was also statistically significant ( P < 0.05).
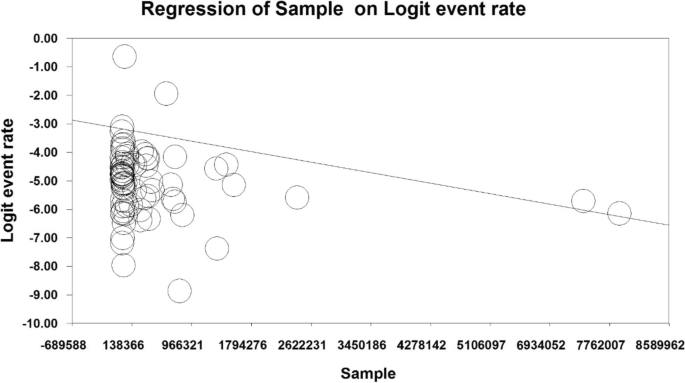
Meta-regression chart of the prevalence of ASD in the world by sample size
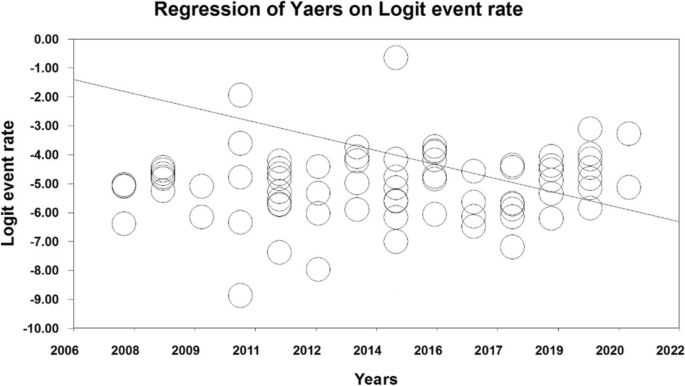
Meta-regression chart of the prevalence of ASD in the world by year
Subgroup analysis
Among the 74 studies, 26 were reviewed in Asia, 4 in Africa, 25 in Europe, 16 in the United States and 3 in Australia. The age of participants in these studies ranged from 0 to 27 years. the prevalence of ASD in Asia, America, Europe, Africa and Australia was 0.4% (95% CI: 0.1–1), 1% (95% CI: 0.8–1.1), 0.5% (95% CI: 0.2–1), 1% (95% CI: 0.3–3.1), 1.7% (95% CI: 0.5–6.1) respectively (Table 2 ) respectively (Table 2 ).
In this study, we performed a systematic review and meta-analysis to provide a general and up-to-date estimate of the prevalence of ASD in different countries. A total of 74 cross-sectional and cohort studies were reviewed, and a total of 30,212,757 patients were assessed. We found that the prevalence of ASD varies from 0.02% in China to 3.66% in Sweden. The differences in estimating ASD prevalence were associated with research methods, screening tools, ASD definition and study population. The Prevalence of ASD in Asia, America, Europe, Africa and Australia was 0.4, 1, 0.5, 1, 1.7% respectively. According to our meta-analysis, the prevalence of ASD globally decreases by increasing the sample size in the world, which is consistent with previous studies [ 14 ] .
The prevalence of ASD worldwide has increased in recent decades [ 71 , 78 , 92 ]. Prevalence estimates also vary widely among studies from different countries, ranging from less than 0.2% in China and Italy to 2.7% in South Korea [ 37 ]. The differences in the prevalence of ASD are probably due to a number of reasons, including the fact that ASD is a spectral disease with different characteristics that even affect the definitions of ASD [ 93 ].
Other reasons for differences in the prevalence of ASD include different levels of awareness in various countries, cultural differences in interpreting children’s behaviours, variabilities in screening tools and diagnostic criteria, the lack of culture-sensitive diagnostic tools, the year of evaluation, and differences in sampling and studied populations (i.e., general population-based or hospital-based sampling) [ 37 , 92 ]. Differences in study designs and protocols can affect the prevalence of ASD estimations, limiting the comparability of recent estimates [ 94 ].
Hansen et al. attributed 60% of the rising trend of ASD during recent years to alterations in diagnostic criteria and the incorporation of outpatients in the ASD registry [ 79 ]. Russell et al. emphasized the importance of improving diagnostic methods, increasing social awareness, and improving ASD-related behaviours by parents and teachers in the timely diagnosis and management of this condition [ 95 ]. A study in Sweden showed that ASD traits do not increase over time, but the number of children diagnosed with ASD increases, so it is concluded that changes in diagnostic tools may be responsible for the increased prevalence [ 96 ].
Our results suggested that differences in ASD prevalence can also be related to the geographical location of studied populations. In this regard, a higher prevalence in American and European countries compared to Asian countries is notable. In a cross-sectional study in Oman in 2011, the prevalence of ASD in children aged 0–14 years was estimated to be 0.14/1000. The low prevalence of ASD in Oman is probably due to underreporting and missed diagnoses [ 41 ].
In the latest study on Omani children, conducted from December 2011 to December 2018, the prevalence was reported as 2.04/1000, 15 times higher than the estimates in 2011. This increase can be attributed to improved diagnostic tools, increased awareness of ASD, better screening programs, and changes in diagnostic criteria. Even if it is almost 15 times higher than the previous study, it is still very low according to many estimates worldwide [ 43 ]. Moreover, the different prevalence of ASD in countries can be related to other socio-cultural and socio-economic factors [ 97 , 98 , 99 ].
According to our results, longitudinal analysis of the data of the same geographical region over the years confirmed an increase in the prevalence of ASD. For example, Randall et al. performed a longitudinal study on children in Australia in 2016 and estimated the prevalence of ASD as 14.1/1000 in 2005–2006 and 25.2/1000 in 2010–2011 [ 91 ], both of which were higher compared to a previously reported estimate (3.92/1000) [ 100 ]. In the most recent study in Australia in 2020, the prevalence of ASD was reported to be 43.6/1000 [ 89 ]. In another longitudinal study in Sweden on children aged 0–17 years, the prevalence of ASD was reported to increase from 4.2/1000 in 2001 to 14.4/1000 in 2011 [ 71 ].
In the United States, the Center for Disease Control and Prevention (CDC) established the ASD and Developmental Disabilities Monitoring Network (ADDM) in 2000 to screen children for ASD. The ADDM network provides the most up-to-date and comprehensive estimate of the prevalence of ASD and other growth disabilities in 8-year-old children, the age with the highest prevalence of ASD among children. Since 2010, the prevalence of ASD has also been estimated in 4-year-old children, and since 2000, these estimates have been updated bi-annually (the most recent estimates being related to 2016). The main advantage of this network is that it uses the same diagnostic criteria and follow-up methods for different groups of patients [ 15 , 61 , 101 , 102 , 103 ]. Although the prevalence of ASD in 8-year-old children in the United States increased from 6.7/1000 in 2000 to 11.3/1000 in 2008, it remained approximately unchanged from 2010 to 2012, but it started to increase again, reaching 16.8/1000 in 2014 and 18.5/1000 in the latest estimate in 2016 [ 15 , 61 , 103 ]. The same increasing trend in the prevalence of ASD has been observed in 4-year-old children, rising from 13.4/1000 in 2010 to 17/1000 in 2014 and descending to 15.6/1000 in 2016 [ 58 , 60 ].
Qiu et al . conducted a systematic review and meta-analysis of 12 studies on the prevalence of ASD until August 2018 in Asia, reporting a widely variable prevalence among countries. Accordingly, ASD prevalence was estimated as 0.51, 0.31, and 0.35% in East, South, and West Asia. The studies showed that the prevalence of ASD was on the rise in Asia, with a higher prevalence in men than in women. According to these 12 studies, the overall prevalence of ASD in Asia was 0.36%, which was lower than the prevalence in Western countries [ 18 ] .
Our results show that there is very limited data on the prevalence of ASD in Africa compared to other parts of the world. Four studies on African communities (Uganda, Nigeria, Somalia, and Libya) were assessed in the present study [ 62 , 63 , 64 , 65 ]. These studies had estimated the prevalence of ASD in a mixed population from urban and rural regions, and most of them had used convenient sampling and extracted data from hospitals and specialized pediatric centres. A shortage in studies on ASD in African countries compared to other regions of the world may be explained by factors such as insufficient psychologists and psychiatrists and a lack of resources for and low interest in researching in this field [ 104 , 105 ].
Gender is a prominent factor affecting ASD prevalence. According to the DSM-5, ASD in men is four times more common than in women [ 1 ]. Based on a comprehensive systematic review, the male to female ratio in children with ASD has been described as three to one [ 106 ], indicating a higher prevalence of ASD in males [ 92 , 107 , 108 ]. Nevertheless, some studies have reported similar ratios for males and females affected with ASD [ 109 , 110 , 111 , 112 ], which may be related to gender differences in presenting clinical symptoms. Generally, girls with ASD show fewer unusual behaviours and are less likely to be diagnosed with ASD [ 113 ]. Several studies based on clinical observations have shown that girls and women with ASD perform better in social communications and display fewer social and communication disorders than boys and men with ASD [ 114 , 115 ].
Girls with ASD represent better speech behaviour and fewer abnormalities in communicational and social skills or show different repetitive and stereotyped activities than boys [ 116 , 117 ]. These social and communication capabilities, which are related to a feature of the female phenotype, can help women adapt to social situations, masking some of the main symptoms of ASD and leading to either misdiagnosis or late diagnosis of ASD in girls [ 118 , 119 ].
The age of diagnosis is another important factor in determining ASD prevalence. While ASD can be diagnosed at 24 months [ 120 ], various studies show that the age of diagnosis is from 36 to 120 months [ 121 , 122 ]. The mean age is reported to be around 55 months [ 121 ]. and in milder forms such as Asperger syndrome, the diagnosis may be delayed until nine or even 11 years of age [ 88 ]. Nonetheless, severe ASD is usually diagnosed in the preschool years [ 123 ]. However, the severity and expression of ASD characteristics vary in patients with ASD, which can influence the time of diagnosis [ 124 ].
There are some limitations to our meta-analysis. First, different diagnostic tools and techniques in the included study may lead to selection bias. Second, the sample size was variable in the included studies, making it difficult to compare. Third, the number of studies was not available due to language limitations.
The dramatic increase in ASD prevalence in recent years has been concerning. In developing countries, accurate and reliable estimates of ASD prevalence are needed so that public health experts and policymakers can develop strategic plans to meet patients’ needs. Early diagnosis and intervention can reduce ASD complications and related disabilities and improve educational performance and cognitive development in children suffering from ASD. Nonetheless, this study cannot draw a definite conclusion whether or not the increasing trend of ASD is real or is just due to altered diagnostic criteria and tools, leading to earlier and more diagnoses of ASD patients. Therefore, it is advisable to employ a common and consistent method in future studies. Because many studies could not be accessed due to language limitations, more research is needed to obtain more reliable information worldwide. There is no information on ASD prevalence in some countries, so more research is required to obtain such data for better global management of ASD.
Availability of data and materials
Datasets are available through the corresponding author upon reasonable request.
Abbreviations
- Autism spectrum disorder
Web of Science
The Diagnostic and Statistical Manual of Mental Disorders,4th edition
International Classification of Disease, 9th revision
Diagnostic and Statistical Manual of Mental Disorder text revision,4th edition
International Classification of Diseases, 10th revision
Diagnostic and Statistical Manual-5
Autism Diagnostic Interview Revised
Autism Diagnostic Observation Schedule
Autism Behavior Checklist
Clancy Autism Behavior Scale
Children Autism Spectrum Test
Checklist for Autism in Toddlers
Autism Spectrum Screening Questionnaire
Social Communication Disorder Checklist
Modified Checklist for Autism in Toddlers
Social Communication Questionnaire
Indian Scale for Assessment of Autism
Autism Quotient-10
Reporting Questionnaire for Children
Strengthening the Reporting of Observational Studies in Epidemiology
Preferred Reporting Items for Systematic Reviews and Meta-Analysis
American Psychiatric Association A, Association AP. Diagnostic and statistical manual of mental disorders: DSM-5. Washington, DC: American psychiatric association; 2013.
Book Google Scholar
Pennington ML, Cullinan D, Southern LB. Defining autism: variability in state education agency definitions of and evaluations for autism spectrum disorders. Autism Res Treat. 2014;2014:327271.
Taylor MJ, Rosenqvist MA, Larsson H, Gillberg C, D’Onofrio BM, Lichtenstein P, et al. Etiology of autism spectrum disorders and autistic traits over time. JAMA Psychiatry. 2020;77(9):936–43.
Article PubMed Google Scholar
Bölte S, Girdler S, Marschik PB. The contribution of environmental exposure to the etiology of autism spectrum disorder. Cell Mol Life Sci. 2019;76(7):1275–97.
Article PubMed CAS Google Scholar
Lord C, Elsabbagh M, Baird G, Veenstra-Vanderweele J. Autism spectrum disorder. Lancet. 2018;392(10146):508–20.
Article PubMed PubMed Central Google Scholar
Bozkurt G, Uysal G, Düzkaya DS. Examination of care burden and stress coping styles of parents of children with autism spectrum disorder. J Pediatr Nurs. 2019;47:142–7.
Cohrs AC, Leslie DL. Depression in parents of children diagnosed with autism spectrum disorder: a claims-based analysis. J Autism Dev Disord. 2017;47(5):1416–22.
Gordon-Lipkin E, Marvin AR, Law JK, Lipkin PH. Anxiety and mood disorder in children with autism spectrum disorder and ADHD. Pediatrics. 2018;141(4):e20171377.
Leigh JP, Du J. Brief report: Forecasting the economic burden of autism in 2015 and 2025 in the United States. J Autism Dev Disord. 2015;45(12):4135–9.
Durkin MS, Maenner MJ, Baio J, Christensen D, Daniels J, Fitzgerald R, et al. Autism spectrum disorder among US children (2002–2010): socioeconomic, racial, and ethnic disparities. Am J Public Health. 2017;107(11):1818–26.
Durkin MS, Wolfe BL. Trends in autism prevalence in the US: A lagging economic indicator? J Autism Dev Disord. 2020;50(3):1095–6.
Nevison CD, Blaxill M. Diagnostic substitution for intellectual disability: a flawed explanation for the rise in autism. J Autism Dev Disord. 2017;47(9):2733–42.
Chiarotti F, Venerosi A. Epidemiology of autism spectrum disorders: a review of worldwide prevalence estimates since 2014. Brain Sci. 2020;10(5):274.
Article PubMed Central Google Scholar
Fombonne E. Epidemiological controversies in autism. Swiss Archives of Neurology. Psychiatr Psychother. 2020;171: w03084
Baio J, Wiggins L, Christensen DL, Maenner MJ, Daniels J, Warren Z, et al. Prevalence of autism spectrum disorder among children aged 8 years—autism and developmental disabilities monitoring network, 11 sites, United States, 2014. MMWR Surveill Summ. 2018;67(6):1.
Xu G, Strathearn L, Liu B, Bao W. Prevalence of autism spectrum disorder among US children and adolescents, 2014-2016. JAMA. 2018;319(1):81–2.
Narzisi A, Posada M, Barbieri F, Chericoni N, Ciuffolini D, Pinzino M, et al. Prevalence of Autism Spectrum Disorder in a large Italian catchment area: a school-based population study within the ASDEU project. Epidemiol Psychiatr Sci. 2020;29:e5
Qiu S, Lu Y, Li Y, Shi J, Cui H, Gu Y, et al. Prevalence of autism spectrum disorder in Asia: A systematic review and meta-analysis. Psychiatry Res. 2020;284:112679.
Alshaigi K, Albraheem R, Alsaleem K, Zakaria M, Jobeir A, Aldhalaan H. Stigmatization among parents of autism spectrum disorder children in Riyadh, Saudi Arabia. Int J Pediatr Adolesc Med. 2020;7(3):140–6.
Boswell K, Zablotsky B, Smith C. Predictors of autism enrollment in public school systems. Except Child. 2014;81(1):96–106.
Article Google Scholar
Imm P, White T, Durkin MS. Assessment of racial and ethnic bias in autism spectrum disorder prevalence estimates from a US surveillance system. Autism. 2019;23(8):1927–35.
Rice CE, Rosanoff M, Dawson G, Durkin MS, Croen LA, Singer A, et al. Evaluating changes in the prevalence of the Autism Spectrum Disorders (ASDs). Public Health Rev. 2012;34(2):1–22.
Moher D, Shamseer L, Clarke M, Ghersi D, Liberati A, Petticrew M, et al. Preferred reporting items for systematic review and meta-analysis protocols (PRISMA-P) 2015 statement. Syst Rev. 2015;4(1):1.
Akhter S, Hussain AE, Shefa J, Kundu GK, Rahman F, Biswas A. Prevalence of Autism Spectrum Disorder (ASD) among the children aged 18–36 months in a rural community of Bangladesh: a cross sectional study. F1000Research. 2018;7:424.
Heys M, Gibbons F, Haworth E, Medeiros E, Tumbahangphe KM, Wickenden M, et al. The estimated prevalence of autism in school-aged children living in rural Nepal using a population-based screening tool. J Autism Dev Disord. 2018;48(10):3483–98.
Raina SK, Chander V, Bhardwaj AK, Kumar D, Sharma S, Kashyap V, et al. Prevalence of autism spectrum disorder among rural, urban, and tribal children (1–10 years of age). J Neurosci Rural Pract. 2017;8(3):368.
Rudra A, Belmonte MK, Soni PK, Banerjee S, Mukerji S, Chakrabarti B. Prevalence of autism spectrum disorder and autistic symptoms in a school-based cohort of children in Kolkata, India. Autism Res. 2017;10(10):1597–605.
Chaaya M, Saab D, Maalouf FT, Boustany R-M. Prevalence of autism spectrum disorder in nurseries in Lebanon: a cross sectional study. J Autism Dev Disord. 2016;46(2):514–22.
Huang JP, Cui SS, Yu H, Hertz-Picciotto I, Qi LH, Zhang X. Prevalence and early signs of autism spectrum disorder (ASD) among 18–36 month old children in Tianjin of China. Biomed Environ Sci. 2014;27(6):453–61.
PubMed Google Scholar
Raz R, Weisskopf MG, Davidovitch M, Pinto O, Levine H. Differences in autism spectrum disorders incidence by sub-populations in Israel 1992–2009: a total population study. J Autism Dev Disord. 2015;45(4):1062–9.
Poovathinal SA, Anitha A, Thomas R, Kaniamattam M, Melempatt N, Anilkumar A, et al. Prevalence of autism spectrum disorders in a semiurban community in south India. Ann Epidemiol. 2016;26(9):663–5.e8.
Raina SK, Kashyap V, Bhardwaj AK, Kumar D, Chander V. Prevalence of autism spectrum disorders among children (1-10 years of age) - findings of a mid-term report from Northwest India. J Postgrad Med. 2015;61(4):243–6.
Article CAS PubMed PubMed Central Google Scholar
Pantelis PC, Kennedy DP. Estimation of the prevalence of autism spectrum disorder in South Korea, revisited. Autism. 2016;20(5):517–27.
Davidovitch M, Hemo B, Manning-Courtney P, Fombonne E. Prevalence and incidence of autism spectrum disorder in an Israeli population. J Autism Dev Disord. 2013;43(4):785–93.
Samadi SA, Mahmoodizadeh A, McConkey R. A national study of the prevalence of autism among five-year-old children in Iran. Autism. 2012;16(1):5–14.
Chien I-C, Lin C-H, Chou Y-J, Chou P. Prevalence and incidence of autism spectrum disorders among national health insurance enrollees in Taiwan from 1996 to 2005. J Child Neurol. 2011;26(7):830–4.
Kim YS, Leventhal BL, Koh Y-J, Fombonne E, Laska E, Lim E-C, et al. Prevalence of autism spectrum disorders in a total population sample. Am J Psychiatr. 2011;168(9):904–12.
Perera H, Wijewardena K, Aluthwelage R. Screening of 18–24-month-old children for autism in a semi-urban community in Sri Lanka. J Trop Pediatr. 2009;55(6):402–5.
Article CAS PubMed Google Scholar
Sun X, Allison C, Matthews FE, Zhang Z, Auyeung B, Baron-Cohen S, et al. Exploring the Underdiagnosis and Prevalence of Autism Spectrum Conditions in Beijing. Autism Res. 2015;8(3):250–60.
Jahan N, Rahman A, Choudhury S, Chowdhury K, Wahab M, Rahman F. Prevalence of mental disorders, mental retardation, epilepsy and substance abuse in children; 2009.
Google Scholar
Al-Farsi YM, Al-Sharbati MM, Al-Farsi OA, Al-Shafaee MS, Brooks DR, Waly MI. Brief report: Prevalence of autistic spectrum disorders in the Sultanate of Oman. J Autism Dev Disord. 2011;41(6):821–5.
Li N, Chen G, Song X, Du W, Zheng X. Prevalence of autism-caused disability among Chinese children: a national population-based survey. Epilepsy Behav. 2011;22(4):786–9.
Al-Mamri W, Idris AB, Dakak S, Al-Shekaili M, Al-Harthi Z, Alnaamani AM, et al. Revisiting the prevalence of autism spectrum disorder among Omani children: a multicentre study. Sultan Qaboos Univ Med J. 2019;19(4):e305.
Alshaban F, Aldosari M, Al-Shammari H, El-Hag S, Ghazal I, Tolefat M, et al. Prevalence and correlates of autism spectrum disorder in Qatar: a national study. J Child Psychol Psychiatry. 2019;60(12):1254–68.
Zhou H, Xu X, Yan W, Zou X, Wu L, Luo X, et al. Prevalence of autism spectrum disorder in China: a nationwide multi-centre population-based study among children aged 6 to 12 years. Neurosci Bull. 2020;36(9):961–71.
Sun X, Allison C, Wei L, Matthews FE, Auyeung B, Wu YY, et al. Autism prevalence in China is comparable to Western prevalence. Mol Autism. 2019;10(1):1–19.
Jin Z, Yang Y, Liu S, Huang H, Jin X. Prevalence of DSM-5 autism spectrum disorder among school-based children aged 3–12 years in Shanghai, China. J Autism Dev Disord. 2018;48(7):2434–43.
Al-Zahrani A. Prevalence and clinical characteristics of autism spectrum disorders in school-age children in Taif-KSA. Int J Med Sci Public Health. 2013;2(3):578–82.
Nicholas JS, Carpenter LA, King LB, Jenner W, Charles JM. Autism spectrum disorders in preschool-aged children: prevalence and comparison to a school-aged population. Ann Epidemiol. 2009;19(11):808–14.
Kogan MD, Blumberg SJ, Schieve LA, Boyle CA, Perrin JM, Ghandour RM, et al. Prevalence of parent-reported diagnosis of autism spectrum disorder among children in the US, 2007. Pediatrics. 2009;124(5):1395–403.
Christensen D, Braun K, Baio J, Bilder D, Charles J, Constantino J, et al. Prevalence and characteristics of autism spectrum disorder among children aged 8 years—autism and developmental disabilities monitoring network, 11 sites, United States, 2012. MMWR Surveillance Summaries. 2016;65(13):1.
Fombonne E, Marcin C, Manero AC, Bruno R, Diaz C, Villalobos M, et al. Prevalence of autism spectrum disorders in Guanajuato, Mexico: the leon survey. J Autism Dev Disord. 2016;46(5):1669–85.
Nicholas JS, Charles JM, Carpenter LA, King LB, Jenner W, Spratt EG. Prevalence and characteristics of children with autism-spectrum disorders. Ann Epidemiol. 2008;18(2):130–6.
Diallo FB, Fombonne É, Kisely S, Rochette L, Vasiliadis H-M, Vanasse A, et al. Prevalence and correlates of autism spectrum disorders in Quebec: Prévalence et corrélats des troubles du spectre de l’autisme au Québec. Can J Psychiatr. 2018;63(4):231–9.
Dekkers LM, Groot NA, Mosquera END, Zúniga IPA, Delfos MF. Prevalence of autism spectrum disorders in Ecuador: a pilot study in Quito. J Autism Dev Disord. 2015;45(12):4165–73.
Montiel-Nava C, Peña JA. Epidemiological findings of pervasive developmental disorders in a Venezuelan study. Autism. 2008;12(2):191–202.
Autism, Investigators DDMNSYP. Prevalence of autism spectrum disorders—autism and developmental disabilities monitoring network, 14 sites, United States, 2008. MMWR Surveill Summ. 2012;61(3):1–19.
Christensen DL, Maenner MJ, Bilder D, Constantino JN, Daniels J, Durkin MS, et al. Prevalence and characteristics of autism spectrum disorder among children aged 4 years—early autism and developmental disabilities monitoring network, seven sites, United States, 2010, 2012, and 2014. MMWR Surveill Summ. 2019;68(2):1.
Autism, Investigators DDMNSYP. Prevalence of autism spectrum disorder among children aged 8 years—autism and developmental disabilities monitoring network, 11 sites, United States, 2010. MMWR Surveill Summ. 2014;63(2):1–21.
Shaw KA, Maenner MJ, Baio J. Early identification of autism spectrum disorder among children aged 4 years—Early Autism and Developmental Disabilities Monitoring Network, six sites, United States, 2016. MMWR Surveill Summ. 2020;69(3):1.
Maenner MJ, Shaw KA, Baio J. Prevalence of autism spectrum disorder among children aged 8 years—autism and developmental disabilities monitoring network, 11 sites, United States, 2016. MMWR Surveill Summ. 2020;69(4):1.
Lagunju I, Bella-Awusah T, Omigbodun O. Autistic disorder in Nigeria: profile and challenges to management. Epilepsy Behav. 2014;39:126–9.
Kakooza-Mwesige A, Ssebyala K, Karamagi C, Kiguli S, Smith K, Anderson MC, et al. Adaptation of the “ten questions” to screen for autism and other neurodevelopmental disorders in Uganda. Autism. 2014;18(4):447–57.
Zeglam A, Maouna A. Prevalence of autistic spectrum disorders in Tripoli, Libya: the need for more research and planned services. East Mediterr Health J. 2012;18(2):184-8.
Hewitt A, Hall-Lande J, Hamre K, Esler AN, Punyko J, Reichle J, et al. Autism spectrum disorder (ASD) prevalence in Somali and non-Somali children. J Autism Dev Disord. 2016;46(8):2599–608.
Kočovská E, Biskupstø R, Gillberg IC, Ellefsen A, Kampmann H, Stórá T, et al. The rising prevalence of autism: a prospective longitudinal study in the Faroe Islands. J Autism Dev Disord. 2012;42(9):1959–66.
Nygren G, Cederlund M, Sandberg E, Gillstedt F, Arvidsson T, Gillberg IC, et al. The prevalence of autism spectrum disorders in toddlers: a population study of 2-year-old Swedish children. J Autism Dev Disord. 2012;42(7):1491–7.
Morales-Hidalgo P, Roigé-Castellví J, Hernández-Martínez C, Voltas N, Canals J. Prevalence and characteristics of autism spectrum disorder among Spanish school-age children. J Autism Dev Disord. 2018;48(9):3176–90.
Fernell E, Gillberg C. Autism spectrum disorder diagnoses in Stockholm preschoolers. Res Dev Disabil. 2010;31(3):680–5.
Skonieczna-Żydecka K, Gorzkowska I, Pierzak-Sominka J, Adler G. The prevalence of autism spectrum disorders in West Pomeranian and Pomeranian regions of Poland. J Appl Res Intellect Disabil. 2017;30(2):283–9.
Idring S, Lundberg M, Sturm H, Dalman C, Gumpert C, Rai D, et al. Changes in prevalence of autism spectrum disorders in 2001–2011: findings from the Stockholm youth cohort. J Autism Dev Disord. 2015;45(6):1766–73.
Saemundsen E, Magnússon P, Georgsdóttir I, Egilsson E, Rafnsson V. Prevalence of autism spectrum disorders in an Icelandic birth cohort. BMJ Open. 2013;3(6):e002748.
Posserud M, Lundervold AJ, Lie SA, Gillberg C. The prevalence of autism spectrum disorders: impact of diagnostic instrument and non-response bias. Soc Psychiatry Psychiatr Epidemiol. 2010;45(3):319–27.
Isaksen J, Diseth TH, Schjølberg S, Skjeldal OH. Observed prevalence of autism spectrum disorders in two Norwegian counties. Eur J Paediatr Neurol. 2012;16(6):592–8.
Mattila M-L, Kielinen M, Linna S-L, Jussila K, Ebeling H, Bloigu R, et al. Autism spectrum disorders according to DSM-IV-TR and comparison with DSM-5 draft criteria: an epidemiological study. J Am Acad Child Adolesc Psychiatry. 2011;50(6):583–92. e11.
van Bakel MME, Delobel-Ayoub M, Cans C, Assouline B, Jouk P-S, Raynaud J-P, et al. Low but increasing prevalence of autism spectrum disorders in a French area from register-based data. J Autism Dev Disord. 2015;45(10):3255–61.
Bachmann CJ, Gerste B, Hoffmann F. Diagnoses of autism spectrum disorders in Germany: time trends in administrative prevalence and diagnostic stability. Autism. 2018;22(3):283–90.
Baron-Cohen S, Scott FJ, Allison C, Williams J, Bolton P, Matthews FE, et al. Prevalence of autism-spectrum conditions: UK school-based population study. Br J Psychiatry. 2009;194(6):500–9.
Hansen SN, Schendel DE, Parner ET. Explaining the increase in the prevalence of autism spectrum disorders: the proportion attributable to changes in reporting practices. JAMA Pediatr. 2015;169(1):56–62.
Parner ET, Schendel DE, Thorsen P. Autism prevalence trends over time in Denmark: changes in prevalence and age at diagnosis. Arch Pediatr Adolesc Med. 2008;162(12):1150–6.
Thomaidis L, Mavroeidi N, Richardson C, Choleva A, Damianos G, Bolias K, et al. Autism Spectrum Disorders in Greece: Nationwide Prevalence in 10–11 Year-Old Children and Regional Disparities. J Clin Med. 2020;9(7):2163.
Van Balkom ID, Bresnahan M, Vogtländer MF, van Hoeken D, Minderaa RB, Susser E, et al. Prevalence of treated autism spectrum disorders in Aruba. J Neurodev Disord. 2009;1(3):197–204.
Surén P, Bakken IJ, Aase H, Chin R, Gunnes N, Lie KK, et al. Autism spectrum disorder, ADHD, epilepsy, and cerebral palsy in Norwegian children. Pediatrics. 2012;130(1):e152–e8.
Fuentes J, Basurko A, Isasa I, Galende I, Muguerza MD, García-Primo P, et al. The ASDEU autism prevalence study in northern Spain. Eur Child Adolesc Psychiatry. 2021;30(4):579–89.
Boilson A, Staines A, Ramirez A, Posada M, Sweeney M. Operationalisation of the European Protocol for Autism Prevalence (EPAP) for autism spectrum disorder prevalence measurement in Ireland. J Autism Dev Disord. 2016;46(9):3054–67.
Linnsand P, Gillberg C, Nilses Å, Hagberg B, Nygren G. A high prevalence of autism spectrum disorder in preschool children in an immigrant, multiethnic population in Sweden: challenges for health care. J Autism Dev Disord. 2021;51(2):538–49.
Taylor B, Jick H, MacLaughlin D. Prevalence and incidence rates of autism in the UK: time trend from 2004–2010 in children aged 8 years. BMJ Open. 2013;3(10):e003219.
Williams E, Thomas K, Sidebotham H, Emond A. Prevalence and characteristics of autistic spectrum disorders in the ALSPAC cohort. Dev Med Child Neurol. 2008;50(9):672–7.
May T, Brignell A, Williams K. Autism spectrum disorder prevalence in children aged 12–13 years from the longitudinal study of Australian children. Autism Res. 2020;13(5):821–7.
Bowden N, Thabrew H, Kokaua J, Audas R, Milne B, Smiler K, et al. Autism spectrum disorder/Takiwātanga: An Integrated Data Infrastructure-based approach to autism spectrum disorder research in New Zealand. Autism. 2020;24(8):2213–27.
Randall M, Sciberras E, Brignell A, Ihsen E, Efron D, Dissanayake C, et al. Autism spectrum disorder: Presentation and prevalence in a nationally representative Australian sample. Aus N Z J Psychiatry. 2016;50(3):243–53.
Elsabbagh M, Divan G, Koh YJ, Kim YS, Kauchali S, Marcín C, et al. Global prevalence of autism and other pervasive developmental disorders. Autism Res. 2012;5(3):160–79.
American Psychiatric Association A. Diagnostic and statistical manual of mental disorders: American Psychiatric Association Washington, DC; 1980.
Fombonne E. Epidemiology of pervasive developmental disorders. Pediatr Res. 2009;65(6):591–8.
Russell G, Collishaw S, Golding J, Kelly SE, Ford T. Changes in diagnosis rates and behavioural traits of autism spectrum disorder over time. BJPsych Open. 2015;1(2):110–5.
Lundström S, Reichenberg A, Anckarsäter H, Lichtenstein P, Gillberg C. Autism phenotype versus registered diagnosis in Swedish children: prevalence trends over 10 years in general population samples. BMJ. 2015;350:h1961.
La Roche MJ, Bush HH, D'Angelo E. The assessment and treatment of autism spectrum disorder: A cultural examination. Pract Innov. 2018;3(2):107.
de Leeuw A, Happé F, Hoekstra RA. A conceptual framework for understanding the cultural and contextual factors on autism across the globe. Autism Res. 2020;13(7):1029–50.
Taiwo T. Organophosphate Exposures, Financial Hardship and Child Neurodevelopmental Outcomes in the CHARGE study. Environ Epidemiolo. 2019;3:196–7.
Icasiano F, Hewson P, Machet P, Cooper C, Marshall A. Childhood autism spectrum disorder in the Barwon region: a community-based study. J Paediatr Child Health. 2004;40(12):696–701.
Rice C. Prevalence of autism spectrum disorders--Autism and developmental disabilities monitoring network, United States, 2006; 2009.
Control CD. Prevention. Prevalence of autism spectrum disorders--autism and developmental disabilities monitoring network, 14 sites, United States, 2002. MMWR Surveill Summ (Washington, DC: 2002). 2007;56(1):12–28.
Christensen DL, Braun KVN, Baio J, Bilder D, Charles J, Constantino JN, et al. Prevalence and characteristics of autism spectrum disorder among children aged 8 years—autism and developmental disabilities monitoring network, 11 sites, United States, 2012. MMWR Surveill Summ. 2018;65(13):1.
Ruparelia K, Abubakar A, Badoe E, Bakare M, Visser K, Chugani DC, et al. Autism spectrum disorders in Africa: current challenges in identification, assessment, and treatment: a report on the International Child Neurology Association Meeting on ASD in Africa, Ghana, April 3-5, 2014. J Child Neurol. 2016;31(8):1018–26.
Abubakar A, Ssewanyana D, Newton CR. A systematic review of research on autism spectrum disorders in Sub-Saharan Africa. Behav Neurol. 2016;2016:3501910.
Loomes R, Hull L, Mandy WPL. What is the male-to-female ratio in autism spectrum disorder? A systematic review and meta-analysis. J Am Acad Child Adolesc Psychiatry. 2017;56(6):466–74.
Hiller RM, Young RL, Weber N. Sex differences in autism spectrum disorder based on DSM-5 criteria: evidence from clinician and teacher reporting. J Abnorm Child Psychol. 2014;42(8):1381–93.
Investigators P, Control CfD, Prevention. Prevalence of autism spectrum disorder among children aged 8 years-autism and developmental disabilities monitoring network, 11 sites, United States, 2010. MMWR Surveill Summ (Washington, DC: 2002). 2014;63(2):1–21.
Harrop C, Gulsrud A, Kasari C. Does gender moderate core deficits in ASD? An investigation into restricted and repetitive behaviors in girls and boys with ASD. J Autism Dev Disord. 2015;45(11):3644–55.
Mussey JL, Ginn NC, Klinger LG. Are males and females with autism spectrum disorder more similar than we thought? Autism. 2017;21(6):733–7.
Messinger DS, Young GS, Webb SJ, Ozonoff S, Bryson SE, Carter A, et al. Early sex differences are not autism-specific: A Baby Siblings Research Consortium (BSRC) study. Mol Autism. 2015;6(1):1–12.
Sutherland R, Hodge A, Bruck S, Costley D, Klieve H. Parent-reported differences between school-aged girls and boys on the autism spectrum. Autism. 2017;21(6):785–94.
Guerra S, Spoto A, Castiello U, Parma V. Sex differences in body ownership in adults with autism spectrum disorder. Front Psychol. 2019;10:168.
Rivet TT, Matson JL. Review of gender differences in core symptomatology in autism spectrum disorders. Res Autism Spectr Disord. 2011;5(3):957–76.
Werling DM, Geschwind DH. Sex differences in autism spectrum disorders. Curr Opin Neurol. 2013;26(2):146–53.
Head AM, McGillivray JA, Stokes MA. Gender differences in emotionality and sociability in children with autism spectrum disorders. Mol Autism. 2014;5(1):19.
Lai M-C, Lombardo MV, Auyeung B, Chakrabarti B, Baron-Cohen S. Sex/gender differences and autism: setting the scene for future research. J Am Acad Child Adolesc Psychiatry. 2015;54(1):11–24.
Hiller RM, Young RL, Weber N. Sex differences in pre-diagnosis concerns for children later diagnosed with autism spectrum disorder. Autism. 2016;20(1):75–84.
Dworzynski K, Ronald A, Bolton P, Happé F. How different are girls and boys above and below the diagnostic threshold for autism spectrum disorders? J Am Acad Child Adolesc Psychiatry. 2012;51(8):788–97.
Zwaigenbaum L, Bryson S, Garon N. Early identification of autism spectrum disorders. Behav Brain Res. 2013;251:133–46.
Brett D, Warnell F, McConachie H, Parr JR. Factors affecting age at ASD diagnosis in UK: no evidence that diagnosis age has decreased between 2004 and 2014. J Autism Dev Disord. 2016;46(6):1974–84.
Daniels AM, Mandell DS. Explaining differences in age at autism spectrum disorder diagnosis: a critical review. Autism. 2014;18(5):583–97.
Wiggins LD, Baio J, Rice C. Examination of the time between first evaluation and first autism spectrum diagnosis in a population-based sample. J Dev Behav Pediatr. 2006;27(2):S79–87.
Barbaro J, Dissanayake C. Autism spectrum disorders in infancy and toddlerhood: a review of the evidence on early signs, early identification tools, and early diagnosis. J Dev Behav Pediatr. 2009;30(5):447–59.
Download references
Acknowledgements
By Student Research Committee of Kermanshah University of Medical Sciences.
Not applicable.
Author information
Authors and affiliations.
Department of Biostatistics, School of Health, Kermanshah University of Medical Sciences, Kermanshah, Iran
Nader Salari
Department of Psychiatric Nursing, School of Nursing and Midwifery, Urmia University of Medical Sciences, Urmia, Iran
Shabnam Rasoulpoor
Student research committee, Kermanshah University of Medical Sciences, Kermanshah, Iran
Shna Rasoulpoor
Department of Biology, Faculty of Science, University Putra Malaysia, Serdang, Selangor, Malaysia
Shamarina Shohaimi
Department of Genetics and Molecular Biology, School of Medicine, Isfahan University of Medical Sciences, Isfahan, Iran
Sima Jafarpour
Department of Psychiatry, Substance Abuse Prevention Research Center, Kermanshah University of Medical Sciences, Kermanshah, Iran
Nasrin Abdoli
Sleep Disorders Research Center, Kermanshah University of Medical Sciences, Kermanshah, Iran
Behnam Khaledi-Paveh
Cellular and Molecular Research Center, Gerash University of Medical Sciences, Gerash, Iran
Masoud Mohammadi
You can also search for this author in PubMed Google Scholar
Contributions
NS and SHR1 contributed to the design, MM statistical analysis and participated in most of the study steps. MM and SHR2 and SHSH and BKH and SJ and NA prepared the manuscript. All authors have read and approved the content of the manuscript.
Corresponding author
Correspondence to Masoud Mohammadi .
Ethics declarations
Ethics approval and consent to participate, consent for publication, competing interests.
The authors declare that they have no conflict of interest.
Additional information
Publisher’s note.
Springer Nature remains neutral with regard to jurisdictional claims in published maps and institutional affiliations.
Rights and permissions
Open Access This article is licensed under a Creative Commons Attribution 4.0 International License, which permits use, sharing, adaptation, distribution and reproduction in any medium or format, as long as you give appropriate credit to the original author(s) and the source, provide a link to the Creative Commons licence, and indicate if changes were made. The images or other third party material in this article are included in the article's Creative Commons licence, unless indicated otherwise in a credit line to the material. If material is not included in the article's Creative Commons licence and your intended use is not permitted by statutory regulation or exceeds the permitted use, you will need to obtain permission directly from the copyright holder. To view a copy of this licence, visit http://creativecommons.org/licenses/by/4.0/ . The Creative Commons Public Domain Dedication waiver ( http://creativecommons.org/publicdomain/zero/1.0/ ) applies to the data made available in this article, unless otherwise stated in a credit line to the data.
Reprints and permissions
About this article
Cite this article.
Salari, N., Rasoulpoor, S., Rasoulpoor, S. et al. The global prevalence of autism spectrum disorder: a comprehensive systematic review and meta-analysis. Ital J Pediatr 48 , 112 (2022). https://doi.org/10.1186/s13052-022-01310-w
Download citation
Received : 07 October 2021
Accepted : 24 June 2022
Published : 08 July 2022
DOI : https://doi.org/10.1186/s13052-022-01310-w
Share this article
Anyone you share the following link with will be able to read this content:
Sorry, a shareable link is not currently available for this article.
Provided by the Springer Nature SharedIt content-sharing initiative
- systematic review
- meta-analysis
Italian Journal of Pediatrics
ISSN: 1824-7288
- Submission enquiries: Access here and click Contact Us
- General enquiries: [email protected]
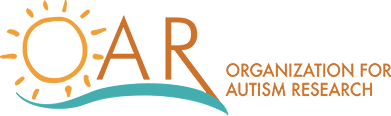
OARacle Newsletter
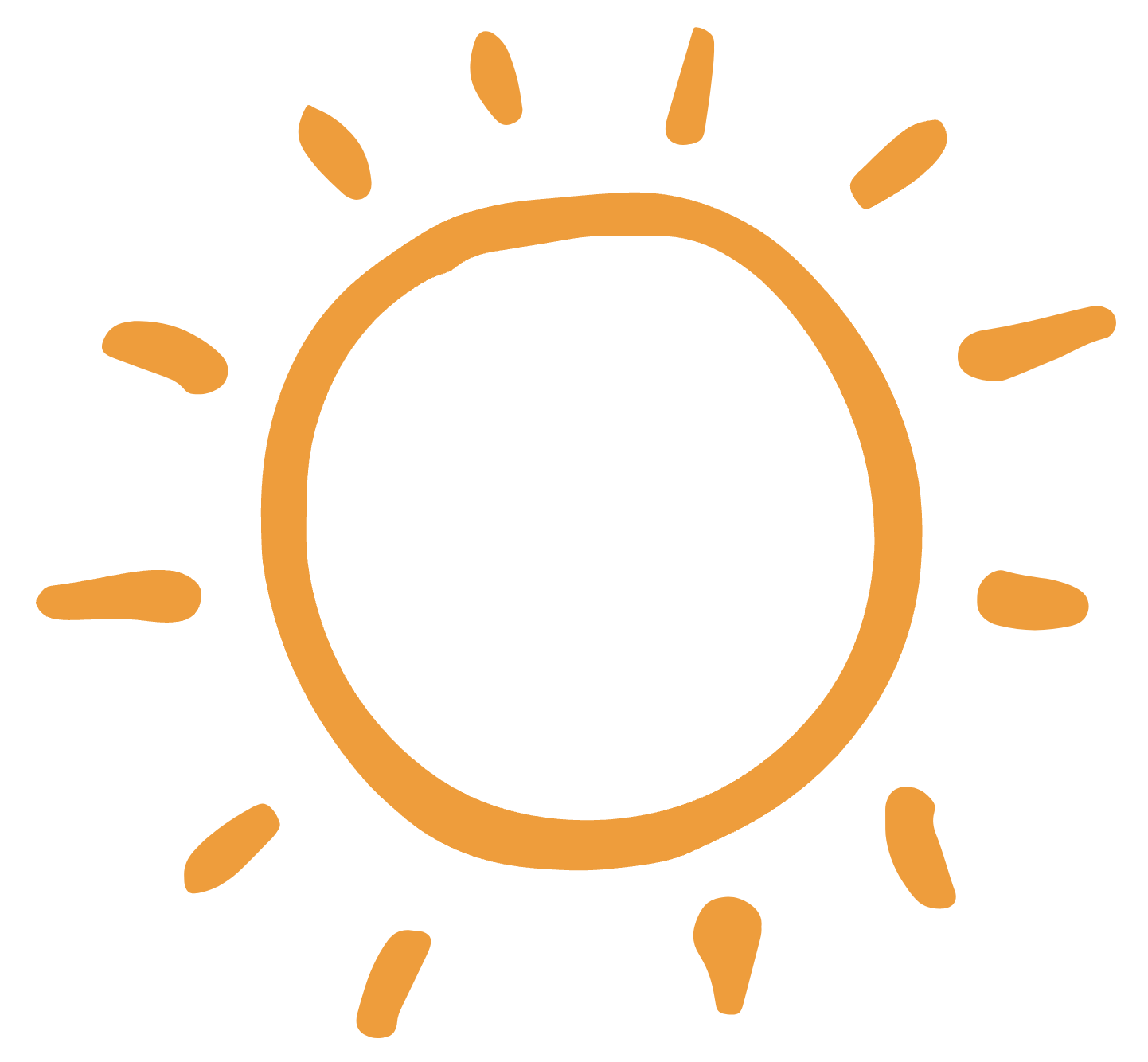
Read the latest issue of the Oaracle
Autism Prevalence Rises
May 02, 2023
By: Sherri Alms
Categories: Research , Families , Community News

In late March, the Centers for Disease Control and Prevention (CDC) reported that the autism prevalence is higher that it was in 2018 . Currently, one in 36 (2.8%) children are identified with autism spectrum disorder, based on 2020 data from 11 communities in the Autism and Developmental Disabilities Monitoring (ADDM) Network. The previous 2018 estimate was one in 44 (2.3%).
The analysis , which was published in the CDC’s Morbidity and Mortality Weekly Report, also found that ASD prevalence has risen by at least 30% since 2018 among Asian, Black, and Hispanic children. Among White children, ASD prevalence was 14.6% higher than in 2018. The report noted that the percentage of 8-year-old Asian or Pacific Islander (3.3%), Hispanic (3.2%), and black (2.9%) children diagnosed with autism was higher than among 8-year-old White children (2.4%) for the first time. That shift may reflect improved screening, awareness, and access to services among historically underserved groups.
The analysis also found that the prevalence of co-occurring intellectual disability is higher among Black autistic children (50.8%) than among Pacific Islander (41.5%), Hispanic (34.9%), Asian (34.8%), or White (31.8%) autistic children. The authors of the analysis posit that the difference could relate to access to services that diagnose and support autistic children.
Boys were diagnosed with autism four times more than girls in the latest analysis. The CDC noted that this is the first ADDM report in which the prevalence of autism among 8-year-old girls has exceeded 1%.
COVID Disruptions
A second report, based on data on 4-year-old children from the 11 communities in the ADDM network, highlighted the effect COVID had on early diagnosis . In the early months of the pandemic, fewer 4-year-old children had an evaluation or were identified with ASD than 8-year-old children when they were the same age.
“The data in this report can help communities better understand how the pandemic impacted early identification of autism in young children and anticipate future needs as these children get older,” said Karen Remley, M.D., director of CDC’s National Center on Birth Defects and Developmental Disabilities.
Sherri Alms is the freelance editor of The OARacle , a role she took on in 2007. She has been a freelance writer and editor for more than 20 years.
Related Posts
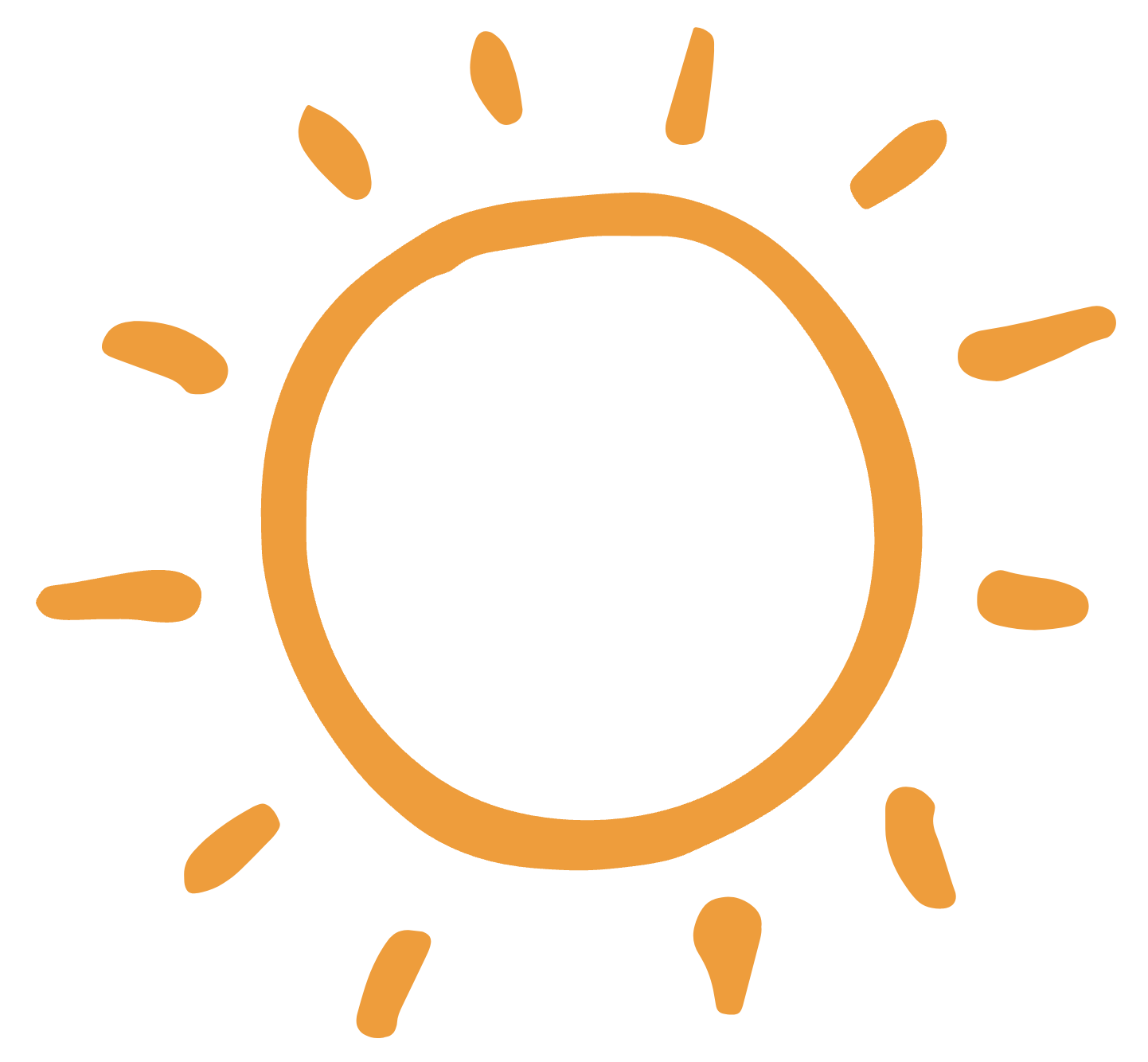
Announcing 2024 Youth Art Contest Winners
Thank you to all the talented artists who participated in OAR’s 2024 Youth Art Contest. With entries from 125 artists across 24 states and…
April 3, 2024
Read More >
IACC Releases 2019-2020 Research Funding Report
In March, the Interagency Autism Coordinating Committee (IACC) released its 2019-2020 IACC Autism Research Portfolio Analysis Report. The…
April 2, 2024
OAR Launches a Library for the Autism Community
OAR’s commitment to providing free resources to information for the autism community has just expanded with our new online…
Our Newsletter
You’ll receive periodic updates and articles from OAR

Factors Associated with Confirmed and Unconfirmed Autism Spectrum Disorder Diagnosis in Children Volunteering for Research
- Original Article
- Published: 12 April 2024
Cite this article
- Susanne W. Duvall ORCID: orcid.org/0000-0002-7202-6555 1 ,
- Rachel K. Greene ORCID: orcid.org/0000-0001-5368-6120 2 ,
- Randi Phelps ORCID: orcid.org/0000-0002-1160-3734 3 , 4 ,
- Tara M. Rutter ORCID: orcid.org/0000-0002-3080-1074 5 ,
- Sheila Markwardt 6 ,
- Julia Grieser Painter 5 ,
- Michaela Cordova ORCID: orcid.org/0000-0002-6860-5159 7 ,
- Beth Calame 5 ,
- Olivia Doyle ORCID: orcid.org/0000-0003-3957-2949 8 ,
- Joel T. Nigg ORCID: orcid.org/0000-0003-0003-3024 9 ,
- Eric Fombonne ORCID: orcid.org/0000-0002-8605-3538 9 &
- Damien Fair ORCID: orcid.org/0000-0001-8602-393X 10
Diagnostic accuracy of autism spectrum disorder (ASD) is crucial to track and characterize ASD, as well as to guide appropriate interventions at the individual level. However, under-diagnosis, over-diagnosis, and misdiagnosis of ASD are still prevalent.
We describe 232 children ( M Age = 10.71 years; 19% female) with community-based diagnoses of ASD referred for research participation. Extensive assessment procedures were employed to confirm ASD diagnosis before study inclusion. The sample was subsequently divided into two groups with either confirmed ASD diagnoses (ASD+) or unconfirmed/inaccurate diagnoses (ASD-). Clinical characteristics differentiating the groups were further analyzed.
47% of children with community-based ASD diagnoses did not meet ASD criteria by expert consensus. ASD + and ASD- groups did not differ in age, gender, ethnicity, or racial make-up. The ASD + group was more likely to have a history of early language delays compared to the ASD- group; however, no group differences in current functional language use were reported by caregivers. The ASD + group scored significantly higher on ADI-R scores and on the ADOS-2 algorithm composite scores and calibrated severity scores (CSSs). The ASD- group attained higher estimated IQ scores and higher rates of psychiatric disorders, including anxiety disorder, disruptive behavior, and mood disorder diagnoses. Broadly, caregiver questionnaires (SRS-2, CCC-2) did not differentiate groups.
Increased reported psychiatric disorders in the ASD- group suggests psychiatric complexity may contribute to community misdiagnosis and possible overdiagnosis of ASD. Clinician-mediated tools (ADI-R, ADOS-2) differentiated ASD + versus ASD- groups, whereas caregiver-reported questionnaires did not.
This is a preview of subscription content, log in via an institution to check access.
Access this article
Price includes VAT (Russian Federation)
Instant access to the full article PDF.
Rent this article via DeepDyve
Institutional subscriptions
Bishop, D. (2006). Children’s communication Checklist—2 U.S. Edition . The Psychological Corporation.
Bishop, S. L., & Lord, C. (2023). Commentary: Best practices and processes for assessment of autism spectrum disorder – the intended role of standardized diagnostic instruments. Journal Child Psychology and Psychiatry , 64 , 834–838. https://doi.org/10.1111/jcpp.13802 .
Article Google Scholar
Blumberg, S. J., Bramlett, M. D., Kogan, M. D., Schieve, L. A., Jones, J. R., & Lu, M. C. (2013). Changes in prevalence of parent-reported autism spectrum disorder in school-aged US children: 2007 to 2011– (Vol. 2012). US Department of Health and Human Services, Centers for Disease Control and Prevention, National Center for Health Statistics. (No. 65.
Capriola-Hall, N. N., McFayden, T., Ollendick, T. H., & White, S. W. (2021). Caution when screening for autism among socially anxious youth. Journal of Autism and Developmental Disorders , 51 (5), 1540–1549. https://doi.org/10.1007/s10803-020-04642-w .
Article PubMed PubMed Central Google Scholar
Center for Disease Control and Prevention (2023). Community Report on Autism.
Cicchetti, D. V., Lord, C., Koenig, K., Klin, A., & Volkmar, F. R. (2008). Reliability of the ADI-R: Multiple examiners evaluate a single case. Journal of Autism and Developmental Disorders , 38 (4), 764–770. https://doi.org/10.1007/s10803-007-0448-3 .
Article PubMed Google Scholar
Constantino, J. N., & Gruber, C. P. (2012). The social responsiveness scale (2nd edition). Los Angeles: Western Psychological Services.
Constantino, J. N., & Todd, R. D. (2000). Genetic structure of reciprocal social behavior. American Journal of Psychiatry , 157 (12), 2043–2045. https://doi.org/10.1176/appi.ajp.157.12.2043 .
Constantino, J. N., Davis, S. A., Todd, R. D., Schindler, M. K., Gross, M. M., Brophy, S. L., & Reich, W. (2003). Validation of a brief quantitative measure of autistic traits: Comparison of the social responsiveness scale with the autism diagnostic interview-revised. Journal of Autism and Developmental Disorders , 33 (4), 427–433. https://doi.org/10.1023/A:1025014929212 .
Centers for Disease Control and Prevention (CDC) Developmental Disabilities Monitoring Network Surveillance Year 2010 Principal Investigators (2014). Prevalence of autism spectrum disorder among children aged 8 years—autism and developmental disabilities monitoring network, 11 sites, United States, 2010. Morbidity and Mortality Weekly Report: Surveillance Summaries , 63 (2), 1–21. https://www.jstor.org/stable/24806108 .
Dolata, J. K., Suarez, S., Calame, B., & Fombonne, E. (2022). Pragmatic language markers of autism diagnosis and severity. Research in Autism Spectrum Disorders , 94 , 101970. https://doi.org/10.1016/j.rasd.2022.101970 .
Duvall, S., Armstrong, K., Shahabuddin, A., Grantz, C., Fein, D., & Lord, C. (2022). A road map for identifying autism spectrum disorder: Recognizing and evaluating characteristics that should raise red or pink flags to guide accurate differential diagnosis. The Clinical Neuropsychologist , 36 (5), 1172–1207. https://doi.org/10.1080/13854046.2021.1921276 .
Fombonne, E. (2018). Editorial: The rising prevalence of autism. Journal of Child Psychology and Psychiatry , 59 (7), 717–720. https://doi.org/10.1111/jcpp.12941 .
Fombonne, E. (2023). Editorial: Is autism overdiagnosed? Journal of Child Psychology and Psychiatry , 64 , 711–714. https://doi.org/10.1111/jcpp.13806 .
Fombonne, E., MacFarlane, H., & Salem, A. C. (2021). Epidemiological surveys of ASD: Advances and remaining challenges. Journal of Autism and Developmental Disorders , 51 (12), 4271–4290. https://doi.org/10.1007/s10803-021-05005-9 .
Gotham, K., Pickles, A., & Lord, C. (2009). Standardizing ADOS scores for a measure of severity in autism spectrum disorders. Journal of Autism and Developmental Disorders , 39 (5), 693–705. https://doi.org/10.1007/s10803-008-0674-3 .
Graf, W. D., Miller, G., Epstein, L. G., & Rapin, I. (2017). The autism epidemic ethical, legal, and social issues in a developmental spectrum disorder. Neurology , 88 (14), 1371–1380. https://doi.org/10.1212/WNL.0000000000003791 .
Greene, R. K., Vasile, I., Bradbury, K. R., Olsen, A., & Duvall, S. W. (2022). Autism Diagnostic Observation schedule (ADOS-2) elevations in a clinical sample of children and adolescents who do not have autism: Phenotypic profiles of false positives. The Clinical Neuropsychologist , 36 (5), 943–959.
Grzadzinski, R., Dick, C., Lord, C., & Bishop, S. (2016). Parent-reported and clinician-observed autism spectrum disorder (ASD) symptoms in children with attention deficit/hyperactivity disorder (ADHD): Implications for practice under DSM-5. Molecular Autism , 7 (1), 1–12. https://doi.org/10.1186/s13229-016-0072-1 .
Guthrie, W., Wallis, K., Bennett, A., Brooks, E., Dudley, J., Gerdes, M., & Miller, J. S. (2019). Accuracy of autism screening in a large pediatric network. Pediatrics , 144 (4). https://doi.org/10.1542/peds.2018-3963 .
Hausman-Kedem, M., Kosofsky, B. E., Ross, G., Yohay, K., Forrest, E., Dennin, M. H., & Ward, M. J. (2018). Accuracy of reported community diagnosis of autism spectrum disorder. Journal of Psychopathology and Behavioral Assessment , 40 (3), 367–375. https://doi.org/10.1007/s10862-018-9642-1 .
Havdahl, K. A., Bal, V. H., Huerta, M., Pickles, A., Øyen, A. S., Stoltenberg, C., & Bishop, S. L. (2016). Multidimensional influences on autism symptom measures: Implications for use in etiological research. Journal of the American Academy of Child & Adolescent Psychiatry , 55 (12), 1054–1063. https://doi.org/10.1016/j.jaac.2016.09.490 .
Hill, A. P., Zuckerman, K., & Fombonne, E. (2015). Epidemiology of autism spectrum disorders. Translational approaches to Autism Spectrum Disorder (pp. 13–38). Springer International Publishing.
Kent, R., & Simonoff, E. (2017). Prevalence of anxiety in autism spectrum disorders. In: Anxiety in Children and Adolescents with Autism Spectrum Disorder. Elsevier, pp 5–32. https://doi.org/10.1016/B978-0-12-805122-1.00002-8 .
Kim, S. H., & Lord, C. (2012). Combining information from multiple sources for the diagnosis of autism spectrum disorders for toddlers and young preschoolers from 12 to 47 months of age. Journal of Child Psychology and Psychiatry , 53 (2), 143–151. https://doi.org/10.1111/j.1469-7610.2011.02458.x .
La Roche, M. J., Bush, H. H., & D’Angelo, E. (2018). The assessment and treatment of autism spectrum disorder: A cultural examination. Practice Innovations , 3 (2), 107–122. https://doi.org/10.1037/pri0000067 .
Laidler, J. R. (2005). US Department of Education Data on Autism Are Not Reliable for Tracking Autism Prevalence Pediatrics , 116(1): e120–e124. doi: https://doi.org/10.1542/peds.2004-2341 .
Lebersfeld, J. B., Swanson, M., Clesi, C. D., & O’Kelley, S. E. (2021). Systematic review and meta-analysis of the clinical utility of the ADOS-2 and the ADI-R in diagnosing autism spectrum disorders in children. Journal of Autism and Developmental Disorders , 51 , 1–14. https://doi.org/10.1007/s10803-020-04839-z .
Lord, C., Rutter, M., DiLavore, P. C., Risi, S., Gotham, K., & Bishop, S. (2012a). Autism diagnostic observation schedule: ADOS-2 . Western Psychological Services.
Lord, C., Rutter, M., DiLavore, P. C., Risi, S., Gotham, K., & Bishop, S. (2012b). ADOS-2. Autism Diagnostic Observation schedule. Manual (part I): Modules 1–4 . Western Psychological Services Los Angeles, CA.
Luciano, C. C., Keller, R., Politi, P., Aguglia, E., Magnano, F., & Burti, L. (2014). Misdiagnosis of high function autism spectrum disorders in adults: An Italian case series. Autism Open Access , 4 (131). https://doi.org/10.4172/2165-7890.1000131 .
Maenner, M. J., Shaw, K. A., Bakian, A. V., Bilder, D. A., Durkin, M. S., Esler, A., Furnier, S. M., Hallas, L., Hall-Lande, J., Hudson, A., Hughes, M. M., Patrick, M., Pierce, K., Poynter, J. N., Salinas, A., Shenouda, J., Vehorn, A., Warren, Z., Constantino, J. N., DiRienzo, M., & Cogswell, M. E. (2021). Prevalence and Characteristics of Autism Spectrum Disorder Among Children Aged 8 Years - Autism and Developmental Disabilities Monitoring Network, 11 Sites, United States, 2018. Morbidity and Mortality Weekly Report. Surveillance summaries (Washington, D.C.: 2002) , 70 (11), 1–16. https://doi.org/10.15585/mmwr.ss7011a1 .
Maenner, M. J., Warren, Z., Williams, A. R., Amoakohene, E., Bakian, A. V., Bilder, D. A., & Shaw, K. A. (2023). Prevalence and characteristics of autism spectrum disorder among children aged 8 years—Autism and Developmental Disabilities Monitoring Network, 11 sites, United States, 2020. MMWR Surveillance Summaries , 72 (2), 1. https://doi.org/10.15585/mmwr.ss7202a1 .
Article PubMed Central Google Scholar
March, J. S. (2012). Manual for the multidimensional anxiety scale for children (MASC 2) . Multi-Health Systems.
Merten, E. C., Cwik, J. C., Margraf, J., & Schneider, S. (2017). Overdiagnosis of mental disorders in children and adolescents (in developed countries). Child and Adolescent Psychiatry and Mental Health , 11 (1), 5. https://doi.org/10.1186/s13034-016-0140-5 .
Pinals, D. A., Hovermale, L., Mauch, D., & Anacker, L. (2022). Persons with intellectual and developmental disabilities in the mental health system: Part 2. Policy and systems considerations. Psychiatric Services , 73 (3), 321–328. https://doi.org/10.1176/appi.ps.201900505 .
Risi, S., Lord, C., Gotham, K., Corsello, C., Chrysler, C., Szatmari, P., & Pickles, A. (2006). Combining information from multiple sources in the diagnosis of autism spectrum disorders. Journal of the American Academy of Child & Adolescent Psychiatry , 45 (9), 1094–1103. https://doi.org/10.1097/01.chi.0000227880.42780.0e .
Rutter, M., Le Couteur, A., & Lord, C. (2003). The ADI-R: Autism diagnostic interview revised: Manual (WPS) . Western Psychological Services.
Sattler, J. M., & Dumont, R. (2004). Assessment of children: WISC-IV and WPPSI-III supplement . J. M. Sattler Publishing Company.
Simonoff, E., Pickles, A., Charman, T., Chandler, S., Loucas, T., & Baird, G. (2008). Psychiatric disorders in children with autism spectrum disorders: Prevalence, comorbidity, and associated factors in a population-derived sample. Journal of the American Academy of Child & Adolescent Psychiatry , 47 (8), 921–929. https://doi.org/10.1097/CHI.0b013e318179964f .
Skellern, C., Schluter, P., & McDowell, M. (2005). From complexity to category: Responding to diagnostic uncertainties of autistic spectrum disorders. Journal of Paediatrics and Child Health , 41 (8), 407–412. https://doi.org/10.1111/j.1440-1754.2005.00634.x .
South, M., Carr, A. W., Stephenson, K. G., Maisel, M. E., & Cox, J. C. (2017). Symptom overlap on the SRS-2 adult self‐report between adults with ASD and adults with high anxiety. Autism Research , 10 (7), 1215–1220. https://doi.org/10.1002/aur.1764 .
Streiner, D. L. (2021). Decision-Making in Psychological Assessment. In The Oxford Handbook of Personality Assessment, Second Edition . https://doi.org/10.1093/oxfordhb/9780190092689.013.32 .
van‘t Hof, M., Tisseur, C., van Berckelear-Onnes, I., van Nieuwenhuyzen, A., Daniels, A. M., Deen, M., Hoek, H. W., & Ester, W. A. (2021). Age at autism spectrum disorder diagnosis: A systematic review and meta-analysis from 2012 to 2019. Autism , 25 (4), 862–873. https://doi.org/10.1177/1362361320971107 .
Van Schalkwyk, G. I., Peluso, F., Qayyum, Z., McPartland, J. C., & Volkmar, F. R. (2015). Varieties of misdiagnosis in ASD: An illustrative case series. Journal of Autism and Developmental Disorders , 45 (4), 911. https://doi.org/10.1007/s10803-014-2239-y .
Ventola, P. E., Kleinman, J., Pandey, J., Barton, M., Allen, S., Green, J., & Fein, D. (2006). Agreement among four diagnostic instruments for autism spectrum disorders in toddlers. Journal of Autism and Developmental Disorders , 36 (7), 839–847. https://doi.org/10.1007/s10803-006-0128-8 .
Wechsler, D. (2003). Wechsler Intelligence Scale for Children–Fourth Edition: Administrative and scoring manual . The Psychological Corporation.
Wiggins, L. D., Baio, J., Schieve, L., Lee, L. C., Nicholas, J., & Rice, C. E. (2012). Retention of autism spectrum diagnoses by community professionals: Findings from the autism and developmental disabilities monitoring network, 2000 and 2006. Journal of Developmental and Behavioral Pediatrics , 33 (5), 387–395. https://doi.org/10.1097/DBP.0b013e3182560b2f .
Woolfenden, S., Sarkozy, V., Ridley, G., & Williams, K. (2012). A systematic review of the diagnostic stability of autism spectrum disorder. Research in Autism Spectrum Disorders , 6 (1), 345–354. https://doi.org/10.1016/j.rasd.2011.06.008 .
Zuckerman, K. E., Mattox, K., Donelan, K., Batbayar, O., Baghaee, A., & Bethell, C. (2013). Pediatrician identification of latino children at risk for autism spectrum disorder. Pediatrics , 132 (3), 445–453. https://doi.org/10.1542/peds.2013-0383 .
Download references
Acknowledgements
Approval was obtained from the institutional review board of Oregon Health & Science University. The procedures used in this study adhere to the tenets of the Declaration of Helsinki. The authors of this study would like to acknowledge all the members of Dr. Damien Fair’s lab for their hard work on this study. We are also extremely grateful to all the families who took the time to participate in this study. This research was supported by the National Institute of Mental Health R01 MH096773 and R00MH091238 (Fair), the Simons Foundation #177894 (Nigg) and the Oregon Health & Science University Pediatric Biostatistics Pilot Program (Duvall). We acknowledge the editorial assistance of the Oregon Clinical & Translational Research Institute, which is supported by the National Center for Advancing Translational Sciences, National Institutes of Health, through Grant Award Number UL1TR002369. Preliminary data were previously presented at the International Society for Autism Research Annual Meeting in Austin, TX, USA in 2022.
Author information
Authors and affiliations.
Departments of Pediatrics and Psychiatry, Institute on Development and Disability, Center for Development and Child Rehabilitation, Oregon Health & Science University, 707 SW Gaines St, Portland, OR, 98239, USA
Susanne W. Duvall
Departments of Pediatrics, Institute on Development and Disability, Center for Development and Child Rehabilitation, Oregon Health & Science University, 707 SW Gaines St, Portland, OR, USA
Rachel K. Greene
Staff Psychologist in the Division of Psychology, Phoenix Children’s Hospital, 1919 E Thomas Rd, Phoenix, AZ, USA
Randi Phelps
Department of Child Health, University of Arizona College of Medicine, 475 N 5th St, Phoenix, AZ, USA
Department of Psychiatry, School of Medicine, Oregon Health & Science University, 3181 S.W. Sam Jackson Rd, Portland, OR, USA
Tara M. Rutter, Julia Grieser Painter & Beth Calame
Biostatistician III, Biostatistics and Design Program, Oregon Health & Science University, 3181 SW Sam Jackson Rd, Portland, OR, 97217, USA
Sheila Markwardt
San Diego State University/UC San Diego Joint Doctoral Program in Clinical Psychology, 6363 Alvarado Ct, San Diego, CA, USA
Michaela Cordova
Department of Psychiatry, Oregon Health & Science University, 3181 SW Sam Jackson Rd, Portland, OR, 97217, USA
Olivia Doyle
Department of Psychiatry, Oregon Health & Science University, 3181 SW Sam Jackson Rd, Portland, OR, 97239, USA
Joel T. Nigg & Eric Fombonne
College of Education and Human Development, Department of Pediatrics, Medical School University of Minnesota, Masonic Institute for the Developing Brain, Professor, Institute of Child Development, 2025 E. River Parkway 7962A, Minneapolis, MN, 55414, USA
Damien Fair
You can also search for this author in PubMed Google Scholar
Corresponding author
Correspondence to Susanne W. Duvall .
Ethics declarations
Author note regarding language.
The authors use both person-first and identity-first language interchangeably within the manuscript to respect diverse opinions within the autism community.
Consent to Participate
Informed consent was obtained from all individual participants included in the study.
Conflict of interest
The authors have no conflicts of interest, financial or non-financial disclosures to report.
Additional information
Publisher’s note.
Springer Nature remains neutral with regard to jurisdictional claims in published maps and institutional affiliations.
Rights and permissions
Springer Nature or its licensor (e.g. a society or other partner) holds exclusive rights to this article under a publishing agreement with the author(s) or other rightsholder(s); author self-archiving of the accepted manuscript version of this article is solely governed by the terms of such publishing agreement and applicable law.
Reprints and permissions
About this article
Duvall, S.W., Greene, R.K., Phelps, R. et al. Factors Associated with Confirmed and Unconfirmed Autism Spectrum Disorder Diagnosis in Children Volunteering for Research. J Autism Dev Disord (2024). https://doi.org/10.1007/s10803-024-06329-y
Download citation
Accepted : 21 March 2024
Published : 12 April 2024
DOI : https://doi.org/10.1007/s10803-024-06329-y
Share this article
Anyone you share the following link with will be able to read this content:
Sorry, a shareable link is not currently available for this article.
Provided by the Springer Nature SharedIt content-sharing initiative
- Autism spectrum disorder
- Community-based diagnosis
- Comorbidity
- Find a journal
- Publish with us
- Track your research
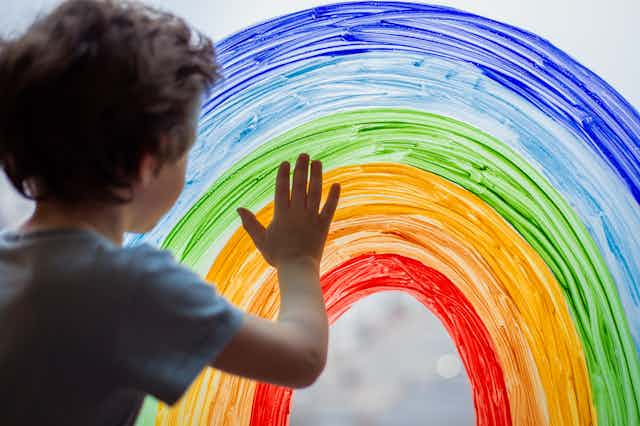
The National Autism Strategy is urgently needed. Does the government’s new draft do enough to help those in crisis?
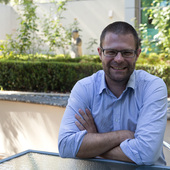
Bennett Chair of Autism, Telethon Kids Institute, The University of Western Australia
Disclosure statement
Andrew Whitehouse receives funding from the National Health and Medical Research Council, the Autism CRC, and the Angela Wright Bennett Foundation. Andrew was a member of the Social Inclusion Working Group that contributed to the National Autism Strategy.
University of Western Australia provides funding as a founding partner of The Conversation AU.
View all partners
The Australian government released its draft National Autism Strategy yesterday. It aims to power a “coordinated national approach, supporting Autistic people at each stage of life”.
The strategy is the fulfilment of an election commitment by the Albanese government, which was met with great optimism by many in the Australian community at the time.
The strategy was co-designed after consultation with more than 2,000 autistic people, families, carers and researchers. It follows the lead of South Australia which released a strategy and appointed an assistant minister for autism in 2022.
Now focus shifts to whether the draft strategy – open for public feedback until the end of May – fulfils the hopes of the community.
Why a national autism strategy?
The prominence of autism in Australia has grown considerably. Increased awareness has been a key factor behind the surge in the prevalence of autism diagnosis in Australia, estimated to be at least 3.2% of school-aged children .
Autistic Australians have among the poorest life outcomes in Australian society. As reported in the draft strategy, the life expectancy of autistic Australians is on average more than 20 years shorter than non-autistic Australians. They are nine times more likely to die of suicide and they experience higher rates of physical and sexual abuse.
There is an urgent need to turn these horrifying statistics around.
Governments contribute to each person’s life through early childhood programs, education, employment, the health and justice systems, to name just a few functions. A whole-of-government strategy is important because it focuses all departments on the same goal.
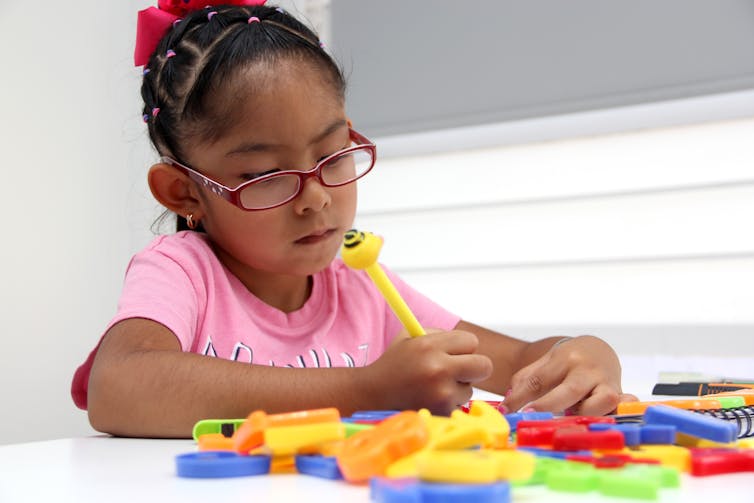
Read more: New national autism guideline will finally give families a roadmap for therapy decisions
Who is involved?
The National Autism Strategy is being developed by the federal government, guided by an oversight council of sector representatives, predominantly members of the autistic community. This is a great strength of the development process, which also involves working groups in key topic areas.
But the fact the National Autism Strategy is being developed independent of state and territory governments constrains it. The strategy can’t include commitments about functions under state and territory control. Unfortunately, it is these areas in most need of urgent action.
Education systems are struggling to meet the demand for the growing number of autistic students coupled with our expectation of inclusive classrooms . There is an acute shortage of safe housing options for generations of autistic Australians who are either in adulthood or approaching it. Autistic Australians are encountering the justice systems at high rates, with evidence they receive longer sentences than non-autistic Australians.
The administration of education policy and public housing rests with the state and territories, and the vast majority of Australian prisoners are convicted under state and territory laws.
The potency of a strategy unable to guide government commitment on these most urgent issues is diluted from the start.
Read more: We don't need a hydrotherapy pool in every school, but we do need quality public education for all kids
What does the strategy include?
The draft strategy proposes 24 commitments across key areas such as:
- social inclusion
- economic inclusion
- services and supports.
The commitments are structured around the human rights of autistic Australians, and their rights to accessible and inclusive environments that meet their needs.
The draft strategy calls for public education campaigns about autistic Australians in the workplace, facilitating meaningful employment opportunities, and improving access to quality and timely supports that nurture autistic identity.
It also calls for greater leadership and active involvement of autistic Australians in related policy and accountability mechanisms.
But there is room for improvement
Even within a scope that is constrained to the roles and responsibilities of the federal government, there were a range of urgent issues not addressed within the draft.
Autism is an area that has historically been plagued by non-evidence based therapies and supports . Improving this evidence base is a key priority to ensure the safety and effectiveness of supports for autistic individuals, but currently the strategy remains silent on this.
Neither does the strategy touch on early childhood education and care (such as child-care subsidies and preschool funding) or aged care, both of which are largely federal responsibilities. Quality support and inclusive policies at these vulnerable and highly impactful times of life are critical to meeting the human rights of autistic Australians.
Even where states and territories have primary jurisdiction, the federal government can still play a major role in leading change. For example, the federal government can strengthen the justice system by creating diversionary programs for autistic individuals, which could avoid situations such as the recent encouragement of a 13-year old autistic boy in his fixation on a terrorist organisation by an undercover federal agent.
Similarly, while education policy is set at the state and territory level, the federal government is a significant contributor to school funding. A strategy that included a commitment to adequate investment for schools to promote inclusive classroom policies would be a system-changing commitment.
Read more: The government says NDIS supports should be 'evidence-based' – but can they be?
Is the draft National Autism Strategy a game-changer?
The draft National Autism Strategy includes an array of commitments that represent a critical step forward for the understanding, inclusion and empowerment of autistic Australians. This is an important document, which marks a celebratory moment for Australia.
However, its title of “National Autism Strategy” implies an ambition the terms of reference mean it could never meet.
Many of the truly thorny challenges that plague autistic Australians – reduced life expectancy, inadequate housing, educational exclusion and underachievement, unemployment and underemployment, experiencing higher rates of physical and sexual abuse – cannot be solved by one government alone.
This work requires the hard grind of all levels of governments working together across multiple generations to integrate and evolve complex systems. Alongside a top-down strategy, the goal of a coordinated approach could be better met by National Cabinet, which brings together the prime minister and the state premiers and territory chief ministers.
All it takes now is the will.
Read more: From deficits to a spectrum, thinking around autism has changed. Now there are calls for a 'profound autism' diagnosis
- Disability coverage

Faculty of Law - Academic Appointment Opportunities

Operations Manager

Senior Education Technologist

Audience Development Coordinator (fixed-term maternity cover)

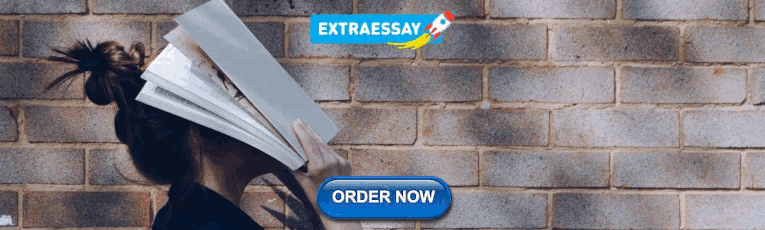
Lecturer (Hindi-Urdu)
- Open access
- Published: 11 April 2024
Teachers and educators’ experiences and perceptions of artificial-powered interventions for autism groups
- Guang Li 1 ,
- Mohammad Amin Zarei 2 ,
- Goudarz Alibakhshi 2 &
- Akram Labbafi 3
BMC Psychology volume 12 , Article number: 199 ( 2024 ) Cite this article
Metrics details
Artificial intelligence-powered interventions have emerged as promising tools to support autistic individuals. However, more research must examine how teachers and educators perceive and experience these AI systems when implemented.
The first objective was to investigate informants’ perceptions and experiences of AI-empowered interventions for children with autism. Mainly, it explores the informants’ perceived benefits and challenges of using AI-empowered interventions and their recommendations for avoiding the perceived challenges.
Methodology
A qualitative phenomenological approach was used. Twenty educators and parents with experience implementing AI interventions for autism were recruited through purposive sampling. Semi-structured and focus group interviews conducted, transcribed verbatim, and analyzed using thematic analysis.
The analysis identified four major themes: perceived benefits of AI interventions, implementation challenges, needed support, and recommendations for improvement. Benefits included increased engagement and personalized learning. Challenges included technology issues, training needs, and data privacy concerns.
Conclusions
AI-powered interventions show potential to improve autism support, but significant challenges must be addressed to ensure effective implementation from an educator’s perspective. The benefits of personalized learning and student engagement demonstrate the potential value of these technologies. However, with adequate training, technical support, and measures to ensure data privacy, many educators will likely find integrating AI systems into their daily practices easier.
Implications
To realize the full benefits of AI for autism, developers must work closely with educators to understand their needs, optimize implementation, and build trust through transparent privacy policies and procedures. With proper support, AI interventions can transform how autistic individuals are educated by tailoring instruction to each student’s unique profile and needs.
Peer Review reports
Introduction
Autism education has become an increasingly important area of focus in recent years due to the rising prevalence of autism spectrum conditions (ASC) among children. The estimated prevalence of ASC has increased from 1 in 10,000 in the 1960s to at least 1 in 100 today [ 1 , 2 , 3 ]. ASC is a neurodevelopmental condition characterized by impaired social interaction and communication abilities and stereotypical or obsessive behavior patterns. These impairments can significantly impact an individual’s social, educational, and employment experiences, leading to poor long-term outcomes and difficulties in social transactions, independent work, and job fulfillment [ 4 , 5 , 6 , 7 , 8 , 9 , 10 ].
The reported prevalence of autism spectrum disorders (ASDs) in developed countries is around 2% [ 11 ]. ASDs typically manifest within the first three years of life. They are characterized by challenges in social interaction, speech and language delays, avoidance of eye contact, difficulty adapting to changes in the environment, display of repetitive behaviors, and differences in learning profiles [ 11 , 12 , 13 ]. Those with ASDs, including children and adults, have a high frequency of anxiety and depression. Neurobiological research has revealed differences in brain development between children with ASDs and neurotypical children [ 14 ]. These excessive connections are thought to be due to reduced pruning of damaged neuronal connections during brain development, resulting in disordered neural patterning across the brain and dysregulation in cognitive function coordination between different brain regions [ 14 , 15 ].
The dominant perspective regarding AI technologies has revolved mainly around understanding these systems as a collection of processes and their corresponding responses, emphasizing autonomy, adaptability, and interactivity [ 16 , 17 , 18 , 19 , 20 , 21 ]. These characteristics are considered fundamental technological focuses that researchers argue should be integral to AI systems. Although autonomy, adaptability, and interactivity are significant, they may only cover some essential criteria for an adequate K-12 education. Specifically, these criteria are about skills taught by human educators, such as B. Self-efficacy, technical skills, and socialization skills. Samuel [ 22 ] emphasizes that AI technologies should replicate human actions and mimic expressions of “human intelligence, cognition, and logic.” This highlights the need to refine features that determine effective AI in education. The recent challenges in education due to the pandemic provide a unique opportunity to examine the demands on stakeholders, including educators, students, and parents [ 23 , 24 , 25 , 26 , 27 ].
The potential of artificial intelligence (AI) to drive developments in education is well-recognized [ 6 , 7 ]. Artificial intelligence is one of the technological advancements which can be used in education. AI encompasses a range of technologies that aim to simulate human intelligence, including machine learning, natural language processing, and computer vision [ 8 ]. These technologies have already been used in various applications, from speech recognition to image classification, and can potentially revolutionize how we think about education. In the context of autism, AI has the potential to provide personalized learning experiences that are tailored to the specific needs of each child [ 8 ]. For example, AI-powered systems can analyze a child’s behavior and responses to stimuli and use this information to adapt the learning materials and activities to suit their needs. Furthermore, AI can also be utilized to support communication and social interaction, which are areas of difficulty for many children with autism [ 9 ].
AI-powered interventions in the context of autism education refer to the utilization of artificial intelligence technologies to create tailored and interactive experiences for individuals on the autism spectrum. These interventions encompass a spectrum of applications, including educational tools, therapeutic programs, and support systems designed to address the unique learning and social communication needs of individuals with autism. AI technologies such as machine learning, natural language processing, and computer vision are employed to analyze and respond to the specific behaviors, preferences, and challenges exhibited by each individual [ 1 , 2 , 3 , 4 , 5 , 6 ]. The goal is to provide personalized and adaptive learning experiences, enhance social interaction skills, and offer targeted support for cognitive and emotional development. Examples of AI-powered interventions include virtual reality scenarios, interactive games, and educational software that can dynamically adjust content based on real-time feedback, creating a more individualized and effective educational approach for children with autism [ 2 , 3 , 4 , 5 ].
Moreover, there is a risk of bias and discrimination in AI-powered interventions for children with autism. For example, if the AI system is trained on data that is not representative of the diverse population of children with autism, it may not be effective for all individuals [ 10 ]. Moreover, there is a risk of perpetuating harmful stereotypes or reinforcing inappropriate behaviors if the AI system is not designed and programmed with ethical considerations (10). Third, there are concerns about data privacy and security when using AI in education for children with autism. For instance, if sensitive personal information is collected and stored by the AI system, there is a risk that it could be misused or accessed by unauthorized parties [ 16 ]. Therefore, it is essential to address these challenges and concerns to fully realize the potential of AI in education for children with autism. By doing so, we can create evidence-based and ethically sound interventions that support personalized learning and social communication skills while mitigating the risks associated with AI-powered education.
The potential of AI in autism education lies in its ability to offer personalized learning experiences, tailoring interventions to the unique needs of each child [ 8 ]. By analyzing a child’s behavior and responses, AI can adapt learning materials, potentially revolutionizing education for children with autism. However, this transformative potential is not without challenges. The risk of bias and discrimination looms large, as AI systems may not be effective if trained on non-representative data, perpetuating harmful stereotypes [ 10 ]. Ethical considerations become paramount, addressing concerns about data privacy and security, which, if overlooked, pose potential risks associated with unauthorized access and misuse of sensitive information [ 16 ]. Bridging the gap between the promise of AI in education and its responsible application is crucial. Therefore, this study aims to explore educators’ experiences and perceptions of AI-powered interventions for autism, shedding light on the nuanced landscape where technological advancements intersect with the delicate realm of autism education.
Research questions
In line with the research gap mentioned in the previous section, the following research questions are raised:
What are the benefits and challenges of using AI-powered interventions to support the learning and social communication skills of children with autism from teachers’ and educators’ perceptions?
How can AI-powered interventions be designed and implemented to ensure that they are culturally and linguistically appropriate for a diverse population of children with autism while also avoiding bias and discrimination in the learning materials and activities?
Review of literature
Theoretical background.
Machine learning is a component of artificial intelligence (AI) wherein models perform tasks autonomously without human intervention. Traditional machine learning models are trained using input data, enabling accurate outcome predictions. Deep learning, a subset of machine learning, employs extensive data to prepare models, achieving similarly high prediction accuracies. Both models are frequently utilized in diagnosing neurological disorders such as autism [ 28 , 29 ], ADHD [ 30 , 31 ], and depression [ 32 , 33 ]. Diagnostic inputs encompass images from computerized tomography (CT), magnetic resonance imaging (MRI), positron emission tomography (PET) scans, or electroencephalogram (EEG) signals.
AI has been instrumental in social skills training for children with autism spectrum disorders (ASDs), aiding in recognizing and responding to social cues. Belpaeme et al. [ 34 ] utilized sensory features (facial expressions, body movements, and voice recordings) as inputs to a machine-learning model implemented in a robot for analyzing autistic children’s behavior and engagement levels during therapy. This study demonstrated the robot’s potential to adapt to interactants, influencing engagement. Another survey by Sanghvi et al. [ 35 ] employed postural expressions, specifically silhouette images of the upper body during chess playing, to analyze the engagement levels of autistic children. The integration of representative data with an affect recognition model suggested the potential for the robot to serve as a game-mate for autistic children in real-world scenarios. Kim et al. [ 36 ] employed audio recordings to assess the emotional states of autistic children, enhancing the robot’s ability to evaluate engagement and modify responses for a more interactive learning environment.
Various studies explored diverse input features such as facial expressions [ 37 ], body movements [ 38 ], and biosignals [ 39 ]. Esteban et al. [ 40 ] investigated facial expressions, direction of look, body posture, and voice tones as input features to a model within the NAO robot for assessing the social engagement of autistic children, showcasing the capability of robots to possess increased autonomy. Rudovic et al. [ 41 ] developed a personalized deep model using coordinated video recordings, audio recordings, and biosignals to assess engagement in autistic children, outperforming non-personalized machine learning solutions. Another study created a hybrid physical education teaching tool using speech recognition and artificial intelligence, achieving a recognition accuracy of over 90% for a voice interactive educational robot. Collectively, these studies affirm that AI holds promise in enhancing social interaction and supportive education for children with mental disorders.
Artificial intelligence and education
The use of AI technology in education has led to increased published studies on the subject, with a reported growing interest and impact of research on AI in education [ 42 ]. AI literacy, which refers to the capacity to comprehend the essential processes and concepts underpinning AI in various products and services, has been discussed in several studies [ 43 , 44 , 45 , 46 , 47 ]. Ng et al. [ 48 ] proposed a four-dimensional AI literacy framework covering knowing and understanding AI, using and applying AI, evaluating and creating AI, and AI ethics.
Recent review papers on AI in education have highlighted several major AI applications, such as intelligent tutoring systems, natural language processing, educational robots, educational data mining, discourse analysis, neural networks, affective computing, and recommender systems [ 22 , 23 , 33 – 34 ]. However, Chen et al. [ 49 ] identified some critical issues in their review paper on AI in education, including a lack of effort in integrating deep learning technologies into educational settings, insufficient use of advanced techniques, and a scarcity of studies that simultaneously employed AI technologies and delved extensively into educational theories. Furthermore, there needs to be more knowledge and discussion on the role of AI in early childhood education (ECE), an area often ignored in cutting-edge research.
Using AI to teach children with ASD
Autism Spectrum Disorder (ASD) is a complex neurodevelopmental disorder that affects communication, social interaction, and behavior (1). The disorder is characterized by various symptoms and severity levels, making it challenging to provide effective interventions for affected individuals [12]. Children with ASD often experience difficulties in learning and require specialized educational interventions to help them achieve their full potential [1]. In recent years, there has been growing interest in the potential of AI to improve the learning outcomes of children with autism [8). AI has the potential to provide personalized learning experiences that are tailored to the specific needs of each child with autism [ 9 ]. For example, AI-powered systems can analyze a child’s behavior and responses to stimuli and use this information to adapt the learning materials and activities to suit their needs [8].
AI can also be used to support communication and social interaction, which are areas of difficulty for many children with autism [10]. Chatbots and virtual assistants can provide a non-judgmental and non-threatening environment for children to practice their social skills while providing feedback and guidance [ 23 ]. These interventions can be particularly valuable for children who struggle with face-to-face interactions or feel uncomfortable in social situations [ 24 ]. Despite the potential benefits of using AI in education for children with autism, several challenges and concerns need to be addressed:
First, there is a lack of consensus on the most effective ways to use AI to support learning for autistic children [ 8 ]. While there have been some promising results from initial studies, more research is needed to determine the most effective methods for using AI to personalize learning and support social communication skills in this population [10]. Second, there is a risk of bias and discrimination in AI-powered interventions for children with autism. For example, if the AI system is trained on data that is not representative of the diverse population of children with autism, it may not be effective for all individuals [ 9 ]. Moreover, there is a risk of perpetuating harmful stereotypes or reinforcing inappropriate behaviors if the AI system is not designed and programmed with ethical considerations [ 23 ]. And, third, there are concerns about data privacy and security when using AI in education for children with autism. For instance, if sensitive personal information is collected and stored by the AI system, there is a risk that it could be misused or accessed by unauthorized parties [10].
Several research studies have investigated the use of AI in education for children with autism. For example, Goodwin and Stone [8] developed an AI-powered system called Maki, which uses natural language processing to provide personalized feedback on social communication skills. The system was effective in improving social communication skills in children with autism. Similarly, Alzoubi et al. [ 50 ] developed an AI-powered system that uses virtual reality to provide social skills training for children with autism. The system was found to be effective in improving social skills and reducing anxiety in children with autism.
Other research studies have explored the potential of AI to improve different aspects of learning for children with autism. For example, Zhang et al. [ 10 ] developed an AI-assisted system that uses computer vision and machine learning to provide personalized feedback on handwriting skills. The system was effective in improving handwriting skills in children with autism. Similarly, Wang et al. [ 51 ] developed an AI-powered system that uses game-based learning to enhance math skills in children with autism.
There have also been efforts to develop AI-powered systems that can assist teachers and parents in providing effective interventions for children with autism. The system effectively improved the quality of interventions offered by teachers and parents. However, there are also concerns about the potential negative impacts of AI on children with autism. For example, some studies have suggested that excessive use of AI-powered interventions could reduce face-to-face interactions and social skills development [9]. Additionally, there are concerns about the potential for AI-powered interventions to replace human teachers and therapists, which could have negative implications for the quality of care provided to children with autism [8].
To address these concerns and maximize the potential benefits of AI for children with autism, it is essential to prioritize ethical considerations and involve stakeholders in designing and implementing AI-powered interventions [ 23 ]. This includes ensuring that AI systems are developed and programmed to avoid bias and discrimination, protecting the privacy and security of personal data, and promoting transparency and accountability in using AI in education for children with autism [ 10 ].
Other studies have investigated using chatbots and virtual assistants to support social communication skills in children with autism. For example, Kocaballi et al. [ 52 ] developed a chatbot called Tess that provides social skills training and support for children with autism. The chatbot was effective in improving social communication skills in children with autism. Similarly, Tanaka et al. [ 53 ] developed a virtual assistant called Miko that uses artificial empathy to support social communication skills in children with autism.
Further studies highlighted the importance of ethical consideration while using AL in education for children with autism. For example, there is a risk of perpetuating harmful stereotypes or reinforcing inappropriate behaviors if the AI system is not designed and programmed with ethical considerations [ 23 ]. Moreover, there is a risk of bias and discrimination if the AI system is trained on data that is not representative of the diverse population of children with autism [9]. Therefore, it is essential to carefully consider the ethical implications of using AI in education for children with autism. In conclusion, utilizing AI in education can transform how we think about learning and support children with autism to achieve their full potential.
Research Methodology
The study used purposive sampling to select 20 informants who met specific criteria. These individuals were parents or educators of autistic children and had valuable experience using AI-powered interventions to improve their children’s learning and social communication skills. They were all Iranian living in Tehran, Iran. 30% ( n = 6) were female and 70% ( n = 14) were male.
The participants in the study encompassed an age range spanning from 29 to 58 years old. Educators teaching experience was above 8 years. Recruitment efforts were conducted through various channels and social media platforms to ensure a diverse and representative sample. Potential participants were fully informed about the study’s purpose, procedures, and possible benefits throughout the recruitment process. They were also told of their rights as participants and the assurance of confidentiality. To confirm their willingness to participate, informants were asked for written consent before formal inclusion in the study.
Data collection
The study used semi-structured interviews and focus groups to collect data from the informants. The researcher developed the interview questions (Appendix), and a panel of three qualitative researchers reviewed their relevance. Interviews were conducted individually, either in person or virtually, and lasted approximately 45–60 min each. Focus groups with 3–5 participants conducted almost or in person were also organized. The duration of the focus group discussions was between 60 and 90 min. During the data collection process, the interviews and focus group sessions were audio-recorded to capture participants’ responses and insights accurately. These recordings were later transcribed verbatim, allowing a comprehensive analysis of the data collected. Through semi-structured interviews and focus groups, the study aimed to obtain complete and detailed information about participants’ experiences and perspectives regarding using AI-assisted interventions to support the learning and social communication skills of children with autism. The semi-structured nature of the interviews allowed for flexibility in exploring different topics while ensuring a consistent data collection framework for all participants. Additionally, the dynamic and interactive nature of the focus groups encouraged group discussions and allowed participants to share and build on one another.
Data analysis
Following the data collection phase, the study thoroughly analyzed the information collected. The audio recordings of the interviews and focus group sessions were transcribed verbatim, resulting in a comprehensive text dataset that captured participants’ responses and insights. The analysis began with a thorough familiarization process in which researchers immersed themselves in the transcribed data to understand participants’ accounts deeply. This immersion allowed researchers to identify recurring themes, patterns, and noteworthy information in the data set. A systematic analysis approach was used to ensure reliability and validity. Data were coded using a combination of inductive and deductive methods. First, an open coding process was conducted in which researchers generated initial codes by closely examining the data and labeling meaningful segments. As the analysis progressed, these codes were refined, grouped, and organized into categories and subcategories, creating a coding framework. After coding, researchers conducted a thematic analysis by identifying overarching themes from the data. The topics represented vital concepts, ideas, and perspectives shared by participants regarding the use of AI-assisted interventions to support the learning and social communication skills of children with autism. Throughout the analysis, the researchers ensured the accuracy and trustworthiness of the findings by employing techniques such as member checking, where participants were allowed to review and validate the interpretations made from their data.
Ethical considerations
The study adhered to ethical guidelines for conducting research with human subjects. Informed consent was obtained from all participants. Participants’ privacy and confidentiality were protected throughout the research process. The study also obtained ethical clearance from a relevant research ethics committee.
The study’s findings were presented in a report summarizing the themes and sub-themes that emerged from the data analysis. The report also provides recommendations for designing and implementing culturally and linguistically appropriate AI-powered interventions for children with autism while avoiding bias and discrimination in the learning materials and activities. The report also includes direct participant quotes to illustrate their experiences and perceptions. The findings are presented based on the order of research questions,
Benefits and challenges of AI-powered interventions
Informants of the study mentioned three benefits and some challenges of AI-empowered intervention for children with autism. Each is explained and exemplified as follows.
Increased engagement and motivation among children with autism
AI-powered interventions can use technologies like robots, virtual reality, and interactive games to provide personalized and engaging experiences for children with autism. Informants believed that AI-powered interventions can effectively increase engagement and motivation among children with autism. For example, educator 1 stated, “Children with autism who interacted with a humanoid robot showed increased engagement and motivation compared to those who received traditional therapy.” Educator 5 said, “By leveraging AI technologies, interventions for children with autism can be tailored to their needs and preferences, providing a more personalized and engaging learning experience. This can lead to improved outcomes and better quality of life for children with autism and their families. This finding is also supported by parent one, who stated, “My son used to struggle with traditional teaching methods, but with AI-powered interventions, he is more engaged and motivated to learn. The technology provides him with immediate feedback, which helps him understand his mistakes and learn from them.”
Customized and individualized interventions that cater to the unique needs of each child
Informants argued that every child with autism is unique, with their own set of strengths and challenges. Therefore, interventions tailored to each child’s specific needs and preferences can be more effective in promoting their development and well-being. This finding echoes the direct quotation by educator 6 who stated, “One size does not fit all when it comes to autism interventions. Each child is unique and requires a personalized approach that takes into account their individual strengths, challenges, and interests.” (Educator 6). Similarly, parent 6 stated, “As a parent, I have learned that the key to helping my child with autism is to focus on his individual needs. By working with his teachers and therapists to develop a personalized intervention plan, we have seen significant progress in his development and well-being.”
Real-time feedback to both children and educators about progress and areas for improvement
Real-time feedback involves providing immediate and ongoing information about a child’s performance and progress in a given activity or intervention. This feedback can reinforce positive behaviors, correct errors, and identify areas where additional support or instruction may be needed. Real-time feedback can be especially beneficial for children with autism, who may benefit from more frequent and targeted feedback to support their learning and development. By providing timely and specific feedback, children with autism can better understand their strengths and areas for improvement, and educators can adjust their interventions and supports accordingly. As an example, one of the educators stated, “Real-time feedback is crucial in helping children with autism learn and grow. By providing immediate and targeted feedback, we can reinforce positive behaviors and help children build new skills.” (Educator 4). Another educator stated, “Real-time feedback is not just important for children but for educators as well. By receiving ongoing feedback about a child’s progress, we can make more informed decisions about the interventions and supports that are most effective for them.“(Educator 8).
The potential for AI-powered interventions to enhance the work of educators and provide them with additional tools and resources
AI-powered interventions have the potential to enhance the work of educators and provide them with additional tools and resources to support the learning and development of children with autism. AI technologies like machine learning algorithms and natural language processing can analyze and interpret data from various sources, including assessment results, behavioral observations, and social communication interactions. This can provide educators with valuable insights and information about each child’s strengths, challenges, and learning needs. Educator 10 stated, “AI-powered interventions can provide educators with powerful tools and resources for supporting autistic children. By analyzing data and providing real-time feedback, these interventions can help educators tailor their teaching strategies and supports to the unique needs of each child.” Educator 3 also stated,” AI-powered interventions have the potential to transform the way we support children with autism in the classroom. By providing educators with insights and information about each child’s learning needs, these interventions can help us deliver more effective and personalized instruction.”
Challenges of AI-powered interventions
The content of interviews with informants was analyzed, and five main themes were extracted. Each is explained and exemplified as follows.
Lack of personalization
Informants stated that while AI-powered interventions have the potential to be personalized, there is a risk that they may not account for the unique needs and preferences of each child. For example, educator 3 stated, “We need to remember that technology is a tool, not a replacement for human interaction.”
Limited access to technology
Not all families and schools can access the necessary technologies for AI-powered interventions. As a parent of a child with autism notes, “Technology can be expensive, and not all families can afford it.”
Difficulty in interpreting and responding to social cues
Children with autism may have trouble analyzing and reacting to social cues, making it challenging to interact with AI technologies. A clinical psychologist notes: “Children with autism may struggle to understand that a robot or virtual character is not a real person, which can limit the effectiveness of AI-powered interventions.”
Ethical concerns
Ethical concerns surrounding using AI technologies with children include privacy, data security, and the potential for misuse or unintended consequences. The Director of Education at one School for Children with Autism notes: “We need to be mindful of the potential risks and unintended consequences of using AI technologies with children with autism.”
Lack of human interaction
While AI-powered interventions can be engaging and interactive, they cannot replace the importance of human interaction in promoting social and emotional development in children with autism. As a parent of a child with autism notes: “Technology can be helpful, but it is important to balance it with real-life experiences and interactions.”
Concerns about the cost and affordability of these interventions
One concern related to using interventions for children with autism is their cost and affordability. Many interventions, such as behavioral and developmental therapies, assistive technologies, and specialized education programs, can be expensive and may not be covered by insurance or other funding sources. This can create barriers for families, particularly those with limited financial resources, in accessing the interventions their child needs to thrive. As Educator 9 stated, “The cost of interventions for children with autism can be a significant burden for families, particularly those with limited financial resources. We must ensure these interventions are accessible and affordable for all families.” Similarly, parent 5 stated, “As a parent of a child with autism, the cost of interventions has been a major concern for our family. Based on our financial limitations, we have had to decide which interventions to prioritize.”
Suggestions for improving the quality of AL-empowered interventions
Interviews with informants were thematically analyzed, and different themes were extracted. Each theme is explained and exemplified as follows.
Using culturally and linguistically appropriate interventions
Participants emphasized the importance of designing and implementing AI-powered interventions that are culturally and linguistically appropriate for a diverse population of children with autism. Some of the suggestions made by participants include:
Ensuring that the language and content of the interventions are culturally sensitive and relevant to the target population.
Incorporating diverse perspectives and experiences into the design and development process.
Providing interventions in multiple languages to accommodate diverse linguistic backgrounds.
Quotations from educators and parents support these suggestions. For instance, educator 1 stated, “Cultural sensitivity is important when designing interventions for children with autism, particularly for those from diverse backgrounds. We need to ensure that the interventions are culturally relevant and take into account the unique needs and experiences of each child.” Similarly, parent 6 stated, “As a parent of a child with autism who comes from a different cultural background, I appreciate interventions that take into account my child’s unique needs and experiences. It’s important to have interventions that are culturally sensitive and relevant.”
Avoiding bias and discrimination
Participants also emphasized the importance of avoiding bias and discrimination in AI-powered interventions’ learning materials and activities. Some of the suggestions made by participants include:
Conducting regular audits of the interventions to identify and address any potential biases or discriminatory content.
Incorporating diverse perspectives and experiences into the design and development process to avoid perpetuating stereotypes.
Providing training and education to educators and developers to ensure that they are aware of and can address potential biases and discrimination.
Quotations from informants support these strategies. As an example, educator 8 stated,
“We need to be careful to avoid stereotypes and biases in the interventions we design and implement. It’s important to be aware of potential biases and to work to address them.” Similarly, parent 7 stated, “To ensure that AI-powered interventions are effective and inclusive, we need to make sure that they are designed with diversity and inclusivity in mind. This means avoiding discrimination and bias in the materials and activities.”
Training educators
Participants discussed the role of educators in implementing AI-powered interventions to support the learning and social communication skills of children with autism. Some of the key findings include:
The importance of providing training and education to educators to ensure that they can effectively implement these interventions.
The need for educators to work collaboratively with parents and other professionals to ensure that the interventions are tailored to the unique needs of each child.
“Educators play a critical role in implementing AI-powered interventions. They need to be trained and educated on how to use these interventions effectively and how to tailor them to the unique needs of each child.” [Educator 3).
We regularly audit the interventions to identify and address potential biases or discriminatory content
Conducting regular audits of interventions for children with autism is an essential step in ensuring that these interventions are effective, evidence-based, and free from biases or discriminatory content. Regular audits help identify areas for improvement, ensure that interventions are aligned with current best practices and ethical guidelines, and promote accountability and transparency in developing and implementing these interventions. Here are two quotations that address the importance of conducting regular audits of interventions for children with autism. To exemplify this finding, the following quotations are presented:
“As educators and researchers, it is our responsibility to ensure that interventions for children with autism are evidence-based, effective, and free from biases or discriminatory content. Regular audits can help us identify and address any areas of concern and promote the highest standards of quality and ethical practice.” (Educator 4). “Regular audits are essential to ensuring that interventions for children with autism are meeting the needs of all children, regardless of their race, ethnicity, gender, or other factors. We must be vigilant in identifying and addressing any biases or discriminatory content that may be present, and work to create interventions that are inclusive and equitable for all children.” (Educator 9).
Involving families and communities in the design and implementation process ensures their voices and perspectives are heard and valued
Involving families and communities in the design and implementation process of interventions for children with autism is crucial to ensuring that their voices and perspectives are heard and valued. Families and communities can provide valuable insights and feedback on the needs and preferences of children with autism and the effectiveness and cultural relevance of interventions. Here are two quotations that address the importance of involving families and communities in the design and implementation process:
“Families and communities are essential partners in the design and implementation of interventions for children with autism. Their insights and feedback can help us create interventions that are effective, culturally relevant, and responsive to the needs of all children.” (Educator 10). “As a parent of a child with autism, I know firsthand the importance of involving families and communities in the design and implementation of interventions. By listening to our voices and perspectives, researchers and educators can create interventions that are more meaningful and effective for our children.” (Parent 8).
Discussion and implications
The present study aimed at exploring the teachers and educators’ experiences and perceptions of artificial intelligence powered interventions for Autism groups. A qualitative research study was employed and interviews were analyzed thematically and different themes were extracted. Participant believed that AI-powered interventions represent a groundbreaking frontier in reshaping the support systems for the learning and social communication skills of children with autism [ 54 ]. Participants also highlighted several noteworthy benefits, with a critical emphasis on the heightened engagement and motivation witnessed among children with autism when exposed to AI-powered interventions [ 1 , 2 , 54 ]. Recognizing the limitations of traditional teaching methods in meeting the distinctive learning needs of these children, AI interventions emerge as a promising avenue [ 1 , 2 ].
The first advantage underscored by participants is the adaptability of AI-powered interventions to provide personalized and individualized support, furnishing real-time feedback to children and educators regarding progress and areas for improvement [ 3 , 4 , 5 ]. This tailored approach aligns seamlessly with the diverse and unique challenges presented by children with autism. However, embracing AI-powered interventions is full of challenges, and participants articulated various concerns [ 55 , 56 ]. Technical glitches and difficulties were identified as potential disruptors of the learning process, prompting apprehensions about an overreliance on technology [ 55 , 56 ]. Moreover, the limited access to technology and resources in specific communities and regions raises concerns about the equitable distribution of intervention benefits [ 55 , 56 ]. Addressing these challenges is imperative to ensure that all children with autism, irrespective of geographical location or socioeconomic status, have equitable access to effective interventions.
The second theme, cultural and linguistic appropriateness, emerged as a primary consideration, with participants highlighting the importance of interventions tailored to the diverse backgrounds of children with autism [ 55 , 56 ]. This includes ensuring that the language and content of interventions are culturally sensitive and relevant, integrating diverse perspectives into the design process, and providing interventions in multiple languages to accommodate linguistic diversity [ 7 , 8 , 9 ]. This finding is consistent with the findings of the previous research which highlighted that language differences can pose significant barriers to accessing autism interventions, highlighting the urgent need for interventions in the child’s native language [ 66 ].
As the third extracted theme “mitigating bias and discrimination in AI-powered interventions” extracted as another critical aspect, necessitating regular audits to identify and rectify potential biases [ 57 ]. The imperative of incorporating diverse perspectives into the design process and providing training to educators and developers to address biases and discrimination was highlighted as crucial [ 10 , 11 ]. This finding confirms the findings of the study that emphasizes the pivotal role of involving families and communities in designing and developing autism interventions to ensure cultural sensitivity and effectiveness [ 67 ].
Despite the above-mentioned potential of AI-powered interventions, the participants concurrently acknowledged the need for further research to evaluate the effectiveness of remote interventions and ensure their cultural and linguistic appropriateness [ 12 , 13 ]. Simultaneously, there are apprehensions and concerns with the potential for these interventions to exacerbate existing disparities in access to care if not implemented equitably. Moreover, challenges have been discerned alongside these benefits, prompting a comprehensive approach to ensure effectiveness, inclusivity, and accessibility [ 55 , 56 ]. Technical glitches, concerns about overreliance on technology, and limited access to resources pose hurdles that need addressing [ 55 , 56 ]. Policymakers must prioritize equitable access, focusing on both technological infrastructure and training programs for educators [ 55 , 56 ].
In addition, ensuring cultural and linguistic appropriateness emerges as a critical consideration in designing and implementing AI-powered interventions [ 55 , 56 ]. Culturally sensitive content, diverse perspectives in development, and multilingual offerings are underscored as essential [ 7 , 8 , 9 ]. Recognizing potential biases and discrimination, participants advocate for regular audits, diversity in development teams, and education on bias mitigation as integral components of ethical AI intervention deployment [ 10 , 11 , 57 ].
AI-powered interventions have emerged as a promising avenue to revolutionize the support for children with autism, offering transformative benefits while presenting challenges that demand careful consideration [ 54 ]. One pivotal advantage emphasized by participants is the heightened engagement and motivation observed among children with autism undergoing AI-powered interventions [ 54 ]. This is particularly noteworthy as traditional teaching methods often need to catch up in meeting the unique learning needs of these children. AI interventions, utilizing technologies such as robots, virtual reality, and interactive games, create personalized and engaging experiences, as reported by educators and parents.
It can also be concluded that transformative potential of AI-powered interventions underscores the need for collaborative efforts among educators, parents, and developers, ensuring effectiveness, inclusivity, and accessibility for all children [ 60 , 61 , 62 , 63 , 64 , 65 ]. The imperative of providing interventions in multiple languages and incorporating diverse perspectives into the design and development process is underscored [ 63 ]. Additionally, including culturally responsive teaching practices alongside AI interventions emerges as a strategy to enhance engagement and outcomes, particularly for children from diverse cultural backgrounds [ 68 ]. Ongoing research, collaborative endeavors, and an unwavering commitment to addressing challenges are imperative to maximize the benefits of AI-powered interventions for children with autism.
It can also be inferred that the collaborative involvement of families and communities is championed to enhance interventions’ impact and cultural sensitivity [ 12 , 13 , 67 ]. Balancing technology with human interaction is deemed crucial, emphasizing the irreplaceable role of personal connections in social and emotional development [ 39 , 41 ]. Moreover, the potential for AI-powered interventions to address access disparities, especially in remote or underserved areas, highlights the importance of further research and evaluation [ 58 , 59 ]. However, concerns persist about exacerbating existing disparities, demanding meticulous attention to cultural, linguistic, and regional nuances.
As another concluding remark, it can be inferred that AI-powered interventions have the potential to revolutionize the way we support the learning and social communication skills of children with autism. These interventions can provide customized and individualized interventions that cater to the unique needs of each child, providing real-time feedback to both children and educators about progress and areas for improvement. AI-powered interventions can also improve access to care for children with autism, particularly for those in remote or underserved areas. The findings suggest that to ensure that AI-powered interventions are culturally and linguistically appropriate for a diverse population of children with autism while also avoiding bias and discrimination in the learning materials and activities, it is essential to incorporate various perspectives and experiences into the design and development process, provide interventions in multiple languages, ensure that the language and content of the interventions are culturally sensitive and relevant, deliver training and education to educators and developers, conduct regular audits of the interventions, involve families and community members in the design and implementation process, and use culturally responsive teaching practices. These efforts can help to address the challenges and considerations of using AI-powered interventions and ensure that all children with autism have access to practical, inclusive, and culturally appropriate interventions.
However, several challenges and considerations need to be taken into account to ensure that these interventions are effective, inclusive, and accessible to all children with autism. These challenges include technical difficulties, overreliance on technology, limited access to technology and resources in specific communities and regions, and the need to design and implement culturally and linguistically appropriate interventions to avoid bias and discrimination.
Finally, one recurring theme is the importance of professional development for educators, which recognizes their critical role in successfully applying AI-powered interventions. Providing educators with technological expertise, cultural sensitivity, and ethical awareness is essential. Furthermore, legislators, educators, and parents must work together to prioritize the financial accessibility of interventions. The ramifications in this complex environment suggest a comprehensive and collaborative strategy. The key to success is overcoming obstacles, adopting technology responsibly, and giving accessibility and inclusivity top priority in intervention and education initiatives. Because technology constantly changes, we must remain committed to ongoing iteration and improvement. Community, parent, and educator feedback loops help us refine AI-powered interventions.
Limitations and suggestions for further studies
The current body of research on AI-powered interventions for children with autism, while promising, grapples with several limitations that warrant careful consideration. Firstly, the generalization of findings remains a challenge, as many studies tend to focus on specific demographic groups or particular manifestations of autism spectrum disorder (ASD). This limits the broader applicability of the insights gained, as the diversity within the autism spectrum may not be comprehensively represented. Additionally, a notable gap exists in understanding the long-term efficacy of AI interventions. While short-term outcomes are frequently explored, there is a scarcity of research delving into the sustained impact of these interventions on the developmental trajectories of children with autism. Longitudinal studies are crucial to elucidating AI-powered approaches’ durability and lasting benefits.
Moreover, the current literature may lack ethnic and cultural diversity, raising concerns about AI interventions’ universal applicability and artistic sensitivity. This underrepresentation hinders our understanding of how these technologies might function across diverse populations. Ethical considerations, although acknowledged, need to be thoroughly examined. Privacy, data security, and potential biases in algorithmic decision-making demand a more in-depth investigation to ensure responsible and equitable use of AI technologies in educational settings.
To address these limitations, future research should prioritize several vital areas. Long-term impact assessments are imperative to ascertain the sustained efficacy of AI interventions over time. Diverse and inclusive studies encompassing a range of ethnicities and cultural backgrounds are essential to validate the universal applicability of these technologies. Robust ethical frameworks should be developed to guide the implementation of AI interventions, addressing privacy, security, and bias concerns. Comparative studies, pitting AI interventions against traditional methods, will offer nuanced insights into their relative advantages and limitations. Family and community involvement in designing and implementing AI interventions should be explored further, recognizing the unique insights these stakeholders bring. Finally, comprehensive cost-benefit analyses are necessary to evaluate the economic aspects of AI interventions, ensuring their affordability and long-term viability in diverse educational settings. In navigating these avenues, researchers can contribute substantively to the responsible and inclusive integration of AI-powered interventions for children with autism.
Data availability
The data will be made available upon request from the corresponding author (Corresponding author: email: [email protected].
Lotter V. Epidemiology of autistic conditions in young children: 1. Prevalence. Social Psychiatry. 1966;1:124–35.
Article Google Scholar
Baird G, Simonoff E, Pickles A, Chandler S, Loucas T, Meldrum D, Charman T. Prevalence of disorders of the autism spectrum in a population cohort of children in South Thames: the special needs and Autism Project (SNAP). Lancet. 2006;368(9531):210–5.
Russell G, Rodgers LR, Ukoumunne OC, Ford T. Prevalence of parent-reported ASD and ADHD in the UK: findings from the Millennium Cohort Study. J Autism Dev Disord. 2014;44:31–40.
Legg H, Tickle A. UK parents’ experiences of their child receiving a diagnosis of autism spectrum disorder: a systematic review of the qualitative evidence. Autism. 2019;23:1897–910.
Howlin P, Moss P, Savage S, Rutter M. Social outcomes in mid- to later adulthood among individuals diagnosed with autism and average nonverbal IQ as children. J Am Acad Child Adolesc Psychiatry. 2013;52:572–81.
Williamson B. Big Data in Education: the Digital Future of Learning, Policy and Practice. Sage; 2017.
Luckin R. Machine learning and Human Intelligence: the Future of Education for the 21st Century. ERIC; 2018.
Goodwin MS, Stone JV. Artificial intelligence, autism and use of technology: a critical analysis. Psychol Cogn Sci Open J. 2018;4(1):12–7. https://doi.org/10.17140/PCSOJ-4-141 .
Lombardo MV, Lai MC, Baron-Cohen S. Big data approaches to decomposing heterogeneity across the autism spectrum. Mol Psychiatry. 2018;24(10):1435–50. https://doi.org/10.1038/s41380-018-0321-0 .
Zhang T, Wright C, Joshi G. Artificial intelligence in autism education: a systematic literature review. Res Dev Disabil. 2021;112., Article 103858. https://doi.org/10.1016/j.ridd.2021.103858 .
Kopp S, Gillberg C. Swedish child and adolescent psychiatric out-patients: a five-year cohort. Eur Child Adolesc Psychiatry. 2003;12:30–5. https://doi.org/10.1007/s00787-003-0309-3 .
Baygin M, Dogan S, Tuncer T, Barua PD, Faust O, Arunkumar N, Abdulhay EW, Palmer EE. Acharya U.R. Automated ASD detection using hybrid deep lightweight features extracted from EEG signals. Comput Biol Med. 2021;134:104548. https://doi.org/10.1016/j.compbiomed.2021.104548 .
Khodatars M, Shoeibi A, Sadeghi D, Ghaasemi N, Jafari M, Moridian P, Khadem A, Alizadehsani R, Zare A, Kong Y, et al. Deep learning for neuroimaging-based diagnosis and rehabilitation of Autism Spectrum disorder: a review. Comput Biol Med. 2021;139:104949. https://doi.org/10.1016/j.compbiomed.2021.104949 .
Tang G, Gudsnuk K, Kuo S-H, Cotrina ML, Rosoklija G, Sosunov A, Sonders MS, Kanter E, Castagna C, Yamamoto A, et al. Loss of mTOR-Dependent Macroautophagy causes autistic-like synaptic pruning deficits. Neuron. 2014;83:1131–43. https://doi.org/10.1016/j.neuron.2014.07.040 .
Article PubMed PubMed Central Google Scholar
Sharif H, Khan RA. A novel framework for automatic detection of Autism: A study on Corpus Callosum and Intracranial Brain Volume. arXiv. 2019.
Ahmad MA, Teredesai A, Eckert C. (2020). Fairness, accountability, transparency in AI at scale: Lessons from national programs. In Proceedings of the 2020 Conference on Fairness, Accountability, and Transparency (pp. 690–690). https://doi.org/10.1145/3351095.3375690 .
Baker T, Smith L, Anissa N. Educ-AI-Tion Rebooted? Exploring the future of Artificial Intelligence in Schools and Colleges. London: Nesta; 2019. Available online at. https://www.nesta.org.uk/report/education-rebooted .
Google Scholar
Chai CS, Lin PY, Jong MSY, Dai Y, Chiu TK, Huang B. (2020b, August). Factors Influencing Students’ Behavioral Intention to Continue Artificial Intelligence Learning. In 2020 International Symposium on Educational Technology (ISET) (pp. 147 150). IEEE. https://doi.org/10.1109/ISET49818.2020.00040 .
Chai CS, Wang X, Xu C. (2020). An extended theory of planned behavior for the modelling of Saudi Arabi secondary school students’ intention to learn. Artificial Intelligence. Mathematics, 8 (11), 2089. https://doi.org/10.3390/math8112089 .
Dai Y, Chai CS, Lin PY, Jong MSY, Guo Y, Qin J. Promoting students’ well-being by developing their readiness for the artificial intelligence age. Sustainability. 2020;12(16):6597. https://doi.org/10.3390/su12166597 .
Dignum V. The role and challenges of education for responsible AI. Lond Rev Educ. 2021;19(1):1–11. https://doi.org/10.14324/LRE.19.1.01 .
Druga S, Vu ST, Likhith E, Qiu T. (2019). Inclusive AI literacy for kids around the world. In Proceedings of FabLearn 2019 (pp. 104–111). https://doi.org/10.1145/3311890.3311904 .
Fourtané S. (2020). Ethics of AI: Benefits and risks of artificial intelligence systems. interesting engineering. Retrieved from.
Samuel J. (2021). A call for proactive policies for informatics and artificial intelligence technologies. Scholars Strategy Network. Available online at: https://scholars.org/contribution/call-proactive-policies-informatics-and (accessed October 2, 2022).
Samuel J, Navazali GG, Rahman M, Esawi E, Samuel Y. COVID-19 public sentiment insights and machine learning for tweets classification. Information. 2020;11(6):314–30. https://doi.org/10.3390/info11060314 .
Samuel J, Kashyap R, Samuel Y, Pelaez A. Adaptive cognitive fit: artificial intelligence augmented management of information facets and representations. Int J Manage. 2022;65(4):102505. https://doi.org/10.1016/j.ijinfomgt.2022.102505 .
Williamson B, Eynon R. Historical threads, missing links, and future directions in AI in education. Learn Media Technol. 2020;45(3):223. https://doi.org/10.1080/17439884.2020.1798995 .
Pham T-H, Vicnesh J, Wei JKE, Oh SL, Arunkumar N, Abdulhay EW, Ciaccio EJ, Acharya UR. Autism Spectrum Disorder Diagnostic System using HOS bispectrum with EEG signals. Int J Environ Res Public Heal. 2020;17:971.
Oh SL, Jahmunah V, Arunkumar N, Abdulhay EW, Gururajan R, Adib N, Ciaccio EJ, Cheong KH, Acharya. U.R. A novel automated autism spectrum disorder detection system. Complex Intell Syst. 2021;7:2399–413.
Dubreuil-Vall L, Ruffini G, Camprodon JA. Deep learning convolutional neural networks discriminate adult ADHD from healthy individuals on the basis of event-related spectral EEG. Front Neurosci. 2020;14:1–12.
Tor HT, Ooi CP, Lim-Ashworth NS, Wei JKE, Jahmunah V, Oh SL, Acharya UR, Fung DSS. Automated detection of conduct disorder and attention deficit hyperactivity disorder using decomposition and nonlinear techniques with EEG signals. Comput Methods Programs Biomed. 2021;200:105941.
Acharya UR, Oh SL, Hagiwara Y, Tan JH, Adeli H, Subha DP. Automated EEG-based screening of depression using deep convolutional neural network. Comput Methods Programs Biomed. 2018;161:103–13.
He L, Cao C. Automated depression analysis using convolutional neural networks from speech. J Biomed Inf. 2018;83:103–11. [CrossRef]Detecting Depression Using a Framework Combining Deep Multimodal Neural Networks with a Purpose-Built Automated Evaluation. Psychol. Assess. 2019, 31, 1019.
Belpaeme T, Baxter PE, Read R, Wood R, Cuayáhuitl H, Kiefer B, Racioppa S, Kruijff-Korbayová I, Athanasopoulos G, Enescu V, et al. Multimodal Child-Robot Interaction: Building Social Bonds J Hum Robot Interact. 2013;1:33–53.
Sanghvi J, Castellano G, Leite I, Pereira A, McOwan PW, Paiva A. Automatic analysis of affective postures and body motion to detect engagement with a game companion. In Proceedings of the 6th International Conference on Human-Robot Interaction—HRI ’11, Lausanne, Switzerland, 6–9 March 2011; pp. 305–312.
Kim JC, Azzi P, Jeon M, Howard AM, Park CH. Audio-based emotion estimation for interactive robotic therapy for children with autism spectrum disorder. In Proceedings of the 2017 14th International Conference on Ubiquitous Robots and Ambient Intelligence (URAI), Jeju, Korea, 28 June–1 July 2017; pp. 39–44.
Anzalone SM, Boucenna S, Ivaldi S, Chetouani M. Evaluating the Engagement with Social Robots. Int J Soc Robot. 2015;7:465–78. [CrossRef].
Colton MB, Ricks DJ, Goodrich MA, Dariush B, Fujimura K, Fujiki M. Toward therapist-in-the-loop assistive robotics for children with autism and specific language impairment. In Proceedings of the 23rd Convention of the Society for the Study of Artificial Intelligence and Simulation of Behaviour, AISB 2009, Edinburgh, UK, 6–9 April 2009.
Hernandez J, Riobo I, Rozga A, Abowd GD, Picard RW. Using electrodermal activity to recognize ease of engagement in children during social interactions. In Proceedings of the 2014 ACM International Joint Conference on Pervasive and Ubiquitous Computing, Seattle, WA, USA, 13–17 September 2014; pp. 307–317.
Esteban PG, Baxter P, Belpaeme T, Billing E, Cai H, Cao H-L, Coeckelbergh M, Costescu C, David D, De Beir A, et al. How to build a Supervised Autonomous System for Robot-enhanced therapy for children with Autism Spectrum Disorder. Paladyn J Behav Robot. 2017;8:18–38.
Rudovic O, Lee J, Dai M, Schuller B, Picard RW. Personalized machine learning for robot perception of affect and engagement in autism therapy. Sci Robot. 2018;3:eaao6760.
Kandlhofer M, Steinbauer G, Hirschmugl-Gaisch S, Huber P. Artificial Intelligence and computer science in education: From kindergarten to university. In 2016 IEEE Frontiers in Education Conference (FIE) (pp. 1–9)., 2016. IEEE.
El Kebir M, Callahan BL, Roseberry K, Masterson T. Ethical considerations for artificial intelligence to support individuals with autism: a review. Autism. 2021;25(4):894–907.
Chen B, Liu Z, Sun C. Artificial intelligence in education: a review. Neurocomputing. 2020;396:3–14.
Burgsteiner H, Gula B. AI literacy: what (and how) teachers should teach about AI. KI–Künstliche Intelligenz. 2016;30(1):89–93.
Kandlhofer M, Steinert M, Wagner M. AI literacy in education: developing critical thinking skills through an AI literacy programme. Eur J Open Distance E-Learning. 2016;19(1):82–93.
Zawacki-Richter O, Marín VI, Bond M, Gouverneur F. Systematic review of research on artificial intelligence applications in higher education–where are the educators? Int J Educational Technol High Educ. 2019;16(1):39.
Ng R, Sui DZ, Chen B. AI literacy for education: a four-dimensional framework. J Educational Comput Res. 2021;59(3):450–66.
Chen B, Wang X, Liu Z. Artificial intelligence in education: recent advances and future directions. Comput Educ. 2022;180:104913.
Alzoubi R, Al-Qudah Z, Al-Sharrah H. Virtual reality-based social skills training for children with autism spectrum disorder. J Med Syst. 2021;45(5):1–10.
Wang Y, Xu H, Luo X, Xie Y, Liu J. The application of artificial intelligence in autism spectrum disorder: a systematic review. J Med Syst. 2021;45(9):1–15.
Kocaballi AB, Velusamy V, Quiroz JC. Chatbots and conversational agents in mental health: a review of the psychiatric landscape. Can J Psychiatry. 2020;65(6):381–7.
Tanaka H, Negoro H, Iwasaka H. Miko, a conversational android for promoting social communication skills in children with autism spectrum disorder. Cyberpsychology Behav Social Netw. 2021;24(3):191–6.
Liang W-C, Chen C-Y, Lee CC. Technology in education for children with autism in the United States: a systematic review and meta-synthesis of qualitative studies. Volume 175. Computers & Education; 2021.
Ienca M, Wangmo T. Ethical considerations for AI in medical education. Med Teach. 2018. https://doi.org/10.1080/0142159X.2018.1539598 .
Keshavarzi S, Ghaznavi G. Artificial intelligence-enabled technologies in autism interventions: a scoping review. J Biomed Inform. 2021;113., Article 103628. https://doi.org/10.1016/j.jbi.2020.103628 .
Gillespie-Lynch K, Kapp SK, Shane-Simpson C, Smith DS, Hutman T. Intersectionality and the study of autism. J Autism Dev Disord. 2017;47(10):3256–60. https://doi.org/10.1007/s10803-017-3168-z .
Leslie DL, Viner RM, Lomax-Smith SC. Bias in machine learning, and how to address it. J Adolesc Health. 2019;65(5):503–5. https://doi.org/10.1016/j.jadohealth.2019.08.010 .
Selbst AD, Barocas S. The intuitive appeal of explainable machines. Fordham Law Rev. 2018;87(3):1085–139.
Whitney DG, von Ameringen M, van der Maesen GP, Skinner SR, Ethans KD. A scoping review of technology-based language interventions for children with autism spectrum disorder. Rev J Autism Dev Disorders. 2019;6(2):124–38. https://doi.org/10.1007/s40489-019-00153-0 .
Avanzo M, Porzio M, Lorenzon L, Milan L, Sghedoni R, Russo G, Mettivier G. Artificial intelligence applications in medical imaging: a review of the medical physics research in Italy. Physica Med. 2021;83:221–41.
Turner Brown C, Watson ZEP, Tolson JF, Kozlowski AM. Perspectives of parenting a child with autism: differences among hispanic, African-American, Asian and caucasian parents. J Family Social Work. 2017;20(1):24–43.
Bortoletto R, Colizzi M. Cannabis Use in Autism: reasons for concern about risk for psychosis. Healthc (Basel). 2022;10(8):1553. https://doi.org/10.3390/healthcare10081553 .
Grynszpan O, Weiss PL, Perez-Diaz F, Gal E. Innovative technology-based interventions for autism spectrum disorders: a meta-analysis. Autism. 2014;18(4):346–61.
Liu X, Wu Q, Zhao W, Luo X. Technology-facilitated diagnosis and treatment of individuals with Autism Spectrum disorder: an Engineering Perspective. Appl Sci. 2017;7(10):1051. https://doi.org/10.3390/app7101051 .
Guldberg K. Evidence-based practice in autism educational research: can we bridge the research and practice gap? Oxf Rev Educ. 2017;43(2):149–61.
Kang-Yi CD, Grinker RR, Beidas R, Agha A, Russell R, Shah SB, Shea K, Mandell DS. Influence of Community-Level Cultural beliefs about autism on families’ and professionals’ care for children. Transcult Psychiatry. 2018;55(5):623–47. https://doi.org/10.1177/1363461518779831 .
Article PubMed Central Google Scholar
Bateman KJ, Wilson SE. Supporting diverse learners with Autism through a culturally responsive visual communication intervention. Intervention School Clin. 2021;56(5):301–7. https://doi.org/10.1177/1053451220963090 .
Download references
Acknowledgements
The authors would like to thank all participants who contributed to the study.
This work was supported by The General Project of Beijing Postdoctoral Research Foundation in 2023, “Research on the Representation of the Tacit Knowledge of High School History Teachers Based on Natural language processing”. (Project No.2023-zz-182)
Author information
Authors and affiliations.
School of History, Capital Normal University, Beijing, China
Allameh Tabataba’i university, Tehran, Iran
Mohammad Amin Zarei & Goudarz Alibakhshi
Maraghe Branch, PhD Candidate of English Language Teaching, Islamic Azad University, Teheran, Iran
Akram Labbafi
You can also search for this author in PubMed Google Scholar
Contributions
GA and AL conceived and designed the concept and wrote the paper, and MAZ collected the data. AL and GL wrote the manuscript. GA, GL, and MAZ edited the manuscript.
Corresponding author
Correspondence to Mohammad Amin Zarei .
Ethics declarations
Ethical approval consent.
The Ethics Committee of Allameh Tabataba’i University approved the study. The ethics committee agreed that the study was conducted based on the principles and guidelines of the institute. The patients/participants provided written informed consent to participate in this study.
Consent for publication
Not applicable.
Competing interests
The authors declare no competing interests.
Additional information
Publisher’s note.
Springer Nature remains neutral with regard to jurisdictional claims in published maps and institutional affiliations.
Electronic supplementary material
Below is the link to the electronic supplementary material.
Supplementary Material 1
Rights and permissions.
Open Access This article is licensed under a Creative Commons Attribution 4.0 International License, which permits use, sharing, adaptation, distribution and reproduction in any medium or format, as long as you give appropriate credit to the original author(s) and the source, provide a link to the Creative Commons licence, and indicate if changes were made. The images or other third party material in this article are included in the article’s Creative Commons licence, unless indicated otherwise in a credit line to the material. If material is not included in the article’s Creative Commons licence and your intended use is not permitted by statutory regulation or exceeds the permitted use, you will need to obtain permission directly from the copyright holder. To view a copy of this licence, visit http://creativecommons.org/licenses/by/4.0/ . The Creative Commons Public Domain Dedication waiver ( http://creativecommons.org/publicdomain/zero/1.0/ ) applies to the data made available in this article, unless otherwise stated in a credit line to the data.
Reprints and permissions
About this article
Cite this article.
Li, G., Zarei, M.A., Alibakhshi, G. et al. Teachers and educators’ experiences and perceptions of artificial-powered interventions for autism groups. BMC Psychol 12 , 199 (2024). https://doi.org/10.1186/s40359-024-01664-2
Download citation
Received : 21 July 2023
Accepted : 15 March 2024
Published : 11 April 2024
DOI : https://doi.org/10.1186/s40359-024-01664-2
Share this article
Anyone you share the following link with will be able to read this content:
Sorry, a shareable link is not currently available for this article.
Provided by the Springer Nature SharedIt content-sharing initiative
- Artificial intelligence
- AI-empowered interventions
- Children with autism
BMC Psychology
ISSN: 2050-7283
- General enquiries: [email protected]
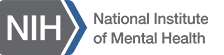
Transforming the understanding and treatment of mental illnesses.
Información en español
Celebrating 75 Years! Learn More >>
- About the Director
- Advisory Boards and Groups
- Strategic Plan
- Offices and Divisions
- Careers at NIMH
- Staff Directories
- Getting to NIMH
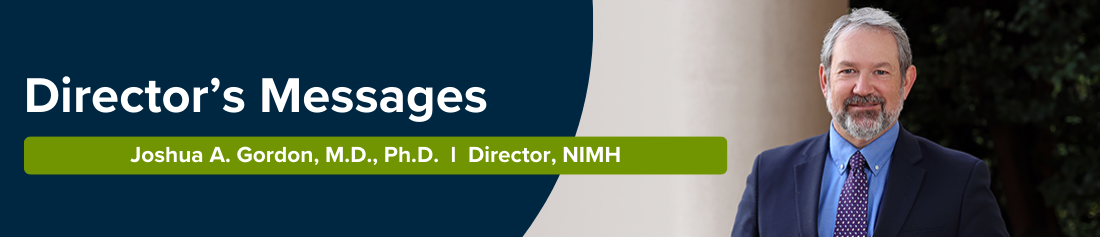
The Importance of Lived Experience Perspectives – Insights From the IACC
Joshua A. Gordon, M.D., Ph.D., and Susan Daniels, Ph.D., HHS National Autism Coordinator and Director of the NIMH Office of National Autism Coordination
April 4, 2024
Follow the NIMH Director on
During National Autism Acceptance Month, NIMH and the NIMH Office of National Autism Coordination celebrate the important contributions of autistic people in our families and our society, and we reaffirm our support for their acceptance, inclusion, and full participation in all aspects of community life. This April, we would like to highlight NIMH’s unique role in federal autism coordination efforts and reflect on how the lived experiences of autistic people and their families have shaped federal autism research, services, and policy.
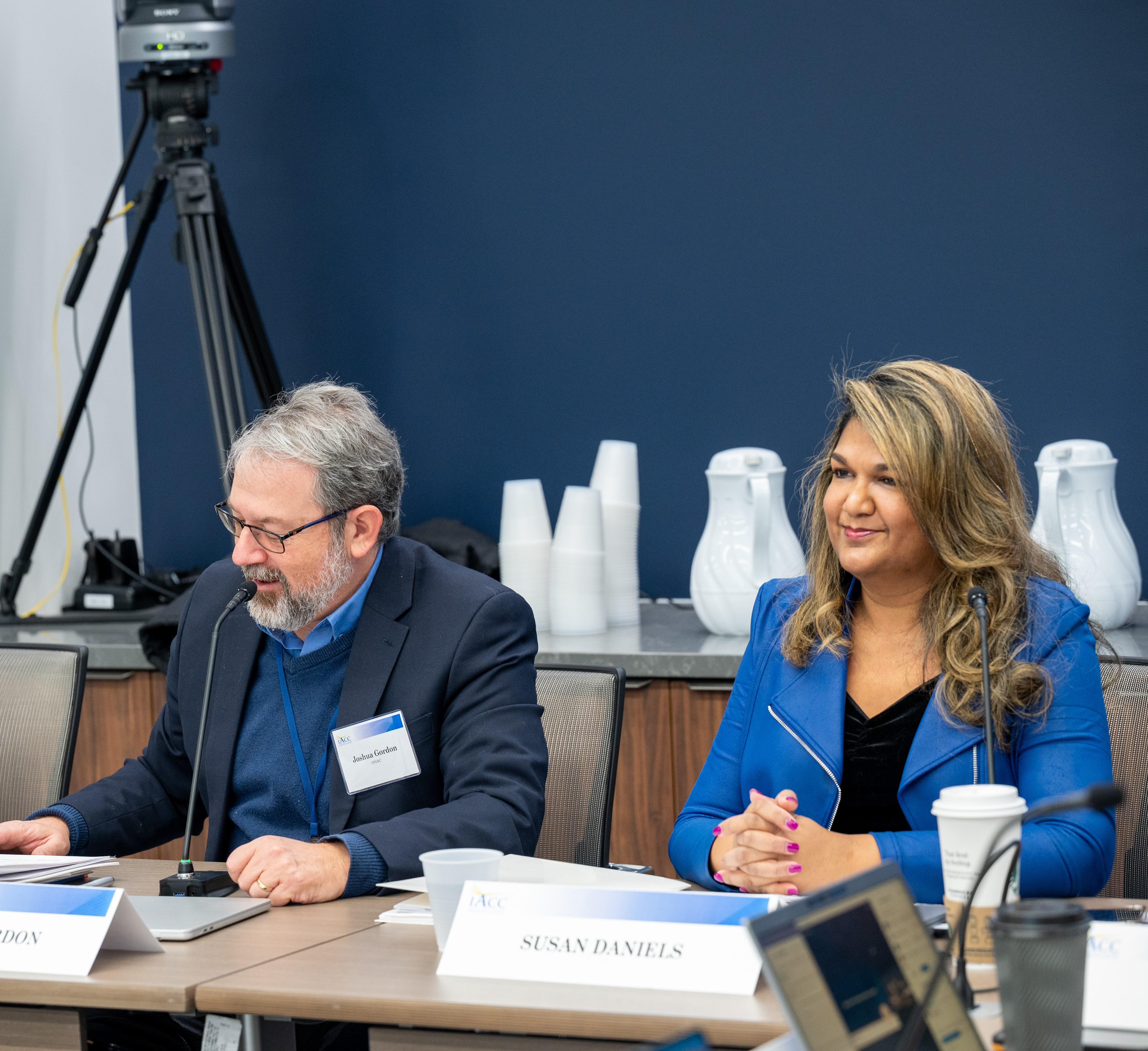
We have the privilege of serving as the Chair and Executive Secretary of the Interagency Autism Coordinating Committee (IACC) . The IACC is a federal advisory committee established by Congress and currently authorized under the Autism CARES Act of 2019. The committee includes federal officials from agencies that support autism research and vital services for people with disabilities, as well as public members, including autistic adults, family members, advocates, researchers, and service providers from diverse communities around the country.
The IACC serves as a forum for community engagement and provides an important point of convergence and collaboration. Federal agency members and public members work together to develop and provide advice that informs the Secretary of the Department of Health and Human Services, federal agencies, Congress, and the President. This advice guides the activities of federal agencies and helps ensure that federal programs are responsive to the needs of the autism community.
Reflecting community needs
In working with the IACC, we have seen how community voices, reflecting the lived experiences of autistic people and their families, can contribute to important advances in federal autism activities. Public input on the co-occurring mental and physical health conditions often experienced by autistic individuals is one such example. These conditions can include seizure disorders, gastrointestinal problems, and disruptions in sleep. They can also include mental disorders and mental health conditions such as anxiety, depression, attention-deficit/hyperactivity disorder (ADHD), self-injury, and suicidal ideation. Many autistic individuals also have learning disabilities or additional developmental conditions and disabilities.
For many people with autism, co-occurring conditions can contribute to lost opportunities and decreased productivity, poor health outcomes, and, in some cases, premature death. Discussions initiated by public members of the IACC, along with public comments received at IACC meetings and at an IACC-sponsored town hall , helped to shape the research objectives on co-occurring conditions in the inaugural 2009 IACC Strategic Plan .
The topic of co-occurring conditions remains an IACC priority today. The 2021-2023 IACC Strategic Plan includes comprehensive recommendations for research investigating the biology underlying co-occurring conditions and autism, as well as interventions and services to address these conditions across the lifespan. Just last year, the IACC issued a Request for Information seeking additional community input on the topic and received responses from more than 1,200 people. Themes and priorities from these responses will be included in the forthcoming IACC Strategic Plan Update , which will focus on the impact of co-occurring conditions on the physical and mental health of people on the autism spectrum. The update aims to further identify opportunities for research and services to improve well-being for autistic people.
Representing diverse experiences
Hearing from people with lived experience has shed light on additional issues important to the autism community, including wandering and elopement, the needs of transition-age youth and adults, and autism in girls and women. Autistic people and family members have also emphasized the breadth of experiences and challenges across the spectrum of ability and disability and the need for a range of personalized tools, interventions, services, and supports rather than a one-size-fits-all approach.
Based on input from autistic people and families from diverse and underserved communities, the IACC has prioritized the need to increase equity and reduce disparities experienced by autistic individuals across race, ethnicity, culture, sex and gender, socioeconomic status, and geographic location, including rural and urban communities. This also includes the need for more researchers and service providers who come from diverse communities and have lived experience with autism and disability.
The 2021-2023 IACC Strategic Plan includes two cross-cutting recommendations – one on equity and disparities and one on sex and gender – to intensify focus on addressing gaps in these areas and increase equity for all autistic people. The committee also continues to support priorities to ensure that autism research and services meet the needs of individuals across the whole spectrum, including those with the highest support needs, and across the full lifespan into older adulthood. Importantly, the strategic plan emphasizes inclusion and acceptance of all autistic people and reducing barriers to their participation in every aspect of community life.
Prioritizing collaboration and inclusion
In all of this work, consideration of diverse viewpoints and experiences from across the autism community and a spirit of cooperation, collaboration, and civility have been crucial. As the autism landscape continues to evolve, collaboration between federal agencies and community members will remain a cornerstone of progress in improving the health and well-being of autistic people and their families.
Community engagement plays an important role across the broad portfolio of federal research, services, and policy activities related to disabilities, mental health, and physical health. Federal agencies gather public input through federal advisory committees; solicit public comments through formal requests for information; and engage individuals with lived experience in grant review panels, community engagement programs, and community-based participatory research. Lived experience perspectives strengthen federal programs and help ensure federal research and services address the issues most important to those whom they serve.
During Autism Acceptance Month, let us honor the contributions of autistic individuals and others with lived experience; strive to ensure that their voices, perspectives, and priorities are heard and represented in federal activities for research, services, and policy; and work toward a more inclusive society for all.
- International edition
- Australia edition
- Europe edition
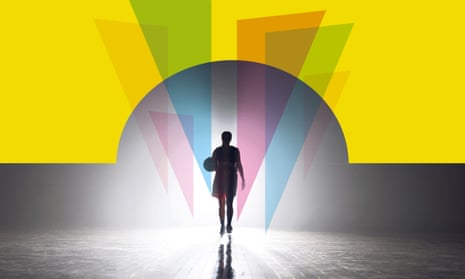
The sudden rise of AuDHD: what is behind the rocketing rates of this life-changing diagnosis?
Just over a decade ago, autism and ADHD were thought to be mutually exclusive. But in recent years, all that has changed
H e had beaten more than 19,000 applicants for a place at medical school, yet Khurram Sadiq was now bunking off his hospital shifts. The 19-year-old felt inexplicably anxious around strangers on the wards and was hiding from his own patients. During lectures he couldn’t focus on what he was being taught. He deemed himself “a goof, a dunce” in contrast to his peers. Sadiq couldn’t motivate himself to revise for his exams and instead found himself panic reading textbooks in the final days. He passed his undergraduate pre-medical exams by the skin of his teeth. That was 30 years ago. In the decades since, Dr Sadiq has qualified as a consultant psychiatrist, been diagnosed with both autism and attention deficit hyperactivity disorder (ADHD), specialised in autism and ADHD psychiatry and met hundreds of patients with struggles similar to his. He is now trying to spread what was once an unbelievable message: that both autism and ADHD can coexist in the same person simultaneously. Just over a decade ago, the two conditions were considered to be mutually exclusive, with the Diagnostic and Statistical Manual of Mental Disorders, often referred to as “psychiatry’s bible”, stating that the diagnosis of one precluded the existence of the other. This wasn’t revised until 2013. “It led to a fork in the road,” says Dr Jessica Eccles, spokesperson for the Royal College of Psychiatrists. “Not only for clinical practice, but also for research and public understanding of these conditions.” Now some specialists believe that the coexistence of both conditions is not just possible, but frequent. One study by researchers at Duke University found that up to half of people diagnosed as autistic also exhibit ADHD symptoms, and that characteristics of autism are present in two-thirds of people with ADHD. “My clinical experience suggests it’s more than three-quarters in both directions,” adds Dr Eccles. Online, the idea that autism and ADHD can coexist is so widely accepted that it has spawned its own label – “AuDHD” – and a groundswell of people who say they recognise its oxymoronic nature, perpetual internal war and rollercoaster of needs. There are tens of thousands of people in AuDHD self-help forums, and millions more watching AuDHD videos. Some of those videos come from Samantha Stein, a British YouTuber. “The fact that you can have both [autism and ADHD] at the same time is kind of paradoxical in nature,” she admits. “You think: ‘How can you be extremely rigid and need routines and structure, but also be completely incapable of maintaining a routine and structure?’” The 38-year-old started making videos on autism after her diagnosis in 2019, then began covering AuDHD after learning that she also had ADHD. “I realised that autistic adults – especially those who are diagnosed late in life – more often than not seem to have ADHD as well,” says Stein. Her first video on the subject, “ 5 signs you have ADHD and autism ”, has now been viewed more than 2m times.
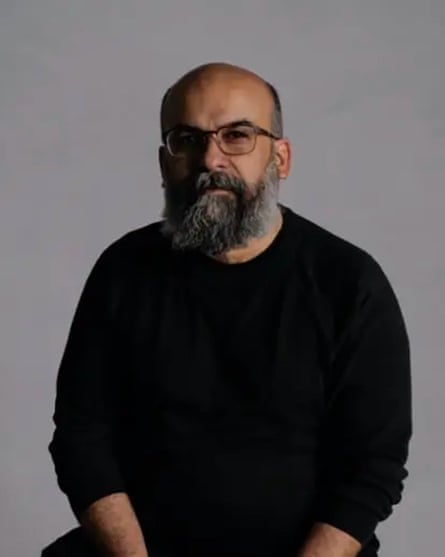
Some critics like to describe ADHD – and more recently autism – as a “fashionable” diagnosis, a misinformed excuse for life’s struggles. It’s almost inevitable that the new AuDHD label will cause a similar backlash. To see just how misguided this is, we must first understand both autism and ADHD. Both are lifelong neurodevelopmental conditions that affect how people think, perceive the world and interact with others, according to Embracing Complexity , an umbrella group of organisations that research neurodiversity.
Autism and ADHD affect people on a spectrum of severity, both are legally recognised as disabilities, and neither are mental illnesses to be “cured”, although the knock-on effects can lead to mental illness . People who experience ways of thinking that diverge from those experienced by the majority of people are described as “ neurodivergent ”. Autism spectrum disorder (ASD) is caused by multiple genetic factors that aren’t yet fully understood . Contrary to misconception, autism doesn’t equate to impaired intelligence, and only around half of people with autism also have a co-occurring intellectual disability. According to the National Autistic Society, autism is characterised by social challenges, repetitive behaviours, over- or under-sensitivity to surroundings and highly focused interests. Autism is experienced in a multitude of ways. To empathise with the autistic trait of oversensitivity, for example, imagine that all your senses are amplified . The hum of your fridge is louder, the overhead lights are brighter, your itchy jumper is pricklier. It’s distracting while you’re trying to work, it’s draining to pretend it isn’t bothering you and you become increasingly stressed as a result. “For me, eating in a canteen is like eating in a nightclub for a neurotypical person,” says Jill Corbyn, who is autistic and the director of support organisation Neurodiverse Connection . “It’s unpleasantly loud, it’s going to distract you from your food, it’s anxiety-inducing.” Additionally, some autistic people may find social situations exhausting or overwhelming, or feel incompetent when they’re unable to decipher the subtleties of interpersonal communication, 60% of which is non-verbal . Charli Clement, 23, explains that while a non-autistic person may rehearse parts of a conversation before a date or a job interview, her autism leads her to “script significantly” before even ordering a drink at a bar.
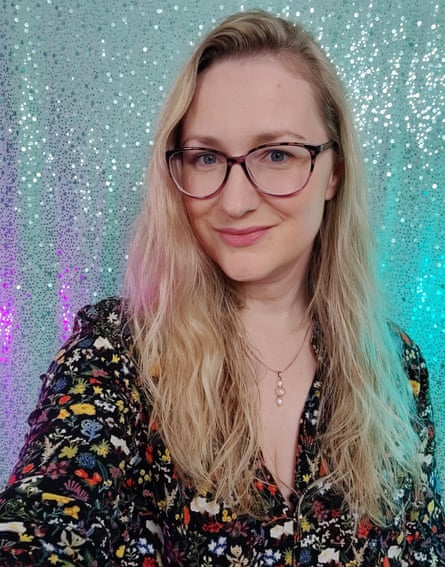
“I try to make sure I’m not doing something that will be perceived as ‘wrong’, so focusing on what the person is saying and what I should be replying is overwhelming,” she says.
Compounding the experience is the feeling many autistic people have that it isn’t “normal” to feel this way and that they must camouflage their discomfort to fit in with the pack. This “masking”, as it’s known, is exhausting, invalidating and can lead to burnout . ADHD is also not fully understood. There’s evidence that the condition, involving an imbalance of neurotransmitters – including dopamine, in the brain – has both genetic and environmental causes. These chemical messengers are responsible for motivation, movement, planning, reward, memory, focus, alertness, impulse control and threat response, among others. People with untreated ADHD, whose reward pathways are therefore more dysregulated, can subsequently experience disordered moods, sleep, eating habits and dysfunction in almost every area of life. Some people with ADHD are like pinballs of external chaos – of lost keys, missed appointments and cluttered homes. Others may appear inattentive, distracted by balls of chaotic thoughts into which they frequently retreat from the world to untangle. ADHD affects people to different degrees. But many say their lives are marred by their brain’s misguided attempts to correct its chemical imbalances . They impulsively dopamine-spike with food, sex, drugs, booze, the internet, people, hobbies and novelty of all shades. “I am a slave to my own brain and it’s tiring,” writes one anonymous person on an ADHD Reddit support group. Another asks: “Do you also feel like a slave to your desires?” She gives the examples of “chasing girls, gambling, chasing men, eating, hobby-hopping, extreme budgeting, falling in love [with] the wrong person, spending extravagantly”.
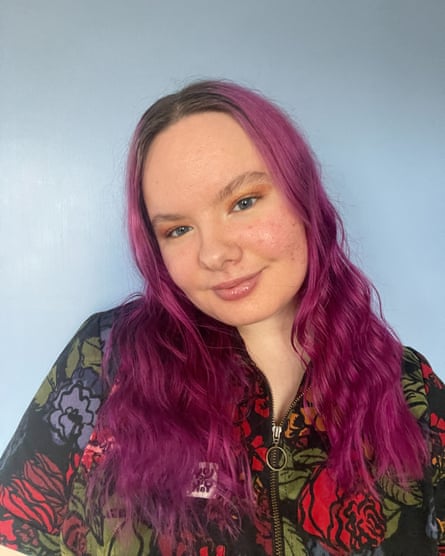
What frequently underpins the external and internal chaos, according to experts and many ADHDers alike, is a pervasive sense of deep shame and the quiet realisation that their potential in life is not being met .
When autism meets ADHD, it’s a curious form of alchemy, according to those who have both. Sometimes the conditions are in conflict; at other times they’re symbiotic. There is no such thing as a perfect 50/50 split, explains Sadiq, and the brain is often “seesawing” between both conditions. This makes the presentation of AuDHD a distinctive condition in its own right, “completely different from pure ADHD or pure ASD”, he adds.
In his Ted Talk, “When Order and Anarchy Live Together”, Sadiq describes the dualities of the condition: “Silence v noise; structure v chaos; repetition v novelty; caution v risk-taking …” Mattia Maurée, a non-binary composer and host of the AuDHD Flourishing podcast, discovered the AuDHD concept after following separate pieces of advice about autism and ADHD that “just weren’t working for me”. “It was like: ‘No, my life is still really, really hard,’” they tell me from Philadelphia. AuDHD is uniquely “cyclical”, says Maurée, with big bursts of energy followed by a crash. “AuDHDers can also be incredibly creative and innovative, maybe because of that brain hyper-connectivity.” Creativity is cited as the most positive AuDHD attribute by everyone I speak to, along with the subtle pairings of traits that “complement each other in a really nice way”, as Stein puts it. “ADHD gives me a love of novelty and a very creative side. And then autism allows me to focus on a topic that I’m really interested in. All of that allows me to be very self-directed.”
The paradoxes of AuDHD can camouflage each other or – on the surface at least – cancel each other out, which is why some AuDHDers experience missed or incorrect diagnoses. In February, Sadiq saw a patient who had been referred to his NHS clinic for an ADHD diagnosis. He realised 15 minutes into the consultation that the patient was autistic. “If I had no lived experience of autism and ADHD I would have missed it completely,” he says. “I would have diagnosed either social anxiety or a personality disorder.” In spite of his expertise, Sadiq is not formally qualified to make an autism diagnosis, and instead he had to refer the patient on to the autism service within the NHS trust. He believes that psychiatrists specialising in autism should also be trained in ADHD and vice versa, because otherwise “they’re going to be missing a lot”.
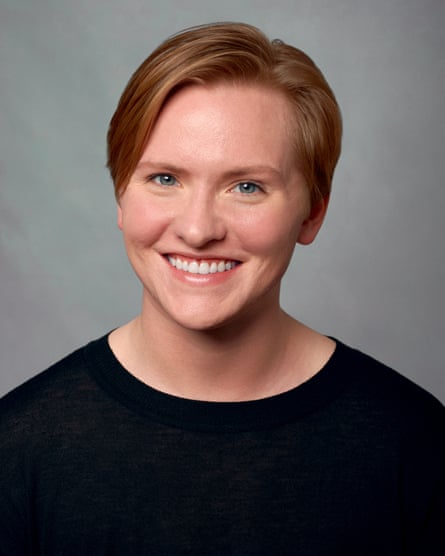
It’s not just the medical profession that needs more coordination. Charities such as ADHD UK and the National Autistic Society also work independently from one another. Legislation such as the government-backed The Buckland Review of Autism Employment, which recently called for employers to boost support for autistic people , scrutinises autism provisions but not ADHD ones. ADHD UK is one of many advocacy groups calling for the Autism Act , which legally compels the government to support autistic people, to be widened in scope to include other forms of neurodiversity. Once a correct dual diagnosis is obtained, there are still complications. ADHD can be successfully managed with medication and behavioural coaching, but some autistic people react badly to this medication. Research indicates that stimulants are overall less tolerable for AuDHDers than they are for people with ADHD, according to the global research platform Embrace Autism, with one report finding that side-effects doubled in those with both conditions.
Another quirk of AuDHD treatment is that in some cases, it’s only after “quietening” someone’s ADHD symptoms that their autism traits come to the fore. This is often when people realise their autistic side for the first time, and it could explain why rates of self-reported autism closely follow those of ADHD. The medical professionals I interviewed for this article were emphatic that ADHD medication cannot cause autism. Instead, Dr Eccles says: “It has just changed the balance of symptoms. The balance of masking has changed.” The prevalence of autism was widely believed to be 1% until last year, when a first of its kind study published in the Lancet found the true rate to be more than double that, with at least 1.2 million autistic people in the UK. The prevalence of ADHD in UK adults is around 4% , according to ADHD UK, and assessment waiting lists for both conditions are increasing year on year, with waits of a decade in some parts of the country for ADHD assessment. When naysayers argue that we are in the midst of an overdiagnosis epidemic, charities often point them to the statistics on suicide, and the fact that the ripple effects of ADHD and autism often lead to mental ill-health. Autistic adults without a learning disability are far more likely to die by suicide. In 2022, researchers from Cambridge and Nottingham University, analysing coroners’ inquest records, concluded that a significant number of people who had died by suicide were likely autistic but undiagnosed. Adults with ADHD, meanwhile, are five times more likely to attempt suicide than their neurotypical peers. Yet AuDHDers have been found to be at even greater risk of suicide than either those with only autism or ADHD, according to an academic study of more than 50,000 people.
For people like Clement, criticism about over-labelling is the least of her concerns. As a teenager she spent time in a psychiatric unit before the nature of her AuDHD was fully realised. “I’d already given myself labels,” she says. “I already thought that I was weird and broken. So having a label that actually made sense and encompassed my experience was so liberating.”
She now works part-time advising psychiatric hospitals on how to ensure their sensory environments are adequate for neurodiverse people.
Other AuDHDers give colourful analogies to describe the epiphany of diagnosis. Before the discovery, I’m told, it’s as if you are trying to fit in and be a horse rather than celebrating the fact that you’re a zebra. It’s like being trapped in a maze in the dark, then suddenly the lights are on and now there’s a way to navigate out.
Stein describes her life as “fundamentally walking parallel to, but never quite included in society”. Her diagnosis, however, “allowed me to look at my life through the lens of far more compassion – as a pretty good autistic person rather than a broken neurotypical person”.
“I think in some ways [AuDHD] can be a very beautiful thing,” she says.
“You just need the right support to be able to access those parts of you. And you need the label to know what the hell is going on in your brain.”
- Health & wellbeing
- Neurodiversity
- Attention deficit hyperactivity disorder
Most viewed
Autism - what could the future hold in store? A platform of hope, learning, opportunity
There are several critical issues facing children and adults with autism and their families. Some of these issues include access to education, support, and resources that can help them become self-reliant, independent, and valued members of their community.
But these issues have changed dramatically over the past 40 years when there were few if any services for children with autism.
Autism was first recognized as a distinct condition by Dr. Leo Kanner in 1943. However, it wasn't until the 1970s and 1980s that autism began to be recognized as a disability by governments and organizations. Although the Individuals with Disabilities Education Act (IDEA) was passed in 1975, autism was not included as a distinct disability category until its 1990 reauthorization. For the first time, children with autism became eligible to receive special education services and accommodations in schools.
According to the U.S. Centers for Disease Control and Prevention (CDC), the prevalence of autism in the United States has risen from one in 150 children in 2000 to one in 36 children in 2023 . There are several factors that may contribute to this increase, including increased awareness and improved diagnostic methods. Additionally, changes in diagnostic criteria and the inclusion of milder cases of autism spectrum disorder may also play a role. Early intervention services are available for toddlers and preschool students diagnosed with autism, but we have seen decreases in the number of children identified with since COVID-19. Yet, the earlier diagnosis allows for initiation of services and support which are critical at a young age. Looking at children and adults ages 5 to 22, 14% of the public school students are receiving special education and 12% of those students have an autism diagnosis.
What happens when you reach adulthood?
It is estimated that around half of adults with autism are employed. Of those, many have only part-time jobs or are doing work for which they're overqualified. There are also many people working as volunteers or in programs outside the mainstream. Others are on waiting lists for adult day programs.
Why is this occurring?
Low expectations: Few schools — and sometimes even families — expect children with autism to find satisfying careers. The exception is if they happen to have extraordinary skills.
Competition and challenges: To secure a job in the general community, people with autism must compete for positions. That can be hard for neurodiverse people whose social skills do not match society's norms. Some autistic adults also find it hard to manage the physical requirements of the workplace, especially if they have sensitivities to light, sound, and other stimuli that they might not have any control over in these settings.
Lack of programs and staff: Most adult day programs were developed for people with intellectual disabilities, not autism. For adults with autism and intellectual disabilities, these programs can be very successful. However, the challenge is finding staff. Based upon regulations, programs can require one staff person per three individuals and some individuals require one-to-one staffing. With many staffing vacancies, we are forced to have waiting lists of adults in need of service. This is a critical problem programs are experiencing across Pennsylvania.
So, what can we expect, hopefully, as we look to the future?
- Earlier identification of children on the autism spectrum. Increased numbers of children under 5 years of age have been identified which allows for earlier intervention.
- Best practices in educating children with autism will be further established through research.
- Increased employment opportunities and adult day programs with higher reimbursement for services.
- Expansion of alternate living arrangements.
More: The toll of COVID on those with autism
More: Candy for a cause: Oil City boy helps autistic brother with vending machine business
As a parent of a child with autism, I truly believe that Erie, Pa., is the very best community in which to live because of the wealth of services and the acceptance that the community has for children and adults with autism and disabilities.
Maureen Barber-Carey, Ed. D., is the executive vice president of the Barber National Institute . She has committed her professional life to ensuring children and adults with disabilities reach their fullest potential. She led the organization in establishing a wide range of research-based programs for children with autism and developmental disabilities. Her motto is “if a child doesn’t learn the way we teach, we must teach the way a child learns.”

An official website of the United States government
The .gov means it’s official. Federal government websites often end in .gov or .mil. Before sharing sensitive information, make sure you’re on a federal government site.
The site is secure. The https:// ensures that you are connecting to the official website and that any information you provide is encrypted and transmitted securely.
- Publications
- Account settings
Preview improvements coming to the PMC website in October 2024. Learn More or Try it out now .
- Advanced Search
- Journal List
- Front Pediatr
Epidemiology of autism spectrum disorders: Global burden of disease 2019 and bibliometric analysis of risk factors
1 Department of Rehabilitation Medicine, Tongji Hospital, Tongji Medical College, Huazhong University of Science and Technology, Wuhan, China
2 World Health Organization Cooperative Training and Research Center in Rehabilitation, Wuhan, China
Ze-Jian Chen
Xiao-dan li.
3 Nursing Department, Tongji Hospital, Tongji Medical College, Huazhong University of Science and Technology, Wuhan, China
Ming-Hui Gu
4 Mrs. T. H. Chan Division of Occupational Science and Occupational Therapy, University of Southern California, Los Angeles, CA, United States
Zhao-Wen Zhou
Gvzalnur yasin.
5 Faculty of Rehabilitation Medicine, College of Xinjiang Uyghur Medicine, Xinjiang, China
6 Division of Physical Therapy Education, College of Allied Health Professions, University of Nebraska Medical Center, Omaha, NE, United States
Xiu-Pan Wei
Xiao-hua han, xiao-lin huang, associated data.
The original contributions presented in the study are included in the article/ Supplementary Material , further inquiries can be directed to the corresponding author/s.
To explore the geographical pattern and temporal trend of autism spectrum disorders (ASD) epidemiology from 1990 to 2019, and perform a bibliometric analysis of risk factors for ASD.
In this study, ASD epidemiology was estimated with prevalence, incidence, and disability-adjusted life-years (DALYs) of 204 countries and territories by sex, location, and sociodemographic index (SDI). Age-standardized rate (ASR) and estimated annual percentage change (EAPC) were used to quantify ASD temporal trends. Besides, the study performed a bibliometric analysis of ASD risk factors since 1990. Publications published were downloaded from the Web of Science Core Collection database, and were analyzed using CiteSpace.
Globally, there were estimated 28.3 million ASD prevalent cases (ASR, 369.4 per 100,000 populations), 603,790 incident cases (ASR, 9.3 per 100,000 populations) and 4.3 million DALYs (ASR, 56.3 per 100,000 populations) in 2019. Increases of autism spectrum disorders were noted in prevalent cases (39.3%), incidence (0.1%), and DALYs (38.7%) from 1990 to 2019. Age-standardized rates and EAPC showed stable trend worldwide over time. A total of 3,991 articles were retrieved from Web of Science, of which 3,590 were obtained for analysis after removing duplicate literatures. “Rehabilitation”, “Genetics & Heredity”, “Nanoscience & Nanotechnology”, “Biochemistry & Molecular biology”, “Psychology”, “Neurosciences”, and “Environmental Sciences” were the hotspots and frontier disciplines of ASD risk factors.
Conclusions
Disease burden and risk factors of autism spectrum disorders remain global public health challenge since 1990 according to the GBD epidemiological estimates and bibliometric analysis. The findings help policy makers formulate public health policies concerning prevention targeted for risk factors, early diagnosis and life-long healthcare service of ASD. Increasing knowledge concerning the public awareness of risk factors is also warranted to address global ASD problem.
Introduction
Autism spectrum disorder (ASD) is a series of neurodevelopmental conditions characterized by deficits in social communication and interaction, and stereotyped, repetitive patterns of sensory–motor behaviors ( 1 ). ASD is associated with heterogeneous symptomatology regarding physical, mental, neurodevelopmental, and functional disorders, which can extend to adulthood and result in a substantial burden on individuals, families, and society ( 2 , 3 ). Although prevalence of autism spectrum disorders in children have been reported in countries and regions such as the USA ( 4 , 5 ), China ( 6 ), India ( 7 ), Europe ( 8 ), and Asia ( 9 ), there is a lack of epidemiological estimates on prevalence, incidence and ASD-related health loss over time with risk factors ( 10 ). In 2016, the Global Research on Developmental Disabilities Collaborators reported the developmental disabilities among children younger than 5 years but not specifically focused on the all-age autism spectrum disorders ( 11 , 12 ). Previous reviews provided global and regional overview of ASD prevalence and commodities, but national-level of ASD burden was not provided ( 13 – 15 ). Moreover, the risk factors leading to ASD were not presented in previous global epidemiological studies for ASD ( 16 ).
The Global Burden of Disease (GBD) database covers epidemiological information on the global, regional and national burden of diseases, injuries, causes of death and risk factors, providing a comprehensive way to investigate the geographical distribution and changes in ASD patterns over time ( 17 ). Analyses of current epidemiologic situations and temporal trends may help policy makers assess the global ASD burden, allocate resources, and formulate relevant policies. This information can further guide diagnosis, prevention, intervention and rehabilitation efforts for collaboration in regions with various degree of socioeconomic development ( 18 – 20 ).
In addition, understanding the status and frontier of the risk factors will facilitate researchers to investigate corresponding prevention approaches. Although many risk factors for ASD have been proposed, the complex causes of autism have made it difficult to link the complicated issue with a definite risk ( 21 ), which is not included in the GBD database yet. Hence, this study aimed to present the epidemiological estimates of autism spectrum disorders in terms of prevalence, incidence, and disability-adjusted life-years (DALYs) from 1990 to 2019 with GBD database. Besides, a bibliometric analysis was conducted to comprehensively analyze the research hotspots of ASD risk factors.
GBD Collaborator Group is a scientific council producing cutting-edge database of the global burden of diseases, injuries, and risk factors ( 17 ). GBD 2019 estimated global epidemiology covering incidence, prevalence, mortality, years lived with disability (YLDs), years of life lost (YLLs), and disability-adjusted life-years (DALYs) in 204 countries and territories grouped into 21 regions. Data utilized in this study were retrieved and publicly available from the Global Health Data Exchange (GHDx) website. The study followed the Guidelines for Accurate and Transparent Health Estimates Reporting (GATHER) recommendations ( 22 ).
Autism spectrum disorders (ASD) are characterized by persistent impairments in social communication, reciprocal interaction, and the presence of restricted, stereotypical behaviors. ASD is a non-fatal but life-long disease commencing in early childhood with overlapping neurodevelopmental causes. In the fifth edition of Diagnostic and Statistical Manual of Mental Disorders, ASD diagnostic criteria eliminated diagnostic subtypes (autistic disorder, Asperger's syndrome, and Pervasive Developmental Disabilities-Not Otherwise Specified), and designated as a single category ( 23 ).
Statistical analysis
Statistical analysis was performed using R software (version 4.1.2). ASD burden was measured using prevalence, incidence, DALYs, and corresponding age-standardized rates in 204 countries and territories, from 1990 to 2019. A team of librarians identified multiple relevant data sources from published literature and websites. Standard methods, such as Bayesian meta-regression tool and regression methods, have been used to estimate prevalence, incidence and DALYs with uncertainty intervals (UIs) of autism spectrum disorders by location, year and sex by the GBD Collaborators using multiple modeling software ( 17 ). Based on the ordered draw of the modeling process, 95% UI, similar with confidence intervals (CI) mathematically, were identified from the epidemiological estimation methodology. The raw data were publicly available from the GBD Results Tool ( https://vizhub.healthdata.org/gbd-results/ ). Age-standardized rate (ASR) and estimated annual percentage change (EAPC) were used to quantify temporal trends of ASD ( 24 ). Specifically, ASR was the sum of the product of the ratio of each age group (most are five years per group) with redistribution and the weight of the selected population group, divided by the total weight of the standard population. As a result, ASRs, including age-standardized prevalence rate (ASPR), age-standardized incidence rate (ASIR) and age-standardized DALY rate, could exclude the interference from variations in age distribution and population quantity. ASR was reported per 100,000 populations annually. Meanwhile, the EAPC point estimation based on ASR was employed to reflect the shifting trends of ASD burden over time. EAPC was calculated with the formula EAPC = 100 × [exp(β) − 1], where β demonstrated the secular trend of ASR in the 30 years. If the EAPC estimation and its 95% confidence interval were both above/below zero, the corresponding ASR was in an increasing/decreasing trend. Otherwise, the changing trend of ASR was deemed to be stable.
Socio-demographic index (SDI) was a comprehensive measurement of educational attainment, lagged distributed income, and total fertility rate to describe socioeconomic development status ( 25 , 26 ). The countries and territories were categorized into five SDI quintiles (low, low-middle, middle, high-middle, and high). Finally, we calculated Pearson's correlation coefficient between EAPCs and ASRs and between EAPCs and SDI values in 2019, to investigate influential factors of ASR change trends ( 27 ).
Risk factor for autism spectrum disorder is multifactorial, such as genetic predispositions or environmental factors, which has not been included in the GBD database. Hence, the current study obtained literature published from January 1, 1990 to November 7, 2022 to make a preliminary bibliometric analysis. The retrieved results were analyzed using CiteSpace (version 6.1 R3), a literature visualization and analysis software developed to identify scientific progress and research frontiers of a certain field ( 28 ). The search strategy included the terms “autism” and “risk factor” from Web of Science. The literature was limited to English language, and finally 3,590 references were obtained after removing duplicates. For visualization, we selected “Top 50 levels of most occurred items from each slice” and “Pruning/pathfinder” to generate keyword clustering.
ASD epidemiology in 2019
Globally, there were estimated 28.3 million (95% UI, 23.5–33.8 million) prevalent cases of autism spectrum disorders, with an age-standardized prevalence rate of 369.4 (95%UI, 305.9 to 441.2 in 2019 ( Table 1 ). ASD was responsible for 603,790 (95% UI, 501,680 to 720,097) incident cases globally, with an age-standardized incidence rate of 9.3 (95% UI, 7.7 to 11.1) in 2019. In addition, ASD accounted for 4.3 million (95% UI, 2.8 to 6.2) DALYs, with an age-standardized rate of 56.3 (95% UI, 36.8 to 81.5) DALYs in 2019 ( Table 2 ). For SDI regions, the Middle SDI region had the highest burden of prevalent cases and DALYs in 2019, while the Low-middle SDI region had the most incident cases. Geographical distribution of the prevalence, incidence and DALYs estimates for autism spectrum disorders in 2019 were presented in Figures 1 , ,2, 2 , and Supplementary Figure S1 .

Global prevalence of autism spectrum disorders in 2019. ( A ) Prevalent cases of autism spectrum disorders by location for both sexes in 2019. ( B ) Age-standardized prevalence rate (ASPR) of autism spectrum disorders by location for both sexes in 2019.

Global incidence of autism spectrum disorder in 2019. ( A ) Incident cases of autism spectrum disorders by location for both sexes in 2019. ( B ) Age-standardized incidence rate (ASIR) of autism spectrum disorders by location for both sexes in 2019.
Number and age-standardized prevalence rate for ASD by global burden of disease region in 1990 and 2019.
ASD, autism spectrum disorders; ASPR, age-standardized prevalence rate; SDI, sociodemographic index.
Number and age-standardized rates of incidence and of DALYs for ASD by global burden of disease region in 1990 and 2019.
ASD, autism spectrum disorders; ASIR, age-standardized incidence rate; DALY, disability-adjusted life year; SDI, sociodemographic index.
Temporal trends of ASD prevalence from 1990 to 2019
From 1990 to 2019, the global ASD prevalent cases increased by 39.3% and the age-standardized prevalence rate has not almost improvement [EAPC = −0.02, 95% CI (−0.03 to −0.01), Supplementary Table S1 ]. Males were more likely to have ASD than females (male to female ratio in ASIR = 3.34:1 in 1990, and 3.23:1 in 2019). At the regional level, the age-standardized prevalence rate of ASD was found to be highest in high-income North America [640.0 (95% UI, 537.7 to 756.4)], high-income Asia Pacific [634.3 (95% UI, 528.8 to 756.7)] and Western Europe [581.3 (95% UI, 488.2 to 686.4)] ( Table 1 ). Subgroup analysis by SDI regions demonstrated that although high SDI region had the most rapid increase in prevalence (ASPR: 539.6 in 1990 and 579.3 in 2019, EAPC = 0.30, 95% CI 0.25 to 0.35) ( Figure 3 , Supplementary Table S1 ). At the level of country or territory, there was wide geographic variation in the ASPR of ASD (range, 215.8 to 739.6). United Kingdom [739.6 (95% UI, 617.2 to 876.3)], Sweden [706.8 (95% UI, 589.1 to 838.5)] and Japan [676.5 (95% UI, 562.8 to 805)] had the three highest ASPR in 2019 ( Figure 1 , Supplementary Tables S1, S5 ).

Global prevalence of autism spectrum disorders (ASD) by sex and socio-demographic index (SDI) quintiles from 1990 to 2019.
Temporal trends of ASD incidence from 1990 to 2019
From 1990 to 2019, the global ASD incidence has not been improved since incident cases increased marginally by 0.1% and the age-standardized prevalence rate increased by 1.1% [EAPC = 0.06, 95% CI (0.04 to 0.07), Supplementary Table S2 ]. Females had fewer ASD incidence than males (1990: 461,633 in males and 141,254 in females; 2019: 459,493 in males and 144,297 in females). At the regional level, the age-standardized incidence rate of ASD was highest in high-income North America 16.4 (95% UI, 13.8 to 19.3), high-income Asia Pacific 15.7 (95% UI, 13.1 to 18.6) and Caribbean 15.2 (95% UI, 13.1 to 17.3) ( Table 2 ). For SDI regions, the high SDI region had the most increase in age-standardized incidence rate from 1990 to 2019 (change = 9%; EAPC = 0.36, 95% CI 0.31 to 0.41, Supplementary Table S2 ). At the level of country or territory, ASIR varied from 5.4 to 18.6. Andorra [18.6 (95% UI, 15.3 to 22.3)], United Kingdom [18.0 (95% UI, 15 to 21.2)] and Sweden [17.1 (95% UI 14.4 to 20.2)] had the three highest age-standardized prevalence rates in 2019 ( Figure 2 , Supplementary Tables S2 and S5 ).
Temporal trends of ASD DALYs from 1990 to 2019
From 1990 to 2019, the global ASD DALYs increased by 38.7% and the age-standardized DALY rate has almost no improvement [EAPC = −0.02, 95% CI (−0.03 to −0.01), Supplementary Table S3 ]. The number of global DALYs in males was higher than in females (1990: 2393774.2 in male and 712134.9 in females; 2019: 3294467.6 in males and 1012147.8 in females). At the regional level, the age-standardized DALYs rate of ASD was found to be highest in high-income Asia Pacific 97.3 (95% UI, 63.6 to 141), high-income North America 96.9 (95% UI, 63.7 to 137.7) and Caribbean 92.9 (95% UI, 62.4 to 131.9) ( Table 2 ). Subgroup analysis by SDI regions demonstrated that although the high SDI region had the most rapid increase in DALYs (age-standardized DALY rate: 82.2 in 1990 and 88.2 in 2019, EAPC = 0.81, 95% CI 0.68 to 0.95, Supplementary Table S3 ). At the level of country or territory, age-standardized incidence DALYs rates varied from 33.3 to 112.3 among the countries and territories. United Kingdom [112.3 (95% UI, 73.7 to 160.5)], Sweden [108.0 (95% UI, 71.2 to 155.3)] and Japan [103.8 (95% UI, 67.7 to 149.7)] showed the highest age-standardized DALYs rates in 2019 ( Supplementary Figure S1 , Supplementary Tables S3 and S4 ).
Correlation between the socio-demographic Index and ASD epidemiology
Pearson's correlation coefficients between ASRs in 1990 and the corresponding EAPC values were not statistically significant ( Supplementary Figure S6 ). We explored the association between SDI in 2019 and EAPC values of ASPR, ASIR, and age-standardized DALY rate among the countries and territories. The results demonstrated that the associations between SDI, and EAPCs of ASIR and age-standardized DALY rate, were not statistically significant. However, the EAPC value of ASPR was positively associated with SDI in 2019 ( Supplementary Figure S7 ). Further, we investigated the correlation between SDI and ASPR, ASIR, and age-standardized DALY rate from 1990 to 2019. As a result, all ASRs were significantly and positively correlated with corresponding SDI values (correlation coefficient of ASPR = 0.672, of ASIR = 0.638, of age-standardized DALY rate = 0.681, P < 0.05) in 21 regions worldwide. For most countries and territories, after a decrease in expected ASRs, these rates increased rapidly when SDI values were higher than 0.4 in 2019 ( Supplementary Figures S8–S10 ).
Bibliometric analysis
A total of 3,991 articles in English were retrieved from Web of Science. After deleting duplicate literatures, 3,590 references were obtained for risk factors of ASD. As presented in Figure 4A , the overall number has been increasing since 1990. Among the countries/territories, USA, England, Canada, China, and Australia make leading contributions to exploring ASD risk factors in the past decades ( Figure 4B ).

( A ) The number of publications on risk factors of ASD since 1990. ( B ) Leading countries or territories that contributed to publications on risk factors of ASD since 1990.
ASD risk factors-research categories
In the current study, we identified the top research categories with citations analyzed by the CiteSpace software. As demonstrated in Figure 5 , research category was represented a cloud of circular nodes, with the areas depicting the number of research literature in each field. Moreover, the node orientations demonstrated the publication's importance. As a result, “#0 Rehabilitation”, “#1 Genetics & Heredity”, “#2 Nanoscience & Nanotechnology”, “#3 Biochemistry & Molecular biology”, “#4/5 Psychology”, “#6 Neurosciences”, and “#7 Environmental Sciences” may have played pivotal roles in the field of ASD risk factors since 1990.

The cluster map of co-occurrence research categories related to risk factors on ASD since 1990.
ASD risk factors-keywords clustering
We use the default setting of CiteSpace (Slice length = “1” year; Select the node type = “Keyword”; Top N = “50”) and a pruning algorithm to cluster the keywords. After processing, top keywords with the strongest co-occurrence frequency were found to understand hotspots in this field since 1990. As shown in the Figure 6 , the Mean Silhouette score was 0.8576, and the Modularity Q score was 0.7409. The emerging clusters revealed that ASD risk factors could be multifaceted and there is no definite conclusion. Hence, we used Burst Keywords analysis to explore the risk factor receiving high attention in the past decades ( Figure 7 ). For example, “Pervasive developmental disorder”, “obstetric complication”, and “perinatal factor” were of the earliest burst. In recent years, “de novo mutation”, “environmental factor”, and “DNA methylation” have been among the most focused keywords.

The cluster map of co-occurrence keywords related to risk factors on ASD since 1990.

The top 30 keywords with the strongest citation bursts related to risk factors on ASD since 1990.
In this study, we presented the epidemiological estimates and temporal trends of autism spectrum disorders based on the GBD database at the global, regional, and national levels from 1990 to 2019. Globally, there were estimated 28.3 million prevalent cases (ASR, 369.4 per 100,000 populations), 603,790 incident cases (ASR, 9.3 per 100,000 populations) and 4.3 million DALYs (ASR, 56.3 per 100,000 populations) in 2019. From 1990 to 2019, the prevalent cases and DALYs of ASD increased by almost 40%, while incidence and the ASRs have not been improved. We found the ASRs were significantly higher in the high SDI region, and the ASRs increased as SDI increased except the low SDI region. Moreover, we identified the hotspots concerning ASD risks factors since 1,900 because it is not provided in the GBD database.
Understanding the epidemiology of ASD burden facilitates tailoring health programs to address this great health challenge. Studies have shown that ASD was the leading cause of disability among all mental disorders in children globally but lack of knowledge concerning its geographical distribution in all-age populations ( 11 , 29 ). A systematic review on ASD prevalence and DALYs found estimated 52 million prevalent cases (ASR, 760) and 7.7 million DALYs (ASR, 58.2) of ASD worldwide in 2010 ( 13 ). However, it is impracticable to compare with our report because of substantial differences in methodologies and data sources. The review included much epidemiological data from high-income countries, lack in low-income and middle-income countries (LMICs), especially Africa, Latin America and Central and Eastern Europe ( 10 , 13 ). At the national level, the age-standardized prevalence rates ranged from 215.8 to 739.6 per 100,000 populations in our study, which were greatly lower than previous reports ( 30 ). The reason for the considerable but not contradictory differences mainly lied in the study population: our study highlighted the all-age populations while most epidemiological studies were only concerned with children and young adolescents. As the incident rate has been increasing but tending to be stable (EAPC = 0.06), the overall ASPR could have been attenuated by the underemphasized age groups ( Supplementary Table S5 ). Besides, data sources could have led to heterogeneous results, such as data derived from health records or parents' report, and from nationally representative population or selected sites ( 5 , 31 , 32 ). Our study also showed a male preponderance in ASRs similar to previous studies, which were related to complicated but unknown mechanisms, including environmental, genetic or epigenetic reasons ( 13 , 33 , 34 ).
Monitoring the temporal trend of ASD burden helps find the emerging challenges and adapt health systems over time. Globally, the ASD prevalent cases and DALYs increased by almost 40% in the past 30 years, while the ASRs and incidence tended to be stable. Such increases may be mainly attributable to the global population growth, instead of change in ASD incidence ( 29 ). As the ASRs were deemed unchanging over time, etiological research and primary prevention for reducing ASD incidence deserve continuous attention for a long time to come ( 30 ). Furthermore, ASD symptoms could extend beyond childhood and persist across lifespan although it was commonly perceived to be childhood disorders ( 35 ). Hence, the epidemiological data in all-age populations, including children, adolescents and adults, should draw attention when considering the increasing burden over time. Meanwhile, medical resources on the topic of early diagnosis and therapy are crucial for secondary prevention of permanent disabilities during golden period sensitive to treatment ( 36 ). Additionally, regions especially those with high prevalent cases, such as China, India and USA, should formulate policies to support long-term healthcare service, education, skills training and vocational assistance for rehabilitation (tertiary prevention) and reducing stigmatization ( 37 , 38 ).
We investigated the correlation between social development status and ASD burden, which may shed a light on ASD prevention and international cooperation to address disease burden. The causes of ASD were unrevealed but usually attributed to complex genetics-environment interactions. According to the results, all ASRs were positively correlated with SDI values from 1990 to 2019. For most countries and territories, after a decrease in expected ASRs, these rates increased rapidly when SDI values were higher than 0.4 in 2019. The positive correlation was underpinned by the view that awareness, concepts, and healthcare service availability of ASDs could be improved with social development ( 39 ). Moreover, environmental exposure, such as air pollution and endocrine-disrupting chemicals, was accompanied with socioeconomic development in the past decades ( 30 ). However, there was a paradox when attributing to socioeconomic status, because ASD burden in low SDI region did not fit the association. This result might be explained by non-etiologic reasons in LMICs, such as public awareness since the diagnosis was based on social and contextual observations ( 40 , 41 ) or etiologic factors such as genetic and environmental risk factors ( 30 ).
ASD is a complex multifactorial disease, and it needs interdisciplinary cooperation to find out the linking causes with the issue, such as Genetic & Heredity, Biochemistry, Public health and Environmental sciences, etc. For example, parental age, environmental exposure, de novo mutations and epigenetic alterations were shown associated with ASD risk ( 1 , 21 , 42 ). After bibliometric analysis, we found literature on risk factors for ASD shows a growing trend since 1999. Moreover, some emerging research directions could provide new perspective on ASD etiology. Previous studies found that allergic diseases are over-represented in ASD and hypothesized that immune dysregulation may have contributed to autism pathogenesis ( 43 ). Furthermore, gut microbiome can modulate gastrointestinal physiology and immune system relevant to ASD symptoms ( 44 ). More specifically, we can inspect the change of research hotspots from keywords with citation burst over time. Earlier bursts indicated that previous studies focused on family history, developmental psychology, and prenatal factors. In recent years, advances in technology have led to further exploration into genetics, immune systems, and environmental factors ( 30 , 45 , 46 ).
This study has several limitations. First, although the GBD provides spatiotemporal estimates of disease burden for geographical location with sparse data, the accuracy and reliability of modeling rely on the quality of data used in the study, thus the epidemiological estimates should be interpreted with cautions ( 17 ). Second, the study included literature only from Web of science. Future study could include articles in multiple databases with various languages to reduce bias. Third, due to the restrictions of data type, further investigation stratified by pathophysiology, etiology, and disease severity should be conducted in future studies ( 47 ). Considering that different ASD phenotypes can be associated with psychiatric, mental, and physical disorders ( 14 , 15 ), GBD models should include monitoring comorbidities to address the overall and life-long needs of individuals with autism spectrum disorders.
Autism spectrum disorders remain a global public health problem. The global prevalent cases and DALYs of ASD increased greatly with population growth, while age-standardized rates and incidence has not been improved from 1990 to 2019. The increased ASRs were associated with higher sociodemographic status except the low SDI region, although which was the main contributor to the rapid increases in prevalent cases and DALYs. The epidemiological findings could help policy makers illustrate the global health challenge to formulate policies and implement measures for prevention from risk factors, early diagnosis, and life-long healthcare service. Increasing knowledge concerning the public awareness, risk factors, diagnostic criteria and interventions are also warranted to reduce ASD burden.
Acknowledgments
Thanks to Institute for Health Metrics and Evaluation staff and the Global Burden of Disease Study collaborators for their work.
Data availability statement
Author contributions.
YAL, ZJC and XDL: conceptualized and designed the study, analyzed the data, drafted the first draft of the manuscript and edited subsequent drafts, and generated all figures and tables. YAL: conducted the bibliometric analysis. MHG, YAL and NX: carried out the initial analyses, and reviewed and revised the manuscript. CG, ZWZ, GY, HYX, XPW, YLL, XHH, and ML: contributed to the interpretation of data, and revised the manuscript critically for important intellectual content. JX and XLH: conceptualized and designed the study, drafted the initial manuscript, and reviewed and revised the manuscript. All authors contributed to the article and approved the submitted version.
Conflict of interest
The authors declare that the research was conducted in the absence of any commercial or financial relationships that could be construed as a potential conflict of interest.
Publisher's note
All claims expressed in this article are solely those of the authors and do not necessarily represent those of their affiliated organizations, or those of the publisher, the editors and the reviewers. Any product that may be evaluated in this article, or claim that may be made by its manufacturer, is not guaranteed or endorsed by the publisher.
Supplementary material
The Supplementary Material for this article can be found online at: https://www.frontiersin.org/articles/10.3389/fped.2022.972809/full#supplementary-material .
Commentary | Why it’s hard to get services for children…
Share this:.
- Click to share on Facebook (Opens in new window)
- Click to share on Twitter (Opens in new window)
Baltimore Sun eNewspaper
- Readers Respond
Commentary | Why it’s hard to get services for children who have autism | GUEST COMMENTARY
April is Autism Acceptance Month, a time to learn, reflect and (I hope) act with and on behalf of the many people who have autism. Despite the increased number of people known to be living with autism, less well-known is just how difficult it can be for them to access beneficial care.
Given the somewhat recent changes in the definition of what constitutes autism, an accepted description (from the American Academy of Child and Adolescent Psychiatry) states that autism is a condition that results from differences in the way that a brain develops. The manifestations of that difference can impact how a person interacts socially and how they behave. Some people with autism can have delays in speech development and some can have a degree of intellectual disability. Because of an increased focus on screening, many people/children with autism are diagnosed before they are 3 years old.
We have recognized that autism is a spectrum with some exhibiting readily identifiable features and others less so. Even though I have been practicing pediatrics for nearly 14 years, I’ve been surprised by my inability to recognize some of autism’s more subtle presentations. This is why screening is important and I make it a point (as is recommended by the American Academy of Pediatrics) to screen all children for autism. It is also why I routinely send more children to a specialist (developmental pediatrician) than I would have had I not used a standardized screening tool. It should also be mentioned that it can be more difficult for certain children (those who have families of modest financial means, those who live in rural areas and/or those who are Black or Latinx) to get diagnosed and evaluated by such specialists.
At the same time that the number of children diagnosed with autism has increased (as I have anecdotally witnessed in my own practice), there has also been an increase in the difficulty of obtaining beneficial care for patients with autism.
Given the increased prevalence of autism, much time and research has gone into determining how best to treat it. One of the most tested and used interventions is Applied Behavior Analysis (ABA) therapy in which therapists work (often individually) with patients to promote more socially normative behaviors. It has helped many (but not all) patients with autism reach more of their social potential.
A cornerstone of child development is that the earlier a deficit is identified and treatment is begun, the easier it is to intervene, improving the likelihood that the child reaches their full potential. Despite this knowledge, it has unfortunately been more difficult to get ABA therapy now than in years past, leading some families and patients to wait months before receiving the care they need. In the past, a simple request/referral from a health care provider was all that was needed for a patient to get therapy. However, providers now often need to complete a multi-step process that sometimes requires prior authorization — something often required for rarely used, expensive or new interventions not covered by insurance. The associated paperwork can lead to delays, lapses in therapy and stress for families who are often already dealing with elevated stress levels at baseline. Additionally, children with autism often need speech, occupational and physical therapy. Each intervention has its special hoops through which providers and families must jump. The challenges facing patients with autism even exist when insurance coverage status and/or the ability to pay has been eliminated (as in the case among military families whose health insurance and therapies are all covered).
All states now have laws mandating insurance coverage of ABA therapy. However, laws are only as good as those who implement them. We must urge our lawmakers to pressure insurance companies to reduce barriers to needed treatment. Additionally, we should apply pressure directly on insurance companies to the same end. As noted above, delays in receiving care reduce the effectiveness of that very care.
So, as we commemorate and work to fully accept people who have autism, let us also make sure that we are increasing our ability to treat those who have it.
Dr. David Myles ([email protected]) is a board-certified general pediatrician at Walter Reed National Military Medical Center, a fellow of the American Academy of Pediatrics and an editorial board member for the journal Pediatrics. He is also a Rockville Council member.
More in Commentary

Commentary | Helping your kids have positive social media experiences | GUEST EDITORIAL

Commentary | David Trone: 90 days in solitary won’t solve America’s criminal justice problem | GUEST COMMENTARY

Commentary | Martin O’Malley is off to a bad start at Social Security | GUEST COMMENTARY

Commentary | Dissatisfied with the current candidates? Soon, we could choose an AI president | GUEST COMMENTARY
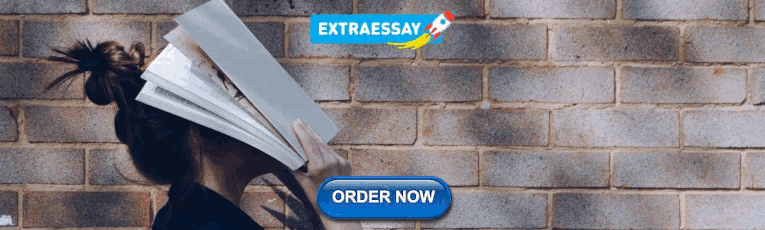
IMAGES
COMMENTS
Data were extracted by two independent researchers. Since 2012, 99 estimates from 71 studies were published indicating a global autism prevalence that ranges within and across regions, with a median prevalence of 100/10,000 (range: 1.09/10,000 to 436.0/10,000). The median male‐to‐female ratio was 4.2.
Prevalence is typically shown as a percent (e.g. 1%) or a proportion (e.g. 1 in 100). Autism Spectrum Disorders (ASDs) are a group of developmental disabilities that can cause significant social, communication and behavioral challenges. CDC is working to find out how many children have ASDs, discover the risk factors, and raise awareness of the ...
Prevalence estimates of autism are essential for informing public policy, raising awareness, and developing research priorities. Using a systematic review, we synthesized estimates of the prevalence of autism worldwide. We examined factors accounting for variability in estimates and critically revie …
Abstract. Problem/Condition: Autism spectrum disorder (ASD). Period Covered: 2020. Description of System: The Autism and Developmental Disabilities Monitoring (ADDM) Network is an active surveillance program that provides estimates of the prevalence of ASD among children aged 8 years.In 2020, there were 11 ADDM Network sites across the United States (Arizona, Arkansas, California, Georgia ...
The average prevalence of autism spectrum disorder in Asia, Europe and North America is estimated at 1% [13, 14]. According to the Centers for ... A systematic review of research on autism spectrum disorders in Sub-Saharan Africa. Behav Neurol. 2016;2016:3501910. [PMC free article] 106. Loomes R, Hull L, Mandy WPL. What is the male-to-female ...
Table 2 Prevalence, Incidence, and DALYs of Autism-Spectrum Disorder in counts and age-standardize rates for both sexes combined in 1990 and 2019, with percentage change between 1990 and 2019 by ...
There was an increase in autism prevalence estimates over time. The prevalence was also significantly higher for children aged between 6 and 12 years compared to children under the age of 5 and over the age of 13 years. ... reported in the scope of previous research: the global pooled point-prevalence of ASD consistently increased from 0.25% ...
We reviewed studies of the prevalence of autism worldwide, considering the impact of geographic, ethnic, and socioeconomic factors on prevalence estimates. Approximately 1/100 children are diagnosed with autism spectrum disorder around the world. Prevalence estimates increased over time and varied greatly within and across sociodemographic groups.
The aim of the current systematic review is to update our previously published estimates of the global preva-lence of autism. Based on 70 estimates, the last system-atic review of global prevalence conducted in 2012 found that the median prevalence of ASD was 62/10,000 chil-dren, with a consistently higher prevalence in boys (Elsabbagh et al ...
Prevalence estimates of autism are essential for informing public policy, raising awareness, and developing research priorities. Using a systematic review, we synthesized estimates of the prevalence of autism worldwide. We examined factors accounting for variability in estimates and critically reviewed evidence relevant for hypotheses about biological or social determinants (viz., biological ...
In 2020, the autism prevalence increased for all of these groups and, for the first time, the prevalence among Asian or Pacific Islander, Black, and Hispanic children was higher than among White children. In addition, higher autism prevalence was associated with lower socioeconomic status—the reverse of what researchers found in earlier reports.
Data were extracted by two independent researchers. Since 2012, 99 estimates from 71 studies were published indicating a global autism prevalence that ranges within and across regions, with a median prevalence of 100/10,000 (range: 1.09/10,000 to 436.0/10,000). The median male-to-female ratio was 4.2.
Background Autism spectrum disorder (ASD) is one of the serious developmental disorders that is usually diagnosed below the age of three years. Although the severity of the disease's symptoms varies from patient to patient, the ability to communicate with others is affected in all forms of ASD. This study aimed to determine the prevalence of ASD in high-risk groups by continent. Methods The ...
Autism spectrum disorders (ASD) are a diverse group of conditions. They are characterised by some degree of difficulty with social interaction and communication. Other characteristics are atypical patterns of activities and behaviours, such as difficulty with transition from one activity to another, a focus on details and unusual reactions to sensations.
00:00. 00:00. The prevalence of autism in the United States has risen steadily since researchers first began tracking it in 2000. The rise has sparked fears of an autism 'epidemic.'. But experts say the bulk of the increase stems from a growing awareness of the condition and changes to its diagnostic criteria.
Autism Prevalence Rises. In late March, the Centers for Disease Control and Prevention (CDC) reported that the autism prevalence is higher that it was in 2018. Currently, one in 36 (2.8%) children are identified with autism spectrum disorder, based on 2020 data from 11 communities in the Autism and Developmental Disabilities Monitoring (ADDM ...
1. Introduction. In the last decades, a large increase in the prevalence of Autism Spectrum Disorder (ASD) has been observed, generating claims about an "epidemic" of autism [1,2].Correct estimates of ASD prevalence rates are important, firstlyin order to determine the economic and health services burden of this condition and to allocate sufficient funding and adequate services for ...
New Research Points to Disparities in Autism Prevalence and Access to Care. April 03, 2023. New research based on data from CDC's Autism and Developmental Disabilities Monitoring Network found that among 8-year-old children, 1 in 36 were identified with autism spectrum disorder in 2020. This is an increase from 1 in 54 in 2016. 1.
The prevalence of autism spectrum disorder (ASD), a neurodevelopmental condition impacting social communication and restricted and repetitive behaviors, has been found to be increasing over recent decades, with current US estimates of 1 in 36 in 8-year-old children and higher rates in males than females (Maenner et al., 2023; Center for Disease Control and Prevention (CDC), 2014 & 2023).
Increased awareness has been a key factor behind the surge in the prevalence of autism diagnosis in Australia, estimated to be at least 3.2% of school-aged children.
Last Reviewed: April 3, 2023. Source: National Center on Birth Defects and Developmental Disabilities, Centers for Disease Control and Prevention. About 1 in 36 of 8-year-old children in 11 communities in the US were identified with autism in 2020. Learn more about CDC's latest ADDM data release.
Autism education has become an increasingly important area of focus in recent years due to the rising prevalence of autism spectrum conditions (ASC) among children. The estimated prevalence of ASC has increased from 1 in 10,000 in the 1960s to at least 1 in 100 today [1,2,3]. ASC is a neurodevelopmental condition characterized by impaired ...
This April, we would like to highlight NIMH's unique role in federal autism coordination efforts and reflect on how the lived experiences of autistic people and their families have shaped federal autism research, services, and policy. Dr. Gordon (left) and Dr. Daniels (right) at the January 2024 IACC meeting.
The prevalence of autism was widely believed to be 1% until last year, when a first of its kind study published in the Lancet found the true rate to be more than double that, with at least 1.2 ...
Since 2012, 99 estimates from 71 studies were published indicating a global autism prevalence that ranges within and across regions, with a median prevalence of 100/10,000 (range: 1.09/10,000 to ...
According to the U.S. Centers for Disease Control and Prevention (CDC), the prevalence of autism in the United States has risen from one in 150 children in 2000 to one in 36 children in 2023.There ...
Although prevalence of autism spectrum disorders in children have been reported in countries and regions such as the USA ... Recent advances in autism research as reflected in DSM-5 criteria for autism spectrum disorder. Annu Rev Clin Psychol. (2015) 11:53-70. 10.1146/annurev-clinpsy-032814-112745 ...
The used experimental procedures have the approval of the Animal Research Ethics Board (AREB), the local authorities of the State of Hamburg (TVA, N007/2018), and the animal care committee of UKE. ... J. L. Daniels, Z. Warren, A. Vehorn, A. Salinas, M. S. Durkin, P. M. Dietz, Prevalence of autism spectrum disorder among children aged 8 years ...
Given the increased prevalence of autism, much time and research has gone into determining how best to treat it. One of the most tested and used interventions is Applied Behavior Analysis (ABA ...
These gaps narrowed over time until ASD prevalence among Black and Hispanic matched prevalence among White children for the first time in 2016 and 2018, respectively (11,12). Similarly, robust associations between autism prevalence and higher socioeconomic status were observed in ADDM Network sites during 2002-2010 (13); however, this association