- Transformation Leaders
- Innovation Experts
- HR, People & Culture
- Latest Articles
- Search Knowledge Base
- The Case For Digital Transformation
- Strategy & Innovation
- People & Change
- Customer Engagement
- Enabling Technologies
- Data & Analytics
- Cyber Security
- Executive Briefing Book Summaries
- Leading Digital Transformation: Podcast Series
- News In Digital: Archive
- Find new business opportunities
- Publish with us
- Meet The Authors
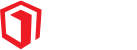
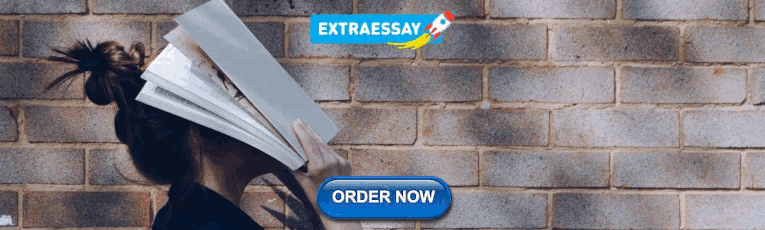
Renault – An industry 4.0 Case Study
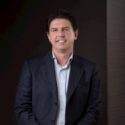
Digital Transformation Practitioner | Innovation Coach | Entrepreneurship | Exponential Technologies | Keynote Speaker
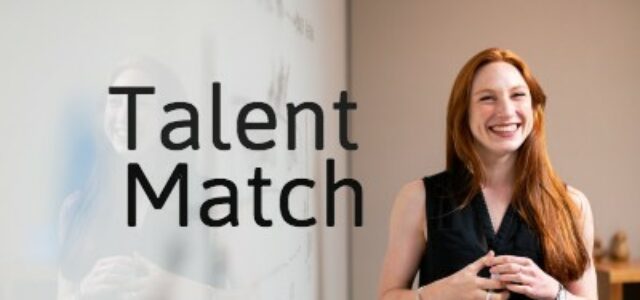
Save time and money securing the right people for maximum impact in your business.
The Digital Economy and the rapid convergence of exponential technologies is forcing many companies to rethink their business models and this is true for automotive companies as well.
Technologies like the Internet of Things , Artificial Intelligence , Blockchain , Robotics or Augmented reality are all converging, and this provides both threats and opportunities for companies like Renault.
We are in an age where autonomous driving is turning from a moonshot a few years back to a reality that will soon hit our streets in most cities. These technology shifts and the convergence of them is also what is shaping the next industrial revolution, i.e. the Industry 4.0.
But not only that, consumer behaviors are changing as well. Vehicle ownership is less on the mind of Millennials that are accustomed to transportation on demand from the likes of Uber or Lyft.
These millennials are also more worried about the impact transportation can have on the environment, they want to see sustainability higher on the agenda for large corporates.
Renault recognizing many of these threats and opportunities decided to embark on a digital transformation journey that is supposed to take the company to new levels.
The Vision: Mobility, Electric Power and Autonomous Driving
It started in 2015, when, with the help of Management Consulting Firm BCG they decided to shape the strategic plan of Renault. The outcome of that effort led to the 2017-2022 strategic plan.
Increase in revenue and productivity are among the usual suspects, when it comes to objectives, but there was also a clear objective to continue strengthening their position in electric vehicles, becoming a more connected and efficient company, leveraging autonomous driving and thinking about new forms of mobility services.
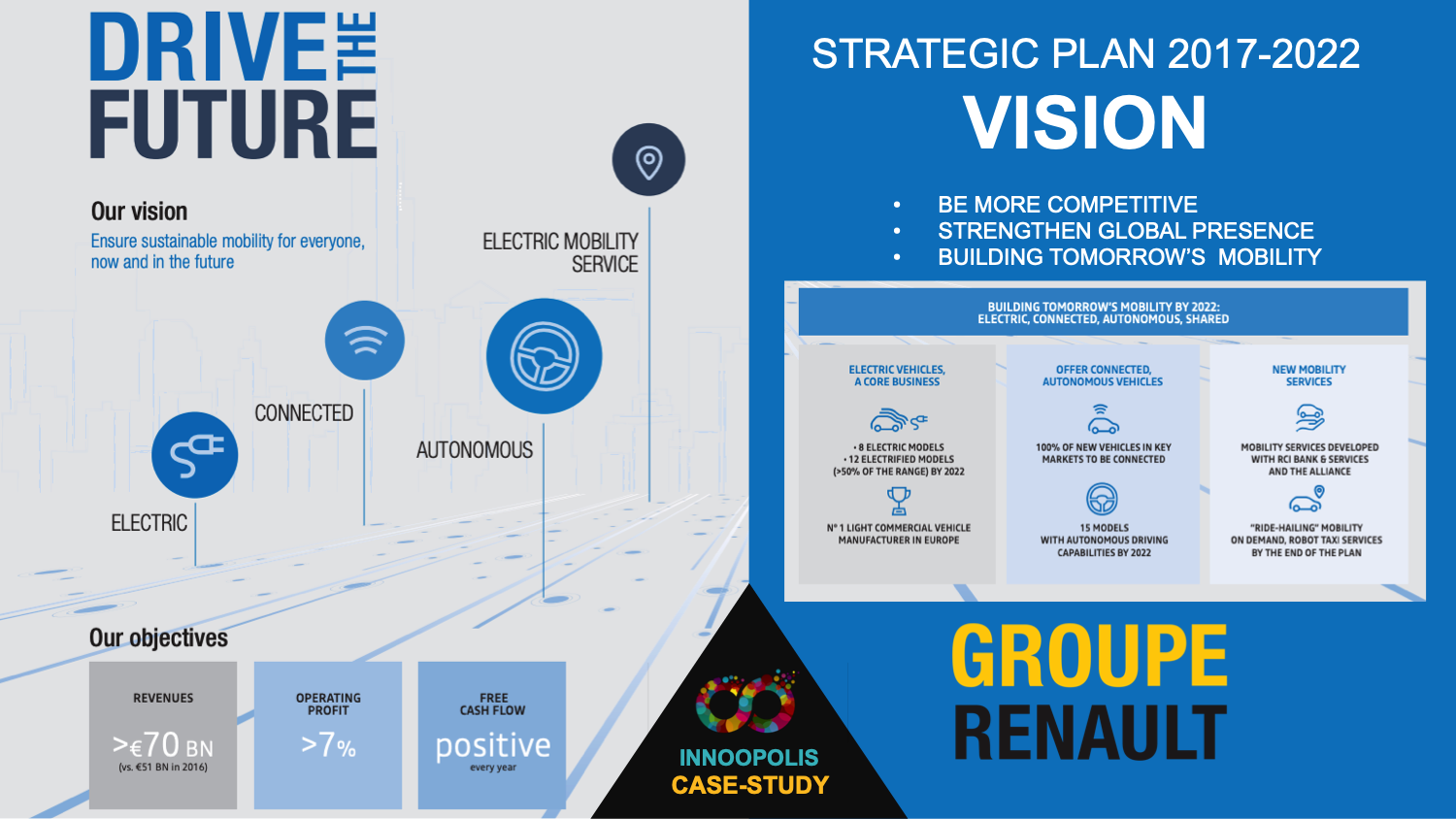
Transforming the company both Upstream and Downstream
In order to execute on this strategy, Renault needed first to understand the impact digital could have on their business. They looked at both upstream and downstream activities to find efficiencies and revenue opportunities.
They found out initially that the cost-saving and optimizations that could be achieved on the design and manufacturing side were close to 300M Euros/Year. Digital and in particular better usage of data, that was still scattered around 2000 legacy applications could provide them with better and faster decisions, increasing productivity.
Getting more of their production equipment connected and getting more of that data to unit managers in real-time was also essential to monetize data. Similarly, they found that becoming more data-driven could also help them to better manage the lifecycle of the customer relationship.
From better managing personalization needs to stronger engagement throughout the lifecycle of the customer relationship, they found out that here also they could make a 300M Euros / Year impact.
Having outlined the vision and developed a long list of use-cases that could impact the business both downstream and upstream, they came up with an overall impact of 600M Euros/Year. They decided then to quickly validate these initial assumptions by going into end-2-end pilots that could measure the real impact.
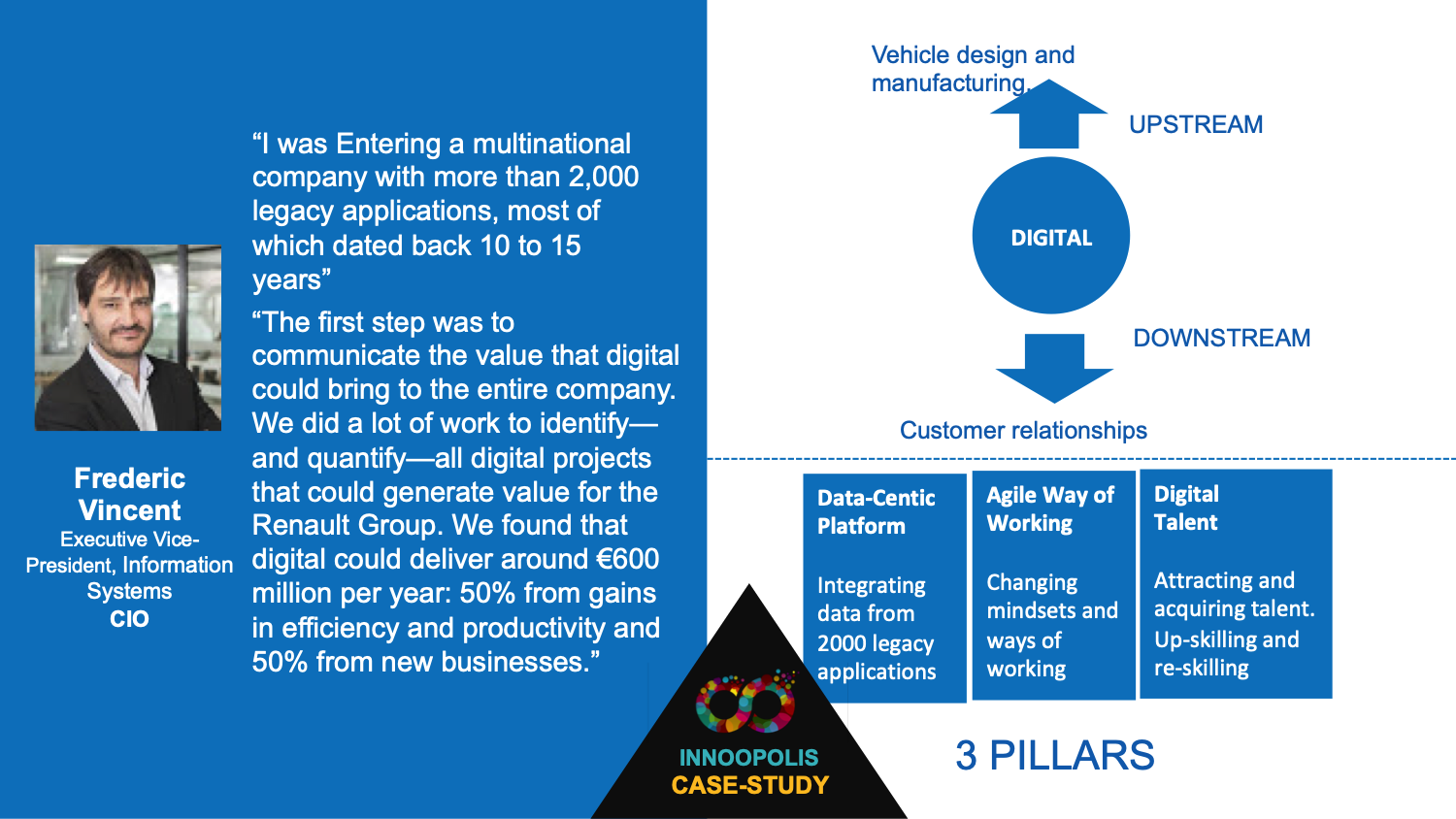
The problem they faced then was a real challenge, they hit a road block. As Frederic Vincent, the “CIO” of the company said in one interview “ the company suffered from lack of internal digital skills, Inconsistent digital methodologies across the company, Heavy governance and processes not adapted to the speed of digital, As well as rigid legacy IT systems .”
Basically for Management, the transformation was not moving fast enough, and the slow down was related to a lack of digital skills and rigid governance. It was essential to change all of this and do it quickly!
Renault Digital, A Digital Center of Excellence
On January 1, 2017, Renault Group decided to set up Renault Digital, an independent, but fully owned subsidiary with the sole objective of supporting the group to achieve its digital transformation objectives.
Renault Digital is the center of excellence that provides all the skills needed to execute digital, agile projects. It consists nowadays of more than 350 employees, with skills and knowledge in Agile methodologies, UX Design, DevOps and Data Science.
Renault Digital works a bit like a startup incubator and that is also the mentality it tries to instil to its staff, but also to the other persons working on their projects, a startup mindset.
For each project request that they get from the business units, they would set up an agile team, that is composed of subject matter experts from the business unit as well as specialists from the centre of excellence.
The projects are then incubated and go-through two distinct phases, an initial framing phase, which aims to narrow down the problem, gather insights and initial hypothesis. This is similar to what you would normally do in initial design thinking workshops, analyzing customer pain points and mapping customer journeys.
In the second phase, they move to an agile development model with the objective to develop the minimum viable product (MVP).
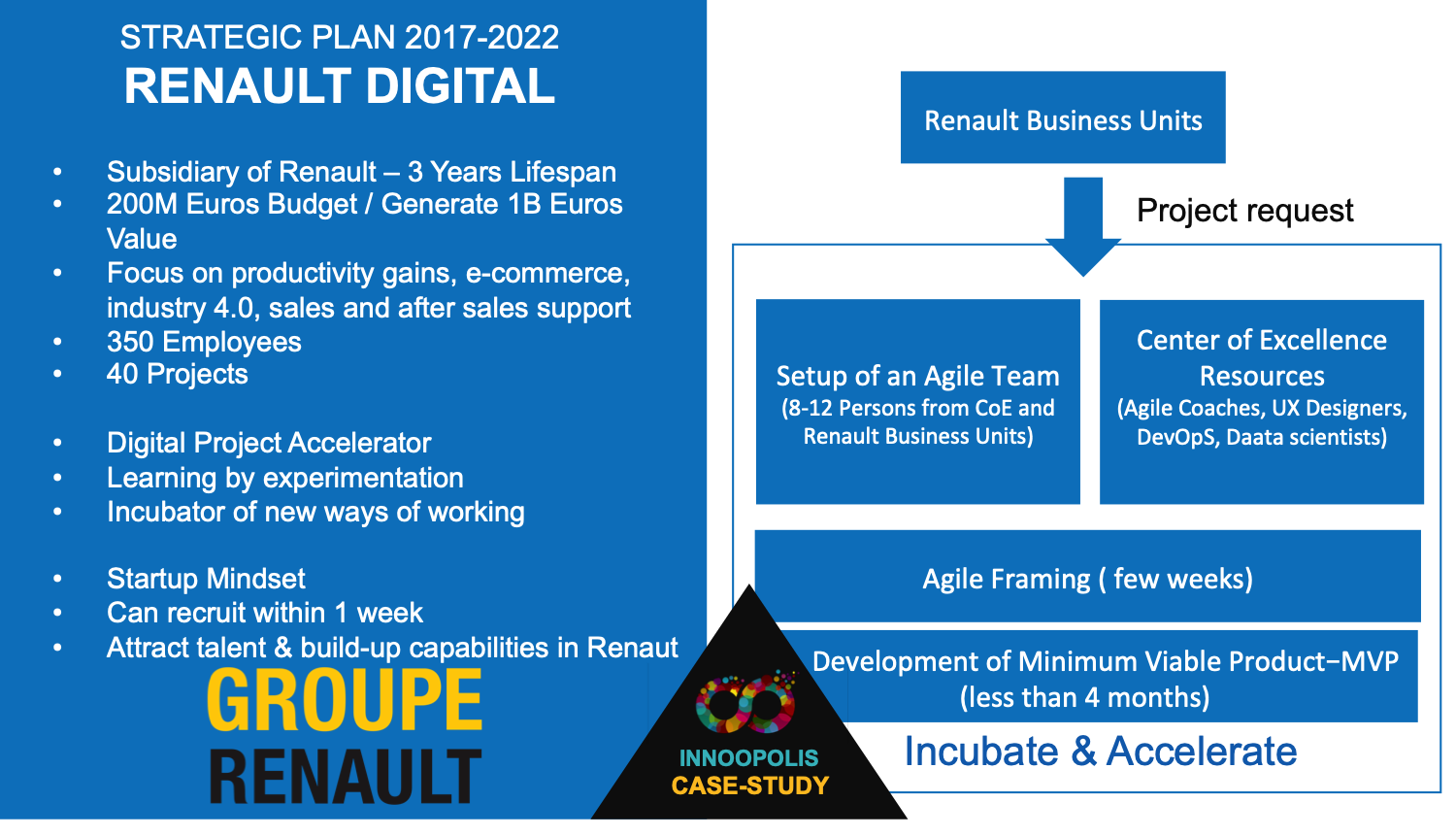
The good thing with this approach is that Renault Digital is really working as a startup and can easily and rapidly recruit new talent when needed.
Secondly, the persons from head office or other business units, that have been incubated for a period of 4-5 months, can now move back to their offices, with a new mindset, they effectively become change agents in the digital transformation.
Industry 4.0 Opportunities
Once Renault set up their Digital Unit, it was important to start building up success stories. Success stories are what helps you set the seeds for a change of culture and since Renault has a lot of staff working in their production plants it was only natural that they tried to find how digital could provide values to their employees.
Project CHUET was this first success story! Basically the challenge plant unit managers were facing back in the days is that they had to manage teams of 10-12 people, but needed to constantly run back and forth to their office computer to get the latest updates on how production had to be tuned, adjusted.
By putting Wi-Fi connectivity in their plants and by developing a bespoke mobile office solution for their unit managers they allowed them to save 45min/Day of ineffective time.
The productivity increase and job satisfaction were notable, now Unit Managers work around with their mobile office tablets and have access to real-time product data whenever they need it.
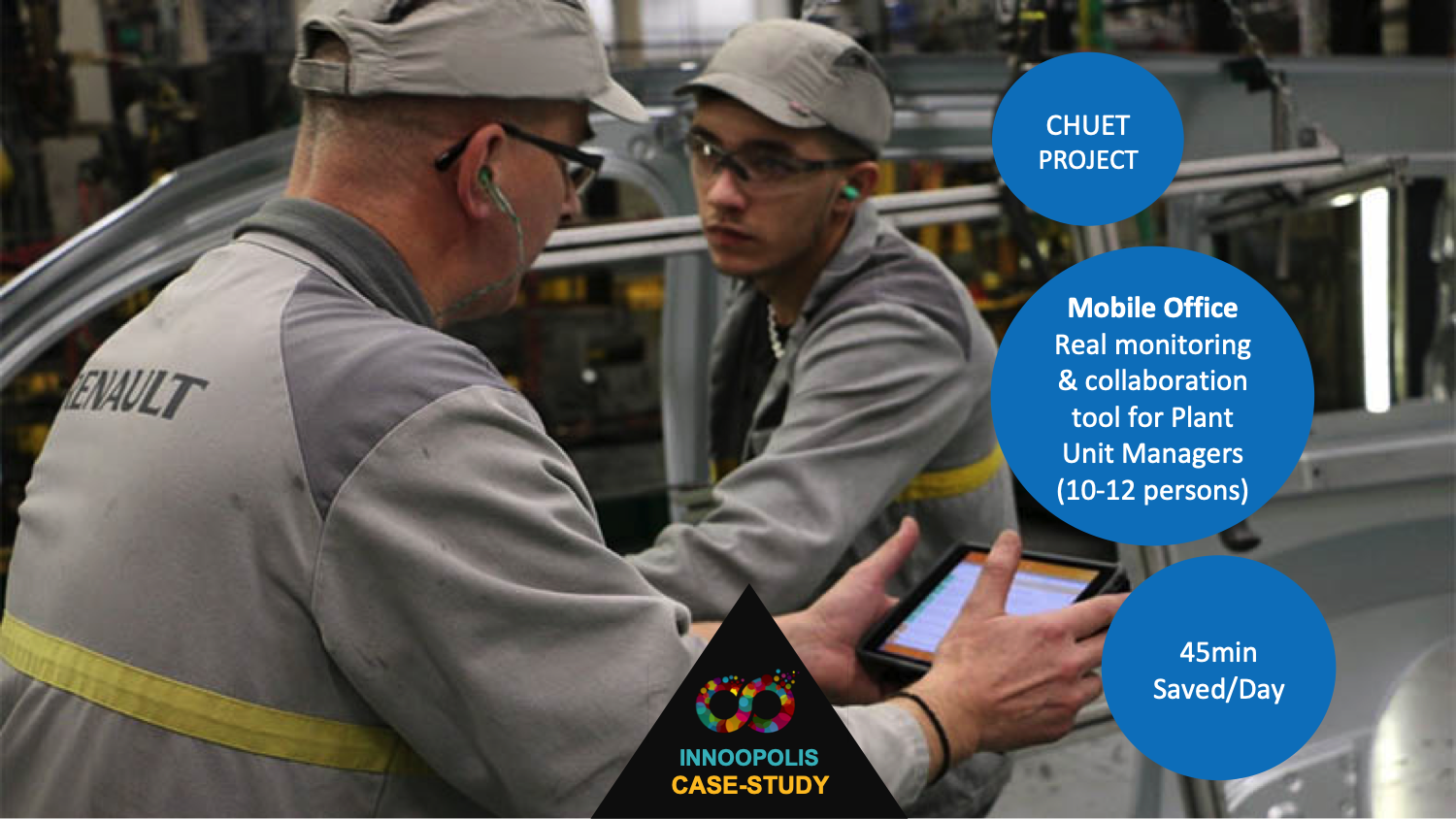
Connecting equipment helped the unit managers, but connecting machines and robots also allowed them to create value in other areas.
Predictive maintenance is one of these areas where expensive downtime due to machine failures can be avoided or at least its probability reduced. Renault has put more than 2000 Lora sensors in place to collect data from their equipment.
In manufacturing plants, robots and automation are of course essential, but with the increasing needs of personalization, with the increasing complexity of the product, some tasks become more complicated and human intervention is often needed to ensure quality.
The collaboration that is needed between robots and humans is such that we are now talking about co-bots (collaborative robots). While the bot takes on heavy lifting and repetitive tasks, the human employee is given more time to perform more complex tasks like quality control.
This new type of collaboration and shift in tasks for plant employees also requires a new type of training, a type of training that is best done with augmented reality and virtual reality tools. Renault is leveraging this type of experiential learning to make sure employees quickly and safely prepared for this new work environment.
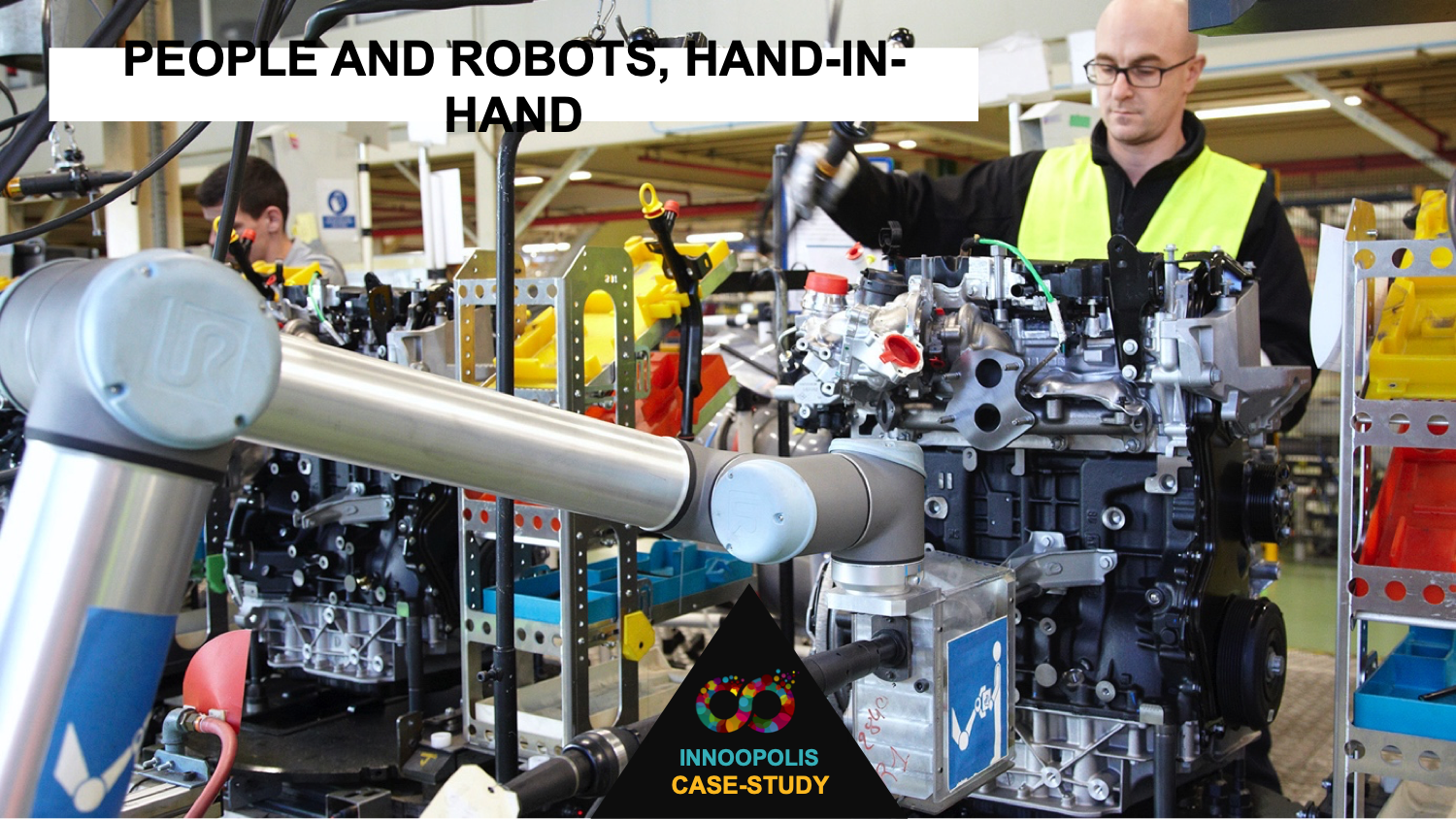
Downstream opportunities
Downstream opportunities are real as well. One of the challenges all auto-makers and dealership face is that they don’t have that long-term permanent relationship with the customer.
Customers typically only come back when a problem arises, and after the warranty is expired they won’t come back until they need a new car… if somebody else didn’t catch their attention first. Connecting cars is the first step, but then it is about building applications that provide engaging experiences and allow to create new value for customers.
By staying connected with its customers Renault expects more sales lead generated through digital, providing better opportunities to achieve its growth ambitions.
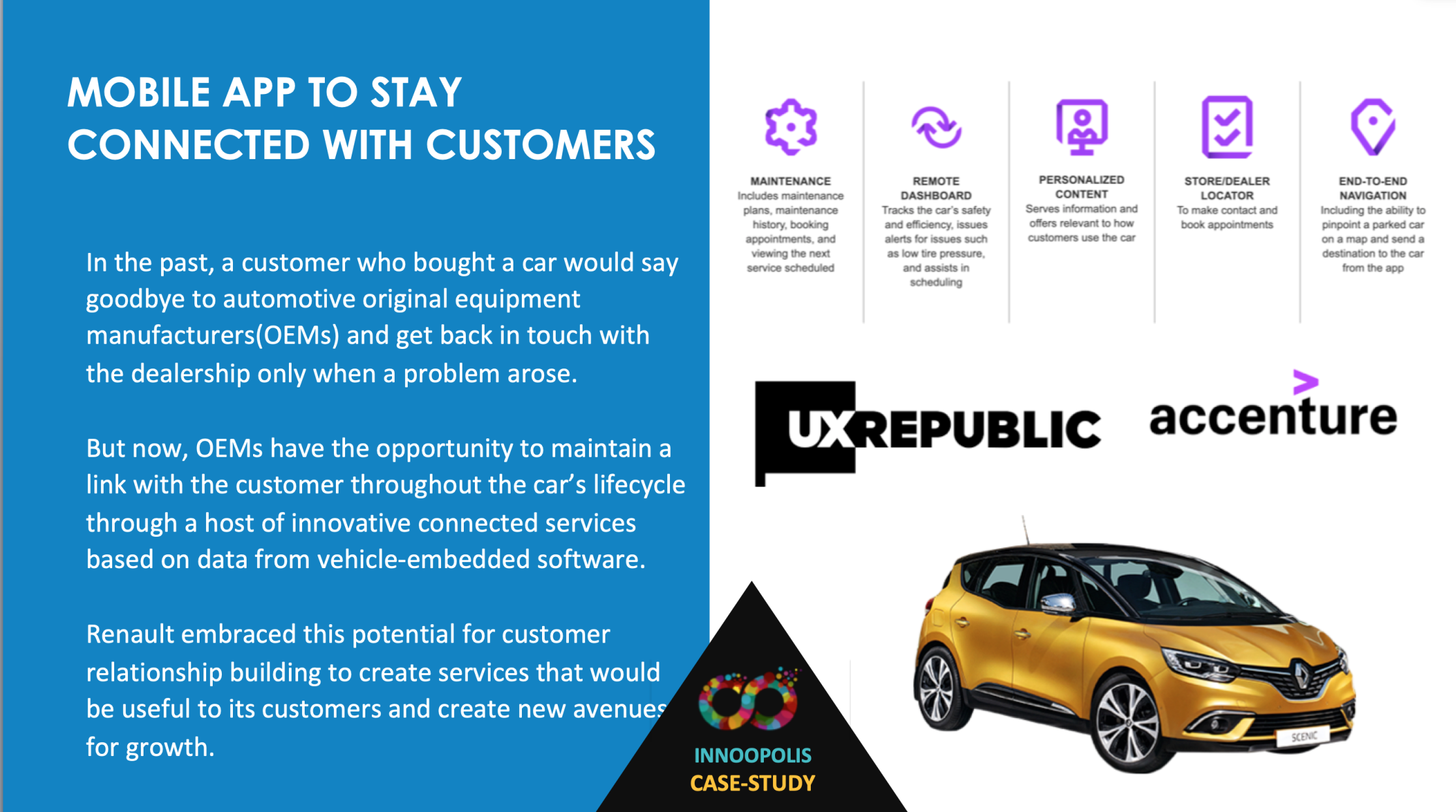
Transformation with a culture change at Heart
Renault Digital has proved to be an immense asset for Renault. Already delivering clear value within 12 months of its inception (through the CHUET project), the ambitions of the group when it comes to the impact of digital have been revised upwards. It is now estimated that the impact will reach 1Billion Euros per year.
But at the end of the day, Renault is aiming to change the entire culture of the company. New leadership mindsets, but also a workforce that becomes increasingly agile.
That focus has also led HR to take a leading role in the transformation of the company. Besides setting various programs to develop digital skills, they also realized that the management style needs to change and that collaboration is becoming more essential to drive agility.
Collaboration and the development of a more aspirational environment are also what is supposed to attract new talent. Ultimately these changes will also lead HR to transform itself. Focus on end-2-end employee journeys, giving more power to employees to manage their careers are all elements that aim to make Renault an attractive employer in a world where digital talent is still scarce.
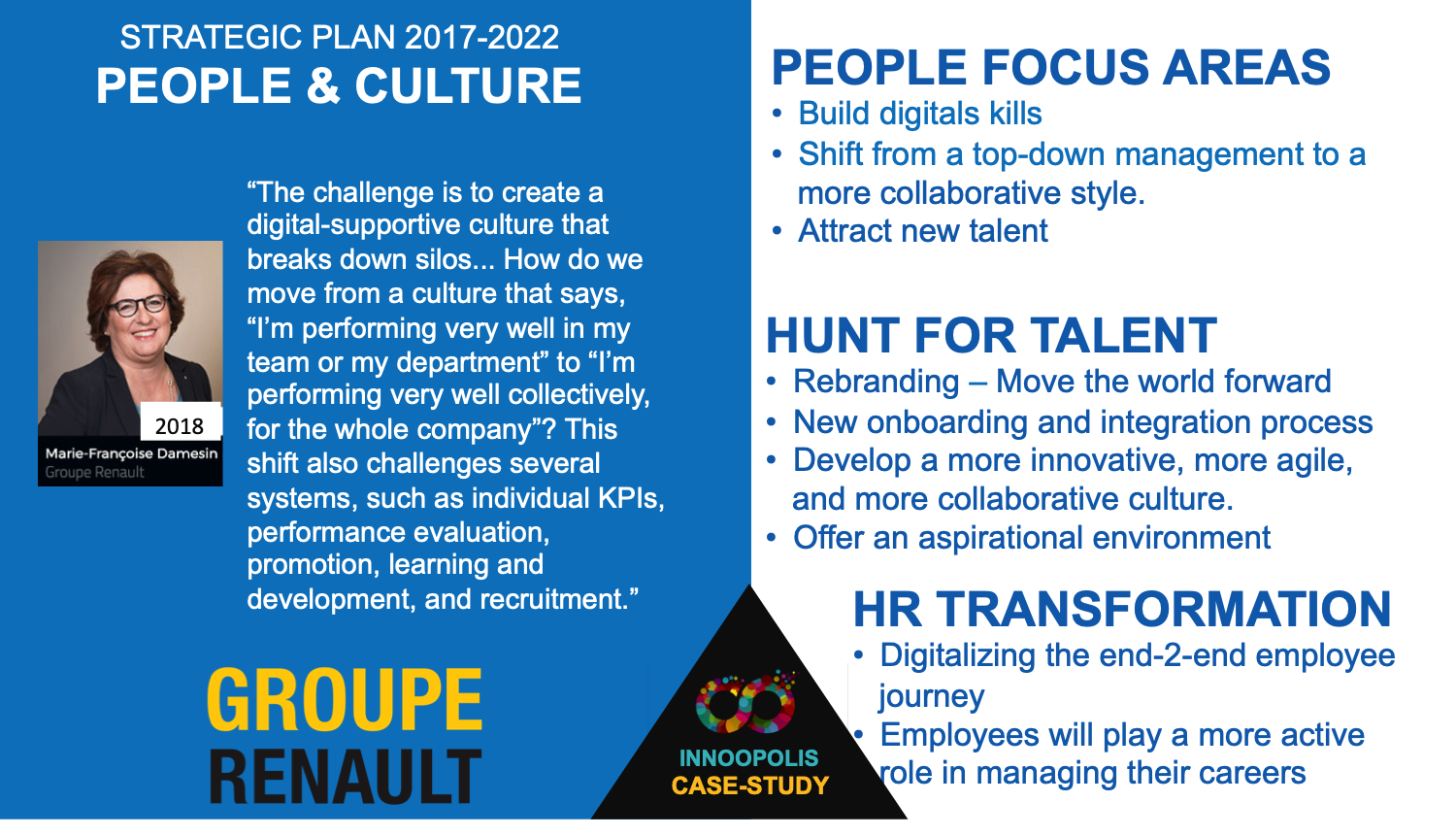
If you have more insights on the Renault Digital Transformation story, then I would love to hear form you to make this story better and more developed.
Do you have similar stories to share? What is the most interesting part of the Renault story?
Disclaimer : This article is based on open public reports, interviews posted in magazines, some of them translated from french. It is an external analysis of the Renault Digital Transformation story to the best of my knowledge. If anything here is inaccurate, please contact me directly and I will update the article.
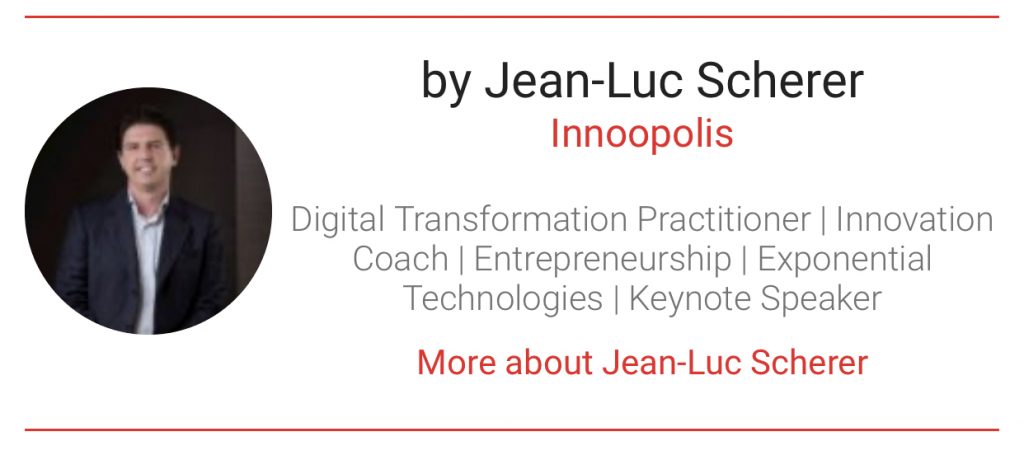
Arrange a Conversation
Article by channel:
Everything you need to know about Digital Transformation
The best articles, news and events direct to your inbox
Read more articles tagged: Featured , Fourth Industrial Revolution
Popular Now
Related articles.
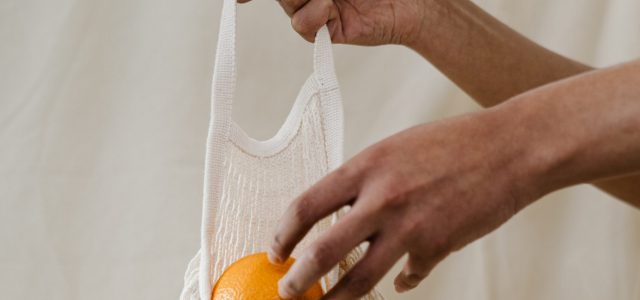
A better way to hire for digital transformation. Quality candidates expertly matched to your business guaranteed to save you time and money .
Industry 4.0 Case Studies (curated)
Curated Set of Case Studies demonstrating problem, solution, and results of implementing Industry 4.0.
- https://www.machinemetrics.com/avalign-case-study requires free login, 10 page pdf article download
- Who – Avalign Technologies medical device company utilized machine metrics for machine and operational data
- Problem – Needed to decrease downtime and increase throughput.
- Solution – deployed measuring, monitoring, dashboards for equipment like grinding, and lathes across 132+ machines at 4 facilities
- Results – 40% increase in OEE, 9.9% increase in throughput, $4.5M capacity utilization increase, 14k hours saved, across 9 months
- Who - BJC healthcare, service provider that operates at multiple hospitals in Missouri and Illinois
- Problem - Inventory tracking is labor intensive and inefficient.
- Solution - RFID tagging to track and manage thousands of medical supplies. Reduced manual labor to maintain inventory.
- Results - Reduce onsite stock by 23% at facilities.
- Who - Bosch Automotive Diesel System factory in Wuxi, China
- Problem - Bottlenecks and production efficiency problems
- Solution - Collect machine monitor sensors' data, combine data sources, and use machine learning analytics to predict equipment failures, thus schedule maintenance before failure.
- Results - Higher uptime durations, contributing to > 10% production output increase in some areas
- Who - Fetch Robotics (California) and DHL distribution center (Netherlands)
- Problem - Improve efficiencies for locating, tracking, and moving inventory in warehouse and logistics facilities
- Solution - Used collaborative Autonomous Mobile Robots (AMRs) to pick and place alongside the workers. AMRs autonomously learning and sharing most efficient travel routes.
- Results - Reduce order cycle time up to 50%, provide up to 2x picking productivity gain.
- Who - Fast Radius Chicago, contract manufacturer with multiple locations.
- Problem - Improve efficiencies for fast turnaround and mass customization of products.
- Solution - Collect data for every part design and identify applications suitable for 3D printing.
- Results - Reduce costs in storing parts through virtual inventory, decrease cycle time.
- Who - Siemens and American pro racing squad Team Penske
- Problem - Speed up the race car development process
- Solution - Create a digital twin, digital representation of a real-world product, machine, process, or system, that allows companies to better understand, analyze and optimize their processes through real-time simulation based on machine learning. Sensors were fitted to a real car and collected data real-time continuously, such as tire pressure, engine control and wind speed, which is then converted into a virtual car model. It’s this model that allows engineers to test different design configurations.
- Results - Reduce testing costs, and time, and ultimately a faster vehicle.
- Who - IBM manufacturing of mainframes, servers, storage, and IT appliances, in Canada, Hungar, mExico and US.
- Problem - Increase efficiencies of visual inspection and product quality. Manual inspections lead to missed defects. Traditional automated inspections also provide too many false positive results.
- Solution - Used IBM Maximo Visual Inspection, an AI-powered computer vision solution to automatically detect quality defects in manufacturing. System was trained using deep learning.
- Results - Up to 5x efficiency gains, 20% reduction in false positives.
- Explained in 7 minute video and implementation of plex.
- Who - Summer Garden Food Manufacturing
- Problem - Not enough productivity and low OEE, no visibility.
- Who - Best Maid food product provider
- Problem - No ERP system - legacy database and excel spreadsheets. Difficulty managing inventory (5 manual audit days/month), Production planning (schedule written day before), challenging for Safe Quality Food Certification.
- Solution - Plex implementation - training, implementation, within 8 months.
- Inventory Management - reduction in raw material inventory of $0.5M, eliminate $50k mislabeled items, eliminate lost 1% due to spoilage.
- Streamline Order to Cash - deliver within 3 days window to avoid penalties (was 1% of orders delayed w/penalties and 50% of Best Maid's revenue)
- Financial management - financial close process now 25% of previously required - was 4 months behind, now closes within 4 weeks of month end.
- Areas with videos, BBC mostly, Logistics, Construction, Public Transport, Manufacturing, Food production, Fieldwork
Please provide the following information to access the course, or click here to learn more about the Industry 4.0 Academy
Industry 4.0 in food processing: drivers, challenges and outcomes
British Food Journal
ISSN : 0007-070X
Article publication date: 4 July 2022
Issue publication date: 19 December 2022
In the age of the Fourth Industrial Revolution, Industry 4.0 can increase the productivity and competitiveness of companies in the international marketplace. The purpose of this article is to investigate the drivers for and outcomes of the adoption of Industry 4.0 technologies in the case of a food processing company located in Italy.
Design/methodology/approach
The present work adopted a case study approach by investigating an Italian food processing company to investigate the drivers, challenges and outcomes of Industry 4.0 adoption in the agri-food sector.
This research highlighted drivers and challenges related to the adoption of different Industry 4.0 technologies. Secondly, it underlined the impacts of Industry 4.0 in terms of firm performance, operations management, human resource management and strategy.
Originality/value
Industry 4.0 technologies remain underexplored from the strategic perspective in the agri-food sector. This article provides preliminary evidence on the digital transformation of food processing companies, with a focus on Industry 4.0. Practical implications for managers, CEOs and entrepreneurs are discussed.
- Industry 4.0
- Digital transformation
- Food processing
Romanello, R. and Veglio, V. (2022), "Industry 4.0 in food processing: drivers, challenges and outcomes", British Food Journal , Vol. 124 No. 13, pp. 375-390. https://doi.org/10.1108/BFJ-09-2021-1056
Emerald Publishing Limited
Copyright © 2022, Rubina Romanello and Valerio Veglio
Published by Emerald Publishing Limited. This article is published under the Creative Commons Attribution (CC BY 4.0) licence. Anyone may reproduce, distribute, translate and create derivative works of this article (for both commercial and non-commercial purposes), subject to full attribution to the original publication and authors. The full terms of this licence may be seen at http://creativecommons.org/licences/by/4.0/legalcode
1. Introduction
Agri-food industry responds to the problem of food needs around the world, entails high levels of employment, strongly contributes to economic growth and has relevant impacts on the natural environment. These motives make urgent to find economically, socially and environmentally sustainable productive solutions for the long term ( Jambrak et al. , 2021 ). From an historical perspective, food industry has traditionally focused on food integrity and safety (until 1950), enhancing flavours (1950–1980) and health (1980–2000), whereas the new evolutionary phase entails the current and future challenge of promoting the “ health of society ” ( Silva et al. , 2018 ; Augusto, 2020 ). Industry 4.0 represents a group of advanced technologies that can have an important role in this scenario. Such technologies can be applied in various business functions, with strong impacts on products, processes, factories and supply chains ( Hasnan and Yusoff, 2018 ; Manavalan and Jayakrishna, 2019 ). For instance, Internet of Things (IoT), automation and robotics when introduced in production plants increase the operational efficiency ( Bortoluzzi et al. , 2020 ). Big data and analytics can be used to categorise data into data-related knowledge that supports strategic decision making at different levels, from operations to marketing ( Ferraris et al. , 2019 ; Rialti et al. , 2019 ). 3D printing is typically used to manufacture small batches of complex or highly customised plastic or metal products at relatively low costs ( Berman, 2012 ; Hannibal and Knight, 2018 ).
The interest for Industry 4.0 has universally increased around the world ( Müller and Kazantsev, 2021 ), with studies emerging in different streams of research and fields of analysis. In agri-food, past studies have suggested that Industry 4.0 could offer practical solutions to typical problems related to quality standards, energy consumption, cost reduction ( Hasnan and Yusoff, 2018 ), but also to mitigate uncertainty and risks characterising the agri-food supply chains ( Lezoche et al. , 2020 ). Agricultural computerisation allows energy saving and sustainability increases, whereas smart drone systems and automatic mobile robots are used to monitor the agricultural produce and to carry field crops ( Secinaro et al. , 2022 ; Trivelli et al. , 2019 ). However, the applications of Industry 4.0 technologies vary according to the different companies and operational contexts, and the agri-food sector has been less investigated compared to manufacturing ( Müller et al. , 2018 ; Manavalan and Jayakrishna, 2019 ). In particular, studies in the agri-food context on Industry 4.0 technologies mainly consist of literature reviews and theoretical papers (e.g. Rana et al. , 2021 ), mostly focussing on technological applications and functionalities in agriculture (e.g. Trivelli et al. , 2019 ; Secinaro et al. , 2022 ). However, empirical research on the influencing factors and outcomes of the adoption and implementation of such technologies in the agri-food sector is scarce. We aim at contributing to filling this gap through a single case approach ( Yin, 2009 ). Our research aims at investigating the outcomes of Industry 4.0 adoption and implementation in the case of an Italian fruit processing company, which represents a novel context of analysis in relation to the topic. The analysis highlights Industry 4.0-related outcomes at the organisational, strategic, human resource management and economic/environmental levels, but also sheds light on relevant factors have influenced this process.
The paper contributes to advance extant agri-food literature by identifying the outcomes of the Industry 4.0 adoption and implementation process in the case of a medium Italian fruit processing company. In doing so, our findings respond to recent calls and contribute to enrich the scarce empirical evidence on Industry 4.0 in the fruit supply chain ( Hasnan and Yusoff, 2018 ; Oltra-Mestre et al. , 2020 ).
2. Literature review
2.1 industry 4.0 adoption and implementation.
The Industry 4.0 paradigm introduces a new phase of technology-driven innovation for firms ( Wee et al. , 2016 ; Culot et al. , 2020 ). Born in the manufacturing context, Industry 4.0 initially identified a group of technological solutions comprising of the internet of Things (IoT), Big Data and analytics (BDA), augmented reality, additive manufacturing, cloud-based systems, simulation, advanced robotics, horizontal and vertical integration, and cybersecurity ( Rüßmann et al. , 2015 ; Trivelli et al. , 2019 ). As there is no universally accepted definition of Industry 4.0, other technologies may be considered depending on the sector, such as blockchain and energy management solutions ( Culot et al. , 2020 ). At present, some consensus was reached on the core and central role of IoT, which is at the base of interconnection among the different Industry 4.0 technologies, but also represents the common element among smart devices, plants and systems ( Culot et al. , 2020 ). Moreover, IoT sets the fundamentals for vertical and horizontal integration, which respectively allow real-time data sharing and analytics throughout different business functions, and extend this possibility across its borders. However, each technology can be implemented in different business functions, leading to distinct outcomes ( Chiarvesio and Romanello, 2018 ).
In the agri-food sector, Trivelli et al. (2019) have identified six clusters of technologies, namely the monitoring, IoT, automation, decision, hardware and laser, underlining the interrelations among them. For instance, the monitoring cluster (e.g. GPS, GIS) is strongly related to the IoT cluster, which refers to the communication of data among machines and devices (e.g. IoT, RFID, sensor networks). Another complementary cluster includes technologies supporting decision making such as artificial intelligence and data mining. In relation to agricultural entrepreneurship, Secinaro et al. (2022) identified macro-topics and themes referring to the value created through new technologies for agri-food entrepreneurs. However, moving from precision agriculture, extant research has scarcely investigated Industry 4.0 applications across other activities of the agri-food supply chain ( Lezoche et al. , 2020 ) and extant works are mainly theoretical. For instance, Rana et al. (2021) have reviewed blockchain applications, whereas Hasnan and Yusoff (2018) have speculated on potential Industry 4.0 applications and impacts in the agri-food supply chain. The scarce empirical agri-food research has so far analysed the opportunities deriving from Industry 4.0 in relation to fresh food products processing in Spain ( Oltra-Mestre et al. , 2020 ), the potential technological innovations in an Italian supply chain of milk, fruit and vegetable products and cereals ( Saetta and Caldarelli, 2020 ), the technical challenges emerged during the implementation in production of Industry 4.0 technologies in the case of a UK SME producing water crackers and biscuits ( Konur et al. , 2021 ) and the barriers and drivers from a supply chain 4.0 perspective by looking at an Australian food and beverage supply chain ( Ali and Aboelmaged, 2022 ). However, there are no studies that specifically address the outcomes of Industry 4.0 integration in the fruit processing to date.
The selection and implementation of Industry 4.0 technologies is not an obstacle-free process ( Wee et al. , 2016 ), and there is no single technological solution fitting all firms' needs ( Lezoche et al. , 2020 ). Extant studies have highlighted the existence of perceived barriers and drivers in this process ( Müller and Kazantsev, 2021 ). Barriers can relate to the lack of finance, technological awareness, collaboration, infrastructure, regulations, knowledge and skills, particularly during the selection of technologies ( Romanello et al. , 2021 ; Manavalan and Jayakrishna, 2019 ). During the implementation process, change management resistance and skills requirements become particularly relevant for small companies ( Ali and Aboelmaged, 2022 ; Romanello and Chiarvesio, 2021 ). Also, some technologies require previous time-consuming processes of knowledge codification and document digitisation that require skilled workforce ( Lezoche et al. , 2020 ). Instead, drivers are strongly related to the expected outcomes ( Ali and Aboelmaged, 2022 ; Lezoche et al. , 2020 ), such as increased productivity, flexibility, mass customisation, environmental sustainability, reduction of time and cost to market, and quality improvements ( Culot et al. , 2020 ). In addition, Industry 4.0 can also influence value creation and capture mechanisms ( Wee et al. , 2016 ; Rüßmann et al. , 2015 ) and product and process innovation ( Oltra-Mestre et al. , 2020 ).
2.2 Industry 4.0 expected outcomes in the agri-food context
Advanced technologies in production are expected to dramatically improve plants' and factories' performance (e.g. operating costs decrease, improved operational efficiency, increased productivity, lowering of production costs), while contributing to reduce electricity, water and heat consumption ( Borowski, 2021 ), making productions more economically and environmentally sustainable ( Borowski, 2021 ; Jambrak et al. , 2021 ). Second, in the agri-food context, robotics and automation merged with interconnected machines are expected to increase the levels of food safety and hygiene, while simplifying maintenance and reducing human injuries ( Saetta and Caldarelli, 2020 ; Rana et al. , 2021 ; Hasnan and Yusoff, 2018 ). In the food processing industry, IoT, alert systems and smart industrial robots endowed with cameras and inspection systems can be used to identify risks and abnormalities, improve food quality ( Manavalan and Jayakrishna, 2019 ) and ensure the fulfilment of food safety standards ( Hasnan and Yusoff, 2018 ). BDA could allow predictive maintenance and diagnosis to service machines and plants ( Saetta and Caldarelli, 2020 ), but also help tracking and forecasting the shelf life of the items and estimating the expiry at real time ( Manavalan and Jayakrishna, 2019 ). Moreover, BDA could help firms finding the right balance between the energy spent and the level of products' transformation and necessary costs, in contrast with the established trend that suggests that companies should try to maximise the shelf life of food products at any cost ( Augusto, 2020 ). In addition, sharing and analysing data across the firm boundaries will increase efficiency, sustainability, flexibility, agility and resilience throughout the supply chain, from farmers to the final customers, contributing to reduce the uncertainty and risks related to products (e.g. shelf life, food quality and safety), processes (e.g. supply lead times, production, resources needed), markets (e.g. demand requirements, price competition) and the environment (e.g. weather, diseases, regulations) in the agri-food sector ( Lezoche et al. , 2020 ). The flexibility and reaction speed of industrial and logistical systems merged with BDA could improve the interpretation of customers' demands and needs in terms of tastes and information accessibility ( Ali and Aboelmaged, 2022 ; Rana et al. , 2021 ; Oltra-Mestre et al. , 2020 ; Hasnan and Yusoff, 2018 ). QR codes and radio frequency identification (RFID) systems can be used to track food materials in the food supply chain, to increase efficiency of stock control ( Saetta and Caldarelli, 2020 ) or to allow consumers to access information on the country of origin and the ingredients included in finished products ( Hasnan and Yusoff, 2018 ). In this respect, blockchain is still unexplored ( Rana et al. , 2021 ), but could effectively enhance data sharing, security, anonymity, trust and decentralisation at all levels ( Lezoche et al. , 2020 ; Saetta and Caldarelli, 2020 ), and could increase products' traceability across the supply chain ( Ali and Aboelmaged, 2022 ; Hasnan and Yusoff, 2018 ; Rana et al. , 2021 ). Blockchain could allow consumers tracking the food's origins and movements across the supply chain, assessing the environmental impact, ethical aspects and quality, with positive returns in terms of consumers' trust, brand reputation and reduced food fraud ( Saetta and Caldarelli, 2020 ). Overall, optimisation through Industry 4.0 is expected to lead to more sustainable data-driven supply chains — supply chain 4.0 ( Lezoche et al. , 2020 ) by reducing supply-demand misalignments ( Ali and Aboelmaged, 2022 ).
Last but not least, 3D printing is now used to manufacture small, customised batches of complex metal or plastic products at lower costs. Food 3D printing is still in an experimentation phase ( Jambrak et al. , 2021 ; Augusto, 2020 ). For instance, Foodink is a 3D printing restaurant offering a gourmet experience of 3D printed food ( http://foodink.io/ ). Although food 3D printing for industrial purposes is considered futuristic, it might be promising as a green and clean production technology to produce customised products for specific customers' needs (e.g. vegan).
3. Research methods
3.1 research context.
Trentofrutta is a food processing company located in Trento with a factory of 38,000 square meters. With 177 employees and a turnover of about 50 million euro, its main activities include: (1) processing fruits into semi-finished products for industry and (2) contract filling of finished products into bottles and pouches.
Initially labelled Cremogem Spa, the firm was established in 1961. Five years later, the firm changed the name and added a new investor, who gradually acquired stakes in the company until he finally became the sole owner in 1971. The company was then acquired in 1988 by a German group, who, in addition to financial capital, endowed the company with a new name, Trentofrutta, and a strategic business plan aimed at extending the fruit processing operations and expanding the business abroad. Nowadays, Trentofrutta belongs to a family group of enterprises, which includes other complementary medium companies focused on specific fruit processing activities.
The firm mainly operates in the business-to-business context with two main business lines. The first and traditional business encompasses the production of fruit and vegetable-based semifinished products for industry. This business line mainly relies on the establishment of long-term relationships with its customers, such as leading players in the international food industry. The second business line is more consumer oriented, as the firm produces finished products in glass and pouch formats as a white brand for leading beverage and baby food brands and large-scale Italian and European distribution clients. With this second line of business, the firm sells products mainly in Germany, Italy, UK and Holland.
Trentofrutta relies on fruit purchasing, while the rest of the chain is short and internalised (from fruit processing to pouch filling and packaging). The short chain sourcing reflects the decision to ensure high levels of safety and quality and makes the business more environmentally and economically sustainable. Fruit is sourced nationally (except from tropical fruits). The firm has established sustainable fruit supply chains, particularly for biological product lines, while it has a “controlled” chain where firm specialists and agronomists perform strict controls on the use of pesticides among suppliers' and agriculturists' activities. This is fundamental to ensure that semi-finished processed fruit can be used for baby food purposes. Over the years, the firm's policy relied on selecting suppliers and establishing long-term relationships with reputed suppliers (e.g. cooperative firms with long-lasting traditions).
The firm stands out for investments made in digitalisation and Industry 4.0 over the last five years, also in consideration to its medium size. Some implementation processes are still in progress, such as the introduction of additional digital measurement systems in production, new internal data sharing systems, predictive maintenance tools and other innovative technical solutions. Table 1 describes the evolution of investments in digital transformation over the last years.
Since 2015, the firm experienced a change in its vision strategy and the owner and the CEO initiated the digital transformation and firm repositioning in the marketplace. First, they fostered and spread a strong customer orientation, e.g. by developing a new packaging design to respond to customers' requirements. Second, they initiated a process to reposition the firm in a medium-high market segment by introducing the pouch production lines, which were considered more profitable and appealing for customers. Since then, the digital strategy was planned and developed for group enterprises, by leveraging synergies both during the selection and implementation of technologies. As illustrated in Table 2 , the digitalisation process started in 2015 with the new management software and information and communication technology systems, followed by advanced robotics and IoT in one plant, a brand-new Industry 4.0 plant in 2017 and big data collection and analytics implemented over the last two years.
Group firms have implemented the same management software and ICT system, which allowed the interconnection of all business functions within the organisation. Production supervision is supported by laptops and screens through which simply checklist activities and uncompleted tasks. Employees use a key card to access departments and record activity developments, which leads to an automatic generation of production and human resource activity reports. Currently, the firm is introducing the use of iPads in production. Through these investments, vertical integration within the company has been successfully achieved. Vertical integration merged with Industry 4.0 in production allow big data collection and analytics at different organisational levels, from orders to production planning and outbound logistics.
A second group of Industry 4.0 investments pertains to production. In addition to existing production lines for glass filling, in 2015 the top management implemented a continuous and automatised pouch-filling production line (not digitalised). As this choice was rewarding, further investments were made to automatise the palletisation phase and widen the tank after some years. In 2017, exploiting the experience gained through the first implementation, the firm introduced also a second production line for pouch filling, completely automatised, robotised and digitalised in accordance with the Industry 4.0 paradigm. This second production line allows automatic controls also related to quality, hygiene conditions and food safety requirements. In general, the firm also adopts X-ray machines, machines to read QR codes, bar code readers, precision weighting machines and other innovative analytical tools in each production line. 3D printing is used to produce spare highly customised parts or single components for cost and saving purpose.
At the moment, horizontal integration with suppliers (e.g. agriculturists) and customers has not been particularly developed yet. In fact, the level of customers' digitalisation tends to be advanced, whereas suppliers are less prepared for this approach. The firm already digitally stores data on the country of origin of products, but customers and final consumers cannot digitally access information. At present, product, process and firm certifications in addition to regular client audits (through audit, inspections, data requests, complete traceability system) are used to guarantee quality to customers.
3.2 Research design
We adopted a case study methodology ( Yin, 2009 ) to explore relevant factors and outcomes related to the Industry 4.0 adoption and implementation processes, which is a relatively new topic in the agri-food sector ( Hasnan and Yusoff, 2018 ; Oltre-Mestre et al. , 2020 ). We chose the case study approach because it is useful to “understand the dynamics present within single settings” ( Eisenhardt, 1989 , p. 534) and is particularly suitable for theory building purposes, also given the relatively new nature of the phenomenon ( Eisenhardt, 1989 ; Eisenhardt et al. , 2016 ; Lyons, 2005 ; Ferraris et al. , 2019 ). The fruit supply chain has been already adopted as a context of analysis in Italy ( Canavari et al. , 2010 ), but not yet in relation to this research question. Single case studies have the advantage of providing rich descriptions of the object of study and revealing aspects of a phenomenon that has so far been inaccessible ( Eisenhardt, 1989 ; Franceschelli et al. , 2018 ; Yin, 2009 ). We followed a theoretical sampling to choose the single case study. Through an initial screening of agri-food companies located in Trentino Alto Adige, we identified and purposefully selected Trentofrutta, a fruit processing company located in Trento (Italy), because it has an ongoing digital strategy and an ongoing plan of Industry 4.0 adoption since 2015. This company represented a single information-rich case, which we considered unique and revelatory ( Eisenhardt, 1989 ; Eisenhardt and Graebner, 2007 ), and, given the ongoing digital strategy, guaranteed the initial assessment of outcomes. It provided an extensive description of what is happening in this particular context of analysis ( Welch et al. , 2011 ), reconnecting and enriching previous evidence on the fruit supply chain in Italy ( Canavari et al. , 2010 ).
3.3 Data collection and analysis
We chose to collect primary data through in-depth interviews with key managers of the company due to the exploratory nature of our study ( Eisenhardt, 1989 ). We gave voice to informants, who were treated as knowledgeable actors. After consulting a general literature on the topic, we developed an interview schedule based on open questions on the Industry 4.0 adoption to allow the raise of novel insights and facilitate theory building ( Goulding, 2002 ). As Industry 4.0, we included technologies proposed by Rüßmann et al. (2015) and blockchain following agri-food studies ( Lezoche et al. , 2020 ; Rana et al. , 2021 ). The interview protocol consisted of five steps. The first in-depth interview was conducted with the Integrated Management System Manager in May 2021. It lasted about 90 min, was recorded and literally transcribed into text. As our objective was to preserve flexibility to adjust interview protocol based on informants responses ( Goulding, 2002 ), iterative interviews were then collected with the respondent to solve and verify unclear issues and integrate data collection. The second interview was collected in July, the third in August and the fourth in September 2021. The fifth step consisted of collecting feedbacks and integrations with the CEO, production director, and human resource and financial director in August/September 2021. We stopped collecting data when we reached theoretical saturation, hence seeing that additional interviews brought minimal incremental learning ( Eisenhardt, 1989 ). Each respondent separately and individually read the case analysis and findings to ensure fidelity of interpretation and for validation purposes ( Goulding, 2002 ). To avoid retrospective bias, we used different sources to gather data for triangulation purposes, including archival documents (e.g. balance sheets, company policy declarations), websites and press releases. Interviews transcripts and archival data were organised in a firm dossier. We used qualitative techniques to analyse data ( Eisenhardt, 1989 ; Miles et al. , 2020 ). After removing data unrelated to the object of this study, we coded data based on a priori codes aligned with the research question ( Miles et al. , 2020 ). According to Miles et al. (2020) , during data analysis, other codes emerge inductively compared to the initial list. Then, we proceeded with data theming by clustering data according to commonalities, and this facilitated the synthesising and emergence of concepts and relationships between variables ( Eisenhardt, 1989 ; Eisenhardt et al. , 2016 ). This analysis led to the creation of tables and figure included in this article.
4. Findings
The outcomes of digitalisation and Industry 4.0 technologies were observed at different levels of the organisation, as illustrated in Figure 1 . The Industry 4.0 technologies positively influenced product quality, process effectiveness and strategic planning capacities.
4.1 Economic and environmental outcomes
The photovoltaic system on the roofs allow to meet average internal energetic needs. We have four steam generators inside the thermal power station and a water purification system. We also self-produce what we need (e.g. spare parts) through 3d printing for instance. This is what characterise our company: we exploit internal technologies and employees’ skills to solve problems, also looking at economic and environmental sustainability. All these plants are interconnected and allow data analytics and energy consumption optimisation. (Third interview)
Compared to the more traditional production line, the Industry 4.0 plant for pouch filling enabled better controls of production processes and throughput time and lead time reduction . Compared to the non-digitalised pouch filling, the Industry 4.0 plant has a lower average operating cost. Automation and digitalisation partially reduced workforce. Compared to the first line, this production plant also includes robots and automatic systems performing 100% controls on quality and hygiene conditions. This improved hygiene conditions in the continuous line , while initial positive impacts were noted on food quality and safety .
4.2 Organisational outcomes
The organisational level emerges in relation to vertical integration across business functions and Industry 4.0 in production, which allowed rapid information and communication flows within the firm and across its borders (e.g. with customers). Digitalisation through the new management software increased flexibility and problem-solving capacity within the organisation, even if requiring organisational and cultural changes as a counterbalance. For instance, digitalisation processes required resource- and time-consuming procedures of knowledge codification. Also, some settings of the new management software were initially perceived as cumbersome and required to be customised and tailored on the necessities of the organisation. This was made possible by the department created ad hoc to support the digital transformation of the firm.
A new business function was created to serve digitalisation purposes: an internal engineering department specialised on technological advancements, in addition to the engineering department for internal maintenance of plants. Both departments benefit from the support of the ICT department, which is located in the group company but serves the whole group. These departments and the digital knowledge and skills accumulated allowed the incremental implementation of other technologies (e.g. BDA), while containing the costs and expenses. This team designed and customised the lines according to specific production requirements and was highly receptive to technological advances.
4.3 Human resource management
As regards the human resource management level, the adoption of Industry 4.0 influenced recruitment processes (new employees, new skills, women, young), current job positions and tasks' characteristics (fewer manual activities, less physical effort, more supervising activities, expected less bureaucracy) and training activities aimed at upskilling/reskilling personnel (organisational culture, digital skills, etc.).
The Industry 4.0 production line employees several women particularly in supervisory roles and as “forewomen”, whereas the less digitalised business-to-business production line still sees a higher percentage of men. Also, digitalisation and Industry 4.0 led to create new supervisory roles, which were often assumed by females. (Third Interview)
The aim is to dramatically reduce bureaucracy for employees, who would be extremely satisfied if they do not need to fill out paper documents anymore (e.g. vacation plan, activity log), and most operations’ results would be digitally generated. (First interview)
Training activities encompassed the strong upskilling and reskilling of personnel, in addition to a program of digital-skills training activities for new employees. In the Industry 4.0 plant, several employees were moved from operational to supervisory activities, and specific training activities were necessary to upskill human resources. Since 2015, the top managers accompanied digitalisation with specific training activities and hired an HR manager, who supervises teams, assesses employees' competences and skills in relation to the assigned tasks, and identifies appropriate training paths. This activity was also supported by an external psychologist, who helped employees to win resistance to change.
4.4 Strategic outcomes
Each working hour is detailed in terms of order, working activity, and task, giving top management the opportunity to effectively calculate and analyse the cost of each activity and order. Big data collection and analytics has revealed an exceptional instrument to optimise planning and production, and – overall – to support decision making at the corporate level. (Third interview)
The digitalisation of processes enables the generation of statistics that are used to: (1) accurately plan production and resource allocation among the two business lines, (2) to plan maintenance activities and (3) to support strategic decision making at the corporate level. Top management members use big data analytics to better forecast demand trends and align strategic capacity requirements , and, eventually, mitigate risks related to demand decreases and agriculture uncertainty. This approach allows the organisation to rapidly respond to market requests . Further developments are expected from the additional investments made in data elaboration, statistics generation and digital solutions to extract strategic value from data. Figure 2 describes associations among Industry 4.0 technologies and outcomes.
5. Discussion: drivers, barriers and strategies related to industry 4.0
Our results do not deviate too much from manufacturing studies, which had shown that SMEs carefully select and incrementally adopt Industry 4.0 in line with their strategic purposes ( Kane et al. , 2015 ; Chiarvesio and Romanello, 2018 ). Likewise, we found that the adoption of some Industry 4.0 technologies (e.g. new management system, IoT in production) paved the way for other related technologies (e.g. Industry 4.0 plant, BDA) ( Bortoluzzi et al. , 2020 ). For instance, the new management system first laid the foundations for vertical integration, whereas the repeated introduction of IoT in production created the conditions to deeply integrate and interconnect the different business functions and perform BDA to support strategic decisions. This technological integration also opens the way for a horizontal integration with customers in the next future (see Table 3 ). Although barriers emerged during the selection and implementation process (as highlighted in Table 3 ), the firm clearly navigated the digitalisation process in an incremental but effective way thanks to the vision and commitment of the top managers. After almost seven years, Trentofrutta has an advanced level of digitalisation and Industry 4.0 and initial positive returns have clearly emerged.
The digitalisation process was made possible by the clear vision who jointly had the owner and the CEO under competitive pressures stemming from the market changes. In line with past evidence (e.g. Romanello et al. , 2021 ), it also depended on the top managers' attitudes and level of technology awareness that led them to carefully develop a digital strategy to incrementally implement Industry 4.0, also in consideration of the novelty of technologies and the lack of best practices in this sector.
The successful implementation was achieved by a learning-by-doing process through which the firm increased its digital skills and found the most suitable technological applications for the business. For instance, BDA were initially adopted to optimise planning and production, but were then found more useful to support strategic decision making. The trial-and-error approach to Industry 4.0 has been found also in the manufacturing context, showing that SME can incur into slowdowns and challenges during this process and may require huge amounts of time and resources before positive returns on performance are obtained ( Müller et al. , 2018 ; Bortoluzzi et al. , 2020 ). Even in our case study, although the selection phase was facilitated by the clear vision of top managers, visualising ex ante the positive impacts of each technological application remained an issue. However, the alignment between digitalisation and the firm strategy represented a key aspect to rapidly overcome emerging challenges, successfully implement technologies and obtain early positive outcomes ( Kane et al. , 2015 ; Bortoluzzi et al. , 2020 ).
Apart from limited public support for training and some national tax benefits, the firm self-financed technological investments. This represented an important economic commitment for the company in consideration to its size and the unpredictability of outcomes. The success was also due to the synergies created among the group companies. Trentofrutta partially benefited from the support of the group company, who shared the ICT department's resources, digital skills and experience, which are invaluable resources in the digitalisation process that is per se knowledge- and skill demanding. In this sense, belonging to a group of enterprises partially compensated the limitations of being a relatively resource-constrained medium company and was determinant to overcome the knowledge and skills requirements. In contrast, medium size somehow represented an advantage in the integration of technology throughout the organisation, as large companies tend to have established organisational routines and large flows of stored information that require complex processes to be codified and digitalised.
The implementation of each technology entailed change management issues, which were faced through specific training activities and psychological support. Specific human resource management strategies were deployed to improve inter-organisational communication about the goals and the expected impacts of digitalisation. Also, the reskilling and upskilling of personnel and the hiring of digitally skilled workers were necessary to support this process. For instance, 3D printer is now used to internally manufacture spare/maintenance parts at lower costs and there are four permanent employees able to use additive manufacturing.
Horizontal integration with customers may be required by clients in the future, while integrating with suppliers could be advisable to optimise costs and achieve digital food traceability in the supply chain. IoT could be used to digitally certify and track country of origin, quality, hygiene conditions, facilitating both the fulfilment of law requirements related to contaminants in baby food and facilitate communication with customers and final consumers. Although there are already Artificial Intelligence-based software products specifically developed for food traceability, this approach would require an additional cultural change in the organisation and within the supply chain. This might represent a qualitative leap for the company, which – despite the strict procedures already in place – could oversimplify procedures and improve controls on externalised phases (agriculture). However, most suppliers are not prepared for such level of technological complexity. Additionally, sharing data across the supply chain would require complete transparency among members, while the use of blockchain would entail that each firm assumes the responsibility for potential mistakes as data are encrypted, shared and unmodifiable ( Saetta and Caldarelli, 2020 ; Rana et al. , 2021 ). This might represent the future for this industry leading to a better alignment between supply and demand, but remains a complex process at the moment.
6. Conclusions and implications for theory, practice and policymakers
This work illustrates the successful case of a firm digital transformation. Our study provides empirical evidence on the Industry 4.0 outcomes in a food processing company, underlining that the adoption process was not straightforward because both facilitating and hindering factors emerged throughout the adoption and implementation processes. To be successful, the digital transformation required the total commitment of the top management, who was responsive in developing prompt strategies to overcome the challenges emerged during the implementation process. This is particularly relevant in consideration of the long time-elapse from the initial adoption of technologies and the time when positive impacts emerge. Although this result is aligned with recent manufacturing studies, our research shows that this incremental process is valuable from a long-term perspective as some specific technology-outcomes are expected to be particularly relevant in food-processing, such as the IoT for customer-information traceability or the integration of information flows across the firm boundaries to better align demand and supply. Our firm-level study complements extant works that have so far adopted an agri-food supply chain perspective ( Lezoche et al. , 2020 ; Manavalan and Jayakrishna, 2019 ; Rana et al. , 2021 ) and identifies categories of outcomes that could be tested in different agri-food contexts and larger samples.
From practitioners' perspective, our study suggests that managers must handle the process of implementation of Industry 4.0 by carefully addressing the psychological drawbacks of changes introduced in the organisation. In line with recent reports ( Eurofound, 2021 ), our study shows the importance of two core aspects. First, the early communication with employees, piloting and psychological support, and the human resource management strategies that allowed to overcoming change resistance and foster/source the skills necessary to the process. Second, the alignment between the firm and digital strategy ( Kane et al. , 2015 ), which allowed to pursue clear strategic goals and reach satisfying performance returns in a relatively short time.
Due to the various complexities, in our view, firms would be more incentivised to adopt Industry 4.0 if the market formally recognised these efforts, e.g. through an Industry 4.0 certification. Sectoral certification agencies might evaluate the possibility to formally recognise a value added to companies investing in Industry 4.0 technologies aimed at digitalising traceability, food quality, food safety, hygienic conditions, employee safety, and guaranteeing data accessibility and transparency. For instance, sharing data across the supply chain using blockchain entails a high level of responsibility that should be counterbalanced by profits or additional value recognised by customers or final consumers. This might be an interesting aspect to be considered by policymakers operating in this sector.
From a policymaking perspective, public support and innovation policies are, indeed, a driver of technological adoption, such as the lack of digital infrastructure represents a barrier ( Eurofound, 2021 ). Supporting firms both financially and through infrastructure investments is important, while thus remembering that advanced technologies that increase firm productivity tend to reduce the need for low-skilled employees. This explicit support to productivity factors should at least be in part compensated by policies that are centred on human resources. As highlighted by Akyazi et al. (2020) , future skill requirements in agri-food will mainly relate to data analysis, the use of digital communication tools and Industry 4.0, technologies critical thinking and decision making, and complex information processing and interpretation. Supporting training related to Industry 4.0, and course to re-skill and upskill human resources should be core in policy programs, such as specific educational programs should be centred on the skills requirements emerging in this sector.
Some limitations must be considered when interpreting our findings. This single case study has an exploratory purpose, rather than generalisability of findings. This work focuses on a fruit processing company, whereas future studies are needed to investigate the outcomes of Industry 4.0 among companies operating in different positions of the agri-food supply chain, because mechanisms and outcomes could largely vary depending on production and product types.
Industry 4.0 outcomes
Industry 4.0 technologies and related outcomes
Investments and employees of Trentofrutta over the last seven years
Drivers, barriers and strategies in relation to Industry 4.0
Akyazi , T. , Goti , A. , Oyarbide , A. , Alberdi , E. and Bayon , F. ( 2020 ), “ A guide for the food industry to meet the future skills requirements emerging with industry 4.0 ”, Foods , Vol. 9 No. 4 , p. 492 .
Ali , I. and Aboelmaged , M.G.S. ( 2022 ), “ Implementation of supply chain 4.0 in the food and beverage industry: perceived drivers and barriers ”, International Journal of Productivity and Performance Management , Vol. 71 No. 4 , pp. 1426 - 1443 , doi: 10.1108/IJPPM-07-2020-0393 .
Augusto , P.E. ( 2020 ), “ Challenges, trends and opportunities in food processing ”, Current Opinion in Food Science , Vol. 35 , pp. 72 - 78 .
Berman , B. ( 2012 ), “ 3-D printing: the new industrial revolution ”, Business Horizons , Vol. 55 No. 2 , pp. 155 - 162 .
Borowski , P.F. ( 2021 ), “ Innovative processes in managing an enterprise from the energy and food sector in the era of industry 4.0 ”, Processes , Vol. 9 No. 2 , p. 381 .
Bortoluzzi , G. , Chiarvesio , M. , Romanello , R. , Tabacco , R. and Veglio , V. ( 2020 ), “ Industry 4.0 technologies and the servitization strategy: a good match? ”, Sinergie Italian Journal of Management , Vol. 38 No. 1 , pp. 55 - 72 .
Canavari , M. , Centonze , R. , Hingley , M. and Spadoni , R. ( 2010 ), “ Traceability as part of competitive strategy in the fruit supply chain ”, British Food Journal , Vol. 112 No. 2 , pp. 171 - 186 , doi: 10.1108/00070701011018851 .
Chiarvesio , M. and Romanello , R. ( 2018 ), “ International Business in the Information and Digital Age ” in van Tulder , R. , Verbeke , A. and Piscitello , L. (Eds) , Progress in International Business Research , Emerald Publishing Limited , Bingley , Vol. 13 , pp. 357 - 378 . https://doi.org/10.1108/S1745-886220180000013015 .
Culot , G. , Nassimbeni , G. , Orzes , G. and Sartor , M. ( 2020 ), “ Behind the definition of Industry 4.0: analysis and open questions ”, International Journal of Production Economics , Vol. 226 , p. 107617 .
Eisenhardt , K.M. ( 1989 ), “ Building theories from case study research ”, Academy of Management Review , Vol. 14 No. 4 , pp. 532 - 550 .
Eisenhardt , K.M. and Graebner , M.E. ( 2007 ), “ Theory building from cases: opportunities and challenges ”, Academy of Management Journal , Vol. 50 No. 1 , pp. 25 - 32 .
Eisenhardt , K.M. , Graebner , M.E. and and Sonenshein , S. ( 2016 ), “ Grand challenges and inductive methods: rigor without rigor mortis ”, Academy of Management Journal , Vol. 59 No. 4 , pp. 1113 - 1123 .
Eurofound ( 2021 ), Digitisation in the Workplace , Publications Office of the European Union , Luxembourg , available at: https://www.eurofound.europa.eu/sites/default/files/ef_publication/field_ef_document/ef21001en.pdf .
Ferraris , A. , Mazzoleni , A. , Devalle , A. and Couturier , J. ( 2019 ), “ Big data analytics capabilities and knowledge management: impact on firm performance ”, Management Decision , Vol. 57 No. 8 , pp. 1923 - 1936 , doi: 10.1108/MD-07-2018-0825 .
Foodink , available at: http://foodink.io/ .
Franceschelli , M.V. , Santoro , G. and Candelo , E. ( 2018 ), “ Business model innovation for sustainability: a food start-up case study ”, British Food Journal , Vol. 120 No. 10 , pp. 2483 - 2494 , doi: 10.1108/BFJ-01-2018-0049 .
Goulding , C. ( 2002 ), Grounded Theory: A Practical Guide for Management, Business and Market Researchers , Sage Publications , London, Thousand Oaks, New Delhi .
Hannibal , M. and Knight , G. ( 2018 ), “ Additive manufacturing and the global factory: disruptive technologies and the location of international business ”, International Business Review , Vol. 27 No. 6 , pp. 1116 - 1127 .
Hasnan , N.Z.N. and Yusoff , Y.M. ( 2018 ), “ Short review: application areas of Industry 4.0 technologies in food processing sector ”, 2018 IEEE Student Conference on Research and Development (SCOReD) , IEEE , pp. 1 - 6 .
Jambrak , A.R. , Nutrizio , M. , Djekić , I. , Pleslić , S. and Chemat , F. ( 2021 ), “ Internet of Nonthermal food processing technologies (IoNTP): food industry 4.0 and sustainability ”, Applied Sciences , Vol. 11 No. 2 , p. 686 .
Kane , G.C. , Palmer , D. , Phillips , A.N. , Kiron , D. and Buckley , N. ( 2015 ), “ Strategy, not technology, drives digital transformation ”, MIT Sloan Management Review and Deloitte University Press , Vol. 14 , pp. 1 - 25 .
Konur , S. , Lan , Y. , Thakker , D. , Morkyani , G. , Polovina , N. and Sharp , J. ( 2021 ), “ Towards design and implementation of Industry 4.0 for food manufacturing ”, Neural Computing and Applications , pp. 1 - 13 , doi: 10.1007/s00521-021-05726-z .
Lezoche , M. , Hernandez , J.E. , Díaz , M.D.M.E.A. , Panetto , H. and Kacprzyk , J. ( 2020 ), “ Agri-food 4.0: a survey of the supply chains and technologies for the future agriculture ”, Computers in Industry , Vol. 117 , pp. 103 - 187 .
Lyons , H. ( 2005 ), “ Food industry case studies: a suitable medium for publication ”, British Food Journal , Vol. 107 No. 9 , pp. 702 - 713 , doi: 10.1108/00070700510615080 .
Manavalan , E. and Jayakrishna , K. ( 2019 ), “ A review of Internet of Things (IoT) embedded sustainable supply chain for Industry 4.0 requirements ”, Computers and Industrial Engineering , Vol. 127 , pp. 925 - 953 .
Miles , B.M. , Huberman , A. and Saldana , J. ( 2020 ), Qualitative Data Analysis: A Methods Sourcebook , 4th ed. , Sage Publishing , Los Angeles .
Müller , J.M. and Kazantsev , N. ( 2021 ), Industry 4.0 in SMEs across the Globe: Drivers, Barriers, and Opportunities , CRC Press for Taylor & Francis , Boca Raton, Florida .
Müller , J.M. , Buliga , O. and Voigt , K.I. ( 2018 ), “ Fortune favours the prepared: how SMEs approach business model innovations in Industry 4.0 ”, Technological Forecasting and Social Change , Vol. 132 , pp. 2 - 17 .
Oltra-Mestre , M.J. , Hargaden , V. , Coughlan , P. and Segura-García del Río , B. ( 2020 ), “ Innovation in the agri-food sector: exploiting opportunities for industry 4.0 ”, Creativity and Innovation Management , Vol. 30 No. 1 , pp. 198 - 210 , doi: 10.1111/caim.12418 .
Rana , R.L. , Tricase , C. and De Cesare , L. ( 2021 ), “ Blockchain technology for a sustainable agri-food supply chain ”, British Food Journal , Vol. 123 No. 11 , pp. 3471 - 3485 , doi: 10.1108/BFJ-09-2020-0832 .
Rialti , R. , Zollo , L. , Ferraris , A. and Alon , I. ( 2019 ), “ Big data analytics capabilities and performance: evidence from a moderated multi-mediation model ”, Technological Forecasting and Social Change , Vol. 149 December 2019 , p. 119781 .
Romanello , R. and Chiarvesio , M. ( 2021 ), “ The adoption of Industry 4.0 technologies: the case of Italian manufacturing SMEs’ in Industry 4.0 ”, in Müller , J.M. and Kazantsev , N. (Eds), SMEs across the Globe: Drivers, Barriers, and Opportunities , Taylor & Francis .
Romanello , R. , Kazantsev , N. , Adamczuk , O. and Müller , J.M. ( 2021 ), “ Industry 4.0 experience in SMEs: an international overview of barriers, drivers and opportunities ”, in Müller , J.M. and Kazantsev , N. (Eds), SMEs across the Globe: Drivers, Barriers, and Opportunities , Taylor & Francis .
Rüßmann , M. , Lorenz , M. , Gerbert , P. , Waldner , M. , Justus , J. , Engel , P. and Harnisch , M. ( 2015 ), “ Industry 4.0: the future of productivity and growth in manufacturing industries ”, Boston Consulting Group , Vol. 9 No. 1 , pp. 54 - 89 .
Saetta , S. and Caldarelli , V. ( 2020 ), “ How to increase the sustainability of the agri-food supply chain through innovations in 4.0 perspective: a first case study analysis ”, Procedia Manufacturing , Vol. 42 , pp. 333 - 336 .
Secinaro , S. , Dal Mas , F. , Massaro , M. and Calandra , D. ( 2022 ), “ Exploring agricultural entrepreneurship and new technologies: academic and practitioners’ views ”, British Food Journal , Vol. 124 No. 7 , pp. 2096 - 2113 , doi: 10.1108/BFJ-08-2021-0905 .
Silva , V.L. , Sereno , A.M. and do Amaral Sobral , P.J. ( 2018 ), “ Food Industry and processing technology: on time to harmonize technology and social drivers ”, Food Engineering Reviews , Vol. 10 No. 1 , pp. 1 - 13 .
Trivelli , L. , Apicella , A. , Chiarello , F. , Rana , R. , Fantoni , G. and Tarabella , A. ( 2019 ), “ From precision agriculture to Industry 4.0 ”, British Food Journal , Vol. 121 No. 8 , pp. 1730 - 1743 .
Wee , D. , Breunig , M. , Kelly , R. and Mathis , R. ( 2016 ), “ Industry 4.0 after the initial hype. Where manu- facturers are finding value and how they can best capture it ”, McKinsey Digital , pp. 1 - 36 .
Welch , C. , Piekkari , R. , Plakoyiannaki , E. and Paavilainen-Mäntymäki , E. ( 2011 ), “ Theorising from case studies: towards a pluralist future for international business research ”, Journal of International Business Studies , Vol. 42 No. 5 , pp. 740 - 762 , doi: 10.1057/jibs.2010.55 .
Yin , R.K. ( 2009 ), Case Study Research: Design and Methods , Vol. 5 , Sage , Thousand Oaks, CA .
Further reading
Porter , M.E. and Heppelmann , J.E. ( 2015 ), “ How smart, connected products are transforming companies ”, Harvard Business Review , Vol. 93 No. 10 , pp. 96 - 114 .
Acknowledgements
The authors are grateful to the participating firm Trentofrutta Spa. The authors would like to kindly thank the CEO Luca Copat, the HR and Accounting Director Mara Benvenuti, the Head of Production Luca Bortolotti and the Integrated Management System Manager Giulia Rossini for their precious time dedicated to this research. The authors also thank Francesco Crisci for his kind support.
Corresponding author
Related articles, we’re listening — tell us what you think, something didn’t work….
Report bugs here
All feedback is valuable
Please share your general feedback
Join us on our journey
Platform update page.
Visit emeraldpublishing.com/platformupdate to discover the latest news and updates
Questions & More Information
Answers to the most commonly asked questions here

An official website of the United States government
The .gov means it’s official. Federal government websites often end in .gov or .mil. Before sharing sensitive information, make sure you’re on a federal government site.
The site is secure. The https:// ensures that you are connecting to the official website and that any information you provide is encrypted and transmitted securely.
- Publications
- Account settings
Preview improvements coming to the PMC website in October 2024. Learn More or Try it out now .
- Advanced Search
- Journal List
- Materials (Basel)

Industry 4.0 and Digitalisation in Healthcare
Vladimir v. popov.
1 Department of Materials Science and Engineering, Tel Aviv University, Ramat Aviv, Tel Aviv 6997801, Israel
2 Higher School of Engineering, Ural Federal University, 620002 Ekaterinburg, Russia; [email protected]
Elena V. Kudryavtseva
3 Obstetrics and Gynecology Department, Ural State Medical University, 620000 Ekaterinburg, Russia; moc.liamg@9102duke
Nirmal Kumar Katiyar
4 School of Engineering, London South Bank University, 103 Borough Road, London SE1 0AA, UK; ku.ca.ubsl@nramuk (N.K.K.); ku.ca.ubsl@sleog (S.G.)
Andrei Shishkin
5 Rudolfs Cimdins Riga Biomaterials Innovations and Development Centre of RTU, Institute of General Chemical Engineering, Faculty of Materials Science and Applied Chemistry, Riga Technical University, 1007 Riga, Latvia; [email protected]
Stepan I. Stepanov
Saurav goel.
6 Department of Mechanical Engineering, University of Petroleum and Energy Studies, Dehradun 248007, India
Associated Data
Not applicable.
Industry 4.0 in healthcare involves use of a wide range of modern technologies including digitisation, artificial intelligence, user response data (ergonomics), human psychology, the Internet of Things, machine learning, big data mining, and augmented reality to name a few. The healthcare industry is undergoing a paradigm shift thanks to Industry 4.0, which provides better user comfort through proactive intervention in early detection and treatment of various diseases. The sector is now ready to make its next move towards Industry 5.0, but certain aspects that motivated this review paper need further consideration. As a fruitful outcome of this review, we surveyed modern trends in this arena of research and summarised the intricacies of new features to guide and prepare the sector for an Industry 5.0-ready healthcare system.
1. Introduction
The Fourth Industrial Revolution, otherwise known as Industry 4.0, is advancing healthcare to unprecedented comfort levels on the foundation of digitisation, artificial intelligence, and 5G telecommunication [ 1 , 2 ]. In this context, Table 1 summarise various definitions used currently in the context of Industry 4.0 to explain many of its subsystems. These factors have helped in many ways to combat the ongoing crisis the world is facing in the wake of the COVID-19 pandemic [ 3 , 4 , 5 , 6 ].
Definitions of critical elements in an Industry 4.0 system.
Different digital projects have been developed globally by incorporating digital diagnostic systems which have significantly improved agility in X-ray and MRI investigations. This has, in turn, allowed quick diagnosis of patients’ healthcare data retrospectively as well as clinical anamnesis to provide prompt feedback [ 7 , 8 ]. A question worthy to be asked at this stage is: what is next? The answer to this question primarily drove this review. As shown in Figure 1 , the review begins by providing an insight into the interoperable development of the current ecosystem involving people, industry, business, and the government, which forms the backbone of Industry 4.0 in sharp contrast to the previous industrial revolutions. In modern times, machines have become sufficiently intelligent to make decisions in real time and to feed those decisions through cloud-based technologies [ 9 ] using neural networks [ 10 , 11 ] and decision-support systems [ 12 ]. Figure 2 shows the core components and essential elements of an Industry 4.0 system.

Evolution of industrial developments over time.

Building blocks of an Industry 4.0 system.
In fact, the use of deep neural networks has enabled AI to make unprecedented improvements to quality of learning. For example, working with Alexa, Google Search, and Yandex Disc has helped learning over time and the more these tools are used, the more the system becomes trained.
There are numerous examples of use of Internet-of-Things (IoT)-enabled systems which can be seen in day-to-day life. An Amazon store without cash registers or cashiers with the capability to charge users simply based on their body movements is an excellent example, while another involving the use of IoT include Uber, Ola, and GetTaxi. Recently, Lv et al. [ 59 ] investigated the issue of quality service and network loading for next generation IoT systems. Additionally, environmental aspects of Industry 4.0 are now also being explored [ 2 , 60 ].
Table 2 highlights state-of-the-art use of advanced technologies in healthcare and medicine revealed by different research papers. This review paper is the first to highlight the prospects of the Industrial Internet of Things (IIoT) in the healthcare sector. Table 2 highlights the novelty of this review paper vis-a-vis increasing interest of the scientific community in this area.
The key technologies discussed in the recent review publications (adapted and expanded from [ 15 ]). Here, “V” stands for the presence, and “X” for the absence of discussion on the relevant topics that make up an Industrial 4.0 system.
Recently, Austin et al. [ 7 ] investigated collaboration between academia, SMEs and digital health industries for the promotion of innovative digital solutions in healthcare. Qadri et al. [ 15 ] presented an extensive review of IoT applications in healthcare with careful articulation of the previous literature in this field. They introduced the term H-IoT (Healthcare IoT) to emphasize the importance of IoT in the field of healthcare and medicine. Marques et al. [ 62 ] presented a review on IoT applications in healthcare highlighting the need of medical professionals, students, and engineers. They discussed the advantages of IoT platforms in achieving personalized healthcare and developing smart devices for diagnosis and monitoring. They also pointed out the limitations on social readiness [ 62 ]. Hau et al. [ 69 ] showed how the digital tools of Industry 4.0 could be used to combat COVID-19 pandemic. Von Eiff et al. [ 51 ], in their short review, discussed prospects of digitalisation in healthcare. Their work partly discussed digital development and the use of Industry 4.0 tools in medicine development.
From this brief discussion, the importance of Industry 4.0 in the healthcare sector is obvious. Thus, this review highlights state-of-the-art digitalisation of medicine and healthcare and alludes to the sharp transition this sector is facing while moving towards Industry 5.0. This review also aims to discuss the trends in digital medicine and healthcare and to provide future directions in this area.
2. Ingredients of an Industry 4.0 Healthcare System
2.1. internet of things (iot).
IoT is a term that refers to any device with network access [ 13 , 26 , 70 , 71 ]. Modern devices/objects/networks of objects/systems are equipped with sensors, software, and network equipment. The network equipment and these sensors are capable of compiling and processing data arrays using internet [ 2 , 70 , 72 , 73 , 74 , 75 ] protocols.
5G has made an enormous impact on IoT technology and economy due to its superior level of connectivity and improved functionality. The key 5G technology drivers are superfast broadband, ultra-reliable low latency communication, massive machine-type communications, high reliability/availability, and efficient energy usage [ 73 , 76 , 77 , 78 ].
The main area of applications of 5G-enabled IoT are the tracking of goods and materials, asset monitoring, remote data collection, self-service systems, remote service delivery systems, real-time market data, and flexible pricing models [ 71 , 79 ]. As per the review of Likens et al. [ 80 ], it would appear as shown in Figure 3 that the Internet of Things will lead to be the most promising techniques that will change the gamut for industries and academia in the post-Fourth Industrial Revolution era.

Leading position of IoT in Industry 4.0 structure [ 80 ].
Most modern industries utilise modelling and simulations for process monitoring, control, diagnosis, optimisation, and design. Industry 4.0 and massive digitisation have made it possible to collect and process large arrays of data, resulting in the development of data-driven decisions and modelling tools [ 81 ]. It is worth mentioning that data-driven, statistical, or empirical models do not require broad initial knowledge about the studied system, but strongly rely on the presence of data collected from the process [ 82 ]. Modern simulation tools are used for predicting natural disasters which might lead to many victims (e.g., tsunami) [ 83 , 84 , 85 , 86 ]. A new trigger for modelling advancement re-emerged in recent years due to the development of machine learning techniques and a variety of Industry 4.0 technologies. Big data and modern modelling and analytical tools provide new horizons even to address old legacy issues and open new scenarios for realising innovative ideas.
2.2. Artificial Intelligence
Artificial intelligence (AI) allows computers to learn from their own experience, adapt to given parameters, and perform tasks that were previously only possible for humans. In most AI implementations such as computer chess players or self-driving cars, the role of deep learning and natural language processing is critical. AI allows automation of repetitive learning and searching processes using data acquisition to identify trends. Forms of AI in use today include digital assistants, chatbots, deep learning, and machine learning [ 16 , 17 , 18 , 19 , 20 , 21 , 22 , 23 , 87 , 88 , 89 ].
2.3. Big Data Analytics (BDA)
Big data analytics (BDA) is one of the key components of Industry 4.0. Big data technology deals with large arrays of data, enabling the derivation of information relevant for rapid decision-making. The derived data is transformed into the relevant goal-oriented knowledge to help achieve agility in problem solving [ 47 , 48 ]. The successful application of BDA in online trade can be seen through AliExpress, Amazon, and eBay. Technologies for image data are also rapidly developing enabling target recognition, photo filtering, and stereoscopic three-dimensional (3D) contents [ 90 , 91 , 92 , 93 ].
2.4. Digital Manufacturing and Advanced Materials Processing
One of the main outcomes of the advances in digital manufacturing is 3D printing technology, also called additive manufacturing (AM) [ 29 , 30 , 31 , 32 , 33 ]. AM enables the processing of polymers, ceramics, glass, and metallic alloys. Using approaches such as the Design for Additive Manufacturing (DfAM) [ 94 ] and Materials Design by Additive Manufacturing (MaDe-by-AM) [ 95 ], novel materials can now be manufactured with ease, which includes tailored composition as well as structural and functionally graded materials [ 32 , 35 , 36 , 37 , 38 , 39 , 96 , 97 , 98 ]. By shape and composition complexity, the design of new porous materials and metamaterials can also be fabricated. Moreover, the flexibility of maneuvering the printing head allows on-site printing of freeform shapes, which are potentially useful to develop custom-sized implants or prostheses [ 31 , 99 , 100 ]. Digitalisation of industrial manufacturing is developing due to the implementation of design strategies for new materials development [ 95 , 96 , 101 , 102 ]. Now, these additive technologies allow the printing of concrete buildings/structures [ 103 ]. Additive manufacturing of concrete structures is much more promising for fast construction in complex natural environments compared to other techniques [ 104 , 105 , 106 ].
2.5. Green Aspects of Industry 4.0
Among other aspects, the environmental aspects of Industry 4.0 deserve a special mention. Some of those aspects in relation to food-water-energy nexus are highlighted below:
- Global energy shortage and depletion of raw materials (energy crisis) [ 107 , 108 , 109 ];
- Reduction of arable land, decrease in soil fertility, and food shortage (food crisis) [ 110 ];
- Depleting availability of clean water [ 111 ]
- Catastrophic state of the environment (ecological crisis) [ 60 , 112 , 113 , 114 ].
- Main spheres of life such as industry, transport, the fuel and energy complex, the economy, public administration, and security have taken new forms. This is due to the penetration of digital technologies into everyday life and the development of alternative energy and electrical vehicles [ 115 ].
- Modern industrial development cannot proceed without efficient re-use and recycling procedures [ 116 , 117 , 118 , 119 ].
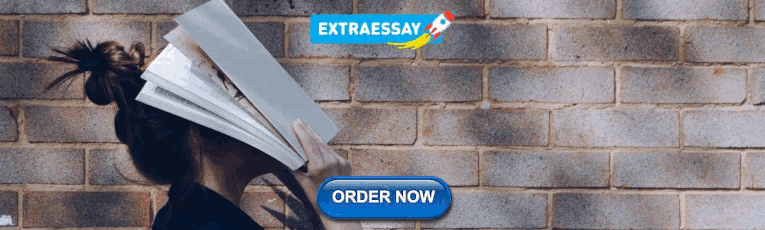
3. Digitalisation in Medicine
The term Medicine 4.0 is closely related to Industry 4.0; it describes the fourth stage in the development of medicine. Modern medicines which emerged around 150 years ago are undergoing a digital journey with the help of robotics, internet and artificial intelligence. The introduction of AI systems in medicine is one of the most important modern trends in world healthcare. Modern medical treatments cannot achieve their full potential without using advanced computing technologies. AI technologies are fundamentally changing the global healthcare system, allowing a radical redesign of the system of medical diagnostics, the development of new drugs, advanced analysis, testing, and treatment to enable advances in the field of transplantation surgeries [ 50 , 51 , 120 , 121 ]. Computational simulation using finite element analysis (FEA) is a crucial part of the digitalisation process in medicine [ 122 , 123 ]. FEA allows medical engineers/industrial designers to study many inter-related concepts including, for instance, device stability and durability (e.g., predicting end-of-life of patient-specific implants). FEA enables modelling of stresses within a material under different thermodynamic conditions [ 124 ]. In an FEA model, the part is simulated and analyzed using representative physical behavior [ 122 , 125 , 126 , 127 ]. Such an approach demonstrates weak areas of the part, and it allows enhancement of the design. Digitalisation and AI generally improve the quality of healthcare services while reducing costs for medical clinics. Figure 4 highlights key technologies enabling digitalisation of medicine.

Digitalisation in medicine—main technologies.
3.1. On-Demand Healthcare
According to Fox et al. [ 128 ], consumers are increasingly using online platforms to obtain medical information due to the following reasons:
- 47% wish to know more about their doctor.
- 38% would like to check a hospital and its medical facilities.
- 77% would prefer online medical appointments.
In the new regime of digital economy, medical professionals, just like freelance professionals, can provide their skills, talents, and expertise directly to the patients.
Several healthcare companies provide an online marketplace that connects medical workers directly with the required medical facilities. This results in a much more effective way to provide on-demand medical procedures and services to consumers. In turn, healthcare workers have now become a part of the digital healthcare industry providing patient-oriented treatments [ 129 ].
3.2. Telemedicine
Telemedicine is a rather modern trend that became especially popular during the COVID-19 pandemic [ 130 ]. Such an approach enables support and care of patients in a non-crucial state. Telemedicine minimise the number of contacts between ill patients. Moreover, such educational support is important for chronic patients, and to prevent diseases [ 131 ]. According to the data of John Hopkins, before the first global lockdown in March 2020, the number of televisits was approximately 50–70 per month. By May 2020, this number radically increased to 94,000. Moreover, after healthcare services were broadly reopened, the number of monthly televisits remained about 35,000 [ 132 ].
Technologically, this kind of telecommunication provided the direct transmission of medical information in various formats (medical history, laboratory data, X-ray images, CT scan results, video images, ultrasound, etc.), as well as real-time video conferencing between medical institutions or a doctor and patients.
The use of telemedicine enabled the provision of consultative medical services in those areas where patients do not have the opportunity to receive the help of focused specialists directly at a medical institution. Telemedicine is of no less importance even in developed countries. With its incorporation, treatment costs have significantly reduced, the quality of diagnostics has improved, and remote monitoring of health has become accessible. This is especially important for elderly patients and patients with chronic diseases. For example, St. Luke University Health Network in Pennsylvania regularly hosts video conferencing to help elderly patients. They recognize that this social group is less likely to use mobile applications and is more comfortable with technologies that target desktops or laptops.
According to the Global Telemedicine Market Outlook, the global telemedicine market reached USD 56.2 billion in 2020 and is expected to reach USD 175.5 billion by 2026. The annual growth rate is about 19.2% [ 133 ]. Patient telemonitoring accounts for the main share of 32–48% of the world market (see Table 3 ). The leading countries in terms of spending on telemedicine and the development of the telemedicine technology market are China and the United States [ 133 ].
Telemedicine directions.
The global telemedicine market can be segmented according to several criteria, including:
- The nature of remote interaction (clinic–clinic, clinic–patient’s home);
- Technological parameters of interaction (monitoring systems, communication and communication channels, measuring instruments and sensors, video conferencing systems, databases, mobile and “wearable” technologies);
- Purpose of application (medical education, diagnostics, monitoring, consultation, treatment).
Depending on these, different approaches to the design and development of software solutions are augmented and, accordingly, different tools are used. However, as these segments are closely intertwined, the developer must have skills and expertise in a wide variety of development areas, including experience with embedded solutions, mobile cloud technologies, and protocols specific to the medical industry. It can be concluded that for telemedicine technologies to flourish, it is crucial to provide:
- Remote interaction of medical workers with each other, with patients, and/or their legal representatives;
- Identification and authentication of specified persons;
- Documenting their actions during consultation and remote medical monitoring of patient’s health.
3.3. Data Privacy & Cybersecurity in Medicine and Healthcare
With progress in big data and its advancement into medical innovation, there are potential risks to patient data privacy [ 134 ]. Healthcare is a prime target for cyberattacks, and even with continued investment in cyber security, critical vulnerabilities remain in many of the medical devices that hospitals rely on to treat patients [ 135 ]. Modern healthcare requires advanced solutions that reduce risks due to cyberattacks. Alongside this, GDPR patient sensitive data also needs to be protected so that the privacy of patients is not compromised.
For healthcare organisations, it is extremely important to ensure proper handling of patient data not only according to GDPR, but also because it is crucial for transparency with patients [ 136 ]. It can be said that the narrow scope of data privacy laws can be an issue. That can be traced to the U.S. health data privacy law (HIPAA), which regulates data between healthcare professionals and patients, but not un-identified data. For example, the data shared with a fitness trainer, tracking from smartphones, and data from various apps can be considered unprotected.
However, the relevant laws have become more and more effective in medical data privacy. The European Union’s General Data Protection Regulation, which passed in 2016, is a good example. EU law now requires data processors and controllers to provide users with their own data, clearly disclose data collection, set high-privacy defaults, and more.
Healthcare gadgets are not fully unprotected, similar to other IoT devices, and that affects data privacy and even the safety of the device. Risks and threats always exist. The trends in the rapid growth of the audience facing cyberattacks can be explained by the growing usage of electronic medical records and an increase in the amount of medical equipment and IoT devices connected to hospital networks. Additionally, the spread of viruses that interfere with the work of not only computers, but also medical devices is a continual problem. However, to eliminate such issues, cybersecurity is actively regulated by healthcare- and government-associated organizations such as the FDA [ 137 ]. This results in reduced risk of potential cybersecurity threats in legally marketed healthcare devices. It can be concluded that there is a necessity of regulated procedures to protect patient data [ 138 ].
3.4. Big Data Analytics (BDA) in Healthcare
BDA in healthcare enables improved diagnostic practice efficiency. Moreover, even therapeutic treatment based on BDA is much more accurate. It is especially relevant for cases involving tumours, including hard cancer pathologies. The main points of extreme importance in this regard are timely diagnosis and accurate choice of treatment [ 50 , 121 , 139 ].
BDA is advantageous for genetic analysis to compute genetic pathology and generate possible problem-oriented knowledge [ 121 , 140 ]. It should be considered that even superfast computers may take hours to do an intense data analysis which can now be accelerated using GPU computing [ 141 ].
BDA helps to decrease the rate of medication errors and to predict future admission rates [ 50 ]. It can be concluded that BDA helps to make healthcare more predictive. Predictive analysis about burden on healthcare systems and admission loading allows healthcare providers to make services more effective and to optimize resources for active deployment.
It should also be considered that predictive analysis could help companies to smoothly mobilise manpower resources by predicting possible outbreaks of colds/flu that could lead to manpower shortages.
Pharmaceutical manufacturers are looking to gain access to patient health data and are striking deals with technical companies knowledgeable in BDA, a tool that creates new possibilities for understanding how drugs work in real life. One recent example is the Roche deal announced in 2018, wherein the company acquired all shares of Flatiron Health, a clinical data collection company for cancer patients, by paying USD 2 billion [ 142 ]. Examining real-world evidence allows pharmaceutical manufacturers to prove the usefulness and value of their drugs. The most active research in this area is carried out in the fields of oncology, heart disease, and respiratory disorders. Actual drug use data is collected outside of traditional randomized clinical trials, which are today’s gold standard for drug evaluation. Neural networks are now effectively used for the development of automated drug discovery. Researchers and medicinal chemists work together to identify problematic issues and create more proficient models for newer drug design [ 143 ]. Advances in interdisciplinary fields that combine computational and genomic technologies are expected to lead to newer horizons in personalized medicine [ 121 , 140 , 144 , 145 ].
In the United States, the Human Microbiome Project was launched simultaneously with the renowned Human Genome Project [ 146 ]. During its implementation, a special Data Analysis and Coordination Center was created within the framework of the US National Institutes of Health. A joint Chinese–European project MetaHit is being implemented, where active research is being carried out in this direction.
3.5. Augmented Reality and Virtual Reality (AR & VR)
Smart glasses with AR functionality allow warehouse workers to achieve a higher level of accuracy. For critical life applications like aircraft production, AR helps manufacturers to precisely assemble and repair planes and to achieve improved reliability during repairs [ 147 ]. VR is transforming healthcare and changing the way patients are being treated. For millions of people suffering from chronic pain, VR is a working alternative to drug medication. VR is a safe and efficient treatment for pain and a powerful rehabilitation instrument for anxiety, post-traumatic syndrome, stress, strokes [ 54 ]. Healthcare professionals and medical students use VR simulations for improving their skills and for complex surgery planning. The global virtual and augmented reality healthcare market is expected to reach USD 5.1 billion by 2025 [ 148 ]. Recently, Nevada Spine Clinic surgeons performed a posterior lumbar fusion procedure using a Medtronic Mazor X robotic platform and an xvision augmented reality headset [ 149 ]. This is usually a rather invasive and time-consuming operation, lasting six to seven hours, but in this case, using the xvision headset in tandem with the Mazor X robotic platform, the surgery took less than two hours. The xvision headset allows the surgeon and their team to locate implants more accurately, which would have otherwise taken longer time. Before the procedure, the orthopedic surgeon and neurological surgeon used a robotic platform to carefully plan the exact placement of the implant and screw system. During surgery, an augmented reality headset allows the surgeon to refer to a 3D anatomical plan that has been previously created. As a result, the entire process becomes minimally invasive and much more efficient. The incidence of complications and the recovery time of patients reduced sharply due to the reduction in the time spent in the operation theatre, as did the minor damage to soft tissues compared to that with the open access.
In late September 2021, Kinomatic launched a virtual reality-based scaffolding platform that allows surgeons to develop customized plans for knee and hip arthroplasty [ 150 ]. This platform can work with any implant system and can be adapted for a variety of surgical techniques and surgical approaches. The company’s patented VR app allows surgeons to view and manipulate surgical plans in unparalleled detail. The platform supports preoperative modeling for knee and hip arthroplasty using preferred implants, surgical techniques, and specifications. Patients receive high-resolution computed tomography, which is then converted into an accurate 3D model of the patient’s joint after completion of the simulation. The surgeon can determine the exact size and orientation of the implant which will most accurately recreate the natural anatomy of the joint.
VR headsets are used for sports and fitness promotion, and these help children with autism to learn how to orient in the real world [ 151 ]. Application of VR training for patients with autism results in improvement of daily living skills [ 151 ]. VR and AR possess the potential to help older adults to overcome mobility issues and cognitive ability and socialisation limitations [ 124 ]. It was recently shown how the translational potential of VR can be used to reduce suicide risk [ 152 ]. VR factors like dissociation and derealisation allowed the simulation of the experience of a suicide opportunity and to reduce this risk. Virtual reality not only helps humans, but also enables investigation of the behavior and environment of animals [ 55 ].
3.6. VR Experiments
Development in computational technologies have led to new ways of supporting research and development work, which are now also regarded as “virtual experiments”. They are quickly emerging and rapidly developing tools within different applications of virtual reality (VR), including medicine [ 153 ]. One can briefly define “VR experiment” (VRE) as an advanced tool of computer modelling. Due to the advantages of modern technology in computation and visualisation, VRE has already started to play a significant role in cases where real experiments are extremely dangerous or prohibitively costly. One such case is related to research in safety, medicine and healthcare. In that aspect, VR experiments can be regarded as non-intrusive and non-invasive. This method is based on the development and utilisation of complex, advanced models of the human body and its parts. These models are further used for testing various “what if” scenarios in both static and dynamic cases, making predictions about how the human body will react to different situations, and how various equipment designed to prevent injuries or help with surgeries will work. Today, such models have become so sophisticated that one can speak about “digital twins” [ 154 , 155 ], and the results of modelling are coming close to experimentation. One of the examples of true success stories in the development of advanced digital twins and virtual experimentation in medical and safety research is modern achievement in the study of traumatic brain injuries and in the related development of modern protection helmets [ 156 , 157 , 158 , 159 , 160 ].
Unfortunately, computer modelling in general and virtual experimentation in particular are not free of problems. One of these problems is that a model cannot be tested from within a model. Further developments in this area are represented by “surrogate twins”. These are body part surrogates (physical models) manufactured using additive manufacturing, another modern technology enabled by digitisation [ 57 , 58 , 161 ]. Such surrogates can have the same shapes and outlines as their digital counterparts, as they use the same CAD files (see Figure 5 ). Properties of materials used in the surrogate mimic soft human tissues and bones and can be exactly characterized. Surrogates often have encapsulated sensors and experiments in realistic conditions are performed with them. Experiments with surrogate twins are also performed without endangering humans, and collected data are equally useful for both research and development and for cross-validation of both digital and physical models. Significant progress in the application of surrogate twins is achieved in the area of safety research and studies of injury mechanisms [ 57 , 58 , 160 , 161 ].

Example of body part surrogate for VRE. Photo courtesy of Prof. A. Koptyug, SportsTech Research Center, Mid Sweden University, Sweden.
These methods harness the power of digitisation, from scanning shapes to making surrogates of differing complexity with embedded sensors using additive manufacturing and synthetic materials mimicking different tissues. Such an innovative approach allows the design of new devices with a high degree of efficacy. The application of surrogate twins is helpful in both civil and military areas.
3.7. Wearable Medical Devices
Wearable medical devices are a modern trend in healthcare. They help to collect health data to monitor patients’ health. These devices provide day-to-day health data and are active monitoring tools compared to once-a-year or once-a-month clinical checkups.
Medical companies are investing in wearable smart devices that can provide up-to-date monitoring of high-risk patients, preventing major health episodes. According to a recent report, the wearable medical device market is expected to reach USD 46.6 million by 2025, a spectacular jump from approximately USD 8 million in 2017 [ 162 ]. Some of the most common of devices include:
- Smart watches with heart rate sensors and exercise tracking.
- Sweat meters used by diabetics to monitor blood sugar levels.
- Smart patches to measure hydration levels, body temperature, heart rate, and other biometric parameters.
- Oximeters measure the amount of oxygen in the blood, which is relevant to patients with respiratory illnesses, e.g., asthma.
- Headphones to monitor blood pressure.
- Biosensors in modern devices are able to not only read pulses and measure steps and calories, but also measure hydration, electrolyte levels, blood pressure; obtain electrocardiogram (ECG) results; and determine muscle load, strength, and fatigue level.
There are many tangible benefits for healthcare companies in spreading the use of wearable devices:
- These devices make patients themselves responsible for monitoring their actions leading to a certain health state.
- People are incentivized to engage in more sports and remain fit by actively monitoring their results, thus setting new goals. Such practices decrease the risk of obesity and reduce the burden on the healthcare system.
- Relevant medical information is on demand for insurance companies to assess the real risks to patients’ health.
- Patients who use technologies for preventive treatment and monitoring are offered discounts on their health insurance.
3.8. 3D Imaging and Prototyping
The whole complex of assisted three-dimensional techniques is used in 3D prototyping as described below:
- 3D visualisation/medical image processing of a medical problem using medical computed tomography, MRI, and X-ray examination tools [ 120 , 139 ]. Machine learning can be used for medical image processing. After the required features from a particular medical case are extracted, this data can be processed for accurate decisionmaking [ 99 ].
- 3D-modelling using modern digital tools like Magics by Materialise and analogues. A complex model of a damaged area could be realized for further surgical planning. This is especially relevant for complex cancer cases where a surgeon needs to attempt resection from multiple sides of a tumor.
- 3D-planning digital systems enable online communication between the surgeon and the engineer responsible for 3D printing of the implant.
- 3D-prototyping using polymer printers improves the accuracy of custom-designed metal implants [ 26 , 100 , 146 ].
- 3D-printing of organs and tissues, also called bio-printing, is a rapidly developing application for meeting the demands of modern transplantology [ 163 , 164 ].
3D printing is used for additive manufacturing of patient-specific metal/ceramics implants; for stereolithography of drug delivery systems; for polymer/metal-based individual prosthesis; and for individual surgeons’ tool designs [ 121 ].
3.9. Machine Learning & Deep Learning
In biomedicine, machine learning and deep learning are used to simulate human knowledge and for complex analysis of special medical data and biomedical and biophysical processes in the human body [ 15 ]. Here, AI works for the systematisation of assisted behavioral processes using complex machine learning (see Table 4 ).
Areas leveraging AI in medicine.
The main goal of AI in biomedicine is to establish relationships between patients’ health, diagnostics, selected treatment program, and follow-up outcomes. Due to AI, the effectiveness of diagnostics and selected therapeutic treatment could be radically improved [ 25 ]. Machine learning for such diagnostic tools as electrocardiography (ECG) and electroencephalography (EEG) supports doctors in screening and recognition of the disease, and possible risks reduction [ 165 ]. The use of machine learning enables the processing of the extracted data, such as heart rate, pulse, intervals, variability from ECG/EEG data, etc. A combination of these parameters can help to identify the existence of heart-related diseases [ 166 ].
The diagnosis of cancerous tumours by applying AI (deep learning, image classification, object recognition) to MRI images is not inferior to the conclusions of highly qualified radiologists in terms of accuracy. AI algorithms are also an effective solution for proper patient-oriented and patient-specific drug selection.
The implementation of novel digital tools is sometimes referred to collectively as the concept of “hospital 4.0”, emphasizing the digital character of progress in medicine [ 50 , 51 , 121 ]. Areas of using AI in medicine can be specified as follows:
- At the design level: predicting diseases, identifying groups of patients with high risk of disease, organizing preventive measures.
- At the production level: automation and optimisation of processes in hospitals, automation and improvement of diagnostic accuracy.
- At the promotion level: price management, risk reduction for patients.
- At the level of service delivery: adaptation of therapy and the composition of drugs for each individual patient, the use of virtual assistants for planning a patient’s route in a polyclinic or hospital.
However, at present, the healthcare community has no unanimous opinion of whether digitalisation in medicine is necessary and helpful for all healthcare industries, or if it is being forced on the medical community because of general trends. This means that deep academic research on this topic is in demand by health care specialists [ 51 ]. The limitations of digitisation stem from massive hardware requirements. Traditionally, mechanical parts such as the bolts and nuts of an instrument can simply be taken off and replaced, but with electronics this is not the case. Hardware now very quickly becomes obsolete, and software or firmware updates are no longer available after their intended design period. Moreover, the electronic circuits themselves become dated, as they can no longer function at the same speeds as newer hardware. Consequently, despite having the so-called “right to repair”, the repair of digital hardware is almost impossible and is not cost-effective even if pursued. This grim situation is leading the accelerated generation of electronic waste and these aspects require serious consideration in view of the growing focus on a “net zero” economy.
In the post-4th Industrial Revolution era, the term “5th Industrial Revolution” started to be used in scientific reported data [ 167 ]. In healthcare, the terms “hospital 4.0” and “medicine 4.0” are gaining popularity by highlighting a new era in medicine- and healthcare-assisted spheres. The main trends in technological and economic development that can be observed are that the advent of the Industrial Internet of Things is a very specific economic and technological evolution that requires new action and will enable incredible development. Now, business strategy and administration need to be customer-oriented through new relationships provided by IIoT. It is also noteworthy that artificial intelligence, big data analytics, and robotics will enable the very fibre of everyday life, especially in safety-critical applications. Additionally, “virtual experiments” and experiments with body part surrogates applied for medicine and healthcare represent the true power of digitisation, from scanning shapes to making surrogates of different complexity with embedded sensors using additive manufacturing and synthetic materials to mimic different tissues. With the use of finite element modeling, “virtual experiments” can achieve newer horizons hitherto unrealised.
In the time to come, newer forms of prescription will become the most exciting medical advances. Tablets with support for microscopic sensors can provide doctors with the best information about the condition of a patient internal organs. In this regard, digital techniques including 3D printing and digital health devices (IoT) will pave the way for patient-specific, need-oriented, and predictive/provision-based approaches for all spectra of the medical industry. BDA will lead various areas of medical digitalisation, having a significant influence on the healthcare industry’s evolution and development. According to analysts’ forecasts, the market volume of the Internet of Things in medicine will exceed USD 158 billion by 2022. The average market growth rate (CAGR) in the period from 2016 to 2022 was estimated by Market Research Engine experts to be 30.8% [ 168 ]. This review identifies the breakthrough technologies of digitalised healthcare as important ingredients.
In the area of big data and modern modelling, the medical industry would benefit from digital tools tailoring existing technology to the needs of the industry, healthcare professionals, and patients.
Among the factors contributing to the increase in medical IoT costs on a global scale are the growing number of chronic diseases, the introduction of favourable initiatives by governments in various countries and the evolution of artificial intelligence technologies. Current assessments suggest that further integration of digital instruments and technologies will improve the efficiency of the healthcare system, the development of patient-oriented innovations, the transformation of business models, and new workspace organization.
It is necessary to note that the digital technologies discussed in this paper relate to the most successful industrial application up to now. However, both international cooperation and organizational efforts are required for deeper research and further digitalisation. Moreover, it is important to mention that higher awareness from society on digitalisation and Industry 4.0 technologies through improved education and development of “digital” professionals will be of great importance.
Future research will cover the aforementioned technologies related to digitalisation in medicine and healthcare, including virtual and VR experiments, biological additive manufacturing, development of cybersecurity, and pandemic predictive big data analysis.
Acknowledgments
We greatly acknowledge the financial support provided by the UKRI via Grants No. EP/L016567/1, EP/S013652/1, EP/S036180/1, EP/T001100/1 and EP/T024607/1, Transformation Foundation Industries NetworkPlus feasibility study award to LSBU (EP/V026402/1), the Royal Academy of Engineering via Grants No. IAPP18-19\295 and TSP1332, EURAMET EMPIR A185 (2018), the EU Cost Action (CA15102, CA18125, CA18224 and CA16235) and the Newton Fellowship award from the Royal Society (NIF\R1\191571). Wherever applicable, the work made use of Isambard Bristol, UK supercomputing service accessed by a Resource Allocation Panel (RAP) grant as well as ARCHER2 resources (Project e648).
Author Contributions
Conceptualization and methodology, V.V.P., E.V.K. and S.G.; resources, V.V.P., E.V.K., A.S., S.I.S. and S.G.; writing—original draft preparation, V.V.P., E.V.K., N.K.K., A.S., S.I.S. and S.G.; writing—review and editing, V.V.P., N.K.K. and S.G.; visualization, V.V.P., N.K.K. and S.G.; supervision, V.V.P., E.V.K. and S.G. All authors have read and agreed to the published version of the manuscript.
This research received no external funding.
Institutional Review Board Statement
Informed consent statement, data availability statement, conflicts of interest.
The authors declare no conflict of interest.
Publisher’s Note: MDPI stays neutral with regard to jurisdictional claims in published maps and institutional affiliations.
- Verify Documents, Clients & Products
- Offices & Labs
- Our Services
- Our Company
- Sustainability
- Insights & Resources
What are you looking for?
Some topics you might be interested in, industry 4.0: building a more secure connected world with iec 62443.
Manufacturing and infrastructure are changing with the adoption of Industry 4.0 – the digitalization of processes and systems to improve supply chain management, drive efficiencies and optimize cost-effectiveness. However, integrating the Internet of Things (IoT) and cloud technologies into value chains also opens them up to the risk of cyberattack.
Vulnerabilities
The latest industrial revolution involves the integration of smart technology into everything from energy systems, processes and factories to urban infrastructures and transportation systems. The goal is better efficiency, improved sustainability and interoperability, enhanced reliability and greater cost-effectiveness for asset owners, system integrators, product suppliers and other stakeholders.
Set against these desired outcomes is an increased risk of cyberattack. Where once, a criminal would need direct access to a system to control it, the inherent vulnerabilities of a connected system mean they can now potentially take control without leaving their home.
Cyberattacks are on the rise in all sectors, with attacks on manufacturing doubling in 2022. 1 If an attack is successful but kept ‘in-house,’ it will, at the very least, offset any potential benefits accrued from the introduction of IoT solutions.
However, in other cases, the impact can be enormous, spreading beyond the directly affected organization to affect whole communities. An example of this is the US Colonial Pipeline shutdown in 2021. The attack was discovered in the early hours of May 7, 2021, when a ransom note was found in the company’s IT system. Hackers had used DarkSide ransomware to access the company’s systems through an outdated VPN. Data was then encrypted, putting the organization’s whole operational technology (OT) network at risk, including the 5,500-mile pipeline.
In addition to the impact on the company, this attack also led to states declaring emergencies, rapid fuel price rises and supply shortages after consumers panicked and stockpiled fuel. In the longer term, it also highlighted vulnerabilities in relation to industrial control systems (ICS) and OT networks and instilled a sense of urgency around the need to take industrial cybersecurity seriously. 2
Industrial drivers
Business continuity is a major driver for the industrial sector. Disruption caused by a cyberattack can be significant in terms of lost business and damage to infrastructure and reputation.
At the same time, the regulatory landscape is evolving, with authorities now looking for evidence of IoT cybersecurity management, e.g. European Union (EU) Radio Equipment Directive (RED) in 2024. IoT also falls under the provisions of a wide variety of other legislation and standards, including the NIS2 Directive, Cyber Security Act and Cyber Resilience Act in the EU and NIST 8425 in the US.
Developers can mitigate the risk of cyberattacks on their systems while ensuring regulatory compliance, enabling market differentiation by certifying their products against the most widely recognized industrial IoT standard, IEC 62443.
This series of standards originated in 2002 when the International Society of Automation (ISA) established the Industrial Automation and Control System Security standards committee (ISA99). Originally known as the ISA99 standards, they were renumbered in 2010 as the ANSI/ISA-62443 series and submitted to and used by International Electrotechnical Commission (IEC) working groups. In 2021, the IEC approved the IEC 62443 family of standards as 'horizontal standards,' meaning they are the foundation for addressing cybersecurity when any sector-specific standard is developed.
The IEC 62443 series provides critical infrastructure agencies and industrial sectors with established guidelines for ensuring secure industrial automation and control systems (IACS). They set best practices for security and provide a way to assess the level of security performance, bridging the gap between operations and information technology, as well as between process safety and cybersecurity.
The series is organized into four parts:
- Part 1 – General – covers topics common to the whole series of standards (terminology, concepts, models, etc.)
- Part 2 – Policies and procedures – focuses on methods and processes associated with IACS security
- Part 3 – System – system-level requirements
- Part 4 – Components and requirements – provides detailed requirements for IACS products
As a whole, they:
- Define organizational and technical requirements for manufacturers, integrators, operators and industry
- Target people, processes, systems, solutions and components across all industries and facilities
- Support tailored security solutions
- Offer varying levels of security assurance
- Deliver a repeatable, holistic approach to the issue of cybersecurity
SGS Solution
SGS Brightsight provides manufacturers and developers with a full range of cybersecurity testing and certification solutions covering the full range of products and systems, from cybersecurity management system components and industrial routers to data diodes, software applications, embedded devices, host devices and network devices.
Our dedicated IEC 62443 services include:
- Workshops and training sessions that provide a comprehensive look at the standard, its framework and technical security requirements
- Developer support
- Pre-evaluation to reduce the potential for failure during formal security evaluations
- Security evaluation against:
- IEC 62443 2-4 – IACS policy and procedures assessment
- IEC 62443-3-3 – System integration assessment. Examples include SCADA systems, consisting of multiple sensors, control units, HMIs and software applications
- IEC 62443 4-1 – assesses secure development procedures implemented by product manufacturers
- IEC 62443-4-2 – assesses the security capabilities of individual system components. Examples include local programmable logic controllers (PLCs) and the control unit on a building’s smart lights
- Certification via our IECEE-accredited certification body (SGS Fimko in Finland)
Learn more about SGS Brightsight .
Enjoyed this article?
Find more news and updates in our Consumer Compact newsletter >
Delivered direct to your inbox
Subscribe to Consumer Compact >
1 Why cybersecurity risks matter - and how to raise security | World Economic Forum
2 How the Colonial Pipeline attack instilled urgency in cybersecurity | Cybersecurity Dive
© SGS Société Générale de Surveillance SA.
For further information, please contact:
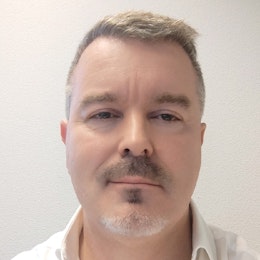
Get insights on international developments, new regulations, case studies and updates on SGS activities.
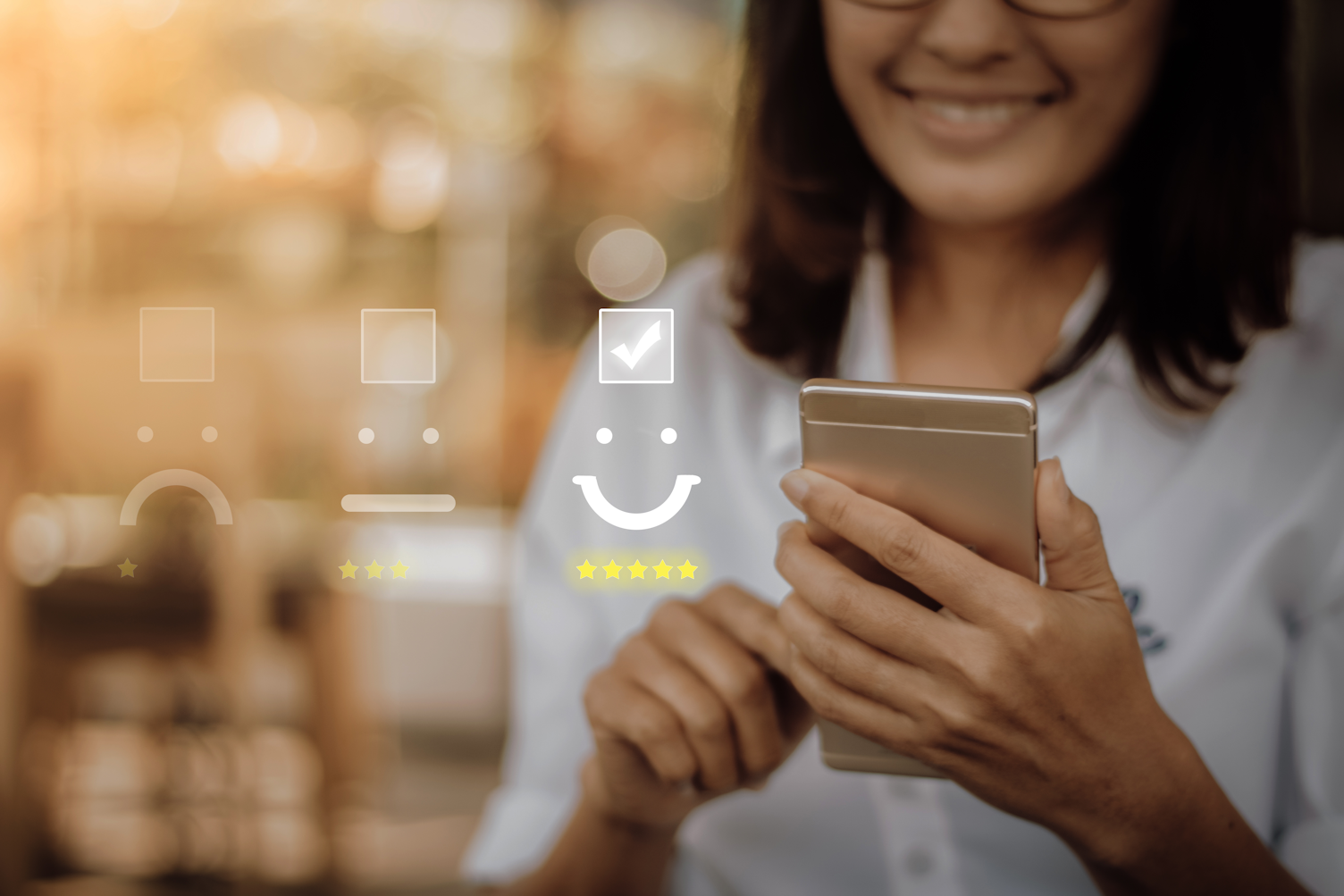
Related Links
Read more in our consumer compact newsletter.
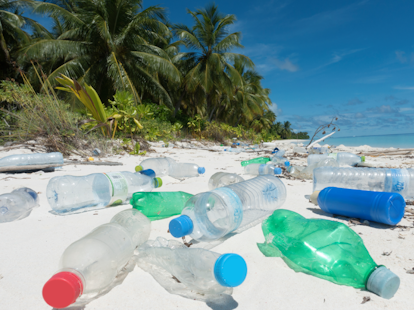
Meeting the Challenge of Sustainability in Plastics
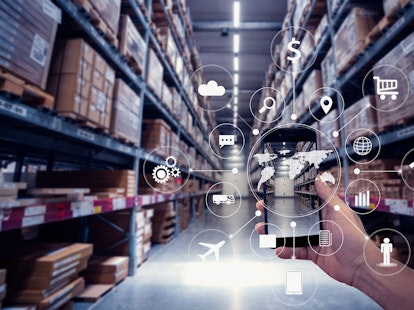
Why Fashion Companies Need Traceability in Their Supply Chains
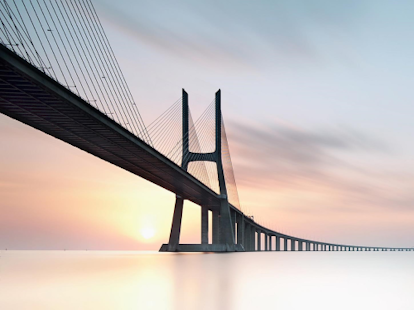
Understanding the EU General Product Safety Regulation: Commonly Asked Questions
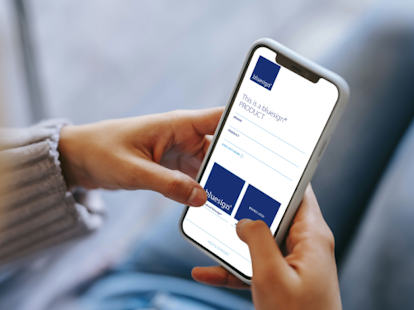
EU Digital Product Passport: Putting Power into Consumer’s Hands When Buying Clothes
News & insights.
- SGS Canada Inc.
+1 905 364 3771
6755 Mississauga Road, Suite 204,
Mississauga, Ontario, Canada
Perspectives on industry 4.0 awareness among undergraduate IT students in IRAQ: University of Anbar as a case study.
Ieee account.
- Change Username/Password
- Update Address
Purchase Details
- Payment Options
- Order History
- View Purchased Documents
Profile Information
- Communications Preferences
- Profession and Education
- Technical Interests
- US & Canada: +1 800 678 4333
- Worldwide: +1 732 981 0060
- Contact & Support
- About IEEE Xplore
- Accessibility
- Terms of Use
- Nondiscrimination Policy
- Privacy & Opting Out of Cookies
A not-for-profit organization, IEEE is the world's largest technical professional organization dedicated to advancing technology for the benefit of humanity. © Copyright 2024 IEEE - All rights reserved. Use of this web site signifies your agreement to the terms and conditions.
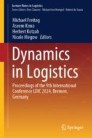
International Conference on Dynamics in Logistics
LDIC 2024: Dynamics in Logistics pp 409–418 Cite as
Enhancing Product Development Through Industry 4.0 Requirements: Willingness to Pay Considerations in a Case Study in Food Processing Machine
- Bruno Turmina Guedes 8 ,
- Diego de Castro Fettermann 8 &
- Enzo Morosini Frazzon 8
- Conference paper
- First Online: 03 April 2024
Part of the book series: Lecture Notes in Logistics ((LNLO))
Industry 4.0 represents a novel paradigm centered around digital factories, capable of integrating information technologies and machines with intelligent products. In this context, this article addresses the added monetary value resulting from adopting Industry 4.0 technologies in the development of a scraped surface heat exchanger equipment. This research aims to estimate the added value of a technology-based redesign of a food processing machine, considering the willingness to pay. The methodology employed to evaluate the integration of these technologies into the product is based on the Stated Preference (SP). The findings reveal a hierarchy among the enhancement opportunities that Industry 4.0 technologies bring to the product. Consequently, in this case, incorporating features from Industry 4.0 that encompass the maintenance aspects contributes significantly to the product’s value.
- Industry 4.0
- Product Development
- Stated Preference
This is a preview of subscription content, log in via an institution .
Brettel, M., et al.: How virtualization, decentralization and network building change the manufacturing landscape: an Industry 4.0 perspective. Int. J. Mech., Ind. Sci. Eng. 8 (1), 37–44 (2014)
Google Scholar
Göran, A., et al.: Cloud manufacturing–a critical review of recent development and future trends. Int. J. Comput. Integr. Manuf. 30 (4–5), 347–380 (2017)
Oliver, F., et al.: Cloud manufacturing as a sustainable process manufacturing route. J. Manuf. Syst. 47 , 53–68 (2018)
Article Google Scholar
Kusiak, A.: Smart manufacturing. Int. J. Prod. Res. 56 (1–2), 508–517 (2018)
Sameer, M., et al.: A critical review of smart manufacturing & Industry 4.0 maturity models: implications for small and medium-sized enterprises (SMEs). J. Manuf. Syst. 49 , 194–214 (2018)
Philipp, O., Lukas, B., Thomas, F.: The smart factory as a key construct of Industry 4.0: a systematic literature review. Int. J. Prod. Econ. 221 , 107476 (2020)
Hugh, B., et al.: The industrial internet of things (IIoT): An analysis framework. Comput. Ind. 101 , 1–12 (2018)
Lee, J., Bagheri, B., Jin, C.: Introduction to cyber manufacturing. Manuf. Lett. 8 , 11–15 (2016)
Frank, A.G., Dalenogare, L.S., Ayala, N.F.: Industry 4.0 technologies: Implementation patterns in manufacturing companies. Int. J. Prod. Econ. 210 , 15–26 (2019)
Li, J.-Q., et al.: Industrial internet: A survey on the enabling technologies, applications, and challenges. IEEE Commun. Surv. Tutorials 19 (3), 1504–1526 (2017)
Lasi, H., kemper, H.G.: Industry 4.0. Bus. Inf. Syst. Eng. 6 (4), 239–242 (2014)
Thames, L., Schaefer, S.: Software-defined cloud manufacturing for industry 4.0. Procedia CIRP 52 , 12–17 (2016)
Meindl, B., Ayala, N.F., Mendonça, J., Frank, A.G.: The four smarts of industry 4.0: evolution of ten years of research and future perspectives. Technol. Forecast. Soc. Change 168 , 120784 (2021)
Culot, G., et al.: Behind the definition of industry 4.0: analysis and open questions. Int. J. Prod. Econ., 107617 (2020)
Schumacher, A., Erol, S., Sihn, W.: A maturity model for assessing industry 4.0 readiness and maturity of manufacturing enterprises. Procedia CIRP 52 , 161–166 (2016)
Ford, M.: Industry 4.0: who benefits? SMT Surface Mount Techn. Mag. 30 (7), 52–55 (2015)
Mulley, C., Ho, C., Ho, L., Hensher, D., Rose, J.: Will bus travellers walk further for a more frequent service? An international study using a stated preference approach. Transp. Policy 69 , 88–97 (2018)
Kim, J., Park, S.Y., Lee, J.: Do people really want renewable energy? Who wants renewable energy? Discrete choice model of reference-dependent preference in South Korea. Energy Policy 120 , 761–770 (2018)
Calegari, L.P., et al.: A conjoint analysis to consumer choice in Brazil: defining device attributes for recognizing customized foods characteristics. Food Res. Int. 109 , 1–13 (2018)
Fettermann, D.C., et al.: Configuration of a smart meter for Brazilian customers. Energy Policy 139 , 111309 (2020)
Fettermann, D.C., et al.: Getting smarter about household energy: the who and what of demand for smart meters. Build. Res. Inf. 49 (1), 100–112 (2021)
Caussade, S., et al.: Assessing the influence of design dimensions on stated choice experiment estimates. Transp. Res. Part B: Methodol. 39 (7), 621–640 (2005)
Tabachnick, B.G., Fidell, L.S., Ullman, J.B.: Using Multivariate Statistics. Pearson, Boston (2007)
Hensher, D., Rose, J., Greene, W.: Applied Choice Analysis. Cambridge University Press, Cambridge (2015)
Book Google Scholar
Mcfadden, D.: Contingent valuation and social choice. Am. J. Agr. Econ. 76 (4), 689–708 (1994)
Krinsky, I., Robb, A.L.: On approximating the statistical properties of elasticities: a correction. Rev. Econ. Stat. 72 (1), 189–190 (1990)
Bliemer, M., Rose, J.: Confidence intervals of willingness-to-pay for random coefficient logit models. Transp. Res. Part B: Methodol. 58 , 199–214 (2013)
Download references
Author information
Authors and affiliations.
Federal University of Santa Catarina, Florianópolis, Brazil
Bruno Turmina Guedes, Diego de Castro Fettermann & Enzo Morosini Frazzon
You can also search for this author in PubMed Google Scholar
Corresponding author
Correspondence to Bruno Turmina Guedes .
Editor information
Editors and affiliations.
Production Systems and Logistics, University of Bremen, Bremen, Germany
Michael Freitag
Global Supply Chain Management, University of Bremen, Bremen, Germany
Aseem Kinra
Crowley Center for Transportation and Logistics, University of North Florida, Jacksonville, FL, USA
Herbert Kotzab
Combinatorial Optimization and Logistics, University of Bremen, Bremen, Germany
Nicole Megow
Rights and permissions
Reprints and permissions
Copyright information
© 2024 The Author(s), under exclusive license to Springer Nature Switzerland AG
About this paper
Cite this paper.
Turmina Guedes, B., de Castro Fettermann, D., Morosini Frazzon, E. (2024). Enhancing Product Development Through Industry 4.0 Requirements: Willingness to Pay Considerations in a Case Study in Food Processing Machine. In: Freitag, M., Kinra, A., Kotzab, H., Megow, N. (eds) Dynamics in Logistics. LDIC 2024. Lecture Notes in Logistics. Springer, Cham. https://doi.org/10.1007/978-3-031-56826-8_31
Download citation
DOI : https://doi.org/10.1007/978-3-031-56826-8_31
Published : 03 April 2024
Publisher Name : Springer, Cham
Print ISBN : 978-3-031-56825-1
Online ISBN : 978-3-031-56826-8
eBook Packages : Engineering Engineering (R0)
Share this paper
Anyone you share the following link with will be able to read this content:
Sorry, a shareable link is not currently available for this article.
Provided by the Springer Nature SharedIt content-sharing initiative
- Publish with us
Policies and ethics
- Find a journal
- Track your research
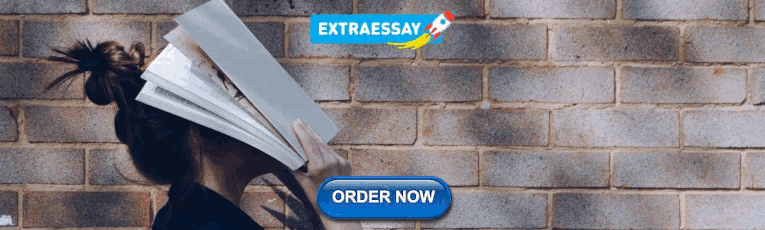
IMAGES
VIDEO
COMMENTS
Renault Digital is the center of excellence that provides all the skills needed to execute digital, agile projects. It consists nowadays of more than 350 employees, with skills and knowledge in Agile methodologies, UX Design, DevOps and Data Science. Renault Digital works a bit like a startup incubator and that is also the mentality it tries to ...
In the past five years, a select group of companies have started pulling ahead in their efforts to implement Industry 4.0 across their manufacturing networks.Leading manufacturers are now realizing significant value from data and analytics, AI, and machine learning (ML). However, a large majority remain stuck in pilot purgatory, struggling to capture the full potential of their transformation ...
The transition to industry 4.0 has impacted factories, but it also affects the entire value chain. In this sense, human-centred factors play a core role in transitioning to sustainable manufacturing processes and consumption. The awareness of human roles in Industry 4.0 is increasing, as evidenced by active work in developing methods, exploring influencing factors, and proving the ...
The purpose of this paper is to provide an analysis of diverse case studies of Industry 4.0. Industry 4.0 represents the fourth Industrial revolution which illustrates the structure of the organization that controls the production of the products in the entire life cycle. Industry 4.0 is a term that can be given to the combination of humans and technology, when is mixed give a digital way of ...
Increasing Industry 4.0 adoption and impact among advanced industry companies. Beginning with 16 sites in 2018, the GLN has grown to 103 sites as of 2022. Nearly 60 percent of the current lighthouses are companies in AI (Exhibit 1). 1. Industry 4.0 has helped these companies make strides across KPIs that drive growth.
1. Introduction. Industry 4.0 (I4.0) represents an ongoing transformational phase for manufacturing organisations aiming to fully interlink their business functions and production systems with data from the entire lifecycle or End-to-End Digital Integration (Liao et al. Citation 2017; Castelo-Branco, Cruz-Jesus, and Oliveira Citation 2019).The implementation of I4.0 has been conceptualised not ...
Sanders, A, C Elangeswaran and J Wulfsberg [2016] Industry 4.0 implies lean manufacturing: Research activities in industry 4.0 function as enablers for lean manufacturing. Journal of Industrial Engineering and Management , 9 (3), 811-833.
Finally, industry 4.0 is changing the technological reality of companies. The smart industry emerges as a new standard for digital systems, information technology and internet solutions. The impacts of industry 4.0 are multifaceted and complex for all organizations and economies.
Executive summary. With an estimated value creation potential for manufacturers and suppliers of USD 3.7 trillion in 2025,1 high hopes are set on Industry 4.0 to bring the next industrial revolution to discrete manufac-turing. Yet, only about 30 percent of companies are capturing value from Industry 4.0 solutions at scale today.
The Industry 4.0 is becoming a hot topic. In this paper, we revisit Industry 4.0 from the perspectives of its purposes, features, and key performance indicators. We present a reference roadmap for advancing an Industry 4.0 project from plan to implementation. We present the case study of steel industry 4.0 and lessons. In addition, we give suggestions directing the advancement of Industry 4.0.
Industry 4.0 has an important and long-term strategic impact on global industrial development, as it signals a growing demand for research on the issues, challenges, and solutions related to the design, implementation, and management of smart manufacturing systems [].In this way, it represents a technology based opportunity to change the way companies generate value for their customers [].
Curated Set of Case Studies demonstrating problem, solution, and results of implementing Industry 4.0.
Abstract. The interest towards Industry 4.0 is growing since its inception. Its introduction has many impacts on the whole supply chain and requires companies to rethink the way they design and manage their supply chain. In particular, companies usually are not able to develop innovation by exclusively relying only on their internal resources.
2.3 The case-study cluster describing how to implement Industry 4.0. The next four articles study how Industry 4.0 can be implemented in practice and what the challenges are during implementation (Hermann et al., 2020; Johansson et al., 2020; Veile et al., 2020; Schott et al., 2020). These articles study cases of companies that implemented ...
The purpose of this article is to investigate the drivers for and outcomes of the adoption of Industry 4.0 technologies in the case of a food processing company located in Italy.,The present work adopted a case study approach by investigating an Italian food processing company to investigate the drivers, challenges and outcomes of Industry 4.0 ...
For this purpose, the study conducts a systematic review of extant literature to identify Industry 4.0 functions for sustainable innovation and applies interpretive structural modeling to devise the promised roadmap. The results offer interesting insights into Industry 4.0 applications for sustainable innovation.
Abstract Industrial Internet is an important component of Industry 4.0. It aims to help manufacturers achieve intelligent management and decision making. ... As such, this paper reviews Industry 4.0 and Industrial Internet and presents a case study about how Sany Heavy Industry applying systems thinking in implementing Industrial Internet and ...
Case study results and future plans are summarized in conclusion. Transforming into a Smart Factory and implementing Industry 4.0 technology is an extensive and long-term process.
Abstract. The transition to industry 4.0 has impacted factories, but it also affects the entire value chain. In this sense, human-centred factors play a core role in transitioning to sustainable manufacturing processes and consumption. The awareness of human roles in Industry 4.0 is increasing, as evidenced by active work in developing methods ...
Actual trends in the industry can be described by the Industry 4.0 manifest. Characteristic features of Industry 4.0 components' communication are interoperability and an ability of autonomous communication with other components. This article examines state-of-art technologies which could provide an environment for such communication and proposes a study of Industrial Communication Platform ...
5 A Case of Human Factors in Implementing New Technology. The challenges of introducing Industry 4.0 in an organization are not purely technical. The effort to change processes through digital technologies in a traditional work environment is often met with strong resistance from the humans affected by the change.
Abstract. Industry 4.0 in healthcare involves use of a wide range of modern technologies including digitisation, artificial intelligence, user response data (ergonomics), human psychology, the Internet of Things, machine learning, big data mining, and augmented reality to name a few. The healthcare industry is undergoing a paradigm shift thanks ...
Industry 4.0: Building a More Secure Connected World with IEC 62443 Consumer Compact Electrical and Electronics March 25, 2024 Manufacturing and infrastructure are changing with the adoption of Industry 4.0 - the digitalization of processes and systems to improve supply chain management, drive efficiencies and optimize cost-effectiveness.
Written by highly qualified authors, Topics in Artificial Intelligence Applied to Industry 4.0 explores sample topics such as: Quantum machine learning, neural network implementation, and cloud and data analytics for effective analysis of industrial data Computer vision, emerging networking technologies, industrial data spaces, and an industry ...
The recent era of technology, Industry 4.0 finds its way to the field of manufacturing based on advanced technology such as artificial intelligence, internet of things, augmented reality, robotics, and more. These technologies can truly lead a massive transformation in production and manufacturing processes. This study aims to understand the extent to which undergraduate students in the field ...
The development of Industry 4.0 seeks to optimize operational efficiency, enhance productivity, and foster automation within production systems . Although Industry 4.0 originates from the high-tech strategy developed in Germany, it still needs a precise and widely accepted definition .
In the era of Industry 3.0, product fault detection systems became important auxiliary systems for factories. These systems efficiently monitor product quality, and as such, substantial amounts of capital were invested in their development. However, with the arrival of Industry 4.0, high-volume low-mix production modes are gradually being replaced by low-volume high-mix production modes ...