Join thousands of product people at Insight Out Conf on April 11. Register free.
Insights hub solutions
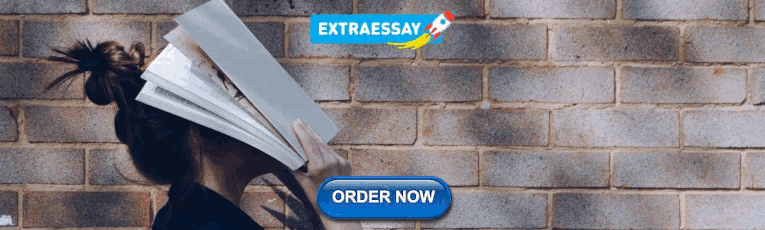
Analyze data
Uncover deep customer insights with fast, powerful features, store insights, curate and manage insights in one searchable platform, scale research, unlock the potential of customer insights at enterprise scale.
Featured reads
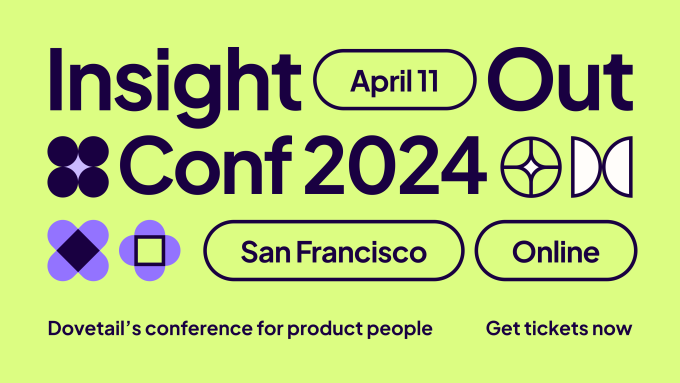
Inspiration
Three things to look forward to at Insight Out
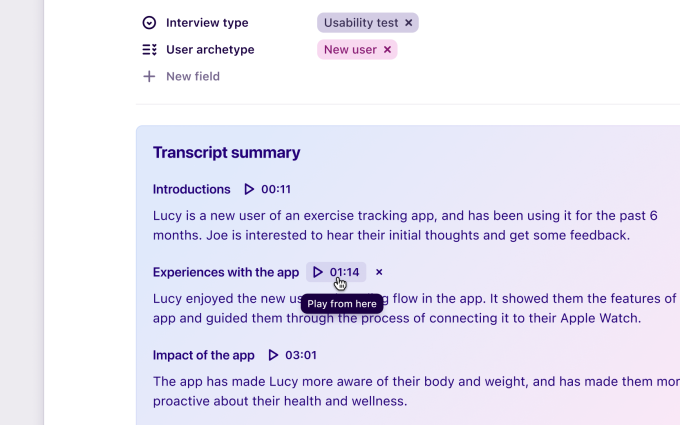
Tips and tricks
Make magic with your customer data in Dovetail
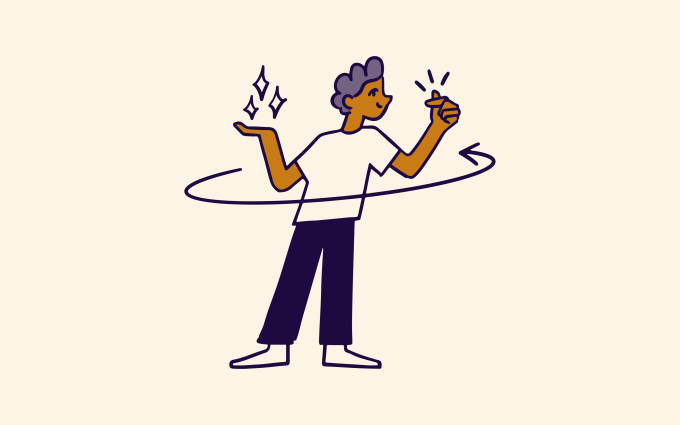
Four ways Dovetail helps Product Managers master continuous product discovery
Events and videos
© Dovetail Research Pty. Ltd.
What is causal research design?
Last updated
14 May 2023
Reviewed by
Examining these relationships gives researchers valuable insights into the mechanisms that drive the phenomena they are investigating.
Organizations primarily use causal research design to identify, determine, and explore the impact of changes within an organization and the market. You can use a causal research design to evaluate the effects of certain changes on existing procedures, norms, and more.
This article explores causal research design, including its elements, advantages, and disadvantages.
Analyze your causal research
Dovetail streamlines causal research analysis to help you uncover and share actionable insights
- Components of causal research
You can demonstrate the existence of cause-and-effect relationships between two factors or variables using specific causal information, allowing you to produce more meaningful results and research implications.
These are the key inputs for causal research:
The timeline of events
Ideally, the cause must occur before the effect. You should review the timeline of two or more separate events to determine the independent variables (cause) from the dependent variables (effect) before developing a hypothesis.
If the cause occurs before the effect, you can link cause and effect and develop a hypothesis .
For instance, an organization may notice a sales increase. Determining the cause would help them reproduce these results.
Upon review, the business realizes that the sales boost occurred right after an advertising campaign. The business can leverage this time-based data to determine whether the advertising campaign is the independent variable that caused a change in sales.
Evaluation of confounding variables
In most cases, you need to pinpoint the variables that comprise a cause-and-effect relationship when using a causal research design. This uncovers a more accurate conclusion.
Co-variations between a cause and effect must be accurate, and a third factor shouldn’t relate to cause and effect.
Observing changes
Variation links between two variables must be clear. A quantitative change in effect must happen solely due to a quantitative change in the cause.
You can test whether the independent variable changes the dependent variable to evaluate the validity of a cause-and-effect relationship. A steady change between the two variables must occur to back up your hypothesis of a genuine causal effect.
- Why is causal research useful?
Causal research allows market researchers to predict hypothetical occurrences and outcomes while enhancing existing strategies. Organizations can use this concept to develop beneficial plans.
Causal research is also useful as market researchers can immediately deduce the effect of the variables on each other under real-world conditions.
Once researchers complete their first experiment, they can use their findings. Applying them to alternative scenarios or repeating the experiment to confirm its validity can produce further insights.
Businesses widely use causal research to identify and comprehend the effect of strategic changes on their profits.
- How does causal research compare and differ from other research types?
Other research types that identify relationships between variables include exploratory and descriptive research .
Here’s how they compare and differ from causal research designs:
Exploratory research
An exploratory research design evaluates situations where a problem or opportunity's boundaries are unclear. You can use this research type to test various hypotheses and assumptions to establish facts and understand a situation more clearly.
You can also use exploratory research design to navigate a topic and discover the relevant variables. This research type allows flexibility and adaptability as the experiment progresses, particularly since no area is off-limits.
It’s worth noting that exploratory research is unstructured and typically involves collecting qualitative data . This provides the freedom to tweak and amend the research approach according to your ongoing thoughts and assessments.
Unfortunately, this exposes the findings to the risk of bias and may limit the extent to which a researcher can explore a topic.
This table compares the key characteristics of causal and exploratory research:
Descriptive research
This research design involves capturing and describing the traits of a population, situation, or phenomenon. Descriptive research focuses more on the " what " of the research subject and less on the " why ."
Since descriptive research typically happens in a real-world setting, variables can cross-contaminate others. This increases the challenge of isolating cause-and-effect relationships.
You may require further research if you need more causal links.
This table compares the key characteristics of causal and descriptive research.
Causal research examines a research question’s variables and how they interact. It’s easier to pinpoint cause and effect since the experiment often happens in a controlled setting.
Researchers can conduct causal research at any stage, but they typically use it once they know more about the topic.
In contrast, causal research tends to be more structured and can be combined with exploratory and descriptive research to help you attain your research goals.
- How can you use causal research effectively?
Here are common ways that market researchers leverage causal research effectively:
Market and advertising research
Do you want to know if your new marketing campaign is affecting your organization positively? You can use causal research to determine the variables causing negative or positive impacts on your campaign.
Improving customer experiences and loyalty levels
Consumers generally enjoy purchasing from brands aligned with their values. They’re more likely to purchase from such brands and positively represent them to others.
You can use causal research to identify the variables contributing to increased or reduced customer acquisition and retention rates.
Could the cause of increased customer retention rates be streamlined checkout?
Perhaps you introduced a new solution geared towards directly solving their immediate problem.
Whatever the reason, causal research can help you identify the cause-and-effect relationship. You can use this to enhance your customer experiences and loyalty levels.
Improving problematic employee turnover rates
Is your organization experiencing skyrocketing attrition rates?
You can leverage the features and benefits of causal research to narrow down the possible explanations or variables with significant effects on employees quitting.
This way, you can prioritize interventions, focusing on the highest priority causal influences, and begin to tackle high employee turnover rates.
- Advantages of causal research
The main benefits of causal research include the following:
Effectively test new ideas
If causal research can pinpoint the precise outcome through combinations of different variables, researchers can test ideas in the same manner to form viable proof of concepts.
Achieve more objective results
Market researchers typically use random sampling techniques to choose experiment participants or subjects in causal research. This reduces the possibility of exterior, sample, or demography-based influences, generating more objective results.
Improved business processes
Causal research helps businesses understand which variables positively impact target variables, such as customer loyalty or sales revenues. This helps them improve their processes, ROI, and customer and employee experiences.
Guarantee reliable and accurate results
Upon identifying the correct variables, researchers can replicate cause and effect effortlessly. This creates reliable data and results to draw insights from.
Internal organization improvements
Businesses that conduct causal research can make informed decisions about improving their internal operations and enhancing employee experiences.
- Disadvantages of causal research
Like any other research method, casual research has its set of drawbacks that include:
Extra research to ensure validity
Researchers can't simply rely on the outcomes of causal research since it isn't always accurate. There may be a need to conduct other research types alongside it to ensure accurate output.
Coincidence
Coincidence tends to be the most significant error in causal research. Researchers often misinterpret a coincidental link between a cause and effect as a direct causal link.
Administration challenges
Causal research can be challenging to administer since it's impossible to control the impact of extraneous variables .
Giving away your competitive advantage
If you intend to publish your research, it exposes your information to the competition.
Competitors may use your research outcomes to identify your plans and strategies to enter the market before you.
- Causal research examples
Multiple fields can use causal research, so it serves different purposes, such as.
Customer loyalty research
Organizations and employees can use causal research to determine the best customer attraction and retention approaches.
They monitor interactions between customers and employees to identify cause-and-effect patterns. That could be a product demonstration technique resulting in higher or lower sales from the same customers.
Example: Business X introduces a new individual marketing strategy for a small customer group and notices a measurable increase in monthly subscriptions.
Upon getting identical results from different groups, the business concludes that the individual marketing strategy resulted in the intended causal relationship.
Advertising research
Businesses can also use causal research to implement and assess advertising campaigns.
Example: Business X notices a 7% increase in sales revenue a few months after a business introduces a new advertisement in a certain region. The business can run the same ad in random regions to compare sales data over the same period.
This will help the company determine whether the ad caused the sales increase. If sales increase in these randomly selected regions, the business could conclude that advertising campaigns and sales share a cause-and-effect relationship.
Educational research
Academics, teachers, and learners can use causal research to explore the impact of politics on learners and pinpoint learner behavior trends.
Example: College X notices that more IT students drop out of their program in their second year, which is 8% higher than any other year.
The college administration can interview a random group of IT students to identify factors leading to this situation, including personal factors and influences.
With the help of in-depth statistical analysis, the institution's researchers can uncover the main factors causing dropout. They can create immediate solutions to address the problem.
Is a causal variable dependent or independent?
When two variables have a cause-and-effect relationship, the cause is often called the independent variable. As such, the effect variable is dependent, i.e., it depends on the independent causal variable. An independent variable is only causal under experimental conditions.
What are the three criteria for causality?
The three conditions for causality are:
Temporality/temporal precedence: The cause must precede the effect.
Rationality: One event predicts the other with an explanation, and the effect must vary in proportion to changes in the cause.
Control for extraneous variables: The covariables must not result from other variables.
Is causal research experimental?
Causal research is mostly explanatory. Causal studies focus on analyzing a situation to explore and explain the patterns of relationships between variables.
Further, experiments are the primary data collection methods in studies with causal research design. However, as a research design, causal research isn't entirely experimental.
What is the difference between experimental and causal research design?
One of the main differences between causal and experimental research is that in causal research, the research subjects are already in groups since the event has already happened.
On the other hand, researchers randomly choose subjects in experimental research before manipulating the variables.
Get started today
Go from raw data to valuable insights with a flexible research platform
Editor’s picks
Last updated: 21 December 2023
Last updated: 16 December 2023
Last updated: 17 February 2024
Last updated: 19 November 2023
Last updated: 5 March 2024
Last updated: 15 February 2024
Last updated: 11 March 2024
Last updated: 12 December 2023
Last updated: 6 March 2024
Last updated: 10 April 2023
Last updated: 20 December 2023
Latest articles
Related topics, log in or sign up.
Get started for free
Chapter 10 Rhetorical Modes
10.8 cause and effect, learning objectives.
- Determine the purpose and structure of cause and effect in writing.
- Understand how to write a cause-and-effect essay.
The Purpose of Cause and Effect in Writing
It is often considered human nature to ask, “why?” and “how?” We want to know how our child got sick so we can better prevent it from happening in the future, or why our colleague a pay raise because we want one as well. We want to know how much money we will save over the long term if we buy a hybrid car. These examples identify only a few of the relationships we think about in our lives, but each shows the importance of understanding cause and effect.
A cause is something that produces an event or condition; an effect is what results from an event or condition. The purpose of the cause-and-effect essay is to determine how various phenomena relate in terms of origins and results. Sometimes the connection between cause and effect is clear, but often determining the exact relationship between the two is very difficult. For example, the following effects of a cold may be easily identifiable: a sore throat, runny nose, and a cough. But determining the cause of the sickness can be far more difficult. A number of causes are possible, and to complicate matters, these possible causes could have combined to cause the sickness. That is, more than one cause may be responsible for any given effect. Therefore, cause-and-effect discussions are often complicated and frequently lead to debates and arguments.
Use the complex nature of cause and effect to your advantage. Often it is not necessary, or even possible, to find the exact cause of an event or to name the exact effect. So, when formulating a thesis, you can claim one of a number of causes or effects to be the primary, or main, cause or effect. As soon as you claim that one cause or one effect is more crucial than the others, you have developed a thesis.
Consider the causes and effects in the following thesis statements. List a cause and effect for each one on your own sheet of paper.
- The growing childhood obesity epidemic is a result of technology.
- Much of the wildlife is dying because of the oil spill.
- The town continued programs that it could no longer afford, so it went bankrupt.
- More young people became politically active as use of the Internet spread throughout society.
- While many experts believed the rise in violence was due to the poor economy, it was really due to the summer-long heat wave.
Write three cause-and-effect thesis statements of your own for each of the following five broad topics.
- Health and nutrition
The Structure of a Cause-and-Effect Essay
The cause-and-effect essay opens with a general introduction to the topic, which then leads to a thesis that states the main cause, main effect, or various causes and effects of a condition or event.
The cause-and-effect essay can be organized in one of the following two primary ways:
- Start with the cause and then talk about the effects.
- Start with the effect and then talk about the causes.
For example, if your essay were on childhood obesity, you could start by talking about the effect of childhood obesity and then discuss the cause or you could start the same essay by talking about the cause of childhood obesity and then move to the effect.
Regardless of which structure you choose, be sure to explain each element of the essay fully and completely. Explaining complex relationships requires the full use of evidence, such as scientific studies, expert testimony, statistics, and anecdotes.
Because cause-and-effect essays determine how phenomena are linked, they make frequent use of certain words and phrases that denote such linkage. See Table 10.4 “Phrases of Causation” for examples of such terms.
Table 10.4 Phrases of Causation
The conclusion should wrap up the discussion and reinforce the thesis, leaving the reader with a clear understanding of the relationship that was analyzed.
Be careful of resorting to empty speculation. In writing, speculation amounts to unsubstantiated guessing. Writers are particularly prone to such trappings in cause-and-effect arguments due to the complex nature of finding links between phenomena. Be sure to have clear evidence to support the claims that you make.
Look at some of the cause-and-effect relationships from Note 10.83 “Exercise 2”. Outline the links you listed. Outline one using a cause-then-effect structure. Outline the other using the effect-then-cause structure.
Writing a Cause-and-Effect Essay
Choose an event or condition that you think has an interesting cause-and-effect relationship. Introduce your topic in an engaging way. End your introduction with a thesis that states the main cause, the main effect, or both.
Organize your essay by starting with either the cause-then-effect structure or the effect-then-cause structure. Within each section, you should clearly explain and support the causes and effects using a full range of evidence. If you are writing about multiple causes or multiple effects, you may choose to sequence either in terms of order of importance. In other words, order the causes from least to most important (or vice versa), or order the effects from least important to most important (or vice versa).
Use the phrases of causation when trying to forge connections between various events or conditions. This will help organize your ideas and orient the reader. End your essay with a conclusion that summarizes your main points and reinforces your thesis. See Chapter 15 “Readings: Examples of Essays” to read a sample cause-and-effect essay.
Choose one of the ideas you outlined in Note 10.85 “Exercise 3” and write a full cause-and-effect essay. Be sure to include an engaging introduction, a clear thesis, strong evidence and examples, and a thoughtful conclusion.
Key Takeaways
- The purpose of the cause-and-effect essay is to determine how various phenomena are related.
- The thesis states what the writer sees as the main cause, main effect, or various causes and effects of a condition or event.
- Start with the cause and then talk about the effect.
- Start with the effect and then talk about the cause.
- Strong evidence is particularly important in the cause-and-effect essay due to the complexity of determining connections between phenomena.
- Phrases of causation are helpful in signaling links between various elements in the essay.
- Successful Writing. Authored by : Anonymous. Provided by : Anonymous. Located at : http://2012books.lardbucket.org/books/successful-writing/ . License : CC BY-NC-SA: Attribution-NonCommercial-ShareAlike
- Bipolar Disorder
- Therapy Center
- When To See a Therapist
- Types of Therapy
- Best Online Therapy
- Best Couples Therapy
- Best Family Therapy
- Managing Stress
- Sleep and Dreaming
- Understanding Emotions
- Self-Improvement
- Healthy Relationships
- Student Resources
- Personality Types
- Verywell Mind Insights
- 2023 Verywell Mind 25
- Mental Health in the Classroom
- Editorial Process
- Meet Our Review Board
- Crisis Support
How to Write a Great Hypothesis
Hypothesis Format, Examples, and Tips
Kendra Cherry, MS, is a psychosocial rehabilitation specialist, psychology educator, and author of the "Everything Psychology Book."
:max_bytes(150000):strip_icc():format(webp)/IMG_9791-89504ab694d54b66bbd72cb84ffb860e.jpg)
Amy Morin, LCSW, is a psychotherapist and international bestselling author. Her books, including "13 Things Mentally Strong People Don't Do," have been translated into more than 40 languages. Her TEDx talk, "The Secret of Becoming Mentally Strong," is one of the most viewed talks of all time.
:max_bytes(150000):strip_icc():format(webp)/VW-MIND-Amy-2b338105f1ee493f94d7e333e410fa76.jpg)
Verywell / Alex Dos Diaz
- The Scientific Method
Hypothesis Format
Falsifiability of a hypothesis, operational definitions, types of hypotheses, hypotheses examples.
- Collecting Data
Frequently Asked Questions
A hypothesis is a tentative statement about the relationship between two or more variables. It is a specific, testable prediction about what you expect to happen in a study.
One hypothesis example would be a study designed to look at the relationship between sleep deprivation and test performance might have a hypothesis that states: "This study is designed to assess the hypothesis that sleep-deprived people will perform worse on a test than individuals who are not sleep-deprived."
This article explores how a hypothesis is used in psychology research, how to write a good hypothesis, and the different types of hypotheses you might use.
The Hypothesis in the Scientific Method
In the scientific method , whether it involves research in psychology, biology, or some other area, a hypothesis represents what the researchers think will happen in an experiment. The scientific method involves the following steps:
- Forming a question
- Performing background research
- Creating a hypothesis
- Designing an experiment
- Collecting data
- Analyzing the results
- Drawing conclusions
- Communicating the results
The hypothesis is a prediction, but it involves more than a guess. Most of the time, the hypothesis begins with a question which is then explored through background research. It is only at this point that researchers begin to develop a testable hypothesis. Unless you are creating an exploratory study, your hypothesis should always explain what you expect to happen.
In a study exploring the effects of a particular drug, the hypothesis might be that researchers expect the drug to have some type of effect on the symptoms of a specific illness. In psychology, the hypothesis might focus on how a certain aspect of the environment might influence a particular behavior.
Remember, a hypothesis does not have to be correct. While the hypothesis predicts what the researchers expect to see, the goal of the research is to determine whether this guess is right or wrong. When conducting an experiment, researchers might explore a number of factors to determine which ones might contribute to the ultimate outcome.
In many cases, researchers may find that the results of an experiment do not support the original hypothesis. When writing up these results, the researchers might suggest other options that should be explored in future studies.
In many cases, researchers might draw a hypothesis from a specific theory or build on previous research. For example, prior research has shown that stress can impact the immune system. So a researcher might hypothesize: "People with high-stress levels will be more likely to contract a common cold after being exposed to the virus than people who have low-stress levels."
In other instances, researchers might look at commonly held beliefs or folk wisdom. "Birds of a feather flock together" is one example of folk wisdom that a psychologist might try to investigate. The researcher might pose a specific hypothesis that "People tend to select romantic partners who are similar to them in interests and educational level."
Elements of a Good Hypothesis
So how do you write a good hypothesis? When trying to come up with a hypothesis for your research or experiments, ask yourself the following questions:
- Is your hypothesis based on your research on a topic?
- Can your hypothesis be tested?
- Does your hypothesis include independent and dependent variables?
Before you come up with a specific hypothesis, spend some time doing background research. Once you have completed a literature review, start thinking about potential questions you still have. Pay attention to the discussion section in the journal articles you read . Many authors will suggest questions that still need to be explored.
To form a hypothesis, you should take these steps:
- Collect as many observations about a topic or problem as you can.
- Evaluate these observations and look for possible causes of the problem.
- Create a list of possible explanations that you might want to explore.
- After you have developed some possible hypotheses, think of ways that you could confirm or disprove each hypothesis through experimentation. This is known as falsifiability.
In the scientific method , falsifiability is an important part of any valid hypothesis. In order to test a claim scientifically, it must be possible that the claim could be proven false.
Students sometimes confuse the idea of falsifiability with the idea that it means that something is false, which is not the case. What falsifiability means is that if something was false, then it is possible to demonstrate that it is false.
One of the hallmarks of pseudoscience is that it makes claims that cannot be refuted or proven false.
A variable is a factor or element that can be changed and manipulated in ways that are observable and measurable. However, the researcher must also define how the variable will be manipulated and measured in the study.
For example, a researcher might operationally define the variable " test anxiety " as the results of a self-report measure of anxiety experienced during an exam. A "study habits" variable might be defined by the amount of studying that actually occurs as measured by time.
These precise descriptions are important because many things can be measured in a number of different ways. One of the basic principles of any type of scientific research is that the results must be replicable. By clearly detailing the specifics of how the variables were measured and manipulated, other researchers can better understand the results and repeat the study if needed.
Some variables are more difficult than others to define. How would you operationally define a variable such as aggression ? For obvious ethical reasons, researchers cannot create a situation in which a person behaves aggressively toward others.
In order to measure this variable, the researcher must devise a measurement that assesses aggressive behavior without harming other people. In this situation, the researcher might utilize a simulated task to measure aggressiveness.
Hypothesis Checklist
- Does your hypothesis focus on something that you can actually test?
- Does your hypothesis include both an independent and dependent variable?
- Can you manipulate the variables?
- Can your hypothesis be tested without violating ethical standards?
The hypothesis you use will depend on what you are investigating and hoping to find. Some of the main types of hypotheses that you might use include:
- Simple hypothesis : This type of hypothesis suggests that there is a relationship between one independent variable and one dependent variable.
- Complex hypothesis : This type of hypothesis suggests a relationship between three or more variables, such as two independent variables and a dependent variable.
- Null hypothesis : This hypothesis suggests no relationship exists between two or more variables.
- Alternative hypothesis : This hypothesis states the opposite of the null hypothesis.
- Statistical hypothesis : This hypothesis uses statistical analysis to evaluate a representative sample of the population and then generalizes the findings to the larger group.
- Logical hypothesis : This hypothesis assumes a relationship between variables without collecting data or evidence.
A hypothesis often follows a basic format of "If {this happens} then {this will happen}." One way to structure your hypothesis is to describe what will happen to the dependent variable if you change the independent variable .
The basic format might be: "If {these changes are made to a certain independent variable}, then we will observe {a change in a specific dependent variable}."
A few examples of simple hypotheses:
- "Students who eat breakfast will perform better on a math exam than students who do not eat breakfast."
- Complex hypothesis: "Students who experience test anxiety before an English exam will get lower scores than students who do not experience test anxiety."
- "Motorists who talk on the phone while driving will be more likely to make errors on a driving course than those who do not talk on the phone."
Examples of a complex hypothesis include:
- "People with high-sugar diets and sedentary activity levels are more likely to develop depression."
- "Younger people who are regularly exposed to green, outdoor areas have better subjective well-being than older adults who have limited exposure to green spaces."
Examples of a null hypothesis include:
- "Children who receive a new reading intervention will have scores different than students who do not receive the intervention."
- "There will be no difference in scores on a memory recall task between children and adults."
Examples of an alternative hypothesis:
- "Children who receive a new reading intervention will perform better than students who did not receive the intervention."
- "Adults will perform better on a memory task than children."
Collecting Data on Your Hypothesis
Once a researcher has formed a testable hypothesis, the next step is to select a research design and start collecting data. The research method depends largely on exactly what they are studying. There are two basic types of research methods: descriptive research and experimental research.
Descriptive Research Methods
Descriptive research such as case studies , naturalistic observations , and surveys are often used when it would be impossible or difficult to conduct an experiment . These methods are best used to describe different aspects of a behavior or psychological phenomenon.
Once a researcher has collected data using descriptive methods, a correlational study can then be used to look at how the variables are related. This type of research method might be used to investigate a hypothesis that is difficult to test experimentally.
Experimental Research Methods
Experimental methods are used to demonstrate causal relationships between variables. In an experiment, the researcher systematically manipulates a variable of interest (known as the independent variable) and measures the effect on another variable (known as the dependent variable).
Unlike correlational studies, which can only be used to determine if there is a relationship between two variables, experimental methods can be used to determine the actual nature of the relationship—whether changes in one variable actually cause another to change.
A Word From Verywell
The hypothesis is a critical part of any scientific exploration. It represents what researchers expect to find in a study or experiment. In situations where the hypothesis is unsupported by the research, the research still has value. Such research helps us better understand how different aspects of the natural world relate to one another. It also helps us develop new hypotheses that can then be tested in the future.
Some examples of how to write a hypothesis include:
- "Staying up late will lead to worse test performance the next day."
- "People who consume one apple each day will visit the doctor fewer times each year."
- "Breaking study sessions up into three 20-minute sessions will lead to better test results than a single 60-minute study session."
The four parts of a hypothesis are:
- The research question
- The independent variable (IV)
- The dependent variable (DV)
- The proposed relationship between the IV and DV
Castillo M. The scientific method: a need for something better? . AJNR Am J Neuroradiol. 2013;34(9):1669-71. doi:10.3174/ajnr.A3401
Nevid J. Psychology: Concepts and Applications. Wadworth, 2013.
By Kendra Cherry, MSEd Kendra Cherry, MS, is a psychosocial rehabilitation specialist, psychology educator, and author of the "Everything Psychology Book."

Want to create or adapt books like this? Learn more about how Pressbooks supports open publishing practices.
10.8 Cause and Effect
Learning objectives.
- Determine the purpose and structure of cause and effect in writing.
- Understand how to write a cause-and-effect essay.
The Purpose of Cause and Effect in Writing
It is often considered human nature to ask, “why?” and “how?” We want to know how our child got sick so we can better prevent it from happening in the future, or why our colleague a pay raise because we want one as well. We want to know how much money we will save over the long term if we buy a hybrid car. These examples identify only a few of the relationships we think about in our lives, but each shows the importance of understanding cause and effect.
A cause is something that produces an event or condition; an effect is what results from an event or condition. The purpose of the cause-and-effect essay is to determine how various phenomena relate in terms of origins and results. Sometimes the connection between cause and effect is clear, but often determining the exact relationship between the two is very difficult. For example, the following effects of a cold may be easily identifiable: a sore throat, runny nose, and a cough. But determining the cause of the sickness can be far more difficult. A number of causes are possible, and to complicate matters, these possible causes could have combined to cause the sickness. That is, more than one cause may be responsible for any given effect. Therefore, cause-and-effect discussions are often complicated and frequently lead to debates and arguments.
Use the complex nature of cause and effect to your advantage. Often it is not necessary, or even possible, to find the exact cause of an event or to name the exact effect. So, when formulating a thesis, you can claim one of a number of causes or effects to be the primary, or main, cause or effect. As soon as you claim that one cause or one effect is more crucial than the others, you have developed a thesis.
Consider the causes and effects in the following thesis statements. List a cause and effect for each one on your own sheet of paper.
- The growing childhood obesity epidemic is a result of technology.
- Much of the wildlife is dying because of the oil spill.
- The town continued programs that it could no longer afford, so it went bankrupt.
- More young people became politically active as use of the Internet spread throughout society.
- While many experts believed the rise in violence was due to the poor economy, it was really due to the summer-long heat wave.
Write three cause-and-effect thesis statements of your own for each of the following five broad topics.
- Health and nutrition
The Structure of a Cause-and-Effect Essay
The cause-and-effect essay opens with a general introduction to the topic, which then leads to a thesis that states the main cause, main effect, or various causes and effects of a condition or event.
The cause-and-effect essay can be organized in one of the following two primary ways:
- Start with the cause and then talk about the effects.
- Start with the effect and then talk about the causes.
For example, if your essay were on childhood obesity, you could start by talking about the effect of childhood obesity and then discuss the cause or you could start the same essay by talking about the cause of childhood obesity and then move to the effect.
Regardless of which structure you choose, be sure to explain each element of the essay fully and completely. Explaining complex relationships requires the full use of evidence, such as scientific studies, expert testimony, statistics, and anecdotes.
Because cause-and-effect essays determine how phenomena are linked, they make frequent use of certain words and phrases that denote such linkage. See Table 10.4 “Phrases of Causation” for examples of such terms.
Table 10.4 Phrases of Causation
The conclusion should wrap up the discussion and reinforce the thesis, leaving the reader with a clear understanding of the relationship that was analyzed.
Be careful of resorting to empty speculation. In writing, speculation amounts to unsubstantiated guessing. Writers are particularly prone to such trappings in cause-and-effect arguments due to the complex nature of finding links between phenomena. Be sure to have clear evidence to support the claims that you make.
Look at some of the cause-and-effect relationships from Note 10.83 “Exercise 2” . Outline the links you listed. Outline one using a cause-then-effect structure. Outline the other using the effect-then-cause structure.
Writing a Cause-and-Effect Essay
Choose an event or condition that you think has an interesting cause-and-effect relationship. Introduce your topic in an engaging way. End your introduction with a thesis that states the main cause, the main effect, or both.
Organize your essay by starting with either the cause-then-effect structure or the effect-then-cause structure. Within each section, you should clearly explain and support the causes and effects using a full range of evidence. If you are writing about multiple causes or multiple effects, you may choose to sequence either in terms of order of importance. In other words, order the causes from least to most important (or vice versa), or order the effects from least important to most important (or vice versa).
Use the phrases of causation when trying to forge connections between various events or conditions. This will help organize your ideas and orient the reader. End your essay with a conclusion that summarizes your main points and reinforces your thesis. See Chapter 15 “Readings: Examples of Essays” to read a sample cause-and-effect essay.
Choose one of the ideas you outlined in Note 10.85 “Exercise 3” and write a full cause-and-effect essay. Be sure to include an engaging introduction, a clear thesis, strong evidence and examples, and a thoughtful conclusion.
Key Takeaways
- The purpose of the cause-and-effect essay is to determine how various phenomena are related.
- The thesis states what the writer sees as the main cause, main effect, or various causes and effects of a condition or event.
The cause-and-effect essay can be organized in one of these two primary ways:
- Start with the cause and then talk about the effect.
- Start with the effect and then talk about the cause.
- Strong evidence is particularly important in the cause-and-effect essay due to the complexity of determining connections between phenomena.
- Phrases of causation are helpful in signaling links between various elements in the essay.
Writing for Success Copyright © 2015 by University of Minnesota is licensed under a Creative Commons Attribution-NonCommercial-ShareAlike 4.0 International License , except where otherwise noted.
- cognitive sophistication
- tolerance of diversity
- exposure to higher levels of math or science
- age (which is currently related to educational level in many countries)
- social class and other variables.
- For example, suppose you designed a treatment to help people stop smoking. Because you are really dedicated, you assigned the same individuals simultaneously to (1) a "stop smoking" nicotine patch; (2) a "quit buddy"; and (3) a discussion support group. Compared with a group in which no intervention at all occurred, your experimental group now smokes 10 fewer cigarettes per day.
- There is no relationship among two or more variables (EXAMPLE: the correlation between educational level and income is zero)
- Or that two or more populations or subpopulations are essentially the same (EXAMPLE: women and men have the same average science knowledge scores.)
- the difference between two and three children = one child.
- the difference between eight and nine children also = one child.
- the difference between completing ninth grade and tenth grade is one year of school
- the difference between completing junior and senior year of college is one year of school
- In addition to all the properties of nominal, ordinal, and interval variables, ratio variables also have a fixed/non-arbitrary zero point. Non arbitrary means that it is impossible to go below a score of zero for that variable. For example, any bottom score on IQ or aptitude tests is created by human beings and not nature. On the other hand, scientists believe they have isolated an "absolute zero." You can't get colder than that.
How to Write a Hypothesis
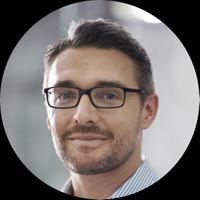
If I [do something], then [this] will happen.
This basic statement/formula should be pretty familiar to all of you as it is the starting point of almost every scientific project or paper. It is a hypothesis – a statement that showcases what you “think” will happen during an experiment. This assumption is made based on the knowledge, facts, and data you already have.
How do you write a hypothesis? If you have a clear understanding of the proper structure of a hypothesis, you should not find it too hard to create one. However, if you have never written a hypothesis before, you might find it a bit frustrating. In this article from EssayPro - custom essay writing services , we are going to tell you everything you need to know about hypotheses, their types, and practical tips for writing them.
Hypothesis Definition
According to the definition, a hypothesis is an assumption one makes based on existing knowledge. To elaborate, it is a statement that translates the initial research question into a logical prediction shaped on the basis of available facts and evidence. To solve a specific problem, one first needs to identify the research problem (research question), conduct initial research, and set out to answer the given question by performing experiments and observing their outcomes. However, before one can move to the experimental part of the research, they should first identify what they expect to see for results. At this stage, a scientist makes an educated guess and writes a hypothesis that he or she is going to prove or refute in the course of their study.
Get Help With Writing a Hypothesis Now!
Head on over to EssayPro. We can help you with editing and polishing up any of the work you speedwrite.
A hypothesis can also be seen as a form of development of knowledge. It is a well-grounded assumption put forward to clarify the properties and causes of the phenomena being studied.
As a rule, a hypothesis is formed based on a number of observations and examples that confirm it. This way, it looks plausible as it is backed up with some known information. The hypothesis is subsequently proved by turning it into an established fact or refuted (for example, by pointing out a counterexample), which allows it to attribute it to the category of false statements.
As a student, you may be asked to create a hypothesis statement as a part of your academic papers. Hypothesis-based approaches are commonly used among scientific academic works, including but not limited to research papers, theses, and dissertations.
Note that in some disciplines, a hypothesis statement is called a thesis statement. However, its essence and purpose remain unchanged – this statement aims to make an assumption regarding the outcomes of the investigation that will either be proved or refuted.
Characteristics and Sources of a Hypothesis
Now, as you know what a hypothesis is in a nutshell, let’s look at the key characteristics that define it:
- It has to be clear and accurate in order to look reliable.
- It has to be specific.
- There should be scope for further investigation and experiments.
- A hypothesis should be explained in simple language—while retaining its significance.
- If you are making a relational hypothesis, two essential elements you have to include are variables and the relationship between them.
The main sources of a hypothesis are:
- Scientific theories.
- Observations from previous studies and current experiences.
- The resemblance among different phenomena.
- General patterns that affect people’s thinking process.
Types of Hypothesis
Basically, there are two major types of scientific hypothesis: alternative and null.
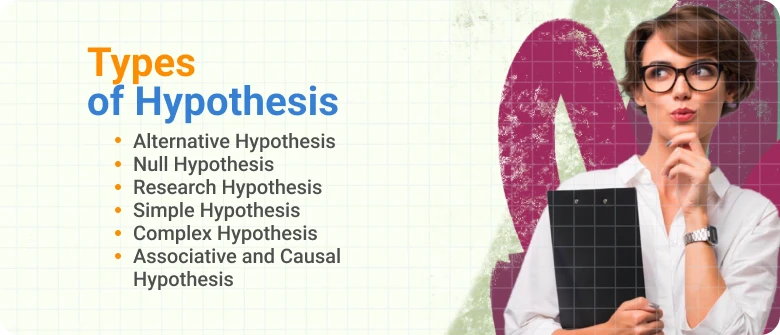
- Alternative Hypothesis
This type of hypothesis is generally denoted as H1. This statement is used to identify the expected outcome of your research. According to the alternative hypothesis definition, this type of hypothesis can be further divided into two subcategories:
- Directional — a statement that explains the direction of the expected outcomes. Sometimes this type of hypothesis is used to study the relationship between variables rather than comparing between the groups.
- Non-directional — unlike the directional alternative hypothesis, a non-directional one does not imply a specific direction of the expected outcomes.
Now, let’s see an alternative hypothesis example for each type:
Directional: Attending more lectures will result in improved test scores among students. Non-directional: Lecture attendance will influence test scores among students.
Notice how in the directional hypothesis we specified that the attendance of more lectures will boost student’s performance on tests, whereas in the non-directional hypothesis we only stated that there is a relationship between the two variables (i.e. lecture attendance and students’ test scores) but did not specify whether the performance will improve or decrease.
- Null Hypothesis
This type of hypothesis is generally denoted as H0. This statement is the complete opposite of what you expect or predict will happen throughout the course of your study—meaning it is the opposite of your alternative hypothesis. Simply put, a null hypothesis claims that there is no exact or actual correlation between the variables defined in the hypothesis.
To give you a better idea of how to write a null hypothesis, here is a clear example: Lecture attendance has no effect on student’s test scores.
Both of these types of hypotheses provide specific clarifications and restatements of the research problem. The main difference between these hypotheses and a research problem is that the latter is just a question that can’t be tested, whereas hypotheses can.
Based on the alternative and null hypothesis examples provided earlier, we can conclude that the importance and main purpose of these hypotheses are that they deliver a rough description of the subject matter. The main purpose of these statements is to give an investigator a specific guess that can be directly tested in a study. Simply put, a hypothesis outlines the framework, scope, and direction for the study. Although null and alternative hypotheses are the major types, there are also a few more to keep in mind:
Research Hypothesis — a statement that is used to test the correlation between two or more variables.
For example: Eating vitamin-rich foods affects human health.
Simple Hypothesis — a statement used to indicate the correlation between one independent and one dependent variable.
For example: Eating more vegetables leads to better immunity.
Complex Hypothesis — a statement used to indicate the correlation between two or more independent variables and two or more dependent variables.
For example: Eating more fruits and vegetables leads to better immunity, weight loss, and lower risk of diseases.
Associative and Causal Hypothesis — an associative hypothesis is a statement used to indicate the correlation between variables under the scenario when a change in one variable inevitably changes the other variable. A causal hypothesis is a statement that highlights the cause and effect relationship between variables.
Be sure to read how to write a DBQ - this article will expand your understanding.
Add a secret ingredient to your hypothesis
Help of a professional writer.
Hypothesis vs Prediction
When speaking of hypotheses, another term that comes to mind is prediction. These two terms are often used interchangeably, which can be rather confusing. Although both a hypothesis and prediction can generally be defined as “guesses” and can be easy to confuse, these terms are different. The main difference between a hypothesis and a prediction is that the first is predominantly used in science, while the latter is most often used outside of science.
Simply put, a hypothesis is an intelligent assumption. It is a guess made regarding the nature of the unknown (or less known) phenomena based on existing knowledge, studies, and/or series of experiments, and is otherwise grounded by valid facts. The main purpose of a hypothesis is to use available facts to create a logical relationship between variables in order to provide a more precise scientific explanation. Additionally, hypotheses are statements that can be tested with further experiments. It is an assumption you make regarding the flow and outcome(s) of your research study.
A prediction, on the contrary, is a guess that often lacks grounding. Although, in theory, a prediction can be scientific, in most cases it is rather fictional—i.e. a pure guess that is not based on current knowledge and/or facts. As a rule, predictions are linked to foretelling events that may or may not occur in the future. Often, a person who makes predictions has little or no actual knowledge of the subject matter he or she makes the assumption about.
Another big difference between these terms is in the methodology used to prove each of them. A prediction can only be proven once. You can determine whether it is right or wrong only upon the occurrence or non-occurrence of the predicted event. A hypothesis, on the other hand, offers scope for further testing and experiments. Additionally, a hypothesis can be proven in multiple stages. This basically means that a single hypothesis can be proven or refuted numerous times by different scientists who use different scientific tools and methods.
To give you a better idea of how a hypothesis is different from a prediction, let’s look at the following examples:
Hypothesis: If I eat more vegetables and fruits, then I will lose weight faster.
This is a hypothesis because it is based on generally available knowledge (i.e. fruits and vegetables include fewer calories compared to other foods) and past experiences (i.e. people who give preference to healthier foods like fruits and vegetables are losing weight easier). It is still a guess, but it is based on facts and can be tested with an experiment.
Prediction: The end of the world will occur in 2023.
This is a prediction because it foretells future events. However, this assumption is fictional as it doesn’t have any actual grounded evidence supported by facts.
Based on everything that was said earlier and our examples, we can highlight the following key takeaways:
- A hypothesis, unlike a prediction, is a more intelligent assumption based on facts.
- Hypotheses define existing variables and analyze the relationship(s) between them.
- Predictions are most often fictional and lack grounding.
- A prediction is most often used to foretell events in the future.
- A prediction can only be proven once – when the predicted event occurs or doesn’t occur.
- A hypothesis can remain a hypothesis even if one scientist has already proven or disproven it. Other scientists in the future can obtain a different result using other methods and tools.
We also recommend that you read about some informative essay topics .
Now, as you know what a hypothesis is, what types of it exist, and how it differs from a prediction, you are probably wondering how to state a hypothesis. In this section, we will guide you through the main stages of writing a good hypothesis and provide handy tips and examples to help you overcome this challenge:
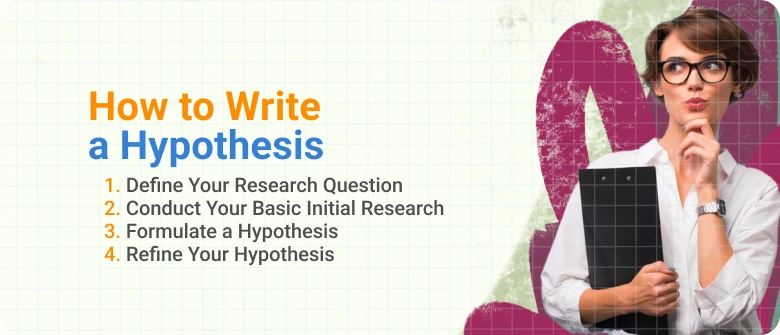
1. Define Your Research Question
Here is one thing to keep in mind – regardless of the paper or project you are working on, the process should always start with asking the right research question. A perfect research question should be specific, clear, focused (meaning not too broad), and manageable.
Example: How does eating fruits and vegetables affect human health?
2. Conduct Your Basic Initial Research
As you already know, a hypothesis is an educated guess of the expected results and outcomes of an investigation. Thus, it is vital to collect some information before you can make this assumption.
At this stage, you should find an answer to your research question based on what has already been discovered. Search for facts, past studies, theories, etc. Based on the collected information, you should be able to make a logical and intelligent guess.
3. Formulate a Hypothesis
Based on the initial research, you should have a certain idea of what you may find throughout the course of your research. Use this knowledge to shape a clear and concise hypothesis.
Based on the type of project you are working on, and the type of hypothesis you are planning to use, you can restate your hypothesis in several different ways:
Non-directional: Eating fruits and vegetables will affect one’s human physical health. Directional: Eating fruits and vegetables will positively affect one’s human physical health. Null: Eating fruits and vegetables will have no effect on one’s human physical health.
4. Refine Your Hypothesis
Finally, the last stage of creating a good hypothesis is refining what you’ve got. During this step, you need to define whether your hypothesis:
- Has clear and relevant variables;
- Identifies the relationship between its variables;
- Is specific and testable;
- Suggests a predicted result of the investigation or experiment.
In case you need some help with your essay, leave us a notice ' pay someone to write my essay ' and we'll help asap. We also provide nursing writing services .
Hypothesis Examples
Following a step-by-step guide and tips from our essay writers for hire , you should be able to create good hypotheses with ease. To give you a starting point, we have also compiled a list of different research questions with one hypothesis and one null hypothesis example for each:
Ask Pros to Make a Perfect Hypothesis for You!
Sometimes, coping with a large academic load is just too much for a student to handle. Papers like research papers and dissertations can take too much time and effort to write, and, often, a hypothesis is a necessary starting point to get the task on track. Writing or editing a hypothesis is not as easy as it may seem. However, if you need help with forming it, the team at EssayPro is always ready to come to your rescue! If you’re feeling stuck, or don’t have enough time to cope with other tasks, don’t hesitate to send us you rewrite my essay for me or any other request.
Related Articles
.webp)
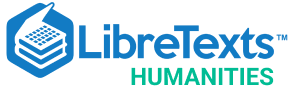
- school Campus Bookshelves
- menu_book Bookshelves
- perm_media Learning Objects
- login Login
- how_to_reg Request Instructor Account
- hub Instructor Commons
- Download Page (PDF)
- Download Full Book (PDF)
- Periodic Table
- Physics Constants
- Scientific Calculator
- Reference & Cite
- Tools expand_more
- Readability
selected template will load here
This action is not available.
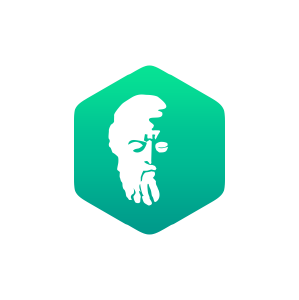
12.1: The Purpose of Cause and Effect in Writing
- Last updated
- Save as PDF
- Page ID 6297
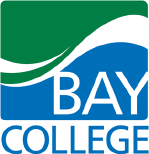
- Amber Kinonen, Jennifer McCann, Todd McCann, & Erica Mead
- Bay College Library
It is often considered human nature to ask, “why?” and “how?” We want to know how our child got sick so we can better prevent it from happening in the future, or why our colleague received a pay raise because we want one as well. We want to know how much money we will save over the long term if we buy a hybrid car. These examples identify only a few of the relationships we think about in our lives, but each shows the importance of understanding cause and effect.
A cause is something that produces an event or condition; an effect is what results from an event or condition. The purpose of the cause-and-effect essay is to determine how various phenomena relate in terms of origins and results. Sometimes the connection between cause and effect is clear, but often determining the exact relationship between the two is very difficult. For example, the following effects of a cold may be easily identifiable: a sore throat, runny nose, and a cough. However, determining the cause of the sickness can be far more difficult. A number of causes are possible, and to complicate matters, these possible causes could have combined to cause the sickness. That is, more than one cause may be responsible for any given effect. Therefore, cause-and-effect discussions are often complicated and frequently lead to debates and arguments.
Use the complex nature of cause and effect to your advantage. Often it is not necessary, or even possible, to find the exact cause of an event or to name the exact effect. So, when formulating a thesis, you can claim one of a number of causes or effects to be the primary, or main, cause or effect. As soon as you claim that one cause or one effect is more significant than the others, you have developed a thesis.

Writing a Strong Hypothesis Statement
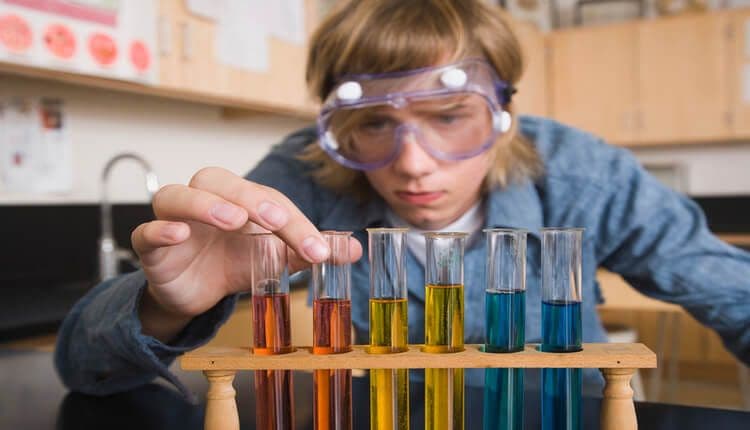
All good theses begins with a good thesis question. However, all great theses begins with a great hypothesis statement. One of the most important steps for writing a thesis is to create a strong hypothesis statement.
What is a hypothesis statement?
A hypothesis statement must be testable. If it cannot be tested, then there is no research to be done.
Simply put, a hypothesis statement posits the relationship between two or more variables. It is a prediction of what you think will happen in a research study. A hypothesis statement must be testable. If it cannot be tested, then there is no research to be done. If your thesis question is whether wildfires have effects on the weather, “wildfires create tornadoes” would be your hypothesis. However, a hypothesis needs to have several key elements in order to meet the criteria for a good hypothesis.
In this article, we will learn about what distinguishes a weak hypothesis from a strong one. We will also learn how to phrase your thesis question and frame your variables so that you are able to write a strong hypothesis statement and great thesis.
What is a hypothesis?
A hypothesis statement posits, or considers, a relationship between two variables.
As we mentioned above, a hypothesis statement posits or considers a relationship between two variables. In our hypothesis statement example above, the two variables are wildfires and tornadoes, and our assumed relationship between the two is a causal one (wildfires cause tornadoes). It is clear from our example above what we will be investigating: the relationship between wildfires and tornadoes.
A strong hypothesis statement should be:
- A prediction of the relationship between two or more variables
A hypothesis is not just a blind guess. It should build upon existing theories and knowledge . Tornadoes are often observed near wildfires once the fires reach a certain size. In addition, tornadoes are not a normal weather event in many areas; they have been spotted together with wildfires. This existing knowledge has informed the formulation of our hypothesis.
Depending on the thesis question, your research paper might have multiple hypothesis statements. What is important is that your hypothesis statement or statements are testable through data analysis, observation, experiments, or other methodologies.
Formulating your hypothesis
One of the best ways to form a hypothesis is to think about “if...then” statements.
Now that we know what a hypothesis statement is, let’s walk through how to formulate a strong one. First, you will need a thesis question. Your thesis question should be narrow in scope, answerable, and focused. Once you have your thesis question, it is time to start thinking about your hypothesis statement. You will need to clearly identify the variables involved before you can begin thinking about their relationship.
One of the best ways to form a hypothesis is to think about “if...then” statements . This can also help you easily identify the variables you are working with and refine your hypothesis statement. Let’s take a few examples.
If teenagers are given comprehensive sex education, there will be fewer teen pregnancies .
In this example, the independent variable is whether or not teenagers receive comprehensive sex education (the cause), and the dependent variable is the number of teen pregnancies (the effect).
If a cat is fed a vegan diet, it will die .
Here, our independent variable is the diet of the cat (the cause), and the dependent variable is the cat’s health (the thing impacted by the cause).
If children drink 8oz of milk per day, they will grow taller than children who do not drink any milk .
What are the variables in this hypothesis? If you identified drinking milk as the independent variable and growth as the dependent variable, you are correct. This is because we are guessing that drinking milk causes increased growth in the height of children.
Refining your hypothesis
Do not be afraid to refine your hypothesis throughout the process of formulation.
Do not be afraid to refine your hypothesis throughout the process of formulation. A strong hypothesis statement is clear, testable, and involves a prediction. While “testable” means verifiable or falsifiable, it also means that you are able to perform the necessary experiments without violating any ethical standards. Perhaps once you think about the ethics of possibly harming some cats by testing a vegan diet on them you might abandon the idea of that experiment altogether. However, if you think it is really important to research the relationship between a cat’s diet and a cat’s health, perhaps you could refine your hypothesis to something like this:
If 50% of a cat’s meals are vegan, the cat will not be able to meet its nutritional needs .
Another feature of a strong hypothesis statement is that it can easily be tested with the resources that you have readily available. While it might not be feasible to measure the growth of a cohort of children throughout their whole lives, you may be able to do so for a year. Then, you can adjust your hypothesis to something like this:
I f children aged 8 drink 8oz of milk per day for one year, they will grow taller during that year than children who do not drink any milk .
As you work to narrow down and refine your hypothesis to reflect a realistic potential research scope, don’t be afraid to talk to your supervisor about any concerns or questions you might have about what is truly possible to research.
What makes a hypothesis weak?
We noted above that a strong hypothesis statement is clear, is a prediction of a relationship between two or more variables, and is testable. We also clarified that statements, which are too general or specific are not strong hypotheses. We have looked at some examples of hypotheses that meet the criteria for a strong hypothesis, but before we go any further, let’s look at weak or bad hypothesis statement examples so that you can really see the difference.
Bad hypothesis 1: Diabetes is caused by witchcraft .
While this is fun to think about, it cannot be tested or proven one way or the other with clear evidence, data analysis, or experiments. This bad hypothesis fails to meet the testability requirement.
Bad hypothesis 2: If I change the amount of food I eat, my energy levels will change .
This is quite vague. Am I increasing or decreasing my food intake? What do I expect exactly will happen to my energy levels and why? How am I defining energy level? This bad hypothesis statement fails the clarity requirement.
Bad hypothesis 3: Japanese food is disgusting because Japanese people don’t like tourists .
This hypothesis is unclear about the posited relationship between variables. Are we positing the relationship between the deliciousness of Japanese food and the desire for tourists to visit? or the relationship between the deliciousness of Japanese food and the amount that Japanese people like tourists? There is also the problematic subjectivity of the assessment that Japanese food is “disgusting.” The problems are numerous.
The null hypothesis and the alternative hypothesis
The null hypothesis, quite simply, posits that there is no relationship between the variables.
What is the null hypothesis?
The hypothesis posits a relationship between two or more variables. The null hypothesis, quite simply, posits that there is no relationship between the variables. It is often indicated as H 0 , which is read as “h-oh” or “h-null.” The alternative hypothesis is the opposite of the null hypothesis as it posits that there is some relationship between the variables. The alternative hypothesis is written as H a or H 1 .
Let’s take our previous hypothesis statement examples discussed at the start and look at their corresponding null hypothesis.
H a : If teenagers are given comprehensive sex education, there will be fewer teen pregnancies .
H 0 : If teenagers are given comprehensive sex education, there will be no change in the number of teen pregnancies .
The null hypothesis assumes that comprehensive sex education will not affect how many teenagers get pregnant. It should be carefully noted that the null hypothesis is not always the opposite of the alternative hypothesis. For example:
If teenagers are given comprehensive sex education, there will be more teen pregnancies .
These are opposing statements that assume an opposite relationship between the variables: comprehensive sex education increases or decreases the number of teen pregnancies. In fact, these are both alternative hypotheses. This is because they both still assume that there is a relationship between the variables . In other words, both hypothesis statements assume that there is some kind of relationship between sex education and teen pregnancy rates. The alternative hypothesis is also the researcher’s actual predicted outcome, which is why calling it “alternative” can be confusing! However, you can think of it this way: our default assumption is the null hypothesis, and so any possible relationship is an alternative to the default.
Step-by-step sample hypothesis statements
Now that we’ve covered what makes a hypothesis statement strong, how to go about formulating a hypothesis statement, refining your hypothesis statement, and the null hypothesis, let’s put it all together with some examples. The table below shows a breakdown of how we can take a thesis question, identify the variables, create a null hypothesis, and finally create a strong alternative hypothesis.
Once you have formulated a solid thesis question and written a strong hypothesis statement, you are ready to begin your thesis in earnest. Check out our site for more tips on writing a great thesis and information on thesis proofreading and editing services.
Editor’s pick
Get free updates.
Subscribe to our newsletter for regular insights from the research and publishing industry!
Review Checklist
Start with a clear thesis question
Think about “if-then” statements to identify your variables and the relationship between them
Create a null hypothesis
Formulate an alternative hypothesis using the variables you have identified
Make sure your hypothesis clearly posits a relationship between variables
Make sure your hypothesis is testable considering your available time and resources
What makes a hypothesis strong? +
A hypothesis is strong when it is testable, clear, and identifies a potential relationship between two or more variables.
What makes a hypothesis weak? +
A hypothesis is weak when it is too specific or too general, or does not identify a clear relationship between two or more variables.
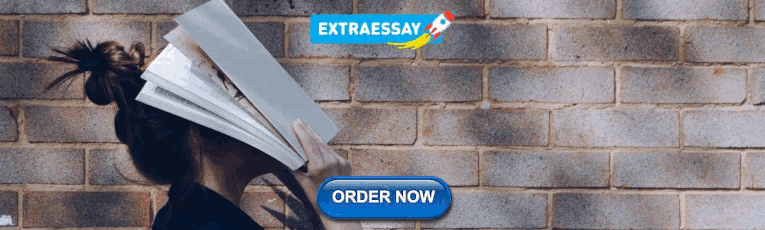
What is the null hypothesis? +
The null hypothesis posits that the variables you have identified have no relationship.
Fastest Nurse Insight Engine
- MEDICAL ASSISSTANT
- Abdominal Key
- Anesthesia Key
- Basicmedical Key
- Otolaryngology & Ophthalmology
- Musculoskeletal Key
- Obstetric, Gynecology and Pediatric
- Oncology & Hematology
- Plastic Surgery & Dermatology
- Clinical Dentistry
- Radiology Key
- Thoracic Key
- Veterinary Medicine
- Gold Membership
Cause and Effect, Hypothesis Testing and Estimation
html xmlns=”http://www.w3.org/1999/xhtml”> 14 Cause and Effect, Hypothesis Testing and Estimation Key points Assertions about cause and effect are probabilistic, not definitive. We make cause-effect predictions in daily life. Cause-effect predictions in healthcare research are the same as those in daily life. Quantitative researchers are concerned with independent variables, dependent variables and intervening variables. Researchers manipulate independent variables, observe any changes to dependent variables and attempt to account for intervening variables. Hypotheses are explicit statements of the predicted relationship between independent and dependent variables. Directional hypotheses are made only when there is reason to think a relationship operates only in one direction. Adequate statistical power is essential to the safe acceptance of the null hypothesis. Hypothesis testing is an all-or-nothing statement of related- ness, but estimation emphasises the extent of a relationship. Introduction The examination of cause and effect is one of the most misunderstood concepts in healthcare research. It has been characterised, variously as mechanistic, unrealistic and over-simple. However, all these assertions about examining cause and effect are themselves based on a version of cause and effect testing and the methods employed to do it (most notably, the randomised controlled trial) which are quite different from those actually practiced by quantitative researchers. Specifically, those who reject methods which seek to establish cause and effect usually do so because they assume that quantitative researchers are claiming the right to a single, definitive answer. However, this is not so. In fact, quantitative researchers are at pains, in their writing, their research methods and even their statistical procedures (which are all about probability not fact) to recognise that their answers to cause and effect questions are always simply the most likely answer given the current state of our knowledge. It is explicitly acknowledged that many different factors influence cause and effect relationships, and that not all of them can be controlled or even identified. The best that can be achieved is to be as careful as possible in taking account of as many factors that we can control or perceive. As a consequence, it is never claimed that a certain intervention (say, a certain wound care procedure) is always the approach of choice for a particular patient problem (a pressure ulcer), only that it is most likely to be effective. The habit, amongst many researchers and clinicians, of describing a particular intervention as effective is merely a shorthand for this expression of a likely relationship between a given cause (e.g. treatment) and effect (e.g. patient improvement). This habit has probably given rise to considerable misunderstanding of the nature of scientific ‘fact’ in quantitative research. Issues of cause and effect are never definitively answered Describing a treatment as effective is a shorthand for saying it is most likely to be effective, given the current state of our knowledge. It is always acknowledged that there are many influencing factors that cannot be known. Cause and effect relationships in daily life Nevertheless, quantitative researchers do say that we live in a cause-effect world. With all the qualifications noted above, it really is contended that, by and large, doing certain things has consequences for patient care and that these can be reliably demonstrated time and time again. However, this is not a stance which is peculiar to quantitative researchers in particular, or even to researchers in general. In Chapter 6, we showed how sampling is a normal everyday activity carried out by all of us. Exactly the same is true for the assessment of cause and effect relationships. Awareness of cause and effect is basic to human life, and, arguably, to the survival of all life. For example, at the level of the other animal species, it is well demonstrated that organisms recognise and avoid aversive stimuli (e.g. pain, loud noises). This is a simple recognition of cause and effect relationships – performing such and such an activity leads to (causes) pain or discomfort and is therefore best avoided. In our own lives, we make such decisions all the time. Every morning I open my window and look at the sky. If it is cloudy, I predict that it will rain and take an umbrella with me to the station. In doing so, I am making a cause-effect prediction: ‘The presence of dark clouds results in rain’. Now, strictly speaking, clouds do not cause rain, but I am unaware of the actual processes which do and would, in any case, be unable to measure them without sophisticated equipment. This does not matter, because it is merely an issue of measurement. From my point of view, I have repeatedly tested the assertion that certain kinds of cloudy skies are followed by rain, and this had led to my making the cause-effect link. Nor does it matter if, on a particular occasion, I am proved wrong. What matters is that, most of the time, this cause-effect relationship holds true. Indeed, if it did not, I would soon stop making this prediction and stop carrying my umbrella. I would then be responding to a criterion I had set for myself according to which I would regard my prediction as having been verified or falsified. This brings us again to the notion that cause and effect relationships are almost always probabilistic. I also will try to take into account other aspects of the weather (did it rain yesterday, what kind of shape are the clouds, how dark are they, what is the temperature) which, according to my level of knowledge, I will include in my assessment of the likelihood of rain today. This leads us to the assertion that cause and effect predictions are complex. This is recognised just as much in research as it is in this everyday example, and we certainly do not expect healthcare research to be less complicated than our everyday decision making. Cause-effect is part of daily life In everyday life, we make cause-effect predictions all the time. In doing this, we recognise that we are making probability predictions. Often, we take many complex issues into consideration in making such predictions in important as well as mundane situations. Strangely, we have no problem in doing so – only when this kind of decision is applied to our research or clinical work do people see this as problematic. Examining cause and effect in healthcare Therefore, the kinds of cause and effect predictions we make in healthcare can be just as rich, probabilistic and complex as those we make intuitively in everyday life. The methods used to tease out these complexities are often painstaking and complex because, in research, we want to ensure that the processes we have used to arrive at our decisions are transparent to all, in a way which we do not have to do with our own everyday decisions. We want to do this so that others can scrutinise and criticise our work with a clear understanding of what we have done and why we have done it. If necessary, we want to attempt to replicate our work (repeat it using exactly the same methods in a different context). This has given rise to a formal language, which is perhaps unfortunate, but is no different than the verbal shorthand health professionals use to communicate rapidly with each other. The actual processes are little different from the way in which we, as a society, have arrived at our shared knowledge that dark clouds are usually followed by rain. Variables In asserting and testing cause-effect relationships, we are basically describing and predicting relationships between variables. Variables are any entities in the world which are not fixed, but are subject to change. There are three types of variables to concern us: independent variables, dependent variables and intervening variables. The independent variable is the thing which we, as researchers, vary or manipulate in some way. Usually, this is a treatment of some sort. So, if we give one group of patients a relaxation exercise prior to surgery and give another group a premedication sedative, this difference in treatment is the independent variable. It is said to vary over two levels (relaxation and pre- medication). If we introduce a further group (treatment as normal), the independent variable will then vary over three levels. The use of the word ‘level’ does not, however, imply that one treatment is more valuable than another, and the term experimental condition is sometimes used interchangeably with level. Thus, relaxation, premedication and treatment as normal are three different experimental conditions in the above example. Levels and conditions – the same, but different Strictly speaking, levels and conditions are not the same. For example, if we tested two interacting independent variables (exercise regime and intensity of exercise), we could have several levels of each variable: regime (running versus circuit training); intensity (half an hour daily versus an hour every 2 days). So, each variable here consists of two levels. There are four conditions (half an hour daily running, hour bi daily running, half an hour daily circuit, hour bi daily circuit). So, levels refer only to different conditions within a single variable, whilst conditions can be used to describe levels across conditions! Remember, all this is just jargon. It is useful to know the language, so that you can better understand written reports, but it does not materially affect the actual conducting of research. By contrast with the independent variable, the dependent variable is not manipulated by the researcher. Instead, changes in this variable are regarded as being dependent on the action of the independent variable. In the example above, one possible dependent variable might be patient anxiety, and we would expect the amount of anxiety to differ between the three levels of the independent variable. If this turned out to be the case, we would conclude that these differences were the result of or, dependent upon, the actions of the different levels of the independent variable. This is the essence of the investigation of cause-effect relationships in quantitative research. The relationship between independent and dependent variables Changes which we measure in the dependent variable are regarded as the result of (dependent on) our manipulations of the independent variable. In clinical practice, this is like concluding that changes in patient well-being are the result of treatment.
Share this:
- Click to share on Twitter (Opens in new window)
- Click to share on Facebook (Opens in new window)
Related posts:
- Choosing Methodological Approaches
- Introduction to Healthcare Research for Evidence-Based Practice
- A Pragmatic Approach to Qualitative Data Analysis
- The Role of Statistics
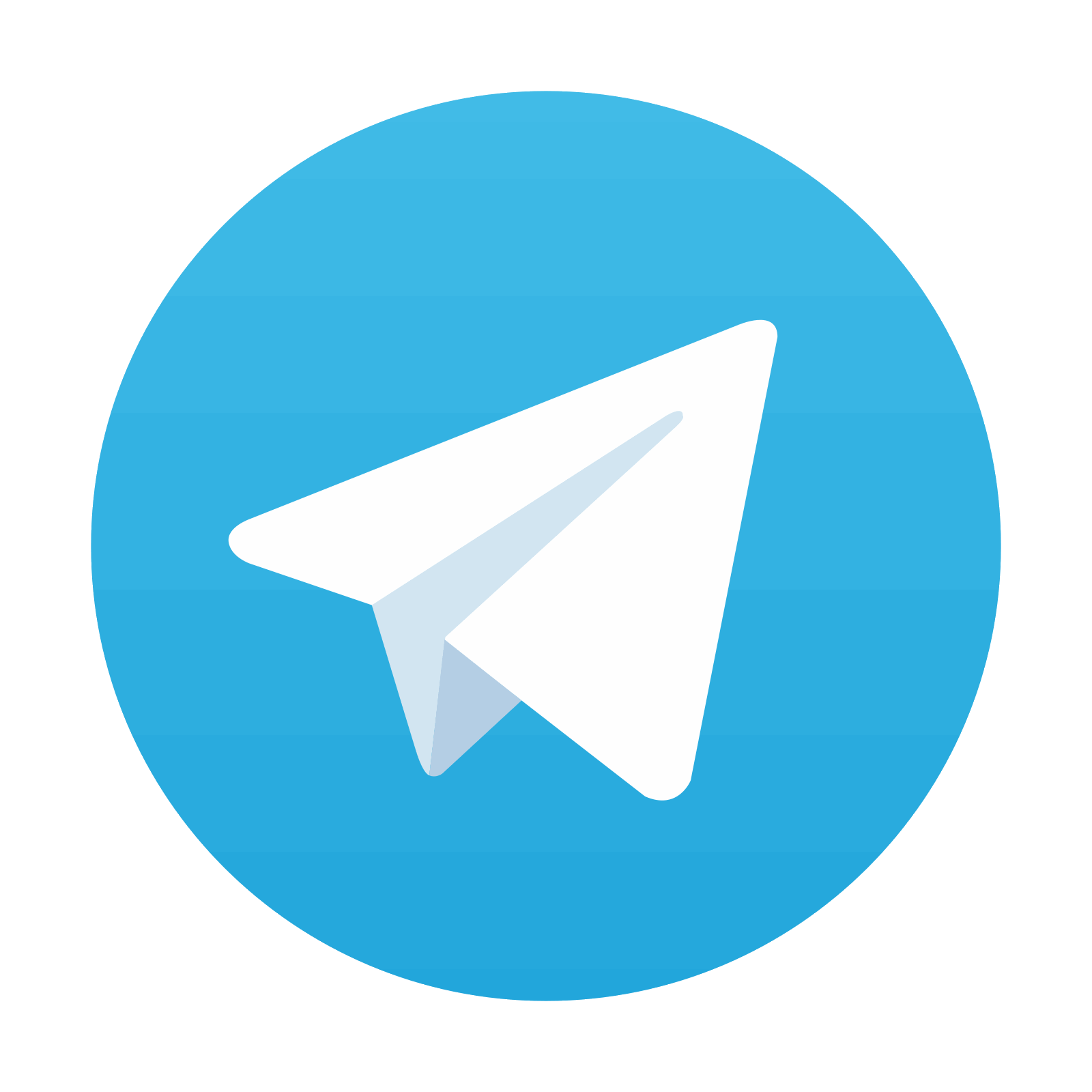
Stay updated, free articles. Join our Telegram channel
Comments are closed for this page.

Full access? Get Clinical Tree
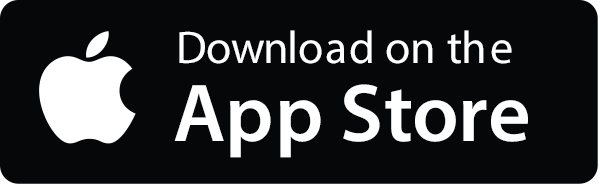
What Is a Hypothesis? (Science)
If...,Then...
Angela Lumsden/Getty Images
- Scientific Method
- Chemical Laws
- Periodic Table
- Projects & Experiments
- Biochemistry
- Physical Chemistry
- Medical Chemistry
- Chemistry In Everyday Life
- Famous Chemists
- Activities for Kids
- Abbreviations & Acronyms
- Weather & Climate
- Ph.D., Biomedical Sciences, University of Tennessee at Knoxville
- B.A., Physics and Mathematics, Hastings College
A hypothesis (plural hypotheses) is a proposed explanation for an observation. The definition depends on the subject.
In science, a hypothesis is part of the scientific method. It is a prediction or explanation that is tested by an experiment. Observations and experiments may disprove a scientific hypothesis, but can never entirely prove one.
In the study of logic, a hypothesis is an if-then proposition, typically written in the form, "If X , then Y ."
In common usage, a hypothesis is simply a proposed explanation or prediction, which may or may not be tested.
Writing a Hypothesis
Most scientific hypotheses are proposed in the if-then format because it's easy to design an experiment to see whether or not a cause and effect relationship exists between the independent variable and the dependent variable . The hypothesis is written as a prediction of the outcome of the experiment.
- Null Hypothesis and Alternative Hypothesis
Statistically, it's easier to show there is no relationship between two variables than to support their connection. So, scientists often propose the null hypothesis . The null hypothesis assumes changing the independent variable will have no effect on the dependent variable.
In contrast, the alternative hypothesis suggests changing the independent variable will have an effect on the dependent variable. Designing an experiment to test this hypothesis can be trickier because there are many ways to state an alternative hypothesis.
For example, consider a possible relationship between getting a good night's sleep and getting good grades. The null hypothesis might be stated: "The number of hours of sleep students get is unrelated to their grades" or "There is no correlation between hours of sleep and grades."
An experiment to test this hypothesis might involve collecting data, recording average hours of sleep for each student and grades. If a student who gets eight hours of sleep generally does better than students who get four hours of sleep or 10 hours of sleep, the hypothesis might be rejected.
But the alternative hypothesis is harder to propose and test. The most general statement would be: "The amount of sleep students get affects their grades." The hypothesis might also be stated as "If you get more sleep, your grades will improve" or "Students who get nine hours of sleep have better grades than those who get more or less sleep."
In an experiment, you can collect the same data, but the statistical analysis is less likely to give you a high confidence limit.
Usually, a scientist starts out with the null hypothesis. From there, it may be possible to propose and test an alternative hypothesis, to narrow down the relationship between the variables.
Example of a Hypothesis
Examples of a hypothesis include:
- If you drop a rock and a feather, (then) they will fall at the same rate.
- Plants need sunlight in order to live. (if sunlight, then life)
- Eating sugar gives you energy. (if sugar, then energy)
- White, Jay D. Research in Public Administration . Conn., 1998.
- Schick, Theodore, and Lewis Vaughn. How to Think about Weird Things: Critical Thinking for a New Age . McGraw-Hill Higher Education, 2002.
- Null Hypothesis Definition and Examples
- Definition of a Hypothesis
- What Are the Elements of a Good Hypothesis?
- Six Steps of the Scientific Method
- What Are Examples of a Hypothesis?
- Understanding Simple vs Controlled Experiments
- Scientific Method Flow Chart
- Scientific Method Vocabulary Terms
- What Is a Testable Hypothesis?
- Null Hypothesis Examples
- What 'Fail to Reject' Means in a Hypothesis Test
- How To Design a Science Fair Experiment
- What Is an Experiment? Definition and Design
- Hypothesis Test for the Difference of Two Population Proportions
- How to Conduct a Hypothesis Test
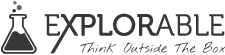
- Foundations
- Write Paper
Search form
- Experiments
- Anthropology
- Self-Esteem
- Social Anxiety

- Experiments >
- Cause and Effect
Establishing Cause and Effect
Cause and effect is one of the most commonly misunderstood concepts in science and is often misused by lawyers, the media, politicians and even scientists themselves, in an attempt to add legitimacy to research.
This article is a part of the guide:
- Experimental Research
- Pretest-Posttest
- Third Variable
- Research Bias
- Independent Variable
Browse Full Outline
- 1 Experimental Research
- 2.1 Independent Variable
- 2.2 Dependent Variable
- 2.3 Controlled Variables
- 2.4 Third Variable
- 3.1 Control Group
- 3.2 Research Bias
- 3.3.1 Placebo Effect
- 3.3.2 Double Blind Method
- 4.1 Randomized Controlled Trials
- 4.2 Pretest-Posttest
- 4.3 Solomon Four Group
- 4.4 Between Subjects
- 4.5 Within Subject
- 4.6 Repeated Measures
- 4.7 Counterbalanced Measures
- 4.8 Matched Subjects
The basic principle of causality is determining whether the results and trends seen in an experiment are actually caused by the manipulation or whether some other factor may underlie the process.
Unfortunately, the media and politicians often jump upon scientific results and proclaim that it conveniently fits their beliefs and policies. Some scientists, fixated upon 'proving' that their view of the world is correct, leak their results to the press before allowing the peer review process to check and validate their work.
Some examples of this are rife in alternative therapy, when a group of scientists announces that they have found the next healthy superfood or that a certain treatment cured swine flu. Many of these claims deviate from the scientific process and pay little heed to cause and effect, diluting the claims of genuine researchers in the field.
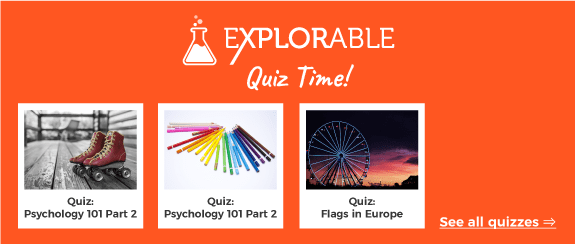
What is Cause and Effect? - The Temporal Issue
The key principle of establishing cause and effect is proving that the effects seen in the experiment happened after the cause.
This seems to be an extremely obvious statement, but that is not always the case. Natural phenomena are complicated and intertwined, often overlapping and making it difficult to establish a natural order. Think about it this way: in an experiment to study the effects of depression upon alcohol consumption, researchers find that people who suffer from higher levels of depression drink more, and announce that this correlation shows that depression drives people to drink.
However, is this necessarily the case? Depression could be the cause that makes people drink more but it is equally possible that heavy consumption of alcohol, a depressant, makes people more depressed. This type of classic 'chicken and egg' argument makes establishing causality one of the most difficult aspects of scientific research . It is also one of the most important factors, because it can misdirect scientists. It also leaves the research open to manipulation by interest groups, who will take the results and proclaim them as a truth.
With the above example, an alcoholic drink manufacturer could use the second interpretation to claim that alcohol is not a factor in depression and that the responsibility is upon society to ensure that people do not become depressed. An anti-alcohol group, on the other hand, could claim that alcohol is harmful and use the results to lobby for harsher drinking laws. The same research leads to two different interpretations and, the answer given to the media can depend upon who funds the work.
Unfortunately, most of the general public are not scientists and cannot be expected to filter every single news item that they read for quality or delve into which group funded research. Even respected and trusted newspapers, journals and internet resources can fall into the causality trap, so marketing groups can influence perceptions.
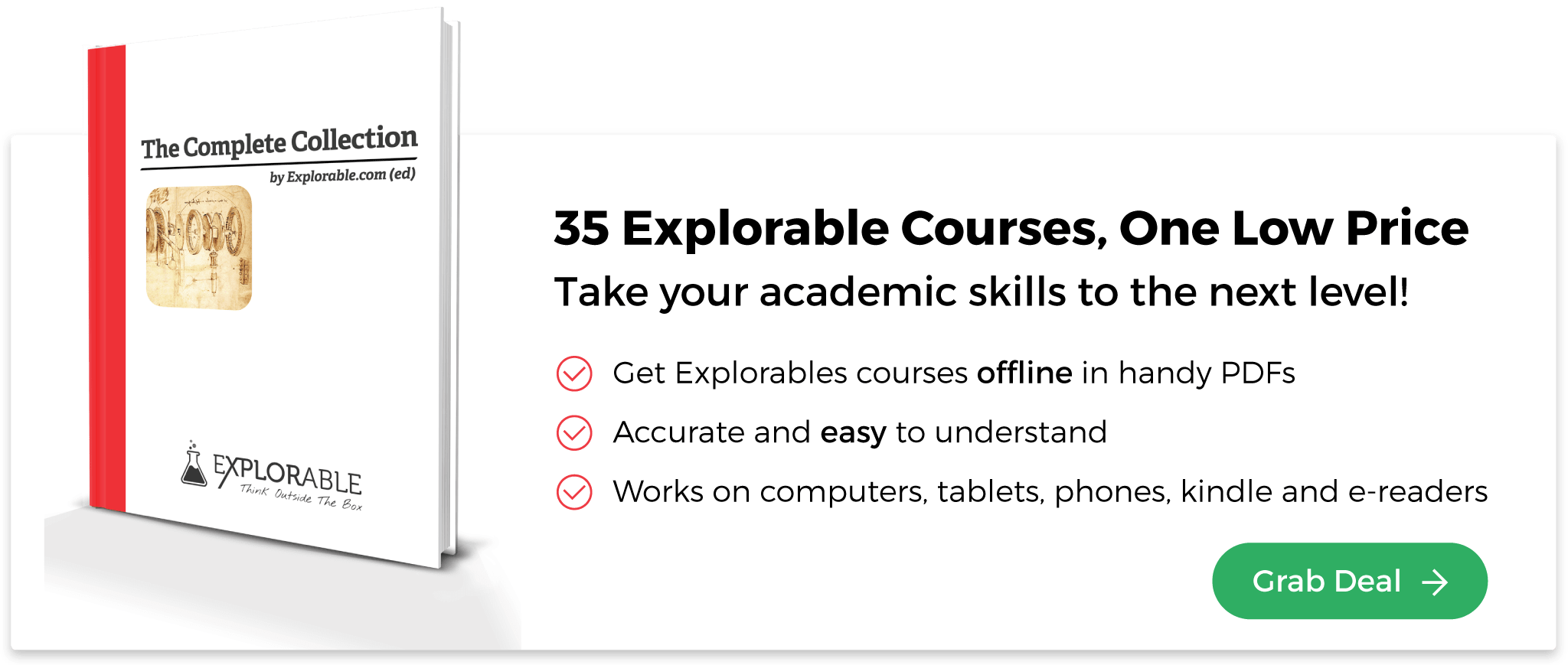
What is Cause and Effect? - The Danger of Alternative Explanations
The other problem with causality is that a researcher cannot always guarantee that their particular manipulation of a variable was the sole reason for the perceived trends and correlation.
In a complex experiment, it is often difficult to isolate and neutralize the influence of confounding variables . This makes it exceptionally difficult for the researcher to state that their treatment is the sole cause, so any research program must contain measures to establish the cause and effect relationship.
In the physical sciences, such as physics and chemistry, it is fairly easy to establish causality, because a good experimental design can neutralize any potentially confounding variables. Sociology, at the other extreme, is exceptionally prone to causality issues, because individual humans and social groups vary so wildly and are subjected to a wide range of external pressures and influences.
For results to have any meaning, a researcher must make causality the first priority, simply because it can have such a devastating effect upon validity. Most experiments with some validity issues can be salvaged, and produce some usable data. An experiment with no established cause and effect, on the other hand, will be practically useless and a waste of resources.
How to Establish Cause and Effect
The first thing to remember with causality, especially in the non-physical sciences, is that it is impossible to establish complete causality.
However, the magical figure of 100% proof of causality is what every researcher must strive for, to ensure that a group of their peers will accept the results. The only way to do this is through a strong and well-considered experimental design, often containing pilot studies to establish cause and effect before plowing on with a complex and expensive study.
The temporal factor is usually the easiest aspect to neutralize, simply because most experiments involve administering a treatment and then observing the effects, giving a linear temporal relationship. In experiments that use historical data, as with the drinking/depression example, this can be a little more complex. Most researchers performing such a program will supplement it with a series of individual case studies, and interviewing a selection of the participants , in depth, will allow the researchers to find the order of events.
For example, interviewing a sample of the depressed heavy drinkers will establish whether they felt that they were depressed before they started drinking or if the depression came later. The process of establishing cause and effect is a matter of ensuring that the potential influence of 'missing variables' is minimized.
One notable example, by the researchers Balnaves and Caputi, looked at the academic performance of university students and attempted to find a correlation with age. Indeed, they found that older, more mature students performed significantly better. However, as they pointed out, you cannot simply say that age causes the effect of making people into better students. Such a simplistic assumption is called a spurious relationship, the process of 'leaping to conclusions.'
In fact, there is a whole host of reasons why a mature student performs better: they have more life experience and confidence, and many feel that it is their last chance to succeed; my graduation year included a 75-year-old man, and nobody studied harder! Mature students may well have made a great financial sacrifice, so they are a little more determined to succeed. Establishing cause and effect is extremely difficult in this case, so the researchers interpreted the results very carefully.
Another example is the idea that because people who eat a lot of extra virgin olive oil live for longer, olive oil makes people live longer. While there is some truth behind this, you have to remember that most regular olive oil eaters also eat a Mediterranean diet, have active lifestyles, and generally less stress. These also have a strong influence, so any such research program should include studies into the effect of these - this is why a research program is not always a single experiment but often a series of experiments.
History Threats and Their Influence Upon Cause and Effect
One of the biggest threats to internal validity through incorrect application of cause and effect is the 'history' threat.
This is where another event actually caused the effect noticed, rather than your treatment or manipulation. Most researchers perform a pre-test upon a group, administer the treatment and then measure the post-test results ( pretest-posttest-design ). If the results are better, it is easy to assume that the treatment caused the result, but this is not necessarily the case.
For example, take the case of an educational researcher wishing to measure the effect of a new teaching method upon the mathematical aptitude of students. They pre-test, teach the new program for a few months and then posttest. Results improve, and they proclaim that their program works.
However, the research was ruined by a historical threat: during the course of the research, a major television network released a new educational series called 'Maths made Easy,' which most of the students watched. This influenced the results and compromised the validity of the experiment.
Fortunately, the solution to this problem is easy: if the researcher uses a two group pretest-posttest design with a control group , the control group will be equally influenced by the historical event, so the researcher can still establish a good baseline. There are a number of other 'single group' threats, but establishing a good control driven study largely eliminates these threats to causality.
Social Threats and Their Influence Upon Cause and Effect
Social threats are a big problem for social researchers simply because they are one of the most difficult of the threats to minimize. These types of threats arise from issues within the participant groups or the researchers themselves. In an educational setting, with two groups of children, one treated and one not, there are a number of potential issues.
- Diffusion or Imitation of Treatment: With this threat, information travels between groups and smoothes out any differences in the results. In a school, for example, students mix outside classes and may swap information or coach the control group about some of the great new study techniques that they have learned. It is practically impossible and extremely unfair to expect students not to mix, so this particular threat is always an issue.
- Compensatory Rivalry: Quite simply, this is where the control group becomes extremely jealous of the treatment group. They might think that the research is unfair, because their fellow students are earning better grades. As a result, they try much harder to show that they are equally as clever, reducing the difference between the two groups.
- Demoralization and Resentment: This jealousy may have the opposite effect and manifest as a built up resentment that the other group is receiving favorable treatment. The control group , quite simply, gives up and does not bother trying and their grades plummet. This makes the educational program appear to be much more successful than it really is.
- Compensatory Equalization of Treatment: This type of social threat arises from the attitude of the researchers or external contributors. If, for example, teachers and parents perceive that there is some unfairness in the system, they might try to compensate, by giving extra tuition or access to better teaching resources. This can easily cause compensatory rivalry, too, if a teacher spurs on the control group to try harder and outdo the others.
These social effects are extremely difficult to minimize without creating other threats to internal validity .
For example, using different schools is one idea, but this can lead to other internal validity issues, especially because the participant groups cannot be randomized. In reality, this is why most social research programs incorporate a variety of different methods and include more than one experiment, to establish the potential level of these threats and incorporate them into the interpretation of the data.
Cause and Effect - The Danger of Multiple Group Threats
Multiple group threats are a danger to causality caused by differences between two or more groups of participants. The main example of this is selection bias , or assignment bias, where the two groups are assigned unevenly, perhaps leaving one group with a larger proportion of high achievers. This will skew the results and mask the effects of the entire experiment.
While there are other types of multiple group threat, they are all subtypes of selection bias and involve the two groups receiving different treatment. If the groups are selected from different socio-economic backgrounds, or one has a much better teacher, this can skew the results. Without going into too much detail, the only way to reduce the influence of multiple group threats is through randomization , matched pairs designs or another assignment type.
As can be seen, establishing cause and effect is one of the most important factors in designing a robust research experiment. One of the best ways to learn about causality is through experience and analysis - every time you see some innovative research or findings in the media, think about what the results are trying to tell you and whether the researchers are justified in drawing their conclusions .
This does not have to be restricted to 'hard' science, because political researchers are the worst habitual offenders. Archaeology, economics and market research are other areas where cause and effect is important, so should provide some excellent examples of how to establish cause and effect.
- Psychology 101
- Flags and Countries
- Capitals and Countries
Martyn Shuttleworth (Sep 20, 2009). Establishing Cause and Effect. Retrieved Apr 01, 2024 from Explorable.com: https://explorable.com/cause-and-effect
You Are Allowed To Copy The Text
The text in this article is licensed under the Creative Commons-License Attribution 4.0 International (CC BY 4.0) .
This means you're free to copy, share and adapt any parts (or all) of the text in the article, as long as you give appropriate credit and provide a link/reference to this page.
That is it. You don't need our permission to copy the article; just include a link/reference back to this page. You can use it freely (with some kind of link), and we're also okay with people reprinting in publications like books, blogs, newsletters, course-material, papers, wikipedia and presentations (with clear attribution).
Want to stay up to date? Follow us!
Get all these articles in 1 guide.
Want the full version to study at home, take to school or just scribble on?
Whether you are an academic novice, or you simply want to brush up your skills, this book will take your academic writing skills to the next level.
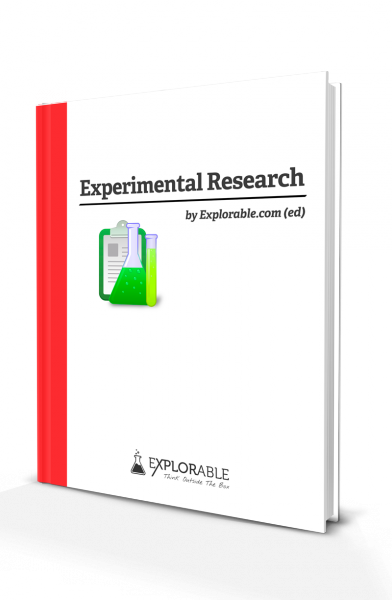
Download electronic versions: - Epub for mobiles and tablets - For Kindle here - For iBooks here - PDF version here
Save this course for later
Don't have time for it all now? No problem, save it as a course and come back to it later.
Footer bottom
- Privacy Policy
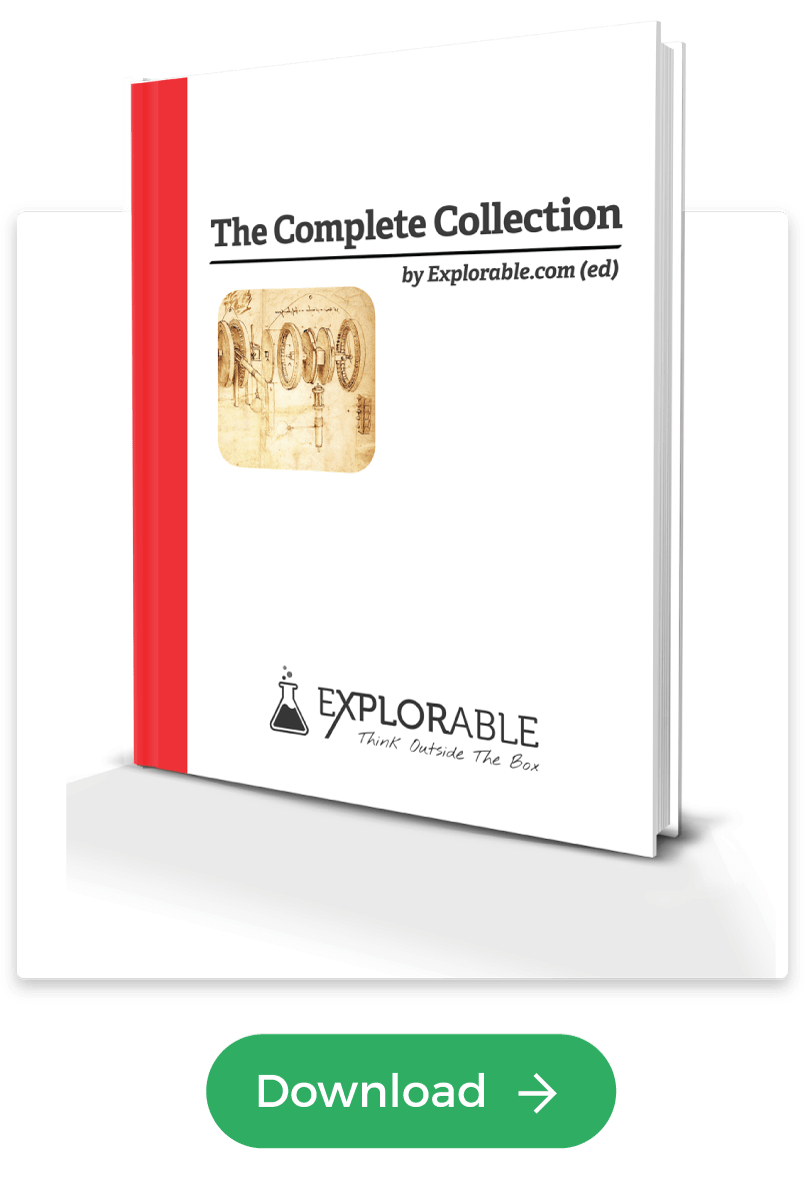
- Subscribe to our RSS Feed
- Like us on Facebook
- Follow us on Twitter
Bivariate Analysis: Associations, Hypotheses, and Causal Stories
- Open Access
- First Online: 04 October 2022
Cite this chapter
You have full access to this open access chapter
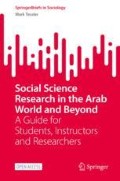
- Mark Tessler 2
Part of the book series: SpringerBriefs in Sociology ((BRIEFSSOCY))
2677 Accesses
Every day, we encounter various phenomena that make us question how, why, and with what implications they vary. In responding to these questions, we often begin by considering bivariate relationships, meaning the way that two variables relate to one another. Such relationships are the focus of this chapter.
Download chapter PDF
3.1 Description, Explanation, and Causal Stories
There are many reasons why we might be interested in the relationship between two variables. Suppose we observe that some of the respondents interviewed in Arab Barometer surveys and other surveys report that they have thought about emigrating, and we are interested in this variable. We may want to know how individuals’ consideration of emigration varies in relation to certain attributes or attitudes. In this case, our goal would be descriptive , sometimes described as the mapping of variance. Our goal may also or instead be explanation , such as when we want to know why individuals have thought about emigrating.
Description
Description means that we seek to increase our knowledge and refine our understanding of a single variable by looking at whether and how it varies in relation to one or more other variables. Descriptive information makes a valuable contribution when the structure and variance of an important phenomenon are not well known, or not well known in relation to other important variables.
Returning to the example about emigration, suppose you notice that among Jordanians interviewed in 2018, 39.5 percent of the 2400 men and women interviewed reported that they have considered the possibility of emigrating.
Our objective may be to discover what these might-be migrants look like and what they are thinking. We do this by mapping the variance of emigration across attributes and orientations that provide some of this descriptive information, with the descriptions themselves each expressed as bivariate relationships. These relationships are also sometimes labeled “associations” or “correlations” since they are not considered causal relationships and are not concerned with explanation.
Of the 39.5 percent of Jordanians who told interviewers that they have considered emigrating, 57.3 percent are men and 42.7 percent are women. With respect to age, 34 percent are age 29 or younger and 19.2 percent are age 50 or older. It might have been expected that a higher percentage of respondents age 29 or younger would have considered emigrating. In fact, however, 56 percent of the 575 men and women in this age category have considered emigrating. And with respect to destination, the Arab country most frequently mentioned by those who have considered emigration is the UAE, named by 17 percent, followed by Qatar at 10 percent and Saudi Arabia at 9.8 percent. Non-Arab destinations were mentioned more frequently, with Turkey named by 18.1 percent, Canada by 21.1 percent, and the U.S. by 24.2 percent.
With the variables sex, age, and prospective destination added to the original variable, which is consideration of emigration, there are clearly more than two variables under consideration. But the variables are described two at a time and so each relationship is bivariate.
These bivariate relationships, between having considered emigration on the one hand and sex, age, and prospective destination on the other, provide descriptive information that is likely to be useful to analysts, policymakers, and others concerned with emigration. They tell, or begin to tell, as noted above, what might-be migrants look like and what they are thinking. Still additional insight may be gained by adding descriptive bivariate relationships for Jordanians interviewed in a different year to those interviewed in 2018. In addition, of course, still more information and possibly a more refined understanding, may be gained by examining the attributes and orientations of prospective emigrants who are citizens of other Arab (and perhaps also non-Arab) countries.
With a focus on description, these bivariate relationships are not constructed to shed light on explanation, that is to contribute to causal stories that seek to account for variance and tell why some individuals but not others have considered the possibility of emigrating. In fact, however, as useful as bivariate relationships that provide descriptive information may be, researchers usually are interested as much if not more in bivariate relationships that express causal stories and purport to provide explanations.
Explanation and Causal Stories
There is a difference in the origins of bivariate relationships that seek to provide descriptive information and those that seek to provide explanatory information. The former can be thought to be responding to what questions: What characterizes potential emigrants? What do they look like? What are their thoughts about this or that subject? If the objective is description, a researcher collects and uses her data to investigate the relationship between two variables without a specific and firm prediction about the relationship between them. Rather, she simply wonders about the “what” questions listed above and believes that finding out the answers will be instructive. In this case, therefore, she selects the bivariate relationships to be considered based on what she thinks it will be useful to know, and not based on assessing the accuracy of a previously articulated causal story that specifies the strength and structure of the effect that one variable has on the other.
A researcher is often interested in causal stories and explanation, however, and this does usually begin with thinking about the relationship between two variables, one of which is the presumed cause and the other the presumed effect. The presumed cause is the independent variable, and the presumed effect is the dependent variable . Offering evidence that there is a strong relationship between two variables is not sufficient to demonstrate that the variables are likely to be causally related, but it is a necessary first step. In this respect it is a point of departure for the fuller, probably multivariate analysis, required to persuasively argue that a relationship is likely to be causal. In addition, as discussed in Chap. 4 , multivariate analysis often not only strengthens the case for inferring that a relationship is causal, but also provides a more elaborate and more instructive causal story. The foundation, however, on which a multivariate analysis aimed at causal inference is built, is a bivariate relationship composed of a presumed independent variable and a presumed dependent variable.
A hypothesis that posits a causal relationship between two variables is not the same as a causal story, although the two are of course closely connected. The former specifies a presumed cause, a presumed determinant of variance on the dependent variable. It probably also specifies the structure of the relationship, such as linear as opposed to non-linear, or positive (also called direct) as opposed to negative (also called inverse).
On the other hand, a causal story describes in more detail what the researcher believes is actually taking place in the relationship between the variables in her hypothesis; and accordingly, why she thinks this involves causality. A causal story provides a fuller account of operative processes, processes that the hypothesis references but does not spell out. These processes may, for example, involve a pathway or a mechanism that tells how it is that the independent variable causes and thus accounts for some of the variance on the dependent variable. Expressed yet another way, the causal story describes the researcher’s understandings, or best guesses, about the real world, understandings that have led her to believe, and then propose for testing, that there is a causal connection between her variables that deserves investigation. The hypothesis itself does not tell this story; it is rather a short formulation that references and calls attention to the existence, or hypothesized existence, of a causal story. Research reports present the causal story as well as the hypothesis, as the hypothesis is often of limited interpretability without the causal story.
A causal story is necessary for causal inference. It enables the researcher to formulate propositions that purport to explain rather than merely describe or predict. There may be a strong relationship between two variables, and if this is the case, it will be possible to predict with relative accuracy the value, or score, of one variable from knowledge of the value, or score, of the other variable. Prediction is not explanation, however. To explain, or attribute causality, there must be a causal story to which a hypothesized causal relationship is calling attention.
An instructive illustration is provided by a recent study of Palestinian participation in protest activities that express opposition to Israeli occupation. Footnote 1 There is plenty of variance on the dependent variable: There are many young Palestinians who take part in these activities, and there are many others who do not take part. Education is one of the independent variables that the researcher thought would be an important determinant of participation, and so she hypothesized that individuals with more education would be more likely to participate in protest activities than individuals with less education.
But why would the researcher think this? The answer is provided by the causal story. To the extent that this as yet untested story is plausible, or preferably, persuasive, at least in the eyes of the investigator, it gives the researcher a reason to believe that education is indeed a determinant of participation in protest activities in Palestine. By spelling out in some detail how and why the hypothesized independent variable, education in this case, very likely impacts a person’s decision about whether or not to protest, the causal story provides a rationale for the researcher’s hypothesis.
In the case of Palestinian participation in protest activities, another investigator offered an insightful causal story about the ways that education pushes toward greater participation, with emphasis on its role in communication and coordination. Footnote 2 Schooling, as the researcher theorizes and subsequently tests, integrates young Palestinians into a broader institutional environment that facilitates mass mobilizations and lowers informational and organizational barriers to collective action. More specifically, she proposes that those individuals who have had at least a middle school education, compared to those who have not finished middle school, have access to better and more reliable sources of information, which, among other things, enables would-be protesters to assess risks. More schooling also makes would-be protesters better able to forge inter-personal relationships and establish networks that share information about needs, opportunities, and risks, and that in this way facilitate engaging in protest activities in groups, rather than on an individual basis. This study offers some additional insights to be discussed later.
The variance motivating the investigation of a causal story may be thought of as the “variable of interest,” and it may be either an independent variable or a dependent variable. It is a variable of interest because the way that it varies poses a question, or puzzle, that a researcher seeks to investigate. It is the dependent variable in a bivariate relationship if the researcher seeks to know why this variable behaves, or varies, as it does, and in pursuit of this objective, she will seek to identify the determinants and drivers that account for this variance. The variable of interest is an independent variable in a particular research project if the researcher seeks to know what difference it makes—on what does its variance have an impact, of what other variable or variables is it a driver or determinant.
The variable in which a researcher is initially interested, that is to say the variable of interest, can also be both a dependent variable and an independent variable. Returning to the variable pertaining to consideration of emigration, but this time with country as the unit of analysis, the variance depicted in Table 3.1 provides an instructive example. The data are based on Arab Barometer surveys conducted in 2018–2019, and the table shows that there is substantial variation across twelve countries. Taking the countries together, the mean percentage of citizens that have thought about relocating to another country is 30.25 percent. But in fact, there is very substantial variation around this mean. Kuwait is an outlier, with only 8 percent having considered emigration. There are also countries in which only 21 percent or 22 percent of the adult population have thought about this, figures that may be high in absolute terms but are low relative to other Arab countries. At the other end of the spectrum are countries in which 45 percent or even 50 percent of the citizens report having considered leaving their country and relocating elsewhere.
The very substantial variance shown in Table 3.1 invites reflection on both the causes and the consequences of this country-level variable, aggregate thinking about emigration. As a dependent variable, the cross-country variance brings the question of why the proportion of citizens that have thought about emigrating is higher in some countries than in others; and the search for an answer begins with the specification of one or more bivariate relationships, each of which links this dependent variable to a possible cause or determinant. As an independent variable, the cross-country variance brings the question of what difference does it make—of what is it a determinant or driver and what are the consequences for a country if more of its citizens, rather than fewer, have thought about moving to another country.
3.2 Hypotheses and Formulating Hypotheses
Hypotheses emerge from the research questions to which a study is devoted. Accordingly, a researcher interested in explanation will have something specific in mind when she decides to hypothesize and then evaluate a bivariate relationship in order to determine whether, and if so how, her variable of interest is related to another variable. For example, if the researcher’s variable of interest is attitude toward gender equality and one of her research questions asks why some people support gender equality and others do not, she might formulate the hypothesis below to see if education provides part of the answer.
Hypothesis 1. Individuals who are better educated are more likely to support gender equality than are individuals who are less well-educated.
The usual case, and the preferred case, is for an investigator to be specific about the research questions she seeks to answer, and then to formulate hypotheses that propose for testing part of the answer to one or more of these questions. Sometimes, however, a researcher will proceed without formulating specific hypotheses based on her research questions. Sometimes she will simply look at whatever relationships between her variable of interest and a second variable her data permit her to identify and examine, and she will then follow up and incorporate into her study any findings that turn out to be significant and potentially instructive. This is sometimes described as allowing the data to “speak.” When this hit or miss strategy of trial and error is used in bivariate and multivariate analysis, findings that are significant and potentially instructive are sometimes described as “grounded theory.” Some researchers also describe the latter process as “inductive” and the former as “deductive.”
Although the inductive, atheoretical approach to data analysis might yield some worthwhile findings that would otherwise have been missed, it can sometimes prove misleading, as you may discover relationships between variables that happened by pure chance and are not instructive about the variable of interest or research question. Data analysis in research aimed at explanation should be, in most cases, preceded by the formulation of one or more hypotheses. In this context, when the focus is on bivariate relationships and the objective is explanation rather than description, each hypothesis will include a dependent variable and an independent variable and make explicit the way the researcher thinks the two are, or probably are, related. As discussed, the dependent variable is the presumed effect; its variance is what a hypothesis seeks to explain. The independent variable is the presumed cause; its impact on the variance of another variable is what the hypothesis seeks to determine.
Hypotheses are usually in the form of if-then, or cause-and-effect, propositions. They posit that if there is variance on the independent variable, the presumed cause, there will then be variance on the dependent variable, the presumed effect. This is because the former impacts the latter and causes it to vary.
An illustration of formulating hypotheses is provided by a study of voting behavior in seven Arab countries: Algeria, Bahrain, Jordan, Lebanon, Morocco, Palestine, and Yemen. Footnote 3 The variable of interest in this individual-level study is electoral turnout, and prominent among the research questions is why some citizens vote and others do not. The dependent variable in the hypotheses proposed in response to this question is whether a person did or did not vote in the country’s most recent parliamentary election. The study initially proposed a number of hypotheses, which include the two listed here and which would later be tested with data from Arab Barometer surveys in the seven countries in 2006–2007. We will return to this illustration later in this chapter.
Hypothesis 1: Individuals who have used clientelist networks in the past are more likely to turn out to vote than are individuals who have not used clientelist networks in the past.
Hypothesis 2: Individuals with a positive evaluation of the economy are more likely to vote than are individuals with a negative evaluation of the economy.
Another example pertaining to voting, which this time is hypothetical but might be instructively tested with Arab Barometer data, considers the relationship between perceived corruption and turning out to vote at the individual level of analysis.
The normal expectation in this case would be that perceptions of corruption influence the likelihood of voting. Even here, however, competing causal relationships are plausible. More perceived corruption might increase the likelihood of voting, presumably to register discontent with those in power. But greater perceived corruption might also actually reduce the likelihood of voting, presumably in this case because the would-be voter sees no chance that her vote will make a difference. But in this hypothetical case, even the direction of the causal connection might be ambiguous. If voting is complicated, cumbersome, and overly bureaucratic, it might be that the experience of voting plays a role in shaping perceptions of corruption. In cases like this, certain variables might be both independent and dependent variables, with causal influence pushing in both directions (often called “endogeneity”), and the researcher will need to carefully think through and be particularly clear about the causal story to which her hypothesis is designed to call attention.
The need to assess the accuracy of these hypotheses, or any others proposed to account for variance on a dependent variable, will guide and shape the researcher’s subsequent decisions about data collection and data analysis. Moreover, in most cases, the finding produced by data analysis is not a statement that the hypothesis is true or that the hypothesis is false. It is rather a statement that the hypothesis is probably true or it is probably false. And more specifically still, when testing a hypothesis with quantitative data, it is often a statement about the odds, or probability, that the researcher will be wrong if she concludes that the hypothesis is correct—if she concludes that the independent variable in the hypothesis is indeed a significant determinant of the variance on the dependent variable. The lower the probability of being wrong, of course, the more confident a researcher can be in concluding, and reporting, that her data and analysis confirm her hypothesis.
Exercise 3.1
Hypotheses emerge from the research questions to which a study is devoted. Thinking about one or more countries with which you are familiar: (a) Identify the independent and dependent variables in each of the example research questions below. (b) Formulate at least one hypothesis for each question. Make sure to include your expectations about the directionality of the relationship between the two variables; is it positive/direct or negative/inverse? (c) In two or three sentences, describe a plausible causal story to which each of your hypotheses might call attention.
Does religiosity affect people’s preference for democracy?
Does preference for democracy affect the likelihood that a person will vote? Footnote 4
Exercise 3.2
Since its establishment in 2006, the Arab Barometer has, as of spring 2022, conducted 68 social and political attitude surveys in the Middle East and North Africa. It has conducted one or more surveys in 16 different Arab countries, and it has recorded the attitudes, values, and preferences of more than 100,000 ordinary citizens.
The Arab Barometer website ( arabbarometer.org ) provides detailed information about the Barometer itself and about the scope, methodology, and conduct of its surveys. Data from the Barometer’s surveys can be downloaded in either SPSS, Stata, or csv format. The website also contains numerous reports, articles, and summaries of findings.
In addition, the Arab Barometer website contains an Online Data Analysis Tool that makes it possible, without downloading any data, to find the distribution of responses to any question asked in any country in any wave. The tool is found in the “Survey Data” menu. After selecting the country and wave of interest, click the “See Results” tab to select the question(s) for which you want to see the response distributions. Click the “Cross by” tab to see the distributions of respondents who differ on one of the available demographic attributes.
The charts below present, in percentages, the response distributions of Jordanians interviewed in 2018 to two questions about gender equality. Below the charts are questions that you are asked to answer. These questions pertain to formulating hypotheses and to the relationship between hypotheses and causal stories.
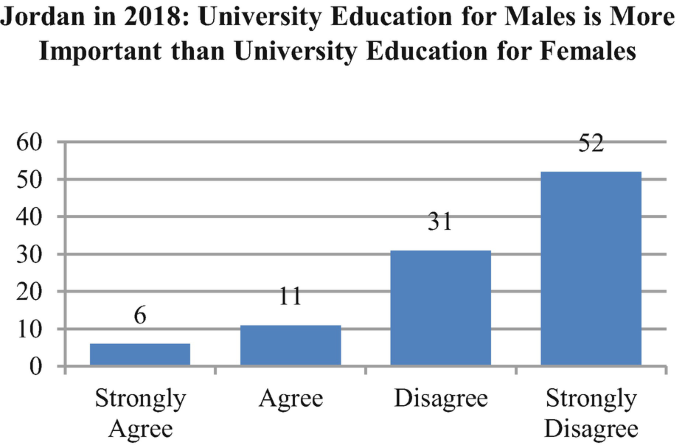
For each of the two distributions, do you think (hypothesize) that the attitudes of Jordanian women are:
About the same as those of Jordanian men
More favorable toward gender equality than those of Jordanian men
Less favorable toward gender equality than those of Jordanian men
For each of the two distributions, do you think (hypothesize) that the attitudes of younger Jordanians are:
About the same as those of older Jordanians
More favorable toward gender equality than those of older Jordanians
Less favorable toward gender equality than those of older Jordanians
Restate your answers to Questions 1 and 2 as hypotheses.
Give the reasons for your answers to Questions 1 and 2. In two or three sentences, make explicit the presumed causal story on which your hypotheses are based.
Using the Arab Barometer’s Online Analysis Tool, check to see whether your answers to Questions 1 and 2 are correct. For those instances in which an answer is incorrect, suggest in a sentence or two a causal story on which the correct relationship might be based.
In which other country surveyed by the Arab Barometer in 2018 do you think the distributions of responses to the questions about gender equality are very similar to the distributions in Jordan? What attributes of Jordan and the other country informed your selection of the other country?
In which other country surveyed by the Arab Barometer in 2018 do you think the distributions of responses to the questions about gender equality are very different from the distributions in Jordan? What attributes of Jordan and the other country informed your selection of the other country?
Using the Arab Barometer’s Online Analysis Tool, check to see whether your answers to Questions 6 and 7 are correct. For those instances in which an answer is incorrect, suggest in a sentence or two a causal story on which the correct relationship might be based.
We will shortly return to and expand the discussion of probabilities and of hypothesis testing more generally. First, however, some additional discussion of hypothesis formulation is in order. Three important topics will be briefly considered. The first concerns the origins of hypotheses; the second concerns the criteria by which the value of a particular hypothesis or set of hypotheses should be evaluated; and the third, requiring a bit more discussion, concerns the structure of the hypothesized relationship between an independent variable and a dependent variable, or between any two variables that are hypothesized to be related.
Origins of Hypotheses
Where do hypotheses come from? How should an investigator identify independent variables that may account for much, or at least some, of the variance on a dependent variable that she has observed and in which she is interested? Or, how should an investigator identify dependent variables whose variance has been determined, presumably only in part, by an independent variable whose impact she deems it important to assess.
Previous research is one place the investigator may look for ideas that will shape her hypotheses and the associated causal stories. This may include previous hypothesis-testing research, and this can be particularly instructive, but it may also include less systematic and structured observations, reports, and testimonies. The point, very simply, is that the investigator almost certainly is not the first person to think about, and offer information and insight about, the topic and questions in which the researcher herself is interested. Accordingly, attention to what is already known will very likely give the researcher some guidance and ideas as she strives for originality and significance in delineating the relationship between the variables in which she is interested.
Consulting previous research will also enable the researcher to determine what her study will add to what is already known—what it will contribute to the collective and cumulative work of researchers and others who seek to reduce uncertainty about a topic in which they share an interest. Perhaps the researcher’s study will fill an important gap in the scientific literature. Perhaps it will challenge and refine, or perhaps even place in doubt, distributions and explanations of variance that have thus far been accepted. Or perhaps her study will produce findings that shed light on the generalizability or scope conditions of previously accepted variable relationships. It need not do any of these things, but that will be for the researcher to decide, and her decision will be informed by knowledge of what is already known and reflection on whether and in what ways her study should seek to add to that body of knowledge.
Personal experience will also inform the researcher’s search for meaningful and informative hypotheses. It is almost certainly the case that a researcher’s interest in a topic in general, and in questions pertaining to this topic in particular, have been shaped by her own experience. The experience itself may involve many different kinds of connections or interactions, some more professional and work-related and some flowing simply and perhaps unintentionally from lived experience. The hypotheses about voting mentioned earlier, for example, might be informed by elections the researcher has witnessed and/or discussions with friends and colleagues about elections, their turnout, and their fairness. Or perhaps the researcher’s experience in her home country has planted questions about the generalizability of what she has witnessed at home.
All of this is to some extent obvious. But the take-away is that an investigator should not endeavor to set aside what she has learned about a topic in the name of objectivity, but rather, she should embrace whatever personal experience has taught her as she selects and refines the puzzles and propositions she will investigate. Should it happen that her experience leads her to incorrect or perhaps distorted understandings, this will be brought to light when her hypotheses are tested. It is in the testing that objectivity is paramount. In hypothesis formation, by contrast, subjectivity is permissible, and, in fact, it may often be unavoidable.
A final arena in which an investigator may look for ideas that will shape her hypotheses overlaps with personal experience and is also to some extent obvious. This is referenced by terms like creativity and originality and is perhaps best captured by the term “sociological imagination.” The take-away here is that hypotheses that deserve attention and, if confirmed, will provide important insights, may not all be somewhere out in the environment waiting to be found, either in the relevant scholarly literature or in recollections about relevant personal experience. They can and sometimes will be the product of imagination and wondering, of discernments that a researcher may come upon during moments of reflection and deliberation.
As in the case of personal experience, the point to be retained is that hypothesis formation may not only be a process of discovery, of finding the previous research that contains the right information. Hypothesis formation may also be a creative process, a process whereby new insights and proposed original understandings are the product of an investigator’s intellect and sociological imagination.
Crafting Valuable Hypotheses
What are the criteria by which the value of a hypothesis or set of hypotheses should be evaluated? What elements define a good hypothesis? Some of the answers to these questions that come immediately to mind pertain to hypothesis testing rather than hypothesis formation. A good hypothesis, it might be argued, is one that is subsequently confirmed. But whether or not a confirmed hypothesis makes a positive contribution depends on the nature of the hypothesis and goals of the research. It is possible that a researcher will learn as much, and possibly even more, from findings that lead to rejection of a hypothesis. In any event, findings, whatever they may be, are valuable only to the extent that the hypothesis being tested is itself worthy of study.
Two important considerations, albeit somewhat obvious ones, are that a hypothesis should be non-trivial and non-obvious. If a proposition is trivial, suggesting a variable relationship with little or no significance, discovering whether and how the variables it brings together are related will not make a meaningful contribution to knowledge about the determinants and/or impact of the variance at the heart of the researcher’s concern. Few will be interested in findings, however rigorously derived, about a trivial proposition. The same is true of an obvious hypothesis, obvious being an attribute that makes a proposition trivial. As stated, these considerations are themselves somewhat obvious, barely deserving mention. Nevertheless, an investigator should self-consciously reflect on these criteria when formulating hypotheses. She should be sure that she is proposing variable relationships that are neither trivial nor obvious.
A third criterion, also somewhat obvious but nonetheless essential, has to do with the significance and salience of the variables being considered. Will findings from research about these variables be important and valuable, and perhaps also useful? If the primary variable of interest is a dependent variable, meaning that the primary goal of the research is to account for variance, then the significance and salience of the dependent variable will determine the value of the research. Similarly, if the primary variable of interest is an independent variable, meaning that the primary goal of the research is to determine and assess impact, then the significance and salience of the independent variable will determine the value of the research.
These three criteria—non-trivial, non-obvious, and variable importance and salience—are not very different from one another. They collectively mean that the researcher must be able to specify why and how the testing of her hypothesis, or hypotheses, will make a contribution of value. Perhaps her propositions are original or innovative; perhaps knowing whether they are true or false makes a difference or will be of practical benefit; perhaps her findings add something specific and identifiable to the body of existing scholarly literature on the subject. While calling attention to these three connected and overlapping criteria might seem unnecessary since they are indeed somewhat obvious, it remains the case that the value of a hypothesis, regardless of whether or not it is eventually confirmed, is itself important to consider, and an investigator should, therefore, know and be able to articulate the reasons and ways that consideration of her hypothesis, or hypotheses, will indeed be of value.
Hypothesizing the Structure of a Relationship
Relevant in the process of hypothesis formation are, as discussed, questions about the origins of hypotheses and the criteria by which the value of any particular hypothesis or set of hypotheses will be evaluated. Relevant, too, is consideration of the structure of a hypothesized variable relationship and the causal story to which that relationship is believed to call attention.
The point of departure in considering the structure of a hypothesized variable relationship is an understanding that such a relationship may or may not be linear. In a direct, or positive, linear relationship, each increase in the independent variable brings a constant increase in the dependent variable. In an inverse, or negative, linear relationship, each increase in the independent variable brings a constant decrease in the dependent variable. But these are only two of the many ways that an independent variable and a dependent variable may be related, or hypothesized to be related. This is easily illustrated by hypotheses in which level of education or age is the independent variable, and this is relevant in hypothesis formation because the investigator must be alert to and consider the possibility that the variables in which she is interested are in fact related in a non-linear way.
Consider, for example, the relationship between age and support for gender equality, the latter measured by an index based on several questions about the rights and behavior of women that are asked in Arab Barometer surveys. A researcher might expect, and might therefore want to hypothesize, that an increase in age brings increased support for, or alternatively increased opposition to, gender equality. But these are not the only possibilities. Likely, perhaps, is the possibility of a curvilinear relationship, in which case increases in age bring increases in support for gender equality until a person reaches a certain age, maybe 40, 45, or 50, after which additional increases in age bring decreases in support for gender equality. Or the researcher might hypothesize that the curve is in the opposite direction, that support for gender equality initially decreases as a function of age until a particular age is reached, after which additional increases in age bring an increase in support.
Of course, there are also other possibilities. In the case of education and gender equality, for example, increased education may initially have no impact on attitudes toward gender equality. Individuals who have not finished primary school, those who have finished primary school, and those who have gone somewhat beyond primary school and completed a middle school program may all have roughly the same attitudes toward gender equality. Thus, increases in education, within a certain range of educational levels, are not expected to bring an increase or a decrease in support for gender equality. But the level of support for gender equality among high school graduates may be higher and among university graduates may be higher still. Accordingly, in this hypothetical illustration, an increase in education does bring increased support for gender equality but only beginning after middle school.
A middle school level of education is a “floor” in this example. Education does not begin to make a difference until this floor is reached, and thereafter it does make a difference, with increases in education beyond middle school bringing increases in support for gender equality. Another possibility might be for middle school to be a “ceiling.” This would mean that increases in education through middle school would bring increases in support for gender equality, but the trend would not continue beyond middle school. In other words, level of education makes a difference and appears to have explanatory power only until, and so not after, this ceiling is reached. This latter pattern was found in the study of education and Palestinian protest activity discussed earlier. Increases in education through middle school brought increases in the likelihood that an individual would participate in demonstrations and protests of Israeli occupation. However, additional education beyond middle school was not associated with greater likelihood of taking part in protest activities.
This discussion of variation in the structure of a hypothesized relationship between two variables is certainly not exhaustive, and the examples themselves are straightforward and not very complicated. The purpose of the discussion is, therefore, to emphasize that an investigator must be open to and think through the possibility and plausibility of different kinds of relationships between her two variables, that is to say, relationships with different structures. Bivariate relationships with several different kinds of structures are depicted visually by the scatter plots in Fig. 3.4 .
These possibilities with respect to structure do not determine the value of a proposed hypothesis. As discussed earlier, the value of a proposed relationship depends first and foremost on the importance and salience of the variable of interest. Accordingly, a researcher should not assume that the value of a hypothesis varies as a function of the degree to which it posits a complicated variable relationship. More complicated hypotheses are not necessarily better or more correct. But while she should not strive for or give preference to variable relationships that are more complicated simply because they are more complicated, she should, again, be alert to the possibility that a more complicated pattern does a better job of describing the causal connection between the two variables in the place and time in which she is interested.
This brings the discussion of formulating hypotheses back to our earlier account of causal stories. In research concerned with explanation and causality, a hypothesis for the most part is a simplified stand-in for a causal story. It represents the causal story, as it were. Expressing this differently, the hypothesis states the causal story’s “bottom line;” it posits that the independent variable is a determinant of variance on the dependent variable, and it identifies the structure of the presumed relationship between the independent variable and the dependent variable. But it does not describe the interaction between the two variables in a way that tells consumers of the study why the researcher believes that the relationship involves causality rather than an association with no causal implications. This is left to the causal story, which will offer a fuller account of the way the presumed cause impacts the presumed effect.
3.3 Describing and Visually Representing Bivariate Relationships
Once a researcher has collected or otherwise obtained data on the variables in a bivariate relationship she wishes to examine, her first step will be to describe the variance on each of the variables using the univariate statistics described in Chap. 2 . She will need to understand the distribution on each variable before she can understand how these variables vary in relation to one another. This is important whether she is interested in description or wishes to explore a bivariate causal story.
Once she has described each one of the variables, she can turn to the relationship between them. She can prepare and present a visual representation of this relationship, which is the subject of the present section. She can also use bivariate statistical tests to assess the strength and significance of the relationship, which is the subject of the next section of this chapter.
Contingency Tables
Contingency tables are used to display the relationship between two categorical variables. They are similar to the univariate frequency distributions described in Chap. 2 , the difference being that they juxtapose the two univariate distributions and display the interaction between them. Also called cross-tabulation tables, the cells of the table may present frequencies, row percentages, column percentages, and/or total percentages. Total frequencies and/or percentages are displayed in a total row and a total column, each one of which is the same as the univariate distribution of one of the variables taken alone.
Table 3.2 , based on Palestinian data from Wave V of the Arab Barometer, crosses gender and the average number of hours watching television each day. Frequencies are presented in the cells of the table. In the cell showing the number of Palestinian men who do not watch television at all, row percentage, column percentage, and total percentage are also presented. Note that total percentage is based on the 10 cells showing the two variables taken together, which are summed in the lower right-hand cell. Thus, total percent for this cell is 342/2488 = 13.7. Only frequencies are given in the other cells of the table; but in a full table, these four figures – frequency, row percent, column percent and total percent – would be given in every cell.
Exercise 3.3
Compute the row percentage, the column percentage, and the total percentage in the cell showing the number of Palestinian women who do not watch television at all.
Describe the relationship between gender and watching television among Palestinians that is shown in the table. Do the television watching habits of Palestinian men and women appear to be generally similar or fairly different? You might find it helpful to convert the frequencies in other cells to row or column percentages.
Stacked Column Charts and Grouped Bar Charts
Stacked column charts and grouped bar charts are used to visually describe how two categorical variables, or one categorical and one continuous variable, relate to one another. Much like contingency tables, they show the percentage or count of each category of one variable within each category of the second variable. This information is presented in columns stacked on each other or next to each other. The charts below show the number of male Palestinians and the number of female Palestinians who watch television for a given number of hours each day. Each chart presents the same information as the other chart and as the contingency table shown above (Fig. 3.1 ).
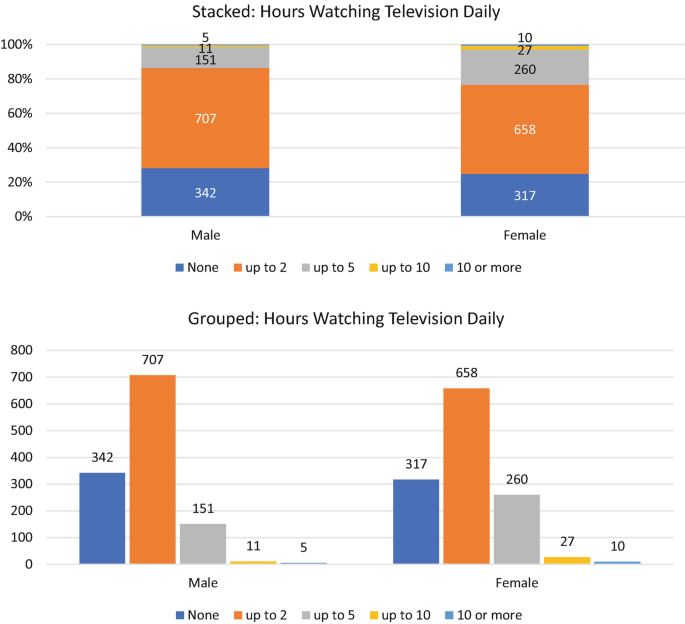
Stacked column charts and grouped bar charts comparing Palestinian men and Palestinian women on hours watching television
Box Plots and Box and Whisker Plots
Box plots, box and whisker plots, and other types of plots can also be used to show the relationship between one categorical variable and one continuous variable. They are particularly useful for showing how spread out the data are. Box plots show five important numbers in a variable’s distribution: the minimum value; the median; the maximum value; and the first and third quartiles (Q1 and Q2), which represent, respectively, the number below which are 25 percent of the distribution’s values and the number below which are 75 percent of the distribution’s values. The minimum value is sometimes called the lower extreme, the lower bound, or the lower hinge. The maximum value is sometimes called the upper extreme, the upper bound, or the upper hinge. The middle 50 percent of the distribution, the range between Q1 and Q3 that represents the “box,” constitutes the interquartile range (IQR). In box and whisker plots, the “whiskers” are the short perpendicular lines extending outside the upper and lower quartiles. They are included to indicate variability below Q1 and above Q3. Values are usually categorized as outliers if they are less than Q1 − IQR*1.5 or greater than Q3 + IQR*1.5. A visual explanation of a box and whisker plot is shown in Fig. 3.2a and an example of a box plot that uses actual data is shown in Fig. 3.2b .
The box plot in Fig. 3.2b uses Wave V Arab Barometer data from Tunisia and shows the relationship between age, a continuous variable, and interpersonal trust, a dichotomous categorical variable. The line representing the median value is shown in bold. Interpersonal trust, sometimes known as generalized trust, is an important personal value. Previous research has shown that social harmony and prospects for democracy are greater in societies in which most citizens believe that their fellow citizens for the most part are trustworthy. Although the interpersonal trust variable is dichotomous in Fig. 3.2b , the variance in interpersonal trust can also be measured by a set of ordered categories or a scale that yields a continuous measure, the latter not being suitable for presentation by a box plot. Figure 3.2b shows that the median age of Tunisians who are trusting is slightly higher than the median age of Tunisians who are mistrustful of other people. Notice also that the box plot for the mistrustful group has an outlier.
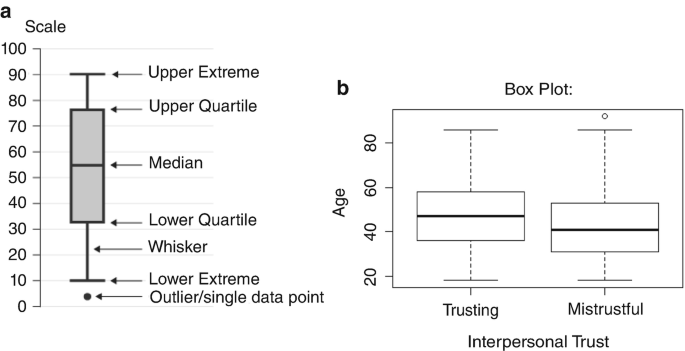
( a ) A box and whisker plot. ( b ) Box plot comparing the ages of trusting and mistrustful Tunisians in 2018
Line plots may be used to visualize the relationship between two continuous variables or a continuous variable and a categorical variable. They are often used when time, or a variable related to time, is one of the two variables. If a researcher wants to show whether and how a variable changes over time for more than one subgroup of the units about which she has data (looking at men and women separately, for example), she can include multiple lines on the same plot, with each line showing the pattern over time for a different subgroup. These lines will generally be distinguished from each other by color or pattern, with a legend provided for readers.
Line plots are a particularly good way to visualize a relationship if an investigator thinks that important events over time may have had a significant impact. The line plot in Fig. 3.3 shows the average support for gender equality among men and among women in Tunisia from 2013 to 2018. Support for gender equality is a scale based on four questions related to gender equality in the three waves of the Arab Barometer. An answer supportive of gender equality on a question adds +.5 to the scale and an answer unfavorable to gender equality adds −.5 to the scale. Accordingly, a scale score of 2 indicates maximum support for gender equality and a scale score of −2 indicates maximum opposition to gender equality.
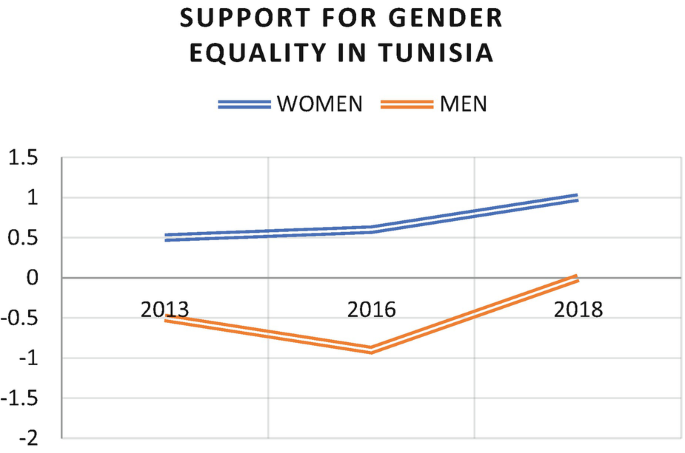
Line plot showing level of support for gender equality among Tunisian women and men in 2013, 2016, and 2018
Scatter Plots
Scatter plots are used to visualize a bivariate relationship when both variables are numerical. The independent variable is put on the x-axis, the horizontal axis, and the dependent variable is put on the y-axis, the vertical axis. Each data point becomes a dot in the scatter plot’s two-dimensional field, with its precise location being the point at which its value on the x-axis intersects with its value on the y-axis. The scatter plot shows how the variables are related to one another, including with respect to linearity, direction, and other aspects of structure. The scatter plots in Fig. 3.4 illustrate a strong positive linear relationship, a moderately strong negative linear relationship, a strong non-linear relationship, and a pattern showing no relationship. Footnote 5 If the scatter plot displays no visible and clear pattern, as in the lower left hand plot shown in Fig. 3.4 , the scatter plot would indicate that the independent variable, by itself, has no meaningful impact on the dependent variable.
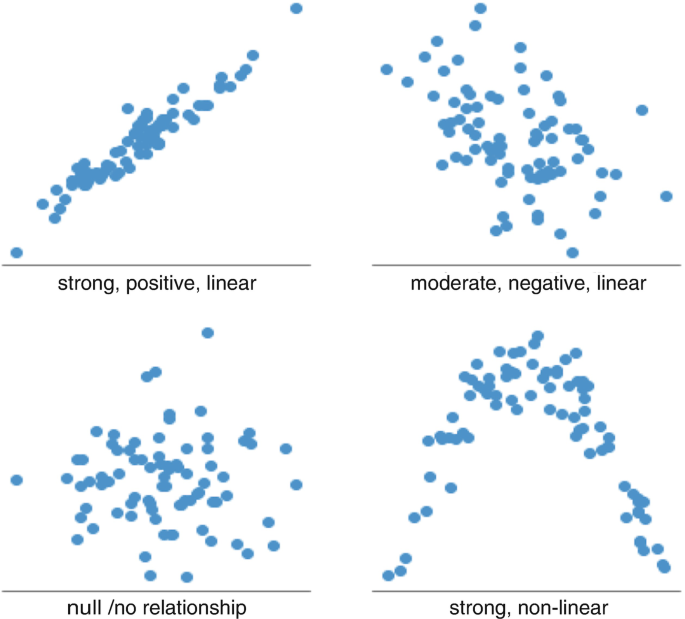
Scatter plots showing bivariate relationships with different structures
Scatter plots are also a good way to identify outliers—data points that do not follow a pattern that characterizes most of the data. These are also called non-scalar types. Figure 3.5 shows a scatter plot with outliers.
Outliers can be informative, making it possible, for example, to identify the attributes of cases for which the measures of one or both variables are unreliable and/or invalid. Nevertheless, the inclusion of outliers may not only distort the assessment of measures, raising unwarranted doubts about measures that are actually reliable and valid for the vast majority of cases, they may also bias bivariate statistics and make relationships seem weaker than they really are for most cases. For this reason, researchers sometimes remove outliers prior to testing a hypothesis. If one does this, it is important to have a clear definition of what is an outlier and to justify the removal of the outlier, both using the definition and perhaps through substantive analysis. There are several mathematical formulas for identifying outliers, and researchers should be aware of these formulas and their pros and cons if they plan to remove outliers.
If there are relatively few outliers, perhaps no more than 5–10 percent of the cases, it may be justifiable to remove them in order to better discern the relationship between the independent variable and the dependent variable. If outliers are much more numerous, however, it is probably because there is not a significant relationship between the two variables being considered. The researcher might in this case find it instructive to introduce a third variable and disaggregate the data. Disaggregation will be discussed in Chap. 4 .
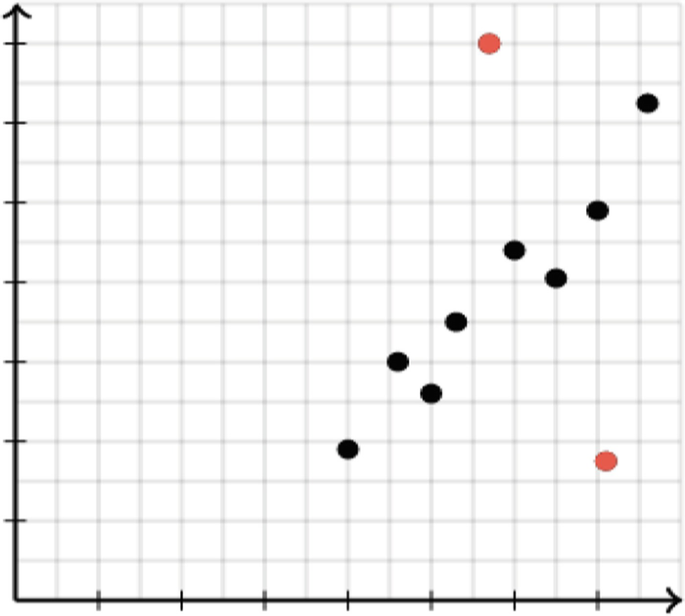
A scatter plot with outliers marked in red
Exercise 3.4 Exploring Hypotheses through Visualizing Data: Exercise with the Arab Barometer Online Analysis Tool
Go to the Arab Barometer Online Analysis Tool ( https://www.arabbarometer.org/survey-data/data-analysis-tool/ )
Select Wave V and a country that interests you
Select “See Results”
Select “Social, Cultural and Religious topics”
Select “Religion: frequency: pray”
Questions: What does the distribution of this variable look like? How would you describe the variance?
Click on “Cross by,” then
Select “Show all variables”
Select “Kind of government preferable” and click
Select “Options,” then “Show % over Row total,” then “Apply”
Questions: Does there seem to be a relationship between religiosity and preference for democracy? If so, what might explain the relationship you observe—what is a plausible causal story? Is it consistent with the hypothesis you wrote for Exercise 3.1?
What other variables could be used to measure religiosity and preference for democracy? Explore your hypothesis using different items from the list of Arab Barometer variables
Do these distributions support the previous results you found? Do you learn anything additional about the relationship between religiosity and preference for democracy?
Now it is your turn to explore variables and variable relationships that interest you!
Pick two variables that interest you from the list of Arab Barometer variables. Are they continuous or categorical? Ordinal or nominal? (Hint: Most Arab Barometer variables are categorical, even if you might be tempted to think of them as continuous. For example, age is divided into the ordinal categories 18–29, 30–49, and 50 and more.)
Do you expect there to be a relationship between the two variables? If so, what do you think will be the structure of that relationship, and why?
Select the wave (year) and the country that interest you
Select one of your two variables of interest
Click on “Cross by,” and then select your second variable of interest.
On the left side of the page, you’ll see a contingency table. On the right side at the top, you’ll see several options to graphically display the relationship between your two variables. Which type of graph best represents the relationship between your two variables of interest?
Do the two variables seem to be independent of each other, or do you think there might be a relationship between them? Is the relationship you see similar to what you had expected
3.4 Probabilities and Type I and Type II Errors
As in visual presentations of bivariate relationships, selecting the appropriate measure of association or bivariate statistical test depends on the types of the two variables. The data on both variables may be categorical; the data on both may be continuous; or the data may be categorical on one variable and continuous on the other variable. These characteristics of the data will guide the way in which our presentation of these measures and tests is organized. Before briefly describing some specific measures of association and bivariate statistical tests, however, it is necessary to lay a foundation by introducing a number of terms and concepts. Relevant here are the distinction between population and sample and the notions of the null hypothesis, of Type I and Type II errors, and of probabilities and confidence intervals. As concepts, or abstractions, these notions may influence the way a researcher thinks about drawing conclusions about a hypothesis from qualitative data, as was discussed in Chap. 2 . In their precise meaning and application, however, these terms and concepts come into play when hypothesis testing involves the statistical analysis of quantitative data.
To begin, it is important to distinguish between, on the one hand, the population of units—individuals, countries, ethnic groups, political movements, or any other unit of analysis—in which the researcher is interested and about which she aspires to advance conclusions and, on the other hand, the units on which she has actually acquired the data to be analyzed. The latter, the units on which she actually has data, is her sample. In cases where the researcher has collected or obtained data on all of the units in which she is interested, there is no difference between the sample and the population, and drawing conclusions about the population based on the sample is straightforward. Most often, however, a researcher does not possess data on all of the units that make up the population in which she is interested, and so the possibility of error when making inferences about the population based on the analysis of data in the sample requires careful and deliberate consideration.
This concern for error is present regardless of the size of the sample and the way it was constructed. The likelihood of error declines as the size of the sample increases and thus comes closer to representing the full population. It also declines if the sample was constructed in accordance with random or other sampling procedures designed to maximize representation. It is useful to keep these criteria in mind when looking at, and perhaps downloading and using, Arab Barometer data. The Barometer’s website gives information about the construction of each sample. But while it is possible to reduce the likelihood of error when characterizing the population from findings based on the sample, it is not possible to eliminate entirely the possibility of erroneous inference. Accordingly, a researcher must endeavor to make the likelihood of this kind of error as small as possible and then decide if it is small enough to advance conclusions that apply to the population as well as the sample.
The null hypothesis, frequently designated as H0, is a statement to the effect that there is no meaningful and significant relationship between the independent variable and the dependent variable in a hypothesis, or indeed between two variables even if the relationship between them has not been formally specified in a hypothesis and does not purport to be causal or explanatory. The null hypothesis may or may not be stated explicitly by an investigator, but it is nonetheless present in her thinking; it stands in opposition to the hypothesized variable relationship. In a point and counterpoint fashion, the hypothesis, H1, posits that the variables are significantly related, and the null hypothesis, H0, replies and says no, they are not significantly related. It further says that they are not related in any meaningful way, neither in the way proposed in H1 nor in any other way that could be proposed.
Based on her analysis, the researcher needs to determine whether her findings permit rejecting the null hypothesis and concluding that there is indeed a significant relationship between the variables in her hypothesis, concluding in effect that the research hypothesis, H1, has been confirmed. This is most relevant and important when the investigator is basing her analysis on some but not all of the units to which her hypothesis purports to apply—when she is analyzing the data in her sample but seeks to advance conclusions that apply to the population in which she is interested. The logic here is that the findings produced by an analysis of some of the data, the data she actually possesses, may be different than the findings her analysis would hypothetically produce were she able to use data from very many more, or ideally even all, of the units that make up her population of interest.
This means, of course, that there will be uncertainty as the researcher adjudicates between H0 and H1 on the basis of her data. An analysis of these data may suggest that there is a strong and significant relationship between the variables in H1. And the stronger the relationship, the more unlikely it is that the researcher’s sample is a subset of a population characterized by H0 and that, therefore, the researcher may consider H1 to have been confirmed. Yet, it remains at least possible that the researcher’s sample, although it provides strong support for H1, is actually a subset of a population characterized by the null hypothesis. This may be unlikely, but it is not impossible, and so, therefore, to consider H1 to have been confirmed is to run the risk, at least a small risk, of what is known as a Type I error. A Type I error is made when a researcher accepts a research hypothesis that is actually false, when she judges to be true a hypothesis that does not characterize the population of which her sample is a subset. Because of the possibility of a Type I error, even if quite unlikely, researchers will often write something like “We can reject the null hypothesis,” rather than “We can confirm our hypothesis.”
Another analysis related to voter turnout provides a ready illustration. In the Arab Barometer Wave V surveys in 12 Arab countries, Footnote 6 13,899 respondents answered a question about voting in the most recent parliamentary election. Of these, 46.6 percent said they had voted, and the remainder, 53.4 percent, said they had not voted in the last parliamentary election. Footnote 7 Seeking to identify some of the determinants of voting—the attitudes and experiences of an individual that increase the likelihood that she will vote, the researcher might hypothesize that a judgment that the country is going in the right direction will push toward voting. More formally:
H1. An individual who believes that her country is going in the right direction is more likely to vote in a national election than is an individual who believes her country is going in the wrong direction.
Arab Barometer surveys provide data with which to test this proposition, and in fact there is a difference associated with views about the direction in which the country is going. Among those who judged that their country is going in the right direction, 52.4 percent voted in the last parliamentary election. By contrast, among those who judged that their country is going in the wrong direction, only 43.8 percent voted in the last parliamentary election.
This illustrates the choice a researcher faces when deciding what to conclude from a study. Does the analysis of her data from a subset of her population of interest confirm or not confirm her hypothesis? In this example, based on Arab Barometer data, the findings are in the direction of her hypothesis, and differences in voting associated with views about the direction the country is going do not appear to be trivial. But are these differences big enough to justify the conclusion that judgements about the country’s path going forward are a determinant of voting, one among others of course, in the population from which her sample was drawn? In other words, although this relationship clearly characterizes the sample, it is unclear whether it characterizes the researcher’s population of interest, the population from which the sample was drawn.
Unless the researcher can gather data on the entire population of eligible voters, or at least almost all of this population, it is not possible to entirely eliminate uncertainty when the researcher makes inferences about the population of voters based on findings from the subset, or sample, of voters on which she has data. She can either conclude that her findings are sufficiently strong and clear to propose that the pattern she has observed characterizes the population as well, and that H1 is therefore confirmed; or she can conclude that her findings are not strong enough to make such an inference about the population, and that H1, therefore, is not confirmed. Either conclusion could be wrong, and so there is a chance of error no matter which conclusion the researcher advances.
The terms Type I error and Type II error are often used to designate the possible error associated with each of these inferences about the population based on the sample. Type I error refers to the rejection of a true null hypothesis. This means, in other words, that the investigator could be wrong if she concludes that her finding of a strong, or at least fairly strong, relationship between her variables characterizes Arab voters in the 12 countries in general, and if she thus judges H1 to have been confirmed when the population from which her sample was drawn is in fact characterized by H0. Type II error refers to acceptance of a false null hypothesis. This means, in other words, that the investigator could be wrong if she concludes that her finding of a somewhat weak relationship, or no relationship at all, between her variables characterizes Arab voters in the 12 countries in general, and that she thus judges H0 to be true when the population from which her sample was drawn is in fact characterized by H1.
In statistical analyses of quantitative data, decisions about whether to risk a Type I error or a Type II error are usually based on probabilities. More specifically, they are based on the probability of a researcher being wrong if she concludes that the variable relationship—or hypothesis in most cases—that characterizes her data, meaning her sample, also characterizes the population on which the researcher hopes her sample and data will shed light. To say this in yet another way, she computes the odds that her sample does not represent the population of which it is a subset; or more specifically still, she computes the odds that from a population that is characterized by the null hypothesis she could have obtained, by chance alone, a subset of the population, her sample, that is not characterized by the null hypothesis. The lower the odds, or probability, the more willing the researcher will be to risk a Type I error.
There are numerous statistical tests that are used to compute such probabilities. The nature of the data and the goals of the analysis will determine the specific test to be used in a particular situation. Most of these tests, frequently called tests of significance or tests of statistical significance, provide output in the form of probabilities, which always range from 0 to 1. The lower the value, meaning the closer to 0, the less likely it is that a researcher has collected and is working with data that produce findings that differ from what she would find were she to somehow have data on the entire population. Another way to think about this is the following:
If the researcher provisionally assumes that the population is characterized by the null hypothesis with respect to the variable relationship under study, what is the probability of obtaining from that population, by chance alone, a subset or sample that is not characterized by the null hypothesis but instead shows a strong relationship between the two variables;
The lower the probability value, meaning the closer to 0, the less likely it is that the researcher’s data, which support H1, have come from a population that is characterized by H0;
The lower the probability that her sample could have come from a population characterized by H0, the lower the possibility that the researcher will be wrong, that she will make a Type I error, if she rejects the null hypothesis and accepts that the population, as well as her sample, is characterized by H1;
When the probability value is low, the chance of actually making a Type I error is small. But while small, the possibility of an error cannot be entirely eliminated.
If it helps you to think about probability and Type I and Type II error, imagine that you will be flipping a coin 100 times and your goal is to determine whether the coin is unbiased, H0, or biased in favor of either heads or tails, H1. How many times more than 50 would heads have to come up before you would be comfortable concluding that the coin is in fact biased in favor of heads? Would 60 be enough? What about 65? To begin to answer these questions, you would want to know the odds of getting 60 or 65 heads from a coin that is actually unbiased, a coin that would come up heads and come up tails roughly the same number of times if it were flipped many more than 100 times, maybe 1000 times, maybe 10,000. With this many flips, would the ratio of heads to tails even out. The lower the odds, the less likely it is that the coin is unbiased. In this analogy, you can think of the mathematical calculations about an unbiased coin’s odds of getting heads as the population, and your actual flips of the coin as the sample.
But exactly how low does the probability of a Type I error have to be for a researcher to run the risk of rejecting H0 and accepting that her variables are indeed related? This depends, of course, on the implications of being wrong. If there are serious and harmful consequences of being wrong, of accepting a research hypothesis that is actually false, the researcher will reject H0 and accept H1 only if the odds of being wrong, of making a Type I error, are very low.
There are some widely used probability values, which define what are known as “confidence intervals,” that help researchers and those who read their reports to think about the likelihood that a Type I error is being made. In the social sciences, rejecting H0 and running the risk of a Type I error is usually thought to require a probability value of less than .05, written as p < .05. The less stringent value of p < .10 is sometimes accepted as sufficient for rejecting H0, although such a conclusion would be advanced with caution and when the consequences of a Type I error are not very harmful. Frequently considered safer, meaning that the likelihood of accepting a false hypothesis is lower, are p < .01 and p < .001. The next section introduces and briefly describes some of the bivariate statistics that may be used to calculate these probabilities.
3.5 Measures of Association and Bivariate Statistical Tests
The following section introduces some of the bivariate statistical tests that can be used to compute probabilities and test hypotheses. The accounts are not very detailed. They will provide only a general overview and refresher for readers who are already fairly familiar with bivariate statistics. Readers without this familiarity are encouraged to consult a statistics textbook, for which the accounts presented here will provide a useful guide. While the account below will emphasize calculating these test statistics by hand, it is also important to remember that they can be calculated with the assistance of statistical software as well. A discussion of statistical software is available in Appendix 4.
Parametric and Nonparametric Statistics
Parametric and nonparametric are two broad classifications of statistical procedures. A parameter in statistics refers to an attribute of a population. For example, the mean of a population is a parameter. Parametric statistical tests make certain assumptions about the shape of the distribution of values in a population from which a sample is drawn, generally that it is normally distributed, and about its parameters, that is to say the means and standard deviations of the assumed distributions. Nonparametric statistical procedures rely on no or very few assumptions about the shape or parameters of the distribution of the population from which the sample was drawn. Chi-squared is the only nonparametric statistical test among the tests described below.
Degrees of Freedom
Degrees of freedom (df) is the number of values in the calculation of a statistic that are free to vary. Statistical software programs usually give degrees of freedom in the output, so it is generally unnecessary to know the number of the degrees of freedom in advance. It is nonetheless useful to understand what degrees of freedom represent. Consistent with the definition above, it is the number of values that are not predetermined, and thus are free to vary, within the variables used in a statistical test.
This is illustrated by the contingency tables below, which are constructed to examine the relationship between two categorical variables. The marginal row and column totals are known since these are just the univariate distributions of each variable. df = 1 for Table 3.3a , which is a 4-cell table. You can enter any one value in any one cell, but thereafter the values of all the other three cells are determined. Only one number is not free to vary and thus not predetermined. df = 2 for Table 3.3b , which is a 6-cell table. You can enter any two values in any two cells, but thereafter the values of all the other cells are determined. Only two numbers are free to vary and thus not predetermined. For contingency tables, the formula for calculating df is:
Chi-Squared
Chi-squared, frequently written X 2 , is a statistical test used to determine whether two categorical variables are significantly related. As noted, it is a nonparametric test. The most common version of the chi-squared test is the Pearson chi-squared test, which gives a value for the chi-squared statistic and permits determining as well a probability value, or p-value. The magnitude of the statistic and of the probability value are inversely correlated; the higher the value of the chi-squared statistic, the lower the probability value, and thus the lower the risk of making a Type I error—of rejecting a true null hypothesis—when asserting that the two variables are strongly and significantly related.
The simplicity of the chi-squared statistic permits giving a little more detail in order to illustrate several points that apply to bivariate statistical tests in general. The formula for computing chi-squared is given below, with O being the observed (actual) frequency in each cell of a contingency table for two categorical variables and E being the frequency that would be expected in each cell if the two variables are not related. Put differently, the distribution of E values across the cells of the two-variable table constitutes the null hypothesis, and chi-squared provides a number that expresses the magnitude of the difference between an investigator’s actual observed values and the values of E.
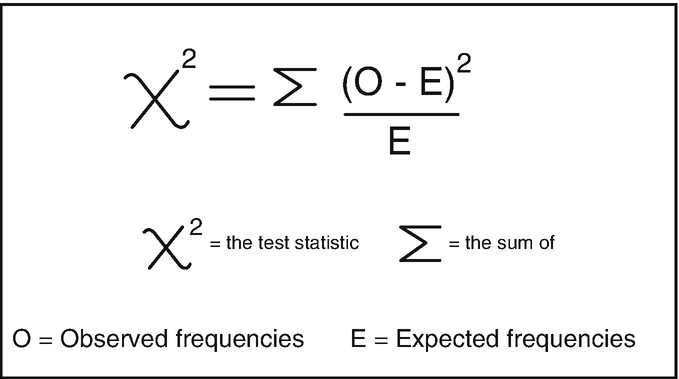
The computation of chi-squared involves the following procedures, which are illustrated using the data in Table 3.4 .
The values of O in the cells of the table are based on the data collected by the investigator. For example, Table 3.4 shows that of the 200 women on whom she collected information, 85 are majoring in social science.
The value of E for each cell is computed by multiplying the marginal total of the column in which the cell is located by the marginal total of the row in which the cell is located divided by N, N being the total number of cases. For the female students majoring in social science in Table 3.4 , this is: 200 * 150/400 = 30,000/400 = 75. For the female students majoring in math and natural science in Table 3.4 , this is: 200 * 100/400 = 20,000/400 = 50.
The difference between the value of O and the value of E is computed for each cell using the formula for chi-squared. For the female students majoring in social science in Table 3.4 , this is: (85–75) 2 /75 = 10 2 /75 = 100/75 = 1.33. For the female students majoring in math and natural science, the value resulting from the application of the chi-squared is: (45–50) 2 /50 = 5 2 /75 = 25/75 = .33.
The values in each cell of the table resulting from the application of the chi-squared formula are summed (Σ). This chi-squared value expresses the magnitude of the difference between a distribution of values indicative of the null hypothesis and what the investigator actually found about the relationship between gender and field of study. In Table 3.4 , the cell for female students majoring in social science adds 1.33 to the sum of the values in the eight cells, the cell for female students majoring in math and natural science adds .33 to the sum, and so forth for the remaining six cells.
A final point to be noted, which applies to many other statistical tests as well, is that the application of chi-squared and other bivariate (and multivariate) statistical tests yields a value with which can be computed the probability that an observed pattern does not differ from the null hypothesis and that a Type I error will be made if the null hypothesis is rejected and the research hypothesis is judged to be true. The lower the probability, of course, the lower the likelihood of an error if the null hypothesis is rejected.
Prior to the advent of computer assisted statistical analysis, the value of the statistic and the number of degrees of freedom were used to find the probability value in a table of probability values in an appendix in most statistics books. At present, however, the probability value, or p-value, and also the degrees of freedom, are routinely given as part of the output when analysis is done by one of the available statistical software packages.
Table 3.5 shows the relationship between economic circumstance and trust in the government among 400 ordinary citizens in a hypothetical country. The observed data were collected to test the hypothesis that greater wealth pushes people toward greater trust and less wealth pushes people toward lesser trust. In the case of all three patterns, the probability that the null hypothesis is true is very low. All three patterns have the same high chi-squared value and low probability value. Thus, the chi-squared and p-values show only that the patterns all differ significantly from what would be expected were the null hypothesis true. They do not show whether the data support the hypothesized variable relationship or any other particular relationship.
As the three patterns in Table 3.5 show, variable relationships with very different structures can yield similar or even identical statistical test and probability values, and thus these tests provide only some of the information a researcher needs to draw conclusions about her hypothesis. To draw the right conclusion, it may also be necessary for the investigator to “look at” her data. For example, as Table 3.5 suggests, looking at a tabular or visual presentation of the data may also be needed to draw the proper conclusion about how two variables are related.
How would you describe the three patterns shown in the table, each of which differs significantly from the null hypothesis? Which pattern is consistent with the research hypothesis? How would you describe the other two patterns? Try to visualize a plot of each pattern.
Pearson Correlation Coefficient
The Pearson correlation coefficient, more formally known as the Pearson product-moment correlation, is a parametric measure of linear association. It gives a numerical representation of the strength and direction of the relationship between two continuous numerical variables. The coefficient, which is commonly represented as r , will have a value between −1 and 1. A value of 1 means that there is a perfect positive, or direct, linear relationship between the two variables; as one variable increases, the other variable consistently increases by some amount. A value of −1 means that there is a perfect negative, or inverse, linear relationship; as one variable increases, the other variable consistently decreases by some amount. A value of 0 means that there is no linear relationship; as one variable increases, the other variable neither consistently increases nor consistently decreases.
It is easy to think of relationships that might be assessed by a Pearson correlation coefficient. Consider, for example, the relationship between age and income and the proposition that as age increases, income consistently increases or consistently decreases as well. The closer a coefficient is to 1 or −1, the greater the likelihood that the data on which it is based are not the subset of a population in which age and income are unrelated, meaning that the population of interest is not characterized by the null hypothesis. Coefficients very close to 1 or −1 are rare; although it depends on the number of units on which the researcher has data and also on the nature of the variables. Coefficients higher than .3 or lower than −.03 are frequently high enough, in absolute terms, to yield a low probability value and justify rejecting the null hypothesis. The relationship in this case would be described as “statistically significant.”
Exercise 3.5
Estimating Correlation Coefficients from scatter plots
Look at the scatter plots in Fig. 3.4 and estimate the correlation coefficient that the bivariate relationship shown in each scatter plot would yield.
Explain the basis for each of your estimates of the correlation coefficient.
Spearman’s Rank-Order Correlation Coefficient
The Spearman’s rank-order correlation coefficient is a nonparametric version of the Pearson product-moment correlation . Spearman’s correlation coefficient, (ρ, also signified by r s ) measures the strength and direction of the association between two ranked variables.
Bivariate Regression
Bivariate regression is a parametric measure of association that, like correlation analysis, assesses the strength and direction of the relationship between two variables. Also, like correlation analysis, regression assumes linearity. It may give misleading results if used with variable relationships that are not linear.
Regression is a powerful statistic that is widely used in multivariate analyses. This includes ordinary least squares (OLS) regression, which requires that the dependent variable be continuous and assumes linearity; binary logistic regression, which may be used when the dependent variable is dichotomous; and ordinal logistic regression, which is used with ordinal dependent variables. The use of regression in multivariate analysis will be discussed in the next chapter. In bivariate analysis, regression analysis yields coefficients that indicate the strength and direction of the relationship between two variables. Researchers may opt to “standardize” these coefficients. Standardized coefficients from a bivariate regression are the same as the coefficients produced by Pearson product-moment correlation analysis.
The t-test, also sometimes called a “difference of means” test, is a parametric statistical test that compares the means of two variables and determines whether they are different enough from each other to reject the null hypothesis and risk a Type I error. The dependent variable in a t-test must be continuous or ordinal—otherwise the investigator cannot calculate a mean. The independent variable must be categorical since t-tests are used to compare two groups.
An example, drawing again on Arab Barometer data, tests the relationship between voting and support for democracy. The hypothesis might be that men and women who voted in the last parliamentary election are more likely than men and women who did not vote to believe that democracy is suitable for their country. Whether a person did or did not vote would be the categorical independent variable, and the dependent variable would be the response to a question like, “To what extent do you think democracy is suitable for your country?” The question about democracy asked respondents to situate their views on a 11-point scale, with 0 indicating completely unsuitable and 10 indicating completely suitable.
Focusing on Tunisia in 2018, Arab Barometer Wave V data show that the mean response on the 11-point suitability question is 5.11 for those who voted and 4.77 for those who did not vote. Is this difference of .34 large enough to be statistically significant? A t-test will determine the probability of getting a difference of this magnitude from a population of interest, most likely all Tunisians of voting age, in which there is no difference between voters and non-voters in views about the suitability of democracy for Tunisia. In this example, the t-test showed p < .086. With this p-value, which is higher than the generally accepted standard of .05, a researcher cannot with confidence reject the null hypotheses, and she is unable, therefore, to assert that the proposed relationship has been confirmed.
This question can also be explored at the country level of analysis with, for example, regime type as the independent variable. In this illustration, the hypothesis is that citizens of monarchies are more likely than citizens of republics to believe that democracy is suitable for their country. Of course, a researcher proposing this hypothesis would also advance an associated causal story that provides the rationale for the hypothesis and specifies what is really being tested. To test this proposition, an investigator might merge data from surveys in, say, three monarchies, perhaps Morocco, Jordan, and Kuwait, and then also merge data from surveys in three republics, perhaps Algeria, Egypt, and Iraq. A t-test would then be used to compare the means of people in republics and people in monarchies and give the p-value.
A similar test, the Wilcoxon-Mann-Whitney test, is a nonparametric test that does not require that the dependent variable be normally distributed.
Analysis of variance, or ANOVA, is closely related to the t-test. It may be used when the dependent variable is continuous and the independent variable is categorical. A one-way ANOVA compares the mean and variance values of a continuous dependent variable in two or more categories of a categorical independent variable in order to determine if the latter affects the former.
ANOVA calculates the F-ratio based on the variance between the groups and the variance within each group. The F-ratio can then be used to calculate a p-value. However, if there are more than two categories of the independent variable, the ANOVA test will not indicate which pairs of categories differ enough to be statistically significant, making it necessary, again, to look at the data in order to draw correct conclusions about the structure of the bivariate relationships. Two-way ANOVA is used when an investigator has more than two variables.
Table 3.6 presents a summary list of the visual representations and bivariate statistical tests that have been discussed. It reminds readers of the procedures that can be used when both variables are categorical, when both variables are numerical/continuous, and when one variable is categorical and one variable is numerical/continuous.
Bivariate Statistics and Causal Inference
It is important to remember that bivariate statistical tests only assess the association or correlation between two variables. The tests described above can help a researcher estimate how much confidence her hypothesis deserves and, more specifically, the probability that any significant variable relationships she has found characterize the larger population from which her data were drawn and about which she seeks to offer information and insight.
The finding that two variables in a hypothesized relationship are related to a statistically significant degree is not evidence that the relationship is causal, only that the independent variable is related to the dependent variable. The finding is consistent with the causal story that the hypothesis represents, and to that extent, it offers support for this story. Nevertheless, there are many reasons why an observed statistically significant relationship might be spurious. The correlation might, for example, reflect the influence of one or more other and uncontrolled variables. This will be discussed more fully in the next chapter. The point here is simply that bivariate statistics do not, by themselves, address the question of whether a statistically significant relationship between two variables is or is not a causal relationship.
Only an Introductory Overview
As has been emphasized throughout, this chapter seeks only to offer an introductory overview of the bivariate statistical tests that may be employed when an investigator seeks to assess the relationship between two variables. Additional information will be presented in Chap. 4 . The focus in Chap. 4 will be on multivariate analysis, on analyses involving three or more variables. In this case again, however, the chapter will provide only an introductory overview. The overviews in the present chapter and the next provide a foundation for understanding social statistics, for understanding what statistical analyses involve and what they seek to accomplish. This is important and valuable in and of itself. Nevertheless, researchers and would-be researchers who intend to incorporate statistical analyses into their investigations, perhaps to test hypotheses and decide whether to risk a Type I error or a Type II error, will need to build on this foundation and become familiar with the contents of texts on social statistics. If this guide offers a bird’s eye view, researchers who implement these techniques will also need to expose themselves to the view of the worm at least once.
Chapter 2 makes clear that the concept of variance is central and foundational for much and probably most data-based and quantitative social science research. Bivariate relationships, which are the focus of the present chapter, are building blocks that rest on this foundation. The goal of this kind of research is very often the discovery of causal relationships, relationships that explain rather than merely describe or predict. Such relationships are also frequently described as accounting for variance. This is the focus of Chap. 4 , and it means that there will be, first, a dependent variable, a variable that expresses and captures the variance to be explained, and then, second, an independent variable, and possibly more than one independent variable, that impacts the dependent variable and causes it to vary.
Bivariate relationships are at the center of this enterprise, establishing the empirical pathway leading from the variance discussed in Chap. 2 to the causality discussed in Chap. 4 . Finding that there is a significant relationship between two variables, a statistically significant relationship, is not sufficient to establish causality, to conclude with confidence that one of the variables impacts the other and causes it to vary. But such a finding is necessary.
The goal of social science inquiry that investigates the relationship between two variables is not always explanation. It might be simply to describe and map the way two variables interact with one another. And there is no reason to question the value of such research. But the goal of data-based social science research is very often explanation; and while the inter-relationships between more than two variables will almost always be needed to establish that a relationship is very likely to be causal, these inter-relationships can only be examined by empirics that begin with consideration of a bivariate relationship, a relationship with one variable that is a presumed cause and one variable that is a presumed effect.
Against this background, with the importance of two-variable relationships in mind, the present chapter offers a comprehensive overview of bivariate relationships, including but not only those that are hypothesized to be causally related. The chapter considers the origin and nature of hypotheses that posit a particular relationship between two variables, a causal relationship if the larger goal of the research is explanation and the delineation of a causal story to which the hypothesis calls attention. This chapter then considers how a bivariate relationship might be described and visually represented, and thereafter it discusses how to think about and determine whether the two variables actually are related.
Presenting tables and graphs to show how two variables are related and using bivariate statistics to assess the likelihood that an observed relationship differs significantly from the null hypothesis, the hypothesis of no relationship, will be sufficient if the goal of the research is to learn as much as possible about whether and how two variables are related. And there is plenty of excellent research that has this kind of description as its primary objective, that makes use for purposes of description of the concepts and procedures introduced in this chapter. But there is also plenty of research that seeks to explain, to account for variance, and for this research, use of these concepts and procedures is necessary but not sufficient. For this research, consideration of a two-variable relationship, the focus of the present chapter, is a necessary intermediate step on a pathway that leads from the observation of variance to explaining how and why that variance looks and behaves as it does.
Dana El Kurd. 2019. “Who Protests in Palestine? Mobilization Across Class Under the Palestinian Authority.” In Alaa Tartir and Timothy Seidel, eds. Palestine and Rule of Power: Local Dissent vs. International Governance . New York: Palgrave Macmillan.
Yael Zeira. 2019. The Revolution Within: State Institutions and Unarmed Resistance in Palestine . New York: Cambridge University Press.
Carolina de Miguel, Amaney A. Jamal, and Mark Tessler. 2015. “Elections in the Arab World: Why do citizens turn out?” Comparative Political Studies 48, (11): 1355–1388.
Question 1: Independent variable is religiosity; dependent variable is preference for democracy. Example of hypothesis for Question 1: H1. More religious individuals are more likely than less religious individuals to prefer democracy to other political systems. Question 2: Independent variable is preference for democracy; dependent variable is turning out to vote. Example of hypothesis for Question 2: H2. Individuals who prefer democracy to other political systems are more likely than individuals who do not prefer democracy to other political systems to turn out to vote.
Mike Yi. “A complete Guide to Scatter Plots,” posted October 16, 2019 and seen at https://chartio.com/learn/charts/what-is-a-scatter-plot/
The countries are Algeria, Egypt, Iraq, Jordan, Kuwait, Lebanon, Libya, Morocco, Palestine, Sudan, Tunisia, and Yemen. The Wave V surveys were conducted in 2018–2019.
Not considered in this illustration are the substantial cross-country differences in voter turnout. For example, 63.6 of the Lebanese respondents reported voting, whereas in Algeria the proportion who reported voting was only 20.3 percent. In addition to testing hypotheses about voting in which the individual is the unit of analysis, country could also be the unit of analysis, and hypotheses seeking to account for country-level variance in voting could be formulated and tested.
Author information
Authors and affiliations.
Department of Political Science, University of Michigan, Ann Arbor, MI, USA
Mark Tessler
You can also search for this author in PubMed Google Scholar
Rights and permissions
Open Access This chapter is licensed under the terms of the Creative Commons Attribution 4.0 International License ( http://creativecommons.org/licenses/by/4.0/ ), which permits use, sharing, adaptation, distribution and reproduction in any medium or format, as long as you give appropriate credit to the original author(s) and the source, provide a link to the Creative Commons license and indicate if changes were made.
The images or other third party material in this chapter are included in the chapter's Creative Commons license, unless indicated otherwise in a credit line to the material. If material is not included in the chapter's Creative Commons license and your intended use is not permitted by statutory regulation or exceeds the permitted use, you will need to obtain permission directly from the copyright holder.
Reprints and permissions
Copyright information
© 2023 The Author(s)
About this chapter
Tessler, M. (2023). Bivariate Analysis: Associations, Hypotheses, and Causal Stories. In: Social Science Research in the Arab World and Beyond. SpringerBriefs in Sociology. Springer, Cham. https://doi.org/10.1007/978-3-031-13838-6_3
Download citation
DOI : https://doi.org/10.1007/978-3-031-13838-6_3
Published : 04 October 2022
Publisher Name : Springer, Cham
Print ISBN : 978-3-031-13837-9
Online ISBN : 978-3-031-13838-6
eBook Packages : Social Sciences Social Sciences (R0)
Share this chapter
Anyone you share the following link with will be able to read this content:
Sorry, a shareable link is not currently available for this article.
Provided by the Springer Nature SharedIt content-sharing initiative
- Publish with us
Policies and ethics
- Find a journal
- Track your research
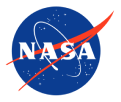
The Causes of Climate Change
Human activities are driving the global warming trend observed since the mid-20th century.
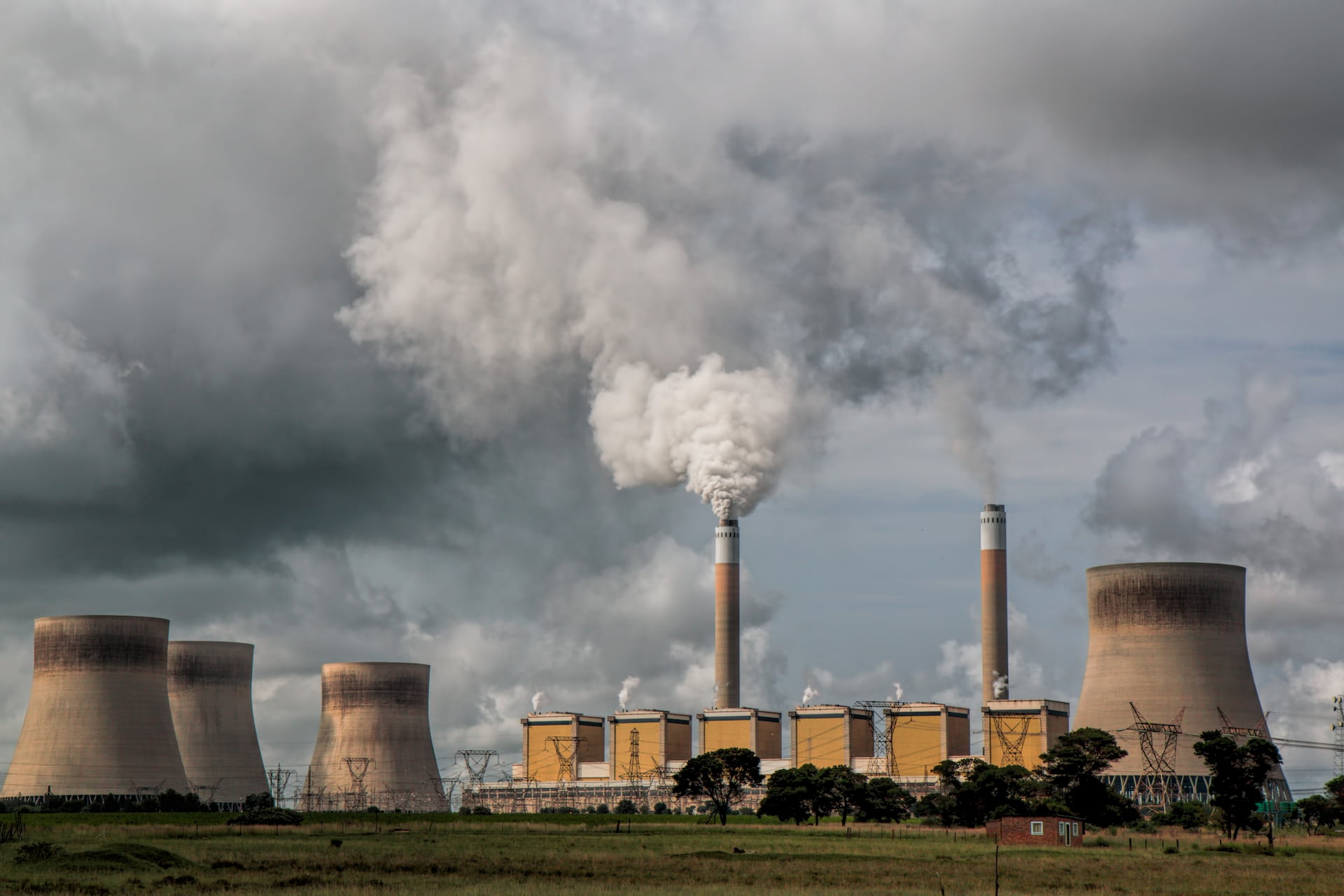
- The greenhouse effect is essential to life on Earth, but human-made emissions in the atmosphere are trapping and slowing heat loss to space.
- Five key greenhouse gases are carbon dioxide, nitrous oxide, methane, chlorofluorocarbons, and water vapor.
- While the Sun has played a role in past climate changes, the evidence shows the current warming cannot be explained by the Sun.
Increasing Greenhouses Gases Are Warming the Planet
Scientists attribute the global warming trend observed since the mid-20 th century to the human expansion of the "greenhouse effect" 1 — warming that results when the atmosphere traps heat radiating from Earth toward space.
Life on Earth depends on energy coming from the Sun. About half the light energy reaching Earth's atmosphere passes through the air and clouds to the surface, where it is absorbed and radiated in the form of infrared heat. About 90% of this heat is then absorbed by greenhouse gases and re-radiated, slowing heat loss to space.
Four Major Gases That Contribute to the Greenhouse Effect
Carbon dioxide.
A vital component of the atmosphere, carbon dioxide (CO 2 ) is released through natural processes (like volcanic eruptions) and through human activities, such as burning fossil fuels and deforestation.
Like many atmospheric gases, methane comes from both natural and human-caused sources. Methane comes from plant-matter breakdown in wetlands and is also released from landfills and rice farming. Livestock animals emit methane from their digestion and manure. Leaks from fossil fuel production and transportation are another major source of methane, and natural gas is 70% to 90% methane.
Nitrous Oxide
A potent greenhouse gas produced by farming practices, nitrous oxide is released during commercial and organic fertilizer production and use. Nitrous oxide also comes from burning fossil fuels and burning vegetation and has increased by 18% in the last 100 years.
Chlorofluorocarbons (CFCs)
These chemical compounds do not exist in nature – they are entirely of industrial origin. They were used as refrigerants, solvents (a substance that dissolves others), and spray can propellants.
FORCING: Something acting upon Earth's climate that causes a change in how energy flows through it (such as long-lasting, heat-trapping gases - also known as greenhouse gases). These gases slow outgoing heat in the atmosphere and cause the planet to warm.
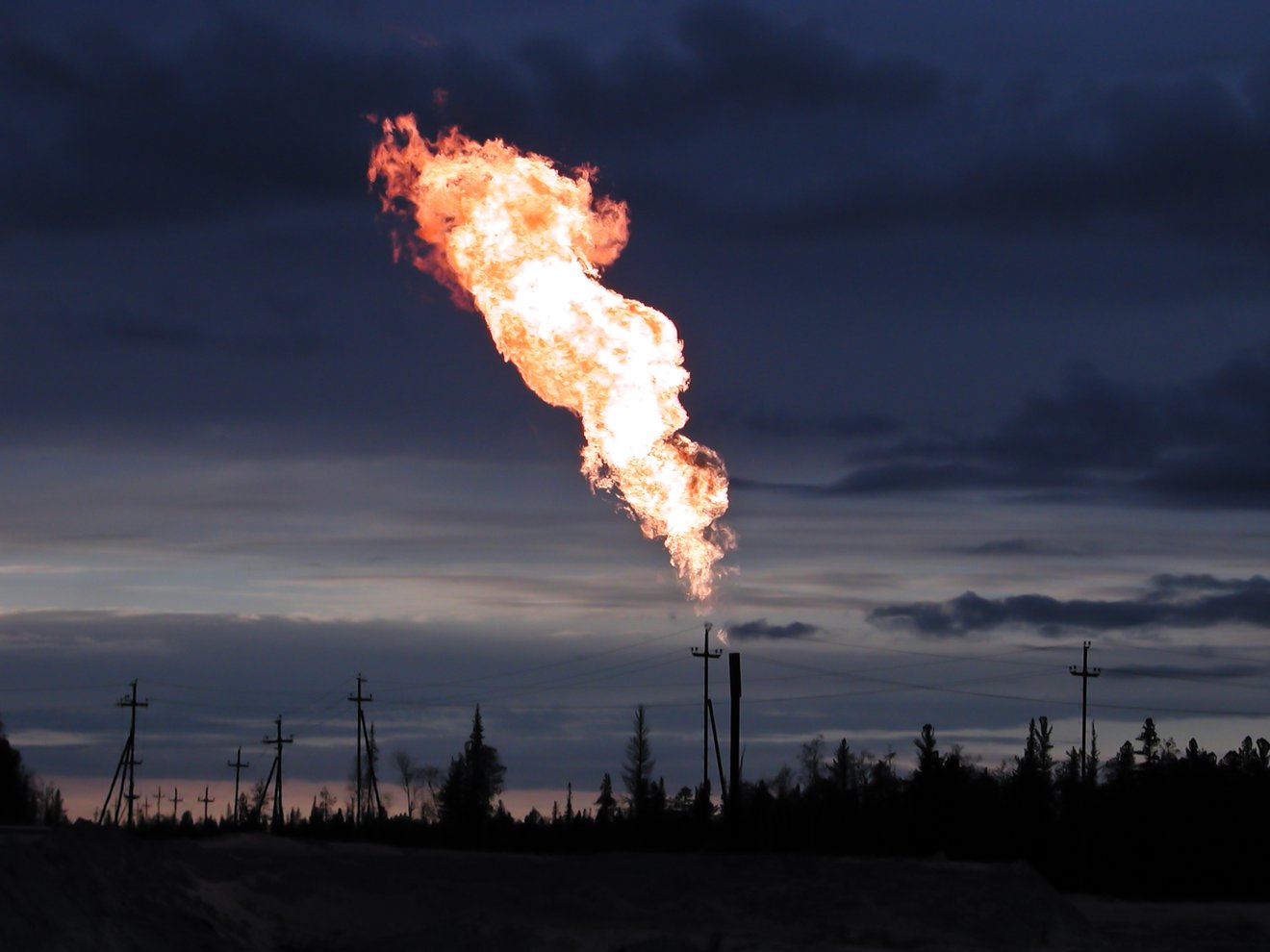
Another Gas That Contributes to the Greenhouse Effect:
Water vapor.
Water vapor is the most abundant greenhouse gas, but because the warming ocean increases the amount of it in our atmosphere, it is not a direct cause of climate change. Credit: John Fowler on Unsplash
FEEDBACKS: A process where something is either amplified or reduced as time goes on, such as water vapor increasing as Earth warms leading to even more warming.
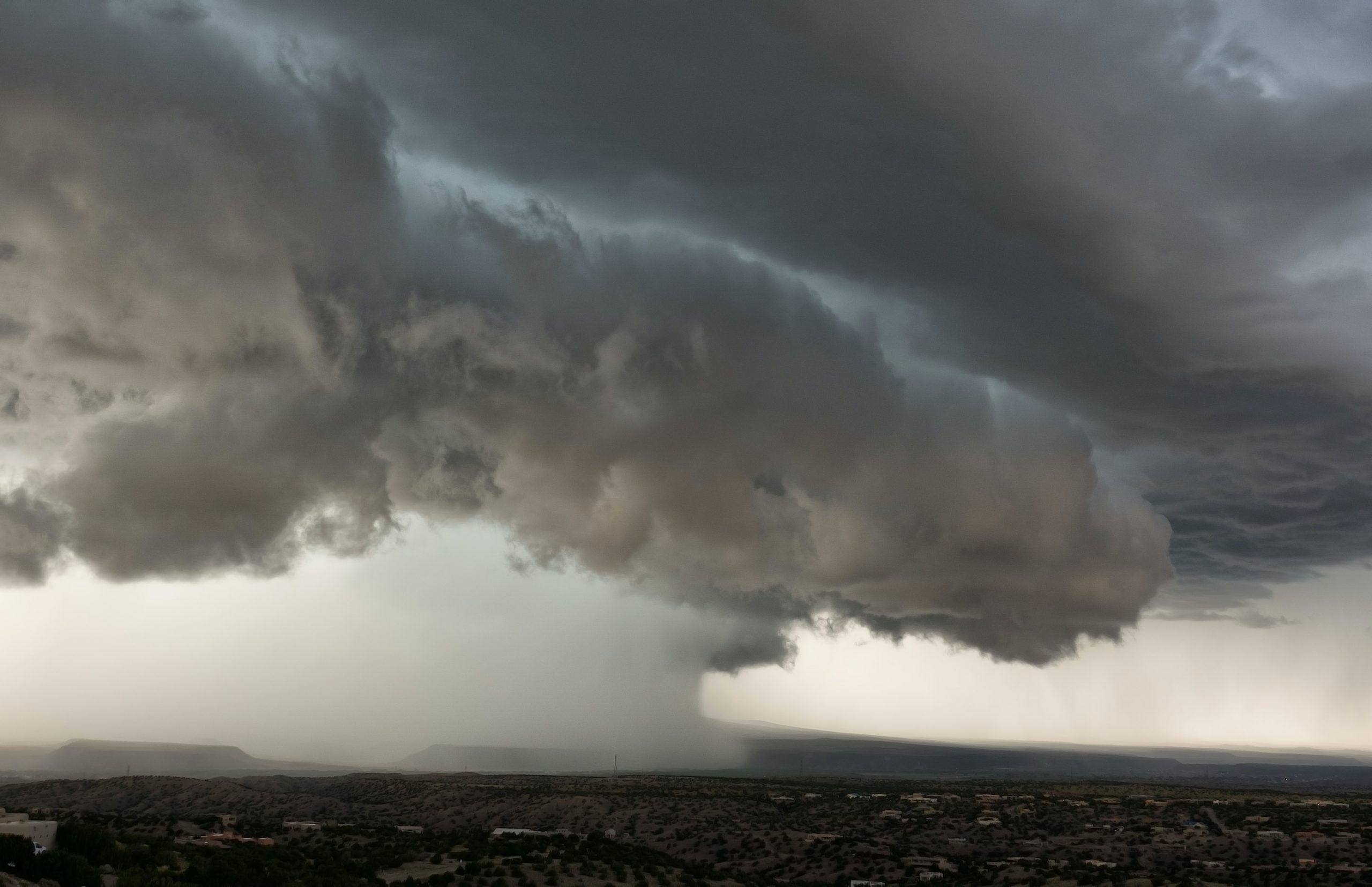
Human Activity Is the Cause of Increased Greenhouse Gas Concentrations
Over the last century, burning of fossil fuels like coal and oil has increased the concentration of atmospheric carbon dioxide (CO 2 ). This increase happens because the coal or oil burning process combines carbon with oxygen in the air to make CO 2 . To a lesser extent, clearing of land for agriculture, industry, and other human activities has increased concentrations of greenhouse gases.
The industrial activities that our modern civilization depends upon have raised atmospheric carbon dioxide levels by nearly 50% since 1750 2 . This increase is due to human activities, because scientists can see a distinctive isotopic fingerprint in the atmosphere.
In its Sixth Assessment Report, the Intergovernmental Panel on Climate Change, composed of scientific experts from countries all over the world, concluded that it is unequivocal that the increase of CO 2 , methane, and nitrous oxide in the atmosphere over the industrial era is the result of human activities and that human influence is the principal driver of many changes observed across the atmosphere, ocean, cryosphere and biosphere.
"Since systematic scientific assessments began in the 1970s, the influence of human activity on the warming of the climate system has evolved from theory to established fact."
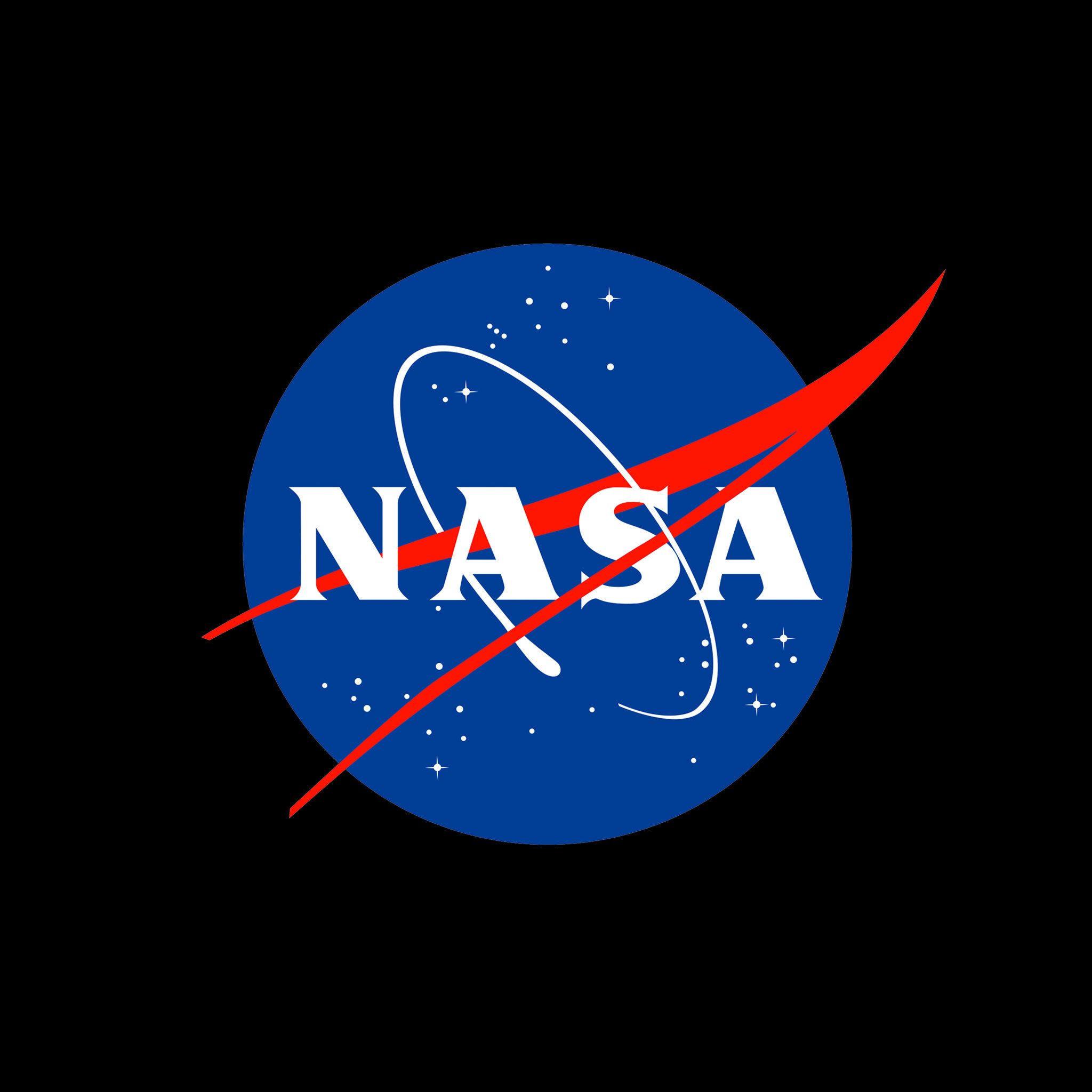
Intergovernmental Panel on Climate Change
The panel's AR6 Working Group I (WGI) Summary for Policymakers report is online at https://www.ipcc.ch/report/ar6/wg1/ .
Evidence Shows That Current Global Warming Cannot Be Explained by Solar Irradiance
Scientists use a metric called Total Solar Irradiance (TSI) to measure the changes in energy the Earth receives from the Sun. TSI incorporates the 11-year solar cycle and solar flares/storms from the Sun's surface.
Studies show that solar variability has played a role in past climate changes. For example, a decrease in solar activity coupled with increased volcanic activity helped trigger the Little Ice Age.
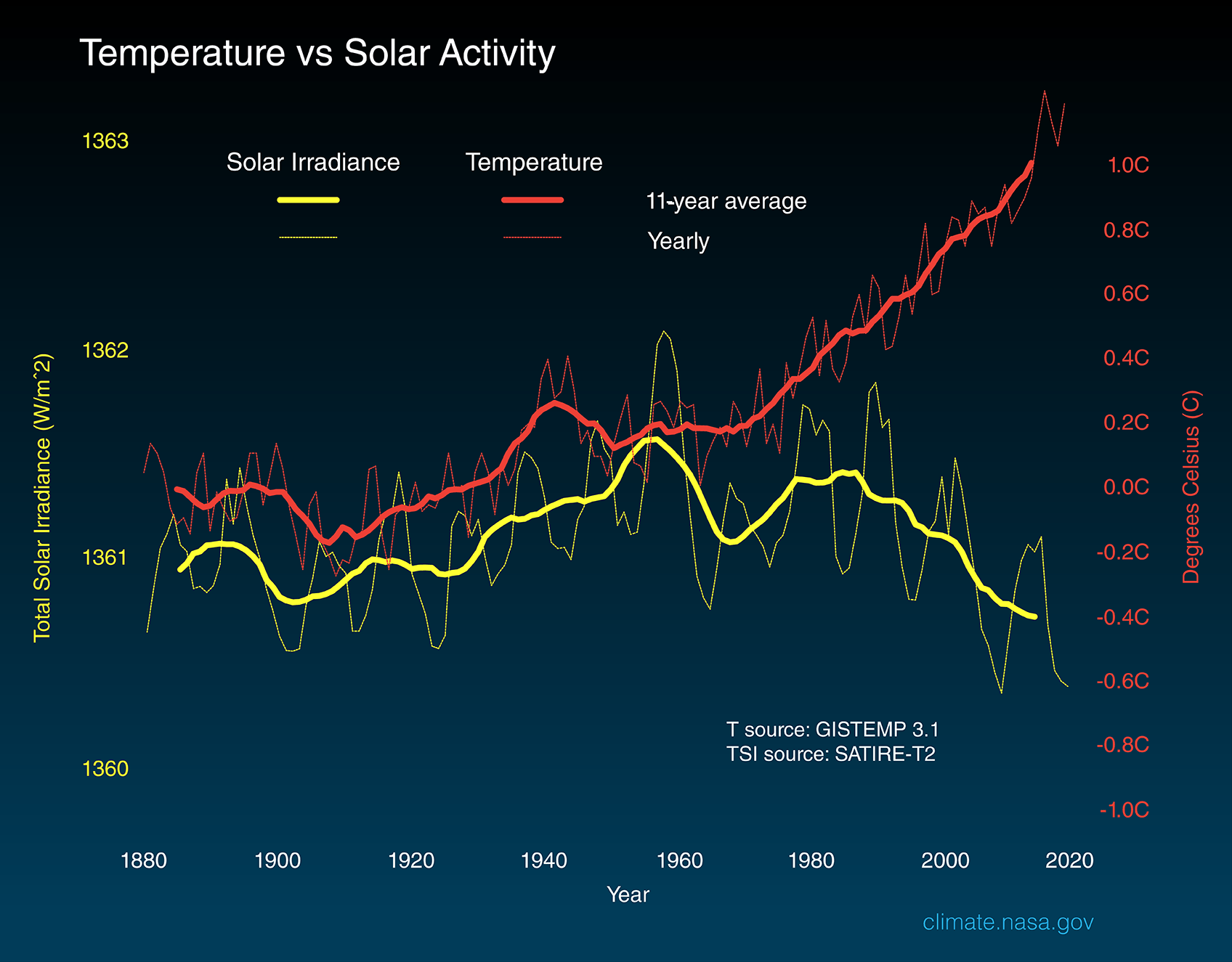
But several lines of evidence show that current global warming cannot be explained by changes in energy from the Sun:
- Since 1750, the average amount of energy from the Sun either remained constant or decreased slightly 3 .
- If a more active Sun caused the warming, scientists would expect warmer temperatures in all layers of the atmosphere. Instead, they have observed a cooling in the upper atmosphere and a warming at the surface and lower parts of the atmosphere. That's because greenhouse gases are slowing heat loss from the lower atmosphere.
- Climate models that include solar irradiance changes can’t reproduce the observed temperature trend over the past century or more without including a rise in greenhouse gases.
1. IPCC 6 th Assessment Report, WG1, Summary for Policy Makers, Sections A, “ The Current State of the Climate ”
IPCC 6 th Assessment Report, WG1, Technical Summary, Sections TS.1.2, TS.2.1 and TS.3.1
2. P. Friedlingstein, et al., 2022: “Global Carbon Budget 2022”, Earth System Science Data ( 11 Nov 2022): 4811–4900. https://doi.org/10.5194/essd-14-4811-2022
3. IPCC 6 th Assessment Report, WG1, Chapter 2, Section 2.2.1, “ Solar and Orbital Forcing ” IPCC 6 th Assessment Report, WG1, Chapter 7, Sections 7.3.4.4, 7.3.5.2, Figure 7.6, “ Solar ” M. Lockwood and W.T. Ball, Placing limits on long-term variations in quiet-Sun irradiance and their contribution to total solar irradiance and solar radiative forcing of climate,” Proceedings of the Royal Society A , 476, issue 2228 (24 June 2020): https://doi 10.1098/rspa.2020.0077
Header image credit: Pixabay/stevepb Four Major Gases image credit: Adobe Stock/Ilya Glovatskiy
Discover More Topics From NASA
Explore Earth Science

Earth Science in Action
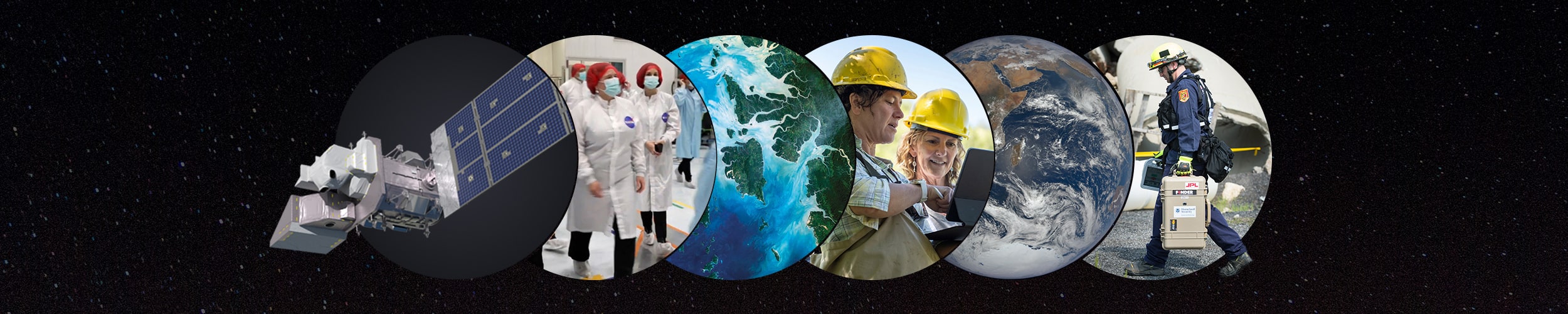
Earth Science Data
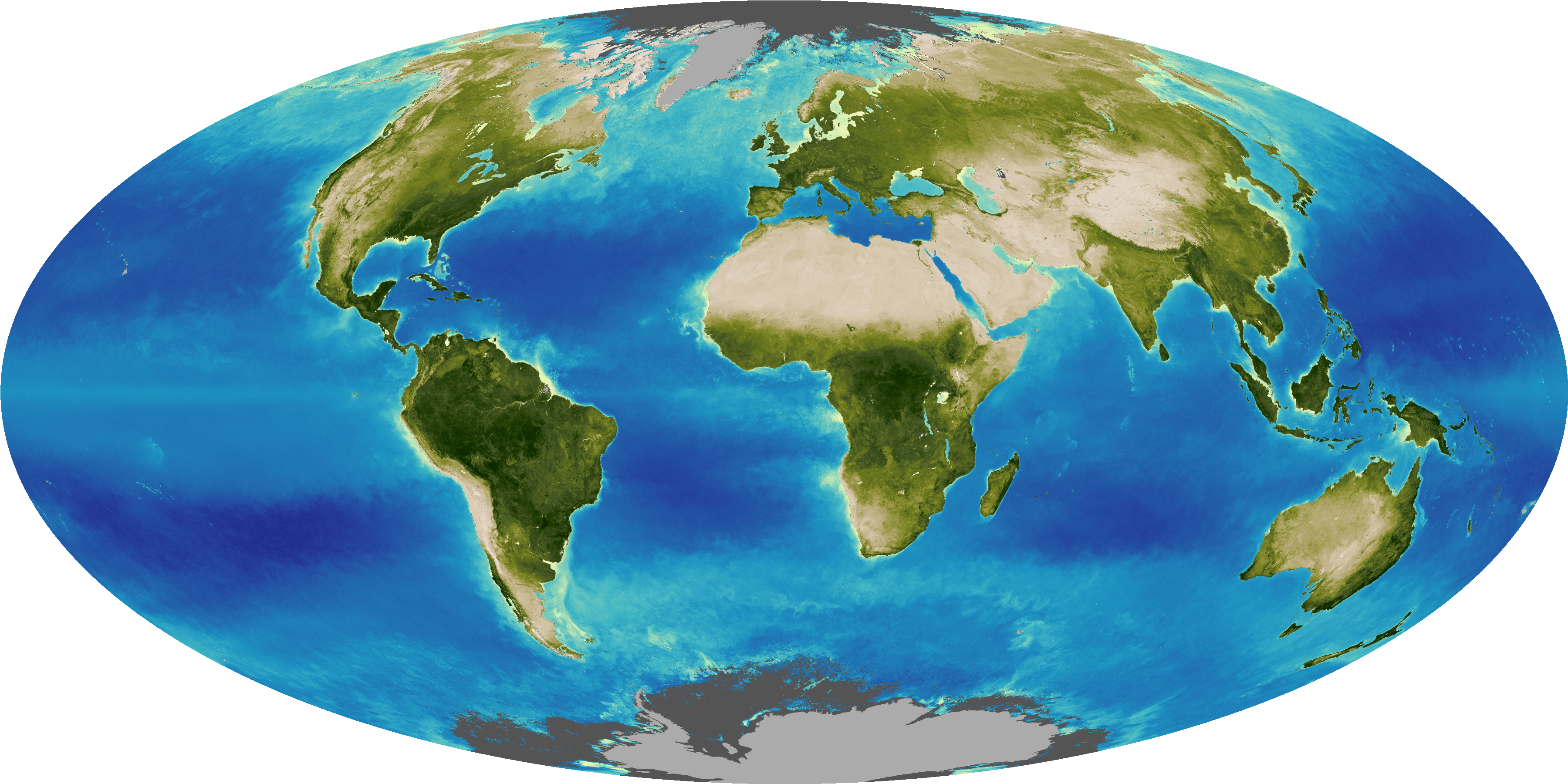
Facts About Earth

Watch CBS News
Who owns the ship that struck the Francis Scott Key Bridge in Baltimore?
By Megan Cerullo
Edited By Anne Marie Lee
Updated on: March 26, 2024 / 5:05 PM EDT / CBS News
The collapse of Baltimore's Francis Scott Key Bridge on Tuesday after being struck by a cargo ship has raised questions about who owns and manages the ship, as well as on the potential impact on one the busiest ports in the U.S.
Called the Dali, the 948-foot vessel that hit the bridge is managed by Synergy Marine Group, a Singapore-based company with over 660 ships under management worldwide, according to its website . The group said the ship was operated by charter vessel company Synergy Group and chartered by Danish shipping giant Maersk at the time of the incident, which sent vehicles and people tumbling into the Patapsco River.
"We are horrified by what has happened in Baltimore, and our thoughts are with all of those affected," Maersk said in a statement to CBS News on Tuesday, in which it also confirmed the ship was carrying cargo for Maersk customers. The company had no crew or personnel aboard the ship.
The Dali, which can carry up to 10,000 twenty-foot equivalent units, or TEUs, was carrying nearly 4,700 containers at the time of the collision. It was operated by a 22-person, Indian crew. It was not immediately clear what kind of cargo the ship was carrying.
Who owns and manages the Dali?
The Dali is owned by Grace Ocean Private, a Singapore-based company that provides water transportation services. The ship was chartered by Danish container shipping company Maersk at the time of the collision.
Synergy Marine, founded in 2006, provides a range of ship management services, including managing ships' technical components and their crews and overseeing safety, according to S&P Capital IQ. Its parent company, Unity Group Holdings International, an investment holding company, was founded in 2008 and is based in Hong Kong.
Where was the ship headed?
The outbound ship had left Baltimore and was headed for Colombo, the capital of Sri Lanka, Synergy Marine Group said in a press release .
How busy is the Port of Baltimore?
In 2023, the Port of Baltimore handled a record 52.3 million tons of foreign cargo, worth $80 billion, according to the office of Maryland Gov. Wes Moore. The port is also a significant provider of local jobs.
The top port in the U.S. for sugar and gypsum imports, it is the ninth busiest U.S. port by the total volume and value of foreign cargo handled. All vessel traffic into and out of the facility is currently suspended, although the port remains open and trucks continue to be processed within its terminals, according to a statement released by Port of Baltimore officials.
What is the potential local economic impact?
Directly, the port supports 15,300 jobs, while another 140,000 in the area are related to port activities. The jobs provide a combined $3.3 billion in personal income, according to a CBS News report . The Port of Baltimore said Tuesday that it is unclear how long ship traffic will be suspended.
The disaster also caused chaos for local drivers. The Maryland Transportation Authority said all lanes were closed in both directions on I-695, with traffic being detoured to I-95 and I-895.
How could the bridge collapse affect consumers and businesses?
Experts say the bridge collapse could cause significant supply chain disruptions.
"While Baltimore is not one of the largest U.S. East Coast ports, it still imports and exports more than 1 million containers each year, so there is the potential for this to cause significant disruption to supply chains," Emily Stausbøll, a market analyst at Xeneta, an ocean and air freight analytics platform, said in a statement.
She added that freight services from Asia to the East Coast in the U.S. have already been hampered by drought in the Panama Canal, as well as risks related to conflict in the Red Sea. Nearby ports, including those in New York, New Jersey and Virginia, will be relied on to handle more shipments if Baltimore remains inaccessible.
Whether ocean freight shipping rates will rise dramatically, potentially affecting consumers as retailers pass along higher costs, will depend on how much extra capacity the alternate ports can handle, Stausbøll said. "However, there is only so much port capacity available and this will leave supply chains vulnerable to any further pressure."
Marty Durbin, senior vice president of policy at the U.S. Chamber of Commerce, said that the bridge is a critical connector of "people, businesses, and communities."
"Unfortunately, its prolonged closure will likely disrupt commercial activities and supply chains that rely on the bridge and Port of Baltimore each day," he said in a statement.
What other industries could be affected?
Trucking companies could be severely affected by the disaster.
"Aside from the obvious tragedy, this incident will have significant and long-lasting impacts on the region," American Trucking Associations spokesperson Jessica Gail said, calling Key Bridge and Baltimore's port "critical components'' of the nation's infrastructure.
Gail noted that 1.3 million trucks cross the bridge every year — 3,600 a day. Trucks that carry hazardous materials will now have to make 30 miles of detours around Baltimore because they are prohibited from using the city's tunnels, she said, adding to delays and increasing fuel costs.
"Time-wise, it's going to hurt us a lot," added Russell Brehm, the terminal manager in Baltimore for Lee Transport, which trucks hazardous materials such as petroleum products and chemicals. The loss of the bridge will double to two hours the time it takes Lee to get loads from its terminal in Baltimore's Curtis Bay to the BJ's gasoline station in the waterfront neighborhood of Canton, he estimated.
Cruise operators are also being affected. A Carnival cruise ship that set off Sunday for the Bahamas had been scheduled to return to Baltimore on March 31. Carnival said Tuesday that it is "currently evaluating options for Carnival Legend's scheduled return on Sunday." The company also has cruises scheduled to set sail from Baltimore through the summer.
Norwegian Cruise Line last year introduced new routes departing from the Port of Baltimore. Its sailings are scheduled for late this year. The company said the Key Bridge collapse doesn't immediately require it to reroute any ships.
Who will pay to rebuild the bridge?
President Biden said Tuesday that the federal government, with congressional support, would pay to rebuild the bridge.
"We're going to work with our partners in Congress to make sure the state gets the support it needs. It's my intention that the federal government will pay for the entire cost of reconstructing that bridge," Biden said in comments from the White House. "And I expect the Congress to support my effort. This is going to take some time. The people of Baltimore can count on us though, to stick with them, at every step of the way, till the port is reopened and the bridge is rebuilt."
—The Associated Press contributed to this report.
- Francis Scott Key Bridge
- Bridge Collapse
- Patapsco River

Megan Cerullo is a New York-based reporter for CBS MoneyWatch covering small business, workplace, health care, consumer spending and personal finance topics. She regularly appears on CBS News Streaming to discuss her reporting.
More from CBS News
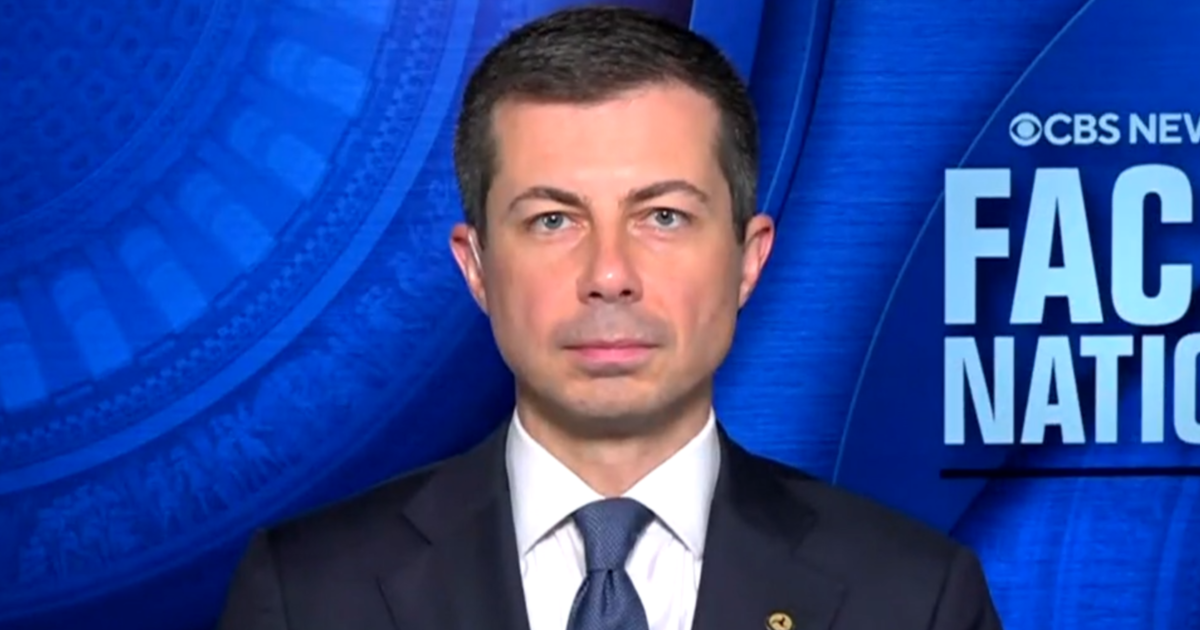
Pete Buttigieg says "we don't fully know" conditions for Baltimore bridge repair
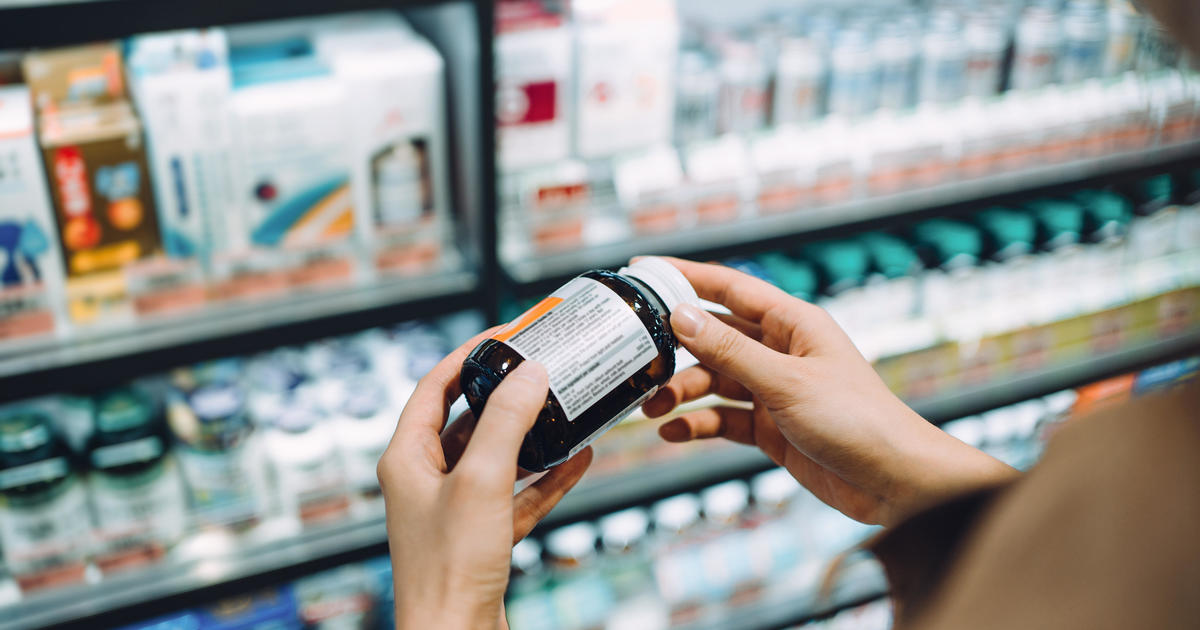
What consumers should know about health supplement linked to 5 deaths
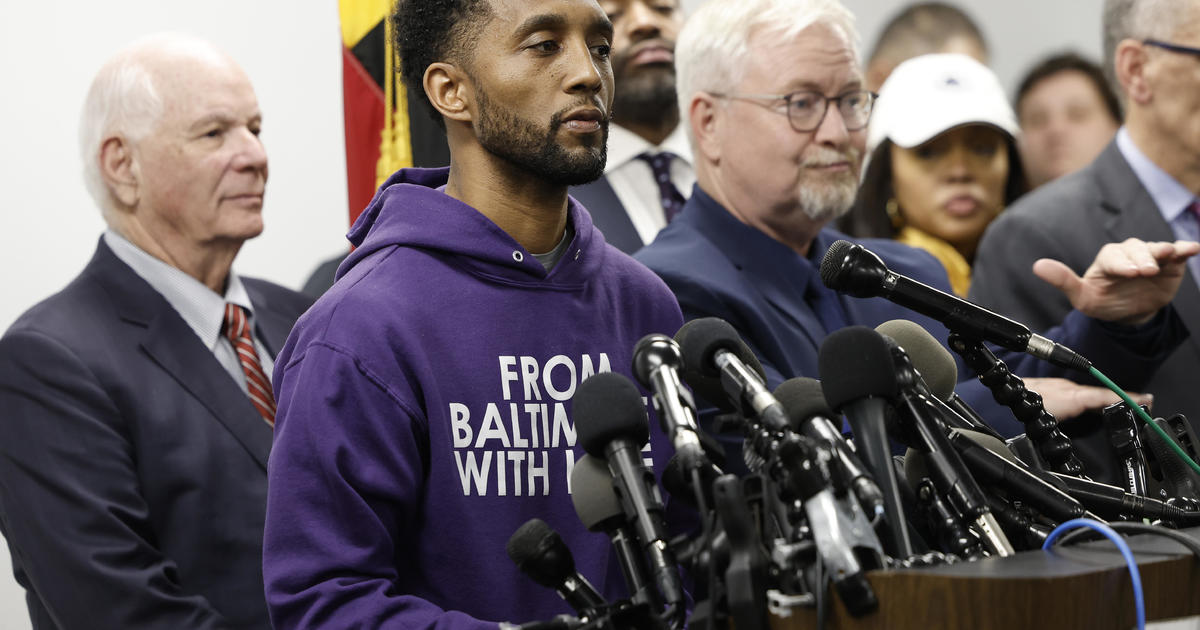
Transcript: Baltimore Mayor Brandon Scott on "Face the Nation," March 31, 2024
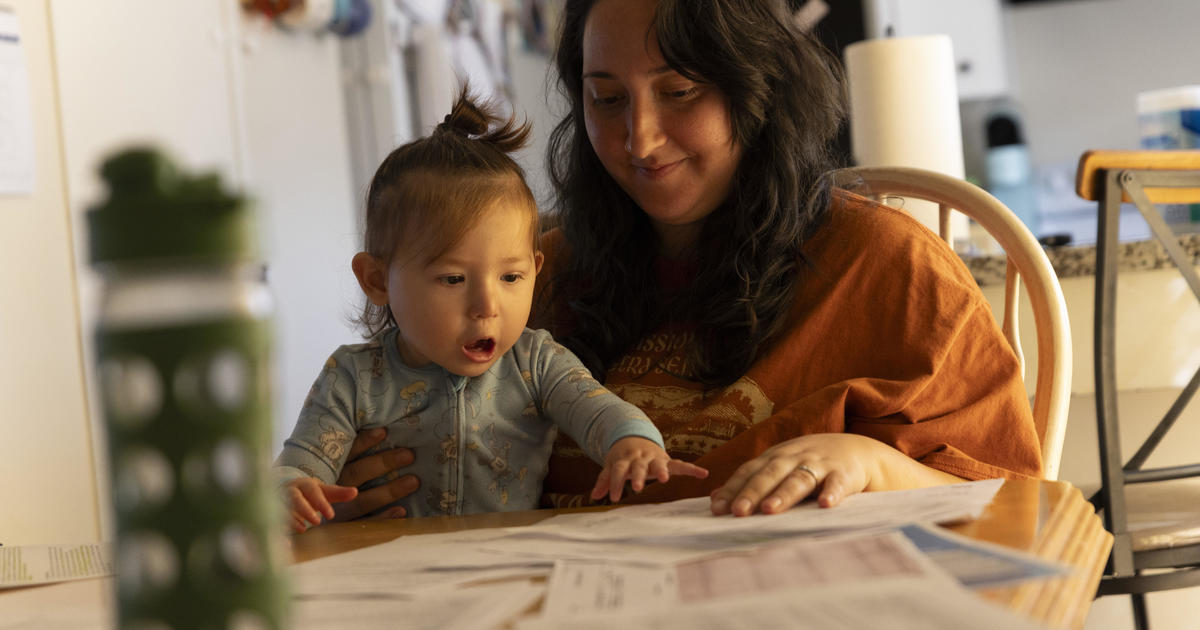
Was her baby's air-ambulance ride not medically necessary?
Advertisement
‘A Lot of Chaos’: Bridge Collapse Creates Upheaval at Largest U.S. Port for Car Trade
A bridge collapse closed Baltimore’s port, an important trade hub that ranks first in the nation by the volume of automobiles and light trucks it handles.
- Share full article
Shipping in the Port of Baltimore
Monthly cargo handled by the Port of Baltimore

By Peter Eavis and Jenny Gross
- March 26, 2024
The Baltimore bridge disaster on Tuesday upended operations at one of the nation’s busiest ports, with disruptions likely to be felt for weeks by companies shipping goods in and out of the country — and possibly by consumers as well.
The upheaval will be especially notable for auto makers and coal producers for whom Baltimore has become one of the most vital shipping destinations in the United States.
As officials began to investigate why a nearly 1,000-foot cargo ship ran into the Francis Scott Key Bridge in the middle of the night, companies that transport goods to suppliers and stores scrambled to get trucks to the other East Coast ports receiving goods diverted from Baltimore. Ships sat idle elsewhere, unsure where and when to dock.
“It’s going to cause a lot of chaos,” said Paul Brashier, vice president for drayage and intermodal at ITS Logistics.
The closure of the Port of Baltimore is the latest hit to global supply chains, which have been strained by monthslong crises at the Panama Canal, which has had to slash traffic because of low water levels; and the Suez Canal, which shipping companies are avoiding because of attacks by the Houthis on vessels in the Red Sea.
The auto industry now faces new supply headaches.
Last year, 570,000 vehicles were imported through Baltimore, according to Sina Golara, an assistant professor of supply chain management at Georgia State University. “That’s a huge amount,” he said, equivalent to nearly a quarter of the current inventory of new cars in the United States.
The Baltimore port handled a record amount of foreign cargo last year, and it was the 17th biggest port in the nation overall in 2021, ranked by total tons, according to Bureau of Transportation Statistics.
Baltimore Ranks in the Top 20 U.S. Ports
Total trade in 2021 in millions of tons
Baltimore ranks first in the United States for the volume of automobiles and light trucks it handles, and for vessels that carry wheeled cargo, including farm and construction machinery, according to a statement by Gov. Wes Moore of Maryland last month.
The incident is another stark reminder of the vulnerability of the supply chains that transport consumer products and commodities around the world.
The extent of the disruption depends on how long it takes to reopen shipping channels into the port of Baltimore. Experts estimate it could take several weeks.
Baltimore is not a leading port for container ships, and other ports can likely absorb traffic that was headed to Baltimore, industry officials said.
Stephen Edwards, the chief executive of the Port of Virginia, said it was expecting a vessel on Tuesday that was previously bound for Baltimore, and that others would soon follow. “Between New York and Virginia, we have sufficient capacity to handle all this cargo,” Mr. Edwards said, referring to container ships.
“Shipping companies are very agile,” said Jean-Paul Rodrigue, a professor in the department of maritime business administration at Texas A&M University-Galveston. “In two to three days, it will be rerouted.”
But other types of cargo could remain snarled.
Alexis Ellender, a global analyst at Kpler, a commodities analytics firm, said he expected the port closure to cause significant disruption of U.S. exports of coal. Last year, about 23 million metric tons of coal exports were shipped from the port of Baltimore, about a quarter of all seaborne U.S. coal shipments. About 12 vessel had been expected to leave the port of Baltimore in the next week or so carrying coal, according to Kpler.
He noted that it would not make a huge dent on the global market, but he added that “the impact is significant for the U.S. in terms of loss of export capacity.”
“You may see coal cargoes coming from the mines being rerouted to other ports instead,” he said, with a port in Norfolk, Va., the most likely.
If auto imports are reduced by Baltimore’s closure, inventories could run low, particularly for models that are in high demand.
“We are initiating discussions with our various transportation providers on contingency plans to ensure an uninterrupted flow of vehicles to our customers and will continue to carefully monitor this situation,” Stellantis, which owns Chrysler, Dodge, Jeep and Ram, said in a statement.
Other ports have the capacity to import cars, but there may not be enough car transporters at those ports to handle the new traffic.
“You have to make sure the capacity exists all the way in the supply chain — all the way to the dealership,” said Mr. Golara, the Georgia State professor.
A looming battle is insurance payouts, once legal liability is determined. The size of the payout from the insurer is likely to be significant and will depend on factors including the value of the bridge, the scale of loss of life compensation owed to families of people who died, the damage to the vessel and disruption to the port.
The ship’s insurer, Britannia P&I Club, part of a global group of insurers, said in a statement that it was “working closely with the ship manager and relevant authorities to establish the facts and to help ensure that this situation is dealt with quickly and professionally.”
The port has also increasingly catered to large container ships like the Dali, the 948-foot-long cargo vessel carrying goods for the shipping giant Maersk that hit a pillar of the bridge around 1:30 a.m. on Tuesday. The Dali had spent two days in Baltimore’s port before setting off toward the 1.6-mile Francis Scott Key Bridge.
State-owned terminals, managed by the Maryland Port Administration, and privately owned terminals in Baltimore transported a record 52.3 million tons of foreign cargo in 2023, worth $80 billion.
Materials transported in large volumes through the city’s port include coal, coffee and sugar. It was the ninth-busiest port in the nation last year for receiving foreign cargo, in terms of volume and value.
The bridge’s collapse will also disrupt cruises traveling in and out of Baltimore. Norwegian Cruise Line last year began a new fall and winter schedule calling at the Port of Baltimore.
An earlier version of this article misstated the Port of Baltimore’s rank among U.S. ports. It was the nation’s 17th biggest port by total tons in 2021, not the 20th largest.
How we handle corrections
Peter Eavis reports on business, financial markets, the economy and companies across different sectors. More about Peter Eavis
Jenny Gross is a reporter for The Times in London covering breaking news and other topics. More about Jenny Gross
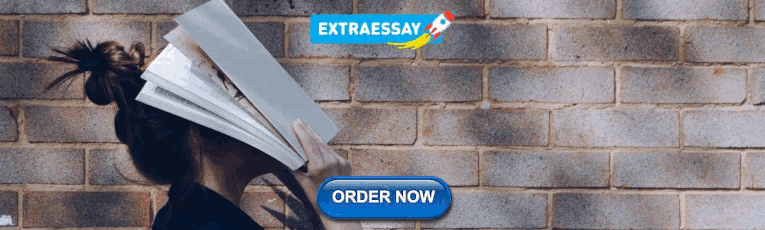
IMAGES
VIDEO
COMMENTS
A hypothesis is a statement that can be tested by scientific research. If you want to test a relationship between two or more variables, you need to write hypotheses. ... the independent variable is exposure to the sun - the assumed cause. The dependent variable is the level of happiness - the assumed effect. Prevent plagiarism. Run a free ...
A causal hypothesis is a statement that predicts a cause-and-effect relationship between variables in a study. It serves as a guide to study design, data collection, and interpretation of results. This thesis statement segment aims to provide you with clear examples of causal hypotheses across diverse fields, along with a step-by-step guide and ...
A causal hypothesis proposes a cause-and-effect interaction between variables. Example: " Long-term alcohol use causes liver damage." Note that some of the types of research hypothesis mentioned above might overlap. The types of hypothesis chosen will depend on the research question and the objective of the study. Research hypothesis examples
Step 5: Phrase your hypothesis in three ways. To identify the variables, you can write a simple prediction in if … then form. The first part of the sentence states the independent variable and the second part states the dependent variable. If a first-year student starts attending more lectures, then their exam scores will improve.
Ideally, the cause must occur before the effect. You should review the timeline of two or more separate events to determine the independent variables (cause) from the dependent variables (effect) before developing a hypothesis. If the cause occurs before the effect, you can link cause and effect and develop a hypothesis.
A hypothesis is an educated guess or prediction of what will happen. In science, a hypothesis proposes a relationship between factors called variables. ... then statement to establish cause and effect on the variables. If you make a change to the independent variable, then the dependent variable will respond. Here's an example of a hypothesis:
A hypothesis states a presumed relationship between two variables in a way that can be tested with empirical data. It may take the form of a cause-effect statement, or an "if x,...then y" statement. The cause is called the independent variable; and the effect is called the dependent variable. Relationships can be of several forms: linear, or ...
statement, whether or not it asserts a direct cause-and-effect relationship. A hypothesis about possible correlation does not stipulate the cause and effect per se, only stating that 'A is related to B'. Causal . relationships can be more difficult to verify than correlations, because
Hill's Criteria of Causation. Determining whether a causal relationship exists requires far more in-depth subject area knowledge and contextual information than you can include in a hypothesis test. In 1965, Austin Hill, a medical statistician, tackled this question in a paper* that's become the standard.
The purpose of the cause-and-effect essay is to determine how various phenomena are related. The thesis states what the writer sees as the main cause, main effect, or various causes and effects of a condition or event. The cause-and-effect essay can be organized in one of these two primary ways: Start with the cause and then talk about the effect.
A hypothesis is a tentative statement about the relationship between two or more variables. Find hypothesis examples and how to format your research hypothesis. ... In a study exploring the effects of a particular drug, the hypothesis might be that researchers expect the drug to have some type of effect on the symptoms of a specific illness ...
The purpose of the cause-and-effect essay is to determine how various phenomena relate in terms of origins and results. Sometimes the connection between cause and effect is clear, but often determining the exact relationship between the two is very difficult. For example, the following effects of a cold may be easily identifiable: a sore throat ...
An hypothesis may describe whether or not a relationship exists, ... Again, some type of cause and effect is usually present in the hypothesis. ... One example is a Likert scale. Respondents are given a statement, such as "I like the Big Bang Theory" then asked if they:
Revised on June 22, 2023. Correlation means there is a statistical association between variables. Causation means that a change in one variable causes a change in another variable. In research, you might have come across the phrase "correlation doesn't imply causation.". Correlation and causation are two related ideas, but understanding ...
Associative and Causal Hypothesis — an associative hypothesis is a statement used to indicate the correlation between variables under the scenario when a change in one variable inevitably changes the other variable. A causal hypothesis is a statement that highlights the cause and effect relationship between variables.
The purpose of the cause-and-effect essay is to determine how various phenomena relate in terms of origins and results. Sometimes the connection between cause and effect is clear, but often determining the exact relationship between the two is very difficult. For example, the following effects of a cold may be easily identifiable: a sore throat ...
In our hypothesis statement example above, the two variables are wildfires and tornadoes, and our assumed relationship between the two is a causal one (wildfires cause tornadoes). It is clear from our example above what we will be investigating: the relationship between wildfires and tornadoes. A strong hypothesis statement should be: Clear
Directional hypotheses are made only when there is reason to think a relationship operates only in one direction. Adequate statistical power is essential to the safe acceptance of the null hypothesis. Hypothesis testing is an all-or-nothing statement of related- ness, but estimation emphasises the extent of a relationship.
A hypothesis (plural hypotheses) is a proposed explanation for an observation. The definition depends on the subject. In science, a hypothesis is part of the scientific method. It is a prediction or explanation that is tested by an experiment. Observations and experiments may disprove a scientific hypothesis, but can never entirely prove one.
Establishing Cause and Effect. A central goal of most research is the identification of causal relationships, or demonstrating that a particular independent variable (the cause) has an effect on the dependent variable of interest (the effect). The three criteria for establishing cause and effect - association, time ordering (or temporal precedence), and non-spuriousness - are familiar to ...
The key principle of establishing cause and effect is proving that the effects seen in the experiment happened after the cause. This seems to be an extremely obvious statement, but that is not always the case. Natural phenomena are complicated and intertwined, often overlapping and making it difficult to establish a natural order.
As discussed, the dependent variable is the presumed effect; its variance is what a hypothesis seeks to explain. The independent variable is the presumed cause; its impact on the variance of another variable is what the hypothesis seeks to determine. Hypotheses are usually in the form of if-then, or cause-and-effect, propositions.
Technically, a "consensus" is a general agreement of opinion, but the scientific method steers us away from this to an objective framework. In science, facts or observations are explained by a hypothesis (a statement of a possible explanation for some natural phenomenon), which can then be tested and retested until it is refuted (or disproved).
In its Sixth Assessment Report, the Intergovernmental Panel on Climate Change, composed of scientific experts from countries all over the world, concluded that it is unequivocal that the increase of CO 2, methane, and nitrous oxide in the atmosphere over the industrial era is the result of human activities and that human influence is the principal driver of many changes observed across the ...
This study proposes a new method to evaluate the effect of WD from a data quality (DQ) perspective and identifies the root causes of WD failures at the operational level by information stream analysis based on an extended value stream mapping 4.0 (VSM4.0). The method is developed through a Design Science Research (DSR) approach and tested in an ...
The collapse of Baltimore's Francis Scott Key Bridge on Tuesday after being struck by a cargo ship has raised questions about who owns and manages the ship, as well as on the potential impact on ...
Alexis Ellender, a global analyst at Kpler, a commodities analytics firm, said he expected the port closure to cause significant disruption of U.S. exports of coal.