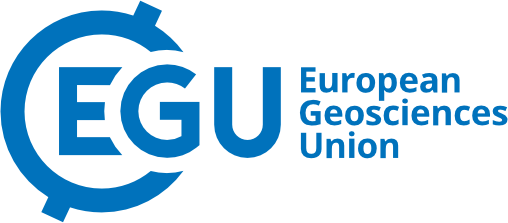
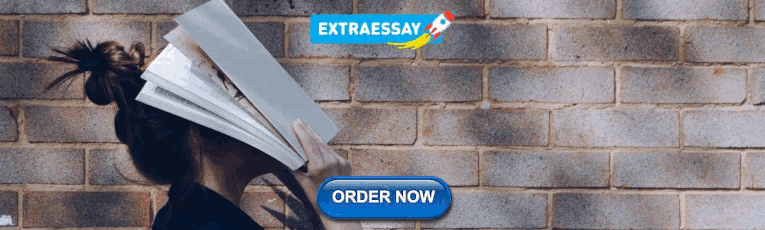
Preprint
- Preprint hess-2018-480
The Kerala flood of 2018: combined impact of extreme rainfall and reservoir storage
Abstract. Extreme precipitation events and flooding that cause losses to human lives and infrastructure have increased under the warming climate. In August 2018, the state of Kerala (India) witnessed large-scale flooding, which affected millions of people and caused 400 or more deaths. Here, we examine the return period of extreme rainfall and the potential role of reservoirs in the recent flooding in Kerala. We show that Kerala experienced 53 % above normal rainfall during the monsoon season (till August 21st) of 2018. Moreover, 1, 2, and 3-day extreme rainfall in Kerala during August 2018 had return periods of 75, 200, and 100 years. Six out of seven major reservoirs were at more than 90 % of their full capacity on August 8, 2018, before extreme rainfall in Kerala. Extreme rainfall at 1–15 days durations in August 2018 in the catchments upstream of the three major reservoirs (Idukki, Kakki, and Periyar) had the return period of more than 500 years. Extreme rainfall and almost full reservoirs resulted in a significant release of water in a short-span of time. Therefore, above normal seasonal rainfall (before August 8, 2018), high reservoir storage, and unprecedented extreme rainfall in the catchments where reservoirs are located worsened the flooding in Kerala. Reservoir operations need be improved using a skillful forecast of extreme rainfall at the longer lead time (4–7 days).
- Preprint (PDF, 1270 KB)
- Supplement (18683 KB)
- Preprint (1270 KB)
- Metadata XML

https://doi.org/10.5194/hess-2018-480-supplement
- HTML: 8,856
- Total: 12,523
- Supplement: 388
- EndNote: 91
Viewed (geographical distribution)
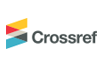
43 citations as recorded by crossref.
- Assessment of extreme rainfall events over Kerala using EVA and NCUM-G model forecasts V. Abhijith et al. 10.1007/s12040-023-02171-5
- Flood impact assessment using field investigations and post-flood survey A. Mathew et al. 10.1007/s12040-021-01657-4
- Landslide susceptibility investigation for Idukki district of Kerala using regression analysis and machine learning S. Jones et al. 10.1007/s12517-021-07156-6
- Persistent Scatterer Interferometry in the post-event monitoring of the Idukki Landslides J. Jennifer et al. 10.1080/10106049.2020.1778101
- Endemism-based butterfly conservation: insights from a study in Southern Western Ghats, India M. Anto et al. 10.1186/s41936-021-00221-2
- Spatiotemporal dependence of soil moisture and precipitation over India A. Manoj J et al. 10.1016/j.jhydrol.2022.127898
- Microplastic pollution of Calicut beach - Contributing factors and possible impacts A. Kumar & G. Varghese 10.1016/j.marpolbul.2021.112492
- Identifying Flood Prediction using Machine Learning Techniques M. Helen Joyice et al. 10.38124/ijisrt/IJISRT24MAR112
- Real-time flood forecasting using an integrated hydrologic and hydraulic model for the Vamsadhara and Nagavali basins, Eastern India G. Venkata Rao et al. 10.1007/s11069-023-06366-3
- Probabilistic Mapping of August 2018 Flood of Kerala, India, Using Space-Borne Synthetic Aperture Radar S. Sherpa et al. 10.1109/JSTARS.2020.2970337
- Increase in Population Exposure Due to Dry and Wet Extremes in India Under a Warming Climate R. Kumar & V. Mishra 10.1029/2020EF001731
- Vulnerability and resilience of the tourism sector in India: Effects of natural disasters and internal conflict M. Barbhuiya & D. Chatterjee 10.1016/j.tmp.2019.100616
- Increased flood risk in Indian sub-continent under the warming climate H. Ali et al. 10.1016/j.wace.2019.100212
- A diagnostic study of extreme precipitation over Kerala during August 2018 Y. Viswanadhapalli et al. 10.1002/asl.941
- Assessment of land surface temperature dynamics over the Bharathapuzha River Basin, India J. John et al. 10.1007/s11600-021-00593-7
- Impacts of Flood on Water Quality of Periyar River and Remediation Using Natural Fibers P. Ayyappan Vasantha et al. 10.1080/15440478.2022.2057382
- Modeling the concurrent impact of extreme rainfall and reservoir storage on Kerala floods 2018: a Copula approach A. Anandalekshmi et al. 10.1007/s40808-019-00635-6
- Investigation on the Failure Mechanism of Rainfall-Induced Long-Runout Landslide at Upputhode, Kerala State of India R. Andrewwinner & S. Chandrasekaran 10.3390/land10111212
- Association of Flood Risk Patterns with Waterborne Bacterial Diseases in Malaysia N. Shafii et al. 10.3390/w15112121
- Combined signatures of atmospheric drivers, soil moisture, and moisture source on floods in Narmada River basin, India J. Nanditha et al. 10.1007/s00382-022-06244-x
- Evaluating the long-term trends of the climatic variables over three humid tropical basins in Kerala, India A. Abraham & S. Kundapura 10.1007/s12517-022-10056-y
- Development of flood susceptibility map using a GIS-based AHP approach: a novel case study on Idukki district, India Z. Khan & B. Jhamnani 10.1080/14498596.2023.2236051
- SAR based flood risk analysis: A case study Kerala flood 2018 N. Pramanick et al. 10.1016/j.asr.2021.07.003
- Epizootics of epizootic ulcerative syndrome among estuarine fishes of Kerala, India, under post-flood conditions T. Sumithra et al. 10.3354/dao03465
- Assessment of Nonstationarity and Uncertainty in Precipitation Extremes of a River Basin Under Climate Change S. Ansa Thasneem et al. 10.1007/s10666-021-09752-y
- C-band polarimetric Doppler Weather Radar observations during an extreme precipitation event and associated dynamics over Peninsular India K. Subrahmanyam & K. Kumar 10.1007/s11069-022-05426-4
- Simulation of an extreme rainfall event over Mumbai using a regional climate model: a case study M. Pant et al. 10.1007/s00703-021-00845-7
- Country‐wide flood exposure analysis using Sentinel‐1 synthetic aperture radar data: Case study of 2019 Iran flood S. Sherpa & M. Shirzaei 10.1111/jfr3.12770
- Coordination and control – limits in standard representations of multi-reservoir operations in hydrological modeling C. Rougé et al. 10.5194/hess-25-1365-2021
- Sensitivity analysis applied to two extreme rainfall events over Kerala using TIGGE ensembles B. George & G. Kutty 10.1007/s00703-022-00863-z
- Evaluating the 2018 extreme flood hazard events in Kerala, India P. Lal et al. 10.1080/2150704X.2020.1730468
- Interaction of a Low-Pressure System, an Offshore Trough, and Mid-Tropospheric Dry Air Intrusion: The Kerala Flood of August 2018 V. Kumar et al. 10.3390/atmos11070740
- Impacts of a massive flood event on the physico-chemistry and water quality of river Pampa in Western Ghats of India A. Saha et al. 10.1080/03067319.2020.1843026
- Deciphering the extreme rainfall scenario over Indian landmass using satellite observations, reanalysis and model forecast: Case studies U. Saha et al. 10.1016/j.atmosres.2020.104943
- Role of Extreme Precipitation and Initial Hydrologic Conditions on Floods in Godavari River Basin, India S. Garg & V. Mishra 10.1029/2019WR025863
- Flood 2018 and the status of reservoir-induced seismicity in Kerala, India S. Ramasamy et al. 10.1007/s11069-019-03741-x
- Bias-Corrected Extended-Range Forecast Over India for Hydrological Applications During Monsoon 2020 P. Kumar et al. 10.1007/s00024-022-02998-x
- The anomalous weather parameters that lead to the extreme rainfall of Kerala in August 2018 S. Suneela et al. 10.1007/s00703-023-00974-1
- Impact of Time Step Size on Different Cumulus Parameterization Schemes in the Numerical Simulation of a Heavy Rainfall Event Over Tamil Nadu, India K. Singh et al. 10.1007/s00024-021-02896-8
- Constructing a complete landslide inventory dataset for the 2018 monsoon disaster in Kerala, India, for land use change analysis L. Hao et al. 10.5194/essd-12-2899-2020
- Assessment of the impact of flood on groundwater hydrochemistry and its suitability for drinking and irrigation in the River Periyar Lower Basin, India A. Krishnakumar et al. 10.1007/s11356-021-17596-y
- Flooding study in the desert climate zone: case study of M’zab valley (Algeria) O. Gueciouer Teffah et al. 10.1080/23249676.2022.2030253
- Eliciting social themes of flood mitigation and community engagement studies through text mining T. Egbelakin et al. 10.1108/IJBPA-02-2023-0022
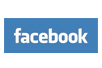
Vimal Mishra
Saran aaadhar, rahul kumar, dushmanta ranjan pattanaik, amar deep tiwari.
Click through the PLOS taxonomy to find articles in your field.
For more information about PLOS Subject Areas, click here .
Loading metrics
Open Access
Peer-reviewed
Research Article
Flood inundation mapping- Kerala 2018; Harnessing the power of SAR, automatic threshold detection method and Google Earth Engine
Roles Conceptualization, Data curation, Formal analysis, Investigation, Methodology, Project administration, Resources, Software, Validation, Visualization, Writing – original draft, Writing – review & editing
* E-mail: [email protected]
Affiliation International Centre for Integrated Mountain Development, Dehradun, India

Roles Formal analysis, Methodology, Supervision, Writing – review & editing
Affiliation Indian Institute of Remote Sensing, Indian Space Research Organization, Dehradun, India
Roles Writing – original draft, Writing – review & editing
Roles Formal analysis, Methodology, Resources, Writing – review & editing
Roles Writing – review & editing
Affiliation Earth System Science Center, University of Alabama, Huntsville, Alabama, United States of America
Roles Visualization
- Varun Tiwari,
- Vinay Kumar,
- Mir Abdul Matin,
- Amrit Thapa,
- Walter Lee Ellenburg,
- Nishikant Gupta,
- Sunil Thapa
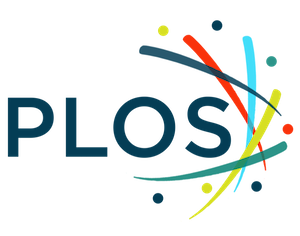
- Published: August 19, 2020
- https://doi.org/10.1371/journal.pone.0237324
- Reader Comments
Flood inundation maps provide valuable information towards flood risk preparedness, management, communication, response, and mitigation at the time of disaster, and can be developed by harnessing the power of satellite imagery. In the present study, Sentinel-1 Synthetic Aperture RADAR (SAR) data and Otsu method were utilized to map flood inundation areas. Google Earth Engine (GEE) was used for implementing Otsu algorithm and processing Sentinel—1 SAR data. The results were assessed by (i) calculating a confusion matrix; (ii) comparing the submerge water areas of flooded (Aug 2018), non-flooded (Jan 2018) and previous year’s flooded season (Aug 2016, Aug 2017), and (iii) analyzing historical rainfall patterns to understand the flood event. The overall accuracy for the Sentinel-1 SAR flood inundation maps of 9th and 21st August 2018 was observed as 94.3% and 94.1% respectively. The submerged area (region under water) classified significant flooding as compared to the non-flooded (January 2018) and previous year’s same season (August 2015–2017) classified outputs. Summing up, observations from Sentinel-1 SAR data using Otsu algorithm in GEE can act as a powerful tool for mapping flood inundation areas at the time of disaster, and enhance existing efforts towards saving lives and livelihoods of communities, and safeguarding infrastructure and businesses.
Citation: Tiwari V, Kumar V, Matin MA, Thapa A, Ellenburg WL, Gupta N, et al. (2020) Flood inundation mapping- Kerala 2018; Harnessing the power of SAR, automatic threshold detection method and Google Earth Engine. PLoS ONE 15(8): e0237324. https://doi.org/10.1371/journal.pone.0237324
Editor: Guy J-P. Schumann, Bristol University/Remote Sensing Solutions Inc., UNITED STATES
Received: January 7, 2020; Accepted: July 24, 2020; Published: August 19, 2020
Copyright: © 2020 Tiwari et al. This is an open access article distributed under the terms of the Creative Commons Attribution License , which permits unrestricted use, distribution, and reproduction in any medium, provided the original author and source are credited.
Data Availability: All relevant data are within the paper and its Supporting Information files.
Funding: The authors received no specific funding for this work.
Competing interests: The authors have declared that no competing interests exist.
Introduction
Any populated areas which normally fall in tropical regions are prone to floods which is considered as one of the hazardous natural disasters. Heavy rainfall enhances the accumulation of water in the catchments and overflow of water flashing beyond its normal confines are termed as floods. Flood usually varies from place to place and depends on numerous factors such as its severity and time of occurrence. Water inundation due to this disastrous event causes significant damage to human lives, properties, agricultural lands and infrastructure, subsequently affecting the economy [ 1 ]. Flood mitigation planning and management requires knowledge of land use as well as accurate identification and mapping of flood prone areas. Critical and timely estimation for monitoring inundated areas during floods can play a significant role in effective and prompt response for risk assessment. This also provides valuable information to the policy cum decision makers for sustainable management and future preparedness [ 2 ]. Generation of real/near real time accurate water inundation maps are required to see the flow of flood water in the affected areas during the time of disaster. Monitoring the flood extent with the help of space based sensors are more effective in providing near real time information as compared to the ground based techniques.
Space based sensors either optical or synthetic aperture radar (SAR) have shown their potential for monitoring and mapping flood or water inundated areas [ 3 , 4 ]. SAR sensors can penetrate through clouds and heavy rain, thus makes them capable of acquiring data during floods while optical sensors such as multispectral are unable to do the same [ 5 ]. Earlier studies have shown that SAR datasets are preferably being used for mapping and monitoring the flood extent. [ 6 – 11 ]. The techniques used for flood mapping are broadly categorized as (i) conventional and machine learning based classification approach [ 12 – 14 ]; and (ii) threshold based approach [ 15 , 16 ]. The first approach requires ample training data from the field which is difficult to collect during or at the time of floods. Hence, these approaches are least recommended for near real time preparation of flood maps [ 17 , 18 ]. However, threshold based techniques (manual as well as automatic) seem to be more effective for flood map generation. Manual threshold detection techniques are often difficult and challenging, as manually deriving the threshold requires knowledge of a few number of training samples [ 19 – 21 ]. This technique also leads to generation of less-accurate and inconsistent water inundated maps. This happens due to variability in the manually selected threshold values for separating water and non-water pixels [ 16 ].
However, an automatic threshold based approach detects the threshold values automatically for separating water and non-water pixels without the use of any training samples [ 22 , 23 ]. This approach computes image statistics for deriving the optimum thresholds to classify water and non-water pixels. Image tiling and split selection, Kittler and Illingworth’s technique, Global minimum threshold method, Quality index thresholds, Split combination and Otsu image threshold are some of the well-known automatic threshold based techniques used for different applications [ 24 – 27 ]. The Otsu image threshold method is the most widely adopted approach used for flood mapping [ 23 , 28 , 29 ]. This method is based on an adaptive threshold method which measures to evaluate the between-class variance of a threshold at a given level computed from a normalized image histogram. This method is less complex and requires least user interaction making it suitable for large SAR image scenes. Tests of this Otsu algorithm were conducted on eight large scenes of Radarsat-2 data for near-real time detection of water bodies and monitoring their dynamic changes [ 29 ]. An operational and robust framework was proposed for precise flood mapping by conducting tests in the large SAR scenes, for classifying flood and non-flood areas. This Otsu method is capable of providing a more precise flood map using Sentinel-1 SAR scenes for large areas [ 23 ]. Monitoring real time water extent during large-scale flood events also requires data from several sources (social media, remote sensing and topographic data). Rapid estimation of flood extent based on the information shared in real-time using social media such as geo-tagged photographs helps in validation of inundation for flood extent [ 30 ].
Various remote sensing based models with field observation have been used for monitoring and mapping of Kerala 2018 extreme flood events. Hunt et al., explored the circumstances which majorly caused this extreme flood by using Weather Research and Forecasting (WRF) model in combination with a hydrological model (WRF-Hydro, run at 125 m resolution) [ 31 ]. Haider et al., used downscaled future and historic climate projections from Coupled Model Inter comparison Project (CMIP5) and the Noah-MP land surface hydrological model, to simulate and observe the projected changes in extreme precipitation and flood events [ 32 ]. The extreme precipitation and runoff conditions observations made by Mishra et al. using the Variable Infiltration Capacity (VIC) model, have shown that the 2018 extreme flood event was unprecedented in the last seven decades from 1951–2018 [ 33 ]. The simulation results by Viswanadhapalli et al., suggested that the high convective instability and transport of mid-tropospheric moisture from the Bay of Bengal were the driving factors behind the heavy rainfall over Kerala in 2018 [ 34 ]. The change in water levels of reservoir storage during extreme rainfall of Kerala in 2018 were also discussed by Ramasamy et al., Anandalekshmi et al.,. The existing six major reservoirs that serve the state would have needed to have 34% more capacity to handle the heavy rainfall, and controlled release might have helped in alleviating the flood disasters [ 35 , 36 ]. These studies suggested that extreme heavy rainfall was the major factor behind this disastrous event and projected climate change could intensify these effects in the near future.
Most of the studies on Kerala flood focused on a hydrological modeling-based approach to study and analyze the reasons behind the flood event; whereas limited focus was on generating near real time water inundation maps. The hydrological model based approach are not suitable as it requires an up-to-date and accurate DEM, AWS (Automatic weather station) data as well as computing infrastructure which is often not available. Lal et al., Vishnu et al., and SK et al., utilized SAR data (Sentinel-1 & ALOS-2 PALSAR) along with a manual threshold-based, conventional and machine learning based classification approaches to prepare flood extent maps and damage assessment [ 37 – 39 ]. Though these studies have shown good results, they used the approach for map generation which requires ample training sample points. Also, the entire process was performed on the desktop based data processing tools which requires enormous time for downloading and processing data. This limits the timely response for providing the flood maps to different decision making agencies for immediate and adequate action. Considering this above methods in a desktop based environment are not suitable for providing rapid processing services critical for a flood response. On the other hand the cloud-based image processing platform utilizing automatic threshold methods are the possible solution. Google Earth Engine (GEE) is a cloud-based image processing platform enabling the rapid processing of such big datasets covering a large area. The GEE has publically made available numerous satellite image collections and provides image analysis functionality at large spatial scales [ 40 ]. In the existing approaches for flood mapping in Kerala no study has been performed which combines openly accessible SAR (Sentinel-1) data and automatic threshold detection techniques in a cloud computing platform (GEE).
The main objective of this study was to evaluate the potential of automatic threshold detection techniques using large SAR scenes in cloud computing platform for rapid and precise mapping of flood affected areas in Kerala, India. In this study, time-series Sentinel-1 SAR data of pre and post flood events and Otsu algorithm [ 41 , 42 ] were utilized in GEE for mapping flood extent or water inundated areas. Rainfall time series analysis was also performed to understand the long term rainfall trend over Kerala.
Materials and methods
The study area selected was the state of Kerala ( Fig 1 ), situated in the southwest part of India (10.8505° N, 76.2711° E with elevation of 54 m Mean Sea Level). The area was severely affected by floods in July-August 2018 which caused substantial loss of lives, and property worth USD 5.8 billion [ 43 ]. The major cities affected were: Chengannur, Pandanad, Edanad, Aranmula, Kozhencherry, Ayiroor, Ranni, Pandalam, Kuttanad, Malappuram, Aluva, Chalakudy, Thiruvalla, Eraviperoor, Vallamkulam, N. Paravur and Cochin.
- PPT PowerPoint slide
- PNG larger image
- TIFF original image
Shapefile reprinted from GADM database under a CC BY license, with permission from Global Administrative Areas ( www.gadm.org ), original copyright 2018. The figure was made with ArcGIS 10.3 under a CC BY license, with permission from ESRI ( www.esri.com ).
https://doi.org/10.1371/journal.pone.0237324.g001
The satellite dataset utilized in the study was Sentinel 1 SAR Vertically transmitted Vertically receive (VV) Polarization data ( Table 1 ). VV was preferred over vertically transmitted Horizontally receive (VH), as VV polarization was acquired with medium incident angle and previous research have shown it being most suitable for flood inundation mapping [ 44 – 47 ]. Sentinel-2 optical data and Climate Hazards Group Infra-Red Precipitation with Station data (CHIRPS) rainfall data of the flooded season (refer Table 1 ) were also utilized for validation of the results.
https://doi.org/10.1371/journal.pone.0237324.t001
Methodology
The flow chart of the detailed methodology adopted in the present study is shown in Fig 2 . The methodology was divided into the following steps:
https://doi.org/10.1371/journal.pone.0237324.g002
Preprocessing of Sentinel 1 VV SAR images
Sentinel-1 images suffer from erroneous noise, (i.e. rigorous geometric, radiometric corrections, thermal, and speckle) [ 48 ]. Any satellite data requires rigorous pre-processing before it can be utilized for any application.
GEE provides Sentinel-1 VV polarization Ground Range Detected (GRD) images which are already preprocessed using Sentinel-1 algorithm [ 48 ]. The preprocessing includes (i) Orbital file correction to eliminate the orbital noise; (ii) Thermal noise correction to eliminate the noise in the data produced by the sensors during data acquisition process onboard the satellite [ 49 ]. Thermal noise can affect the quality of the data in the areas having low mean signal response detected by the SAR system like lakes, standing water, rivers, etc., (iii) Radiometric calibration, to calibrate RADAR reflectivity (DN) to backscattering coefficient (physical units) which is mainly performed to compare the SAR images of different acquisition dates; and (iv) Terrain correction, to convert Sentinel-1 SLC (Single look complex) data from slant range geometry to a map coordinate system, and to rectify the distortions like foreshortening, layover, or shadowing effects. [ 50 ].
The Lee speckle filter with kernel window size 5x5 was applied in GEE environment to remove speckle noise from the dataset. [ 14 , 51 ]
Classification of water and non-water areas
After preprocessing of Sentinel-1 VV SAR images, the data was classified into two major classes: water and non-water, to determine the water inundated areas. Otsu’s automatic threshold detection method was used to determine optimum threshold for this separation. The Otsu method is typically used for deriving threshold for optical data [ 52 ]. However, some recent studies have suggested that the Otsu method can also be applied in SAR data for separating water and non-water classes [ 53 , 54 ].
The Otsu’s method is a clustering based thresholding method used for separating two classes i.e. foreground from background. The technique assumes that the distribution of image pixel intensities follows a bi-modal histogram, and separates those pixels into two classes (e.g. foreground and background). The optimum threshold value is determined by minimizing the weighted sum of within-class (intra-class) variances of the foreground and background pixels. The mathematical algorithm of Otsu method is described as follows:
ρ = criteria function
ω 0 = Probabilities of class occurrence for background
ω 1 = Probabilities of class occurrence for foreground
μ 0 = Class mean of background
μ 1 = Class mean of foreground
μ T = Total mean grey level of the image
k = Threshold value
Eqs 8 and 9 defines the probabilities of class occurrence and the class mean levels.
Validation and calculation of water inundated areas
The validation of the classified map was done through calculating a confusion matrix. The Sentinel -1 SAR classified images of 9 th and 21 st August 2018 were validated utilizing corresponding Sentinel-2 optical images, acquired on the 10 th and 20 th August 2018. The optical Sentinel 2 image acquired on 10 th and 20 th August were mostly affected with cloud cover therefore, these images were first preprocessed to remove clouds/ bad pixels. The cloud masking utilizes Sentinel-2 Band QA60, a quality flag band, to identify and mask out flagged cloud and cirrus pixels. After that, reference samples were randomly identified and collected using visual interpretation approach, for water and non-water class for the area that were not masked out due to cloud cover. The areas which were masked out due to cloud cover in sentinel-2 data, the reference samples were collected using sentinel-1 data.
The collected reference samples (from sentinel-2) were overlaid on Sentinel-1 SAR images of 9 th and 21 st August 2018. The backscatter values from water and non-water class were extracted and examined. It was observed that water class (Backscatter value = -23.53±2.44 db) exhibits low backscatter than non-water class (Backscatter value = -8.78±1.49 db) as shown in Fig 3 . Then the same rule was utilized for collecting reference samples from Sentinel -1 SAR data (random sampling) for the areas which were affected by clouds. The aptness of the Otsu’s method was assessed by deriving not only the overall accuracy, but also individual class accuracies. The validation was also performed by (i) comparing the area submerged under water in the classified images of flooded (August 2018), non-flooded (January 2018) and previous year’s same month data (August of 2015, 2016 and 2017); and (ii) analyzing the rainfall data for confirming the flood event.
https://doi.org/10.1371/journal.pone.0237324.g003
Analyzing the rainfall data
Climate Hazards Group Infra-Red Precipitation with Station data (CHIRPS) is a quasi-global rainfall dataset prepared by blending satellite imagery with in-situ station data [ 55 ]. It has daily temporal resolution with 0.05 degree spatial resolution. Studies has been done in the past to evaluate the accuracy of the CHIRPS data over Indian region. Gupta, V. et al., [ 56 ] reported higher correlation with station data (>0.80) and lower systematic error (<10%) of CHIRPS rainfall over Kerala region. Similar results were also observed by Prakash, S. et al., [ 57 ]. This signifies that the CHIRPS rainfall has good potential for hydro metrological studies in this region. The linear trend analysis was performed to study the overall rainfall trend over the study area. Cumulative rainfall plot is used to analyze the variation of precipitation during monsoon season June, July and August (JJA) between 2015 and 2018. This period was selected to comply with the inundation analysis. Trend analysis during JJA (1981–2018) was also performed to monitor the long term rainfall pattern. The selected period of rainfall data was based on the recommendation of World Meteorological Organization (WMO)—WMO recommends a period of at least 30 years for the assessment of climatic change [ 58 ]. The advantage of having climatic data for longer period enables us to perform comparison of trends across different flood effected locations.
Results and discussion
Time series backscatter profile was also examined at the flooded location as shown in Fig 4 . The backscattered vs. time curve was smoothed utilizing Fourier transform smoothening technique [ 59 ]. Since water behaves as a smooth surface, the graph shows sudden decrease in the backscatter signal at the time of the flood (August 2018). Changes in the backscatter value describe the occurrence of the flood event in August 2018. The August 2018 flood event was also reported in the news [ 60 , 61 ]. The low backscatter values (function of surface roughness) in the month of August 2018 were due to the increase in water inundation (mainly due to the flood event) which again went up in September 2018 because of the decrease in water inundation (post flood event).
https://doi.org/10.1371/journal.pone.0237324.g004
Water inundation maps from 2015–2018 and different seasons of 2018 were developed as depicted in Figs 5 and 6 respectively. Our analysis clearly show that the area submerged under water increased in the classified map of August 2018 as compared to the maps of the same month from 2015 to 2017 ( Table 2 ). Similarly, in the classified images, water covered areas observed were less in pre-flood and post flood acquired data of 2018 (January-April and September) ( Fig 6 and Table 2 ).
https://doi.org/10.1371/journal.pone.0237324.g005
https://doi.org/10.1371/journal.pone.0237324.g006
https://doi.org/10.1371/journal.pone.0237324.t002
Fig 7 shows the graph between the different years (August 2015–2018) and the area submerged under water; and Fig 5 depicts a graph between different seasons (2018) and the area covered by water. It can be interpreted from the above graphs that the extent of the area in August 2018 is maximum as compared to other years (2015–2017) and seasons (January-September 2018). This clearly indicates the occurrence of a flood event in August 2018.
https://doi.org/10.1371/journal.pone.0237324.g007
The accuracy assessment was performed on the 9 th and 21 st August 2018 flood maps. The overall accuracy of the classification was found to be 94.73% and 94.71% with a kappa coefficient of 0.87 and 0.88 respectively. The individual class accuracy of the classification is depicted in Fig 8 . High classification accuracy demonstrates the potential of the Otsu’s algorithm for classifying the water and non-water pixels.
https://doi.org/10.1371/journal.pone.0237324.g008
Sentinel-1 SAR operates in C band with its penetration capability ranges between ~5.6cm. Presence of clouds and rain droplets with thickness more than 7.5 cm at the time of data acquisition attenuates signal which result in noisy sentinel-1 images. This may be the reason for misclassification. However, using L or X band sensors can overcome this kind of uncertainty as these sensors have high penetration capability then C band.
The variation of the backscatter values was also examined using non-water sample points used in the validation. The backscatter response of the non-water sample points were examined for the month of Aug. 2015–2018 as depicted in Fig 9 . It was observed that during the flood event (Aug. 2018), the backscatter values of the non-water class exhibits low backscatter values with a mean and average deviation value of -23.52 ± 2.14 db respectively. Contradictory, the backscatter values of the non-water class in non-flood year’s shows low backscattering i.e. -7.45 db ≤ Mean backscatter≤ -6.23 db and average deviation of ±1.8 db respectively. In addition to inundation delineation of the 2018 flood event, cumulative rainfall for the Kerala district was analyzed to provide further context to the magnitude of the flooding event. Fig 10 shows the cumulative rainfall during JJA for four different locations which were severely affected by floods. It was observed that all the locations received more amount of rainfall during 2018 as compared to other years (2015–2018).
https://doi.org/10.1371/journal.pone.0237324.g009
https://doi.org/10.1371/journal.pone.0237324.g010
Cumulative rainfall during 2018 was calculated to be 1.5 times higher than the average of cumulative rainfall for the period 2015–2018. This clearly signifies that rainfall was one of the driving factors for the flood event. Hunt et al., [ 31 ] have done the reservoirs capacity assessment of the six major reservoir of Kerala using WRF hydrological model where they depict that flooding event would have not taken place if the capacity of the six reservoirs would have been 34% more.
Further analysis was performed to detect long term (1981–2018) rainfall trend during JJA. Fig 11a depicts the spatial variability of annual monsoon trend of rainfall over Kerala state from 1981–2018. It was observed that majority of the locations in Kerala have shown positive trends. Statistically significant trend (confidence level >95%) was extracted and illustrated in Fig 11b . It was observed that major flood affected locations (described in methods and material section) in Kerala have shown statistically significant increasing trend of JJA rainfall from 1981–2018. The increasing trend varies between 5 to 20 mm per year. Similar findings were depicted in Ali et al., [ 32 ] and Sharmila S, [ 62 ] where surface hydrological model was utilized to observed and projected changes in extreme precipitation and flood events. Their study demonstrate that the multi-day flood events are projected to increase with a faster rate in the future than the single day events.
a) Pixel wise trend analysis of annual monsoon rainfall from 1981–2018 over Kerala, India; b) Pixel wise signification trend analysis (95% confidence level.) of annual monsoon rainfall from 1981–2018 over Kerala, India. Shapefile reprinted from GADM database under a CC BY license, with permission from Global Administrative Areas ( www.gadm.org ), original copyright 2018. The figure was made with ArcGIS 10.3 under a CC BY license, with permission from ESRI ( www.esri.com ).
https://doi.org/10.1371/journal.pone.0237324.g011
Conclusions and future recommendations
Swift and accurate estimation of water inundated areas can provide valuable and timely information during flood events. In this study, we assessed the Otsu thresolding approach using Sentinel-1 SAR for mapping flood areas. The analyses were done on the GEE platform. The conclusions that can be drawn from this study are as follows: (i) currently available Sentinel-1 SAR allows data retrieval in all weather conditions therefore, has a good potential for mapping water inundated areas at the time of floods specifically in Kerala where more flood are likely to occur in near future ( Fig 11a & 11b ); (ii) Otsu’s method is capable of automatically detecting thresholds for separating water and non-water pixels without requiring user dependent parameter (reference points from the ground) unlike supervised machine learning methods; and (iii) the GEE platform can be used for processing and analysis of the inundation maps without the need for downloading data or high computing hardware. The automated and on-the-fly performance at acceptable accuracy level proves the utility and potential of GEE applications for rapid response in flood inundation mapping. While this paper highlights a proof of concept, further analysis is needed to explore the VH Polarization and band ratio techniques such as VV/VH. Since the Otsu method has been implemented and tested in GEE environment, the method can be tried on other areas for mapping real time flood events without requirement of ground sample points, except for validation. Though Otsu have shown good performance with far and wide case studies for flood mapping. It has limitations, Otsu assumed to have bimodal histogram distribution and also assumed to possess a deep and sharp valley between two peaks. In the study area if the object area is small compared with the background area, the histogram no longer exhibits bimodality. Therefore, Two-dimensional Otsu’s method can be consider as it performs better image segmentation in noisy images [ 63 ]. Additionally, how information can be utilized and shared through social media could be explored for further validation and dissemination of the findings for rapid response as described in Babu et al., [ 64 ].
It is important to note that the natural occurrence and impact of floods can be magnified by socio-economic development, including encroachment on the floodplains and inadequate drainage management [ 65 ]. In addition, traditional knowledge of dealing with floods has been forgotten, which have the potential to offer site-specific knowledge and critical guidance to planners and decision makers. Flooding causes economic and livelihood losses especially in developing countries where low-income earners undergo great stress. Losses due to floods reduces the asset base of households, communities and societies through the destruction of standing crops, dwellings, infrastructure, machinery and buildings, in addition to tragic loss of life. Despite the rich natural resources and water, people living in these areas are poorer as compared to the rest of the country, and this is primarily due to the annual floods and related devastation. The resulting financial burden is often back-breaking for the communities. The pressure to maintain the day to day expenses, education of children, rituals, health amongst these disasters, increasing poverty, and few available adaptive mechanisms has sometimes led to migration from such areas. Flood and migration have direct relation as higher the flood, higher is the migration rate. It is important to note that floods impact different sections of society differentially, and requires a thorough understanding of flood-related issues. Floods have adverse impacts such as the risk to life and property in the coming years, both because floods are likely to be more frequent, and because increase in population is likely to result in more people settling in areas vulnerable to flooding. An increase in floods is likely to influence prevalence of water-related diseases and contaminate water sources whereas, the insufficient water for hygiene purposes during dry periods is likely to increase the risk of water-washed diseases. Given the projected changes in precipitation, it is likely that there is going to be serious costs for communities and sectors here. In view of the above, there is an urgent need for actions to assist communities and dependent sectors to adapt. Therefore, the various heavily water-dependent sectors—agriculture, industry, energy, navigation and water supply and sanitation—need to cooperate to work towards achieving a resilient State.
Supporting information
https://doi.org/10.1371/journal.pone.0237324.s001
Acknowledgments
The authors express great gratitude to David Molden, Director General and Eklabya Sharma, Deputy Director General of ICIMOD for their overall guidance.
The authors would also like to thank reviewers for reviewing the article and for providing the valuable suggestions to improve the manuscript. The views and interpretations in this publication are those of the author’s and they are not necessarily attributable to their organizations.
- View Article
- Google Scholar
- 18. Kumar R. Flood Inundation and Hazard Mapping of 2017 Floods in the Rapti River Basin Using Sentinel-1A Synthetic Aperture Radar Images. In: Kumar P, Rani M, Chandra Pandey P, Sajjad H, Chaudhary BS, editors. Applications and Challenges of Geospatial Technology [Internet]. Cham: Springer International Publishing; 2019 [cited 2019 Jan 13]. p. 77–98. http://link.springer.com/10.1007/978-3-319-99882-4_6
- 20. Liu C. Analysis of Sentinel-1 SAR data for mapping standing water in the Twente region.:47.
- 26. Miasnikov AD, Rome JE, Haralick RM. A hierarchical projection pursuit clustering algorithm. In: Proceedings of the 17th International Conference on Pattern Recognition, 2004 ICPR 2004 [Internet]. Cambridge, UK: IEEE; 2004 [cited 2019 Dec 10]. p. 268–271 Vol.1. http://ieeexplore.ieee.org/document/1334104/
- 43. G R. Flood damages in Kerala pegged at Rs 40,000 crore. Times of India [Internet]. 2018 Sep 13 [cited 2020 Mar 13]; https://timesofindia.indiatimes.com/city/thiruvananthapuram/kerala-lost-rs-40000-cr-due-to-floods-report-will-be-submitted-to-centre/articleshow/65783705.cms
- PubMed/NCBI
- 48. Filipponi F. Sentinel-1 GRD Preprocessing Workflow. Proceedings. 2019 Jun 4;18(1):11.
- 61. Jose T. Post-Disaster Needs Assessment: Kerala, India [Internet]. United Nations Developement Programme; 2018 Oct. https://reliefweb.int/report/india/kerala-post-disaster-needs-assessment-floods-and-landslides-august-2018-october-2018
- 63. Yi-bing L, Ting M, Ao L. Two-Dimensional Otsu Image Segmentation Algorithm Based on the Particle Swarm Optimization Algorithm. In: Deng W, editor. Future Control and Automation [Internet]. Berlin, Heidelberg: Springer Berlin Heidelberg; 2012 [cited 2020 Mar 9]. p. 115–20. (Lecture Notes in Electrical Engineering; vol. 172). http://link.springer.com/10.1007/978-3-642-31006-5_14
- 65. Dutta D, Babel MS, Gupta AD. 2004-CB01NSY-Dutta Final Report submitted to APN.:214.
Advertisement
Spatial Mapping of the Flood-Affected Regions of Northern Kerala: A Case Study of 2018 Kerala Floods
- Research Article
- Published: 30 January 2022
- Volume 50 , pages 677–688, ( 2022 )
Cite this article
- K. Chithra 1 ,
- B. V. Binoy ORCID: orcid.org/0000-0002-6397-1096 1 &
- P. Bimal 1
804 Accesses
2 Citations
Explore all metrics
Flood mapping studies gained popularity in recent years with the availability of high-resolution SAR imagery. Satellite images in the visible spectrum cannot be used for disaster management applications due to inherent cloud cover during adverse weather conditions. The cloud penetrating capabilities and all-weather operation make radar images more suitable for flood mapping applications than optical images. The Kerala floods of 2018 were the worst disaster the state experienced in a century after the 1924 floods. It has resulted in widespread destruction and damages all around the state. Even though few flood inundation studies are based on the Kerala floods, they all concentrate on Central Kerala. Previous studies have not investigated detailed flood mapping of Northern Kerala using remote sensing techniques. This research paper explores the extent of flooding in Kozhikode, Malappuram and Wayanad districts of Northern Kerala by applying the thresholding method on radar images. The inundation extent of August 2018 is compared with the water-covered area of 2017 August to determine the extent of flooding. The current research also analyses the level of flood influence at the village level by using a spatial approach. The study reveals that the low-lying regions of the Malappuram district are the worse affected in the study area, with a few villages being severely flooded, with more than 10% of the geographical area inundated during the peak time. The flood inundation maps can be used to study the causative factors by applying modeling approaches.
This is a preview of subscription content, log in via an institution to check access.
Access this article
Price includes VAT (Russian Federation)
Instant access to the full article PDF.
Rent this article via DeepDyve
Institutional subscriptions
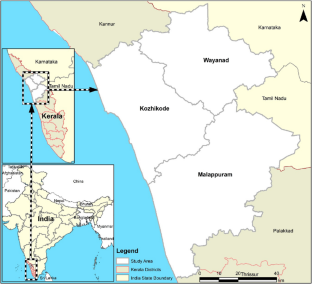
Similar content being viewed by others
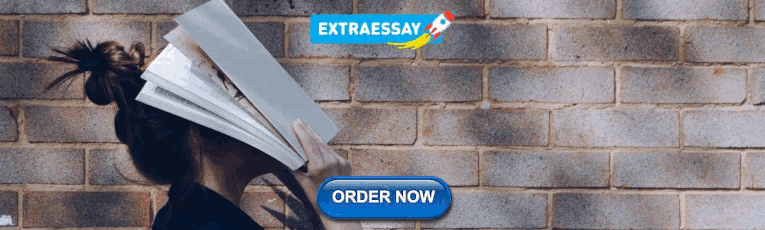
Flood Inundation Mapping Using Earth Observation Data in the Po River (North of Italy)
Flood hazard and risk assessment of 2014 floods in kashmir valley: a space-based multisensor approach.
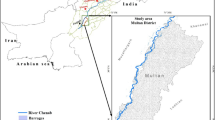
Riverine flood mapping and impact assessment using remote sensing technique: a case study of Chenab flood-2014 in Multan district, Punjab, Pakistan
Agarwal, R. (2018). Lesson learned from killer floods in kerala: time for retrospection. Management and Economics Research Journal, 4 (2), 268. https://doi.org/10.18639/MERJ.2018.04.735013
Article Google Scholar
Agnihotri, A. K., et al. (2019). Flood inundation mapping and monitoring using SAR data and its impact on Ramganga River in Ganga basin. Environmental Monitoring and Assessment, 191 (12), 760. https://doi.org/10.1007/s10661-019-7903-4
Anandalekshmi, A., et al. (2019). Modeling the concurrent impact of extreme rainfall and reservoir storage on Kerala floods 2018: A Copula approach. Modeling Earth Systems and Environment, 5 (4), 1283–1296. https://doi.org/10.1007/s40808-019-00635-6
Anusha, N., & Bharathi, B. (2019). Change detection and flood water mapping using sentinel-1A synthetic aperture radar images. Journal of Computational and Theoretical Nanoscience, 16 (8), 3544–3549. https://doi.org/10.1166/jctn.2019.8321
Arabindoo, P. (2016). Unprecedented natures? An anatomy of the Chennai floods. City, 20 (6), 800–821. https://doi.org/10.1080/13604813.2016.1239410
Berezowski, T., Bielinski, T., & Osowicki, J. (2020). Flooding extent mapping for synthetic aperture radar time series using river gauge observations. IEEE Journal of Selected Topics in Applied Earth Observations and Remote Sensing, 13 , 2626–2638. https://doi.org/10.1109/JSTARS.2020.2995888
Borah, S. B., et al. (2018). Flood inundation mapping and monitoring in Kaziranga National Park, Assam using Sentinel-1 SAR data. Environmental Monitoring and Assessment, 190 (9), 520. https://doi.org/10.1007/s10661-018-6893-y
Census of India (2011).
Cho, C., et al. (2016). Anthropogenic footprint of climate change in the June 2013 northern India flood. Climate Dynamics, 46 (3–4), 797–805. https://doi.org/10.1007/s00382-015-2613-2
Cian, F., Marconcini, M., & Ceccato, P. (2018). Normalized Difference Flood Index for rapid flood mapping: Taking advantage of EO big data. Remote Sensing of Environment, 209 (March), 712–730. https://doi.org/10.1016/j.rse.2018.03.006
Clement, M. A., Kilsby, C. G., & Moore, P. (2018). Multi-temporal synthetic aperture radar flood mapping using change detection. Journal of Flood Risk Management, 11 (2), 152–168. https://doi.org/10.1111/jfr3.12303
Dube, A., et al. (2014). Forecasting the heavy rainfall during Himalayan flooding—June 2013. Weather and Climate Extremes, 4 , 22–34. https://doi.org/10.1016/j.wace.2014.03.004
Elhag, M., & Abdurahman, S. G. (2020). Advanced remote sensing techniques in flash flood delineation in Tabuk City, Saudi Arabia. Natural Hazards, 103 (3), 3401–3413. https://doi.org/10.1007/s11069-020-04135-0
Ezzine, A., et al. (2018). Evaluation of Sentinel-1 data for flood mapping in the upstream of Sidi Salem dam (Northern Tunisia). Arabian Journal of Geosciences, 11 (8), 170. https://doi.org/10.1007/s12517-018-3505-7
Ezzine, A., et al. (2020). Flood mapping using hydraulic modeling and Sentinel-1 image: Case study of Medjerda Basin, northern Tunisia. The Egyptian Journal of Remote Sensing and Space Science, 23 (3), 303–310. https://doi.org/10.1016/j.ejrs.2020.03.001
Goumehei, E., et al. (2019). Surface water body detection in polarimetric sar data using contextual complex wishart classification. Water Resources Research, 55 (8), 7047–7059. https://doi.org/10.1029/2019WR025192
Gupta, K. (2007). Urban flood resilience planning and management and lessons for the future: A case study of Mumbai, India. Urban Water Journal, 4 (3), 183–194. https://doi.org/10.1080/15730620701464141
Hajaj, P. M. T., & Yarrakula, K. (2019). Application of synthetic aperture radar imagery for spatio-temporal assessment of flood in Nagavali River. Indian Journal of Ecology, 46 (3), 521–524.
Google Scholar
Hiep, N. Q. et al. (2019) ‘A method for constructing reservoir area-storage-elevation curve using sentinel-1 radar remote sensing image’, in 40th Asian Conference on Remote Sensing (ACRS 2019) , pp. 1–10.
Hunt, K. M. R., & Menon, A. (2020). The 2018 Kerala floods: A climate change perspective. Climate Dynamics, 54 (3–4), 2433–2446. https://doi.org/10.1007/s00382-020-05123-7
Iglesias, R. et al. (2018) ‘Towards Unsupervised Flood Mapping Generation Using Automatic Thresholding and Classification Aproaches’, in IGARSS 2018 - 2018 IEEE International Geoscience and Remote Sensing Symposium . IEEE, pp. 4927–4930. doi: https://doi.org/10.1109/IGARSS.2018.8519111 .
Jacinth Jennifer, J., Saravanan, S., & Abijith, D. (2020). Integration of SAR and multi-spectral imagery in flood inundation mapping – a case study on Kerala floods 2018. ISH Journal of Hydraulic Engineering . https://doi.org/10.1080/09715010.2020.1791265
Jensen, J. R. (2018) Introductory Digital Image Processing: A Remote Sensing Perspective . Prentice Hall (Prentice Hall series in geographic information science). Available at: https://books.google.co.in/books?id=FvIsAQAAMAAJ .
Jones, S., et al. (2021). Landslide susceptibility investigation for Idukki district of Kerala using regression analysis and machine learning. Arabian Journal of Geosciences, 14 (10), 838. https://doi.org/10.1007/s12517-021-07156-6
Joseph, J. K., et al. (2020). Community resilience mechanism in an unexpected extreme weather event: An analysis of the Kerala floods of 2018, India. International Journal of Disaster Risk Reduction, 49 (June), 101741. https://doi.org/10.1016/j.ijdrr.2020.101741
Joy, J., Kanga, S., & Singh, S. K. (2019). Kerala flood 2018: Flood mapping by participatory GIS approach, Meloor Panchayat. International Journal on Emerging Technologies, 10 (1), 197–205.
Kiran, K. S., Manjusree, P., & Viswanadham, M. (2019). Sentinel-1 SAR data preparation for extraction of flood footprints-A case study. Disaster Advances, 12 (12), 10–20.
Kotal, S. D., Roy, S. Sen., & Bhowmik, S. K. R. (2014). Catastrophic heavy rainfall episode over Uttarakhand during 16–18 June 2013 – observational aspects. Current Science, 107 (2), 234–245.
Krishna Vanama, V. S. and Rao, Y. S. (2019) ‘Change Detection Based Flood Mapping of 2015 Flood Event of Chennai City Using Sentinel-1 SAR Images’, in IGARSS 2019 - 2019 IEEE International Geoscience and Remote Sensing Symposium . IEEE, pp. 9729–9732. doi: https://doi.org/10.1109/IGARSS.2019.8899282 .
Kumar, P., Rani, M., Pandey, P. C., Sajjad, H., & Chaudhary, B. S. (Eds.). (2019). Applications and challenges of geospatial technology: potential and future trends . Cham: Springer International Publishing. https://doi.org/10.1007/978-3-319-99882-4
Book Google Scholar
Kuntla, S. K., & Manjusree, P. (2020). Development of an automated tool for delineation of flood footprints from sar imagery for rapid disaster response: A case study. Journal of the Indian Society of Remote Sensing, 48 (6), 935–944. https://doi.org/10.1007/s12524-020-01125-4
Lal, P., et al. (2020). Evaluating the 2018 extreme flood hazard events in Kerala, India. Remote Sensing Letters, 11 (5), 436–445. https://doi.org/10.1080/2150704X.2020.1730468
Liang, J., & Liu, D. (2019). (2020) ‘A local thresholding approach to flood water delineation using Sentinel-1 SAR imagery.’ ISPRS Journal of Photogrammetry and Remote Sensing, 159 , 53–62. https://doi.org/10.1016/j.isprsjprs.2019.10.017
Manjusree, P., et al. (2012). Optimization of threshold ranges for rapid flood inundation mapping by evaluating backscatter profiles of high incidence angle SAR images. International Journal of Disaster Risk Science, 3 (2), 113–122. https://doi.org/10.1007/s13753-012-0011-5
Martha, T. R., et al. (2015). Landslides triggered by the June 2013 extreme rainfall event in parts of Uttarakhand state, India. Landslides, 12 (1), 135–146. https://doi.org/10.1007/s10346-014-0540-7
Meghanadh, D. et al. (2020) ‘Rapid flood mapping using Sentinel-1A images: A case study of flood in panamaram, Kerala’, in IGARSS 2020 - 2020 IEEE International Geoscience and Remote Sensing Symposium . IEEE, pp. 6883–6885. doi: https://doi.org/10.1109/IGARSS39084.2020.9324674 .
Mehrabi, A. (2021). Monitoring the Iran Pol-e-Dokhtar flood extent and detecting its induced ground displacement using sentinel 1 imagery techniques. Natural Hazards, 105 (3), 2603–2617. https://doi.org/10.1007/s11069-020-04414-w
Mishra, A. K. (2021). Observing a severe flooding over southern part of India in monsoon season of 2019. Journal of Earth System Science, 130 (1), 2. https://doi.org/10.1007/s12040-020-01509-7
Mishra, A. K., & Nagaraju, V. (2019). Space-based monitoring of severe flooding of a southern state in India during south-west monsoon season of 2018. Natural Hazards, 97 (2), 949–953. https://doi.org/10.1007/s11069-019-03673-6
Mishra, V., & Shah, H. L. (2018). Hydroclimatological perspective of the Kerala Flood of 2018. Journal of the Geological Society of India, 92 (5), 645–650. https://doi.org/10.1007/s12594-018-1079-3
Mohammadi, A., Kamran, K. V., Karimzadeh, S., Shahabi, H., & Al-Ansari, N. (2020). Flood detection and susceptibility mapping using sentinel-1 time series, alternating decision trees, and bag-ADTree models. Complexity, 2020 , 1–21. https://doi.org/10.1155/2020/4271376
Mohandas, S., et al. (2020). NWP perspective of the extreme precipitation and flood event in Kerala (India) during August 2018. Dynamics of Atmospheres and Oceans, 91 (June), 101158. https://doi.org/10.1016/j.dynatmoce.2020.101158
Nagendra, N. P., Narayanamurthy, G., & Moser, R. (2020). Management of humanitarian relief operations using satellite big data analytics: The case of Kerala floods. Annals of Operations Research . https://doi.org/10.1007/s10479-020-03593-w
Neeraj, S., Mannakkara, S., & Wilkinson, S. (2021). Build back better concepts for resilient recovery: A case study of India’s 2018 flood recovery. International Journal of Disaster Resilience in the Built Environment, 12 (3), 280–294. https://doi.org/10.1108/IJDRBE-05-2020-0044
Otsu, N. (1979). A threshold selection method from gray-level histograms. IEEE Transactions on Systems, Man, and Cybernetics, 9 (1), 62–66. https://doi.org/10.1109/TSMC.1979.4310076
Pambudi, R. A. et al. (2020) ‘Flood Inundation Mapping of Cempaka Tropical Cyclone Effect in Gunung Sewu Karst Landscape Using Sentinel 1’, E3S Web of Conferences . Edited by B. Warsito, Sudarno, and T. Triadi Putranto, 202, p. 03030. doi: https://doi.org/10.1051/e3sconf/202020203030 .
Perrou, T., Garioud, A., & Parcharidis, I. (2018). Use of Sentinel-1 imagery for flood management in a reservoir-regulated river basin. Frontiers of Earth Science, 12 (3), 506–520. https://doi.org/10.1007/s11707-018-0711-2
Plank, S., et al. (2017). Mapping of flooded vegetation by means of polarimetric Sentinel-1 and ALOS-2/PALSAR-2 imagery. International Journal of Remote Sensing, 38 (13), 3831–3850. https://doi.org/10.1080/01431161.2017.1306143
Prasad, A. K., & Singh, R. P. (2005). Extreme rainfall event of July 25–27, 2005 over Mumbai, West Coast, India. Journal of the Indian Society of Remote Sensing, 33 (3), 365.
Rana, V. K., & Suryanarayana, T. M. (2019). Evaluation of SAR speckle filter technique for inundation mapping. Remote Sensing Applications: Society and Environment, 16 (October), 100271. https://doi.org/10.1016/j.rsase.2019.100271
Ranjan, R., Sarma, H. and Saharia, N. (2018) ‘Finding out the needy one from Tweets: An analysis using #kerelafloods’, in 2018 3rd International Conference on Contemporary Computing and Informatics (IC3I) . IEEE, pp. 184–187. doi: https://doi.org/10.1109/IC3I44769.2018.9007270 .
Sadek, M., et al. (2020). ‘Low-cost solutions for assessment of flash flood impacts using sentinel-1/2 data fusion and hydrologic/hydraulic modeling: Wadi El-Natrun Region Egypt. Advances in Civil Engineering, 2020 , 1–21. https://doi.org/10.1155/2020/1039309
Sahoo, S. N., & Sreeja, P. (2017). Development of flood inundation maps and quantification of flood risk in an urban catchment of Brahmaputra River. ASCE-ASME Journal of Risk and Uncertainty in Engineering Systems, Part a: Civil Engineering, 3 (1), 1–11. https://doi.org/10.1061/AJRUA6.0000822
Samuele, D. P., Filippo, S., & Enrico, B.-M. (2021). Multi-temporal mapping of flood damage to crops using sentinel-1 imagery: A case study of the Sesia River (October 2020). Remote Sensing Letters, 12 (5), 459–469. https://doi.org/10.1080/2150704X.2021.1890262
Sarkar, S., & Singh, R. P. (2017). June 19 2015 rainfall event over mumbai: some observational analysis. Journal of the Indian Society of Remote Sensing, 45 (1), 185–192. https://doi.org/10.1007/s12524-016-0572-7
Schumann, G. J. P., & Moller, D. K. (2015). Microwave remote sensing of flood inundation. Physics and Chemistry of the Earth, Parts a/b/c, 83–84 , 84–95. https://doi.org/10.1016/j.pce.2015.05.002
Sharifi, A. (2020). Flood mapping using relevance vector machine and sar data: a case study from Aqqala, Iran. Journal of the Indian Society of Remote Sensing, 48 (9), 1289–1296. https://doi.org/10.1007/s12524-020-01155-y
Sherpa, S. F., et al. (2020). Probabilistic mapping of august 2018 flood of kerala, india, using space-borne synthetic aperture radar. IEEE Journal of Selected Topics in Applied Earth Observations and Remote Sensing, 13 , 896–913. https://doi.org/10.1109/JSTARS.2020.2970337
Study report: Kerala floods of August 2018 (2018).
Sudheer, K. P., et al. (2019). Role of dams on the floods of august 2018 in Periyar River Basin, Kerala. Current Science, 116 (5), 780. https://doi.org/10.18520/cs/v116/i5/780-794
Sumith, S. S. and John, C. M. (2017) ‘Analysing the capability of sentinel-1 SAR data for flood monitoring and mapping in Idukki dam Reservoir, Southern Western Ghats of India’, in 38th Asian Conference on Remote Sensing - Space Applications: Touching Human Lives, ACRS 2017 , pp. 1–8.
Surampudi, S., & Yarrakula, K. (2020). Mapping and assessing spatial extent of floods from multitemporal synthetic aperture radar images: A case study on Brahmaputra River in Assam State, India. Environmental Science and Pollution Research, 27 (2), 1521–1532. https://doi.org/10.1007/s11356-019-06849-6
Tavus, B., et al. (2018). Considerations on the use of sentinel-1 data in flood mapping in urban areas: Ankara (Turkey) 2018 Floods. The International Archives of the Photogrammetry, Remote Sensing and Spatial Information Sciences,. https://doi.org/10.5194/isprs-archives-XLII-5-575-2018
Thakur, P. K., et al. (2020). Synergistic use of remote sensing, GIS and hydrological models for study of august 2018 Kerala Floods. The International Archives of the Photogrammetry, Remote Sensing and Spatial Information Sciences,. https://doi.org/10.5194/isprs-archives-XLIII-B3-2020-1263-2020
Tiwari, V. et al. (2020) ‘Flood inundation mapping- Kerala 2018; Harnessing the power of SAR, automatic threshold detection method and Google Earth Engine’, PLOS ONE . Edited by G. J.-P. Schumann, 15(8), e0237324. doi: https://doi.org/10.1371/journal.pone.0237324 .
Tripathi, A., Attri, L., & Tiwari, R. K. (2021). Spaceborne C-band SAR remote sensing–based flood mapping and runoff estimation for 2019 flood scenario in Rupnagar, Punjab, India. Environmental Monitoring and Assessment, 193 (3), 110. https://doi.org/10.1007/s10661-021-08902-9
Tripathi, G., et al. (2020). Flood inundation mapping and impact assessment using multi-temporal optical and SAR Satellite Data: A case study of 2017 Flood in Darbhanga District, Bihar, India. Water Resources Management, 34 (6), 1871–1892. https://doi.org/10.1007/s11269-020-02534-3
Twele, A., et al. (2016). Sentinel-1-based flood mapping: A fully automated processing chain. International Journal of Remote Sensing, 37 (13), 2990–3004. https://doi.org/10.1080/01431161.2016.1192304
United Nations Development Programme (2018) Kerala post disaster needs assessment: Floods and landslides-August 2018 . Available at: https://reliefweb.int/report/india/kerala-post-disaster-needs-assessment-floods-and-landslides-august-2018 .
Vanama, V. S. K., et al. (2021a). Inundation mapping of Kerala flood event in 2018 using ALOS-2 and temporal Sentinel-1 SAR images. Current Science, 120 (5), 915–925. https://doi.org/10.18520/cs/v120/i5/915-925
Vanama, V. S. K., Rao, Y. S., & Bhatt, C. M. (2021b). Change detection based flood mapping using multi-temporal Earth Observation satellite images: 2018 flood event of Kerala, India. European Journal of Remote Sensing, 54 (1), 42–58. https://doi.org/10.1080/22797254.2020.1867901
Vishnu, C. L., et al. (2019). Satellite-based assessment of the August 2018 flood in parts of Kerala, India. Geomatics, Natural Hazards and Risk, 10 (1), 758–767. https://doi.org/10.1080/19475705.2018.1543212
Download references
Acknowledgements
This research is undertaken as part of the DST–NRDMS project funded by the Department of Science and Technology, GOI. The authors thank the funding agency for providing the necessary guidance and financial support. We are grateful to Copernicus Open Access Hub and European Space Agency for providing Sentinel-1 data freely.
Author information
Authors and affiliations.
Department of Architecture and Planning, National Institute of Technology Calicut, Kozhikode, India
K. Chithra, B. V. Binoy & P. Bimal
You can also search for this author in PubMed Google Scholar
Corresponding author
Correspondence to B. V. Binoy .
Ethics declarations
Conflict of interest.
The authors declare that there exists no conflict of interest with any individual or organization in the present work.
Additional information
Publisher's note.
Springer Nature remains neutral with regard to jurisdictional claims in published maps and institutional affiliations.
About this article
Chithra, K., Binoy, B.V. & Bimal, P. Spatial Mapping of the Flood-Affected Regions of Northern Kerala: A Case Study of 2018 Kerala Floods. J Indian Soc Remote Sens 50 , 677–688 (2022). https://doi.org/10.1007/s12524-021-01485-5
Download citation
Received : 29 September 2021
Accepted : 18 December 2021
Published : 30 January 2022
Issue Date : April 2022
DOI : https://doi.org/10.1007/s12524-021-01485-5
Share this article
Anyone you share the following link with will be able to read this content:
Sorry, a shareable link is not currently available for this article.
Provided by the Springer Nature SharedIt content-sharing initiative
- Flood mapping
- 2018 Kerala floods
- Thresholding method
- Find a journal
- Publish with us
- Track your research
- 0 Shopping Cart
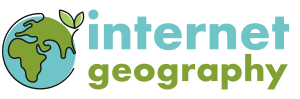
Rivers Revision
Kerala Flood 2018 Quiz
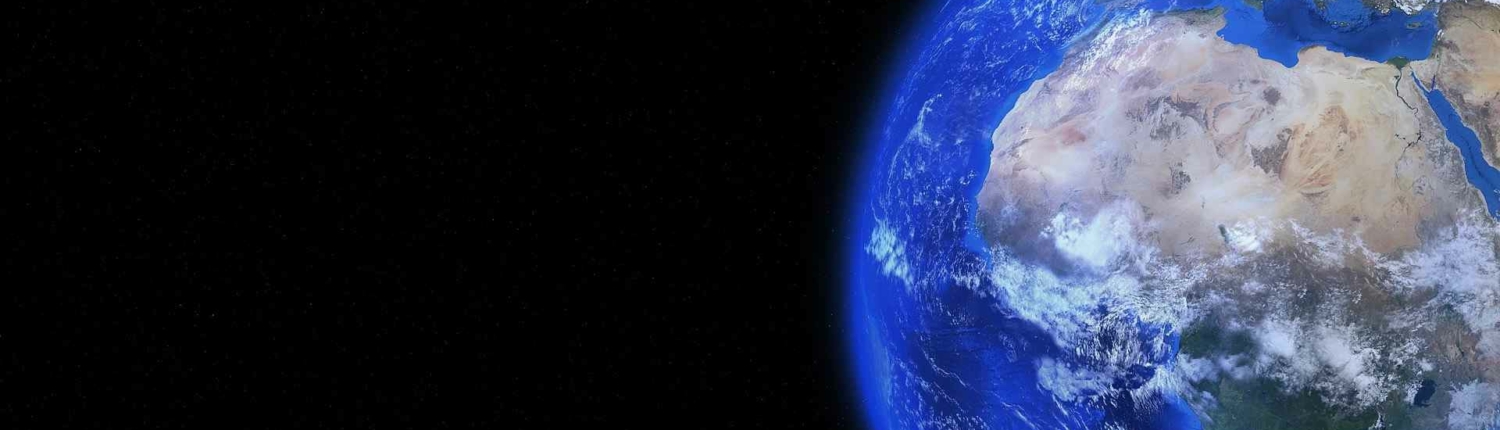
You are here: GCSE Geography Interactive Revision > Rivers > Multiple Choice Quiz
What is Kerala?
Kerala is a state in which country, where in india is kerala located, true or false kerala has the highest population of all of india's states..
Kerala has the 13th largest population in India.
What is the climate of Kerala?
Identify the mountain range in eastern kerala., true or false the indian state of kerala receives some of india’s highest rainfall during the monsoon season., what is the average rate of rainfall in kerala during the monsoon season, how much rain fell in kerala during the 2018 monsoon season, how much more rain fell during august 2018 compared to the august average, true or false between august 1 and 19, the state received 758.6 mm of precipitation, compared to the average of 287.6 mm, or 164% more., true or false the unprecedented rainfall was caused by a spell of high pressure over the region..
The unprecedented rainfall was caused by a spell of low pressure over the region.
True or false? The unprecedented rainfall was caused by a spell of low pressure over the region. As a result, there was a perfect confluence of the south-west monsoon wind system and the two low-pressure systems formed over the Bay of Bengal and Odisha. The low-pressure regions pull in the moist south-west monsoon winds, increasing their speed, as they then hit the Western Ghats, travel skywards, and form rain-bearing clouds.
True or false downpours on already saturated land led to more surface run-off causing landslides and widespread flooding., how many dams on kerala's 41 rivers flowing into the arabian sea were opened after being overwhelmed, true or false the opening of dams on kerala's rivers caused water treatment plants to be submerged, damaging their motors., what was the depth of flood water in the worst affected areas, how many people died as the result of flooding in the state of kerala during the 2018 floods, how did many of the victims of the flood die, how many people were made homeless by the flooding, true or false parts of kerala’s commercial capital, cochin, were underwater, snarling up roads and leaving railways across the state impassable. in addition, the state’s airport, which domestic and overseas tourists use, was closed, causing significant disruption., true or false schools in seven of kerala's fouteen districts were closed.
Schools in all 14 districts of Kerala were closed.
How many emergency relief camps were set up in the area?
True or false more than 83,000km of roads were damaged and the total recovery cost was estimated to be between £2.2bn and $2.7bn., which of the following are examples of responses to the flooding in kerala.
Please select 4 correct answers
True or false? In the wake of the disaster, the UAE, Qatar and the Maldives came forward with offers of financial aid amounting to nearly £82m. The United Arab Emirates promised $100m (£77m) of this aid.
True or false since 2004, india declined to accept international aid donations..
The main reason for this is to protect its image as a newly industrialised country; it does not need to rely on other countries for financial help.
Which technology company provided a donation platform to allow donors to make donations securely?
Google partners with the Center for Disaster Philanthropy (CDP), an intermediary organisation that specialises in distributing your donations to local nonprofits that work in the affected region to ensure funds reach those who need them the most.
Share this:
- Click to share on Twitter (Opens in new window)
- Click to share on Facebook (Opens in new window)
- Click to share on Pinterest (Opens in new window)
- Click to email a link to a friend (Opens in new window)
- Click to share on WhatsApp (Opens in new window)
- Click to print (Opens in new window)
Please Support Internet Geography
If you've found the resources on this site useful please consider making a secure donation via PayPal to support the development of the site. The site is self-funded and your support is really appreciated.
Search Internet Geography
Top posts and pages.

Latest Blog Entries

Pin It on Pinterest
- Click to share
- Print Friendly
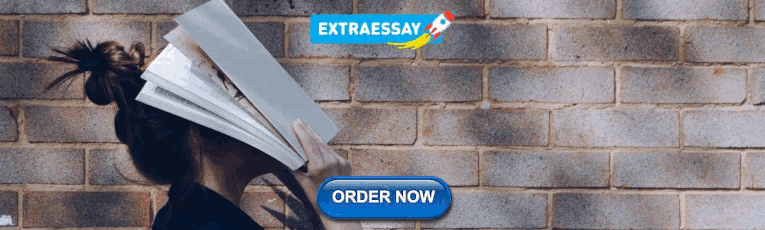
IMAGES
VIDEO
COMMENTS
Effects. In some areas, floodwater was between 3-4.5m deep. Floods in the southern Indian state of Kerala have killed more than 410 people since June 2018 in what local officials said was the worst flooding in 100 years. Many of those who died had been crushed under debris caused by landslides.
Floodplain zoning policies influence how land on, or close to, floodplains are used. River restoration involves removing any hard-engineering strategies and restoring the river to its original course. This can involve making the river less straight which can slow the flow of the river. Floods can bring both advantages and disadvantages to an ...
Specifically in this project, we study the "KeralaGram" group on Telegram, which had 15,000 users at the time of the 2018 flood and was focused on issues/events/news related to the state of Kerala. While Twitter has been extensively used for social sensing, the use of Telegram is less common. Most relevant Telegram research involves either ...
Kerala is located on the southwestern part of the Malabar Coast in India. It covers a territory of 38,863 km 2 (15,005 square miles) and borders Tamil Nadu, Karnataka, Lakshadweep Sea, and Lakshadweep group of Islands (UT) (Azzali et al., 2021, 120).The state, due to its geographical location along the coast and slopes of the Western Ghats, is highly vulnerable to the effects of natural ...
In August 2018, the Indian state of Kerala received an extended period of very heavy rainfall as a result of a low-pressure system near the beginning of the month being followed several days later by a monsoon depression. The resulting floods killed over 400 people and displaced a million more. Here, a high resolution setup (4 km) of the Weather Research and Forecasting (WRF) model is used in ...
For example, the state's literacy rate is 94%, compared to the national average of 73%. The country's average life expec-tancy at birth was 68.8 years in 2013 -2017, whereas Kerala at the same time had 75.2 year life expectancy (RBI, 2020, 15 -16). Among all the states, it has the highest life expectancy at birth.
Flooding is a major hazard that is responsible for substantial damage and risks to human health. worldwide. The 2018 ood event in Kerala, India, killed 433 people and displaced more than 1 ...
Geography Case Studies - A wide selection of geography case studies to support you with GCSE Geography revision, homework and research. ... The Great Floods of 2000; Kerala flood case study; Rocks, Resources and Scenery. ... Please Support Internet Geography. If you've found the resources on this site useful please consider making a secure ...
The 2018 Kerala flood damage data were collected and processed to train and test the selected machine learning models to classify the buildings to different flood damage states. Parameters such as flood depth, flood duration, floor area, age of the building, roof material, and wall material were considered in this study for flood damage prediction.
Abstract. Extreme precipitation events and flooding that cause losses to human lives and infrastructure have increased under the warming climate. In August 2018, the state of Kerala (India) witnessed large-scale flooding, which affected millions of people and caused 400 or more deaths. Here, we examine the return period of extreme rainfall and the potential role of reservoirs in the recent ...
Abstract. Kerala experienced extremely heavy rainfall from 8th to 11th August 2019, which caused a widespread flood throughout the state and disrupted thousands of lives as well as a substantial ...
More than 10% of the geographical area is flooded in the villages of Edappal, Nannambra, Nannamukku, Kuttippu-ram, Thirunavaya, Irimbiliyam and Marancheri in the August 21 imagery. The hilly district of Wayanad is the second most affected in the study area. The major flood-affected regions are represented in Fig. 6.
A short span of abnormal precipitation in the year 2018 has ravaged the southwestern state of Kerala. It has resulted in widespread flooding and landslides with more than 400 casualties. The present study has implemented the SAR (Synthetic Aperture Radar)-based Sentinel 1C band satellite data. The researchers have analyzed the remotely sensed ...
The hydro-climatological perspective of Kerala floods [12,25], combined effect of extreme rainfall and reservoir storage [26], role of dams on the Periyar floods [38], case study on Kakki ...
Kerala experienced extremely heavy rainfall from 8th to 11th August 2019, which caused a widespread flood throughout the state and disrupted thousands of lives as well as a substantial amount of property. The novel approach of utilising the observed and merged satellite rainfall products along with remotely sensed optical and microwave images was applied to monitor the event. The results ...
Fig. 6 A view from Munnar after the 1924 floods [59] Social Inclusivity: A Case Study on Community Resilience … 115. 2.2 August 2018 Kerala Flood. From June to August 2018, Kerala has received ...
Flood inundation maps provide valuable information towards flood risk preparedness, management, communication, response, and mitigation at the time of disaster, and can be developed by harnessing the power of satellite imagery. In the present study, Sentinel-1 Synthetic Aperture RADAR (SAR) data and Otsu method were utilized to map flood inundation areas. Google Earth Engine (GEE) was used for ...
The main causes of floods are typically climatic forces. Flooding is affected by rainfall intensity, seasonal variations in discharge, relief, and urbanisation. Prevention and prediction improvements have involved moving from 'hard engineering' to 'soft engineering' methods and utilising technology for better forecasting.
Flood mapping studies gained popularity in recent years with the availability of high-resolution SAR imagery. Satellite images in the visible spectrum cannot be used for disaster management applications due to inherent cloud cover during adverse weather conditions. The cloud penetrating capabilities and all-weather operation make radar images more suitable for flood mapping applications than ...
Kerala Flood 2018 Quiz - Test your knowledge of the 2018 Kerala floods with this interactive online quiz. ... Please Support Internet Geography. If you've found the resources on this site useful please consider making a secure donation via PayPal to support the development of the site. The site is self-funded and your support is really ...