
An official website of the United States government
The .gov means it’s official. Federal government websites often end in .gov or .mil. Before sharing sensitive information, make sure you’re on a federal government site.
The site is secure. The https:// ensures that you are connecting to the official website and that any information you provide is encrypted and transmitted securely.
- Publications
- Account settings
Preview improvements coming to the PMC website in October 2024. Learn More or Try it out now .
- Advanced Search
- Journal List
- HHS Author Manuscripts

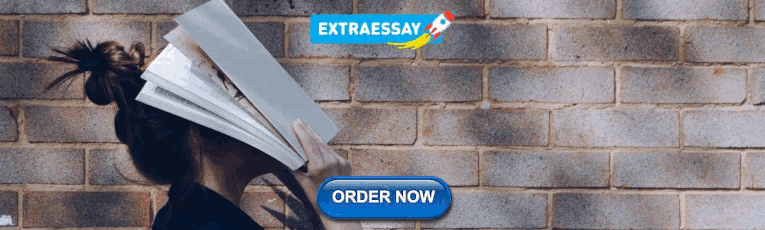
The Origins of Higher-Order Thinking Lie in Children’s Spontaneous Talk Across the Pre-School Years
Rebecca r. frausel.
a The University of Chicago, United States of America
Catriona Silvey
b University College London, United Kingdom
Cassie Freeman
c The College Board, United States of America
Natalie Dowling
Lindsey e. richland.
d University of California Irvine, United States of America
Susan C. Levine
Steve raudenbush, susan goldin-meadow.
Higher-order thinking is relational reasoning in which multiple representations are linked together, through inferences, comparisons, abstractions, and hierarchies. We examine the development of higher-order thinking in 64 preschool-aged children, observed from 14 to 58 months in naturalistic situations at home. We used children’s spontaneous talk about and with relations (i.e., higher-order thinking talk, or HOTT) as a window onto their higher-order thinking skills. We find that surface HOTT, in which relations between representations are more immediate and easily perceptible, appears before—and is far more frequent than— structure HOTT, in which relations between representations are more abstract and less easy to perceive. Child-specific factors (including early vocabulary and gesture use, first-born status, and family income) predict differences in children’s onset (i.e., age of acquisition) of HOTT and its trajectory of use across development. Although HOTT utterances tend to be longer and more syntactically complex than non-HOTT utterances, HOTT frequently appears in non-complex utterances, and a substantial proportion of children achieve complex utterance onset prior to the onset of HOTT. This finding suggests that complex language is neither necessary nor sufficient for HOTT to occur; other factors above and beyond complex linguistic skills are involved in the onset and use of higher-order thinking. Finally, we found that the trajectory of HOTT, particularly structure HOTT—but not complex utterances—during the preschool period predicts standardized outcome measures of inference and analogy skills in grade school, which underscores the crucial role that this kind of early talk plays for later outcomes.
1. Introduction
As children acquire language, they also develop the ability to use higher-order thinking, which is the cognitive capacity to make inferences and generalizations, use classifications and taxonomies, and broadly go beyond the information given ( Bruner, 1973 ; Resnick, 1987 ). It is a crucial part of children’s emerging cognitive development, and higher-order thinking is increasingly highlighted as a focal goal for education in the 21 st century ( Koenig, 2015 ).
Despite its importance, little is known about the developmental trajectory of children’s higher-order thinking communication in naturalistic contexts (as opposed to experimental contexts, where far more is known), nor about individual variation in its use. In addition, although the human capacities for both generative, symbolic language and higher-order cognitive skills have been argued to differentiate humans from other animals ( Gentner, 2003 ; Penn, Holyoak, & Povinelli, 2008 ; Gentner & Christie, 2008 ), the relations between their development are not well understood.
In this paper, we examine the early foundations of higher-order thinking by examining children’s engagement in talk about and with relations, which we define as higher-order thinking talk (HOTT). We use longitudinal observations of 64 children, videotaped at home every 4 months from 14 to 58 months, to determine the age in development when children begin to regularly display higher-order thinking in their spontaneous talk (their HOTT onset), and we chart the trajectory of this talk over time ( Section 3.1 ). We also explore child-specific factors (e.g., first-born status, family income, early vocabulary and gesture use) that have the potential to influence the onset and developmental trajectory of HOTT ( Section 3.2 ). Furthermore, because HOTT utterances may be longer and more syntactically complex than non-HOTT utterances, we investigated the extent to which HOTT use can be disentangled from complex language use ( Section 3.3 ). Finally, we ask whether children’s early HOTT is related to their performance on standardized measures of higher-order thinking (including verbal and non-verbal analogical reasoning and text-based inferencing ability) administered years later during grade school ( Section 3.4 ). If so, this relation would validate the role of HOTT as an early index of, and potential training opportunity for, children’s higher-order thinking.
1.1. Defining Higher-Order Thinking
Higher-order thinking is, in the words of educational psychologist Lauren Resnick (1987) , “difficult to define but easy to recognize when it occurs” (pg. 44). There are as many different ways to define it as there are researchers studying it, but what most definitions have in common is that higher-order thinking involves rearranging or extending knowledge in novel ways. As Lewis and Smith (1993) say, “Higher-order thinking occurs when a person takes new information and information stored in memory and interrelates and/or rearranges and extends this information to achieve a purpose or find possible answers in perplexing situations” (pg. 136). In this paper, we use the definition of higher-order thinking offered by Resnick (1987) : Higher-order thinking involves “elaborating the given material, making inferences beyond what is explicitly presented, building adequate representations, [and] analyzing and constructing relationships” (pg. 45).
More specifically, we operationalize higher-order thinking as talk in which an individual’s utterance (a unit of speech; see the Methods section) includes reference to an inference or explanation, a comparison, an abstraction/generalization, or a hierarchy/taxonomic relationship. We identified these four types of higher-order thinking on the basis of literature reviews and pilot analyses. Together, they constitute a broad category of speech we call ‘higher-order thinking talk,’ or HOTT. We also differentiate between HOTT that references more immediate and perceivable relationships (surface) versus deeper, underlying relationships (structure), a distinction that the literature on reasoning and transfer has clarified to require different levels of skill (see Bransford, Brown, & Cocking, 1999 ; Gentner & Markman, 1997 ; Gick & Holyoak, 1983 ).
1.2. Importance of Higher-Order Thinking
The ability to use higher-order thinking is increasingly recognized as critical to academic and employment success in the 21 st century ( Common Core State Standards Initiative, 2010 ; National Research Council, 2001 ; 2007 ; 2012 ; 2013 ). As technology has advanced, today’s students have fingertip access to wide Internet knowledge and information resources. At the same time, students are increasingly challenged by how best to attend to and use this information, and to organize it effectively so it can be applied to novel situations.
This dilemma is acute: students in the United States, one of the wealthiest and most well-connected countries in the world, frequently lag behind their international peers in standardized assessments of math and science ( Gonzales, 2001 ; Mullis, Martin, Gonzalez, & Chrostowski, 2004 ). Differences in teaching instruction can partially explain these gaps ( Richland, Zur, & Holyoak, 2007 ; Hiebert et al., 2003 ). For example, although American teachers use conceptually rich problems and comparisons at similar rates as teachers from higher-achieving regions such as Hong Kong or Japan, they differ in their use of visuo-spatial supports that draw students’ attention to relevant abstract relations ( Richland, Zur, & Holyoak, 2007 ). Richland and colleagues (2007) suggest that these differences might reflect different cultural orientations to relational reasoning. Developing the ability to attend to relations between representations may therefore be fundamental to supporting abstract thought and developing domain-general thinking skills, which are critical areas of concern for the modern student.
1.3. Developmental Origins of Higher-Order Thinking
Educational and developmental psychologists question when in development children ‘start’ using higher-order thinking (e.g., Walker & Gopnik, 2014 ). We take the position, however, that there is no one particular moment of onset, as children’s capacity for complex relational thinking depends on domain, context, and the specific demands of the task at hand; for example, whether or not the tasks use language. Studies using looking-time or simple physical problem-solving tasks suggest that higher-order and relational reasoning capacity at a basic level emerges very early in development. Pre-linguistic infants as young as 7 months demonstrate generalization of the same-different relation, and generalize this relation to novel pairs ( Ferry, Hespos, & Gentner, 2015 ). Paradigms exploring early causal thinking similarly show that young infants grapple with relational thinking ( Wang & Baillargeon, 2008 ). For example, 13-month-olds learned to transfer a pulling relation (where a cloth could be pulled to reach a toy) to a novel situation ( Chen, Sanchez, & Campbell, 1997 ). The fact that pre-linguistic infants can identify and transfer relationships provides some evidence that higher-order reasoning cannot be entirely dependent on language.
Researchers have found evidence of relational reasoning in experimental tasks that use language beginning around 2 years of age ( Loewenstein & Gentner, 2005 ; Christie & Gentner, 2014 ), suggesting this might also be the age at which children first use higher-order thinking in their spontaneous talk. By ages 3–4, children are much more reliable in their skills even when assessed with language-based tasks ( Richland, Morrison, & Holyoak, 2006 ), and are able to use higher-order relational reasoning when they have adequate knowledge of a task and the task demands are minimized.
Although children begin cultivating higher-order reasoning abilities very early in development, at this young age, they are still challenged by increasing relational complexity, and show susceptibility to errors related to perceptually-similar foils ( Richland, Morrison, & Holyoak, 2006 ). Early in development, children largely attend to surface-level perceptual relationships between representations (as in surface HOTT) when these are salient and available. For example, children might state that a plant stem is similar to a drinking straw because both are long and skinny. As they grow and become more knowledgeable, and as their cognitive resources expand, children more often attend to deeper functional similarities (as in structure HOTT). They begin to make adult-like judgments such as explaining that a plant stem is similar to a drinking straw because both are used to deliver nourishment to a living thing, and both use differential pressure to move liquid up the shaft ( Gentner, 1988 ). This change has been frequently described as the ‘relational shift’ ( Gentner, 1988 ; Gentner & Ratterman, 1991 ), although the nature of children’s developmental changes in relational reasoning is debated (e.g., see Goswami, 2002; Simms, Frausel & Richland, 2018 ). Whatever the cause of the change, the early prioritization of salient object-level and perceptual information may pave the way for more complex and abstract relational structures later in development.
At the same time that children are developing these more robust higher-order skills, they are simultaneously acquiring more complex language abilities. Next, we discuss relations between language and higher-order thinking development, including how specific language features (e.g., vocabulary, syntax) may be useful, or even necessary, for higher-order reasoning.
1.4. The Role of Language in Higher-Order and Relational Reasoning
Gentner and Goldin-Meadow (2003) described three models for how language could support thinking: (1) the language as lens view, or linguistic determinism, which theorizes that language shapes speakers’ perceptions of the world; (2) the language as category shift view, or linguistic relativism, which theorizes that while conceptual categories are universal, language can influence their boundaries; and (3) the language as toolkit view. Our approach best fits under this third view, which postulates that language provides concepts and strategies to support representation and reasoning skills, but does not supplant them. Language may serve to ‘bootstrap’ relational reasoning, and provide the tools for children to extract and formulate relational representations in the world. Consequently, language may also serve as a ‘bottleneck,’ preventing children who lack adequate linguistic skills from engaging in and communicating their higher-order thoughts.
1.4.1. Evidence from Experiments
The experimental literature has provided some indication that language is interrelated with reasoning proficiency. One well-documented phenomenon is that adding useful lexical items to task instructions can improve children’s proficiency. For instance, providing spatial ( in , on , under ) and relational ( top , middle , bottom ) words made 3- and 4-year-olds more proficient at a spatial mapping task ( Loewenstein & Gentner, 2005 ). Children given a challenging mapping task perform better even when provided with more abstract relational labels— Daddy , Mommy , and Baby —that convey monotonic changes in size ( Gentner & Ratterman, 1991 ; Ratterman & Gentner, 1998 ; see also Christie & Gentner, 2014 ). These studies suggest that relational reasoning can be supported by the introduction of linguistic labels denoting relational concepts; the use of relational language may invite children to form deeper relational representations.
1.4.2. Evidence from Naturalistic Studies
We turn next to the relatively few studies of spontaneous language and higher-order thinking development. Özçalışkan, Goldin-Meadow, Gentner, and Mylander (2009) asked whether being exposed to a conventional linguistic system—one that contains explicit terms to highlight comparison such as ‘like’—is essential for children to make different types of similarity comparisons. Özçalışkan and colleagues (2009) compared spontaneous comparisons made by 1-to-3-year-old typically-developing children to spontaneous comparisons made by 2-to-4-year-old deaf children who lacked exposure to a usable language model. The hearing losses of the deaf children prevented them from benefiting from spoken linguistic input, and their hearing parents had not exposed them to sign language. Nonetheless, these deaf children and their hearing families invented gesture systems called homesigns to communicate with their hearing family members ( Feldman, Goldin-Meadow, & Gleitman, 1978 ; Goldin-Meadow, 2003 ). These homesign systems are structured in language-like ways ( Goldin-Meadow & Mylander, 1984 ), but, importantly for our purposes, lack an explicit comparison term, such as ‘like.’
Özçalışkan and colleagues (2009) found that the homesigners expressed similarity relations in their gestures (e.g., point at cat + point at tiger; point at train + point at car), even though none had spontaneously developed a sign for the term ‘like.’ However, their comparisons were always between objects from the same superordinate category that shared multiple features (as in the cat-tiger and train-car examples), and thus were more limited in scope. In contrast, the typically-developing children not only used these broad comparisons, but also used more focused comparisons between objects from different superordinate categories that revolved around a single feature (e.g., highlighting the similarity in color between a red apple and a red book, or the similarity in shape between a lollipop and a balloon). Interestingly, they did so only after acquiring the word ‘like’ around 30 months.
The fact that the typically-developing children began producing these more focused comparisons, based on specific features, after they acquired the word ‘like’—and that the homesigners who lacked the word ‘like’ did not produce focused comparisons—suggests that learning and using a word for similarity may be instrumental in expressing more complex relationships between representations. Certain lexical items may be necessary for children to engage in higher-order reasoning, as when making comparisons. However, less is known about whether other aspects of language (e.g., certain types of syntactic structures) facilitate higher-order thinking use.
1.5. The Current Study
Despite the importance of higher-order and relational reasoning, and its development in tandem with other important linguistic skills in early childhood, the emergence of higher-order cognitive skills in everyday home contexts is not well understood (though see Callanan & Oakes, 1992 , who used diary records from parents to record how preschoolers’ causal questions in everyday interaction contributes to their understanding of causal knowledge structures). The current project bridges this gap through analysis of an unusually rich set of longitudinal data on children’s early talk and later thinking and reasoning skills. Sixty-four typically-developing, monolingual English-acquiring children were videotaped in their homes every 4 months between 14 and 58 months while engaging in regular, everyday activities, yielding over 1,000 hours of video. These same children were visited years later in grade school and administered standardized reasoning outcome measures.
Although early language is known to vary by socioeconomic status (SES) and to predict differences in children’s long-term school and career success, researchers have not yet examined whether these same disparities are present for higher-order reasoning development. Furthermore, little previous research has examined how the development of higher-order reasoning is related to the development of complex language abilities—in part, because the majority of studies of children’s thinking and reasoning have been conducted cross-sectionally using experimenter-derived tasks (e.g., Loewenstein & Gentner, 2005 ; Richland, Morrison, & Holyoak, 2006 ). Moreover, studies of language development using naturalistic data (e.g., Hart & Risley, 1995 ; Huttenlocher, Haight, Bryk, Seltzer, & Lyons, 1991 ) have largely examined differences in language use, such as vocabulary diversity and syntactic complexity. Little previous research has explored the nature and complexity of the thinking embedded in the spontaneous talk produced by children early in development.
Despite these traditionally distinct research pathways, the spontaneous use of conceptually and linguistically complex language in early childhood may have long-term impacts on children’s later thinking and reasoning skills. The spontaneous home talk collected and coded in this study spans from 14 months to 58 months, a crucial period in which language emerges and grows in complexity, and which also represents a period of great growth in children’s thinking and reasoning development. These data thus allow for new insights into the development of children’s thinking and reasoning as expressed through their early language.
1.6. Research Questions
This paper addresses four research questions.
1.6.1. How do children from 14–58 months vary in their onset and developmental trajectory of spontaneous higher-order thinking talk (HOTT)?
Based on the experimental literature (e.g., Loewenstein & Gentner, 2005 ; Christie & Gentner, 2014 ), we predict that HOTT will emerge with regularity around the beginning of the second year of life. Furthermore, we predict that children will vary widely in their onset and trajectory of HOTT, just as they exhibit great variability in their early lexicons ( Huttenlocher, et al., 1991 ), syntax ( Huttenlocher, Vasilyeva, Cymerman, & Levine, 2002 ), and other linguistic skills. Finally, we predict that, due to the different levels of skill required when identifying deeper, more abstract relationships in contrast to simpler, more immediate relationships, onset of structure HOTT will occur later in development than onset of surface HOTT.
1.6.2. What child-specific factors, such as family income, birth order, and early language use, are associated with the onset and trajectory of HOTT?
We expect that HOTT might be another language area, similar to vocabulary (e.g., Hart & Risley, 1995 ), where we find socioeconomic disparities. Children whose parents have higher incomes may use HOTT earlier and more often overall. In addition, we might expect birth order to influence input and use, since the oldest or only child may receive more individualized input from his or her parents, resulting in earlier or more frequent HOTT use. Finally, early language use might influence HOTT. Children with larger vocabularies early in development, or who use a wider variety of gestures to communicate, may elicit richer input from their caregivers, which may result in earlier onset of HOTT and more HOTT use across development.
1.6.3. What is the relation between HOTT and complex language?
Previous research has focused on relations between higher-order thinking and vocabulary. We expand this inquiry to explore two related questions concerning the extent to which HOTT use can be disentangled from complex language use.
Our first question is whether HOTT utterances are longer and more complex than non-HOTT utterances. To answer this question, we assessed the linguistic form (utterance length and verbs per utterance) of surface HOTT, structure HOTT, and non-HOTT utterances. If HOTT utterances are comprised primarily of complex utterances (which we define as utterances containing two or more verbs), this would suggest that our measure of HOTT largely reduces to being another measure of language complexity. Furthermore, it would suggest that describing complex relationships in the world necessarily requires speakers to use complex language—not just certain kinds of words, but lengthier speech and more complex syntax. If, however, HOTT appears in non-complex utterances as well as complex utterances, this would suggest that HOTT and complex language, although perhaps related, are not redundant. Complex language may provide speakers with strategies to support complex representation and reasoning, but may not be necessary for it to occur.
Our second question is whether the onset of HOTT coincides with the onset of complex utterances. Complex utterances allow the expression of two or more propositions within a single utterance, and thus have the potential to foster an understanding of relations between those propositions; children may start using HOTT at the same time they start using complex language. But if onset of complex language precedes onset of HOTT, we would have evidence that, although having complex language may relate to higher-order thinking development, complex language alone is not sufficient. The ability to identify and articulate relations between representations in the world using HOTT may call upon additional cognitive and linguistic skills beyond the ability to construct a complex utterance.
1.6.4. How is HOTT use from 14–58 months related to reasoning outcomes in grade school, including analogical reasoning and inferencing ability?
We explore, for the first time, whether higher-order thinking skills are related across development, as are skills in domains such as math (e.g., Mazzocco, Feigenson, & Halberda, 2011 ; Stevenson & Newman, 1986 ; Case, Griffin, & Kelly, 1999 ). Finding that relationships across development are weak would suggest that factors later in development (e.g., schooling) are needed to explain individual differences in higher-order thinking outcomes.
Overall, the current manuscript focuses on research questions pertaining to identifying HOTT as an important characteristic of children’s early spontaneous language, mapping its developmental trajectory and relations to individual characteristics such as family income, and assessing its predictive validity for future higher-order thinking skills. Determining contributions and mechanisms underlying these patterns, including the role of parent HOTT input, is beyond the scope of this manuscript, though future analyses will be important in exploring these questions.
2.1. Participants
Sixty-four typically-developing children and their primary caregiver(s) participated as part of a larger study of language development ( Goldin-Meadow, et al., 2014 ). Participants were recruited from the greater Chicago area. In order to recruit children and families, direct mailings were sent to approximately 5,000 families living in targeted zip codes, and advertisements were placed in a free, monthly parenting magazine. Responding parents were asked to confirm that they were raising their children in an English-only language environment (approximately 85–90% English, based on parent report). Given that they met this criterion, families were then interviewed for information on their background characteristics in order to create a sample that was demographically representative of the greater Chicago area, as reported in the 2000 U.S. Census.
The resulting final sample has 31 girls and 33 boys, including 34 first-born or only children. The sample was ethnically diverse, including 14 African American, 9 Latino, 35 White, and 6 children of mixed race. At the beginning of the study period, 5 families reported incomes of less than $15,000; 13 had incomes between $15,000 and $34,999; 8 had incomes between $35,000 and $49,999; 13 had incomes between $50,000 and $74,999; 11 had incomes between $75,000 and $99,000, and 14 reported incomes greater than $100,000. Using the midpoint of each income category as an estimate, the sample had an average income of $61,000 ( SD = $32,000).
Parents were asked to report who was primarily responsible for childcare. This person was asked to be home during filming of the home visits. The majority of children ( n = 56) had the mother as the primary caregiver, two children had the father as the primary caregiver, and six families reported that both parents equally shared the role (referred to as dual caregivers). Primary caregivers had an average of 15.6 years ( SD = 2.2 years, range 10 to 18 years) of education, the equivalent of slightly less than a Bachelor’s degree (the mother’s education level was used for the dual caregiver families).
2.2. Procedure
Children were videotaped interacting with the primary caregiver(s) during twelve 90-minute home visits conducted every 4 months from 14 to 58 months. This age range was selected because it represents the period during which children first began to produce language until they entered school. Parents and children were recorded engaging in ordinary, everyday activities such as playing with toys, reading books, and having meals. Not all participants completed every session; on average, subjects completed 11.3 sessions ( SD = 1.8 sessions, range 4 to 12 sessions). Out of a possible 768 session visits (64 subjects x 12 visits each), a total of 726 visits were completed; i.e., only 5.5% of visits were missing.
In addition, children were visited in their homes annually or bi-annually beginning in grade school and continuing through late adolescence. We examine whether children’s early HOTT is related to longer-term reasoning outcomes. The reasoning outcome measures are standardized measures of text-based inferencing given to children at age 9 and verbal and non-verbal analogy given at age 11. When children were 10, their parents completed IQ measures, which we use as a covariate. These measures will be discussed below in Section 2.6 , Standardized Measures.
2.2.1. Transcription of Speech in Spontaneous Interactions
All spontaneous speech by children was transcribed, including all dictionary words, onomatopoeic sounds (e.g., woof-woof), and evaluative sounds (e.g., uh-oh). Ritualized or memorized speech, such as song (e.g., singing the ABC’s) and prayer (e.g., reciting the Lord’s Prayer), was not transcribed. Although a small number of utterances consisting of verbatim reading from books was initially transcribed ( n = 375), these utterances were removed from analyses to more accurately capture children’s use of spontaneous language. Transcribed speech was divided into utterances, defined as any sequence of words preceded and followed by a pause, a change in intonational pattern, or a change in conversational turn ( Rowe & Goldin-Meadow, 2009 ; Rowe, 2012 ). A total of 368,509 child utterances were analyzed, distributed across the 726 visit transcripts.
One out of every three transcripts was checked for transcription agreement; agreement was calculated at the utterance level and the word level, and transcribers had to be at least 90% in agreement for both measures. Ten minutes of each video (randomly selected from the whole video) were transcribed by a second coder. If the first 10 minutes was not at least 90% in agreement, a second 10 minutes were transcribed by the second coder. If the transcript was still not at least 90% in agreement, the transcript was sent back to the coder to be re-transcribed. After re-transcription, another 10 minutes would be transcribed by the second coder. This process continued until all reliability transcripts were at least 90% in agreement for both words and utterance boundaries.
2.3. Coding Higher-Order Thinking Talk in Spontaneous Interactions
Each parent and child utterance was coded for the presence of HOTT, although this paper only examines child utterances. HOTT is talk that indexes two or more representations and constructs a bridge or link between them. HOTT was coded when the child’s utterance contained both representations and the link between them; for example, “They’re laughing because he fell down.” In this example, the representations indexed are two events—people laughing and a person falling down—and the link between them, the word ‘because,’ implies causality. HOTT was also coded when the child responded to a HOTT-eliciting question; for example, a parent asks, “Why were they laughing?” and the child replies, “Because he fell down,” where the question provides one representation and the response provides the second representation. HOTT was also coded when the child asked the HOTT-eliciting question; for example, if the child asks, “Why were they laughing?”
Reliability analyses were performed for parent and child speech combined due to the interdependent nature of the coding, which relied on surrounding talk. Ninety-seven transcripts (approximately 8 from each time point), constituting 13.3% of the transcripts, were coded by two or more people. The mean interrater percent agreement for identification of utterances as HOTT or not was 98.1% (range: 96.0–99.3%).
We also computed Cohen’s kappa ( Cohen, 1968 ), which assesses the reliability of assigning observations to mutually exclusive categories while correcting for chance agreement. It has values ranging from −1 to 1, though Cohen (1968) notes that values less than 0 are unlikely in practice, so it generally ranges from 0 to 1; values 0.40–0.59 are regarded as moderate, values 0.60–0.79 are regarded as substantial, and values over 0.80 are regarded as almost perfect ( McHugh, 2012 ). The mean Cohen’s kappa for identification of utterances as HOTT or not was 0.81 (range 0.73–0.87). Disagreements were resolved through discussion or by the more experienced coders.
2.3.1. Coding the Four Types of HOTT
For this study, four types of links or relationships between representations were included in HOTT: inference, comparison, abstraction, and hierarchy. Although many types of relations could be considered ‘higher-order,’ these four related skills are particularly useful for educational application ( Anderson, Greeno, Reder, & Simon, 2000 ; Dumas, Alexander, & Grossnickle, 2013 ; Halford, Wilson, & Phillips, 2010 ; Speed, 2010 ). The four types of HOTT that we coded are defined and illustrated below. Due to the relative infrequency of HOTT within spontaneous discourse, we analyze the types of HOTT as a combined set.
We calculated reliability for each HOTT type independently. Mean interrater percent agreement was 99.3% (range 99.1–99.7%; M kappa = 0.86; range kappa 0.79–0.92) for inferences; 99.4% (range 99.0–99.9%; M kappa = 0.71; range kappa 0.58–0.82) for comparisons; 99.8% (range 99.4–99.9%; M kappa = 0.62, range kappa 0.41–0.81) for abstractions; and 99.9% (range 99.8–100%; M kappa = 0.72; range kappa 0.40–1.0) for hierarchies.
Inference is defined as deriving a conclusion not otherwise given by using known (or logical) premises. The bridge between representations in inferences is cause-and-effect, a conditional, or speculation based on reasoning. For example, one 54-month-old child said, “If I didn’t have teeth, then I couldn’t eat candy.” This utterance arose as part of a conversation about the child’s favorite part of her body. In this example, the child uses conditional reasoning to infer, in a situation where she had no teeth [representation 1], what might happen—she would be unable to eat candy [representation 2].
Comparison:
Comparison is defined as demonstrating similarities or differences between entities by analogy or by example. The bridge between representations in comparisons is of similarity or difference. For example, the utterance, “A tornado is a like a mean monster” indexes the representations of ‘tornado’ and ‘monster’ and links them through the word “like.” An utterance such as, “The blue stick is longer than the red stick” also illustrates a comparison between two representations—the blue stick and the red stick—based on a featural difference (in this example, length).
Abstraction:
Abstraction is defined as pointing out mental frameworks or models that could facilitate thinking, or making definitions that attempt to describe the meaning of a word or concept, beyond giving a label. In abstractions, representations are bridged through generalizations or definitions. One sample abstraction utterance is, “Every Halloween you can be something new.” Here the two representations, ‘Halloween’ and ‘what you can be,’ are linked through the use of the term “every,” invoking a generalization about Halloween. Abstractions can also take the form of generalizing or generic statements that ascribe defining characteristics to a concept, such as “Big kids carry their own plates.” In this example, carrying one’s own plates is defined as a quality of big kids. Abstractions could also take the form of word definitions, as in the utterance, “‘Spa’ means there’s a new place that has a bath.”
Finally, hierarchy is defined as an arrangement of categories with a superordinate or subordinate framework. An utterance such as, “A hammer is a type of tool,” demonstrates a hierarchical relationship by indexing the representations of ‘hammer’ and ‘tool,’ which are linked through the use of the word ‘type,’ stating that hammers belong to a broader category of tools. More rarely, families used more abstract classifications to denote hierarchies, as in the utterance, “Killer whales are in the dolphin family.”
2.3.2. Coding Surface and Structure HOTT
In addition to coding the type of higher-order relationship, each HOTT utterance was coded for conceptual complexity. Surface HOTT is defined as a single-level mapping where the relationship between the referents is not complex, and not dependent on a deep understanding of the referents indexed; the types of correspondences identified were often easy to perceive and more immediate ( Richland & Simms, 2015 ). For example, a surface inference is, “You knocked it so it fell down,” and a surface comparison is, “Those are both red.” In contrast, structure HOTT is defined as more complex mapping at a systemic level, and requires a deeper understanding of the ideas being linked. The types of correspondences identified are often relatively abstract ( Richland & Simms, 2015 ). For example, a structure inference is, “She’s sad because she misses her momma,” and a structure comparison is, “I want to be brave like Piglet.” Appendix A contains additional criteria and further examples of surface and structure HOTT for each of the four types of HOTT.
Utterances that contain multiple types of HOTT were automatically coded as structure. For example, one child describes a drink she was having at a restaurant by saying, “It was just like Coke because it was spicy.” She illustrates the similarity between the unknown drink and Coke using the word ‘like,’ and justifies their similarity using the word ‘because.’ Multiple types were found in only 3% of children’s HOTT utterances; the remaining 97% of HOTT utterances contained only one type of relationship.
Two coders coded the surface/structure distinction on all HOTT utterances after achieving a mean 98.3% agreement ( κmean = 0.84) on 126 transcripts (which comprised 17% of the 726 transcripts in the corpus). Appendix B reports the frequency of each HOTT type across development. As noted above, for the remainder of this paper, we collapse across HOTT types, and instead focus our analyses on the two levels of HOTT complexity (surface vs. structure).
2.4. Coding Language Complexity in Spontaneous Interactions
2.4.1. utterance length.
The number of words in each utterance was coded to calculate mean length of utterance (in words rather than morphemes; MLU-w) for each child at each session ( Demir, Rowe, Heller, Goldin-Meadow, & Levine, 2015 ). We calculate the average MLU-w for surface HOTT, structure HOTT, and non-HOTT utterances at each timepoint in the corpus.
2.4.2. Syntactic Complexity
Each word of each utterance was also tagged for part of speech using CLAN tools ( MacWhinney, 2000 ). The number of verbs in each utterance was used as a measure of syntactic complexity, because it correlated strongly ( r = .88, p < 0.001) with number of clauses per utterance that were coded manually on a subset of the data. Any utterance containing two or more verbs was classified as a complex utterance.
2.5. Early Child Word and Gesture Types in Spontaneous Interactions as Covariates
2.5.1. child word types at 14 months.
We used the number of different word types children produced at 14 months to control for early vocabulary and linguistic skill. Child word types at 14 months ranged from 0 to 59 ( M = 14.0, SD = 14.5).
2.5.2. Child Gesture Types at 14 Months
The spontaneous communicative gestures that children produce can serve as an early index of variation in subsequent linguistic skill ( Iverson, Capirci, Volterra, & Goldin-Meadow, 2008 ; Rowe & Goldin-Meadow, 2009 ). Therefore, in addition to speech transcription, gestures were isolated from parents’ and children’s motor behavior, and lexical meanings were attributed to the gestures using coding criteria reported in previous studies ( Goldin-Meadow & Mylander, 1984 ). We use the number of gesture types that children produced at 14 months as another indicator of early linguistic skill. Gesture types was defined as the number of different meanings conveyed by gesture. We counted each conventional and representational gesture used by children associated with a different meaning (e.g., shake head no to convey no ; arms flapping to convey flying ), as well as each deictic gesture that indicated a different object as a distinct gesture type. Child gesture types at 14 months ranged from 4 to 54 ( M = 21.7, SD = 12.5).
2.6. Standardized Measures
2.6.1. child higher-order thinking outcome measures.
At ages 9 and 11, participants were administered four standardized outcome measures of higher-order thinking in their homes, including text-based inferencing ability and analogical reasoning. Ten children dropped out of the study in the early period and thus were not given any of the outcome measures. Out of the 54 participants who took the outcome measures, 48 had all four measures; two children were each missing a single measure (one from age 9 and one from age 11), and four were missing both measures at age 11. The four measures are described below.
Diagnostic Assessment of Reading Comprehension:
To assess inferential ability, participants took the Diagnostic Assessment of Reading Comprehension (DARC; August, Francis, & Calderón, 2002 ) at age 9. The DARC has 164 multiple choice items designed to test reading comprehension components, including text memory, text inferencing, knowledge access, knowledge integration, and inference, while controlling for background knowledge. Fifty-three children completed this measure. The mean was 33.9 questions correct ( SD = 7.1, range 14 to 45) on the 45 questions that tested knowledge integration and text inferencing (components of higher-order thinking).
Gates-MacGinitie:
To assess inferencing in a school-like task, and to complement the DARC, we administered the Gates-MacGinitie (GM; MacGinitie, MacGinitie, Maria, & Dreyer, 2002 ). This norm-referenced assessment contains vocabulary and reading comprehension sections with both literal and inferential questions, and is widely used in school settings. We administered the measure to the participants in both the fall and spring at ages 8 and 9. For this paper, the age 9 fall and spring scores were analyzed (averaged together, after finding that these two outcome measures were highly correlated; r = 0.80, p < 0.001) in order to complement the DARC, also administered at age 9. Fifty participants completed this outcome measure both times, and four children completed either the fall or spring outcome measure (3 were missing the fall score, and 1 was missing the spring score). The mean score was 512.1 ( SD = 39.7; range 425.5 to 590.5) for the 54 children.
Ravens Progressive Matrices:
The Ravens Progressive Matrices (Ravens; Raven, Raven, & Court, 2004 ), a nonverbal measure of relational reasoning, was administered at age 11. The 60 items on this measure have complex black-and-white patterns that participants must use to determine the missing item that completes the visual pattern. The mean score on the Raven’s was 44.5 ( SD = 7.0, range 28 to 58) for the 49 children who completed the measure.
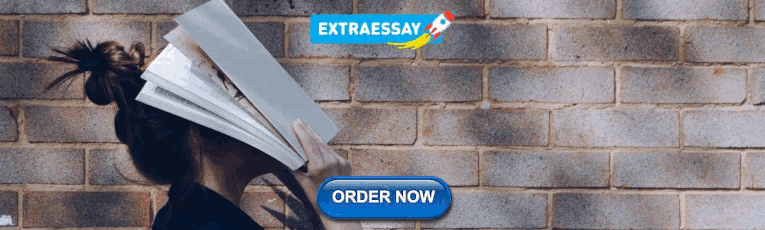
Woodcock-Johnson III Verbal Analogies:
Participants took the Woodcock-Johnson III Verbal Analogies (WJ-VA; Woodcock, McGrew, & Mather, 2001 ) subtest at age 11. Analogical reasoning in this measure involves both vocabulary knowledge and relational reasoning in 13 items. The mean performance on the WJ-VA was 9.3 ( SD = 1.9, range 5 to 13) for the 50 children who completed the age 11 visit.
2.6.2. Parent IQ Measures Used as Covariates
When children were 10 years old, mothers completed the Wechsler Abbreviated Scale of Intelligence, which is linked to the Wechsler Adult Intelligence Scale-III used to determine IQ scores. There are two scales: Verbal (WASI-V) and Perceptual (WASI-P). Of the 64 participants, 14 (22%) were missing these measures.
The verbal comprehension component (WASI-V) contains vocabulary and similarities subtests, with 55 items, yielding t -scores and scaled scores based on age ( Maccow, 2011 ). The WASI-V mean score for the 50 participants who completed it was 112.1 ( SD = 16.6, range 80 to 149).
The perceptual reasoning component contained matrix reasoning and block design subtests with 43 items, yielding t -scores and scaled scores based on age ( Maccow, 2011 ). For the 50 participants who completed the WASI-P, the mean score was 103.7 ( SD =13.4, range 67 to 133).
2.7. Analytic Approach
We use several statistical analyses, all of which used the utterance as the elementary unit of analysis, to address our research questions. This is an exploratory study (as this is the first paper on this specific phenomenon using a naturalistic sample), so any statistical associations should be taken as suggestive rather than definitive. For some descriptive analyses, we transformed raw numbers of utterances to number of HOTT utterances per hour to capture variations in session length when transcripts were not exactly 90 minutes. On average, sessions were 88.6 minutes long ( SD = 4.8 minutes, range 44 to 97 minutes). In the models, we used session length (in hours) as a time variable to account for differences in session length. We use HOTT and age as within-subjects factors, and child-specific factors (such as family income, first-born status, and early child word and gesture types) as between-subjects factors in our hierarchical linear growth models to describe early HOTT development in children.
3. Results and Discussion
In order to ensure reader clarity, and due to the complex nature of these longitudinal data and analyses, we have integrated some discussion of the results with the presentation of the data themselves. We report analyses to address each of our four research questions in turn.
3.1. Onset and Growth of HOTT Between 14 and 58 Months
We first report the frequency of HOTT and non-HOTT utterances produced each hour across early childhood. As a baseline, Figure 1a presents the mean number of child utterances produced per hour that do not contain HOTT from 14 to 58 months. Children greatly increase the quantity of speech they produce, but tend to level out at around 400–450 utterances per hour at 34 months (the slight reduction in quantity of utterances between 38 and 58 months may reflect less direct interaction between children and others in the home as they age). Figure 1b presents the mean number of HOTT utterances produced per hour between 14 and 58 months (note that the scale is different from the scale in Figure 1a ). Children increase their HOTT during this period, but generally level off at 46 months, when they are producing approximately 20 HOTT utterances per hour.

Mean number of (a) non-HOTT utterances and (b) HOTT utterances produced during the spontaneous interactions from 14 to 58 months. Dashed line in both graphs represents the mean age of HOTT onset. Error bars represent ±2 standard errors.
Age of onset of HOTT was coded as the age at which a child produced at least one HOTT utterance in two consecutive sessions (e.g., a child who produced no HOTT utterances at 14- and 18-months, but did produce HOTT utterances at 22- and 26-months, was given a HOTT onset of 22 months). Using this criterion, the mean age of HOTT onset was 27.0 months ( SD = 6.7 months, range 14 to 42 months) 1 , indicated with a dashed line on both graphs. Given the 4-month gap between sessions we cannot be more precise, but HOTT appears to emerge in spontaneous speech at approximately 23–27 months, which is in line with prior literature indicating that children begin showing evidence of relational reasoning in language-based tasks around the second year of life (e.g. Loewenstein & Gentner, 2005 ; Christie & Gentner, 2014 ). Note that the onset of HOTT occurs several months after the steep increase in non-HOTT utterances, suggesting that the ability to produce HOTT calls upon cognitive skills that go beyond language production.
Figure 2 presents the mean number of surface and structure HOTT utterances produced during the 14–58 month period 1. All children produced surface HOTT utterances, and the onset of surface HOTT was almost identical to overall HOTT onset ( M = 27.7, SD = 6.8, range = 14 to 42 months; indicated with the dark gray dashed line in Figure 2 ), simply because the majority of HOTT utterances early in development were surface. In contrast, only 54 of the 60 relevant children 2 used structure HOTT two sessions in a row and thus achieved the criterion for onset. 3 For these children, the mean age of structure HOTT onset was 34.7 months ( SD = 7.8, range 14 to 54 months; indicated with the light gray dashed line in Figure 2 ).

Mean number of surface and structure HOTT utterances produced during the spontaneous interactions from 14 to 58 months. Dashed lines represent the mean onset age of surface HOTT (in dark gray) and structure HOTT (in light gray). Error bars represent ±2 standard errors.
Onset of structure HOTT was significantly later than onset of surface HOTT ( t (53) = −7.1, p < 0.001), but the two onsets were correlated ( r = 0.50, p < 0.001). At an individual level, 43 of the 54 children with measurable surface and structure HOTT onsets (80%) began producing surface HOTT before structure HOTT. Eight children (15%) had surface and structure HOTT onset within the same session. Only three children (5%) had structure HOTT onset prior to surface HOTT onset; these children produced only a small number of structure HOTT (fewer than three utterances per session).
3.2. Using Child-Specific Factors to Predict the Onset and Trajectory of HOTT
3.2.1. predicting hott onset.
We next examined relationships between the onset of surface and structure HOTT and child-specific factors, including parent characteristics (family income, education, and WASI-V and WASI-P scores), child characteristics (gender and first-born status), and early child language covariates (word and gesture types at 14 months). Table 1 presents the data. Using Pearson’s correlations, we found a significant relation between family income and HOTT onset—children from higher-income families began using both levels of HOTT earlier than children from lower-income families. Note, however, that early child language use—both word types and gesture types—relates to surface HOTT onset, but not structure HOTT onset, which is particularly interesting given that the two HOTT onsets strongly correlate.
Pearson’s correlations between child-specific factors and age of onset of children’s surface and structure HOTT.
Using a series of simple two-sample t -tests, we also investigated whether there were differences in surface or structure HOTT onset between boys and girls, and between first-born and later-born children. We found no significant differences (0.19 < p < 0.78), suggesting that boys and girls, and first- and later-born children, do not differ in the age at which they begin using surface or structure HOTT.
3.2.2. Predicting HOTT Trajectories from 14 to 58 Months
We next examine relationships between the trajectory (i.e., intercept, slope, and acceleration) of surface and structure HOTT and child-specific factors (e.g., family income, first-born status, early child word and gesture types). This analysis allowed us to move beyond simply onset or overall quantity of use, but rather rates of growth and acceleration. We used a two-level, hierarchical linear model (HLM) with number of child HOTT utterances at a given age as a Poisson outcome (appropriate when examining counts of rare events whose average rate is small and always positive, and where the distribution is positively skewed) and session length in hours as a time variable. In longitudinal HLM models, time points (level 1) are nested within individuals (level 2); the level 1 model accounts for variation over time within each child (i.e., within-subjects), and the level 2 model represents variation between children (i.e., between-subjects) ( Raudenbush & Bryk, 2002 , chapter 10). At level 1, we include age in months centered at 36 months, the middle of the 14–58 month period. A quadratic term for age was also used to examine differences among individuals in rates of change. A cubic age term was tested but did not improve the model, and thus is not included in the model that follows:
In this model, the outcome ( Y ti ) at level 1 is the number of child HOTT utterances for child i at time t . The λ ti term represents the latent event rate per hour and the l ti term represents the session length in hours, for child i at time t . The η ti term is the link function, and represents the natural logarithm of child’s rate of HOTT utterances. Finally, the a ti term represents the child’s age in months, which has been centered at 36 months. Because we centered age at the middle of the study period, the intercept ( π 0 ), or status, represents average use at 36 months, as well as average use over the entire study period, and the growth term ( π 1 ) represents both the velocity at 36 months, as well as the average velocity over the entire study period (the acceleration term, π 2 , is not impacted by centering). Centering at the middle of the study period enables the intercept and growth to have conceptual significance, and also represents approximately the mean onset of structure HOTT (34.5 months). At level 2, the intercept ( π 0 ), growth ( π 1 ), and acceleration ( π 2 ) terms are predicted by each p in n different child-specific factors ( C i ). The three trajectory parameters vary by the individual i , as each parameter has an r i random effect term.
Figure 3 presents plots of empirical trajectories (left graphs) for surface and structure HOTT, along with unconditional (i.e., with no child-specific factors) predicted trajectories after exponentiating log transformations (right graphs), demonstrating great variability in children’s use, growth, and rate of change of growth of HOTT. The steep drop-off at the end of the study period in the predicted graphs reflects a decline in children’s total number of utterances (and HOTT utterances) at the end of the study period (see Figure 1 above).

Plots of empirical (a,c) and predicted (b,d) trajectories from quadratic model (after exponentiating log transformations) for surface (a,b) and structure (c,d) HOTT. Thick black lines in left (empirical) graphs represent mean usage, and thick black lines in right (predicted) graphs represent the average fitted growth trajectories from the quadratic models.
We modeled surface and structure HOTT trajectories separately. After running the unconditional growth models, we added child-specific factors at level 2 stepwise to the models. The level 2 variables that we tested include family income, parent education, parent verbal and perceptual IQ, child gender, child first-born status, and child word and gesture types at 14-months. We assessed the ability of these variables to explain variation between individuals in intercept, growth, and acceleration of surface and structure HOTT. The estimates of the fixed and random effects are reported in Table 2 (for surface HOTT) and Table 3 (for structure HOTT).
A series of hierarchical linear models predicting child surface HOTT over development. We report fixed effects with robust standard errors. CWT14 = Child Word Types at 14 months.
A series of hierarchical linear models predicting child structure HOTT over development. We report fixed effects with robust standard errors. CGT14 = Child Gesture Types at 14 months.
For surface HOTT, we first entered income to the model to examine whether income-based disparities present in other measures of early child language (e.g., vocabulary development) are likewise present in HOTT development. Early child word types were next added, to examine effects of children’s early linguistic skill. Child status as the first- or only-born child in his or her family was added next, which could reflect differences in individualized input that first- or only-born children, in contrast to later-born children, receive from caregivers. Model comparison tests found that including other characteristics—child gender, child gesture types at 14 months, parent verbal and perceptual IQ, and parent education—did not explain any additional variation in surface HOTT trajectories (all p ’s > 0.50).
The final model for surface HOTT (Model 4 in Table 2 ) shows that income, child word types, and child first-born status all relate to surface HOTT use at 36 months (intercept), and child word types at 14 months relates to both how surface HOTT changes over development (growth), as well as its rate of change (acceleration).
We next modeled structure HOTT ( Table 3 ). As with surface HOTT, adding family income to the model explained variation in children’s structure HOTT use at 36 months. There were no effects of income on structure HOTT growth or acceleration. Because child word types at 14 months predicts surface HOTT, it was added next to the structure model. This model (not shown) marginally improved fit over a model including only income, ( χ 2 (3) = 8.99, p = 0.08), showing that child word types at 14 months also predicts structure HOTT at 36 months, with income remaining significant. Neither income nor child word types predicts growth or acceleration rates of structure HOTT.
Because child word types only marginally improved model fit, we compared a model containing income against another model including income and child gesture types at 14 months (which serves as an earlier index of children’s language abilities than word types). The model containing income and child gesture types at 14 months was a significant improvement over the model containing only income ( χ 2 (3) = 13.06, p = 0.005). As a result, we use child gesture types at 14 months as a proxy for children’s early language abilities to model trajectories of structure HOTT.
Child first-born status was next added to the model, which predicted the intercept (along with income and child gesture types at 14 months). Model comparison tests showed that no other level 2 variables significantly improved model fit (all p ’s > 0.50). Thus, the final model for child structure HOTT (Model 4 in Table 3 ) includes family income, child gesture types at 14, and child first-born status as predictors of the intercept, growth, and acceleration.
Figure 4 depicts model graphs (after exponentiating log transformations), showing predicted trajectories in surface and structure HOTT use over development for different kinds of hypothetical children: those at the 25 th and 75 th percentiles of income, and the 25 th and 75 th percentiles of child word types at 14 months (for surface HOTT) and child gesture types at 14 months (for structure HOTT). These figures show the income-based disparities in child surface and structure HOTT trajectories. Although producing a greater variety of word types at 14 months does not interact with income-based disparities in surface HOTT, producing a greater variety of gesture types at 14 months does interact with income-based disparities in structure HOTT. Lower-income children who are in the upper quartile with respect to gesture types have a structure HOTT trajectory that is comparable to upper-income children who are in the lower quartile with respect to gesture types (see two middle lines in right graph).

Model graphs (after exponentiating log transformations) of (a) surface HOTT and (b) structure HOTT over the early period, as a function of family income and 14-month child word types for surface HOTT, and family income and14-month child gesture types for structure HOTT.
3.3. Relations Between Complex Language and HOTT Development
Our findings thus far indicate significant variation among children in the age at which they begin to produce HOTT (onset) and their change over development (trajectory). Next, we examine two questions concerning the extent to which HOTT use can be disentangled from complex language use. First, we examine whether HOTT utterances differ systematically from non-HOTT utterances in terms of linguistic complexity. Second, we examine whether the onset of complex language precedes the onset of HOTT.
3.3.1. Length and Syntactic Complexity of HOTT and Non-HOTT Utterances
We compared children’s surface and structure HOTT utterances to non-HOTT utterances on two measures of linguistic complexity: mean length of utterance in words and number of verbs per utterance (see Table 4 , columns 1 and 2; see Appendix C for results reported for each child age). These results indicate that HOTT utterances are, on average, linguistically more complex than non-HOTT utterances, and that structure HOTT utterances are, on average, linguistically more complex than surface HOTT utterances. Thus, describing relationships in the world using HOTT is associated with using more complex language, and patterns are stronger when describing more conceptually complex relationships as in structure HOTT.
Mean length of utterance, mean number of verbs per utterance, and proportion complex utterances for non-HOTT, surface HOTT, and structure HOTT utterances produced over 14–58 months.
Given the strong association between HOTT and complex language, a potential concern is that the development of complex language is all we are measuring. To address this concern, we investigated the proportion of HOTT utterances expressed using complex utterances , defined as utterances containing two or more verbs (see Table 4 , column 3; see Appendix C for results by child age). Here, too, we find that a greater proportion of structure HOTT utterances contain two or more verbs than surface HOTT utterances, which, in turn, contain a greater proportion of two or more verbs than non-HOTT utterances. It is important to note that even for structure HOTT, two-thirds of utterances are non-complex (i.e., they contain one or even no verbs), which means that it is both possible, and relatively common, for children to produce HOTT using non-complex utterances. These findings suggest that HOTT does not reduce to being just another measure of language complexity, and that HOTT can be produced without complex language.
3.3.2. Onset of Complex Utterances Relative to Onset of HOTT
Next, we investigated whether the onset of HOTT preceded the onset of complex utterances. We coded children’s age of onset of complex utterances (using the same criterion described earlier for HOTT onset, i.e., use of complex utterances in two consecutive sessions). On average, children began using complex utterances at 25.4 months ( SD = 5.32 months, range 14 to 42 months), which was significantly earlier than the onset of surface HOTT (27.7 months; t (62) = 2.47, p = 0.016). On average, 2.2 months elapsed between children’s complex utterance onset and their surface HOTT onset ( SD = 7.1, range −16 to 16). On an individual level, 30 children (48%) had complex utterance onset before using surface HOTT; 19 (30%) had their complex utterance and surface HOTT onsets during the same session; only 14 (22%) had their complex utterance onset after the onset of surface HOTT. One child who dropped out of the study at 26 months had no surface HOTT onset, but he did have a measurable complex utterance onset at 22 months.
Onset of complex utterances (25.4 months) was also earlier than onset of structure HOTT (34.7 months; t (53) = 9.12, p < 0.001). On average, 9.4 months elapsed between children’s complex utterance onset and their onset of structure HOTT ( SD = 7.6, range −8 to 24). Among the 54 children with a measurable structure HOTT onset, 47 children (87%) had complex utterance onset before structure HOTT onset; 3 children (6%) had complex utterance onset during the same session in which they had structure HOTT onset; and only 4 children (8%) had complex utterance onset after their structure HOTT onset. Taken together, these findings suggest that the onset of HOTT does not reduce to the onset of complex language. The onset of HOTT, particularly structure HOTT, requires additional cognitive and/or language skills above and beyond the ability to construct a complex utterance.
3.3.3. Trajectories of Complex Utterances Relative to Trajectories of HOTT
We next analyzed the developmental trajectories of complex utterances, and compared them to the developmental trajectories of surface and structure HOTT. First, we determined the child-specific factors that relate to development of complex utterances, using the same procedure described in Section 3.2.2 . We found that child word types at 14 months predicts the intercept and growth of complex utterances ( β intercept = 0.018, SE intercept = 0.007; β growth = −0.0008, SE growth = 0.003), and family income predicts the intercept ( β intercept = 0.008, SE intercept = 0.0003); no other child-specific factors predicted the complex utterance trajectories nor improved model fit (all p ’s > 0.50).
Next, we extracted empirical Bayes estimates ( Raudenbush & Bryk, 2002 ; Rowe, Raudenbush, & Goldin-Meadow, 2012 ) for each individual child’s intercept, growth, and acceleration of the three types of speech: complex utterances, surface HOTT (derived from Model 4 in Table 2 ) and structure HOTT (derived from Model 4 in Table 3 ). We performed Pearson’s correlations among the trajectory parameters, which are reported in Table 5 . This table demonstrates that the trajectories for complex utterances positively correlate with the trajectories for both surface and structure HOTT. Children who use more, and grow more quickly in their use of, complex utterances also tend to use more, and grow more quickly in their use of, surface and structure HOTT. These findings underscore the tight relation between language form (complex utterances with two or more verbs) and language content (HOTT). These findings also set the stage for our analyses in section 3.4.2 , where we investigate whether children’s early complex utterance trajectories predict grade-school higher-order thinking outcomes as well as the early HOTT trajectories.
Correlations among empirical Bayes estimates for complex utterances, surface HOTT, and structure HOTT trajectory parameters. Italicized cells highlight correlations among parameters within the same type of speech (e.g., complex utterances). Bolded cells highlight correlations between the same parameter (e.g., intercept) among different types of speech.
3.4. Does HOTT during 14–58 Months Predict Higher-Order Thinking in Grade School?
In this section, we examine the relation between early spontaneous HOTT and later higher-order thinking outcomes, as assessed by standardized measures of text-based inferencing ability at age 9 (DARC and GM), and non-verbal (Ravens) and verbal (WJ-VA) analogical reasoning ability at age 11. We first report correlations among standardized higher-order thinking outcomes (1–4), early child language covariates (5–6), and parent characteristics (7–10) (see Table 6 ). After finding that parent education and income (9–10) correlated strongly with many of these measures, we also performed partial correlations among the remaining measures (1–8) controlling for parent income and education (reported in the lower left of Table 6 ).
Pearson’s correlations among outcomes and child-specific factors. Cells on lower left report partial correlations controlling for parent income and education. DARC = Diagnostic Assessment of Reading Criteria. GM = Gates-MacGinitie. WJ-VA = Woodcock-Johnson Verbal Analogies. Ravens = Ravens Progressive Matrices. WASI-P = Wechsler Adult Intelligence Scale, Perceptual. WASI-V = Wechsler Adult Intelligence Scale, Verbal. Significant values ( p < 0.05) are bolded.
This table shows that the four standardized higher-order thinking outcomes (1–4) are all related, suggesting we are tapping a unified skill area. Children’s word and gesture types at 14 months are both related to the verbal higher-order thinking outcomes (1–3), but not to the nonverbal outcome (i.e., Ravens, 4). Turning to parent and family characteristics (7–10), we see that family income, parent education, and parent IQ correlate with the higher-order thinking outcomes. However, even after controlling for family income and parent education (see lower left portion of Table 6 ), the higher-order thinking outcomes are still generally related to each other, and early word types and gesture types still significantly relate to the higher-order thinking outcomes.
3.4.1. Using HOTT Onset to Predict Higher-Order Thinking Outcomes in Grade School
Next, we explored whether the onset of surface HOTT, structure HOTT, or complex utterances relates to later higher-order thinking assessed in grade school at ages 9 and 11. Table 7 presents the findings, revealing that HOTT onset was a significant predictor of standardized higher-order thinking outcomes, with some variation due to the role of language contributions. Higher performance on the DARC is associated with earlier onset of surface and structure HOTT, as well as complex utterances. The GM is only associated with earlier surface and structure HOTT, and is not predicted by earlier onset of complex utterances. The non-verbal analogical reasoning test, the Ravens, is associated only with earlier surface HOTT onset, while the WJ-VA is weakly associated with an earlier surface HOTT onset, but is most strongly predicted by the complex utterance onset. Together, these findings provide the first set of data showing that early HOTT is related to later standardized higher order thinking outcomes, particularly for outcomes that have greater reliance on language skills.
Pearson’s correlations between grade-school higher-order thinking outcomes and children’s age of onset of surface and structure HOTT.
3.4.2. Using HOTT Trajectories to Predict Higher-Order Thinking Outcomes in Grade School
We next evaluated whether children’s trajectories of surface and/or structure HOTT use across development predict later standardized higher-order thinking outcomes at ages 9 and 11. We used empirical Bayes estimates (as described in section 3.3.3 ), derived from Model 4 in Table 2 (including family income, child word types at 14 months, and child first-born status to predict surface HOTT) and Model 4 in Table 3 (including family income, child gesture types at 14 months, and child first-born status to predict structure HOTT) to estimate each child’s individual intercept (i.e., status), growth, and acceleration of surface and structure HOTT. These trajectory parameters were then used to predict the grade school higher-order thinking outcomes in a series of simple linear regressions.
We follow the procedure outlined in Rowe, Goldin-Meadow, and Raudenbush (2012) , and fit several models containing one or two of the trajectory parameter estimates at a time. Our aim is to determine which aspects, if any, of children’s trajectories of surface and/or structure HOTT use relate to the grade school higher-order thinking outcomes. We did not fit a model including all three parameters because of the collinearity between the intercept, growth, and acceleration ( Rowe, Raudenbush, & Goldin-Meadow, 2012 ; see also Table 5 ). In all models, we include the controls used to derive the original parameters: for surface HOTT, family income, child word types at 14 months, and child first-born status; and for structure HOTT, family income, child gesture types at 14 months, and child first-born status. In Table 8 , we report results for each of the four outcomes in columns (a) for surface HOTT and (b) for structure HOTT, for (1) the control-only model and (2) the ‘best’ model using the HOTT growth trajectory parameters (see Appendix D for additional models that were run).
A series of regression models using (a) surface and (b) structure HOTT parameters (and controls) to predict grade school higher-order thinking as assessed by standardized measures. All coefficients are standardized. Bolded numbers highlight best overall model for each outcome. DARC = Diagnostic Assessment of Reading Criteria. GM = Gates-MacGinitie. WJ-VA = Woodcock-Johnson Verbal Analogies. Ravens = Ravens Progressive Matrices. CWT14 = Child Word Types at 14 months. CGT14 = Child Gesture Types at 14 months.
These models show that the controls associated with surface HOTT (family income, child word types at 14 months, and child first-born status) on their own explain 14–27% of the variation in children’s grade school higher-order thinking outcomes. The controls associated with structure HOTT (family income, child gesture types at 14 months, first-born status) offer slightly more explanatory power, and explain 18–33% of the variation in children’s grade school higher-order thinking outcomes.
For the DARC, the surface HOTT trajectory parameters explain no additional variation in children’s performance, as the model reported in the table illustrates. However, including the structure HOTT trajectory parameters accounts for up to an additional 7.8% of variation, although only in the model containing the intercept and acceleration were any parameters—in this case, the intercept—statistically significant ( p = 0.033). We highlight this model as the ‘best’ overall model for the DARC, which represents a marginal improvement ( p = 0.088) over the control-only model.
For the GM, the pattern of results for surface HOTT and structure HOTT were largely parallel; the best models contained the intercept (either alone, or with growth or acceleration) or both the growth and acceleration. However, the surface HOTT trajectory parameters only explain up to 6.7% additional variation between children beyond the controls (as when including the growth and acceleration), whereas the structure HOTT parameters explained up to 12.9% additional variation beyond the controls (as when including the intercept and acceleration). This latter model, which we highlight as the ‘best’ overall model for the GM, represents a significant improvement ( p = 0.006) over the control-only model.
Interestingly, for the Ravens, the ‘best’ model contains the surface, and not the structure, HOTT trajectory parameters. Including the structure HOTT trajectory parameters only explained up to an additional 2.3% of variation beyond the controls; including the surface HOTT growth ( p = 0.024) and acceleration ( p = 0.087) explains an additional 9.7% of variation between children above and beyond the controls (which represents a marginal improvement over the control-only model, p = 0.077). Trajectories of HOTT that involve less verbal complexity, i.e., surface HOTT, may play a more central role for nonverbal measures of analogical reasoning such as the Ravens.
Finally, for the WJ-VA, the structure HOTT parameters explained up to 12.2% additional variation above and beyond the controls, with each individual parameter offering some explanatory power in different models; the ‘best’ model, as reported in the table, contains both the intercept ( p = 0.026) and growth ( p = 0.028) of structure HOTT, and offered significantly more explanatory power ( p = 0.018) beyond the control-only model. The surface HOTT trajectory parameters, in contrast, only explained up to 5.8% additional variation beyond the controls (as when including the intercept and growth), with no significant effects of the surface HOTT trajectory parameters and no improvement to model fit ( p = 0.185).
These results suggest that the rate at which children use, grow, and change in their use of HOTT, particularly structure HOTT, between 14 and 58 months is associated with both text-based inferencing and analogy performance up to five years later. Early structure HOTT during the preschool years is particularly important in predicting language-based measures of higher-order thinking in grade school; early surface HOTT during these years is better at predicting non-verbal analogical reasoning, at least as it was measured by the Ravens.
3.4.3. Using Complex Utterance Trajectories to Predict Higher-Order Thinking Outcomes in Grade School
Given the tight relation between the use of complex utterances and HOTT, we tested whether the complex utterance trajectory parameters (using empirical Bayes estimates extracted from the model for complex utterance trajectories, described in Section 3.3.3 ) explain additional variation in later higher-order thinking above and beyond the variation accounted for by child-specific factors (family income and child word types at 14 months), using the same procedure outlined above.
The analyses demonstrate that, despite the strong correlations observed between the complex utterance and HOTT trajectory parameters, the complex utterance trajectory parameters do not explain additional variation in later higher-order thinking above and beyond the variation accounted for by the child-specific factors. Furthermore, no complex utterance parameters were significant. For example, the controls—family income ( β standardized = 0.297, p = 0.029) and child word types at 14 months ( β standardized = 0.193, p = 0.151)—explained 12.5% of variation between children’s DARC scores ( F (50,2) = 3.59, p = 0.035). Including the complex utterance growth and acceleration, although producing a significant model ( F (48,2) = 2.57, p = 0.050) and explaining an additional 5.1% of DARC-score variation beyond the controls, did not significantly improve model fit ( p = 0.24). Furthermore, none of the complex utterance trajectory parameters were significant (all p ’s > 0.27); in this model, only family income remained significant ( β standardized = 0.332, p = 0.016).
The model just described represents the largest relative improvement over the control-only models when including the complex utterance trajectory parameters; the findings from the other models are reported in Appendix D . Ultimately, this suggests, that although the ability to produce complex utterances may facilitate children’s expression of HOTT, it is not the linguistic form itself that predicts later higher-order thinking outcomes, it is the higher-order content expressed in that form.
4. General Discussion
4.1. mechanisms underlying the onset and trajectory of hott.
Our study is the first to describe how children use higher-order thinking in spontaneous talk at home during the preschool years. We found that HOTT emerged as a regular feature in children’s speech at around 23–27 months, first as surface HOTT in which relations between representations are immediate and easily perceptible. The onset of structure HOTT, in which the relations between representations are more abstract and less easy to perceive, emerged significantly later, at around 30–34 months. At every age, surface HOTT was more common than structure HOTT, suggesting that identifying and expressing deeper relations between representations is challenging for children during this period.
We also found that children vary in the age at which they first produce HOTT, as well as in the trajectories they follow during the 14–58 month period. As in other aspects of language, family income was related to both the onset of HOTT and its intercept; children from higher-income families began using HOTT earlier and used more HOTT at 36 months, but did not differ from their lower-income peers in HOTT growth and acceleration. In addition to income, child status as the first-born or only child was a significant predictor of the HOTT intercept. Although these data do not pinpoint the mechanism through which first-born and only children use more HOTT at 36 months, the findings are compatible with the hypothesis that first-born and only children are exposed to more adult conversation—and those conversations may be better fit to their level of understanding—than those later-born children experience. There was no effect of other family traits—parental education, verbal IQ, or perceptual IQ—on child HOTT development, nor did boys and girls differ in HOTT onset or trajectories across development.
Child word types produced at 14 months correlated with surface HOTT onset and predicted surface HOTT intercept, growth, and acceleration, and child gesture types at 14 months predicted structure HOTT intercept. These findings highlight the importance of early language and gesture use in the development of higher-level cognitive skills, suggesting a larger repertoire of early vocabulary and gesture may support children’s burgeoning higher-order thinking skills.
Moreover, structure HOTT utterances, and to a lesser extent surface HOTT utterances, were longer and syntactically more complex (i.e., they contained more verbs) than non-HOTT utterances. Thus, HOTT is associated with using more complex language. This may be because using longer utterances makes it easier to index two representations and the link or bridge between them, and also because utterances with multiple verbs allow for multiple propositions to appear within the same utterance, and consequently are an ideal vehicle for HOTT. At the same time, the majority of children’s surface and structure HOTT utterances were expressed using one or even no verbs, providing evidence that, although using complex language may provide speakers with strategies for describing relations between representations in the world, it is not necessary for complex reasoning to occur.
Furthermore, the onset of complex utterances preceded by several months the onset of both surface and structure HOTT. The ability to produce complex utterances may foster a deeper understanding between ideas in talk, but this ability clearly is not sufficient for the onset and use of HOTT, suggesting that other factors above and beyond linguistic skills (such as executive functions, e.g., Richland & Burchinal, 2013 ; Simms, Frausel, & Richland, 2018 ) are involved.
4.2. The Function of Early HOTT with Respect to Later Higher-Order Thinking
Our early measures of HOTT predicted children’s performance on later outcome measures of higher-order thinking as assessed by standardized measures, above and beyond the variation explained by child-specific factors. This finding underscores the relevance of these pre-school talk measures, particularly structure HOTT, for later higher-order reasoning outcomes. HOTT onset was correlated with the four grade school higher-order thinking outcomes, and the trajectories of structure HOTT in the early period predicted the verbal-based higher-order thinking tasks at ages 9 and 11. This finding suggests that it is not just the amount of HOTT produced when children are young, but also how they change across development in their ability to produce HOTT, that may affect their future school success.
Finally, our findings suggest that HOTT development is related to, but not redundant with, complex language proficiency. That is, although the trajectories of surface and structure HOTT over the 14–58 month period strongly correlate with the trajectories of complex utterances, it was the trajectories of structure HOTT (and to a lesser extent surface HOTT), not the trajectories of complex utterances, that predicted performance on standardized measures of higher-order thinking taken in grade school. Thus, although complex linguistic skills may support higher-order thinking talk across the preschool years, it appears that the content of that talk, not its form , is what paves the way for higher-order thinking in grade school.
4.3. Study Limitations
This study has several limitations. Given that this is the first analysis of naturally-occurring and spontaneous higher-order thinking in the talk of young pre-school aged children, the results are intended to be exploratory rather than confirmatory. We set out to describe the development of higher-order thinking and generate hypotheses about developmental relations between higher-order thinking and language development. Thus, the statistical associations described in this paper should be taken as suggestive rather than definitive, particularly given the correlational nature of the data.
Another limitation is that it is not clear whether these findings will generalize to other populations. All of the participants were typically-developing monolingual English speakers; it is therefore not clear whether the same patterns will be found in other populations of children, such as bilingual children or children with specific language impairments.
Finally, the observational nature of this study is based on the assumption that the sample of children’s experiences captured on the videotapes is representative of the children’s cumulative experiences. Although we told the families to do what they would typically do during the time of day of our visits, the presence of the experimenter might have led the families to behave in ways that are not typical. Moreover, the 90-minute taping sessions might have constrained the types of talk that children and their parents produce. Nevertheless, most studies of video data find that videotaped subjects are unable to maintain unusual patterns of behavior for extended periods of time ( Jewitt, 2012 ), and we conducted these 90-minute sessions three times per year for four years, so the tapes are likely to have captured a range of typical experiences within each home context. Regardless, this limitation is important to consider, particularly when interpreting the onset results.
4.4. Theoretical and Practical Implications
In spite of these limitations, our study has theoretical and practical implications. This paper increases our theoretical grasp of the relation between language form (complex utterances) and content (higher-order thinking talk). Our findings suggest that complex language—lengthier utterances and more complex syntax—is neither necessary nor sufficient for higher-order thinking talk to occur. Although complex language provides tools and strategies that support children’s relational representation and reasoning skills, it is not redundant with these skills. The ability to identify and articulate relational representations in the world calls upon additional cognitive skills (e.g., executive functions, content knowledge) beyond complex linguistic skills.
The current research also enhances our understanding of the origins of abstract higher-order and relational reasoning. While the majority of analyses on children’s early language environments focus on individual differences in language abilities and the implications of these differences for later outcomes, our findings explore individual differences in thinking , as expressed through early language, and show that these differences are related to later higher-order thinking skills. Children who regularly engage in the deeper, relational thinking represented by higher-order thinking talk may be at the cutting edge of the development of a ‘relational mindset’ ( Vendetti, Wu, & Holyoak, 2014 ; Simms & Richland, 2019 ), and more attuned to identifying abstract relations in the world.
Our findings also have practical implications, particularly with regard to the finding of relations between early spontaneous HOTT and later inferencing and analogy outcomes in grade school. These correlational findings can guide researchers in developing and testing interventions that support children’s early higher-order thinking talk at home and in school, to test the causal relation between early HOTT and later higher-order thinking. Developing interventions that can effectively support the development of higher-order thinking is of vital concern given the importance of higher-order thinking to academic success, particularly in the 21 st century.
Moreover, the income-related disparities observed with HOTT onset and use suggests intervening on HOTT during the early years may help close income-related achievement gaps. Interventions with low-SES families have been successful in increasing the quantity and quality of the language that families use ( Engle et al., 2011 ; Marulis & Neuman, 2010 ; Roberts & Kaiser, 2011 ). Our findings highlight the importance of focusing not only on the form of the language that parents use, but also on its content . In fact, interventions have successfully heightened parents’ use of decontextualized talk (Leech, Wei, Harring, & Rowe, 2016), where parents are encouraged to discuss ideas and events removed from the present environment. It may be the case that interventions targeting families’ use of HOTT could enhance children’s early reasoning and thinking skills, potentially affecting their later success in school.
4.5. Future Research
This paper presents the first look at child HOTT in spontaneous conversations at home prior to school entry. Our next step will be to examine the linguistic models for HOTT that parents provide to their children. The children in our study were scaffolded and supported by questions, prompts, and statements from their parents (e.g. Crowley, Callanan, Jipson, Galco, Topping, & Shrager, 2001 ). We plan to examine how parents use HOTT in spontaneous speech with their children, and whether parent linguistic input, including parent use of HOTT, is associated with children’s early HOTT and later higher order thinking. If so, we plan to examine the timing of input—whether early parent HOTT input is more important for later child higher-order thinking abilities than later input; whether later input is more important than early input; or whether there are cumulative effects of parent HOTT input.
In addition, there may be certain contexts in which children and their parents are particularly likely to invoke HOTT. For example, personal narrative, talk in which individuals recount stories of personal experience about past or future events (e.g., Rowe, 2012 ; Demir et al., 2015 ), may serve as a rich ‘breeding ground’ for HOTT, particularly inferences and comparisons. Personal narrative talk is structured in story-like forms, and theoretically defined ‘good’ narratives require storytellers to coherently link story elements in a cause-and-effect framework ( Stein & Albro, 1997 ); essentially, to make inferences. Additionally, parents in informal conversational contexts have been shown to enhance their children’s overall comprehension of novel scientific concepts by using analogy or comparisons to link their children’s past experiences to the concepts they are discussing ( Valle & Callanan, 2006 ). Personal narrative talk may encourage the use of higher-order thinking. Future research will examine whether certain types of HOTT are more likely to be invoked in particular speech contexts such as personal narrative.
Finally, the broader study of language development from which this study’s typically-developing (TD) participants come has a parallel sample of children with early brain injuries (BI), who were also observed in spontaneous interactions with their parents from 14- to 58-months. Other work (e.g., Rowe, Levine, Fisher, & Goldin-Meadow, 2009 ; Demir, et al., 2015 ; Özçalışkan, Levine, & Goldin-Meadow, 2013 ) has examined differences and similarities between the BI and TD children in terms of spontaneous language and gesture use and relations to later outcomes. For example, Rowe, et al. (2009) found that the linguistic input children received from their parents played the same role in BI and TD children with respect to vocabulary development, but played a different, and more central, role for BI than TD children with respect to syntactic development. Future work will examine whether TD and BI children differ in their use of HOTT over development, and whether the relations between early HOTT and later higher-order thinking for BI children are similar to, or different from, the relations reported here for TD children.
4.6. Conclusion
In sum, we have demonstrated that spontaneously-produced higher-order thinking in children’s early talk grows over development from 14–58 months, and that it becomes increasingly more conceptually and linguistically complex. Furthermore, early production of HOTT is associated with higher performance on standardized measures of higher-order thinking in grade school. Our findings highlight how studies of children’s language development based on naturalistic data can explore not only differences in language development and outcomes, but also the nature and complexity of the thinking embedded in children’s early talk. Moreover, our findings suggest that intervening to support early talk about and with relations may lead to increases in children’s later relational reasoning and higher-order thinking.
Acknowledgements:
We thank the participating families for sharing their children’s language and reasoning development with us; the research assistants who helped to collect, transcribe, and code the data; and Kristi Schonwald, Jodi Khan, Michael Hochman, Richard Williams, Jason Voigt, and Nick Pentella for administrative and technical assistance.
Funding : The research reported in this publication was supported by the Eunice Kennedy Shriver National Institute of Child Health & Human Development of the National Institutes of Health [Award P01HD040605]; by the Institute of Education Sciences [Award R05A190467]; by a grant from the Spencer Foundation; and by a grant from the Successful Pathways from School to Work initiative of the University of Chicago, funded by the Hymen Milgrom Supporting Organization. The content is solely the responsibility of the authors and does not necessarily represent the official views of the National Institutes of Health, the Institute of Education Sciences, or the Department of Education.
Appendix ACriteria Used to Distinguish Between Surface and Structure HOTT for Each of the Four Types of HOTT
1. inference:.
Surface inferences discuss relationships that are more cut-and-dry, that are more easily perceived, that refer to physical sensations, or that are based on knowledge of concurrent or immediate events (e.g., “You bumped it so it fell down”). Structure inferences describe relationships where the cause-and-effect are separated in time; where the inference contains multiple, complex, or unstated links; where the speaker brings in outside knowledge; or where the speaker uses theory of mind or evidence that is not immediately obvious (e.g., “This little girl is sad because she misses her mom”).
2. Comparison:
Surface comparisons concern basic parallels and differences, and easily perceivable features such as color (e.g., “Those are both red”), while structure comparisons describe deeper underlying characteristics such as function or emotional states (e.g., “I want to be brave like Piglet”), or that compare along multiple dimensions, such as size and shape.
3. Abstraction:
Surface abstractions discuss simple associations, such as a letter with its sound, or an animal with the noise it makes (e.g., “Sheeps go ‘baaa’”), or providing the English translation for a foreign word. Structure abstractions describe associations that are more complex, including describing concepts or defining words (e.g., “Winking is looking with one eye”).
4. Hierarchy:
Surface hierarchies were coded when people create their own categories that could apply in multiple situations (e.g., describing cars as different ‘kinds’ when differentiating them by features such as size or color), or when people are providing descriptions that happen to use hierarchical language (e.g., “I’m going to make a kitty kind of puppet show”). Other utterances with hierarchies were coded as structure, as long as both the superordinate and subordinate category were provided (e.g., “A tiger is a kind of animal”).
Appendix BFrequency of HOTT Types across Development
This section describes describe development trends among the four types of HOTT. Figure B.1 shows the mean number of utterances produced per hour each of the four HOTT types across development. Inferences were by far the most commonly used type of higher-order reasoning, and comparisons were used second most frequently. At the end of the study period at 58 months, children used an average of 13.6 inferences per hour ( SD = 8.5, range 2 to 47), and 4.4 comparisons per hour ( SD = 3.5, range 0 to 16). Abstractions and hierarchies, in contrast, were used very rarely; even at 58 months, children only used, on average, two or fewer abstractions per hour ( M = 1.8, SD = 2.4, range 0 to 12), and one or fewer hierarchies per hour ( M = 0.60, SD = 0.87, range 0 to 3). However, all four types of HOTT became more common as children developed.
Figure B.1.

Mean number of inferences, comparisons, abstractions, and hierarchies produced by children between 14 and 58 months per hour. Error bars represent ±2 standard errors.
In Figure B.2 , we report the proportion of each HOTT utterance type categorized as surface or structure for each child visit age. At 14-months, the only HOTT type used was abstractions, of which an average of 87.5% of children’s utterances were surface. By 18-months, children were using each of the four HOTT types, although most utterances were surface (however, one hierarchy utterance produced by one child was structure-level). Across development, the majority of inference and comparison HOTT utterances were surface, although as children grew, an increasingly greater proportion were categorized as structure. At 58 months, 20% of inferences were structure, and 12% of comparisons were structure. In contrast, most abstractions were structure; more than 50% of abstraction utterances were structure-level starting around 34 months, and by 58 months, about 75% of abstraction utterances were structure. The proportion of hierarchy utterances categorized as surface or structure varied across development, likely due to the small number of hierarchy utterances produced by children.
Figure B.2.

Mean proportion of (a) inference, (b) comparison, (c) abstraction, and (d) hierarchy utterances categorized as surface (gray) or structure (black) for children between 14 and 58 months. Error bars represent ±2 standard errors.
Appendix CComplex Language and HOTT Across Development
This section reports our analyses of the linguistic complexity of children’s HOTT utterances over development. For each child at each session, we calculated their mean length of utterance in words and mean number of verbs in their non-HOTT, surface HOTT, and structure HOTT utterances, which we then averaged across each session. Figures C.1 (mean length of utterance) and C.2 (verbs per utterance) report the mean child value at each visit age.
Our findings suggest that children’s HOTT utterances were indistinguishable from their non-HOTT utterances in terms of length for the first four sessions (14–26 months) (see Figure C.1 ). At 30- and 34-months, non-HOTT utterances were shorter than HOTT utterances, but there was no difference between surface and structure HOTT. Beginning at 38-months, structure HOTT utterances were, on average, longer than surface HOTT utterances, which in turn were longer than non-HOTT, with the gap between the three increasing over time. By session 12 (58 months), structure HOTT was on average 7.97 words long (95% CI [7.2, 8.7]), while surface HOTT was on average 6.6 words long (95% CI [6.1, 7.1]), and non-HOTT was on average 3.5 words long (95% CI [3.36, 3.60]). Estimates for structure HOTT are less precise because there are fewer structure HOTT utterances.
Figure C.1.

Mean utterance length in words for structure HOTT, surface HOTT, and non-HOTT speech over sessions. Error bars represent ±2 standard errors.
Turning to number of verbs per utterance, our measure of syntactic complexity, we see that HOTT utterances are not more syntactically complex than non-HOTT utterances at and before 34 months (see Figure C.2 ). However, from 38 months onwards, surface and structure HOTT utterances begin to grow in complexity, with the gap between the three increasing over development. Structure HOTT utterances reach 1.38 verbs per utterance (95% CI [1.24, 1.52]), on average, by 58 months, compared to an average of 1.08 verbs per utterance (95% CI [0.98, 1.17]) for surface HOTT utterances, and 0.625 verbs per utterance (95% CI [0.60, 0.65]) for non-HOTT utterances. These results suggest that from the age of around 38 months and onward, using HOTT is, on average, regularly associated with using more complex language, and that structure HOTT generally involves more complex language than surface HOTT.
Figure C.2.

Mean number of verbs per utterance for structure HOTT, surface HOTT, and non-HOTT speech over sessions. Error bars represent ±2 standard errors.
Next we report the mean proportion of utterances (non-HOTT, surface HOTT, and structure HOTT) that are complex, or that contain two or more verbs. The results demonstrate that even though HOTT utterances are, on average, more complex than non-HOTT, the majority of HOTT utterances are not complex (i.e., contain one or no verbs) (See Figure C.3 ). This pattern holds for both surface HOTT (27.5% complex at 58 months; 95% CI [23.4, 31.7]) and structure HOTT (38.0% complex at 58 months; 95% CI [30.5, 45.6])). As expected, the percentage of complex forms in HOTT utterances is higher than the baseline rate for non-HOTT utterances (7.6% complex at 58 months; 95% CI [6.9, 8.4]). Nevertheless, across development, it is both possible, and relatively common, to produce HOTT without producing complex language.
Figure C.3.

Mean proportion of utterances that were complex utterances (defined as containing 2 or more verbs), by speech type (Structure HOTT, Surface HOTT, and non-HOTT). Error bars represent ±2 standard errors.
We also examined by individuals the HOTT utterances produced at 54–58 months to assess the proportional complexity of HOTT utterances in the most mature state that we examined. On average, 25.6% of surface HOTT utterances were complex (with a minimum of 0% and a maximum of 82.8%), while 38.4% of structure HOTT utterances were complex (with a minimum of 0% and a maximum of 100%). 4 Even though the proportion of complex utterances was higher than the baseline rate for non-HOTT utterances (7.5% on average, with a minimum of 1.1% and a maximum of 18.2%), the average child was still producing almost two-thirds of their structure HOTT utterances using one or no verbs.
Table C.1 shows examples, all drawn from session 12 (58 months), of each type and level of HOTT expressed using utterances that are complex or non-complex.
Examples of HOTT of each type and level expressed in non-complex utterances (with 0 or 1 verbs, top two rows) and complex utterances (with 2 or more verbs, bottom two rows). N/A = no examples found in corpus.
Appendix DPredicting Grade School Higher-Order Thinking Outcomes Using Surface HOTT, Structure HOTT, and Complex Utterance Trajectory Parameters
A series of regression models using surface HOTT trajectory parameters (and controls) to predict grade school higher-order thinking as assessed by standardized measures. All coefficients are standardized. Bolded cells highlight ‘best’ overall model for Ravens (as described in text). DARC = Diagnostic Assessment of Reading Criteria. GM = Gates-MacGinitie. WJ-VA = Woodcock-Johnson Verbal Analogies. Ravens = Ravens Progressive Matrices. CWT14 = Child Word Types at 14 months. Acc. = Surface HOTT Acceleration.
A series of regression models using structure HOTT trajectory parameters (and controls) to predict grade school higher-order thinking as assessed by standardized measures. All coefficients are standardized. Bolded numbers highlight ‘best’ overall model for DARC, GM, and WJ-VA (as described in text). DARC = Diagnostic Assessment of Reading Criteria. GM = Gates-MacGinitie. WJ-VA = Woodcock-Johnson Verbal Analogies. Ravens = Ravens Progressive Matrices. CGT14 = Child Gesture Types at 14 months. Acc. = Structure HOTT Acceleration.
A series of regression models using complex utterance trajectory parameters (and controls) to predict grade school higher-order thinking as assessed by standardized measures. All coefficients are standardized. Bolded numbers highlight model described in text. DARC = Diagnostic Assessment of Reading Criteria. GM = Gates-MacGinitie. WJ-VA = Woodcock-Johnson Verbal Analogies. Ravens = Ravens Progressive Matrices. CWT14 = Child Word Types at 14 months. Acc. = Complex Utterance Acceleration.
The authors report that they have no conflict of interest to declare.
1 One child is eliminated from the HOTT onset analysis and the surface onset analyses because he dropped out of the study at 26-months, before his age of HOTT onset could be determined.
2 Four children dropped out of the study before structure HOTT onset could be established: one child in addition to the one described in the previous footnote, who both dropped out after the 26-month visit, and two who dropped out after the 34-month visit. Three of these children never produced any structure HOTT at all.
3 Six children with sufficient data nevertheless did not meet our criterion for structure HOTT onset and were coded as ‘no measurable onset.’ Their structure HOTT onset likely occurred outside the study period (14–58 months), a phenomenon known as ‘censoring.’
4 Across the two sessions, there are six occasions where participants’ structure HOTT utterance are 100% complex. However, these participants produced 3 or fewer structure HOTT utterances, suggesting this high value is an artifact of small samples rather than a deterministic relationship. Eliminating these six occasions results in an individual’s average complexity of structure HOTT utterances of 34.7%, ranging from 0% to 85.6%.
- Anderson JR, Greeno JG, Reder LM, & Simon HA (2000). Perspectives on learning, thinking, and activity . Educational Researcher , 29 ( 4 ), 11–13. [ Google Scholar ]
- August D, Francis DJ, & Calderón M (2002). Diagnostic assessment of reading comprehension (DARC) . Washington, D.C.: Center for Applied Linguistics. [ Google Scholar ]
- Bransford JD, Brown RL, & Cocking RR (1999). How people learn: Brain, mind, experience, and school . Washington, D.C.: National Academy Press. [ Google Scholar ]
- Bruner J (1973). The relevance of education . New York, NY: W.W. Norton & Company. [ Google Scholar ]
- Callanan MA, & Oakes LM (1992). Preschoolers’ questions and parents’ explanations: Causal thinking in everyday activity . Cognitive Development , 7 ( 2 ), 213–233. [ Google Scholar ]
- Case R, Griffin S, & Kelly WM (1999). Socioeconomic gradients in mathematical ability and their responsiveness to intervention during early childhood In Keating DP and Hertzman C (Eds.), Developmental health and the wealth of nations: Social, biological, and educational dynamics (pp. 125–152) . New York, NY: Guilford Press. [ Google Scholar ]
- Chen Z, Sanchez RP, & Campbell T (1997). From beyond to within their grasp: The rudiments of analogical problem solving in 10-and 13-month-olds . Developmental Psychology , 33 ( 5 ), 790–801. [ PubMed ] [ Google Scholar ]
- Christie S, & Gentner D (2014). Language helps children succeed on a classic analogy task . Cognitive Science , 38 ( 2 ), 383–397. [ PubMed ] [ Google Scholar ]
- Cohen J (1968). Weighted kappa: Nominal scale agreement provision for scaled disagreement or partial credit . Psychological Bulletin , 70 ( 4 ), 213–220. [ PubMed ] [ Google Scholar ]
- Common Core State Standards Initiative. (2010). Common core state standards for mathematics . Washington, D.C: National Governors Association Center for Best Practices and the Council of Chief State School Officers. [ Google Scholar ]
- Crowley K, Callanan MA, Jipson JL, Galco J, Topping K, & Shrager J (2001). Shared scientific thinking in everyday parent-child activity . Science Education , 85 ( 6 ), 712–732. [ Google Scholar ]
- Demir ÖE, Rowe ML, Heller G, Goldin-Meadow S, & Levine SC (2015). Vocabulary, syntax, and narrative development in typically developing children and children with early unilateral brain injury: Early parental talk about the “there-and-then” matters . Developmental Psychology , 51 ( 2 ), 161–175. [ PMC free article ] [ PubMed ] [ Google Scholar ]
- Dumas D, Alexander PA, & Grossnickle EM (2013). Relational reasoning and its manifestations in the educational context: A systematic review of the literature . Educational Psychology Review , 25 ( 3 ), 391–427. [ Google Scholar ]
- Engle PL, Fernald LCH, Alderman H, Behrman O’Gara C, Yousafzai A, Cabral de Mello M, Hidrobo M, Ulkuer N, Ertem I, Iltus S, & the Global Child Development Steering Group (2011). Strategies for reducing inequalities and improving developmental outcomes for young children in low-income and middle-income countries . The Lancet , 378 ( 9799 ), 1339–1353. [ PubMed ] [ Google Scholar ]
- Feldman H, Goldin-Meadow S, & Gleitman L (1978). Beyond Herodotus: The creation of language by linguistically deprived deaf children In Lock A (Ed), Action, symbol, and gesture: The emergence of language (pp. 351–414) . New York, NY: Academic Press. [ Google Scholar ]
- Ferry AL, Hespos SJ, & Gentner D (2015). Prelinguistic relational concepts: Investigating analogical processing in infants . Child Development , 86 ( 5 ), 1386–1405. [ PubMed ] [ Google Scholar ]
- Gentner D (1988). Metaphor as structure mapping: The relational shift . Child development , 59 ( 1 ), 47–59. [ Google Scholar ]
- Gentner D (2003). Why we’re so smart In Gentner D and Goldin-Meadow S (Eds), Language in mind: Advances in the study of language and thought (pp. 195–235). MIT Press. [ Google Scholar ]
- Gentner D, & Christie S (2008). Relational language supports relational cognition in humans and apes . Behavioral and Brain Sciences , 31 ( 2 ), 136–137. [ Google Scholar ]
- Gentner D, & Goldin-Meadow S (2003). Whither Whorf In Gentner D and Goldin-Meadow S (Eds.), Language in mind: Advances in the study of language and cognition (pp. 3–14). Cambridge, MA: MIT Press. [ Google Scholar ]
- Gentner D, & Markman A (1997). Structure mapping in analogy and similarity . American Psychologist , 52 ( 1 ), 45–56. [ Google Scholar ]
- Gentner D, & Ratterman MJ (1991). Language and the career of similarity In Gelman SA and Byrnes JP (Eds.), Perspectives on language and thought: Interrelations in development (pp. 225–277) . New York: Cambridge University Press. [ Google Scholar ]
- Gick ML, & Holyoak KJ (1983). Schema induction and analogical transfer . Cognitive Psychology , 15 ( 1 ), 1–38. [ Google Scholar ]
- Goldin-Meadow S (2003). The resilience of language: What gesture creation in deaf children can tell us about how all children learn language . New York, NY: Psychology Press. [ Google Scholar ]
- Goldin-Meadow S, Levine SC, Hedges LV, Huttenlocher J, Raudenbush S, & Small S (2014). New evidence about language and cognitive development based on a longitudinal study: Hypotheses for intervention , American Psychologist , 69 ( 6 ), 588–599. [ PMC free article ] [ PubMed ] [ Google Scholar ]
- Goldin-Meadow S, & Mylander C (1984). Gestural communication in deaf children: The effects and noneffects of parental input on early language development . Monographs of the Society for Research in Child Development , 49 (serial no. 207 ). [ PubMed ] [ Google Scholar ]
- Gonzales PA (2001). Pursuing excellence: Comparisons of international eighth-grade mathematics and science achievement from a US perspective, 1995 and 1999: Initial findings from the Third International Mathematics and Science Study-Repeat . DIANE Publishing. [ Google Scholar ]
- Goswami U (1992). Analogical reasoning in children . Erlbaum. [ Google Scholar ]
- Halford GS, Wilson WH, & Phillips S (2010). Relational knowledge: The foundation of higher cognition . Trends in Cognitive Sciences , 14 ( 11 ), 497–505. [ PubMed ] [ Google Scholar ]
- Hart B, & Risley TR (1995). Meaningful differences in the everyday experiences of young children . Baltimore, MD: Paul H. Brookes Publishing. [ Google Scholar ]
- Hiebert J, Gallimore R, Garnier H, Givvin KB, Hollingsworth H, Jacobs J, Chui AM-Y, Wearne D, Smith M, Kersting N, Manaster A, Tseng E, Etterbeek W, Manaster C, Gonzales P, & Stigler JW (2003). Teaching mathematics in seven countries: Results from the TIMSS 1999 Video Study (NCES 2003–013) . Washington, D.C.: U.S. Department of Education, National Center for Education Statistics. [ Google Scholar ]
- Huttenlocher J, Haight W, Bryk A, Seltzer M, & Lyons T (1991). Early vocabulary growth: Relation to language input and gender . Developmental Psychology , 27 ( 2 ), 236–248. [ Google Scholar ]
- Huttenlocher J, Vasilyeva M, Cymerman E, & Levine S (2002). Language input and child syntax . Cognitive Psychology , 45 ( 3 ), 337–374. [ PubMed ] [ Google Scholar ]
- Iverson JM, Capirci O, Volterra V, & Goldin-Meadow S (2008). Learning to talk in a gesture-rich world: Early communication in Italian vs. American children . First Language , 28 ( 2 ), 164–181. [ PMC free article ] [ PubMed ] [ Google Scholar ]
- Jewitt C (2012). An introduction to using video for research . National Centre for Research Methods Working Paper (unpublished) . Retrieved from http://eprints.ncrm.ac.uk/2259/4/NCRM_workingpaper_0312.pdf
- Koenig JA (2015). Assessing 21st Century Skills: Summary of a Workshop . Retrieved August 5, 2019 from https://www.learntechlib.org/p/159080/ [ PubMed ]
- Leech K, Wei R, Harring JR, & Rowe ML (2018). A brief parent-focused intervention to improve preschoolers’ conversational skills and school readiness . Developmental psychology , 54 ( 1 ), 15–28. [ PubMed ] [ Google Scholar ]
- Lewis A, & Smith D (1993). Defining higher order thinking . Theory Into Practice , 32 ( 3 ), 131–137. [ Google Scholar ]
- Loewenstein J, & Gentner D (2005). Relational language and the development of relational mapping . Cognitive Psychology , 50 ( 4 ), 315–353. [ PubMed ] [ Google Scholar ]
- Maccow G (2011). Overview of WASI-II . [PDF Slides] Retrieved from http://images.pearsonclinical.com/images/ca/Webinars/WASI-II-Webinar-Handout-12-8-2011.pdf
- MacGinitie WH, MacGinitie RK, Maria K, & Dreyer LG (2002). Gates MacGinitie Reading Tests - Technical report (4th ed.). Rolling Meadows, IL: Riverside. [ Google Scholar ]
- MacWhinney B (2000). The CHILDES Project: Tools for Analyzing Talk (3rd Edition). Mahwah, NJ: Lawrence Erlbaum Associates. [ Google Scholar ]
- Marulis LM, & Neuman SB (2010). The effects of vocabulary intervention on young children’s word learning: A meta-analysis . Review of Educational Research , 80 ( 3 ), 300–335. [ Google Scholar ]
- Mazzocco MM, Feigenson L, & Halberda J (2011). Preschoolers’ precision of the approximate number system predicts later school mathematics performance . PLoS One , 6 ( 9 ), e23749. [ PMC free article ] [ PubMed ] [ Google Scholar ]
- McHugh ML (2012). Interrater reliability: the kappa statistic . Biochemia Medica , 22 ( 3 ), 276–282. [ PMC free article ] [ PubMed ] [ Google Scholar ]
- Mullis IV, Martin MO, Gonzalez EJ, & Chrostowski SJ (2004). TIMSS 2003 International Mathematics Report: Findings from IEA’s Trends in International Mathematics and Science Study at the Fourth and Eighth Grades . TIMSS & PIRLS International Study Center. [ Google Scholar ]
- National Research Council. (2001). Adding it up: Helping children learn mathematics In Kilpatrick J, Swafford J, and Findell B (Eds.), Mathematics learning study committee, center for education, division of behavioral and social sciences, and education . Washington, D.C.: National Academies Press. [ Google Scholar ]
- National Research Council (2007). Rising above the gathering storm: Energizing and employing America for a brighter economic future . Washington, D.C.: National Academies Press. [ Google Scholar ]
- National Research Council (2012). Education for life and work: Developing transferable knowledge and skills in the 21st century . Washington, D.C.: National Academies Press. [ Google Scholar ]
- National Research Council. (2013). Next Generation Science Standards: For states, by states . Washington, D.C.: National Academies Press. [ PubMed ] [ Google Scholar ]
- Özçalışkan Ş, Goldin-Meadow S, Gentner D, & Mylander C (2009). Does language about similarity play a role in fostering similarity comparison in children? Cognition , 112 ( 2 ), 217–228. [ PMC free article ] [ PubMed ] [ Google Scholar ]
- Özçalışkan Ş, Levine SC, & Goldin-Meadow S (2013). Gesturing with an injured brain: how gesture helps children with early brain injury learn linguistic constructions . Journal of Child Language , 40 ( 1 ), 69–105. [ PMC free article ] [ PubMed ] [ Google Scholar ]
- Penn DC, Holyoak KJ, & Povinelli DJ (2008). Darwin’s mistake: Explaining the discontinuity between human and nonhuman minds . Behavioral and Brain Sciences , 31 ( 2 ), 109–130. [ PubMed ] [ Google Scholar ]
- Ratterman MJ, & Gentner D (1998). More evidence for a relational shift in the development of analogy: Children’s performance on a causal mapping task . Cognitive Development , 13 ( 4 ), 453–478. [ Google Scholar ]
- Raudenbush SW, & Bryk AS (2002). Hierarchical linear models: Applications and data analysis methods . New York, NY: Sage Publications. [ Google Scholar ]
- Raven J, Raven JC, and Court JH (2000, updated 2004). Manual for Raven’s Progressive Matrices and Vocabulary Scales. Section 3: The Standard Progressive Matrices . San Antonio, TX: The Psychological Corporation. [ Google Scholar ]
- Resnick LB (1987). Education and learning to think . Washington, D.C.: National Academy Press. [ Google Scholar ]
- Richland LE, & Burchinal MR (2013). Early executive function predicts reasoning development . Psychological Science , 24 ( 1 ), 87–92. [ PubMed ] [ Google Scholar ]
- Richland LE, Morrison RG, & Holyoak KJ (2006). Children’s development of analogical reasoning: Insights from scene analogy problems . Journal of Experimental Child Psychology , 94 ( 3 ), 249–273. [ PubMed ] [ Google Scholar ]
- Richland LE, & Simms N (2015). Analogy, higher order thinking, and education . Wiley Interdisciplinary Reviews: Cognitive Science , 6 ( 2 ), 177–192. [ PubMed ] [ Google Scholar ]
- Richland LE, Zur O, & Holyoak KJ (2007). Cognitive supports for analogies in the mathematics classroom . Science , 316 ( 5828 ), 1128–1129. [ PubMed ] [ Google Scholar ]
- Roberts MY, & Kaiser AP (2011). The effectiveness of parent-implemented language interventions: A meta-analysis . American Journal of Speech-Language Pathology , 20 ( 3 ), 180–199. [ PubMed ] [ Google Scholar ]
- Rowe ML (2012). A longitudinal investigation of the role of quantity and quality of child-directed speech in vocabulary development . Child Development , 83 ( 5 ), 1762–1774. [ PMC free article ] [ PubMed ] [ Google Scholar ]
- Rowe ML, & Goldin-Meadow S (2009). Differences in early gesture explain SES disparities in child vocabulary size at school entry . Science , 323 ( 5916 ), 951–953. [ PMC free article ] [ PubMed ] [ Google Scholar ]
- Rowe ML, Levine SC, Fisher J, & Goldin-Meadow S (2009). Does linguistic input play the same role in language learning for children with and without early brain injury? Developmental Psychology , 45 ( 1 ), 90–102. [ PMC free article ] [ PubMed ] [ Google Scholar ]
- Rowe ML, Raudenbush SW, & Goldin-Meadow S (2012). The pace of vocabulary growth helps predict later vocabulary skill . Child Development , 83 ( 2 ), 508–525. [ PMC free article ] [ PubMed ] [ Google Scholar ]
- Simms NK, Frausel RR, & Richland LE (2018). Working memory predicts children’s analogical reasoning . Journal of experimental child psychology , 166 , 160–177. [ PubMed ] [ Google Scholar ]
- Simms NK, & Richland LE (2019). Generating relations elicits a relational mindset in children . Cognitive Science , 43 ( 10 ), e12795. [ PubMed ] [ Google Scholar ]
- Stein NL, & Albro ER (1997). Building complexity and coherence: Children’s use of goal-structured knowledge in telling stories In Bamberg M (Ed.), Narrative development: Six approaches (pp. 5–44) . Lawrence Erlbaum Associates. [ Google Scholar ]
- Speed A (2010). Abstract relational categories, graded persistence, and prefrontal cortical representation . Cognitive Neuroscience , 1 ( 2 ), 126–137 [ PubMed ] [ Google Scholar ]
- Stevenson HW, & Newman RS (1986). Long-term prediction of achievement and attitudes in mathematics and reading . Child Development , 57 ( 3 ), 646–659. [ PubMed ] [ Google Scholar ]
- Valle A, & Callanan MA (2006). Similarity comparisons and relational analogies in parent-child conversations about science topics . Merrill-Palmer Quarterly , 52 ( 1 ), 96–124. [ Google Scholar ]
- Vendetti MS, Wu A, & Holyoak KJ (2014). Far-out thinking: Generating solutions to distant analogies promotes relational thinking . Psychological science , 25 ( 4 ), 928–933. [ PubMed ] [ Google Scholar ]
- Walker CM, & Gopnik A (2014). Toddlers infer higher-order relational principles in causal learning . Psychological Science , 25 ( 1 ), 161–169. [ PubMed ] [ Google Scholar ]
- Wang SH, & Baillargeon R (2008). Can infants be “taught” to attend to a new physical variable in an event category? The case of height in covering events . Cognitive Psychology , 56 ( 4 ), 284–326. [ PMC free article ] [ PubMed ] [ Google Scholar ]
- Woodcock RW, McGrew KS, & Mather N (2001). Woodcock–Johnson Tests of Cognitive Abilities (3rd Edition). Itasca, IL: Riverside. [ Google Scholar ]
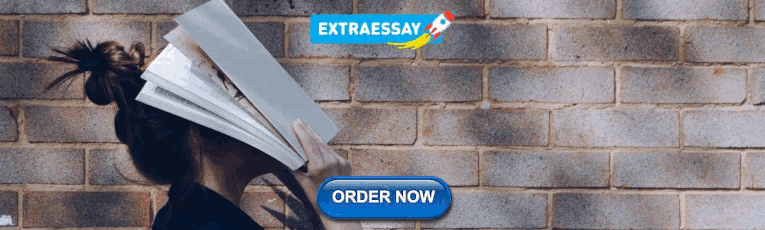
IMAGES
VIDEO
COMMENTS
Higher-order thinking is relational reasoning in which multiple representations are linked together, through inferences, comparisons, abstractions, and hierarchies. We examine the development of higher-order thinking in 64 preschool-aged children, observed from 14 to 58 months in naturalistic situations at home.