- Subject List
- Take a Tour
- For Authors
- Subscriber Services
- Publications
- African American Studies
- African Studies
- American Literature
- Anthropology
- Architecture Planning and Preservation
- Art History
- Atlantic History
- Biblical Studies
- British and Irish Literature
- Childhood Studies
- Chinese Studies
- Cinema and Media Studies
- Communication
- Criminology
- Environmental Science
- Evolutionary Biology
- International Law
- International Relations
- Islamic Studies
- Jewish Studies
- Latin American Studies
- Latino Studies
- Linguistics
- Literary and Critical Theory
- Medieval Studies
- Military History
- Political Science
- Public Health
- Renaissance and Reformation
- Social Work
- Urban Studies
- Victorian Literature
- Browse All Subjects
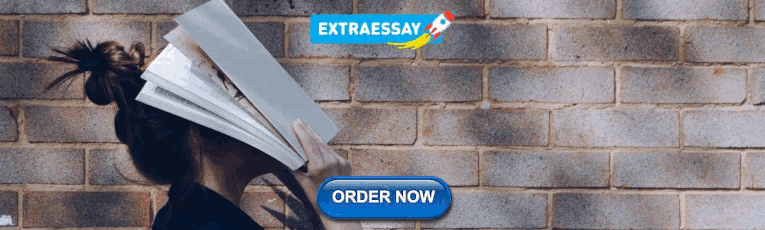
How to Subscribe
- Free Trials
In This Article Expand or collapse the "in this article" section Quantitative Research Designs in Educational Research
Introduction, general overviews.
- Survey Research Designs
- Correlational Designs
- Other Nonexperimental Designs
- Randomized Experimental Designs
- Quasi-Experimental Designs
- Single-Case Designs
- Single-Case Analyses
Related Articles Expand or collapse the "related articles" section about
About related articles close popup.
Lorem Ipsum Sit Dolor Amet
Vestibulum ante ipsum primis in faucibus orci luctus et ultrices posuere cubilia Curae; Aliquam ligula odio, euismod ut aliquam et, vestibulum nec risus. Nulla viverra, arcu et iaculis consequat, justo diam ornare tellus, semper ultrices tellus nunc eu tellus.
- Methodologies for Conducting Education Research
- Mixed Methods Research
- Multivariate Research Methodology
- Qualitative Data Analysis Techniques
- Qualitative, Quantitative, and Mixed Methods Research Sampling Strategies
- Researcher Development and Skills Training within the Context of Postgraduate Programs
- Single-Subject Research Design
- Social Network Analysis
- Statistical Assumptions
Other Subject Areas
Forthcoming articles expand or collapse the "forthcoming articles" section.
- Gender, Power, and Politics in the Academy
- Girls' Education in the Developing World
- Non-Formal & Informal Environmental Education
- Find more forthcoming articles...
- Export Citations
- Share This Facebook LinkedIn Twitter
Quantitative Research Designs in Educational Research by James H. McMillan , Richard S. Mohn , Micol V. Hammack LAST REVIEWED: 29 July 2020 LAST MODIFIED: 24 July 2013 DOI: 10.1093/obo/9780199756810-0113
The field of education has embraced quantitative research designs since early in the 20th century. The foundation for these designs was based primarily in the psychological literature, and psychology and the social sciences more generally continued to have a strong influence on quantitative designs until the assimilation of qualitative designs in the 1970s and 1980s. More recently, a renewed emphasis on quasi-experimental and nonexperimental quantitative designs to infer causal conclusions has resulted in many newer sources specifically targeting these approaches to the field of education. This bibliography begins with a discussion of general introductions to all quantitative designs in the educational literature. The sources in this section tend to be textbooks or well-known sources written many years ago, though still very relevant and helpful. It should be noted that there are many other sources in the social sciences more generally that contain principles of quantitative designs that are applicable to education. This article then classifies quantitative designs primarily as either nonexperimental or experimental but also emphasizes the use of nonexperimental designs for making causal inferences. Among experimental designs the article distinguishes between those that include random assignment of subjects, those that are quasi-experimental (with no random assignment), and those that are single-case (single-subject) designs. Quasi-experimental and nonexperimental designs used for making causal inferences are becoming more popular in education given the practical difficulties and expense in conducting well-controlled experiments, particularly with the use of structural equation modeling (SEM). There have also been recent developments in statistical analyses that allow stronger causal inferences. Historically, quantitative designs have been tied closely to sampling, measurement, and statistics. In this bibliography there are important sources for newer statistical procedures that are needed for particular designs, especially single-case designs, but relatively little attention to sampling or measurement. The literature on quantitative designs in education is not well focused or comprehensively addressed in very many sources, except in general overview textbooks. Those sources that do include the range of designs are introductory in nature; more advanced designs and statistical analyses tend to be found in journal articles and other individual documents, with a couple exceptions. Another new trend in educational research designs is the use of mixed-method designs (both quantitative and qualitative), though this article does not emphasize these designs.
For many years there have been textbooks that present the range of quantitative research designs, both in education and the social sciences more broadly. Indeed, most of the quantitative design research principles are much the same for education, psychology, and other social sciences. These sources provide an introduction to basic designs that are used within the broader context of other educational research methodologies such as qualitative and mixed-method. Examples of these textbooks written specifically for education include Johnson and Christensen 2012 ; Mertens 2010 ; Arthur, et al. 2012 ; and Creswell 2012 . An example of a similar text written for the social sciences, including education that is dedicated only to quantitative research, is Gliner, et al. 2009 . In these texts separate chapters are devoted to different types of quantitative designs. For example, Creswell 2012 contains three quantitative design chapters—experimental, which includes both randomized and quasi-experimental designs; correlational (nonexperimental); and survey (also nonexperimental). Johnson and Christensen 2012 also includes three quantitative design chapters, with greater emphasis on quasi-experimental and single-subject research. Mertens 2010 includes a chapter on causal-comparative designs (nonexperimental). Often survey research is addressed as a distinct type of quantitative research with an emphasis on sampling and measurement (how to design surveys). Green, et al. 2006 also presents introductory chapters on different types of quantitative designs, but each of the chapters has different authors. In this book chapters extend basic designs by examining in greater detail nonexperimental methodologies structured for causal inferences and scaled-up experiments. Two additional sources are noted because they represent the types of publications for the social sciences more broadly that discuss many of the same principles of quantitative design among other types of designs. Bickman and Rog 2009 uses different chapter authors to cover topics such as statistical power for designs, sampling, randomized controlled trials, and quasi-experiments, and educational researchers will find this information helpful in designing their studies. Little 2012 provides a comprehensive coverage of topics related to quantitative methods in the social, behavioral, and education fields.
Arthur, James, Michael Waring, Robert Coe, and Larry V. Hedges, eds. 2012. Research methods & methodologies in education . Thousand Oaks, CA: SAGE.
Readers will find this book more of a handbook than a textbook. Different individuals author each of the chapters, representing quantitative, qualitative, and mixed-method designs. The quantitative chapters are on the treatment of advanced statistical applications, including analysis of variance, regression, and multilevel analysis.
Bickman, Leonard, and Debra J. Rog, eds. 2009. The SAGE handbook of applied social research methods . 2d ed. Thousand Oaks, CA: SAGE.
This handbook includes quantitative design chapters that are written for the social sciences broadly. There are relatively advanced treatments of statistical power, randomized controlled trials, and sampling in quantitative designs, though the coverage of additional topics is not as complete as other sources in this section.
Creswell, John W. 2012. Educational research: Planning, conducting, and evaluating quantitative and qualitative research . 4th ed. Boston: Pearson.
Creswell presents an introduction to all major types of research designs. Three chapters cover quantitative designs—experimental, correlational, and survey research. Both the correlational and survey research chapters focus on nonexperimental designs. Overall the introductions are complete and helpful to those beginning their study of quantitative research designs.
Gliner, Jeffrey A., George A. Morgan, and Nancy L. Leech. 2009. Research methods in applied settings: An integrated approach to design and analysis . 2d ed. New York: Routledge.
This text, unlike others in this section, is devoted solely to quantitative research. As such, all aspects of quantitative designs are covered. There are separate chapters on experimental, nonexperimental, and single-subject designs and on internal validity, sampling, and data-collection techniques for quantitative studies. The content of the book is somewhat more advanced than others listed in this section and is unique in its quantitative focus.
Green, Judith L., Gregory Camilli, and Patricia B. Elmore, eds. 2006. Handbook of complementary methods in education research . Mahwah, NJ: Lawrence Erlbaum.
Green, Camilli, and Elmore edited forty-six chapters that represent many contemporary issues and topics related to quantitative designs. Written by noted researchers, the chapters cover design experiments, quasi-experimentation, randomized experiments, and survey methods. Other chapters include statistical topics that have relevance for quantitative designs.
Johnson, Burke, and Larry B. Christensen. 2012. Educational research: Quantitative, qualitative, and mixed approaches . 4th ed. Thousand Oaks, CA: SAGE.
This comprehensive textbook of educational research methods includes extensive coverage of qualitative and mixed-method designs along with quantitative designs. Three of twenty chapters focus on quantitative designs (experimental, quasi-experimental, and single-case) and nonexperimental, including longitudinal and retrospective, designs. The level of material is relatively high, and there are introductory chapters on sampling and quantitative analyses.
Little, Todd D., ed. 2012. The Oxford handbook of quantitative methods . Vol. 1, Foundations . New York: Oxford Univ. Press.
This handbook is a relatively advanced treatment of quantitative design and statistical analyses. Multiple authors are used to address strengths and weaknesses of many different issues and methods, including advanced statistical tools.
Mertens, Donna M. 2010. Research and evaluation in education and psychology: Integrating diversity with quantitative, qualitative, and mixed methods . 3d ed. Thousand Oaks, CA: SAGE.
This textbook is an introduction to all types of educational designs and includes four chapters devoted to quantitative research—experimental and quasi-experimental, causal comparative and correlational, survey, and single-case research. The author’s treatment of some topics is somewhat more advanced than texts such as Creswell 2012 , with extensive attention to threats to internal validity for some of the designs.
back to top
Users without a subscription are not able to see the full content on this page. Please subscribe or login .
Oxford Bibliographies Online is available by subscription and perpetual access to institutions. For more information or to contact an Oxford Sales Representative click here .
- About Education »
- Meet the Editorial Board »
- Academic Achievement
- Academic Audit for Universities
- Academic Freedom and Tenure in the United States
- Action Research in Education
- Adjuncts in Higher Education in the United States
- Administrator Preparation
- Adolescence
- Advanced Placement and International Baccalaureate Courses
- Advocacy and Activism in Early Childhood
- African American Racial Identity and Learning
- Alaska Native Education
- Alternative Certification Programs for Educators
- Alternative Schools
- American Indian Education
- Animals in Environmental Education
- Art Education
- Artificial Intelligence and Learning
- Assessing School Leader Effectiveness
- Assessment, Behavioral
- Assessment, Educational
- Assessment in Early Childhood Education
- Assistive Technology
- Augmented Reality in Education
- Beginning-Teacher Induction
- Bilingual Education and Bilingualism
- Black Undergraduate Women: Critical Race and Gender Perspe...
- Blended Learning
- Case Study in Education Research
- Changing Professional and Academic Identities
- Character Education
- Children’s and Young Adult Literature
- Children's Beliefs about Intelligence
- Children's Rights in Early Childhood Education
- Citizenship Education
- Civic and Social Engagement of Higher Education
- Classroom Learning Environments: Assessing and Investigati...
- Classroom Management
- Coherent Instructional Systems at the School and School Sy...
- College Admissions in the United States
- College Athletics in the United States
- Community Relations
- Comparative Education
- Computer-Assisted Language Learning
- Computer-Based Testing
- Conceptualizing, Measuring, and Evaluating Improvement Net...
- Continuous Improvement and "High Leverage" Educational Pro...
- Counseling in Schools
- Critical Approaches to Gender in Higher Education
- Critical Perspectives on Educational Innovation and Improv...
- Critical Race Theory
- Crossborder and Transnational Higher Education
- Cross-National Research on Continuous Improvement
- Cross-Sector Research on Continuous Learning and Improveme...
- Cultural Diversity in Early Childhood Education
- Culturally Responsive Leadership
- Culturally Responsive Pedagogies
- Culturally Responsive Teacher Education in the United Stat...
- Curriculum Design
- Data Collection in Educational Research
- Data-driven Decision Making in the United States
- Deaf Education
- Desegregation and Integration
- Design Thinking and the Learning Sciences: Theoretical, Pr...
- Development, Moral
- Dialogic Pedagogy
- Digital Age Teacher, The
- Digital Citizenship
- Digital Divides
- Disabilities
- Distance Learning
- Distributed Leadership
- Doctoral Education and Training
- Early Childhood Education and Care (ECEC) in Denmark
- Early Childhood Education and Development in Mexico
- Early Childhood Education in Aotearoa New Zealand
- Early Childhood Education in Australia
- Early Childhood Education in China
- Early Childhood Education in Europe
- Early Childhood Education in Sub-Saharan Africa
- Early Childhood Education in Sweden
- Early Childhood Education Pedagogy
- Early Childhood Education Policy
- Early Childhood Education, The Arts in
- Early Childhood Mathematics
- Early Childhood Science
- Early Childhood Teacher Education
- Early Childhood Teachers in Aotearoa New Zealand
- Early Years Professionalism and Professionalization Polici...
- Economics of Education
- Education For Children with Autism
- Education for Sustainable Development
- Education Leadership, Empirical Perspectives in
- Education of Native Hawaiian Students
- Education Reform and School Change
- Educational Statistics for Longitudinal Research
- Educator Partnerships with Parents and Families with a Foc...
- Emotional and Affective Issues in Environmental and Sustai...
- Emotional and Behavioral Disorders
- Environmental and Science Education: Overlaps and Issues
- Environmental Education
- Environmental Education in Brazil
- Epistemic Beliefs
- Equity and Improvement: Engaging Communities in Educationa...
- Equity, Ethnicity, Diversity, and Excellence in Education
- Ethical Research with Young Children
- Ethics and Education
- Ethics of Teaching
- Ethnic Studies
- Evidence-Based Communication Assessment and Intervention
- Family and Community Partnerships in Education
- Family Day Care
- Federal Government Programs and Issues
- Feminization of Labor in Academia
- Finance, Education
- Financial Aid
- Formative Assessment
- Future-Focused Education
- Gender and Achievement
- Gender and Alternative Education
- Gender-Based Violence on University Campuses
- Gifted Education
- Global Mindedness and Global Citizenship Education
- Global University Rankings
- Governance, Education
- Grounded Theory
- Growth of Effective Mental Health Services in Schools in t...
- Higher Education and Globalization
- Higher Education and the Developing World
- Higher Education Faculty Characteristics and Trends in the...
- Higher Education Finance
- Higher Education Governance
- Higher Education Graduate Outcomes and Destinations
- Higher Education in Africa
- Higher Education in China
- Higher Education in Latin America
- Higher Education in the United States, Historical Evolutio...
- Higher Education, International Issues in
- Higher Education Management
- Higher Education Policy
- Higher Education Research
- Higher Education Student Assessment
- High-stakes Testing
- History of Early Childhood Education in the United States
- History of Education in the United States
- History of Technology Integration in Education
- Homeschooling
- Inclusion in Early Childhood: Difference, Disability, and ...
- Inclusive Education
- Indigenous Education in a Global Context
- Indigenous Learning Environments
- Indigenous Students in Higher Education in the United Stat...
- Infant and Toddler Pedagogy
- Inservice Teacher Education
- Integrating Art across the Curriculum
- Intelligence
- Intensive Interventions for Children and Adolescents with ...
- International Perspectives on Academic Freedom
- Intersectionality and Education
- Knowledge Development in Early Childhood
- Leadership Development, Coaching and Feedback for
- Leadership in Early Childhood Education
- Leadership Training with an Emphasis on the United States
- Learning Analytics in Higher Education
- Learning Difficulties
- Learning, Lifelong
- Learning, Multimedia
- Learning Strategies
- Legal Matters and Education Law
- LGBT Youth in Schools
- Linguistic Diversity
- Linguistically Inclusive Pedagogy
- Literacy Development and Language Acquisition
- Literature Reviews
- Mathematics Identity
- Mathematics Instruction and Interventions for Students wit...
- Mathematics Teacher Education
- Measurement for Improvement in Education
- Measurement in Education in the United States
- Meta-Analysis and Research Synthesis in Education
- Methodological Approaches for Impact Evaluation in Educati...
- Mindfulness, Learning, and Education
- Motherscholars
- Multiliteracies in Early Childhood Education
- Multiple Documents Literacy: Theory, Research, and Applica...
- Museums, Education, and Curriculum
- Music Education
- Narrative Research in Education
- Native American Studies
- Note-Taking
- Numeracy Education
- One-to-One Technology in the K-12 Classroom
- Online Education
- Open Education
- Organizing for Continuous Improvement in Education
- Organizing Schools for the Inclusion of Students with Disa...
- Outdoor Play and Learning
- Outdoor Play and Learning in Early Childhood Education
- Pedagogical Leadership
- Pedagogy of Teacher Education, A
- Performance Objectives and Measurement
- Performance-based Research Assessment in Higher Education
- Performance-based Research Funding
- Phenomenology in Educational Research
- Philosophy of Education
- Physical Education
- Podcasts in Education
- Policy Context of United States Educational Innovation and...
- Politics of Education
- Portable Technology Use in Special Education Programs and ...
- Post-humanism and Environmental Education
- Pre-Service Teacher Education
- Problem Solving
- Productivity and Higher Education
- Professional Development
- Professional Learning Communities
- Program Evaluation
- Programs and Services for Students with Emotional or Behav...
- Psychology Learning and Teaching
- Psychometric Issues in the Assessment of English Language ...
- Qualitative, Quantitative, and Mixed Methods Research Samp...
- Qualitative Research Design
- Quantitative Research Designs in Educational Research
- Queering the English Language Arts (ELA) Writing Classroom
- Race and Affirmative Action in Higher Education
- Reading Education
- Refugee and New Immigrant Learners
- Relational and Developmental Trauma and Schools
- Relational Pedagogies in Early Childhood Education
- Reliability in Educational Assessments
- Religion in Elementary and Secondary Education in the Unit...
- Researcher Development and Skills Training within the Cont...
- Research-Practice Partnerships in Education within the Uni...
- Response to Intervention
- Restorative Practices
- Risky Play in Early Childhood Education
- Scale and Sustainability of Education Innovation and Impro...
- Scaling Up Research-based Educational Practices
- School Accreditation
- School Choice
- School Culture
- School District Budgeting and Financial Management in the ...
- School Improvement through Inclusive Education
- School Reform
- Schools, Private and Independent
- School-Wide Positive Behavior Support
- Science Education
- Secondary to Postsecondary Transition Issues
- Self-Regulated Learning
- Self-Study of Teacher Education Practices
- Service-Learning
- Severe Disabilities
- Single Salary Schedule
- Single-sex Education
- Social Context of Education
- Social Justice
- Social Pedagogy
- Social Science and Education Research
- Social Studies Education
- Sociology of Education
- Standards-Based Education
- Student Access, Equity, and Diversity in Higher Education
- Student Assignment Policy
- Student Engagement in Tertiary Education
- Student Learning, Development, Engagement, and Motivation ...
- Student Participation
- Student Voice in Teacher Development
- Sustainability Education in Early Childhood Education
- Sustainability in Early Childhood Education
- Sustainability in Higher Education
- Teacher Beliefs and Epistemologies
- Teacher Collaboration in School Improvement
- Teacher Evaluation and Teacher Effectiveness
- Teacher Preparation
- Teacher Training and Development
- Teacher Unions and Associations
- Teacher-Student Relationships
- Teaching Critical Thinking
- Technologies, Teaching, and Learning in Higher Education
- Technology Education in Early Childhood
- Technology, Educational
- Technology-based Assessment
- The Bologna Process
- The Regulation of Standards in Higher Education
- Theories of Educational Leadership
- Three Conceptions of Literacy: Media, Narrative, and Gamin...
- Tracking and Detracking
- Traditions of Quality Improvement in Education
- Transformative Learning
- Transitions in Early Childhood Education
- Tribally Controlled Colleges and Universities in the Unite...
- Understanding the Psycho-Social Dimensions of Schools and ...
- University Faculty Roles and Responsibilities in the Unite...
- Using Ethnography in Educational Research
- Value of Higher Education for Students and Other Stakehold...
- Virtual Learning Environments
- Vocational and Technical Education
- Wellness and Well-Being in Education
- Women's and Gender Studies
- Young Children and Spirituality
- Young Children's Learning Dispositions
- Young Children's Working Theories
- Privacy Policy
- Cookie Policy
- Legal Notice
- Accessibility
Powered by:
- [66.249.64.20|45.133.227.243]
- 45.133.227.243
- Technical Support
- Find My Rep
You are here
Quantitative Research in Education A Primer
- Wayne K. Hoy - Ohio State University, USA
- Curt M. Adams - University of Oklahoma, USA
- Description
“ The book provides a reference point for beginning educational researchers to grasp the most pertinent elements of designing and conducting research… ”
— Megan Tschannen-Moran, The College of William & Mary
Quantitative Research in Education: A Primer, Second Edition is a brief and practical text designed to allay anxiety about quantitative research. Award-winning authors Wayne K. Hoy and Curt M. Adams first introduce readers to the nature of research and science, and then present the meaning of concepts and research problems as they dispel notions that quantitative research is too difficult, too theoretical, and not practical. Rich with concrete examples and illustrations, the Primer emphasizes conceptual understanding and the practical utility of quantitative methods while teaching strategies and techniques for developing original research hypotheses.
The Second Edition includes suggestions for empirical investigation and features a new section on self-determination theory, examples from the latest research, a concluding chapter illustrating the practical applications of quantitative research, and much more. This accessible Primer is perfect for students and researchers who want a quick understanding of the process of scientific inquiry and who want to learn how to effectively create and test ideas.
See what’s new to this edition by selecting the Features tab on this page. Should you need additional information or have questions regarding the HEOA information provided for this title, including what is new to this edition, please email [email protected] . Please include your name, contact information, and the name of the title for which you would like more information. For information on the HEOA, please go to http://ed.gov/policy/highered/leg/hea08/index.html .
For assistance with your order: Please email us at [email protected] or connect with your SAGE representative.
SAGE 2455 Teller Road Thousand Oaks, CA 91320 www.sagepub.com
“This text will definitely be useful in providing students with a solid orientation to research design particularly in quantitative research”
“Precision, precision, precision! I think this is a must have companion text for graduate students who have to complete a thesis or dissertation. The author does an outstanding job of cataloging and describing difficult research methods terms in a clear and concise way.”
“Greatest strength is the comprehensiveness of the treatment”
“A reference point for beginning educational researchers to grasp the most pertinent elements of designing and conducting research”
Provides all the essential information for quantitative research in a concise book.
A book on research in education but quite well can be accommodated into other social science areas. A great easy to follow publication especially if someone is new to statistical analysis.
There are two strong chapters in this publication that are clearer and more relevant that the sources presently being used by my students. Chapter 3 is particularly well written and clear and builds a progression in terms of understanding statistics. Chapter 4 is also effective however I would probably place this before Chapter 3. In terms of detail there is probably too much in Chapter 4 on Hypothesis whereas Chapter 3 could be developed perhaps by the inclusion of more examples.
Very helpful book that provides a basis for students undertaking education based research.
For those that are interested in doing research that is quantitative in nature, this book is useful, although we tend to advise a more qualitative approach. Therefore I can see myself dipping in and out of this book as it provides some good explanations and there is follow through. I would have welcomed more working examples as this would have concretised everything a lot more.
This is a good supplement to the research methods module, especially for those students who are entering into the field of education. The quantitative methods discussed are also transferrable to other subjects.
NEW TO THIS EDITION:
- A new chapter devoted to the practical applications of education research uses the concepts of collective trust, organizational climate, and improvement science to illustrate the utility of a quantitative approach. It also offers guidelines for analyzing and improving the practice of research in education.
- New hypotheses found in a variety of research studies are available for readers to analyze and diagram.
- A new section on self-determination theory has been added to demonstrate the relation between theory and practice.
- A new section on self-regulatory climate gives readers an opportunity to explore an exciting new area that they are likely to encounter in practice.
- A conceptual description of Hierarchical Linear Modeling (HLM) has been added to help readers understand statistical data organized at more than one level.
KEY FEATURES:
- Education-specific concrete examples bring concepts to life and engage readers with relevant, meaningful illustrations.
- Check Your Understanding exercises and questions assess the reader’s ability to understand, value, and apply the content of the chapter.
- Strat egies and techniques for generating hypotheses help readers understand the process of creating their own hypotheses.
- Key Terms are highlighted in the text when they first appear and then summarized in a list at the end of the chapter to help reinforce key concepts.
- A Glossary concisely and clearly defines all the key terms in the text so readers have immediate access to ideas and concepts needing review.
- Charts throughout the text allow readers to select appropriate statistical techniques for given scenarios.
- The Diagramming Table (in Chapter 4) enables readers to diagram and dissect hypotheses by ensuring the key elements of a hypothesis are considered, analyzed, and understood.
- An Elements of a Proposal section (Appendix A) gives readers directions for developing a quantitative research plan and motivates readers to get started—the most difficult step for many.
- The A Few Writing Tips section (Appendix B) lists a number of salient writing suggestions to help readers avoid common mistakes found in formal writing.
Sample Materials & Chapters
For instructors, select a purchasing option.
Quantitative research in education : Journals
- Computers and education "Computers & Education aims to increase knowledge and understanding of ways in which digital technology can enhance education, through the publication of high-quality research, which extends theory and practice."
- Journal of educational and behavioral statistics "Cosponsored by the American Statistical Association, the Journal of Educational and Behavioral Statistics (JEBS) publishes articles that are original and useful to those applying statistical approaches to problems and issues in educational or behavioral research. Typical papers present new methods of analysis."
- Research in higher education "Research in Higher Education publishes studies that examine issues pertaining to postsecondary education. The journal is open to studies using a wide range of methods, but has particular interest in studies that apply advanced quantitative research methods to issues in postsecondary education or address postsecondary education policy issues."
- << Previous: Recent print books
- Next: Databases >>
- Background information
- Recent e-books
- Recent print books
- Connect to Stanford e-resources
- Last Updated: Jan 23, 2024 12:46 PM
- URL: https://guides.library.stanford.edu/quantitative_research_in_ed
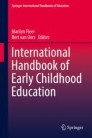
International Handbook of Early Childhood Education pp 295–316 Cite as
Current Approaches in Quantitative Research in Early Childhood Education
- Linda J. Harrison 3 &
- Cen Wang 3
11k Accesses
1 Citations
Part of the book series: Springer International Handbooks of Education ((SIHE))
Research in early childhood education has witnessed an increasing demand for high-quality, large-scale quantitative studies. This chapter discusses the contributions of quantitative research to early childhood education, summarises its defining features and addresses the strengths and limitations of different techniques and approaches. It provides an overview of new directions and state-of-the-art approaches in quantitative research, outlined under four key topic areas: identifying and understanding naturalistic groups (i.e., chi-square analysis, analysis of variance, cluster analysis), identifying mechanisms (i.e., correlation, regression analysis, structural equation modelling), identifying causation (i.e. randomised controlled trial, regression discontinuity) and identifying trajectories and patterns of change in individual learning, development and wellbeing (i.e. latent growth curve modelling, growth mixture modelling). Each section explains the selected research methods and illustrates these with recent examples drawn from early childhood quantitative research conducted in Australia, Canada, Germany, the United States and Chile.
- Postpositivist approaches in early childhood research
- Statistical analysis in early childhood research
- Testing causation in intervention studies
- Trajectories of learning and development
- Quantitative research in early childhood
This is a preview of subscription content, log in via an institution .
Buying options
- Available as PDF
- Read on any device
- Instant download
- Own it forever
- Available as EPUB and PDF
- Compact, lightweight edition
- Dispatched in 3 to 5 business days
- Free shipping worldwide - see info
- Durable hardcover edition
Tax calculation will be finalised at checkout
Purchases are for personal use only
Ahnert, L., Harwardt-Heinecke, E., Kappler, G., Eckstein-Madry, T., & Milatz, A. (2012). Student-teacher relationships and classroom climate in first grade: How do they relate to students’ stress regulation? Attachment and Human Development, 14 , 249–263. doi: 10.1080/14616734.2012.673277 .
Article Google Scholar
Brownell, M. D, Ekuma, O., C. Nickel, Chartier, M., Koseva, I., & Santos, R. G., (2016). A population-based analysis of factors that predict early language and cognitive development. Early Childhood Research Quarterly, 35 , 6–18. doi: 10.1016/j.ecresq.2015.10.0040885-2006
Bryman, A. (2012). Social research methods . New York: Oxford University Press.
Google Scholar
Cole, D. A., & Maxwell, S. E. (2003). Testing meditational models with longitudinal data: Questions and tips in the use of structural equation modeling. Journal of Abnormal Psychology, 112 , 558–577. doi: 10.1037/0021-843X.112.4.558 .
Council of Australian Governments [COAG]. (2009). Investing in the early years – a national early childhood development strategy . Canberra: Commonwealth of Australia.
Feng, X., Shaw, D. S., & Moilanen, K. L. (2011). Parental negative control moderates the shyness-emotion regulation pathway to school-age internalizing symptoms. Journal of Abnormal Child Psychology, 39 , 425–436. doi: 10.1007/s10802-010-9469-z .
Field, A. (2005). Discovering statistics using SPSS (2nd ed.). London: Sage Publications.
Gray, M., & Sanson, A. (2005). Growing up in Australia: The longitudinal study of Australian children. Family Matters, 72 , 4–9 https://aifs.gov.au/sites/default/files/mg(2).pdf .
Janus, M., Harrison, L. J, Goldfeld, S., Guhn, M., & Brinkman, S. (2016). International research utilizing the Early Development Instrument (EDI) as a measure of early child development: Introduction. Early Childhood Research Quarterly, 35 , 1–5. doi: 10.1016/j.ecresq.2015.12.0070885-2006 .
Jung, T., & Wickrama, K. A. S. (2008). An introduction to latent class growth analysis and growth mixture modeling. Social and Personality Psychology Compass, 2 , 302–317. doi: 10.1111/j.1751-9004. 2007.00054.x .
Kline, R. B. (2011). Principles and practice of. structural equation modeling (3rd ed.). New York: Guilford.
Love, J. M., Kisker, E. E., Ross, C., Raikes, H., Constantine, J., Boller, K., … & Vogel, C. (2005). The effectiveness of Early Head Start for 3-year-old children and their parents: Lessons for policy and programs. Developmental Psychology, 41 (6), 885–901.
Mackenzie, N. & Knipe, S. (2006). Research dilemmas: Paradigms, methods and methodologies. Issues in. Educational Research, 16 . Accessed online: http://www.iier.org.au/iier16/mackenzie.html
Magnusson, D., & Bergman, L. R. (1988). Individual and variablebased approaches to longitudinal research on early risk factors. In M. Rutter (Ed.), Studies of psychosocial risk (pp. 45–61). New York: Cambridge University Press.
McLeod, S., Verdon, S., & Kneebone, L. B. (2014). Celebrating young Indigenous Australian children’s speech and language competence. Early Childhood Research Quarterly, 29 , 118–131. doi: 10.1016/j.ecresq. 2013.11.003 .
Meredith, M, & Perkoski, E. (2015). Regression discontinuity design. Emerging trends in the social and behavioral sciences: An interdisciplinary, searchable, and linkable resource. Wiley Online Library. Retrieved from http://onlinelibrary.wiley.com/doi/10.1002/9781118900772.etrds0278/abstract
Mertens, D. M. (2010). Research and evaluation in education and psychology: Integrating diversity with quantitative, qualitative, and mixed. methods (3rd ed.). Los Angeles: Sage.
Murnane, R., & Willett, J. (2010). Methods matter . Oxford: Oxford University Press.
Muthén, B., & Muthén, L. K. (2000). Integrating person-centered and variable-centered analyses: Growth mixture modeling with latent trajectory classes. Alcoholism: Clinical and Experimental Research, 24 (6), 882–891.
NSW Education (2015). NSW Government launches groundbreaking new study. http://exar.nsw.gov.au/nsw-government-launches-groundbreaking-new-study-in-early-education-practices/
Office of Child Care. (2014). A foundation for quality improvement systems: State licensing, preschool, and QRIS program quality standards . Washington, DC: Department of Health and Human Services.
Pianta, R. (2001). Student–teacher relationship scale–short form . Lutz: Psychological Assessment Resources.
Puma, M., Bell, S., Cook, R., Heid, C., Shapiro, G., Broene, P., … & Spier, E. (2010). Head start impact study. Final Report. Washington, DC: Administration for Children & Families, United States Department of Health and Human Services.
Punch, L. (2003). Survey research: The basics . London: Sage Publications.
Book Google Scholar
Silva, P. A., & Stanton, W. R. (Eds.). (1996). From child to adult: The Dunedin multidisciplinary health and development study . Oxford University Press.
Singer, J. D., & Willett, J. B. (2003). Applied longitudinal data analysis. Modeling change and event occurrence . New York: Oxford University Press.
Spilt, J. L., Hughes, J. N., Wu, J.-Y., & Kwok, O.-M. (2012). Dynamics of teacher-student relationships: Stability and change across elementary school and the influence on children’s academic success. Child Development, 83 , 1180–1195. doi: 10.1111/j.1467-8624. 2012.01761.x .
Sylva, K., Melhuish, E., Sammons, P., Siraj-Blatchford, I., & Taggart, B. (Eds.). (2010). Early childhood matters: Evidence from the effective pre-school and primary education project . Routledge.
Verdon, S., McLeod, S., & Winsler, A. (2014). Language maintenance and loss in a population study of young Australian children. Early Childhood Research Quarterly, 29 , 168–181.
Weiland, C., & Yoshikawa, H. (2013). Impacts of a prekindergarten program on children’s mathematics, language, literacy, executive function, and emotional skills. Child Development, 84 (6), 2112–2130.
Wetter, E. K., & El-Sheikh, M. (2012). Trajectories of children’s internalizing symptoms: The role of maternal internalizing symptoms, respiratory sinus arrhythmia and child sex. Journal of Child Psychology and Psychiatry, 53 , 168–177. doi: 10.1111/j.1469-7610. 2011.02470.x .
Williams, K. E., Barrett, M. S., Welch, G. F., Abad, V., & Broughton, M. (2015). Associations between early shared music activities in the home and later child outcomes: Findings from the Longitudinal Study of Australian Children. Early Childhood Research Quarterly, 31 , 113–124.
Yoshikawa, H., Leyva, D., Snow, C. E., Treviño, E., Barata, M., Weiland, C., … & Arbour, M. C. (2015). Experimental impacts of a teacher professional development program in Chile on preschool classroom quality and child outcomes. Developmental Psychology, 51 (3), 309–322.
Download references
Author information
Authors and affiliations.
Research Institute for Professional Practice, Learning and Education, Faculty of Arts and Education, Charles Sturt University, Bathurst, NSW, Australia
Linda J. Harrison & Cen Wang
You can also search for this author in PubMed Google Scholar
Corresponding author
Correspondence to Linda J. Harrison .
Editor information
Editors and affiliations.
Faculty of Education, Monash University, Melbourne, Victoria, Australia
Marilyn Fleer
Department of Theory & Research in Education, VU Amsterdam, Amsterdam, The Netherlands
Bert van Oers
Rights and permissions
Reprints and permissions
Copyright information
© 2018 Springer Science+Business Media B.V.
About this chapter
Cite this chapter.
Harrison, L.J., Wang, C. (2018). Current Approaches in Quantitative Research in Early Childhood Education. In: Fleer, M., van Oers, B. (eds) International Handbook of Early Childhood Education. Springer International Handbooks of Education. Springer, Dordrecht. https://doi.org/10.1007/978-94-024-0927-7_12
Download citation
DOI : https://doi.org/10.1007/978-94-024-0927-7_12
Publisher Name : Springer, Dordrecht
Print ISBN : 978-94-024-0925-3
Online ISBN : 978-94-024-0927-7
eBook Packages : Education Education (R0)
Share this chapter
Anyone you share the following link with will be able to read this content:
Sorry, a shareable link is not currently available for this article.
Provided by the Springer Nature SharedIt content-sharing initiative
- Publish with us
Policies and ethics
- Find a journal
- Track your research
Qualitative vs. Quantitative Research: Comparing the Methods and Strategies for Education Research
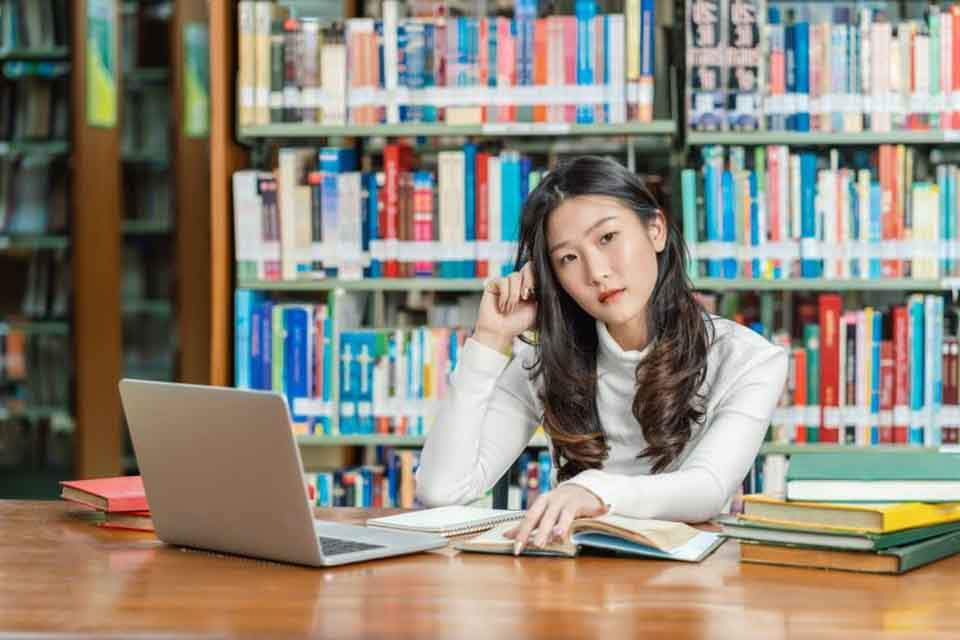
No matter the field of study, all research can be divided into two distinct methodologies: qualitative and quantitative research. Both methodologies offer education researchers important insights.
Education research assesses problems in policy, practices, and curriculum design, and it helps administrators identify solutions. Researchers can conduct small-scale studies to learn more about topics related to instruction or larger-scale ones to gain insight into school systems and investigate how to improve student outcomes.
Education research often relies on the quantitative methodology. Quantitative research in education provides numerical data that can prove or disprove a theory, and administrators can easily share the number-based results with other schools and districts. And while the research may speak to a relatively small sample size, educators and researchers can scale the results from quantifiable data to predict outcomes in larger student populations and groups.
Qualitative vs. Quantitative Research in Education: Definitions
Although there are many overlaps in the objectives of qualitative and quantitative research in education, researchers must understand the fundamental functions of each methodology in order to design and carry out an impactful research study. In addition, they must understand the differences that set qualitative and quantitative research apart in order to determine which methodology is better suited to specific education research topics.
Generate Hypotheses with Qualitative Research
Qualitative research focuses on thoughts, concepts, or experiences. The data collected often comes in narrative form and concentrates on unearthing insights that can lead to testable hypotheses. Educators use qualitative research in a study’s exploratory stages to uncover patterns or new angles.
Form Strong Conclusions with Quantitative Research
Quantitative research in education and other fields of inquiry is expressed in numbers and measurements. This type of research aims to find data to confirm or test a hypothesis.
Differences in Data Collection Methods
Keeping in mind the main distinction in qualitative vs. quantitative research—gathering descriptive information as opposed to numerical data—it stands to reason that there are different ways to acquire data for each research methodology. While certain approaches do overlap, the way researchers apply these collection techniques depends on their goal.
Interviews, for example, are common in both modes of research. An interview with students that features open-ended questions intended to reveal ideas and beliefs around attendance will provide qualitative data. This data may reveal a problem among students, such as a lack of access to transportation, that schools can help address.
An interview can also include questions posed to receive numerical answers. A case in point: how many days a week do students have trouble getting to school, and of those days, how often is a transportation-related issue the cause? In this example, qualitative and quantitative methodologies can lead to similar conclusions, but the research will differ in intent, design, and form.
Taking a look at behavioral observation, another common method used for both qualitative and quantitative research, qualitative data may consider a variety of factors, such as facial expressions, verbal responses, and body language.
On the other hand, a quantitative approach will create a coding scheme for certain predetermined behaviors and observe these in a quantifiable manner.
Qualitative Research Methods
- Case Studies : Researchers conduct in-depth investigations into an individual, group, event, or community, typically gathering data through observation and interviews.
- Focus Groups : A moderator (or researcher) guides conversation around a specific topic among a group of participants.
- Ethnography : Researchers interact with and observe a specific societal or ethnic group in their real-life environment.
- Interviews : Researchers ask participants questions to learn about their perspectives on a particular subject.
Quantitative Research Methods
- Questionnaires and Surveys : Participants receive a list of questions, either closed-ended or multiple choice, which are directed around a particular topic.
- Experiments : Researchers control and test variables to demonstrate cause-and-effect relationships.
- Observations : Researchers look at quantifiable patterns and behavior.
- Structured Interviews : Using a predetermined structure, researchers ask participants a fixed set of questions to acquire numerical data.
Choosing a Research Strategy
When choosing which research strategy to employ for a project or study, a number of considerations apply. One key piece of information to help determine whether to use a qualitative vs. quantitative research method is which phase of development the study is in.
For example, if a project is in its early stages and requires more research to find a testable hypothesis, qualitative research methods might prove most helpful. On the other hand, if the research team has already established a hypothesis or theory, quantitative research methods will provide data that can validate the theory or refine it for further testing.
It’s also important to understand a project’s research goals. For instance, do researchers aim to produce findings that reveal how to best encourage student engagement in math? Or is the goal to determine how many students are passing geometry? These two scenarios require distinct sets of data, which will determine the best methodology to employ.
In some situations, studies will benefit from a mixed-methods approach. Using the goals in the above example, one set of data could find the percentage of students passing geometry, which would be quantitative. The research team could also lead a focus group with the students achieving success to discuss which techniques and teaching practices they find most helpful, which would produce qualitative data.
Learn How to Put Education Research into Action
Those with an interest in learning how to harness research to develop innovative ideas to improve education systems may want to consider pursuing a doctoral degree. American University’s School of Education Online offers a Doctor of Education (EdD) in Education Policy and Leadership that prepares future educators, school administrators, and other education professionals to become leaders who effect positive changes in schools. Courses such as Applied Research Methods I: Enacting Critical Research provides students with the techniques and research skills needed to begin conducting research exploring new ways to enhance education. Learn more about American’ University’s EdD in Education Policy and Leadership .
What’s the Difference Between Educational Equity and Equality?
EdD vs. PhD in Education: Requirements, Career Outlook, and Salary
Top Education Technology Jobs for Doctorate in Education Graduates
American University, EdD in Education Policy and Leadership
Edutopia, “2019 Education Research Highlights”
Formplus, “Qualitative vs. Quantitative Data: 15 Key Differences and Similarities”
iMotion, “Qualitative vs. Quantitative Research: What Is What?”
Scribbr, “Qualitative vs. Quantitative Research”
Simply Psychology, “What’s the Difference Between Quantitative and Qualitative Research?”
Typeform, “A Simple Guide to Qualitative and Quantitative Research”
Request Information
- Research article
- Open access
- Published: 06 February 2017
Blended learning effectiveness: the relationship between student characteristics, design features and outcomes
- Mugenyi Justice Kintu ORCID: orcid.org/0000-0002-4500-1168 1 , 2 ,
- Chang Zhu 2 &
- Edmond Kagambe 1
International Journal of Educational Technology in Higher Education volume 14 , Article number: 7 ( 2017 ) Cite this article
750k Accesses
217 Citations
37 Altmetric
Metrics details
This paper investigates the effectiveness of a blended learning environment through analyzing the relationship between student characteristics/background, design features and learning outcomes. It is aimed at determining the significant predictors of blended learning effectiveness taking student characteristics/background and design features as independent variables and learning outcomes as dependent variables. A survey was administered to 238 respondents to gather data on student characteristics/background, design features and learning outcomes. The final semester evaluation results were used as a measure for performance as an outcome. We applied the online self regulatory learning questionnaire for data on learner self regulation, the intrinsic motivation inventory for data on intrinsic motivation and other self-developed instruments for measuring the other constructs. Multiple regression analysis results showed that blended learning design features (technology quality, online tools and face-to-face support) and student characteristics (attitudes and self-regulation) predicted student satisfaction as an outcome. The results indicate that some of the student characteristics/backgrounds and design features are significant predictors for student learning outcomes in blended learning.
Introduction
The teaching and learning environment is embracing a number of innovations and some of these involve the use of technology through blended learning. This innovative pedagogical approach has been embraced rapidly though it goes through a process. The introduction of blended learning (combination of face-to-face and online teaching and learning) initiatives is part of these innovations but its uptake, especially in the developing world faces challenges for it to be an effective innovation in teaching and learning. Blended learning effectiveness has quite a number of underlying factors that pose challenges. One big challenge is about how users can successfully use the technology and ensuring participants’ commitment given the individual learner characteristics and encounters with technology (Hofmann, 2014 ). Hofmann adds that users getting into difficulties with technology may result into abandoning the learning and eventual failure of technological applications. In a report by Oxford Group ( 2013 ), some learners (16%) had negative attitudes to blended learning while 26% were concerned that learners would not complete study in blended learning. Learners are important partners in any learning process and therefore, their backgrounds and characteristics affect their ability to effectively carry on with learning and being in blended learning, the design tools to be used may impinge on the effectiveness in their learning.
This study tackles blended learning effectiveness which has been investigated in previous studies considering grades, course completion, retention and graduation rates but no studies regarding effectiveness in view of learner characteristics/background, design features and outcomes have been done in the Ugandan university context. No studies have also been done on how the characteristics of learners and design features are predictors of outcomes in the context of a planning evaluation research (Guskey, 2000 ) to establish the effectiveness of blended learning. Guskey ( 2000 ) noted that planning evaluation fits in well since it occurs before the implementation of any innovation as well as allowing planners to determine the needs, considering participant characteristics, analyzing contextual matters and gathering baseline information. This study is done in the context of a plan to undertake innovative pedagogy involving use of a learning management system (moodle) for the first time in teaching and learning in a Ugandan university. The learner characteristics/backgrounds being investigated for blended learning effectiveness include self-regulation, computer competence, workload management, social and family support, attitude to blended learning, gender and age. We investigate the blended learning design features of learner interactions, face-to-face support, learning management system tools and technology quality while the outcomes considered include satisfaction, performance, intrinsic motivation and knowledge construction. Establishing the significant predictors of outcomes in blended learning will help to inform planners of such learning environments in order to put in place necessary groundwork preparations for designing blended learning as an innovative pedagogical approach.
Kenney and Newcombe ( 2011 ) did their comparison to establish effectiveness in view of grades and found that blended learning had higher average score than the non-blended learning environment. Garrison and Kanuka ( 2004 ) examined the transformative potential of blended learning and reported an increase in course completion rates, improved retention and increased student satisfaction. Comparisons between blended learning environments have been done to establish the disparity between academic achievement, grade dispersions and gender performance differences and no significant differences were found between the groups (Demirkol & Kazu, 2014 ).
However, blended learning effectiveness may be dependent on many other factors and among them student characteristics, design features and learning outcomes. Research shows that the failure of learners to continue their online education in some cases has been due to family support or increased workload leading to learner dropout (Park & Choi, 2009 ) as well as little time for study. Additionally, it is dependent on learner interactions with instructors since failure to continue with online learning is attributed to this. In Greer, Hudson & Paugh’s study as cited in Park and Choi ( 2009 ), family and peer support for learners is important for success in online and face-to-face learning. Support is needed for learners from all areas in web-based courses and this may be from family, friends, co-workers as well as peers in class. Greer, Hudson and Paugh further noted that peer encouragement assisted new learners in computer use and applications. The authors also show that learners need time budgeting, appropriate technology tools and support from friends and family in web-based courses. Peer support is required by learners who have no or little knowledge of technology, especially computers, to help them overcome fears. Park and Choi, ( 2009 ) showed that organizational support significantly predicts learners’ stay and success in online courses because employers at times are willing to reduce learners’ workload during study as well as supervisors showing that they are interested in job-related learning for employees to advance and improve their skills.
The study by Kintu and Zhu ( 2016 ) investigated the possibility of blended learning in a Ugandan University and examined whether student characteristics (such as self-regulation, attitudes towards blended learning, computer competence) and student background (such as family support, social support and management of workload) were significant factors in learner outcomes (such as motivation, satisfaction, knowledge construction and performance). The characteristics and background factors were studied along with blended learning design features such as technology quality, learner interactions, and Moodle with its tools and resources. The findings from that study indicated that learner attitudes towards blended learning were significant factors to learner satisfaction and motivation while workload management was a significant factor to learner satisfaction and knowledge construction. Among the blended learning design features, only learner interaction was a significant factor to learner satisfaction and knowledge construction.
The focus of the present study is on examining the effectiveness of blended learning taking into consideration learner characteristics/background, blended learning design elements and learning outcomes and how the former are significant predictors of blended learning effectiveness.
Studies like that of Morris and Lim ( 2009 ) have investigated learner and instructional factors influencing learning outcomes in blended learning. They however do not deal with such variables in the contexts of blended learning design as an aspect of innovative pedagogy involving the use of technology in education. Apart from the learner variables such as gender, age, experience, study time as tackled before, this study considers social and background aspects of the learners such as family and social support, self-regulation, attitudes towards blended learning and management of workload to find out their relationship to blended learning effectiveness. Identifying the various types of learner variables with regard to their relationship to blended learning effectiveness is important in this study as we embark on innovative pedagogy with technology in teaching and learning.
Literature review
This review presents research about blended learning effectiveness from the perspective of learner characteristics/background, design features and learning outcomes. It also gives the factors that are considered to be significant for blended learning effectiveness. The selected elements are as a result of the researcher’s experiences at a Ugandan university where student learning faces challenges with regard to learner characteristics and blended learning features in adopting the use of technology in teaching and learning. We have made use of Loukis, Georgiou, and Pazalo ( 2007 ) value flow model for evaluating an e-learning and blended learning service specifically considering the effectiveness evaluation layer. This evaluates the extent of an e-learning system usage and the educational effectiveness. In addition, studies by Leidner, Jarvenpaa, Dillon and Gunawardena as cited in Selim ( 2007 ) have noted three main factors that affect e-learning and blended learning effectiveness as instructor characteristics, technology and student characteristics. Heinich, Molenda, Russell, and Smaldino ( 2001 ) showed the need for examining learner characteristics for effective instructional technology use and showed that user characteristics do impact on behavioral intention to use technology. Research has dealt with learner characteristics that contribute to learner performance outcomes. They have dealt with emotional intelligence, resilience, personality type and success in an online learning context (Berenson, Boyles, & Weaver, 2008 ). Dealing with the characteristics identified in this study will give another dimension, especially for blended learning in learning environment designs and add to specific debate on learning using technology. Lin and Vassar, ( 2009 ) indicated that learner success is dependent on ability to cope with technical difficulty as well as technical skills in computer operations and internet navigation. This justifies our approach in dealing with the design features of blended learning in this study.
Learner characteristics/background and blended learning effectiveness
Studies indicate that student characteristics such as gender play significant roles in academic achievement (Oxford Group, 2013 ), but no study examines performance of male and female as an important factor in blended learning effectiveness. It has again been noted that the success of e- and blended learning is highly dependent on experience in internet and computer applications (Picciano & Seaman, 2007 ). Rigorous discovery of such competences can finally lead to a confirmation of high possibilities of establishing blended learning. Research agrees that the success of e-learning and blended learning can largely depend on students as well as teachers gaining confidence and capability to participate in blended learning (Hadad, 2007 ). Shraim and Khlaif ( 2010 ) note in their research that 75% of students and 72% of teachers were lacking in skills to utilize ICT based learning components due to insufficient skills and experience in computer and internet applications and this may lead to failure in e-learning and blended learning. It is therefore pertinent that since the use of blended learning applies high usage of computers, computer competence is necessary (Abubakar & Adetimirin, 2015 ) to avoid failure in applying technology in education for learning effectiveness. Rovai, ( 2003 ) noted that learners’ computer literacy and time management are crucial in distance learning contexts and concluded that such factors are meaningful in online classes. This is supported by Selim ( 2007 ) that learners need to posses time management skills and computer skills necessary for effectiveness in e- learning and blended learning. Self-regulatory skills of time management lead to better performance and learners’ ability to structure the physical learning environment leads to efficiency in e-learning and blended learning environments. Learners need to seek helpful assistance from peers and teachers through chats, email and face-to-face meetings for effectiveness (Lynch & Dembo, 2004 ). Factors such as learners’ hours of employment and family responsibilities are known to impede learners’ process of learning, blended learning inclusive (Cohen, Stage, Hammack, & Marcus, 2012 ). It was also noted that a common factor in failure and learner drop-out is the time conflict which is compounded by issues of family , employment status as well as management support (Packham, Jones, Miller, & Thomas, 2004 ). A study by Thompson ( 2004 ) shows that work, family, insufficient time and study load made learners withdraw from online courses.
Learner attitudes to blended learning can result in its effectiveness and these shape behavioral intentions which usually lead to persistence in a learning environment, blended inclusive. Selim, ( 2007 ) noted that the learners’ attitude towards e-learning and blended learning are success factors for these learning environments. Learner performance by age and gender in e-learning and blended learning has been found to indicate no significant differences between male and female learners and different age groups (i.e. young, middle-aged and old above 45 years) (Coldwell, Craig, Paterson, & Mustard, 2008 ). This implies that the potential for blended learning to be effective exists and is unhampered by gender or age differences.
Blended learning design features
The design features under study here include interactions, technology with its quality, face-to-face support and learning management system tools and resources.
Research shows that absence of learner interaction causes failure and eventual drop-out in online courses (Willging & Johnson, 2009 ) and the lack of learner connectedness was noted as an internal factor leading to learner drop-out in online courses (Zielinski, 2000 ). It was also noted that learners may not continue in e- and blended learning if they are unable to make friends thereby being disconnected and developing feelings of isolation during their blended learning experiences (Willging & Johnson, 2009). Learners’ Interactions with teachers and peers can make blended learning effective as its absence makes learners withdraw (Astleitner, 2000 ). Loukis, Georgious and Pazalo (2007) noted that learners’ measuring of a system’s quality, reliability and ease of use leads to learning efficiency and can be so in blended learning. Learner success in blended learning may substantially be affected by system functionality (Pituch & Lee, 2006 ) and may lead to failure of such learning initiatives (Shrain, 2012 ). It is therefore important to examine technology quality for ensuring learning effectiveness in blended learning. Tselios, Daskalakis, and Papadopoulou ( 2011 ) investigated learner perceptions after a learning management system use and found out that the actual system use determines the usefulness among users. It is again noted that a system with poor response time cannot be taken to be useful for e-learning and blended learning especially in cases of limited bandwidth (Anderson, 2004 ). In this study, we investigate the use of Moodle and its tools as a function of potential effectiveness of blended learning.
The quality of learning management system content for learners can be a predictor of good performance in e-and blended learning environments and can lead to learner satisfaction. On the whole, poor quality technology yields no satisfaction by users and therefore the quality of technology significantly affects satisfaction (Piccoli, Ahmad, & Ives, 2001 ). Continued navigation through a learning management system increases use and is an indicator of success in blended learning (Delone & McLean, 2003 ). The efficient use of learning management system and its tools improves learning outcomes in e-learning and blended learning environments.
It is noted that learner satisfaction with a learning management system can be an antecedent factor for blended learning effectiveness. Goyal and Tambe ( 2015 ) noted that learners showed an appreciation to Moodle’s contribution in their learning. They showed positivity with it as it improved their understanding of course material (Ahmad & Al-Khanjari, 2011 ). The study by Goyal and Tambe ( 2015 ) used descriptive statistics to indicate improved learning by use of uploaded syllabus and session plans on Moodle. Improved learning is also noted through sharing study material, submitting assignments and using the calendar. Learners in the study found Moodle to be an effective educational tool.
In blended learning set ups, face-to-face experiences form part of the blend and learner positive attitudes to such sessions could mean blended learning effectiveness. A study by Marriot, Marriot, and Selwyn ( 2004 ) showed learners expressing their preference for face-to-face due to its facilitation of social interaction and communication skills acquired from classroom environment. Their preference for the online session was only in as far as it complemented the traditional face-to-face learning. Learners in a study by Osgerby ( 2013 ) had positive perceptions of blended learning but preferred face-to-face with its step-by-stem instruction. Beard, Harper and Riley ( 2004 ) shows that some learners are successful while in a personal interaction with teachers and peers thus prefer face-to-face in the blend. Beard however dealt with a comparison between online and on-campus learning while our study combines both, singling out the face-to-face part of the blend. The advantage found by Beard is all the same relevant here because learners in blended learning express attitude to both online and face-to-face for an effective blend. Researchers indicate that teacher presence in face-to-face sessions lessens psychological distance between them and the learners and leads to greater learning. This is because there are verbal aspects like giving praise, soliciting for viewpoints, humor, etc and non-verbal expressions like eye contact, facial expressions, gestures, etc which make teachers to be closer to learners psychologically (Kelley & Gorham, 2009 ).
Learner outcomes
The outcomes under scrutiny in this study include performance, motivation, satisfaction and knowledge construction. Motivation is seen here as an outcome because, much as cognitive factors such as course grades are used in measuring learning outcomes, affective factors like intrinsic motivation may also be used to indicate outcomes of learning (Kuo, Walker, Belland, & Schroder, 2013 ). Research shows that high motivation among online learners leads to persistence in their courses (Menager-Beeley, 2004 ). Sankaran and Bui ( 2001 ) indicated that less motivated learners performed poorly in knowledge tests while those with high learning motivation demonstrate high performance in academics (Green, Nelson, Martin, & Marsh, 2006 ). Lim and Kim, ( 2003 ) indicated that learner interest as a motivation factor promotes learner involvement in learning and this could lead to learning effectiveness in blended learning.
Learner satisfaction was noted as a strong factor for effectiveness of blended and online courses (Wilging & Johnson, 2009) and dissatisfaction may result from learners’ incompetence in the use of the learning management system as an effective learning tool since, as Islam ( 2014 ) puts it, users may be dissatisfied with an information system due to ease of use. A lack of prompt feedback for learners from course instructors was found to cause dissatisfaction in an online graduate course. In addition, dissatisfaction resulted from technical difficulties as well as ambiguous course instruction Hara and Kling ( 2001 ). These factors, once addressed, can lead to learner satisfaction in e-learning and blended learning and eventual effectiveness. A study by Blocker and Tucker ( 2001 ) also showed that learners had difficulties with technology and inadequate group participation by peers leading to dissatisfaction within these design features. Student-teacher interactions are known to bring satisfaction within online courses. Study results by Swan ( 2001 ) indicated that student-teacher interaction strongly related with student satisfaction and high learner-learner interaction resulted in higher levels of course satisfaction. Descriptive results by Naaj, Nachouki, and Ankit ( 2012 ) showed that learners were satisfied with technology which was a video-conferencing component of blended learning with a mean of 3.7. The same study indicated student satisfaction with instructors at a mean of 3.8. Askar and Altun, ( 2008 ) found that learners were satisfied with face-to-face sessions of the blend with t-tests and ANOVA results indicating female scores as higher than for males in the satisfaction with face-to-face environment of the blended learning.
Studies comparing blended learning with traditional face-to-face have indicated that learners perform equally well in blended learning and their performance is unaffected by the delivery method (Kwak, Menezes, & Sherwood, 2013 ). In another study, learning experience and performance are known to improve when traditional course delivery is integrated with online learning (Stacey & Gerbic, 2007 ). Such improvement as noted may be an indicator of blended learning effectiveness. Our study however, delves into improved performance but seeks to establish the potential of blended learning effectiveness by considering grades obtained in a blended learning experiment. Score 50 and above is considered a pass in this study’s setting and learners scoring this and above will be considered to have passed. This will make our conclusions about the potential of blended learning effectiveness.
Regarding knowledge construction, it has been noted that effective learning occurs where learners are actively involved (Nurmela, Palonen, Lehtinen & Hakkarainen, 2003 , cited in Zhu, 2012 ) and this may be an indicator of learning environment effectiveness. Effective blended learning would require that learners are able to initiate, discover and accomplish the processes of knowledge construction as antecedents of blended learning effectiveness. A study by Rahman, Yasin and Jusoff ( 2011 ) indicated that learners were able to use some steps to construct meaning through an online discussion process through assignments given. In the process of giving and receiving among themselves, the authors noted that learners learned by writing what they understood. From our perspective, this can be considered to be accomplishment in the knowledge construction process. Their study further shows that learners construct meaning individually from assignments and this stage is referred to as pre-construction which for our study, is an aspect of discovery in the knowledge construction process.
Predictors of blended learning effectiveness
Researchers have dealt with success factors for online learning or those for traditional face-to-face learning but little is known about factors that predict blended learning effectiveness in view of learner characteristics and blended learning design features. This part of our study seeks to establish the learner characteristics/backgrounds and design features that predict blended learning effectiveness with regard to satisfaction, outcomes, motivation and knowledge construction. Song, Singleton, Hill, and Koh ( 2004 ) examined online learning effectiveness factors and found out that time management (a self-regulatory factor) was crucial for successful online learning. Eom, Wen, and Ashill ( 2006 ) using a survey found out that interaction, among other factors, was significant for learner satisfaction. Technical problems with regard to instructional design were a challenge to online learners thus not indicating effectiveness (Song et al., 2004 ), though the authors also indicated that descriptive statistics to a tune of 75% and time management (62%) impact on success of online learning. Arbaugh ( 2000 ) and Swan ( 2001 ) indicated that high levels of learner-instructor interaction are associated with high levels of user satisfaction and learning outcomes. A study by Naaj et al. ( 2012 ) indicated that technology and learner interactions, among other factors, influenced learner satisfaction in blended learning.
Objective and research questions of the current study
The objective of the current study is to investigate the effectiveness of blended learning in view of student satisfaction, knowledge construction, performance and intrinsic motivation and how they are related to student characteristics and blended learning design features in a blended learning environment.
Research questions
What are the student characteristics and blended learning design features for an effective blended learning environment?
Which factors (among the learner characteristics and blended learning design features) predict student satisfaction, learning outcomes, intrinsic motivation and knowledge construction?
Conceptual model of the present study
The reviewed literature clearly shows learner characteristics/background and blended learning design features play a part in blended learning effectiveness and some of them are significant predictors of effectiveness. The conceptual model for our study is depicted as follows (Fig. 1 ):
Conceptual model of the current study
Research design
This research applies a quantitative design where descriptive statistics are used for the student characteristics and design features data, t-tests for the age and gender variables to determine if they are significant in blended learning effectiveness and regression for predictors of blended learning effectiveness.
This study is based on an experiment in which learners participated during their study using face-to-face sessions and an on-line session of a blended learning design. A learning management system (Moodle) was used and learner characteristics/background and blended learning design features were measured in relation to learning effectiveness. It is therefore a planning evaluation research design as noted by Guskey ( 2000 ) since the outcomes are aimed at blended learning implementation at MMU. The plan under which the various variables were tested involved face-to-face study at the beginning of a 17 week semester which was followed by online teaching and learning in the second half of the semester. The last part of the semester was for another face-to-face to review work done during the online sessions and final semester examinations. A questionnaire with items on student characteristics, design features and learning outcomes was distributed among students from three schools and one directorate of postgraduate studies.
Participants
Cluster sampling was used to select a total of 238 learners to participate in this study. Out of the whole university population of students, three schools and one directorate were used. From these, one course unit was selected from each school and all the learners following the course unit were surveyed. In the school of Education ( n = 70) and Business and Management Studies ( n = 133), sophomore students were involved due to the fact that they have been introduced to ICT basics during their first year of study. Students of the third year were used from the department of technology in the School of Applied Sciences and Technology ( n = 18) since most of the year two courses had a lot of practical aspects that could not be used for the online learning part. From the Postgraduate Directorate ( n = 17), first and second year students were selected because learners attend a face-to-face session before they are given paper modules to study away from campus.
The study population comprised of 139 male students representing 58.4% and 99 females representing 41.6% with an average age of 24 years.
Instruments
The end of semester results were used to measure learner performance. The online self-regulated learning questionnaire (Barnard, Lan, To, Paton, & Lai, 2009 ) and the intrinsic motivation inventory (Deci & Ryan, 1982 ) were applied to measure the constructs on self regulation in the student characteristics and motivation in the learning outcome constructs. Other self-developed instruments were used for the other remaining variables of attitudes, computer competence, workload management, social and family support, satisfaction, knowledge construction, technology quality, interactions, learning management system tools and resources and face-to-face support.
Instrument reliability
Cronbach’s alpha was used to test reliability and the table below gives the results. All the scales and sub-scales had acceptable internal consistency reliabilities as shown in Table 1 below:
Data analysis
First, descriptive statistics was conducted. Shapiro-Wilk test was done to test normality of the data for it to qualify for parametric tests. The test results for normality of our data before the t- test resulted into significant levels (Male = .003, female = .000) thereby violating the normality assumption. We therefore used the skewness and curtosis results which were between −1.0 and +1.0 and assumed distribution to be sufficiently normal to qualify the data for a parametric test, (Pallant, 2010 ). An independent samples t -test was done to find out the differences in male and female performance to explain the gender characteristics in blended learning effectiveness. A one-way ANOVA between subjects was conducted to establish the differences in performance between age groups. Finally, multiple regression analysis was done between student variables and design elements with learning outcomes to determine the significant predictors for blended learning effectiveness.
Student characteristics, blended learning design features and learning outcomes ( RQ1 )
A t- test was carried out to establish the performance of male and female learners in the blended learning set up. This was aimed at finding out if male and female learners do perform equally well in blended learning given their different roles and responsibilities in society. It was found that male learners performed slightly better ( M = 62.5) than their female counterparts ( M = 61.1). An independent t -test revealed that the difference between the performances was not statistically significant ( t = 1.569, df = 228, p = 0.05, one tailed). The magnitude of the differences in the means is small with effect size ( d = 0.18). A one way between subjects ANOVA was conducted on the performance of different age groups to establish the performance of learners of young and middle aged age groups (20–30, young & and 31–39, middle aged). This revealed a significant difference in performance (F(1,236 = 8.498, p < . 001).
Average percentages of the items making up the self regulated learning scale are used to report the findings about all the sub-scales in the learner characteristics/background scale. Results show that learner self-regulation was good enough at 72.3% in all the sub-scales of goal setting, environment structuring, task strategies, time management, help-seeking and self-evaluation among learners. The least in the scoring was task strategies at 67.7% and the highest was learner environment structuring at 76.3%. Learner attitude towards blended learning environment is at 76% in the sub-scales of learner autonomy, quality of instructional materials, course structure, course interface and interactions. The least scored here is attitude to course structure at 66% and their attitudes were high on learner autonomy and course interface both at 82%. Results on the learners’ computer competences are summarized in percentages in the table below (Table 2 ):
It can be seen that learners are skilled in word processing at 91%, email at 63.5%, spreadsheets at 68%, web browsers at 70.2% and html tools at 45.4%. They are therefore good enough in word processing and web browsing. Their computer confidence levels are reported at 75.3% and specifically feel very confident when it comes to working with a computer (85.7%). Levels of family and social support for learners during blended learning experiences are at 60.5 and 75% respectively. There is however a low score on learners being assisted by family members in situations of computer setbacks (33.2%) as 53.4% of the learners reported no assistance in this regard. A higher percentage (85.3%) is reported on learners getting support from family regarding provision of essentials for learning such as tuition. A big percentage of learners spend two hours on study while at home (35.3%) followed by one hour (28.2%) while only 9.7% spend more than three hours on study at home. Peers showed great care during the blended learning experience (81%) and their experiences were appreciated by the society (66%). Workload management by learners vis-à-vis studying is good at 60%. Learners reported that their workmates stand in for them at workplaces to enable them do their study in blended learning while 61% are encouraged by their bosses to go and improve their skills through further education and training. On the time spent on other activities not related to study, majority of the learners spend three hours (35%) while 19% spend 6 hours. Sixty percent of the learners have to answer to someone when they are not attending to other activities outside study compared to the 39.9% who do not and can therefore do study or those other activities.
The usability of the online system, tools and resources was below average as shown in the table below in percentages (Table 3 ):
However, learners became skilled at navigating around the learning management system (79%) and it was easy for them to locate course content, tools and resources needed such as course works, news, discussions and journal materials. They effectively used the communication tools (60%) and to work with peers by making posts (57%). They reported that online resources were well organized, user friendly and easy to access (71%) as well as well structured in a clear and understandable manner (72%). They therefore recommended the use of online resources for other course units in future (78%) because they were satisfied with them (64.3%). On the whole, the online resources were fine for the learners (67.2%) and useful as a learning resource (80%). The learners’ perceived usefulness/satisfaction with online system, tools, and resources was at 81% as the LMS tools helped them to communicate, work with peers and reflect on their learning (74%). They reported that using moodle helped them to learn new concepts, information and gaining skills (85.3%) as well as sharing what they knew or learned (76.4%). They enjoyed the course units (78%) and improved their skills with technology (89%).
Learner interactions were seen from three angles of cognitivism, collaborative learning and student-teacher interactions. Collaborative learning was average at 50% with low percentages in learners posting challenges to colleagues’ ideas online (34%) and posting ideas for colleagues to read online (37%). They however met oftentimes online (60%) and organized how they would work together in study during the face-to-face meetings (69%). The common form of communication medium frequently used by learners during the blended learning experience was by phone (34.5%) followed by whatsapp (21.8%), face book (21%), discussion board (11.8%) and email (10.9%). At the cognitive level, learners interacted with content at 72% by reading the posted content (81%), exchanging knowledge via the LMS (58.4%), participating in discussions on the forum (62%) and got course objectives and structure introduced during the face-to-face sessions (86%). Student-teacher interaction was reported at 71% through instructors individually working with them online (57.2%) and being well guided towards learning goals (81%). They did receive suggestions from instructors about resources to use in their learning (75.3%) and instructors provided learning input for them to come up with their own answers (71%).
The technology quality during the blended learning intervention was rated at 69% with availability of 72%, quality of the resources was at 68% with learners reporting that discussion boards gave right content necessary for study (71%) and the email exchanges containing relevant and much needed information (63.4%) as well as chats comprising of essential information to aid the learning (69%). Internet reliability was rated at 66% with a speed considered averagely good to facilitate online activities (63%). They however reported that there was intermittent breakdown during online study (67%) though they could complete their internet program during connection (63.4%). Learners eventually found it easy to download necessary materials for study in their blended learning experiences (71%).
Learner extent of use of the learning management system features was as shown in the table below in percentage (Table 4 ):
From the table, very rarely used features include the blog and wiki while very often used ones include the email, forum, chat and calendar.
The effectiveness of the LMS was rated at 79% by learners reporting that they found it useful (89%) and using it makes their learning activities much easier (75.2%). Moodle has helped learners to accomplish their learning tasks more quickly (74%) and that as a LMS, it is effective in teaching and learning (88%) with overall satisfaction levels at 68%. However, learners note challenges in the use of the LMS regarding its performance as having been problematic to them (57%) and only 8% of the learners reported navigation while 16% reported access as challenges.
Learner attitudes towards Face-to-face support were reported at 88% showing that the sessions were enjoyable experiences (89%) with high quality class discussions (86%) and therefore recommended that the sessions should continue in blended learning (89%). The frequency of the face-to-face sessions is shown in the table below as preferred by learners (Table 5 ).
Learners preferred face-to-face sessions after every month in the semester (33.6%) and at the beginning of the blended learning session only (27.7%).
Learners reported high intrinsic motivation levels with interest and enjoyment of tasks at 83.7%, perceived competence at 70.2%, effort/importance sub-scale at 80%, pressure/tension reported at 54%. The pressure percentage of 54% arises from learners feeling nervous (39.2%) and a lot of anxiety (53%) while 44% felt a lot of pressure during the blended learning experiences. Learners however reported the value/usefulness of blended learning at 91% with majority believing that studying online and face-to-face had value for them (93.3%) and were therefore willing to take part in blended learning (91.2%). They showed that it is beneficial for them (94%) and that it was an important way of studying (84.3%).
Learner satisfaction was reported at 81% especially with instructors (85%) high percentage reported on encouraging learner participation during the course of study 93%, course content (83%) with the highest being satisfaction with the good relationship between the objectives of the course units and the content (90%), technology (71%) with a high percentage on the fact that the platform was adequate for the online part of the learning (76%), interactions (75%) with participation in class at 79%, and face-to-face sessions (91%) with learner satisfaction high on face-to-face sessions being good enough for interaction and giving an overview of the courses when objectives were introduced at 92%.
Learners’ knowledge construction was reported at 78% with initiation and discovery scales scoring 84% with 88% specifically for discovering the learning points in the course units. The accomplishment scale in knowledge construction scored 71% and specifically the fact that learners were able to work together with group members to accomplish learning tasks throughout the study of the course units (79%). Learners developed reports from activities (67%), submitted solutions to discussion questions (68%) and did critique peer arguments (69%). Generally, learners performed well in blended learning in the final examination with an average pass of 62% and standard deviation of 7.5.
Significant predictors of blended learning effectiveness ( RQ 2)
A standard multiple regression analysis was done taking learner characteristics/background and design features as predictor variables and learning outcomes as criterion variables. The data was first tested to check if it met the linear regression test assumptions and results showed the correlations between the independent variables and each of the dependent variables (highest 0.62 and lowest 0.22) as not being too high, which indicated that multicollinearity was not a problem in our model. From the coefficients table, the VIF values ranged from 1.0 to 2.4, well below the cut off value of 10 and indicating no possibility of multicollinearity. The normal probability plot was seen to lie as a reasonably straight diagonal from bottom left to top right indicating normality of our data. Linearity was found suitable from the scatter plot of the standardized residuals and was rectangular in distribution. Outliers were no cause for concern in our data since we had only 1% of all cases falling outside 3.0 thus proving the data as a normally distributed sample. Our R -square values was at 0.525 meaning that the independent variables explained about 53% of the variance in overall satisfaction, motivation and knowledge construction of the learners. All the models explaining the three dependent variables of learner satisfaction, intrinsic motivation and knowledge construction were significant at the 0.000 probability level (Table 6 ).
From the table above, design features (technology quality and online tools and resources), and learner characteristics (attitudes to blended learning, self-regulation) were significant predictors of learner satisfaction in blended learning. This means that good technology with the features involved and the learner positive attitudes with capacity to do blended learning with self drive led to their satisfaction. The design features (technology quality, interactions) and learner characteristics (self regulation and social support), were found to be significant predictors of learner knowledge construction. This implies that learners’ capacity to go on their work by themselves supported by peers and high levels of interaction using the quality technology led them to construct their own ideas in blended learning. Design features (technology quality, online tools and resources as well as learner interactions) and learner characteristics (self regulation), significantly predicted the learners’ intrinsic motivation in blended learning suggesting that good technology, tools and high interaction levels with independence in learning led to learners being highly motivated. Finally, none of the independent variables considered under this study were predictors of learning outcomes (grade).
In this study we have investigated learning outcomes as dependent variables to establish if particular learner characteristics/backgrounds and design features are related to the outcomes for blended learning effectiveness and if they predict learning outcomes in blended learning. We took students from three schools out of five and one directorate of post-graduate studies at a Ugandan University. The study suggests that the characteristics and design features examined are good drivers towards an effective blended learning environment though a few of them predicted learning outcomes in blended learning.
Student characteristics/background, blended learning design features and learning outcomes
The learner characteristics, design features investigated are potentially important for an effective blended learning environment. Performance by gender shows a balance with no statistical differences between male and female. There are statistically significant differences ( p < .005) in the performance between age groups with means of 62% for age group 20–30 and 67% for age group 31 –39. The indicators of self regulation exist as well as positive attitudes towards blended learning. Learners do well with word processing, e-mail, spreadsheets and web browsers but still lag below average in html tools. They show computer confidence at 75.3%; which gives prospects for an effective blended learning environment in regard to their computer competence and confidence. The levels of family and social support for learners stand at 61 and 75% respectively, indicating potential for blended learning to be effective. The learners’ balance between study and work is a drive factor towards blended learning effectiveness since their management of their workload vis a vis study time is at 60 and 61% of the learners are encouraged to go for study by their bosses. Learner satisfaction with the online system and its tools shows prospect for blended learning effectiveness but there are challenges in regard to locating course content and assignments, submitting their work and staying on a task during online study. Average collaborative, cognitive learning as well as learner-teacher interactions exist as important factors. Technology quality for effective blended learning is a potential for effectiveness though features like the blog and wiki are rarely used by learners. Face-to-face support is satisfactory and it should be conducted every month. There is high intrinsic motivation, satisfaction and knowledge construction as well as good performance in examinations ( M = 62%, SD = 7.5); which indicates potentiality for blended learning effectiveness.
Significant predictors of blended learning effectiveness
Among the design features, technology quality, online tools and face-to-face support are predictors of learner satisfaction while learner characteristics of self regulation and attitudes to blended learning are predictors of satisfaction. Technology quality and interactions are the only design features predicting learner knowledge construction, while social support, among the learner backgrounds, is a predictor of knowledge construction. Self regulation as a learner characteristic is a predictor of knowledge construction. Self regulation is the only learner characteristic predicting intrinsic motivation in blended learning while technology quality, online tools and interactions are the design features predicting intrinsic motivation. However, all the independent variables are not significant predictors of learning performance in blended learning.
The high computer competences and confidence is an antecedent factor for blended learning effectiveness as noted by Hadad ( 2007 ) and this study finds learners confident and competent enough for the effectiveness of blended learning. A lack in computer skills causes failure in e-learning and blended learning as noted by Shraim and Khlaif ( 2010 ). From our study findings, this is no threat for blended learning our case as noted by our results. Contrary to Cohen et al. ( 2012 ) findings that learners’ family responsibilities and hours of employment can impede their process of learning, it is not the case here since they are drivers to the blended learning process. Time conflict, as compounded by family, employment status and management support (Packham et al., 2004 ) were noted as causes of learner failure and drop out of online courses. Our results show, on the contrary, that these factors are drivers for blended learning effectiveness because learners have a good balance between work and study and are supported by bosses to study. In agreement with Selim ( 2007 ), learner positive attitudes towards e-and blended learning environments are success factors. In line with Coldwell et al. ( 2008 ), no statistically significant differences exist between age groups. We however note that Coldwel, et al dealt with young, middle-aged and old above 45 years whereas we dealt with young and middle aged only.
Learner interactions at all levels are good enough and contrary to Astleitner, ( 2000 ) that their absence makes learners withdraw, they are a drive factor here. In line with Loukis (2007) the LMS quality, reliability and ease of use lead to learning efficiency as technology quality, online tools are predictors of learner satisfaction and intrinsic motivation. Face-to-face sessions should continue on a monthly basis as noted here and is in agreement with Marriot et al. ( 2004 ) who noted learner preference for it for facilitating social interaction and communication skills. High learner intrinsic motivation leads to persistence in online courses as noted by Menager-Beeley, ( 2004 ) and is high enough in our study. This implies a possibility of an effectiveness blended learning environment. The causes of learner dissatisfaction noted by Islam ( 2014 ) such as incompetence in the use of the LMS are contrary to our results in our study, while the one noted by Hara and Kling, ( 2001 ) as resulting from technical difficulties and ambiguous course instruction are no threat from our findings. Student-teacher interaction showed a relation with satisfaction according to Swan ( 2001 ) but is not a predictor in our study. Initiating knowledge construction by learners for blended learning effectiveness is exhibited in our findings and agrees with Rahman, Yasin and Jusof ( 2011 ). Our study has not agreed with Eom et al. ( 2006 ) who found learner interactions as predictors of learner satisfaction but agrees with Naaj et al. ( 2012 ) regarding technology as a predictor of learner satisfaction.
Conclusion and recommendations
An effective blended learning environment is necessary in undertaking innovative pedagogical approaches through the use of technology in teaching and learning. An examination of learner characteristics/background, design features and learning outcomes as factors for effectiveness can help to inform the design of effective learning environments that involve face-to-face sessions and online aspects. Most of the student characteristics and blended learning design features dealt with in this study are important factors for blended learning effectiveness. None of the independent variables were identified as significant predictors of student performance. These gaps are open for further investigation in order to understand if they can be significant predictors of blended learning effectiveness in a similar or different learning setting.
In planning to design and implement blended learning, we are mindful of the implications raised by this study which is a planning evaluation research for the design and eventual implementation of blended learning. Universities should be mindful of the interplay between the learner characteristics, design features and learning outcomes which are indicators of blended learning effectiveness. From this research, learners manifest high potential to take on blended learning more especially in regard to learner self-regulation exhibited. Blended learning is meant to increase learners’ levels of knowledge construction in order to create analytical skills in them. Learner ability to assess and critically evaluate knowledge sources is hereby established in our findings. This can go a long way in producing skilled learners who can be innovative graduates enough to satisfy employment demands through creativity and innovativeness. Technology being less of a shock to students gives potential for blended learning design. Universities and other institutions of learning should continue to emphasize blended learning approaches through installation of learning management systems along with strong internet to enable effective learning through technology especially in the developing world.
Abubakar, D. & Adetimirin. (2015). Influence of computer literacy on post-graduates’ use of e-resources in Nigerian University Libraries. Library Philosophy and Practice. From http://digitalcommons.unl.edu/libphilprac/ . Retrieved 18 Aug 2015.
Ahmad, N., & Al-Khanjari, Z. (2011). Effect of Moodle on learning: An Oman perception. International Journal of Digital Information and Wireless Communications (IJDIWC), 1 (4), 746–752.
Google Scholar
Anderson, T. (2004). Theory and Practice of Online Learning . Canada: AU Press, Athabasca University.
Arbaugh, J. B. (2000). How classroom environment and student engagement affect learning in internet-basedMBAcourses. Business Communication Quarterly, 63 (4), 9–18.
Article Google Scholar
Askar, P. & Altun, A. (2008). Learner satisfaction on blended learning. E-Leader Krakow , 2008.
Astleitner, H. (2000) Dropout and distance education. A review of motivational and emotional strategies to reduce dropout in web-based distance education. In Neuwe Medien in Unterricht, Aus-und Weiterbildung Waxmann Munster, New York.
Barnard, L., Lan, W. Y., To, Y. M., Paton, V. O., & Lai, S. (2009). Measuring self regulation in online and blended learning environments’. Internet and Higher Education, 12 (1), 1–6.
Beard, L. A., Harper, C., & Riley, G. (2004). Online versus on-campus instruction: student attitudes & perceptions. TechTrends, 48 (6), 29–31.
Berenson, R., Boyles, G., & Weaver, A. (2008). Emotional intelligence as a predictor for success in online learning. International Review of Research in open & Distance Learning, 9 (2), 1–16.
Blocker, J. M., & Tucker, G. (2001). Using constructivist principles in designing and integrating online collaborative interactions. In F. Fuller & R. McBride (Eds.), Distance education. Proceedings of the Society for Information Technology & Teacher Education International Conference (pp. 32–36). ERIC Document Reproduction Service No. ED 457 822.
Cohen, K. E., Stage, F. K., Hammack, F. M., & Marcus, A. (2012). Persistence of master’s students in the United States: Developing and testing of a conceptual model . USA: PhD Dissertation, New York University.
Coldwell, J., Craig, A., Paterson, T., & Mustard, J. (2008). Online students: Relationships between participation, demographics and academic performance. The Electronic Journal of e-learning, 6 (1), 19–30.
Deci, E. L., & Ryan, R. M. (1982). Intrinsic Motivation Inventory. Available from selfdeterminationtheory.org/intrinsic-motivation-inventory/ . Accessed 2 Aug 2016.
Delone, W. H., & McLean, E. R. (2003). The Delone and McLean model of information systems success: A Ten-year update. Journal of Management Information Systems, 19 (4), 9–30.
Demirkol, M., & Kazu, I. Y. (2014). Effect of blended environment model on high school students’ academic achievement. The Turkish Online Journal of Educational Technology, 13 (1), 78–87.
Eom, S., Wen, H., & Ashill, N. (2006). The determinants of students’ perceived learning outcomes and satisfaction in university online education: an empirical investigation’. Decision Sciences Journal of Innovative Education, 4 (2), 215–235.
Garrison, D. R., & Kanuka, H. (2004). Blended learning: Uncovering its transformative potential in higher education. Internet and Higher Education, 7 (2), 95–105.
Goyal, E., & Tambe, S. (2015). Effectiveness of Moodle-enabled blended learning in private Indian Business School teaching NICHE programs. The Online Journal of New Horizons in Education, 5 (2), 14–22.
Green, J., Nelson, G., Martin, A. J., & Marsh, H. (2006). The causal ordering of self-concept and academic motivation and its effect on academic achievement. International Education Journal, 7 (4), 534–546.
Guskey, T. R. (2000). Evaluating Professional Development . Thousands Oaks: Corwin Press.
Hadad, W. (2007). ICT-in-education toolkit reference handbook . InfoDev. from http://www.infodev.org/en/Publication.301.html . Retrieved 04 Aug 2015.
Hara, N. & Kling, R. (2001). Student distress in web-based distance education. Educause Quarterly. 3 (2001).
Heinich, R., Molenda, M., Russell, J. D., & Smaldino, S. E. (2001). Instructional Media and Technologies for Learning (7th ed.). Englewood Cliffs: Prentice-Hall.
Hofmann, J. (2014). Solutions to the top 10 challenges of blended learning. Top 10 challenges of blended learning. Available on cedma-europe.org .
Islam, A. K. M. N. (2014). Sources of satisfaction and dissatisfaction with a learning management system in post-adoption stage: A critical incident technique approach. Computers in Human Behaviour, 30 , 249–261.
Kelley, D. H. & Gorham, J. (2009) Effects of immediacy on recall of information. Communication Education, 37 (3), 198–207.
Kenney, J., & Newcombe, E. (2011). Adopting a blended learning approach: Challenges, encountered and lessons learned in an action research study. Journal of Asynchronous Learning Networks, 15 (1), 45–57.
Kintu, M. J., & Zhu, C. (2016). Student characteristics and learning outcomes in a blended learning environment intervention in a Ugandan University. Electronic Journal of e-Learning, 14 (3), 181–195.
Kuo, Y., Walker, A. E., Belland, B. R., & Schroder, L. E. E. (2013). A predictive study of student satisfaction in online education programs. International Review of Research in Open and Distributed Learning, 14 (1), 16–39.
Kwak, D. W., Menezes, F. M., & Sherwood, C. (2013). Assessing the impact of blended learning on student performance. Educational Technology & Society, 15 (1), 127–136.
Lim, D. H., & Kim, H. J. (2003). Motivation and learner characteristics affecting online learning and learning application. Journal of Educational Technology Systems, 31 (4), 423–439.
Lim, D. H., & Morris, M. L. (2009). Learner and instructional factors influencing learner outcomes within a blended learning environment. Educational Technology & Society, 12 (4), 282–293.
Lin, B., & Vassar, J. A. (2009). Determinants for success in online learning communities. International Journal of Web-based Communities, 5 (3), 340–350.
Loukis, E., Georgiou, S. & Pazalo, K. (2007). A value flow model for the evaluation of an e-learning service. ECIS, 2007 Proceedings, paper 175.
Lynch, R., & Dembo, M. (2004). The relationship between self regulation and online learning in a blended learning context. The International Review of Research in Open and Distributed Learning, 5 (2), 1–16.
Marriot, N., Marriot, P., & Selwyn. (2004). Accounting undergraduates’ changing use of ICT and their views on using the internet in higher education-A Research note. Accounting Education, 13 (4), 117–130.
Menager-Beeley, R. (2004). Web-based distance learning in a community college: The influence of task values on task choice, retention and commitment. (Doctoral dissertation, University of Southern California). Dissertation Abstracts International, 64 (9-A), 3191.
Naaj, M. A., Nachouki, M., & Ankit, A. (2012). Evaluating student satisfaction with blended learning in a gender-segregated environment. Journal of Information Technology Education: Research, 11 , 185–200.
Nurmela, K., Palonen, T., Lehtinen, E. & Hakkarainen, K. (2003). Developing tools for analysing CSCL process. In Wasson, B. Ludvigsen, S. & Hoppe, V. (eds), Designing for change in networked learning environments (pp 333–342). Dordrecht, The Netherlands, Kluwer.
Osgerby, J. (2013). Students’ perceptions of the introduction of a blended learning environment: An exploratory case study. Accounting Education, 22 (1), 85–99.
Oxford Group, (2013). Blended learning-current use, challenges and best practices. From http://www.kineo.com/m/0/blended-learning-report-202013.pdf . Accessed on 17 Mar 2016.
Packham, G., Jones, P., Miller, C., & Thomas, B. (2004). E-learning and retention key factors influencing student withdrawal. Education and Training, 46 (6–7), 335–342.
Pallant, J. (2010). SPSS Survival Mannual (4th ed.). Maidenhead: OUP McGraw-Hill.
Park, J.-H., & Choi, H. J. (2009). Factors influencing adult learners’ decision to drop out or persist in online learning. Educational Technology & Society, 12 (4), 207–217.
Picciano, A., & Seaman, J. (2007). K-12 online learning: A survey of U.S. school district administrators . New York, USA: Sloan-C.
Piccoli, G., Ahmad, R., & Ives, B. (2001). Web-based virtual learning environments: a research framework and a preliminary assessment of effectiveness in basic IT skill training. MIS Quarterly, 25 (4), 401–426.
Pituch, K. A., & Lee, Y. K. (2006). The influence of system characteristics on e-learning use. Computers & Education, 47 (2), 222–244.
Rahman, S. et al, (2011). Knowledge construction process in online learning. Middle East Journal of Scientific Research, 8 (2), 488–492.
Rovai, A. P. (2003). In search of higher persistence rates in distance education online programs. Computers & Education, 6 (1), 1–16.
Sankaran, S., & Bui, T. (2001). Impact of learning strategies and motivation on performance: A study in Web-based instruction. Journal of Instructional Psychology, 28 (3), 191–198.
Selim, H. M. (2007). Critical success factors for e-learning acceptance: Confirmatory factor models. Computers & Education, 49 (2), 396–413.
Shraim, K., & Khlaif, Z. N. (2010). An e-learning approach to secondary education in Palestine: opportunities and challenges. Information Technology for Development, 16 (3), 159–173.
Shrain, K. (2012). Moving towards e-learning paradigm: Readiness of higher education instructors in Palestine. International Journal on E-Learning, 11 (4), 441–463.
Song, L., Singleton, E. S., Hill, J. R., & Koh, M. H. (2004). Improving online learning: student perceptions of useful and challenging characteristics’. Internet and Higher Education, 7 (1), 59–70.
Stacey, E., & Gerbic, P. (2007). Teaching for blended learning: research perspectives from on-campus and distance students. Education and Information Technologies, 12 , 165–174.
Swan, K. (2001). Virtual interactivity: design factors affecting student satisfaction and perceived learning in asynchronous online courses. Distance Education, 22 (2), 306–331.
Article MathSciNet Google Scholar
Thompson, E. (2004). Distance education drop-out: What can we do? In R. Pospisil & L. Willcoxson (Eds.), Learning Through Teaching (Proceedings of the 6th Annual Teaching Learning Forum, pp. 324–332). Perth, Australia: Murdoch University.
Tselios, N., Daskalakis, S., & Papadopoulou, M. (2011). Assessing the acceptance of a blended learning university course. Educational Technology & Society, 14 (2), 224–235.
Willging, P. A., & Johnson, S. D. (2009). Factors that influence students’ decision to drop-out of online courses. Journal of Asynchronous Learning Networks, 13 (3), 115–127.
Zhu, C. (2012). Student satisfaction, performance and knowledge construction in online collaborative learning. Educational Technology & Society, 15 (1), 127–137.
Zielinski, D. (2000). Can you keep learners online? Training, 37 (3), 64–75.
Download references
Authors’ contribution
MJK conceived the study idea, developed the conceptual framework, collected the data, analyzed it and wrote the article. CZ gave the technical advice concerning the write-up and advised on relevant corrections to be made before final submission. EK did the proof-reading of the article as well as language editing. All authors read and approved the final manuscript.
Competing interests
The authors declare that they have no competing interests.
Author information
Authors and affiliations.
Mountains of the Moon University, P.O. Box 837, Fort Portal, Uganda
Mugenyi Justice Kintu & Edmond Kagambe
Vrije Universiteit Brussel, Pleinlaan 2, Brussels, 1050, Ixelles, Belgium
Mugenyi Justice Kintu & Chang Zhu
You can also search for this author in PubMed Google Scholar
Corresponding author
Correspondence to Mugenyi Justice Kintu .
Rights and permissions
Open Access This article is distributed under the terms of the Creative Commons Attribution 4.0 International License ( http://creativecommons.org/licenses/by/4.0/ ), which permits unrestricted use, distribution, and reproduction in any medium, provided you give appropriate credit to the original author(s) and the source, provide a link to the Creative Commons license, and indicate if changes were made.
Reprints and permissions
About this article
Cite this article.
Kintu, M.J., Zhu, C. & Kagambe, E. Blended learning effectiveness: the relationship between student characteristics, design features and outcomes. Int J Educ Technol High Educ 14 , 7 (2017). https://doi.org/10.1186/s41239-017-0043-4
Download citation
Received : 13 July 2016
Accepted : 23 November 2016
Published : 06 February 2017
DOI : https://doi.org/10.1186/s41239-017-0043-4
Share this article
Anyone you share the following link with will be able to read this content:
Sorry, a shareable link is not currently available for this article.
Provided by the Springer Nature SharedIt content-sharing initiative
- Blended learning effectiveness
- Learner characteristics
- Design features
- Learning outcomes and significant predictors
- Privacy Policy
Buy Me a Coffee

Home » Quantitative Research – Methods, Types and Analysis
Quantitative Research – Methods, Types and Analysis
Table of Contents

Quantitative Research
Quantitative research is a type of research that collects and analyzes numerical data to test hypotheses and answer research questions . This research typically involves a large sample size and uses statistical analysis to make inferences about a population based on the data collected. It often involves the use of surveys, experiments, or other structured data collection methods to gather quantitative data.
Quantitative Research Methods

Quantitative Research Methods are as follows:
Descriptive Research Design
Descriptive research design is used to describe the characteristics of a population or phenomenon being studied. This research method is used to answer the questions of what, where, when, and how. Descriptive research designs use a variety of methods such as observation, case studies, and surveys to collect data. The data is then analyzed using statistical tools to identify patterns and relationships.
Correlational Research Design
Correlational research design is used to investigate the relationship between two or more variables. Researchers use correlational research to determine whether a relationship exists between variables and to what extent they are related. This research method involves collecting data from a sample and analyzing it using statistical tools such as correlation coefficients.
Quasi-experimental Research Design
Quasi-experimental research design is used to investigate cause-and-effect relationships between variables. This research method is similar to experimental research design, but it lacks full control over the independent variable. Researchers use quasi-experimental research designs when it is not feasible or ethical to manipulate the independent variable.
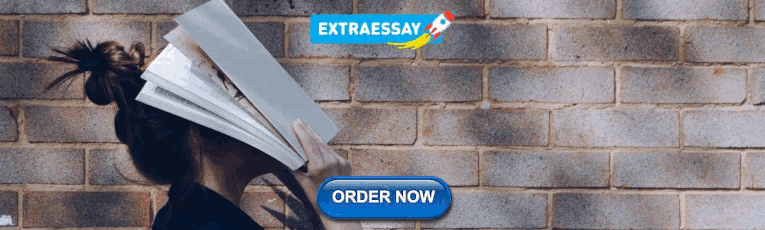
Experimental Research Design
Experimental research design is used to investigate cause-and-effect relationships between variables. This research method involves manipulating the independent variable and observing the effects on the dependent variable. Researchers use experimental research designs to test hypotheses and establish cause-and-effect relationships.
Survey Research
Survey research involves collecting data from a sample of individuals using a standardized questionnaire. This research method is used to gather information on attitudes, beliefs, and behaviors of individuals. Researchers use survey research to collect data quickly and efficiently from a large sample size. Survey research can be conducted through various methods such as online, phone, mail, or in-person interviews.
Quantitative Research Analysis Methods
Here are some commonly used quantitative research analysis methods:
Statistical Analysis
Statistical analysis is the most common quantitative research analysis method. It involves using statistical tools and techniques to analyze the numerical data collected during the research process. Statistical analysis can be used to identify patterns, trends, and relationships between variables, and to test hypotheses and theories.
Regression Analysis
Regression analysis is a statistical technique used to analyze the relationship between one dependent variable and one or more independent variables. Researchers use regression analysis to identify and quantify the impact of independent variables on the dependent variable.
Factor Analysis
Factor analysis is a statistical technique used to identify underlying factors that explain the correlations among a set of variables. Researchers use factor analysis to reduce a large number of variables to a smaller set of factors that capture the most important information.
Structural Equation Modeling
Structural equation modeling is a statistical technique used to test complex relationships between variables. It involves specifying a model that includes both observed and unobserved variables, and then using statistical methods to test the fit of the model to the data.
Time Series Analysis
Time series analysis is a statistical technique used to analyze data that is collected over time. It involves identifying patterns and trends in the data, as well as any seasonal or cyclical variations.
Multilevel Modeling
Multilevel modeling is a statistical technique used to analyze data that is nested within multiple levels. For example, researchers might use multilevel modeling to analyze data that is collected from individuals who are nested within groups, such as students nested within schools.
Applications of Quantitative Research
Quantitative research has many applications across a wide range of fields. Here are some common examples:
- Market Research : Quantitative research is used extensively in market research to understand consumer behavior, preferences, and trends. Researchers use surveys, experiments, and other quantitative methods to collect data that can inform marketing strategies, product development, and pricing decisions.
- Health Research: Quantitative research is used in health research to study the effectiveness of medical treatments, identify risk factors for diseases, and track health outcomes over time. Researchers use statistical methods to analyze data from clinical trials, surveys, and other sources to inform medical practice and policy.
- Social Science Research: Quantitative research is used in social science research to study human behavior, attitudes, and social structures. Researchers use surveys, experiments, and other quantitative methods to collect data that can inform social policies, educational programs, and community interventions.
- Education Research: Quantitative research is used in education research to study the effectiveness of teaching methods, assess student learning outcomes, and identify factors that influence student success. Researchers use experimental and quasi-experimental designs, as well as surveys and other quantitative methods, to collect and analyze data.
- Environmental Research: Quantitative research is used in environmental research to study the impact of human activities on the environment, assess the effectiveness of conservation strategies, and identify ways to reduce environmental risks. Researchers use statistical methods to analyze data from field studies, experiments, and other sources.
Characteristics of Quantitative Research
Here are some key characteristics of quantitative research:
- Numerical data : Quantitative research involves collecting numerical data through standardized methods such as surveys, experiments, and observational studies. This data is analyzed using statistical methods to identify patterns and relationships.
- Large sample size: Quantitative research often involves collecting data from a large sample of individuals or groups in order to increase the reliability and generalizability of the findings.
- Objective approach: Quantitative research aims to be objective and impartial in its approach, focusing on the collection and analysis of data rather than personal beliefs, opinions, or experiences.
- Control over variables: Quantitative research often involves manipulating variables to test hypotheses and establish cause-and-effect relationships. Researchers aim to control for extraneous variables that may impact the results.
- Replicable : Quantitative research aims to be replicable, meaning that other researchers should be able to conduct similar studies and obtain similar results using the same methods.
- Statistical analysis: Quantitative research involves using statistical tools and techniques to analyze the numerical data collected during the research process. Statistical analysis allows researchers to identify patterns, trends, and relationships between variables, and to test hypotheses and theories.
- Generalizability: Quantitative research aims to produce findings that can be generalized to larger populations beyond the specific sample studied. This is achieved through the use of random sampling methods and statistical inference.
Examples of Quantitative Research
Here are some examples of quantitative research in different fields:
- Market Research: A company conducts a survey of 1000 consumers to determine their brand awareness and preferences. The data is analyzed using statistical methods to identify trends and patterns that can inform marketing strategies.
- Health Research : A researcher conducts a randomized controlled trial to test the effectiveness of a new drug for treating a particular medical condition. The study involves collecting data from a large sample of patients and analyzing the results using statistical methods.
- Social Science Research : A sociologist conducts a survey of 500 people to study attitudes toward immigration in a particular country. The data is analyzed using statistical methods to identify factors that influence these attitudes.
- Education Research: A researcher conducts an experiment to compare the effectiveness of two different teaching methods for improving student learning outcomes. The study involves randomly assigning students to different groups and collecting data on their performance on standardized tests.
- Environmental Research : A team of researchers conduct a study to investigate the impact of climate change on the distribution and abundance of a particular species of plant or animal. The study involves collecting data on environmental factors and population sizes over time and analyzing the results using statistical methods.
- Psychology : A researcher conducts a survey of 500 college students to investigate the relationship between social media use and mental health. The data is analyzed using statistical methods to identify correlations and potential causal relationships.
- Political Science: A team of researchers conducts a study to investigate voter behavior during an election. They use survey methods to collect data on voting patterns, demographics, and political attitudes, and analyze the results using statistical methods.
How to Conduct Quantitative Research
Here is a general overview of how to conduct quantitative research:
- Develop a research question: The first step in conducting quantitative research is to develop a clear and specific research question. This question should be based on a gap in existing knowledge, and should be answerable using quantitative methods.
- Develop a research design: Once you have a research question, you will need to develop a research design. This involves deciding on the appropriate methods to collect data, such as surveys, experiments, or observational studies. You will also need to determine the appropriate sample size, data collection instruments, and data analysis techniques.
- Collect data: The next step is to collect data. This may involve administering surveys or questionnaires, conducting experiments, or gathering data from existing sources. It is important to use standardized methods to ensure that the data is reliable and valid.
- Analyze data : Once the data has been collected, it is time to analyze it. This involves using statistical methods to identify patterns, trends, and relationships between variables. Common statistical techniques include correlation analysis, regression analysis, and hypothesis testing.
- Interpret results: After analyzing the data, you will need to interpret the results. This involves identifying the key findings, determining their significance, and drawing conclusions based on the data.
- Communicate findings: Finally, you will need to communicate your findings. This may involve writing a research report, presenting at a conference, or publishing in a peer-reviewed journal. It is important to clearly communicate the research question, methods, results, and conclusions to ensure that others can understand and replicate your research.
When to use Quantitative Research
Here are some situations when quantitative research can be appropriate:
- To test a hypothesis: Quantitative research is often used to test a hypothesis or a theory. It involves collecting numerical data and using statistical analysis to determine if the data supports or refutes the hypothesis.
- To generalize findings: If you want to generalize the findings of your study to a larger population, quantitative research can be useful. This is because it allows you to collect numerical data from a representative sample of the population and use statistical analysis to make inferences about the population as a whole.
- To measure relationships between variables: If you want to measure the relationship between two or more variables, such as the relationship between age and income, or between education level and job satisfaction, quantitative research can be useful. It allows you to collect numerical data on both variables and use statistical analysis to determine the strength and direction of the relationship.
- To identify patterns or trends: Quantitative research can be useful for identifying patterns or trends in data. For example, you can use quantitative research to identify trends in consumer behavior or to identify patterns in stock market data.
- To quantify attitudes or opinions : If you want to measure attitudes or opinions on a particular topic, quantitative research can be useful. It allows you to collect numerical data using surveys or questionnaires and analyze the data using statistical methods to determine the prevalence of certain attitudes or opinions.
Purpose of Quantitative Research
The purpose of quantitative research is to systematically investigate and measure the relationships between variables or phenomena using numerical data and statistical analysis. The main objectives of quantitative research include:
- Description : To provide a detailed and accurate description of a particular phenomenon or population.
- Explanation : To explain the reasons for the occurrence of a particular phenomenon, such as identifying the factors that influence a behavior or attitude.
- Prediction : To predict future trends or behaviors based on past patterns and relationships between variables.
- Control : To identify the best strategies for controlling or influencing a particular outcome or behavior.
Quantitative research is used in many different fields, including social sciences, business, engineering, and health sciences. It can be used to investigate a wide range of phenomena, from human behavior and attitudes to physical and biological processes. The purpose of quantitative research is to provide reliable and valid data that can be used to inform decision-making and improve understanding of the world around us.
Advantages of Quantitative Research
There are several advantages of quantitative research, including:
- Objectivity : Quantitative research is based on objective data and statistical analysis, which reduces the potential for bias or subjectivity in the research process.
- Reproducibility : Because quantitative research involves standardized methods and measurements, it is more likely to be reproducible and reliable.
- Generalizability : Quantitative research allows for generalizations to be made about a population based on a representative sample, which can inform decision-making and policy development.
- Precision : Quantitative research allows for precise measurement and analysis of data, which can provide a more accurate understanding of phenomena and relationships between variables.
- Efficiency : Quantitative research can be conducted relatively quickly and efficiently, especially when compared to qualitative research, which may involve lengthy data collection and analysis.
- Large sample sizes : Quantitative research can accommodate large sample sizes, which can increase the representativeness and generalizability of the results.
Limitations of Quantitative Research
There are several limitations of quantitative research, including:
- Limited understanding of context: Quantitative research typically focuses on numerical data and statistical analysis, which may not provide a comprehensive understanding of the context or underlying factors that influence a phenomenon.
- Simplification of complex phenomena: Quantitative research often involves simplifying complex phenomena into measurable variables, which may not capture the full complexity of the phenomenon being studied.
- Potential for researcher bias: Although quantitative research aims to be objective, there is still the potential for researcher bias in areas such as sampling, data collection, and data analysis.
- Limited ability to explore new ideas: Quantitative research is often based on pre-determined research questions and hypotheses, which may limit the ability to explore new ideas or unexpected findings.
- Limited ability to capture subjective experiences : Quantitative research is typically focused on objective data and may not capture the subjective experiences of individuals or groups being studied.
- Ethical concerns : Quantitative research may raise ethical concerns, such as invasion of privacy or the potential for harm to participants.
About the author
Muhammad Hassan
Researcher, Academic Writer, Web developer
You may also like

Questionnaire – Definition, Types, and Examples

Case Study – Methods, Examples and Guide

Observational Research – Methods and Guide

Qualitative Research Methods

Explanatory Research – Types, Methods, Guide

Survey Research – Types, Methods, Examples
Advertisement

- Previous Issue
- Previous Article
- Next Article
Clarifying the Research Purpose
Methodology, measurement, data analysis and interpretation, tools for evaluating the quality of medical education research, research support, competing interests, quantitative research methods in medical education.
Submitted for publication January 8, 2018. Accepted for publication November 29, 2018.
- Split-Screen
- Article contents
- Figures & tables
- Supplementary Data
- Peer Review
- Open the PDF for in another window
- Cite Icon Cite
- Get Permissions
- Search Site
John T. Ratelle , Adam P. Sawatsky , Thomas J. Beckman; Quantitative Research Methods in Medical Education. Anesthesiology 2019; 131:23–35 doi: https://doi.org/10.1097/ALN.0000000000002727
Download citation file:
- Ris (Zotero)
- Reference Manager
There has been a dramatic growth of scholarly articles in medical education in recent years. Evaluating medical education research requires specific orientation to issues related to format and content. Our goal is to review the quantitative aspects of research in medical education so that clinicians may understand these articles with respect to framing the study, recognizing methodologic issues, and utilizing instruments for evaluating the quality of medical education research. This review can be used both as a tool when appraising medical education research articles and as a primer for clinicians interested in pursuing scholarship in medical education.

Image: J. P. Rathmell and Terri Navarette.
There has been an explosion of research in the field of medical education. A search of PubMed demonstrates that more than 40,000 articles have been indexed under the medical subject heading “Medical Education” since 2010, which is more than the total number of articles indexed under this heading in the 1980s and 1990s combined. Keeping up to date requires that practicing clinicians have the skills to interpret and appraise the quality of research articles, especially when serving as editors, reviewers, and consumers of the literature.
While medical education shares many characteristics with other biomedical fields, substantial particularities exist. We recognize that practicing clinicians may not be familiar with the nuances of education research and how to assess its quality. Therefore, our purpose is to provide a review of quantitative research methodologies in medical education. Specifically, we describe a structure that can be used when conducting or evaluating medical education research articles.
Clarifying the research purpose is an essential first step when reading or conducting scholarship in medical education. 1 Medical education research can serve a variety of purposes, from advancing the science of learning to improving the outcomes of medical trainees and the patients they care for. However, a well-designed study has limited value if it addresses vague, redundant, or unimportant medical education research questions.
What is the research topic and why is it important? What is unknown about the research topic? Why is further research necessary?
What is the conceptual framework being used to approach the study?
What is the statement of study intent?
What are the research methodology and study design? Are they appropriate for the study objective(s)?
Which threats to internal validity are most relevant for the study?
What is the outcome and how was it measured?
Can the results be trusted? What is the validity and reliability of the measurements?
How were research subjects selected? Is the research sample representative of the target population?
Was the data analysis appropriate for the study design and type of data?
What is the effect size? Do the results have educational significance?
Fortunately, there are steps to ensure that the purpose of a research study is clear and logical. Table 1 2–5 outlines these steps, which will be described in detail in the following sections. We describe these elements not as a simple “checklist,” but as an advanced organizer that can be used to understand a medical education research study. These steps can also be used by clinician educators who are new to the field of education research and who wish to conduct scholarship in medical education.
Steps in Clarifying the Purpose of a Research Study in Medical Education

Literature Review and Problem Statement
A literature review is the first step in clarifying the purpose of a medical education research article. 2 , 5 , 6 When conducting scholarship in medical education, a literature review helps researchers develop an understanding of their topic of interest. This understanding includes both existing knowledge about the topic as well as key gaps in the literature, which aids the researcher in refining their study question. Additionally, a literature review helps researchers identify conceptual frameworks that have been used to approach the research topic. 2
When reading scholarship in medical education, a successful literature review provides background information so that even someone unfamiliar with the research topic can understand the rationale for the study. Located in the introduction of the manuscript, the literature review guides the reader through what is already known in a manner that highlights the importance of the research topic. The literature review should also identify key gaps in the literature so the reader can understand the need for further research. This gap description includes an explicit problem statement that summarizes the important issues and provides a reason for the study. 2 , 4 The following is one example of a problem statement:
“Identifying gaps in the competency of anesthesia residents in time for intervention is critical to patient safety and an effective learning system… [However], few available instruments relate to complex behavioral performance or provide descriptors…that could inform subsequent feedback, individualized teaching, remediation, and curriculum revision.” 7
This problem statement articulates the research topic (identifying resident performance gaps), why it is important (to intervene for the sake of learning and patient safety), and current gaps in the literature (few tools are available to assess resident performance). The researchers have now underscored why further research is needed and have helped readers anticipate the overarching goals of their study (to develop an instrument to measure anesthesiology resident performance). 4
The Conceptual Framework
Following the literature review and articulation of the problem statement, the next step in clarifying the research purpose is to select a conceptual framework that can be applied to the research topic. Conceptual frameworks are “ways of thinking about a problem or a study, or ways of representing how complex things work.” 3 Just as clinical trials are informed by basic science research in the laboratory, conceptual frameworks often serve as the “basic science” that informs scholarship in medical education. At a fundamental level, conceptual frameworks provide a structured approach to solving the problem identified in the problem statement.
Conceptual frameworks may take the form of theories, principles, or models that help to explain the research problem by identifying its essential variables or elements. Alternatively, conceptual frameworks may represent evidence-based best practices that researchers can apply to an issue identified in the problem statement. 3 Importantly, there is no single best conceptual framework for a particular research topic, although the choice of a conceptual framework is often informed by the literature review and knowing which conceptual frameworks have been used in similar research. 8 For further information on selecting a conceptual framework for research in medical education, we direct readers to the work of Bordage 3 and Irby et al. 9
To illustrate how different conceptual frameworks can be applied to a research problem, suppose you encounter a study to reduce the frequency of communication errors among anesthesiology residents during day-to-night handoff. Table 2 10 , 11 identifies two different conceptual frameworks researchers might use to approach the task. The first framework, cognitive load theory, has been proposed as a conceptual framework to identify potential variables that may lead to handoff errors. 12 Specifically, cognitive load theory identifies the three factors that affect short-term memory and thus may lead to communication errors:
Conceptual Frameworks to Address the Issue of Handoff Errors in the Intensive Care Unit

Intrinsic load: Inherent complexity or difficulty of the information the resident is trying to learn ( e.g. , complex patients).
Extraneous load: Distractions or demands on short-term memory that are not related to the information the resident is trying to learn ( e.g. , background noise, interruptions).
Germane load: Effort or mental strategies used by the resident to organize and understand the information he/she is trying to learn ( e.g. , teach back, note taking).
Using cognitive load theory as a conceptual framework, researchers may design an intervention to reduce extraneous load and help the resident remember the overnight to-do’s. An example might be dedicated, pager-free handoff times where distractions are minimized.
The second framework identified in table 2 , the I-PASS (Illness severity, Patient summary, Action list, Situational awareness and contingency planning, and Synthesis by receiver) handoff mnemonic, 11 is an evidence-based best practice that, when incorporated as part of a handoff bundle, has been shown to reduce handoff errors on pediatric wards. 13 Researchers choosing this conceptual framework may adapt some or all of the I-PASS elements for resident handoffs in the intensive care unit.
Note that both of the conceptual frameworks outlined above provide researchers with a structured approach to addressing the issue of handoff errors; one is not necessarily better than the other. Indeed, it is possible for researchers to use both frameworks when designing their study. Ultimately, we provide this example to demonstrate the necessity of selecting conceptual frameworks to clarify the research purpose. 3 , 8 Readers should look for conceptual frameworks in the introduction section and should be wary of their omission, as commonly seen in less well-developed medical education research articles. 14
Statement of Study Intent
After reviewing the literature, articulating the problem statement, and selecting a conceptual framework to address the research topic, the final step in clarifying the research purpose is the statement of study intent. The statement of study intent is arguably the most important element of framing the study because it makes the research purpose explicit. 2 Consider the following example:
This study aimed to test the hypothesis that the introduction of the BASIC Examination was associated with an accelerated knowledge acquisition during residency training, as measured by increments in annual ITE scores. 15
This statement of study intent succinctly identifies several key study elements including the population (anesthesiology residents), the intervention/independent variable (introduction of the BASIC Examination), the outcome/dependent variable (knowledge acquisition, as measure by in In-training Examination [ITE] scores), and the hypothesized relationship between the independent and dependent variable (the authors hypothesize a positive correlation between the BASIC examination and the speed of knowledge acquisition). 6 , 14
The statement of study intent will sometimes manifest as a research objective, rather than hypothesis or question. In such instances there may not be explicit independent and dependent variables, but the study population and research aim should be clearly identified. The following is an example:
“In this report, we present the results of 3 [years] of course data with respect to the practice improvements proposed by participating anesthesiologists and their success in implementing those plans. Specifically, our primary aim is to assess the frequency and type of improvements that were completed and any factors that influence completion.” 16
The statement of study intent is the logical culmination of the literature review, problem statement, and conceptual framework, and is a transition point between the Introduction and Methods sections of a medical education research report. Nonetheless, a systematic review of experimental research in medical education demonstrated that statements of study intent are absent in the majority of articles. 14 When reading a medical education research article where the statement of study intent is absent, it may be necessary to infer the research aim by gathering information from the Introduction and Methods sections. In these cases, it can be useful to identify the following key elements 6 , 14 , 17 :
Population of interest/type of learner ( e.g. , pain medicine fellow or anesthesiology residents)
Independent/predictor variable ( e.g. , educational intervention or characteristic of the learners)
Dependent/outcome variable ( e.g. , intubation skills or knowledge of anesthetic agents)
Relationship between the variables ( e.g. , “improve” or “mitigate”)
Occasionally, it may be difficult to differentiate the independent study variable from the dependent study variable. 17 For example, consider a study aiming to measure the relationship between burnout and personal debt among anesthesiology residents. Do the researchers believe burnout might lead to high personal debt, or that high personal debt may lead to burnout? This “chicken or egg” conundrum reinforces the importance of the conceptual framework which, if present, should serve as an explanation or rationale for the predicted relationship between study variables.
Research methodology is the “…design or plan that shapes the methods to be used in a study.” 1 Essentially, methodology is the general strategy for answering a research question, whereas methods are the specific steps and techniques that are used to collect data and implement the strategy. Our objective here is to provide an overview of quantitative methodologies ( i.e. , approaches) in medical education research.
The choice of research methodology is made by balancing the approach that best answers the research question against the feasibility of completing the study. There is no perfect methodology because each has its own potential caveats, flaws and/or sources of bias. Before delving into an overview of the methodologies, it is important to highlight common sources of bias in education research. We use the term internal validity to describe the degree to which the findings of a research study represent “the truth,” as opposed to some alternative hypothesis or variables. 18 Table 3 18–20 provides a list of common threats to internal validity in medical education research, along with tactics to mitigate these threats.
Threats to Internal Validity and Strategies to Mitigate Their Effects

Experimental Research
The fundamental tenet of experimental research is the manipulation of an independent or experimental variable to measure its effect on a dependent or outcome variable.
True Experiment
True experimental study designs minimize threats to internal validity by randomizing study subjects to experimental and control groups. Through ensuring that differences between groups are—beyond the intervention/variable of interest—purely due to chance, researchers reduce the internal validity threats related to subject characteristics, time-related maturation, and regression to the mean. 18 , 19
Quasi-experiment
There are many instances in medical education where randomization may not be feasible or ethical. For instance, researchers wanting to test the effect of a new curriculum among medical students may not be able to randomize learners due to competing curricular obligations and schedules. In these cases, researchers may be forced to assign subjects to experimental and control groups based upon some other criterion beyond randomization, such as different classrooms or different sections of the same course. This process, called quasi-randomization, does not inherently lead to internal validity threats, as long as research investigators are mindful of measuring and controlling for extraneous variables between study groups. 19
Single-group Methodologies
All experimental study designs compare two or more groups: experimental and control. A common experimental study design in medical education research is the single-group pretest–posttest design, which compares a group of learners before and after the implementation of an intervention. 21 In essence, a single-group pre–post design compares an experimental group ( i.e. , postintervention) to a “no-intervention” control group ( i.e. , preintervention). 19 This study design is problematic for several reasons. Consider the following hypothetical example: A research article reports the effects of a year-long intubation curriculum for first-year anesthesiology residents. All residents participate in monthly, half-day workshops over the course of an academic year. The article reports a positive effect on residents’ skills as demonstrated by a significant improvement in intubation success rates at the end of the year when compared to the beginning.
This study does little to advance the science of learning among anesthesiology residents. While this hypothetical report demonstrates an improvement in residents’ intubation success before versus after the intervention, it does not tell why the workshop worked, how it compares to other educational interventions, or how it fits in to the broader picture of anesthesia training.
Single-group pre–post study designs open themselves to a myriad of threats to internal validity. 20 In our hypothetical example, the improvement in residents’ intubation skills may have been due to other educational experience(s) ( i.e. , implementation threat) and/or improvement in manual dexterity that occurred naturally with time ( i.e. , maturation threat), rather than the airway curriculum. Consequently, single-group pre–post studies should be interpreted with caution. 18
Repeated testing, before and after the intervention, is one strategy that can be used to reduce the some of the inherent limitations of the single-group study design. Repeated pretesting can mitigate the effect of regression toward the mean, a statistical phenomenon whereby low pretest scores tend to move closer to the mean on subsequent testing (regardless of intervention). 20 Likewise, repeated posttesting at multiple time intervals can provide potentially useful information about the short- and long-term effects of an intervention ( e.g. , the “durability” of the gain in knowledge, skill, or attitude).
Observational Research
Unlike experimental studies, observational research does not involve manipulation of any variables. These studies often involve measuring associations, developing psychometric instruments, or conducting surveys.
Association Research
Association research seeks to identify relationships between two or more variables within a group or groups (correlational research), or similarities/differences between two or more existing groups (causal–comparative research). For example, correlational research might seek to measure the relationship between burnout and educational debt among anesthesiology residents, while causal–comparative research may seek to measure differences in educational debt and/or burnout between anesthesiology and surgery residents. Notably, association research may identify relationships between variables, but does not necessarily support a causal relationship between them.
Psychometric and Survey Research
Psychometric instruments measure a psychologic or cognitive construct such as knowledge, satisfaction, beliefs, and symptoms. Surveys are one type of psychometric instrument, but many other types exist, such as evaluations of direct observation, written examinations, or screening tools. 22 Psychometric instruments are ubiquitous in medical education research and can be used to describe a trait within a study population ( e.g. , rates of depression among medical students) or to measure associations between study variables ( e.g. , association between depression and board scores among medical students).
Psychometric and survey research studies are prone to the internal validity threats listed in table 3 , particularly those relating to mortality, location, and instrumentation. 18 Additionally, readers must ensure that the instrument scores can be trusted to truly represent the construct being measured. For example, suppose you encounter a research article demonstrating a positive association between attending physician teaching effectiveness as measured by a survey of medical students, and the frequency with which the attending physician provides coffee and doughnuts on rounds. Can we be confident that this survey administered to medical students is truly measuring teaching effectiveness? Or is it simply measuring the attending physician’s “likability”? Issues related to measurement and the trustworthiness of data are described in detail in the following section on measurement and the related issues of validity and reliability.
Measurement refers to “the assigning of numbers to individuals in a systematic way as a means of representing properties of the individuals.” 23 Research data can only be trusted insofar as we trust the measurement used to obtain the data. Measurement is of particular importance in medical education research because many of the constructs being measured ( e.g. , knowledge, skill, attitudes) are abstract and subject to measurement error. 24 This section highlights two specific issues related to the trustworthiness of data: the validity and reliability of measurements.
Validity regarding the scores of a measurement instrument “refers to the degree to which evidence and theory support the interpretations of the [instrument’s results] for the proposed use of the [instrument].” 25 In essence, do we believe the results obtained from a measurement really represent what we were trying to measure? Note that validity evidence for the scores of a measurement instrument is separate from the internal validity of a research study. Several frameworks for validity evidence exist. Table 4 2 , 22 , 26 represents the most commonly used framework, developed by Messick, 27 which identifies sources of validity evidence—to support the target construct—from five main categories: content, response process, internal structure, relations to other variables, and consequences.
Sources of Validity Evidence for Measurement Instruments

Reliability
Reliability refers to the consistency of scores for a measurement instrument. 22 , 25 , 28 For an instrument to be reliable, we would anticipate that two individuals rating the same object of measurement in a specific context would provide the same scores. 25 Further, if the scores for an instrument are reliable between raters of the same object of measurement, then we can extrapolate that any difference in scores between two objects represents a true difference across the sample, and is not due to random variation in measurement. 29 Reliability can be demonstrated through a variety of methods such as internal consistency ( e.g. , Cronbach’s alpha), temporal stability ( e.g. , test–retest reliability), interrater agreement ( e.g. , intraclass correlation coefficient), and generalizability theory (generalizability coefficient). 22 , 29
Example of a Validity and Reliability Argument
This section provides an illustration of validity and reliability in medical education. We use the signaling questions outlined in table 4 to make a validity and reliability argument for the Harvard Assessment of Anesthesia Resident Performance (HARP) instrument. 7 The HARP was developed by Blum et al. to measure the performance of anesthesia trainees that is required to provide safe anesthetic care to patients. According to the authors, the HARP is designed to be used “…as part of a multiscenario, simulation-based assessment” of resident performance. 7
Content Validity: Does the Instrument’s Content Represent the Construct Being Measured?
To demonstrate content validity, instrument developers should describe the construct being measured and how the instrument was developed, and justify their approach. 25 The HARP is intended to measure resident performance in the critical domains required to provide safe anesthetic care. As such, investigators note that the HARP items were created through a two-step process. First, the instrument’s developers interviewed anesthesiologists with experience in resident education to identify the key traits needed for successful completion of anesthesia residency training. Second, the authors used a modified Delphi process to synthesize the responses into five key behaviors: (1) formulate a clear anesthetic plan, (2) modify the plan under changing conditions, (3) communicate effectively, (4) identify performance improvement opportunities, and (5) recognize one’s limits. 7 , 30
Response Process Validity: Are Raters Interpreting the Instrument Items as Intended?
In the case of the HARP, the developers included a scoring rubric with behavioral anchors to ensure that faculty raters could clearly identify how resident performance in each domain should be scored. 7
Internal Structure Validity: Do Instrument Items Measuring Similar Constructs Yield Homogenous Results? Do Instrument Items Measuring Different Constructs Yield Heterogeneous Results?
Item-correlation for the HARP demonstrated a high degree of correlation between some items ( e.g. , formulating a plan and modifying the plan under changing conditions) and a lower degree of correlation between other items ( e.g. , formulating a plan and identifying performance improvement opportunities). 30 This finding is expected since the items within the HARP are designed to assess separate performance domains, and we would expect residents’ functioning to vary across domains.
Relationship to Other Variables’ Validity: Do Instrument Scores Correlate with Other Measures of Similar or Different Constructs as Expected?
As it applies to the HARP, one would expect that the performance of anesthesia residents will improve over the course of training. Indeed, HARP scores were found to be generally higher among third-year residents compared to first-year residents. 30
Consequence Validity: Are Instrument Results Being Used as Intended? Are There Unintended or Negative Uses of the Instrument Results?
While investigators did not intentionally seek out consequence validity evidence for the HARP, unanticipated consequences of HARP scores were identified by the authors as follows:
“Data indicated that CA-3s had a lower percentage of worrisome scores (rating 2 or lower) than CA-1s… However, it is concerning that any CA-3s had any worrisome scores…low performance of some CA-3 residents, albeit in the simulated environment, suggests opportunities for training improvement.” 30
That is, using the HARP to measure the performance of CA-3 anesthesia residents had the unintended consequence of identifying the need for improvement in resident training.
Reliability: Are the Instrument’s Scores Reproducible and Consistent between Raters?
The HARP was applied by two raters for every resident in the study across seven different simulation scenarios. The investigators conducted a generalizability study of HARP scores to estimate the variance in assessment scores that was due to the resident, the rater, and the scenario. They found little variance was due to the rater ( i.e. , scores were consistent between raters), indicating a high level of reliability. 7
Sampling refers to the selection of research subjects ( i.e. , the sample) from a larger group of eligible individuals ( i.e. , the population). 31 Effective sampling leads to the inclusion of research subjects who represent the larger population of interest. Alternatively, ineffective sampling may lead to the selection of research subjects who are significantly different from the target population. Imagine that researchers want to explore the relationship between burnout and educational debt among pain medicine specialists. The researchers distribute a survey to 1,000 pain medicine specialists (the population), but only 300 individuals complete the survey (the sample). This result is problematic because the characteristics of those individuals who completed the survey and the entire population of pain medicine specialists may be fundamentally different. It is possible that the 300 study subjects may be experiencing more burnout and/or debt, and thus, were more motivated to complete the survey. Alternatively, the 700 nonresponders might have been too busy to respond and even more burned out than the 300 responders, which would suggest that the study findings were even more amplified than actually observed.
When evaluating a medical education research article, it is important to identify the sampling technique the researchers employed, how it might have influenced the results, and whether the results apply to the target population. 24
Sampling Techniques
Sampling techniques generally fall into two categories: probability- or nonprobability-based. Probability-based sampling ensures that each individual within the target population has an equal opportunity of being selected as a research subject. Most commonly, this is done through random sampling, which should lead to a sample of research subjects that is similar to the target population. If significant differences between sample and population exist, those differences should be due to random chance, rather than systematic bias. The difference between data from a random sample and that from the population is referred to as sampling error. 24
Nonprobability-based sampling involves selecting research participants such that inclusion of some individuals may be more likely than the inclusion of others. 31 Convenience sampling is one such example and involves selection of research subjects based upon ease or opportuneness. Convenience sampling is common in medical education research, but, as outlined in the example at the beginning of this section, it can lead to sampling bias. 24 When evaluating an article that uses nonprobability-based sampling, it is important to look for participation/response rate. In general, a participation rate of less than 75% should be viewed with skepticism. 21 Additionally, it is important to determine whether characteristics of participants and nonparticipants were reported and if significant differences between the two groups exist.
Interpreting medical education research requires a basic understanding of common ways in which quantitative data are analyzed and displayed. In this section, we highlight two broad topics that are of particular importance when evaluating research articles.
The Nature of the Measurement Variable
Measurement variables in quantitative research generally fall into three categories: nominal, ordinal, or interval. 24 Nominal variables (sometimes called categorical variables) involve data that can be placed into discrete categories without a specific order or structure. Examples include sex (male or female) and professional degree (M.D., D.O., M.B.B.S., etc .) where there is no clear hierarchical order to the categories. Ordinal variables can be ranked according to some criterion, but the spacing between categories may not be equal. Examples of ordinal variables may include measurements of satisfaction (satisfied vs . unsatisfied), agreement (disagree vs . agree), and educational experience (medical student, resident, fellow). As it applies to educational experience, it is noteworthy that even though education can be quantified in years, the spacing between years ( i.e. , educational “growth”) remains unequal. For instance, the difference in performance between second- and third-year medical students is dramatically different than third- and fourth-year medical students. Interval variables can also be ranked according to some criteria, but, unlike ordinal variables, the spacing between variable categories is equal. Examples of interval variables include test scores and salary. However, the conceptual boundaries between these measurement variables are not always clear, as in the case where ordinal scales can be assumed to have the properties of an interval scale, so long as the data’s distribution is not substantially skewed. 32
Understanding the nature of the measurement variable is important when evaluating how the data are analyzed and reported. Medical education research commonly uses measurement instruments with items that are rated on Likert-type scales, whereby the respondent is asked to assess their level of agreement with a given statement. The response is often translated into a corresponding number ( e.g. , 1 = strongly disagree, 3 = neutral, 5 = strongly agree). It is remarkable that scores from Likert-type scales are sometimes not normally distributed ( i.e. , are skewed toward one end of the scale), indicating that the spacing between scores is unequal and the variable is ordinal in nature. In these cases, it is recommended to report results as frequencies or medians, rather than means and SDs. 33
Consider an article evaluating medical students’ satisfaction with a new curriculum. Researchers measure satisfaction using a Likert-type scale (1 = very unsatisfied, 2 = unsatisfied, 3 = neutral, 4 = satisfied, 5 = very satisfied). A total of 20 medical students evaluate the curriculum, 10 of whom rate their satisfaction as “satisfied,” and 10 of whom rate it as “very satisfied.” In this case, it does not make much sense to report an average score of 4.5; it makes more sense to report results in terms of frequency ( e.g. , half of the students were “very satisfied” with the curriculum, and half were not).
Effect Size and CIs
In medical education, as in other research disciplines, it is common to report statistically significant results ( i.e. , small P values) in order to increase the likelihood of publication. 34 , 35 However, a significant P value in itself does necessarily represent the educational impact of the study results. A statement like “Intervention x was associated with a significant improvement in learners’ intubation skill compared to education intervention y ( P < 0.05)” tells us that there was a less than 5% chance that the difference in improvement between interventions x and y was due to chance. Yet that does not mean that the study intervention necessarily caused the nonchance results, or indicate whether the between-group difference is educationally significant. Therefore, readers should consider looking beyond the P value to effect size and/or CI when interpreting the study results. 36 , 37
Effect size is “the magnitude of the difference between two groups,” which helps to quantify the educational significance of the research results. 37 Common measures of effect size include Cohen’s d (standardized difference between two means), risk ratio (compares binary outcomes between two groups), and Pearson’s r correlation (linear relationship between two continuous variables). 37 CIs represent “a range of values around a sample mean or proportion” and are a measure of precision. 31 While effect size and CI give more useful information than simple statistical significance, they are commonly omitted from medical education research articles. 35 In such instances, readers should be wary of overinterpreting a P value in isolation. For further information effect size and CI, we direct readers the work of Sullivan and Feinn 37 and Hulley et al. 31
In this final section, we identify instruments that can be used to evaluate the quality of quantitative medical education research articles. To this point, we have focused on framing the study and research methodologies and identifying potential pitfalls to consider when appraising a specific article. This is important because how a study is framed and the choice of methodology require some subjective interpretation. Fortunately, there are several instruments available for evaluating medical education research methods and providing a structured approach to the evaluation process.
The Medical Education Research Study Quality Instrument (MERSQI) 21 and the Newcastle Ottawa Scale-Education (NOS-E) 38 are two commonly used instruments, both of which have an extensive body of validity evidence to support the interpretation of their scores. Table 5 21 , 39 provides more detail regarding the MERSQI, which includes evaluation of study design, sampling, data type, validity, data analysis, and outcomes. We have found that applying the MERSQI to manuscripts, articles, and protocols has intrinsic educational value, because this practice of application familiarizes MERSQI users with fundamental principles of medical education research. One aspect of the MERSQI that deserves special mention is the section on evaluating outcomes based on Kirkpatrick’s widely recognized hierarchy of reaction, learning, behavior, and results ( table 5 ; fig .). 40 Validity evidence for the scores of the MERSQI include its operational definitions to improve response process, excellent reliability, and internal consistency, as well as high correlation with other measures of study quality, likelihood of publication, citation rate, and an association between MERSQI score and the likelihood of study funding. 21 , 41 Additionally, consequence validity for the MERSQI scores has been demonstrated by its utility for identifying and disseminating high-quality research in medical education. 42

Kirkpatrick’s hierarchy of outcomes as applied to education research. Reaction = Level 1, Learning = Level 2, Behavior = Level 3, Results = Level 4. Outcomes become more meaningful, yet more difficult to achieve, when progressing from Level 1 through Level 4. Adapted with permission from Beckman and Cook, 2007. 2
The Medical Education Research Study Quality Instrument for Evaluating the Quality of Medical Education Research

The NOS-E is a newer tool to evaluate the quality of medication education research. It was developed as a modification of the Newcastle-Ottawa Scale 43 for appraising the quality of nonrandomized studies. The NOS-E includes items focusing on the representativeness of the experimental group, selection and compatibility of the control group, missing data/study retention, and blinding of outcome assessors. 38 , 39 Additional validity evidence for NOS-E scores includes operational definitions to improve response process, excellent reliability and internal consistency, and its correlation with other measures of study quality. 39 Notably, the complete NOS-E, along with its scoring rubric, can found in the article by Cook and Reed. 39
A recent comparison of the MERSQI and NOS-E found acceptable interrater reliability and good correlation between the two instruments 39 However, noted differences exist between the MERSQI and NOS-E. Specifically, the MERSQI may be applied to a broad range of study designs, including experimental and cross-sectional research. Additionally, the MERSQI addresses issues related to measurement validity and data analysis, and places emphasis on educational outcomes. On the other hand, the NOS-E focuses specifically on experimental study designs, and on issues related to sampling techniques and outcome assessment. 39 Ultimately, the MERSQI and NOS-E are complementary tools that may be used together when evaluating the quality of medical education research.
Conclusions
This article provides an overview of quantitative research in medical education, underscores the main components of education research, and provides a general framework for evaluating research quality. We highlighted the importance of framing a study with respect to purpose, conceptual framework, and statement of study intent. We reviewed the most common research methodologies, along with threats to the validity of a study and its measurement instruments. Finally, we identified two complementary instruments, the MERSQI and NOS-E, for evaluating the quality of a medical education research study.
Bordage G: Conceptual frameworks to illuminate and magnify. Medical education. 2009; 43(4):312–9.
Cook DA, Beckman TJ: Current concepts in validity and reliability for psychometric instruments: Theory and application. The American journal of medicine. 2006; 119(2):166. e7–166. e116.
Franenkel JR, Wallen NE, Hyun HH: How to Design and Evaluate Research in Education. 9th edition. New York, McGraw-Hill Education, 2015.
Hulley SB, Cummings SR, Browner WS, Grady DG, Newman TB: Designing clinical research. 4th edition. Philadelphia, Lippincott Williams & Wilkins, 2011.
Irby BJ, Brown G, Lara-Alecio R, Jackson S: The Handbook of Educational Theories. Charlotte, NC, Information Age Publishing, Inc., 2015
Standards for Educational and Psychological Testing (American Educational Research Association & American Psychological Association, 2014)
Swanwick T: Understanding medical education: Evidence, theory and practice, 2nd edition. Wiley-Blackwell, 2013.
Sullivan GM, Artino Jr AR: Analyzing and interpreting data from Likert-type scales. Journal of graduate medical education. 2013; 5(4):541–2.
Sullivan GM, Feinn R: Using effect size—or why the P value is not enough. Journal of graduate medical education. 2012; 4(3):279–82.
Tavakol M, Sandars J: Quantitative and qualitative methods in medical education research: AMEE Guide No 90: Part II. Medical teacher. 2014; 36(10):838–48.
Support was provided solely from institutional and/or departmental sources.
The authors declare no competing interests.
Citing articles via
Most viewed, email alerts, related articles, social media, affiliations.
- ASA Practice Parameters
- Online First
- Author Resource Center
- About the Journal
- Editorial Board
- Rights & Permissions
- Online ISSN 1528-1175
- Print ISSN 0003-3022
- Anesthesiology
- ASA Monitor

- Terms & Conditions Privacy Policy
- Manage Cookie Preferences
- © Copyright 2024 American Society of Anesthesiologists
This Feature Is Available To Subscribers Only
Sign In or Create an Account
Study Tracks Shifts in Student Mental Health During College
Dartmouth study followed 200 students all four years, including through the pandemic.
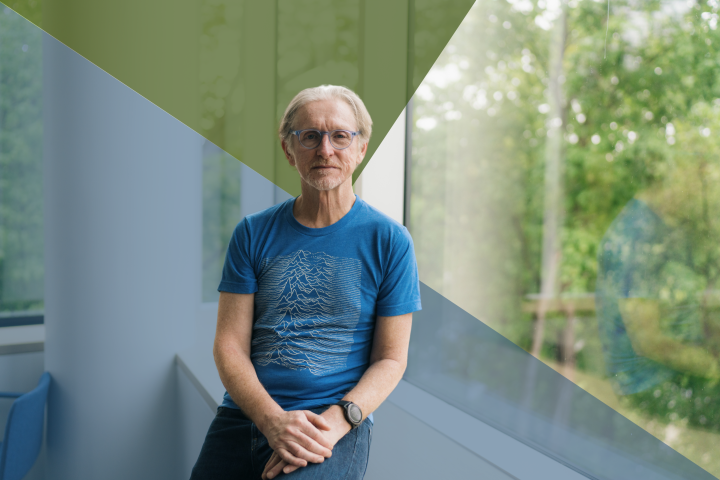
Phone App Uses AI to Detect Depression From Facial Cues
A four-year study by Dartmouth researchers captures the most in-depth data yet on how college students’ self-esteem and mental health fluctuates during their four years in academia, identifying key populations and stressors that the researchers say administrators could target to improve student well-being.
The study also provides among the first real-time accounts of how the coronavirus pandemic affected students’ behavior and mental health. The stress and uncertainty of COVID-19 resulted in long-lasting behavioral changes that persisted as a “new normal” even as the pandemic diminished, including students feeling more stressed, less socially engaged, and sleeping more.
The researchers tracked more than 200 Dartmouth undergraduates in the classes of 2021 and 2022 for all four years of college. Students volunteered to let a specially developed app called StudentLife tap into the sensors that are built into smartphones. The app cataloged their daily physical and social activity, how long they slept, their location and travel, the time they spent on their phone, and how often they listened to music or watched videos. Students also filled out weekly behavioral surveys, and selected students gave post-study interviews.
The study—which is the longest mobile-sensing study ever conducted—is published in the Proceedings of the ACM on Interactive, Mobile, Wearable and Ubiquitous Technologies .
The researchers will present it at the Association of Computing Machinery’s UbiComp/ISWC 2024 conference in Melbourne, Australia, in October.
These sorts of tools will have a tremendous impact on projecting forward and developing much more data-driven ways to intervene and respond exactly when students need it most.
The team made their anonymized data set publicly available —including self-reports, surveys, and phone-sensing and brain-imaging data—to help advance research into the mental health of students during their college years.
Andrew Campbell , the paper’s senior author and Dartmouth’s Albert Bradley 1915 Third Century Professor of Computer Science, says that the study’s extensive data reinforces the importance of college and university administrators across the country being more attuned to how and when students’ mental well-being changes during the school year.
“For the first time, we’ve produced granular data about the ebb and flow of student mental health. It’s incredibly dynamic—there’s nothing that’s steady state through the term, let alone through the year,” he says. “These sorts of tools will have a tremendous impact on projecting forward and developing much more data-driven ways to intervene and respond exactly when students need it most.”
First-year and female students are especially at risk for high anxiety and low self-esteem, the study finds. Among first-year students, self-esteem dropped to its lowest point in the first weeks of their transition from high school to college but rose steadily every semester until it was about 10% higher by graduation.
“We can see that students came out of high school with a certain level of self-esteem that dropped off to the lowest point of the four years. Some said they started to experience ‘imposter syndrome’ from being around other high-performing students,” Campbell says. “As the years progress, though, we can draw a straight line from low to high as their self-esteem improves. I think we would see a similar trend class over class. To me, that’s a very positive thing.”
Female students—who made up 60% of study participants—experienced on average 5% greater stress levels and 10% lower self-esteem than male students. More significantly, the data show that female students tended to be less active, with male students walking 37% more often.
Sophomores were 40% more socially active compared to their first year, the researchers report. But these students also reported feeling 13% more stressed during their second year than during their first year as their workload increased, they felt pressure to socialize, or as first-year social groups dispersed.
One student in a sorority recalled that having pre-arranged activities “kind of adds stress as I feel like I should be having fun because everyone tells me that it is fun.” Another student noted that after the first year, “students have more access to the whole campus and that is when you start feeling excluded from things.”
In a novel finding, the researchers identify an “anticipatory stress spike” of 17% experienced in the last two weeks of summer break. While still lower than mid-academic year stress, the spike was consistent across different summers.
In post-study interviews, some students pointed to returning to campus early for team sports as a source of stress. Others specified reconnecting with family and high school friends during their first summer home, saying they felt “a sense of leaving behind the comfort and familiarity of these long-standing friendships” as the break ended, the researchers report.
“This is a foundational study,” says Subigya Nepal , first author of the study and a PhD candidate in Campbell’s research group. “It has more real-time granular data than anything we or anyone else has provided before. We don’t know yet how it will translate to campuses nationwide, but it can be a template for getting the conversation going.”
The depth and accuracy of the study data suggest that mobile-sensing software could eventually give universities the ability to create proactive mental-health policies specific to certain student populations and times of year, Campbell says.
For example, a paper Campbell’s research group published in 2022 based on StudentLife data showed that first-generation students experienced lower self-esteem and higher levels of depression than other students throughout their four years of college.
“We will be able to look at campus in much more nuanced ways than waiting for the results of an annual mental health study and then developing policy,” Campbell says. “We know that Dartmouth is a small and very tight-knit campus community. But if we applied these same methods to a college with similar attributes, I believe we would find very similar trends.”
Weathering the pandemic
When students returned home at the start of the coronavirus pandemic, the researchers found that self-esteem actually increased during the pandemic by 5% overall and by another 6% afterward when life returned closer to what it was before. One student suggested in their interview that getting older came with more confidence. Others indicated that being home led to them spending more time with friends talking on the phone, on social media, or streaming movies together.
The data show that phone usage—measured by the duration a phone was unlocked—indeed increased by nearly 33 minutes, or 19%, during the pandemic, while time spent in physical activity dropped by 52 minutes, or 27%. By 2022, phone usage fell from its pandemic peak to just above pre-pandemic levels, while engagement in physical activity had recovered to exceed the pre-pandemic period by three minutes.
Despite reporting higher self-esteem, students’ feelings of stress increased by more than 10% during the pandemic. By the end of the study in June 2022, stress had fallen by less than 2% of its pandemic peak, indicating that the experience had a lasting impact on student well-being, the researchers report.
In early 2021, as students returned to campus, their reunion with friends and community was tempered by an overwhelming concern about the still-rampant coronavirus. “There was the first outbreak in winter 2021 and that was terrifying,” one student recalls. Another student adds: “You could be put into isolation for a long time even if you did not have COVID. Everyone was afraid to contact-trace anyone else in case they got mad at each other.”
Female students were especially concerned about the coronavirus, on average 13% more than male students. “Even though the girls might have been hanging out with each other more, they are more aware of the impact,” one female student reported. “I actually had COVID and exposed some friends of mine. All the girls that I told tested as they were worried. They were continually checking up to make sure that they did not have it and take it home to their family.”
Students still learning remotely had social levels 16% higher than students on campus, who engaged in activity an average of 10% less often than when they were learning from home. However, on-campus students used their phones 47% more often. When interviewed after the study, these students reported spending extended periods of time video-calling or streaming movies with friends and family.
Social activity and engagement had not yet returned to pre-pandemic levels by the end of the study in June 2022, recovering by a little less than 3% after a nearly 10% drop during the pandemic. Similarly, the pandemic correlates with students sticking closer to home, with their distance traveled nearly cut in half during the pandemic and holding at that level since then.
Campbell and several of his fellow researchers are now developing a smartphone app known as MoodCapture that uses artificial intelligence paired with facial-image processing software to reliably detect the onset of depression before the user even knows something is wrong.
Morgan Kelly can be reached at [email protected] .
- Mental Health and Wellness
- Innovation and Impact
- Arts and Sciences
- Class of 2021
- Class of 2022
- Department of Computer Science
- Guarini School of Graduate and Advanced Studies
- Mental Health
Dartmouth Symposium Highlights AI’s Innovations in Medicine
It’s conspiratorial nonsense.
Research Articles
Vol. 28 No. 1
Amplifying Community Partner Voices in Rural Community Service-Learning Partnerships
- Lauren R. Paulson
- Caitlyn Davis
This mixed-methods study delves into rural community service-learning (CSL) partnerships, shedding light on the complexities and dynamics of collaboration between colleges and rural communities. Through quantitative surveys and qualitative interviews, the research amplifies the voices of rural community partners, emphasizing the crucial role of trust, communication, and reciprocity. Challenges such as staff demands and organizational mismatches underscore the need for rural institutions to better prepare students and allocate resources to support their community partners effectively. The study advocates for transformative CSL approaches that prioritize community needs and nurture long-lasting collaborations. By providing insights into the impact of CSL on rural partners and organizations, this research offers valuable recommendations for improving future practices and fostering meaningful engagement in both rural and urban settings.
Login Register
Forgot your password?

Medical students’ and educators’ opinions of teleconsultation in practice and undergraduate education: a UK-based mixed-methods study
- Find this author on Google Scholar
- Find this author on PubMed
- Search for this author on this site
- ORCID record for Lisa-Christin Wetzlmair
- ORCID record for Andrew O’Malley
- For correspondence: [email protected]
- Info/History
- Preview PDF
Introduction As information and communication technology continues to shape the healthcare landscape, future medical practitioners need to be equipped with skills and competencies that ensure safe, high-quality, and person-centred healthcare in a digitised healthcare system. This study investigated undergraduate medical students’ and medical educators’ opinions of teleconsultation practice in general and their opinions of teleconsultation education.
Methods This study used a cross-sectional, mixed-methods approach, utilising the additional coverage design to sequence and integrate qualitative and quantitative data. An online questionnaire was sent out to all medical schools in the UK, inviting undergraduate medical students and medical educators to participate. Questionnaire participants were given the opportunity to take part in a qualitative semi-structured interview. Descriptive and correlation analyses and a thematic analysis were conducted.
Results A total of 248 participants completed the questionnaire and 23 interviews were conducted. Saving time and the reduced risks of transmitting infectious diseases were identified as common advantages of using teleconsultation. However, concerns about confidentiality and accessibility to services were expressed by students and educators. Eight themes were identified from the thematic analysis. The themes relevant to teleconsultation practice were (1) The benefit of teleconsultations, (2) A second-best option, (3) Patient choice, (4) Teleconsultations differ from in-person interactions, and (5) Impact on the healthcare system. The themes relevant to teleconsultation education were (6) Considerations and reflections on required skills, (7) Learning and teaching content, and (8) The future of teleconsultation education.
Discussion The results of this study have implications for both medical practice and education. Patient confidentiality, safety, respecting patients’ preferences, and accessibility are important considerations for implementing teleconsultations in practice. Education should focus on assessing the appropriateness of teleconsultations, offering accessible and equal care, and developing skills for effective communication and clinical reasoning. High-quality teleconsultation education can influence teleconsultation practice.
Competing Interest Statement
The authors have declared no competing interest.
Funding Statement
The author(s) received no specific funding for this work.
Author Declarations
I confirm all relevant ethical guidelines have been followed, and any necessary IRB and/or ethics committee approvals have been obtained.
The details of the IRB/oversight body that provided approval or exemption for the research described are given below:
Data collection was initiated after the initial ethics approval received from the School of Medicine at the University of St Andrews (approval code MD15263). Additional approval and permission to undertake the research was provided by the UK Medical Schools Council (MSC), and individual UK medical schools upon request.
I confirm that all necessary patient/participant consent has been obtained and the appropriate institutional forms have been archived, and that any patient/participant/sample identifiers included were not known to anyone (e.g., hospital staff, patients or participants themselves) outside the research group so cannot be used to identify individuals.
I understand that all clinical trials and any other prospective interventional studies must be registered with an ICMJE-approved registry, such as ClinicalTrials.gov. I confirm that any such study reported in the manuscript has been registered and the trial registration ID is provided (note: if posting a prospective study registered retrospectively, please provide a statement in the trial ID field explaining why the study was not registered in advance).
I have followed all appropriate research reporting guidelines, such as any relevant EQUATOR Network research reporting checklist(s) and other pertinent material, if applicable.
Data Availability
Research data underpinning the PhD thesis are available from 9 May 2028 at https://doi.org/10.17630/84eb74f3-e316-4618-a112-19b2f24377ac
https://doi.org/10.17630/84eb74f3-e316-4618-a112-19b2f24377ac
View the discussion thread.
Thank you for your interest in spreading the word about medRxiv.
NOTE: Your email address is requested solely to identify you as the sender of this article.

Citation Manager Formats
- EndNote (tagged)
- EndNote 8 (xml)
- RefWorks Tagged
- Ref Manager
- Tweet Widget
- Facebook Like
- Google Plus One
Subject Area
- Medical Education
- Addiction Medicine (316)
- Allergy and Immunology (617)
- Anesthesia (159)
- Cardiovascular Medicine (2274)
- Dentistry and Oral Medicine (279)
- Dermatology (201)
- Emergency Medicine (369)
- Endocrinology (including Diabetes Mellitus and Metabolic Disease) (798)
- Epidemiology (11572)
- Forensic Medicine (10)
- Gastroenterology (677)
- Genetic and Genomic Medicine (3575)
- Geriatric Medicine (336)
- Health Economics (615)
- Health Informatics (2303)
- Health Policy (913)
- Health Systems and Quality Improvement (863)
- Hematology (335)
- HIV/AIDS (751)
- Infectious Diseases (except HIV/AIDS) (13147)
- Intensive Care and Critical Care Medicine (755)
- Medical Education (359)
- Medical Ethics (100)
- Nephrology (388)
- Neurology (3346)
- Nursing (191)
- Nutrition (506)
- Obstetrics and Gynecology (651)
- Occupational and Environmental Health (644)
- Oncology (1756)
- Ophthalmology (524)
- Orthopedics (209)
- Otolaryngology (284)
- Pain Medicine (223)
- Palliative Medicine (66)
- Pathology (437)
- Pediatrics (1001)
- Pharmacology and Therapeutics (422)
- Primary Care Research (405)
- Psychiatry and Clinical Psychology (3056)
- Public and Global Health (5983)
- Radiology and Imaging (1221)
- Rehabilitation Medicine and Physical Therapy (713)
- Respiratory Medicine (810)
- Rheumatology (367)
- Sexual and Reproductive Health (350)
- Sports Medicine (315)
- Surgery (386)
- Toxicology (50)
- Transplantation (170)
- Urology (142)
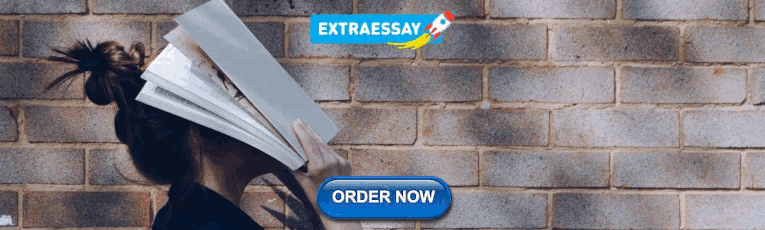
IMAGES
VIDEO
COMMENTS
The. quantitative research methods in education emphasise basic group designs. for research and evaluation, analytic metho ds for exploring re lationships. between categorical and continuous ...
In this study. we aim to analyze the advantages and limitations of homework, based on questionnaires survey. that measure teachers' perception of the importance, volume, typology, purposes, degree ...
The purpose of this quantitative study was to investigate the relationship between certain types of school structures and the effectiveness of teacher leaders. The study focused on teachers who lead from within their classrooms, as opposed to those who have left the classroom to take on different responsibilities. The types of school structures
Introduction. The field of education has embraced quantitative research designs since early in the 20th century. The foundation for these designs was based primarily in the psychological literature, and psychology and the social sciences more generally continued to have a strong influence on quantitative designs until the assimilation of qualitative designs in the 1970s and 1980s.
Quantitative research in the social sciences is undergoing a change. After years of scholarship on the oppressive history of quantitative methods, quantitative scholars are grappling with the ways that our preferred methodology reinforces social injustices (Zuberi, 2001).Among others, the emerging fields of CritQuant (critical quantitative studies) and QuantCrit (quantitative critical race ...
Educational research has a strong tradition of employing state-of-the-art statistical and psychometric (psychological measurement) techniques. Commonly referred to as quantitative methods, these techniques cover a range of statistical tests and tools. The Sage encyclopedia of educational research, measurement, and evaluation by Bruce B. Frey (Ed.)
2 DOING QUANTITATIVE RESEARCH IN EDUCATION WITH SPSS 8725 AR.qxd 25/08/2010 16:36 Page 2. seen as the most important part of quantitative studies. This is a bit of a misconception, as, while using the right data analysis tools obviously mat- ... studies, ethnographic research) which are quite different, they are used by researchers with quite ...
Features. Preview. "The book provides a reference point for beginning educational researchers to grasp the most pertinent elements of designing and conducting research…". —Megan Tschannen-Moran, The College of William & Mary. Quantitative Research in Education: A Primer, Second Edition is a brief and practical text designed to allay ...
David Gibson (Ed.) Publication Date: 2020. The book aims to advance global knowledge and practice in applying data science to transform higher education learning and teaching to improve personalization, access and effectiveness of education for all. Currently, higher education institutions and involved stakeholders can derive multiple benefits ...
This book presents a clear and straightforward guide for all those seeking to conduct quantitative research in the field of education, using primary research data samples. It provides educational researchers with the tools they can work with to achieve results efficiently.
Research in higher education. "Research in Higher Education publishes studies that examine issues pertaining to postsecondary education. The journal is open to studies using a wide range of methods, but has particular interest in studies that apply advanced quantitative research methods to issues in postsecondary education or address ...
This chapter starts from the observation that there is a limited presence of quantitative research published in leading adult education journals such as Adult Education Quarterly, Studies in Continuing Education and International Journal of Lifelong Learning. This observation was also discussed by Fejes and Nylander (2015, see also Chap. 7 ).
Quantitative Research Excellence: Study Design and Reliable and Valid Measurement of Variables. Laura J. Duckett, BSN, MS, PhD, ... Designing and Conducting Research in Education. 2008. SAGE Knowledge. Entry . Quasi-Experimental Research. Show details Hide details. Geneva D. Haertel. Encyclopedia of Curriculum Studies.
Abstract. Research in early childhood education has witnessed an increasing demand for high-quality, large-scale quantitative studies. This chapter discusses the contributions of quantitative research to early childhood education, summarises its defining features and addresses the strengths and limitations of different techniques and approaches.
Educators use qualitative research in a study's exploratory stages to uncover patterns or new angles. Form Strong Conclusions with Quantitative Research. Quantitative research in education and other fields of inquiry is expressed in numbers and measurements. This type of research aims to find data to confirm or test a hypothesis.
The research must be situated in the context of school education. 4. The research should encompass both the operationalization and measurement of teacher digital competences. 5. The research should adopt a quantitative or mixed-methods approach. Exclusion criteria: 1. Theoretical reviews of competency models and frameworks without empirical ...
The study has utilized a quantitative research approach with a descriptive research design. ... their work from home arrangement while checking students' output is the primary task they carry in ...
Existing international research on methods in education policy has focused mainly on qualitative data (e.g., Levinson et al., 2020; Owen, 2014; Saarinen, 2008), while research on education policy regarding accountability, data use, and the role of numbers focuses more on quantitative data (e.g., Gorard, 2001).
Quantitative education research provides numerical data that can prove or disprove a theory, and administrators can easily share the quantitative findings with other academics and districts. While the study may be based on relative sample size, educators and researchers can extrapolate the results from quantitative data to predict outcomes for ...
This research applies a quantitative design where descriptive statistics are used for the student characteristics and design features data, t-tests for the age and gender variables to determine if they are significant in blended learning effectiveness and regression for predictors of blended learning effectiveness. ... An exploratory case study ...
Education Research: Quantitative research is used in education research to study the effectiveness of teaching methods, assess student learning outcomes, and identify factors that influence student success. Researchers use experimental and quasi-experimental designs, as well as surveys and other quantitative methods, to collect and analyze data
The Medical Education Research Study Quality Instrument (MERSQI) 21 ... This article provides an overview of quantitative research in medical education, underscores the main components of education research, and provides a general framework for evaluating research quality. We highlighted the importance of framing a study with respect to purpose ...
IJQRE aims to enhance the practice and theory of quantitative research in education. In this journal, "education" is defined in the broadest sense of the word, to include settings outside the school. IJQRE publishes peer-reviewed, empirical research employing a variety of quantitative methods and approaches, including but not limited to surveys, cross sectional studies, longitudinal ...
The increasing use of quasi-experimental research designs (QEDs) in education, brought into focus following the "credibility revolution" (Angrist & Pischke, 2010) in economics, which sought to use data to empirically test theoretical assertions, has indeed improved causal claims in education (Loeb et al., 2017).However, more recently, scholars, practitioners, and policymakers have ...
The team made their anonymized data set publicly available—including self-reports, surveys, and phone-sensing and brain-imaging data—to help advance research into the mental health of students during their college years.. Andrew Campbell, the paper's senior author and Dartmouth's Albert Bradley 1915 Third Century Professor of Computer Science, says that the study's extensive data ...
This mixed-methods study delves into rural community service-learning (CSL) partnerships, shedding light on the complexities and dynamics of collaboration between colleges and rural communities. Through quantitative surveys and qualitative interviews, the research amplifies the voices of rural community partners, emphasizing the crucial role of trust, communication, and reciprocity.
This research aims to explore the paradigm of applying learning by doing to create active learning in Islamic education. A combination of both quantitative and qualitative methods was used in this study. Data collection begins with qualitative data and continues with quantitative data. This flow is also known as exploratory sequential design.
This study investigated undergraduate medical students' and medical educators' opinions of teleconsultation practice in general and their opinions of teleconsultation education. Methods: This study used a cross-sectional, mixed-methods approach, utilising the additional coverage design to sequence and integrate qualitative and quantitative ...
The purpose of this study is to investigate the relationship between Arabic Language Proficiency (ALP) and expatriate adjustment (EA), and job performance (JP) in Saudi Arabia. In addition, the moderating role of personal and environmental factors is investigated. This investigation employs a mixed-methods research design. The intended audience is the personnel of Saudi Arabia's Higher ...
Chow J. C., Eckholm E. (2018). Do published studies yield larger effect sizes than unpublished studies in education and special education? A meta-review. Educational ... Therrien W. J. (2017). Null effects and publication bias in special education research. Behavioral Disorders, 42(4 ... quantitative; research methodology; meta-analysis;