Skip to content
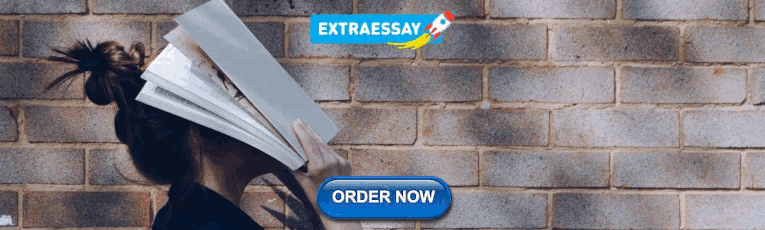
Global Skin Diseases Research Consortium (GSDRC)
The Global Skin Diseases Research Center (GSDRC) was established to foster collaboration among academic partners that actively maintain institutional skin research centers, which support basic, translational and/or clinical skin research. Current GSDRC member institutions include the following:
Columbia University Medical Center Skin Disease Resource-Based Center (epiCURE) Department of Dermatology New York, NY USA https://epicure.cumc.columbia.edu/
University of Cologne Collaborative Research Center (CRC 829) “Molecular Mechanisms Regulating Skin Homeostasis” Cologne, Germany http://sfb829.uni-koeln.de/
Keio University School of Medicine Department of Dermatology Tokyo, Japan https://keio.pure.elsevier.com/en/organisations/1540-department-of-dermatology
University of Colorado Anschutz Medical Center Anschutz Medical Campus Skin Diseases Research Center http://www.ucdenver.edu/academics/colleges/medicalschool/departments/Dermatology/research/SDRC/Pages/default.aspx
Stanford University Genetic Skin Disease Center Stanford Medicine Dermatology Redwood City, CA http://www.med.stanford.edu/dermatology/resources/gsdc.html
Institute of Medical Biology Skin Biology Cluster A*STAR Singapore https://www.a-star.edu.sg/imb
The long-term goal of the GSDRC is to provide global opportunities to spur innovation, create novel approaches and insights into cutaneous biology, disease pathogenesis, and clinical dermatology by coordinating multidisciplinary research aimed at discovering cutting-edge translational approaches to improve the care of patients with skin disease and to train the scientific leaders of tomorrow. Another key mission of the GSDRC is to lead the development of a global view of dermatologic research. The consortium encompasses a unique international resource for training and networking opportunities for PhD and MD/PhD students and post-doctoral fellows.
The GSDRC framework for accomplishing these goals includes:
Symposia supported by the six Institutions
Summer school training programs for PhD and MD/PhD students and postdocs
Exchange of PhD and MD/PhD students and postdocs to learn new techniques and approaches, and to promote collaborative projects across Institutions
Exchange of faculty for short periods
Establish a web-based inventory to promote exchange of mouse models, other animal models, disease-specific iPS cells, reagents and protocols
Annual in-person meetings at SID and ESDR
At Columbia University, our Skin Disease Resource Center dramatically increased the size, scope and stature of dermatologic research at the Medical Center. Without question, over the last twenty years, Columbia’s SDRC was the nucleus around which CUIMC built a national and international reputation as a center of excellence in dermatologic research. Columbia’s SDRC supports three main research themes:
- Genetics and immunology to discover novel treatment strategies for skin and hair disorders.
- Skin cancer and stem cells to develop novel, mechanism-driven targeted prevention and treatment strategies for these cancers.
Our Skin Disease Resource-Based Center, epiCURE , is designed to eliminate barriers to translational research and accelerate the progress of investigation in skin disease research to rapidly advance from basepairs to bedside through three Research Cores. These are:
- Skin Immunity, Integrity, and Disease Core (SIND): Facilitate high-quality skin-focused translational research through in vivo and ex vivo models that aim to mimic disease relevant aspects of human skin.
- Skin Stem Cell Imaging and Manipulation Core (SCIM) : Provides an array of histological, immunohistochemical, and molecular-based tools to characterize gross and microscopic morphology, functional features and gene expression in skin of human and genetically engineered laboratory animals.
- Translational and Precision Medicine Resources Core (TRAP) : Offers cutting-edge approaches to assist investigators in biomarker discovery, assessment and validation.
David Bickers
United States
Areas of Focus
Skin Diseases
Thank you for visiting nature.com. You are using a browser version with limited support for CSS. To obtain the best experience, we recommend you use a more up to date browser (or turn off compatibility mode in Internet Explorer). In the meantime, to ensure continued support, we are displaying the site without styles and JavaScript.
- View all journals
- My Account Login
- Explore content
- About the journal
- Publish with us
- Sign up for alerts
- Open access
- Published: 05 March 2021
AI-based localization and classification of skin disease with erythema
- Ha Min Son 1 ,
- Wooho Jeon 1 ,
- Jinhyun Kim 2 ,
- Chan Yeong Heo 3 ,
- Hye Jin Yoon 1 ,
- Ji-Ung Park ORCID: orcid.org/0000-0002-6403-4918 2 &
- Tai-Myoung Chung 1
Scientific Reports volume 11 , Article number: 5350 ( 2021 ) Cite this article
14k Accesses
12 Citations
Metrics details
- Health care
- Mathematics and computing
- Medical research
- Signs and symptoms
Although computer-aided diagnosis (CAD) is used to improve the quality of diagnosis in various medical fields such as mammography and colonography, it is not used in dermatology, where noninvasive screening tests are performed only with the naked eye, and avoidable inaccuracies may exist. This study shows that CAD may also be a viable option in dermatology by presenting a novel method to sequentially combine accurate segmentation and classification models. Given an image of the skin, we decompose the image to normalize and extract high-level features. Using a neural network-based segmentation model to create a segmented map of the image, we then cluster sections of abnormal skin and pass this information to a classification model. We classify each cluster into different common skin diseases using another neural network model. Our segmentation model achieves better performance compared to previous studies, and also achieves a near-perfect sensitivity score in unfavorable conditions. Our classification model is more accurate than a baseline model trained without segmentation, while also being able to classify multiple diseases within a single image. This improved performance may be sufficient to use CAD in the field of dermatology.
Similar content being viewed by others
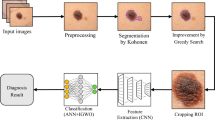
Skin cancer diagnosis (SCD) using Artificial Neural Network (ANN) and Improved Gray Wolf Optimization (IGWO)
Wanqi Lai, Meixia Kuang, … Sangkeum Lee
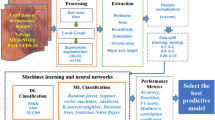
Refining skin lesions classification performance using geometric features of superpixels
Simona Moldovanu, Mihaela Miron, … Luminita Moraru
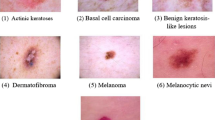
Skin lesion classification of dermoscopic images using machine learning and convolutional neural network
Bhuvaneshwari Shetty, Roshan Fernandes, … Kuruva Lakshmanna
Introduction
Computer-aided diagnosis (CAD) is a computer-based system that is used in the medical imaging field to aid healthcare workers in their diagnoses 1 . CAD has become a mainstream tool in several medical fields such as mammography and colonography 1 , 2 . However, in dermatology, although skin disease is a common disease, one in which early detection and classification is crucial for the successful treatment and recovery of patients, dermatologists perform most noninvasive screening tests only with the naked eye. This may result in avoidable diagnostic inaccuracies as a result of human error, as the detection of the disease can be easily overlooked. Furthermore, classification of a disease is difficult due to the strong similarities between common skin disease symptoms. Therefore, it would be beneficial to exploit the strengths of CAD using artificial intelligence techniques, in order to improve the accuracy of dermatology diagnosis. This paper shows that CAD may be a viable option in the field of dermatology using state-of-the-art deep learning models.
The segmentation and classification of skin diseases has been gaining attention in the field of artificial intelligence because of its promising results. Two of the more prominent approaches for skin disease segmentation and classification are clustering algorithms and support vector machines (SVMs). Clustering algorithms generally have the advantage of being flexible, easy to implement, with the ability to generalize features that have a similar statistical variance. Trabelsi et al. 3 experimented with various clustering algorithms, such as fuzzy c-means, improved fuzzy c-means, and K-means, achieving approximately 83% true positive rates in segmenting a skin disease. Rajab et al. 4 implemented an ISODATA clustering algorithm to find the optimal threshold for the segmentation of skin lesions. An inherent disadvantage of clustering a skin disease is its lack of robustness against noise. Clustering algorithms rely on the identification of a centroid that can generalize a cluster of data. Noisy data, or the presence of outliers, can significantly degrade the performance of these algorithms. Therefore, with noisy datasets, caused by images with different types of lighting, non-clustering algorithms may be preferred; however, Keke et al. 5 implemented an improved version of the fuzzy clustering algorithm using the RGB, HSV, and LAB color spaces to create a model that is more robust to noisy data. SVMs have gained attention for their effectiveness in high-dimensional data and their capability to decipher “…subtle patterns in noisy and complex datasets” 6 . Lu et al. 7 segmented erythema in the skin using the radial basis kernel function that allows SVMs to separate nonlinear hyperplanes. Sumithra et al. 8 combined a linear SVM with a k-NN classifier to segment and classify five different classes of skin lesions. Maglogiannis et al. 9 implemented a threshold on the RGB value for segmentation and used an SVM for classification. Although more robust than clustering algorithms, SVMs are more reliant on the preprocessing of data for feature extraction. Without preprocessing that allows a clear definition of hyperplanes, SVMs may also underperform.
Owing to the disadvantages of these traditional approaches, convolution neural networks (CNNs) have gained popularity because of their ability to extract high-level features with minimal preprocessing 10 . CNNs can expand the advantages of SVMs, such as robustness in noisy datasets without the need for optimal preprocessing, by capturing image context and extracting high-level features through down-sampling. CNNs can interpret the pixels of an image within its own image-level context, as opposed to viewing each pixel in a dataset-level context. However, although down-sampling allows CNNs to view an image in its own context, it degrades the resolution of the image. Although context is gained, the location of a target is lost through down-sampling. This is not a problem for classification, but causes some difficulty for segmentation, as both the context and location of the target are essential for optimal performance. To solve this, up-sampling is needed, which works in a manner opposite to that of down-sampling, in the sense that it increases the resolution of the image. While down-sampling takes a matrix and decreases it to a smaller feature map, up-sampling takes a feature map and increases it to a larger matrix. By learning to accurately create a higher-resolution image, CNNs can determine the location of the targets to segment. Thus, for segmentation, we use a combination of down-sampling and up-sampling, whereas for classification, we use only down-sampling. To further leverage the advantages of CNNs, skip-connections were introduced, which provided a solution to the degradation problem that occurs when CNN models become too large and complex. We implement skip-connections in both segmentation and classification models. In the segmentation model, blocks of equal feature numbers are connected between the down and up-sampling sections. In the classification model, these skip-connections exist in the form of inverted residual blocks. This allows our models to grow in complexity without any performance degradation.
In this paper, we present a method to sequentially combine two separate models to solve a larger problem. In the past, skin disease models have been applied to either segmentation or classification. In this study, we sequentially combine both models by using the output of a segmentation model as input to a classification model. In addition, although past studies of non-CNN segmentation models used innovative preprocessing methods, recent CNN developments have focused more on the architecture of the model than on the preprocessing of data. As such, we apply an innovative preprocessing method to the data of our CNN segmentation model. The methods described above lack the ability to localize and classify multiple diseases within one image; however, we have developed a method to address this problem. Our objective is two-fold. First, we show that CAD can be used in the field of dermatology. Second, we show that state-of-the-art models can be used with current computing power to solve a wider range of complex problems than previously imagined. We begin by explaining the results of our experimentation, followed by a discussion of our findings, a more detailed description of our methodology, and finally, the conclusions that can be drawn from our study.
Results and discussion
Figure 1 shows the schematic flow of our study. We started with the original image. We preprocessed this image by decomposing it into its hemoglobin and melanin constituents. These images were then input to the U-Net to generate the segmented output. We drew contours around each cluster and used a convex hull algorithm to draw rectangles around these clusters and crop them as individual images. These cropped images were used as input to the EfficientNet, which generated a prediction along with the confidence rate.
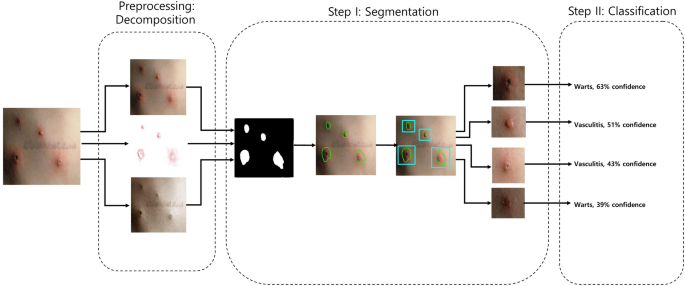
source implementation of the U-Net (v0.1.2) is available at: https://github.com/qubvel/segmentation_models.pytorch .
Schematic flow. From left to right, the original is first decomposed into hemoglobin and melanin images. All three images are input to the U-Net which outputs a black-and-white mask image. This mask image is used to draw contours each cluster. A convex hull algorithm is applied to crop each cluster. Each cluster is input to the EfficientNet, which generates a prediction alongside the confidence rate. An open-
Table 1 shows the results of the test data for segmentation on our Dermnet dataset. The K-means clustering algorithm showed sub-optimal performance, owing to its limitations with noisy data. The SVM method showed a significant improvement in performance, that was attributed to the advantages of using SVMs to extract information from decomposition, rather than clustering algorithms. Even without the extra information, the U-Net trained without decomposition outperformed the previous two methods in terms of sensitivity. The U-Net model was also trained with decomposition and showed the highest sensitivity rate.
In our results, we focused on the sensitivity metric because our objective was to assess the viability of using CAD with skin images. Although our U-Net model was not as good as the SVM model in terms of the specificity rate, it showed the best sensitivity rate, thus satisfying the objective of our study. In addition, we included the Dice coefficient and Hausdorff distance to demonstrate the performance of our methods with greater transparency. Our method showed clear improvements considering these alternative metrics. A major contributing factor 7 to the underperformance of other methods is that performance of the SVM algorithm deteriorated when the images contained differences in lighting and shade. The K-means clustering method 3 was also affected by the lighting and shade in the images. As our data had a significant mix of shade and lighting, the CNN was able to generalize the data better by learning to use the context of the image.
In any classification problem, it is important to set the baseline performance. We set our baseline to be the accuracy rate of the data without segmentation. The original image was input into the EfficientNet without going through the U-Net to determine the baseline accuracy rate. We compared this to the accuracy rate of the model trained to classify segmented images. Figure 2 shows the accuracy rates for the classification of our Dermnet dataset. We observed similar accuracy in the baseline model with and without contextual segmentation. The performance did not decrease when compared with the baseline. Thus, as we gained knowledge of the location of the disease without degrading the performance, we may say that the classification model was successfully implemented.
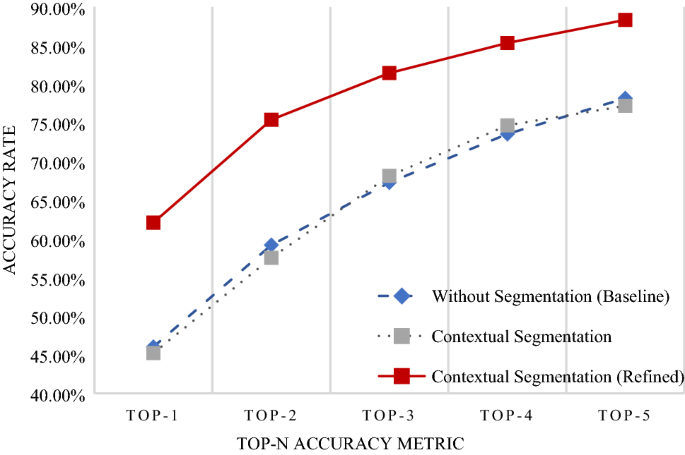
source implementation of the EfficientNet (v0.7.0) is available at https://github.com/lukemelas/EfficientNet-PyTorch .
Accuracy rate for classification. The x-axis represents the Top-n accuracy metric, while the y-axis represents accuracy. The blue line is the accuracy of the model trained without segmentation. Images did not enter the U-Net before entering the EfficientNet. The gray line represents the accuracy of the model trained with segmentation. Images were segmented and cropped through the U-Net before entering the EfficientNet. The red line represents the accuracy of the model trained with segmentation and refined data. Images were segmented, cropped, and verified to ensure that segmentation had been done correctly before entering the EfficientNet. An open-
However, we were also aware that the accuracy may have decreased due to false positives caused by areas such as the lips, which have similar characteristics to erythema. Hence, a separate model was trained with refined data, where we went through each image and excluded those that were incorrectly segmented. This improved accuracy substantially, as shown in Figure 2 . In addition, Table 2 shows additional metrics of the area under the curve (AUC), specificity, sensitivity, and F1-score. These values are weighted averages according to the number of data contained in each class. The AUC and specificity scores are high across all methods owing to the positive correlation of these metrics with the number of classes in a classification problem. Therefore, the more meaningful metrics in this dataset are the sensitivity and F1-score. The refined segmentation method demonstrated the highest performance considering these metrics, similar to the trend shown with the accuracy metric.
This was a result of an improved performance when there is a smaller area to search for the disease. Because we segmented only the abnormal areas of the skin, the EfficientNet model showed better performance compared to images with a larger ratio of normal skin. Thus, we can learn about the location of the disease that is present in an image and improve performance by training a CNN model to focus on particular subsections of the images. Figure 3 shows a visual representation of this claim using an implementation of the Grad-CAM method 11 . Activation, which is the intensity with which a model focuses on an area, is represented on a rainbow colormap. Red represents areas of highest activation, while violet represents areas of lowest activation. When trained with unsegmented data, our model focused on an area larger than that of abnormal skin. The area of activation was highest around the erythema, although there were other areas of high activation. In these cases, the model utilized the shapes of body parts for classification. This decreases performance because skin disease can appear in virtually any part of body and there is a lack of data required to form an association between the probability of a skin disease based on the body part. When trained with contextually segmented data, however, our model correctly focused only on erythema. The area of activation was highest around the erythema, while areas of low activation were demonstrated elsewhere. Not only does this add validity to our reported results, but this is also a justification for the inclusion of the segmentation phase before the classification phase because there were clear improvements in all metrics regarding the use of the U-Net before the EfficientNet.
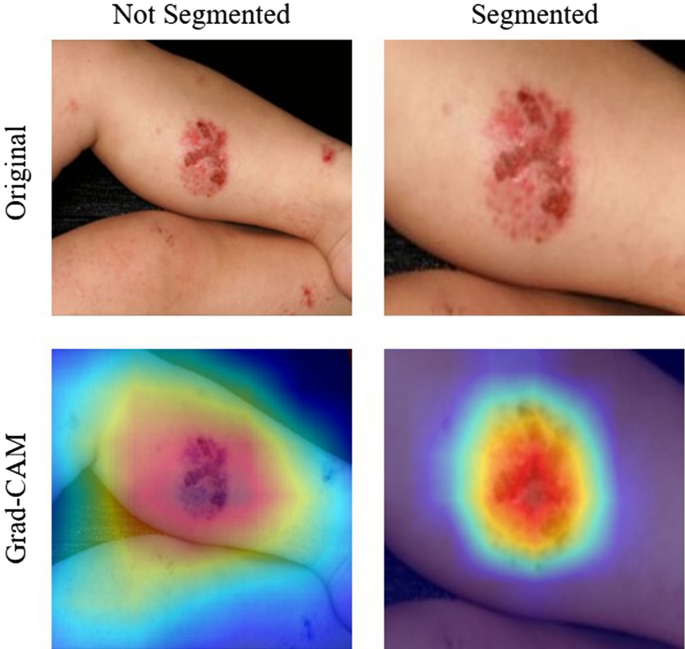
Grad-CAM results for unsegmented and segmented images in our Dermnet dataset. The top row shows the original input images. The left image shows the unsegmented image and the right image shows the segmented image. The bottom row shows the result of Grad-CAM 11 . The left image of Grad-CAM for the unsegmented image shows that the EfficientNet model focused on a larger surface other than erythema. The right image of Grad-CAM for the segmented image shows than that the EfficientNet model correctly focused mostly on erythema.
Table 3 shows the results of the test data for segmentation on our three independent dermatoscopic image datasets—ISIC2016 12 , ISIC2017 13 , and HAM10000 14 . These datasets are some of the few publicly available datasets that had segmentation maps and classification labels. We use these datasets to verify our methods with data from independent sources. One major difference with the dermatoscopic image datasets is that they are obtained using a special dermatoscopic device. This eliminates noise in the form of background and non-skin areas, in addition to limiting the number of disease and fixing the location of skin disease within an image. This was shown to decrease the significance of our method.
With the ISIC2016 and ISIC2017 datasets, the performance of the less-complex K-means clustering algorithm and SVM method showed similar trends to that of our Dermnet dataset. The performance was sub-optimal, owing to the noise present in the form of varying skin and lesion colors. With the HAM10000 dataset, however, the K-means clustering algorithm outperformed the SVM method in terms of the specificity and Hausdorff distance. This performance is a result of a more statistically similar training and testing set, as they were user-defined and created after stratifying the labels. Regardless of this, the less complex methods showed sub-optimal performances with all datasets.
Across all three datasets, the U-Net models outperformed previous models in all metrics. One interesting tendency is the small performance discrepancy between the U-Net models with and without decomposition. The U-Net model without decomposition occasionally outperformed the U-Net with decomposition. This was attributed to the skin lesion being mostly fixed at the center of the image. The hemoglobin and melanin constituents aid the U-Net model to ignore areas of non-skin and to focus on areas of skin with abnormal intensities. Therefore, this did not add significant information.
Table 4 shows the results of the test data for classification on the three dermatoscopic image datasets. With the ISIC2016 dataset, the Without Segmentation method showed the highest performance in all metrics. With the ISIC2017 dataset, the Refined Contextual Segmentation method showed the highest performance by a minimal margin. With the HAM10000 dataset, the Without Segmentation method showed the highest performance in all but one category. In short, with dermatoscopic images, models trained without segmentation learned to generalize skin lesions most effectively.
This was a result of an improved performance when the location of the skin lesion is mostly fixed. The segmentation phase aids models to ignore areas of normal skin and to focus on areas of disease. With dermatoscopic images, this information is insignificant, as the location of the disease is static. Figure 4 shows a visual representation of this. The Grad-CAM images show that with both non-segmented and segmented images, the models correctly focused on the skin disease. Because of this, the segmentation phase only decreased the resolution of the image without providing useful information, thus decreasing the performance of the model.
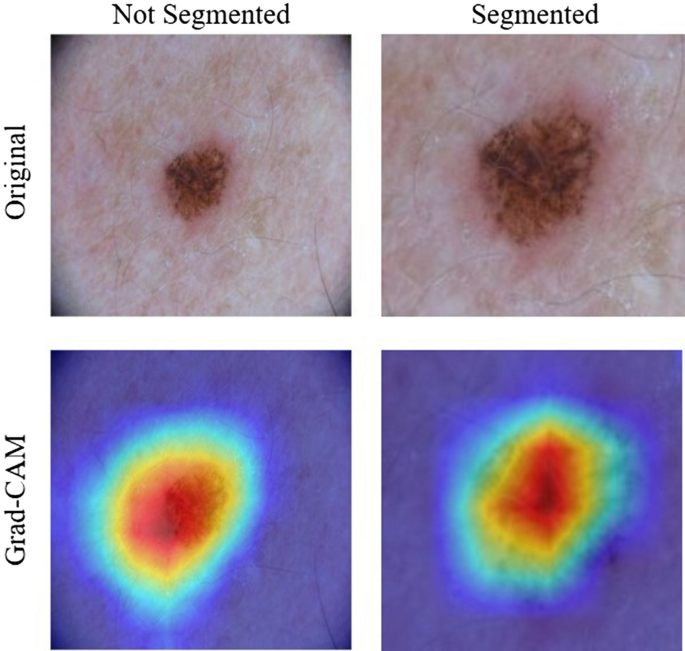
Grad-CAM results for unsegmented and segmented images in the ISIC2017 dataset. For both images of Grad-CAM, the EfficientNet model correctly focused mostly on erythema.
The main contribution of our study is researching the viability of CAD in the field of dermatology. This is achieved through the increase in the classification performance of skin disease images, owing to the increase in performance of segmentation. However, our model is most effective with camera images of skin diseases with erythema, which is a limitation of our study. We chose to focus on camera images and erythema because these images are very accessible, and erythema is one of the most common symptoms of skin disease. In addition, currently we only classify diseases into 18 categories due to the limitations of the data. In the future, we plan to create a more comprehensive skin disease classification model, and this seems to be viable if enough data can be obtained. In addition, we plan to work on a method to help dermatologists with time-series analysis of patients. This seems viable with the accumulation of data through CAD.
Analysis methodology
Our 2-phase analysis model for localization and classification is shown via the pseudocode in Algorithm 1 and visually in Fig. 5 . We decomposed the original image into its hemoglobin and melanin constituents using preprocessing, to help our model extract valuable information from data that would have been otherwise unavailable. We provide these images as input to our segmentation model, the U-Net, which generated a segmented image. This segmented image was then analyzed for clusters, which were subsequently cropped and input to our classification model, the EfficientNet, which then produced a classified label, thus completing our analysis model.
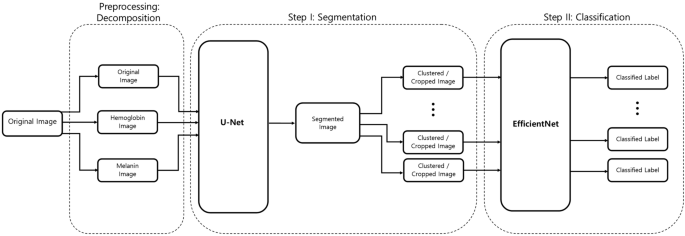
Two-phase analysis model. The original image primarily enters a preprocessing stage, where normalization and decomposition occur. Afterwards, the first step is segmentation, where cluster of abnormal skin are segmented and cropped. The second step is classification, where each cluster is classified into its corresponding class.
The data for training and testing were obtained from Dermnet NZ, an archive of skin disease information launched and maintained by a group of dermatologists from New Zealand. The site provides open source images with labels. We selected 18 top-level categories (Table 5 ) each of which included enough data, besides including erythema as one of its common symptoms. Using a web crawler, we gathered a total of 15,851 images. Among the images obtained through Dermnet, the erythema of 100 images was masked by dermatologists, to be used as a ground truth. For segmentation, 60 images were used for training, and 40 images were used for testing. For classification, 13,473 images were used for training, and 2,378 images were used for testing. In addition, the test set for classification was split before segmentation cropping to prevent the subsections of one image from appearing in both the training and testing sets. Table 6 shows the distribution of data in greater detail. We chose the 100 images for segmentation in a balanced manner from each class, to minimize any bias that could occur during the classification phase.
One of the significant merits of the Dermnet dataset is that it was created and is maintained by a diverse group of dermatologists. The images in each top-level category are independent as they are images of different patients at distinct locations taken with varying devices. This is evident in the diverse resolutions, lighting, and aspect ratios of the images. Regardless, it would be optimal to possess a similar dataset from an entirely separate association to truly validate the performance of our model. However, as there are strict regulations regarding the use of data in our private institutions, we utilize publicly available datasets. These datasets were chosen based on the availability of both a segmentation map and a classification label.
ISIC2016 12 , ISIC2017 13 , and HAM10000 14 are datasets that have been used in previous AI competitions. They were provided as challenges for both segmentation and classification, and they therefore possess segmentation maps and classification labels. Table 7 shows a detailed distribution of these datasets. As the ISIC2016 and ISIC2017 datasets also provided a separate test dataset, these datasets were preserved and used for testing. For the HAM10000 dataset, we stratified the dataset according to the classification label, and created a balanced 50% split between the train and test data. There is no separate segmentation dataset, as each image contained a segmentation map. Therefore, all images are used in the training and testing for both segmentation and classification.
There is one significant difference between these datasets and our Dermnet dataset. The images in these datasets were obtained with a special dermatoscopic device. These devices create high-resolution images with the skin disease located near the center. Therefore, these devices create images similar to the Dermnet dataset images after our segmentation phase. Thus, it is doubtful that our method will demonstrate an improved performance with the dermatoscopic images.
For all datasets, the testing dataset is unused for validation until the end of training. This is done to verify that our models learn to generalize unseen images. We take a three-fold cross-validation approach with training data for validation during training. We generate three replicas of each dataset and create a unique 90-to-10 training and validation set. With each replica, we use a grid search algorithm to test different combinations of hyperparameters. Lastly, we train our model using the entire training set and select our hyperparameters based on the cross-validation stage. Training and testing were performed on a single GTX Titan V and four Intel Xeon Gold 5115 processors. We now explain each section of our analysis model in more detail.
Preprocessing: decomposition
The main constituents of the skin that are visible to humans are melanin and hemoglobin. These constituents provide valuable information for the segmentation of abnormal skin. To ensure that our model can learn to use these features, we used independent component analysis (ICA) to extract the melanin and hemoglobin constituents 7 , 15 , 16 . Assuming that these components are linearly separable, the separated linear vectors can be represented by the following formula 7 :
where \({d}^{m}\) and \({d}^{h}\) represent the density vectors of melanin and hemoglobin, respectively, \({q}_{x,y}^{m}\) and \({q}_{x,y}^{h}\) represent the quantity of these components, and \(\Delta\) represents values that are caused by other colors. As shown in 7 , by applying ICA, we can decompose skin as
where \(\stackrel{-}{D}\) represents the estimated values of \({d}^{m}\) and \({d}^{h}\) , and \({I}_{x,y}\) represents the decomposed result. Figure 6 shows an example of one of these decompositions.
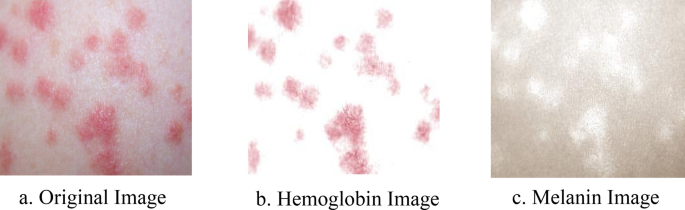
Decomposed result of skin. The original image is decomposed into its hemoglobin and melanin constituents through ICA.
Segmentation
The U-Net 17 , as shown in Fig. 7 , is an architecture created by CNNs, that has attracted attention for accurate biomedical image segmentation through the combination of down-sampling, up-sampling, and skip connections. Its name is attributed to the shape of its architecture, the first half of the ‘U’ representing down-sampling. Here, the context and key features of the input images are gained at the cost of a decrease in resolution. The second half of the ‘U’ represents up-sampling. Here, the resolution is increased to gain knowledge of the location of the target segment. To combat degradation due to the complexity of the model, skip connections are added to each up-sampling block.
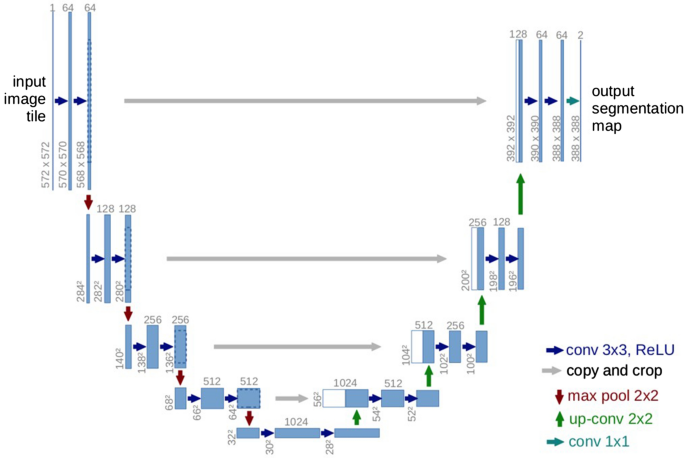
U-Net architecture. A fully CNN network, comprised of down-sampling, up-sampling, and skip connections 17 .
Although in the original paper 17 , the resolutions of input and output were different, that is, 572 × 572 and 388 × 388 pixels, respectively, we chose to keep our input and output resolution consistent at 304 × 304 pixels. This was done because the images in our dataset were not large enough to warrant the tiling strategy required for extremely large images. Thus, zero-padding allowed us to keep the input and output resolutions consistent, thereby allowing the retention of information present on the border of our images.
Using the decomposed images, in one instance, we input three images, namely, the original, the hemoglobin, and the melanin images, to our U-Net and obtained a single black-and-white mask image as output as shown in Fig. 8 . In this image, a black pixel represented normal skin, and a white pixel represented abnormal skin. Using the mask image, we used a simple contour-finding algorithm to draw an outline around clusters of erythema. We then used a convex hull algorithm to draw rectangles around the contours. The dimensions and locations of these rectangles were then used to crop the original image. These cropped images of each cluster were saved as individual pictures. We added padding to each cluster to create a larger and squarer image, as the performance of classification can suffer due to clusters being too small or not evenly shaped. Figure 9 shows contours and rectangles around each cluster showing how each cluster was cropped.
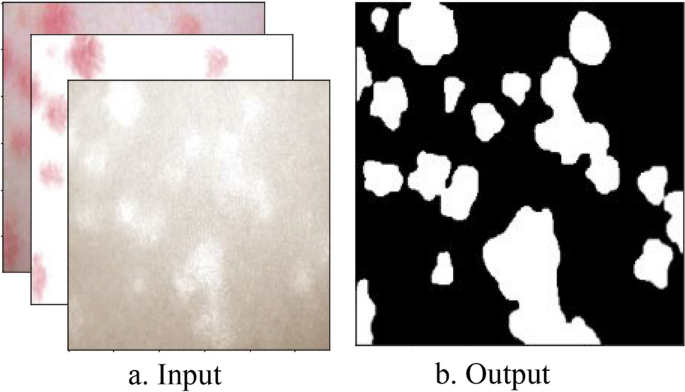
Input and Output of the U-Net. The inputs of the U-Net are the original, hemoglobin, and melanin images obtained from the preprocessing step. The output of the U-Net is a single masked image.
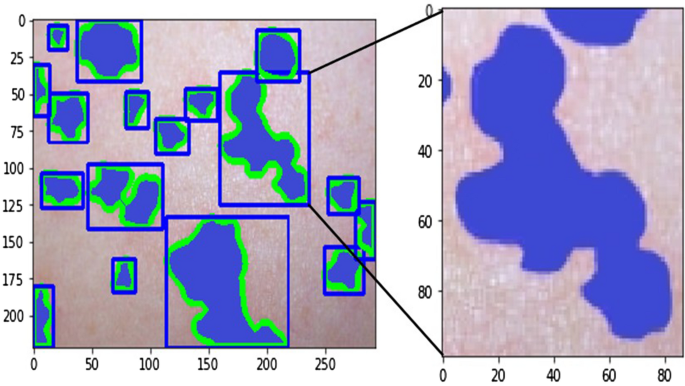
Contour finding algorithm applied to output of U-Net. Clusters of abnormal skin are identified through a contour finding algorithm. Each cluster is cropped in the shape of a rectangle through a convex hull algorithm used to surround each contour.
After generating three replicas of our dataset, we create a unique 90-to-10 training and validation set. With each replica, we perform a grid search algorithm to find the optimal hyperparameters. For the loss function, we test the Binary Cross-Entropy and Dice Coefficient Loss . For the optimizer, we test Adam with learning rates of 1e−4, 5e−5, and 1e−5; RMSprop with learning rates of 1e−4, 5e−5, and 1e−5; and SGD with a momentum of 0.9 and learning rates of 1e−1, 5e−2, and 5e−2. For the number of epochs, we test with 40, 60, and 80 epochs and decrease the learning rate by a factor of 0.1 every 20 epochs. After testing with the replicas, we use the full training set for training with the hyperparameters: Binary Cross-Entropy, Adam with a learning rate of 5e−4, a weight decay of 5e−4, 60 epochs, and a decrease in learning rate by a factor of 0.1 every 20 epochs.
As our main objective was to demonstrate the viability of CAD, the performance was mostly determined using pixel-level sensitivity rather than the Intersection over Union or the Dice coefficient metrics that are often used to measure segmentation performance. Moreover, we mainly focused on the true positive rates of segmentation, represented by the sensitivity metric. This is because our aim was to create a screening test method to help healthcare workers make a more accurate diagnosis by preventing abnormal skin from being overlooked. Nevertheless, we also measured the performance of our model using the specificity, Dice coefficient, and Hausdorff distance to provide a more complete performance comparison. We measured these metrics by comparing the output from our U-Net model to an image that was masked by professional dermatologists. Going through each pixel, if a pixel of the U-Net output was black and the pixel of the dermatologist-masked image at the same location was black, this is seen as a true negative. If both were white, this was seen as a true positive. If the U-Net output was black but the dermatologist mask was white, this was seen as a false negative, and the converse was a false positive. The equations for sensitivity, specificity, and Dice coefficient metric can be represented by the following formulas:
The Hausdorff distance (HD) is used to measure the dissimilarity between the predicted segmentation masks the and ground truth. The Hausdorff distance can be calculated by the formula 18 :
where \(h\left(X,Y\right)=\underset{x\in X}{\mathrm{max}}\underset{y\in Y}{\mathrm{min}}\Vert x-y\Vert.\)
We use an implementation of the method presented 18 to calculate the Hausdorff distance between the output and ground truth.
Classification
EfficientNets 18 were introduced in late 2019 as a state-of-the-art model for image classification. Rather than scaling a CNN model without balance between the depth, width, and resolution of the image at hand, EfficientNets were developed by scaling a baseline model in a methodical manner. This allows for an efficient increase in accuracy rates without unreasonable amounts of required memory and floating-point operations (FLOPS) through the optimization of the following formulas 18 :
Here, d , w , and r represent the depth, width, and resolution of the scaled model, and \(\widehat{H}, \widehat{W},\widehat{C},\widehat{\mathcal{F}},\widehat{L}\) represent the parameters of the optimized baseline model. Thus, in summary, the goal of the EfficientNet model, namely, \(N(d,w,r)\) , is to produce maximum accuracy in a classification problem. The model is represented by the product of its variable-weighted parameters, represented as \(\underset{i=1\dots s}{\odot }{\widehat{\mathcal{F}}}_{i}^{d\bullet \widehat{{L}_{i}}}({X}_{<r\bullet {\widehat{H}}_{i},r\bullet {\widehat{W}}_{i}, w\bullet {\widehat{C}}_{i}>})\) . The memory usage, \(Memory\left(N\right)\) , and required computational performance, \(FLOPS\left(N\right)\) , for the model must be less than that of the target.
The original paper 19 presents eight different models, ranging from EfficientNet-B0 through EfficientNet-B7, each increasing in complexity. Table 8 shows the accuracy and training time per epoch of each of these models trained on unsegmented images. There are sharp increases in training time between the EfficientNet-B4 and EfficientNet-B7 models, as we were forced to use smaller batch sizes during training owing to the increased number of trainable parameters and the limited memory in our GPU. In addition, as we employ a grid search algorithm, many models must be trained for many epochs. Therefore, a lower training time is desirable. After testing these models with our dataset and hardware, we chose to implement the EfficientNet-B4 model as it used substantial memory and training time without losing excessive complexity. We applied transfer learning to the segmented and cropped images from the previous section and classified them into 18 different classes.
We further improved the performance by using the Synthetic Minority Oversampling Technique 20 library, as a more balanced dataset was needed for training. In addition, because our segmentation model required more data to better generalize erythema, there were clusters of normal skin that were cropped and included in different classes. It was observed that this confused the model, as similar images were seen throughout different classes. To combat this, we refined the data by going through each image and excluding certain images that were either too small or incorrectly segmented images.
We created replicas of the training set and performed a grid search algorithm, as in the method utilized in the segmentation phase. For the loss function, we tested the Categorical Cross-Entropy and Focal Loss . For the optimizer, we test Adam with learning rates of 1e−4, 5e−5, and 1e−5; RMSprop with learning rates of 1e−4, 5e−5, and 1e−5; and SGD with a momentum of 0.9 and learning rates of 1e−1, 5e−2, and 5e−2. For the number of epochs, we test with 40 epochs, 60 epochs, and 80 epochs and decrease the learning rate by a factor of 0.1 every 20 epochs. After testing with the replicas, we used the full training set for training with the hyperparameters: Categorical Cross-Entropy, Adam with a learning rate of 1e−5, a weight decay of 5e−4, 80 epochs, and a decrease in learning rate by a factor of 0.1 every 20 epochs. The AUC is calculated by taking the integral of the curve created by points at different sensitivity and specificity thresholds. In addition, specificity, sensitivity, and the F1-score can be represented by the following formulas:
For all performance metrics, scores are calculated individually for each class present in the dataset. The scores are then weighted and averaged according to the number of data points in a class corresponding to the entire dataset.
Ethics declarations
This study was exempted from the approval by the Institutional Review Board of Seoul National University Boramae Medical Center (No. 07-2020-148). The informed consent was waived by the Institutional Review Board of Seoul National University Boramae Medical Center because patient records/information was anonymized and de-identified prior to analysis. All experiments were performed in accordance with the relevant guidelines and regulations.
We have shown that even without a large dataset and high-quality images, it is possible to achieve sufficient accuracy rates. In addition, we have shown that current state-of-the-art CNN models can outperform models created by previous research, through proper data preprocessing, self-supervised learning, transfer learning, and special CNN architecture techniques. Furthermore, with accurate segmentation, we gain knowledge of the location of the disease, which is useful in the preprocessing of data used in classification, as it allows the CNN model to focus on the area of interest. Lastly, unlike previous studies, our method provides a solution to classify multiple diseases within a single image. With higher quality and a larger quantity of data, it will be viable to use state-of-the-art models to enable the use of CAD in the field of dermatology.
Data availability
The dataset used for segmentation is available upon request from the corresponding author for academic use. The dataset used for classification is available on Dermnet for academic use ( https://dermnetnz.org/ ).
Code availability
The code for the development of a mobile app is available on ( https://github.com/sonhamin/dermtools ). The code regarding the analysis model is available upon request from the corresponding author for academic use.
Doi, K. Computer-aided diagnosis in medical imaging: Historical review, current status and future potential. Comput. Med. Imaging Graph. 31 , 198–211. https://doi.org/10.1016/j.compmedimag.2007.02.002 (2007).
Article PubMed PubMed Central Google Scholar
Yoshida, H. & Dachman, A. H. Computer-aided diagnosis for CT colonography. Semin. Ultrasound CT MRI 25 , 419–431. https://doi.org/10.1053/j.sult.2004.07.002 (2004).
Article Google Scholar
Trabelsi, O., Tlig, L., Sayadi, M. & Fnaiech, F., Skin disease analysis and tracking based on image segmentation. 2013 International Conference on Electrical Engineering and Software Applications , Hammamet, 1–7. https://doi.org/10.1109/ICEESA.2013.6578486 (2013).
Rajab, M. I., Woolfson, M. S. & Morgan, S. P. Application of region-based segmentation and neural network edge detection to skin lesions. Comput. Med. Imaging Graph. 28 , 61–68. https://doi.org/10.1016/S0895-6111(03)00054-5 (2004).
Article CAS PubMed Google Scholar
Keke, S., Peng, Z. & Guohui, L., Study on skin color image segmentation used by fuzzy-c-means arithmetic. In 2010 Seventh International Conference on Fuzzy Systems and Knowledge Discovery , Yantai, 612–615. https://doi.org/10.1109/FSKD.2010.5569451 (2010).
Hongmao, S. Quantitative Structure-Activity Relationships: Promise, Validations, and Pitfalls in A Practical Guide to Rational Drug Design 163–192 (Woodhead Publishing, Sawston, 2016). https://doi.org/10.1016/B978-0-08-100098-4.00005-3 .
Book Google Scholar
Lu, J., Manton, J. H., Kazmierczak E. & Sinclair, R., Erythema detection in digital skin images. In 2010 IEEE International Conference on Image Processing , Hong Kong, 2545–2548. https://doi.org/10.1109/ICIP.2010.5653524 (2010).
Sumithra, R., Suhil, M. & Guru, D. S. Segmentation and classification of skin lesions for disease diagnosis. Proced. Comput. Sci. 45 , 76–85. https://doi.org/10.1016/j.procs.2015.03.090 (2015).
Maglogiannis, I., Zafiropoulos, E. & Kyranoudis, C. Intelligent segmentation and classification of pigmented skin lesions in dermatological images in Advances in Artificial Intelligence. SETN 2006. In Lecture Notes in Computer Science Vol. 3955 (eds Antoniou, G. et al. ) 214–223 (Springer, Berlin, 2006). https://doi.org/10.1007/11752912_23 .
Chapter Google Scholar
Albawi, S., Mohammed, T. A. & Al-Zawi, S., Understanding of a convolutional neural network. In 2017 International Conference on Engineering and Technology (ICET) , Antalya, 1–6. https://doi.org/10.1109/ICEngTechnol.2017.8308186 (2017).
Selvaraju, R. et al. Grad-CAM: Visual explanations from deep networks via gradient-based localization. Int. J. Comput. Vis. 128 , 336–359. https://doi.org/10.1007/s11263-019-01228-7 (2019).
Gutman, D., Codella, N., Celebi, E., Helba, B., Marchettic, M., Mishra, N., & Halpern, A., Skin Lesion Analysis toward Melanoma Detection: A Challenge at the International Symposium on Biomedical Imaging (ISBI) 2016, hosted by the International Skin Imaging Collaboration (ISIC). https://arxiv.org/abs/1605.01397 (2016).
Codella, N., Gutman, D., Celebi, ME., Helba, B., Marchetti, MA., Dusza, S., Kalloo, A., Liopyris, K., Mishra, N., Kittler, H., & Halpern, A., Skin Lesion Analysis Toward Melanoma Detection: A Challenge at the 2017 International Symposium on Biomedical Imaging (ISBI), Hosted by the International Skin Imaging Collaboration (ISIC). https://arxiv.org/abs/1710.05006 (2017).
Tschandl, P., Rosendahl, C. & Kittler, H. The HAM10000 dataset, a large collection of multi-source dermatoscopic images of common pigmented skin lesions. Sci. Data 5 , 180161. https://doi.org/10.1038/sdata.2018.161 (2018).
Tsumura, N., Haneishi, H. & Miyake, Y. Independent-component analysis of skin color image. J. Opt. Soc. Am. A 16 , 2169–2176. https://doi.org/10.1364/JOSAA.16.002169 (1999).
Article CAS ADS Google Scholar
Hyvärinen, A. & Oja, E. Independent component analysis: Algorithms and applications. Neural Netw. 13 , 411–430. https://doi.org/10.1016/S0893-6080(00)00026-5 (2000).
Article PubMed Google Scholar
Ronneberger, O., Fischer, P. & Brox, T. U-net: Convolutional networks for biomedical image segmentation. Medical image computing and computer-assisted intervention—MICCAI 2015. MICCAI 2015. In Lecture Notes in Computer Science Vol. 9351 (eds Navab, N. et al. ) 234–241 (Springer, Berlin, 2015). https://doi.org/10.1007/978-3-319-24574-4_28 .
Taha, A. & Hanbury, A. An efficient algorithm for calculating the exact hausdorff distance. IEEE Trans. Pattern Anal. Mach. Intell. 37 (11), 2153–2163. https://doi.org/10.1109/TPAMI.2015.2408351 (2015).
Tan, M. & Le, Q., Efficientnet: Rethinking model scaling for convolutional neural networks, in ICML , 6105–6114. https://arxiv.org/abs/1905.11946 (2019).
Chawla, N., Bowyer, K., Hall, L. & Kegelmeyer, W. SMOTE: Synthetic minority over-sampling technique. J. Artif. Intell. Res. 16 , 321–357. https://doi.org/10.1613/jair.953 (2002).
Article MATH Google Scholar
Download references
Acknowledgements
This work was supported by the Institute of Information and communications Technology Planning and Evaluation (IITP) grant funded by the Korea government (MSIT) (no. 2020-0-00990, Platform Development and Proof of High Trust and Low Latency Processing for Heterogeneous·Atypical·Large Scaled Data in 5G-IoT Environment).
Author information
Authors and affiliations.
Department of Computer Science and Engineering, Sungkyunkwan University College of Computing, Sungkyunkwan University, 2044 Seobu-ro, Jangan-gu, Suwon, 16419, Republic of Korea
Ha Min Son, Wooho Jeon, Hye Jin Yoon & Tai-Myoung Chung
Department of Plastic and Reconstructive Surgery, Seoul National University Boramae Hospital, Seoul National University College of Medicine, 5 Gil 20, Borame-Road, Dongjak-Gu, Seoul, 07061, Republic of Korea
Jinhyun Kim & Ji-Ung Park
Department of Plastic and Reconstructive Surgery, Seoul National University Bundang Hospital, Seoul National University College of Medicine, Seongnam, 13619, Korea
Chan Yeong Heo
You can also search for this author in PubMed Google Scholar
Contributions
H.M.S. analyzed the results and wrote the full manuscript. H.M.S. and W.J. conducted the research and experiments. J.K. and J.P. created detailed masked images, allowing the research for segmentation. H.M.S and H.J.Y. are developing an app based on the analysis model of this study. J.P. and T.C. provided invaluable guidance throughout the research, experiment, analysis of results, and writing of the manuscript. All authors have reviewed the manuscript.
Corresponding authors
Correspondence to Ji-Ung Park or Tai-Myoung Chung .
Competing interests
The authors declare no competing interests.
Additional information
Publisher's note.
Springer Nature remains neutral with regard to jurisdictional claims in published maps and institutional affiliations.
Rights and permissions
Open Access This article is licensed under a Creative Commons Attribution 4.0 International License, which permits use, sharing, adaptation, distribution and reproduction in any medium or format, as long as you give appropriate credit to the original author(s) and the source, provide a link to the Creative Commons licence, and indicate if changes were made. The images or other third party material in this article are included in the article's Creative Commons licence, unless indicated otherwise in a credit line to the material. If material is not included in the article's Creative Commons licence and your intended use is not permitted by statutory regulation or exceeds the permitted use, you will need to obtain permission directly from the copyright holder. To view a copy of this licence, visit http://creativecommons.org/licenses/by/4.0/ .
Reprints and permissions
About this article
Cite this article.
Son, H.M., Jeon, W., Kim, J. et al. AI-based localization and classification of skin disease with erythema. Sci Rep 11 , 5350 (2021). https://doi.org/10.1038/s41598-021-84593-z
Download citation
Received : 21 August 2020
Accepted : 15 February 2021
Published : 05 March 2021
DOI : https://doi.org/10.1038/s41598-021-84593-z
Share this article
Anyone you share the following link with will be able to read this content:
Sorry, a shareable link is not currently available for this article.
Provided by the Springer Nature SharedIt content-sharing initiative
This article is cited by
Psoriasis severity classification based on adaptive multi-scale features for multi-severity disease.
- Cho-I. Moon
Scientific Reports (2023)
Automatic Diagnosis of Melanoma Based on EfficientNet and Patch Strategy
- Jinyong Cheng
- Zhenlu Liang
International Journal of Computational Intelligence Systems (2023)
By submitting a comment you agree to abide by our Terms and Community Guidelines . If you find something abusive or that does not comply with our terms or guidelines please flag it as inappropriate.
Quick links
- Explore articles by subject
- Guide to authors
- Editorial policies
Sign up for the Nature Briefing newsletter — what matters in science, free to your inbox daily.

- Frontiers in Cell and Developmental Biology
- Molecular and Cellular Pathology
- Research Topics
Recent Advances in Skin Disorders: From Basic Mechanisms to Therapies
Total Downloads
Total Views and Downloads
About this Research Topic
As the largest organ in multicellular organisms, the skin acts as the first line of defense against external stimuli such as mechanical damage, UV irradiation, and pathogen infection, all of which can cause an imbalance of tissue homeostasis and result in the development and progression of skin disorders ...
Keywords : inflammation, biomaterials, nanoparticle, skin disorders, peptides, cell migration
Important Note : All contributions to this Research Topic must be within the scope of the section and journal to which they are submitted, as defined in their mission statements. Frontiers reserves the right to guide an out-of-scope manuscript to a more suitable section or journal at any stage of peer review.
Topic Editors
Topic coordinators, recent articles, submission deadlines.
Submission closed.
Participating Journals
Total views.
- Demographics
No records found
total views article views downloads topic views
Top countries
Top referring sites, about frontiers research topics.
With their unique mixes of varied contributions from Original Research to Review Articles, Research Topics unify the most influential researchers, the latest key findings and historical advances in a hot research area! Find out more on how to host your own Frontiers Research Topic or contribute to one as an author.
- Open access
- Published: 24 November 2021
Opportunities and Challenges: Classification of Skin Disease Based on Deep Learning
- Bin Zhang 1 , 2 ,
- Xue Zhou 1 , 2 ,
- Yichen Luo 1 , 2 ,
- Hao Zhang 1 , 2 ,
- Huayong Yang 1 , 2 ,
- Jien Ma 3 &
- Liang Ma ORCID: orcid.org/0000-0002-6242-1850 1 , 2
Chinese Journal of Mechanical Engineering volume 34 , Article number: 112 ( 2021 ) Cite this article
11k Accesses
20 Citations
1 Altmetric
Metrics details
Deep learning has become an extremely popular method in recent years, and can be a powerful tool in complex, prior-knowledge-required areas, especially in the field of biomedicine, which is now facing the problem of inadequate medical resources. The application of deep learning in disease diagnosis has become a new research topic in dermatology. This paper aims to provide a quick review of the classification of skin disease using deep learning to summarize the characteristics of skin lesions and the status of image technology. We study the characteristics of skin disease and review the research on skin disease classification using deep learning. We analyze these studies using datasets, data processing, classification models, and evaluation criteria. We summarize the development of this field, illustrate the key steps and influencing factors of dermatological diagnosis, and identify the challenges and opportunities at this stage. Our research confirms that a skin disease recognition method based on deep learning can be superior to professional dermatologists in specific scenarios and has broad research prospects.
1 Introduction
Skin lesions are a common disease that cause suffering, some of which can have serious consequences, for millions of people globally [ 1 ]. Because of its complexity, diversity, and similarity, skin disease can only be diagnosed by dermatologists with long-term clinical experience and is rarely reproducible. It is likely to be misdiagnosed by an inexperienced dermatologist, which can exacerbate the condition and impede appropriate treatment. Thus, it is necessary to provide a quick and reliable method to assist patients and dermatologists in data processing and judgment.
Advances in deep learning have influenced numerous scientific and industrial fields and have realized significant achievements with inspiration from the human nervous system. With the rapid development of deep learning in biomedical data processing, numerous specialists have adopted this technique to acquire more precise and accurate data. With the rapid increase in the amount of available biomedical data including images, medical records, and omics, deep learning has achieved considerable success in a number of medical image processing problems [ 2 , 3 , 4 ]. In this regard, deep learning is expected to influence the roles of image experts in biomedical diagnosis owing to its ability to perform quick and accurate assessments. This paper presents the characteristics of skin lesions, overviews image techniques, generalizes the developments in deep learning for skin disease classification, and discusses the limitations and direction of automatic diagnosis.
2 Features of Skin Disease
The skin is the largest organ of the human body; in adults, it can typically weigh 3.6 kg and cover 2 m 2 [ 5 ]. Skin guards the body against extremes of temperature, damaging sunlight, and harmful chemicals. As a highly organized structure, it consists of the epidermis, dermis, and hypodermis, providing the functions of protection, sensation, and thermoregulation [ 6 ]. The epidermis, the outermost layer of the skin, provides an excellent aegis to avoid environmental aggression. The dermis, beneath the epidermis, contains tough connective tissue, hair follicles, and sweat glands, which leads to the differentiation of skin appearance [ 7 ]. There are numerous causes of skin disease, including physical factors such as light, temperature, and friction, and biological factors such as insect bites, allergic diseases, and even viral infections. Environmental and genetic factors can also lead to the occurrence of skin diseases. In lesion imaging, complicating difficulties can include variations in skin tone, presence of artifacts such as hair, air bubbles, non-uniform lighting, and the physical location of the lesion. Moreover, the majority of lesions vary in terms of color, texture, shape, size, and location in an image frame [ 8 ]. There are 5.4 million new skin cancer patients in America every year. As of 2014, there were 420 million people globally suffering from skin disease, including nearly 150 million people in China, the population of which accounts for 22% of the world’s population, yet medical resources account for only 2%. Influenced by the living environment, areas with reduced economic development and poverty are more prone to skin disease. The high cost of treatment, repeated illness occurrences, and delays in treatment have focused attention on the requirement for healthy survival and social development. The high cost of treatment, repeated illness occurrences, and delays in treatment have brought challenges to the healthy survival and social development.
The accurate diagnosis of a particular skin disease can be a challenging task, mainly for the following reasons. First, there are numerous kinds of dermatoses, nearly 3000 recorded in the literature. Stanford University has developed an algorithm to demonstrate generalizable classification with a new dermatologist-labeled dataset of 129450 clinical images divided into 2032 categories [ 9 ]. Figure 1 displays a subset of the full taxonomy; this has been organized clinically and visually by medical experts. Secondly, the complex manifestation of the disease is also a major challenge for doctors. Morphological differences in the appearance of skin lesions directly influence the diagnosis mainly as there can be relatively poor contrast between different skin diseases, which cannot be distinguished without considerable experience. Finally, for different skin diseases, the lesions can be overly similar to be distinguished using only visual information. Different diseases can have similar manifestations and the same disease can have different manifestations in different people, body parts, and disease periods [ 10 ]. Figure 2 displays sample images demonstrating the difficulty in distinguishing between malignant and benign lesions, which share several visual features. Unlike benign skin diseases, malignant diseases, if not treated promptly, can lead serious consequences. Melanoma [ 11 ], for example, is one of the major and most fatal skin cancers. The five-year survival rate of melanoma can be greater than 98% if found in time; this figure in those where spread has occurred demonstrates a significant drop to 17% [ 12 ]. In 2015, there were 3.1 million active cases, representing approximately 70% of skin cancer deaths worldwide [ 13 , 14 ].
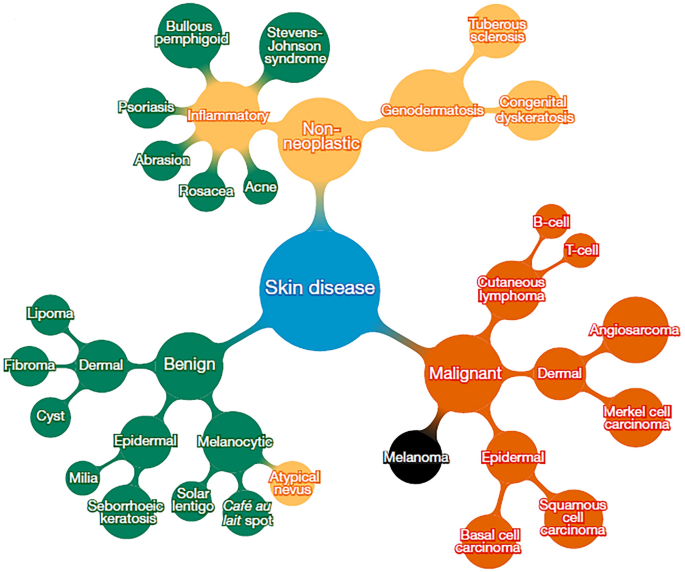
Reproduced with permission from Ref. [ 9 ] and credit (CC BY 4.0))
Subset of top of tree-structured taxonomy of skin disease (
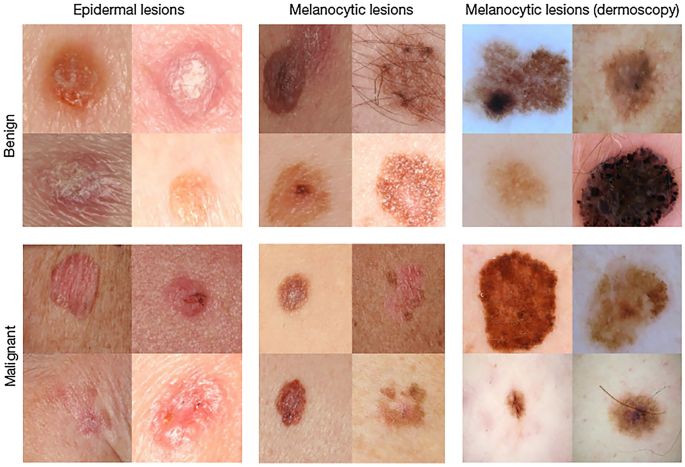
Malignant and benign sample images from two disease classes (
The diagnosis of skin disease relies on clinical experience and visual perception. However, human visual diagnosis is subjective and lacks accuracy and repeatability, which is not found in computerized skin-image analysis systems. The use of these systems enables inexperienced operators to prescreen patients [ 15 ]. Compared with other diseases or applications such as industrial fault diagnosis, the visual manifestation of skin disease is more prominent, facilitating the significant value of deep learning in image recognition with visual sensitivity. Through the study of large detailed images, dermatology can become one of the most suitable medical fields for telemedicine and artificial intelligence (AI). Using imaging methods, it could be possible for deep learning to assist or even replace dermatologists in the diagnosis of skin disease in the near future.
3 Image Methods
Deep learning is a class of machine learning that automatically learns hierarchical features of data using multiple layers composed of simple and nonlinear modules. It transforms the data into representations that are important for discriminating the data [ 16 ]. As early as 1998, the LeNet network was proposed for handwritten digital recognition [ 17 ]. However, owing to the lack of computational power, it was difficult to support the required computation. Until 2012, this method was successfully applied and overwhelmingly outperformed previous machine learning methods for visual recognition tasks at a competitive challenge in the ImageNet Large Scale Visual Recognition Challenge (ILSVRC) [ 18 , 19 ]. This was a breakthrough that used convolutional networks to virtually halve the error rate for object recognition, and precipitated the rapid adoption of deep learning by the computer vision community [ 16 ]. Since then, deep learning algorithms have undergone considerable development because of the improved capabilities of hardware such as graphics processing units (GPUs). Different models, such as ZFNet [ 20 ], VGG [ 21 ], GoogLeNet [ 22 ], and ResNet [ 23 ], have been proposed. The top-5 error rate in ImageNet dropped from 16.4% in 2012 to 2.25% in 2017 (Figure 3 ); correspondingly, that of humans was approximately 5%. It has dramatically improved tasks in different scientific and industrial fields including not only computer vision but also speech recognition, drug discovery, clinical surgery, and bioinformatics [ 24 , 25 , 26 ].
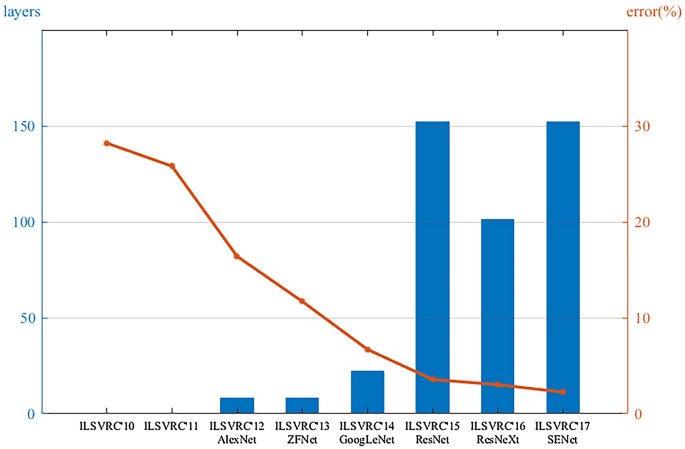
Deeper networks, lower error rates
The structure of a convolutional neural network (CNN), which is a representative deep learning algorithm, is displayed in Figure 4 . The actual model is similar to this figure, in addition to deeper layers and more convolution kernels. A CNN is a type of “feedforward neural network” inspired by human visual perception mechanisms, and can learn a large number of mappings between inputs and outputs without any precise mathematical expression between them. The first convolutional filter of the CNN is used to detect low-order features such as edges, angles, and curves. As the convolutional layer increases, the detected features become more complex [ 20 ]. The pooling layer, or named subsampling layer, converts a window into a pixel by taking the maximum or average value [ 27 ], which can reduce the size of the feature map. After the image passes the last fully connected layer, the model maps the learned distributed feature to the sample mark space and provides the final classification type. The layout of the CNN is similar to the biological neural network, with sparse structures and shared weights, which can reduce the number of parameters and improve the fitting effect to prevent overfitting. Deep CNNs demonstrate the potential for variable tasks across numerous fine-grained object categories and have unique advantages in the field of image recognition.
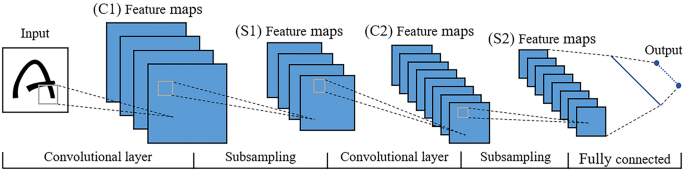
CNN architecture and principles
The selection of a suitable model is crucial. The GoogLeNet model, with a structure called inception (Figure 5 ), is proposed which can not only maintain the sparsity of the network structure but can also use the high computational performance of the dense matrix [ 22 ]. GoogLeNet has been learned and used by numerous researchers because of its excellent performance. Therefore, the Google team has further explored and improved it, resulting in an upgraded version of GoogLeNet, Inception v3 [ 28 ], which has become the first choice for current research. With Google’s Inception v3 CNN architecture pretrained to a high-level accuracy on the 1000 object class of ImageNet, researchers can remove the final classification layer from the network, retrain it with their own dataset, and fine-tune the parameters across all the layers.
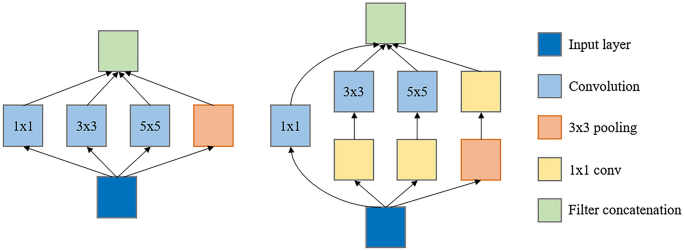
Inception module: ( a ) Inception module, naïve version; ( b ) Inception module with dimension reductions
Google’s TensorFlow [ 29 ], Caffe [ 30 ], and Theano [ 31 ] deep learning frameworks can be used for training. Theano is a Python library and optimizing compiler for manipulating and evaluating mathematical expressions. It pioneered the trend of using symbolic graphs for programming a network; however, it lacks a low-level interface and the inefficiency of the Python interpreter limits its usage. Caffe’s ConvNet implementation with numerous extensions being actively added is excellent; however, its support for recurrent networks and language modeling in general is poor. If both CPU and GPU supports are required, additional functions must be implemented. Specifying a new network is fairly easy in TensorFlow using a symbolic graph of vector operations; however, it has a major weakness in terms of modeling flexibility. It has a clean, modular architecture with multiple frontends and execution platforms, and the library can be compiled on Advanced RISC Machines (ARM).
Deep learning has been gradually applied to medical image data, as medical image analysis approaches are considerably similar to computer vision techniques [ 32 ]. Although numerous studies were initially undertaken using relatively small datasets and a pretrained deep learning model as a feasibility study, a robust validation of the medical application is required [ 33 , 34 , 35 ]. Hence, big data from medical images have been collected to validate the feasibility of medical applications [ 9 , 36 ]. For example, Google researchers collected large datasets consisting of more than 120,000 retinal fundus images for diagnosing diabetic retinopathy and demonstrated high sensitivity and specificity for detection [ 37 ].
Owing to the development of hardware and advancement of the algorithms, deep learning now includes considerably more functionality than could previously be imagined. Researchers are now more likely to predict and distinguish what is difficult to diagnose with complex mechanisms and similar characterizations [ 38 , 39 ]. Deep learning is a powerful machine learning algorithm for classification while extracting low- to high-level features [ 40 , 41 ]. A key difference in deep learning compared to other diagnostic methods is its self-learning nature. The neural network is not designed by humans; rather, it is designed by the data itself. Table 1 presents several published achievements on disease diagnosis using pictures or clinical images, which proves that deep learning can be compared with professional specialists in certain fields. Furthermore, many researchers have indicated interest in mobile diagnostics that allow the use of mobile technology. Smartphones with sufficient computing power and fast development to extend the versatility and utility could be used to scan, calculate, analyze anytime and anywhere to detect skin disease [ 42 , 43 , 44 ]. Researchers have developed such a system based on AI that allows users to install apps on their smartphones and analyze and judge suspicious lesions on the body by taking a picture [ 45 ].
4 Skin Disease Classification Using Deep Learning
Using the deep learning technique, the pattern recognition of images can be performed automatically once the program is established. Images can be input to a CNN with high fidelity and important features can be automatically obtained. Therefore, information extraction from images prior to the learning process is not necessary with this technique. In shallow layers, simple features such as the edges within the images are learned. At deep layers near the output layer, more complex high-order features are learned [ 56 ]. Different researchers, institutions, and challenges are working on the automatic diagnosis of skin disease, and different deep learning methods have been developed for the recognition of dermatological disease; these have been proven to be effective in numerous fields [ 57 ]. For example, the International Skin Imaging Collaboration (ISIC) is a challenge that focuses on the automatic analysis of skin lesions. The goal of the challenge (started in 2017) is to support the research and development of algorithms for the automated diagnosis of melanoma including lesion segmentation, dermoscopic feature detection within a lesion, and classification of melanoma [ 58 , 59 ], which is also the main goal in the field of dermatology [ 60 ]. In general, this method is a modeling framework that can learn the functional mapping from the input images to output. The input image is a preprocessed image; the output image is a segmentation mask. The network structure involves a series of convolution and pooling layers, followed by a fully connected layer, followed by a series of unpooling and disconnection operations [ 61 ].
The diagnosis of skin diseases typically consists of four components: image acquisition, image preprocessing, feature extraction and classification, and evaluation of the criteria. Image acquisition is the basis for skin classification, and more images typically indicate greater accuracy and better adaptability (for the data size of selected projects, please refer to Table 2 ). Preprocessing is used to crop and zoom the images and segment lesions for better training. Feature extraction mainly acquires the features of the skin lesions through color, texture, and boundary information. The evaluation of the results is the final step, which is used to judge whether the classification model is reasonable and achieves its objective.
4.1 Image Acquisition
Deep learning requires a large number of images to extract disease features. These datasets are typically available from the Internet, open dermatology databases, and hospitals in collaboration with research units, and are labeled by professional dermatologists after removing blurry and distant images. An excellent dataset should be composed of dermoscopic images. Dermoscopy is a non-invasive skin imaging technology that can observe the skin structure at the junction of the epidermis and dermis, and clearly indicate the nature, distribution, arrangement, edge, and shape of pigmented skin lesions. Because of the uncertainty of imaging conditions, such as shooting angle, illumination, and storage pixels, the imaging effect of non-dermoscopic images can be influenced. Selected published datasets are listed in Table 3 covering more than a dozen kind of skin diseases, among which melanoma has the greatest probability of occurrence. However, owing to the lack of a unified standard for skin disease images, the labeling of images is time-consuming and labor-intensive, which significantly limits the size of the current public datasets. Therefore, numerous studies have combined multiple datasets for use [ 43 , 63 ].
4.2 Image Preprocessing
Effective image quality can improve the generalization ability of a model. Preprocessing can reduce irrelevant information in the image, improve the intensity of the relevant information, simplify the data, and improve the reliability. The general image preprocessing process is as follows:
Image segmentation. Skin lesion segmentation is the essential step for the majority of classification tasks. Accurate segmentation contributes to the accuracy, computation time, and error rate of subsequent lesion classification [ 71 , 72 ]. It is crucial for image analysis for the following two reasons. First, the border of a lesion provides important information for accurate diagnosis, including numerous clinical features such as asymmetry and border irregularity. Secondly, the extraction of other important clinical features such as atypical dots and color variegation critically depends on the accuracy of the border detection [ 8 , 73 ]. Given a inputted dermoscopic image (Figure 6 a), the goal of the segmentation process is to generate a two-dimensional mask (Figure 6 b) that provides an accurate separation between the lesion area and surrounding healthy skin [ 74 ].
Resize. Lesions frequently occupy a relatively small area, although skin images can be considerably large [ 75 , 76 ]. Before this task, images for a deep learning network should be preprocessed because the resolution of the original lesion images is typically overly large, which entails a high computation cost [ 77 ]. Accurate skin lesion segmentation enhances its capability by incorporating a multiscale contextual information integration scheme [ 62 ]. To avoid distorting the shape of the skin lesion, the images should be cropped to the center area first and then proportionally resized. Images are frequently resized to 224×224 or 227×227 pixels through scaling and clipping [ 78 ], which is the appropriate size after combining the amount of calculation and information density.
Normalization. The image data are mapped to the interval of [0,1] or [−1,1] in the same dimension. The essence of normalization is a kind of linear transformation that does not cause “failure” after changing the data. Conversely, it can improve the performance of the data, accelerate the solution speed of gradient descent, and enhance the convergence speed of the model.
Data augmentation. Owing to privacy and professional equipment problems, it is difficult to collect sufficient data in the process of skin disease identification. A data set that is overly small can easily lead to overfitting owing to the lack of learning ability of the model, which makes the network model lack generalization ability. A method called data augmentation is adopted to expand the dataset to meet the requirements of deep learning for big data, such as rotation, random cropping, and noise [ 79 ]. Figure 7 displays several methods of image processing by which the image database can be extended to meet the training requirements.
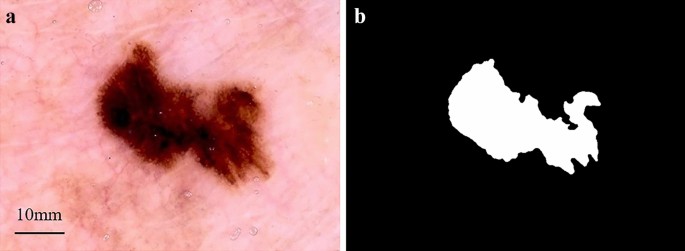
Skin lesion segmentation: ( a ) Dermoscopic image input; ( b ) Binary mask output
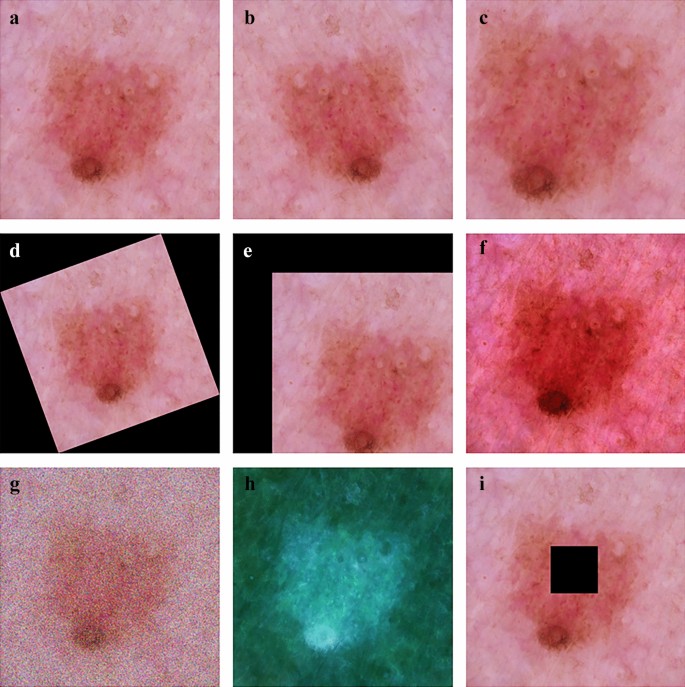
Data argumentation: ( a ) Original image; ( b ) Flip; ( c ) Random crop; ( d ) Rotation; ( e ) Shift; ( f ) Color jittering; ( g ) Noise; ( h ) Standardization; and ( i ) Paste
4.3 Feature Extraction and Classification
Early detection of lesions is a crucial step in the field of skin cancer treatment. There is a significant benefit if this can be achieved without penetrating the body. Feature extraction of skin disease is an important tool that can be used to properly analyze and explore an image [ 80 ]. Feature extraction can be simply viewed as a dimensionality reduction process; that is, converting picture data into a vector of a certain dimension with picture features. Before deep learning, this was typically determined manually by dermatologists or researchers after investigating a large number of digital skin lesion images. A well-known method for feature extraction is based on the ABCD rule of dermoscopy. ABCD stands for asymmetry, border structure, color variation, and lesion diameter. It defines the basis for disease diagnosis [ 81 ]. The extracted and fused traits such as color, texture, and Histogram of Oriented Gradient (HOG) are applied subsequently with a serial-based method. The fused features are selected afterwards by implementing a novel Boltzman entropy method [ 82 ], which can be used for the early detection. However, this typically has enormous randomness and depends on the quantity and quality of the pictures, as well as the experience of the dermatologists.
From a classification perspective, feature extraction has numerous benefits: (i) reducing classifier complexity for better generalization, (ii) improving prediction accuracy, (iii) reducing training and testing time, and (iv) enhancing the understanding and visualization of the data. The mechanism of neural networks is considerably different from that of traditional methods. Visualization indicates that the first layers are essentially calculating edge gradients and other simple operations such as SIFT [ 83 ] and HOG [ 84 ]. The folded layers combine the local patterns into a more global pattern, ultimately resulting in a more powerful feature extractor. In a study using nearly 130000 clinical dermatology images, 21 certified dermatologists tested the skin lesion classification with a single CNN, directly using pixels and image labels for end-to-end training; this had an accuracy of 0.96 for carcinoma [ 9 ]. Subsequently, researchers used deep learning to develop an automated classification system for 12 skin disorders by learning the abnormal characteristics of a malignancy and determined visual explanations from the deep network [ 47 ]. A third study combined deep learning with traditional methods such as hand-coded feature extraction and sparse coding to create a collection for melanoma detection that could yield higher performance than expert dermatologists. These results and others [ 85 , 86 , 87 ] confirm that deep learning has significant potential to reduce doctors’ repetitive work. Despite problems, it would be a significant advance if AI could reliably simulate experienced dermatologists.
4.4 Evaluation Criteria and Benchmarking
Evaluation and criterion, typically based on the following three points, reliability, time consumption, and training and validation are vital in this field [ 88 ]. Researchers [ 73 , 89 , 90 ] have used all three criteria to develop and design methods and techniques for detecting and diagnosing skin disease. Others [ 71 , 91 , 92 ] have used only two criteria, reliability, and training and validation to evaluate and discuss the different types of classifiers.
Numerous studies have demonstrated that acceptable reliability, time complexity, and error rates within a dataset cannot be achieved at the same time; hence, researchers must establish different standards. Once one of them is selected, the performance of the others diminishes [ 90 , 93 ]. Consequently, conflicts among dermatological evaluation criteria pose a serious challenge to dermatological classification methods. These requirements must be considered during the evaluation and benchmarking. The dermatological classification method should standardize the requirements and objectives and use a programmatic process in research, evaluation, and benchmarking. Moreover, new flexible evaluations should address all conflicting standards and issues [ 94 ].
Despite the conflicts, important criteria are the key goals for evaluation and benchmarking. It is necessary to develop appropriate procedures for these goals while increasing the importance of specific evaluation criteria and decreasing other standards [ 95 ]. When evaluating the results obtained using the diagnostic model, researchers must consider the quality of the dataset used to build the model and choose the parameters that can adjust that model. The time complexity and error rate in the dataset have proven to be important in the field of dermatology, which, with more consideration during the evaluation process, can optimize the consistency of the results [ 63 ]. In general, the goal is to obtain a balanced classifier for sensitivity and specificity.
5 Limitations and Prospect
5.1 limitations.
In general, the advantage of AI is that it can help doctors perform tedious repetitive tasks. For example, if sufficient blood is scanned, an AI-powered microscope can detect low-density infections in micrographs of standard, field-prepared thick blood films, which is considered to be time-consuming, difficult, and tedious owing to the low density and small parasite size and abundance of similar non-parasite objects [ 49 ]. The requirement for staff training and purchase of expensive equipment for creating dermoscopic images can be replaced by software using CNNs [ 96 ]. In the future, the clinical application of deep learning for the diagnosis of other diseases can be investigated. Transfer learning could be useful in developing CNN models for relatively rare diseases. Models could also evolve such that they require fewer preprocessing steps. In addition to these topics, a deeper understanding of the reconstruction kernel or image thickness could lead to improved deep learning model performance. Positive effects should continue to grow owing to the emergence of higher precision scanners and image reconstruction techniques [ 56 ]. However, we must realize that although AI has the ability to defeat humans in several specific fields, in general the performance of AI is considerably less than acceptable in the majority of cases [ 97 ]. The main reasons for this are as follows.
Medicine is an area that is not yet fully understood. Information is not completely transparent. The characteristics of dermatology determine that the majority of the data cannot be obtained. At the same time, the AI technology route is immature, the identification accuracy of which must be improved owing to the uncertainty of manual diagnosis. There is no strict correspondence between the symptoms and results of a disease and no clear boundary between the different diseases. Thus, the use of deep learning for disease diagnosis continues to require considerable effort.
Before systematic debugging, extensive simulation, and robust validation, flawed algorithms could harm patients, which could lead to medical ethical issues, and therefore require forward-looking scrutiny and stricter regulation [ 98 ]. As a “black box”, the principle of deep learning is unexplained at this stage, which could result in unpredictable system output. Moreover, it is possible that humans could not truly understand how a machine functions, even though it is actually inspired by humans [ 99 , 100 ]. Hence, whether or not patient care can be accepted using an opaque algorithm remains a point of discussion.
There is a problem with the change in the error rate value in a dataset, which is caused by the change in the size of the dataset used in different skin cancer experiments. Therefore, the lack of a standard dataset can lead to serious problems; the error rate values are considered in many experiments. In addition, the collection of datasets for numerous studies depends on individual research, leading to unnecessary effort and time. When the actual class is manually marked and compared to the predicted class to calculate one of the parameter matrices, pixels are lost when the background is cut from the skin cancer image using Adobe Photoshop [ 101 ]. At this point, the process influences the results of all the parameter reliability groups (matrices, relationships, and behaviors), which are considered controversial. High reliability and low rate of time complexity cannot be achieved simultaneously, which is reflected in the training process and is influenced by conflicts between different standards, leading to considerable challenges [ 93 , 102 ]. A method that works for the detection of one skin lesion could possibly not work for the detection of others [ 103 ]. Numerous different training and test sets have been used to evaluate the proposed methods. Moreover, for the parameters in the training and evaluation, different researchers are interested in different parts. This lack of uniformity and standardization across all papers makes a fair comparison virtually impossible [ 50 , 104 ]. Although these indicators in the literature have been widely criticized, studies continue to use them to evaluate the application to skin cancer and other image processing fields.
The data used for evaluation are frequently overly small to allow a convincing statement regarding a system’s performance to be made. Although it is not impossible to collect an abundance of relevant data through the Internet in this information age, this information, with significant uncertainty, apparently cannot meet the requirements of independent and identical distribution, which is one of the important prerequisites for deep learning to be successfully applied. For certain rare diseases and minorities, only a limited number of images are available for training. To date, a large number of algorithms have demonstrated prejudice against minority groups, which could cause a greater gap in health service between the “haves” and the “have-nots” [ 105 ]. Numerous cases are required for the training process using deep learning techniques. In addition, although the deep learning technique has been successfully applied to other tasks, the developed models in skin are valid in only specific dedicated diseases and are not applicable to common situations. Diagnosing dermatology is a complex process that, in addition to image recognition, must be supplemented by other means such as palpation, smell, temperature change, and microscopy.
5.2 Prospect
Deep learning has made considerable progress in the field of skin disease recognition. More attempts and explorations in the future can be considered in the following aspects.
Establishment of standardized skin disease image dataset
A large amount of data is the basis of skin disease recognition and the premise of acceptable generalization ability of the network model. However, the number of images, disease types, image size, and shooting and processing methods of the published datasets are considerably different, which leads to the confusion of different studies and the loss of the ability to quantitatively describe different models, Moreover, it is difficult to collect images of certain rare diseases. As mentioned above, there are numerous kinds of skin diseases; however, only approximately 20 datasets are available, including less than 20 kinds of skin diseases. There is an urgent requirement to expand access to medical images. For example, Indian researchers have trained neural networks to analyze images from “handheld imaging devices” instead of stationary dermatoscope devices to provide more prospects for early and correct diagnosis [ 106 ]. However, a public database that allows the collection of a sufficient number of labeled datasets is likely necessary to truly represent projections of the population.
Interpretability of skin disease recognition
The progress of deep learning in skin disease recognition depends on a highly nonlinear model and parameter adjustment technology. However, the majority of the neural networks are “black box” models, and their internal decision-making process is difficult to understand. This “end-to-end” decision-making mode leads to the weak explanatory power of deep learning. The internal logic of deep learning is not clear, which makes the diagnosis results of the model less convincing. The interpretability research of skin disease classification could allow the owner of the system to clearly know the behavior and boundary of the system, and ensure the reliability and safety of the system. Moreover, it could monitor the moral problems and violations caused by training data deviation and provide a superior mechanism to follow the requirements within an organization to solve the bias and audit problems caused by AI [ 107 ].
Intelligent diagnosis and treatment of skin diseases
Deep learning can be used to address the increasing number of patients with skin disease and relieve the pressure of limited dermatologists. With the popularity of mobile phones, mobile computers, and wearable devices, a skin disease recognition system based on deep learning can be expected to be available to intelligent devices to serve more people. Using a mobile device camera, users can upload their own photos of the affected area to the cloud recognition system and download the diagnosis results at any time. Through simple communication with the “skin manager”, diagnosis suggestions and possible treatment methods could be available. Furthermore, the “skin manager” could monitor the user’s skin condition and provide real-time protection methods and treatment suggestions.
Computer diagnostic systems can assist trained dermatologists rather than replace them. These systems can also be useful for untrained general practitioners or telemedicine clinics. For health systems, improving workflows could increase efficiency and reduce medical errors. Hospitals could make use of large-scale data and recommend data sharing with a cloud-based platform, thus facilitating multihospital collaboration [ 108 ]. For patients, it should be possible to enjoy the medical resources of the top hospitals in big cities in remote and less modernized areas by telemedicine or enabling them to process their own data [ 109 ].
6 Conclusions
The potential benefits of deep learning solutions for skin disease are tremendous and there is an unparalleled advantage in reducing the repetitive work of dermatologists and pressure on medical resources. Accurate detection is a tedious task that inevitably increases the demand for a reliable automated detection process that can be adopted routinely in the diagnostic process by expert and non-expert clinicians. Deep learning is a comprehensive subject that requires a wide range of knowledge in engineering, information, computer science, and medicine. With the continuous development of the above fields, deep learning is undergoing rapid development and has attracted the attention of numerous countries. Powered by more affordable solutions, software that can quickly collect and meaningfully process massive data, and hardware that can accomplish what people cannot, it is evident that deep learning for the identification of skin disease is a potential technique in the foreseeable future.
T Tarver. Cancer Facts & Figures 2012. American Cancer Society (ACS). Journal of Consumer Health on the Internet , 2012, 16(3): 366-367.
G M Weber, K D Mandl, I S Kohane. Finding the missing link for big biomedical data. Jama , 2014, 311(24): 2479.
Google Scholar
D-M Filimon, A Albu. Skin diseases diagnosis using artificial neural networks. 2014 IEEE 9th IEEE International Symposium on Applied Computational Intelligence and Informatics (SACI) , IEEE, 2014: 189-194, https://doi.org/10.1109/SACI.2014.6840059 .
A Serener, S Serte. Geographic variation and ethnicity in diabetic retinopathy detection via deeplearning. Turkish Journal of Electrical Engineering and Computer Sciences , 2020, 28(2): 664-678.
B Zhang, Y Luo, L Ma, et al. 3D bioprinting: an emerging technology full of opportunities and challenges. Bio-Design and Manufacturing , 2018, 1(1): 2-13.
S Pathan, K G Prabhu, P Siddalingaswamy. Techniques and algorithms for computer aided diagnosis of pigmented skin lesions—A review. Biomedical Signal Processing and Control , 2018, 39: 237-262.
A Paradisi, S Tabolli, B Didona, et al. Markedly reduced incidence of melanoma and nonmelanoma skin cancer in a nonconcurrent cohort of 10,040 patients with vitiligo. Journal of the American Academy of Dermatology , 2014, 71(6): 1110-1116.
M E Celebi, Q Wen, H Iyatomi, et al. A state-of-the-art survey on lesion border detection in dermoscopy images. Dermoscopy Image Analysis , 2015: 97-129.
A Esteva, B Kuprel, R A Novoa, et al. Dermatologist-level classification of skin cancer with deep neural networks. Nature , 2017, 542(7639): 115.
A Steiner, H Pehamberger, K Wolff. Improvement of the diagnostic accuracy in pigmented skin lesions by epiluminescent light microscopy. Anticancer Research , 1987, 7(3): 433-434.
S Joseph, J R Panicker. Skin lesion analysis system for melanoma detection with an effective hair segmentation method. 2016 International Conference in Information Science (ICIS) , IEEE, 2016: 91-96, https://doi.org/10.1109/infosci.2016.7845307 .
P Zaenker, L Lo, R Pearce, et al. A diagnostic autoantibody signature for primary cutaneous melanoma. Oncotarget , 2018, 9(55): 30539.
C Barata, M Ruela, M Francisco, et al. Two systems for the detection of melanomas in dermoscopy images using texture and color features. IEEE Systems Journal , 2014, 8(3): 965-979.
T Vos, C Allen, M Arora, et al. Global, regional, and national incidence, prevalence, and years lived with disability for 310 diseases and injuries, 1990–2015: A systematic analysis for the Global Burden of Disease Study 2015. The Lancet , 2016, 388(10053): 1545-1602.
P Wang, S Wang. Computer-aided CT image processing and modeling method for tibia microstructure. Bio-Design and Manufacturing , 2020, 3(1): 71-82.
Y LeCun, Y Bengio, G Hinton. Deep learning. Nature , 2015, 521(7553): 436.
Y LeCun, L Bottou, Y Bengio, et al. Gradient-based learning applied to document recognition. Proceedings of the IEEE , 1998, 86(11): 2278-2324.
O Russakovsky, J Deng, H Su, et al. Imagenet large scale visual recognition challenge. International Journal of Computer Vision , 2015, 115(3): 211-252.
MathSciNet Google Scholar
A Krizhevsky, I Sutskever, G E Hinton. Imagenet classification with deep convolutional neural networks. Advances in Neural Information Processing Systems , 2012, 25: 1097-1105.
M D Zeiler, R Fergus. Visualizing and understanding convolutional networks. European Conference on Computer Vision , Springer, Cham, 2014: 818-833.
K Simonyan, A Zisserman. Very deep convolutional networks for large-scale image recognition. arXiv preprint arXiv:1409.1556 , 2014.
C Szegedy, W Liu, Y Jia, et al. Going deeper with convolutions. Proceedings of the IEEE Conference on Computer Vision and Pattern Recognition , 2015: 1-9. https://doi.org/10.1109/CVPR.2015.7298594 .
K He, X Zhang, S Ren, et al. Deep residual learning for image recognition. 2016 IEEE Conference on Computer Vision and Pattern Recognition (CVPR) , 2016: 770-778, https://doi.org/10.1109/CVPR.2016.90 .
Article Google Scholar
B Alipanahi, A Delong, M T Weirauch, et al. Predicting the sequence specificities of DNA- and RNA-binding proteins by deep learning. Nature Biotechnology , 2015, 33(8): 831.
J Zhou, O G Troyanskaya. Predicting effects of noncoding variants with deep learning–based sequence model. Nature Methods , 2015, 12(10): 931.
A Shademan, R S Decker, J D Opfermann, et al. Supervised autonomous robotic soft tissue surgery. Science Translational Medicine , 2016, 8(337): 337ra64-337ra64.
S Kaymak, A Serener. Automated age-related macular degeneration and diabetic macular edema detection on OCT images using deep learning. 2018 IEEE 14th International Conference on Intelligent Computer Communication and Processing (ICCP) , IEEE, 2018, https://doi.org/10.1109/ICCP.2018.8516635 .
C Szegedy, V Vanhoucke, S Ioffe, et al. Rethinking the inception architecture for computer vision. Proceedings of the IEEE conference on computer vision and pattern recognition , 2016: 2818-2826, https://doi.org/10.1109/CVPR.2016.308 .
M Abadi, A Agarwal, P Barham, et al. TensorFlow: Large-scale machine learning on heterogeneous distributed systems. arXiv preprint arXiv:1603.04467, 2016.
Y Jia, E Shelhamer, J Donahue, et al. Caffe: Convolutional architecture for fast feature embedding. Proceedings of the 22nd ACM International Conference on Multimedia , 2014: 675-678, https://doi.org/10.1145/2647868.2654889 .
F Bastien, P Lamblin, R Pascanu, et al. Theano: new features and speed improvements. arXiv preprint arXiv:1211.5590 , 2012.
H Choi. Deep learning in nuclear medicine and molecular imaging: current perspectives and future directions. Nuclear Medicine and Molecular Imaging , 2018, 52(2): 109-118.
N Tajbakhsh, J Y Shin, S R Gurudu, et al. Convolutional neural networks for medical image analysis: Full training or fine tuning? IEEE Transactions on Medical Imaging , 2016, 35(5): 1299-1312.
Y Xu, T Mo, Q Feng, et al. Deep learning of feature representation with multiple instance learning for medical image analysis. 2014 IEEE International Conference on Acoustics, Speech and Signal Processing (ICASSP) , IEEE, 2014: 1626-1630, https://doi.org/10.1109/ICASSP.2014.6853873 .
E Long, H Lin, Z Liu, et al. An artificial intelligence platform for the multihospital collaborative management of congenital cataracts. Nature Biomedical Engineering , 2017, 1(2): 0024.
P Rajpurkar, J Irvin, K Zhu, et al. Chexnet: Radiologist-level pneumonia detection on chest x-rays with deep learning. arXiv preprint arXiv:1711.05225, 2017.
V Gulshan, L Peng, M Coram, et al. Development and validation of a deep learning algorithm for detection of diabetic retinopathy in retinal fundus photographs. Jama , 2016, 316(22): 2402-2410.
S F Weng, J Reps, J Kai, et al. Can machine-learning improve cardiovascular risk prediction using routine clinical data? PloS One , 2017, 12(4): e0174944.
H C Hazlett, H Gu, B C Munsell, et al. Early brain development in infants at high risk for autism spectrum disorder. Nature , 2017, 542(7641): 348.
S Sarraf, G Tofighi. Classification of alzheimer's disease structural MRI data by deep learning convolutional neural networks. arXiv preprint arXiv:1607.06583 , 2016.
N Amoroso, M La Rocca, S Bruno, et al. Brain structural connectivity atrophy in Alzheimer's disease. arXiv preprint arXiv:1709.02369 , 2017.
L Rosado, M Ferreira. A prototype for a mobile-based system of skin lesion analysis using supervised classification. 2013 2nd Experiment International Conference (exp. at'13) , IEEE, 2013: 156-157, https://doi.org/10.1109/ExpAt.2013.6703051 .
J Hagerty, J Stanley, H Almubarak, et al. Deep learning and handcrafted method fusion: Higher diagnostic accuracy for melanoma dermoscopy images. IEEE Journal of Biomedical and Health Informatics , 2019: 1-1, https://doi.org/10.1109/JBHI.2019.2891049 .
Andres, Diaz-Pinto, Sandra, et al. CNNs for automatic glaucoma assessment using fundus images: an extensive validation. Biomedical Engineering Online , 2019, 18(1), https://doi.org/10.1186/s12938-019-0649-y .
Y Li, L Shen. Skin lesion analysis towards melanoma detection using deep learning network. Sensors , 2018, 18(2): 556.
Y Gurovich, Y Hanani, O Bar, et al. Identifying facial phenotypes of genetic disorders using deep learning. Nature Medicine , 2019, 25(1): 60.
S S Han, M S Kim, W Lim, et al. Classification of the clinical images for benign and malignant cutaneous tumors using a deep learning algorithm. Journal of Investigative Dermatology , 2018, 138(7): 1529-1538.
H Haenssle, C Fink, R Schneiderbauer, et al. Man against machine: Diagnostic performance of a deep learning convolutional neural network for dermoscopic melanoma recognition in comparison to 58 dermatologists. Annals of Oncology , 2018, 29(8): 1836-1842, 2018.
C Mehanian, M Jaiswal, C Delahunt, et al. Computer-automated malaria diagnosis and quantitation using convolutional neural networks. 2017 IEEE International Conference on Computer Vision Workshop (ICCVW) , IEEE, https://doi.org/10.1109/ICCVW.2017.22 .
M Poostchi, K Silamut, R Maude, et al. Image analysis and machine learning for detecting malaria. Translational Research the Journal of Laboratory & Clinical Medicine , 2018, 194: 36-55.
Z I Attia, S Kapa, F Lopez-Jimenez, et al. Screening for cardiac contractile dysfunction using an artificial intelligence–enabled electrocardiogram. Nature Medicine , 2019, 25(1): 70.
A Y Hannun, P Rajpurkar, M Haghpanahi, et al. Cardiologist-level arrhythmia detection and classification in ambulatory electrocardiograms using a deep neural network. Nature Medicine , 2019, 25(1): 65.
J Zhang, Y Xie, Y Xia, et al. Attention residual learning for skin lesion classification. IEEE Transactions on Medical Imaging , 2019: 1-1, https://doi.org/10.1109/TMI.2019.2893944 .
Y Fujisawa, Y Otomo, Y Ogata, et al. Deep‐learning‐based, computer‐aided classifier developed with a small dataset of clinical images surpasses board‐certified dermatologists in skin tumour diagnosis. British Journal of Dermatology , 2019, 180(61), https://doi.org/10.1111/bjd.16924 .
A Rezvantalab, H Safigholi, S Karimijeshni. Dermatologist level dermoscopy skin cancer classification using different deep learning convolutional neural networks algorithms. arXiv preprint arXiv:1810.10348 , 2018.
K Yasaka, H Akai, A Kunimatsu, et al. Deep learning with convolutional neural network in radiology. Japanese Journal of Radiology , 2018: 1-16.
A Khamparia, P K Singh, P Rani, et al. An internet of health things‐driven deep learning framework for detection and classification of skin cancer using transfer learning. Transactions on Emerging Telecommunications Technologies , 2020.
D Gutman, N C Codella, E Celebi, et al. Skin lesion analysis toward melanoma detection: A challenge at the international symposium on biomedical imaging (ISBI) 2016, hosted by the international skin imaging collaboration (ISIC). arXiv preprint arXiv:1605.01397 , 2016.
L Bi, J Kim, E Ahn, et al. Automatic skin lesion analysis using large-scale dermoscopy images and deep residual networks. arXiv preprint arXiv:1703.04197 , 2017.
S Serte, H Demirel. Gabor wavelet-based deep learning for skin lesion classification. Computers in Biology and Medicine , 2019, 113: 103423.
N C Codella, Q-B Nguyen, S Pankanti, et al. Deep learning ensembles for melanoma recognition in dermoscopy images. IBM Journal of Research and Development, 2017, 61(4/5): 5:1-5:15.
L Yu, H Chen, Q Dou, et al. Automated melanoma recognition in dermoscopy images via very deep residual networks. IEEE Transactions on Medical Imaging , 2017, 36(4): 994-1004.
X Fan, M Dai, C Liu, et al. Effect of image noise on the classification of skin lesions using deep convolutional neural networks. Tsinghua Science and Technology , 2020, 25(3): 425-434.
M Combalia, N Codella, V Rotemberg, et al. BCN20000: Dermoscopic Lesions in the Wild, arXiv preprint arXiv:1908.02288, 2019.
P Tschandl, C Rosendahl, H Kittler. The HAM10000 dataset, a large collection of multi-source dermatoscopic images of common pigmented skin lesions. Scientific Data , 2018, 5(1): 1-9.
ISIC Project-ISIC Archive. Accessed: May 23, 2021. Available: https://www.isic-archive.com .
N Codella, D Gutman, M E Celebi, et al. Skin lesion analysis toward melanoma detection: A challenge at the 2017 International Symposium on Biomedical Imaging (ISBI), Hosted by the International Skin Imaging Collaboration (ISIC), 2018 IEEE 15th International Symposium on Biomedical Imaging (ISBI 2018), 2018: 168-172, https://doi.org/10.1109/ISBI.2018.8363547 .
Y Yang, Y Ge, L Guo, et al. Development and validation of two artificial intelligence models for diagnosing benign, pigmented facial skin lesions. Skin Research and Technology , 2020, https://doi.org/10.1111/srt.12911 .
Derm101 Image Library. Accessed: Jan. 12, 2019. Available: https://www.derm101.com/image librarv/.
Dermnet-Skin Disease Altas. Accessed: Dec. 31, 2018. Available: http://www.dermnet.com/ .
H Mhaske, D Phalke. Melanoma skin cancer detection and classification based on supervised and unsupervised learning. 2013 International Conference on Circuits, Controls and Communications (CCUBE) , 2013: 1-5, https://doi.org/10.1109/CCUBE.2013.6718539 .
I G Díaz. Incorporating the knowledge of dermatologists to convolutional neural networks for the diagnosis of skin lesions. IEEE Journal of Biomedical and Health Informatics , 2017, https://doi.org/10.1109/JBHI.2018.2806962 .
O Abuzaghleh, B D Barkana, M Faezipour. Automated skin lesion analysis based on color and shape geometry feature set for melanoma early detection and prevention. IEEE Long Island Systems, Applications and Technology (LISAT) Conference , 2014: 1-6, https://doi.org/10.1109/LISAT.2014.6845199 .
A Pennisi, D D Bloisi, D Nardi, et al. Skin lesion image segmentation using Delaunay Triangulation for melanoma detection. Computerized Medical Imaging and Graphics , 2016, 52: 89-103.
D D Gómez, C Butakoff, B K Ersboll, et al. Independent histogram pursuit for segmentation of skin lesions. IEEE Transactions on Biomedical Engineering , 2008, 55(1): 157-161.
S Kaymak, P Esmaili, A Serener. Deep learning for two-step classification of malignant pigmented skin lesions. 2018 14th Symposium on Neural Networks and Applications (NEUREL) , 2018:1-6.
H Balazs. Skin lesion classification with ensembles of deep convolutional neural networks. Journal of Biomedical Informatics , 2018, 86: S1532046418301618-.
A Mahbod, G Schaefer, C Wang, et al. Transfer learning using a multi-scale and multi-network ensemble for skin lesion classification. Computer Methods and Programs in Biomedicine , 2020, 193: 105475.
A G Howard. Some improvements on deep convolutional neural network based image classification, arXiv preprint arXiv:1312.5402, 2013.
W Paja, M Wrzesień. Melanoma important features selection using random forest approach. 2013 6th International Conference on Human System Interactions (HSI) , 2013: 415-418, https://doi.org/10.1109/HSI.2013.6577857 .
F Nachbar, W Stolz, T Merkle, et al. The ABCD rule of dermatoscopy: High prospective value in the diagnosis of doubtful melanocytic skin lesions. Journal of the American Academy of Dermatology , 1994, 30(4): 551-559.
M Nasir, M Attique Khan, M Sharif, et al. An improved strategy for skin lesion detection and classification using uniform segmentation and feature selection based approach. Microscopy Research and Technique , 2018, 81(6): 528-543.
D G Lowe. Method and apparatus for identifying scale invariant features in an image and use of same for locating an object in an image: US, US6711293. 2004-3-23.
N Dalal, B Triggs. Histograms of oriented gradients for human detection. 2005 IEEE Computer Society Conference on Computer Vision and Pattern Recognition (CVPR'05), 2005, 1: 886-893, https://doi.org/10.1109/CVPR.2005.177 .
L Ballerini, R B Fisher, B Aldridge, et al. A color and texture based hierarchical K-NN approach to the classification of non-melanoma skin lesions, color medical image analysis . Dordrecht: Springer, 2013.
C Leo, V Bevilacqua, L Ballerini, et al. Hierarchical classification of ten skin lesion classes. Proc. SICSA Dundee Medical Image Analysis Workshop , 2015.
K Shimizu, H Iyatomi, M E Celebi, et al. Four-class classification of skin lesions with task decomposition strategy. IEEE Transactions on Biomedical Engineering , 2015, 62(1): 274-283.
A Zaidan, B Zaidan, O Albahri, et al. A review on smartphone skin cancer diagnosis apps in evaluation and benchmarking: Coherent taxonomy, open issues and recommendation pathway solution. Health and Technology , 2018: 1-16.
T-T Do, Y Zhou, H Zheng, et al. Early melanoma diagnosis with mobile imaging. Conf. Proc. IEEE Eng. Med. Biol. Soc. , 2014: 6752-6757, https://doi.org/10.1109/EMBC.2014.6945178 .
A Masood, A Al-Jumaily, K Anam. Self-supervised learning model for skin cancer diagnosis. 2015 7th International IEEE/EMBS Conference on Neural Engineering (NER) , 2015: 1012-1015, https://doi.org/10.1109/NER.2015.7146798 .
M F Duarte, T E Matthews, W S Warren, et al. Melanoma classification from Hidden Markov tree features. 2012 IEEE International Conference on Acoustics, Speech and Signal Processing (ICASSP) , 2012: 685-688, https://doi.org/10.1109/ICASSP.2012.6287976 .
K Phillips, O Fosu, I Jouny. Mobile melanoma detection application for android smart phones. 2015 41st Annual Northeast Biomedical Engineering Conference (NEBEC) , 2015: 1-2, https://doi.org/10.1109/NEBEC.2015.7117184 .
F Topfer, S Dudorov, J Oberhammer. Millimeter-wave near-field probe designed for high-resolution skin cancer diagnosis. IEEE Transactions on Microwave Theory & Techniques , 2015, 63(6): 2050-2059.
I Valavanis, K Moutselos, I Maglogiannis, et al. Inference of a robust diagnostic signature in the case of Melanoma: Gene selection by information gain and Gene Ontology tree exploration. 13th IEEE International Conference on BioInformatics and BioEngineering , 2013: 1-4, https://doi.org/10.1109/BIBE.2013.6701618 .
P Sabouri, H GholamHosseini, T Larsson, et al. A cascade classifier for diagnosis of melanoma in clinical images. 2014 36th Annual International Conference of the IEEE Engineering in Medicine and Biology Society , 2014: 6748-6751, https://doi.org/10.1109/EMBC.2014.6945177 .
M Efimenko, A Ignatev, K Koshechkin. Review of medical image recognition technologies to detect melanomas using neural networks. BMC Bioinformatics, 2020, 21(11): 1-7.
H L Semigran, D M Levine, S Nundy, et al. Comparison of physician and computer diagnostic accuracy. Jama Intern. Med. , 2016, 176(12): 1860-1861.
C Ross, I Swetlitz. IBM’s Watson supercomputer recommended ‘unsafe and incorrect’ cancer treatments, internal documents show, Stat News , 2018, https://www.statnews.com/2018/07/25/ibm-watson-recommended-unsafe-incorrect-treatments .
D Castelvecchi. Can we open the black box of AI? Nature News , 2016, 538(7623): 20.
D Weinberger, Our machines now have knowledge we’ll never understand, Backchannel , 2017, https://www.wired.com/story/our-machines-now-have-knowledge-well-never-understand .
A Körner, R Garland, Z Czajkowska, et al. Supportive care needs and distress in patients with non-melanoma skin cancer: Nothing to worry about? European Journal of Oncology Nursing , 2016, 20: 150-155.
O Malyuskin, V Fusco. Resonance microwave reflectometry for early stage skin cancer identification. 2015 9th European Conference on Antennas and Propagation (EuCAP) , 2015: 1-6.
S Serte, A Serener, F Al‐Turjman. Deep learning in medical imaging: A brief review. Trans. Emerging Tel. Tech. , 2020: e4080.
C M Doran, R Ling, J Byrnes, et al. Benefit cost analysis of three skin cancer public education mass-media campaigns implemented in New South Wales, Australia. Plos One , 2016, 11(1): e0147665.
A P Miller. Want less-biased decisions? Use algorithms. Harvard Business Review , 2018.
Gautam, Diwakar, Ahmed, et al. Machine learning-based diagnosis of melanoma using macro images. International Journal for Numerical Methods in Biomedical Engineering , 2018, 34(5): e2953.1.
W Fang, Y Li, H Zhang, et al. On the throughput-energy tradeoff for data transmission between cloud and mobile devices. Information Sciences , 2014, 283: 79-93, https://doi.org/10.1016/j.ins.2014.06.022 .
J He, S L Baxter, J Xu, et al. The practical implementation of artificial intelligence technologies in medicine. Nature Medicine , 2019, 25(1): 30.
E J Topol. High-performance medicine: the convergence of human and artificial intelligence. Nature Medicine , 2019, 25(1): 44-56.
Download references
Acknowledgements
Not applicable.
Supported by Key Research and Development Projects of Zhejiang Province of China (Grant No. 2017C01054), National Key Research and Development Program of China (Grant No. 2018YFA0703000), National Natural Science Foundation of China (Grant No. 51875518), and Fundamental Research Funds for the Central Universities of China (Grant Nos. 2019XZZX003-02, 2019FZA4002).
Author information
Authors and affiliations.
State Key Laboratory of Fluid Power and Mechatronic Systems, Zhejiang University, Hangzhou, 310058, China
Bin Zhang, Xue Zhou, Yichen Luo, Hao Zhang, Huayong Yang & Liang Ma
School of Mechanical Engineering, Zhejiang University, Hangzhou, 310058, China
College of Electrical Engineering, Zhejiang University, Hangzhou, 310027, China
You can also search for this author in PubMed Google Scholar
Contributions
BZ was in charge of the whole trial; XZ wrote the manuscript; YCL assisted with structure and language of the manuscript. HZ designed the experiments; HYY assisted with experimental setup; LM and JEM jointly supervised this work; All authors read and approved the final manuscript.
Authors’ information
Bin Zhang received his PhD degree in Engineering from Zhejiang University, China , in 2009. Currently, he is a researcher at School of Mechanical Engineering, Zhejiang University, China . His research interests include intelligent digital hydraulics and biological manufacturing based on fluid extrusion.
Xue Zhou received his B.S. degree in mechanical design and manufacturing from Xiamen University, China , in 2017. He is currently a PhD candidate at State Key Laboratory of Fluid Power Transmission and Control, Zhejiang University, China . His research mainly focuses on in vivo 3D printing and skin wound detection and repair.
Yichen Luo received his B.S. degree from Southeast University, China , in 2015. He is currently a PhD candidate at State Key Laboratory of Fluid Power Transmission and Control, Zhejiang University, China . His research mainly interests include 3D bioprinting and biological manufacturing.
Hao Zhang received his master degree from Zhejiang University, China , in 2019. Her research interests include deep learning and skin disease classification.
Huayong Yang received his PhD degree from the University of Bath, United Kingdom , in 1988. He is the dean of School of Mechanical Engineering, Zhejiang University, China . He was elected as an academician of the Chinese Academy of Engineering in 2013. His research mainly focuses on energy saving of fluid power and electromechanical systems and 3D printing of biological organs.
Jien Ma received her PhD degree from Zhejiang University, China , in 2009. She is currently a professor at College of Electrical Engineering, Zhejiang University, China . Her research interests include electrician theory and new energy technology.
Liang Ma received his PhD degree from University of Washington, USA , 2012. He is currently as an associate professor at School of Mechanical Engineering, Zhejiang University, China. His research interests include organ bioprinting and Microfluidic chip.
Corresponding author
Correspondence to Liang Ma .
Ethics declarations
Competing interests.
The authors declare there is no competing interests.
Rights and permissions
Open Access This article is licensed under a Creative Commons Attribution 4.0 International License, which permits use, sharing, adaptation, distribution and reproduction in any medium or format, as long as you give appropriate credit to the original author(s) and the source, provide a link to the Creative Commons licence, and indicate if changes were made. The images or other third party material in this article are included in the article's Creative Commons licence, unless indicated otherwise in a credit line to the material. If material is not included in the article's Creative Commons licence and your intended use is not permitted by statutory regulation or exceeds the permitted use, you will need to obtain permission directly from the copyright holder. To view a copy of this licence, visit http://creativecommons.org/licenses/by/4.0/ .
Reprints and permissions
About this article
Cite this article.
Zhang, B., Zhou, X., Luo, Y. et al. Opportunities and Challenges: Classification of Skin Disease Based on Deep Learning. Chin. J. Mech. Eng. 34 , 112 (2021). https://doi.org/10.1186/s10033-021-00629-5
Download citation
Received : 10 November 2020
Revised : 16 May 2021
Accepted : 08 October 2021
Published : 24 November 2021
DOI : https://doi.org/10.1186/s10033-021-00629-5
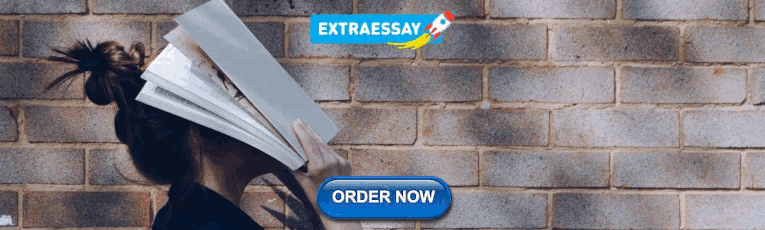
Share this article
Anyone you share the following link with will be able to read this content:
Sorry, a shareable link is not currently available for this article.
Provided by the Springer Nature SharedIt content-sharing initiative
- Skin disease
- Image method
- Deep learning
- Disease classification
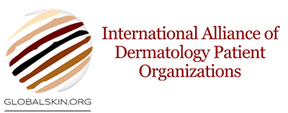
Member Login
- Sign Up for Newsletter
- About GRIDD
- GRIDD Study
- Phase 3 and 4
- Phases & Outcomes
- Resources & Publications
- Meet The Team
- Get Involved
- Science Behind GRIDD

GlobalSkin's GRIDD Study: A Wrap-up Report
GlobalSkin has successfully concluded its Global Research on the Impact of Dermatological Diseases (GRIDD) Study, a novel patient-led research initiative which it launched on June 5, 2023 in collaboration with Cardiff University (UK) and University Medical Centre Hamburg-Eppendorf (Germany).
The GRIDD Study gathered global data about the real impact of conditions of the skin, hair, nails, and mucosa. The objective of collecting these insights is to help reshape global perspectives on dermatological diseases, stimulate research efforts, optimize healthcare system spending, and inform future policies for improved patient outcomes. At the heart of the study was the new Patient-Reported Impact of Dermatological Diseases (PRIDD) measure, a tool we developed with more than 6000 patients over the past five years to capture and quantify patient experiences.
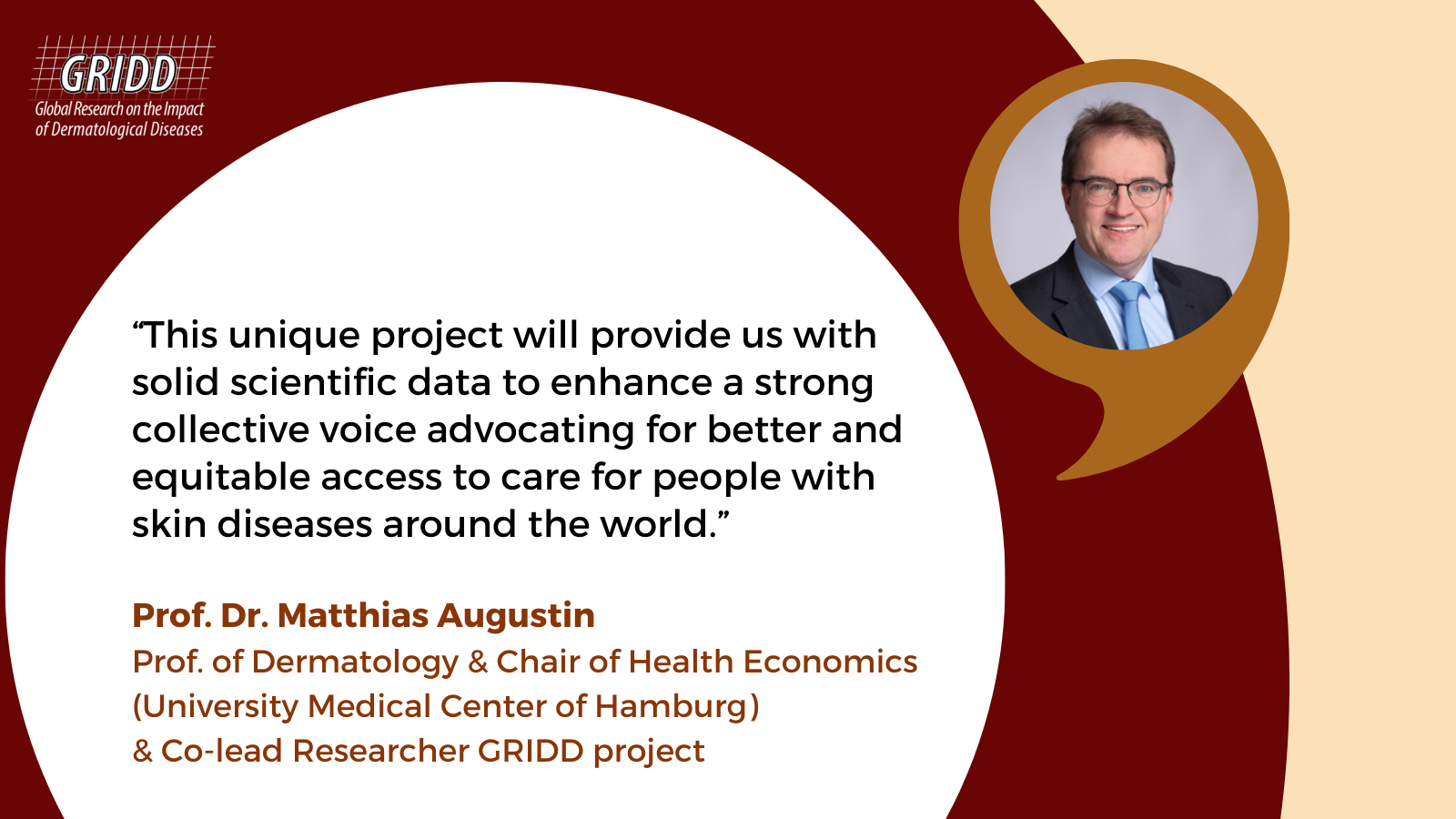
- Privacy Policy
- Terms of Service
- Social Media Disclaimer
Copyright 2018- 2024 International Alliance of Dermatology Patient Organizations. All right reserved.
- Vision & Mission
- Collaborations
- Board & Committees
- Partnerships
- Member List
- Communities
- Member Impact Fund
- Patient Expert Scholarship
- World Atopic Eczema Day Fund
- Rare Disease Day Fund
- Publications
- Events Listing
- Workshop Series
- GlobalSkin 2019 Conference
- GlobalSkin 2021 Conference
- GlobalSkin 2023 Conference
Login
Sign in to your account
- Forgot your username?
- Forgot your password?

Fostering skin and epithelial biology research.
Since 2009, the Skin Biology & Diseases Resource-Based Center (formerly the Skin Disease Research Center) has promoted outstanding bench and clinical research in cutaneous biology by providing high-quality technical services, enhancing education and communication among our members and facilitating translational research. We are funded through the NIH National Institute of Arthritis and Musculoskeletal and Skin Diseases.
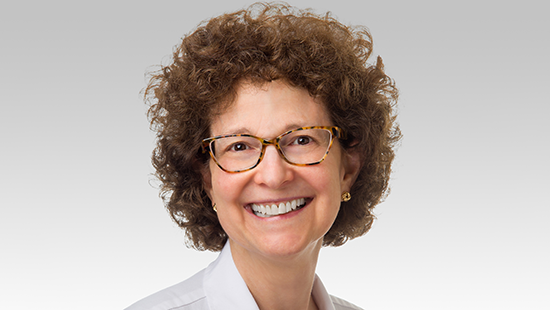
Our team of expert scientists provide outstanding and cost-effective services. We are eager to partner with you in the development of your projects.”
Read her message
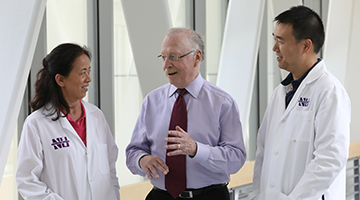
Enrichment Programs
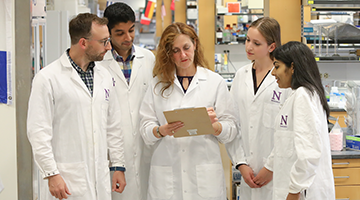
Access our three shared cores, which offer a number of resource to assist our scientists in their investigations.
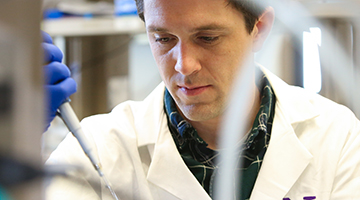
Meet Our Team
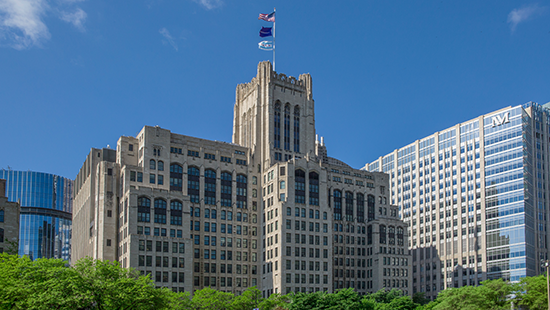
We welcome your questions and comments. Get in touch with our staff, or browse our member list to get an in-depth look at our scientists' backgrounds and latest research endeavors.
Contact Us Meet Our Members
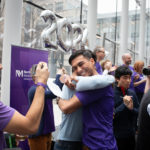

Skin Diseases Research Center
Mission and vision.
The mission of the Skin Diseases Research Center (SDRC) is to add value to our investigators through strength of focus on uniting basic and translational skin science. The vision of the SDRC is:
- To enhance career development and to fuel the projects of new and experienced investigators with a rich matrix of resources in order to speed the progress and enhance the quality of skin diseases research
- To generate a new knowledge base that will have a significant and sustained impact on cutaneous biology aimed at improving the quality of life of patients with skin disease
- To integrate the components and activities of the Skin Diseases Research Center (SDRC)
- To manage the fiscal operations
- To coordinate the core facilities and pilot and feasibility awarded programs
- To implement an enrichment program
- To foster new SDRC initiatives
- To translate innovative basic research to patients with skin diseases
- To facilitate utilization of genomics and informatics for skin diseases
The SDRC at Case Western Reserve University / University Hospitals (UH) Health Systems promotes cooperative interaction among investigators engaged directly in skin diseases research with those conducting state-of-the-art biomedical research in other disciplines that have relevance to skin disease. The center's primary goal is to integrate groups of investigators from Case Western Reserve, UH, and other institutions into a cohesive unit that will advance research relevant to the diagnosis, pathogenesis and treatment of skin diseases. The center has attracted a number of outstanding scientists and has been instrumental in developing new technologies and encouraging their timely transfer to the clinical setting. The SDRC has also developed informative educational programs in dermatologic diseases for its investigators, physicians and the public.
Major programs include:
- a pilot and feasibility grants program,
- core facilities to support research,
- a visiting lectureship program,
- special national and regional educational programs,
- a scholars exchange program,
- and a minority student and fellow research program.
SDRC Membership
The strong record of achievement of the SDRC at Case Western Reserve and UH is due to the accomplishments of the individual investigators and the synergistic interactions fostered by the center grant. When the SDRC was originally funded in 1988, its membership was 13 (four from the Department of Dermatology, ten from five other departments). At the present time it is comprised of more than 70 members from 34 different departments and centers within Case Western Reserve, University Hospitals of Cleveland, the Cleveland VA Medical Center, the Lerner Research Institute of Cleveland Clinic and Henry Ford Hospital in Detroit. SDRC investigators in the aggregate average approximately $10 million annually to funded research projects relevant to skin.
Taken together, the research base of the SDRC at Case Western Reserve has breadth and depth, is multidisciplinary and interrelated, and provides an excellent, vibrant platform for advancing the interests of patients with skin disease through the SDRC mechanisms.
Members of our university community are encouraged to participate in the center's enrichment programs (i.e., seminar series, visiting scholars' program, P&F research-in-progress lunches, etc.) and are offered the opportunity to submit proposals to the Pilot & Feasibility Study Program (P&FS). A major benefit of membership in the SDRC is unrestricted access to the core facilities.
The primary criterion for membership in the SDRC is a commitment to research applications in skin diseases. Interdisciplinary collaborations involving skin-related research are also looked upon favorably. Membership in the SDRC requires approval by the director with the concurrence of the executive committee.
The guidelines for membership are as follows:
- A member of the Department of Dermatology who is actively engaged in research.
- A non-member of the Department of Dermatology with a funded research project directly relevant to dermatology or the SDRC's cores.
- A non-member of the Department of Dermatology who has been awarded an SDRC P&F grant.
- A non-member of the Department of Dermatology who has a productive collaboration with a member of the Department of Dermatology on a skin-related project.
Continued membership requires:
- Ongoing productivity in skin-related research leading to the publication of peer-reviewed articles and acquisition of extramural grants.
- Participation in SDRC-related activities including its cores, committees, and enrichment program activities
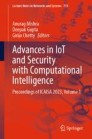
International Conference on Advances in IoT and Security with AI
ICAISA 2023: Advances in IoT and Security with Computational Intelligence pp 147–162 Cite as
Skin Disease Classification and Detection by Deep Learning and Machine Learning Approaches
- Summi Goindi ORCID: orcid.org/0009-0007-4851-1212 12 ,
- Khushal Thakur ORCID: orcid.org/0000-0002-9896-8428 12 &
- Divneet Singh Kapoor ORCID: orcid.org/0000-0002-3562-6148 12
- Conference paper
- First Online: 23 September 2023
139 Accesses
Part of the book series: Lecture Notes in Networks and Systems ((LNNS,volume 755))
One of the most common diseases around the globe is dermatological problem. Despite being frequent, its diagnosis is very challenging because of the complexity of its skin tone, colour and hair existence. This research offers a method to automatically forecast the many types of skin illnesses using a variety of computer vision-based approaches (deep learning). With the many skin photos, the system uses deep learning techniques to train itself. One of the main goals of this approach is improving the accuracy with which skin illness can be predicted and vast majority of the population is healthier. The most common causes of skin disorders include allergies, bacteria, mycosis, viruses, etc. The rapid development of medical and laser technologies that are founded on photonics has made it possible to identify skin issues in a manner that is both timely and precise. Such diagnoses can only be made with expensive, specialized medical equipment. As a result, deep learning algorithms help diagnose skin problems before they become severe. The categorization of skin conditions relies heavily on extracting features. Deep learning algorithms have substantially reduced the requirement for labour-intensive manual operations such as data restoration and extraction of features for classifications.
- Skin disease
- Dermatologically
- Deep learning
This is a preview of subscription content, log in via an institution .
Buying options
- Available as PDF
- Read on any device
- Instant download
- Own it forever
- Available as EPUB and PDF
- Compact, lightweight edition
- Dispatched in 3 to 5 business days
- Free shipping worldwide - see info
Tax calculation will be finalised at checkout
Purchases are for personal use only
Deepalakshmi P, Lavanya K, Srinivasu PN (2021) Plant leaf disease detection using CNN algorithm. Int J Inf Syst Model Des (IJISMD) 12(1):1–21
Article Google Scholar
Anitha J (2018) Identification of melanoma in dermoscopy images using image processing algorithms. In: International conference on control, power, communication and computing technologies (ICCPCCT). IEEE, pp 553–557
Google Scholar
Roy K, Chaudhuri SS, Ghosh S, Dutta SK, Chakraborty P, Sarkar R (2019) Skin disease detection based on different segmentation techniques. In: International conference on opto-electronics and applied optics (Optronix). IEEE, pp 1–5
Kotian AL, Deepa K (2017) Detection and classification of skin diseases by image analysis using MATLAB. Int J Emerg Res Manag Technol 6(5):779–784
Sundaramurthy S, Saravanabhavan C, Kshirsagar P (2020) Prediction and Classification of rheumatoid arthritis using ensemble machine learning approaches. In: Proceedings of the 2020 international conference on decision aid sciences and application (DASA). Sakheer, Bahrain, pp 17–21
Skin Disease. https://www.medanta.org/patient-education-blog/everything-about-common-skin-disorders/
Ronneberger O, Fischer P, Brox T (2015) U-net: convolutional networks for biomedical image segmentation. In: International conference on medical image computing and computer-assisted intervention. Springer, Cham, pp 234–241
Yu Z, Jiang X, Zhou F, Qin J, Ni D, Chen S, Wang T (2018) Melanoma recognition in dermoscopy images via aggregated deep convolutional features. IEEE Trans Biomed Eng 66(4):1006–1016
Phillips A, Teo I, Lang J (2019) Segmentation of prognostic tissue structures in cutaneous melanoma using whole slide images. In: Proceedings of the IEEE/CVF conference on computer vision and pattern recognition workshops
Reddy VJ, Nagalakshmi TJ (2019) Skin disease detection using artificial neural network. Indian J Public Health Res Dev 10(11)
Jana E, Subban R, Saraswathi S (2017) Research on skin cancer cell detection using image processing. In: 2017 IEEE international conference on computational intelligence and computing research (ICCIC). IEEE, pp 1–8
Zaqout I (2019) Diagnosis of skin lesions based on dermoscopic images using image processing techniques. Pattern Recognit Sel Methods Appl
Kumar VB, Kumar SS, Saboo V (2016) Dermatological disease detection using image processing and machine learning. In: 2016 Third international conference on artificial intelligence and pattern recognition (AIPR). IEEE, pp 1–6
Khan MA, Javed MY, Sharif M, Saba T, Rehman A (2019) Multi-model deep neural network-based features extraction and optimal selection approach for skin lesion classification. In: 2019 international conference on computer and information sciences (ICCIS). IEEE, pp 1–7
Amarathunga AA, Ellawala EP, Abeysekar GN, Amalraj CR (2015) Expert system for diagnosis of skin diseases. Int J Sci Technol Res 4(01):174–178
Chakraborty S, Mali K, Chatterjee S, Anand S, Basu A, Banerjee S, Bhattacharya A et al (2017) Image based skin disease detection using hybrid neural network coupled bag-of-features. In: 2017 IEEE 8th annual ubiquitous computing, electronics and mobile communication conference (UEMCON). IEEE, pp 242–246
Arifin MS, Kibria MG, Firoze A, Amini MA, Yan H (2012) Dermatological disease diagnosis using color-skin images. In: 2012 international conference on machine learning and cybernetics, vol 5. IEEE, pp 1675–1680
Premaladha J, Sujitha S, Priya ML, Ravichandran KS (2014) A survey on melanoma diagnosis using image processing and soft computing techniques. Res J Inf Technol 6(2):65–80
Manerkar MS, Snekhalatha U, Harsh S, Saxena J, Sarma SP, Anburajan M (2016) Automated skin disease segmentation and classification using multi-class SVM classifier
Barati E, Saraee MH, Mohammadi A, Adibi N, Ahmadzadeh MR (2011) A survey on utilization of data mining approaches for dermatological (skin) diseases prediction. J Sel Areas Health Inform (JSHI) 2(3):1–11
Lopez AR, Giro-i-Nieto X, Burdick J, Marques O (2017) Skin lesion classification from dermoscopic images using deep learning techniques. In: 2017 13th IASTED international conference on biomedical engineering (BioMed). IEEE, pp 49–54
Zhang X, Wang S, Liu J, Tao C (2018) Towards improving diagnosis of skin diseases by combining deep neural network and human knowledge. BMC Med Inform Decis Mak 18(2):69–76
Shi X, Dou Q, Xue C, Qin J, Chen H, Heng PA (2019) An active learning approach for reducing annotation cost in skin lesion analysis. In: International workshop on machine learning in medical imaging. Springer, Cham, pp 628–636
Chatterjee S, Dey D, Munshi S, Gorai S (2019) Extraction of features from cross correlation in space and frequency domains for classification of skin lesions. Biomed Signal Process Control 53:101581
Monisha M, Suresh A, Rashmi MR (2019) Artificial intelligence-based skin classification using GMM. J Med Syst 43(1):1–8
Esteva A, Kuprel B, Novoa RA, Ko J, Swetter SM, Blau HM, Thrun S (2017) Dermatologist-level classification of skin cancer with deep neural networks. Nature 542(7639):115–118
Data Science. https://www.medanta.org/patient-education-blog/everything-about-common-skin-disorders/
Brinker TJ, Hekler A, Utikal JS, Grabe N, Schadendorf D, Klode J, Von Kalle C (2018) Skin cancer classification using convolutional neural networks: systematic review. J Med Internet Res 20(10):e11936
Kulhalli R, Savadikar C, Garware B (2019) A hierarchical approach to skin lesion classification. In: Proceedings of the ACM India joint international conference on data science and management of data. pp 245–250
Download references
Author information
Authors and affiliations.
Chandigarh University, Gharuan, Mohali, Punjab, India
Summi Goindi, Khushal Thakur & Divneet Singh Kapoor
You can also search for this author in PubMed Google Scholar
Corresponding author
Correspondence to Summi Goindi .
Editor information
Editors and affiliations.
Department of Electronics, Deen Dayal Upadhyaya College, University of Delhi, New Delhi, India
Anurag Mishra
Department of Computer Science and Engineering, MNNIT Allahabad, Prayagraj, India
Deepak Gupta
Faculty of Science and Technology, University of Canberra, Bruce, ACT, Australia
Girija Chetty
Rights and permissions
Reprints and permissions
Copyright information
© 2023 The Author(s), under exclusive license to Springer Nature Singapore Pte Ltd.
About this paper
Cite this paper.
Goindi, S., Thakur, K., Kapoor, D.S. (2023). Skin Disease Classification and Detection by Deep Learning and Machine Learning Approaches. In: Mishra, A., Gupta, D., Chetty, G. (eds) Advances in IoT and Security with Computational Intelligence. ICAISA 2023. Lecture Notes in Networks and Systems, vol 755. Springer, Singapore. https://doi.org/10.1007/978-981-99-5085-0_16
Download citation
DOI : https://doi.org/10.1007/978-981-99-5085-0_16
Published : 23 September 2023
Publisher Name : Springer, Singapore
Print ISBN : 978-981-99-5084-3
Online ISBN : 978-981-99-5085-0
eBook Packages : Intelligent Technologies and Robotics Intelligent Technologies and Robotics (R0)
Share this paper
Anyone you share the following link with will be able to read this content:
Sorry, a shareable link is not currently available for this article.
Provided by the Springer Nature SharedIt content-sharing initiative
- Publish with us
Policies and ethics
- Find a journal
- Track your research
- Introduction to Genomics
- Educational Resources
- Policy Issues in Genomics
- The Human Genome Project
- Funding Opportunities
- Funded Programs & Projects
- Division and Program Directors
- Scientific Program Analysts
- Contact by Research Area
- News & Events
- Research Areas
- Research investigators
- Research Projects
- Clinical Research
- Data Tools & Resources
- Genomics & Medicine
- Family Health History
- For Patients & Families
- For Health Professionals
- Jobs at NHGRI
- Training at NHGRI
- Funding for Research Training
- Professional Development Programs
- NHGRI Culture
- Social Media
- Broadcast Media
- Image Gallery
- Press Resources
- Organization
- NHGRI Director
- Mission & Vision
- Policies & Guidance
- Institute Advisors
- Strategic Vision
- Leadership Initiatives
- Diversity, Equity, and Inclusion
- Partner with NHGRI
- Staff Search
NIH scientists find treatment for rare genetic skin disorder
- Share on Facebook
- Submit to Reddit
- Share on LinkedIn
Genome sequencing reveals genetic basis for disabling pansclerotic morphea, a severe inflammatory disease.
Researchers at the National Institutes of Health and their colleagues have identified genomic variants that cause a rare and severe inflammatory skin disorder, known as disabling pansclerotic morphea, and have found a potential treatment. Scientists discovered that people with the disorder have an overactive version of a protein called STAT4, which regulates inflammation and wound healing. The work also identified a drug that targets an important feedback loop controlled by the STAT4 protein and significantly improves symptoms in these patients. The results were published in the New England Journal of Medicine .
The study was led by researchers at the National Human Genome Research Institute (NHGRI), part of NIH, in collaboration with researchers from the University of California, San Diego (UCSD) and the University of Pittsburgh. Researchers from the National Institute of Arthritis and Musculoskeletal and Skin Diseases and the National Institute of Allergy and Infectious Diseases, both part of NIH, also participated in the study.
Only a handful of patients have been diagnosed with disabling pansclerotic morphea, a disorder first described in the medical literature around 100 years ago. The disorder causes severe skin lesions and poor wound healing, leading to deep scarring of all layers of the skin and muscles. The muscles eventually harden and break down while the joints stiffen, leading to reduced mobility. Because the disorder is so rare, its genetic cause had not been identified until now.
“Researchers previously thought that this disorder was caused by the immune system attacking the skin,” said Sarah Blackstone, a predoctoral fellow within NHGRI's Inflammatory Disease Section, a medical student at the University of South Dakota and co-first author of the study. “However, we found that this is an oversimplification, and that both skin and the immune system play an active role in disabling pansclerotic morphea.”
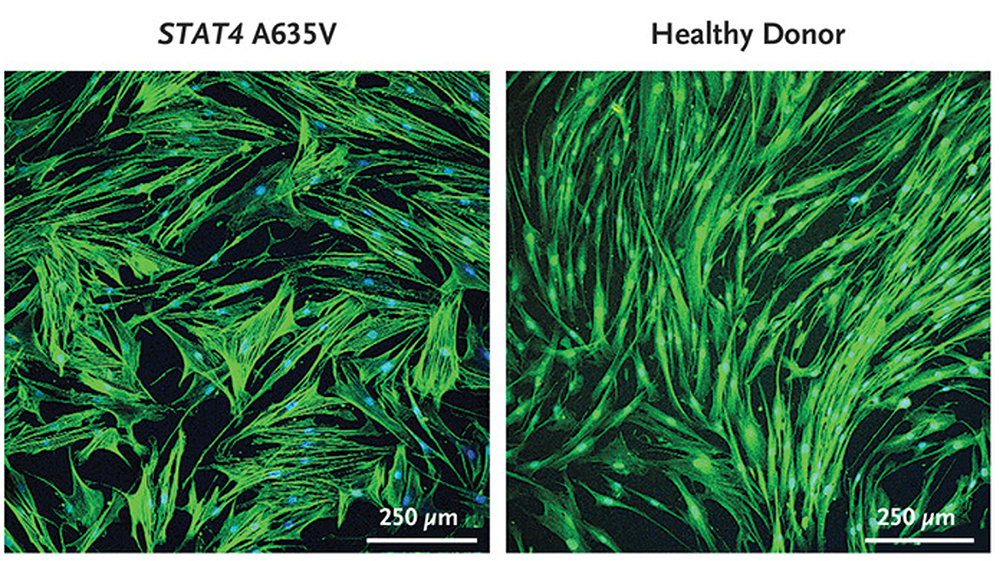
The researchers used genome sequencing to study four individuals with disabling pansclerotic morphea and found that all four have genomic variants in the STAT4 gene. The STAT4 gene encodes a type of protein that helps turn genes on and off, known as a transcription factor. The STAT4 protein not only plays a role in fighting infections but also controls important aspects of wound-healing in the skin.
The findings of this study open doors for JAK inhibitors to be a potential treatment for other inflammatory skin disorders or disorders related to tissue scarring, whether it is scarring of the lungs, liver or bone marrow.
The scientists found that the STAT4 genomic variants result in an overactive STAT4 protein in these four patients, creating a positive feedback loop of inflammation and impaired wound-healing that worsens over time. To stop this harmful feedback loop, they targeted another protein in the inflammatory pathway that interacts with the STAT4 molecule and is called Janus kinase, also known as JAK. When the researchers treated the patients with a JAK-inhibiting drug called ruxolitinib, the patients’ rashes and ulcers dramatically improved.
“So far, there has not been a standard treatment for this disorder because it’s so rare and not well-understood. However, our study gives an important new treatment option for these patients,” said Blackstone.
Existing treatments for disabling pansclerotic morphea are designed to halt the progression of the disorder, but previous therapies have been mostly ineffective, often with severe side effects. People with the disorder typically don’t live more than 10 years after their diagnosis.
The study suggests that ruxolitinib could be an effective treatment for patients with this disorder. Ruxolitinib is part of a broader class of drugs called JAK inhibitors, which are commonly used to treat arthritis, eczema, ulcerative colitis and other chronic inflammatory diseases.
“The findings of this study open doors for JAK inhibitors to be a potential treatment for other inflammatory skin disorders or disorders related to tissue scarring, whether it is scarring of the lungs, liver or bone marrow,” said Dan Kastner, M.D., Ph.D. , an NIH distinguished investigator, head of NHGRI’s Inflammatory Disease Section and a senior author of the paper.
“We hope to continue studying other molecules in this pathway and how they are altered in patients with disabling pansclerotic morphea and related conditions to find clues to understanding a broader array of more common diseases,” said Lori Broderick, M.D., Ph.D., a senior author of the paper and an associate professor at UCSD.
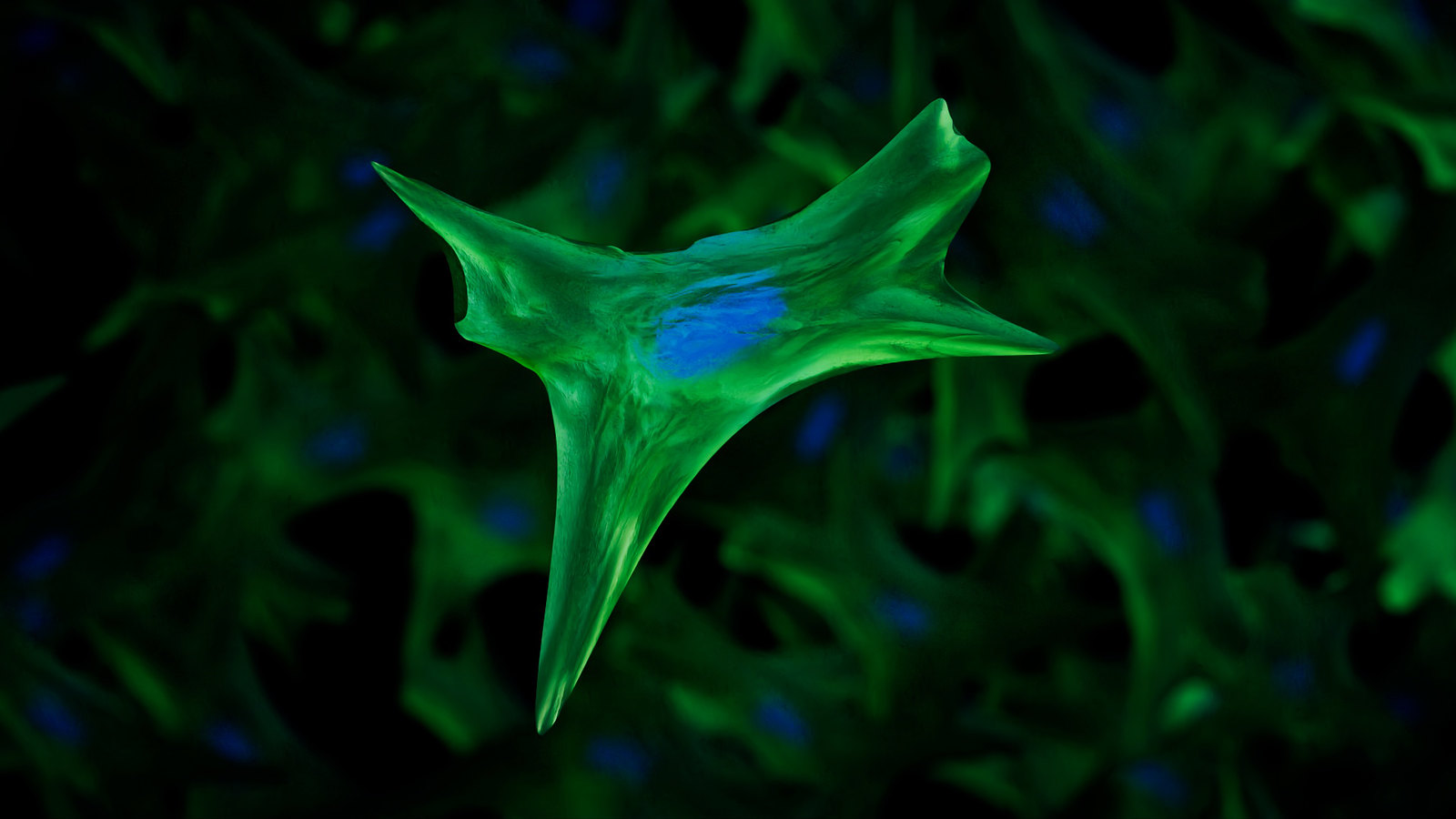
About NHGRI and NIH
About the National Human Genome Research Institute (NHGRI): At NHGRI, we are focused on advances in genomics research. Building on our leadership role in the initial sequencing of the human genome, we collaborate with the world's scientific and medical communities to enhance genomic technologies that accelerate breakthroughs and improve lives. By empowering and expanding the field of genomics, we can benefit all of humankind. For more information about NHGRI and its programs, visit www.genome.gov . About the National Institutes of Health (NIH): NIH, the nation's medical research agency, includes 27 Institutes and Centers and is a component of the U.S. Department of Health and Human Services. NIH is the primary federal agency conducting and supporting basic, clinical, and translational medical research, and is investigating the causes, treatments, and cures for both common and rare diseases. For more information about NIH and its programs, visit www.nih.gov .
Press Contact
Last updated: June 1, 2023
Watch CBS News
Parkinson's can be detected through skin, study says
Skin Biopsy Might Help Diagnose Parkinson's or Other Brain Disorders
Skin Biopsy Might Help Diagnose Parkinson's or Other Brain Disorders
By Dennis Thompson HealthDay Reporter

THURSDAY, March 21, 2024 (HealthDay News) -- Folks can learn their risk for Parkinson’s disease and other related brain disorders through a simple skin biopsy, a new study says.
Skin tests can detect an abnormal form of alpha-synuclein, a protein that is the hallmark of Parkinson’s disease and similar degenerative brain illnesses, researchers say.
This simple test could be a reliable and convenient tool to help doctors accurately diagnose patients at risk for these brain diseases linked to abnormal alpha-synuclein, researchers conclude in the Journal of the American Medical Association .
“Too often patients experience delays in diagnosis or are misdiagnosed due to the complexity of these diseases,” lead researcher Dr. Christopher Gibbons , a neurologist at Beth Israel Deaconess Medical Center in Boston, said in a news release.
U.S. Cities With the Most Homelessness

Diseases related to alpha-synuclein abnormalities include Parkinson’s, dementia with Lewy bodies (DLB), multiple system atrophy (MSA), and pure autonomic failure (PAF), researchers said in background notes.
These diseases – referred to as synucleinopathies – affect an estimated 2.5 million people in the United States.
For the study, researchers recruited 428 people who had been diagnosed with one of the synucleinopathies. Each person had three 3-millimeter skin biopsies taken from their neck, knee and ankle.
Researchers found abnormal alpha-synuclein in 93% of people with Parkinson’s; 96% of people with dementia with Lewy bodies; and 98% of people with multiple system atrophy, results show. All patients with PAF had the abnormal protein in their skin samples.
By comparison, only about 3% of a group of healthy people tested positive for abnormal alpha-synuclein, researchers said.
“While we have been aware of the presence of alpha-synuclein in (skin) nerves for many years, we were thrilled with the accuracy of this diagnostic test,” senior researcher Dr. Roy Freeman , director of the Center for Autonomic and Peripheral Nerve Disorders at Beth Israel Deaconess Medical Center, said in a news release.
“Parkinson’s disease and its subgroup of progressive neurodegenerative diseases show gradual progression, but alpha-synuclein is present in the skin even at the earliest stages,” Freeman added.
In addition to catching these brain diseases earlier, the skin test also could accelerate the development of effective drugs to treat them, researchers said.
“Enrolling the right patients in clinical trials for these complex diseases is of utmost importance,” Freeman said. “Identifying the relevant biomarker in a patient and tracking it over the course of a clinical trial is an essential component of drug development in the neurodegeneration field.”
More information
The Mayo Clinic has more about the synucleinopathies .
SOURCE: Beth Israel Deaconess Medical Center, news release, March 19, 2024
Copyright © 2024 HealthDay . All rights reserved.
Join the Conversation
Tags: Parkinson's disease
America 2024

Health News Bulletin
Stay informed on the latest news on health and COVID-19 from the editors at U.S. News & World Report.
Sign in to manage your newsletters »
Sign up to receive the latest updates from U.S News & World Report and our trusted partners and sponsors. By clicking submit, you are agreeing to our Terms and Conditions & Privacy Policy .
You May Also Like
The 10 worst presidents.
U.S. News Staff Feb. 23, 2024

Cartoons on President Donald Trump
Feb. 1, 2017, at 1:24 p.m.

Photos: Obama Behind the Scenes
April 8, 2022

Photos: Who Supports Joe Biden?
March 11, 2020
House GOP Sees Fresh Drama
Cecelia Smith-Schoenwalder March 22, 2024

The GOP’s Trump-Inspired Money Woes
Susan Milligan March 22, 2024

House Passes $1.2T Funding Package

What to Know: $1.2T Spending Bill
Cecelia Smith-Schoenwalder March 21, 2024

New York Poised to Seize Trump Holdings
Lauren Camera March 21, 2024

Who Could Be Trump’s VP?

Parkinson’s Disease Can Now Be Detected Through the Skin
Debi Lucas had a tremor in her arm. Her feet froze when she tried to walk and she fell into her coffee table, busting her lip.
She went to a neurologist who thought she had Parkinson’s disease. Doctors normally diagnose the neurodegenerative condition by symptoms. Lucas, 59, had them.
But the neurologist, Dr. Jason Crowell, couldn’t be sure. The symptoms might be related to a traumatic brain injury Lucas suffered in a car accident decades earlier, he thought. Or they might be from her medications.
To find an answer, Crowell turned to a new test: a skin biopsy that can detect an abnormal protein people with Parkinson’s have inside their nerves. He took samples of skin near her ankle, knee and shoulder and sent them to a lab.
The results confirmed that Lucas has Parkinson’s. The diagnosis was scary, but Lucas finally knew what was causing her symptoms. “I was glad to have a name on it,” she said.
The test sped her diagnosis, said Crowell, a movement-disorders neurologist at the Norton Neuroscience Institute in Louisville, Ky. “It just gives me more confidence,” he said.
The skin test is an important part of progress researchers are making against Parkinson’s, the second-most common age-related neurodegenerative condition, which is on the rise and a major driver of disability, dementia and death. The test Lucas received, made by CND Life Sciences, a medical technology company in Scottsdale, Ariz., is one of a few in use or development to allow doctors to diagnose Parkinson’s based on biology rather than symptoms that can take years to appear.
“The skin test basically is a window into the brain,” said Dr. Joseph Jankovic, professor of neurology at Baylor College of Medicine in Houston, where the test is used to diagnose patients and conduct research.
The test accurately detected the abnormal alpha-synuclein protein in 93% of people who had already been diagnosed by their symptoms with Parkinson’s, according to a study published Wednesday in the JAMA. It detected the protein at high rates in participants with similar disorders, including dementia with Lewy bodies.
The test can help doctors rule out diseases with similar symptoms that might be treated differently or have different prognoses. Patients with symptoms of Parkinson’s are often misdiagnosed, according to data from autopsies, said Dr. Christopher Gibbons, a neurologist at Beth Israel Deaconess Medical Center in Boston and the study’s lead author. He is also an adviser to CND Life Sciences. More than 20% of participants in the study had received a wrong diagnosis, he said.
Another test finds the protein in spinal fluid. Researchers are also working on tests using blood, nasal swabs and tears. The goal is to detect the protein in people years before they develop symptoms and treat them with drugs that could slow or stop the disease, said Dr. Todd Levine, chief medical officer of CND Life Sciences. Studies on early detection with the skin test are under way, he said.
“What we’re hoping for is within the next few years, we’ll have some disease-modifying therapies,” he said.
The spinal-fluid test can detect alpha-synuclein in people with a sleep behavior disorder that is a precursor to Parkinson’s, said Dr. Russell Lebovitz, chief executive officer of Amprion, the test’s maker. “We can give a very clear and very early diagnosis,” he said.
The skin test costs just under $1,500 but is usually covered mostly or all by insurance, Levine said. The spinal-fluid test is $1,500 if billed to insurance, or $995 for patients who pay on their own.
People at risk of Parkinson’s could be screened using tests, said Mark Frasier, chief scientific officer at the Michael J. Fox Foundation, which provided early funding and worked with Amprion on the spinal-fluid test, and is funding development of others.
“It is a progressive disorder where brain cells are lost over time,” he said. “If you intervene at the earliest possible stage…you’re likely to see a more positive outcome.”
The tests also help with research, he said.
Parkinson’s occurs when cells deep in the brain that produce dopamine, which coordinates movement, become impaired or die. Symptoms include tremors, stiffness, slow movements and problems with balance. Patients also have non-motor complications such as depression, sleep disorders and pain. The disease usually occurs in older people, more often men. It is on the rise as the population ages, because the risk the body will produce abnormal proteins increases as it gets older.
But young people develop it, too. Actor Michael J. Fox was diagnosed at age 29.
There is no cure, but drugs and other treatments help relieve symptoms. Companies are working on treatments that target alpha-synuclein or other ways to slow Parkinson’s. The precise role that alpha-synuclein plays in the disease isn’t clear.
Not all patients need the test, said Dr. Joy Antonelle de Marcaida, who uses it as medical director of the David & Rhoda Chase Family Movement Disorders Center at Hartford Healthcare. The diagnosis is clear for many from symptoms, she said.
But others have subtler symptoms or other possible explanations, and it can take time to diagnose them. “We used to have to tell them, come back in six months, a year and I’ll look again,” she said.
Michael Dale learned six months ago that he is in the early stages of Parkinson’s. The 77-year-old, who lives in Houston, had balance problems and tremors. A neurologist at Baylor suggested he get the skin test. It was like a ballpoint pen pushing into his skin, he said.
Now Dale is doing exercises and monitoring his symptoms. “There’s a lot of anxiety about what the future holds,” he said.
He’s glad to know his diagnosis. “You know what’s going on and you can kind of prepare,” he said.
Write to Betsy McKay at [email protected]
MTA Missing Math Clock
Release: as part of new “project women’s health” initiative, gottheimer announces legislation to combat rare diseases impacting women.
Multi-front effort to boost clinical trials and find treatments and cures
Women often left out or underrepresented in clinical trials
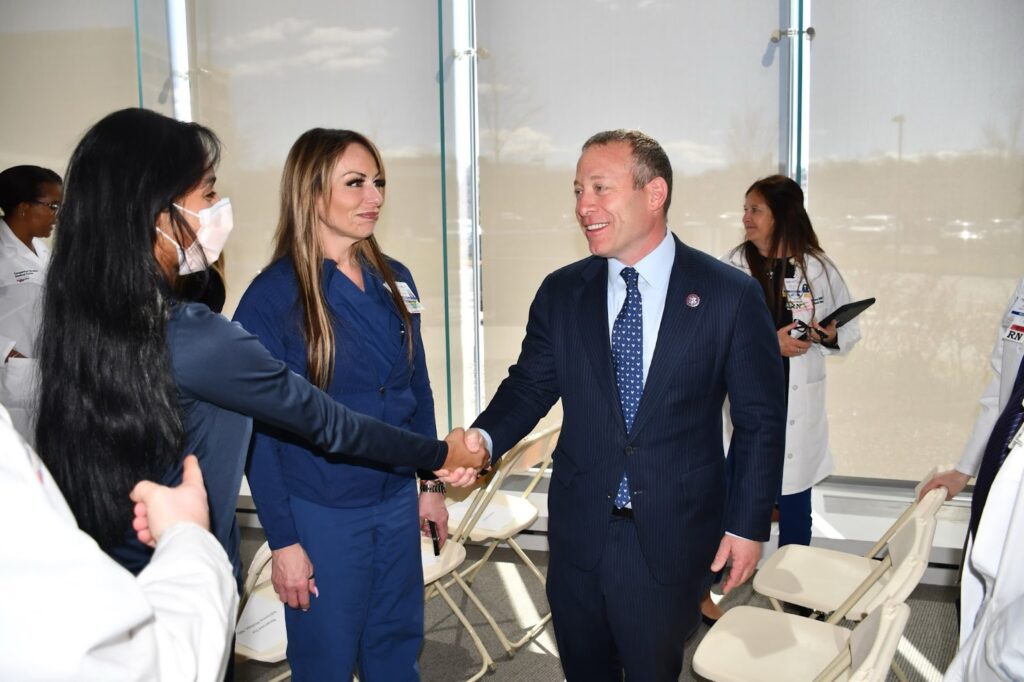
Above: Gottheimer at Cooperman Barnabas Medical Center.
LIVINGSTON, NJ — Today, March 19, 2024, U.S. Congressman Josh Gottheimer (NJ-5) announced new legislation, the Securing Equal Access to Research, Care, and Health, or SEARCH Act, to help fund new efforts to increase the number of women in clinical research trials, leading to treatments and cures for rare diseases and blood disorders which affect millions of women in New Jersey and across our nation. The legislation is part of Gottheimer’s new, multi-front legislative initiative: “Project Women’s Health.”
Video of the announcement can be found here .
Gottheimer also highlighted his support for the President’s State of the Union Address earlier this month, which called on Congress to provide $12 billion to research health conditions and diseases that disproportionately affect women. In Congress, Gottheimer plans to work to help make this investment a reality.
“There is a clear gap in research, awareness, diagnosis, management, treatment, and cures of diseases and disorders affecting women. We must address the inequality between men and women in research, clinical trials, and awareness. It must be taken seriously and fixed and there’s no better time than the present,” said Congressman Josh Gottheimer (NJ-5), Rare Disease Caucus Member. “Even with all of the challenges we are facing, I remain optimistic and hopeful. I hope that you are, too. Built on collaborative work with experts and advocates, I’m confident that the SEARCH Act will ultimately lead to life changing medication, treatment, and cures for women impacted by rare diseases and bleeding disorders.”
“The Congressman is an advocate for ensuring equal access to care for all residents in New Jersey. He is focused on addressing disparities and improving healthcare outcomes across the state. We share a deep commitment with the Congressman to the most vulnerable among us,” said RWJBarnabas Health President and CEO Mark E. Manigan. “While we’re very proud of the awesome clinical services we offer here at Cooper and across the system, we are equally proud of that commitment to the most vulnerable as this state’s largest provider of charity care and as the state’s largest providers of care to beneficiaries to the medicare program by two times.”
“Thank you congressman for giving me the opportunity to speak this morning and for leading the charge on the SEARCH Act. It’s an important piece of legislation that champions the health of women, especially those affected by rare disease. The importance of the SEARCH act goes beyond my professional role and in fact connects deeply and is personal to me. In 1999, my family’s life changed forever when my niece who was ten years old at the time was diagnosed with Friedreich’s ataxia — a rare, debilitating, degenerative, neuromuscular disease. Sadly, in 2020, my niece’s battle ended just months after her 30th birthday… just last year… almost three years to the day after she died, the FDA approved a new medication to slow the progression for Friedreich’s ataxia. A breakthrough that arrived too late for my niece, but marked a significant step forward for others. The SEARCH Act represents a beacon of hope for women suffering from rare diseases,” said RWJBarnabas Health Vice President of Women’s Services Suzanne Spernal.
“I have witnessed the disparities women face and have faced for decades. Women are overlooked, misdiagnosed, or not diagnosed at all and it has become clear that legislation of this kind is not only necessary but critical for women, not only with bleeding disorders but also for women in the rare disease community at large,” said Hemophilia Association of New Jersey Executive Director Stephanie Lapidow.
“Although a disease may be rare, thus impacting a small number of people, it does not change the profound impact a condition has on those afflicted, as well as their families. Not uncommonly, due to the limited understanding of these diseases and the small number of people affected, funding for research is often scarce, making it difficult to find treatments,” said RWJBarnabas Director of Cell Therapy and Bone Marrow Transplantation Northern Regions Dr. Adrienne Phillips . “For the individual or family with a disease, getting to a diagnosis may be a long and frustrating road fraught with prolonged diagnosis and treatment delays.”
Challenges Facing Women’s Health Related to Rare Diseases and Blood Disorders:
- Since 1994, fewer than 4% of adults in the United States participate in clinical trials despite increasingly prolonged recruitment periods.
- Up to 85% of clinical trials fail to recruit or retain a sufficient sample size, leading to failures to meet targets in four out of every five trials. For rare diseases more commonly impacting women, like rett syndrome and multiple sclerosis, it can be even harder to start and maintain proper trials.
- The CDC estimates that up to 1 in 100 women and girls in the United States — more than 1.5 million Americans — have a bleeding disorder, many of whom aren’t even aware of their condition.
- The most common bleeding disorder affecting women is von Willebrand disease, or VWD, which results from a deficiency or defect in the body’s ability to produce a certain protein that helps blood clot.
- Reports indicate that women are directed to a hospital and specialists later than men following the onset of symptoms, which delays diagnosis and care. This can often lead to a rapid progression of the disease.
Gottheimer’s New Legislation, the Securing Equal Access to Research, Care, and Health or SEARCH Act will:
- There are many diseases that disproportionately impact women and are often swept under the research and funding mat.
- Fund recruitment campaigns for women in NIH clinical research trials for rare diseases by increasing advertising at hospitals, doctors’ offices, health centers, and healthcare clinics. This can have a direct and immediate impact on helping Jersey families.
- Require the CDC and HHS to increase the number of women in federal bleeding disorder programs that provide funding for research, surveillance, prevention, and services through public awareness campaigns.
- Create a task force between the HHS, NIH, FDA, CMS, and the private sector , including hospitals and labs in New Jersey, to produce a report on the rare diseases that disproportionately impact women, helping ensure more funding goes toward diseases and conditions that impact women.
Gottheimer was joined by RWJBarnabas Health President and CEO Mark E. Manigan, Cooperman Barnabas Medical Center President and CEO Richard L. Davis, RWJBarnabas Health Senior Vice President Dr. Balpreet Grewal-Virk, RWJBarnabas Health Vice President of Women’s Services, Director of Cell Therapy and Bone Marrow Transplantation Northern Regions Adrienne Phillips, and Hemophilia Association of New Jersey Executive Director Stephanie Lapidow.
Below: Gottheimer with rare disease doctors, nurses, and advocates.
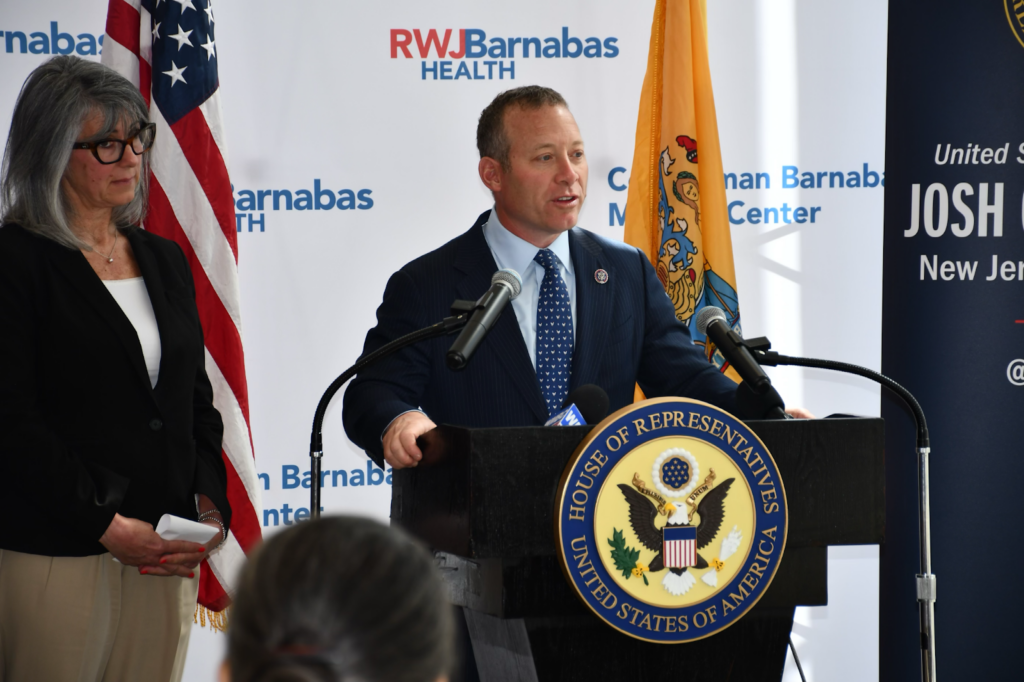
Gottheimer’s remarks as prepared for delivery:
Good afternoon, and thank you again to Mark and Rick, Dr. Phillips, Stephanie, and Suzanne. It’s great to be back here in Livingston at Cooperman Barnabas Medical Center. I say back because I was born here and grew up down the road in North Caldwell. I also had surgery here after I broke my elbow as a kid. I can’t tell you the exact room. It’s a little different than what I remember — and the hospital looks fantastic. I do remember my mom sneaking me apple pancakes from the Ritz diner.
Before I begin, I want to thank all our nurses, doctors, frontline health care workers, our great North Jersey bio and life sciences leaders, and of course our rare disease researchers and advocates for the incredible work they do each and every day for our families. I cannot thank all of you enough for everything you do for our community to help improve the health and lives of countless Americans.
There are a few reasons it’s appropriate that we’re here this morning. March not only is it Women’s History Month, but it’s Blood Disorder Month, and it was just recently Rare Disease week.
Just yesterday, recognizing the importance of this month, the President signed an Executive order directing federal agencies to deploy federal funds to research health conditions and diseases that disproportionately affect women. As a member of the Rare Disease Caucus in Congress, I couldn’t agree more.
That is why today, I’m here to announce new legislation to help fund a new effort to increase the number of women in clinical research trials, leading to treatments and cures for rare diseases and blood disorders which affect millions of women across our nation.
It’s all part of my new, multi-front legislative initiative project women’s health which builds on my years of work on this front.
When it comes to research on rare and blood diseases, including those that disproportionately affect women, there is a shortage of women in clinical trials. My new legislation — the Securing Equal Access to Research, Care, and Health or SEARCH Act — will take that on.
As a quick refresher, a rare disease is one that impacts fewer than 200,000 people and far too often has no FDA-approved treatments. We just heard from Suzanne about her niece who was diagnosed with Friedreich Ataxia and sadly passed away at the age of 30, just three years before an FDA medication was approved to help delay the effects of her condition. As Suzanne mentioned, if we had had more investments for recruitment and public awareness campaigns for clinical trials, maybe the medication to delay the effects of her niece’s condition would have been discovered in time.
Personally, I lost my mom, of blessed memory, to a rare disease, Sarcoidosis, four and a half years ago.
While we are making progress, and have had some big breakthroughs, it’s far too slow, and we still have a long way to go. In fact, 95 percent of the more than 10,000 rare diseases that impact more than 30 million Americans have no FDA-approved treatment.
Rare disease research is critical. Not only can it lead to a cure or treatment for those afflicted, but the research can also be a gateway to breakthroughs for other treatments and cures.
Here in New Jersey, we are lucky to have some of the best, hospitals and medical facilities, labs, and R&D in the country, the best doctors, medical school graduates, nurses, and life sciences leaders.
With fourteen of the world’s top twenty research-based biopharmaceutical companies here in our state, we not only have cutting-edge hospitals, but our pharmaceutical and medical device companies have led the way on some of the biggest medical breakthroughs. New Jersey ranks second in the nation for cancer medicines in development and first for heart and stroke drugs and development. In 2017, 50 percent of all new FDA approvals came from companies with a New Jersey footprint.
What’s great is we already have some success stories from rare disease and bleeding disorder research.
In 2019, there were new approvals for the first triple combination therapy for patients with cystic fibrosis and a new gene therapy to treat pediatric patients under two-years-old with spinal muscular atrophy.
And just last year, the FDA approved gene therapies for hemophilia A and hemophilia B — an important advancement in providing treatment options and reducing the need for ongoing, routine therapy.
And Allergan, right here in New Jersey, announced FDA approval for an existing drug application that can now be used to treat lower-limb spasticity in children ages two to seventeen.
I want us to double down, so that we expand in the years ahead. We can lead the way in helping to develop the next cures for orphan diseases and blood disorders too commonly leaving women without the support and treatments they need.
And when we find those cures, it’ll help the amazing medical professionals — like here at Cooperman Barnabas Medical Center — care for women too often left without the help they need.
As Suzanne intimated, there are clear challenges and obstacles — especially when it comes to research.
First, participation in clinical trials is poor. Since 1994, fewer than four percent of adults in the United States participate in clinical trials despite increasingly prolonged recruitment periods. What’s worse, up to 85 percent of clinical trials fail to recruit or retain a sufficient sample size, leading to failures to meet targets in four out of every five trials. For rare diseases more commonly impacting women, like rett syndrome and multiple sclerosis, it can be even harder to start and maintain proper trials.
Second, like rare diseases, women with bleeding disorders have often been underrecognized and underdiagnosed. The CDC even estimates that up to 1 of every 100 women and girls in the United States — more than 1.5 million Americans — have a bleeding disorder, many of whom aren’t even aware of their condition. The most common bleeding disorder affecting women is von Willebrand disease, or VWD, which results from a deficiency or defect in the body’s ability to produce a certain protein that helps blood clot. Women with bleeding disorders have historically been excluded from research.
Third, because women tend to have rare diseases diagnosed later than men, bias in cures or treatments start early and leave lasting impacts. Reports indicate that women are directed to a hospital and specialists later than men following the onset of symptoms, which delays diagnosis and care. This can often lead to a rapid progression of the disease.
The bottom line: There is a clear gap in research, awareness, diagnosis, management, treatment, and cures of diseases and disorders affecting women. It must be taken seriously and fixed. We must do more to provide hope and support to the families who struggle every day to manage a rare disease or bleeding disorder — or any disease, for that matter. We must address the inequality between men and women in research, clinical trials, and awareness. And there’s no better time than the present.
That’s why, today, as I mentioned earlier, I’m here with medical professionals, advocates, and family members of those taken from rare diseases to announce the Securing Equal Access to Research, Care, and Health or SEARCH Act. This new legislation I’m introducing in Congress will support new research and clinical studies — and hopefully new treatments and cures — for diseases impacting women, including rare diseases and blood disorders.
First, the legislation will require the National Institute of Health, or NIH, to produce an action plan within 180 days to highlight the rare disease and health complications that uniquely impact women. As we laid out today, there are many diseases like blood disorders that disproportionately impact women and are often swept under the research and funding mat.
Second, the SEARCH Act will fund recruitment campaigns for women in NIH clinical research trials for rare diseases by increasing advertising at hospitals, doctors’ offices, health centers, and healthcare clinics. This can have a direct and immediate impact on helping Jersey families.
Third, the new legislation requires the CDC and HHS to increase the number of women in federal bleeding disorder programs that provide funding for research, surveillance, prevention, and services through public awareness campaigns.
Fourth, the SEARCH Act will create a task force between the HHS, NIH, FDA, CMS, and the private sector, including hospital and labs in New Jersey, to produce a report on the rare diseases that disproportionately impact women, helping ensure more funding goes toward diseases and conditions that impact women.
Finally, in his State of the Union Address earlier this month, the President called on Congress to provide $12 billion to research health conditions and diseases that disproportionately affect women. In Congress, I plan to work overtime in the coming months to make this investment a reality. We must pass legislation to create a new fund for Women’s Health Research at the NIH that will support a nationwide network of cutting-edge research centers in women’s health care.
Today, we’re here as part of my new, legislative fight on multiple fronts to protect women’s health care – whether that’s about research, clinical trials, treatments, and finding cures, or doing everything we can to protect a woman’s fundamental right to make her own personal, private healthcare decisions.
Even with all of the challenges we are facing, I remain optimistic and hopeful. I hope that you are, too. Built on collaborative work with experts and advocates, I’m confident that the SEARCH Act will ultimately lead to life changing medication, treatment, and cures for women impacted by rare diseases and bleeding disorders.
In the greatest country in the world, if we can come together to solve problems and support women’s health, I know that our best days will always be ahead of us. Once again, thank you for joining me here today.
May God bless you, your families, and may God continue to bless the United States of America.
Recent Posts
Release: gottheimer claws back $2.3 million for north jersey firefighters.
Above: Gottheimer with North Jersey firefighters. NORTH JERSEY — Today, March 22, 2024, U.S. Congressman Josh Gottheimer (NJ-5) announced that more than $2.3 million in American Rescue Plan Firefighter Grants (ARPFFG) secured by a federal investment have been awarded to local and regional fire departments across North Jersey. These investments will help ensure firefighters have the […]
RELEASE: Gottheimer, Kean Lead Bipartisan Letter Thanking Germany for Supporting Israel’s right to Self-Defense
WASHINGTON, D.C. — U.S. Representatives Josh Gottheimer (NJ-5) and Tom Kean (NJ-7) led a letter signed by a bipartisan group of more than twenty Members of Congress thanking German Chancellor Olaf Scholz for announcing his country’s intention to intervene on Israel’s behalf and for supporting Israel’s right to self-defense in the International Court of Justice […]
RELEASE: Gottheimer-led Bipartisan Legislation Overwhelmingly Passes House to Protect Americans from TikTok
Controlled by Chinese Government Disinformation and Data Mining Machine Used to Undermine Our Democracy WASHINGTON, D.C. — Today, March 13, 2024, bipartisan legislation co-led by U.S. Representative Josh Gottheimer (NJ-5), with Representative Mike Gallagher (WI-8) and Raja Krishnamoorthi (IL-8), and the House Select Committee on the Strategic Competition Between the United States and the Chinese Communist […]

An official website of the United States government
The .gov means it’s official. Federal government websites often end in .gov or .mil. Before sharing sensitive information, make sure you’re on a federal government site.
The site is secure. The https:// ensures that you are connecting to the official website and that any information you provide is encrypted and transmitted securely.
- Publications
- Account settings
Preview improvements coming to the PMC website in October 2024. Learn More or Try it out now .
- Advanced Search
- Journal List
- Indian Dermatol Online J
- v.5(1); Jan-Mar 2014
Skin diseases and conditions among students of a medical college in southern India
Nitin joseph.
Department of Community Medicine, Kasturba Medical College, Manipal University, Mangalore, India
Ganesh S Kumar
1 Department of Community Medicine, JIPMER, Puducherry, India
Maria Nelliyanil
2 Department of Community Medicine, A. J. Institute of Medical Sciences, Mangalore, India
Introduction:
Skin diseases are a common problem among young adults. There is paucity of data about it among medical students. This study aimed to find out the pattern of skin disorders and to describe their association with various socio-demographic factors among medical students.
Materials and Methods:
This cross-sectional study was conducted in June 2011 in a medical college in Mangalore, Karnataka. Two-hundred and seventy eight medical students were chosen from the 4 th , 6 th and 8 th semester through convenient sampling method. Data on hair and skin morbidities suffered over past 1 year and its associated factors were collected using a self-administered questionnaire.
Most of the participants 171 (61.5%) were of the age group 20-21 years and majority were females 148 (53.2%). The most common hair/skin morbidities suffered in the past one year were acne 185 (66.6%), hair loss 165 (59.3%), and sun tan 147 (52.9%). Fungal infection ( P = 0.051) and severe type of acne ( P = 0.041) were seen significantly more among males while hair morbidities like hair loss ( P = 0.003), split ends of hairs ( P < 0.0001) and dandruff ( P =0.006) were seen significantly more among female students. Patterned baldness ( P = 0.018) and sun tan ( P < 0.0001) were significantly more among non-Mangalorean students than native Mangaloreans. Presence of dandruff was significantly associated with hair loss ( P = 0.039) and usage of sunscreen was found to protect from developing sun tans ( P = 0.049).
Conclusion:
Skin disorders, particularly the cosmetic problems are very common among medical students. Gender and place of origin were found to significantly influence the development of certain morbidities.
INTRODUCTION
Skin diseases are a major health problem affecting a high proportion of the population in India.[ 1 ] Skin diseases can place a heavy emotional and psychological burden on patients that may be far worse than the physical impact.[ 2 ] Increased consciousness especially among the youth of their body and beauty further aggravates their anxiety.[ 3 ]
Many factors determine the pattern and prevalence of cutaneous diseases among the youth such as gender, race, personal hygiene, quality of skin care, environmental milieu and diet.[ 4 ] In some instances, patients appear to produce their skin lesions as an outlet for nervous tensions arising from interpersonal conflicts and/or unresolved emotional problems.[ 5 ]
Even though dermatology is characterized by an enormous range of disease/reaction patterns, prevalence surveys suggest that the bulk of skin diseases belong to fewer than ten categories.[ 6 ] Such observations are useful in developing educational and preventive health programs for the benefit of university students. Their proper management at earlier stages with education of students is important to prevent disfiguring complications and psychological sequelae later in life.[ 3 ]
However, very few studies have been carried out in India to find out the problem of skin diseases and that especially among the medical students. The reason for this negligence could be the low mortality rate of the majority of skin diseases in comparison with other diseases. This has also resulted in international health policy makers and local decision makers to make dermatological morbidities a low priority.[ 7 ] Another concern is that the benefits of public health interventions in reducing the prevalence, morbidity and mortality of skin diseases may be underestimated.[ 8 ] Thus there is a need for more studies with respect to dermatological morbidities in a developing country like India. With this background, this study was carried out to find out the pattern and severity of skin disorders and to describe their association with various socio-demographic factors among medical students of a private medical college in Mangalore city of south India.
MATERIALS AND METHODS
This cross-sectional study was done in June 2011. The ethical approval for conducting this study was obtained from institutional ethics clearance committee. A sample size of 278 was determined using a confidence level of 95%, with 15% degree of precision of the expected proportion and an estimated minimum prevalence of 40%. These students were chosen from the 4 th , 6 th and 8 th semester through convenient sampling method so that the sample will have a balanced representation of 2 nd , 3 rd and final phase medical students of the institution.
The students were briefed about the objective of the study and written informed consent was taken for participation. A pre-tested self-administered semi-structured questionnaire was used for data collection. The face validity of this questionnaire was done by an expert in dermatology who reviewed the contents of the questionnaire. The questionnaire was subjected to a pilot trial on 10 students before it was distributed in its final form. Reliability of the questionnaire was assessed using Cronbach's Alpha the value of which was 0.82 indicating good internal consistency. Questions on the presence of any skin morbidities suffered by the student participants in the past 1 year were asked.
Additionally questions like frequency of face wash in a day, usage of facial cleansing products, frequency of head and body bath in a week, frequency of usage of hair shampoo in a week, usage of sunscreen lotions, moisturizers or cosmetics, frequency of changing into new clothes, habit of sharing linen with friends and promptness in seeking dermatologist consultation for skin ailments were asked to assess the quality of skin care.
Life style habits were assessed based on amount of water consumed in a day, frequency of eating fatty or oily food stuffs in a week, frequency of consumption of fruits and vegetables in a week, smoking habits and recreation habits like swimming.
Each response for the question meant to assess quality of skin care and life style habits were given scores from 0 to 2. Scores from 0 to 11 for questions deciding quality of skin care meant poor, 12-22 meant good level of skin care. Similarly scores from 0 to 5 for questions deciding life style meant poor and 6-10 meant good level of lifestyle habits.
The data entry and analysis were done using Statistical Package for Social Sciences software package (SPSS Inc., Chicago, IL) version 16. Chi-square test was used to find out the association of socio-demographic variables with the presence of skin morbidities, quality of skin care and life style habits P < 0.05 was taken as statistically significant association.
Mean age of participants was 20.35 ± 1.23 years [ Table 1 ].
Age, gender and place distribution of students

Of the 278 students, 69 (24.8%) had fair skin, 120 (43.2%) had wheatish skin, 74 (26.6%) had brown skin and 15 (5.4%) had dark skin. The one- year-period prevalence of various skin morbidities showed acne to be the commonest skin morbidity in 185 (66.5%) cases followed by sun tan in 147 (52.9%) cases. Among the hair morbidities commonest was hair loss seen in 165 (59.3%) cases followed by dandruff seen in 129 (46.4%) cases [ Table 2 ].
Association between various hair/skin morbidities among students with gender

Fungal infection was seen significantly among a greater proportion of males while among females the significant morbidities were hair loss, split end of hairs and dandruff [ Table 2 ].
Patterned baldness and sun tan were seen significantly more among greater proportion of non-Mangaloreans than native Mangaloreans [ Table 3 ].
Association between various hair/skin morbidities among students with place of origin ( n =278)

White/black heads were seen significantly more among females while papular and pustular types of acne were seen significantly more among a greater proportion of males. The proportion of cases with pustular type of acne was 30 (10.8%) [ Table 4 ].
Association between gender with type and duration of acne

Of the 278 students with morbidities, 236 (84.9%) had good quality skin care and the rest had poor quality skin care. 108 (83.1%) males and 128 (86.5%) females reported good quality skin care ( P = 0.428). Among the participants with good quality skin care, 161 (68.2%) reported presence of morbidities whereas among participants with poor quality skin care, 24 (57.1%) reported presence of morbidities ( P = 0.161).
Of the 278 students with morbidities, 236 (84.9%) had good life style habits and the rest had poor life style habits. One hundred and seven (82.3%) males and 129 (87.2%) females reported good life style habits ( P = 0.259). Among the participants with good life-style habits, 162 (68.6%) reported presence of morbidities, whereas among participants with poor life-style habits, 23 (54.8%) reported presence of morbidities ( P = 0.079). Out of 129 cases with history of dandruff, hair loss was present in 85 (65.9%) cases ( P = 0.039).
Usage of sunscreen in hot sun was associated with significant reduction in proportion of cases with sun tan among the participants [ Table 5 ].
Association between presence of sun tan with usage of sun screen among students

It has been found that one- fourth of us (or more) suffer from at least one skin disease, a situation that constitutes a significant global burden of disease.[ 9 ] Economic burden of skin diseases is enormous and added to this easy visibility of dermatological illness has led to deterioration in the quality of life resulting in social handicap.[ 10 , 11 ]
In certain parts of the world, it was observed that the mortality rate and disability-adjusted life years due to skin diseases were at par with certain communicable and non-communicable diseases.[ 7 ] In a regression model, skin diseases as well as rheumatism was more strongly associated with feeling depressed than asthma, diabetes and angina pectoris.[ 12 ] Considering their significant impact on the individual, the family, the social life of patients and their heavy economical burden, the public health importance of these diseases is underappreciated.[ 8 ] This study too has shown that various types of skin morbidities are common among medical students. It has been reported that younger adults suffer more social problems as a result of skin problems than older adults.[ 12 ] Thus control of skin morbidities will definitely lead to improvement in the quality of life of young adults. In this study the most common morbidity reported was acne followed by hair loss which was also supported by other studies.[ 3 , 13 ]
Acne has been incriminated with sweating and hot weather, which is very compatible with the hot and humid climatic conditions prevailing in Mangalore.[ 14 ] The proportion of severe acne cases in this study was 10.8% which was more than the observation of 5.4% made in the Sindh based study.[ 3 ] Studies carried out in other countries have found that acne is a disfiguring disease and it should not be looked at as trivial,[ 15 ] as it may seriously affect the patient's life.[ 16 ] Screening adolescents for conditions like acne may be of great importance because it affects their image in the society and because of the wide armamentarium of therapy which is available.[ 17 ]
Hair loss was the next most common problem, which is very much global in nature. The true magnitude of problem is difficult to establish from this study as the data on the hair density and thickness in our subjects was lacking. There was significant association of dandruff as a risk factor for hair loss in this study which was similar to the findings of other studies.[ 3 , 18 ] However, in the absence of any apparent systemic or local cause for generalized hair loss, it can be assumed that constitutional factors or micro-deficiency of iron, vitamins and proteins may be the cause of hair loss in these subjects.[ 19 , 20 ]
Hair loss culminating in baldness is another sensitive issue among adolescents as they are invariably sensitive regarding their external features and thus may be easily withdrawn psychologically and avoid social activities due to androgenetic alopecia and this tends to affect girls more than boys.[ 21 ] In this study almost a quarter of students had baldness with greater proportion observed among males.
Increased tanning of skin was the third most common morbidity. This was understandable as 68% of the participants had fair or wheatish skin. This skin type is prone to tanning on sun exposure. Being less aware of the tanning effect of sun light and not using personal protective measures while outdoors must have promoted tanning and darkening in these subjects.[ 22 ]
Fungal infections were reported by more than a third of our participants in the past 1 year. Previous studies have reported that periods of high humidity (50-80%) and elevated temperatures reaching up to 35°C are ideal for fungal infections.[ 17 ] This probably could explain the reason behind a number of cases with fungal infections among students in Mangalore.
In a study carried out among university students in Sindh, Pakistan acne was seen in 59.5%, hair loss in 59%, pigmentary disorders in 36.3%, dandruff in 26.1% and fungal infection in 4.9% of the cases. All these observations made were lower than our findings. The study also found pruritis among 2.3% of the cases and eczema among 2.1% of the cases.[ 3 ] In another study carried out among 1279 university medical students by Roodsari et al ., 91.7% students had skin morbidities. Here acne was seen in 56%, hair loss (evaluated only in females) in 14%, dandruff in 11%, hand eczema in 10%, seborrheic dermatitis in 9% and pityriasis versicolor in 8% cases.[ 13 ] But for acne which is easily identifiable, the other skin morbidities were higher in this study than ours probably because disease identification in the former study was done by dermatologists unlike our study where it was self-reported by students. An Icelandic study found that the prevalence of urticaria was significantly higher among the medical students and was seen in 41% of students.[ 23 ] These variations in morbidities among students of same age group in different parts of the world could be due to racial, genetic and environmental variations.
In this study acne was found to be slightly more and hair problems was seen significantly more among females, which was similar to the findings of a study done among university students in Lebanon where both acne and hair problems were significantly more among females.[ 17 ]
Although there was no significant difference between the proportion of males and females with acne in the present study, the type of acne differed significantly between the two groups. White/black heads were seen significantly more among females while papule and pustule were seen significantly more males. This was similar to the observation made in another study carried out in New Zealand where severe type of acne was seen more among males.[ 24 ] Severity of this condition among males could be because of hormonal factors.[ 25 ]
Fungal infection seen significantly more among males in this study could be due to their lesser quality of skin care and life style habits in comparison to females. Other cutaneous disorders like pyoderma, folliculitis, scabies and pediculosis were not seen in this study. The reason for absence of these bacterial and parasitic infections could probably be that very few participants in this study had poor quality of skin care or hygiene. No cases of eczema, hyper pigmentary lesions like melasma, hypopigmentary lesions like vitiligo, nail disorders or skin cancers were reported by any of the participants.
Sun tans were seen significantly more among a greater proportion of non-Mangaloreans than native Mangaloreans. This could probably be explained by the non-adjustment to the hot and humid conditions of Mangalore among the outstation students. It was also observed that the users of sunscreen had significantly less cases of sun tans compared to non-users, signifying the importance of spreading awareness about the usage of such protective methods.
Limitations
The present study may not be generalized to other population groups because of different factors associated with different skin morbidities. It may not reveal the true burden of skin disorders among young adults as much as a population-based study. Also as these morbidities were self-reported there may be a possibility of recall bias. In this study, quality of skin care was assessed based on frequency of activities like face wash or body bath or based on the frequency of usage of hair shampoo or sunscreen lotions or moisturizers or cosmetics. Since the quality of these activities or products as well as its proper application on the body was not enquired, it could be a limitation in estimating the true quality of skin care.
Moreover it was difficult to differentiate between the physiological and pathological conditions in hair loss. The most important drawback of this study was that few skin morbidities might have been diagnosed by medical students themselves without actually consulting a dermatologist leading to inaccurate self-reported diagnosis. Hence more of such studies from a broader socioeconomic spectrum are required, which need to be suitably supported with dermatological examination of study subjects.
From the findings of one- year- period prevalence of various skin disorders we conclude that skin morbidities are very common among medical students, particularly cosmetic problems like acne, hair loss and skin tan. Severe types of acne and fungal infections were significantly more among males whereas hair morbidities were significantly more among females. Patterned baldness and sun tans were seen significantly more among non-Mangalorean students than native Mangaloreans. This emphasizes the need to popularize the importance of personal protective measures like usage of sun screens among students. Establishment of registries for specific skin diseases, particularly for those with a high disease burden will also help in good case accountability stressing importance to dermatological public health.
ACKNOWLEDGMENTS
The authors of this study would like to thank M.B.B.S students, Ms. Monica N, Mr. Ishan Parashar, Ms. Hemashri, Ms. Supraja Subramanian, Ms. Liya Susan Peter, Ms. Anupriya Dalmiya and Ms. Akanksha Bansal of K.M.C Mangalore for their help in data collection. We also thank Dr. Mohan Kudur, Associate Professor, Department of Dermatology, Venereology and Leprology, Srinivas Institute of Medical sciences and Research Centre, Mangalore for his help and support.
Source of Support: Nil
Conflict of Interest: None declared
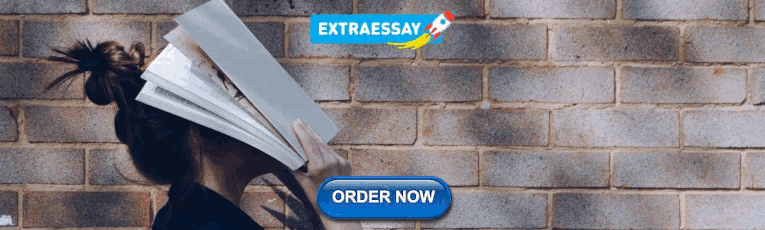
IMAGES
VIDEO
COMMENTS
The Global Skin Diseases Research Center (GSDRC) was established to foster collaboration among academic partners that actively maintain institutional skin research centers, which support basic, translational and/or clinical skin research. Current GSDRC member institutions include the following: The long-term goal of the GSDRC is to provide ...
This study explores the impact of skin diseases on psychosocial measures of well-being and HROQL. In this study, participants with history of any skin diseases had a mean EQ-5D index score of 0.89. This is equivalent to people suffering from migraine headaches and is lower than the mean score (0.95) obtained from a large representative sample ...
Methods. Data on the skin and subcutaneous diseases were obtained from the Global Burden of Disease Study 2019. The incidence, disability-adjusted life years (DALYs), and deaths due to skin and subcutaneous diseases in 204 countries and regions from 1990 to 2019 were analyzed and stratified by sex, age, geographical location, and sociodemographic index (SDI).
MobileNet V2 is used in classifying the type of skin disease, and LSTM is used to enhance the performance of the model by maintaining the state information of the features that it comes across in the previous generation of the image classification. 3.1. MobileNet Architecture Model for Image Classification.
Except for the test group in Project 3, all of the train and test groups in the Projects (2,3, and 4) from Taxonomy 2 have a higher precision on prediction of skin disease than the corresponding ...
AI-based localization and classification of skin disease with erythema. Ha Min Son, Wooho Jeon, Jinhyun Kim, Chan Yeong Heo, Hye Jin Yoon, Ji-Ung Park &. Tai-Myoung Chung. Scientific Reports 11 ...
The Penn Skin Biology and Disease Resource-based Center ("Penn SBDRC") is a hub for skin investigators across the University of Pennsylvania, Philadelphia, and beyond. Leveraging the excellence of Penn Dermatology's research and clinical programs, the Penn SBDRC was established in 2015 to enhance collaboration, bring new technologies to ...
This Research Topic may include, but is not limited to, the subtopics listed below: • Development of biomaterials, nanoparticle, or stem-cell-based therapeutic strategies for skin diseases; • Molecular/cellular regulatory mechanisms of various skin diseases; • Side effects of current treatments for skin disorders; • Discovery of novel ...
Deep learning has become an extremely popular method in recent years, and can be a powerful tool in complex, prior-knowledge-required areas, especially in the field of biomedicine, which is now facing the problem of inadequate medical resources. The application of deep learning in disease diagnosis has become a new research topic in dermatology. This paper aims to provide a quick review of the ...
GlobalSkin has successfully concluded its Global Research on the Impact of Dermatological Diseases (GRIDD) Study, a novel patient-led research initiative which it launched on June 5, 2023 in collaboration with Cardiff University (UK) and University Medical Centre Hamburg-Eppendorf (Germany). The GRIDD Study gathered global data about the real ...
Skin diseases are the fourth most common cause of all human disease, affecting almost one-third of the world's population, yet their burden is often underestimated, despite their visibility. 1, 2 Burden of disease is a concept that was first developed in the 1990s by the Harvard School of Public Health, the World Bank and the World Health Organization (WHO) to describe death and loss of ...
Since 2009, the Skin Biology & Diseases Resource-Based Center (formerly the Skin Disease Research Center) has promoted outstanding bench and clinical research in cutaneous biology by providing high-quality technical services, enhancing education and communication among our members and facilitating translational research.
Skin is the largest organ of the body which provides protection, regulates the body fluids and temperature, and enables sense of the external environment. 1 Skin diseases are the most common cause of all human illnesses which affects almost 900 million people in the world at any time. 2 According to the global burden of disease project, skin ...
The vision of the SDRC is: To enhance career development and to fuel the projects of new and experienced investigators with a rich matrix of resources in order to speed the progress and enhance the quality of skin diseases research. To generate a new knowledge base that will have a significant and sustained impact on cutaneous biology aimed at ...
Many researchers have worked on skin disease classification for the last three decades. The area is so significant and has become a hot research topic. Even though many research papers are done on skin disease detection and classification, there is still a gap to be filled.
partition algorithm was designed to classify a given skin lesion. to be malignant, benign or non-neoplastic. Also, 9-w ay disease. partition was performed to classify a given lesion into one. the ...
Molecular Research and Treatment of Skin Diseases. The intention of this Special Issue is to highlight current treatment options to target the cause, as well as disease-associated complications, of skin diseases, including a group of monogenetic skin disorders referred to as genodermatoses. The clinical heterogeneity of genodermatoses is ...
Skin disease classification is the last step in the CAD method for skin disease diagnosis. Based on their objectives, several classification algorithms may produce binary, trinary or N4 types of skin disease categorization results. Numerous deep learning algorithms have been created in order to summarize skin disease photographs.
Skin Disorders Research Project/Presentation Anatomy & Physiology Fall 2015 Purpose: Everyone should have an understanding of some common skin diseases, disorders and rashes that you may encounter during your life. In addition, you will gather information and organize it so you can educate your classmates on your disorder.
The burden of skin disease is regarded as a multidimensional concept that comprehends psychological, social and economic significance of the skin disease at the sufferers and their households and on society. It is a contamination that takes place in humans of all ages. Skin is regularly broken due to the fact it's far a touchy a part of the body.
NHGRI Press Office. Email: [email protected]. Phone: (301) 402-0911. Last updated: June 1, 2023. Researchers at the National Institutes of Health and their colleagues have identified genomic variants that cause a rare and severe inflammatory skin disorder, known as disabling pansclerotic morphea, and have found a potential treatment.
There is more evidence that Parkinson's disease can be diagnosed through the skin, according to a new study published in the Journal of the American Medical Association. Dr. Christopher Gibbons, a ...
Skin tests can detect an abnormal form of alpha-synuclein, a protein that is the hallmark of Parkinson's disease and similar degenerative brain illnesses, researchers say.
The Global Burden of Disease project has shown that skin diseases continue to be the 4 th leading cause of nonfatal disease burden world-wide. However, research efforts and funding do not match with the relative disability of skin diseases. International and national efforts, such as the WHO List of Essential Medicines, are critical towards ...
The skin test costs just under $1,500 but is usually covered mostly or all by insurance, Levine said. The spinal-fluid test is $1,500 if billed to insurance, or $995 for patients who pay on their own.
Above: Gottheimer at Cooperman Barnabas Medical Center. LIVINGSTON, NJ — Today, March 19, 2024, U.S. Congressman Josh Gottheimer (NJ-5) announced new legislation, the Securing Equal Access to Research, Care, and Health, or SEARCH Act, to help fund new efforts to increase the number of women in clinical research trials, leading to treatments and cures for rare diseases and blood disorders ...
Materials and Methods: This cross-sectional study was conducted in June 2011 in a medical college in Mangalore, Karnataka. Two-hundred and seventy eight medical students were chosen from the 4 th, 6 th and 8 th semester through convenient sampling method. Data on hair and skin morbidities suffered over past 1 year and its associated factors were collected using a self-administered questionnaire.