- Privacy Policy
Buy Me a Coffee

Home » Quantitative Research – Methods, Types and Analysis
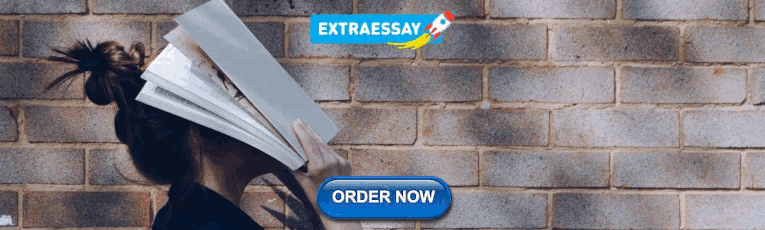
Quantitative Research – Methods, Types and Analysis
Table of Contents

Quantitative Research
Quantitative research is a type of research that collects and analyzes numerical data to test hypotheses and answer research questions . This research typically involves a large sample size and uses statistical analysis to make inferences about a population based on the data collected. It often involves the use of surveys, experiments, or other structured data collection methods to gather quantitative data.
Quantitative Research Methods

Quantitative Research Methods are as follows:
Descriptive Research Design
Descriptive research design is used to describe the characteristics of a population or phenomenon being studied. This research method is used to answer the questions of what, where, when, and how. Descriptive research designs use a variety of methods such as observation, case studies, and surveys to collect data. The data is then analyzed using statistical tools to identify patterns and relationships.
Correlational Research Design
Correlational research design is used to investigate the relationship between two or more variables. Researchers use correlational research to determine whether a relationship exists between variables and to what extent they are related. This research method involves collecting data from a sample and analyzing it using statistical tools such as correlation coefficients.
Quasi-experimental Research Design
Quasi-experimental research design is used to investigate cause-and-effect relationships between variables. This research method is similar to experimental research design, but it lacks full control over the independent variable. Researchers use quasi-experimental research designs when it is not feasible or ethical to manipulate the independent variable.
Experimental Research Design
Experimental research design is used to investigate cause-and-effect relationships between variables. This research method involves manipulating the independent variable and observing the effects on the dependent variable. Researchers use experimental research designs to test hypotheses and establish cause-and-effect relationships.
Survey Research
Survey research involves collecting data from a sample of individuals using a standardized questionnaire. This research method is used to gather information on attitudes, beliefs, and behaviors of individuals. Researchers use survey research to collect data quickly and efficiently from a large sample size. Survey research can be conducted through various methods such as online, phone, mail, or in-person interviews.
Quantitative Research Analysis Methods
Here are some commonly used quantitative research analysis methods:
Statistical Analysis
Statistical analysis is the most common quantitative research analysis method. It involves using statistical tools and techniques to analyze the numerical data collected during the research process. Statistical analysis can be used to identify patterns, trends, and relationships between variables, and to test hypotheses and theories.
Regression Analysis
Regression analysis is a statistical technique used to analyze the relationship between one dependent variable and one or more independent variables. Researchers use regression analysis to identify and quantify the impact of independent variables on the dependent variable.
Factor Analysis
Factor analysis is a statistical technique used to identify underlying factors that explain the correlations among a set of variables. Researchers use factor analysis to reduce a large number of variables to a smaller set of factors that capture the most important information.
Structural Equation Modeling
Structural equation modeling is a statistical technique used to test complex relationships between variables. It involves specifying a model that includes both observed and unobserved variables, and then using statistical methods to test the fit of the model to the data.
Time Series Analysis
Time series analysis is a statistical technique used to analyze data that is collected over time. It involves identifying patterns and trends in the data, as well as any seasonal or cyclical variations.
Multilevel Modeling
Multilevel modeling is a statistical technique used to analyze data that is nested within multiple levels. For example, researchers might use multilevel modeling to analyze data that is collected from individuals who are nested within groups, such as students nested within schools.
Applications of Quantitative Research
Quantitative research has many applications across a wide range of fields. Here are some common examples:
- Market Research : Quantitative research is used extensively in market research to understand consumer behavior, preferences, and trends. Researchers use surveys, experiments, and other quantitative methods to collect data that can inform marketing strategies, product development, and pricing decisions.
- Health Research: Quantitative research is used in health research to study the effectiveness of medical treatments, identify risk factors for diseases, and track health outcomes over time. Researchers use statistical methods to analyze data from clinical trials, surveys, and other sources to inform medical practice and policy.
- Social Science Research: Quantitative research is used in social science research to study human behavior, attitudes, and social structures. Researchers use surveys, experiments, and other quantitative methods to collect data that can inform social policies, educational programs, and community interventions.
- Education Research: Quantitative research is used in education research to study the effectiveness of teaching methods, assess student learning outcomes, and identify factors that influence student success. Researchers use experimental and quasi-experimental designs, as well as surveys and other quantitative methods, to collect and analyze data.
- Environmental Research: Quantitative research is used in environmental research to study the impact of human activities on the environment, assess the effectiveness of conservation strategies, and identify ways to reduce environmental risks. Researchers use statistical methods to analyze data from field studies, experiments, and other sources.
Characteristics of Quantitative Research
Here are some key characteristics of quantitative research:
- Numerical data : Quantitative research involves collecting numerical data through standardized methods such as surveys, experiments, and observational studies. This data is analyzed using statistical methods to identify patterns and relationships.
- Large sample size: Quantitative research often involves collecting data from a large sample of individuals or groups in order to increase the reliability and generalizability of the findings.
- Objective approach: Quantitative research aims to be objective and impartial in its approach, focusing on the collection and analysis of data rather than personal beliefs, opinions, or experiences.
- Control over variables: Quantitative research often involves manipulating variables to test hypotheses and establish cause-and-effect relationships. Researchers aim to control for extraneous variables that may impact the results.
- Replicable : Quantitative research aims to be replicable, meaning that other researchers should be able to conduct similar studies and obtain similar results using the same methods.
- Statistical analysis: Quantitative research involves using statistical tools and techniques to analyze the numerical data collected during the research process. Statistical analysis allows researchers to identify patterns, trends, and relationships between variables, and to test hypotheses and theories.
- Generalizability: Quantitative research aims to produce findings that can be generalized to larger populations beyond the specific sample studied. This is achieved through the use of random sampling methods and statistical inference.
Examples of Quantitative Research
Here are some examples of quantitative research in different fields:
- Market Research: A company conducts a survey of 1000 consumers to determine their brand awareness and preferences. The data is analyzed using statistical methods to identify trends and patterns that can inform marketing strategies.
- Health Research : A researcher conducts a randomized controlled trial to test the effectiveness of a new drug for treating a particular medical condition. The study involves collecting data from a large sample of patients and analyzing the results using statistical methods.
- Social Science Research : A sociologist conducts a survey of 500 people to study attitudes toward immigration in a particular country. The data is analyzed using statistical methods to identify factors that influence these attitudes.
- Education Research: A researcher conducts an experiment to compare the effectiveness of two different teaching methods for improving student learning outcomes. The study involves randomly assigning students to different groups and collecting data on their performance on standardized tests.
- Environmental Research : A team of researchers conduct a study to investigate the impact of climate change on the distribution and abundance of a particular species of plant or animal. The study involves collecting data on environmental factors and population sizes over time and analyzing the results using statistical methods.
- Psychology : A researcher conducts a survey of 500 college students to investigate the relationship between social media use and mental health. The data is analyzed using statistical methods to identify correlations and potential causal relationships.
- Political Science: A team of researchers conducts a study to investigate voter behavior during an election. They use survey methods to collect data on voting patterns, demographics, and political attitudes, and analyze the results using statistical methods.
How to Conduct Quantitative Research
Here is a general overview of how to conduct quantitative research:
- Develop a research question: The first step in conducting quantitative research is to develop a clear and specific research question. This question should be based on a gap in existing knowledge, and should be answerable using quantitative methods.
- Develop a research design: Once you have a research question, you will need to develop a research design. This involves deciding on the appropriate methods to collect data, such as surveys, experiments, or observational studies. You will also need to determine the appropriate sample size, data collection instruments, and data analysis techniques.
- Collect data: The next step is to collect data. This may involve administering surveys or questionnaires, conducting experiments, or gathering data from existing sources. It is important to use standardized methods to ensure that the data is reliable and valid.
- Analyze data : Once the data has been collected, it is time to analyze it. This involves using statistical methods to identify patterns, trends, and relationships between variables. Common statistical techniques include correlation analysis, regression analysis, and hypothesis testing.
- Interpret results: After analyzing the data, you will need to interpret the results. This involves identifying the key findings, determining their significance, and drawing conclusions based on the data.
- Communicate findings: Finally, you will need to communicate your findings. This may involve writing a research report, presenting at a conference, or publishing in a peer-reviewed journal. It is important to clearly communicate the research question, methods, results, and conclusions to ensure that others can understand and replicate your research.
When to use Quantitative Research
Here are some situations when quantitative research can be appropriate:
- To test a hypothesis: Quantitative research is often used to test a hypothesis or a theory. It involves collecting numerical data and using statistical analysis to determine if the data supports or refutes the hypothesis.
- To generalize findings: If you want to generalize the findings of your study to a larger population, quantitative research can be useful. This is because it allows you to collect numerical data from a representative sample of the population and use statistical analysis to make inferences about the population as a whole.
- To measure relationships between variables: If you want to measure the relationship between two or more variables, such as the relationship between age and income, or between education level and job satisfaction, quantitative research can be useful. It allows you to collect numerical data on both variables and use statistical analysis to determine the strength and direction of the relationship.
- To identify patterns or trends: Quantitative research can be useful for identifying patterns or trends in data. For example, you can use quantitative research to identify trends in consumer behavior or to identify patterns in stock market data.
- To quantify attitudes or opinions : If you want to measure attitudes or opinions on a particular topic, quantitative research can be useful. It allows you to collect numerical data using surveys or questionnaires and analyze the data using statistical methods to determine the prevalence of certain attitudes or opinions.
Purpose of Quantitative Research
The purpose of quantitative research is to systematically investigate and measure the relationships between variables or phenomena using numerical data and statistical analysis. The main objectives of quantitative research include:
- Description : To provide a detailed and accurate description of a particular phenomenon or population.
- Explanation : To explain the reasons for the occurrence of a particular phenomenon, such as identifying the factors that influence a behavior or attitude.
- Prediction : To predict future trends or behaviors based on past patterns and relationships between variables.
- Control : To identify the best strategies for controlling or influencing a particular outcome or behavior.
Quantitative research is used in many different fields, including social sciences, business, engineering, and health sciences. It can be used to investigate a wide range of phenomena, from human behavior and attitudes to physical and biological processes. The purpose of quantitative research is to provide reliable and valid data that can be used to inform decision-making and improve understanding of the world around us.
Advantages of Quantitative Research
There are several advantages of quantitative research, including:
- Objectivity : Quantitative research is based on objective data and statistical analysis, which reduces the potential for bias or subjectivity in the research process.
- Reproducibility : Because quantitative research involves standardized methods and measurements, it is more likely to be reproducible and reliable.
- Generalizability : Quantitative research allows for generalizations to be made about a population based on a representative sample, which can inform decision-making and policy development.
- Precision : Quantitative research allows for precise measurement and analysis of data, which can provide a more accurate understanding of phenomena and relationships between variables.
- Efficiency : Quantitative research can be conducted relatively quickly and efficiently, especially when compared to qualitative research, which may involve lengthy data collection and analysis.
- Large sample sizes : Quantitative research can accommodate large sample sizes, which can increase the representativeness and generalizability of the results.
Limitations of Quantitative Research
There are several limitations of quantitative research, including:
- Limited understanding of context: Quantitative research typically focuses on numerical data and statistical analysis, which may not provide a comprehensive understanding of the context or underlying factors that influence a phenomenon.
- Simplification of complex phenomena: Quantitative research often involves simplifying complex phenomena into measurable variables, which may not capture the full complexity of the phenomenon being studied.
- Potential for researcher bias: Although quantitative research aims to be objective, there is still the potential for researcher bias in areas such as sampling, data collection, and data analysis.
- Limited ability to explore new ideas: Quantitative research is often based on pre-determined research questions and hypotheses, which may limit the ability to explore new ideas or unexpected findings.
- Limited ability to capture subjective experiences : Quantitative research is typically focused on objective data and may not capture the subjective experiences of individuals or groups being studied.
- Ethical concerns : Quantitative research may raise ethical concerns, such as invasion of privacy or the potential for harm to participants.
About the author
Muhammad Hassan
Researcher, Academic Writer, Web developer
You may also like

Case Study – Methods, Examples and Guide

Qualitative Research – Methods, Analysis Types...

Descriptive Research Design – Types, Methods and...

Qualitative Research Methods

Basic Research – Types, Methods and Examples

Exploratory Research – Types, Methods and...

Quantitative Data Analysis 101
The lingo, methods and techniques, explained simply.
By: Derek Jansen (MBA) and Kerryn Warren (PhD) | December 2020
Quantitative data analysis is one of those things that often strikes fear in students. It’s totally understandable – quantitative analysis is a complex topic, full of daunting lingo , like medians, modes, correlation and regression. Suddenly we’re all wishing we’d paid a little more attention in math class…
The good news is that while quantitative data analysis is a mammoth topic, gaining a working understanding of the basics isn’t that hard , even for those of us who avoid numbers and math . In this post, we’ll break quantitative analysis down into simple , bite-sized chunks so you can approach your research with confidence.
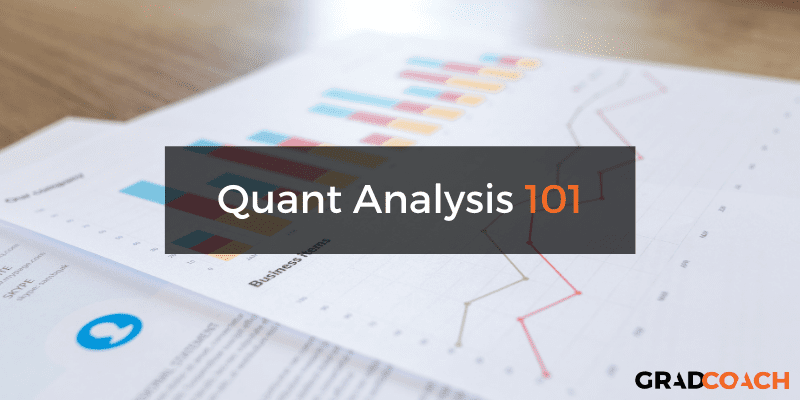
Overview: Quantitative Data Analysis 101
- What (exactly) is quantitative data analysis?
- When to use quantitative analysis
- How quantitative analysis works
The two “branches” of quantitative analysis
- Descriptive statistics 101
- Inferential statistics 101
- How to choose the right quantitative methods
- Recap & summary
What is quantitative data analysis?
Despite being a mouthful, quantitative data analysis simply means analysing data that is numbers-based – or data that can be easily “converted” into numbers without losing any meaning.
For example, category-based variables like gender, ethnicity, or native language could all be “converted” into numbers without losing meaning – for example, English could equal 1, French 2, etc.
This contrasts against qualitative data analysis, where the focus is on words, phrases and expressions that can’t be reduced to numbers. If you’re interested in learning about qualitative analysis, check out our post and video here .
What is quantitative analysis used for?
Quantitative analysis is generally used for three purposes.
- Firstly, it’s used to measure differences between groups . For example, the popularity of different clothing colours or brands.
- Secondly, it’s used to assess relationships between variables . For example, the relationship between weather temperature and voter turnout.
- And third, it’s used to test hypotheses in a scientifically rigorous way. For example, a hypothesis about the impact of a certain vaccine.
Again, this contrasts with qualitative analysis , which can be used to analyse people’s perceptions and feelings about an event or situation. In other words, things that can’t be reduced to numbers.
How does quantitative analysis work?
Well, since quantitative data analysis is all about analysing numbers , it’s no surprise that it involves statistics . Statistical analysis methods form the engine that powers quantitative analysis, and these methods can vary from pretty basic calculations (for example, averages and medians) to more sophisticated analyses (for example, correlations and regressions).
Sounds like gibberish? Don’t worry. We’ll explain all of that in this post. Importantly, you don’t need to be a statistician or math wiz to pull off a good quantitative analysis. We’ll break down all the technical mumbo jumbo in this post.
Need a helping hand?
As I mentioned, quantitative analysis is powered by statistical analysis methods . There are two main “branches” of statistical methods that are used – descriptive statistics and inferential statistics . In your research, you might only use descriptive statistics, or you might use a mix of both , depending on what you’re trying to figure out. In other words, depending on your research questions, aims and objectives . I’ll explain how to choose your methods later.
So, what are descriptive and inferential statistics?
Well, before I can explain that, we need to take a quick detour to explain some lingo. To understand the difference between these two branches of statistics, you need to understand two important words. These words are population and sample .
First up, population . In statistics, the population is the entire group of people (or animals or organisations or whatever) that you’re interested in researching. For example, if you were interested in researching Tesla owners in the US, then the population would be all Tesla owners in the US.
However, it’s extremely unlikely that you’re going to be able to interview or survey every single Tesla owner in the US. Realistically, you’ll likely only get access to a few hundred, or maybe a few thousand owners using an online survey. This smaller group of accessible people whose data you actually collect is called your sample .
So, to recap – the population is the entire group of people you’re interested in, and the sample is the subset of the population that you can actually get access to. In other words, the population is the full chocolate cake , whereas the sample is a slice of that cake.
So, why is this sample-population thing important?
Well, descriptive statistics focus on describing the sample , while inferential statistics aim to make predictions about the population, based on the findings within the sample. In other words, we use one group of statistical methods – descriptive statistics – to investigate the slice of cake, and another group of methods – inferential statistics – to draw conclusions about the entire cake. There I go with the cake analogy again…
With that out the way, let’s take a closer look at each of these branches in more detail.
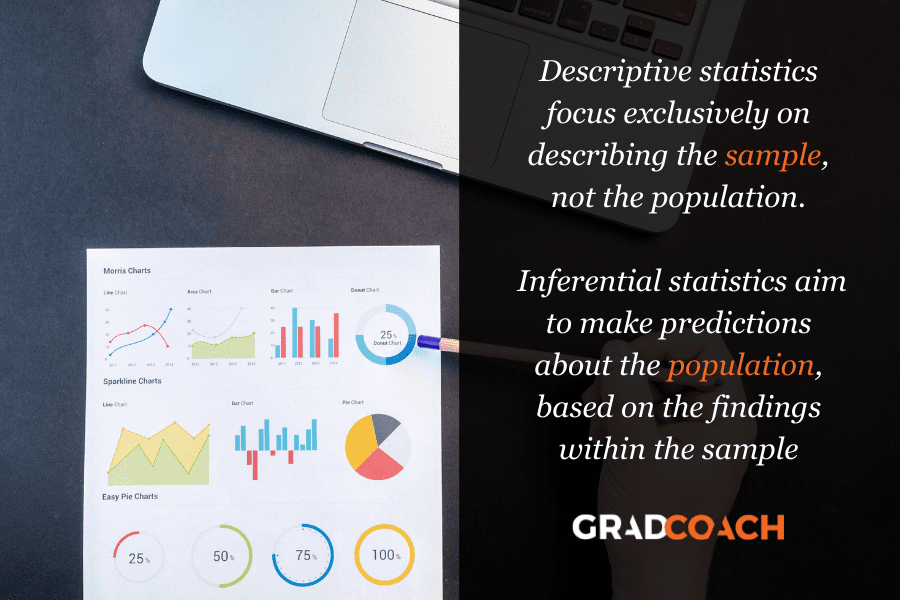
Branch 1: Descriptive Statistics
Descriptive statistics serve a simple but critically important role in your research – to describe your data set – hence the name. In other words, they help you understand the details of your sample . Unlike inferential statistics (which we’ll get to soon), descriptive statistics don’t aim to make inferences or predictions about the entire population – they’re purely interested in the details of your specific sample .
When you’re writing up your analysis, descriptive statistics are the first set of stats you’ll cover, before moving on to inferential statistics. But, that said, depending on your research objectives and research questions , they may be the only type of statistics you use. We’ll explore that a little later.
So, what kind of statistics are usually covered in this section?
Some common statistical tests used in this branch include the following:
- Mean – this is simply the mathematical average of a range of numbers.
- Median – this is the midpoint in a range of numbers when the numbers are arranged in numerical order. If the data set makes up an odd number, then the median is the number right in the middle of the set. If the data set makes up an even number, then the median is the midpoint between the two middle numbers.
- Mode – this is simply the most commonly occurring number in the data set.
- In cases where most of the numbers are quite close to the average, the standard deviation will be relatively low.
- Conversely, in cases where the numbers are scattered all over the place, the standard deviation will be relatively high.
- Skewness . As the name suggests, skewness indicates how symmetrical a range of numbers is. In other words, do they tend to cluster into a smooth bell curve shape in the middle of the graph, or do they skew to the left or right?
Feeling a bit confused? Let’s look at a practical example using a small data set.
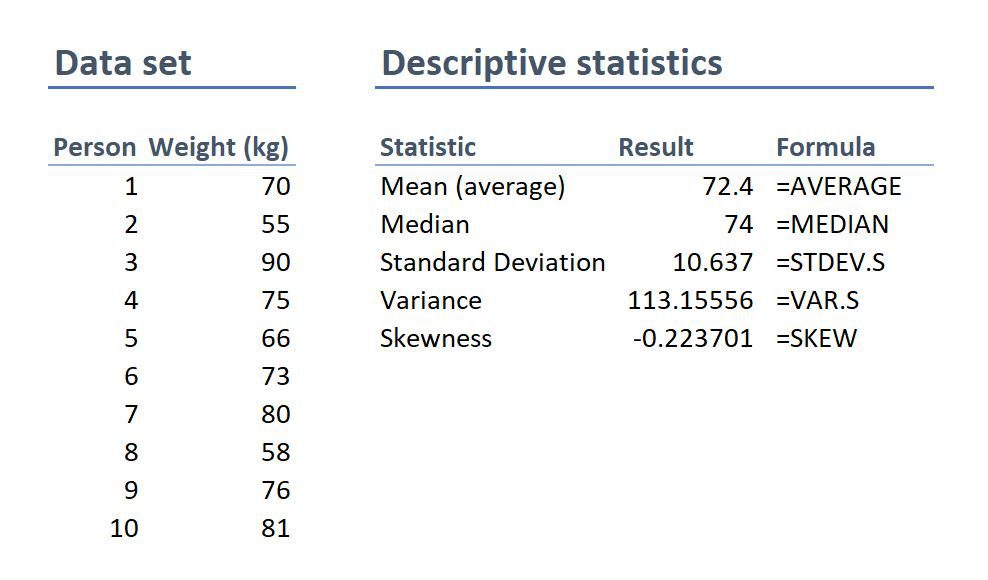
On the left-hand side is the data set. This details the bodyweight of a sample of 10 people. On the right-hand side, we have the descriptive statistics. Let’s take a look at each of them.
First, we can see that the mean weight is 72.4 kilograms. In other words, the average weight across the sample is 72.4 kilograms. Straightforward.
Next, we can see that the median is very similar to the mean (the average). This suggests that this data set has a reasonably symmetrical distribution (in other words, a relatively smooth, centred distribution of weights, clustered towards the centre).
In terms of the mode , there is no mode in this data set. This is because each number is present only once and so there cannot be a “most common number”. If there were two people who were both 65 kilograms, for example, then the mode would be 65.
Next up is the standard deviation . 10.6 indicates that there’s quite a wide spread of numbers. We can see this quite easily by looking at the numbers themselves, which range from 55 to 90, which is quite a stretch from the mean of 72.4.
And lastly, the skewness of -0.2 tells us that the data is very slightly negatively skewed. This makes sense since the mean and the median are slightly different.
As you can see, these descriptive statistics give us some useful insight into the data set. Of course, this is a very small data set (only 10 records), so we can’t read into these statistics too much. Also, keep in mind that this is not a list of all possible descriptive statistics – just the most common ones.
But why do all of these numbers matter?
While these descriptive statistics are all fairly basic, they’re important for a few reasons:
- Firstly, they help you get both a macro and micro-level view of your data. In other words, they help you understand both the big picture and the finer details.
- Secondly, they help you spot potential errors in the data – for example, if an average is way higher than you’d expect, or responses to a question are highly varied, this can act as a warning sign that you need to double-check the data.
- And lastly, these descriptive statistics help inform which inferential statistical techniques you can use, as those techniques depend on the skewness (in other words, the symmetry and normality) of the data.
Simply put, descriptive statistics are really important , even though the statistical techniques used are fairly basic. All too often at Grad Coach, we see students skimming over the descriptives in their eagerness to get to the more exciting inferential methods, and then landing up with some very flawed results.
Don’t be a sucker – give your descriptive statistics the love and attention they deserve!
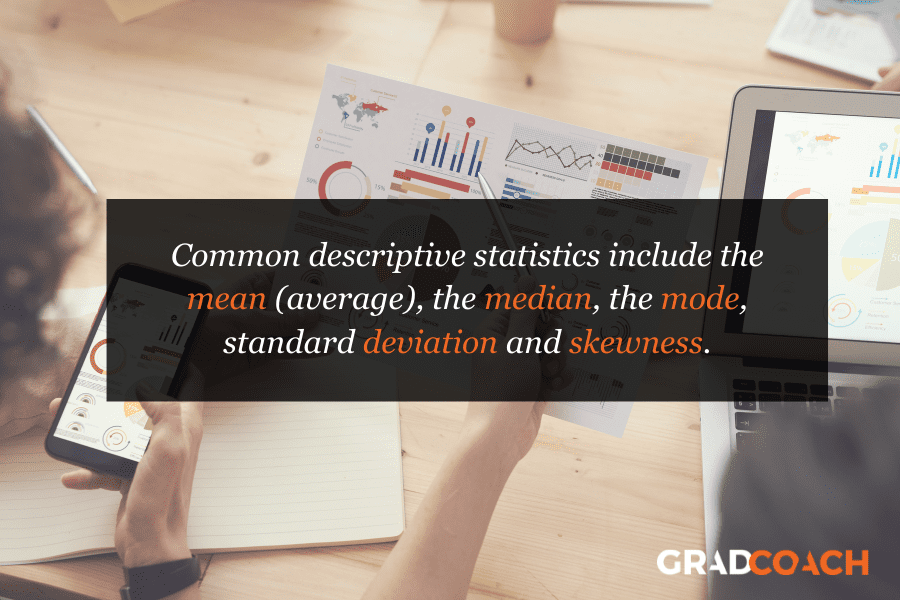
Branch 2: Inferential Statistics
As I mentioned, while descriptive statistics are all about the details of your specific data set – your sample – inferential statistics aim to make inferences about the population . In other words, you’ll use inferential statistics to make predictions about what you’d expect to find in the full population.
What kind of predictions, you ask? Well, there are two common types of predictions that researchers try to make using inferential stats:
- Firstly, predictions about differences between groups – for example, height differences between children grouped by their favourite meal or gender.
- And secondly, relationships between variables – for example, the relationship between body weight and the number of hours a week a person does yoga.
In other words, inferential statistics (when done correctly), allow you to connect the dots and make predictions about what you expect to see in the real world population, based on what you observe in your sample data. For this reason, inferential statistics are used for hypothesis testing – in other words, to test hypotheses that predict changes or differences.
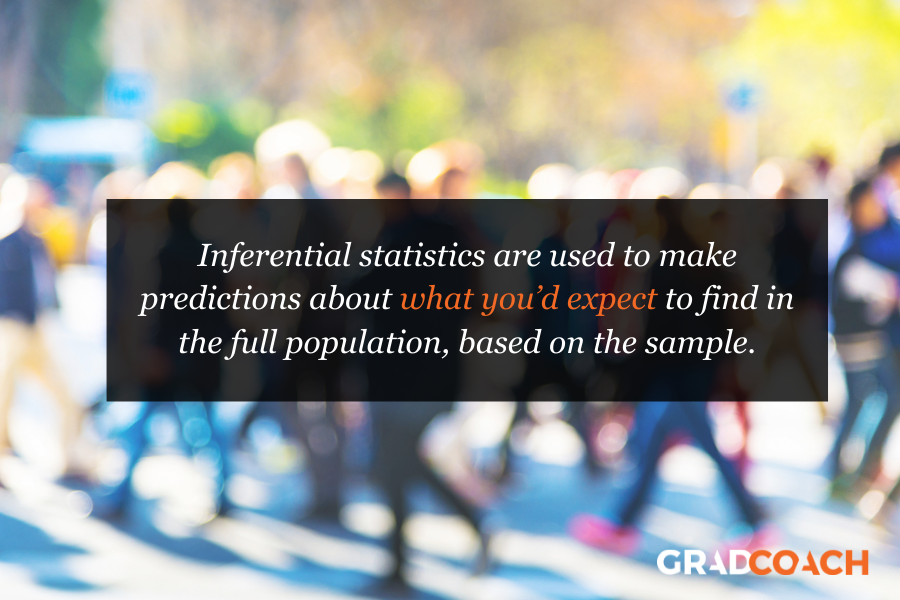
Of course, when you’re working with inferential statistics, the composition of your sample is really important. In other words, if your sample doesn’t accurately represent the population you’re researching, then your findings won’t necessarily be very useful.
For example, if your population of interest is a mix of 50% male and 50% female , but your sample is 80% male , you can’t make inferences about the population based on your sample, since it’s not representative. This area of statistics is called sampling, but we won’t go down that rabbit hole here (it’s a deep one!) – we’ll save that for another post .
What statistics are usually used in this branch?
There are many, many different statistical analysis methods within the inferential branch and it’d be impossible for us to discuss them all here. So we’ll just take a look at some of the most common inferential statistical methods so that you have a solid starting point.
First up are T-Tests . T-tests compare the means (the averages) of two groups of data to assess whether they’re statistically significantly different. In other words, do they have significantly different means, standard deviations and skewness.
This type of testing is very useful for understanding just how similar or different two groups of data are. For example, you might want to compare the mean blood pressure between two groups of people – one that has taken a new medication and one that hasn’t – to assess whether they are significantly different.
Kicking things up a level, we have ANOVA, which stands for “analysis of variance”. This test is similar to a T-test in that it compares the means of various groups, but ANOVA allows you to analyse multiple groups , not just two groups So it’s basically a t-test on steroids…
Next, we have correlation analysis . This type of analysis assesses the relationship between two variables. In other words, if one variable increases, does the other variable also increase, decrease or stay the same. For example, if the average temperature goes up, do average ice creams sales increase too? We’d expect some sort of relationship between these two variables intuitively , but correlation analysis allows us to measure that relationship scientifically .
Lastly, we have regression analysis – this is quite similar to correlation in that it assesses the relationship between variables, but it goes a step further to understand cause and effect between variables, not just whether they move together. In other words, does the one variable actually cause the other one to move, or do they just happen to move together naturally thanks to another force? Just because two variables correlate doesn’t necessarily mean that one causes the other.
Stats overload…
I hear you. To make this all a little more tangible, let’s take a look at an example of a correlation in action.
Here’s a scatter plot demonstrating the correlation (relationship) between weight and height. Intuitively, we’d expect there to be some relationship between these two variables, which is what we see in this scatter plot. In other words, the results tend to cluster together in a diagonal line from bottom left to top right.
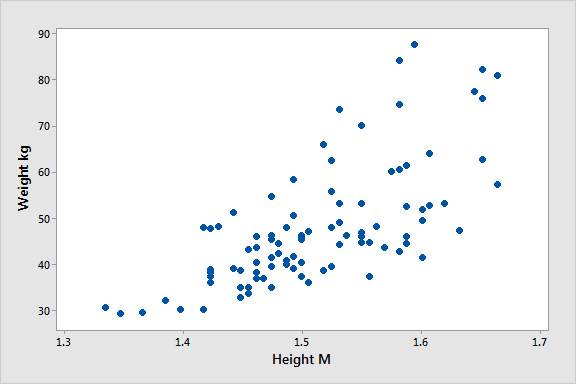
As I mentioned, these are are just a handful of inferential techniques – there are many, many more. Importantly, each statistical method has its own assumptions and limitations.
For example, some methods only work with normally distributed (parametric) data, while other methods are designed specifically for non-parametric data. And that’s exactly why descriptive statistics are so important – they’re the first step to knowing which inferential techniques you can and can’t use.
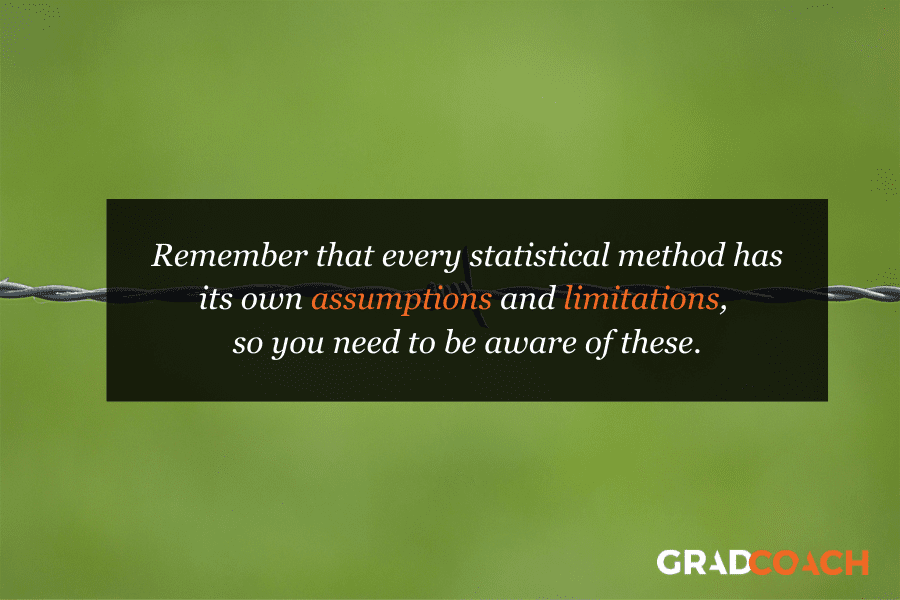
How to choose the right analysis method
To choose the right statistical methods, you need to think about two important factors :
- The type of quantitative data you have (specifically, level of measurement and the shape of the data). And,
- Your research questions and hypotheses
Let’s take a closer look at each of these.
Factor 1 – Data type
The first thing you need to consider is the type of data you’ve collected (or the type of data you will collect). By data types, I’m referring to the four levels of measurement – namely, nominal, ordinal, interval and ratio. If you’re not familiar with this lingo, check out the video below.
Why does this matter?
Well, because different statistical methods and techniques require different types of data. This is one of the “assumptions” I mentioned earlier – every method has its assumptions regarding the type of data.
For example, some techniques work with categorical data (for example, yes/no type questions, or gender or ethnicity), while others work with continuous numerical data (for example, age, weight or income) – and, of course, some work with multiple data types.
If you try to use a statistical method that doesn’t support the data type you have, your results will be largely meaningless . So, make sure that you have a clear understanding of what types of data you’ve collected (or will collect). Once you have this, you can then check which statistical methods would support your data types here .
If you haven’t collected your data yet, you can work in reverse and look at which statistical method would give you the most useful insights, and then design your data collection strategy to collect the correct data types.
Another important factor to consider is the shape of your data . Specifically, does it have a normal distribution (in other words, is it a bell-shaped curve, centred in the middle) or is it very skewed to the left or the right? Again, different statistical techniques work for different shapes of data – some are designed for symmetrical data while others are designed for skewed data.
This is another reminder of why descriptive statistics are so important – they tell you all about the shape of your data.
Factor 2: Your research questions
The next thing you need to consider is your specific research questions, as well as your hypotheses (if you have some). The nature of your research questions and research hypotheses will heavily influence which statistical methods and techniques you should use.
If you’re just interested in understanding the attributes of your sample (as opposed to the entire population), then descriptive statistics are probably all you need. For example, if you just want to assess the means (averages) and medians (centre points) of variables in a group of people.
On the other hand, if you aim to understand differences between groups or relationships between variables and to infer or predict outcomes in the population, then you’ll likely need both descriptive statistics and inferential statistics.
So, it’s really important to get very clear about your research aims and research questions, as well your hypotheses – before you start looking at which statistical techniques to use.
Never shoehorn a specific statistical technique into your research just because you like it or have some experience with it. Your choice of methods must align with all the factors we’ve covered here.
Time to recap…
You’re still with me? That’s impressive. We’ve covered a lot of ground here, so let’s recap on the key points:
- Quantitative data analysis is all about analysing number-based data (which includes categorical and numerical data) using various statistical techniques.
- The two main branches of statistics are descriptive statistics and inferential statistics . Descriptives describe your sample, whereas inferentials make predictions about what you’ll find in the population.
- Common descriptive statistical methods include mean (average), median , standard deviation and skewness .
- Common inferential statistical methods include t-tests , ANOVA , correlation and regression analysis.
- To choose the right statistical methods and techniques, you need to consider the type of data you’re working with , as well as your research questions and hypotheses.
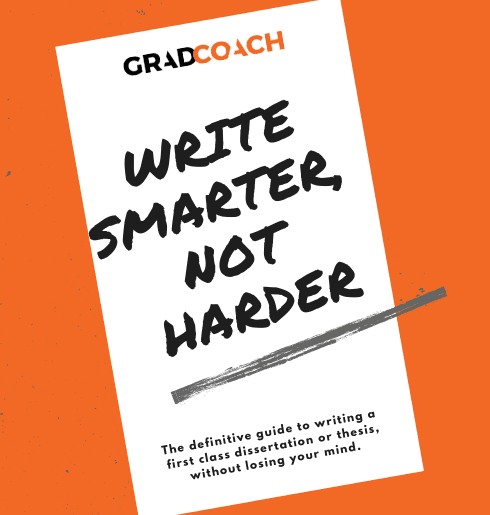
Psst… there’s more (for free)
This post is part of our dissertation mini-course, which covers everything you need to get started with your dissertation, thesis or research project.
You Might Also Like:
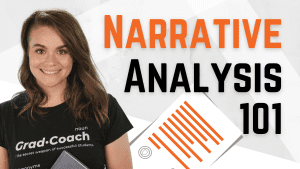
73 Comments
Hi, I have read your article. Such a brilliant post you have created.
Thank you for the feedback. Good luck with your quantitative analysis.
Thank you so much.
Thank you so much. I learnt much well. I love your summaries of the concepts. I had love you to explain how to input data using SPSS
Amazing and simple way of breaking down quantitative methods.
This is beautiful….especially for non-statisticians. I have skimmed through but I wish to read again. and please include me in other articles of the same nature when you do post. I am interested. I am sure, I could easily learn from you and get off the fear that I have had in the past. Thank you sincerely.
Send me every new information you might have.
i need every new information
Thank you for the blog. It is quite informative. Dr Peter Nemaenzhe PhD
It is wonderful. l’ve understood some of the concepts in a more compréhensive manner
Your article is so good! However, I am still a bit lost. I am doing a secondary research on Gun control in the US and increase in crime rates and I am not sure which analysis method I should use?
Based on the given learning points, this is inferential analysis, thus, use ‘t-tests, ANOVA, correlation and regression analysis’
Well explained notes. Am an MPH student and currently working on my thesis proposal, this has really helped me understand some of the things I didn’t know.
I like your page..helpful
wonderful i got my concept crystal clear. thankyou!!
This is really helpful , thank you
Thank you so much this helped
Wonderfully explained
thank u so much, it was so informative
THANKYOU, this was very informative and very helpful
This is great GRADACOACH I am not a statistician but I require more of this in my thesis
Include me in your posts.
This is so great and fully useful. I would like to thank you again and again.
Glad to read this article. I’ve read lot of articles but this article is clear on all concepts. Thanks for sharing.
Thank you so much. This is a very good foundation and intro into quantitative data analysis. Appreciate!
You have a very impressive, simple but concise explanation of data analysis for Quantitative Research here. This is a God-send link for me to appreciate research more. Thank you so much!
Avery good presentation followed by the write up. yes you simplified statistics to make sense even to a layman like me. Thank so much keep it up. The presenter did ell too. i would like more of this for Qualitative and exhaust more of the test example like the Anova.
This is a very helpful article, couldn’t have been clearer. Thank you.
Awesome and phenomenal information.Well done
The video with the accompanying article is super helpful to demystify this topic. Very well done. Thank you so much.
thank you so much, your presentation helped me a lot
I don’t know how should I express that ur article is saviour for me 🥺😍
It is well defined information and thanks for sharing. It helps me a lot in understanding the statistical data.
I gain a lot and thanks for sharing brilliant ideas, so wish to be linked on your email update.
Very helpful and clear .Thank you Gradcoach.
Thank for sharing this article, well organized and information presented are very clear.
VERY INTERESTING AND SUPPORTIVE TO NEW RESEARCHERS LIKE ME. AT LEAST SOME BASICS ABOUT QUANTITATIVE.
An outstanding, well explained and helpful article. This will help me so much with my data analysis for my research project. Thank you!
wow this has just simplified everything i was scared of how i am gonna analyse my data but thanks to you i will be able to do so
simple and constant direction to research. thanks
This is helpful
Great writing!! Comprehensive and very helpful.
Do you provide any assistance for other steps of research methodology like making research problem testing hypothesis report and thesis writing?
Thank you so much for such useful article!
Amazing article. So nicely explained. Wow
Very insightfull. Thanks
I am doing a quality improvement project to determine if the implementation of a protocol will change prescribing habits. Would this be a t-test?
The is a very helpful blog, however, I’m still not sure how to analyze my data collected. I’m doing a research on “Free Education at the University of Guyana”
tnx. fruitful blog!
So I am writing exams and would like to know how do establish which method of data analysis to use from the below research questions: I am a bit lost as to how I determine the data analysis method from the research questions.
Do female employees report higher job satisfaction than male employees with similar job descriptions across the South African telecommunications sector? – I though that maybe Chi Square could be used here. – Is there a gender difference in talented employees’ actual turnover decisions across the South African telecommunications sector? T-tests or Correlation in this one. – Is there a gender difference in the cost of actual turnover decisions across the South African telecommunications sector? T-tests or Correlation in this one. – What practical recommendations can be made to the management of South African telecommunications companies on leveraging gender to mitigate employee turnover decisions?
Your assistance will be appreciated if I could get a response as early as possible tomorrow
This was quite helpful. Thank you so much.
wow I got a lot from this article, thank you very much, keep it up
Thanks for yhe guidance. Can you send me this guidance on my email? To enable offline reading?
Thank you very much, this service is very helpful.
Every novice researcher needs to read this article as it puts things so clear and easy to follow. Its been very helpful.
Wonderful!!!! you explained everything in a way that anyone can learn. Thank you!!
I really enjoyed reading though this. Very easy to follow. Thank you
Many thanks for your useful lecture, I would be really appreciated if you could possibly share with me the PPT of presentation related to Data type?
Thank you very much for sharing, I got much from this article
This is a very informative write-up. Kindly include me in your latest posts.
Very interesting mostly for social scientists
Thank you so much, very helpfull
You’re welcome 🙂
woow, its great, its very informative and well understood because of your way of writing like teaching in front of me in simple languages.
I have been struggling to understand a lot of these concepts. Thank you for the informative piece which is written with outstanding clarity.
very informative article. Easy to understand
Beautiful read, much needed.
Always greet intro and summary. I learn so much from GradCoach
Quite informative. Simple and clear summary.
I thoroughly enjoyed reading your informative and inspiring piece. Your profound insights into this topic truly provide a better understanding of its complexity. I agree with the points you raised, especially when you delved into the specifics of the article. In my opinion, that aspect is often overlooked and deserves further attention.
Absolutely!!! Thank you
Submit a Comment Cancel reply
Your email address will not be published. Required fields are marked *
Save my name, email, and website in this browser for the next time I comment.
- Print Friendly
Quantitative Data Analysis: A Comprehensive Guide
By: Ofem Eteng Published: May 18, 2022
Related Articles
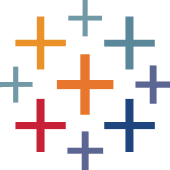
A healthcare giant successfully introduces the most effective drug dosage through rigorous statistical modeling, saving countless lives. A marketing team predicts consumer trends with uncanny accuracy, tailoring campaigns for maximum impact.
Table of Contents
These trends and dosages are not just any numbers but are a result of meticulous quantitative data analysis. Quantitative data analysis offers a robust framework for understanding complex phenomena, evaluating hypotheses, and predicting future outcomes.
In this blog, we’ll walk through the concept of quantitative data analysis, the steps required, its advantages, and the methods and techniques that are used in this analysis. Read on!
What is Quantitative Data Analysis?
Quantitative data analysis is a systematic process of examining, interpreting, and drawing meaningful conclusions from numerical data. It involves the application of statistical methods, mathematical models, and computational techniques to understand patterns, relationships, and trends within datasets.
Quantitative data analysis methods typically work with algorithms, mathematical analysis tools, and software to gain insights from the data, answering questions such as how many, how often, and how much. Data for quantitative data analysis is usually collected from close-ended surveys, questionnaires, polls, etc. The data can also be obtained from sales figures, email click-through rates, number of website visitors, and percentage revenue increase.
Quantitative Data Analysis vs Qualitative Data Analysis
When we talk about data, we directly think about the pattern, the relationship, and the connection between the datasets – analyzing the data in short. Therefore when it comes to data analysis, there are broadly two types – Quantitative Data Analysis and Qualitative Data Analysis.
Quantitative data analysis revolves around numerical data and statistics, which are suitable for functions that can be counted or measured. In contrast, qualitative data analysis includes description and subjective information – for things that can be observed but not measured.
Let us differentiate between Quantitative Data Analysis and Quantitative Data Analysis for a better understanding.
Data Preparation Steps for Quantitative Data Analysis
Quantitative data has to be gathered and cleaned before proceeding to the stage of analyzing it. Below are the steps to prepare a data before quantitative research analysis:
- Step 1: Data Collection
Before beginning the analysis process, you need data. Data can be collected through rigorous quantitative research, which includes methods such as interviews, focus groups, surveys, and questionnaires.
- Step 2: Data Cleaning
Once the data is collected, begin the data cleaning process by scanning through the entire data for duplicates, errors, and omissions. Keep a close eye for outliers (data points that are significantly different from the majority of the dataset) because they can skew your analysis results if they are not removed.
This data-cleaning process ensures data accuracy, consistency and relevancy before analysis.
- Step 3: Data Analysis and Interpretation
Now that you have collected and cleaned your data, it is now time to carry out the quantitative analysis. There are two methods of quantitative data analysis, which we will discuss in the next section.
However, if you have data from multiple sources, collecting and cleaning it can be a cumbersome task. This is where Hevo Data steps in. With Hevo, extracting, transforming, and loading data from source to destination becomes a seamless task, eliminating the need for manual coding. This not only saves valuable time but also enhances the overall efficiency of data analysis and visualization, empowering users to derive insights quickly and with precision
Hevo is the only real-time ELT No-code Data Pipeline platform that cost-effectively automates data pipelines that are flexible to your needs. With integration with 150+ Data Sources (40+ free sources), we help you not only export data from sources & load data to the destinations but also transform & enrich your data, & make it analysis-ready.
Start for free now!
Now that you are familiar with what quantitative data analysis is and how to prepare your data for analysis, the focus will shift to the purpose of this article, which is to describe the methods and techniques of quantitative data analysis.
Methods and Techniques of Quantitative Data Analysis
Quantitative data analysis employs two techniques to extract meaningful insights from datasets, broadly. The first method is descriptive statistics, which summarizes and portrays essential features of a dataset, such as mean, median, and standard deviation.
Inferential statistics, the second method, extrapolates insights and predictions from a sample dataset to make broader inferences about an entire population, such as hypothesis testing and regression analysis.
An in-depth explanation of both the methods is provided below:
- Descriptive Statistics
- Inferential Statistics
1) Descriptive Statistics
Descriptive statistics as the name implies is used to describe a dataset. It helps understand the details of your data by summarizing it and finding patterns from the specific data sample. They provide absolute numbers obtained from a sample but do not necessarily explain the rationale behind the numbers and are mostly used for analyzing single variables. The methods used in descriptive statistics include:
- Mean: This calculates the numerical average of a set of values.
- Median: This is used to get the midpoint of a set of values when the numbers are arranged in numerical order.
- Mode: This is used to find the most commonly occurring value in a dataset.
- Percentage: This is used to express how a value or group of respondents within the data relates to a larger group of respondents.
- Frequency: This indicates the number of times a value is found.
- Range: This shows the highest and lowest values in a dataset.
- Standard Deviation: This is used to indicate how dispersed a range of numbers is, meaning, it shows how close all the numbers are to the mean.
- Skewness: It indicates how symmetrical a range of numbers is, showing if they cluster into a smooth bell curve shape in the middle of the graph or if they skew towards the left or right.
2) Inferential Statistics
In quantitative analysis, the expectation is to turn raw numbers into meaningful insight using numerical values, and descriptive statistics is all about explaining details of a specific dataset using numbers, but it does not explain the motives behind the numbers; hence, a need for further analysis using inferential statistics.
Inferential statistics aim to make predictions or highlight possible outcomes from the analyzed data obtained from descriptive statistics. They are used to generalize results and make predictions between groups, show relationships that exist between multiple variables, and are used for hypothesis testing that predicts changes or differences.
There are various statistical analysis methods used within inferential statistics; a few are discussed below.
- Cross Tabulations: Cross tabulation or crosstab is used to show the relationship that exists between two variables and is often used to compare results by demographic groups. It uses a basic tabular form to draw inferences between different data sets and contains data that is mutually exclusive or has some connection with each other. Crosstabs help understand the nuances of a dataset and factors that may influence a data point.
- Regression Analysis: Regression analysis estimates the relationship between a set of variables. It shows the correlation between a dependent variable (the variable or outcome you want to measure or predict) and any number of independent variables (factors that may impact the dependent variable). Therefore, the purpose of the regression analysis is to estimate how one or more variables might affect a dependent variable to identify trends and patterns to make predictions and forecast possible future trends. There are many types of regression analysis, and the model you choose will be determined by the type of data you have for the dependent variable. The types of regression analysis include linear regression, non-linear regression, binary logistic regression, etc.
- Monte Carlo Simulation: Monte Carlo simulation, also known as the Monte Carlo method, is a computerized technique of generating models of possible outcomes and showing their probability distributions. It considers a range of possible outcomes and then tries to calculate how likely each outcome will occur. Data analysts use it to perform advanced risk analyses to help forecast future events and make decisions accordingly.
- Analysis of Variance (ANOVA): This is used to test the extent to which two or more groups differ from each other. It compares the mean of various groups and allows the analysis of multiple groups.
- Factor Analysis: A large number of variables can be reduced into a smaller number of factors using the factor analysis technique. It works on the principle that multiple separate observable variables correlate with each other because they are all associated with an underlying construct. It helps in reducing large datasets into smaller, more manageable samples.
- Cohort Analysis: Cohort analysis can be defined as a subset of behavioral analytics that operates from data taken from a given dataset. Rather than looking at all users as one unit, cohort analysis breaks down data into related groups for analysis, where these groups or cohorts usually have common characteristics or similarities within a defined period.
- MaxDiff Analysis: This is a quantitative data analysis method that is used to gauge customers’ preferences for purchase and what parameters rank higher than the others in the process.
- Cluster Analysis: Cluster analysis is a technique used to identify structures within a dataset. Cluster analysis aims to be able to sort different data points into groups that are internally similar and externally different; that is, data points within a cluster will look like each other and different from data points in other clusters.
- Time Series Analysis: This is a statistical analytic technique used to identify trends and cycles over time. It is simply the measurement of the same variables at different times, like weekly and monthly email sign-ups, to uncover trends, seasonality, and cyclic patterns. By doing this, the data analyst can forecast how variables of interest may fluctuate in the future.
- SWOT analysis: This is a quantitative data analysis method that assigns numerical values to indicate strengths, weaknesses, opportunities, and threats of an organization, product, or service to show a clearer picture of competition to foster better business strategies
How to Choose the Right Method for your Analysis?
Choosing between Descriptive Statistics or Inferential Statistics can be often confusing. You should consider the following factors before choosing the right method for your quantitative data analysis:
1. Type of Data
The first consideration in data analysis is understanding the type of data you have. Different statistical methods have specific requirements based on these data types, and using the wrong method can render results meaningless. The choice of statistical method should align with the nature and distribution of your data to ensure meaningful and accurate analysis.
2. Your Research Questions
When deciding on statistical methods, it’s crucial to align them with your specific research questions and hypotheses. The nature of your questions will influence whether descriptive statistics alone, which reveal sample attributes, are sufficient or if you need both descriptive and inferential statistics to understand group differences or relationships between variables and make population inferences.
Pros and Cons of Quantitative Data Analysis
1. Objectivity and Generalizability:
- Quantitative data analysis offers objective, numerical measurements, minimizing bias and personal interpretation.
- Results can often be generalized to larger populations, making them applicable to broader contexts.
Example: A study using quantitative data analysis to measure student test scores can objectively compare performance across different schools and demographics, leading to generalizable insights about educational strategies.
2. Precision and Efficiency:
- Statistical methods provide precise numerical results, allowing for accurate comparisons and prediction.
- Large datasets can be analyzed efficiently with the help of computer software, saving time and resources.
Example: A marketing team can use quantitative data analysis to precisely track click-through rates and conversion rates on different ad campaigns, quickly identifying the most effective strategies for maximizing customer engagement.
3. Identification of Patterns and Relationships:
- Statistical techniques reveal hidden patterns and relationships between variables that might not be apparent through observation alone.
- This can lead to new insights and understanding of complex phenomena.
Example: A medical researcher can use quantitative analysis to pinpoint correlations between lifestyle factors and disease risk, aiding in the development of prevention strategies.
1. Limited Scope:
- Quantitative analysis focuses on quantifiable aspects of a phenomenon , potentially overlooking important qualitative nuances, such as emotions, motivations, or cultural contexts.
Example: A survey measuring customer satisfaction with numerical ratings might miss key insights about the underlying reasons for their satisfaction or dissatisfaction, which could be better captured through open-ended feedback.
2. Oversimplification:
- Reducing complex phenomena to numerical data can lead to oversimplification and a loss of richness in understanding.
Example: Analyzing employee productivity solely through quantitative metrics like hours worked or tasks completed might not account for factors like creativity, collaboration, or problem-solving skills, which are crucial for overall performance.
3. Potential for Misinterpretation:
- Statistical results can be misinterpreted if not analyzed carefully and with appropriate expertise.
- The choice of statistical methods and assumptions can significantly influence results.
This blog discusses the steps, methods, and techniques of quantitative data analysis. It also gives insights into the methods of data collection, the type of data one should work with, and the pros and cons of such analysis.
Gain a better understanding of data analysis with these essential reads:
- Data Analysis and Modeling: 4 Critical Differences
- Exploratory Data Analysis Simplified 101
- 25 Best Data Analysis Tools in 2024
Carrying out successful data analysis requires prepping the data and making it analysis-ready. That is where Hevo steps in.
Want to give Hevo a try? Sign Up for a 14-day free trial and experience the feature-rich Hevo suite first hand. You may also have a look at the amazing Hevo price , which will assist you in selecting the best plan for your requirements.
Share your experience of understanding Quantitative Data Analysis in the comment section below! We would love to hear your thoughts.
Ofem is a freelance writer specializing in data-related topics, who has expertise in translating complex concepts. With a focus on data science, analytics, and emerging technologies.
No-code Data Pipeline for your Data Warehouse
- Data Analysis
- Data Warehouse
- Quantitative Data Analysis
Continue Reading
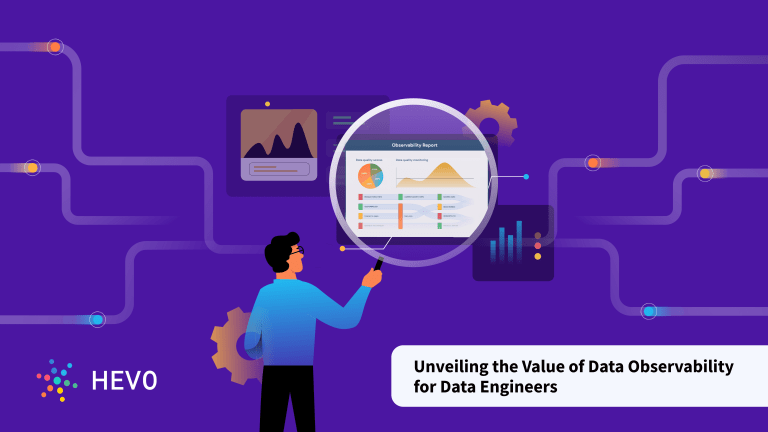
Kyle Kirwan
The Data Engineer’s Crystal Ball: How Data Observability Helps You See What’s Coming
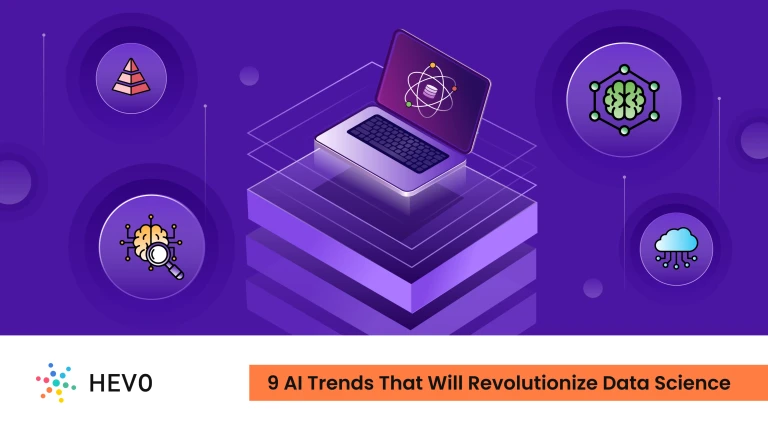
Shane Barker
9 AI Trends That Will Revolutionize Data Science
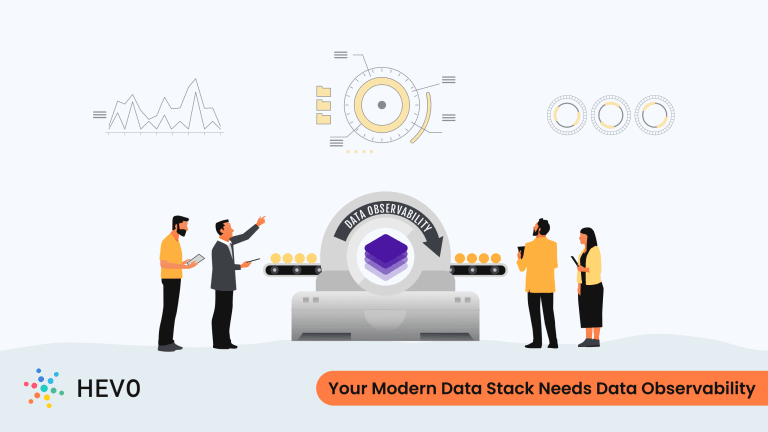
Loretta Jones
Data ‘Poka-Yoking’ With Data Observability for the Modern Data Stack
I want to read this e-book.
Have a language expert improve your writing
Run a free plagiarism check in 10 minutes, automatically generate references for free.
- Knowledge Base
- Methodology
- What Is Quantitative Research? | Definition & Methods
What Is Quantitative Research? | Definition & Methods
Published on 4 April 2022 by Pritha Bhandari . Revised on 10 October 2022.
Quantitative research is the process of collecting and analysing numerical data. It can be used to find patterns and averages, make predictions, test causal relationships, and generalise results to wider populations.
Quantitative research is the opposite of qualitative research , which involves collecting and analysing non-numerical data (e.g. text, video, or audio).
Quantitative research is widely used in the natural and social sciences: biology, chemistry, psychology, economics, sociology, marketing, etc.
- What is the demographic makeup of Singapore in 2020?
- How has the average temperature changed globally over the last century?
- Does environmental pollution affect the prevalence of honey bees?
- Does working from home increase productivity for people with long commutes?
Table of contents
Quantitative research methods, quantitative data analysis, advantages of quantitative research, disadvantages of quantitative research, frequently asked questions about quantitative research.
You can use quantitative research methods for descriptive, correlational or experimental research.
- In descriptive research , you simply seek an overall summary of your study variables.
- In correlational research , you investigate relationships between your study variables.
- In experimental research , you systematically examine whether there is a cause-and-effect relationship between variables.
Correlational and experimental research can both be used to formally test hypotheses , or predictions, using statistics. The results may be generalised to broader populations based on the sampling method used.
To collect quantitative data, you will often need to use operational definitions that translate abstract concepts (e.g., mood) into observable and quantifiable measures (e.g., self-ratings of feelings and energy levels).
Prevent plagiarism, run a free check.
Once data is collected, you may need to process it before it can be analysed. For example, survey and test data may need to be transformed from words to numbers. Then, you can use statistical analysis to answer your research questions .
Descriptive statistics will give you a summary of your data and include measures of averages and variability. You can also use graphs, scatter plots and frequency tables to visualise your data and check for any trends or outliers.
Using inferential statistics , you can make predictions or generalisations based on your data. You can test your hypothesis or use your sample data to estimate the population parameter .
You can also assess the reliability and validity of your data collection methods to indicate how consistently and accurately your methods actually measured what you wanted them to.
Quantitative research is often used to standardise data collection and generalise findings . Strengths of this approach include:
- Replication
Repeating the study is possible because of standardised data collection protocols and tangible definitions of abstract concepts.
- Direct comparisons of results
The study can be reproduced in other cultural settings, times or with different groups of participants. Results can be compared statistically.
- Large samples
Data from large samples can be processed and analysed using reliable and consistent procedures through quantitative data analysis.
- Hypothesis testing
Using formalised and established hypothesis testing procedures means that you have to carefully consider and report your research variables, predictions, data collection and testing methods before coming to a conclusion.
Despite the benefits of quantitative research, it is sometimes inadequate in explaining complex research topics. Its limitations include:
- Superficiality
Using precise and restrictive operational definitions may inadequately represent complex concepts. For example, the concept of mood may be represented with just a number in quantitative research, but explained with elaboration in qualitative research.
- Narrow focus
Predetermined variables and measurement procedures can mean that you ignore other relevant observations.
- Structural bias
Despite standardised procedures, structural biases can still affect quantitative research. Missing data , imprecise measurements or inappropriate sampling methods are biases that can lead to the wrong conclusions.
- Lack of context
Quantitative research often uses unnatural settings like laboratories or fails to consider historical and cultural contexts that may affect data collection and results.
Quantitative research deals with numbers and statistics, while qualitative research deals with words and meanings.
Quantitative methods allow you to test a hypothesis by systematically collecting and analysing data, while qualitative methods allow you to explore ideas and experiences in depth.
In mixed methods research , you use both qualitative and quantitative data collection and analysis methods to answer your research question .
Data collection is the systematic process by which observations or measurements are gathered in research. It is used in many different contexts by academics, governments, businesses, and other organisations.
Operationalisation means turning abstract conceptual ideas into measurable observations.
For example, the concept of social anxiety isn’t directly observable, but it can be operationally defined in terms of self-rating scores, behavioural avoidance of crowded places, or physical anxiety symptoms in social situations.
Before collecting data , it’s important to consider how you will operationalise the variables that you want to measure.
Reliability and validity are both about how well a method measures something:
- Reliability refers to the consistency of a measure (whether the results can be reproduced under the same conditions).
- Validity refers to the accuracy of a measure (whether the results really do represent what they are supposed to measure).
If you are doing experimental research , you also have to consider the internal and external validity of your experiment.
Hypothesis testing is a formal procedure for investigating our ideas about the world using statistics. It is used by scientists to test specific predictions, called hypotheses , by calculating how likely it is that a pattern or relationship between variables could have arisen by chance.
Cite this Scribbr article
If you want to cite this source, you can copy and paste the citation or click the ‘Cite this Scribbr article’ button to automatically add the citation to our free Reference Generator.
Bhandari, P. (2022, October 10). What Is Quantitative Research? | Definition & Methods. Scribbr. Retrieved 20 March 2024, from https://www.scribbr.co.uk/research-methods/introduction-to-quantitative-research/
Is this article helpful?
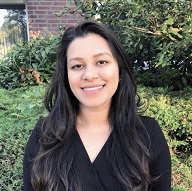
Pritha Bhandari
No internet connection.
All search filters on the page have been cleared., your search has been saved..
- All content
- Dictionaries
- Encyclopedias
- Expert Insights
- Foundations
- How-to Guides
- Journal Articles
- Little Blue Books
- Little Green Books
- Project Planner
- Tools Directory
- Sign in to my profile My Profile
- Sign in Signed in
- My profile My Profile
- Offline Playback link
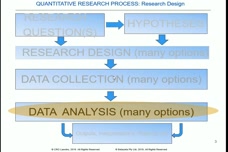
Have you created a personal profile? sign in or create a profile so that you can create alerts, save clips, playlists and searches.
Introduction to the Main Groups of Quantitative Data Analysis Methods
- Watching now: Chapter 1: Data Analysis Techniques Start time: 00:00:00 End time: 00:04:33
Video Type: Tutorial
(Academic). (2021). Introduction to the main groups of quantitative data analysis methods [Video]. Sage Research Methods. https:// doi. org/10.4135/9781529695342
"Introduction to the Main Groups of Quantitative Data Analysis Methods." In Sage Video . : Betazeta Pty Ltd ATF Lawoko Holdings Trust, 2021. Video, 00:04:33. https:// doi. org/10.4135/9781529695342.
, 2021. Introduction to the Main Groups of Quantitative Data Analysis Methods , Sage Video. [Streaming Video] London: Sage Publications Ltd. Available at: <https:// doi. org/10.4135/9781529695342 & gt; [Accessed 24 Mar 2024].
Introduction to the Main Groups of Quantitative Data Analysis Methods . Online video clip. SAGE Video. London: SAGE Publications, Ltd., 30 Jan 2024. doi: https:// doi. org/10.4135/9781529695342. 24 Mar 2024.
Introduction to the Main Groups of Quantitative Data Analysis Methods [Streaming video]. 2021. doi:10.4135/9781529695342. Accessed 03/24/2024
Please log in from an authenticated institution or log into your member profile to access the email feature.
- Sign in/register
Add this content to your learning management system or webpage by copying the code below into the HTML editor on the page. Look for the words HTML or </>. Learn More about Embedding Video icon link (opens in new window)
Sample View:
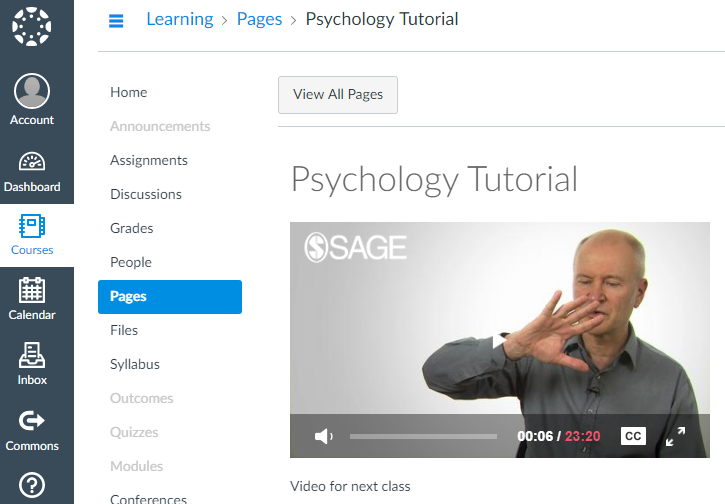
- Download PDF opens in new window
- icon/tools/download-video icon/tools/video-downloaded Download video Downloading... Video downloaded
Dr. Charles Lawoko provides a high-level overview of four common groups of data analysis techniques in quantitative research: exploratory data analysis, dependence techniques, interdependence techniques, and prediction techniques.
Chapter 1: Data Analysis Techniques
- Start time: 00:00:00
- End time: 00:04:33
- Product: Sage Research Methods Video: Quantitative and Mixed Methods
- Type of Content: Tutorial
- Title: Introduction to the Main Groups of Quantitative Data Analysis Methods
- Publisher: Betazeta Pty Ltd ATF Lawoko Holdings Trust
- Series: Introduction to Quantitative Research Methods
- Publication year: 2021
- Online pub date: January 30, 2024
- Discipline: Business and Management , Political Science and International Relations , Social Work , Communication and Media Studies , Nursing , Geography , History , Counseling and Psychotherapy , Mathematics , Criminology and Criminal Justice , Medicine , Dentistry , Public Health , Psychology , Health , Economics , Social Policy and Public Policy , Technology , Education , Computer Science , Engineering , Anthropology , Science , Sociology , Marketing
- Methods: Quantitative data analysis
- Duration: 00:04:33
- DOI: https:// doi. org/10.4135/9781529695342
- Keywords: dependence , descriptive statistics , exploratory data analysis , interdependence , prediction (methodology) Show all Show less
Sign in to access this content
Get a 30 day free trial, more like this, sage recommends.
We found other relevant content for you on other Sage platforms.
Have you created a personal profile? Login or create a profile so that you can save clips, playlists and searches
Navigating away from this page will delete your results
Please save your results to "My Self-Assessments" in your profile before navigating away from this page.
Sign in to my profile
Sign up for a free trial and experience all Sage Learning Resources have to offer.
You must have a valid academic email address to sign up.
Get off-campus access
- View or download all content my institution has access to.
Sign up for a free trial and experience all Sage Research Methods has to offer.
- view my profile
- view my lists
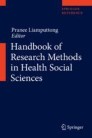
Handbook of Research Methods in Health Social Sciences pp 27–49 Cite as
Quantitative Research
- Leigh A. Wilson 2 , 3
- Reference work entry
- First Online: 13 January 2019
3995 Accesses
4 Citations
Quantitative research methods are concerned with the planning, design, and implementation of strategies to collect and analyze data. Descartes, the seventeenth-century philosopher, suggested that how the results are achieved is often more important than the results themselves, as the journey taken along the research path is a journey of discovery. High-quality quantitative research is characterized by the attention given to the methods and the reliability of the tools used to collect the data. The ability to critique research in a systematic way is an essential component of a health professional’s role in order to deliver high quality, evidence-based healthcare. This chapter is intended to provide a simple overview of the way new researchers and health practitioners can understand and employ quantitative methods. The chapter offers practical, realistic guidance in a learner-friendly way and uses a logical sequence to understand the process of hypothesis development, study design, data collection and handling, and finally data analysis and interpretation.
- Quantitative
- Epidemiology
- Data analysis
- Methodology
- Interpretation
This is a preview of subscription content, log in via an institution .
Buying options
- Available as PDF
- Read on any device
- Instant download
- Own it forever
- Available as EPUB and PDF
- Durable hardcover edition
- Dispatched in 3 to 5 business days
- Free shipping worldwide - see info
Tax calculation will be finalised at checkout
Purchases are for personal use only
Babbie ER. The practice of social research. 14th ed. Belmont: Wadsworth Cengage; 2016.
Google Scholar
Descartes. Cited in Halverston, W. (1976). In: A concise introduction to philosophy, 3rd ed. New York: Random House; 1637.
Doll R, Hill AB. The mortality of doctors in relation to their smoking habits. BMJ. 1954;328(7455):1529–33. https://doi.org/10.1136/bmj.328.7455.1529 .
Article Google Scholar
Liamputtong P. Research methods in health: foundations for evidence-based practice. 3rd ed. Melbourne: Oxford University Press; 2017.
McNabb DE. Research methods in public administration and nonprofit management: quantitative and qualitative approaches. 2nd ed. New York: Armonk; 2007.
Merriam-Webster. Dictionary. http://www.merriam-webster.com . Accessed 20th December 2017.
Olesen Larsen P, von Ins M. The rate of growth in scientific publication and the decline in coverage provided by Science Citation Index. Scientometrics. 2010;84(3):575–603.
Pannucci CJ, Wilkins EG. Identifying and avoiding bias in research. Plast Reconstr Surg. 2010;126(2):619–25. https://doi.org/10.1097/PRS.0b013e3181de24bc .
Petrie A, Sabin C. Medical statistics at a glance. 2nd ed. London: Blackwell Publishing; 2005.
Portney LG, Watkins MP. Foundations of clinical research: applications to practice. 3rd ed. New Jersey: Pearson Publishing; 2009.
Sheehan J. Aspects of research methodology. Nurse Educ Today. 1986;6:193–203.
Wilson LA, Black DA. Health, science research and research methods. Sydney: McGraw Hill; 2013.
Download references
Author information
Authors and affiliations.
School of Science and Health, Western Sydney University, Penrith, NSW, Australia
Leigh A. Wilson
Faculty of Health Science, Discipline of Behavioural and Social Sciences in Health, University of Sydney, Lidcombe, NSW, Australia
You can also search for this author in PubMed Google Scholar
Corresponding author
Correspondence to Leigh A. Wilson .
Editor information
Editors and affiliations.
Pranee Liamputtong
Rights and permissions
Reprints and permissions
Copyright information
© 2019 Springer Nature Singapore Pte Ltd.
About this entry
Cite this entry.
Wilson, L.A. (2019). Quantitative Research. In: Liamputtong, P. (eds) Handbook of Research Methods in Health Social Sciences. Springer, Singapore. https://doi.org/10.1007/978-981-10-5251-4_54
Download citation
DOI : https://doi.org/10.1007/978-981-10-5251-4_54
Published : 13 January 2019
Publisher Name : Springer, Singapore
Print ISBN : 978-981-10-5250-7
Online ISBN : 978-981-10-5251-4
eBook Packages : Social Sciences Reference Module Humanities and Social Sciences Reference Module Business, Economics and Social Sciences
Share this entry
Anyone you share the following link with will be able to read this content:
Sorry, a shareable link is not currently available for this article.
Provided by the Springer Nature SharedIt content-sharing initiative
- Publish with us
Policies and ethics
- Find a journal
- Track your research
- Skip to main content
- Skip to primary sidebar
- Skip to footer
- QuestionPro

- Solutions Industries Gaming Automotive Sports and events Education Government Travel & Hospitality Financial Services Healthcare Cannabis Technology Use Case NPS+ Communities Audience Contactless surveys Mobile LivePolls Member Experience GDPR Positive People Science 360 Feedback Surveys
- Resources Blog eBooks Survey Templates Case Studies Training Help center

Home Market Research
Data Analysis in Research: Types & Methods
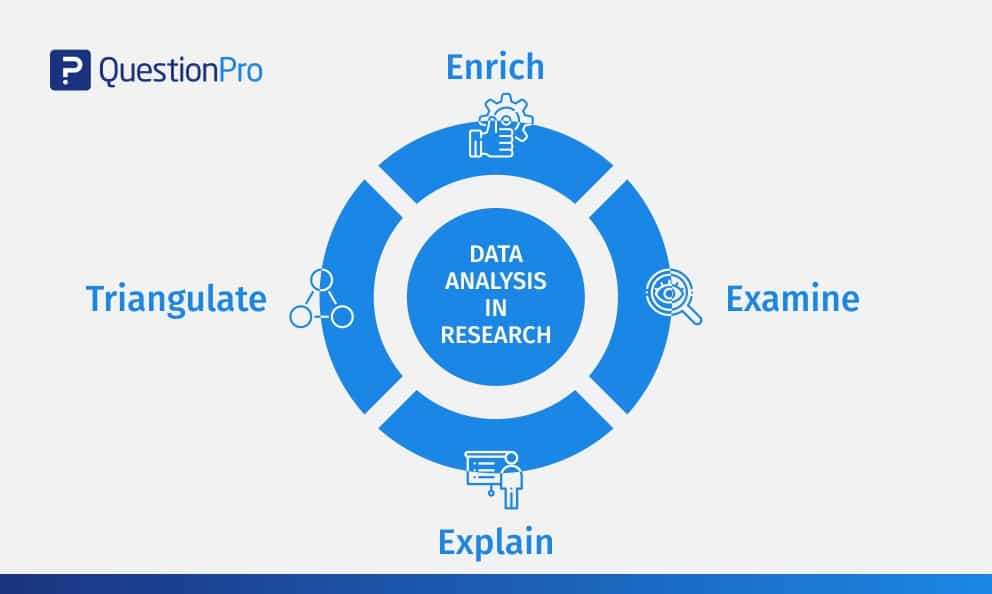
Content Index
Why analyze data in research?
Types of data in research, finding patterns in the qualitative data, methods used for data analysis in qualitative research, preparing data for analysis, methods used for data analysis in quantitative research, considerations in research data analysis, what is data analysis in research.
Definition of research in data analysis: According to LeCompte and Schensul, research data analysis is a process used by researchers to reduce data to a story and interpret it to derive insights. The data analysis process helps reduce a large chunk of data into smaller fragments, which makes sense.
Three essential things occur during the data analysis process — the first is data organization . Summarization and categorization together contribute to becoming the second known method used for data reduction. It helps find patterns and themes in the data for easy identification and linking. The third and last way is data analysis – researchers do it in both top-down and bottom-up fashion.
LEARN ABOUT: Research Process Steps
On the other hand, Marshall and Rossman describe data analysis as a messy, ambiguous, and time-consuming but creative and fascinating process through which a mass of collected data is brought to order, structure and meaning.
We can say that “the data analysis and data interpretation is a process representing the application of deductive and inductive logic to the research and data analysis.”
Researchers rely heavily on data as they have a story to tell or research problems to solve. It starts with a question, and data is nothing but an answer to that question. But, what if there is no question to ask? Well! It is possible to explore data even without a problem – we call it ‘Data Mining’, which often reveals some interesting patterns within the data that are worth exploring.
Irrelevant to the type of data researchers explore, their mission and audiences’ vision guide them to find the patterns to shape the story they want to tell. One of the essential things expected from researchers while analyzing data is to stay open and remain unbiased toward unexpected patterns, expressions, and results. Remember, sometimes, data analysis tells the most unforeseen yet exciting stories that were not expected when initiating data analysis. Therefore, rely on the data you have at hand and enjoy the journey of exploratory research.
Create a Free Account
Every kind of data has a rare quality of describing things after assigning a specific value to it. For analysis, you need to organize these values, processed and presented in a given context, to make it useful. Data can be in different forms; here are the primary data types.
- Qualitative data: When the data presented has words and descriptions, then we call it qualitative data . Although you can observe this data, it is subjective and harder to analyze data in research, especially for comparison. Example: Quality data represents everything describing taste, experience, texture, or an opinion that is considered quality data. This type of data is usually collected through focus groups, personal qualitative interviews , qualitative observation or using open-ended questions in surveys.
- Quantitative data: Any data expressed in numbers of numerical figures are called quantitative data . This type of data can be distinguished into categories, grouped, measured, calculated, or ranked. Example: questions such as age, rank, cost, length, weight, scores, etc. everything comes under this type of data. You can present such data in graphical format, charts, or apply statistical analysis methods to this data. The (Outcomes Measurement Systems) OMS questionnaires in surveys are a significant source of collecting numeric data.
- Categorical data: It is data presented in groups. However, an item included in the categorical data cannot belong to more than one group. Example: A person responding to a survey by telling his living style, marital status, smoking habit, or drinking habit comes under the categorical data. A chi-square test is a standard method used to analyze this data.
Learn More : Examples of Qualitative Data in Education
Data analysis in qualitative research
Data analysis and qualitative data research work a little differently from the numerical data as the quality data is made up of words, descriptions, images, objects, and sometimes symbols. Getting insight from such complicated information is a complicated process. Hence it is typically used for exploratory research and data analysis .
Although there are several ways to find patterns in the textual information, a word-based method is the most relied and widely used global technique for research and data analysis. Notably, the data analysis process in qualitative research is manual. Here the researchers usually read the available data and find repetitive or commonly used words.
For example, while studying data collected from African countries to understand the most pressing issues people face, researchers might find “food” and “hunger” are the most commonly used words and will highlight them for further analysis.
LEARN ABOUT: Level of Analysis
The keyword context is another widely used word-based technique. In this method, the researcher tries to understand the concept by analyzing the context in which the participants use a particular keyword.
For example , researchers conducting research and data analysis for studying the concept of ‘diabetes’ amongst respondents might analyze the context of when and how the respondent has used or referred to the word ‘diabetes.’
The scrutiny-based technique is also one of the highly recommended text analysis methods used to identify a quality data pattern. Compare and contrast is the widely used method under this technique to differentiate how a specific text is similar or different from each other.
For example: To find out the “importance of resident doctor in a company,” the collected data is divided into people who think it is necessary to hire a resident doctor and those who think it is unnecessary. Compare and contrast is the best method that can be used to analyze the polls having single-answer questions types .
Metaphors can be used to reduce the data pile and find patterns in it so that it becomes easier to connect data with theory.
Variable Partitioning is another technique used to split variables so that researchers can find more coherent descriptions and explanations from the enormous data.
LEARN ABOUT: Qualitative Research Questions and Questionnaires
There are several techniques to analyze the data in qualitative research, but here are some commonly used methods,
- Content Analysis: It is widely accepted and the most frequently employed technique for data analysis in research methodology. It can be used to analyze the documented information from text, images, and sometimes from the physical items. It depends on the research questions to predict when and where to use this method.
- Narrative Analysis: This method is used to analyze content gathered from various sources such as personal interviews, field observation, and surveys . The majority of times, stories, or opinions shared by people are focused on finding answers to the research questions.
- Discourse Analysis: Similar to narrative analysis, discourse analysis is used to analyze the interactions with people. Nevertheless, this particular method considers the social context under which or within which the communication between the researcher and respondent takes place. In addition to that, discourse analysis also focuses on the lifestyle and day-to-day environment while deriving any conclusion.
- Grounded Theory: When you want to explain why a particular phenomenon happened, then using grounded theory for analyzing quality data is the best resort. Grounded theory is applied to study data about the host of similar cases occurring in different settings. When researchers are using this method, they might alter explanations or produce new ones until they arrive at some conclusion.
LEARN ABOUT: 12 Best Tools for Researchers
Data analysis in quantitative research
The first stage in research and data analysis is to make it for the analysis so that the nominal data can be converted into something meaningful. Data preparation consists of the below phases.
Phase I: Data Validation
Data validation is done to understand if the collected data sample is per the pre-set standards, or it is a biased data sample again divided into four different stages
- Fraud: To ensure an actual human being records each response to the survey or the questionnaire
- Screening: To make sure each participant or respondent is selected or chosen in compliance with the research criteria
- Procedure: To ensure ethical standards were maintained while collecting the data sample
- Completeness: To ensure that the respondent has answered all the questions in an online survey. Else, the interviewer had asked all the questions devised in the questionnaire.
Phase II: Data Editing
More often, an extensive research data sample comes loaded with errors. Respondents sometimes fill in some fields incorrectly or sometimes skip them accidentally. Data editing is a process wherein the researchers have to confirm that the provided data is free of such errors. They need to conduct necessary checks and outlier checks to edit the raw edit and make it ready for analysis.
Phase III: Data Coding
Out of all three, this is the most critical phase of data preparation associated with grouping and assigning values to the survey responses . If a survey is completed with a 1000 sample size, the researcher will create an age bracket to distinguish the respondents based on their age. Thus, it becomes easier to analyze small data buckets rather than deal with the massive data pile.
LEARN ABOUT: Steps in Qualitative Research
After the data is prepared for analysis, researchers are open to using different research and data analysis methods to derive meaningful insights. For sure, statistical analysis plans are the most favored to analyze numerical data. In statistical analysis, distinguishing between categorical data and numerical data is essential, as categorical data involves distinct categories or labels, while numerical data consists of measurable quantities. The method is again classified into two groups. First, ‘Descriptive Statistics’ used to describe data. Second, ‘Inferential statistics’ that helps in comparing the data .
Descriptive statistics
This method is used to describe the basic features of versatile types of data in research. It presents the data in such a meaningful way that pattern in the data starts making sense. Nevertheless, the descriptive analysis does not go beyond making conclusions. The conclusions are again based on the hypothesis researchers have formulated so far. Here are a few major types of descriptive analysis methods.
Measures of Frequency
- Count, Percent, Frequency
- It is used to denote home often a particular event occurs.
- Researchers use it when they want to showcase how often a response is given.
Measures of Central Tendency
- Mean, Median, Mode
- The method is widely used to demonstrate distribution by various points.
- Researchers use this method when they want to showcase the most commonly or averagely indicated response.
Measures of Dispersion or Variation
- Range, Variance, Standard deviation
- Here the field equals high/low points.
- Variance standard deviation = difference between the observed score and mean
- It is used to identify the spread of scores by stating intervals.
- Researchers use this method to showcase data spread out. It helps them identify the depth until which the data is spread out that it directly affects the mean.
Measures of Position
- Percentile ranks, Quartile ranks
- It relies on standardized scores helping researchers to identify the relationship between different scores.
- It is often used when researchers want to compare scores with the average count.
For quantitative research use of descriptive analysis often give absolute numbers, but the in-depth analysis is never sufficient to demonstrate the rationale behind those numbers. Nevertheless, it is necessary to think of the best method for research and data analysis suiting your survey questionnaire and what story researchers want to tell. For example, the mean is the best way to demonstrate the students’ average scores in schools. It is better to rely on the descriptive statistics when the researchers intend to keep the research or outcome limited to the provided sample without generalizing it. For example, when you want to compare average voting done in two different cities, differential statistics are enough.
Descriptive analysis is also called a ‘univariate analysis’ since it is commonly used to analyze a single variable.
Inferential statistics
Inferential statistics are used to make predictions about a larger population after research and data analysis of the representing population’s collected sample. For example, you can ask some odd 100 audiences at a movie theater if they like the movie they are watching. Researchers then use inferential statistics on the collected sample to reason that about 80-90% of people like the movie.
Here are two significant areas of inferential statistics.
- Estimating parameters: It takes statistics from the sample research data and demonstrates something about the population parameter.
- Hypothesis test: I t’s about sampling research data to answer the survey research questions. For example, researchers might be interested to understand if the new shade of lipstick recently launched is good or not, or if the multivitamin capsules help children to perform better at games.
These are sophisticated analysis methods used to showcase the relationship between different variables instead of describing a single variable. It is often used when researchers want something beyond absolute numbers to understand the relationship between variables.
Here are some of the commonly used methods for data analysis in research.
- Correlation: When researchers are not conducting experimental research or quasi-experimental research wherein the researchers are interested to understand the relationship between two or more variables, they opt for correlational research methods.
- Cross-tabulation: Also called contingency tables, cross-tabulation is used to analyze the relationship between multiple variables. Suppose provided data has age and gender categories presented in rows and columns. A two-dimensional cross-tabulation helps for seamless data analysis and research by showing the number of males and females in each age category.
- Regression analysis: For understanding the strong relationship between two variables, researchers do not look beyond the primary and commonly used regression analysis method, which is also a type of predictive analysis used. In this method, you have an essential factor called the dependent variable. You also have multiple independent variables in regression analysis. You undertake efforts to find out the impact of independent variables on the dependent variable. The values of both independent and dependent variables are assumed as being ascertained in an error-free random manner.
- Frequency tables: The statistical procedure is used for testing the degree to which two or more vary or differ in an experiment. A considerable degree of variation means research findings were significant. In many contexts, ANOVA testing and variance analysis are similar.
- Analysis of variance: The statistical procedure is used for testing the degree to which two or more vary or differ in an experiment. A considerable degree of variation means research findings were significant. In many contexts, ANOVA testing and variance analysis are similar.
- Researchers must have the necessary research skills to analyze and manipulation the data , Getting trained to demonstrate a high standard of research practice. Ideally, researchers must possess more than a basic understanding of the rationale of selecting one statistical method over the other to obtain better data insights.
- Usually, research and data analytics projects differ by scientific discipline; therefore, getting statistical advice at the beginning of analysis helps design a survey questionnaire, select data collection methods, and choose samples.
LEARN ABOUT: Best Data Collection Tools
- The primary aim of data research and analysis is to derive ultimate insights that are unbiased. Any mistake in or keeping a biased mind to collect data, selecting an analysis method, or choosing audience sample il to draw a biased inference.
- Irrelevant to the sophistication used in research data and analysis is enough to rectify the poorly defined objective outcome measurements. It does not matter if the design is at fault or intentions are not clear, but lack of clarity might mislead readers, so avoid the practice.
- The motive behind data analysis in research is to present accurate and reliable data. As far as possible, avoid statistical errors, and find a way to deal with everyday challenges like outliers, missing data, data altering, data mining , or developing graphical representation.
LEARN MORE: Descriptive Research vs Correlational Research The sheer amount of data generated daily is frightening. Especially when data analysis has taken center stage. in 2018. In last year, the total data supply amounted to 2.8 trillion gigabytes. Hence, it is clear that the enterprises willing to survive in the hypercompetitive world must possess an excellent capability to analyze complex research data, derive actionable insights, and adapt to the new market needs.
LEARN ABOUT: Average Order Value
QuestionPro is an online survey platform that empowers organizations in data analysis and research and provides them a medium to collect data by creating appealing surveys.
MORE LIKE THIS
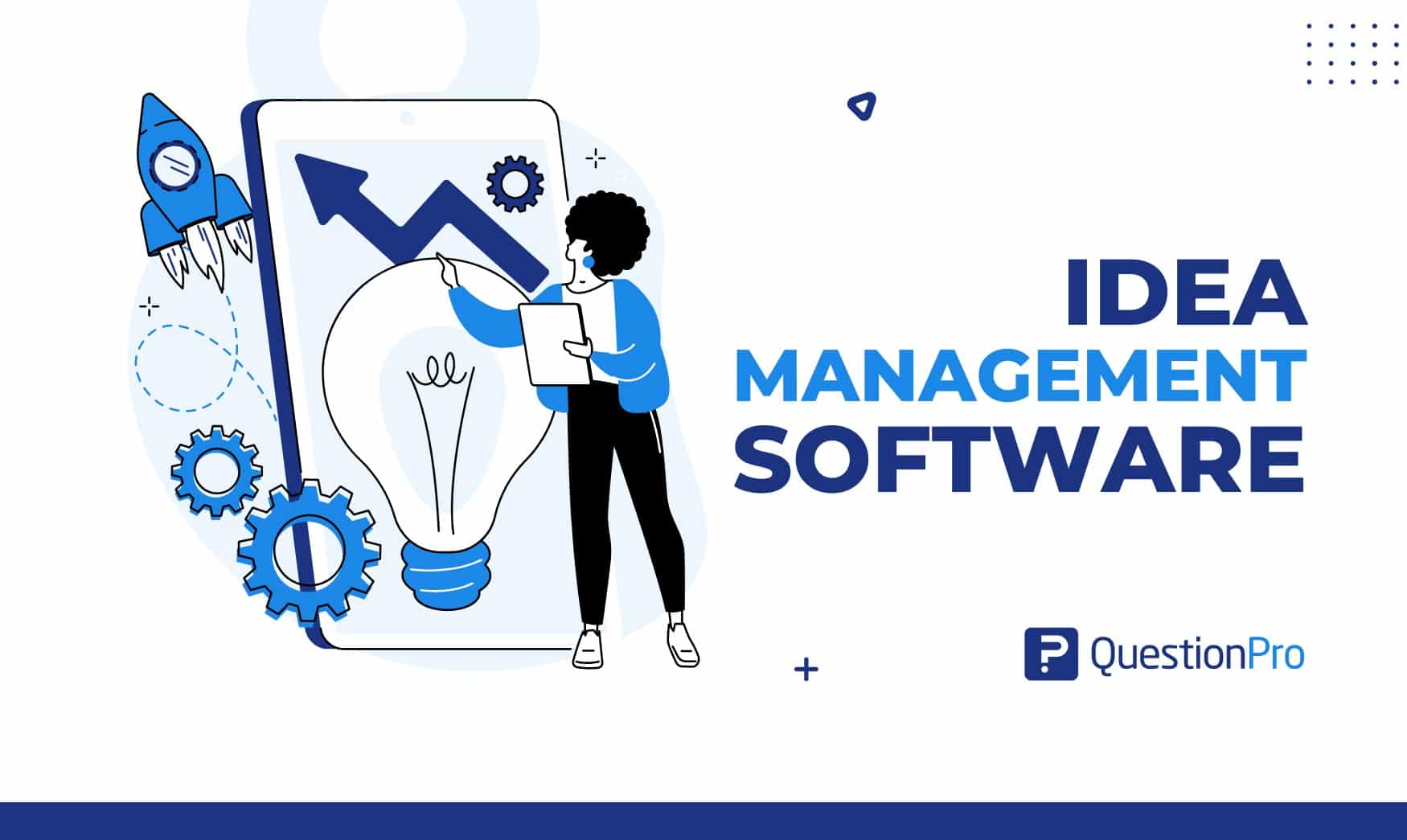
Unlocking Creativity With 10 Top Idea Management Software
Mar 23, 2024
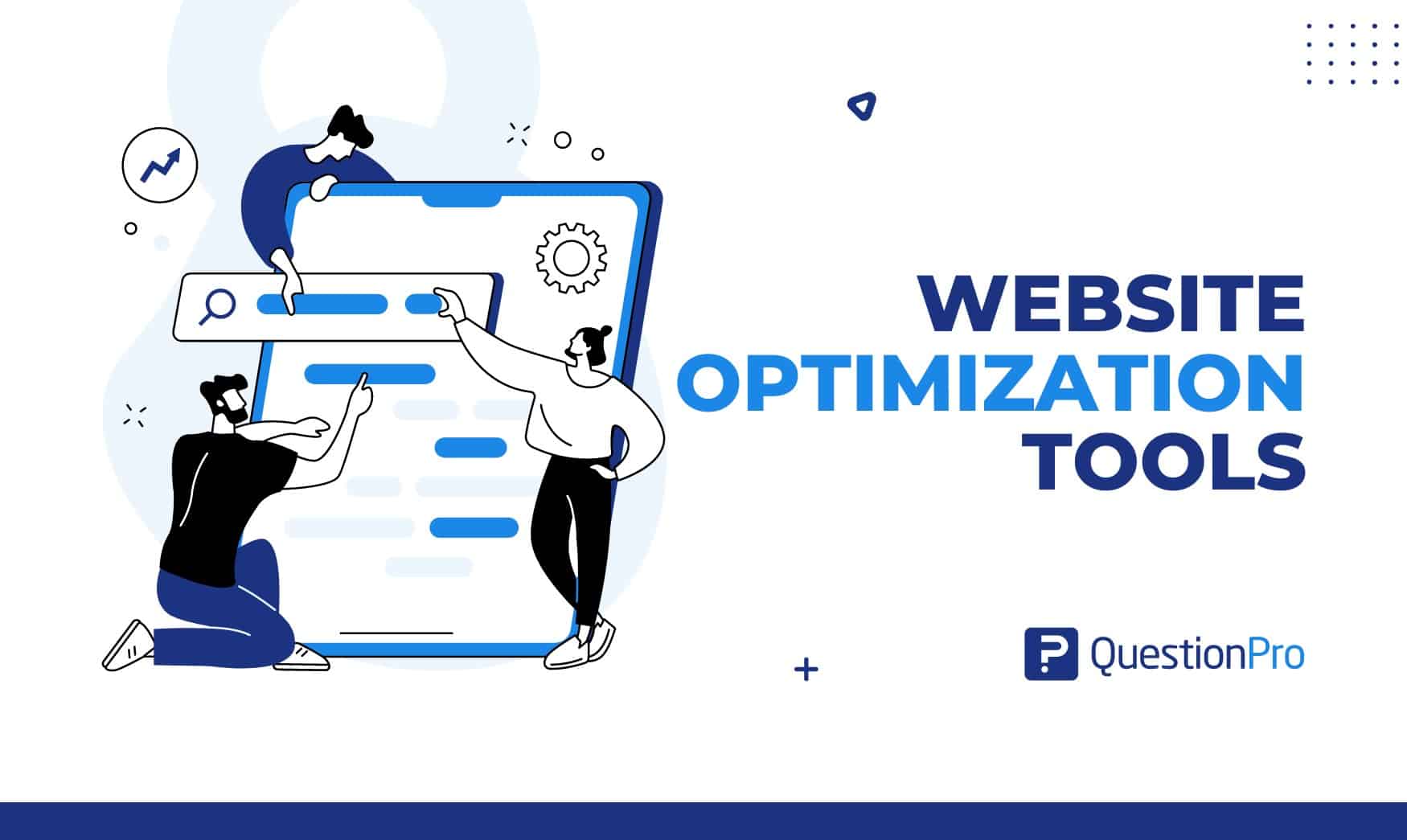
20 Best Website Optimization Tools to Improve Your Website
Mar 22, 2024
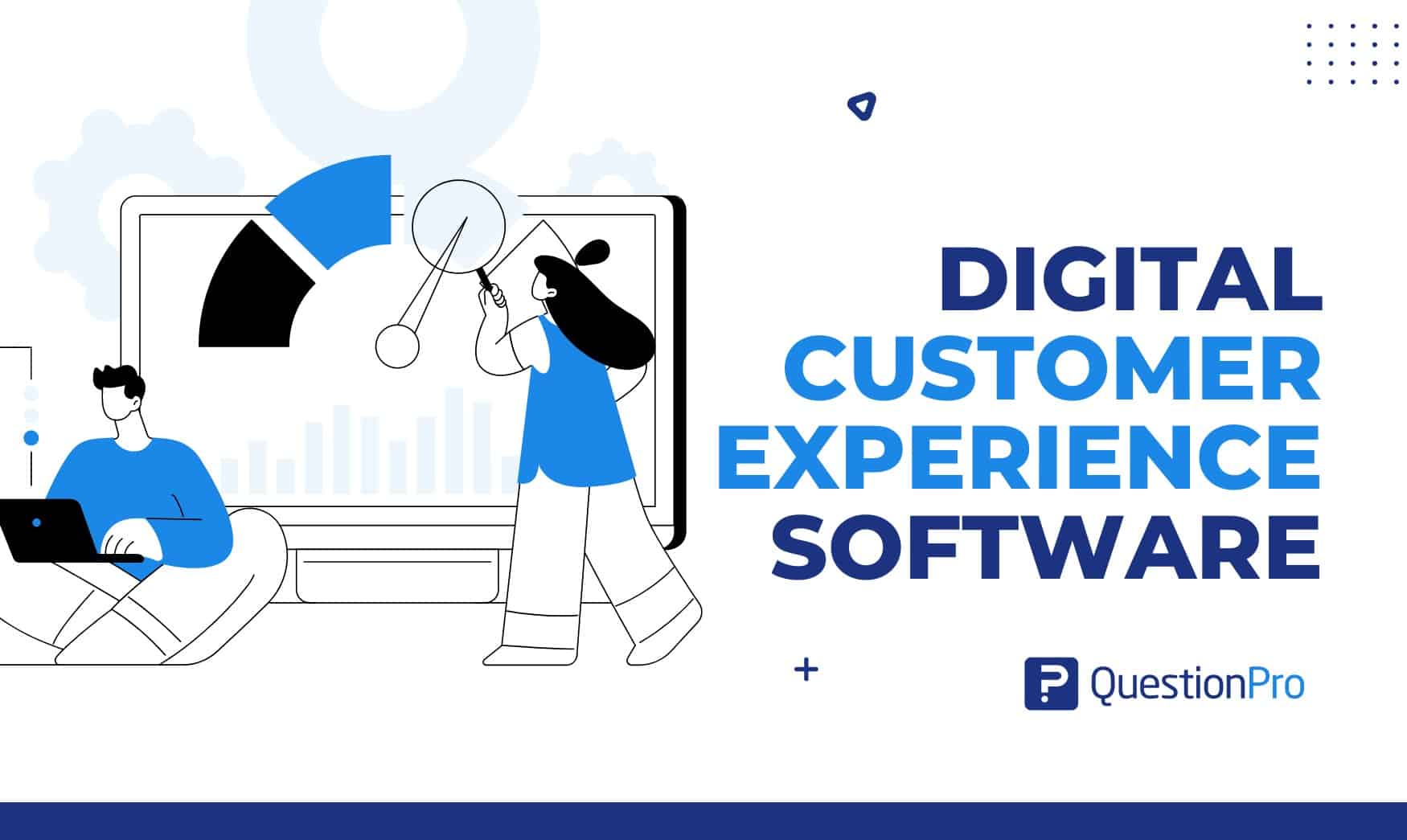
15 Best Digital Customer Experience Software of 2024
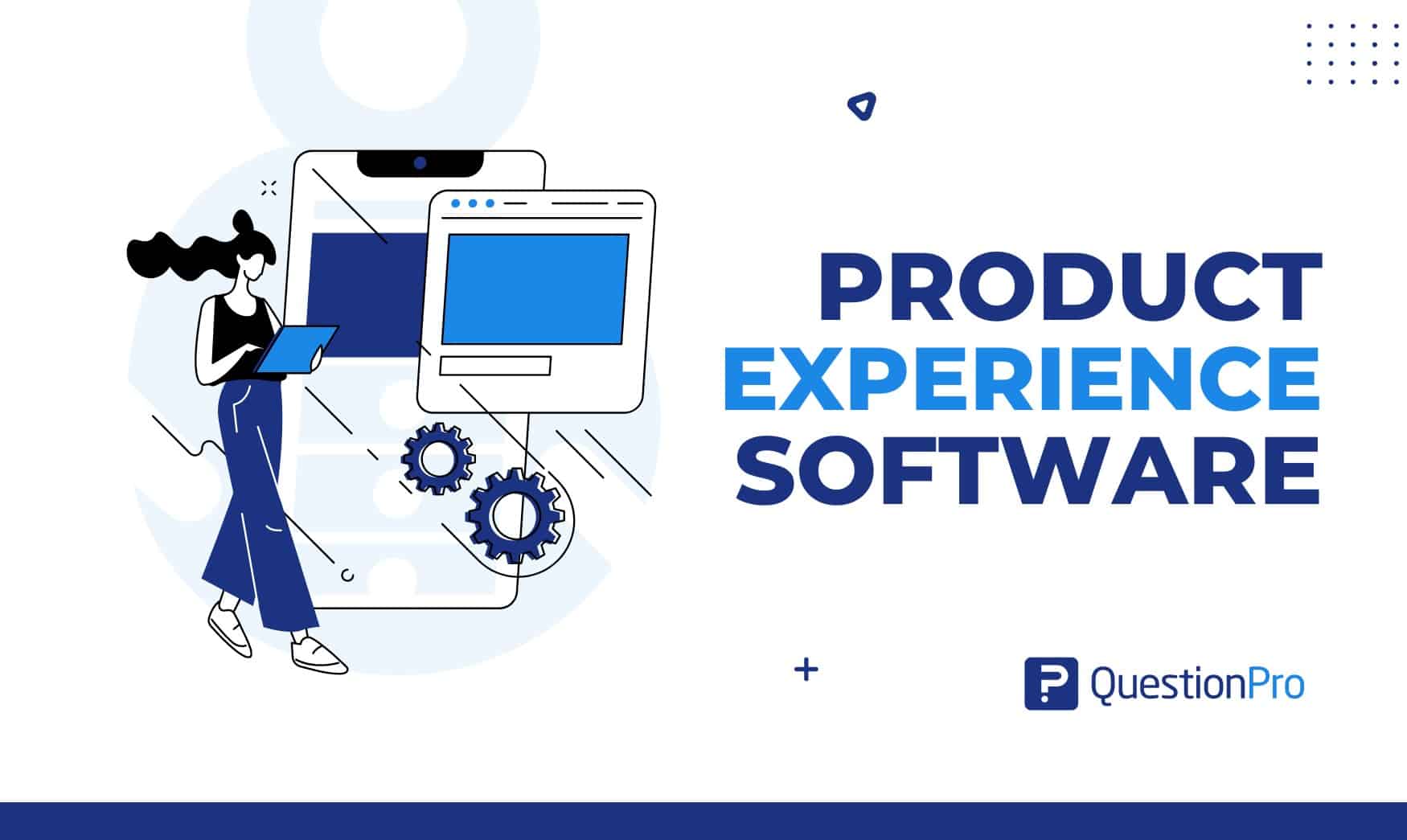
15 Best Product Experience Software of 2024
Other categories.
- Academic Research
- Artificial Intelligence
- Assessments
- Brand Awareness
- Case Studies
- Communities
- Consumer Insights
- Customer effort score
- Customer Engagement
- Customer Experience
- Customer Loyalty
- Customer Research
- Customer Satisfaction
- Employee Benefits
- Employee Engagement
- Employee Retention
- Friday Five
- General Data Protection Regulation
- Insights Hub
- Life@QuestionPro
- Market Research
- Mobile diaries
- Mobile Surveys
- New Features
- Online Communities
- Question Types
- Questionnaire
- QuestionPro Products
- Release Notes
- Research Tools and Apps
- Revenue at Risk
- Survey Templates
- Training Tips
- Uncategorized
- Video Learning Series
- What’s Coming Up
- Workforce Intelligence
Have a language expert improve your writing
Run a free plagiarism check in 10 minutes, generate accurate citations for free.
- Knowledge Base
Methodology
- Qualitative vs. Quantitative Research | Differences, Examples & Methods
Qualitative vs. Quantitative Research | Differences, Examples & Methods
Published on April 12, 2019 by Raimo Streefkerk . Revised on June 22, 2023.
When collecting and analyzing data, quantitative research deals with numbers and statistics, while qualitative research deals with words and meanings. Both are important for gaining different kinds of knowledge.
Common quantitative methods include experiments, observations recorded as numbers, and surveys with closed-ended questions.
Quantitative research is at risk for research biases including information bias , omitted variable bias , sampling bias , or selection bias . Qualitative research Qualitative research is expressed in words . It is used to understand concepts, thoughts or experiences. This type of research enables you to gather in-depth insights on topics that are not well understood.
Common qualitative methods include interviews with open-ended questions, observations described in words, and literature reviews that explore concepts and theories.
Table of contents
The differences between quantitative and qualitative research, data collection methods, when to use qualitative vs. quantitative research, how to analyze qualitative and quantitative data, other interesting articles, frequently asked questions about qualitative and quantitative research.
Quantitative and qualitative research use different research methods to collect and analyze data, and they allow you to answer different kinds of research questions.
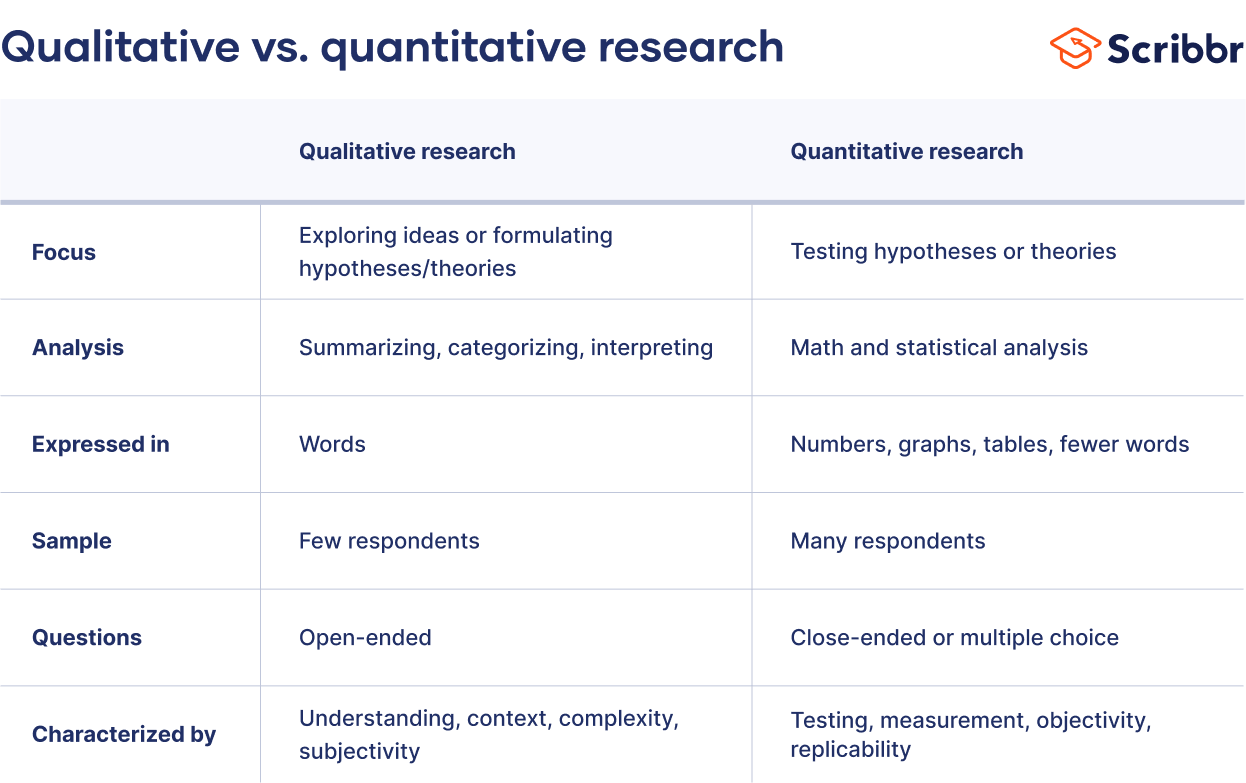
Quantitative and qualitative data can be collected using various methods. It is important to use a data collection method that will help answer your research question(s).
Many data collection methods can be either qualitative or quantitative. For example, in surveys, observational studies or case studies , your data can be represented as numbers (e.g., using rating scales or counting frequencies) or as words (e.g., with open-ended questions or descriptions of what you observe).
However, some methods are more commonly used in one type or the other.
Quantitative data collection methods
- Surveys : List of closed or multiple choice questions that is distributed to a sample (online, in person, or over the phone).
- Experiments : Situation in which different types of variables are controlled and manipulated to establish cause-and-effect relationships.
- Observations : Observing subjects in a natural environment where variables can’t be controlled.
Qualitative data collection methods
- Interviews : Asking open-ended questions verbally to respondents.
- Focus groups : Discussion among a group of people about a topic to gather opinions that can be used for further research.
- Ethnography : Participating in a community or organization for an extended period of time to closely observe culture and behavior.
- Literature review : Survey of published works by other authors.
A rule of thumb for deciding whether to use qualitative or quantitative data is:
- Use quantitative research if you want to confirm or test something (a theory or hypothesis )
- Use qualitative research if you want to understand something (concepts, thoughts, experiences)
For most research topics you can choose a qualitative, quantitative or mixed methods approach . Which type you choose depends on, among other things, whether you’re taking an inductive vs. deductive research approach ; your research question(s) ; whether you’re doing experimental , correlational , or descriptive research ; and practical considerations such as time, money, availability of data, and access to respondents.
Quantitative research approach
You survey 300 students at your university and ask them questions such as: “on a scale from 1-5, how satisfied are your with your professors?”
You can perform statistical analysis on the data and draw conclusions such as: “on average students rated their professors 4.4”.
Qualitative research approach
You conduct in-depth interviews with 15 students and ask them open-ended questions such as: “How satisfied are you with your studies?”, “What is the most positive aspect of your study program?” and “What can be done to improve the study program?”
Based on the answers you get you can ask follow-up questions to clarify things. You transcribe all interviews using transcription software and try to find commonalities and patterns.
Mixed methods approach
You conduct interviews to find out how satisfied students are with their studies. Through open-ended questions you learn things you never thought about before and gain new insights. Later, you use a survey to test these insights on a larger scale.
It’s also possible to start with a survey to find out the overall trends, followed by interviews to better understand the reasons behind the trends.
Qualitative or quantitative data by itself can’t prove or demonstrate anything, but has to be analyzed to show its meaning in relation to the research questions. The method of analysis differs for each type of data.
Analyzing quantitative data
Quantitative data is based on numbers. Simple math or more advanced statistical analysis is used to discover commonalities or patterns in the data. The results are often reported in graphs and tables.
Applications such as Excel, SPSS, or R can be used to calculate things like:
- Average scores ( means )
- The number of times a particular answer was given
- The correlation or causation between two or more variables
- The reliability and validity of the results
Analyzing qualitative data
Qualitative data is more difficult to analyze than quantitative data. It consists of text, images or videos instead of numbers.
Some common approaches to analyzing qualitative data include:
- Qualitative content analysis : Tracking the occurrence, position and meaning of words or phrases
- Thematic analysis : Closely examining the data to identify the main themes and patterns
- Discourse analysis : Studying how communication works in social contexts
If you want to know more about statistics , methodology , or research bias , make sure to check out some of our other articles with explanations and examples.
- Chi square goodness of fit test
- Degrees of freedom
- Null hypothesis
- Discourse analysis
- Control groups
- Mixed methods research
- Non-probability sampling
- Quantitative research
- Inclusion and exclusion criteria
Research bias
- Rosenthal effect
- Implicit bias
- Cognitive bias
- Selection bias
- Negativity bias
- Status quo bias
Quantitative research deals with numbers and statistics, while qualitative research deals with words and meanings.
Quantitative methods allow you to systematically measure variables and test hypotheses . Qualitative methods allow you to explore concepts and experiences in more detail.
In mixed methods research , you use both qualitative and quantitative data collection and analysis methods to answer your research question .
The research methods you use depend on the type of data you need to answer your research question .
- If you want to measure something or test a hypothesis , use quantitative methods . If you want to explore ideas, thoughts and meanings, use qualitative methods .
- If you want to analyze a large amount of readily-available data, use secondary data. If you want data specific to your purposes with control over how it is generated, collect primary data.
- If you want to establish cause-and-effect relationships between variables , use experimental methods. If you want to understand the characteristics of a research subject, use descriptive methods.
Data collection is the systematic process by which observations or measurements are gathered in research. It is used in many different contexts by academics, governments, businesses, and other organizations.
There are various approaches to qualitative data analysis , but they all share five steps in common:
- Prepare and organize your data.
- Review and explore your data.
- Develop a data coding system.
- Assign codes to the data.
- Identify recurring themes.
The specifics of each step depend on the focus of the analysis. Some common approaches include textual analysis , thematic analysis , and discourse analysis .
A research project is an academic, scientific, or professional undertaking to answer a research question . Research projects can take many forms, such as qualitative or quantitative , descriptive , longitudinal , experimental , or correlational . What kind of research approach you choose will depend on your topic.
Cite this Scribbr article
If you want to cite this source, you can copy and paste the citation or click the “Cite this Scribbr article” button to automatically add the citation to our free Citation Generator.
Streefkerk, R. (2023, June 22). Qualitative vs. Quantitative Research | Differences, Examples & Methods. Scribbr. Retrieved March 21, 2024, from https://www.scribbr.com/methodology/qualitative-quantitative-research/
Is this article helpful?
Raimo Streefkerk
Other students also liked, what is quantitative research | definition, uses & methods, what is qualitative research | methods & examples, mixed methods research | definition, guide & examples, "i thought ai proofreading was useless but..".
I've been using Scribbr for years now and I know it's a service that won't disappoint. It does a good job spotting mistakes”
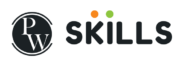
Quantitative Data Analysis: Types, Analysis & Examples
Analysis of Quantitative data enables you to transform raw data points, typically organised in spreadsheets, into actionable insights. Refer to the article to know more!
Analysis of Quantitative Data : Data, data everywhere — it’s impossible to escape it in today’s digitally connected world. With business and personal activities leaving digital footprints, vast amounts of quantitative data are being generated every second of every day. While data on its own may seem impersonal and cold, in the right hands it can be transformed into valuable insights that drive meaningful decision-making. In this article, we will discuss analysis of quantitative data types and examples!
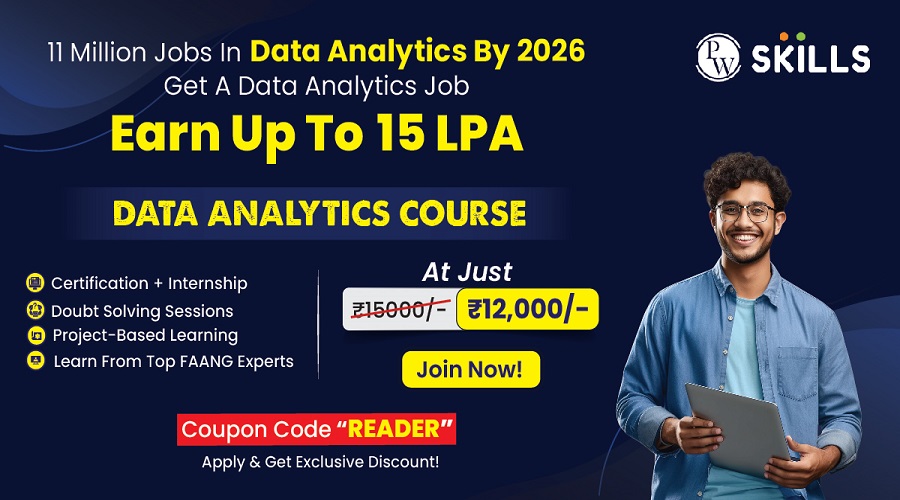
If you are looking to acquire hands-on experience in quantitative data analysis, look no further than Physics Wallah’s Data Analytics Course . And as a token of appreciation for reading this blog post until the end, use our exclusive coupon code “READER” to get a discount on the course fee.
Table of Contents
What is the Quantitative Analysis Method?
Quantitative Analysis refers to a mathematical approach that gathers and evaluates measurable and verifiable data. This method is utilized to assess performance and various aspects of a business or research. It involves the use of mathematical and statistical techniques to analyze data. Quantitative methods emphasize objective measurements, focusing on statistical, analytical, or numerical analysis of data. It collects data and studies it to derive insights or conclusions.
In a business context, it helps in evaluating the performance and efficiency of operations. Quantitative analysis can be applied across various domains, including finance, research, and chemistry, where data can be converted into numbers for analysis.
Also Read: Analysis vs. Analytics: How Are They Different?
What is the Best Analysis for Quantitative Data?
The “best” analysis for quantitative data largely depends on the specific research objectives, the nature of the data collected, the research questions posed, and the context in which the analysis is conducted. Quantitative data analysis encompasses a wide range of techniques, each suited for different purposes. Here are some commonly employed methods, along with scenarios where they might be considered most appropriate:
1) Descriptive Statistics:
- When to Use: To summarize and describe the basic features of the dataset, providing simple summaries about the sample and measures of central tendency and variability.
- Example: Calculating means, medians, standard deviations, and ranges to describe a dataset.
2) Inferential Statistics:
- When to Use: When you want to make predictions or inferences about a population based on a sample, testing hypotheses, or determining relationships between variables.
- Example: Conducting t-tests to compare means between two groups or performing regression analysis to understand the relationship between an independent variable and a dependent variable.
3) Correlation and Regression Analysis:
- When to Use: To examine relationships between variables, determining the strength and direction of associations, or predicting one variable based on another.
- Example: Assessing the correlation between customer satisfaction scores and sales revenue or predicting house prices based on variables like location, size, and amenities.
4) Factor Analysis:
- When to Use: When dealing with a large set of variables and aiming to identify underlying relationships or latent factors that explain patterns of correlations within the data.
- Example: Exploring underlying constructs influencing employee engagement using survey responses across multiple indicators.
5) Time Series Analysis:
- When to Use: When analyzing data points collected or recorded at successive time intervals to identify patterns, trends, seasonality, or forecast future values.
- Example: Analyzing monthly sales data over several years to detect seasonal trends or forecasting stock prices based on historical data patterns.
6) Cluster Analysis:
- When to Use: To segment a dataset into distinct groups or clusters based on similarities, enabling pattern recognition, customer segmentation, or data reduction.
- Example: Segmenting customers into distinct groups based on purchasing behavior, demographic factors, or preferences.
The “best” analysis for quantitative data is not one-size-fits-all but rather depends on the research objectives, hypotheses, data characteristics, and contextual factors. Often, a combination of analytical techniques may be employed to derive comprehensive insights and address multifaceted research questions effectively. Therefore, selecting the appropriate analysis requires careful consideration of the research goals, methodological rigor, and interpretative relevance to ensure valid, reliable, and actionable outcomes.
Analysis of Quantitative Data in Quantitative Research
Analyzing quantitative data in quantitative research involves a systematic process of examining numerical information to uncover patterns, relationships, and insights that address specific research questions or objectives. Here’s a structured overview of the analysis process:
1) Data Preparation:
- Data Cleaning: Identify and address errors, inconsistencies, missing values, and outliers in the dataset to ensure its integrity and reliability.
- Variable Transformation: Convert variables into appropriate formats or scales, if necessary, for analysis (e.g., normalization, standardization).
2) Descriptive Statistics:
- Central Tendency: Calculate measures like mean, median, and mode to describe the central position of the data.
- Variability: Assess the spread or dispersion of data using measures such as range, variance, standard deviation, and interquartile range.
- Frequency Distribution: Create tables, histograms, or bar charts to display the distribution of values for categorical or discrete variables.
3) Exploratory Data Analysis (EDA):
- Data Visualization: Generate graphical representations like scatter plots, box plots, histograms, or heatmaps to visualize relationships, distributions, and patterns in the data.
- Correlation Analysis: Examine the strength and direction of relationships between variables using correlation coefficients.
4) Inferential Statistics:
- Hypothesis Testing: Formulate null and alternative hypotheses based on research questions, selecting appropriate statistical tests (e.g., t-tests, ANOVA, chi-square tests) to assess differences, associations, or effects.
- Confidence Intervals: Estimate population parameters using sample statistics and determine the range within which the true parameter is likely to fall.
5) Regression Analysis:
- Linear Regression: Identify and quantify relationships between an outcome variable and one or more predictor variables, assessing the strength, direction, and significance of associations.
- Multiple Regression: Evaluate the combined effect of multiple independent variables on a dependent variable, controlling for confounding factors.
6) Factor Analysis and Structural Equation Modeling:
- Factor Analysis: Identify underlying dimensions or constructs that explain patterns of correlations among observed variables, reducing data complexity.
- Structural Equation Modeling (SEM): Examine complex relationships between observed and latent variables, assessing direct and indirect effects within a hypothesized model.
7) Time Series Analysis and Forecasting:
- Trend Analysis: Analyze patterns, trends, and seasonality in time-ordered data to understand historical patterns and predict future values.
- Forecasting Models: Develop predictive models (e.g., ARIMA, exponential smoothing) to anticipate future trends, demand, or outcomes based on historical data patterns.
8) Interpretation and Reporting:
- Interpret Results: Translate statistical findings into meaningful insights, discussing implications, limitations, and conclusions in the context of the research objectives.
- Documentation: Document the analysis process, methodologies, assumptions, and findings systematically for transparency, reproducibility, and peer review.
Also Read: Learning Path to Become a Data Analyst in 2024
Analysis of Quantitative Data Examples
Analyzing quantitative data involves various statistical methods and techniques to derive meaningful insights from numerical data. Here are some examples illustrating the analysis of quantitative data across different contexts:
How to Write Data Analysis in Quantitative Research Proposal?
Writing the data analysis section in a quantitative research proposal requires careful planning and organization to convey a clear, concise, and methodologically sound approach to analyzing the collected data. Here’s a step-by-step guide on how to write the data analysis section effectively:
Step 1: Begin with an Introduction
- Contextualize : Briefly reintroduce the research objectives, questions, and the significance of the study.
- Purpose Statement : Clearly state the purpose of the data analysis section, outlining what readers can expect in this part of the proposal.
Step 2: Describe Data Collection Methods
- Detail Collection Techniques : Provide a concise overview of the methods used for data collection (e.g., surveys, experiments, observations).
- Instrumentation : Mention any tools, instruments, or software employed for data gathering and its relevance.
Step 3 : Discuss Data Cleaning Procedures
- Data Cleaning : Describe the procedures for cleaning and pre-processing the data.
- Handling Outliers & Missing Data : Explain how outliers, missing values, and other inconsistencies will be managed to ensure data quality.
Step 4 : Present Analytical Techniques
- Descriptive Statistics : Outline the descriptive statistics that will be calculated to summarize the data (e.g., mean, median, mode, standard deviation).
- Inferential Statistics : Specify the inferential statistical tests or models planned for deeper analysis (e.g., t-tests, ANOVA, regression).
Step 5: State Hypotheses & Testing Procedures
- Hypothesis Formulation : Clearly state the null and alternative hypotheses based on the research questions or objectives.
- Testing Strategy : Detail the procedures for hypothesis testing, including the chosen significance level (e.g., α = 0.05) and statistical criteria.
Step 6 : Provide a Sample Analysis Plan
- Step-by-Step Plan : Offer a sample plan detailing the sequence of steps involved in the data analysis process.
- Software & Tools : Mention any specific statistical software or tools that will be utilized for analysis.
Step 7 : Address Validity & Reliability
- Validity : Discuss how you will ensure the validity of the data analysis methods and results.
- Reliability : Explain measures taken to enhance the reliability and replicability of the study findings.
Step 8 : Discuss Ethical Considerations
- Ethical Compliance : Address ethical considerations related to data privacy, confidentiality, and informed consent.
- Compliance with Guidelines : Ensure that your data analysis methods align with ethical guidelines and institutional policies.
Step 9 : Acknowledge Limitations
- Limitations : Acknowledge potential limitations in the data analysis methods or data set.
- Mitigation Strategies : Offer strategies or alternative approaches to mitigate identified limitations.
Step 10 : Conclude the Section
- Summary : Summarize the key points discussed in the data analysis section.
- Transition : Provide a smooth transition to subsequent sections of the research proposal, such as the conclusion or references.
Step 11 : Proofread & Revise
- Review : Carefully review the data analysis section for clarity, coherence, and consistency.
- Feedback : Seek feedback from peers, advisors, or mentors to refine your approach and ensure methodological rigor.
What are the 4 Types of Quantitative Analysis?
Quantitative analysis encompasses various methods to evaluate and interpret numerical data. While the specific categorization can vary based on context, here are four broad types of quantitative analysis commonly recognized:
- Descriptive Analysis: This involves summarizing and presenting data to describe its main features, such as mean, median, mode, standard deviation, and range. Descriptive statistics provide a straightforward overview of the dataset’s characteristics.
- Inferential Analysis: This type of analysis uses sample data to make predictions or inferences about a larger population. Techniques like hypothesis testing, regression analysis, and confidence intervals fall under this category. The goal is to draw conclusions that extend beyond the immediate data collected.
- Time-Series Analysis: In this method, data points are collected, recorded, and analyzed over successive time intervals. Time-series analysis helps identify patterns, trends, and seasonal variations within the data. It’s particularly useful in forecasting future values based on historical trends.
- Causal or Experimental Research: This involves establishing a cause-and-effect relationship between variables. Through experimental designs, researchers manipulate one variable to observe the effect on another variable while controlling for external factors. Randomized controlled trials are a common method within this type of quantitative analysis.
Each type of quantitative analysis serves specific purposes and is applied based on the nature of the data and the research objectives.
Also Read: AI and Predictive Analytics: Examples, Tools, Uses, Ai Vs Predictive Analytics
Steps to Effective Quantitative Data Analysis
Quantitative data analysis need not be daunting; it’s a systematic process that anyone can master. To harness actionable insights from your company’s data, follow these structured steps:
Step 1 : Gather Data Strategically
Initiating the analysis journey requires a foundation of relevant data. Employ quantitative research methods to accumulate numerical insights from diverse channels such as:
- Interviews or Focus Groups: Engage directly with stakeholders or customers to gather specific numerical feedback.
- Digital Analytics: Utilize tools like Google Analytics to extract metrics related to website traffic, user behavior, and conversions.
- Observational Tools: Leverage heatmaps, click-through rates, or session recordings to capture user interactions and preferences.
- Structured Questionnaires: Deploy surveys or feedback mechanisms that employ close-ended questions for precise responses.
Ensure that your data collection methods align with your research objectives, focusing on granularity and accuracy.
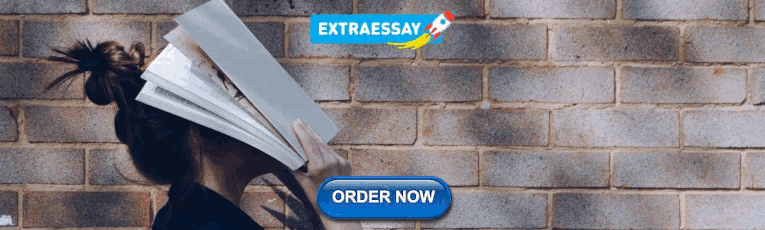
Step 2 : Refine and Cleanse Your Data
Raw data often comes with imperfections. Scrutinize your dataset to identify and rectify:
- Errors and Inconsistencies: Address any inaccuracies or discrepancies that could mislead your analysis.
- Duplicates: Eliminate repeated data points that can skew results.
- Outliers: Identify and assess outliers, determining whether they should be adjusted or excluded based on contextual relevance.
Cleaning your dataset ensures that subsequent analyses are based on reliable and consistent information, enhancing the credibility of your findings.
Step 3 : Delve into Analysis with Precision
With a refined dataset at your disposal, transition into the analytical phase. Employ both descriptive and inferential analysis techniques:
- Descriptive Analysis: Summarize key attributes of your dataset, computing metrics like averages, distributions, and frequencies.
- Inferential Analysis: Leverage statistical methodologies to derive insights, explore relationships between variables, or formulate predictions.
The objective is not just number crunching but deriving actionable insights. Interpret your findings to discern underlying patterns, correlations, or trends that inform strategic decision-making. For instance, if data indicates a notable relationship between user engagement metrics and specific website features, consider optimizing those features for enhanced user experience.
Step 4 : Visual Representation and Communication
Transforming your analytical outcomes into comprehensible narratives is crucial for organizational alignment and decision-making. Leverage visualization tools and techniques to:
- Craft Engaging Visuals: Develop charts, graphs, or dashboards that encapsulate key findings and insights.
- Highlight Insights: Use visual elements to emphasize critical data points, trends, or comparative metrics effectively.
- Facilitate Stakeholder Engagement: Share your visual representations with relevant stakeholders, ensuring clarity and fostering informed discussions.
Tools like Tableau, Power BI, or specialized platforms like Hotjar can simplify the visualization process, enabling seamless representation and dissemination of your quantitative insights.
Also Read: Top 10 Must Use AI Tools for Data Analysis [2024 Edition]
Statistical Analysis in Quantitative Research
Statistical analysis is a cornerstone of quantitative research, providing the tools and techniques to interpret numerical data systematically. By applying statistical methods, researchers can identify patterns, relationships, and trends within datasets, enabling evidence-based conclusions and informed decision-making. Here’s an overview of the key aspects and methodologies involved in statistical analysis within quantitative research:
- Mean, Median, Mode: Measures of central tendency that summarize the average, middle, and most frequent values in a dataset, respectively.
- Standard Deviation, Variance: Indicators of data dispersion or variability around the mean.
- Frequency Distributions: Tabular or graphical representations that display the distribution of data values or categories.
- Hypothesis Testing: Formal methodologies to test hypotheses or assumptions about population parameters using sample data. Common tests include t-tests, chi-square tests, ANOVA, and regression analysis.
- Confidence Intervals: Estimation techniques that provide a range of values within which a population parameter is likely to lie, based on sample data.
- Correlation and Regression Analysis: Techniques to explore relationships between variables, determining the strength and direction of associations. Regression analysis further enables prediction and modeling based on observed data patterns.
3) Probability Distributions:
- Normal Distribution: A bell-shaped distribution often observed in naturally occurring phenomena, forming the basis for many statistical tests.
- Binomial, Poisson, and Exponential Distributions: Specific probability distributions applicable to discrete or continuous random variables, depending on the nature of the research data.
4) Multivariate Analysis:
- Factor Analysis: A technique to identify underlying relationships between observed variables, often used in survey research or data reduction scenarios.
- Cluster Analysis: Methodologies that group similar objects or individuals based on predefined criteria, enabling segmentation or pattern recognition within datasets.
- Multivariate Regression: Extending regression analysis to multiple independent variables, assessing their collective impact on a dependent variable.
5) Data Modeling and Forecasting:
- Time Series Analysis: Analyzing data points collected or recorded at specific time intervals to identify patterns, trends, or seasonality.
- Predictive Analytics: Leveraging statistical models and machine learning algorithms to forecast future trends, outcomes, or behaviors based on historical data.
If this blog post has piqued your interest in the field of data analytics, then we highly recommend checking out Physics Wallah’s Data Analytics Course . This course covers all the fundamental concepts of quantitative data analysis and provides hands-on training for various tools and software used in the industry.
With a team of experienced instructors from different backgrounds and industries, you will gain a comprehensive understanding of a wide range of topics related to data analytics. And as an added bonus for being one of our dedicated readers, use the coupon code “ READER ” to get an exclusive discount on this course!
For Latest Tech Related Information, Join Our Official Free Telegram Group : PW Skills Telegram Group
Analysis of Quantitative Data FAQs
What is quantitative data analysis.
Quantitative data analysis involves the systematic process of collecting, cleaning, interpreting, and presenting numerical data to identify patterns, trends, and relationships through statistical methods and mathematical calculations.
What are the main steps involved in quantitative data analysis?
The primary steps include data collection, data cleaning, statistical analysis (descriptive and inferential), interpretation of results, and visualization of findings using graphs or charts.
What is the difference between descriptive and inferential analysis?
Descriptive analysis summarizes and describes the main aspects of the dataset (e.g., mean, median, mode), while inferential analysis draws conclusions or predictions about a population based on a sample, using statistical tests and models.
How do I handle outliers in my quantitative data?
Outliers can be managed by identifying them through statistical methods, understanding their nature (error or valid data), and deciding whether to remove them, transform them, or conduct separate analyses to understand their impact.
Which statistical tests should I use for my quantitative research?
The choice of statistical tests depends on your research design, data type, and research questions. Common tests include t-tests, ANOVA, regression analysis, chi-square tests, and correlation analysis, among others.
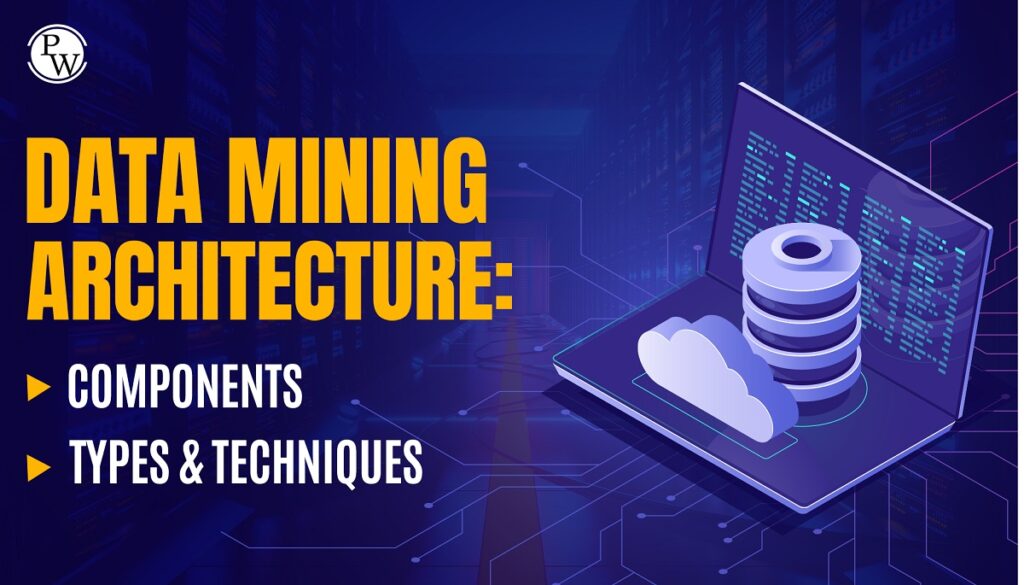
Data Mining Architecture: Components, Types & Techniques
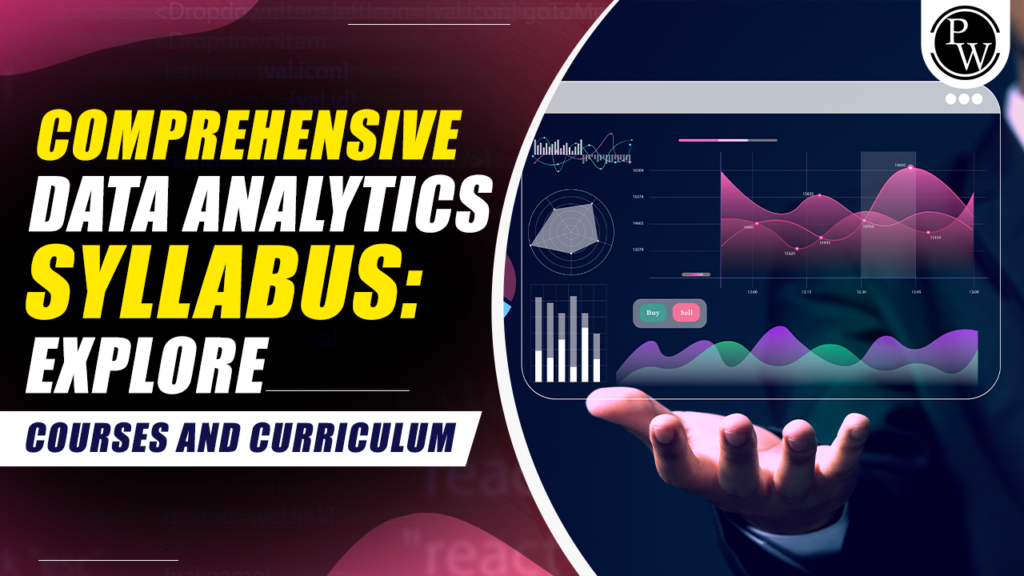
Comprehensive Data Analytics Syllabus: Courses and Curriculum
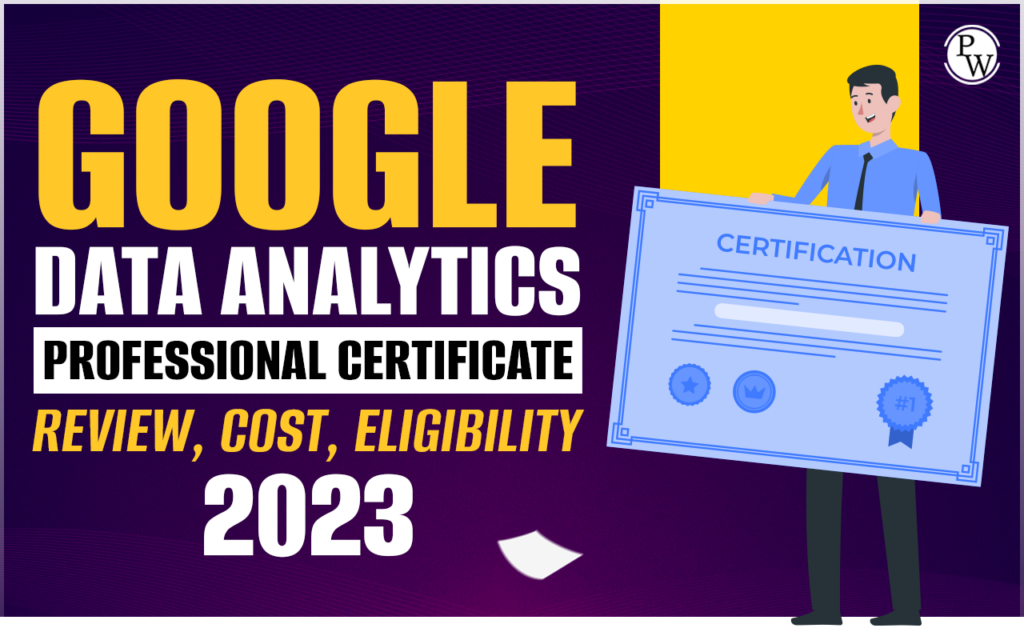
Google Data Analytics Professional Certificate Review, Cost, Eligibility 2023

Types of Quantitative Research | An Absolute Guide for Beginners
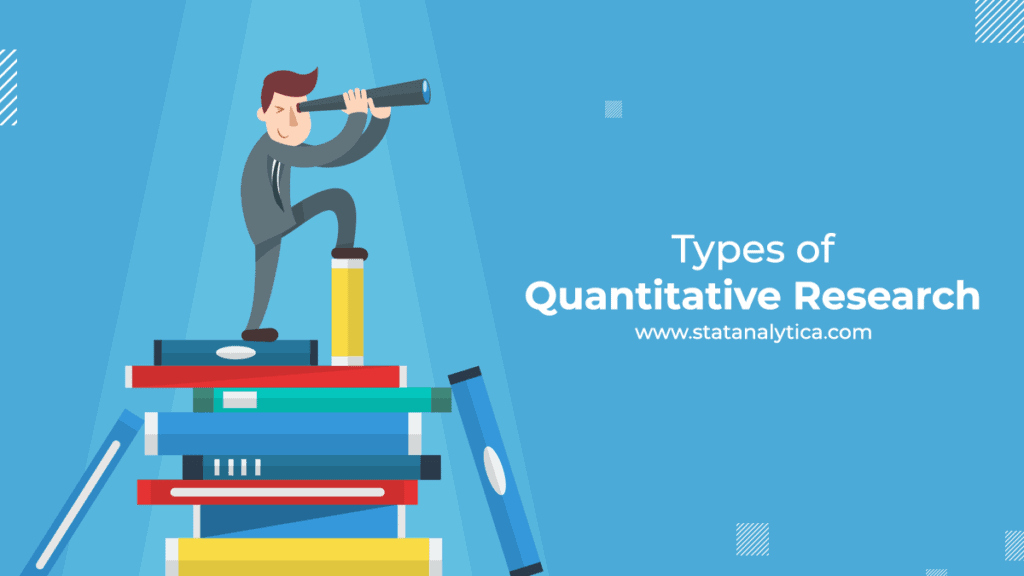
It does not matter which discipline a person belongs to. You have to come across the quantitative research data once or multiple times in life. Most people often come across one or multiple questionnaires or surveys.
Let’s take an example of quantitative research. A survey is conducted to know how much time a shopkeeper takes to attend to the customer. And how many times he/she walks into the shop.
Here, the survey is conducted using different questions. Such as how much time the shopkeeper takes to attend to the customer. And how many times the customer comes in the shop, etc.
The aim of these surveys is to draw the most relevant analytical conclusions. That helps in understanding the targeted audience.
There are various types of quantitative research that a company uses. It is used to understand the product’s demand within the market.
In this blog, we have given the necessary details about what quantitative research is. And its types.
So, let’s move on to the details.
What is quantitative research?
Table of Contents
Quantitative research is one of the systematic techniques. It is used to collect the data using the sampling for quantitative methods. For example , online polls , questionnaires, and online surveys. You can seamlessly integrate them into your WordPress website using a versatile WordPress voting plugin , providing an interactive and engaging way to collect valuable insights from your audience.
The data is collected from both existing and potential users and represented numerically.
Quantitative research also used to measure the variables, analyze, and register the relationships between the variable studies with a numerical system’s help.
In quantitative research, the information is collected via structured research. And the outcomes reflect or represent the population.
Where we use quantitative research?
Quantitative researchers use different tools. The tools are used to collect numeric data in terms of numbers and statistics. This data is represented in non-textual forms, such as charts, figures, and tables.
Moreover, the researchers can take the non-numerical data to examine the information.
Quantitative research is using in several areas, such as:
- gender studies,
- demography,
- community health,
- psychology,
- education, and so on.
What are the 5 types of quantitative research?
Survey research.
The survey is one of the primary statistic methods. It is used for different types of quantitative research. The aim of this is to provide a comprehensive description of the characteristics of the specific population or group.
Both large and small organizations also apply the offline and online survey research method. This helps to know their users and understand the product and merchandise views.
There are numerous methods to manage survey research. It can be done on the phone, in person, or by email or mails.
Descriptive research
It describes the present status of all the selected or identified variables. The basic objective of descriptive research is to describe and evaluate the people’s present status, conditions, settings, or events.
Descriptive research is considered to be one of the important types of quantitative research.
The most common descriptive questions start with the “How much..,” “what is the…,” “what is a percentage of…,” and these kinds of questions.
Let’s take an example of this survey. An Exit poll is a descriptive survey that includes questions like: “ Which candidate will win this election?”
Moreover, the demographic segmentation survey might be like this: “ How many students between the 18-25 age do study at night?”
Experimental research
This is one of the types of quantitative research, as its name suggests that it is based on single or multiple theories.
It terms to be the true experiments that utilize the scientific technique to verify the cause-effect relations within the group of variables.
Therefore, more than one theory is used to conduct the particular research. An example of experimental research is “ the effect of the particular dose and treatment on breast cancer.”
The use of experimental research can be implemented in various fields. And these fields are sociology, physics, biology, chemistry, medicine, and so on.
Correlational research
It is used for establishing relationships among two close entities. And determining the relational impact on each other.
For such cases, a researcher requires a minimum of two different groups. Additionally, this research approaches and recognizes the patterns and trends in the data without going far into the observation to analyze various trends and patterns.
An example of correlational research is the correlation between self-esteem and intelligence.
Suppose your favorite ice-cream truck has a specific jingle, and the truck is coming to your area. The more would be the sound of the jingle, the more closer the ice-cream truck would be.
But, if two ice-cream trucks are coming in your area, you can easily know which sounds are from your favorite ice-cream truck. This is what you are not taught in your classroom, but you can relate the fact in your mind on your own.
Moreover, it depends on your intelligence that you can quickly recognize without anybody’s help. This is what the correlation research method is.
Causal-comparative research
It is one of the scientific methods that apply to summarize the cause and effect equations among different variables. In causal relationships, a single variable is based on the complementary experimental variable.
The experimenters do not manipulate the independent variable. But, the impact of independent variables over the dependent variables can be measured in causal-comparative research.
Let’s take some examples. The impact of divorce of the parents on their children. The impact of sports activity on the participants, and so on.
- What is probability and types of Probability Sampling
- Types of statistical analysis
- Types of statistical terms
Why do I select different types of quantitative research over qualitative research?
It has been seen that quantitative research prefers over qualitative research. The reasons for it can be like quantitative research can be done fast, scientific, acceptable, objective, and focused.
Apart from this, there are several reasons to select different types of quantitative research. Let’s check each of them one by one.
Deal with the larger sample data
The types of quantitative research results depend on the large sample size. This sample size represents the population. The lager is the sample size; the more valid results will be drawn.
The types of quantitative research results depend on the large sample size. This sample size represents the population. The lager is the sample size; the more valid results will be drawn.
Control-sensitive
It has been seen that researchers have more control over the data collection methods. This data is also different from the experiments.
Researchers use different types of quantitative research. It is used to establish facts, make predictions, and test the previous hypotheses.
The relatable aims for finding evidence that may or may not support an existing hypothesis. By testing and validating the constructed theories, it can give reasons why a phenomenon has occurred.
Generalizable
A project can generalize the concepts more accurately. It also analyzes the casual relationship, and predicts results. Moreover, the findings can generalize when the selection processes are designed. And the sample represents the population study.
Arrange in simple analytical ways
The data is being collected in the form of statistics and numbers. Further, it is arranged in charts, tables, figures, or another non-textual form.
The methods of data collection that use a quantitative research method are comparatively quick (such as telephone interviews). Some companies even use the best landline phone service for more professionalism and speed. Some companies even use a special telephone service for more professionalism and speed. Moreover, the data analysis is also comparatively less time-consuming (as it does use statistical software).
Consistent with data
Using the different types of quantitative research, you can easily get data. This data is reliable, precise, and consistent, numerical, and quantitative.
More structured
The researchers use different tools to get structured quantitative researched data. The tools can be equipment or questionnaires for collecting numerical data.
The repeatable and replica methods are usually done in research studies. This leads to high reliability.
Decision-making
The data taken from quantitative research like demographics, market size, and user preferences can help provide information on business decisions.
So, what are the methods of quantitative research?
The quantitative research method features objective calculations and mathematical, statistical, or numerical analysis. The data is collected by questionnaires, polls, and surveys for analysis.
The quantitative research method mainly focuses on collecting numerical data. This data generalize across the set of people so that a specific phenomenon can be explained.
Researchers who use the quantitative research method try to identify and separate the variables. These variables separate within a study framework, seek relationships, correlation, and casualties.
After this, the quantitative researchers try to control the system in which the information is being gathered. This helps in avoiding the variables’ risk by which the accurate relationships can be identified.
What is the methodology for the quantitative research designs?
The structure of various types of quantitative research depends on the scientific method.
This utilizes deductive reasoning, in which the researchers: find out the hypothesis. Gather the information. Uses it for further investigation to prove whether the hypothesis is true or not. Once the analysis is done, share your summary.
Therefore, a basic procedure is followed for the quantitative research design:
- Make the observations related to something, which is new and unexplained. Analyzing the present theory that is surrounded by issues or problems.
- Hypothesizing the observations’ explanations.
- Predict the result depends on the hypothesis studies by formulating the plan for your prediction test control.
- Gather and process the information. If the prediction is right, move to the next step; otherwise, return to step 2. Get new hypotheses that depend on the new knowledge and situations.
- Finally, verify the findings of the sample on different factors. Make the conclusions. Represent the outcomes in a well-structured manner to your audience.
Now, let’s check your knowledge regarding types of quantitative research!
Now, you have studied different types of quantitative research. Let’s check what you have learned.
Take a quiz regarding the different quantitative research. Select the correct quantitative research type to the given statement.
- Do people think working from home is a great option to enhance the employee’s productivity with longer commutes?
- Descriptive Research
- Experimental Research
- Correlational Research
- Causal-Comparative Research
- How frequently do employees get the chance of traveling for a holiday?
- What is the primary difference between senior citizens and Millennials regarding smartphone usage?
- How has the Covid-19 modified profession for white-collar employees?
- Does the management method of the car shop owners foretell the work satisfaction of car sales associates?
There are various types of quantitative research . And researchers use different kinds of scientific tools to collect numeric data.
It has been observed that the quantitative research survey questions are must. So that the participants can have an easy and effective medium to responses.
Hope you easily understand the details mentioned in the blog. If you still have any queries, comment in the below section, and we will help you in the best possible way. Are you looking for statistics help for students ? Get the best help from our experts to clear all your doubts.
Frequently Asked Questions
Where is quantitative research used.
The main use of quantitative research is for quantifying the problem by forming numerical data. (Moreover, this data must transform into useful statistics.) The quantitative research is used to quantify opinions, attitudes, behaviors, and another defined variable. By this, you can generalize the results from a greater population sample.
What are the steps in quantitative research?
There are 11 steps to following in Quantitative Research, and these are:
Theory. Hypothesis. Research design. Operationalizing concepts. Selection of a research site(s). Selection of respondents. Data collection. Processing data. Data analysis. Findings and conclusions. Writing the findings in a well-structured manner.
What are the 7 characteristics of quantitative research?
The 7 major characteristics of quantitative research methods are as follows:
Practice Standardized Research Instruments. Contain Measurable Variables. Data representation in Graphs, Tables, or Figures. Use a Repeatable Method. Work on Measuring Devices. Allows a Normal Population Distribution. Can Predict Outcomes.
Related Posts
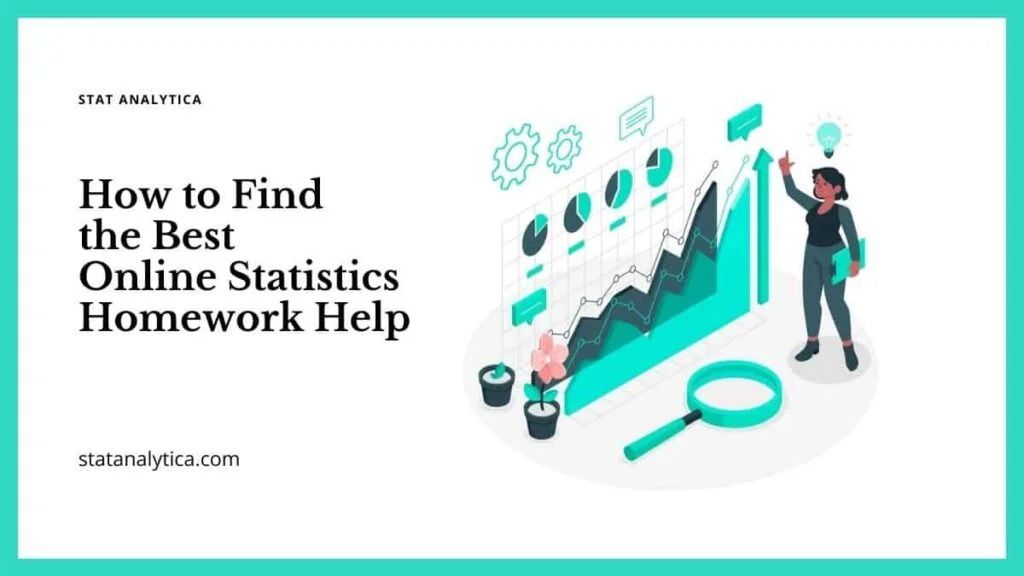
How to Find the Best Online Statistics Homework Help
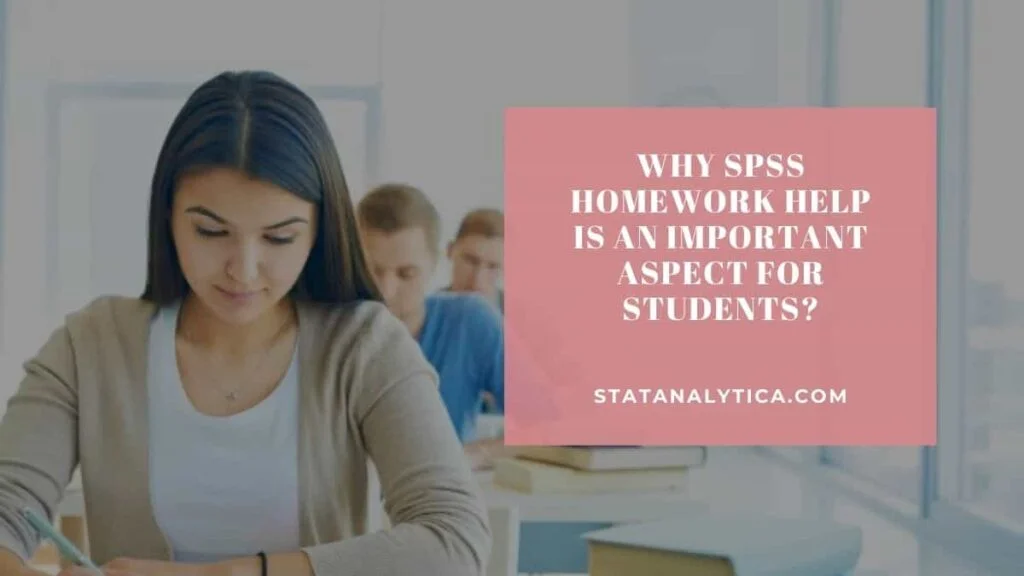
Why SPSS Homework Help Is An Important aspect for Students?
- (855) 776-7763
Training Maker
All Products
Qualaroo Insights
ProProfs.com
- Sign Up Free
Do you want a free Survey Software?
We have the #1 Online Survey Maker Software to get actionable user insights.
A Comprehensive Guide to Quantitative Research: Types, Characteristics, Methods & Examples
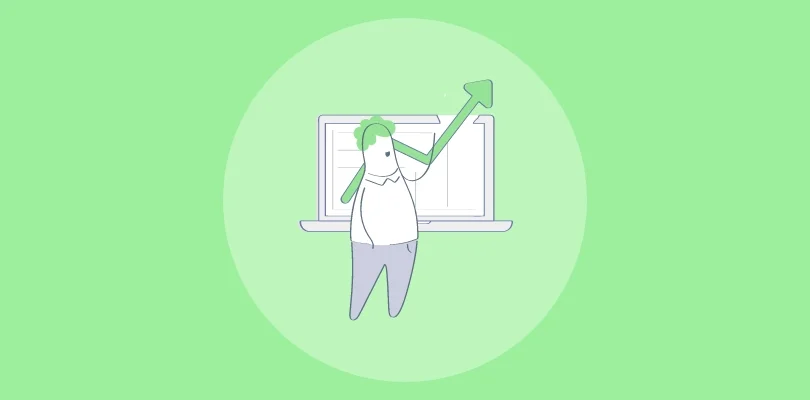
Step into the fascinating world of quantitative research, where numbers reveal extraordinary insights!
By gathering and studying data in a systematic way, quantitative research empowers us to understand our ever-changing world better. It helps understand a problem or an already-formed hypothesis by generating numerical data. The results don’t end here, as you can process these numbers to get actionable insights that aid decision-making.
You can use quantitative research to quantify opinions, behaviors, attitudes, and other definitive variables related to the market, customers, competitors, etc. The research is conducted on a larger sample population to draw predictive, average, and pattern-based insights.
Here, we delve into the intricacies of this research methodology, exploring various quantitative methods, their advantages, and real-life examples that showcase their impact and relevance.
Ready to embark on a journey of discovery and knowledge? Let’s go!
What Is Quantitative Research?
Quantitative research is a method that uses numbers and statistics to test theories about customer attitudes and behaviors. It helps researchers gather and analyze data systematically to gain valuable insights and draw evidence-based conclusions about customer preferences and trends.
Researchers use online surveys , questionnaires , polls , and quizzes to question a large number of people to obtain measurable and bias-free data.
In technical terms, quantitative research is mainly concerned with discovering facts about social phenomena while assuming a fixed and measurable reality.
Offering numbers and stats-based insights, this research methodology is a crucial part of primary research and helps understand how well an organizational decision is going to work out.
Let’s consider an example.
Suppose your qualitative analysis shows that your customers are looking for social media-based customer support . In that case, quantitative analysis will help you see how many of your customers are looking for this support.
If 10% of your customers are looking for such a service, you might or might not consider offering this feature. But, if 40% of your regular customers are seeking support via social media, then it is something you just cannot overlook.
Characteristics of Quantitative Research
Quantitative research clarifies the fuzziness of research data from qualitative research analysis. With numerical insights, you can formulate a better and more profitable business decision.
Hence, quantitative research is more readily contestable, sharpens intelligent discussion, helps you see the rival hypotheses, and dynamically contributes to the research process.
Let us have a quick look at some of its characteristics.
- Measurable Variables
The data collection methods in quantitative research are structured and contain items requiring measurable variables, such as age, number of family members, salary range, highest education, etc.
These structured data collection methods comprise polls, surveys, questionnaires, etc., and may have questions like the ones shown in the following image:
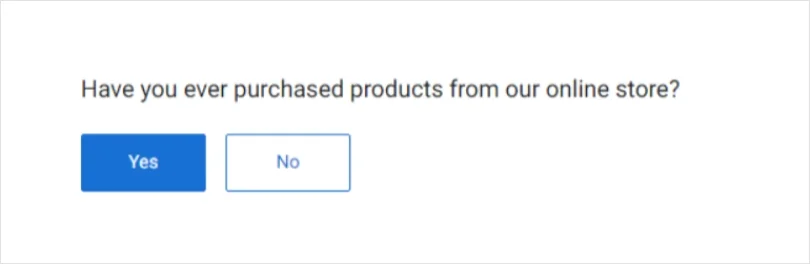
As you can see, all the variables are measurable. This ensures that the research is in-depth and provides less erroneous data for reliable, actionable insights.
- Sample Size
No matter what data analysis methods for quantitative research are being used, the sample size is kept such that it represents the target market.
As the main aim of the research methodology is to get numerical insights, the sample size should be fairly large. Depending on the survey objective and scope, it might span hundreds of thousands of people.
- Normal Population Distribution
To maintain the reliability of a quantitative research methodology, we assume that the population distribution curve is normal.
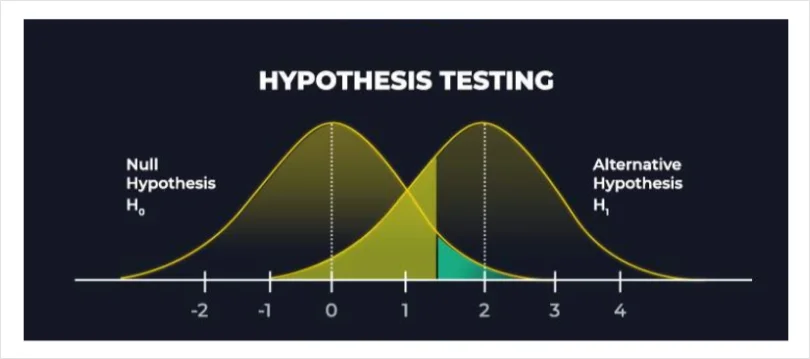
This type of population distribution curve is preferred over a non-normal distribution as the sample size is large, and the characteristics of the sample vary with its size.
This requires adhering to the random sampling principle to avoid the researcher’s bias in result interpretation. Any bias can ruin the fairness of the entire process and defeats the purpose of research.
- Well-Structured Data Representation
Data analysis in quantitative research produces highly structured results and can form well-defined graphical representations. Some common examples include tables, figures, graphs, etc., that combine large blocks of data.
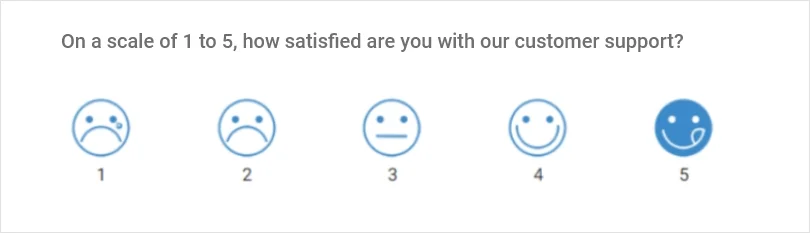
This way, you can discover hidden data trends, relationships, and differences among various measurable variables. This can help researchers understand the survey data and formulate actionable insights for decision-making.
- Predictive Outcomes
Quantitative analysis of data can also be used for estimations and prediction outcomes. You can construct if-then scenarios and analyze the data for the identification of any upcoming trends or events.
However, this requires advanced analytics and involves complex mathematical computations. So, it is mostly done via quantitative research tools that come with advanced analytics capabilities.
8 Best Practices to Conduct Quantitative Research
Here are some best practices to keep in mind while conducting quantitative research:
1. Define Research Objectives
There can be many ways to collect data via quantitative research methods that are chosen as per the research objective and scope. These methods allow you to build your own observations regarding any hypotheses – unknown, entirely new, or unexplained.
You can hypothesize a proof and build a prediction of outcomes supporting the same. You can also create a detailed stepwise plan for data collection, analysis, and testing.
Below, we explore quantitative research methods and discuss some examples to enhance your understanding of them.
2. Keep Your Questions Simple
The surveys are meant to reach people en-masse, and that includes a wide demographic range with recipients from all walks of life. Asking simple questions will ensure that they grasp what’s being asked easily.
Read More: Proven Tips to Avoid Leading and Loaded Questions in Your Survey
3. Develop a Solid Research Design
Choose an appropriate research design that aligns with your objectives, whether it’s experimental, quasi-experimental, or correlational. You also need to pay attention to the sample size and sampling technique such that it represents the target population accurately.
4. Use Reliable & Valid Instruments
It’s crucial to select or develop measurement instruments such as questionnaires, scales, or tests that have been validated and are reliable. Before proceeding with the main study, pilot-test these instruments on a small sample to assess their effectiveness and make any necessary improvements.
5. Ensure Data Quality
Implement data collection protocols to minimize errors and bias during data gathering. Double-check data entries and cleaning procedures to eliminate any inconsistencies or missing values that may affect the accuracy of your results. For instance, you might regularly cross-verify data entries to identify and correct any discrepancies.
6. Employ Appropriate Data Analysis Techniques
Select statistical methods that match the nature of your data and research questions. Whether it’s regression analysis, t-tests, ANOVA, or other techniques, using the right approach is important for drawing meaningful conclusions. Utilize software tools like SPSS or R for data analysis to ensure the accuracy and reproducibility of your findings.
7. Interpret Results Objectively
Present your findings in a clear and unbiased manner. Avoid making unwarranted causal claims, especially in correlational studies. Instead, focus on describing the relationships and patterns observed in your data.
8. Address Ethical Considerations
Prioritize ethical considerations throughout your research process. Obtain informed consent from participants, ensuring their voluntary participation and confidentiality of data. Comply with ethical guidelines and gain approval from a governing body if necessary.
Read More: How to Find Survey Participants & Respondents
Types of Quantitative Research Methods
Quantitative research is usually conducted using two methods. They are-
- Primary quantitative research methods
- Secondary quantitative research methods
1. Primary Methods
Primary quantitative research is the most popular way of conducting market research. The differentiating factor of this method is that the researcher relies on collecting data firsthand instead of relying on data collected from previous research.
There are multiple types of primary quantitative research. They can be distinguished based on three distinctive aspects, which are:
A. Techniques & Types of Studies:
- Survey Research
Surveys are the easiest, most common, and one of the most sought-after quantitative research techniques. The main aim of a survey is to widely gather and describe the characteristics of a target population or customers. Surveys are the foremost quantitative method preferred by both small and large organizations.
They help them understand their customers, products, and other brand offerings in a proper manner.
Surveys can be conducted using various methods, such as online polls, web-based surveys, paper questionnaires, phone calls, or face-to-face interviews. Survey research allows organizations to understand customer opinions, preferences, and behavior, making it crucial for market research and decision-making.
You can watch this quick video to learn more about creating surveys.
Surveys are of two types:
- Cross-Sectional Surveys Cross-sectional surveys are used to collect data from a sample of the target population at a specific point in time. Researchers evaluate various variables simultaneously to understand the relationships and patterns within the data.
- Cross-sectional surveys are popular in retail, small and medium-sized enterprises (SMEs), and healthcare industries, where they assess customer satisfaction, market trends, and product feedback.
- Longitudinal Surveys Longitudinal surveys are conducted over an extended period, observing changes in respondent behavior and thought processes.
- Researchers gather data from the same sample multiple times, enabling them to study trends and developments over time. These surveys are valuable in fields such as medicine, applied sciences, and market trend analysis.
Surveys can be distributed via various channels. Some of the most popular ones are listed below:
- Email: Sending surveys via email is a popular and effective method. People recognize your brand, leading to a higher response rate. With ProProfs Survey Maker’s in-mail survey-filling feature, you can easily send out and collect survey responses.
- Embed on a website: Boost your response rate by embedding the survey on your website. When visitors are already engaged with your brand, they are more likely to take the survey.
- Social media: Take advantage of social media platforms to distribute your survey. People familiar with your brand are likely to respond, increasing your response numbers.
- QR codes: QR codes store your survey’s URL, and you can print or publish these codes in magazines, signs, business cards, or any object to make it easy for people to access your survey.
- SMS survey: Collect a high number of responses quickly with SMS surveys. It’s a time-effective way to reach your target audience.
Read More: 24 Different Types of Survey Methods With Examples
2. Correlational Research:
Correlational research aims to establish relationships between two or more variables.
Researchers use statistical analysis to identify patterns and trends in the data, but it does not determine causality between the variables. This method helps understand how changes in one variable may impact another.
Examples of correlational research questions include studying the relationship between stress and depression, fame and money, or classroom activities and student performance.
3. Causal-Comparative Research:
Causal-comparative research, also known as quasi-experimental research, seeks to determine cause-and-effect relationships between variables.
Researchers analyze how an independent variable influences a dependent variable, but they do not manipulate the independent variable. Instead, they observe and compare different groups to draw conclusions.
Causal-comparative research is useful in situations where it’s not ethical or feasible to conduct true experiments.
Examples of questions for this type of research include analyzing the effect of training programs on employee performance, studying the influence of customer support on client retention, investigating the impact of supply chain efficiency on cost reduction, etc.
4. Experimental Research:
Experimental research is based on testing theories to validate or disprove them. Researchers conduct experiments and manipulate variables to observe their impact on the outcomes.
This type of research is prevalent in natural and social sciences, and it is a powerful method to establish cause-and-effect relationships. By randomly assigning participants to experimental and control groups, researchers can draw more confident conclusions.
Examples of experimental research include studying the effectiveness of a new drug, the impact of teaching methods on student performance, or the outcomes of a marketing campaign.
B. Data collection methodologies
After defining research objectives, the next significant step in primary quantitative research is data collection. This involves using two main methods: sampling and conducting surveys or polls.
Sampling methods:
In quantitative research, there are two primary sampling methods: Probability and Non-probability sampling.
Probability Sampling
In probability sampling, researchers use the concept of probability to create samples from a population. This method ensures that every individual in the target audience has an equal chance of being selected for the sample.
There are four main types of probability sampling:
- Simple random sampling: Here, the elements or participants of a sample are selected randomly, and this technique is used in studies that are conducted over considerably large audiences. It works well for large target populations.
- Stratified random sampling: In this method, the entire population is divided into strata or groups, and the sample members get chosen randomly from these strata only. It is always ensured that different segregated strata do not overlap with each other.
- Cluster sampling: Here, researchers divide the population into clusters, often based on geography or demographics. Then, random clusters are selected for the sample.
- Systematic sampling: In this method, only the starting point of the sample is randomly chosen. All the other participants are chosen using a fixed interval. Researchers calculate this interval by dividing the size of the study population by the target sample size.
Non-probability Sampling
Non-probability sampling is a method where the researcher’s knowledge and experience guide the selection of samples. This approach doesn’t give all members of the target population an equal chance of being included in the sample.
There are five non-probability sampling models:
- Convenience sampling: The elements or participants are chosen on the basis of their nearness to the researcher. The people in close proximity can be studied and analyzed easily and quickly, as there is no other selection criterion involved. Researchers simply choose samples based on what is most convenient for them.
- Consecutive sampling: Similar to convenience sampling, researchers select samples one after another over a significant period. They can opt for a single participant or a group of samples to conduct quantitative research in a consecutive manner for a significant period of time. Once this is over, they can conduct the research from the start.
- Quota sampling: With quota sampling, researchers use their understanding of target traits and personalities to form groups (strata). They then choose samples from each stratum based on their own judgment.
- Snowball sampling: This method is used where the target audiences are difficult to contact and interviewed for data collection. Researchers start with a few participants and then ask them to refer others, creating a snowball effect.
- Judgmental sampling: In judgmental sampling, researchers rely solely on their experience and research skills to handpick samples that they believe will be most relevant to the study.
Read More: Data Collection Methods: Definition, Types & Examples
C. Data analysis techniques
To analyze the quantitative data accurately, you’ll need to use specific statistical methods such as:
- SWOT Analysis: This stands for Strengths, Weaknesses, Opportunities, and Threats analysis. Organizations use SWOT analysis to evaluate their performance internally and externally. It helps develop effective improvement strategies.
- Conjoint Analysis: This market research method uncovers how individuals make complex purchasing decisions. It involves considering trade-offs in their daily activities when choosing from a list of product/service options.
- Cross-tabulation: A preliminary statistical market analysis method that reveals relationships, patterns, and trends within various research study parameters.
- TURF Analysis: Short for Totally Unduplicated Reach and Frequency Analysis, this method helps analyze the reach and frequency of favorable communication sources. It provides insights into the potential of a target market.
- By using these statistical techniques and inferential statistics methods like confidence intervals and margin of error, you can draw meaningful insights from your primary quantitative research that you can use in making informed decisions.
II. Secondary Quantitative Research Methods
- Secondary quantitative research, also known as desk research, is a valuable method that uses existing data, called secondary data.
- Instead of collecting new data, researchers analyze and combine already available information to enhance their research. This approach involves gathering quantitative data from various sources such as the internet, government databases, libraries, and research reports.
- Secondary quantitative research plays a crucial role in validating data collected through primary quantitative research. It helps reinforce or challenge existing findings.
Here are five commonly used secondary quantitative research methods:
A. Data Available on the Internet:
The Internet has become a vast repository of data, making it easier for researchers to access a wealth of information. Online databases, websites, and research repositories provide valuable quantitative data for researchers to analyze and validate their primary research findings.
B. Government and Non-Government Sources:
Government agencies and non-government organizations often conduct extensive research and publish reports. These reports cover a wide range of topics, providing researchers with reliable and comprehensive data for quantitative analysis.
C. Public Libraries:
While less commonly used in the digital age, public libraries still hold valuable research reports, historical data, and publications that can contribute to quantitative research.
D. Educational Institutions:
Educational institutions frequently conduct research on various subjects. Their research reports and publications can serve as valuable sources of information for researchers, validating and supporting primary quantitative research outcomes.
E. Commercial Information Sources:
Commercial sources such as local newspapers, journals, magazines, and media outlets often publish relevant data on economic trends, market research, and demographic analyses. Researchers can access this data to supplement their own findings and draw better conclusions.
Advantages of Quantitative Research Methods
Quantitative research data is often standardized and can be easily used to generalize findings for making crucial business decisions and uncover insights to supplement the qualitative research findings.
Here are some core benefits this research methodology offers.
Direct Result Comparison
As the studies can be replicated for different cultural settings and different times, even with different groups of participants, they tend to be extremely useful. Researchers can compare the results of different studies in a statistical manner and arrive at comprehensive conclusions for a broader understanding.
Replication
Researchers can repeat the study by using standardized data collection protocols over well-structured data sets. They can also apply tangible definitions of abstract concepts to arrive at different conclusions for similar research objectives with minor variations.
Large Samples
As the research data comes from large samples, the researchers can process and analyze the data via highly reliable and consistent analysis procedures. They can arrive at well-defined conclusions that can be used to make the primary research more thorough and reliable.
Hypothesis Testing
This research methodology follows standardized and established hypothesis testing procedures. So, you have to be careful while reporting and analyzing your research data , and the overall quality of results gets improved.
Proven Examples of Quantitative Research Methods
Below, we discuss two excellent examples of quantitative research methods that were used by highly distinguished business and consulting organizations. Both examples show how different types of analysis can be performed with qualitative approaches and how the analysis is done once the data is collected.
1. STEP Project Global Consortium / KPMG 2019 Global Family Business survey
This research utilized quantitative methods to identify ways that kept the family businesses sustainably profitable with time.
The study also identified the ways in which the family business behavior changed with demographic changes and had “why” and “how” questions. Their qualitative research methods allowed the KPMG team to dig deeper into the mindsets and perspectives of the business owners and uncover unexpected research avenues as well.
It was a joint effort in which STEP Project Global Consortium collected 26 cases, and KPMG collected 11 cases.
The research reached the stage of data analysis in 2020, and the analysis process spanned over 4 stages.
The results, which were also the reasons why family businesses tend to lose their strength with time, were found to be:
- Family governance
- Family business legacy
2. EY Seren Teams Research 2020
This is yet another commendable example of qualitative research where the EY Seren Team digs into the unexplored depths of human behavior and how it affected their brand or service expectations.
The research was done across 200+ sources and involved in-depth virtual interviews with people in their homes, exploring their current needs and wishes. It also involved diary studies across the entire UK customer base to analyze human behavior changes and patterns.
The study also included interviews with professionals and design leaders from a wide range of industries to explore how COVID-19 transformed their industries. Finally, quantitative surveys were conducted to gain insights into the EY community after every 15 days.
The insights and results were:
- A culture of fear, daily resilience, and hopes for a better world and a better life – these were the macro trends.
- People felt massive digitization to be a resourceful yet demanding aspect as they have to adapt every day.
- Some people wished to have a new world with lots of possibilities, and some were looking for a new purpose.
Enhance Your Quantitative Research With Cutting-Edge Software
While no single research methodology can produce 100% reliable results, you can always opt for a hybrid research method by opting for the methods that are most relevant to your objective.
This understanding comes gradually as you learn how to implement the correct combination of qualitative and quantitative research methods for your research projects. For the best results, we recommend investing in smart, efficient, and scalable research tools that come with delightful reporting and advanced analytics to make every research initiative a success.
These software tools, such as ProProfs Survey Maker, come with pre-built survey templates and question libraries and allow you to create a high-converting survey in just a few minutes.
So, choose the best research partner, create the right research plan, and gather insights that drive sustainable growth for your business.
About the author
Jared cornell.
Jared is a customer support expert. He has been published in CrazyEgg , Foundr , and CXL . As a customer support executive at ProProfs, he has been instrumental in developing a complete customer support system that more than doubled customer satisfaction. You can connect and engage with Jared on Twitter , Facebook , and LinkedIn .
Popular Posts in This Category
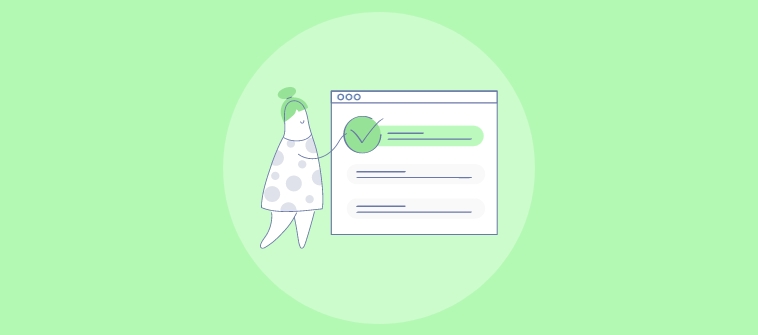
Client Onboarding Questionnaire: Best Practices & 20+ Questions
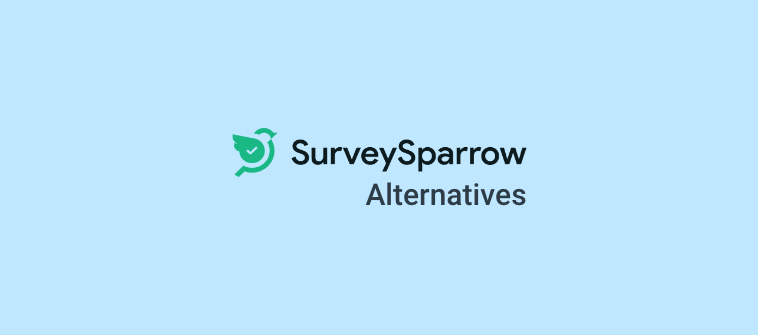
11 Best SurveySparrow Alternatives & Competitors in 2024
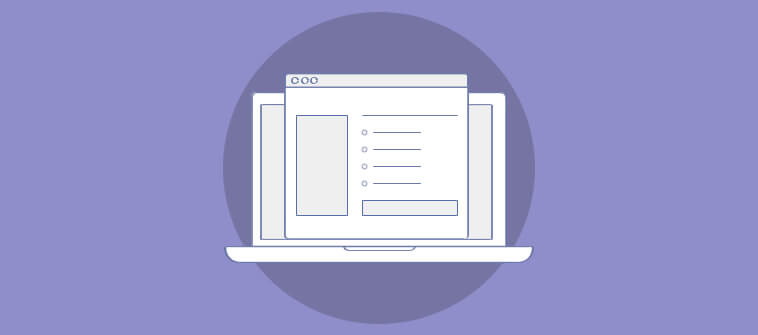
Create an Online Survey in 8 Easy Steps: A Detailed Guide
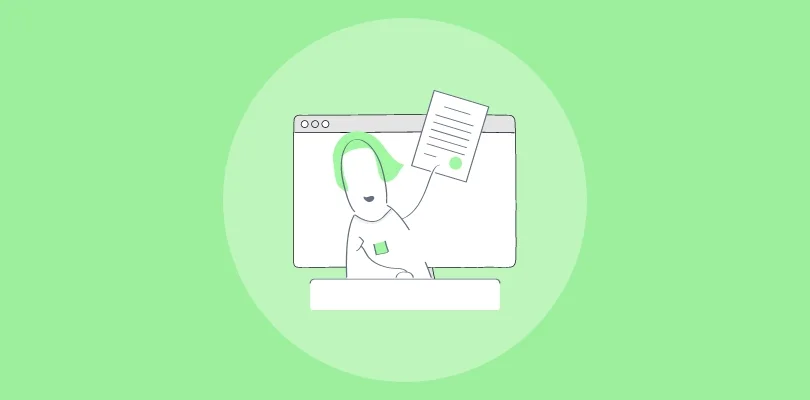
16 Advantages & Disadvantages of Questionnaires
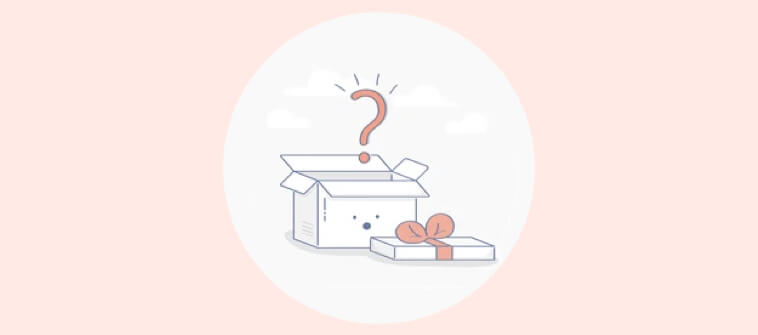
How Many Questions Should Be Asked in a Survey?
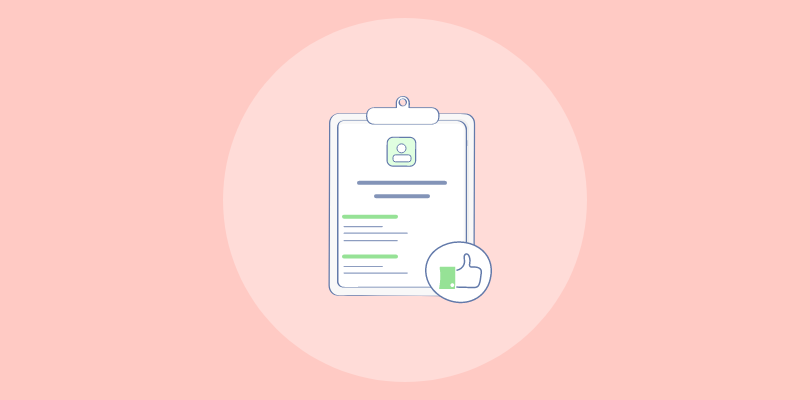
Create the Perfect Employee Evaluation Form: Templates and Detailed Guide

An official website of the United States government
The .gov means it’s official. Federal government websites often end in .gov or .mil. Before sharing sensitive information, make sure you’re on a federal government site.
The site is secure. The https:// ensures that you are connecting to the official website and that any information you provide is encrypted and transmitted securely.
- Publications
- Account settings
Preview improvements coming to the PMC website in October 2024. Learn More or Try it out now .
- Advanced Search
- Journal List
- Indian J Anaesth
- v.60(9); 2016 Sep
Basic statistical tools in research and data analysis
Zulfiqar ali.
Department of Anaesthesiology, Division of Neuroanaesthesiology, Sheri Kashmir Institute of Medical Sciences, Soura, Srinagar, Jammu and Kashmir, India
S Bala Bhaskar
1 Department of Anaesthesiology and Critical Care, Vijayanagar Institute of Medical Sciences, Bellary, Karnataka, India
Statistical methods involved in carrying out a study include planning, designing, collecting data, analysing, drawing meaningful interpretation and reporting of the research findings. The statistical analysis gives meaning to the meaningless numbers, thereby breathing life into a lifeless data. The results and inferences are precise only if proper statistical tests are used. This article will try to acquaint the reader with the basic research tools that are utilised while conducting various studies. The article covers a brief outline of the variables, an understanding of quantitative and qualitative variables and the measures of central tendency. An idea of the sample size estimation, power analysis and the statistical errors is given. Finally, there is a summary of parametric and non-parametric tests used for data analysis.
INTRODUCTION
Statistics is a branch of science that deals with the collection, organisation, analysis of data and drawing of inferences from the samples to the whole population.[ 1 ] This requires a proper design of the study, an appropriate selection of the study sample and choice of a suitable statistical test. An adequate knowledge of statistics is necessary for proper designing of an epidemiological study or a clinical trial. Improper statistical methods may result in erroneous conclusions which may lead to unethical practice.[ 2 ]
Variable is a characteristic that varies from one individual member of population to another individual.[ 3 ] Variables such as height and weight are measured by some type of scale, convey quantitative information and are called as quantitative variables. Sex and eye colour give qualitative information and are called as qualitative variables[ 3 ] [ Figure 1 ].

Classification of variables
Quantitative variables
Quantitative or numerical data are subdivided into discrete and continuous measurements. Discrete numerical data are recorded as a whole number such as 0, 1, 2, 3,… (integer), whereas continuous data can assume any value. Observations that can be counted constitute the discrete data and observations that can be measured constitute the continuous data. Examples of discrete data are number of episodes of respiratory arrests or the number of re-intubations in an intensive care unit. Similarly, examples of continuous data are the serial serum glucose levels, partial pressure of oxygen in arterial blood and the oesophageal temperature.
A hierarchical scale of increasing precision can be used for observing and recording the data which is based on categorical, ordinal, interval and ratio scales [ Figure 1 ].
Categorical or nominal variables are unordered. The data are merely classified into categories and cannot be arranged in any particular order. If only two categories exist (as in gender male and female), it is called as a dichotomous (or binary) data. The various causes of re-intubation in an intensive care unit due to upper airway obstruction, impaired clearance of secretions, hypoxemia, hypercapnia, pulmonary oedema and neurological impairment are examples of categorical variables.
Ordinal variables have a clear ordering between the variables. However, the ordered data may not have equal intervals. Examples are the American Society of Anesthesiologists status or Richmond agitation-sedation scale.
Interval variables are similar to an ordinal variable, except that the intervals between the values of the interval variable are equally spaced. A good example of an interval scale is the Fahrenheit degree scale used to measure temperature. With the Fahrenheit scale, the difference between 70° and 75° is equal to the difference between 80° and 85°: The units of measurement are equal throughout the full range of the scale.
Ratio scales are similar to interval scales, in that equal differences between scale values have equal quantitative meaning. However, ratio scales also have a true zero point, which gives them an additional property. For example, the system of centimetres is an example of a ratio scale. There is a true zero point and the value of 0 cm means a complete absence of length. The thyromental distance of 6 cm in an adult may be twice that of a child in whom it may be 3 cm.
STATISTICS: DESCRIPTIVE AND INFERENTIAL STATISTICS
Descriptive statistics[ 4 ] try to describe the relationship between variables in a sample or population. Descriptive statistics provide a summary of data in the form of mean, median and mode. Inferential statistics[ 4 ] use a random sample of data taken from a population to describe and make inferences about the whole population. It is valuable when it is not possible to examine each member of an entire population. The examples if descriptive and inferential statistics are illustrated in Table 1 .
Example of descriptive and inferential statistics

Descriptive statistics
The extent to which the observations cluster around a central location is described by the central tendency and the spread towards the extremes is described by the degree of dispersion.
Measures of central tendency
The measures of central tendency are mean, median and mode.[ 6 ] Mean (or the arithmetic average) is the sum of all the scores divided by the number of scores. Mean may be influenced profoundly by the extreme variables. For example, the average stay of organophosphorus poisoning patients in ICU may be influenced by a single patient who stays in ICU for around 5 months because of septicaemia. The extreme values are called outliers. The formula for the mean is

where x = each observation and n = number of observations. Median[ 6 ] is defined as the middle of a distribution in a ranked data (with half of the variables in the sample above and half below the median value) while mode is the most frequently occurring variable in a distribution. Range defines the spread, or variability, of a sample.[ 7 ] It is described by the minimum and maximum values of the variables. If we rank the data and after ranking, group the observations into percentiles, we can get better information of the pattern of spread of the variables. In percentiles, we rank the observations into 100 equal parts. We can then describe 25%, 50%, 75% or any other percentile amount. The median is the 50 th percentile. The interquartile range will be the observations in the middle 50% of the observations about the median (25 th -75 th percentile). Variance[ 7 ] is a measure of how spread out is the distribution. It gives an indication of how close an individual observation clusters about the mean value. The variance of a population is defined by the following formula:

where σ 2 is the population variance, X is the population mean, X i is the i th element from the population and N is the number of elements in the population. The variance of a sample is defined by slightly different formula:

where s 2 is the sample variance, x is the sample mean, x i is the i th element from the sample and n is the number of elements in the sample. The formula for the variance of a population has the value ‘ n ’ as the denominator. The expression ‘ n −1’ is known as the degrees of freedom and is one less than the number of parameters. Each observation is free to vary, except the last one which must be a defined value. The variance is measured in squared units. To make the interpretation of the data simple and to retain the basic unit of observation, the square root of variance is used. The square root of the variance is the standard deviation (SD).[ 8 ] The SD of a population is defined by the following formula:

where σ is the population SD, X is the population mean, X i is the i th element from the population and N is the number of elements in the population. The SD of a sample is defined by slightly different formula:

where s is the sample SD, x is the sample mean, x i is the i th element from the sample and n is the number of elements in the sample. An example for calculation of variation and SD is illustrated in Table 2 .
Example of mean, variance, standard deviation

Normal distribution or Gaussian distribution
Most of the biological variables usually cluster around a central value, with symmetrical positive and negative deviations about this point.[ 1 ] The standard normal distribution curve is a symmetrical bell-shaped. In a normal distribution curve, about 68% of the scores are within 1 SD of the mean. Around 95% of the scores are within 2 SDs of the mean and 99% within 3 SDs of the mean [ Figure 2 ].

Normal distribution curve
Skewed distribution
It is a distribution with an asymmetry of the variables about its mean. In a negatively skewed distribution [ Figure 3 ], the mass of the distribution is concentrated on the right of Figure 1 . In a positively skewed distribution [ Figure 3 ], the mass of the distribution is concentrated on the left of the figure leading to a longer right tail.

Curves showing negatively skewed and positively skewed distribution
Inferential statistics
In inferential statistics, data are analysed from a sample to make inferences in the larger collection of the population. The purpose is to answer or test the hypotheses. A hypothesis (plural hypotheses) is a proposed explanation for a phenomenon. Hypothesis tests are thus procedures for making rational decisions about the reality of observed effects.
Probability is the measure of the likelihood that an event will occur. Probability is quantified as a number between 0 and 1 (where 0 indicates impossibility and 1 indicates certainty).
In inferential statistics, the term ‘null hypothesis’ ( H 0 ‘ H-naught ,’ ‘ H-null ’) denotes that there is no relationship (difference) between the population variables in question.[ 9 ]
Alternative hypothesis ( H 1 and H a ) denotes that a statement between the variables is expected to be true.[ 9 ]
The P value (or the calculated probability) is the probability of the event occurring by chance if the null hypothesis is true. The P value is a numerical between 0 and 1 and is interpreted by researchers in deciding whether to reject or retain the null hypothesis [ Table 3 ].
P values with interpretation

If P value is less than the arbitrarily chosen value (known as α or the significance level), the null hypothesis (H0) is rejected [ Table 4 ]. However, if null hypotheses (H0) is incorrectly rejected, this is known as a Type I error.[ 11 ] Further details regarding alpha error, beta error and sample size calculation and factors influencing them are dealt with in another section of this issue by Das S et al .[ 12 ]
Illustration for null hypothesis

PARAMETRIC AND NON-PARAMETRIC TESTS
Numerical data (quantitative variables) that are normally distributed are analysed with parametric tests.[ 13 ]
Two most basic prerequisites for parametric statistical analysis are:
- The assumption of normality which specifies that the means of the sample group are normally distributed
- The assumption of equal variance which specifies that the variances of the samples and of their corresponding population are equal.
However, if the distribution of the sample is skewed towards one side or the distribution is unknown due to the small sample size, non-parametric[ 14 ] statistical techniques are used. Non-parametric tests are used to analyse ordinal and categorical data.
Parametric tests
The parametric tests assume that the data are on a quantitative (numerical) scale, with a normal distribution of the underlying population. The samples have the same variance (homogeneity of variances). The samples are randomly drawn from the population, and the observations within a group are independent of each other. The commonly used parametric tests are the Student's t -test, analysis of variance (ANOVA) and repeated measures ANOVA.
Student's t -test
Student's t -test is used to test the null hypothesis that there is no difference between the means of the two groups. It is used in three circumstances:

where X = sample mean, u = population mean and SE = standard error of mean

where X 1 − X 2 is the difference between the means of the two groups and SE denotes the standard error of the difference.
- To test if the population means estimated by two dependent samples differ significantly (the paired t -test). A usual setting for paired t -test is when measurements are made on the same subjects before and after a treatment.
The formula for paired t -test is:

where d is the mean difference and SE denotes the standard error of this difference.
The group variances can be compared using the F -test. The F -test is the ratio of variances (var l/var 2). If F differs significantly from 1.0, then it is concluded that the group variances differ significantly.
Analysis of variance
The Student's t -test cannot be used for comparison of three or more groups. The purpose of ANOVA is to test if there is any significant difference between the means of two or more groups.
In ANOVA, we study two variances – (a) between-group variability and (b) within-group variability. The within-group variability (error variance) is the variation that cannot be accounted for in the study design. It is based on random differences present in our samples.
However, the between-group (or effect variance) is the result of our treatment. These two estimates of variances are compared using the F-test.
A simplified formula for the F statistic is:

where MS b is the mean squares between the groups and MS w is the mean squares within groups.
Repeated measures analysis of variance
As with ANOVA, repeated measures ANOVA analyses the equality of means of three or more groups. However, a repeated measure ANOVA is used when all variables of a sample are measured under different conditions or at different points in time.
As the variables are measured from a sample at different points of time, the measurement of the dependent variable is repeated. Using a standard ANOVA in this case is not appropriate because it fails to model the correlation between the repeated measures: The data violate the ANOVA assumption of independence. Hence, in the measurement of repeated dependent variables, repeated measures ANOVA should be used.
Non-parametric tests
When the assumptions of normality are not met, and the sample means are not normally, distributed parametric tests can lead to erroneous results. Non-parametric tests (distribution-free test) are used in such situation as they do not require the normality assumption.[ 15 ] Non-parametric tests may fail to detect a significant difference when compared with a parametric test. That is, they usually have less power.
As is done for the parametric tests, the test statistic is compared with known values for the sampling distribution of that statistic and the null hypothesis is accepted or rejected. The types of non-parametric analysis techniques and the corresponding parametric analysis techniques are delineated in Table 5 .
Analogue of parametric and non-parametric tests

Median test for one sample: The sign test and Wilcoxon's signed rank test
The sign test and Wilcoxon's signed rank test are used for median tests of one sample. These tests examine whether one instance of sample data is greater or smaller than the median reference value.
This test examines the hypothesis about the median θ0 of a population. It tests the null hypothesis H0 = θ0. When the observed value (Xi) is greater than the reference value (θ0), it is marked as+. If the observed value is smaller than the reference value, it is marked as − sign. If the observed value is equal to the reference value (θ0), it is eliminated from the sample.
If the null hypothesis is true, there will be an equal number of + signs and − signs.
The sign test ignores the actual values of the data and only uses + or − signs. Therefore, it is useful when it is difficult to measure the values.
Wilcoxon's signed rank test
There is a major limitation of sign test as we lose the quantitative information of the given data and merely use the + or – signs. Wilcoxon's signed rank test not only examines the observed values in comparison with θ0 but also takes into consideration the relative sizes, adding more statistical power to the test. As in the sign test, if there is an observed value that is equal to the reference value θ0, this observed value is eliminated from the sample.
Wilcoxon's rank sum test ranks all data points in order, calculates the rank sum of each sample and compares the difference in the rank sums.
Mann-Whitney test
It is used to test the null hypothesis that two samples have the same median or, alternatively, whether observations in one sample tend to be larger than observations in the other.
Mann–Whitney test compares all data (xi) belonging to the X group and all data (yi) belonging to the Y group and calculates the probability of xi being greater than yi: P (xi > yi). The null hypothesis states that P (xi > yi) = P (xi < yi) =1/2 while the alternative hypothesis states that P (xi > yi) ≠1/2.
Kolmogorov-Smirnov test
The two-sample Kolmogorov-Smirnov (KS) test was designed as a generic method to test whether two random samples are drawn from the same distribution. The null hypothesis of the KS test is that both distributions are identical. The statistic of the KS test is a distance between the two empirical distributions, computed as the maximum absolute difference between their cumulative curves.
Kruskal-Wallis test
The Kruskal–Wallis test is a non-parametric test to analyse the variance.[ 14 ] It analyses if there is any difference in the median values of three or more independent samples. The data values are ranked in an increasing order, and the rank sums calculated followed by calculation of the test statistic.
Jonckheere test
In contrast to Kruskal–Wallis test, in Jonckheere test, there is an a priori ordering that gives it a more statistical power than the Kruskal–Wallis test.[ 14 ]
Friedman test
The Friedman test is a non-parametric test for testing the difference between several related samples. The Friedman test is an alternative for repeated measures ANOVAs which is used when the same parameter has been measured under different conditions on the same subjects.[ 13 ]
Tests to analyse the categorical data
Chi-square test, Fischer's exact test and McNemar's test are used to analyse the categorical or nominal variables. The Chi-square test compares the frequencies and tests whether the observed data differ significantly from that of the expected data if there were no differences between groups (i.e., the null hypothesis). It is calculated by the sum of the squared difference between observed ( O ) and the expected ( E ) data (or the deviation, d ) divided by the expected data by the following formula:

A Yates correction factor is used when the sample size is small. Fischer's exact test is used to determine if there are non-random associations between two categorical variables. It does not assume random sampling, and instead of referring a calculated statistic to a sampling distribution, it calculates an exact probability. McNemar's test is used for paired nominal data. It is applied to 2 × 2 table with paired-dependent samples. It is used to determine whether the row and column frequencies are equal (that is, whether there is ‘marginal homogeneity’). The null hypothesis is that the paired proportions are equal. The Mantel-Haenszel Chi-square test is a multivariate test as it analyses multiple grouping variables. It stratifies according to the nominated confounding variables and identifies any that affects the primary outcome variable. If the outcome variable is dichotomous, then logistic regression is used.
SOFTWARES AVAILABLE FOR STATISTICS, SAMPLE SIZE CALCULATION AND POWER ANALYSIS
Numerous statistical software systems are available currently. The commonly used software systems are Statistical Package for the Social Sciences (SPSS – manufactured by IBM corporation), Statistical Analysis System ((SAS – developed by SAS Institute North Carolina, United States of America), R (designed by Ross Ihaka and Robert Gentleman from R core team), Minitab (developed by Minitab Inc), Stata (developed by StataCorp) and the MS Excel (developed by Microsoft).
There are a number of web resources which are related to statistical power analyses. A few are:
- StatPages.net – provides links to a number of online power calculators
- G-Power – provides a downloadable power analysis program that runs under DOS
- Power analysis for ANOVA designs an interactive site that calculates power or sample size needed to attain a given power for one effect in a factorial ANOVA design
- SPSS makes a program called SamplePower. It gives an output of a complete report on the computer screen which can be cut and paste into another document.
It is important that a researcher knows the concepts of the basic statistical methods used for conduct of a research study. This will help to conduct an appropriately well-designed study leading to valid and reliable results. Inappropriate use of statistical techniques may lead to faulty conclusions, inducing errors and undermining the significance of the article. Bad statistics may lead to bad research, and bad research may lead to unethical practice. Hence, an adequate knowledge of statistics and the appropriate use of statistical tests are important. An appropriate knowledge about the basic statistical methods will go a long way in improving the research designs and producing quality medical research which can be utilised for formulating the evidence-based guidelines.
Financial support and sponsorship
Conflicts of interest.
There are no conflicts of interest.
Qualitative vs Quantitative Research Methods & Data Analysis
Saul Mcleod, PhD
Editor-in-Chief for Simply Psychology
BSc (Hons) Psychology, MRes, PhD, University of Manchester
Saul Mcleod, PhD., is a qualified psychology teacher with over 18 years of experience in further and higher education. He has been published in peer-reviewed journals, including the Journal of Clinical Psychology.
Learn about our Editorial Process
Olivia Guy-Evans, MSc
Associate Editor for Simply Psychology
BSc (Hons) Psychology, MSc Psychology of Education
Olivia Guy-Evans is a writer and associate editor for Simply Psychology. She has previously worked in healthcare and educational sectors.
On This Page:
What is the difference between quantitative and qualitative?
The main difference between quantitative and qualitative research is the type of data they collect and analyze.
Quantitative research collects numerical data and analyzes it using statistical methods. The aim is to produce objective, empirical data that can be measured and expressed in numerical terms. Quantitative research is often used to test hypotheses, identify patterns, and make predictions.
Qualitative research, on the other hand, collects non-numerical data such as words, images, and sounds. The focus is on exploring subjective experiences, opinions, and attitudes, often through observation and interviews.
Qualitative research aims to produce rich and detailed descriptions of the phenomenon being studied, and to uncover new insights and meanings.
Quantitative data is information about quantities, and therefore numbers, and qualitative data is descriptive, and regards phenomenon which can be observed but not measured, such as language.
What Is Qualitative Research?
Qualitative research is the process of collecting, analyzing, and interpreting non-numerical data, such as language. Qualitative research can be used to understand how an individual subjectively perceives and gives meaning to their social reality.
Qualitative data is non-numerical data, such as text, video, photographs, or audio recordings. This type of data can be collected using diary accounts or in-depth interviews and analyzed using grounded theory or thematic analysis.
Qualitative research is multimethod in focus, involving an interpretive, naturalistic approach to its subject matter. This means that qualitative researchers study things in their natural settings, attempting to make sense of, or interpret, phenomena in terms of the meanings people bring to them. Denzin and Lincoln (1994, p. 2)
Interest in qualitative data came about as the result of the dissatisfaction of some psychologists (e.g., Carl Rogers) with the scientific study of psychologists such as behaviorists (e.g., Skinner ).
Since psychologists study people, the traditional approach to science is not seen as an appropriate way of carrying out research since it fails to capture the totality of human experience and the essence of being human. Exploring participants’ experiences is known as a phenomenological approach (re: Humanism ).
Qualitative research is primarily concerned with meaning, subjectivity, and lived experience. The goal is to understand the quality and texture of people’s experiences, how they make sense of them, and the implications for their lives.
Qualitative research aims to understand the social reality of individuals, groups, and cultures as nearly as possible as participants feel or live it. Thus, people and groups are studied in their natural setting.
Some examples of qualitative research questions are provided, such as what an experience feels like, how people talk about something, how they make sense of an experience, and how events unfold for people.
Research following a qualitative approach is exploratory and seeks to explain ‘how’ and ‘why’ a particular phenomenon, or behavior, operates as it does in a particular context. It can be used to generate hypotheses and theories from the data.
Qualitative Methods
There are different types of qualitative research methods, including diary accounts, in-depth interviews , documents, focus groups , case study research , and ethnography.
The results of qualitative methods provide a deep understanding of how people perceive their social realities and in consequence, how they act within the social world.
The researcher has several methods for collecting empirical materials, ranging from the interview to direct observation, to the analysis of artifacts, documents, and cultural records, to the use of visual materials or personal experience. Denzin and Lincoln (1994, p. 14)
Here are some examples of qualitative data:
Interview transcripts : Verbatim records of what participants said during an interview or focus group. They allow researchers to identify common themes and patterns, and draw conclusions based on the data. Interview transcripts can also be useful in providing direct quotes and examples to support research findings.
Observations : The researcher typically takes detailed notes on what they observe, including any contextual information, nonverbal cues, or other relevant details. The resulting observational data can be analyzed to gain insights into social phenomena, such as human behavior, social interactions, and cultural practices.
Unstructured interviews : generate qualitative data through the use of open questions. This allows the respondent to talk in some depth, choosing their own words. This helps the researcher develop a real sense of a person’s understanding of a situation.
Diaries or journals : Written accounts of personal experiences or reflections.
Notice that qualitative data could be much more than just words or text. Photographs, videos, sound recordings, and so on, can be considered qualitative data. Visual data can be used to understand behaviors, environments, and social interactions.
Qualitative Data Analysis
Qualitative research is endlessly creative and interpretive. The researcher does not just leave the field with mountains of empirical data and then easily write up his or her findings.
Qualitative interpretations are constructed, and various techniques can be used to make sense of the data, such as content analysis, grounded theory (Glaser & Strauss, 1967), thematic analysis (Braun & Clarke, 2006), or discourse analysis.
For example, thematic analysis is a qualitative approach that involves identifying implicit or explicit ideas within the data. Themes will often emerge once the data has been coded.
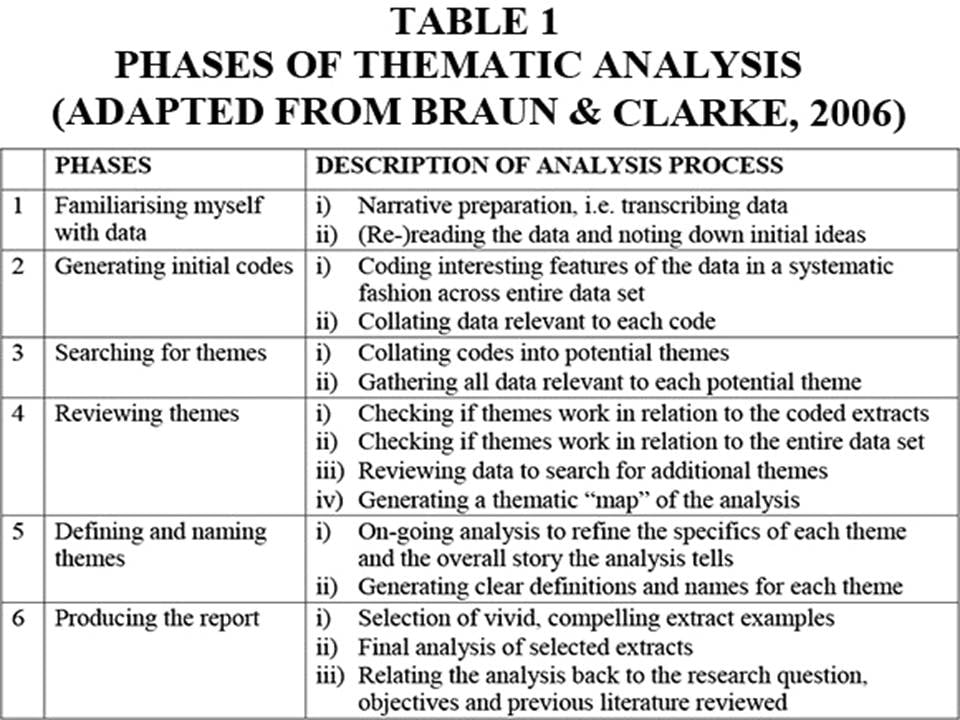
Key Features
- Events can be understood adequately only if they are seen in context. Therefore, a qualitative researcher immerses her/himself in the field, in natural surroundings. The contexts of inquiry are not contrived; they are natural. Nothing is predefined or taken for granted.
- Qualitative researchers want those who are studied to speak for themselves, to provide their perspectives in words and other actions. Therefore, qualitative research is an interactive process in which the persons studied teach the researcher about their lives.
- The qualitative researcher is an integral part of the data; without the active participation of the researcher, no data exists.
- The study’s design evolves during the research and can be adjusted or changed as it progresses. For the qualitative researcher, there is no single reality. It is subjective and exists only in reference to the observer.
- The theory is data-driven and emerges as part of the research process, evolving from the data as they are collected.
Limitations of Qualitative Research
- Because of the time and costs involved, qualitative designs do not generally draw samples from large-scale data sets.
- The problem of adequate validity or reliability is a major criticism. Because of the subjective nature of qualitative data and its origin in single contexts, it is difficult to apply conventional standards of reliability and validity. For example, because of the central role played by the researcher in the generation of data, it is not possible to replicate qualitative studies.
- Also, contexts, situations, events, conditions, and interactions cannot be replicated to any extent, nor can generalizations be made to a wider context than the one studied with confidence.
- The time required for data collection, analysis, and interpretation is lengthy. Analysis of qualitative data is difficult, and expert knowledge of an area is necessary to interpret qualitative data. Great care must be taken when doing so, for example, looking for mental illness symptoms.
Advantages of Qualitative Research
- Because of close researcher involvement, the researcher gains an insider’s view of the field. This allows the researcher to find issues that are often missed (such as subtleties and complexities) by the scientific, more positivistic inquiries.
- Qualitative descriptions can be important in suggesting possible relationships, causes, effects, and dynamic processes.
- Qualitative analysis allows for ambiguities/contradictions in the data, which reflect social reality (Denscombe, 2010).
- Qualitative research uses a descriptive, narrative style; this research might be of particular benefit to the practitioner as she or he could turn to qualitative reports to examine forms of knowledge that might otherwise be unavailable, thereby gaining new insight.
What Is Quantitative Research?
Quantitative research involves the process of objectively collecting and analyzing numerical data to describe, predict, or control variables of interest.
The goals of quantitative research are to test causal relationships between variables , make predictions, and generalize results to wider populations.
Quantitative researchers aim to establish general laws of behavior and phenomenon across different settings/contexts. Research is used to test a theory and ultimately support or reject it.
Quantitative Methods
Experiments typically yield quantitative data, as they are concerned with measuring things. However, other research methods, such as controlled observations and questionnaires , can produce both quantitative information.
For example, a rating scale or closed questions on a questionnaire would generate quantitative data as these produce either numerical data or data that can be put into categories (e.g., “yes,” “no” answers).
Experimental methods limit how research participants react to and express appropriate social behavior.
Findings are, therefore, likely to be context-bound and simply a reflection of the assumptions that the researcher brings to the investigation.
There are numerous examples of quantitative data in psychological research, including mental health. Here are a few examples:
Another example is the Experience in Close Relationships Scale (ECR), a self-report questionnaire widely used to assess adult attachment styles .
The ECR provides quantitative data that can be used to assess attachment styles and predict relationship outcomes.
Neuroimaging data : Neuroimaging techniques, such as MRI and fMRI, provide quantitative data on brain structure and function.
This data can be analyzed to identify brain regions involved in specific mental processes or disorders.
For example, the Beck Depression Inventory (BDI) is a clinician-administered questionnaire widely used to assess the severity of depressive symptoms in individuals.
The BDI consists of 21 questions, each scored on a scale of 0 to 3, with higher scores indicating more severe depressive symptoms.
Quantitative Data Analysis
Statistics help us turn quantitative data into useful information to help with decision-making. We can use statistics to summarize our data, describing patterns, relationships, and connections. Statistics can be descriptive or inferential.
Descriptive statistics help us to summarize our data. In contrast, inferential statistics are used to identify statistically significant differences between groups of data (such as intervention and control groups in a randomized control study).
- Quantitative researchers try to control extraneous variables by conducting their studies in the lab.
- The research aims for objectivity (i.e., without bias) and is separated from the data.
- The design of the study is determined before it begins.
- For the quantitative researcher, the reality is objective, exists separately from the researcher, and can be seen by anyone.
- Research is used to test a theory and ultimately support or reject it.
Limitations of Quantitative Research
- Context: Quantitative experiments do not take place in natural settings. In addition, they do not allow participants to explain their choices or the meaning of the questions they may have for those participants (Carr, 1994).
- Researcher expertise: Poor knowledge of the application of statistical analysis may negatively affect analysis and subsequent interpretation (Black, 1999).
- Variability of data quantity: Large sample sizes are needed for more accurate analysis. Small-scale quantitative studies may be less reliable because of the low quantity of data (Denscombe, 2010). This also affects the ability to generalize study findings to wider populations.
- Confirmation bias: The researcher might miss observing phenomena because of focus on theory or hypothesis testing rather than on the theory of hypothesis generation.
Advantages of Quantitative Research
- Scientific objectivity: Quantitative data can be interpreted with statistical analysis, and since statistics are based on the principles of mathematics, the quantitative approach is viewed as scientifically objective and rational (Carr, 1994; Denscombe, 2010).
- Useful for testing and validating already constructed theories.
- Rapid analysis: Sophisticated software removes much of the need for prolonged data analysis, especially with large volumes of data involved (Antonius, 2003).
- Replication: Quantitative data is based on measured values and can be checked by others because numerical data is less open to ambiguities of interpretation.
- Hypotheses can also be tested because of statistical analysis (Antonius, 2003).
Antonius, R. (2003). Interpreting quantitative data with SPSS . Sage.
Black, T. R. (1999). Doing quantitative research in the social sciences: An integrated approach to research design, measurement and statistics . Sage.
Braun, V. & Clarke, V. (2006). Using thematic analysis in psychology . Qualitative Research in Psychology , 3, 77–101.
Carr, L. T. (1994). The strengths and weaknesses of quantitative and qualitative research : what method for nursing? Journal of advanced nursing, 20(4) , 716-721.
Denscombe, M. (2010). The Good Research Guide: for small-scale social research. McGraw Hill.
Denzin, N., & Lincoln. Y. (1994). Handbook of Qualitative Research. Thousand Oaks, CA, US: Sage Publications Inc.
Glaser, B. G., Strauss, A. L., & Strutzel, E. (1968). The discovery of grounded theory; strategies for qualitative research. Nursing research, 17(4) , 364.
Minichiello, V. (1990). In-Depth Interviewing: Researching People. Longman Cheshire.
Punch, K. (1998). Introduction to Social Research: Quantitative and Qualitative Approaches. London: Sage
Further Information
- Designing qualitative research
- Methods of data collection and analysis
- Introduction to quantitative and qualitative research
- Checklists for improving rigour in qualitative research: a case of the tail wagging the dog?
- Qualitative research in health care: Analysing qualitative data
- Qualitative data analysis: the framework approach
- Using the framework method for the analysis of
- Qualitative data in multi-disciplinary health research
- Content Analysis
- Grounded Theory
- Thematic Analysis
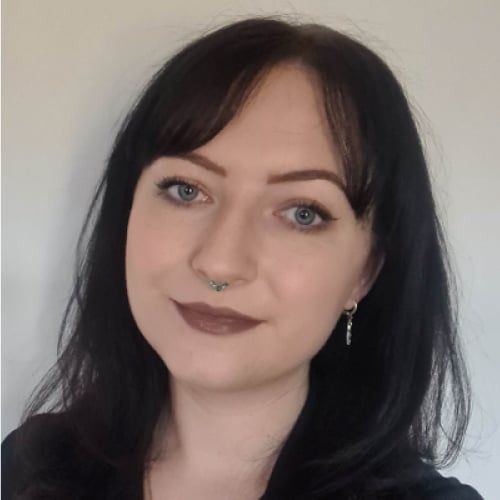
- Open access
- Published: 24 March 2024
Integrative multi-omics analysis identifies genetically supported druggable targets and immune cell specificity for myasthenia gravis
- Jiao Li 1 , 2 , 3 na1 ,
- Fei Wang 1 , 3 na1 ,
- Zhen Li 1 ,
- Jingjing Feng 1 ,
- Jinming Han 1 ,
- Jiangwei Xia 1 ,
- Chen Zhang 1 ,
- Yilai Han 1 ,
- Teng Chen 1 ,
- Yinan Zhao 1 ,
- Sirui Zhou 4 ,
- Yuwei Da 1 ,
- Guoliang Chai 1 , 2 &
- Junwei Hao ORCID: orcid.org/0000-0002-3075-5434 1 , 2 , 3
Journal of Translational Medicine volume 22 , Article number: 302 ( 2024 ) Cite this article
Metrics details
Myasthenia gravis (MG) is a chronic autoimmune disorder characterized by fluctuating muscle weakness. Despite the availability of established therapies, the management of MG symptoms remains suboptimal, partially attributed to lack of efficacy or intolerable side-effects. Therefore, new effective drugs are warranted for treatment of MG.
By employing an analytical framework that combines Mendelian randomization (MR) and colocalization analysis, we estimate the causal effects of blood druggable expression quantitative trait loci (eQTLs) and protein quantitative trait loci (pQTLs) on the susceptibility of MG. We subsequently investigated whether potential genetic effects exhibit cell-type specificity by utilizing genetic colocalization analysis to assess the interplay between immune-cell-specific eQTLs and MG risk.
We identified significant MR results for four genes ( CDC42BPB, CD226, PRSS36, and TNFSF12 ) using cis- eQTL genetic instruments and three proteins (CTSH, PRSS8, and CPN2) using cis- pQTL genetic instruments. Six of these loci demonstrated evidence of colocalization with MG susceptibility (posterior probability > 0.80). We next undertook genetic colocalization to investigate cell-type-specific effects at these loci. Notably, we identified robust evidence of colocalization, with a posterior probability of 0.854, linking CTSH expression in T H 2 cells and MG risk.
Conclusions
This study provides crucial insights into the genetic and molecular factors associated with MG susceptibility, singling out CTSH as a potential candidate for in-depth investigation and clinical consideration. It additionally sheds light on the immune-cell regulatory mechanisms related to the disease. However, further research is imperative to validate these targets and evaluate their feasibility for drug development.
Myasthenia gravis (MG) is a chronic autoimmune disorder of the neuromuscular junction [ 1 ]. The clinical hallmark of MG is muscle weakness associated with fatigability, which can lead to potentially life-threatening exacerbations, such as myasthenic crisis, which affects around 15% of individuals with MG, remains the leading cause of mortality among patients [ 2 , 3 ]. MG is a rare disease with an annual incidence of roughly 10–29 cases per 1 million people and a prevalence ranging from 100 cases to around 350 cases per 1 million people [ 2 ]. Unlike congenital myasthenic syndromes, characterized by mutations in different genes encoding molecules important in the neuromuscular junction cause major changes in function and are inherited in classic mendelian patterns [ 4 ], it can be broadly stated that MG is a complex disorder resulting from the interplay of genetic and environmental factors, triggering autoimmune responses [ 5 ]. The underlying genetic pathogenesis is evidenced by the high disease concordance among identical twins [ 6 ], and associations with genes in the major histocompatibility complex (MHC) locus have been recognized for more than 30 years [ 7 ]. Over the past few decades, the emergence of genome-wide association studies (GWAS) has identified multiple susceptibility variants beyond the MHC with MG risk, yielding an estimated heritability of 25.6% [ 8 ]. In a recent GWAS encompassing 1,873 MG patients and 36,370 healthy individuals, identified significant associations in the CHRNA1 and CHRNB1 genes, as well as confirmed the previous association signals at PTPN22 , HLA-DQA1/HLA-B and TNFRSF11A [ 9 ]. These discoveries shed light on the intricate genetic landscape of MG and provide valuable insights into its underlying mechanisms.
The goal of MG treatment is to achieve complete remission or minimal manifestation status with minimal side effects and eventually to avoid a myasthenic crisis [ 10 ]. Despite the availability of standard therapies, including acetylcholinesterase inhibitors, steroids, steroid-sparing immunosuppressants, and thymectomy, symptoms of MG are unsatisfactorily treated in up to half of individuals over the course of their disease [ 11 ]. A significant proportion of patients heavily rely on corticosteroid administration, which can result in severe side effects, including infections, osteoporosis, diabetes, glaucoma, and other complications [ 11 ]. Furthermore, some patients exhibit inadequate response to conventional treatment, with approximately 10–20% of MG patients classified as having "refractory" MG, emphasizing the pressing demand for innovative therapeutic solutions. Although there are several novel treatment options for MG, the therapeutic aim of complete remission only be achieved in a subset of patients [ 12 ], indicating that new safe and effective immunotherapies are desperately needed.
The conventional process of drug discovery and development is a time-consuming and costly endeavor. The integration of genomics into the drug discovery process has become indispensable, providing a vital avenue for expediting the development of novel therapeutic targets [ 13 ]. The combination of molecular quantitative trait locus (molQTL) studies, such as gene expression or protein quantitative trait loci (eQTLs or pQTLs), with genome-wide association (GWAS) data allows for the identification of target genes associated with risk variants through causal inference [ 14 ]. One approach is through drug target Mendelian randomization (MR), a statistical genetic methodology that leverages genetic variants as instrumental variables to assess the causal relationship between an exposure (like genetically predicted druggable gene expression or protein levels) and a specific outcome (such as MG risk). This approach employs genetic data to simulate the design of a randomized controlled trial (RCT) without requiring a drug intervention (Additional file 1 : Figure S1) [ 15 ]. By synergistically amalgamating diverse data sources [ 16 , 17 , 18 , 19 , 20 , 21 , 22 ] (Additional file 2 : Table S1) and employing rigorous MR and colocalization analyses, this study endeavor strives to identify potential repurposing opportunities for MG, delving into their potential implications in MG susceptibility. Subsequently, further investigation was conducted on the MR associations that exhibited statistical significance and provided evidence for colocalization, aiming to identify immune-cell-specific effects.
Identification of actionable druggable genes
The druggable genome was defined as described in Finan et al. as a selection of genes [ 23 ], which included 4479 genes and divided into 3 Tiers based on druggability levels: Tier 1 contains genes encoding targets of approved or clinical trial drugs, Tier 2 genes encoding targets with high sequence similarity to Tier 1 proteins or targeted by small drug-like molecules, and Tier 3 contains genes encoding secreted and extracellular proteins, genes belonging to the main druggable gene families, and genes encoding proteins with more restricted similarity to Tier 1 targets. After removing duplicate and non-autosomal genes, 4300 of 4479 druggable genes were retained in the subsequent analysis (Additional file 2 : Table S2).
Selection of eQTL genetic instruments for drug target gene expression
To simulate exposure to the corresponding drugs, we sought publicly accessible eQTL data for gene expression that encode these druggable proteins. Publicly available data from the eQTLGen consortium ( https://eqtlgen.org/ , n = 31,684) was utilized to identify common (minor allele frequency > 1%) single-nucleotide variants (SNVs) associated with the expression of drug target genes in blood [ 16 ]. We retrieved the complete cis- eQTL results (distance between SNV and gene is < 1 Mb, FDR < 0.05) and allele frequency data from the consortium. The SNPs that were associated with gene expression ( cis- eQTL P < 5 × 10 –8 ) were selected as genetic instrumental variables (IVs). To obtain independent IVs, we conducted LD clumping using the TwoSampleMR R package with genotype data of Europeans from the 1000 Genomes were used as a reference panel. In a 10 Mb window, if LD values (r 2 ) of two or more SNPs were smaller than 0.01, these SNPs were considered independent IVs.
Deriving pQTL genetic determinants of circulating protein levels
We obtained pQTL data from six large-scale genome-wide proteomic GWAS studies, namely the ARIC study [ 17 ], the INTEVAL study [ 18 ], the KORA F4 study [ 19 ], the IMPROVE study [ 20 ], the AGES-Reykjavik study [ 21 ], and the Framingham Heart study (FHS) [ 22 ]. Each of these studies undertook proteomic profiling using either SomaLogic SomaScans or O-link proximal extension assays. We restricted proposed instrumental variants to cis- pQTLs for druggable proteins, used a P value threshold of 5 × 10 –8 . For proteins derived from the ARIC study [ 17 ], we utilized the sentinel cis- pQTL specific to each protein as it was available. Regarding proteins from the other five studies [ 18 , 19 , 20 , 21 , 22 ], we employed lead variants categorized as tier 1 instrumental variants according to Zheng et al [ 24 ], which were associated with fewer than five proteins and exhibited no heterogeneity across the studies.
Immune-cell-type-specific eQTL data
The immune-cell-specific RNA expression and eQTL data were acquired from the Database of Immune Cell Expression (DICE, https://dice-database.org/) [ 25 ], which included eQTLs from 15 different immune cell types from 91 healthy subjects. The presented cell types account for over 60% of all circulating mononuclear cells, consisting of three innate immune cell types (Classical monocytes, Non-classical monocytes, NK cells), four adaptive immune cell types that have not encountered cognate antigen in the periphery (Naive B cells, Naive CD4 + T cells, Naive CD8 + T cells, and Naive T REG T cells), six CD4 + memory or more differentiated T cell subsets (T H 1, T H 1/17, T H 17, T H 2, follicular T FH , and Memory T REG cells), and two activated cell types (Activated Naive CD4 + cells and Activated Naive CD8 + T cells) [ 25 ]. We used these datasets specifically for follow-up analyses of genetically predicted effects identified to evaluate cell-type specificity.
GWAS summary statistics of MG
For the primary analysis, the largest MG GWAS reported by Chia et al. was used in this study. Briefly, Chia et al. performed a large-scale GWAS analysis on MG, which included 1,873 patients and 36,370 controls [ 9 ] ( https://www.ebi.ac.uk/gwas/ , GWAS Catalog ID: GCST90093061). In this study, the diagnosis of MG relied on standard clinical criteria, including characteristic fatigable weakness, supported by electrophysiological and/or pharmacological abnormalities. Notably, the study is limited to anti-acetylcholine receptor antibodies (anti-AChR) positive cases, and individuals testing positive for antibodies to muscle-specific kinase (anti-MuSK) were excluded. We summarized the genome-wide significant loci identified in the MG GWAS conducted by Chia et al. in Additional file 2 : Table S3. To explore age-dependent genetic heterogeneity in MG, we utilized summary statistics from early-onset MG (GWAS Catalog ID: GCST90093465; 595 cases vs. 2,718 controls, aged 40 years or younger) and late-onset MG (GWAS Catalog ID: GCST90093466; 1,278 cases vs. 33,652 controls) separately. For external validation, summary statistics were obtained from the UK Biobank ( http://www.nealelab.is/uk-biobank ) (224 MG cases vs. 417,332 controls) and FinnGen Biobank ( https://r10.finngen.fi/pheno/G6_MYASTHENIA ) (461 MG cases vs. 408,430 controls). The MG phenotype was identified through questionnaires completed by the participants, and data on MG subtypes were not applicable. Detailed information on various GWAS datasets is provided in Table 1 . To ensure data integrity, we removed SNPs with duplicate or missing identification (rsID) from the dataset for subsequent analysis.
SNP-based heritability calculation
We used Linkage Disequilibrium Score (LDSC) Regression ( https://github.com/bulik/ldsc ) to estimate the SNP-based heritability (h 2 ) of each of the trait, representing the proportion of phenotypic variance that is explained by all common genetic variants included in the analysis. Also, we used single-trait LDSC to estimate genomic inflation factors λGC, used to evaluate the polygenicity and confounding because of population stratification or cryptic relatedness. To minimize bias caused by low interpolation quality, we restricted this analysis to haplotype map 3 SNPs.
Mendelian randomization analysis
The two-sample MR approach was based on the following assumptions: (i) the genetic variants used as an instrumental variable are associated with target exposure, i.e. gene expression levels and protein levels; (ii) there are no unmeasured confounders of the associations between genetic variants and outcome; (iii) the genetic variants are associated with the outcome only through changes in the exposure, i.e. no pleiotropy. We therefore used a curated genotype–phenotype database (PhenoScanner) [ 26 ] to search for associations between variants used to instrument each drug target and other traits that may represent pleiotropic pathways. We used fixed-effects, inverse-variance-weighted MR for proposed instruments that contain more than one variant, and Wald ratio for proposed instruments with one variant. Steiger filtering was applied to exclude variants that were potentially influenced by reverse causation [ 27 ]. For proposed instruments with multiple variants, we assessed the heterogeneity across variant-level MR estimates using the Cochrane Q method (mr_heterogeneity option in TwoSampleMR package). Benjamini–Hochberg false discovery rate (FDR) of 0.05 was applied to select best MR estimates with robust signals. The findings of our MR studies are presented as MR estimates (β) or odds ratio (OR) and 95% confidence interval (CI) for the risk of MG per genetically predicted 1-standard deviation (SD) increase in blood gene expression or circulating protein level. We conducted MR analyses using the TwoSampleMR ( https://mrcieu.github.io/TwoSampleMR/ ) package.
Colocalization analysis
While MR largely mitigates bias from confounding, linkage disequilibrium (LD) between SNPs might be an important source of noncausal associations. For statistically significant MR results, coloc ( https://github.com/cran/coloc ) was used to evaluate the probability of QTL and MG loci sharing a single causal variant [ 28 ], which assess potential confounding by LD. To determine the posterior probability of each genomic locus containing a single variant affecting both the gene/protein and the MG risk, we analyzed all SNPs within 1 Mb of the cis- eQTL/ cis- pQTL. Moreover, we evaluated whether each genomic locus harbored a causal variant that influenced both disease risk and the variability in gene expression across the 15 cell-type-specific datasets individually. Assuming a solitary causal variant, four hypotheses can be outlined: H0, proposing the lack of causal variants for both traits; H1, positing the existence of a causal variant for trait 1; H2, suggesting a causal variant for trait 2; H3, postulating two distinct causal variants for traits 1 and 2; and H4, proposing a shared causal variant between the two traits. Statistically significant MR hits with posterior probability for hypothesis 4 (PPH4) > 0.8 (the probability of a shared causal variant) were investigated. Visualization of colocalization results was performed using the LocusCompareR R package [ 29 ].
Overall analysis plan
The study design is illustrated in Fig. 1 . Initially, we identified druggable proteins as described in Finan et al. [ 23 ]. These proteins include targets of approved and clinical-phase drugs, proteins resembling approved drug targets and proteins accessible to monoclonal antibodies or drug-like small molecules in vivo. Next, we selected independent genetic variants that act locally on gene expression from eQTLGen Consortium or specifically influence plasma levels of the proteins from six large proteomic GWASs of individuals of European ancestry. The primary analysis involved retrieving summary statistics from the largest MG GWAS dataset of European ancestry [ 9 ]. In addition, we conducted a subgroup analysis using GWAS summaries for early-onset MG and late-onset MG separately. Employing the single-trait LDSC method, the SNP heritability for MG was estimated at 0.075 (SE = 0.015), while early-onset MG showed a heritability of 0.3649 (SE = 0.135), and late-onset MG exhibited a heritability of 0.078 (SE = 0.015) (Table 1 ). Then, genetic colocalization was conducted on MR results that surpassed our significance threshold after accounting for multiple testing, to mitigate potential confounding by LD. Afterward, we performed external replication using MG GWAS summary statistics from the FinnGen Consortium and UK Biobank. Subsequently, we explored whether putative genetic effects may be cell type specific by employing genetic colocalization to assess the interplay between immune-cell-specific eQTLs and MG risk.
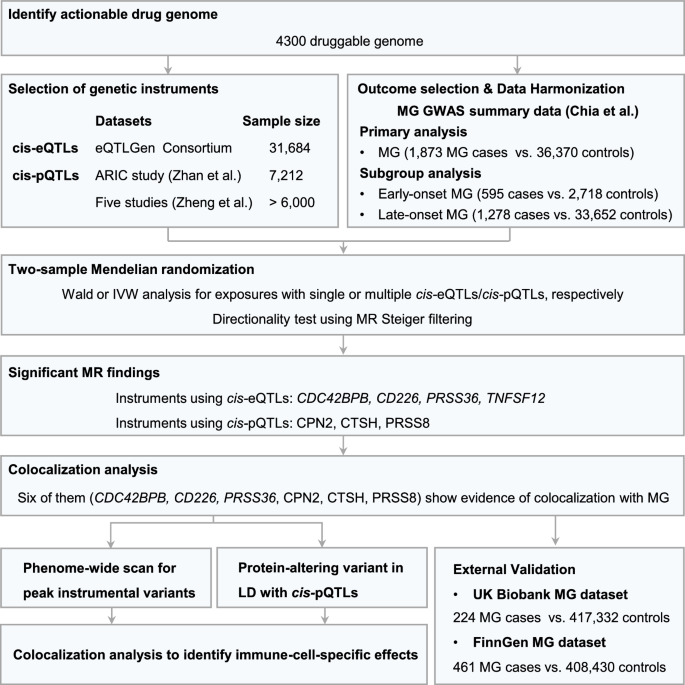
Flow diagram of study design. Using a variety of data sources, this study examined the instruments proposed for actionable druggable proteins, specifically cis- pQTL and cis- eQTL, against MG GWAS summary statistics. Subsequently, further investigation was conducted on the MR associations that exhibited statistical significance and provided evidence for colocalization, aiming to identify immune-cell-specific effects
MR analysis with blood gene expression and MG outcome
We used two-sample MR to systematically evaluate the evidence for the causal effects of druggable gene expression on MG outcome. Using cis- eQTLs as proposed instruments available from the eQTLGen Consortium, four genes ( CDC42BPB , TNFSF12 , CD226 and PRSS36 ) showed significant MR results (Table 2 , Fig. 2 ). Specifically, we found that a 1 standard deviation (SD) increased blood expression of CDC42BPB (OR = 1.694; 95% CI 1.361–2.108; P = 2.347 × 10 –6 ), TNFSF12 (OR = 1.433; 95% CI 1.214–1.691; P = 2.119 × 10 –5 ), and PRSS36 (OR = 3.186; 95% CI 1.805–5.624; P = 6.401 × 10 –5 ) were significantly associated with increased MG susceptibility, whereas increased CD226 gene expression (OR = 0.652; 95% CI 0.528–0.804; P = 6.205 × 10 –5 ) in blood were associated with decreased MG risk ( cis- eQTL instruments in Additional file 2 : Table S4, full MR results in Additional file 2 : Table S5, MR scatter plot shown in Additional file 1 : Figure S2). We employed Cochran's Q test to assess potential heterogeneity in the IVW results, estimating MR effects across each eQTL and none of the MR signals exhibited evidence of significant heterogeneity. In the replication phase, our study employed GWAS summary data from the UK and FinnGen Biobank datasets. Although MR analysis did not identify any genetically predicted gene expression causally linked to MG risk after FDR correction (full MR results in Additional file 2 : Table S5), we observed similar pattern for CD226 expression with MG susceptibility (OR = 0.595; 95% CI 0.403–0.877; P = 8.754 × 10 –03 ) (Additional file 1 : Figure S3).
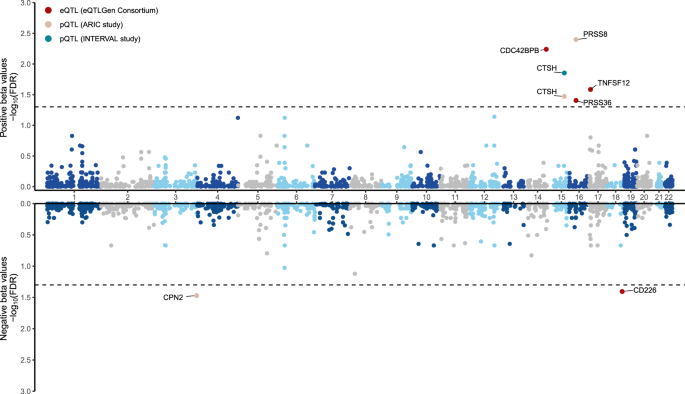
Miami plot with circles representing the MR results for gene/protein on MG. FDR, P value (FDR adjust); Black dashed line indicates the threshold for significance (FDR < 0.05) threshold. The x axis is the chromosome and gene start position of each MR finding in the cis region. The y axis represents the − log 10 FDR of the MR findings. MR findings with positive effects (increased level of gene expression/protein associated with increasing the MG risk) are represented by filled circles on the top half of the plot; Conversely, MR findings suggesting a negative effect, implying a reduced level of gene expression or protein linked to an elevated risk of MG, are illustrated in the lower half of the plot
In the subgroup analysis discerning early-onset and late-onset MG, coherent patterns emerged (Fig. 3 ), For instance, a 1 SD increase in genetically predicted CDC42BPB expression levels was associated with elevated risk for both early-onset MG (OR = 2.065; 95% CI 1.322–3.225; P = 1.433 × 10 –3 ) and late-onset MG (OR = 1.578; 95% CI 1.191–2.092; P = 1.506 × 10 –3 ) risk. Furthermore, pertaining to CD226 and TNFSF12 , subgroup MR findings revealed a relationship between CD226 levels and late-onset MG (OR = 0.621; 95% CI 0.451–0.854; P = 3.412 × 10 –3 ), as well as an association between TNFSF12 levels and late-onset MG (OR = 1.533; 95% CI 1.243–1.891; P = 6.570 × 10 –5 ), while no statistically significant MR estimates were observed in the context of early-onset MG.
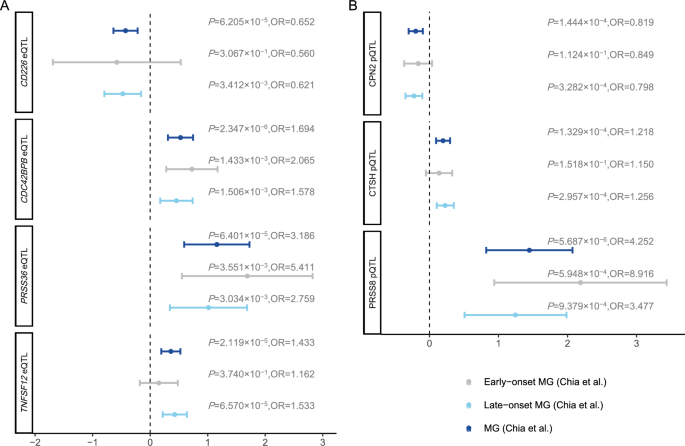
Forest plot showing MR estimate for genetically proxied gene and protein expression on MG and its subgroups. Forest plot showing MR estimate (95% CI) from two sample MR analyses. P are unadjusted. CI confidence interval. MR estimates of significant MR results used cis- eQTL instruments on MG and its subgroups. MR estimates of significant MR results used cis- pQTL instruments on MG and its subgroups
We then performed genetic colocalization analyses to evaluate the probability of shared single causal variants between MG loci and eQTLs. Three loci ( CDC42BPB , CD226 , and PRSS36 ) exhibited profiles suggesting causal relationships, except for TNFSF12 (Fig. 4 , Additional file 2 : Table S6). Furthermore, we investigated the associations between index cis- eQTLs (specifically, rs1790974 for CD226 levels, rs10143668 for CDC42BPB levels, and rs78924645 for PRSS36 levels) and more than 5,000 other diseases, traits or proteins levels as documented in PhenoScanner [ 26 ] (Additional file 1 : Figure S4), which suggest potential horizontal pleiotropic effects in our MR analysis.
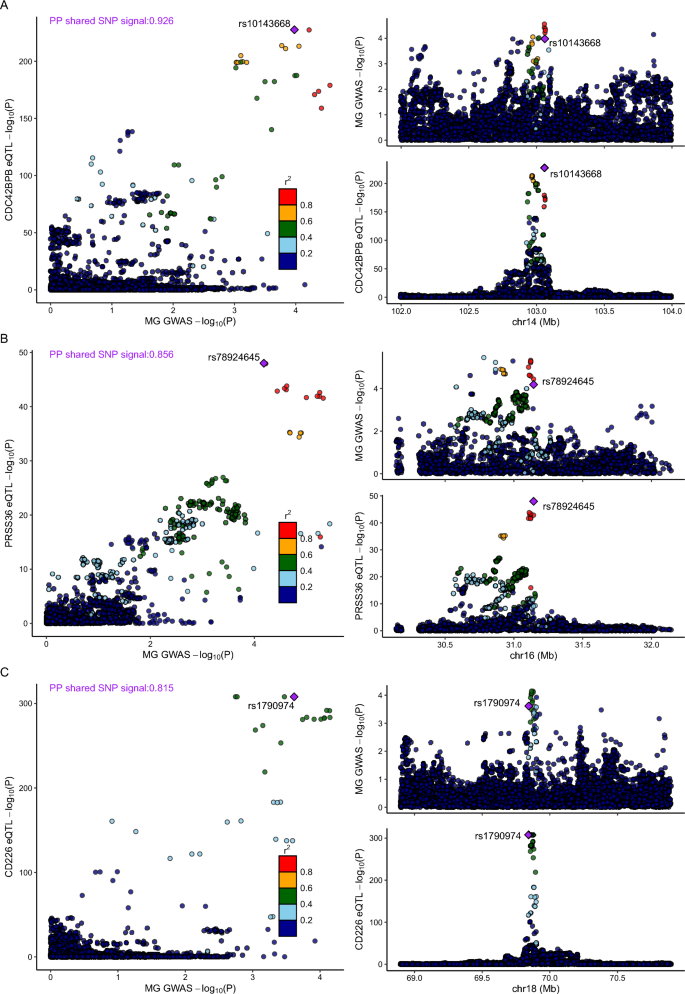
LocusCompare plot depicting colocalization of the top SNP associated with eQTL surrounding CDC42BPB ( A ), PRSS36 ( B ) and CD226 ( C ) and MG GWAS. The top right plots show the association results in the MG GWAS; the bottom right plots represent the corresponding eQTL results; the left plot shows the colocalization of genetic association and eQTL signals. The SNP indicated by the purple diamond is the SNP for which the European LD information is shown
MR analysis of circulating proteome identifies actionable targets in MG
Using proposed cis- pQTL instruments, MR analyses revealed significant associations between the levels of three circulating proteins (PRSS8, CTSH, CPN2) and MG susceptibility in the primary analysis, after FDR correction ( cis- pQTL instruments in Additional file 2 : Table S7, and full MR results in Additional file 2 : Table S8), which exhibit compelling evidence of colocalization with MG risk (Fig. 5 , Additional file 2 : Table S9). Notably, among these proteins, circulating CTSH exhibited associations that were replicated in two independent proteomic studies (Fig. 2 , Table 2 ). The estimated associations with MG per 1 SD increase in the genetically predicted circulating CTSH level were consistent between the INTERVAL (OR = 1.257; 95% CI 1.125–1.405; P = 5.49 × 10 –4 ) and ARIC (OR = 1.218; 95% CI 1.101–1.348; P = 1.330 × 10 –4 ) studies and displayed strong evidence of colocalization (Fig. 5 ). Despite the MR analysis failing to identify any genetically predicted circulating protein levels associated with MG susceptibility using the proposed cis- pQTL instruments and GWAS summary data from the FinnGen and UK Biobank datasets after FDR correction, a persistent association for Cathepsin H (CTSH) abundance abundance with MG was evident. This sustained association suggests an elevated risk of MG across three MG outcomes. A SD increase in genetically predicted circulating CTSH abundance was found to be associated with an elevated risk of MG (minimum OR = 1.218; 95% CI 1.101–1.348; P = 1.329 × 10 –4 ), and this trend was consistently observed in both the UK Biobank (OR = 1.275; 95% CI 1.003–1.620; P = 4.713 × 10 –2 ) and FinnGen Biobank (OR = 1.185; 95% CI 1.017–1.381; P = 2.942 × 10 –2 ) datasets (Additional file 1 : Figure S3).
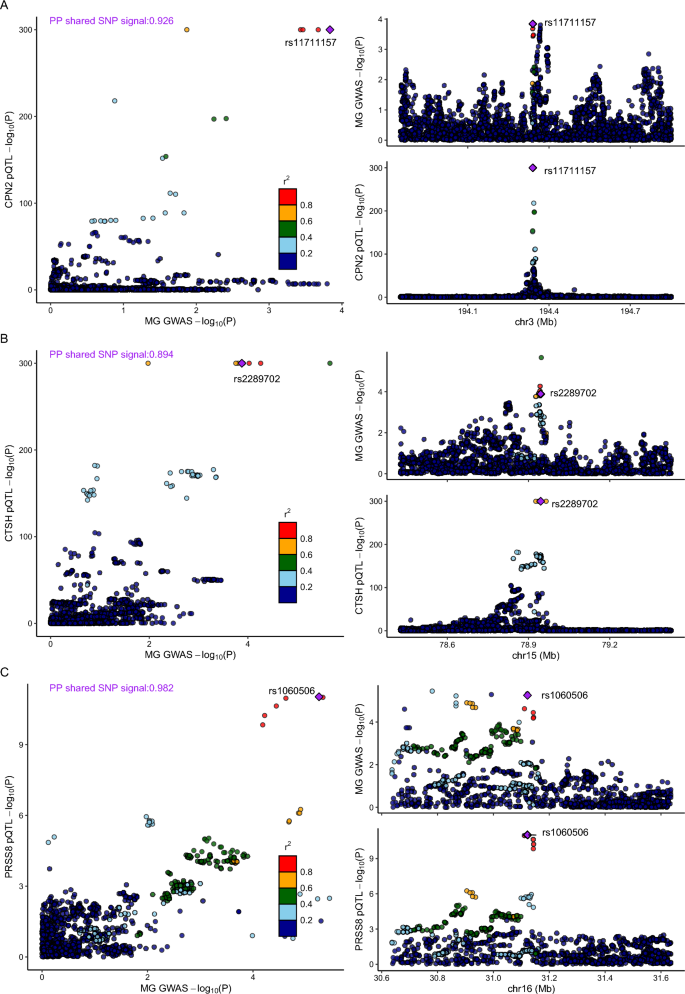
LocusCompare plot depicting colocalization of the top SNP associated with pQTL surrounding CPN2 ( A ), CTSH ( B ) and PRSS8 ( C )and MG GWAS. The top right plots show the association results in the MG GWAS; the bottom right plots represent the corresponding pQTL results; the left plot shows the colocalization of genetic association and eQTL signals. The SNP indicated by the purple diamond is the SNP for which the European LD information is shown
Additionally, subgroup analyses can provide further insights into the nuanced relationships between these proteins and MG susceptibility (Fig. 3 ). Genetically predicted circulating CTSH abundance was associated with an increased risk of late-onset MG (OR = 1.256; 95% CI 1.110–1.421; P = 2.957 × 10 –4 ). Also, genetically predicted elevation in circulating PRSS8 abundance is associated with an increased risk of MG (OR = 4.252; 95% CI 2.275–7.945; P = 5.69 × 10 –6 ), as well as heightened risks for both early-onset MG (OR = 8.916; 95% CI 2.558–31.083; P = 5.948 × 10 –6 ) and late-onset MG (OR = 3.477; 95% CI 1.662–7.275; p = 9.379 × 10 –4 ).Conversely, a genetically proxied increase in circulating CPN2 levels demonstrated an association with decreased MG risk (OR = 0.819; 95% CI 0.739–0.908; P = 1.444 × 10 −4 ) and also late-onset MG (OR = 0.798; 95% CI 0.706–0.903; P = 3.282 × 10– 04 ). Due to the limited number of cis- acting pQTLs available for each protein, applying classic sensitivity methods to test MR assumptions becomes challenging.
We also searched in the PhenoScanner database to assess potentially pleiotropic effects of the cis-pQTLs of MR-prioritized proteins by testing the association of cis-pQTLs with other diseases or traits [ 26 ] (Additional file 1 : Figure S4). We observed no evidence of pleiotropic effects for the cis-pQTL associated with CPN2 (rs11711157). We discovered an association between the CTSH cis- pQTL (rs34593439) and narcolepsy, a condition for which immune-mediated dysregulation has been considered as one of the potential causes [ 30 ]. Also, we found that PRSS8 cis- pQTL (rs1060506) was associated with a broad spectrum of weight-related traits, including whole body fat mass and body fat percentage, among others, which was consistent with the findings for cis- eQTL for PRSS36 . This suggests that our MR estimate of the effect of the above-mentioned proteins on MG susceptibility may have been biased by the stated confounders.
Identifying immune-cell-specific effects
Given the pivotal role of immune cells in the pathogenesis of MG, we aim to investigate whether potential genetic effects exhibit specificity toward certain cell types. Leveraging immune cell eQTL datasets from the DICE database, we conducted a comprehensive genetic colocalization analysis for each of the six identified loci ( CDC42BPB , CD226 , PRSS36 , PRSS8 , CTSH , and CPN2 ) from the preceding analysis. Remarkably, we identified robust evidence of colocalization, with a posterior probability of 0.854, linking CTSH expression in T H 2 cells and MG risk (Additional file 2 : Table S10, Additional file 1 : Figure S5). T H 2 cells are renowned for their capacity to produce cytokines that stimulate B cell activation and differentiation of B cells, essential for antibody production [ 31 ]. Furthermore, while analyzing data from other immune cell subsets, we observed less pronounced evidence specific to individual cell types (Fig. 6 ). The identification of a colocalization signal within a cell type relevant to MG pathogenesis suggests a potential direct contribution of the genetic variant to disease development by influencing gene expression within that specific cell subset.
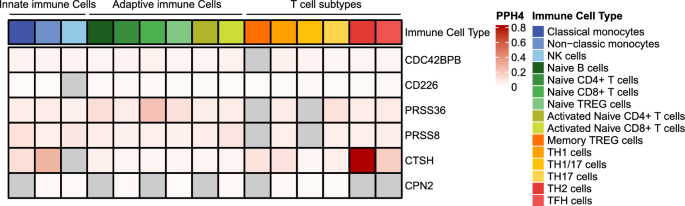
Colocalization results between GWAS and eQTLs across immune cell types. PPH4 of shared genetic signal between GWAS and eQTLs for MG across different immune cell types
Gaining an in-depth understanding of the intricate relationship between genetic discoveries and pharmaceutical targets holds paramount importance in effectively translating GWAS findings into clinical applications [ 32 ]. Our study assumes pioneering significance as the first to center on actionable druggable genes, unlocking the potential of drug repurposing strategies within the domain of MG treatment. Notably, we identified three genes ( CDC42BPB , CD226 , and PRSS36 ) and three proteins (PRSS8, CTSH, and CPN2) as having significant MR results using cis-eQTL and cis-pQTL genetic instruments, which also exhibit compelling evidence of colocalization with MG. Additionally, through a meticulous exploration of immune cell-specific effects, this study may shed light on mechanistic insights underlying the loci associated with MG.
Our MR analysis establishes a compelling link between genetic variants associated with increased CDC42BPB gene expression and an elevated MG susceptibility. This finding underscores the potential of CDC42BPB inhibitors as a promising avenue for therapeutic intervention. CDC42BPB is a serine/threonine protein kinase intricately involved in regulating actin cytoskeleton dynamics and cell contraction. Several small molecules or biological inhibitors of CDC42BPB , such as SR-7826, BDP8900 and BDP9066 [ 33 ], have exhibited antitumor activity. However, a comprehensive understanding of the mechanistic underpinnings through which elevated CDC42BPB contributes to the pathophysiology of MG necessitates further investigation. Conversely, we observed a protective effect associated with increased CD226 gene expression against MG. CD226 , also known as DNAX accessory molecule-1 (DNAM-1), is a member of the immunoglobulin superfamily and is expressed on various immune cells, including natural killer (NK) and T cells [ 34 ]. CD226 , along with the inhibitory receptors TIGIT and CD96, constitutes cell-surface receptor family 3, binding to nectin and nectin-like proteins [ 35 ]. Prior research emphasizes the potent roles of this receptor family in regulating tumor immunity [ 36 ]. These findings underscore the importance of maintaining the expression of the activating receptor CD226, in orchestrating effective immune responses. Genetic polymorphisms within the CD226 gene have been associated with serval autoimmune diseases, including multiple sclerosis [ 37 ]. Building on these insights, we hypothesize that targeted manipulation of CDC42BPB and CD226 expression could potentially serve as a potent therapeutic strategy for managing MG. Nonetheless, a comprehensive validation of these proposed therapeutic targets necessitates further rigorous investigation.
Our study emphasizes a consistent and robust association between genetically predicted circulating Cathepsin H abundance and an elevated risk of MG across diverse datasets, including the UK Biobank and FinnGen Biobank. The subgroup analyses further revealed nuanced insights, particularly the association between genetically predicted circulating CTSH abundance and an increased risk of late-onset MG. Additionally, the posterior probability that CTSH expression levels in T H 2 cells and MG outcomes shared a single causal signal in the 1-Mb locus around the cis-pQTL, rs12148472, was 0.8854 for MG susceptibility (Additional file 1 : Figure S5). This observation implies that CTSH abundance might serve as a biomarker or contribute to the biological mechanisms underlying MG. CTSH encodes cathepsin H, a member of the papain-like cysteine proteases that are involved in major histocompatibility complex class II antigen presentation. Notably, CTSH has garnered attention for its involvement in type 1 diabetes [ 38 ] and narcolepsy risk [ 39 ], both of which prominently feature autoimmune components. Worth noting is the fact that other members of the cathepsin family, such as cathepsin S and cathepsin K, have received more attention as drug targets for autoimmune diseases. For example, cathepsin S inhibitors have been investigated for their potential in treating diseases like and multiple sclerosis [ 40 ].
Furthermore, our study provided robust genetic evidence supporting a potential causal role of increased CPN2 protein levels in reducing the risk of MG. CPN2, also known as Carboxypeptidase N Subunit 2, forms a complex with enzymatically active small subunits (CPN1). CPN plays a pivotal role as a zinc metalloprotease responsible for cleaving and partially inactivating anaphylactic peptides, specifically complement component 3a (C3a) and C5a, within the classical and lectin pathways of complement activation [ 41 ]. Protein–protein interaction studies using the STRING database ( https://string-db.org/ ) have shown interactions between CPN2 and C5 (Additional file 1 : Figure S6). It is widely recognized that dysregulated complement activation is a primary pathogenic mechanism in MG. Notably, C5 inhibitors, such as Eculizumab, have proven effective in preventing complement-dependent membrane attacks at the neuromuscular junction, presenting a promising avenue for the treatment of MG [ 42 ]. Future research is warranted to investigate the potential therapeutic implications of CPN2 in MG.
A significant hurdle in our analytical pursuit lay in discerning whether the association with MG risk stemmed from PRSS36, PRSS8, or both entities, given the substantial correlation between the cis- eQTL instrument for PRSS36 (rs78924645) and cis- pQTL instrument (rs1060506) for PRSS8 (r 2 = 0.93 in 1000 Genomes Project European ancestry participants). Considering that both PRSS36 and PRSS8 belong to the serine protease family, a group of proteolytic enzymes involved in diverse biological processes, we regard them as potential drug targets for MG. It is worth noting that PRSS8 inhibits TLR4-mediated inflammation in human and mouse models of inflammatory bowel disease [ 43 ], thereby implicating its plausible relevance to TLR4-mediated inflammation pathways in the context of MG. As such, the identification of PRSS36 and PRSS8 as putative targets holds promise; however, the trajectory towards their validation as credible therapeutic avenues warrants further inquiry, coupled with a comprehensive assessment of their viability for subsequent drug development endeavors.
Genetic variation controls transcriptional regulation in a cell type-specific manner to regulate immune pathways [ 44 , 45 ]. The cell-type specificity of the effects elicited by common genetic variants on both gene and protein expression engenders a pronounced reliance on the precise cellular contexts. In navigating this intricacy, the relationships underpinning eQTL and pQTL manifest a profound dependence on the distinct cell types under scrutiny. In this study, we embarked on a comprehensive exploration, utilizing genetic colocalization analysis, to delineate the interplay between immune-cell-specific eQTLs and MG susceptibility, thereby unearthing the underlying molecular mechanisms. This approach yielded valuable insights into the genetic orchestration governing CTSH expression across an array of distinct cell types. Of note is the robust colocalization unveiled between CTSH expression within T H 2 cells and the propensity for MG risk, signifying a putative involvement of CTSH expression within this cellular subtype in driving the genesis and advancement of MG. This revelatory aspect of our study underscores the imperative of acknowledging and integrating cell type heterogeneity within the realm of future research pursuits aimed at identifying potential therapeutic targets for MG. This newfound awareness stands poised to wield transformative influence in guiding the trajectory of future scientific explorations and therapeutic innovations targeting MG and other akin disorders.
The current study notably complements and extends previous efforts by employing key approaches to protect against potential biases, strengthen causal inference and enhance understanding of potential mechanisms. With our rigorous instrument selection process that used comprehensive datasets on gene expression and plasma protein levels, we have facilitated a thorough evaluation of actionable drug targets, notably including the previously unexplored CTSH. Our study, enriched with multi-omics data, has successfully overlooked targets that do not align with the potentially druggable gene targets prioritized in the Chia et al. study, as indicated by the Priority Index analysis [ 9 , 46 ]. It is crucial to underscore that the peak cis- eQTL or cis- pQTL identified at each locus did not attain the genome-wide significance level threshold. Consequently, these loci were not deemed significantly associated with MG in Chia et al.'s study. This constitutes a noteworthy extension to previous study, emphasizing our pivotal role in contributing novel insights that surpass the confines of earlier investigations.
Several limitations need to be considered in our study. Firstly, only a small proportion of genes/proteins may be effectively instrumented by multiple SNPs, with the majority being instrumented by only two or one SNP. This limitation restricts our ability to conduct sensitivity analyses. Secondly, there is a potential concern related to the presence of epitope-binding artifacts caused by coding variants. These artifacts may introduce spurious signals, potentially leading to false-positive cis-pQTLs. Thirdly, our study is confined to AchR-positive MG cases, so caution is advised when extrapolating our results to patients with anti-MuSK and other autoantibodies. Additionally, although the GWAS summary data integrated into our study already constitute the most extensive MG GWAS dataset currently available, the limited number of cases in both the primary and replication datasets constrained our ability to replicate the MR estimates observed in the discovery cohort. Therefore, it is crucial to conduct further research with larger and more diverse populations, especially including non-European individuals and patients with anti-MuSK and other autoantibodies.
Overall, this study contributes valuable insights into the genetic and molecular factors associated with MG susceptibility, with CTSH emerging as a potential candidate for further investigation and clinical consideration. Moreover, by laying bare the intricacies of cell-specific genetic influences on gene and protein expression, our investigation serves as a clarion call for a nuanced consideration of cellular contexts in the quest for unraveling the underlying mechanisms of complex diseases like MG. It is imperative to underscore, however, that a requisite next step involves undertaking further studies, encompassing rigorous functional analyses, to definitively validate the viability of these targets and to ascertain their appropriateness for subsequent drug development endeavors.
Availability of data and materials
The eQTL data from eQTLGen consortium were obtained from https://www.eqtlgen.org/ . The eQTL data from DICE were obtained from https://dice-database.org/ . The ARIC pQTL summary statistics used in the paper are freely downloaded from ARIC website ( http://nilanjanchatterjeelab.org/pwas/ ). Summary statistics for the MG GWAS are publicly available for download from the GWAS catalog. Data processing was completed using R software (version 3.6.3), with packages TwoSampleMR (version 0.5.4), coloc (version 4.0.4), dplyr (version 1.0.0), readr (version 1.3.1), tidyverse (version 1.3.0), forestplot (version 1.9), plyr (version 1.8.6), devtools (version 2.3.0), remotes (version 2.1.1).
Gilhus NE, Tzartos S, Evoli A, et al. Myasthenia gravis. Nat Rev Dis Primers. 2019;5(1):30. https://doi.org/10.1038/s41572-019-0079-y .
Article PubMed Google Scholar
Punga AR, Maddison P, Heckmann JM, et al. Epidemiology, diagnostics, and biomarkers of autoimmune neuromuscular junction disorders. Lancet Neurol. 2022;21(2):176–88. https://doi.org/10.1016/s1474-4422(21)00297-0 .
Zhang C, Wang F, Long Z, et al. Mortality of myasthenia gravis: a national population-based study in China. Ann Clin Transl Neurol. 2023;10(7):1095–105. https://doi.org/10.1002/acn3.51792 .
Article PubMed PubMed Central Google Scholar
Finsterer J. Congenital myasthenic syndromes. Orphanet J Rare Dis. 2019;14(1):57. https://doi.org/10.1186/s13023-019-1025-5 .
Avidan N, Le Panse R, Berrih-Aknin S, et al. Genetic basis of myasthenia gravis - a comprehensive review. J Autoimmun. 2014;52:146–53. https://doi.org/10.1016/j.jaut.2013.12.001 .
Article CAS PubMed Google Scholar
Ramanujam R, Pirskanen R, Ramanujam S, et al. Utilizing twins concordance rates to infer the predisposition to myasthenia gravis. Twin Res Hum Genet. 2011;14(2):129–36. https://doi.org/10.1375/twin.14.2.129 .
Zhong H, Zhao C, Luo S. HLA in myasthenia gravis: from superficial correlation to underlying mechanism. Autoimmun Rev. 2019;18(9):102349. https://doi.org/10.1016/j.autrev.2019.102349 .
Renton AE, Pliner HA, Provenzano C, et al. A genome-wide association study of myasthenia gravis. JAMA Neurol. 2015;72(4):396–404. https://doi.org/10.1001/jamaneurol.2014.4103 .
Chia R, Saez-Atienzar S, Murphy N, et al. Identification of genetic risk loci and prioritization of genes and pathways for myasthenia gravis: a genome-wide association study. Proc Natl Acad Sci U S A. 2022. https://doi.org/10.1073/pnas.2108672119 .
Iorio R. Myasthenia gravis: the changing treatment landscape in the era of molecular therapies. Nat Rev Neurol. 2024. https://doi.org/10.1038/s41582-023-00916-w .
Verschuuren JJ, Palace J, Murai H, et al. Advances and ongoing research in the treatment of autoimmune neuromuscular junction disorders. Lancet Neurol. 2022;21(2):189–202. https://doi.org/10.1016/s1474-4422(21)00463-4 .
Narayanaswami P, Sanders DB, Wolfe G, et al. International consensus guidance for management of myasthenia gravis: 2020 Update. Neurology. 2021;96(3):114–22. https://doi.org/10.1212/wnl.0000000000011124 .
Namba S, Konuma T, Wu KH, et al. A practical guideline of genomics-driven drug discovery in the era of global biobank meta-analysis. Cell Genom. 2022;2(10):100190. https://doi.org/10.1016/j.xgen.2022.100190 .
Article CAS PubMed PubMed Central Google Scholar
Kreitmaier P, Katsoula G, Zeggini E. Insights from multi-omics integration in complex disease primary tissues. Trends in genetics : TIG. 2023;39(1):46–58. https://doi.org/10.1016/j.tig.2022.08.005 .
Davey SG. Capitalizing on Mendelian randomization to assess the effects of treatments. J R Soc Med. 2007;100(9):432–5. https://doi.org/10.1177/014107680710000923 .
Article Google Scholar
Võsa U, Claringbould A, Westra H-J, et al. Large-scale cis- and trans-eQTL analyses identify thousands of genetic loci and polygenic scores that regulate blood gene expression. Nat Genet. 2021;53(9):1300–10. https://doi.org/10.1038/s41588-021-00913-z .
Zhang J, Dutta D, Köttgen A, et al. Plasma proteome analyses in individuals of European and African ancestry identify cis-pQTLs and models for proteome-wide association studies. Nat Genet. 2022;54(5):593–602. https://doi.org/10.1038/s41588-022-01051-w .
Sun BB, Maranville JC, Peters JE, et al. Genomic atlas of the human plasma proteome. Nature. 2018;558(7708):73–9. https://doi.org/10.1038/s41586-018-0175-2 .
Suhre K, Arnold M, Bhagwat AM, et al. Connecting genetic risk to disease end points through the human blood plasma proteome. Nat Commun. 2017;8:14357. https://doi.org/10.1038/ncomms14357 .
Folkersen L, Fauman E, Sabater-Lleal M, et al. Mapping of 79 loci for 83 plasma protein biomarkers in cardiovascular disease. PLoS Genet. 2017;13(4):e1006706. https://doi.org/10.1371/journal.pgen.1006706 .
Emilsson V, Ilkov M, Lamb JR, et al. Co-regulatory networks of human serum proteins link genetics to disease. Science. 2018;361(6404):769–73. https://doi.org/10.1126/science.aaq1327 .
Yao C, Chen G, Song C, et al. Genome-wide mapping of plasma protein QTLs identifies putatively causal genes and pathways for cardiovascular disease. Nat Commun. 2018;9(1):3268. https://doi.org/10.1038/s41467-018-05512-x .
Finan C, Gaulton A, Kruger FA, et al. The druggable genome and support for target identification and validation in drug development. Sci Trans Med. 2017. https://doi.org/10.1126/scitranslmed.aag1166 .
Zheng J, Haberland V, Baird D, et al. Phenome-wide Mendelian randomization mapping the influence of the plasma proteome on complex diseases. Nat Genet. 2020;52(10):1122–31. https://doi.org/10.1038/s41588-020-0682-6 .
Schmiedel BJ, Singh D, Madrigal A, et al. Impact of genetic polymorphisms on human immune cell gene expression. Cell. 2018;175(6):1701-15.e16. https://doi.org/10.1016/j.cell.2018.10.022 .
Staley JR, Blackshaw J, Kamat MA, et al. PhenoScanner: a database of human genotype-phenotype associations. Bioinformatics. 2016;32(20):3207–9. https://doi.org/10.1093/bioinformatics/btw373 .
Hemani G, Tilling K, Davey SG. Orienting the causal relationship between imprecisely measured traits using GWAS summary data. PLoS Genet. 2017;13(11):e1007081. https://doi.org/10.1371/journal.pgen.1007081 .
Wallace C. Eliciting priors and relaxing the single causal variant assumption in colocalisation analyses. PLoS Genet. 2020;16(4):e1008720. https://doi.org/10.1371/journal.pgen.1008720 .
Liu B, Gloudemans MJ, Rao AS, et al. Abundant associations with gene expression complicate GWAS follow-up. Nat Genet. 2019;51(5):768–9. https://doi.org/10.1038/s41588-019-0404-0 .
Faraco J, Lin L, Kornum BR, et al. ImmunoChip study implicates antigen presentation to T cells in narcolepsy. PLoS Genet. 2013;9(2):e1003270. https://doi.org/10.1371/journal.pgen.1003270 .
Walker JA, McKenzie ANJ. T(H)2 cell development and function. Nat Rev Immunol. 2018;18(2):121–33. https://doi.org/10.1038/nri.2017.118 .
Carss KJ, Deaton AM, Del Rio-Espinola A, et al. Using human genetics to improve safety assessment of therapeutics. Nat Rev Drug Discovery. 2023;22(2):145–62. https://doi.org/10.1038/s41573-022-00561-w .
East MP, Asquith CRM. CDC42BPA/MRCKα: a kinase target for brain, ovarian and skin cancers. Nat Rev Drug Discov. 2021;20(3):167. https://doi.org/10.1038/d41573-021-00023-9 .
Du X, de Almeida P, Manieri N, et al. CD226 regulates natural killer cell antitumor responses via phosphorylation-mediated inactivation of transcription factor FOXO1. Proc Natl Acad Sci U S A. 2018;115(50):E11731–40. https://doi.org/10.1073/pnas.1814052115 .
Bi J. CD226: a potent driver of antitumor immunity that needs to be maintained. Cell Mol Immunol. 2022;19(9):969–70. https://doi.org/10.1038/s41423-020-00633-0 .
Weulersse M, Asrir A, Pichler AC, et al. Eomes-dependent loss of the co-activating receptor CD226 restrains CD8(+) T cell anti-tumor functions and limits the efficacy of cancer immunotherapy. Immunity. 2020;53(4):824-39.e10. https://doi.org/10.1016/j.immuni.2020.09.006 .
Piédavent-Salomon M, Willing A, Engler JB, et al. Multiple sclerosis associated genetic variants of CD226 impair regulatory T cell function. Brain. 2015;138(Pt 11):3263–74. https://doi.org/10.1093/brain/awv256 .
Fløyel T, Brorsson C, Nielsen LB, et al. CTSH regulates β-cell function and disease progression in newly diagnosed type 1 diabetes patients. Proc Natl Acad Sci U S A. 2014;111(28):10305–10. https://doi.org/10.1073/pnas.1402571111 .
Ollila HM, Sharon E, Lin L, et al. Narcolepsy risk loci outline role of T cell autoimmunity and infectious triggers in narcolepsy. Nat Commun. 2023;14(1):2709. https://doi.org/10.1038/s41467-023-36120-z .
Link JO, Zipfel S. Advances in cathepsin S inhibitor design. Curr Opin Drug Discov Devel. 2006;9(4):471–82.
CAS PubMed Google Scholar
Morser J, Shao Z, Nishimura T, et al. Carboxypeptidase B2 and N play different roles in regulation of activated complements C3a and C5a in mice. J Thromb Haemost. 2018;16(5):991–1002. https://doi.org/10.1111/jth.13964 .
DeHart-McCoyle M, Patel S, Du X. New and emerging treatments for myasthenia gravis. BMJ Med. 2023;2(1):e000241. https://doi.org/10.1136/bmjmed-2022-000241 .
Sugitani Y, Nishida A, Inatomi O, et al. Sodium absorption stimulator prostasin (PRSS8) has an anti-inflammatory effect via downregulation of TLR4 signaling in inflammatory bowel disease. J Gastroenterol. 2020;55(4):408–17. https://doi.org/10.1007/s00535-019-01660-z .
Yazar S, Alquicira-Hernandez J, Wing K, et al. Single-cell eQTL mapping identifies cell type-specific genetic control of autoimmune disease. Science. 2022;376(6589):eabf3041. https://doi.org/10.1126/science.abf3041 .
Onuora S. Single-cell RNA sequencing sheds light on cell-type specific gene expression in immune cells. Nat Rev Rheumatol. 2022;18(7):363. https://doi.org/10.1038/s41584-022-00802-7 .
Chia R, Saez-Atienzar S, Drachman DB, et al. Implications of CHRNB1 and ERBB2 in the pathobiology of myasthenia gravis. Proc Natl Acad Sci U S A. 2022;119(36):e2209096119. https://doi.org/10.1073/pnas.2209096119 .
Download references
Acknowledgements
The authors gratefully thank the International Myasthenia Gravis Genomics Consortium, the UK Biobank and the FinnGen study for providing publicly accessible MG GWAS summary statistics for this analysis. Special thanks are extended to the Chinese Institute for Brain Research (Beijing) for their assistance. Thanks for the support from the Chinese Institutes for Medical Research, Beijing. We extend our sincere appreciation to the authors of the referenced QTL projects for their generous and open sharing of data. We express our gratitude to all the patients and families whose voluntary decision to donate samples has made our research possible.
This study was supported by the National Natural Science Foundation of China (81825008), the National Key Research and Development Program of China (2021YFA1101403), the Beijing Municipal Public Welfare Development and Reform Pilot Project for Medical Research Institutes (JYY2023-7), the Project for Innovation and Development of Beijing Municipal Geriatric Medical Research Center (11000023T000002041657), and the Project of Construction and Support for High-level Innovative Teams of Beijing Municipal Institutions (BPHR20220112), Youth Beijing Scholar NO.020, Dengfeng Talent Program (DFL20220701), the China Postdoctoral Science Foundation (2023M732407), and the Beijing Postdoctoral Research Foundation (2023-ZZ-004). The funders played no role in the design and conduct of the study; collection, management, analysis, and interpretation of the data; preparation, review, or approval of the manuscript; and decision to submit the manuscript for publication.
Author information
Jiao Li and Fei Wang have contributed equally to this work.
Authors and Affiliations
Department of Neurology, Xuanwu Hospital, National Center for Neurological Disorders, Capital Medical University, Beijing, 100053, China
Jiao Li, Fei Wang, Zhen Li, Jingjing Feng, Yi Men, Jinming Han, Jiangwei Xia, Chen Zhang, Yilai Han, Teng Chen, Yinan Zhao, Yuwei Da, Guoliang Chai & Junwei Hao
Beijing Municipal Geriatric Medical Research Center, Beijing, China
Jiao Li, Guoliang Chai & Junwei Hao
Key Laboratory for Neurodegenerative Diseases of Ministry of Education, Beijing, China
Jiao Li, Fei Wang & Junwei Hao
Department of Human Genetics, McGill University, Montréal, QC, Canada
You can also search for this author in PubMed Google Scholar
Contributions
JH and GC had full access to all the data in the study and take responsibility for the integrity of the data and the accuracy of the data analysis. JL and FW contributed equally to this work, and contributed equally to this work. Concept and design: JL, GC, JH. Drafting of the manuscript: JL, FW, ZL. Critical revision of the manuscript for important intellectual content: JH, YM. Statistical analysis: JL, FW, JF, JX. Obtained funding: JL, GC, JH. Administrative, technical, or material support: CZ, YH, TC, YZ, SZ. Supervision: YD, GC, JH. All authors approved the final version of the manuscript.
Corresponding authors
Correspondence to Guoliang Chai or Junwei Hao .
Ethics declarations
Ethics approval and consent to participate.
This study is covered under the Xuanwu Hospital, Capital Medical University, Human Research Ethics Committee approval ([2022]-148).
Consent for publication
Not applicable.
Competing interests
The authors declare that the research was conducted in the absence of any commercial or financial relationships that could be construed as a potential competing interests.
Additional information
Publisher's note.
Springer Nature remains neutral with regard to jurisdictional claims in published maps and institutional affiliations.
Supplementary Information
Additional file 1: figure s1..
Mendelian Randomization (MR) vs. Randomized controlled trial (RCT). Figure S2. Scatter plots of significant results from genetically predicted gene expression on MG risk in the primary analysis. Figure S3. Forest plot showing MR estimate for genetically proxied gene and protein expression on MG outcome across different datasets. Figure S4. PhenoScanner disease/trait annotation of the index eQTL/pQTL instrument with other traits. Figure S5. LocusCompare plot depicting colocalization of the top SNP associated with eQTL surrounding CTSH in T H 2 cell and MG GWAS. Figure S6. Protein–protein interaction of CPN2 using the STRING database ( https://string-db.org/ ).
Additional file 2: Table S1.
Basic Characteristics of eQTL Databases, pQTL Studies, and GWAS Datasets in the study. Table S2. An overview of druggable proteins and the coverage of genes/proteins in eQTLGen and pQTL studies. Table S3. Genome-wide significant loci in MG GWAS from Chia et al. Table S4. The cis- eQTLs instruments used for drug target gene expression on MG risk in the primary analysis. The outcome is MG GWAS from Chia et al. (1,873 patients and 36,370 controls). Table S5. MR full results using cis- eQTLs on MG risk across different datasets. Table S6. Colocalization results using cis-eQTLs on MG across different datasets. Table S7. The cis- pQTLs instruments used for protein expression on MG risk in the primary analysis. The outcome is MG GWAS from Chia et al. (1,873 patients and 36,370 controls). Table S8. MR full results of proteins using sentinel cis- pQTLs from six large proteomic studies on MG risk across different datasets. Table S9. Colocalization results using cis- pQTLs on MG risk across different datasets. Table S10. Colocalization results for genes/proteins using eQTL from the DICE database.
Rights and permissions
Open Access This article is licensed under a Creative Commons Attribution 4.0 International License, which permits use, sharing, adaptation, distribution and reproduction in any medium or format, as long as you give appropriate credit to the original author(s) and the source, provide a link to the Creative Commons licence, and indicate if changes were made. The images or other third party material in this article are included in the article's Creative Commons licence, unless indicated otherwise in a credit line to the material. If material is not included in the article's Creative Commons licence and your intended use is not permitted by statutory regulation or exceeds the permitted use, you will need to obtain permission directly from the copyright holder. To view a copy of this licence, visit http://creativecommons.org/licenses/by/4.0/ . The Creative Commons Public Domain Dedication waiver ( http://creativecommons.org/publicdomain/zero/1.0/ ) applies to the data made available in this article, unless otherwise stated in a credit line to the data.
Reprints and permissions
About this article
Cite this article.
Li, J., Wang, F., Li, Z. et al. Integrative multi-omics analysis identifies genetically supported druggable targets and immune cell specificity for myasthenia gravis. J Transl Med 22 , 302 (2024). https://doi.org/10.1186/s12967-024-04994-2
Download citation
Received : 28 September 2023
Accepted : 12 February 2024
Published : 24 March 2024
DOI : https://doi.org/10.1186/s12967-024-04994-2
Share this article
Anyone you share the following link with will be able to read this content:
Sorry, a shareable link is not currently available for this article.
Provided by the Springer Nature SharedIt content-sharing initiative
- Myasthenia gravis
- Actionable druggable genome
- Mendelian randomization
- Genetic colocalization
- Cell-type specificity
Journal of Translational Medicine
ISSN: 1479-5876
- Submission enquiries: Access here and click Contact Us
- General enquiries: [email protected]
Subscribe to the PwC Newsletter
Join the community, edit social preview.

Add a new code entry for this paper
Remove a code repository from this paper, mark the official implementation from paper authors, add a new evaluation result row, remove a task, add a method, remove a method, edit datasets, quantitative analysis of ai-generated texts in academic research: a study of ai presence in arxiv submissions using ai detection tool.
9 Feb 2024 · Arslan Akram · Edit social preview
Many people are interested in ChatGPT since it has become a prominent AIGC model that provides high-quality responses in various contexts, such as software development and maintenance. Misuse of ChatGPT might cause significant issues, particularly in public safety and education, despite its immense potential. The majority of researchers choose to publish their work on Arxiv. The effectiveness and originality of future work depend on the ability to detect AI components in such contributions. To address this need, this study will analyze a method that can see purposely manufactured content that academic organizations use to post on Arxiv. For this study, a dataset was created using physics, mathematics, and computer science articles. Using the newly built dataset, the following step is to put originality.ai through its paces. The statistical analysis shows that Originality.ai is very accurate, with a rate of 98%.
Code Edit Add Remove Mark official
Tasks edit add remove, datasets edit, results from the paper edit add remove, methods edit add remove.
10 March Madness tips from a certified bracket master

As you stare at your blank March Madness bracket, don’t focus on the 9,223,372,036,854,775,808 different ways this men’s basketball tournament could play out, or worry about which teams are going to be the talk of the country for their stunning first-round upsets. We are going to walk through the best way to construct a bracket so it is the last one standing in your office or family pool — and help you answer the questions that actually matter.
March Madness

The approach outlined here is similar to the one I used last year to correctly identify six of the Elite Eight teams — San Diego State, Kansas State, Miami, Texas, Connecticut and Gonzaga, none of which were No. 1 seeds. That approach also helped me predict that fourth-seeded Connecticut would make the Final Four, and that fifth-seeded San Diego State would reach the title game, picks that helped plenty of my readers win their pools. If you followed the “People’s Bracket” last year — the most popular picks for every round in ESPN’s contest — you would have finished with 45 points in standard scoring systems, with only one Elite Eight team and none of the Final Four. My bracket finished with 96 points.
To build our strategy, we’re going to incorporate historical trends, make some educated guesses based on analytics and lean on betting markets to point us in the right direction. Some of these tips may be new to you, but rest assured this is the correct path to take — and a far more productive blueprint than making picks based on school colors, mascots or the advice of noisy TV pundits.
And for more advice, see also my annual Perfect Bracket ; the most likely first-round upsets ; the best bets to win it all that you can trust; and the most vulnerable top seeds .
Don’t start filling out your bracket with Round 1; start with the Elite Eight or Final Four
Most people sit down with their brackets, start at the 1 vs. 16 matchup in the top left corner and work their way through that region until they come to the Final Four. We’re not most people. We start with the teams we think will reach the Elite Eight or the Final Four and work backward to reduce the number of decisions we need to make.
Why? Because according to a March 2020 study in the Journal of Quantitative Analysis in Sports (“ Models for generating NCAA men’s basketball tournament bracket pools ”), bracket generators that start by selecting the teams that reach the Elite Eight or Final Four tend to outperform generators that start with the round of 64 or the national champion. It’s also the “ best for balancing initial pick risk against the number of decisions ,” per Sheldon H. Jacobson, a computer science professor at Illinois and one of the authors of the paper.
You can find my full “Perfect Bracket” here , but here’s a spoiler: No. 4 seed Auburn looks awfully enticing. The Tigers enter the tournament as the fourth-best team in the country, per analyst Ken Pomeroy’s ratings, which adjust margin of victory for tempo and strength of schedule. The Tigers also have one of the best defenses in the country. They’re in a powerhouse East Region with No. 1 seed Connecticut, No. 2 seed Iowa State and No. 3 seed Illinois, but that just means fewer of your competitors focus on them.
Look for value in the Elite Eight
According to data from ESPN’s past tournament pools, No. 1 and No. 2 seeds are typically well represented in the public’s Elite Eight. An average No. 1 seed is advanced to that round by 63 percent of entrants, while an average No. 2 seed is found in the Elite Eight on nearly half of all brackets. That tells us we should look elsewhere for our choices when possible, especially if we’re entering larger pools.
Specifically, look for highly rated teams in Pomeroy’s rankings that have been under-seeded in the tournament. You could also target lower-seeded teams that have a high consensus rating, using analyst Ken Massey’s aggregation of dozens of rating methods, relative to the field. In addition to Auburn, a team to consider is No. 5 Saint Mary’s in the West Region. The Gaels won the West Coast Conference regular season and tournament titles, reeled off 16 straight wins at one point this year and rank 20th in Pomeroy’s ratings.
Be selective picking upsets
Validation from picking upsets — defined here as a win by a team at least two seed lines below the losing team — is wonderful. I should know; I had fifth-seeded San Diego State in the title game last year , as you might have heard. Yet there probably aren’t as many of these upsets as you think, even in the early rounds. Since 1985, when the men’s field expanded to 64 teams, there have been, on average, 12 of these upsets per tournament .
Sometimes there are more — there were 14 last year, in a historically wacky tournament — and sometimes there are fewer — there were only four in 2007. As you would expect, the deeper you get into the tournament, the fewer such upsets occur. And obviously, if two lower-seeded teams meet in a later round, one will advance, even if it might not be a huge upset by seed.
So how do you decide which teams are capable of busting brackets? If you are comfortable with sports betting, check out the point spreads for each individual game and find lower-seeded teams that are either small underdogs or favored outright. Some of those this season include No. 10 Drake (which is favored over No. 7 Washington State), No. 11 New Mexico (which is favored over No. 6 Clemson) and No. 10 Nevada (which is favored over No. 7 Dayton), although there are also other appealing first-round upset candidates . And No. 11 Oregon is just a slight underdog against No. 6 South Carolina.
You could also check out the consensus rankings and make decisions accordingly. Historically speaking, the higher-rated team wins approximately 67 percent of the time, giving you a good indicator of a few potential upsets to target.
The size of your pool will tell you how much risk to take
If you are in a small pool — say, 25 people or less — you want to minimize your risk. That means not picking a large number of surprises, especially in the late rounds, and focusing on the favorites.
As the pool size gets bigger, it’s necessary to take more calculated risks to make your bracket unique. In other words, if you pick a favorite to win the title in a big pool — meaning you aren’t guaranteed much of anything even if your team wins — you need to be contrarian elsewhere, like picking a Cinderella team to reach the Final Four or taking more risks in the early rounds. If you decide to make riskier plays in the later rounds — the Elite Eight and beyond — you can play it safer leading up to the Sweet 16. Last year’s successful Perfect Bracket predicted that 12 of the top 16 seeds would advance to the Sweet 16; the riskier picks later on carried the bracket to success.
Don’t buy into the 12-seed mystique. Focus on the No. 11 seeds instead
You are going to hear a lot about how appealing the No. 12 seeds are, and how often they upset No. 5 seeds in the first round. That used to be the case, but lately there’s a better strategy. Since 2011, when the field expanded to 68 teams, No. 12 seeds have an 18-30 record against No. 5 seeds in the round of 64. The No. 11 seeds, by comparison, are 25-23 in their matchups against the No. 6 seeds — and often with a whole lot less hype. My top first-round upset picks this year include No. 11 New Mexico over No. 6 Clemson and No. 11 N.C. State over No. 6 Texas Tech.
Believe in at least one ‘First Four’ team
The First Four games open the tournament, pitting the last four automatic qualifiers against each other and the last four at-large teams against each other. The winners of the First Four games advance into the field of 64. They don’t all succeed there, but from 2011 to 2023, only once (in 2019) has an at-large First Four team failed to win a game in the 64-team bracket.
The most famous example is VCU steamrolling into the Final Four as a No. 11 seed in 2011. A decade later, UCLA went from the First Four to Final Four after beating No. 1 Michigan in the Elite Eight. La Salle (2013), Tennessee (2014) and Syracuse (2018) are other First Four teams with multiple wins in the 64-field tournament.
Last year got even crazier, when Fairleigh Dickinson became the first automatic qualifier to go from a First Four win to claiming a game in the round of 64. They did it in historic fashion, upsetting No. 1 Purdue to become just the second No. 16 seed to reach the round of 32.
And because these teams aren’t penciled into the 64-team grid on Sunday night, many bracket-pickers avoid them — which can give you an even bigger edge. This year, the “First Four” teams include four No. 10 seeds: Virginia plays Colorado State, and Boise State faces Colorado. Take a close look at the winners of those two games.
Favor teams that did well in conference tournaments
There was a time when you wanted your eventual title team to have won its just-concluded conference tournament, but that’s no longer necessary. From 1999 to 2010, eight out of 12 national champions previously won their conference tournaments. In the 12 tournaments since, just four conference champions won the national championship.
However, every national championship-winning team since 1985 — with the exception of UCLA in 1995 and Arizona in 1997, which didn’t have a conference tournament — has lasted at least to the semifinal round in its conference tournament. So plan on avoiding teams that made an early exit, at least for your national title pick. This year, such teams include No. 3 seed Creighton, No. 4 seed Duke, No. 4 seed Kansas and three teams from the SEC: No. 2 seed Tennessee, No. 3 seed Kentucky and No. 4 seed Alabama. Kansas at least had an excuse; Kevin McCullar Jr. and Hunter Dickinson missed the Jayhawks’ loss to Cincinnati because of injuries, and Parker Braun wasn’t 100 percent.
Focus on the statistics that matter
There are 68 teams in the tournament after thousands of games played this season, producing a variety of statistics indicating which teams will win or lose a particular matchup. Most of these are irrelevant. Instead, investigate the essentials of shooting, rebounding, creating turnovers and getting to the free throw line, also known as the four factors for offense and defense.
For upset candidates, offensive rebounding just might be the most important. Those rebounds provide teams with the extra possessions that are crucial to pulling off a March upset. Since 2011, in three-quarters of NCAA tournament upsets, the worse seed had the better offensive rebounding rate during the game in question. Some of the best offensive rebounding teams that are lower-seeded teams in this tournament include No. 16 Longwood (12th), No. 12 UAB (22nd) and No. 15 Saint Peter’s (23rd). Other strong offensive rebounding teams that could be under the radar include No. 9 seed Texas A&M, No. 5 seed Saint Mary’s and No. 7 seed Florida.
Don’t just guess at the tiebreaker total
The tiebreaker most often used — total points scored in the championship game — is often treated as an afterthought. It doesn’t have to be .
Since 1985, when the men’s tournament expanded to 64 teams, the national title game has averaged 145 total points when decided in regulation. The four overtime games in that span averaged 157 total points. The most total points scored in regulation was in 1990, when UNLV beat Duke, 103-73 (176). The fewest total points came in 2011, when Connecticut beat Butler, 53-41 (94).
How many points you choose should be influenced by which teams you have in the final, since pace of play and offensive efficiency help determine how many points a team might score. Here’s a quick list of some of the most frequent matchups in the Elite Eight and beyond and the average total points scored in those contests since 2011 — but look at the averages of your chosen teams. I will have a guide to picking the tiebreaker later this week.
Know how to spot a potential championship team
Success leaves clues, and we have a lot of data on how an eventual championship team usually performs leading up to the tournament. My colleague Matt Bonesteel outlines some of those clues in his annual best bets column — which included eventual national champion Connecticut as one of the five most likely winners last year — and there are some other guidelines you can follow. Since the field expanded to 68 teams in 2011, for example, every national champion except two — No. 7 seed Connecticut in 2014 and Connecticut again as a No. 4 seed last year — was a No. 1, 2 or 3 seed. Since 1985, when the field expanded to 64 teams, all but five of the 38 winners were a No. 1, 2 or 3 seed and 24 of the 38 (63 percent) were No. 1 seeds.
And all but four of the past 19 winners have had their individual Simple Rating System , a schedule-adjusted margin of victory rating that is expressed in points per game, rank in the top four nationally. Connecticut just missed last year, at No. 5. The top four schools in SRS this year are Houston, Arizona, Purdue and Connecticut.
You could also look at teams with similar profiles and see how far they advanced in the tournament, a technique that helped me identify San Diego State last year. For example, this year’s Auburn squad, a No. 4 seed, is similar to teams that have won a robust 2.3 games per tournament , on average (the same as No. 2 seeds from 2011 to 2023 ). This Auburn team also has similar performance metrics as runner-up Texas Tech in 2019 plus Florida in 2017 and Houston in 2022, two Elite Eight teams.
The NCAA tournament is back. Get caught up with our March Madness cheat sheet .
The brackets: Defending national champion U-Conn. returns as the top overall seed in the men’s bracket . In the women’s tournament , undefeated South Carolina leads the way. Read our picks for the biggest snubs and surprises in the tournament field.
Analysis from our team: Looking to win your bracket pool? These tips from our resident bracket master can help. If you want help picking a national champion, these are our favorites and best bets to win the tournament, plus tips for selecting your Final Four . Take a look at some of the teams we think are most likely to pull an upset .
- The perfect bracket to win your March Madness men’s pool 48 minutes ago The perfect bracket to win your March Madness men’s pool 48 minutes ago
- These four sleepers could find their way to the women’s Final Four 1 hour ago These four sleepers could find their way to the women’s Final Four 1 hour ago
- These are the NCAA men’s tournament’s most vulnerable top seeds 2 hours ago These are the NCAA men’s tournament’s most vulnerable top seeds 2 hours ago

Help | Advanced Search
Mathematical Physics
Title: spectral analysis of lattice schrödinger-type operators associated with the nonstationary anderson model and intermittency.
Abstract: The research explores a high irregularity, commonly referred to as intermittency, of the solution to the non-stationary parabolic Anderson problem: \begin{equation*} \frac{\partial u}{\partial t} = \varkappa \mathcal{L}u(t,x) + \xi_{t}(x)u(t,x) \end{equation*} with the initial condition \(u(0,x) \equiv 1\), where \((t,x) \in [0,\infty)\times \mathbb{Z}^d\). Here, \(\varkappa \mathcal{L}\) denotes a non-local Laplacian, and \(\xi_{t}(x)\) is a correlated white noise potential. The observed irregularity is intricately linked to the upper part of the spectrum of the multiparticle Schrödinger equations for the moment functions \(m_p(t,x_1,x_2,\cdots,x_p) = \langle u(t,x_1)u(t,x_2)\cdots u(t,x_p)\rangle\). In the first half of the paper, a weak form of intermittency is expressed through moment functions of order $p\geq 3$ and established for a wide class of operators $\varkappa \mathcal{L}$ with a positive-definite correlator $B=B(x))$ of the white noise. In the second half of the paper, the strong intermittency is studied. It relates to the existence of a positive eigenvalue for the lattice Schrödinger type operator with the potential $B$. This operator is associated with the second moment $m_2$. Now $B$ is not necessarily positive-definite, but $\sum B(x)\geq 0$.
Submission history
Access paper:.
- Download PDF
- HTML (experimental)
- Other Formats

References & Citations
- Google Scholar
- Semantic Scholar
BibTeX formatted citation

Bibliographic and Citation Tools
Code, data and media associated with this article, recommenders and search tools.
- Institution
arXivLabs: experimental projects with community collaborators
arXivLabs is a framework that allows collaborators to develop and share new arXiv features directly on our website.
Both individuals and organizations that work with arXivLabs have embraced and accepted our values of openness, community, excellence, and user data privacy. arXiv is committed to these values and only works with partners that adhere to them.
Have an idea for a project that will add value for arXiv's community? Learn more about arXivLabs .
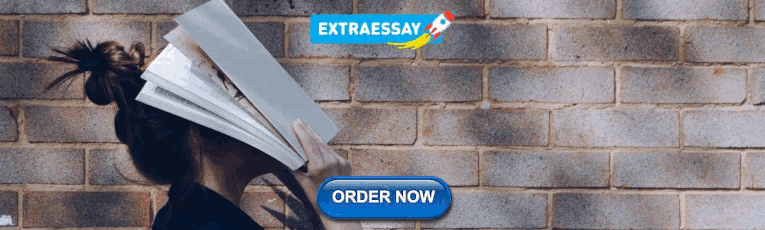
IMAGES
VIDEO
COMMENTS
Quantitative Research. Quantitative research is a type of research that collects and analyzes numerical data to test hypotheses and answer research questions.This research typically involves a large sample size and uses statistical analysis to make inferences about a population based on the data collected.
Revised on June 22, 2023. Quantitative research is the process of collecting and analyzing numerical data. It can be used to find patterns and averages, make predictions, test causal relationships, and generalize results to wider populations. Quantitative research is the opposite of qualitative research, which involves collecting and analyzing ...
The two "branches" of quantitative analysis. As I mentioned, quantitative analysis is powered by statistical analysis methods.There are two main "branches" of statistical methods that are used - descriptive statistics and inferential statistics.In your research, you might only use descriptive statistics, or you might use a mix of both, depending on what you're trying to figure out.
Quantitative research is the process of collecting and analyzing numerical data to describe, predict, or control variables of interest. This type of research helps in testing the causal relationships between variables, making predictions, and generalizing results to wider populations. The purpose of quantitative research is to test a predefined ...
Image Source. Quantitative data analysis is a systematic process of examining, interpreting, and drawing meaningful conclusions from numerical data. It involves the application of statistical methods, mathematical models, and computational techniques to understand patterns, relationships, and trends within datasets.
5. Efficiency: Quantitative methods often allow for efficient data collection and analysis. Surveys, experiments, and statistical software tools facilitate the processing of large amounts of data, making it feasible to study complex phenomena within a reasonable timeframe. Limitations of Quantitative Methods: 1.
Quantitative research is the opposite of qualitative research, which involves collecting and analysing non-numerical data (e.g. text, video, or audio). Quantitative research is widely used in the natural and social sciences: biology, chemistry, psychology, economics, sociology, marketing, etc. Quantitative research question examples.
Quantitative data analysis serves as part of an essential process of evidence-making in health and social sciences. It is adopted for any types of research question and design whether it is descriptive, explanatory, or causal. However, compared with qualitative counterpart, quantitative data analysis has less flexibility.
Statistical analysis means investigating trends, patterns, and relationships using quantitative data. It is an important research tool used by scientists, governments, businesses, and other organizations. To draw valid conclusions, statistical analysis requires careful planning from the very start of the research process. You need to specify ...
Quantitative research is a research strategy that focuses on quantifying the collection and analysis of data. It is formed from a deductive approach where emphasis is placed on the testing of theory, shaped by empiricist and positivist philosophies.. Associated with the natural, applied, formal, and social sciences this research strategy promotes the objective empirical investigation of ...
Quantitative research is a type of research that focuses on collecting and analyzing numerical data to answer research questions. There are two main methods used to conduct quantitative research: 1. Primary Method. There are several methods of primary quantitative research, each with its own strengths and limitations.
End time: 00:04:33. Product: Sage Research Methods Video: Quantitative and Mixed Methods. Type of Content: Tutorial. Title: Introduction to the Main Groups of Quantitative Data Analysis Methods. Publisher: Betazeta Pty Ltd ATF Lawoko Holdings Trust. Series: Introduction to Quantitative Research Methods. Publication year: 2021.
Quantitative research methods are concerned with the planning, design, and implementation of strategies to collect and analyze data. Descartes, the seventeenth-century philosopher, suggested that how the results are achieved is often more important than the results themselves, as the journey taken along the research path is a journey of discovery. . High-quality quantitative research is ...
LEARN ABOUT: Steps in Qualitative Research. Methods used for data analysis in quantitative research. After the data is prepared for analysis, researchers are open to using different research and data analysis methods to derive meaningful insights. For sure, statistical analysis plans are the most favored to analyze numerical data.
A research question is what a study aims to answer after data analysis and interpretation. The answer is written in length in the discussion section of the paper. ... Research questions and hypotheses are crucial components to any type of research, whether quantitative or qualitative. These questions should be developed at the very beginning of ...
Use quantitative research if you want to confirm or test something (a theory or hypothesis) Use qualitative research if you want to understand something (concepts, thoughts, experiences) For most research topics you can choose a qualitative, quantitative or mixed methods approach. Which type you choose depends on, among other things, whether ...
Employ quantitative research methods to accumulate numerical insights from diverse channels such as: ... Statistical Analysis in Quantitative Research. Statistical analysis is a cornerstone of quantitative research, providing the tools and techniques to interpret numerical data systematically. By applying statistical methods, researchers can ...
Key points regarding survey research:In the survey research, the users raised various queries; therefore, the quantitative analysis was also done on the same basis.For conducting the survey research analysis, longitudinal and cross-sectional surveys are performed.The longitudinal survey research applies to the population at different time durations.
Learn what quantitative research is, its types, and the different methodologies it uses for researching data sets with examples. (855) 776-7763; Get a Demo ... Data analysis in quantitative research produces highly structured results and can form well-defined graphical representations. Some common examples include tables, figures, graphs, etc ...
Research in which collected data is converted into numbers or numerical data is quantitative research. It is widely used in surveys, demographic studies, census information, marketing, and other studies that use numerical data to analyze results. Primary quantitative research yields results that are objective, statistical, and unbiased.
Abstract. Statistical methods involved in carrying out a study include planning, designing, collecting data, analysing, drawing meaningful interpretation and reporting of the research findings. The statistical analysis gives meaning to the meaningless numbers, thereby breathing life into a lifeless data. The results and inferences are precise ...
Qualitative research aims to produce rich and detailed descriptions of the phenomenon being studied, and to uncover new insights and meanings. Quantitative data is information about quantities, and therefore numbers, and qualitative data is descriptive, and regards phenomenon which can be observed but not measured, such as language.
Descriptive and inferential are the two general types of statistical analyses in quantitative research. Descriptive includes simple calculations of central tendency (mean, median and mode), spread (quartile ranges, standard deviation and variance) and frequency distributions displayed in graphs. Inferential includes more complex calculations of ...
Unfortunately, research about clinical characteristics and management of COMISA based on quantitative evidence is lacking. Method Standard procedures for literature retrieval, selection and quality assessment, data extraction, analysis, and interpretation were conducted step by step.
Background Myasthenia gravis (MG) is a chronic autoimmune disorder characterized by fluctuating muscle weakness. Despite the availability of established therapies, the management of MG symptoms remains suboptimal, partially attributed to lack of efficacy or intolerable side-effects. Therefore, new effective drugs are warranted for treatment of MG. Methods By employing an analytical framework ...
For this study, a dataset was created using physics, mathematics, and computer science articles. Using the newly built dataset, the following step is to put originality.ai through its paces. The statistical analysis shows that Originality.ai is very accurate, with a rate of 98%. PDF Abstract
March 18, 2024 at 6:05 a.m. EDT. Readers thanked Neil Greenberg for his winning picks in 2023. (Cece Pascual/The Washington Post) 13 min. As you stare at your blank March Madness bracket, don't ...
CHICAGO, March 18, 2024 — An analysis of over 20,000 U.S. adults found that people who limited their eating across less than 8 hours per day, a time-restricted eating plan, were more likely to die from cardiovascular disease compared to people who ate across 12-16 hours per day, according to preliminary research presented at the American ...
Abstract: The research explores a high irregularity, commonly referred to as intermittency, ... Download a PDF of the paper titled Spectral Analysis of Lattice Schr\"{o}dinger-Type Operators Associated with the Nonstationary Anderson Model and Intermittency, by Dan Han and 2 other authors. Download PDF; HTML (experimental)