
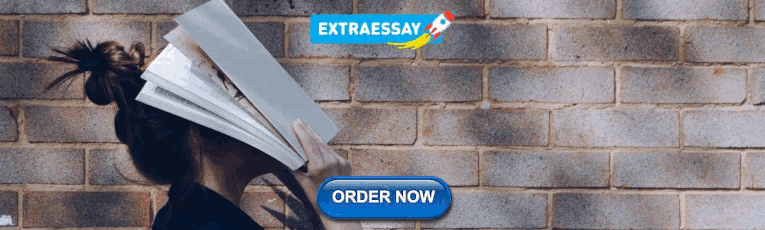
Research Design 101
Everything You Need To Get Started (With Examples)
By: Derek Jansen (MBA) | Reviewers: Eunice Rautenbach (DTech) & Kerryn Warren (PhD) | April 2023
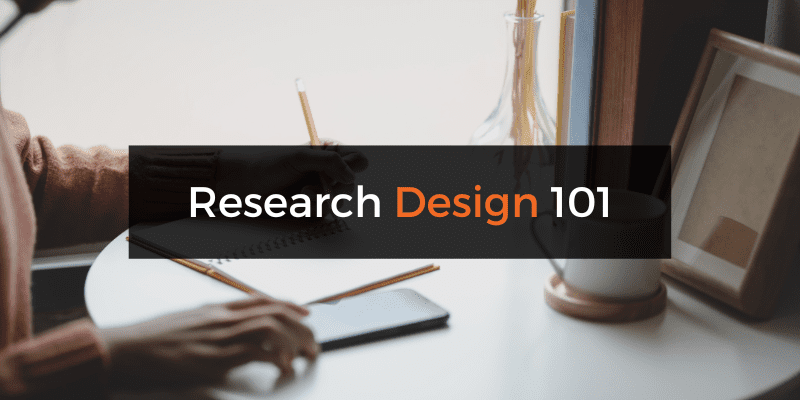
Navigating the world of research can be daunting, especially if you’re a first-time researcher. One concept you’re bound to run into fairly early in your research journey is that of “ research design ”. Here, we’ll guide you through the basics using practical examples , so that you can approach your research with confidence.
Overview: Research Design 101
What is research design.
- Research design types for quantitative studies
- Video explainer : quantitative research design
- Research design types for qualitative studies
- Video explainer : qualitative research design
- How to choose a research design
- Key takeaways
Research design refers to the overall plan, structure or strategy that guides a research project , from its conception to the final data analysis. A good research design serves as the blueprint for how you, as the researcher, will collect and analyse data while ensuring consistency, reliability and validity throughout your study.
Understanding different types of research designs is essential as helps ensure that your approach is suitable given your research aims, objectives and questions , as well as the resources you have available to you. Without a clear big-picture view of how you’ll design your research, you run the risk of potentially making misaligned choices in terms of your methodology – especially your sampling , data collection and data analysis decisions.
The problem with defining research design…
One of the reasons students struggle with a clear definition of research design is because the term is used very loosely across the internet, and even within academia.
Some sources claim that the three research design types are qualitative, quantitative and mixed methods , which isn’t quite accurate (these just refer to the type of data that you’ll collect and analyse). Other sources state that research design refers to the sum of all your design choices, suggesting it’s more like a research methodology . Others run off on other less common tangents. No wonder there’s confusion!
In this article, we’ll clear up the confusion. We’ll explain the most common research design types for both qualitative and quantitative research projects, whether that is for a full dissertation or thesis, or a smaller research paper or article.
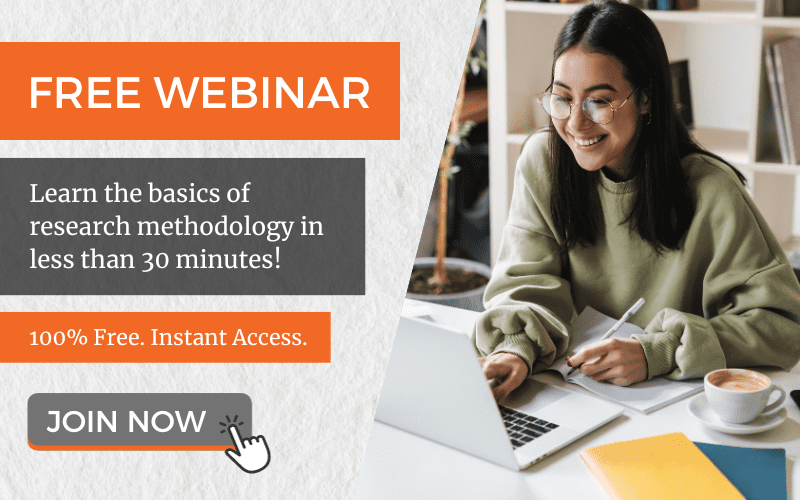
Research Design: Quantitative Studies
Quantitative research involves collecting and analysing data in a numerical form. Broadly speaking, there are four types of quantitative research designs: descriptive , correlational , experimental , and quasi-experimental .
Descriptive Research Design
As the name suggests, descriptive research design focuses on describing existing conditions, behaviours, or characteristics by systematically gathering information without manipulating any variables. In other words, there is no intervention on the researcher’s part – only data collection.
For example, if you’re studying smartphone addiction among adolescents in your community, you could deploy a survey to a sample of teens asking them to rate their agreement with certain statements that relate to smartphone addiction. The collected data would then provide insight regarding how widespread the issue may be – in other words, it would describe the situation.
The key defining attribute of this type of research design is that it purely describes the situation . In other words, descriptive research design does not explore potential relationships between different variables or the causes that may underlie those relationships. Therefore, descriptive research is useful for generating insight into a research problem by describing its characteristics . By doing so, it can provide valuable insights and is often used as a precursor to other research design types.
Correlational Research Design
Correlational design is a popular choice for researchers aiming to identify and measure the relationship between two or more variables without manipulating them . In other words, this type of research design is useful when you want to know whether a change in one thing tends to be accompanied by a change in another thing.
For example, if you wanted to explore the relationship between exercise frequency and overall health, you could use a correlational design to help you achieve this. In this case, you might gather data on participants’ exercise habits, as well as records of their health indicators like blood pressure, heart rate, or body mass index. Thereafter, you’d use a statistical test to assess whether there’s a relationship between the two variables (exercise frequency and health).
As you can see, correlational research design is useful when you want to explore potential relationships between variables that cannot be manipulated or controlled for ethical, practical, or logistical reasons. It is particularly helpful in terms of developing predictions , and given that it doesn’t involve the manipulation of variables, it can be implemented at a large scale more easily than experimental designs (which will look at next).
That said, it’s important to keep in mind that correlational research design has limitations – most notably that it cannot be used to establish causality . In other words, correlation does not equal causation . To establish causality, you’ll need to move into the realm of experimental design, coming up next…
Need a helping hand?
Experimental Research Design
Experimental research design is used to determine if there is a causal relationship between two or more variables . With this type of research design, you, as the researcher, manipulate one variable (the independent variable) while controlling others (dependent variables). Doing so allows you to observe the effect of the former on the latter and draw conclusions about potential causality.
For example, if you wanted to measure if/how different types of fertiliser affect plant growth, you could set up several groups of plants, with each group receiving a different type of fertiliser, as well as one with no fertiliser at all. You could then measure how much each plant group grew (on average) over time and compare the results from the different groups to see which fertiliser was most effective.
Overall, experimental research design provides researchers with a powerful way to identify and measure causal relationships (and the direction of causality) between variables. However, developing a rigorous experimental design can be challenging as it’s not always easy to control all the variables in a study. This often results in smaller sample sizes , which can reduce the statistical power and generalisability of the results.
Moreover, experimental research design requires random assignment . This means that the researcher needs to assign participants to different groups or conditions in a way that each participant has an equal chance of being assigned to any group (note that this is not the same as random sampling ). Doing so helps reduce the potential for bias and confounding variables . This need for random assignment can lead to ethics-related issues . For example, withholding a potentially beneficial medical treatment from a control group may be considered unethical in certain situations.
Quasi-Experimental Research Design
Quasi-experimental research design is used when the research aims involve identifying causal relations , but one cannot (or doesn’t want to) randomly assign participants to different groups (for practical or ethical reasons). Instead, with a quasi-experimental research design, the researcher relies on existing groups or pre-existing conditions to form groups for comparison.
For example, if you were studying the effects of a new teaching method on student achievement in a particular school district, you may be unable to randomly assign students to either group and instead have to choose classes or schools that already use different teaching methods. This way, you still achieve separate groups, without having to assign participants to specific groups yourself.
Naturally, quasi-experimental research designs have limitations when compared to experimental designs. Given that participant assignment is not random, it’s more difficult to confidently establish causality between variables, and, as a researcher, you have less control over other variables that may impact findings.
All that said, quasi-experimental designs can still be valuable in research contexts where random assignment is not possible and can often be undertaken on a much larger scale than experimental research, thus increasing the statistical power of the results. What’s important is that you, as the researcher, understand the limitations of the design and conduct your quasi-experiment as rigorously as possible, paying careful attention to any potential confounding variables .
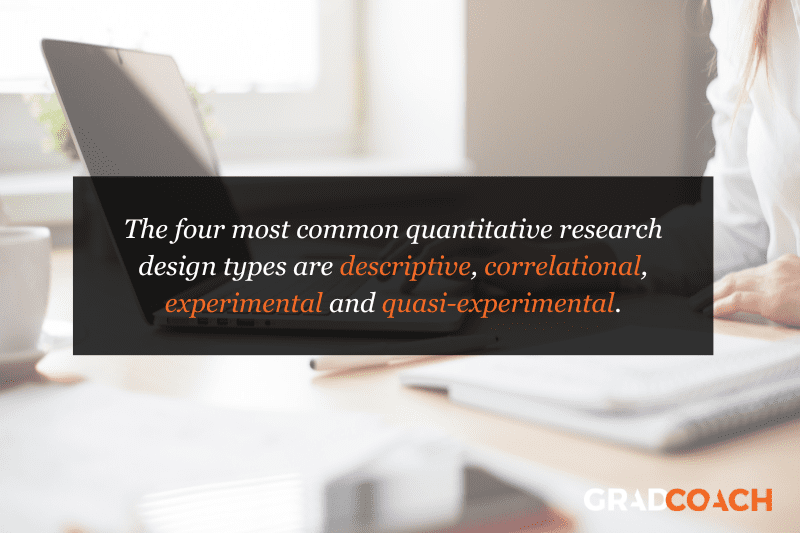
Research Design: Qualitative Studies
There are many different research design types when it comes to qualitative studies, but here we’ll narrow our focus to explore the “Big 4”. Specifically, we’ll look at phenomenological design, grounded theory design, ethnographic design, and case study design.
Phenomenological Research Design
Phenomenological design involves exploring the meaning of lived experiences and how they are perceived by individuals. This type of research design seeks to understand people’s perspectives , emotions, and behaviours in specific situations. Here, the aim for researchers is to uncover the essence of human experience without making any assumptions or imposing preconceived ideas on their subjects.
For example, you could adopt a phenomenological design to study why cancer survivors have such varied perceptions of their lives after overcoming their disease. This could be achieved by interviewing survivors and then analysing the data using a qualitative analysis method such as thematic analysis to identify commonalities and differences.
Phenomenological research design typically involves in-depth interviews or open-ended questionnaires to collect rich, detailed data about participants’ subjective experiences. This richness is one of the key strengths of phenomenological research design but, naturally, it also has limitations. These include potential biases in data collection and interpretation and the lack of generalisability of findings to broader populations.
Grounded Theory Research Design
Grounded theory (also referred to as “GT”) aims to develop theories by continuously and iteratively analysing and comparing data collected from a relatively large number of participants in a study. It takes an inductive (bottom-up) approach, with a focus on letting the data “speak for itself”, without being influenced by preexisting theories or the researcher’s preconceptions.
As an example, let’s assume your research aims involved understanding how people cope with chronic pain from a specific medical condition, with a view to developing a theory around this. In this case, grounded theory design would allow you to explore this concept thoroughly without preconceptions about what coping mechanisms might exist. You may find that some patients prefer cognitive-behavioural therapy (CBT) while others prefer to rely on herbal remedies. Based on multiple, iterative rounds of analysis, you could then develop a theory in this regard, derived directly from the data (as opposed to other preexisting theories and models).
Grounded theory typically involves collecting data through interviews or observations and then analysing it to identify patterns and themes that emerge from the data. These emerging ideas are then validated by collecting more data until a saturation point is reached (i.e., no new information can be squeezed from the data). From that base, a theory can then be developed .
As you can see, grounded theory is ideally suited to studies where the research aims involve theory generation , especially in under-researched areas. Keep in mind though that this type of research design can be quite time-intensive , given the need for multiple rounds of data collection and analysis.
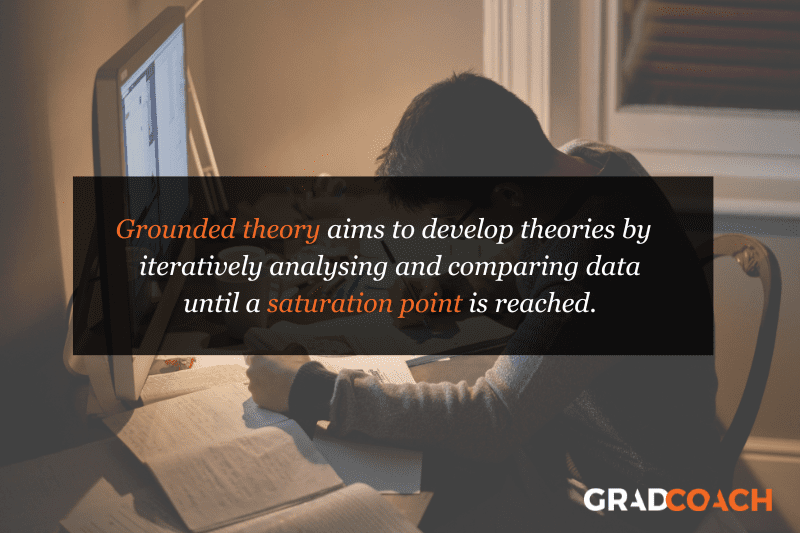
Ethnographic Research Design
Ethnographic design involves observing and studying a culture-sharing group of people in their natural setting to gain insight into their behaviours, beliefs, and values. The focus here is on observing participants in their natural environment (as opposed to a controlled environment). This typically involves the researcher spending an extended period of time with the participants in their environment, carefully observing and taking field notes .
All of this is not to say that ethnographic research design relies purely on observation. On the contrary, this design typically also involves in-depth interviews to explore participants’ views, beliefs, etc. However, unobtrusive observation is a core component of the ethnographic approach.
As an example, an ethnographer may study how different communities celebrate traditional festivals or how individuals from different generations interact with technology differently. This may involve a lengthy period of observation, combined with in-depth interviews to further explore specific areas of interest that emerge as a result of the observations that the researcher has made.
As you can probably imagine, ethnographic research design has the ability to provide rich, contextually embedded insights into the socio-cultural dynamics of human behaviour within a natural, uncontrived setting. Naturally, however, it does come with its own set of challenges, including researcher bias (since the researcher can become quite immersed in the group), participant confidentiality and, predictably, ethical complexities . All of these need to be carefully managed if you choose to adopt this type of research design.
Case Study Design
With case study research design, you, as the researcher, investigate a single individual (or a single group of individuals) to gain an in-depth understanding of their experiences, behaviours or outcomes. Unlike other research designs that are aimed at larger sample sizes, case studies offer a deep dive into the specific circumstances surrounding a person, group of people, event or phenomenon, generally within a bounded setting or context .
As an example, a case study design could be used to explore the factors influencing the success of a specific small business. This would involve diving deeply into the organisation to explore and understand what makes it tick – from marketing to HR to finance. In terms of data collection, this could include interviews with staff and management, review of policy documents and financial statements, surveying customers, etc.
While the above example is focused squarely on one organisation, it’s worth noting that case study research designs can have different variation s, including single-case, multiple-case and longitudinal designs. As you can see in the example, a single-case design involves intensely examining a single entity to understand its unique characteristics and complexities. Conversely, in a multiple-case design , multiple cases are compared and contrasted to identify patterns and commonalities. Lastly, in a longitudinal case design , a single case or multiple cases are studied over an extended period of time to understand how factors develop over time.
As you can see, a case study research design is particularly useful where a deep and contextualised understanding of a specific phenomenon or issue is desired. However, this strength is also its weakness. In other words, you can’t generalise the findings from a case study to the broader population. So, keep this in mind if you’re considering going the case study route.
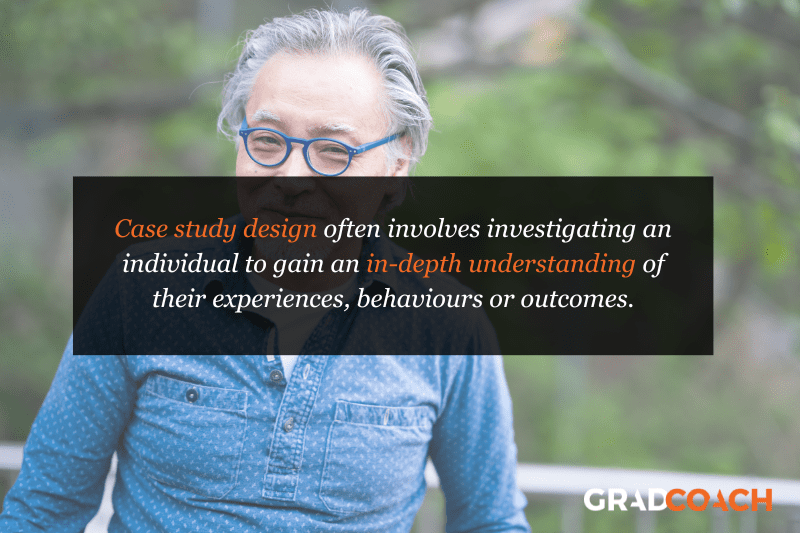
How To Choose A Research Design
Having worked through all of these potential research designs, you’d be forgiven for feeling a little overwhelmed and wondering, “ But how do I decide which research design to use? ”. While we could write an entire post covering that alone, here are a few factors to consider that will help you choose a suitable research design for your study.
Data type: The first determining factor is naturally the type of data you plan to be collecting – i.e., qualitative or quantitative. This may sound obvious, but we have to be clear about this – don’t try to use a quantitative research design on qualitative data (or vice versa)!
Research aim(s) and question(s): As with all methodological decisions, your research aim and research questions will heavily influence your research design. For example, if your research aims involve developing a theory from qualitative data, grounded theory would be a strong option. Similarly, if your research aims involve identifying and measuring relationships between variables, one of the experimental designs would likely be a better option.
Time: It’s essential that you consider any time constraints you have, as this will impact the type of research design you can choose. For example, if you’ve only got a month to complete your project, a lengthy design such as ethnography wouldn’t be a good fit.
Resources: Take into account the resources realistically available to you, as these need to factor into your research design choice. For example, if you require highly specialised lab equipment to execute an experimental design, you need to be sure that you’ll have access to that before you make a decision.
Keep in mind that when it comes to research, it’s important to manage your risks and play as conservatively as possible. If your entire project relies on you achieving a huge sample, having access to niche equipment or holding interviews with very difficult-to-reach participants, you’re creating risks that could kill your project. So, be sure to think through your choices carefully and make sure that you have backup plans for any existential risks. Remember that a relatively simple methodology executed well generally will typically earn better marks than a highly-complex methodology executed poorly.
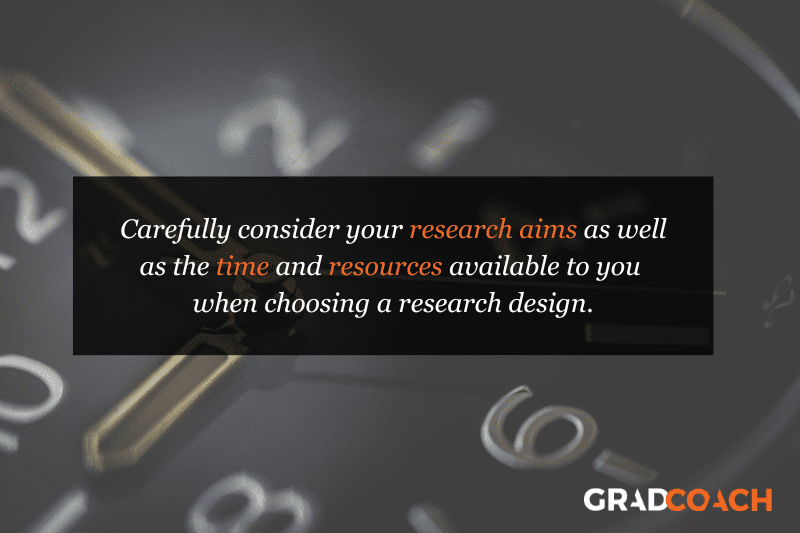
Recap: Key Takeaways
We’ve covered a lot of ground here. Let’s recap by looking at the key takeaways:
- Research design refers to the overall plan, structure or strategy that guides a research project, from its conception to the final analysis of data.
- Research designs for quantitative studies include descriptive , correlational , experimental and quasi-experimenta l designs.
- Research designs for qualitative studies include phenomenological , grounded theory , ethnographic and case study designs.
- When choosing a research design, you need to consider a variety of factors, including the type of data you’ll be working with, your research aims and questions, your time and the resources available to you.
If you need a helping hand with your research design (or any other aspect of your research), check out our private coaching services .
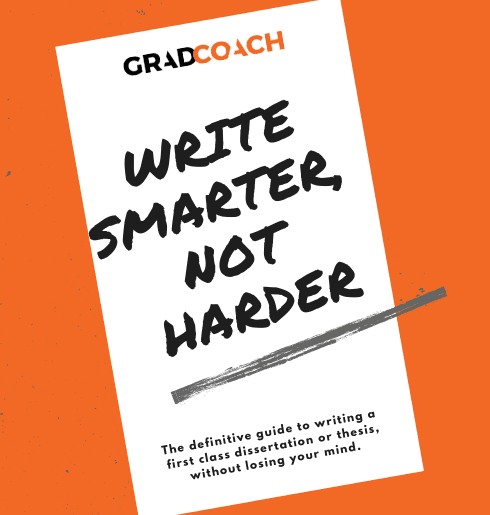
Psst… there’s more (for free)
This post is part of our dissertation mini-course, which covers everything you need to get started with your dissertation, thesis or research project.
You Might Also Like:
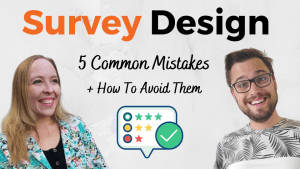
Is there any blog article explaining more on Case study research design? Is there a Case study write-up template? Thank you.
Thanks this was quite valuable to clarify such an important concept.
Thanks for this simplified explanations. it is quite very helpful.
This was really helpful. thanks
Thank you for your explanation. I think case study research design and the use of secondary data in researches needs to be talked about more in your videos and articles because there a lot of case studies research design tailored projects out there.
Please is there any template for a case study research design whose data type is a secondary data on your repository?
This post is very clear, comprehensive and has been very helpful to me. It has cleared the confusion I had in regard to research design and methodology.
This post is helpful, easy to understand, and deconstructs what a research design is. Thanks
how to cite this page
Thank you very much for the post. It is wonderful and has cleared many worries in my mind regarding research designs. I really appreciate .
Submit a Comment Cancel reply
Your email address will not be published. Required fields are marked *
Save my name, email, and website in this browser for the next time I comment.
- Print Friendly
Have a language expert improve your writing
Run a free plagiarism check in 10 minutes, automatically generate references for free.
- Knowledge Base
- Methodology
- What Is a Conceptual Framework? | Tips & Examples
What Is a Conceptual Framework? | Tips & Examples
Published on 4 May 2022 by Bas Swaen and Tegan George. Revised on 18 March 2024.
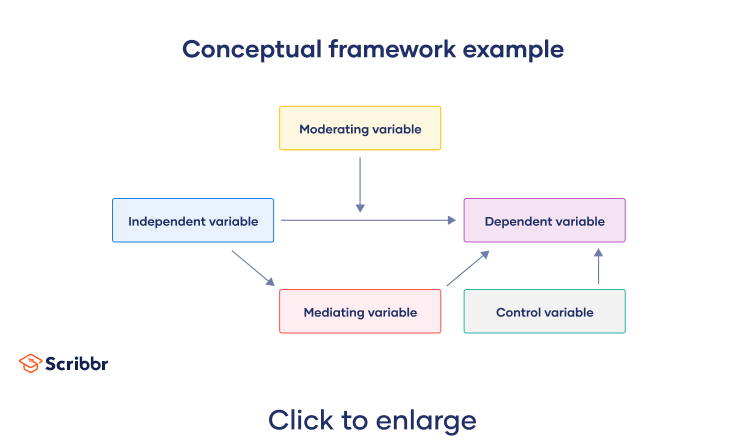
A conceptual framework illustrates the expected relationship between your variables. It defines the relevant objectives for your research process and maps out how they come together to draw coherent conclusions.
Keep reading for a step-by-step guide to help you construct your own conceptual framework.
Table of contents
Developing a conceptual framework in research, step 1: choose your research question, step 2: select your independent and dependent variables, step 3: visualise your cause-and-effect relationship, step 4: identify other influencing variables, frequently asked questions about conceptual models.
A conceptual framework is a representation of the relationship you expect to see between your variables, or the characteristics or properties that you want to study.
Conceptual frameworks can be written or visual and are generally developed based on a literature review of existing studies about your topic.
Your research question guides your work by determining exactly what you want to find out, giving your research process a clear focus.
However, before you start collecting your data, consider constructing a conceptual framework. This will help you map out which variables you will measure and how you expect them to relate to one another.
In order to move forward with your research question and test a cause-and-effect relationship, you must first identify at least two key variables: your independent and dependent variables .
- The expected cause, ‘hours of study’, is the independent variable (the predictor, or explanatory variable)
- The expected effect, ‘exam score’, is the dependent variable (the response, or outcome variable).
Note that causal relationships often involve several independent variables that affect the dependent variable. For the purpose of this example, we’ll work with just one independent variable (‘hours of study’).
Now that you’ve figured out your research question and variables, the first step in designing your conceptual framework is visualising your expected cause-and-effect relationship.
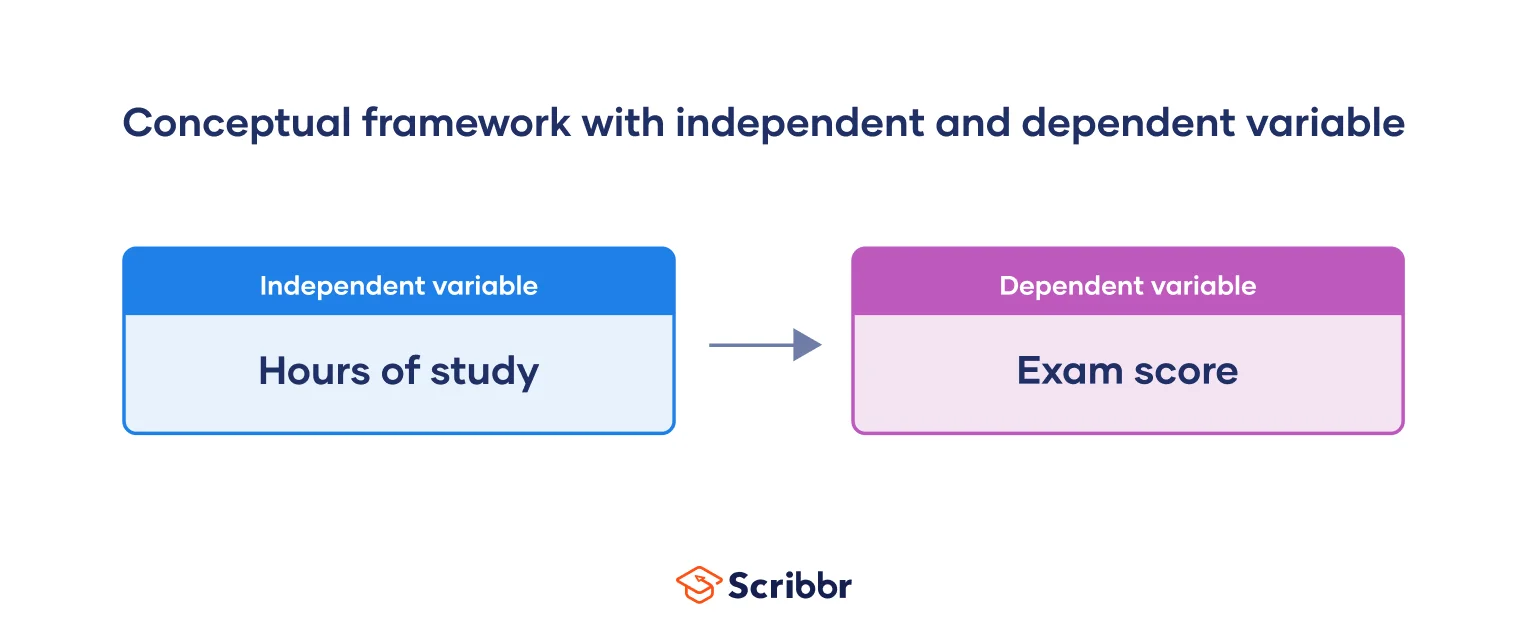
It’s crucial to identify other variables that can influence the relationship between your independent and dependent variables early in your research process.
Some common variables to include are moderating, mediating, and control variables.
Moderating variables
Moderating variable (or moderators) alter the effect that an independent variable has on a dependent variable. In other words, moderators change the ‘effect’ component of the cause-and-effect relationship.
Let’s add the moderator ‘IQ’. Here, a student’s IQ level can change the effect that the variable ‘hours of study’ has on the exam score. The higher the IQ, the fewer hours of study are needed to do well on the exam.
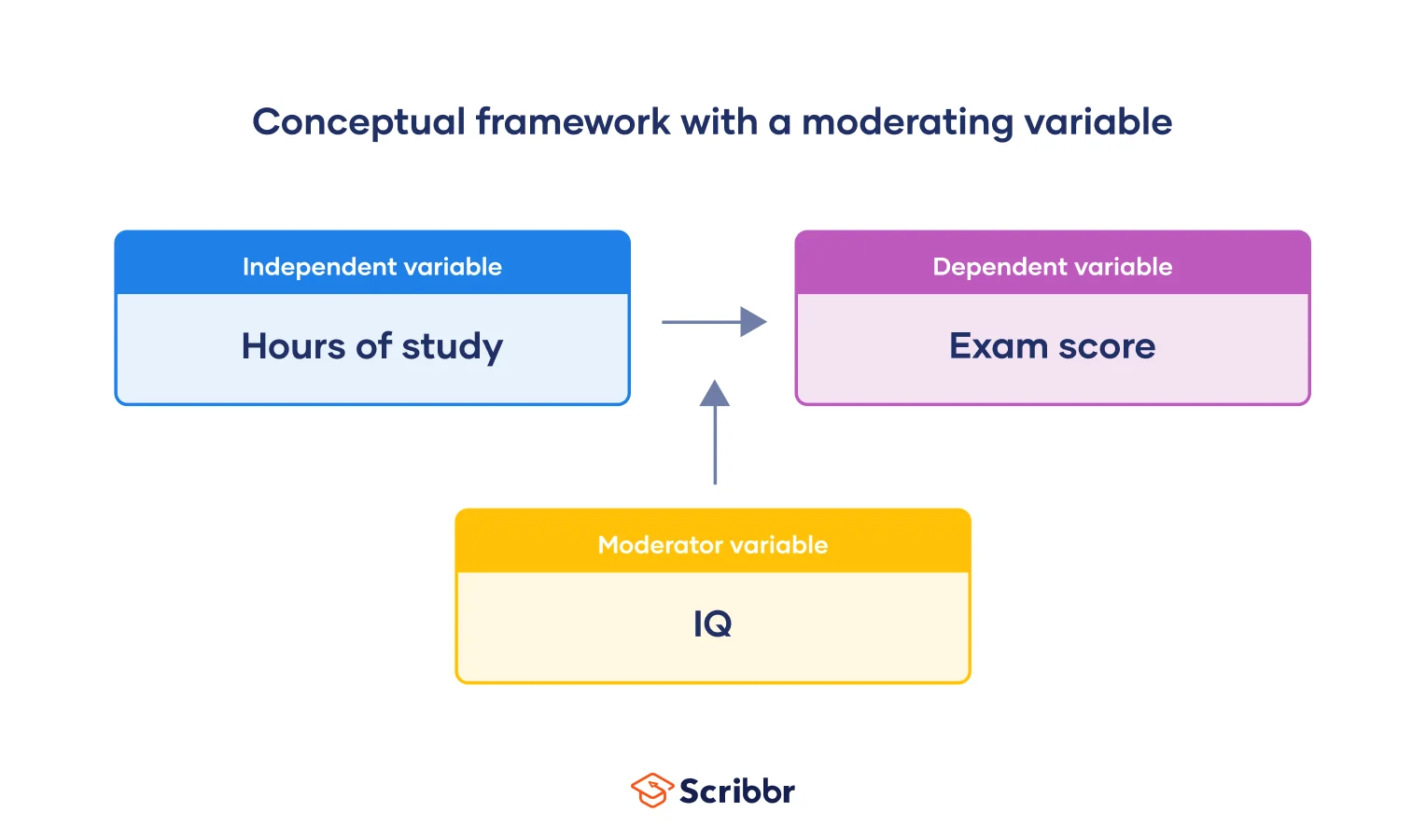
Let’s take a look at how this might work. The graph below shows how the number of hours spent studying affects exam score. As expected, the more hours you study, the better your results. Here, a student who studies for 20 hours will get a perfect score.
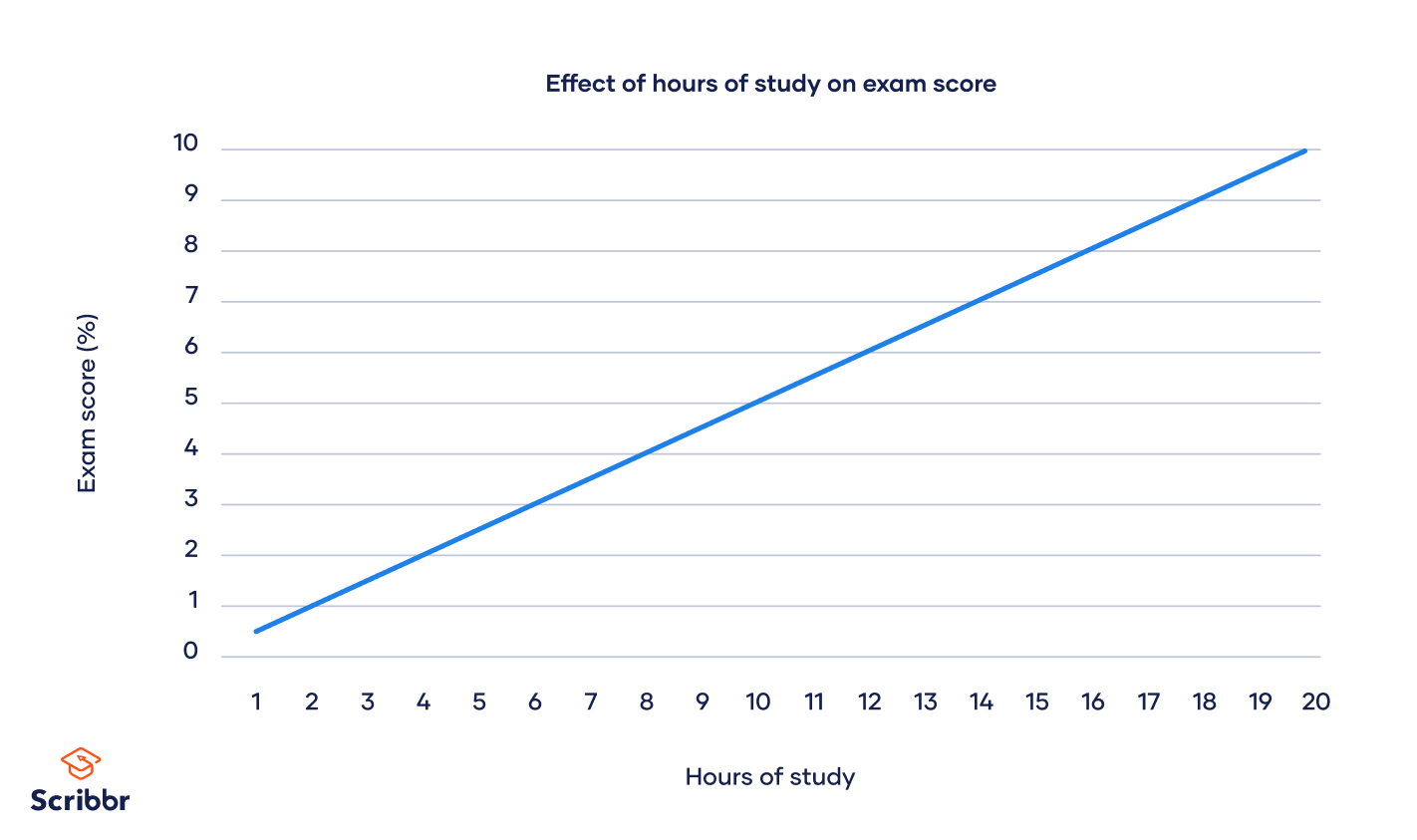
But the graph looks different when we add our ‘IQ’ moderator of 120. A student with this IQ will achieve a perfect score after just 15 hours of study.
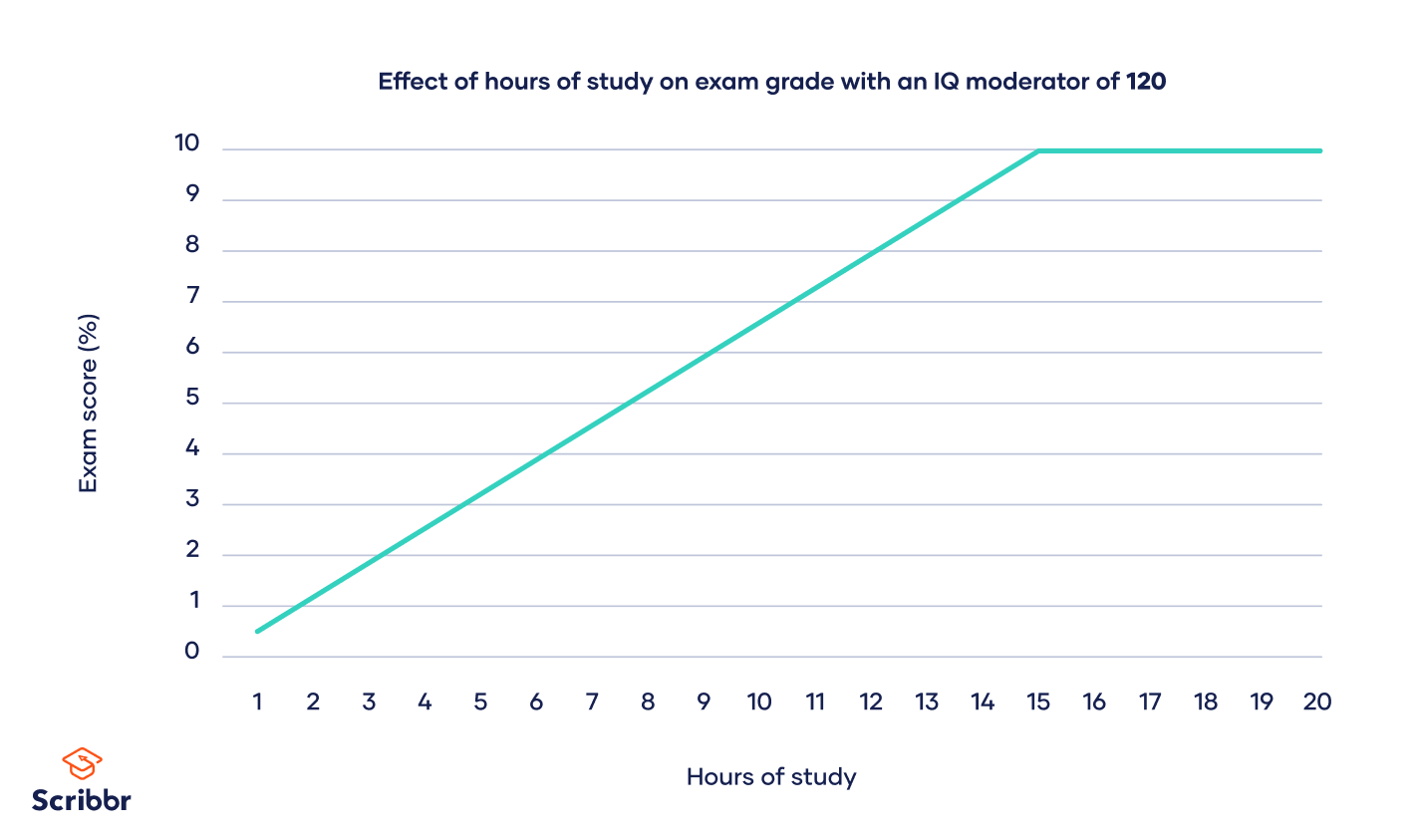
Below, the value of the ‘IQ’ moderator has been increased to 150. A student with this IQ will only need to invest five hours of study in order to get a perfect score.
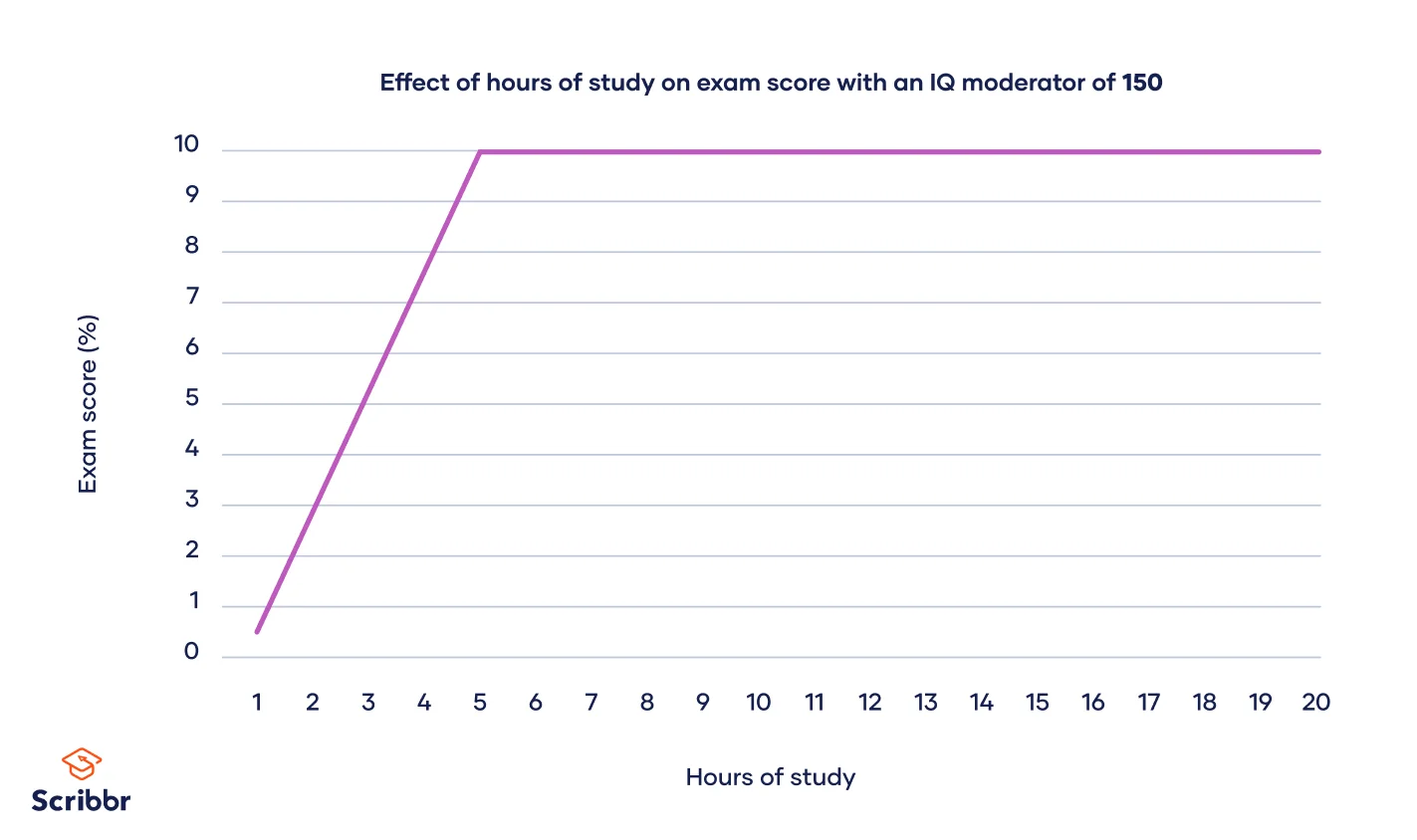
Here, we see that a moderating variable does indeed change the cause-and-effect relationship between two variables.
Mediating variables
Now we’ll expand the framework by adding a mediating variable . Mediating variables link the independent and dependent variables, allowing the relationship between them to be better explained.
Here’s how the conceptual framework might look if a mediator variable were involved:
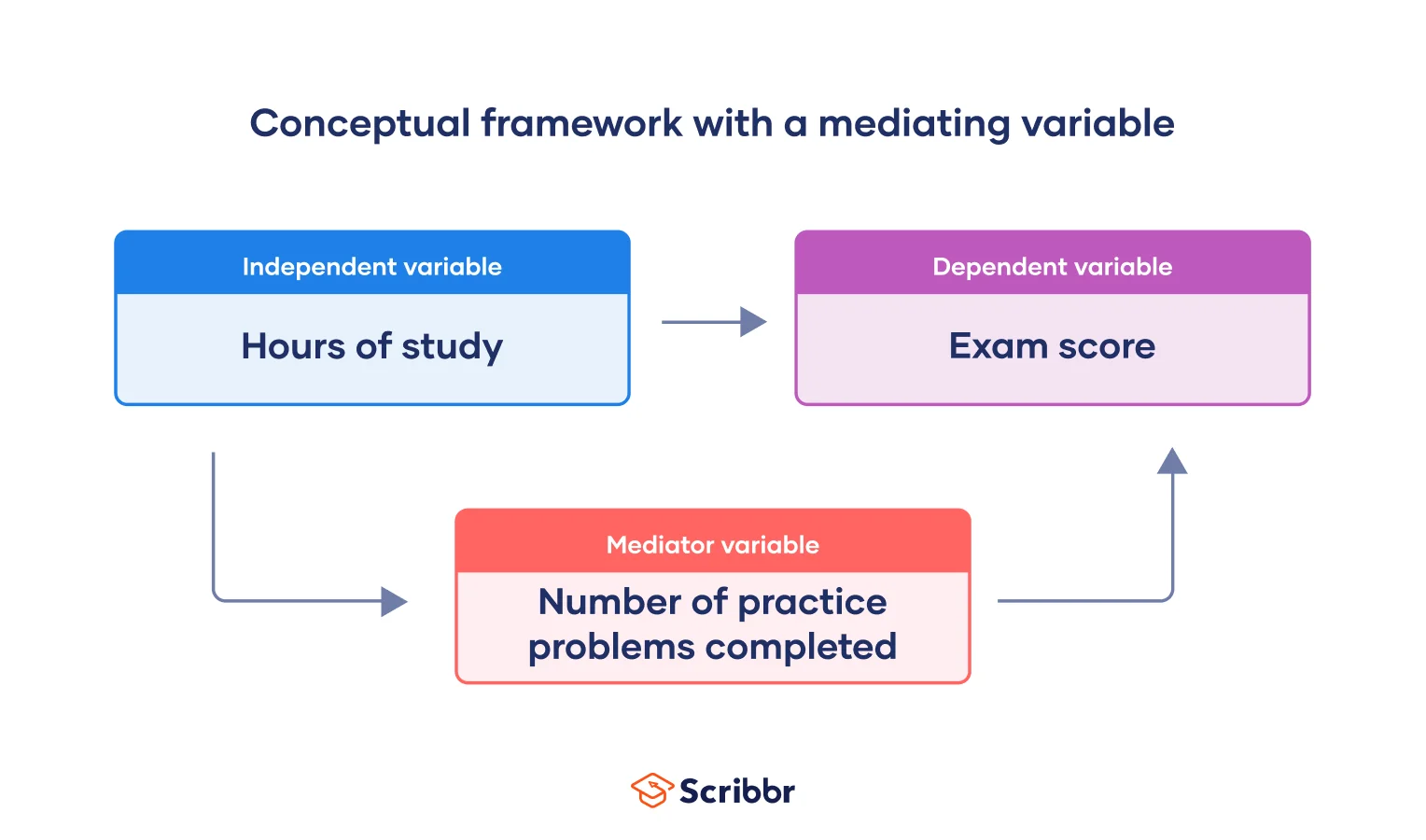
In this case, the mediator helps explain why studying more hours leads to a higher exam score. The more hours a student studies, the more practice problems they will complete; the more practice problems completed, the higher the student’s exam score will be.
Moderator vs mediator
It’s important not to confuse moderating and mediating variables. To remember the difference, you can think of them in relation to the independent variable:
- A moderating variable is not affected by the independent variable, even though it affects the dependent variable. For example, no matter how many hours you study (the independent variable), your IQ will not get higher.
- A mediating variable is affected by the independent variable. In turn, it also affects the dependent variable. Therefore, it links the two variables and helps explain the relationship between them.
Control variables
Lastly, control variables must also be taken into account. These are variables that are held constant so that they don’t interfere with the results. Even though you aren’t interested in measuring them for your study, it’s crucial to be aware of as many of them as you can be.
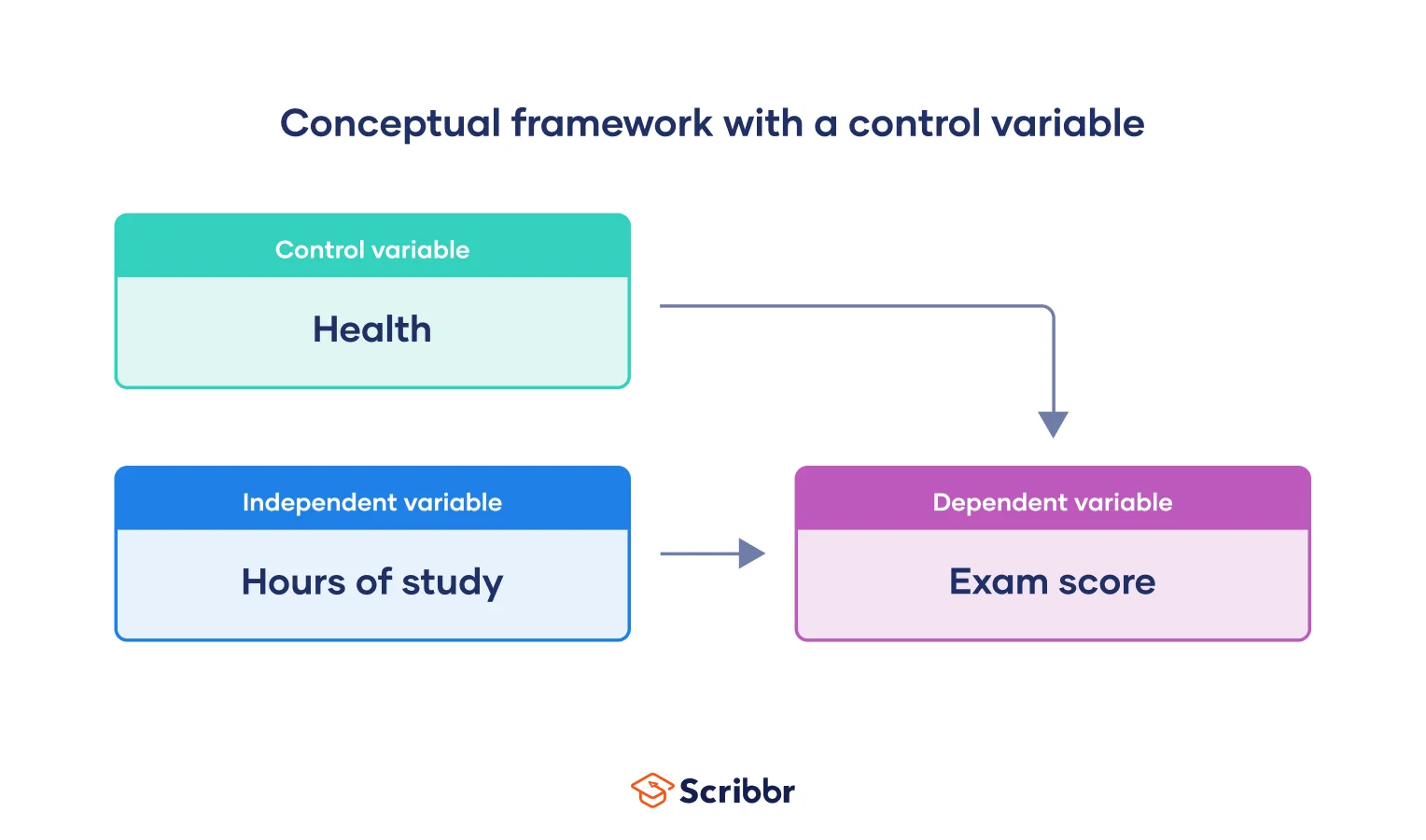
A mediator variable explains the process through which two variables are related, while a moderator variable affects the strength and direction of that relationship.
No. The value of a dependent variable depends on an independent variable, so a variable cannot be both independent and dependent at the same time. It must be either the cause or the effect, not both.
Yes, but including more than one of either type requires multiple research questions .
For example, if you are interested in the effect of a diet on health, you can use multiple measures of health: blood sugar, blood pressure, weight, pulse, and many more. Each of these is its own dependent variable with its own research question.
You could also choose to look at the effect of exercise levels as well as diet, or even the additional effect of the two combined. Each of these is a separate independent variable .
To ensure the internal validity of an experiment , you should only change one independent variable at a time.
A control variable is any variable that’s held constant in a research study. It’s not a variable of interest in the study, but it’s controlled because it could influence the outcomes.
A confounding variable , also called a confounder or confounding factor, is a third variable in a study examining a potential cause-and-effect relationship.
A confounding variable is related to both the supposed cause and the supposed effect of the study. It can be difficult to separate the true effect of the independent variable from the effect of the confounding variable.
In your research design , it’s important to identify potential confounding variables and plan how you will reduce their impact.
Cite this Scribbr article
If you want to cite this source, you can copy and paste the citation or click the ‘Cite this Scribbr article’ button to automatically add the citation to our free Reference Generator.
Swaen, B. & George, T. (2024, March 18). What Is a Conceptual Framework? | Tips & Examples. Scribbr. Retrieved 9 April 2024, from https://www.scribbr.co.uk/research-methods/conceptual-frameworks/
Is this article helpful?
Other students also liked
Mediator vs moderator variables | differences & examples, independent vs dependent variables | definition & examples, what are control variables | definition & examples.
- How it works
How to Write a Research Design – Guide with Examples
Published by Alaxendra Bets at August 14th, 2021 , Revised On October 3, 2023
A research design is a structure that combines different components of research. It involves the use of different data collection and data analysis techniques logically to answer the research questions .
It would be best to make some decisions about addressing the research questions adequately before starting the research process, which is achieved with the help of the research design.
Below are the key aspects of the decision-making process:
- Data type required for research
- Research resources
- Participants required for research
- Hypothesis based upon research question(s)
- Data analysis methodologies
- Variables (Independent, dependent, and confounding)
- The location and timescale for conducting the data
- The time period required for research
The research design provides the strategy of investigation for your project. Furthermore, it defines the parameters and criteria to compile the data to evaluate results and conclude.
Your project’s validity depends on the data collection and interpretation techniques. A strong research design reflects a strong dissertation , scientific paper, or research proposal .
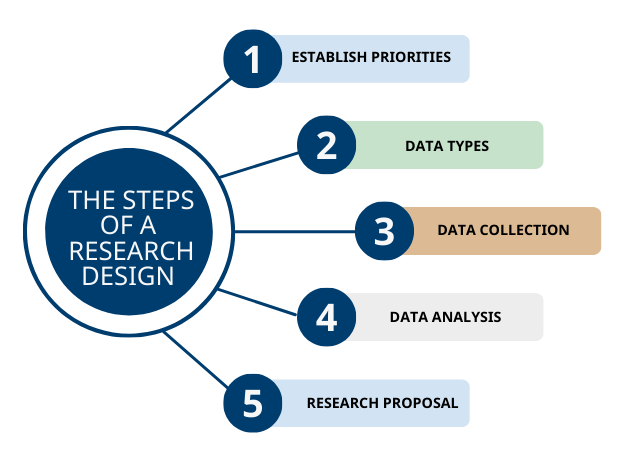
Step 1: Establish Priorities for Research Design
Before conducting any research study, you must address an important question: “how to create a research design.”
The research design depends on the researcher’s priorities and choices because every research has different priorities. For a complex research study involving multiple methods, you may choose to have more than one research design.
Multimethodology or multimethod research includes using more than one data collection method or research in a research study or set of related studies.
If one research design is weak in one area, then another research design can cover that weakness. For instance, a dissertation analyzing different situations or cases will have more than one research design.
For example:
- Experimental research involves experimental investigation and laboratory experience, but it does not accurately investigate the real world.
- Quantitative research is good for the statistical part of the project, but it may not provide an in-depth understanding of the topic .
- Also, correlational research will not provide experimental results because it is a technique that assesses the statistical relationship between two variables.
While scientific considerations are a fundamental aspect of the research design, It is equally important that the researcher think practically before deciding on its structure. Here are some questions that you should think of;
- Do you have enough time to gather data and complete the write-up?
- Will you be able to collect the necessary data by interviewing a specific person or visiting a specific location?
- Do you have in-depth knowledge about the different statistical analysis and data collection techniques to address the research questions or test the hypothesis ?
If you think that the chosen research design cannot answer the research questions properly, you can refine your research questions to gain better insight.
Step 2: Data Type you Need for Research
Decide on the type of data you need for your research. The type of data you need to collect depends on your research questions or research hypothesis. Two types of research data can be used to answer the research questions:
Primary Data Vs. Secondary Data
Qualitative vs. quantitative data.
Also, see; Research methods, design, and analysis .
Need help with a thesis chapter?
- Hire an expert from ResearchProspect today!
- Statistical analysis, research methodology, discussion of the results or conclusion – our experts can help you no matter how complex the requirements are.
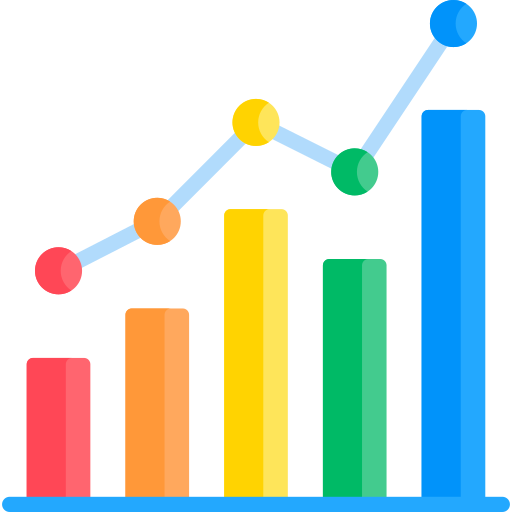
Step 3: Data Collection Techniques
Once you have selected the type of research to answer your research question, you need to decide where and how to collect the data.
It is time to determine your research method to address the research problem . Research methods involve procedures, techniques, materials, and tools used for the study.
For instance, a dissertation research design includes the different resources and data collection techniques and helps establish your dissertation’s structure .
The following table shows the characteristics of the most popularly employed research methods.
Research Methods
Step 4: Procedure of Data Analysis
Use of the correct data and statistical analysis technique is necessary for the validity of your research. Therefore, you need to be certain about the data type that would best address the research problem. Choosing an appropriate analysis method is the final step for the research design. It can be split into two main categories;
Quantitative Data Analysis
The quantitative data analysis technique involves analyzing the numerical data with the help of different applications such as; SPSS, STATA, Excel, origin lab, etc.
This data analysis strategy tests different variables such as spectrum, frequencies, averages, and more. The research question and the hypothesis must be established to identify the variables for testing.
Qualitative Data Analysis
Qualitative data analysis of figures, themes, and words allows for flexibility and the researcher’s subjective opinions. This means that the researcher’s primary focus will be interpreting patterns, tendencies, and accounts and understanding the implications and social framework.
You should be clear about your research objectives before starting to analyze the data. For example, you should ask yourself whether you need to explain respondents’ experiences and insights or do you also need to evaluate their responses with reference to a certain social framework.
Step 5: Write your Research Proposal
The research design is an important component of a research proposal because it plans the project’s execution. You can share it with the supervisor, who would evaluate the feasibility and capacity of the results and conclusion .
Read our guidelines to write a research proposal if you have already formulated your research design. The research proposal is written in the future tense because you are writing your proposal before conducting research.
The research methodology or research design, on the other hand, is generally written in the past tense.
How to Write a Research Design – Conclusion
A research design is the plan, structure, strategy of investigation conceived to answer the research question and test the hypothesis. The dissertation research design can be classified based on the type of data and the type of analysis.
Above mentioned five steps are the answer to how to write a research design. So, follow these steps to formulate the perfect research design for your dissertation .
ResearchProspect writers have years of experience creating research designs that align with the dissertation’s aim and objectives. If you are struggling with your dissertation methodology chapter, you might want to look at our dissertation part-writing service.
Our dissertation writers can also help you with the full dissertation paper . No matter how urgent or complex your need may be, ResearchProspect can help. We also offer PhD level research paper writing services.
Frequently Asked Questions
What is research design.
Research design is a systematic plan that guides the research process, outlining the methodology and procedures for collecting and analysing data. It determines the structure of the study, ensuring the research question is answered effectively, reliably, and validly. It serves as the blueprint for the entire research project.
How to write a research design?
To write a research design, define your research question, identify the research method (qualitative, quantitative, or mixed), choose data collection techniques (e.g., surveys, interviews), determine the sample size and sampling method, outline data analysis procedures, and highlight potential limitations and ethical considerations for the study.
How to write the design section of a research paper?
In the design section of a research paper, describe the research methodology chosen and justify its selection. Outline the data collection methods, participants or samples, instruments used, and procedures followed. Detail any experimental controls, if applicable. Ensure clarity and precision to enable replication of the study by other researchers.
How to write a research design in methodology?
To write a research design in methodology, clearly outline the research strategy (e.g., experimental, survey, case study). Describe the sampling technique, participants, and data collection methods. Detail the procedures for data collection and analysis. Justify choices by linking them to research objectives, addressing reliability and validity.
You May Also Like
To help students organise their dissertation proposal paper correctly, we have put together detailed guidelines on how to structure a dissertation proposal.
Make sure that your selected topic is intriguing, manageable, and relevant. Here are some guidelines to help understand how to find a good dissertation topic.
Repository of ten perfect research question examples will provide you a better perspective about how to create research questions.
USEFUL LINKS
LEARNING RESOURCES
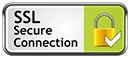
COMPANY DETAILS
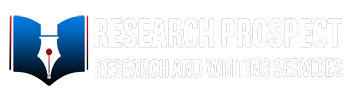
- How It Works
- USC Libraries
- Research Guides
Organizing Your Social Sciences Research Paper
- Types of Research Designs
- Purpose of Guide
- Design Flaws to Avoid
- Independent and Dependent Variables
- Glossary of Research Terms
- Reading Research Effectively
- Narrowing a Topic Idea
- Broadening a Topic Idea
- Extending the Timeliness of a Topic Idea
- Academic Writing Style
- Applying Critical Thinking
- Choosing a Title
- Making an Outline
- Paragraph Development
- Research Process Video Series
- Executive Summary
- The C.A.R.S. Model
- Background Information
- The Research Problem/Question
- Theoretical Framework
- Citation Tracking
- Content Alert Services
- Evaluating Sources
- Primary Sources
- Secondary Sources
- Tiertiary Sources
- Scholarly vs. Popular Publications
- Qualitative Methods
- Quantitative Methods
- Insiderness
- Using Non-Textual Elements
- Limitations of the Study
- Common Grammar Mistakes
- Writing Concisely
- Avoiding Plagiarism
- Footnotes or Endnotes?
- Further Readings
- Generative AI and Writing
- USC Libraries Tutorials and Other Guides
- Bibliography
Introduction
Before beginning your paper, you need to decide how you plan to design the study .
The research design refers to the overall strategy and analytical approach that you have chosen in order to integrate, in a coherent and logical way, the different components of the study, thus ensuring that the research problem will be thoroughly investigated. It constitutes the blueprint for the collection, measurement, and interpretation of information and data. Note that the research problem determines the type of design you choose, not the other way around!
De Vaus, D. A. Research Design in Social Research . London: SAGE, 2001; Trochim, William M.K. Research Methods Knowledge Base. 2006.
General Structure and Writing Style
The function of a research design is to ensure that the evidence obtained enables you to effectively address the research problem logically and as unambiguously as possible . In social sciences research, obtaining information relevant to the research problem generally entails specifying the type of evidence needed to test the underlying assumptions of a theory, to evaluate a program, or to accurately describe and assess meaning related to an observable phenomenon.
With this in mind, a common mistake made by researchers is that they begin their investigations before they have thought critically about what information is required to address the research problem. Without attending to these design issues beforehand, the overall research problem will not be adequately addressed and any conclusions drawn will run the risk of being weak and unconvincing. As a consequence, the overall validity of the study will be undermined.
The length and complexity of describing the research design in your paper can vary considerably, but any well-developed description will achieve the following :
- Identify the research problem clearly and justify its selection, particularly in relation to any valid alternative designs that could have been used,
- Review and synthesize previously published literature associated with the research problem,
- Clearly and explicitly specify hypotheses [i.e., research questions] central to the problem,
- Effectively describe the information and/or data which will be necessary for an adequate testing of the hypotheses and explain how such information and/or data will be obtained, and
- Describe the methods of analysis to be applied to the data in determining whether or not the hypotheses are true or false.
The research design is usually incorporated into the introduction of your paper . You can obtain an overall sense of what to do by reviewing studies that have utilized the same research design [e.g., using a case study approach]. This can help you develop an outline to follow for your own paper.
NOTE : Use the SAGE Research Methods Online and Cases and the SAGE Research Methods Videos databases to search for scholarly resources on how to apply specific research designs and methods . The Research Methods Online database contains links to more than 175,000 pages of SAGE publisher's book, journal, and reference content on quantitative, qualitative, and mixed research methodologies. Also included is a collection of case studies of social research projects that can be used to help you better understand abstract or complex methodological concepts. The Research Methods Videos database contains hours of tutorials, interviews, video case studies, and mini-documentaries covering the entire research process.
Creswell, John W. and J. David Creswell. Research Design: Qualitative, Quantitative, and Mixed Methods Approaches . 5th edition. Thousand Oaks, CA: Sage, 2018; De Vaus, D. A. Research Design in Social Research . London: SAGE, 2001; Gorard, Stephen. Research Design: Creating Robust Approaches for the Social Sciences . Thousand Oaks, CA: Sage, 2013; Leedy, Paul D. and Jeanne Ellis Ormrod. Practical Research: Planning and Design . Tenth edition. Boston, MA: Pearson, 2013; Vogt, W. Paul, Dianna C. Gardner, and Lynne M. Haeffele. When to Use What Research Design . New York: Guilford, 2012.
Action Research Design
Definition and Purpose
The essentials of action research design follow a characteristic cycle whereby initially an exploratory stance is adopted, where an understanding of a problem is developed and plans are made for some form of interventionary strategy. Then the intervention is carried out [the "action" in action research] during which time, pertinent observations are collected in various forms. The new interventional strategies are carried out, and this cyclic process repeats, continuing until a sufficient understanding of [or a valid implementation solution for] the problem is achieved. The protocol is iterative or cyclical in nature and is intended to foster deeper understanding of a given situation, starting with conceptualizing and particularizing the problem and moving through several interventions and evaluations.
What do these studies tell you ?
- This is a collaborative and adaptive research design that lends itself to use in work or community situations.
- Design focuses on pragmatic and solution-driven research outcomes rather than testing theories.
- When practitioners use action research, it has the potential to increase the amount they learn consciously from their experience; the action research cycle can be regarded as a learning cycle.
- Action research studies often have direct and obvious relevance to improving practice and advocating for change.
- There are no hidden controls or preemption of direction by the researcher.
What these studies don't tell you ?
- It is harder to do than conducting conventional research because the researcher takes on responsibilities of advocating for change as well as for researching the topic.
- Action research is much harder to write up because it is less likely that you can use a standard format to report your findings effectively [i.e., data is often in the form of stories or observation].
- Personal over-involvement of the researcher may bias research results.
- The cyclic nature of action research to achieve its twin outcomes of action [e.g. change] and research [e.g. understanding] is time-consuming and complex to conduct.
- Advocating for change usually requires buy-in from study participants.
Coghlan, David and Mary Brydon-Miller. The Sage Encyclopedia of Action Research . Thousand Oaks, CA: Sage, 2014; Efron, Sara Efrat and Ruth Ravid. Action Research in Education: A Practical Guide . New York: Guilford, 2013; Gall, Meredith. Educational Research: An Introduction . Chapter 18, Action Research. 8th ed. Boston, MA: Pearson/Allyn and Bacon, 2007; Gorard, Stephen. Research Design: Creating Robust Approaches for the Social Sciences . Thousand Oaks, CA: Sage, 2013; Kemmis, Stephen and Robin McTaggart. “Participatory Action Research.” In Handbook of Qualitative Research . Norman Denzin and Yvonna S. Lincoln, eds. 2nd ed. (Thousand Oaks, CA: SAGE, 2000), pp. 567-605; McNiff, Jean. Writing and Doing Action Research . London: Sage, 2014; Reason, Peter and Hilary Bradbury. Handbook of Action Research: Participative Inquiry and Practice . Thousand Oaks, CA: SAGE, 2001.
Case Study Design
A case study is an in-depth study of a particular research problem rather than a sweeping statistical survey or comprehensive comparative inquiry. It is often used to narrow down a very broad field of research into one or a few easily researchable examples. The case study research design is also useful for testing whether a specific theory and model actually applies to phenomena in the real world. It is a useful design when not much is known about an issue or phenomenon.
- Approach excels at bringing us to an understanding of a complex issue through detailed contextual analysis of a limited number of events or conditions and their relationships.
- A researcher using a case study design can apply a variety of methodologies and rely on a variety of sources to investigate a research problem.
- Design can extend experience or add strength to what is already known through previous research.
- Social scientists, in particular, make wide use of this research design to examine contemporary real-life situations and provide the basis for the application of concepts and theories and the extension of methodologies.
- The design can provide detailed descriptions of specific and rare cases.
- A single or small number of cases offers little basis for establishing reliability or to generalize the findings to a wider population of people, places, or things.
- Intense exposure to the study of a case may bias a researcher's interpretation of the findings.
- Design does not facilitate assessment of cause and effect relationships.
- Vital information may be missing, making the case hard to interpret.
- The case may not be representative or typical of the larger problem being investigated.
- If the criteria for selecting a case is because it represents a very unusual or unique phenomenon or problem for study, then your interpretation of the findings can only apply to that particular case.
Case Studies. Writing@CSU. Colorado State University; Anastas, Jeane W. Research Design for Social Work and the Human Services . Chapter 4, Flexible Methods: Case Study Design. 2nd ed. New York: Columbia University Press, 1999; Gerring, John. “What Is a Case Study and What Is It Good for?” American Political Science Review 98 (May 2004): 341-354; Greenhalgh, Trisha, editor. Case Study Evaluation: Past, Present and Future Challenges . Bingley, UK: Emerald Group Publishing, 2015; Mills, Albert J. , Gabrielle Durepos, and Eiden Wiebe, editors. Encyclopedia of Case Study Research . Thousand Oaks, CA: SAGE Publications, 2010; Stake, Robert E. The Art of Case Study Research . Thousand Oaks, CA: SAGE, 1995; Yin, Robert K. Case Study Research: Design and Theory . Applied Social Research Methods Series, no. 5. 3rd ed. Thousand Oaks, CA: SAGE, 2003.
Causal Design
Causality studies may be thought of as understanding a phenomenon in terms of conditional statements in the form, “If X, then Y.” This type of research is used to measure what impact a specific change will have on existing norms and assumptions. Most social scientists seek causal explanations that reflect tests of hypotheses. Causal effect (nomothetic perspective) occurs when variation in one phenomenon, an independent variable, leads to or results, on average, in variation in another phenomenon, the dependent variable.
Conditions necessary for determining causality:
- Empirical association -- a valid conclusion is based on finding an association between the independent variable and the dependent variable.
- Appropriate time order -- to conclude that causation was involved, one must see that cases were exposed to variation in the independent variable before variation in the dependent variable.
- Nonspuriousness -- a relationship between two variables that is not due to variation in a third variable.
- Causality research designs assist researchers in understanding why the world works the way it does through the process of proving a causal link between variables and by the process of eliminating other possibilities.
- Replication is possible.
- There is greater confidence the study has internal validity due to the systematic subject selection and equity of groups being compared.
- Not all relationships are causal! The possibility always exists that, by sheer coincidence, two unrelated events appear to be related [e.g., Punxatawney Phil could accurately predict the duration of Winter for five consecutive years but, the fact remains, he's just a big, furry rodent].
- Conclusions about causal relationships are difficult to determine due to a variety of extraneous and confounding variables that exist in a social environment. This means causality can only be inferred, never proven.
- If two variables are correlated, the cause must come before the effect. However, even though two variables might be causally related, it can sometimes be difficult to determine which variable comes first and, therefore, to establish which variable is the actual cause and which is the actual effect.
Beach, Derek and Rasmus Brun Pedersen. Causal Case Study Methods: Foundations and Guidelines for Comparing, Matching, and Tracing . Ann Arbor, MI: University of Michigan Press, 2016; Bachman, Ronet. The Practice of Research in Criminology and Criminal Justice . Chapter 5, Causation and Research Designs. 3rd ed. Thousand Oaks, CA: Pine Forge Press, 2007; Brewer, Ernest W. and Jennifer Kubn. “Causal-Comparative Design.” In Encyclopedia of Research Design . Neil J. Salkind, editor. (Thousand Oaks, CA: Sage, 2010), pp. 125-132; Causal Research Design: Experimentation. Anonymous SlideShare Presentation; Gall, Meredith. Educational Research: An Introduction . Chapter 11, Nonexperimental Research: Correlational Designs. 8th ed. Boston, MA: Pearson/Allyn and Bacon, 2007; Trochim, William M.K. Research Methods Knowledge Base. 2006.
Cohort Design
Often used in the medical sciences, but also found in the applied social sciences, a cohort study generally refers to a study conducted over a period of time involving members of a population which the subject or representative member comes from, and who are united by some commonality or similarity. Using a quantitative framework, a cohort study makes note of statistical occurrence within a specialized subgroup, united by same or similar characteristics that are relevant to the research problem being investigated, rather than studying statistical occurrence within the general population. Using a qualitative framework, cohort studies generally gather data using methods of observation. Cohorts can be either "open" or "closed."
- Open Cohort Studies [dynamic populations, such as the population of Los Angeles] involve a population that is defined just by the state of being a part of the study in question (and being monitored for the outcome). Date of entry and exit from the study is individually defined, therefore, the size of the study population is not constant. In open cohort studies, researchers can only calculate rate based data, such as, incidence rates and variants thereof.
- Closed Cohort Studies [static populations, such as patients entered into a clinical trial] involve participants who enter into the study at one defining point in time and where it is presumed that no new participants can enter the cohort. Given this, the number of study participants remains constant (or can only decrease).
- The use of cohorts is often mandatory because a randomized control study may be unethical. For example, you cannot deliberately expose people to asbestos, you can only study its effects on those who have already been exposed. Research that measures risk factors often relies upon cohort designs.
- Because cohort studies measure potential causes before the outcome has occurred, they can demonstrate that these “causes” preceded the outcome, thereby avoiding the debate as to which is the cause and which is the effect.
- Cohort analysis is highly flexible and can provide insight into effects over time and related to a variety of different types of changes [e.g., social, cultural, political, economic, etc.].
- Either original data or secondary data can be used in this design.
- In cases where a comparative analysis of two cohorts is made [e.g., studying the effects of one group exposed to asbestos and one that has not], a researcher cannot control for all other factors that might differ between the two groups. These factors are known as confounding variables.
- Cohort studies can end up taking a long time to complete if the researcher must wait for the conditions of interest to develop within the group. This also increases the chance that key variables change during the course of the study, potentially impacting the validity of the findings.
- Due to the lack of randominization in the cohort design, its external validity is lower than that of study designs where the researcher randomly assigns participants.
Healy P, Devane D. “Methodological Considerations in Cohort Study Designs.” Nurse Researcher 18 (2011): 32-36; Glenn, Norval D, editor. Cohort Analysis . 2nd edition. Thousand Oaks, CA: Sage, 2005; Levin, Kate Ann. Study Design IV: Cohort Studies. Evidence-Based Dentistry 7 (2003): 51–52; Payne, Geoff. “Cohort Study.” In The SAGE Dictionary of Social Research Methods . Victor Jupp, editor. (Thousand Oaks, CA: Sage, 2006), pp. 31-33; Study Design 101. Himmelfarb Health Sciences Library. George Washington University, November 2011; Cohort Study. Wikipedia.
Cross-Sectional Design
Cross-sectional research designs have three distinctive features: no time dimension; a reliance on existing differences rather than change following intervention; and, groups are selected based on existing differences rather than random allocation. The cross-sectional design can only measure differences between or from among a variety of people, subjects, or phenomena rather than a process of change. As such, researchers using this design can only employ a relatively passive approach to making causal inferences based on findings.
- Cross-sectional studies provide a clear 'snapshot' of the outcome and the characteristics associated with it, at a specific point in time.
- Unlike an experimental design, where there is an active intervention by the researcher to produce and measure change or to create differences, cross-sectional designs focus on studying and drawing inferences from existing differences between people, subjects, or phenomena.
- Entails collecting data at and concerning one point in time. While longitudinal studies involve taking multiple measures over an extended period of time, cross-sectional research is focused on finding relationships between variables at one moment in time.
- Groups identified for study are purposely selected based upon existing differences in the sample rather than seeking random sampling.
- Cross-section studies are capable of using data from a large number of subjects and, unlike observational studies, is not geographically bound.
- Can estimate prevalence of an outcome of interest because the sample is usually taken from the whole population.
- Because cross-sectional designs generally use survey techniques to gather data, they are relatively inexpensive and take up little time to conduct.
- Finding people, subjects, or phenomena to study that are very similar except in one specific variable can be difficult.
- Results are static and time bound and, therefore, give no indication of a sequence of events or reveal historical or temporal contexts.
- Studies cannot be utilized to establish cause and effect relationships.
- This design only provides a snapshot of analysis so there is always the possibility that a study could have differing results if another time-frame had been chosen.
- There is no follow up to the findings.
Bethlehem, Jelke. "7: Cross-sectional Research." In Research Methodology in the Social, Behavioural and Life Sciences . Herman J Adèr and Gideon J Mellenbergh, editors. (London, England: Sage, 1999), pp. 110-43; Bourque, Linda B. “Cross-Sectional Design.” In The SAGE Encyclopedia of Social Science Research Methods . Michael S. Lewis-Beck, Alan Bryman, and Tim Futing Liao. (Thousand Oaks, CA: 2004), pp. 230-231; Hall, John. “Cross-Sectional Survey Design.” In Encyclopedia of Survey Research Methods . Paul J. Lavrakas, ed. (Thousand Oaks, CA: Sage, 2008), pp. 173-174; Helen Barratt, Maria Kirwan. Cross-Sectional Studies: Design Application, Strengths and Weaknesses of Cross-Sectional Studies. Healthknowledge, 2009. Cross-Sectional Study. Wikipedia.
Descriptive Design
Descriptive research designs help provide answers to the questions of who, what, when, where, and how associated with a particular research problem; a descriptive study cannot conclusively ascertain answers to why. Descriptive research is used to obtain information concerning the current status of the phenomena and to describe "what exists" with respect to variables or conditions in a situation.
- The subject is being observed in a completely natural and unchanged natural environment. True experiments, whilst giving analyzable data, often adversely influence the normal behavior of the subject [a.k.a., the Heisenberg effect whereby measurements of certain systems cannot be made without affecting the systems].
- Descriptive research is often used as a pre-cursor to more quantitative research designs with the general overview giving some valuable pointers as to what variables are worth testing quantitatively.
- If the limitations are understood, they can be a useful tool in developing a more focused study.
- Descriptive studies can yield rich data that lead to important recommendations in practice.
- Appoach collects a large amount of data for detailed analysis.
- The results from a descriptive research cannot be used to discover a definitive answer or to disprove a hypothesis.
- Because descriptive designs often utilize observational methods [as opposed to quantitative methods], the results cannot be replicated.
- The descriptive function of research is heavily dependent on instrumentation for measurement and observation.
Anastas, Jeane W. Research Design for Social Work and the Human Services . Chapter 5, Flexible Methods: Descriptive Research. 2nd ed. New York: Columbia University Press, 1999; Given, Lisa M. "Descriptive Research." In Encyclopedia of Measurement and Statistics . Neil J. Salkind and Kristin Rasmussen, editors. (Thousand Oaks, CA: Sage, 2007), pp. 251-254; McNabb, Connie. Descriptive Research Methodologies. Powerpoint Presentation; Shuttleworth, Martyn. Descriptive Research Design, September 26, 2008; Erickson, G. Scott. "Descriptive Research Design." In New Methods of Market Research and Analysis . (Northampton, MA: Edward Elgar Publishing, 2017), pp. 51-77; Sahin, Sagufta, and Jayanta Mete. "A Brief Study on Descriptive Research: Its Nature and Application in Social Science." International Journal of Research and Analysis in Humanities 1 (2021): 11; K. Swatzell and P. Jennings. “Descriptive Research: The Nuts and Bolts.” Journal of the American Academy of Physician Assistants 20 (2007), pp. 55-56; Kane, E. Doing Your Own Research: Basic Descriptive Research in the Social Sciences and Humanities . London: Marion Boyars, 1985.
Experimental Design
A blueprint of the procedure that enables the researcher to maintain control over all factors that may affect the result of an experiment. In doing this, the researcher attempts to determine or predict what may occur. Experimental research is often used where there is time priority in a causal relationship (cause precedes effect), there is consistency in a causal relationship (a cause will always lead to the same effect), and the magnitude of the correlation is great. The classic experimental design specifies an experimental group and a control group. The independent variable is administered to the experimental group and not to the control group, and both groups are measured on the same dependent variable. Subsequent experimental designs have used more groups and more measurements over longer periods. True experiments must have control, randomization, and manipulation.
- Experimental research allows the researcher to control the situation. In so doing, it allows researchers to answer the question, “What causes something to occur?”
- Permits the researcher to identify cause and effect relationships between variables and to distinguish placebo effects from treatment effects.
- Experimental research designs support the ability to limit alternative explanations and to infer direct causal relationships in the study.
- Approach provides the highest level of evidence for single studies.
- The design is artificial, and results may not generalize well to the real world.
- The artificial settings of experiments may alter the behaviors or responses of participants.
- Experimental designs can be costly if special equipment or facilities are needed.
- Some research problems cannot be studied using an experiment because of ethical or technical reasons.
- Difficult to apply ethnographic and other qualitative methods to experimentally designed studies.
Anastas, Jeane W. Research Design for Social Work and the Human Services . Chapter 7, Flexible Methods: Experimental Research. 2nd ed. New York: Columbia University Press, 1999; Chapter 2: Research Design, Experimental Designs. School of Psychology, University of New England, 2000; Chow, Siu L. "Experimental Design." In Encyclopedia of Research Design . Neil J. Salkind, editor. (Thousand Oaks, CA: Sage, 2010), pp. 448-453; "Experimental Design." In Social Research Methods . Nicholas Walliman, editor. (London, England: Sage, 2006), pp, 101-110; Experimental Research. Research Methods by Dummies. Department of Psychology. California State University, Fresno, 2006; Kirk, Roger E. Experimental Design: Procedures for the Behavioral Sciences . 4th edition. Thousand Oaks, CA: Sage, 2013; Trochim, William M.K. Experimental Design. Research Methods Knowledge Base. 2006; Rasool, Shafqat. Experimental Research. Slideshare presentation.
Exploratory Design
An exploratory design is conducted about a research problem when there are few or no earlier studies to refer to or rely upon to predict an outcome . The focus is on gaining insights and familiarity for later investigation or undertaken when research problems are in a preliminary stage of investigation. Exploratory designs are often used to establish an understanding of how best to proceed in studying an issue or what methodology would effectively apply to gathering information about the issue.
The goals of exploratory research are intended to produce the following possible insights:
- Familiarity with basic details, settings, and concerns.
- Well grounded picture of the situation being developed.
- Generation of new ideas and assumptions.
- Development of tentative theories or hypotheses.
- Determination about whether a study is feasible in the future.
- Issues get refined for more systematic investigation and formulation of new research questions.
- Direction for future research and techniques get developed.
- Design is a useful approach for gaining background information on a particular topic.
- Exploratory research is flexible and can address research questions of all types (what, why, how).
- Provides an opportunity to define new terms and clarify existing concepts.
- Exploratory research is often used to generate formal hypotheses and develop more precise research problems.
- In the policy arena or applied to practice, exploratory studies help establish research priorities and where resources should be allocated.
- Exploratory research generally utilizes small sample sizes and, thus, findings are typically not generalizable to the population at large.
- The exploratory nature of the research inhibits an ability to make definitive conclusions about the findings. They provide insight but not definitive conclusions.
- The research process underpinning exploratory studies is flexible but often unstructured, leading to only tentative results that have limited value to decision-makers.
- Design lacks rigorous standards applied to methods of data gathering and analysis because one of the areas for exploration could be to determine what method or methodologies could best fit the research problem.
Cuthill, Michael. “Exploratory Research: Citizen Participation, Local Government, and Sustainable Development in Australia.” Sustainable Development 10 (2002): 79-89; Streb, Christoph K. "Exploratory Case Study." In Encyclopedia of Case Study Research . Albert J. Mills, Gabrielle Durepos and Eiden Wiebe, editors. (Thousand Oaks, CA: Sage, 2010), pp. 372-374; Taylor, P. J., G. Catalano, and D.R.F. Walker. “Exploratory Analysis of the World City Network.” Urban Studies 39 (December 2002): 2377-2394; Exploratory Research. Wikipedia.
Field Research Design
Sometimes referred to as ethnography or participant observation, designs around field research encompass a variety of interpretative procedures [e.g., observation and interviews] rooted in qualitative approaches to studying people individually or in groups while inhabiting their natural environment as opposed to using survey instruments or other forms of impersonal methods of data gathering. Information acquired from observational research takes the form of “ field notes ” that involves documenting what the researcher actually sees and hears while in the field. Findings do not consist of conclusive statements derived from numbers and statistics because field research involves analysis of words and observations of behavior. Conclusions, therefore, are developed from an interpretation of findings that reveal overriding themes, concepts, and ideas. More information can be found HERE .
- Field research is often necessary to fill gaps in understanding the research problem applied to local conditions or to specific groups of people that cannot be ascertained from existing data.
- The research helps contextualize already known information about a research problem, thereby facilitating ways to assess the origins, scope, and scale of a problem and to gage the causes, consequences, and means to resolve an issue based on deliberate interaction with people in their natural inhabited spaces.
- Enables the researcher to corroborate or confirm data by gathering additional information that supports or refutes findings reported in prior studies of the topic.
- Because the researcher in embedded in the field, they are better able to make observations or ask questions that reflect the specific cultural context of the setting being investigated.
- Observing the local reality offers the opportunity to gain new perspectives or obtain unique data that challenges existing theoretical propositions or long-standing assumptions found in the literature.
What these studies don't tell you
- A field research study requires extensive time and resources to carry out the multiple steps involved with preparing for the gathering of information, including for example, examining background information about the study site, obtaining permission to access the study site, and building trust and rapport with subjects.
- Requires a commitment to staying engaged in the field to ensure that you can adequately document events and behaviors as they unfold.
- The unpredictable nature of fieldwork means that researchers can never fully control the process of data gathering. They must maintain a flexible approach to studying the setting because events and circumstances can change quickly or unexpectedly.
- Findings can be difficult to interpret and verify without access to documents and other source materials that help to enhance the credibility of information obtained from the field [i.e., the act of triangulating the data].
- Linking the research problem to the selection of study participants inhabiting their natural environment is critical. However, this specificity limits the ability to generalize findings to different situations or in other contexts or to infer courses of action applied to other settings or groups of people.
- The reporting of findings must take into account how the researcher themselves may have inadvertently affected respondents and their behaviors.
Historical Design
The purpose of a historical research design is to collect, verify, and synthesize evidence from the past to establish facts that defend or refute a hypothesis. It uses secondary sources and a variety of primary documentary evidence, such as, diaries, official records, reports, archives, and non-textual information [maps, pictures, audio and visual recordings]. The limitation is that the sources must be both authentic and valid.
- The historical research design is unobtrusive; the act of research does not affect the results of the study.
- The historical approach is well suited for trend analysis.
- Historical records can add important contextual background required to more fully understand and interpret a research problem.
- There is often no possibility of researcher-subject interaction that could affect the findings.
- Historical sources can be used over and over to study different research problems or to replicate a previous study.
- The ability to fulfill the aims of your research are directly related to the amount and quality of documentation available to understand the research problem.
- Since historical research relies on data from the past, there is no way to manipulate it to control for contemporary contexts.
- Interpreting historical sources can be very time consuming.
- The sources of historical materials must be archived consistently to ensure access. This may especially challenging for digital or online-only sources.
- Original authors bring their own perspectives and biases to the interpretation of past events and these biases are more difficult to ascertain in historical resources.
- Due to the lack of control over external variables, historical research is very weak with regard to the demands of internal validity.
- It is rare that the entirety of historical documentation needed to fully address a research problem is available for interpretation, therefore, gaps need to be acknowledged.
Howell, Martha C. and Walter Prevenier. From Reliable Sources: An Introduction to Historical Methods . Ithaca, NY: Cornell University Press, 2001; Lundy, Karen Saucier. "Historical Research." In The Sage Encyclopedia of Qualitative Research Methods . Lisa M. Given, editor. (Thousand Oaks, CA: Sage, 2008), pp. 396-400; Marius, Richard. and Melvin E. Page. A Short Guide to Writing about History . 9th edition. Boston, MA: Pearson, 2015; Savitt, Ronald. “Historical Research in Marketing.” Journal of Marketing 44 (Autumn, 1980): 52-58; Gall, Meredith. Educational Research: An Introduction . Chapter 16, Historical Research. 8th ed. Boston, MA: Pearson/Allyn and Bacon, 2007.
Longitudinal Design
A longitudinal study follows the same sample over time and makes repeated observations. For example, with longitudinal surveys, the same group of people is interviewed at regular intervals, enabling researchers to track changes over time and to relate them to variables that might explain why the changes occur. Longitudinal research designs describe patterns of change and help establish the direction and magnitude of causal relationships. Measurements are taken on each variable over two or more distinct time periods. This allows the researcher to measure change in variables over time. It is a type of observational study sometimes referred to as a panel study.
- Longitudinal data facilitate the analysis of the duration of a particular phenomenon.
- Enables survey researchers to get close to the kinds of causal explanations usually attainable only with experiments.
- The design permits the measurement of differences or change in a variable from one period to another [i.e., the description of patterns of change over time].
- Longitudinal studies facilitate the prediction of future outcomes based upon earlier factors.
- The data collection method may change over time.
- Maintaining the integrity of the original sample can be difficult over an extended period of time.
- It can be difficult to show more than one variable at a time.
- This design often needs qualitative research data to explain fluctuations in the results.
- A longitudinal research design assumes present trends will continue unchanged.
- It can take a long period of time to gather results.
- There is a need to have a large sample size and accurate sampling to reach representativness.
Anastas, Jeane W. Research Design for Social Work and the Human Services . Chapter 6, Flexible Methods: Relational and Longitudinal Research. 2nd ed. New York: Columbia University Press, 1999; Forgues, Bernard, and Isabelle Vandangeon-Derumez. "Longitudinal Analyses." In Doing Management Research . Raymond-Alain Thiétart and Samantha Wauchope, editors. (London, England: Sage, 2001), pp. 332-351; Kalaian, Sema A. and Rafa M. Kasim. "Longitudinal Studies." In Encyclopedia of Survey Research Methods . Paul J. Lavrakas, ed. (Thousand Oaks, CA: Sage, 2008), pp. 440-441; Menard, Scott, editor. Longitudinal Research . Thousand Oaks, CA: Sage, 2002; Ployhart, Robert E. and Robert J. Vandenberg. "Longitudinal Research: The Theory, Design, and Analysis of Change.” Journal of Management 36 (January 2010): 94-120; Longitudinal Study. Wikipedia.
Meta-Analysis Design
Meta-analysis is an analytical methodology designed to systematically evaluate and summarize the results from a number of individual studies, thereby, increasing the overall sample size and the ability of the researcher to study effects of interest. The purpose is to not simply summarize existing knowledge, but to develop a new understanding of a research problem using synoptic reasoning. The main objectives of meta-analysis include analyzing differences in the results among studies and increasing the precision by which effects are estimated. A well-designed meta-analysis depends upon strict adherence to the criteria used for selecting studies and the availability of information in each study to properly analyze their findings. Lack of information can severely limit the type of analyzes and conclusions that can be reached. In addition, the more dissimilarity there is in the results among individual studies [heterogeneity], the more difficult it is to justify interpretations that govern a valid synopsis of results. A meta-analysis needs to fulfill the following requirements to ensure the validity of your findings:
- Clearly defined description of objectives, including precise definitions of the variables and outcomes that are being evaluated;
- A well-reasoned and well-documented justification for identification and selection of the studies;
- Assessment and explicit acknowledgment of any researcher bias in the identification and selection of those studies;
- Description and evaluation of the degree of heterogeneity among the sample size of studies reviewed; and,
- Justification of the techniques used to evaluate the studies.
- Can be an effective strategy for determining gaps in the literature.
- Provides a means of reviewing research published about a particular topic over an extended period of time and from a variety of sources.
- Is useful in clarifying what policy or programmatic actions can be justified on the basis of analyzing research results from multiple studies.
- Provides a method for overcoming small sample sizes in individual studies that previously may have had little relationship to each other.
- Can be used to generate new hypotheses or highlight research problems for future studies.
- Small violations in defining the criteria used for content analysis can lead to difficult to interpret and/or meaningless findings.
- A large sample size can yield reliable, but not necessarily valid, results.
- A lack of uniformity regarding, for example, the type of literature reviewed, how methods are applied, and how findings are measured within the sample of studies you are analyzing, can make the process of synthesis difficult to perform.
- Depending on the sample size, the process of reviewing and synthesizing multiple studies can be very time consuming.
Beck, Lewis W. "The Synoptic Method." The Journal of Philosophy 36 (1939): 337-345; Cooper, Harris, Larry V. Hedges, and Jeffrey C. Valentine, eds. The Handbook of Research Synthesis and Meta-Analysis . 2nd edition. New York: Russell Sage Foundation, 2009; Guzzo, Richard A., Susan E. Jackson and Raymond A. Katzell. “Meta-Analysis Analysis.” In Research in Organizational Behavior , Volume 9. (Greenwich, CT: JAI Press, 1987), pp 407-442; Lipsey, Mark W. and David B. Wilson. Practical Meta-Analysis . Thousand Oaks, CA: Sage Publications, 2001; Study Design 101. Meta-Analysis. The Himmelfarb Health Sciences Library, George Washington University; Timulak, Ladislav. “Qualitative Meta-Analysis.” In The SAGE Handbook of Qualitative Data Analysis . Uwe Flick, editor. (Los Angeles, CA: Sage, 2013), pp. 481-495; Walker, Esteban, Adrian V. Hernandez, and Micheal W. Kattan. "Meta-Analysis: It's Strengths and Limitations." Cleveland Clinic Journal of Medicine 75 (June 2008): 431-439.
Mixed-Method Design
- Narrative and non-textual information can add meaning to numeric data, while numeric data can add precision to narrative and non-textual information.
- Can utilize existing data while at the same time generating and testing a grounded theory approach to describe and explain the phenomenon under study.
- A broader, more complex research problem can be investigated because the researcher is not constrained by using only one method.
- The strengths of one method can be used to overcome the inherent weaknesses of another method.
- Can provide stronger, more robust evidence to support a conclusion or set of recommendations.
- May generate new knowledge new insights or uncover hidden insights, patterns, or relationships that a single methodological approach might not reveal.
- Produces more complete knowledge and understanding of the research problem that can be used to increase the generalizability of findings applied to theory or practice.
- A researcher must be proficient in understanding how to apply multiple methods to investigating a research problem as well as be proficient in optimizing how to design a study that coherently melds them together.
- Can increase the likelihood of conflicting results or ambiguous findings that inhibit drawing a valid conclusion or setting forth a recommended course of action [e.g., sample interview responses do not support existing statistical data].
- Because the research design can be very complex, reporting the findings requires a well-organized narrative, clear writing style, and precise word choice.
- Design invites collaboration among experts. However, merging different investigative approaches and writing styles requires more attention to the overall research process than studies conducted using only one methodological paradigm.
- Concurrent merging of quantitative and qualitative research requires greater attention to having adequate sample sizes, using comparable samples, and applying a consistent unit of analysis. For sequential designs where one phase of qualitative research builds on the quantitative phase or vice versa, decisions about what results from the first phase to use in the next phase, the choice of samples and estimating reasonable sample sizes for both phases, and the interpretation of results from both phases can be difficult.
- Due to multiple forms of data being collected and analyzed, this design requires extensive time and resources to carry out the multiple steps involved in data gathering and interpretation.
Burch, Patricia and Carolyn J. Heinrich. Mixed Methods for Policy Research and Program Evaluation . Thousand Oaks, CA: Sage, 2016; Creswell, John w. et al. Best Practices for Mixed Methods Research in the Health Sciences . Bethesda, MD: Office of Behavioral and Social Sciences Research, National Institutes of Health, 2010Creswell, John W. Research Design: Qualitative, Quantitative, and Mixed Methods Approaches . 4th edition. Thousand Oaks, CA: Sage Publications, 2014; Domínguez, Silvia, editor. Mixed Methods Social Networks Research . Cambridge, UK: Cambridge University Press, 2014; Hesse-Biber, Sharlene Nagy. Mixed Methods Research: Merging Theory with Practice . New York: Guilford Press, 2010; Niglas, Katrin. “How the Novice Researcher Can Make Sense of Mixed Methods Designs.” International Journal of Multiple Research Approaches 3 (2009): 34-46; Onwuegbuzie, Anthony J. and Nancy L. Leech. “Linking Research Questions to Mixed Methods Data Analysis Procedures.” The Qualitative Report 11 (September 2006): 474-498; Tashakorri, Abbas and John W. Creswell. “The New Era of Mixed Methods.” Journal of Mixed Methods Research 1 (January 2007): 3-7; Zhanga, Wanqing. “Mixed Methods Application in Health Intervention Research: A Multiple Case Study.” International Journal of Multiple Research Approaches 8 (2014): 24-35 .
Observational Design
This type of research design draws a conclusion by comparing subjects against a control group, in cases where the researcher has no control over the experiment. There are two general types of observational designs. In direct observations, people know that you are watching them. Unobtrusive measures involve any method for studying behavior where individuals do not know they are being observed. An observational study allows a useful insight into a phenomenon and avoids the ethical and practical difficulties of setting up a large and cumbersome research project.
- Observational studies are usually flexible and do not necessarily need to be structured around a hypothesis about what you expect to observe [data is emergent rather than pre-existing].
- The researcher is able to collect in-depth information about a particular behavior.
- Can reveal interrelationships among multifaceted dimensions of group interactions.
- You can generalize your results to real life situations.
- Observational research is useful for discovering what variables may be important before applying other methods like experiments.
- Observation research designs account for the complexity of group behaviors.
- Reliability of data is low because seeing behaviors occur over and over again may be a time consuming task and are difficult to replicate.
- In observational research, findings may only reflect a unique sample population and, thus, cannot be generalized to other groups.
- There can be problems with bias as the researcher may only "see what they want to see."
- There is no possibility to determine "cause and effect" relationships since nothing is manipulated.
- Sources or subjects may not all be equally credible.
- Any group that is knowingly studied is altered to some degree by the presence of the researcher, therefore, potentially skewing any data collected.
Atkinson, Paul and Martyn Hammersley. “Ethnography and Participant Observation.” In Handbook of Qualitative Research . Norman K. Denzin and Yvonna S. Lincoln, eds. (Thousand Oaks, CA: Sage, 1994), pp. 248-261; Observational Research. Research Methods by Dummies. Department of Psychology. California State University, Fresno, 2006; Patton Michael Quinn. Qualitiative Research and Evaluation Methods . Chapter 6, Fieldwork Strategies and Observational Methods. 3rd ed. Thousand Oaks, CA: Sage, 2002; Payne, Geoff and Judy Payne. "Observation." In Key Concepts in Social Research . The SAGE Key Concepts series. (London, England: Sage, 2004), pp. 158-162; Rosenbaum, Paul R. Design of Observational Studies . New York: Springer, 2010;Williams, J. Patrick. "Nonparticipant Observation." In The Sage Encyclopedia of Qualitative Research Methods . Lisa M. Given, editor.(Thousand Oaks, CA: Sage, 2008), pp. 562-563.
Philosophical Design
Understood more as an broad approach to examining a research problem than a methodological design, philosophical analysis and argumentation is intended to challenge deeply embedded, often intractable, assumptions underpinning an area of study. This approach uses the tools of argumentation derived from philosophical traditions, concepts, models, and theories to critically explore and challenge, for example, the relevance of logic and evidence in academic debates, to analyze arguments about fundamental issues, or to discuss the root of existing discourse about a research problem. These overarching tools of analysis can be framed in three ways:
- Ontology -- the study that describes the nature of reality; for example, what is real and what is not, what is fundamental and what is derivative?
- Epistemology -- the study that explores the nature of knowledge; for example, by what means does knowledge and understanding depend upon and how can we be certain of what we know?
- Axiology -- the study of values; for example, what values does an individual or group hold and why? How are values related to interest, desire, will, experience, and means-to-end? And, what is the difference between a matter of fact and a matter of value?
- Can provide a basis for applying ethical decision-making to practice.
- Functions as a means of gaining greater self-understanding and self-knowledge about the purposes of research.
- Brings clarity to general guiding practices and principles of an individual or group.
- Philosophy informs methodology.
- Refine concepts and theories that are invoked in relatively unreflective modes of thought and discourse.
- Beyond methodology, philosophy also informs critical thinking about epistemology and the structure of reality (metaphysics).
- Offers clarity and definition to the practical and theoretical uses of terms, concepts, and ideas.
- Limited application to specific research problems [answering the "So What?" question in social science research].
- Analysis can be abstract, argumentative, and limited in its practical application to real-life issues.
- While a philosophical analysis may render problematic that which was once simple or taken-for-granted, the writing can be dense and subject to unnecessary jargon, overstatement, and/or excessive quotation and documentation.
- There are limitations in the use of metaphor as a vehicle of philosophical analysis.
- There can be analytical difficulties in moving from philosophy to advocacy and between abstract thought and application to the phenomenal world.
Burton, Dawn. "Part I, Philosophy of the Social Sciences." In Research Training for Social Scientists . (London, England: Sage, 2000), pp. 1-5; Chapter 4, Research Methodology and Design. Unisa Institutional Repository (UnisaIR), University of South Africa; Jarvie, Ian C., and Jesús Zamora-Bonilla, editors. The SAGE Handbook of the Philosophy of Social Sciences . London: Sage, 2011; Labaree, Robert V. and Ross Scimeca. “The Philosophical Problem of Truth in Librarianship.” The Library Quarterly 78 (January 2008): 43-70; Maykut, Pamela S. Beginning Qualitative Research: A Philosophic and Practical Guide . Washington, DC: Falmer Press, 1994; McLaughlin, Hugh. "The Philosophy of Social Research." In Understanding Social Work Research . 2nd edition. (London: SAGE Publications Ltd., 2012), pp. 24-47; Stanford Encyclopedia of Philosophy . Metaphysics Research Lab, CSLI, Stanford University, 2013.
Sequential Design
- The researcher has a limitless option when it comes to sample size and the sampling schedule.
- Due to the repetitive nature of this research design, minor changes and adjustments can be done during the initial parts of the study to correct and hone the research method.
- This is a useful design for exploratory studies.
- There is very little effort on the part of the researcher when performing this technique. It is generally not expensive, time consuming, or workforce intensive.
- Because the study is conducted serially, the results of one sample are known before the next sample is taken and analyzed. This provides opportunities for continuous improvement of sampling and methods of analysis.
- The sampling method is not representative of the entire population. The only possibility of approaching representativeness is when the researcher chooses to use a very large sample size significant enough to represent a significant portion of the entire population. In this case, moving on to study a second or more specific sample can be difficult.
- The design cannot be used to create conclusions and interpretations that pertain to an entire population because the sampling technique is not randomized. Generalizability from findings is, therefore, limited.
- Difficult to account for and interpret variation from one sample to another over time, particularly when using qualitative methods of data collection.
Betensky, Rebecca. Harvard University, Course Lecture Note slides; Bovaird, James A. and Kevin A. Kupzyk. "Sequential Design." In Encyclopedia of Research Design . Neil J. Salkind, editor. (Thousand Oaks, CA: Sage, 2010), pp. 1347-1352; Cresswell, John W. Et al. “Advanced Mixed-Methods Research Designs.” In Handbook of Mixed Methods in Social and Behavioral Research . Abbas Tashakkori and Charles Teddle, eds. (Thousand Oaks, CA: Sage, 2003), pp. 209-240; Henry, Gary T. "Sequential Sampling." In The SAGE Encyclopedia of Social Science Research Methods . Michael S. Lewis-Beck, Alan Bryman and Tim Futing Liao, editors. (Thousand Oaks, CA: Sage, 2004), pp. 1027-1028; Nataliya V. Ivankova. “Using Mixed-Methods Sequential Explanatory Design: From Theory to Practice.” Field Methods 18 (February 2006): 3-20; Bovaird, James A. and Kevin A. Kupzyk. “Sequential Design.” In Encyclopedia of Research Design . Neil J. Salkind, ed. Thousand Oaks, CA: Sage, 2010; Sequential Analysis. Wikipedia.
Systematic Review
- A systematic review synthesizes the findings of multiple studies related to each other by incorporating strategies of analysis and interpretation intended to reduce biases and random errors.
- The application of critical exploration, evaluation, and synthesis methods separates insignificant, unsound, or redundant research from the most salient and relevant studies worthy of reflection.
- They can be use to identify, justify, and refine hypotheses, recognize and avoid hidden problems in prior studies, and explain data inconsistencies and conflicts in data.
- Systematic reviews can be used to help policy makers formulate evidence-based guidelines and regulations.
- The use of strict, explicit, and pre-determined methods of synthesis, when applied appropriately, provide reliable estimates about the effects of interventions, evaluations, and effects related to the overarching research problem investigated by each study under review.
- Systematic reviews illuminate where knowledge or thorough understanding of a research problem is lacking and, therefore, can then be used to guide future research.
- The accepted inclusion of unpublished studies [i.e., grey literature] ensures the broadest possible way to analyze and interpret research on a topic.
- Results of the synthesis can be generalized and the findings extrapolated into the general population with more validity than most other types of studies .
- Systematic reviews do not create new knowledge per se; they are a method for synthesizing existing studies about a research problem in order to gain new insights and determine gaps in the literature.
- The way researchers have carried out their investigations [e.g., the period of time covered, number of participants, sources of data analyzed, etc.] can make it difficult to effectively synthesize studies.
- The inclusion of unpublished studies can introduce bias into the review because they may not have undergone a rigorous peer-review process prior to publication. Examples may include conference presentations or proceedings, publications from government agencies, white papers, working papers, and internal documents from organizations, and doctoral dissertations and Master's theses.
Denyer, David and David Tranfield. "Producing a Systematic Review." In The Sage Handbook of Organizational Research Methods . David A. Buchanan and Alan Bryman, editors. ( Thousand Oaks, CA: Sage Publications, 2009), pp. 671-689; Foster, Margaret J. and Sarah T. Jewell, editors. Assembling the Pieces of a Systematic Review: A Guide for Librarians . Lanham, MD: Rowman and Littlefield, 2017; Gough, David, Sandy Oliver, James Thomas, editors. Introduction to Systematic Reviews . 2nd edition. Los Angeles, CA: Sage Publications, 2017; Gopalakrishnan, S. and P. Ganeshkumar. “Systematic Reviews and Meta-analysis: Understanding the Best Evidence in Primary Healthcare.” Journal of Family Medicine and Primary Care 2 (2013): 9-14; Gough, David, James Thomas, and Sandy Oliver. "Clarifying Differences between Review Designs and Methods." Systematic Reviews 1 (2012): 1-9; Khan, Khalid S., Regina Kunz, Jos Kleijnen, and Gerd Antes. “Five Steps to Conducting a Systematic Review.” Journal of the Royal Society of Medicine 96 (2003): 118-121; Mulrow, C. D. “Systematic Reviews: Rationale for Systematic Reviews.” BMJ 309:597 (September 1994); O'Dwyer, Linda C., and Q. Eileen Wafford. "Addressing Challenges with Systematic Review Teams through Effective Communication: A Case Report." Journal of the Medical Library Association 109 (October 2021): 643-647; Okoli, Chitu, and Kira Schabram. "A Guide to Conducting a Systematic Literature Review of Information Systems Research." Sprouts: Working Papers on Information Systems 10 (2010); Siddaway, Andy P., Alex M. Wood, and Larry V. Hedges. "How to Do a Systematic Review: A Best Practice Guide for Conducting and Reporting Narrative Reviews, Meta-analyses, and Meta-syntheses." Annual Review of Psychology 70 (2019): 747-770; Torgerson, Carole J. “Publication Bias: The Achilles’ Heel of Systematic Reviews?” British Journal of Educational Studies 54 (March 2006): 89-102; Torgerson, Carole. Systematic Reviews . New York: Continuum, 2003.
- << Previous: Purpose of Guide
- Next: Design Flaws to Avoid >>
- Last Updated: Apr 11, 2024 10:59 AM
- URL: https://libguides.usc.edu/writingguide
Open Access is an initiative that aims to make scientific research freely available to all. To date our community has made over 100 million downloads. It’s based on principles of collaboration, unobstructed discovery, and, most importantly, scientific progression. As PhD students, we found it difficult to access the research we needed, so we decided to create a new Open Access publisher that levels the playing field for scientists across the world. How? By making research easy to access, and puts the academic needs of the researchers before the business interests of publishers.
We are a community of more than 103,000 authors and editors from 3,291 institutions spanning 160 countries, including Nobel Prize winners and some of the world’s most-cited researchers. Publishing on IntechOpen allows authors to earn citations and find new collaborators, meaning more people see your work not only from your own field of study, but from other related fields too.
Brief introduction to this section that descibes Open Access especially from an IntechOpen perspective
Want to get in touch? Contact our London head office or media team here
Our team is growing all the time, so we’re always on the lookout for smart people who want to help us reshape the world of scientific publishing.
Home > Books > Cyberspace
Research Design and Methodology
Submitted: 23 January 2019 Reviewed: 08 March 2019 Published: 07 August 2019
DOI: 10.5772/intechopen.85731
Cite this chapter
There are two ways to cite this chapter:
From the Edited Volume
Edited by Evon Abu-Taieh, Abdelkrim El Mouatasim and Issam H. Al Hadid
To purchase hard copies of this book, please contact the representative in India: CBS Publishers & Distributors Pvt. Ltd. www.cbspd.com | [email protected]
Chapter metrics overview
30,677 Chapter Downloads
Impact of this chapter
Total Chapter Downloads on intechopen.com

Total Chapter Views on intechopen.com
Overall attention for this chapters
There are a number of approaches used in this research method design. The purpose of this chapter is to design the methodology of the research approach through mixed types of research techniques. The research approach also supports the researcher on how to come across the research result findings. In this chapter, the general design of the research and the methods used for data collection are explained in detail. It includes three main parts. The first part gives a highlight about the dissertation design. The second part discusses about qualitative and quantitative data collection methods. The last part illustrates the general research framework. The purpose of this section is to indicate how the research was conducted throughout the study periods.
- research design
- methodology
- data sources
Author Information
Kassu jilcha sileyew *.
- School of Mechanical and Industrial Engineering, Addis Ababa Institute of Technology, Addis Ababa University, Addis Ababa, Ethiopia
*Address all correspondence to: [email protected]
1. Introduction
Research methodology is the path through which researchers need to conduct their research. It shows the path through which these researchers formulate their problem and objective and present their result from the data obtained during the study period. This research design and methodology chapter also shows how the research outcome at the end will be obtained in line with meeting the objective of the study. This chapter hence discusses the research methods that were used during the research process. It includes the research methodology of the study from the research strategy to the result dissemination. For emphasis, in this chapter, the author outlines the research strategy, research design, research methodology, the study area, data sources such as primary data sources and secondary data, population consideration and sample size determination such as questionnaires sample size determination and workplace site exposure measurement sample determination, data collection methods like primary data collection methods including workplace site observation data collection and data collection through desk review, data collection through questionnaires, data obtained from experts opinion, workplace site exposure measurement, data collection tools pretest, secondary data collection methods, methods of data analysis used such as quantitative data analysis and qualitative data analysis, data analysis software, the reliability and validity analysis of the quantitative data, reliability of data, reliability analysis, validity, data quality management, inclusion criteria, ethical consideration and dissemination of result and its utilization approaches. In order to satisfy the objectives of the study, a qualitative and quantitative research method is apprehended in general. The study used these mixed strategies because the data were obtained from all aspects of the data source during the study time. Therefore, the purpose of this methodology is to satisfy the research plan and target devised by the researcher.
2. Research design
The research design is intended to provide an appropriate framework for a study. A very significant decision in research design process is the choice to be made regarding research approach since it determines how relevant information for a study will be obtained; however, the research design process involves many interrelated decisions [ 1 ].
This study employed a mixed type of methods. The first part of the study consisted of a series of well-structured questionnaires (for management, employee’s representatives, and technician of industries) and semi-structured interviews with key stakeholders (government bodies, ministries, and industries) in participating organizations. The other design used is an interview of employees to know how they feel about safety and health of their workplace, and field observation at the selected industrial sites was undertaken.
Hence, this study employs a descriptive research design to agree on the effects of occupational safety and health management system on employee health, safety, and property damage for selected manufacturing industries. Saunders et al. [ 2 ] and Miller [ 3 ] say that descriptive research portrays an accurate profile of persons, events, or situations. This design offers to the researchers a profile of described relevant aspects of the phenomena of interest from an individual, organizational, and industry-oriented perspective. Therefore, this research design enabled the researchers to gather data from a wide range of respondents on the impact of safety and health on manufacturing industries in Ethiopia. And this helped in analyzing the response obtained on how it affects the manufacturing industries’ workplace safety and health. The research overall design and flow process are depicted in Figure 1 .

Research methods and processes (author design).
3. Research methodology
To address the key research objectives, this research used both qualitative and quantitative methods and combination of primary and secondary sources. The qualitative data supports the quantitative data analysis and results. The result obtained is triangulated since the researcher utilized the qualitative and quantitative data types in the data analysis. The study area, data sources, and sampling techniques were discussed under this section.
3.1 The study area
According to Fraenkel and Warren [ 4 ] studies, population refers to the complete set of individuals (subjects or events) having common characteristics in which the researcher is interested. The population of the study was determined based on random sampling system. This data collection was conducted from March 07, 2015 to December 10, 2016, from selected manufacturing industries found in Addis Ababa city and around. The manufacturing companies were selected based on their employee number, established year, and the potential accidents prevailing and the manufacturing industry type even though all criterions were difficult to satisfy.
3.2 Data sources
3.2.1 primary data sources.
It was obtained from the original source of information. The primary data were more reliable and have more confidence level of decision-making with the trusted analysis having direct intact with occurrence of the events. The primary data sources are industries’ working environment (through observation, pictures, and photograph) and industry employees (management and bottom workers) (interview, questionnaires and discussions).
3.2.2 Secondary data
Desk review has been conducted to collect data from various secondary sources. This includes reports and project documents at each manufacturing sectors (more on medium and large level). Secondary data sources have been obtained from literatures regarding OSH, and the remaining data were from the companies’ manuals, reports, and some management documents which were included under the desk review. Reputable journals, books, different articles, periodicals, proceedings, magazines, newsletters, newspapers, websites, and other sources were considered on the manufacturing industrial sectors. The data also obtained from the existing working documents, manuals, procedures, reports, statistical data, policies, regulations, and standards were taken into account for the review.
In general, for this research study, the desk review has been completed to this end, and it had been polished and modified upon manuals and documents obtained from the selected companies.
4. Population and sample size
4.1 population.
The study population consisted of manufacturing industries’ employees in Addis Ababa city and around as there are more representative manufacturing industrial clusters found. To select representative manufacturing industrial sector population, the types of the industries expected were more potential to accidents based on random and purposive sampling considered. The population of data was from textile, leather, metal, chemicals, and food manufacturing industries. A total of 189 sample sizes of industries responded to the questionnaire survey from the priority areas of the government. Random sample sizes and disproportionate methods were used, and 80 from wood, metal, and iron works; 30 from food, beverage, and tobacco products; 50 from leather, textile, and garments; 20 from chemical and chemical products; and 9 from other remaining 9 clusters of manufacturing industries responded.
4.2 Questionnaire sample size determination
A simple random sampling and purposive sampling methods were used to select the representative manufacturing industries and respondents for the study. The simple random sampling ensures that each member of the population has an equal chance for the selection or the chance of getting a response which can be more than equal to the chance depending on the data analysis justification. Sample size determination procedure was used to get optimum and reasonable information. In this study, both probability (simple random sampling) and nonprobability (convenience, quota, purposive, and judgmental) sampling methods were used as the nature of the industries are varied. This is because of the characteristics of data sources which permitted the researchers to follow the multi-methods. This helps the analysis to triangulate the data obtained and increase the reliability of the research outcome and its decision. The companies’ establishment time and its engagement in operation, the number of employees and the proportion it has, the owner types (government and private), type of manufacturing industry/production, types of resource used at work, and the location it is found in the city and around were some of the criteria for the selections.
The determination of the sample size was adopted from Daniel [ 5 ] and Cochran [ 6 ] formula. The formula used was for unknown population size Eq. (1) and is given as

where n = sample size, Z = statistic for a level of confidence, P = expected prevalence or proportion (in proportion of one; if 50%, P = 0.5), and d = precision (in proportion of one; if 6%, d = 0.06). Z statistic ( Z ): for the level of confidence of 95%, which is conventional, Z value is 1.96. In this study, investigators present their results with 95% confidence intervals (CI).
The expected sample number was 267 at the marginal error of 6% for 95% confidence interval of manufacturing industries. However, the collected data indicated that only 189 populations were used for the analysis after rejecting some data having more missing values in the responses from the industries. Hence, the actual data collection resulted in 71% response rate. The 267 population were assumed to be satisfactory and representative for the data analysis.
4.3 Workplace site exposure measurement sample determination
The sample size for the experimental exposure measurements of physical work environment has been considered based on the physical data prepared for questionnaires and respondents. The response of positive were considered for exposure measurement factors to be considered for the physical environment health and disease causing such as noise intensity, light intensity, pressure/stress, vibration, temperature/coldness, or hotness and dust particles on 20 workplace sites. The selection method was using random sampling in line with purposive method. The measurement of the exposure factors was done in collaboration with Addis Ababa city Administration and Oromia Bureau of Labour and Social Affair (AACBOLSA). Some measuring instruments were obtained from the Addis Ababa city and Oromia Bureau of Labour and Social Affair.
5. Data collection methods
Data collection methods were focused on the followings basic techniques. These included secondary and primary data collections focusing on both qualitative and quantitative data as defined in the previous section. The data collection mechanisms are devised and prepared with their proper procedures.
5.1 Primary data collection methods
Primary data sources are qualitative and quantitative. The qualitative sources are field observation, interview, and informal discussions, while that of quantitative data sources are survey questionnaires and interview questions. The next sections elaborate how the data were obtained from the primary sources.
5.1.1 Workplace site observation data collection
Observation is an important aspect of science. Observation is tightly connected to data collection, and there are different sources for this: documentation, archival records, interviews, direct observations, and participant observations. Observational research findings are considered strong in validity because the researcher is able to collect a depth of information about a particular behavior. In this dissertation, the researchers used observation method as one tool for collecting information and data before questionnaire design and after the start of research too. The researcher made more than 20 specific observations of manufacturing industries in the study areas. During the observations, it found a deeper understanding of the working environment and the different sections in the production system and OSH practices.
5.1.2 Data collection through interview
Interview is a loosely structured qualitative in-depth interview with people who are considered to be particularly knowledgeable about the topic of interest. The semi-structured interview is usually conducted in a face-to-face setting which permits the researcher to seek new insights, ask questions, and assess phenomena in different perspectives. It let the researcher to know the in-depth of the present working environment influential factors and consequences. It has provided opportunities for refining data collection efforts and examining specialized systems or processes. It was used when the researcher faces written records or published document limitation or wanted to triangulate the data obtained from other primary and secondary data sources.
This dissertation is also conducted with a qualitative approach and conducting interviews. The advantage of using interviews as a method is that it allows respondents to raise issues that the interviewer may not have expected. All interviews with employees, management, and technicians were conducted by the corresponding researcher, on a face-to-face basis at workplace. All interviews were recorded and transcribed.
5.1.3 Data collection through questionnaires
The main tool for gaining primary information in practical research is questionnaires, due to the fact that the researcher can decide on the sample and the types of questions to be asked [ 2 ].
In this dissertation, each respondent is requested to reply to an identical list of questions mixed so that biasness was prevented. Initially the questionnaire design was coded and mixed up from specific topic based on uniform structures. Consequently, the questionnaire produced valuable data which was required to achieve the dissertation objectives.
The questionnaires developed were based on a five-item Likert scale. Responses were given to each statement using a five-point Likert-type scale, for which 1 = “strongly disagree” to 5 = “strongly agree.” The responses were summed up to produce a score for the measures.
5.1.4 Data obtained from experts’ opinion
The data was also obtained from the expert’s opinion related to the comparison of the knowledge, management, collaboration, and technology utilization including their sub-factors. The data obtained in this way was used for prioritization and decision-making of OSH, improving factor priority. The prioritization of the factors was using Saaty scales (1–9) and then converting to Fuzzy set values obtained from previous researches using triangular fuzzy set [ 7 ].
5.1.5 Workplace site exposure measurement
The researcher has measured the workplace environment for dust, vibration, heat, pressure, light, and noise to know how much is the level of each variable. The primary data sources planned and an actual coverage has been compared as shown in Table 1 .

Planned versus actual coverage of the survey.
The response rate for the proposed data source was good, and the pilot test also proved the reliability of questionnaires. Interview/discussion resulted in 87% of responses among the respondents; the survey questionnaire response rate obtained was 71%, and the field observation response rate was 90% for the whole data analysis process. Hence, the data organization quality level has not been compromised.
This response rate is considered to be representative of studies of organizations. As the study agrees on the response rate to be 30%, it is considered acceptable [ 8 ]. Saunders et al. [ 2 ] argued that the questionnaire with a scale response of 20% response rate is acceptable. Low response rate should not discourage the researchers, because a great deal of published research work also achieves low response rate. Hence, the response rate of this study is acceptable and very good for the purpose of meeting the study objectives.
5.1.6 Data collection tool pretest
The pretest for questionnaires, interviews, and tools were conducted to validate that the tool content is valid or not in the sense of the respondents’ understanding. Hence, content validity (in which the questions are answered to the target without excluding important points), internal validity (in which the questions raised answer the outcomes of researchers’ target), and external validity (in which the result can generalize to all the population from the survey sample population) were reflected. It has been proved with this pilot test prior to the start of the basic data collections. Following feedback process, a few minor changes were made to the originally designed data collect tools. The pilot test made for the questionnaire test was on 10 sample sizes selected randomly from the target sectors and experts.
5.2 Secondary data collection methods
The secondary data refers to data that was collected by someone other than the user. This data source gives insights of the research area of the current state-of-the-art method. It also makes some sort of research gap that needs to be filled by the researcher. This secondary data sources could be internal and external data sources of information that may cover a wide range of areas.
Literature/desk review and industry documents and reports: To achieve the dissertation’s objectives, the researcher has conducted excessive document review and reports of the companies in both online and offline modes. From a methodological point of view, literature reviews can be comprehended as content analysis, where quantitative and qualitative aspects are mixed to assess structural (descriptive) as well as content criteria.
A literature search was conducted using the database sources like MEDLINE; Emerald; Taylor and Francis publications; EMBASE (medical literature); PsycINFO (psychological literature); Sociological Abstracts (sociological literature); accident prevention journals; US Statistics of Labor, European Safety and Health database; ABI Inform; Business Source Premier (business/management literature); EconLit (economic literature); Social Service Abstracts (social work and social service literature); and other related materials. The search strategy was focused on articles or reports that measure one or more of the dimensions within the research OSH model framework. This search strategy was based on a framework and measurement filter strategy developed by the Consensus-Based Standards for the Selection of Health Measurement Instruments (COSMIN) group. Based on screening, unrelated articles to the research model and objectives were excluded. Prior to screening, researcher (principal investigator) reviewed a sample of more than 2000 articles, websites, reports, and guidelines to determine whether they should be included for further review or reject. Discrepancies were thoroughly identified and resolved before the review of the main group of more than 300 articles commenced. After excluding the articles based on the title, keywords, and abstract, the remaining articles were reviewed in detail, and the information was extracted on the instrument that was used to assess the dimension of research interest. A complete list of items was then collated within each research targets or objectives and reviewed to identify any missing elements.
6. Methods of data analysis
Data analysis method follows the procedures listed under the following sections. The data analysis part answered the basic questions raised in the problem statement. The detailed analysis of the developed and developing countries’ experiences on OSH regarding manufacturing industries was analyzed, discussed, compared and contrasted, and synthesized.
6.1 Quantitative data analysis
Quantitative data were obtained from primary and secondary data discussed above in this chapter. This data analysis was based on their data type using Excel, SPSS 20.0, Office Word format, and other tools. This data analysis focuses on numerical/quantitative data analysis.
Before analysis, data coding of responses and analysis were made. In order to analyze the data obtained easily, the data were coded to SPSS 20.0 software as the data obtained from questionnaires. This task involved identifying, classifying, and assigning a numeric or character symbol to data, which was done in only one way pre-coded [ 9 , 10 ]. In this study, all of the responses were pre-coded. They were taken from the list of responses, a number of corresponding to a particular selection was given. This process was applied to every earlier question that needed this treatment. Upon completion, the data were then entered to a statistical analysis software package, SPSS version 20.0 on Windows 10 for the next steps.
Under the data analysis, exploration of data has been made with descriptive statistics and graphical analysis. The analysis included exploring the relationship between variables and comparing groups how they affect each other. This has been done using cross tabulation/chi square, correlation, and factor analysis and using nonparametric statistic.
6.2 Qualitative data analysis
Qualitative data analysis used for triangulation of the quantitative data analysis. The interview, observation, and report records were used to support the findings. The analysis has been incorporated with the quantitative discussion results in the data analysis parts.
6.3 Data analysis software
The data were entered using SPSS 20.0 on Windows 10 and analyzed. The analysis supported with SPSS software much contributed to the finding. It had contributed to the data validation and correctness of the SPSS results. The software analyzed and compared the results of different variables used in the research questionnaires. Excel is also used to draw the pictures and calculate some analytical solutions.
7. The reliability and validity analysis of the quantitative data
7.1 reliability of data.
The reliability of measurements specifies the amount to which it is without bias (error free) and hence ensures consistent measurement across time and across the various items in the instrument [ 8 ]. In reliability analysis, it has been checked for the stability and consistency of the data. In the case of reliability analysis, the researcher checked the accuracy and precision of the procedure of measurement. Reliability has numerous definitions and approaches, but in several environments, the concept comes to be consistent [ 8 ]. The measurement fulfills the requirements of reliability when it produces consistent results during data analysis procedure. The reliability is determined through Cranach’s alpha as shown in Table 2 .

Internal consistency and reliability test of questionnaires items.
K stands for knowledge; M, management; T, technology; C, collaboration; P, policy, standards, and regulation; H, hazards and accident conditions; PPE, personal protective equipment.
7.2 Reliability analysis
Cronbach’s alpha is a measure of internal consistency, i.e., how closely related a set of items are as a group [ 11 ]. It is considered to be a measure of scale reliability. The reliability of internal consistency most of the time is measured based on the Cronbach’s alpha value. Reliability coefficient of 0.70 and above is considered “acceptable” in most research situations [ 12 ]. In this study, reliability analysis for internal consistency of Likert-scale measurement after deleting 13 items was found similar; the reliability coefficients were found for 76 items were 0.964 and for the individual groupings made shown in Table 2 . It was also found internally consistent using the Cronbach’s alpha test. Table 2 shows the internal consistency of the seven major instruments in which their reliability falls in the acceptable range for this research.
7.3 Validity
Face validity used as defined by Babbie [ 13 ] is an indicator that makes it seem a reasonable measure of some variables, and it is the subjective judgment that the instrument measures what it intends to measure in terms of relevance [ 14 ]. Thus, the researcher ensured, in this study, when developing the instruments that uncertainties were eliminated by using appropriate words and concepts in order to enhance clarity and general suitability [ 14 ]. Furthermore, the researcher submitted the instruments to the research supervisor and the joint supervisor who are both occupational health experts, to ensure validity of the measuring instruments and determine whether the instruments could be considered valid on face value.
In this study, the researcher was guided by reviewed literature related to compliance with the occupational health and safety conditions and data collection methods before he could develop the measuring instruments. In addition, the pretest study that was conducted prior to the main study assisted the researcher to avoid uncertainties of the contents in the data collection measuring instruments. A thorough inspection of the measuring instruments by the statistician and the researcher’s supervisor and joint experts, to ensure that all concepts pertaining to the study were included, ensured that the instruments were enriched.
8. Data quality management
Insight has been given to the data collectors on how to approach companies, and many of the questionnaires were distributed through MSc students at Addis Ababa Institute of Technology (AAiT) and manufacturing industries’ experience experts. This made the data quality reliable as it has been continually discussed with them. Pretesting for questionnaire was done on 10 workers to assure the quality of the data and for improvement of data collection tools. Supervision during data collection was done to understand how the data collectors are handling the questionnaire, and each filled questionnaires was checked for its completeness, accuracy, clarity, and consistency on a daily basis either face-to-face or by phone/email. The data expected in poor quality were rejected out of the acting during the screening time. Among planned 267 questionnaires, 189 were responded back. Finally, it was analyzed by the principal investigator.
9. Inclusion criteria
The data were collected from the company representative with the knowledge of OSH. Articles written in English and Amharic were included in this study. Database information obtained in relation to articles and those who have OSH area such as interventions method, method of accident identification, impact of occupational accidents, types of occupational injuries/disease, and impact of occupational accidents, and disease on productivity and costs of company and have used at least one form of feedback mechanism. No specific time period was chosen in order to access all available published papers. The questionnaire statements which are similar in the questionnaire have been rejected from the data analysis.
10. Ethical consideration
Ethical clearance was obtained from the School of Mechanical and Industrial Engineering, Institute of Technology, Addis Ababa University. Official letters were written from the School of Mechanical and Industrial Engineering to the respective manufacturing industries. The purpose of the study was explained to the study subjects. The study subjects were told that the information they provided was kept confidential and that their identities would not be revealed in association with the information they provided. Informed consent was secured from each participant. For bad working environment assessment findings, feedback will be given to all manufacturing industries involved in the study. There is a plan to give a copy of the result to the respective study manufacturing industries’ and ministries’ offices. The respondents’ privacy and their responses were not individually analyzed and included in the report.
11. Dissemination and utilization of the result
The result of this study will be presented to the Addis Ababa University, AAiT, School of Mechanical and Industrial Engineering. It will also be communicated to the Ethiopian manufacturing industries, Ministry of Labor and Social Affair, Ministry of Industry, and Ministry of Health from where the data was collected. The result will also be availed by publication and online presentation in Google Scholars. To this end, about five articles were published and disseminated to the whole world.
12. Conclusion
The research methodology and design indicated overall process of the flow of the research for the given study. The data sources and data collection methods were used. The overall research strategies and framework are indicated in this research process from problem formulation to problem validation including all the parameters. It has laid some foundation and how research methodology is devised and framed for researchers. This means, it helps researchers to consider it as one of the samples and models for the research data collection and process from the beginning of the problem statement to the research finding. Especially, this research flow helps new researchers to the research environment and methodology in particular.
Conflict of interest
There is no “conflict of interest.”
- 1. Aaker A, Kumar VD, George S. Marketing Research. New York: John Wiley & Sons Inc; 2000
- 2. Saunders M, Lewis P, Thornhill A. Research Methods for Business Student. 5th ed. Edinburgh Gate: Pearson Education Limited; 2009
- 3. Miller P. Motivation in the Workplace. Work and Organizational Psychology. Oxford: Blackwell Publishers; 1991
- 4. Fraenkel FJ, Warren NE. How to Design and Evaluate Research in Education. 4th ed. New York: McGraw-Hill; 2002
- 5. Danniel WW. Biostatist: A Foundation for Analysis in the Health Science. 7th ed. New York: John Wiley & Sons; 1999
- 6. Cochran WG. Sampling Techniques. 3rd ed. New York: John Wiley & Sons; 1977
- 7. Saaty TL. The Analytical Hierarchy Process. Pittsburg: PWS Publications; 1990
- 8. Sekaran U, Bougie R. Research Methods for Business: A Skill Building Approach. 5th ed. New Delhi: John Wiley & Sons, Ltd; 2010. pp. 1-468
- 9. Luck DJ, Rubin RS. Marketing Research. 7th ed. New Jersey: Prentice-Hall International; 1987
- 10. Wong TC. Marketing Research. Oxford, UK: Butterworth-Heinemann; 1999
- 11. Cronbach LJ. Coefficient alpha and the internal structure of tests. Psychometrika. 1951; 16 :297-334
- 12. Tavakol M, Dennick R. Making sense of Cronbach’s alpha. International Journal of Medical Education. 2011; 2 :53-55. DOI: 10.5116/ijme.4dfb.8dfd
- 13. Babbie E. The Practice of Social Research. 12th ed. Belmont, CA: Wadsworth; 2010
- 14. Polit DF, Beck CT. Generating and Assessing Evidence for Nursing Practice. 8th ed. Williams and Wilkins: Lippincott; 2008
© 2019 The Author(s). Licensee IntechOpen. This chapter is distributed under the terms of the Creative Commons Attribution 3.0 License , which permits unrestricted use, distribution, and reproduction in any medium, provided the original work is properly cited.
Continue reading from the same book
Edited by Evon Abu-Taieh
Published: 17 June 2020
By Sabína Gáliková Tolnaiová and Slavomír Gálik
1001 downloads
By Carlos Pedro Gonçalves
1540 downloads
By Konstantinos-George Thanos, Andrianna Polydouri, A...
1040 downloads
Have a language expert improve your writing
Run a free plagiarism check in 10 minutes, generate accurate citations for free.
- Knowledge Base
- Dissertation
Theoretical Framework Example for a Thesis or Dissertation
Published on October 14, 2015 by Sarah Vinz . Revised on July 18, 2023 by Tegan George.
Your theoretical framework defines the key concepts in your research, suggests relationships between them, and discusses relevant theories based on your literature review .
A strong theoretical framework gives your research direction. It allows you to convincingly interpret, explain, and generalize from your findings and show the relevance of your thesis or dissertation topic in your field.
Instantly correct all language mistakes in your text
Upload your document to correct all your mistakes in minutes
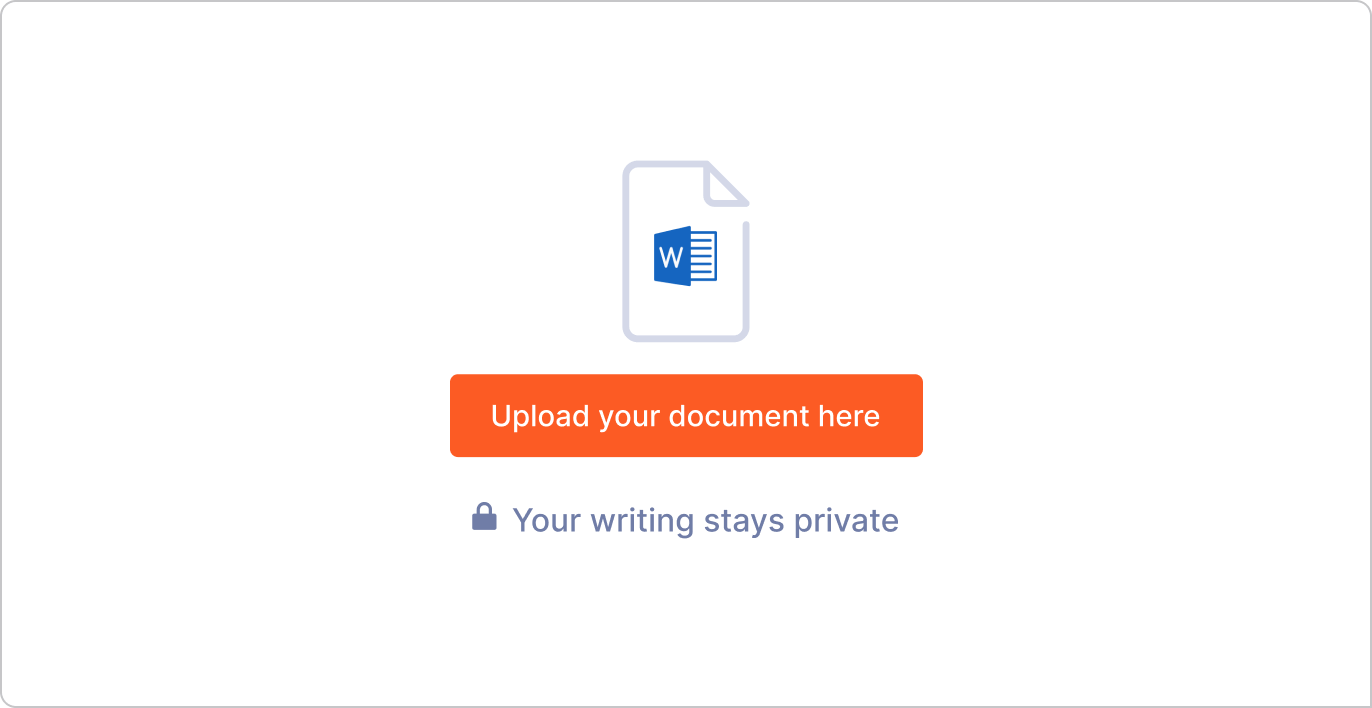
Table of contents
Sample problem statement and research questions, sample theoretical framework, your theoretical framework, other interesting articles.
Your theoretical framework is based on:
- Your problem statement
- Your research questions
- Your literature review
A new boutique downtown is struggling with the fact that many of their online customers do not return to make subsequent purchases. This is a big issue for the otherwise fast-growing store.Management wants to increase customer loyalty. They believe that improved customer satisfaction will play a major role in achieving their goal of increased return customers.
To investigate this problem, you have zeroed in on the following problem statement, objective, and research questions:
- Problem : Many online customers do not return to make subsequent purchases.
- Objective : To increase the quantity of return customers.
- Research question : How can the satisfaction of the boutique’s online customers be improved in order to increase the quantity of return customers?
The concepts of “customer loyalty” and “customer satisfaction” are clearly central to this study, along with their relationship to the likelihood that a customer will return. Your theoretical framework should define these concepts and discuss theories about the relationship between these variables.
Some sub-questions could include:
- What is the relationship between customer loyalty and customer satisfaction?
- How satisfied and loyal are the boutique’s online customers currently?
- What factors affect the satisfaction and loyalty of the boutique’s online customers?
As the concepts of “loyalty” and “customer satisfaction” play a major role in the investigation and will later be measured, they are essential concepts to define within your theoretical framework .
Here's why students love Scribbr's proofreading services
Discover proofreading & editing
Below is a simplified example showing how you can describe and compare theories in your thesis or dissertation . In this example, we focus on the concept of customer satisfaction introduced above.
Customer satisfaction
Thomassen (2003, p. 69) defines customer satisfaction as “the perception of the customer as a result of consciously or unconsciously comparing their experiences with their expectations.” Kotler & Keller (2008, p. 80) build on this definition, stating that customer satisfaction is determined by “the degree to which someone is happy or disappointed with the observed performance of a product in relation to his or her expectations.”
Performance that is below expectations leads to a dissatisfied customer, while performance that satisfies expectations produces satisfied customers (Kotler & Keller, 2003, p. 80).
The definition of Zeithaml and Bitner (2003, p. 86) is slightly different from that of Thomassen. They posit that “satisfaction is the consumer fulfillment response. It is a judgement that a product or service feature, or the product of service itself, provides a pleasurable level of consumption-related fulfillment.” Zeithaml and Bitner’s emphasis is thus on obtaining a certain satisfaction in relation to purchasing.
Thomassen’s definition is the most relevant to the aims of this study, given the emphasis it places on unconscious perception. Although Zeithaml and Bitner, like Thomassen, say that customer satisfaction is a reaction to the experience gained, there is no distinction between conscious and unconscious comparisons in their definition.
The boutique claims in its mission statement that it wants to sell not only a product, but also a feeling. As a result, unconscious comparison will play an important role in the satisfaction of its customers. Thomassen’s definition is therefore more relevant.
Thomassen’s Customer Satisfaction Model
According to Thomassen, both the so-called “value proposition” and other influences have an impact on final customer satisfaction. In his satisfaction model (Fig. 1), Thomassen shows that word-of-mouth, personal needs, past experiences, and marketing and public relations determine customers’ needs and expectations.
These factors are compared to their experiences, with the interplay between expectations and experiences determining a customer’s satisfaction level. Thomassen’s model is important for this study as it allows us to determine both the extent to which the boutique’s customers are satisfied, as well as where improvements can be made.
Figure 1 Customer satisfaction creation
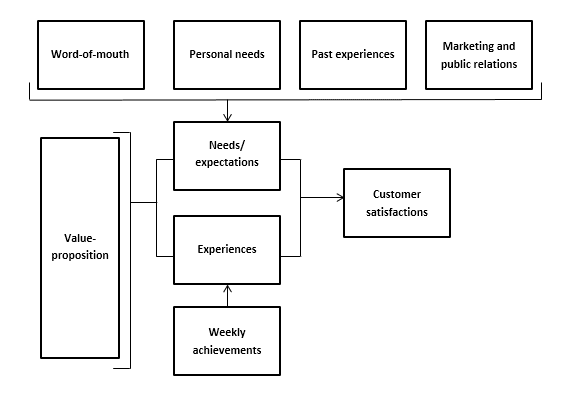
Of course, you could analyze the concepts more thoroughly and compare additional definitions to each other. You could also discuss the theories and ideas of key authors in greater detail and provide several models to illustrate different concepts.
If you want to know more about AI for academic writing, AI tools, or research bias, make sure to check out some of our other articles with explanations and examples or go directly to our tools!
Research bias
- Anchoring bias
- Halo effect
- The Baader–Meinhof phenomenon
- The placebo effect
- Nonresponse bias
- Deep learning
- Generative AI
- Machine learning
- Reinforcement learning
- Supervised vs. unsupervised learning
(AI) Tools
- Grammar Checker
- Paraphrasing Tool
- Text Summarizer
- AI Detector
- Plagiarism Checker
- Citation Generator
Cite this Scribbr article
If you want to cite this source, you can copy and paste the citation or click the “Cite this Scribbr article” button to automatically add the citation to our free Citation Generator.
Vinz, S. (2023, July 18). Theoretical Framework Example for a Thesis or Dissertation. Scribbr. Retrieved April 11, 2024, from https://www.scribbr.com/dissertation/theoretical-framework-example/
Is this article helpful?
Sarah's academic background includes a Master of Arts in English, a Master of International Affairs degree, and a Bachelor of Arts in Political Science. She loves the challenge of finding the perfect formulation or wording and derives much satisfaction from helping students take their academic writing up a notch.
Other students also liked
What is a theoretical framework | guide to organizing, how to write a literature review | guide, examples, & templates, what is a research methodology | steps & tips, "i thought ai proofreading was useless but..".
I've been using Scribbr for years now and I know it's a service that won't disappoint. It does a good job spotting mistakes”

Experimental Research Design — 6 mistakes you should never make!
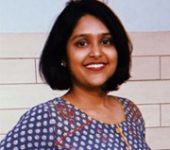
Since school days’ students perform scientific experiments that provide results that define and prove the laws and theorems in science. These experiments are laid on a strong foundation of experimental research designs.
An experimental research design helps researchers execute their research objectives with more clarity and transparency.
In this article, we will not only discuss the key aspects of experimental research designs but also the issues to avoid and problems to resolve while designing your research study.
Table of Contents
What Is Experimental Research Design?
Experimental research design is a framework of protocols and procedures created to conduct experimental research with a scientific approach using two sets of variables. Herein, the first set of variables acts as a constant, used to measure the differences of the second set. The best example of experimental research methods is quantitative research .
Experimental research helps a researcher gather the necessary data for making better research decisions and determining the facts of a research study.
When Can a Researcher Conduct Experimental Research?
A researcher can conduct experimental research in the following situations —
- When time is an important factor in establishing a relationship between the cause and effect.
- When there is an invariable or never-changing behavior between the cause and effect.
- Finally, when the researcher wishes to understand the importance of the cause and effect.
Importance of Experimental Research Design
To publish significant results, choosing a quality research design forms the foundation to build the research study. Moreover, effective research design helps establish quality decision-making procedures, structures the research to lead to easier data analysis, and addresses the main research question. Therefore, it is essential to cater undivided attention and time to create an experimental research design before beginning the practical experiment.
By creating a research design, a researcher is also giving oneself time to organize the research, set up relevant boundaries for the study, and increase the reliability of the results. Through all these efforts, one could also avoid inconclusive results. If any part of the research design is flawed, it will reflect on the quality of the results derived.
Types of Experimental Research Designs
Based on the methods used to collect data in experimental studies, the experimental research designs are of three primary types:
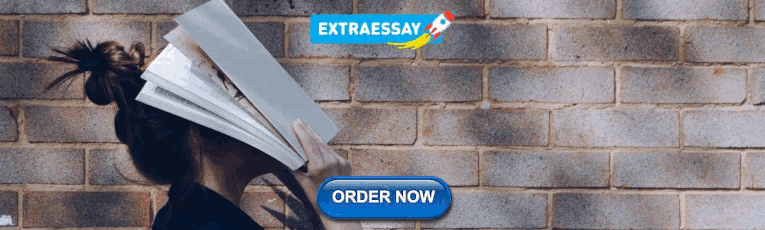
1. Pre-experimental Research Design
A research study could conduct pre-experimental research design when a group or many groups are under observation after implementing factors of cause and effect of the research. The pre-experimental design will help researchers understand whether further investigation is necessary for the groups under observation.
Pre-experimental research is of three types —
- One-shot Case Study Research Design
- One-group Pretest-posttest Research Design
- Static-group Comparison
2. True Experimental Research Design
A true experimental research design relies on statistical analysis to prove or disprove a researcher’s hypothesis. It is one of the most accurate forms of research because it provides specific scientific evidence. Furthermore, out of all the types of experimental designs, only a true experimental design can establish a cause-effect relationship within a group. However, in a true experiment, a researcher must satisfy these three factors —
- There is a control group that is not subjected to changes and an experimental group that will experience the changed variables
- A variable that can be manipulated by the researcher
- Random distribution of the variables
This type of experimental research is commonly observed in the physical sciences.
3. Quasi-experimental Research Design
The word “Quasi” means similarity. A quasi-experimental design is similar to a true experimental design. However, the difference between the two is the assignment of the control group. In this research design, an independent variable is manipulated, but the participants of a group are not randomly assigned. This type of research design is used in field settings where random assignment is either irrelevant or not required.
The classification of the research subjects, conditions, or groups determines the type of research design to be used.
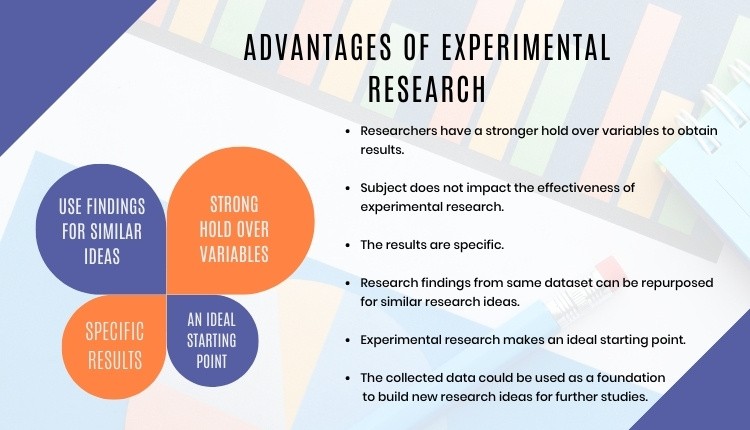
Advantages of Experimental Research
Experimental research allows you to test your idea in a controlled environment before taking the research to clinical trials. Moreover, it provides the best method to test your theory because of the following advantages:
- Researchers have firm control over variables to obtain results.
- The subject does not impact the effectiveness of experimental research. Anyone can implement it for research purposes.
- The results are specific.
- Post results analysis, research findings from the same dataset can be repurposed for similar research ideas.
- Researchers can identify the cause and effect of the hypothesis and further analyze this relationship to determine in-depth ideas.
- Experimental research makes an ideal starting point. The collected data could be used as a foundation to build new research ideas for further studies.
6 Mistakes to Avoid While Designing Your Research
There is no order to this list, and any one of these issues can seriously compromise the quality of your research. You could refer to the list as a checklist of what to avoid while designing your research.
1. Invalid Theoretical Framework
Usually, researchers miss out on checking if their hypothesis is logical to be tested. If your research design does not have basic assumptions or postulates, then it is fundamentally flawed and you need to rework on your research framework.
2. Inadequate Literature Study
Without a comprehensive research literature review , it is difficult to identify and fill the knowledge and information gaps. Furthermore, you need to clearly state how your research will contribute to the research field, either by adding value to the pertinent literature or challenging previous findings and assumptions.
3. Insufficient or Incorrect Statistical Analysis
Statistical results are one of the most trusted scientific evidence. The ultimate goal of a research experiment is to gain valid and sustainable evidence. Therefore, incorrect statistical analysis could affect the quality of any quantitative research.
4. Undefined Research Problem
This is one of the most basic aspects of research design. The research problem statement must be clear and to do that, you must set the framework for the development of research questions that address the core problems.
5. Research Limitations
Every study has some type of limitations . You should anticipate and incorporate those limitations into your conclusion, as well as the basic research design. Include a statement in your manuscript about any perceived limitations, and how you considered them while designing your experiment and drawing the conclusion.
6. Ethical Implications
The most important yet less talked about topic is the ethical issue. Your research design must include ways to minimize any risk for your participants and also address the research problem or question at hand. If you cannot manage the ethical norms along with your research study, your research objectives and validity could be questioned.
Experimental Research Design Example
In an experimental design, a researcher gathers plant samples and then randomly assigns half the samples to photosynthesize in sunlight and the other half to be kept in a dark box without sunlight, while controlling all the other variables (nutrients, water, soil, etc.)
By comparing their outcomes in biochemical tests, the researcher can confirm that the changes in the plants were due to the sunlight and not the other variables.
Experimental research is often the final form of a study conducted in the research process which is considered to provide conclusive and specific results. But it is not meant for every research. It involves a lot of resources, time, and money and is not easy to conduct, unless a foundation of research is built. Yet it is widely used in research institutes and commercial industries, for its most conclusive results in the scientific approach.
Have you worked on research designs? How was your experience creating an experimental design? What difficulties did you face? Do write to us or comment below and share your insights on experimental research designs!
Frequently Asked Questions
Randomization is important in an experimental research because it ensures unbiased results of the experiment. It also measures the cause-effect relationship on a particular group of interest.
Experimental research design lay the foundation of a research and structures the research to establish quality decision making process.
There are 3 types of experimental research designs. These are pre-experimental research design, true experimental research design, and quasi experimental research design.
The difference between an experimental and a quasi-experimental design are: 1. The assignment of the control group in quasi experimental research is non-random, unlike true experimental design, which is randomly assigned. 2. Experimental research group always has a control group; on the other hand, it may not be always present in quasi experimental research.
Experimental research establishes a cause-effect relationship by testing a theory or hypothesis using experimental groups or control variables. In contrast, descriptive research describes a study or a topic by defining the variables under it and answering the questions related to the same.

good and valuable
Very very good
Good presentation.
Rate this article Cancel Reply
Your email address will not be published.
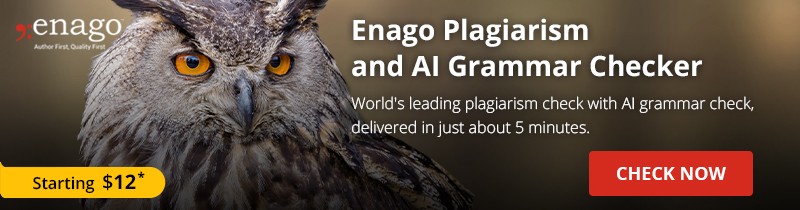
Enago Academy's Most Popular Articles
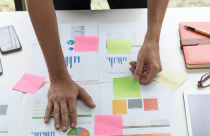
- Publishing Research
- Reporting Research
How to Optimize Your Research Process: A step-by-step guide
For researchers across disciplines, the path to uncovering novel findings and insights is often filled…
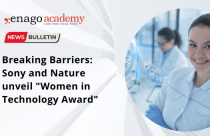
- Industry News
- Trending Now
Breaking Barriers: Sony and Nature unveil “Women in Technology Award”
Sony Group Corporation and the prestigious scientific journal Nature have collaborated to launch the inaugural…
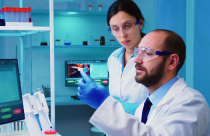
Achieving Research Excellence: Checklist for good research practices
Academia is built on the foundation of trustworthy and high-quality research, supported by the pillars…
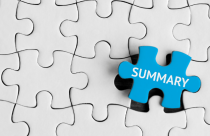
- Promoting Research
Plain Language Summary — Communicating your research to bridge the academic-lay gap
Science can be complex, but does that mean it should not be accessible to the…
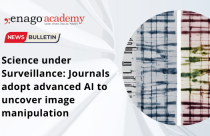
Science under Surveillance: Journals adopt advanced AI to uncover image manipulation
Journals are increasingly turning to cutting-edge AI tools to uncover deceitful images published in manuscripts.…
Choosing the Right Analytical Approach: Thematic analysis vs. content analysis for…
Comparing Cross Sectional and Longitudinal Studies: 5 steps for choosing the right…
Research Recommendations – Guiding policy-makers for evidence-based decision making

Sign-up to read more
Subscribe for free to get unrestricted access to all our resources on research writing and academic publishing including:
- 2000+ blog articles
- 50+ Webinars
- 10+ Expert podcasts
- 50+ Infographics
- 10+ Checklists
- Research Guides
We hate spam too. We promise to protect your privacy and never spam you.
I am looking for Editing/ Proofreading services for my manuscript Tentative date of next journal submission:
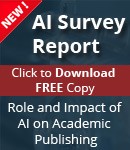
What should universities' stance be on AI tools in research and academic writing?

An official website of the United States government
The .gov means it’s official. Federal government websites often end in .gov or .mil. Before sharing sensitive information, make sure you’re on a federal government site.
The site is secure. The https:// ensures that you are connecting to the official website and that any information you provide is encrypted and transmitted securely.
- Publications
- Account settings
Preview improvements coming to the PMC website in October 2024. Learn More or Try it out now .
- Advanced Search
- Journal List
- J Korean Med Sci
- v.37(16); 2022 Apr 25

A Practical Guide to Writing Quantitative and Qualitative Research Questions and Hypotheses in Scholarly Articles
Edward barroga.
1 Department of General Education, Graduate School of Nursing Science, St. Luke’s International University, Tokyo, Japan.
Glafera Janet Matanguihan
2 Department of Biological Sciences, Messiah University, Mechanicsburg, PA, USA.
The development of research questions and the subsequent hypotheses are prerequisites to defining the main research purpose and specific objectives of a study. Consequently, these objectives determine the study design and research outcome. The development of research questions is a process based on knowledge of current trends, cutting-edge studies, and technological advances in the research field. Excellent research questions are focused and require a comprehensive literature search and in-depth understanding of the problem being investigated. Initially, research questions may be written as descriptive questions which could be developed into inferential questions. These questions must be specific and concise to provide a clear foundation for developing hypotheses. Hypotheses are more formal predictions about the research outcomes. These specify the possible results that may or may not be expected regarding the relationship between groups. Thus, research questions and hypotheses clarify the main purpose and specific objectives of the study, which in turn dictate the design of the study, its direction, and outcome. Studies developed from good research questions and hypotheses will have trustworthy outcomes with wide-ranging social and health implications.
INTRODUCTION
Scientific research is usually initiated by posing evidenced-based research questions which are then explicitly restated as hypotheses. 1 , 2 The hypotheses provide directions to guide the study, solutions, explanations, and expected results. 3 , 4 Both research questions and hypotheses are essentially formulated based on conventional theories and real-world processes, which allow the inception of novel studies and the ethical testing of ideas. 5 , 6
It is crucial to have knowledge of both quantitative and qualitative research 2 as both types of research involve writing research questions and hypotheses. 7 However, these crucial elements of research are sometimes overlooked; if not overlooked, then framed without the forethought and meticulous attention it needs. Planning and careful consideration are needed when developing quantitative or qualitative research, particularly when conceptualizing research questions and hypotheses. 4
There is a continuing need to support researchers in the creation of innovative research questions and hypotheses, as well as for journal articles that carefully review these elements. 1 When research questions and hypotheses are not carefully thought of, unethical studies and poor outcomes usually ensue. Carefully formulated research questions and hypotheses define well-founded objectives, which in turn determine the appropriate design, course, and outcome of the study. This article then aims to discuss in detail the various aspects of crafting research questions and hypotheses, with the goal of guiding researchers as they develop their own. Examples from the authors and peer-reviewed scientific articles in the healthcare field are provided to illustrate key points.
DEFINITIONS AND RELATIONSHIP OF RESEARCH QUESTIONS AND HYPOTHESES
A research question is what a study aims to answer after data analysis and interpretation. The answer is written in length in the discussion section of the paper. Thus, the research question gives a preview of the different parts and variables of the study meant to address the problem posed in the research question. 1 An excellent research question clarifies the research writing while facilitating understanding of the research topic, objective, scope, and limitations of the study. 5
On the other hand, a research hypothesis is an educated statement of an expected outcome. This statement is based on background research and current knowledge. 8 , 9 The research hypothesis makes a specific prediction about a new phenomenon 10 or a formal statement on the expected relationship between an independent variable and a dependent variable. 3 , 11 It provides a tentative answer to the research question to be tested or explored. 4
Hypotheses employ reasoning to predict a theory-based outcome. 10 These can also be developed from theories by focusing on components of theories that have not yet been observed. 10 The validity of hypotheses is often based on the testability of the prediction made in a reproducible experiment. 8
Conversely, hypotheses can also be rephrased as research questions. Several hypotheses based on existing theories and knowledge may be needed to answer a research question. Developing ethical research questions and hypotheses creates a research design that has logical relationships among variables. These relationships serve as a solid foundation for the conduct of the study. 4 , 11 Haphazardly constructed research questions can result in poorly formulated hypotheses and improper study designs, leading to unreliable results. Thus, the formulations of relevant research questions and verifiable hypotheses are crucial when beginning research. 12
CHARACTERISTICS OF GOOD RESEARCH QUESTIONS AND HYPOTHESES
Excellent research questions are specific and focused. These integrate collective data and observations to confirm or refute the subsequent hypotheses. Well-constructed hypotheses are based on previous reports and verify the research context. These are realistic, in-depth, sufficiently complex, and reproducible. More importantly, these hypotheses can be addressed and tested. 13
There are several characteristics of well-developed hypotheses. Good hypotheses are 1) empirically testable 7 , 10 , 11 , 13 ; 2) backed by preliminary evidence 9 ; 3) testable by ethical research 7 , 9 ; 4) based on original ideas 9 ; 5) have evidenced-based logical reasoning 10 ; and 6) can be predicted. 11 Good hypotheses can infer ethical and positive implications, indicating the presence of a relationship or effect relevant to the research theme. 7 , 11 These are initially developed from a general theory and branch into specific hypotheses by deductive reasoning. In the absence of a theory to base the hypotheses, inductive reasoning based on specific observations or findings form more general hypotheses. 10
TYPES OF RESEARCH QUESTIONS AND HYPOTHESES
Research questions and hypotheses are developed according to the type of research, which can be broadly classified into quantitative and qualitative research. We provide a summary of the types of research questions and hypotheses under quantitative and qualitative research categories in Table 1 .
Research questions in quantitative research
In quantitative research, research questions inquire about the relationships among variables being investigated and are usually framed at the start of the study. These are precise and typically linked to the subject population, dependent and independent variables, and research design. 1 Research questions may also attempt to describe the behavior of a population in relation to one or more variables, or describe the characteristics of variables to be measured ( descriptive research questions ). 1 , 5 , 14 These questions may also aim to discover differences between groups within the context of an outcome variable ( comparative research questions ), 1 , 5 , 14 or elucidate trends and interactions among variables ( relationship research questions ). 1 , 5 We provide examples of descriptive, comparative, and relationship research questions in quantitative research in Table 2 .
Hypotheses in quantitative research
In quantitative research, hypotheses predict the expected relationships among variables. 15 Relationships among variables that can be predicted include 1) between a single dependent variable and a single independent variable ( simple hypothesis ) or 2) between two or more independent and dependent variables ( complex hypothesis ). 4 , 11 Hypotheses may also specify the expected direction to be followed and imply an intellectual commitment to a particular outcome ( directional hypothesis ) 4 . On the other hand, hypotheses may not predict the exact direction and are used in the absence of a theory, or when findings contradict previous studies ( non-directional hypothesis ). 4 In addition, hypotheses can 1) define interdependency between variables ( associative hypothesis ), 4 2) propose an effect on the dependent variable from manipulation of the independent variable ( causal hypothesis ), 4 3) state a negative relationship between two variables ( null hypothesis ), 4 , 11 , 15 4) replace the working hypothesis if rejected ( alternative hypothesis ), 15 explain the relationship of phenomena to possibly generate a theory ( working hypothesis ), 11 5) involve quantifiable variables that can be tested statistically ( statistical hypothesis ), 11 6) or express a relationship whose interlinks can be verified logically ( logical hypothesis ). 11 We provide examples of simple, complex, directional, non-directional, associative, causal, null, alternative, working, statistical, and logical hypotheses in quantitative research, as well as the definition of quantitative hypothesis-testing research in Table 3 .
Research questions in qualitative research
Unlike research questions in quantitative research, research questions in qualitative research are usually continuously reviewed and reformulated. The central question and associated subquestions are stated more than the hypotheses. 15 The central question broadly explores a complex set of factors surrounding the central phenomenon, aiming to present the varied perspectives of participants. 15
There are varied goals for which qualitative research questions are developed. These questions can function in several ways, such as to 1) identify and describe existing conditions ( contextual research question s); 2) describe a phenomenon ( descriptive research questions ); 3) assess the effectiveness of existing methods, protocols, theories, or procedures ( evaluation research questions ); 4) examine a phenomenon or analyze the reasons or relationships between subjects or phenomena ( explanatory research questions ); or 5) focus on unknown aspects of a particular topic ( exploratory research questions ). 5 In addition, some qualitative research questions provide new ideas for the development of theories and actions ( generative research questions ) or advance specific ideologies of a position ( ideological research questions ). 1 Other qualitative research questions may build on a body of existing literature and become working guidelines ( ethnographic research questions ). Research questions may also be broadly stated without specific reference to the existing literature or a typology of questions ( phenomenological research questions ), may be directed towards generating a theory of some process ( grounded theory questions ), or may address a description of the case and the emerging themes ( qualitative case study questions ). 15 We provide examples of contextual, descriptive, evaluation, explanatory, exploratory, generative, ideological, ethnographic, phenomenological, grounded theory, and qualitative case study research questions in qualitative research in Table 4 , and the definition of qualitative hypothesis-generating research in Table 5 .
Qualitative studies usually pose at least one central research question and several subquestions starting with How or What . These research questions use exploratory verbs such as explore or describe . These also focus on one central phenomenon of interest, and may mention the participants and research site. 15
Hypotheses in qualitative research
Hypotheses in qualitative research are stated in the form of a clear statement concerning the problem to be investigated. Unlike in quantitative research where hypotheses are usually developed to be tested, qualitative research can lead to both hypothesis-testing and hypothesis-generating outcomes. 2 When studies require both quantitative and qualitative research questions, this suggests an integrative process between both research methods wherein a single mixed-methods research question can be developed. 1
FRAMEWORKS FOR DEVELOPING RESEARCH QUESTIONS AND HYPOTHESES
Research questions followed by hypotheses should be developed before the start of the study. 1 , 12 , 14 It is crucial to develop feasible research questions on a topic that is interesting to both the researcher and the scientific community. This can be achieved by a meticulous review of previous and current studies to establish a novel topic. Specific areas are subsequently focused on to generate ethical research questions. The relevance of the research questions is evaluated in terms of clarity of the resulting data, specificity of the methodology, objectivity of the outcome, depth of the research, and impact of the study. 1 , 5 These aspects constitute the FINER criteria (i.e., Feasible, Interesting, Novel, Ethical, and Relevant). 1 Clarity and effectiveness are achieved if research questions meet the FINER criteria. In addition to the FINER criteria, Ratan et al. described focus, complexity, novelty, feasibility, and measurability for evaluating the effectiveness of research questions. 14
The PICOT and PEO frameworks are also used when developing research questions. 1 The following elements are addressed in these frameworks, PICOT: P-population/patients/problem, I-intervention or indicator being studied, C-comparison group, O-outcome of interest, and T-timeframe of the study; PEO: P-population being studied, E-exposure to preexisting conditions, and O-outcome of interest. 1 Research questions are also considered good if these meet the “FINERMAPS” framework: Feasible, Interesting, Novel, Ethical, Relevant, Manageable, Appropriate, Potential value/publishable, and Systematic. 14
As we indicated earlier, research questions and hypotheses that are not carefully formulated result in unethical studies or poor outcomes. To illustrate this, we provide some examples of ambiguous research question and hypotheses that result in unclear and weak research objectives in quantitative research ( Table 6 ) 16 and qualitative research ( Table 7 ) 17 , and how to transform these ambiguous research question(s) and hypothesis(es) into clear and good statements.
a These statements were composed for comparison and illustrative purposes only.
b These statements are direct quotes from Higashihara and Horiuchi. 16
a This statement is a direct quote from Shimoda et al. 17
The other statements were composed for comparison and illustrative purposes only.
CONSTRUCTING RESEARCH QUESTIONS AND HYPOTHESES
To construct effective research questions and hypotheses, it is very important to 1) clarify the background and 2) identify the research problem at the outset of the research, within a specific timeframe. 9 Then, 3) review or conduct preliminary research to collect all available knowledge about the possible research questions by studying theories and previous studies. 18 Afterwards, 4) construct research questions to investigate the research problem. Identify variables to be accessed from the research questions 4 and make operational definitions of constructs from the research problem and questions. Thereafter, 5) construct specific deductive or inductive predictions in the form of hypotheses. 4 Finally, 6) state the study aims . This general flow for constructing effective research questions and hypotheses prior to conducting research is shown in Fig. 1 .

Research questions are used more frequently in qualitative research than objectives or hypotheses. 3 These questions seek to discover, understand, explore or describe experiences by asking “What” or “How.” The questions are open-ended to elicit a description rather than to relate variables or compare groups. The questions are continually reviewed, reformulated, and changed during the qualitative study. 3 Research questions are also used more frequently in survey projects than hypotheses in experiments in quantitative research to compare variables and their relationships.
Hypotheses are constructed based on the variables identified and as an if-then statement, following the template, ‘If a specific action is taken, then a certain outcome is expected.’ At this stage, some ideas regarding expectations from the research to be conducted must be drawn. 18 Then, the variables to be manipulated (independent) and influenced (dependent) are defined. 4 Thereafter, the hypothesis is stated and refined, and reproducible data tailored to the hypothesis are identified, collected, and analyzed. 4 The hypotheses must be testable and specific, 18 and should describe the variables and their relationships, the specific group being studied, and the predicted research outcome. 18 Hypotheses construction involves a testable proposition to be deduced from theory, and independent and dependent variables to be separated and measured separately. 3 Therefore, good hypotheses must be based on good research questions constructed at the start of a study or trial. 12
In summary, research questions are constructed after establishing the background of the study. Hypotheses are then developed based on the research questions. Thus, it is crucial to have excellent research questions to generate superior hypotheses. In turn, these would determine the research objectives and the design of the study, and ultimately, the outcome of the research. 12 Algorithms for building research questions and hypotheses are shown in Fig. 2 for quantitative research and in Fig. 3 for qualitative research.

EXAMPLES OF RESEARCH QUESTIONS FROM PUBLISHED ARTICLES
- EXAMPLE 1. Descriptive research question (quantitative research)
- - Presents research variables to be assessed (distinct phenotypes and subphenotypes)
- “BACKGROUND: Since COVID-19 was identified, its clinical and biological heterogeneity has been recognized. Identifying COVID-19 phenotypes might help guide basic, clinical, and translational research efforts.
- RESEARCH QUESTION: Does the clinical spectrum of patients with COVID-19 contain distinct phenotypes and subphenotypes? ” 19
- EXAMPLE 2. Relationship research question (quantitative research)
- - Shows interactions between dependent variable (static postural control) and independent variable (peripheral visual field loss)
- “Background: Integration of visual, vestibular, and proprioceptive sensations contributes to postural control. People with peripheral visual field loss have serious postural instability. However, the directional specificity of postural stability and sensory reweighting caused by gradual peripheral visual field loss remain unclear.
- Research question: What are the effects of peripheral visual field loss on static postural control ?” 20
- EXAMPLE 3. Comparative research question (quantitative research)
- - Clarifies the difference among groups with an outcome variable (patients enrolled in COMPERA with moderate PH or severe PH in COPD) and another group without the outcome variable (patients with idiopathic pulmonary arterial hypertension (IPAH))
- “BACKGROUND: Pulmonary hypertension (PH) in COPD is a poorly investigated clinical condition.
- RESEARCH QUESTION: Which factors determine the outcome of PH in COPD?
- STUDY DESIGN AND METHODS: We analyzed the characteristics and outcome of patients enrolled in the Comparative, Prospective Registry of Newly Initiated Therapies for Pulmonary Hypertension (COMPERA) with moderate or severe PH in COPD as defined during the 6th PH World Symposium who received medical therapy for PH and compared them with patients with idiopathic pulmonary arterial hypertension (IPAH) .” 21
- EXAMPLE 4. Exploratory research question (qualitative research)
- - Explores areas that have not been fully investigated (perspectives of families and children who receive care in clinic-based child obesity treatment) to have a deeper understanding of the research problem
- “Problem: Interventions for children with obesity lead to only modest improvements in BMI and long-term outcomes, and data are limited on the perspectives of families of children with obesity in clinic-based treatment. This scoping review seeks to answer the question: What is known about the perspectives of families and children who receive care in clinic-based child obesity treatment? This review aims to explore the scope of perspectives reported by families of children with obesity who have received individualized outpatient clinic-based obesity treatment.” 22
- EXAMPLE 5. Relationship research question (quantitative research)
- - Defines interactions between dependent variable (use of ankle strategies) and independent variable (changes in muscle tone)
- “Background: To maintain an upright standing posture against external disturbances, the human body mainly employs two types of postural control strategies: “ankle strategy” and “hip strategy.” While it has been reported that the magnitude of the disturbance alters the use of postural control strategies, it has not been elucidated how the level of muscle tone, one of the crucial parameters of bodily function, determines the use of each strategy. We have previously confirmed using forward dynamics simulations of human musculoskeletal models that an increased muscle tone promotes the use of ankle strategies. The objective of the present study was to experimentally evaluate a hypothesis: an increased muscle tone promotes the use of ankle strategies. Research question: Do changes in the muscle tone affect the use of ankle strategies ?” 23
EXAMPLES OF HYPOTHESES IN PUBLISHED ARTICLES
- EXAMPLE 1. Working hypothesis (quantitative research)
- - A hypothesis that is initially accepted for further research to produce a feasible theory
- “As fever may have benefit in shortening the duration of viral illness, it is plausible to hypothesize that the antipyretic efficacy of ibuprofen may be hindering the benefits of a fever response when taken during the early stages of COVID-19 illness .” 24
- “In conclusion, it is plausible to hypothesize that the antipyretic efficacy of ibuprofen may be hindering the benefits of a fever response . The difference in perceived safety of these agents in COVID-19 illness could be related to the more potent efficacy to reduce fever with ibuprofen compared to acetaminophen. Compelling data on the benefit of fever warrant further research and review to determine when to treat or withhold ibuprofen for early stage fever for COVID-19 and other related viral illnesses .” 24
- EXAMPLE 2. Exploratory hypothesis (qualitative research)
- - Explores particular areas deeper to clarify subjective experience and develop a formal hypothesis potentially testable in a future quantitative approach
- “We hypothesized that when thinking about a past experience of help-seeking, a self distancing prompt would cause increased help-seeking intentions and more favorable help-seeking outcome expectations .” 25
- “Conclusion
- Although a priori hypotheses were not supported, further research is warranted as results indicate the potential for using self-distancing approaches to increasing help-seeking among some people with depressive symptomatology.” 25
- EXAMPLE 3. Hypothesis-generating research to establish a framework for hypothesis testing (qualitative research)
- “We hypothesize that compassionate care is beneficial for patients (better outcomes), healthcare systems and payers (lower costs), and healthcare providers (lower burnout). ” 26
- Compassionomics is the branch of knowledge and scientific study of the effects of compassionate healthcare. Our main hypotheses are that compassionate healthcare is beneficial for (1) patients, by improving clinical outcomes, (2) healthcare systems and payers, by supporting financial sustainability, and (3) HCPs, by lowering burnout and promoting resilience and well-being. The purpose of this paper is to establish a scientific framework for testing the hypotheses above . If these hypotheses are confirmed through rigorous research, compassionomics will belong in the science of evidence-based medicine, with major implications for all healthcare domains.” 26
- EXAMPLE 4. Statistical hypothesis (quantitative research)
- - An assumption is made about the relationship among several population characteristics ( gender differences in sociodemographic and clinical characteristics of adults with ADHD ). Validity is tested by statistical experiment or analysis ( chi-square test, Students t-test, and logistic regression analysis)
- “Our research investigated gender differences in sociodemographic and clinical characteristics of adults with ADHD in a Japanese clinical sample. Due to unique Japanese cultural ideals and expectations of women's behavior that are in opposition to ADHD symptoms, we hypothesized that women with ADHD experience more difficulties and present more dysfunctions than men . We tested the following hypotheses: first, women with ADHD have more comorbidities than men with ADHD; second, women with ADHD experience more social hardships than men, such as having less full-time employment and being more likely to be divorced.” 27
- “Statistical Analysis
- ( text omitted ) Between-gender comparisons were made using the chi-squared test for categorical variables and Students t-test for continuous variables…( text omitted ). A logistic regression analysis was performed for employment status, marital status, and comorbidity to evaluate the independent effects of gender on these dependent variables.” 27
EXAMPLES OF HYPOTHESIS AS WRITTEN IN PUBLISHED ARTICLES IN RELATION TO OTHER PARTS
- EXAMPLE 1. Background, hypotheses, and aims are provided
- “Pregnant women need skilled care during pregnancy and childbirth, but that skilled care is often delayed in some countries …( text omitted ). The focused antenatal care (FANC) model of WHO recommends that nurses provide information or counseling to all pregnant women …( text omitted ). Job aids are visual support materials that provide the right kind of information using graphics and words in a simple and yet effective manner. When nurses are not highly trained or have many work details to attend to, these job aids can serve as a content reminder for the nurses and can be used for educating their patients (Jennings, Yebadokpo, Affo, & Agbogbe, 2010) ( text omitted ). Importantly, additional evidence is needed to confirm how job aids can further improve the quality of ANC counseling by health workers in maternal care …( text omitted )” 28
- “ This has led us to hypothesize that the quality of ANC counseling would be better if supported by job aids. Consequently, a better quality of ANC counseling is expected to produce higher levels of awareness concerning the danger signs of pregnancy and a more favorable impression of the caring behavior of nurses .” 28
- “This study aimed to examine the differences in the responses of pregnant women to a job aid-supported intervention during ANC visit in terms of 1) their understanding of the danger signs of pregnancy and 2) their impression of the caring behaviors of nurses to pregnant women in rural Tanzania.” 28
- EXAMPLE 2. Background, hypotheses, and aims are provided
- “We conducted a two-arm randomized controlled trial (RCT) to evaluate and compare changes in salivary cortisol and oxytocin levels of first-time pregnant women between experimental and control groups. The women in the experimental group touched and held an infant for 30 min (experimental intervention protocol), whereas those in the control group watched a DVD movie of an infant (control intervention protocol). The primary outcome was salivary cortisol level and the secondary outcome was salivary oxytocin level.” 29
- “ We hypothesize that at 30 min after touching and holding an infant, the salivary cortisol level will significantly decrease and the salivary oxytocin level will increase in the experimental group compared with the control group .” 29
- EXAMPLE 3. Background, aim, and hypothesis are provided
- “In countries where the maternal mortality ratio remains high, antenatal education to increase Birth Preparedness and Complication Readiness (BPCR) is considered one of the top priorities [1]. BPCR includes birth plans during the antenatal period, such as the birthplace, birth attendant, transportation, health facility for complications, expenses, and birth materials, as well as family coordination to achieve such birth plans. In Tanzania, although increasing, only about half of all pregnant women attend an antenatal clinic more than four times [4]. Moreover, the information provided during antenatal care (ANC) is insufficient. In the resource-poor settings, antenatal group education is a potential approach because of the limited time for individual counseling at antenatal clinics.” 30
- “This study aimed to evaluate an antenatal group education program among pregnant women and their families with respect to birth-preparedness and maternal and infant outcomes in rural villages of Tanzania.” 30
- “ The study hypothesis was if Tanzanian pregnant women and their families received a family-oriented antenatal group education, they would (1) have a higher level of BPCR, (2) attend antenatal clinic four or more times, (3) give birth in a health facility, (4) have less complications of women at birth, and (5) have less complications and deaths of infants than those who did not receive the education .” 30
Research questions and hypotheses are crucial components to any type of research, whether quantitative or qualitative. These questions should be developed at the very beginning of the study. Excellent research questions lead to superior hypotheses, which, like a compass, set the direction of research, and can often determine the successful conduct of the study. Many research studies have floundered because the development of research questions and subsequent hypotheses was not given the thought and meticulous attention needed. The development of research questions and hypotheses is an iterative process based on extensive knowledge of the literature and insightful grasp of the knowledge gap. Focused, concise, and specific research questions provide a strong foundation for constructing hypotheses which serve as formal predictions about the research outcomes. Research questions and hypotheses are crucial elements of research that should not be overlooked. They should be carefully thought of and constructed when planning research. This avoids unethical studies and poor outcomes by defining well-founded objectives that determine the design, course, and outcome of the study.
Disclosure: The authors have no potential conflicts of interest to disclose.
Author Contributions:
- Conceptualization: Barroga E, Matanguihan GJ.
- Methodology: Barroga E, Matanguihan GJ.
- Writing - original draft: Barroga E, Matanguihan GJ.
- Writing - review & editing: Barroga E, Matanguihan GJ.
Thank you for visiting nature.com. You are using a browser version with limited support for CSS. To obtain the best experience, we recommend you use a more up to date browser (or turn off compatibility mode in Internet Explorer). In the meantime, to ensure continued support, we are displaying the site without styles and JavaScript.
- View all journals
- My Account Login
- Explore content
- About the journal
- Publish with us
- Sign up for alerts
- Open access
- Published: 11 April 2024
Design principles for integrating science practices with conceptual understanding: an example from a digital learning environment on microbial resistance to antibiotics
- Constantinos P. Constantinou ORCID: orcid.org/0000-0003-3183-4131 1 &
- Eliza Rybska ORCID: orcid.org/0000-0003-2778-1313 2
Humanities and Social Sciences Communications volume 11 , Article number: 501 ( 2024 ) Cite this article
Metrics details
- Science, technology and society
We aim to illustrate the use of generic design principles to attain the integrated promotion of conceptual understanding and science competences in online enquiry-oriented learning environments. Engaging students in the development of competences related to science practices in unison with nurturing rigorous understanding of the mechanisms underpinning phenomena is thought to be valuable both for making science education more relevant to students’ interests and for attaining meaningful learning outcomes. We describe the design and development of a learning environment on the socio-scientific issue of microbial resistance to antibiotics. Our effort is situated in reflective enquiry, a framework for teaching and learning in science. The learning environment seeks to promote argumentation skills and conceptual understanding of evolutionary adaptation, in unison. On the basis of theoretical grounds, we have chosen to work with five design principles, integration of epistemic practices, making evidence-based inferences, competence-oriented design, authentic and relevant context and scaffolding. The first part of the study presents the design principles that have been embedded in the learning environment and the second part provides a description of the learning environment linking the various features to the corresponding design principles. Finally, we discuss the implications of this study for research and teaching practice.
Introduction
The design and development of learning environments with the potential to improve the quality of science learning is an important mechanism for science education reform. In this context, the need to promote the development of competences relating to scientific practice in unison with conceptual understanding has been identified as an important priority (Hazelkorn et al., 2015 ; National Research Council, 2012 ). Researchers have formulated design frameworks and principles based on findings from education, psychology, and learning science. Recently, emphasis has been placed on technology-enhanced or online learning environments due to the inherent advantages of interactivity, monitoring, and feedback (Shi et al., 2021 ; De Jong, 2019 ). The attention of researchers has also turned to research on technology-enhanced or online learning environments that are designed in the framework of an enquiry-oriented learning strategy (Constantinou et al., 2018 ; Cai et al., 2021 ), often in the context of socio-scientific issues (SSI) to enhance relevance to students’ interests and aspirations (Hansson et al., 2011 ; Hernández-Ramos et al., 2021 ). SSIs often relate to value-laden, controversial, socially relevant, real-world problems that are informed by science (Sadler et al., 2007 ) and are constrained by missing knowledge (Chiappetta et al., 1998 ). Engaging students to work with socio-scientific issues can be motivating and challenging because ‘they embed evidence of cognitive and moral dissonance, where scientific evidence, real-time data collection, analysis, interpretation, and investigation become entangled with socio-political decisions, and where a public understanding of science is tantamount to life and death decisions at global, national, regional, and personal levels’ (Zeidler and Sadler, 2023 ).
In this paper, we present the principles employed in designing an online enquiry-oriented learning environment on the topical issue of microbial resistance as an example of a socio-scientific issue. Microbial resistance to antibiotics or anti-microbial resistance has emerged as a challenge of global concern with implications for public health, the safety of medical procedures and institutions, as well as home hygiene routines (Ferri et al., 2017 ; Bloomfield and Ackerley, 2023 ).
The value of this paper relates to how pedagogical principles can be applied in design for learning but also in how they can be used to promote the combined promotion of competencies and conceptual understanding. This approach holds enormous potential in promoting a paradigm shift in science education with substantial improvements in the quality of learning outcomes (Papadouris and Constantinou, 2017 ).
Theoretical background
Social constructivism posits that learner construction of knowledge is attained through social interaction, interpretation, and understanding. Learning emerges through active knowledge construction, which is evaluated through the development of consensus between individuals (Adams, 2006 ).
Consistent with this, enquiry-based teaching and learning is a complex process of sense-making and constructing coherent conceptual models where students approach the study of phenomena by formulating questions, gathering evidence, and investigating to find answers. Through this framework, students collaboratively build new understandings, meanings, and knowledge, communicate their learning to others, and apply their learning productively in unfamiliar situations. Enquiry-based science education engages students in: i) authentic, problem-based learning activities, where there may not be one correct answer; ii) experimental procedures, experiments, and ‘hands-on’ activities, including searching for information; iii) self-regulated learning facilitated by teaching sequences where the emergence of student autonomy is emphasised; and iv) discursive argumentation, negotiation of ideas and communication with peers (‘talking science’) (Hazelkorn et al., 2015 ; Constantinou et al., 2018 ).
Within this paradigm, nurturing the development of competences and coherent conceptual understanding in unison has received special attention. It is a challenging educational goal that is nevertheless thought to be worthwhile in promoting rigorous learning, critical thinking, and creativity (Papadouris et al., 2018 ). Educational standards have come to emphasise competences, such as argumentation, scientific modelling, problem-solving and technological design, as robust educational goals that serve to enculturate a new generation into the values and practices of science and technology (NRC, 2012 ).
To make it possible for educational efforts to respond to the challenges of meeting these goals for rigorous learning, tools in the form of structured learning environments, guidelines for teaching and formative assessment instruments to provide feedback for learning progression are of paramount importance. The formulation of design principles for the development of such educational artefacts can be a useful strategy both for consistency and replication (Quinton, 2010 ).
Design principles for an enquiry-oriented learning environment
In this section, we present the pedagogical tenets that have guided and informed the design of an enquiry-oriented learning environment on ‘Microbial Resistance.’ The tenets utilised in the design were derived from social constructivism and the framework of enquiry-based teaching and learning for the purpose of designing a learning environment that facilitates the attainment of targeted learning outcomes on a topic of socio-scientific interest. Figure 1 presents the theoretical framework and the principles that emerged for the design of the learning environment.
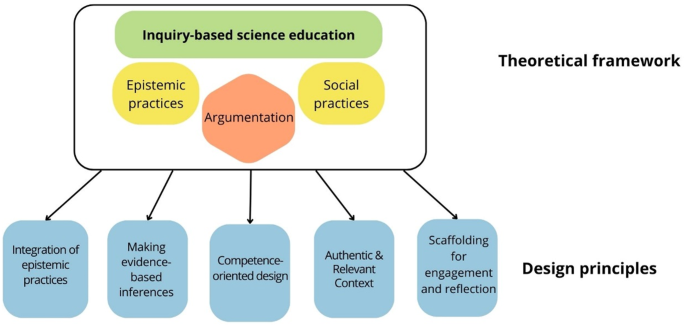
Formulation of theoretically grounded principles for the design of the learning environment.
Effective learning through the integration of epistemic practices
In recent decades, there is increasing emphasis on educational reform that promotes shifts away from the notions of learning as the transmission of knowledge and science as the accumulation of uncontroversial facts. Instead, the science education research community strives to promote and facilitate the study and understanding of science as a human enterprise (Driver et al., 2000 ; NRC, 2000 ). Within this effort, enquiry–based learning has gained substantial support as a learning approach that focuses not only on understanding of content but also on cognitive processes that students engage in during the exploration of a scientific topic thus also promoting the development of scientific practices (Constantinou et al., 2018 ). Scientific practices have been proposed as an integrative set of competences, which emerge as young people engage with active sense-making, investigation, problem-solving and project work with scientific issues of contemporary relevance (Kuhn et al., 2017 ).
Singer et al. ( 2000 ) included enquiry as one of the design frameworks that guided their development of several sets of curriculum materials enhanced with the use of appropriate learning technologies. In our endeavour, we regarded enquiry as the core teaching-learning framework and developed around it by employing design principles that originated from educational psychology and education studies addressing the issue of how people learn by constructing meaning, retaining knowledge and actively using it in co-creation in response to a driving question or a problem-based challenge. Following recent emphasis on the development of learning environments that engage students in authentic enquiry tenets, we expanded our focus to incorporate two other elements of scientific enquiry that are often neglected: epistemic and social practices (Grandy and Duschl, 2007 ; Chinn and Malhotra, 2002 ). Thus, the designed learning environment on Microbial Resistance to Antibiotics entails key aspects of enquiry, such as examining and analysing data, interpreting data, and drawing conclusions, all of which have been integrated under the prominent scientific practice of argumentation (Sandoval and Reiser, 2004 ; Jiménez-Aleixandre and Crujeiras, 2017 ; Sandoval and Millwood, 2007 ; Kolstø and Ratcliffe, 2007 ). A significant part of scientists’ time is devoted to reading other scientists’ communications, building on each other’s work and exchanging well thought and grounded arguments, to support their theories or to evaluate other scientists’ claims (Chinn and Malhotra, 2002 ). The practice of argumentation is a fundamental tenet of scientific enquiry and should also be considered a significant goal in teaching and learning science. Nurturing argumentation competence as an integral part of science instruction is one way of promoting students’ epistemological development (Kuhn, 1991 ; Duschl and Osborne, 2002 ; Iordanou and Constantinou, 2015 ). Argumentation also provides a bridge to enhance the relevance of science learning for resolving issues that concern students’ daily lives. At times of open access to scientific and other information and the widespread use of AI tools, students need to be able to identify and critically evaluate information of relevance to make informed decisions on any issue that concerns them and they also need to have the capacity to communicate those decisions in a clear, understandable and persuasive manner according to the audience (Zeidler, 2014 ; Valladares, 2021 ).
Sandoval and Reiser ( 2004 ) coined a term for an equivalent overarching principle used in the development of their learning environments and scaffolding tools. They referred to their key principle as ‘grounding process in the products’ and, for their learning environments, the focus (product) was scientific explanations. According to Reiser et al., ( 2001 ), while students are grappling with data to construct explanations, they employ various cognitive and social skills that are important for science learning. This principle is also in line with Jonassen’s ( 1999 , p. 222–223) ‘model’ for designing learning environments. At the heart of his model is the case or problem driving the learning, which is subdivided into three important components, context, representation, and manipulative space. The manipulative space of the ‘problem’ is the mindful engagement of the student in manipulating an epistemic artefact, e.g., a simulation (or physical objects) or developing a coherent argument.
Our own efforts sought to establish a view of science as a human construct and this was facilitated by promoting conceptual understanding and the competence of argumentation in unison. In Sandoval and Reiser’s ( 2004 ) terms, the ‘product’ of our study, was the written arguments constructed by the students themselves in an effort to support their claims with valid and sufficient evidence identified from resources provided within the learning environment. As shown in Fig. 1 , IBSE and scientific practices create a space for making connections to the nature of science as part of teaching and learning science. We have emphasised making evidence-based inferences as a crucial element that allows students to develop a sustained interest in science and their competence in making informed decisions.
Making evidence-based inferences
The overt emphasis on ‘ making evidence-based inferences ’ originates from the design principle formulated by Sandoval and Reiser ( 2004 ), which they termed as ‘link evidence to causal claims’. This also relates to the difficulty that students often encounter in grappling with data and in backing their hypotheses/claims with appropriate evidence. Kuhn ( 1991 ) found that students and adults had particular difficulty in coordinating evidence to claims. Most of the participants in her studies supplied pseudo-evidence, which reiterates the theory and thus cannot be accepted as ‘genuine’ evidence. Kuhn’s ( 2010 ) interpretation of the use of pseudo-evidence is that students fail to conceptualise the different epistemological statuses between data and explanation. Sandoval and Reiser ( 2004 , p. 351) also agree with this position stating that students ‘view explanations as being embodied in data’ and consider this view as being impregnated by instruction of science in schools that is based on the ‘over-objectification of data’. Thus, it is imperative for designed curricula and learning environments to include explicit activities and scaffolding that help students overcome those difficulties. Digital learning environments offer an additional advantage when it comes to data due to the multiple data representations that can be supported by technology, aiding students in employing their creativity in interpreting the available evidence (Krajcik et al., 2000 ).
In addition to spotlighting the different epistemological status of data and explanations, which is vital in the construction of causal explanations and cogent arguments, it is also important to include activities that engage students in evaluating data. Using credibility criteria, students can formulate judgements about data sources and about the completeness and validity of the evidence itself (Nicolaidou et al., 2011 ). These types of activities offer guidance to students throughout their investigation in recognising relevant and reliable data.
The creation of learning environments that help students to develop their abilities to reason from evidence and participate in scientific argumentation is recognised as a priority in science education (AAAS, 1993 ; NRC, 1996 , 2001 , 2007 ). According to Duschl and Gitomer ( 1997 ), this involves prioritising competences such as ‘the development of thinking, reasoning, and problem-solving skills to prepare students to participate in the generation and evaluation of scientific knowledge claims, explanations, models, and experimental designs’ (p. 38).
Competence-oriented design
Since the beginning of the XXI century, a concerted effort by many educational researchers has been made to promote argumentation in the science classroom (Driver et al., 2000 ; Duschl and Osborne, 2002 ; Kuhn and Udell, 2003 ). This effort has been promoted from four distinct educational perspectives at the same time: understanding the nature of science (Driver et al., 2000 ; Sandoval and Reiser, 2004 ), engagement in scientific public debates on critical issues for promoting citizenship (Erduran et al., 2004 ), development of cognitive skills (Jiménez-Aleixandre and Erduran, 2007 ) and scientific literacy (Cavagnetto, 2010 ). Argumentation can refer either to written arguments, rhetorical argumentation when someone formulates a line of reasoning to support a claim (argument as a product), or social, dialogical argumentation when two or more people engage in a debate (argument as a process) (Wegerif, 2019 ). These two types of argumentation operate on a similar set of skills (Kuhn, 2005 ; p.113; Driver et al., 2000 ). The practice of argumentation strengthens efforts to develop scientific literacy through active participation in evidence-based communication and also highlights the connections between enquiry-based science and developing an understanding of the nature of science.
Students encounter numerous difficulties while formulating arguments or participating in argumentative discourse. Students’ difficulties include coordinating evidence with claims (Kuhn, 1991 ), focusing on superficial evidence (Zeidler et al., 2009 ), supplying insufficient evidence (Sandoval and Millwood, 2005 ), providing reasoning that does not adequately connect the evidence with the claim (Bell, 2000 ), and handling cognitive overload in argumentative discourse (Kuhn, 2010 ). These research studies demonstrate the complexity of argumentation competence and suggest explicit teaching and extended engagement of students in aspects of argumentation (Kuhn and Udell, 2003 ; Larson et al., 2009 ).
In an effort to support students in garnering their resources to overcome these difficulties, our learning objectives aimed for students to: 1) formulate arguments that integrate elements of Toulmin’s ( 1958 ) model (claim, data, warrant, backing, rebuttal), 2) recognise the different roles of data and warrants, 3) evaluate evidence and elaborate their reasoning with an explicit intent to connect the data with their claims, 4) evaluate arguments constructed by their peers, and 5) refine their arguments based on the received comments.
The adoption of epistemic and social practices as educational goals encouraged us to seek an authentic context for students’ scientific inquiries that would be conducive to placing emphasis on the development of coherent and conceptual models but would also be topical and appreciated as relevant to students’ interests.
Authentic and relevant context: a socioscientific approach
The context of a set of curriculum materials or learning environment is critical for its success. Various researchers have devoted time, effort and space in their work to exemplify the multifaceted role of context. Linn et al., ( 2004 ) treat context under the design principle of ‘making science accessible’. Singer et al., ( 2000 ) include ‘context’ in their design principles and state that curriculum context needs to be meaningful and challenging for the learner. Meyers and Nulty ( 2009 , p.567) claim that students’ learning improves when teaching and learning materials are authentic, real-world and relevant to students’ lives.
The issue, problem, or question guiding the whole learning process should be authentic and adequately ill-structured, thus allowing students to make the associations and connections required for knowledge transfer into unfamiliar and complex real-world problems. Authenticity has a diverse meaning. The somewhat oversimplified and circumscribed meaning sometimes ascribed to authentic is a personally relevant or interesting topic. The more widely accepted definition refers to activities that engage students ‘on the same type of cognitive challenges as those in the real world’ (Jonassen, 1999 , p. 221). The essence is that science thinking and co-creation should be situated in real, important, and complex issues that are at the centre of attention and are meaningful to students. Nonetheless, the transfer of real-world, scientifically-based problems into the classroom requires adaptation to make them suitable for the students. Adaptation is the modification of the entire content (e.g. enclosed information, data representation, and methods) to match the cognitive level and prior knowledge of the students (Crawford, 2012 ) and to support constructive pathways for sense-making and co-creation. Building on students’ existing knowledge, ideas and experiences is not a new construct; it has been around for a long time, but it continues to receive significant attention as an important priority in design guidelines (Driver, 1989 ; Krajcik et al., 2000 ).
Contextualising a learning material or environment with a problem that is personally relevant to students encourages engagement and motivation (Jonassen, 1999 ; Herrington et al., 2004 ). Edelson, Gordin and Pea ( 1999 ) recognise student motivation (content-focused motivation) as the first that learning materials’ designers should address by creating legitimate interest. Students’ interaction with a learning environment or curriculum could be further enhanced by framing it with a pedagogical scenario. For example, in Kyza et al., ( 2011 ) a scenario was used to increase the appeal of the investigation. So, after students completed their investigation and had constructed their product (explanation), they had to communicate their findings on the issue of what caused the sudden death of a large number of flamingos in a Salt Lake , by writing a final report for use by the Fisheries Department. Similarly, in mission-driven learning environments, students, acting as scientists, are scaffolded to address a socio-scientific issue using evidence and tools for processing that evidence (De Jong et al., 2012 ). Using a pedagogical scenario strengthens the connections that students make with socio-scientific issues and creates a sense of ownership for their learning as a mission.
Scaffolding for engagement and reflection
Scaffolding , at its core, is any process that guides and assists a learner to activate their cognitive and epistemological resources and to exert the perseverance needed to overcome difficulties that make the completion of a task unattainable (Quintana et al., 2004 ). Providing appropriate scaffolding is integral to the design of learning materials and relies on design principles such as making thinking visible and offering tools for co-ordinating theory with evidence. Education reform can be advanced significantly by the development of learning materials that are challenging and engaging and also require students to employ substantive content knowledge, higher-order skills and processes. In this effort, it becomes imperative to anticipate student difficulties and plan for interactive resources in advance, on how to resolve them.
Scaffolding is separated into two types depending on ‘who’ is the source of the assistance provided to the students: teacher (or peer)-enhanced scaffolding and technology-enhanced scaffolding. It is also possible to blend combinations of the two in a learning intervention. Teacher (or peer)-enhanced scaffolding taking place face-to-face or online is concerned with the different approaches of human collaboration that may support students in accomplishing the task at hand. Technology-enhanced scaffolding can take various forms depending on the design purpose. Examples of scaffolding (software) tools include maps, graphic representations, reminders, prompts, templates and reflection tools. Despite the differences between the two types of scaffolding, they share the same underlying principle, which involves nudging students to activate their resources and bringing them to bear on the task at hand as well as helping students overcome learning impasses and reach goals that they never thought possible (Quintana et al., 2004 ; Lund, 2004 ). Raes et al. ( 2012 ) investigated the effect of different types of scaffolding in a web-based learning project and their findings support combined scaffolding as the approach that facilitates both knowledge acquisition and development of metacognitive skills. However, the challenge when designing scaffolding is to find the balance between under- and over-scaffolding; it is important for students to have opportunities to learn through failure, to have the required support in order not to feel incompetent, and also to work with gradually faded scaffolding in a way that enhances emergent autonomy (Guzdial, 1994 ).
In our study, we employed the framework for designing scaffolding that was proposed by Quintana et al., 2004 , p. 341). The framework revolves around three processes: sense-making (basic operations for testing hypothesis and interpreting data), process management (strategic decisions involved in controlling the enquiry process), and articulation and reflection (process of constructing, evaluating and articulating what has been learned). Specifically, emphasis was given to creating opportunities for students to externalise their thinking and reflect on what they have been working on, reducing the complexity of tasks or concepts and social scaffolds to support constructive peer-collaboration. Collaborative learning implicates students in cognitive activities, such as explanation and argumentation, in their attempt to co-construct knowledge, develop consensus, and co-create (Dillenbourg, 1999 ).
Design methodology
The iterative methodology we used in the design and development of the learning environment, consists of a series of processes shown in Fig. 2 (Papadouris and Constantinou, 2009 ). The first process includes the formulation of learning objectives. These need to be a) consistent with students’ conceptual and cognitive resources, and b) aligned with the conceptualisation of what is involved in learning in science. The second process draws on empirical investigations of students’ initial understandings and difficulties regarding the specified learning objectives. The third process focuses on the development of the teaching/learning sequence, largely drawing on inputs from the previous two processes. The last process, which is also empirical in nature, includes the enactment of the designed teaching/learning sequence in classroom settings and the collection of data on students’ learning outcomes. The findings from this field-testing are fed back to the design and development process so as to undertake refinements to the teaching design. This last process is vitally important - it allows for understanding how the designed ‘learning materials’ actually perform in the context in which they are intended.
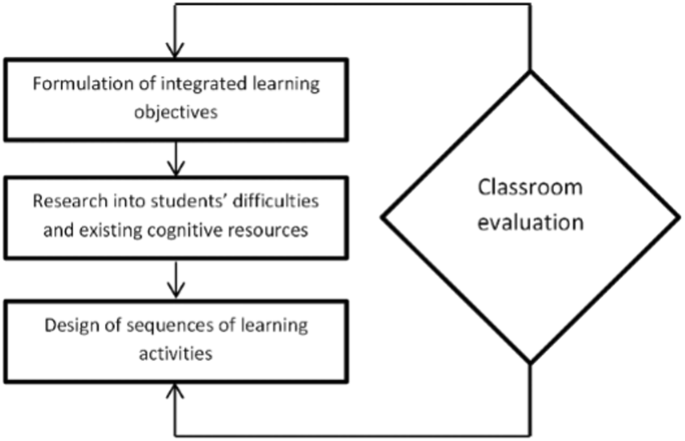
The methodology employed in the design and development of the learning environment.
The designed learning environment on ‘Microbial Resistance to Antibiotics’.
Target audience
The microbial resistance learning environment is designed to address the needs of high school students. The phenomenon of microbial resistance is quite complex, drawing from different domains of biology, including physiology, immunology and microbiology, population genetics as well as chemistry. However, the learning environment could be adapted to a different age range by varying the breadth and depth of understanding that students are expected to attain. The designed learning environment is also suitable for use in teacher education. Pre-service teachers benefit additionally from such exposure by identifying and understanding the integration of innovative features in the design.
Addressing the principle of ‘Science in Context’
The design principle of ‘ science in context ’ was employed by framing the entire environment within the topical SSI of antimicrobial resistance. SSIs have been extensively used for contextualising learning environments. The prominent position they hold in science education is due to the suitable frames they offer for promoting scientific literacy for active citizenship (Hazelkorn et al., 2015 ). Working with SSIs presents a number of affordances for students. Firstly, making informed decisions on relevant issues is grounded in scientific evidence and understanding. Secondly, SSIs have a local dimension, which helps in relating to students’ experiences but also extends beyond local, to national and global levels. Furthermore, SSIs provide a context for engaging students in cost-benefit analysis also requiring from them to bring together ideas from different domains, such as science, politics and economics, (Tal et al., 2011 ). Finally, the moral dimensions of these issues motivate students to engage in constructive discourse and enhance their need for augmenting their content knowledge (Zeidler and Nichols, 2009 ). Therefore, SSIs constitute an effective context for engaging students and keeping them motivated for the extended period of time needed for robust knowledge construction. Several educational researchers have reported that students engaging in discourse around SSIs show improved conceptual understanding (Klosterman and Sadler, 2010 ; Sadler, 2011 ; Theobald et al., 2015 ), informal reasoning (Sadler and Zeidler, 2005 ), reflective judgement (Zeidler et al., 2009 ; Liu et al., 2015 ), and argumentation skills (Jimenez-Aleixandre, 2002 ; Zohar and Nemet, 2002 ).
Sadler ( 2009 ; p.13), also recommends SSIs ‘because they provide opportunities for students and teachers to engage with science in meaningful and relevant ways’ and offer opportunities for students to understand and appreciate ‘how science affects their lives and the lives of others’ (p. 15).
The context topic of microbial resistance
Microbial resistance is the resistance of microorganisms to antimicrobial drugs that were originally effective for treating infections caused by them (WHO, 2014 ). However, the term is used more commonly to describe or refer to bacteria resistant to antibiotics. Resistant bacteria are created by random mutations or when resistant traits are exchanged between bacteria. In an antibiotic-rich environment, resistant bacteria are favoured by natural selection and thrive over time. Therefore, misuse and overuse of antibiotics and general antimicrobial agents create an environment that helps resistant microbes to emerge and prevail over sensitive ones. There is overwhelming evidence attesting to increasing use of antibiotics, including through over-prescription and overconsumption, leading to an increasing prevalence of resistant bugs (Morrison and Zembower, 2020 ; van de Sande-Bruinsma et al., 2008 ). Overconsumption and misuse of antimicrobials by the general public are due to false beliefs regarding infections and antimicrobial agents. Thus, a vital step toward the containment of microbial resistance is education (Sosa et al., 2010 ; WHO, 2001 ). Part of educating the lay public is educating students to be in a position to make informed decisions about the use of antibiotics.
Despite the fact that microbial resistance attracts significant attention in the health industry, often due to persistent strains of resistant bacteria turning up in hospitals, a substantial percentage of the general public is unaware of this issue. It is important that students are informed and helped to understand the need to safeguard against infectious disease and to appreciate the importance of efforts extend the longevity of existing antibiotics and discover new ones.
Addressing the principles of integrating epistemic and social practices
In our learning environment students were engaged in rhetorical argumentation. Working in groups for the full duration of the intervention, they had to co-construct valid arguments supporting their standpoints. Argumentation entails the ability to formulate and evaluate claims. In the intervention, students first learn how to construct sound arguments and after they have accrued the required knowledge and skills, they proceed to evaluate arguments constructed by their peers. The learning objectives and the pacing of activities for the development of argumentation skills emerged by combining theoretical knowledge on formulating and evaluating arguments and associate students’ difficulties as presented in the research literature.
The learning environment examines the complex SSI of microbial resistance and aims to successfully combine and promote the development of a) argumentation skills and b) conceptual understanding of concepts pertaining to the topic of microbial resistance.
Conceptually, our main priority was for students to acknowledge microbial resistance as a natural selection process and develop understanding of the basic evolution mechanisms. Evolution is a core idea in the Life Sciences. Students have great difficulty understanding both evolution (Neubrand and Harms, 2017 , Archila and Molina, 2020 ) and microbial resistance (Fonseca et al., 2012 ; Richard et al., 2017 ). The ‘example’ of microbial resistance is conducive to promoting students’ understanding due to the fast generation times of bacteria and the relevance of the SSI to public health. The implementation of the design principles in the learning environment Microbial Resistance to Antibiotics is shown in Table 1 .
Apart from evolution, for students to develop a coherent conception of microbial resistance, it was important to differentiate between the main types of microbes causing infections, to recognise the important and beneficial role of certain bacteria, viruses (eg. Bacteriophage) and fungi, to understand the human body’s defence mechanisms (immune system), and the main types of microbial medication available for fighting infections. The learning environment included information and structured activities on all these issues.
Description of the learning environment
The microbial resistance learning environment was developed in STOCHASMOS (Kyza and Constantinou, 2007 ), a web-based platform designed to promote enquiry-based learning. The STOCHASMOS platform is organised into two main parts: the Inquiry Environment and the Reflective Workspace. The Inquiry Environment contains all the information that students are given access to in relation to the issue/problem under study. In the Reflective Workspace the designers create templates, which help students gather and synthesise important information to attain the learning objectives. The Reflective Workspace is used by the students as a co-creation environment to collaboratively develop their project in response to the driving question or the mission declared from the start. Following is a description of the Inquiry Environment and Reflective Workspace with reference to the design principles presented in the first section of this paper.
Our first design principle has been embodied in the learning environment by scaffolding the development of conceptual understanding and argumentation competence in unison. Typically, scientific literacy in schools is restricted to scientific ideas and concepts which are presented as separable and unambiguous facts, stripped from their context, often as the work of a single individual. Argumentation activities engage students in collaborative discourse and constructive criticism, during the consensus-building process, which resembles the practices scientists follow for the justification and verification of scientific knowledge. Contemporary views of science learning manifest the integration of conceptual understanding with other significant components of science learning, such as argumentation. The following paragraphs exemplify the way conceptual understanding and argumentation were integrated into the Inquiry Environment, as well as the remaining design principles presented in the first section, through the unfolding of the learning environment.
The first dimension Taking a Role (Fig. 3 ) of the Inquiry Environment introduces the microbial resistance issue through newspaper clippings and presents the students’ mission, which is to co-design communication products (posters, brochures and short videos) aiming to inform the lay public and stakeholders (doctors, pharmacists, nursing and other medical personnel) on the issue of microbial resistance. In addition to contextualising the learning environment with the SSI of microbial resistance, a scenario was used to enhance students’ motivation. In the framing scenario, students undertake the role of employees at a communication firm, who work in design teams to develop an awareness campaign to raise public awareness and engage health professionals in taking a more active role in pre-empting the issue of microbial resistance and mitigating its implications for public health. The students’ mission was formulated to facilitate co-creation and to maintain their interest for the extended period of time required to attain understanding of microbial resistance and to learn to formulate robust and well-supported arguments.
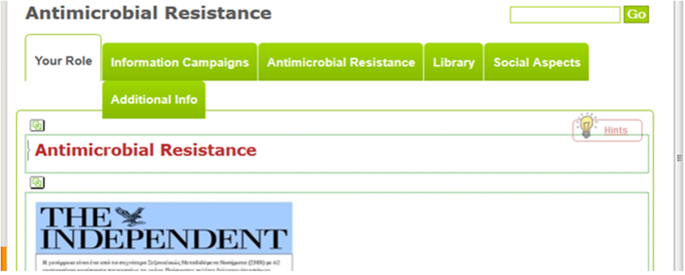
Image of the online Inquiry Environment on Microbial Resistance.
The second dimension, ‘ Information Campaigns ’, includes guidelines that help students develop an effective awareness campaign. Particular emphasis was placed on how to adapt their approach and content according to the target audience: lay public and stakeholders, including health professionals. This dimension also incorporates explicit teaching/learning activities for cultivating argumentation skills.
The ‘ Microbial Resistance ’ dimension scaffolds students to build an initial understanding of the issue of microbial resistance and to recognise the need for raising public awareness, thus giving ‘true’ purpose and meaning to the mission. The importance of this major public health issue is manifest through the presentation of authentic evidence, including data from public health monitoring websites showing the increasing detection of antibiotic resistant microbes around the world, interviews from the scientific community, patients and the general public. After students have covered the argumentation explicit activities, they are in a better position to recognise, appreciate and assess the value of various types of evidence portrayed under this section and the rest of the learning environment. Students have to evaluate the evidence while in the process of formulating their final arguments that underpin their campaign messages.
The ‘ Library ’ dimension encloses all the background information needed by the students to develop deep understanding of the biological phenomenon of microbial resistance. The Library covers the following concepts: microorganisms and infections, the immune system, evolution and natural selection, and the function of antibiotics. The scope of this dimension is not to get students to develop deep conceptual understanding on each of the individual concepts covered in the various sections (sub-tabs) of this dimension. Instead, the Library provides a more elaborative overview of these concepts to assist students in formulating a complete picture of microbial resistance and select what information is more relevant for their mission and delve more deeply into it. This dimension interweaves conceptual understanding and argumentation skills by providing students with biological facts that can be employed as pieces of data (evidence) in their arguments. More significantly, students develop their reasoning by conceptualising the causal mechanisms that create and feed the predominance of resistant bacteria. To scientifically warrant their claims, students have to come to terms with fundamental concepts covered in the Library.
The fifth dimension, ‘ Social Aspects ’, focuses on various erroneous behaviours of both target groups (lay public and public health stakeholders) at a national and international level, such as antibiotic consumption for the production of food products, over-prescription, overconsumption and inappropriate storage of antibiotics. The dimension also contains real data in diverse formats (e.g. text, graphs, tables) that illustrate the increasing trends in antibiotic consumption. Students are encouraged to use the platform tools to manipulate and interpret the supplied data to reach conclusions regarding a range of issues in relation to microbial resistance, such as exploring connections between the increasing trend in antibiotic consumption and the increasing percentages of multi-resistant bacteria, or other social and economic ramifications of microbial resistance. The final section (sub-tab) of this dimension is entitled ‘ Science and Technology ’. Through the design, development and the mass production stages of antibiotic development, students come to realise the interconnection and interrelationships between scientific research and technological development.
The learning environment is designed in such a way that students can go back and forth between the various dimensions and cross-reference their working hypotheses and their arguments as they are being developed. By undertaking the mission to develop an awareness campaign students are encouraged to work through the first three dimensions in an almost sequential manner. This is supported by the fact that the dimensions have been sequenced in a way that responds to the challenge at hand: what are the main steps in developing an effective campaign? Why is an awareness campaign on microbial resistance necessary? What arguments can be developed to support the information that will go out to different stakeholder groups? Normally, the subsection enclosing the argumentation activities is not made available to students until they have covered the introductory section on ‘ Microbial Resistance ’. This is intended to safeguard that students have developed a grasp of the basic ideas before they engage with the process of formulating claims and evaluating what evidence is relevant to their claims and how can it be used to support each claim.
Regarding the ‘Library ’ dimension, students become engaged in collaborative activities that cover every section before moving to the next. The intended purpose is for students to grapple with concepts in a sequence that facilitates understanding and also encourages connections. For example, students can better understand the defence mechanisms our immune system has in place for the different ‘enemies’, after they learn to identify a few key characteristics of the various types of microbes. After students have covered the ‘ Library ’ dimension and have gained information on how to formulate structurally complete (based on Toulmin’s model) and valid arguments, they move freely back and forth guided by their mission, in efforts to construct concise and robust arguments for their awareness campaigns. An overview of the activity sequence for the entire learning environment is presented in Fig. 4 .
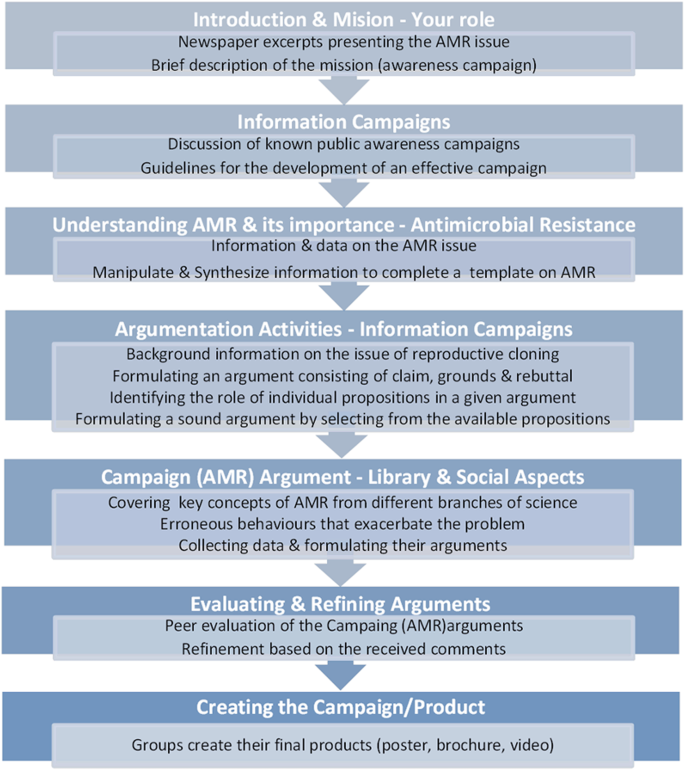
Activity Sequence embedded in the Learning Environment.
Argumentation activities
To nurture the argumentation competence, we designed an initial set of activities for explicit teaching of the structural features and quality characteristics of an argument, followed by exploratory enquiry activities with embedded peer-assessment. To encourage students to appreciate the value of ‘ making evidence-based inferences ’, we included multiple opportunities to discuss the distinct epistemological features of evidence and explanations (data and warrants using Toulmin’s terminology) and to formulate or review evidence-based arguments. We also discussed explicitly the ‘value’ of internal consistency in the reasoning that connects evidence to claim.
The argumentation activities also place emphasis on structure, following Toulmin’s model (1958), as well as the quality of the argument, by stressing the relative value of various types of evidence, the need for scientifically accepted explanations to link evidence to claim, and the coherence and cohesion of the argument as a whole.
Students are assessed and given feedback on the different elements of a robust argument and the quality aspects using a different SSI, that of reproductive cloning. The aim is for students to attempt transfer of what they have learned from the context of cloning to the context of cloning to the context of microbial resistance. It is important to stress here that the information on reproductive cloning were made available to students through the Inquiry Environment but the first two activities were developed in the Reflective Workspace of the STOCHAMOS platform.
The introductory activity was the formulation of an argument including all the essential/main structural components (claim, data, warrant), as well as rebuttal. Students studied all the relevant information and watched two short documentaries on the subject of cloning and then had to complete a template where the questions guided them to state their claim and provide evidence supporting it. Following this, students had to think as someone holding an opposing position and provide supporting evidence and then to challenge those evidence. The second activity was based on a template providing an argument against reproductive cloning and students were asked to identify the function/role of the individual propositions. The final activity was prompting students to formulate the strongest argument possible by selecting from the available choices of individual propositions. For each structural component, students were given two options and according to their previous selection, one of the propositions was qualitatively stronger. After completing the task students had to compare their arguments with the one formulated according to the teacher’s selection and discuss advantages and disadvantages of their choices. Once students had completed those argumentation activities, explaining explicitly a robust argument’s structure and content, they had to construct their own sound argument on the issue of microbial resistance and evaluate an argument created by their peers.
Scaffolding used in the learning environment
Inquiry environment scaffolding.
To support students in their endeavour in the Inquiry Environment two scaffolding tools embedded in STOCHASMOS platform were utilised, hints and glossary. The glossary helps students to sustain focus in their activities without being distracted by difficult terminology. When a term has been entered in the glossary database of the environment, the word appears in the text with a green colour and upon mouseover the definition appears on a pop-up window. Hints were provided in the learning environment for two purposes: a) to direct students’ attention on specific sections that were important for completing their mission and b) to provide explanations for concepts or phenomena. For example, while students were working on the argumentation activities on the provided information, the terms gene and genome emerged. Genetic concepts are usually confusing to students (Smith et al., 2008 ) and contrasting the two terms might enable students to distinguish them. Moreover, different representation formats were employed to support students; particularly useful was the embedment of multimedia representations. In biology where we are dealing with abstract concepts and phenomena it is important to connect verbal description with a picture, or even better, with a multimedia representation. According to Yarden and Yarden ( 2013 , p. 95) multimedia representations ‘are most likely to lead to meaningful learning’.
In the learning environment, a blended approach was used regarding scaffolding. Hence software scaffolding co-existed with teacher-enhanced scaffolding or human support (Lund, 2004 ). In pre-determined points of the Inquiry Environment, students were directed to discuss with one of the members of the teaching staff. The interaction of the staff with the students was based on the Socratic method. During the semi-Socratic discussions, students acquired knowledge was used to advance their understanding. Students’ engagement with this type of discourse, where they are not given answers but are guided through questions to reach to the next level of understanding, helps them process information and improves their thinking and reasoning skills.
Workspace scaffolding
All the environments hosted in the STOCHASMOS platform incorporate the Reflective Workspace Environment. In the Workspace, using pre-designed templates, students gather and synthesise data from the Inquiry Environment. The templates provide scaffolding to students in selecting appropriate data and to synthesise them according to the requirements of the task. In the Microbial Resistance Learning environment, six template pages were created and used by the students; three pages were devoted to argumentation, two pages to microbial resistance and one page was for the construction of the awareness campaign.
The argumentation template pages are sequencing the development of argumentation skills (see 3.3.4. Argumentation Activities). The first template guides students in a step-by-step process to formulate arguments comprising the main elements of Toulmin’s model. The second template support students in comprehending the different role and epistemology of the main elements and the final template engages students in peer-discourse and teacher-discourse, in their groups, by juxtaposing two arguments, one formulated by them and the other by the teacher. This template examines both vital aspects in constructing robust arguments, structure and quality of the individual elements. In all three template pages there are discussions taking place to improve students’ epistemologies of what counts as good evidence (data) and good explanations (warrants).
Regarding the microbial resistance templates, these focused on enhancing students’ understanding on microbial resistance as a SSI and as a biological phenomenon based on natural selection. The last template supported students in formulating the argument that would constitute their awareness campaign. The template helped students to collect and organise their data and then to formulate an argument congruent to Toulmin’s model. This template was also used to scaffold peer-discourse between the groups. The groups were paired and exchanged their templates. Students had to evaluate each other’s arguments based on three criteria: structure, content and language adaptation according to the target audience, and quality of the content. Criteria were given to students in a checklist format to help them provide their peers with constructive feedback.
The Reflective Workspace environment was designed to scaffold ongoing reflection. When learning materials are contextualised with complex authentic problems, students need additional support to meet our expectations. Students need support to collect and organise data, to synthesise findings, and to determine how to proceed. By articulating their thinking in the pre-designed templates, students reflect on their progress; what has been understood and what needs revising. Reflection was also supported by the structured discussions, organised around the templates, with the teacher.
This study focused on the design and development of an online enquiry-based environment on the topical socio-scientific issue of microbial resistance to antibiotics. Emphasis was given on the design principles embedded in the learning environment which emerged from research focusing on promoting enquiry learning in order to change the way science is taught in schools. This study exemplified the following design principles: science in context , integration of epistemic practices , evidence based-inferences, competence-oriented design and scaffolding for student engagement and reflection . These design principles were adopted and adapted from existing literature in designing curriculum materials and learning environments in the subject of science.
The way the design principles have been presented at the beginning of the article seeks to provide, aspiring designers of learning materials, with basic tenets that could be modified to fit their purposes. Hence, the description of each tenet starts by revealing the reasons behind the selection, what we were trying to accomplish and why this is important regarding our knowledge on how students learn and the challenges (barriers) that impede their learning. Following this, there is an overview of how it was exemplified in the learning environment. In the description of the learning environment it becomes more apparent how each principle was adapted and applied in the design. Therefore, one of the aims of the article is to support teachers and other educators that undertake the task of designing activities and learning sequences to elicit certain features that would benefit their teaching practice and also to reflect on their own designs and how to evaluate them.
Furthermore, it is important to stress here the significant role the enactment of the learning material and the school and classroom settings have in the achievement of the learning outcomes. Enquiry-based learning needs to be also facilitated by the classroom culture. Students need to learn to collaborate, to exchange ideas and to construct on each other’s knowledge. To transform classroom culture demands a radical change in the role the teacher adopts in the classroom and the teaching approaches. The teacher needs to act as a guide, as a facilitator and as an equal member of the learning community of the classroom. Hence, reformed learning materials have to be accompanied by reformed classroom cultures (Reiser et al., 2001 ).
Regarding educational research, the article describes development of a learning environment that has innovative features and utilises a SSI that is at the forefront of the interests of the scientific community and public health policymakers and deserves wider public attention. The SSI of antimicrobial resistance, to our knowledge, has not been used as a context in a digital science learning environment. Examples of other socio-scientific topics that have been employed in the past as contexts for learning environments include, cloning, genetically modified organisms, global warming and other environmental and ecological issues. Furthermore, following the designed principles presented here, we developed an environment that is in accordance with the conceptual framework proposed by the National Research Council Committee (NRC, 2012 ). The proposed framework comprises three dimensions, practices, cross-cutting concepts and core ideas in the science disciplines that need to be woven together to facilitate students’ learning (NRC, 2012 , p. 29). From the major practices included in the NRC report, the microbial resistance learning environment promotes the development of the argumentation competence. Also, in the context of microbial resistance, students cover the core idea of evolutionary adaptation of microbes. The combination of formulating arguments and the framing issue of microbial resistance engage students in exploring causal relationships, which is one of the crosscutting concepts identified in current educational policy priorities.
Data availability
No data were generated or analysed for the purposes of the work reported in this article. The article describes the principles for creating a digital learning environment and how they were enacted in a platform for supporting reflective enquiry in science. Hence data sharing is not applicable to this research.
Adams P (2006) Exploring social constructivism: theories and practicalities. Education 34(3):243–257. https://doi.org/10.1080/03004270600898893
Article Google Scholar
American Association for the Advancement of Science (AAAS) (1993) Project 2061: benchmarks for science literacy. New York: Oxford University Press. http://www.project2061.org/publications/bsl/online/index.php
Archila PA, Molina J (2020) Evolution and creationism: views of students in a Colombian university—findings from 7 years of data using a three-question survey. Res Sci Educ 50(4):1619–1638. https://doi.org/10.1007/s11165-018-9746-3
Bell P (2000) Scientific arguments as learning artifacts: designing for learning from the web with KIE. Int J Sci Educ 22(8):797–817. https://doi.org/10.1080/095006900412284
Bloomfield SF, Ackerley LM (2023) Developing resilience against the threat of infectious diseases and anti-microbial resistance: putting targeted hygiene into practice in home and everyday lives. Public Health Pract, 100362. https://doi.org/10.1016/j.puhip.2023.100362
Cai S, Niu X, Wen Y, Li J (2021) Interaction analysis of teachers and students in inquiry class learning based on augmented reality by iFIAS and LSA. Interact Learn Environ 31(9):5551–5567. https://doi.org/10.1080/10494820.2021.2012808
Cavagnetto AR (2010) Argument to foster scientific literacy a review of argument interventions in K–12 science contexts. Rev Educ Res 80(3):336–371. https://doi.org/10.3102/0034654310376953
Chiappetta E, Koballa T, Collette A (1998) Science instruction in the middle and secondary schools, 4th ed Upper Saddle River, NJ: Merrill
Chinn CA, Malhotra BA (2002) Epistemologically authentic inquiry in schools: a theoretical framework for evaluating inquiry tasks. Sci Educ 86(2):175–218. https://doi.org/10.1002/sce.10001
Constantinou CP, Tsivitanidou OE, Rybska E (2018) What is inquiry-based science teaching and learning? In: Constantinou CP, Tsivitanidou O, Gray P, Rybska E, Louca L (eds) Professional development for inquiry-based science teaching and learning, Springer International Publishing, p 1–23
Crawford BA (2012) Moving the essence of inquiry into the classroom: engaging teachers and students in authentic science. In: Tan KCD, Kim M (eds) Issues and challenges in science education research moving forward, Springer Netherlands, p 25–42. https://doi.org/10.1007/978-94-007-3980-2_13
Dillenbourg P (1999) What do you mean by collaborative learning? In: Dillenbourg P (ed) Collaborative-learning: cognitive and computational approaches, Elsevier, Oxford, p 1–19
Driver R (1989) Students’ conceptions and the learning of science. Int J Sci Educ 11(5):481–490. https://doi.org/10.1080/0950069890110501
Driver R, Newton P, Osborne J (2000) Establishing the norms of scientific argumentation in classrooms Sci Educ 84(3):287–312. https://doi.org/10.1002/%SICI%1098-237X%200005%84%3A33.0.CO;2-A
Duschl RA, Gitomer DH (1997) Strategies and challenges to changing the focus of assessment and instruction in science classrooms. Educ Assess 4(1):37–73. https://doi.org/10.1207/s15326977ea0401_2
Duschl RA, Osborne J (2002) Supporting and promoting argumentation discourse in science education. Stud Sci Educ 38(1):39–72. https://doi.org/10.1080/03057260208560187
De Jong T (2019) Moving towards engaged learning in STEM domains; there is no simple answer, but clearly a road ahead. J Comput Assist Learn 35(2):153–167. https://doi.org/10.1111/jcal.12337
De Jong T, Weinberger A, Girault I, Kluge A, Lazonder AW, Pedaste M, Zacharia ZC (2012) Using scenarios to design complex technology-enhanced learning environments. Educ Technol Res. Dev 60(5):883–901. https://doi.org/10.1007/s11423-012-9258-1
Edelson DC, Gordin DN, Pea RD (1999) Addressing the challenges of inquiry-based learning through technology and curriculum design. J Learn Sci 8(3-4):391–450. https://doi.org/10.1080/10508406.1999.9672075
Erduran S, Simon S, Osborne J (2004) TAPping into argumentation: developments in the application for studying science of Toulmin’s Argument Pattern for studying science discourse. Sci Educ 88(6):915–933. https://doi.org/10.1002/sce.20012
Ferri M, Ranucci E, Romagnoli P, Giaccone V (2017) Antimicrobial resistance: a global emerging threat to public health systems. Crit Rev Food Sci Nutr 57(13):2857–2876. https://doi.org/10.1080/10408398.2015.1077192
Article CAS PubMed Google Scholar
Fonseca MJ, Santos CL, Costa P, Lencastre L, Tavares F (2012) Increasing awareness about antibiotic use and resistance: a hands-on project for high school students. PLoS One 7(9):e44699. https://doi.org/10.1371/journal.pone.0044699
Article ADS PubMed PubMed Central Google Scholar
Grandy R, Duschl RA (2007) Reconsidering the character and role of inquiry in school science: analysis of a conference. Sci Educ 16(2):141–166. https://doi.org/10.1007/s11191-005-2865-z
Guzdial M (1994) Software‐realized scaffolding to facilitate programming for science learning. Interact Learn Environ 4(1):001–044. https://doi.org/10.1080/1049482940040101
Hansson L, Redfors A, Rosberg M (2011) Students’ socio-scientific reasoning in an astrobiological context during work with a digital learning environment. J Sci Educ Technol 20:388–402. https://doi.org/10.1007/s10956-010-9260-5
Hazelkorn E, Ryan Ch, Beernaert Y, Constantinou CP, Deca L, Grangeat M, Karikorpi M, Lazoudis A, Pintó Casulleras R, Welzel-Breuer, M (2015) Science education for responsible citizenship, directorate-general for research and innovation, science with and for society, European Commission, EUR 26893, Brussels, Belgium. https://doi.org/10.2777/12626
Hernández-Ramos J, Pernaa J, Cáceres-Jensen L, Rodríguez-Becerra J (2021) The effects of using socio-scientific issues and technology in problem-based learning: a systematic review. Educ Sci 11(10):640. https://doi.org/10.3390/educsci11100640
Herrington J, Reeves TC, Oliver R, Woo Y (2004) Designing authentic activities in web-based courses. J Comput High Educ 16(1):3–29. https://doi.org/10.1007/BF02960280
Iordanou K, Constantinou CP (2015) Supporting use of evidence in argumentation through practice in argumentation and reflection in the context of SOCRATES learning environment. Sci Educ 99(2):282–311. https://doi.org/10.1002/sce.21152
Jimenez-Aleixandre M (2002) Knowledge producers or knowledge consumers? Argumentation and decision-making about environmental management. Int J Sci Educ 24(11):1171–1190. https://doi.org/10.1080/09500690210134857
Jiménez-Aleixandre MP, Crujeiras B (2017) Epistemic practices and scientific practices in science education. In: Taber KS, Akpan B (eds), Science education: an international course companion, Rotterdam: The Netherlands: Sense Publishers, p 69–80
Jiménez-Aleixandre MP, Erduran S (2007) Argumentation in science education: an overview. In: Jiménez-Aleixandre MP, Erduran S (eds) Argumentation in science education: perspectives from classroom-based research, Dordrecht, Netherlands: Springer, p 3–27
Jonassen DH (1999) Designing constructivist learning environments. Instructional design theories and models: a new paradigm of instructional theory, Vol. II, Mahwah, NJ: Lawrence Erlbaum Associates, p 215–239
Klosterman ML, Sadler TD (2010) Multi‐level assessment of scientific content knowledge gains associated with socioscientific issues‐based instruction. Int J Sci Educ 32(8):1017–1043. https://doi.org/10.1080/09500690902894512
Kolstø SD, Ratcliffe M (2007) Social aspects of argumentation. In: Erduran S, Jiménez-Aleixandre MP (eds) Argumentation in science education: perspectives from classroom-based research, Dordrecht: Springer Netherlands, p 117–136
Krajcik J, Blumenfeld P, Marx R, Soloway E (2000) Instructional, curricular, and technological supports for inquiry in science classrooms. In: Minstell J, Van Zee E, (eds) Inquiry into inquiry: science learning and teaching, American Association for the Advancement of Science Press, Washington, D.C., p 283–315
Kuhn D (1991) The skills of argument. Cambridge University Press
Kuhn D (2005) Education for thinking. Harvard University Press
Kuhn D (2010) Teaching and learning science as argument. Sci Educ 94:810–824. https://doi.org/10.1002/sce.20395
Kuhn D, Arvidsson TS, Lesperance R, Corprew R (2017) Can engaging in science practices promote deep understanding of them? Sci Educ 101(2):232–250. https://doi.org/10.1002/sce.21263
Kuhn D, Udell W (2003) The development of argument skills. Child Dev 74(5):1245–1260. https://doi.org/10.1111/1467-8624.00605
Article PubMed Google Scholar
Kyza EA, Constantinou CP (2007) STOCHASMOS: a web-based platform for reflective, inquiry-based teaching and learning [s/w]. Cyprus: LSG. Retrieved from http://www.stochasmos.org/media/Public%20website/STOCHASMOSManual_English_version.pdf . ( http://www.webcitation.org/6W6kRZGzn )
Kyza EA, Constantinou CP, Spanoudis G (2011) Sixth graders’ co-construction of explanations of a disturbance in an ecosystem: exploring relationships between grouping, reflective scaffolding, and evidence-based explanations. Int J Sci Educ 33(18):2489–2525. https://doi.org/10.1080/09500693.2010.550951
Larson AA, Britt MA, Kurby CA (2009) Improving students’ evaluation of informal arguments. J Exp Educ 77(4):339–366. https://doi.org/10.3200/JEXE.77.4.339-366
Article PubMed PubMed Central Google Scholar
Linn MC, Davies EA, Eylon B-S (2004) The scaffolded knowledge integration framework for instruction, In: Linn MC, Davies EA, Bell P (eds) Internet environments for science education, Mahwah, NJ: Lawrence Erlbaum Associates. p 47–72
Liu S, Roehrig G, Bhattacharya D, Varma K (2015) In-service teachers’ attitudes, knowledge and classroom teaching of global climate change. Sci Educ 24(1):12–22. https://digitalcommons.unl.edu/cgi/viewcontent.cgi?article=2015&context=natrespapers Papers in Natural Resources. 1000. Retrieved from Accessed 23 Oct 2023
Google Scholar
Lund K (2004) Human support in CSCL. In: Strijbos JW, Kirschner PA, Martens RL (eds) What we know about CSCL. Computer-supported collaborative learning series, vol 3. Springer, Dordrecht, p 245–259. https://doi.org/10.1007/1-4020-7921-4_7
Meyers NM, Nulty DD (2009) How to use (five) curriculum design principles to align authentic learning environments, assessment, students’ approaches to thinking and learning outcomes. Assess Eval High Educ 34(5):565–577. https://doi.org/10.1080/02602930802226502
Morrison L, Zembower TR (2020) Antimicrobial resistance. Gastrointest Endosc Clin 30(4):619–635. https://doi.org/10.1016/j.giec.2020.06.004
National Research Council (NRC) (1996) National Science Education Standards. The National Academies Press, Washington, DC. https://doi.org/10.17226/4962
National Research Council (NRC) (2000) Inquiry and the national education standards: a guide for teaching and learning. The National Academies Press, Washington, DC
National Research Council (NRC) (2001) Classroom Assessment and the National Science Education Standards. The National Academies Press, Washington, DC. https://doi.org/10.17226/9847
National Research Council (NRC) (2007) Taking Science to School: Learning and Teaching Science in Grades K-8. The National Academies Press, Washington, DC. https://doi.org/10.17226/11625
National Research Council (NRC) (2012) A framework for K-12 science education: practices, crosscutting concepts, and core ideas, committee on a conceptual framework for new K-12 science education standards. The National Academies Press, Washington, DC
Neubrand C, Harms U (2017) Tackling the difficulties in learning evolution: effects of adaptive self-explanation prompts. J Biol Educ 51(4):336–348. https://doi.org/10.1080/00219266.2016.1233129
Nicolaidou I, Kyza EA, Terzian F, Hadjichambis A, Kafouris D (2011) A framework for scaffolding students’ assessment of the credibility of evidence. J Res Sci Teach 48(7):711–744. https://doi.org/10.1002/tea.20420
Papadouris N, Constantinou CP (2009) A methodology for integrating computer‐based learning tools in science curricula. J Curric Stud 41(4):521–538. https://doi.org/10.1080/00220270802123946
Papadouris N, Constantinou CP (2017) Integrating the epistemic and ontological aspects of content knowledge in science teaching and learning. Int J Sci Educ 39(6):663–682. https://doi.org/10.1080/09500693.2017.1299950
Papadouris N, Vokos S, Constantinou CP (2018) The pursuit of a ‘better’ explanation as an organizing framework for science teaching and learning. Sci Educ 102(2):219–237. https://doi.org/10.1002/sce.21326
Quintana C, Reiser BJ, Davis EA, Krajcik J, Fretz E, Duncan RG, Soloway E (2004) A scaffolding design framework for software to support science inquiry. J Learn Sci 13(3):337–386. https://doi.org/10.1207/s15327809jls1303_4
Quinton SR (2010) Principles of effective learning environment design. In: Ebner M, Schiefner M (Eds) Looking toward the future of technology-enhanced education: ubiquitous learning and the digital native, IGI Global, p 327–352. https://doi.org/10.4018/978-1-61520-678-0.ch019
Raes A, Schellens T, De Wever B, Vanderhoven E (2012) Scaffolding information problem-solving in web-based collaborative inquiry learning. Comput Educ 59(1):82–94. https://doi.org/10.1016/j.compedu.2011.11.010
Reiser BJ, Tabak I, Sandoval WA, Smith BK, Steinmuller F, Leone AJ (2001) BGuILE: strategic and conceptual scaffolds for scientific inquiry in biology classrooms. In: Carver SM, Klahr D (eds) Cognition and instruction: twenty-five years of progress, Mahwah, NJ: Lawrence Erlbaum Associates, p 263–305
Richard M, Coley JD, Tanner KD (2017) Investigating undergraduate students’ use of intuitive reasoning and evolutionary knowledge in explanations of antibiotic resistance. CBE—Life Sci Educ, 16(3), ar55. https://doi.org/10.1187/cbe.16-11-0317
Sadler TD (2009) Situated learning in science education: socio‐scientific issues as contexts for practice. Stud Sci Educ 45(1):1–42. https://doi.org/10.1080/03057260802681839
Sadler TD (2011) Situating socio-scientific issues in classrooms as a means of achieving goals of science education. In: Sadler TD (ed) Socio-scientific Issues in the classroom, contemporary trends and issues in science education, vol 39, Springer, Dordrecht, p 1–9. https://doi.org/10.1007/978-94-007-1159-4_1
Sadler TD, Barab SA, Scott B (2007) What do students gain by engaging in socioscientific inquiry? Res Sci Educ 37:371–391. https://doi.org/10.1007/s11165-006-9030-9
Sadler TD, Zeidler DL (2005) Patterns of informal reasoning in the context of socioscientific decision making. J Res Sci Teach 42(1):112–138. https://doi.org/10.1002/tea.20042
Sandoval WA, Millwood KA (2005) The quality of students’ use of evidence in written scientific explanations. Cognit Instr 23(1):23–55. https://doi.org/10.1207/s1532690xci2301_2
Sandoval WA, Millwood KA (2007) What can argumentation tell us about epistemology? In: Erduran S, Jiménez-Aleixandre MP (eds) Argumentation in science education: perspectives from classroom-based research, Dordrecht: Springer Netherlands, p 71–88
Sandoval WA, Reiser BJ (2004) Explanation‐driven inquiry: Integrating conceptual and epistemic scaffolds for scientific inquiry. Sci Educ 88(3):345–372. https://doi.org/10.1002/sce.10130
Shi Y, Zhang J, Yang H, Yang HH (2021) Effects of interactive whiteboard-based instruction on students’ cognitive learning outcomes: a meta-analysis. Interact Learn Environ 29(2):283–300. https://doi.org/10.1080/10494820.2020.1769683
Singer J, Marx RW, Krajcik J, Clay Chambers J (2000) Constructing extended inquiry projects: Curriculum materials for science education reform. Educ Psychol 35(3):165–178. https://doi.org/10.1207/S15326985EP3503_3
Smith MK, Wood WB, Knight JK (2008) The genetics concept assessment: a new concept inventory for gauging student understanding of genetics. CBE Life Sci Educ 7(4):422–430. https://doi.org/10.1187/cbe.08-08-0045
Sosa A, Byarugaba D, Amabile-Cuevas C, Hsueh P, Kariuki S, Okeke I (2010) Antimicrobial resistance in developing countries, Springer. https://doi.org/10.1007/978-0-387-89370-9
Tal T, Kali Y, Magid S, Madhok JJ (2011) Enhancing the authenticity of a web-based module for teaching simple inheritance. In: Sadler T (ed) Socio-scientific issues in the classroom. Contemporary trends and issues in science education, vol 39. Springer, Dordrecht, p 11–38. https://doi.org/10.1007/978-94-007-1159-4_2
Theobald EJ, Crowe A, HilleRisLambers J, Wenderoth MP, Freeman S (2015) Women learn more from local than global examples of the biological impacts of climate change. Front Ecol Environ 13(3):132–137. https://doi.org/10.1890/140261
Toulmin S (1958) The uses of argument. Cambridge University Press, Cambridge
van de Sande-Bruinsma N, Grundmann H, Verloo D, Tiemersma E, Ferech M (2008) Antimicrobial drug use and resistance in Europe. Emerg Infect Dis 14(11):1722–1730. https://doi.org/10.3201/eid1411.070467
Valladares L (2021) Scientific literacy and social transformation: critical perspectives about science participation and emancipation. Sci Educ 30(3):557–587. https://doi.org/10.1007/s11191-021-00205-2
Wegerif R (2019) Dialogic education. In: Noblit GW (ed) Oxford research encyclopedia of education. Oxford University Press. https://doi.org/10.1093/acrefore/9780190264093.013.396
World Health Organisation (WHO) (2001) Global strategy for containment of microbial resistance, retrieved from http://www.who.int/drugresistance/WHO_Global_Strategy_English.pdf Accessed 23 Oct 2023
World Health Organisation (WHO) (2014) Microbial resistance: global report on surveillance, retrieved from http://www.who.int/drugresistance/documents/surveillancereport/en/ Accessed 23 Oct 2023
Yarden H, Yarden A (2013) Learning and teaching biotechnology methods using animations, In: Treagust D, Tsui C-Y (eds) Multiple representations in biological education. Book Series: Models and modeling in science education, Vol 7, Dordrecht: Springer Netherlands, p 93–108. https://doi.org/10.1007/978-94-007-4192-8_6
Zeidler DL (2014) Socioscientific issues as a curriculum emphasis. Theory, research, and practice. In: Lederman NG, Abell SK (eds) Handbook of research on science education, vol 2, Routledge, p 714–740
Zeidler DL, Nichols BH (2009) Socioscientific issues: theory and practice. J Elem Sci Educ 21(2):49–58. https://doi.org/10.1007/BF03173684
Zeidler DL, Sadler TD, Applebaum S, Callahan BE (2009) Advancing reflective judgment through socioscientific issues. J Res Sci Teach 46(1):74–101. https://doi.org/10.1002/tea.20281
Zeidler DL, Sadler TD (2023) Exploring and expanding the frontiers of socioscientific issues. In: Lederman NG, Zeidler DL, Lederman JS (eds) Handbook of research on science education Routledge, p 899–929
Zohar A, Nemet F (2002) Fostering students’ knowledge and argumentation skills through dilemmas in human genetics. J Res Sci Teach 39(1):35–62. https://doi.org/10.1002/tea.10008
Download references
Acknowledgements
We would like to acknowledge substantial contributions by Ms Argyro Scholinaki and Dr Nicos Papadouris to both of whom we are most grateful. This work has received support from the Microbial Resistance Project (AΝΘΡΩΠΙΣΤΙΚΕΣ/ΠΑΙΔΙ/0311/26), which was co-funded by the European Regional Development Fund and the Republic of Cyprus through the Research Promotion Foundation and the Partnerships for Science Education (PAFSE) project which has received support from the European Commission, DG Research, under H2020, Grant Agreement No. 10100646 - PAFSE - H2020-SwafS-2018-2020.
Author information
Authors and affiliations.
Learning in Science Group, Department of Educational Sciences, University of Cyprus, Nicosia, Cyprus
Constantinos P. Constantinou
Laboratory of Nature Education and Conservation, Adam Mickiewicz University, Poznań, Poland
Eliza Rybska
You can also search for this author in PubMed Google Scholar
Contributions
CC initiated the formulation of the design principles and set the frame for the design enactment. CC and ER contributed to the elaboration of the ideas and the writing of the manuscript. The authors of this study participated voluntarily, collectively contributing to the conception of the main ideas and the writing process. This collaborative effort underscores the shared commitment to advancing our understanding of digital learning environments and their purposeful design for enhancing meaningful interactions and the quality of educational experiences.
Corresponding author
Correspondence to Constantinos P. Constantinou .
Ethics declarations
Competing interests.
The authors declare no competing interests.
Ethical approval
This study did not involve human participants, their data or any form of biological material. Hence no ethical approval was required to conduct this research.
Informed consent
This article does not contain any studies with human participants performed by any of the authors.
Additional information
Publisher’s note Springer Nature remains neutral with regard to jurisdictional claims in published maps and institutional affiliations.
Rights and permissions
Open Access This article is licensed under a Creative Commons Attribution 4.0 International License, which permits use, sharing, adaptation, distribution and reproduction in any medium or format, as long as you give appropriate credit to the original author(s) and the source, provide a link to the Creative Commons licence, and indicate if changes were made. The images or other third party material in this article are included in the article’s Creative Commons licence, unless indicated otherwise in a credit line to the material. If material is not included in the article’s Creative Commons licence and your intended use is not permitted by statutory regulation or exceeds the permitted use, you will need to obtain permission directly from the copyright holder. To view a copy of this licence, visit http://creativecommons.org/licenses/by/4.0/ .
Reprints and permissions
About this article
Cite this article.
Constantinou, C.P., Rybska, E. Design principles for integrating science practices with conceptual understanding: an example from a digital learning environment on microbial resistance to antibiotics. Humanit Soc Sci Commun 11 , 501 (2024). https://doi.org/10.1057/s41599-024-03022-4
Download citation
Received : 16 November 2023
Accepted : 28 March 2024
Published : 11 April 2024
DOI : https://doi.org/10.1057/s41599-024-03022-4
Share this article
Anyone you share the following link with will be able to read this content:
Sorry, a shareable link is not currently available for this article.
Provided by the Springer Nature SharedIt content-sharing initiative
Quick links
- Explore articles by subject
- Guide to authors
- Editorial policies

- Victor Yocco
- Apr 9, 2024
Connecting With Users: Applying Principles Of Communication To UX Research
- 30 min read
- UX , User Research , Communication
- Share on Twitter , LinkedIn
About The Author
Victor is a Philadelphia based researcher, author, and speaker. His book Design for the Mind , is available from Manning Publications. Victor frequently writes … More about Victor ↬
Email Newsletter
Weekly tips on front-end & UX . Trusted by 200,000+ folks.
Communication is in everything we do. We communicate with users through our research, our design, and, ultimately, the products and services we offer. UX practitioners and those working on digital product teams benefit from understanding principles of communication and their application to our craft. Treating our UX processes as a mode of communication between users and the digital environment can help unveil in-depth, actionable insights.
In this article, I’ll focus on UX research. Communication is a core component of UX research , as it serves to bridge the gap between research insights, design strategy, and business outcomes. UX researchers, designers, and those working with UX researchers can apply key aspects of communication theory to help gather valuable insights, enhance user experiences, and create more successful products.
Fundamentals of Communication Theory
Communications as an academic field encompasses various models and principles that highlight the dynamics of communication between individuals and groups. Communication theory examines the transfer of information from one person or group to another. It explores how messages are transmitted, encoded, and decoded, acknowledges the potential for interference (or ‘noise’), and accounts for feedback mechanisms in enhancing the communication process.
In this article, I will focus on the Transactional Model of Communication . There are many other models and theories in the academic literature on communication. I have included references at the end of the article for those interested in learning more.
The Transactional Model of Communication (Figure 1) is a two-way process that emphasizes the simultaneous sending and receiving of messages and feedback . Importantly, it recognizes that communication is shaped by context and is an ongoing, evolving process. I’ll use this model and understanding when applying principles from the model to UX research. You’ll find that much of what is covered in the Transactional Model would also fall under general best practices for UX research, suggesting even if we aren’t communications experts, much of what we should be doing is supported by research in this field.
Understanding the Transactional Model
Let’s take a deeper dive into the six key factors and their applications within the realm of UX research:
- Sender: In UX research, the sender is typically the researcher who conducts interviews, facilitates usability tests, or designs surveys. For example, if you’re administering a user interview, you are the sender who initiates the communication process by asking questions.
- Receiver: The receiver is the individual who decodes and interprets the messages sent by the sender. In our context, this could be the user you interview or the person taking a survey you have created. They receive and process your questions, providing responses based on their understanding and experiences.
- Message: This is the content being communicated from the sender to the receiver. In UX research, the message can take various forms, like a set of survey questions, interview prompts, or tasks in a usability test.
- Channel: This is the medium through which the communication flows. For instance, face-to-face interviews, phone interviews, email surveys administered online, and usability tests conducted via screen sharing are all different communication channels. You might use multiple channels simultaneously, for example, communicating over voice while also using a screen share to show design concepts.
- Noise: Any factor that may interfere with the communication is regarded as ‘noise.’ In UX research, this could be complex jargon that confuses respondents in a survey, technical issues during a remote usability test, or environmental distractions during an in-person interview.
- Feedback: The communication received by the receiver, who then provides an output, is called feedback. For example, the responses given by a user during an interview or the data collected from a completed survey are types of feedback or the physical reaction of a usability testing participant while completing a task.
Applying the Transactional Model of Communication to Preparing for UX Research
We can become complacent or feel rushed to create our research protocols. I think this is natural in the pace of many workplaces and our need to deliver results quickly. You can apply the lens of the Transactional Model of Communication to your research preparation without adding much time. Applying the Transactional Model of Communication to your preparation should:
- Improve Clarity The model provides a clear representation of communication, empowering the researcher to plan and conduct studies more effectively.
- Minimize misunderstanding By highlighting potential noise sources, user confusion or misunderstandings can be better anticipated and mitigated.
- Enhance research participant participation With your attentive eye on feedback, participants are likely to feel valued, thus increasing active involvement and quality of input.
You can address the specific elements of the Transactional Model through the following steps while preparing for research:
Defining the Sender and Receiver
In UX research, the sender can often be the UX researcher conducting the study, while the receiver is usually the research participant. Understanding this dynamic can help researchers craft questions or tasks more empathetically and efficiently. You should try to collect some information on your participant in advance to prepare yourself for building a rapport.
For example, if you are conducting contextual inquiry with the field technicians of an HVAC company, you’ll want to dress appropriately to reflect your understanding of the context in which your participants (receivers) will be conducting their work. Showing up dressed in formal attire might be off-putting and create a negative dynamic between sender and receiver.
Message Creation
The message in UX research typically is the questions asked or tasks assigned during the study. Careful consideration of tenor, terminology, and clarity can aid data accuracy and participant engagement. Whether you are interviewing or creating a survey, you need to double-check that your audience will understand your questions and provide meaningful answers. You can pilot-test your protocol or questionnaire with a few representative individuals to identify areas that might cause confusion.
Using the HVAC example again, you might find that field technicians use certain terminology in a different way than you expect, such as asking them about what “tools” they use to complete their tasks yields you an answer that doesn’t reflect digital tools you’d find on a computer or smartphone, but physical tools like a pipe and wrench.
Choosing the Right Channel
The channel selection depends on the method of research. For instance, face-to-face methods might use physical verbal communication, while remote methods might rely on emails, video calls, or instant messaging. The choice of the medium should consider factors like tech accessibility, ease of communication, reliability, and participant familiarity with the channel. For example, you introduce an additional challenge (noise) if you ask someone who has never used an iPhone to test an app on an iPhone.
Minimizing Noise
Noise in UX research comes in many forms, from unclear questions inducing participant confusion to technical issues in remote interviews that cause interruptions. The key is to foresee potential issues and have preemptive solutions ready.
Facilitating Feedback
You should be prepared for how you might collect and act on participant feedback during the research. Encouraging regular feedback from the user during UX research ensures their understanding and that they feel heard. This could range from asking them to ‘think aloud’ as they perform tasks or encouraging them to email queries or concerns after the session. You should document any noise that might impact your findings and account for that in your analysis and reporting.
Track Your Alignment to the Framework
You can track what you do to align your processes with the Transactional Model prior to and during research using a spreadsheet. I’ll provide an example of a spreadsheet I’ve used in the later case study section of this article. You should create your spreadsheet during the process of preparing for research, as some of what you do to prepare should align with the factors of the model.
You can use these tips for preparation regardless of the specific research method you are undertaking. Let’s now look closer at a few common methods and get specific on how you can align your actions with the Transactional Model.
Applying the Transactional Model to Common UX Research Methods
UX research relies on interaction with users. We can easily incorporate aspects of the Transactional Model of Communication into our most common methods. Utilizing the Transactional Model in conducting interviews, surveys, and usability testing can help provide structure to your process and increase the quality of insights gathered.
Interviews are a common method used in qualitative UX research. They provide the perfect method for applying principles from the Transactional Model. In line with the Transactional Model, the researcher (sender) sends questions (messages) in-person or over the phone/computer medium (channel) to the participant (receiver), who provides answers (feedback) while contending with potential distraction or misunderstanding (noise). Reflecting on communication as transactional can help remind us we need to respect the dynamic between ourselves and the person we are interviewing. Rather than approaching an interview as a unidirectional interrogation, researchers need to view it as a conversation.
Applying the Transactional Model to conducting interviews means we should account for a number of facts to allow for high-quality communication. Note how the following overlap with what we typically call best practices.
Asking Open-ended Questions
To truly harness a two-way flow of communication, open-ended questions, rather than close-ended ones, are crucial. For instance, rather than asking, “Do you use our mobile application?” ask, “Can you describe your use of our mobile app?”. This encourages the participant to share more expansive and descriptive insights, furthering the dialogue.
Actively Listening
As the success of an interview relies on the participant’s responses, active listening is a crucial skill for UX researchers. The researcher should encourage participants to express their thoughts and feelings freely. Reflective listening techniques , such as paraphrasing or summarizing what the participant has shared, can reinforce to the interviewee that their contributions are being acknowledged and valued. It also provides an opportunity to clarify potential noise or misunderstandings that may arise.
Being Responsive
Building on the simultaneous send-receive nature of the Transactional Model, researchers must remain responsive during interviews. Providing non-verbal cues (like nodding) and verbal affirmations (“I see,” “Interesting”) lets participants know their message is being received and understood, making them feel comfortable and more willing to share.
We should always attempt to account for noise in advance, as well as during our interview sessions. Noise, in the form of misinterpretations or distractions, can disrupt effective communication. Researchers can proactively reduce noise by conducting a dry run in advance of the scheduled interviews . This helps you become more fluent at going through the interview and also helps identify areas that might need improvement or be misunderstood by participants. You also reduce noise by creating a conducive interview environment, minimizing potential distractions, and asking clarifying questions during the interview whenever necessary.
For example, if a participant uses a term the researcher doesn’t understand, the researcher should politely ask for clarification rather than guessing its meaning and potentially misinterpreting the data.
Additional forms of noise can include participant confusion or distraction. You should let participants know to ask if they are unclear on anything you say or do. It’s a good idea to always ask participants to put their smartphones on mute. You should only provide information critical to the process when introducing the interview or tasks. For example, you don’t need to give a full background of the history of the product you are researching if that isn’t required for the participant to complete the interview. However, you should let them know the purpose of the research, gain their consent to participate, and inform them of how long you expect the session to last.
Strategizing the Flow
Researchers should build strategic thinking into their interviews to support the Transaction Model. Starting the interview with less intrusive questions can help establish rapport and make the participant more comfortable, while more challenging or sensitive questions can be left for later when the interviewee feels more at ease.
A well-planned interview encourages a fluid dialogue and exchange of ideas. This is another area where conducting a dry run can help to ensure high-quality research. You and your dry-run participants should recognize areas where questions aren’t flowing in the best order or don’t make sense in the context of the interview, allowing you to correct the flow in advance.
While much of what the Transactional Model informs for interviews already aligns with common best practices, the model would suggest we need to have a deeper consideration of factors that we can sometimes give less consideration when we become overly comfortable with interviewing or are unaware of the implications of forgetting to address the factors of context considerations, power dynamics, and post-interview actions.
Context Considerations
You need to account for both the context of the participant, e.g., their background, demographic, and psychographic information, as well as the context of the interview itself. You should make subtle yet meaningful modifications depending on the channel you are conducting an interview.
For example, you should utilize video and be aware of your facial and physical responses if you are conducting an interview using an online platform, whereas if it’s a phone interview, you will need to rely on verbal affirmations that you are listening and following along, while also being mindful not to interrupt the participant while they are speaking.
Power Dynamics
Researchers need to be aware of how your role, background, and identity might influence the power dynamics of the interview. You can attempt to address power dynamics by sharing research goals transparently and addressing any potential concerns about bias a participant shares.
We are responsible for creating a safe and inclusive space for our interviews. You do this through the use of inclusive language, listening actively without judgment, and being flexible to accommodate different ways of knowing and expressing experiences. You should also empower participants as collaborators whenever possible . You can offer opportunities for participants to share feedback on the interview process and analysis. Doing this validates participants’ experiences and knowledge and ensures their voices are heard and valued.
Post-Interview Actions
You have a number of options for actions that can close the loop of your interviews with participants in line with the “feedback” the model suggests is a critical part of communication. Some tactics you can consider following your interview include:
- Debriefing Dedicate a few minutes at the end to discuss the participant’s overall experience, impressions, and suggestions for future interviews.
- Short surveys Send a brief survey via email or an online platform to gather feedback on the interview experience.
- Follow-up calls Consider follow-up calls with specific participants to delve deeper into their feedback and gain additional insight if you find that is warranted.
- Thank you emails Include a “feedback” section in your thank you email, encouraging participants to share their thoughts on the interview.
You also need to do something with the feedback you receive. Researchers and product teams should make time for reflexivity and critical self-awareness.
As practitioners in a human-focused field, we are expected to continuously examine how our assumptions and biases might influence our interviews and findings. “
We shouldn’t practice our craft in a silo. Instead, seeking feedback from colleagues and mentors to maintain ethical research practices should be a standard practice for interviews and all UX research methods.
By considering interviews as an ongoing transaction and exchange of ideas rather than a unidirectional Q&A, UX researchers can create a more communicative and engaging environment. You can see how models of communication have informed best practices for interviews. With a better knowledge of the Transactional Model, you can go deeper and check your work against the framework of the model.
The Transactional Model of Communication reminds us to acknowledge the feedback loop even in seemingly one-way communication methods like surveys. Instead of merely sending out questions and collecting responses, we need to provide space for respondents to voice their thoughts and opinions freely. When we make participants feel heard, engagement with our surveys should increase, dropouts should decrease, and response quality should improve.
Like other methods, surveys involve the researcher(s) creating the instructions and questionnaire (sender), the survey, including any instructions, disclaimers, and consent forms (the message), how the survey is administered, e.g., online, in person, or pen and paper (the channel), the participant (receiver), potential misunderstandings or distractions (noise), and responses (feedback).
Designing the Survey
Understanding the Transactional Model will help researchers design more effective surveys. Researchers are encouraged to be aware of both their role as the sender and to anticipate the participant’s perspective as the receiver. Begin surveys with clear instructions, explaining why you’re conducting the survey and how long it’s estimated to take. This establishes a more communicative relationship with respondents right from the start. Test these instructions with multiple people prior to launching the survey.
Crafting Questions
The questions should be crafted to encourage feedback and not just a simple yes or no. You should consider asking scaled questions or items that have been statistically validated to measure certain attributes of users.
For example, if you were looking deeper at a mobile banking application, rather than asking, “Did you find our product easy to use?” you would want to break that out into multiple aspects of the experience and ask about each with a separate question such as “On a scale of 1–7, with 1 being extremely difficult and 7 being extremely easy, how would you rate your experience transferring money from one account to another?” .
Reducing ‘noise,’ or misunderstandings, is crucial for increasing the reliability of responses. Your first line of defense in reducing noise is to make sure you are sampling from the appropriate population you want to conduct the research with. You need to use a screener that will filter out non-viable participants prior to including them in the survey. You do this when you correctly identify the characteristics of the population you want to sample from and then exclude those falling outside of those parameters.
Additionally, you should focus on prioritizing finding participants through random sampling from the population of potential participants versus using a convenience sample, as this helps to ensure you are collecting reliable data.
When looking at the survey itself, there are a number of recommendations to reduce noise. You should ensure questions are easily understandable, avoid technical jargon, and sequence questions logically. A question bank should be reviewed and tested before being finalized for distribution.
For example, question statements like “Do you use and like this feature?” can confuse respondents because they are actually two separate questions: do you use the feature, and do you like the feature? You should separate out questions like this into more than one question.
You should use visual aids that are relevant whenever possible to enhance the clarity of the questions. For example, if you are asking questions about an application’s “Dashboard” screen, you might want to provide a screenshot of that page so survey takers have a clear understanding of what you are referencing. You should also avoid the use of jargon if you are surveying a non-technical population and explain any terminology that might be unclear to participants taking the survey.
The Transactional Model suggests active participation in communication is necessary for effective communication . Participants can become distracted or take a survey without intending to provide thoughtful answers. You should consider adding a question somewhere in the middle of the survey to check that participants are paying attention and responding appropriately, particularly for longer surveys.
This is often done using a simple math problem such as “What is the answer to 1+1?” Anyone not responding with the answer of “2” might not be adequately paying attention to the responses they are providing and you’d want to look closer at their responses, eliminating them from your analysis if deemed appropriate.
Encouraging Feedback
While descriptive feedback questions are one way of promoting dialogue, you can also include areas where respondents can express any additional thoughts or questions they have outside of the set question list. This is especially useful in online surveys, where researchers can’t immediately address participant’s questions or clarify doubts.
You should be mindful that too many open-ended questions can cause fatigue , so you should limit the number of open-ended questions. I recommend two to three open-ended questions depending on the length of your overall survey.
Post-Survey Actions
After collecting and analyzing the data, you can send follow-up communications to the respondents. Let them know the changes made based on their feedback, thank them for their participation, or even share a summary of the survey results. This fulfills the Transactional Model’s feedback loop and communicates to the respondent that their input was received, valued, and acted upon.
You can also meet this suggestion by providing an email address for participants to follow up if they desire more information post-survey. You are allowing them to complete the loop themselves if they desire.
Applying the transactional model to surveys can breathe new life into the way surveys are conducted in UX research. It encourages active participation from respondents, making the process more interactive and engaging while enhancing the quality of the data collected. You can experiment with applying some or all of the steps listed above. You will likely find you are already doing much of what’s mentioned, however being explicit can allow you to make sure you are thoughtfully applying these principles from the field communication.
Usability Testing
Usability testing is another clear example of a research method highlighting components of the Transactional Model. In the context of usability testing, the Transactional Model of Communication’s application opens a pathway for a richer understanding of the user experience by positioning both the user and the researcher as sender and receiver of communication simultaneously.
Here are some ways a researcher can use elements of the Transactional Model during usability testing:
Task Assignment as Message Sending
When a researcher assigns tasks to a user during usability testing, they act as the sender in the communication process. To ensure the user accurately receives the message, these tasks need to be clear and well-articulated. For example, a task like “Register a new account on the app” sends a clear message to the user about what they need to do.
You don’t need to tell them how to do the task, as usually, that’s what we are trying to determine from our testing, but if you are not clear on what you want them to do, your message will not resonate in the way it is intended. This is another area where a dry run in advance of the testing is an optimal solution for making sure tasks are worded clearly.
Observing and Listening as Message Receiving
As the participant interacts with the application, concept, or design, the researcher, as the receiver, picks up on verbal and nonverbal cues. For instance, if a user is clicking around aimlessly or murmuring in confusion, the researcher can take these as feedback about certain elements of the design that are unclear or hard to use. You can also ask the user to explain why they are giving these cues you note as a way to provide them with feedback on their communication.
Real-time Interaction
The transactional nature of the model recognizes the importance of real-time interaction. For example, if during testing, the user is unsure of what a task means or how to proceed, the researcher can provide clarification without offering solutions or influencing the user’s action. This interaction follows the communication flow prescribed by the transactional model. We lose the ability to do this during unmoderated testing; however, many design elements are forms of communication that can serve to direct users or clarify the purpose of an experience (to be covered more in article two).
In usability testing, noise could mean unclear tasks, users’ preconceived notions, or even issues like slow software response. Acknowledging noise can help researchers plan and conduct tests better. Again, carrying out a pilot test can help identify any noise in the main test scenarios, allowing for necessary tweaks before actual testing. Other forms of noise can be less obvious but equally intrusive. For example, if you are conducting a test using a Macbook laptop and your participant is used to a PC, there is noise you need to account for, given their unfamiliarity with the laptop you’ve provided.
The fidelity of the design artifact being tested might introduce another form of noise. I’ve always advocated testing at any level of fidelity, but you should note that if you are using “Lorem Ipsum” or black and white designs, this potentially adds noise.
One of my favorite examples of this was a time when I was testing a financial services application, and the designers had put different balances on the screen; however, the total for all balances had not been added up to the correct total. Virtually every person tested noted this discrepancy, although it had nothing to do with the tasks at hand. I had to acknowledge we’d introduced noise to the testing. As at least one participant noted, they wouldn’t trust a tool that wasn’t able to total balances correctly.
Under the Transactional Model’s guidance, feedback isn’t just final thoughts after testing; it should be facilitated at each step of the process. Encouraging ‘think aloud’ protocols , where the user verbalizes their thoughts, reactions, and feelings during testing, ensures a constant flow of useful feedback.
You are receiving feedback throughout the process of usability testing, and the model provides guidance on how you should use that feedback to create a shared meaning with the participants. You will ultimately summarize this meaning in your report. You’ll later end up uncovering if this shared meaning was correctly interpreted when you design or redesign the product based on your findings.
We’ve now covered how to apply the Transactional Model of Communication to three common UX Research methods. All research with humans involves communication. You can break down other UX methods using the Model’s factors to make sure you engage in high-quality research.
Analyzing and Reporting UX Research Data Through the Lens of the Transactional Model
The Transactional Model of Communication doesn’t only apply to the data collection phase (interviews, surveys, or usability testing) of UX research. Its principles can provide valuable insights during the data analysis process.
The Transactional Model instructs us to view any communication as an interactive, multi-layered dialogue — a concept that is particularly useful when unpacking user responses. Consider the ‘message’ components: In the context of data analysis, the messages are the users’ responses. As researchers, thinking critically about how respondents may have internally processed the survey questions, interview discussion, or usability tasks can yield richer insights into user motivations.
Understanding Context
Just as the Transactional Model emphasizes the simultaneous interchange of communication, UX researchers should consider the user’s context while interpreting data. Decoding the meaning behind a user’s words or actions involves understanding their background, experiences, and the situation when they provide responses.
Deciphering Noise
In the Transactional Model, noise presents a potential barrier to effective communication. Similarly, researchers must be aware of snowballing themes or frequently highlighted issues during analysis. Noise, in this context, could involve patterns of confusion, misunderstandings, or consistently highlighted problems by users. You need to account for this, e.g., the example I provided where participants constantly referred to the incorrect math on static wireframes.
Considering Sender-Receiver Dynamics
Remember that as a UX researcher, your interpretation of user responses will be influenced by your understandings, biases, or preconceptions, just as the responses were influenced by the user’s perceptions. By acknowledging this, researchers can strive to neutralize any subjective influence and ensure the analysis remains centered on the user’s perspective. You can ask other researchers to double-check your work to attempt to account for bias.
For example, if you come up with a clear theme that users need better guidance in the application you are testing, another researcher from outside of the project should come to a similar conclusion if they view the data; if not, you should have a conversation with them to determine what different perspectives you are each bringing to the data analysis.
Reporting Results
Understanding your audience is crucial for delivering a persuasive UX research presentation. Tailoring your communication to resonate with the specific concerns and interests of your stakeholders can significantly enhance the impact of your findings. Here are some more details:
- Identify Stakeholder Groups Identify the different groups of stakeholders who will be present in your audience. This could include designers, developers, product managers, and executives.
- Prioritize Information Prioritize the information based on what matters most to each stakeholder group. For example, designers might be more interested in usability issues, while executives may prioritize business impact.
- Adapt Communication Style Adjust your communication style to align with the communication preferences of each group. Provide technical details for developers and emphasize user experience benefits for executives.
Acknowledging Feedback
Respecting this Transactional Model’s feedback loop, remember to revisit user insights after implementing design changes. This ensures you stay user-focused, continuously validating or adjusting your interpretations based on users’ evolving feedback. You can do this in a number of ways. You can reconnect with users to show them updated designs and ask questions to see if the issues you attempted to resolve were resolved.
Another way to address this without having to reconnect with the users is to create a spreadsheet or other document to track all the recommendations that were made and reconcile the changes with what is then updated in the design. You should be able to map the changes users requested to updates or additions to the product roadmap for future updates. This acknowledges that users were heard and that an attempt to address their pain points will be documented.
Crucially, the Transactional Model teaches us that communication is rarely simple or one-dimensional. It encourages UX researchers to take a more nuanced, context-aware approach to data analysis, resulting in deeper user understanding and more accurate, user-validated results.
By maintaining an ongoing feedback loop with users and continually refining interpretations, researchers can ensure that their work remains grounded in real user experiences and needs. “
Tracking Your Application of the Transactional Model to Your Practice
You might find it useful to track how you align your research planning and execution to the framework of the Transactional Model. I’ve created a spreadsheet to outline key factors of the model and used this for some of my work. Demonstrated below is an example derived from a study conducted for a banking client that included interviews and usability testing. I completed this spreadsheet during the process of planning and conducting interviews. Anonymized data from our study has been furnished to show an example of how you might populate a similar spreadsheet with your information.
You can customize the spreadsheet structure to fit your specific research topic and interview approach. By documenting your application of the transactional model, you can gain valuable insights into the dynamic nature of communication and improve your interview skills for future research.
You can use the suggested columns from this table as you see fit, adding or subtracting as needed, particularly if you use a method other than interviews. I usually add the following additional Columns for logistical purposes:
- Date of Interview,
- Participant ID,
- Interview Format (e.g., in person, remote, video, phone).
By incorporating aspects of communication theory into UX research, UX researchers and those who work with UX researchers can enhance the effectiveness of their communication strategies, gather more accurate insights, and create better user experiences. Communication theory provides a framework for understanding the dynamics of communication, and its application to UX research enables researchers to tailor their approaches to specific audiences, employ effective interviewing techniques, design surveys and questionnaires, establish seamless communication channels during usability testing, and interpret data more effectively.
As the field of UX research continues to evolve, integrating communication theory into research practices will become increasingly essential for bridging the gap between users and design teams, ultimately leading to more successful products that resonate with target audiences.
As a UX professional, it is important to continually explore and integrate new theories and methodologies to enhance your practice . By leveraging communication theory principles, you can better understand user needs, improve the user experience, and drive successful outcomes for digital products and services.
Integrating communication theory into UX research is an ongoing journey of learning and implementing best practices. Embracing this approach empowers researchers to effectively communicate their findings to stakeholders and foster collaborative decision-making, ultimately driving positive user experiences and successful design outcomes.
References and Further Reading
- The Mathematical Theory of Communication (PDF), Shannon, C. E., & Weaver, W.
- From organizational effectiveness to relationship indicators: Antecedents of relationships, public relations strategies, and relationship outcomes , Grunig, J. E., & Huang, Y. H.
- Communication and persuasion: Psychological studies of opinion change, Hovland, C. I., Janis, I. L., & Kelley, H. H. (1953). Yale University Press
- Communication research as an autonomous discipline, Chaffee, S. H. (1986). Communication Yearbook, 10, 243-274
- Interpersonal Communication: Everyday Encounters (PDF), Wood, J. (2015)
- Theories of Human Communication , Littlejohn, S. W., & Foss, K. A. (2011)
- McQuail’s Mass Communication Theory (PDF), McQuail, D. (2010)
- Bridges Not Walls: A Book About Interpersonal Communication , Stewart, J. (2012)
Smashing Newsletter
Tips on front-end & UX, delivered weekly in your inbox. Just the things you can actually use.
Front-End & UX Workshops, Online
With practical takeaways, live sessions, video recordings and a friendly Q&A.
TypeScript in 50 Lessons
Everything TypeScript, with code walkthroughs and examples. And other printed books.
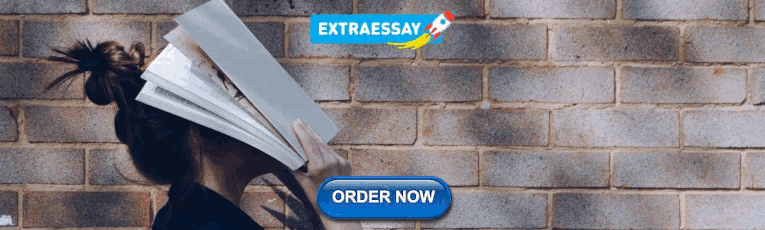
IMAGES
VIDEO
COMMENTS
A research design is a strategy for answering your research question using empirical data. Creating a research design means making decisions about: Your overall research objectives and approach. Whether you'll rely on primary research or secondary research. Your sampling methods or criteria for selecting subjects. Your data collection methods.
A theoretical framework guides the research process like a roadmap for the study, so you need to get this right. Theoretical framework 1,2 is the structure that supports and describes a theory. A theory is a set of interrelated concepts and definitions that present a systematic view of phenomena by describing the relationship among the variables for explaining these phenomena.
Research design refers to the overall plan, structure or strategy that guides a research project, from its conception to the final analysis of data. Research designs for quantitative studies include descriptive, correlational, experimental and quasi-experimenta l designs. Research designs for qualitative studies include phenomenological ...
Step 2: Choose a type of research design. Within both qualitative and quantitative approaches, there are several types of research design to choose from. Each type provides a framework for the overall shape of your research. Types of quantitative research designs. Quantitative designs can be split into four main types.
The purpose of research design is to plan and structure a research study in a way that enables the researcher to achieve the desired research goals with accuracy, validity, and reliability. Research design is the blueprint or the framework for conducting a study that outlines the methods, procedures, techniques, and tools for data collection ...
A research design is the plan or framework used to conduct a research study. It involves outlining the overall approach and methods that will be used to collect and analyze data in order to answer research questions or test hypotheses. ... Qualitative research design types and qualitative research design examples ...
What is Research Design? At its core, research design is the framework that outlines the structure and methodology of a study. It's the roadmap that guides researchers from hypothesis formulation to data collection and analysis. A well-designed study ensures that the research objectives are met efficiently and effectively. Types of Research ...
A conceptual framework illustrates the expected relationship between your variables. It defines the relevant objectives for your research process and maps out how they come together to draw coherent conclusions. Tip. You should construct your conceptual framework before you begin collecting your data.
Step 2: Data Type you Need for Research. Decide on the type of data you need for your research. The type of data you need to collect depends on your research questions or research hypothesis. Two types of research data can be used to answer the research questions: Primary Data Vs. Secondary Data.
framework is a generative source of thinking, planning, conscious action, and reflection throughout the research process. A conceptual framework makes the case for why a study is significant and relevant and for how the study design (including data collection and analysis methods) appropri - ately and rigorously answers the research questions.
Research study design is a framework, or the set of methods and procedures used to collect and analyze data on variables specified in a particular research problem. Research study designs are of many types, each with its advantages and limitations. The type of study design used to answer a particular research question is determined by the ...
Before beginning your paper, you need to decide how you plan to design the study.. The research design refers to the overall strategy and analytical approach that you have chosen in order to integrate, in a coherent and logical way, the different components of the study, thus ensuring that the research problem will be thoroughly investigated. It constitutes the blueprint for the collection ...
Design a scientific study to objectively gather quantitative data under ... ences, not simple points of view. For example, in the 1950s and 1960s, studies of the psychological stability of homosexuals found that they were ... research emerged in the past century as a useful framework for social science research, but its history has not been the ...
2. Research design. The research design is intended to provide an appropriate framework for a study. A very significant decision in research design process is the choice to be made regarding research approach since it determines how relevant information for a study will be obtained; however, the research design process involves many interrelated decisions [].
The first kind, "Research into design" studies the design product post hoc and the MIR framework suits the interdisciplinary study of such a product. In contrast, "Research for design" generates knowledge that feeds into the noun and the verb 'design', which means it precedes the design (ing).
Theoretical Framework. Definition: Theoretical framework refers to a set of concepts, theories, ideas, and assumptions that serve as a foundation for understanding a particular phenomenon or problem. It provides a conceptual framework that helps researchers to design and conduct their research, as well as to analyze and interpret their findings.
Theoretical Framework Example for a Thesis or Dissertation. Published on October 14, 2015 by Sarah Vinz . Revised on July 18, 2023 by Tegan George. Your theoretical framework defines the key concepts in your research, suggests relationships between them, and discusses relevant theories based on your literature review.
Reeves et al. is an example of an article that proposed a conceptual framework about graduate teaching assistant professional development evaluation and research. The authors used existing literature to create a novel framework that filled a gap in current research and practice related to the training of graduate teaching assistants.
A research framework is a set of concepts, principles, and guidelines that shape and guide your research process. It helps you to define your research problem, objectives, questions, hypotheses ...
Experimental research design is a framework of protocols and procedures created to conduct experimental research with a scientific approach using two sets of variables. Herein, the first set of variables acts as a constant, used to measure the differences of the second set. ... The best example of experimental research methods is quantitative ...
INTRODUCTION. Scientific research is usually initiated by posing evidenced-based research questions which are then explicitly restated as hypotheses.1,2 The hypotheses provide directions to guide the study, solutions, explanations, and expected results.3,4 Both research questions and hypotheses are essentially formulated based on conventional theories and real-world processes, which allow the ...
National Research Council (NRC) (2012) A framework for K-12 science education: practices, crosscutting concepts, and core ideas, committee on a conceptual framework for new K-12 science education ...
Communication is a core component of UX research, as it serves to bridge the gap between research insights, design strategy, and business outcomes. UX researchers, designers, and those working with UX researchers can apply key aspects of communication theory to help gather valuable insights, enhance user experiences, and create more successful ...