Accessibility Links
- Skip to content
- Skip to search IOPscience
- Skip to Journals list
- Accessibility help
- Accessibility Help
Click here to close this panel.
Purpose-led Publishing is a coalition of three not-for-profit publishers in the field of physical sciences: AIP Publishing, the American Physical Society and IOP Publishing.
Together, as publishers that will always put purpose above profit, we have defined a set of industry standards that underpin high-quality, ethical scholarly communications.
We are proudly declaring that science is our only shareholder.
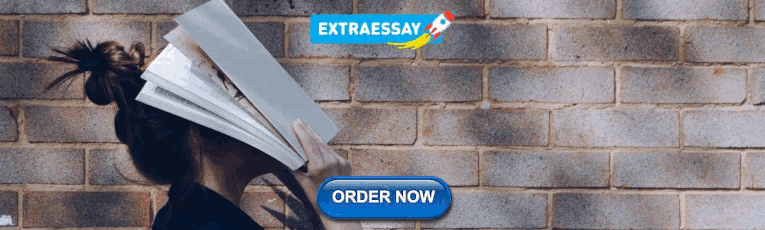
Design and Implementation Data Warehouse in Insurance Company
Ryan Ari Setyawan 1 , Eko Prasetyo 2 and Abba Suganda Girsang 2
Published under licence by IOP Publishing Ltd Journal of Physics: Conference Series , Volume 1175 , 1st International Conference on Advance and Scientific Innovation 23–24 April 2018, Medan, Indonesia Citation Ryan Ari Setyawan et al 2019 J. Phys.: Conf. Ser. 1175 012072 DOI 10.1088/1742-6596/1175/1/012072
Article metrics
2092 Total downloads
Share this article
Author e-mails.
Author affiliations
1 Department of Informatics Engineering, Janabadra University, Yogyakarta, Indonesia 55231.
2 Computer Science Department, Binus Graduate Program-Master of Computer Science, Bina Nusantara University, Jakarta, Indonesia.
Buy this article in print
Insurance company are certainly has a rich of data from their business process. One of the data is related to business process sales. From this sales data can be used to analyze the condition of a company whether the condition of the company is in a good condition or not. The purpose of this research is to develop a technique to analyze this data from the company. The method used to implement the data analysis in this paper is to design a data warehouse. Use ninestep methodology as the method for design data warehouse and Pentaho as a tool for ETL (Extract, Transform, Load), OLAP analysis and reporting data, the results of this research concluded that the implementation of data warehouse for perform data analysis and reporting better and more effective.
Export citation and abstract BibTeX RIS
Content from this work may be used under the terms of the Creative Commons Attribution 3.0 licence . Any further distribution of this work must maintain attribution to the author(s) and the title of the work, journal citation and DOI.
You are using an outdated browser. Please, upgrade to a different browser or install Google Chrome Frame to experience this site.
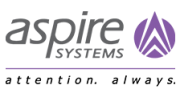
- Producteering
- Usability Engineering
- Integration
- Banking and Finance
- Big Data and Analytics
- Collaboration
- User Experience
- Cyber Security
- Data and Analytics
- ERP – Automobile
- ERP – Real Estate
- ERP Implementation
- ERP Support
- Financials Cloud
- Managed Services
- Robotic Process Automation
- Request for information
Building a Data Warehouse and its benefits to the Insurance Industry

“As of 2022, over 60% of all corporate data is stored in the cloud. This is up from 30% in 2015”
Data warehouse is an essential tool for businesses looking to make better use of their data. In the insurance industry, cloud data warehouse can help organizations store and analyse vast amounts of data to gain insights into their operations, customers, and markets.
Steps to implement data warehouse tools in your organisation:
- Define your data requirements :
The first step in implementing data warehouse is to identify the data that needs to be stored and analysed. In the insurance industry, this could include data on policyholders, claims, premiums, underwriting, and risk assessment.
- Choose an appropriate data warehouse platform :
There are many data warehouse tools available, and each has its strengths and weaknesses. Insurance companies should evaluate different options based on their specific needs, such as scalability, security, and cost.
- Build the data warehouse :
Once the desired cloud data warehouse platform is chosen, the next step is to build the data warehouse. This involves creating a database schema and data structures that can efficiently store and retrieve data.
- Extract and load the data :
The next step is to extract data from various sources, such as legacy systems, spreadsheets, and databases, and load it into the data warehouse. This can be a complex process that requires careful planning and execution.
- Analyse the data :
With the data warehouse in place, insurance companies can begin to analyse their data. This could include building dashboards and reports to visualize data, creating predictive models to identify trends and patterns, and performing ad-hoc analysis to answer specific questions.
- Make data-driven decisions :
The ultimate goal of building a data warehouse is to help companies make better decisions. In the insurance industry, this could involve identifying new market opportunities, improving risk assessment, and enhancing customer satisfaction.
The following are the key steps that insurers should take when implementing data warehousing in the insurance industry:
Understand the data needs of the business:
Before embarking on a data warehousing project, it is crucial to understand the specific data requirements of the business. This involves understanding existing business processes and identifying any new or existing data sources that could be leveraged for improved decision-making.
Choose the right platform:
Insurers need to decide which platform is best suited for their specific needs, considering factors such as scalability, performance, cost, and integration capabilities.
Develop an efficient data architecture:
Insurers must design an efficient data architecture that ensures the security, availability, and scalability of the warehouse. This includes developing a standard data model and setting up processes for data ingestion and storage.
Implement data governance policies:
Data governance is essential to ensure the accuracy and integrity of the data stored in the warehouse. This involves setting policies related to data security, privacy, and quality.
Leverage analytics:
Once the data is stored in the warehouse, insurers can leverage analytics tools to gain insights into customer behaviour, market trends, and financial performance.
Implementing an effective data warehouse requires careful planning and consideration. However, when done right, it can bring many benefits such as improved customer service, increased operational efficiency, reduced costs, and better decision-making.
Benefits of data warehouse implementation in Insurance sector:
- Improved Data Integration :
Data warehousing allows the integration of data from various sources, such as policy administration systems, claims systems, underwriting systems, and financial systems. By integrating data from these different sources, insurers can gain a holistic view of their business, identify trends and patterns, and make better-informed decisions.
- Enhanced Reporting and Analytics:
With data warehousing, insurers can perform advanced analytics and reporting on their data. This can help insurers to identify new business opportunities, optimize their underwriting processes, improve their claims management, and detect fraud.
- Better Risk Management :
Data warehousing can help insurers to identify and manage risks more effectively. By analysing historical data, insurers can identify patterns of risky behaviour and take proactive measures to mitigate potential losses.
- Improved Customer Insights :
With data warehousing, insurers can gain a deeper understanding of their customers. By analysing customer data, insurers can identify customer preferences, behaviours, and needs, and develop more targeted marketing and customer service strategies.
- Increased Operational Efficiency :
Data warehousing can help insurers to streamline their operations by reducing the time and effort required to access and analyse data. By centralizing data in a single location, insurers can also reduce the risk of data errors and inconsistencies.
Data warehouse: a positive disruption in the realm of insurance business
There is little to no question that the world is witnessing a remarkable revolution in the realms of technology and industries. Now that you know how to put data warehousing to good use, you can give a new lease of life to the business data that have been unnoticed and hidden somewhere laying across your organization. It is important to note that implementing an effective data warehouse requires careful planning and consideration. However, when done right with the right technological partner such as Aspire Systems, rest assured that the implementation results in bringing up a plethora of benefits such as improved customer service, increased operational efficiency, reduced costs, and better decision making. For insurers looking to get the most out of their data, investing in a robust data warehouse system is a worthwhile endeavour at that.
Why still thinking…!!!! Looking for Data Warehouse services? click the below link.
- Recent Posts
- Building a Data Warehouse and its benefits to the Insurance Industry - April 26, 2023
Leave a Reply Cancel reply
You must be logged in to post a comment.
© Copyrights 2024 Aspire Systems
Careers | In the News | Terms of Use | Privacy | Site Map

Careers | In the News
Terms of Use | Privacy | Site Map | Contact Us
� Copyrights 2024 Aspire Systems
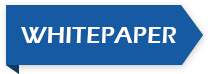
please enter your email address to access the whitepaper
Kindly fill in your details.
- Subscribe to exclusive whitepapers and newsletter
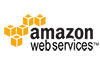
Sign up now and get FREE access to articles, newsletter, infographics, videos and blogs
The Data Warehouse Toolkit: The Complete Guide to Dimensional Modeling by
Get full access to The Data Warehouse Toolkit: The Complete Guide to Dimensional Modeling and 60K+ other titles, with a free 10-day trial of O'Reilly.
There are also live events, courses curated by job role, and more.
Chapter 15. Insurance
We will bring together concepts from nearly all the previous chapters to build a data warehouse for a property and casualty insurance company in this final case study. If you are from the insurance industry and jumped directly to this chapter for a quick fix, please accept our apology, but this material depends heavily on ideas from the earlier chapters. You'll need to turn back to the beginning of the book to have this chapter make any sense.
As has been our standard procedure, this chapter is launched with background information for a business case. While the requirements unfold, we'll draft the data warehouse bus matrix, much like we would in a real-life requirements analysis effort. We'll then design a series of dimensional models by overlaying the core techniques learned thus far in a manner similar to the overlay of overhead transparencies.
Chapter 15 reviews the following concepts:
Requirements-driven approach to dimensional design
Value-chain implications
Data warehouse bus matrix
Complementary transaction, periodic snapshot, and accumulating snapshot schemas
Four-step design process for dimensional models
Dimension role-playing
Handling of slowly changing dimension attributes
Minidimensions for dealing with large, more rapidly changing dimension attributes
Multivalued dimension attributes
Degenerate dimensions for operational control numbers
Audit dimensions to track data lineage
Heterogeneous products with attributes and facts that vary by line of business ...
Get The Data Warehouse Toolkit: The Complete Guide to Dimensional Modeling now with the O’Reilly learning platform.
O’Reilly members experience books, live events, courses curated by job role, and more from O’Reilly and nearly 200 top publishers.
Don’t leave empty-handed
Get Mark Richards’s Software Architecture Patterns ebook to better understand how to design components—and how they should interact.
It’s yours, free.
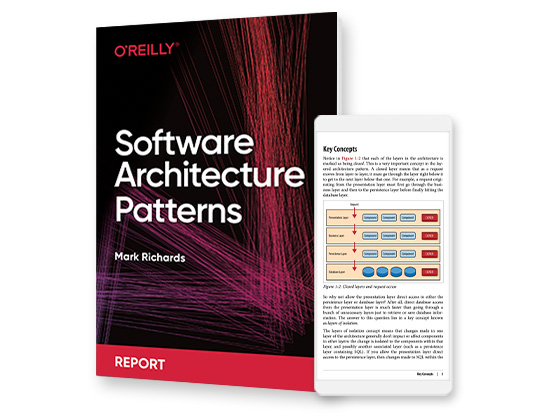
Check it out now on O’Reilly
Dive in for free with a 10-day trial of the O’Reilly learning platform—then explore all the other resources our members count on to build skills and solve problems every day.
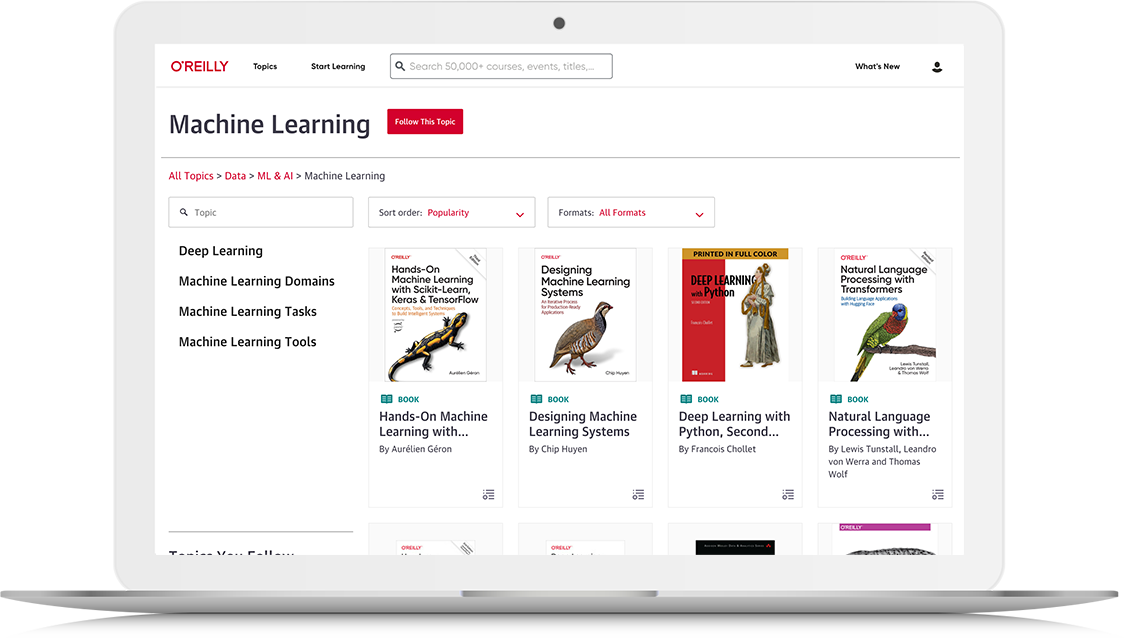
What every insurance leader should know about cloud
The increasing use of digital tools and services, as well as the corresponding surge in data generated from digital interactions, has made technology a crucial competitive capability for insurance carriers. In that context, cloud has emerged as a generational opportunity, with leading carriers already using it to serve customers better, faster, and more efficiently.
About the authors
This article is a collaborative effort by Sanjay Kaniyar, Mathew Lee , Ani Majumder, Binu Sudhakaran, and Steve Van Kuiken , representing views from McKinsey Digital and McKinsey’s Financial Services and Operations practices.
Insurers join most organizations across all sectors in expecting to significantly ramp up adoption and migrate a growing share of their compute environment to public cloud within the next five years (Exhibit 1). That intention is reflected in the projected 32 percent annual growth in cloud services by 2025. 1 laaS + PaaS revenue, Gartner 2018 and 2020 reports; McKinsey analysis.
The most important thing to understand about cloud, however, is that it’s not a more efficient way to operate IT, but a force multiplier for generating value for the business. This reality is why it is critical for business leaders, particularly business unit CEOs and business unit heads, to understand the value at stake and what it takes to capture it. Insurers that use the cloud effectively can unlock such desirable capabilities as providing omnichannel experiences for customers, developing a diverse portfolio of integrated services, and rolling out solutions at unprecedented speeds.
Business unit CEOs understand the nuances of the business and have accountability for identifying and driving the change. They should therefore act as orchestrators of the cloud migration and coaches for the rest of the business leadership in setting bold aspirations and establishing the organizational model that enables the business to harness cloud’s full value.
Through deep discussions with insurance business unit leaders and years of experience helping them migrate to the cloud , we have found that the business units most effective in capturing cloud’s value focus on two key areas: understanding where the value in cloud lies and building a partnership between business and IT.
Would you like to learn more about Cloud by McKinsey ?
Be clear about where the value is in cloud.
Most insurers still vastly undervalue cloud’s potential. Our research shows that the EBITDA run-rate impact of cloud on the insurance sector will be $70 billion to $110 billion by 2030—in the top five of all sectors analyzed. When looking at EBITDA impact as a percentage of 2030 EBITDA, insurance is the top-ranked of all sectors, at 43–70 percent. 2 “ Cloud’s trillion-dollar prize is up for grabs ,” McKinsey Quarterly , February 26, 2021.
This value comes from two sources. 3 McKinsey analysis also identified value from a third source: pioneering, which is the use of advanced but nascent technologies that can extend cloud’s value. These developments, however, are not yet mature enough for their effect to be accurately calculated. The first is rejuvenation , which focuses on using cloud to lower costs and risk across IT and core operations. It can be predominantly driven by IT teams.
The second source of value is innovation , which focuses on harnessing the cloud to accelerate or enable the development of new revenue streams. That includes, for example, faster time to market or new-product development—using advanced analytics, IoT, and automation at scale. A close partnership between IT and business leadership is needed to drive the innovation. One insurance carrier, for example, announced a new direct-to-consumer business targeting gig-economy workers and retired baby boomers, which was made possible by a host of cloud-native services including AI-based chatbots, data services, and automated or digitized workflows.
As in most sectors, the value of cloud-facilitated innovation in the insurance industry dwarfs what’s achievable through rejuvenation.
By understanding the hierarchy of value , insurance companies can move past the proverbial low-hanging fruit, the most accessible benefits of cloud that require limited business engagement (Exhibit 2). Only a select few companies, where business leaders have led the cloud transformation in tandem with technology leaders, have been able to capture the full potential of cloud.
Those carriers that have effectively tapped the hierarchy of cloud value have realized significant benefits, including the following:
- Faster time to market. New capabilities, business features, and products can be more quickly developed, tested, and launched in cloud than in traditional environments. This advantage is particularly acute for early movers, who can respond to market changes much more quickly than their non-cloud competitors. For example, when a large, global property-and-casualty (P&C) company adopted a cloud-based policy administration platform, it was able to bring new specialty insurance products to market within three months.
- Lower cost to serve. Cloud technologies and tools enable better asset utilization and more-flexible operating models. These make existing revenue pools more profitable and help businesses access opportunities that were previously not economically viable. One investment management company developed a “cloud-native” record-keeping solution, which has improved efficiency, scalability, durability, and automation. It now has a 100 percent cloud-native architecture that can reduce the cost of computing by 30 percent and deploy workloads up to 20 times faster with greater resiliency.
- Economies of scale. Cloud can easily scale either up or down as needed, unlike on-premises data centers. Cloud provides the compute power that is needed to fully understand and make use of incredibly large data sets, such as tens of millions of claims data points. One wealth management firm is using cloud-based data and analytics algorithms to achieve industry-leading rollover rates and improving the lifetime value of clients by delivering nudges based on key life events, such as likelihood to terminate and retirement.
- Access to advanced capabilities through cloud services. Advanced cloud capabilities allow companies to generate insights that previously demanded intensive resources to develop. For example, machine learning services can be used to identify potentially fraudulent activity much faster. Tools are available that enable companies and their partners easier access to regulatory information, helping them to stay compliant at lower cost. One financial-services company has moved all servicing to cloud to use a host of cloud-native tools. This has enabled it to serve smaller customers than it could before. Costs per call are much lower because agents are far more productive when they rely on completely automated “transactional interactions,” such as the use of advanced interactive voice response, chatbots, and self-service tools. Customer satisfaction scores have increased, and agents can now spend more time with customers for more complex transactions and interactions. Insurance companies have found 12 cloud capabilities particularly relevant and useful to their business (Exhibit 3).
To increase the cloud’s business impact, major institutions are negotiating transformative partnerships with cloud service providers (CSPs) to make better use of cloud technologies.
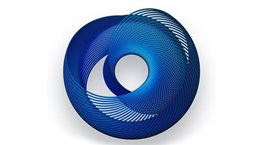
Cloud by McKinsey Insights
Build a close working relationship between it and the business side early in the cloud journey.
In our experience, cloud transformations are most successful when they are joint efforts between business and IT, rather than purely IT-led initiatives. Such collaborations are more likely to direct efforts efficiently at the sources of business value and at business outcomes aligned to the institution’s overall goals.
In successful business–IT partnerships, IT leaders and business unit heads have worked closely with each other to educate the business—and the board —about cloud in the following ways:
- Incorporate cloud topics into strategy discussions by laying out the ways that business goals can be enabled and accelerated through cloud services—for example, by harnessing AI to improve the accuracy of predictive analytics on insurance needs. In this way, both the business and IT can build a practical understanding of cloud technologies and their business benefits. IT leaders should develop a portfolio of examples detailing how their peers leverage cloud. These activities are critical from a capability-building, business-adoption, and change-management point of view and will also help the leaders across the business visualize the “art of the possible.”
- Build excitement about the cloud and its possibilities through a joint immersion program for IT and business leaders (Exhibit 4). These immersion sessions could serve as jumping-off points for new and existing portfolios of cloud-enabled initiatives as well as lay the foundations for effective business–IT collaboration. They might include “go-and-see” visits where business leaders can learn from their peers in other institutions who have gone through similar cloud journeys. The most effective immersion programs also include a cloud learning curriculum for business leaders, with a focus on the business impact of cloud. This will help them to gain a deeper understanding of why cloud matters and how it can enable and accelerate the business value of transformation initiatives in each business domain.
- Encourage the business unit leadership team to be the change agents and evangelists for cloud within their organization and communicate the value that cloud can unlock within their areas. Successful cloud journeys involve selecting and training leaders in the business to, for example, think in terms of operating expenditures in calculating cloud spend and work with teams to develop products, and to become cloud champions to help the rest of the organization understand the business value of cloud. They also call on functional teams, such as operations, marketing, and legal, to highlight the value of cloud for their areas and how it can enhance their functions.
At one large, global life and retirement company, the CIO orchestrated a three-month cloud immersion program for the company’s top 100 business and IT leaders. This senior leadership team started off by learning the basics of cloud through field and forum exercises, went on “go-and-see” visits to two companies further along in their cloud journey, and targeted sessions with CSPs to learn about practical use cases from other industries in such specific functional areas as marketing and customer analytics. Coming out of these sessions, the leadership team identified the key cloud capabilities most relevant for them. For each capability, they assigned a go-to person, or “navigator,” from within the leadership team to help the rest of the organization quickly and efficiently advance in that capability.
It is critical for business leaders to understand the potential of cloud and inspire senior leadership to think about the “art of the possible.” It is the first step in what is often an intense learning and change-management process, but making that investment now, before individual companies are overtaken by faster-acting competitors, is essential for companies to compete effectively and sustainably.
Sanjay Kaniyar is a partner in McKinsey’s Boston office, where Binu Sudhakaran is an associate partner. Mathew Lee is a partner in the Miami office, Ani Majumder is a partner in the New York office, and Steve Van Kuiken is a senior partner in the New Jersey office.
The authors wish to thank Ritesh Agarwal, Ramnath Balasubramanian, Sven Blumberg, Mark Gu, James Kaplan, Krish Krishnakanthan, and Neha Sahgal for their contributions to this article.
Explore a career with us
Related articles.

Cloud’s trillion-dollar prize is up for grabs
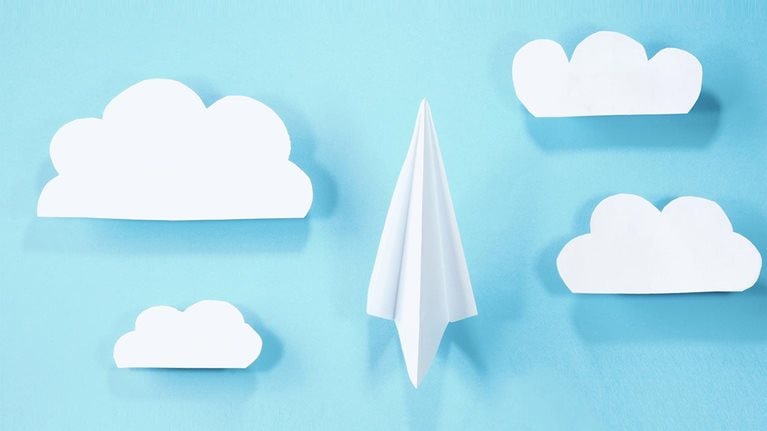
Four ways boards can shape the cloud agenda
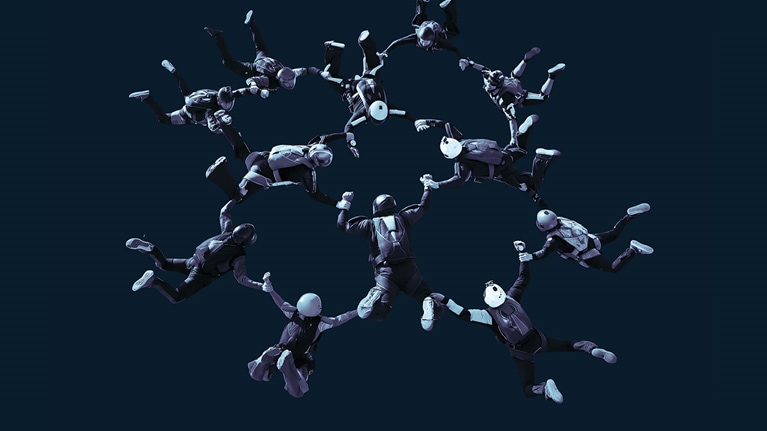
Three actions CEOs can take to get value from cloud computing
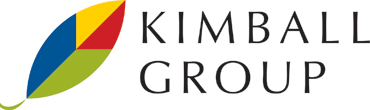
- Data Warehouse Insurance
- By Ralph Kimball
- December 1, 1995

Insurance is an important and growing sector for the data warehousing market. Several factors have come together in the last year or two to make data warehouses for large insurance companies both possible and extremely necessary. Insurance companies generate several complicated transactions that must be analyzed in many different ways. Until recently, it wasn’t practical to consider storing hundreds of millions — or even billions — of transactions for online access. With the advent of powerful SMP and MPP Unix processors and powerful database query software, these big complicated databases have begun to enter the comfort zone for data warehousing. At the same time, the insurance industry is under incredible pressure to reduce costs. Costs in this business come almost entirely from claims or “losses,” as the insurance industry more accurately describes them.
The design of a big insurance data warehouse must deal with several issues common to all insurance companies. This month, I use InsureCo as a case study to illustrate these issues and show how to resolve them in a data warehouse environment. InsureCo is the pseudonym of a major insurance company that offers automobile, homeowner’s, and personal property insurance to about two million customers. InsureCo has annual revenues of more than $2 billion. My company designed InsureCo’s corporate data warehouse for analyzing all claims across all its lines of business, with history in some cases stretching back more than 15 years.
The first step at InsureCo was to spend two weeks interviewing prospective end users in claims analysis, claims processing, field operations, fraud and security management, finance, and marketing. We talked to more than 50 users, ranging from individual contributors to senior management. From each group of users we elicited descriptions of what they did in a typical day, how they measured the success of what they did, and how they thought they could understand their businesses better. We did not ask them what they wanted in a computerized database. It was our job to design, not theirs.
From these interviews we found three major themes that profoundly affected our design. First, to understand their claims in detail, the users needed to see every possible transaction. This precluded presenting summary data only. Many end-user analyses required the slicing and dicing of the huge pool of transactions.
Second, the users needed to view the business in monthly intervals. Claims needed to be grouped by month, and compared at month’s end to other months of the same year, or to months in previous years. This conflicted with the need to store every transaction, because it was impractical to roll-up complex sequences of transactions just to get monthly premiums and monthly claims payments. Third, we needed to deal with the heterogeneous nature of InsureCo’s lines of business. The facts recorded for an automobile accident claim are different than those recorded for a homeowner’s fire loss claim or for a burglary claim.
These data conflicts arise in many different industries, and are familiar themes for data warehouse designers. The conflict between the detailed transaction view and the monthly snapshot view almost always requires that you build both kinds of tables in the data warehouse. We call these the transaction views and monthly snapshot views of a business. Note that we are not referring to SQL views here, but to physical tables. The need to analyze the entire business across all products (lines of business in InsureCo’s case) versus the need to analyze a specific product with unique measures is called the “heterogeneous products” problem. At InsureCo, we first tackled the transaction and monthly snapshot views of the business by carefully dimensionalizing the base-level claims processing transactions. Every claims processing transaction was able to fit into the star join schema.
This structure is characteristic of transaction-level data warehouse schemas. The central transaction-level fact table consists almost entirely of keys. Transaction fact tables typically have only one additive fact, which we call Amount. The interpretation of the Amount field depends on the transaction type, which is identified in the transaction dimension. The Time dimension is actually two instances of the same dimension table connecting to the fact table to provide independent constraints on the Transaction Date and the Effective Date.
This transaction-level star join schema provided an extremely powerful way for InsureCo to analyze claims. The number of claimants, the timing of claims, the timing of payments made, and the involvement of third parties, such as witnesses and lawyers, were all easily derived from this view of the data. Strangely enough, it was somewhat difficult to derive “claim-to-date” measures, such as monthly snapshots, because of the need to crawl through every detailed transaction from the beginning of history. The solution was to add to InsureCo’s data warehouse a monthly snapshot version of the data. The monthly snapshot removed some of the dimensions, while adding more facts.
The grain of this monthly snapshot fact table was the monthly activity of each claimant’s claim against InsureCo’s insured party. Several of the transaction schema dimensions were suppressed in this monthly snapshot, including Effective Date, Employee, Third Party, and Transaction Type. However, it was important to add a Status dimension to the monthly snapshot so that InsureCo could quickly find all open, closed, and reopened claims. The list of additive, numeric facts was expanded to include several useful measures. These include the amount of the reserve set aside to pay for a claim, amounts paid and received during the month, and an overall count of the transaction activity for this claim. This monthly snapshot schema was extremely useful at InsureCo as a way to rapidly analyze the month-to-month changes in claims and exposure to loss. Monthly snapshot tables were very flexible because interesting summaries could be added as facts, almost at will. Of course, we could never add enough summary buckets to do away with the need for the transaction schema itself. There are hundreds of detailed measures, representing combinations and counts and timings of interesting transactions, all of which would be suppressed if we didn’t preserve the detailed transaction history.
After dispensing with the first big representation problem, we faced the problem of how to deal with heterogeneous products. This problem arose primarily in the monthly snapshot fact table, in which we wanted to store additional monthly summary measures specific to each line of business. These additional measures included automobile coverage, homeowner’s fire coverage, and personal article loss coverage. After talking to the insurance specialists in each line of business, we realized that there were at least 10 custom facts for each line of business. Logically, our fact table design could be extended to include the custom facts for each line of business, but physically we had a disaster on our hands.
Because the custom facts for each line of business were incompatible with each other, for any given monthly snapshot record, most of the fact table was filled with nulls. Only the custom facts for the particular line of business were populated in any given record. The answer was to separate physically the monthly snapshot fact table by coverage type. We ended up with a single core monthly snapshot schema, and a series of custom monthly snapshot schemas, one for each coverage type.
A key element of this design was the repetition of the core facts in each of the custom schemas. This is sometimes hard for a database designer to accept, but it is very important. The core schema is the one InsureCo uses when analyzing the business across different coverage types. Those kinds of analyses use only the core table. InsureCo uses the Automobile Custom schema when analyzing the automobile segment of the business. When performing detailed analyses within the automobile line of business, for example, it is important to avoid linking to the core fact table to get the core measures such as amounts paid and amounts received. In these large databases, it is very dangerous to access more than one fact table at a time. It is far better, in this case, to repeat a little of the data in order to keep the users’ queries confined to single fact tables.
The data warehouse we built at InsureCo is a classic example of a large data warehouse that has to accommodate the conflicting needs for detailed transaction history, high-level monthly summaries, company-wide views, and individual lines of business. We used standard data warehouse design techniques, including transaction views and monthly snapshot views, as well as heterogeneous product schemas to address InsureCo’s needs. This dimensional data warehouse gives the company many interesting ways to view its data.
About the Author: Ralph Kimball
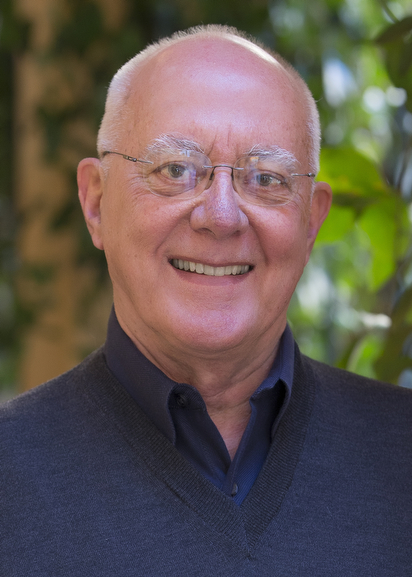
Ralph Kimball is the founder of the Kimball Group and Kimball University where he has taught data warehouse design to more than 10,000 students. He is known for the best selling series of Toolkit books. He started with a Ph.D. in man-machine systems from Stanford in 1973 and has spent nearly four decades designing systems for users that are simple and fast.
Data Consulting & Analytics Experts

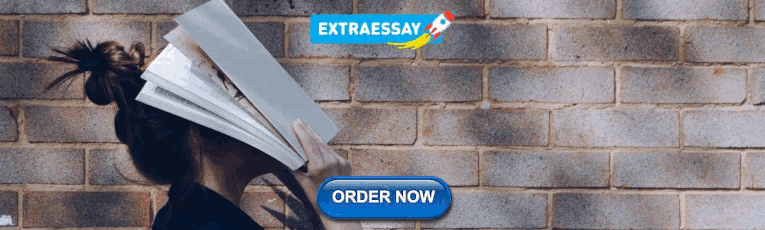
Select a Region
Choose the region closest to you for relevant content and targeted services
Loading metrics
Open Access
Good practices for clinical data warehouse implementation: A case study in France
* E-mail: [email protected]
Affiliations Mission Data, Haute Autorité de Santé, Saint-Denis, France, Inria, Soda team, Palaiseau, France

Affiliation Mission Data, Haute Autorité de Santé, Saint-Denis, France
Affiliations Univ. Lille, CHU Lille, ULR 2694—METRICS: Évaluation des Technologies de santé et des Pratiques médicales, Lille, France, Fédération régionale de recherche en psychiatrie et santé mentale (F2RSM Psy), Hauts-de-France, Saint-André-Lez-Lille, France
Affiliation Sorbonne Université, Inserm, Université Sorbonne Paris-Nord, Laboratoire d’informatique médicale et d’ingénierie des connaissances en e-Santé, LIMICS, France
- Matthieu Doutreligne,
- Adeline Degremont,
- Pierre-Alain Jachiet,
- Antoine Lamer,
- Xavier Tannier
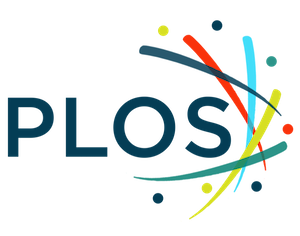
Published: July 6, 2023
- https://doi.org/10.1371/journal.pdig.0000298
- Reader Comments
29 Sep 2023: Doutreligne M, Degremont A, Jachiet PA, Lamer A, Tannier X (2023) Correction: Good practices for clinical data warehouse implementation: A case study in France. PLOS Digital Health 2(9): e0000369. https://doi.org/10.1371/journal.pdig.0000369 View correction
Real-world data (RWD) bears great promises to improve the quality of care. However, specific infrastructures and methodologies are required to derive robust knowledge and brings innovations to the patient. Drawing upon the national case study of the 32 French regional and university hospitals governance, we highlight key aspects of modern clinical data warehouses (CDWs): governance, transparency, types of data, data reuse, technical tools, documentation, and data quality control processes. Semi-structured interviews as well as a review of reported studies on French CDWs were conducted in a semi-structured manner from March to November 2022. Out of 32 regional and university hospitals in France, 14 have a CDW in production, 5 are experimenting, 5 have a prospective CDW project, 8 did not have any CDW project at the time of writing. The implementation of CDW in France dates from 2011 and accelerated in the late 2020. From this case study, we draw some general guidelines for CDWs. The actual orientation of CDWs towards research requires efforts in governance stabilization, standardization of data schema, and development in data quality and data documentation. Particular attention must be paid to the sustainability of the warehouse teams and to the multilevel governance. The transparency of the studies and the tools of transformation of the data must improve to allow successful multicentric data reuses as well as innovations in routine care.
Author summary
Reusing routine care data does not come free of charges. Attention must be paid to the entire life cycle of the data to create robust knowledge and develop innovation. Building upon the first overview of CDWs in France, we document key aspects of the collection and organization of routine care data into homogeneous databases: governance, transparency, types of data, data reuse main objectives, technical tools, documentation, and data quality control processes. The landscape of CDWs in France dates from 2011 and accelerated in the late 2020, showing a progressive but still incomplete homogenization. National and European projects are emerging, supporting local initiatives in standardization, methodological work, and tooling. From this sample of CDWs, we draw general recommendations aimed at consolidating the potential of routine care data to improve healthcare. Particular attention must be paid to the sustainability of the warehouse teams and to the multilevel governance. The transparency of the data transformation tools and studies must improve to allow successful multicentric data reuses as well as innovations for the patient.
Citation: Doutreligne M, Degremont A, Jachiet P-A, Lamer A, Tannier X (2023) Good practices for clinical data warehouse implementation: A case study in France. PLOS Digit Health 2(7): e0000298. https://doi.org/10.1371/journal.pdig.0000298
Editor: Dukyong Yoon, Yonsei University College of Medicine, REPUBLIC OF KOREA
Copyright: © 2023 Doutreligne et al. This is an open access article distributed under the terms of the Creative Commons Attribution License , which permits unrestricted use, distribution, and reproduction in any medium, provided the original author and source are credited.
Funding: MD, AD, PAJ salaries were funded by the French Haute Autorité de Santé (HAS). XT received fundings to participate in interviews and participate to the article redaction. AL received no fundings for this study. The funders validated the study original idea and the study conclusions. The funders had no role in study design, data collection and analysis, decision to publish, or preparation of the manuscript.
Competing interests: I have read the journal’s policy and the authors of this manuscript have the following competing interests: The first author did a (non-paid) visiting in Leo Anthony Celi’s lab during the first semester of 2023.
Introduction
Real-world data.
Health information systems (HIS) are increasingly collecting routine care data [ 1 – 7 ]. This source of real-world data (RWD) [ 8 ] bears great promises to improve the quality of care. On the one hand, the use of this data translates into direct benefits—primary uses—for the patient by serving as the cornerstone of the developing personalized medicine [ 9 , 10 ]. They also bring indirect benefits—secondary uses—by accelerating and improving knowledge production: on pathologies [ 11 ], on the conditions of use of health products and technologies [ 12 , 13 ], on the measures of their safety [ 14 ], efficacy or usefulness in everyday practice [ 15 ]. They can also be used to assess the organizational impact of health products and technologies [ 16 , 17 ].
In recent years, health agencies in many countries have conducted extensive work to better support the generation and use of real-life data [ 8 , 17 – 19 ]. Study programs have been launched by regulatory agencies: the DARWIN EU program by the European Medicines Agency and the Real World Evidence Program by the Food and Drug Administration [ 20 ].
Clinical data warehouse
In practice, the possibility of mobilizing these routinely collected data depends very much on their degree of concentration, in a gradient that goes from centralization in a single, homogenous HIS to fragmentation in a multitude of HIS with heterogeneous formats. The structure of the HIS reflects the governance structure. Thus, the ease of working with these data depends heavily on the organization of the healthcare actors. The 2 main sources of RWD are insurance claims—more centralized—and clinical data—more fragmented.
Claims data is often collected by national agencies into centralized repositories. In South Korea, the government agency responsible for healthcare system performance and quality (HIRA) is connected to the HIS of all healthcare stakeholders. HIRA data consists of national insurance claims [ 21 ]. England has a centralized healthcare system under the National Health Service (NHS). Despite not having detailed clinical data, this allowed the NHS to merge claims data with detailed data from 2 large urban medicine databases, corresponding to the 2 major software publishers [ 22 ]. This data is currently accessed through Opensafely, a first platform focused on Coronavirus Disease 2019 (COVID-19) research [ 23 ]. In the United States, even if scattered between different insurance providers, claims are pooled into large databases such as Medicare, Medicaid, or IBM MarketScan. Lastly, in Germany, the distinct federal claims have been centralized only very recently [ 24 ].
Clinical data on the other hand, tends to be distributed among many entities, that made different choices, without common management or interoperability. But large institutional data-sharing networks begin to emerge. South Korea very recently launched an initiative to build a national wide data network focused on intensive care. United States is building Chorus4ai, an analysis platform pooling data from 14 university hospitals [ 25 ]. To unlock the potential of clinical data, the German Medical Informatics Initiative [ 26 ] created 4 consortia in 2018. They aim at developing technical and organizational solutions to improve the consistency of clinical data.
Israel stands out as one of the rare countries that pooled together both claims and clinical data at a large scale: half of the population depends on 1 single healthcare provider and insurer [ 27 ].
An infrastructure is needed to pool data data from 1 or more medical information systems—whatever the organizational framework—to homogeneous formats, for management, research, or care reuses [ 28 , 29 ]. Fig 1 illustrates for a CDW, the 4 phases of data flow from the various sources that make up the HIS:
- Collection and copying of original sources.
- Integration of sources into a unique database.
- Deduplication of identifiers.
- Standardization: A unique data model, independent of the software models harmonizes the different sources in a common schema, possibly with common nomenclatures.
- Pseudonymization: Removal of directly identifying elements.
- Provision of subpopulation data sets and transformed datamarts for primary and secondary reuse.
- Usages thanks to dedicated applications and tools accessing the datamarts and data sets.
In France, the national insurer collects all hospital activity and city care claims into a unique reimbursement database [ 13 ]. However, clinical data is historically scattered at each care site in numerous HISs. Several hospitals deployed efforts for about 10 years to create CDWs from electronic medical records [ 30 – 39 ]. This work has accelerated recently, with the beginning of CDWs structuring at the regional and national levels. Regional cooperation networks are being set up—such as the Ouest Data Hub [ 40 ]. In July 2022, the Ministry of Health opened a 50 million euros call for projects to set up and strengthen a network of hospital CDWs coordinated with the national platform, the Health Data Hub by 2025.
- PPT PowerPoint slide
- PNG larger image
- TIFF original image
CDW: Four steps of data flow from the Hospital Information System: (1) collection, (2) transformations, and (3) provisioning. CDW, clinical data warehouse.
https://doi.org/10.1371/journal.pdig.0000298.g001
Based on an overview of university hospital CDWs in France, this study makes general recommendations for properly leveraging the potential of CDWs to improve healthcare. It focuses on: governance, transparency, types of data, data reuse, technical tools, documentation, and data quality control processes.
Material and methods
Interviews were conducted from March to November 2022 with 32 French regional and university hospitals, both with existing and prospective CDWs.
Ethics statement
This work has been authorized by the board of the French High Authority of Health (HAS). Every interviewed participant was asked by email for their participation and informed on the possible forms of publication: a French official report and an international publication. Furthermore, at each interview, every participant has been asked for their agreement before recording the interview. Only 1 participant refused the video to be recorded.
Semi-structured interviews were conducted on the following themes: the initiation and construction of the CDWs, the current status of the project and the studies carried out, opportunities and obstacles, and quality criteria for observational research. S1 Table lists all interviewed people with their team title. The complete form, with the precised questions, is available in S2 Table .
The interview form was sent to participants in advance and then used as a support to conduct the interviews. The interviews lasted 90 min and were recorded for reference.
Quantitative methods
Three tables detailed the structured answers in S1 Text . The first 2 tables deal with the characteristics of the actors and those of the data warehouses. We completed them based on the notes taken during the interviews, the recordings, and by asking the participants for additional information. The third table focuses on ongoing studies in the CDWs. We collected the list of these studies from the dedicated reporting portals, which we found for 8 out of 14 operational CDWs. We developed a classification of studies, based on the typology of retrospective studies described by the OHDSI research network [ 41 ]. We enriched this typology by comparing it with the collected studies resulting in the 6 following categories:
- Outcome frequency : Incidence or prevalence estimation for a medically well-defined target population.
- Population characterization : Characterization of a specific set of covariates. Feasibility and prescreening studies belong to this category [ 42 ].
- Risk factors : Identification of covariates most associated with a well-defined clinical target (disease course, care event). These studies look at association study without quantifying the causal effect of the factors on the outcome of interest.
- Treatment effect : Evaluation of the effect of a well-defined intervention on a specific outcome target. These studies intend to show a causal link between these 2 variables [ 43 ].
- Development of diagnostic and prognostic algorithms : Improve or automate a diagnostic or prognostic process, based on clinical data from a given patient. This can take the form of a risk, a preventive score, or the implementation of a diagnostic assistance system. These studies are part of the individualized medicine approach, with the goal of inferring relevant information at the level of individual patient’s files.
- Medical informatics : Methodological or tool oriented. These studies aim to improve the understanding and capacity for action of researchers and clinicians. They include the evaluation of a decision support tool, the extraction of information from unstructured data, or automatic phenotyping methods.
Studies were classified according to this nomenclature based on their title and description.
Fig 2 summarizes the development state of progress of CDWs in France. Out of 32 regional and university hospitals in France, 14 have a CDW in production, 5 are experimenting, 5 have a prospective CDW project, 8 did not have any CDW project at the time of writing. The results are described for all projects that are at least in the prospective stage minus the 3 that we were unable to interview after multiple reminders (Orléans, Metz, and Caen), resulting in a denominator of 21 university hospitals.
Base map and data from OpenStreetMap and OpenStreetMap Foundation. Link to the base layer of the map: https://github.com/mapnik/mapnik . CDW, clinical data warehouse.
https://doi.org/10.1371/journal.pdig.0000298.g002
Fig 3 shows the history of the implementation of CDWs. A distinction must be made between the first works—in blue—, which systematically precede the regulatory authorization—in green—from the French Commission on Information Technology and Liberties (CNIL).
CDW, clinical data warehouse.
https://doi.org/10.1371/journal.pdig.0000298.g003
The CDWs have so far been initiated by 1 or 2 people from the hospital world with an academic background in bioinformatics, medical informatics, or statistics. The sustainability of the CDW is accompanied by the construction of a cooperative environment between different actors: Medical Information Department (MID), Information Systems Department (IT), Clinical Research Department (CRD), clinical users, and the support of the management or the Institutional Medical Committee. It is also accompanied by the creation of a team, or entity, dedicated to the maintenance and implementation of the CDW. More recent initiatives, such as those of the HCL (Hospitals of the city of Lyon) or the Grand-Est region, are distinguished by an initial, institutional, and high-level support.
The CDW has a federating potential for the different business departments of the hospital with the active participation of the CRD, the IT Department, and the MID. Although there is always an operational CDW team, the human resources allocated to it vary greatly: from half a full-time equivalent to 80 people for the AP-HP, with a median of 6.0 people. The team systematically includes a coordinating physician. It is multidisciplinary with skills in public health, medical informatics, informatics (web service, database, network, infrastructure), data engineering, and statistics.
Historically, the first CDWs were based on in-house solution development. More recently, private actors are offering their services for the implementation and implementation of CDWs (15/21). These services range from technical expertise in order to build up the data flows and data cleaning up to the delivery of a platform integrating the different stages of data processing.
Management of studies
Before starting, projects are systematically analyzed by a scientific and ethical committee. A local submission and follow-up platform is often mentioned (12/21), but its functional scope is not well defined. It ranges from simple authorization of the project to the automatic provision of data into a Trusted Research Environment (TRE) [ 44 ]. The processes for starting a new project on the CDW are always communicated internally but rarely documented publicly (8/21).
Transparency
Ongoing studies in CDWs are unevenly referenced publicly on hospital websites. Some institutions have comprehensive study portals, while others list only a dozen studies on their public site while mentioning several hundreds ongoing projects during interviews. In total, we found 8 of these portals out of 14 CDWs in production. Uses other than ongoing scientific studies are very rarely documented. The publication of the list of ongoing studies is very heterogeneous and fragmented between several sources: clinicaltrials.gov, the mandatory project portal of the Health Data Hub [ 45 ] or the website of the hospital data warehouse.
Strong dependance to the HIS.
CDW data reflect the HIS used on a daily basis by hospital staff. Stakeholders point out that the quality of CDW data and the amount of work required for rapid and efficient reuse are highly dependent on the source HIS. The possibility of accessing data from an HIS in a structured and standardized format greatly simplifies its integration into the CDW and then its reuse.
Categories of data.
Although the software landscape is varied across the country, the main functionalities of HIS are the same. We can therefore conduct an analysis of the content of the CDWs, according to the main categories of common data present in the HIS.
The common base for all CDWs is constituted by data from the Patient Administrative Management software (patient identification, hospital movements) and the billing codes. Then, data flows are progressively developed from the various softwares that make up the HIS. The goal is to build a homogeneous data schema, linking the sources together, controlled by the CDW team. The prioritization of sources is done through thematic projects, which feed the CDW construction process. These projects improve the understanding of the sources involved, by confronting the CDW team with the quality issues present in the data.
Table 1 presents the different ratio of data categories integrated in French CDWs. Structured biology and texts are almost always integrated (20/21 and 20/21). The texts contain a large amount of information. They constitute unstructured data and are therefore more difficult to use than structured tables. Other integrated sources are the hospital drug circuit (prescriptions and administration, 16/21), Intense Care Unit (ICU, 2/21), or nurse forms (4/21). Imaging is rarely integrated (4/21), notably for reasons of volume. Genomic data are well identified, but never integrated, even though they are sometimes considered important and included in the CDW work program.
https://doi.org/10.1371/journal.pdig.0000298.t001
Data reuse.
Today, the main use put forward for the constitution of CDWs is that of scientific research.
The studies are mainly observational (non-interventional). Fig 4 presents the distribution of the 6 categories defined in Quantitative methods for 231 studies collected on the study portals of 9 hospitals. The studies focus first on population characterization (25%), followed by the development of decision support processes (24%), the study of risk factors (18%), and the treatment effect evaluations (16%).
https://doi.org/10.1371/journal.pdig.0000298.g004
The CDWs are used extensively for internal projects such as student theses (at least in 9/21) and serve as an infrastructure for single-service research: their great interest being the de-siloing of different information systems. For most of the institutions interviewed, there is still a lack of resources and maturity of methods and tools for conducting inter-institutional research (such as in the Grand-Ouest region of France) or via European calls for projects (EHDEN). These 2 research networks are made possible by supra-local governance and a common data schema, respectively, eHop [ 46 ] and OMOP [ 47 ]. The Paris hospitals, thanks to its regional coverage and the choice of OMOP, is also well advanced in multicentric research. At the same time, the Grand-Est region is building a network of CDW based on the model of the Grand-Ouest region, also using eHop.
CDW are used for monitoring and management (16/21).
The CDW have sometimes been initiated to improve and optimize billing coding (4/21). The clinical texts gathered in the same database are queried using keywords to facilitate the structuring of information. The data are then aggregated into indicators, some of which are reported at the national level. The construction of indicators from clinical data can also be used for the administrative management of the institution. Finally, closer to the clinic, some actors state that the CDW could also be used to provide regular and appropriate feedback to healthcare professionals on their practices. This feedback would help to increase the involvement and interest of healthcare professionals in CDW projects. The CDW is sometimes of interest for health monitoring (e.g., during COVID-19) or pharmacovigilance (13/21).
Strong interest for CDW in the context of care (13/21).
Some CDWs develop specific applications that provide new functionalities compared to care software. Search engines can be used to query all the hospital’s data gathered in the CDW, without data compartmentalization between different softwares. Dedicated interfaces can then offer a unified view of the history of a patient’s data, with inter-specialty transversality, which is particularly valuable in internal medicine. These cross-disciplinary search tools also enable healthcare professionals to conduct rapid searches in all the texts, for example, to find similar patients [ 32 ]. Uses for prevention, automation of repetitive tasks, and care coordination are also highlighted. Concrete examples are the automatic sorting of hospital prescriptions by order of complexity or the setting up of specialized channels for primary or secondary prevention.
Technical architecture
The technical architecture of modern CDWs has several layers:
- Data processing: connection and export of source data, diverse transformation (cleaning, aggregation, filtering, standardization).
- Data storage: database engines, file storage (on file servers or object storage), indexing engines to optimize certain queries.
- Data exposure: raw data, APIs, dashboards, development and analysis environments, specific web applications.
Supplementary cross-functional components ensure the efficient and secure operation of the platform: identity and authorization management, activity logging, automated administration of servers and applications.
The analysis environment (Jupyterhub or RStudio datalabs) is a key component of the platform, as it allows data to be processed within the CDW infrastructure. A few CDWs had such operational datalab at the time of our study (6/21) and almost all of them have decided to provide it to researchers. Currently, clinical research teams are still often working on data extractions in less secure environments.
Data quality, standard formats
Quality tools..
Systematic data quality monitoring processes are being built in some CDWs. Often (8/21), scripts are run at regular intervals to detect technical anomalies in data flows. Rare data quality investigation tools, in the form of dashboards, are beginning to be developed internally (3/21). Theoretical reflections are underway on the possibility of automating data consistency checks, for example, demographic or temporal. Some facilities randomly pull records from the EHR to compare them with the information in the CDW.
Standard format.
No single standard data model stands out as being used by all CDWs. All are aware of the existence of the OMOP (research standard) [ 47 ] and HL7 FHIR (communication standard) models [ 48 ]. Several CDWs consider the OMOP model to be a central part of the warehouse, particularly for research purposes (9/21). This tendency has been encouraged by the European call for projects EHDEN, launched by the OHDSI research consortium, the originator of this data model. In the Grand-Ouest region of France, the CDWs use the eHop warehouse software. The latter uses a common data model also named eHop. This model will be extended with the future warehouse network of the Grand Est region also choosing this solution. Including this grouping and the other establishments that have chosen eHop, this model includes 12 establishments out of the 32 university hospitals. This allows eHop adopters to launch ambitious interregional projects. However, eHop does not define a standard nomenclature to be used in its model and is not aligned with emerging international standards.
Documentation.
Half of the CDWs have put in place documentation accessible within the organization on data flows, the meaning and proper use of qualified data (10/21 mentioned). This documentation is used by the team that develops and maintains the warehouse. It is also used by users to understand the transformations performed on the data. However, it is never publicly available. No schema of the data once it has been transformed and prepared for analysis is published.
Principal findings
We give the first overview of the CDWs in university hospitals of France with 32 hospitals reviewed. The implementation of CDW dates from 2011 and accelerated in the late 2020. Today, 24 of the university hospitals have an ongoing CDW project. From this case study, some general considerations can be drawn that should be valuable to all healthcare system implementing CDWs on a national scale.
As the CDW becomes an essential component of data management in the hospital, the creation of an autonomous internal team dedicated to data architecture, process automation, and data documentation should be encouraged [ 44 ]. This multidisciplinary team should develop an excellent knowledge of the data collection process and potential reuses in order to qualify the different flows coming from the source IS, standardize them towards a homogenous schema and harmonize the semantics. It should have a sound knowledge of public health, as well as the technical and statistical skills to develop high-quality software that facilitates data reuse.
The resources specific to the warehouse are rare and often taken from other budgets or from project-based credits. While this is natural for an initial prototyping phase, it does not seem adapted to the perennial and transversal nature of the tool. As a research infrastructure of growing importance, it must have the financial and organizational means to plan for the long term.
The governance of the CDW has multiple layers: local within the university hospital, interregional, and national/international. The first level allow to ensure the quality of data integration as well as the pertinence of data reuse by clinicians themselves. The interregional level is well adapted for resources mutualization and collaboration. Finally, the national and international levels assure coordination, encourage consensus for committing choices such as metadata or interoperability, and provide financial, technical, and regulatory support.
Health technology assessment agencies advocate for public registration of comparative observational study protocols before conducting the analysis [ 8 , 17 , 49 ]. They often refer to clinicaltrials.gov as potential but not ideal registration portal for observational studies. The research community advocates for public registrations of all observational studies [ 50 , 51 ]. More recently, it emphasizes the need for more easy data access and the publication of study code [ 29 , 52 , 53 ]. We embrace these recommendations and we point to the unfortunate duplication of these study reporting systems in France. One source could be favored at the national level and the second one automatically fed from the reference source, by agreeing on common metadata.
From a patient’s perspective, there is currently no way to know if their personal data is included for a specific project. Better patient information about the reuse of their data is needed to build trust over the long term. A strict minimum is the establishment and update of the declarative portals of ongoing studies at each institution.
Data and data usage
When using CDW, the analyst has not defined the data collection process and is generally unaware of the context in which the information is logged. This new dimension of medical research requires a much greater development of data science skills to change the focus from the implementation of the statistical design to the data engineering process. Data reuse requires more effort to prepare the data and document the transformations performed.
The more heterogeneous a HIS system is, the less qualitative would be the CDW built on top of it. There is a need for increasing interoperability, to help EHR vendors interfacing the different hospital softwares, thus facilitating CDW development. One step in this direction would be the open source publication of HIS data schema and vocabularies. At the analysis level, international recommendations insist on the need for common data formats [ 52 , 54 ]. However, there is still a lack of adoption of research standards from hospital CDWs to conduct robust studies across multiple sites. Building open-source tools on top of these standards such as those of OHDSI [ 41 ] could foster their adoption. Finally, in many clinical domains, sufficient sample size is hard to obtain without international data-sharing collaborations. Thus, more incitation is needed to maintain and update the terminology mappings between local nomenclatures and international standards.
Many ongoing studies concern the development of decision support processes whose goal is to save time for healthcare professionals. These are often research projects, not yet integrated into routine care. The analysis of study portals and the interviews revealed that data reuse oriented towards primary care is still rare and rarely supported by appropriate funding. The translation from research to clinical practice takes time and need to be supported on the long run to yield substantial results.
Tools, methods, and data formats of CDW lack harmonization due to the strong technical innovation and the presence of many actors. As suggested by the recent report on the use of data for research in the UK [ 44 ], it would be wise to focus on a small number of model technical platforms.
These platforms should favor open-source solutions to assure transparency by default, foster collaboration and consensus, and avoid technological lock-in of the hospitals.
Data quality and documentation
Quality is not sufficiently considered as a relevant scientific topic itself. However, it is the backbone of all research done within a CDW. In order to improve the quality of the data with respect to research uses, it is necessary to conduct continuous studies dedicated to this topic [ 52 , 54 – 56 ]. These studies should contribute to a reflection on methodologies and standard tools for data quality, such as those developed by the OHDSI research network [ 41 ].
Finally, there is a need for open-source publication of research code to ensure quality retrospective research [ 55 , 57 ]. Recent research in data analysis has shown that innumerable biases can lurk in training data sets [ 58 , 59 ]. Open publication of data schemas is considered an indispensable prerequisite for all data science and artificial intelligence uses [ 58 ]. Inspired by data set cards [ 58 ] and data set publication guides, it would be interesting to define a standard CDW card documenting the main data flows.
Limitations
The interviews were conducted in a semi-structured manner within a limited time frame. As a result, some topics were covered more quickly and only those explicitly mentioned by the participants could be recorded. The uneven existence of study portals introduces a bias in the recording of the types of studies conducted on CDW. Those with a transparency portal already have more maturity in use cases.
For clarity, our results are focused on the perimeter of university hospitals. We have not covered the exhaustive healthcare landscape in France. CDW initiatives also exist in primary care, in smaller hospital groups and in private companies.
Conclusions
The French CDW ecosystem is beginning to take shape, benefiting from an acceleration thanks to national funding, the multiplication of industrial players specializing in health data and the beginning of a supra-national reflection on the European Health Data Space [ 60 ]. However, some points require special attention to ensure that the potential of the CDW translates into patient benefits.
The priority is the creation and perpetuation of multidisciplinary warehouse teams capable of operating the CDW and supporting the various projects. A combination of public health, data engineering, data stewardship, statistics, and IT competences is a prerequisite for the success of the CDW. The team should be the privileged point of contact for data exploitation issues and should collaborate closely with the existing hospital departments.
The constitution of a multilevel collaboration network is another priority. The local level is essential to structure the data and understand its possible uses. Interregional, national, and international coordination would make it possible to create thematic working groups in order to stimulate a dynamic of cooperation and mutualization.
A common data model should be encouraged, with precise metadata allowing to map the integrated data, in order to qualify the uses to be developed today from the CDWs. More broadly, open-source documentation of data flows and transformations performed for quality enhancement would require more incentives to unleash the potential for innovation for all health data reusers.
Finally, the question of expanding the scope of the data beyond the purely hospital domain must be asked. Many risk factors and patient follow-up data are missing from the CDWs, but are crucial for understanding pathologies. Combining city data and hospital data would provide a complete view of patient care.
Supporting information
S1 table. list of interviewed stakeholders with their teams..
https://doi.org/10.1371/journal.pdig.0000298.s001
S2 Table. Interview form.
https://doi.org/10.1371/journal.pdig.0000298.s002
S1 Text. Study data tables.
https://doi.org/10.1371/journal.pdig.0000298.s003
Acknowledgments
We want to thanks all participants and experts interviewed for this study. We also want to thanks other people that proof read the manuscript for external review: Judith Fernandez (HAS), Pierre Liot (HAS), Bastien Guerry (Etalab), Aude-Marie Lalanne Berdouticq (Institut Santé numérique en Société), Albane Miron de L’Espinay (ministère de la Santé et de la Prévention), and Caroline Aguado (ministère de la Santé et de la Prévention). We also thank Gaël Varoquaux for his support and advice.
- View Article
- PubMed/NCBI
- Google Scholar

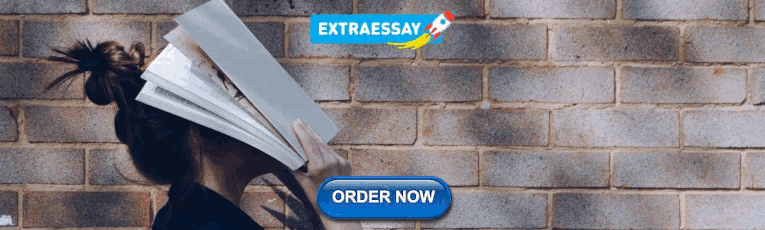
IMAGES
VIDEO
COMMENTS
CASE STUDY Data Warehouse Accelerates Compliance, Powers Business Intelligence at Insurance Company Challenges An insurance company began building a data warehouse as the keystone in its MAR compliance program. After a rocky year and a half, the effort completely stalled and the company retained Princeton Consultants to get it back on track.
Use ninestep methodology as the method for design data warehouse and Pentaho as a tool for ETL (Extract, Transform, Load), OLAP analysis and reporting data, the results of this research concluded that the implementation of data warehouse for perform data analysis and reporting better and more effective. Export citation and abstract BibTeX RIS.
The data warehouse has the highest adoption of data solutions, used by 54% of organizations. (Flexera 2021) Data Warehousing for Insurance: Creating a Single Source of Truth. Insurance companies generate and receive large amounts of data from various business functions and subsidiaries that are stored in disparate systems and in a variety of ...
Phase 1—Define aspiration and set vision. The first step in shaping a "data as a business" strategy is for an organization's senior leaders to define a compelling aspiration for the new business. Given the enormous economic potential the data hold, the aspiration should be bold and include business-backed, strategic use cases.
The stock-portfolio data for life and property and casualty insurance, all accounting entries (e.g. premiums, benefits, commissions, expenses) and the data concerning our sales organization and customers are regularly infiled. The data from life insurance is completely transformed from the ODD-level to the Central Warehouse.
The 7 Advantages of a Data Warehouse for Analytics and Reporting. Empowers everyone throughout the organization with the information they need to make correct decisions. Enables self-service analytics for diving deeper into the data. Illuminates data and business process issues that need improving.
in practice: An insurance case study Received (in revised form): 28th April, 2003 Edith N. Pula ... data warehouse and marketing database build, data analysis and data mining for customer segmentation and scoring, profitability and risk management (including Basel II) and integrated customer campaign communications, including contact ...
data warehouse. This combined data resource is called the logical data warehouse. As with all complex databases it is vital that a single data model is used to understand, access and govern. IBM Insurance Information Warehouse is ideally suited to this task of data warehouse augmentation as it is implementation neutral and can be deployed on a ...
An event-driven Data pipeline system is scheduled to initiate on new data arrival. Data transformation and consolidation into an Azure Cloud-based data warehouse. Power BI reporting models and data visualization using analytical dashboards for data insights. Shareable data reports in multiple formats. An end-to-end automated solution to save ...
A Data Warehouse is the main repository of an organisation's historical data, its corporate memory. This paper presents a case description into the new IT strategies in general and Data Warehouse ...
The first step in implementing data warehouse is to identify the data that needs to be stored and analysed. In the insurance industry, this could include data on policyholders, claims, premiums, underwriting, and risk assessment. There are many data warehouse tools available, and each has its strengths and weaknesses.
The Data Warehouse Toolkit: The Complete Guide to Dimensional Modeling by. Chapter 15. Insurance. We will bring together concepts from nearly all the previous chapters to build a data warehouse for a property and casualty insurance company in this final case study. If you are from the insurance industry and jumped directly to this chapter for a ...
Organized all data informationsquelle into one dimensionally modeled data warehouses to support reporting across many subject areas and enable safety of partner data feeds. Implemented a colored insurance data ingestion built, allowing rapid consumption out an unlimited number of datasources while reducing the manual effort mandatory.
Most insurers still vastly undervalue cloud's potential. Our research shows that the EBITDA run-rate impact of cloud on the insurance sector will be $70 billion to $110 billion by 2030—in the top five of all sectors analyzed. When looking at EBITDA impact as a percentage of 2030 EBITDA, insurance is the top-ranked of all sectors, at 43-70 ...
data such as Internet of Things (IoT) data, online data, or bank account / credit card data in order to perform more sophisticated and comprehensive analysis, in a process that is commonly known as 'data enrichment.' The data used by insurance firms in the different stages of the insurance value chain may include personal data. 3 (e.g. 2
The design of a big insurance data warehouse must deal with several issues common to all insurance companies. This month, I use InsureCo as a case study to illustrate these issues and show how to resolve them in a data warehouse environment. InsureCo is the pseudonym of a major insurance company that offers automobile, homeowner's, and ...
Pekin Insurance dramatically gashes costs and democratizes data for speeds decision-making and business agility by migrating yours data to Snowflake's Cloud-based Data Warehouse. Business Need Pekin Indemnity was struggling to derive to right value for the data and reports outstanding to significant data accessibility limitations with their ...
This leading medical insurance company is a reputable organization with a long-standing history of providing comprehensive medical insurance to clients all over Australia. The company has a team of highly experienced professionals and maintains local offices throughout the country. In 2020, the company engaged Altis Consulting to provide a detailed architecture plan for an implementation […]
Author summary Reusing routine care data does not come free of charges. Attention must be paid to the entire life cycle of the data to create robust knowledge and develop innovation. Building upon the first overview of CDWs in France, we document key aspects of the collection and organization of routine care data into homogeneous databases: governance, transparency, types of data, data reuse ...
The goal of this book is to provide a one-stop shop for dimensional modeling techniques. The book is authored by Ralph Kimball and Margy Ross, known worldwide as educators, consultants, and influential thought leaders in data warehousing and business intelligence. The book begins with a primer on data warehousing, business intelligence, and ...
Case Study. European Insurance Company. 2. the encryption solution had to work with the . insurer's data integration software, Informatica . PowerCenter. This is used to extract data from . various sources, transform it, and load it into a new system, such as a data warehouse. Solution. To address these challenges, the insurer turned
Based on my prior experience as Data Engineer and Analyst, I will explain Data Warehousing and Dimensional modeling using an e-Wallet case study. — Manoj. Data Warehouse. A data warehouse is a large collection of business-related historical data that would be used to make business decisions.
A centralised, single-source data warehouse has enabled this insurer to reduce closing cycles by five days with a higher level of confidence and significantly improved data quality. Staff previously assigned to consolidating data sources were retrained to leverage this data via new business intelligence solutions.