- Vol 20, No 1 (2021)
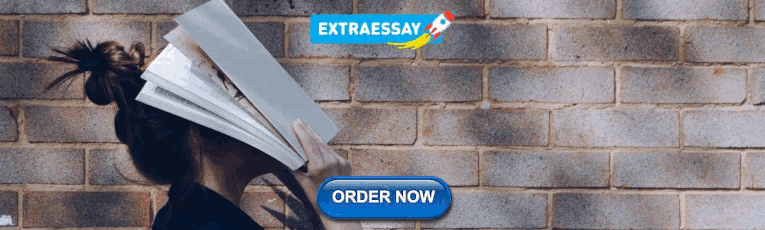
Cryptocurrencies in Modern Finance: A Literature Review
The focus on cryptocurrencies in the finance and banking sectors is gaining momentum. In this paper, we investigate the role of cryptocurrencies in modern finance. We apply a narrative literature review method to synthesize prior research and draw insights into the opportunities and challenges of leveraging cryptocurrencies. The results indicate that cryptocurrencies offer businesses and individuals’ lower transaction costs, higher efficiencies, increased security and privacy, meaningful diversification benefits, alternative financing solutions, and financial inclusion.Challenges exist related to the integration of cryptocurrencies in modern finance. These include the lack of regulatory standards, the risk of criminal activity, high energy and environmental costs, regulatory bans and usage restrictions, security and privacy concerns, and the high volatility of cryptocurrencies.The current review is useful for scholars and managers, including those seeking to have a more balanced understanding of these emerging financial instruments.
JEL Classification: E42, F30, F65, G21, G23
How to Cite:
Rejeb, A., Rejeb, K., & Keogh, J. G. (2021). Cryptocurrencies in Modern Finance: a Literature Review. Etikonomi , 20(1), 93 – 118. https://doi.org/10.15408/etk.v20i1.16911.
Adhami, S., Giudici, G., & Martinazzi, S. (2018). Why Do Businesses Go Crypto? An Empirical Analysis of Initial Coin Offerings. Journal of Economics and Business, 100, 64–75. https://doi.org/10.1016/j.jeconbus.2018.04.001
Afzal, A., & Asif, A. (2019). Cryptocurrencies, Blockchain and Regulation: A Review. The Lahore Journal of Economics, 24(1), 103–130.
Alam, N., & Zameni, A. P. (2019). Existing Regulatory Frameworks of Cryptocurrency and the Shari’ah Alternative. In Billah, M (eds). Halal Cryptocurrency Management, 179–194. London: Palgrave Macmillan.
Alonso, N., & Luis, S. (2019). Activities and Operations with Cryptocurrencies and Their Taxation Implications: The Spanish Case. Laws, 8(3), 1-13.
Alonso-Monsalve, S., Suárez-Cetrulo, A. L., Cervantes, A., & Quintana, D. (2020). Convolution on Neural Networks for High-Frequency Trend Prediction of Cryptocurrency Exchange Rates using Technical indicators. Expert Systems with Applications, 149, 113250. https://doi.org/10.1016/j.eswa.2020.113250
Amsden, R., & Schweizer, D. (2018). Are Blockchain Crowdsales the New “Gold Rush”? Success Determinants of Initial Coin Offerings, SSRN Scholarly Paper ID 3163849.
Aslan, A., & Sensoy, A. (2020). Intraday Efficiency-Frequency Nexus in The Cryptocurrency Markets. Finance Research Letters, 35, 101298. https://doi.org/10.1016/j.frl.2019.09.013
Auer, R. (2019). Beyond the Doomsday Economics of “Proof-of-Work” in Cryptocurrencies. SSRN Scholarly Paper ID 3331413.
Avdeychik, V., & Capozzi, J. (2018). SEC’s Division of Investment Management Voices Concerns Over Registered Funds Investing in Cryptocurrencies and Cryptocurrency-Related Products. Journal of Investment Compliance, 19(2), 8–12.
Babkin, A. V., Burkaltseva, D., Pshenichnikov, W., & Tyulin, A. (2017). Cryptocurrency and Blockchain-Technology in Digital Economy: Development Genesis. St. Petersburg State Polytechnical University Journal. Economics, 67(5), 9–22.
Bação, P., Duarte, A. P., Sebastião, H., & Redzepagic, S. (2018). Information Transmission Between Cryptocurrencies: Does Bitcoin Rule the Cryptocurrency World? Scientific Annals of Economics and Business, 65(2), 97–117.
Baldimtsi, F., Kiayias, A., & Samari, K. (2017). Watermarking Public-Key Cryptographic Functionalities and Implementations. In Nguyen, P. Q., & Zhou, J. (Eds.). Information Security, 173–191. Berlin: Springer.
Baldwin, J. (2018). In Digital We Trust: Bitcoin Discourse, Digital Currencies, and Decentralized Network Fetishism. Palgrave Communications, 4(1), 1–10. https://doi.org/10.1057/s41599-018-0065-0
Bartos, J. (2015). Does Bitcoin follow the hypothesis of efficient market? International Journal of Economic Sciences, 4(2), 10–23.
Baumöhl, E. (2019). Are Cryptocurrencies Connected to Forex? A Quantile Cross-Spectral Approach. Finance Research Letters, 29, 363–372. https://doi.org/10.1016/j.frl.2018.09.002
Baur, A. W., Bühler, J., Bick, M., & Bonorden, C. S. (2015). Cryptocurrencies as a Disruption? Empirical Findings on User Adoption and Future Potential of Bitcoin and Co. In Janssen, M., Mäntymäki, M., Hidders, J., Klievink, B., Lamersdorf, W., van Loenen, B., & Zuiderwijk, A. (Eds.), Open and Big Data Management and Innovation, 63–80. Berlin: Springer International Publishing.
Baur, D. G., Hong, K., & Lee, A. D. (2018). Bitcoin: Medium of Exchange or Speculative Assets? Journal of International Financial Markets, Institutions and Money, 54, 177–189. https://doi.org/10.1016/j.intfin.2017.12.004
Bech, M. L., & Garratt, R. (2017). Central Bank Cryptocurrencies. SSRN Scholarly Paper ID 3041906.
Berg, C., Davidson, S., & Potts, J. (2019). Blockchain Technology as Economic Infrastructure: Revisiting the Electronic Markets Hypothesis. Frontiers in Blockchain, 2. https://doi.org/10.3389/fbloc.2019.00022
Boell, S. K., & Cecez-Kecmanovic, D. (2015). On being ‘systematic’ in literature reviews. In Willcocks, L. P ., Sauer, C., & Lacity, M. C. (Eds.), Formulating Research Methods for Information Systems: Volume 2, 48–78. London: Palgrave Macmillan UK.
Bonneau, J., Miller, A., Clark, J., Narayanan, A., Kroll, J. A., & Felten, E. W. (2015). SoK: Research Perspectives and Challenges for Bitcoin and Cryptocurrencies. 2015 IEEE Symposium on Security and Privacy, 104–121. https://doi.org/10.1109/SP.2015.14
Bouri, E., Azzi, G., & Dyhrberg, A. H. (2017). On the Return-Volatility Relationship in The Bitcoin Market Around the Price Crash of 2013. Economics - The Open-Access, Open-Assessment E-Journal, 11, 1–16.
Bouri, E., Gupta, R., Lau, C. K. M., Roubaud, D., & Wang, S. (2018). Bitcoin and global financial stress: A copula-based approach to dependence and causality in the quantiles. The Quarterly Review of Economics and Finance, 69, 297–307.
Bouri, E., Molnár, P., Azzi, G., Roubaud, D., & Hagfors, L. I. (2017). On The Hedge and Safe Haven Properties of Bitcoin: Is It Really More than a Diversifier? Finance Research Letters, 20, 192–198. https://doi.org/10.1016/j.frl.2016.09.025
Briere, M., Oosterlinck, K., & Szafarz, A. (2013). Virtual currency, tangible return: Portfolio diversification with bitcoins ULB–Universite Libre de Bruxelles. Working Papers CEB, 13-031.
Brito, J., Shadab, H. B., & Castillo O’Sullivan, A. (2015). Bitcoin Financial Regulation: Securities, Derivatives, Prediction Markets, and Gambling. SSRN Scholarly Paper ID 2423461.
Buhalis, D., Harwood, T., Bogicevic, V., Viglia, G., Beldona, S., & Hofacker, C. (2019). Technological Disruptions in Services: Lessons from Tourism and Hospitality. Journal of Service Management, 30(4), 484–506.
Bulut, A. (2018). Cryptocurrencies in the New Economy. Journal of International Trade, Logistics and Law, 4(2), 45–52.
Bunjaku, F., Gorgieva-Trajkovska, O., & Miteva-Kacarski, E. (2017). Cryptocurrencies – Advantages and Disadvantages. Journal of Economics, 2(1), 31-39.
Burniske, C., & White, A. (2017). Bitcoin: Ringing the bell for a new asset class. Ark Invest (January 2017) Https://Research. Ark-Invest. Com/Hubfs/1_Download_Files_ARK-Invest/White_Papers/Bitcoin-Ringing-The-Bell-For-A-New-Asset-Class. Pdf.
Button, S. (2018). Cryptocurrency and Blockchains in Emerging Economies. Software Quality Professional, 20(3).
Calcaterra, C., Kaal, W. A., & Rao, V. (2020). Stable Cryptocurrencies. Washington University Journal of Law & Policy, 61, 193-228.
Campbell-Verduyn, M.. (2017). Conclusion: Towards a Block Age or Blockages of Global Governance? In Tendulkar, S (Ed). Bitcoin and Beyond, 178–197. London: Routledge.
Catalini, C., & Gans, J. S. (2016). Some Simple Economics of the Blockchain. https://doi.org/10.2139/ssrn.2874598
Cennamo, C., Marchesi, C., & Meyer, T. (2020). Two Sides of The Same Coin? Decentralized Versus Proprietary Blockchains and The Performance of Digital Currencies. Academy of Management Discoveries, 6(3). https://doi.org/10.5465/amd.2019.0044.
Cerqueti, R., Giacalone, M., & Mattera, R. (2020). Skewed Non-Gaussian GARCH Models for Cryptocurrencies Volatility Modelling. Information Sciences, 527, 1–26. https://doi.org/10.1016/j.ins.2020.03.075
Charfeddine, L., Benlagha, N., & Maouchi, Y. (2020). Investigating the Dynamic Relationship Between Cryptocurrencies and Conventional Assets: Implications for Financial Investors. Economic Modelling, 85, 198-217.
Chapron, G. (2017). The Environment Needs Cryptogovernance. Nature News, 545(7655), 403–405.
Chohan, U. W. (2017). The Double Spending Problem and Cryptocurrencies. SSRN Scholarly Paper ID 3090174.
Clark, B., & Burstall, R. (2018). Blockchain, IP and The Pharma Industry—How Distributed Ledger Technologies Can Help Secure the Pharma Supply Chain. Journal of Intellectual Property Law & Practice, 13(7), 531–533.
Cocco, L., Concas, G., & Marchesi, M. (2017). Using an artificial financial market for studying a cryptocurrency market. Journal of Economic Interaction and Coordination, 12(2), 345–365. https://doi.org/10.1007/s11403-015-0168-2
Conti, M., Sandeep Kumar, E., Lal, C., & Ruj, S. (2018). A Survey on Security and Privacy Issues of Bitcoin. IEEE Communications Surveys Tutorials, 20(4), 3416–3452. https://doi.org/10.1109/COMST.2018.2842460
Corbet, S., Lucey, B., Urquhart, A., & Yarovaya, L. (2019). Cryptocurrencies as a Financial Asset: A Systematic Analysis. International Review of Financial Analysis, 62, 182–199. https://doi.org/10.1016/j.irfa.2018.09.003
Courtois, N. T., Grajek, M., & Naik, R. (2014). The Unreasonable Fundamental Incertitudes Behind Bitcoin Mining. ArXiv:1310.7935 [Cs]. http://arxiv.org/abs/1310.7935
Cronin, P., Ryan, F., & Coughlan, M. (2008). Undertaking a Literature Review: A Step-by-Step Approach. British Journal of Nursing, 17(1), 38–43.
Crosby, M., Pattanayak, P., Verma, S., & Kalyanaraman, V. (2016). Blockchain technology: Beyond bitcoin. Applied Innovation, 2(6–10), 7-19.
Dashkevich, N., Counsell, S., & Destefanis, G. (2020). Blockchain Application for Central Banks: A Systematic Mapping Study. IEEE Access, 8, 139918–139952.
Demidenko, D. S., Malevskaia-Malevich, E. D., & Dubolazova, Y. A. (2018). ISO as a Real Source of Funding. Pricing issues. 2018 International Conference on Information Networking (ICOIN), 622–625. https://doi.org/10.1109/ICOIN.2018.8343193
Dimitrova, V., Fernández-Martínez, M., Sánchez-Granero, M. A., & Segovia, J. E. T. (2019). Some Comments on Bitcoin Market (In)Efficiency. PLOS ONE, 14(7), e0219243. https://doi.org/10.1371/journal.pone.0219243
Dodd, N. (2018). The Social Life of Bitcoin. Theory, Culture and Society, 35(3), 35–56. https://doi.org/10.1177/0263276417746464
Dorfleitner, G., & Lung, C. (2018). Cryptocurrencies from The Perspective of Euro Investors: A Re-examination of Diversification Benefits and a New Day-of-The-Week Effect. Journal of Asset Management, 19(7), 472–494.
Dostov, V., & Shust, P. (2014). Cryptocurrencies: An unconventional Challenge to The AML/CFT Regulators? Journal of Financial Crime, 21(3), 249–263.
Duque, J. J. (2020). State Involvement in Cryptocurrencies. A Potential World Money? The Japanese Political Economy, 46(1), 65–82.
Dyhrberg, A. H., Foley, S., & Svec, J. (2018). How Investible is Bitcoin? Analyzing The Liquidity and Transaction Costs of Bitcoin Markets. Economics Letters, 171, 140–143. https://doi.org/10.1016/j.econlet.2018.07.032
Fabian, B., Ermakova, T., & Sander, U. (2016). Anonymity in Bitcoin—The Users’ Perspective. ICIS 2016 Proceedings.
Fadeyi, O., Krejcar, O., Maresova, P., Kuca, K., Brida, P., & Selamat, A. (2020). Opinions on Sustainability of Smart Cities in the Context of Energy Challenges Posed by Cryptocurrency Mining. Sustainability, 12(1), 169. https://doi.org/10.3390/su12010169
Fantacci, L. (2019). Cryptocurrencies and the Denationalization of Money. International Journal of Political Economy, 48(2), 105–126. https://doi.org/10.1080/08911916.2019.1624319
Flori, A. (2019). Cryptocurrencies In Finance: Review and Applications. International Journal of Theoretical and Applied Finance, 22(5), 1–22.
Foley, S., Karlsen, J. R., & Putniņš, T. J. (2019). Sex, Drugs, and Bitcoin: How Much Illegal Activity Is Financed through Cryptocurrencies? The Review of Financial Studies, 32(5), 1798–1853. https://doi.org/10.1093/rfs/hhz015
García-Medina, A., & Hernández, J. B. (2020). Network Analysis of Multivariate Transfer Entropy of Cryptocurrencies in Times of Turbulence. Entropy, 22(7), 760-762. https://doi.org/10.3390/e22070760
Glaser, F., & Bezzenberger, L. (2015). Beyond Cryptocurrencies—A Taxonomy of Decentralized Consensus Systems. SSRN Scholarly Paper ID 2605803.
Gott, M., Ward, S., Gardiner, C., Cobb, M., & Ingleton, C. (2011). A Narrative Literature Review of The Evidence Regarding The Economic Impact of Avoidable hospitalizations Amongst Palliative Care Patients in The UK. Progress in Palliative Care, 19(6), 291–298. https://doi.org/10.1179/1743291X11Y.0000000014
Guadamuz, A., & Marsden, C. (2015). Blockchains and Bitcoin: Regulatory Responses to Cryptocurrencies. First Monday, 20(12). https://doi.org/10.5210/fm.v20i12.6198
Gurrib, I., Kweh, Q. L., Nourani, M., & Ting, I. W. K. (2019). Are Cryptocurrencies Affected by Their Asset Class Movements or News Announcements? Malaysian Journal of Economic Studies, 56(2), 201–225. https://doi.org/10.22452/MJES.vol56no2.2
Härdle, W. K., Harvey, C. R., & Reule, R. C. G. (2020). Understanding Cryptocurrencies. Journal of Financial Econometrics, 18(2), 181–208. https://doi.org/10.1093/jjfinec/nbz033
Harvey, J., & Branco-Illodo, I. (2020). Why Cryptocurrencies Want Privacy: A Review of Political Motivations and Branding Expressed in “Privacy Coin” Whitepapers. Journal of Political Marketing, 19(1–2), 107–136.
Hashemi Joo, M., Nishikawa, Y., & Dandapani, K. (2019). Cryptocurrency, a Successful Application of Blockchain Technology. Managerial Finance, 46(6), 715–733.
Hayes, A. (2015). A Cost of Production Model for Bitcoin. Working Papers No. 1505. New School for Social Research, Department of Economics.
Herskind, L., Katsikouli, P., & Dragoni, N. (2020). Privacy and Cryptocurrencies—A Systematic Literature Review. IEEE Access, 8, 54044–54059.
Hong, K. (2017). Bitcoin as an alternative investment vehicle. Information Technology and Management, 18(4), 265–275. https://doi.org/10.1007/s10799-016-0264-6
Houy, N. (2014). The economics of Bitcoin transaction fees. Working Papers Halshs-00951358.
Hsieh, Y.-Y., Vergne, J.-P., Anderson, P., Lakhani, K., & Reitzig, M. (2018). Bitcoin and the Rise of Decentralized Autonomous Organizations. Journal of Organization Design, 7(1), 14. https://doi.org/10.1186/s41469-018-0038-1
Hu, A., Parlour, C. A., & Rajan, U. (2018). Cryptocurrencies: Stylized Facts on a New Investible Instrument. SSRN Scholarly Paper ID 3182113.
Hudson, R., & Urquhart, A. (2019). Technical Trading and Cryptocurrencies. Annals of Operations Research, 297, 191-220. https://doi.org/10.1007/s10479-019-03357-1.
Hughes, S., & Middlebrook, S. (2014). Regulating Cryptocurrencies in the United States: Current Issues and Future Directions. William Mitchell Law Review, 40(2), 814–848.
Ibba, S., Pinna, A., Baralla, G., & Marchesi, M. (2018). ICOs Overview: Should Investors Choose an ICO Developed with the Lean Startup Methodology? In Garbajosa, J., Wang, X., & Aguiar, A. (Eds.), Agile Processes in Software Engineering and Extreme Programming, 293–308. Berlin: Springer International Publishing.
Jaag, C., & Bach, C. (2015). Cryptocurrencies: New Opportunities for Postal Financial Services. Working Paper No. 0052.
Kaponda, K. (2019). An Investigation into the State of Cryptocurrencies and Regulatory Challenges in Zambia. SSRN Scholarly Paper ID 3433153.
Karpan, A. (2019). Cryptocurrencies and Blockchain Technology. New York: Greenhaven Publishing LLC.
Katsiampa, P. (2017). Volatility Estimation for Bitcoin: A Comparison of GARCH Models. Economics Letters, 158, 3–6. https://doi.org/10.1016/j.econlet.2017.06.023
Keogh, J. G., Dube, L., Rejeb, A., Hand, K. J., Khan, N., & Dean, K. (2020). The Future Food Chain: Digitization as an Enabler of Society 5.0. In Detwiler, D. (Ed.), Building the Future of Food Safety Technology. Netherlands: Elsevier.
Keogh, J. G., Rejeb, A., Khan, N., Dean, K., & Hand, K. J. (2020). Blockchain and GS1 Standards in the Food Chain: A Review of the Possibilities and Challenges. In Detwiler, D. (Ed.), Building the Future of Food Safety Technology. Netherlands: Elsevier.
Kerr, J. (2018). How Can Legislators Protect Sport from the Integrity Threat Posed by Cryptocurrencies? The International Sports Law Journal, 18(1), 79–97.
Kfir, I. (2020). Cryptocurrencies, National Security, Crime and Terrorism. Comparative Strategy, 39(2), 113–127. https://doi.org/10.1080/01495933.2020.1718983
Kim, S. (2018). Chapter Two—Blockchain for a Trust Network Among Intelligent Vehicles. In Raj, P., & Deka, G. C. (Eds.), Advances in Computers, Vol. 111, 43–68. Netherlands: Elsevier.
Kolber, A. J. (2018). Not-So-Smart Blockchain Contracts and Artificial Responsibility. Stanford Technology Law Review, 21, 198. https://heinonline.org/HOL/Page?handle=hein.journals/stantlr21&id=198&div=&collection=
Korpela, K., Hallikas, J., & Dahlberg, T. (2017). Digital Supply Chain Transformation toward Blockchain Integration. Proceedings of the 50th Hawaii International Conference on System Sciences.
Lanko, A., Vatin, N., & Kaklauskas, A. (2018). Application of RFID Combined with Blockchain Technology in Logistics of Construction Materials. MATEC Web of Conferences, 170, 03032. https://doi.org/10.1051/matecconf/201817003032
Larios-Hernández, G. J. (2017). Blockchain Entrepreneurship Opportunity in The Practices of The Unbanked. Business Horizons, 60(6), 865–874.
Lerer, M., & McGarrigle, C. (2018). Art in the Age of Financial Crisis. Visual Resources, 34(1–2), 1–12. https://doi.org/10.1080/01973762.2018.1455355
Lim, I.-K., Kim, Y.-H., Lee, J.-G., Lee, J.-P., Nam-Gung, H., & Lee, J.-K. (2014). The Analysis and Countermeasures on Security Breach of Bitcoin. In Murgante, B., Misra, S., Rocha, A. M. A. C., Torre, C., Rocha, J. G., Falcão, M. I., Taniar, D., Apduhan, B. O., & Gervasi, O. (Eds.), Computational Science and Its Applications – ICCSA 2014, 720–732. Berlin: Springer International Publishing.
Lu, Q., Xu, X., Liu, Y., Weber, I., Zhu, L., & Zhang, W. (2019). uBaaS: A Unified Blockchain as a Service Platform. Future Generation Computer Systems, 101, 564–575.
Marian, O. (2013). Are Cryptocurrencies Super Tax Havens? Michigan Law Review First Impressions, 112(1), 38–48. https://repository.law.umich.edu/mlr_fi/vol112/iss1/2
Marquez-Velazquez, A. (2010). The Report of the Stiglitz Commission: A Summary and Comment. SSRN Scholarly Paper ID 2196125.
Maurer, B., Nelms, T. C., & Swartz, L. (2013). “When Perhaps the Real Problem is Money Itself!”: The Practical Materiality of Bitcoin. Social Semiotics, 23(2), 261–277. https://doi.org/10.1080/10350330.2013.777594
Mendoza-Tello, J. C., Mora, H., Pujol-López, F. A., & Lytras, M. D. (2018). Social Commerce as a Driver to Enhance Trust and Intention to Use Cryptocurrencies for Electronic Payments. IEEE Access, 6, 50737–50751.
Mendoza-Tello, J. C., Mora, H., Pujol-López, F. A., & Lytras, M. D. (2019). Disruptive Innovation of Cryptocurrencies in Consumer Acceptance and Trust. Information Systems and E-Business Management, 17(2), 195–222.
Michelman, P. (2017). Seeing Beyond the Blockchain Hype. MIT Sloan Management Review, 58(4), 17-20.
Miller, P. (2016). Chapter 1—The Cryptocurrency Enigma. In Sammons, J. (Ed.), Digital Forensics, 1–25. Syngress. https://doi.org/10.1016/B978-0-12-804526-8.00001-0
Milne, A. (2018). Cryptocurrencies from an Austrian Perspective. In Godart-van der Kroon, A., & Vonlanthen, P. (Eds.), Banking and Monetary Policy from the Perspective of Austrian Economics, 223–257. Berlin: Springer International Publishing.
Momtaz, P. P. (2019). The Pricing and Performance of Cryptocurrency. The European Journal of Finance, 1–14. https://doi.org/10.1080/1351847X.2019.1647259
Nakamoto, S. (2008). Bitcoin: A Peer-to-Peer Electronic Cash System. Satoshi Nakamoto Institute Working Paper. Retrieved from: http://nakamotoinstitute.org/bitcoin/
Ng, D., & Griffin, P. (2018). The wider impact of a national cryptocurrency. Global Policy, 1–18. https://ink.library.smu.edu.sg/lkcsb_research/5880
Nica, O., Piotrowska, K., & Schenk-Hoppé, K. R. (2017). Cryptocurrencies: Economic Benefits and Risks. SSRN Scholarly Paper ID 3059856.
Omane-Adjepong, M., & Alagidede, I. P. (2020). High- and Low-Level Chaos in The Time and Frequency Market Returns of Leading Cryptocurrencies and Emerging Assets. Chaos, Solitons & Fractals, 132, 109563. https://doi.org/10.1016/j.chaos.2019.109563
Omane-Adjepong, M., Alagidede, P., & Akosah, N. K. (2019). Wavelet Time-Scale Persistence Analysis of Cryptocurrency Market Returns and Volatility. Physica A: Statistical Mechanics and Its Applications, 514, 105–120.
Peters, G. W., Panayi, E., & Chapelle, A. (2015). Trends in Crypto-Currencies and Blockchain Technologies: A Monetary Theory and Regulation Perspective. Journal of Financial Perspectives, 3(3), 92-113.
Pinna, A., Tonelli, R., Orrú, M., & Marchesi, M. (2018). A Petri Nets Model for Blockchain Analysis. The Computer Journal, 61(9), 1374–1388.
Platanakis, E., Sutcliffe, C., & Urquhart, A. (2018). Optimal vs Naïve Diversification in Cryptocurrencies. Economics Letters, 171, 93–96.
Polasik, M., Piotrowska, A. I., Wisniewski, T. P., Kotkowski, R., & Lightfoot, G. (2015). Price Fluctuations and the Use of Bitcoin: An Empirical Inquiry. International Journal of Electronic Commerce, 20(1), 9–49. https://doi.org/10.1080/10864415.2016.1061413
Pournader, M., Shi, Y., Seuring, S., & Koh, S. C. L. (2020). Blockchain Applications in Supply Chains, Transport and Logistics: A Systematic Review of the Literature. International Journal of Production Research, 58(7), 2063–2081.
Raymaekers, W. (2015). Cryptocurrency Bitcoin: Disruption, Challenges and Opportunities. Journal of Payments Strategy & Systems, 9(1), 30–46.
Rehman, M. H. ur, Salah, K., Damiani, E., & Svetinovic, D. (2019). Trust in Blockchain Cryptocurrency Ecosystem. IEEE Transactions on Engineering Management, 1–17.
Rejeb, A. (2018a). Blockchain Potential in Tilapia Supply Chain in Ghana. Acta Technica Jaurinensis, 11(2), 104–118.
Rejeb, A. (2018b). Halal Meat Supply Chain Traceability Based on HACCP , Blockchain and Internet of Things. Acta Technica Jaurinensis, 11(4), 1–30. https://doi.org/10.14513/actatechjaur.v11.n1.000
Rejeb, A., & Bell, L. (2019). Potentials of Blockchain for Healthcare: Case of Tunisia. World Scientific News, 136, 173–193.
Rejeb, A., Keogh, J. G., & Treiblmaier, H. (2019a). Leveraging the Internet of Things and Blockchain Technology in Supply Chain Management. Future Internet, 11(7), 161. https://doi.org/10.3390/fi11070161
Rejeb, A., Keogh, J. G., & Treiblmaier, H. (2019b). The impact of blockchain on medical tourism. WeB2019 Workshop on E-Business, 1–12.
Rejeb, A., & Rejeb, K. (2019). Blockchain Technology in Tourism: Applications and Possibilities. World Scientific News, 137, 119–144.
Rejeb, A., & Rejeb, K. (2020). Blockchain and supply chain sustainability. Logforum, 16(3), 363–372. https://doi.org/10.17270/J.LOG.2020.467
Rejeb, A., Sűle, E., & Keogh, J. G. (2018). Exploring new technologies in procurement. Transport & Logistics: The International Journal, 18(45), 76–86.
Ricciardi, V. (2004). A Risk Perception Primer: A Narrative Research Review of the Risk Perception Literature in Behavioral Accounting and Behavioral Finance. SSRN Scholarly Paper ID 566802.
Richards, T., & Briefing, A. B. E. (2018). Cryptocurrencies and Distributed Ledger Technology. Australian Business Economists Briefing, Sydney, 26.
Rowan, S., Clear, M., Gerla, M., Huggard, M., & Goldrick, C. M. (2017). Securing Vehicle to Vehicle Communications using Blockchain through Visible Light and Acoustic Side-Channels. ArXiv:1704.02553 [Cs]. http://arxiv.org/abs/1704.02553
Scharding, T. (2019). National Currency, World Currency, Cryptocurrency: A Fichtean Approach to the Ethics of Bitcoin. Business and Society Review, 124(2), 219–238.
Searing, J. M., & MacLeod, D. (2019). Cryptocurrency Gift Strategies for Not-for-Profits: Here’s What Organizations Should Consider as They Ponder Whether and How to Accept Donations of Virtual Currency. Journal of Accountancy, 227(2), 34-36.
Shahzad, F., Xiu, G., Wang, J., & Shahbaz, M. (2018). An Empirical Investigation on the Adoption of Cryptocurrencies Among the People of Mainland China. Technology in Society, 55, 33–40. https://doi.org/10.1016/j.techsoc.2018.05.006
Sharma, A. K., & Kumar, S. (2010). Economic Value Added (EVA)—Literature Review and Relevant Issues. International Journal of Economics and Finance, 2(2), 200-220.
Sharma, D. K., Pant, S., Sharma, M., & Brahmachari, S. (2020). Chapter 13 - Cryptocurrency Mechanisms for Blockchains: Models, Characteristics, Challenges, and Applications. In Krishnan, S., Balas, V. E., Julie, E. G., Robinson, Y. H., Balaji, S., & Kumar, R. (Eds.), Handbook of Research on Blockchain Technology, 323–348. Academic Press. https://doi.org/10.1016/B978-0-12-819816-2.00013-7
Söderlund, P., & Kestilä-Kekkonen, E. (2014). Economic Voting in Finland Before and After an Economic Crisis. Acta Politica, 49(4), 395–412.
Srokosz, W., & Kopciaski, T. (2015). Legal and Economic Analysis of The Cryptocurrencies Impact on The Financial System Stability. Journal of Teaching and Education, 4(2), 619–627.
Sudzina, F. (2018). Distribution of Foreign Aid in Cryptocurrencies: Initial Considerations. International Advances in Economic Research, 24(4), 387–388.
Symitsi, E., & Chalvatzis, K. J. (2018). Return, Volatility and Shock Spillovers of Bitcoin with Energy and Technology Companies. Economics Letters, 170, 127–130. https://doi.org/10.1016/j.econlet.2018.06.012
Tama, B. A., Kweka, B. J., Park, Y., & Rhee, K.-H. (2017). A Critical Review of Blockchain and Its Current Applications. 2017 International Conference on Electrical Engineering and Computer Science (ICECOS), 109–113.
Till, B. M., Peters, A. W., Afshar, S., & Meara, J. G. (2017). From Blockchain Technology to Global Health Equity: Can Cryptocurrencies Finance Universal Health Coverage? BMJ Global Health, 2(4), e000570. https://doi.org/10.1136/bmjgh-2017-000570
Trautman, L. J. (2014). Virtual Currencies; Bitcoin & What Now After Liberty Reserve, Silk Road, and Mt. Gox? Richmond Journal of Law and Technology, 20(4), 1-108.
Treiblmaier, H. (2018). The Impact of The Blockchain on The Supply Chain: A Theory-Based Research Framework and a Call for Action. Supply Chain Management: An International Journal, 23(6), 545–559. https://doi.org/10.1108/SCM-01-2018-0029
Treiblmaier, H. (2019). Combining Blockchain Technology and the Physical Internet to Achieve Triple Bottom Line Sustainability: A Comprehensive Research Agenda for Modern Logistics and Supply Chain Management. Logistics, 3(1), 1–13.
Treiblmaier, H., Rejeb, A., & Strebinger, A. (2020). Blockchain as a Driver for Smart City Development: Application Fields and a Comprehensive Research Agenda. Smart Cities, 3(3), 853–872. https://doi.org/10.3390/smartcities3030044
Truby, J. (2018). Decarbonizing Bitcoin: Law and Policy Choices for Reducing the Energy Consumption of Blockchain Technologies and Digital Currencies. Energy Research & Social Science, 44, 399–410.
Tucker, T. (2013, December 5). Bitcoin’s Volatility Problem: Why Today’s Selloff Won’t Be the Last. Bloomberg.Com. https://www.bloomberg.com/news/articles/2013-12-05/bitcoins-volatility-problem-why-todays-selloff-wont-be-the-last
Uddin, M. A., Stranieri, A., Gondal, I., & Balasurbramanian, V. (2019). A Lightweight Blockchain Based Framework for Underwater IoT. Electronics, 8(12), 1552. https://doi.org/10.3390/electronics8121552
Vandezande, N. (2017). Virtual Currencies Under EU Anti-money Laundering Law. Computer Law & Security Review, 33(3), 341–353.
Vaz, J., & Brown, K. (2020). Sustainable Development and Cryptocurrencies as Private Money. Journal of Industrial and Business Economics, 47(1), 163–184. https://doi.org/10.1007/s40812-019-00139-5
Vidal-Tomás, D., Ibáñez, A. M., & Farinós, J. E. (2019). Weak efficiency of the cryptocurrency market: A market portfolio approach. Applied Economics Letters, 26(19), 1627–1633. https://doi.org/10.1080/13504851.2019.1591583
Vincent, O., & Evans, O. (2019). Can Cryptocurrency, Mobile Phones, and Internet Herald Sustainable Financial Sector Development in Emerging Markets? Journal of Transnational Management, 24(3), 259–279.
Volosovych, S., & Baraniuk, Y. (2018). Tax control of cryptocurrency transactions in Ukraine. Banks and Bank Systems, 13(2), 89–106. https://www.ceeol.com/search/article-detail?id=696926
Wang, G. (2019). Marx’s Monetary Theory and Its Practical Value. China Political Economy, 2(2), 182–200. https://doi.org/10.1108/CPE-10-2019-0026
Wang, J., Wu, P., Wang, X., & Shou, W. (2017). The Outlook of Blockchain Technology for Construction Engineering Management. Frontiers of Engineering Management, 4(1), 67–75. https://doi.org/10.15302/J-FEM-2017006
Wang, Y., Singgih, M., Wang, J., & Rit, M. (2019). Making Sense of Blockchain Technology: How Will It Transform Supply Chains? International Journal of Production Economics, 211, 221–236. https://doi.org/10.1016/j.ijpe.2019.02.002
Wei, Q., Li, S., Li, W., Li, H., & Wang, M. (2019). Decentralized Hierarchical Authorized Payment with Online Wallet for Blockchain. In Biagioni, E. S., Zheng, Y., & Cheng, S (Eds.), Wireless Algorithms, Systems, and Applications, 358–369. Berlin: Springer.
Wet, J. H. v H. D. (2005). EVA versus traditional accounting measures of performance as drivers of shareholder value—A comparative analysis. Meditari : Research Journal of the School of Accounting Sciences, 13(2), 1–16.
Wetherell, M., & Potter, J. (1992). Mapping the Language of Racism: Discourse and the Legitimation of Exploitation. London and New York: Harvester Wheatsheaf and Columbia University Press.
Wilson, C. (2019). Cryptocurrencies: The Future of Finance? In Yu, F-L. T., & Kwan, D. S. (Eds.). Contemporary Issues in International Political Economy, 359–394. Berlin: Springer.
Yalaman, G. Ö., & Yıldırım, H. (2019). Cryptocurrency and Tax Regulation: Global Challenges for Tax Administration. In Hacioglu, U (Ed.), Blockchain Economics and Financial Market Innovation: Financial Innovations in the Digital Age, 407–422. Berlin: Springer International Publishing.
Zhang, J. Y. (2017). The Rise of Market Concentration and Rent Seeking in the Financial Sector. John M. Olin Center for Law, Economics, and Business Fellows’ Discussion Paper Series.
Zook, M. A., & Blankenship, J. (2018). New Spaces of Disruption? The Failures of Bitcoin and the Rhetorical Power of Algorithmic Governance. Geoforum, 96, 248–255. https://doi.org/10.1016/j.geoforum.2018.08.023
- There are currently no refbacks.
Abderahman Rejeb Doctoral School of Regional Sciences and Business Administration, Széchenyi István University, 9026 Gyor Hungary
Karim Rejeb Higher Institute of Computer Science of El Manar, 2, Rue Abou Raïhan El Bayrouni, 2080 Ariana Tunisia
John G. Keogh Henley Business School, University of Reading‚ Greenlands, Henley-on-Thames, RG9 3AU, UK United Kingdom
- Other Journals
- For Readers
- For Authors
- For Librarians
- Focus and Scope
- Section Policies
- Peer Review Process
- Open Access Policy
Submissions
- Online Submissions
- Author Guidelines
- Copyright Notice
- Privacy Statement
- Journal Sponsorship
- About this Publishing System
ISSN: 2461-0771
To read this content please select one of the options below:
Please note you do not have access to teaching notes, cryptocurrency adoption: a systematic literature review and bibliometric analysis.
EuroMed Journal of Business
ISSN : 1450-2194
Article publication date: 17 May 2022
Issue publication date: 16 August 2022
Cryptocurrencies put consumers at the heart of a potential revolution by shifting central authority to a distributed peer-to-peer monetary system. This study aims to perform a systematic literature review and bibliometric analysis within the topic of cryptocurrencies and consumer trust.
Design/methodology/approach
The Web of Science database has been selected, and the analyses performed allowed us to identify five research trends obtained from the bibliographic coupling analysis: (1) Understanding consumer's (non)acceptance of cryptocurrencies, (2) Ethical aspects and trust in cryptocurrencies, (3) Blockchain technology as a trust-free technology, (4) The blockchain/trust economy, and (5) Blockchain technology: challenging trust.
Findings uncover the intellectual structure in the field of cryptocurrencies and consumers' trust and offer insights on the pros and cons of consumers' willingness to trust the digital currency.
Originality/value
The study proved a great gap in the current literature in linking cryptocurrencies and trust theories in a consumer context. The authors also outline several gaps that allowed us to propose future research guidelines.
- Cryptocurrency
- Consumer trust
- Systematic literature review
- Bibliometric analysis
Acknowledgements
This work is supported by national funding’s of FCT - Fundação para a Ciência e a Tecnologia, I.P., in the project UIDB/04005/2020.
Sousa, A. , Calçada, E. , Rodrigues, P. and Pinto Borges, A. (2022), "Cryptocurrency adoption: a systematic literature review and bibliometric analysis", EuroMed Journal of Business , Vol. 17 No. 3, pp. 374-390. https://doi.org/10.1108/EMJB-01-2022-0003
Emerald Publishing Limited
Copyright © 2022, Emerald Publishing Limited
Related articles
We’re listening — tell us what you think, something didn’t work….
Report bugs here
All feedback is valuable
Please share your general feedback
Join us on our journey
Platform update page.
Visit emeraldpublishing.com/platformupdate to discover the latest news and updates
Questions & More Information
Answers to the most commonly asked questions here
- Search Menu
- Browse content in A - General Economics and Teaching
- Browse content in A1 - General Economics
- A11 - Role of Economics; Role of Economists; Market for Economists
- A13 - Relation of Economics to Social Values
- A14 - Sociology of Economics
- Browse content in C - Mathematical and Quantitative Methods
- Browse content in C0 - General
- C02 - Mathematical Methods
- Browse content in C1 - Econometric and Statistical Methods and Methodology: General
- C10 - General
- C11 - Bayesian Analysis: General
- C12 - Hypothesis Testing: General
- C13 - Estimation: General
- C14 - Semiparametric and Nonparametric Methods: General
- C15 - Statistical Simulation Methods: General
- C18 - Methodological Issues: General
- Browse content in C2 - Single Equation Models; Single Variables
- C21 - Cross-Sectional Models; Spatial Models; Treatment Effect Models; Quantile Regressions
- C22 - Time-Series Models; Dynamic Quantile Regressions; Dynamic Treatment Effect Models; Diffusion Processes
- C23 - Panel Data Models; Spatio-temporal Models
- Browse content in C3 - Multiple or Simultaneous Equation Models; Multiple Variables
- C32 - Time-Series Models; Dynamic Quantile Regressions; Dynamic Treatment Effect Models; Diffusion Processes; State Space Models
- C38 - Classification Methods; Cluster Analysis; Principal Components; Factor Models
- Browse content in C4 - Econometric and Statistical Methods: Special Topics
- C45 - Neural Networks and Related Topics
- Browse content in C5 - Econometric Modeling
- C50 - General
- C51 - Model Construction and Estimation
- C52 - Model Evaluation, Validation, and Selection
- C53 - Forecasting and Prediction Methods; Simulation Methods
- C55 - Large Data Sets: Modeling and Analysis
- C58 - Financial Econometrics
- Browse content in C6 - Mathematical Methods; Programming Models; Mathematical and Simulation Modeling
- C61 - Optimization Techniques; Programming Models; Dynamic Analysis
- C62 - Existence and Stability Conditions of Equilibrium
- C65 - Miscellaneous Mathematical Tools
- Browse content in C7 - Game Theory and Bargaining Theory
- C70 - General
- C72 - Noncooperative Games
- C73 - Stochastic and Dynamic Games; Evolutionary Games; Repeated Games
- C78 - Bargaining Theory; Matching Theory
- Browse content in C8 - Data Collection and Data Estimation Methodology; Computer Programs
- C81 - Methodology for Collecting, Estimating, and Organizing Microeconomic Data; Data Access
- Browse content in C9 - Design of Experiments
- C91 - Laboratory, Individual Behavior
- C92 - Laboratory, Group Behavior
- C93 - Field Experiments
- Browse content in D - Microeconomics
- Browse content in D0 - General
- D03 - Behavioral Microeconomics: Underlying Principles
- Browse content in D1 - Household Behavior and Family Economics
- D10 - General
- D11 - Consumer Economics: Theory
- D12 - Consumer Economics: Empirical Analysis
- D14 - Household Saving; Personal Finance
- D15 - Intertemporal Household Choice: Life Cycle Models and Saving
- D18 - Consumer Protection
- Browse content in D2 - Production and Organizations
- D20 - General
- D21 - Firm Behavior: Theory
- D22 - Firm Behavior: Empirical Analysis
- D23 - Organizational Behavior; Transaction Costs; Property Rights
- D24 - Production; Cost; Capital; Capital, Total Factor, and Multifactor Productivity; Capacity
- D25 - Intertemporal Firm Choice: Investment, Capacity, and Financing
- Browse content in D3 - Distribution
- D30 - General
- D31 - Personal Income, Wealth, and Their Distributions
- Browse content in D4 - Market Structure, Pricing, and Design
- D40 - General
- D43 - Oligopoly and Other Forms of Market Imperfection
- D44 - Auctions
- D47 - Market Design
- D49 - Other
- Browse content in D5 - General Equilibrium and Disequilibrium
- D50 - General
- D51 - Exchange and Production Economies
- D52 - Incomplete Markets
- D53 - Financial Markets
- Browse content in D6 - Welfare Economics
- D60 - General
- D61 - Allocative Efficiency; Cost-Benefit Analysis
- D62 - Externalities
- Browse content in D7 - Analysis of Collective Decision-Making
- D70 - General
- D71 - Social Choice; Clubs; Committees; Associations
- D72 - Political Processes: Rent-seeking, Lobbying, Elections, Legislatures, and Voting Behavior
- D73 - Bureaucracy; Administrative Processes in Public Organizations; Corruption
- D74 - Conflict; Conflict Resolution; Alliances; Revolutions
- D78 - Positive Analysis of Policy Formulation and Implementation
- Browse content in D8 - Information, Knowledge, and Uncertainty
- D80 - General
- D81 - Criteria for Decision-Making under Risk and Uncertainty
- D82 - Asymmetric and Private Information; Mechanism Design
- D83 - Search; Learning; Information and Knowledge; Communication; Belief; Unawareness
- D84 - Expectations; Speculations
- D85 - Network Formation and Analysis: Theory
- D86 - Economics of Contract: Theory
- D87 - Neuroeconomics
- Browse content in D9 - Micro-Based Behavioral Economics
- D90 - General
- D91 - Role and Effects of Psychological, Emotional, Social, and Cognitive Factors on Decision Making
- D92 - Intertemporal Firm Choice, Investment, Capacity, and Financing
- Browse content in E - Macroeconomics and Monetary Economics
- Browse content in E0 - General
- E00 - General
- E03 - Behavioral Macroeconomics
- Browse content in E1 - General Aggregative Models
- E17 - Forecasting and Simulation: Models and Applications
- Browse content in E2 - Consumption, Saving, Production, Investment, Labor Markets, and Informal Economy
- E20 - General
- E21 - Consumption; Saving; Wealth
- E22 - Investment; Capital; Intangible Capital; Capacity
- E23 - Production
- E24 - Employment; Unemployment; Wages; Intergenerational Income Distribution; Aggregate Human Capital; Aggregate Labor Productivity
- Browse content in E3 - Prices, Business Fluctuations, and Cycles
- E30 - General
- E31 - Price Level; Inflation; Deflation
- E32 - Business Fluctuations; Cycles
- E37 - Forecasting and Simulation: Models and Applications
- Browse content in E4 - Money and Interest Rates
- E40 - General
- E41 - Demand for Money
- E42 - Monetary Systems; Standards; Regimes; Government and the Monetary System; Payment Systems
- E43 - Interest Rates: Determination, Term Structure, and Effects
- E44 - Financial Markets and the Macroeconomy
- E47 - Forecasting and Simulation: Models and Applications
- Browse content in E5 - Monetary Policy, Central Banking, and the Supply of Money and Credit
- E50 - General
- E51 - Money Supply; Credit; Money Multipliers
- E52 - Monetary Policy
- E58 - Central Banks and Their Policies
- Browse content in E6 - Macroeconomic Policy, Macroeconomic Aspects of Public Finance, and General Outlook
- E60 - General
- E61 - Policy Objectives; Policy Designs and Consistency; Policy Coordination
- E62 - Fiscal Policy
- E63 - Comparative or Joint Analysis of Fiscal and Monetary Policy; Stabilization; Treasury Policy
- E64 - Incomes Policy; Price Policy
- E65 - Studies of Particular Policy Episodes
- E66 - General Outlook and Conditions
- Browse content in E7 - Macro-Based Behavioral Economics
- E71 - Role and Effects of Psychological, Emotional, Social, and Cognitive Factors on the Macro Economy
- Browse content in F - International Economics
- Browse content in F0 - General
- F02 - International Economic Order and Integration
- Browse content in F1 - Trade
- F14 - Empirical Studies of Trade
- Browse content in F2 - International Factor Movements and International Business
- F21 - International Investment; Long-Term Capital Movements
- F22 - International Migration
- F23 - Multinational Firms; International Business
- Browse content in F3 - International Finance
- F30 - General
- F31 - Foreign Exchange
- F32 - Current Account Adjustment; Short-Term Capital Movements
- F33 - International Monetary Arrangements and Institutions
- F34 - International Lending and Debt Problems
- F36 - Financial Aspects of Economic Integration
- F37 - International Finance Forecasting and Simulation: Models and Applications
- F38 - International Financial Policy: Financial Transactions Tax; Capital Controls
- Browse content in F4 - Macroeconomic Aspects of International Trade and Finance
- F40 - General
- F41 - Open Economy Macroeconomics
- F42 - International Policy Coordination and Transmission
- F43 - Economic Growth of Open Economies
- F44 - International Business Cycles
- F47 - Forecasting and Simulation: Models and Applications
- Browse content in F5 - International Relations, National Security, and International Political Economy
- F51 - International Conflicts; Negotiations; Sanctions
- Browse content in F6 - Economic Impacts of Globalization
- F63 - Economic Development
- F65 - Finance
- Browse content in G - Financial Economics
- Browse content in G0 - General
- G00 - General
- G01 - Financial Crises
- G02 - Behavioral Finance: Underlying Principles
- Browse content in G1 - General Financial Markets
- G10 - General
- G11 - Portfolio Choice; Investment Decisions
- G12 - Asset Pricing; Trading volume; Bond Interest Rates
- G13 - Contingent Pricing; Futures Pricing
- G14 - Information and Market Efficiency; Event Studies; Insider Trading
- G15 - International Financial Markets
- G17 - Financial Forecasting and Simulation
- G18 - Government Policy and Regulation
- G19 - Other
- Browse content in G2 - Financial Institutions and Services
- G20 - General
- G21 - Banks; Depository Institutions; Micro Finance Institutions; Mortgages
- G22 - Insurance; Insurance Companies; Actuarial Studies
- G23 - Non-bank Financial Institutions; Financial Instruments; Institutional Investors
- G24 - Investment Banking; Venture Capital; Brokerage; Ratings and Ratings Agencies
- G28 - Government Policy and Regulation
- G29 - Other
- Browse content in G3 - Corporate Finance and Governance
- G30 - General
- G31 - Capital Budgeting; Fixed Investment and Inventory Studies; Capacity
- G32 - Financing Policy; Financial Risk and Risk Management; Capital and Ownership Structure; Value of Firms; Goodwill
- G33 - Bankruptcy; Liquidation
- G34 - Mergers; Acquisitions; Restructuring; Corporate Governance
- G35 - Payout Policy
- G38 - Government Policy and Regulation
- G39 - Other
- Browse content in G4 - Behavioral Finance
- G40 - General
- G41 - Role and Effects of Psychological, Emotional, Social, and Cognitive Factors on Decision Making in Financial Markets
- Browse content in G5 - Household Finance
- G50 - General
- G51 - Household Saving, Borrowing, Debt, and Wealth
- G52 - Insurance
- G53 - Financial Literacy
- Browse content in H - Public Economics
- H0 - General
- Browse content in H1 - Structure and Scope of Government
- H11 - Structure, Scope, and Performance of Government
- H19 - Other
- Browse content in H2 - Taxation, Subsidies, and Revenue
- H22 - Incidence
- H24 - Personal Income and Other Nonbusiness Taxes and Subsidies; includes inheritance and gift taxes
- H25 - Business Taxes and Subsidies
- H26 - Tax Evasion and Avoidance
- Browse content in H3 - Fiscal Policies and Behavior of Economic Agents
- H31 - Household
- Browse content in H4 - Publicly Provided Goods
- H40 - General
- H41 - Public Goods
- Browse content in H5 - National Government Expenditures and Related Policies
- H50 - General
- H52 - Government Expenditures and Education
- H53 - Government Expenditures and Welfare Programs
- H54 - Infrastructures; Other Public Investment and Capital Stock
- H55 - Social Security and Public Pensions
- H56 - National Security and War
- H57 - Procurement
- Browse content in H6 - National Budget, Deficit, and Debt
- H63 - Debt; Debt Management; Sovereign Debt
- Browse content in H7 - State and Local Government; Intergovernmental Relations
- H70 - General
- H72 - State and Local Budget and Expenditures
- H74 - State and Local Borrowing
- H75 - State and Local Government: Health; Education; Welfare; Public Pensions
- Browse content in H8 - Miscellaneous Issues
- H81 - Governmental Loans; Loan Guarantees; Credits; Grants; Bailouts
- Browse content in I - Health, Education, and Welfare
- Browse content in I1 - Health
- I11 - Analysis of Health Care Markets
- I12 - Health Behavior
- I13 - Health Insurance, Public and Private
- I18 - Government Policy; Regulation; Public Health
- Browse content in I2 - Education and Research Institutions
- I22 - Educational Finance; Financial Aid
- I23 - Higher Education; Research Institutions
- I28 - Government Policy
- Browse content in I3 - Welfare, Well-Being, and Poverty
- I30 - General
- I38 - Government Policy; Provision and Effects of Welfare Programs
- Browse content in J - Labor and Demographic Economics
- Browse content in J0 - General
- J00 - General
- Browse content in J1 - Demographic Economics
- J11 - Demographic Trends, Macroeconomic Effects, and Forecasts
- J12 - Marriage; Marital Dissolution; Family Structure; Domestic Abuse
- J13 - Fertility; Family Planning; Child Care; Children; Youth
- J15 - Economics of Minorities, Races, Indigenous Peoples, and Immigrants; Non-labor Discrimination
- J16 - Economics of Gender; Non-labor Discrimination
- J18 - Public Policy
- Browse content in J2 - Demand and Supply of Labor
- J20 - General
- J21 - Labor Force and Employment, Size, and Structure
- J22 - Time Allocation and Labor Supply
- J23 - Labor Demand
- J24 - Human Capital; Skills; Occupational Choice; Labor Productivity
- J26 - Retirement; Retirement Policies
- J28 - Safety; Job Satisfaction; Related Public Policy
- Browse content in J3 - Wages, Compensation, and Labor Costs
- J30 - General
- J31 - Wage Level and Structure; Wage Differentials
- J32 - Nonwage Labor Costs and Benefits; Retirement Plans; Private Pensions
- J33 - Compensation Packages; Payment Methods
- J38 - Public Policy
- Browse content in J4 - Particular Labor Markets
- J41 - Labor Contracts
- J44 - Professional Labor Markets; Occupational Licensing
- J45 - Public Sector Labor Markets
- J46 - Informal Labor Markets
- J49 - Other
- Browse content in J5 - Labor-Management Relations, Trade Unions, and Collective Bargaining
- J51 - Trade Unions: Objectives, Structure, and Effects
- J52 - Dispute Resolution: Strikes, Arbitration, and Mediation; Collective Bargaining
- Browse content in J6 - Mobility, Unemployment, Vacancies, and Immigrant Workers
- J61 - Geographic Labor Mobility; Immigrant Workers
- J62 - Job, Occupational, and Intergenerational Mobility
- J63 - Turnover; Vacancies; Layoffs
- J64 - Unemployment: Models, Duration, Incidence, and Job Search
- J65 - Unemployment Insurance; Severance Pay; Plant Closings
- J68 - Public Policy
- Browse content in J7 - Labor Discrimination
- J71 - Discrimination
- Browse content in J8 - Labor Standards: National and International
- J88 - Public Policy
- Browse content in K - Law and Economics
- Browse content in K1 - Basic Areas of Law
- K12 - Contract Law
- Browse content in K2 - Regulation and Business Law
- K22 - Business and Securities Law
- K23 - Regulated Industries and Administrative Law
- Browse content in K3 - Other Substantive Areas of Law
- K31 - Labor Law
- K32 - Environmental, Health, and Safety Law
- K34 - Tax Law
- K35 - Personal Bankruptcy Law
- Browse content in K4 - Legal Procedure, the Legal System, and Illegal Behavior
- K42 - Illegal Behavior and the Enforcement of Law
- Browse content in L - Industrial Organization
- Browse content in L1 - Market Structure, Firm Strategy, and Market Performance
- L10 - General
- L11 - Production, Pricing, and Market Structure; Size Distribution of Firms
- L13 - Oligopoly and Other Imperfect Markets
- L14 - Transactional Relationships; Contracts and Reputation; Networks
- L15 - Information and Product Quality; Standardization and Compatibility
- Browse content in L2 - Firm Objectives, Organization, and Behavior
- L21 - Business Objectives of the Firm
- L22 - Firm Organization and Market Structure
- L23 - Organization of Production
- L24 - Contracting Out; Joint Ventures; Technology Licensing
- L25 - Firm Performance: Size, Diversification, and Scope
- L26 - Entrepreneurship
- L29 - Other
- Browse content in L3 - Nonprofit Organizations and Public Enterprise
- L33 - Comparison of Public and Private Enterprises and Nonprofit Institutions; Privatization; Contracting Out
- Browse content in L4 - Antitrust Issues and Policies
- L43 - Legal Monopolies and Regulation or Deregulation
- L44 - Antitrust Policy and Public Enterprises, Nonprofit Institutions, and Professional Organizations
- Browse content in L5 - Regulation and Industrial Policy
- L51 - Economics of Regulation
- Browse content in L6 - Industry Studies: Manufacturing
- L66 - Food; Beverages; Cosmetics; Tobacco; Wine and Spirits
- Browse content in L8 - Industry Studies: Services
- L81 - Retail and Wholesale Trade; e-Commerce
- L85 - Real Estate Services
- L86 - Information and Internet Services; Computer Software
- Browse content in L9 - Industry Studies: Transportation and Utilities
- L92 - Railroads and Other Surface Transportation
- L94 - Electric Utilities
- Browse content in M - Business Administration and Business Economics; Marketing; Accounting; Personnel Economics
- Browse content in M0 - General
- M00 - General
- Browse content in M1 - Business Administration
- M12 - Personnel Management; Executives; Executive Compensation
- M13 - New Firms; Startups
- M14 - Corporate Culture; Social Responsibility
- M16 - International Business Administration
- Browse content in M2 - Business Economics
- M20 - General
- M21 - Business Economics
- Browse content in M3 - Marketing and Advertising
- M30 - General
- M31 - Marketing
- M37 - Advertising
- Browse content in M4 - Accounting and Auditing
- M40 - General
- M41 - Accounting
- M42 - Auditing
- M48 - Government Policy and Regulation
- Browse content in M5 - Personnel Economics
- M51 - Firm Employment Decisions; Promotions
- M52 - Compensation and Compensation Methods and Their Effects
- M54 - Labor Management
- Browse content in N - Economic History
- Browse content in N1 - Macroeconomics and Monetary Economics; Industrial Structure; Growth; Fluctuations
- N10 - General, International, or Comparative
- N12 - U.S.; Canada: 1913-
- Browse content in N2 - Financial Markets and Institutions
- N20 - General, International, or Comparative
- N21 - U.S.; Canada: Pre-1913
- N22 - U.S.; Canada: 1913-
- N23 - Europe: Pre-1913
- N24 - Europe: 1913-
- N25 - Asia including Middle East
- N27 - Africa; Oceania
- Browse content in N3 - Labor and Consumers, Demography, Education, Health, Welfare, Income, Wealth, Religion, and Philanthropy
- N32 - U.S.; Canada: 1913-
- Browse content in N4 - Government, War, Law, International Relations, and Regulation
- N43 - Europe: Pre-1913
- Browse content in N7 - Transport, Trade, Energy, Technology, and Other Services
- N71 - U.S.; Canada: Pre-1913
- Browse content in N8 - Micro-Business History
- N80 - General, International, or Comparative
- N82 - U.S.; Canada: 1913-
- Browse content in O - Economic Development, Innovation, Technological Change, and Growth
- Browse content in O1 - Economic Development
- O11 - Macroeconomic Analyses of Economic Development
- O12 - Microeconomic Analyses of Economic Development
- O13 - Agriculture; Natural Resources; Energy; Environment; Other Primary Products
- O16 - Financial Markets; Saving and Capital Investment; Corporate Finance and Governance
- O17 - Formal and Informal Sectors; Shadow Economy; Institutional Arrangements
- Browse content in O2 - Development Planning and Policy
- O23 - Fiscal and Monetary Policy in Development
- Browse content in O3 - Innovation; Research and Development; Technological Change; Intellectual Property Rights
- O30 - General
- O31 - Innovation and Invention: Processes and Incentives
- O32 - Management of Technological Innovation and R&D
- O33 - Technological Change: Choices and Consequences; Diffusion Processes
- O34 - Intellectual Property and Intellectual Capital
- O35 - Social Innovation
- O38 - Government Policy
- Browse content in O4 - Economic Growth and Aggregate Productivity
- O40 - General
- O43 - Institutions and Growth
- Browse content in O5 - Economywide Country Studies
- O53 - Asia including Middle East
- Browse content in P - Economic Systems
- Browse content in P1 - Capitalist Systems
- P16 - Political Economy
- P18 - Energy: Environment
- Browse content in P2 - Socialist Systems and Transitional Economies
- P26 - Political Economy; Property Rights
- Browse content in P3 - Socialist Institutions and Their Transitions
- P31 - Socialist Enterprises and Their Transitions
- P34 - Financial Economics
- P39 - Other
- Browse content in P4 - Other Economic Systems
- P43 - Public Economics; Financial Economics
- P48 - Political Economy; Legal Institutions; Property Rights; Natural Resources; Energy; Environment; Regional Studies
- Browse content in Q - Agricultural and Natural Resource Economics; Environmental and Ecological Economics
- Browse content in Q0 - General
- Q02 - Commodity Markets
- Browse content in Q3 - Nonrenewable Resources and Conservation
- Q31 - Demand and Supply; Prices
- Q32 - Exhaustible Resources and Economic Development
- Browse content in Q4 - Energy
- Q40 - General
- Q41 - Demand and Supply; Prices
- Q42 - Alternative Energy Sources
- Q43 - Energy and the Macroeconomy
- Browse content in Q5 - Environmental Economics
- Q50 - General
- Q51 - Valuation of Environmental Effects
- Q53 - Air Pollution; Water Pollution; Noise; Hazardous Waste; Solid Waste; Recycling
- Q54 - Climate; Natural Disasters; Global Warming
- Q56 - Environment and Development; Environment and Trade; Sustainability; Environmental Accounts and Accounting; Environmental Equity; Population Growth
- Browse content in R - Urban, Rural, Regional, Real Estate, and Transportation Economics
- Browse content in R0 - General
- R00 - General
- Browse content in R1 - General Regional Economics
- R10 - General
- R11 - Regional Economic Activity: Growth, Development, Environmental Issues, and Changes
- R12 - Size and Spatial Distributions of Regional Economic Activity
- Browse content in R2 - Household Analysis
- R20 - General
- R21 - Housing Demand
- R23 - Regional Migration; Regional Labor Markets; Population; Neighborhood Characteristics
- Browse content in R3 - Real Estate Markets, Spatial Production Analysis, and Firm Location
- R30 - General
- R31 - Housing Supply and Markets
- R32 - Other Spatial Production and Pricing Analysis
- R33 - Nonagricultural and Nonresidential Real Estate Markets
- R38 - Government Policy
- Browse content in R4 - Transportation Economics
- R41 - Transportation: Demand, Supply, and Congestion; Travel Time; Safety and Accidents; Transportation Noise
- Browse content in R5 - Regional Government Analysis
- R51 - Finance in Urban and Rural Economies
- Browse content in Z - Other Special Topics
- Browse content in Z1 - Cultural Economics; Economic Sociology; Economic Anthropology
- Z11 - Economics of the Arts and Literature
- Z13 - Economic Sociology; Economic Anthropology; Social and Economic Stratification
- Advance articles
- Editor's Choice
- Author Guidelines
- Submission Site
- Open Access
- About The Review of Financial Studies
- Editorial Board
- Advertising and Corporate Services
- Journals Career Network
- Self-Archiving Policy
- Dispatch Dates
- Terms and Conditions
- Journals on Oxford Academic
- Books on Oxford Academic
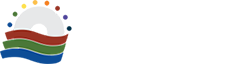
Article Contents
1. data and basic characteristics, 2. cryptocurrency-specific factors, 3. exposures to other assets, 4. additional results, 5. conclusion, acknowledgement, risks and returns of cryptocurrency.
- Article contents
- Figures & tables
- Supplementary Data
Yukun Liu, Aleh Tsyvinski, Risks and Returns of Cryptocurrency, The Review of Financial Studies , Volume 34, Issue 6, June 2021, Pages 2689–2727, https://doi.org/10.1093/rfs/hhaa113
- Permissions Icon Permissions
We establish that cryptocurrency returns are driven and can be predicted by factors that are specific to cryptocurrency markets. Cryptocurrency returns are exposed to cryptocurrency network factors but not cryptocurrency production factors. We construct the network factors to capture the user adoption of cryptocurrencies and the production factors to proxy for the costs of cryptocurrency production. Moreover, there is a strong time-series momentum effect, and proxies for investor attention strongly forecast future cryptocurrency returns.
Cryptocurrency is a recent phenomenon that is receiving significant attention. On the one hand, it is based on a fundamentally new technology, the potential of which is not fully understood. On the other hand, at least in the current form, it fulfills similar functions as other, more traditional assets. Extensive academic attention has focused on developing theoretical models of cryptocurrencies. The theoretical literature on cryptocurrencies has suggested a number of factors that are potentially important in the valuation of cryptocurrencies. The first group of papers builds models stressing the network effect of cryptocurrency adoption (e.g., Pagnotta and Buraschi 2018 ; Biais et al. 2018 ; Cong, Li, and Wang 2019 ) and emphasizes the price dynamics induced by the positive externality of the network effect. The second group of papers focuses on the production side of the coins—the miners’ problem (e.g., Cong, He, and Li 2018 ; Sockin and Xiong 2019 )—and shows that the evolution of cryptocurrency prices is linked to the marginal cost of production. The third group of papers ties the movements of cryptocurrency prices to those of traditional asset classes such as fiat money (e.g., Athey et al. 2016 ; Schilling and Uhlig 2019 ; Jermann 2018 ). There is also a growing literature on the empirical regularities of cryptocurrencies. Borri (2019) shows that individual cryptocurrencies are exposed to cryptomarket tail-risks. Makarov and Schoar (2020) find that cryptocurrency markets exhibit periods of potential arbitrage opportunites across exchanges. Griffin and Shams (2020) study Bitcoin price manipulation. Our paper is the first comprehensive analysis of cryptocurrencies through the lens of empirical asset pricing. Its contribution is twofold. First, it tests the mechanisms and predictions of the existing theoretical models. Second, it establishes a set of basic asset pricing facts for this asset class, which provides a common benchmark that the current and future models of cryptocurrencies should take into consideration.
We start by constructing an index of cryptocurrency (or coin) market returns. This index is the value-weighted returns of all the coins with capitalizations of more than 1 million USD (1,707 coins in total) and covers the period of January 1, 2011, to December 31, 2018. We now describe some basic statistical properties of this index. During the sample period, the averages of the coin market returns at the daily, weekly, and monthly frequencies are 0.46%, 3.44%, and 20.44%, respectively. The daily, weekly, and monthly standard deviations of the coin market returns are 5.46%, 16.50%, and 70.80%, respectively. The coin market returns have positive skewness and kurtosis. We observe that the mean and standard deviation of the coin market returns are an order of magnitude higher than those of the stock returns during the same period. The Sharpe ratios at the daily and weekly levels are about 60% and 90% higher, and the Sharpe ratio at the monthly level is comparable to those of stocks. The returns have positive skewness increasing with the frequencies from daily to monthly. The returns experience high probabilities of extreme losses and gains. For example, an extreme loss of the daily 20% negative return on the coin market happens with a probability of 0.48%, while an extreme gain of the same size occurs with a probability of 0.89%.
We next turn to examine the relationship between the coin market returns and the main cryptocurrency-specific factors that are proposed in the theoretical literature. We formulate and investigate potential drivers and predictors for cryptocurrency returns. Specifically, we construct cryptocurrency network factors, cryptocurrency production factors, cryptocurrency momentum, proxies for average and negative investor attention, and proxies for cryptocurrency valuation ratios. For each of these factors, we aim to provide a number of possible empirical measures, as there are no canonical ways to define them in the cryptocurrency market.
We consider five measures to capture the cryptocurrency network effect. Consistent with the cryptocurrency models based on the network effect, 1 we find that the coin market returns are positively and significantly exposed to cryptocurrency network growth. Furthermore, we show that the evolution of cryptocurrency prices not only reflects current cryptocurrency adoption but also contains information about expected future network growth.
We then study the implications of the cryptocurrency models based on the miners’ production problem. 2 We construct production factors of cryptocurrency to proxy for the cost of mining and test the relationship between these production factors and cryptocurrency prices. To the first approximation, mining a cryptocurrency requires two inputs: electricity and computer power. We separately construct eight proxies for electricity costs and six proxies for computing costs. For electricity, we use time-varying and location-specific measures of the price, consumption, and generation of electricity in the United States and China (including Sichuan province, which hosts the largest mining farm in the world). For proxies of computing costs, we use the prices of Bitmain Antminer, one of the common Bitcoin mining equipments, as our primary measure. We also consider indirect measures—the stock returns of the companies that are major manufacturers of mining chips. Overall, we find that the coin market returns are not significantly exposed to the cryptocurrency production factors.
The existing theoretical models of cryptocurrencies have a number of implications for the predictability of cryptocurrency returns. Some papers argue that the evolution of cryptocurrency prices should follow a martingale, and thus cryptocurrency returns are not predictable (e.g., Schilling and Uhlig 2019 ). Other papers argue that, in dynamic cryptocurrency valuation models, cryptocurrency returns could potentially be predicted by momentum, investor attention, and cryptocurrency valuation ratios (e.g., Cong, Li, and Wang 2019 ; Sockin and Xiong 2019 ). We show that momentum and investor attention strongly predict future cryptocurrency cumulative returns, but cryptocurrency valuation ratios do not.
First, we show that there is a significant time-series momentum phenomenon in the cryptocurrency market. We find that the current coin market returns predict cumulative future coin market returns from one week to eight weeks ahead. For example, a one-standard-deviation increase in the current coin market returns predicts a 3.30% increase in the weekly returns over the next week. Grouping weekly returns by terciles, we find that the top terciles outperform the bottom terciles over the one- to four-week horizons. For example, at the one-week horizon, the average return of the top tercile is 8.01% per week with a t -statistic of 4.30, while the average return of the bottom tercile is only 1.10% per week with a t -statistic of 0.92. The time-series momentum results are valid both in sample and out of sample.
Second, we construct proxies for investor attention with Google searches and show that high investor attention predicts high future returns over the one- to six-week horizons. For example, a one-standard-deviation increase in the investor attention measure yields a 3.0% increase in the 1-week-ahead future coin market returns. At the one-week horizon, the average return of the investor attention tercile is 6.53% per week with a t -statistic of 3.82, while the average return of the bottom tercile is only 0.43% per week with a t -statistic of 0.42. Another proxy for investor attention we construct is Twitter post counts, and we reach similar results with the Twitter measure. Additionally, we construct a proxy for negative investor attention and show that relatively high negative investor attention negatively predicts future cumulative coin market returns.
Research on the equity market (e.g., Hong, Lim, and Stein 2000 ; Hou, Xiong, and Peng 2009 ) shows that there is a strong interaction between momentum and investor attention. Sockin and Xiong (2019) also show that investor attention can generate momentum in the cryptocurrency market, and in their model, the momentum effect disappears controlling for investor attention. We investigate whether there is a similar interaction between momentum and investor attention in the cryptocurrency market. We find that investor attention is high during and after periods of high coin market returns. However, in a bivariate coin market predictability regression with both variables, we show that the two effects do not subsume each other. Finally, we test whether the magnitude of the momentum effect is different during periods of high investor attention and vice versa. In contrast to the equity market, we show that there is limited interaction between cryptocurrency momentum and investor attention.
Moreover, we test whether the cryptocurrency valuation ratios similar to those in the financial markets can predict future coin market returns. In the equity market, the fundamental-to-market ratios are commonly referred to as valuation ratios and are measured as the ratio of the book value of equity to the market value of equity or some other ratio of fundamental value to market value. It is more difficult to define a similar measure of the fundamental value for cryptocurrency. In their dynamic cryptocurrency asset pricing model, Cong, Li, and Wang (2019) show that the cryptocurrency fundamental-to-value ratio, defined as the number of user adoptions over market capitalization, negatively predicts future cryptocurrency returns. Motivated by the theoretical model and studies of other financial markets, we construct six cryptocurrency valuation ratios and test the return predictability of these valuation ratios. Although the coefficient estimates are consistently negative, none of the six cryptocurrency valuation ratios predict future cumulative coin market returns significantly.
Another approach to study what cryptocurrencies represent is to examine the exposures of cryptocurrency returns to other asset classes. In other words, we assess how investors and markets value current and future prospects of cryptocurrencies. The theoretical literature and the community of cryptocurrency have proposed various narratives for what cryptocurrencies represent. Schilling and Uhlig (2019) argue that, in an endowment economy where fiat money and cryptocurrency coexist and compete, the cryptocurrency returns comove with the price evolution of the fiat money. Athey et al. (2016) emphasize the importance of currency exchange rates on cryptocurrency prices. Another popular narrative is that cryptocurrency is “digital gold” and represents a new way to store value. Specifically, we study whether major cryptocurrencies comove with currencies, commodities, stocks, and macroeconomic factors. In contrast to these popular explanations, we find that the exposures of cryptocurrencies to these traditional assets are low. Overall, there is little evidence, in the view of the markets, behind the narrative that there are similarities between cryptocurrencies and these traditional assets.
We note several additional results. First, we acknowledge that we have a short time series and that there is much uncertainty and learning about cryptocurrencies during the sample period. We show that our main results are similar for the first half and the second half of the sample. Second, we discuss the relationship between the cryptocurrency time-series momentum and cross-sectional momentum. Third, we investigate the importance of regulative events in affecting cryptocurrency prices, and show that negative regulative events but not positive regulative events significantly affect cryptocurrency prices. Fourth, we examine the importance of speculative interests in driving cryptocurrency prices. We show that cryptocurrency returns are higher when speculative interests increase, but the coefficient estimates are only marginally significant. Fifth, we construct a direct measure of cryptocurrency investor sentiment and show that the expected coin market return is higher when investor sentiment is high. In the multivariate regressions with the sentiment, investor attention, and momentum measures, all three variables are statistically significant in predicting future cryptocurrency returns. Sixth, we test the role of beauty contests in the cryptocurrency market. Motivated by Biais and Bossaerts (1998) , we use the volume-volatility ratio to capture the degree of disagreement in the cryptocurrency market and show that cryptocurrency return is high when the current volume-volatility ratio is high. Seventh, we conduct a VAR analysis with the coin market returns and the different measures of coin network growth measures. Eighth, we test the effect of production factors with an alternative specification. Lastly, we examine the subsample results based on cryptocurrency characteristics.
Our paper uses standard textbook empirical asset pricing tools and methods, the discussion of which we mostly omit for conciseness. Our findings on momentum are related to a series of papers such as Jegadeesh and Titman (1993) , Moskowitz and Grinblatt (1999) , Moskowitz, Ooi, and Pedersen (2012) , Asness, Moskowitz, and Pedersen (2013) , and Daniel and Moskowitz (2016) . Da, Engelberg, and Gao (2011) use Google searches to proxy for investor attention.
Yermack (2015) is one of the first papers that brought academic attention to the field of cryptocurrency. Several recent articles document individual facts related to cryptocurrency investment. Stoffels (2017) studies cross-sectional cryptocurrency momentum. Hu, Parlour, and Rajan (2018) show that individual cryptocurrency returns correlate with Bitcoin returns. Borri (2019) shows that individual cryptocurrencies are exposed to cryptomarket tail-risks. Makarov and Schoar (2020) and Borri and Shakhnov (2018) find that cryptocurrency markets exhibit periods of potential arbitrage opportunites across exchanges. Griffin and Shams (2020) study Bitcoin price manipulation. Corbet et al. (2019) studies cryptocurrencies as a financial asset. Moreover, a number of recent papers develop models of cryptocurrencies (see, e.g., Weber 2016 ; Huberman, Leshno, and Moallemi 2017 ; Biais et al. 2018 ; Chiu and Koeppl 2017 ; Cong and He 2019 ; Cong, Li, and Wang 2019 ; Cong, He, and Li 2018 ; Sockin and Xiong 2019 ; Saleh 2018 ; Schilling and Uhlig 2019 ; Jermann 2018 ; Abadi and Brunnermeier 2018 ; Routledge and Zetlin-Jones 2018 ).
We collect trading data of all cryptocurrencies available from Coinmarketcap.com. Coinmarketcap.com is a leading source of cryptocurrency price and volume data. It aggregates information from over 200 major exchanges and provides daily data on opening, closing, high, and low prices, as well as volume and market capitalization (in dollars) for most of the cryptocurrencies. 3 For each cryptocurrency on the website, Coinmarketcap.com calculates its price by taking the volume-weighted average of all prices reported at each market. A cryptocurrency needs to meet a list of criteria to be listed, such as being traded on a public exchange with an application programming interface (API) that reports the last traded price and the last 24-hour trading volume, and having a nonzero trading volume on at least one supported exchange so that a price can be determined. Coinmarketcap.com lists both active and defunct cryptocurrencies, thus alleviating concerns about survivorship bias.
We first construct a coin market return as the value-weighted return of all the underlying coins. We use daily close prices to construct daily coin market returns. The weekly and monthly coin market returns are calculated from the daily coin market returns. We require the coins to have information on price, volume, and market capitalization. We further exclude coins with market capitalizations of less than 1,000,000 USD. For earlier years that are not covered by Coinmarketcap.com, we splice the coin market returns with Bitcoin returns from earlier years. The data of the earlier year Bitcoin returns are from CoinDeck and span from January 1, 2011, to April 29, 2013. We start from January 1, 2011, because there was not much liquidity and trading before that date. Altogether, the index of the coin market return covers the period from January 1, 2011, to December 31, 2018.
We use four primary measures to proxy for the network effect of user adoption: the number of wallet users, the number of active addresses, the number of transaction count, and the number of payment count. The data of wallet users are from Blockchain.info. We obtain data on active addresses, transaction count, and payment count from Coinmetrics.io. We use seven primary production factors to proxy for the cost of mining: the average price of electricity in the United States, the net generation of electricity of all sectors in the United States, the total electricity consumption of all sectors in the United States, the average price of electricity in China, and the average price of electricity in Sichuan province. We obtain data on the average price of electricity in the United States, the net generation of electricity of all sectors in the United States, and the total electricity consumption of all sectors in the United States from the U.S. Energy Information Administration. We obtain data on the average price of electricity in China and the average price of electricity in Sichuan province from the National Bureau of Statistics of China and the Price Monitoring Center, NDRC. Our primary computing cost data are the prices of Bitmain Antminer. We extract the Bitmain Antminer data from Keepa.com. The data for Bitmain Antminer start from September 2015.
Google search data series are downloaded from Google. Twitter post counts for the word “Bitcoin” are downloaded from Crimson Hexagon. 4 The spot exchange rates in units of U.S. dollars per foreign currency are from the Federal Reserve Bank of St. Louis. We focus on five major currencies: Australian dollar, Canadian dollar, euro, Singaporean dollar, and U.K. pound. The spot prices of precious metals are from several sources. The gold and silver prices are from the London Bullion Market Association (LBMA). Platinum prices are from the London Platinum and Palladium Market (LPPM).
Aggregate and individual stock returns are from CRSP. Detailed SIC three-digit industry return data series are constructed using individual stock returns. Chinese stock return data are from CSMAR. We build the value-weighted aggregate Chinese stock returns and detailed CIC (China Industry Classification) industry return data series from the individual stocks. The data series of Chinese stock returns last until December 2016. The return series of the 155 anomalies are downloaded from Andrew Chen’s website. 5
We obtain data on the Fama-French three-factor, Carhart four-factor, Fama-French five-factor, and Fama-French six-factor models from Kenneth French’s website. We also collect the return series of Fama-French 30 industries, Europe, Japan, AsiaExJapan, and North America from Kenneth French’s website.
The macroeconomic data series are from the website of the Federal Reserve Bank of St. Louis. Nondurable consumption is defined as the sum of personal consumption expenditures: nondurable goods, and personal consumption expenditures: services.
Stock market prices, dividends, and earnings, as well as the three-month Treasury bill rates, are from Robert Shiller’s website. Using these data series, we construct the stock market price-to-dividend ratio (pd), price-to-earnings ratio (pe), and the relative bill rate (tbill). The relative bill rate is defined as the three-month Treasury bill rate minus its 12 month backward moving average. Credit spread (credit) is defined as the yield spread between BAA corporate bonds and AAA corporate bonds. Term spread (term) is defined as the yield spread between the 10-year Treasury and 3-month Treasury. Data series on the BAA corporate yield, AAA corporate yield, 10-year Treasury yield, and 3-month Treasury yield are from the Federal Reserve Bank of St. Louis’s website.
We now document the main statistical properties of the time series for the coin market returns. Figure 1 shows the return distributions of coin market returns and coin market log returns at daily, weekly, and monthly frequencies. Figure 2 plots the price movements of the coin market compared with those of the three major cryptocurrencies. There are strong comovements across the three major cryptocurrencies. Table 1 compares the properties of the coin market returns with those of Bitcoin returns, Ethereum returns, Ripple returns, and stock market returns.

Coin market return distributions
This figure plots the distributions of daily, weekly, and monthly cryptocurrency returns and log returns.

Cryptocurrency market returns and major coins
This figure plots the cryptocurrency market returns against Bitcoin, Ethereum, and Ripple. The figures show the value of investment over time for one dollar of investment at the starting point of the graphs. The Bitcoin graph starts at April 29, 2013. The Ethereum graph starts at August 8, 2015. The Ripple graph starts at August 5, 2013.
Summary statistics
This table documents the summary statistics of the coin market returns (CMKT). Panel A reports the daily, weekly, and monthly summary statistics of the coin market index and compares them with returns for Bitcoin, Ethereum, Ripple, and the stock market. The mean, standard deviation, t -statistics, Sharpe ratio, skewness, kurtosis, and the percentage of obervations that are positive are reported. Panel B reports the percentage of extreme events based on the daily coin market index returns. The coin market returns, the Bitcoin returns, and the stock market returns are from January 1, 2011, to December 31, 2018. The Ethereum returns are from August 8, 2015, to December 31, 2018. The Ripple returns are from August 5, 2013 to December 31, 2018.
Table 1 shows the statistics of the coin market returns at the daily, weekly, and monthly frequencies compared with those of the stock market returns. Both the average and the standard deviation of the coin market returns are very high. At the daily frequency, the mean return is 0.46% and the standard deviation is 5.46%; at the weekly frequency, the mean return is 3.44% and the standard deviation is 16.50%; at the monthly frequency, the mean return is 20.44% and the standard deviation is 70.80%. Both the means and the standard deviations are an order of magnitude higher than those for the stock market returns. These facts are broadly known.
The Sharpe ratios of the coin market returns are 0.08 at the daily frequency, 0.21 at the weekly frequency, and 0.29 at the monthly frequency. At the daily and weekly frequencies, the Sharpe ratios of the coin market are about 60% and 90% higher than those of the stock market for the comparable time period. At the monthly frequency, the Sharpe ratio is similar to that of the stock market for the comparable time period.
We compare the characteristics of the coin market returns to those of the Bitcoin, Ripple, and Ethereum returns. Note that the Ripple return series starts on August 4, 2013, and the Ethereum return series starts on August 7, 2015. For the Bitcoin returns, the Sharpe ratios are 0.08 at the daily frequency, 0.21 at the weekly frequency, and 0.29 at the monthly frequency. For Ethereum, the Sharpe ratios are 0.08 at the daily frequency, 0.20 at the weekly frequency, and 0.36 at the monthly frequency. The Ethereum returns have a higher mean and standard deviation than the coin market returns. For the Ripple returns, the Sharpe ratios are 0.07 at the daily frequency, 0.13 at the weekly frequency, and 0.24 at the monthly frequency. The Ripple returns have a markedly higher mean and standard deviation compared with those of the coin market returns. The Sharpe ratios of Ripple returns are lower than those of the coin market returns at all three frequencies.
The coin market returns are positively skewed at all frequencies, in contrast to the stock returns, which are negatively skewed. The skewness increases from 0.74 at the daily frequency to 1.74 at the weekly frequency, and to 4.37 at the monthly frequency. The corresponding kurtosis is 15.52 at the daily frequency, 10.22 at the weekly frequency, and 26.54 at the monthly frequency. All three of the major cryptocurrencies have positive skewness and high kurtosis. The coin market returns have high probabilities of exceptional negative and positive daily returns. For example, the probability of a –20% daily return is almost 0.5%, and the probability of a 20% daily return is almost 0.9%.
In the Internet Appendix , we also show the mean, standard deviation, and Sharpe ratios of the returns on different days of the week. In contrast to the stocks, there is no pronounced Monday effect. However, the returns are lower on Saturdays: the average Sunday coin market return is 0.28% with a Sharpe ratio of 0.05, compared with a 0.46% daily average with a Sharpe ratio of 0.08; the average Sunday Bitcoin is 0.29% with a Sharpe ratio of 0.06, compared with a 0.46% daily average with a Sharpe ratio of 0.08; the average Sunday Ethereum is 0.25% with a Sharpe ratio of 0.03, compared with a 0.60% daily average with a Sharpe ratio of 0.08; and the average Sunday Ethereum is –0.15% with a Sharpe ratio of –0.02, compared with a 0.53% daily average with a Sharpe ratio of 0.07. While the coin market and Bitcoin returns are somewhat lower on Sundays, the returns on Saturday are consistently lower.
The theoretical literature has proposed a number of cryptocurrency-specific factors as drivers of cryptocurrency prices and as predictors of cryptocurrency returns. In this section, we develop and investigate the implications of cryptocurrency-specific factors. We first construct cryptocurrency network and production factors. We find that the coin market returns are strongly exposed to the network factors but not the production factors. Then, we test if cryptocurrency returns are predictable by studying whether different cryptocurrency-specific factors can predict future coin market returns. We consider momentum, proxies for investor attention, and proxies for cryptocurrency valuation ratios. All of these variables are specific to the cryptocurrency markets. We find that momentum and proxies for investor attention can account for future coin market returns, and thus strongly reject the notion that cryptocurrency prices are a martingale.
2.1 Network factors
The theoretical literature on cryptocurrency has emphasized the importance of network factors in the valuation of cryptocurrencies (e.g., Cong, Li, and Wang 2019 ; Sockin and Xiong 2019 ; Pagnotta and Buraschi 2018 ; Biais et al. 2018 ). In particular, the network effect of user adoption can potentially play a central role in the valuation of cryptocurrencies. Because users’ adoption of cryptocurrencies generates positive network externality, cryptocurrency prices respond to user adoptions. Hence, variations in user adoptions of the cryptocurrency network could contribute to movements in cryptocurrency prices.
We construct network factors of cryptocurrency and test whether these factors can account for variations in cryptocurrency prices. We use four measures to proxy for the network effect: the number of wallet users, the number of active addresses, the number of transaction count, and the number of payment count. 6 Thus, we measure cryptocurrency network growth using the wallet user growth, active address growth, transaction count growth, and payment count growth. We also construct a composite measure by taking the first principal component of the four primary measures, which we denote as |$PC^{network}$| . Panel A of Table 2 reports the correlation across the network factors we consider. The four primary measures correlate with each other positively, with correlations ranging from 0.17 to 0.77. The first principal component of the four demand factors strongly correlates with all four of the primary measures. The first principal component has correlations of 0.45, 0.88, 0.88, and 0.90 with the wallet user growth measure, the active address growth measure, the transaction count growth measure, and the payment count growth measure, respectively.
Cryptocurrency return loadings to network factors
This table reports the factor loadings of the coin market returns on the network factors. The network factors include wallet user growth, active address growth, transaction count growth, payment count growth, and the first principal component of the four primary measures. Panel A shows the correlation matrix of the variables. Panel B reports the loadings of the coin market returns on the network factors. The standard t -statistic is reported in parentheses. *, **, and *** denote significance levels at the 10%, 5%, and 1% levels based on the standard t -statistics. The data frequency is monthly.
We regress the coin market returns on each of the four measures of changes in the cryptocurrency network and the composite measure. Panel B of Table 2 presents the results using the network factors. The coin market returns positively correlate with all four of the individual cryptocurrency network factors and the composite measure. The coefficient on the wallet user growth measure is significant at the 10% level, and the three other coefficients are significant at the 1% level. The |$R^2$| s range from 5% for the wallet user growth measure to 30% for the active address growth measure. The |$R^2$| s using the composite measure is 19%. Consistent with the theoretical models, these results suggest that the network factors that measure the network effect of user adoptions are important drivers of cryptocurrency prices.
Moreover, in a dynamic cryptocurrency pricing model with the network effect, cryptocurrency prices not only reflect current cryptocurrency adoption but also contain information about expected future network growth—a key mechanism of Cong, Li, and Wang (2019) . We test this model implication by examining whether current coin market returns contain information about future cryptocurrency network growth. In particular, we predict cumulative future cryptocurrency adoption growth over different horizons using current coin market returns. We investigate cumulative future cryptocurrency adoption growth from one-month to eight-month horizons. We use cumulative wallet user growth, active address growth, transaction count growth, and payment count growth to capture cryptocurrency adoption growth.
Consistent with the prediction that cryptocurrency returns reflect expected future cryptocurrency adoptions, we find that coin market returns positively predict future cryptocurrency adoption growth as shown in Table 3 . Specifically, coin market returns positively and statistically significantly predict cumulative wallet user growth at all the horizons. Coin market returns positively and statistically significantly predict cumulative active address growth and cumulative payment count growth for the first three periods and two periods, respectively, and cease to be significant afterward. The coin market returns positively predict cumulative transaction count growth for the first five periods, but the predictability is not statistically significant. The only exception is the transaction growth measure: there is an insignificant, negative effect on transaction growth over the long horizons. A possible explanation for the negative effect is congestion, as it becomes very expensive to transact in Bitcoin when there is congestion, which deters many of the smaller transactions that would have occurred otherwise (e.g., Easley, O’Hara, and Basu 2019 ).
Predicting future network growth
This table reports the results of predicting cumulative future coin network growth with coin market returns. The network factors include wallet user growth, active address growth, transaction count growth, and payment count growth. Data are monthly. The t -statistics are reported in parentheses and are Newey-West adjusted with |$n-1$| lags. *, **, and *** denote significance levels at the 10%, 5%, and 1% levels. The data frequency is weekly.
2.2 Production factors
Several papers have argued that the costs of mining are essential for the infrastructure and security of cryptocurrencies (e.g., Sockin and Xiong 2019 ; Abadi and Brunnermeier 2018 ; Cong, He, and Li 2018 ). Notably, Sockin and Xiong (2019) show that, in a general equilibrium model with cryptocurrency production, the prices of the cryptocurrency are intimately linked to the marginal cost of mining.
We construct production factors of cryptocurrency to proxy for the cost of mining and test the relationship between these production factors and cryptocurrency prices. To the first approximation, mining a cryptocurrency requires two inputs: electricity and computer power. We separately construct proxies for electricity costs and computing costs. We first discuss our proxies for electricity costs. For electricity, we use seven primary measures. Three of the seven primary measures are U.S.-related: (i) average price of electricity in the United States, (ii) net generation of electricity of all sectors in the United States, and (iii) total electricity consumption of all sectors in the United States. The other four measures are China-related: (i) average price of electricity in China, (ii) electricity generation in China, (iii) average price of electricity in Sichuan province, and (iv) electricity generation in Sichuan province. We include the China proxies, because electricity supply is location specific and because China is considered to have the largest coin-mining operation among all countries. 7 We include Sichuan province proxies because Sichuan province hosts the largest mining farm in the world. Similarly, we also construct a composite measure as the first principal component of these seven primary measures. We denote the composite measure as |$PC^{elec}$| .
Panel A of Table 4 presents the correlation matrix of the electricity factors. Except for the two electricity price measures in China, the other five primary measures positively and strongly correlate with one another. Electricity prices in China are under strict government control. Unsurprisingly, they have low correlations with other electricity measures. The first principal component of the seven electricity factors strongly and positively correlates with most of the seven primary factors. The correlations are 0.76, 0.93, 0.88, 0.71, and 0.77 with the U.S. electricity price growth measure, the net U.S. generation growth measure, the U.S. electricity consumption growth measure, the China generation growth measure, and the Sichuan generation growth measure, respectively. The correlation between the first principal component and the China electricity price growth measure is –0.15, and the correlation between the first principal component and the Sichuan electricity price growth measure is 0.18. Panel B of Table 4 presents the electricity factor results for the coin market returns. Somewhat surprisingly, the coin market returns are not statistically significantly exposed to any of these production factor proxies. The |$R^2$| s of these regressions are low.
Cryptocurrency return loadings to electricity factors
This table reports the factor loadings of the coin market returns on the production factors that relate to electricity costs. Panel A shows the correlation matrix of the production factors. Panel B reports the factor loadings of the coin market returns on the production factors. Standard t -statistics are reported in parentheses. *, **, and *** denote significance levels at the 10%, 5%, and 1% levels based on the standard t -statistics. The data frequency is monthly.
For proxies of computing costs, we use as our primary measure the prices of Bitmain Antminer, a major piece of Bitcoin mining equipment. We also consider the excess stock returns of the companies that are major manufacturers of either GPU mining chips (Nvidia Corporation and Advanced Micro Devices, Inc.) or ASIC mining chips (Taiwan Semiconductor Manufacturing Company, Limited, and Advanced Semiconductor Engineering, Inc.). 8 We construct a composite measure as the first principal component of these five primary computing factors. We denote the composite measure as |$PC^{comp}$| .
Panel A of Table 5 presents the correlation matrix of the computing factors. Most of the pairs are positively correlated. The correlation between Antminer price growth and Nvidia return is –0.03, and the correlation between Antminer price growth and AMD return is –0.15. The first principal component is positively correlated with the four return measures and has a low correlation with the Antminer price growth measure. Panel B of Table 5 presents the computing factor results for the coin market returns. The coin market returns have insignificant loadings on the four excess return measures. The coin market returns have some loadings on the Antminer price growth measure, but they are only significant at the 10% level. The coin market returns are not significantly exposed to the first principal component.
Cryptocurrency return loadings to computing factors
This table reports the factor loadings of the coin market returns on the production factors that relate to computing costs. Panel A shows the correlation matrix of the production factors. Panel B reports the factor loadings of the coin market returns on the production factors. Standard t -statistics are reported in parentheses. *, **, and *** denote significance levels at the 10%, 5%, and 1% levels based on the standard t -statistics. The data frequency is monthly.
The model of Sockin and Xiong (2019) primarily concerns utility tokens. Therefore, we conduct our analyses on production factors on Bitcoin, Ethereum, and Ripple, respectively. Because Ethereum and Ripple are utility tokens, while Bitcoin is not, we expect to find that Ethereum and Ripple load significantly on the production factors. We show the results in the Internet Appendix . There is some evidence that Bitcoin returns are exposed to the Bitmain Antminer price growth, but Bitcoin and Ripple returns do not load significantly on these production factors. Overall, there is limited evidence that the computing factors are important drivers of cryptocurrency returns.
Lastly, we test the lead-lag effects between the changes in production factors and cryptocurrency returns to account for possible anticipation effects. We document the results in the Internet Appendix . We show that the one-month-ahead coin market returns are not significantly exposed to most of the production factors. The only exception is the changes in the average price of electricity in the United States, but the significant level is negative and only at the 10% level. However, we find that the current coin market returns positively predict some of the future production factors. In particular, the coin market returns positively and statistically significantly predict future changes in the average price of electricity in the United States, net generation of electricity of all sectors in the United States, total electricity consumption of all sectors in the United States, electricity generation in Sichuan province, and the first principal component of the production factors. Interestingly, we find that the results are stronger for the U.S.-based measures relative to the China-based measures. This is consistent with the fact that electricity prices and generation are heavily regulated in China. These results are consistent with a potential anticipation effect of production costs in the cryptocurrency market.
2.3 Are cryptocurrency returns predictable?
In this section, we test whether the coin market returns are predictable. The existing theoretical models of cryptocurrencies provide various predictions on the predictability of cryptocurrency returns. Schilling and Uhlig (2019) argue that the evolution of cryptocurrency prices should follow a martingale, and thus cryptocurrency returns are not predictable. Other papers predict that, in dynamic cryptocurrency valuation models, cryptocurrency returns could potentially be predicted by momentum, investor attention, and cryptocurrency valuation ratios (e.g., Cong, Li, and Wang 2019 ; Sockin and Xiong 2019 ). Motivated by the existing theoretical development and empirical findings in the financial markets, we test whether the cryptocurrency returns are predictable by momentum, investor attention, and proxies for cryptocurrency valuation ratios.
2.3.1 Cryptocurrency momentum
One of the most studied asset pricing regularities is momentum (e.g., Jegadeesh and Titman 1993 ; Moskowitz and Grinblatt 1999 ). As discussed in Cong, Li, and Wang (2019) , the network effect of user adoption generates a positive externality that is not immediately incorporated into cryptocurrency prices. This channel can potentially lead to a momentum effect in cryptocurrency returns. In their model, Sockin and Xiong (2019) generate momentum in the cryptocurrency market through investor attention—a mechanism similar to De Long et al. (1990) .
In this section, we start by establishing that there is strong evidence of time-series momentum at various time horizons. Panel A in Table 6 documents the time-series momentum results in the regression setting. Specifically, we regress cumulative future coin market returns on current coin market returns from the one-week to eight-week horizons. The current coin market returns positively and statistically significantly predict cumulative future coin market returns at all eight horizons. The results are significant at the 5% level for the one-week to five-week horizons and are significant at the 10% level from the six-week to eight-week horizons. For example, a one-standard-deviation increase in the current coin market return leads to increases in cumulative future coin market returns of 3.30%, 9 8.09%, 13.37%, and 17.66% increases at the one-week, two-week, three-week, and four-week horizons, respectively. Specifically, the one-week-ahead weekly return is that of buying the underlying coin market index at 11:59:59 UTD Sunday and selling the underlying coin market index at 11:59:59 UTD one week later. In the Internet Appendix , we also report the results based on noncumulative returns. The current coin market returns positively and significantly predict one-week- to five-week-ahead returns. The current coin market returns positively but insignificantly predict six-week- and seven-week-ahead returns. The current coin market returns negatively but insignificantly predict eight-week-ahead returns, suggesting some potential long-term reversal effect.
Time-series momentum
This table reports the time-series momentum results. Panel A shows the regression results, and panel B shows the results based on grouping weekly coin market returns into terciles. The first part of panel B reports results for the whole sample. The second part of panel B uses the first two years of data to determine the tercile cutoffs and examine the out-of-sample time-series momentum performance. The t -statistics are reported in parentheses and are Newey-West adjusted with |$n-1$| lags. *, **, and *** denote significance levels at the 10%, 5%, and 1% levels. The data frequency is weekly.
In the first part of panel B in Table 6 , we estimate the magnitude of the time-series momentum by grouping weekly returns into terciles and evaluating their performance going forward. We find that the top terciles outperform the bottom terciles at the one- to four-week horizons, consistent with the time-series regression results presented earlier. For example, at the one-week horizon, the average return of the top tercile is 8.01% per week with a t -statistic of 4.30, while the average return of the bottom tercile is 1.10% per week with a t -statistic of 0.92. The difference between the top and bottom terciles is 6.91% at the one-week horizon. At the two-week horizon, the average of the cumulative coin market returns of the top tercile is 16.22%, and that of the bottom tercile is only 3.59%. The difference between the top and bottom terciles is 12.63%. In the additional results section, we restrict our sample to 2014 onward. Again, we find a strong and significant momentum effect of somewhat smaller magnitude. 10
In the second part of panel B in Table 6 , we use the first two years of data to determine the tercile cutoffs and study the out-of-sample time-series momentum performance. We find a strong and significant momentum effect for the out-of-sample tests. For example, at the one-week horizon, the average return of the top tercile is 6.42%, and that of the bottom tercile is 0.80%. The difference between the top and bottom terciles is 5.62%, which is economically large and slightly smaller than the in-sample result of 6.91%.
Additionally, we test whether the time-series momentum effect is linked to network externalities, as suggested in Cong, Li, and Wang (2019) . In their dynamic cryptocurrency valuation model, the momentum effect is generated by the positive externality of the network effect that is not incorporated into cryptocurrency prices immediately. That is, their model implies that controlling for cryptocurrency adoption growth would subsume the time-series momentum effect. In Table 7 , we show that there is evidence that cryptocurrency adoption growth positively predicts future coin market returns. However, controlling for cryptocurrency adoption growth does not subsume the time-series momentum effect documented presented earlier.
Momentum and network effect
This table reports the results that compare coin market return predictability of momentum and network effect. The table reports the results of predicting cumulative future coin market returns with current coin market returns and each of the network factors. The Newey-West adjusted t -statistics with |$n-1$| lags are reported in parentheses. *, **, and *** denote significance levels at the 10%, 5%, and 1% levels. The data frequency is weekly.
2.3.2 Cryptocurrency investor attention
The theoretical literature of cryptocurrencies has also suggested that investor attention could potentially be linked to future cryptocurrency returns (e.g., Sockin and Xiong 2019 ). In this section, we investigate the role of investor attention in predicting cryptocurrency returns. Specifically, we construct the deviation of Google searches for the word “Bitcoin” in a given week compared with the average of those in the preceding four weeks. We standardize the Google search measure to have a mean of zero and a standard deviation of one. We use Google searches for the word “Bitcoin” to proxy for investor attention of the cryptocurrency market because Bitcoin is by far the largest and most visible cryptocurrency available. In panel A of Table 8 , we report the results of regressing cumulative future coin market returns from one-week to eight-week horizons on the Google search measure. The Google search measure statistically significantly predicts the one-week to six-week ahead cumulative coin market returns at the 5% level. The coefficient estimates of the seven-week and eight-week horizons are positive but are no longer statistically significant. A one-standard-deviation increase in searches leads to increases in weekly returns of about 3% for the one-week ahead cumulative coin market returns and about 5% for the two-week-ahead cumulative coin market returns. 11 In the Internet Appendix , we also report results based on noncumulative returns. The current coin market returns positively and significantly predict one-week- to four-week-ahead returns. The current coin market returns positively but insignificantly predict five-week-ahead returns. The current coin market returns negatively but insignificantly predict six-, seven-, and eight-week-ahead returns.
Google searches
This table reports the time-series Google search results. Panel A shows the regression results, and panel B shows the results based on grouping weekly coin market returns into terciles. The first part of panel B reports results for the whole sample. The second part of panel B uses the first two years of data to determine the tercile cutoffs and examine the out-of-sample time-series performance. The Google search measure is constructed as the Google search data for the word “Bitcoin” minus its average of the previous four weeks, and then normalized to have a mean of zero and a standard deviation of one. The t -statistics are reported in parentheses and are Newey-West adjusted with |$n-1$| lags. *, **, and *** denote significance levels at the 10%, 5%, and 1% levels. The data frequency is weekly.
In the first part of panel B in Table 8 , we investigate the return predictability of the Google search measures by grouping them into terciles and evaluating their performance going forward. Consistent with the regression results, we find that the top tercile outperforms the bottom tercile in terms of cumulative coin market returns at the one- to four-week-ahead horizons. For example, at the one-week horizon, the average return of the top tercile is 6.53% per week with a t -statistic of 3.82, while the average return of the bottom tercile is 0.43% per week with a t -statistic of 0.42. The difference between the top and bottom terciles is 6.09% at the one-week horizon. At the two-week horizon, the average of the cumulative coin market returns of the top tercile is 13.95% with a t -statistic of 4.89, and that of the bottom tercile is only 0.02% with a t -statistic of 0.01. The difference between the top and bottom terciles is 13.93%. In the additional results section, we restrict our sample to 2014 onward and find similar return predictive power of the Google search measures.
In the second part of panel B in Table 8 , we use the first two years of data to determine the tercile cutoffs and study the out-of-sample effect of investor attention, and we find a strong positive investor attention effect as well. For example, at the one-week horizon, the average return of the top tercile is 6.12%, and that of the bottom tercile is 0.70%. The difference between the top and the bottom terciles is 5.42%, which is economically large and slightly smaller than the in-sample estimate of 6.09%.
2.3.3 Negative investor attention
We have shown that unconditionally investor attention positively predicts cryptocurrency returns. However, not all investor attention is positive. For example, in their model, Sockin and Xiong (2019) differentiate positive investor attention and negative investor attention, and show that negative investor attention is followed by cryptocurrency price depreciation in the future.
In this section, we investigate whether negative investor attention predicts cryptocurrency returns. We construct a ratio between Google searches for the phrase “Bitcoin hack” and searches for the word “Bitcoin” to proxy for negative investor attention. We standardize the measure to have a mean of zero and a standard deviation of one. Panel A of Table 9 shows the results of the predictive regressions. The ratio negatively and significantly predicts one- to six-week-ahead cumulative coin market returns. For example, a one-standard-deviation increase in the ratio leads to a 2% decrease of coin market returns in the next week. Panel B of Table 9 reports the in-sample and out-of-sample return predictability of the negative investor attention measures by grouping them into terciles and evaluating their performance going forward. Consistent with the regression results, we find strong negative return predictability results of the negative investor attention measures.
Bitcoin hack
This table reports the time-series Bitcoin hack results. Panel A reports the regression results, and panel B reports the sorting results. The Bitcoin hack measure is constructed as the ratio between Google searches for the phrase “Bitcoin hack” and searches for the word “Bitcoin,” and then normalized to have a mean of zero and a standard deviation of one. Results are based on weekly data. The t -statistics are reported in parentheses and are Newey-West adjusted with |$n-1$| lags. *, **, and *** denote significance levels at the 10%, 5%, and 1% levels. The data frequency is weekly.
Another way to see the results on the investor attention is that our measures of investor attentions proxy for speculative interest and sentiment in cryptocurrencies. Positive investor sentiment is followed by cryptocurrency price appreciation, and negative investor sentiment is followed by depreciation. We further investigate these issues in Section 4 .
2.3.4 Interaction between momentum and attention
We have shown that there are strong effects of time-series momentum and investor attention in the cryptocurrency market. The equity market research (e.g., Hong, Lim, and Stein 2000 ; Hou, Xiong, and Peng 2009 ) shows that there is a strong relationship between momentum and investor attention. It is possible that these two results capture the same underlying phenomenon. For example, Sockin and Xiong (2019) propose a potential channel to generate momentum. In their model, momentum arises because users have incorrect expectations about future prices—a mechanism similar to De Long et al. (1990) . Their model suggests that cryptocurrency momentum and investor attention could potentially arise from the same underlying mechanism. The cryptocurrency momentum and investor attention results could also interact with each other. For example, the cryptocurrency time-series momentum effect may be weaker at times of high investor attention, because there is little information leakage at times of high investor attention.
First, we show that the current investor attention of cryptocurrencies is indeed associated with current and past coin market performance. We regress the current deviation in the Google searches on the contemporaneous and the coin market returns of the previous four weeks. Table 10 documents the results. We find that the deviations in Google searches are positively and significantly associated with contemporaneous and the previous week’s coin market returns. The Google search measures do not significantly correlate with past coin market returns beyond one week. Intuitively, these results suggest that investor attention is elevated after superior cryptocurrency market performance.
Google searches and past returns
This table reports the relationships between the Google search measure and past coin market returns. The Google search measure is constructed as the Google search data for the word “Bitcoin” minus its average of the previous four weeks, and then normalized to have a mean of zero and a standard deviation of one. The standard t -statistic is reported in parentheses, and the bootstrapped t -statistic is reported in brackets. *, **, and *** denote significance levels at the 10%, 5%, and 1% levels based on the standard t -statistics. The data frequency is weekly.
We further test the interaction between the time-series momentum and the investor attention phenomena. The results are reported in Table 11 . In the first test of Table 11 , we regress cumulative future coin market returns on current coin market returns and Google search measures. We find that the coefficients to the current coin market returns are significant for all the horizons, and the coefficients to the Google search measures are significant from the one-week to the five-week horizons. The magnitudes of the coefficients are similar to the standalone estimates. For example, the one-week-ahead coefficients under the univariate regressions are 0.20 and 0.03 for the current coin market returns and the Google search measures, respectively, while they are 0.18 and 0.03 under the bivariate regressions. These results show that the time-series momentum and the investor attention results do not subsume each other.
Interaction between momentum and attention
This table reports the predictive regressions of future cumulative coin market returns on momentum, attention, and the interaction of the two. The indicator variable |$1_{\{Google>0\}}$| equals one if the current Google search measure is above the sample mean and zero otherwise. The indicator variable |$1_{\{R>0\}}$| equals one if the current coin market return is positive and zero otherwise. Results are based on weekly returns. The Newey-West adjusted t -statistics with |$n-1$| lags are reported in parentheses. *, **, and *** denote significance levels at the 10%, 5%, and 1% levels. The data frequency is weekly.
In the second test of Table 11 , we test the performance of the time-series momentum result when investor attention is high. We construct an indicator variable, |$1_{\{Google>0\}}$| , that equals one if the current Google search measure is above the sample mean and zero otherwise. We regress the cumulative future coin market returns from one-week to eight-week horizons to the current coin market return, the indicator variable, and the interaction term. The interaction term is not significant at any of the eight horizons, suggesting that the magnitude of the time-series momentum effect is similar for high and low investor attention periods. In the third test of Table 11 , we test the performance of the investor attention result when the current coin market return is high. We construct an indicator variable, |$1_{\{R>0\}}$| , that equals one if the current coin market return is positive and zero otherwise. We regress the cumulative future coin market returns from one-week to eight-week horizons to current Google search measure, the indicator variable, and the interaction term. The interaction term is not significant at any of the eight horizons, suggesting that the magnitude of the investor attention effect is similar for high and low coin market return periods.
Furthermore, we study the cross-section of time-series momentum for high- and low-attention coins. We collect Google attention data for the 10 largest cryptocurrencies from the beginning of 2014 to the end of 2018. The sample period is shorter for this analysis, because before 2014, there are very few cryptocurrencies and the data for alternative coins are hard to get. The list of coins is Bitcoin, Ethereum, Ripple, Litecoin, Tether, Bitcoin-Cash, Tezos, Binance-coin, Monero, and Cardano. At a given point in time, we group the existing coins into two subsamples based on the Google attention data—a group of high-attention coins and a group of low-attention coins. We construct the value-weighted returns of the high-attention group and the low-attention group, separately, and test the time-series momentum strategy effect in each subgroup.
We regress the future cumulative returns on current returns for each of the subsamples and report the results in the Internet Appendix . We find that in this sample, the time-series momentum effect is stronger for the relatively low-attention coins. In particular, the coefficient estimates for both the high-attention and the low-attention subgroups are positive, suggesting that there are time-series momentum effects for both groups. However, the coefficient estimates for the high-attention subgroup is not statistically significant, while the coefficient estimates for the low-attention subgroup is statistically significant up to six weeks out. The magnitudes of the coefficient estimates are also much larger for the low-attention subgroup relative to the high-attention subgroup. The results are consistent with the “underreaction” mechanism of momentum.
2.3.5 Cryptocurrency valuation ratio
Additionally, we test whether the cryptocurrency valuation ratios similar to those in the financial markets can predict future coin market returns. In the equity market, the fundamental-to-market ratios are commonly referred to as valuation ratios and are measured as the ratio of the book value to the market value of equity or some other fundamental value to market value (e.g., dividend-to-price; earnings-to-price). Another value measure used in the literature that has been shown to correlate highly with fundamental-to-market value is the negative of the long-term cumulative past returns (e.g., De Bondt and Thaler 1985 , 1987 ; Fama and French 1996 ; and Moskowitz 2015 ). It is more difficult to define a similar measure of fundamental value for cryptocurrency. However, in their dynamic cryptocurrency asset pricing model, Cong, Li, and Wang (2019) argue that the cryptocurrency fundamental-to-value ratio can be defined as the number of user adoptions over market capitalization, which negatively predicts future cryptocurrency returns.
The market value of cryptocurrency is readily available. However, there is no direct measure of fundamental value for the cryptocurrencies. In its essence, value is a measure of the gap between the market value and the fundamental value of an asset. Because of the lack of a standard “book” value measure of the cryptocurrency market, we use an array of different proxies to capture the idea of fundamental value. We proxy the fundamental-to-market ratio by a number of value measures motivated by the finance literature. The first one is the long-term past performance measure: the negative of the past 100-week cumulative coin market return. The other four measures aim to proxy the cryptocurrency fundamental-to-market value directly: the user-to-market ratio, the address-to-market ratio, the transaction-to-market ratio, and the payment-to-market ratio. The idea of these four measures is to use some measures of the “book” value of the underlying cryptocurrency market and scale by the current market capitalization. The user base of the cryptocurrency market seems to capture the concept of “book” value in the financial markets. This is consistent with the theoretical literature of the cryptocurrency market that emphasizes the notion of the network effect, which can be proxied by the current user base of the cryptocurrencies. On the other hand, the market value of the cryptocurrency provides a market assessment of the current value of the complete cryptocurrency infrastructure. Therefore, the user base-to-market value measure can capture the notion of fundamental-to-market ratio in the financial markets. In panel A of Table 12 , we report the correlations across the different valuation ratios in the cryptocurrency market. The five primary measures are highly correlated with one another, with correlations ranging from 0.73 to 0.91. The first principal component measure for the five fundamental-to-market ratios has correlations of 0.91, 0.91, 0.96, 0.93, and 0.93 with the long-term past returns, the wallet user-to-market ratio, the active address-to-market ratio, the transaction-to-market ratio, and the payment-to-market ratio, respectively.
Cryptocurrency valuation ratio
This table reports the predictive regressions of coin market returns on proxies for cryptocurrency market fundamental-to-market ratio. The proxies for cryptocurrency valuation ratio include the (negative) past 100-week cumulative coin market returns, the user-to-market ratio, the address-to-market ratio, the transaction-to-market ratio, payment-to-market ratio, and the first principal component of the previous five proxies. The ratios are estimated using the cointegration method. Results are based on weekly returns. The Newey-West adjusted t -statistics with |$n-1$| lags are reported in parentheses. *, **, and *** denote significance levels at the 10%, 5%, and 1% levels. The data frequency is weekly.
We regress the coin market returns on the lagged cryptocurrency fundamental-to-market ratios, and the results are reported in panel B of Table 12 . We document the regression results from one-week to eight-week horizons. Although the coefficient estimates are consistently negative, none of the five standalone fundamental-to-market ratios predict future coin market returns significantly over any horizon. The principal component measure also fails to predict future coin market returns over these horizons. Overall, there is a very weak relationship between the future coin market returns and the current cryptocurrency fundamental-to-value ratio.
Both the cryptocurrency literature and the community have debated the nature of cryptocurrencies. For example, Schilling and Uhlig (2019) show that, in an endowment economy with both fiat money and cryptocurrency, the evolution of cryptocurrency prices is linked to that of the fiat money. Athey et al. (2016) emphasize the importance of fiat money risks of cryptocurrencies. The cryptocurrency community has proposed that cryptocurrencies are “digital gold” and serve the purpose of the traditional precious metal commodity. Moreover, Schilling and Uhlig (2019) argue that cryptocurrency returns can have exposure to macroeconomic risks such as monetary policies. In this section, we evaluate these claims by examining the relationship between cryptocurrency returns and traditional asset returns such as currency, commodity, and equity.
3.1 Currency and commodity factor loadings
In an endowment economy where fiat money and cryptocurrency coexist and compete with each other, Schilling and Uhlig (2019) show that the evolution of cryptocurrency prices is correlated with the that of the fiat money prices. Athey et al. (2016) also emphasize the importance of fiat money risks of cryptocurrencies. We test this prediction by investigating the cryptocurrency exposures to traditional currencies. Columns (1) to (6) of Table 13 show the coin market returns’ exposures to the traditional currency returns. For currency returns, we consider five major currencies: Australian dollar, Canadian dollar, euro, Singaporean dollar, and U.K. pound. The exposures of the coin market returns to these major currencies are not statistically significant, and the alpha estimates barely change. We further test cryptocurrency exposures on currency factors as in Lustig, Roussanov, and Verdelhan (2011) instead of individual major currency returns. 12 Columns (7) to (9) of Table 13 report the coin market returns’ exposures to these currency factors. Consistent with the results on individual currency returns, we find that the coin market returns do not have significant exposures to the currency factors. We conclude that there is no consistent evidence of systematic currency exposures in cryptocurrencies.
Currency loadings of coin market returns
This table reports the factor loadings of the coin market returns on returns of different currencies and currency factors. The currencies include Australian dollar, Canadian dollar, euro, Singapore dollar, and U.K. pound. The currency factors are based on Lustig, Roussanov, and Verdelhan (2011) . The returns are in percentage. The results are based on monthly returns. The standard t -statistic is reported in parentheses, and the bootstrapped t -statistic is reproted in brackets. *, **, and *** denote significance levels at the 10%, 5%, and 1% levels based on the standard t -statistics. The data frequency is monthly.
Another popular narrative around cryptocurrencies is that cryptocurrencies serve the same purpose as traditional precious metal commodities. That is, cryptocurrencies are “digital gold.” If the investors of cryptocurrencies hold this belief, we would expect to find that the returns of cryptocurrencies comove with the returns of the traditional precious metal commodities. We test the precious metal commodity exposures of the coin market returns and report the results in Table 14 . For precious metal commodities, we consider gold, platinum, and silver. The exposures of the coin market return to these three major commodities are not statistically significant. Overall, we conclude that there is no consistent evidence of systematic precious metal commodity exposures in cryptocurrencies.
Commodity loadings of coin market returns
This table reports the factor loadings of the coin market returns on returns of different precious metal commodities. The commodities include gold, platinum, and silver. Returns are in percentage. The standard t -statistic is reported in parentheses and the bootstrapped t -statistic is reproted in brackets. *, **, and *** denote significance levels at the 10%, 5%, and 1% levels based on the standard t -statistics. The data frequency is monthly.
3.2 Equity factor loadings
We document the common stock factor exposures of the coin market returns in the Internet Appendix . For the equity risk factors, we choose the Capital Asset Pricing Model (CAPM), Fama-French three-factor, Carhart four-factor, Fama-French five-factor, and Fama-French six-factor models. 13 The alphas for all of the considered models are statistically significant. The average return of the period is 20.44% per month. The CAPM-adjusted alpha decreases to 17.53% per month—a reduction of about 14%. The CAPM beta is large at 3.15 but not statistically significant. The betas are statistically significant at the 10% level only for the five-factor and six-factor models. The corresponding alphas are 15.32% and 15.00% per month for the five-factor model and six-factor model, respectively. The exposures to the other factors are not statistically significant. The exposures to the SMB (small-minus-big) factor are negative but not stable across the specifications: the magnitude of the coefficient decreases when five-factor and six-factor models are considered. The exposures to the HML (high-minus-low) factor are negative and have consistent magnitudes and signs; this suggests that the coin market returns may comove more with growth rather than with value firms. The exposures to the RMW (robust-minus-weak) factor are positive and are estimated slightly more accurately than other statistically not significant factors; this suggests that the coin market returns comove more with high-profit rather than low-profit firms. The point estimates on the MOM (momentum) and CMA (conservative-minus-aggressive) factors are very inaccurate. 14
3.2.1 Exploring the factor zoo
The finance literature has documented more than a hundred factors for predicting the cross-section of stock returns (see e.g., summarizes in Feng, Giglio, and Xiu 2017 and Chen and Velikov 2017 ). To investigate whether any of those factors may be important in pricing cryptocurrencies, we estimate the loadings of the 155 common factors from Andrew Chen’s website. One caveat is that this data set ends at the end of 2016 and thus does not cover the most recent return experiences. We report the results in the Internet Appendix due to the large number of factors. We find that only four out of the 155 factors are significant, but those four factors do not form any discernible patterns.
3.3 Macroeconomic factors
We further examine the macroeconomic factor exposures of the coin market returns. For macroeconomic factors, we consider the nondurable consumption growth, durable consumption growth, industrial production growth, and personal income growth. We document the results in the Internet Appendix . We find that the coin market returns do not significantly load on these macroeconomic factors. We further investigate the three major cryptocurrencies individually. For Bitcoin and Ripple, all of the exposures are not statistically significant. For Ethereum, notably, the durable consumption growth factor has a significant loading.
4.1 Short sample
We have eight years of coin market return data spanning from the beginning of 2011 to the end of 2018. The short sample is a potential barrier to study cryptocurrency that we cannot avoid. Moreover, there is a great deal of uncertainty and learning about cryptocurrencies during the period. As argued by Pástor and Veronesi (2003) , it takes time for investors to fully learn and understand emerging technologies, which can lead to price bubbles.
One approach we take to partially address these concerns is to break the sample into two halves and check whether our results are stable for these subsamples. During the first half of the sample, there are considerably more uncertainty and learning about cryptocurrency as an asset class. We document these results in the Internet Appendix . We find that the directions of all of the results are the same for the first and second halves of the sample. The magnitudes of the results are also comparable between the two subsamples. There is potentially still a lot of uncertainty and learning about cryptocurrencies today, but the assumption we need for the subsample tests is relatively mild: the uncertainty has decreased from the first half of the sample to the second half of the sample. The analysis on the volatility of the coin market returns also supports this assumption. We find that the standard deviation of coin market returns decreased significantly from the first half to the second half of the sample period. The figure in the Internet Appendix shows a significant decrease in the volatility of the coin market returns over time.
4.2 Time-series momentum and cross-sectional momentum
We study the relationship between time-series momentum and cross-sectional momentum. It is difficult to directly compare the time-series momentum and cross-section momentum results. The time-series momentum is a phenomenon on the aggregate coin market returns, while the cross-sectional momentum results are neutral in terms of the aggregate performance of the coin market. We use two different methods to test the relationships between the time-series momentum and the cross-sectional momentum results. In the first method, we use coin market returns to predict the cross-sectional cryptocurrency momentum. This approach gives us a sense about whether the cross-sectional momentum effect is stronger when the time-series momentum is on a positive trajectory. We report the results in the Internet Appendix . The coin market returns do not significantly predict future cumulative cross-sectional momentum returns. This result suggests that the profitable periods of the cryptocurrency time-series momentum and cross-sectional momentum are different.
In the second method, we follow the approach similar to Moskowitz, Ooi, and Pedersen (2012) and construct a portfolio version of the time-series momentum. For our set of instruments, we use one of the following: largest three coins, largest five coins, and largest ten coins. For each instrument and month, we consider whether the excess return over the past three weeks is positive or negative and go long the instrument if positive and short if negative. We hold the position for one week, so there is no overlapping sample. The unadjusted excess returns are positive and significant at the 1% level for all three of the specifications. The economic magnitudes of the excess returns are large, ranging from 3.17% for the top three coins to 4.62% for the top ten coins. Controlling for the coin market returns, the economic magnitudes of the excess returns barely change. Controlling for the cryptocurrency cross-sectional momentum as constructed in Liu, Tsyvinski, and Wu (2019) , the magnitudes of the spreads decrease but remain highly statistically significant. It is not surprising that the magnitudes of the spreads decrease after controlling for the cross-sectional momentum because the excess returns of the strategy contain information about the cross-sectional momentum by construction. However, there is additional information coming from the construction similar to Moskowitz, Ooi, and Pedersen (2012) , which is evidenced by the fact that the magnitudes of the spreads remain positive and statistically significant after controlling for the cross-sectional momentum. Finally, we also test whether the strategies contain information above and beyond the cryptocurrency three-factor model in Liu, Tsyvinski, and Wu (2019) . After controlling for the three-factor model, the magnitudes of the spreads further decrease but still remain positive and significant at the 5% level for the top five and top ten coins and at the 10% level for the top three coins.
4.3 Regulations
A potentially important determinant of cryptocurrency valuation is regulations. To test whether cryptocurrency regulations are important determinants of cryptocurrency valuations, we follow the method of Auer and Claessens (2018) and Shanaev et al. (2019) and determine 120 regulative events. We further categorize these regulative events into positive and negative events based on Auer and Claessens (2018) . We document the list of regulative events and the results in the Internet Appendix . We find that the contemporaneous cryptocurrency returns are lower during the days of regulative events. However, we find that the cryptocurrency returns respond to negative regulative events but not to positive regulative events.
4.4 Speculative interest and sentiment
In this section, we test whether speculation and investor sentiment may be important drivers of cryptocurrency prices. We extract the speculative shares of cryptocurrency usage from Coindesk.com. We test whether the cryptocurrency returns strongly respond to the contemporaneous and expectations of future speculative share growth. We further control for the network growth rates as discussed earlier to examine whether the results of network effects are driven by variations in speculative interests. We document the results on speculative interests in the Internet Appendix . We find some evidence that the cryptocurrency returns positively load on the contemporaneous speculative share growth, but the coefficient estimates are not significant. In the bivariate regressions, we show that the loadings of the cryptocurrency returns to the contemporaneous network growth remain positive and statistically significant. Furthermore, we show that the coin market returns positively forecast future speculative share growth. The coefficients are significant at the three-month and eight-month horizons. These results show that current coin market returns also contain information about expectations of future speculative share growth.
To test the effect of sentiment, we construct a measure that is directly aimed to capture investor sentiment. 15 The measure of cryptocurrency sentiment is defined as the log ratio between the count of positive and the count of negative phrases of cryptocurrencies in Google searches. The positive and negative phrases are described in the corresponding table in the Internet Appendix . Therefore, when the measure is high, investor sentiment is more positive and vice versa. We test whether the sentiment measure predicts future cryptocurrency returns, and compare that to the measures of investor attention and momentum. We find that the sentiment measure positively and significantly predicts future cryptocurrency returns. However, this result is distinct from the investor attention and cryptocurrency momentum results. All three variables are statistically significant in predicting future cryptocurrency returns in the multivariate regressions.
4.5 Beauty contests
One potential theoretical explanation of high volatility in financial markets may be that they are represented by the Keynesian beauty contest model. In this section, we aim to test the role of beauty contests in the cryptocurrency markets. We need a time-varying measure of the degrees of disagreement among the cryptocurrency investors. Ideally, we would like to have the expectations of individual cryptocurrency investors. Practically, this is not feasible due to data limitation at this time. We take a different route and measure the dispersion of investor expectations using the ratio between cryptocurrency volume and return volatility. This choice is motivated by Biais and Bossaerts (1998) , who show theoretically that the volume-volatility ratio summarizes the degree of disagreement among the investors and discriminates between genuine disagreement and mere Bayesian learning with agreeing agents. We test empirically whether the coin market returns respond to the volume-volatility ratio contemporaneously and whether the volume-volatility ratio predicts future coin market returns. We summarize these results in the Internet Appendix . We find that the coin market return is higher when the current volume-volatility ratio is higher. This result is consistent with the idea that investors tend to bid the price up when there is a lot of disagreement in the cryptocurrency market. The flip side is that the volume-volatility ratio does not predict future cumulative coin market returns over any horizon.
4.6 VAR analysis
One concern about the network factor analysis is that the contemporaneous correlations between the network size/activity factors and coin market returns might be mechanical and not truly capture the value of network externalities. To address the concern, we conduct a bivariate VAR analysis with the coin market returns and different coin network growth measures. The results are documented in the Internet Appendix .
To differentiate the network effects from the potential mechanical effects, we examine how changes in the network factors affect valuations in the future—that is, what is the cumulative permanent return associated with a shock to network size/activity factors. The bottom-left graph of each panel shows the associated impulse response function. We find that a shock to the wallet user growth, active address growth, and transaction count growth factors positively predict the coin market returns in the future and that there is not any reversal effect. The effects tend to concentrate on the first couple of weeks. In particular, the wallet user growth and active address growth factors positively and statistically significantly predict coin market returns in the future. The payment count growth measure is the only exception, but the point estimate is not significant. In terms of the cumulative effect, the cumulative return responses to one standard deviation of network factor shock are about 4% based on the changes in wallet user growth, about 2% based on the active address growth, and about 0.45% based on the transaction count growth.
Moreover, there is a bidirectional relationship between the network factors and the cryptocurrency returns. Consistent with the results in Table 3 in the paper, the VAR shows that the coin market returns positively and significantly predict future network growth based on all four specifications. The top-right graph of each panel shows the associated impulse response function. In the bivariate VAR framework, the approach accounts for this bidirectional effect, that returns may affect trading decisions and therefore affect the network size. Additionally, the bivariate VAR again reveals a coin market momentum effect in all four specifications. The top-left graph of each panel shows the associated impulse response function. The VAR approach also helps account for the momentum effect in the cumulative effect of network size/activity factors on the valuation of cryptocurrencies. Overall, the VAR suggests some evidence that a positive shock to the network size/activity factors leads to a permanent increase in the valuations of the coin market in the future. In terms of the timing of the effects, the impulse response functions suggest that it can take a few weeks for the impulse responses to decay to zero.
4.7 Additional production factor test
When the electricity price increases, the return to mining should decrease. However, the reduction in the return to mining would force some miners to exit, which leads to a higher probability of any given miner receiving the reward plus fees and a reduction of the difficulty of the cryptographic puzzles. These two effects would endogenously restore the profitability of the mining. Therefore, the shocks to electricity prices or computing power do not necessarily affect the marginal cost of mining because a change in these costs would affect the profitability of miners, causing an adjustment in the number of miners and therefore an adjustment in the required computing effort to restore profitability (e.g., Easley, O’Hara, and Basu 2019 ).
To address this effect, we include another set of tests. We regress the coin market returns on the number of Bitcoins given as a block reward, controlling for the price of Bitcoins and the fees paid. The rationale of the test is the following: the price of Bitcoin is endogenous, the fees paid could be endogenous as they are driven by network usage, but the number of Bitcoins given as a block reward is exogenous and deterministically changes through time according to the Bitcoin protocol. Therefore, the exogenous variation, controlling for the price of Bitcoin and the fees, could be used to identify the effects of mining costs.
Column (1) of the table documents the baseline specification. The coefficient of interest is in the changes of the number of Bitcoins given as a block reward, or |$\Delta Gen\ Coin$| . We find that, although the coefficient estimate is positive, it is not statistically significant. Column (2) uses next-month changes of the number of Bitcoins given as a block reward to test any anticipation effect. The point estimate is again positive but not statistically significant. Column (3) includes both the current and next-month changes in the number of Bitcoins given as a block reward as the independent variables, and both coefficient estimates are not significant. Columns (4) to (6) repeat the exercises of columns (1) to (3) but include the first principal component of the production factor, and the results are similar. Columns (7) to (12) repeat the exercises but control for the level of fees instead of changes in fees. We find that the coefficient estimates of |$\Delta Gen\ Coin$| and |$\Delta Gen\ Coin_{+1}$| are not statistically significant, consistent with results in columns (1) to (6).
Easley, O’Hara, and Basu (2019) argue that fee is not the only endogenous variable in the mining process, and that the confirmation time is also endogenously determined as a function of miner competition. Therefore, we also control for the confirmation time in our analysis and examine the results. Columns (13) to (24) report the results controlling for the confirmation time. In particular, columns (13) to (18) control for the changes of confirmation time, and columns (19) to (24) control for the level of confirmation time. We find that the coefficient estimates of |$\Delta Gen\ Coin$| and |$\Delta Gen\ Coin_{+1}$| remain statistically insignificant. Overall, we do not find a significant effect of the exogenous variation in the number of Bitcoins given as a block reward, controlling for the price of Bitcoin and the fees, on the cryptocurrency valuations.
4.8 Subsample by cryptocurrency characteristics
In this section, we consider a number of cryptocurrency characteristics: (i) whether the cryptocurrency is based on Proof-of-Work (PoW) or Proof-of-Stake (PoS), (ii) whether the cryptocurrency is minable, (iii) whether the cryptocurrency is built on an Ethereum blockchain, (iv) whether the cryptocurrency is a stable coin, and (v) whether the cryptocurrency is a smart contract. Based on each characteristic, we form a value-weighted portfolio of all the underlying cryptocurrencies. In the untabulated results, we look at the loadings of returns for each subgroup on the network factors, production factors, currency factors, commodity factors, equity factors, and macroeconomic factors.
First, we examine the loadings on the network factors. We find that the returns for the subgroups generally load positively on the network factors. Based on the first principal component of the four primary measures, we show that the returns of all six subgroups load positively on the network factors. In particular, the returns of PoW, minable coins, Ethereum blockchain coins, and stable coins positively and statistically significantly expose to the first principal component. On the other hand, the returns of PoS and smart contract coins do not statistically significantly expose to the first principal component. Then, we turn to the loadings on the production factors. We find that the returns for most of the subgroups do not significantly load on the production factors. We conclude that the factor exposures of the subgroup returns are largely consistent with the aggregate coin market returns. Lastly, we turn to the loadings of the returns for subgroups on the currency, commodity, equity, and macroeconomic factors. In general, we find that the returns of the subgroups have low exposures to these factor models. We conclude that the factor exposures of the subgroup returns are largely consistent with the aggregate coin market returns.
We find that cryptocurrency returns strongly respond to cryptocurrency network factors, as suggested by the theoretical literature. However, our empirical results do not support the notion that the evolution of cryptocurrency prices is linked to cryptocurrency production factors. At the same time, the returns of cryptocurrency can be predicted by two factors specific to its markets: momentum and investor attention. In contrast to the equity market, we show that the momentum result and the investor attention result are distinct phenomena and that there is only limited interaction between them. Moreover, cryptocurrency returns have low exposures to traditional asset classes such as currencies, commodities, and stocks, and to macroeconomic factors.
Authors have furnished an Internet Appendix, which is available on the Oxford University Press Web site next to the link to the final published paper online.
We thank Andrew Atkeson, Nicola Borri, Eduardo Davila, Stefano Giglio, William Goetzmann, Andrew Karolyi, Ye Li, Stephen Roach, Robert Shiller, Michael Sockin, and Jessica Wachter for their comments. Colton Conley provided outstanding research assistance. Supplementary data can be found on The Review of Financial Studies web site.
1 See, e.g., Cong, Li, and Wang (2019) , Pagnotta and Buraschi (2018) , and Biais et al. (2018) .
2 See, e.g., Cong, He, and Li (2018) and Sockin and Xiong (2019) .
3 Some coins are not tracked by the website because the coins’ exchanges do not provide accessible APIs.
4 We thank William Goetzmann for kindly sharing the Twitter post count data with us.
5 One of the 156 anomalies does not exist during the sample period. The database ends at December 2016.
6 Because Bitcoin is by far the largest and well-known cryptocurrency available, we use Bitcoin network data. The tests in the paper use coin market returns; in the Internet Appendix , we show that our results are qualitatively similar using Bitcoin returns.
7 See Jasper Pickering and Fraser Moore, “How China Become a Haven for People Looking to Cash in on the Bitcoin Gold Rush,” Business Insider, December 12, 2017.
8 See Shanthi Rexaline, “The Companies Behind the Chips That Power Cryptocurrency Minning,” Benzinga, February 2, 2018.
9 The 3.30% weekly return is calculated by multiplying a one-standard-deviation increase of coin market returns (16.50%) and the coefficient estimate (0.20).
10 Stoffels (2017) documents that a cross-sectional momentum strategy based on 15 cryptocurrencies generates abnormal returns during the period between 2016 and 2017.
11 Wang and Vergne (2017) use the level of newspaper mentions of Bitcoin to proxy for the “buzz” of Bitcoin. They document that high “buzz” predicts low Bitcoin returns in the future. Mai et al. (2016) use the level of Twitter post counts to predict Bitcoin returns.
12 We thank Nicola Borri for providing us with the up-to-date currency factors.
13 The Fama-French three-factor model is based on Fama and French (1993) and Fama and French (1996) . The Carhart four-factor model is based on Carhart (1997) . The Fama-French five-factor model is based on Fama and French (2016) . The Fama-French six-factor model is based on Fama and French (2017) .
14 Stoffels (2017) and Gilbert and Loi (2018) examine cryptocurrency loadings on the CAPM and Fama-French three-factor models.
15 Chen et al. (2019) classify cryptocurrency-related positive and negative words of StockTwits and Reddit.
Abadi, J. , and Brunnermeier M. . 2018 . Blockchain economics . Working Paper , Princeton University .
Google Scholar
Google Preview
Asness, C. , Moskowitz T. J. , and Pedersen L. H. . 2013 . Value and momentum everywhere . Journal of Finance 68 : 929 – 985 .
Athey, S. , Parashkevov I. , Sarukkai V. , and Xia J. . 2016 . Bitcoin pricing, adoption, and usage: Theory and evidence . Working Paper , Stanford University .
Auer, R. , and Claessens S. . 2018 . Regulating cryptocurrencies: Assessing market reactions . BIS Quarterly Review 51 – 65 .
Biais, B. , Bisiere C. , Bouvard M. , Casamatta C. , Menkveld A. J. . 2018 . Equilibrium bitcoin pricing . Working Paper , Toulouse School of Economics .
Biais, B. , and Bossaerts P. . 1998 . Asset prices and trading volume in a beauty contest . Review of Economic Studies 65 : 307 – 340 .
Bianchi, D. 2017 . Cryptocurrencies as an asset class: An empirical assessment . Working Paper , Warwick University .
Borri, N. 2019 . Conditional tail-risk in cryptocurrency markets . Journal of Empirical Finance 50 : 1 – 19 .
Borri, N. , and Shakhnov K. . 2018 . Cryptomarket discounts . Working Paper , LUISS University .
Carhart, M. M. 1997 . On persistence in mutual fund performance . Journal of Finance 52 : 57 – 87 .
Chen, A. , and Velikov M. . 2017 . Accounting for the anomaly zoo: A trading cost perspective . Working Paper , Penn State University .
Chen, C. Y. , Despres R. , Guo L. , and Renault T. . 2019 . What makes cryptocurrencies special? Investor sentiment and return predictability during the bubble . Working Paper .
Chiu, J. , and Koeppl T. V. . 2017 . The economics of cryptocurrencies–bitcoin and beyond . Working Paper , Queen’s University .
Cong, L. W. , and He Z. . 2019 . Blockchain disruption and smart contracts . Review of Financial Studies 32 : 1754 – 1797 .
Cong, L. W. , He Z. , and Li J. . 2018 . Decentralized mining in centralized pools . Working Paper , University of Chicago .
Cong, L. W. , Li Y. , and Wang N. . 2019 . Tokenomics: Dynamic adoption and valuation . Working Paper , Columbia University .
Corbet, S. , Lucey B. M. , Urquhart A. , and Yarovaya L. . 2019 . Cryptocurrencies as a financial asset: A systematic analysis . International Review of Financial Analysis 62 : 182 – 199 .
Da, Z. , Engelberg J. , and Gao P. . 2011 . In search of attention . Journal of Finance 66 : 1461 – 1499 .
Daniel, K. , and Moskowitz T. J. . 2016 . Momentum crashes . Journal of Financial Economics 122 : 221 – 247 .
De Long, J. B. , Shleifer A. , Summers L. H. , and Waldmann R. J. . 1990 . Noise trader risk in financial markets . Journal of Political Economy 98 : 703 – 738 .
De Bondt, W. F. , and Thaler R. . 1985 . Does the stock market overreact? Journal of Finance 40 : 793 – 805 .
De Bondt, W. F. , and Thaler R. . 1987 . Further evidence on investor overreaction and stock market seasonality . The Journal of Finance 42 : 557 – 581 .
Easley, D. , O’Hara M. , and Basu S. . 2019 . From mining to markets: The evolution of bitcoin transaction fees . Journal of Financial Economics 134 : 91 – 109 .
Fama, E. F. , and French K. R. . 1993 . Common risk factors in the returns on stocks and bonds . Journal of Financial Economics 33 : 3 – 56 .
Fama, E. F. , and French K. R. . 1996 . Multifactor explanations of asset pricing anomalies . Journal of Finance 51 : 55 – 84 .
Fama, E. F. , and French K. R. . 2016 . Dissecting anomalies with a five-factor model . Review of Financial Studies 29 : 69 – 103 .
Fama, E. F. , and French K. R. . 2017 . Choosing factors . Working Paper , University of Chicago .
Feng, G. , Giglio S. , and Xiu D. . 2017 . Taming the factor zoo . Working Paper , University of Chicago .
Gilbert, S. , and Loi H. . 2018 . Digital currency risk . International Journal of Economics and Finance 10 : 108 .
Griffin, J. M. , and Shams A. . 2020 . Is bitcoin really un-tethered? Journal of Finance 52 : 57 – 87 .
Hong, H. , Lim T. , and Stein J. C. . 2000 . Bad news travels slowly: Size, analyst coverage, and the profitability of momentum strategies . Journal of Finance 55 : 265 – 295 .
Hou, K. , Xiong W. , and Peng L. . 2009 . A tale of two anomalies: The implications of investor attention for price and earnings momentum . Working Paper , Princeton University .
Hu, A. , Parlour C. A. , and Rajan U. . 2018 . Cryptocurrencies: Stylized facts on a new investible instrument . Working Paper , University of California , Berkeley .
Huberman, G. , Leshno J. D. , and Moallemi C. C. . 2017 . Monopoly without a monopolist: An economic analysis of the bitcoin payment system . Working Paper , University of Chicago .
Jegadeesh, N. , and Titman S. . 1993 . Returns to buying winners and selling losers: Implications for stock market efficiency . Journal of Finance 48 : 65 – 91 .
Jermann, U. J. 2018 . Bitcoin and Cagan’s model of hyperinflation . Working Paper , University of Pennsylvania .
Liu, Y. , Tsyvinski A. , and Wu X. . 2019 . Common Risk Factors in Cryptocurrency . Working Paper , Yale University .
Lustig, H. , Roussanov N. , and Verdelhan A. . 2011 . Common risk factors in currency markets . Review of Financial Studies 24 : 3731 – 3777 .
Mai, F. , Bai Q. , Shan Z. , Wang X. S. , and Chiang R. . 2016 . The impacts of social media on Bitcoin performance . Working Paper , University of Cincinnati .
Makarov, I. , and Schoar A. . 2020 . Trading and arbitrage in cryptocurrency markets . Journal of Financial Economics 135 : 293 – 319 .
Moskowitz, T. J. 2015 . Asset pricing and sports betting . Working Paper , Yale University .
Moskowitz, T. J. , and Grinblatt M. . 1999 . Do industries explain momentum? Journal of Finance 54 : 1249 – 1290 .
Moskowitz, T. J. , Ooi Y. H. , and Pedersen L. H. . 2012 . Time series momentum . Journal of Financial Economics 104 : 228 – 250 .
Pagnotta, E. , and Buraschi A. . 2018 . An equilibrium valuation of bitcoin and decentralized network assets . Working Paper , Imperial College Business School .
Pástor, L. and P. Veronesi. 2003 . Stock valuation and learning about profitability . Journal of Finance 58 : 1749 – 1789 .
Routledge, B. , and Zetlin-Jones A. . 2018 . Currency stability using blockchain technology . Working Paper , Carnegie Mellon University .
Saleh, F. 2018 . Blockchain without waste: Proof-of-stake . Working Paper , McGill University .
Schilling, L. , and Uhlig H. . 2019 . Some simple bitcoin economics . Journal of Monetary Economics 106 : 16 – 26 .
Shanaev, S. , Sharma S. , Shuraeva A. , and Ghimire B. . 2019 . Taming the blockchain beast? Regulatory implications for the cryptocurrency market . Working Paper , University of London .
Sockin, M. , and Xiong W. . 2019 . A model of cryptocurrencies . Working Paper , Princeton University .
Stoffels, J. 2017 . Asset pricing of cryptocurrencies and momentum based patterns . Working Paper , Erasmus School of Economics .
Wang, S. , and Vergne J. . 2017 . Buzz factor or innovation potential: What explains cryptocurrencies’ returns? PloS One 12 .
Weber, W. E. 2016 . A Bitcoin standard: Lessons from the gold standard . Working Paper , Bank of Canada .
Yermack, D. 2015 . Is Bitcoin a real currency? An economic appraisal. In Handbook of Digital Currency , ed. Chuen D. L. K. , 31 – 43 . Amsterdam : Elsevier .
Supplementary data
Email alerts, citing articles via.
- Recommend to your Library
Affiliations
- Online ISSN 1465-7368
- Print ISSN 0893-9454
- Copyright © 2024 Society for Financial Studies
- About Oxford Academic
- Publish journals with us
- University press partners
- What we publish
- New features
- Open access
- Institutional account management
- Rights and permissions
- Get help with access
- Accessibility
- Advertising
- Media enquiries
- Oxford University Press
- Oxford Languages
- University of Oxford
Oxford University Press is a department of the University of Oxford. It furthers the University's objective of excellence in research, scholarship, and education by publishing worldwide
- Copyright © 2024 Oxford University Press
- Cookie settings
- Cookie policy
- Privacy policy
- Legal notice
This Feature Is Available To Subscribers Only
Sign In or Create an Account
This PDF is available to Subscribers Only
For full access to this pdf, sign in to an existing account, or purchase an annual subscription.
- Open access
- Published: 01 January 2022
A bibliometric review of cryptocurrencies: how have they grown?
- Francisco Javier García-Corral ORCID: orcid.org/0000-0003-1184-2185 1 ,
- José Antonio Cordero-García 2 ,
- Jaime de Pablo-Valenciano 3 &
- Juan Uribe-Toril 3
Financial Innovation volume 8 , Article number: 2 ( 2022 ) Cite this article
17k Accesses
37 Citations
Metrics details
With the development of new technologies, some concepts become relevant in the economic area, as is the case with cryptocurrencies, in general, or Bitcoin and Ethereum, in particular. Due to the impact of these tools, a detailed bibliometric study that allows us to obtain all information about cryptocurrencies must be conducted. This study will help scientific production by specifying the development and lines of related research that have been followed and are currently being followed. We have used Tableau, R (Bibliometrix R Package), and VOSviewer software to analyze the information. These have been combined to create and review unified metadata from the Web of Science (WoS) and Scopus databases. The bibliometric analysis shows 771 articles on the WoS database and 648 articles on Scopus published between 2010 and early 2019. They present the most relevant articles, research areas, countries, institutions, authors, journals, and trends during the last few years. In conclusion, the number of publications has grown in the last 3 years. The analysis shows the evolution of blockchain technology used in this type of cryptocurrency. The review of this period marks a possible end to the historical part of cryptocurrencies, thereby opening the current topic to its multiple applications.
Introduction
In the last decade, secondary payment methods other than legal tender have been developed to boost the market (Corrons 2017 ). Lietaer and Hallsmith ( 2006 ) defined one of these payment mechanisms as an agreement to use more than just legal tender as a means of exchange to link unused sources to unmet needs. In particular, a series of complementary currencies incorporated into the economic world are mentioned. Although these new supplementary payment methods are not listed in any global database, more than 6000 types are presumed to exist. Among them, new electronic payment methods have recently been incorporated, including virtual currencies or cryptocurrencies. Although complementary currencies have been used for a longer period, by historical amount and weight, the central focus of this study is the most innovative cryptocurrencies.
A broad spectrum of terminology are coined to differentiate between these cryptocurrencies, ranging from virtual complementary currency to electronic currency and its derivative, cryptocurrency (Dai 1998 ). The first currency to become popular was Bitcoin, which was founded in 2008 by Satoshi Nakamoto. Although previous attempts at virtual currencies, such as E-gold in 1996 or Liberty Reverse in 2006, have been made, Bitcoin was the first to exist in the global socio-economic sphere (Garcia et al. 2014 ).
During these cryptocurrencies’ short period of existence, they have been and are studied by a wide variety of disciplines, as they incorporate a number of innovative technologies, such as blockchain, cryptography, and smart contracts (Xu et al. 2019 ). Several studies have characterized cryptocurrencies as having a volatile future (Urquhart 2016 ; Katsiampa 2017 ; Chu et al. 2017 ; Conrad et al. 2018 ; Bouri et al. 2019 ) and initially presented them as non-perishable albeit secure. However, these promising technologies have kept them (Zheng et al. 2018 ; Zulfiqar and Gulzar 2021 ). The globalization process to which they are subjected, together with the lack of legal regulation, indicate that they have been used in multiple forms as the primary component (Gomá-Garcés 2014 ; Zimmer 2017 ). They are also the subject of much discussion and debate by entities, such as the European Central Bank ( 2012 ) seeking to better define them as a means of exchange and a unit of value accepted by a virtual community.
This article aims to contribute to the extant literature by conducting a bibliometric analysis of the main currencies, as the number of publications on this subject is increasing. Therefore, a review of the materials published in this interdisciplinary area must be incorporated. Moreover, this methodology is applied in multiple areas of knowledge from the mapping analysis of bibliographic information obtained from high-impact databases.
First, we will focus on Bitcoin and Ethereum as the main currencies and the concept of cryptocurrency. The results obtained are intended to inform about a specific field of study and its evolution and productivity. In addition, they help identify, analyze, and organize the main elements of the search focus to show the evolution of trends in the subject. Finally, the results seek to establish whether major changes occur in the lines of research to determine whether the theoretical part is more irrelevant. In this case, the new lines of research will be more practical, changing their orientation and making the previous publications more historical-theoretical.
This method has been used in several studies with similar themes. However, unlike previous studies (Table 1 ), the present study considered three keywords, along with a new temporal division in the discussion. Exclusively and to increase the importance of this article, this study will include the results of “cryptocurrency, Bitcoin and Ethereum,” thus covering a broader index of results with economic topics from the Web of Science (WoS) and Scopus databases. This differentiates it from the works related to blockchain only as a concept that does not come into discussion, from those that analyze Bitcoin only (e.g., Merediz-Sola et al. 2019 ; Orastean et al. 2019 ; Shen et al. 2020 ), or those that only examine one database (Dabbagh et al. 2019 ).
This study begins with an introduction and a literature review on alternative forms of payment and their different concepts and interpretations. Then, it explains which selected payment systems have the greatest impact. The methodology of the bibliometric analysis and the sources used to extract the data during the search process are then presented. Subsequently, the results are presented independently, followed by a discussion on the future of these tools with more up-to-date data until 2020. Finally, the last section concludes with both definitive comments and potential research streams from the data analysis.
The concept
Cryptocurrencies are a form of digital exchange that ensures that transactions are made through a robust encryption process, which, in turn, controls the number of stocks (Luu et al. 2016 ). This is a recent phenomenon gaining momentum in a volatile and fluctuating economic world (Ciaian et al. 2016 ) and has experienced significant growth, despite not being considered an official form of debt cancellation (Dwyer 2015 ). Due to the decentralized nature of cryptocurrencies, they cannot be used as a substitute for legal currency (Nakamoto 2008 ) even if they were created to be used as such, thus making them an unconventional currency. The creation and management of currencies are controlled by non-governmental entities (Kim 2015 ); hence, although they are considered a promising alternative for the future, they have various detractors who prefer to use them as a form of speculation (Baur et al. 2018 ; Krugman 2018 ; Zhang et al. 2021 ). The decentralized structure without regulated activity makes them a novel option to the traditional financial system (Franco 2014 ). Thus, although they start from a totally negative configuration, they have a series of advantages: cheaper transaction costs due to the absence of intermediaries; reduction of transaction times as these are carried out via the Internet; the suppression of intermediaries as unnecessary financial agents in this series of transactions; or their globality (Kostakis and Giotitsas 2014 ; Koblitz and Menezes 2016 ).
In addition, individuals have freedom to develop this type of currency; consequently, multiple currencies have been created for specific purposes (Kondor et al. 2014 ) and have become standard payment mechanisms (Fabian 2016 ). They are used globally in a society that views its transactions between direct parties and perceives them as being more straightforward and negotiable because monetary conversion is not needed (Kristoufek 2013 ).
Privacy and security
Originally, virtual currencies emerged as a means of digital exchange that guaranteed their security, integrity, and balance due to a higher level of protection created by users. In exchange for compensation, these individuals help with security work by processing algorithms (Van Alstyne 2014 ; Urquhart 2018 ). That is, the security mechanisms of this payment method arise from the users themselves who maintain and protect the base fabric by providing computing power (Böhme et al. 2015 ). Mathematically speaking, the security of an electronic currency or the blockchain can be compromised, but the cost required to achieve this would be high, depending on the algorithm and its creation protocol (Xu 2016 ; Khan and Salah 2018 ; Zhang et al. 2019 ).
Transactions carried out with these currencies are direct between users and generally anonymous (Miers et al. 2013 ), compared with those carried out with legal currency in which payments are made through banking networks. Therefore, anonymity has been a key factor since their very inception (Ober et al. 2013 ). Although the development of cryptocurrency has not always been equal and not all types of cryptocurrencies operate the same, the complexity of violating anonymity is equal to the breach of their security (Wang et al. 2018 ). Privacy and protection are mechanisms that, although considered strong, need to be improved to add new functionality as they progress in their use because their standardization makes them attractive to hackers (Conti et al. 2018 ; Feng et al. 2019 ).
Blockchain setup and maintenance
Electronic currencies are created through mining, an incentive process in which transactions are verified and new units are created and added to the core of existing ones (Eyal and Sirer 2013 ). The miners are responsible for collecting the latest transactions into blocks and finding a solution to the algorithm of each currency. As a reward, a fixed amount of that currency is acquired by these miners (Böhme et al. 2015 ; Bonneah et al. 2015 ). The solution to the algorithm changes continually and depends on previous results to perform the next calculation in the sequence. This means that, as time goes by, the difficulty in finding a solution will become greater, and its cost increases (Eyal and Sirer 2013 ; Giungato et al. 2017 ). Thus, the process has been affected because the investment cost does not exceed the profits offered (Kristoufek 2015 ; Cocco and Marchesi 2016 ).
All the information related to the cryptocurrency is recorded on the blockchain, a digital book shared on the network and responsible for collecting all the transactions carried out with the cryptocurrency in two parts (i.e., input and output) (Franco 2014 ). These exchanges or transactions are called blocks and are encoded and linked with others (Böhme et al. 2015 ). Blockchain information is stored on participating devices and is open access (Zyskind et al. 2015 ), making the exchange process transparent and immune to modifications (unalterable) (Brandvold et al. 2015 ). Once the data are verified, they can no longer be edited without the community’s consent. This recent technology in cryptocurrencies can be used for multiple purposes (Sikorski et al. 2017 ; Kuo et al. 2017 ; Lee 2017 ) and is one of the most dynamic elements of the economy (Yin et al. 2017 ).
Due to the simplicity of use (Selgin 2013 ) and the lack of regulation, particularly concerning taxation (Follador 2017 ), virtual currencies have been linked to numerous unregulated activities, including criminal acts, and may contribute to further price distortion (Barratt et al. 2013 ; Hardy and Norgaard 2016 ; Foley et al. 2019 ; Griffin and Shams 2020 ). Another problem with these currencies is their high level of volatility, losses, and a lack of widespread acceptance among the general public, which could indicate their inefficiency (Nadarajah and Chu 2017 ; Klein et al. 2018 ). Although volatility can mean both a risk and an opportunity (Brière et al. 2013 ), it is an intrinsic part of the currency (Bariviera 2017 ) and virtually impossible to predict (Balcilar et al. 2017 ). Recent studies have found that short-term bubbles limit the ability to profit from these tools; however, investments in these currencies are not limited, leaving only conjectures about obtaining economic benefits (Li et al. 2018 ). The continuous variations and collapse in the exchange of distributed volume generate large fluctuations in prices (Navas-Navarro 2015 ; Polaski et al. 2015 ) that denote the inefficiency of this market (Urquhart 2016 ; Zhang et al. 2018 ; Neslihanoglu 2021 ). It is an exchange mechanism whose real value starts from zero (Van Alstyne 2014 ; Cheah and Fry 2015 ). Although their permanence is currently being discussed as a matter of general interest, research has posited that the life cycle of cryptocurrencies increases, as they stabilize (Bariviera et al. 2017 ).
The market and the protocols
Many virtual currencies have currently been given a relative value, based on different variables, to the different legal tender currencies (Table 2 ). All belong to a version of the protocol, depending on their application. Thus, we find that Bitcoin uses version 1.0 of the blockchain, whereas other alternatives, such as Ethereum, use version 2.0. The latest version, called version 3.0, is part of an extension of the applications used. Bitcoin and Ethereum have been chosen as the most relevant currencies based on their original protocols, which share several characteristics, such as mining or their structure; however, differences also exist between them (Table 3 ).
Bitcoin is the pioneering platform of the blockchain concept based on a peer-to-peer exchange that does not rely on traditional transaction schemes in which central authorities or banks carry out transactions. Bitcoin can be defined as a form of cryptocurrency or payment system based on cryptographic evidence whose unit is bitcoin (Nakamoto 2008 ) and has unique characteristics that have defined the properties of these currencies (Phillip et al. 2018 ). Having evolved from the Blockchain 1.0 protocol, Bitcoin is currently the most valuable and central axis of cryptocurrency studies (Jang and Lee 2018 ). However, it has shared its weight with those of recent creation.
Meanwhile, Ethereum is an open-source, decentralized platforms whose purpose is to create the most significant smart contract agreements (Luu et al. 2016 ). It is a framework for the execution of contracts and useful automated computer applications (Bhargavan et al. 2016 ), without the need to trust third parties. It is currently considered one of the most complex networks under review. We have chosen to analyze Ethereum in this study because it is one of the pioneering and most stable cryptocurrencies 2.0.
Research methodology
The bibliometric analysis is responsible for reviewing different bibliographic material to organize the relevant information on a specific topic. It is also a way of presenting scientific publications that seek to assess the status of a given topic and the quality and influence of authors and sources (Van Raan 2014 ).
For the elaboration of the present study, we have followed a series of systematic stages. First, we established a list of research questions oriented for this study, which helped delimit the most important words, the search pages, and the chosen period, marking the direction of the work. Once the main theme had been structured and created, the first results were filtered, delimiting the research toward a total number of 1455 scientific articles distributed among the WoS and Scopus databases. With the obtained metadata, we then proceeded creating our own database which has been used for the present analysis.
Research questions
We formulate research questions that can help us identify the volume of articles to predict future patterns and determine future lines of work to focus on. These questionnaires will also make us easier to determine which papers and publication venues to publicize our research. Lastly, these questions will help establish the relevance of the field at a general level and help find possible new funding or coordinated research avenues among the agents involved. We thus present the following research questions:
Q1 : What is the distribution of publications on cryptocurrencies, especially Bitcoin and Ethereum, in relation to their citations?
Q2 : What areas of publications have the highest impact?
Q3 : Which articles are the most influential in this technology according to the number of citations, and where are they located?
Q4 : Which are the most relevant and related countries and institutions?
Data extraction
This study analyzed cryptocurrency, and the sources are the WoS and Scopus databases that include the largest number of academic journals and publications. It also analyzes the most frequently published authors, the most common or relevant topics, the number of publications by country, and the language used for the largest number of publications.
Two noteworthy sources have been chosen to solidify their documentary strength (Manterola et al. 2005 ). This study’s validity study depends on whether the subject area or the topic being researched is included in the sources of information. For many years, WoS was the only database designed as an international and multidisciplinary tool. Subsequently, Scopus was developed to compensate for the limitations of its predecessor, and to date, it is a more extensive database.
Based on several assumptions, the analysis is structured as follows: First, the parameters of the study were chosen or defined to select the appropriate databases from which to extract the data. Second, the corresponding search criteria were adjusted, and the bibliographic information categories were compiled. Finally, the extracted material was coded and used to create a combined database, and the extracted data were analyzed and contrasted.
The words selected for the search were “cryptocurrency,” “Bitcoin,” or “Ethereum.” This selection covered both the generic concept of electronic currency and the two types of pioneering and best-known currencies in the protocol’s respective version. The period selected was from 2010, the date of the first publication, to 2018, using the years 2019–2021 to check whether the published articles influenced future research trends. This is because, looking at all the data, we determine a turning point at which publications begin to double the number of the previous year (Fig. 1 ). From the aforementioned search criteria, we selected the filter for scientific articles as these were considered to be the most representative.
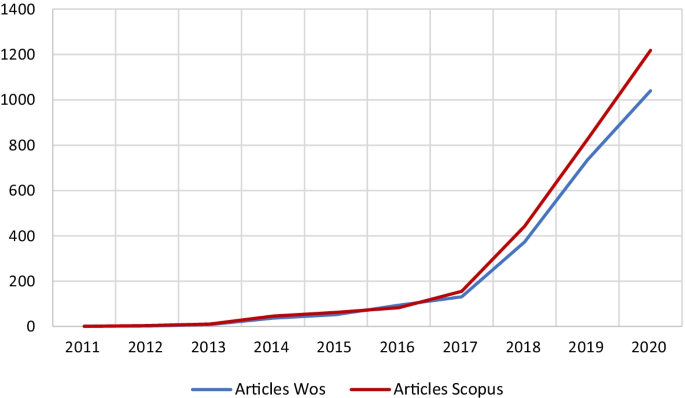
Source : Own compilation
Annual scientific production.
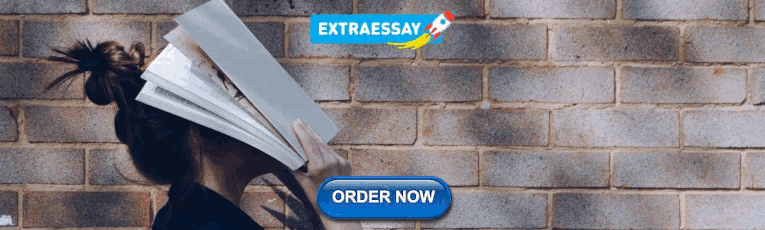
Documents selection and data analysis
We have used three different indicators for the selection of documents: quantity, quality, and the structural form and the relationship between publications. Quantity shows the productivity index in terms of the number of publications. Meanwhile, quality shows which publications have the greatest impact according to the total number of citations received by a given text. Of the three, the two central ones of this text will be quantity and quality. These lead to the development and identification of successive rankings that will be displayed in various tables.
After selecting the documents to be used, we created three databases, that is, an individual one for each platform for comparison and a common unified one for specific analyses. For this, we have used three software packages: Tableau, R (Bibliometrix R Package), and VOSviewer.
The coding process was conducted by building a database using different variables that store information about each article, thereby extracting the productivity related to this research field.
Finally, after selecting the questions and extracting and preparing the data, we conducted an analysis consisting of the number of publications and their incidence, a selection of research areas, a distribution by country, institutions and journals, a more detailed section dedicated to their authors, and a summary of the trends.
To achieve a global view of the productivity in this field of research, this study’s results encompass the articles published during a given period and include information about their respective languages, countries, institutions, journals, and authors. As we have mentioned, the WoS and Scopus database search applies from 2010 to the end of 2018 because from 2019 onwards, the number of publications has multiplied, especially those related to the term blockchain, which may mislead the results (Fig. 2 ).
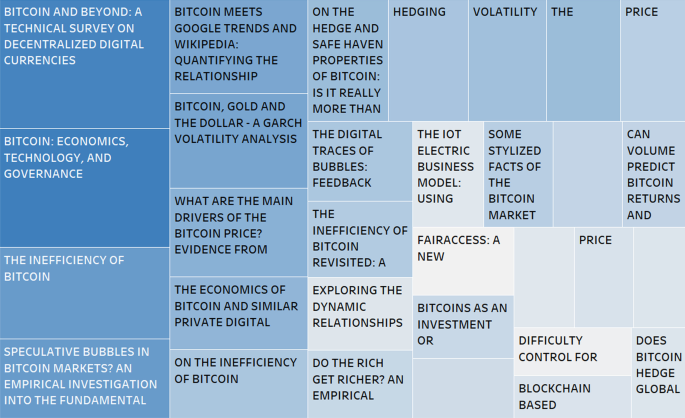
Most Cited articles in both databases.
Initial approach
The following data show the evolutionary state of the cryptocurrencies up to the present. As mentioned, the referenced sources are the WoS and Scopus databases, in which WoS is considered the pivotal source because of its greater seniority.
The first section analyzes the sample. Applying the corresponding search filters, we found 684 documents on the WoS database and 771 items on Scopus. Of these combined results, 407 documents appeared in both databases. The search in the two databases utilized the same period and began receiving content relevant to this study at almost the same time. Although the search is delimited by years, we focus on the starting year 2010 because of an anomalous result in Scopus in 1952 that coined the term Ethereum in an investigation by Dr. H. Greiner in the area of medicine. After excluding this search result, both bases coincide in the date of publication of articles, thus establishing this criterion equally.
Publications that included keywords, such as “Bitcoin,” “Ethereum,” or “Cryptocurrency,” appeared in 2011. Thereafter, the number of publications that included these keywords doubled annually. The recent creation of the aforementioned cryptocurrencies and their low impact indicate no related publications during the first years. Since 2011, when a single publication appeared in both databases, the results have increased exponentially. Figure 2 highlights that the trajectory followed by both databases is similar in terms of total publications, although with internal differences. If the set of publications is analyzed, Scopus includes a larger number than WoS, except for 2016, in which this trend is reversed.
The first publication included in WoS is “On Bitcoin and Red Balloons” (Babaioff et al. 2012 ), which talks about getting a reward in a node “competition.” Meanwhile, on Scopus, the first article is “Bitcoin: A bit too far?” (Jacobs 2011 ), which deals with issues internal to the currency. Although both publications received a low number of citations, the article “Bitcoin: A bit too far?” obtained a total of 10 citations compared to the two citations received by the article on WoS.
In terms of citations on both platforms, the most significant articles practically coincide, making it more relevant even with the creation of a common database that combines both sources (Fig. 2 ). In a separate analysis, both databases would show concordance in two of the three articles. Moreover, both articles would be in WoS and Scopus, although in different ranking positions. The article “Bitcoin: Economics, Technology, and Governance” (Böhme et al. 2015 ) is ranked first in WoS with 139 citations, whereas in Scopus, it is ranked third with a total of 207 citations. The article that ranked second on WoS is “Bitcoin and Beyond: A Technical Survey on Decentralized Digital Currencies” (Tschorsch and Scheuermann 2016 ), with 133 citations; however, this article is ranked first in Scopus, with a total of 225 citations. Meanwhile, the article “Where is current research on Blockchain technology?—A systematic review” (Yli-Huumo et al. 2016 ) ranks second on Scopus, with a total of 210 mentions, but it did not have any citations on WoS. Finally, the third-ranked article on WoS, that is, “Speculative bubbles in Bitcoin markets? An empirical investigation into the fundamental value of Bitcoin” (Cheah and Fry 2015 ), has 109 citations.
Apart from the articles ranked first, the number of citations on Scopus is higher than on WoS (Fig. 3 ). The average number of citations per article is also higher, that is, 19 on Scopus compared to 15 on WoS, even though WoS contains a larger number of documents on the topic. Although both databases commence with articles without citations, the ends of the diagram show a greater number of atypical results in Scopus.
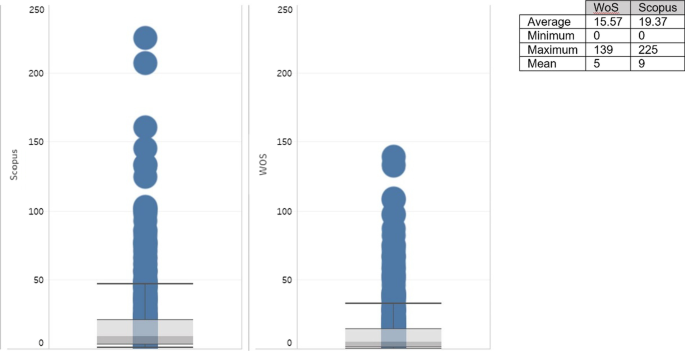
Comparison of citations.
As can be seen, the results are quite similar, both being in an equal position. The country variable in both also shows a homogeneous growth and with similar results. The most significant distinction can be found in the total number of citations if the results are distributed over the years with a significantly higher number of citations on WoS. This is because although the number of articles is lower, the variables of authors and journals are higher (Table 4 ).
Distribution by area of research
When comparing the databases, our search results show that the main areas of knowledge are information technology and economics (Table 5 ). Although WoS had 100 fewer results when the same number of research areas were considered, the wide range of classified thematic areas contained within WoS is greater than the classification in Scopus, and thus the articles are distributed across a wider range of subjects.
Using WoS as a reference, we use areas of economic knowledge, such as economics and business finance, in the ranking. The total sum of these articles is 282, which is similar to the second category in Scopus, which encompasses Economics, Econometrics, and Finance. The remaining positions in the ranking are related to computer science, systems, and telecommunications, almost half of those included in the list. The remaining articles are distributed among multiple categories, that is, a total of 76 different research areas include the terms Bitcoin, Ethereum or Cryptocurrency, although only 14 of these are specifically listed in the table. In contrast, Scopus directly links computer-related articles and ranks them first. Next, the economic and social sciences are ranked second and third with the remaining articles being linked, to a greater extent, to computer sciences, such as engineering and mathematics; and the social sciences with business and management. Once the threshold of the eighth theme is crossed, a greater diversity of topics begins to be seen.
The results of both the databases and the many thematic areas denote the wide variety of applications that technologies derived from electronic currencies have. Although the keywords are based on economics, the standardized use of technologies born from cryptocurrencies, most notably digital ledgers or blockchain, means that the distribution of themes is very widespread. The blockchain shows a positive evolution in databases, such as WoS, with a total of 692 results solely in articles in a period of just three years. The term “blockchain” did not receive citations until 2015, the year in which its development really took off. Hence, its importance is evident when compared to the origins in Bitcoin, because it has managed to equal the same number of articles in half the number of years.
Distribution by country
In terms of geographical distribution, an apparent growing trend toward research on this topic originates from the Asian continent, apart from the time factor (Figs. 4 and 5 ). That said, the principal language used is still English, and virtually all articles appear in the two databases published in this language. Other articles were published in Russian, Spanish, and Turkish in WoS, whereas the most used languages were Chinese, Russian, and German in Scopus. Note that although both databases consider Russian to an influential language, as a geographical region, Russia is not featured as one of the most influential countries in terms of the number of publications.
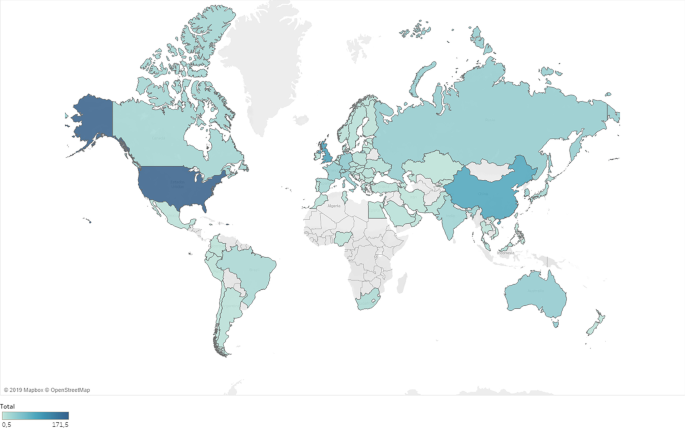
Geographical distribution. Mercator projection Map. Landmasses appear larger the farther they are from the poles. The projection, however, maintains constant bearings for navigation.
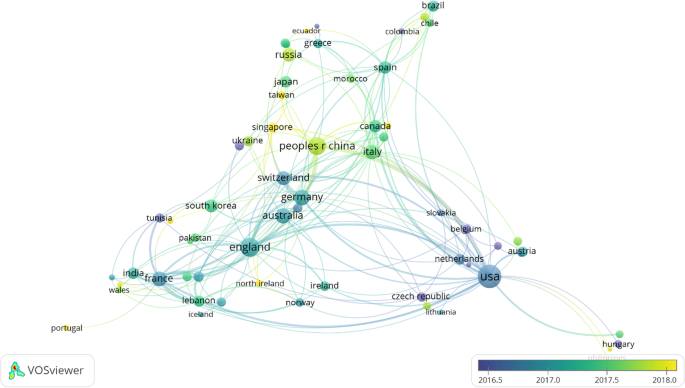
Grouping by country and year.
In a more detailed comparison, both databases show similar results with respect to the first four and the last two ranked countries (Table 6 ). Both databases show the USA, UK and China leading the ranking. These countries also account for the largest number of articles and citations together with the highest H-indexes. The databases also coincide with respect to the countries ranked last, with the possible exception of India, which in Scopus, is ranked sixth. Specifically, considering the ranking in terms of the number of articles published, the results from both databases practically coincide, whereas the results are more disparate in terms of the total number of citations. The discrepancy mentioned earlier in India can only be highlighted in the number of articles. Regarding the total number of citations, the rankings of Russia and Spain stand out for different reasons. In the case of Russia, the total number of citations is much lower than expected given the number of articles published. In contrast, Spain obtained a number of citations that would place it in several higher positions compared to the number of published articles; the h-index is clearly higher than that obtained in the classification.
If we develop the content dealt with in each country in a more important way, taking a total of 5 words as the focus of studies, we can see how the USA has always studied bitcoin, deriving from it the concept of currency, blockchain, innovation, and economy together with security. For its part, and also taking bitcoin as a central focus, England has added the volatility of these currencies together with their technology, such as blockchain, to its most relevant words. China is next, giving the same importance to bitcoin as to the blockchain, deriving two lines of research from which the main concern of bitcoin comes from its inefficiency and prices; however, the blockchain mentions security and smart contracts. Germany and Australia are next on the list, but the main focus is on bitcoin, but it is much shorter in terms of secondary issues, just mentioning economics and blockchain. Meanwhile, Russia remains with bitcoin and cryptocurrencies in general and, if the number of keywords is lowered as a concurrence, China appears as another result, being the only ones to mention another place directly.
Institutions
The most pivotal institution related to electronic currencies that focuses on Bitcoin and Ethereum is the University of London with a total of 24 and 14 articles in WoS and Scopus, respectively (Table 7 ). This institution is followed by PDX Currency Corp in WoS, with 17 published articles, although no citations are related to them. Again, in terms of number of published articles, the next ranked institutions are the University College London with 14 articles and Eidgenössische Technische Hochschule Zürich (ETH Zurich) and the University of California System with 13 articles each. They have also attracted a large number of citations. Except for ETH Zurich, the aforementioned institutions are all English-speaking, which coincides with the high number of publications in that language.
In contrast, Scopus shows a greater spatial distribution with respect to institutions. Although the first result coincides with the aforementioned results from WoS, the institutions appearing next in the ranking are Montpellier Business School, Chinese Academy of Sciences, ETH Zurich and Holy Spirit Univ Kaslik located, respectively, in France, China, Switzerland and Lebanon. The articles published by these institutions have a higher number of references compared with more prominent institutions listed in WoS.
The results show that WoS has a greater concentration of English and American institutions as a central pillar, bringing together a core of English-speaking institutions that makes up 40% of the total. In contrast, Scopus has a more varied distribution. The central focus of the five institutions of each essential database has always been on issues related to bitcoin as a core, with publications on its volatility, hedge, and economics deriving from it. In a more minor way this time, the concept of the blockchain appears. To conclude this section, we created a cluster map of institutions. As suggested by Fig. 6 and given the recent development of the topic, the links and relationships between institutions are scarce, with only a suggestion of a rapprochement between Asian entities.
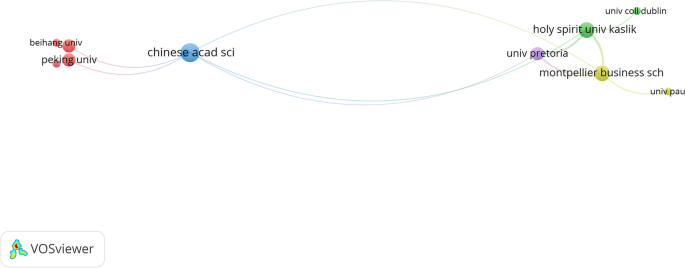
Cluster map of institutions.
The journals with the highest number of publications in WoS and Scopus are Economics Letters and IEEE Access with a total of 29 and 28 publications, respectively in the case of Economic Letters and 26 and 30 in the case of IEEE Access. They both clearly have a high H-Index along with a large total number of accumulated citations. Two sources appear in the third position of the ranking of both databases, albeit without any associated citations. They are Digital Currency Challenge Shaping Online Payment Systems through US Financial Regulations and Economist United Kingdom with 17 and 21 articles, respectively from USA and UK. This phenomenon of not receiving any citations is repeated in the WoS ranking with the fourth ranked journal, Palgrave Pivot, and in Scopus with the seventh ranked journal, Technology Review.
In the sample provided, only five journals are considered global publications in Table 8 for both databases. This is evidence of the disparity between the two sources because, aside from the two journals mentioned in the previous paragraph, Finance Research Letters, PLOS One, and Physica A: Statistical Mechanics and its Applications are the only journals listed in both sources. Although there are no other concurrences, the basic scheme observed is remarkably similar because the coincident entities do so in almost an equal number of the ranking, whereas the remaining journals coincide approximately in the number of articles. The number of publications in these journals is always related to economics, inefficiency, volatility, and gold, leaving blockchain and security as secondary topics.
As a final comparison, Table 9 shows the authors ordered according to the index of publications on the topic. The 17 articles by P.C. Mullan, which appear solely in WoS, can be highlighted as an anomalous result, as they have received no citations. This can be linked to the previous section on publications, as these articles are contained in a manual. Regarding the rest of authors, E. Bouri and D. Roubaud stand out with nine articles each, published in 2017 and 2018. Both authors have collaborated extensively and had many citations, well above the average of other authors, although not in all articles.
Based solely on the total number of publications, and focusing on the most influential authors, the distribution of authors in both databases is quite similar. Regarding the field to which the authors belong, the most important ones come from Business & Economics, Computer Science and Environmental Sciences & Ecology. However, in a cluster analysis (Figs. 7 and 8 ) and using the two databases as the basis for the analysis, we determine that the relationship between them changes. In both cases, the grouping has been generated using the same basic parameters that, together with the greater distribution among the Scopus institutions, shows broader results with six central nuclei versus the two mere nuclei in WoS.
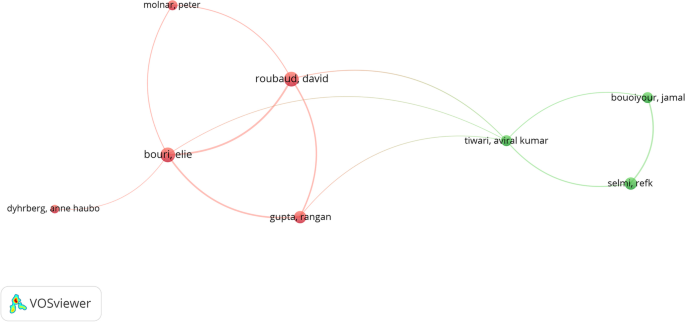
Author cluster on WoS.
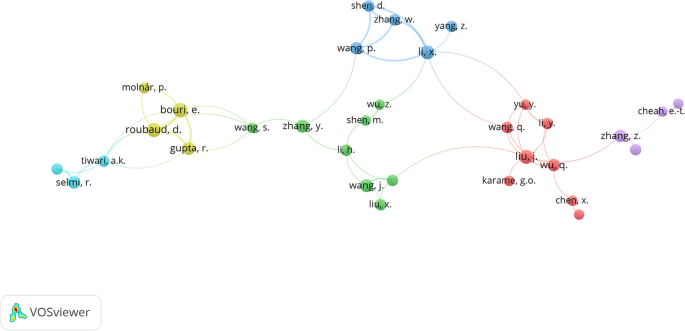
Author cluster on Scopus.
Figure 9 shows the evolution of the scientific production achieved by the most relevant authors, taking WoS as a reference to observe their trajectory. The circles on the cluster map represent the number of articles, and the color represents the intensity of the citations received during the year. This would show how the most important publications were produced in WoS during 2017, coinciding precisely with the beginning of the increase in scientific publications.
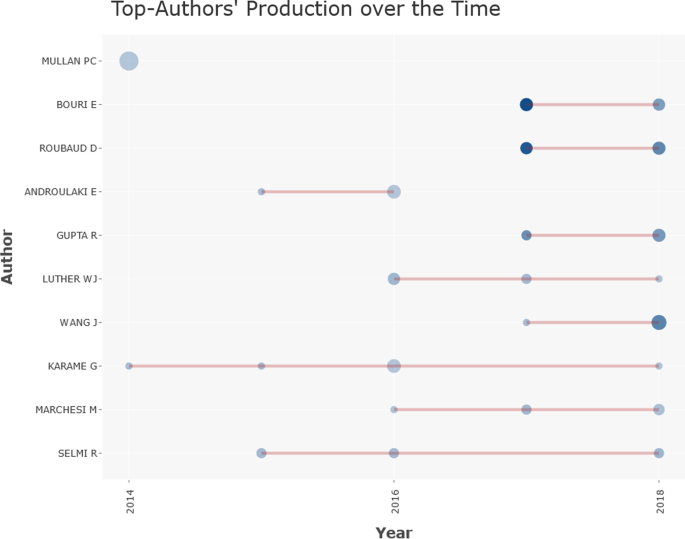
Top authors’ production.
Trend analysis
Based on the content of all the articles, we can identify the most common terms and those with the greatest impact related to electronic currencies. Using the VOSviewer software and R (Bibliometrix package), we compiled a series of large clusters indicating the frequency and evolution of the keywords (Figs. 10 and 11 ), combined with a three-field plot of top Keywords Plus, Sources, and Author Keywords (Fig. 12 ). Notably, the wide variety of terms in Scopus is due to a higher index of publications, even if some of them have not been followed up.
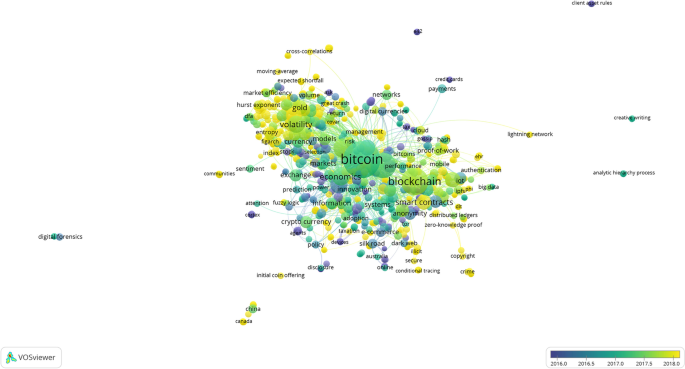
Keywords on WoS.
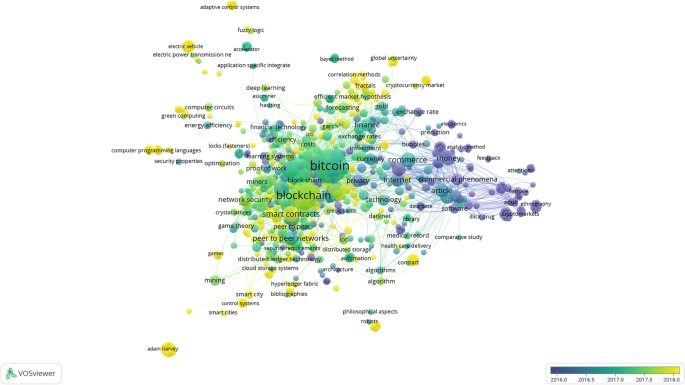
Keywords on Scopus.
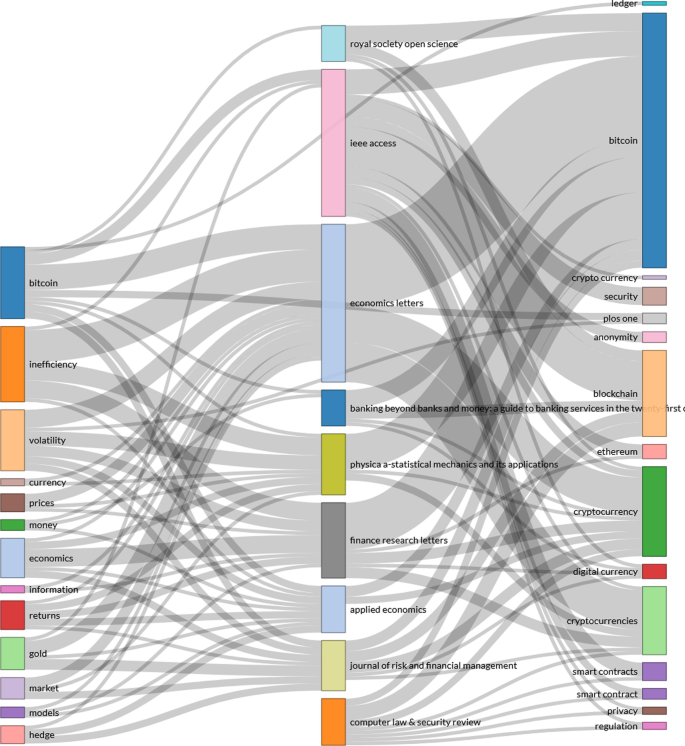
Three-fields plot of top Keywords plus, sources, and Author keywords.
The results of both graphs show similarities in terms of key concepts that are maintained over time. The secondary issues continue to have Bitcoin as the central focus, drifting toward the concepts of blockchain, money, and security. Remarkably, although the term Ethereum has been used as a study keyword, it does not appear directly in the cluster figures, although the derivative terms, such as Smart contracts, appear as the purpose of this type of currency.
The concept of security appears directly related to electronic currency, and hence, the fact that it is not reflected in any type of legal regulation is conspicuous, given the complexity of these payment mechanisms. If the latest publications and texts taken from conferences are incorporated, changes are made to the graph that had not been previously considered, such as security becoming an impactful mainstay of the topic. This is due to the standardization and greater acceptance of these types of currencies that had even been temporarily banned in countries, such as China ( 2019 ), which is now one of the largest producers of articles related to the subject, coming to appear in the keywords of both databases, although the current situation in China is complex, as its uses have recently been limited (China 2021 ).
Returning to the concept of security, we determine that the term crime appears close due to the increase in publications related to criminal acts, such as money laundering processes, darknet shops, or payment to ransomware, that in the last three years has doubled the number of publications (Turner et al. 2019 ; Albrecht et al. 2019 ). This terminology is related to the illicit and dark web keywords that evolve from the concept of anonymity.
To finish with the new trends section, we compiled a Sankey diagram (Fig. 12 ). The diagram shows the relationship between sources (center), Keywords Plus (left), and Author Keywords (right), which is especially useful for locating the topic in each of the journals (Riehmann et al. 2005 ). The size of the nodes represents the frequency of the item and the lines show the connections between them. The use of Keywords Plus and Authors' keywords shows a difference to be considered, as Keywords Plus are more effective than words given by authors in bibliometric analyses even if they are less representative of the article’s content. (Zhang et al. 2016 ).
We can argue that Economics Letters relates its publications to a greater number of terms, such as inefficiency, volatility, or market, covering more topics or characteristics because of connector flows. These are in turn closely related to the words “electronic currencies, bitcoin and smart contracts” as the authors’ keywords. Therefore, although this first node mentions more topics, they are all related to the economic world, leaving in the background the importance of applied technologies, such as blockchain. The publications of the second most influential node, IEEE Access , are closely related to the concepts of “inefficiency, Bitcoin, and volatility,” with special interest in the authors' words “bitcoin, security, blockchain, smart contracts, privacy and privacy regulation.” Therefore, the authors of these publications can focus more on the financial applications that arise from blockchain networks than on developing the currencies themselves. This perspective seems to be shared by four other sources (i.e., Computer Law & Security Review, Journal of Risk and Financial Management, Banking Beyond Banks and Money, and Royal Society Open Science ), whereas Applied Economics and Finance Research Letters follow the trend of the first node.
Using 2020 as a deadline, we can see that the aforementioned trends are the ones that have finally concentrated on these publication types. The conceptual structure map (Fig. 13 ) of the MCA keyword plus method shows two main clusters in different colors that coincide with the driving themes of these publications (Fig. 14 ). This word clustering allows us to identify from today the groups with the same meaning and their relationships. Porter's derivation algorithm has been used to reduce the number of words used in a root form, but this time, from the authors’ keywords with similar results. In both cases, a maximum of 250 words per term has been applied. Both show that regardless of the analysis used and keywords, the central topics are Bitcoin and the blockchain network, which creates and supports the need for a separate bibliometric review of different areas to check the trends in them in the future. This situation is repeated in the different analyses conducted on the subject regardless of the basis used, clearly showing a separation between technology and economy (Merediz-Sola et al. 2019; Shen et al. 2020 ).
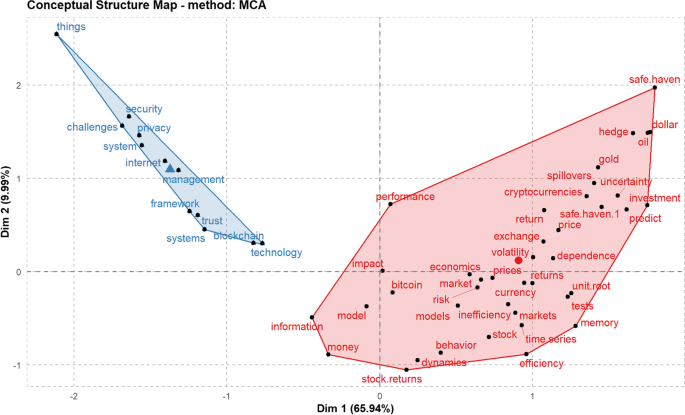
Conceptual structure map using MCA method.
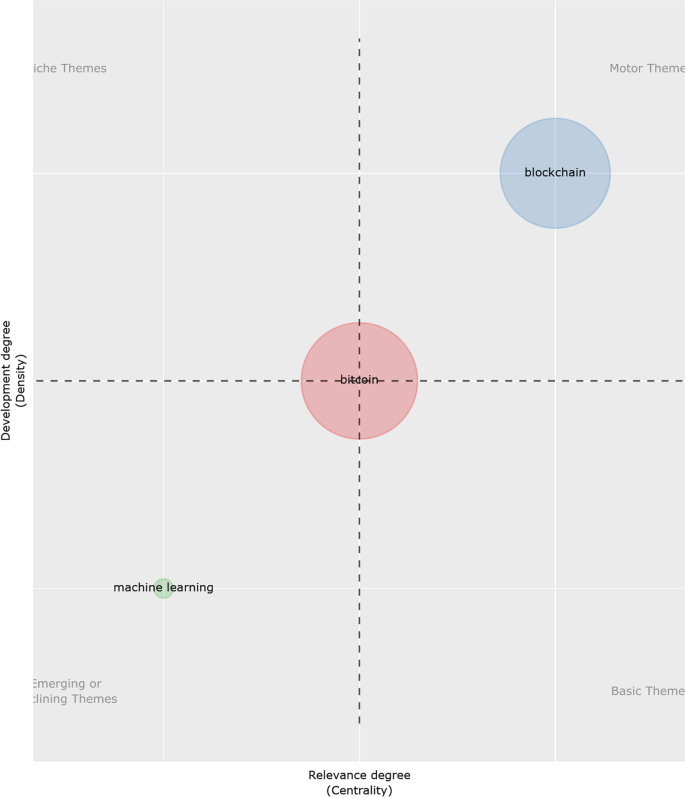
Thematic map using porter's derivation algorithm method.
Figure 15 shows a thematic division into four different periods. This is conducted to clarify how the same area has been clearly divided into two distinct interconnected branches since 2017–2018, creating the aforementioned economic-technological division. Although the concern for cryptocurrencies is related to their value in the market, technological evolution has opened up new lines of research thanks to its multiple applications, such as machine learning.
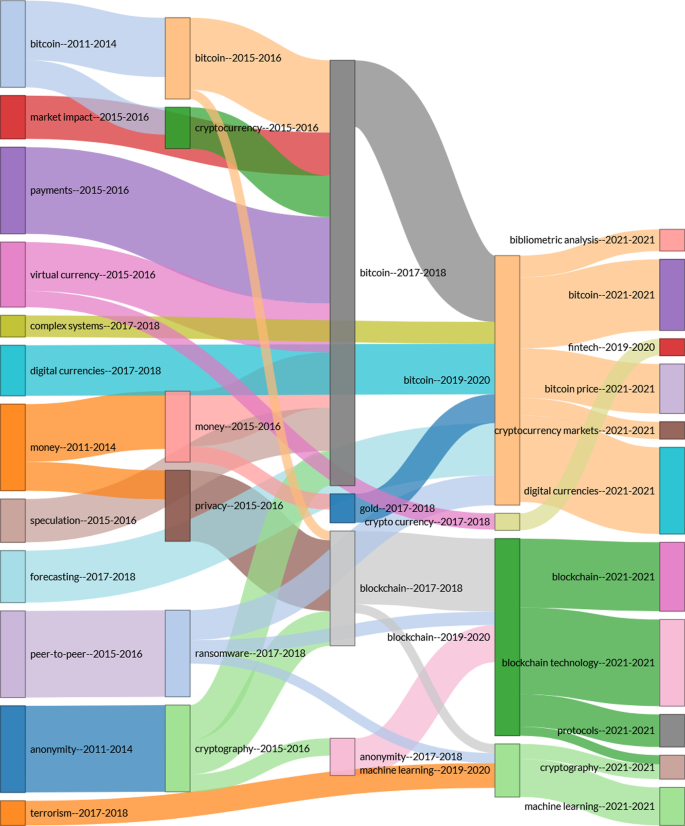
Topic evolution research (2010–2020).
At this point, some questions arise:
What should be the way forward for cryptocurrency research?
Cryptocurrencies will continue to be published, following the concepts of volatility, decentralization, and efficiency as characteristics, along with the smart contract as an application that initiated the 2.0 protocol. Especially in this context, the concept of efficiency or inefficiency should be emphasized in a broader sense, given that the cost of maintaining certain global networks based on peer-to-peer technology is starting to cause survival problems and requires optimizations that were already foreseen (Courtois et al. 2014 ). For example, Bitcoin power grid consumed approximately 2.55 GW of electricity in 2018, which is comparable to the consumption of countries, such as Ireland and its 3.1 GW (de Vries 2018 ). The hash rate, or the computing power needed to keep the network stable and the technology moving forward, is its main strength and weakness. The network will be more secure the higher the ratio is held, but more complex to mine and more computational and energy intensive. For example, some markets are currently affected by the COVID-19 pandemic, among other reasons. Of these, and in direct relation to cryptocurrencies, we must highlight the lack of stock and increase in computer components (mostly graphic cards) used for mining algorithms (Allan 2021 ; Faulkner 2021 ). We have recently seen mining farms using laptops in parallel due to their lower power consumption or companies, such as Nvidia Corporation ( 2021 ), launching versions exclusively for these purposes. The use of these technologies is promising but uncertain based on their overall cost alone (Li et al. 2019 ).
Should these tools be separated generally from the technology created at the level of future research?
As mentioned before, a constant relationship has both a technological and an economic side. Undoubtedly, the impact of technology and its multiple applications will keep them together, so this separation will not materialize. Although cryptocurrencies have led the path, as shown in Fig. 15 , blockchain technology is the main topic that will eventually leave Bitcoin and Ethereum as basic or niche topics, as Shen et al. ( 2020 ) concluded.
Can the technology created be applied to more business issues, and can they benefit from it?
Above all, the Blockchain network is the pioneering technology that has appeared in a number of publications on cryptocurrencies (Yli-Huumo et al. 2016 ). Since 2016, several authors, such as Yu Zhang, Young-Sik Jeong, K.K.R. Choo or J.H. Park have established this trend, with the highest number of mentions of blockchain appearing in late 2020. Blockchain is a disruptive technology that can be used in all subject areas. This multiplicity of uses made necessary a systematic review, with special attention to business and economics (Xu et al. 2019 ). This suggests that we should take into account the application of the base technology and its potential applications at the business level (Zhao et al. 2016 ). Moreover, the cryptocurrency technology should be considered.
Based on the blockchain network analysis, this technology has great potential and offers many opportunities for the business area (Xu et al. 2019 ). The blockchain encryption system allows, for example, conducting secure and reliable financial transactions quickly, thanks to the distribution on independent nodes. The system also makes the data more difficult to falsify since it must be exchanged from multiple nodes simultaneously and allows the realization of smart contracts. Furthermore, it keeps the information more accessible because, as long as a node is still online, the information can be accessed; it does not have a single source server (Felin and Lakhani 2018 ; Gatteschi et al. 2018 ; Tönniseen et al. 2018 ; Chang et al. 2019 ).
This study has reviewed an 8-year international search related to cryptocurrency due to bibliometric analysis of the WoS and Scopus databases.
The results show the positive evolution both in terms of the number of articles published and citations, with a growing number of publications and relevance in recent years. Comparing the evolution of both databases, we determine that WoS contains a greater number of citations received, whereas the Scopus database includes a greater number of articles. The main topics or research areas that contain the concepts related to cryptocurrencies are computer science and economics. If we delve further into the number of research areas in both databases, limiting the criteria to articles only, the enormous amount of categorical division seems to indicate that it is an interdisciplinary branch. However, on closer inspection, this perception changes because the majority of knowledge areas are related to the aforementioned sciences (i.e., computer science and economics). The subsequent thematic areas are legal sciences, criminology, philosophy, and physics.
The countries with the greatest number of publications are the USA, UK, and China, with the latter appearing alongside Canada in analyzing the most relevant keywords. The constant evolution of the regulatory framework regarding cryptocurrencies has generated various controversies at a global level. One notable case is in China, where after the general ban on Bitcoin trading in 2017, the Hangzhou Internet Court recently granted it a new status as a virtual asset. Hangzhou Internet Court was responsible for making cryptocurrencies public and reversing the ban without being considered fiat money. Meanwhile, the most used language for communications is English, coinciding with the native language of two of the countries with the highest rate of published articles. In contrast, although Chinese is not the language with the highest number of publications, China is one of the most often recurrent keywords in the last three years, making it a country showing the most interest in the subject. The authors’ cluster analysis also demonstrates the high participation rate they acquire.
A more in-depth analysis confirms that the main journals and authors belonging to the ranking also belong to the countries with the highest number of publications, to clarify any doubts that may arise from this new phenomenon. The number of outstanding journals and authors is increasing, but note that, especially when referring to authors, the wide participation of the Asian continent is prevalent if Scopus references are taken into account and even if the journals are English-speaking.
From the keywords obtained from the documents, the most frequent topics in the world of cryptocurrencies can be linked and recognized. Although WoS mainly contains words related to Bitcoin, Blockchain, and the volatility of these cryptocurrencies, Scopus publications focus on Bitcoin, Blockchain, and the technological aspects derived from them. Due to the importance of Blockchain technology, the publications on this topic have doubled in the last two years. A basic analysis of the theme shows a total of 550 articles in 2018, whereas the figure exceeds 1100 2019 in WoS. Scopus in turn shows results of approximately 650 and 1370. This is evidence of new lines of research among which stand out, blockchain appearing on both platforms as noteworthy, and Smart Contracts as an alternative to the conclusion of classic contracts that had been conducted.
At this point, and after starting to look at the reviews, especially of the most important keywords or the evolution in the discussion, we can see how the theory and background of cryptocurrencies has begun to conclude the publications on cryptocurrencies, leaving practical research as a new line of research. This opens the way to other interdisciplinary studies, especially after the controversy over the lack of regularization and harmonization in matters, such as legislative issues. Internally, these currencies are constantly revising and evolving to rectify the problems they previously had. Thus, the current information about them will be transformed by version periods, closing the chapter on version 1.0 and analyzing the modifications corresponding to version 2.0.
Finally, despite this study’s contribution, it also has some limitations. First, the field of study is based solely on two of the most influential academic databases (WoS and Scopus). Second, the type of document included in the analysis has been limited to articles. Given the recent creation of the topic and trying to cover the largest possible field of study, expanding the results with Google Scholar as a third data source or using a wide range of publication types could yield a larger document count, which in turn could change the results, especially concerning the keywords used. If the subject were focused on documents from Google Scholar, but the type of publication was not delimited, some 7750 total documents would be obtained. The following will be included in the top 10 publications: “Blockchain technology: Beyond bitcoin,” followed by “Zerocash: Decentralized anonymous payments from bitcoin,” and “The inefficiency of Bitcoin.” Although in different positions, all these articles are well placed in the two databases considered in this study. However, if the document type were to be extended, the existing procedural paper with the same time limitation as the articles in WoS amounts to 875, which, together with 684 articles, would add up to a total of 1559 of the 1678 results obtained. Scopus would yield a total of 1281 and 771, respectively, showing that 83.2% of the 2467 total results without applying filters are of both classes. In this way, an analysis of almost all the elements could be conducted.
Availability of data and materials
The datasets analyzed during the current study are available on the following websites: Price: https://coinmarketcap.com , Web of Science: http://wos.fecyt.es/ , Scopus: https://www.scopus.com/
Abbreviations
Average citation
First publication
Journal citation reports
Last publication
Position in the ranking
Research area scopus
Research area WoS
Scimago Journal Rank
Total cites
Web of Science
Albrecht C, Duffin KM, Hawkins S, Rocha VMM (2019) The use of cryptocurrencies in the money laundering process 0. J Money Laund Control 22(2):210–216. https://doi.org/10.1108/JMLC-12-2017-0074
Article Google Scholar
Allan D (2021) Processor and graphics card stock woes could last into 2022, if chip shortage prediction is right. https://www.techradar.com/news/processor-and-graphics-card-stock-woes-could-last-into-2022-if-chip-shortage-prediction-is-right . Accessed 24 Feb 2021
Babaioff M, Dobzinski S, Oren S, Zohar A (2012) On bitcoin and red balloons. In: 13th ACM conference on electronic commerce (EC '12), Association for Computing Machinery, New York, NY, USA, pp 56–73
Balcilar M, Bouri E, Gupta R, Roubaud D (2017) Can volume predict Bitcoin returns and volatility? A quantiles-based approach. Econ Model 64:74–81
Bariviera AF, Basgall MJ, Hasperué W, Naiouf M (2017) Some stylized facts of the bitcoin market. Physica A 484:82–90. https://doi.org/10.1016/j.physa.2017.04.159
Bariviera AF (2017) The inefficiency of Bitcoin revisited: a dynamic approach. Econ Let 161(C):1–4.
Barratt MJ, Lenton S, Allen M (2013) Internet content regulation, public drug websites and the growth in hidden Internet services. Drugs Educ Prevent Policy 20(3):195–202. https://doi.org/10.3109/09687637.2012.745828
Baur DG, Hong K, Lee AD (2018) Bitcoin: Medium of exchange or speculative assets? J Int Finan Markets Inst Money 54:177–189
Bhargavan K, Delignat-Lavaud A, Fournet C et al. (2016) Formal verification of smart contracts short paper. In: 2016 ACM workshop on programming languages and analysis for security (PLAS'16), Vienna, Austria, Oct 2016, pp 91–96. https://doi.org/10.1145/2993600.2993611
Böhme R, Chirstin N, Edelman B (2015) Bitcoin: economics, technology, and governance. J Econ Perspect 29(2):213–238. https://doi.org/10.1257/jep.29.2.213
Bonneau J, Miller A, Clark J et al. (2015) Sok: research perspectives and challenges for bitcoin and cryptocurrencies. Secur Privacy IEEE, pp 104–121.
Bouri E, Keung Marco Lau C, Lucey B, Roubaud D (2019) Trading volume and the predictability of return and volatility in the cryptocurrency market. Financ Res Lett 29:340–346. https://doi.org/10.1016/j.frl.2018.08.015
Brandvold M, Molnár P, Vagstad K, Andreas Valstad OC (2015) Price discovery on Bitcoin exchanges. J Int Finan Markets Inst Money 36:18–35
Brière M, Oosterlinck K, Szafarz A (2013) Virtual currency, tangible return: port-folio diversication with bitcoins, tangible return: portfolio Diversication with Bitcoins. J Asset Manag 16(6):365–373. https://doi.org/10.1057/jam.2015.5
Chang SE, Chen Y, Lu M (2019) Supply chain re-engineering using blockchain technology: a case of smart contract based tracking process. Technol Forecast Soc Chang 144:1–11. https://doi.org/10.1016/j.techfore.2019.03.015
Cheah ET, Fry J (2015) Speculative bubbles in Bitcoin markets? An empirical investigation into the fundamental value of Bitcoin. Econ Lett 130:32–36. https://doi.org/10.1016/j.econlet.2015.02.029
China (2019) Bitcoin's virtual property attributes recognized for the first time by the judiciary. http://m.caijing.com.cn/api/show?contentid=4603403 . Accessed 10 Jan 2020
China (2021) Bitcoin tumbles below $30,000 on China crypto-crackdown. https://www.bbc.com/news/business-57549543 . Accessed 30 June 2021
Chu J, Chan S, Nadarajah S, Osterrieder J (2017) GARCH modelling of cryptocurrencies. J Risk Financ Manag 10:17. https://doi.org/10.3390/jrfm10040017
Ciaian P, Rajcaniova M, Kancs D (2016) The economics of Bitcoin price formation. Appl Econ 48(19):1799–1815. https://doi.org/10.1080/00036846.2015.1109038
Cocco L, Marchesi M (2016) Modeling and simulation of the economics of mining in the bitcoin market. PLoS ONE 11(10):e0164603.
Conrad C, Custovic A, Ghysels E (2018) Long- and short-term cryptocurrency volatility components: a GARCH-MIDAS Analysis. J Risk Financ Manag 11:23. https://doi.org/10.3390/jrfm11020023
Conti M, Sandeep Kumar E, Lal C, Ruj S (2018) A survey on security and privacy issues of bitcoin. IEEE Commun Surv Tutorials 20(4):3416–3452. https://doi.org/10.1109/COMST.2018.2842460
Corrons A (2017) Monedas complementarias: dinero con valores. RIO 18:109–34
Courtois NT, Grajek M, Naik R (2014) Optimizing SHA256 in bitcoin mining. Cryptography and security systems. In: CSS 2014. Communications in computer and information science, vol 448. https://doi.org/10.1007/978-3-662-44893-9_12
Dabbagh M, Sookhak M, Safa NS (2019) The evolution of blockchain: a bibliometric study. IEEE Access 7:19212–19221. https://doi.org/10.1109/ACCESS.2019.2895646
Dai W (1998) B-money. Available via DIALOG. http://www.weidai.com/bmoney.txt . Accessed 20 Dec 2018
de Vries A (2018) Bitcoin’s growing energy problem. Joule 2(5):801–805. https://doi.org/10.1016/j.joule.2018.04.016
Dwyer GP (2015) The economics of bitcoin and similar private digital currencies. J Financ Stab 17:81–91. https://doi.org/10.1016/j.jfs.2014.11.006
Eyal I, Sirer EG (2013) Majority is not enough: bitcoin mining is vulnerable. Comput Sci 8437:436–454
Google Scholar
Fabian ML (2016) Analyzing the bitcoin network: the first four years. Future Internet. https://doi.org/10.3390/fi8010007
Faulkner C (2021) Nvidia made $5 billion during a GPU shortage and expects to do it again in Q1. https://www.theverge.com/2021/2/24/22299505/nvidia-earnings-q4-2021-quarterly-gpu-shortage-revenue . Accessed 24 Feb 2021
Felin T, Lakhani K (2018) What problems will you solve with Blockchain? MIT Sloan Manag Rev 60(1):32–3+.
Feng Q, He D, Zeadally S, Khurram Khan M, Kumar N (2019) A survey on privacy protection in blockchain system. J Netw Comput Appl 126:45–58. https://doi.org/10.1016/j.jnca.2018.10.020
Foley S, Karlsen J, Putnins T (2019) Sex, drugs, and bitcoin: how much illegal activity is financed through cryptocurrencies? Rev Financ Stud 32(5):1798–1853. https://doi.org/10.1093/rfs/hhz015
Follador GB (2017) Criptomoedas e competência tributária. Revista brasileira de políticas públicas 7(3)
Franco P (2014) Understanding bitcoin: cryptography, engineering, and economics. Wiley, New York
Book Google Scholar
Garcia D, Tessone CJ, Mavrodiev P, Perony N (2014) The digital traces of bubbles: feedback cycles between socio-economic signals in the Bitcoin economy. J R Soc Interface 11(99)
Gatteschi V, Lamberti F, Demartini C, Pranteda C, Santamaría V (2018) To blockchain or not to blockchain: that is the question. IT Professional 20(2):62–74. https://doi.org/10.1109/MITP.2018.021921652
Giungato P, Rana R, Tarabella A, Tricase C (2017) SoK: current trends in sustainability of bitcoins and related blockchain technology. Sustainability 9(12):2214
Gomá-Garcés I (2014) Bitcoin, una aproximación jurídica al fenómeno de la desmaterialización y privatización del dinero. Available via DIALOG. https://studylib.es/doc/8030153/bitcoin.-una-aproximaci%C3%B3n-jur%C3%ADdica-al-fen%C3%B3meno-de-la . Accessed 3 Feb 2019
Griffin J, Shams A (2020) Is bitcoin really untethered? J Financ 75(4):1913–1964
Hardy R, Norgaard J (2016) Reputation in the Internet black market: an empirical and theoretical analysis of the Deep Web. J Inst Econ 12(3):515–539. https://doi.org/10.1017/S1744137415000454
Jacobs E (2011) Bitcoin: a bit too far? J Internet Bank Commerce, 16
Jang H, Lee J (2018) An empirical study on modeling and prediction of Bitcoin prices with Bayesian neural networks based on Blockchain information. IEEE Access 6:5427–5437. https://doi.org/10.1109/ACCESS.2017.2779181
Katsiampa P (2017) Volatility estimation for bitcoin: a comparison of GARCH models. Econ Lett 158:3–6. https://doi.org/10.1016/j.econlet.2017.06.023
Khan MA, Salah K (2018) IoT security: review, blockchain solutions, and open challenges. Future Gener Comput Syst Int J Sci 82:395–411. https://doi.org/10.1016/j.future.2017.11.022
Kim T (2015) The predecessors of bitcoin and their implications for the prospect of virtual currencies. PLoS ONE 10(4):e0123071. https://doi.org/10.1371/journal.pone.0123071
Klein T, Thu HP, Walther T (2018) Bitcoin is not the New Gold—a comparison of volatility, correlation, and portfolio performance. Int Rev Financ Anal 59:105–116. https://doi.org/10.1016/j.irfa.2018.07.010
Koblitz N, Menezes AJ (2016) Cryptocash, cryptocurrencies, and cryptocontracts. Des Codes Crypt 78(1):87–102. https://doi.org/10.1007/s10623-015-0148-5
Kondor D, Pósfai M, Csabai I, Vattay G (2014) Do the rich get richer? An empirical analysis of the bitcoin transaction network. PLoS ONE 9(5):e97205. Corrected and republished from: Do the Rich Get Richer? An Empirical Analysis of the Bitcoin Transaction Network. PLoS ONE 9(2):e86197
Kostakis V, Giotitsas C (2014) The (A)political economy of bitcoin. Triplec Comun Capit Crit 12(2):431–440
Kristoufek L (2013) BitCoin meets Google Trends and Wikipedia: quantifying the relationship between phenomena of the Internet era. Sci Rep 3:3415
Kristoufek L (2015) What are the main drivers of the bitcoin price? Evidence from wavelet coherence analysis. PLoS ONE 10(4):e0123923. https://doi.org/10.1371/journal.pone.0123923
Krugman P (2018) Transaction costs and tethers: why I’m a crypto skeptic. In: NewYorkTimes. https://www.nytimes.com/2018/07/31/opinion/transaction-costs-and-tethers-why-im-a-crypto-skeptic.html . Accessed 4 May 2019
Kuo T, Kim H, Ohno-Machado L (2017) Blockchain distributed ledger technologies for biomedical and health care applications. J Am Med Inform Assoc 24(6):1211–1220
Lee J (2017) BIDaaS: blockchain based ID as a service. IEEE Access 6:2274–2278
Li J, Li N, Peng J, Cui H, Wu Z (2019) Energy consumption of cryptocurrency mining: a study of electricity consumption in mining cryptocurrencies. Energy 168:160–168. https://doi.org/10.1016/j.energy.2018.11.046
Li T, Shin D, Wang B (2018) Cryptocurrency pump-and-dump schemes, unpublished manuscript, University of North Carolina. SSRN: https://ssrn.com/abstract=3267041 or http://dx.doi.org/ https://doi.org/10.2139/ssrn.3267041
Lietaer BA, Hallsmith W (2006) Community currency guide. Available via DIALOG. http://community-currency.info/wp-content/uploads/2014/12/lietaer-hallsmith-community-currency-guide.pdf . Accessed 5 Nov 2018
Luu L, Chu DH, Olickel H, Saxena P, Hobor A (2016) Making smart contracts smarter. In: 2016 ACM SIGSAC conference on computer and communications security, pp 254–269. https://doi.org/10.1145/2976749.2978309
Manterola C, Pineda V, Vial M, Losada H (2005) ¿Es el factor de impacto un índice apropiado para determinar el grado de evidencia de estudios sobre procedimientos terapéuticos en revistas quirúrgicas? Cir Esp 78(2):96–99
Merediz-Solà I, Bariviera A (2019) A bibliometric analysis of bitcoin scientific production. Res Int Bus Financ 50:294–305. https://doi.org/10.1016/j.ribaf.2019.06.008
Miers, I, Garman C, Green M et al. (2013) Zerocoin: anonymous distributed e-cash from bitcoin. In: 2012 IEEE symposium on security and privacy, pp 397–411. https://doi.org/10.1109/SP.2013.34
Nadarajah S, Chu J (2017) On the inefficiency of Bitcoin. Econ Let 150(C):6–9
Nakamoto S (2008) Bitcoin: a peer-to-peer electronic cash system. Available via DIALOG. http://bitcoin.org/bitcoin.pdf . Accessed 30 Dec 2018
Navas-Navarro S (2015) Un mercado financiero floreciente: el del dinero virtual no regulado (Especial atención a los BITCOINS). Rev CESCO De Derecho De Consumo 13:79–115
Neslihanoglu S (2021) Linearity extensions of the market model: a case of the top 10 cryptocurrency prices during the pre-COVID-19 and COVID-19 periods. Financ Innov 7:38. https://doi.org/10.1186/s40854-021-00247-z
Nvidia Corporation (2021). https://www.nvidia.com/en-us/cmp/ . Accessed 24 Feb 2021
Ober M, Katzenbeisser S, Hamacher K (2013) Structure and anonymity of the bitcoin transaction graph. Future Internet 5(2):237–250. https://doi.org/10.3390/fi5020237
Orastean R, Silvia CM, Sava R (2019) Bitcoin in the scientific literature—a bibliometric study. Stud Bus Econ 14(3):160–174. https://doi.org/10.2478/sbe-2019-0051
Phillip A, Chan JSK, Peiris S (2018) A new look at cryptocurrencies. Econ Lett 163:6–9. https://doi.org/10.1016/j.econlet.2017.11.020
Polasik M, Piotrowska AI, Wisniewski TP, Kotkowski R, Lightfoot J (2015) Price fluctuations and the use of bitcoin: an empirical inquiry. Int J Electron Commer 20(1):9–49. https://doi.org/10.1080/10864415.2016.1061413
Van Raan AF (2014) Advances in bibliometric analysis: research performance assessment and science mapping. In: Blockmans W, Engwall L, Weaire D (eds) Bibliometrics. Use and abuse in the review of research performance. Wenner-Gren International Series, Portland Press, pp 17–28
Riehmann P, Hanfler M, Froehlich B (2005) Interactive Sankey diagrams. In: IEEE symposium on information visualization. INFOVIS 2005. Minneapolis, MN, USA, 2005, pp 233–240. https://doi.org/10.1109/INFVIS.2005.1532152
European Central Bank (2012) Virtual Currency Schemes. DIALOG. https://www.ecb.europa.eu/pub/pdf/other/virtualcurrencyschemes201210en.pdf . Accessed 9 Jan 2019
Selgin G (2013) Synthetic commodity money. In: SSRN https://doi.org/10.2139/ssrn.2000118 . Accessed 16 May 2019
Shen C, Chang LC, Su, TC (2020) Research development of Bitcoin: a network and concept linking analysis. Library Hi Tech, vol ahead-of-print No. ahead-of-print. https://doi.org/10.1108/LHT-10-2019-0210
Sikorski J, Haughton J, Kraft M (2017) Blockchain technology in the chemical industry: machine-to-machine electricity market. Appl Energy 195:234–246
Tönnissen S, Teuteberg F (2018) Using blockchain technology for business processes in purchasing—concept and case study-based evidence. In: Abramowicz W, Paschke A (eds) Business information systems. BIS 2018. Lecture notes in business information processing 320. https://doi.org/10.1007/978-3-319-93931-5_18
Tschorsch F, Scheuermann N (2016) Bitcoin and beyond: a technical survey on decentralized digital currencies. IEEE Commun Surv Tutorials 18(3):2084–2123. https://doi.org/10.1109/COMST.2016.2535718
Turner AB, McCombie S, Uhlmann AJ (2019) A target-centric intelligence approach to WannaCry 2.0. J Money Laund Control 22(4):646–665. https://doi.org/10.1108/JMLC-01-2019-0005
Urquhart A (2016) The inefficiency of bitcoin. Econ Lett 148:80–82. https://doi.org/10.1016/j.econlet.2016.09.019
Urquhart A (2018) What causes the attention of bitcoin? Econ Lett 166:40–44. https://doi.org/10.1016/j.econlet.2018.02
Van Alstyne M (2014) Why bitcoin has value. Commun ACM 57(5):30–32. https://doi.org/10.1145/2594288
Wang Q, Li X, Yu Y (2018) Anonymity for bitcoin from secure escrow address. IEEE Access 6:12336–12341. https://doi.org/10.1109/ACCESS.2017.2787563
Xu JJ (2016) Are blockchains immune to all malicious attacks? Financ Innov 2:25. https://doi.org/10.1186/s40854-016-0046-5
Xu M, Chen X, Kou G (2019) A systematic review of blockchain. Financ Innov 5:27. https://doi.org/10.1186/s40854-019-0147-z
Yin W, Wen Q, Li W, Huan Z, Jin Z (2017) An anti-quantum transaction authentication approach in blockchain. IEEE Access 6:5393–5401
Yli-Huumo J, Ko D, Choi S, Park S, Smolander K (2016) Where is current research on blockchain technology—a systematic review. PLoS ONE 11(10):1–27
Zhang J, Yu Q, Zheng F, Long C, Lu Z, Duan Z (2016) Comparing keywords plus of WOS and author keywords: a case study of patient adherence research. J Assoc Inf Sci Technol 67(4):967–972. https://doi.org/10.1002/asi.23437
Zhang W, Wang P, Li X, Shen D (2018) The inefficiency of cryptocurrency and its cross-correlation with Dow Jones Industrial Average. Physica A 510:658–670. https://doi.org/10.1016/j.physa.2018.07.032
Zhang R, Xue R, Li L (2019) Security and privacy on blockchain. ACM Comput Surv 52(3):51. https://doi.org/10.1145/3316481
Zhang X, Lu F, Tao R, Wang S (2021) The time-varying causal relationship between the Bitcoin market and internet attention. Financ Innov 7:66. https://doi.org/10.1186/s40854-021-00275-9
Zhao JL, Fan S, Yan J (2016) Overview of business innovations and research opportunities in blockchain and introduction to the special issue. Financ Innov 2:28. https://doi.org/10.1186/s40854-016-0049-2
Zheng ZB, Xie SA, Dai HN, Chen XP, Wang HM (2018) Blockchain challenges and opportunities: a survey International. J Web Grid Serv 14(4):352–375. https://doi.org/10.1504/IJWGS.2018.095647
Zimmer Z (2017) Bitcoin and potosi silver historical perspectives on cryptocurrency. Technol Cult 58(2):307–334. https://doi.org/10.1353/tech.2017.0038
Zulfiqar N, Gulzar S (2021) Implied volatility estimation of bitcoin options and the stylized facts of option pricing. Financ Innov 7:67. https://doi.org/10.1186/s40854-021-00280-y
Zyskind G, Nathan O, Pentland A (2015) Decentralizing privacy: using blockchain to protect personal data. In: 2015 IEEE security and privacy workshops, San Jose, CA, pp 180–184
Download references
Acknowledgements
Not applicable.
No funding is declared.
Author information
Authors and affiliations.
Research Group: Almeria Group of Applied Economy (SEJ 147), University of Almeria, Carretera Sacramento s/n, 04120, La Cañada de San Urbano, Almería, Spain
Francisco Javier García-Corral
Department of Law, Financial and Tax Law, University of Almeria, Carretera Sacramento s/n, 04120, La Cañada de San Urbano, Almería, Spain
José Antonio Cordero-García
Department of Business and Economics, Applied Economic Area, University of Almería, Carretera Sacramento s/n, 04120, La Cañada de San Urbano, Almería, Spain
Jaime de Pablo-Valenciano & Juan Uribe-Toril
You can also search for this author in PubMed Google Scholar
Contributions
FJGC contributed by retrieving literature, conducting data analysis and writing the paper. JdPV contributed by retrieving literature and participated in its design and coordination. JUT contributed conducting data analysis and revised the paper. JACG revised the paper and helped to write the manuscript. All authors read and approved the final manuscript.
Corresponding author
Correspondence to Francisco Javier García-Corral .
Ethics declarations
Competing interests.
The authors declare that they have no competing interests.
Additional information
Publisher's note.
Springer Nature remains neutral with regard to jurisdictional claims in published maps and institutional affiliations.
Rights and permissions
Open Access This article is licensed under a Creative Commons Attribution 4.0 International License, which permits use, sharing, adaptation, distribution and reproduction in any medium or format, as long as you give appropriate credit to the original author(s) and the source, provide a link to the Creative Commons licence, and indicate if changes were made. The images or other third party material in this article are included in the article's Creative Commons licence, unless indicated otherwise in a credit line to the material. If material is not included in the article's Creative Commons licence and your intended use is not permitted by statutory regulation or exceeds the permitted use, you will need to obtain permission directly from the copyright holder. To view a copy of this licence, visit http://creativecommons.org/licenses/by/4.0/ .
Reprints and permissions
About this article
Cite this article.
García-Corral, F.J., Cordero-García, J.A., de Pablo-Valenciano, J. et al. A bibliometric review of cryptocurrencies: how have they grown?. Financ Innov 8 , 2 (2022). https://doi.org/10.1186/s40854-021-00306-5
Download citation
Received : 06 August 2020
Accepted : 14 November 2021
Published : 01 January 2022
DOI : https://doi.org/10.1186/s40854-021-00306-5
Share this article
Anyone you share the following link with will be able to read this content:
Sorry, a shareable link is not currently available for this article.
Provided by the Springer Nature SharedIt content-sharing initiative
- Cryptocurrency
- Bibliometric analysis
- Business and economics
Privacy and Cryptocurrencies—A Systematic Literature Review
Ieee account.
- Change Username/Password
- Update Address
Purchase Details
- Payment Options
- Order History
- View Purchased Documents
Profile Information
- Communications Preferences
- Profession and Education
- Technical Interests
- US & Canada: +1 800 678 4333
- Worldwide: +1 732 981 0060
- Contact & Support
- About IEEE Xplore
- Accessibility
- Terms of Use
- Nondiscrimination Policy
- Privacy & Opting Out of Cookies
A not-for-profit organization, IEEE is the world's largest technical professional organization dedicated to advancing technology for the benefit of humanity. © Copyright 2024 IEEE - All rights reserved. Use of this web site signifies your agreement to the terms and conditions.
Bitcoin in the economics and finance literature: a survey
- Original Article
- Published: 03 June 2021
- Volume 1 , article number 88 , ( 2021 )
Cite this article
- Parthajit Kayal ORCID: orcid.org/0000-0001-7741-5800 1 &
- Purnima Rohilla 1
14k Accesses
24 Citations
231 Altmetric
28 Mentions
Explore all metrics
This paper provides a review of the literature on key matters related to the popular cryptocurrency Bitcoin. Another key motivation of this paper is to understand the underlying principle of this digital currency from the economic and financial point of view. For the survey to be comprehensive, the paper is categorized into varied themes: price dynamics, volatility, bubble dynamics, mode of recognition in the financial market, efficiency, economics, social media and investor sentiment, and lastly regulation and legality. We argue that Bitcoin is still in an embryonic phase and needs to evolve with time especially keeping in pace with technological advancements. It should be robust to get accepted as an alternative currency and be able to prevent any fraudulent exploitation.
Similar content being viewed by others
The Role of Bitcoin in the Monetary System: Its Development and the Possible Future
The digital agenda of virtual currencies: can bitcoin become a global currency.
Pavel Ciaian, Miroslava Rajcaniova & d’Artis Kancs
Beware the Middleman: Empirical Analysis of Bitcoin-Exchange Risk
Avoid common mistakes on your manuscript.
Introduction
Bitcoin is a cryptocurrency (or digital currency) formulated on the concept of “peer-to-peer” (P2P) network (Nakamoto 2008 ). This invention is credited to a pseudonymous software developer or maybe a hacker named “Satoshi Nakamoto". The key intention was to create a transaction system free from intervention by any central or monetary authority, be based on a mathematical algorithm instead of “third-party trust", payments can be done electronically in a protected, verifiable and incontrovertible way. The application of this idea implies a payment system that all transactions happen directly between the owner and the receiver and is broadcast through a P2P network. Despite the information being public, the identity of the user is anonymous. The coin is mined to collect information in the form of “blocks” and each block contains information with respect to the current transaction and the previous block. This links every current block to the first block. All recorded transactions on the block are verified by the principle of Proof-of-Work. It is almost impossible to change the Bitcoin software unless the majority of participants agree to do so. A hacker or user with criminal intention requires enormous computational power to alter this arrangement. Do such fundamentally strong principles make this a true-blue system? The academic literature has a varying response to this question. The aim of this paper to consolidate evaluations and interpretations of various academicians who have probed into the existence and working of the Bitcoin payment system. The motivation behind this literature survey is to integrate the existing research work in this area and possibly present it as a starting point for future researchers to use this study as a preliminary pathfinder. We aim to address some central ideas like price and volatility dynamics; economics and efficiency of the system; and financial aspects and regulation. Kindly note that this work does not regard technicalities of blockchain or mining strategies which serve the working of the Bitcoin payment system. The key focus is to address the questions from economics and finance belief through simplified discussion. Therefore, the themes of our work are chosen to be in line with Economic and Finance theory and literature. We have tried to cover all the important work published at the time of completion of this work. The major criteria we have used for paper selection are minimum of 10 citations and quality of the journal it is published with. Most of the journals are graded as B and above as per the Australian Business Deans Council ranking. However, we have made exceptions for some working papers if they had a high citation. Subsequently, the paper has been structured as follows: “ Price dynamics ” draws attention to volatility that exists in the Bitcoin market. It also addresses a key question on speculation of Bitcoin being a mere bubble. “ Volatility and bubbles ” elucidates the economics behind the entire system and reviews whether the system is efficient or not. “ Economics and efficiency of Bitcoin ” analyses the crucial “Asset vs Currency” debate that keeps contouring around the Bitcoin. It also throws light on the speculative hedging and investment virtues with Bitcoin. “ Bitcoin as currency vs asset ” calls attention to the impact of social media on investor sentiments and the traceable consumer behavior towards the Bitcoin. “ Social media and investor sentiment ” focuses on the regulatory and legal aspects of the Bitcoin system and the threats it faces in terms of cyber-crimes. Finally, the paper presents conclusions and the scope for future work.
- Price dynamics
Why does Bitcoin exactly have any value? It is not backed by any regulatory authority nor does it have any asset backing. It is operating in a virtual space through some complicated mathematical algorithm like in some science fiction. It is not, yet a very widely accepted payment system. It has been associated with some evasions in terms of legality. And yet, it continues to survive to show comprehensive price fluctuations ever since its formal existence in the virtual market. Prices of any commodity are determined by basic economic indicators or drivers such as utility, supply, demand, and scarcity. These factors do determine the price of Bitcoin but are also with several other factors which might be very absurd to be considered for any other fiat currency. For example, a Google search of Dollar will not impact the value and volume of it, but it can possibly drive the prices of a cryptocurrency (Aalborg et al. 2019 ).
An extensive amount of academic research has been done to determine what gives Bitcoin its value or what are the factors behind its constantly fluctuating prices. The demand–supply theory is the most common principle used in literature to determine the price of Bitcoin. “Inelastic demand and tight supply” result in soaring prices of Bitcoin as found by Blundell-Wignall ( 2014 ). The price determination model used is based on the “medium of exchange” role of Bitcoin. They form that the demand curve and supply functions are derived from the advantages or benefits of using Bitcoin. However, the prices can fall to zero if these benefits are taken away by the government or the coins are hampered by fraudulent activities or if a better alternative emerges in the market. The transaction volume also proves to be a significant demand driving ingredient implying that the transactional needs of users drive up the prices. The supply-side variables, on the other hand, prove to be insignificant in driving the prices of this unregulated contemporary currency. This phenomenon has been explained by Polasik et al. ( 2015 ) that it is because the bitcoin is governed by a mathematical algorithm and any expected future change is already reflected in the current prices. Kristoufek ( 2015 ) conducts a similar analysis by constructing a demand variable as a trade exchange ratio and incorporating the method of wavelength coherent analysis. The study finds that the price leads the Trade exchange ratio in the short run but not in the longer run. It means that Bitcoin does appreciate in the long run when used for more non-exchange transactions while in the short run the price boosts the exchange-based transactions. The supply-side factor remains difficult to be determined as the future money supply is also already reflected in the current prices because of a known algorithm. This relationship between the expected future supply of Bitcoin and its prices remains trivial (Polasik et al. 2015 ).
Using Economic Freedom Index, Viglione ( 2015 ) studies the role of governance and other related factors in determining the price of Bitcoin as measured by the willingness of users to pay a premium. This work exhibits that real interest rates, tax burden, and investment freedom across different countries is significant in determining Bitcoin prices. In contrast, inflation rates and monetary freedom across boundaries have no impact on Bitcoin prices.
Price information can often be taken from the exchanges the Bitcoins are trading on as noted by Brandvold et al. ( 2015 ). They find that Mt. Gox dominated the price discovery and sharing of information Bitcoin (Mt. Gox has now ceased to exist due to malicious activities). They also document that other smaller exchanges, such as Btc-e, are better at providing price information during market shock.
Bitcoin being a digital currency needs to be analyzed from a further perspective than just as an ordinary currency. Both the traditional determinants of currency prices and factors specific to digital currency are analyzed by Ciaian and Rajcaniova ( 2016 ). They examine if Bitcoin has the characteristics such as a medium of exchange, a unit of account and a store of value. They argue that the attractiveness of Bitcoin is the main driver of its’ price and Bitcoin cannot compete with standard currencies due to its speculative nature.
Later Athey et al. ( 2016 ) build a model to examine the equilibrium prices under two different scenarios. First in the absence of an investor and second by including an investor with certain characteristics in the model. They demonstrate the existence of a unique equilibrium for Bitcoin prices defined by economic forces (supply and demand) and show that the inclusion of an investor in the model gives a dynamic equilibrium. In their explanation, the fluctuation In Bitcoin prices is due to associated market expectations. However, Griffin and Shams ( 2020 ) observe that Bitcoin price reflects much more than just standard supply/demand and fundamental news. They argue that price manipulation has substantial distortive effects on Bitcoin. In a theoretical work, Bouoiyour et al. ( 2016 ) use the technique of Empirical Mode Decomposition to extract Intrinsic Mode Function (IMF) of time series data. The model first decomposes the time series into different IMFs arranging it from high to low frequency and then composes these IMFs into the fluctuating process. The findings exhibit that the slowly fluctuating (long term) components are more influential on Bitcoin price than swiftly fluctuating (short term) components. This varying short-run versus long-run results are also reflected in the empirical study by Wang et al. ( 2016 ). They find stock price index, oil prices, and daily traded volume to have a stable relationship with Bitcoin price in the long term but in the short-run it gets dynamic. A change in stock price index significantly affects Bitcoin prices as the investors tend to shift in and out of the market when experiencing variations. Changes in oil prices and trading volume, on the other hand, do not have much impact. The Bitcoin price is positively related to trading volume but negatively related to the stock price index, and the oil price. Bitcoin prices were further studied to see with respect to Bitcoin futures by Baur and Dimpfl ( 2019 ) and by trading platform by Pagnottoni and Dimpfl ( 2019 ). The former observes that Bitcoin spot price leads the futures price and later finds Chinese platforms like OKCoin and BTC China are the leader in price discovery of Bitcoin. Further, Kapor and Olmo ( 2019 ) show that Bitcoin futures and spot log-price have predictive drives for the Bitcoin spot price.
With increasing popularity and acceptance in the era of IoT (internet of things), Bitcoin is gaining more attention and more scrutiny. Being operated on an unregulated mechanism makes it highly vulnerable to manipulations. This issue has been highlighted by Gandal et al. ( 2018 ) and Griffin and Shams ( 2020 ). Authors of the former work set up an association between suspicious trading and resulting price fluctuations. Their study pivots around the much controversial suspicious activity on Mt. Gox in early 2014 and finds that Bitcoin prices rose an approximately 80% of the days on which suspicious trading activity was recorded, while it rose on a comparatively lesser number of days, 55% in which no such suspicious activity was observed. Looking at intraday price dynamics, Eross et al. ( 2019 ) observe that over time, Bitcoin returns have increased, while trading volume, liquidity and volatility varied markedly.
Price clustering is a phenomenon where prices tend to congregate around some specific set of values, usually whole digits. This phenomenon has been examined by Urquhart ( 2017 ) to check whether the cluster at a round number is being approached by rising or falling prices, or other relevant conditions that might influence the reaction. The price reaction, after round numbers show that in one, two, three, five, and ten days before a round number from rising prices, the returns are positively and statistically significant. However, there is no significant evidence of a return pattern after round numbers, but there is evidence of an insignificant next day negative reaction after round numbers. Further, clustering increases as prices and volume increase. In a recent finding, Alaoui et al. ( 2019 ) observe that changes in the Bitcoin prices and trading volume mutually interact in a nonlinear way. Looking at geographically defined markets, Panagiotidis et al. ( 2019 ) observe increased impact of Asian markets on bitcoin compared to other geographical markets.
However, most of these studies have contrasting findings due to differences in methodologies. Further results are also time-dependent and far from being stable due to the lack of long-term data.
Volatility and bubbles
Price fluctuations, if are continuously going through bouts of sudden rise and fall can lead to volatility in the Bitcoin market. The volatile market can give higher returns and alternatively pull-down invested capital to zero in the worst-case scenario.
This hype around the volatility of Bitcoin and whether its existence is substantial is audited by Sapuric and Kokkinaki ( 2014 ) by comparing its exchange rate with other established currencies. They use three parameters: change in the exchange rate, annualized volatility, and adjusted returns to show that the acclaimed volatility of Bitcoin in the literature is because it ignores the trading volume. The Bitcoin has relatively lower trading volume due to which the Bitcoin exchange rate faces unavoidable shocks and hence, so does its price. A study by Bouoiyour and Selmi ( 2015 ) examines extreme volatilities of Bitcoin prices. They state that bad or negative news has a greater effect on the volatility of Bitcoin prices than good or positive news and is highly driven by presumptions of the market participants. Consistent with a similar view, Aysan et al. ( 2019 ) examine the predictive power of global geopolitical risks (GPR index) on Bitcoin returns and volatility. They show that Bitcoin returns and volatility are negatively and positively related to GPR index. Further, Su et al. ( 2020 ) find the Bitcoin market to be a leading indicator of the global geopolitical situation. Given that Bitcoin prices reflect global geopolitical risk and investors react sharply to this risk, Chevapatrakul and Mascia ( 2019 ) report overreaction in the Bitcoin market and argue that Bitcoin investors overreact during days of sharp declines and sharp rallies.
There has been a widespread debate among academicians whether the Bitcoin is an asset or a currency or a commodity. We explore the literature in wider detail in later sections but highlight the common ground between the three in terms of volatility and stability. Dyhrberg ( 2016a ) draws a parallel between Bitcoin, currency, and gold. They argue that Bitcoin lies somewhere between a commodity and a currency as it acts like a currency due to its medium of exchange virtue and its reaction to federal fund rates. Taking into account the hedging capabilities of Bitcoin or its reactions to the news, they claim that Bitcoin mirrors gold. Klein et al. ( 2018 ) also do a comparative analysis of Bitcoin with gold and other precious metals and find a contrasting result. They find a similarity between Bitcoin and gold (and other precious metals) in terms of response to market shocks: both have an asymmetric response. However, from a market linkage point of view, the study exhibits that Bitcoin behaves completely diverse from Gold. During market distress, while the Gold remains relatively steady Bitcoin tends to decline amidst facing market shocks. It leads us to look at the safe haven properties of Bitcoin.
The safe-haven property of Bitcoin is examined by Bouri et al. ( 2016 ) by studying the relationship between change in price returns and volatility using a daily database of prices divided into two phases i.e., before and after the 2013 crash. They show that there exists a positive relation between return shocks and volatility in the pre-crash period which reflected upon the safe haven property of Bitcoin. However, the post-crash period witnessed a negative relationship thereby suggesting that Bitcoin lost its credibility as a safe investment and hedging instrument among the investors. However, in a recent study, Bouri et al. ( 2020 ) examine gold, commodities, and Bitcoin as safe havens for stocks to find Bitcoin to be superior over gold and commodities. However, looking at the safe haven characteristics of Bitcoin during the recent coronavirus pandemic, two separate studies (Chen et al. 2020 ; Conlon and McGee 2020 ) find Bitcoin not to be a safe haven during market turbulence. A similar observation was made by Matkovskyy and Jalan ( 2019 ) as they find risk-averse investors moving away from Bitcoin markets to safe financial assets during crisis periods. Bouoiyour and Selmi ( 2016 ) also, study the volatility trend of Bitcoin using price data over two sub-periods: from 2010 to 14 and from 2015 to 16. Their findings reveal that the first period to be explosive due to high volatility while the second period is relatively stable. In addition, they also report that price dynamics is more responsive to bad news than it is to the good news (similar to the findings of Bouoiyour and Selmi 2015 ).
Post any economic or financial crash, there is widespread panic among the investors, and their opinions about the market take a back seat. A feared investor sentiment is not good for the market progression as it can further deteriorate the conditions. The investor sentiment does affect the volatility of Bitcoin and has been empirically examined by Bukovina and Martiček ( 2016 ) for the period 2013–2015, i.e., right after the crash of 2013. They find that the impact of sentiment is higher during the periods of high volatility and bubble period especially, for positive sentiment. Bariviera et al. ( 2017 ) makes a comparison of Bitcoin with standard currencies and focuses on the analysis of returns from 2011 to 2017. Their study shows that Bitcoin faces large volatility. However, the volatility is getting reduced and stabilized over time. A similar observation is made by Kayal and Balasubramanian ( 2021 ) in a very recent study.
In another comparative analysis against standard currencies, Baur and Dimplf ( 2017 ) examine the volatility of Bitcoin and find it to be thirty times more volatile than other currencies (US dollars, Euro and Yen). They declare Bitcoin as unfit to be used as currency since the high volatility feature adversely affects its store of the value property. But since Bitcoin is characterized by historical price movements and expected future returns, it can still act as an investment instrument. In a comparison with major currency exchange, Pichl and Kaizoji ( 2017 ) find Bitcoin prices to be more volatile than EUR-USD exchange. They also highlight that Bitcoin experience a higher number of bubbles and crashes.
Balcilar et al. ( 2017 ) analyse the causal relationship between trading volume and Bitcoin return and volatility for the period of 2011 and 2016 by incorporating structural breaks as well as non-linearity in prices. The study demonstrates that the volume can predict the returns when the market is in normal mode i.e., around the median of the conditional distribution. But it, cannot predict the volatility. However, when the market is bullish or bearish i.e., either performing too bad or too good, the volume has no role in predicting even the returns. In this case, it can be better predicted by past lags of returns. A similar observation was made in a recent work by Aalborg et al. ( 2019 ). They observe trading volume partly explain the volatility.
A few studies claim that the crash of 2013 was due to a bundle of reason such as a negative sentiment among investors, speculative trading, suspicious trading, etc. Blau ( 2017 ), however, finds that speculative trading was not a reason behind the crash of 2013 and neither was it responsible for the high volatility of the Bitcoin price during the period. Contrary to many other researchers, the author dispute that there was no existence of a bubble and that Bitcoin could be viable as a currency rather than just being a speculative instrument.
By now, we know that Bitcoin is a sensitive instrument. Its price can fluctuate and tends to be highly volatile. The price crash of 2013 hints at the possibility of the presence of bubbles in the Bitcoin market which eventually came down crashing. A quite a few academicians believe that Bitcoin is nothing but a mere bubble. The price crash of 2013 was simply a foretaste of what lies ahead. Remember the housing market bubble and its consequences? The Bitcoin market might be on its path to face a similar bitter end. Given that it is establishing and gaining popularity among investors especially the newbies, a bubble burst will be shattering. Here are a few pieces of evidence from the literature for and against the claim of the Bitcoin market being a bubble.
The role of social interactions in creating price bubbles in the Bitcoin market has been analysed by Garcia et al. ( 2014 ). The study uses tries to quantify and analyse four socio-economic signals: price on online exchanges, volume of word-of-mouth communication in online social media, the volume of information search, and user base growth. They document that the word of mouth and expanding Bitcoin user base are significant influent on the existence of a pricing bubble. This could be explained as follows: the media reports a price increase which further triggers search activities among investors. It increases investors’ interest to buy Bitcoin thereby increasing the demand and finally resulting in a surge price. This attracts new investors thus increasing the user base. Another important finding is that user search activity responds more to negative shocks (bad news). Such a search spike can result in a price drop as well. A similar claim is made by Gronwald ( 2019 ) as the author explains the movements in Bitcoin prices by demand shocks for Bitcoin. A study by Cheah and Fry ( 2015 ) finds that the ‘Bitcoin prices do exhibit rich and volatile market dynamics and that the Bitcoin market is subjected to speculative bubbles for the period between 2010 and 2014. The studies identify that a bubble existed just before the crash of 2013. This was contradicted by the findings of Blau ( 2017 ). Another confirmation of the existence of bubbles during 2013 was done by Cheung et al. ( 2015 ). The study uses the Phillips et al. ( 2013 ) method which is robust in detecting bubbles to find evidence of a number of bubbles (a combination of short-lived and long-lasting). Using a similar method, Corbett et al. ( 2018 ) also examine the existence of a price bubble in the Bitcoin market as well as attempt to date stamp them. They analyse the data using an ex ante method which detects exuberance in asset price series during periods of inflation. Further, they develop a dating strategy to identify the origination and termination points of bubbles using backward regression techniques. As per the finding’s Bitcoin does show bubble behavior around the turning point of 2013/14. However, the method fails to prove the much recent price breakouts (around 2017) to be a bubble. A similar observation was made in recent work by Chaim and Laurini ( 2019 ) as they claim Bitcoin was in the bubble phase during early 2013 to mid-2014, but, interestingly, not in late 2017. In a recent work, Geuder et al. ( 2019 ) examine the Bitcoin prices during the years 2016–2018. They underline that Bitcoin’s bubble behavior is common and reoccurring. However, they also did not find any evidence of bubble behaviour after the time point December 6, 2017. Further, Cagli ( 2019 ) observes Bitcoin along with other cryptocurrencies to exhibit explosive behaviour.
Fry and Cheah ( 2016 ) incorporate econophysics to build models for financial bubbles and crashes using statistical physics and mathematical finance. The study asserts evidence of a negative bubble around the year 2014 in the largest cryptocurrency markets, i.e., Bitcoin and Ripple. It also finds a very evident spillover from the Ripple to Bitcoin which is held responsible for the recent price falls of Bitcoin. This has highlighted the concern over the stiff competition among cryptocurrencies especially for Bitcoin from more flexible options available in markets. In another study, Fry ( 2018 ) builds rationale models for Bitcoin and other cryptocurrencies to incorporate heavy tail probabilities and the probability of a complete collapse in asset prices. The model is based on the assumption that the intrinsic rate of return and the intrinsic level of risk are asymptotically constant. The study also exhibits a link between number of Google searches and degree of speculation using Google Relative Search Trends. Further, the author observes that the number of searches declines as the bubble nears its end.
Economics and efficiency of Bitcoin
Bitcoin has presented itself as a highly innovative and attractive digital currency. A major difficulty among new users or researchers is to understand its design aspects and the economics behind its existence. It has only been a decade since it came into existence. There is extensive literature trying to examine whether it is an efficient means of payment.
First, it is important to understand the economics behind Bitcoin. This virtual currency has absolutely no central backing or point of trust. Its feature of being decentralized attracts individuals who want a “freely traded currency” and stay away from any intermediators such as the bank, or the government (Barber et al. 2012 ; Bohme et al. 2015 ). Both these papers outline a few more similarities such as the incentivized system of Bitcoin, fixed or predictable supply and anonymity. The fixed supply of Bitcoin as noted before is due to its creation through mathematical algorithms. However, fixed, supply poses some major problems which can create an imbalance in the macroeconomy if the transaction volume increases indefinitely. Further, Bitcoin may have a fixed supply but the digital currency market is not fixed overall. There are more than two thousand digital currencies available to date and new currencies are getting launched often.
As noted by Yermack ( 2015 ) and Ali et al. ( 2014 ), a fixed supply will lead to deflation which will, in turn, lead to high welfare destroying volatility. It will be a difficult task indeed to match the variation in demand. Ali et al. ( 2014 ) propose that a more flexible system is required to respond to varying demands. One way is to have an adjustable growth rate of currency supply and another is a decentralized voting mechanism. While some researchers predict a possibility of deflation, Lo and Wang ( 2014 ) throw light on a possible scenario of hyperinflation if the central bank chooses to oversupply currency. These possible scenarios of deflation and inflation are ruled out by Iwamura et al. ( 2014b ) as they argue that fixed supply will only negatively impact the profitability of mining activity but not lead to a deflation-like situation.
Although the supply of Bitcoin is fixed and will converge to a finite limit, it is indestructible. Evans ( 2014 ) refers to Bitcoin and other digital currencies as a long-lived asset of which the value at any point in time reflects its expectations about the future value and a change in this expectation can change the value further. The study also claims that even though Bitcoin appears as highly volatile in the short-run, it will stabilize over a longer period of time. The participants collect more information about demand and supply as well as utility being derived from the Bitcoin use and hence become more efficient. This has been further emphasized by Dwyer ( 2015 ) and also confirms the existence of equilibrium in the Bitcoin market to agree with the theoretical results of Marimon et al. (2012). The theoretical framework by Dwyer ( 2015 ) lists out the demand and supply factors of Bitcoin in a structured way and, its usage in terms of exchangeability with goods and services in comparison with its competing currencies. The determination of equilibrium has been of keen interest among the scholars and as seen earlier, it is constructed on varying factors of the market. Another such model constructed by Chiu and Koeppl ( 2017 ) gives more holistic requirements for an equilibrium to exist in the Bitcoin market. They scheme the conditions to be inclusive of buyer’s utility and miner’s utility and prove that the equilibrium exists when there is a sufficiently large number of buyers. Schilling and Uhlig ( 2019 ) construct an equilibrium state and find that the Central banks via appropriate monetary policies keep the value of Dollar constant and the production/supply of Bitcoin is by default decentralized through its algorithmic mechanism.
How well, the Bitcoin market follows key economic principles to determine the efficiency of the same. Most of the literature has established that the Bitcoin market is volatile due to the fact it is in its nascent stage. In the longer run, the price stabilizes, volatilities dampen and the existence of bubble diminishes. The entire Bitcoin transaction mechanism is based on blockchain technology and more or less every form of technology evolves over time to get better. A direct implication of this noted by Li and Wang ( 2017 ) is that the mining technology gets better in the long run thereby reducing the mining difficulty and making the entire process more efficient. An older study by Houy ( 2014 ) analyses the economics of Bitcoin transaction fees and finds that efficiency is enhanced by implementing transaction fee and limited block size in mining.
Apart from understanding the economics of Bitcoin for analysing its efficiency, it is also important for formulating the underlying guidelines of financial reporting of Bitcoin transactions. Although Tan and Low ( 2017 ) find that Bitcoin transaction requires no new accounting principle but requires an authoritative interpretation of existing accounting principles with respect to Bitcoin.
The existing literature also highlights the inefficiency aspect of Bitcoin. A two-period analysis by Urquhart ( 2016 ) displays that the Bitcoin market is an overall inefficient market but, on its path, to become an efficient one as it matures over the longer run. A similar result is obtained by Bariviera ( 2017 ), Kurihara and Fukushima ( 2017 ), Nadarajah and Chu ( 2017 ) and Sensoy ( 2019 ).
Bitcoin as currency vs asset
The blockchain technology makes every transaction of Bitcoin safe, verified and almost anonymous. Bitcoin is unregulated and the supply is predictable due to the application of a mathematical algorithm. The user base has expanded since its inception and has a long way to go (given it survives all the hardships). Any financial instrument can have two fundamental uses: a store of value and a medium of exchange. The question arises which of the two dominates Bitcoin. The “Asset vs Currency” debate has been a hot topic of discussion among scholars. Since the Bitcoin price is characterized by high price fluctuations, users may use it only as a speculative instrument. Users can buy Bitcoins and sell them when the exchange rate rises and thereby earning high returns.
Before formerly answering whether Bitcoin is used as an asset or currency, it is imperative to learn whether existing research has given it a green signal to be qualified as an uncompromising currency. The ability of Bitcoins to avoid the interference brought by banks, centralized payment systems and governments has been discussed by Maurer et al. ( 2013 ) as they refer to two main features of “privatisation” of identities that transacting Bitcoins and its “digital metallism." To them, Bitcoin also promises solidity, materiality, stability, anonymity, and community. The “proof-of-work” principle has been scrutinized by Becker et al. ( 2013 ) and points out that Bitcoin payment system is secure when no single party controls more than 50% of the network's computing power. It does criticize that electricity consumption needed to carry out high computational problem leaves behind a carbon footprint.
Carrick ( 2016 ) considers Bitcoin as highly effective for transactions and can be used in conjunction with fiat currencies i.e., it is not a substitute but a compliment. Bitcoin can be balanced out between being a medium of exchange and a speculative instrument depending upon the risk-holding capacity of users (Luther and White 2014 ). Bitcoin has been referred to as gold in a virtual environment by Rogojanu and Badea ( 2014 ). They have listed the advantages and disadvantages of Bitcoin keeping in mind the ideal properties of a currency given by the Nobel Prize Laureate FA Hayek. The advantages of Bitcoin include tie savings, business flexibility, cost minimisation, avoids third-party commissions, does not generate inflation, the anonymity of traders, and escapes central intervention. The major disadvantages subsume extreme volatility of price, uncontrolled transaction, large speculative attacks that can cause negative effects, limited confidence in Bitcoin, and increased vulnerability of cyber theft. Resembling pros and cons have been quested by Richter et al. ( 2015 ) and Lo and Wang ( 2014 ). They address the shortcomings in the huge hard drive space required to store the entire blockchain and the resource cost of mining constantly growing. Legitimacy issues of the Bitcoin financial system have been discussed in workings of Weber ( 2014 ) which draws attention to the failure of Bitcoin to fulfill multifunctionality of currency and stability. In another allied study by Weber ( 2014 ), the author recommends the need to create a mass demand for Bitcoin to have a parallel economy and later serve the instability and deflationary pressure issues. Testing against standard definitions of money, Kubat ( 2015 ) does not pass Bitcoin to be an alternative currency and asserts that it cannot function as a store value of money. Further, Bjerg ( 2016 ) hails Bitcoin as a digital Ponzi scheme down the road if it fails to prove itself as cheap, efficient, ingenious, democratic, and a stable payment system. Findings in agreement with the above have been enumerated in the workings of Ciaian et al. ( 2016 ) and Luther ( 2016 ).
A few characteristics of Bitcoin which make it a good currency substitute are discussed by Chowdhury and Mendelson ( 2013 ). Bitcoin has a lower transaction cost and is an inexpensive fund transfer system. It allows remittances at less cost (Folkinshteyn et al. 2015 ) and helps improve access to financial services. Moreover, it has no strict capital controls and no intervention by a central authority, thus no scope of reversal of transactions. However, it lacks liquidity and yet to achieve a widespread user base. Being decentralized, there is absolutely no guarantee of any help or resort in case of a failure and is thereby difficult to safeguard it from various types of risk. It is such drawbacks that limit people from using it as a hardcore currency. The economic rationales are required in the long run for bitcoin to become a currency or asset (Iwamura et al. 2014a ; b ).
New users generally do not intend to spend Bitcoin currency for buying goods and services as indicated in studies by Glaser et al. ( 2014 ) and is instead more widely used as speculative instruments (Kajtazia and Moro 2019 ). This finding is further supported by Baur et al. ( 2016 , 2018 ) The latter study analyses the statistical property's Bitcoin and compares it with other assets such as stocks, commodities, and bonds in both financially stable and unstable periods. The author finds Bitcoin to be uncorrelated with other traditional assets. It is mostly held by users for speculative and investment purposes.
This leads to another interesting insight. If the majority of users use it for speculative purposes, can there be the one influencing the Bitcoin price and returns? This has been examined by Baek and Elbeck ( 2015 ). They show that the returns are independent of external economic factors. It is the market participants that internally drive the market returns which make it a front runner as a speculative instrument.
What drives users to invest in Bitcoin or use it as a speculative instrument? It is highly volatile and some argue it to be a bubble and yet there is a huge rise in the number of users. A finance principle will tell us that high volatility implies high returns. Brière et al. ( 2015 ) advocate that high risk needs to be compensated by high returns. They also find that Bitcoin is weakly correlated with other assets. Bouri et al. ( 2017 ) define a diversifier as one, which has a weak positive correlation with other assets such as gold. Thus, the inclusion of Bitcoin in the portfolio makes it well diversified. In a more detailed work, Bedi and Nashier ( 2020 ) examine Bitcoin’s investment credentials through a cross-currency analysis. They find that Bitcoin exhibits striking variation in terms of the diversification benefits. While analysing Bitcoin price behaviour during the recent coronavirus pandemic, Conlon and McGee ( 2020 ) find Bitcoin substantially increases portfolio downside risk, especially during market turbulence.
Apart from being useful as a diversifying instrument, it is also utilized as a hedging device. Gold is a common hedging instrument used widely and Bitcoin is often studied compared to the same, in terms of hedging and volatility. The hedging capabilities of Bitcoin have been analysed by Dyhrberg ( 2016b ) against Financial Times Stock Exchange Index and the American dollar. This study indicates that Bitcoin can find a good spot in the list of assets used for hedging. In a recent study, Akhtaruzzaman et al. ( 2020 ) observe that Bitcoin can be used to hedge the risk against industry sectors and bond index. Pal and Mitra ( 2019 ) also examine Bitcoin’s hedging effectiveness. Adding further to the literature, Fang et al. ( 2019 ) notice that Bitcoin’s hedging effectiveness against global equities and global bonds becomes prominent if we consider the level of global economic policy uncertainty. Further, Bitcoin derivative instruments are also found to be hedging effective (Alexander et al. 2020 ). In contrast, Beneki et al. ( 2019 ) argue that Bitcoin’s hedging abilities have reduced significantly over the recent periods due to increased policy uncertainty. In contrast, Chan et al. ( 2019 ) find Bitcoin to be an effective strong dynamic hedge using frequency dependence approach on monthly data for the period of 2010–2017.
Bitcoin has also been assessed against energy commodities by Bouri et al. ( 2017 ) since electricity is a key input in Bitcoin transactions and can be expected to have different results from non-energy commodities such as gold. The analysis is done in two sub-periods (before and after the 2013 crash) comparing Bitcoin index with energy and non-energy commodity indices. They find that Bitcoin shows hedging as well as safe-haven capabilities for both the indices for the entire period and before the crash period but only as a diversifier in the post-crash period. The literature proposes that Bitcoin is an attractive investment choice especially, in diversification and hedging. Recent work like Giudici and Abu-Hashish ( 2019 ), Symitsia and Chalvatzis ( 2019 ), Kilber et al. ( 2019 ), Urquhart and Zhang ( 2019 ), and Wang Et Al. ( 2019 ) also confirm the same. Symitsi and Chalvatzis ( 2018 ) observe the presence of spillover effects from Bitcoin to energy-technology companies. The study also detects bi-directional asymmetric shock spillovers. In the short run, there is a spillover from technology stocks to Bitcoin while in the longer run Bitcoin volatility affects the energy companies. The investible feature is confirmed by Dyhrberg et al. ( 2018 ) in their analysis of transaction cost and intraday trading patterns on the Bitcoin-USD exchange rate. The findings are especially in favour of retail size traders. A very recent study by Zeng et al. ( 2020 ) further observes spillover effects (asymmetric) between Bitcoin and conventional assets.
- Social media and investor sentiment
Unlike our usual currency, Bitcoin is not driven by any central monetary authority. It is free from regulatory norms and has no government intervention. Instead, the Bitcoin market is user-driven. It has a value till the users think it has some worth or can be converted to currency at a higher return. Thus, it can be said to be driven by future expectations of the Bitcoin holders and future investors. As noted in previous sections, the Bitcoin supply and demand are independent of macroeconomic factors in contrast to standard currencies. The supply is fixed or controlled by mathematical algorithm and demand depends on the future expectations on Bitcoin returns. Bitcoin being a digital currency cannot be isolated from the protagonist of its existence: the internet. In this digital era, an individual feeds his curiosity through web browsing as it gives an instant answer. A high interest in Bitcoin could imply enormous web searches on the internet and this interest influences the expectations of users and thereby the Bitcoin price (or demand and supply). This is a slippery road as a rational person will react positively to good news and negatively to a bad one. Thus, a piece of false or fake news can blow out easily and thereby causing unrest in the Bitcoin market.
Academicians have analyzed the role of media, social media and internet in driving the Bitcoin price or causing high volatility in the market. A bidirectional relationship between web searches (Google and Wikipedia) and prices of Bitcoin was examined by Kristoufek ( 2013 ). The interest of users was captured by internet searches to find that web queries influenced prices and prices, in turn, influenced the number of searches. Another study incorporating Google searches done by Yelowitz and Wilson ( 2015 ) find that computer programming and illegal activity search terms are positively correlated with Bitcoin interest. A similar price analysis based on the popularity of Bitcoin was done by Polasik et al. ( 2015 ). The key determinant of this result is the tone score used in the sentiment analysis. It implies that if the tone of an article is on a praising note the prices are observed to be increasing while the tone is denouncing the prices went down. The findings also present that the return increased as Google searches increased and when the number of articles on Bitcoin in the newspaper appeared more frequently. Contributions of impacts of social media on the Bitcoin market are more broadly analyzed by Feng Mai et al. ( 2015 ). They believe that social media can provide key insights into the general public’s acceptance of Bitcoin. The study observes that a bullish post predicts positive returns, and a bearish post predicts negative returns. A higher disagreement among the public reflected in the comments leads to a higher exchange trading volume. Further, transaction volumes can be predicted using the messages and comments posted online. Garcia and Schweitzer ( 2015 ) highlight the scope of profit-making through social media signals by combining statistical analysis and back setting server. The study uses the framework to incorporate Google searches, Twitter feeds and opinion polarization (to echo the emotions), and opinions to predict financial returns and derive large profits. Dastgir et al. ( 2019 ) observe a bi-directional causal relationship between Bitcoin attention (measured by the Google Trends search queries) and Bitcoin returns. Shen et al. ( 2019 ) find the number of tweets to be a significant driver of Bitcoin’s trading volume and realized volatility.
The application of users’ comment analysis is also validated by Kim et al. ( 2016 ) to predict future prices and the effective number of transactions among users. In another analysis by Kim et al. ( 2017 ), a model based on deep learning was developed to predict Bitcoin count and prices. Twitter has been interpreted as a ‘virtual trading floor that emotionally reflects Bitcoin’s market movement’ in a study by Kaminski ( 2014 ). The study applies static Pearson correlation methods and Granger causality test to a possible relationship between Twitter posts and Bitcoin market indicators. While the former confirms a moderate correlation, the latter test rejects any statistical significance for Twitter signals as a predictor of Bitcoin market indicators. A link between the number of Google searches and the degree of speculation using Google Relative Search Trends was specified by Fry ( 2018 ). A recent study by Chen et al. ( 2020 ) focuses on the fear sentiment caused by the coronavirus pandemic. They find Bitcoin to be impacted negatively as returns fell with high trading volume during the pandemic. Further, they argue that Bitcoin does not act as a safe haven during the pandemic. Other similar works like Shahzad et al. ( 2019 ), Smales ( 2019 ), Shahzad et al. ( 2020 ), and Wu et al. ( 2019 ) draw similar conclusions as they find Bitcoin is not a strong safe-haven instrument.
Regulation, legality and cybercrime
Bitcoin works on a decentralized mechanism that allows it to be isolated from any intervention by a regulatory authority. It does overcome the difficulty of transport and storage compared to standard currency. However, the latter still carries more liquidity and trust among people. Bitcoin has been endorsed to be anonymous in terms of the identity of users and each transaction is irreversible (Bradbury, 2013 ). The literature, however, demonstrates that Bitcoin is not completely but anonymous as Bitcoin transaction history is freely available when one joins the peer-to-peer network to ensure the prevention of double-spending. This has been explained by Reid and Harrigan ( 2013 ) also. They point this out along with other features such as several inputs and outputs and multiple public keys. Further scrutiny of the network structure used in Bitcoin transactions has led them to conclude that there is a possibility of associating several public keys with each other and thus arising a possibility of knowing the activities of users. This semi-anonymous feature is supported in a study by Androulaki et al. ( 2013 ) that analyses the genuineness of Bitcoin system by simulating in a university setting to mimic its working with real-world scenarios. It uses the adversarial model and behavior-based clustering techniques to find that it is possible to unveil up to 40% of Bitcoin user profiles. A solution to this quasi, anonymity has been given by Bonneau et al. ( 2014 ) wherein they propose a concept of “Mixcoin” to achieve cryptographic accountability, randomized mixing fees, and an adaptation of mix networks to Bitcoin. The Bitcoin exchange and its associated risk have been mentioned in studies of Moore and Christin ( 2013 ) and Li and Wang ( 2017 ). It is evident that investors face a massive exchange rate-related risk and users with a criminal intention will try to avoid popular exchanges as they are more scrutinized.
Anonymity feature has led this nascent currency in the world of the dark web in recent times and help criminals carry out illegal activities and money laundering. The infamous Silk Road incident was one such evidence. The findings of Stokes ( 2012 ), Moser et al. ( 2013 ), Meiklejohn et al. ( 2013 ), and Blundell-Wignalli ( 2014 ) recognize the threat of money laundering which comes as a ramification of Bitcoin design and can attract criminals. A common proposition that finds a place in all these papers is to enhance the transaction-related regulations to curb money laundering and criminal activities. All the papers recommend not to put a complete ban on Bitcoin as that could hinder the technological advancement in this IoT era. The standing of imposing regulations has been mentioned by Brito et al. ( 2014 ) and Pieters and Vivanco ( 2017 ) in their studies. Brito et al. ( 2014 ) while focusing on the legal treatment of traditional securities and Bitcoin-backed derivatives, conclude that financial regulators should consider excluding certain Bitcoin-related financial transactions from regulatory eye watch. They should rather come with measures to enhance resilience and adaptation. Similarly, Pieters and Vivanco ( 2017 ) conclude that standard financial regulations can have a quite significant impact on the Bitcoin market. Risk model used in the study by Moser et al. ( 2014 ) helps predict the risk of a transaction getting blacklisted by spotting a bad transaction using an open-source knowledge base of the Bitcoin blockchain. This information is available to be overserved by all participants thus making the system more transparent.
There has been repeated mention of two major incidents that portray the downsides, and the immense risk associated with the Bitcoin system: the crash of Mt. Gox and The Silk Road episode. These two have been discussed at length by Trautman ( 2014 ) and are valuable a read to get a grasp of these infamous events. Given that the Bitcoin system has managed to be in constant news for defaulting, whether as a bursting bubble or dodging illicit activities, a few major countries have decided to prohibit this cryptocurrency. A Government has the power to prevent an ancillary currency in countries’ economy if it enforces severe penalties (Hendrickson and Luther, 2017 ). Therefore, the government should lead public expectation to keep the confidence to authority and help in reducing the speculation behavior of Bitcoin (and other cryptocurrencies) to stabilize the market (Li et al. 2019 ).
Bitcoin is thus still in an embryonic phase and needs to evolve with time especially keeping in pace with technological advancements. It should be robust to get accepted as an alternative currency and be able to prevent any fraudulent exploitation.
This paper systematized the growing research on Bitcoin published. It has segmented the publications on the basis of various elements of economics and finance such as price, demand and supply, market efficiency, volatility and returns, and investment prospects and regulatory aspects. It also highlights the impact of social media on these factors.
There are a few limitations to this paper. Our study only takes into account research from economics and finance perspective. Technological aspects such as blockchain and mining strategies are not considered. The focus is only on Bitcoin. There are other cryptocurrencies that are merging in the digital market. This study can be extended later to take them into account. The study might have missed out on a few important publications for the reason that it cannot be analyzed from Economics and Finance point of view. Another reason being that new studies keep emerging very frequently and some will become available later.
Based on our research, we enumerate a few observations and recommendations on the basis of gaps observed in the existing research. We notice that most of the findings are methodology and data frequency-dependent. Variations in the results are also observed due to differences in time periods and lack of longer periods of data. Therefore, an exhaustive study can be undertaken to discuss the limitations of different methodologies and its’ implications on the results. There are very few country-specific studies conducted. Further analysis can be directed to incorporate geographical boundaries and differences in Bitcoin behavior across them (if any). Regulation norms also vary across different countries and can be analysed. Research focusing on regulatory and legality aspects fails to suggest suitable solutions to make Bitcoin a safer and widely acceptable cryptocurrency to avoid illegal activities. The application of Bitcoin (or cryptocurrency) for the upliftment of economies and financial inclusion needs more exploration. The market for Bitcoin is very dynamic. The prices see a swift change and hence the intrinsic value also changes. Thus, the research needs to be timely updated to analyse the changing trends. The study can be extended to include other areas such as technical aspects and also take in other emerging cryptocurrencies.
Data availability statement
This study does not use any data. This work is a survey of academic research papers. All the papers used in this work are summarized in tables.
Aalborg HA, Molnár P, de Vries JE (2019) What can explain the price, volatility and trading volume of Bitcoin? Financ Res Lett 29:255–265
Article Google Scholar
Akhtaruzzaman M, Sensoy A, Corbet S (2020) The influence of bitcoin on portfolio diversification and design. Financ Res Lett 37:101344
Alexander C, Choi J, Park H, Sohn S (2020) BitMEX bitcoin derivatives: Price discovery, informational efficiency, and hedging effectiveness. J Futur Mark 40(1):23–43
Ali R, Barrdear J, Clews R, Southgate J (2014) The economics of digital currencies. Bank Engl Q Bull 54:276–286
Androulaki E, Karame GO, Roeschlin M, Scherer T, Capkun S (2013) Evaluating user privacy in Bitcoin. International conference on financial cryptography and data security. Springer, Berlin, pp 34–51
Chapter Google Scholar
Athey S, Parashkevov I, Sarukkai V, Xia J (2016) Bitcoin pricing, adoption, and usage: theory and evidence (No. 17- 033). IDEAS working paper series from RePEc. St. Louis. Retrieved from https://papers.ssrn.com/sol3/papers.cfm?abstract_id=2826674
Aysan AF, Demir E, Gozgor G, Lau CKM (2019) Effects of the geopolitical risks on Bitcoin returns and volatility. Res Int Bus Financ 47:511–518
Baek C, Elbeck M (2015) Bitcoins as an investment or speculative vehicle? A first look. Appl Econ Lett 22(1):30–34
Balcilar M, Bouri E, Gupta R, Roubaud D (2017) Can volume predict Bitcoin returns and volatility? A quantiles-based approach. Econ Model 64:74–81
Barber S, Boyen X, Shi E, Uzun E (2012) Bitter to better—how to make Bitcoin a better currency. International conference on financial cryptography and data security. Springer, Berlin, pp 399–414
Bariviera AF (2017) The inefficiency of Bitcoin revisited: a dynamic approach. Econ Lett 161:1–4
Bariviera AF, Basgall MJ, Hasperué W, Naiouf M (2017) Some stylized facts of the Bitcoin market. Phys A 484:82–90
Baur DG, Dimpfl T (2017) Realized Bitcoin volatility. SSRN 2949754:1–26
Google Scholar
Baur DG, Dimpfl T (2019) Price discovery in bitcoin spot or futures? J Futur Mark 39(7):803–817
Baur DG, Hong KJ, Lee AD (2016) Bitcoin–currency or asset? Melbourne Business School. SSRN J. https://doi.org/10.2139/ssrn.2561183
Baur DG, Hong K, Lee AD (2018) Bitcoin: medium of exchange or speculative assets? J Int Finan Markets Inst Money 54:177–189
Becker J, Breuker D, Heide T, Holler J, Rauer HP, Böhme R (2013) Can we afford integrity by proof-of-work? Scenarios inspired by the Bitcoin currency. The economics of information security and privacy. Springer, Berlin, pp 135–156
Bedi P, Nashier T (2020) On the investment credentials of bitcoin: a cross-currency perspective. Res Int Bus Financ 51:101087
Beneki C, Koulis A, Kyriazis NA, Papadamou S (2019) Investigating volatility transmission and hedging properties between Bitcoin and Ethereum. Res Int Bus Financ 48:219–227
Bjerg O (2016) How is Bitcoin money? Theory Cult Soc 33(1):53–72
Blau BM (2017) Price dynamics and speculative trading in Bitcoin. Res Int Bus Financ 41:493–499
Blundell-Wignall A (2014) The Bitcoin question: currency versus trust-less transfer technology (No. 37). OECD Publishing, Paris
Böhme R, Christin N, Edelman B, Moore T (2015) Bitcoin: economics, technology, and governance. J Econ Perspect 29(2):213–238
Bonneau J, Narayanan A, Miller A, Clark J, Kroll JA, Felten EW (2014) Mixcoin: anonymity for Bitcoin with accountable mixes. International conference on financial cryptography and data security. Springer, Berlin, pp 486–504
Bouoiyour J, Selmi R (2015) Bitcoin price: is it really that new round of volatility can be on way? Technical report, CATT, University of Pau, France, ESC, Tunis Business School of Tunis, Tunisia, mPRA Paper No. 65580. http://mpra.ub.unimuenchen.de/65580/
Bouoiyour J, Selmi R (2016) Bitcoin: a beginning of a new phase. Econ Bull 36(3):1430–1440
Bouoiyour J, Selmi R, Tiwari AK, Olayeni OR (2016) What drives Bitcoin price. Econ Bull 36(2):843–850
Bouri E, Azzi G, Dyhrberg AH (2016) On the return-volatility relationship in the Bitcoin market around the price crash of 2013. SSRN J. https://doi.org/10.2139/ssrn.2869855
Bouri E, Jalkh N, Molnár P, Roubaud D (2017) Bitcoin for energy commodities before and after the December 2013 crash: diversifier, hedge or safe haven? Appl Econ 49(50):5063–5073
Bouri E, Shahzad SJH, Roubaud D, Kristoufek L, Lucey B (2020) Bitcoin, gold, and commodities as safe havens for stocks: new insight through wavelet analysis. Q Rev Econ Financ 77:156–164
Bradbury D (2013) The problem with Bitcoin. Comput Fraud Sec 2013(11):5–8
Brandvold M, Molnár P, Vagstad K, Valstad OCA (2015) Price discovery on Bitcoin exchanges. J Int Finan Markets Inst Money 36:18–35
Briere M, Oosterlinck K, Szafarz A (2015) Virtual currency, tangible return: portfolio diversification with Bitcoin. J Asset Manag 16(6):365–373
Brito J, Shadab HB, Castillo O’Sullivan A (2014) Bitcoin financial regulation: Securities, derivatives, prediction markets, and gambling. Columbia Science and Technology Law Review. SSRN J. https://doi.org/10.2139/ssrn.2423461
Bukovina J, Marticek M (2016) Sentiment and Bitcoin volatility (No. 2016-58). Mendel University in Brno, Faculty of Business and Economics.
Cagli EC (2019) Explosive behavior in the prices of Bitcoin and altcoins. Financ Res Lett 29:398–403
Carrick J (2016) Bitcoin as a complement to emerging market currencies. Emerg Mark Financ Trade 52(10):2321–2334
Chaim P, Laurini MP (2019) Is Bitcoin a bubble? Phys A 517:222–232
Chan WH, Le M, Wu YW (2019) Holding Bitcoin longer: the dynamic hedging abilities of Bitcoin. Q Rev Econ Financ 71:107–113
Cheah ET, Fry J (2015) Speculative bubbles in Bitcoin markets? An empirical investigation into the fundamental value of Bitcoin. Econ Lett 130:32–36
Chen C, Liu L, Zhao N (2020) Fear sentiment, uncertainty, and bitcoin price dynamics: The case of COVID-19. Emerg Mark Financ Trade 56(10):2298–2309
Cheung A, Roca E, Su JJ (2015) Crypto-currency bubbles: an application of the Phillips–Shi–Yu (2013) methodology on Mt. Gox Bitcoin Prices. Appl Econ 47(23):2348–2358
Chevapatrakul T, Mascia DV (2019) Detecting overreaction in the Bitcoin market: a quantile autoregression approach. Financ Res Lett 30:371–377
Chiu J, Koeppl TV (2017) The economics of cryptocurrencies–Bitcoin and beyond. SSRN J. https://doi.org/10.2139/ssrn.3048124
Chowdhury A, Mendelson BK (2013) Virtual currency and the financial system: the case of Bitcoin (No. 2013–09). Marquette University, Center for Global and Economic Studies and Department of Economics.
Ciaian P, Rajcaniova M (2016) The digital agenda of virtual currencies: Can Bitcoin become a global currency? ISEB 14(4):883–919
Ciaian P, Rajcaniova M, Kancs DA (2016) The economics of Bitcoin price formation. Appl Econ 48(19):1799–1815
Conlon T, McGee R (2020) Safe haven or risky hazard? Bitcoin during the COVID-19 bear market. Financ Res Lett 35:101607
Corbet S, Lucey B, Yarovaya L (2018) Datestamping the Bitcoin and Ethereum bubbles. Financ Res Lett 26:81–88
Dastgir S, Demir E, Downing G, Gozgor G, Lau CKM (2019) The causal relationship between Bitcoin attention and Bitcoin returns: evidence from the Copula-based Granger causality test. Financ Res Lett 28:160–164
Dwyer GP (2015) The economics of Bitcoin and similar private digital currencies. J Financ Stab 17:81–91
Dyhrberg AH (2016a) Bitcoin, gold and the dollar–A GARCH volatility analysis. Financ Res Lett 16:85–92
Dyhrberg AH (2016b) Hedging capabilities of Bitcoin. Is it the virtual gold? Financ Res Lett 16:139–144
Dyhrberg AH, Foley S, Svec J (2018) How investible is Bitcoin? Analyzing the liquidity and transaction costs of Bitcoin markets. Econ Lett 171:140–143
El Alaoui M, Bouri E, Roubaud D (2019) Bitcoin price–volume: a multifractal cross-correlation approach. Financ Res Lett. https://doi.org/10.1016/j.frl.2018.12.011
Eross A, McGroarty F, Urquhart A, Wolfe S (2019) The intraday dynamics of bitcoin. Res Int Bus Financ 49:71–81
Evans DS (2014) Economic aspects of Bitcoin and other decentralized public-ledger currency platforms. SSRN J. https://doi.org/10.2139/ssrn.2424516
Fang L, Bouri E, Gupta R, Roubaud D (2019) Does global economic uncertainty matter for the volatility and hedging effectiveness of Bitcoin? Int Rev Financ Anal 61:29–36
Folkinshteyn, D., Lennon, M. M., & Reilly, T. (2015). The Bitcoin mirage: an oasis of financial remittance. J Strateg Int Stud Forthcom
Fry J (2018) Booms, busts and heavy-tails: the story of Bitcoin and cryptocurrency markets? Econ Lett 171:225–229
Fry J, Cheah ET (2016) Negative bubbles and shocks in cryptocurrency markets. Int Rev Financ Anal 47:343–352
Gandal N, Hamrick JT, Moore T, Oberman T (2018) Price manipulation in the Bitcoin ecosystem. J Monet Econ 95:86–96
Garcia D, Schweitzer F (2015) Social signals and algorithmic trading of Bitcoin. R Soc Open Sci 2(9):150288
Garcia D, Tessone CJ, Mavrodiev P, Perony N (2014) The digital traces of bubbles: feedback cycles between socio-economic signals in the Bitcoin economy. J R Soc Interface 11(99):20140623
Geuder J, Kinateder H, Wagner NF (2019) Cryptocurrencies as financial bubbles: the case of Bitcoin. Financ Res Lett. https://doi.org/10.1016/j.frl.2018.11.011
Giudici P, Abu-Hashish I (2019) What determines bitcoin exchange prices? A network VAR approach. Financ Res Lett 28:309–318
Glaser F, Zimmermann K, Haferkorn M, Weber MC, Siering M (2014) Bitcoin-asset or currency? Revealing users' hidden intentions. In: Proceedings of the 22nd European conference on information systems (ECIS 2014); Tel Aviv, Israel
Griffin JM, Shams A (2020) Is Bitcoin really untethered? J Financ 75(4):1913–1964
Gronwald M (2019) Is Bitcoin a Commodity? On price jumps, demand shocks, and certainty of supply. J Int Money Financ 97:86–92
Hendrickson JR, Luther WJ (2017) Banning Bitcoin. J Econ Behav Organ 141:188–195
Houy N (2014) The economics of Bitcoin transaction fees. GATE WP, 1407, Groupe d’Analyse et de Théorie Economique, Lyon
Iwamura M, Kitamura Y, Matsumoto T (2014a) Is Bitcoin the only cryptocurrency in the town? Economics of cryptocurrency and Friedrich A. Hayek. SSRN J. https://doi.org/10.2139/ssrn.2405790
Iwamura M, Kitamura Y, Matsumoto T, Saito K (2014b) Can we stabilize the price of a cryptocurrency? Understanding the design of Bitcoin and its potential to compete with Central Bank money. SSRN J. https://doi.org/10.2139/ssrn.2519367
Kajtazi A, Moro A (2019) The role of bitcoin in well diversified portfolios: a comparative global study. Int Rev Financ Anal 61:143–157
Kaminski J (2014) Nowcasting the Bitcoin market with twitter signals. Cambridge. Retrieved from http://arxiv.org/abs/1406.7577
Kapar B, Olmo J (2019) An analysis of price discovery between Bitcoin futures and spot markets. Econ Lett 174:62–64
Kayal P, Balasubramanian G (2021) Excess volatility in bitcoin: extreme value volatility estimation. IIM Kozhikode Soc Manag Rev. https://doi.org/10.1177/2277975220987686
Kim YB, Kim JG, Kim W, Im JH, Kim TH, Kang SJ, Kim CH (2016) Predicting fluctuations in cryptocurrency transactions based on user comments and replies. PLoS ONE 11(8):e0161197
Kim YB, Lee J, Park N, Choo J, Kim JH, Kim CH (2017) When Bitcoin encounters information in an online forum: Using text mining to analyse user opinions and predict value fluctuation. PLoS ONE 12(5):e0177630
Kliber A, Marszałek P, Musiałkowska I, Świerczyńska K (2019) Bitcoin: safe haven, hedge or diversifier? Perception of bitcoin in the context of a country’s economic situation—a stochastic volatility approach. Phys A 524:246–257
Kristoufek L (2013) Bitcoin meets google trends and wikipedia: quantifying the relationship between phenomena of the Internet era. Sci Rep 3:3415
Kristoufek L (2015) What are the main drivers of the Bitcoin price? Evidence from wavelet coherence analysis. PLoS ONE 10(4):e0123923
Kubát M (2015) Virtual currency Bitcoin in the scope of money definition and store of value. Proced Econ Financ 30:409–416
Kurihara Y, Fukushima A (2017) The market efficiency of Bitcoin: a weekly anomaly perspective. J Appl Financ Bank 7(3):57
Klein T, Thu HP, Walther T (2018) Bitcoin is not the New Gold: a comparison of volatility, correlation, and portfolio performance. Int Rev Financ Anal 59:105–116
Li X, Wang CA (2017) The technology and economic determinants of cryptocurrency exchange rates: the case of Bitcoin. Decis Support Syst 95:49–60
Li ZZ, Tao R, Su CW, Lobonţ OR (2019) Does Bitcoin bubble burst? Qual Quant 53(1):91–105
Lo S, Wang JC (2014) Bitcoin as money? vol 14–4. Current Policy Perspectives, Boston
Luther WJ (2016) Bitcoin and the future of digital payments. Indep Rev 20(3):397–404
Luther WJ, White LH (2014) Can Bitcoin become a major currency? SSRN J. https://doi.org/10.2139/ssrn.2446604
Mai F, Bai Q, Shan J, Wang XS, Chiang RH (2015) The impacts of social media on Bitcoin performance. SSRN J. https://doi.org/10.2139/ssrn.2545957
Matkovskyy R, Jalan A (2019) From financial markets to Bitcoin markets: A fresh look at the contagion effect. Financ Res Lett 31:93–97
Marimon R, Nicolini JP, Teles P (2012) Money is an experience good: competition and trust in the private provision of money. J Monetary Econ 59(8):815–825
Maurer B, Nelms TC, Swartz L (2013) “When perhaps the real problem is money itself!”: the practical materiality of Bitcoin. Soc Semiot 23(2):261–277
Meiklejohn S, Pomarole M, Jordan G, Levchenko K, McCoy D, Voelker GM, Savage S (2013) A fistful of Bitcoins: characterizing payments among men with no names. In: Proceedings of the 2013 Conference on Internet Measurement Conference, ACM, pp 127–140
Moore T, Christin N (2013) Beware the middleman: Empirical analysis of Bitcoin-exchange risk. International conference on financial cryptography and data security. Springer, Berlin, pp 25–33
Möser M, Böhme R, Breuker D (2013) An inquiry into money laundering tools in the Bitcoin ecosystem. In: 2013 APWG eCrime Researchers Summit, IEEE, pp 1–14
Möser M, Böhme R, Breuker D (2014) Towards risk scoring of Bitcoin transactions. International conference on financial cryptography and data security. Springer, Berlin, pp 16–32
Nadarajah S, Chu J (2017) On the inefficiency of Bitcoin. Econ Lett 150:6–9
Nakamoto S (2008) Bitcoin: a peer-to-peer electronic cash system. http://bitcoin.org/bitcoin.pdf , Retrieved 21 Dec 2010
Phillips PC, Shi S, Yu J (2015) Testing for multiple bubbles: historical episodes of exuberance and collapse in the S&P 500. Int Econ Rev 56(4):1043–1078
Pagnottoni P, Dimpfl T (2019) Price discovery on Bitcoin markets. Digit Financ 1(1):139–161
Pal D, Mitra SK (2019) Hedging bitcoin with other financial assets. Financ Res Lett 30:30–36
Panagiotidis T, Stengos T, Vravosinos O (2019) The effects of markets, uncertainty and search intensity on bitcoin returns. Int Rev Financ Anal 63:220–242
Pichl L, Kaizoji T (2017) Volatility analysis of bitcoin. Quant Financ Econ 1:474–485
Pieters G, Vivanco S (2017) Financial regulations and price inconsistencies across Bitcoin markets. Inf Econ Policy 39:1–14
Polasik M, Piotrowska AI, Wisniewski TP, Kotkowski R, Lightfoot G (2015) Price fluctuations and the use of Bitcoin: an empirical inquiry. Int J Electron Commer 20(1):9–49
Reid F, Harrigan M (2013) An analysis of anonymity in the Bitcoin system. Security and privacy in social networks. Springer, New York, pp 197–223
Richter C, Kraus S, Bouncken RB (2015) Virtual currencies like Bitcoin as a paradigm shift in the field of transactions. Int Bus Econ Res J 14(4):575
Rogojanu A, Badea L (2014) The issue of competing currencies. Case study—Bitcoin. Theor Appl Econ XXI(1):103–114
Sapuric S, Kokkinaki A (2014) Bitcoin is volatile! Isn’t that right? International conference on business information systems. Springer, Cham, pp 255–265
Schilling L, Uhlig H (2019) Some simple bitcoin economics. J Monet Econ 106:16–26
Sensoy A (2019) The inefficiency of Bitcoin revisited: a high-frequency analysis with alternative currencies. Financ Res Lett 28:68–73
Shahzad SJH, Bouri E, Roubaud D, Kristoufek L, Lucey B (2019) Is Bitcoin a better safe-haven investment than gold and commodities? Int Rev Financ Anal 63:322–330
Shahzad SJH, Bouri E, Roubaud D, Kristoufek L (2020) Safe haven, hedge and diversification for G7 stock markets: gold versus bitcoin. Econ Model 87:212–224
Shen D, Urquhart A, Wang P (2019) Does twitter predict Bitcoin? Econ Lett 174:118–122
Smales LA (2019) Bitcoin as a safe haven: Is it even worth considering? Financ Res Lett 30:385–393
Stokes R (2012) Virtual money laundering: the case of Bitcoin and the Linden dollar. Inform Commun Technol Law 21(3):221–236
Su CW, Qin M, Tao R, Shao XF, Albu LL, Umar M (2020) Can Bitcoin hedge the risks of geopolitical events? Technol Forecast Soc Change 159:120182
Symitsi E, Chalvatzis KJ (2018) Return, volatility and shock spillovers of Bitcoin with energy and technology companies. Econ Lett 170:127–130
Symitsi E, Chalvatzis KJ (2019) The economic value of Bitcoin: a portfolio analysis of currencies, gold, oil and stocks. Res Int Bus Financ 48:97–110
Tan BS, Low KY (2017) Bitcoin–its economics for financial reporting. Aust Acc Rev 27(2):220–227
Trautman, LJ (2014) Virtual currencies; Bitcoin and what now after Liberty Reserve, Silk Road, and Mt. Gox?. Richmond J Law Technol 20(4):108
Urquhart A (2016) The inefficiency of Bitcoin. Econ Lett 148:80–82
Urquhart A (2017) Price clustering in Bitcoin. Econ Lett 159:145–148
Urquhart A, Zhang H (2019) Is Bitcoin a hedge or safe haven for currencies? An intraday analysis. Int Rev Financ Anal 63:49–57
Viglione R (2015) Does governance have a role in pricing? Cross-country evidence from Bitcoin markets. SSRN Electr J. https://doi.org/10.2139/ssrn.2666243
Wang J, Xue Y, Liu M (2016) An analysis of Bitcoin price based on VEC model. International conference on economics and management innovations. Atlantis Press, Paris
Wang GJ, Xie C, Wen D, Zhao L (2019) When Bitcoin meets economic policy uncertainty (EPU): Measuring risk spillover effect from EPU to Bitcoin. Financ Res Lett. https://doi.org/10.1016/j.frl.2018.12.028
Weber B (2014) Bitcoin and the legitimacy crisis of money. Camb J Econ 40(1):17–41
Wu S, Tong M, Yang Z, Derbali A (2019) Does gold or Bitcoin hedge economic policy uncertainty? Financ Res Lett 31:171–178
Yelowitz A, Wilson M (2015) Characteristics of Bitcoin users: an analysis of Google search data. Appl Econ Lett 22(13):1030–1036
Yermack D (2015) Is Bitcoin a real currency? An economic appraisal. Handbook of digital currency. Academic Press, London, pp 31–43
Zeng T, Yang M, Shen Y (2020) Fancy Bitcoin and conventional financial assets: Measuring market integration based on connectedness networks. Econ Model 90:209–220
Download references
This study was not funded.
Author information
Authors and affiliations.
Madras School of Economics (MSE), Gandhi Mandapam Road, Behind Government Data Centre, Kottur, Chennai, 600025, India
Parthajit Kayal & Purnima Rohilla
You can also search for this author in PubMed Google Scholar
Corresponding author
Correspondence to Parthajit Kayal .
Ethics declarations
Conflict of interest.
Parthajit Kayal declares that he has no conflict of interest. Purnima Rohilla declares that she has no conflict of interest.
Rights and permissions
Reprints and permissions
About this article
Kayal, P., Rohilla, P. Bitcoin in the economics and finance literature: a survey. SN Bus Econ 1 , 88 (2021). https://doi.org/10.1007/s43546-021-00090-5
Download citation
Received : 02 August 2020
Accepted : 18 May 2021
Published : 03 June 2021
DOI : https://doi.org/10.1007/s43546-021-00090-5
Share this article
Anyone you share the following link with will be able to read this content:
Sorry, a shareable link is not currently available for this article.
Provided by the Springer Nature SharedIt content-sharing initiative
- Cryptocurrency
- Bubble dynamics
- Find a journal
- Publish with us
- Track your research
- Today’s average refinance rates
- Today’s refinance interest rates
Current refinance rate trends
What to know about 2024 refinance rate trends, what to know about refinancing, choosing the right refinance type and term, does refinancing make sense, refi rates gradually ease for homeowners: mortgage refinance rates on march 29, 2024.
If you're shopping for the best refinance rate, keep an eye on the mortgage market over the next few months.

Katherine Watt
Katherine Watt is a CNET Money writer focusing on mortgages, home equity and banking. She previously wrote about personal finance for NextAdvisor. Based in New York, Katherine graduated summa cum laude from Colgate University with a bachelor's degree in English literature.
Laura Michelle Davis
Laura is a professional nitpicker and good-humored troubleshooter with over 10 years of experience in print and digital publishing. Before becoming an editor with CNET, she worked as an English teacher, Spanish medical interpreter, copy editor and proofreader. She is a fearless but flexible defender of both grammar and weightlifting, and firmly believes that technology should serve the people. Her first computer was a Macintosh Plus.
CNET staff -- not advertisers, partners or business interests -- determine how we review the products and services we cover. If you buy through our links, we may get paid.
- 30-year fixed-rate 6.88% (-0.09)
- 15-year fixed-rate 6.34% (-0.13)
- 30-year fixed-rate jumbo 7.01% (-0.08)
- 5/1 ARM 6.51% (+0.00)
- 10-year fixed-rate 6.28% (-0.12)
- 30-year fixed-rate refinance 6.88% (-0.12)
- 15-year fixed-rate refinance 6.38% (-0.11)
- 10-year fixed refinance 6.30% (-0.13)
Today's rates
Today’s average refinance rates, today’s refinance interest rates.
Refinance rates are still high, but your personal interest rate will depend on your credit history, financial profile and application.
Average refinance rates reported by lenders across the US as of March 29, 2024. We track refinance rate trends using information from Bankrate.
Mortgage refinance rates change every day. Experts recommend shopping around to make sure you’re getting the lowest rate. By entering your information below, you can get a custom quote from one of CNET’s partner lenders.
About these rates: Like CNET, Bankrate is owned by Red Ventures. This tool features partner rates from lenders that you can use when comparing multiple mortgage rates.
A vast majority of US homeowners already have mortgages with a rate below 6%. Because mortgage refinance rates have been averaging above 6.5% over the past several months, households are choosing to hold on to their existing mortgages instead of swapping them out with a new home loan.
If rates fell to 6%, at least a third of borrowers who took out mortgages in 2023 could reduce their rate by a full percentage point through a refinance, according to BlackKnight .
Refinancing in today’s market could make sense if you have a rate above 8%, said Logan Mohtashami , lead analyst at HousingWire. “However, with all refinancing options, it’s a personal financial choice because of the cost that goes with the loan process,” he said.
Mortgage rates have been sky-high over the last two years, largely as a result of the Federal Reserve’s aggressive attempt to tame inflation by spiking interest rates. Experts say that decelerating inflation and the Fed’s projected interest rate cuts should help stabilize mortgage interest rates by the end of 2024. But the timing of Fed cuts will depend on incoming economic data and the response of the market.
For homeowners looking to refinance, remember that you can’t time the economy: Interest rates fluctuate on an hourly, daily and weekly basis, and are influenced by an array of factors. Your best move is to keep an eye on day-to-day rate changes and have a game plan on how to capitalize on a big enough percentage drop, said Matt Graham of Mortgage News Daily.
When you refinance your mortgage, you take out another home loan that pays off your initial mortgage. With a traditional refinance, your new home loan will have a different term and/or interest rate. With a cash-out refinance, you’ll tap into your equity with a new loan that’s bigger than your existing mortgage balance, allowing you to pocket the difference in cash.
Refinancing can be a great financial move if you score a low rate or can pay off your home loan in less time, but consider whether it’s the right choice for you. Reducing your interest rate by 1% or more is an incentive to refinance, allowing you to cut your monthly payment significantly.
The rates advertised online often require specific conditions for eligibility. Your personal interest rate will be influenced by market conditions as well as your specific credit history, financial profile and application. Having a high credit score, a low credit utilization ratio and a history of consistent and on-time payments will generally help you get the best interest rates.
30-year fixed-rate refinance
The average rate for a 30-year fixed refinance loan is currently 6.84%, a decrease of 16 basis points over this time last week. (A basis point is equivalent to 0.01%.) A 30-year fixed refinance will typically have lower monthly payments than a 15-year or 10-year refinance, but it will take you longer to pay off and typically cost you more in interest over the long term.
15-year fixed-rate refinance
The average rate for a 15-year fixed refinance loan is currently 6.36%, a decrease of 13 basis points compared to one week ago. Though a 15-year fixed refinance will most likely raise your monthly payment compared to a 30-year loan, you’ll save more money over time because you’re paying off your loan quicker. Also, 15-year refinance rates are typically lower than 30-year refinance rates, which will help you save more in the long run.
10-year fixed-rate refinance
The average 10-year fixed refinance rate right now is 6.21%, a decrease of 19 basis points compared to one week ago. A 10-year refinance typically has the lowest interest rate but the highest monthly payment of all refinance terms. A 10-year refinance can help you pay off your house much quicker and save on interest, but make sure you can afford the steeper monthly payment.
To get the best refinance rates, make your application as strong as possible by getting your finances in order, using credit responsibly and monitoring your credit regularly. And don’t forget to speak with multiple lenders and shop around.
Homeowners usually refinance to save money, but there are other reasons to do so. Here are the most common reasons homeowners refinance:
- To get a lower interest rate: If you can secure a rate that’s at least 1% lower than the one on your current mortgage, it could make sense to refinance.
- To switch the type of mortgage: If you have an adjustable-rate mortgage and want greater security, you could refinance to a fixed-rate mortgage.
- To eliminate mortgage insurance: If you have an FHA loan that requires mortgage insurance, you can refinance to a conventional loan once you have 20% equity.
- To change the length of a loan term: Refinancing to a longer loan term could lower your monthly payment. Refinancing to a shorter term will save you interest in the long run.
- To tap into your equity through a cash-out refinance: If you replace your mortgage with a larger loan, you can receive the difference in cash to cover a large expense.
- To take someone off the mortgage: In case of divorce, you can apply for a new home loan in just your name and use the funds to pay off your existing mortgage.
Recommended Articles
Compare current refinance rates in march 2024, refinancing a mortgage: how it works, 30-year refinance rates for march 2024, 15-year mortgage refinance rates for march 2024, how to refinance your home, how does a cash-out refinance work, va refinance rates for march 2024.
CNET editors independently choose every product and service we cover. Though we can’t review every available financial company or offer, we strive to make comprehensive, rigorous comparisons in order to highlight the best of them. For many of these products and services, we earn a commission. The compensation we receive may impact how products and links appear on our site.
Writers and editors and produce editorial content with the objective to provide accurate and unbiased information. A separate team is responsible for placing paid links and advertisements, creating a firewall between our affiliate partners and our editorial team. Our editorial team does not receive direct compensation from advertisers.
CNET Money is an advertising-supported publisher and comparison service. We’re compensated in exchange for placement of sponsored products and services, or when you click on certain links posted on our site. Therefore, this compensation may impact where and in what order affiliate links appear within advertising units. While we strive to provide a wide range of products and services, CNET Money does not include information about every financial or credit product or service.
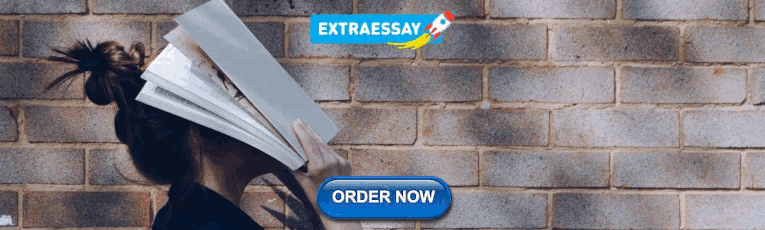
IMAGES
VIDEO
COMMENTS
Link. A narrative literature review is a methodological approach that aims to establish a . ... cryptocurrency implementation often utilizes the proof-of-work mechanism to record all .
Abstract. Bitcoin was first introduced by Nakamoto,(2008), as a peer-to- peer electronic system to do online payments from one party to the other without the intervention of a financial institution.
This review aims to analyze and synthesize the literature produced so far on investor behavior in the cryptocurrency market. We use VOSviewer 1.6.17 software to perform a bibliometric analysis and elaborate a systematic literature review on investor behavior in the cryptocurrency market on a sample of 166 papers published in journals ranked in the ABS 2021 journal list, considering the ...
This review provides a comprehensive and consolidated view of cryptocurrency pricing and maps the significant influential factors.,This paper is the first to systematically and comprehensively review the relevant literature on cryptocurrency to identify the factors of pricing fluctuation.
The second issue, widely debated in the cryptocurrency literature, is whether cryptocurrencies have a fundamental own value. Dwyer ( 2015 ) conjectures the limitation of the quantity produced can create an equilibrium in which a digital currency has a positive value: this limitation is a form of commitment, replacing the implicit obligation of ...
This study contributes to the unconsolidated cryptocurrency literature, with a systematic literature review focused on cryptocurrency market microstructure. We searched Web of Science database and focused only on journals listed on 2021 ABS list. Our final sample comprises 138 research papers. We employed a quantitative and an integrative analysis, and revealed complex network associations ...
We apply a narrative literature review method to synthesize prior research and draw insights into the opportunities and challenges of leveraging cryptocurrencies. ... Alam, N., & Zameni, A. P. (2019). Existing Regulatory Frameworks of Cryptocurrency and the Shari'ah Alternative. In Billah, M (eds). Halal Cryptocurrency Management, 179-194 ...
This study aims to perform a systematic literature review and bibliometric analysis within the topic of cryptocurrencies and consumer trust.,The Web of Science database has been selected, and the analyses performed allowed us to identify five research trends obtained from the bibliographic coupling analysis: (1) Understanding consumer's (non ...
Such a growth pace could soon create interoperability problems due to the heterogeneity of cryptocurrency applications (Tschorsch and Scheuermann, 2016, ... To provide a transparent, reproducible and scientific literature review of blockchain-based applications, the process suggested by Briner and Denyer (2012) ...
Table 2 identifies the key characteristics of the fifty-two quantitative investigations of cryptocurrency markets. The research papers are sub-categorised in the same manner with data reported to include the dependent variable of the investigated methodology and indeed the type of methodology used, the rationale behind its use, the frequency and source the data used, the selected control ...
The Sharpe ratios at the daily and weekly levels are about 60% and 90% higher, and the Sharpe ratio at the monthly level is comparable to those of stocks. The returns have positive skewness increasing with the frequencies from daily to monthly. The returns experience high probabilities of extreme losses and gains.
In this work, a systematic review of the literature [2, 42] is carried out regarding the use of cryptocurrencies, the general vulnerabilities they face, and the cryptocurrency-related crimes that it is possible to commit with them.To achieve our goals, we have proposed the following questions to be answered during our study:
In this paper, we investigate the role of cryptocurrencies in modern finance. We apply a narrative literature review method to synthesize prior research and draw insights into the opportunities and challenges of leveraging cryptocurrencies. The results indicate that cryptocurrencies offer businesses and individuals' lower transaction costs ...
This multiplicity of uses made necessary a systematic review, with special attention to business and economics (Xu et al. 2019). This suggests that we should take into account the application of the base technology and its potential applications at the business level (Zhao et al. 2016). Moreover, the cryptocurrency technology should be considered.
This study aims to systematically analyze and synthesize the literature produced thus far on cryptocurrency investment. We use a systematic review process supported by VOSviewer bibliographic coupling to review 482 papers published in the ABS 2021 journal list, considering all different areas of knowledge. This paper contributes an in-depth systematic analysis on the unconsolidated topic of ...
In this work, we perform a systematic literature review on the realm of privacy for electronic currencies. We present the development of digital money from electronic cash to cryptocurrencies and focus on the techniques that are employed to enhance user-privacy. ... Furthermore, we present flaws of the current cryptocurrency systems, which ...
Given the surge of literature on cryptocurrencies (both academic and practitioner), the aim of this brief thematic review is to bring to light several areas of interest where both generalist and specialist attention is required and warranted. It synthesizes and summarizes this salient literature on cryptocurrencies thematically with a view to ...
Cryptocurrencies and the Economy: A Review of the Literature 9 Above are the top ten cryptocurrency markets and their worth in dollars. The cryptocurrency market is huge and there is an immense amount of money to be made by taxing cryptocurrency revenue. Digital currencies are taxable by United States law. The Internal Revenue Service issued
This paper provides a review of the literature on key matters related to the popular cryptocurrency Bitcoin. Another key motivation of this paper is to understand the underlying principle of this digital currency from the economic and financial point of view. For the survey to be comprehensive, the paper is categorized into varied themes: price dynamics, volatility, bubble dynamics, mode of ...
S. Alzahrani and T. U. Daim, "Analysis of the Cryptocurrency Adoption Decision: Literature Review," 2019 Portland International Conference on Management of Engineering and Technology (PICMET), Portland, OR, USA, 2019, pp. 1-11. This Article is brought to you for free and open access. It has been accepted for inclusion in Engineering and
This study conducted a systematic review regarding the association between cryptocurrency and the stock market. This study used bibliometric and content analysis covering 151 articles from 2008 to November 2021. Using VOSviewer software, we explored the influential aspects of the literature, such as the prominent institutions, authors ...
The focus on cryptocurrencies in the finance and banking sectors is gaining momentum. In this paper, we investigate the role of cryptocurrencies in modern finance. We apply a narrative literature ...
In this section, we review various methodologies in the field of cryptocurrency fraud detection, ranging from traditional machine learning algorithms to advanced graph-based neural networks. Table 1 provides a detailed overview of these methodologies and categorizes them into two main types: traditional approaches and graph-based techniques.
6.41%. -0.19. Average refinance rates reported by lenders across the US as of March 29, 2024. We track refinance rate trends using information from Bankrate. Mortgage refinance rates change every ...