What Is Universal Representation And Why Is It Important?
This content was paid for by an advertiser. It was produced by our commercial team and did not involve HuffPost editorial staff.
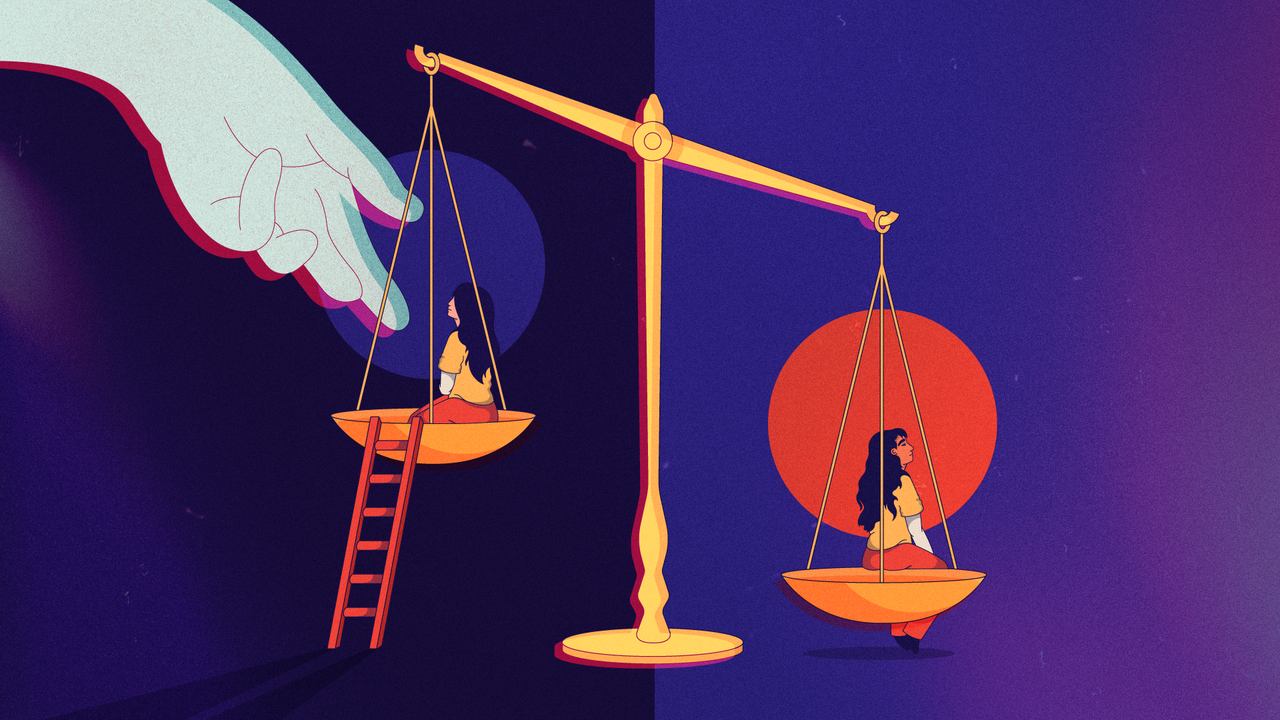
Imagine arriving to the US, unable to speak the language. Now imagine being forced to appear in court, alone, to defend your case against deportation. Could you do it and succeed?
The likely answer is no. You’d need the aid of an attorney who knows how to navigate immigration law. If you’re an immigrant with no money to pay for one, you’re out of luck. But with universal representation, all that could change.
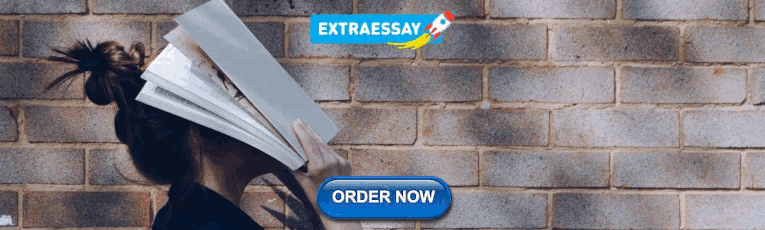
What is universal representation?
With universal representation , if you’re an immigrant facing deportation, you’d be entitled to a lawyer who’d represent you throughout the legal process if you cannot afford one yourself.
What’s the big deal?
While people facing criminal charges are entitled to government-funded representation, people in immigration court are not. If they are unable to finance a lawyer, they are forced to appear in court alone. These people often face deportation, as they are unable to defend themselves before a judge. In the more than 2.1 million cases pending in immigration courts as of February 2023, over 1.3 million people — or 59% of immigrants in deportation proceedings — lack legal representation and must defend themselves against deportation. This figure includes adults, families, and unaccompanied children.
Why is universal representation important?
The right to a lawyer is an integral part of the US criminal justice system — so shouldn’t that right also be granted to immigrants facing deportation, for whom the stakes are just as high?
When immigrants go unrepresented in deportation cases, they are unable to defend themselves in front of a judge. As a result, they can be deported simply because they cannot afford an attorney — even if they have a legal right to remain in the US.
Universal representation can prevent the separation of families, stop the deportation of immigrants, and get people out of detention centers and back into their communities.
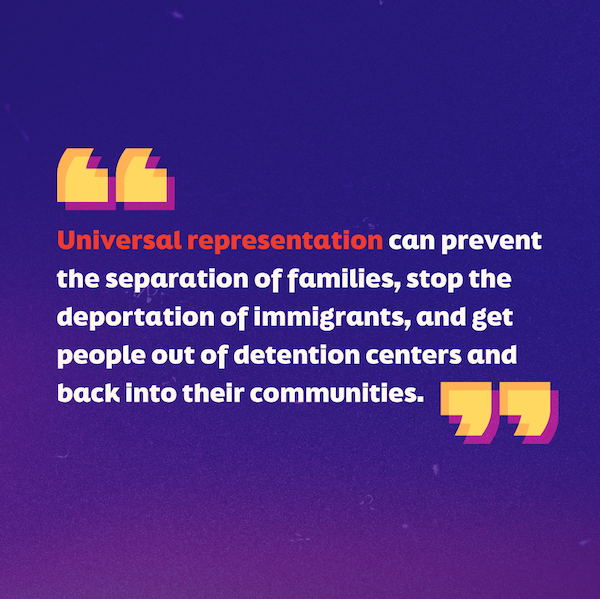
Raina and Ana’s Story
Both from Mexico, Raina and Ana , whose names have been changed to protect their identities, fled their country to escape violence. When they arrived, they were taken to a detention center, where they were instructed to sign papers written in English. Because Raina and Ana did not speak the language, and because neither a translator nor an attorney were provided to them, they were unaware that by signing, they were waiving their right to seek asylum, which meant they would be sent back to Mexico.
“They just said, ‘Sign it here, sign it here, sign it here,’” Ana recalled.
When Halinka Zolcik, an accredited representative of Prisoners’ Legal Services of New York, took on their case, Raina and Ana’s situation improved.
“After I started working with these ladies, [ICE] started being careful,” Zolcik said. “It 1,000% changed how they were treated.”
With Zolcik’s help, Raina and Ana’s orders of deportation were reversed, and they were able to complete their asylum applications.
Raina and Ana’s experience illustrates the importance of universal representation. With an attorney to guide them, they not only were able to navigate the legal system with fairness, respect, and clarity, but they were also able to forge a clear path to citizenship.
What’s being done about this?
Organizations such as the Vera Institute of Justice are fighting for the federal right to universal representation. In fact, their Advancing Universal Representation initiative has supported numerous programs at the state and local levels. Among those is the New York Immigrant Family Unity Project (NYIFUP) , a statewide defender program that provides lawyers for immigrants. It is the first and largest public defender program in the country.
Vera has also launched the Fairness to Freedom Campaign alongside the National Partnership for New Americans (NPNA) . This campaign pushes for legislation that establishes the federal right to government-funded legal representation for those who cannot afford a lawyer. The Fairness to Freedom Act of 2023 was introduced in Congress in April by U.S. Sens. Kirsten Gillibrand and Cory Booker and U.S. Reps. Norma Torres, Pramila Jayapal, and Grace Meng.
What can you do?
Anyone can join in the fight for universal representation . A first step is as easy as learning more about the issue. You can also get involved with organizations like Vera .
All facts via Vera Institute of Justice .
This article was paid for by Vera Institute of Justice and created by HuffPost’s Branded Creative Team. HuffPost editorial staff did not participate in the creation of this content.
More In Impact

Universal Design for Learning (UDL)
- UDL Principle: Representation
- UDL Principle: Action & Expression
- UDL Principle: Engagement
- UDL Syllabus Rubric
- UDL Rubric for Online Instruction
- UDL FIG, 2015-16
- UDL Trainings
Provide Multiple Means of Representation
Learners differ in the ways that they perceive and comprehend information that is presented to them. For example, those with sensory disabilities (e.g., blindness or deafness); learning disabilities (e.g., dyslexia); language or cultural differences, and so forth may all require different ways of approaching content. Others may simply grasp information quicker or more efficiently through visual or auditory means rather than printed text. Also learning, and transfer of learning, occurs when multiple representations are used, because it allows students to make connections within, as well as between, concepts. In short, there is not one means of representation that will be optimal for all learners; providing options for representation is essential. For greater detail, please refer to the CAST UDL Guidelines on Representation.
Provide Options for Perception
Learning is impossible if information is imperceptible to the learner, and difficult when information is presented in formats that require extraordinary effort or assistance. To reduce barriers to learning, it is important to ensure that key information is equally perceptible to all learners by:
- providing the same information through different modalities (e.g., through vision, hearing, or touch);
- providing information in a format that will allow for adjustability by the user (e.g., text that can be enlarged, sounds that can be amplified)
Such multiple representations not only ensure that information is accessible to learners with particular sensory and perceptual disabilities, but also easier to access and comprehend for many others.
Provide Options for Language, Mathematical Expression & Symbols
Learners vary in their facility with different forms of representation – both linguistic and non-linguistic. Vocabulary that may sharpen and clarify concepts for one learner may be opaque and foreign to another.
An equals sign (=) might help some learners understand that the two sides of the equation need to be balanced, but might cause confusion to a student who does not understand what it means.
A graph that illustrates the relationship between two variables may be informative to one learner and inaccessible or puzzling to another.
A picture or image that carries meaning for some learners may carry very different meanings for learners from differing cultural or familial backgrounds.
As a result, inequalities arise when information is presented to all learners through a single form of representation. An important instructional strategy is to ensure that alternative representations are provided not only for accessibility, but for clarity and comprehensibility across all learners.
Provide Options for Comprehension
The purpose of education is not to make information accessible, but rather to teach learners how to transform accessible information into useable knowledge . Decades of cognitive science research have demonstrated that the capability to transform accessible information into useable knowledge is not a passive process but an active one.
Constructing useable knowledge, knowledge that is accessible for future decision-making, depends not upon merely perceiving information, but upon active “information processing skills” like selective attending, integrating new information with prior knowledge, strategic categorization, and active memorization.
Individuals differ greatly in their skills in information processing and in their access to prior knowledge through which they can assimilate new information. Proper design and presentation of information – the responsibility of any curriculum or instructional methodology - can provide the scaffolds necessary to ensure that all learners have access to knowledge.
Representation Takeaway Strategy: Graphic Organizers
What: Graphic Organizers (GOs) are visual representations of knowledge, concepts, thoughts, or ideas. GOs entered the realm of education in the late twentieth century as ways of helping students to organize their thoughts (as a sort of pre-writing exercise). For example, a student is asked, "What were the causes of the French Revolution?" The student places the question in the middle of a sheet of paper. Branching off of this, the student jots down her ideas, such as "poor harvests," "unfairness of the Old Regime," etc. Branching off of these are more of the student's thoughts, such as "the nobles paid no taxes" branching from "unfairness of the Old Regime."
Why : Positive effects on higher order knowledge but not on facts (Robinson & Kiewra, 1995); Quiz scores higher using partially complete GO (Robinson et al., 2006). In addition, GOs have been known to help:
· relieve learner boredom
· enhances recall
· provide motivation
· create interest
· clarify information
· assist in organizing thoughts
· promote understanding
How : Advanced organizers, Venn diagrams , concept /spider/story maps, flowcharts , hierarchies , etc.
1. Provide completed GO to students (Learn by viewing)
2. Students construct their own GO (Learn by doing)
3. Students finalize partially complete GO (scaffolding)
- << Previous: Principles
- Next: UDL Principle: Action & Expression >>
- Last Updated: Feb 6, 2024 1:40 PM
- URL: https://mtsac.libguides.com/udl
Advertisement
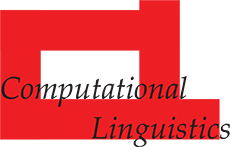
- Previous Article
1. Introduction
2. discourse representation structures, 3. computational formats, 4. crosslingual semantic parser, 5. experiments, 7. related work, 8. conclusion, acknowledgments, universal discourse representation structure parsing.
- Cite Icon Cite
- Open the PDF for in another window
- Permissions
- Article contents
- Figures & tables
- Supplementary Data
- Peer Review
- Search Site
Jiangming Liu , Shay B. Cohen , Mirella Lapata , Johan Bos; Universal Discourse Representation Structure Parsing. Computational Linguistics 2021; 47 (2): 445–476. doi: https://doi.org/10.1162/coli_a_00406
Download citation file:
- Ris (Zotero)
- Reference Manager
We consider the task of crosslingual semantic parsing in the style of Discourse Representation Theory (DRT) where knowledge from annotated corpora in a resource-rich language is transferred via bitext to guide learning in other languages. We introduce 𝕌niversal Discourse Representation Theory (𝕌DRT), a variant of DRT that explicitly anchors semantic representations to tokens in the linguistic input. We develop a semantic parsing framework based on the Transformer architecture and utilize it to obtain semantic resources in multiple languages following two learning schemes. The many-to-one approach translates non-English text to English, and then runs a relatively accurate English parser on the translated text, while the one-to-many approach translates gold standard English to non-English text and trains multiple parsers (one per language) on the translations. Experimental results on the Parallel Meaning Bank show that our proposal outperforms strong baselines by a wide margin and can be used to construct (silver-standard) meaning banks for 99 languages.
Recent years have seen a surge of interest in representational frameworks for natural language semantics. These include novel representation schemes such as Abstract Meaning Representation (AMR; Banarescu et al. 2013 ), Universal Conceptual Cognitive Annotation (Abend and Rappoport 2013 ), and Universal Decompositional Semantics (White et al. 2016 ), as well as existing semantic formalisms such as Minimal Recursion Semantics (MRS; Copestake et al. 2005 ), and Discourse Representation Theory (DRT; Kamp and Reyle 1993 ). The availability of annotated corpora (Flickinger, Zhang, and Kordoni 2012 ; May 2016 ; Hershcovich, Abend, and Rappoport 2017 ; Abzianidze et al. 2017 ) has further enabled the development and exploration of various semantic parsing models aiming to map natural language to formal meaning representations.
In this work, we focus on parsing meaning representations in the style of DRT (Kamp 1981 ; Kamp and Reyle 1993 ; Asher and Lascarides 2003 ), a formal semantic theory designed to handle a variety of linguistic phenomena, including anaphora, presuppositions (Van der Sandt 1992 ; Venhuizen et al. 2018 ), and temporal expressions within and across sentences. The basic meaning-carrying units in DRT are Discourse Representation Structures (DRSs), which are recursive, have a model-theoretic interpretation, and can be translated into first-order logic (Kamp and Reyle 1993 ). DRSs are scoped meaning representations—they capture the semantics of negation, modals, quantification, and presupposition triggers.
Although initial attempts at DRT parsing focused on small fragments of English (Johnson and Klein 1986 ; Wada and Asher 1986 ), more recent work has taken advantage of the availability of syntactic treebanks and robust parsers trained on them (Hockenmaier and Steedman 2007 ; Curran, Clark, and Bos 2007 ; Bos 2015 ) or corpora specifically annotated with discourse representation structures upon which DRS parsers can be developed more directly. Examples of such resources are the Redwoods Treebank (Oepen et al. 2002 ; Baldridge and Lascarides 2005b , 2005a ), the Groningen Meaning Bank (Basile et al. 2012 ; Bos et al. 2017 ), and the Parallel Meaning Bank (PMB; Abzianidze et al. 2017 ), which contains annotations for English, German, Dutch, and Italian sentences based on a parallel corpus. Aside from larger-scale resources, renewed interest (Oepen et al. 2020 ) 1 in DRT parsing has been triggered by the realization that document-level semantic analysis is a prerequisite to various applications ranging from machine translation (Kim, Tran, and Ney 2019 ) to machine reading (Gangemi et al. 2017 ; Chen 2018 ) and generation (Basile and Bos 2013 ; Narayan and Gardent 2014 ).
Figure 1(a) shows the DRS corresponding to an example sentence taken from the PMB, and its Italian translation. Conventionally, DRSs are depicted as boxes. Each box comes with a unique label (see b 1 , b 2 , b 3 in the figure) and has two layers. The top layer contains discourse referents (e.g., x 1 , t 1 ), and the bottom layer contains conditions over discourse referents. Each referent or condition belongs to a unique box label, showing the referent or the condition that is interpreted in that box (e.g., b 2 : x 1 and b 2 : person.n.01( x 1 )). The predicates are disambiguated with senses (e.g., n.01 and v.01) provided in WordNet (Fellbaum 1998 ). More details on the DRT formalism are discussed in Section 2.1 .

(a) DRS for English sentence everyone was killed and its Italian translation sono stati uccisi tutti , taken from the Parallel Meaning Bank; (b) Universal Discourse Representation Structures (𝕌DRS) for English; and (c) Italian sentence. The two 𝕌DRSs differ in how they are anchored to the English and Italian sentences (via alignment). Anchors $0 and $2 in (b) refer to English tokens everyone and killed , respectively, while anchors $3 and $2 in (c) refer to Italian tokens tutti and uccisi .
Despite efforts to create crosslingual DRS annotations (Abzianidze et al. 2017 ), the amount of gold-standard data for languages other than English is limited to a few hundred sentences that are useful for evaluation but small-scale for model training. In addition, for many languages, semantic analysis cannot be performed at all due to the lack of annotated data. The creation of such data remains an expensive endeavor requiring expert knowledge, namely, familiarity with the semantic formalism and language at hand. Because it is unrealistic to expect that semantic resources will be developed for many low-resource languages in the near future, previous work has resorted to machine translation and bitexts that are more readily available (Evang and Bos 2016 ; Damonte and Cohen 2018 ; Zhang et al. 2018 ; Conneau et al. 2018 ; Fancellu et al. 2020 ). Crosslingual semantic parsing leverages an existing parser in a source language (e.g., English) together with a machine translation system to learn a semantic parser for a target language (e.g., Italian or Chinese).
In this article, we also aim to develop a crosslingual DRT parser for languages where no gold-standard training data is available. We first propose a variant of the DRT formalism, which explicitly anchors semantic representations to words. Specifically, we introduce 𝕌niversal Discourse Representation Structures (𝕌DRSs) where language-dependent symbols are replaced with anchors referring to tokens (or characters, e.g., in the case of Chinese) of the input sentence. 𝕌DRSs are intended as an alternative representation that abstracts away from decisions regarding concepts in the source language. As shown in Figure 1(b) and Figure 1(c) , “ person ” and “ kill ” are replaced with anchors $0 and $2, corresponding to English tokens everyone and killed , and $3 and $2, corresponding to Italian tokens tutti and uccisi . Also, notice that 𝕌DRSs omit information about word senses (denoted by WordNet synsets, e.g., person.n.01 , time.n.08 in Figure 1(a) ) as the latter cannot be assumed to be the same across languages (see Section 2.2 for further discussion).
Like other related broad-coverage semantic representations (e.g., AMR), DRSs are not directly anchored in the sentences whose meaning they purport to represent. The lack of an explicit linking between sentence tokens and semantic structures makes DRSs less usable for downstream processing tasks and less suitable for crosslingual parsing that relies on semantic and structural equivalences between languages. 𝕌DRSs omit lexical details pertaining to the input sentence and as such are able to capture similarities in the representation of expressions within the same language and across languages.
Our crosslingual parser takes advantage of 𝕌DRSs and state-of-the-art machine translation to develop semantic resources in multiple languages following two learning schemes. The many-to-one approach works by translating non-English text to English, and then running a relatively accurate English DRS parser on the translated text, while the one-to-many approach translates gold-standard English (training data) to non-English text and trains multiple parsers (one per language) on the translations. 2 In this article, we propose (1) 𝕌DRSs to explicitly anchor DRSs to lexical tokens, which we argue is advantageous for both monolingual and crosslingual parsing; (2) a box-to-tree conversion algorithm that is lossless and reversible; and (3) a general crosslingual semantic parsing framework based on the Transformer architecture and its evaluation on the PMB following the one-to-many and many-to-one learning paradigms. We showcase the scalability of the approach by creating a large corpus with (silver-standard) discourse representation annotations in 99 languages.
We first describe the basics of traditional DRSs, and then explain how to obtain 𝕌DRSs based on them. We also highlight the advantages of 𝕌DRSs when they are used for multilingual semantic representations.
2.1 Traditional DRSs
DRSs, the basic meaning-carrying units of DRT, are typically visualized as one or more boxes, which can be nested to represent the semantics of sentences recursively. An example is given in Figure 1(a) . Each box has a label (e.g., b 1 ) and consists of two layers. The top layer contains variables (e.g., x 1 ), and the bottom layer contains conditions over the variables. For example, person.n.01( x 1 ) means that variable x 1 is applied to predicate person.n.01, which in fact is a WordNet synset.
The PMB adopts an extension of DRT that treats presupposition 3 with projection pointers (Venhuizen, Bos, and Brouwer 2013 ), marking how the accommodation site (box) variables and conditions are bounded and interpreted. For example, b 2 : x 1 and b 1 : time.n.08( t 1 ) indicate that variable x 1 and condition time.n.08( t 1 ) should be interpreted within boxes b 2 and b 1 , respectively. The boxes are constructed incrementally by a set of rules mapping syntactic structures to variables and conditions (Kamp and Reyle 1993 ). As shown in Figure 1(a) , the phrase was killed gives rise to temporal variable t 1 and condition time.n.08( t 1 ) and t 1 < now; these temporal markers are located in box b 3 together with the predicate “ kill ,” and are bound by outer box b 4 (not drawn in the figure) which would be created to accommodate any discourse that might continue the current sentence.
2.2 𝕌niversal DRSs
How can DRSs be used to represent meaning across languages? An obvious idea would be to assume that English and non-English languages share identical meaning representations and sense distinctions (aka identical DRSs). For example, the English sentence everyone was killed and the Italian sentence sono stati uccisi tutti would be represented by the same DRS, shown in Figure 1(a) . Unfortunately, this assumption is unrealistic, as sense distinctions can vary widely across languages. 4 For instance, the verb eat/essen has six senses according to the English WordNet (Fellbaum 1998 ) but only one in GermaNet (Hamp and Feldweg 1997 ), and the word good /好 has 23 senses in the English WordNet but 17 senses in the Chinese WordNet (Huang et al. 2010 ). In other words, we cannot assume that there will be a one-to-one correspondence in the senses of the same predicate in any two languages.
Because word sense disambiguation is language-specific, we do not consider it part of the proposed crosslingual meaning representation but assume that DRS operators (e.g., negation) and semantic roles are consistent across languages. We introduce 𝕌DRSs, which replace “DRS tokens,” such as constants and predicates of conditions, with alignments to tokens or spans (e.g., named entities) in the input sentence. An example is shown in Figure 2(b) , where condition b 2 : eat.v.01( e 1 ) is generalized to b 2 : $2.v( e 1 ). Here, $2 corresponds to eating and v denotes the predicates part of speech (i.e., verb). Note that even though senses are not part of the 𝕌DRS representation, parts of speech (for predicates) are because they can provide cues for sense disambiguation across languages.

(a) DRS for sentence Tom is eating an apple ; (b) DRS for sentence Jack is cleaning a car ; (c) 𝕌DRS for both sentences; (d) 𝕌DRS for sentence 汤姆正在吃一个苹果 (Tom is eating an apple) constructed via (c) by substituting indices with their corresponding words. Expressions in brackets make the anchoring explicit (e.g., $4 is anchored to Chinese characters 苹果) and are only shown for ease of understanding, they are not the part of the 𝕌DRS.
𝕌DRS representations abstract semantic structures within the same language and across languages. Monolingually, they are generalizations of sentences with different semantic content but similar syntax. As shown in Figure 2 , Tom is eating an apple and Jack is washing a car are represented by the same 𝕌DRS, which can be viewed as a template describing an event in past tense with an agent and a theme. 𝕌DRSs are more compact representations and advantageous from a modeling perspective; they are easier to generate compared to DRSs since multiple training instances are represented by the same semantic structure. Moreover, 𝕌DRSs can be used to capture basic meaning across languages. The 𝕌DRS in Figure 2(c) can be used to recover the semantics of the sentence “汤姆正在吃一个苹果” (Tom is eating an apple) by substituting index $0 with 汤姆, index $2 with 吃, and index $4 with 苹果 (see Figure 2(d) ).
Link to Knowledge Bases.
An important distinction between 𝕌DRSs and DRSs is that the former do not represent word senses. We view word sense disambiguation as a post-processing step that can enrich 𝕌DRSs with more fine-grained semantic information according to specific tasks and knowledge resources. 𝕌DRSs are agnostic when it comes to sense distinctions. They are compatible with WordNet that exists in multiple languages, 5 and has been used for English DRSs, but also related to resources such as BabelNet (Navigli and Ponzetto 2010 ), ConceptNet (Speer, Chin, and Havasi 2017 ), HowNet (Dong, Dong, and Hao 2006 ), and Wikidata. 6 An example of how 𝕌DRSs can be combined with word senses to provide more detailed meaning representations is shown in Figure 3 .

Illustration of how 𝕌DRSs can enrich deep contextual word representations; word senses can be disambiguated as a post-processing step according to the definitions of various language-specific resources.
Link to Language Models.
As explained earlier, predicates and constants in 𝕌DRSs are anchored (via alignments) to lexical tokens (see 吃.v( e 1 ) in Figure 2(d) ). As a result, 𝕌DRSs represent multiword expressions as a combination of multiple tokens aiming to assign atomic meanings and avoid redundant lexical semantics. For example, in the sentence Tom picked the graphic card up , graphic card corresponds to entity $3–$4.n( x 2 ) and picked up to relation $1–$5.v( x 1 , x 2 ). The link between elements of the semantic representation and words in sentences is advantageous because it renders 𝕌DRSs amenable to further linguistic processing. For example, they could be interfaced with large-scale pretrained models such as BERT (Devlin et al. 2019 ) and GPT (Radford et al. 2019 ), thereby fusing together deep contextual representations and rich semantic symbols (see Figure 3 ). Aside from enriching pretrained models (Wu and He 2019 ; Hardalov, Koychev, and Nakov 2020 ; Kuncoro et al. 2020 ), such representations could further motivate future research on their interpretability (Wu and He 2019 ; Hardalov, Koychev, and Nakov 2020 ; Kuncoro et al. 2020 ; Hewitt and Manning 2019 ; Kulmizev et al. 2020 ).
DRSs are displayed in a box-like format that is intuitive and easy to read but not particularly convenient for modeling purposes. As a result, DRSs are often post-processed in a format that can be straightforwardly handled by modern neural network models (Liu, Cohen, and Lapata 2018 ; van Noord et al. 2018b ; Liu, Cohen, and Lapata 2019a ). In this section, we provide an overview of existing computational formats, prior to describing our own proposed format.
3.1 Clause Format
In the PMB (Abzianidze et al. 2017 ), DRS variables and conditions are converted to clauses. Specifically, variables in the top box layer are converted to clauses by introducing a special condition called “REF.” Figure 4(b) presents the clause format of the DRS in Figure 4(a) ; here, “ b 2 REF x 1 ” indicates that variable x 1 is bound in box b 2 . Analogously, clause “ b 3 kill v.01 e 1 ” corresponds to condition kill.v.01( e 1 ) which is bound in box b 3 , and b 4 TRP t 1 “now” is bound in box b 4 (TRP corresponds to temporal). 7 The mapping from boxes to clauses is not reversible; in other words, it is not straightforward to recover the original box from the clause format and restore the syntactic structure of the original sentence. For instance, the clause format discloses that temporal information is bound to box b 4 , but not which box this information is located in (i.e., b 3 in Figure 4(a) ). Although PMB is released with clause annotations, Algorithm 1 re-implements the conversion procedure of Abzianidze et al. ( 2017 ) to allow for a more direct comparison between clauses and the tree format introduced below.

DRS in box format; (b) DRS in clause format; (c) DRS in tree format proposed in this article.
In Algorithm 1, the function GetVariableBound returns pairs of variables and box labels (indicating where these are bound) by enumerating all nested boxes. 8 The element P [ v ] represents the label of the box bounding variable v . Basic conditions are converted to a clause in lines 9–10, where cond . args is a list of the arguments of the condition (e.g., predicates and referents). Unary complex conditions (i.e., negation, possibility, and necessity) are converted to clauses in lines 11–13, while lines 14–16 show how to convert binary complex conditions (i.e., implication, disjunction, and duplication) to clauses. 9 An example is shown in Figure 4(b) .

3.2 Tree Format
Liu, Cohen, and Lapata ( 2018 ) propose an algorithm that converts DRS boxes to trees, where each DRS box is converted to a subtree and conditions within the box are introduced as children of the subtree. In follow-on work, Liu, Cohen, and Lapata ( 2019a ) define Discourse Representation Tree Structure based on this conversion. Problematically, the algorithm of Liu, Cohen, and Lapata ( 2018 ) simplifies the semantic representation as it does not handle presuppositions, word categories (e.g., n for noun), and senses (e.g., n .01). In this article, we propose an improved box-to-tree conversion algorithm, which is reversible and lossless—that is, it preserves all the information present in the DRS box, as well as the syntactic structure of the original text. Our conversion procedure is described in Algorithm 2. Similar to the box-to-clause algorithm, basic conditions are converted to a tree in lines 10–11, where cond . args is a list of the arguments of the condition (e.g., predicates and referents). Unary complex conditions (i.e., negation, possibility, and necessity) are converted to subtrees in lines 12–15, while lines 16–20 show how to convert binary complex conditions (i.e., implication, disjunction, and duplication) to subtrees.

An example is shown in Figure 4(c) . Basic condition b 2 : person.n.01( x 1 ) is converted to the subtree shown in Figure 5(a) . Binary complex condition → is converted to the subtree shown in Figure 5(b) . Unary complex conditions are converted in a similar way. The final tree can be further linearized to ( b 1 ( → b 1 ( b 2 (Ref b 2 x 1 ) (Pred b 2 x 1 person n.01)) ( b 3 (Ref b 4 t 1 ) (Pred b 4 t 1 time n.08) (TPR b 4 t 1 now) (Ref b 3 e 1 ) (Pred b 3 e 1 kill v.01) (Time b 3 e 1 t 1 ) (Patient b 3 e 1 x 1 )))).

Example of converting a basic condition (a) and a binary complex condition (b) to a tree.
As mentioned earlier, PMB (Abzianidze et al. 2017 ) contains a small number of gold standard DRS annotations in German, Italian, and Dutch. Multilingual DRSs in PMB use English WordNet synsets regardless of the source language. The output of our crosslingual semantic parser is compatible with this assumption, which is also common in related broad-coverage semantic formalisms (Damonte and Cohen 2018 ; Zhang et al. 2018 ). In the following, we present two learning schemes (illustrated schematically in Figure 6 ) for bootstrapping DRT semantic parsers for languages lacking gold standard training data.

4.1 Many-to-one Method
According to the many-to-one approach, target sentences (e.g., in German) are translated to source sentences (e.g., in English) via a machine translation system; and then a relatively accurate source DRS parser (trained on gold-standard data) is adopted to map the target translations to their semantic representation. Figure 6(a) provides an example for the three PMB languages.
An advantage of this method is that labeled training data in the target language is not required. However, the performance of the semantic parser on the target languages is limited by the performance of the semantic parser in the source language; moreover, the crosslingual parser must be interfaced with a machine translation system at run-time, since it only accepts input in the pivot language (such as English).
4.2 One-to-many Method
The one-to-many approach constructs training data for the target languages (see Figure 6(b) ) via machine translation. The translated sentences are paired with gold DRSs (from the source language) and collected as training data for the target languages. An advantage of this method is that the obtained semantic parsers are sensitive to the linguistic aspects of individual languages (and how these correspond to meaning representations). From a modeling perspective, it is also possible to exploit large amounts of unlabeled data in the target language to improve the performance of the semantic parser. Also, notice that the parser is independent of the machine translation engine utilized (sentences need to be translated only once) and the semantic parser developed for the source language. In theory, different parsing models can be used to cater for language-specific properties.
The learning schemes just described are fairly general and compatible with either clause or tree DRS formats, or indeed meaning representation schemes that are not based on DRT. However, the proposed 𝕌DRS representation heavily depends on the order of the tokens in the natural language sentences, and as a result is less suited to the many-to-one method; parallel sentences in different languages might have different word orders and consequently different 𝕌DRSs (recall that the latter are obtained via aligning non-English tokens to English ones). Many-to-one adopts the rather strong assumption that meaning representations are invariant across languages. If the Italian sentence sono stati uccisi tutti is translated as everyone was killed in English, a parser trained on English data would output the 𝕌DRS in Figure 1(b) , while the correct analysis would be Figure 1(c) . The resulting 𝕌DRS would have to be post-processed in order for the indices to accurately correspond to tokens in the source language (in this case Italian). Many-to-one would thus involve the extra step of modifying English 𝕌DRSs back to the 𝕌DRSs of the source language. Because 𝕌DRS indices are anchored to input sentences via word alignments, we would need to use word alignment models for every new language seen at test time, which renders many-to-one for 𝕌DRS representations slightly impractical.
4.3 Semantic Parsing Model
Following previous work on semantic parsing (Dong and Lapata 2016 ; Jia and Liang 2016 ; Liu, Cohen, and Lapata 2018 ; van Noord et al. 2018b ), we adopt a neural sequence-to-sequence model which assumes that trees or clauses can be linearized into PTB-style bracketed sequences and sequences of symbols, respectively. Specifically, our encoder-decoder model builds on the Transformer architecture (Vaswani et al. 2017 ), a highly efficient model that has achieved state-of-the-art performance in machine translation (Vaswani et al. 2017 ), question answering (Yu et al. 2018 ), summarization (Liu, Titov, and Lapata 2019 ), and grounded semantic parsing (Wang et al. 2020 ).
Our DRS parser takes a sequence of tokens, { s 0 , s 1 ,…, s n −1 } as input and outputs their linearized DRS { t 0 , t 1 ,…, t m −1 }, where n is the number of input tokens, and m is the number of the symbols in the output DRS.
4.4 Training
Our models are trained with standard back-propagation that requires a large-scale corpus with gold-standard annotations. The PMB does not contain high-volume annotations for model training in languages other than English (although gold-standard data for development and testing are provided). The situation is unfortunately common when developing multilingual semantic resources that demand linguistic expertise and familiarity with the target meaning representation (discourse representation theory in our case). In such cases, model training can be enhanced by recourse to automatically generated annotations that can be obtained with a trained parser. The quality of these data varies depending on the accuracy of the underlying parser and whether any manual correction has taken place on the output. In this section, we introduce an iterative training method that makes use of auto-standard annotations of varying quality and is model-independent.
Let D a u t o = D 0 , D 1 , … , D m − 1 denote different versions of training data generated automatically; indices denote the quality of the auto-standard data, D 0 has lowest quality, D m −1 has highest quality, and D i (0 ≤ i < m ) is auto-standard data with quality i . The model is first collectively trained on all available data D a u t o and then at each iteration on subset D a u t o / D 1 which excludes the data with the lowest quality D i . So, the model takes advantage of large-scale data for more reliable parameter estimation but is progressively optimized on better quality data. Algorithm 3 provides a sketch of this training procedure. Iterative training is related to self-training, where model predictions are refined by training on progressively more accurate data. In the case of self-training, the model is trained on its own predictions, while in iterative training, the model uses annotations of increasingly better quality. These can be produced by other models, human experts, or a mixture. Because we know a priori the quality of annotations, we can ensure that later model iterations make use of better data.

In this section we describe the data set used in our experiments, as well as details concerning the training and evaluation of our models.
Our experiments were carried out on PMB 2.2.0, which is annotated with DRSs for English (en), German (de), Italian (it), and Dutch (nl). The data set contains gold standard training data for English only, while development and test gold standard data is available in all four languages. The PMB also provides silver and bronze standard training data in all languages. Silver data is only partially checked for correctness, while bronze data is not manually checked in any way. Both types of data were built using Boxer (Bos 2008 ), an open-domain semantic parser that produces DRS representations by capitalizing on the syntactic analysis provided by a robust CCG parser (Curran, Clark, and Bos 2007 ).
5.2 Settings
All models share the same hyperparameters. The dimension of the word embeddings is 300, the Transformer encoder and decoder have 6 layers with a hidden size of 300 and 6 heads; the dimension of position-wise feedforward networks is 4,096. The models were trained to minimize a cross-entropy loss objective with an l 2 regularization term. We used Adam (Kingma and Ba 2014 ) as the learning rate optimizer; the initial learning rate was set to 0.001 with a 0.7 learning rate decay for every 4,000 updates starting after 30,000 updates. The batch size was 2,048 tokens. Our hyperparameter settings follow previous work (van Noord et al. 2018b ; Liu, Cohen, and Lapata 2019b ).
Monolingual Setting.
Our monolingual experiments were conducted on the English portion of the PMB. We used the standard training/test splits provided in the data set. We obtained 𝕌DRSs using the manual alignments from DRS tokens to sentence tokens included in the PMB release. Our models were trained with the iterative training scheme introduced in Section 4.4 using the PMB bronze-, silver- and gold-standard data (we use D 0 to refer to bronze, D 1 denotes silver, and D 2 gold).
Crosslingual Setting.
All crosslingual experiments were conducted using Google Translate’s API, 10 a commercial state-of-the-art system supporting more than a hundred languages (Wu et al. 2016 ). Bronze- and silver-training data for German, Italian, and Dutch are provided with the PBM release. For experiments on other non-English languages, we only used the one-to-many method to create training data for 𝕌DRS parsing ( Section 4.2 ). For this, the original alignments (of meaning constructs to input tokens) in English 𝕌DRSs need to be modified to correspond to tokens in the translated target sentences. We used the GIZA++ toolkit to obtain forward alignments from source to target and backward alignments from target to source (Koehn, Och, and Marcu 2003 ). 𝕌DRSs for which no available alignment for tokens was found were excluded from training. 11 For iterative training, we consider bronze- and silver-training data (if these are available) of lower quality (i.e., D 0 and D 1 , respectively) compared to data constructed by the one-to-many method (i.e., D 2 ).
5.3 Evaluation
We evaluated the output of our semantic parser using Counter (van Noord et al. 2018a ), a recently proposed metric suited for scoped meaning representations. Counter operates over DRSs in clause format and computes precision and recall on matching clauses. DRSs and 𝕌DRSs in tree format can easily revert to boxes, which in turn can be rendered as clauses for evaluation purposes. 12
SPAR is a baseline system that outputs the same DRS for each test instance. 13
SIM-SPAR is a baseline system that outputs the DRS of the most similar sentence in the training set, based on a simple word embedding metric (van Noord et al. 2018b ).
Boxer is a system that outputs the DRSs of sentences according to their supertags and CCG derivations (Bos 2015 ). Each word is assigned a lexical semantic representation according to its supertag category, and the representation of a larger span is obtained by combining the representations of two continuous spans (or words). The semantic representation of the entire sentence is composed based on the underlying CCG derivation.
Graph is a graph neural network that generates DRSs according to a directed acyclic graph grammar (Fancellu et al. 2019 ). Grammar rules are extracted from the training data, and the model learns how to apply these to obtain DRSs.
Transition is a neural transition-based model that incrementally generates DRSs (Evang 2019 ). It repeatedly selects transition actions within a stack-buffer framework. The stack contains the sequence of generated partial DRSs, while the buffer stores incoming words. Transition actions either consume a word in the buffer or merge two partial DRSs to a new DRS. The system terminates when all words are consumed, and only one item remains on top of the stack.
Neural-Boxer is an LSTM-based neural sequence-to-sequence model that outputs DRSs in clause format (van Noord et al. 2018b ).
MultiEnc (van Noord, Toral, and Bos 2019 ) extends Neural-Boxer with multiple encoders representing grammatical (e.g., parts of speech) and syntactic information (e.g., dependency parses). It also outputs DRSs in clause format.
Cls/Tree-Transformer is the Transformer model from Section 4.3 ; it outputs DRSs in clause and tree format using the box-to-tree conversion algorithm introduced in Section 3.2 . For the sake of completeness, we also re-implement LSTM models trained on clauses and trees ( Cls/Tree-LSTM ).
Cls/Tree-m2o uses the many-to-one method to translate non-English sentences into English and parse them using an English Transformer trained on clauses or trees.
Cls/Tree-o2m applies the one-to-many method to construct training data in the target languages for training clause and tree Transformer models.
We first present results on DRS parsing in order to assess the performance of our model on its own and whether differences in the format of the DRS representations make any difference. Having settled the question of which format to use, we next report experiments on 𝕌DRS parsing.
6.1 DRS Parsing
Table 1 summarizes our results on DRS parsing. As can be seen, neural models overwhelmingly outperform comparison baselines. Transformers trained on trees and clauses perform better (by 4.0 F 1 and 4.4 F 1 , respectively) than LSTMs trained on data in the same format. A Transformer trained on trees performs slightly better (by 0.8 F 1 ) than the same model trained on clauses and is overall best among models using DRS-based representations.
English DRS parsing (PMB 2.2.0 test set); results for SPAR, SIM-SPAR, BOXER, Transition, Graph, Neural-BOXER, and MultiEnc are taken from respective papers; best result per metric shown in bold.
Our results on the DRS crosslingual setting are summarized in Table 2 . Many-to-one parsers outperform one-to-many ones, although, the difference is starker for Clauses than for Trees (5.3 vs. 4.6 F 1 points). With the many-to-one strategy, Tree-based representations are overall slightly better on DRS parsing.
DRS parsing results on German, Italian, and Dutch (PMB test set); best result per metric shown in bold.
6.2 𝕌DRS Parsing
Table 3 summarizes our results on the 𝕌DRS monolingual setting. We observe that a Transformer model trained on tree-based representations is better (by 1.3 F 1 ) compared to the same model trained on clauses. This suggests that 𝕌DRS parsing indeed benefits more from tree representations.
English 𝕌DRS parsing results (PMB test set); best result per metric is shown in bold.
Our results on the 𝕌DRS crosslingual setting are shown in Table 4 . Aside from 𝕌DRS parsers trained with the one-to-many strategy (Cls-o2m and Tree-o2m), we also report the performance of monolingual Transformers (clause and tree formats) trained on the silver and bronze standard data sets provided in PMB. All models were evaluated on the gold standard PMB test data. We only report the performance of one-to-many 𝕌DRS parsers because of the post-processing issue discussed in Section 4.2 . Compared to models trained on silver and bronze data, the one-to-many strategy improves performance for both clause and tree formats (by 5.7 F 1 and 5.7 F 1 , on average). Overall, the crosslingual experiments show that we can indeed bootstrap fairly accurate semantic parsers across languages, without any manual annotations on the target language.
𝕌DRS parsing results on German, Italian, and Dutch (PMB test set); best result per metric shown in bold.
6.3 Analysis
In this section, we analyze in more detail the output of our parsers in order to determine which components of the semantic representation are modeled best. We also examine the effect of the iterative training on parsing accuracy.
Fine-grained Evaluation.
C ounter (van Noord et al. 2018a ) provides detailed break-down scores for DRS operators (e.g., negation), Roles (e.g., Agent), Concepts (i.e., predicates), and Synsets (e.g., “n.01”). Table 5 compares the output of our English semantic parsers. For DRS representations, Tree models perform better than Clauses on most components except for adjective and adverb synsets. All models are better at predicting noun senses compared to verbs, adjectives, and adverbs. The clause format is better when it comes to predicting the senses of adverbs. Nevertheless, all models perform poorly on adverbs, which are relatively rare in the PMB. In our crosslingual experiments, we observe that Tree models slightly outperform Clauses across languages. For the sake of brevity, Table 6 only reports a break-down of the results for Trees. Interestingly, we see that the bootstrapping strategies proposed here are a better alternative to just training semantic parsers on PMB’s silver and bronze data (see Tree column in Table 6 ). Moreover, the success of the bootstrapping strategy seems to be consistent among languages, with many-to-one being overwhelmingly better than one-to-many, even though many-to-one fails to predict the adverb synset. Overall, the prediction of synsets is a harder task and indeed performance improves when the model only focuses on operators and semantic roles (compare Tree and Tree-o2m columns in DRS and 𝕌DRS). Without incorporating sense distinctions, 𝕌DRSs are relatively easier to predict. The vocabulary of the meaning constructs is smaller, and it only includes global symbols like semantic role names and DRS operators that are shared across languages, thus making the parsing task simpler.
Fine-grained evaluation (F 1 ) on the English PMB test set by Cls/Tree-Transformer and Cls/Tree-LSTM. Best result per meaning construct shown in bold.
Fine-grained evaluation (F 1 %) on German, Italian, and Dutch (test set); best result per synset shown in bold.
Iterative Training.
Figure 7 shows how prediction accuracy varies with the quality of the training data. The black dotted curve shows the accuracy of a model trained on the combination of bronze-, silver-, and gold-standard data ( D 0 + D 1 + D 2 ), the red dashed curve shows the accuracy of a model trained on the silver- and gold-standard data ( D 1 + D 2 ), and the blue curve shows the accuracy of a model trained only on gold-standard data ( D 2 ). As can be seen, the use of more data leads to a big performance boost (compare the model trained on D 0 + D 1 + D 2 against just D 2 ). We also show what happens after the model converges on D 0 + D 1 + D 2 : Further iterations on D 1 + D 2 slightly improve performance, while a big boost is gained from continually training on gold-standard data ( D 2 ). The relatively small gold-standard data is of high quality but has low coverage; parameter optimization on the combination of bronze-, silver- and gold-standard data enhances model coverage, while fine-grained optimization on gold-standard data increases its accuracy.

The effect of iterative training on model performance (Tree-Transformer, English development set).
Table 7 presents our results, which are clustered according to language family (we report only the performance of Tree 𝕌DRS models for the sake of brevity). All models for all languages used the same settings (see Section 5.2). As can be seen, the majority of languages we experimented with are Indo-European. In this family, the highest F 1 is 81.92 for Danish and Yiddish. In the Austronesian family, our parser performs best for Indonesian (F 1 is 78.20). In the Afro-Asiatic family, Vietnamese achieves the highest F 1 of 79.37. In the Niger-Congo family, the highest F 1 is 77.21 for Swahili. In the Turkic family, our parser performs best for Turkish (F 1 is 74.39). In the Dravidian and Uralic families, Kannada and Estonian obtain the highest F 1 , respectively. The worst parsing performance is obtained for Khmer (F 1 of 61.53), while for the majority of languages our parser is in the 71%–82% ballpark. Perhaps unsurprisingly, better parsing performance correlates with a higher quality of machine translation and statistical word alignments. 14
𝕌DRS parsing results via Tree-o2m for 96 languages individually and on average; languages are grouped per language family and sorted alphabetically; best results in each family are shown in bold.
6.4 Scalability Experiments
We further assessed whether the crosslingual approach advocated in this article scales to multiple languages. We thus obtained 𝕌DRS parsers for all languages supported by Google Translate in addition to German, Italian, and Dutch (99 in total). Specifically, we applied the one-to-many bootstrapping method on the English gold-standard PMB annotations to obtain semantic parsers for 96 additional languages.
Unfortunately, there are no gold-standard annotations to evaluate the performance of these parsers and the effort of creating these for 99 languages would be prohibitive. Rather than focusing on a few languages for which annotations could be procured, we adopt a more approximate but larger-scale evaluation methodology. Damonte and Cohen ( 2018 ) estimate the accuracy of crosslingual AMR parsers following a full-cycle evaluation scheme. The idea is to invert the learning process and bootstrap an English parser from the induced crosslingual parser via back-translation. The resulting English parser is then evaluated against the (English) gold-standard under the assumption that the English parser can be used as a proxy to the score of the crosslingual parser. In our case, we applied the one-to-many method to project non-English annotations back to English, and evaluated the parsers on the PMB gold-standard English test set.
We further investigated the quality of the constructed data sets by extrapolating from experiments on German, Italian, and Dutch for which a gold-standard test set is available. Specifically, using the one-to-many method, we constructed silver-standard test sets and compared these with their gold-standard counterparts provided in the PMB. We first assessed translation quality by measuring the BLEU score (Papineni et al. 2002 ). We also used Counter to evaluate the degree to which silver-standard 𝕌DRSs deviate from gold-standard ones. As shown in Table 8 , the average BLEU (across three languages) is 65.12, while the average F 1 given by Counter is 92.23. These results indicate that the translation quality is rather good, at least for these three languages, and the PMB sentences. Counter scores further show that annotations are transferred relatively accurately, and that silver-standard data is not terribly noisy, where approximately 8% of the annotations deviate from the gold standard.
Comparison between gold-standard 𝕌DRSs and constructed 𝕌DRSs by our methods in German, Italian, and Dutch using BLEU and Counter; standard deviations are shown in parentheses.
In Table 4 , we show the crosslingual 𝕌DRS parsing results on the gold-standard test set in German, Italian, and Dutch, which are close to English. In order to investigate the crosslingual 𝕌DRS parsing in languages that are far from English, we performed 𝕌DRS parsing experiments in Japanese and Chinese, two languages that are typologically distinct from English, in the way concepts are expressed and combined by grammar to generate meaning. For each language, we manually constructed gold standard 𝕌DRS annotations for 50 sentences. Table 9 shows the accuracy of the Chinese and Japanese parsers we obtained following the one-to-many training approach. These two languages have relatively lower scores compared to German, Italian, and Dutch in Table 4 . Our results highlight that translation-based crosslingual methods will be less accurate for target languages with large typological differences from the source.
𝕌DRS parsing results on gold-standard Japanese (ja) and Chinese (zh) test sets.
6.5 Translation Divergence
Our crosslingual methods depend on machine translation and alignments, which can be affected by translation divergences. In this section, we discuss how translation divergences might influence our methods. We focus on the seven types of divergence highlighted in Dorr ( 1994 ) (i.e., promotional, demotional, structural, conflational, categorical, lexical, and thematic divergences) and discuss whether the proposed 𝕌DRS representation can handle them.
Promotional Divergence.
Promotional divergence describes the phenomenon where the logical modifier of a main verb can be changed. For example, consider the English sentence John usually goes home and its Spanish translation Juan suele ir a casa (John tends to go home), where the modifier ( usually ) is realized as an adverbial phrase in English but as a verb ( sueler ) in Spanish. As shown in Figure 8 , to obtain the Spanish 𝕌DRS, the English words are replaced with aligned words. However, the adverbial usually is replaced with the verb suele , which together with the thematic relation, Manner qualifies how the action ir is carried out. The divergence will raise a Category Inconsistency in the 𝕌DRS, which means that the category (or part of speech) of the translation is not consistent with that of the source language.

Example of promotional divergence. (a) 𝕌DRS of English sentence John usually goes home ; (b) word alignments between the two sentences; (c) Incorrect 𝕌DRS of the Spanish translation Juan suele ir a casa , constructed via alignments.
Demotional Divergence.
In demotional divergence, a logical head into an internal argument position can be changed. For example, consider the English sentence I like eating and its German translation Ich esse gern (I eat likingly). Here, the head ( like ) is realized as a verb in English but as an adverbial satellite in German. Figure 9 shows the alignments between the two sentences and their 𝕌DRSs. Similar to promotional divergence, this also leads to Category Inconsistency in the German 𝕌DRS, since gern should be an adverb, not a verb.

Example of demotional divergence. (a) 𝕌DRS of English sentence I like eating ; (b) word alignments between two sentences; (c) incorrect 𝕌DRS of German translation Ich esse gern , constructed via alignments.
Structural Divergence.
Structural divergences are different in that syntactic structure is changed and, as a result, syntactic relations may also become different. For example, for the English sentence John entered the house , the Spanish translation is Juan entró en la casa (John entered in the house), where the noun phrase the house in English becomes a prepositional phrase ( en la casa ) in Spanish. This divergence does not affect the correctness of the Spanish 𝕌DRS (see Figure 10 ). In general, 𝕌DRSs display coarse-grained thematic relations (in this case Destination) abstracting away from how these are realized (e.g., as a noun or prepositional phrase).

Examples of structural divergence. (a) 𝕌DRS of English sentence John entered the house ; (b) word alignments between two sentences; (c) correct 𝕌DRS of Spanish translation Juan entró en la casa , constructed via alignments.
Conflational and Lexical Divergence.
We discuss both types of divergence together. Words or phrases in the source language can be paraphrased using various descriptions in the target language. In conflational divergence, for example, the English sentence I stabbed John is translated into Spanish as Yo le di puñaladas a Juan (I gave knife-wounds to John) using the paraphrase di puñaladas (gave knife-wounds to) to describe the English word stabbed . Analogously, the word broke in the English sentence He broke into the room is aligned to forzó (force) in Spanish ( Juan forzó la entrada al cuarto ). The two words do not have exactly the same meaning, and yet they convey the breaking event in their respective language. We expect these divergences to be resolved with many-to-many word alignments, and yield correct 𝕌DRSs as long as the translations are accurate (see Figures 11 and 12 ).

Example of conflational divergence. (a) 𝕌DRS of English sentence I stabbed John ; (b) word alignments between two sentences; (c) correct 𝕌DRS of Spanish translation Yo le di puñaladas a Juan , constructed via alignments.

Example of lexical divergence. (a) 𝕌DRS of English sentence John broke into the room ; (b) word alignments between two sentences; (c) correct 𝕌DRS of Spanish translation Juan forzó la entrada al cuarto , constructed via alignments.
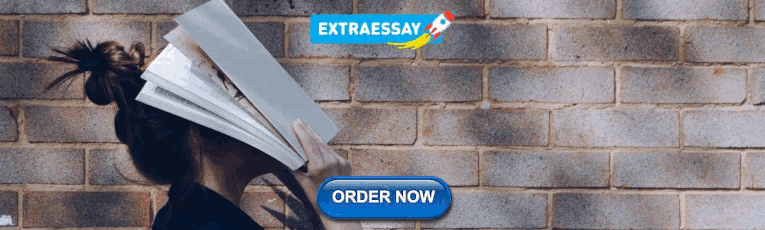
Categorical Divergence.
The lexical categories (or parts of speech) might change due to the translation from the source to the target language. For example, the English sentence I am hungry is translated to German as Ich habe Hunger (I have hunger), where the adjective hungry in English is translated with the noun Hunger in German. As shown in Figure 13 , categorical divergences will often lead to incorrect 𝕌DRSs in the target language.

Example of categorical divergence. (a) 𝕌DRS of English sentence I am hungry ; (b) word alignments between two sentences; (c) incorrect 𝕌DRS of German translation Ich habe Hunger , constructed via alignments.
Thematic Divergence.
Thematic relations are governed by the main verbs of sentences, and it is possible for thematic roles to change in translation. For example, the English sentence I like Mary is translated in Spanish as María me gusta a mí (Mary pleases me), where the English subject ( I ) is changed to an object ( me ) in Spanish. As shown in Figure 14 , although word alignments can capture the semantic correspondence between words in the two sentences, the Spanish 𝕌DRS ends up with the wrong thematic relations showing Thematic Inconsistency .

Example of thematic divergence. (a) 𝕌DRS of English sentence I like Mary ; (b) word alignments between two sentences; (c) incorrect 𝕌DRS of Spanish translation María me gusta a mí , constructed via alignments.
In sum, category and thematic inconsistencies will represent the majority of errors in the construction of 𝕌DRSs in another language from English (via translation and alignments). Category inconsistencies can be addressed with the help of language-specific knowledge bases by learning a function f ( s , c ) = ( s′ , c′ ), where s and c are a translated word and an original category, respectively, and s′ and c′ are the corrected word and category. Addressing thematic inconsistencies is difficult, as it requires comparing verbs between languages in order to decide whether thematic relations must be changed.
In order to estimate how many 𝕌DRSs are incorrect and quantify what types of errors they display, we randomly sampled 50 German, Italian, and Dutch 𝕌DRSs. As shown in Table 10 , we found that alignment errors are the main cause of incorrect 𝕌DRSs. Translation divergences do not occur very frequently, even though we used machine translation systems. We also sampled and analyzed 50 𝕌DRSs in Chinese, a language typologically very different from English. Again, the number of translation divergences is small, which may be due to the fact that sentences in PMB are short and thus relatively simple to translate.
Number of correct and incorrect 𝕌DRSs on a sample of 50 sentences for German, Italian, Dutch, andChinese.
Recent years have seen growing interest in the development of DRT parsing models. Early seminal work (Bos 2008 ) created Boxer, an open-domain DRS semantic parser, which has been instrumental in enabling the development of the Groningen Meaning Bank (Bos et al. 2017 ) and the Parallel Meaning Bank (Abzianidze et al. 2017 ).
Le and Zuidema ( 2012 ) were the first to train a data-driven DRT parser using a graph-based representation leaving anaphora and presupposition aside. The availability of annotated corpora has further allowed the exploration of neural models. Liu, Cohen, and Lapata ( 2018 ) conceptualize DRT parsing as a tree structure prediction problem that they model with a series of encoder-decoder architectures (see also the extensions proposed in Liu, Cohen, and Lapata 2019a ). van Noord et al. ( 2018b ) adapt sequence-to-sequence models with LSTM units to parse DRSs in clause format, also following a graph-based representation. Fancellu et al. ( 2019 ) represent DRSs as direct acyclic graphs and design a DRT parser with an encoder-decoder architecture that takes as input a sentence and outputs a graph using a graph-to-string rewriting system. In addition, their parser exploits various linguistically motivated features based on part-of-speech embeddings, lemmatization, dependency labels, and semantic tags. Our crosslingual strategies can be applied to their work as well. So our own work unifies the proposals of Liu, Cohen, and Lapata ( 2018 ) and van Noord et al. ( 2018b ) under a general modeling framework based on the Transformer architecture, allowing for comparisons between the two, as well as for the development of crosslingual parsers. In addition, we introduce 𝕌DRT, a variant of the DRT formalism that we argue facilitates both monolingual and crosslingual learning.
The idea of leveraging existing English annotations to overcome the resource shortage in other languages by exploiting translational equivalences is by no means new. A variety of methods have been proposed in the literature under the general framework of annotation projection (Yarowsky and Ngai 2001 ; Hwa et al. 2005 ; Padó and Lapata 2005 , 2009 ; Akbik et al. 2015 ; Evang and Bos 2016 ; Damonte and Cohen 2018 ; Zhang et al. 2018 ; Conneau et al. 2018 ), which focuses on projecting existing annotations on source-language text to the target language. While other work focuses on model transfer where model parameters are shared across languages (Cohen, Das, and Smith 2011 ; McDonald, Petrov, and Hall 2011 ; Søgaard 2011 ; Wang and Manning 2014 ), our crosslingual parsers rely on translation systems following two ways commonly adopted in the literature (Conneau et al. 2018 ; Yang et al. 2019 ; Huang et al. 2019 ): translating the training data into each target language (one-to-many) to provide data to train a semantic parser per language, and using a translation system at test time to translate the input sentences to the training language (many-to-one). Our experiments show that the combination of one-to-many and 𝕌DRS representations allows us to speed-up meaning bank creation and the annotation process.
In this article, we introduced 𝕌niversal Discourse Representation Structures as a variant of canonical DRSs; 𝕌DRSs link elements of the DRS structure to tokens in the input sentence and are ideally suited to crosslingual learning; they omit details pertaining to the lexical makeup of sentences and as a result disentangle the problems of translating tokens and semantic parsing. We further proposed a general framework for crosslingual learning based on neural networks and state-of-the-art machine translation and demonstrated it can incorporate various DRT formats (e.g., trees vs. clauses) and is scalable. In the future, we would like to improve the annotation quality of automatically created meaning banks by utilizing human-in-the-loop methods (Zanzotto 2019 ) that leverage machine learning algorithms (e.g., for identifying problematic annotations or automatically correcting obvious mistakes) or crowdsourcing platforms.
We thank the anonymous reviewers for their feedback. We thank Alex Lascarides for her comments. We gratefully acknowledge the support of the European Research Council (Lapata, Liu; award number 681760), the EU H2020 project SUMMA (Cohen, Liu; grant agreement 688139) and Bloomberg (Cohen, Liu). This work was partly funded by the NWO-VICI grant “Lost in Translation – Found in Meaning” (288-89-003).
Details on the IWCS 2019 shared task on Discourse Representation Structure parsing can be found at https://sites.google.com/view/iwcs2019/home .
We use the term many-to-one to emphasize the fact that a semantic parser is trained only once (e.g., in English). In the one-to-many setting, multiple semantic parsers are trained, one per target language. The terms are equivalent to “translate test” (many-to-one) and “translate train” (one-to-many) used in previous work (Conneau et al. 2018 ).
Presupposition is the phenomenon whereby speakers mark linguistically the information that is presupposed or taken for granted rather than being part of the main propositional content of an utterance (Beaver and Guerts 2014 ). Expressions and constructions carrying presuppositions are called “presupposition triggers,” forming a large class including definites and factive verbs.
http://globalwordnet.org/about-gwa/ .
http://globalwordnet.org/resources/wordnets-in-the-world/ .
https://www.wikidata.org/wiki/Wikidata:Main_Page .
For the full list of DRS clauses, see https://pmb.let.rug.nl/drs.php .
Each variable has exactly one bounding box.
We refer to Bos et al. ( 2017 ) for more details on basic and complex conditions in DRS boxes.
https://translate.google.com/toolkit .
Two sentences were discarded for German and 55 for Italian.
𝕌DRSs replace language-specific symbols with anchors without, however, changing the structure of the meaning representation in any way; 𝕌DRSs can be evaluated with Counter in the same way as DRSs.
In PMB (release 2.2.0) this is the DRS for the sentence Tom voted for himself.
We will make the 𝕌DRS data sets for the 96 languages publicly available as a means of benchmarking semantic parsing performance and also in the hope that some of these might be manually corrected.
Email alerts
Related articles, related book chapters, affiliations.
- Online ISSN 1530-9312
- Print ISSN 0891-2017
A product of The MIT Press
Mit press direct.
- About MIT Press Direct
Information
- Accessibility
- For Authors
- For Customers
- For Librarians
- Direct to Open
- Open Access
- Media Inquiries
- Rights and Permissions
- For Advertisers
- About the MIT Press
- The MIT Press Reader
- MIT Press Blog
- Seasonal Catalogs
- MIT Press Home
- Give to the MIT Press
- Direct Service Desk
- Terms of Use
- Privacy Statement
- Crossref Member
- COUNTER Member
- The MIT Press colophon is registered in the U.S. Patent and Trademark Office
This Feature Is Available To Subscribers Only
Sign In or Create an Account
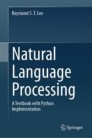
Natural Language Processing pp 95–113 Cite as
Meaning Representation
- Raymond S. T. Lee 2
- First Online: 15 November 2023
923 Accesses
Before the study of semantic analysis, this chapter explores meaning representation, a vital component in NLP before the discussion of semantic and pragmatic analysis. It studies four major meaning representation techniques which include: first-order predicate calculus (FOPC), semantic net, conceptual dependency diagram (CDD), and frame-based representation. After that it explores canonical form and introduces Fillmore’s theory of universal cases followed by predicate logic and inference work using FOPC with live examples.
This is a preview of subscription content, log in via an institution .
Buying options
- Available as PDF
- Read on any device
- Instant download
- Own it forever
- Available as EPUB and PDF
- Durable hardcover edition
- Dispatched in 3 to 5 business days
- Free shipping worldwide - see info
Tax calculation will be finalised at checkout
Purchases are for personal use only
Bender, E. M. (2013) Linguistic Fundamentals for Natural Language Processing: 100 Essentials from Morphology and Syntax (Synthesis Lectures on Human Language Technologies). Morgan & Claypool Publishers
Book Google Scholar
Bender, E. M. and Lascarides, A. (2019) Linguistic Fundamentals for Natural Language Processing II: 100 Essentials from Semantics and Pragmatics (Synthesis Lectures on Human Language Technologies). Springer.
Google Scholar
Best, W., Bryan, K. and Maxim, J. (2000) Semantic Processing: Theory and Practice. Wiley.
Blackburn, P and Bos, J. (2005) Representation and Inference for Natural Language: A First Course in Computational Semantics (Studies in Computational Linguistics). Center for the Study of Language and Information.
Bunt, H. (2013) Computing Meaning: Volume 4 (Text, Speech and Language Technology Book 47). Springer.
Butler, A. (2015) Linguistic Expressions and Semantic Processing: A Practical Approach. Springer.
Book MATH Google Scholar
Cui, Y., Huang, C., Lee, Raymond (2020). AI Tutor: A Computer Science Domain Knowledge Graph-Based QA System on JADE platform. World Academy of Science, Engineering and Technology, Open Science Index 168, International Journal of Industrial and Manufacturing Engineering, 14(12), 543 - 553.
Dijkstra, E. W. and Scholten, C. S. (1989) Predicate Calculus and Program Semantics (Monographs in Computer Science). Springer. Advanced Reasoning Forum.
Doyle, A. C. (2019) The Adventures of Sherlock Holmes (AmazonClassics Edition). AmazonClassics.
Epstein, R. (2012) Predicate Logic. Advanced Reasoning Forum.
Fillmore, C. J. (1968) The Case for Case. In Bach and Harms (Ed.): Universals in Linguistic Theory. New York: Holt, Rinehart, and Winston, 1-88.
Fillmore, C. J. (2020) Form and Meaning in Language, Volume III: Papers on Linguistic Theory and Constructions (Volume 3). Center for the Study of Language and Information.
Goddard, C. (1998) Semantic Analysis: A Practical Introduction (Oxford Textbooks in Linguistics). Oxford University Press.
Goldrei, D. (2005) Propositional and Predicate Calculus: A Model of Argument. Springer.
MATH Google Scholar
Jackson, P. C. (2019) Toward Human-Level Artificial Intelligence: Representation and Computation of Meaning in Natural Language (Dover Books on Mathematics). Dover Publications.
Mazarweh, S. (2010) Fillmore Case Grammar: Introduction to the Theory. GRIN Verlag.
Minsky, M. (1975). A framework for representing knowledge. In P. Winston, Ed., The Psychology of Computer Vision. New York: McGraw-Hill, pp. 211-277.
Parry, W. T. and Hacker, E. A. (1991) Aristotelian logic. Suny Press.
Potts, T. C. (1994) Structures and Categories for the Representation of Meaning. Cambridge University Press.
Schank, R. C. (1972). Conceptual dependency: A theory of natural language processing. Cognitive Psychology, 3, 552–631.
Article Google Scholar
Sowa, J. (1991) Principles of Semantic Networks: Explorations in the Representation of Knowledge (Morgan Kaufmann Series in Representation and Reasoning). Morgan Kaufmann Publication.
Download references
Author information
Authors and affiliations.
United International College, Beijing Normal University-Hong Kong Baptist University, Zhuhai, China
Raymond S. T. Lee
You can also search for this author in PubMed Google Scholar
Rights and permissions
Reprints and permissions
Copyright information
© 2024 The Author(s), under exclusive license to Springer Nature Singapore Pte Ltd.
About this chapter
Cite this chapter.
Lee, R.S.T. (2024). Meaning Representation. In: Natural Language Processing. Springer, Singapore. https://doi.org/10.1007/978-981-99-1999-4_5
Download citation
DOI : https://doi.org/10.1007/978-981-99-1999-4_5
Published : 15 November 2023
Publisher Name : Springer, Singapore
Print ISBN : 978-981-99-1998-7
Online ISBN : 978-981-99-1999-4
eBook Packages : Computer Science Computer Science (R0)
Share this chapter
Anyone you share the following link with will be able to read this content:
Sorry, a shareable link is not currently available for this article.
Provided by the Springer Nature SharedIt content-sharing initiative
- Publish with us
Policies and ethics
- Find a journal
- Track your research

You can help transform the criminal legal system.
Donate now to end inhumane conditions for people behind bars.
Vera Institute of Justice
Advancing universal representation.

In an era of unprecedented and aggressive immigration enforcement, attacks on the immigration system, and anti-immigrant rhetoric, millions of people across the United States are at risk of long-term detention and deportation.
Since 1996, the average daily population of detained immigrants has ballooned from around 9,000 to more than 51,000 projected by the end of 2018. [ ] 1996 figure from Alison Siskin, Immigration-Related Detention: Current Legislative Issues (Washington, DC: Congressional Research Service, 2004), 12 & figure 1, https://perma.cc/R4VN-L7YV . 2018 figure from U.S. Department of Homeland Security (DHS), U.S. Immigration and Customs Enforcement Budget Overview: Fiscal Year 2019 Congressional Justification (Washington, DC: DHS, 2018), 5, https://perma.cc/98A8-EBZY . This dramatic increase in detention is the result of stepped-up enforcement and is the latest in a decades-long push to criminalize immigration, placing immigrant communities under significant duress. Meanwhile, the federal government continues to ratchet up its demands for more detention funding in both private facilities and in contracts with local jails. [ ] See DHS, U.S. Immigration and Customs Enforcement Budget Overview: FY 2019 , 2018.
In response to the visible community impacts of these policies, a growing movement of local, state, and national advocates and leaders are pioneering strategies and policies to protect the rights of immigrants in their neighborhoods. This toolkit offers one proven solution for those who wish to strengthen protections for immigrants: universal legal representation for all at imminent risk of deportation.
While people accused of crimes are entitled to government-funded counsel to assist in their defense, immigrants facing deportation are not.
Despite the high stakes involved when individuals face deportation—permanent separation from their families and their communities, and sometimes life-threatening risks in their countries of origin—immigrants are only entitled to representation paid for by the government in extremely limited circumstances. [ ] Under a court order in the 9 th Circuit, certain detained immigrants who have been deemed mentally incompetent to represent themselves must be provided with counsel. See Franco-Gonzalez v. Holder, 767 F. Supp. 2d 1034, 1056-58 (C.D. Cal 2010).
As a result, many immigrants go unrepresented, facing detention and deportation alone.
In contrast, providing publicly funded universal representation for anyone in immigration proceedings who cannot afford a lawyer (akin to public defense in criminal cases) protects the widely shared American values of due process and fairness. It has also proven highly effective against extended detention and deportation. [ ] For a summary of this research, see Karen Berberich and Nina Siulc, Why Does Representation Matter? (New York: Vera Institute of Justice, 2018), https://perma.cc/NTM6-F8UN . Universal representation injects fairness into the system by giving immigrants the opportunity to access the rights they are entitled to under U.S. law. Universal representation is a crucial last line of defense to keep families and communities together.
The Center for Popular Democracy (CPD), the National Immigration Law Center (NILC), and the Vera Institute of Justice (Vera) work together to expand the national movement for publicly funded universal representation. CPD and NILC provide strategic support to local and state advocacy campaigns. In 2017, Vera launched the Safety and Fairness for Everyone (SAFE) Network in partnership with a diverse group of local jurisdictions, all dedicated to providing publicly funded representation for people facing deportation. Through the SAFE Network, Vera provides strategic support to government partners, legal services providers, and advocates. Collectively, CPD, NILC, and Vera also coordinate at a national level, creating resources and space for advocates advancing universal representation to share, strategize, and learn from one another.
Using This Toolkit
This toolkit is informed by CPD, NILC, and Vera’s experiences advancing the universal representation movement.
These experiences have been guided by the expertise of advocates, organizers, legal services providers, and policymakers across the country who have led publicly funded deportation defense efforts.
The toolkit is intended to equip these stakeholders—advocates, organizers, legal services providers, and policymakers—with strategies to make the case for implementing and sustaining universal representation programs.
The toolkit consists of three parts:
• Module 1 makes the case for universal representation and describes the importance of implementing the universal representation model, even when it starts out on a small scale.
• Module 2 outlines how to build an advocacy campaign advancing universal representation.
• Module 3 describes best practices for implementing local and state universal representation programs and profiles different jurisdictions’ programs.
The Case for Universal Representation
Building the movement, implementing the vision.
On Learning Universal Representations Across Languages
Xiangpeng wei , rongxiang weng , yue hu , luxi xing , heng yu , weihua luo, send feedback.
Enter your feedback below and we'll get back to you as soon as possible. To submit a bug report or feature request, you can use the official OpenReview GitHub repository: Report an issue
BibTeX Record
Not logged in
- Create account
Universal representation (C*-algebra)
Page actions.
- View source
In the theory of C*-algebras , the universal representation of a C*-algebra is a faithful representation which is the direct sum of the GNS representations corresponding to the states of the C*-algebra. The various properties of the universal representation are used to obtain information about the ideals and quotients of the C*-algebra. The close relationship between an arbitrary representation of a C*-algebra and its universal representation can be exploited to obtain several criteria for determining whether a linear functional on the algebra is ultraweakly continuous. The method of using the properties of the universal representation as a tool to prove results about the C*-algebra and its representations is commonly referred to as universal representation techniques in the literature.
- 1.1 States of Φ( A )
- 1.2 Bounded functionals of Φ( A )
- 1.3 Ideals of Φ( A )
- 1.4 Representations of A
- 1.5 Ultraweakly continuous, and singular components
- 2.1 Christensen–Haagerup principle
- 3 References
Formal definition and properties
As the universal representation is faithful, A is *-isomorphic to the C*-subalgebra Φ( A ) of B(H Φ ) .
States of Φ( A )
With τ a state of A , let π τ denote the corresponding GNS representation on the Hilbert space H τ . Using the notation defined here , τ is ω x ∘ π τ for a suitable unit vector x (= x τ ) in H τ . Thus τ is ω y ∘ Φ, where y is the unit vector Σ ρ∈ S ⊕ y ρ in H Φ , defined by y τ =x, y ρ =0(ρ≠τ). Since the mapping τ → τ ∘ Φ −1 takes the state space of A onto the state space of Φ( A ), it follows that each state of Φ( A ) is a vector state .
Bounded functionals of Φ( A )
Let Φ( A ) − denote the weak-operator closure of Φ( A ) in B(H Φ ) . Each bounded linear functional ρ on Φ( A ) is weak-operator continuous and extends uniquely preserving norm, to a weak-operator continuous linear functional ρ on the von Neumann algebra Φ( A ) − . If ρ is hermitian, or positive, the same is true of ρ . The mapping ρ → ρ is an isometric isomorphism from the dual space Φ( A ) * onto the predual of Φ( A ) − . As the set of linear functionals determining the weak topologies coincide, the weak-operator topology on Φ( A ) − coincides with the ultraweak topology. Thus the weak-operator and ultraweak topologies on Φ( A ) both coincide with the weak topology of Φ( A ) obtained from its norm-dual as a Banach space.
Ideals of Φ( A )
If K is a convex subset of Φ( A ), the ultraweak closure of K (denoted by K − )coincides with the strong-operator, weak-operator closures of K in B(H Φ ) . The norm closure of K is Φ( A ) ∩ K − . One can give a description of norm-closed left ideals in Φ( A ) from the structure theory of ideals for von Neumann algebras, which is relatively much more simple. If K is a norm-closed left ideal in Φ( A ), there is a projection E in Φ( A ) − such that
If K is a norm-closed two-sided ideal in Φ( A ), E lies in the center of Φ( A ) − .
Representations of A
If π is a representation of A , there is a projection P in the center of Φ( A ) − and a *-isomorphism α from the von Neumann algebra Φ( A ) − P onto π( A ) − such that π( a ) = α(Φ( a ) P ) for each a in A . This can be conveniently captured in the commutative diagram below :
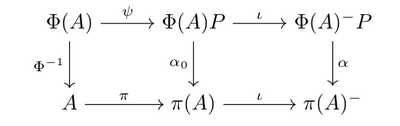
Here ψ is the map that sends a to aP , α 0 denotes the restriction of α to Φ( A ) P , ι denotes the inclusion map.
As α is ultraweakly bicontinuous, the same is true of α 0 . Moreover, ψ is ultraweakly continuous, and is a *-isomorphism if π is a faithful representation.
Ultraweakly continuous, and singular components
Let A be a C*-algebra acting on a Hilbert space H . For ρ in A * and S in Φ( A ) − , let S ρ in A * be defined by S ρ( a ) = ρ∘Φ −1 (Φ( a )S) for all a in A . If P is the projection in the above commutative diagram when π: A → B(H) is the inclusion mapping, then ρ in A * is ultraweakly continuous if and only if ρ = P ρ. A functional ρ in A * is said to be singular if P ρ = 0. Each ρ in A * can be uniquely expressed in the form ρ=ρ u +ρ s , with ρ u ultraweakly continuous and ρ s singular. Moreover, ||ρ||=||ρ u ||+||ρ s || and if ρ is positive, or hermitian, the same is true of ρ u , ρ s .
Applications
Christensen–haagerup principle.
Let f and g be continuous, real-valued functions on C 4m and C 4n , respectively, σ 1 , σ 2 , ..., σ m be ultraweakly continuous, linear functionals on a von Neumann algebra R acting on the Hilbert space H , and ρ 1 , ρ 2 , ..., ρ n be bounded linear functionals on R such that, for each a in R ,
Then the above inequality holds if each ρ j is replaced by its ultraweakly continuous component (ρ j ) u .
- Kadison, Richard, Fundamentals of the Theory of Operator Algebras, Vol. I : Elementary Theory , American Mathematical Society. ISBN :978-0821808191.
- Kadison, Richard, Fundamentals of the Theory of Operator Algebras, Vol. II : Advanced Theory , American Mathematical Society. ISBN :978-0821808207.
- "On an inequality of Haagerup–Pisier" , Journal of Operator Theory 29 (1): 57–67, 1993 , http://www.theta.ro/jot/archive/1993-029-001/1993-029-001-004.html .
- Operator algebras
- C*-algebras
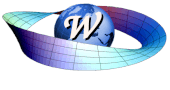
- Add a new article
- Search in all topics
- Search in namespaces
- Search in categories
- Search using prefix
- About HandWiki
- How to edit
- Citation manager
- Formatting articles
- List of categories
- Recent pages
- Recent changes
- Random page
- Support & Donate
- Special pages
- Cite this page
User page tools
- What links here
- Related changes
- Printable version
- Permanent link
- Page information
Other projects
In other languages.

- This page was last edited on 16 November 2021, at 10:40.
- Privacy policy
- Disclaimers
- More from M-W
- To save this word, you'll need to log in. Log In
Definition of universal
(Entry 1 of 2)
Definition of universal (Entry 2 of 2)
- all-round
Examples of universal in a Sentence
These examples are programmatically compiled from various online sources to illustrate current usage of the word 'universal.' Any opinions expressed in the examples do not represent those of Merriam-Webster or its editors. Send us feedback about these examples.
Word History
Middle English, from Anglo-French, from Latin universalis , from universum universe
14th century, in the meaning defined at sense 1
15th century, in the meaning defined at sense 1
Phrases Containing universal
- universal donor
- universal motor
- universal resource locator
- universal suffrage
- universal recipient
- universal joint
- universal grammar
Dictionary Entries Near universal
univerbation
universal affirmation
Cite this Entry
“Universal.” Merriam-Webster.com Dictionary , Merriam-Webster, https://www.merriam-webster.com/dictionary/universal. Accessed 24 Mar. 2024.
Kids Definition
Kids definition of universal, legal definition, legal definition of universal, more from merriam-webster on universal.
Nglish: Translation of universal for Spanish Speakers
Britannica English: Translation of universal for Arabic Speakers
Britannica.com: Encyclopedia article about universal
Subscribe to America's largest dictionary and get thousands more definitions and advanced search—ad free!
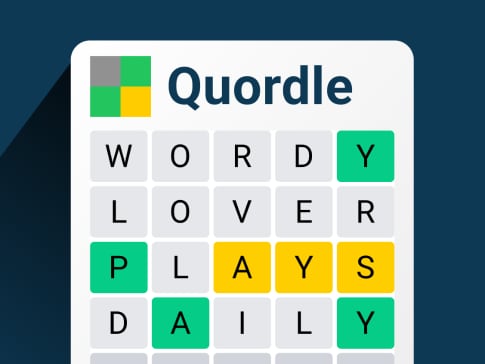
Can you solve 4 words at once?
Word of the day.
See Definitions and Examples »
Get Word of the Day daily email!
Popular in Grammar & Usage
8 grammar terms you used to know, but forgot, homophones, homographs, and homonyms, commonly misspelled words, how to use em dashes (—), en dashes (–) , and hyphens (-), absent letters that are heard anyway, popular in wordplay, the words of the week - mar. 22, 12 words for signs of spring, 9 superb owl words, 'gaslighting,' 'woke,' 'democracy,' and other top lookups, 10 words for lesser-known games and sports, games & quizzes.
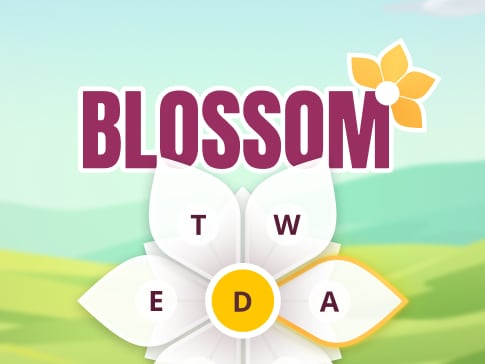
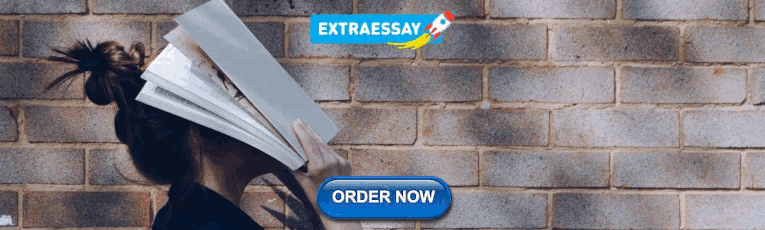
IMAGES
VIDEO
COMMENTS
Universal representation protects against this unfairness. The universal representation model can also help legal service providers attain more balanced caseloads. Cases that lack valid defenses or claims for relief, which demand less time and effort from attorneys, can be resolved quickly and removed from caseloads.
The universal representation system ensures that people with all types of cases and backgrounds have access to expert legal support, preventing prejudice in who receives legal services and promoting fairness for all. Universal representation safeguards due process by allowing all immigrants to access the rights afforded to them under existing law.
In fact, their Advancing Universal Representation initiative has supported numerous programs at the state and local levels. Among those is the New York Immigrant Family Unity Project (NYIFUP), a statewide defender program that provides lawyers for immigrants. It is the first and largest public defender program in the country.
Universal representation injects fairness into the system by giving immigrants the opportunity to access the rights they are entitled to under U.S. law. Universal representation is a crucial last line of defense to keep families and communities together. The Center for Popular Democracy (CPD), the National Immigration Law Center (NILC), and the ...
The universal representation model affects the entire immigration system. While any model of representation provides the opportunity to develop new case law—yielding benefits beyond any one person—it is the ingenuity and dexterity of practice required under universal representation that makes its impact on case law unique.
The universal representation model, brought to the immigration arena in 2013, offered a distinct alternative to the tra-ditional model of merits-focused case selection in immigrant representation.4 It began with the New York Immigrant Rep- resentation Study, a two-part study launched by the Study Group on Immigrant Representation, which was convened by
Using Meaning as Universal Knowledge Representation. Meaning is the building block of human language, uncovered by context. Meaning is core to language because the meaning of a sentence determines ...
In this work we describe various challenges and possible solutions in designing a semantic representation that is universal across a variety of languages. Proceedings of the First International Workshop on Designing Meaning Representations. Huaiyu Zhu, Yunyao Li, and Laura Chiticariu. 2019.
Universal Design for Learning (or UDL) is a way to "improve and optimize teaching and learning for all people based on scientific insights into how humans learn" (CAST, 2015). UDL considers the why, what and how of students' learning. One way to integrate universal design principles into your classroom is to provide learners with multiple ...
a universal meaning representation, and the chal-lenges in resolving these issues. We will describe our tentative solutions and call the attention of the community to these issues. 2 Examples of semantic variations Across different languages, semantic structures are much more uniform than syntactic structures.
Illustration of universal representation learning. In the first stage (a), we learn a task-specific deep network for each task.In the second stage (b), our goal is to learn a multi-task network that shares the feature encoder across all tasks and build multiple task-specific decoders on top of the feature encoder such that it performs well on these tasks compared to task-specific models ...
notations, they must first be able to unlock the meaning that each word or symbol conveys. They are then able to build on this knowledge and create meaning as they learn about sentence structures and mathematical formulas, as well as the meaning of figurative language and idioms. Educators support Guideline 2 by considering the following questions.
A picture or image that carries meaning for some learners may carry very different meanings for learners from differing cultural or familial backgrounds. As a result, inequalities arise when information is presented to all learners through a single form of representation.
growing movement for universal representation. Universal representation programs at a glance Universal representation is predicated on the belief that everyone deserves to be treated with human dignity and respect, including being afforded the right to meaningfully participate in legal proceedings, particularly when their own liberty is at stake.
The basic meaning-carrying units in DRT are Discourse Representation Structures (DRSs), which are recursive, have a model-theoretic interpretation, and can be translated into first-order logic (Kamp and Reyle 1993). DRSs are scoped meaning representations—they capture the semantics of negation, modals, quantification, and presupposition triggers.
We propose a unified look at jointly learning multiple vision tasks and visual domains through universal representations, a single deep neural network. Learning multiple problems simultaneously involves minimizing a weighted sum of multiple loss functions with different magnitudes and characteristics and thus results in unbalanced state of one loss dominating the optimization and poor results ...
However, universal representation among different levels of linguistic units may offer a great convenience when it is needed to handle free text in language hierarchy in a unified way. As well known that, embedding representation for a cer-tain linguistic unit (i.e., word) enables linguistics-meaningful
It studies four major meaning representation techniques which include: first-order predicate calculus (FOPC), semantic net, conceptual dependency diagram (CDD), and frame-based representation. After that it explores canonical form and introduces Fillmore's theory of universal cases followed by predicate logic and inference work using FOPC ...
Download scientific diagram | 4: Illustration of the definition of a universal model, representation and task-solving. from publication: On The Universality of Visual and Multimodal ...
Universal representation injects fairness into the system by giving immigrants the opportunity to access the rights they are entitled to under U.S. law. Universal representation is a crucial last line of defense to keep families and communities together. The Center for Popular Democracy (CPD), the National Immigration Law Center (NILC), and the ...
One-sentence Summary: In this work, we extend pre-trained language models to learn universal representations among multiple languages, and show the effectiveness on cross-lingual understanding and generation. Code Of Ethics: I acknowledge that I and all co-authors of this work have read and commit to adhering to the ICLR Code of Ethics.
Formal definition and properties. Definition. Let A be a C*-algebra with state space S. The representation. Φ := ∑ ρ ∈ S ⊕ π ρ. on the Hilbert space H Φ is known as the universal representation of A. As the universal representation is faithful, A is *-isomorphic to the C*-subalgebra Φ ( A) of B (HΦ) .
universal: [noun] one that is universal: such as. a universal proposition in logic. a predicable of traditional logic. a general concept or term or something in reality to which it corresponds : essence.