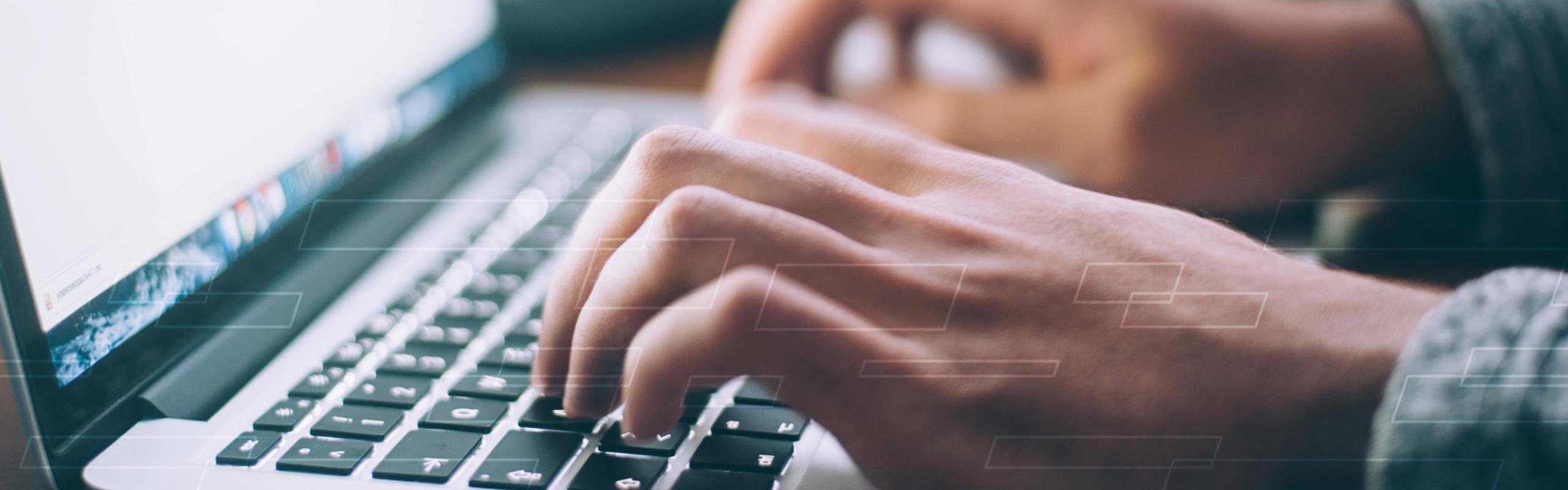
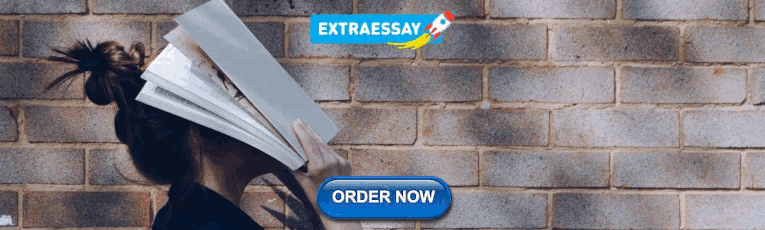
User Preferences
Content preview.
Arcu felis bibendum ut tristique et egestas quis:
- Ut enim ad minim veniam, quis nostrud exercitation ullamco laboris
- Duis aute irure dolor in reprehenderit in voluptate
- Excepteur sint occaecat cupidatat non proident
Keyboard Shortcuts
S.3.3 hypothesis testing examples.
- Example: Right-Tailed Test
- Example: Left-Tailed Test
- Example: Two-Tailed Test
Brinell Hardness Scores
An engineer measured the Brinell hardness of 25 pieces of ductile iron that were subcritically annealed. The resulting data were:
The engineer hypothesized that the mean Brinell hardness of all such ductile iron pieces is greater than 170. Therefore, he was interested in testing the hypotheses:
H 0 : μ = 170 H A : μ > 170
The engineer entered his data into Minitab and requested that the "one-sample t -test" be conducted for the above hypotheses. He obtained the following output:
Descriptive Statistics
$\mu$: mean of Brinelli
Null hypothesis H₀: $\mu$ = 170 Alternative hypothesis H₁: $\mu$ > 170
The output tells us that the average Brinell hardness of the n = 25 pieces of ductile iron was 172.52 with a standard deviation of 10.31. (The standard error of the mean "SE Mean", calculated by dividing the standard deviation 10.31 by the square root of n = 25, is 2.06). The test statistic t * is 1.22, and the P -value is 0.117.
If the engineer set his significance level α at 0.05 and used the critical value approach to conduct his hypothesis test, he would reject the null hypothesis if his test statistic t * were greater than 1.7109 (determined using statistical software or a t -table):
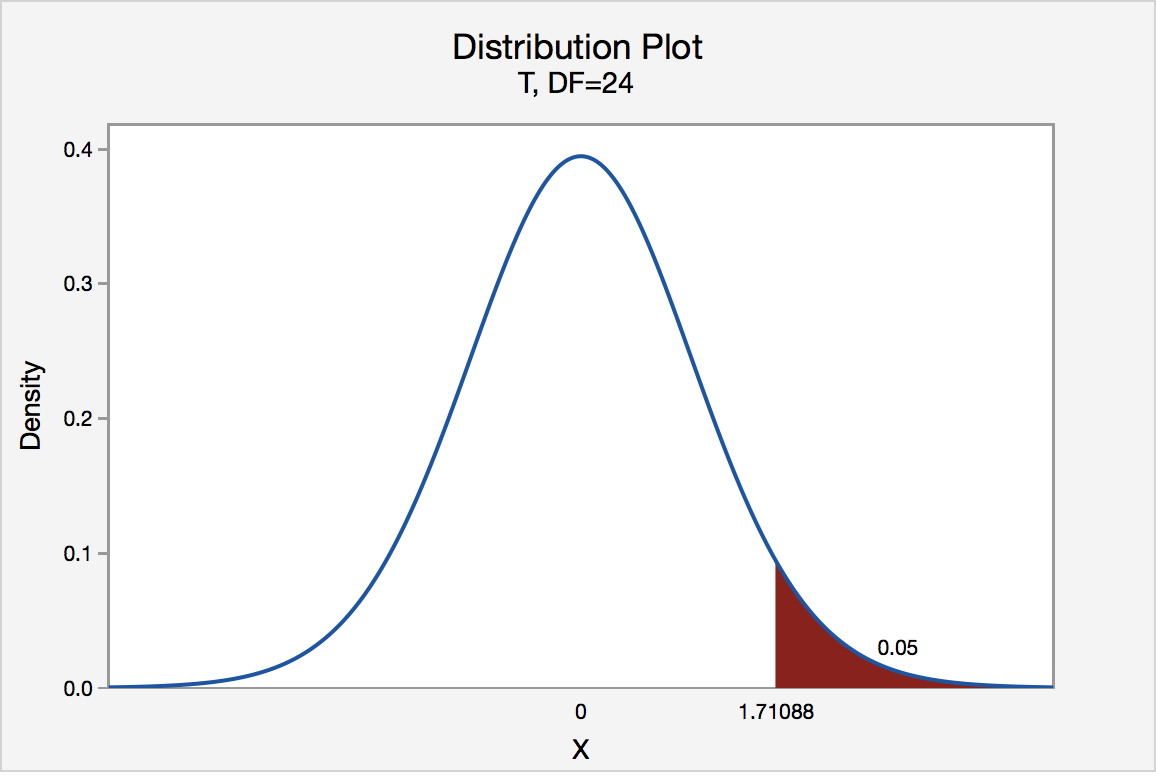
Since the engineer's test statistic, t * = 1.22, is not greater than 1.7109, the engineer fails to reject the null hypothesis. That is, the test statistic does not fall in the "critical region." There is insufficient evidence, at the \(\alpha\) = 0.05 level, to conclude that the mean Brinell hardness of all such ductile iron pieces is greater than 170.
If the engineer used the P -value approach to conduct his hypothesis test, he would determine the area under a t n - 1 = t 24 curve and to the right of the test statistic t * = 1.22:
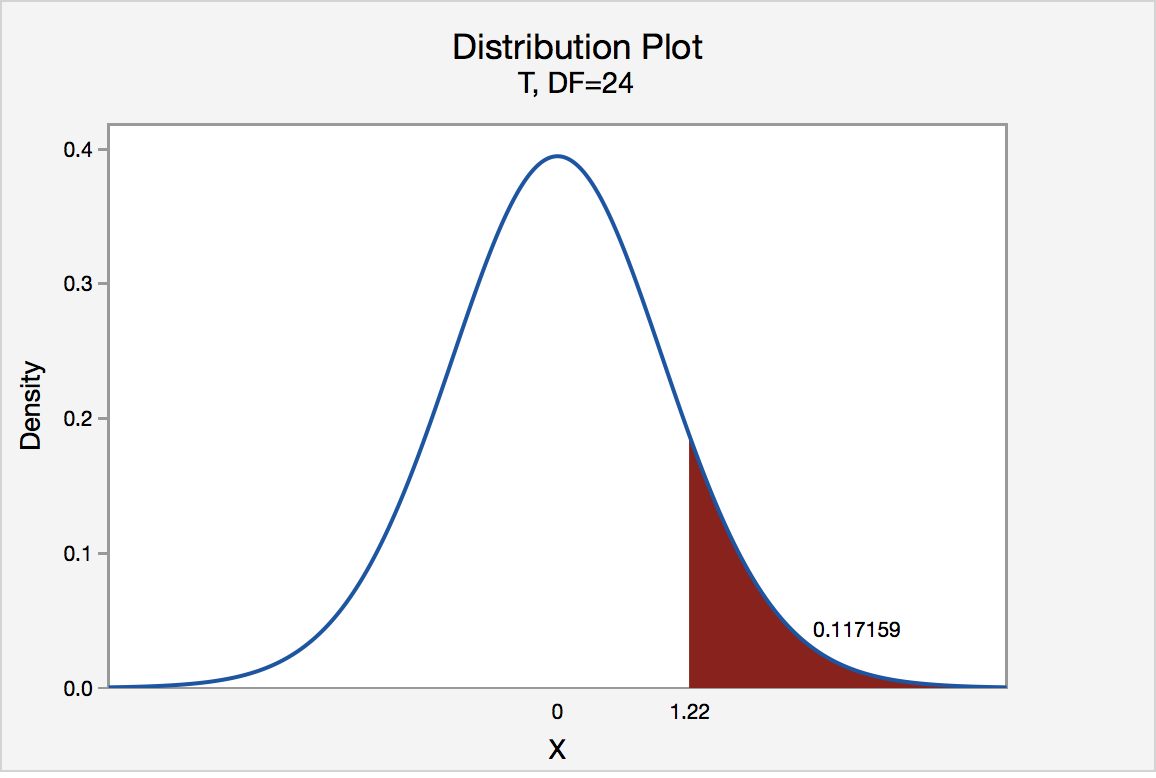
In the output above, Minitab reports that the P -value is 0.117. Since the P -value, 0.117, is greater than \(\alpha\) = 0.05, the engineer fails to reject the null hypothesis. There is insufficient evidence, at the \(\alpha\) = 0.05 level, to conclude that the mean Brinell hardness of all such ductile iron pieces is greater than 170.
Note that the engineer obtains the same scientific conclusion regardless of the approach used. This will always be the case.
Height of Sunflowers
A biologist was interested in determining whether sunflower seedlings treated with an extract from Vinca minor roots resulted in a lower average height of sunflower seedlings than the standard height of 15.7 cm. The biologist treated a random sample of n = 33 seedlings with the extract and subsequently obtained the following heights:
The biologist's hypotheses are:
H 0 : μ = 15.7 H A : μ < 15.7
The biologist entered her data into Minitab and requested that the "one-sample t -test" be conducted for the above hypotheses. She obtained the following output:
$\mu$: mean of Height
Null hypothesis H₀: $\mu$ = 15.7 Alternative hypothesis H₁: $\mu$ < 15.7
The output tells us that the average height of the n = 33 sunflower seedlings was 13.664 with a standard deviation of 2.544. (The standard error of the mean "SE Mean", calculated by dividing the standard deviation 13.664 by the square root of n = 33, is 0.443). The test statistic t * is -4.60, and the P -value, 0.000, is to three decimal places.
Minitab Note. Minitab will always report P -values to only 3 decimal places. If Minitab reports the P -value as 0.000, it really means that the P -value is 0.000....something. Throughout this course (and your future research!), when you see that Minitab reports the P -value as 0.000, you should report the P -value as being "< 0.001."
If the biologist set her significance level \(\alpha\) at 0.05 and used the critical value approach to conduct her hypothesis test, she would reject the null hypothesis if her test statistic t * were less than -1.6939 (determined using statistical software or a t -table):s-3-3
Since the biologist's test statistic, t * = -4.60, is less than -1.6939, the biologist rejects the null hypothesis. That is, the test statistic falls in the "critical region." There is sufficient evidence, at the α = 0.05 level, to conclude that the mean height of all such sunflower seedlings is less than 15.7 cm.
If the biologist used the P -value approach to conduct her hypothesis test, she would determine the area under a t n - 1 = t 32 curve and to the left of the test statistic t * = -4.60:
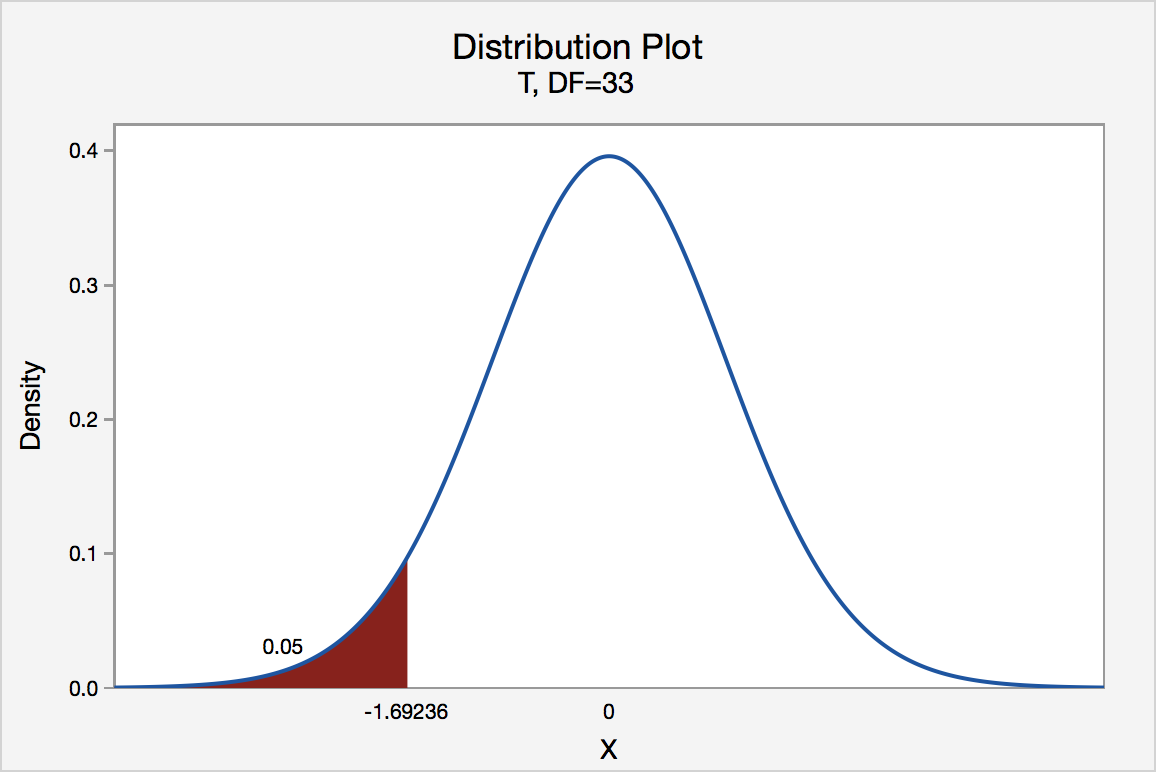
In the output above, Minitab reports that the P -value is 0.000, which we take to mean < 0.001. Since the P -value is less than 0.001, it is clearly less than \(\alpha\) = 0.05, and the biologist rejects the null hypothesis. There is sufficient evidence, at the \(\alpha\) = 0.05 level, to conclude that the mean height of all such sunflower seedlings is less than 15.7 cm.
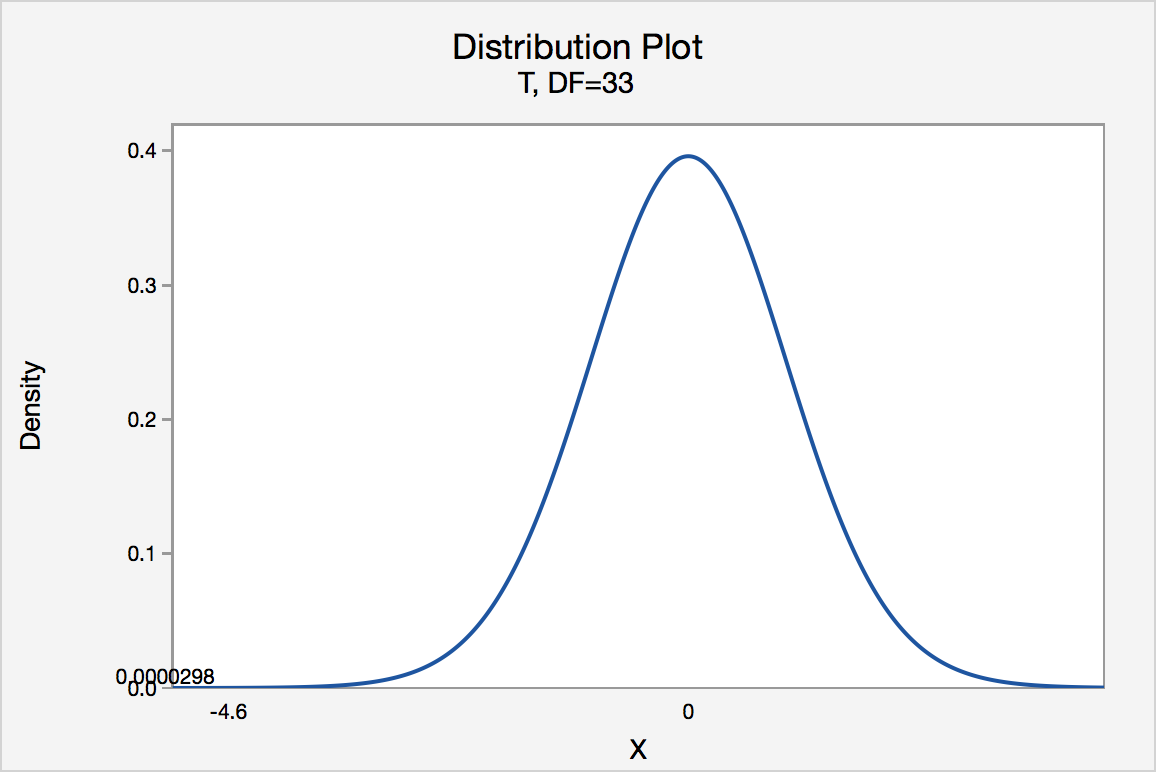
Note again that the biologist obtains the same scientific conclusion regardless of the approach used. This will always be the case.
Gum Thickness
A manufacturer claims that the thickness of the spearmint gum it produces is 7.5 one-hundredths of an inch. A quality control specialist regularly checks this claim. On one production run, he took a random sample of n = 10 pieces of gum and measured their thickness. He obtained:
The quality control specialist's hypotheses are:
H 0 : μ = 7.5 H A : μ ≠ 7.5
The quality control specialist entered his data into Minitab and requested that the "one-sample t -test" be conducted for the above hypotheses. He obtained the following output:
$\mu$: mean of Thickness
Null hypothesis H₀: $\mu$ = 7.5 Alternative hypothesis H₁: $\mu \ne$ 7.5
The output tells us that the average thickness of the n = 10 pieces of gums was 7.55 one-hundredths of an inch with a standard deviation of 0.1027. (The standard error of the mean "SE Mean", calculated by dividing the standard deviation 0.1027 by the square root of n = 10, is 0.0325). The test statistic t * is 1.54, and the P -value is 0.158.
If the quality control specialist sets his significance level \(\alpha\) at 0.05 and used the critical value approach to conduct his hypothesis test, he would reject the null hypothesis if his test statistic t * were less than -2.2616 or greater than 2.2616 (determined using statistical software or a t -table):
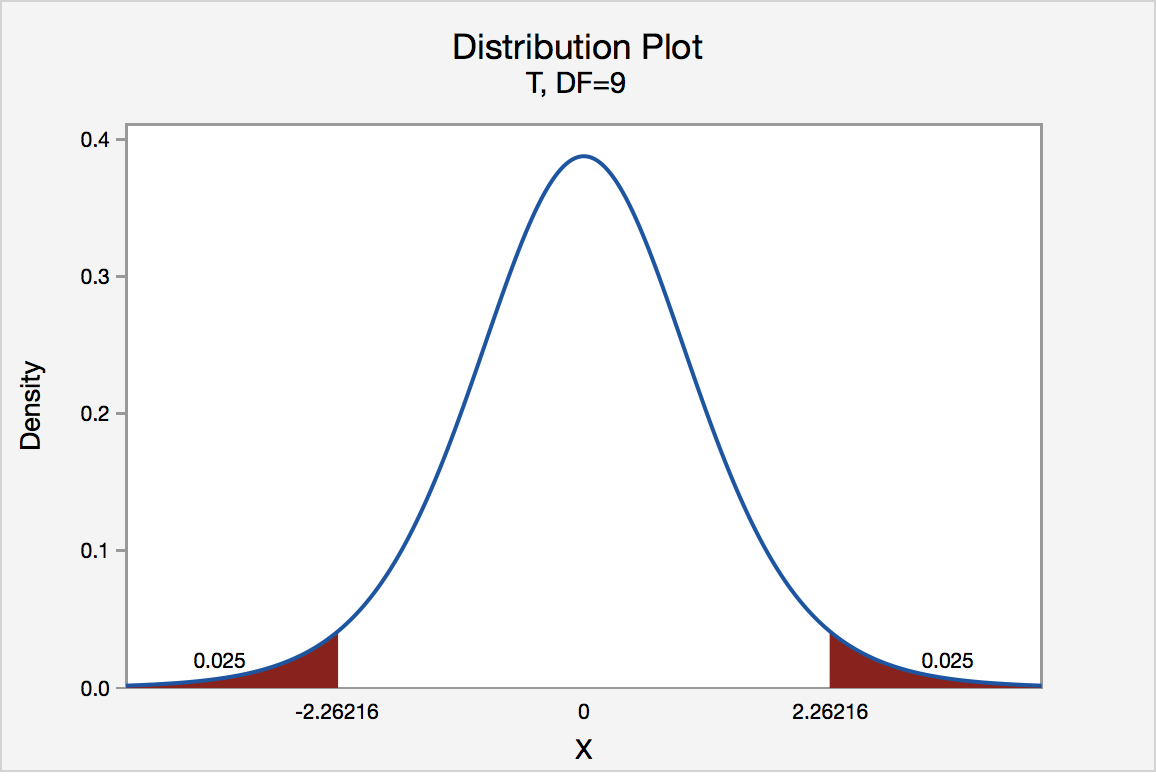
Since the quality control specialist's test statistic, t * = 1.54, is not less than -2.2616 nor greater than 2.2616, the quality control specialist fails to reject the null hypothesis. That is, the test statistic does not fall in the "critical region." There is insufficient evidence, at the \(\alpha\) = 0.05 level, to conclude that the mean thickness of all of the manufacturer's spearmint gum differs from 7.5 one-hundredths of an inch.
If the quality control specialist used the P -value approach to conduct his hypothesis test, he would determine the area under a t n - 1 = t 9 curve, to the right of 1.54 and to the left of -1.54:
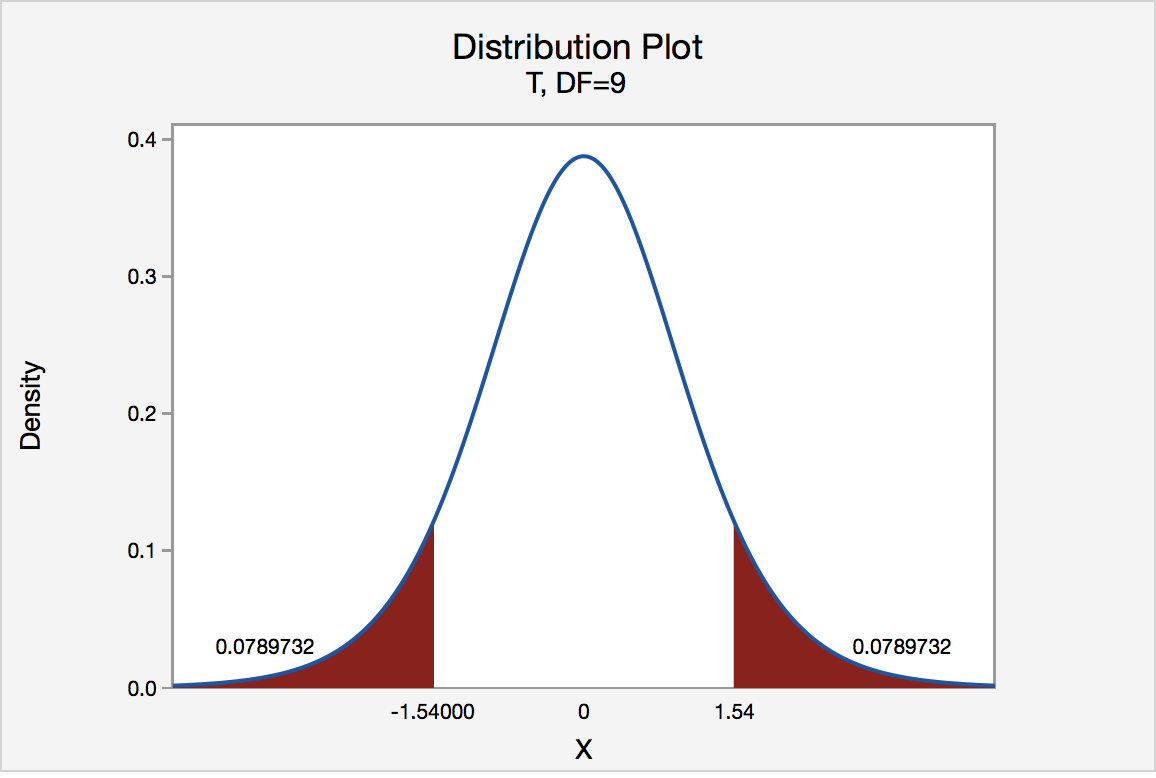
In the output above, Minitab reports that the P -value is 0.158. Since the P -value, 0.158, is greater than \(\alpha\) = 0.05, the quality control specialist fails to reject the null hypothesis. There is insufficient evidence, at the \(\alpha\) = 0.05 level, to conclude that the mean thickness of all pieces of spearmint gum differs from 7.5 one-hundredths of an inch.
Note that the quality control specialist obtains the same scientific conclusion regardless of the approach used. This will always be the case.
In our review of hypothesis tests, we have focused on just one particular hypothesis test, namely that concerning the population mean \(\mu\). The important thing to recognize is that the topics discussed here — the general idea of hypothesis tests, errors in hypothesis testing, the critical value approach, and the P -value approach — generally extend to all of the hypothesis tests you will encounter.
9.4 Full Hypothesis Test Examples
Tests on means, example 9.8.
Jeffrey, as an eight-year old, established a mean time of 16.43 seconds for swimming the 25-yard freestyle, with a standard deviation of 0.8 seconds . His dad, Frank, thought that Jeffrey could swim the 25-yard freestyle faster using goggles. Frank bought Jeffrey a new pair of expensive goggles and timed Jeffrey for 15 25-yard freestyle swims . For the 15 swims, Jeffrey's mean time was 16 seconds. Frank thought that the goggles helped Jeffrey to swim faster than the 16.43 seconds. Conduct a hypothesis test using a preset α = 0.05. Assume that the swim times for the 25-yard freestyle are normal.
Set up the Hypothesis Test:
Since the problem is about a mean, this is a test of a single population mean .
H 0 : μ = 16.43 H a : μ < 16.43
For Jeffrey to swim faster, his time will be less than 16.43 seconds. The "<" tells you this is left-tailed.
Determine the distribution needed:
Random variable: X ¯ X ¯ = the mean time to swim the 25-yard freestyle.
Distribution for the test: X ¯ X ¯ is normal (population standard deviation is known: σ = 0.8)
X ¯ ~ N ( μ , σ X n ) X ¯ ~ N ( μ , σ X n ) Therefore, X ¯ ~ N ( 16.43 , 0.8 15 ) X ¯ ~ N ( 16.43 , 0.8 15 )
μ = 16.43 comes from H 0 and not the data. σ = 0.8, and n = 15.
Calculate the p -value using the normal distribution for a mean:
p -value = P ( x ¯ x ¯ < 16) = 0.0187 where the sample mean in the problem is given as 16.
p -value = 0.0187 (This is called the actual level of significance .) The p -value is the area to the left of the sample mean is given as 16.
μ = 16.43 comes from H 0 . Our assumption is μ = 16.43.
Interpretation of the p -value: If H 0 is true , there is a 0.0187 probability (1.87%)that Jeffrey's mean time to swim the 25-yard freestyle is 16 seconds or less. Because a 1.87% chance is small, the mean time of 16 seconds or less is unlikely to have happened randomly. It is a rare event.
Compare α and the p -value:
α = 0.05 p -value = 0.0187 α > p -value
Make a decision: Since α > α > p -value, reject H 0 .
This indicates that you reject the null hypothesis that the mean time to swim the 25-yard freestyle is at least 16.43 seconds.
Conclusion: At the 5% significance level, there is sufficient evidence that Jeffrey's mean time to swim the 25-yard freestyle is less than 16.43 seconds. Thus, based on the sample data, we conclude that Jeffrey swims faster using the new goggles.
The Type I and Type II errors for this problem are as follows: The Type I error is to conclude that Jeffrey swims the 25-yard freestyle, on average, in less than 16.43 seconds when, in fact, he actually swims the 25-yard freestyle, on average, in at least 16.43 seconds. (Reject the null hypothesis when the null hypothesis is true.)
The Type II error is that there is not evidence to conclude that Jeffrey swims the 25-yard freestyle, on average, in less than 16.43 seconds when, in fact, he actually does swim the 25-yard free-style, on average, in less than 16.43 seconds. (Do not reject the null hypothesis when the null hypothesis is false.)
The mean throwing distance of a football for Marco, a high school quarterback, is 40 yards, with a standard deviation of two yards. The team coach tells Marco to adjust his grip to get more distance. The coach records the distances for 20 throws. For the 20 throws, Marco’s mean distance was 45 yards. The coach thought the different grip helped Marco throw farther than 40 yards. Conduct a hypothesis test using a preset α = 0.05. Assume the throw distances for footballs are normal.
First, determine what type of test this is, set up the hypothesis test, find the p -value, sketch the graph, and state your conclusion.
Example 9.9
Jasmine has just begun her new job on the sales force of a very competitive company. In a sample of 16 sales calls it was found that she closed the contract for an average value of 108 dollars with a standard deviation of 12 dollars. Test at 5% significance that the population mean is at least 100 dollars against the alternative that it is less than 100 dollars. Company policy requires that new members of the sales force must exceed an average of $100 per contract during the trial employment period. Can we conclude that Jasmine has met this requirement at the significance level of 95%?
- H 0 : µ ≤ 100 H a : µ > 100 The null and alternative hypothesis are for the parameter µ because the number of dollars of the contracts is a continuous random variable. Also, this is a one-tailed test because the company has only an interested if the number of dollars per contact is below a particular number not "too high" a number. This can be thought of as making a claim that the requirement is being met and thus the claim is in the alternative hypothesis.
- Test statistic: t c = x ¯ − µ 0 s n = 108 − 100 ( 12 16 ) = 2.67 t c = x ¯ − µ 0 s n = 108 − 100 ( 12 16 ) = 2.67
- Critical value: t a = 1.753 t a = 1.753 with n-1 degrees of freedom= 15
The test statistic is a Student's t because the sample size is below 30; therefore, we cannot use the normal distribution. Comparing the calculated value of the test statistic and the critical value of t t ( t a ) ( t a ) at a 5% significance level, we see that the calculated value is in the tail of the distribution. Thus, we conclude that 108 dollars per contract is significantly larger than the hypothesized value of 100 and thus we cannot accept the null hypothesis. There is evidence that supports Jasmine's performance meets company standards.
It is believed that a stock price for a particular company will grow at a rate of $5 per week with a standard deviation of $1. An investor believes the stock won’t grow as quickly. The changes in stock price is recorded for ten weeks and are as follows: $4, $3, $2, $3, $1, $7, $2, $1, $1, $2. Perform a hypothesis test using a 5% level of significance. State the null and alternative hypotheses, state your conclusion, and identify the Type I errors.
Example 9.10
A manufacturer of salad dressings uses machines to dispense liquid ingredients into bottles that move along a filling line. The machine that dispenses salad dressings is working properly when 8 ounces are dispensed. Suppose that the average amount dispensed in a particular sample of 35 bottles is 7.91 ounces with a variance of 0.03 ounces squared, s 2 s 2 . Is there evidence that the machine should be stopped and production wait for repairs? The lost production from a shutdown is potentially so great that management feels that the level of significance in the analysis should be 99%.
Again we will follow the steps in our analysis of this problem.
STEP 1 : Set the Null and Alternative Hypothesis. The random variable is the quantity of fluid placed in the bottles. This is a continuous random variable and the parameter we are interested in is the mean. Our hypothesis therefore is about the mean. In this case we are concerned that the machine is not filling properly. From what we are told it does not matter if the machine is over-filling or under-filling, both seem to be an equally bad error. This tells us that this is a two-tailed test: if the machine is malfunctioning it will be shutdown regardless if it is from over-filling or under-filling. The null and alternative hypotheses are thus:
STEP 2 : Decide the level of significance and draw the graph showing the critical value.
This problem has already set the level of significance at 99%. The decision seems an appropriate one and shows the thought process when setting the significance level. Management wants to be very certain, as certain as probability will allow, that they are not shutting down a machine that is not in need of repair. To draw the distribution and the critical value, we need to know which distribution to use. Because this is a continuous random variable and we are interested in the mean, and the sample size is greater than 30, the appropriate distribution is the normal distribution and the relevant critical value is 2.575 from the normal table or the t-table at 0.005 column and infinite degrees of freedom. We draw the graph and mark these points.
STEP 3 : Calculate sample parameters and the test statistic. The sample parameters are provided, the sample mean is 7.91 and the sample variance is .03 and the sample size is 35. We need to note that the sample variance was provided not the sample standard deviation, which is what we need for the formula. Remembering that the standard deviation is simply the square root of the variance, we therefore know the sample standard deviation, s, is 0.173. With this information we calculate the test statistic as -3.07, and mark it on the graph.
STEP 4 : Compare test statistic and the critical values Now we compare the test statistic and the critical value by placing the test statistic on the graph. We see that the test statistic is in the tail, decidedly greater than the critical value of 2.575. We note that even the very small difference between the hypothesized value and the sample value is still a large number of standard deviations. The sample mean is only 0.08 ounces different from the required level of 8 ounces, but it is 3 plus standard deviations away and thus we cannot accept the null hypothesis.
STEP 5 : Reach a Conclusion
Three standard deviations of a test statistic will guarantee that the test will fail. The probability that anything is within three standard deviations is almost zero. Actually it is 0.0026 on the normal distribution, which is certainly almost zero in a practical sense. Our formal conclusion would be “ At a 99% level of significance we cannot accept the hypothesis that the sample mean came from a distribution with a mean of 8 ounces” Or less formally, and getting to the point, “At a 99% level of significance we conclude that the machine is under filling the bottles and is in need of repair”.
Try It 9.10
A company records the mean time of employees working in a day. The mean comes out to be 475 minutes, with a standard deviation of 45 minutes. A manager recorded times of 20 employees. The times of working were (frequencies are in parentheses) 460(3); 465(2); 470(3); 475(1); 480(6); 485(3); 490(2).
Conduct a hypothesis test using a 2.5% level of significance to determine if the mean time is more than 475 .
Hypothesis Test for Proportions
Just as there were confidence intervals for proportions, or more formally, the population parameter p of the binomial distribution, there is the ability to test hypotheses concerning p .
The population parameter for the binomial is p . The estimated value (point estimate) for p is p′ where p′ = x/n , x is the number of successes in the sample and n is the sample size.
When you perform a hypothesis test of a population proportion p , you take a simple random sample from the population. The conditions for a binomial distribution must be met, which are: there are a certain number n of independent trials meaning random sampling, the outcomes of any trial are binary, success or failure, and each trial has the same probability of a success p . The shape of the binomial distribution needs to be similar to the shape of the normal distribution. To ensure this, the quantities np′ and nq′ must both be greater than five ( np′ > 5 and nq′ > 5). In this case the binomial distribution of a sample (estimated) proportion can be approximated by the normal distribution with μ = np μ = np and σ = npq σ = npq . Remember that q = 1 – p q = 1 – p . There is no distribution that can correct for this small sample bias and thus if these conditions are not met we simply cannot test the hypothesis with the data available at that time. We met this condition when we first were estimating confidence intervals for p .
Again, we begin with the standardizing formula modified because this is the distribution of a binomial.
Substituting p 0 p 0 , the hypothesized value of p , we have:
This is the test statistic for testing hypothesized values of p , where the null and alternative hypotheses take one of the following forms:
The decision rule stated above applies here also: if the calculated value of Z c shows that the sample proportion is "too many" standard deviations from the hypothesized proportion, the null hypothesis cannot be accepted. The decision as to what is "too many" is pre-determined by the analyst depending on the level of significance required in the test.
Example 9.11
The mortgage department of a large bank is interested in the nature of loans of first-time borrowers. This information will be used to tailor their marketing strategy. They believe that 50% of first-time borrowers take out smaller loans than other borrowers. They perform a hypothesis test to determine if the percentage is the same or different from 50% . They sample 100 first-time borrowers and find 53 of these loans are smaller that the other borrowers. For the hypothesis test, they choose a 5% level of significance.
STEP 1 : Set the null and alternative hypothesis.
H 0 : p = 0.50 H a : p ≠ 0.50
The words "is the same or different from" tell you this is a two-tailed test. The Type I and Type II errors are as follows: The Type I error is to conclude that the proportion of borrowers is different from 50% when, in fact, the proportion is actually 50%. (Reject the null hypothesis when the null hypothesis is true). The Type II error is there is not enough evidence to conclude that the proportion of first time borrowers differs from 50% when, in fact, the proportion does differ from 50%. (You fail to reject the null hypothesis when the null hypothesis is false.)
STEP 2 : Decide the level of significance and draw the graph showing the critical value
The level of significance has been set by the problem at the 5% level. Because this is two-tailed test one-half of the alpha value will be in the upper tail and one-half in the lower tail as shown on the graph. The critical value for the normal distribution at the 95% level of confidence is 1.96. This can easily be found on the student’s t-table at the very bottom at infinite degrees of freedom remembering that at infinity the t-distribution is the normal distribution. Of course the value can also be found on the normal table but you have go looking for one-half of 95 (0.475) inside the body of the table and then read out to the sides and top for the number of standard deviations.
STEP 3 : Calculate the sample parameters and critical value of the test statistic.
The test statistic is a normal distribution, Z, for testing proportions and is:
For this case, the sample of 100 found 53 of these loans were smaller than those of other borrowers. The sample proportion, p′ = 53/100= 0.53 The test question, therefore, is : “Is 0.53 significantly different from .50?” Putting these values into the formula for the test statistic we find that 0.53 is only 0.60 standard deviations away from .50. This is barely off of the mean of the standard normal distribution of zero. There is virtually no difference from the sample proportion and the hypothesized proportion in terms of standard deviations.
STEP 4 : Compare the test statistic and the critical value.
The calculated value is well within the critical values of ± 1.96 standard deviations and thus we cannot reject the null hypothesis. To reject the null hypothesis we need significant evident of difference between the hypothesized value and the sample value. In this case the sample value is very nearly the same as the hypothesized value measured in terms of standard deviations.
STEP 5 : Reach a conclusion
The formal conclusion would be “At a 5% level of significance we cannot reject the null hypothesis that 50% of first-time borrowers take out smaller loans than other borrowers.” Notice the length to which the conclusion goes to include all of the conditions that are attached to the conclusion. Statisticians, for all the criticism they receive, are careful to be very specific even when this seems trivial. Statisticians cannot say more than they know, and the data constrain the conclusion to be within the metes and bounds of the data.
Try It 9.11
A teacher believes that 85% of students in the class will want to go on a field trip to the local zoo. The teacher performs a hypothesis test to determine if the percentage is the same or different from 85%. The teacher samples 50 students and 39 reply that they would want to go to the zoo. For the hypothesis test, use a 1% level of significance.
Example 9.12
Suppose a consumer group suspects that the proportion of households that have three or more cell phones is 30%. A cell phone company has reason to believe that the proportion is not 30%. Before they start a big advertising campaign, they conduct a hypothesis test. Their marketing people survey 150 households with the result that 43 of the households have three or more cell phones.
Here is an abbreviate version of the system to solve hypothesis tests applied to a test on a proportions.
Try It 9.12
Marketers believe that 92% of adults in the United States own a cell phone. A cell phone manufacturer believes that number is actually lower. 200 American adults are surveyed, of which, 174 report having cell phones. Use a 5% level of significance. State the null and alternative hypothesis, find the p -value, state your conclusion, and identify the Type I and Type II errors.
Example 9.13
The National Institute of Standards and Technology provides exact data on conductivity properties of materials. Following are conductivity measurements for 11 randomly selected pieces of a particular type of glass.
1.11; 1.07; 1.11; 1.07; 1.12; 1.08; .98; .98; 1.02; .95; .95 Is there convincing evidence that the average conductivity of this type of glass is greater than one? Use a significance level of 0.05.
Let’s follow a four-step process to answer this statistical question.
- H 0 : μ ≤ 1
- H a : μ > 1
- Plan : We are testing a sample mean without a known population standard deviation with less than 30 observations. Therefore, we need to use a Student's-t distribution. Assume the underlying population is normal.
- Do the calculations and draw the graph .
- State the Conclusions : We cannot accept the null hypothesis. It is reasonable to state that the data supports the claim that the average conductivity level is greater than one.
Try It 9.13
The boiling point of a specific liquid is measured for 15 samples, and the boiling points are obtained as follows:
205; 206; 206; 202; 199; 194; 197; 198; 198; 201; 201; 202; 207; 211; 205
Is there convincing evidence that the average boiling point is greater than 200? Use a significance level of 0.1. Assume the population is normal.
Example 9.14
In a study of 420,019 cell phone users, 172 of the subjects developed brain cancer. Test the claim that cell phone users developed brain cancer at a greater rate than that for non-cell phone users (the rate of brain cancer for non-cell phone users is 0.0340%). Since this is a critical issue, use a 0.005 significance level. Explain why the significance level should be so low in terms of a Type I error.
- H 0 : p ≤ 0.00034
- H a : p > 0.00034
If we commit a Type I error, we are essentially accepting a false claim. Since the claim describes cancer-causing environments, we want to minimize the chances of incorrectly identifying causes of cancer.
- We will be testing a sample proportion with x = 172 and n = 420,019. The sample is sufficiently large because we have np' = 420,019(0.00034) = 142.8, nq' = 420,019(0.99966) = 419,876.2, two independent outcomes, and a fixed probability of success p' = 0.00034. Thus we will be able to generalize our results to the population.
Try It 9.14
In a study of 390,000 moisturizer users, 138 of the subjects developed skin diseases. Test the claim that moisturizer users developed skin diseases at a greater rate than that for non-moisturizer users (the rate of skin diseases for non-moisturizer users is 0.041%). Since this is a critical issue, use a 0.005 significance level. Explain why the significance level should be so low in terms of a Type I error.
This book may not be used in the training of large language models or otherwise be ingested into large language models or generative AI offerings without OpenStax's permission.
Want to cite, share, or modify this book? This book uses the Creative Commons Attribution License and you must attribute OpenStax.
Access for free at https://openstax.org/books/introductory-business-statistics-2e/pages/1-introduction
- Authors: Alexander Holmes, Barbara Illowsky, Susan Dean
- Publisher/website: OpenStax
- Book title: Introductory Business Statistics 2e
- Publication date: Dec 13, 2023
- Location: Houston, Texas
- Book URL: https://openstax.org/books/introductory-business-statistics-2e/pages/1-introduction
- Section URL: https://openstax.org/books/introductory-business-statistics-2e/pages/9-4-full-hypothesis-test-examples
© Dec 6, 2023 OpenStax. Textbook content produced by OpenStax is licensed under a Creative Commons Attribution License . The OpenStax name, OpenStax logo, OpenStax book covers, OpenStax CNX name, and OpenStax CNX logo are not subject to the Creative Commons license and may not be reproduced without the prior and express written consent of Rice University.
Hypothesis Testing
Hypothesis testing is a tool for making statistical inferences about the population data. It is an analysis tool that tests assumptions and determines how likely something is within a given standard of accuracy. Hypothesis testing provides a way to verify whether the results of an experiment are valid.
A null hypothesis and an alternative hypothesis are set up before performing the hypothesis testing. This helps to arrive at a conclusion regarding the sample obtained from the population. In this article, we will learn more about hypothesis testing, its types, steps to perform the testing, and associated examples.
What is Hypothesis Testing in Statistics?
Hypothesis testing uses sample data from the population to draw useful conclusions regarding the population probability distribution . It tests an assumption made about the data using different types of hypothesis testing methodologies. The hypothesis testing results in either rejecting or not rejecting the null hypothesis.
Hypothesis Testing Definition
Hypothesis testing can be defined as a statistical tool that is used to identify if the results of an experiment are meaningful or not. It involves setting up a null hypothesis and an alternative hypothesis. These two hypotheses will always be mutually exclusive. This means that if the null hypothesis is true then the alternative hypothesis is false and vice versa. An example of hypothesis testing is setting up a test to check if a new medicine works on a disease in a more efficient manner.
Null Hypothesis
The null hypothesis is a concise mathematical statement that is used to indicate that there is no difference between two possibilities. In other words, there is no difference between certain characteristics of data. This hypothesis assumes that the outcomes of an experiment are based on chance alone. It is denoted as \(H_{0}\). Hypothesis testing is used to conclude if the null hypothesis can be rejected or not. Suppose an experiment is conducted to check if girls are shorter than boys at the age of 5. The null hypothesis will say that they are the same height.
Alternative Hypothesis
The alternative hypothesis is an alternative to the null hypothesis. It is used to show that the observations of an experiment are due to some real effect. It indicates that there is a statistical significance between two possible outcomes and can be denoted as \(H_{1}\) or \(H_{a}\). For the above-mentioned example, the alternative hypothesis would be that girls are shorter than boys at the age of 5.
Hypothesis Testing P Value
In hypothesis testing, the p value is used to indicate whether the results obtained after conducting a test are statistically significant or not. It also indicates the probability of making an error in rejecting or not rejecting the null hypothesis.This value is always a number between 0 and 1. The p value is compared to an alpha level, \(\alpha\) or significance level. The alpha level can be defined as the acceptable risk of incorrectly rejecting the null hypothesis. The alpha level is usually chosen between 1% to 5%.
Hypothesis Testing Critical region
All sets of values that lead to rejecting the null hypothesis lie in the critical region. Furthermore, the value that separates the critical region from the non-critical region is known as the critical value.
Hypothesis Testing Formula
Depending upon the type of data available and the size, different types of hypothesis testing are used to determine whether the null hypothesis can be rejected or not. The hypothesis testing formula for some important test statistics are given below:
- z = \(\frac{\overline{x}-\mu}{\frac{\sigma}{\sqrt{n}}}\). \(\overline{x}\) is the sample mean, \(\mu\) is the population mean, \(\sigma\) is the population standard deviation and n is the size of the sample.
- t = \(\frac{\overline{x}-\mu}{\frac{s}{\sqrt{n}}}\). s is the sample standard deviation.
- \(\chi ^{2} = \sum \frac{(O_{i}-E_{i})^{2}}{E_{i}}\). \(O_{i}\) is the observed value and \(E_{i}\) is the expected value.
We will learn more about these test statistics in the upcoming section.
Types of Hypothesis Testing
Selecting the correct test for performing hypothesis testing can be confusing. These tests are used to determine a test statistic on the basis of which the null hypothesis can either be rejected or not rejected. Some of the important tests used for hypothesis testing are given below.
Hypothesis Testing Z Test
A z test is a way of hypothesis testing that is used for a large sample size (n ≥ 30). It is used to determine whether there is a difference between the population mean and the sample mean when the population standard deviation is known. It can also be used to compare the mean of two samples. It is used to compute the z test statistic. The formulas are given as follows:
- One sample: z = \(\frac{\overline{x}-\mu}{\frac{\sigma}{\sqrt{n}}}\).
- Two samples: z = \(\frac{(\overline{x_{1}}-\overline{x_{2}})-(\mu_{1}-\mu_{2})}{\sqrt{\frac{\sigma_{1}^{2}}{n_{1}}+\frac{\sigma_{2}^{2}}{n_{2}}}}\).
Hypothesis Testing t Test
The t test is another method of hypothesis testing that is used for a small sample size (n < 30). It is also used to compare the sample mean and population mean. However, the population standard deviation is not known. Instead, the sample standard deviation is known. The mean of two samples can also be compared using the t test.
- One sample: t = \(\frac{\overline{x}-\mu}{\frac{s}{\sqrt{n}}}\).
- Two samples: t = \(\frac{(\overline{x_{1}}-\overline{x_{2}})-(\mu_{1}-\mu_{2})}{\sqrt{\frac{s_{1}^{2}}{n_{1}}+\frac{s_{2}^{2}}{n_{2}}}}\).
Hypothesis Testing Chi Square
The Chi square test is a hypothesis testing method that is used to check whether the variables in a population are independent or not. It is used when the test statistic is chi-squared distributed.
One Tailed Hypothesis Testing
One tailed hypothesis testing is done when the rejection region is only in one direction. It can also be known as directional hypothesis testing because the effects can be tested in one direction only. This type of testing is further classified into the right tailed test and left tailed test.
Right Tailed Hypothesis Testing
The right tail test is also known as the upper tail test. This test is used to check whether the population parameter is greater than some value. The null and alternative hypotheses for this test are given as follows:
\(H_{0}\): The population parameter is ≤ some value
\(H_{1}\): The population parameter is > some value.
If the test statistic has a greater value than the critical value then the null hypothesis is rejected
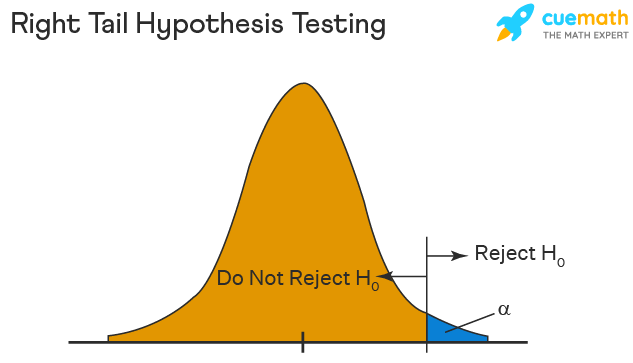
Left Tailed Hypothesis Testing
The left tail test is also known as the lower tail test. It is used to check whether the population parameter is less than some value. The hypotheses for this hypothesis testing can be written as follows:
\(H_{0}\): The population parameter is ≥ some value
\(H_{1}\): The population parameter is < some value.
The null hypothesis is rejected if the test statistic has a value lesser than the critical value.
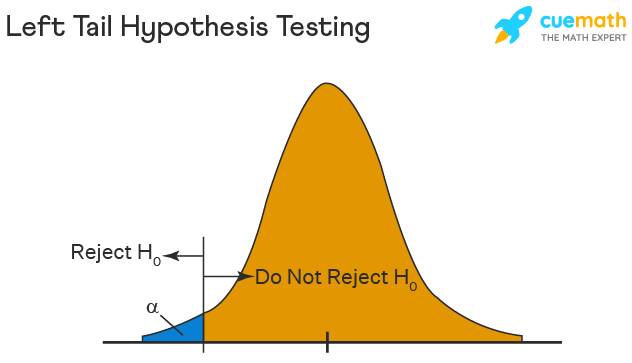
Two Tailed Hypothesis Testing
In this hypothesis testing method, the critical region lies on both sides of the sampling distribution. It is also known as a non - directional hypothesis testing method. The two-tailed test is used when it needs to be determined if the population parameter is assumed to be different than some value. The hypotheses can be set up as follows:
\(H_{0}\): the population parameter = some value
\(H_{1}\): the population parameter ≠ some value
The null hypothesis is rejected if the test statistic has a value that is not equal to the critical value.
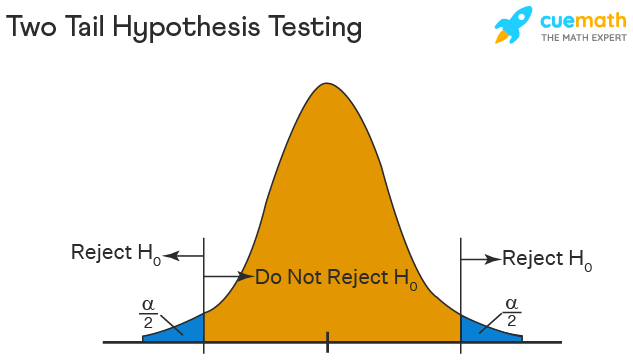
Hypothesis Testing Steps
Hypothesis testing can be easily performed in five simple steps. The most important step is to correctly set up the hypotheses and identify the right method for hypothesis testing. The basic steps to perform hypothesis testing are as follows:
- Step 1: Set up the null hypothesis by correctly identifying whether it is the left-tailed, right-tailed, or two-tailed hypothesis testing.
- Step 2: Set up the alternative hypothesis.
- Step 3: Choose the correct significance level, \(\alpha\), and find the critical value.
- Step 4: Calculate the correct test statistic (z, t or \(\chi\)) and p-value.
- Step 5: Compare the test statistic with the critical value or compare the p-value with \(\alpha\) to arrive at a conclusion. In other words, decide if the null hypothesis is to be rejected or not.
Hypothesis Testing Example
The best way to solve a problem on hypothesis testing is by applying the 5 steps mentioned in the previous section. Suppose a researcher claims that the mean average weight of men is greater than 100kgs with a standard deviation of 15kgs. 30 men are chosen with an average weight of 112.5 Kgs. Using hypothesis testing, check if there is enough evidence to support the researcher's claim. The confidence interval is given as 95%.
Step 1: This is an example of a right-tailed test. Set up the null hypothesis as \(H_{0}\): \(\mu\) = 100.
Step 2: The alternative hypothesis is given by \(H_{1}\): \(\mu\) > 100.
Step 3: As this is a one-tailed test, \(\alpha\) = 100% - 95% = 5%. This can be used to determine the critical value.
1 - \(\alpha\) = 1 - 0.05 = 0.95
0.95 gives the required area under the curve. Now using a normal distribution table, the area 0.95 is at z = 1.645. A similar process can be followed for a t-test. The only additional requirement is to calculate the degrees of freedom given by n - 1.
Step 4: Calculate the z test statistic. This is because the sample size is 30. Furthermore, the sample and population means are known along with the standard deviation.
z = \(\frac{\overline{x}-\mu}{\frac{\sigma}{\sqrt{n}}}\).
\(\mu\) = 100, \(\overline{x}\) = 112.5, n = 30, \(\sigma\) = 15
z = \(\frac{112.5-100}{\frac{15}{\sqrt{30}}}\) = 4.56
Step 5: Conclusion. As 4.56 > 1.645 thus, the null hypothesis can be rejected.
Hypothesis Testing and Confidence Intervals
Confidence intervals form an important part of hypothesis testing. This is because the alpha level can be determined from a given confidence interval. Suppose a confidence interval is given as 95%. Subtract the confidence interval from 100%. This gives 100 - 95 = 5% or 0.05. This is the alpha value of a one-tailed hypothesis testing. To obtain the alpha value for a two-tailed hypothesis testing, divide this value by 2. This gives 0.05 / 2 = 0.025.
Related Articles:
- Probability and Statistics
- Data Handling
Important Notes on Hypothesis Testing
- Hypothesis testing is a technique that is used to verify whether the results of an experiment are statistically significant.
- It involves the setting up of a null hypothesis and an alternate hypothesis.
- There are three types of tests that can be conducted under hypothesis testing - z test, t test, and chi square test.
- Hypothesis testing can be classified as right tail, left tail, and two tail tests.
Examples on Hypothesis Testing
- Example 1: The average weight of a dumbbell in a gym is 90lbs. However, a physical trainer believes that the average weight might be higher. A random sample of 5 dumbbells with an average weight of 110lbs and a standard deviation of 18lbs. Using hypothesis testing check if the physical trainer's claim can be supported for a 95% confidence level. Solution: As the sample size is lesser than 30, the t-test is used. \(H_{0}\): \(\mu\) = 90, \(H_{1}\): \(\mu\) > 90 \(\overline{x}\) = 110, \(\mu\) = 90, n = 5, s = 18. \(\alpha\) = 0.05 Using the t-distribution table, the critical value is 2.132 t = \(\frac{\overline{x}-\mu}{\frac{s}{\sqrt{n}}}\) t = 2.484 As 2.484 > 2.132, the null hypothesis is rejected. Answer: The average weight of the dumbbells may be greater than 90lbs
- Example 2: The average score on a test is 80 with a standard deviation of 10. With a new teaching curriculum introduced it is believed that this score will change. On random testing, the score of 38 students, the mean was found to be 88. With a 0.05 significance level, is there any evidence to support this claim? Solution: This is an example of two-tail hypothesis testing. The z test will be used. \(H_{0}\): \(\mu\) = 80, \(H_{1}\): \(\mu\) ≠ 80 \(\overline{x}\) = 88, \(\mu\) = 80, n = 36, \(\sigma\) = 10. \(\alpha\) = 0.05 / 2 = 0.025 The critical value using the normal distribution table is 1.96 z = \(\frac{\overline{x}-\mu}{\frac{\sigma}{\sqrt{n}}}\) z = \(\frac{88-80}{\frac{10}{\sqrt{36}}}\) = 4.8 As 4.8 > 1.96, the null hypothesis is rejected. Answer: There is a difference in the scores after the new curriculum was introduced.
- Example 3: The average score of a class is 90. However, a teacher believes that the average score might be lower. The scores of 6 students were randomly measured. The mean was 82 with a standard deviation of 18. With a 0.05 significance level use hypothesis testing to check if this claim is true. Solution: The t test will be used. \(H_{0}\): \(\mu\) = 90, \(H_{1}\): \(\mu\) < 90 \(\overline{x}\) = 110, \(\mu\) = 90, n = 6, s = 18 The critical value from the t table is -2.015 t = \(\frac{\overline{x}-\mu}{\frac{s}{\sqrt{n}}}\) t = \(\frac{82-90}{\frac{18}{\sqrt{6}}}\) t = -1.088 As -1.088 > -2.015, we fail to reject the null hypothesis. Answer: There is not enough evidence to support the claim.
go to slide go to slide go to slide
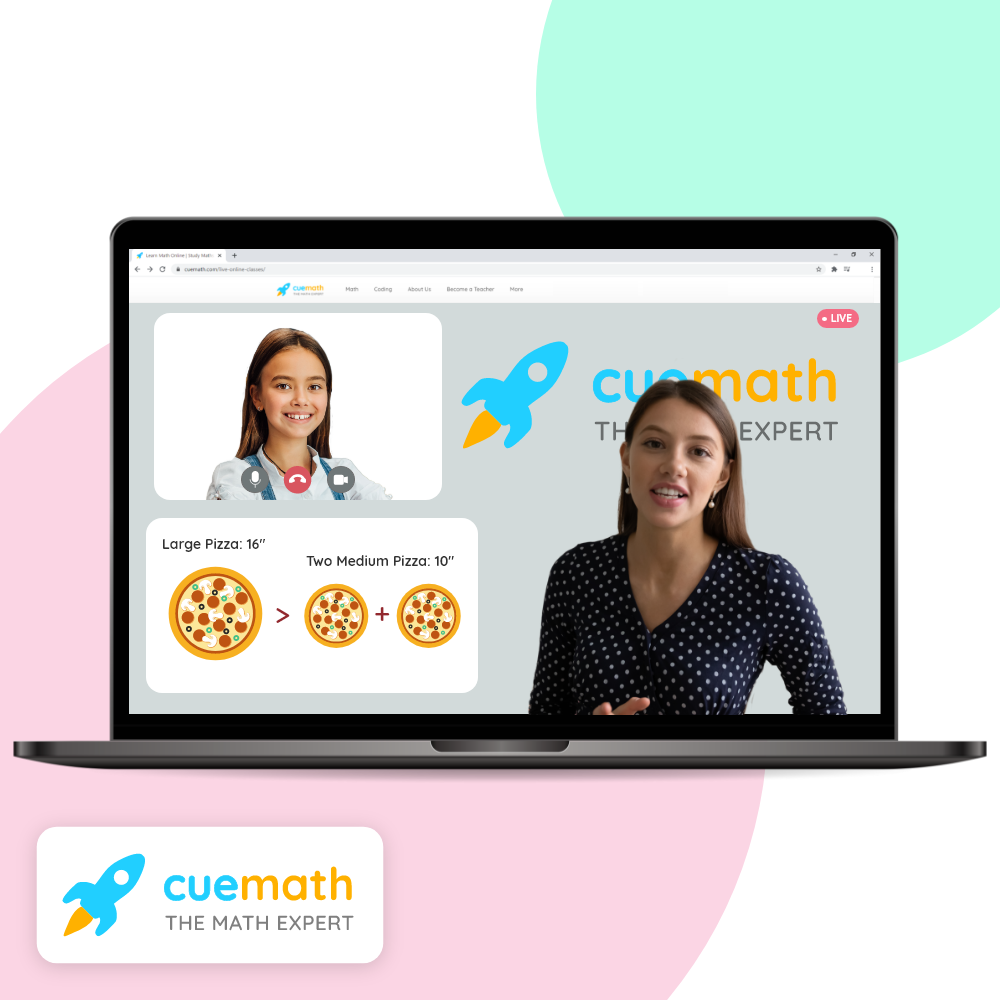
Book a Free Trial Class
FAQs on Hypothesis Testing
What is hypothesis testing.
Hypothesis testing in statistics is a tool that is used to make inferences about the population data. It is also used to check if the results of an experiment are valid.
What is the z Test in Hypothesis Testing?
The z test in hypothesis testing is used to find the z test statistic for normally distributed data . The z test is used when the standard deviation of the population is known and the sample size is greater than or equal to 30.
What is the t Test in Hypothesis Testing?
The t test in hypothesis testing is used when the data follows a student t distribution . It is used when the sample size is less than 30 and standard deviation of the population is not known.
What is the formula for z test in Hypothesis Testing?
The formula for a one sample z test in hypothesis testing is z = \(\frac{\overline{x}-\mu}{\frac{\sigma}{\sqrt{n}}}\) and for two samples is z = \(\frac{(\overline{x_{1}}-\overline{x_{2}})-(\mu_{1}-\mu_{2})}{\sqrt{\frac{\sigma_{1}^{2}}{n_{1}}+\frac{\sigma_{2}^{2}}{n_{2}}}}\).
What is the p Value in Hypothesis Testing?
The p value helps to determine if the test results are statistically significant or not. In hypothesis testing, the null hypothesis can either be rejected or not rejected based on the comparison between the p value and the alpha level.
What is One Tail Hypothesis Testing?
When the rejection region is only on one side of the distribution curve then it is known as one tail hypothesis testing. The right tail test and the left tail test are two types of directional hypothesis testing.
What is the Alpha Level in Two Tail Hypothesis Testing?
To get the alpha level in a two tail hypothesis testing divide \(\alpha\) by 2. This is done as there are two rejection regions in the curve.

Want to create or adapt books like this? Learn more about how Pressbooks supports open publishing practices.
11 Hypothesis Testing with One Sample
Student learning outcomes.
By the end of this chapter, the student should be able to:
- Be able to identify and develop the null and alternative hypothesis
- Identify the consequences of Type I and Type II error.
- Be able to perform an one-tailed and two-tailed hypothesis test using the critical value method
- Be able to perform a hypothesis test using the p-value method
- Be able to write conclusions based on hypothesis tests.
Introduction
Now we are down to the bread and butter work of the statistician: developing and testing hypotheses. It is important to put this material in a broader context so that the method by which a hypothesis is formed is understood completely. Using textbook examples often clouds the real source of statistical hypotheses.
Statistical testing is part of a much larger process known as the scientific method. This method was developed more than two centuries ago as the accepted way that new knowledge could be created. Until then, and unfortunately even today, among some, “knowledge” could be created simply by some authority saying something was so, ipso dicta . Superstition and conspiracy theories were (are?) accepted uncritically.
The scientific method, briefly, states that only by following a careful and specific process can some assertion be included in the accepted body of knowledge. This process begins with a set of assumptions upon which a theory, sometimes called a model, is built. This theory, if it has any validity, will lead to predictions; what we call hypotheses.
As an example, in Microeconomics the theory of consumer choice begins with certain assumption concerning human behavior. From these assumptions a theory of how consumers make choices using indifference curves and the budget line. This theory gave rise to a very important prediction, namely, that there was an inverse relationship between price and quantity demanded. This relationship was known as the demand curve. The negative slope of the demand curve is really just a prediction, or a hypothesis, that can be tested with statistical tools.
Unless hundreds and hundreds of statistical tests of this hypothesis had not confirmed this relationship, the so-called Law of Demand would have been discarded years ago. This is the role of statistics, to test the hypotheses of various theories to determine if they should be admitted into the accepted body of knowledge; how we understand our world. Once admitted, however, they may be later discarded if new theories come along that make better predictions.
Not long ago two scientists claimed that they could get more energy out of a process than was put in. This caused a tremendous stir for obvious reasons. They were on the cover of Time and were offered extravagant sums to bring their research work to private industry and any number of universities. It was not long until their work was subjected to the rigorous tests of the scientific method and found to be a failure. No other lab could replicate their findings. Consequently they have sunk into obscurity and their theory discarded. It may surface again when someone can pass the tests of the hypotheses required by the scientific method, but until then it is just a curiosity. Many pure frauds have been attempted over time, but most have been found out by applying the process of the scientific method.
This discussion is meant to show just where in this process statistics falls. Statistics and statisticians are not necessarily in the business of developing theories, but in the business of testing others’ theories. Hypotheses come from these theories based upon an explicit set of assumptions and sound logic. The hypothesis comes first, before any data are gathered. Data do not create hypotheses; they are used to test them. If we bear this in mind as we study this section the process of forming and testing hypotheses will make more sense.
One job of a statistician is to make statistical inferences about populations based on samples taken from the population. Confidence intervals are one way to estimate a population parameter. Another way to make a statistical inference is to make a decision about the value of a specific parameter. For instance, a car dealer advertises that its new small truck gets 35 miles per gallon, on average. A tutoring service claims that its method of tutoring helps 90% of its students get an A or a B. A company says that women managers in their company earn an average of $60,000 per year.
A statistician will make a decision about these claims. This process is called ” hypothesis testing .” A hypothesis test involves collecting data from a sample and evaluating the data. Then, the statistician makes a decision as to whether or not there is sufficient evidence, based upon analyses of the data, to reject the null hypothesis.
In this chapter, you will conduct hypothesis tests on single means and single proportions. You will also learn about the errors associated with these tests.
Null and Alternative Hypotheses
The actual test begins by considering two hypotheses . They are called the null hypothesis and the alternative hypothesis . These hypotheses contain opposing viewpoints.

Since the null and alternative hypotheses are contradictory, you must examine evidence to decide if you have enough evidence to reject the null hypothesis or not. The evidence is in the form of sample data.
Table 1 presents the various hypotheses in the relevant pairs. For example, if the null hypothesis is equal to some value, the alternative has to be not equal to that value.
NOTE
We want to test whether the mean GPA of students in American colleges is different from 2.0 (out of 4.0). The null and alternative hypotheses are:

We want to test if college students take less than five years to graduate from college, on the average. The null and alternative hypotheses are:
Outcomes and the Type I and Type II Errors
The four possible outcomes in the table are:
Each of the errors occurs with a particular probability. The Greek letters α and β represent the probabilities.

By way of example, the American judicial system begins with the concept that a defendant is “presumed innocent”. This is the status quo and is the null hypothesis. The judge will tell the jury that they can not find the defendant guilty unless the evidence indicates guilt beyond a “reasonable doubt” which is usually defined in criminal cases as 95% certainty of guilt. If the jury cannot accept the null, innocent, then action will be taken, jail time. The burden of proof always lies with the alternative hypothesis. (In civil cases, the jury needs only to be more than 50% certain of wrongdoing to find culpability, called “a preponderance of the evidence”).
The example above was for a test of a mean, but the same logic applies to tests of hypotheses for all statistical parameters one may wish to test.
The following are examples of Type I and Type II errors.
Type I error : Frank thinks that his rock climbing equipment may not be safe when, in fact, it really is safe.
Type II error : Frank thinks that his rock climbing equipment may be safe when, in fact, it is not safe.
Notice that, in this case, the error with the greater consequence is the Type II error. (If Frank thinks his rock climbing equipment is safe, he will go ahead and use it.)
This is a situation described as “accepting a false null”.
Type I error : The emergency crew thinks that the victim is dead when, in fact, the victim is alive. Type II error : The emergency crew does not know if the victim is alive when, in fact, the victim is dead.
The error with the greater consequence is the Type I error. (If the emergency crew thinks the victim is dead, they will not treat him.)
Distribution Needed for Hypothesis Testing
Particular distributions are associated with hypothesis testing.We will perform hypotheses tests of a population mean using a normal distribution or a Student’s t -distribution. (Remember, use a Student’s t -distribution when the population standard deviation is unknown and the sample size is small, where small is considered to be less than 30 observations.) We perform tests of a population proportion using a normal distribution when we can assume that the distribution is normally distributed. We consider this to be true if the sample proportion, p ‘ , times the sample size is greater than 5 and 1- p ‘ times the sample size is also greater then 5. This is the same rule of thumb we used when developing the formula for the confidence interval for a population proportion.
Hypothesis Test for the Mean
Going back to the standardizing formula we can derive the test statistic for testing hypotheses concerning means.

This gives us the decision rule for testing a hypothesis for a two-tailed test:
P-Value Approach
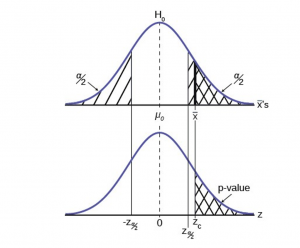
Both decision rules will result in the same decision and it is a matter of preference which one is used.
One and Two-tailed Tests

The claim would be in the alternative hypothesis. The burden of proof in hypothesis testing is carried in the alternative. This is because failing to reject the null, the status quo, must be accomplished with 90 or 95 percent significance that it cannot be maintained. Said another way, we want to have only a 5 or 10 percent probability of making a Type I error, rejecting a good null; overthrowing the status quo.
Figure 5 shows the two possible cases and the form of the null and alternative hypothesis that give rise to them.
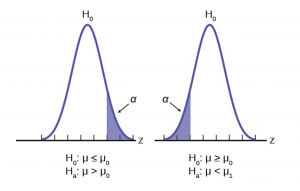
Effects of Sample Size on Test Statistic

Table 3 summarizes test statistics for varying sample sizes and population standard deviation known and unknown.
A Systematic Approach for Testing A Hypothesis
A systematic approach to hypothesis testing follows the following steps and in this order. This template will work for all hypotheses that you will ever test.
- Set up the null and alternative hypothesis. This is typically the hardest part of the process. Here the question being asked is reviewed. What parameter is being tested, a mean, a proportion, differences in means, etc. Is this a one-tailed test or two-tailed test? Remember, if someone is making a claim it will always be a one-tailed test.
- Decide the level of significance required for this particular case and determine the critical value. These can be found in the appropriate statistical table. The levels of confidence typical for the social sciences are 90, 95 and 99. However, the level of significance is a policy decision and should be based upon the risk of making a Type I error, rejecting a good null. Consider the consequences of making a Type I error.
- Take a sample(s) and calculate the relevant parameters: sample mean, standard deviation, or proportion. Using the formula for the test statistic from above in step 2, now calculate the test statistic for this particular case using the parameters you have just calculated.
- Compare the calculated test statistic and the critical value. Marking these on the graph will give a good visual picture of the situation. There are now only two situations:
a. The test statistic is in the tail: Cannot Accept the null, the probability that this sample mean (proportion) came from the hypothesized distribution is too small to believe that it is the real home of these sample data.
b. The test statistic is not in the tail: Cannot Reject the null, the sample data are compatible with the hypothesized population parameter.
- Reach a conclusion. It is best to articulate the conclusion two different ways. First a formal statistical conclusion such as “With a 95 % level of significance we cannot accept the null hypotheses that the population mean is equal to XX (units of measurement)”. The second statement of the conclusion is less formal and states the action, or lack of action, required. If the formal conclusion was that above, then the informal one might be, “The machine is broken and we need to shut it down and call for repairs”.
All hypotheses tested will go through this same process. The only changes are the relevant formulas and those are determined by the hypothesis required to answer the original question.
Full Hypothesis Test Examples
Tests on means.
Jeffrey, as an eight-year old, established a mean time of 16.43 seconds for swimming the 25-yard freestyle, with a standard deviation of 0.8 seconds . His dad, Frank, thought that Jeffrey could swim the 25-yard freestyle faster using goggles. Frank bought Jeffrey a new pair of expensive goggles and timed Jeffrey for 15 25-yard freestyle swims . For the 15 swims, Jeffrey’s mean time was 16 seconds. Frank thought that the goggles helped Jeffrey to swim faster than the 16.43 seconds. Conduct a hypothesis test using a preset α = 0.05.
Solution – Example 6
Set up the Hypothesis Test:
Since the problem is about a mean, this is a test of a single population mean . Set the null and alternative hypothesis:
In this case there is an implied challenge or claim. This is that the goggles will reduce the swimming time. The effect of this is to set the hypothesis as a one-tailed test. The claim will always be in the alternative hypothesis because the burden of proof always lies with the alternative. Remember that the status quo must be defeated with a high degree of confidence, in this case 95 % confidence. The null and alternative hypotheses are thus:
For Jeffrey to swim faster, his time will be less than 16.43 seconds. The “<” tells you this is left-tailed. Determine the distribution needed:
Distribution for the test statistic:
The sample size is less than 30 and we do not know the population standard deviation so this is a t-test and the proper formula is:

Our step 2, setting the level of significance, has already been determined by the problem, .05 for a 95 % significance level. It is worth thinking about the meaning of this choice. The Type I error is to conclude that Jeffrey swims the 25-yard freestyle, on average, in less than 16.43 seconds when, in fact, he actually swims the 25-yard freestyle, on average, in 16.43 seconds. (Reject the null hypothesis when the null hypothesis is true.) For this case the only concern with a Type I error would seem to be that Jeffery’s dad may fail to bet on his son’s victory because he does not have appropriate confidence in the effect of the goggles.
To find the critical value we need to select the appropriate test statistic. We have concluded that this is a t-test on the basis of the sample size and that we are interested in a population mean. We can now draw the graph of the t-distribution and mark the critical value (Figure 6). For this problem the degrees of freedom are n-1, or 14. Looking up 14 degrees of freedom at the 0.05 column of the t-table we find 1.761. This is the critical value and we can put this on our graph.
Step 3 is the calculation of the test statistic using the formula we have selected.

We find that the calculated test statistic is 2.08, meaning that the sample mean is 2.08 standard deviations away from the hypothesized mean of 16.43.
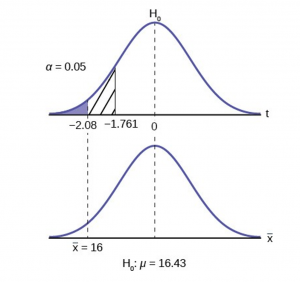
Step 4 has us compare the test statistic and the critical value and mark these on the graph. We see that the test statistic is in the tail and thus we move to step 4 and reach a conclusion. The probability that an average time of 16 minutes could come from a distribution with a population mean of 16.43 minutes is too unlikely for us to accept the null hypothesis. We cannot accept the null.
Step 5 has us state our conclusions first formally and then less formally. A formal conclusion would be stated as: “With a 95% level of significance we cannot accept the null hypothesis that the swimming time with goggles comes from a distribution with a population mean time of 16.43 minutes.” Less formally, “With 95% significance we believe that the goggles improves swimming speed”
If we wished to use the p-value system of reaching a conclusion we would calculate the statistic and take the additional step to find the probability of being 2.08 standard deviations from the mean on a t-distribution. This value is .0187. Comparing this to the α-level of .05 we see that we cannot accept the null. The p-value has been put on the graph as the shaded area beyond -2.08 and it shows that it is smaller than the hatched area which is the alpha level of 0.05. Both methods reach the same conclusion that we cannot accept the null hypothesis.
Jane has just begun her new job as on the sales force of a very competitive company. In a sample of 16 sales calls it was found that she closed the contract for an average value of $108 with a standard deviation of 12 dollars. Test at 5% significance that the population mean is at least $100 against the alternative that it is less than 100 dollars. Company policy requires that new members of the sales force must exceed an average of $100 per contract during the trial employment period. Can we conclude that Jane has met this requirement at the significance level of 95%?
Solution – Example 7
STEP 1 : Set the Null and Alternative Hypothesis.
STEP 2 : Decide the level of significance and draw the graph (Figure 7) showing the critical value.

STEP 3 : Calculate sample parameters and the test statistic.
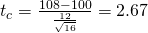
STEP 4 : Compare test statistic and the critical values
STEP 5 : Reach a Conclusion
The test statistic is a Student’s t because the sample size is below 30; therefore, we cannot use the normal distribution. Comparing the calculated value of the test statistic and the critical value of t ( t a ) at a 5% significance level, we see that the calculated value is in the tail of the distribution. Thus, we conclude that 108 dollars per contract is significantly larger than the hypothesized value of 100 and thus we cannot accept the null hypothesis. There is evidence that supports Jane’s performance meets company standards.

Again we will follow the steps in our analysis of this problem.
Solution – Example 8
STEP 1 : Set the Null and Alternative Hypothesis. The random variable is the quantity of fluid placed in the bottles. This is a continuous random variable and the parameter we are interested in is the mean. Our hypothesis therefore is about the mean. In this case we are concerned that the machine is not filling properly. From what we are told it does not matter if the machine is over-filling or under-filling, both seem to be an equally bad error. This tells us that this is a two-tailed test: if the machine is malfunctioning it will be shutdown regardless if it is from over-filling or under-filling. The null and alternative hypotheses are thus:
STEP 2 : Decide the level of significance and draw the graph showing the critical value.
This problem has already set the level of significance at 99%. The decision seems an appropriate one and shows the thought process when setting the significance level. Management wants to be very certain, as certain as probability will allow, that they are not shutting down a machine that is not in need of repair. To draw the distribution and the critical value, we need to know which distribution to use. Because this is a continuous random variable and we are interested in the mean, and the sample size is greater than 30, the appropriate distribution is the normal distribution and the relevant critical value is 2.575 from the normal table or the t-table at 0.005 column and infinite degrees of freedom. We draw the graph and mark these points (Figure 8).
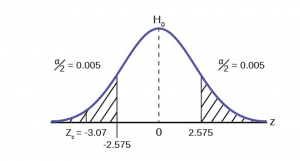
STEP 3 : Calculate sample parameters and the test statistic. The sample parameters are provided, the sample mean is 7.91 and the sample variance is .03 and the sample size is 35. We need to note that the sample variance was provided not the sample standard deviation, which is what we need for the formula. Remembering that the standard deviation is simply the square root of the variance, we therefore know the sample standard deviation, s, is 0.173. With this information we calculate the test statistic as -3.07, and mark it on the graph.

STEP 4 : Compare test statistic and the critical values Now we compare the test statistic and the critical value by placing the test statistic on the graph. We see that the test statistic is in the tail, decidedly greater than the critical value of 2.575. We note that even the very small difference between the hypothesized value and the sample value is still a large number of standard deviations. The sample mean is only 0.08 ounces different from the required level of 8 ounces, but it is 3 plus standard deviations away and thus we cannot accept the null hypothesis.
Three standard deviations of a test statistic will guarantee that the test will fail. The probability that anything is within three standard deviations is almost zero. Actually it is 0.0026 on the normal distribution, which is certainly almost zero in a practical sense. Our formal conclusion would be “ At a 99% level of significance we cannot accept the hypothesis that the sample mean came from a distribution with a mean of 8 ounces” Or less formally, and getting to the point, “At a 99% level of significance we conclude that the machine is under filling the bottles and is in need of repair”.
Media Attributions
- Type1Type2Error
- HypTestFig2
- HypTestFig3
- HypTestPValue
- OneTailTestFig5
- HypTestExam7
- HypTestExam8
Quantitative Analysis for Business Copyright © by Margo Bergman is licensed under a Creative Commons Attribution-NonCommercial-ShareAlike 4.0 International License , except where otherwise noted.
Share This Book
One Hypothesis Testing Example
Starting with this page, we'll begin considering the scenario where we only have sample data; that is, when we refer to df in the following pages, we are using df_sample from the previous page, a random sample of Chicago Airbnb listings from March 2023. Depending on your context, having sample data may be more or less common than obtaining population data. We'll only use our population data to confirm our results and/or demonstrate how inference procedures work.
First, we'll explore how we can test hypotheses. Hypothesis testing allows us to make a decision between two competing theories about our unknown population parameter, allowing us to understand the corresponding population better.
General Motivation and Framework
Hypothesis testing exists to help researchers decide between two competing theories (or statements) about the unknown population parameter. Although we don't know the parameter, we want to use information from our statistic to help guide us to selecting a theory that we have reasonable evidence to support.
For example, suppose that you would like to convince someone that Airbnb hosts have been a host for more than 3 years, on average. We may want to decide between your theory, and another person's theory that this is not true. (We'll work through this example on this page).
What do we do with these theories once we have defined them? One of the theories will serve as a "skeptic's theory". The other theory is one that you hope to persuade the skeptic to believe. How will you successfully persuade the skeptic? The approach used in hypothesis testing is that you will start by assuming the skeptic is actually correct. Then, you'll use your sample to calculate how unlikely (inconsistent) the sample statistic is if the skeptic's theory is correct. If the sample is unlikely enough and meets the skeptic's threshold, then you have successfully persuaded the skeptic to change their mind! If not, the skeptic tells you: "good try, but this isn't convincing enough for me. I'm sticking with my theory."
To help demonstrate the process of hypothesis testing, let's first preview the results of a hypothesis test. Once we've done that, we'll describe the formal steps that we've completed for performing the hypothesis test on the next page. We'll then complete examples and discuss limitations and other implications after this introduction.
Hypothesis Testing Example
How does the process of hypothesis testing actually work?
Suppose that you are trying to convince a skeptic who believes that the population mean time that the host has been on Airbnb for all Chicago Airbnb listings is 4 years (1461 days). You'd like to convince the skeptic that the mean time for hosts on Airbnb is actually longer than 4 years. We can use our sample of Chicago Airbnb listings to calculate the sample mean number of days that a host has been on Airbnb.
We see that for our sample, the average time for hosts to be on Airbnb for the Chicago Airbnb listings was actually much closer to 6 years. Is this different enough from 4 years to convince our skeptic? Our skeptic is quite logical, so we'll need to provide additional evidence to this skeptic to convince them. To do so, we'll adopt the viewpoint that 4 years is in fact the population mean and adjust the data so that this is truly the case (represented in skeptic_data created below).
Now, let's use a resampling scheme in order to determine plausible values for sample means if the skeptic were in fact true.
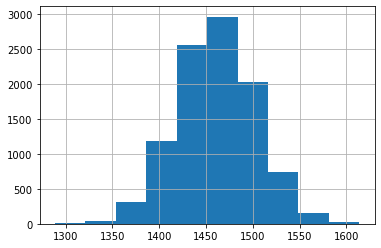
Histogram of a simulated sampling distribution for the median price per night for a Chicago Airbnb.
We see that none of our 10,000 simulated sample means were similar to what we observed from our actual sample (2188 days). That means that if the skeptic were correct, we would not have any samples that had a sample mean close to what we observed in our sample. This would likely convince any skeptic that the population probably doesn't have a mean host time on Airbnb of 4 years.
Testing a Second Skeptic
For illustration purposes, consider that we have a second skeptic who had a different claim about Airbnb owners. Our second skeptic thinks that the population average time on Airbnb was 5 years and 9 months (approximately 2100 days)? We are trying to convince this skeptic that the population mean time is more than 5.75 years.
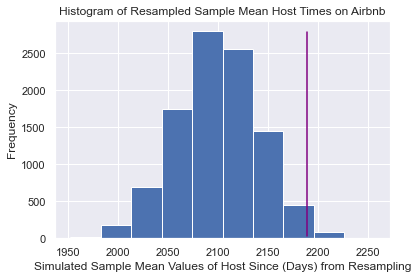
Histogram of the minimum nights required for the population of Chicago Airbnb listings.
Now, in this simulated sampling distribution, there are a few resamples that had similar sample means to our observed sample. What proportion were similar (or larger) than our observed sample mean?
We see that only 1.46% of our resampled sample means provided evidence similar to our observed sample against the skeptic's claim and in favor of our claim. That means our sample is fairly unlikely if the skeptic is correct - it would only happen about 1.5% of the time (less than 2 samples out of 100, or about 15 samples in 1000). Is this enough to convince the skeptic? It depends on your skeptic.
Personally, I'd say this is fairly strong evidence that my sample isn't consistent with the skeptic's claim. If I were a skeptic, I'd want to reevaluate my claim.
Calcworkshop
Hypothesis Testing w/ 21 Step-by-Step Examples!
// Last Updated: October 9, 2020 - Watch Video //
In statistical testing, also referred to as hypothesis testing, our goal is to show the credibility of a claim regarding the population.
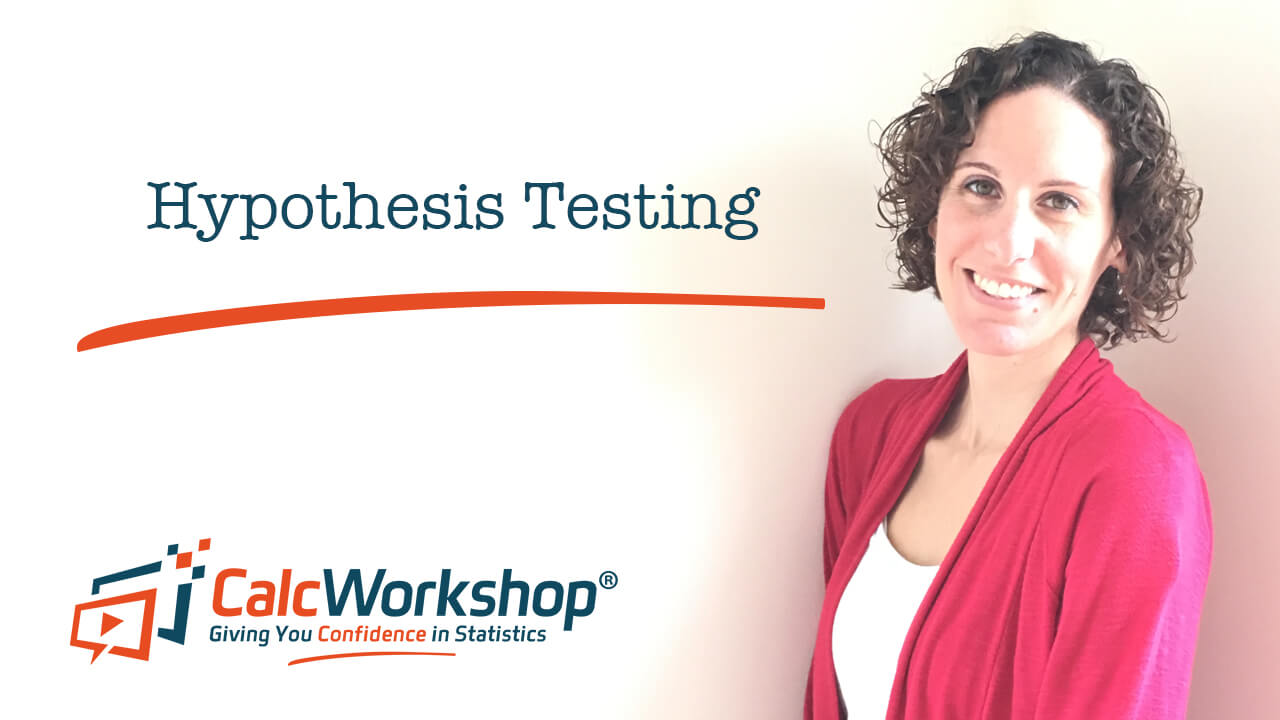
Jenn, Founder Calcworkshop ® , 15+ Years Experience (Licensed & Certified Teacher)
What Is Hypothesis Testing
Now it would be unreasonable to assume that we can test the entire population to determine the feasibility of every claim one might have.
Thus, we need a way to conclude an assumption is true or false by taking an appropriate sample and calculating a relevant statistic.
And knowing that we must expect that there will be some variation between the sample statistic that is calculated and the true population parameter, leads us to the understanding of statistical inferences (hypotheses).
Hypothesis Testing Steps
First, we must identify the parameter of interest.
Remember that a parameter always points to the population so that it will be either a population mean, population proportion, population slope, or some other population parameter.
Types of Hypothesis Tests
Then we will write a declaration of our significance test, which will include a null hypothesis statement and an alternative hypothesis.
The null hypothesis is the expected value of the population parameter, similar to the status quo, whereas the alternative hypothesis is a statement of negation of the null hypothesis as discussed by Penn State .
Next, we will calculate the desired test statistic from our random sample. This test statistic is a numerical quantity that measures the difference between the observed value and the expected value, divided by the standard error, which is the sample standard deviation.
Then we will compare this test statistic with a specified level of significance (alpha), just like we did with confidence intervals.
If the probability of yielding the sample statistic is as extreme or more extreme is smaller than our significance level, then we declare the sample statistic to be significant and reject the null hypothesis in favor of the alternative. In other words, if the probability is inside our shaded critical region then it is considered more extreme; thus, rejecting the hypothesis. But if it is outside the critical region, we will fail to reject our claim in favor of the alternative.

Null and Alternative Hypothesis
Additionally, we will also learn how to determine whether our study calls for a one or two-tailed test.
Type 1 And Type 2 Errors
Now, with all inferences and tests of significance, there is always room for error. A Type I error occurs if we reject the null hypothesis, when in actuality, the null hypothesis is true. Similarly, if we fail to reject the null hypothesis when, in reality, the null hypothesis is false, this is considered a Type II error .

Type 1 Vs. Type 2 Error
Imagine you are in a court of law, where a defendant is presumed innocent until proven guilty. What possible errors could a jury make regarding the outcome of the trial?
First, let’s state the following:
- The Null Hypothesis: The defendant is innocent.
- The Alternative Hypothesis: the defendant is guilty.
Now, a Type I Error would happen if the jury rejects the null hypothesis as false when, in reality, the null hypothesis is true. In other words, the jury finds the defendant guilty of a crime they didn’t commit.
And a Type II Error is when a jury accepts the null hypothesis as true when, in reality, the null hypothesis is false. Meaning, the defendant is found innocent of a crime they did commit.
Let’s look at an example where we put all of these ideas together.
Worked Example
Imagine we have a textile manufacturer investigating a new yarn, which claims it has a thread elongation of 12 kilograms with a standard deviation of 0.5 kilograms.
Using a random sample of 4 specimens, the manufacturer wishes to test the claim that the mean thread elongation is less than 12 kilograms.
Write a hypothesis statement for this scenario and using a normal distribution, find the Type 1 error if the sample mean is less than 11.5 kilograms.

Type 1 Error — Example
As we can see, from the example above, the likelihood of a type I error, where the manufacturer rejects the null hypothesis when the null hypothesis is actually true, is approximately 0.023 or 2.3% likely.
Together, we will look at these two types of error and how they affect decision-making and begin to explore the notion of a probability value and how it helps us determine the validity or falsity of our claim.
Hypothesis Testing – Lesson & Examples (Video)
1 hr 17 min
- Introduction to Video: Statistical Hypotheses
- 00:00:38 – Overview of Hypothesis Testing and determining a correctly stated hypothesis testing problem (Examples #1-7)
- Exclusive Content for Members Only
- 00:14:34 – State the Null Hypothesis and the Alternative Hypothesis for each scenario (Examples #8-12)
- 00:25:46 – Hypothesis Testing Steps and Overview of Type I and Type II errors (Examples #13-14)
- 00:40:32 – Describe a Type 1 and Type 2 error (Examples #15-16)
- 00:46:32 – Overview of p-value and Tails of the Hypothesis Test
- 00:55:55 – Find the probability of a Type I and Type II error (Example #17)
- 01:06:08 – Identify null hypothesis, alternative hypothesis, and state whether the scenario is a one-tail or two-tailed test (Examples #18-21)
- Practice Problems with Step-by-Step Solutions
- Chapter Tests with Video Solutions
Get access to all the courses and over 450 HD videos with your subscription
Monthly and Yearly Plans Available
Get My Subscription Now
Still wondering if CalcWorkshop is right for you? Take a Tour and find out how a membership can take the struggle out of learning math.
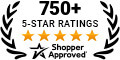

Statistics Made Easy
4 Examples of Hypothesis Testing in Real Life
In statistics, hypothesis tests are used to test whether or not some hypothesis about a population parameter is true.
To perform a hypothesis test in the real world, researchers will obtain a random sample from the population and perform a hypothesis test on the sample data, using a null and alternative hypothesis:
- Null Hypothesis (H 0 ): The sample data occurs purely from chance.
- Alternative Hypothesis (H A ): The sample data is influenced by some non-random cause.
If the p-value of the hypothesis test is less than some significance level (e.g. α = .05), then we can reject the null hypothesis and conclude that we have sufficient evidence to say that the alternative hypothesis is true.
The following examples provide several situations where hypothesis tests are used in the real world.
Example 1: Biology
Hypothesis tests are often used in biology to determine whether some new treatment, fertilizer, pesticide, chemical, etc. causes increased growth, stamina, immunity, etc. in plants or animals.
For example, suppose a biologist believes that a certain fertilizer will cause plants to grow more during a one-month period than they normally do, which is currently 20 inches. To test this, she applies the fertilizer to each of the plants in her laboratory for one month.
She then performs a hypothesis test using the following hypotheses:
- H 0 : μ = 20 inches (the fertilizer will have no effect on the mean plant growth)
- H A : μ > 20 inches (the fertilizer will cause mean plant growth to increase)
If the p-value of the test is less than some significance level (e.g. α = .05), then she can reject the null hypothesis and conclude that the fertilizer leads to increased plant growth.
Example 2: Clinical Trials
Hypothesis tests are often used in clinical trials to determine whether some new treatment, drug, procedure, etc. causes improved outcomes in patients.
For example, suppose a doctor believes that a new drug is able to reduce blood pressure in obese patients. To test this, he may measure the blood pressure of 40 patients before and after using the new drug for one month.
He then performs a hypothesis test using the following hypotheses:
- H 0 : μ after = μ before (the mean blood pressure is the same before and after using the drug)
- H A : μ after < μ before (the mean blood pressure is less after using the drug)
If the p-value of the test is less than some significance level (e.g. α = .05), then he can reject the null hypothesis and conclude that the new drug leads to reduced blood pressure.
Example 3: Advertising Spend
Hypothesis tests are often used in business to determine whether or not some new advertising campaign, marketing technique, etc. causes increased sales.
For example, suppose a company believes that spending more money on digital advertising leads to increased sales. To test this, the company may increase money spent on digital advertising during a two-month period and collect data to see if overall sales have increased.
They may perform a hypothesis test using the following hypotheses:
- H 0 : μ after = μ before (the mean sales is the same before and after spending more on advertising)
- H A : μ after > μ before (the mean sales increased after spending more on advertising)
If the p-value of the test is less than some significance level (e.g. α = .05), then the company can reject the null hypothesis and conclude that increased digital advertising leads to increased sales.
Example 4: Manufacturing
Hypothesis tests are also used often in manufacturing plants to determine if some new process, technique, method, etc. causes a change in the number of defective products produced.
For example, suppose a certain manufacturing plant wants to test whether or not some new method changes the number of defective widgets produced per month, which is currently 250. To test this, they may measure the mean number of defective widgets produced before and after using the new method for one month.
They can then perform a hypothesis test using the following hypotheses:
- H 0 : μ after = μ before (the mean number of defective widgets is the same before and after using the new method)
- H A : μ after ≠ μ before (the mean number of defective widgets produced is different before and after using the new method)
If the p-value of the test is less than some significance level (e.g. α = .05), then the plant can reject the null hypothesis and conclude that the new method leads to a change in the number of defective widgets produced per month.
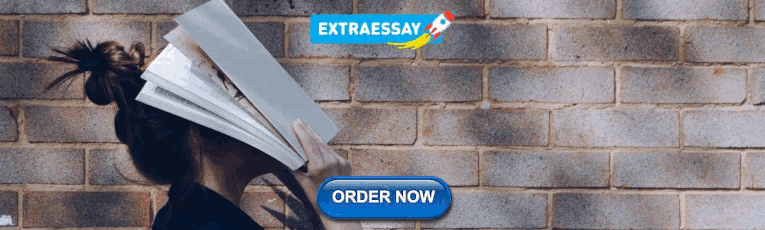
Additional Resources
Introduction to Hypothesis Testing Introduction to the One Sample t-test Introduction to the Two Sample t-test Introduction to the Paired Samples t-test

Published by Zach
Leave a reply cancel reply.
Your email address will not be published. Required fields are marked *

- school Campus Bookshelves
- menu_book Bookshelves
- perm_media Learning Objects
- login Login
- how_to_reg Request Instructor Account
- hub Instructor Commons
- Download Page (PDF)
- Download Full Book (PDF)
- Periodic Table
- Physics Constants
- Scientific Calculator
- Reference & Cite
- Tools expand_more
- Readability
selected template will load here
This action is not available.
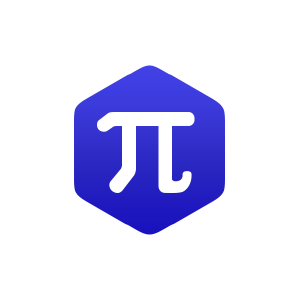
9.5: Additional Information and Full Hypothesis Test Examples
- Last updated
- Save as PDF
- Page ID 113355
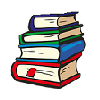
- In a hypothesis test problem, you may see words such as "the level of significance is 1%." The "1%" is the preconceived or preset \(\alpha\).
- The statistician setting up the hypothesis test selects the value of α to use before collecting the sample data.
- If no level of significance is given, a common standard to use is \(\alpha = 0.05\).
- When you calculate the \(p\)-value and draw the picture, the \(p\)-value is the area in the left tail, the right tail, or split evenly between the two tails. For this reason, we call the hypothesis test left, right, or two tailed.
- The alternative hypothesis, \(H_{a}\), tells you if the test is left, right, or two-tailed. It is the key to conducting the appropriate test.
- \(H_{a}\) never has a symbol that contains an equal sign.
- Thinking about the meaning of the \(p\)-value: A data analyst (and anyone else) should have more confidence that he made the correct decision to reject the null hypothesis with a smaller \(p\)-value (for example, 0.001 as opposed to 0.04) even if using the 0.05 level for alpha. Similarly, for a large p -value such as 0.4, as opposed to a \(p\)-value of 0.056 (\(\alpha = 0.05\) is less than either number), a data analyst should have more confidence that she made the correct decision in not rejecting the null hypothesis. This makes the data analyst use judgment rather than mindlessly applying rules.
The following examples illustrate a left-, right-, and two-tailed test.
Example \(\PageIndex{1}\)
\(H_{0}: \mu = 5, H_{a}: \mu < 5\)
Test of a single population mean. \(H_{a}\) tells you the test is left-tailed. The picture of the \(p\)-value is as follows:
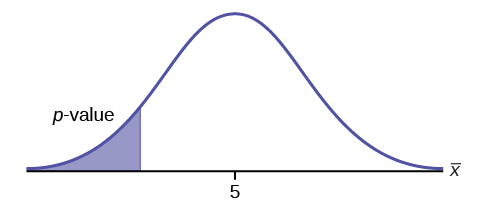
Exercise \(\PageIndex{1}\)
\(H_{0}: \mu = 10, H_{a}: \mu < 10\)
Assume the \(p\)-value is 0.0935. What type of test is this? Draw the picture of the \(p\)-value.
left-tailed test
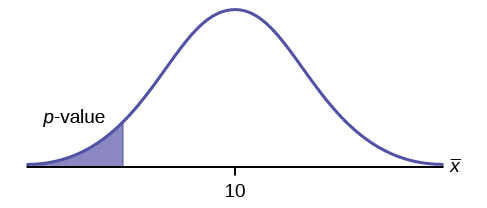
Example \(\PageIndex{2}\)
\(H_{0}: \mu \leq 0.2, H_{a}: \mu > 0.2\)
This is a test of a single population proportion. \(H_{a}\) tells you the test is right-tailed . The picture of the p -value is as follows:
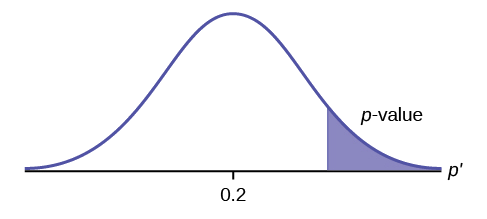
Exercise \(\PageIndex{2}\)
\(H_{0}: \mu \leq 1, H_{a}: \mu > 1\)
Assume the \(p\)-value is 0.1243. What type of test is this? Draw the picture of the \(p\)-value.
right-tailed test
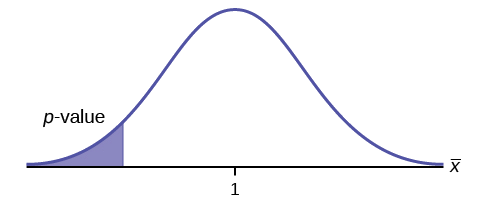
Example \(\PageIndex{3}\)
\(H_{0}: \mu = 50, H_{a}: \mu \neq 50\)
This is a test of a single population mean. \(H_{a}\) tells you the test is two-tailed . The picture of the \(p\)-value is as follows.
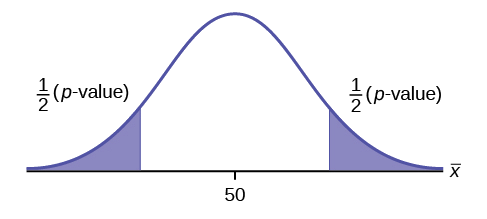
Exercise \(\PageIndex{3}\)
\(H_{0}: \mu = 0.5, H_{a}: \mu \neq 0.5\)
Assume the p -value is 0.2564. What type of test is this? Draw the picture of the \(p\)-value.
two-tailed test
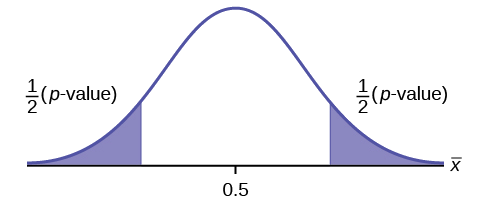
Full Hypothesis Test Examples
Example \(\pageindex{4}\).
Jeffrey, as an eight-year old, established a mean time of 16.43 seconds for swimming the 25-yard freestyle, with a standard deviation of 0.8 seconds . His dad, Frank, thought that Jeffrey could swim the 25-yard freestyle faster using goggles. Frank bought Jeffrey a new pair of expensive goggles and timed Jeffrey for 15 25-yard freestyle swims . For the 15 swims, Jeffrey's mean time was 16 seconds. Frank thought that the goggles helped Jeffrey to swim faster than the 16.43 seconds. Conduct a hypothesis test using a preset α = 0.05. Assume that the swim times for the 25-yard freestyle are normal.
Set up the Hypothesis Test:
Since the problem is about a mean, this is a test of a single population mean .
\(H_{0}: \mu = 16.43, H_{a}: \mu < 16.43\)
For Jeffrey to swim faster, his time will be less than 16.43 seconds. The "\(<\)" tells you this is left-tailed.
Determine the distribution needed:
Random variable: \(\bar{X} =\) the mean time to swim the 25-yard freestyle.
Distribution for the test: \(\bar{X}\) is normal (population standard deviation is known: \(\sigma = 0.8\))
\(\bar{X} - N \left(\mu, \frac{\sigma_{x}}{\sqrt{n}}\right)\) Therefore, \(\bar{X} - N\left(16.43, \frac{0.8}{\sqrt{15}}\right)\)
\(\mu = 16.43\) comes from \(H_{0}\) and not the data. \(\sigma = 0.8\), and \(n = 15\).
Calculate the \(p-\text{value}\) using the normal distribution for a mean:
\(p\text{-value} = P(\bar{x} < 16) = 0.0187\) where the sample mean in the problem is given as 16.
\(p\text{-value} = 0.0187\) (This is called the actual level of significance .) The \(p-\text{value}\) is the area to the left of the sample mean is given as 16.
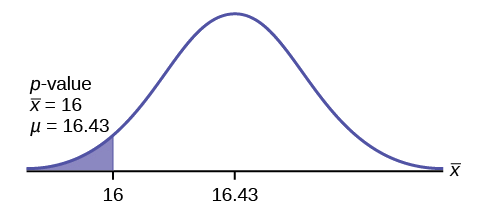
\(\mu = 16.43\) comes from \(H_{0}\). Our assumption is \(\mu = 16.43\).
Interpretation of the \(p-\text{value}\): If \(H_{0}\) is true , there is a 0.0187 probability (1.87%) that Jeffrey's mean time to swim the 25-yard freestyle is 16 seconds or less. Because a 1.87% chance is small, the mean time of 16 seconds or less is unlikely to have happened randomly. It is a rare event.
Compare \(\alpha\) and the \(p-\text{value}\):
\(\alpha = 0.05 p\text{-value} = 0.0187 \alpha > p\text{-value}\)
Make a decision: Since \(\alpha > p\text{-value}\), reject \(H_{0}\).
This means that you reject \(\mu = 16.43\). In other words, you do not think Jeffrey swims the 25-yard freestyle in 16.43 seconds but faster with the new goggles.
Conclusion: At the 5% significance level, we conclude that Jeffrey swims faster using the new goggles. The sample data show there is sufficient evidence that Jeffrey's mean time to swim the 25-yard freestyle is less than 16.43 seconds.
The p -value can easily be calculated.
Press STAT and arrow over to TESTS . Press 1:Z-Test . Arrow over to Stats and press ENTER . Arrow down and enter 16.43 for \(\mu_{0}\) (null hypothesis), .8 for σ , 16 for the sample mean, and 15 for n . Arrow down to \(\mu\) : (alternate hypothesis) and arrow over to \(< \mu_{0}\). Press ENTER . Arrow down to Calculate and press ENTER . The calculator not only calculates the p -value (\(p = 0.0187\)) but it also calculates the test statistic ( z -score) for the sample mean. \(\mu < 16.43\) is the alternative hypothesis. Do this set of instructions again except arrow to Draw (instead of Calculate ). Press ENTER . A shaded graph appears with \(z = -2.08\) (test statistic) and \(p = 0.0187\) (\(p-\text{value}\)). Make sure when you use Draw that no other equations are highlighted in \(Y =\) and the plots are turned off.
When the calculator does a \(Z\)-Test, the Z-Test function finds the p -value by doing a normal probability calculation using the central limit theorem:
\(P(\bar{X} < 16)\) 2nd DISTR normcdf (\((−10^{99},16,16.43,\frac{0.8}{\sqrt{15}})\).
The Type I and Type II errors for this problem are as follows:
The Type I error is to conclude that Jeffrey swims the 25-yard freestyle, on average, in less than 16.43 seconds when, in fact, he actually swims the 25-yard freestyle, on average, in 16.43 seconds. (Reject the null hypothesis when the null hypothesis is true.)
The Type II error is that there is not evidence to conclude that Jeffrey swims the 25-yard free-style, on average, in less than 16.43 seconds when, in fact, he actually does swim the 25-yard free-style, on average, in less than 16.43 seconds. (Do not reject the null hypothesis when the null hypothesis is false.)
Exercise \(\PageIndex{4}\)
The mean throwing distance of a football for a Marco, a high school freshman quarterback, is 40 yards, with a standard deviation of two yards. The team coach tells Marco to adjust his grip to get more distance. The coach records the distances for 20 throws. For the 20 throws, Marco’s mean distance was 45 yards. The coach thought the different grip helped Marco throw farther than 40 yards. Conduct a hypothesis test using a preset \(\alpha = 0.05\). Assume the throw distances for footballs are normal.
First, determine what type of test this is, set up the hypothesis test, find the p -value, sketch the graph, and state your conclusion.
Press STAT and arrow over to TESTS. Press 1: \(Z\)-Test. Arrow over to Stats and press ENTER. Arrow down and enter 40 for \(\mu_{0}\) (null hypothesis), 2 for \(\sigma\), 45 for the sample mean, and 20 for \(n\). Arrow down to \(\mu\): (alternative hypothesis) and set it either as \(<\), \(\neq\), or \(>\). Press ENTER. Arrow down to Calculate and press ENTER. The calculator not only calculates the p -value but it also calculates the test statistic ( z -score) for the sample mean. Select \(<\), \(\neq\), or \(>\) for the alternative hypothesis. Do this set of instructions again except arrow to Draw (instead of Calculate). Press ENTER. A shaded graph appears with test statistic and \(p\)-value. Make sure when you use Draw that no other equations are highlighted in \(Y =\) and the plots are turned off.
Since the problem is about a mean, this is a test of a single population mean.
- \(H_{0}: \mu = 40\)
- \(H_{a}: \mu > 40\)
- \(p = 0.0062\)
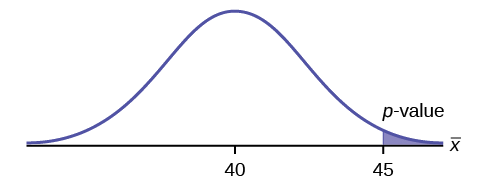
Because \(p < \alpha\), we reject the null hypothesis. There is sufficient evidence to suggest that the change in grip improved Marco’s throwing distance.
Historical Note
The traditional way to compare the two probabilities, \(\alpha\) and the \(p-\text{value}\), is to compare the critical value (\(z\)-score from \(\alpha\)) to the test statistic (\(z\)-score from data). The calculated test statistic for the \(p\)-value is –2.08. (From the Central Limit Theorem, the test statistic formula is \(z = \frac{\bar{x}-\mu_{x}}{\left(\frac{\sigma_{x}}{\sqrt{n}}\right)}\). For this problem, \(\bar{x} = 16\), \(\mu_{x} = 16.43\) from the null hypotheses is, \(\sigma_{x} = 0.8\), and \(n = 15\).) You can find the critical value for \(\alpha = 0.05\) in the normal table (see 15.Tables in the Table of Contents). The \(z\)-score for an area to the left equal to 0.05 is midway between –1.65 and –1.64 (0.05 is midway between 0.0505 and 0.0495). The \(z\)-score is –1.645. Since –1.645 > –2.08 (which demonstrates that \(\alpha > p-\text{value}\)), reject \(H_{0}\). Traditionally, the decision to reject or not reject was done in this way. Today, comparing the two probabilities \(\alpha\) and the \(p\)-value is very common. For this problem, the \(p-\text{value}\), 0.0187 is considerably smaller than \(\alpha = 0.05\). You can be confident about your decision to reject. The graph shows \(\alpha\), the \(p-\text{value}\), and the test statistics and the critical value.
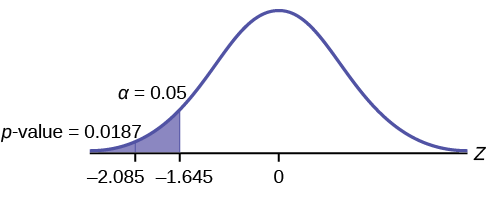
Example \(\PageIndex{5}\)
A college football coach thought that his players could bench press a mean weight of 275 pounds . It is known that the standard deviation is 55 pounds . Three of his players thought that the mean weight was more than that amount. They asked 30 of their teammates for their estimated maximum lift on the bench press exercise. The data ranged from 205 pounds to 385 pounds. The actual different weights were (frequencies are in parentheses) 205(3); 215(3); 225(1); 241(2); 252(2); 265(2); 275(2); 313(2); 316(5); 338(2); 341(1); 345(2); 368(2); 385(1).
Conduct a hypothesis test using a 2.5% level of significance to determine if the bench press mean is more than 275 pounds.
Since the problem is about a mean weight, this is a test of a single population mean.
- \(H_{0}: \mu = 275\)
- \(H_{a}: \mu > 275\)
This is a right-tailed test.
Calculating the distribution needed:
Random variable: \(\bar{X} =\) the mean weight, in pounds, lifted by the football players.
Distribution for the test: It is normal because \(\sigma\) is known.
- \(\bar{X} - N\left(275, \frac{55}{\sqrt{30}}\right)\)
- \(\bar{x} = 286.2\) pounds (from the data).
- \(\sigma = 55\) pounds (Always use \(\sigma\) if you know it.) We assume \(\mu = 275\) pounds unless our data shows us otherwise.
Calculate the p -value using the normal distribution for a mean and using the sample mean as input (see [link] for using the data as input):
\[p\text{-value} = P(\bar{x} > 286.2) = 0.1323.\nonumber \]
Interpretation of the p -value: If \(H_{0}\) is true, then there is a 0.1331 probability (13.23%) that the football players can lift a mean weight of 286.2 pounds or more. Because a 13.23% chance is large enough, a mean weight lift of 286.2 pounds or more is not a rare event.
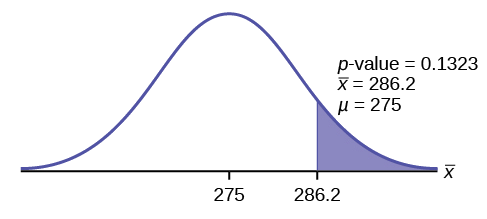
\(\alpha = 0.025 p-value = 0.1323\)
Make a decision: Since \(\alpha < p\text{-value}\), do not reject \(H_{0}\).
Conclusion: At the 2.5% level of significance, from the sample data, there is not sufficient evidence to conclude that the true mean weight lifted is more than 275 pounds.
The \(p-\text{value}\) can easily be calculated.
Put the data and frequencies into lists. Press STAT and arrow over to TESTS . Press 1:Z-Test . Arrow over to Data and press ENTER . Arrow down and enter 275 for \(\mu_{0}\), 55 for \(\sigma\), the name of the list where you put the data, and the name of the list where you put the frequencies. Arrow down to \(\mu\) : and arrow over to \(> \mu_{0}\). Press ENTER . Arrow down to Calculate and press ENTER . The calculator not only calculates the \(p-\text{value}\) (\(p = 0.1331\)), a little different from the previous calculation - in it we used the sample mean rounded to one decimal place instead of the data) but it also calculates the test statistic ( z -score) for the sample mean, the sample mean, and the sample standard deviation. \(\mu > 275\) is the alternative hypothesis. Do this set of instructions again except arrow to Draw (instead of Calculate ). Press ENTER . A shaded graph appears with \(z = 1.112\) (test statistic) and \(p = 0.1331\) (\(p-\text{value})\). Make sure when you use Draw that no other equations are highlighted in \(Y =\) and the plots are turned off.
Example \(\PageIndex{6}\)
Statistics students believe that the mean score on the first statistics test is 65. A statistics instructor thinks the mean score is higher than 65. He samples ten statistics students and obtains the scores 65 65 70 67 66 63 63 68 72 71. He performs a hypothesis test using a 5% level of significance. The data are assumed to be from a normal distribution.
Set up the hypothesis test:
A 5% level of significance means that \(\alpha = 0.05\). This is a test of a single population mean .
\(H_{0}: \mu = 65 H_{a}: \mu > 65\)
Since the instructor thinks the average score is higher, use a "\(>\)". The "\(>\)" means the test is right-tailed.
Random variable: \(\bar{X} =\) average score on the first statistics test.
Distribution for the test: If you read the problem carefully, you will notice that there is no population standard deviation given . You are only given \(n = 10\) sample data values. Notice also that the data come from a normal distribution. This means that the distribution for the test is a student's \(t\).
Use \(t_{df}\). Therefore, the distribution for the test is \(t_{9}\) where \(n = 10\) and \(df = 10 - 1 = 9\).
Calculate the \(p\)-value using the Student's \(t\)-distribution:
\(p\text{-value} = P(\bar{x} > 67) = 0.0396\) where the sample mean and sample standard deviation are calculated as 67 and 3.1972 from the data.
Interpretation of the p -value: If the null hypothesis is true, then there is a 0.0396 probability (3.96%) that the sample mean is 65 or more.
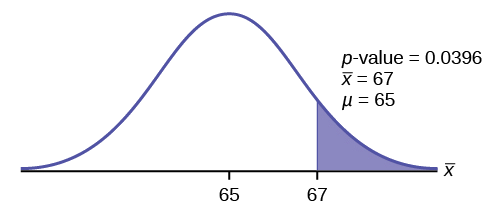
Since \(α = 0.05\) and \(p\text{-value} = 0.0396\). \(\alpha > p\text{-value}\).
This means you reject \(\mu = 65\). In other words, you believe the average test score is more than 65.
Conclusion: At a 5% level of significance, the sample data show sufficient evidence that the mean (average) test score is more than 65, just as the math instructor thinks.
The \(p\text{-value}\) can easily be calculated.
Put the data into a list. Press STAT and arrow over to TESTS . Press 2:T-Test . Arrow over to Data and press ENTER . Arrow down and enter 65 for \(\mu_{0}\), the name of the list where you put the data, and 1 for Freq: . Arrow down to \(\mu\): and arrow over to \(> \mu_{0}\). Press ENTER . Arrow down to Calculate and press ENTER . The calculator not only calculates the \(p\text{-value}\) (p = 0.0396) but it also calculates the test statistic ( t -score) for the sample mean, the sample mean, and the sample standard deviation. \(\mu > 65\) is the alternative hypothesis. Do this set of instructions again except arrow to Draw (instead of Calculate ). Press ENTER . A shaded graph appears with \(t = 1.9781\) (test statistic) and \(p = 0.0396\) (\(p\text{-value}\)). Make sure when you use Draw that no other equations are highlighted in \(Y =\) and the plots are turned off.
Exercise \(\PageIndex{6}\)
It is believed that a stock price for a particular company will grow at a rate of $5 per week with a standard deviation of $1. An investor believes the stock won’t grow as quickly. The changes in stock price is recorded for ten weeks and are as follows: $4, $3, $2, $3, $1, $7, $2, $1, $1, $2. Perform a hypothesis test using a 5% level of significance. State the null and alternative hypotheses, find the p -value, state your conclusion, and identify the Type I and Type II errors.
- \(H_{0}: \mu = 5\)
- \(H_{a}: \mu < 5\)
- \(p = 0.0082\)
Because \(p < \alpha\), we reject the null hypothesis. There is sufficient evidence to suggest that the stock price of the company grows at a rate less than $5 a week.
- Type I Error: To conclude that the stock price is growing slower than $5 a week when, in fact, the stock price is growing at $5 a week (reject the null hypothesis when the null hypothesis is true).
- Type II Error: To conclude that the stock price is growing at a rate of $5 a week when, in fact, the stock price is growing slower than $5 a week (do not reject the null hypothesis when the null hypothesis is false).
Example \(\PageIndex{7}\)
Joon believes that 50% of first-time brides in the United States are younger than their grooms. She performs a hypothesis test to determine if the percentage is the same or different from 50% . Joon samples 100 first-time brides and 53 reply that they are younger than their grooms. For the hypothesis test, she uses a 1% level of significance.
The 1% level of significance means that α = 0.01. This is a test of a single population proportion .
\(H_{0}: p = 0.50\) \(H_{a}: p \neq 0.50\)
The words "is the same or different from" tell you this is a two-tailed test.
Calculate the distribution needed:
Random variable: \(P′ =\) the percent of of first-time brides who are younger than their grooms.
Distribution for the test: The problem contains no mention of a mean. The information is given in terms of percentages. Use the distribution for P′ , the estimated proportion.
\[P' - N\left(p, \sqrt{\frac{p-q}{n}}\right)\nonumber \]
\[P' - N\left(0.5, \sqrt{\frac{0.5-0.5}{100}}\right)\nonumber \]
where \(p = 0.50, q = 1−p = 0.50\), and \(n = 100\)
Calculate the p -value using the normal distribution for proportions:
\[p\text{-value} = P(p′ < 0.47 \space or \space p′ > 0.53) = 0.5485\nonumber \]
where \[x = 53, p' = \frac{x}{n} = \frac{53}{100} = 0.53\nonumber \].
Interpretation of the p-value: If the null hypothesis is true, there is 0.5485 probability (54.85%) that the sample (estimated) proportion \(p'\) is 0.53 or more OR 0.47 or less (see the graph in Figure).
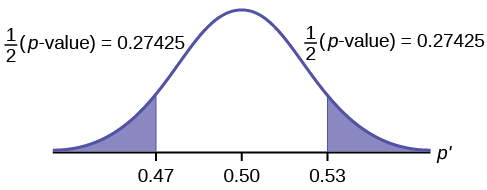
\(\mu = p = 0.50\) comes from \(H_{0}\), the null hypothesis.
\(p′ = 0.53\). Since the curve is symmetrical and the test is two-tailed, the \(p′\) for the left tail is equal to \(0.50 – 0.03 = 0.47\) where \(\mu = p = 0.50\). (0.03 is the difference between 0.53 and 0.50.)
Compare \(\alpha\) and the \(p\text{-value}\):
Since \(\alpha = 0.01\) and \(p\text{-value} = 0.5485\). \(\alpha < p\text{-value}\).
Make a decision: Since \(\alpha < p\text{-value}\), you cannot reject \(H_{0}\).
Conclusion: At the 1% level of significance, the sample data do not show sufficient evidence that the percentage of first-time brides who are younger than their grooms is different from 50%.
Press STAT and arrow over to TESTS . Press 5:1-PropZTest . Enter .5 for \(p_{0}\), 53 for \(x\) and 100 for \(n\). Arrow down to Prop and arrow to not equals \(p_{0}\). Press ENTER . Arrow down to Calculate and press ENTER . The calculator calculates the \(p\text{-value}\) (\(p = 0.5485\)) and the test statistic (\(z\)-score). Prop not equals .5 is the alternate hypothesis. Do this set of instructions again except arrow to Draw (instead of Calculate ). Press ENTER . A shaded graph appears with \(z = 0.6\) (test statistic) and \(p = 0.5485\) (\(p\text{-value}\)). Make sure when you use Draw that no other equations are highlighted in \(Y =\) and the plots are turned off.
The Type I and Type II errors are as follows:
The Type I error is to conclude that the proportion of first-time brides who are younger than their grooms is different from 50% when, in fact, the proportion is actually 50%. (Reject the null hypothesis when the null hypothesis is true).
The Type II error is there is not enough evidence to conclude that the proportion of first time brides who are younger than their grooms differs from 50% when, in fact, the proportion does differ from 50%. (Do not reject the null hypothesis when the null hypothesis is false.)
Exercise \(\PageIndex{7}\)
A teacher believes that 85% of students in the class will want to go on a field trip to the local zoo. She performs a hypothesis test to determine if the percentage is the same or different from 85%. The teacher samples 50 students and 39 reply that they would want to go to the zoo. For the hypothesis test, use a 1% level of significance.
First, determine what type of test this is, set up the hypothesis test, find the \(p\text{-value}\), sketch the graph, and state your conclusion.
Since the problem is about percentages, this is a test of single population proportions.
- \(H_{0} : p = 0.85\)
- \(H_{a}: p \neq 0.85\)
- \(p = 0.7554\)
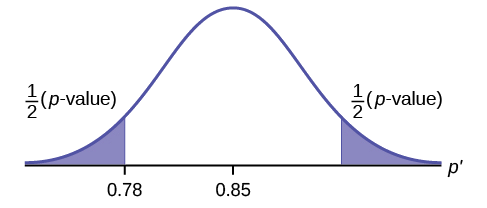
Because \(p > \alpha\), we fail to reject the null hypothesis. There is not sufficient evidence to suggest that the proportion of students that want to go to the zoo is not 85%.
Example \(\PageIndex{8}\)
Suppose a consumer group suspects that the proportion of households that have three cell phones is 30%. A cell phone company has reason to believe that the proportion is not 30%. Before they start a big advertising campaign, they conduct a hypothesis test. Their marketing people survey 150 households with the result that 43 of the households have three cell phones.
\(H_{0}: p = 0.30, H_{a}: p \neq 0.30\)
The random variable is \(P′ =\) proportion of households that have three cell phones.
The distribution for the hypothesis test is \(P' - N\left(0.30, \sqrt{\frac{(0.30 \cdot 0.70)}{150}}\right)\)
Exercise \(\PageIndex{8}\).2
a. The value that helps determine the \(p\text{-value}\) is \(p′\). Calculate \(p′\).
a. \(p' = \frac{x}{n}\) where \(x\) is the number of successes and \(n\) is the total number in the sample.
\(x = 43, n = 150\)
\(p′ = 43150\)
Exercise \(\PageIndex{8}\).3
b. What is a success for this problem?
b. A success is having three cell phones in a household.
Exercise \(\PageIndex{8}\).4
c. What is the level of significance?
c. The level of significance is the preset \(\alpha\). Since \(\alpha\) is not given, assume that \(\alpha = 0.05\).
Exercise \(\PageIndex{8}\).5
d. Draw the graph for this problem. Draw the horizontal axis. Label and shade appropriately.
Calculate the \(p\text{-value}\).
d. \(p\text{-value} = 0.7216\)
Exercise \(\PageIndex{8}\).6
e. Make a decision. _____________(Reject/Do not reject) \(H_{0}\) because____________.
e. Assuming that \(\alpha = 0.05, \alpha < p\text{-value}\). The decision is do not reject \(H_{0}\) because there is not sufficient evidence to conclude that the proportion of households that have three cell phones is not 30%.
Exercise \(\PageIndex{8}\)
Marketers believe that 92% of adults in the United States own a cell phone. A cell phone manufacturer believes that number is actually lower. 200 American adults are surveyed, of which, 174 report having cell phones. Use a 5% level of significance. State the null and alternative hypothesis, find the p -value, state your conclusion, and identify the Type I and Type II errors.
- \(H_{0}: p = 0.92\)
- \(H_{a}: p < 0.92\)
- \(p\text{-value} = 0.0046\)
Because \(p < 0.05\), we reject the null hypothesis. There is sufficient evidence to conclude that fewer than 92% of American adults own cell phones.
- Type I Error: To conclude that fewer than 92% of American adults own cell phones when, in fact, 92% of American adults do own cell phones (reject the null hypothesis when the null hypothesis is true).
- Type II Error: To conclude that 92% of American adults own cell phones when, in fact, fewer than 92% of American adults own cell phones (do not reject the null hypothesis when the null hypothesis is false).
The next example is a poem written by a statistics student named Nicole Hart. The solution to the problem follows the poem. Notice that the hypothesis test is for a single population proportion. This means that the null and alternate hypotheses use the parameter \(p\). The distribution for the test is normal. The estimated proportion \(p′\) is the proportion of fleas killed to the total fleas found on Fido. This is sample information. The problem gives a preconceived \(\alpha = 0.01\), for comparison, and a 95% confidence interval computation. The poem is clever and humorous, so please enjoy it!
Example \(\PageIndex{9}\)
My dog has so many fleas,
They do not come off with ease. As for shampoo, I have tried many types Even one called Bubble Hype, Which only killed 25% of the fleas, Unfortunately I was not pleased.
I've used all kinds of soap, Until I had given up hope Until one day I saw An ad that put me in awe.
A shampoo used for dogs Called GOOD ENOUGH to Clean a Hog Guaranteed to kill more fleas.
I gave Fido a bath And after doing the math His number of fleas Started dropping by 3's! Before his shampoo I counted 42.
At the end of his bath, I redid the math And the new shampoo had killed 17 fleas. So now I was pleased.
Now it is time for you to have some fun With the level of significance being .01, You must help me figure out
Use the new shampoo or go without?
\(H_{0}: p \leq 0.25\) \(H_{a}: p > 0.25\)
In words, CLEARLY state what your random variable \(\bar{X}\) or \(P′\) represents.
\(P′ =\) The proportion of fleas that are killed by the new shampoo
State the distribution to use for the test.
\[N\left(0.25, \sqrt{\frac{(0.25){1-0.25}}{42}}\right)\nonumber \]
Test Statistic: \(z = 2.3163\)
Calculate the \(p\text{-value}\) using the normal distribution for proportions:
\[p\text{-value} = 0.0103\nonumber \]
In one to two complete sentences, explain what the p -value means for this problem.
If the null hypothesis is true (the proportion is 0.25), then there is a 0.0103 probability that the sample (estimated) proportion is 0.4048 \(\left(\frac{17}{42}\right)\) or more.
Use the previous information to sketch a picture of this situation. CLEARLY, label and scale the horizontal axis and shade the region(s) corresponding to the \(p\text{-value}\).
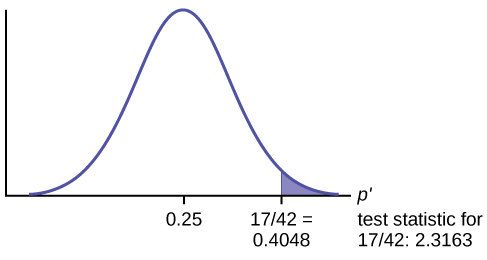
Indicate the correct decision (“reject” or “do not reject” the null hypothesis), the reason for it, and write an appropriate conclusion, using complete sentences.
Conclusion: At the 1% level of significance, the sample data do not show sufficient evidence that the percentage of fleas that are killed by the new shampoo is more than 25%.
Construct a 95% confidence interval for the true mean or proportion. Include a sketch of the graph of the situation. Label the point estimate and the lower and upper bounds of the confidence interval.
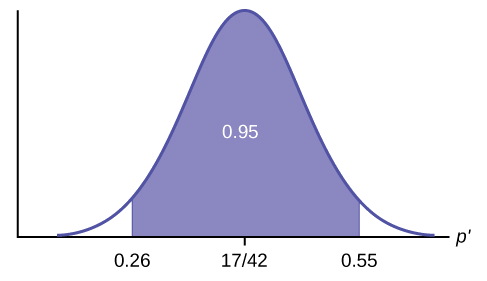
Confidence Interval: (0.26,0.55) We are 95% confident that the true population proportion p of fleas that are killed by the new shampoo is between 26% and 55%.
This test result is not very definitive since the \(p\text{-value}\) is very close to alpha. In reality, one would probably do more tests by giving the dog another bath after the fleas have had a chance to return.
Example \(\PageIndex{10}\)
The National Institute of Standards and Technology provides exact data on conductivity properties of materials. Following are conductivity measurements for 11 randomly selected pieces of a particular type of glass.
1.11; 1.07; 1.11; 1.07; 1.12; 1.08; .98; .98 1.02; .95; .95
Is there convincing evidence that the average conductivity of this type of glass is greater than one? Use a significance level of 0.05. Assume the population is normal.
Let’s follow a four-step process to answer this statistical question.
- \(H_{0}: \mu \leq 1\)
- \(H_{a}: \mu > 1\)
- Plan : We are testing a sample mean without a known population standard deviation. Therefore, we need to use a Student's-t distribution. Assume the underlying population is normal.
- Do the calculations : We will input the sample data into the TI-83 as follows.

4. State the Conclusions : Since the \(p\text{-value} (p = 0.036)\) is less than our alpha value, we will reject the null hypothesis. It is reasonable to state that the data supports the claim that the average conductivity level is greater than one.
Example \(\PageIndex{11}\)
In a study of 420,019 cell phone users, 172 of the subjects developed brain cancer. Test the claim that cell phone users developed brain cancer at a greater rate than that for non-cell phone users (the rate of brain cancer for non-cell phone users is 0.0340%). Since this is a critical issue, use a 0.005 significance level. Explain why the significance level should be so low in terms of a Type I error.
We will follow the four-step process.
- \(H_{0}: p \leq 0.00034\)
- \(H_{a}: p > 0.00034\)
If we commit a Type I error, we are essentially accepting a false claim. Since the claim describes cancer-causing environments, we want to minimize the chances of incorrectly identifying causes of cancer.
- We will be testing a sample proportion with \(x = 172\) and \(n = 420,019\). The sample is sufficiently large because we have \(np = 420,019(0.00034) = 142.8\), \(nq = 420,019(0.99966) = 419,876.2\), two independent outcomes, and a fixed probability of success \(p = 0.00034\). Thus we will be able to generalize our results to the population.

Figure \(\PageIndex{11}\).

Figure \(\PageIndex{12}\).
- Since the \(p\text{-value} = 0.0073\) is greater than our alpha value \(= 0.005\), we cannot reject the null. Therefore, we conclude that there is not enough evidence to support the claim of higher brain cancer rates for the cell phone users.
Example \(\PageIndex{12}\)
According to the US Census there are approximately 268,608,618 residents aged 12 and older. Statistics from the Rape, Abuse, and Incest National Network indicate that, on average, 207,754 rapes occur each year (male and female) for persons aged 12 and older. This translates into a percentage of sexual assaults of 0.078%. In Daviess County, KY, there were reported 11 rapes for a population of 37,937. Conduct an appropriate hypothesis test to determine if there is a statistically significant difference between the local sexual assault percentage and the national sexual assault percentage. Use a significance level of 0.01.
We will follow the four-step plan.
- We need to test whether the proportion of sexual assaults in Daviess County, KY is significantly different from the national average.
- \(H_{0}: p = 0.00078\)
- \(H_{a}: p \neq 0.00078\)

Figure \(\PageIndex{13}\).

Figure \(\PageIndex{14}\).
- Since the \(p\text{-value}\), \(p = 0.00063\), is less than the alpha level of 0.01, the sample data indicates that we should reject the null hypothesis. In conclusion, the sample data support the claim that the proportion of sexual assaults in Daviess County, Kentucky is different from the national average proportion.
The hypothesis test itself has an established process. This can be summarized as follows:
- Determine \(H_{0}\) and \(H_{a}\). Remember, they are contradictory.
- Determine the random variable.
- Determine the distribution for the test.
- Draw a graph, calculate the test statistic, and use the test statistic to calculate the \(p\text{-value}\). (A z -score and a t -score are examples of test statistics.)
- Compare the preconceived α with the p -value, make a decision (reject or do not reject H 0 ), and write a clear conclusion using English sentences.
Notice that in performing the hypothesis test, you use \(\alpha\) and not \(\beta\). \(\beta\) is needed to help determine the sample size of the data that is used in calculating the \(p\text{-value}\). Remember that the quantity \(1 – \beta\) is called the Power of the Test . A high power is desirable. If the power is too low, statisticians typically increase the sample size while keeping α the same.If the power is low, the null hypothesis might not be rejected when it should be.
Assume \(H_{0}: \mu = 9\) and \(H_{a}: \mu < 9\). Is this a left-tailed, right-tailed, or two-tailed test?
This is a left-tailed test.
Exercise \(\PageIndex{9}\)
Assume \(H_{0}: \mu \leq 6\) and \(H_{a}: \mu > 6\). Is this a left-tailed, right-tailed, or two-tailed test?
Exercise \(\PageIndex{10}\)
Assume \(H_{0}: p = 0.25\) and \(H_{a}: p \neq 0.25\). Is this a left-tailed, right-tailed, or two-tailed test?
This is a two-tailed test.
Exercise \(\PageIndex{11}\)
Draw the general graph of a left-tailed test.
Exercise \(\PageIndex{12}\)
Draw the graph of a two-tailed test.
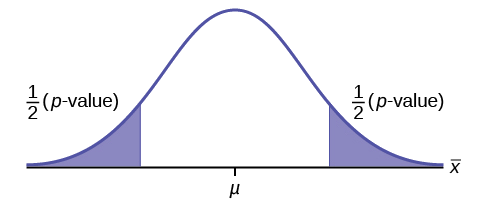
Exercise \(\PageIndex{13}\)
A bottle of water is labeled as containing 16 fluid ounces of water. You believe it is less than that. What type of test would you use?
Exercise \(\PageIndex{14}\)
Your friend claims that his mean golf score is 63. You want to show that it is higher than that. What type of test would you use?
a right-tailed test
Exercise \(\PageIndex{15}\)
A bathroom scale claims to be able to identify correctly any weight within a pound. You think that it cannot be that accurate. What type of test would you use?
Exercise \(\PageIndex{16}\)
You flip a coin and record whether it shows heads or tails. You know the probability of getting heads is 50%, but you think it is less for this particular coin. What type of test would you use?
a left-tailed test
Exercise \(\PageIndex{17}\)
If the alternative hypothesis has a not equals ( \(\neq\) ) symbol, you know to use which type of test?
Exercise \(\PageIndex{18}\)
Assume the null hypothesis states that the mean is at least 18. Is this a left-tailed, right-tailed, or two-tailed test?
Exercise \(\PageIndex{19}\)
Assume the null hypothesis states that the mean is at most 12. Is this a left-tailed, right-tailed, or two-tailed test?
Exercise \(\PageIndex{20}\)
Assume the null hypothesis states that the mean is equal to 88. The alternative hypothesis states that the mean is not equal to 88. Is this a left-tailed, right-tailed, or two-tailed test?
- Data from Amit Schitai. Director of Instructional Technology and Distance Learning. LBCC.
- Data from Bloomberg Businessweek . Available online at www.businessweek.com/news/2011- 09-15/nyc-smoking-rate-falls-to-record-low-of-14-bloomberg-says.html.
- Data from energy.gov. Available online at http://energy.gov (accessed June 27. 2013).
- Data from Gallup®. Available online at www.gallup.com (accessed June 27, 2013).
- Data from Growing by Degrees by Allen and Seaman.
- Data from La Leche League International. Available online at www.lalecheleague.org/Law/BAFeb01.html.
- Data from the American Automobile Association. Available online at www.aaa.com (accessed June 27, 2013).
- Data from the American Library Association. Available online at www.ala.org (accessed June 27, 2013).
- Data from the Bureau of Labor Statistics. Available online at http://www.bls.gov/oes/current/oes291111.htm .
- Data from the Centers for Disease Control and Prevention. Available online at www.cdc.gov (accessed June 27, 2013)
- Data from the U.S. Census Bureau, available online at quickfacts.census.gov/qfd/states/00000.html (accessed June 27, 2013).
- Data from the United States Census Bureau. Available online at www.census.gov/hhes/socdemo/language/.
- Data from Toastmasters International. Available online at http://toastmasters.org/artisan/deta...eID=429&Page=1 .
- Data from Weather Underground. Available online at www.wunderground.com (accessed June 27, 2013).
- Federal Bureau of Investigations. “Uniform Crime Reports and Index of Crime in Daviess in the State of Kentucky enforced by Daviess County from 1985 to 2005.” Available online at http://www.disastercenter.com/kentucky/crime/3868.htm (accessed June 27, 2013).
- “Foothill-De Anza Community College District.” De Anza College, Winter 2006. Available online at research.fhda.edu/factbook/DA...t_da_2006w.pdf.
- Johansen, C., J. Boice, Jr., J. McLaughlin, J. Olsen. “Cellular Telephones and Cancer—a Nationwide Cohort Study in Denmark.” Institute of Cancer Epidemiology and the Danish Cancer Society, 93(3):203-7. Available online at http://www.ncbi.nlm.nih.gov/pubmed/11158188 (accessed June 27, 2013).
- Rape, Abuse & Incest National Network. “How often does sexual assault occur?” RAINN, 2009. Available online at www.rainn.org/get-information...sexual-assault (accessed June 27, 2013).
The Genius Blog
Hypothesis Testing Solved Examples(Questions and Solutions)
Here is a list hypothesis testing exercises and solutions. Try to solve a question by yourself first before you look at the solution.
Question 1 In the population, the average IQ is 100 with a standard deviation of 15. A team of scientists want to test a new medication to see if it has either a positive or negative effect on intelligence, or not effect at all. A sample of 30 participants who have taken the medication has a mean of 140. Did the medication affect intelligence? View Solution to Question 1
A professor wants to know if her introductory statistics class has a good grasp of basic math. Six students are chosen at random from the class and given a math proficiency test. The professor wants the class to be able to score above 70 on the test. The six students get the following scores:62, 92, 75, 68, 83, 95. Can the professor have 90% confidence that the mean score for the class on the test would be above 70. Solution to Question 2
Question 3 In a packaging plant, a machine packs cartons with jars. It is supposed that a new machine would pack faster on the average than the machine currently used. To test the hypothesis, the time it takes each machine to pack ten cartons are recorded. The result in seconds is as follows.
Do the data provide sufficient evidence to conclude that, on the average, the new machine packs faster? Perform the required hypothesis test at the 5% level of significance. Solution to Question 3
Question 4 We want to compare the heights in inches of two groups of individuals. Here are the measurements: X: 175, 168, 168, 190, 156, 181, 182, 175, 174, 179 Y: 120, 180, 125, 188, 130, 190, 110, 185, 112, 188 Solution to Question 4
Question 5 A clinic provides a program to help their clients lose weight and asks a consumer agency to investigate the effectiveness of the program. The agency takes a sample of 15 people, weighing each person in the sample before the program begins and 3 months later. The results a tabulated below
Determine is the program is effective. Solution to Question 5
Question 6 A sample of 20 students were selected and given a diagnostic module prior to studying for a test. And then they were given the test again after completing the module. . The result of the students scores in the test before and after the test is tabulated below.
We want to see if there is significant improvement in the student’s performance due to this teaching method Solution to Question 6
Question 7 A study was performed to test wether cars get better mileage on premium gas than on regular gas. Each of 10 cars was first filled with regular or premium gas, decided by a coin toss, and the mileage for the tank was recorded. The mileage was recorded again for the same cars using other kind of gasoline. Determine wether cars get significantly better mileage with premium gas.
Mileage with regular gas: 16,20,21,22,23,22,27,25,27,28 Mileage with premium gas: 19, 22,24,24,25,25,26,26,28,32 Solution to Question 7
Question 8 An automatic cutter machine must cut steel strips of 1200 mm length. From a preliminary data, we checked that the lengths of the pieces produced by the machine can be considered as normal random variables with a 3mm standard deviation. We want to make sure that the machine is set correctly. Therefore 16 pieces of the products are randomly selected and weight. The figures were in mm: 1193,1196,1198,1195,1198,1199,1204,1193,1203,1201,1196,1200,1191,1196,1198,1191 Examine wether there is any significant deviation from the required size Solution to Question 8
Question 9 Blood pressure reading of ten patients before and after medication for reducing the blood pressure are as follows
Patient: 1,2,3,4,5,6,7,8,9,10 Before treatment: 86,84,78,90,92,77,89,90,90,86 After treatment: 80,80,92,79,92,82,88,89,92,83
Test the null hypothesis of no effect agains the alternate hypothesis that medication is effective. Execute it with Wilcoxon test Solution to Question 9
Question on ANOVA Sussan Sound predicts that students will learn most effectively with a constant background sound, as opposed to an unpredictable sound or no sound at all. She randomly divides 24 students into three groups of 8 each. All students study a passage of text for 30 minutes. Those in group 1 study with background sound at a constant volume in the background. Those in group 2 study with nose that changes volume periodically. Those in group 3 study with no sound at all. After studying, all students take a 10 point multiple choice test over the material. Their scores are tabulated below.
Group1: Constant sound: 7,4,6,8,6,6,2,9 Group 2: Random sound: 5,5,3,4,4,7,2,2 Group 3: No sound at all: 2,4,7,1,2,1,5,5 Solution to Question 10
Question 11 Using the following three groups of data, perform a one-way analysis of variance using α = 0.05.
Solution to Question 11
Question 12 In a packaging plant, a machine packs cartons with jars. It is supposed that a new machine would pack faster on the average than the machine currently used. To test the hypothesis, the time it takes each machine to pack ten cartons are recorded. The result in seconds is as follows.
New Machine: 42,41,41.3,41.8,42.4,42.8,43.2,42.3,41.8,42.7 Old Machine: 42.7,43.6,43.8,43.3,42.5,43.5,43.1,41.7,44,44.1
Perform an F-test to determine if the null hypothesis should be accepted. Solution to Question 12
Question 13 A random sample 500 U.S adults are questioned about their political affiliation and opinion on a tax reform bill. We need to test if the political affiliation and their opinon on a tax reform bill are dependent, at 5% level of significance. The observed contingency table is given below.
Solution to Question 13
Question 14 Can a dice be considered regular which is showing the following frequency distribution during 1000 throws?
Solution to Question 14
Solution to Question 15
Question 16 A newly developed muesli contains five types of seeds (A, B, C, D and E). The percentage of which is 35%, 25%, 20%, 10% and 10% according to the product information. In a randomly selected muesli, the following volume distribution was found.
Lets us decide about the null hypothesis whether the composition of the sample corresponds to the distribution indicated on the packaging at alpha = 0.1 significance level. Solution to Question 16
Question 17 A research team investigated whether there was any significant correlation between the severity of a certain disease runoff and the age of the patients. During the study, data for n = 200 patients were collected and grouped according to the severity of the disease and the age of the patient. The table below shows the result
Let us decided about the correlation between the age of the patients and the severity of disease progression. Solution to Question 17
Question 18 A publisher is interested in determine which of three book cover is most attractive. He interviews 400 people in each of the three states (California, Illinois and New York), and asks each person which of the cover he or she prefers. The number of preference for each cover is as follows:
Do these data indicate that there are regional differences in people’s preferences concerning these covers? Use the 0.05 level of significance. Solution to Question 18
Question 19 Trees planted along the road were checked for which ones are healthy(H) or diseased (D) and the following arrangement of the trees were obtained:
H H H H D D D H H H H H H H D D H H D D D
Test at the = 0.05 significance wether this arrangement may be regarded as random
Solution to Question 19
Question 20 Suppose we flip a coin n = 15 times and come up with the following arrangements
H T T T H H T T T T H H T H H
(H = head, T = tail)
Test at the alpha = 0.05 significance level whether this arrangement may be regarded as random.
Solution to Question 20
kindsonthegenius
You might also like, kolmogorov-smirnov goodness of fit test, welch’s t-test – how and when to use it, how to perform wald-wolfowitz test – testing for homogeneity with run test.
I am really impressed with your writing abilities as well as with the structure to your weblog. Is this a paid subject matter or did you modify it yourself?
Either way stay up the excellent high quality writing, it’s uncommon to look a great blog like this one these days..
Below are given the gain in weights (in lbs.) of pigs fed on two diet A and B Dieta 25 32 30 34 24 14 32 24 30 31 35 25 – – DietB 44 34 22 10 47 31 40 30 32 35 18 21 35 29
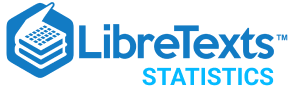
- school Campus Bookshelves
- menu_book Bookshelves
- perm_media Learning Objects
- login Login
- how_to_reg Request Instructor Account
- hub Instructor Commons
- Download Page (PDF)
- Download Full Book (PDF)
- Periodic Table
- Physics Constants
- Scientific Calculator
- Reference & Cite
- Tools expand_more
- Readability
selected template will load here
This action is not available.
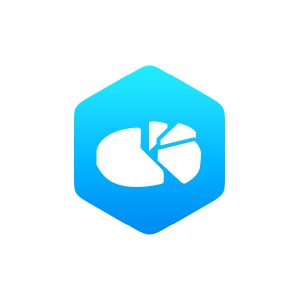
8.2: Hypothesis Test Examples for Means
- Last updated
- Save as PDF
- Page ID 11531
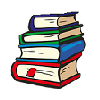
Full Hypothesis Test Examples
Example \(\PageIndex{4}\)
Jeffrey, as an eight-year old, established a mean time of 16.43 seconds for swimming the 25-yard freestyle, with a standard deviation of 0.8 seconds . His dad, Frank, thought that Jeffrey could swim the 25-yard freestyle faster using goggles. Frank bought Jeffrey a new pair of expensive goggles and timed Jeffrey for 15 25-yard freestyle swims . For the 15 swims, Jeffrey's mean time was 16 seconds. Frank thought that the goggles helped Jeffrey to swim faster than the 16.43 seconds. Conduct a hypothesis test using a preset α = 0.05. Assume that the swim times for the 25-yard freestyle are normal.
Set up the Hypothesis Test:
Since the problem is about a mean, this is a test of a single population mean .
\(H_{0}: \mu = 16.43, H_{a}: \mu < 16.43\)
For Jeffrey to swim faster, his time will be less than 16.43 seconds. The "\(<\)" tells you this is left-tailed.
Determine the distribution needed:
Random variable: \(\bar{X} =\) the mean time to swim the 25-yard freestyle.
Distribution for the test: \(\bar{X}\) is normal (population standard deviation is known: \(\sigma = 0.8\))
\(\bar{X} - N \left(\mu, \frac{\sigma_{x}}{\sqrt{n}}\right)\) Therefore, \(\bar{X} - N\left(16.43, \frac{0.8}{\sqrt{15}}\right)\)
\(\mu = 16.43\) comes from \(H_{0}\) and not the data. \(\sigma = 0.8\), and \(n = 15\).
Calculate the \(p-\text{value}\) using the normal distribution for a mean:
\(p\text{-value} = P(\bar{x} < 16) = 0.0187\) where the sample mean in the problem is given as 16.
\(p\text{-value} = 0.0187\) (This is called the actual level of significance .) The \(p-\text{value}\) is the area to the left of the sample mean is given as 16.
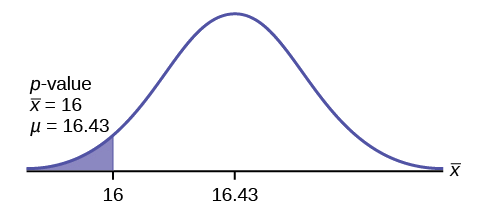
\(\mu = 16.43\) comes from \(H_{0}\). Our assumption is \(\mu = 16.43\).
Interpretation of the \(p-\text{value}\): If \(H_{0}\) is true , there is a 0.0187 probability (1.87%) that Jeffrey's mean time to swim the 25-yard freestyle is 16 seconds or less. Because a 1.87% chance is small, the mean time of 16 seconds or less is unlikely to have happened randomly. It is a rare event.
Compare \(\alpha\) and the \(p-\text{value}\):
\(\alpha = 0.05 p\text{-value} = 0.0187 \alpha > p\text{-value}\)
Make a decision: Since \(\alpha > p\text{-value}\), reject \(H_{0}\).
This means that you reject \(\mu = 16.43\). In other words, you do not think Jeffrey swims the 25-yard freestyle in 16.43 seconds but faster with the new goggles.
Conclusion: At the 5% significance level, we conclude that Jeffrey swims faster using the new goggles. The sample data show there is sufficient evidence that Jeffrey's mean time to swim the 25-yard freestyle is less than 16.43 seconds.
The p -value can easily be calculated.
Press STAT and arrow over to TESTS . Press 1:Z-Test . Arrow over to Stats and press ENTER . Arrow down and enter 16.43 for \(\mu_{0}\) (null hypothesis), .8 for σ , 16 for the sample mean, and 15 for n . Arrow down to \(\mu\) : (alternate hypothesis) and arrow over to \(< \mu_{0}\). Press ENTER . Arrow down to Calculate and press ENTER . The calculator not only calculates the p -value (\(p = 0.0187\)) but it also calculates the test statistic ( z -score) for the sample mean. \(\mu < 16.43\) is the alternative hypothesis. Do this set of instructions again except arrow to Draw (instead of Calculate ). Press ENTER . A shaded graph appears with \(z = -2.08\) (test statistic) and \(p = 0.0187\) (\(p-\text{value}\)). Make sure when you use Draw that no other equations are highlighted in \(Y =\) and the plots are turned off.
When the calculator does a \(Z\)-Test, the Z-Test function finds the p -value by doing a normal probability calculation using the central limit theorem:
\(P(\bar{X} < 16)\) 2nd DISTR normcdf (\((−10^{99},16,16.43,\frac{0.8}{\sqrt{15}})\).
The Type I and Type II errors for this problem are as follows:
The Type I error is to conclude that Jeffrey swims the 25-yard freestyle, on average, in less than 16.43 seconds when, in fact, he actually swims the 25-yard freestyle, on average, in 16.43 seconds. (Reject the null hypothesis when the null hypothesis is true.)
The Type II error is that there is not evidence to conclude that Jeffrey swims the 25-yard free-style, on average, in less than 16.43 seconds when, in fact, he actually does swim the 25-yard free-style, on average, in less than 16.43 seconds. (Do not reject the null hypothesis when the null hypothesis is false.)
Exercise \(\PageIndex{4}\)
The mean throwing distance of a football for a Marco, a high school freshman quarterback, is 40 yards, with a standard deviation of two yards. The team coach tells Marco to adjust his grip to get more distance. The coach records the distances for 20 throws. For the 20 throws, Marco’s mean distance was 45 yards. The coach thought the different grip helped Marco throw farther than 40 yards. Conduct a hypothesis test using a preset \(\alpha = 0.05\). Assume the throw distances for footballs are normal.
First, determine what type of test this is, set up the hypothesis test, find the p -value, sketch the graph, and state your conclusion.
Press STAT and arrow over to TESTS. Press 1: \(Z\)-Test. Arrow over to Stats and press ENTER. Arrow down and enter 40 for \(\mu_{0}\) (null hypothesis), 2 for \(\sigma\), 45 for the sample mean, and 20 for \(n\). Arrow down to \(\mu\): (alternative hypothesis) and set it either as \(<\), \(\neq\), or \(>\). Press ENTER. Arrow down to Calculate and press ENTER. The calculator not only calculates the p -value but it also calculates the test statistic ( z -score) for the sample mean. Select \(<\), \(\neq\), or \(>\) for the alternative hypothesis. Do this set of instructions again except arrow to Draw (instead of Calculate). Press ENTER. A shaded graph appears with test statistic and \(p\)-value. Make sure when you use Draw that no other equations are highlighted in \(Y =\) and the plots are turned off.
Since the problem is about a mean, this is a test of a single population mean.
- \(H_{0}: \mu = 40\)
- \(H_{a}: \mu > 40\)
- \(p = 0.0062\)
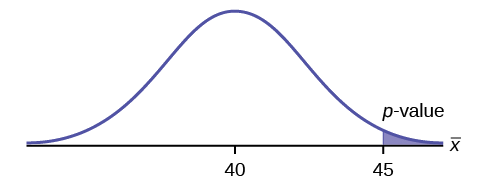
Because \(p < \alpha\), we reject the null hypothesis. There is sufficient evidence to suggest that the change in grip improved Marco’s throwing distance.
Historical Note
The traditional way to compare the two probabilities, \(\alpha\) and the \(p-\text{value}\), is to compare the critical value (\(z\)-score from \(\alpha\)) to the test statistic (\(z\)-score from data). The calculated test statistic for the \(p\)-value is –2.08. (From the Central Limit Theorem, the test statistic formula is \(z = \frac{\bar{x}-\mu_{x}}{\left(\frac{\sigma_{x}}{\sqrt{n}}\right)}\). For this problem, \(\bar{x} = 16\), \(\mu_{x} = 16.43\) from the null hypotheses is, \(\sigma_{x} = 0.8\), and \(n = 15\).) You can find the critical value for \(\alpha = 0.05\) in the normal table (see 15.Tables in the Table of Contents). The \(z\)-score for an area to the left equal to 0.05 is midway between –1.65 and –1.64 (0.05 is midway between 0.0505 and 0.0495). The \(z\)-score is –1.645. Since –1.645 > –2.08 (which demonstrates that \(\alpha > p-\text{value}\)), reject \(H_{0}\). Traditionally, the decision to reject or not reject was done in this way. Today, comparing the two probabilities \(\alpha\) and the \(p\)-value is very common. For this problem, the \(p-\text{value}\), 0.0187 is considerably smaller than \(\alpha = 0.05\). You can be confident about your decision to reject. The graph shows \(\alpha\), the \(p-\text{value}\), and the test statistics and the critical value.
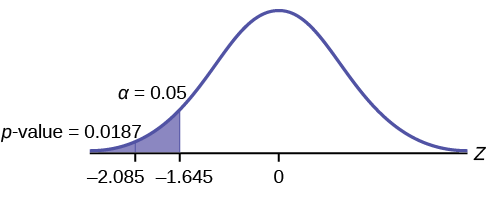
Example \(\PageIndex{5}\)
A college football coach thought that his players could bench press a mean weight of 275 pounds . It is known that the standard deviation is 55 pounds . Three of his players thought that the mean weight was more than that amount. They asked 30 of their teammates for their estimated maximum lift on the bench press exercise. The data ranged from 205 pounds to 385 pounds. The actual different weights were (frequencies are in parentheses) 205(3); 215(3); 225(1); 241(2); 252(2); 265(2); 275(2); 313(2); 316(5); 338(2); 341(1); 345(2); 368(2); 385(1).
Conduct a hypothesis test using a 2.5% level of significance to determine if the bench press mean is more than 275 pounds.
Since the problem is about a mean weight, this is a test of a single population mean.
- \(H_{0}: \mu = 275\)
- \(H_{a}: \mu > 275\)
This is a right-tailed test.
Calculating the distribution needed:
Random variable: \(\bar{X} =\) the mean weight, in pounds, lifted by the football players.
Distribution for the test: It is normal because \(\sigma\) is known.
- \(\bar{X} - N\left(275, \frac{55}{\sqrt{30}}\right)\)
- \(\bar{x} = 286.2\) pounds (from the data).
- \(\sigma = 55\) pounds (Always use \(\sigma\) if you know it.) We assume \(\mu = 275\) pounds unless our data shows us otherwise.
Calculate the p -value using the normal distribution for a mean and using the sample mean as input (see [link] for using the data as input):
\[p\text{-value} = P(\bar{x} > 286.2) = 0.1323.\nonumber \]
Interpretation of the p -value: If \(H_{0}\) is true, then there is a 0.1331 probability (13.23%) that the football players can lift a mean weight of 286.2 pounds or more. Because a 13.23% chance is large enough, a mean weight lift of 286.2 pounds or more is not a rare event.
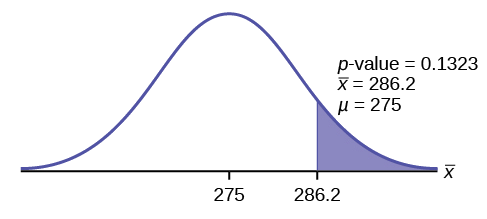
\(\alpha = 0.025 p-value = 0.1323\)
Make a decision: Since \(\alpha < p\text{-value}\), do not reject \(H_{0}\).
Conclusion: At the 2.5% level of significance, from the sample data, there is not sufficient evidence to conclude that the true mean weight lifted is more than 275 pounds.
The \(p-\text{value}\) can easily be calculated.
Put the data and frequencies into lists. Press STAT and arrow over to TESTS . Press 1:Z-Test . Arrow over to Data and press ENTER . Arrow down and enter 275 for \(\mu_{0}\), 55 for \(\sigma\), the name of the list where you put the data, and the name of the list where you put the frequencies. Arrow down to \(\mu\) : and arrow over to \(> \mu_{0}\). Press ENTER . Arrow down to Calculate and press ENTER . The calculator not only calculates the \(p-\text{value})\ (\(p = 0.1331\)), a little different from the previous calculation - in it we used the sample mean rounded to one decimal place instead of the data) but it also calculates the test statistic ( z -score) for the sample mean, the sample mean, and the sample standard deviation. \(\mu > 275\) is the alternative hypothesis. Do this set of instructions again except arrow to Draw (instead of Calculate ). Press ENTER . A shaded graph appears with \(z = 1.112\) (test statistic) and \(p = 0.1331\) (\(p-\text{value})\). Make sure when you use Draw that no other equations are highlighted in \(Y =\) and the plots are turned off.
- Data from Amit Schitai. Director of Instructional Technology and Distance Learning. LBCC.
- Data from Bloomberg Businessweek . Available online at http://www.businessweek.com/news/2011- 09-15/nyc-smoking-rate-falls-to-record-low-of-14-bloomberg-says.html.
- Data from energy.gov. Available online at http://energy.gov (accessed June 27. 2013).
- Data from Gallup®. Available online at www.gallup.com (accessed June 27, 2013).
- Data from Growing by Degrees by Allen and Seaman.
- Data from La Leche League International. Available online at www.lalecheleague.org/Law/BAFeb01.html.
- Data from the American Automobile Association. Available online at www.aaa.com (accessed June 27, 2013).
- Data from the American Library Association. Available online at www.ala.org (accessed June 27, 2013).
- Data from the Bureau of Labor Statistics. Available online at http://www.bls.gov/oes/current/oes291111.htm .
- Data from the Centers for Disease Control and Prevention. Available online at www.cdc.gov (accessed June 27, 2013)
- Data from the U.S. Census Bureau, available online at quickfacts.census.gov/qfd/states/00000.html (accessed June 27, 2013).
- Data from the United States Census Bureau. Available online at www.census.gov/hhes/socdemo/language/.
- Data from Toastmasters International. Available online at http://toastmasters.org/artisan/deta...eID=429&Page=1 .
- Data from Weather Underground. Available online at www.wunderground.com (accessed June 27, 2013).
- Federal Bureau of Investigations. “Uniform Crime Reports and Index of Crime in Daviess in the State of Kentucky enforced by Daviess County from 1985 to 2005.” Available online at http://www.disastercenter.com/kentucky/crime/3868.htm (accessed June 27, 2013).
- “Foothill-De Anza Community College District.” De Anza College, Winter 2006. Available online at research.fhda.edu/factbook/DA...t_da_2006w.pdf.
- Johansen, C., J. Boice, Jr., J. McLaughlin, J. Olsen. “Cellular Telephones and Cancer—a Nationwide Cohort Study in Denmark.” Institute of Cancer Epidemiology and the Danish Cancer Society, 93(3):203-7. Available online at http://www.ncbi.nlm.nih.gov/pubmed/11158188 (accessed June 27, 2013).
- Rape, Abuse & Incest National Network. “How often does sexual assault occur?” RAINN, 2009. Available online at www.rainn.org/get-information...sexual-assault (accessed June 27, 2013).
Contributors and Attributions
Barbara Illowsky and Susan Dean (De Anza College) with many other contributing authors. Content produced by OpenStax College is licensed under a Creative Commons Attribution License 4.0 license. Download for free at http://cnx.org/contents/[email protected] .
- Search Search Please fill out this field.
What Is Hypothesis Testing?
Step 1: define the hypothesis, step 2: set the criteria, step 3: calculate the statistic, step 4: reach a conclusion, types of errors, the bottom line.
- Trading Skills
- Trading Basic Education
Hypothesis Testing in Finance: Concept and Examples
Charlene Rhinehart is a CPA , CFE, chair of an Illinois CPA Society committee, and has a degree in accounting and finance from DePaul University.
:max_bytes(150000):strip_icc():format(webp)/CharleneRhinehartHeadshot-CharleneRhinehart-ca4b769506e94a92bc29e4acc6f0f9a5.jpg)
Your investment advisor proposes you a monthly income investment plan that promises a variable return each month. You will invest in it only if you are assured of an average $180 monthly income. Your advisor also tells you that for the past 300 months, the scheme had investment returns with an average value of $190 and a standard deviation of $75. Should you invest in this scheme? Hypothesis testing comes to the aid for such decision-making.
Key Takeaways
- Hypothesis testing is a mathematical tool for confirming a financial or business claim or idea.
- Hypothesis testing is useful for investors trying to decide what to invest in and whether the instrument is likely to provide a satisfactory return.
- Despite the existence of different methodologies of hypothesis testing, the same four steps are used: define the hypothesis, set the criteria, calculate the statistic, and reach a conclusion.
- This mathematical model, like most statistical tools and models, has limitations and is prone to certain errors, necessitating investors also considering other models in conjunction with this one
Hypothesis or significance testing is a mathematical model for testing a claim, idea or hypothesis about a parameter of interest in a given population set, using data measured in a sample set. Calculations are performed on selected samples to gather more decisive information about the characteristics of the entire population, which enables a systematic way to test claims or ideas about the entire dataset.
Here is a simple example: A school principal reports that students in their school score an average of 7 out of 10 in exams. To test this “hypothesis,” we record marks of say 30 students (sample) from the entire student population of the school (say 300) and calculate the mean of that sample. We can then compare the (calculated) sample mean to the (reported) population mean and attempt to confirm the hypothesis.
To take another example, the annual return of a particular mutual fund is 8%. Assume that mutual fund has been in existence for 20 years. We take a random sample of annual returns of the mutual fund for, say, five years (sample) and calculate its mean. We then compare the (calculated) sample mean to the (claimed) population mean to verify the hypothesis.
This article assumes readers' familiarity with concepts of a normal distribution table, formula, p-value and related basics of statistics.
Different methodologies exist for hypothesis testing, but the same four basic steps are involved:
Usually, the reported value (or the claim statistics) is stated as the hypothesis and presumed to be true. For the above examples, the hypothesis will be:
- Example A: Students in the school score an average of 7 out of 10 in exams.
- Example B: The annual return of the mutual fund is 8% per annum.
This stated description constitutes the “ Null Hypothesis (H 0 ) ” and is assumed to be true – the way a defendant in a jury trial is presumed innocent until proven guilty by the evidence presented in court. Similarly, hypothesis testing starts by stating and assuming a “ null hypothesis ,” and then the process determines whether the assumption is likely to be true or false.
The important point to note is that we are testing the null hypothesis because there is an element of doubt about its validity. Whatever information that is against the stated null hypothesis is captured in the Alternative Hypothesis (H 1 ). For the above examples, the alternative hypothesis will be:
- Students score an average that is not equal to 7.
- The annual return of the mutual fund is not equal to 8% per annum.
In other words, the alternative hypothesis is a direct contradiction of the null hypothesis.
As in a trial, the jury assumes the defendant's innocence (null hypothesis). The prosecutor has to prove otherwise (alternative hypothesis). Similarly, the researcher has to prove that the null hypothesis is either true or false. If the prosecutor fails to prove the alternative hypothesis, the jury has to let the defendant go (basing the decision on the null hypothesis). Similarly, if the researcher fails to prove an alternative hypothesis (or simply does nothing), then the null hypothesis is assumed to be true.
The decision-making criteria have to be based on certain parameters of datasets.
The decision-making criteria have to be based on certain parameters of datasets and this is where the connection to normal distribution comes into the picture.
As per the standard statistics postulate about sampling distribution , “For any sample size n, the sampling distribution of X̅ is normal if the population X from which the sample is drawn is normally distributed.” Hence, the probabilities of all other possible sample mean that one could select are normally distributed.
For e.g., determine if the average daily return, of any stock listed on XYZ stock market , around New Year's Day is greater than 2%.
H 0 : Null Hypothesis: mean = 2%
H 1 : Alternative Hypothesis: mean > 2% (this is what we want to prove)
Take the sample (say of 50 stocks out of total 500) and compute the mean of the sample.
For a normal distribution, 95% of the values lie within two standard deviations of the population mean. Hence, this normal distribution and central limit assumption for the sample dataset allows us to establish 5% as a significance level. It makes sense as, under this assumption, there is less than a 5% probability (100-95) of getting outliers that are beyond two standard deviations from the population mean. Depending upon the nature of datasets, other significance levels can be taken at 1%, 5% or 10%. For financial calculations (including behavioral finance), 5% is the generally accepted limit. If we find any calculations that go beyond the usual two standard deviations, then we have a strong case of outliers to reject the null hypothesis.
Graphically, it is represented as follows:
In the above example, if the mean of the sample is much larger than 2% (say 3.5%), then we reject the null hypothesis. The alternative hypothesis (mean >2%) is accepted, which confirms that the average daily return of the stocks is indeed above 2%.
However, if the mean of the sample is not likely to be significantly greater than 2% (and remains at, say, around 2.2%), then we CANNOT reject the null hypothesis. The challenge comes on how to decide on such close range cases. To make a conclusion from selected samples and results, a level of significance is to be determined, which enables a conclusion to be made about the null hypothesis. The alternative hypothesis enables establishing the level of significance or the "critical value” concept for deciding on such close range cases.
According to the textbook standard definition , “A critical value is a cutoff value that defines the boundaries beyond which less than 5% of sample means can be obtained if the null hypothesis is true. Sample means obtained beyond a critical value will result in a decision to reject the null hypothesis." In the above example, if we have defined the critical value as 2.1%, and the calculated mean comes to 2.2%, then we reject the null hypothesis. A critical value establishes a clear demarcation about acceptance or rejection.
This step involves calculating the required figure(s), known as test statistics (like mean, z-score , p-value , etc.), for the selected sample. (We'll get to these in a later section.)
With the computed value(s), decide on the null hypothesis. If the probability of getting a sample mean is less than 5%, then the conclusion is to reject the null hypothesis. Otherwise, accept and retain the null hypothesis.
There can be four possible outcomes in sample-based decision-making, with regard to the correct applicability to the entire population:
The “Correct” cases are the ones where the decisions taken on the samples are truly applicable to the entire population. The cases of errors arise when one decides to retain (or reject) the null hypothesis based on the sample calculations, but that decision does not really apply for the entire population. These cases constitute Type 1 ( alpha ) and Type 2 ( beta ) errors, as indicated in the table above.
Selecting the correct critical value allows eliminating the type-1 alpha errors or limiting them to an acceptable range.
Alpha denotes the error on the level of significance and is determined by the researcher. To maintain the standard 5% significance or confidence level for probability calculations, this is retained at 5%.
According to the applicable decision-making benchmarks and definitions:
- “This (alpha) criterion is usually set at 0.05 (a = 0.05), and we compare the alpha level to the p-value. When the probability of a Type I error is less than 5% (p < 0.05), we decide to reject the null hypothesis; otherwise, we retain the null hypothesis.”
- The technical term used for this probability is the p-value . It is defined as “the probability of obtaining a sample outcome, given that the value stated in the null hypothesis is true. The p-value for obtaining a sample outcome is compared to the level of significance."
- A Type II error, or beta error, is defined as the probability of incorrectly retaining the null hypothesis, when in fact it is not applicable to the entire population.
A few more examples will demonstrate this and other calculations.
A monthly income investment scheme exists that promises variable monthly returns. An investor will invest in it only if they are assured of an average $180 monthly income. The investor has a sample of 300 months’ returns which has a mean of $190 and a standard deviation of $75. Should they invest in this scheme?
Let’s set up the problem. The investor will invest in the scheme if they are assured of the investor's desired $180 average return.
H 0 : Null Hypothesis: mean = 180
H 1 : Alternative Hypothesis: mean > 180
Method 1: Critical Value Approach
Identify a critical value X L for the sample mean, which is large enough to reject the null hypothesis – i.e. reject the null hypothesis if the sample mean >= critical value X L
P (identify a Type I alpha error) = P(reject H 0 given that H 0 is true),
This would be achieved when the sample mean exceeds the critical limits.
= P (given that H 0 is true) = alpha
Graphically, it appears as follows:
Taking alpha = 0.05 (i.e. 5% significance level), Z 0.05 = 1.645 (from the Z-table or normal distribution table)
= > X L = 180 +1.645*(75/sqrt(300)) = 187.12
Since the sample mean (190) is greater than the critical value (187.12), the null hypothesis is rejected, and the conclusion is that the average monthly return is indeed greater than $180, so the investor can consider investing in this scheme.
Method 2: Using Standardized Test Statistics
One can also use standardized value z.
Test Statistic, Z = (sample mean – population mean) / (std-dev / sqrt (no. of samples).
Then, the rejection region becomes the following:
Z= (190 – 180) / (75 / sqrt (300)) = 2.309
Our rejection region at 5% significance level is Z> Z 0.05 = 1.645.
Since Z= 2.309 is greater than 1.645, the null hypothesis can be rejected with a similar conclusion mentioned above.
Method 3: P-value Calculation
We aim to identify P (sample mean >= 190, when mean = 180).
= P (Z >= (190- 180) / (75 / sqrt (300))
= P (Z >= 2.309) = 0.0084 = 0.84%
The following table to infer p-value calculations concludes that there is confirmed evidence of average monthly returns being higher than 180:
A new stockbroker (XYZ) claims that their brokerage fees are lower than that of your current stock broker's (ABC). Data available from an independent research firm indicates that the mean and std-dev of all ABC broker clients are $18 and $6, respectively.
A sample of 100 clients of ABC is taken and brokerage charges are calculated with the new rates of XYZ broker. If the mean of the sample is $18.75 and std-dev is the same ($6), can any inference be made about the difference in the average brokerage bill between ABC and XYZ broker?
H 0 : Null Hypothesis: mean = 18
H 1 : Alternative Hypothesis: mean <> 18 (This is what we want to prove.)
Rejection region: Z <= - Z 2.5 and Z>=Z 2.5 (assuming 5% significance level, split 2.5 each on either side).
Z = (sample mean – mean) / (std-dev / sqrt (no. of samples))
= (18.75 – 18) / (6/(sqrt(100)) = 1.25
This calculated Z value falls between the two limits defined by:
- Z 2.5 = -1.96 and Z 2.5 = 1.96.
This concludes that there is insufficient evidence to infer that there is any difference between the rates of your existing broker and the new broker.
Alternatively, The p-value = P(Z< -1.25)+P(Z >1.25)
= 2 * 0.1056 = 0.2112 = 21.12% which is greater than 0.05 or 5%, leading to the same conclusion.
Graphically, it is represented by the following:
Criticism Points for the Hypothetical Testing Method:
- A statistical method based on assumptions
- Error-prone as detailed in terms of alpha and beta errors
- Interpretation of p-value can be ambiguous, leading to confusing results
Hypothesis testing allows a mathematical model to validate a claim or idea with a certain confidence level. However, like the majority of statistical tools and models, it is bound by a few limitations. The use of this model for making financial decisions should be considered with a critical eye, keeping all dependencies in mind. Alternate methods like Bayesian Inference are also worth exploring for similar analysis.
Sage Publications. " Introduction to Hypothesis Testing ," Page 13.
Sage Publications. " Introduction to Hypothesis Testing ," Page 11.
Sage Publications. " Introduction to Hypothesis Testing ," Page 7.
Sage Publications. " Introduction to Hypothesis Testing ," Pages 10-11.
:max_bytes(150000):strip_icc():format(webp)/GettyImages-175599141-50515a113a524f5585dedf7846ccce8b.jpg)
- Terms of Service
- Editorial Policy
- Privacy Policy
- Your Privacy Choices
On the power of popular two-sample tests applied to precipitation and discharge series
- ORIGINAL PAPER
- Published: 30 March 2024
Cite this article
- Giuseppe Mascaro 1 , 2
Explore all metrics
Two-sample tests are widely used in hydrologic and climate studies to investigate whether two samples of a variable of interest could be considered drawn from different populations. Despite this, the information on the power (i.e., the probability of correctly rejecting the null hypothesis) of these tests applied to hydroclimatic variables is limited. Here, this need is addressed considering four popular two-sample tests applied to daily and extreme precipitation, and annual peak flow series. The chosen tests assess differences in location (t-Student and Wilcoxon) and distribution (Kolmogorov–Smirnov and likelihood-ratio). The power was quantified through Monte Carlo simulations relying on pairs of realistic samples of the three variables with equal size, generated with a procedure based on suitable parametric distributions and copulas. After showing that differences in sample skewness are monotonically related to differences in spread, power surfaces were built as a function of the relative changes in location and spread of the samples and utilized to interpret three case studies comparing samples of observed precipitation and discharge series in the U.S. It was found that (1) the t-Student applied to the log-transformed samples has the same power as the Wilcoxon test; (2) location (distribution) tests perform better than distribution (location) tests for small (moderate-to-large) differences in spread and skewness; (3) the power is relatively lower (higher) if the differences in location and spread or skewness have concordant (discordant) sign; and (4) the power increases with the sample size but could be quite low for tests applied to extreme precipitation and discharge records that are commonly short. This work provides useful recommendations for selecting and interpreting two-sample tests in a broad range of hydroclimatic applications.
This is a preview of subscription content, log in via an institution to check access.
Access this article
Price includes VAT (Russian Federation)
Instant access to the full article PDF.
Rent this article via DeepDyve
Institutional subscriptions
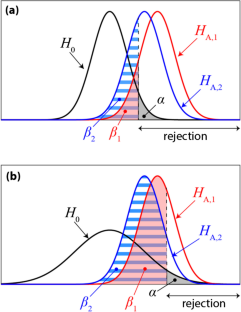
Data availability
Precipitation data from the GHCNd rain gage network are available at: https://www.ncei.noaa.gov/products/land-based-station/global-historical-climatology-network-daily . Discharge data for the HCDN2009 stream gages are available at: https://waterdata.usgs.gov/nwis/sw .
Amorim R, Villarini G (2023) Assessing the performance of parametric and non-parametric tests for trend detection in partial duration time series. J Flood Risk Manag, e12957. https://doi.org/10.1111/JFR3.12957
Angelina A, Gado Djibo A, Seidou O, Seidou Sanda I, Sittichok K (2015) Changes to flow regime on the Niger River at Koulikoro under a changing climate | Modifications du régime d’écoulement du fleuve Niger à Koulikoro sous changement climatique. Hydrol Sci J 60:1709–1723. https://doi.org/10.1080/02626667.2014.916407
Article Google Scholar
Ansh Srivastava N, Mascaro G (2023) Improving the utility of weather radar for the spatial frequency analysis of extreme precipitation. J Hydrol (amst) 624:129902. https://doi.org/10.1016/J.JHYDROL.2023.129902
Bartlett M (1937) Properties of sufficiency and statistical tests. Proc R Soc Lond A Math Phys Sci 160. https://doi.org/10.1098/rspa.1937.0109
Baumgartner D, Kolassa J (2021) Power considerations for Kolmogorov-Smirnov and Anderson-Darling two-sample tests. Commun Stat Simul Comput. https://doi.org/10.1080/03610918.2021.1928193
Beguería S, Angulo-Martínez M, Vicente-Serrano SM, López-Moreno JI, El-Kenawy A (2011) Assessing trends in extreme precipitation events intensity and magnitude using non-stationary peaks-over-threshold analysis: A case study in northeast Spain from 1930 to 2006. Int J Climatol 31:2102–2114. https://doi.org/10.1002/joc.2218
Blanchet J, Ceresetti D, Molinié G, Creutin JD (2016) A regional GEV scale-invariant framework for Intensity–Duration–Frequency analysis. J Hydrol (amst) 540:82–95. https://doi.org/10.1016/j.jhydrol.2016.06.007
van den Brink WP, van den Brink SGJ (1989) A comparison of the power of the t test, Wilcoxon’s test, and the approximate permutation test for the two-sample location problem. Br J Math Stat Psychol 42:183–189. https://doi.org/10.1111/j.2044-8317.1989.tb00907.x
Chu PS. Chen YR, Schroeder TA (2010) Changes in precipitation extremes in the Hawaiian Islands in a warming climate. J Clim, 23. https://doi.org/10.1175/2010JCLI3484.1
Collings BJ, Hamilton MA (1988) Estimating the power of the two-sample Wilcoxon test for location shift. Biometrics 44:847–860. https://doi.org/10.2307/2531596
Article CAS Google Scholar
Cressie NAC, Whitford HJ (1986) How to Use the Two Sample t‐Test. Biometric J, 28. https://doi.org/10.1002/bimj.4710280202
Deidda R, Hellies M, Langousis A (2021) A critical analysis of the shortcomings in spatial frequency analysis of rainfall extremes based on homogeneous regions and a comparison with a hierarchical boundaryless approach. Stochas Environ Res Risk Assess 35(12):2605–2628. https://doi.org/10.1007/S00477-021-02008-X
England JF Jr, Cohn TA, Faber BA, Stedinger JR, Thomas WO Jr, Veilleux AG, Kiang JE, Mason RR Jr (2018) Guidelines for determining flood flow frequency—Bulletin 17C (ver. 1.1, May 2019): U.S. Geological Survey Techniques and Methods, book 4, chap. B5, p 148. https://doi.org/10.3133/tm4B5
Fagerland MW (2012) T-tests, non-parametric tests, and large studiesa paradox of statistical practice? BMC Med Res Methodol, 12. https://doi.org/10.1186/1471-2288-12-78
Fagerland MW, Sandvik L (2009) Performance of five two-sample location tests for skewed distributions with unequal variances. Contemp Clin Trials, 30. https://doi.org/10.1016/j.cct.2009.06.007
Farris S, Deidda R, Viola F, Mascaro G (2021) On the role of serial correlation and field significance in detecting changes in extreme precipitation frequency. Water Resour Res, e2021WR030172. https://doi.org/10.1029/2021WR030172
Feltovich N (2003) Nonparametric tests of differences in medians: Comparison of the Wilcoxon-Mann-Whitney and robust rank-order tests. Exp Econ 6:273–297. https://doi.org/10.1023/A:1026273319211
Freidlin B, Gastwirth JL (2000) Should the Median Test be Retired from General Use? Am Statistic, 54. https://doi.org/10.1080/00031305.2000.10474539
Gretton A, Sejdinovic D, Strathmann H, Balakrishnan S, Pontil M, Fukumizu K, Sriperumbudur BK (2012) Optimal kernel choice for large-scale two-sample tests. Adv Neural Inform Process Syste 25
Griffis VW, Stedinger JR (2007) Log-Pearson Type 3 Distribution and Its Application in Flood Frequency Analysis. I: Distribution Characteristics. J Hydrol Eng 12. https://doi.org/10.1061/(asce)1084-0699(2007)12:5(482 ).
Hoenig JM, Heisey DM (2001) The Abuse of Power. Am Stat 55:19–24. https://doi.org/10.1198/000313001300339897
Hosking JRM (1990) L-Moments: Analysis and Estimation of Distributions Using Linear Combinations of Order Statistics. J Roy Stat Soc: Ser B (methodol) 52:105–124. https://doi.org/10.1111/J.2517-6161.1990.TB01775.X
Hosking JRM, Wallis JR (1997) Regional Frequency Analysis. Cambridge University Press. https://doi.org/10.1017/CBO9780511529443
Karl TR, Koss WJ (1984) Regional and national monthly, seasonal, and annual temperature weighted by area, 1895–1983. Historical Climatol Series 3–3
Knighton JO, Walter MT (2016) Critical rainfall statistics for predicting watershed flood responses: rethinking the design storm concept. Hydrol Process 30:3788–3803. https://doi.org/10.1002/hyp.10888
Knoben WJM, Woods RA, Freer JE (2018) A Quantitative Hydrological Climate Classification Evaluated With Independent Streamflow Data. Water Resour Res 54:5088–5109. https://doi.org/10.1029/2018WR022913
Kruskal WH (1957) Historical Notes on the Wilcoxon Unpaired Two-Sample Test. J Am Stat Assoc 52(279):356–360. https://doi.org/10.1080/01621459.1957.10501395
Kunkel KE, Easterling DR, Kristovich DAR, Gleason B, Stoecker L, Smith R (2012) Meteorological Causes of the Secular Variations in Observed Extreme Precipitation Events for the Conterminous United States. J Hydrometeorol 13:1131–1141. https://doi.org/10.1175/JHM-D-11-0108.1
Kunkel TR, Karl MF, Squires X, Yin ST, Stegall, and D. R. Easterling, (2020) Precipitation extremes: Trends and relationships with average precipitation and precipitable water in the contiguous United States. J Appl Meteorol Climatol 59(125–142):2020. https://doi.org/10.1175/JAMC-D-19-0185.1
Lee ET, Desu MM, Gehan EA (1975) A Monte Carlo study of the power of some two-sample tests. Biometrika 62:425–432. https://doi.org/10.1093/biomet/62.2.425
Mascaro G (2020) Comparison of local, regional, and scaling models for rainfall intensity–duration–frequency analysis. J Appl Meteorol Climatol 59:1519–1536. https://doi.org/10.1175/JAMC-D-20-0094.1
Mascaro G, Papalexiou SM, Wright DB (2023) Advancing Characterization and Modeling of Space-Time Correlation Structure and Marginal Distribution of Short-Duration Precipitation. Adv Water Resour 177:104451. https://doi.org/10.1016/J.ADVWATRES.2023.104451
Massey FJ (1951) The Kolmogorov-Smirnov Test for Goodness of Fit. J Am Stat Assoc 46:68. https://doi.org/10.2307/2280095
Menne MJ, Durre I, Vose RS, Gleason BE, Houston TG (2012) An overview of the global historical climatology network-daily database. J Atmos Ocean Technol, 29. https://doi.org/10.1175/JTECH-D-11-00103.1
O’Gorman TW (1995) The effect of unequal variances on the power of several two–sample tests. Commun Stat Simul Comput 24:853–867. https://doi.org/10.1080/03610919508813279
Orskaug E, Scheel I, Frigessi A, Guttorp P, Haugen JE, Tveito OE, Haug O (2011) Evaluation of a dynamic downscaling of precipitation over the Norwegian mainland. Tellus, Ser: Dyn Meteorol Oceanograph 63:746–756. https://doi.org/10.1111/j.1600-0870.2011.00525.x
Papalexiou SM (2022) Rainfall Generation Revisited: Introducing CoSMoS-2s and Advancing Copula-Based Intermittent Time Series Modeling. Water Resour Res, 58, e2021WR031641. https://doi.org/10.1029/2021WR031641
Papalexiou SM, Koutsoyiannis D (2012) Entropy based derivation of probability distributions: A case study to daily rainfall. Adv Water Resour. https://doi.org/10.1016/j.advwatres.2011.11.007
Papalexiou SM, Koutsoyiannis D (2013) Battle of extreme value distributions: A global survey on extreme daily rainfall. Water Resour Res 49:187–201. https://doi.org/10.1029/2012WR012557
Papalexiou SM, Koutsoyiannis D (2016) A global survey on the seasonal variation of the marginal distribution of daily precipitation. Adv Water Resour, 94. https://doi.org/10.1016/j.advwatres.2016.05.005
Park J-S, Kang H-S, Lee YS, Kim M-K (2011) Changes in the extreme daily rainfall in South Korea. Int J Climatol 31:2290–2299. https://doi.org/10.1002/joc.2236
Penfield DA (1994) Choosing a two-sample location test. J Exp Educ 62(4):343–360. https://doi.org/10.1080/00220973.1994.9944139
Prosdocimi I, Kjeldsen TR, Svensson C (2014) Non-stationarity in annual and seasonal series of peak flow and precipitation in the UK. Nat Hazard 14:1125–1144. https://doi.org/10.5194/nhess-14-1125-2014
Rasch D, Teuscher F, Guiard V (2007) How robust are tests for two independent samples?. J Stat Plan Inference, 137. https://doi.org/10.1016/j.jspi.2006.04.011
Rauscher SA, O’Brien TA, Piani C, Coppola E, Giorgi F, Collins WD, Lawston PM (2016) A multimodel intercomparison of resolution effects on precipitation: simulations and theory. Clim Dyn 47:2205–2218. https://doi.org/10.1007/s00382-015-2959-5
Roth M, Buishand TA, Jongbloed G, Klein Tank AMG, van Zanten JH (2012) A regional peaks-over-threshold model in a nonstationary climate. Water Resour Res, 48. https://doi.org/10.1029/2012WR012214
Schindler A, Toreti A, Zampieri M, Scoccimarro E, Gualdi S, Fukutome S, Xoplaki E, Luterbacher J (2015) On the internal variability of simulated daily precipitation. J Clim, 28. https://doi.org/10.1175/JCLI-D-14-00745.1
Shao Y, Wu J, Ye J, Liu Y (2015) Frequency analysis and its spatiotemporal characteristics of precipitation extreme events in China during 1951–2010. Theor Appl Climatol 121:775–787. https://doi.org/10.1007/s00704-015-1481-3
Shen SSP, Wied O, Weithmann A, Regele T, Bailey BA, Lawrimore JH (2016) Six temperature and precipitation regimes of the contiguous United States between 1895 and 2010: a statistical inference study. Theor Appl Climatol 125:197–211. https://doi.org/10.1007/s00704-015-1502-2
Slack JR, Landwehr JM (1992) Hydro-Climatic Data Network (HCDN); a U.S. Geological Survey streamflow data set for the United States for the study of climate variations, pp 1874–1988. https://doi.org/10.3133/ofr92129
Student, 1908 The Probable Error of a Mean. Biometrika, 6. https://doi.org/10.2307/2331554
Sugahara S, da Rocha RP, Ynoue RY, da Silveira RB (2015) Statistical detection of spurious variations in daily raingauge data caused by changes in observation practices, as applied to records from various parts of the world. Int J Climatol 35:2922–2933. https://doi.org/10.1002/joc.4183
Sýkorová P, Huth R (2020) The applicability of the Hess–Brezowsky synoptic classification to the description of climate elements in Europe. Theor Appl Climatol, 142. https://doi.org/10.1007/s00704-020-03375-1
Thober S, Samaniego L (2014) Robust ensemble selection by multivariate evaluation of extreme precipitation and temperature characteristics. J Geophys Res 119:594–613. https://doi.org/10.1002/2013JD020505
Totaro V, Gioia A, Iacobellis V (2020) Numerical investigation on the power of parametric and nonparametric tests for trend detection in annual maximum series. Hydrol Earth Syst Sci 24:473–488. https://doi.org/10.5194/HESS-24-473-2020
Vogel RM, Thomas WO, McMahon TA (1993) Flood‐Flow Frequency Model Selection in Southwestern United States. J Water Resour Plan Manag, 119. https://doi.org/10.1061/(asce)0733-9496(1993)119:3(353)
Vogel RM, Rosner A, Kirshen PH (2013) Brief Communication: Likelihood of societal preparedness for global change: trend detection. Nat Hazard 13:1773–1778. https://doi.org/10.5194/nhess-13-1773-2013
Wilks DS (2006) On “Field Significance” and the False Discovery Rate. J Appl Meteorol Climatol 45:1181–1189. https://doi.org/10.1175/JAM2404.1
Wilks (2011) Statistical methods in the atmospheric sciences. Academic Press, International Geophysics Series, p 676
Google Scholar
Wilks DS (2016) “The Stippling Shows Statistically Significant Grid Points”: How Research Results are Routinely Overstated and Overinterpreted, and What to Do about It. Bull Am Meteorol Soc 97:2263–2273. https://doi.org/10.1175/BAMS-D-15-00267.1
Xu ZX, Takeuchi K, Ishidaira H (2003) Monotonic trend and step changes in Japanese precipitation. J Hydrol (amst) 279:144–150. https://doi.org/10.1016/S0022-1694(03)00178-1
Zaghloul M, Papalexiou SM, Elshorbagy A, Coulibaly P (2020) Revisiting flood peak distributions: A pan-Canadian investigation. Adv Water Resour 145:103720. https://doi.org/10.1016/J.ADVWATRES.2020.103720
Download references
Acknowledgements
The author thanks the Editor and three anonymous reviewers whose comments greatly helped to improve the quality of the manuscript. The MATLAB codes used for the analyses could be shared upon request to the author.
This work has been supported by the National Science Foundation (NSF) awards #2212702: “CAS-Climate: A Novel Process-Driven Method for Flood Frequency Analysis Based on Mixed Distributions” and #2221803: “Collaborative Research: CAS—Climate: Improving Nonstationary Intensity-Duration-Frequency Analysis of Extreme Precipitation by Advancing Knowledge on the Generating Mechanisms”.
Author information
Authors and affiliations.
School of Sustainable Engineering and the Built Environment, Arizona State University, Tempe, AZ, USA
Giuseppe Mascaro
Center for Hydrologic Innovations, Arizona State University, Tempe, AZ, USA
You can also search for this author in PubMed Google Scholar
Contributions
Giuseppe Mascaro performed all the work.
Corresponding author
Correspondence to Giuseppe Mascaro .
Ethics declarations
Competing interests.
The authors declare no competing interests.
Additional information
Publisher's note.
Springer Nature remains neutral with regard to jurisdictional claims in published maps and institutional affiliations.
Electronic supplementary material
Below is the link to the electronic supplementary material.
Supplementary file1 (DOCX 1223 KB)
Appendix 1: summary of two-sample statistical tests.
In the following, we provide a summary of null hypothesis H 0 , test statistics, and null distribution used to derive the p -value of the two-sample statistical tests used in the paper. Let \({{\varvec{x}}}_{1}=\left\{{x}_{\mathrm{1,1}},{x}_{\mathrm{1,2}},\dots , {x}_{1,{n}_{1}}\right\}\) and \({{\varvec{x}}}_{2}=\left\{{x}_{\mathrm{2,1}},{x}_{\mathrm{2,2}},\dots , {x}_{2,{n}_{2}}\right\}\) be two independent samples with size n 1 and n 2 , respectively. The Student t -test (t-S) evaluates the null hypothesis H 0 of equal mean between the two samples. The t-S test statistic is \(z= \frac{{\overline{x} }_{1}-{\overline{x} }_{2}}{{\left[\frac{{s}_{1}^{2}}{{n}_{1}}+\frac{{s}_{2}^{2}}{{n}_{2}}\right]}^{1/2}}\) , where \({\overline{x} }_{1}\) and \({\overline{x} }_{2}\) are the sample means, and \({s}_{1}^{2}\) and \({s}_{2}^{2}\) are the sample variances. The null distribution for z is the t distribution with degrees of freedom \(\nu ={\text{min}}\left({n}_{1},{n}_{2}\right)-1\) for small sample sizes while, for moderately large sizes (as the case of our records), the null distribution of z is well modeled by the standard Gaussian. The t-S test is two-sided. The Wilcoxon (Wi) test is a non-parametric test whose H 0 is that both samples have equal median. The statistic is \(U={R}_{1}-\frac{{n}_{1}}{2}({n}_{1}+1)\) , where R 1 is the sum of the ranks of the first sample and n 1 is its size, while the null distribution of U is Gaussian for sample sizes larger than 10. The Kolmogorov–Smirnov (KS) test is also a non-parametric test that investigates the null hypothesis H 0 that the samples come from the same distribution; its statistic is \({D}_{s}=\underset{x}{{\text{max}}}\left|{F}_{1}\left(x\right)-{F}_{2}\left(x\right)\right|\) , where F 1 ( x ) and F 2 ( x ) are the empirical cumulative distribution functions of the first and second samples, respectively; the p value is computed through the Kolmogorov distribution or other approximations. The likelihood ratio (LR) test has the same H 0 as the Kolmogorov–Smirnov test and requires assuming parametric forms for the distribution of samples 1 and 2 and the two samples combined. Let \({G}_{1}\left(x;{\widehat{{\varvec{\theta}}}}_{1}\right)\) , \({G}_{2}\left(x;{\widehat{{\varvec{\theta}}}}_{2}\right)\) , and \({G}_{0}\left(x;{\widehat{{\varvec{\theta}}}}_{0}\right)\) be such distributions with parameters \({\widehat{{\varvec{\theta}}}}_{1}\) , \({\widehat{{\varvec{\theta}}}}_{2}\) , and \({\widehat{{\varvec{\theta}}}}_{0}\) estimated on the corresponding samples \({{\varvec{x}}}_{1}\) , \({{\varvec{x}}}_{2}\) , and \({{\varvec{x}}}_{0}=\left\{{{\varvec{x}}}_{1},{{\varvec{x}}}_{2}\right\}.\) The test statistic is \({\Lambda }^{*}=2[{L}_{1}\left({\widehat{{\varvec{\theta}}}}_{1};{{\varvec{x}}}_{1}\right)+{L}_{2}\left({\widehat{{\varvec{\theta}}}}_{2};{{\varvec{x}}}_{2}\right)-{L}_{0}\left({\widehat{{\varvec{\theta}}}}_{0};{{\varvec{x}}}_{0}\right)]\) , where \({L}_{k}\left({\widehat{{\varvec{\theta}}}}_{{\varvec{k}}};{{\varvec{x}}}_{{\varvec{k}}}\right)\) is the log-likelihood of the corresponding distribution \({G}_{k}\left(x;{\widehat{{\varvec{\theta}}}}_{{\varvec{k}}}\right)\) , with k = 0, 1, and 2. The null distribution is the χ 2 with degrees of freedom ν = m 1 + m 2 – m 0 , where m k is the number of parameters of the k -th distribution.
Appendix 2: Parametric probability distributions and multivariate distributions of their parameters
The cumulative distribution function (CDF) of the Burr Type XII ( \(\mathcal{B}\mathcal{r}{\text{XII}}\) ), generalized extreme value ( \(\mathcal{G}\mathcal{E}\mathcal{V}\) ) and Pearson Type 3 ( \(\mathcal{P}3\) ) distributions are:
The \(\mathcal{B}\mathcal{r}{\text{XII}}\) distribution has two shape parameters, γ 1 > 0 and γ 2 > 0, and one scale parameter, θ > 0, and is defined for x ≥ 0. The \(\mathcal{G}\mathcal{E}\mathcal{V}\) distribution has a shape parameter, k ∈ (-∞, + ∞), a location parameter, μ ∈ (-∞, + ∞), and a scale parameter, σ > 0; it is defined in the sets
\(-\infty <x<\infty\) if k = 0, \(\mu -\frac{\sigma }{k}\le x<\infty\) if k > 0, and \(-\infty <x\le \mu -\frac{\sigma }{k}\) if k < 0. The \(\mathcal{P}3\) distribution has a shape parameter, α > 0, a scale parameter, β ∈ (-∞, + ∞), and a location parameter, ξ ∈ (-∞, + ∞); it is defined in the sets \(\xi \le x<\infty\) if the skewness coefficient γ > 0, and \(-\infty <x\le \xi\) if γ < 0. In equation (A3), \({\text{G}}\left(\bullet ,\bullet \right)\) and \(\Gamma \left(\bullet \right)\) are the incomplete and complete gamma functions, respectively. The Log-Pearson Type 3 ( \(\mathcal{L}\mathcal{P}3\) ) is the \(\mathcal{P}3\) distribution where x is the log-transformed value of the original sample (here, the APF series).
Parameters of the \(\mathcal{B}\mathcal{r}{\text{XII}}\) were estimated using the numerical procedure proposed by Zaghloul et al. ( 2020 ) based on the method of L -moments. Parameters of the \(\mathcal{G}\mathcal{E}\mathcal{V}\) and \(\mathcal{L}\mathcal{P}3\) were also estimated with the method of L -moments following Hosking and Wallis ( 1997 ). For the \(\mathcal{G}\mathcal{E}\mathcal{V}\) , a bias correction of the shape parameter was applied to account for the short sample size using the empirical relations proposed by Papalexiou and Koutsoyiannis ( 2013 ) and recently applied by Ansh Srivastava and Mascaro ( 2023 ). For each distribution, copulas were used to model the multivariate distribution of the parameters. For the \(\mathcal{B}\mathcal{r}{\text{XII}}\) distribution, we used a three-dimensional Gaussian copula with marginal distributions given by the Generalized Exponential Type 4 ( \(\mathcal{G}\mathcal{E}4\) ; Papalexiou 2022 ) for γ 1 , the Generalized Gamma ( \(\mathcal{G}\mathcal{G}\) ) for γ 2 , and the empirical CDF for β . For the \(\mathcal{G}\mathcal{E}\mathcal{V}\) distribution, we used a bidimensional Gaussian copula for σ and μ with their empirical CDFs adopted as marginal distributions. No significant relationship was found between k and the other two parameters; therefore, after generating a correlated pair of σ and μ , the synthetic value of k was randomly drawn from the empirical CDF of the observed estimates. Finally, for the \(\mathcal{L}\mathcal{P}3\) distribution, a three-dimensional Gaussian copula was used separately for the cases of positive and negative skewness coefficients with marginal distributions given by the \(\mathcal{B}\mathcal{r}{\text{XII}}\) for α and β , and the empirical CDF for ξ . For all three distributions, the selected copulas captured quite well the dependence structure among the parameters, as shown through the scatterplots, histograms, and values of the Spearman rank correlation coefficients reported in Figs. S1 . S2 , and S3 .
Rights and permissions
Springer Nature or its licensor (e.g. a society or other partner) holds exclusive rights to this article under a publishing agreement with the author(s) or other rightsholder(s); author self-archiving of the accepted manuscript version of this article is solely governed by the terms of such publishing agreement and applicable law.
Reprints and permissions
About this article
Mascaro, G. On the power of popular two-sample tests applied to precipitation and discharge series. Stoch Environ Res Risk Assess (2024). https://doi.org/10.1007/s00477-024-02709-z
Download citation
Accepted : 12 March 2024
Published : 30 March 2024
DOI : https://doi.org/10.1007/s00477-024-02709-z
Share this article
Anyone you share the following link with will be able to read this content:
Sorry, a shareable link is not currently available for this article.
Provided by the Springer Nature SharedIt content-sharing initiative
- Two-sample tests
- Precipitation
- Find a journal
- Publish with us
- Track your research
Help | Advanced Search
Mathematics > Statistics Theory
Title: the sample complexity of simple binary hypothesis testing.
Abstract: The sample complexity of simple binary hypothesis testing is the smallest number of i.i.d. samples required to distinguish between two distributions $p$ and $q$ in either: (i) the prior-free setting, with type-I error at most $\alpha$ and type-II error at most $\beta$; or (ii) the Bayesian setting, with Bayes error at most $\delta$ and prior distribution $(\alpha, 1-\alpha)$. This problem has only been studied when $\alpha = \beta$ (prior-free) or $\alpha = 1/2$ (Bayesian), and the sample complexity is known to be characterized by the Hellinger divergence between $p$ and $q$, up to multiplicative constants. In this paper, we derive a formula that characterizes the sample complexity (up to multiplicative constants that are independent of $p$, $q$, and all error parameters) for: (i) all $0 \le \alpha, \beta \le 1/8$ in the prior-free setting; and (ii) all $\delta \le \alpha/4$ in the Bayesian setting. In particular, the formula admits equivalent expressions in terms of certain divergences from the Jensen--Shannon and Hellinger families. The main technical result concerns an $f$-divergence inequality between members of the Jensen--Shannon and Hellinger families, which is proved by a combination of information-theoretic tools and case-by-case analyses. We explore applications of our results to robust and distributed (locally-private and communication-constrained) hypothesis testing.
Submission history
Access paper:.
- HTML (experimental)
- Other Formats
References & Citations
- Google Scholar
- Semantic Scholar
BibTeX formatted citation

Bibliographic and Citation Tools
Code, data and media associated with this article, recommenders and search tools.
- Institution
arXivLabs: experimental projects with community collaborators
arXivLabs is a framework that allows collaborators to develop and share new arXiv features directly on our website.
Both individuals and organizations that work with arXivLabs have embraced and accepted our values of openness, community, excellence, and user data privacy. arXiv is committed to these values and only works with partners that adhere to them.
Have an idea for a project that will add value for arXiv's community? Learn more about arXivLabs .
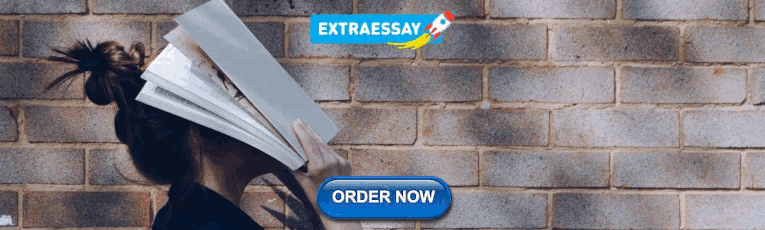
IMAGES
VIDEO
COMMENTS
If the biologist set her significance level \(\alpha\) at 0.05 and used the critical value approach to conduct her hypothesis test, she would reject the null hypothesis if her test statistic t* were less than -1.6939 (determined using statistical software or a t-table):s-3-3. Since the biologist's test statistic, t* = -4.60, is less than -1.6939, the biologist rejects the null hypothesis.
There are 5 main steps in hypothesis testing: State your research hypothesis as a null hypothesis and alternate hypothesis (H o) and (H a or H 1 ). Collect data in a way designed to test the hypothesis. Perform an appropriate statistical test. Decide whether to reject or fail to reject your null hypothesis. Present the findings in your results ...
A teacher believes that 85% of students in the class will want to go on a field trip to the local zoo. The teacher performs a hypothesis test to determine if the percentage is the same or different from 85%. The teacher samples 50 students and 39 reply that they would want to go to the zoo. For the hypothesis test, use a 1% level of significance.
This page titled 9.E: Hypothesis Testing with One Sample (Exercises) is shared under a CC BY 4.0 license and was authored, remixed, and/or curated by OpenStax via source content that was edited to the style and standards of the LibreTexts platform; a detailed edit history is available upon request. These are homework exercises to accompany the ...
Hypothesis testing is a technique that is used to verify whether the results of an experiment are statistically significant. It involves the setting up of a null hypothesis and an alternate hypothesis. There are three types of tests that can be conducted under hypothesis testing - z test, t test, and chi square test.
Likelihood ratio. In the likelihood ratio test, we reject the null hypothesis if the ratio is above a certain value i.e, reject the null hypothesis if L(X) > 𝜉, else accept it. 𝜉 is called the critical ratio.. So this is how we can draw a decision boundary: we separate the observations for which the likelihood ratio is greater than the critical ratio from the observations for which it ...
Unit test. Significance tests give us a formal process for using sample data to evaluate the likelihood of some claim about a population value. Learn how to conduct significance tests and calculate p-values to see how likely a sample result is to occur by random chance. You'll also see how we use p-values to make conclusions about hypotheses.
Developing a hypothesis (with example) Step 1. Ask a question. Writing a hypothesis begins with a research question that you want to answer. The question should be focused, specific, and researchable within the constraints of your project. Example: Research question.
A hypothesis test can help determine if a difference in the estimated proportions reflects a difference in the population proportions. 10.5: Matched or Paired Samples When using a hypothesis test for matched or paired samples, the following characteristics should be present: Simple random sampling is used. Sample sizes are often small.
Alternative Hypothesis: This is a statement that the population parameter (such as the proportion, mean, standard deviation, or variance) is somehow different the value involved in the Null Hypothesis. For our examples, "somehow different" will involve the use of < <, > >, or ≠ ≠. In simpler terms, the Alternative Hypothesis is a ...
Example 3: Public Opinion About President Step 1. Determine the null and alternative hypotheses. Null hypothesis: There is no clear winning opinion on this issue; the proportions who would answer yes or no are each 0.50. Alternative hypothesis: Fewer than 0.50, or 50%, of the population would answer yes to this question.
9.1: Prelude to Hypothesis Testing. A statistician will make a decision about claims via a process called "hypothesis testing." A hypothesis test involves collecting data from a sample and evaluating the data. Then, the statistician makes a decision as to whether or not there is sufficient evidence, based upon analysis of the data, to reject ...
Full Hypothesis Test Examples Tests on Means. Example 6. Jeffrey, as an eight-year old, established a mean time of 16.43 seconds for swimming the 25-yard freestyle, with a standard deviation of 0.8 seconds. His dad, Frank, thought that Jeffrey could swim the 25-yard freestyle faster using goggles.
To help demonstrate the process of hypothesis testing, let's first preview the results of a hypothesis test. Once we've done that, we'll describe the formal steps that we've completed for performing the hypothesis test on the next page. We'll then complete examples and discuss limitations and other implications after this introduction.
Worked Example. Imagine we have a textile manufacturer investigating a new yarn, which claims it has a thread elongation of 12 kilograms with a standard deviation of 0.5 kilograms. Using a random sample of 4 specimens, the manufacturer wishes to test the claim that the mean thread elongation is less than 12 kilograms.
In statistics, hypothesis tests are used to test whether or not some hypothesis about a population parameter is true. To perform a hypothesis test in the real world, researchers will obtain a random sample from the population and perform a hypothesis test on the sample data, using a null and alternative hypothesis:. Null Hypothesis (H 0): The sample data occurs purely from chance.
It tests the null hypothesis that the population variances are equal (called homogeneity of variance or homoscedasticity). Suppose the resulting p-value of Levene's test is less than the significance level (typically 0.05).In that case, the obtained differences in sample variances are unlikely to have occurred based on random sampling from a population with equal variances.
A statistics instructor thinks the mean score is higher than 65. He samples ten statistics students and obtains the scores 65 65 70 67 66 63 63 68 72 71. He performs a hypothesis test using a 5% level of significance. The data are assumed to be from a normal distribution. Answer. Set up the hypothesis test:
Simple hypothesis testing. Niels has a Magic 8 -Ball, which is a toy used for fortune-telling or seeking advice. To consult the ball, you ask the ball a question and shake it. One of 5 different possible answers then appears at random in the ball. Niels sensed that the ball answers " Ask again later " too frequently.
In a hypothesis test problem, you may see words such as "the level of significance is 1%." The "1%" is the preconceived or preset \(\alpha\). The statistician setting up the hypothesis test selects the value of α to use before collecting the sample data. If no level of significance is given, a common standard to use is \(\alpha = 0.05\).
View Solution to Question 1. Question 2. A professor wants to know if her introductory statistics class has a good grasp of basic math. Six students are chosen at random from the class and given a math proficiency test. The professor wants the class to be able to score above 70 on the test. The six students get the following scores:62, 92, 75 ...
Full Hypothesis Test Examples. Example 8.2.4 8.2. 4. Jeffrey, as an eight-year old, established a mean time of 16.43 seconds for swimming the 25-yard freestyle, with a standard deviation of 0.8 seconds. His dad, Frank, thought that Jeffrey could swim the 25-yard freestyle faster using goggles.
Hypothesis or significance testing is a mathematical model for testing a claim, idea or hypothesis about a parameter of interest in a given population set, using data measured in a sample set.
Two-sample tests are widely used in hydrologic and climate studies to investigate whether two samples of a variable of interest could be considered drawn from different populations. Despite this, the information on the power (i.e., the probability of correctly rejecting the null hypothesis) of these tests applied to hydroclimatic variables is limited. Here, this need is addressed considering ...
Title: The Sample Complexity of Simple Binary Hypothesis Testing Authors: Ankit Pensia , Varun Jog , Po-Ling Loh View a PDF of the paper titled The Sample Complexity of Simple Binary Hypothesis Testing, by Ankit Pensia and 2 other authors
By making use of the wealth of knowledge that already exists in the literature on quantum hypothesis testing, we characterize the sample complexity of binary quantum hypothesis testing in the symmetric and asymmetric settings, and we provide bounds on the sample complexity of multiple quantum hypothesis testing.