Click through the PLOS taxonomy to find articles in your field.
For more information about PLOS Subject Areas, click here .
Loading metrics
Open Access
Peer-reviewed
Research Article
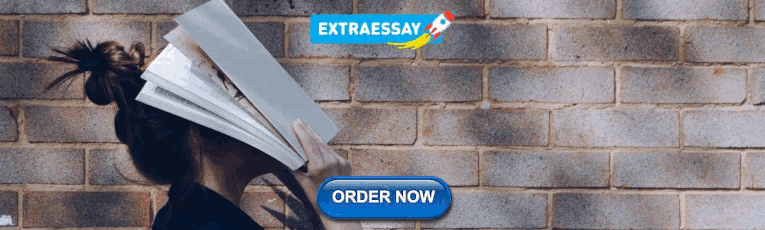
Research on corn production efficiency and influencing factors of typical farms: Based on data from 12 corn-producing countries from 2012 to 2019
Roles Conceptualization, Data curation, Investigation, Methodology, Resources, Software, Writing – original draft
Affiliation Institute of Agricultural Economics and Development, Chinese Academy of Agricultural Sciences, Beijing, China

Roles Conceptualization, Funding acquisition, Validation, Visualization, Writing – review & editing
* E-mail: [email protected]
- Jiamei Wang,
- Xiangdong Hu
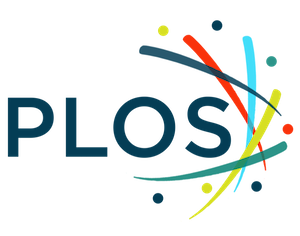
- Published: July 9, 2021
- https://doi.org/10.1371/journal.pone.0254423
- Reader Comments
Globally, corn is characterised by high production and high export concentrations, yet the world is experiencing an unprecedented, huge change in this regard. Ensuring the global supply of corn, and thereby the energy and food security of nations has become particularly important. To understand the importance of corn production as an influencing mechanism of global food supplies, the present study researched the corn production of typical farms in major corn-producing and importing countries around the world. I selected the corn input and output data of 18 typical farms in 12 countries from 2012 to 2019, used the data envelopment analysis (DEA) model to calculate the technical efficiency of corn production, and built a tobit model to explore the impact of farming methods, input elements, supporting services, and other factors on efficiency. The study established that the average comprehensive technical efficiency of corn production on a typical farm was 0.863, and the average loss was 13.7%. In addition, it concluded that intensive tillage and conservation tillage have high technical efficiency. It also demonstrated that the proportion of mechanical labour and technical efficiency is in a ‘U’-shaped relationship, among others.
Citation: Wang J, Hu X (2021) Research on corn production efficiency and influencing factors of typical farms: Based on data from 12 corn-producing countries from 2012 to 2019. PLoS ONE 16(7): e0254423. https://doi.org/10.1371/journal.pone.0254423
Editor: Carlos Alberto Zúniga-González, Universidad Nacional Autonoma de Nicaragua Leon, NICARAGUA
Received: March 26, 2021; Accepted: June 25, 2021; Published: July 9, 2021
Copyright: © 2021 Wang, Hu. This is an open access article distributed under the terms of the Creative Commons Attribution License , which permits unrestricted use, distribution, and reproduction in any medium, provided the original author and source are credited.
Data Availability: All relevant data are within the paper and its Supporting Information files.
Funding: This research is supported by Science and Technology Innovation Engineering Talent Project of Chinese Academy of Agricultural Sciences (ASTIP-IAED-2021-RC-05) and Science and Technology Innovation Project of Chinese Academy of Agricultural Sciences (ASTIP-IAED-2021- 01). The funders had no role in study design, data collection and analysis, decision to publish, or preparation of the manuscript.
Competing interests: The authors have declared that no competing interests exist.
Introduction
Corn is one of the most widely-planted crops in the world. It is grown in more than 170 regions globally. Corn production is highly concentrated in certain regions like North America, Asia, and South America. According to the United States Department of Agriculture, in 2020, corn production in the United States (US), China, Brazil, and Argentina accounted for 64.63% of global production [ 1 ]. In addition to holding inventory, a portion of the corn produced is consumed domestically, while the rest is exported. Corn exports and production are also highly concentrated. The main corn exporting countries are the US, Brazil, Argentina, and Ukraine. During 2020–2021, the cumulative corn exports of these four countries accounted for 88.12% of global exports [ 1 ]. This indicates that, although China is a major corn producer, it is not a major corn exporter. Global corn production is showing a slight downward trend, and the growth rate of consumption is higher than that of production. Global corn consumption is also highly concentrated. The US and China are the two largest corn consumers. In 2020, China’s corn consumption reached 279 million tons, an increase of 2 million tons from the previous year [ 1 ]. In recent years, the destocking speed of corn in China has accelerated, and nearly 260 million tons of stock have been consumed in 4 years [ 2 ]. With the continuous consumption of temporarily-stored corn, the overall corn supply has tightened, and the gap between supply and demand has gradually increased. According to UN Comtrade, China’s corn imports reached 11.3 million tons in 2020, a yearly increase of 135.73% [ 3 ]. China is a large corn consumer, most of which is from domestic production and a small part of it is from imports, but the import volume is showing an upward trend. Ukraine and the US are the main sources of China’s corn imports. In 2020, China’s corn imports from these two countries accounted for 94.20% of the total imports [ 3 ].
Grain production has achieved bumper harvests for 17 consecutive years, since China attaches great importance to food security, corn production has increased steadily in the past five years. However, there are still major problems in China’s corn production. From the perspective of supply and demand, the rapid development of animal husbandry has caused China’s corn consumption to exceed corn production since 2016, resulting in a large amount of imported corn. According to UN Comtrade, in terms of imports, corn reached 3.52 million tons in 2018, a yearly increase of 24.38%. In 2019, corn imports reached 4.79 million tons, a yearly increase of 36.08%. Simultaneously, since 2008, corn exports have reduced to 270,000 tons, a yearly decrease of 94.5%. Corn exports have been below 300,000 tons in the past 12 years [ 3 ]. Regarding production costs, China’s demographic dividend period has passed, and labour costs have increased significantly; land costs, seeds, fertilisers, and other agricultural materials costs have also increased, increasing the total cost of corn. From a pecuniary point of view, due to China’s policy of supporting the food market for many years, coupled with the increase in corn production costs, the phenomenon of domestic and foreign corn prices is inverted. Effectively ensuring corn production, increasing corn productivity, and ensuring food security have become particularly important. This study examines the production technology efficiency of 12 major corn-producing countries around the world from a macro perspective, analyses the main influencing factors, explores its influence mechanism, and proposes policy recommendations for improving China’s corn production capacity.
Literature review
Agricultural growth mainly depends on the improvement of production efficiency, which is a key indicator of agricultural progress [ 4 ]. Improvements in technical efficiency are necessary to increase food production and release production potential [ 5 ]. To effectively improve technical efficiency, it is required to accurately estimate technical efficiency, analyse important factors affecting corn production efficiency, and propose targeted policy recommendations [ 6 ]. This article reviews the research on the technical efficiency of corn and other important agricultural products. One is to compare and sort out the methods of measuring efficiency in existing research, the other is to provide reference for the methods used in their own research programs, and the third is to compare and verify the existing research results with their own conclusions. Many scholars use certain methods to measure the technical efficiency of corn production, which can be roughly divided into two categories: parametric and non-parametric methods. One is to use the non-parametric data envelopment analysis (DEA) method to measure technical efficiency. Zhang, Meng and Gao [ 7 ] calculated the average technical efficiency of corn production in the Xiliao River Basin to be 0.88, based on the DEA of investment-oriented BCC model. They established that, compared with the traditional technology, the use of the non-film shallow drip irrigation technology improves the technical efficiency. Gao, Liu and Dai [ 8 ] conducted efficiency calculations on nine major corn-producing areas in Xinjiang in 2006. Two output indicators and two input indicators are used. The output indicators are mainly corn yield and output value per unit area. The input indicators use the working price and material cost per hectare in turn. Pei and Zhou [ 9 ] used the DEA method to evaluate corn production efficiency in Heilongjiang Province in 2014. The study established that the overall efficiency of corn production in Heilongjiang was 78.80%. Koc, Gul and Parlakay [ 10 ] measured through the DEA model that the technical efficiency of corn farms in the Eastern Mediterranean region of Turkey is 81%. Mulwa, Emrouznejad and Muhammad [ 11 ] used the DEA model to measure the technical efficiency of corn production in western Kenya and determined that the production efficiency was only 67.70%, which is technically inefficient. However, agricultural training can reduce this inefficiency. The extension agents organize farmer training sessions to inform of farmer field schools to inform farmers about modern farming methods. DEA is used to model efficiencies as an explicit function of seed, fertiliser, family labour, hired labour and hired capital costs. This study constructs a meta-frontier for the two regions.
Another way is to use a parametric method to measure technical efficiency using the stochastic frontier model. Based on the stochastic frontier analysis, at the end of the 20th century and the beginning of the 21st century, the average technical efficiency of corn production in China was above 0.8 [ 12 , 13 ]. Moreover, there are obvious gaps in technical efficiency between regions, and the degree of technology utilisation is different [ 14 ]. The application of scientific research and development in corn production has an advancing effect on corn production, and technological progress is conducive to improving the efficiency of corn production technology [ 15 ]. By 2015, technical efficiency had slightly improved, stabilising above 0.9 [ 16 ]. Abdallah and Awal [ 17 ] reported that the technical efficiency of Ghana’s corn production from 2001 to 2004 was 53%. A Cobb-Douglas production function was used as the functional form of the stochastic frontier production function to define the relationship between outputs and inputs. Chiona, Kalinda and Tembo [ 18 ] conducted a stochastic frontier analysis of the technical efficiency of smallholder corn growers in the central province of Zambia and measured an average technical efficiency of 50%. This study specifies the stochastic frontier production function using the flexible translog specification. A likelihood test was conducted that the translog stochastic frontier production function can be reduced to a Cobb Douglas. Using the stochastic frontier method, Siaw et al. [ 19 ] measured the average technical efficiency of corn production in Ghana to be 74% and established that agricultural credit can increase technical efficiency by 8%. Some scholars use DEA and stochastic frontier method to measure the technical efficiency of corn production at the same time, and find that the efficiency calculated by DEA method is higher than that by stochastic frontier method. Hassan et al. [ 20 ] measured the technical efficiency of corn production in Nigeria from 1971 to 2010. The efficiencies measured by the stochastic frontier method and DEA were 64.1% and 87.7%, respectively. The average level of corn production efficiency in Indonesia calculated by Asmara using the stochastic frontier method was 0.78, and the technical efficiency calculated using the DEA method was 0.91 [ 21 ].
Technical efficiency measurement of other important agricultural products. The above summarizes the measurement of the transnational technical efficiency of corn. Some scholars have also measured the technical efficiency of other agricultural products. Here I mainly review the measurement of the efficiency of soybean, wheat, cotton, and coffee bulk agricultural products. For soybean varieties, some scholars use the super-logarithmic stochastic frontier model to measure the efficiency. Otitoju and Arene [ 22 ] measured the technical efficiency of soybean production in Benue State, Nigeria as 0.73, and Asodina et al. [ 23 ] measured 0.58 in Upper West Region, Ghana. For wheat varieties, Tuna and Oren [ 24 ] used DEA to measure the technical efficiency of wheat production in south-eastern Anatolia, Turkey to be 0.78. Some scholars use the stochastic frontier production function to measure the efficiency of wheat production technology. Jaime and Salazar [ 25 ] measured the efficiency of Chile as 0.6, and Kamruzzaman and Islam [ 26 ] measured the efficiency of Dinajpur District, Bangladesh as 0.7. Ojo [ 27 ] measured the technical efficiency of food crops in Swaziland to be 0.77. Some scholars used DEA to measure the technical efficiency of other agricultural products. Poudel, Yamamoto and Johnson [ 28 ] used DEA to measure the technical efficiency of coffee production in Nepal as 0.83. Gul [ 29 ] measured the cotton technical efficiency in Turkey to be 0.89. The above-mentioned scholars’ technical efficiency measurement and use methods of corn and other agricultural products in different regions are shown in Table 1 . More about the measurement method of efficiency, from the beginning of Farrell to the improvement and innovation of the original method by later scholars, is presented by Zuniga who carried out a detailed and systematic combing [ 30 ].
- PPT PowerPoint slide
- PNG larger image
- TIFF original image
https://doi.org/10.1371/journal.pone.0254423.t001
Numerous scholars have researched the factors affecting corn production efficiency from different perspectives. Miho [ 31 ] studied corn planting efficiency in two areas of Tanzania and established that there is a positive relationship between the number of people who can work in the family and technical inefficiency. Production input inhibits the improvement of technical efficiency, and good living conditions can promote efficiency. Olarinde [ 32 ] established that the main determinants of the technical efficiency of maize planting in Nigeria include extension services, farming experience, and farm distance. Boundeth, Nanseki and Takeuchi [ 33 ] established that the technical inefficiency of corn growers in the northern provinces of Laos decreases with an increase in farm size. When studying the factors affecting corn production technology in China, Liu et al. [ 16 ] determined that the power of agricultural machinery has a positive effect on the technical efficiency of corn production and that the basic conditions of agriculture will affect the loss of technical efficiency. They proposed an increase in machinery investment to promote mechanised production. Wang and Wu [ 34 ] established that the use of biochemical inputs such as chemical fertilisers and the use of mechanical agricultural materials improved the technical efficiency of corn production and proposed increasing chemical fertiliser subsidies and promoting large-scale production. Jia and Xia [ 35 ] established that the scale efficiency and the corn planting area indicated an ‘inverted U-shaped relationship’. It can be seen that promoting the scale operation of grain is not the bigger the better. The government should give the guidance and standardization to farmers and make them seek the appropriate degree of scale operation.
Based on the above literature, it can be determined that many scholars use non-parametric analysis methods to measure efficiency in addition to parametric analysis methods. The parametric analysis method has higher requirements for the correct construction of the model and the selection of variables. Scholars have mostly used the envelope analysis method and linear programming to measure efficiency. Concurrently, it can also be established that most scholars focused on the perspective of corn production in a certain country, region, province, or state. They rarely explored the technical efficiency and factors influencing corn production across multiple countries. Therefore, in this study, the corn input and output data of 18 typical farms in 12 countries worldwide from 2012 to 2019 were selected. I used the DEA model to measure technical efficiency and the tobit model, considering the measured technical efficiency as the dependent variable, to explore the impact of the farming system, business scale, and quantity and structure of factor inputs on the efficiency of corn production technology.
Materials and methods
Model settings.
The production unit that needs to be measured in DEA is called a decision-making unit (DMU). There are n units that measure efficiency, denoted as DMU j , each of which has m types of inputs denoted as x i , and q types of outputs denoted as y r . The current DMU to be measured is denoted as DMU K , and the linear combination coefficient of the DMU is represented by λ. With a certain input, technical efficiency is the ratio of the actual output of a production unit to the production frontier. Under the assumption that the return to scale is constant, the production frontier can be represented by the OB ray in Fig 1 , and B is the only effective production unit. In the case of variable returns to scale, the production frontier is a curve formed by MABD that is convex to the left.
https://doi.org/10.1371/journal.pone.0254423.g001
Tobit model.
To further study the influencing factors of technical efficiency, the technical efficiency measured by DEA was used as the dependent variable, and the influencing factors were regressed. As the efficiency value of a typical farm calculated by DEA is truncated data between 0 and 1, estimating it using the ordinary least squares method will cause bias and inconsistency. The tobit model can effectively reduce the deviation [ 38 , 39 ] and is suitable for analysing the factors influencing technical efficiency [ 40 ]. Therefore, this study uses the tobit model, which is expressed as formula ( 2 ), and the maximum likelihood estimation method is used for the regression analysis. The data used in this study are from 2012 to 2019, which are short panel data. Due to the lack of sufficient statistics for individual heterogeneity, the fixed-effects tobit model cannot perform a maximum likelihood estimation, and the regression results are usually biased [ 41 ]. The use of the random effects estimation is effective [ 42 ]; therefore, this study adopts the tobit model of random effects.
Data source
The data used in this study are sourced from agri-benchmark, a global, non-profit network of agricultural economists, advisors, producers and specialists. It is managed by the Thünen Institute and global networks under the German Federal Ministry of Food and Agriculture. According to agri-benchmark, the standard definition of a typical farm is that a region or country has medium-scale and large-scale farms to reflect the average management level of most farms, that is, the average profit level. The areas where the selected typical farms are located are the intensive and main production areas of a particular crop. The cost-benefit data of a typical farm are collected in a comprehensive group with the participation of farmers and consultants, and standard questionnaires are issued to ensure that each figure reflects a typical situation.
This study selects corn input and output data of typical farms in 12 countries, that is, Argentina, Brazil, and Uruguay (South America), Russia, France, Ukraine, Bulgaria, Poland, Czech Republic, and Hungary (Europe), as well as the US (North America) and South Africa (Africa) including 18 typical farms such as AR330ZN, AR700SBA, AR900WBA, BR65PR, BR1300MT, US700IA, US1300ND, etc. from 2012 to 2019. The first two digits of the farm code represent the country, the number represents the size of the farm, and the last few alphabets represent the area where the farm is located. Consider US700IA as an example, which means a 700-hectare farm in Iowa, USA.
Variable selection and descriptive analysis
Based on the scholarly research of Yang and Lu [ 43 ], Zhao, Wang and Zhang [ 44 ], and Xiao and Zhao [ 45 ], this study selected corn yield per unit area as the output indicator, and land, labour, machinery and fuel, construction costs, and other miscellaneous expenses as input indicators. The land input is the corn planting and operating area of the farm, and the labour input is the amount of labour per unit area of corn production. Machinery and fuel inputs are machinery and fuel costs, and construction costs include depreciation, repair, and financial costs. Other miscellaneous expenses include inventory insurance premiums and taxes, consulting fees, and accounting costs. A descriptive analysis of the corn input and output indicators for typical farms is presented in Table 2 .
https://doi.org/10.1371/journal.pone.0254423.t002
Liu et al. [ 46 ] used the comprehensive technical efficiency measured by DEA as the dependent variable and land, machinery, and seed prices as independent variables to study the factors affecting corn production efficiency. They established that land cost, machinery cost, and seed price affect comprehensive technical efficiency. All of these factors have a positive impact. Tian and Zhu [ 39 ] used grain-sown area, labour input (indicated by agriculture, forestry, animal husbandry, and fishery), and fertiliser application as explanatory variables when studying the factors affecting food production efficiency in China. The results showed that chemical fertilisers and machinery promoted the efficiency of food production. The main factors for the improvement, the grain-sown area, and labour input have no significant impact on efficiency.
The technical efficiency measured by the DEA model was selected as the dependent variable, and farming methods, input elements, supporting services, and other factors were used as independent variables to perform the random-effects tobit model regression analysis. The statistical descriptions of the explanatory variables are presented in Table 3 . The farming system is divided into five methods: no-tillage, conservation farming (reducing stubble and covering seeds), conservation farming (covering seeds), intensive farming, traditional farming, and deep farming. I constructed dummy variables for the farming system. The proportion of mechanical labour is the proportion of mechanical labour to total labour, which is used to reflect the degree of mechanisation. To explore the influence of the degree of mechanisation on technical efficiency, the square term of the proportion of mechanical labour was added to the constructed model. The proportion of hired workers indicates the ratio of the number of employees to the total labour force. The total labour force includes family and hired labour. Land cost refers to the cost of renting a unit area of land or the opportunity cost of land. Drying costs refer to the costs incurred when drying corn. Insurance premium is the cost of purchasing agricultural insurance for farms. Consultation fees refer to the expenses incurred by consulting experts in agricultural production. The addition of time-trend variables reduces the impact of time on technical efficiency. Regional dummy variables were set up, and North America was used as the benchmark group to compare regional technical efficiency differences with South America, Europe, and Africa.
https://doi.org/10.1371/journal.pone.0254423.t003
Results and discussion
The use of the DEA model for efficiency measurement needs higher requirements for the selection of input items, and the difference in the selection of input items directly leads to differences in the results of the efficiency calculation. After the input items were determined, multiple collinear tests were performed to ensure that the selected input items were not redundant. Using Stata to test this, the results show that the input variables selected by the DEA model are all less than 10, with an average value of 2.85, indicating that the input variables selected by the DEA model are not redundant. A multicollinearity test was also performed on the explanatory variables selected by the tobit model. The results indicated that the variance expansion factors of the selected explanatory variables were all less than 10, with an average value of 2.02, indicating that there was no multicollinearity in the variable settings of the tobit model [ 47 , 48 ].
Technical efficiency and decomposition of corn production in typical farms
DEAP2.1 software was used to calculate the technical efficiency of 18 typical farms, which is shown in Table 4 . The average level of technical efficiency of corn production was 0.863, and the average loss of efficiency was 13.7%. Table 3 shows that the comprehensive technical efficiency of nine typical farms is 1, indicating that half of the farms in the research group have corn production at the forefront of production, and corn production is DEA-effective. These farms are in Europe and South America, of which three are in Argentina, one is in Brazil, and the others are in the Czech Republic, Poland, Russia, Ukraine, and Uruguay. The comprehensive technical efficiency of US1215INC farms in Bulgaria, Hungary, and the US was approximately 0.5. This is because the pure technical efficiency of corn production is low, which reduces the contribution of scale efficiency. From the perspective of scale efficiency, the average level is 0.939, which is relatively high. However, half of the farms are still in a state of ineffective scale. Farms in Bulgaria, Brazil, Hungary, and South Africa are in a state of increasing returns to scale, and five farms in France and the US are experiencing diminishing returns to scale.
https://doi.org/10.1371/journal.pone.0254423.t004
Based on the two aspects of the main corn-producing countries and the production technology being at the forefront, the corn production conditions in the US, Brazil, and Argentina are analysed.
The pure technical efficiency of corn production in two typical farms in the US is 1, the scale efficiency is above 0.9, and the returns to scale are both in a diminishing stage. As the main agricultural business entity in the US, a large number of family farms have a long history of development. In 2010, the number of family farms in the US reached more than 1.9 million. On average, each family farm has a large area of arable land. The development of family farms requires a higher degree of mechanisation. Therefore, the US attaches great importance to the research and development of agricultural science and technology. The use of agricultural technology reduces labour input and saves transaction costs propagated by hired labour, which is an important reason for maintaining a high level of agricultural production efficiency [ 49 – 51 ]. Furthermore, the US government has always attached great importance to agricultural protection. Due to the surplus of agricultural production, the return to scale of farm corn production is in a diminishing stage. To avoid excessive production by farmers, the government has issued several compensation policies to encourage farmers to participate in fallow programs to adjust supply and protect farmers’ income [ 52 ].
The comprehensive technical efficiency, pure technical efficiency, and scale efficiency of the BR1300MT farms in Brazil and three farms in Argentina are all 1, and the return to scale remains unchanged.
Natural conditions. Brazil, Uruguay, and Argentina are all located in South America. Corn growth has more intensive water requirement. Abundant rainfall and vertical and horizontal river networks provide irrigation conditions, and corn has become the main crop in these areas.
Government support. The Brazilian government has issued several policies to support the soybean planting industry. Brazil convened soybean farmers across the country to form farm consortiums, which purchase materials for soybean planting and production in a unified manner and provide farmers with financing, product transportation, and storage services [ 53 ]. It is beneficial to reducing procurement costs, promoting the consistency of soybean product quality, and achieving large-scale mass production. Soybean varieties grown in Brazil were introduced in the US and transferred to the country for research and breeding. The new varieties cultivated by scientific research institutions based on the country’s soil and climatic conditions are more adaptable to the country’s natural conditions and more suitable for growth in the tropics and subtropics. Consequently, the yield of corn improved [ 54 ]. The Argentine government has always attached great importance to the research, development, and promotion of agricultural science and technology [ 55 ]. It has established several scientific research institutions to promote the development of agricultural science and technology, establish extension stations to provide farmers with agricultural knowledge, train the use of new technologies, promote the implementation and transformation of scientific research results [ 56 ], and establish specialised corn production areas; production specialisation has greatly improved the production efficiency of corn. The export strategy has been formulated to focus on reducing production costs and increasing output per unit area. Consequently, its competitiveness in international trade has improved [ 56 ].
Influencing factors of corn production technology efficiency
The regression results of the tobit model are presented in Table 5 .
https://doi.org/10.1371/journal.pone.0254423.t005
Regional difference.
The North American region represented by the US was considered a benchmark. The regression results indicate that the average technical efficiency level of South America does not show a significant difference from that of North America. At the 1% level, the technical efficiency of Europe and Africa is significantly lower than that of North America to varying degrees. Among them, Africa, represented by South Africa, has the lowest technical efficiency. North America, represented by the US, and South America, represented by Brazil and Argentina, have the highest maize production efficiency. The US, Brazil, and Argentina are the main corn producers and exporters. In addition to having a unique natural resource endowment, government agencies have invested heavily in science and technology and issued several policies to protect the interests of farmers and promote and guarantee the effective production of corn [ 56 ].
Time difference.
At the 1% significance level, the time variable had a positive effect on technical efficiency. This shows that over time, the technical efficiency of agricultural production is constantly increasing, and the level of agricultural technology is constantly improving. The advancement of agricultural production technology relies on economic growth. In the exogenous technological progress growth model, the technological factor is regarded as a function of time.
Tillage system.
The regression coefficients of deep tillage and no-tillage did not pass the significance test, indicating that the technical efficiency of deep tillage and no-tillage did not show obvious differences compared with conservation tillage (reducing stubble and covering seeds). At a significance level of 1%, seed mulching and intensive farming have higher technical efficiency than conservation tillage, and seed-covering farming modes have higher technical efficiency. Covering seeds can replace fallow in summer, protect soil organic carbon, and have beneficial effects on maintaining soil fertility and improving soil quality, thereby effectively disposing biological waste [ 57 ]. Conservation tillage integrated mulch technology can effectively coordinate crop yield, water consumption, and reducing carbon emissions, which can increase the water use efficiency of corn and improve corn production efficiency [ 58 ].
Supporting service.
The input of the drying fee reflects that typical farms dry harvested corn. However, not all production entities dry corn, but the harvested corn has a high moisture content. If stored improperly, the corn will deteriorate and produce mildew, resulting in food waste and economic losses [ 59 ]. In the regression results, the drying fee has a significant promoting effect on the improvement of technical efficiency, indicating that the drying treatment of corn significantly reduced the loss of harvested fruits, promoted agricultural efficiency, and improved corn production efficiency.
The farm insurance premium did not pass the significance test in the regression results, indicating that agricultural insurance did not fully promote the production of planting entities. Due to the risks of agricultural production that are difficult to control, the agricultural insurance market system is unsophisticated [ 60 ], the implementation of insurance is arbitrary, and the protection of agricultural production is not enough; therefore, the interests of farmers have not been effectively protected [ 61 ]. Expert consulting fees have a significant inhibitory effect on corn production efficiency at the 1% level. On the one hand, the methods used to collect farmers’ knowledge are flawed, resulting in inaccurate or incomplete information. The use of inaccurate information or misunderstandings between farmers and scientists result in the development and extension of unprofitable, unsustainable or inappropriate management recommendations [ 62 ]. On the other hand, a small number of experts are far from the reality of agricultural production and fail to propose practical solutions to farmers. Moreover, farmers are restricted by their level, unable to achieve effective docking between farmers and experts, and have not played a role in improving agricultural production efficiency [ 63 ].
Regarding input factors, at a significance level of 1%, land cost has a positive impact on technical efficiency, but the impact is relatively small. This indicates that when the cost of land input factors increases, it will curb the input of land factors, which in turn will increase the utilisation rate of existing land and promote an increase in land productivity. They cope with the increase in the cost of production materials by increasing the output per unit area of land and promoting the improvement of production technology. The regression coefficient of the proportion of hired workers is significantly positive at the 1% level, indicating that it is difficult to improve technical efficiency by relying solely on family labour. The increase in the proportion of hired labour in labour input has a positive effect on the development of agricultural production. Additionally, as agricultural production reaches a certain stage, the demand for labour will inevitably increase. The limited family labour force has led to a large demand for hired labour, which has met the expansion of agricultural production and stimulate technical efficiency.
At a significance level of 5%, the proportion of mechanical labour can promote technical efficiency. Due to the continuous increase in labour costs, the main body of production has increased the use of machinery [ 64 ], shortened the operation time of unit agricultural production, substantially increased labour productivity [ 65 ], and improved corn production efficiency. However, at the 1% significance level, the regression coefficient of the square term of the proportion of mechanical labour is negative, indicating that there is an inverted U-shaped relationship between the proportion of mechanical labour and technical efficiency. When the proportion of mechanical labour exceeds the critical value, continuing to invest in machinery will lead to a decline in technical efficiency, which is due to the use of machinery restricted by topographical characteristics and agricultural production conditions [ 66 ]. Plain areas are easier to mechanise than hilly areas. Small machinery can be used in hilly areas. Excessive machinery investment leads to low agricultural production efficiency and economic losses. The use of machinery also has requirements for the extent of the entire land. It is difficult for these fragmented lands to implement large-scale mechanised production. Agricultural operations still rely mostly on human labour. The proportion of machinery investment is too high, and it is difficult for them to be effective, resulting in technical inefficiency.
Conclusions
The main conclusions of this study are as follows: first, the overall level of technical efficiency of corn production on family farms in Argentina, the US, Ukraine, and other countries is at a relatively high level and is at the forefront of production technology. However, some farms are affected by the low efficiency of pure technology. Technical inefficiency reached 50%, and the average loss of technical efficiency was 13.7%. Second, among the five farming modes, seed-covering, intensive farming, and conservation farming have higher production efficiency than no-tillage and deep tillage. The increase in the cost of production factors of land and labour forces has caused the continuous improvement of technology, thereby increasing the efficiency of corn production. Third, corn drying can promote production efficiency. Farm insurance premiums and expert consultation fees have not played a role in stabilising agricultural production and promoting crop management. The transformation of the economic benefits of agricultural production equipment services is still lacking. Finally, the input and use of machinery are within a certain threshold, which improve labour productivity and promote the development efficiency of corn planting agriculture. However, if the mechanical input exceeds the turning point, it will cause redundancy in the input of elements, resulting in waste and loss of efficiency.
The findings of this study are as follows. First, to achieve the goal of improving corn production efficiency, reducing biological waste, and realizing green and economical production, it is necessary to innovate the production and farming mode of corn, promote the diversification of production methods, and develop a protection farming method that combines with bean crops. Second, improving and refining the construction of agricultural insurance regulations and systems, effectively protecting farmers’ interests, and sharing the risks faced by agricultural production for farmers, are all conducive to improving the efficiency of food production and its sustainability. Third, it is high time to promote the landing and transformation of agricultural scientific research results, strengthen the training and support of scientific research institutions, experts and scholars to production entities, and earnestly take farmers as the main resource for agricultural production ideas. Fourth, it is imperative to consider to promote land circulation and reduce fragmented land to create conditions for mechanised agricultural production and provide basic guarantees for modern agricultural production. Simultaneously, various types of machinery should be developed as well as a technical strategy that combines large-scale and small-scale machinery. For areas where it is difficult to operate large-scale machinery, small-scale agricultural machinery with characteristics of adapting to local conditions should be considered. Finally, strengthen the construction and improvement of the agricultural service system and provide farmers with various forms of information services so that agricultural products can better meet the market demand. At present, there is a large gap between socialised service funds and unreasonable use. Agricultural socialised services must be considered with high importance to effectively serve both farmers and agriculture, ensure agricultural production, and improve economic benefits.
Supporting information
S1 table. corn production input and output indicators in typical farms for 2012–2019..
https://doi.org/10.1371/journal.pone.0254423.s001
S2 Table. Model variable settings and descriptive statistics.
https://doi.org/10.1371/journal.pone.0254423.s002
Acknowledgments
The authors thank agri-benchmark for providing the data. We would like to thank the editor and three reviewers whose comments helped to greatly improve this manuscript.
- 1. USDA, ERS. International Baseline Data. 2021 International Long-Term Projections to 2030. [2021 May 10]. Available from: https://www.ers.usda.gov/data-products/international-baseline-data/
- 2. China. Database: BRIC Agricultural Database [Internet]. [updated 2020 Sep 11]. Available from: http://www.agdata.cn/
- 3. United nations, Department of economic and social affairs. Trade statistics. Database: UN Comtrade [Internet]. Available from: https://comtrade.un.org/data/
- View Article
- Google Scholar
- PubMed/NCBI
- 50. Saeidirad MH. Mechanization of saffron production. In: Koocheki A, Khajeh-Hosseini M, editors. Saffron. 2020; 187–204. https://doi.org/10.1016/b978-0-12-818638-1.00011–3
- 52. USDA Expands and Renews Conservation Reserve Program in Effort to Boost Enrollment and Address Climate Change. USDA Press. 2021 April 21. Available from: https://www.usda.gov/media/press-releases/2021/04/21/usda-expands-and-renews-conservation-reserve-program-effort-boost
- 54. Government of Brazil. Schedule strategic 2010–2015 corn and sorghum. Available from: https://www.gov.br/agricultura/pt-br/assuntos/camaras-setoriais-tematicas/agendas/arquivos/milho.pdf
- 55. USDA. Agricultural Biotechnology Annual– 2020. [2020 December 11]. Available from: https://apps.fas.usda.gov/newgainapi/api/Report/DownloadReportByFileName?fileName=Agricultural%20Biotechnology%20Annual_Amman_Jordan_10-20-2020
Open Access is an initiative that aims to make scientific research freely available to all. To date our community has made over 100 million downloads. It’s based on principles of collaboration, unobstructed discovery, and, most importantly, scientific progression. As PhD students, we found it difficult to access the research we needed, so we decided to create a new Open Access publisher that levels the playing field for scientists across the world. How? By making research easy to access, and puts the academic needs of the researchers before the business interests of publishers.
We are a community of more than 103,000 authors and editors from 3,291 institutions spanning 160 countries, including Nobel Prize winners and some of the world’s most-cited researchers. Publishing on IntechOpen allows authors to earn citations and find new collaborators, meaning more people see your work not only from your own field of study, but from other related fields too.
Brief introduction to this section that descibes Open Access especially from an IntechOpen perspective
Want to get in touch? Contact our London head office or media team here
Our team is growing all the time, so we’re always on the lookout for smart people who want to help us reshape the world of scientific publishing.
Home > Books > Corn - Production and Human Health in Changing Climate
Corn Productivity: The Role of Management and Biotechnology
Submitted: 13 February 2018 Reviewed: 07 April 2018 Published: 05 November 2018
DOI: 10.5772/intechopen.77054
Cite this chapter
There are two ways to cite this chapter:
From the Edited Volume
Corn - Production and Human Health in Changing Climate
Edited by Amanullah and Shah Fahad
To purchase hard copies of this book, please contact the representative in India: CBS Publishers & Distributors Pvt. Ltd. www.cbspd.com | [email protected]
Chapter metrics overview
1,684 Chapter Downloads
Impact of this chapter
Total Chapter Downloads on intechopen.com

Total Chapter Views on intechopen.com
Overall attention for this chapters
The last few decades have seen a rapid increase in corn production, making corn the most important cereal in the world. This evolution is due in large part to rapid productivity growth for corn. Both improved genetics and improved farm management have contributed to large increases in corn yield. The paper reviews how genetics, biotechnology and management have interacted to increase agricultural productivity and reduce farm risk exposure. It documents the stellar performance of corn in terms of productivity growth. It also discusses the recent evolution of corn markets and evaluates the prospects for the future.
- productivity
- biotechnology
Author Information
Jean-paul chavas *.
- Department of Agricultural and Applied Economics, University of Wisconsin, USA
Paul D. Mitchell
- Renk Agribusiness Institute, University of Wisconsin, USA
*Address all correspondence to: [email protected]
1. Introduction
Corn ( Zea mays ), also called maize or field corn, is the most important cereal in the world, with annual global production exceeding that of wheat and rice. In 2017, corn production accounted for 41% of total grain production in the world [ 1 ]. While corn is a staple food in parts of the world, it has many uses, including animal feed, biofuel and sweetener. This chapter provides an overview of the evolving role of corn in agriculture.
Corn was first domesticated in southern Mexico about 9000 years ago [ 2 , 3 ]. Its closest wild relative is teosinte, a wild grass of Mexico, Guatemala and Honduras. A major puzzle is the great genetic differences between teosinte and corn, indicating how key mutations and human selection contributed to genetic evolution [ 4 ]. After the Columbian exchange, corn production spread throughout the world. Corn is a highly productive crop with the ability to exploit available soil nutrients. As a C4 plant, corn has some photosynthetic advantages in capturing solar energy in warm weather compared to C3 crops such as wheat, rice and soybean. Due to its high productivity under various climate conditions, corn is now the largest grain crop in the world [ 1 ]. Favorable agro-climatic conditions in the US “Corn Belt” have made the US the largest corn producer. In 2017, corn production in the US accounted for 35% of world corn production [ 1 ].
The rise of corn as the most important cereal in the world has been associated with important improvements in its productivity [ 5 ]. Figure 1 illustrates the evolution of the average corn yield on US farms from 1870 to 2017 [ 6 ]. Figure 1 shows that corn productivity was basically stagnant before 1940: during the period 1870–1940, US average corn yields stayed within a narrow range between 20 and 30 bu/acre. (between 1200 and 1900 kg/ha) 1 Starting in 1940, a period of fast and steady rise in corn productivity began and continues to this time. US average corn yield increased from 28.9 bu/acre (1.81 metric tons/ha) in 1940 to 176.6 bu/acre (11.1 metric tons/ha) in 2017 [ 6 ]. This amazing achievement means that a given area of land can produce 6.1 times more corn in 2017 than in 1940, which corresponds to an average annual growth rate of 2.35%, reflecting the rapid technological progress sustained over the last seven decades. This achievement raises two questions. First, what are the sources of this growth in corn productivity? Second, is it likely to continue in the future? Below, we discuss the role played by two key drivers of corn productivity: improved genetics and improved management. We also consider the corn market and its evolving prices. Finally, we reflect on what may come next. Some evidence suggests that agricultural productivity growth may be slowing down, raising concerns about our ability to feed a growing world population (e.g., [ 7 ]). We ponder these prospects as they apply to corn production.

Figure 1.
Historical corn yield, US. Source: The corn yield is measured in dollar per bushel, as reported by USDA-NASS [ 6 ].
2. Corn productivity
Genetic selection has been a very important driver of agricultural productivity. The process started some 9000 years ago in Mexico when corn was first “selected” and evolved from its wild ancestor [ 2 ]. Over the centuries, accidental mutations and some intentional selections contributed to beneficial changes [ 3 ]. But as Figure 1 indicates, the rate of genetic improvement was very slow before 1940. Genetic selection was then based mostly on traditional breeding methods trying to combine desirable characteristics of each parent into the progeny. Applied to crops, farmers used selective breeding to pass on desirable traits while omitting undesirable ones. The desirable traits included higher yield and better quality as well as improved adaptation to local agro-climatic and ecological conditions. When applied by farmers, the selection intensity was low, generating slow genetic changes.
The early part of the twentieth century saw the rise of modern genetics and its applications to plant breeding. The discovery of hybrid vigor led to the development of hybrid seed corn and rapid improvements in corn productivity [ 5 , 8 ]. The higher corn yields stimulated the rapid adoption of hybrid seed corn among US farmers [ 8 , 9 ]. The new corn hybrids also contributed to the development of a seed corn industry that focused on refined genetic selection [ 10 ]. The increased intensity of genetic selection contributed to the development of improved varieties that were better at capturing soil nutrients and more resistant to diseases [ 5 ]. As Figure 1 shows, the result has been decades of genetic improvements and rapid and sustained growth in corn yields.
Starting in the 1980s, progress in biotechnology revolutionized genetic selection. The identification of genes and the refinements in gene transfer 2 technologies opened new opportunities for genetic selection. Eventually, this process led to the development of genetically engineered (GE) corn hybrids that, along with the patenting of GE seeds, stimulated the growth of biotechnology in agriculture. The first GE corn hybrids became commercially available in the US in 1996, with US farmers rapidly adopting the technology. In 2017, more than 90% of all corn planted in the US was GE [ 12 ]. The rapid adoption of GE corn in the US led to significant productivity improvements [ 13 ]. Over the last two decades, the adoption of GE seed in agriculture has proceeded around the world, though at different rates depending on each country’s regulations [ 14 ].
Two major types of GE traits are currently available in the hybrid seed corn market: those providing insect resistance (IR) (commercially available in corn in 1996) and those providing herbicide tolerance (HT) (commercially available for corn in 1998). Hybrid seed corn contains these traits either singly or combined as stacks or pyramids, so that a single hybrid is both IR to multiple pests and HT to more than one herbicide.
In the US, currently available IR traits involve gene transfers from the soil bacterium Bacillus thuringiensis (Bt) so that hybrids express insecticidal proteins in their tissues that help control specific insect pests. Bt corn hybrids in the US focus on two pests that have had significant adverse effects on corn yield: European corn borer ( Ostrinia nubilalis ) and corn rootworm, a complex of four closely related species ( Diabrotica spp.). European corn borer larvae feed on corn plant tissues, including tunneling through corn stalks and ear shanks, which not only disrupts plant functions and so causes direct yield loss, but also causes plant lodging and ear drops, causing additional yield loss. Corn rootworm larvae feed on corn roots, which disrupts water and nutrient uptake by the plant and so causes direct yield loss, and also causes plant lodging. Both pests have historically caused significant damage to corn plants, reduced corn yield and are somewhat difficult to control using conventional insecticides [ 15 ].
Bt corn has proven more effective in controlling European corn borer and corn rootworm than conventional insecticides, thus increasing harvested yields. In addition, farmer adoption in the US of Bt corn has reduced the aggregate use of insecticides [ 16 ]. The rapid adoption of IR Bt corn in the US reflects that US farmers have obtained significant productivity benefits from this technology [ 12 , 13 ].
HT corn hybrids simplify herbicide-based weed management by allowing application of herbicides on the crop without causing crop damage. Weed management without HT hybrids is managerially more complicated since several weed species look similar when they are small at the time when farmers must make herbicide decisions, but different species commonly require different herbicides for effective control. The earliest and still most popular HT hybrid is tolerant of the herbicide glyphosate, though other types of HT hybrids have been available. As a broad-spectrum herbicide, glyphosate controls a wide range of weed species, so that farmers do not need to know the specific weed species in their fields and which herbicides provide effective control. As a result, farmers rapidly adopted glyphosate tolerant corn hybrids and glyphosate quickly become the most commonly used corn herbicide, with glyphosate used on approximately 75% of US corn acres since 2008 [ 17 ]. In US, farmer adoption of HT hybrids has reduced the aggregate use of herbicides [ 16 ]. In addition, HT varieties facilitate farmer adoption of reduced tillage and no-till systems, which not only reduces soil erosion, but also lowers labor and fuel requirements [ 18 ]. Features such as these have made GE corn attractive to US farmers, contributing to their rapid adoption [ 12 , 13 ].
3. The role of management
While improved genetics have contributed greatly to increasing corn productivity over the last 70 years, other factors also played a role. Duvick [ 5 ] has noted that corn productivity per plant has not changed much over the last few decades, suggesting that, under favorable conditions, the efficiency of photosynthesis for corn (as a C4 plan) has not improved. If so, what is the source of corn productivity growth? Duvick [ 5 ] argued that most of the historical increases in US corn yields are due to increases in plant density. Thus, corn productivity gains have come from the interactions between the plant and its environment, along with improvements in farm management and cultural practices. Over the years, new corn hybrids have been selected to be more resistant to lodging and more tolerant of biotic stress (pest damage, weed competition, disease) and abiotic stress (adverse weather, poor soil conditions). These genetic changes have interacted with improved management practices, including fertilizer use, irrigation, tillage system, weed control, pest management and crop rotation. Fertilizer applications remedy soil nutrient scarcity, as corn yield is very responsive to nitrogen [ 5 ]. When available, irrigation alleviates soil water scarcity and drought. Pest and weed populations can be (at least partially) controlled and suppressed by tillage, crop rotations and by the use of pesticides (insecticides and herbicides). Crop rotation had been used by farmers for centuries to reduce pest and weed infestation and to restore soil fertility [ 19 , 20 , 21 ].
The hypothesis that management and genetic biotechnology interacted in generating recent corn productivity gains have been investigated by Chavas and Shi [ 22 ] and Chavas et al. [ 23 ]. They found evidence of the important role of management and of interaction effects between technology and management. First, they documented how biotechnology has been a major driver of improved corn productivity over the last decade. They also explored how the benefit of GE traits can vary with agro-climatic conditions. Second, they showed how GE hybrids provide enhanced control of pest damages, thus reducing exposure to both risk and downside risk (the provability of facing low yields). Reducing risk exposure is a major part of the benefits of GE technology [ 24 ]. Importantly these GE benefits can go beyond the farm if the suppression of pest population is regional [ 25 ]. Third, Chavas and Shi [ 22 ] and Chavas et al. [ 23 ] showed how crop rotation and GE technology provide alternative ways to control pest populations, indicating that they behave as substitutes in the corn production process. Fourth, they reported the presence of synergy between biotechnology and plant density as they affect corn productivity. By improving pest control, GE hybrids make it possible to obtain greater productivity from higher plant density, evidence that the observed growth in corn productivity has been the outcome of important synergies between genetics and improved management.
4. Corn markets
In a market economy, technological progress affects producers, consumers and prices. Figure 2 presents the evolution of US corn prices ($/bu) over the period 1947–2017, reporting both nominal prices and real prices [ 6 ]. Real prices are nominal prices adjusted for inflation by dividing by the US Consumer Price Index (CPI), in this case with 1983 normalized to 1. Figure 2 shows that the nominal price of corn has gone from $1.52/bu ($59.8/metric ton) in 1950 to $3.36/bu ($132.3/metric ton) in 2017, corresponding to an average increase of +1.19% per year. It also shows that the real price of corn has gone from $6.30 to $1.37/bu, corresponding to an average decline of −2.25% per year. 3 This sharp decline in real price means that, holding purchasing power constant, an individual can buy 4.6 times more corn in 2017 than in 1950. This dramatic change mostly arises from productivity gains. Indeed, the rate of change in the real corn price (−2.25% per year) almost perfectly matches the rate of change in yield reported earlier (+2.35% per year).

Figure 2.
Historical price of corn, US. Source: The nominal corn price is the price received by farmers ($/bu) as reported by USDA-NASS [ 6 ]. The real price of corn is the nominal price divided by the consumer price index ( CPI ) as reported by BLS, with CP I 1983 = 1 .
In general, technological progress improves the aggregate welfare of society by allowing the production of greater outputs at lower cost (less resource use). But productivity growth can also have important distributional effects. In the corn sector, rapid technological progress has reduced cost and stimulated supply, which in turn has pushed market prices down. As just noted, the observed decrease in real prices reported in Figure 2 can be attributed in large part to technological progress in the corn industry. It indicates that most of the benefits of productivity gains are actually captured by consumers in the form of expanded quantities produced and lower market prices. As most corn is not directly consumed by people, but used for livestock feed and more recently fuel, these consumer gains arise from lower prices for meat, dairy products, eggs and fuel. But these lower (real) market prices contribute to declining farm revenue.
Interestingly, technological progress in agriculture may not benefit farmers at the aggregate—if the lower output price due to increased productivity generates a decline in revenue that exceeds the reduction in production cost. 4 This process is called the technology treadmill or Cochrane’s treadmill after the originator of the theory [ 27 ]. Early adopters of new productive technologies benefit by reducing their cost of production, but later adopters will lose if, as supply expands, the output price declines more than the decrease in production costs. The treadmill occurs because, even if farmers in aggregate are made worse off by the new technology, farmers individually still have an incentive to adopt the new technology to reduce their cost of production in a race to outrun the decline in real prices [ 28 , 29 ].
Globally, about 5% of the calories consumed per person come directly from corn, but this demand varies across countries. In much of Latin America, corn is mostly used for direct human consumption. For example, 33% of the calories consumed per person in Mexico come directly from corn. 5 In the US (and many other countries), corn is used mainly as livestock feed, an important input in the production of meat (beef, pork, and poultry), dairy and eggs. As a result, the demand for corn is a derived demand, with meat, dairy and eggs being the final consumer good.
Corn also has other uses such as for making sweeteners and ethanol. Derived demands for these corn products depend in part on government policy. For example, the US has a protectionist policy toward sugar, so sugar import restrictions have increased the domestic price of sugar [ 30 ]. The higher US sugar price has stimulated the search for sugar substitutes in the US, including corn sweeteners. This policy increases demand for corn, with more than 5% of US corn production used for sweeteners, and contributes to a higher corn price, which benefits US farmers but costs US consumers [ 30 ].
The US ethanol policy has an even larger impact. The rapid development of the US ethanol industry after 2000 is closely associated with government policies supporting the production of biofuel [ 31 ]. Ethanol subsidies, restrictions on ethanol imports and mandates for blending ethanol with gasoline have greatly stimulated the production of corn-based ethanol, leading the US ethanol industry to consume almost one third of US corn production. Over the last 15 years, US biofuel policy has greatly stimulated the demand for corn and affected agricultural markets. Roberts and Schlenker [ 26 ] estimated that US ethanol policy has increased world food prices by about 30%. This large effect is due to a price-inelastic demand for food and a diversion of land away from producing feed/food toward producing biofuel. In general, farmers have benefited from higher food prices, but the policy has significant distributional consequences, as consumers pay significantly more for food. Using “consumer surplus” as a measure of consumer welfare, Roberts and Schlenker [ 26 ] estimated that US ethanol policy contributed to a loss in world consumer welfare of $180 billion per year. The debate about the economics and policy of corn-based biofuel continues [ 32 , 33 ].
5. Prospects for the future
Over the last several decades, productivity growth in the corn sector has been stellar, which is good news in a world where feeding a growing world population is challenging. There are current concerns that agricultural productivity growth may be slowing down (e.g., [ 7 , 34 ]). So far, such concerns do not seem to apply to corn, since US average corn yields continue to climb at a steady rate, and Chavas et al. [ 13 ] provide evidence that biotechnology has helped increase corn productivity growth over the last two decades. Despite these continual productivity gains, challenges still exist, chief among them are resistance and climate change.
The stellar productivity gains from commercially applying biotechnology in corn have focused on improving insect and weed management, which has created selection pressure on many pest species to evolve resistance to control. Even if farmers follow resistance management practices, pests have and will continue to evolve resistance—these practices only slow the rate of resistance evolution, they do not stop it.
Western corn rootworm ( Diabrotica virgifera virgifera ) evolved resistance to rootworm Bt corn within a few years of commercial release [ 35 ]. Rootworm Bt hybrids still have value to farmers, but their continued use requires that companies pyramid multiple rootworm traits together and that farmers use additional management practices such as crop rotation and conventional insecticides [ 36 ]. Companies have also responded by developing alternative GE traits to manage corn rootworm. Potentially the most promising is RNA interference (RNAi), which uses biotechnology so that crops create double-stranded RNA segments that interfere with transcription of specific segments of RNA found in only the target species [ 37 , 38 , 39 ]. The first US commercialization of RNAi in corn received EPA approval in 2017. 6 Also, corn has been genetically engineered to express insecticidal proteins from non-Bt bacteria and shows excellent activity for control of corn rootworm larvae [ 40 ].
Weed control in corn (as with many crops) is important, with potential yield losses without control exceeding 50% [ 41 ]. Over the last few decades, herbicide resistant weed populations have continued to develop and spread globally [ 42 ]. HT seeds do not directly cause the development of herbicide resistant weeds, as herbicide resistant weeds have evolved in regions such as Western Australia where HT crops are not used [ 42 ]. Rather, HT crops contribute by encouraging farmers to rely on fewer herbicides modes of action and less tillage, which accelerate the development and spread of resistant weed populations [ 43 , 44 ]. Problems with herbicide resistant weeds continue to develop and spread globally, which is worrisome because no new herbicide modes of action have become commercially available since the early 1990s and weed populations resistant to multiple modes of action having been documented [ 45 , 46 ]. How weed control in corn and other crops will evolve over the next few decades to address herbicide resistant weeds and the possible role that GE hybrids and biotechnology will play is unclear. The race between insects and weeds and our ability to develop technologies and management schemes will continue to impact agricultural productivity. Maintaining our lead in this race will require R&D investments and continued innovations in the future.
Climate change presents another challenge for agricultural productivity, with studies documenting impacts on corn yields [ 47 ]. Adaptation to climate change is a rising concern [ 48 , 49 ]. Some regions will gain and some will lose productivity as climate patterns evolve and crop production shifts among regions. US farmers generally see agricultural adaptation to climate change as a private problem. They expect to respond with managerial changes, such as adjusting crops, using irrigation, modifying leases and using crop insurance, while seed companies will breed varieties and hybrids adapted to new climates [ 50 , 51 ]. Breeding will certainly be important for corn, since hybrids must be adapted to new photoperiods when changing latitudes. Also, seed companies have commercialized drought-resistant corn hybrids, but these and other traits providing yield gains under extreme conditions tend to be quantitative or polygenic and can imply productivity tradeoffs [ 52 , 53 , 54 ].
Despite these and other emerging challenges, several promising opportunities exist to continue the productivity gains for corn and agriculture more broadly, among them microbial seed treatments and gene editing techniques. Seed treatments have been used in crop production for some time, fungicides to protect seeds during storage, so that in the US all corn seed (both GE and conventional) uses fungicide seed treatments. More recently, insecticidal seed treatments became widely used in corn production, particularly neonicotinoid seed treatments, to control below-ground and early season insect pests. In the US, more than 90% of corn planted area uses neonicotinoid seed treatment [ 17 , 55 ]. In addition to insecticidal properties, neonicotinoids have demonstrated plant grower regulator effects in the laboratory and are associated with increased early season vigor in the field [ 56 ].
These chemical seed treatments have contributed to observed corn yield productivity, but significant research focus has moved to microbial seed treatments, soil microbes and fungi that increase yields. These seed treatments improve the rhizosphere around crop seedlings and plants through a variety of mechanisms, such as increasing nutrient availability, controlling diseases or nematodes, or supplying plant growth hormones [ 57 ]. Though some microbial seed treatments have been commercialized, including for corn, research needs still exist before widespread commercialization and achievement of their potential can occur [ 58 ]. An interesting possibility is to engineer microbes or fungi to enhance soil microbes for agricultural use.
A variety of gene editing techniques have recently been developed (e.g., CRISPR/Cas9, TALENs, ZFNs) with agricultural applications only beginning to be realized. The cost of using gene editing techniques is relatively low compared to gene-transfer technology. Also, gene editing is likely to face lower regulatory burden, as it does not require gene transfer across species. Public acceptance exists for therapeutic human health applications and some agricultural applications as well [ 59 , 60 ]. Applications to crops could include pest and pathogen control, as well as improved tolerance to abiotic stresses such as extreme heat or cold and drought, helping crop production adapt to climate change and increases in extreme weather events. Furthermore, gene editing could include the possibility of increasing the efficiency of photosynthesis in crops. Besides applications to crops directly, gene editing could be applied to other key organisms, such as to engineer soil microbes to develop new or more effective microbial seed treatments. Similarly, gene editing can be used to engineer gene drives in order to introgress select genes into populations in order to suppress or eliminate pest populations or to make herbicide-resistant weed populations susceptible to herbicides [ 61 , 62 ]. Given the economic importance of corn and its existing research and commercial infrastructure, corn seems likely to be at the frontier of the next wave of such innovations in agriculture.
- 1. USDA. World Agricultural Supply and Demand Estimates. USDA, Economics, Statistics and Market Information System. WASDE-575, March 8, 2018. Available from: https://www.usda.gov/oce/commodity/wasde/latest.pdf
- 2. Matsuoka Y, Vigouroux Y, Goodman MM, Sanchez J, Buckler GE, Doebley J. A single domestication for maize shown by multilocus microsatellite genotyping. Proceedings of the National Academy of Sciences. 2002; 99 :6080-6084
- 3. Piperno DR. The origins of plant cultivation and domestication in the new world tropics: Patterns, process, and new developments. Current Anthropology. 2011; 52 :453-470
- 4. Hufford MB, Lubinksy P, Pyhäjärvi T, Devengenzo MT, Ellstrand NC, Ross-Ibarra J. The genomic signature of crop-wild introgression in maize. PLoS Genetics. 2013; 9 :e1003477. DOI: 10.1371/journal.pgen.1003477
- 5. Duvick D. The contribution of breeding to yield advances in maize. Advances in Agronomy. 2005; 86 :84-145
- 6. USDA-NASS. National Agricultural Statistical Service. 2018. Available from: https://www.nass.usda.gov/Statistics_by_Subject/index.php?sector=CROPS
- 7. Ray DK, Ramankutty N, Mueller ND, West PC, Foley JA. Recent patterns of crop yield growth and stagnation. Nature Communications. 2012; 3 :1293. DOI: 10.1038/ncomms2296
- 8. Crow JF. 90 years ago: The beginning of hybrid maize. Genetics. 1998; 148 :923-928
- 9. Griliches Z. Hybrid corn: An exploration in the economics of technological change. Econometrica. 1957; 25 :501-522
- 10. Troyer AF. Background of U.S. hybrid corn. Crop Science. 1999; 39 :601-626
- 11. Simonson AB, Servin JA, Skophammer RG, Herbold CW, Rivera MC, Lake JA. Decoding the genomic tree of life. Proceedings of the National Academy of Sciences. 2005; 102 :6608-6613. DOI: 10.1073/pnas.0501996102
- 12. USDA-ERS. Economic Research Service. Washington, DC. 2018. Available from: www.ers.usda.gov/data-products/adoption-of-genetically-engineered-crops-in-the-us.aspx#.U9XFj7EvfNg
- 13. Chavas JP, Shi G, Nehring R, Stiegert K. The effects of biotechnology on productivity and input demands in U.S. Agriculture. Journal of Agricultural and Applied Economics. 2018 (forthcoming)
- 14. Qaim M. The economics of genetically modified crops. Annual Review of Resource Economics. 2009; 1 :665-694
- 15. Gray ME, Sappington TW, Miller NJ, Moeser J, Bohn MO. Adaptation and invasiveness of western corn rootworm: Intensifying research on a worsening pest. Annual Review of Entomology. 2009; 54 :303-321. DOI: 10.1146/annurev.ento.54.110807.090434
- 16. Perry ED, Ciliberto F, Hennessy DA, Moschini G. Genetically engineered crops and pesticide use in U.S. maize and soybeans. Science Advances. 2016; 2 :e1600850. DOI: 10.1126/sciadv.1600850
- 17. Mitchell PD. Methods and assumptions for estimating the impact of neonicotinoid insecticides on pest management practices and costs for U.S. corn, soybean, wheat, cotton and sorghum farmers. In: AgInfomatics Research Report. Madison, WI; 2014. 96 p. Available from: http://aginfomatics.com/index.html
- 18. National Academies of Sciences, Engineering, and Medicine. Genetically Engineered Crops: Experiences and Prospects. Washington, DC: The National Academies Press; 2016. DOI: 10.17226/23395
- 19. Anderson RL. Are some crops synergistic to following crops? Agronomy Journal. 2005; 97 :7-10
- 20. Anderson RL. Corn tolerance to weed interference varies with preceding crop. Weed Technology. 2011; 25 :486-491
- 21. Bullock DG. Crop rotation. Critical Reviews in Plant Sciences. 1992; 11 :309-326
- 22. Chavas JP, Shi G. An economic analysis of risk, management and agricultural technology. Journal of Agricultural and Resource Economics. 2015; 40 :63-79
- 23. Chavas JP, Shi G, Lauer J. The effects of GM technology on maize yield. Crop Science. 2014; 54 :1331-1335
- 24. Hurley TM, Mitchell PD, Rice ME. Risk and the value of Bt corn. American Journal of Agricultural Economics. 2004; 86 :345-358
- 25. Hutchison WD, Burkness EC, Mitchell PD, Moon RD, Leslie TW, Fleischer SJ, Abrahamson M, Hamilton KL, Steffey KL, Gray ME, Hellmich RL, Kaster LV, Hunt TE, Wright RJ, Pecinovsky K, Rabaey TL, Flood BR, Raun ES. Areawide suppression of European corn borer with Bt maize reaps savings to non-Bt maize growers. Science. 2010; 330 :222-225
- 26. Roberts MJ, Schlenker W. Identifying supply and demand elasticities of agricultural commodities: Implications for the US ethanol mandate. American Economic Review. 2013; 103 :2265-2295
- 27. Cochrane WW. Farm Prices: Myth and Reality. St. Paul: University of Minnesota Press; 1958
- 28. Gabre-Madhin E, Barrett CB, Dorosh P. Technological Change and Price Effects in Agriculture: Conceptual and Comparative Perspectives Discussion Paper. Washington, D.C.: International Food Policy Research Institute; May 2002
- 29. Levins RA, Cochrane WW. The treadmill revisited. Land Economics. 1996; 72 :550-553
- 30. Beghin JC, El Osta B, Cherlow JR, Mohanty S. The cost of the US sugar program revisited. Contemporary Economic Policy. 2003; 21 :106-116
- 31. Tyner WE. The US ethanol and biofuels boom: Its origins, current status, and future prospects. Bioscience. 2008; 58 :646-653
- 32. Cui J, Lapan H, Moschini G, Cooper J. Welfare impacts of alternative biofuel and energy policies. American Journal of Agricultural Economics. 2011; 93 :1235-1256
- 33. Tao L, Aden A. The economics of current and future biofuels. In: Tomes D, Lakshmanan P, Songstad D, editors. Biofuels. NY: Springer; 2011
- 34. Alston JM, Babcock BA, Pardey PG. The shifting patterns of agricultural production and productivity worldwide. In: CARD Books. Ames, IA: Midwest Agribusiness Trace Research and Information Center; Vol. 2. 2010. Available from: https://lib.dr.iastate.edu/card_books/2
- 35. Gassmann AJ, Petzold-Maxwell JL, Keweshan RS, Dunbar MW. Field-evolved resistance to Bt maize by western corn rootworm. PLoS One. 2011; 6 (7):e22629
- 36. Andow DA, Pueppke SG, Schaafsma AW, Gassman AJ, Sappington TW, Meinke LJ, Mitchell PD, Hurley TM, Hellmich RL, Porter RP. Early detection and mitigation of resistance to Bt maize by western corn rootworm (Coleoptera: Chrysomelidae). Journal of Economic Entomology. 2015; 109 :1-12
- 37. Baum JA, Bogaert T, Clinton W, Heck G, Feldmann P, Ilagan O, Johnson S, Plaetinck G, Munyikwa T, Pleau M, Vaughn T, Roberts J. Control of coleopteran insect pests through RNA interference. Nature Biotechnology. 2007; 25 :1322-1326. DOI: 10.1038/nbt1359
- 38. Hu X, Richtman N, Zhao J, Duncan K, Niu X, Procyk L, Oneal M, Kernodle B, Steimel J, Crane V, Sandahl G, Ritland J, Howard R, Presnail J, Lu A, Wu G. Discovery of midgut genes for the RNA interference control of corn rootworm. Scientific Reports. 2016; 6 :30542. DOI: 10.1038/srep30542
- 39. Niu X, Kassa A, Hu X, Robeson J, McMahon M, Richtman N, Steimel J, Kernodle B, Crane V, Sandahl G, Ritland J, Presnail J, Lu A, Wu G. Control of western corn rootworm ( Diabrotica virgifera virgifera ) reproduction through plant-mediated RNA interference. Scientific Reports. 2017; 7 :12591. DOI: 10.1038/s41598-017-12638-3
- 40. Sampson K, Zaitseva J, Stauffer M, Vande Berg B, Guo R, Tomso D, McNulty B, Desai N, Balasubramanian D. Discovery of a novel insecticidal protein from Chromobacterium piscinae , with activity against western corn rootworm, Diabrotica virgifera virgifera . Journal of Invertebrate Pathology. 2017; 142 :34-43
- 41. Soltani N, Dille JA, Burke I, Everman W, VanGessel M, Davis V, Sikkema P. Potential corn yield losses from weeds in North America. Weed Technology. 2016; 30 :979-984
- 42. Pannell DJ, Tillie P, Rodríguez-Cerezo E, Ervin D, Frisvold GB. Herbicide resistance: Economic and environmental challenges. AgBioforum. 2016; 19 :136-155
- 43. Dong F, Mitchell PD, Davis V, Recker R. Impact of atrazine prohibition on the sustainability of weed management in Wisconsin corn production. Pest Management Science. 2016; 73 . DOI: 10.1002/ps.4298
- 44. Norsworthy JK, Ward S, Shaw D, Llewellyn R, Nichols R, Webster T, et al. Reducing the risks of herbicide resistance: Best management practices and recommendations. Weed Science. 2012; 60 (sp1):31-62
- 45. Duke S. Why have no new herbicide modes of action appeared in recent years? Pest Management Science. 2012; 68 :505-512
- 46. Varanasi VJ, Godar A, Currie R, Dille A, Thompson C, Stahlman P, Jugulam M. Field-evolved resistance to four modes of action of herbicides in a single kochia ( Kochia scoparia L. Schrad.) population. Pest Management Science. 2015; 71 :1207-1212
- 47. Schlenker W, Roberts MJ. Nonlinear temperature effects indicate severe damages to U.S. crop yields under climate change. Proceedings of the National Academy of Sciences. 2009; 106 :15594-15598
- 48. Nelson GC, Valin H, Sands RD, et al. Climate change effects on agriculture: Economic responses to biophysical shocks. Proceedings of the National Academy of Sciences. 2014; 11 :3274-3279. DOI: 10.1073/pnas.1222465110
- 49. Vermeulen SJ, Aggarwal PK, Ainslie A, et al. Options for support to agriculture and food security under climate change. Environmental Science and Policy. 2012; 15 :136-144
- 50. Arbuckle JG, Prokopy LS, Haigh T, Hobbs J, Knoot T, Knutson C, Loy A, Mase AS, et al. Climate change beliefs, concerns, and attitudes toward adaptation and mitigation among farmers in the Midwestern United States. Climatic Change. 2013; 117 :943-950. DOI: 10.1007/s10584-013-0707-6
- 51. Rejesus RM, Mutuc-Hensley M, Mitchell PD, Coble KH, Knight TO. U.S. agricultural producer perceptions of climate change. Journal of Agricultural and Applied Economics. 2013; 45 :701-718
- 52. Gaffney J, Schussler J, Löffler C, Cai W, Paszkiewicz S, Messina C, Groeteke J, Keaschall J, Cooper M. Industry-scale evaluation of maize hybrids selected for increased yield in drought-stress conditions of the US corn belt. Crop Science. 2015; 55 :1608-1618
- 53. Nemali KS, Bonin C, Dohleman F, Stephens M, Reeves W, Nelson D, Castiglioni P, et al. Physiological responses related to increased grain yield under drought in the first biotechnology-derived drought-tolerant maize. Plant, Cell and Environment. 2015; 38 :1866-1880
- 54. Varshney RK, Bansal K, Aggarwal P, Datta S, Craufurd P. Agricultural biotechnology for crop improvement in a variable climate: Hope or hype? Trends in Plant Science. 2011; 16 :363-371
- 55. Douglas MR, Tooker JF. Large-scale deployment of seed treatments has driven rapid increase in use of neonicotinoid insecticides and preemptive pest management in U.S. field crops. Environmental Science and Technology. 2013; 49 (2015):5088-5097. DOI: 10.1021/es506141g
- 56. Macedo WR, de Camargo e Castro PR. Thiamethoxam: Molecule moderator of growth, metabolism and production of spring wheat. Pesticide Biochemistry and Physiology. 2011; 100 :299-304
- 57. Dubey KK, Kumar P. Microbes in crop improvement: Future challenges and perspective. In: Prasad R, Singh Gill S, Tuteja N, editors. Crop Improvement through Microbial Biotechnology. Amsterdam: Elsevier; 2018. pp. 415-425. DOI: 10.1016/B978-0-444-63987-5.00021-9
- 58. O’Callaghan M. Microbial inoculation of seed for improved crop performance: Issues and opportunities. Applied Microbiology and Biotechnology. 2016; 100 :5729-5746. DOI: 10.1007/s00253-016-7590-9
- 59. Jones MS, Brown Z, Delborne J, Mitchell P, Elsensohn J. U.S. public attitudes and uncertainties about gene drive insects. 2018. In review
- 60. Scheufele DA, Xenos MA, Howell EL, Rose KM, Brossard D, Hardy BW. U.S. attitudes on human genome editing. Science. 2017; 357 :553-554. DOI: 10.1126/science.aan3708
- 61. Esvelt KM, Smidler AL, Catteruccia F, Church GM. Concerning RNA-guided gene drives for the alteration of wild populations. eLife. 2014; 3 :20131071. DOI: 10.7554/eLife.03401
- 62. Mitchell PD, Brown Z, McRoberts N. Economic issues to consider for gene drives. Journal of Responsible Innovation. 2017; 5 :S180-S202. DOI: 10.1080/23299460.2017.1407914
- 1 bushel of corn equals 25.40 kg and 1 acre of land equals 0.4047 hectare. Thus, 1 bu/acre = 62.77 kg/hectare.
- We now know that horizontal gene transfers across species are not uncommon and that they played an important role in the evolution of life (e.g., [11]).
- The difference is due to inflation, the average US inflation rate between 1950 and 2017 being +3.44% per year.
- This can take place when the demand is highly price-inelastic, i.e., when the output price decline is “large enough” to imply a substantial decline in revenue that swamps the decrease in cost. This scenario is relevant as the demand for food in general and for corn in particular tends to be highly price-inelastic (e.g., [26]).
- These estimates from https://www.nationalgeographic.com/what-the-world-eats/ based on UN FAOSTAT data for 2011.
- Official US EPA news release: https://www.epa.gov/newsreleases/epa-registers-innovative-tool-control-corn-rootworm.
© 2018 The Author(s). Licensee IntechOpen. This chapter is distributed under the terms of the Creative Commons Attribution 3.0 License , which permits unrestricted use, distribution, and reproduction in any medium, provided the original work is properly cited.
Continue reading from the same book
Edited by Dr. Amanullah
Published: 10 October 2018
By Bañuelos-Pineda Jacinto, Gómez-Rodiles Carmen Ceci...
1431 downloads
By Haq Nawaz, Saima Muzaffar, Momna Aslam and Shakeel...
2529 downloads
By Arturo Navarro, Andrea Torres, Fernanda Fernández-...
1539 downloads
Advertisement
Multiple Utilization Ways of Corn By-Products for Biomaterial Production with Bio-Refinery Concept; a Review
- Published: 10 June 2023
- Volume 5 , article number 7 , ( 2023 )
Cite this article
- Haymanot Enawgaw 1 , 2 ,
- Tamrat Tesfaye 1 , 2 ,
- Kelem Tiessasie Yilma 1 , 2 &
- Derseh Yilie Limeneh 1 , 3
379 Accesses
2 Citations
Explore all metrics
Agricultural crops generate large amount of by-products such as straws, stalks, husks, leaves, etc. Corn is one of the three mostly grown crops with huge by-products after harvesting the kernel. Only 5% of the corn plant is the kernel that is used as a food component, while 95% of the plant is by-products (cobs, stalks, leaves, and husks). Most of these by-products contains useful lignocellulosic components that can be converted into valuable products. However, conventionally these by-products are considered as wastes and are mainly used for animal feed, and/or get burned. But now a day, researchers are focused on the utilization of these biomasses for the development of valuable biodegradable products. The use of these by-products in the preparation of biomaterials helps for sustainable eco-friendly material production by substituting synthetic materials conventionally produced from fossil fuels. This review focuses on the possibilities for using these by-products to prepare biomaterials such as plastics, hydrogels, fibers, composites, etc.
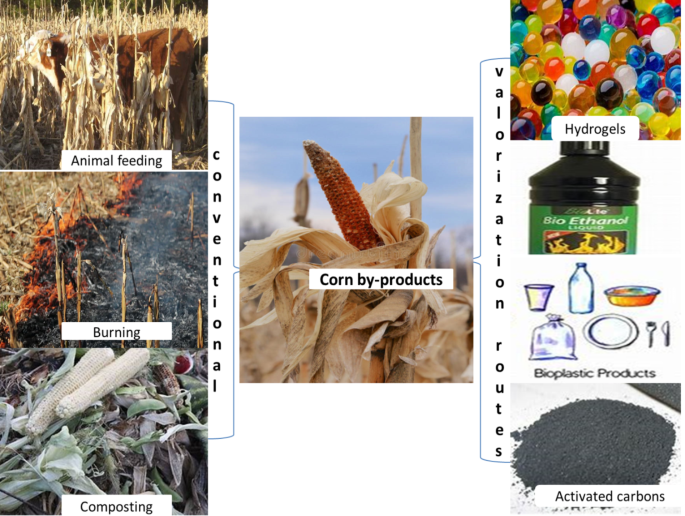
Graphical abstract
This is a preview of subscription content, log in via an institution to check access.
Access this article
Price includes VAT (Russian Federation)
Instant access to the full article PDF.
Rent this article via DeepDyve
Institutional subscriptions
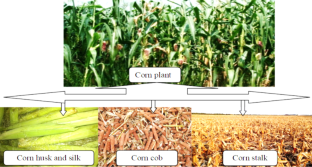
Similar content being viewed by others
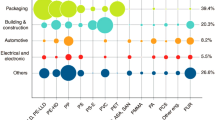
Bioplastic from Renewable Biomass: A Facile Solution for a Greener Environment
Gerardo Coppola, Maria Teresa Gaudio, … Sudip Chakraborty
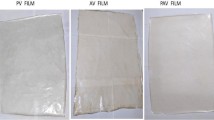
Synthesis and characterization of eco-friendly bioplastic from low-cost plant resources
Akshaya Krishnamurthy & Pavithra Amritkumar
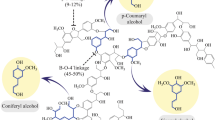
Transforming lignin into value-added products: Perspectives on lignin chemistry, lignin-based biocomposites, and pathways for augmenting ligninolytic enzyme production
Subhashree Rath, Deepak Pradhan, … Hrudayanath Thatoi
Data Availability
Data sharing is not applicable to this article as no datasets were generated or analysed during the current study.
Ahmad A, Azam T (2019) Water Purification Technologies. Bottled and packaged water. Woodhead Publishing, pp 83–120
Ajila CM, Brar SK, Verma M, Tyagi RD, Godbout S, Valéro JR (2012) Bio-processing of agro-byproducts to animal feed. Crit Rev Biotechnol 32(4):382–400
Article CAS Google Scholar
Akguuml M, Tozluolu A (2010) Alkaline-ethanol pulping of cotton stalks. Sci Res essays 5(10):1068–1074
Google Scholar
Akinyemi AB, Afolayan JO, Oluwatobi EO (2016) Some properties of composite corn cob and sawdust particle boards. Constr Build Mater 127:436–441
Article Google Scholar
Albuquerque PB, Malafaia CB (2018) Perspectives on the production, structural characteristics and potential applications of bioplastics derived from polyhydroxyalkanoates. Int J Biol Macromol 107:615–625
Ali A, Ahmed S (2018) Recent advances in edible polymer based hydrogels as a sustainable alternative to conventional polymers. J Agric Food Chem 66(27):6940–6967
Ali M, Byrd M, Jameel H (2001) November. Soda-AQ pulping of cotton stalks. In Proceedings, 2001 TAPPI Pulping Conference, Seattle
Antunes F, Marçal S, Taofiq O, Morais MMB, Freitas A, Ferreira ACCFR, I. and, Pintado M (2020) Valorization of mushroom by-products as a source of value-added compounds and potential applications. Molecules, 25(11), p.2672
Anukam AI, Goso BP, Okoh OO, Mamphweli SN (2017) Studies on characterization of corn cob for application in a gasification process for energy production. Journal of Chemistry , 2017
Aravamuthan RG (2004) Pulping. Chemical Pulping. Encyclopedia of Forest Sciences
Arvanitoyannis IS, Tserkezou P (2008) Corn and rice waste: a comparative and critical presentation of methods and current and potential uses of treated waste. Int J Food Sci Technol 43(6):958–988
Babbar N, Dejonghe W, Gatti M, Sforza S, Elst K (2016) Pectic oligosaccharides from agricultural by-products: production, characterization and health benefits. Crit Rev Biotechnol 36(4):594–606
Bajpai P (2018) Biermann’s Handbook of Pulp and Paper: volume 1: raw material and pulp making. Elsevier
Barbash V, Trembus I, Nagorna J (2012) Pulp obtaining from corn stalks. Chemistry&Chemical Technol 6(1):83–87
CAS Google Scholar
Barcelos MC, Ramos CL, Kuddus M, Rodriguez-Couto S, Srivastava N, Ramteke PW, Mishra PK, Molina G (2020) Enzymatic potential for the valorization of agro-industrial by-products. Biotechnol Lett 42:1799–1827
Bayram B, Ozkan G, Kostka T, Capanoglu E, Esatbeyoglu T (2021) Valorization and application of fruit and vegetable wastes and by-products for food packaging materials. Molecules, 26(13), p.4031
Bian H, Tu P, Chen JY (2020) Fabrication of all-cellulose nanocomposites from Corn Stalk. Journal of the Science of Food and Agriculture.
Bilo F, Pandini S, Sartore L, Depero LE, Gargiulo G, Bonassi A, Federici S, Bontempi E (2018) A sustainable bioplastic obtained from rice straw. J Clean Prod 200:357–368
Biswas A, Saha BC, Lawton JW, Shogren RL, Willett JL (2006) Process for obtaining cellulose acetate from agricultural by-products. Carbohydr Polym 64(1):134–137
Buwalda SJ (2020) Bio-based composite hydrogels for biomedical applications. Multifunctional Materials , 3 (2), p.022001
Byun Y, Kim YT (2014) Bioplastics for food packaging: chemistry and physics. Innovations in food packaging. Academic Press, pp 353–368
Cao Y, Li H, Zhang Y, Zhang J, He J (2010) Structure and properties of novel regenerated cellulose films prepared from cornhusk cellulose in room temperature ionic liquids. J Appl Polym Sci 116(1):547–554
Chang C, Zhang L (2011) Cellulose-based hydrogels: Present status and application prospects. Carbohydr Polym 84(1):40–53
Chen YW, Hassan SB, Yahya M, Lee HV (2018) Novel superabsorbent cellulose-based hydrogels: present status, synthesis, characterization, and application prospects. Cellulose-based superabsorbent hydrogels. Springer, New York, pp 1–41
Chen Z, Chen Z, Yi J, Feng D (2019) Preparation Method of Corn Stalk Fiber Material and Its Performance Investigation in Asphalt Concrete. Sustainability , 11 (15), p.4050
Cheng WM, Hu XM, Wang DM, Liu GH (2015) Preparation and characteristics of corn straw-Co-AMPS-Co-AA superabsorbent hydrogel. Polymers 7(11):2431–2445
Chirani N, Gritsch L, Motta FL, Fare S (2015) History and applications of hydrogels. Journal of biomedical sciences , 4 (2)
de Oliveira JP, Bruni GP, Lima KO, Halal E, da Rosa SLM, Dias GS, A.R.G. and, da Zavareze R (2017) E., Cellulose fibers extracted from rice and oat husks and their application in hydrogel. Food chemistry , 221 , pp.153–160
Demitri C, Scalera F, Madaghiele M, Sannino A, Maffezzoli A (2013) Potential of cellulose-based superabsorbent hydrogels as water reservoir in agriculture. International Journal of Polymer Science , 2013
Duquette D, Dumont MJ (2019) Comparative studies of chemical crosslinking reactions and applications of bio-based hydrogels. Polym Bull 76(5):2683–2710
Dutta N, Usman M, Luo G, Zhang S (2022) An insight into valorization of lignocellulosic biomass by optimization with the combination of hydrothermal (HT) and biological techniques: a review. Sustainable Chem 3(1):35–55
Ek M, Gellerstedt G, Henriksson G (eds) (2009) Pulping chemistry and technology, vol 2. Walter de Gruyter
Enawgaw H, Tesfaye T, Yilma KT, Limeneh DY (2021) Synthesis of a cellulose-co-amps hydrogel for personal hygiene applications using cellulose extracted from corncobs. Gels, 7(4), p.236
Engler RE (2012) The complex interaction between marine debris and toxic chemicals in the ocean. Environ Sci Technol 46(22):12302–12315
Ezeoha SL, Ezenwanne JN (2013) Production of biodegradable plastic packaging film from cassava starch. IOSR J Eng 3(10):14–20
Fahim IS, Chbib H, Mahmoud HM (2019) The synthesis, production & economic feasibility of manufacturing PLA from agricultural waste. Sustainable Chemistry and Pharmacy , 12 , p.100142
Fahnhorst GW, Hoye TR (2019) Superabsorbent Poly (isoprenecarboxylate) hydrogels from glucose. ACS Sustainable Chemistry & Engineering 7(8):7491–7495
Farris S, Schaich KM, Liu L, Piergiovanni L, Yam KL (2009) Development of polyion-complex hydrogels as an alternative approach for the production of bio-based polymers for food packaging applications: a review. Trends in food science & technology 20(8):316–332
Ferreira-Santos P, Zanuso E, Genisheva Z, Rocha CM, Teixeira JA (2020) Green and sustainable valorization of bioactive phenolic compounds from pinus by-products. Molecules, 25(12), p.2931
Fu LH, Qi C, Ma MG, Wan P (2019) Multifunctional cellulose-based hydrogels for biomedical applications. J Mater Chem B 7(10):1541–1562
Galanakis CM (2013) Emerging technologies for the production of nutraceuticals from agricultural by-products: a viewpoint of opportunities and challenges. Food Bioprod Process 91(4):575–579
Getachew A, Woldesenbet F (2016) Production of biodegradable plastic by polyhydroxybutyrate (PHB) accumulating bacteria using low cost agricultural waste material. BMC Res Notes 9(1):1–9
Gómez-García R, Campos DA, Aguilar CN, Madureira AR, Pintado M (2021) Valorisation of food agro-industrial by-products: From the past to the present and perspectives. Journal of Environmental Management, 299, p.113571
Govind A, Nishitha K (2023) Plastic and its side Effects on Humans–A review article. Asian Pac J Environ Cancer 6(1):81–85
Gu S, Xiong J, Kou W, Yi K, Zhao Y, Lun X, Zhang D (2011) Comparison of the physical-chemical properties of corn stalk by expansion and chemical pretreatments and their effect on the yield of biogas. In International Conference on New Technology of Agricultural (pp. 737–741)
Gupta GK, Mondal MK (2020) Bioenergy generation from agricultural wastes and enrichment of end products. Refining biomass residues for sustainable energy and bioproducts. Academic Press, pp 337–356
Ha TLB, Quan TM, Vu DN (2013) Naturally derived biomaterials: preparation and application. In Regenerative medicine and tissue engineering . IntechOpen
Haile A, Gebino G, Tesfaye T, Mengie W, Ayele M, Abuhay A, Yilie D (2021) Utilization of non-wood biomass for pulp manufacturing in paper industry: case of Ethiopia. Biomass Conversion and Biorefinery, pp 1–19
Han Q, Gao X, Zhang H, Chen K, Peng L, Jia Q (2019) Preparation and comparative assessment of regenerated cellulose films from corn (Zea mays) stalk pulp fines in DMAc/LiCl solution. Carbohydrate polymers , 218 , pp.315–323
Hasdiana H, Ayuddin A (2017) September. Quality Improvement of Corn Husk as Raw Material for Textile Products. In 1st International Conference on Social, Applied Science and Technology in Home Economics (ICONHOMECS 2017) . Atlantis Press
Heise K, Kirsten M, Schneider Y, Jaros D, Keller H, Rohm H, Kalbitz K, Fischer S (2019) From Agricultural Byproducts to Value-Added materials: wheat straw-based hydrogels as Soil Conditioners? ACS Sustainable Chemistry & Engineering 7(9):8604–8612
Hu H, Liang W, Zhang Y, Wu S, Yang Q, Wang Y, Zhang M, Liu Q (2018) Multipurpose use of a Corncob Biomass for the production of Polysaccharides and the fabrication of a Biosorbent. ACS Sustainable Chemistry & Engineering 6(3):3830–3839
Huang HJ, Ramaswamy S, Tschirner UW, Ramarao BV (2010) Separation and purification processes for lignocellulose-to-bioalcohol production. Bioalcohol production. Woodhead Publishing, pp 246–277
Huda S, Yang Y (2008) Chemically extracted cornhusk fibers as reinforcement in light-weight poly (propylene) composites. Macromol Mater Eng 293(3):235–243
Husseien M, Amer AA, El-Maghraby A, Hamedallah N (2009) A comprehensive characterization of corn stalk and study of carbonized corn stalk in dye and gas oil sorption. J Anal Appl Pyrol 86(2):360–363
Ibrahim MIJ, Sapuan SM, Zainudin ES, Zuhri MYM (2020) Preparation and characterization of cornhusk/sugar palm fiber reinforced cornstarch-based hybrid composites. J Mater Res Technol 9(1):200–211
Insam H, De Bertoldi M (2007) Microbiology of the composting process. Waste management series, vol 8. Elsevier, pp 25–48
Iwuozor KO, Emenike EC, Ighalo JO, Eshiemogie S, Omuku PE, Adeniyi AG (2022) Valorization of sugar industry’s by-products: a perspective. Sugar Tech 24(4):1052–1078
Jabeen N, Majid I, Nayik GA (2015) Bioplastics and food packaging: A review. Cogent Food & Agriculture , 1 (1), p.1117749
Jahan MS, Russel MAN, Shamim SAN, Mostafa AI, Quaiyyum MA (2004) Alkaline pulp of corn stalks. TIANJIN KEJI DAXUE XUEBAO, 19, p.95
Jain R, Tiwari A (2015) Biosynthesis of planet friendly bioplastics using renewable carbon source. Journal of Environmental Health Science and Engineering , 13 (1), p.11
Jantip S, Suwanruji P (2011) Preparation and properties of microcrystalline cellulose from corn residues. Advanced materials research, vol 332. Trans Tech Publications Ltd, pp 1781–1784
Kabir SF, Sikdar PP, Haque B, Bhuiyan MR, Ali A, Islam MN (2018) Cellulose-based hydrogel materials: Chemistry, properties and their prospective applications. Prog Biomater 7(3):153–174
Kaliyan N, Morey RV (2010) Densification characteristics of corn cobs. Fuel Process Technol 91(5):559–565
Kayra N, Aytekin A (2019) Synthesis of cellulose-based hydrogels: Preparation, formation, mixture, and modification. Cellulose-based superabsorbent hydrogels , pp.407–434
Kaźmierczak J, Nowicki P, Pietrzak R (2013) Sorption properties of activated carbons obtained from corn cobs by chemical and physical activation. Adsorption 19(2–4):273–281
Keziah VS, Gayathri R, Priya VV (2018) Biodegradable plastic production from corn starch. Drug Invention Today 10(7):1315–1317
Klein M, Poverenov E (2020) Natural biopolymer-based hydrogels for use in food and agriculture. J Sci Food Agric 100(6):2337–2347
Kumar H, Christopher LP (2017) Recent trends and developments in dissolving pulp production and application. Cellulose 24:2347–2365
Kurakake M, Kisaka W, Ouchi K, Komaki T (2001) Pretreatment with ammonia water for enzymatic hydrolysis of corn husk, bagasse, and switchgrass. Appl Biochem Biotechnol 90(3):251–259
Latibari AJ, Hossein MA, Hossienpour R (2011) Application of alkaline sulfite pulping on corn stalks. BioResources 6(1):48–58
Lebreton LC, Van Der Zwet J, Damsteeg JW, Slat B, Andrady A, Reisser J (2017) River plastic emissions to the world’s oceans. Nature communications , 8 , p.15611
Li S, Chen G (2020) Agricultural waste-derived superabsorbent hydrogels: Preparation, performance, and socioeconomic impacts. Journal of Cleaner Production , 251 , p.119669
Li P, Cai D, Luo Z, Qin P, Chen C, Wang Y, Zhang C, Wang Z, Tan T (2016) Effect of acid pretreatment on different parts of corn stalk for second generation ethanol production. Bioresource technology , 206 , pp.86–92
Liu Y, Lv X, Bao J, Xie J, Tang X, Che J, Ma Y, Tong J (2019) Characterization of silane treated and untreated natural cellulosic fibre from corn stalk waste as potential reinforcement in polymer composites. Carbohydrate polymers , 218 , pp.179–187
Luo Z, Li P, Cai D, Chen Q, Qin P, Tan T, Cao H (2017) Comparison of performances of corn fiber plastic composites made from different parts of corn stalk. Ind Crops Prod 95:521–527
Lv GJ, Wu SB, Lou R (2010) Characteristics of corn stalk hemicellulose pyrolysis in a tubular reactor. BioResources 5(4):2051–2062
Ma D, Zhu B, Cao B, Wang J, Zhang J (2017) Fabrication of the novel hydrogel based on waste corn stalk for removal of methylene blue dye from aqueous solution. Appl Surf Sci 422:944–952
Marichelvam MK, Jawaid M, Asim M (2019) Corn and rice starch-based bio-plastics as alternative packaging materials. Fibers , 7 (4), p.32
Mendes CADC, Adnet FADO, Leite MCAM, Furtado CG, Sousa AMFD (2015) Chemical, physical, mechanical, thermal and morphological characterization of corn husk residue. Cellul Chem Technol 49(9–10):727–735
Obi FO, Ugwuishiwu BO, Nwakaire JN (2016) Agricultural waste concept, generation, utilization and management. Nigerian J Technol 35(4):957–964
Omidian H, Park K, Rocca JG (2007) Recent developments in superporous hydrogels. J Pharm Pharmacol 59(3):317–327
Organisation for Economic Co-operation and Development (2018) OECD-FAO agricultural outlook 2018–2027. OECD Publishing
Oyeleke SB, Jibrin NM (2009) Production of bioethanol from guinea cornhusk and millet husk. Afr J Microbiol Res 3(4):147–152
Partanen P, Hultman J, Paulin L, Auvinen P, Romantschuk M (2010) Bacterial diversity at different stages of the composting process. BMC Microbiol 10(1):1–11
Peelman N, Ragaert P, De Meulenaer B, Adons D, Peeters R, Cardon L, Van Impe F, Devlieghere F (2013) Application of bioplastics for food packaging. Trends in Food Science & Technology 32(2):128–141
Pittman CU Jr, Mohan D, Eseyin A, Li Q, Ingram L, Hassan EBM, Mitchell B, Guo H, Steele PH (2012) Characterization of bio-oils produced from fast pyrolysis of corn stalks in an auger reactor. Energy & Fuels 26(6):3816–3825
Pointner M, Kuttner P, Obrlik T, Jager A, Kahr H (2014) Composition of corncobs as a substrate for fermentation of biofuels. Agron Res 12(2):391–396
Priyadi P, Mangiring W (2019) Characteristics of Corn Cobs Waste activated Carbon for slow release Micro fertilizer carrier. SAINS TANAH-Journal of Soil Science and Agroclimatology 16(2):147–158
Ranganathan N, Bensingh J, Abdul Kader R, M. and, Nayak SK (2018) Synthesis and properties of hydrogels prepared by various polymerization reaction systems. Springer International Publishing: Cham, Switzerland
Ranum P, Peña-Rosas JP, Garcia‐Casal MN (2014) Global maize production, utilization, and consumption. Ann N Y Acad Sci 1312(1):105–112
Ratanakamnuan U, Manorom W, Inthasai P (2013) Preparation of biodegradable film from esterified corn husk cellulose. In Advanced Materials Research (Vol. 701, pp. 229–233). Trans Tech Publications Ltd
Reddy N, Yang Y (2005) Properties and potential applications of natural cellulose fibers from cornhusks. Green Chem 7(4):190–195
Romaní A, Rocha CM, Michelin M, Domingues L, Teixeira JA (2020) Valorization of lignocellulosic-based wastes. Current developments in Biotechnology and Bioengineering. Elsevier, pp 383–410
Ruan Z, Wang X, Liu Y, Liao W (2019) Corn. Integrated Processing Technologies for Food and Agricultural By-Products. Academic Press, pp 59–72
Samer M (2019) Bioplastics production from agricultural crop residues. Agricultural Eng International: CIGR J 21(3):190–194
Shiferaw B, Prasanna BM, Hellin J, Bänziger M (2011) Crops that feed the world 6. Past successes and future challenges to the role played by maize in global food security. Food security , 3 (3), p.307
Singh TA, Sharma M, Sharma M, Sharma GD, Passari AK, Bhasin S (2022) Valorization of agro-industrial residues for production of commercial biorefinery products. Fuel, 322, p.124284
Song M, Jin B, Xiao R, Yang L, Wu Y, Zhong Z, Huang Y (2013) The comparison of two activation techniques to prepare activated carbon from corn cob. Biomass Bioenergy 48:250–256
Song B, Liang H, Sun R, Peng P, Jiang Y, She D (2020) Hydrogel synthesis based on lignin/sodium alginate and application in agriculture. Int J Biol Macromol 144:219–230
Sorek N, Yeats TH, Szemenyei H, Youngs H, Somerville CR (2014) The implications of lignocellulosic biomass chemical composition for the production of advanced biofuels. Bioscience 64(3):192–201
Sriroth K, Sangseethong K (2005) June. Biodegradable plastics from cassava starch. In II International Symposium on Sweetpotato and Cassava: Innovative Technologies for Commercialization 703 (pp. 145–152)
Sulaiman MA, Adetifa BO, Adekomaya SO, Lawal NS, Adama OO (2019) Experimental characterization of Maize Cob and Stalk Based Pellets for Energy Use. Eng J 23(6):117–128
Tsai WT, Chang CY, Wang SY, Chang CF, Chien SF, Sun HF (2001) Utilization of agricultural waste corn cob for the preparation of carbon adsorbent. J Environ Sci Health Part B 36(5):677–686
Tsakana M, Rucevska L (2020) Baseline report on plastic waste. Seychelles.
Venturin B, Camargo AF, Scapini T, Mulinari J, Bonatto C, Bazoti S, Siqueira DP, Colla LM, Alves SL Jr, Bender JP, Steinmetz RLR (2018) Effect of pretreatments on corn stalk chemical properties for biogas production purposes. Bioresour Technol 266:116–124
Wan T, Xiong L, Huang R, Zhao Q, Tan X, Qin L, Hu J (2014) Structure and properties of corn stalk-composite superabsorbent. Polym Bull 71(2):371–383
Wang W, Themelis NJ, Sun K, Bourtsalas AC, Huang Q, Zhang Y, Wu Z (2019) Current influence of China’s ban on plastic waste imports. Waste Disposal & Sustainable Energy 1(1):67–78
Wang Y, Li S, Ma L, Dong S, Liu L (2020) Corn stalk as starting material to prepare a novel adsorbent via SET-LRP and its adsorption performance for Pb (II) and Cu (II). Royal Society open science , 7 (3), p.191811
Winarti C, Kurniati M, Arif AB, Sasmitaloka KS (2018) December. Cellulose-Based Nanohydrogel from Corncob with Chemical Crosslinking Methods. In IOP Conference Series: Earth and Environmental Science (Vol. 209, No. 1, p. 012043). IOP Publishing
Xiao S, Gao R, Gao L, Li J (2016) Poly (vinyl alcohol) films reinforced with nanofibrillated cellulose (NFC) isolated from corn husk by high intensity ultrasonication. Carbohydr Polym 136:1027–1034
Xu F, Yu J, Tesso T, Dowell F, Wang D (2013) Qualitative and quantitative analysis of lignocellulosic biomass using infrared techniques: a mini-review. Appl Energy 104:801–809
Yanti Y, Yayota M (2017) Agricultural by-products as feed for ruminants in tropical area: nutritive value and mitigating methane emission. Reviews in Agricultural Science 5:65–76
Young M, Boland M, Hofstrand D (2009) Current issues in ethanol production. Agricultural Marketing Resource Center, Iowa State University , http://www.agmrc.org/media/cms/EthanolPaperRev_049574599F3A9.pdf Retrieved, 7.
Zhang Y, Ghaly AE, Li B (2012) Physical properties of corn residues. Am J Biochem Biotechnol 8(2):44–53
Zhang H, Chen K, Gao X, Han Q, Peng L (2019) Improved thermal stability of regenerated cellulose films from corn (Zea mays) stalk pith using facile preparation with low-concentration zinc chloride dissolving. Carbohydr Polym 217:190–198
Zoghlami A, Paës G (2019) Lignocellulosic biomass: understanding recalcitrance and predicting hydrolysis. Front Chem 7:874
Download references
Acknowledgements
Not applicable.
No fund is received to do this paper.
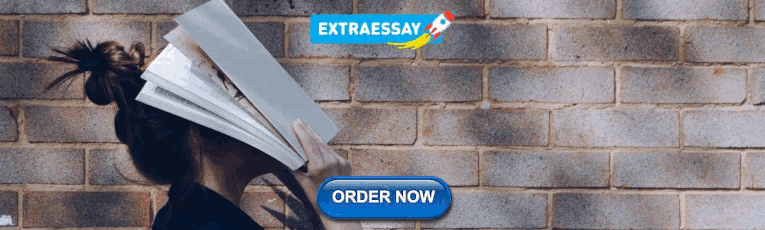
Author information
Authors and affiliations.
Bio-refinery Research Center, Ethiopian Institute of Textile and Fashion Technology, Bahir Dar University, Bahir Dar, Ethiopia
Haymanot Enawgaw, Tamrat Tesfaye, Kelem Tiessasie Yilma & Derseh Yilie Limeneh
Textile Chemistry Research Center, Ethiopian Institute of Textile and Fashion Technology, Bahir Dar University, Bahir Dar, Ethiopia
Haymanot Enawgaw, Tamrat Tesfaye & Kelem Tiessasie Yilma
Textile Production Research Center, Ethiopian Institute of Textile and Fashion Technology, Bahir Dar University, Bahir Dar, Ethiopia
Derseh Yilie Limeneh
You can also search for this author in PubMed Google Scholar
Contributions
HE and KTY wrote the main draft of the document about the beneficiation of corn by-products for different uses. TT drafted the outline of the document that the manuscript should contain. DYL helped in editing the manuscript. All authors read and approved the final manuscript.
Corresponding authors
Correspondence to Haymanot Enawgaw or Tamrat Tesfaye .
Ethics declarations
Competing interests.
The authors declare that they have no competing interests.
Additional information
Publisher’s note.
Springer Nature remains neutral with regard to jurisdictional claims in published maps and institutional affiliations.
Rights and permissions
Springer Nature or its licensor (e.g. a society or other partner) holds exclusive rights to this article under a publishing agreement with the author(s) or other rightsholder(s); author self-archiving of the accepted manuscript version of this article is solely governed by the terms of such publishing agreement and applicable law.
Reprints and permissions
About this article
Enawgaw, H., Tesfaye, T., Yilma, K.T. et al. Multiple Utilization Ways of Corn By-Products for Biomaterial Production with Bio-Refinery Concept; a Review. Mater Circ Econ 5 , 7 (2023). https://doi.org/10.1007/s42824-023-00078-6
Download citation
Received : 13 April 2023
Revised : 01 June 2023
Accepted : 01 June 2023
Published : 10 June 2023
DOI : https://doi.org/10.1007/s42824-023-00078-6
Share this article
Anyone you share the following link with will be able to read this content:
Sorry, a shareable link is not currently available for this article.
Provided by the Springer Nature SharedIt content-sharing initiative
- Corn By-Products
- Valorization
- Bio-Materials
- Bio-Degradability
- Bio-Hydrogels
- Bio-Plastics
- Find a journal
- Publish with us
- Track your research
bt corn Recently Published Documents
Total documents.
- Latest Documents
- Most Cited Documents
- Contributed Authors
- Related Sources
- Related Keywords
Assessing the effects of Bt maize on the non-target pest Rhopalosiphum maidis by demographic and life-history measurement endpoints
Abstract The most commercialized Bt maize plants in Europe were transformed with genes which express a truncated form of the insecticidal delta-endotoxin (Cry1Ab) from the soil bacterium Bacillus thuringiensis (Bt) specifically against Lepidoptera. Studies on the effect of transgenic maize on non-target arthropods have mainly converged on beneficial insects. However, considering the worldwide extensive cultivation of Bt maize, an increased availability of information on their possible impact on non-target pests is also required. In this study, the impact of Bt-maize on the non-target corn leaf aphid, Rhopalosiphum maidis, was examined by comparing biological traits and demographic parameters of two generations of aphids reared on transgenic maize with those on untransformed near-isogenic plants. Furthermore, free and bound phenolics content on transgenic and near-isogenic plants were measured. Here we show an increased performance of the second generation of R. maidis on Bt-maize that could be attributable to indirect effects, such as the reduction of defense against pests due to unintended changes in plant characteristics caused by the insertion of the transgene. Indeed, the comparison of Bt-maize with its corresponding near-isogenic line strongly suggests that the transformation could have induced adverse effects on the biosynthesis and accumulation of free phenolic compounds. In conclusion, even though there is adequate evidence that aphids performed better on Bt-maize than on non-Bt plants, aphid economic damage has not been reported in commercial Bt corn fields in comparison to non-Bt corn fields. Nevertheless, Bt-maize plants can be more easily exploited by R. maidis, possibly due to a lower level of secondary metabolites present in their leaves. The recognition of this mechanism increases our knowledge concerning how insect-resistant genetically modified plants impact on non-target arthropods communities, including tritrophic web interactions, and can help support a sustainable use of genetically modified crops.
Bt corn growing information system in Cagayan Province, Philippines: An analysis for enhanced extension delivery service
The adoption of Bacillus thuringiensis (Bt) corn gene has increased production and agricultural information exchange plays a vital role towards sustained production growth. The study examined the information system by assessing the usefulness of information, frequency of consultations and strength of information exchange in Bt corn production in Cagayan Province, Philippines. Using descriptive correlation design, it was revealed that both technical and economic information from all sources were rated useful. Technical information on seed variety, land preparation, weather forecast, soil management and fertilizer application are frequently utilized while on the economic information only current market prices was frequently used. Frequency of Consultation with personal information sources gained the highest mean value of 5.02 signifying a two to three times exchange of information. Public information sources obtained an average mean of 2.80 which means four or five times contact in a year. This reveals the gap between the corn growers and public information sources. For the mass media sources, both radio (4.56) and television (4.72) are frequently used at two to three times a month. Personal information sources showed strong (224.25 IS) exchange of information between and among the Bt corn growers which comprise their communication network. Total information scores from public and mass media information sources revealed weak exchange of information. The total information score is significantly correlated with membership to organization, years of membership, nature of membership, land ownership and position in the farm. Total information score from friends, radio and newspaper showed significant relationship with income. Thus, information exchange with friends, listening to radio and reading newspaper had a significant influence on the increase of corn growers’ income.
Intercropped Bt and non‐Bt corn with ruzigrass ( Urochloa ruziziensis ) as a tool to resistance management of Spodoptera frugiperda ( JE Smith , 1797) ( Lepidoptera: Noctuidae )
Facing bt toxins growing up: developmental changes of susceptibility to bt corn hybrids in fall armyworm populations and the implications for resistance management, populations of helicoverpa zea (boddie) in the southeastern united states are commonly resistant to cry1ab, but still susceptible to vip3aa20 expressed in mir 162 corn.
The corn earworm, Helicoverpa zea (Boddie), is a major pest targeted by pyramided Bacillus thuringiensis (Bt) corn and cotton in the U.S. Cry1Ab is one of the first insecticidal toxins used in Bt crops, while Vip3A is a relatively new toxin that has recently been incorporated into Cry corn with event MIR 162 and Cry cotton varieties to generate pyramided Bt traits targeting lepidopteran pests including H. zea. The objectives of this study were to determine the current status and distribution of the Cry1Ab resistance, and evaluate the susceptibility to Vip3Aa20 expressed in MIR 162 corn in H. zea in the southeastern U.S. During 2018 and 2019, 32 H. zea populations were collected from non-Bt corn (19 populations), Cry corn (12), and Cry/Vip3A cotton (1) across major corn areas in seven southeastern states of the U.S. Susceptibility of these populations to Cry1Ab and Vip3Aa20 was determined using diet-overlay bioassays. Compared to a known susceptible insect strain, 80% of the field populations were 13- to >150-fold resistant to Cry1Ab, while their response to Vip3Aa20 ranged from >11-fold more susceptible to 9-fold more tolerant. Mean susceptibility to each Bt toxin was not significantly different between the two groups of the populations collected from non-Bt and Bt crops, as well as between the two groups of the populations collected during 2018 and 2019. The results show that resistance to Cry1Ab in H. zea is widely distributed across the region. However, the Cry1Ab-resistant populations are not cross-resistant to Vip3Aa20, and H. zea in the region is still susceptible to the Vip3Aa20 toxin. Vip3Aa20 concentrations between 5 and 10 µg/cm2 may be used as diagnostic concentrations for susceptibility monitoring in future. Additional studies are necessary to elucidate the impact of the selection with Bt corn on resistance evolution in H. zea to Vip3A cotton in the U.S.
When the average hides the risk of Bt-corn pollen on non-target Lepidoptera: Application to Aglais io in Catalonia
Conventional soil management may promote nutrients that lure an insect pest to a toxic crop.
Abstract Slow and consistent nutrient release by organic fertilizers can improve plant nutrient balance and defenses, leading to herbivore avoidance of organically managed crops in favor of conventional crops with weaker defenses. We propose that this relative attraction to conventional plants, coupled with the use of genetically modified, insecticidal crops (Bt), has created an unintentional attract-and-kill system. We sought to determine whether Bt and non-Bt corn Zea mays L. plants grown in soil collected from five paired organic and conventional fields differed in attractiveness to European corn borer [Ostrinia nubilalis (Hübner)] moths, by conducting ovipositional choice and flight tunnel assays. We then examined the mechanisms driving the observed differences in attraction by comparing soil nutrient profiles, soil microbial activity, plant nutrition, and plant volatile profiles. Finally, we assessed whether European corn borer abundance near corn fields differed based on soil management. European corn borer preferred plants grown in conventional soil but did not discriminate between Bt and non-Bt corn. Organic management and more alkaline soil were associated with an increased soil magnesium:potassium ratio, which increased plant magnesium, and were linked to reduced European corn borer oviposition. There was an inconsistent trend for higher European corn borer moth activity near conventional fields. Our results extend the mineral balance hypothesis describing conventional plant preference by showing that it can also improve attraction to plants with genetically inserted toxins. Unintentional attract (to conventional) and (Bt) kill is a plausible scenario for pest declines in response to Bt corn adoption, but this effect may be obscured by variation in other management practices and landscape characteristics.
Survival and Plant Injury of Cry2Ab2-susceptible and Resistant Sugarcane Borer (Lepidoptera: Crambidae) on Bt Corn Containing Single or Pyramided Transgenes
Susceptibility of fall armyworms (spodoptera frugiperda j.e.) from mexico and puerto rico to bt proteins.
Fall armyworm is one of the main pests of conventional and Bacillus thuringiensis (Bt) corn in many countries in the Americas, Africa, Asia and in Australia. We conducted diet-overlay bioassays to determine the status of susceptibility to four Bt proteins (Cry1A.105, Cry2Ab2, Cry1F and Cry1Ac) in three different populations of fall armyworm from Mexico, and one population from Puerto Rico. Bioassays showed that fall armyworms from Puerto Rico were resistant to Cry1F with a resistance ratio 50 (RR50) higher than 10,000 ng/cm2 and to Cry1Ac with a RR50 = 12.2 ng/cm2, displaying the highest median lethal concentration (LC50) values to all Bt proteins tested. The effective concentration 50 (EC50) values further confirmed the loss of susceptibility to Cry1F and Cry1Ac in this population. However, LC50 and EC50 results with Cry1A.105 and Cry2Ab2 revealed that fall armyworm from Puerto Rico remained largely susceptible to these two proteins. The Mexican populations were highly susceptible to all the Bt proteins tested and displayed the lowest LC50 and EC50 values to all Bt proteins. Our results suggest that Cry1F and Cry1Ac resistance is stable in fall armyworm from Puerto Rico. However, this population remains susceptible to Cry1A.105 and Cry2Ab2. Results with Mexican fall armyworms suggest that possible deployment of Bt corn in Mexico will not be immediately challenged by Bt-resistant genes in those regions.
Author Correction: The Impact of Bt Corn on Aflatoxin-Related Insurance Claims in the United States
An amendment to this paper has been published and can be accessed via a link at the top of the paper.
Export Citation Format
Share document.
This page has been archived and is no longer updated
Use and Impact of Bt Maize

Most people are familiar with the hungry caterpillar in the vegetable garden or the elusive beetle in the pantry, and contending with these unwelcomed pests is a longstanding problem. Ever since humans started farming they have shared part of their harvest with insects. Growers of maize, Zea mays (corn), are challenged with a number of pests, but the most important are lepidopteran larvae (i.e., caterpillars) that are stalk borers, ear or leaf feeders, and coleopteran larvae (i.e., beetle grubs) that feed on roots. The European corn borer , Ostrinia nubilalis , for example, was nicknamed the "billion dollar bug" because it cost growers over a billion dollars annually in insecticides and lost crop yields (Figure 1). Most maize growers rely on traditional crop protection practices to manage these insects, including cultural , biological or chemical (insecticide) methods or a balance of these methods that aims to minimize environmental impact called integrated pest management (IPM) (Hellmich et al. 2008). However, in 1996 USA growers were introduced to commercial maize that was genetically engineered (GE) with resistance to European corn borer and other lepidopteran maize pests. In 2003 another GE maize was introduced that killed corn rootworm larvae (beetle grubs), especially larvae of the western corn rootworm , Diabrotica virgifera virgifera , another "billion dollar bug" (Figure 2). These GE plants produce crystal (Cry) proteins or toxins derived from the soil bacterium, Bacillus thuringiensis (Bt), hence the common name "Bt maize". Bt maize has revolutionized pest control in a number of countries, but there still are questions about its use and impact. This article will focus on the opportunities and challenges presented by Bt maize as they relate to current insect-resistant products.

What is Bt Maize?
There are a number of Cry toxins that are categorized by their spectrum of activity. For maize pests, primary Cry proteins are Cry1 and Cry2 for Lepidoptera and Cry3 proteins for Coleoptera (Schnepf et al. 1998). Maize can be genetically engineered to produce these specific Cry toxins. As such, aspects of Bt maize are similar to host plant resistance traits such as DIMBOA (2,4-dihydroxy-7-methoxy-1,4-benzoxazin-3-one) , which at high levels reduces damage by European corn borer (Klun et al. 1967). Seed providers often combine or stack traits for Lepidoptera and Coleoptera control into the same plant. Also, different types of Bt toxins targeted for the same insects are often combined into more effective plant protectants called pyramids . This multiple toxin approach is useful for managing insect resistance to Bt maize.
Why is Bt Maize Popular with Growers?
Reduced use of insecticides, protected yields, improved grain quality, why is bt maize not accepted by some people, possible effects on non-target organisms, maize gene flow, insect resistance.
Successful control of insects by Bt maize has many scientists concerned that overuse of Bt maize could produce pests resistant to Bt toxins. Field-evolved resistance to Bt maize has occurred for two moth species: fall armyworm, Spodoptera frugiperda , in Puerto Rico, and African stem borer, Busseola fusca , in South Africa, so this concern is warranted. Various strategies have been proposed for managing insect resistance to Bt maize, but currently the high-dose/refuge (HDR) strategy is the most commonly recommended (Bates et al. 2005, Tabashnik 1994). With this strategy, insects that feed on the Bt maize are exposed to an extremely high dose of toxin; and this is complemented with refuges, usually non-Bt maize, that provide a population of susceptible insects that are not exposed to Bt toxin (Figure 3). Consequently, rare resistant moths that develop on Bt maize, instead of mating with each other, mate with individuals among the overwhelming number of susceptible moths from the refuge (Gould 1998, Tabashnik & Croft 1982). This process essentially dilutes resistance genes and maintains a population of susceptible insects. The HDR strategy should be effective as long as plants express a high dose of the toxin, genes conferring resistance are rare, and there are many insects from the refuge available to mate randomly with resistant insects (Gould 1998). Sometimes convincing growers to plant non-Bt maize refuges is a challenge because it requires careful planning of where to plant the refuge and could reduce yields. Currently in the USA refuge recommendations range from 5–20 percent, depending on region of the country and type of Bt maize.
Pyramided maize that produces two Bt proteins with different modes of action targeted for the same insect has reduced refuges because the two-toxin "redundant killing" reduces the chances that insects will evolve resistance. A challenge with the HDR strategy is Bt maize is not high dose for many common maize pests. For example, lepidopteran Bt maize is not high dose for fall armyworm, S. frugiperda , corn earworm, Helicoverpa zea , and cutworm species (family Noctuidae), while coleopteran Bt maize is not high dose for corn rootworm ( Diabrotica spp.). Both instances of field resistance, fall armyworm, S. frugiperda , in Puerto Rico, and African stem borer, B. fusca , in South Africa, involved species that were only moderately susceptible to Bt maize. But it is unclear if resistance evolution was due to lack of high dose or insufficient refuge.
Compatibility with Other Control Methods
Although Bt maize is an important tool for growers, it cannot completely replace other pest control tactics. Insecticides, for example, may be necessary to control secondary insect pests. Host plant resistance is important to maintain because if pests become resistant to Bt toxins it will be needed as a backup method of pest control. Finally, Bt maize should be especially compatible with biological control because reduced use of insecticides should lead to an increase in beneficial insects (Naranjo 2009). In general, traditional pest management practices must be maintained in order to avoid reliance on a single tactic.
Active ingredient : Substance in a pesticide that is biologically active.
Biological control : Control of a pest by the introduction of a natural enemy (predator, parasite or pathogen).
Coleoptera : The insect order comprising beetles.
Crystal (Cry) proteins : Proteinacous inclusions produced by many strains of Bacillus thuringiensis during spore formation that have insecticidal activity.
Cultural control : Management techniques used in agricultural to reduce pest populations, such as crop rotation.
Deoxynivalenol : Mycotoxin produced by the fungus Fusarium graminearum and other fungi that occurs mainly in grains such as wheat, barley, oats and maize, also known as vomitoxin.
DIMBOA (2,4-dihydroxy-7-methoxy-1,4-benzoxazin-3-one) : Naturally occurring compound (hydroxamic acid) present in maize and other related grasses that serves as a defense against insects, fungi and bacteria.
Entomologist : Person who studies insects.
European corn borer : Stem-boring insect native to Europe that was accidently introduced into North America in the first decade of the 20th century. Larval stage is a pest of grain, particularly maize.
Fumonisin : Toxins produced by species of Fusarium molds that frequently occur in maize and other crops. Certain types are harmful to humans.
Gene flow : Transfer of genes from one population to another within the same species.
Genetic engineering : Insertion of a modified gene or gene from another organism using various recombinant DNA methods.
High-dose/refuge strategy : Tactic used to delay insect resistance to a genetically modified (GM) crop that involves planting non-GM plants (refuge) to produce susceptible insects. In order for the strategy to be effective the toxin dose in the plant must be sufficiently high to kill offspring derived from susceptible and resistant insects (i.e., heterozygous individuals).
Host plant resistance : Natural defenses plants evolved to reduce the impact of herbivores. Plant breeders often select these traits to reduce losses due to pests.
Insect resistance : Many insects have evolved resistance to insecticides, which can result in crop failures. Likewise insects can evolve resistance to GM plants.
Integrated pest management : A pest management strategy that uses a variety of methods usually biological control, host plant resistance, cultural control, and minimal use of pesticides to control crop pests.
Lepidoptera : An order of insects that includes butterflies and moths.
Leukoencephalomalacia : Disease of the central nervous system that affects horses, mules and donkeys, commonly called "Moldy Corn Poisoning".
Mycotoxins : A toxin produced by a fungus.
Natural insecticides : An insecticide produced from plant extracts, e.g., pyrethrum is produced from the flower chrysanthemum.
Open-pollinated : Pollination occurs by natural mechanisms such as insects, birds or wind. Maize is wind pollinated.
Plant pyramid : Plants with two or more Bt toxins targeted against the same pest.
Pulmonary edema : Abnormal build up of fluid in air sacs of lungs.
Western corn rootworm : A beetle from the family Chrysomelidae that is a major pest of maize in the United States. The main economic damage occurs when larvae feed on maize roots that can lead to plant lodging, harvesting difficulties and yield loss. In 1992 this beetle was discovered in Europe.
References and Recommended Reading
Bates, S. L. et al. Insect resistance management in GM crops: Past, present and future. Nature Biotechnology 23 , 57-62 (2005). doi:10.1038/nbt1056
Brookes, G. & Barfoot, P. Global impact of biotech crops: Environmental effects, 1996-2008. AgBioForum 13 , 76-94 (2010).
Candolfi, M. P. et al. A faunistic approach to assess potential side-effects of genetically modified Bt-corn on non-target arthropods under field conditions. Biocontrol Science and Technology 14 , 129-170 (2004).
Dively, G. P. et al. Effects on monarch butterfly larvae (Lepidoptera: Danaidae) after continuous exposure to Cry1Ab-expressing corn during anthesis. Environmental Entomology 33 , 1116-1125 (2004). doi:10.1603/0046-225x-33.4.1116
Dowd, P. F. Indirect reduction of ear molds and associated mycotoxins in Bacillus thuringiensis corn under controlled and open field conditions: Utility and limitations. Journal of Economic Entomology 93 , 1669-1679 (2000).
Federici, B. A. "Case study: Bt crops - a novel mode of insect control," in Genetically Modified Crops: Assessing Safety , ed. K. T. Atherton (Taylor & Francis, 2002) 164-200.
Gómez-Barbero, M., Berbel, J., & Rodríguez-Cerezo, E. Bt corn in Spain - the performance of the EU's first GM crop. Nature Biotechnology 26 , 384-386 (2008). doi:10.1038/nbt0408-384
Gould, F. Sustainability of transgenic insecticidal cultivars: Integrating pest genetics and ecology. Annual Review of Entomology 43 , 701-726 (1998).
Hellmich, R. L. et al. "The present and future role of insect-resistant genetically modified maize in IPM," in Integration of Insect-Resistant Genetically Modified Crops within IPM Programs , eds. J. Romeis, A. M. Shelton, & G. G. Kennedy (Springer, 2008) 119-158.
Hellmich, R. L. et al . Monarch larvae sensitivity to Bacillus thuringiensis -purified proteins and pollen. Proceedings of the National Academy of Sciences of the United States of America 98 , 11925-11930 (2001).
Hutchison, W. D. et al . Areawide suppression of European corn borer with Bt maize reaps savings to non-Bt maize growers. Science 330 , 222-225 (2010). doi:10.1126/science.1190242
James, C. Global review of commercialized transgenic crops: 2002 Feature: Bt maize. ISAAA Briefs No. 29 . (ISAAA, 2003) xiii + 182 pp. ( article )
Jesse, L. C. H., & Obrycki, J. J. Field deposition of Bt transgenic corn pollen: Lethal effects on the monarch butterfly. Oecologia 125 , 241-248 (2000).
Klun, J. A., Tipton, C. L., & Brindley, T. A. 2,4-dihydroxy-7-methoxy-1, 4-benzoxazin-3-one (DIMBOA), an active agent in the resistance of maize to the European corn borer. Journal of Economic Entomology 60 , 1529-1533 (1967).
Losey, J. E., Rayor, L. S., & Carter, M. E. Transgenic pollen harms monarch larvae. Nature 399 , 214 (1999).
Messeguer, J. Gene flow assessment in transgenic plants. Plant Cell Tissue and Organ Culture 73 , 201-212 (2003).
Munkvold, G. P., Hellmich, R. L., & Rice, L. G. Comparison of fumonisin concentrations in kernels of transgenic Bt maize hybrids and nontransgenic hybrids. Plant Disease 83 , 130-138 (1999).
Naranjo, S. E. Impacts of Bt crops on non-target invertebrates and insecticide use pattern. CAB Reviews: Perspectives in Agriculture, Veterinary Science, Nutrition and Natural Resources 11 , 1-23 (2009). doi: 10.1079/PAVSNNR20094011 ( link )
O'Callaghan, M. et al. Effects of plants genetically modified for insect resistance on nontarget organisms. Annual Review of Entomology 50 , 271-292 (2005). doi:10.1146/annurev.ento.50.071803.130352
Pilcher, C. D., Rice, M. E., & Obrycki, J. J. Impact of transgenic Bacillus thuringiensis corn and crop phenology on five nontarget arthropods. Environmental Entomology 34 , 1302-1316 (2005).
Pilcher, C. D. et al. Biotechnology and the European corn borer: Measuring historical farmer perceptions and adoption of transgenic Bt corn as a pest management strategy. Journal of Economic Entomology 95 , 878-892 (2002).
Rice, M. E. Transgenic rootworm corn: Assessing potential agronomic, economic, and environmental benefits. Plant Health Progress (2004). doi:10.1094/PHP-2004-0301-01-RV
Romeis, J. et al. Assessment of risk of insect-resistant transgenic crops to nontarget arthropods. Nature Biotechnology 26 , 203-208 (2008). doi:10.1038/nbt1381
Schnepf, E. et al. Bacillus thuringiensis and its pesticidal crystal proteins. Microbiology and Molecular Biology Reviews 62 , 775-806 (1998).
Sears, M. K. et al. Impact of Bt corn pollen on monarch butterfly populations: A risk assessment. Proceedings of the National Academy of Sciences of the United States of America 98 , 11937-11942 (2001).
Stanley-Horn, D. E. et al. Assessing the impact of Cry1Ab-expressing corn pollen on monarch butterfly larvae in field studies. Proceedings of the National Academy of Sciences of the United States of America 98 , 11931-11936 (2001).
Storer, N. P., Dively, G. P., & Herman, R. A. "Landscape effects of insect-resistant genetically modified crops," in Integration of Insect-Resistant Genetically Modified Crops within IPM Programs , eds. J. Romeis, A. M. Shelton, & G. G. Kennedy (Springer, 2008) 273-302.
Tabashnik, B. E. Evolution of resistance to Bacillus thuringiensis . Annual Review of Entomology 39 , 47-79 (1994).
Tabashnik, B. E., & Croft, B. A. Managing pesticide resistance in crop-arthropod complexes - Interactions between biological and operational factors. Environmental Entomology 11 , 1137-1144 (1982).
Wu, F. Mycotoxin reduction in Bt corn: Potential economic, health, and regulatory impacts. Transgenic Research 15 , 277-289 (2006). doi:10.1007/s11248-005-5237-1
Wu, F., Miller, J. D., & Casman, E. A. The economic impact of Bt corn resulting from mycotoxin reduction. Journal of Toxicology —Toxin Reviews 23 , 397-424 (2004). doi:10.1081/txr-200027872
Flag Inappropriate


Email your Friend
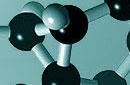
- | Lead Editor: Sanjai J. Parikh

Within this Subject (20)
- Introductory (4)
- Intermediate (10)
- Advanced (6)
Other Topic Rooms
- Ecosystem Ecology
- Physiological Ecology
- Population Ecology
- Community Ecology
- Global and Regional Ecology
- Conservation and Restoration
- Animal Behavior
- Teach Ecology
- Earth's Climate: Past, Present, and Future
- Terrestrial Geosystems
- Marine Geosystems
- Scientific Underpinnings
- Paleontology and Primate Evolution
- Human Fossil Record
- The Living Primates

© 2014 Nature Education
- Press Room |
- Terms of Use |
- Privacy Notice |

Visual Browse

An official website of the United States government
The .gov means it’s official. Federal government websites often end in .gov or .mil. Before sharing sensitive information, make sure you’re on a federal government site.
The site is secure. The https:// ensures that you are connecting to the official website and that any information you provide is encrypted and transmitted securely.
- Publications
- Account settings
Preview improvements coming to the PMC website in October 2024. Learn More or Try it out now .
- Advanced Search
- Journal List
- Plants (Basel)

Classification of Corn Diseases from Leaf Images Using Deep Transfer Learning
Mohammad fraiwan.
1 Department of Computer Engineering, Jordan University of Science and Technology, Irbid 22110, Jordan
Esraa Faouri
Natheer khasawneh.
2 Department of Software Engineering, Jordan University of Science and Technology, Irbid 22110, Jordan
Associated Data
Not applicable.
Corn is a mass-produced agricultural product that plays a major role in the food chain and many agricultural products in addition to biofuels. Furthermore, households in poor countries may depend on small-scale corn cultivation for their basic needs. However, corn crops are vulnerable to diseases, which greatly affects farming yields. Moreover, extreme weather conditions and unseasonable temperatures can accelerate the spread of diseases. The pervasiveness and ubiquity of technology have allowed for the deployment of technological innovations in many areas. Particularly, applications powered by artificial intelligence algorithms have established themselves in many disciplines relating to image, signal, and sound recognition. In this work, we target the application of deep transfer learning in the classification of three corn diseases (i.e., Cercospora leaf spot, common rust, and northern leaf blight) in addition to the healthy plants. Using corn leaf image as input and convolutional neural networks models, no preprocessing or explicit feature extraction was required. Transfer learning using well-established and well-designed deep learning models was performed and extensively evaluated using multiple scenarios for splitting the data. In addition, the experiments were repeated 10 times to account for variability in picking random choices. The four classes were discerned with a mean accuracy of 98.6%. This and the other performance metrics exhibit values that make it feasible to build and deploy applications that can aid farmers and plant pathologists to promptly and accurately perform disease identification and apply the correct remedies.
1. Introduction
Plant diseases are one of the major factors in the destruction of agricultural crops, with an estimated global average yield loss of 2000 billion US dollars each year [ 1 , 2 ]. Plants are adversely affected by infection, which may be fungal, viral, or bacterial. These diseases attack leaves, fruits, or branches and may appear in the form of changes in color, shape, or edges. Thus, it is imperative to investigate possible fast detection, control, and treatment options. Traditional naked eye inspection is the first line of defense toward disease diagnosis [ 3 ], but it is prone to errors [ 4 , 5 ]. Furthermore, farmers can employ the wrong type of treatment method or chemical [ 6 ]. It generally needs a plant pathologist to analyze and discover the type of infection, which may be costly, not prompt enough, or unavailable [ 7 , 8 ].
Corn, or maze, is a worldwide mass-produced agricultural crop. In addition to being consumed directly as food, it forms the basis for many other products such as cooking oil, starch, flour, sugar, biofuel, alcohol, and animal feed. Corn grows in varying environmental conditions and it is considered one of the most important crops characterized by high genetic diversity and production potential, along with rice and wheat [ 9 ]. The global corn production reached 1.15 billion tons in 2020 [ 10 ]. It is naturally exposed to many diseases that can attack various parts of the plant including the leaves, trunk, and fruit at all growth stages. This has a direct impact on the yield of corn harvest and consequently can lead to great financial loss. On a global scale, depleted agricultural production of staple crops such as corn can lead to food shortages, hunger, or even famine [ 11 ]. Diseases that infect corn leaves during the growth process are the most dangerous of these maladies. Hence, this work considers three common leaf diseases [ 12 ]: Cercospora leaf spot, common rust, and northern leaf blight.
Cercospora leaf spot is caused by a two types of fungi, Cercospora zeae-maydis and Cercospora zeina, which inflect damage to the color and shape of the leaves and cause reduction in productivity. These fungi live on the soil surface and need a moist and warm environment and cause necrotic lesions of brown to grey color. Common treatments include the use of appropriate agent, but they must be conducted before the start of grain formation [ 13 , 14 ]. Northern Leaf blight is another type of fungal infection caused by the Exserohilum turcicum fungus. It can lead to great loss in agricultural crops if it attacks during the growth and reproduction stages. Moreover, it is exacerbated by certain weather conditions. The disease appears on the leaves in the form of dark brown spots with a straight rectangular shape. It is treated by the right corresponding chemical agent [ 15 , 16 ]. Common rust is caused by a fungus called Puccinia sorghi, and appears as dark blisters of reddish-brown color on both the upper and lower surfaces of corn leaves. It is treated with the help of the appropriate chemicals [ 17 , 18 , 19 ].
Technological advances have powered many agricultural innovations. More specifically, artificial intelligence (AI) and deep learning algorithms can use plant images to drive decision-making applications. Deep learning is based upon neural networks that are comprised of a number of layers far greater than the input, output and hidden layers. Convolutional neural networks (CNNs) are a subclass of deep learning networks that are concerned with finding features and relationships in images through a sequence of convolution, pooling and rectified linear unit (ReLU) layers that feed to a fully connected layer. This layer combines the various features discovered by the former layers. CNNs have been shown to be effective in discerning features regardless of many changes that can affect the input images [ 20 ]. In designing CNNs-based systems, researchers can build the network structure from scratch. Alternatively, existing reliable and well-established models can be reused and their learned knowledge can be transferred to other applications in what is known as transfer learning. Deep transfer learning adapts existing models with retraining partially or fully in a manner that fits the new application. It has the advantages of detecting generic features (e.g., colors, borders) with earlier layers and customizing later layers for specific applications.
Recently, several research works in AI-based corn disease detection and classification were conducted. Padilla et al. [ 21 ] designed a system using Raspberry Pi to capture and process corn leaf images and classify the type of disease exhibited by those leaves. They used CNN implemented using the multiprocessing programing library OpenMP. However, the paper does not include a description of the CNN architecture. The highest accuracy that they reported was 93% for the classification of leaf blight. However, the rust and leaf spot diseases were detected with an 89% accuracy. Panigrahi et al. [ 22 ] designed a CNN architecture that is nine layers deep. They reported an accuracy of 98.78% and an F1-score of 98.7%. However, little details are provided about the experimental setup in terms of how randomness in splitting the data is managed and how to guard against lucky choices. Recently, Amin et al. [ 23 ] fused features from two CNN models, EfficientNetB0, and DenseNet121, to produce a more representative feature map and hence superior performance. They experimented with multiple fusion techniques and compared the performance of their approach, which produced a superior accuracy of 98.56%, to that of the ResNet152 and Inceptionv3 models. However, the reported performance may have been skewed by the authors’ choice to increase the size of the dataset by augmentation. Subramanian et al. [ 10 ] used transfer learning and employed four CNN models: ResNet50, VGG16, Inceptionv3, and Xception. They tweaked the models’ hyperparameters and measured the accuracy for individual disease classes, which ranged from 83.92% to 99.9%. Xu et al. [ 24 ] modified an AlexNet CNN by adding a new convolution layer and an inception module. They compared their model with transfer learning based on VGGNet-16, DenseNet, ResNet-50 and AlexNet. Their modified model achieved a 93.28% accuracy.
The research landscape on the use of AI in agriculture and specifically in disease identification is ripe for more innovation and further confirmative studies. The work in this paper evaluates transfer learning using a wide range of CNN models to classify corn diseases. Instead of designing a CNN model from scratch, the work employs efficient well-known models that had undergone extensive evaluation to gain their place in the literature. Furthermore, the approach makes deployment and implementation easier by not requiring explicit feature extraction or elaborate image preprocessing. The contributions of this paper are as follows:
- Using leaf images as input, develop deep transfer learning models for the classification of corn diseases. Three such diseases were considered in this study: Cercospora leaf spot, common rust, and northern leaf blight. In addition, a fourth healthy class was included.
- Implement transfer learning with ten deep convolutional neural network models for the classification of leaf images into four classes.
- Evaluate the performance of the various models using multiple metrics that cover many aspects of the detection and classification capabilities. Moreover, the training and valuations times were reported. The results show that wrapping such models in mobile and smartphone devices can aid farmers to quickly and correctly identify diseases.
The remainder of this paper is organized as follows. Section 2 describes the dataset, deep learning models, the experimental setup and performance metrics. The results are described and discussed in Section 3 . In Section 4 , we present our conclusions.
2. Materials and Methods
Given a dataset of corn leaf images representing a number of diseases and a healthy state, the goal of this work is to classify leaf images into the correct state. The methods in this work follow the transfer learning approach, where generically pre-trained deep learning models are customized to the new application (e.g., number of classes, and type of output) and re-trained on the new data. In transfer learning, initial (i.e., early) layers detect common low-level features (e.g., colors, edges, blobs) and later layers learn domain-specific features. Transfer learning utilizes this knowledge, and enables faster training in comparison to randomly setting initial weights. In addition, it makes it possible to learn from smaller number of images. Deep transfer learning has been shown to be useful and effective in a diverse set of applications from many disciplines [ 25 ]. The general steps followed in this work are shown in Figure 1 . In the next few subsections, we go through each step in detail.

The general steps taken to build the corn disease classification model.
2.1. Dataset
The dataset is comprised of 3852 corn leaf images divided into four folders each representing one class of four possible disease states, as follows: 513 Cercospora leaf spot, 1192 common rust, 985 northern leaf blight, and 1162 healthy [ 26 ]. The leaf images are in JPEG format with a size of 256 × 256 pixels. Each file contains an image of one leaf only. Samples of the images of the three diseases and healthy leaves are shown in Figure 2 .

Sample images from the three disease classes and the healthy one: ( a ) Cercospora leaf spot. ( b ) Common rust. ( c ) Northern leaf blight. ( d ) Healthy.
2.2. Convolutional Neural Network Models
The CNN architecture is more elaborate than the traditional multilayer perceptron (MLP) networks (i.e., artificial neural networks (ANN)). MLP networks generally rely upon explicitly extracted numerical features performed through signal/image processing techniques. However, in themselves, they are unable to capture correlations and relative positions between various parts of the image. In other words, minor changes in scale, orientation, or positions can significantly degrade their performance. On the other hand, deep learning CNN involves building a network of layers that apply filters of various scales on the image to extract features. Subsequent layers reduce the dimensionality of the data for a lower overhead, aggregate information from the various feature maps, and improve the learning process by the introduction on non-linearity. The neural networks are comprised of three layers: input, output, and hidden. On the other hand, deep learning involves a far greater number of layers, which enables the capturing of input features and details at various scales. CNNs have been shown throughout the literature to be exceptionally well at handling image-based classification problems [ 20 ].
A plethora of custom CNN models have been proposed in the literature to handle specific applications. Furthermore, technology companies (e.g., Google Inc., Mountain View, CA, USA) have made some complex well-designed generic deep learning CNN models. These models were trained using large datasets (e.g., ImageNet [ 27 ]) to classify 1000 different image categories. Building on the credibility and power of these models, it is possible to utilize their architecture and learned parameters in a process called transfer learning. This approach offers the benefit of the network structure design and the learned model values. Retraining later parts of their network will employ the network structure in learning new task-related features and benefit from earlier layers in detecting non-application specific features (e.g., colors, boundaries) [ 28 , 29 ]. In this research, ten public CNN models were utilized through transfer learning to classify corn diseases based on leaf images. These were: DarkNet-53 [ 30 ], DenseNet-201 [ 31 ], GoogLeNet [ 32 ], Inceptionv3 [ 33 ], MobileNetv2, ResNet-18, ResNet-101 [ 34 ], ShuffleNet [ 35 ], SqueezeNet [ 36 ], and Xception [ 37 ].
The differences between these models stem from variations in the structural construction of the connections, parameter optimization, type of connections, designing new block types and operations, width, and depths [ 38 ]. GoogLeNet is a small spatial exploitation-based CNN, which examines the surrounding of image pixels (i.e., spatial locality). Thus, the granularity level can be controlled by using filters of the appropriate sizes. This model was the first to introduce splitting, merging, and transformation of features by introducing the inception block. ShuffleNet improved on the computational complexity of GoogLeNet via the process of channel shuffle. The ResNet101(18) and Incpetionv3 models are depth-based CNNs. The premise of such designs is that extremely large number of neuros in one layer can capture the input features with great accuracy, but at the expense of excessive computational cost. On the other hand, a large number of layers would produce the same performance but with greatly reduced overhead. The ResNet introduced the concept of residual learning and used skip connections (i.e., bypass pathways) without incurring more data or parameters. The Inceptionv3 model used small asymmetric filers to improve the computational cost over large symmetric filters. The DenseNet-201, as the name suggests, is a densely-connected network with the goal of solving the vanishing gradient problem with such large number of feed-forward cross-layer connections. The Xception model is width-based multi-connection CNN, which is focused on and utilized the importance of the width of the architecture through parallel processing within the network layers. SqueezeNet uses feature maps and suppresses the less important features using the SE-block, which performs two operations: squeeze (i.e., suppress) and excitation. The MobileNetv2 model was designed for resource-limited mobile devices. This was accomplished by reducing the number of trainable parameters using the depth-wise separable convolution operations. Table 1 shows the number of parameters as calculated from the pre-trained models, the depth of each model in layers, and the required input size.
The depth in terms of number of layers, the number of parameters, and the required input size for each model.
2.3. Performance Evaluation Setup
For a fair comparison, the training parameters were set to the same values for all models. The Number of epochs was set to 10. It was possible to set the minimum batch size to 16 based on the available system memory. The learning rate was 0.003, and the stochastic gradient descent with momentum (SGDM) was used in network training [ 39 ].
The dataset was split using three separate different ratios (i.e., 50/50, 70/30, and 90/10). The larger training sets will provide better learning for the CNN models. Augmentation was performed to introduce variety into the dataset. However, the size of the dataset did not increase as only the augmented images were used and the originals were discarded. The augmentations operations were: 1. scaling in the range [0.9, 1.1]; 2. X-Y translation using range [−30, 30] pixels; 3. Random reflection over the horizontal access was performed.
The models were implemented and evaluated using MATLAB R2021a software running on an HP OMEN 30L desktop GT13 with 64 GB RAM, NVIDIA ® GeForce RTX TM 3080 GPU, Intel ® Core TM i7-10700K CPU @ 3.80 GHz, and 1TB SSD.
2.4. Performance Evaluation Metrics
The metrics used to evaluate the performance of the CNN models are shown in Equations ( 1 )–( 5 ). In these equations, T P represent the true positive (i.e., a leaf image correctly classified in one of the nine disease states), F N represent the false negative (i.e., a leaf image classified as healthy but in reality it is drawn from one of the disease classes), F P represent the false positive (i.e., a healthy leaf image wrongly classified as representing a disease), and T N represent the true negative (i.e., a healthy image classified correctly as such). R e c a l l (i.e., true positive rate (TPR) or sensitivity) measures the ability of the model to identify a leaf image as belonging to the correct disease class out of all the positive images, which is affected by the existence of false negatives. Moreover, S p e c i f i c i t y measures the ability of the model to identify a leaf image as belonging to the healthy class, which is affected by the existence of false positives. High sensitivity indicates that the model easily recognizes leaf images as representing a disease, but may include a large number of false positives. P r e c i s i o n measures the ratio of false positives to all cases identified as positive (i.e., false positives included). The A c c u r a c y measures the ratio of the sum of true positives and true negatives to the total number of testing images. However, since different classes have different number of images (i.e., class imbalance), the F1 score is considered a more reliable measure of the model classification performance [ 40 ].
3. Results and Discussion
Different deep learning models may achieve varying classification performance, require a wide range of training/validation times, or favor certain classes at the expense of others. Moreover, some models may benefit from more training data, while others may be unable to generalize to new data. Furthermore, the performance evaluation needs to account for random choices for the images chosen to be in each subset (e.g., training). Repeating the experiments until favourable results are produced will not provide a faithful reflection of the actual performance. In addition, AI models may be susceptible to overfitting/underfitting. It should be noted that, although fine-tuning certain parameters may improve the results, no such experimentation was performed and standard recommendations from the Matlab R2021a software libraries were followed.
The dataset was split into three different setups: 50/50, 70/30, and 90/10. This will reveal if complex models may be able to learn better from more data in comparison to the smaller ones. In addition, it will help reveal any overfitting or underfitting models. Table 2 displays the average overall F1 score, precision, recall, specificity, and accuracy using 50% of the data for training in addition to the standard deviation (SD) of the accuracy. The results represent the average of 10 runs. The performance of the models was comparably close (i.e., 1.6% difference in F1 score between the best and worst performing models). The highest F1 score and accuracy were 96.5% and 97.5%, respectively. These were achieved by the Inceptionv3 and ResNet101 models.
The mean overall F1 score, precision, recall, specificity, and accuracy using 50% of the data for training. The results are an average of 10 runs.
Further insight into the results is provided by the sample confusion matrices of the Inceptionv3 and ResNet101 models shown in Figure 3 . This shows that the major source of errors in Inceptionv3 is caused by classifying some of the images displaying northern leaf blight as Cercospora leaf spot. ResNet101 behaved somewhat similarly, but the misclassification was in both ways for the two aforementioned disease classes. Such errors may be explained by the sample of wrongly classified images shown in Figure 4 . To the untrained eye, these two images seem to represent the same disease. However, the dataset provides them in different disease classes. This may be an error in the dataset but only professional pathologists can confirm the gold standard. In this work, the classification in the public dataset was followe.

Sample confusion matrices for the Inceptionv3 and ResNet101 models using 50% of the data for training: ( a ) Inceptionv3. ( b ) ResNet101.

A sample of wrongly classified images along with the class probability.
Increasing the size of the training data subset provide results that corroborate and confirm the good performance that was achieved. Table 3 and Table 4 show the mean overall F1 score, precision, recall, specificity, and accuracy for all models using 70% and 90% of the data for testing, respectively. The Inceptionv3 could not keep up with the lead. Instead, the DarkNet-53 benefitted better from more training data and achieved an F1 score of 97.5% using 70% of the data for training, and 98.0% using 90% of the data of training. The corresponding accuracy values were 98.2% and 98.6%, respectively. Moreover, with an increase size of the training dataset, it exhibited less fluctuation in the accuracy over different runs.
The mean overall F1 score, precision, recall, specificity, and accuracy using 70% of the data for training. The results are an average of 10 runs.
The mean overall F1 score, precision, recall, specificity, and accuracy using 90% of the data for training. The results are an average of 10 runs.
A similar source of classification errors persists when increasing the size of the training data from 50% to 70%. Figure 5 shows a sample confusion matrix for the DarkNet-53 model using 70% of the data for training. Again, the major source of errors is caused by classifying northern leaf blight as Cercospora leaf spot. On the other hand, increasing the training data to 90% of the dataset seemed to reduce such misclassification. This is displayed in Figure 6 , which displays a sample confusion matrix for DarkNet-53 and using 90% of the data for training. Much of the errors are rectified, but those remaining are still caused by the northern leaf blight–Cercospora leaf spot misclassification and vice versa. Moreover, the lesser absolute number of errors may be caused by having fewer testing data (i.e., 10% as opposed to 30%), but this is perhaps ruled out as the accuracies in the matrices are within 1% of each other. In other words, relative number show a better performance with more training data. Figure 7 shows samples of correctly classified images along with the corresponding class probability.

A sample confusion matrix for the DarkNet-53 model and using 70% of the data for training.

A sample confusion matrix for the DarkNet-53 model and using 90% of the data for training.

A sample of correctly classified images along with the class probability.
Table 5 shows the mean training and validation times for all the models and data split strategies. The table shows roughly three tiers of training/validation speeds: the fast tier comprised of SqueezeNet, GoogLeNet and ResNet18, the very slow tier of DenseNet-201 and Xceptionv, and the medium speed tier containing Inceptionv3, MobileNetv2, ResNet101, ShuffleNet and DarkNet-53. ResNet18 model presents the best compromise in terms of training time (152–195.3 s) and classification performance (96.9–97.8% accuracy). It should be noted, however, that training times do not generally affect the ability to deploy the model. This is because training is conducted offline. Once the model is set with the appropriate weights, it performs classification quickly in individual image testing. Moreover, applications wrapping around the model, does not perform any kind of update to the classification model during deployment. Table 6 shows the inference times per image in milliseconds. The ResNet18 is the model with the fastest inference and represents a good compromise in terms of speed versus accuracy.
The mean training and validation times for all algorithms and all data split strategies. All times are in seconds.
The mean ± SD inference times per image for all algorithms. All times are in milliseconds.
The results show that there does not seem any correlation between the input size and having high accuracy in comparison to other models. The models with the largest input size did not achieve the best accuracy, nor they had the longest training/run time. For example, Xception had the longest training time, but inception had somewhat average training time. DenseNet-201 had the highest inference times and DarkNet-53 produced the best accuracy. Moreover, the inference times do not seem to be correlated with the input size.
The agricultural discipline holds a huge potential for great innovation using artificial intelligence. In the context of this work, image-based object detection and classification applications based on deep learning are promising to revolutionize many avenues of research. Nonetheless, several studies have recently been conducted in classifying corn diseases. Padilla et al. [ 21 ] developed a system using Raspberry Pi as the hardware platform. Using OpenMP for faster performance, they showed that it is possible to deploy CNN-based applications in practice using limited hardware Amin et al. [ 23 ] used different fusion techniques to exploit the synergy of two CNN models: EfficientNetB0 and DenseNet121. However, such an approach may add to the already high computational and memory requirements of deep learning algorithms. Xu et al. [ 24 ] and Panigrahi et al. [ 22 ] designed their own CNN, with the former modifying AlexNet and the later building a new network from scratch. Although excellent performance was reported, it requires extensive and multiple separate research studies to confirm the validity and performance of new designs in comparison to well-established models. Subramanian et al. [ 10 ] used transfer learning with VGG16, ResNet50, InceptionV3, and Xception models, and reported an accuracy range of 83.92% to 99.9% using a dataset of 18,888 images. Table 7 shows a summary of the related literature to identify and classify corn disease.
A summary of the related literature to identify and classify corn diseases.
4. Conclusions
Corn is a staple agricultural product that is important in feeding hundreds of millions of people worldwide through largescale as well as hobby farming. Moreover, it forms the basis for many industrial products and biofuels. However, climate change and its consequences in terms of drought, extreme weather conditions, and unseasonable temperatures have put huge strains on the global agricultural output. Furthermore, plant diseases can cause devastation to corn yield and significant financial losses. These factors make it more pressing to deploy technological innovations at various farming scales to protect plants and aid farmers in disease identification and control. In this work, we aimed at using deep learning artificial intelligence algorithms to classify common corn diseases from leaf images. Transfer learning from well-designed and well-evaluated convolutional network models was used to identify the type of disease out of four possible cases (i.e., three diseases and one healthy state). The performance evaluation results show great promise in developing and deploying commercial applications that satisfy the accuracy and ease-of-use requirements. Such efforts will go a long way in helping farmers tackle corn diseases and preserve their livelihood.
The research in this paper can be expanded further by collecting more images of the four classes in the dataset. More data will help develop systems that are more suitable to daily life usage scenarios. For example, in real life, input images may have any type of background, which is not necessarily the same or unified as in the current dataset. Furthermore, more types of corn diseases can be included (e.g., Stewart’s Bacterial Wilt). Moreover, if athe application is ready for field deployment, then it would be useful to incrementally update the deep learning models to increase accuracy and reliability, and counter the phenomena of catastrophic forgetting, which is suffered by such models.
Abbreviations
The following abbreviations are used in this manuscript:
Funding Statement
This research received no external funding.
Author Contributions
Conceptualization, M.F.; methodology, M.F. and E.F.; software, M.F. and E.F.; validation, M.F. and E.F.; formal analysis, M.F.; investigation, M.F., E.F. and N.K.; resources, M.F. and N.K.; data curation, M.F. and E.F.; writing—original draft preparation, M.F. and E.F.; writing—review and editing, M.F., and N.K.; supervision, M.F. and N.K.; project administration, M.F. All authors have read and agreed to the published version of the manuscript.
Institutional Review Board Statement
Informed consent statement, data availability statement, conflicts of interest.
The authors declare no conflict of interest.
Publisher’s Note: MDPI stays neutral with regard to jurisdictional claims in published maps and institutional affiliations.
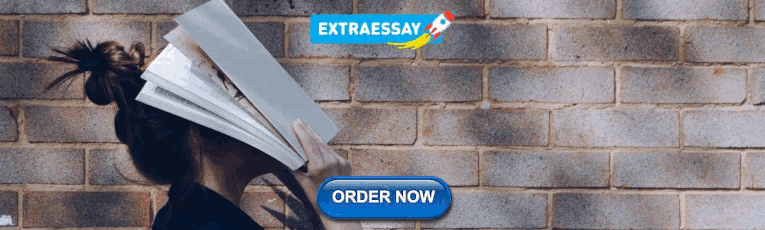
IMAGES
VIDEO
COMMENTS
Globally, corn is characterised by high production and high export concentrations, yet the world is experiencing an unprecedented, huge change in this regard. Ensuring the global supply of corn, and thereby the energy and food security of nations has become particularly important. To understand the importance of corn production as an influencing mechanism of global food supplies, the present ...
I selected the corn input and output data of 18 typical farms in 12 countries from 2012 to 2019, used the data envelopment analysis (DEA) model to calculate the technical efficiency of corn ...
Based on the current research, adding liquid organic fertilizer made from T. diversifolia and A. conyzoides at a rate of 2.250 mL per plant led to the best growth and yield of sweet corn.
This review is a summary of the sweet corn research published during the five years preceding 2021. Discover the world's research. 25+ million members; 160+ million publication pages;
Since its domestication some 9,000 years ago, maize (Zea mays L.; corn) has played an increasing and diverse role in global agri-food systems. Global maize production has surged in the past few decades, propelled by rising demand and a combination of technological advances, yield increases and area expansion. Maize is already the leading cereal in terms of production volume and is set to ...
The last few decades have seen a rapid increase in corn production, making corn the most important cereal in the world. This evolution is due in large part to rapid productivity growth for corn. Both improved genetics and improved farm management have contributed to large increases in corn yield. The paper reviews how genetics, biotechnology and management have interacted to increase ...
Feature papers represent the most advanced research with significant potential for high impact in the field. A Feature Paper should be a substantial original Article that involves several techniques or approaches, provides an outlook for future research directions and describes possible research applications. ... and corn armyworm as the ...
1 Successfully Establishing Corn in Cover Crops: Year 2 2019 Research Report to the Kentucky Corn Promotion Council 1Dan Quinn, 1Hanna Poffenbarger, 2Kiersten Wise, and 1had Lee 1Department of Plant and Soil Sciences, University of Kentucky, Lexington, KY 40546 2Department of Plant and Soil Sciences, University of Kentucky, Princeton, KY 42445 PH: (859) 257-3203; Email: [email protected]
This paper describes in detail the processing and higher-value utilization of the five main by-products: corn gluten meal, corn husks, corn steep liquor, corn germ, and fuel ethanol by-product. The utilization status of corn processing by-products was discussed roundly, and the development trend of corn processing by-products in China and other ...
It is currently the subject of intense debate. An open-pollinated crop, corn is known for its promiscuity—making it more prone to gene flow risks than other crops. Genetic contamination takes on a whole new meaning when the escapable trait could produce proteins to treat diabetes or a hepatitis B vaccine.
trend. This research is important because it can be used to justify producers' adoption of traited corn varieties, help analyze producers' returns on investments, and further explain the increasing trend in U.S. average corn yields. Similar research has been done in this area examining the effects of drought tolerant traits
This research trial assessed corn yield differences in response to the inclusion of Proven40 OS seed treatment across multiple N fertilizer rates. All plots received a 2x2 starter nitrogen
In 2019, corn imports reached 4.79 million tons, a yearly increase of 36.08%. Simultaneously, since 2008, corn exports have reduced to 270,000 tons, a yearly decrease of 94.5%. Corn exports have been below 300,000 tons in the past 12 years . Regarding production costs, China's demographic dividend period has passed, and labour costs have ...
General appearance of the root systems in sweet corn plants in experiment 6, cv. 'Butter Sweet' at harvest time for direct sowing and of 17, 25 and 33 days-old transplants (from left to right ...
About half of the corn plant is corn stover. It means that the mass ratio of corn stover to corn grain is 1:1 [15].Corn stover is the most abundant agricultural residue in the United States [16].A study performed by the U.S. Department of Agriculture and the U.S. Department of Energy in 2005 estimated that by 2030 between 170 and 256 million dry tons of corn stover could be harvested ...
This paper is a comprehensive review of biomaterial preparation such as bioplastics, bio-hydrogels, and others from corn by-products (husk, stalk, ... Preparation and properties of microcrystalline cellulose from corn residues. Advanced materials research, vol 332. Trans Tech Publications Ltd, pp 1781-1784.
Corn Fields. Abstract The most commercialized Bt maize plants in Europe were transformed with genes which express a truncated form of the insecticidal delta-endotoxin (Cry1Ab) from the soil bacterium Bacillus thuringiensis (Bt) specifically against Lepidoptera. Studies on the effect of transgenic maize on non-target arthropods have mainly ...
This paper aims to make a comprehensive review on the previously established studies regarding preparation, characterization, and properties of different parts of the corn plant. In addition, the paper discusses the effect of fiber treatment and the addition of cross-linking and plasticizers to corn starch-based biocomposites for different ...
Corn provides a healthy and impressive amount. of fiber when eaten either on the cob or in the form of popcorn. Corn provides an. impressive 7 grams of fiber per 100 grams of serving. Fiber helps ...
Interestingly, due to the introduction of Bt maize, recent research suggests there has been an areawide suppression of European corn borer populations (Hutchison et al. 2010). This is beneficial ...
A wide range of research has been conducted to understand the genetics and biochemistry of starch synthesis. ... used by food companies as a stabilizer and thickener. Moreover, it is used in the textile, adhesive, corrugating, and paper industries. Corn with a wx1 mutant allele was discovered in the early 1900s . A single recessive gene ...
In this research paper will be made from corn husk. The use of corn husk material can reduce newsprint production costs because it uses cheap and waste materials and can help overcome the problem of corn husk waste. 2 Research Methods The main ingredient in this research is corn husk. Other supporting materials used are
In this work, bioplastic was produced using corn starch, rice husk with. glycerol as plasticizer. MA TERIALS AND METHODS. Collection of Raw Materials: Corn starch was. collected from the local ...
Corn is a mass-produced agricultural product that plays a major role in the food chain and many agricultural products in addition to biofuels. Furthermore, households in poor countries may depend on small-scale corn cultivation for their basic needs. ... The research in this paper can be expanded further by collecting more images of the four ...