ORIGINAL RESEARCH article
Why knowledge sharing in scientific research teams is difficult to sustain: an interpretation from the interactive perspective of knowledge hiding behavior.

- 1 School of Economics and Management, Dalian University of Technology, Dalian, China
- 2 Institute of China Innovation and Entrepreneurship Education, Wenzhou Medical University, Wenzhou, China
Efficient knowledge sharing is an important support for the continuous innovation and sustainable development of scientific research teams. However, in realistic management situations, the knowledge sharing of scientific research teams always appears to be unsustainable, and the reasons for this are the subject of considerable debate. In this study, an attempt was made to explore the interactive mechanism of knowledge hiding behaviors in scientific research teams between individual and collective knowledge hiding behaviors and its impact on knowledge sharing by adopting grounded theory to comprehensively understand this situation. The results show that knowledge hiding behavior in the scientific research team is a two-phase interactive process and is capable of affecting sustainable knowledge sharing by reducing the supply of knowledge, creating a poor knowledge sharing atmosphere, and forming an interpersonal distrust relationship. This research may provide a strong basis for a deeper understanding of the interaction mechanism of knowledge hiding behavior and its impact on knowledge sharing.
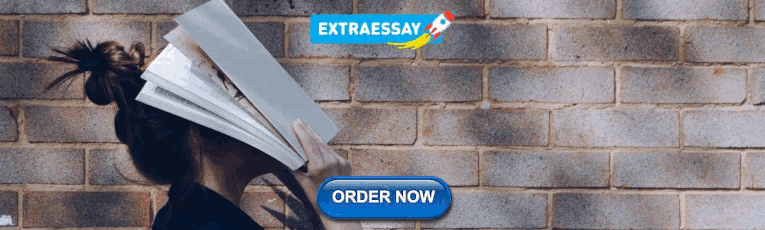
Introduction
Currently, human society has spanned from the era of the industrial economy to the era of the knowledge economy ( Kim and Mauborgne, 1998 ). The creation, dissemination, and use of knowledge, information, and data are restructuring traditional economic development forms into knowledge-based development. Knowledge has become an “intangible asset” that promotes sustainable development and continuous innovation in various organizations ( Grunwald, 2004 ). In this macro background, the scientific research team, as an important organizational form, is facing increasing competition and assessment pressures and demands for innovation ( Peltokorpi and Hasu, 2016 ). Notably, knowledge has become the core resource, which has gradually replaced traditional scientific research funds, equipment, and venues, for the survival and competition of the current scientific research teams. The sustainable development of scientific research teams and the output of high-quality scientific research achievements are inseparable from the continuous acquisition of new knowledge and efficient knowledge utilization in the knowledge economy ( Johnson, 2017 ). Therefore, how to continuously acquire new knowledge and effectively use the existing knowledge mastered by scientific research teams has become the core issue and key practical problem that the knowledge management of the scientific team must address ( Gloet, 2006 ). Relevant research results have shown that effective knowledge acquisition and utilization within the scientific research team are the key to restricting the success of implementing knowledge management and further verifying that good knowledge sharing among scientific research team members, which plays an important role in scientific decision-making, improves the effectiveness and efficiency of the scientific team’s knowledge management. Therefore, knowledge sharing among members of the scientific research team can inevitably lead to a significant increase in the efficiency of the scientific research team in using and creating knowledge. Based on this, the scientific research team can promote knowledge flow, sharing, and collaborative knowledge creation among knowledge workers of the scientific research team by specifying appropriate knowledge sharing incentive strategies, which can lay a strong foundation for the sustainable development of the scientific research team ( Zhuge, 2002 ; Sung and Choi, 2012 ).
However, in practical knowledge sharing scenarios in scientific research teams, one of the most common behaviors, namely, knowledge hiding, is widespread and makes it difficult to sustain knowledge sharing within scientific research teams ( Huo et al., 2016 ). Existing research on knowledge hiding has investigated its antecedents and consequences, but the exploration and interpretation of the characteristics and the interaction between the targets and the perpetrators of knowledge hiding are still limited ( Connelly and Zweig, 2015 ; Connelly et al., 2019 ). From this point of view, Connelly and Zweig (2015) pointed out that knowledge hiding behavior can be a mutual influence between targets and perpetrators. Zhao et al. (2019) proved that leader–member exchange (LMX) may affect how much they hide knowledge from their colleagues. These studies reveal that knowledge hiding behavior interacts among individuals. However, it is still unclear whether this interaction exists between individuals and teams. In other words, the exact interaction mechanisms of knowledge hiding behavior in organizations or research teams are still unclear.
Therefore, the first aim of this study is to explore (1) the interaction mechanism of the individual and the collective knowledge hiding behavior. In addition, considering the full range of the outcomes of knowledge hiding has yet to be examined ( Connelly et al., 2019 ), such as how knowledge hiding and its interaction between individuals and the team affect knowledge sharing. The second aim of this paper is to reveal (2) the relationship between knowledge hiding and knowledge sharing within scientific research teams.
Our theoretical views will make significant contributions to research on knowledge hiding and knowledge sharing. First, we expanded the research on knowledge hiding behavior from the traditional dyadic level to the collective level and constructed a cross-level interactive cycle model between individual knowledge hiding and collective knowledge hiding in research teams, which deepened the academic community’s understanding of hierarchical interaction on knowledge hiding behavior and broadened the perspective of knowledge hiding behavior research. Second, we identified two new influencing factors that affect the relationship between knowledge hiding behavior and knowledge sharing: reducing knowledge supply and forming a poor knowledge sharing atmosphere. These findings enrich the research on the antecedent factors of knowledge sharing and deepen the academic and practical understanding of the relationship between knowledge hiding and knowledge sharing. Moreover, from a practical point of view, our research helps explain how knowledge hiding behavior forms cross-level interactions, which in turn affects knowledge sharing within the research team and what management measures can be used to solve these problems.
Literature Review
Knowledge sharing.
Existing studies on knowledge sharing have discussed from multiple dimensions and formed two different research paradigms. The first research paradigm is a technology-centric paradigm ( McElroy, 2000 ). It is characterized by technology as an important factor for knowledge sharing. This paradigm can be further divided into the communication and tool perspectives. From the perspective of communication, scholars believe that knowledge sharing is an effective way of interaction and communication ( Mei et al., 2004 ) and can also be enhanced by the communities of practices ( Manuti et al., 2017 ). Active communication between knowledge holders and receivers contributes to knowledge sharing, leading to the effective management of knowledge within the organization. From the tool perspective, knowledge management researchers pay significant attention to the form of knowledge sharing media and the impact of knowledge sharing, such as the impact of IT technology, practical seminars, and teams on knowledge sharing ( Choi et al., 2010 ). The second research paradigm is based on the people-centric paradigm, that is, the “interpersonal interaction” and “interpersonal relations” as entry points, focusing on the role of interpersonal structure and relationships on knowledge sharing. This paradigm can be further refined into an interactive perspective, learning perspective, power perspective, market perspective, and social exchange perspective. The interactive perspective was represented by Japanese scholars, such as Nonaka and Takeuchi (1995) , focusing on exploring the interaction between tacit and explicit knowledge. The learning perspective focuses on the connotation of knowledge sharing from the perspective of individual learning and development, team learning, collective development ( Zellmer-Bruhn and Gibson, 2006 ; Manuti et al., 2017 ), and the learning climate/culture ( Watkins and Marsick, 2003 ). The power perspective is characterized by team members’ ownership of private knowledge, which is regarded as a kind of power resource, and the process of re-sharing private knowledge is also a process of allocating power relations among people ( Robert et al., 2009 ). Furthermore, the market perspective defines knowledge as a unique, exclusive resource that can be bought, sold, and exchanged in the research team ( Nonaka and von Krogh, 2009 ). The social exchange perspective regards knowledge sharing as an exchange ( Liu et al., 2011 ). Under these two paradigms, scholars have carried out extensive discussions with the objective of promoting knowledge sharing; however, the discussion on the obstacles to knowledge sharing is relatively limited ( Connelly et al., 2012 ). Therefore, it is necessary to appropriately improve the current discussion from the perspective of obstacles to knowledge sharing. Considering that although knowledge hiding behavior is not necessarily a kind of behavior that harms the organization’s knowledge sharing, to a certain extent, its impact on the sustainability of knowledge sharing is obvious. Therefore, exploration of the obstacles to knowledge sharing from the perspective of knowledge hiding behavior is desirable.
Knowledge Hiding Behavior
In 2012, the concept of knowledge hiding behavior was proposed to describe an intentional attempt by an individual to withhold or conceal knowledge that has been requested by another person ( Connelly et al., 2012 ). Once the knowledge hiding behavior was proposed, it immediately attracted significant attention of scholars in the field of knowledge management and organizational behavior. At present, the current research on knowledge hiding behavior mainly focuses on its antecedents and consequences ( Connelly et al., 2019 ). From the perspective of antecedents, previous studies have shown that knowledge hiding behavior is affected by many different factors, such as intellectual psychological ownership and territorial behavior ( Peng, 2013 ), complexity of knowledge, relevance of knowledge and tasks ( Connelly et al., 2012 ), high distrust and competitiveness ( Hernaus et al., 2019 ), dark triad psychological traits ( Pan et al., 2018 ) and Big Five personality ( Anand and Jain, 2014 ), task interdependence ( Gagné et al., 2019 ), LMX ( Zhao et al., 2019 ), and performance-proven goal orientation ( Zhu et al., 2019 ). From the perspective of its consequences, studies have shown that knowledge hiding behavior can cause greater interpersonal distrust ( Connelly et al., 2012 ), the deterioration of interpersonal relationships ( Connelly and Zweig, 2015 ), the reduction individual and team creativity ( Bogilović et al., 2017 ; Rhee and Choi, 2017 ), the reduction of psychological safety ( Jiang et al., 2019 ), and so forth. Although these studies provide important information to understand knowledge hiding behavior, most of the published articles focus on dyadic levels. As suggested by Černe et al. (2015) , it is clear that research on knowledge hiding behavior need not only focused on dyadic levels but also on collective knowledge hiding behavior. In addition, research focusing on the difference and interaction mechanism between individual and collective knowledge hiding behaviors within scientific research teams has not yet been adequately discovered, and research on how this behavioral interaction affects knowledge sharing requires further improvement. Therefore, it is necessary to explore the interaction mechanism from the perspective of the social interaction of knowledge hiding behavior and further interpret the impact on knowledge sharing. Specifically, it is valuable to explore how the knowledge hiding behavior of individual members within the scientific research team is transferred to the scientific research team, and how the collective knowledge hiding behavior of the scientific research team affects the individual members. Further, undeniably, more systematic explorations are still required to reveal the impact of this interaction on the knowledge sharing of scientific research teams, which has important theoretical and practical value for supplementing and improving research on knowledge hiding behavior and knowledge sharing.
Research Design
Research method.
The qualitative method was used because the main objectives of the study were to answer “how and why” questions. In addition, qualitative methods allow for an overall understanding of the complex phenomenon under investigation by allowing researchers to carry out an empirical inquiry that investigates a bounded contemporary phenomenon within a real-life context ( Creswell, 2013 ). Furthermore, among the qualitative research methods, grounded theory is considered to be one of the most important qualitative research methods in the field of philosophy and social sciences because of its special research question presentation methods and rigorous data analysis methods ( Corbin and Strauss, 1990 ; Strauss and Corbin, 1997 ). In recent years, the grounded theory research method has been widely used in the field of organizational behavior and knowledge management research ( Benoliel, 1996 ; Danielsson et al., 2019 ), which provides good support for the use of grounded theory in our study. Furthermore, related research also noted that the grounded theory research method is, in particular, suitable for analyzing micro-behavior and social interaction processes, which is mostly in line with what we are concerned with. Therefore, this study utilized the grounded theory research method to explore the interaction mechanism of knowledge hiding behaviors in scientific research teams and carried out further research ( McCann and Polacsek, 2018 ).
Thus far, grounded theory has been classified into three main types that are connected yet different: the original version of the grounded theory originally proposed by Glaser and Strauss (1967) , that is, the classic (original) grounded theory, the proceduralized version, and the constructivist’s approach to grounded theory ( Charmaz, 1996 ; Strauss and Corbin, 1997 ). There are some differences among the three schools with respect to the process of epistemology and coding. The proceduralized version based on hermeneutics is more suitable for this study. This is not only because this research paradigm is the most widely used, but also because the proceduralized version of grounded theory provides a standardized analysis technique that will play an important role in analyzing and predicting specific behaviors. Based on the above-mentioned discussion, this research follows the research paradigm of the proceduralized version of grounded theory to guide the corresponding qualitative data collection and analysis.
Sampling and Research Sample Selection
In grounded theory research, the following three common sampling methods are involved: theoretical sampling, objective sampling, and selective sampling ( Sandelowski, 1995 ; Robinson, 2014 ). Among them, theoretical sampling is known to develop the theory. Researchers are often unsure who the next sample is during the research process, and the sampling object is entirely driven by the theory. In contrast, objective sampling is known for selecting rich case data and conducting in-depth research. In objective sampling, researchers can identify in-depth events to achieve in-depth discussions on research-related issues. Selective sampling can solve several problems, such as researchers’ time constraints and research frame limitations, and can enhance the feasibility of research. Based on the research objective of this study, as well as the feasibility and convenience of the study, objective and selective sampling were selected. That is, scientific research teams and members who are interested in the interaction of knowledge hiding behaviors and have the time and experience to provide the most detailed information on knowledge hiding behaviors were selected as the sampling objects of this research. Finally, through classroom recruitment, friend introduction, and active visits, interactive interview information was collected on the knowledge hiding behaviors of 31 research team members in 9 research teams from different disciplines, including innovation management, history, chemistry, and molecular biology. We chose participants who were nested in teams, since we needed to analyze the collective knowledge hiding behavior at the team (collective) level. In this way, the triangle verification of the discourse among different members of the same team ensures the reliability and validity of data collection at the team level.
The interviewees were mainly in the age range of 24 to 56, including 22 males and 9 females. The interviewees’ work experience was between 0 and 28 years. All respondents had bachelor’s degrees and 14 had doctorates. In order to effectively implement triangular verification of the data, the study also collected the work diaries of some employees to supplement and verify the interview data. In addition, for interviewees, they were compensated with a gift that was worth 200 RMB.
Collection of Qualitative Research Data
Semi-structured, open, face-to-face interviews were primarily conducted to collect data on the interaction mechanism of the cross-level knowledge hiding behavior of scientific research teams and their impact on knowledge sharing. Interviews are one of the most commonly used research methods in qualitative research, and face-to-face interview is the most commonly used research method among interview methods ( Gillham, 2000 ). Moreover, face-to-face interview can also enable researchers to capture variation of details in the facial expressions of the interviewees, as well as sound performance and body movements, which can provide relevant information for grounded theory research. This research focuses on the cross-level interaction of knowledge hiding behavior within scientific research teams and its impact on knowledge sharing, which needs to fully collect the ideas of scientific team members when making interactive decisions on knowledge hiding behavior. Therefore, semi-structured, open, face-to-face interviews are more suitable for this research. In order to improve the efficiency of the interviews, we established the outline of an interview, as presented in Table 1 . The interview themes mainly focused on “the status quo of knowledge hiding behavior of scientific research team members,” “the interactive process and mechanism of knowledge hiding behavior in scientific research teams,” “the intervention of knowledge hiding behavior in scientific research teams,” and “the impact of knowledge hiding behavior interactions on knowledge sharing in scientific research teams.”
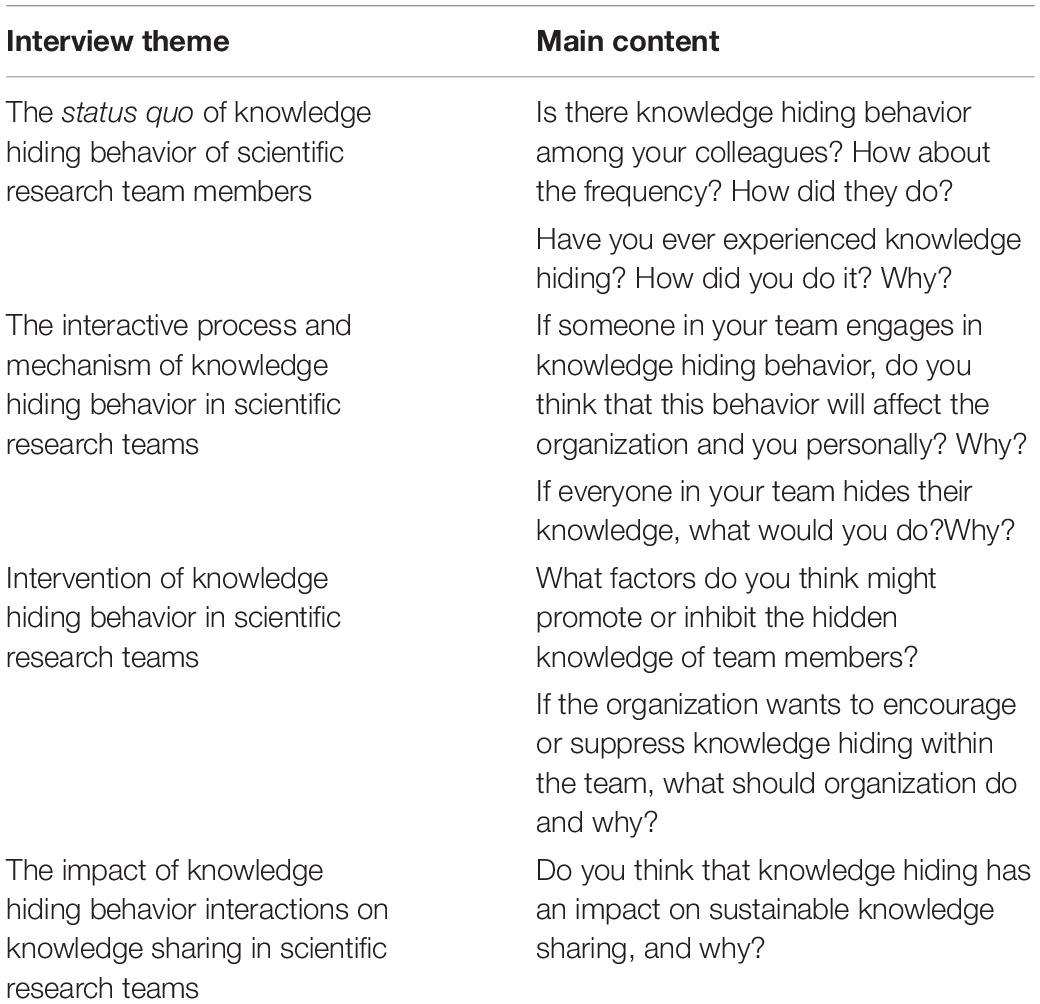
Table 1. Outline of an interview.
Considering that the respondents of the knowledge hiding behavior of the scientific research team may perceive social desirability ( Furnham, 1986 ) as a relatively negative concept, knowledge hiding behavior may cause social desirability when a third person is involved. In order to protect the participants and obtain interview data with good reliability and validity, face-to-face interviews were conducted and completed by the authors of this article alone. The authors communicated with the interviewees about the interview themes in advance via telephone or WeChat . In the actual implementation of the interview, the interview was carried out according to the outline but was not limited to the outline. The interview was generally selected by the interviewee. Usually, a quieter, independent office was selected. In addition, the authors protected the information of each interviewee, and it would not be mentioned by any third person. Different interviewees within the same team were also independent of each other. The average interview time length was 47 min. Finally, when 31 members of the scientific research team were interviewed, 82 pages of transcripts were created. The data and theory became saturated, and we stopped collecting the interview data.
Research Data Analysis
After data collection, we entered the data analysis stage. As mentioned above, we drew on the analysis thought of the proceduralized version of grounded theory, which includes open, axial, and selective coding for our data analysis. It is important to mention that Excel was used to organize the coding and to better observe the relationship between different categories.
Open Coding
Open coding was the first step in our study, and it is a process of analyzing interview data word by word and sentence by sentence, and refining meaningful concepts ( Pieterse, 2011 ). This study followed the principle of “live coding,” which involved the extraction of as much of the interviewee’s original words as possible. In our study, two authors independently coded the interview data. After the authors’ independent coding, 624 “live codings” were obtained. Subsequently, the authors merged the same codes, discussed and determined the different codes together, and finally formed the relevant “live coding,” in this case, about 500, for further categorization. The categorization process is a process of sorting and categorizing concepts. Considering the disciplinary attributes of research, standard concepts in the fields of management and organizational behavior were selected to represent “live coding.” Table 2 presents the results of the categorization in this research. According to Table 2 , the main concepts included in this study mainly comprise individual knowledge hiding behavior, collective knowledge hiding behavior, individual status, work interdependence, herd mentality, imitative learning, collectivist orientation, team identification, leaders’ supervision, purpose of assessment, poor knowledge sharing atmosphere, reduced knowledge supply, interpersonal distrust, and knowledge sharing.
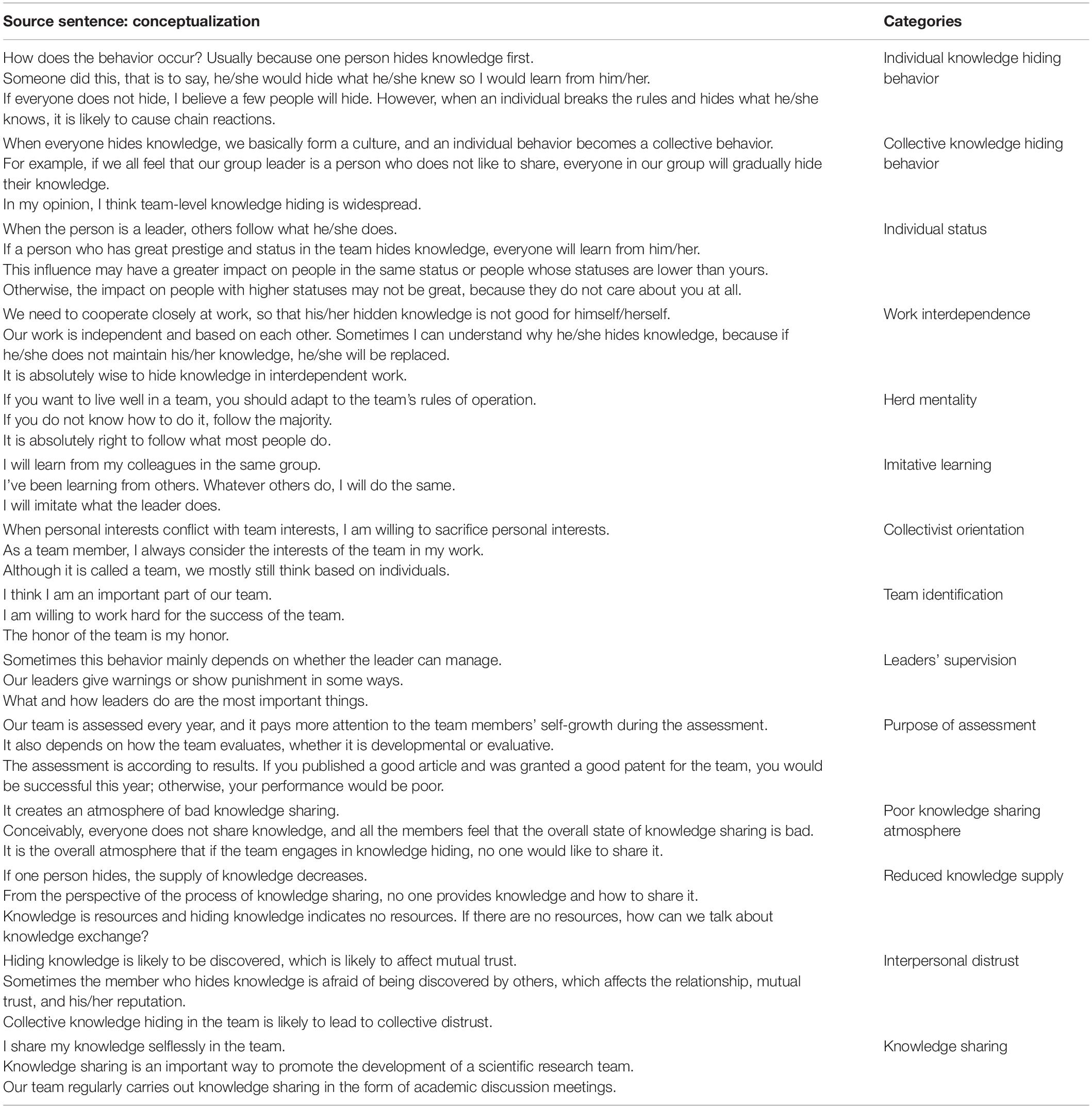
Table 2. Results of categorization.
It is noteworthy that unlike the general idea of data analysis, this study followed the general data analysis strategy of grounded theory research; thus, the analysis was carried out quickly after data collection, so that on the one hand, the understanding error during analysis can be smaller and the analysis can truly reflect the interviewees’ thoughts. On the other hand, it can also provide support for the next cycle of data collection and analysis. Finally, after completing the interviews with the 31 members of the scientific research team, there were no more new concepts and relations among the concepts, and the theory was essentially saturated.
Axial Coding
After the open coding is completed, the study enters the axial coding stage. Axial coding is a process of organizing related categories around an “axis,” and it is also a process of deepening the cognition of scattered categories ( Kendall, 1999 ). The primary goal in this stage is to develop the theory comprehensively. In order to achieve this goal, all categories were reorganized based on conceptual levels, dimensions, and characteristics through self-questioning. Moreover, through the common paradigm model of axial coding, the correlation between the various categories was analyzed, and the category levels, dimensions, and features were then classified. The classification results are presented in Table 3 .
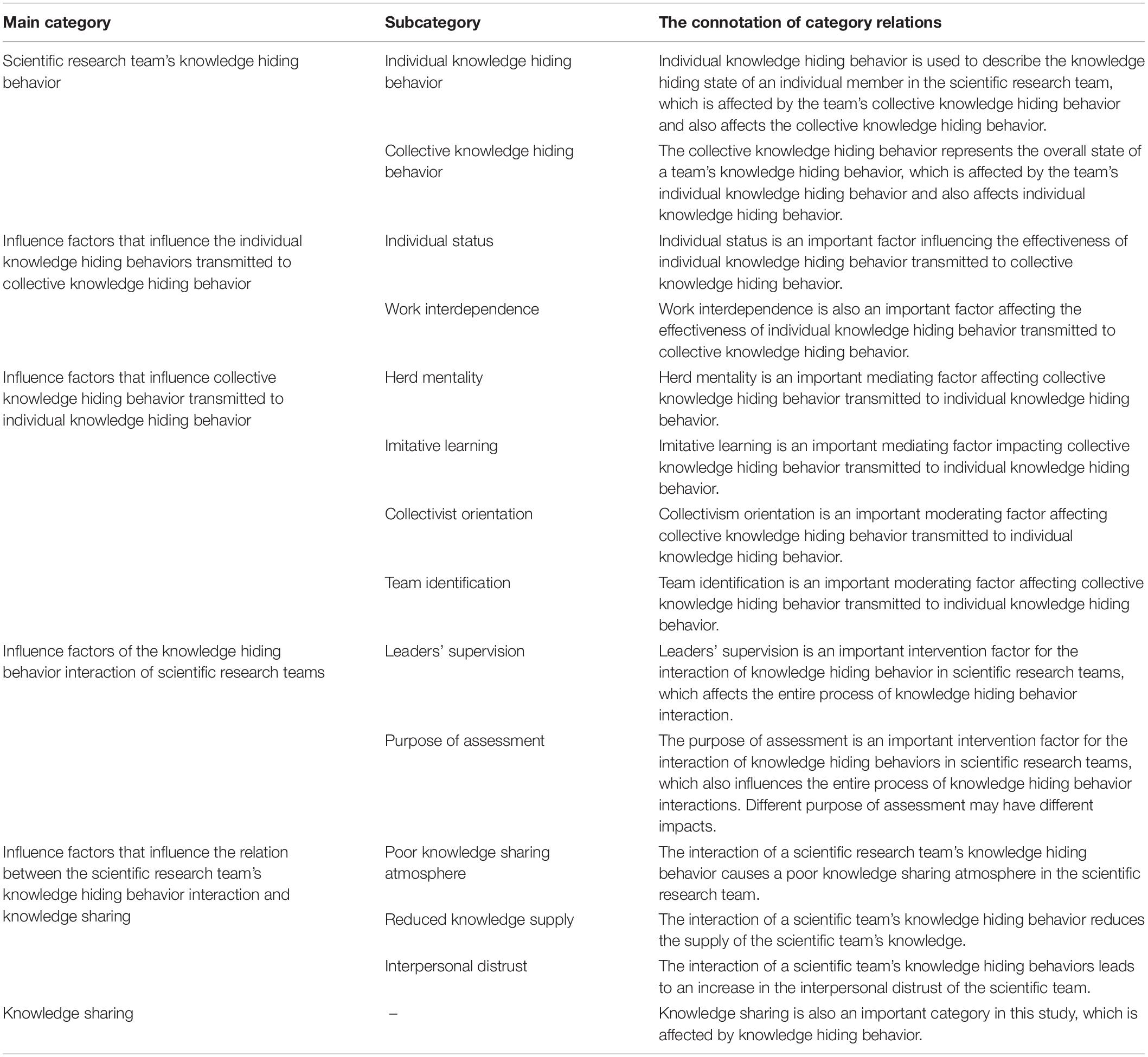
Table 3. Results of axial coding.
(1) The formation of the main category of knowledge hiding behavior of the scientific research team: The interview data show that there are two types of knowledge hiding behaviors observed during the knowledge hiding behavior cross-level interaction within scientific research teams. One is for the individual members, namely, individual knowledge hiding behavior. For example, the interview data show, “Someone did this, that is to say he/she would hide what he/she knew, so I would learn from him/her.” The second is the collective knowledge hiding behavior of the team. Collective knowledge hiding behavior refers to the total amount of knowledge hiding that occurs within the team that is relevant, rather than the dispersion or variability in the hiding that occurs ( Černe et al., 2015 ). The interview data, “When everyone hides knowledge, we basically form a culture, and an individual behavior becomes a collective behavior,” also indicate that individual knowledge hiding behavior is the inducement of collective knowledge hiding behavior, which often leads to the diffusion of individual knowledge hiding to collective knowledge hiding. Conversely, when collective knowledge hiding behavior appears, members in the team may consciously follow the overall norms of the team, thereby strengthening or promoting the generation of individual knowledge hiding behaviors.
(2) The formation of the category of influence factors that influence individual knowledge hiding behavior transmitted to collective knowledge hiding behavior: The interview data show that individual knowledge hiding behavior is transferred to collective knowledge hiding behavior, and this transmission is often affected by the status of the individual and the interdependence of the team work. For example, the interviewee said, “If a person who has great prestige and status in the team hides knowledge, everyone will learn from him/her.” Another interviewee said, “Our work is independent and based on each other. Sometimes I can understand why he/she hides knowledge because if he/she does not maintain his/her knowledge, he/she will be replaced.”
(3) The formation of the category of influence factors that influence collective knowledge hiding behavior transmitted to individual knowledge hiding behavior: the interview data also show that collective knowledge hiding behavior is transmitted to individual knowledge hiding behavior, and this transmission is often affected by four influencing factors, namely, herd mentality, imitative learning, collectivism orientation, and team identification. For example, one interviewee said, “It is absolutely right to follow what most people do.” Another interviewee indicated the importance of imitative learning: “I’ve been learning from others. Whatever others do, I will do the same.” Each factor plays a significantly different role, among which imitative learning and herd mentality play a mediating role in collective knowledge hiding behaviors transmitted to individual knowledge hiding behaviors; however, collectivism orientation and team identification play a moderating role.
(4) The formation of the category of interactive influence factors for the knowledge hiding behavior of the scientific research team: The interview data show that the scientific research team’s knowledge hiding behavior is a two-stage model that includes the individual knowledge hiding behavior transmitted to the collective knowledge hiding behavior and the collective knowledge hiding behavior transmitted to the individual knowledge hiding behavior. In the two-stage model, each has its own influencing factors. Moreover, there are also some factors that can directly affect the two stages. The interview data reveal that leaders’ supervision and the purpose of assessment are the most important factors influencing the social interaction of knowledge hiding behaviors in scientific research teams. For example, the interviewees say, “Our leaders give warnings or show punishment in some ways,” and “The assessment is according to results. If you published a good article and was granted a good patent for the team, you would be successful this year, otherwise your performance would be poor.”
(5) The formation of the categories of influence factors that influence the relationship between the scientific research team’s knowledge hiding behavior interaction and knowledge sharing: interview materials indicate that the scientific knowledge team’s knowledge hiding behavior interaction can negatively impact knowledge sharing. The impact mainly works through the following three aspects, namely, the poor knowledge sharing atmosphere (interview materials: Conceivably, everyone does not share knowledge, and all the members feel that the overall state of knowledge sharing is bad ), reduction of the supply of knowledge (interview materials: Knowledge is resources and hiding knowledge indicates no resources. If there are no resources, how can we talk about knowledge exchange? ), and interpersonal distrust (interview material: Collective knowledge hiding in the team is likely to lead to collective distrust ).
(6) Knowledge sharing: the interview data show that knowledge sharing is also an important concept in this study. Through the analysis of interview data, we found that there is no other concept that can be classified into a category with knowledge sharing; thus, we regard it as an independent category.
Selective Coding
Selective coding is the process of systematically collating qualitative data and information and realizing theoretical construction and development. Moreover, it is also the ultimate foothold for considering the data analysis of grounded theory ( Hernandez, 2009 ). Its main objective is to sort out the scattered main categories, discover the relationship between the main categories, further condense the core categories of research, and finally construct a complete theoretical process around the core categories. When grounded theory is utilized for analysis, the selection of core categories often has its own specific criteria. In order to determine the core category of this research, we again reviewed the research results of open coding and axial coding, and we kept asking the following questions: “What is the relationship between the main categories?”; “Which problem is the core of the research that is at the center position of the materials?”; “Which problem can provide the abstract expression of all main and sub-categories?”; and “Which category can be changed without changing the interviewees?” In the process of looking for the answers to these four questions by studying the interview records, the authors gradually discovered and clarified the core category of this research: “The interactive mechanism of knowledge hiding behaviors in scientific research teams between individual knowledge hiding behavior and collective knowledge hiding behavior and its impact on knowledge sharing.”
After the core category was determined, it was necessary to describe and depict the complex relationship between various categories in the form of a “story line” ( Corbin and Strauss, 1990 ; Strauss and Corbin, 1997 ). The process of the complete description and depiction of a “story” is the process of final theoretical development. The relationship between the various categories is presented in Table 4 and Figure 1 .
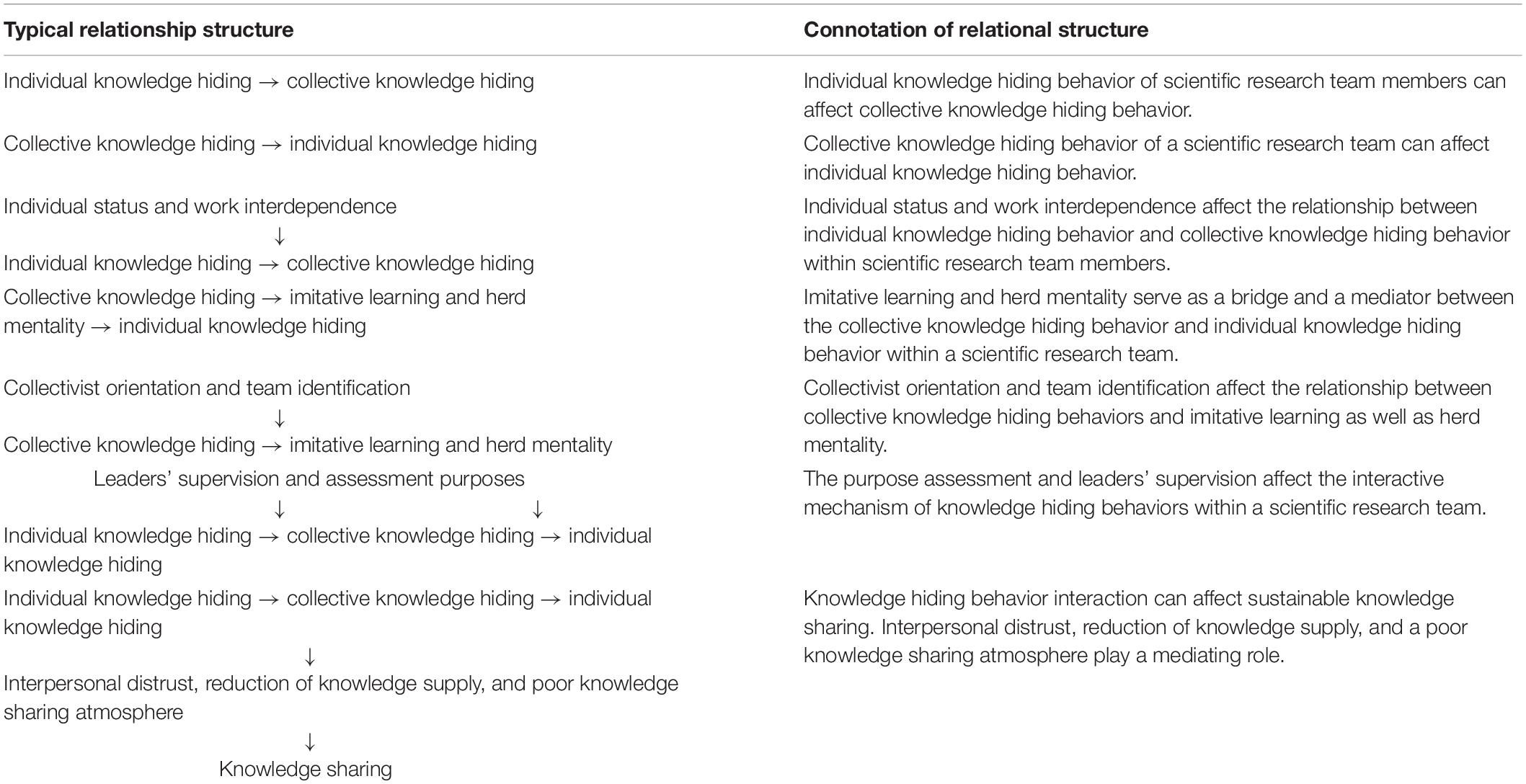
Table 4. Results of selective coding.
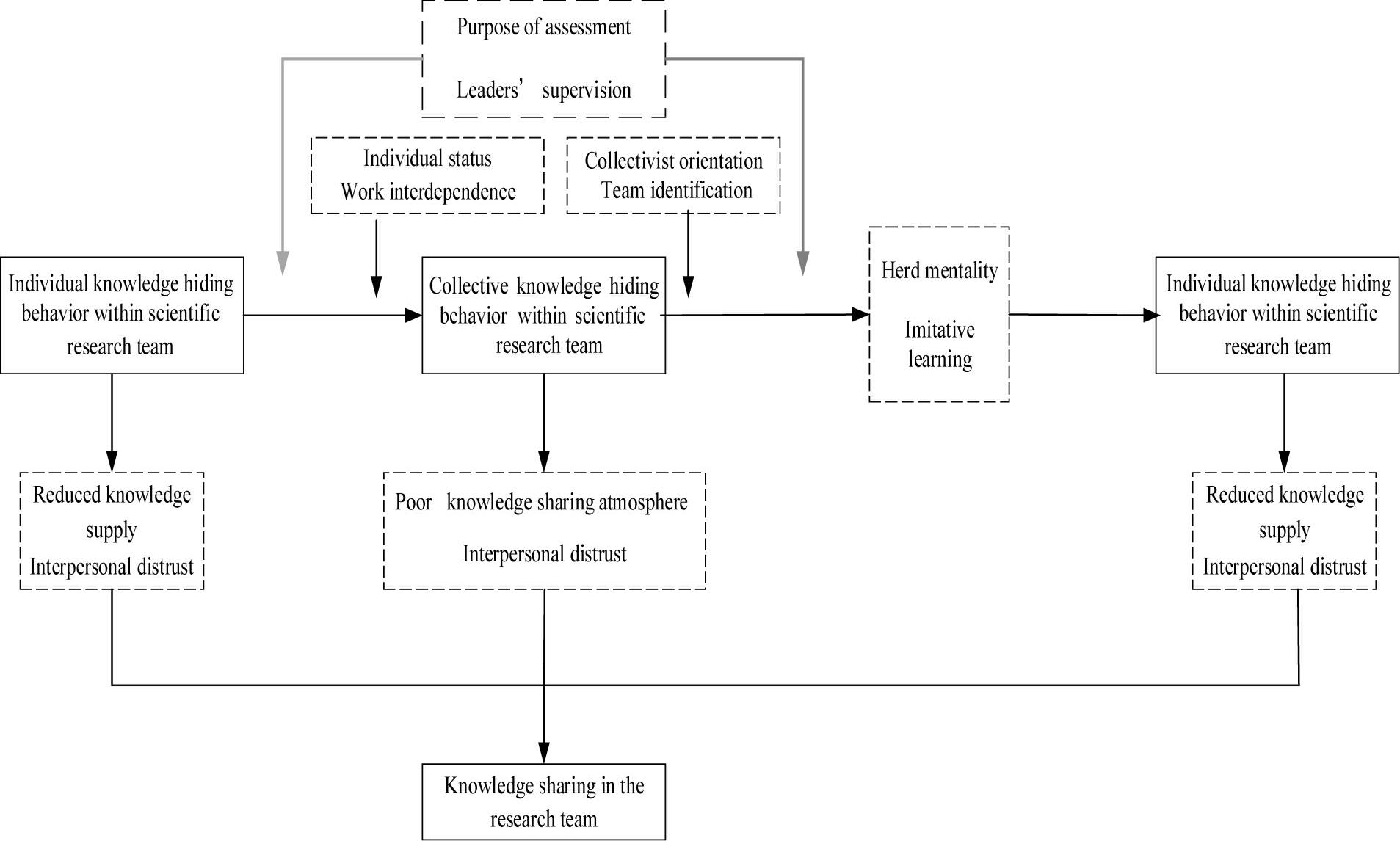
Figure 1. Theoretical model of a scientific research team’s knowledge hiding behavior interaction and its impact on knowledge sharing.
By integrating the research process and conclusion of the steps of the axis coding and selective coding mentioned above, we further tried to integrate the specific pairs of selective coding to build a conceptual model of the interactive mechanism of knowledge hiding behaviors in scientific research teams and its impact on knowledge sharing (see Figure 1 ).
This study indicates that the scientific research team’s knowledge hiding behavior interaction is a two-stage interaction model. The first stage is “the interaction of the scientific research team’s individual knowledge hiding behavior and collective knowledge hiding behavior.” In this stage, the individual’s knowledge hiding behavior spreads to the collective knowledge hiding behavior, and the effectiveness of the diffusion is affected by individual status and work interdependence. Our interview record supports this view: “When everyone hides knowledge, we basically form a culture, and an individual behavior becomes a collective behavior.” This is also similar to the related research on the “bad apple” effect by related scholars ( Gino et al., 2009 ). Our study also indicates that if the status of an individual with knowledge concealment is higher, the behavior is more likely to be imitated by team members; in contrast, the impact will be smaller, which is similar to the study of status or power. Our interview record also supports this view: “If a person who has great prestige and status in the team hides knowledge, everyone will learn from him/her.” This is consistent with the theoretical assumption of knowledge as power ( Mudambi and Navarra, 2004 ). Moreover, our research also finds that work interdependence also influences the excessive transfer of individual knowledge hiding behavior to collective knowledge hiding behavior, which can be supported by the interviewee. For example, one interviewee said, “Our work is independent and based on each other. Sometimes I can understand why he/she hides knowledge because if he/she does not maintain his/her knowledge, he/she will be replaced.” This is in line with the general assumptions of social learning theory ( Bandura, 1977 ) and social interaction theory ( Ben-Sira, 1976 ).
The second stage is the “interaction between the collective knowledge hiding behavior and the individual knowledge hiding behavior of the scientific research team.” At this stage, the knowledge hiding behavior of the scientific research team is also transmitted to the individual, which is consistent with the behavior of peer influence discussed by related scholars in the field of organizational behavior ( Gino et al., 2009 ). The study also found that this contagious process is mediated by herd mentality and imitative learning. Herd mentality is an important concept in the field of psychological research ( Vishwanath and Scamurra, 2007 ). Team members with herd mentality are more likely to accept team-level influences. Our interview record supports this view: “If you do not know how to do it, follow the majority.” However, imitative learning is an important way for team members to learn organizational behaviors. The conclusions of the study are consistent with the relevant results of the social learning theory. Furthermore, interviewees also indicated that the mediating role of herd mentality and imitative learning is also affected by collectivist orientation and team identification. The more obvious the collectivist orientation, the more the team identification, the stronger their relationship, and vice versa.
Moreover, the study also found that the magnitude of the effect of the two stages is affected by the leaders’ supervision and purpose of assessment. The interview data indicate that leaders’ supervision is likely to cut off the influence of the individual knowledge hiding behavior on the collective knowledge hiding behavior, and it can also cut off the influence of the collective knowledge hiding behavior on the individual knowledge hiding behavior. This result is consistent with previous research conclusions on unethical behavior and organizational behaviors, such as corruption. Furthermore, the objective of different types of assessments can also prevent or promote the interaction of cross-level knowledge hiding behavior. This also shows inner consistency in the conclusions of scholars referring to the relationship between knowledge hiding behavior and creativity ( Černe et al., 2014 ).
The study found that the knowledge hiding behavior interaction has a negative impact on knowledge sharing. The impact is reflected in the following three factors. First, under the influence of the “bad apple” when the individual knowledge hiding behavior occurs in a scientific research team, the first manifestation is the reduction of individual knowledge supply, which is consistent with the findings of research on social exchange theory; that is, the most important reason for the failure of knowledge sharing is insufficient knowledge supply. This view is supported by the interviewee. For example, an interviewee said, “Knowledge is resources, and hiding knowledge indicates no resources. If there are no resources, how can we talk about knowledge exchange?” Second, interpersonal distrust is another important factor affecting the relationship between knowledge hiding behavior and knowledge sharing, which is supported by the interview record: “Sometimes the member who hides knowledge is afraid of being discovered by others, which affects the relationship, mutual trust, and his/her reputation.” This result is similar to the research findings of Connelly et al. (2012) . That is, knowledge hiding behavior generates a state of interpersonal distrust and further affects knowledge exchange among members and leads to the failure of knowledge sharing. In addition, poor knowledge sharing atmosphere is a third important factor. In other words, a scientific research team with a high level of team knowledge hiding behaviors naturally creates a poor knowledge sharing atmosphere. When a poor knowledge sharing atmosphere is created within a team, knowledge sharing is difficult to sustain. Our interview records support this view, such as the following: “Conceivably, everyone does not share knowledge, and all the members feel that the overall state of knowledge sharing is bad”.
Theoretical Contributions and Practical Implications
This research makes a significant contribution to the existing studies on knowledge hiding behavior by constructing a cross-level interactive cycle model between individual knowledge hiding behavior and collective knowledge hiding behavior within the scientific research team. Current studies show that knowledge hiding behavior not only occurs at the dyadic level but also occurs in teams and forms collective-level knowledge hiding behavior ( Černe et al., 2015 ). However, to date, research on the interactive relationship between individual-level knowledge hiding behavior and collective-level knowledge hiding behavior is still limited ( Connelly et al., 2019 ). Therefore, this research attempts to fill this gap. Our research shows that individual knowledge hiding behavior in scientific research teams will promote the formation of knowledge hiding behavior at the collective level, and its role is mainly affected by the individual status of the knowledge hiding person and the work interdependence within the team; that is, the higher the status of the implementer of knowledge hiding behavior, the easier it is for individual knowledge hiding behavior to form collective knowledge hiding behavior. Different from the role of individual status, the interview data show the influence of work interdependence on two different directions between individual and collective knowledge hiding. Similarly, collective knowledge hiding behavior will also promote the formation of individual knowledge hiding behavior, and this role is mainly played through the mediating effect of herd mentality and imitative learning. In addition, the research results show that the role of herd mentality and imitative learning will be affected by collectivism orientation and team identification; thus, the higher the collectivism orientation and team identification of individuals in the team, the more vulnerable individuals are to collective knowledge hiding behavior, thereby producing individual knowledge hiding behavior. Moreover, we have also identified two important influencing factors of the cross-level interaction cycle of individual knowledge hiding behavior and collective knowledge hiding behavior, that is, leaders’ supervision and the purpose of assessment.
This research is also helpful in understanding the relationship between different levels of knowledge hiding behavior and sustainable knowledge sharing within the research team. Current studies have fully demonstrated the importance of knowledge sharing and extensively discussed the antecedents of knowledge sharing ( Anand and Jain, 2014 ; Pan et al., 2018 ; Hernaus et al., 2019 ). Among these antecedents, scholars have noticed that knowledge hiding behavior weakens the sustainable knowledge sharing behavior within the team and verified the important role of distrust, which is consistent with our research conclusions ( Connelly et al., 2012 ); that is, interpersonal distrust is an important factor affecting the relationship between knowledge hiding behavior and knowledge sharing. In addition, we have also identified another two important factors that affect knowledge hiding behavior and knowledge sharing; that is, knowledge hiding behavior affects knowledge sharing within the team by reducing knowledge supply and forming a poor knowledge sharing atmosphere. These research findings will deepen our understanding of the antecedents of knowledge sharing as well as the relationship between knowledge hiding behavior and knowledge sharing.
Our study also has several implications for scientific research team managers. First, the research finds that individual knowledge hiding behavior will promote the formation of collective knowledge hiding behavior. Therefore, when individual knowledge hiding behavior takes place in teams, it is necessary to intervene in the individual knowledge hiding behavior in time to hinder the formation of collective knowledge hiding behavior. In addition, compared with individuals with low team status, scientific research team managers also need to pay more attention to knowledge hiding individuals with higher team status. Second, the study found that collective knowledge hiding behavior will affect individual knowledge hiding behavior. This effect depends on the imitative learning and herd mentality of individual team members. Further research also found that collectivist orientation and team identification are key regulatory factors that affect imitative learning and herd mentality. Therefore, when a team is in a state of high collective knowledge hiding behavior, team managers need to take diversified measures to cultivate the individuality of team members; create a good atmosphere of innovation and fault tolerance and thereby weaken the collective orientation, team identification, imitative learning, and herd mentality; and ultimately prevent the spread of collective knowledge hiding behavior to individual knowledge hiding behavior. Third, our research also found that the leader’s supervision and the purpose of assessment are important factors that affect the cross-level interaction cycle of individual knowledge hiding behavior and collective knowledge hiding behavior. Therefore, managers of scientific research teams should be mindful of knowledge hiding behavior to timely discover, intervene, and take charge of knowledge hiding behavior. Furthermore, scientific research team managers should also set a reasonable purpose for assessment. Through adjustments to the purpose of assessment, scientific research team managers could hinder the cross-level interaction between individual knowledge hiding behavior and collective knowledge hiding behavior in a timely manner. Finally, the research results show that knowledge hiding behavior mainly weakens sustainable knowledge sharing within the team by reducing knowledge supply, forming interpersonal distrust, and establishing a poor knowledge sharing atmosphere. Therefore, team managers need to formulate good knowledge sharing incentive policies to increase the supply of knowledge and reduce the impact of knowledge hiding on knowledge sharing. In addition, team managers should make efforts to establish trust relationships within the scientific research team. Managers also need to take measures to build effective knowledge sharing policies and constitute knowledge sharing information systems in order to promote the team to form a good knowledge sharing atmosphere and further weaken the influence of knowledge hiding behavior on sustainable knowledge sharing.
Limitations and Suggestions for Future Research
Undeniably, this article has certain limitations. First, this study does not focus on the knowledge interaction between different individuals or different scientific research teams during the implementation of knowledge hiding behavior. This is mainly attributed to the fact that this study mainly explores from the perspective of the individual-collective interaction. Nonetheless, the interaction of individual-to-individual knowledge hiding behaviors exists, and related research studies have been conducted by Connelly et al. (2012) and Connelly and Zweig (2015) . Moreover, the research samples in this study are mainly based on Chinese scientific research teams, which have certain geographical limitations, and the distribution of samples can be further expanded in the future. Moreover, related scholars have pointed out that knowledge hiding behavior can be divided into different specific types, and the impact of different specific types of knowledge hiding behaviors may vary; however, the differences among specific knowledge hiding behaviors were not explored herein. More explorations can be conducted in the future to divide knowledge hiding behaviors into different types and to explore the differences in the interaction mechanisms between different types of individual knowledge hiding behavior and collective knowledge hiding behavior and the differences in their impact on knowledge sharing. In addition, our findings and conclusions are mainly based on interview data and are still subjective compared to quantitative research. Therefore, it is necessary to conduct quantitative research in the future to increase the reliability and validity of the research results.
Based on the research objectives, 31 representative members of 9 scientific research teams were selected to participate in semi-structured interviews to collect qualitative data on knowledge hiding behavior. Subsequently, the qualitative data were obtained through three necessary steps, namely, open coding, axis coding, and selective coding, to gradually reveal the interactive process of knowledge hiding behaviors in scientific research teams between individual knowledge hiding behavior and collective knowledge hiding behavior and to clarify the complex influence on knowledge sharing. The results show that the scientific research team’s knowledge hiding behavior interaction is a two-stage cross-level interaction model. The effect of the first stage is influenced by individual status and work interdependence; however, the effect of the second stage is influenced by herd mentality, imitative learning, collectivism orientation, and team identification. Furthermore, the study also found that the purpose of assessment and leaders’ supervision affect both phases simultaneously, resulting in effective intervention or inhibition. Additionally, the results also revealed that knowledge hiding behavior can affect the sustainable knowledge sharing of the research team by reducing the supply of knowledge, creating a poor knowledge sharing atmosphere, and forming an interpersonal distrust relationship. This research can also provide a basis for a deeper understanding of the interaction mechanism of knowledge hiding behavior and its impact on knowledge sharing.
Data Availability Statement
The raw data supporting the conclusions of this article will be made available by the authors, without undue reservation, to any qualified researcher.
Ethics Statement
This study was approved by the Institutional Review Board of Institute of China Innovation and Entrepreneurship Education at Wenzhou Medical University and each participant signed the informed written consent.
Author Contributions
FL contributed to research design, data analysis, and manuscript writing. YL provided efforts on research design. PW contributed to research design and manuscript writing and provided quality assurance of the research. All authors contributed to the article and approved the submitted version.
This study was supported by Zhejiang Philosophy and Social Sciences Key Research Base [Institute of China Innovation and Entrepreneurship Education of Wenzhou Medical University (Number 20JDZD042)].
Conflict of Interest
The authors declare that the research was conducted in the absence of any commercial or financial relationships that could be construed as a potential conflict of interest.
Acknowledgments
We are indebted to the reviewers, the editor, and Editage for language assistance.
Anand, P., and Jain, K. (2014). Big five personality types & knowledge hiding behaviour: a theoretical framework. Arch. Bus. Res. 2, 47–56. doi: 10.14738/abr.25.355
CrossRef Full Text | Google Scholar
Bandura, A. (1977). Social Learning Theory. Englewood Cliffs, NJ: Prentice-Hall.
Google Scholar
Benoliel, J. Q. (1996). Grounded theory and nursing knowledge. Qual. Health Res. 6, 406–428. doi: 10.1177/104973239600600308
Ben-Sira, Z. (1976). The function of the professional’s affective behavior in client satisfaction: a revised approach to social interaction theory. J. Health Soc. Behav. 17, 3–11. doi: 10.2307/2136462
Bogilović, S., Černe, M., and Škerlavaj, M. (2017). Hiding behind a mask?Cultural intelligence, knowledge hiding, and individual and team creativity. Eur. J. Work Organ. Psychol. 26, 710–723. doi: 10.1080/1359432X.2017.1337747
Černe, M., Babic, K., Connelly, C. E., and Skerlavaj, M. (2015). Team-level knowledge hiding, social leader-member exchange, and prosocial motivation. Acad. Manage. Annu. Meeting Proc. 1:16302. doi: 10.5465/ambpp.2015.16302abstract
Černe, M., Nerstad, C. G. L., Dysvik, A., and Škerlavaj, M. (2014). What goes around comes around: knowledge hiding, perceived motivational climate, and creativity. Acad. Manage. J. 57, 172–192. doi: 10.5465/amj.2012.0122
Charmaz, K. (1996). “The search for meanings – grounded theory,” in Rethinking Methods in Psychology , eds J. A. Smith, R. Harre, and L. Van Langenhove (London: Sage).
Choi, S. Y., Lee, H., and Yoo, Y. (2010). The impact of information technology and transactive memory systems on knowledge sharing, application, and team performance: a field study. MIS Q. 34, 833–854. doi: 10.2307/25750708
Connelly, C. E., Černe, M., Dysvik, A., and Škerlavaj, M. (2019). Understanding knowledge hiding in organizations. J. Organ. Behav. 40, 779–782. doi: 10.1002/job.2407
Connelly, C. E., and Zweig, D. (2015). How perpetrators and targets construe knowledge hiding in organizations. Eur. J. Work Organ. Psychol. 24, 479–489. doi: 10.1080/1359432X.2014.931325
Connelly, C. E., Zweig, D., Webster, J., and Trougakos, J. P. (2012). Knowledge hiding in organizations. J. Organ. Behav. 33, 64–88. doi: 10.1002/job.737
Corbin, J. M., and Strauss, A. (1990). Grounded theory research: procedures, canons, and evaluative criteria. Qual. Sociol. 13, 3–21. doi: 10.1007/BF00988593
Creswell, J. W. (2013). Steps in Conducting a Scholarly Mixed Methods Study. In Discipline-based Education Research Speaker Series. Paper 48. Available online at https://digitalcommons.unl.edu/cgi/viewcontent.cgi?article=1047&context=dberspeakers (accessed January 21, 2017).
Danielsson, L., Elf, M., and Hensing, G. (2019). Strategies to keep working among workers with common mental disorders–a grounded theory study. Disabil. Rehabil. 41, 786–795. doi: 10.1080/09638288.2017.1408711
PubMed Abstract | CrossRef Full Text | Google Scholar
Furnham, A. (1986). Response bias, social desirability and dissimulation. Pers. Individ. Differ. 7, 385–400. doi: 10.1016/0191-8869(86)90014-0
Gagné, M., Tian, A. W., Soo, C., Zhang, B., Ho, K. S. B., and Hosszu, K. (2019). Different motivations for knowledge sharing and hiding: the role of motivating work design. J. Organ. Behav. 40, 783–799. doi: 10.1002/job.2364
Gillham, B. (2000). The Research Interview. London: Continuum.
Gino, F., Ayal, S., and Ariely, D. (2009). Contagion and differentiation in unethical behavior: the effect of one bad apple on the barrel. Psychol. Sci. 20, 393–398. doi: 10.1111/j.1467-9280.2009.02306.x
Glaser, B., and Strauss, A. (1967). The Discovery of Grounded Theory. Chicago, IL: Aldine.
Gloet, M. (2006). Knowledge management and the links to HRM: developing leadership and management capabilities to support sustainability. Manage. Res. News 29, 402–413. doi: 10.1108/01409170610690862
Grunwald, A. (2004). Strategic knowledge for sustainable development: the need for reflexivity and learning at the interface between science and society. Int. J. Foresight Innov. Policy 1, 150–167. doi: 10.1504/ijfip.2004.004619
Hernandez, C. A. (2009). Theoretical coding in grounded theory methodology. Ground Theory Rev. 8, 51–59.
Hernaus, T., Černe, M., Connelly, C., Poloski Vokic, N., and Škerlavaj, M. (2019). Evasive knowledge hiding in academia: when competitive individuals are asked to collaborate. J. Knowl. Manag. 23, 597–618. doi: 10.1108/JKM-11-2017-0531
Huo, W., Cai, Z., Luo, J., Men, C., and Jia, R. (2016). Antecedents and intervention mechanisms: a multi-level study of R&D team’s knowledge hiding behavior. J. Knowl. Manag. 20, 880–897. doi: 10.1108/JKM-11-2015-0451
Jiang, Z., Hu, X., Wang, Z., and Jiang, X. (2019). Knowledge hiding as a barrier to thriving: the mediating role of psychological safety and moderating role of organizational cynicism. J. Organ. Behav. 40, 800–818. doi: 10.1002/job.2358
Johnson, M. P. (2017). Knowledge acquisition and development in sustainability-oriented small and medium-sized enterprises: exploring the practices, capabilities, and cooperation. J. Clean. Prod. 142, 3769–3781. doi: 10.1016/j.jclepro.2016.10.087
Kendall, J. (1999). Axial coding and the grounded theory controversy. Western J. Nurs. Res. 21, 743–757. doi: 10.1177/019394599902100603
Kim, W. C., and Mauborgne, R. (1998). Procedural justice, strategic decision making, and the knowledge economy. Strateg. Manage. J. 19, 323–338. doi: 10.1002/(sici)1097-0266(199804)19:4<323::aid-smj976>3.0.co;2-f
Liu, Y., Keller, R. T., and Shih, H. A. (2011). The impact of team-member exchange, differentiation, team commitment, and knowledge sharing on R&D project team performance. R D Manage. 41, 274–287. doi: 10.1111/j.1467-9310.2011.00636.x
Manuti, A., Impedovo, M. A., and De Palma, P. D. (2017). Managing social and human capital in organizations: communities of practices as strategic tools for individual and organizational development. J. Workplace Learn. 29, 217–234. doi: 10.1108/JWL-07-2016-0062
McCann, T., and Polacsek, M. (2018). Understanding, choosing and applying grounded theory: Part 1. Nurse Res. 26, 36–41. doi: 10.7748/nr.2018.e1592
McElroy, M. W. (2000). Integrating complexity theory, knowledge management and organizational learning. J. Knowl. Manag. 4, 195–203. doi: 10.1108/13673270010377652
Mei, Y. M., Lee, S. T., and Al-Hawamdeh, S. (2004). Formulating a communication strategy for effective knowledge sharing. J. Inf. Sci. 30, 12–22. doi: 10.1177/0165551504041674
Mudambi, R., and Navarra, P. (2004). Is knowledge power? Knowledge flows, subsidiary power and rent-seeking within MNCs. J. Int. Bus. Stud. 35, 385–406. doi: 10.1057/palgrave.jibs.8400093
Nonaka, I., and Takeuchi, H. (1995). The Knowledge-creating Company: How Japanese Companies Create the Dynamics of Innovation. Oxford: Oxford university press.
Nonaka, I., and von Krogh, G. (2009). Tacit knowledge and knowledge conversion: controversy and advancement in organizational knowledge creation theory. Organ. Sci. 20, 635–652. doi: 10.1287/orsc.1080.0412
Pan, W., Zhang, Q., Teo, T. S. H., and Lim, V. K. G. (2018). The dark triad and knowledge hiding. Int. J. Inf. Manage. 42, 36–48. doi: 10.1016/j.ijinfomgt.2018.05.008
Peltokorpi, V., and Hasu, M. (2016). Transactive memory systems in research team innovation: a moderated mediation analysis. J. Eng. Technol. Manage. 39, 1–12. doi: 10.1016/j.jengtecman.2015.11.001
Peng, H. (2013). Why and when do people hide knowledge? J. Knowl. Manag. 17, 398–415. doi: 10.1108/JKM-12-2012-0380
Pieterse, H. J. C. (2011). An open coding analytical model of sermons on poverty with Matthew 25:31-46 as sermon text. Acta Theologica 31, 95–112. doi: 10.4314/actat.v31i1.6
Rhee, Y. W., and Choi, J. N. (2017). Knowledge management behavior and individual creativity: goal orientations as antecedents and in-group social status as moderating contingency. J. Organ. Behav. 38, 813–832. doi: 10.1002/job.2168
Robert, L. Jr., Denis, A., and Hung, Y. T. (2009). Individual swift trust and knowledge-based trust in face-to-face and virtual team members. J. Manage. Inform. Syst . 26, 241–279. doi: 10.2753/MIS0742-1222260210
Robinson, O. C. (2014). Sampling in interview-based qualitative research: a theoretical and practical guide. Qual. Res. Psychol. 11, 25–41. doi: 10.1080/14780887.2013.801543
Sandelowski, M. (1995). Sample size in qualitative research. Res. Nurs. Health 18, 179–183. doi: 10.1002/nur.4770180211
Strauss, A. L., and Corbin, J. (1997). Grounded Theory in Practice. Thousands Oaks, CA: Sage.
Sung, S. Y., and Choi, J. N. (2012). Effects of team knowledge management on the creativity and financial performance of organizational teams. Organ. Behav. Hum. Decis. Process. 118, 4–13. doi: 10.1016/j.obhdp.2012.01.001
Vishwanath, A., and Scamurra, D. S. (2007). Barriers to the adoption of electronic health records: using concept mapping to develop a comprehensive empirical model. Health Inform. J. 13, 119–134. doi: 10.1177/1460458207076468
Watkins, K. E., and Marsick, V. J. (2003). Summing Up: demonstrating the value of an Organization’s learning culture. Adv. Dev. Hum. Resour. 5, 129–131. doi: 10.1177/1523422303005002001
Zellmer-Bruhn, M., and Gibson, C. (2006). Multinational organization context: implications for team learning and performance. Acad. Manage. J. 49, 501–518. doi: 10.5465/AMJ.2006.21794668
Zhao, H., Liu, W., Li, J., and Yu, X. (2019). Leader–member exchange, organizational identification, and knowledge hiding: the moderating role of relative leader–member exchange. J. Organ. Behav. 40, 834–848. doi: 10.1002/job.2359
Zhu, Y., Chen, T., Wang, M., Jin, Y., and Wang, Y. (2019). Rivals or allies: how performance-prove goal orientation influences knowledge hiding. J. Organ. Behav. 40, 849–868. doi: 10.1002/job.2372
Zhuge, H. (2002). A knowledge flow model for peer-to-peer team knowledge sharing and management. Expert Syst. Appl. 23, 23–30. doi: 10.1016/S0957-4174(02)00024-6
Keywords : sustainable development, knowledge sharing, knowledge hiding, behavioral interaction, research teams
Citation: Liu F, Lu Y and Wang P (2020) Why Knowledge Sharing in Scientific Research Teams Is Difficult to Sustain: An Interpretation From the Interactive Perspective of Knowledge Hiding Behavior. Front. Psychol. 11:537833. doi: 10.3389/fpsyg.2020.537833
Received: 25 February 2020; Accepted: 22 September 2020; Published: 08 December 2020.
Reviewed by:
Copyright © 2020 Liu, Lu and Wang. This is an open-access article distributed under the terms of the Creative Commons Attribution License (CC BY) . The use, distribution or reproduction in other forums is permitted, provided the original author(s) and the copyright owner(s) are credited and that the original publication in this journal is cited, in accordance with accepted academic practice. No use, distribution or reproduction is permitted which does not comply with these terms.
*Correspondence: Peng Wang, [email protected]
Disclaimer: All claims expressed in this article are solely those of the authors and do not necessarily represent those of their affiliated organizations, or those of the publisher, the editors and the reviewers. Any product that may be evaluated in this article or claim that may be made by its manufacturer is not guaranteed or endorsed by the publisher.
To read this content please select one of the options below:
Please note you do not have access to teaching notes, the influence of knowledge sharing on innovation.
European Business Review
ISSN : 0955-534X
Article publication date: 18 May 2010
The purpose of this paper is to explore the effects of knowledge sharing on innovation. Two forms of knowledge sharing are examined, knowledge donating and knowledge collecting. In particular, the effects of knowledge donating and collecting on ambidexterity in organizations are also studied, with ambidexterity defined as the simultaneous achievement of exploratory and exploitative innovation.
Design/methodology/approach
Primary data are collected via a questionnaire designed to measure the relationship between knowledge sharing and innovation. Data which were collected from 246 middle and top‐level managers in Turkey was explored by multiple regression analysis.
The results showed that knowledge collecting had a significant effect on all types of innovation and ambidexterity, whereas knowledge donating, involving donating inside and outside the group, did not have any effect on exploratory innovation. It was also observed that in‐group knowledge donating affected both exploitative innovation and ambidexterity.
Research limitations/implications
This paper is limited to Turkish managers. Hence, impact of culture should be considered in future studies. It is advised that future research should be designed for different countries in order to conduct a comparative study.
Practical implications
These results provide some information that is useful to decision makers and managers who are in charge of directing innovation strategies in organizations. The study also emphasizes the importance of effective knowledge management that can improve innovativeness in the organizations.
Originality/value
Studies comprising the relationship between knowledge sharing and innovation types are not abundant in the academic literature. So, the paper provides practical information to a relatively unexplored area.
- Knowledge sharing
- Organizational innovation
- Knowledge capture
Kamaşak, R. and Bulutlar, F. (2010), "The influence of knowledge sharing on innovation", European Business Review , Vol. 22 No. 3, pp. 306-317. https://doi.org/10.1108/09555341011040994
Emerald Group Publishing Limited
Copyright © 2010, Emerald Group Publishing Limited
Related articles
We’re listening — tell us what you think, something didn’t work….
Report bugs here
All feedback is valuable
Please share your general feedback
Join us on our journey
Platform update page.
Visit emeraldpublishing.com/platformupdate to discover the latest news and updates
Questions & More Information
Answers to the most commonly asked questions here

An official website of the United States government
The .gov means it’s official. Federal government websites often end in .gov or .mil. Before sharing sensitive information, make sure you’re on a federal government site.
The site is secure. The https:// ensures that you are connecting to the official website and that any information you provide is encrypted and transmitted securely.
- Publications
- Account settings
Preview improvements coming to the PMC website in October 2024. Learn More or Try it out now .
- Advanced Search
- Journal List
- Health Res Policy Syst

Knowledge sharing in global health research – the impact, uptake and cost of open access to scholarly literature
Elise smith.
1 École de Bibliothéconomie et des Sciences de l’Information, Université de Montréal, Montréal, QC Canada
2 Sciences Humaines Appliquées (Option Bioéthique), Médecine Sociale et Préventive, Montreal, QC Canada
Stefanie Haustein
Philippe mongeon.
3 School of Information Studies, McGill University, Montréal, QC Canada
Valéry Ridde
4 École de Santé Publique, Département de Médecine Sociale et Préventive, Université de Montréal, Montréal, QC Canada
6 Université de Montréal Public Health Research Institute (Institut de Recherche en Santé Publique (IRSPUM)), Université de Montréal, Montréal, QC Canada
Vincent Larivière
5 Observatoire des Sciences et des Technologies (OST - CIRST), Université du Québec à Montréal, Montréal, QC Canada
Associated Data
The data analysed during the current study is available online [ 42 ].
In 1982, the Annals of Virology published a paper showing how Liberia has a highly endemic potential of Ebola warning health authorities of the risk for potential outbreaks; this journal is only available by subscription. Limiting the accessibility of such knowledge may have reduced information propagation toward public health actors who were indeed surprised by and unprepared for the 2014 epidemic. Open access (OA) publication can allow for increased access to global health research (GHR). Our study aims to assess the use, cost and impact of OA diffusion in the context of GHR.
A total of 3366 research articles indexed under the Medical Heading Subject Heading “Global Health” published between 2010 and 2014 were retrieved using PubMed to (1) quantify the uptake of various types of OA, (2) estimate the article processing charges (APCs) of OA, and (3) analyse the relationship between different types of OA, their scholarly impact and gross national income per capita of citing countries.
Most GHR publications are not available directly on the journal’s website (69%). Further, 60.8% of researchers do not self-archive their work even when it is free and in keeping with journal policy. The total amount paid for APCs was estimated at US$1.7 million for 627 papers, with authors paying on average US$2732 per publication; 94% of APCs were paid to journals owned by the ten most prominent publication houses from high-income countries. Researchers from low- and middle-income countries are generally citing less expensive types of OA, while researchers in high-income countries are citing the most expensive OA.
Conclusions
Although OA may help in building global research capacity in GHR, the majority of publications remain subscription only. It is logical and cost-efficient for institutions and researchers to promote OA by self-archiving publications of restricted access, as it not only allows research to be cited by a broader audience, it also augments citation rates. Although OA does not ensure full knowledge transfer from research to practice, limiting public access can negatively impact implementation and outcomes of health policy and reduce public understanding of health issues.
The 2014 Ebola outbreak proved disastrous for nations such as Guinea, Sierra Leone and Liberia, which were already rife with civil unrest [ 1 ]. Yet, in 1982, knowledge that Liberia had a high potential of endemic Ebola had been published in the Annals of Virology [ 2 ]. Local public health institutions and officials were most likely unaware of these findings as they remained hidden behind a paywall in a subscription only journal. This may have contributed to the lack of preventative measures which could have mitigated the severity and magnitude of the eventual outbreak [ 3 ]. During the outbreak, understanding the evolution of the epidemic from an epidemiological standpoint was, in itself, difficult given the lack of investment in data collection, sharing and management [ 4 ].
As mentioned by WHO in their report entitled Research for Universal Health Coverage [ 5 ], accessible knowledge is an important first step in the translation of knowledge from research to policymakers and stakeholders in low- and middle-income countries (LMICs). Although access to knowledge would not of itself have prevented or averted the Ebola epidemic, better informed health officials might have taken timely preventive measures and been better equipped to mitigate risks during and after the outbreak [ 6 ]. Actually, in the recent Zika virus outbreak, research is more readily accessible, providing evidence-based knowledge faster to mitigate immediate and future harms [ 7 ]. This may be due to consensus reached by important stakeholders ( British Medical Journal , the Nature journals, the New England Journal of Medicine , and the seven PLoS journals) during the 2015 WHO consultation promoting sharing of data, results and pre-prints during public health emergencies [ 8 ].
As international healthcare research has evolved over the past several decades, so has the sharing of knowledge. Prior to the 1990s, the involvement of multiple international collaborators was much less prevalent and usually limited to matters of complex disease control (e.g. smallpox) [ 9 ]. Generally, the scope of ‘public health’ was determined by the resource capacity and geographical reach of a specific country or community [ 10 ]. It was common practice for researchers from high-income countries (HICs) to study health issues in LMICs. However, this type of research in the field of ‘international health’ was exclusive in that it rarely included or considered the interests and needs of researchers and communities in LMICs [ 11 ]. Knowledge remained with HIC research groups and was published in subscription journals, held behind ‘paywalls’ – expensive subscriptions or toll access, affordable mainly for HIC researchers and/or institutions. Restricting access to knowledge from public health research that can have direct influence in life or death contexts remains a serious social justice concern [ 12 ].
The contemporary approach to global health research (GHR) promotes partnerships that meaningfully include researchers and communities from LMICs [ 13 ]. Research ethics has developed benchmarks for ethical global research to minimise exploitation of local players by including them and giving them fair recognition in collaborative research partnerships [ 14 ]. Mutual capacity building is encouraged so that researchers from both HICs and LMICs may learn from each other [ 15 , 16 ]. These partnerships can facilitate knowledge translation among the diverse actors in LMIC health research, including researchers, non-governmental organisations and healthcare providers [ 17 ]. The intended outcome is greater health equity on a global scale among people and nations [ 10 ]. Since researchers in GHR are called upon to work in a collaborative fashion for health equity, sharing knowledge on a global scale is of central importance [ 6 , 16 , 18 ].
There are different ways to increase access to published knowledge. In LMICs, there are programmes such as the Health Internetwork Access to Research Initiative (HINARI), an initiative put together by WHO in collaboration with journal publishers, which provides greater access to many different research resources including e-books, textbooks and up to 14,000 journals, many of which are subscription based [ 19 ]. HINARI’s goal is to contribute to improving world health [ 19 ]. HINARI promotes ideals central to GHR, such as furthering equity in research access, but it does have important practical limitations. While HINARI provides free access to research institutions in low-income countries, it still requires that medium-income countries pay ‘low cost fees’ (US$1500 per year) for full access to HINARI resources [ 20 ]. Although these fees are indeed lower than the full price for HINARI resources through subscriptions or toll access, certain institutions – notably those that play many other roles such as healthcare provision and health prevention and promotion – have competing claims for limited funding, may not prioritise research funding within their institution, or may simply not have the necessary funding.
Moreover, since HINARI is a voluntary programme, publishers may choose to opt out or restrict free access status to specific countries; this creates uneven and uncertain access for users. This instability was well exemplified in 2011, when five publishers withdrew free access to more than 2500 biomedical and health journals including Elsevier’s Lancet journals from Bangladesh [ 21 ]. Although free access was soon reinstated after public outcry, the sustainability of such initiatives led by for-profit publishers remains questionable [ 22 ]. In fact, a similar withdrawal of access to various publishers in Nigeria in 2013 and 2014 has had the effect of reducing HINARI users in Nigeria [ 23 ]. It is noteworthy that the subscription journals are published almost exclusively by Western publishers such as Wiley, Taylor & Francis, Springer Nature, and Elsevier; the consequence of this is a financially induced knowledge inequality focused in the places where the research could have the highest impact.
Open access (OA) is another method where scholarly content is freely available online to all readers. Research has shown that OA is associated with higher citation rates [ 12 , 24 – 26 ], likely as a consequence of wider accessibility. One main issue of certain high-profile OA journals is the existence of significant article processing fees (APCs) paid by authors, which may disadvantage researchers who are unable to cover these costs, emphasising the already significant inequity in research dissemination [ 27 ]. Although many funding institutions or universities may cover APCs [ 28 ], this is not systematically the case, especially in LMICs. To offset this financial barrier, certain journals offer OA waivers for researchers in low-income countries and in certain middle-income countries [ 29 ]; however, criteria to obtain waivers differ based on the journal.
It must be noted at the outset that the OA model has also led to the creation of a number of ‘deceptive publication practices’ often referred to as ‘predatory journals’ that do not follow standard peer-review process and often lack quality and transparency [ 12 ]. To ensure a level of quality control, journals do traditionally have an important role in managing the peer-review system where experts critically review research before it is made public. Regardless of the journal model – whether subscription based or OA – a certain level of peer-review is seen as essential [ 30 ].
Free access to knowledge may also be provided when researchers self-archive their papers, as we often see in public or institutional repositories. The copyright transfer agreements of many journals allow for the archiving of pre-prints and/or post-prints of journal articles, a practice termed ‘green OA’. Journal policies that do not allow for self-archiving often have an embargo period during which they control access to peer-reviewed articles for a specific range of time (generally 6 months to 1 year in journals publishing GHR papers); the impetus behind such embargos is to require institutions to purchase and thus fund subscription-based journals.
Over the last few decades, many studies have analysed the evolution of the OA availability of papers [ 31 – 36 ]. When one combines all different forms of OA, 50% of all biomedical research papers published between 2004 and 2011 were freely available in 2013 [ 24 ]. The same study shows that for the field of Public Health and Health Services, of which GHR can be considered a subfield, the share of OA is slightly higher, with 57.2% [ 24 ]. Given the importance of worldwide knowledge access in GHR, one might expect OA to be more prevalent in this field than in others. However, some may consider APCs to be simply too costly. The goal of this article is to (1) quantify the uptake of various types of OA used in GHR research, (2) calculate the financial costs of such practices from the authors’ point of view (paying for APCs), and (3) assess the impact of different OA models as indicated through citation analysis. Although there exist many other elements that influence the use of OA in GHR, such as journal prestige, general awareness, funder requirements and availability of repositories, these aspects are outside the scope of this specific research. Since the main goal of this paper is to assess the differences between different types of OA models, the comparison of subscription costs paid by university libraries and OA costs known as APCs paid by the author(s) is beyond the scope of this paper.
The PubMed search engine was used to retrieve all research articles indexed under the Medical Subject Heading (MeSH) ‘Global Health’ from 2010 to 2014 [ 37 ]. From 1978 to 2014, research in global health was indexed under ‘International Health’; however, with the increase of institutions, research and journals in GHR [ 38 ], ‘International Health’ was replaced by ‘Global Health’ in 2015. This modification can be explained by the historical shift described at the outset of this paper, in which researchers wished to create global partnerships based on global equity. The analysis is based on 3366 GHR journal articles published in 909 journals. OA availability was defined at journal level (Table 1 ) as well as paper level (Table 2 ).
Definition of access categories at the journal level
APC article processing charge, OA open access
Definition of access categories at the paper level
The OA status of a journal was determined using the Directory of Open Access Journals, Ulrich’s Periodicals Directory and journal lists from Elsevier, Sage, Springer Nature, Taylor & Francis, and Wiley-Blackwell. Due to conflicting and missing information, the status of each journal was verified and APCs retrieved from the journal websites. APCs were collected in or converted to USD. If APCs were not provided in USD, currencies were converted using the mean of weekly historical conversion rates between 1 January 2010 and 31 December 2014 using OANDA [ 39 ]. To understand who benefits financially from OA, we identified publishers of journals (as seen in [ 40 ]). The SHERPA RoMEO database was used to determine whether self-archiving was formally supported or not. Self-archiving may come in different forms, including pre-print (i.e. before peer-review), post-print (i.e. after peer-review) or in the final PDF formatted by the journal publishers. We did not verify which type of manuscript versions were shared (e.g. pre-print, post-print), we only distinguished between journals that did or did not allow self-archiving.
At the paper level, OA availability was determined using the journal level information together with PubMed search as well as a manual Google search for self-archived versions of articles published in subscription journals. Since the main criteria is ‘availability’ of research, we included articles online from various platforms such as institutional repositories (e.g. university, research centre), publicly based repository (e.g. PubMed Central, Europe PMC, SciElo, BIREME), venture-capital based online social networking sites that allows self-archiving (e.g. academia.edu, researchgate.net), and private pages owned by researchers. Since many closed access journals allow for self-archiving, we compared which toll access papers could be self-archived but were not.
A citation analysis was carried out at the paper level to compare the scholarly impact of different types of access categories. Citations were obtained from the Web of Science (WoS), thus restricting the set of papers from 3366 to 2655 papers. These citations were then normalised by year. A normalised citation rate of 1.0 thus indicates that a paper (or a set of papers) was cited according to the expected average citation rate for the set of GHR papers published in a particular year. A citation rate above 1 indicates impact above average and a citation rate below 1 indicates impact below average.
Using institutional affiliations of authors appearing on citing articles, an analysis was conducted to explore whether certain accessibility categories are cited more or less predominantly by various countries depending on their socio-economic context. Countries were categorised into (1) low-, (2) lower middle-, (3) upper middle- or (4) high-income countries [ 41 ]. A citing paper written by authors from different countries was assigned once per World Bank Atlas (WBA) country group. Countries were retrieved manually for 374 citing papers, for which the WoS did not include any address information. Two citing papers were excluded because the authors’ addresses could not be determined and 50 citations from Guadeloupe, Netherlands-Antilles, Palestine and Reunion were excluded because they were not classified by the WBA. The analysis of citing countries was normalised in a way that each paper was weighted equally (as a percentage of citing papers) regardless of their actual number of citations, as we aimed to evaluate how the distribution of countries changed per category. In other words, the over- and under-representation of a WBA group among citations per access category was calculated as the average percentage per paper per access category divided by the overall average percentage of that WBA group. Research was conducted using publicly available data and, as such, is not considered ‘research on human subjects’; such research is exempted from institutional review board ethics approval. Datasets used for this study are available online [ 42 ].
Results and Discussion
Uptake of oa.
From 2010 to 2014, 909 journals published at least one paper indexed as GHR. Figure 1 provides an overview of the frequency of different access categories at the journal (Fig. 1a ) and paper level (Fig. 1b ). Including both those with and without APCs, 18.8% journals and 18.6% papers are available through gold OA. While there are less gold OA journals with an APC, the percentage of papers published in these periodicals (12.0%) exceeds those in gold OA journals without an APC (8.6%), most likely because they are more renowned and have impact factors (IFs) (such as Lancet Global Health , PLOS Medicine and Global Health Action ). The majority of journals (64.2%) are hybrid, meaning that authors can choose to publish research in closed access without financial cost or provide an APC and make the paper OA; still, only 6.8% of papers make use of the hybrid OA option. If immediate OA access is sought via the publisher, GHR researchers seem to choose gold journals over hybrid ones. On the paper level, 69.2% of all GHR publications are not available for free on the publishers’ website. However, 27.2% of toll accessed papers are self-archived (also called green OA) leaving a total of 42.0% of GHR papers only accessible through subscription or toll access.

Percentage of journals ( a ) and articles ( b ) per type of access category
According to the archiving policy recorded by RoMEO, 84.0% of the 700 subscription and hybrid journals allow green OA, while 7.4% explicitly prohibit it (8.9% of the 700 subscription and hybrid journals were not graded by RoMEO). Among RoMEO-graded subscription and hybrid journals, we determined that 733 papers were self-archived in accordance to journal policy and 1139 were not self-archived, despite authors having the possibility of doing so according to the journal policy. This shows that 60.8% of papers that could have been self-archived were not. In a field where OA seems of practical and ethical importance for the sharing of knowledge promoting health equity, it is surprising that researchers do not make their papers available when they are legally able to do so without any cost; this suggest that authors might not be aware of green OA policies.
Figure 2 presents the total APCs required for GHR publications over the 2010–2014 period, by publisher and OA category. The total fees amounted to US$1.7 million for 627 gold OA (APC) and hybrid papers; on average, authors paid US$2732 (SD = US$1090) to make their publication freely available on the publisher’s website. These APCs can be explained by many factors, such as the high scholarly capital associated with publishing in journals of big publishers (which are generally hybrid), as well as the presence of an oligopoly in the academic publishing system [ 40 ]. Such oligopolistic conditions create a limited market, reducing economic competition between publication houses and giving little incentive to decrease prices. More specifically, according to our findings, 93.4% of APCs were paid to journals owned by the 10 most prominent publishing companies. Elsevier alone accounts for 22.8% of the total APCs and charged the highest average gold APCs (on average US$4435 for 69 papers) among all publishers in the GHR set. Their APCs for 26 hybrid fees were lower and close to the GHR average at US$3271 per paper; nevertheless, Elsevier’s hybrid uptake remained low at 3.5%.

Sum of gold and hybrid article processing charges per publisher
Mean OA fees in hybrid journals (US$3240 per paper) are higher than those in gold OA journals (US$2452), which is somewhat surprising given that the former group of journals already has revenues from subscriptions [ 43 ], while APCs are the main source of revenues of the latter. Historically, hybrid journals have justified this double income stream as a way to reduce subscription fees proportionally with the uptake of OA [ 44 ]. However, this fee reduction has been questioned given the lack of transparency of journal costs and the growing fees of both APCs and subscriptions [ 45 ]. This lack of transparency augments the possibility of a phenomenon of ‘double dipping’, in which journals profit from both revenue streams – APCs and subscriptions – without readjusting the price based on APC uptake [ 46 ]. Even though APCs are getting considerably expensive, they continue to be promoted by many important stakeholders and funders making gold and hybrid OA publishing a growing business [ 47 ].
Impact of OA
Figure 3 demonstrates the number of papers and the mean number of citations per type of OA. Articles categorised as delayed, green and hybrid OA are cited above average while toll access and gold OA papers are cited below average. Of particular interest is the difference between green OA (1.5) and toll access (0.7), which shows that self-archived papers receive more than twice as many citations as those hidden behind a paywall, which corroborates previous findings obtained in other fields [ 48 , 49 ]. It should be noted that the green OA articles available on PubMed Central were cited more (1.9) than those deposited on other platforms (1.3).

Citation impact and number of papers per access category
Hybrid articles were cited 37% more than the average GHR paper and twice as much as toll access articles, which supports the previous findings that OA broadens citation impact. However, one has to keep in mind the paramount fees for hybrid publishing, while self-archiving comes at no charge to the author and a higher increase in impact. Although proper IT infrastructure and human resources are necessary to ensure an organised, indexed and sustainable repository, studies show that such costs are meager compared to subscription or gold and hybrid OA (e.g. [ 35 , 50 ]). Articles published in gold OA journals remained cited 40% below the average GHR paper, with no difference between APC and non-APC journals. The fact that the impact of gold OA papers is lower than those published in subscription journals (green, hybrid, toll) can be partly explained by the fact that prestigious journals are largely subscription journals, while many gold OA journals are younger and, thus, are not as prestigious. Journal prestige is an important confounding factor that limits this type of study [ 51 ]. Results for delayed OA and other papers are based on as few as 49 and 16 papers, respectively. Given this limited number, results are inconclusive.
Usage of GHR papers varied according to the socioeconomic situation of countries (Fig. 4 ). Indeed, 3.1% of the 42,479 citing WBA category-cited paper combinations came from low-income countries, 8.5% from lower middle-income, 20.0% from upper middle-income and 68.4% from HICs. Such underrepresentation of researchers from LMICs is well-known [ 52 , 53 ]. Analysing the average share of citing countries per paper, researchers from low-income countries were, on average, 29.0% and 46.9% more likely to cite papers from gold OA journals with and without an APC and 8.6% more likely to cite a green OA paper, while they were underrepresented on papers citing hybrid (–37.4%) and toll access papers (–15.0%). The underrepresentation of LMICs on papers citing hybrid papers show that, even with availability on the publisher’s website, such articles are rarely considered by LMIC researchers. This may simply be the result of subscription journals not traditionally being accessible and thus researchers are not in the habit of searching in such resources. The results for HICs suggest that the type of access has less influence on HIC authors. However, they are underrepresented on papers citing articles published in gold OA journals with (–16.8) and without (–5.8) APCs, which might again be explained by the lower prestige of these journals in comparison to many traditional subscription-based journals.

Over- and underrepresentation of citing countries by World Bank Atlas (WBA) country classification (a, low-income countries; b, lower middle-income; c, upper middle-income; d, high-income countries) and access category. Numbers in parentheses represent the number of papers cited by each country category as well as the number of citing WBA category-cited paper combination
Limitations
The first limitation of this study pertains to the calculation of green OA articles at the paper level. After we compiled the number of PubMed articles that were freely available in green OA format, we performed a manual search on Google to assess other articles that may be freely accessible. We used the author’s name and the title of the article, and then looked at the top ten results to find a green OA article. We found many articles on institutional repositories, publicly based repositories and social media sites. However, there may be some available research that was simply not found by our search method.
The second limitation is regarding the calculation of costs (APCs) paid by authors. In cost calculations, we did not include waivers or institutional discounts. Waivers can be given to cover part or all of APCs where funding is limited, especially in the case where a researcher is affiliated to an institution in a LMIC. Waivers are generally granted on a case by case basis and are not made public. Institutional discounts were also not included in our calculation because they are quite variable based on institution and year. In our study, APC costs were gathered in 2016, but APCs were paid before that time and thus may have been different – most likely slightly lower due to inflation. Given all these factors, the total APCs may be slightly overestimated herein.
The third limitation pertains to the categorisation of citing papers written by authors from different countries once per WBA country group. This decision was made because it is not possible to know how much contribution each author made to the paper. We could (1) fractionalise by number of authors or country group according to WBA classification or (2) count once for each author or country group. Since we analyse on the country level (or country group according to WBA) we do not wish to risk punishing papers with multiple authors that are in more than one country groups.
The fourth limitation is that we decided to compare average citation rate of different access levels without controlling for IF. We decided not to include the IF as it is a flawed indicator already overused in the scientific community [ 54 ]. It is also discipline-specific and not readily comparable between fields. Additionally, in this specific study, the majority of the 909 journals published only one or a few papers; such an analysis would be performed on very small amounts of data. However, herein, we did make the logical assumption that closed journals will most likely have more prestige because prestige is built with time, something that is not yet acquired with novel OA journals. Yet, there is no empirical evidence-based research to validate this last assumption.
This paper explored publication practices of GHR researchers, a field where sharing of knowledge is inherent to its mission of equity in healthcare and essential to its collaborative nature. Regardless of this emphasis on sharing, our research shows that 42.0% of scholarly articles are not freely available online even if many funders, scholars and universities promote some form of OA (mainly green or gold). While it is understandable that researchers gravitate towards traditional, highly reputable journals, it remains sobering to note that only 39.2% of papers published in journals that allow green OA, which comes at no cost for the authors, were in fact self-archived. Findings clearly show that self-archiving does not only promote knowledge sharing but also increases the impact of research. Many reasons could explain this behaviour, such as a lack of knowledge of journals’ self-archiving policies, lack of appropriate user-friendly self-archiving platforms, lack of time or general unawareness of the advantages of green OA (i.e. such as increased impact). Researchers may think that publication in traditional closed (paywalled) journals are sufficient because of initiatives such as HINARI, which provide a certain level of free or low cost access to research for LMIC researchers.
Despite increased access provided by HINARI, LMIC researchers are still underrepresented in citing subscription journals. Our study supports the claim that increased access through green and gold OA is reaching underrepresented researchers more so than subscription journal articles. As such, it provides more research capability that is at the centre of GHR. When researchers are to publish their work in an accessible format it is important to choose an OA type that best suits their needs and not assume that the most expensive APC has the best impact, reach and citability. In fact, hybrid OA journals, which have the most expensive APCs, were the most underrepresented in LMICs. It remains unclear why APCs for hybrid journals remain higher than gold APCs given the fact that these journals also ask for subscription fees.
Since the APCs are mainly paid to the ten same publishers creating an oligopoly, there is little incentive to keep APCs low. This oligopoly may also run much deeper than costs; it creates an important inequity in publication. Although publishers may wish to include researchers from LMICs through waivers, they have not really included LMICs in the publication industry itself. After witnessing significant inequities and issues related to exploitation in global health, the impetus behind GHR was to provide a space for equal partnerships. Broadening these partnerships to the publication industry, which is a significant gatekeeper in research, may provide for a stronger voice for researchers in LMICs with the goal of reducing power inequities in global health more broadly.
Acknowledgements
The authors would like to thank Ilya Razykov for his help in determining the green OA status of papers and finding missing author addresses.
Availability of data and materials
Abbreviations, authors’ contributions.
All authors contributed to the study design. ES, SH, PM and FS completed data collection. ES and SH analysed the data and wrote the initial draft of the article. All authors provided critical commentary. ES and SH contributed equally to this research. All authors read and approved the final manuscript.
Ethics approval and consent to participate
All data was publically accessible and exempt from any institutional board review on human subjects.
Consent for publication
Not applicable.
Competing interests
The authors declare that they have no competing interests.
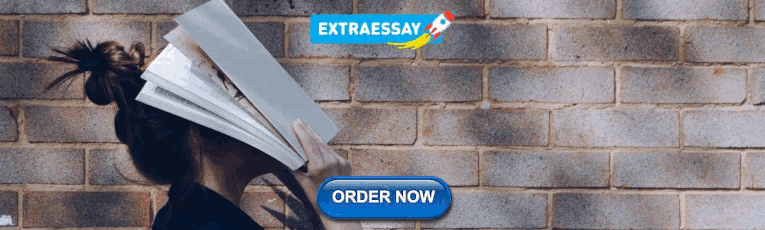
Publisher’s Note
Springer Nature remains neutral with regard to jurisdictional claims in published maps and institutional affiliations.
Contributor Information
Elise Smith, Email: [email protected] .
Stefanie Haustein, Email: [email protected] .
Philippe Mongeon, Email: [email protected] .
Fei Shu, Email: [email protected] .
Valéry Ridde, Email: [email protected] .
Vincent Larivière, Email: [email protected] .
Thank you for visiting nature.com. You are using a browser version with limited support for CSS. To obtain the best experience, we recommend you use a more up to date browser (or turn off compatibility mode in Internet Explorer). In the meantime, to ensure continued support, we are displaying the site without styles and JavaScript.
- View all journals
- Explore content
- About the journal
- Publish with us
- Sign up for alerts
- Perspective
- Published: 06 March 2024
Artificial intelligence and illusions of understanding in scientific research
- Lisa Messeri ORCID: orcid.org/0000-0002-0964-123X 1 na1 &
- M. J. Crockett ORCID: orcid.org/0000-0001-8800-410X 2 , 3 na1
Nature volume 627 , pages 49–58 ( 2024 ) Cite this article
21k Accesses
3 Citations
708 Altmetric
Metrics details
- Human behaviour
- Interdisciplinary studies
- Research management
- Social anthropology
Scientists are enthusiastically imagining ways in which artificial intelligence (AI) tools might improve research. Why are AI tools so attractive and what are the risks of implementing them across the research pipeline? Here we develop a taxonomy of scientists’ visions for AI, observing that their appeal comes from promises to improve productivity and objectivity by overcoming human shortcomings. But proposed AI solutions can also exploit our cognitive limitations, making us vulnerable to illusions of understanding in which we believe we understand more about the world than we actually do. Such illusions obscure the scientific community’s ability to see the formation of scientific monocultures, in which some types of methods, questions and viewpoints come to dominate alternative approaches, making science less innovative and more vulnerable to errors. The proliferation of AI tools in science risks introducing a phase of scientific enquiry in which we produce more but understand less. By analysing the appeal of these tools, we provide a framework for advancing discussions of responsible knowledge production in the age of AI.
This is a preview of subscription content, access via your institution
Access options
Access Nature and 54 other Nature Portfolio journals
Get Nature+, our best-value online-access subscription
24,99 € / 30 days
cancel any time
Subscribe to this journal
Receive 51 print issues and online access
185,98 € per year
only 3,65 € per issue
Rent or buy this article
Prices vary by article type
Prices may be subject to local taxes which are calculated during checkout
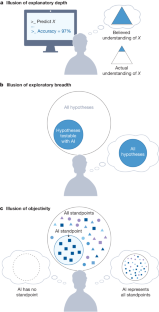
Similar content being viewed by others
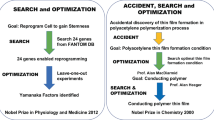
Nobel Turing Challenge: creating the engine for scientific discovery
Hiroaki Kitano
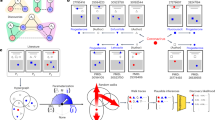
Accelerating science with human-aware artificial intelligence
Jamshid Sourati & James A. Evans
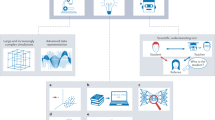
On scientific understanding with artificial intelligence
Mario Krenn, Robert Pollice, … Alán Aspuru-Guzik
Crabtree, G. Self-driving laboratories coming of age. Joule 4 , 2538–2541 (2020).
Article CAS Google Scholar
Wang, H. et al. Scientific discovery in the age of artificial intelligence. Nature 620 , 47–60 (2023). This review explores how AI can be incorporated across the research pipeline, drawing from a wide range of scientific disciplines .
Article CAS PubMed ADS Google Scholar
Dillion, D., Tandon, N., Gu, Y. & Gray, K. Can AI language models replace human participants? Trends Cogn. Sci. 27 , 597–600 (2023).
Article PubMed Google Scholar
Grossmann, I. et al. AI and the transformation of social science research. Science 380 , 1108–1109 (2023). This forward-looking article proposes a variety of ways to incorporate generative AI into social-sciences research .
Gil, Y. Will AI write scientific papers in the future? AI Mag. 42 , 3–15 (2022).
Google Scholar
Kitano, H. Nobel Turing Challenge: creating the engine for scientific discovery. npj Syst. Biol. Appl. 7 , 29 (2021).
Article PubMed PubMed Central Google Scholar
Benjamin, R. Race After Technology: Abolitionist Tools for the New Jim Code (Oxford Univ. Press, 2020). This book examines how social norms about race become embedded in technologies, even those that are focused on providing good societal outcomes .
Broussard, M. More Than a Glitch: Confronting Race, Gender, and Ability Bias in Tech (MIT Press, 2023).
Noble, S. U. Algorithms of Oppression: How Search Engines Reinforce Racism (New York Univ. Press, 2018).
Bender, E. M., Gebru, T., McMillan-Major, A. & Shmitchell, S. On the dangers of stochastic parrots: can language models be too big? in Proc. 2021 ACM Conference on Fairness, Accountability, and Transparency 610–623 (Association for Computing Machinery, 2021). One of the first comprehensive critiques of large language models, this article draws attention to a host of issues that ought to be considered before taking up such tools .
Crawford, K. Atlas of AI: Power, Politics, and the Planetary Costs of Artificial Intelligence (Yale Univ. Press, 2021).
Johnson, D. G. & Verdicchio, M. Reframing AI discourse. Minds Mach. 27 , 575–590 (2017).
Article Google Scholar
Atanasoski, N. & Vora, K. Surrogate Humanity: Race, Robots, and the Politics of Technological Futures (Duke Univ. Press, 2019).
Mitchell, M. & Krakauer, D. C. The debate over understanding in AI’s large language models. Proc. Natl Acad. Sci. USA 120 , e2215907120 (2023).
Kidd, C. & Birhane, A. How AI can distort human beliefs. Science 380 , 1222–1223 (2023).
Birhane, A., Kasirzadeh, A., Leslie, D. & Wachter, S. Science in the age of large language models. Nat. Rev. Phys. 5 , 277–280 (2023).
Kapoor, S. & Narayanan, A. Leakage and the reproducibility crisis in machine-learning-based science. Patterns 4 , 100804 (2023).
Hullman, J., Kapoor, S., Nanayakkara, P., Gelman, A. & Narayanan, A. The worst of both worlds: a comparative analysis of errors in learning from data in psychology and machine learning. In Proc. 2022 AAAI/ACM Conference on AI, Ethics, and Society (eds Conitzer, V. et al.) 335–348 (Association for Computing Machinery, 2022).
Rudin, C. Stop explaining black box machine learning models for high stakes decisions and use interpretable models instead. Nat. Mach. Intell. 1 , 206–215 (2019). This paper articulates the problems with attempting to explain AI systems that lack interpretability, and advocates for building interpretable models instead .
Crockett, M. J., Bai, X., Kapoor, S., Messeri, L. & Narayanan, A. The limitations of machine learning models for predicting scientific replicability. Proc. Natl Acad. Sci. USA 120 , e2307596120 (2023).
Article CAS PubMed PubMed Central Google Scholar
Lazar, S. & Nelson, A. AI safety on whose terms? Science 381 , 138 (2023).
Article PubMed ADS Google Scholar
Collingridge, D. The Social Control of Technology (St Martin’s Press, 1980).
Wagner, G., Lukyanenko, R. & Paré, G. Artificial intelligence and the conduct of literature reviews. J. Inf. Technol. 37 , 209–226 (2022).
Hutson, M. Artificial-intelligence tools aim to tame the coronavirus literature. Nature https://doi.org/10.1038/d41586-020-01733-7 (2020).
Haas, Q. et al. Utilizing artificial intelligence to manage COVID-19 scientific evidence torrent with Risklick AI: a critical tool for pharmacology and therapy development. Pharmacology 106 , 244–253 (2021).
Article CAS PubMed Google Scholar
Müller, H., Pachnanda, S., Pahl, F. & Rosenqvist, C. The application of artificial intelligence on different types of literature reviews – a comparative study. In 2022 International Conference on Applied Artificial Intelligence (ICAPAI) https://doi.org/10.1109/ICAPAI55158.2022.9801564 (Institute of Electrical and Electronics Engineers, 2022).
van Dinter, R., Tekinerdogan, B. & Catal, C. Automation of systematic literature reviews: a systematic literature review. Inf. Softw. Technol. 136 , 106589 (2021).
Aydın, Ö. & Karaarslan, E. OpenAI ChatGPT generated literature review: digital twin in healthcare. In Emerging Computer Technologies 2 (ed. Aydın, Ö.) 22–31 (İzmir Akademi Dernegi, 2022).
AlQuraishi, M. AlphaFold at CASP13. Bioinformatics 35 , 4862–4865 (2019).
Jumper, J. et al. Highly accurate protein structure prediction with AlphaFold. Nature 596 , 583–589 (2021).
Article CAS PubMed PubMed Central ADS Google Scholar
Lee, J. S., Kim, J. & Kim, P. M. Score-based generative modeling for de novo protein design. Nat. Computat. Sci. 3 , 382–392 (2023).
Gómez-Bombarelli, R. et al. Design of efficient molecular organic light-emitting diodes by a high-throughput virtual screening and experimental approach. Nat. Mater. 15 , 1120–1127 (2016).
Krenn, M. et al. On scientific understanding with artificial intelligence. Nat. Rev. Phys. 4 , 761–769 (2022).
Extance, A. How AI technology can tame the scientific literature. Nature 561 , 273–274 (2018).
Hastings, J. AI for Scientific Discovery (CRC Press, 2023). This book reviews current and future incorporation of AI into the scientific research pipeline .
Ahmed, A. et al. The future of academic publishing. Nat. Hum. Behav. 7 , 1021–1026 (2023).
Gray, K., Yam, K. C., Zhen’An, A. E., Wilbanks, D. & Waytz, A. The psychology of robots and artificial intelligence. In The Handbook of Social Psychology (eds Gilbert, D. et al.) (in the press).
Argyle, L. P. et al. Out of one, many: using language models to simulate human samples. Polit. Anal. 31 , 337–351 (2023).
Aher, G., Arriaga, R. I. & Kalai, A. T. Using large language models to simulate multiple humans and replicate human subject studies. In Proc. 40th International Conference on Machine Learning (eds Krause, A. et al.) 337–371 (JMLR.org, 2023).
Binz, M. & Schulz, E. Using cognitive psychology to understand GPT-3. Proc. Natl Acad. Sci. USA 120 , e2218523120 (2023).
Ornstein, J. T., Blasingame, E. N. & Truscott, J. S. How to train your stochastic parrot: large language models for political texts. Github , https://joeornstein.github.io/publications/ornstein-blasingame-truscott.pdf (2023).
He, S. et al. Learning to predict the cosmological structure formation. Proc. Natl Acad. Sci. USA 116 , 13825–13832 (2019).
Article MathSciNet CAS PubMed PubMed Central ADS Google Scholar
Mahmood, F. et al. Deep adversarial training for multi-organ nuclei segmentation in histopathology images. IEEE Trans. Med. Imaging 39 , 3257–3267 (2020).
Teixeira, B. et al. Generating synthetic X-ray images of a person from the surface geometry. In Proc. IEEE Conference on Computer Vision and Pattern Recognition 9059–9067 (Institute of Electrical and Electronics Engineers, 2018).
Marouf, M. et al. Realistic in silico generation and augmentation of single-cell RNA-seq data using generative adversarial networks. Nat. Commun. 11 , 166 (2020).
Watts, D. J. A twenty-first century science. Nature 445 , 489 (2007).
boyd, d. & Crawford, K. Critical questions for big data. Inf. Commun. Soc. 15 , 662–679 (2012). This article assesses the ethical and epistemic implications of scientific and societal moves towards big data and provides a parallel case study for thinking about the risks of artificial intelligence .
Jolly, E. & Chang, L. J. The Flatland fallacy: moving beyond low–dimensional thinking. Top. Cogn. Sci. 11 , 433–454 (2019).
Yarkoni, T. & Westfall, J. Choosing prediction over explanation in psychology: lessons from machine learning. Perspect. Psychol. Sci. 12 , 1100–1122 (2017).
Radivojac, P. et al. A large-scale evaluation of computational protein function prediction. Nat. Methods 10 , 221–227 (2013).
Bileschi, M. L. et al. Using deep learning to annotate the protein universe. Nat. Biotechnol. 40 , 932–937 (2022).
Barkas, N. et al. Joint analysis of heterogeneous single-cell RNA-seq dataset collections. Nat. Methods 16 , 695–698 (2019).
Demszky, D. et al. Using large language models in psychology. Nat. Rev. Psychol. 2 , 688–701 (2023).
Karjus, A. Machine-assisted mixed methods: augmenting humanities and social sciences with artificial intelligence. Preprint at https://arxiv.org/abs/2309.14379 (2023).
Davies, A. et al. Advancing mathematics by guiding human intuition with AI. Nature 600 , 70–74 (2021).
Peterson, J. C., Bourgin, D. D., Agrawal, M., Reichman, D. & Griffiths, T. L. Using large-scale experiments and machine learning to discover theories of human decision-making. Science 372 , 1209–1214 (2021).
Ilyas, A. et al. Adversarial examples are not bugs, they are features. Preprint at https://doi.org/10.48550/arXiv.1905.02175 (2019)
Semel, B. M. Listening like a computer: attentional tensions and mechanized care in psychiatric digital phenotyping. Sci. Technol. Hum. Values 47 , 266–290 (2022).
Gil, Y. Thoughtful artificial intelligence: forging a new partnership for data science and scientific discovery. Data Sci. 1 , 119–129 (2017).
Checco, A., Bracciale, L., Loreti, P., Pinfield, S. & Bianchi, G. AI-assisted peer review. Humanit. Soc. Sci. Commun. 8 , 25 (2021).
Thelwall, M. Can the quality of published academic journal articles be assessed with machine learning? Quant. Sci. Stud. 3 , 208–226 (2022).
Dhar, P. Peer review of scholarly research gets an AI boost. IEEE Spectrum spectrum.ieee.org/peer-review-of-scholarly-research-gets-an-ai-boost (2020).
Heaven, D. AI peer reviewers unleashed to ease publishing grind. Nature 563 , 609–610 (2018).
Conroy, G. How ChatGPT and other AI tools could disrupt scientific publishing. Nature 622 , 234–236 (2023).
Nosek, B. A. et al. Replicability, robustness, and reproducibility in psychological science. Annu. Rev. Psychol. 73 , 719–748 (2022).
Altmejd, A. et al. Predicting the replicability of social science lab experiments. PLoS ONE 14 , e0225826 (2019).
Yang, Y., Youyou, W. & Uzzi, B. Estimating the deep replicability of scientific findings using human and artificial intelligence. Proc. Natl Acad. Sci. USA 117 , 10762–10768 (2020).
Youyou, W., Yang, Y. & Uzzi, B. A discipline-wide investigation of the replicability of psychology papers over the past two decades. Proc. Natl Acad. Sci. USA 120 , e2208863120 (2023).
Rabb, N., Fernbach, P. M. & Sloman, S. A. Individual representation in a community of knowledge. Trends Cogn. Sci. 23 , 891–902 (2019). This comprehensive review paper documents the empirical evidence for distributed cognition in communities of knowledge and the resultant vulnerabilities to illusions of understanding .
Rozenblit, L. & Keil, F. The misunderstood limits of folk science: an illusion of explanatory depth. Cogn. Sci. 26 , 521–562 (2002). This paper provided an empirical demonstration of the illusion of explanatory depth, and inspired a programme of research in cognitive science on communities of knowledge .
Hutchins, E. Cognition in the Wild (MIT Press, 1995).
Lave, J. & Wenger, E. Situated Learning: Legitimate Peripheral Participation (Cambridge Univ. Press, 1991).
Kitcher, P. The division of cognitive labor. J. Philos. 87 , 5–22 (1990).
Hardwig, J. Epistemic dependence. J. Philos. 82 , 335–349 (1985).
Keil, F. in Oxford Studies In Epistemology (eds Gendler, T. S. & Hawthorne, J.) 143–166 (Oxford Academic, 2005).
Weisberg, M. & Muldoon, R. Epistemic landscapes and the division of cognitive labor. Philos. Sci. 76 , 225–252 (2009).
Sloman, S. A. & Rabb, N. Your understanding is my understanding: evidence for a community of knowledge. Psychol. Sci. 27 , 1451–1460 (2016).
Wilson, R. A. & Keil, F. The shadows and shallows of explanation. Minds Mach. 8 , 137–159 (1998).
Keil, F. C., Stein, C., Webb, L., Billings, V. D. & Rozenblit, L. Discerning the division of cognitive labor: an emerging understanding of how knowledge is clustered in other minds. Cogn. Sci. 32 , 259–300 (2008).
Sperber, D. et al. Epistemic vigilance. Mind Lang. 25 , 359–393 (2010).
Wilkenfeld, D. A., Plunkett, D. & Lombrozo, T. Depth and deference: when and why we attribute understanding. Philos. Stud. 173 , 373–393 (2016).
Sparrow, B., Liu, J. & Wegner, D. M. Google effects on memory: cognitive consequences of having information at our fingertips. Science 333 , 776–778 (2011).
Fisher, M., Goddu, M. K. & Keil, F. C. Searching for explanations: how the internet inflates estimates of internal knowledge. J. Exp. Psychol. Gen. 144 , 674–687 (2015).
De Freitas, J., Agarwal, S., Schmitt, B. & Haslam, N. Psychological factors underlying attitudes toward AI tools. Nat. Hum. Behav. 7 , 1845–1854 (2023).
Castelo, N., Bos, M. W. & Lehmann, D. R. Task-dependent algorithm aversion. J. Mark. Res. 56 , 809–825 (2019).
Cadario, R., Longoni, C. & Morewedge, C. K. Understanding, explaining, and utilizing medical artificial intelligence. Nat. Hum. Behav. 5 , 1636–1642 (2021).
Oktar, K. & Lombrozo, T. Deciding to be authentic: intuition is favored over deliberation when authenticity matters. Cognition 223 , 105021 (2022).
Bigman, Y. E., Yam, K. C., Marciano, D., Reynolds, S. J. & Gray, K. Threat of racial and economic inequality increases preference for algorithm decision-making. Comput. Hum. Behav. 122 , 106859 (2021).
Claudy, M. C., Aquino, K. & Graso, M. Artificial intelligence can’t be charmed: the effects of impartiality on laypeople’s algorithmic preferences. Front. Psychol. 13 , 898027 (2022).
Snyder, C., Keppler, S. & Leider, S. Algorithm reliance under pressure: the effect of customer load on service workers. Preprint at SSRN https://doi.org/10.2139/ssrn.4066823 (2022).
Bogert, E., Schecter, A. & Watson, R. T. Humans rely more on algorithms than social influence as a task becomes more difficult. Sci Rep. 11 , 8028 (2021).
Raviv, A., Bar‐Tal, D., Raviv, A. & Abin, R. Measuring epistemic authority: studies of politicians and professors. Eur. J. Personal. 7 , 119–138 (1993).
Cummings, L. The “trust” heuristic: arguments from authority in public health. Health Commun. 29 , 1043–1056 (2014).
Lee, M. K. Understanding perception of algorithmic decisions: fairness, trust, and emotion in response to algorithmic management. Big Data Soc. 5 , https://doi.org/10.1177/2053951718756684 (2018).
Kissinger, H. A., Schmidt, E. & Huttenlocher, D. The Age of A.I. And Our Human Future (Little, Brown, 2021).
Lombrozo, T. Explanatory preferences shape learning and inference. Trends Cogn. Sci. 20 , 748–759 (2016). This paper provides an overview of philosophical theories of explanatory virtues and reviews empirical evidence on the sorts of explanations people find satisfying .
Vrantsidis, T. H. & Lombrozo, T. Simplicity as a cue to probability: multiple roles for simplicity in evaluating explanations. Cogn. Sci. 46 , e13169 (2022).
Johnson, S. G. B., Johnston, A. M., Toig, A. E. & Keil, F. C. Explanatory scope informs causal strength inferences. In Proc. 36th Annual Meeting of the Cognitive Science Society 2453–2458 (Cognitive Science Society, 2014).
Khemlani, S. S., Sussman, A. B. & Oppenheimer, D. M. Harry Potter and the sorcerer’s scope: latent scope biases in explanatory reasoning. Mem. Cognit. 39 , 527–535 (2011).
Liquin, E. G. & Lombrozo, T. Motivated to learn: an account of explanatory satisfaction. Cogn. Psychol. 132 , 101453 (2022).
Hopkins, E. J., Weisberg, D. S. & Taylor, J. C. V. The seductive allure is a reductive allure: people prefer scientific explanations that contain logically irrelevant reductive information. Cognition 155 , 67–76 (2016).
Weisberg, D. S., Hopkins, E. J. & Taylor, J. C. V. People’s explanatory preferences for scientific phenomena. Cogn. Res. Princ. Implic. 3 , 44 (2018).
Jerez-Fernandez, A., Angulo, A. N. & Oppenheimer, D. M. Show me the numbers: precision as a cue to others’ confidence. Psychol. Sci. 25 , 633–635 (2014).
Kim, J., Giroux, M. & Lee, J. C. When do you trust AI? The effect of number presentation detail on consumer trust and acceptance of AI recommendations. Psychol. Mark. 38 , 1140–1155 (2021).
Nguyen, C. T. The seductions of clarity. R. Inst. Philos. Suppl. 89 , 227–255 (2021). This article describes how reductive and quantitative explanations can generate a sense of understanding that is not necessarily correlated with actual understanding .
Fisher, M., Smiley, A. H. & Grillo, T. L. H. Information without knowledge: the effects of internet search on learning. Memory 30 , 375–387 (2022).
Eliseev, E. D. & Marsh, E. J. Understanding why searching the internet inflates confidence in explanatory ability. Appl. Cogn. Psychol. 37 , 711–720 (2023).
Fisher, M. & Oppenheimer, D. M. Who knows what? Knowledge misattribution in the division of cognitive labor. J. Exp. Psychol. Appl. 27 , 292–306 (2021).
Chromik, M., Eiband, M., Buchner, F., Krüger, A. & Butz, A. I think I get your point, AI! The illusion of explanatory depth in explainable AI. In 26th International Conference on Intelligent User Interfaces (eds Hammond, T. et al.) 307–317 (Association for Computing Machinery, 2021).
Strevens, M. No understanding without explanation. Stud. Hist. Philos. Sci. A 44 , 510–515 (2013).
Ylikoski, P. in Scientific Understanding: Philosophical Perspectives (eds De Regt, H. et al.) 100–119 (Univ. Pittsburgh Press, 2009).
Giudice, M. D. The prediction–explanation fallacy: a pervasive problem in scientific applications of machine learning. Preprint at PsyArXiv https://doi.org/10.31234/osf.io/4vq8f (2021).
Hofman, J. M. et al. Integrating explanation and prediction in computational social science. Nature 595 , 181–188 (2021). This paper highlights the advantages and disadvantages of explanatory versus predictive approaches to modelling, with a focus on applications to computational social science .
Shmueli, G. To explain or to predict? Stat. Sci. 25 , 289–310 (2010).
Article MathSciNet Google Scholar
Hofman, J. M., Sharma, A. & Watts, D. J. Prediction and explanation in social systems. Science 355 , 486–488 (2017).
Logg, J. M., Minson, J. A. & Moore, D. A. Algorithm appreciation: people prefer algorithmic to human judgment. Organ. Behav. Hum. Decis. Process. 151 , 90–103 (2019).
Nguyen, C. T. Cognitive islands and runaway echo chambers: problems for epistemic dependence on experts. Synthese 197 , 2803–2821 (2020).
Breiman, L. Statistical modeling: the two cultures. Stat. Sci. 16 , 199–215 (2001).
Gao, J. & Wang, D. Quantifying the benefit of artificial intelligence for scientific research. Preprint at arxiv.org/abs/2304.10578 (2023).
Hanson, B. et al. Garbage in, garbage out: mitigating risks and maximizing benefits of AI in research. Nature 623 , 28–31 (2023).
Kleinberg, J. & Raghavan, M. Algorithmic monoculture and social welfare. Proc. Natl Acad. Sci. USA 118 , e2018340118 (2021). This paper uses formal modelling methods to demonstrate that when companies all rely on the same algorithm to make decisions (an algorithmic monoculture), the overall quality of those decisions is reduced because valuable options can slip through the cracks, even when the algorithm performs accurately for individual companies .
Article MathSciNet CAS PubMed PubMed Central Google Scholar
Hofstra, B. et al. The diversity–innovation paradox in science. Proc. Natl Acad. Sci. USA 117 , 9284–9291 (2020).
Hong, L. & Page, S. E. Groups of diverse problem solvers can outperform groups of high-ability problem solvers. Proc. Natl Acad. Sci. USA 101 , 16385–16389 (2004).
Page, S. E. Where diversity comes from and why it matters? Eur. J. Soc. Psychol. 44 , 267–279 (2014). This article reviews research demonstrating the benefits of cognitive diversity and diversity in methodological approaches for problem solving and innovation .
Clarke, A. E. & Fujimura, J. H. (eds) The Right Tools for the Job: At Work in Twentieth-Century Life Sciences (Princeton Univ. Press, 2014).
Silva, V. J., Bonacelli, M. B. M. & Pacheco, C. A. Framing the effects of machine learning on science. AI Soc. https://doi.org/10.1007/s00146-022-01515-x (2022).
Sassenberg, K. & Ditrich, L. Research in social psychology changed between 2011 and 2016: larger sample sizes, more self-report measures, and more online studies. Adv. Methods Pract. Psychol. Sci. 2 , 107–114 (2019).
Simon, A. F. & Wilder, D. Methods and measures in social and personality psychology: a comparison of JPSP publications in 1982 and 2016. J. Soc. Psychol. https://doi.org/10.1080/00224545.2022.2135088 (2022).
Anderson, C. A. et al. The MTurkification of social and personality psychology. Pers. Soc. Psychol. Bull. 45 , 842–850 (2019).
Latour, B. in The Social After Gabriel Tarde: Debates and Assessments (ed. Candea, M.) 145–162 (Routledge, 2010).
Porter, T. M. Trust in Numbers: The Pursuit of Objectivity in Science and Public Life (Princeton Univ. Press, 1996).
Lazer, D. et al. Meaningful measures of human society in the twenty-first century. Nature 595 , 189–196 (2021).
Knox, D., Lucas, C. & Cho, W. K. T. Testing causal theories with learned proxies. Annu. Rev. Polit. Sci. 25 , 419–441 (2022).
Barberá, P. Birds of the same feather tweet together: Bayesian ideal point estimation using Twitter data. Polit. Anal. 23 , 76–91 (2015).
Brady, W. J., McLoughlin, K., Doan, T. N. & Crockett, M. J. How social learning amplifies moral outrage expression in online social networks. Sci. Adv. 7 , eabe5641 (2021).
Article PubMed PubMed Central ADS Google Scholar
Barnes, J., Klinger, R. & im Walde, S. S. Assessing state-of-the-art sentiment models on state-of-the-art sentiment datasets. In Proc. 8th Workshop on Computational Approaches to Subjectivity, Sentiment and Social Media Analysis (eds Balahur, A. et al.) 2–12 (Association for Computational Linguistics, 2017).
Gitelman, L. (ed.) “Raw Data” is an Oxymoron (MIT Press, 2013).
Breznau, N. et al. Observing many researchers using the same data and hypothesis reveals a hidden universe of uncertainty. Proc. Natl Acad. Sci. USA 119 , e2203150119 (2022). This study demonstrates how 73 research teams analysing the same dataset reached different conclusions about the relationship between immigration and public support for social policies, highlighting the subjectivity and uncertainty involved in analysing complex datasets .
Gillespie, T. in Media Technologies: Essays on Communication, Materiality, and Society (eds Gillespie, T. et al.) 167–194 (MIT Press, 2014).
Leonelli, S. Data-Centric Biology: A Philosophical Study (Univ. Chicago Press, 2016).
Wang, A., Kapoor, S., Barocas, S. & Narayanan, A. Against predictive optimization: on the legitimacy of decision-making algorithms that optimize predictive accuracy. ACM J. Responsib. Comput. , https://doi.org/10.1145/3636509 (2023).
Athey, S. Beyond prediction: using big data for policy problems. Science 355 , 483–485 (2017).
del Rosario Martínez-Ordaz, R. Scientific understanding through big data: from ignorance to insights to understanding. Possibility Stud. Soc. 1 , 279–299 (2023).
Nussberger, A.-M., Luo, L., Celis, L. E. & Crockett, M. J. Public attitudes value interpretability but prioritize accuracy in artificial intelligence. Nat. Commun. 13 , 5821 (2022).
Zittrain, J. in The Cambridge Handbook of Responsible Artificial Intelligence: Interdisciplinary Perspectives (eds. Voeneky, S. et al.) 176–184 (Cambridge Univ. Press, 2022). This article articulates the epistemic risks of prioritizing predictive accuracy over explanatory understanding when AI tools are interacting in complex systems.
Shumailov, I. et al. The curse of recursion: training on generated data makes models forget. Preprint at arxiv.org/abs/2305.17493 (2023).
Latour, B. Science In Action: How to Follow Scientists and Engineers Through Society (Harvard Univ. Press, 1987). This book provides strategies and approaches for thinking about science as a social endeavour .
Franklin, S. Science as culture, cultures of science. Annu. Rev. Anthropol. 24 , 163–184 (1995).
Haraway, D. Situated knowledges: the science question in feminism and the privilege of partial perspective. Fem. Stud. 14 , 575–599 (1988). This article acknowledges that the objective ‘view from nowhere’ is unobtainable: knowledge, it argues, is always situated .
Harding, S. Objectivity and Diversity: Another Logic of Scientific Research (Univ. Chicago Press, 2015).
Longino, H. E. Science as Social Knowledge: Values and Objectivity in Scientific Inquiry (Princeton Univ. Press, 1990).
Daston, L. & Galison, P. Objectivity (Princeton Univ. Press, 2007). This book is a historical analysis of the shifting modes of ‘objectivity’ that scientists have pursued, arguing that objectivity is not a universal concept but that it shifts alongside scientific techniques and ambitions .
Prescod-Weinstein, C. Making Black women scientists under white empiricism: the racialization of epistemology in physics. Signs J. Women Cult. Soc. 45 , 421–447 (2020).
Mavhunga, C. What Do Science, Technology, and Innovation Mean From Africa? (MIT Press, 2017).
Schiebinger, L. The Mind Has No Sex? Women in the Origins of Modern Science (Harvard Univ. Press, 1991).
Martin, E. The egg and the sperm: how science has constructed a romance based on stereotypical male–female roles. Signs J. Women Cult. Soc. 16 , 485–501 (1991). This case study shows how assumptions about gender affect scientific theories, sometimes delaying the articulation of what might be considered to be more accurate descriptions of scientific phenomena .
Harding, S. Rethinking standpoint epistemology: What is “strong objectivity”? Centen. Rev. 36 , 437–470 (1992). In this article, Harding outlines her position on ‘strong objectivity’, by which clearly articulating one’s standpoint can lead to more robust knowledge claims .
Oreskes, N. Why Trust Science? (Princeton Univ. Press, 2019). This book introduces the reader to 20 years of scholarship in science and technology studies, arguing that the tools the discipline has for understanding science can help to reinstate public trust in the institution .
Rolin, K., Koskinen, I., Kuorikoski, J. & Reijula, S. Social and cognitive diversity in science: introduction. Synthese 202 , 36 (2023).
Hong, L. & Page, S. E. Problem solving by heterogeneous agents. J. Econ. Theory 97 , 123–163 (2001).
Sulik, J., Bahrami, B. & Deroy, O. The diversity gap: when diversity matters for knowledge. Perspect. Psychol. Sci. 17 , 752–767 (2022).
Lungeanu, A., Whalen, R., Wu, Y. J., DeChurch, L. A. & Contractor, N. S. Diversity, networks, and innovation: a text analytic approach to measuring expertise diversity. Netw. Sci. 11 , 36–64 (2023).
AlShebli, B. K., Rahwan, T. & Woon, W. L. The preeminence of ethnic diversity in scientific collaboration. Nat. Commun. 9 , 5163 (2018).
Campbell, L. G., Mehtani, S., Dozier, M. E. & Rinehart, J. Gender-heterogeneous working groups produce higher quality science. PLoS ONE 8 , e79147 (2013).
Nielsen, M. W., Bloch, C. W. & Schiebinger, L. Making gender diversity work for scientific discovery and innovation. Nat. Hum. Behav. 2 , 726–734 (2018).
Yang, Y., Tian, T. Y., Woodruff, T. K., Jones, B. F. & Uzzi, B. Gender-diverse teams produce more novel and higher-impact scientific ideas. Proc. Natl Acad. Sci. USA 119 , e2200841119 (2022).
Kozlowski, D., Larivière, V., Sugimoto, C. R. & Monroe-White, T. Intersectional inequalities in science. Proc. Natl Acad. Sci. USA 119 , e2113067119 (2022).
Fehr, C. & Jones, J. M. Culture, exploitation, and epistemic approaches to diversity. Synthese 200 , 465 (2022).
Nakadai, R., Nakawake, Y. & Shibasaki, S. AI language tools risk scientific diversity and innovation. Nat. Hum. Behav. 7 , 1804–1805 (2023).
National Academies of Sciences, Engineering, and Medicine et al. Advancing Antiracism, Diversity, Equity, and Inclusion in STEMM Organizations: Beyond Broadening Participation (National Academies Press, 2023).
Winner, L. Do artifacts have politics? Daedalus 109 , 121–136 (1980).
Eubanks, V. Automating Inequality: How High-Tech Tools Profile, Police, and Punish the Poor (St. Martin’s Press, 2018).
Littmann, M. et al. Validity of machine learning in biology and medicine increased through collaborations across fields of expertise. Nat. Mach. Intell. 2 , 18–24 (2020).
Carusi, A. et al. Medical artificial intelligence is as much social as it is technological. Nat. Mach. Intell. 5 , 98–100 (2023).
Raghu, M. & Schmidt, E. A survey of deep learning for scientific discovery. Preprint at arxiv.org/abs/2003.11755 (2020).
Bishop, C. AI4Science to empower the fifth paradigm of scientific discovery. Microsoft Research Blog www.microsoft.com/en-us/research/blog/ai4science-to-empower-the-fifth-paradigm-of-scientific-discovery/ (2022).
Whittaker, M. The steep cost of capture. Interactions 28 , 50–55 (2021).
Liesenfeld, A., Lopez, A. & Dingemanse, M. Opening up ChatGPT: Tracking openness, transparency, and accountability in instruction-tuned text generators. In Proc. 5th International Conference on Conversational User Interfaces 1–6 (Association for Computing Machinery, 2023).
Chu, J. S. G. & Evans, J. A. Slowed canonical progress in large fields of science. Proc. Natl Acad. Sci. USA 118 , e2021636118 (2021).
Park, M., Leahey, E. & Funk, R. J. Papers and patents are becoming less disruptive over time. Nature 613 , 138–144 (2023).
Frith, U. Fast lane to slow science. Trends Cogn. Sci. 24 , 1–2 (2020). This article explains the epistemic risks of a hyperfocus on scientific productivity and explores possible avenues for incentivizing the production of higher-quality science on a slower timescale .
Stengers, I. Another Science is Possible: A Manifesto for Slow Science (Wiley, 2018).
Lake, B. M. & Baroni, M. Human-like systematic generalization through a meta-learning neural network. Nature 623 , 115–121 (2023).
Feinman, R. & Lake, B. M. Learning task-general representations with generative neuro-symbolic modeling. Preprint at arxiv.org/abs/2006.14448 (2021).
Schölkopf, B. et al. Toward causal representation learning. Proc. IEEE 109 , 612–634 (2021).
Mitchell, M. AI’s challenge of understanding the world. Science 382 , eadm8175 (2023).
Sartori, L. & Bocca, G. Minding the gap(s): public perceptions of AI and socio-technical imaginaries. AI Soc. 38 , 443–458 (2023).
Download references
Acknowledgements
We thank D. S. Bassett, W. J. Brady, S. Helmreich, S. Kapoor, T. Lombrozo, A. Narayanan, M. Salganik and A. J. te Velthuis for comments. We also thank C. Buckner and P. Winter for their feedback and suggestions.
Author information
These authors contributed equally: Lisa Messeri, M. J. Crockett
Authors and Affiliations
Department of Anthropology, Yale University, New Haven, CT, USA
Lisa Messeri
Department of Psychology, Princeton University, Princeton, NJ, USA
M. J. Crockett
University Center for Human Values, Princeton University, Princeton, NJ, USA
You can also search for this author in PubMed Google Scholar
Contributions
The authors contributed equally to the research and writing of the paper.
Corresponding authors
Correspondence to Lisa Messeri or M. J. Crockett .
Ethics declarations
Competing interests.
The authors declare no competing interests.
Peer review
Peer review information.
Nature thanks Cameron Buckner, Peter Winter and the other, anonymous, reviewer(s) for their contribution to the peer review of this work.
Additional information
Publisher’s note Springer Nature remains neutral with regard to jurisdictional claims in published maps and institutional affiliations.
Rights and permissions
Springer Nature or its licensor (e.g. a society or other partner) holds exclusive rights to this article under a publishing agreement with the author(s) or other rightsholder(s); author self-archiving of the accepted manuscript version of this article is solely governed by the terms of such publishing agreement and applicable law.
Reprints and permissions
About this article
Cite this article.
Messeri, L., Crockett, M.J. Artificial intelligence and illusions of understanding in scientific research. Nature 627 , 49–58 (2024). https://doi.org/10.1038/s41586-024-07146-0
Download citation
Received : 31 July 2023
Accepted : 31 January 2024
Published : 06 March 2024
Issue Date : 07 March 2024
DOI : https://doi.org/10.1038/s41586-024-07146-0
Share this article
Anyone you share the following link with will be able to read this content:
Sorry, a shareable link is not currently available for this article.
Provided by the Springer Nature SharedIt content-sharing initiative
This article is cited by
Ai is no substitute for having something to say.
Nature Reviews Physics (2024)
Perché gli scienziati si fidano troppo dell'intelligenza artificiale - e come rimediare
Nature Italy (2024)
Why scientists trust AI too much — and what to do about it
Nature (2024)
By submitting a comment you agree to abide by our Terms and Community Guidelines . If you find something abusive or that does not comply with our terms or guidelines please flag it as inappropriate.
Quick links
- Explore articles by subject
- Guide to authors
- Editorial policies
Sign up for the Nature Briefing newsletter — what matters in science, free to your inbox daily.

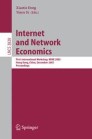
International Workshop on Internet and Network Economics
WINE 2005: Internet and Network Economics pp 816–825 Cite as
Study on Improving Efficiency of Knowledge Sharing in Knowledge-Intensive Organization
- Lingling Zhang 18 ,
- Jun Li 19 &
- Yong Shi 20
- Conference paper
2050 Accesses
3 Citations
Part of the book series: Lecture Notes in Computer Science ((LNISA,volume 3828))
Knowledge sharing is an important part in knowledge management. The value of knowledge only can be seen in its transfer, sharing and utilizing. The transfer of individual tacit knowledge to organizational capacity can improve the competitiveness of a organization. However, there are a lot of barriers in knowledge sharing, especially in those knowledge-intensive organizations, where knowledge is very important for individuals to keep advantage, so they are usually unwilling to share it with others, or ‘contribute’ their personal knowledge to the organization. At present, most studies are emphasizing on how to create a good physical environment and platform to improve it. In this paper, we’ll analyze it using game theory. We think that to improve the efficiency of knowledge sharing efficiency, motivation mechanism and physical platform must be improved simultaneously, especially in knowledge-intensive organization, motivation mechanism is more important. Then, to problem of ‘prisoner’ dilemma’ between individuals knowledge sharing, this paper will show how to design motivation method to improve efficiency of it.
- Efficiency of Knowledge Sharing
- Game theory
- Knowledge- Intensive Organization
This is a preview of subscription content, log in via an institution .
Buying options
- Available as PDF
- Read on any device
- Instant download
- Own it forever
- Compact, lightweight edition
- Dispatched in 3 to 5 business days
- Free shipping worldwide - see info
Tax calculation will be finalised at checkout
Purchases are for personal use only
Unable to display preview. Download preview PDF.
Bell, D.: The Coming of Post-industrial Society. Basic Books, New York (1973)
Google Scholar
Castells, M.: The Information Age: Economy, Society and Culture. The Risk of the Network Society, vol. 1. Blackwell, Oxford (1996)
Kallinikos, J.: Organizations in an age of information Lund. Academia Adacta (1996)
Kought, B., Zander, U.: Knowledge of the firm, Combinative capabilityes, and the replication of technology. Organization Science 3, 383–397 (1992)
Article Google Scholar
Dixon, N.M.: Common Knowledge: How Organization Thrive by Sharing What They Know. Harvard Business School Press, Boston (2000)
Lynne, M.M.: Toward a Theory of Knowledge Reuse: Types of Knowledge Reuse Situations and Factors in Reuse Success. Journal of Management Information System 18(1) (2001)
Barson, R.J., Foster, G., Struck, T.: Inter - and Intra -Organizational Barriers to Sharing Knowledge in the Extended Supply - Chain (2000), http://www.corma.net/download/e2000.pdf
Davenport, T.H., Prusak, L.: Working Knowledge. Harvard Business School Press, Boston (1998)
Cummings, J.: Knowledge Sharing : A Review of the Literature (2003), Doc UNID View For JavaSearch/D9E389E7414BE9DE 85256DC600572CA0/ $ file/ knowledge, http://lnweb18.worldbank.org/oed/oeddoclib.nsf/
Arrow, K.J.: The economic implications of learning by doing. Review of Economic Studies 29, 155–173 (1962)
Romer, P.M.: Increasing returns and long run growth. Journal of Political Economy 94(5), 1002–1037 (1986)
Article MathSciNet Google Scholar
Foss, N.J.: Knowledge-based approaches to the theory of the firm: A paradox in managing in the west and Japan. In: Lorange, P., Chakravarthy, B., Roos, J., Van de Ven, A. (eds.) Implementing Strategic Process: Change, Learning, and Co-operation, pp. 117–144. Basil Blackwell, Oxford (1996)
Leonard-Barton, D.: Core capabilities and core rigidities: A Paradox in managing new product development. Strategic Management Journal 13, 111–125 (1992)
Gupta, A.K., Govindarajan, V.: Knowledge flows and structure of control within multinational corporations. The Academy of Management Review 16, 768–792 (1991)
Nonaka, I., Takeuchi, Y.: Knowledge Creating Organization. Oxford University Press, New York (1995)
Nonaka, I., Nonno, N., Toyama, R.: Emergence of “Ba”. In: Nonaka, I., Nishinguchi, T. (eds.) Knowledge Emergence, pp. 13–29. Oxford Universty Press (2001)
Download references
Author information
Authors and affiliations.
Management School of Graduate University of Chinese Academy of Sciences, Associate Professor, Chinese Academy of Sciences Research Center on Data Technology and Knowledge Economy, 100080, Beijing, China
Lingling Zhang
Management School of Graduate University of Chinese Academy of Sciences, Ph.D Student, Chinese Academy of Sciences Research Center on Data Technology and Knowledge Economy, 100080, Beijing, China
Graduate University of Chinese Academy of Sciences, Professor, Chinese Academy of Sciences Research Center on Data Technology and Knowledge Economy, 100080, Beijing, China
You can also search for this author in PubMed Google Scholar
Editor information
Editors and affiliations.
Department of Computer Science, City University of Hong Kong, Tat Chee Avenue, Kowloon ,, Hong Kong
Xiaotie Deng
Stanford University, Stanford, CA
Rights and permissions
Reprints and permissions
Copyright information
© 2005 Springer-Verlag Berlin Heidelberg
About this paper
Cite this paper.
Zhang, L., Li, J., Shi, Y. (2005). Study on Improving Efficiency of Knowledge Sharing in Knowledge-Intensive Organization. In: Deng, X., Ye, Y. (eds) Internet and Network Economics. WINE 2005. Lecture Notes in Computer Science, vol 3828. Springer, Berlin, Heidelberg. https://doi.org/10.1007/11600930_83
Download citation
DOI : https://doi.org/10.1007/11600930_83
Publisher Name : Springer, Berlin, Heidelberg
Print ISBN : 978-3-540-30900-0
Online ISBN : 978-3-540-32293-1
eBook Packages : Computer Science Computer Science (R0)
Share this paper
Anyone you share the following link with will be able to read this content:
Sorry, a shareable link is not currently available for this article.
Provided by the Springer Nature SharedIt content-sharing initiative
- Publish with us
Policies and ethics
- Find a journal
- Track your research
Help | Advanced Search
Computer Science > Computation and Language
Title: uni-smart: universal science multimodal analysis and research transformer.
Abstract: In scientific research and its application, scientific literature analysis is crucial as it allows researchers to build on the work of others. However, the fast growth of scientific knowledge has led to a massive increase in scholarly articles, making in-depth literature analysis increasingly challenging and time-consuming. The emergence of Large Language Models (LLMs) has offered a new way to address this challenge. Known for their strong abilities in summarizing texts, LLMs are seen as a potential tool to improve the analysis of scientific literature. However, existing LLMs have their own limits. Scientific literature often includes a wide range of multimodal elements, such as molecular structure, tables, and charts, which are hard for text-focused LLMs to understand and analyze. This issue points to the urgent need for new solutions that can fully understand and analyze multimodal content in scientific literature. To answer this demand, we present Uni-SMART (Universal Science Multimodal Analysis and Research Transformer), an innovative model designed for in-depth understanding of multimodal scientific literature. Through rigorous quantitative evaluation across several domains, Uni-SMART demonstrates superior performance over leading text-focused LLMs. Furthermore, our exploration extends to practical applications, including patent infringement detection and nuanced analysis of charts. These applications not only highlight Uni-SMART's adaptability but also its potential to revolutionize how we interact with scientific literature.
Submission history
Access paper:.
- Download PDF
- HTML (experimental)
- Other Formats

References & Citations
- Google Scholar
- Semantic Scholar
BibTeX formatted citation

Bibliographic and Citation Tools
Code, data and media associated with this article, recommenders and search tools.
- Institution
arXivLabs: experimental projects with community collaborators
arXivLabs is a framework that allows collaborators to develop and share new arXiv features directly on our website.
Both individuals and organizations that work with arXivLabs have embraced and accepted our values of openness, community, excellence, and user data privacy. arXiv is committed to these values and only works with partners that adhere to them.
Have an idea for a project that will add value for arXiv's community? Learn more about arXivLabs .
Academia.edu no longer supports Internet Explorer.
To browse Academia.edu and the wider internet faster and more securely, please take a few seconds to upgrade your browser .
- We're Hiring!
- Help Center
Knowledge sharing
- Most Cited Papers
- Most Downloaded Papers
- Newest Papers
- Save to Library
- Last »
- Knowledge Management Follow Following
- Boundary-Spanning Knowledge Sharing Follow Following
- Formats Follow Following
- Knowledge organization Follow Following
- Knowledge Transfer Follow Following
- Technical Systems (IT, web, etc.) for Managing Knowledge Follow Following
- Knowledge types Follow Following
- Innovation and Knowledge Management Follow Following
- Knowledge Deployment & Distributions Follow Following
- Forms Follow Following
Enter the email address you signed up with and we'll email you a reset link.
- Academia.edu Publishing
- We're Hiring!
- Help Center
- Find new research papers in:
- Health Sciences
- Earth Sciences
- Cognitive Science
- Mathematics
- Computer Science
- Academia ©2024
- Share full article
Advertisement
Supported by
In One Key A.I. Metric, China Pulls Ahead of the U.S.: Talent
China has produced a huge number of top A.I. engineers in recent years. New research shows that, by some measures, it has already eclipsed the United States.

By Paul Mozur and Cade Metz
Paul Mozur reported from Taipei, Taiwan, and Cade Metz from San Francisco.
When it comes to the artificial intelligence that powers chatbots like ChatGPT, China lags behind the United States . But when it comes to producing the scientists behind a new generation of humanoid technologies, China is pulling ahead.
New research shows that China has by some metrics eclipsed the United States as the biggest producer of A.I. talent, with the country generating almost half the world’s top A.I. researchers. By contrast, about 18 percent come from U.S. undergraduate institutions, according to the study , from MacroPolo, a think tank run by the Paulson Institute, which promotes constructive ties between the United States and China.
The findings show a jump for China, which produced about one-third of the world’s top talent three years earlier. The United States, by contrast, remained mostly the same. The research is based on the backgrounds of researchers whose papers were published at 2022’s Conference on Neural Information Processing Systems. NeurIPS, as it is known, is focused on advances in neural networks , which have anchored recent developments in generative A.I.
The talent imbalance has been building for the better part of a decade. During much of the 2010s, the United States benefited as large numbers of China’s top minds moved to American universities to complete doctoral degrees. A majority of them stayed in the United States. But the research shows that trend has also begun to turn, with growing numbers of Chinese researchers staying in China.
What happens in the next few years could be critical as China and the United States jockey for primacy in A.I. — a technology that can potentially increase productivity, strengthen industries and drive innovation — turning the researchers into one of the most geopolitically important groups in the world.
Generative A.I. has captured the tech industry in Silicon Valley and in China, causing a frenzy in funding and investment. The boom has been led by U.S. tech giants such as Google and start-ups like OpenAI. That could attract China’s researchers, though rising tensions between Beijing and Washington could also deter some, experts said.
(The New York Times has sued OpenAI and Microsoft for copyright infringement of news content related to A.I. systems.)
China has nurtured so much A.I. talent partly because it invested heavily in A.I. education. Since 2018, the country has added more than 2,000 undergraduate A.I. programs, with more than 300 at its most elite universities, said Damien Ma, the managing director of MacroPolo, though he noted the programs were not heavily focused on the technology that had driven breakthroughs by chatbots like ChatGPT.
“A lot of the programs are about A.I. applications in industry and manufacturing, not so much the generative A.I. stuff that’s come to dominate the American A.I. industry at the moment,” he said.
While the United States has pioneered breakthroughs in A.I., most recently with the uncanny humanlike abilities of chatbots , a significant portion of that work was done by researchers educated in China.
Researchers originally from China now make up 38 percent of the top A.I. researchers working in the United States, with Americans making up 37 percent, according to the research. Three years earlier, those from China made up 27 percent of top talent working in the United States, compared with 31 percent from the United States.
“The data shows just how critical Chinese-born researchers are to the United States for A.I. competitiveness,” said Matt Sheehan, a fellow at the Carnegie Endowment for International Peace who studies Chinese A.I.
He added that the data seemed to show the United States was still attractive. “We’re the world leader in A.I. because we continue to attract and retain talent from all over the world, but especially China,” he said.
Pieter Abbeel, a professor at the University of California, Berkeley, and a founder of Covariant , an A.I. and robotics start-up, said working alongside large numbers of Chinese researchers was taken for granted inside the leading American companies and universities.
“It’s just a natural state of affairs,” he said.
In the past, U.S. defense officials were not too concerned about A.I. talent flows from China, partly because many of the biggest A.I. projects did not deal with classified data and partly because they reasoned that it was better to have the best minds available. That so much of the leading research in A.I. is published openly also held back worries.
Despite bans introduced by the Trump administration that prohibit entry to the United States for students from some military-linked universities in China and a relative slowdown in the flow of Chinese students into the country during Covid, the research showed large numbers of the most promising A.I. minds continued coming to the United States to study.
But this month, a Chinese citizen who was an engineer at Google was charged with trying to transfer A.I. technology — including critical microchip architecture — to a Beijing-based company that paid him in secret , according to a federal indictment.
The substantial numbers of Chinese A.I. researchers working in the United States now present a conundrum for policymakers, who want to counter Chinese espionage while not discouraging the continued flow of top Chinese computer engineers into the United States, according to experts focused on American competitiveness.
“Chinese scholars are almost leading the way in the A.I. field,” said Subbarao Kambhampati, a professor and researcher of A.I. at Arizona State University. If policymakers try to bar Chinese nationals from research in the United States, he said, they are “shooting themselves in the foot.”
The track record of U.S. policymakers is mixed. A policy by the Trump administration aimed at curbing Chinese industrial espionage and intellectual property theft has since been criticized for errantly prosecuting a number of professors. Such programs, Chinese immigrants said, have encouraged some to stay in China.
For now, the research showed, most Chinese who complete doctorates in the United States stay in the country, helping to make it the global center of the A.I. world. Even so, the U.S. lead has begun to slip, to hosting about 42 percent of the world’s top talent, down from about 59 percent three years ago, according to the research.
Paul Mozur is the global technology correspondent for The Times, based in Taipei. Previously he wrote about technology and politics in Asia from Hong Kong, Shanghai and Seoul. More about Paul Mozur
Cade Metz writes about artificial intelligence, driverless cars, robotics, virtual reality and other emerging areas of technology. More about Cade Metz
Intermittent fasting linked to higher risk of cardiovascular death, research suggests
Intermittent fasting, a diet pattern that involves alternating between periods of fasting and eating, can lower blood pressure and help some people lose weight , past research has indicated.
But an analysis presented Monday at the American Heart Association’s scientific sessions in Chicago challenges the notion that intermittent fasting is good for heart health. Instead, researchers from Shanghai Jiao Tong University School of Medicine in China found that people who restricted food consumption to less than eight hours per day had a 91% higher risk of dying from cardiovascular disease over a median period of eight years, relative to people who ate across 12 to 16 hours.
It’s some of the first research investigating the association between time-restricted eating (a type of intermittent fasting) and the risk of death from cardiovascular disease.
The analysis — which has not yet been peer-reviewed or published in an academic journal — is based on data from the Centers for Disease Control and Prevention’s National Health and Nutrition Examination Survey collected between 2003 and 2018. The researchers analyzed responses from around 20,000 adults who recorded what they ate for at least two days, then looked at who had died from cardiovascular disease after a median follow-up period of eight years.
However, Victor Wenze Zhong, a co-author of the analysis, said it’s too early to make specific recommendations about intermittent fasting based on his research alone.
“Practicing intermittent fasting for a short period such as 3 months may likely lead to benefits on reducing weight and improving cardiometabolic health,” Zhong said via email. But he added that people “should be extremely cautious” about intermittent fasting for longer periods of time, such as years.
Intermittent fasting regimens vary widely. A common schedule is to restrict eating to a period of six to eight hours per day, which can lead people to consume fewer calories, though some eat the same amount in a shorter time. Another popular schedule is the "5:2 diet," which involves eating 500 to 600 calories on two nonconsecutive days of the week but eating normally for the other five.
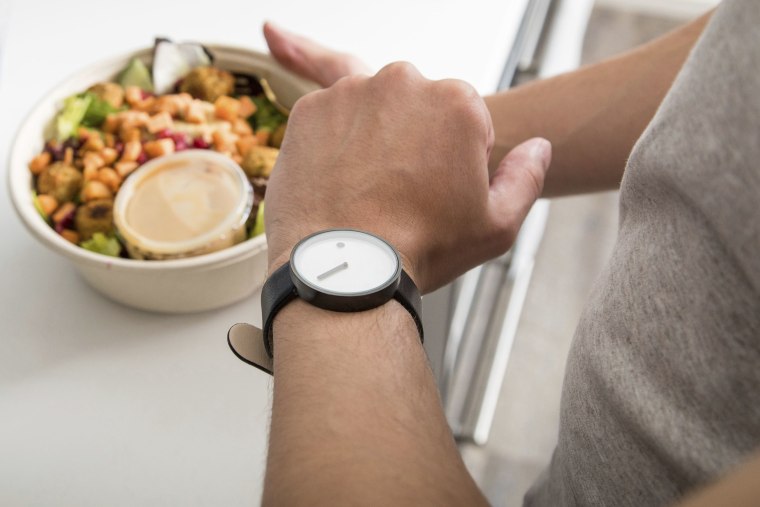
Zhong said it’s not clear why his research found an association between time-restricted eating and a risk of death from cardiovascular disease. He offered an observation, though: People who limited their eating to fewer than eight hours per day had less lean muscle mass than those who ate for 12 to 16 hours. Low lean muscle mass has been linked to a higher risk of cardiovascular death .
Cardiovascular and nutrition experts who were not involved in the analysis offered several theories about what might explain the results.
Dr. Benjamin Horne, a research professor at Intermountain Health in Salt Lake City, said fasting can increase stress hormones such as cortisol and adrenaline, since the body doesn’t know when to expect food next and goes into survival mode. That added stress may raise the short-term risk of heart problems among vulnerable groups, he said, particularly elderly people or those with chronic health conditions.
Horne’s research has shown that fasting twice a week for four weeks, then once a week for 22 weeks may increase a person’s risk of dying after one year but decrease their 10-year risk of chronic disease.
“In the long term, what it does is reduces those risk factors for heart disease and reduces the risk factors for diabetes and so forth — but in the short term, while you’re actually doing it, your body is in a state where it’s at a higher risk of having problems,” he said.
Even so, Horne added, the analysis “doesn’t change my perspective that there are definite benefits from fasting, but it’s a cautionary tale that we need to be aware that there are definite, potentially major, adverse effects.”
Intermittent fasting gained popularity about a decade ago, when the 5:2 diet was touted as a weight loss strategy in the U.K. In the years to follow, several celebrities espoused the benefits of an eight-hour eating window for weight loss, while some Silicon Valley tech workers believed that extreme periods of fasting boosted productivity . Some studies have also suggested that intermittent fasting might help extend people’s lifespans by warding off disease .
However, a lot of early research on intermittent fasting involved animals. In the last seven years or so, various clinical trials have investigated potential benefits for humans, including for heart health.
“The purpose of intermittent fasting is to cut calories, lose weight,” said Penny Kris-Etherton, emeritus professor of nutritional sciences at Penn State University and a member of the American Heart Association nutrition committee. “It’s really how intermittent fasting is implemented that’s going to explain a lot of the benefits or adverse associations.”
Dr. Francisco Lopez-Jimenez, a cardiologist at Mayo Clinic, said the timing of when people eat may influence the effects they see.
“I haven’t met a single person or patient that has been practicing intermittent fasting by skipping dinner,” he said, noting that people more often skip breakfast, a schedule associated with an increased risk of heart disease and death .
The new research comes with limitations: It relies on people’s memories of what they consumed over a 24-hour period and doesn’t consider the nutritional quality of the food they ate or how many calories they consumed during an eating window.
So some experts found the analysis too narrow.
“It’s a retrospective study looking at two days’ worth of data, and drawing some very big conclusions from a very limited snapshot into a person’s lifestyle habits,” said Dr. Pam Taub, a cardiologist at UC San Diego Health.
Taub said her patients have seen “incredible benefits” from fasting regimens.
“I would continue doing it,” she said. “For people that do intermittent fasting, their individual results speak for themselves. Most people that do intermittent fasting, the reason they continue it is they see a decrease in their weight. They see a decrease in blood pressure. They see an improvement in their LDL cholesterol.”
Kris-Etherton, however, urged caution: “Maybe consider a pause in intermittent fasting until we have more information or until the results of the study can be better explained,” she said.

Aria Bendix is the breaking health reporter for NBC News Digital.
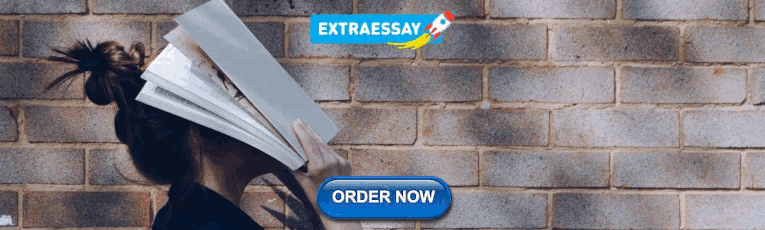
IMAGES
VIDEO
COMMENTS
With knowledge sharing, information flows through effective communication, information searching, information learning, or those who need information (Kurata et al., 2017). Knowledge sharing is basically considered as information that can be obtained by other employees within the organization . When the results of the research are analyzed, it ...
Therefore, this paper reviews the concepts of knowledge sharing and the related factors that affect knowledge sharing. Finally, this paper proposes future research directions about knowledge ...
Knowledge management activities as well as knowledge sharing practices enhance the performance of the organization by achieving its mission. Therefore, this article aims to analyze and recognize the aspects that promote and inhibit KM and knowledge sharing within organizations. This study conducts a systematic literature review and selects 57 research articles from 2000 to 2020, which includes ...
Abstract. The success of knowledge management initiatives depends on knowledge sharing. This paper reviews qualitative and quantitative studies of individual-level knowledge sharing. Based on the literature review we developed a framework for understanding knowledge sharing research. The framework identifies five areas of emphasis of knowledge ...
A Literature Review on Knowledge Sharing. Tingting Zheng. Business, Sociology. 2017. TLDR. This paper reviews the concepts of knowledge sharing and the related factors that affect knowledge sharing, and proposes future research directions about knowledge sharing. Expand. 53. Highly Influenced.
Abstract: The main objective of this paper is to bring together scattered literature on knowledge sharing, and analyse them to provide a better understanding of the concept and to suggest emerging directions for future research. The review went through three stages: setting the review protocol, administering the review, and reporting the review.
This paper aims to summarize the findings from past research on knowledge-sharing outcomes in organizations and to suggest promising directions for future research. Design/methodology/approach: We conducted a systematic literature review that consisted of three main phases: defining a review protocol, conducting the review and reporting the review.
In tertiary institutions, KM core activities include Knowledge acquisition, knowledge management, knowledge utilisation and knowledge sharing to promote a positive learning system (Diab, 2021 ...
The success of knowledge management initiatives depends on knowledge sharing. This paper reviews qualitative and quantitative studies of individual-level knowledge sharing. Based on the literature review we developed a framework for understanding knowledge sharing research. The framework identifies five areas of emphasis of knowledge sharing research: organizational context, interpersonal and ...
Knowledge sharing is an activity through which knowledge, namely, information, skills, or expertise, is exchanged between people, friends, families, communities or organizations. Online knowledge sharing activities are flourishing with the advent of social media and digital life. However, despite of the importance of the online knowledge sharing methods and mechanisms, there is not any ...
However, in realistic management situations, the knowledge sharing of scientific research teams always appears to be unsustainable, and the reasons for this are the subject of considerable debate. ... 2019), such as how knowledge hiding and its interaction between individuals and the team affect knowledge sharing. The second aim of this paper ...
Built upon the prior research, this paper constructs the cost-benefit matrix of knowledge sharing within online group based on relationship strength according to the interactive principles and treatment methods under different relationships and combining with the characteristics of knowledge sharing in virtual communities, and then applies ...
Knowledge sharing is a key challenge for modern organizations, especially in periods of uncertainty such as that of the current pandemic. This conceptual paper argues that intergenerational learning (IGL) is a useful intervention to address the challenge of knowledge sharing in the New Ways of Working. We conducted an integrated literature ...
The paper measured KSE through perceived instructor support of knowledge sharing instructor support (IS), perceived information and communication technology (ICT) support of knowledge sharing and perceived ability to share knowledge (ASK).,A survey questionnaire was used as an instrument for data collection.
Second, this review provides an organizing framework for previous knowledge sharing research and identifies emerging theoretical and methodological issues and future research needs. The framework shown in Fig. 1 was based on the review of the literature and provides a structure for the paper.
The method employed in this study was a systematic review that covered publications on knowledge sharing and innovation from 1973, when the first article relating the two topics was published, to 2017. The survey was based on 7,991 articles from the Scopus and ISI Web of Science databases using VantagePoint 11.0 software.
The importance of knowledge sharing between students is reviewed in relation to the current higher education landscape, especially in relation to graduate attributes and employability. A framework for the classification of arrangements for knowledge sharing is presented as a spectrum that ranges between formal and informal approaches.
- The purpose of this paper is to explore the effects of knowledge sharing on innovation. Two forms of knowledge sharing are examined, knowledge donating and knowledge collecting. ... It is advised that future research should be designed for different countries in order to conduct a comparative study., - These results provide some ...
This paper explored publication practices of GHR researchers, a field where sharing of knowledge is inherent to its mission of equity in healthcare and essential to its collaborative nature. Regardless of this emphasis on sharing, our research shows that 42.0% of scholarly articles are not freely available online even if many funders, scholars ...
An additional benefit of sharing research materials is that it facilitates the replication of published research. Replication efforts are critical for understanding the validity of the current scientific record, as they tell us about the stability of our accumulated knowledge over time (McEwan et al., Citation 2018 ).
Universities need to be aware of the impact of knowledge management (KM) in order to become world-class academic institutions. This research fills an unexplored gap in regards to the impact of KM enablers (i.e. trust, knowledge self-efficacy, reciprocal benefits, top management support, organizational rewards, organizational culture, KM system infrastructure and KM system quality, openness ...
This paper provided an empirical demonstration of the illusion of explanatory depth, and inspired a programme of research in cognitive science on communities of knowledge.
Knowledge sharing is an important part in knowledge management. ... organization, motivation mechanism is more important. Then, to problem of 'prisoner' dilemma' between individuals knowledge sharing, this paper will show how to design motivation method to improve efficiency of it. ... Chinese Academy of Sciences Research Center on Data ...
From magazine articles to peer-reviewed papers and case laws, Google Scholar can provide cutting-edge research for free. It's one of Google's lesser-known search tools—but it's invaluable if you ...
In scientific research and its application, scientific literature analysis is crucial as it allows researchers to build on the work of others. However, the fast growth of scientific knowledge has led to a massive increase in scholarly articles, making in-depth literature analysis increasingly challenging and time-consuming. The emergence of Large Language Models (LLMs) has offered a new way to ...
11. Knowledge Management , Research Methodology , Knowledge sharing , Engineering Design. Academic Paper of Policy Analyst Functional Position. This academic paper was written by academia of FISIP UGM and Policy Analyst Development Center of National Institute for Public Administration Republic Indonesia.
China has produced a huge number of top A.I. engineers in recent years. New research shows that, by some measures, it has already eclipsed the United States. By Paul Mozur and Cade Metz Paul Mozur ...
Horne's research has shown that fasting twice a week for four weeks, then once a week for 22 weeks may increase a person's risk of dying after one year but decrease their 10-year risk of ...
President Biden on Monday will issue an executive order to bolster women's health research, while federal agencies are announcing new steps to close long-standing gender gaps in clinical trials and care.. Why it matters: They're among the first concrete steps the White House is taking on a new women's health research initiative led by first lady Jill Biden, and they come shortly after the ...
The Department will continue to assist our external partners through webinars, resources, and updates on the Knowledge Center.We also welcome our partners to continue to submit questions related to the 2024-25 FAFSA launch using the Contact Customer Support form in FSA's Partner Connect Help Center. To submit a question, please enter your name, email address, topic, and question.